- Privacy Policy

Home » Quantitative Research – Methods, Types and Analysis
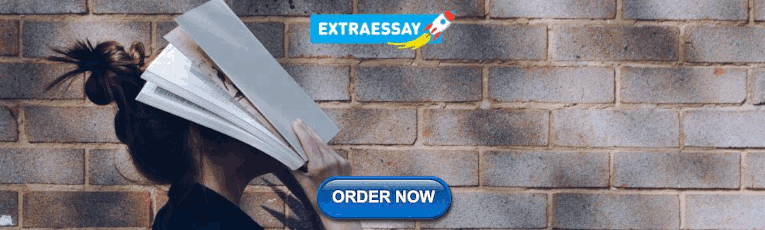
Quantitative Research – Methods, Types and Analysis
Table of Contents

Quantitative Research
Quantitative research is a type of research that collects and analyzes numerical data to test hypotheses and answer research questions . This research typically involves a large sample size and uses statistical analysis to make inferences about a population based on the data collected. It often involves the use of surveys, experiments, or other structured data collection methods to gather quantitative data.
Quantitative Research Methods

Quantitative Research Methods are as follows:
Descriptive Research Design
Descriptive research design is used to describe the characteristics of a population or phenomenon being studied. This research method is used to answer the questions of what, where, when, and how. Descriptive research designs use a variety of methods such as observation, case studies, and surveys to collect data. The data is then analyzed using statistical tools to identify patterns and relationships.
Correlational Research Design
Correlational research design is used to investigate the relationship between two or more variables. Researchers use correlational research to determine whether a relationship exists between variables and to what extent they are related. This research method involves collecting data from a sample and analyzing it using statistical tools such as correlation coefficients.
Quasi-experimental Research Design
Quasi-experimental research design is used to investigate cause-and-effect relationships between variables. This research method is similar to experimental research design, but it lacks full control over the independent variable. Researchers use quasi-experimental research designs when it is not feasible or ethical to manipulate the independent variable.
Experimental Research Design
Experimental research design is used to investigate cause-and-effect relationships between variables. This research method involves manipulating the independent variable and observing the effects on the dependent variable. Researchers use experimental research designs to test hypotheses and establish cause-and-effect relationships.
Survey Research
Survey research involves collecting data from a sample of individuals using a standardized questionnaire. This research method is used to gather information on attitudes, beliefs, and behaviors of individuals. Researchers use survey research to collect data quickly and efficiently from a large sample size. Survey research can be conducted through various methods such as online, phone, mail, or in-person interviews.
Quantitative Research Analysis Methods
Here are some commonly used quantitative research analysis methods:
Statistical Analysis
Statistical analysis is the most common quantitative research analysis method. It involves using statistical tools and techniques to analyze the numerical data collected during the research process. Statistical analysis can be used to identify patterns, trends, and relationships between variables, and to test hypotheses and theories.
Regression Analysis
Regression analysis is a statistical technique used to analyze the relationship between one dependent variable and one or more independent variables. Researchers use regression analysis to identify and quantify the impact of independent variables on the dependent variable.
Factor Analysis
Factor analysis is a statistical technique used to identify underlying factors that explain the correlations among a set of variables. Researchers use factor analysis to reduce a large number of variables to a smaller set of factors that capture the most important information.
Structural Equation Modeling
Structural equation modeling is a statistical technique used to test complex relationships between variables. It involves specifying a model that includes both observed and unobserved variables, and then using statistical methods to test the fit of the model to the data.
Time Series Analysis
Time series analysis is a statistical technique used to analyze data that is collected over time. It involves identifying patterns and trends in the data, as well as any seasonal or cyclical variations.
Multilevel Modeling
Multilevel modeling is a statistical technique used to analyze data that is nested within multiple levels. For example, researchers might use multilevel modeling to analyze data that is collected from individuals who are nested within groups, such as students nested within schools.
Applications of Quantitative Research
Quantitative research has many applications across a wide range of fields. Here are some common examples:
- Market Research : Quantitative research is used extensively in market research to understand consumer behavior, preferences, and trends. Researchers use surveys, experiments, and other quantitative methods to collect data that can inform marketing strategies, product development, and pricing decisions.
- Health Research: Quantitative research is used in health research to study the effectiveness of medical treatments, identify risk factors for diseases, and track health outcomes over time. Researchers use statistical methods to analyze data from clinical trials, surveys, and other sources to inform medical practice and policy.
- Social Science Research: Quantitative research is used in social science research to study human behavior, attitudes, and social structures. Researchers use surveys, experiments, and other quantitative methods to collect data that can inform social policies, educational programs, and community interventions.
- Education Research: Quantitative research is used in education research to study the effectiveness of teaching methods, assess student learning outcomes, and identify factors that influence student success. Researchers use experimental and quasi-experimental designs, as well as surveys and other quantitative methods, to collect and analyze data.
- Environmental Research: Quantitative research is used in environmental research to study the impact of human activities on the environment, assess the effectiveness of conservation strategies, and identify ways to reduce environmental risks. Researchers use statistical methods to analyze data from field studies, experiments, and other sources.
Characteristics of Quantitative Research
Here are some key characteristics of quantitative research:
- Numerical data : Quantitative research involves collecting numerical data through standardized methods such as surveys, experiments, and observational studies. This data is analyzed using statistical methods to identify patterns and relationships.
- Large sample size: Quantitative research often involves collecting data from a large sample of individuals or groups in order to increase the reliability and generalizability of the findings.
- Objective approach: Quantitative research aims to be objective and impartial in its approach, focusing on the collection and analysis of data rather than personal beliefs, opinions, or experiences.
- Control over variables: Quantitative research often involves manipulating variables to test hypotheses and establish cause-and-effect relationships. Researchers aim to control for extraneous variables that may impact the results.
- Replicable : Quantitative research aims to be replicable, meaning that other researchers should be able to conduct similar studies and obtain similar results using the same methods.
- Statistical analysis: Quantitative research involves using statistical tools and techniques to analyze the numerical data collected during the research process. Statistical analysis allows researchers to identify patterns, trends, and relationships between variables, and to test hypotheses and theories.
- Generalizability: Quantitative research aims to produce findings that can be generalized to larger populations beyond the specific sample studied. This is achieved through the use of random sampling methods and statistical inference.
Examples of Quantitative Research
Here are some examples of quantitative research in different fields:
- Market Research: A company conducts a survey of 1000 consumers to determine their brand awareness and preferences. The data is analyzed using statistical methods to identify trends and patterns that can inform marketing strategies.
- Health Research : A researcher conducts a randomized controlled trial to test the effectiveness of a new drug for treating a particular medical condition. The study involves collecting data from a large sample of patients and analyzing the results using statistical methods.
- Social Science Research : A sociologist conducts a survey of 500 people to study attitudes toward immigration in a particular country. The data is analyzed using statistical methods to identify factors that influence these attitudes.
- Education Research: A researcher conducts an experiment to compare the effectiveness of two different teaching methods for improving student learning outcomes. The study involves randomly assigning students to different groups and collecting data on their performance on standardized tests.
- Environmental Research : A team of researchers conduct a study to investigate the impact of climate change on the distribution and abundance of a particular species of plant or animal. The study involves collecting data on environmental factors and population sizes over time and analyzing the results using statistical methods.
- Psychology : A researcher conducts a survey of 500 college students to investigate the relationship between social media use and mental health. The data is analyzed using statistical methods to identify correlations and potential causal relationships.
- Political Science: A team of researchers conducts a study to investigate voter behavior during an election. They use survey methods to collect data on voting patterns, demographics, and political attitudes, and analyze the results using statistical methods.
How to Conduct Quantitative Research
Here is a general overview of how to conduct quantitative research:
- Develop a research question: The first step in conducting quantitative research is to develop a clear and specific research question. This question should be based on a gap in existing knowledge, and should be answerable using quantitative methods.
- Develop a research design: Once you have a research question, you will need to develop a research design. This involves deciding on the appropriate methods to collect data, such as surveys, experiments, or observational studies. You will also need to determine the appropriate sample size, data collection instruments, and data analysis techniques.
- Collect data: The next step is to collect data. This may involve administering surveys or questionnaires, conducting experiments, or gathering data from existing sources. It is important to use standardized methods to ensure that the data is reliable and valid.
- Analyze data : Once the data has been collected, it is time to analyze it. This involves using statistical methods to identify patterns, trends, and relationships between variables. Common statistical techniques include correlation analysis, regression analysis, and hypothesis testing.
- Interpret results: After analyzing the data, you will need to interpret the results. This involves identifying the key findings, determining their significance, and drawing conclusions based on the data.
- Communicate findings: Finally, you will need to communicate your findings. This may involve writing a research report, presenting at a conference, or publishing in a peer-reviewed journal. It is important to clearly communicate the research question, methods, results, and conclusions to ensure that others can understand and replicate your research.
When to use Quantitative Research
Here are some situations when quantitative research can be appropriate:
- To test a hypothesis: Quantitative research is often used to test a hypothesis or a theory. It involves collecting numerical data and using statistical analysis to determine if the data supports or refutes the hypothesis.
- To generalize findings: If you want to generalize the findings of your study to a larger population, quantitative research can be useful. This is because it allows you to collect numerical data from a representative sample of the population and use statistical analysis to make inferences about the population as a whole.
- To measure relationships between variables: If you want to measure the relationship between two or more variables, such as the relationship between age and income, or between education level and job satisfaction, quantitative research can be useful. It allows you to collect numerical data on both variables and use statistical analysis to determine the strength and direction of the relationship.
- To identify patterns or trends: Quantitative research can be useful for identifying patterns or trends in data. For example, you can use quantitative research to identify trends in consumer behavior or to identify patterns in stock market data.
- To quantify attitudes or opinions : If you want to measure attitudes or opinions on a particular topic, quantitative research can be useful. It allows you to collect numerical data using surveys or questionnaires and analyze the data using statistical methods to determine the prevalence of certain attitudes or opinions.
Purpose of Quantitative Research
The purpose of quantitative research is to systematically investigate and measure the relationships between variables or phenomena using numerical data and statistical analysis. The main objectives of quantitative research include:
- Description : To provide a detailed and accurate description of a particular phenomenon or population.
- Explanation : To explain the reasons for the occurrence of a particular phenomenon, such as identifying the factors that influence a behavior or attitude.
- Prediction : To predict future trends or behaviors based on past patterns and relationships between variables.
- Control : To identify the best strategies for controlling or influencing a particular outcome or behavior.
Quantitative research is used in many different fields, including social sciences, business, engineering, and health sciences. It can be used to investigate a wide range of phenomena, from human behavior and attitudes to physical and biological processes. The purpose of quantitative research is to provide reliable and valid data that can be used to inform decision-making and improve understanding of the world around us.
Advantages of Quantitative Research
There are several advantages of quantitative research, including:
- Objectivity : Quantitative research is based on objective data and statistical analysis, which reduces the potential for bias or subjectivity in the research process.
- Reproducibility : Because quantitative research involves standardized methods and measurements, it is more likely to be reproducible and reliable.
- Generalizability : Quantitative research allows for generalizations to be made about a population based on a representative sample, which can inform decision-making and policy development.
- Precision : Quantitative research allows for precise measurement and analysis of data, which can provide a more accurate understanding of phenomena and relationships between variables.
- Efficiency : Quantitative research can be conducted relatively quickly and efficiently, especially when compared to qualitative research, which may involve lengthy data collection and analysis.
- Large sample sizes : Quantitative research can accommodate large sample sizes, which can increase the representativeness and generalizability of the results.
Limitations of Quantitative Research
There are several limitations of quantitative research, including:
- Limited understanding of context: Quantitative research typically focuses on numerical data and statistical analysis, which may not provide a comprehensive understanding of the context or underlying factors that influence a phenomenon.
- Simplification of complex phenomena: Quantitative research often involves simplifying complex phenomena into measurable variables, which may not capture the full complexity of the phenomenon being studied.
- Potential for researcher bias: Although quantitative research aims to be objective, there is still the potential for researcher bias in areas such as sampling, data collection, and data analysis.
- Limited ability to explore new ideas: Quantitative research is often based on pre-determined research questions and hypotheses, which may limit the ability to explore new ideas or unexpected findings.
- Limited ability to capture subjective experiences : Quantitative research is typically focused on objective data and may not capture the subjective experiences of individuals or groups being studied.
- Ethical concerns : Quantitative research may raise ethical concerns, such as invasion of privacy or the potential for harm to participants.
About the author
Muhammad Hassan
Researcher, Academic Writer, Web developer
You may also like

Observational Research – Methods and Guide

Correlational Research – Methods, Types and...

Case Study – Methods, Examples and Guide

Mixed Methods Research – Types & Analysis

Questionnaire – Definition, Types, and Examples

Research Methods – Types, Examples and Guide
Educational resources and simple solutions for your research journey
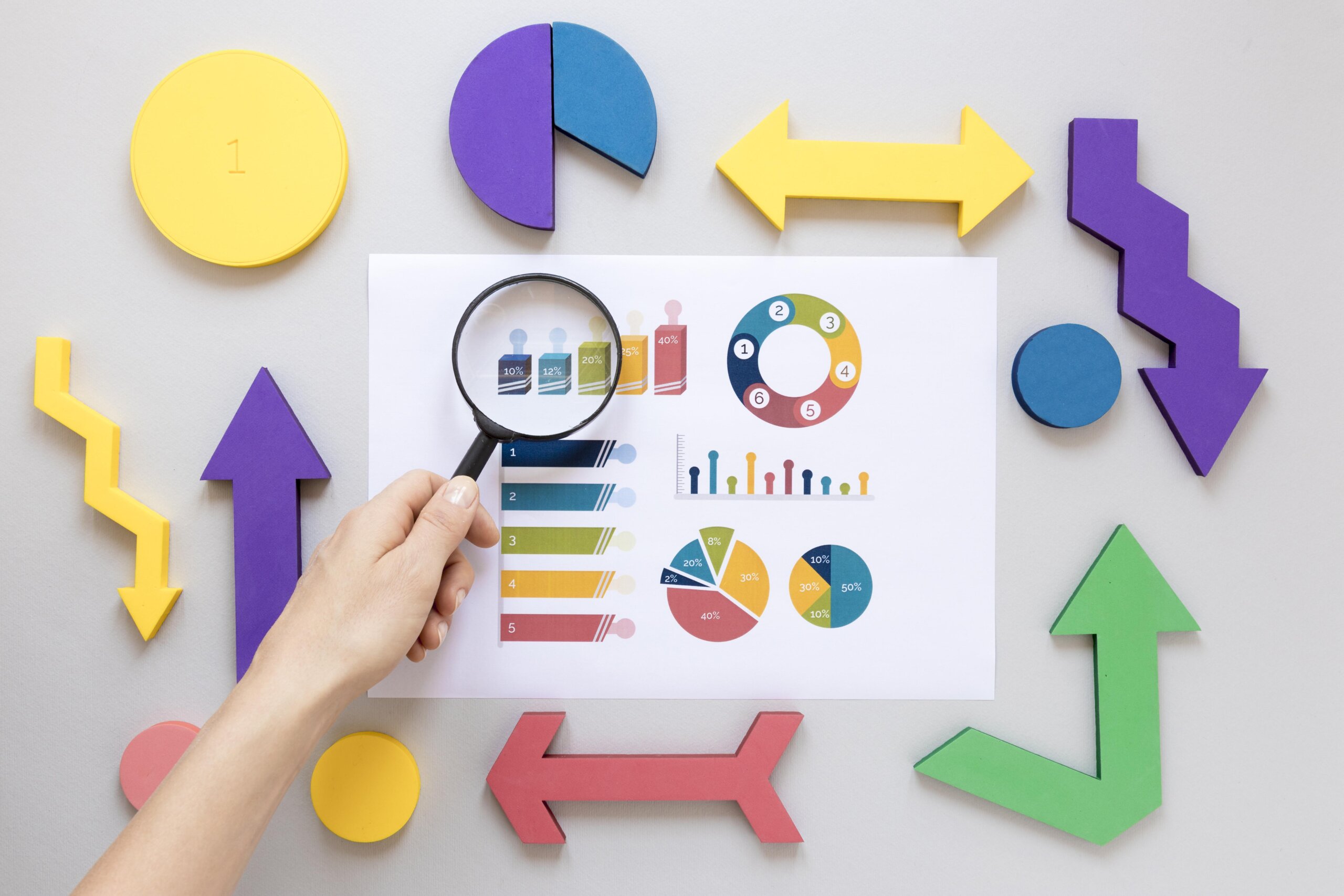
What is Quantitative Research? Definition, Methods, Types, and Examples

If you’re wondering what is quantitative research and whether this methodology works for your research study, you’re not alone. If you want a simple quantitative research definition , then it’s enough to say that this is a method undertaken by researchers based on their study requirements. However, to select the most appropriate research for their study type, researchers should know all the methods available.
Selecting the right research method depends on a few important criteria, such as the research question, study type, time, costs, data availability, and availability of respondents. There are two main types of research methods— quantitative research and qualitative research. The purpose of quantitative research is to validate or test a theory or hypothesis and that of qualitative research is to understand a subject or event or identify reasons for observed patterns.
Quantitative research methods are used to observe events that affect a particular group of individuals, which is the sample population. In this type of research, diverse numerical data are collected through various methods and then statistically analyzed to aggregate the data, compare them, or show relationships among the data. Quantitative research methods broadly include questionnaires, structured observations, and experiments.
Here are two quantitative research examples:
- Satisfaction surveys sent out by a company regarding their revamped customer service initiatives. Customers are asked to rate their experience on a rating scale of 1 (poor) to 5 (excellent).
- A school has introduced a new after-school program for children, and a few months after commencement, the school sends out feedback questionnaires to the parents of the enrolled children. Such questionnaires usually include close-ended questions that require either definite answers or a Yes/No option. This helps in a quick, overall assessment of the program’s outreach and success.

Table of Contents
What is quantitative research ? 1,2

The steps shown in the figure can be grouped into the following broad steps:
- Theory : Define the problem area or area of interest and create a research question.
- Hypothesis : Develop a hypothesis based on the research question. This hypothesis will be tested in the remaining steps.
- Research design : In this step, the most appropriate quantitative research design will be selected, including deciding on the sample size, selecting respondents, identifying research sites, if any, etc.
- Data collection : This process could be extensive based on your research objective and sample size.
- Data analysis : Statistical analysis is used to analyze the data collected. The results from the analysis help in either supporting or rejecting your hypothesis.
- Present results : Based on the data analysis, conclusions are drawn, and results are presented as accurately as possible.
Quantitative research characteristics 4
- Large sample size : This ensures reliability because this sample represents the target population or market. Due to the large sample size, the outcomes can be generalized to the entire population as well, making this one of the important characteristics of quantitative research .
- Structured data and measurable variables: The data are numeric and can be analyzed easily. Quantitative research involves the use of measurable variables such as age, salary range, highest education, etc.
- Easy-to-use data collection methods : The methods include experiments, controlled observations, and questionnaires and surveys with a rating scale or close-ended questions, which require simple and to-the-point answers; are not bound by geographical regions; and are easy to administer.
- Data analysis : Structured and accurate statistical analysis methods using software applications such as Excel, SPSS, R. The analysis is fast, accurate, and less effort intensive.
- Reliable : The respondents answer close-ended questions, their responses are direct without ambiguity and yield numeric outcomes, which are therefore highly reliable.
- Reusable outcomes : This is one of the key characteristics – outcomes of one research can be used and replicated in other research as well and is not exclusive to only one study.
Quantitative research methods 5
Quantitative research methods are classified into two types—primary and secondary.
Primary quantitative research method:
In this type of quantitative research , data are directly collected by the researchers using the following methods.
– Survey research : Surveys are the easiest and most commonly used quantitative research method . They are of two types— cross-sectional and longitudinal.
->Cross-sectional surveys are specifically conducted on a target population for a specified period, that is, these surveys have a specific starting and ending time and researchers study the events during this period to arrive at conclusions. The main purpose of these surveys is to describe and assess the characteristics of a population. There is one independent variable in this study, which is a common factor applicable to all participants in the population, for example, living in a specific city, diagnosed with a specific disease, of a certain age group, etc. An example of a cross-sectional survey is a study to understand why individuals residing in houses built before 1979 in the US are more susceptible to lead contamination.
->Longitudinal surveys are conducted at different time durations. These surveys involve observing the interactions among different variables in the target population, exposing them to various causal factors, and understanding their effects across a longer period. These studies are helpful to analyze a problem in the long term. An example of a longitudinal study is the study of the relationship between smoking and lung cancer over a long period.
– Descriptive research : Explains the current status of an identified and measurable variable. Unlike other types of quantitative research , a hypothesis is not needed at the beginning of the study and can be developed even after data collection. This type of quantitative research describes the characteristics of a problem and answers the what, when, where of a problem. However, it doesn’t answer the why of the problem and doesn’t explore cause-and-effect relationships between variables. Data from this research could be used as preliminary data for another study. Example: A researcher undertakes a study to examine the growth strategy of a company. This sample data can be used by other companies to determine their own growth strategy.

– Correlational research : This quantitative research method is used to establish a relationship between two variables using statistical analysis and analyze how one affects the other. The research is non-experimental because the researcher doesn’t control or manipulate any of the variables. At least two separate sample groups are needed for this research. Example: Researchers studying a correlation between regular exercise and diabetes.
– Causal-comparative research : This type of quantitative research examines the cause-effect relationships in retrospect between a dependent and independent variable and determines the causes of the already existing differences between groups of people. This is not a true experiment because it doesn’t assign participants to groups randomly. Example: To study the wage differences between men and women in the same role. For this, already existing wage information is analyzed to understand the relationship.
– Experimental research : This quantitative research method uses true experiments or scientific methods for determining a cause-effect relation between variables. It involves testing a hypothesis through experiments, in which one or more independent variables are manipulated and then their effect on dependent variables are studied. Example: A researcher studies the importance of a drug in treating a disease by administering the drug in few patients and not administering in a few.
The following data collection methods are commonly used in primary quantitative research :
- Sampling : The most common type is probability sampling, in which a sample is chosen from a larger population using some form of random selection, that is, every member of the population has an equal chance of being selected. The different types of probability sampling are—simple random, systematic, stratified, and cluster sampling.
- Interviews : These are commonly telephonic or face-to-face.
- Observations : Structured observations are most commonly used in quantitative research . In this method, researchers make observations about specific behaviors of individuals in a structured setting.
- Document review : Reviewing existing research or documents to collect evidence for supporting the quantitative research .
- Surveys and questionnaires : Surveys can be administered both online and offline depending on the requirement and sample size.
The data collected can be analyzed in several ways in quantitative research , as listed below:
- Cross-tabulation —Uses a tabular format to draw inferences among collected data
- MaxDiff analysis —Gauges the preferences of the respondents
- TURF analysis —Total Unduplicated Reach and Frequency Analysis; helps in determining the market strategy for a business
- Gap analysis —Identify gaps in attaining the desired results
- SWOT analysis —Helps identify strengths, weaknesses, opportunities, and threats of a product, service, or organization
- Text analysis —Used for interpreting unstructured data
Secondary quantitative research methods :
This method involves conducting research using already existing or secondary data. This method is less effort intensive and requires lesser time. However, researchers should verify the authenticity and recency of the sources being used and ensure their accuracy.
The main sources of secondary data are:
- The Internet
- Government and non-government sources
- Public libraries
- Educational institutions
- Commercial information sources such as newspapers, journals, radio, TV
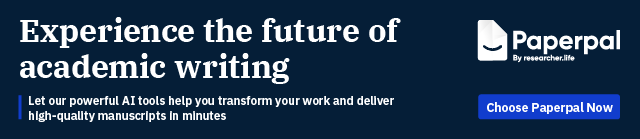
When to use quantitative research 6
Here are some simple ways to decide when to use quantitative research . Use quantitative research to:
- recommend a final course of action
- find whether a consensus exists regarding a particular subject
- generalize results to a larger population
- determine a cause-and-effect relationship between variables
- describe characteristics of specific groups of people
- test hypotheses and examine specific relationships
- identify and establish size of market segments
A research case study to understand when to use quantitative research 7
Context: A study was undertaken to evaluate a major innovation in a hospital’s design, in terms of workforce implications and impact on patient and staff experiences of all single-room hospital accommodations. The researchers undertook a mixed methods approach to answer their research questions. Here, we focus on the quantitative research aspect.
Research questions : What are the advantages and disadvantages for the staff as a result of the hospital’s move to the new design with all single-room accommodations? Did the move affect staff experience and well-being and improve their ability to deliver high-quality care?
Method: The researchers obtained quantitative data from three sources:
- Staff activity (task time distribution): Each staff member was shadowed by a researcher who observed each task undertaken by the staff, and logged the time spent on each activity.
- Staff travel distances : The staff were requested to wear pedometers, which recorded the distances covered.
- Staff experience surveys : Staff were surveyed before and after the move to the new hospital design.
Results of quantitative research : The following observations were made based on quantitative data analysis:
- The move to the new design did not result in a significant change in the proportion of time spent on different activities.
- Staff activity events observed per session were higher after the move, and direct care and professional communication events per hour decreased significantly, suggesting fewer interruptions and less fragmented care.
- A significant increase in medication tasks among the recorded events suggests that medication administration was integrated into patient care activities.
- Travel distances increased for all staff, with highest increases for staff in the older people’s ward and surgical wards.
- Ratings for staff toilet facilities, locker facilities, and space at staff bases were higher but those for social interaction and natural light were lower.
Advantages of quantitative research 1,2
When choosing the right research methodology, also consider the advantages of quantitative research and how it can impact your study.
- Quantitative research methods are more scientific and rational. They use quantifiable data leading to objectivity in the results and avoid any chances of ambiguity.
- This type of research uses numeric data so analysis is relatively easier .
- In most cases, a hypothesis is already developed and quantitative research helps in testing and validatin g these constructed theories based on which researchers can make an informed decision about accepting or rejecting their theory.
- The use of statistical analysis software ensures quick analysis of large volumes of data and is less effort intensive.
- Higher levels of control can be applied to the research so the chances of bias can be reduced.
- Quantitative research is based on measured value s, facts, and verifiable information so it can be easily checked or replicated by other researchers leading to continuity in scientific research.
Disadvantages of quantitative research 1,2
Quantitative research may also be limiting; take a look at the disadvantages of quantitative research.
- Experiments are conducted in controlled settings instead of natural settings and it is possible for researchers to either intentionally or unintentionally manipulate the experiment settings to suit the results they desire.
- Participants must necessarily give objective answers (either one- or two-word, or yes or no answers) and the reasons for their selection or the context are not considered.
- Inadequate knowledge of statistical analysis methods may affect the results and their interpretation.
- Although statistical analysis indicates the trends or patterns among variables, the reasons for these observed patterns cannot be interpreted and the research may not give a complete picture.
- Large sample sizes are needed for more accurate and generalizable analysis .
- Quantitative research cannot be used to address complex issues.
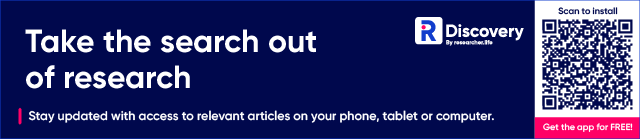
Frequently asked questions on quantitative research
Q: What is the difference between quantitative research and qualitative research? 1
A: The following table lists the key differences between quantitative research and qualitative research, some of which may have been mentioned earlier in the article.
Purpose and design | ||
Research question | ||
Sample size | Large | Small |
Data | ||
Data collection method | Experiments, controlled observations, questionnaires and surveys with a rating scale or close-ended questions. The methods can be experimental, quasi-experimental, descriptive, or correlational. | Semi-structured interviews/surveys with open-ended questions, document study/literature reviews, focus groups, case study research, ethnography |
Data analysis |
Q: What is the difference between reliability and validity? 8,9
A: The term reliability refers to the consistency of a research study. For instance, if a food-measuring weighing scale gives different readings every time the same quantity of food is measured then that weighing scale is not reliable. If the findings in a research study are consistent every time a measurement is made, then the study is considered reliable. However, it is usually unlikely to obtain the exact same results every time because some contributing variables may change. In such cases, a correlation coefficient is used to assess the degree of reliability. A strong positive correlation between the results indicates reliability.
Validity can be defined as the degree to which a tool actually measures what it claims to measure. It helps confirm the credibility of your research and suggests that the results may be generalizable. In other words, it measures the accuracy of the research.
The following table gives the key differences between reliability and validity.
Importance | Refers to the consistency of a measure | Refers to the accuracy of a measure |
Ease of achieving | Easier, yields results faster | Involves more analysis, more difficult to achieve |
Assessment method | By examining the consistency of outcomes over time, between various observers, and within the test | By comparing the accuracy of the results with accepted theories and other measurements of the same idea |
Relationship | Unreliable measurements typically cannot be valid | Valid measurements are also reliable |
Types | Test-retest reliability, internal consistency, inter-rater reliability | Content validity, criterion validity, face validity, construct validity |
Q: What is mixed methods research? 10
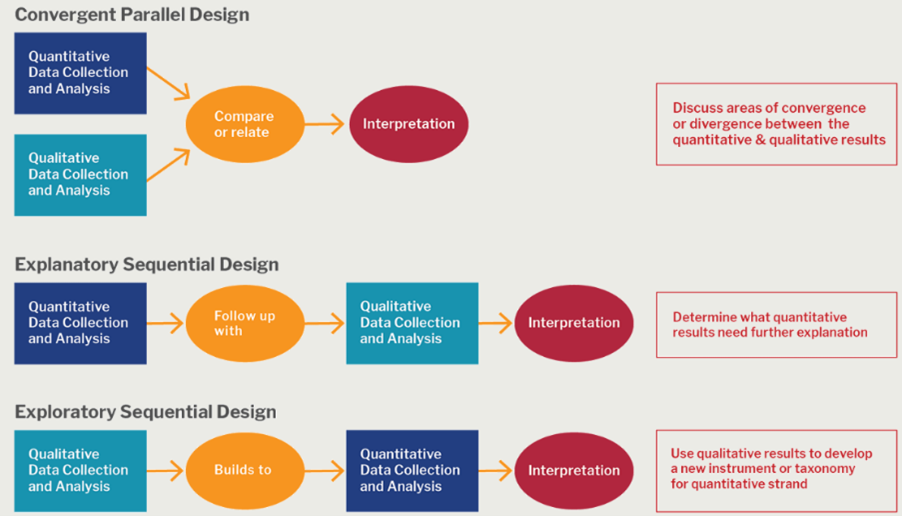
A: A mixed methods approach combines the characteristics of both quantitative research and qualitative research in the same study. This method allows researchers to validate their findings, verify if the results observed using both methods are complementary, and explain any unexpected results obtained from one method by using the other method. A mixed methods research design is useful in case of research questions that cannot be answered by either quantitative research or qualitative research alone. However, this method could be more effort- and cost-intensive because of the requirement of more resources. The figure 3 shows some basic mixed methods research designs that could be used.
Thus, quantitative research is the appropriate method for testing your hypotheses and can be used either alone or in combination with qualitative research per your study requirements. We hope this article has provided an insight into the various facets of quantitative research , including its different characteristics, advantages, and disadvantages, and a few tips to quickly understand when to use this research method.
References
- Qualitative vs quantitative research: Differences, examples, & methods. Simply Psychology. Accessed Feb 28, 2023. https://simplypsychology.org/qualitative-quantitative.html#Quantitative-Research
- Your ultimate guide to quantitative research. Qualtrics. Accessed February 28, 2023. https://www.qualtrics.com/uk/experience-management/research/quantitative-research/
- The steps of quantitative research. Revise Sociology. Accessed March 1, 2023. https://revisesociology.com/2017/11/26/the-steps-of-quantitative-research/
- What are the characteristics of quantitative research? Marketing91. Accessed March 1, 2023. https://www.marketing91.com/characteristics-of-quantitative-research/
- Quantitative research: Types, characteristics, methods, & examples. ProProfs Survey Maker. Accessed February 28, 2023. https://www.proprofssurvey.com/blog/quantitative-research/#Characteristics_of_Quantitative_Research
- Qualitative research isn’t as scientific as quantitative methods. Kmusial blog. Accessed March 5, 2023. https://kmusial.wordpress.com/2011/11/25/qualitative-research-isnt-as-scientific-as-quantitative-methods/
- Maben J, Griffiths P, Penfold C, et al. Evaluating a major innovation in hospital design: workforce implications and impact on patient and staff experiences of all single room hospital accommodation. Southampton (UK): NIHR Journals Library; 2015 Feb. (Health Services and Delivery Research, No. 3.3.) Chapter 5, Case study quantitative data findings. Accessed March 6, 2023. https://www.ncbi.nlm.nih.gov/books/NBK274429/
- McLeod, S. A. (2007). What is reliability? Simply Psychology. www.simplypsychology.org/reliability.html
- Reliability vs validity: Differences & examples. Accessed March 5, 2023. https://statisticsbyjim.com/basics/reliability-vs-validity/
- Mixed methods research. Community Engagement Program. Harvard Catalyst. Accessed February 28, 2023. https://catalyst.harvard.edu/community-engagement/mmr
Editage All Access is a subscription-based platform that unifies the best AI tools and services designed to speed up, simplify, and streamline every step of a researcher’s journey. The Editage All Access Pack is a one-of-a-kind subscription that unlocks full access to an AI writing assistant, literature recommender, journal finder, scientific illustration tool, and exclusive discounts on professional publication services from Editage.
Based on 22+ years of experience in academia, Editage All Access empowers researchers to put their best research forward and move closer to success. Explore our top AI Tools pack, AI Tools + Publication Services pack, or Build Your Own Plan. Find everything a researcher needs to succeed, all in one place – Get All Access now starting at just $14 a month !
Related Posts

How to Write a Dissertation: A Beginner’s Guide

Back to School – Lock-in All Access Pack for a Year at the Best Price
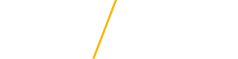
- Chapter Four: Quantitative Methods (Part 1)
Once you have chosen a topic to investigate, you need to decide which type of method is best to study it. This is one of the most important choices you will make on your research journey. Understanding the value of each of the methods described in this textbook to answer different questions allows you to be able to plan your own studies with more confidence, critique the studies others have done, and provide advice to your colleagues and friends on what type of research they should do to answer questions they have. After briefly reviewing quantitative research assumptions, this chapter is organized in three parts or sections. These parts can also be used as a checklist when working through the steps of your study. Specifically, part 1 focuses on planning a quantitative study (collecting data), part two explains the steps involved in doing a quantitative study, and part three discusses how to make sense of your results (organizing and analyzing data).
- Chapter One: Introduction
- Chapter Two: Understanding the distinctions among research methods
- Chapter Three: Ethical research, writing, and creative work
- Chapter Four: Quantitative Methods (Part 2 - Doing Your Study)
- Chapter Four: Quantitative Methods (Part 3 - Making Sense of Your Study)
- Chapter Five: Qualitative Methods (Part 1)
- Chapter Five: Qualitative Data (Part 2)
- Chapter Six: Critical / Rhetorical Methods (Part 1)
- Chapter Six: Critical / Rhetorical Methods (Part 2)
- Chapter Seven: Presenting Your Results
Quantitative Worldview Assumptions: A Review
In chapter 2, you were introduced to the unique assumptions quantitative research holds about knowledge and how it is created, or what the authors referred to in chapter one as "epistemology." Understanding these assumptions can help you better determine whether you need to use quantitative methods for a particular research study in which you are interested.
Quantitative researchers believe there is an objective reality, which can be measured. "Objective" here means that the researcher is not relying on their own perceptions of an event. S/he is attempting to gather "facts" which may be separate from people's feeling or perceptions about the facts. These facts are often conceptualized as "causes" and "effects." When you ask research questions or pose hypotheses with words in them such as "cause," "effect," "difference between," and "predicts," you are operating under assumptions consistent with quantitative methods. The overall goal of quantitative research is to develop generalizations that enable the researcher to better predict, explain, and understand some phenomenon.
Because of trying to prove cause-effect relationships that can be generalized to the population at large, the research process and related procedures are very important for quantitative methods. Research should be consistently and objectively conducted, without bias or error, in order to be considered to be valid (accurate) and reliable (consistent). Perhaps this emphasis on accurate and standardized methods is because the roots of quantitative research are in the natural and physical sciences, both of which have at their base the need to prove hypotheses and theories in order to better understand the world in which we live. When a person goes to a doctor and is prescribed some medicine to treat an illness, that person is glad such research has been done to know what the effects of taking this medicine is on others' bodies, so s/he can trust the doctor's judgment and take the medicines.
As covered in chapters 1 and 2, the questions you are asking should lead you to a certain research method choice. Students sometimes want to avoid doing quantitative research because of fear of math/statistics, but if their questions call for that type of research, they should forge ahead and use it anyway. If a student really wants to understand what the causes or effects are for a particular phenomenon, they need to do quantitative research. If a student is interested in what sorts of things might predict a person's behavior, they need to do quantitative research. If they want to confirm the finding of another researcher, most likely they will need to do quantitative research. If a student wishes to generalize beyond their participant sample to a larger population, they need to be conducting quantitative research.
So, ultimately, your choice of methods really depends on what your research goal is. What do you really want to find out? Do you want to compare two or more groups, look for relationships between certain variables, predict how someone will act or react, or confirm some findings from another study? If so, you want to use quantitative methods.
A topic such as self-esteem can be studied in many ways. Listed below are some example RQs about self-esteem. Which of the following research questions should be answered with quantitative methods?
- Is there a difference between men's and women's level of self- esteem?
- How do college-aged women describe their ups and downs with self-esteem?
- How has "self-esteem" been constructed in popular self-help books over time?
- Is there a relationship between self-esteem levels and communication apprehension?
What are the advantages of approaching a topic like self-esteem using quantitative methods? What are the disadvantages?
For more information, see the following website: Analyse This!!! Learning to analyse quantitative data
Answers: 1 & 4
Quantitative Methods Part One: Planning Your Study
Planning your study is one of the most important steps in the research process when doing quantitative research. As seen in the diagram below, it involves choosing a topic, writing research questions/hypotheses, and designing your study. Each of these topics will be covered in detail in this section of the chapter.
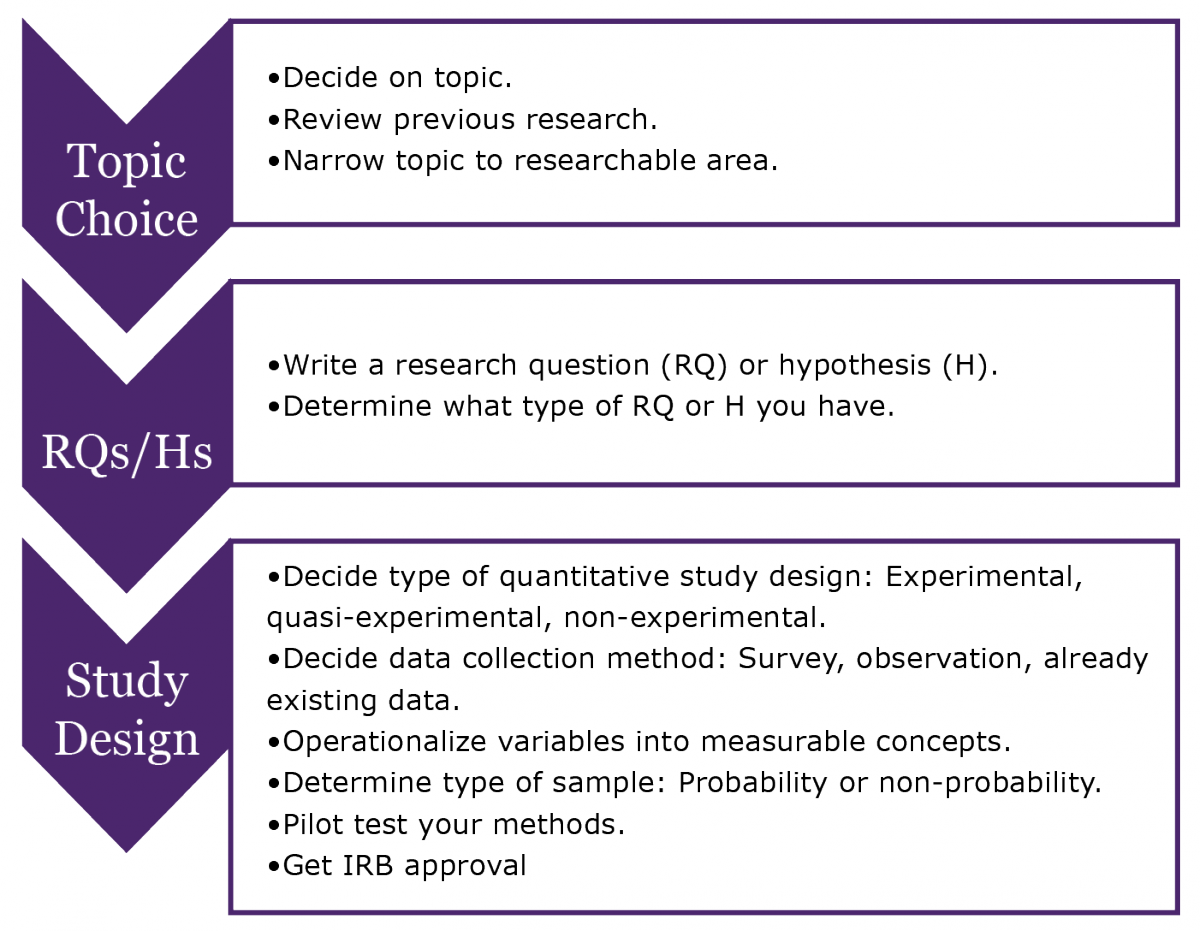
Topic Choice
Decide on topic.
How do you go about choosing a topic for a research project? One of the best ways to do this is to research something about which you would like to know more. Your communication professors will probably also want you to select something that is related to communication and things you are learning about in other communication classes.
When the authors of this textbook select research topics to study, they choose things that pique their interest for a variety of reasons, sometimes personal and sometimes because they see a need for more research in a particular area. For example, April Chatham-Carpenter studies adoption return trips to China because she has two adopted daughters from China and because there is very little research on this topic for Chinese adoptees and their families; she studied home vs. public schooling because her sister home schools, and at the time she started the study very few researchers had considered the social network implications for home schoolers (cf. http://www.uni.edu/chatham/homeschool.html ).
When you are asked in this class and other classes to select a topic to research, think about topics that you have wondered about, that affect you personally, or that know have gaps in the research. Then start writing down questions you would like to know about this topic. These questions will help you decide whether the goal of your study is to understand something better, explain causes and effects of something, gather the perspectives of others on a topic, or look at how language constructs a certain view of reality.
Review Previous Research
In quantitative research, you do not rely on your conclusions to emerge from the data you collect. Rather, you start out looking for certain things based on what the past research has found. This is consistent with what was called in chapter 2 as a deductive approach (Keyton, 2011), which also leads a quantitative researcher to develop a research question or research problem from reviewing a body of literature, with the previous research framing the study that is being done. So, reviewing previous research done on your topic is an important part of the planning of your study. As seen in chapter 3 and the Appendix, to do an adequate literature review, you need to identify portions of your topic that could have been researched in the past. To do that, you select key terms of concepts related to your topic.
Some people use concept maps to help them identify useful search terms for a literature review. For example, see the following website: Concept Mapping: How to Start Your Term Paper Research .
Narrow Topic to Researchable Area
Once you have selected your topic area and reviewed relevant literature related to your topic, you need to narrow your topic to something that can be researched practically and that will take the research on this topic further. You don't want your research topic to be so broad or large that you are unable to research it. Plus, you want to explain some phenomenon better than has been done before, adding to the literature and theory on a topic. You may want to test out what someone else has found, replicating their study, and therefore building to the body of knowledge already created.
To see how a literature review can be helpful in narrowing your topic, see the following sources. Narrowing or Broadening Your Research Topic and How to Conduct a Literature Review in Social Science
Research Questions & Hypotheses
Write Your Research Questions (RQs) and/or Hypotheses (Hs)
Once you have narrowed your topic based on what you learned from doing your review of literature, you need to formalize your topic area into one or more research questions or hypotheses. If the area you are researching is a relatively new area, and no existing literature or theory can lead you to predict what you might find, then you should write a research question. Take a topic related to social media, for example, which is a relatively new area of study. You might write a research question that asks:
"Is there a difference between how 1st year and 4th year college students use Facebook to communicate with their friends?"
If, however, you are testing out something you think you might find based on the findings of a large amount of previous literature or a well-developed theory, you can write a hypothesis. Researchers often distinguish between null and alternative hypotheses. The alternative hypothesis is what you are trying to test or prove is true, while the null hypothesis assumes that the alternative hypothesis is not true. For example, if the use of Facebook had been studied a great deal, and there were theories that had been developed on the use of it, then you might develop an alternative hypothesis, such as: "First-year students spend more time on using Facebook to communicate with their friends than fourth-year students do." Your null hypothesis, on the other hand, would be: "First-year students do not spend any more time using Facebook to communication with their friends than fourth-year students do." Researchers, however, only state the alternative hypothesis in their studies, and actually call it "hypothesis" rather than "alternative hypothesis."
Process of Writing a Research Question/Hypothesis.
Once you have decided to write a research question (RQ) or hypothesis (H) for your topic, you should go through the following steps to create your RQ or H.
Name the concepts from your overall research topic that you are interested in studying.
RQs and Hs have variables, or concepts that you are interested in studying. Variables can take on different values. For example, in the RQ above, there are at least two variables – year in college and use of Facebook (FB) to communicate. Both of them have a variety of levels within them.
When you look at the concepts you identified, are there any concepts which seem to be related to each other? For example, in our RQ, we are interested in knowing if there is a difference between first-year students and fourth-year students in their use of FB, meaning that we believe there is some connection between our two variables.
- Decide what type of a relationship you would like to study between the variables. Do you think one causes the other? Does a difference in one create a difference in the other? As the value of one changes, does the value of the other change?
Identify which one of these concepts is the independent (or predictor) variable, or the concept that is perceived to be the cause of change in the other variable? Which one is the dependent (criterion) variable, or the one that is affected by changes in the independent variable? In the above example RQ, year in school is the independent variable, and amount of time spent on Facebook communicating with friends is the dependent variable. The amount of time spent on Facebook depends on a person's year in school.
If you're still confused about independent and dependent variables, check out the following site: Independent & Dependent Variables .
Express the relationship between the concepts as a single sentence – in either a hypothesis or a research question.
For example, "is there a difference between international and American students on their perceptions of the basic communication course," where cultural background and perceptions of the course are your two variables. Cultural background would be the independent variable, and perceptions of the course would be your dependent variable. More examples of RQs and Hs are provided in the next section.
APPLICATION: Try the above steps with your topic now. Check with your instructor to see if s/he would like you to send your topic and RQ/H to him/her via e-mail.
Types of Research Questions/Hypotheses
Once you have written your RQ/H, you need to determine what type of research question or hypothesis it is. This will help you later decide what types of statistics you will need to run to answer your question or test your hypothesis. There are three possible types of questions you might ask, and two possible types of hypotheses. The first type of question cannot be written as a hypothesis, but the second and third types can.
Descriptive Question.
The first type of question is a descriptive question. If you have only one variable or concept you are studying, OR if you are not interested in how the variables you are studying are connected or related to each other, then your question is most likely a descriptive question.
This type of question is the closest to looking like a qualitative question, and often starts with a "what" or "how" or "why" or "to what extent" type of wording. What makes it different from a qualitative research question is that the question will be answered using numbers rather than qualitative analysis. Some examples of a descriptive question, using the topic of social media, include the following.
"To what extent are college-aged students using Facebook to communicate with their friends?"
"Why do college-aged students use Facebook to communicate with their friends?"
Notice that neither of these questions has a clear independent or dependent variable, as there is no clear cause or effect being assumed by the question. The question is merely descriptive in nature. It can be answered by summarizing the numbers obtained for each category, such as by providing percentages, averages, or just the raw totals for each type of strategy or organization. This is true also of the following research questions found in a study of online public relations strategies:
"What online public relations strategies are organizations implementing to combat phishing" (Baker, Baker, & Tedesco, 2007, p. 330), and
"Which organizations are doing most and least, according to recommendations from anti- phishing advocacy recommendations, to combat phishing" (Baker, Baker, & Tedesco, 2007, p. 330)
The researchers in this study reported statistics in their results or findings section, making it clearly a quantitative study, but without an independent or dependent variable; therefore, these research questions illustrate the first type of RQ, the descriptive question.
Difference Question/Hypothesis.
The second type of question is a question/hypothesis of difference, and will often have the word "difference" as part of the question. The very first research question in this section, asking if there is a difference between 1st year and 4th year college students' use of Facebook, is an example of this type of question. In this type of question, the independent variable is some type of grouping or categories, such as age. Another example of a question of difference is one April asked in her research on home schooling: "Is there a difference between home vs. public schoolers on the size of their social networks?" In this example, the independent variable is home vs. public schooling (a group being compared), and the dependent variable is size of social networks. Hypotheses can also be difference hypotheses, as the following example on the same topic illustrates: "Public schoolers have a larger social network than home schoolers do."
Relationship/Association Question/Hypothesis.
The third type of question is a relationship/association question or hypothesis, and will often have the word "relate" or "relationship" in it, as the following example does: "There is a relationship between number of television ads for a political candidate and how successful that political candidate is in getting elected." Here the independent (or predictor) variable is number of TV ads, and the dependent (or criterion) variable is the success at getting elected. In this type of question, there is no grouping being compared, but rather the independent variable is continuous (ranges from zero to a certain number) in nature. This type of question can be worded as either a hypothesis or as a research question, as stated earlier.
Test out your knowledge of the above information, by answering the following questions about the RQ/H listed below. (Remember, for a descriptive question there are no clear independent & dependent variables.)
- What is the independent variable (IV)?
- What is the dependent variable (DV)?
- What type of research question/hypothesis is it? (descriptive, difference, relationship/association)
- "Is there a difference on relational satisfaction between those who met their current partner through online dating and those who met their current partner face-to-face?"
- "How do Fortune 500 firms use focus groups to market new products?"
- "There is a relationship between age and amount of time spent online using social media."
Answers: RQ1 is a difference question, with type of dating being the IV and relational satisfaction being the DV. RQ2 is a descriptive question with no IV or DV. RQ3 is a relationship hypothesis with age as the IV and amount of time spent online as the DV.
Design Your Study
The third step in planning your research project, after you have decided on your topic/goal and written your research questions/hypotheses, is to design your study which means to decide how to proceed in gathering data to answer your research question or to test your hypothesis. This step includes six things to do. [NOTE: The terms used in this section will be defined as they are used.]
- Decide type of study design: Experimental, quasi-experimental, non-experimental.
- Decide kind of data to collect: Survey/interview, observation, already existing data.
- Operationalize variables into measurable concepts.
- Determine type of sample: Probability or non-probability.
- Decide how you will collect your data: face-to-face, via e-mail, an online survey, library research, etc.
- Pilot test your methods.
Types of Study Designs
With quantitative research being rooted in the scientific method, traditional research is structured in an experimental fashion. This is especially true in the natural sciences, where they try to prove causes and effects on topics such as successful treatments for cancer. For example, the University of Iowa Hospitals and Clinics regularly conduct clinical trials to test for the effectiveness of certain treatments for medical conditions ( University of Iowa Hospitals & Clinics: Clinical Trials ). They use human participants to conduct such research, regularly recruiting volunteers. However, in communication, true experiments with treatments the researcher controls are less necessary and thus less common. It is important for the researcher to understand which type of study s/he wishes to do, in order to accurately communicate his/her methods to the public when describing the study.
There are three possible types of studies you may choose to do, when embarking on quantitative research: (a) True experiments, (b) quasi-experiments, and (c) non-experiments.
For more information to read on these types of designs, take a look at the following website and related links in it: Types of Designs .
The following flowchart should help you distinguish between the three types of study designs described below.
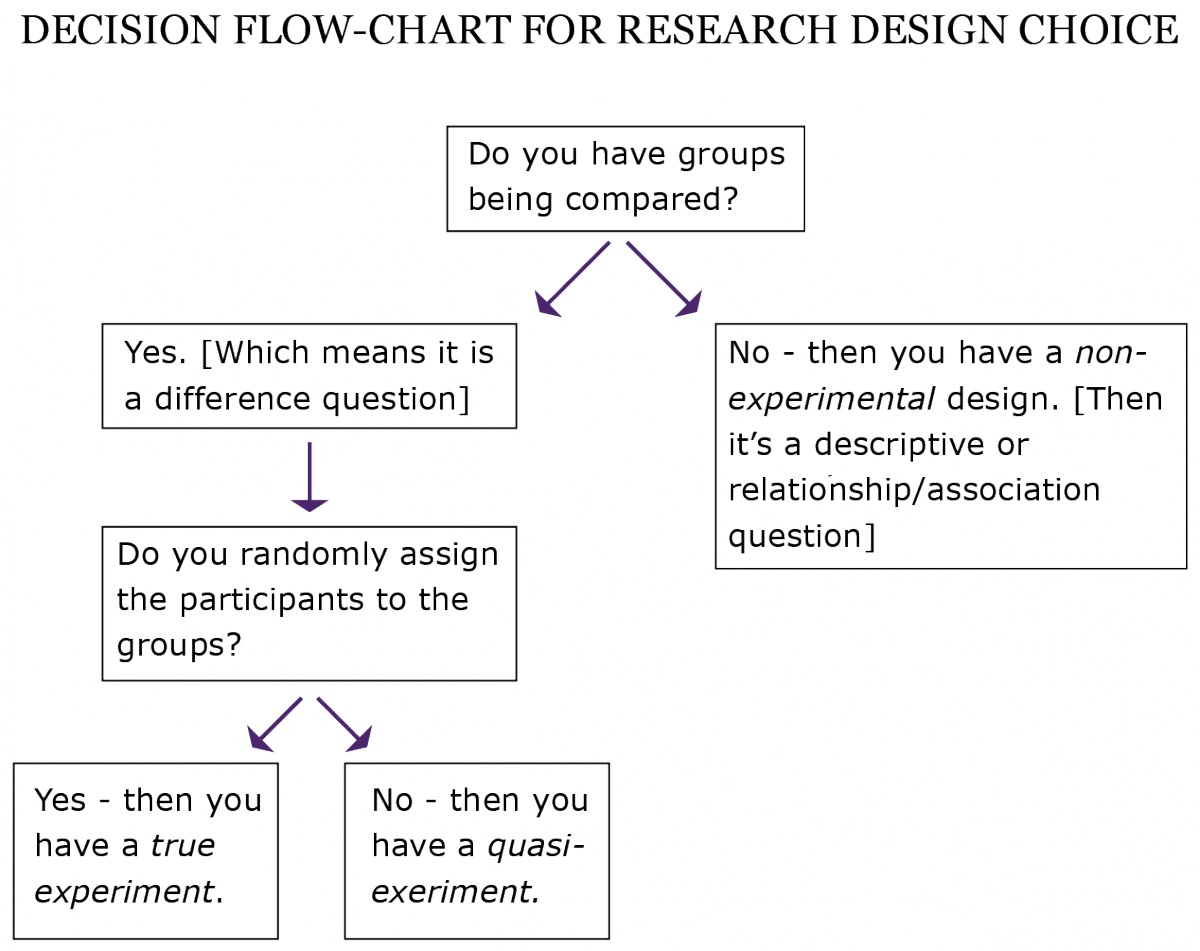
True Experiments.
The first two types of study designs use difference questions/hypotheses, as the independent variable for true and quasi-experiments is nominal or categorical (based on categories or groupings), as you have groups that are being compared. As seen in the flowchart above, what distinguishes a true experiment from the other two designs is a concept called "random assignment." Random assignment means that the researcher controls to which group the participants are assigned. April's study of home vs. public schooling was NOT a true experiment, because she could not control which participants were home schooled and which ones were public schooled, and instead relied on already existing groups.
An example of a true experiment reported in a communication journal is a study investigating the effects of using interest-based contemporary examples in a lecture on the history of public relations, in which the researchers had the following two hypotheses: "Lectures utilizing interest- based examples should result in more interested participants" and "Lectures utilizing interest- based examples should result in participants with higher scores on subsequent tests of cognitive recall" (Weber, Corrigan, Fornash, & Neupauer, 2003, p. 118). In this study, the 122 college student participants were randomly assigned by the researchers to one of two lecture video viewing groups: a video lecture with traditional examples and a video with contemporary examples. (To see the results of the study, look it up using your school's library databases).
A second example of a true experiment in communication is a study of the effects of viewing either a dramatic narrative television show vs. a nonnarrative television show about the consequences of an unexpected teen pregnancy. The researchers randomly assigned their 367 undergraduate participants to view one of the two types of shows.
Moyer-Gusé, E., & Nabi, R. L. (2010). Explaining the effects of narrative in an entertainment television program: Overcoming resistance to persuasion. Human Communication Research, 36 , 26-52.
A third example of a true experiment done in the field of communication can be found in the following study.
Jensen, J. D. (2008). Scientific uncertainty in news coverage of cancer research: Effects of hedging on scientists' and journalists' credibility. Human Communication Research, 34, 347-369.
In this study, Jakob Jensen had three independent variables. He randomly assigned his 601 participants to 1 of 20 possible conditions, between his three independent variables, which were (a) a hedged vs. not hedged message, (b) the source of the hedging message (research attributed to primary vs. unaffiliated scientists), and (c) specific news story employed (of which he had five randomly selected news stories about cancer research to choose from). Although this study was pretty complex, it does illustrate the true experiment in our field since the participants were randomly assigned to read a particular news story, with certain characteristics.
Quasi-Experiments.
If the researcher is not able to randomly assign participants to one of the treatment groups (or independent variable), but the participants already belong to one of them (e.g., age; home vs. public schooling), then the design is called a quasi-experiment. Here you still have an independent variable with groups, but the participants already belong to a group before the study starts, and the researcher has no control over which group they belong to.
An example of a hypothesis found in a communication study is the following: "Individuals high in trait aggression will enjoy violent content more than nonviolent content, whereas those low in trait aggression will enjoy violent content less than nonviolent content" (Weaver & Wilson, 2009, p. 448). In this study, the researchers could not assign the participants to a high or low trait aggression group since this is a personality characteristic, so this is a quasi-experiment. It does not have any random assignment of participants to the independent variable groups. Read their study, if you would like to, at the following location.
Weaver, A. J., & Wilson, B. J. (2009). The role of graphic and sanitized violence in the enjoyment of television dramas. Human Communication Research, 35 (3), 442-463.
Benoit and Hansen (2004) did not choose to randomly assign participants to groups either, in their study of a national presidential election survey, in which they were looking at differences between debate and non-debate viewers, in terms of several dependent variables, such as which candidate viewers supported. If you are interested in discovering the results of this study, take a look at the following article.
Benoit, W. L., & Hansen, G. J. (2004). Presidential debate watching, issue knowledge, character evaluation, and vote choice. Human Communication Research, 30 (1), 121-144.
Non-Experiments.
The third type of design is the non-experiment. Non-experiments are sometimes called survey designs, because their primary way of collecting data is through surveys. This is not enough to distinguish them from true experiments and quasi-experiments, however, as both of those types of designs may use surveys as well.
What makes a study a non-experiment is that the independent variable is not a grouping or categorical variable. Researchers observe or survey participants in order to describe them as they naturally exist without any experimental intervention. Researchers do not give treatments or observe the effects of a potential natural grouping variable such as age. Descriptive and relationship/association questions are most often used in non-experiments.
Some examples of this type of commonly used design for communication researchers include the following studies.
- Serota, Levine, and Boster (2010) used a national survey of 1,000 adults to determine the prevalence of lying in America (see Human Communication Research, 36 , pp. 2-25).
- Nabi (2009) surveyed 170 young adults on their perceptions of reality television on cosmetic surgery effects, looking at several things: for example, does viewing cosmetic surgery makeover programs relate to body satisfaction (p. 6), finding no significant relationship between those two variables (see Human Communication Research, 35 , pp. 1-27).
- Derlega, Winstead, Mathews, and Braitman (2008) collected stories from 238 college students on reasons why they would disclose or not disclose personal information within close relationships (see Communication Research Reports, 25 , pp. 115-130). They coded the participants' answers into categories so they could count how often specific reasons were mentioned, using a method called content analysis , to answer the following research questions:
RQ1: What are research participants' attributions for the disclosure and nondisclosure of highly personal information?
RQ2: Do attributions reflect concerns about rewards and costs of disclosure or the tension between openness with another and privacy?
RQ3: How often are particular attributions for disclosure/nondisclosure used in various types of relationships? (p. 117)
All of these non-experimental studies have in common no researcher manipulation of an independent variable or even having an independent variable that has natural groups that are being compared.
Identify which design discussed above should be used for each of the following research questions.
- Is there a difference between generations on how much they use MySpace?
- Is there a relationship between age when a person first started using Facebook and the amount of time they currently spend on Facebook daily?
- Is there a difference between potential customers' perceptions of an organization who are shown an organization's Facebook page and those who are not shown an organization's Facebook page?
[HINT: Try to identify the independent and dependent variable in each question above first, before determining what type of design you would use. Also, try to determine what type of question it is – descriptive, difference, or relationship/association.]
Answers: 1. Quasi-experiment 2. Non-experiment 3. True Experiment
Data Collection Methods
Once you decide the type of quantitative research design you will be using, you will need to determine which of the following types of data you will collect: (a) survey data, (b) observational data, and/or (c) already existing data, as in library research.
Using the survey data collection method means you will talk to people or survey them about their behaviors, attitudes, perceptions, and demographic characteristics (e.g., biological sex, socio-economic status, race). This type of data usually consists of a series of questions related to the concepts you want to study (i.e., your independent and dependent variables). Both of April's studies on home schooling and on taking adopted children on a return trip back to China used survey data.
On a survey, you can have both closed-ended and open-ended questions. Closed-ended questions, can be written in a variety of forms. Some of the most common response options include the following.
Likert responses – for example: for the following statement, ______ do you strongly agree agree neutral disagree strongly disagree
Semantic differential – for example: does the following ______ make you Happy ..................................... Sad
Yes-no answers for example: I use social media daily. Yes / No.
One site to check out for possible response options is http://www.360degreefeedback.net/media/ResponseScales.pdf .
Researchers often follow up some of their closed-ended questions with an "other" category, in which they ask their participants to "please specify," their response if none of the ones provided are applicable. They may also ask open-ended questions on "why" a participant chose a particular answer or ask participants for more information about a particular topic. If the researcher wants to use the open-ended question responses as part of his/her quantitative study, the answers are usually coded into categories and counted, in terms of the frequency of a certain answer, using a method called content analysis , which will be discussed when we talk about already-existing artifacts as a source of data.
Surveys can be done face-to-face, by telephone, mail, or online. Each of these methods has its own advantages and disadvantages, primarily in the form of the cost in time and money to do the survey. For example, if you want to survey many people, then online survey tools such as surveygizmo.com and surveymonkey.com are very efficient, but not everyone has access to taking a survey on the computer, so you may not get an adequate sample of the population by doing so. Plus you have to decide how you will recruit people to take your online survey, which can be challenging. There are trade-offs with every method.
For more information on things to consider when selecting your survey method, check out the following website:
Selecting the Survey Method .
There are also many good sources for developing a good survey, such as the following websites. Constructing the Survey Survey Methods Designing Surveys
Observation.
A second type of data collection method is observation . In this data collection method, you make observations of the phenomenon you are studying and then code your observations, so that you can count what you are studying. This type of data collection method is often called interaction analysis, if you collect data by observing people's behavior. For example, if you want to study the phenomenon of mall-walking, you could go to a mall and count characteristics of mall-walkers. A researcher in the area of health communication could study the occurrence of humor in an operating room, for example, by coding and counting the use of humor in such a setting.
One extended research study using observational data collection methods, which is cited often in interpersonal communication classes, is John Gottman's research, which started out in what is now called "The Love Lab." In this lab, researchers observe interactions between couples, including physiological symptoms, using coders who look for certain items found to predict relationship problems and success.
Take a look at the YouTube video about "The Love Lab" at the following site to learn more about the potential of using observation in collecting data for a research study: The "Love" Lab .
Already-Existing Artifacts.
The third method of quantitative data collection is the use of already-existing artifacts . With this method, you choose certain artifacts (e.g., newspaper or magazine articles; television programs; webpages) and code their content, resulting in a count of whatever you are studying. With this data collection method, researchers most often use what is called quantitative content analysis . Basically, the researcher counts frequencies of something that occurs in an artifact of study, such as the frequency of times something is mentioned on a webpage. Content analysis can also be used in qualitative research, where a researcher identifies and creates text-based themes but does not do a count of the occurrences of these themes. Content analysis can also be used to take open-ended questions from a survey method, and identify countable themes within the questions.
Content analysis is a very common method used in media studies, given researchers are interested in studying already-existing media artifacts. There are many good sources to illustrate how to do content analysis such as are seen in the box below.
See the following sources for more information on content analysis. Writing Guide: Content Analysis A Flowchart for the Typical Process of Content Analysis Research What is Content Analysis?
With content analysis and any method that you use to code something into categories, one key concept you need to remember is inter-coder or inter-rater reliability , in which there are multiple coders (at least two) trained to code the observations into categories. This check on coding is important because you need to check to make sure that the way you are coding your observations on the open-ended answers is the same way that others would code a particular item. To establish this kind of inter-coder or inter-rater reliability, researchers prepare codebooks (to train their coders on how to code the materials) and coding forms for their coders to use.
To see some examples of actual codebooks used in research, see the following website: Human Coding--Sample Materials .
There are also online inter-coder reliability calculators some researchers use, such as the following: ReCal: reliability calculation for the masses .
Regardless of which method of data collection you choose, you need to decide even more specifically how you will measure the variables in your study, which leads us to the next planning step in the design of a study.
Operationalization of Variables into Measurable Concepts
When you look at your research question/s and/or hypotheses, you should know already what your independent and dependent variables are. Both of these need to be measured in some way. We call that way of measuring operationalizing a variable. One way to think of it is writing a step by step recipe for how you plan to obtain data on this topic. How you choose to operationalize your variable (or write the recipe) is one all-important decision you have to make, which will make or break your study. In quantitative research, you have to measure your variables in a valid (accurate) and reliable (consistent) manner, which we discuss in this section. You also need to determine the level of measurement you will use for your variables, which will help you later decide what statistical tests you need to run to answer your research question/s or test your hypotheses. We will start with the last topic first.
Level of Measurement
Level of measurement has to do with whether you measure your variables using categories or groupings OR whether you measure your variables using a continuous level of measurement (range of numbers). The level of measurement that is considered to be categorical in nature is called nominal, while the levels of measurement considered to be continuous in nature are ordinal, interval, and ratio. The only ones you really need to know are nominal, ordinal, and interval/ratio.

Nominal variables are categories that do not have meaningful numbers attached to them but are broader categories, such as male and female, home schooled and public schooled, Caucasian and African-American. Ordinal variables do have numbers attached to them, in that the numbers are in a certain order, but there are not equal intervals between the numbers (e.g., such as when you rank a group of 5 items from most to least preferred, where 3 might be highly preferred, and 2 hated). Interval/ratio variables have equal intervals between the numbers (e.g., weight, age).
For more information about these levels of measurement, check out one of the following websites. Levels of Measurement Measurement Scales in Social Science Research What is the difference between ordinal, interval and ratio variables? Why should I care?
Validity and Reliability
When developing a scale/measure or survey, you need to be concerned about validity and reliability. Readers of quantitative research expect to see researchers justify their research measures using these two terms in the methods section of an article or paper.
Validity. Validity is the extent to which your scale/measure or survey adequately reflects the full meaning of the concept you are measuring. Does it measure what you say it measures? For example, if researchers wanted to develop a scale to measure "servant leadership," the researchers would have to determine what dimensions of servant leadership they wanted to measure, and then create items which would be valid or accurate measures of these dimensions. If they included items related to a different type of leadership, those items would not be a valid measure of servant leadership. When doing so, the researchers are trying to prove their measure has internal validity. Researchers may also be interested in external validity, but that has to do with how generalizable their study is to a larger population (a topic related to sampling, which we will consider in the next section), and has less to do with the validity of the instrument itself.
There are several types of validity you may read about, including face validity, content validity, criterion-related validity, and construct validity. To learn more about these types of validity, read the information at the following link: Validity .
To improve the validity of an instrument, researchers need to fully understand the concept they are trying to measure. This means they know the academic literature surrounding that concept well and write several survey questions on each dimension measured, to make sure the full idea of the concept is being measured. For example, Page and Wong (n.d.) identified four dimensions of servant leadership: character, people-orientation, task-orientation, and process-orientation ( A Conceptual Framework for Measuring Servant-Leadership ). All of these dimensions (and any others identified by other researchers) would need multiple survey items developed if a researcher wanted to create a new scale on servant leadership.
Before you create a new survey, it can be useful to see if one already exists with established validity and reliability. Such measures can be found by seeing what other respected studies have used to measure a concept and then doing a library search to find the scale/measure itself (sometimes found in the reference area of a library in books like those listed below).
Reliability . Reliability is the second criterion you will need to address if you choose to develop your own scale or measure. Reliability is concerned with whether a measurement is consistent and reproducible. If you have ever wondered why, when taking a survey, that a question is asked more than once or very similar questions are asked multiple times, it is because the researchers one concerned with proving their study has reliability. Are you, for example, answering all of the similar questions similarly? If so, the measure/scale may have good reliability or consistency over time.
Researchers can use a variety of ways to show their measure/scale is reliable. See the following websites for explanations of some of these ways, which include methods such as the test-retest method, the split-half method, and inter-coder/rater reliability. Types of Reliability Reliability
To understand the relationship between validity and reliability, a nice visual provided below is explained at the following website (Trochim, 2006, para. 2). Reliability & Validity
Self-Quiz/Discussion:
Take a look at one of the surveys found at the following poll reporting sites on a topic which interests you. Critique one of these surveys, using what you have learned about creating surveys so far.
http://www.pewinternet.org/ http://pewresearch.org/ http://www.gallup.com/Home.aspx http://www.kff.org/
One of the things you might have critiqued in the previous self-quiz/discussion may have had less to do with the actual survey itself, but rather with how the researchers got their participants or sample. How participants are recruited is just as important to doing a good study as how valid and reliable a survey is.
Imagine that in the article you chose for the last "self-quiz/discussion" you read the following quote from the Pew Research Center's Internet and American Life Project: "One in three teens sends more than 100 text messages a day, or 3000 texts a month" (Lenhart, 2010, para.5). How would you know whether you could trust this finding to be true? Would you compare it to what you know about texting from your own and your friends' experiences? Would you want to know what types of questions people were asked to determine this statistic, or whether the survey the statistic is based on is valid and reliable? Would you want to know what type of people were surveyed for the study? As a critical consumer of research, you should ask all of these types of questions, rather than just accepting such a statement as undisputable fact. For example, if only people shopping at an Apple Store were surveyed, the results might be skewed high.
In particular, related to the topic of this section, you should ask about the sampling method the researchers did. Often, the researchers will provide information related to the sample, stating how many participants were surveyed (in this case 800 teens, aged 12-17, who were a nationally representative sample of the population) and how much the "margin of error" is (in this case +/- 3.8%). Why do they state such things? It is because they know the importance of a sample in making the case for their findings being legitimate and credible. Margin of error is how much we are confident that our findings represent the population at large. The larger the margin of error, the less likely it is that the poll or survey is accurate. Margin of error assumes a 95% confidence level that what we found from our study represents the population at large.
For more information on margin of error, see one of the following websites. Answers.com Margin of Error Stats.org Margin of Error Americanresearchgroup.com Margin of Error [this last site is a margin of error calculator, which shows that margin of error is directly tied to the size of your sample, in relationship to the size of the population, two concepts we will talk about in the next few paragraphs]
In particular, this section focused on sampling will talk about the following topics: (a) the difference between a population vs. a sample; (b) concepts of error and bias, or "it's all about significance"; (c) probability vs. non-probability sampling; and (d) sample size issues.
Population vs. Sample
When doing quantitative studies, such as the study of cell phone usage among teens, you are never able to survey the entire population of teenagers, so you survey a portion of the population. If you study every member of a population, then you are conducting a census such as the United States Government does every 10 years. When, however, this is not possible (because you do not have the money the U.S. government has!), you attempt to get as good a sample as possible.
Characteristics of a population are summarized in numerical form, and technically these numbers are called parameters . However, numbers which summarize the characteristics of a sample are called statistics .
Error and Bias
If a sample is not done well, then you may not have confidence in how the study's results can be generalized to the population from which the sample was taken. Your confidence level is often stated as the margin of error of the survey. As noted earlier, a study's margin of error refers to the degree to which a sample differs from the total population you are studying. In the Pew survey, they had a margin of error of +/- 3.8%. So, for example, when the Pew survey said 33% of teens send more than 100 texts a day, the margin of error means they were 95% sure that 29.2% - 36.8% of teens send this many texts a day.
Margin of error is tied to sampling error , which is how much difference there is between your sample's results and what would have been obtained if you had surveyed the whole population. Sample error is linked to a very important concept for quantitative researchers, which is the notion of significance . Here, significance does not refer to whether some finding is morally or practically significant, it refers to whether a finding is statistically significant, meaning the findings are not due to chance but actually represent something that is found in the population. Statistical significance is about how much you, as the researcher, are willing to risk saying you found something important and be wrong.
For the difference between statistical significance and practical significance, see the following YouTube video: Statistical and Practical Significance .
Scientists set certain arbitrary standards based on the probability they could be wrong in reporting their findings. These are called significance levels and are commonly reported in the literature as p <.05 or p <.01 or some other probability (or p ) level.
If an article says a statistical test reported that p < .05 , it simply means that they are most likely correct in what they are saying, but there is a 5% chance they could be wrong and not find the same results in the population. If p < .01, then there would be only a 1% chance they were wrong and would not find the same results in the population. The lower the probability level, the more certain the results.
When researchers are wrong, or make that kind of decision error, it often implies that either (a) their sample was biased and was not representative of the true population in some way, or (b) that something they did in collecting the data biased the results. There are actually two kinds of sampling error talked about in quantitative research: Type I and Type II error. Type 1 error is what happens when you think you found something statistically significant and claim there is a significant difference or relationship, when there really is not in the actual population. So there is something about your sample that made you find something that is not in the actual population. (Type I error is the same as the probability level, or .05, if using the traditional p-level accepted by most researchers.) Type II error happens when you don't find a statistically significant difference or relationship, yet there actually is one in the population at large, so once again, your sample is not representative of the population.
For more information on these two types of error, check out the following websites. Hypothesis Testing: Type I Error, Type II Error Type I and Type II Errors - Making Mistakes in the Justice System
Researchers want to select a sample that is representative of the population in order to reduce the likelihood of having a sample that is biased. There are two types of bias particularly troublesome for researchers, in terms of sampling error. The first type is selection bias , in which each person in the population does not have an equal chance to be chosen for the sample, which happens frequently in communication studies, because we often rely on convenience samples (whoever we can get to complete our surveys). The second type of bias is response bias , in which those who volunteer for a study have different characteristics than those who did not volunteer for the study, another common challenge for communication researchers. Volunteers for a study may very well be different from persons who choose not to volunteer for a study, so that you have a biased sample by relying just on volunteers, which is not representative of the population from which you are trying to sample.
Probability vs. Non-Probability Sampling
One of the best ways to lower your sampling error and reduce the possibility of bias is to do probability or random sampling. This means that every person in the population has an equal chance of being selected to be in your sample. Another way of looking at this is to attempt to get a representative sample, so that the characteristics of your sample closely approximate those of the population. A sample needs to contain essentially the same variations that exist in the population, if possible, especially on the variables or elements that are most important to you (e.g., age, biological sex, race, level of education, socio-economic class).
There are many different ways to draw a probability/random sample from the population. Some of the most common are a simple random sample , where you use a random numbers table or random number generator to select your sample from the population.
There are several examples of random number generators available online. See the following example of an online random number generator: http://www.randomizer.org/ .
A systematic random sample takes every n-th number from the population, depending on how many people you would like to have in your sample. A stratified random sample does random sampling within groups, and a multi-stage or cluster sample is used when there are multiple groups within a large area and a large population, and the researcher does random sampling in stages.
If you are interested in understanding more about these types of probability/random samples, take a look at the following website: Probability Sampling .
However, many times communication researchers use whoever they can find to participate in their study, such as college students in their classes since these people are easily accessible. Many of the studies in interpersonal communication and relationship development, for example, used this type of sample. This is called a convenience sample. In doing so, they are using a non- probability or non-random sample. In these types of samples, each member of the population does not have an equal opportunity to be selected. For example, if you decide to ask your facebook friends to participate in an online survey you created about how college students in the U.S. use cell phones to text, you are using a non-random type of sample. You are unable to randomly sample the whole population in the U.S. of college students who text, so you attempt to find participants more conveniently. Some common non-random or non-probability samples are:
- accidental/convenience samples, such as the facebook example illustrates
- quota samples, in which you do convenience samples within subgroups of the population, such as biological sex, looking for a certain number of participants in each group being compared
- snowball or network sampling, where you ask current participants to send your survey onto their friends.
For more information on non-probability sampling, see the following website: Nonprobability Sampling .
Researchers, such as communication scholars, often use these types of samples because of the nature of their research. Most research designs used in communication are not true experiments, such as would be required in the medical field where they are trying to prove some cause-effect relationship to cure or alleviate symptoms of a disease. Most communication scholars recognize that human behavior in communication situations is much less predictable, so they do not adhere to the strictest possible worldview related to quantitative methods and are less concerned with having to use probability sampling.
They do recognize, however, that with either probability or non-probability sampling, there is still the possibility of bias and error, although much less with probability sampling. That is why all quantitative researchers, regardless of field, will report statistical significance levels if they are interested in generalizing from their sample to the population at large, to let the readers of their work know how confident they are in their results.
Size of Sample
The larger the sample, the more likely the sample is going to be representative of the population. If there is a lot of variability in the population (e.g., lots of different ethnic groups in the population), a researcher will need a larger sample. If you are interested in detecting small possible differences (e.g., in a close political race), you need a larger sample. However, the bigger your population, the less you have to increase the size of your sample in order to have an adequate sample, as is illustrated by an example sample size calculator such as can be found at http://www.raosoft.com/samplesize.html .
Using the example sample size calculator, see how you might determine how large of a sample you might need in order to study how college students in the U.S. use texting on their cell phones. You would have to first determine approximately how many college students are in the U.S. According to ANEKI, there are a little over 14,000,000 college students in the U.S. ( Countries with the Most University Students ). When inputting that figure into the sample size calculator below (using no commas for the population size), you would need a sample size of approximately 385 students. If the population size was 20,000, you would need a sample of 377 students. If the population was only 2,000, you would need a sample of 323. For a population of 500, you would need a sample of 218.
It is not enough, however, to just have an adequate or large sample. If there is bias in the sampling, you can have a very bad large sample, one that also does not represent the population at large. So, having an unbiased sample is even more important than having a large sample.
So, what do you do, if you cannot reasonably conduct a probability or random sample? You run statistics which report significance levels, and you report the limitations of your sample in the discussion section of your paper/article.
Pilot Testing Methods
Now that we have talked about the different elements of your study design, you should try out your methods by doing a pilot test of some kind. This means that you try out your procedures with someone to try to catch any mistakes in your design before you start collecting data from actual participants in your study. This will save you time and money in the long run, along with unneeded angst over mistakes you made in your design during data collection. There are several ways you might do this.
You might ask an expert who knows about this topic (such as a faculty member) to try out your experiment or survey and provide feedback on what they think of your design. You might ask some participants who are like your potential sample to take your survey or be a part of your pilot test; then you could ask them which parts were confusing or needed revising. You might have potential participants explain to you what they think your questions mean, to see if they are interpreting them like you intended, or if you need to make some questions clearer.
The main thing is that you do not just assume your methods will work or are the best type of methods to use until you try them out with someone. As you write up your study, in your methods section of your paper, you can then talk about what you did to change your study based on the pilot study you did.
Institutional Review Board (IRB) Approval
The last step of your planning takes place when you take the necessary steps to get your study approved by your institution's review board. As you read in chapter 3, this step is important if you are planning on using the data or results from your study beyond just the requirements for your class project. See chapter 3 for more information on the procedures involved in this step.
Conclusion: Study Design Planning
Once you have decided what topic you want to study, you plan your study. Part 1 of this chapter has covered the following steps you need to follow in this planning process:
- decide what type of study you will do (i.e., experimental, quasi-experimental, non- experimental);
- decide on what data collection method you will use (i.e., survey, observation, or already existing data);
- operationalize your variables into measureable concepts;
- determine what type of sample you will use (probability or non-probability);
- pilot test your methods; and
- get IRB approval.
At that point, you are ready to commence collecting your data, which is the topic of the next section in this chapter.
Have a language expert improve your writing
Run a free plagiarism check in 10 minutes, automatically generate references for free.
- Knowledge Base
- Methodology
- What Is Quantitative Research? | Definition & Methods
What Is Quantitative Research? | Definition & Methods
Published on 4 April 2022 by Pritha Bhandari . Revised on 10 October 2022.
Quantitative research is the process of collecting and analysing numerical data. It can be used to find patterns and averages, make predictions, test causal relationships, and generalise results to wider populations.
Quantitative research is the opposite of qualitative research , which involves collecting and analysing non-numerical data (e.g. text, video, or audio).
Quantitative research is widely used in the natural and social sciences: biology, chemistry, psychology, economics, sociology, marketing, etc.
- What is the demographic makeup of Singapore in 2020?
- How has the average temperature changed globally over the last century?
- Does environmental pollution affect the prevalence of honey bees?
- Does working from home increase productivity for people with long commutes?
Table of contents
Quantitative research methods, quantitative data analysis, advantages of quantitative research, disadvantages of quantitative research, frequently asked questions about quantitative research.
You can use quantitative research methods for descriptive, correlational or experimental research.
- In descriptive research , you simply seek an overall summary of your study variables.
- In correlational research , you investigate relationships between your study variables.
- In experimental research , you systematically examine whether there is a cause-and-effect relationship between variables.
Correlational and experimental research can both be used to formally test hypotheses , or predictions, using statistics. The results may be generalised to broader populations based on the sampling method used.
To collect quantitative data, you will often need to use operational definitions that translate abstract concepts (e.g., mood) into observable and quantifiable measures (e.g., self-ratings of feelings and energy levels).
Research method | How to use | Example |
---|---|---|
Control or manipulate an to measure its effect on a dependent variable. | To test whether an intervention can reduce procrastination in college students, you give equal-sized groups either a procrastination intervention or a comparable task. You compare self-ratings of procrastination behaviors between the groups after the intervention. | |
Ask questions of a group of people in-person, over-the-phone or online. | You distribute with rating scales to first-year international college students to investigate their experiences of culture shock. | |
(Systematic) observation | Identify a behavior or occurrence of interest and monitor it in its natural setting. | To study college classroom participation, you sit in on classes to observe them, counting and recording the prevalence of active and passive behaviors by students from different backgrounds. |
Secondary research | Collect data that has been gathered for other purposes e.g., national surveys or historical records. | To assess whether attitudes towards climate change have changed since the 1980s, you collect relevant questionnaire data from widely available . |
Prevent plagiarism, run a free check.
Once data is collected, you may need to process it before it can be analysed. For example, survey and test data may need to be transformed from words to numbers. Then, you can use statistical analysis to answer your research questions .
Descriptive statistics will give you a summary of your data and include measures of averages and variability. You can also use graphs, scatter plots and frequency tables to visualise your data and check for any trends or outliers.
Using inferential statistics , you can make predictions or generalisations based on your data. You can test your hypothesis or use your sample data to estimate the population parameter .
You can also assess the reliability and validity of your data collection methods to indicate how consistently and accurately your methods actually measured what you wanted them to.
Quantitative research is often used to standardise data collection and generalise findings . Strengths of this approach include:
- Replication
Repeating the study is possible because of standardised data collection protocols and tangible definitions of abstract concepts.
- Direct comparisons of results
The study can be reproduced in other cultural settings, times or with different groups of participants. Results can be compared statistically.
- Large samples
Data from large samples can be processed and analysed using reliable and consistent procedures through quantitative data analysis.
- Hypothesis testing
Using formalised and established hypothesis testing procedures means that you have to carefully consider and report your research variables, predictions, data collection and testing methods before coming to a conclusion.
Despite the benefits of quantitative research, it is sometimes inadequate in explaining complex research topics. Its limitations include:
- Superficiality
Using precise and restrictive operational definitions may inadequately represent complex concepts. For example, the concept of mood may be represented with just a number in quantitative research, but explained with elaboration in qualitative research.
- Narrow focus
Predetermined variables and measurement procedures can mean that you ignore other relevant observations.
- Structural bias
Despite standardised procedures, structural biases can still affect quantitative research. Missing data , imprecise measurements or inappropriate sampling methods are biases that can lead to the wrong conclusions.
- Lack of context
Quantitative research often uses unnatural settings like laboratories or fails to consider historical and cultural contexts that may affect data collection and results.
Quantitative research deals with numbers and statistics, while qualitative research deals with words and meanings.
Quantitative methods allow you to test a hypothesis by systematically collecting and analysing data, while qualitative methods allow you to explore ideas and experiences in depth.
In mixed methods research , you use both qualitative and quantitative data collection and analysis methods to answer your research question .
Data collection is the systematic process by which observations or measurements are gathered in research. It is used in many different contexts by academics, governments, businesses, and other organisations.
Operationalisation means turning abstract conceptual ideas into measurable observations.
For example, the concept of social anxiety isn’t directly observable, but it can be operationally defined in terms of self-rating scores, behavioural avoidance of crowded places, or physical anxiety symptoms in social situations.
Before collecting data , it’s important to consider how you will operationalise the variables that you want to measure.
Reliability and validity are both about how well a method measures something:
- Reliability refers to the consistency of a measure (whether the results can be reproduced under the same conditions).
- Validity refers to the accuracy of a measure (whether the results really do represent what they are supposed to measure).
If you are doing experimental research , you also have to consider the internal and external validity of your experiment.
Hypothesis testing is a formal procedure for investigating our ideas about the world using statistics. It is used by scientists to test specific predictions, called hypotheses , by calculating how likely it is that a pattern or relationship between variables could have arisen by chance.
Cite this Scribbr article
If you want to cite this source, you can copy and paste the citation or click the ‘Cite this Scribbr article’ button to automatically add the citation to our free Reference Generator.
Bhandari, P. (2022, October 10). What Is Quantitative Research? | Definition & Methods. Scribbr. Retrieved 3 September 2024, from https://www.scribbr.co.uk/research-methods/introduction-to-quantitative-research/
Is this article helpful?
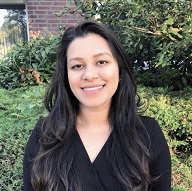
Pritha Bhandari
Research Methodologies
Quantitative research methodologies.
- Qualitative Research Methodologies
- Systematic Reviews
- Finding Articles by Methodology
- Design Your Research Project
Library Help
What is quantitative research.
Quantitative methodologies use statistics to analyze numerical data gathered by researchers to answer their research questions. Quantitative methods can be used to answer questions such as:
- What are the relationships between two or more variables?
- What factors are at play in an environment that might affect the behavior or development of the organisms in that environment?
Quantitative methods can also be used to test hypotheses by conducting quasi-experimental studies or designing experiments.
Independent and Dependent Variables
In quantitative research, a variable is something (an intervention technique, a pharmaceutical, a temperature, etc.) that changes. There are two kinds of variables: independent variables and dependent variables . In the simplest terms, the independent variable is whatever the researchers are using to attempt to make a change in their dependent variable.
Independent Variable(s) | Dependent Variable |
---|---|
A new cancer-treating drug being tested in different dosage strengths | The number of detectable cancer cells in a patient or cell sample |
Different genres of music* | Plant growth within a specific time frame |
* This is a real, repeatable experiment you can try on your plants.
Correlational
Researchers will compare two sets of numbers to try and identify a relationship (if any) between two things.
- Köse S., & Murat, M. (2021). Examination of the relationship between smartphone addiction and cyberchondria in adolescents. Archives of Psychiatric Nursing, 35(6): 563-570.
- Pilger et al. (2021). Spiritual well-being, religious/spiritual coping and quality of life among the elderly undergoing hemodialysis: a correlational study. Journal of Religion, Spirituality & Aging, 33(1): 2-15.
Descriptive
Researchers will attempt to quantify a variety of factors at play as they study a particular type of phenomenon or action. For example, researchers might use a descriptive methodology to understand the effects of climate change on the life cycle of a plant or animal.
- Lakshmi, E. (2021). Food consumption pattern and body mass index of adolescents: A descriptive study. International Journal of Nutrition, Pharmacology, Neurological Diseases, 11(4), 293–297.
- Lin, J., Singh, S., Sha, L., Tan, W., Lang, D., Gašević, D., & Chen, G. (2022). Is it a good move? Mining effective tutoring strategies from human–human tutorial dialogues. Future Generation Computer Systems, 127, 194–207.
Experimental
To understand the effects of a variable, researchers will design an experiment where they can control as many factors as possible. This can involve creating control and experimental groups. The experimental group will be exposed to the variable to study its effects. The control group provides data about what happens when the variable is absent. For example, in a study about online teaching, the control group might receive traditional face-to-face instruction while the experimental group would receive their instruction virtually.
- Jinzhang Jia, Yinuo Chen, Guangbo Che, Jinchao Zhu, Fengxiao Wang, & Peng Jia. (2021). Experimental study on the explosion characteristics of hydrogen-methane premixed gas in complex pipe networks. Scientific Reports, 11(1), 1–11.
- Sasaki, R. et al. (2021). Effects of cryotherapy applied at different temperatures on inflammatory pain during the acute phase of arthritis in rats. Physical Therapy, 101(2), 1–9.
Quasi-Experimental/Quasi-Comparative
Researchers will attempt to determine what (if any) effect a variable can have. These studies may have multiple independent variables (causes) and multiple dependent variables (effects), but this can complicate researchers' efforts to find out if A can cause B or if X, Y, and Z are also playing a role.
- Jafari, A., Alami, A., Charoghchian, E., Delshad Noghabi, A., & Nejatian, M. (2021). The impact of effective communication skills training on the status of marital burnout among married women. BMC Women’s Health, 21(1), 1-10.
- Phillips, S. W., Kim, D.-Y., Sobol, J. J., & Gayadeen, S. M. (2021). Total recall?: A quasi-experimental study of officer’s recollection in shoot - don’t shoot simulators. Police Practice and Research, 22(3), 1229–1240.
Surveys can be considered a quantitative methodology if the researchers require their respondents to choose from pre-determined responses.
- Harries et al. (2021). Effects of the COVID-19 pandemic on medical students: A multicenter quantitative study. BMC Medical Education, 21(14), 1-8.
- Call : 801.863.8840
- Text : 801.290.8123
- In-Person Help
- Email a Librarian
- Make an Appointment
- << Previous: Home
- Next: Qualitative Research Methodologies >>
- Last Updated: Jun 20, 2024 3:16 PM
- URL: https://uvu.libguides.com/methods

Home » Types of Quantitative Research Methods
Types of Quantitative Research Methods
Quantitative Research is essentially about numbers and statistical analysis. It’s how we turn observations into data that we can measure and analyze in a structured way. This type of research allows us to quantify behaviors, opinions, or phenomena and translate that into figures and statistics that can be used to uncover patterns, test theories, or make predictions.
Table of Contents

Now, let’s explore the main types of Quantitative Research Methods:
Imagine you have a big jar of jellybeans, and you want to know which color is the most popular. So, you ask everyone in your class to pick their favorite color. That’s like doing a survey! You’re asking lots of people questions to gather lots of number-based information or data.
Experiments
This is like doing a science experiment in class. You might change one thing, like the amount of sunlight a plant gets, to see how it affects something else, like how tall the plant grows. You’re trying different things and watching what happens, keeping track of the results with numbers.
Observational Studies
Imagine you’re bird watching, and you’re writing down every time a bird comes to the feeder and what type it is. You’re not changing anything; you’re just watching and noting down numbers and facts.
Longitudinal Studies
Think of this like taking a picture of the same tree every day for a whole year. You’re trying to see how the tree changes over time, from winter to spring, and all the way back to winter again. You’re collecting data over a long period to see trends or changes.
Cross-Sectional Studies
This is like taking a single snapshot of a crowded park. You’re getting a lot of information all at once, but just for one point in time. It’s like a group photo that tells you who was there at that moment, but not what happens before or after.
Correlational Studies
Now, imagine you noticed that the taller your classmates are, the bigger their shoe size. You start to think there’s a connection between height and shoe size. So, you measure everyone’s height and shoe size to see if taller people really do have bigger feet, looking for a pattern or correlation.
Each of these tools helps you collect different kinds of number-based information for solving different kinds of mysteries. Just like a detective, you need to pick the right tool for the job to find the clues you need!
You may also like

Types of Mixed Research Methods

What is Mixed Research Methods

Quantitative Research Methods

Types of Qualitative Research Methods

Qualitative Research Methods

Types of Research Methods
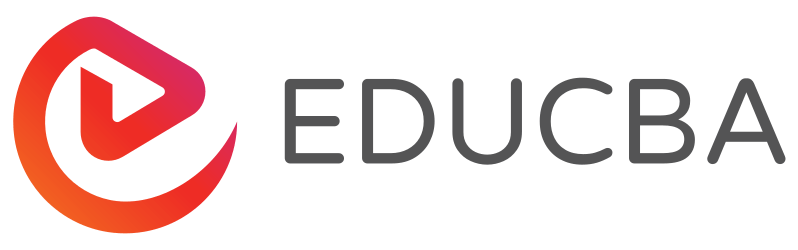
Types of Quantitative Research
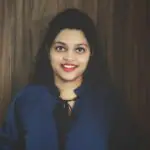
Introduction to Quantitative Research
Quantitative research is outlined as a scientific investigation of phenomena by gathering quantitative information and activity applied mathematics, or procedure techniques.
Quantitative research is a research method where you gather and analyze numerical data to understand and explain various phenomena. The different types of quantitative research are survey, descriptive, experiential, correlational, and causal-comparative.
It focuses on using mathematical and statistical techniques to understand and investigate the subject. This is why the collected information must be in numerical form.
Start Your Free Data Science Course
Hadoop, Data Science, Statistics & others
Different Types of Quantitative Research
The following are the different types of Quantitative research types with the description of each.
1. Survey Research
Survey Research is one of the most common types of quantitative research techniques. In this research method, you can use surveys to collect numerical data from a particular group or population. After that, you can analyze the data and explain the characteristics of the group. Both small and large organizations typically employ it for a proper understanding of their customers and to understand the merchandise and product views. It is of two types:
- Cross-sectional: In a cross-sectional survey, you collect information from a large group of people at a specific time. These are mostly useful in retail stores, education, etc.
- Longitudinal: In a longitudinal survey, you collect information from a small group of people over a long period of time, usually years. These are useful in medical trials and applied sciences.
2. Descriptive Research
Descriptive research aims to explain and understand the current state of things like people, places, conditions, or events. Here, the researcher’s goal is to gather general observational data without exploring the reasons behind them. Also, you don’t need to begin with a hypothesis; instead, you gather data first and then create a hypothesis if needed. Importantly, descriptive research doesn’t try to prove or disprove the hypothesis. It is mainly about creating a research hypothesis.
3. Experimental Research
Experimental research, as the name suggests, uses the scientific method to establish the cause-effect relationship among a group of variables using experiments. Researchers can use multiple theories to conduct this research. The major components of experimental research are:
- A comparison group of participants who are randomly selected and assigned to experimental and control groups.
- An independent variable, referred to as the experimental variable that can be applied to the experimental group.
- A dependent variable, referred to as the effect or posttest variable that can be measured in an identical manner for all groups.
4. Correlational Research
Correlational research establishes a relationship between two close entities and determines how one impacts the other. For this, a researcher needs at least two separate groups. This type of research will recognize trends and patterns in data, but it does not go so far in its analysis to observe the different patterns.
5. Casual-Comparative Research
Causal-comparative research is employed to conclude the cause-effect equation between two or more variables, where one variable depends on the opposite experimental variable. The experimenter does not manipulate the independent variable and then measures the effects of the independent variable on the dependent variable.
Types of Quantitative Research Infographic
Here is a detailed infographic explaining the different types of quantitative research.
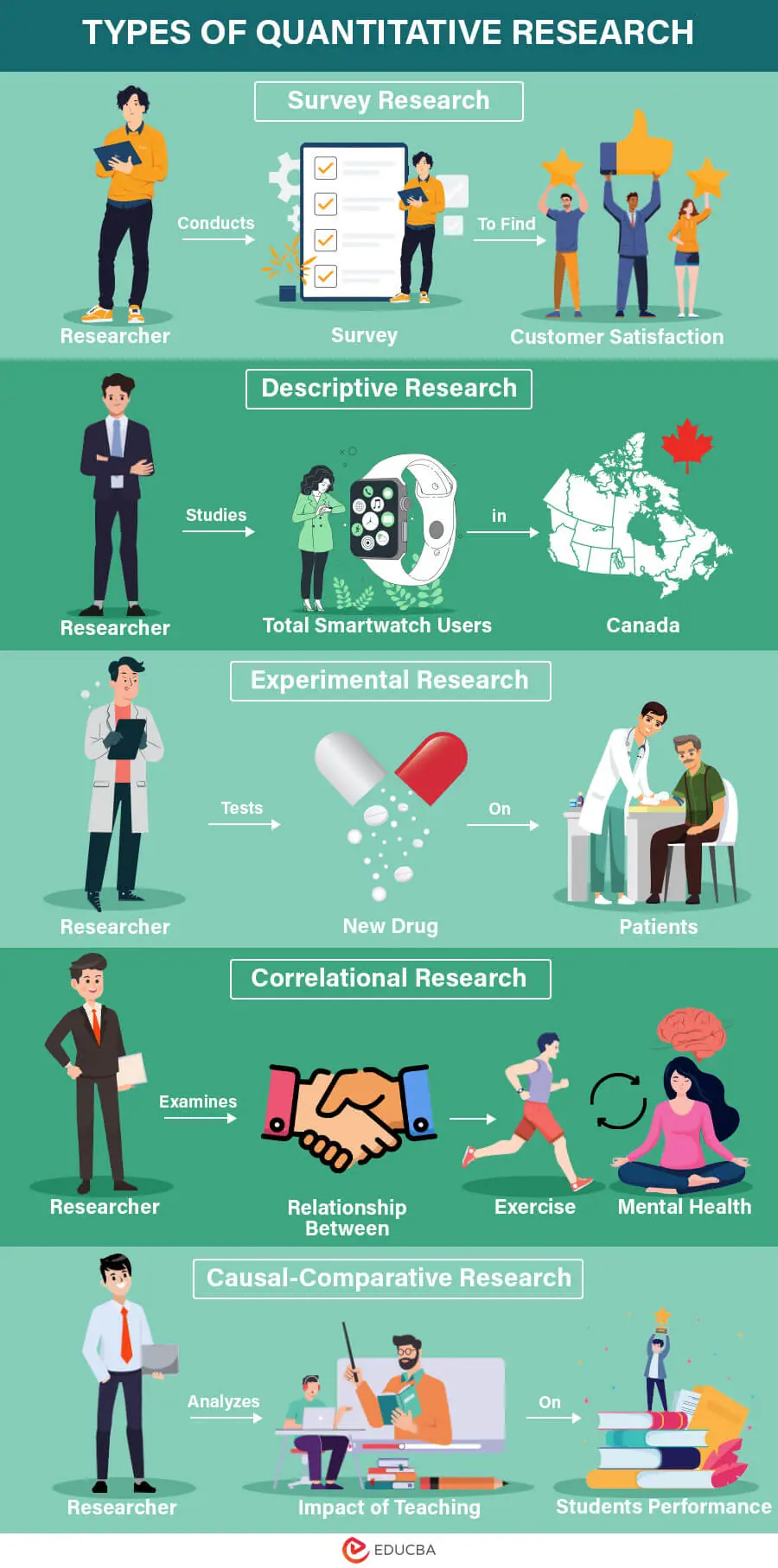
Characteristics
Here are the common characteristics of different types of quantitative research.
1. Numerical Data: Quantitative research primarily deals with numerical data, which can be quantified and measured. Researchers use structured instruments like surveys, experiments, or observations to collect this data.
2. Large Sample Sizes: Quantitative research typically involves large sample sizes to ensure statistical validity and generalize findings to a broader population. This allows for greater confidence in the results.
3. Statistical Analysis: Statistical analysis plays a central role in quantitative research. Researchers use statistical methods to analyze and interpret the data, identify patterns and relationships, and draw conclusions. Common statistical techniques include regression analysis, correlation analysis, t-tests, ANOVA, and chi-squared tests.
4. Structured Research Design: Quantitative research often employs a structured and pre-defined research design. Researchers develop hypotheses, select variables, and design experiments or surveys before data collection begins. This structured approach helps maintain consistency and rigor in the research process.
5. Generalizability: Quantitative research often aims to generalize findings to a larger population. When conducted correctly, quantitative studies can provide insights that are applicable beyond the specific sample studied.
6. Closed-Ended Questions: Surveys and questionnaires used in quantitative research often employ closed-ended questions with predetermined response options. This facilitates data analysis and allows for comparisons between participants.
Here is a step-by-step process of performing different types of quantitative research.
Step 1: Clearly define your research problem or question. Step 2: Conduct a literature review to identify relevant studies and theories related to your research topic. Step 3: Develop specific hypotheses or research questions that you aim to answer through your research. Step 4: Decide on the research design (e.g., experimental, cross-sectional, longitudinal) and your target population, and select a representative sample. Also, develop a data collection plan and choose appropriate data collection methods (e.g., surveys, experiments, observations). Step 5: Choose valid and reliable measurement instruments (e.g., questionnaires, scales) to collect data. Make sure to test your measurement tools before actual research. Step 6: Administer your selected data collection methods to your sample population. Step 7: Select appropriate statistical techniques (e.g., descriptive statistics, inferential statistics) based on your research design and hypotheses. Analyze the data to test your hypotheses or answer your research questions. Step 8: Interpret the statistical findings and summarize them to determine if they support or refute your hypothesis. Step 9: Prepare a research report or paper that includes the research process, methodology, results, and conclusions to share your findings.
Following are some of the common uses of various types of quantitative research.
Analyzing consumer preferences and trends | Conducting a survey to determine which smartphone brand is most popular among young adults. | |
Testing cause-and-effect relationships | Conducting a clinical trial to determine the efficacy of a new drug in treating a specific medical condition. | |
Statistical analysis for decision-making | Analyzing sales data to identify the most profitable products for a retail company. | |
Assessing the effectiveness of programs or products | Evaluating the impact of a training program on employee productivity in a corporate setting. | |
Identifying patterns and forecasting future trends | Using historical data to predict future market trends and investment opportunities. | |
Quantifying potential risks and mitigations | Assessing the associated with a particular . | |
Studying disease prevalence and treatment outcomes | Investigating the relationship between smoking and lung cancer using patient data. | |
Measuring student performance and learning outcomes | Analyzing standardized test scores to assess the effectiveness of a new teaching method. | |
Evaluating investment opportunities and risks | Calculating the return on investment (ROI) for a potential real estate investment. |
Advantages and Disadvantages
Some of the key advantages and disadvantages of types of quantitative research are:
Quantitative research offers objectivity by relying on numerical data and reducing subjectivity. | Sampling issues, such as bias, can impact the reliability of findings. |
It enables generalizability by collecting data from large, representative samples. | The approach may reduce validity by not capturing all aspects of a problem. |
The structured approach allows other researchers to duplicate the study. | It lacks depth as it may oversimplify complex phenomena. |
It is efficient for studying large populations or conducting surveys. | Large-scale projects can be costly and resource-intensive. |
Final Thoughts
Quantitative research is an effective method that helps us build statistically reliable insights across numerous fields. However, researchers should select the appropriate quantitative approach considering the limitations and benefits of each method. Moreover, new emerging technologies like artificial intelligence (AI) can simplify data collection, analysis, and visualization of research outcomes.
Frequently Asked Questions (FAQs)
Q1. What are the methods of data analysis in quantitative research? Answer: Quantitative research uses different ways to analyze data. It uses descriptive stats, like averages and spreads, to summarize data. It also uses inferential statistics, like tests and regression, to check ideas and connections. For more complex situations, it uses multivariate methods like ANOVA and factor analysis to look at how different things interact. Researchers often use special software like SPSS or R to do these analyses.
Q2. How to interpret data in quantitative research? Answer: Interpreting quantitative data involves looking at the statistical results and assessing the present relationships or trends. You must also discuss if the data is applicable in both theoretical and practical sense. It is also crucial to present results clearly with tables and graphs and think about any problems or biases in collecting data. When interpreting, stay objective and let the data guide you, avoiding making guesses or assumptions.
Q3. What is quantitative vs. qualitative research? Answer: Quantitative research involves collecting and analyzing numerical data to uncover patterns, relationships, and trends. It relies on structured surveys, experiments, and statistical methods to draw objective conclusions. In contrast, qualitative research focuses on exploring the depth and meaning of phenomena through non-numerical data, such as interviews, observations, and open-ended questions. It aims to understand the underlying context and subjective experiences.
Recommended Articles
This is a guide to Types of Quantitative Research. Here, we also discuss the introduction and different types of quantitative research, which include survey, descriptive, experimental research, etc. You may also have a look at the following articles to learn more –
- Types of Research Methodology
- Quantitative Research Examples
- Descriptive Research
- Advantages and Disadvantages of Qualitative Research

*Please provide your correct email id. Login details for this Free course will be emailed to you
By signing up, you agree to our Terms of Use and Privacy Policy .
Forgot Password?
This website or its third-party tools use cookies, which are necessary to its functioning and required to achieve the purposes illustrated in the cookie policy. By closing this banner, scrolling this page, clicking a link or continuing to browse otherwise, you agree to our Privacy Policy
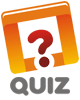
Explore 1000+ varieties of Mock tests View more
Submit Next Question
Early-Bird Offer: ENROLL NOW
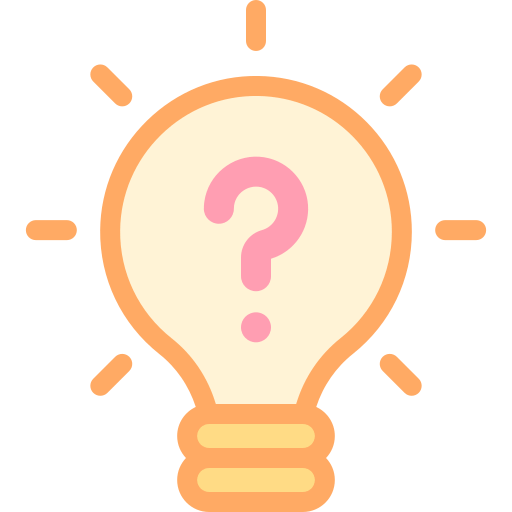
- (855) 776-7763
Training Maker
All Products
Qualaroo Insights
ProProfs.com
- Get Started Free
FREE. All Features. FOREVER!
Try our Forever FREE account with all premium features!
What Is Quantitative Research? Types, Characteristics & Methods

Market Research Specialist
Emma David, a seasoned market research professional, specializes in employee engagement, survey administration, and data management. Her expertise in leveraging data for informed decisions has positively impacted several brands, enhancing their market position.
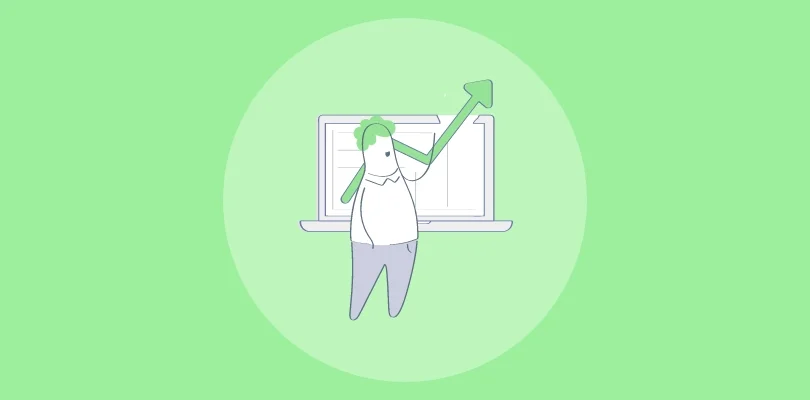
Step into the fascinating world of quantitative research , where numbers reveal extraordinary insights!
By gathering and studying data in a systematic way, quantitative research empowers us to understand our ever-changing world better. It helps understand a problem or an already-formed hypothesis by generating numerical data. The results don’t end here, as you can process these numbers to get actionable insights that aid decision-making.
You can use quantitative research to quantify opinions, behaviors, attitudes, and other definitive variables related to the market, customers, competitors, etc. The research is conducted on a larger sample population to draw predictive, average, and pattern-based insights.
Here, we delve into the intricacies of this research methodology, exploring various quantitative methods, their advantages, and real-life examples that showcase their impact and relevance.
Ready to embark on a journey of discovery and knowledge? Let’s go!
What Is Quantitative Research?
Quantitative research is a method that uses numbers and statistics to test theories about customer attitudes and behaviors. It helps researchers gather and analyze data systematically to gain valuable insights and draw evidence-based conclusions about customer preferences and trends.
Researchers use online surveys, questionnaires , polls , and quizzes to question a large number of people to obtain measurable and bias-free data.
In technical terms, quantitative research is mainly concerned with discovering facts about social phenomena while assuming a fixed and measurable reality.
Offering numbers and stats-based insights, this research methodology is a crucial part of primary research and helps understand how well an organizational decision is going to work out.
Let’s consider an example.
Suppose your qualitative analysis shows that your customers are looking for social media-based customer support. In that case, quantitative analysis will help you see how many of your customers are looking for this support.
If 10% of your customers are looking for such a service, you might or might not consider offering this feature. But, if 40% of your regular customers are seeking support via social media, then it is something you just cannot overlook.
Characteristics of Quantitative Research
Quantitative research clarifies the fuzziness of research data from qualitative research analysis. With numerical insights, you can formulate a better and more profitable business decision.
Hence, quantitative research is more readily contestable, sharpens intelligent discussion, helps you see the rival hypotheses, and dynamically contributes to the research process.
Let us have a quick look at some of its characteristics.
1. Measurable Variables
The data collection methods in quantitative research are structured and contain items requiring measurable variables, such as age, number of family members, salary range, highest education, etc.
These structured data collection methods comprise polls, surveys, questionnaires, etc., and may have questions like the ones shown in the following image:
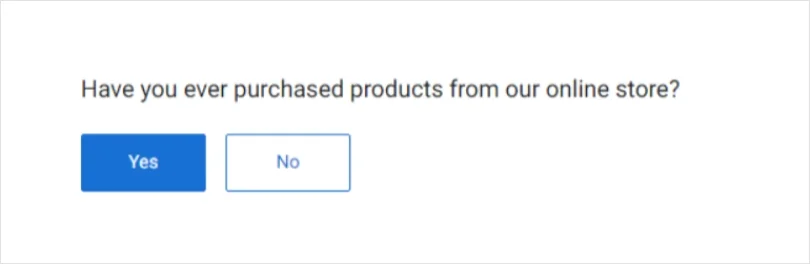
As you can see, all the variables are measurable. This ensures that the research is in-depth and provides less erroneous data for reliable, actionable insights.
2. Sample Size
No matter what data analysis methods for quantitative research are being used, the sample size is kept such that it represents the target market.
As the main aim of the research methodology is to get numerical insights, the sample size should be fairly large. Depending on the survey objective and scope, it might span hundreds of thousands of people.
3. Normal Population Distribution
To maintain the reliability of a quantitative research methodology, we assume that the population distribution curve is normal.
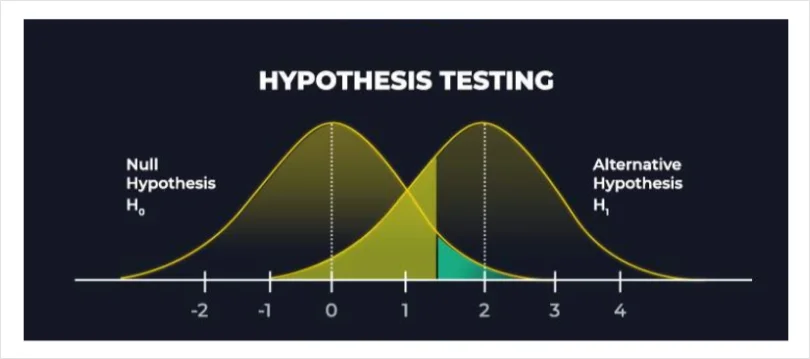
This type of population distribution curve is preferred over a non-normal distribution as the sample size is large, and the characteristics of the sample vary with its size.
This requires adhering to the random sampling principle to avoid the researcher’s bias in result interpretation. Any bias can ruin the fairness of the entire process and defeats the purpose of research.
4. Well-Structured Data Representation
Data analysis in quantitative research produces highly structured results and can form well-defined graphical representations. Some common examples include tables, figures, graphs, etc., that combine large blocks of data.
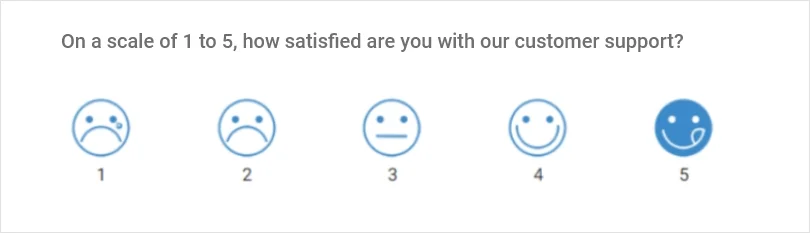
This way, you can discover hidden data trends, relationships, and differences among various measurable variables. This can help researchers understand the survey data and formulate actionable insights for decision-making.
5. Predictive Outcomes
Quantitative analysis of data can also be used for estimations and prediction outcomes. You can construct if-then scenarios and analyze the data for the identification of any upcoming trends or events.
However, this requires advanced analytics and involves complex mathematical computations. So, it is mostly done via quantitative research tools that come with advanced analytics capabilities.
Types of Quantitative Research Methods
Quantitative research is usually conducted using two methods. They are-
- Primary quantitative research methods
- Secondary quantitative research methods
1. Primary quantitative research methods
Primary quantitative research is the most popular way of conducting market research. The differentiating factor of this method is that the researcher relies on collecting data firsthand instead of relying on data collected from previous research.
There are multiple types of primary quantitative research. They can be distinguished based on three distinctive aspects, which are:
1.1. Techniques & Types of Studies:
- Survey Research
Surveys are the easiest, most common, and one of the most sought-after quantitative research techniques. The main aim of a survey is to widely gather and describe the characteristics of a target population or customers. Surveys are the foremost quantitative method preferred by both small and large organizations.
They help them understand their customers, products, and other brand offerings in a proper manner.
Surveys can be conducted using various methods, such as online polls, web-based surveys, paper questionnaires, phone calls, or face-to-face interviews. Survey research allows organizations to understand customer opinions, preferences, and behavior, making it crucial for market research and decision-making.
You can watch this quick video to learn more about creating surveys.
Watch: How to Create a Survey Using ProProfs Survey Maker
Surveys are of two types:
- Cross-Sectional Surveys Cross-sectional surveys are used to collect data from a sample of the target population at a specific point in time. Researchers evaluate various variables simultaneously to understand the relationships and patterns within the data.
- Cross-sectional surveys are popular in retail, small and medium-sized enterprises (SMEs), and healthcare industries, where they assess customer satisfaction, market trends, and product feedback.
- Longitudinal Surveys Longitudinal surveys are conducted over an extended period, observing changes in respondent behavior and thought processes.
- Researchers gather data from the same sample multiple times, enabling them to study trends and developments over time. These surveys are valuable in fields such as medicine, applied sciences, and market trend analysis.
Surveys can be distributed via various channels. Some of the most popular ones are listed below:
- Email: Sending surveys via email is a popular and effective method. People recognize your brand, leading to a higher response rate. With ProProfs Survey Maker’s in-mail survey-filling feature, you can easily send out and collect survey responses.
- Embed on a website: Boost your response rate by embedding the survey on your website. When visitors are already engaged with your brand, they are more likely to take the survey.
- Social media: Take advantage of social media platforms to distribute your survey. People familiar with your brand are likely to respond, increasing your response numbers.
- QR codes: QR codes store your survey’s URL, and you can print or publish these codes in magazines, signs, business cards, or any object to make it easy for people to access your survey.
- SMS survey: Collect a high number of responses quickly with SMS surveys. It’s a time-effective way to reach your target audience.
1.2. Correlational Research:
Correlational research aims to establish relationships between two or more variables.
Researchers use statistical analysis to identify patterns and trends in the data, but it does not determine causality between the variables. This method helps understand how changes in one variable may impact another.
Examples of correlational research questions include studying the relationship between stress and depression, fame and money, or classroom activities and student performance.
1.3. Causal-Comparative Research:
Causal-comparative research, also known as quasi-experimental research, seeks to determine cause-and-effect relationships between variables.
Researchers analyze how an independent variable influences a dependent variable, but they do not manipulate the independent variable. Instead, they observe and compare different groups to draw conclusions.
Causal-comparative research is useful in situations where it’s not ethical or feasible to conduct true experiments.
Examples of questions for this type of research include analyzing the effect of training programs on employee performance, studying the influence of customer support on client retention, investigating the impact of supply chain efficiency on cost reduction, etc.
1.4. Experimental Research:
Experimental research is based on testing theories to validate or disprove them. Researchers conduct experiments and manipulate variables to observe their impact on the outcomes.
This type of research is prevalent in natural and social sciences, and it is a powerful method to establish cause-and-effect relationships. By randomly assigning participants to experimental and control groups, researchers can draw more confident conclusions.
Examples of experimental research include studying the effectiveness of a new drug, the impact of teaching methods on student performance, or the outcomes of a marketing campaign.
2. Data collection methodologies
After defining research objectives, the next significant step in primary quantitative research is data collection. This involves using two main methods: sampling and conducting surveys or polls.
2.1Sampling methods:
In quantitative research, there are two primary sampling methods: Probability and Non-probability sampling.
2.2Probability Sampling
In probability sampling, researchers use the concept of probability to create samples from a population. This method ensures that every individual in the target audience has an equal chance of being selected for the sample.
There are four main types of probability sampling:
- Simple random sampling: Here, the elements or participants of a sample are selected randomly, and this technique is used in studies that are conducted over considerably large audiences. It works well for large target populations.
- Stratified random sampling: In this method, the entire population is divided into strata or groups, and the sample members get chosen randomly from these strata only. It is always ensured that different segregated strata do not overlap with each other.
- Cluster sampling: Here, researchers divide the population into clusters, often based on geography or demographics. Then, random clusters are selected for the sample.
- Systematic sampling: In this method, only the starting point of the sample is randomly chosen. All the other participants are chosen using a fixed interval. Researchers calculate this interval by dividing the size of the study population by the target sample size.
2.3Non-probability Sampling
Non-probability sampling is a method where the researcher’s knowledge and experience guide the selection of samples. This approach doesn’t give all members of the target population an equal chance of being included in the sample.
There are five non-probability sampling models:
- Convenience sampling: The elements or participants are chosen on the basis of their nearness to the researcher. The people in close proximity can be studied and analyzed easily and quickly, as there is no other selection criterion involved. Researchers simply choose samples based on what is most convenient for them.
- Consecutive sampling: Similar to convenience sampling, researchers select samples one after another over a significant period. They can opt for a single participant or a group of samples to conduct quantitative research in a consecutive manner for a significant period of time. Once this is over, they can conduct the research from the start.
- Quota sampling: With quota sampling, researchers use their understanding of target traits and personalities to form groups (strata). They then choose samples from each stratum based on their own judgment.
- Snowball sampling: This method is used where the target audiences are difficult to contact and interviewed for data collection. Researchers start with a few participants and then ask them to refer others, creating a snowball effect.
- Judgmental sampling: In judgmental sampling, researchers rely solely on their experience and research skills to handpick samples that they believe will be most relevant to the study.
3. Data analysis techniques
To analyze the quantitative data accurately, you’ll need to use specific statistical methods such as:
- SWOT Analysis: This stands for Strengths, Weaknesses, Opportunities, and Threats analysis. Organizations use SWOT analysis to evaluate their performance internally and externally. It helps develop effective improvement strategies.
- Conjoint Analysis: This market research method uncovers how individuals make complex purchasing decisions. It involves considering trade-offs in their daily activities when choosing from a list of product/service options.
- Cross-tabulation: A preliminary statistical market analysis method that reveals relationships, patterns, and trends within various research study parameters.
- TURF Analysis: Short for Totally Unduplicated Reach and Frequency Analysis, this method helps analyze the reach and frequency of favorable communication sources. It provides insights into the potential of a target market.
- By using these statistical techniques and inferential statistics methods like confidence intervals and margin of error, you can draw meaningful insights from your primary quantitative research that you can use in making informed decisions.
2. Secondary Quantitative Research Methods
- Secondary quantitative research, also known as desk research, is a valuable method that uses existing data, called secondary data.
- Instead of collecting new data, researchers analyze and combine already available information to enhance their research. This approach involves gathering quantitative data from various sources such as the internet, government databases, libraries, and research reports.
- Secondary quantitative research plays a crucial role in validating data collected through primary quantitative research. It helps reinforce or challenge existing findings.
Here are five commonly used secondary quantitative research methods:
A. Data Available on the Internet:
The Internet has become a vast repository of data, making it easier for researchers to access a wealth of information. Online databases, websites, and research repositories provide valuable quantitative data for researchers to analyze and validate their primary research findings.
B. Government and Non-Government Sources:
Government agencies and non-government organizations often conduct extensive research and publish reports. These reports cover a wide range of topics, providing researchers with reliable and comprehensive data for quantitative analysis.
C. Public Libraries:
While less commonly used in the digital age, public libraries still hold valuable research reports, historical data, and publications that can contribute to quantitative research.
D. Educational Institutions:
Educational institutions frequently conduct research on various subjects. Their research reports and publications can serve as valuable sources of information for researchers, validating and supporting primary quantitative research outcomes.
E. Commercial Information Sources:
Commercial sources such as local newspapers, journals, magazines, and media outlets often publish relevant data on economic trends, market research, and demographic analyses. Researchers can access this data to supplement their own findings and draw better conclusions.
Advantages of Quantitative Research Methods
Quantitative research data is often standardized and can be easily used to generalize findings for making crucial business decisions and uncover insights to supplement the qualitative research findings.
Here are some core benefits this research methodology offers.
Direct Result Comparison
As the studies can be replicated for different cultural settings and different times, even with different groups of participants, they tend to be extremely useful. Researchers can compare the results of different studies in a statistical manner and arrive at comprehensive conclusions for a broader understanding.
Replication
Researchers can repeat the study by using standardized data collection protocols over well-structured data sets. They can also apply tangible definitions of abstract concepts to arrive at different conclusions for similar research objectives with minor variations.
Large Samples
As the research data comes from large samples, the researchers can process and analyze the data via highly reliable and consistent analysis procedures. They can arrive at well-defined conclusions that can be used to make the primary research more thorough and reliable.
Hypothesis Testing
This research methodology follows standardized and established hypothesis testing procedures. So, you have to be careful while reporting and analyzing your research data , and the overall quality of results gets improved.
Proven Examples of Quantitative Research Methods
Below, we discuss two excellent examples of quantitative research methods that were used by highly distinguished business and consulting organizations. Both examples show how different types of analysis can be performed with qualitative approaches and how the analysis is done once the data is collected.
1. STEP Project Global Consortium / KPMG 2019 Global Family Business survey
This research utilized quantitative methods to identify ways that kept the family businesses sustainably profitable with time.
The study also identified the ways in which the family business behavior changed with demographic changes and had “why” and “how” questions. Their qualitative research methods allowed the KPMG team to dig deeper into the mindsets and perspectives of the business owners and uncover unexpected research avenues as well.
It was a joint effort in which STEP Project Global Consortium collected 26 cases, and KPMG collected 11 cases.
The research reached the stage of data analysis in 2020, and the analysis process spanned over 4 stages.
The results, which were also the reasons why family businesses tend to lose their strength with time, were found to be:
- Family governance
- Family business legacy
2. EY Seren Teams Research 2020
This is yet another commendable example of qualitative research where the EY Seren Team digs into the unexplored depths of human behavior and how it affected their brand or service expectations.
The research was done across 200+ sources and involved in-depth virtual interviews with people in their homes, exploring their current needs and wishes. It also involved diary studies across the entire UK customer base to analyze human behavior changes and patterns.
The study also included interviews with professionals and design leaders from a wide range of industries to explore how COVID-19 transformed their industries. Finally, quantitative surveys were conducted to gain insights into the EY community after every 15 days.
The insights and results were:
- A culture of fear, daily resilience, and hopes for a better world and a better life – these were the macro trends.
- People felt massive digitization to be a resourceful yet demanding aspect as they have to adapt every day.
- Some people wished to have a new world with lots of possibilities, and some were looking for a new purpose.
8 Best Practices to Conduct Quantitative Research
Here are some best practices to keep in mind while conducting quantitative research:
1. Define Research Objectives
There can be many ways to collect data via quantitative research methods that are chosen as per the research objective and scope. These methods allow you to build your own observations regarding any hypotheses – unknown, entirely new, or unexplained.
You can hypothesize a proof and build a prediction of outcomes supporting the same. You can also create a detailed stepwise plan for data collection, analysis, and testing.
Below, we explore quantitative research methods and discuss some examples to enhance your understanding of them.
2. Keep Your Questions Simple
The surveys are meant to reach people en-masse, and that includes a wide demographic range with recipients from all walks of life. Asking simple questions will ensure that they grasp what’s being asked easily.
3. Develop a Solid Research Design
Choose an appropriate research design that aligns with your objectives, whether it’s experimental, quasi-experimental, or correlational. You also need to pay attention to the sample size and sampling technique such that it represents the target population accurately.
4. Use Reliable & Valid Instruments
It’s crucial to select or develop measurement instruments such as questionnaires, scales, or tests that have been validated and are reliable. Before proceeding with the main study, pilot-test these instruments on a small sample to assess their effectiveness and make any necessary improvements.
5. Ensure Data Quality
Implement data collection protocols to minimize errors and bias during data gathering. Double-check data entries and cleaning procedures to eliminate any inconsistencies or missing values that may affect the accuracy of your results. For instance, you might regularly cross-verify data entries to identify and correct any discrepancies.
6. Employ Appropriate Data Analysis Techniques
Select statistical methods that match the nature of your data and research questions. Whether it’s regression analysis, t-tests, ANOVA, or other techniques, using the right approach is important for drawing meaningful conclusions. Utilize software tools like SPSS or R for data analysis to ensure the accuracy and reproducibility of your findings.
7. Interpret Results Objectively
Present your findings in a clear and unbiased manner. Avoid making unwarranted causal claims, especially in correlational studies. Instead, focus on describing the relationships and patterns observed in your data.
8. Address Ethical Considerations
Prioritize ethical considerations throughout your research process. Obtain informed consent from participants, ensuring their voluntary participation and confidentiality of data. Comply with ethical guidelines and gain approval from a governing body if necessary.
Enhance Your Quantitative Research With Cutting-Edge Software
While no single research methodology can produce 100% reliable results, you can always opt for a hybrid research method by opting for the methods that are most relevant to your objective.
This understanding comes gradually as you learn how to implement the correct combination of qualitative and quantitative research methods for your research projects. For the best results, we recommend investing in smart, efficient, and scalable research tools that come with delightful reporting and advanced analytics to make every research initiative a success.
These software tools, such as ProProfs Survey Maker, come with pre-built survey templates and question libraries and allow you to create a high-converting survey in just a few minutes.
So, choose the best research partner, create the right research plan, and gather insights that drive sustainable growth for your business.
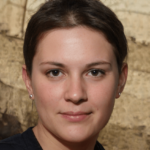
About the author
Emma David is a seasoned market research professional with 8+ years of experience. Having kick-started her journey in research, she has developed rich expertise in employee engagement, survey creation and administration, and data management. Emma believes in the power of data to shape business performance positively. She continues to help brands and businesses make strategic decisions and improve their market standing through her understanding of research methodologies.
Related Posts
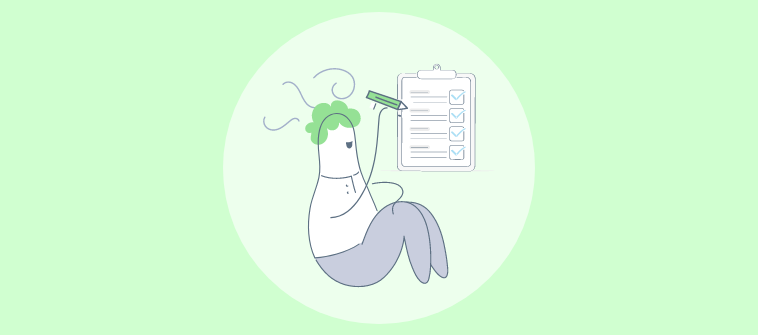
40+ Mental Health Survey Questions With Templates
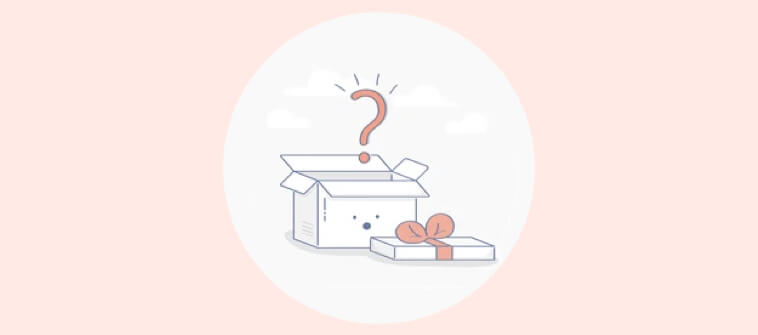
How Many Questions Should Be Asked in a Survey?
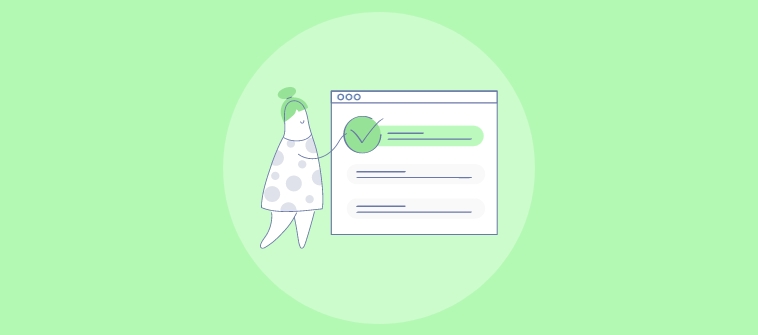
Client Onboarding Questionnaire: Best Practices & 20+ Questions
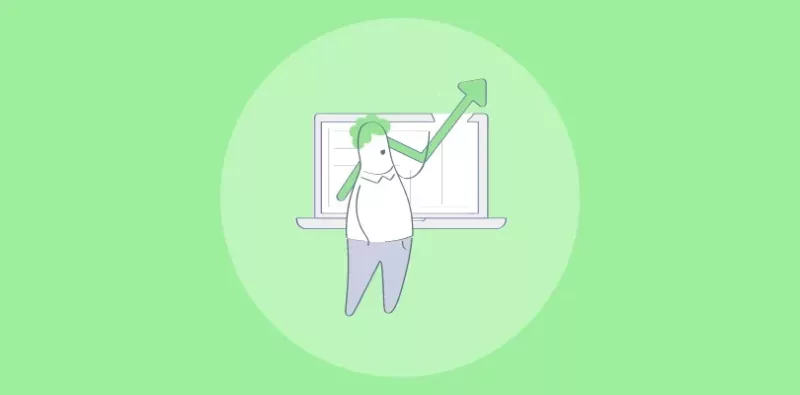
Qualitative Research Methods: Types, Examples, and Analysis
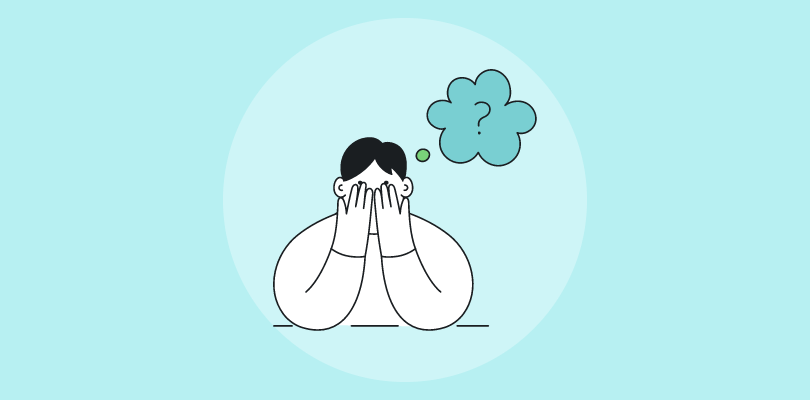
70+ Mental Health Survey Questionnaire for Students
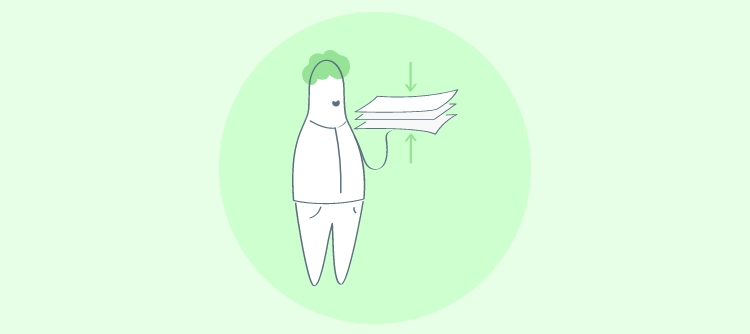
Data Collection Methods: Definition, Types & Examples
An Overview of Quantitative Research Methods
- August 2023
- INTERNATIONAL JOURNAL OF MULTIDISCIPLINARY RESEARCH AND ANALYSIS 06(08)

- Veritas University College
Discover the world's research
- 25+ million members
- 160+ million publication pages
- 2.3+ billion citations

- Christofer D. Sta. Ana
- Isaiah M. Makhetha

- James C. Royo
- Regine L. Generalao
- Muhammad Ali Zia
- Abdul Rasheed
- Jennifer Davis-Adesegha
- asmaa Yaseen
- Mogeda Mohamed Mehany
- Ahmed Mohamed Ali
- Safaa mohamed Adam

- David E. McNabb
- H. Johnson Nenty
- Clifford Woody

- Annie W. Ward
- Connie B. Comer
- EDUC PSYCHOL MEAS
- Kenneth D. Hopkins

- Alan Bryman
- Robert E. Stake
- A Thornhill
- Recruit researchers
- Join for free
- Login Email Tip: Most researchers use their institutional email address as their ResearchGate login Password Forgot password? Keep me logged in Log in or Continue with Google Welcome back! Please log in. Email · Hint Tip: Most researchers use their institutional email address as their ResearchGate login Password Forgot password? Keep me logged in Log in or Continue with Google No account? Sign up
Have a language expert improve your writing
Run a free plagiarism check in 10 minutes, generate accurate citations for free.
- Knowledge Base
Methodology
- Qualitative vs. Quantitative Research | Differences, Examples & Methods
Qualitative vs. Quantitative Research | Differences, Examples & Methods
Published on April 12, 2019 by Raimo Streefkerk . Revised on June 22, 2023.
When collecting and analyzing data, quantitative research deals with numbers and statistics, while qualitative research deals with words and meanings. Both are important for gaining different kinds of knowledge.
Common quantitative methods include experiments, observations recorded as numbers, and surveys with closed-ended questions.
Quantitative research is at risk for research biases including information bias , omitted variable bias , sampling bias , or selection bias . Qualitative research Qualitative research is expressed in words . It is used to understand concepts, thoughts or experiences. This type of research enables you to gather in-depth insights on topics that are not well understood.
Common qualitative methods include interviews with open-ended questions, observations described in words, and literature reviews that explore concepts and theories.
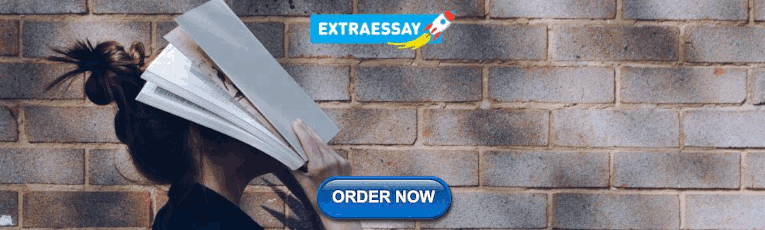
Table of contents
The differences between quantitative and qualitative research, data collection methods, when to use qualitative vs. quantitative research, how to analyze qualitative and quantitative data, other interesting articles, frequently asked questions about qualitative and quantitative research.
Quantitative and qualitative research use different research methods to collect and analyze data, and they allow you to answer different kinds of research questions.
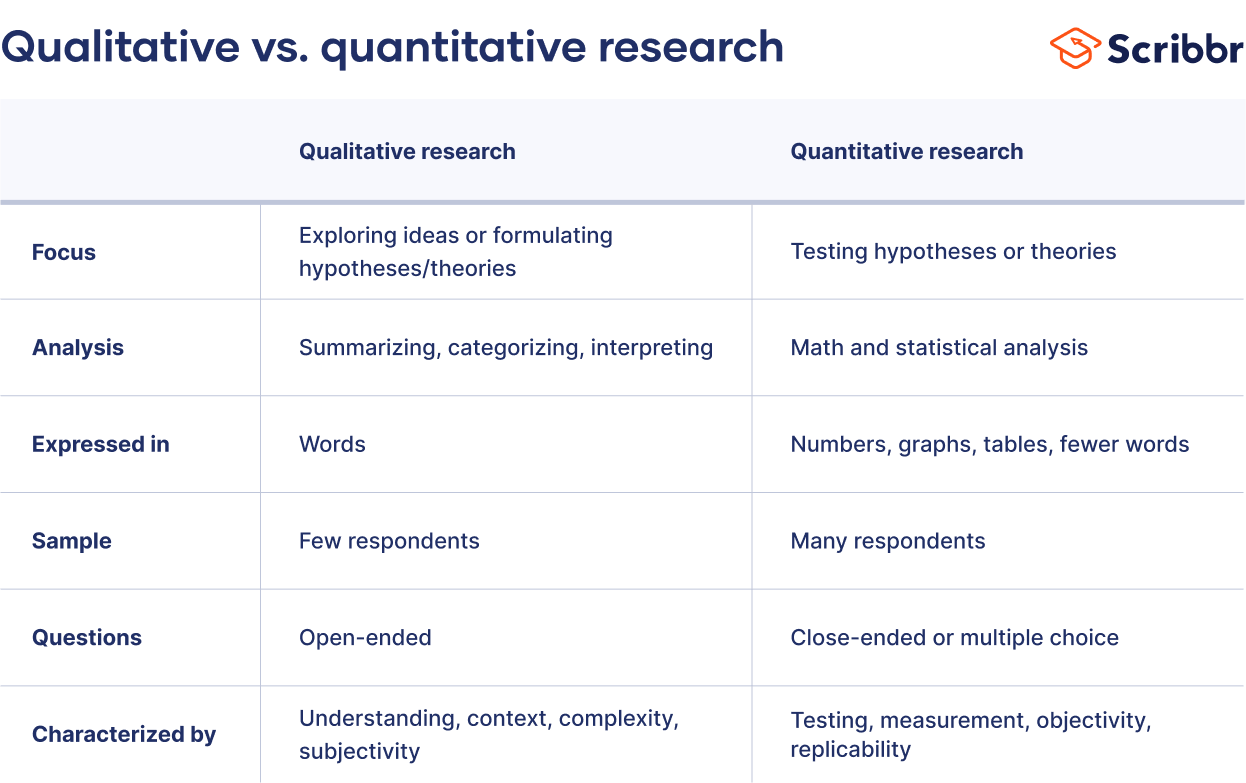
Quantitative and qualitative data can be collected using various methods. It is important to use a data collection method that will help answer your research question(s).
Many data collection methods can be either qualitative or quantitative. For example, in surveys, observational studies or case studies , your data can be represented as numbers (e.g., using rating scales or counting frequencies) or as words (e.g., with open-ended questions or descriptions of what you observe).
However, some methods are more commonly used in one type or the other.
Quantitative data collection methods
- Surveys : List of closed or multiple choice questions that is distributed to a sample (online, in person, or over the phone).
- Experiments : Situation in which different types of variables are controlled and manipulated to establish cause-and-effect relationships.
- Observations : Observing subjects in a natural environment where variables can’t be controlled.
Qualitative data collection methods
- Interviews : Asking open-ended questions verbally to respondents.
- Focus groups : Discussion among a group of people about a topic to gather opinions that can be used for further research.
- Ethnography : Participating in a community or organization for an extended period of time to closely observe culture and behavior.
- Literature review : Survey of published works by other authors.
A rule of thumb for deciding whether to use qualitative or quantitative data is:
- Use quantitative research if you want to confirm or test something (a theory or hypothesis )
- Use qualitative research if you want to understand something (concepts, thoughts, experiences)
For most research topics you can choose a qualitative, quantitative or mixed methods approach . Which type you choose depends on, among other things, whether you’re taking an inductive vs. deductive research approach ; your research question(s) ; whether you’re doing experimental , correlational , or descriptive research ; and practical considerations such as time, money, availability of data, and access to respondents.
Quantitative research approach
You survey 300 students at your university and ask them questions such as: “on a scale from 1-5, how satisfied are your with your professors?”
You can perform statistical analysis on the data and draw conclusions such as: “on average students rated their professors 4.4”.
Qualitative research approach
You conduct in-depth interviews with 15 students and ask them open-ended questions such as: “How satisfied are you with your studies?”, “What is the most positive aspect of your study program?” and “What can be done to improve the study program?”
Based on the answers you get you can ask follow-up questions to clarify things. You transcribe all interviews using transcription software and try to find commonalities and patterns.
Mixed methods approach
You conduct interviews to find out how satisfied students are with their studies. Through open-ended questions you learn things you never thought about before and gain new insights. Later, you use a survey to test these insights on a larger scale.
It’s also possible to start with a survey to find out the overall trends, followed by interviews to better understand the reasons behind the trends.
Qualitative or quantitative data by itself can’t prove or demonstrate anything, but has to be analyzed to show its meaning in relation to the research questions. The method of analysis differs for each type of data.
Analyzing quantitative data
Quantitative data is based on numbers. Simple math or more advanced statistical analysis is used to discover commonalities or patterns in the data. The results are often reported in graphs and tables.
Applications such as Excel, SPSS, or R can be used to calculate things like:
- Average scores ( means )
- The number of times a particular answer was given
- The correlation or causation between two or more variables
- The reliability and validity of the results
Analyzing qualitative data
Qualitative data is more difficult to analyze than quantitative data. It consists of text, images or videos instead of numbers.
Some common approaches to analyzing qualitative data include:
- Qualitative content analysis : Tracking the occurrence, position and meaning of words or phrases
- Thematic analysis : Closely examining the data to identify the main themes and patterns
- Discourse analysis : Studying how communication works in social contexts
If you want to know more about statistics , methodology , or research bias , make sure to check out some of our other articles with explanations and examples.
- Chi square goodness of fit test
- Degrees of freedom
- Null hypothesis
- Discourse analysis
- Control groups
- Mixed methods research
- Non-probability sampling
- Quantitative research
- Inclusion and exclusion criteria
Research bias
- Rosenthal effect
- Implicit bias
- Cognitive bias
- Selection bias
- Negativity bias
- Status quo bias
Quantitative research deals with numbers and statistics, while qualitative research deals with words and meanings.
Quantitative methods allow you to systematically measure variables and test hypotheses . Qualitative methods allow you to explore concepts and experiences in more detail.
In mixed methods research , you use both qualitative and quantitative data collection and analysis methods to answer your research question .
The research methods you use depend on the type of data you need to answer your research question .
- If you want to measure something or test a hypothesis , use quantitative methods . If you want to explore ideas, thoughts and meanings, use qualitative methods .
- If you want to analyze a large amount of readily-available data, use secondary data. If you want data specific to your purposes with control over how it is generated, collect primary data.
- If you want to establish cause-and-effect relationships between variables , use experimental methods. If you want to understand the characteristics of a research subject, use descriptive methods.
Data collection is the systematic process by which observations or measurements are gathered in research. It is used in many different contexts by academics, governments, businesses, and other organizations.
There are various approaches to qualitative data analysis , but they all share five steps in common:
- Prepare and organize your data.
- Review and explore your data.
- Develop a data coding system.
- Assign codes to the data.
- Identify recurring themes.
The specifics of each step depend on the focus of the analysis. Some common approaches include textual analysis , thematic analysis , and discourse analysis .
A research project is an academic, scientific, or professional undertaking to answer a research question . Research projects can take many forms, such as qualitative or quantitative , descriptive , longitudinal , experimental , or correlational . What kind of research approach you choose will depend on your topic.
Cite this Scribbr article
If you want to cite this source, you can copy and paste the citation or click the “Cite this Scribbr article” button to automatically add the citation to our free Citation Generator.
Streefkerk, R. (2023, June 22). Qualitative vs. Quantitative Research | Differences, Examples & Methods. Scribbr. Retrieved September 6, 2024, from https://www.scribbr.com/methodology/qualitative-quantitative-research/
Is this article helpful?
Raimo Streefkerk
Other students also liked, what is quantitative research | definition, uses & methods, what is qualitative research | methods & examples, mixed methods research | definition, guide & examples, "i thought ai proofreading was useless but..".
I've been using Scribbr for years now and I know it's a service that won't disappoint. It does a good job spotting mistakes”
- Skip to main content
- Skip to primary sidebar
- Skip to footer
- QuestionPro

- Solutions Industries Gaming Automotive Sports and events Education Government Travel & Hospitality Financial Services Healthcare Cannabis Technology Use Case AskWhy Communities Audience Contactless surveys Mobile LivePolls Member Experience GDPR Positive People Science 360 Feedback Surveys
- Resources Blog eBooks Survey Templates Case Studies Training Help center

Home Market Research
Quantitative Research: What It Is, Practices & Methods
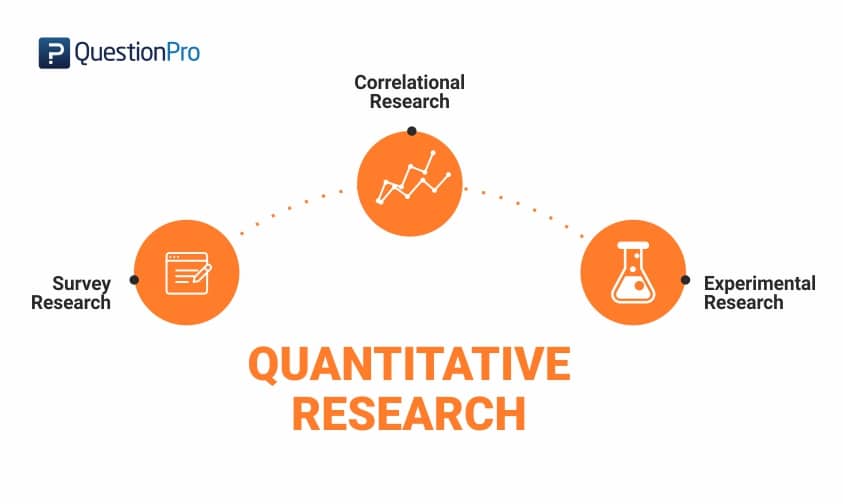
Quantitative research involves analyzing and gathering numerical data to uncover trends, calculate averages, evaluate relationships, and derive overarching insights. It’s used in various fields, including the natural and social sciences. Quantitative data analysis employs statistical techniques for processing and interpreting numeric data.
Research designs in the quantitative realm outline how data will be collected and analyzed with methods like experiments and surveys. Qualitative methods complement quantitative research by focusing on non-numerical data, adding depth to understanding. Data collection methods can be qualitative or quantitative, depending on research goals. Researchers often use a combination of both approaches to gain a comprehensive understanding of phenomena.
What is Quantitative Research?
Quantitative research is a systematic investigation of phenomena by gathering quantifiable data and performing statistical, mathematical, or computational techniques. Quantitative research collects statistically significant information from existing and potential customers using sampling methods and sending out online surveys , online polls , and questionnaires , for example.
One of the main characteristics of this type of research is that the results can be depicted in numerical form. After carefully collecting structured observations and understanding these numbers, it’s possible to predict the future of a product or service, establish causal relationships or Causal Research , and make changes accordingly. Quantitative research primarily centers on the analysis of numerical data and utilizes inferential statistics to derive conclusions that can be extrapolated to the broader population.
An example of a quantitative research study is the survey conducted to understand how long a doctor takes to tend to a patient when the patient walks into the hospital. A patient satisfaction survey can be administered to ask questions like how long a doctor takes to see a patient, how often a patient walks into a hospital, and other such questions, which are dependent variables in the research. This kind of research method is often employed in the social sciences, and it involves using mathematical frameworks and theories to effectively present data, ensuring that the results are logical, statistically sound, and unbiased.
Data collection in quantitative research uses a structured method and is typically conducted on larger samples representing the entire population. Researchers use quantitative methods to collect numerical data, which is then subjected to statistical analysis to determine statistically significant findings. This approach is valuable in both experimental research and social research, as it helps in making informed decisions and drawing reliable conclusions based on quantitative data.
Quantitative Research Characteristics
Quantitative research has several unique characteristics that make it well-suited for specific projects. Let’s explore the most crucial of these characteristics so that you can consider them when planning your next research project:
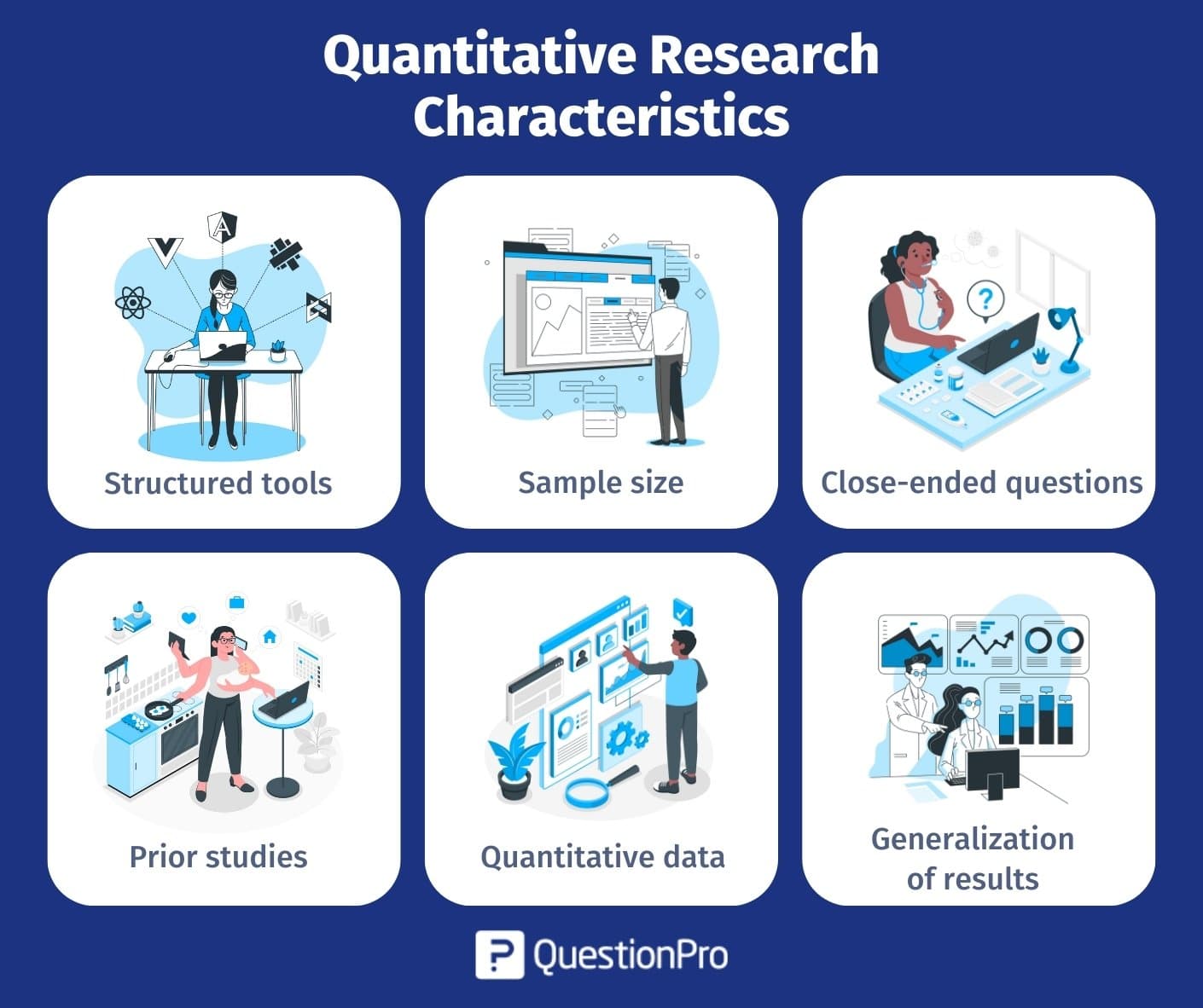
- Structured tools: Quantitative research relies on structured tools such as surveys, polls, or questionnaires to gather quantitative data . Using such structured methods helps collect in-depth and actionable numerical data from the survey respondents, making it easier to perform data analysis.
- Sample size: Quantitative research is conducted on a significant sample size representing the target market . Appropriate Survey Sampling methods, a fundamental aspect of quantitative research methods, must be employed when deriving the sample to fortify the research objective and ensure the reliability of the results.
- Close-ended questions: Closed-ended questions , specifically designed to align with the research objectives, are a cornerstone of quantitative research. These questions facilitate the collection of quantitative data and are extensively used in data collection processes.
- Prior studies: Before collecting feedback from respondents, researchers often delve into previous studies related to the research topic. This preliminary research helps frame the study effectively and ensures the data collection process is well-informed.
- Quantitative data: Typically, quantitative data is represented using tables, charts, graphs, or other numerical forms. This visual representation aids in understanding the collected data and is essential for rigorous data analysis, a key component of quantitative research methods.
- Generalization of results: One of the strengths of quantitative research is its ability to generalize results to the entire population. It means that the findings derived from a sample can be extrapolated to make informed decisions and take appropriate actions for improvement based on numerical data analysis.
Quantitative Research Methods
Quantitative research methods are systematic approaches used to gather and analyze numerical data to understand and draw conclusions about a phenomenon or population. Here are the quantitative research methods:
- Primary quantitative research methods
- Secondary quantitative research methods
Primary Quantitative Research Methods
Primary quantitative research is the most widely used method of conducting market research. The distinct feature of primary research is that the researcher focuses on collecting data directly rather than depending on data collected from previously done research. Primary quantitative research design can be broken down into three further distinctive tracks and the process flow. They are:
A. Techniques and Types of Studies
There are multiple types of primary quantitative research. They can be distinguished into the four following distinctive methods, which are:
01. Survey Research
Survey Research is fundamental for all quantitative outcome research methodologies and studies. Surveys are used to ask questions to a sample of respondents, using various types such as online polls, online surveys, paper questionnaires, web-intercept surveys , etc. Every small and big organization intends to understand what their customers think about their products and services, how well new features are faring in the market, and other such details.
By conducting survey research, an organization can ask multiple survey questions , collect data from a pool of customers, and analyze this collected data to produce numerical results. It is the first step towards collecting data for any research. You can use single ease questions . A single-ease question is a straightforward query that elicits a concise and uncomplicated response.
This type of research can be conducted with a specific target audience group and also can be conducted across multiple groups along with comparative analysis . A prerequisite for this type of research is that the sample of respondents must have randomly selected members. This way, a researcher can easily maintain the accuracy of the obtained results as a huge variety of respondents will be addressed using random selection.
Traditionally, survey research was conducted face-to-face or via phone calls. Still, with the progress made by online mediums such as email or social media, survey research has also spread to online mediums.There are two types of surveys , either of which can be chosen based on the time in hand and the kind of data required:
Cross-sectional surveys: Cross-sectional surveys are observational surveys conducted in situations where the researcher intends to collect data from a sample of the target population at a given point in time. Researchers can evaluate various variables at a particular time. Data gathered using this type of survey is from people who depict similarity in all variables except the variables which are considered for research . Throughout the survey, this one variable will stay constant.
- Cross-sectional surveys are popular with retail, SMEs, and healthcare industries. Information is garnered without modifying any parameters in the variable ecosystem.
- Multiple samples can be analyzed and compared using a cross-sectional survey research method.
- Multiple variables can be evaluated using this type of survey research.
- The only disadvantage of cross-sectional surveys is that the cause-effect relationship of variables cannot be established as it usually evaluates variables at a particular time and not across a continuous time frame.
Longitudinal surveys: Longitudinal surveys are also observational surveys , but unlike cross-sectional surveys, longitudinal surveys are conducted across various time durations to observe a change in respondent behavior and thought processes. This time can be days, months, years, or even decades. For instance, a researcher planning to analyze the change in buying habits of teenagers over 5 years will conduct longitudinal surveys.
- In cross-sectional surveys, the same variables were evaluated at a given time, and in longitudinal surveys, different variables can be analyzed at different intervals.
- Longitudinal surveys are extensively used in the field of medicine and applied sciences. Apart from these two fields, they are also used to observe a change in the market trend analysis , analyze customer satisfaction, or gain feedback on products/services.
- In situations where the sequence of events is highly essential, longitudinal surveys are used.
- Researchers say that when research subjects need to be thoroughly inspected before concluding, they rely on longitudinal surveys.
02. Correlational Research
A comparison between two entities is invariable. Correlation research is conducted to establish a relationship between two closely-knit entities and how one impacts the other, and what changes are eventually observed. This research method is carried out to give value to naturally occurring relationships, and a minimum of two different groups are required to conduct this quantitative research method successfully. Without assuming various aspects, a relationship between two groups or entities must be established.
Researchers use this quantitative research design to correlate two or more variables using mathematical analysis methods. Patterns, relationships, and trends between variables are concluded as they exist in their original setup. The impact of one of these variables on the other is observed, along with how it changes the relationship between the two variables. Researchers tend to manipulate one of the variables to attain the desired results.
Ideally, it is advised not to make conclusions merely based on correlational research. This is because it is not mandatory that if two variables are in sync that they are interrelated.
Example of Correlational Research Questions :
- The relationship between stress and depression.
- The equation between fame and money.
- The relation between activities in a third-grade class and its students.
03. Causal-comparative Research
This research method mainly depends on the factor of comparison. Also called quasi-experimental research , this quantitative research method is used by researchers to conclude the cause-effect equation between two or more variables, where one variable is dependent on the other independent variable. The independent variable is established but not manipulated, and its impact on the dependent variable is observed. These variables or groups must be formed as they exist in the natural setup. As the dependent and independent variables will always exist in a group, it is advised that the conclusions are carefully established by keeping all the factors in mind.
Causal-comparative research is not restricted to the statistical analysis of two variables but extends to analyzing how various variables or groups change under the influence of the same changes. This research is conducted irrespective of the type of relationship that exists between two or more variables. Statistical analysis plan is used to present the outcome using this quantitative research method.
Example of Causal-Comparative Research Questions:
- The impact of drugs on a teenager. The effect of good education on a freshman. The effect of substantial food provision in the villages of Africa.
04. Experimental Research
Also known as true experimentation, this research method relies on a theory. As the name suggests, experimental research is usually based on one or more theories. This theory has yet to be proven before and is merely a supposition. In experimental research, an analysis is done around proving or disproving the statement. This research method is used in natural sciences. Traditional research methods are more effective than modern techniques.
There can be multiple theories in experimental research. A theory is a statement that can be verified or refuted.
After establishing the statement, efforts are made to understand whether it is valid or invalid. This quantitative research method is mainly used in natural or social sciences as various statements must be proved right or wrong.
- Traditional research methods are more effective than modern techniques.
- Systematic teaching schedules help children who struggle to cope with the course.
- It is a boon to have responsible nursing staff for ailing parents.
B. Data Collection Methodologies
The second major step in primary quantitative research is data collection. Data collection can be divided into sampling methods and data collection using surveys and polls.
01. Data Collection Methodologies: Sampling Methods
There are two main sampling methods for quantitative research: Probability and Non-probability sampling .
Probability sampling: A theory of probability is used to filter individuals from a population and create samples in probability sampling . Participants of a sample are chosen by random selection processes. Each target audience member has an equal opportunity to be selected in the sample.
There are four main types of probability sampling:
- Simple random sampling: As the name indicates, simple random sampling is nothing but a random selection of elements for a sample. This sampling technique is implemented where the target population is considerably large.
- Stratified random sampling: In the stratified random sampling method , a large population is divided into groups (strata), and members of a sample are chosen randomly from these strata. The various segregated strata should ideally not overlap one another.
- Cluster sampling: Cluster sampling is a probability sampling method using which the main segment is divided into clusters, usually using geographic segmentation and demographic segmentation parameters.
- Systematic sampling: Systematic sampling is a technique where the starting point of the sample is chosen randomly, and all the other elements are chosen using a fixed interval. This interval is calculated by dividing the population size by the target sample size.
Non-probability sampling: Non-probability sampling is where the researcher’s knowledge and experience are used to create samples. Because of the researcher’s involvement, not all the target population members have an equal probability of being selected to be a part of a sample.
There are five non-probability sampling models:
- Convenience sampling: In convenience sampling , elements of a sample are chosen only due to one prime reason: their proximity to the researcher. These samples are quick and easy to implement as there is no other parameter of selection involved.
- Consecutive sampling: Consecutive sampling is quite similar to convenience sampling, except for the fact that researchers can choose a single element or a group of samples and conduct research consecutively over a significant period and then perform the same process with other samples.
- Quota sampling: Using quota sampling , researchers can select elements using their knowledge of target traits and personalities to form strata. Members of various strata can then be chosen to be a part of the sample as per the researcher’s understanding.
- Snowball sampling: Snowball sampling is conducted with target audiences who are difficult to contact and get information. It is popular in cases where the target audience for analysis research is rare to put together.
- Judgmental sampling: Judgmental sampling is a non-probability sampling method where samples are created only based on the researcher’s experience and research skill .
02. Data collection methodologies: Using surveys & polls
Once the sample is determined, then either surveys or polls can be distributed to collect the data for quantitative research.
Using surveys for primary quantitative research
A survey is defined as a research method used for collecting data from a pre-defined group of respondents to gain information and insights on various topics of interest. The ease of survey distribution and the wide number of people it can reach depending on the research time and objective makes it one of the most important aspects of conducting quantitative research.
Fundamental levels of measurement – nominal, ordinal, interval, and ratio scales
Four measurement scales are fundamental to creating a multiple-choice question in a survey. They are nominal, ordinal, interval, and ratio measurement scales without the fundamentals of which no multiple-choice questions can be created. Hence, it is crucial to understand these measurement levels to develop a robust survey.
Use of different question types
To conduct quantitative research, close-ended questions must be used in a survey. They can be a mix of multiple question types, including multiple-choice questions like semantic differential scale questions , rating scale questions , etc.
Survey Distribution and Survey Data Collection
In the above, we have seen the process of building a survey along with the research design to conduct primary quantitative research. Survey distribution to collect data is the other important aspect of the survey process. There are different ways of survey distribution. Some of the most commonly used methods are:
- Email: Sending a survey via email is the most widely used and effective survey distribution method. This method’s response rate is high because the respondents know your brand. You can use the QuestionPro email management feature to send out and collect survey responses.
- Buy respondents: Another effective way to distribute a survey and conduct primary quantitative research is to use a sample. Since the respondents are knowledgeable and are on the panel by their own will, responses are much higher.
- Embed survey on a website: Embedding a survey on a website increases a high number of responses as the respondent is already in close proximity to the brand when the survey pops up.
- Social distribution: Using social media to distribute the survey aids in collecting a higher number of responses from the people that are aware of the brand.
- QR code: QuestionPro QR codes store the URL for the survey. You can print/publish this code in magazines, signs, business cards, or on just about any object/medium.
- SMS survey: The SMS survey is a quick and time-effective way to collect a high number of responses.
- Offline Survey App: The QuestionPro App allows users to circulate surveys quickly, and the responses can be collected both online and offline.
Survey example
An example of a survey is a short customer satisfaction (CSAT) survey that can quickly be built and deployed to collect feedback about what the customer thinks about a brand and how satisfied and referenceable the brand is.
Using polls for primary quantitative research
Polls are a method to collect feedback using close-ended questions from a sample. The most commonly used types of polls are election polls and exit polls . Both of these are used to collect data from a large sample size but using basic question types like multiple-choice questions.
C. Data Analysis Techniques
The third aspect of primary quantitative research design is data analysis . After collecting raw data, there must be an analysis of this data to derive statistical inferences from this research. It is important to relate the results to the research objective and establish the statistical relevance of the results.
Remember to consider aspects of research that were not considered for the data collection process and report the difference between what was planned vs. what was actually executed.
It is then required to select precise Statistical Analysis Methods , such as SWOT, Conjoint, Cross-tabulation, etc., to analyze the quantitative data.
- SWOT analysis: SWOT Analysis stands for the acronym of Strengths, Weaknesses, Opportunities, and Threat analysis. Organizations use this statistical analysis technique to evaluate their performance internally and externally to develop effective strategies for improvement.
- Conjoint Analysis: Conjoint Analysis is a market analysis method to learn how individuals make complicated purchasing decisions. Trade-offs are involved in an individual’s daily activities, and these reflect their ability to decide from a complex list of product/service options.
- Cross-tabulation: Cross-tabulation is one of the preliminary statistical market analysis methods which establishes relationships, patterns, and trends within the various parameters of the research study.
- TURF Analysis: TURF Analysis , an acronym for Totally Unduplicated Reach and Frequency Analysis, is executed in situations where the reach of a favorable communication source is to be analyzed along with the frequency of this communication. It is used for understanding the potential of a target market.
Inferential statistics methods such as confidence interval, the margin of error, etc., can then be used to provide results.
Secondary Quantitative Research Methods
Secondary quantitative research or desk research is a research method that involves using already existing data or secondary data. Existing data is summarized and collated to increase the overall effectiveness of the research.
This research method involves collecting quantitative data from existing data sources like the internet, government resources, libraries, research reports, etc. Secondary quantitative research helps to validate the data collected from primary quantitative research and aid in strengthening or proving, or disproving previously collected data.
The following are five popularly used secondary quantitative research methods:
- Data available on the internet: With the high penetration of the internet and mobile devices, it has become increasingly easy to conduct quantitative research using the internet. Information about most research topics is available online, and this aids in boosting the validity of primary quantitative data.
- Government and non-government sources: Secondary quantitative research can also be conducted with the help of government and non-government sources that deal with market research reports. This data is highly reliable and in-depth and hence, can be used to increase the validity of quantitative research design.
- Public libraries: Now a sparingly used method of conducting quantitative research, it is still a reliable source of information, though. Public libraries have copies of important research that was conducted earlier. They are a storehouse of valuable information and documents from which information can be extracted.
- Educational institutions: Educational institutions conduct in-depth research on multiple topics, and hence, the reports that they publish are an important source of validation in quantitative research.
- Commercial information sources: Local newspapers, journals, magazines, radio, and TV stations are great sources to obtain data for secondary quantitative research. These commercial information sources have in-depth, first-hand information on market research, demographic segmentation, and similar subjects.
Quantitative Research Examples
Some examples of quantitative research are:
- A customer satisfaction template can be used if any organization would like to conduct a customer satisfaction (CSAT) survey . Through this kind of survey, an organization can collect quantitative data and metrics on the goodwill of the brand or organization in the customer’s mind based on multiple parameters such as product quality, pricing, customer experience, etc. This data can be collected by asking a net promoter score (NPS) question , matrix table questions, etc. that provide data in the form of numbers that can be analyzed and worked upon.
- Another example of quantitative research is an organization that conducts an event, collecting feedback from attendees about the value they see from the event. By using an event survey , the organization can collect actionable feedback about the satisfaction levels of customers during various phases of the event such as the sales, pre and post-event, the likelihood of recommending the organization to their friends and colleagues, hotel preferences for the future events and other such questions.
What are the Advantages of Quantitative Research?
There are many advantages to quantitative research. Some of the major advantages of why researchers use this method in market research are:
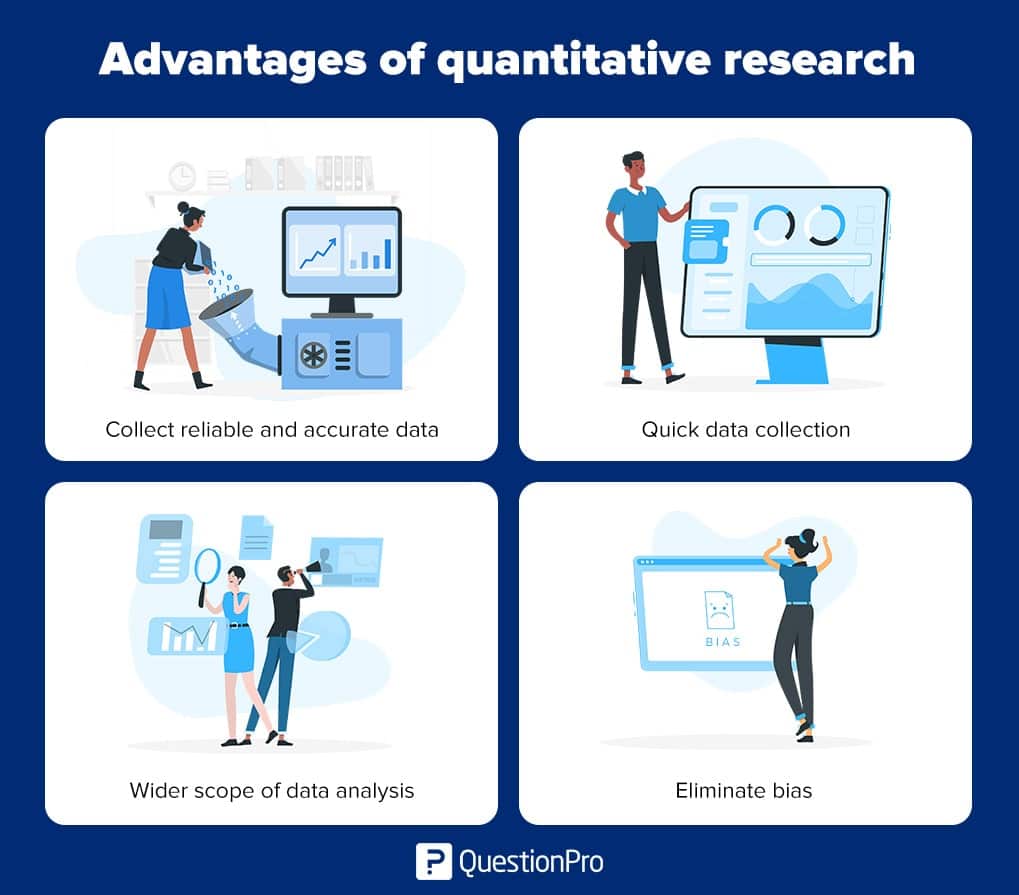
Collect Reliable and Accurate Data:
Quantitative research is a powerful method for collecting reliable and accurate quantitative data. Since data is collected, analyzed, and presented in numbers, the results obtained are incredibly reliable and objective. Numbers do not lie and offer an honest and precise picture of the conducted research without discrepancies. In situations where a researcher aims to eliminate bias and predict potential conflicts, quantitative research is the method of choice.
Quick Data Collection:
Quantitative research involves studying a group of people representing a larger population. Researchers use a survey or another quantitative research method to efficiently gather information from these participants, making the process of analyzing the data and identifying patterns faster and more manageable through the use of statistical analysis. This advantage makes quantitative research an attractive option for projects with time constraints.
Wider Scope of Data Analysis:
Quantitative research, thanks to its utilization of statistical methods, offers an extensive range of data collection and analysis. Researchers can delve into a broader spectrum of variables and relationships within the data, enabling a more thorough comprehension of the subject under investigation. This expanded scope is precious when dealing with complex research questions that require in-depth numerical analysis.
Eliminate Bias:
One of the significant advantages of quantitative research is its ability to eliminate bias. This research method leaves no room for personal comments or the biasing of results, as the findings are presented in numerical form. This objectivity makes the results fair and reliable in most cases, reducing the potential for researcher bias or subjectivity.
In summary, quantitative research involves collecting, analyzing, and presenting quantitative data using statistical analysis. It offers numerous advantages, including the collection of reliable and accurate data, quick data collection, a broader scope of data analysis, and the elimination of bias, making it a valuable approach in the field of research. When considering the benefits of quantitative research, it’s essential to recognize its strengths in contrast to qualitative methods and its role in collecting and analyzing numerical data for a more comprehensive understanding of research topics.
Best Practices to Conduct Quantitative Research
Here are some best practices for conducting quantitative research:
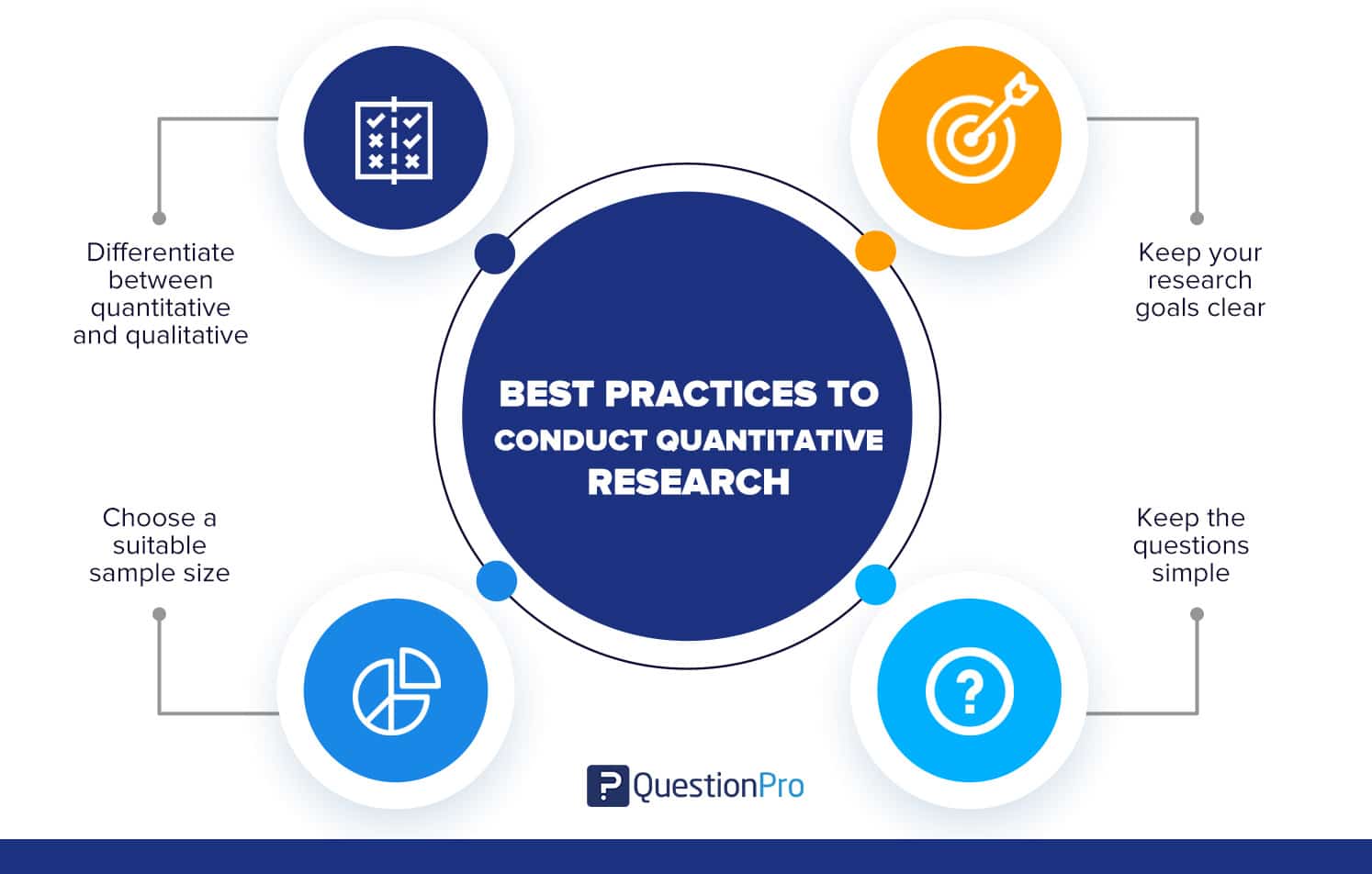
- Differentiate between quantitative and qualitative: Understand the difference between the two methodologies and apply the one that suits your needs best.
- Choose a suitable sample size: Ensure that you have a sample representative of your population and large enough to be statistically weighty.
- Keep your research goals clear and concise: Know your research goals before you begin data collection to ensure you collect the right amount and the right quantity of data.
- Keep the questions simple: Remember that you will be reaching out to a demographically wide audience. Pose simple questions for your respondents to understand easily.
Quantitative Research vs Qualitative Research
Quantitative research and qualitative research are two distinct approaches to conducting research, each with its own set of methods and objectives. Here’s a comparison of the two:
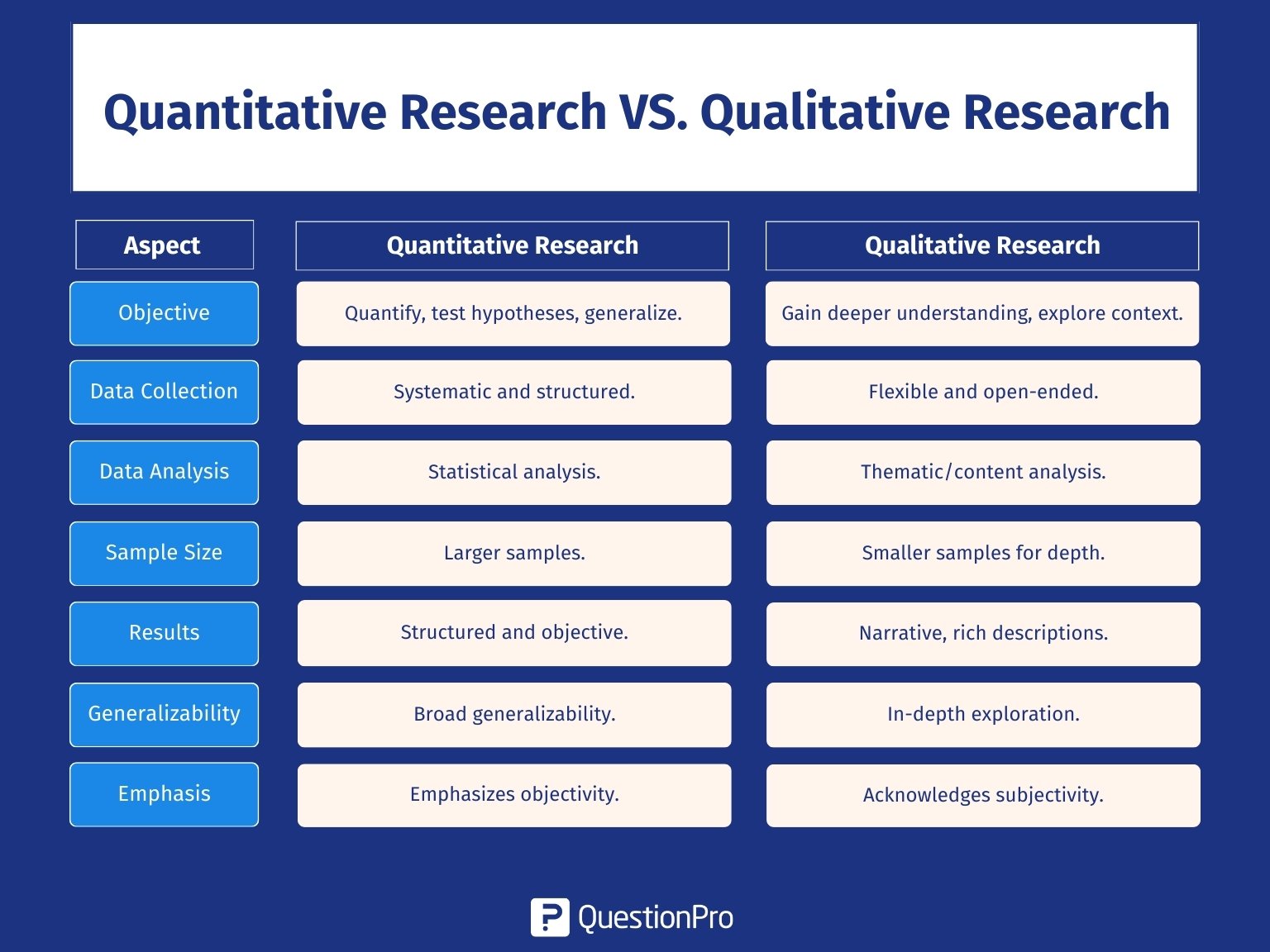
Quantitative Research
- Objective: The primary goal of quantitative research is to quantify and measure phenomena by collecting numerical data. It aims to test hypotheses, establish patterns, and generalize findings to a larger population.
- Data Collection: Quantitative research employs systematic and standardized approaches for data collection, including techniques like surveys, experiments, and observations that involve predefined variables. It is often collected from a large and representative sample.
- Data Analysis: Data is analyzed using statistical techniques, such as descriptive statistics, inferential statistics, and mathematical modeling. Researchers use statistical tests to draw conclusions and make generalizations based on numerical data.
- Sample Size: Quantitative research often involves larger sample sizes to ensure statistical significance and generalizability.
- Results: The results are typically presented in tables, charts, and statistical summaries, making them highly structured and objective.
- Generalizability: Researchers intentionally structure quantitative research to generate outcomes that can be helpful to a larger population, and they frequently seek to establish causative connections.
- Emphasis on Objectivity: Researchers aim to minimize bias and subjectivity, focusing on replicable and objective findings.
Qualitative Research
- Objective: Qualitative research seeks to gain a deeper understanding of the underlying motivations, behaviors, and experiences of individuals or groups. It explores the context and meaning of phenomena.
- Data Collection: Qualitative research employs adaptable and open-ended techniques for data collection, including methods like interviews, focus groups, observations, and content analysis. It allows participants to express their perspectives in their own words.
- Data Analysis: Data is analyzed through thematic analysis, content analysis, or grounded theory. Researchers focus on identifying patterns, themes, and insights in the data.
- Sample Size: Qualitative research typically involves smaller sample sizes due to the in-depth nature of data collection and analysis.
- Results: Findings are presented in narrative form, often in the participants’ own words. Results are subjective, context-dependent, and provide rich, detailed descriptions.
- Generalizability: Qualitative research does not aim for broad generalizability but focuses on in-depth exploration within a specific context. It provides a detailed understanding of a particular group or situation.
- Emphasis on Subjectivity: Researchers acknowledge the role of subjectivity and the researcher’s influence on the Research Process . Participant perspectives and experiences are central to the findings.
Researchers choose between quantitative and qualitative research methods based on their research objectives and the nature of the research question. Each approach has its advantages and drawbacks, and the decision between them hinges on the particular research objectives and the data needed to address research inquiries effectively.
Quantitative research is a structured way of collecting and analyzing data from various sources. Its purpose is to quantify the problem and understand its extent, seeking results that someone can project to a larger population.
Companies that use quantitative rather than qualitative research typically aim to measure magnitudes and seek objectively interpreted statistical results. So if you want to obtain quantitative data that helps you define the structured cause-and-effect relationship between the research problem and the factors, you should opt for this type of research.
At QuestionPro , we have various Best Data Collection Tools and features to conduct investigations of this type. You can create questionnaires and distribute them through our various methods. We also have sample services or various questions to guarantee the success of your study and the quality of the collected data.
Quantitative research is a systematic and structured approach to studying phenomena that involves the collection of measurable data and the application of statistical, mathematical, or computational techniques for analysis.
Quantitative research is characterized by structured tools like surveys, substantial sample sizes, closed-ended questions, reliance on prior studies, data presented numerically, and the ability to generalize findings to the broader population.
The two main methods of quantitative research are Primary quantitative research methods, involving data collection directly from sources, and Secondary quantitative research methods, which utilize existing data for analysis.
1.Surveying to measure employee engagement with numerical rating scales. 2.Analyzing sales data to identify trends in product demand and market share. 4.Examining test scores to assess the impact of a new teaching method on student performance. 4.Using website analytics to track user behavior and conversion rates for an online store.
1.Differentiate between quantitative and qualitative approaches. 2.Choose a representative sample size. 3.Define clear research goals before data collection. 4.Use simple and easily understandable survey questions.
MORE LIKE THIS
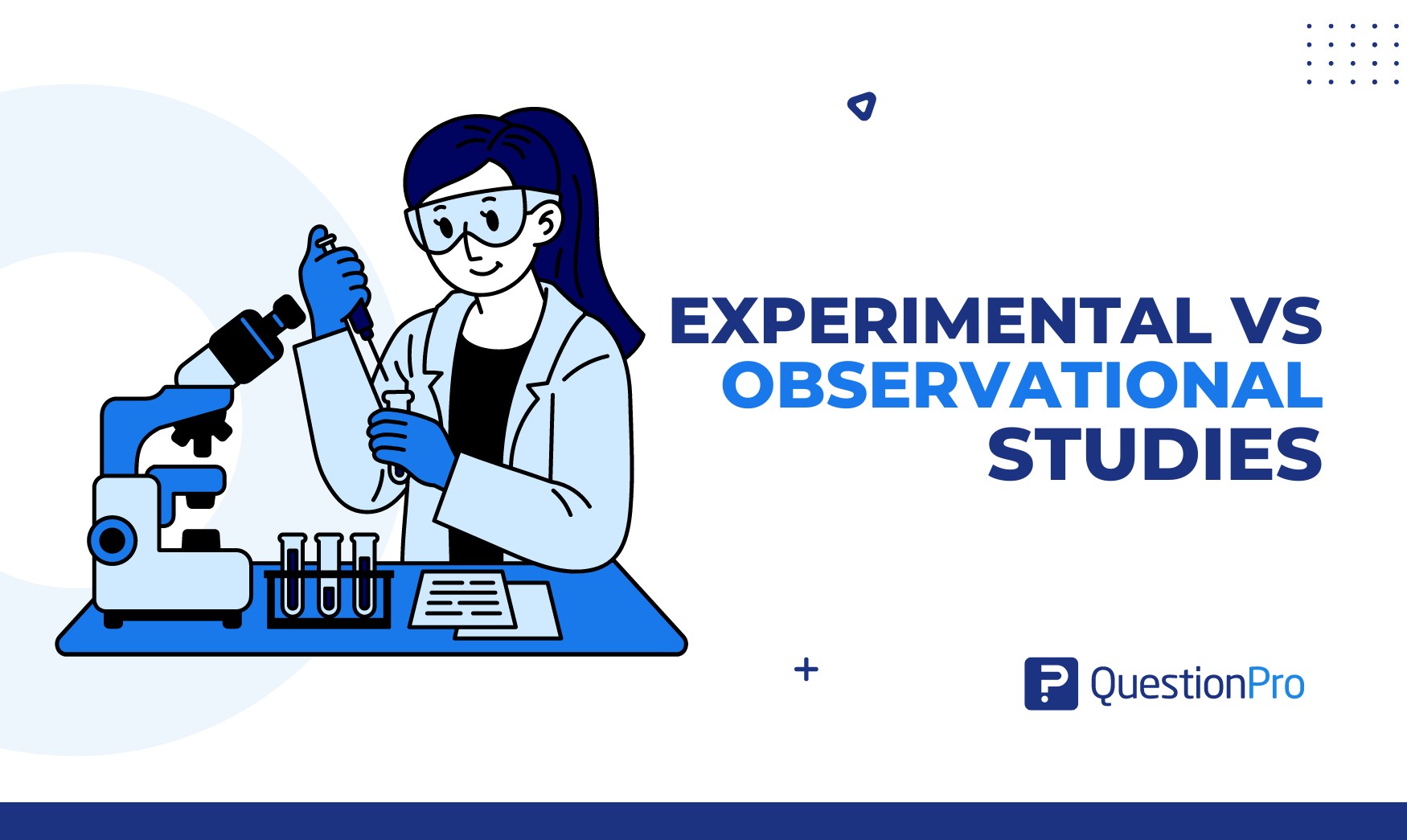
Experimental vs Observational Studies: Differences & Examples
Sep 5, 2024
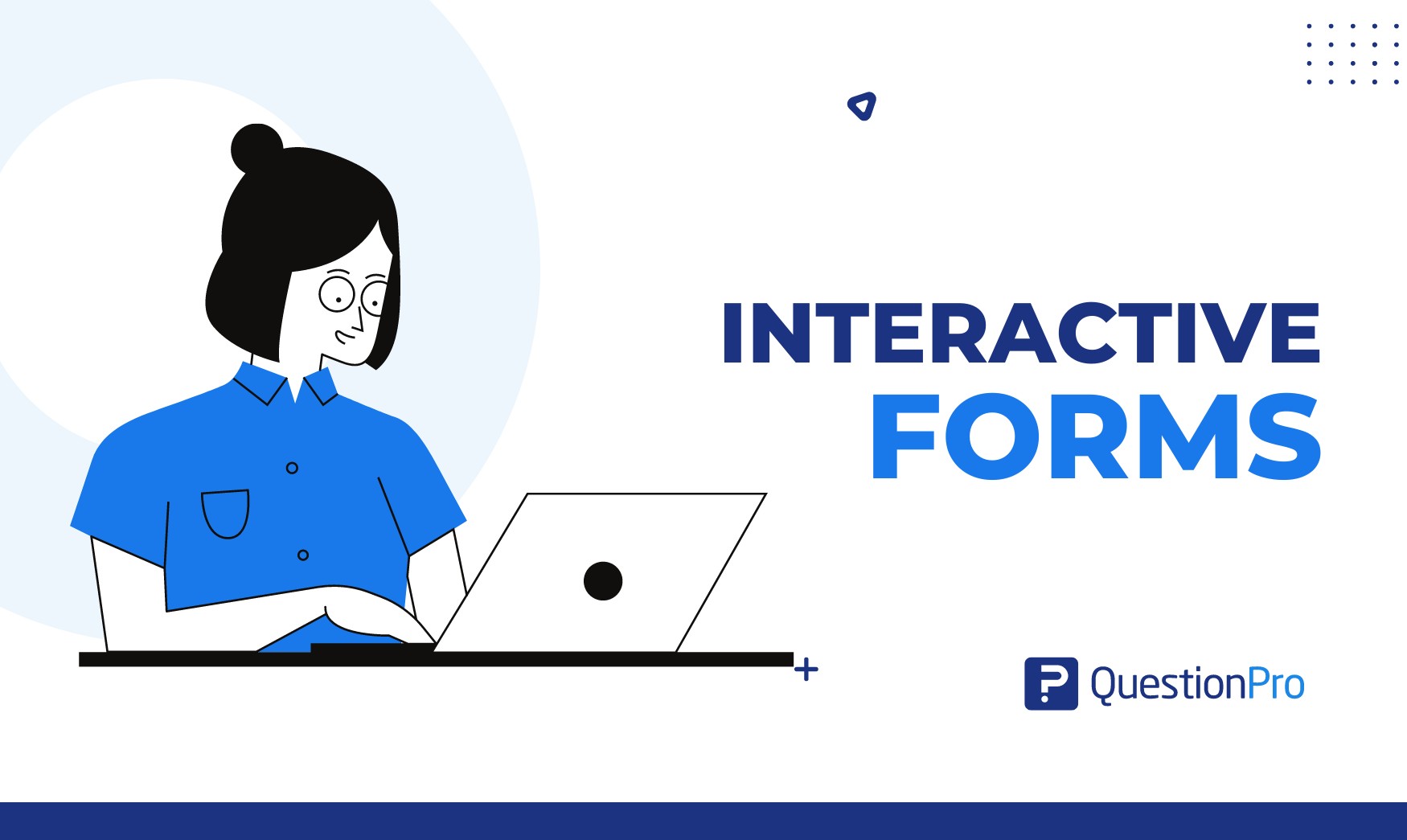
Interactive Forms: Key Features, Benefits, Uses + Design Tips
Sep 4, 2024
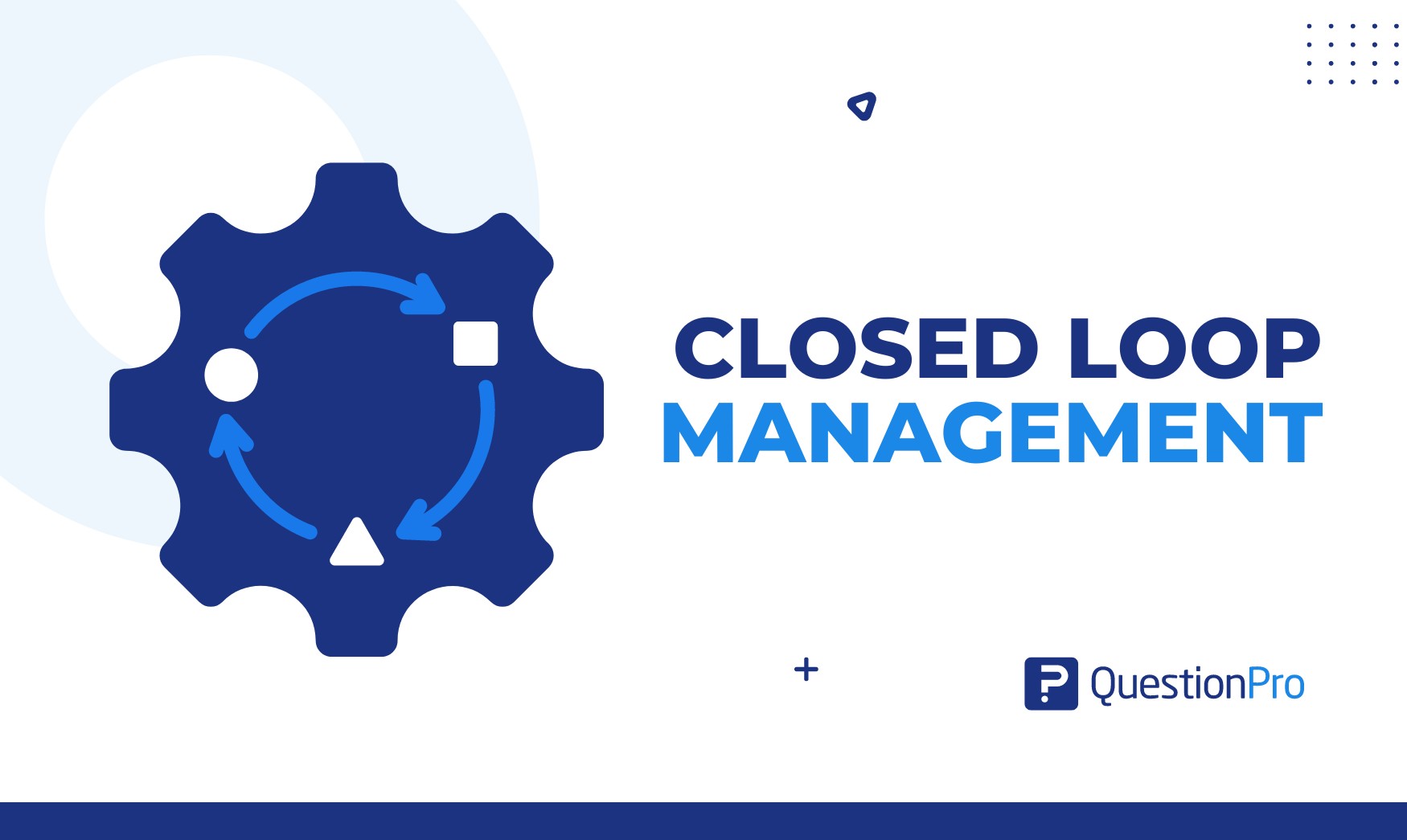
Closed-Loop Management: The Key to Customer Centricity
Sep 3, 2024
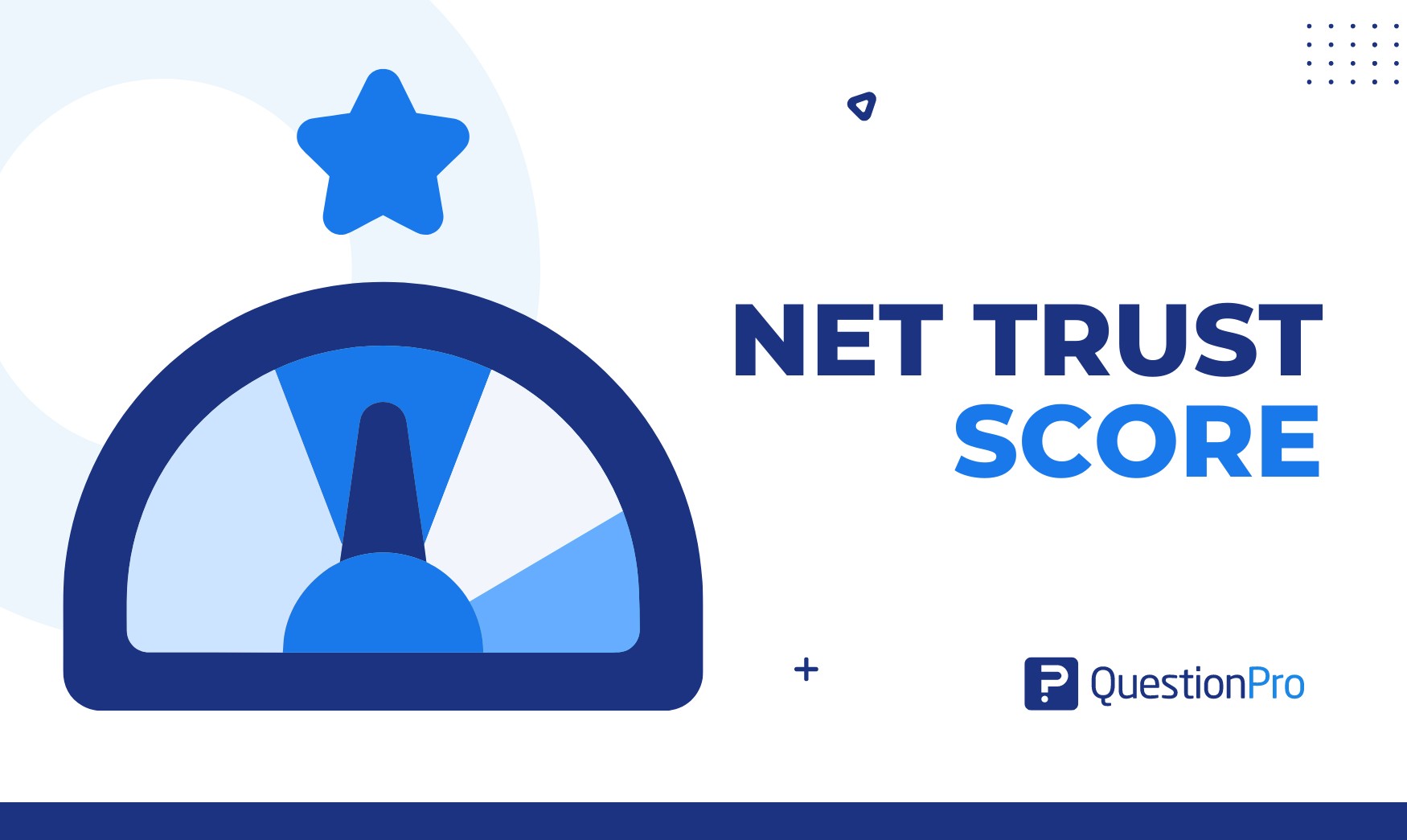
Net Trust Score: Tool for Measuring Trust in Organization
Sep 2, 2024
Other categories
- Academic Research
- Artificial Intelligence
- Assessments
- Brand Awareness
- Case Studies
- Communities
- Consumer Insights
- Customer effort score
- Customer Engagement
- Customer Experience
- Customer Loyalty
- Customer Research
- Customer Satisfaction
- Employee Benefits
- Employee Engagement
- Employee Retention
- Friday Five
- General Data Protection Regulation
- Insights Hub
- Life@QuestionPro
- Market Research
- Mobile diaries
- Mobile Surveys
- New Features
- Online Communities
- Question Types
- Questionnaire
- QuestionPro Products
- Release Notes
- Research Tools and Apps
- Revenue at Risk
- Survey Templates
- Training Tips
- Tuesday CX Thoughts (TCXT)
- Uncategorized
- What’s Coming Up
- Workforce Intelligence

An official website of the United States government
The .gov means it’s official. Federal government websites often end in .gov or .mil. Before sharing sensitive information, make sure you’re on a federal government site.
The site is secure. The https:// ensures that you are connecting to the official website and that any information you provide is encrypted and transmitted securely.
- Publications
- Account settings
Preview improvements coming to the PMC website in October 2024. Learn More or Try it out now .
- Advanced Search
- Journal List
- J Korean Med Sci
- v.37(16); 2022 Apr 25

A Practical Guide to Writing Quantitative and Qualitative Research Questions and Hypotheses in Scholarly Articles
Edward barroga.
1 Department of General Education, Graduate School of Nursing Science, St. Luke’s International University, Tokyo, Japan.
Glafera Janet Matanguihan
2 Department of Biological Sciences, Messiah University, Mechanicsburg, PA, USA.
The development of research questions and the subsequent hypotheses are prerequisites to defining the main research purpose and specific objectives of a study. Consequently, these objectives determine the study design and research outcome. The development of research questions is a process based on knowledge of current trends, cutting-edge studies, and technological advances in the research field. Excellent research questions are focused and require a comprehensive literature search and in-depth understanding of the problem being investigated. Initially, research questions may be written as descriptive questions which could be developed into inferential questions. These questions must be specific and concise to provide a clear foundation for developing hypotheses. Hypotheses are more formal predictions about the research outcomes. These specify the possible results that may or may not be expected regarding the relationship between groups. Thus, research questions and hypotheses clarify the main purpose and specific objectives of the study, which in turn dictate the design of the study, its direction, and outcome. Studies developed from good research questions and hypotheses will have trustworthy outcomes with wide-ranging social and health implications.
INTRODUCTION
Scientific research is usually initiated by posing evidenced-based research questions which are then explicitly restated as hypotheses. 1 , 2 The hypotheses provide directions to guide the study, solutions, explanations, and expected results. 3 , 4 Both research questions and hypotheses are essentially formulated based on conventional theories and real-world processes, which allow the inception of novel studies and the ethical testing of ideas. 5 , 6
It is crucial to have knowledge of both quantitative and qualitative research 2 as both types of research involve writing research questions and hypotheses. 7 However, these crucial elements of research are sometimes overlooked; if not overlooked, then framed without the forethought and meticulous attention it needs. Planning and careful consideration are needed when developing quantitative or qualitative research, particularly when conceptualizing research questions and hypotheses. 4
There is a continuing need to support researchers in the creation of innovative research questions and hypotheses, as well as for journal articles that carefully review these elements. 1 When research questions and hypotheses are not carefully thought of, unethical studies and poor outcomes usually ensue. Carefully formulated research questions and hypotheses define well-founded objectives, which in turn determine the appropriate design, course, and outcome of the study. This article then aims to discuss in detail the various aspects of crafting research questions and hypotheses, with the goal of guiding researchers as they develop their own. Examples from the authors and peer-reviewed scientific articles in the healthcare field are provided to illustrate key points.
DEFINITIONS AND RELATIONSHIP OF RESEARCH QUESTIONS AND HYPOTHESES
A research question is what a study aims to answer after data analysis and interpretation. The answer is written in length in the discussion section of the paper. Thus, the research question gives a preview of the different parts and variables of the study meant to address the problem posed in the research question. 1 An excellent research question clarifies the research writing while facilitating understanding of the research topic, objective, scope, and limitations of the study. 5
On the other hand, a research hypothesis is an educated statement of an expected outcome. This statement is based on background research and current knowledge. 8 , 9 The research hypothesis makes a specific prediction about a new phenomenon 10 or a formal statement on the expected relationship between an independent variable and a dependent variable. 3 , 11 It provides a tentative answer to the research question to be tested or explored. 4
Hypotheses employ reasoning to predict a theory-based outcome. 10 These can also be developed from theories by focusing on components of theories that have not yet been observed. 10 The validity of hypotheses is often based on the testability of the prediction made in a reproducible experiment. 8
Conversely, hypotheses can also be rephrased as research questions. Several hypotheses based on existing theories and knowledge may be needed to answer a research question. Developing ethical research questions and hypotheses creates a research design that has logical relationships among variables. These relationships serve as a solid foundation for the conduct of the study. 4 , 11 Haphazardly constructed research questions can result in poorly formulated hypotheses and improper study designs, leading to unreliable results. Thus, the formulations of relevant research questions and verifiable hypotheses are crucial when beginning research. 12
CHARACTERISTICS OF GOOD RESEARCH QUESTIONS AND HYPOTHESES
Excellent research questions are specific and focused. These integrate collective data and observations to confirm or refute the subsequent hypotheses. Well-constructed hypotheses are based on previous reports and verify the research context. These are realistic, in-depth, sufficiently complex, and reproducible. More importantly, these hypotheses can be addressed and tested. 13
There are several characteristics of well-developed hypotheses. Good hypotheses are 1) empirically testable 7 , 10 , 11 , 13 ; 2) backed by preliminary evidence 9 ; 3) testable by ethical research 7 , 9 ; 4) based on original ideas 9 ; 5) have evidenced-based logical reasoning 10 ; and 6) can be predicted. 11 Good hypotheses can infer ethical and positive implications, indicating the presence of a relationship or effect relevant to the research theme. 7 , 11 These are initially developed from a general theory and branch into specific hypotheses by deductive reasoning. In the absence of a theory to base the hypotheses, inductive reasoning based on specific observations or findings form more general hypotheses. 10
TYPES OF RESEARCH QUESTIONS AND HYPOTHESES
Research questions and hypotheses are developed according to the type of research, which can be broadly classified into quantitative and qualitative research. We provide a summary of the types of research questions and hypotheses under quantitative and qualitative research categories in Table 1 .
Quantitative research questions | Quantitative research hypotheses |
---|---|
Descriptive research questions | Simple hypothesis |
Comparative research questions | Complex hypothesis |
Relationship research questions | Directional hypothesis |
Non-directional hypothesis | |
Associative hypothesis | |
Causal hypothesis | |
Null hypothesis | |
Alternative hypothesis | |
Working hypothesis | |
Statistical hypothesis | |
Logical hypothesis | |
Hypothesis-testing | |
Qualitative research questions | Qualitative research hypotheses |
Contextual research questions | Hypothesis-generating |
Descriptive research questions | |
Evaluation research questions | |
Explanatory research questions | |
Exploratory research questions | |
Generative research questions | |
Ideological research questions | |
Ethnographic research questions | |
Phenomenological research questions | |
Grounded theory questions | |
Qualitative case study questions |
Research questions in quantitative research
In quantitative research, research questions inquire about the relationships among variables being investigated and are usually framed at the start of the study. These are precise and typically linked to the subject population, dependent and independent variables, and research design. 1 Research questions may also attempt to describe the behavior of a population in relation to one or more variables, or describe the characteristics of variables to be measured ( descriptive research questions ). 1 , 5 , 14 These questions may also aim to discover differences between groups within the context of an outcome variable ( comparative research questions ), 1 , 5 , 14 or elucidate trends and interactions among variables ( relationship research questions ). 1 , 5 We provide examples of descriptive, comparative, and relationship research questions in quantitative research in Table 2 .
Quantitative research questions | |
---|---|
Descriptive research question | |
- Measures responses of subjects to variables | |
- Presents variables to measure, analyze, or assess | |
What is the proportion of resident doctors in the hospital who have mastered ultrasonography (response of subjects to a variable) as a diagnostic technique in their clinical training? | |
Comparative research question | |
- Clarifies difference between one group with outcome variable and another group without outcome variable | |
Is there a difference in the reduction of lung metastasis in osteosarcoma patients who received the vitamin D adjunctive therapy (group with outcome variable) compared with osteosarcoma patients who did not receive the vitamin D adjunctive therapy (group without outcome variable)? | |
- Compares the effects of variables | |
How does the vitamin D analogue 22-Oxacalcitriol (variable 1) mimic the antiproliferative activity of 1,25-Dihydroxyvitamin D (variable 2) in osteosarcoma cells? | |
Relationship research question | |
- Defines trends, association, relationships, or interactions between dependent variable and independent variable | |
Is there a relationship between the number of medical student suicide (dependent variable) and the level of medical student stress (independent variable) in Japan during the first wave of the COVID-19 pandemic? |
Hypotheses in quantitative research
In quantitative research, hypotheses predict the expected relationships among variables. 15 Relationships among variables that can be predicted include 1) between a single dependent variable and a single independent variable ( simple hypothesis ) or 2) between two or more independent and dependent variables ( complex hypothesis ). 4 , 11 Hypotheses may also specify the expected direction to be followed and imply an intellectual commitment to a particular outcome ( directional hypothesis ) 4 . On the other hand, hypotheses may not predict the exact direction and are used in the absence of a theory, or when findings contradict previous studies ( non-directional hypothesis ). 4 In addition, hypotheses can 1) define interdependency between variables ( associative hypothesis ), 4 2) propose an effect on the dependent variable from manipulation of the independent variable ( causal hypothesis ), 4 3) state a negative relationship between two variables ( null hypothesis ), 4 , 11 , 15 4) replace the working hypothesis if rejected ( alternative hypothesis ), 15 explain the relationship of phenomena to possibly generate a theory ( working hypothesis ), 11 5) involve quantifiable variables that can be tested statistically ( statistical hypothesis ), 11 6) or express a relationship whose interlinks can be verified logically ( logical hypothesis ). 11 We provide examples of simple, complex, directional, non-directional, associative, causal, null, alternative, working, statistical, and logical hypotheses in quantitative research, as well as the definition of quantitative hypothesis-testing research in Table 3 .
Quantitative research hypotheses | |
---|---|
Simple hypothesis | |
- Predicts relationship between single dependent variable and single independent variable | |
If the dose of the new medication (single independent variable) is high, blood pressure (single dependent variable) is lowered. | |
Complex hypothesis | |
- Foretells relationship between two or more independent and dependent variables | |
The higher the use of anticancer drugs, radiation therapy, and adjunctive agents (3 independent variables), the higher would be the survival rate (1 dependent variable). | |
Directional hypothesis | |
- Identifies study direction based on theory towards particular outcome to clarify relationship between variables | |
Privately funded research projects will have a larger international scope (study direction) than publicly funded research projects. | |
Non-directional hypothesis | |
- Nature of relationship between two variables or exact study direction is not identified | |
- Does not involve a theory | |
Women and men are different in terms of helpfulness. (Exact study direction is not identified) | |
Associative hypothesis | |
- Describes variable interdependency | |
- Change in one variable causes change in another variable | |
A larger number of people vaccinated against COVID-19 in the region (change in independent variable) will reduce the region’s incidence of COVID-19 infection (change in dependent variable). | |
Causal hypothesis | |
- An effect on dependent variable is predicted from manipulation of independent variable | |
A change into a high-fiber diet (independent variable) will reduce the blood sugar level (dependent variable) of the patient. | |
Null hypothesis | |
- A negative statement indicating no relationship or difference between 2 variables | |
There is no significant difference in the severity of pulmonary metastases between the new drug (variable 1) and the current drug (variable 2). | |
Alternative hypothesis | |
- Following a null hypothesis, an alternative hypothesis predicts a relationship between 2 study variables | |
The new drug (variable 1) is better on average in reducing the level of pain from pulmonary metastasis than the current drug (variable 2). | |
Working hypothesis | |
- A hypothesis that is initially accepted for further research to produce a feasible theory | |
Dairy cows fed with concentrates of different formulations will produce different amounts of milk. | |
Statistical hypothesis | |
- Assumption about the value of population parameter or relationship among several population characteristics | |
- Validity tested by a statistical experiment or analysis | |
The mean recovery rate from COVID-19 infection (value of population parameter) is not significantly different between population 1 and population 2. | |
There is a positive correlation between the level of stress at the workplace and the number of suicides (population characteristics) among working people in Japan. | |
Logical hypothesis | |
- Offers or proposes an explanation with limited or no extensive evidence | |
If healthcare workers provide more educational programs about contraception methods, the number of adolescent pregnancies will be less. | |
Hypothesis-testing (Quantitative hypothesis-testing research) | |
- Quantitative research uses deductive reasoning. | |
- This involves the formation of a hypothesis, collection of data in the investigation of the problem, analysis and use of the data from the investigation, and drawing of conclusions to validate or nullify the hypotheses. |
Research questions in qualitative research
Unlike research questions in quantitative research, research questions in qualitative research are usually continuously reviewed and reformulated. The central question and associated subquestions are stated more than the hypotheses. 15 The central question broadly explores a complex set of factors surrounding the central phenomenon, aiming to present the varied perspectives of participants. 15
There are varied goals for which qualitative research questions are developed. These questions can function in several ways, such as to 1) identify and describe existing conditions ( contextual research question s); 2) describe a phenomenon ( descriptive research questions ); 3) assess the effectiveness of existing methods, protocols, theories, or procedures ( evaluation research questions ); 4) examine a phenomenon or analyze the reasons or relationships between subjects or phenomena ( explanatory research questions ); or 5) focus on unknown aspects of a particular topic ( exploratory research questions ). 5 In addition, some qualitative research questions provide new ideas for the development of theories and actions ( generative research questions ) or advance specific ideologies of a position ( ideological research questions ). 1 Other qualitative research questions may build on a body of existing literature and become working guidelines ( ethnographic research questions ). Research questions may also be broadly stated without specific reference to the existing literature or a typology of questions ( phenomenological research questions ), may be directed towards generating a theory of some process ( grounded theory questions ), or may address a description of the case and the emerging themes ( qualitative case study questions ). 15 We provide examples of contextual, descriptive, evaluation, explanatory, exploratory, generative, ideological, ethnographic, phenomenological, grounded theory, and qualitative case study research questions in qualitative research in Table 4 , and the definition of qualitative hypothesis-generating research in Table 5 .
Qualitative research questions | |
---|---|
Contextual research question | |
- Ask the nature of what already exists | |
- Individuals or groups function to further clarify and understand the natural context of real-world problems | |
What are the experiences of nurses working night shifts in healthcare during the COVID-19 pandemic? (natural context of real-world problems) | |
Descriptive research question | |
- Aims to describe a phenomenon | |
What are the different forms of disrespect and abuse (phenomenon) experienced by Tanzanian women when giving birth in healthcare facilities? | |
Evaluation research question | |
- Examines the effectiveness of existing practice or accepted frameworks | |
How effective are decision aids (effectiveness of existing practice) in helping decide whether to give birth at home or in a healthcare facility? | |
Explanatory research question | |
- Clarifies a previously studied phenomenon and explains why it occurs | |
Why is there an increase in teenage pregnancy (phenomenon) in Tanzania? | |
Exploratory research question | |
- Explores areas that have not been fully investigated to have a deeper understanding of the research problem | |
What factors affect the mental health of medical students (areas that have not yet been fully investigated) during the COVID-19 pandemic? | |
Generative research question | |
- Develops an in-depth understanding of people’s behavior by asking ‘how would’ or ‘what if’ to identify problems and find solutions | |
How would the extensive research experience of the behavior of new staff impact the success of the novel drug initiative? | |
Ideological research question | |
- Aims to advance specific ideas or ideologies of a position | |
Are Japanese nurses who volunteer in remote African hospitals able to promote humanized care of patients (specific ideas or ideologies) in the areas of safe patient environment, respect of patient privacy, and provision of accurate information related to health and care? | |
Ethnographic research question | |
- Clarifies peoples’ nature, activities, their interactions, and the outcomes of their actions in specific settings | |
What are the demographic characteristics, rehabilitative treatments, community interactions, and disease outcomes (nature, activities, their interactions, and the outcomes) of people in China who are suffering from pneumoconiosis? | |
Phenomenological research question | |
- Knows more about the phenomena that have impacted an individual | |
What are the lived experiences of parents who have been living with and caring for children with a diagnosis of autism? (phenomena that have impacted an individual) | |
Grounded theory question | |
- Focuses on social processes asking about what happens and how people interact, or uncovering social relationships and behaviors of groups | |
What are the problems that pregnant adolescents face in terms of social and cultural norms (social processes), and how can these be addressed? | |
Qualitative case study question | |
- Assesses a phenomenon using different sources of data to answer “why” and “how” questions | |
- Considers how the phenomenon is influenced by its contextual situation. | |
How does quitting work and assuming the role of a full-time mother (phenomenon assessed) change the lives of women in Japan? |
Qualitative research hypotheses | |
---|---|
Hypothesis-generating (Qualitative hypothesis-generating research) | |
- Qualitative research uses inductive reasoning. | |
- This involves data collection from study participants or the literature regarding a phenomenon of interest, using the collected data to develop a formal hypothesis, and using the formal hypothesis as a framework for testing the hypothesis. | |
- Qualitative exploratory studies explore areas deeper, clarifying subjective experience and allowing formulation of a formal hypothesis potentially testable in a future quantitative approach. |
Qualitative studies usually pose at least one central research question and several subquestions starting with How or What . These research questions use exploratory verbs such as explore or describe . These also focus on one central phenomenon of interest, and may mention the participants and research site. 15
Hypotheses in qualitative research
Hypotheses in qualitative research are stated in the form of a clear statement concerning the problem to be investigated. Unlike in quantitative research where hypotheses are usually developed to be tested, qualitative research can lead to both hypothesis-testing and hypothesis-generating outcomes. 2 When studies require both quantitative and qualitative research questions, this suggests an integrative process between both research methods wherein a single mixed-methods research question can be developed. 1
FRAMEWORKS FOR DEVELOPING RESEARCH QUESTIONS AND HYPOTHESES
Research questions followed by hypotheses should be developed before the start of the study. 1 , 12 , 14 It is crucial to develop feasible research questions on a topic that is interesting to both the researcher and the scientific community. This can be achieved by a meticulous review of previous and current studies to establish a novel topic. Specific areas are subsequently focused on to generate ethical research questions. The relevance of the research questions is evaluated in terms of clarity of the resulting data, specificity of the methodology, objectivity of the outcome, depth of the research, and impact of the study. 1 , 5 These aspects constitute the FINER criteria (i.e., Feasible, Interesting, Novel, Ethical, and Relevant). 1 Clarity and effectiveness are achieved if research questions meet the FINER criteria. In addition to the FINER criteria, Ratan et al. described focus, complexity, novelty, feasibility, and measurability for evaluating the effectiveness of research questions. 14
The PICOT and PEO frameworks are also used when developing research questions. 1 The following elements are addressed in these frameworks, PICOT: P-population/patients/problem, I-intervention or indicator being studied, C-comparison group, O-outcome of interest, and T-timeframe of the study; PEO: P-population being studied, E-exposure to preexisting conditions, and O-outcome of interest. 1 Research questions are also considered good if these meet the “FINERMAPS” framework: Feasible, Interesting, Novel, Ethical, Relevant, Manageable, Appropriate, Potential value/publishable, and Systematic. 14
As we indicated earlier, research questions and hypotheses that are not carefully formulated result in unethical studies or poor outcomes. To illustrate this, we provide some examples of ambiguous research question and hypotheses that result in unclear and weak research objectives in quantitative research ( Table 6 ) 16 and qualitative research ( Table 7 ) 17 , and how to transform these ambiguous research question(s) and hypothesis(es) into clear and good statements.
Variables | Unclear and weak statement (Statement 1) | Clear and good statement (Statement 2) | Points to avoid |
---|---|---|---|
Research question | Which is more effective between smoke moxibustion and smokeless moxibustion? | “Moreover, regarding smoke moxibustion versus smokeless moxibustion, it remains unclear which is more effective, safe, and acceptable to pregnant women, and whether there is any difference in the amount of heat generated.” | 1) Vague and unfocused questions |
2) Closed questions simply answerable by yes or no | |||
3) Questions requiring a simple choice | |||
Hypothesis | The smoke moxibustion group will have higher cephalic presentation. | “Hypothesis 1. The smoke moxibustion stick group (SM group) and smokeless moxibustion stick group (-SLM group) will have higher rates of cephalic presentation after treatment than the control group. | 1) Unverifiable hypotheses |
Hypothesis 2. The SM group and SLM group will have higher rates of cephalic presentation at birth than the control group. | 2) Incompletely stated groups of comparison | ||
Hypothesis 3. There will be no significant differences in the well-being of the mother and child among the three groups in terms of the following outcomes: premature birth, premature rupture of membranes (PROM) at < 37 weeks, Apgar score < 7 at 5 min, umbilical cord blood pH < 7.1, admission to neonatal intensive care unit (NICU), and intrauterine fetal death.” | 3) Insufficiently described variables or outcomes | ||
Research objective | To determine which is more effective between smoke moxibustion and smokeless moxibustion. | “The specific aims of this pilot study were (a) to compare the effects of smoke moxibustion and smokeless moxibustion treatments with the control group as a possible supplement to ECV for converting breech presentation to cephalic presentation and increasing adherence to the newly obtained cephalic position, and (b) to assess the effects of these treatments on the well-being of the mother and child.” | 1) Poor understanding of the research question and hypotheses |
2) Insufficient description of population, variables, or study outcomes |
a These statements were composed for comparison and illustrative purposes only.
b These statements are direct quotes from Higashihara and Horiuchi. 16
Variables | Unclear and weak statement (Statement 1) | Clear and good statement (Statement 2) | Points to avoid |
---|---|---|---|
Research question | Does disrespect and abuse (D&A) occur in childbirth in Tanzania? | How does disrespect and abuse (D&A) occur and what are the types of physical and psychological abuses observed in midwives’ actual care during facility-based childbirth in urban Tanzania? | 1) Ambiguous or oversimplistic questions |
2) Questions unverifiable by data collection and analysis | |||
Hypothesis | Disrespect and abuse (D&A) occur in childbirth in Tanzania. | Hypothesis 1: Several types of physical and psychological abuse by midwives in actual care occur during facility-based childbirth in urban Tanzania. | 1) Statements simply expressing facts |
Hypothesis 2: Weak nursing and midwifery management contribute to the D&A of women during facility-based childbirth in urban Tanzania. | 2) Insufficiently described concepts or variables | ||
Research objective | To describe disrespect and abuse (D&A) in childbirth in Tanzania. | “This study aimed to describe from actual observations the respectful and disrespectful care received by women from midwives during their labor period in two hospitals in urban Tanzania.” | 1) Statements unrelated to the research question and hypotheses |
2) Unattainable or unexplorable objectives |
a This statement is a direct quote from Shimoda et al. 17
The other statements were composed for comparison and illustrative purposes only.
CONSTRUCTING RESEARCH QUESTIONS AND HYPOTHESES
To construct effective research questions and hypotheses, it is very important to 1) clarify the background and 2) identify the research problem at the outset of the research, within a specific timeframe. 9 Then, 3) review or conduct preliminary research to collect all available knowledge about the possible research questions by studying theories and previous studies. 18 Afterwards, 4) construct research questions to investigate the research problem. Identify variables to be accessed from the research questions 4 and make operational definitions of constructs from the research problem and questions. Thereafter, 5) construct specific deductive or inductive predictions in the form of hypotheses. 4 Finally, 6) state the study aims . This general flow for constructing effective research questions and hypotheses prior to conducting research is shown in Fig. 1 .

Research questions are used more frequently in qualitative research than objectives or hypotheses. 3 These questions seek to discover, understand, explore or describe experiences by asking “What” or “How.” The questions are open-ended to elicit a description rather than to relate variables or compare groups. The questions are continually reviewed, reformulated, and changed during the qualitative study. 3 Research questions are also used more frequently in survey projects than hypotheses in experiments in quantitative research to compare variables and their relationships.
Hypotheses are constructed based on the variables identified and as an if-then statement, following the template, ‘If a specific action is taken, then a certain outcome is expected.’ At this stage, some ideas regarding expectations from the research to be conducted must be drawn. 18 Then, the variables to be manipulated (independent) and influenced (dependent) are defined. 4 Thereafter, the hypothesis is stated and refined, and reproducible data tailored to the hypothesis are identified, collected, and analyzed. 4 The hypotheses must be testable and specific, 18 and should describe the variables and their relationships, the specific group being studied, and the predicted research outcome. 18 Hypotheses construction involves a testable proposition to be deduced from theory, and independent and dependent variables to be separated and measured separately. 3 Therefore, good hypotheses must be based on good research questions constructed at the start of a study or trial. 12
In summary, research questions are constructed after establishing the background of the study. Hypotheses are then developed based on the research questions. Thus, it is crucial to have excellent research questions to generate superior hypotheses. In turn, these would determine the research objectives and the design of the study, and ultimately, the outcome of the research. 12 Algorithms for building research questions and hypotheses are shown in Fig. 2 for quantitative research and in Fig. 3 for qualitative research.

EXAMPLES OF RESEARCH QUESTIONS FROM PUBLISHED ARTICLES
- EXAMPLE 1. Descriptive research question (quantitative research)
- - Presents research variables to be assessed (distinct phenotypes and subphenotypes)
- “BACKGROUND: Since COVID-19 was identified, its clinical and biological heterogeneity has been recognized. Identifying COVID-19 phenotypes might help guide basic, clinical, and translational research efforts.
- RESEARCH QUESTION: Does the clinical spectrum of patients with COVID-19 contain distinct phenotypes and subphenotypes? ” 19
- EXAMPLE 2. Relationship research question (quantitative research)
- - Shows interactions between dependent variable (static postural control) and independent variable (peripheral visual field loss)
- “Background: Integration of visual, vestibular, and proprioceptive sensations contributes to postural control. People with peripheral visual field loss have serious postural instability. However, the directional specificity of postural stability and sensory reweighting caused by gradual peripheral visual field loss remain unclear.
- Research question: What are the effects of peripheral visual field loss on static postural control ?” 20
- EXAMPLE 3. Comparative research question (quantitative research)
- - Clarifies the difference among groups with an outcome variable (patients enrolled in COMPERA with moderate PH or severe PH in COPD) and another group without the outcome variable (patients with idiopathic pulmonary arterial hypertension (IPAH))
- “BACKGROUND: Pulmonary hypertension (PH) in COPD is a poorly investigated clinical condition.
- RESEARCH QUESTION: Which factors determine the outcome of PH in COPD?
- STUDY DESIGN AND METHODS: We analyzed the characteristics and outcome of patients enrolled in the Comparative, Prospective Registry of Newly Initiated Therapies for Pulmonary Hypertension (COMPERA) with moderate or severe PH in COPD as defined during the 6th PH World Symposium who received medical therapy for PH and compared them with patients with idiopathic pulmonary arterial hypertension (IPAH) .” 21
- EXAMPLE 4. Exploratory research question (qualitative research)
- - Explores areas that have not been fully investigated (perspectives of families and children who receive care in clinic-based child obesity treatment) to have a deeper understanding of the research problem
- “Problem: Interventions for children with obesity lead to only modest improvements in BMI and long-term outcomes, and data are limited on the perspectives of families of children with obesity in clinic-based treatment. This scoping review seeks to answer the question: What is known about the perspectives of families and children who receive care in clinic-based child obesity treatment? This review aims to explore the scope of perspectives reported by families of children with obesity who have received individualized outpatient clinic-based obesity treatment.” 22
- EXAMPLE 5. Relationship research question (quantitative research)
- - Defines interactions between dependent variable (use of ankle strategies) and independent variable (changes in muscle tone)
- “Background: To maintain an upright standing posture against external disturbances, the human body mainly employs two types of postural control strategies: “ankle strategy” and “hip strategy.” While it has been reported that the magnitude of the disturbance alters the use of postural control strategies, it has not been elucidated how the level of muscle tone, one of the crucial parameters of bodily function, determines the use of each strategy. We have previously confirmed using forward dynamics simulations of human musculoskeletal models that an increased muscle tone promotes the use of ankle strategies. The objective of the present study was to experimentally evaluate a hypothesis: an increased muscle tone promotes the use of ankle strategies. Research question: Do changes in the muscle tone affect the use of ankle strategies ?” 23
EXAMPLES OF HYPOTHESES IN PUBLISHED ARTICLES
- EXAMPLE 1. Working hypothesis (quantitative research)
- - A hypothesis that is initially accepted for further research to produce a feasible theory
- “As fever may have benefit in shortening the duration of viral illness, it is plausible to hypothesize that the antipyretic efficacy of ibuprofen may be hindering the benefits of a fever response when taken during the early stages of COVID-19 illness .” 24
- “In conclusion, it is plausible to hypothesize that the antipyretic efficacy of ibuprofen may be hindering the benefits of a fever response . The difference in perceived safety of these agents in COVID-19 illness could be related to the more potent efficacy to reduce fever with ibuprofen compared to acetaminophen. Compelling data on the benefit of fever warrant further research and review to determine when to treat or withhold ibuprofen for early stage fever for COVID-19 and other related viral illnesses .” 24
- EXAMPLE 2. Exploratory hypothesis (qualitative research)
- - Explores particular areas deeper to clarify subjective experience and develop a formal hypothesis potentially testable in a future quantitative approach
- “We hypothesized that when thinking about a past experience of help-seeking, a self distancing prompt would cause increased help-seeking intentions and more favorable help-seeking outcome expectations .” 25
- “Conclusion
- Although a priori hypotheses were not supported, further research is warranted as results indicate the potential for using self-distancing approaches to increasing help-seeking among some people with depressive symptomatology.” 25
- EXAMPLE 3. Hypothesis-generating research to establish a framework for hypothesis testing (qualitative research)
- “We hypothesize that compassionate care is beneficial for patients (better outcomes), healthcare systems and payers (lower costs), and healthcare providers (lower burnout). ” 26
- Compassionomics is the branch of knowledge and scientific study of the effects of compassionate healthcare. Our main hypotheses are that compassionate healthcare is beneficial for (1) patients, by improving clinical outcomes, (2) healthcare systems and payers, by supporting financial sustainability, and (3) HCPs, by lowering burnout and promoting resilience and well-being. The purpose of this paper is to establish a scientific framework for testing the hypotheses above . If these hypotheses are confirmed through rigorous research, compassionomics will belong in the science of evidence-based medicine, with major implications for all healthcare domains.” 26
- EXAMPLE 4. Statistical hypothesis (quantitative research)
- - An assumption is made about the relationship among several population characteristics ( gender differences in sociodemographic and clinical characteristics of adults with ADHD ). Validity is tested by statistical experiment or analysis ( chi-square test, Students t-test, and logistic regression analysis)
- “Our research investigated gender differences in sociodemographic and clinical characteristics of adults with ADHD in a Japanese clinical sample. Due to unique Japanese cultural ideals and expectations of women's behavior that are in opposition to ADHD symptoms, we hypothesized that women with ADHD experience more difficulties and present more dysfunctions than men . We tested the following hypotheses: first, women with ADHD have more comorbidities than men with ADHD; second, women with ADHD experience more social hardships than men, such as having less full-time employment and being more likely to be divorced.” 27
- “Statistical Analysis
- ( text omitted ) Between-gender comparisons were made using the chi-squared test for categorical variables and Students t-test for continuous variables…( text omitted ). A logistic regression analysis was performed for employment status, marital status, and comorbidity to evaluate the independent effects of gender on these dependent variables.” 27
EXAMPLES OF HYPOTHESIS AS WRITTEN IN PUBLISHED ARTICLES IN RELATION TO OTHER PARTS
- EXAMPLE 1. Background, hypotheses, and aims are provided
- “Pregnant women need skilled care during pregnancy and childbirth, but that skilled care is often delayed in some countries …( text omitted ). The focused antenatal care (FANC) model of WHO recommends that nurses provide information or counseling to all pregnant women …( text omitted ). Job aids are visual support materials that provide the right kind of information using graphics and words in a simple and yet effective manner. When nurses are not highly trained or have many work details to attend to, these job aids can serve as a content reminder for the nurses and can be used for educating their patients (Jennings, Yebadokpo, Affo, & Agbogbe, 2010) ( text omitted ). Importantly, additional evidence is needed to confirm how job aids can further improve the quality of ANC counseling by health workers in maternal care …( text omitted )” 28
- “ This has led us to hypothesize that the quality of ANC counseling would be better if supported by job aids. Consequently, a better quality of ANC counseling is expected to produce higher levels of awareness concerning the danger signs of pregnancy and a more favorable impression of the caring behavior of nurses .” 28
- “This study aimed to examine the differences in the responses of pregnant women to a job aid-supported intervention during ANC visit in terms of 1) their understanding of the danger signs of pregnancy and 2) their impression of the caring behaviors of nurses to pregnant women in rural Tanzania.” 28
- EXAMPLE 2. Background, hypotheses, and aims are provided
- “We conducted a two-arm randomized controlled trial (RCT) to evaluate and compare changes in salivary cortisol and oxytocin levels of first-time pregnant women between experimental and control groups. The women in the experimental group touched and held an infant for 30 min (experimental intervention protocol), whereas those in the control group watched a DVD movie of an infant (control intervention protocol). The primary outcome was salivary cortisol level and the secondary outcome was salivary oxytocin level.” 29
- “ We hypothesize that at 30 min after touching and holding an infant, the salivary cortisol level will significantly decrease and the salivary oxytocin level will increase in the experimental group compared with the control group .” 29
- EXAMPLE 3. Background, aim, and hypothesis are provided
- “In countries where the maternal mortality ratio remains high, antenatal education to increase Birth Preparedness and Complication Readiness (BPCR) is considered one of the top priorities [1]. BPCR includes birth plans during the antenatal period, such as the birthplace, birth attendant, transportation, health facility for complications, expenses, and birth materials, as well as family coordination to achieve such birth plans. In Tanzania, although increasing, only about half of all pregnant women attend an antenatal clinic more than four times [4]. Moreover, the information provided during antenatal care (ANC) is insufficient. In the resource-poor settings, antenatal group education is a potential approach because of the limited time for individual counseling at antenatal clinics.” 30
- “This study aimed to evaluate an antenatal group education program among pregnant women and their families with respect to birth-preparedness and maternal and infant outcomes in rural villages of Tanzania.” 30
- “ The study hypothesis was if Tanzanian pregnant women and their families received a family-oriented antenatal group education, they would (1) have a higher level of BPCR, (2) attend antenatal clinic four or more times, (3) give birth in a health facility, (4) have less complications of women at birth, and (5) have less complications and deaths of infants than those who did not receive the education .” 30
Research questions and hypotheses are crucial components to any type of research, whether quantitative or qualitative. These questions should be developed at the very beginning of the study. Excellent research questions lead to superior hypotheses, which, like a compass, set the direction of research, and can often determine the successful conduct of the study. Many research studies have floundered because the development of research questions and subsequent hypotheses was not given the thought and meticulous attention needed. The development of research questions and hypotheses is an iterative process based on extensive knowledge of the literature and insightful grasp of the knowledge gap. Focused, concise, and specific research questions provide a strong foundation for constructing hypotheses which serve as formal predictions about the research outcomes. Research questions and hypotheses are crucial elements of research that should not be overlooked. They should be carefully thought of and constructed when planning research. This avoids unethical studies and poor outcomes by defining well-founded objectives that determine the design, course, and outcome of the study.
Disclosure: The authors have no potential conflicts of interest to disclose.
Author Contributions:
- Conceptualization: Barroga E, Matanguihan GJ.
- Methodology: Barroga E, Matanguihan GJ.
- Writing - original draft: Barroga E, Matanguihan GJ.
- Writing - review & editing: Barroga E, Matanguihan GJ.
4 Different Types of Quantitative Research
When you think of research, you probably think of hitting the book (or web), grabbing a bunch of facts, and taking some notes. Unfortunately, this is a good way to pull false information and ruin a report or study.
Qualitative vs Quantitative
Table of Contents
It’s a good idea to first understand the difference between quantitative and qualitative research. Qualitative tends to use a narrow pool of subjects for more precise information. Longitudinal studies (observing a test group over a long period of time) is an important form of qualitative research.
See Also: Different Types of Educational Research
Four Types of Quantitative Research
Now that we have an idea of what quantitative research is, let’s take a moment to look at some different kinds of quantitative research is and how they might suit your needs in work, school, or everyday life.
1. Causal-Comparative Research
This method of research is based on Mill’s Methods of Agreement and Disagreement (two of the five methods introduced by philosopher John Stuart Mill).
The idea of agreement is that when a single factor is present in all groups and those suffer the same effect, that factor is the cause. Disagreement follows the opposite logic, where the only exception to a factor is directly related to the only exception in the results, that factor is the cause. The University of Hong Kong illustrates Mill’s Methods beautifully.
2. Correlational Research
As the name implies, this form of research takes two or more variables and examines how they correlate with one another. Two or more groups are used to observe the variables and their effects without interfering with those variables. The goal of correlative research is to find out how certain variables may predict other variables and their potential relationships.
An example of a correlated study was performed by W.H. Decker in 1987 and measured the correlation between a manager’s perceived sense of humor and the positive effects on employees’ job satisfaction. Note that humor is subjective, thus it could not be proven as entirely causal (some employees might not react to the same joke in the same way or even at all).
3. Experimental Research
This method is essentially the scientific method you learned in school. It takes one or more hypotheses and test them to reach a true/false/inconclusive result. It often uses probability in its final results.
See Also: 7 Different Types of Ecology
4. Survey Research
Perhaps the most common form of quantitative research, surveys use one or more groups as the test pool. The individuals in these groups must be picked randomly unless a specific factor is involved (for example, when the survey is related to smoking habits, you’ll want smokers to respond).
To keep surveys as random as possible, data is usually collected in a public place where passersby are asked to fill out a form if they qualify.
As mentioned, surveys quite often use questionnaire forms to collect data. These forms may use yes/no answers or ratings to gain more precise results. Interviews are also a common tool, although sampling polls are also effective in specific situations (such as feedback for customer service quality).
The results are often very precise and the broader the pool of respondents used, the more accurate the results will likely be.
Two famous examples of survey research are US election polls and the Kinsey Report (a periodical report on human sexuality released by the Kinsey Institute ).
How to Perform Quantitative Research
As with all forms of research, there is an organized series of steps involved in quantitative research that are easy to learn and master but cannot be skipped at any point. To perform your research, simply follow the steps in order.
We’ll pretend to be a small family clothing business so you can see these steps in action.
Step 1: Define the Problem & Choose a Method (AKA The Question)
Once you know the question and have a theory, decide which quantitative method best suits your needs. Make a list of the materials, subjects, and other factors you’ll need to perform the research.
We want to add to our upcoming summer line but will halter tops or sports bras be more popular this year? The weather is supposed to be hot, so we think looser halter tops might fare better.
Conversely, sports bras may prove more popular if local women intend to be more active. To find out which type of top would be better, we decided to perform a survey at the local university and mall.
Step 2: Gather the Data
Once you’ve gathered your tools, perform the research and record all relevant results. In the event you have multiple test groups, be sure to keep the results separate for comparison purposes later.
As quantitative research is mathematically-based, the majority of data should be based on numbers. Make note of any unexpected variables that arise, as these could skew the result or require further testing to account for.
Our survey teams quickly completed research at both locations and have compiled the survey papers into separate marked folders. These were then turned over to my husband (the marketing expert) to examine. The teams both reported seeing trends that will help give a better picture, although they also had a lot of comments that may affect the final results.
Step 3: Analysis and Conclusion
33 of the college girls chose halter tops, and of those 24 mentioned their boyfriends as a deciding factor. 14 quoted morning jogs or aerobics/yoga as the reason for preferring sports bras. 3 were undecided. 49 of these were in the 18-24 age range with one professor participating.
After looking at the data, we found 60% of women planned to wear halter tops this summer. Another 33% wanted sports bras. Because of the close split, we should aim to produce halter tops this year and, if early summer profits permit, make a smaller quantity of sports bras by mid-summer.
1 thought on “4 Different Types of Quantitative Research”
excellent work
Leave a Comment Cancel reply

Types of Quantitative Research | An Absolute Guide for Beginners
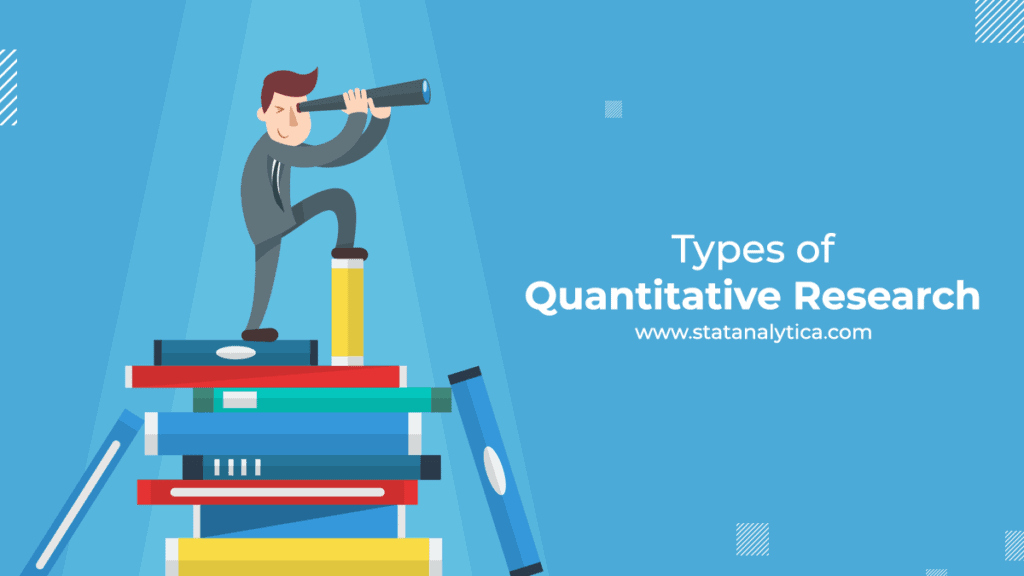
It does not matter which discipline a person belongs to. You have to come across the quantitative research data once or multiple times in life. Most people often come across one or multiple questionnaires or surveys.
Let’s take an example of quantitative research. A survey is conducted to know how much time a shopkeeper takes to attend to the customer. And how many times he/she walks into the shop.
Here, the survey is conducted using different questions. Such as how much time the shopkeeper takes to attend to the customer. And how many times the customer comes in the shop, etc.
The aim of these surveys is to draw the most relevant analytical conclusions. That helps in understanding the targeted audience.
There are various types of quantitative research that a company uses. It is used to understand the product’s demand within the market.
In this blog, we have given the necessary details about what quantitative research is. And its types.
So, let’s move on to the details.
What is quantitative research?
Table of Contents
Quantitative research is one of the systematic techniques. It is used to collect the data using the sampling for quantitative methods. For example , online polls , questionnaires, and online surveys. You can seamlessly integrate them into your WordPress website using a versatile WordPress voting plugin , providing an interactive and engaging way to collect valuable insights from your audience.
The data is collected from both existing and potential users and represented numerically.
Quantitative research also used to measure the variables, analyze, and register the relationships between the variable studies with a numerical system’s help.
In quantitative research, the information is collected via structured research. And the outcomes reflect or represent the population.
Where we use quantitative research?
Quantitative researchers use different tools. The tools are used to collect numeric data in terms of numbers and statistics. This data is represented in non-textual forms, such as charts, figures, and tables.
Moreover, the researchers can take the non-numerical data to examine the information.
Quantitative research is using in several areas, such as:
- gender studies,
- demography,
- community health,
- psychology,
- education, and so on.
What are the 5 types of quantitative research?
Survey research.
The survey is one of the primary statistic methods. It is used for different types of quantitative research. The aim of this is to provide a comprehensive description of the characteristics of the specific population or group.
Both large and small organizations also apply the offline and online survey research method. This helps to know their users and understand the product and merchandise views.
There are numerous methods to manage survey research. It can be done on the phone, in person, or by email or mails.
In the survey research, the users raised various queries; therefore, the quantitative analysis was also done on the same basis. For conducting the survey research analysis, longitudinal and cross-sectional surveys are performed.The survey research applies to the population at different time durations. It utilizes in The survey research applies to the specific or targeted population over a particular period of time. This use in researching the field of , and much more. |
Descriptive research
It describes the present status of all the selected or identified variables. The basic objective of descriptive research is to describe and evaluate the people’s present status, conditions, settings, or events.
Descriptive research is considered to be one of the important types of quantitative research.
The most common descriptive questions start with the “How much..,” “what is the…,” “what is a percentage of…,” and these kinds of questions.
Let’s take an example of this survey. An Exit poll is a descriptive survey that includes questions like: “ Which candidate will win this election?”
Moreover, the demographic segmentation survey might be like this: “ How many students between the 18-25 age do study at night?”
The researchers in descriptive research do not start the research with the hypothesis. But, it is mostly developed once the information is collected.The systematic collection of data requires a careful selection of measurements and units of each variable. |
Experimental research
This is one of the types of quantitative research, as its name suggests that it is based on single or multiple theories.
It terms to be the true experiments that utilize the scientific technique to verify the cause-effect relations within the group of variables.
Therefore, more than one theory is used to conduct the particular research. An example of experimental research is “ the effect of the particular dose and treatment on breast cancer.”
The use of experimental research can be implemented in various fields. And these fields are sociology, physics, biology, chemistry, medicine, and so on.
A dependent variable in experimental research refers to the posttest variable. Or effect that measures identically for all the groups.An independent variable in experimental research refers to the experimental variable. It applies to the particular experimental group. |
Correlational research
It is used for establishing relationships among two close entities. And determining the relational impact on each other.
For such cases, a researcher requires a minimum of two different groups. Additionally, this research approaches and recognizes the patterns and trends in the data without going far into the observation to analyze various trends and patterns.
An example of correlational research is the correlation between self-esteem and intelligence.
Suppose your favorite ice-cream truck has a specific jingle, and the truck is coming to your area. The more would be the sound of the jingle, the more closer the ice-cream truck would be.
But, if two ice-cream trucks are coming in your area, you can easily know which sounds are from your favorite ice-cream truck. This is what you are not taught in your classroom, but you can relate the fact in your mind on your own.
Moreover, it depends on your intelligence that you can quickly recognize without anybody’s help. This is what the correlation research method is.
Sometimes, these types of quantitative research are considered in the category of As it is not a single variable that is manipulated within the studies.The cause-effect relationship is not considered as the observational research type.The different subjects classroom activity and achievement of students in the schools. These are some of the basic examples of correlational research. |
Causal-comparative research
It is one of the scientific methods that apply to summarize the cause and effect equations among different variables. In causal relationships, a single variable is based on the complementary experimental variable.
The experimenters do not manipulate the independent variable. But, the impact of independent variables over the dependent variables can be measured in causal-comparative research.
Let’s take some examples. The impact of divorce of the parents on their children. The impact of sports activity on the participants, and so on.
The analysis of casual-comparatives is not limited to the applied maths of two or more variables. But can extend to analyzing various groups and variables. These types of quantitative research work on the comparison process.When the conclusions and analysis are made of various variables, the unknown and far-famed variables can affect the outcomes. |
- What is probability and types of Probability Sampling
- Types of statistical analysis
- Types of statistical terms
Why do I select different types of quantitative research over qualitative research?
It has been seen that quantitative research prefers over qualitative research. The reasons for it can be like quantitative research can be done fast, scientific, acceptable, objective, and focused.
Apart from this, there are several reasons to select different types of quantitative research. Let’s check each of them one by one.
Deal with the larger sample data
The types of quantitative research results depend on the large sample size. This sample size represents the population. The lager is the sample size; the more valid results will be drawn.
The types of quantitative research results depend on the large sample size. This sample size represents the population. The lager is the sample size; the more valid results will be drawn.
Control-sensitive
It has been seen that researchers have more control over the data collection methods. This data is also different from the experiments.
Researchers use different types of quantitative research. It is used to establish facts, make predictions, and test the previous hypotheses.
The relatable aims for finding evidence that may or may not support an existing hypothesis. By testing and validating the constructed theories, it can give reasons why a phenomenon has occurred.
Generalizable
A project can generalize the concepts more accurately. It also analyzes the casual relationship, and predicts results. Moreover, the findings can generalize when the selection processes are designed. And the sample represents the population study.
Arrange in simple analytical ways
The data is being collected in the form of statistics and numbers. Further, it is arranged in charts, tables, figures, or another non-textual form.
The methods of data collection that use a quantitative research method are comparatively quick (such as telephone interviews). Some companies even use the best landline phone service for more professionalism and speed. Some companies even use a special telephone service for more professionalism and speed. Moreover, the data analysis is also comparatively less time-consuming (as it does use statistical software).
Consistent with data
Using the different types of quantitative research, you can easily get data. This data is reliable, precise, and consistent, numerical, and quantitative.
More structured
The researchers use different tools to get structured quantitative researched data. The tools can be equipment or questionnaires for collecting numerical data.
The repeatable and replica methods are usually done in research studies. This leads to high reliability.
Decision-making
The data taken from quantitative research like demographics, market size, and user preferences can help provide information on business decisions.
So, what are the methods of quantitative research?
The quantitative research method features objective calculations and mathematical, statistical, or numerical analysis. The data is collected by questionnaires, polls, and surveys for analysis.
The quantitative research method mainly focuses on collecting numerical data. This data generalize across the set of people so that a specific phenomenon can be explained.
Researchers who use the quantitative research method try to identify and separate the variables. These variables separate within a study framework, seek relationships, correlation, and casualties.
After this, the quantitative researchers try to control the system in which the information is being gathered. This helps in avoiding the variables’ risk by which the accurate relationships can be identified.
What is the methodology for the quantitative research designs?
The structure of various types of quantitative research depends on the scientific method.
This utilizes deductive reasoning, in which the researchers: find out the hypothesis. Gather the information. Uses it for further investigation to prove whether the hypothesis is true or not. Once the analysis is done, share your summary.
Therefore, a basic procedure is followed for the quantitative research design:
- Make the observations related to something, which is new and unexplained. Analyzing the present theory that is surrounded by issues or problems.
- Hypothesizing the observations’ explanations.
- Predict the result depends on the hypothesis studies by formulating the plan for your prediction test control.
- Gather and process the information. If the prediction is right, move to the next step; otherwise, return to step 2. Get new hypotheses that depend on the new knowledge and situations.
- Finally, verify the findings of the sample on different factors. Make the conclusions. Represent the outcomes in a well-structured manner to your audience.
Now, let’s check your knowledge regarding types of quantitative research!
Now, you have studied different types of quantitative research. Let’s check what you have learned.
Take a quiz regarding the different quantitative research. Select the correct quantitative research type to the given statement.
- Do people think working from home is a great option to enhance the employee’s productivity with longer commutes?
- Descriptive Research
- Experimental Research
- Correlational Research
- Causal-Comparative Research
The employee with longer commutes Working in-office and from home |
- How frequently do employees get the chance of traveling for a holiday?
Working person Number of times employees get the chance to travel during the holiday |
- What is the primary difference between senior citizens and Millennials regarding smartphone usage?
Seniors and Millennials Time consumed on smartphone usage |
- How has the Covid-19 modified profession for white-collar employees?
White-collar employees Work types and status |
- Does the management method of the car shop owners foretell the work satisfaction of car sales associates?
Car shop owners & sales associates Management method & work satisfaction |
There are various types of quantitative research . And researchers use different kinds of scientific tools to collect numeric data.
It has been observed that the quantitative research survey questions are must. So that the participants can have an easy and effective medium to responses.
Hope you easily understand the details mentioned in the blog. If you still have any queries, comment in the below section, and we will help you in the best possible way. Are you looking for statistics help for students ? Get the best help from our experts to clear all your doubts.
Frequently Asked Questions
Where is quantitative research used.
The main use of quantitative research is for quantifying the problem by forming numerical data. (Moreover, this data must transform into useful statistics.) The quantitative research is used to quantify opinions, attitudes, behaviors, and another defined variable. By this, you can generalize the results from a greater population sample.
What are the steps in quantitative research?
There are 11 steps to following in Quantitative Research, and these are:
Theory. Hypothesis. Research design. Operationalizing concepts. Selection of a research site(s). Selection of respondents. Data collection. Processing data. Data analysis. Findings and conclusions. Writing the findings in a well-structured manner.
What are the 7 characteristics of quantitative research?
The 7 major characteristics of quantitative research methods are as follows:
Practice Standardized Research Instruments. Contain Measurable Variables. Data representation in Graphs, Tables, or Figures. Use a Repeatable Method. Work on Measuring Devices. Allows a Normal Population Distribution. Can Predict Outcomes.
Related Posts
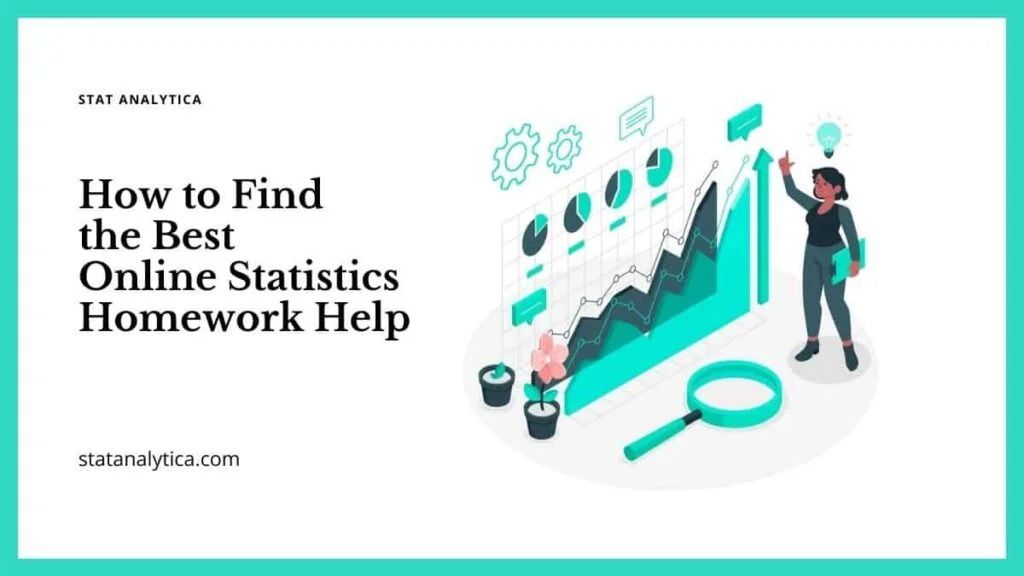
How to Find the Best Online Statistics Homework Help
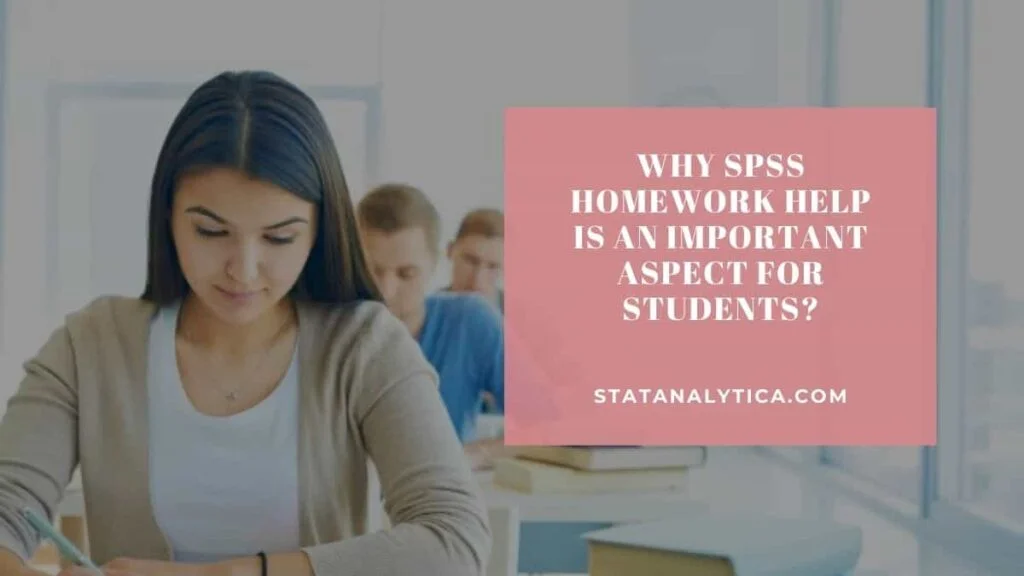
Why SPSS Homework Help Is An Important aspect for Students?
We Trust in Human Precision
20,000+ Professional Language Experts Ready to Help. Expertise in a variety of Niches.
API Solutions
- API Pricing
- Cost estimate
- Customer loyalty program
- Educational Discount
- Non-Profit Discount
- Green Initiative Discount1
Value-Driven Pricing
Unmatched expertise at affordable rates tailored for your needs. Our services empower you to boost your productivity.

- Special Discounts
- Enterprise transcription solutions
- Enterprise translation solutions
- Transcription/Caption API
- AI Transcription Proofreading API
Trusted by Global Leaders
GoTranscript is the chosen service for top media organizations, universities, and Fortune 50 companies.
GoTranscript
One of the Largest Online Transcription and Translation Agencies in the World. Founded in 2005.
Speaker 1: Welcome to this overview of quantitative research methods. This tutorial will give you the big picture of quantitative research and introduce key concepts that will help you determine if quantitative methods are appropriate for your project study. First, what is educational research? Educational research is a process of scholarly inquiry designed to investigate the process of instruction and learning, the behaviors, perceptions, and attributes of students and teachers, the impact of institutional processes and policies, and all other areas of the educational process. The research design may be quantitative, qualitative, or a mixed methods design. The focus of this overview is quantitative methods. The general purpose of quantitative research is to explain, predict, investigate relationships, describe current conditions, or to examine possible impacts or influences on designated outcomes. Quantitative research differs from qualitative research in several ways. It works to achieve different goals and uses different methods and design. This table illustrates some of the key differences. Qualitative research generally uses a small sample to explore and describe experiences through the use of thick, rich descriptions of detailed data in an attempt to understand and interpret human perspectives. It is less interested in generalizing to the population as a whole. For example, when studying bullying, a qualitative researcher might learn about the experience of the victims and the experience of the bully by interviewing both bullies and victims and observing them on the playground. Quantitative studies generally use large samples to test numerical data by comparing or finding correlations among sample attributes so that the findings can be generalized to the population. If quantitative researchers were studying bullying, they might measure the effects of a bully on the victim by comparing students who are victims and students who are not victims of bullying using an attitudinal survey. In conducting quantitative research, the researcher first identifies the problem. For Ed.D. research, this problem represents a gap in practice. For Ph.D. research, this problem represents a gap in the literature. In either case, the problem needs to be of importance in the professional field. Next, the researcher establishes the purpose of the study. Why do you want to do the study, and what do you intend to accomplish? This is followed by research questions which help to focus the study. Once the study is focused, the researcher needs to review both seminal works and current peer-reviewed primary sources. Based on the research question and on a review of prior research, a hypothesis is created that predicts the relationship between the study's variables. Next, the researcher chooses a study design and methods to test the hypothesis. These choices should be informed by a review of methodological approaches used to address similar questions in prior research. Finally, appropriate analytical methods are used to analyze the data, allowing the researcher to draw conclusions and inferences about the data, and answer the research question that was originally posed. In quantitative research, research questions are typically descriptive, relational, or causal. Descriptive questions constrain the researcher to describing what currently exists. With a descriptive research question, one can examine perceptions or attitudes as well as more concrete variables such as achievement. For example, one might describe a population of learners by gathering data on their age, gender, socioeconomic status, and attributes towards their learning experiences. Relational questions examine the relationship between two or more variables. The X variable has some linear relationship to the Y variable. Causal inferences cannot be made from this type of research. For example, one could study the relationship between students' study habits and achievements. One might find that students using certain kinds of study strategies demonstrate greater learning, but one could not state conclusively that using certain study strategies will lead to or cause higher achievement. Causal questions, on the other hand, are designed to allow the researcher to draw a causal inference. A causal question seeks to determine if a treatment variable in a program had an effect on one or more outcome variables. In other words, the X variable influences the Y variable. For example, one could design a study that answered the question of whether a particular instructional approach caused students to learn more. The research question serves as a basis for posing a hypothesis, a predicted answer to the research question that incorporates operational definitions of the study's variables and is rooted in the literature. An operational definition matches a concept with a method of measurement, identifying how the concept will be quantified. For example, in a study of instructional strategies, the hypothesis might be that students of teachers who use Strategy X will exhibit greater learning than students of teachers who do not. In this study, one would need to operationalize learning by identifying a test or instrument that would measure learning. This approach allows the researcher to create a testable hypothesis. Relational and causal research relies on the creation of a null hypothesis, a version of the research hypothesis that predicts no relationship between variables or no effect of one variable on another. When writing the hypothesis for a quantitative question, the null hypothesis and the research or alternative hypothesis use parallel sentence structure. In this example, the null hypothesis states that there will be no statistical difference between groups, while the research or alternative hypothesis states that there will be a statistical difference between groups. Note also that both hypothesis statements operationalize the critical thinking skills variable by identifying the measurement instrument to be used. Once the research questions and hypotheses are solidified, the researcher must select a design that will create a situation in which the hypotheses can be tested and the research questions answered. Ideally, the research design will isolate the study's variables and control for intervening variables so that one can be certain of the relationships being tested. In educational research, however, it is extremely difficult to establish sufficient controls in the complex social settings being studied. In our example of investigating the impact of a certain instructional strategy in the classroom on student achievement, each day the teacher uses a specific instructional strategy. After school, some of the students in her class receive tutoring. Other students have parents that are very involved in their child's academic progress and provide learning experiences in the home. These students may do better because they received extra help, not because the teacher's instructional strategy is more effective. Unless the researcher can control for the intervening variable of extra help, it will be impossible to effectively test the study's hypothesis. Quantitative research designs can fall into two broad categories, experimental and quasi-experimental. Classic experimental designs are those that randomly assign subjects to either a control or treatment comparison group. The researcher can then compare the treatment group to the control group to test for an intervention's effect, known as a between-subject design. It is important to note that the control group may receive a standard treatment or may receive a treatment of any kind. Quasi-experimental designs do not randomly assign subjects to groups, but rather take advantage of existing groups. A researcher can still have a control and comparison group, but assignment to the groups is not random. The use of a control group is not required. However, the researcher may choose a design in which a single group is pre- and post-tested, known as a within-subjects design. Or a single group may receive only a post-test. Since quasi-experimental designs lack random assignment, the researcher should be aware of the threats to validity. Educational research often attempts to measure abstract variables such as attitudes, beliefs, and feelings. Surveys can capture data about these hard-to-measure variables, as well as other self-reported information such as demographic factors. A survey is an instrument used to collect verifiable information from a sample population. In quantitative research, surveys typically include questions that ask respondents to choose a rating from a scale, select one or more items from a list, or other responses that result in numerical data. Studies that use surveys or tests need to include strategies that establish the validity of the instrument used. There are many types of validity that need to be addressed. Face validity. Does the test appear at face value to measure what it is supposed to measure? Content validity. Content validity includes both item validity and sampling validity. Item validity ensures that the individual test items deal only with the subject being addressed. Sampling validity ensures that the range of item topics is appropriate to the subject being studied. For example, item validity might be high, but if all the items only deal with one aspect of the subjects, then sampling validity is low. Content validity can be established by having experts in the field review the test. Concurrent validity. Does a new test correlate with an older, established test that measures the same thing? Predictive validity. Does the test correlate with another related measure? For example, GRE tests are used at many colleges because these schools believe that a good grade on this test increases the probability that the student will do well at the college. Linear regression can establish the predictive validity of a test. Construct validity. Does the test measure the construct it is intended to measure? Establishing construct validity can be a difficult task when the constructs being measured are abstract. But it can be established by conducting a number of studies in which you test hypotheses regarding the construct, or by completing a factor analysis to ensure that you have the number of constructs that you say you have. In addition to ensuring the validity of instruments, the quantitative researcher needs to establish their reliability as well. Strategies for establishing reliability include Test retest. Correlates scores from two different administrations of the same test. Alternate forms. Correlates scores from administrations of two different forms of the same test. Split half reliability. Treats each half of one test or survey as a separate administration and correlates the results from each. Internal consistency. Uses Cronbach's coefficient alpha to calculate the average of all possible split halves. Quantitative research almost always relies on a sample that is intended to be representative of a larger population. There are two basic sampling strategies, random and non-random, and a number of specific strategies within each of these approaches. This table provides examples of each of the major strategies. The next section of this tutorial provides an overview of the procedures in conducting quantitative data analysis. There are specific procedures for conducting the data collection, preparing for and analyzing data, presenting the findings, and connecting to the body of existing research. This process ensures that the research is conducted as a systematic investigation that leads to credible results. Data comes in various sizes and shapes, and it is important to know about these so that the proper analysis can be used on the data. In 1946, S.S. Stevens first described the properties of measurement systems that allowed decisions about the type of measurement and about the attributes of objects that are preserved in numbers. These four types of data are referred to as nominal, ordinal, interval, and ratio. First, let's examine nominal data. With nominal data, there is no number value that indicates quantity. Instead, a number has been assigned to represent a certain attribute, like the number 1 to represent male and the number 2 to represent female. In other words, the number is just a label. You could also assign numbers to represent race, religion, or any other categorical information. Nominal data only denotes group membership. With ordinal data, there is again no indication of quantity. Rather, a number is assigned for ranking order. For example, satisfaction surveys often ask respondents to rank order their level of satisfaction with services or programs. The next level of measurement is interval data. With interval data, there are equal distances between two values, but there is no natural zero. A common example is the Fahrenheit temperature scale. Differences between the temperature measurements make sense, but ratios do not. For instance, 20 degrees Fahrenheit is not twice as hot as 10 degrees Fahrenheit. You can add and subtract interval level data, but they cannot be divided or multiplied. Finally, we have ratio data. Ratio is the same as interval, however ratios, means, averages, and other numerical formulas are all possible and make sense. Zero has a logical meaning, which shows the absence of, or having none of. Examples of ratio data are height, weight, speed, or any quantities based on a scale with a natural zero. In summary, nominal data can only be counted. Ordinal data can be counted and ranked. Interval data can also be added and subtracted, and ratio data can also be used in ratios and other calculations. Determining what type of data you have is one of the most important aspects of quantitative analysis. Depending on the research question, hypotheses, and research design, the researcher may choose to use descriptive and or inferential statistics to begin to analyze the data. Descriptive statistics are best illustrated when viewed through the lens of America's pastimes. Sports, weather, economy, stock market, and even our retirement portfolio are presented in a descriptive analysis. Basic terminology for descriptive statistics are terms that we are most familiar in this discipline. Frequency, mean, median, mode, range, variance, and standard deviation. Simply put, you are describing the data. Some of the most common graphic representations of data are bar graphs, pie graphs, histograms, and box and whisker graphs. Attempting to reach conclusions and make causal inferences beyond graphic representations or descriptive analyses is referred to as inferential statistics. In other words, examining the college enrollment of the past decade in a certain geographical region would assist in estimating what the enrollment for the next year might be. Frequently in education, the means of two or more groups are compared. When comparing means to assist in answering a research question, one can use a within-group, between-groups, or mixed-subject design. In a within-group design, the researcher compares measures of the same subjects across time, therefore within-group, or under different treatment conditions. This can also be referred to as a dependent-group design. The most basic example of this type of quasi-experimental design would be if a researcher conducted a pretest of a group of students, subjected them to a treatment, and then conducted a post-test. The group has been measured at different points in time. In a between-group design, subjects are assigned to one of the two or more groups. For example, Control, Treatment 1, Treatment 2. Ideally, the sampling and assignment to groups would be random, which would make this an experimental design. The researcher can then compare the means of the treatment group to the control group. When comparing two groups, the researcher can gain insight into the effects of the treatment. In a mixed-subjects design, the researcher is testing for significant differences between two or more independent groups while subjecting them to repeated measures. Choosing a statistical test to compare groups depends on the number of groups, whether the data are nominal, ordinal, or interval, and whether the data meet the assumptions for parametric tests. Nonparametric tests are typically used with nominal and ordinal data, while parametric tests use interval and ratio-level data. In addition to this, some further assumptions are made for parametric tests that the data are normally distributed in the population, that participant selection is independent, and the selection of one person does not determine the selection of another, and that the variances of the groups being compared are equal. The assumption of independent participant selection cannot be violated, but the others are more flexible. The t-test assesses whether the means of two groups are statistically different from each other. This analysis is appropriate whenever you want to compare the means of two groups, and especially appropriate as the method of analysis for a quasi-experimental design. When choosing a t-test, the assumptions are that the data are parametric. The analysis of variance, or ANOVA, assesses whether the means of more than two groups are statistically different from each other. When choosing an ANOVA, the assumptions are that the data are parametric. The chi-square test can be used when you have non-parametric data and want to compare differences between groups. The Kruskal-Wallis test can be used when there are more than two groups and the data are non-parametric. Correlation analysis is a set of statistical tests to determine whether there are linear relationships between two or more sets of variables from the same list of items or individuals, for example, achievement and performance of students. The tests provide a statistical yes or no as to whether a significant relationship or correlation exists between the variables. A correlation test consists of calculating a correlation coefficient between two variables. Again, there are parametric and non-parametric choices based on the assumptions of the data. Pearson R correlation is widely used in statistics to measure the strength of the relationship between linearly related variables. Spearman-Rank correlation is a non-parametric test that is used to measure the degree of association between two variables. Spearman-Rank correlation test does not assume any assumptions about the distribution. Spearman-Rank correlation test is used when the Pearson test gives misleading results. Often a Kendall-Taw is also included in this list of non-parametric correlation tests to examine the strength of the relationship if there are less than 20 rankings. Linear regression and correlation are similar and often confused. Sometimes your methodologist will encourage you to examine both the calculations. Calculate linear correlation if you measured both variables, x and y. Make sure to use the Pearson parametric correlation coefficient if you are certain you are not violating the test assumptions. Otherwise, choose the Spearman non-parametric correlation coefficient. If either variable has been manipulated using an intervention, do not calculate a correlation. While linear regression does indicate the nature of the relationship between two variables, like correlation, it can also be used to make predictions because one variable is considered explanatory while the other is considered a dependent variable. Establishing validity is a critical part of quantitative research. As with the nature of quantitative research, there is a defined approach or process for establishing validity. This also allows for the findings transferability. For a study to be valid, the evidence must support the interpretations of the data, the data must be accurate, and their use in drawing conclusions must be logical and appropriate. Construct validity concerns whether what you did for the program was what you wanted to do, or whether what you observed was what you wanted to observe. Construct validity concerns whether the operationalization of your variables are related to the theoretical concepts you are trying to measure. Are you actually measuring what you want to measure? Internal validity means that you have evidence that what you did in the study, i.e., the program, caused what you observed, i.e., the outcome, to happen. Conclusion validity is the degree to which conclusions drawn about relationships in the data are reasonable. External validity concerns the process of generalizing, or the degree to which the conclusions in your study would hold for other persons in other places and at other times. Establishing reliability and validity to your study is one of the most critical elements of the research process. Once you have decided to embark upon the process of conducting a quantitative study, use the following steps to get started. First, review research studies that have been conducted on your topic to determine what methods were used. Consider the strengths and weaknesses of the various data collection and analysis methods. Next, review the literature on quantitative research methods. Every aspect of your research has a body of literature associated with it. Just as you would not confine yourself to your course textbooks for your review of research on your topic, you should not limit yourself to your course texts for your review of methodological literature. Read broadly and deeply from the scholarly literature to gain expertise in quantitative research. Additional self-paced tutorials have been developed on different methodologies and techniques associated with quantitative research. Make sure that you complete all of the self-paced tutorials and review them as often as needed. You will then be prepared to complete a literature review of the specific methodologies and techniques that you will use in your study. Thank you for watching.

Thank you for visiting nature.com. You are using a browser version with limited support for CSS. To obtain the best experience, we recommend you use a more up to date browser (or turn off compatibility mode in Internet Explorer). In the meantime, to ensure continued support, we are displaying the site without styles and JavaScript.
- View all journals
- Explore content
- About the journal
- Publish with us
- Sign up for alerts
- Open access
- Published: 05 September 2024
Quantitative classification evaluation model for tight sandstone reservoirs based on machine learning
- Xinglei Song 1 , 2 ,
- Congjun Feng 1 , 2 ,
- Teng Li 3 , 4 , 5 ,
- Qin Zhang 6 ,
- Xinhui Pan 1 , 2 ,
- Mengsi Sun 7 &
- Yanlong Ge 1 , 2
Scientific Reports volume 14 , Article number: 20712 ( 2024 ) Cite this article
Metrics details
Tight sandstone reservoirs are a primary focus of research on the geological exploration of petroleum. However, many reservoir classification criteria are of limited applicability due to the inherent strong heterogeneity and complex micropore structure of tight sandstone reservoirs. This investigation focused on the Chang 8 tight reservoir situated in the Jiyuan region of the Ordos Basin. High-pressure mercury intrusion experiments, casting thin sections, and scanning electron microscopy experiments were conducted. Image recognition technology was used to extract the pore shape parameters of each sample. Based on the above, through grey relational analysis (GRA), analytic hierarchy process (AHP), entropy weight method (EWM) and comprehensive weight method, the relationship index Q1 between initial productivity and high pressure mercury injection parameters and the relationship index Q2 between initial productivity and pore shape parameters are obtained by fitting. Then a dual-coupled comprehensive quantitative classification prediction model for tight sandstone reservoirs was developed based on pore structure and shape parameters. A quantitative classification study was conducted on the target reservoir, analyzing the correlation between reservoir quality and pore structure and shape parameters, leading to the proposal of favourable exploration areas. The research results showed that when Q1 ≥ 0.5 and Q2 ≥ 0.5, the reservoir was classified as type I. When Q1 > 0.7 and Q2 > 0.57, it was classified as type I 1 , indicating a high-yield reservoir. When 0.32 < Q1 < 0.47 and 0.44 < Q2 < 0.56, was classified as type II. When 0.1 < Q1 < 0.32 and 0.3 < Q2 < 0.44, it was classified as type III. Type I reservoirs exhibit a zigzag pattern in the northwest part of the study area. Thus, the northwest should be prioritized in actual exploration and development. Additionally, the initial productivity of tight sandstone reservoirs showed a positive correlation with the porosity, permeability, sorting coefficient, coefficient of variation, and median radius. Conversely, it demonstrated a negative correlation with the median pressure and displacement pressure. The perimeters of pores, their circularity, and the length of the major axis showed a positive correlation with the porosity, permeability, sorting coefficient, coefficient of variation, and median radius. On the other hand, they exhibited a negative correlation with the median pressure and displacement pressure. This study quantitatively constructed a new classification and evaluation system for tight sandstone reservoirs from the perspective of microscopic pore structure, achieving an overall model accuracy of 93.3%. This model effectively predicts and evaluates tight sandstone reservoirs. It provides new guidance for identifying favorable areas in the study region and other tight sandstone reservoirs.
Similar content being viewed by others
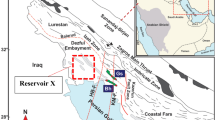
A case study of petrophysical rock typing and permeability prediction using machine learning in a heterogenous carbonate reservoir in Iran
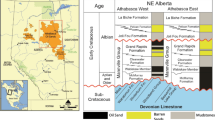
Hierarchical automated machine learning (AutoML) for advanced unconventional reservoir characterization
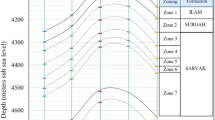
Permeability modelling in a highly heterogeneous tight carbonate reservoir using comparative evaluating learning-based and fitting-based approaches
Introduction.
With the depletion of conventional oil and gas reservoirs, tight oil reservoirs have gradually become a hot topic and a focal point for exploration and development, both domestically and internationally 1 , 2 , 3 , 4 , 5 , 6 , 7 , 8 , 9 . However, tight sandstone oil reservoirs exhibit complex reservoir characteristics, primarily manifested in their deep burial depths, wide distribution, and complex depositional processes. The reservoirs exhibit characteristics of low porosity, poor permeability, and high heterogeneity. The dominant pores are micro- and nano-scale, with narrow and dispersed throats, and are unfavorable for the migration and accumulation of oil and gas 10 , 11 , 12 , 13 , 14 , 15 . These factors necessitate considering the interdependent influences of multiple factors when classifying and evaluating tight sandstone reservoirs, which affects the accuracy of reservoir evaluation and hinders the selection of high-quality reservoirs. Therefore, the rapid and effective classification and evaluation of tight sandstone reservoirs has long been a focal point of scholarly research.
The quality of the reservoir is a key factor that determines the oil and gas production capacity. The classification and evaluation of reservoirs are central to reservoir studies and play a significant role in oilfield development. With the continuous advancement of oilfield development technologies, reservoir classification and evaluation methods have become increasingly diverse, gradually evolving from qualitative to quantitative research and from macro-parameter to micro-parameter evaluation. At present, both domestic and international scholars classify reservoirs using two main methods. The first is the traditional classification and evaluation method, which directly uses indicators such as the lithology, physical properties, pore structure, sedimentary facies, and oil and production experiments for classification. For example, Wei et al. classified the tight sandstone reservoirs of the Sha Creek Formation in the central Sichuan Basin based on the transverse relaxation (T 2 ) distribution of nuclear magnetic resonance 16 . Xu et al. studied the characteristics and controlling factors of tight sandstone using thin-section casting, scanning electron microscopy, X-ray diffraction (XRD), and spontaneous imbibition experiments 17 . Wu et al. analyzed the logging response characteristics using core data and electric imaging logging data and identified the reservoir type with the highest industrial production in the study area 18 . Zhang et al. established classification criteria for the third member of the Quan Formation based on mercury injection curves, core physical properties, and sedimentary facies characteristics 19 . Talib et al. quantitatively characterized tight oil and gas reservoirs through rock physics experiments and seismic inversion profiles 20 .
The second approach to reservoir classification involves initially choosing evaluation parameters that align with the geological conditions of the target area. Subsequently, machine learning techniques such as GRA the AHP, the EWM, and fuzzy analysis are employed to assign weight coefficients to each evaluation parameter. Finally, the reservoir is comprehensively scored. For example, Fang et al. proposed an automatic classification and verification method for reservoir types based on k-means clustering and Bayesian discriminant theory, using core logging and logging data from coring wells, combined with physical characteristics such as reservoir deposition and diagenesis 21 . Li et al. classified the Fuyu reservoir using GRA, Q clustering analysis, and discriminant analysis 22 . Wang et al.combined AHP and EWM, used the multi-factor superposition method, and established a new reservoir classification and evaluation method 23 . Fan et al. quantified the weight of evaluation parameters’ contribution to production by combining the relationships between variables and directional good production using the GRA 24 . Niu et al. proposed a new machine learning framework (GCA-CE-MGPK) for shale reservoirs, achieving efficient and accurate multi-scale evaluation of shale reservoirs 25 . In summary, traditional classification and evaluation methods are costly, inefficient and require extensive experimental data. They are mainly suitable for specific regions, making them inadequate for large-scale reservoir evaluation and prediction. Although machine learning techniques can improve efficiency and reduce costs, their accuracy often depends on the optimization of various mathematical methods, leading to high subjectivity in some models and lower overall precision, failing to meet the practical needs of production. Moreover, previous studies have primarily focused on evaluating single factors, lacking the integration of macro and micro perspectives. Based on these, this study combined multiple machine learning methods to directly link actual oilfield production data with micro-scale pore shape and structure parameters, effectively integrating macro and micro parameters.
Given the significant influence of subjective factors on the classification criteria for the quantitative evaluation of conventional reservoirs, adopting a new method for reservoir evaluation is essential. This study focuses on the Chang 8 tight sandstone reservoir in the Jiyuan area of the Ordos Basin, extracting pore shape parameters from 52 rock samples. Combined with the experimental data of high pressure mercury injection and the actual initial production capacity of the oil field. Through GRA, AHP, EWM and comprehensive weight method, the relationship index Q1 between initial productivity and high pressure mercury injection parameters and the relationship index Q2 between initial productivity and pore shape parameters are obtained by fitting. Then a dual-coupled comprehensive quantitative classification prediction model for tight sandstone reservoirs was developed based on pore structure and shape parameters. A quantitative classification study was conducted on the target reservoir, analyzing the correlation between reservoir quality and pore structure and shape parameters, leading to the proposal of favourable exploration areas. This method effectively combined the subjectivity-influenced AHP with the objectivity-influenced EWM to calculate the comprehensive weight coefficient, mitigating the impact of subjective factors and enhancing the model's accuracy. Validation results indicate that the model has an overall accuracy of 93.3%. Therefore, it was an effective tool for predicting and classifying tight sandstone reservoirs. It is significant for further exploration in the study area and other similar reservoirs.
Geological setting
The Ordos Basin is a large, multi-cycle, cratonic basin that formed on the crystalline basement during the Paleoproterozoic–Mesoproterozoic. The Ordos Basin, the second-largest sedimentary basin in China, has experienced five significant stages of sedimentary evolution. These stages include the middle to late Proterozoic rift valley, the early Paleozoic shallow marine platform, the late Paleozoic nearshore plain, the Mesozoic inland lake basin, and Cenozoic peripheral subsidence. This basin is known for its substantial reserves of oil and gas. The Ordos Basin extends across five provinces and regions, namely, Shaanxi, Gansu, Shanxi, Ningxia, and Inner Mongolia. Geographically, it stretches from the Yin Mountains in the north to the Qinling Mountains in the south, and from the Liupan Mountains in the west to the Lvliang Mountains in the east. The basin’s total area is 25 × 10 4 km 2 , with favorable areas covering 9.9 × 10 4 km 2 . The estimated resource volume is 6.2 × 10 12 m 3 , indicating significant exploration and development potential. Based on the basin’s geological nature, tectonic evolution, and structural pattern, the Ordos Basin can be divided into six primary tectonic units: the northern Shaanxi slope, the Tianhuan Depression, the western thrust fault zone, the Yimeng Uplift, the Weihebei Uplift, and the western Shanxi fold belt. The Jiyuan area, located in the central-western part of the Ordos Basin, covers a total area of 1302 km 2 (Fig. 1 a, c). This area spans the two primary tectonic units of the northern Shaanxi slope and the Tianhuan Depression, exhibiting a gently inclined monocline structure towards the west. Since the Mesozoic, the basin has developed thick fluvio-lacustrine deposits. In the Cenozoic, rift valleys were formed around the basin due to fault subsidence. The overall geological conditions are relatively complex, posing challenges for exploration. However, the area is rich in oil and gas resources, indicating favourable exploration prospects 26 , 27 , 28 , 29 . The proven petroleum geological reserves in this area amount to 800 × 10 6 t, with annual crude oil production of 700 × 10 4 t, making it the oilfield with the largest reserves and production levels in the Ordos Basin from the Mesozoic. Existing exploration results indicate that the Chang 8 oil-bearing formation is one of the most favourable hydrocarbon accumulation zones in the Jiyuan area, with a proven favourable oil-bearing area of 1500 km 2 .
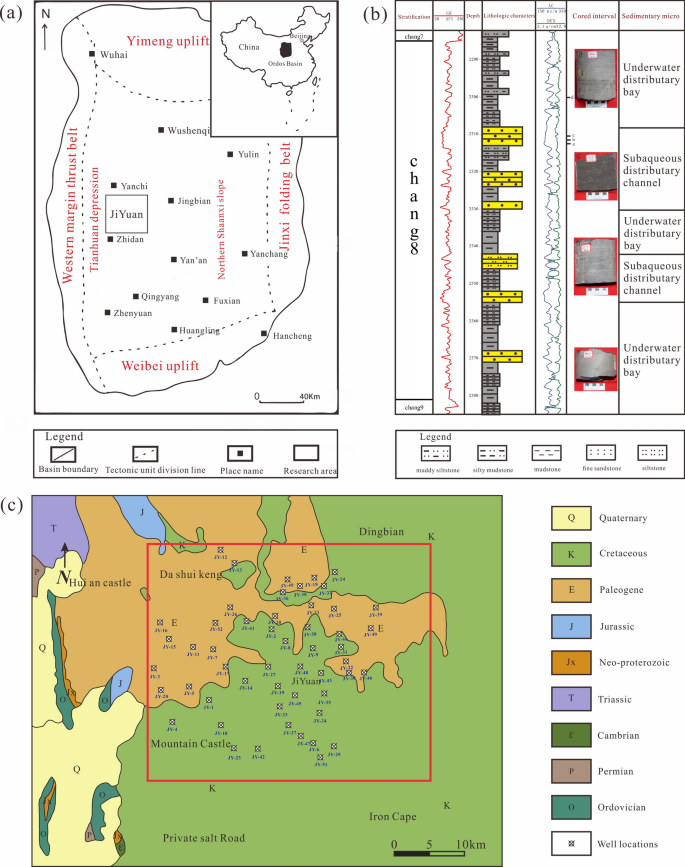
( a ) Location of the study area(modified from Tong 29 ), ( b ) columnar diagram of the Chang 8 formation, ( c ) well location distribution map of the study area.
The Chang 8 reservoir is located in the lower part of the Upper Triassic Yan’an Formation. It is primarily composed of grey sandstone and dark black mudstone interbeds. These sedimentary microfacies are predominantly characterized by subaqueous distributary channels and underwater distributary bays, indicating a deposition pattern typical of a shallow-water deltaic environment (Fig. 1 b). Based on the thin-section identification of the study area (Fig. 2 ), the lithology of the Chang 8 reservoir is predominantly composed of fine-grained feldspathic sandstone, feldspathic lithic sandstone, and a small amount of feldspar sandstone. The detrital components in the study area mainly consist of quartz, feldspar, and detritus. The ranges of contents are as follows: the quartz content is 20.1% to 58.6%, with an average of 31.21%; the feldspar content is 23.56% to 57.62%, with an average of 34.43%; and the detritus content is 6.25% to 29.45%, with an average of 21.38%.
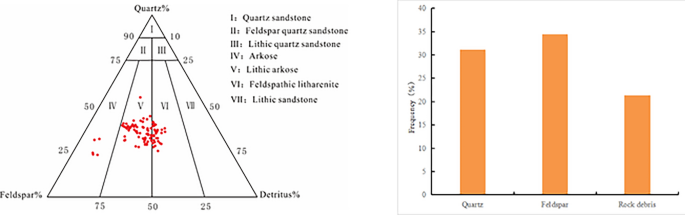
Triangular diagram and detrital composition diagram of the study area. ( a ) Triangular classification diagram of the sandstone in the Chang 8 reservoir, ( b ) histogram of the relative content of detrital components in the Chang 8 reservoir.
Materials and statistical methods
Materials and experiments.
In this study, 52 drilling core samples were obtained from the Chang 8 reservoir in Jiyuan, Ordos Basin, with all samples exhibiting a fine sandstone lithology. The samples underwent oil washing, gas permeability measurements, and the weight method for porosity calculation, allowing the determination of the reservoir’s petrophysical parameters (Table 1 ). The samples' average porosity was 8.23%, between 2.41 and 13.6%. The average permeability was 0.18 × 10 –3 µm 2 , ranging between 0.01 × 10 –3 µm 2 and 1.10 × 10 –3 µm 2 . Subsequently, thin-section casting and scanning electron microscopy experiments were conducted, resulting in 300 photographs. Additionally, high-pressure mercury intrusion was performed on the 52 samples to obtain the micropore throat characteristic parameters.
High pressure mercury intrusion and scanning electron microscopy
High pressure mercury intrusion experiment was used to evaluate the micropore throat characteristics of reservoirs quantitatively. This is achieved by observing the pressure changes during mercury injection into the pores, analyzing the characteristics of the capillary pressure curves, and studying the relationship between the intrusion volume of mercury and these characteristics 30 , 31 . In this experiment, the Auto Pore IV 9530 fully automated mercury porosimeter was utilized, with a pore diameter measurement range of 3 nm to 1100 μm. Continuous mercury injection was employed, with volume accuracy of less than 0.1 μl for both injection and withdrawal. The experimental procedure followed the national standard GB/T29171-2012, and the maximum mercury injection pressure reached 95.39 MPa.
Scanning electron microscopy (SEM) allows for high-resolution morphological observation and analysis of samples, as well as structural and compositional characterization. It also enables direct observation of the development characteristics of the micro-pore throats in the reservoir 32 , 33 , 34 . The experiment employed the Japanese Electron JSM-7500F field emission scanning electron microscope, which achieves a secondary-electron image resolution of 1 nm and magnification ranging from 20 to 300,000 times.
Pore parameter extraction technology
The ImageJ software, initially developed by Wayne Rasband at the National Institutes of Health in the United States, is a powerful open-source image processing system written in Java. It was initially applied in the fields of biomedical and agricultural sciences 35 . Recently, an increasing number of scholars have used it to identify and extract reservoir pores and fracture features 36 , 37 , 38 , 39 . In this study, the ImageJ software was used to process 210 scanning electron microscope images, extracting various pore parameters, including the perimeter, circularity, major axis length, aspect ratio, and solidity.
Statistical methodology
GRA is to address infinite space problems using finite sequences. It aims to evaluate the correlations between various factors within a system and determine the significance of each factor to the target function. This approach helps to avoid the subjective process of manually assigning weights to factor indicators 40 . In recent years, GRA has been applied in production forecasting and development plan optimization for tight sandstone reservoirs 41 , 42 , 43 , 44 . The specific steps are as follows.
Determine the initial sequence:
where X 0 is the reference sequence, X i is the comparative sequence, i is the number of comparative sequences, m is the number of independent variables, and n is the number of samples.
Normalize the data using the extreme value method:
Calculate the gray correlation coefficient:
Obtain the gray correlation coefficient matrix:
where ρ is the resolution coefficient, which takes values between 0 and 1. A smaller resolution coefficient indicates greater differences between the correlation coefficients and stronger discriminatory power. Usually, ρ is set to 0.5.
Determine the correlation degree. Represent the correlation strength between the series using the average of the n correlation coefficients:
where \(\mathop \varepsilon \nolimits_{{\mathop o\nolimits_{i} }}\) represents the correlation degree between the i -th comparative sequence and the reference sequence.
Determine the weights and rank the correlation degrees. Normalize the correlation degrees to obtain the weight W i of each comparative sequence:
AHP is a methodology that categorizes the factors within a complex problem into interconnected and prioritized levels. This approach facilitates the process of making decisions based on multiple criteria. It is primarily used to determine the weighting coefficients for comprehensive evaluations 45 , 46 , 47 . The process is as follows.
Construction of a judgment matrix: a judgment matrix is constructed to compare the importance of different factors:
where A is the matrix of pairwise comparisons, W is the weight vector, and λ max is the maximum eigenvalue.
Calculation of weights: the weight vector W is determined using the sum-product method.
Consistency check:
where n is the number of elements, I c is the consistency index, I R is the random consistency index, I cR is the consistency ratio, and \(\lambda^{\prime } \max\) is the average of the maximum eigenvalues.
If I cR < 0.10, the consistency of the judgment matrix is considered acceptable.
EWM is an objective weighting approach that comprehensively examines the underlying patterns and informational value of unprocessed data. It can determine the uncertainty in variables through entropy values, where larger information content corresponds to smaller uncertainty and smaller entropy, and vice versa. The entropy weighting method is characterized by high accuracy and strong objectivity, and many scholars have applied it to oilfield production with good results 48 , 49 . The basic steps are as follows.
Normalize the data and calculate the information entropy:
where E i is the information entropy of the i th indicator, X ij is the value of the i th indicator on the j th sample, and N is the number of samples.
Calculate the weights:
where W i is the weight of the i th indicator, E i is the information entropy of the i th indicator, and M is the number of indicators.
Comprehensive weight coefficient
Weight coefficients can be used to classify and evaluate the reservoir quality effectively, and several methods are currently available to determine the weight coefficients. These include GRA, the expert evaluation method, Q clustering analysis and discriminant analysis, and factor analysis 50 , 51 , 52 . In this research, a comprehensive weight analysis methodology that integrated AHP and EWM was employed. The key advantage of this approach lies in its amalgamation of the subjective AHP analysis and the objective numerical analysis of EWM. This combination helps to mitigate the influence of subjective factors to a certain extent, thereby enhancing the reliability of the data.
where W iAHP is the weight coefficient obtained from the AHP method, and W iEWM is the weight coefficient obtained from the EWM method.
Results and discussion
Evaluation parameter selection.
Tight sandstone reservoirs are influenced by deposition, tectonics, and diagenesis.. These reservoirs demonstrate significant heterogeneity and an intricate distribution of micropore throats. The pore structure plays a crucial role in governing the storage and flow behaviour of the reservoir, where the different shape parameters of the pores govern the micropore structure of the rock formation 53 , 54 , 55 , 56 , 57 . Considering the characteristics above, this study aimed to provide a quantitative characterization of the reservoir by assessing three key aspects: the pore structure, the physical properties, and the pore shape parameters. Twelve parameters were selected to establish the relationship between the initial production capacity index and the pore structure and shape parameters. The actual initial production capacity of the oilfield was used as the indicator.
Sensitivity parameter selection for pore structure characteristics
The selected 52 samples were subjected to high-pressure mercury intrusion experiments using an Auto Pore IV 9530 automatic mercury porosimeter. The sorting coefficient varied between 1.5 and 2.74, with an average of 2.10. The coefficient of variation ranged between 13.94 and 17.32, with a mean value of 15.54. With an average value of 13.86 MPa, the median pressure varied between 10.5 and 18.79 MPa. The average displacement pressure was 1.23 MPa, ranging between 0.09 and 2.57 MPa. The median radius had a mean value of 0.09 μm and varied from 0.05 to 0.15 μm. With a mean value of 84.52%, the maximum mercury saturation varied from 62.77 to 93.76%. With an average of 34.90%, the mercury withdrawal efficiency varied between 16.7 and 46.6%. Overall, the pore structure of the reservoir in the study area was poor, with uneven sorting and poor connectivity among the pore throats, indicating strong heterogeneity. Correlation analysis was conducted on the initial production and mercury intrusion parameters (Fig. 3 ), and it was found that the correlation between the production capacity and permeability and porosity was the strongest, with correlation coefficients (R 2 ) of 0.91 and 0.75, respectively. This is mainly because porosity plays a crucial role in determining the size of the pore space within a reservoir, while permeability governs its flow capacity. In the context of tight sandstone reservoirs, the reservoir quality often depends on favourable pore permeability. The sorting coefficient and coefficient of variation provide insights into the uniformity of the distribution of the pore throat sizes. Higher values of these parameters indicate an improved pore structure and increased reservoir productivity. The median radius and median pressure indicate the pore permeability of the reservoir. A larger median radius and smaller median pressure indicate a larger pore space and stronger flow capacity, resulting in a larger oil production capacity. Therefore, the median radius positively correlates with production, while the median pressure is inversely correlated. The displacement pressure is inversely correlated with production (R 2 = 0.65). This is because displacement pressure refers to the capillary pressure corresponding to the largest connected pore, and a higher displacement pressure means a higher capillary pressure, making it more difficult for fluid to flow through. This indicates that tight oil has poor flow capacity in the reservoir and is more difficult to accumulate and extract. In conclusion, the initial production capacity is sensitive to the porosity, permeability, sorting coefficient, coefficient of variation, median pressure, median radius, and displacement pressure.
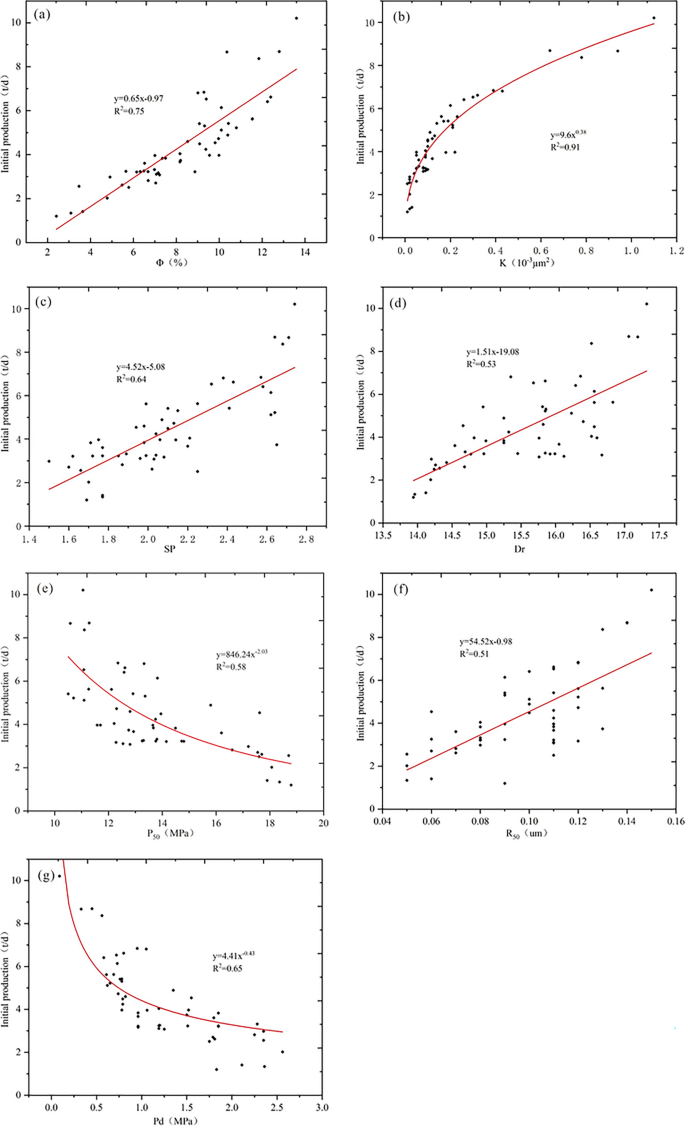
Relationship between initial production and porosity, permeability, selectivity coefficient, coefficient of variation, median pressure, median radius, and displacement pressure.
Selection of pore-shape-sensitive parameters
A total of 210 high-resolution SEM images were captured for the 52 samples. The rock core pores were identified and extracted using ImageJ, obtaining pore shape parameters such as the perimeter, circularity, major axis length, aspect ratio, and solidity (Fig. 4 , Table 2 ). The average values of the identified pore shape parameters for each sample were then calculated. It was found that the pore perimeters of the 52 samples varied between 40.3 and 486.2 μm, with a mean value of 250.5 μm. The circularity ranged between 0.11 and 0.96, with a mean value of 0.31. The major axis lengths of the circumscribed ellipses spanned from 42.52 to 221.19 μm, with an average of 111.67 μm. The aspect ratios ranged from 1.14 to 2.92, and the average value was 2.32. The solidity values ranged between 0.09 and 0.89, with an average of 0.67. In general, the pore shape parameters of the tight sandstone reservoirs exhibited a wide range of variation, with relatively large average perimeters, average major axis lengths of the circumscribed ellipses, aspect ratios, and solidity, and with small average circularity (Fig. 5 ). This indicates that the pore shapes in tight sandstone are diverse, predominantly irregular and elongated, with few circular pores. Pearson correlation analysis was conducted between the most sensitive parameters for the prioritized pore structure characteristics and the extracted pore shape parameters (Fig. 6 ). The absolute value of the correlation coefficient always lies between −1 and 1. In this context, a value closer to 1 indicates a stronger positive relationship between the two independent variables, a value closer to -1 indicates a stronger negative relationship between the independent variables, and a value closer to 0 indicates a weak relationship between the variables. A significant and strong correlation (R 2 > 0.5) observed between the different shape parameters of the pores and the mercury injection parameters. This suggests that the shape parameters of the pores play a crucial role in determining the pore structures of tight sandstone reservoirs. In general, the perimeter, circularity, and major axis length of the pores displayed a positive correlation with the porosity (Φ), permeability (K), sorting coefficient (S p ), coefficient of variation (D r ), and median radius (R50). Conversely, they exhibited a negative correlation with the median pressure (P 50 ) and displacement pressure (Pd). On the other hand, the aspect ratio and solidity of the pores were inversely proportional to the porosity, permeability, sorting coefficient, coefficient of variation, and median radius. However, they were positively correlated with the median pressure and displacement pressure. Among them, there was a strong positive correlation (R 2 = 0.914) between the perimeter and porosity and a relatively strong negative correlation (R 2 = –0.766) with the displacement pressure. A larger pore perimeter results in a greater contact area between the reservoir fluid and the solid, facilitating fluid infiltration and storage. Circularity was strongly positively correlated with permeability (R 2 = 0.927) and negatively correlated with the displacement pressure (R 2 = –0.604). This is because larger circularity indicates a closer approximation to circular pores, which typically exhibit a uniform distribution, resulting in improved connectivity and fluid flow. The major axis length was strongly positively correlated with the permeability and porosity because the major axis length of the circumscribed ellipses of pores affects the connectivity and fluid flow path within the pores. A larger major axis length indicates better connectivity between pores, resulting in a more direct fluid flow path and higher permeability. Moreover, a longer major axis length corresponds to a larger pore size and higher porosity. The aspect ratio exhibited a strong negative correlation with the permeability and selectivity coefficient (R 2 = –0.866, R 2 = –0.754, respectively) and a strong positive correlation with the displacement pressure (R 2 = 0.652). As the aspect ratio increases, the pores become narrower and more uneven, resulting in longer and narrower flow channels, making fluid flow more difficult. As a result, the displacement pressure increases, the selectivity coefficient decreases, and the permeability decreases. Solidity exhibited a strong negative correlation with permeability (R 2 = –0.862) and a positive correlation with the displacement pressure (R 2 = 0.574). As the solidity increases, the pore shape becomes more concave, and the roundness deteriorates, making fluid flow between the pores more difficult. In conclusion, it can be observed that the perimeter, circularity, major axis of the circumscribed ellipse, aspect ratio, and solidity of the pores are sensitive to various parameters of mercury intrusion.
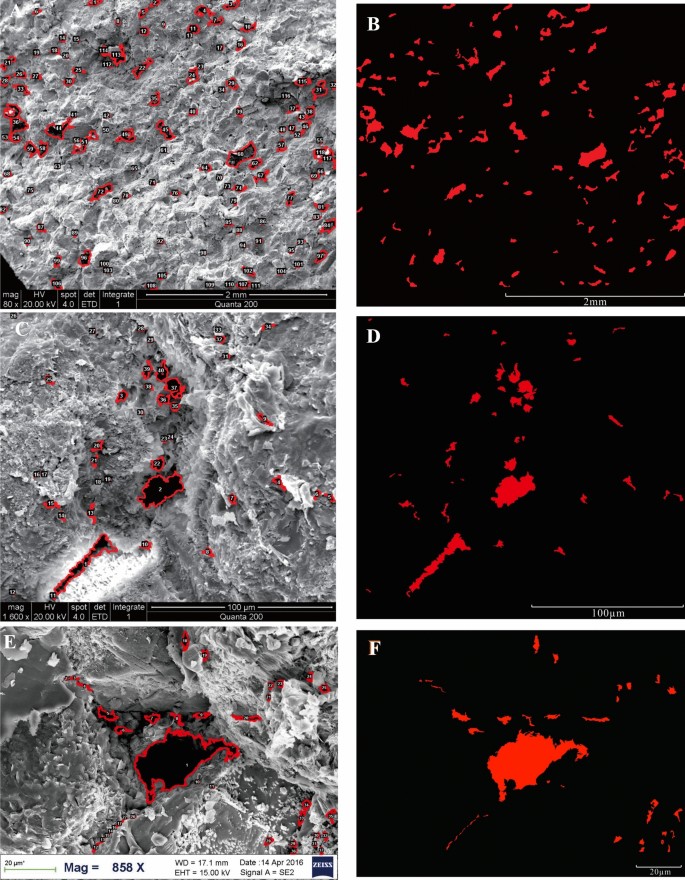
Visualization of pore extraction results for rock samples. ( A ) Pore identification (sample no. 1), ( B ) pore extraction (sample no. 1), ( C ) pore identification (sample no. 10), ( D ) pore extraction (sample no. 10), ( E ) pore identification (sample no. 25), ( F ) pore extraction (sample no. 25).
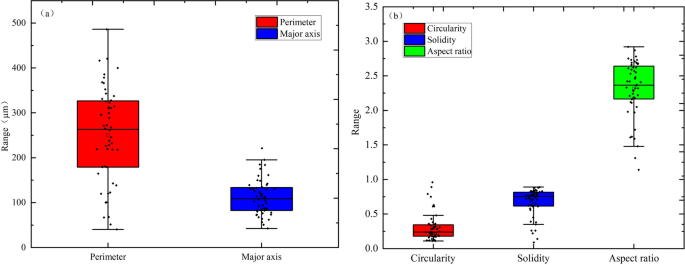
Distribution of pore shape parameters. ( a ) Distribution range of pore perimeter and major axis, ( b ) distribution range of pore circularity, solidity, and aspect ratio.
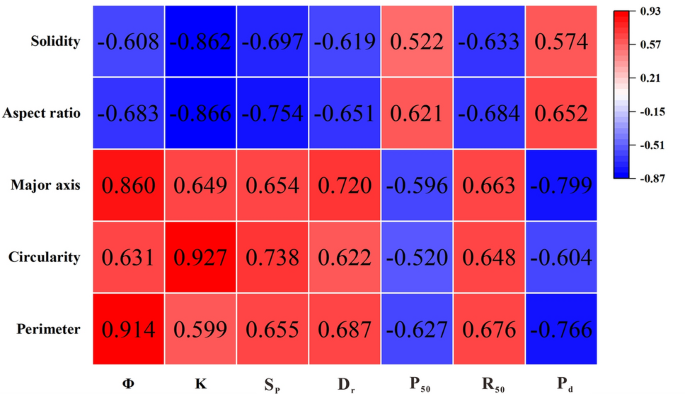
Correlations between pore structural parameters and pore shape parameters.
Reservoir classification evaluation
Quantitative classification prediction formula.
Based on the results of the GRA, AHP, and EWM, a comprehensive quantitative classification prediction formula was constructed using the superposition principle. This formula was then used to classify and evaluate tight sandstone reservoirs.
where Q is the productivity index, a i is the dimensionless weight coefficients of various parameters, b i,N is the dimensionless normalized parameters, and n is the number of parameters.
Determination of weight coefficients
In this study, the initial production rate directly reflecting the reservoir quality was taken as the fundamental sequence. Seven sensitive parameters, namely, the porosity, permeability, sorting coefficient, coefficient of variation, median pressure, median radius, and displacement pressure, were considered as sub-sequences. The principles and steps of GRA were employed to determine the weights of various parameters, thereby assessing the sensitivity of each factor to the initial production rate (Table 3 ). Combining the correlation degree between the sensitive parameters determined by the gray correlation method and the initial productivity. Then, the parameters were compared in pairs, and values were assigned based on the 9-point scale method. The judgment matrix was obtained by pairwise comparisons of the seven sensitive parameters (Table 4 ). Subsequently, the weight coefficients were determined using the weighted product method within the AHP (Table 5 ). Formula ( 14 ) shows that the judgment matrix I cR = 0.093 is less than 0.1, meeting the consistency requirements. Subsequently, the EWM analysis method was employed to conduct an objective analysis of each sensitive parameter, resulting in objective weight indices. The comprehensive weight coefficients were calculated using Eq. ( 17 ) (Table 5 ). The formula for the initial productivity and the mercury intrusion sensitivity parameter can be obtained as follows:
where Φ N is the normalized porosity, K N is the normalized permeability, S P,N is the normalized sorting coefficient, Dr, N is the normalized coefficient of variation, P 50,N is the normalized median pressure, R 50,N is the normalized median radius, and P d,N is the normalized displacement pressure.
Then, using the mercury intrusion parameter as the fundamental sequence, five sensitive parameters related to the pore shape, namely, the perimeter, circularity, major axis length, aspect ratio, and solidity, were considered sub-sequences. The correlation between the mercury intrusion parameters and the pore-shape-sensitive parameters was determined using GRA. The comprehensive weight coefficients for each mercury intrusion parameter were calculated using a combination of the AHP and the EWM (Table 6 ). Based on these weight coefficients, the correlation formulas between each mercury intrusion parameter and the pore shape parameters were obtained as follows:
Combined with Formula ( 19 ), the relationship between the initial productivity and pore shape parameters can be obtained:
where P N is the normalized perimeter, C N is the normalized circularity, M N is the normalized major axis, A N is the normalized aspect ratio, and S N is the normalized solidity.
Classification scheme and feature evaluation
Based on the indices Q1, which relate initial productivity to high-pressure mercury intrusion sensitivity parameters, and Q2, which relate initial productivity to pore shape parameters, a classification and evaluation scheme for the Chang 8 tight sandstone reservoir have been determined. As depicted in Fig. 7 , Q1 for type III reservoirs ranges from 0.1 to 0.31, and Q2 ranges between 0.3 and 0.44. For type II reservoirs, Q1 ranges from 0.32 to 0.47, and Q2 ranges from 0.44 to 0.56. For type I reservoirs, Q1 ≥ 0.5 and Q2 ≥ 0.5. Moreover, type I reservoirs can be further divided into type I 1 , comprising high-yield reservoirs, and type I 2 , comprising high-quality reservoirs, with Q1 > 0.7 and Q2 > 0.57 indicating type I 1 high-yield reservoirs. Type I reservoirs are considered optimal for the Chang 8 formation, with 15 out of 52 samples belonging to this type, accounting for 28.8%. The characteristics associated with this type of reservoir include favourable pore permeability, featuring an average porosity of 11.1% and permeability of 0.4 × 10 –3 µm 2 . Additionally, these reservoirs possess a low displacement pressure of 0.62 MPa, a low median pressure of 11.79 MPa, and a relatively high median radius of 0.12 µm. The reservoir exhibits good pore throat selectivity, characterized by a large sorting coefficient (2.5) and variation coefficient (16.43). The average pore perimeter of the reservoir is relatively long (360.30 µm), with good circularity (0.50) and a small aspect ratio (1.92). This indicates that the pore shape is more regular and almost circular. Generally, type II displays moderate petrophysical characteristics, characterized by an average porosity of 8.43% and permeability of 0.1 × 10 –3 µm 2 . Within this classification, 19 samples contribute to 36.54% of the dataset. Compared to type I, this reservoir type has a somewhat higher average displacement pressure and median pressure (1.11 MPa and 13.48 MPa, respectively). The median radius is lower (0.10 µm), and the average sorting coefficient and coefficient of variation are 2.41 and 16.18, respectively, indicating moderate sorting. The average pore perimeter of this reservoir type is smaller than that of type I (261.61 µm), with smaller circularity (0.26) and a larger aspect ratio (2.41). Compared to type I, the pores of type II reservoirs exhibit irregular and more elongated shapes. Type III exhibits poorer petrophysical properties, with an average permeability of 0.06 × 10 –3 μm 2 and porosity of 5.7%, significantly lower than those of type I and type II. There were 18 samples belonging to this type, accounting for 34.62%. This reservoir type has an average displacement pressure of 1.89 MPa and a median pressure of 16.1 MPa, greater than type II. The median radius is the smallest (0.07 µm). The average sorting coefficient and coefficient of variation are 1.81 and 14.7, respectively, indicating poor pore throat sorting. The average pore perimeter is the smallest (147.37 µm), with the poorest circularity (0.19) and the largest aspect ratio (2.56). This indicates that the pores of type III reservoirs are more elongated and slender, making them unfavorable for fluid flow and leading to poor reservoir permeability. In summary, it can be observed that as the reservoir quality deteriorates, the pore structure becomes increasingly worse, and the pore shapes become more complex and variable.
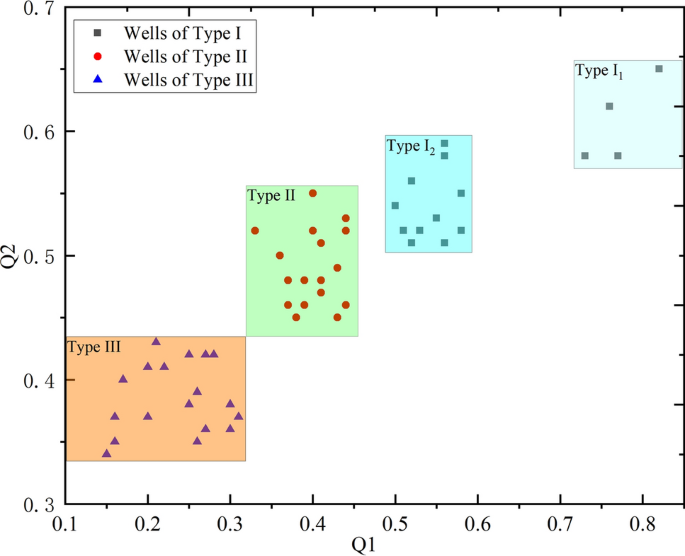
Comprehensive quantitative classification prediction model for the research area of the Chang 8 reservoir.
According to the distribution maps of the well locations and sedimentary microfacies (Figs. 1 c, 8 ), it is observed that type I reservoir wells are mostly found in the northwest of the research region, within the subaqueous distributary channels, exhibiting a zigzag pattern. Most type II reservoir wells are located in the study area's centre, mainly within the middle portions of the subaqueous distributary channel's lateral sand bodies. On the other hand, the relatively poor type III reservoir wells are scattered around the type II reservoirs, with most of them located in the marginal areas adjacent to the interdistributary bay and the edge of the channel’s lateral sand bodies. Therefore, in practical exploration and development, the high-quality reservoirs (type I) in the study area's northwest part should be prioritised.
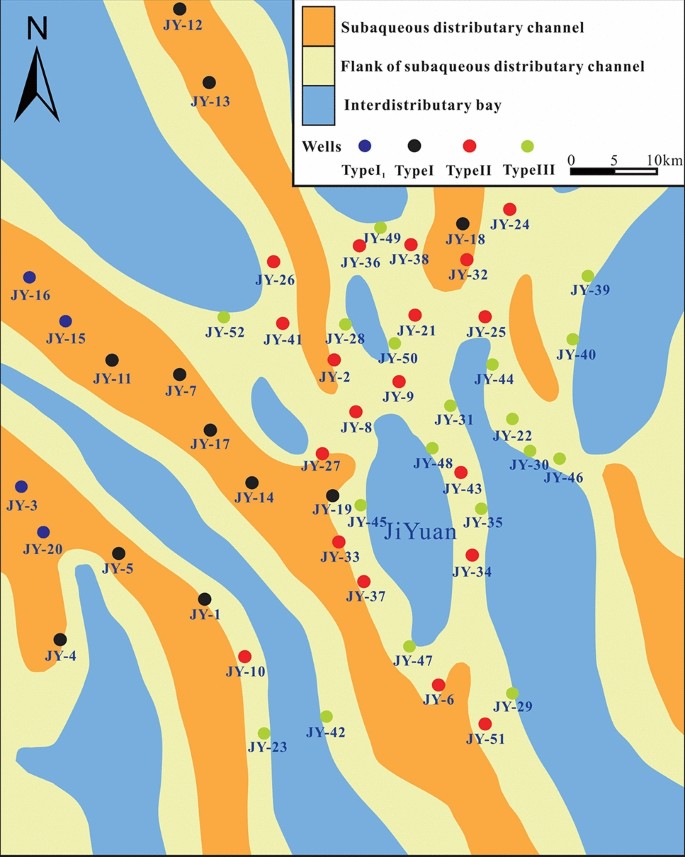
Planar distribution map of comprehensive quantitative classification for the research area of the Chang 8 reservoir.
Additionally, the main reason for the high productivity of type I 1 reservoirs is the higher content of dissolved pores in type I reservoirs. According to Table 7 and Fig. 9 , samples 3, 15, 16, and 20 from type I reservoirs exhibit significant development of feldspar dissolution pores, intergranular pores, and a small number of rock particles that dissolve pores. The average absolute contents of feldspar dissolution and intergranular pores are 1.2% and 5.15%, respectively. The average face rate is 0.8%, higher than the other samples. The greater the development of feldspar dissolution and intergranular pores, the larger the flow channels and storage space they provide, thus improving the reservoir’s porosity and permeability, resulting in high-productivity reservoirs. The pore shape parameters of samples 3, 15, 16, and 20 were compared with those of the other samples (Table 2 ). It was found that these four samples have longer pore perimeters and major axes, larger shape factor (roundness) coefficients, and relatively smaller aspect ratios and concavity. This indicates that high-productivity reservoirs (type I 1 ) have larger pore perimeters, an increased contact area between the pores and reservoir fluids, higher pore circularity, and more circular shapes favourable for fluid flow and storage. Furthermore, as shown in Fig. 8 , the four high-productivity wells (JY-3, JY-15, JY-16, JY-20) are all located on the main channel of the subaqueous distributary channel. Therefore, from a macro perspective, thicker sand bodies may be another reason for their high productivity.
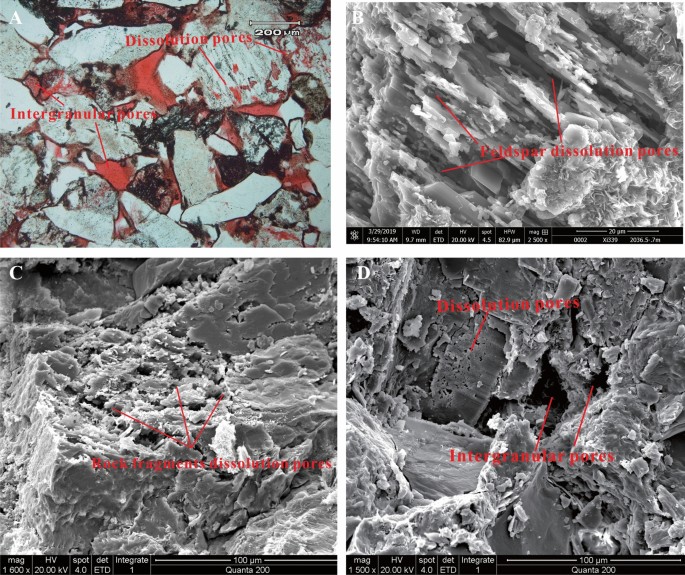
Porosity structure of type I 1 reservoir. ( A ) Intergranular pores, developed dissolution pores (sample no. 3), ( B ) feldspar dissolution pores (sample no. 20), ( C ) rock fragment dissolution pores (sample no. 15), ( D ) intergranular pores, locally developed dissolution pores (sample no. 16).
Model validation
In order to verify the model, 15 coring wells in Jiyuan Chang 8 reservoir were selected. High-pressure mercury intrusion tests, scanning electron microscopy, and thin-section casting experiments were conducted on corresponding samples to extract the pore shape parameters. Next, the comprehensive indices Q1 and Q2, for reservoir categorization, were determined using the GRA, the AHP, and the EWM. Finally, the accuracy of the classification results was compared with that of the existing oil test parameters. As shown in Fig. 10 , three wells were classified as type I reservoirs, with an average initial yield of 5.73 t/d. Six wells were classified as type II reservoirs, with an average initial yield lower than type I at 3.52 t/d. One well was misclassified, deviating from the expected value. Five wells were classified as type III reservoirs, with the lowest average initial yield of 1.32 t/d. The quantitative evaluation of the comprehensive parameters matched the actual production capacity results, demonstrating a high matching rate of 93.3%. Compared to conventional models by other scholars for tight sandstone reservoirs, this model establishes a direct connection between actual oilfield production data, microscale pore shape parameters, and pore structure parameters, leading to quantitative reservoir classification evaluation 58 , 59 , 60 . It demonstrates higher and more stable classification accuracy.
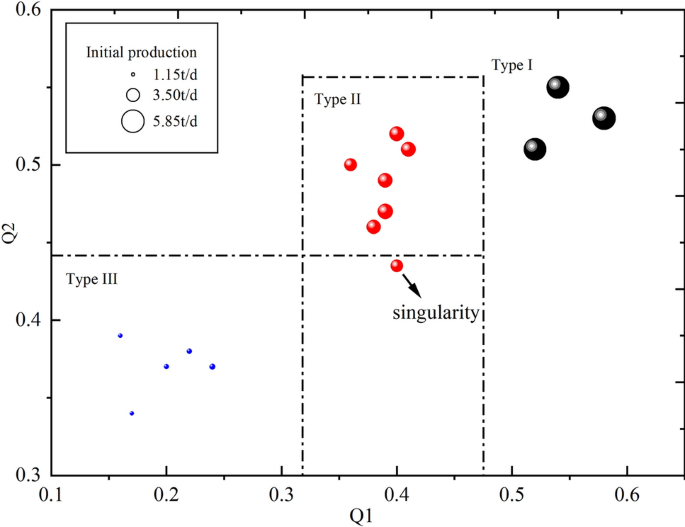
Comparative analysis of the integrated quantitative classification prediction for the Chang 8 reservoir.
Conclusions
Tight sandstone reservoirs display significant heterogeneity and intricate microscopic pore structures, which impact the accuracy of reservoir assessment. This study employed scanning electron microscopy, thin section analysis, and high-pressure mercury intrusion data as samples. It utilized image recognition technology and machine learning methods to develop a novel classification and evaluation system for tight sandstone reservoirs based on microscopic pore structures. This method utilizes minimal experimental data, is cost-effective, demonstrates relatively high model accuracy, and is particularly suitable for tight sandstone reservoirs. The research conclusions are as follows:
By analyzing high pressure mercury parameters, scanning electron microscopy images, and thin sections of the study area in the Chang 8 reservoir, a comprehensive quantitative classification prediction model for tight sandstone reservoirs was established. The model was constructed using twelve sensitive parameters: porosity, permeability, sorting coefficient, coefficient of variation, median pressure, median radius, displacement pressure, pore perimeter, circularity, major axis length, aspect ratio, and solidity, all extracted using image recognition technology.
The case study based on the comprehensive quantitative classification prediction model showed that Q1 ≥ 0.5 and Q2 ≥ 0.5 corresponded to type I reservoirs, while Q1 > 0.7 and Q2 > 0.57 corresponded to type I 1 high-yield reservoirs. When 0.32 < Q1 < 0.47 and 0.44 < Q2 < 0.56, a type II reservoir was identified. When 0.1 < Q1 < 0.32 and 0.3 < Q2 < 0.44, a type III reservoir was identified. Additionally, the presence of high-content dissolution pores, intergranular pores, and larger pore perimeters, as well as higher pore circularity, were the main factors contributing to high-yield reservoirs (type I 1 ). The model was validated, achieving an overall accuracy of 93.3%, which indicates its effectiveness in predicting the classification and evaluation of tight reservoirs.
Reservoir quality is influenced by the pore structure characteristics and shape parameters. In tight sandstone reservoirs, the productivity is positively correlated with the porosity, permeability, sorting coefficient, coefficient of variation, and median radius, but negatively correlated with the median pressure and displacement pressure. The perimeter, circularity, and major axis length of the pores are positively correlated with the porosity, permeability, sorting coefficient, coefficient of variation, and median radius, but negatively correlated with the median pressure and displacement pressure.
Type I reservoir wells were primarily found in the northwest of the research region, within the subaqueous distributary channels, exhibiting a zigzag pattern. The majority of type II reservoir wells were located in the study area's center, mostly within the middle portions of the subaqueous distributary channel’s lateral sand bodies. In contrast, the relatively inferior type III reservoir wells were dispersed among the type II reservoirs, primarily situated in the marginal zones bordering the interdistributary bay and the periphery of the channel’s lateral sand bodies. Therefore, in terms of practical exploration and development, priority should be given to the superior reservoirs (type I) in the northwestern sector of the research region.
The evaluation results of the quantitative classification of tight sandstone reservoirs using machine learning are generally consistent with previous multiparameter conventional evaluation studies. However, this approach effectively integrates macroscopic and microscopic parameters, resulting in higher model accuracy, easier operation, and lower costs. It is particularly suitable for large-scale quality assessments of tight sandstone reservoirs, offering essential guidance for further exploration in the study area and other similar reservoirs.
Data availability
The data that support the findings of this study are available from the corresponding author upon reasonable request.
Abbreviations
Analytic hierarchy process
Grey relational analysis
Entropy weight method
X-ray diffraction
Scanning electron microscopy
Fine-grained lithic feldspar sandstone
Fine-grained feldspar lithic sandstone
Fine-grained feldspar sandstone
Grey correlation analysis, clustering ensemble, and the Kriging model combined with macro geological parameters
Ledingham, & Glen, W. Santigo, pool. California: Geological note. AAPG Bull. 31 (11), 2063–2067 (1947).
Google Scholar
Wang, Q. R., Tao, S. Z. & Guan, P. Progress in research and exploration & development of shale oil in continental basins in China. Nat. Gas Geosci. 31 (3), 417–427 (2020).
Zou, C. N. et al. Geological concepts, characteristics, resource potential and key techniques of unconventional hydro-carbon: On unconventional petroleum geology. Pet. Explor. Dev. 40 (4), 385–399 (2013).
Article Google Scholar
Zou, C. N. et al. Progress in China’s unconventional oil & gas exploration and development and theoretical technologies. Acta Geol. Sin. 89 (6), 979–1007 (2015).
CAS Google Scholar
Zhou, N. et al. Limits and grading evaluation criteria of tight oil reservoirs in typical continental basins of China. Petrol. Explor. Dev. 48 (05), 1089–1100 (2021).
Zhao, W. et al. Types and resource potential of continental shale oil in China and its boundary with tight oil. Petrol. Explor. Dev. 47 (01), 1–11 (2020).
Article CAS Google Scholar
Sun, L. et al. Development characteristics and orientation of tight oil and gas in China. Petrol. Explor. Dev. 46 (06), 1073–1087 (2019).
Xiang, F. et al. Classification evaluation criteria and exploration potential of tight oil resources in key basins of China. J. Nat. Gas Geosci. 4 (6), 309–319 (2019).
Gao, X., Chen, J., Xu, R., Zhen, Z., Zeng, X., Chen, X. & Cui, L. Research progress and prospect of the materials of bipolar plates for proton exchange membrane fuel cells (PEMFCs)[J]. International Journal of Hydrogen Energy . 50 , 711–743 (2024).
Article ADS CAS Google Scholar
Wang, J., Wu, S., Li, Q. & Guo, Q. An investigation into pore structure fractal characteristics in tight oil reservoirs: A case study of the Triassic tight sandstone with ultra-low permeability in the Ordos Basin, China. Arab. J. Geosci. 13 (18), 961 (2020).
Gao, H., Cao, J., Wang, C., He, M., Dou, L., Huang, X. & Li, T. Comprehensive characterization of pore and throat system for tight sandstone reservoirs and associated permeability determination method using SEM, rate controlled mercury and high pressure mercury. J. Petrol. Sci. Eng . 174 (2018).
Gao, H. et al. Effect of pressure pulse stimulation on imbibition displacement within a tight sandstone reservoir with local variations in porosity. Geoenergy Sci. Eng. 226 , 211811 (2023).
Wang, C., Gao, H., Gao, Y. & Fan, H. Influence of pressure on spontaneous imbibition in tight sandstone reservoirs. Energy Fuels 34 (8), 9275–9282 (2020).
Wang, C., Li, T., Gao, H., Zhao, J. & Gao, Y. Quantitative study on the blockage degree of pores due to asphaltene precipitation in low-permeability reservoirs with NMR technique. J. Petrol. Sci. Eng. 163 , 703–711 (2018).
Gao, H. et al. Effects of pore structure and salinity on the imbibition of shale samples using physical simulation and NMR technique: A case from Chang 7 shale, Ordos basin. Simulation. 97 (2), 167–173 (2021).
Wei, H. et al. Classification of tight sandstone reservoirs based on the nuclear magnetic resonance T 2 distribution: A case study on the Shaximiao Formation in Central Sichuan, China. Energy Fuels 36 , 10803–10812 (2022).
Xu, J. et al. Characteristics and controlling factors of tight gas sandstones from the Upper Shanxi and Lower Shihezi Formations in the Northern Sulige Area, Ordos Basin, China. Energy Fuels 37 (20), 15712–15729 (2023).
Wu, X. et al. A novel evaluation method of dolomite reservoir using electrical image logs: The Cambrian dolomites in Tarim Basin, China. Geoenergy Sci. Eng. 233 , 212509 (2024).
Zhang, Q. et al. Comprehensive evaluation and reservoir classification in the Quan 3 member of the Cretaceous Quantou Formation in the Fuxin Uplift, Songliao Basin. Front. Earth Sci. 10 , 1016924 (2022).
Article ADS Google Scholar
Talib, M., Durrani, M. Z. A., Palekar, A. H., Sarosh, B. & Rahman, S. A. Quantitative characterization of unconventional (tight) hydrocarbon reservoir by integrating rock physics analysis and seismic inversion: A case study from the Lower Indus Basin of Pakistan. Acta Geophys. 70 (6), 2715–2731 (2022).
Fang, X., Zhu, G., Yang, Y., Li, F. & Feng, H. Quantitative method of classification and discrimination of a porous carbonate reservoir integrating k-means clustering and Bayesian theory. Acta Geol. Sin. (Beijing) 97 (1), 176–189 (2023).
Li, Y. et al. Microscopic pore-throat grading evaluation in a tight oil reservoir using machine learning: A case study of the Fuyu oil layer in Bayanchagan area, Songliao Basin central depression. Earth Sci. Inform. 14 (2), 601–617 (2021).
Wang, Z. et al. Quantitative evaluation of unconsolidated sandstone heavy oil reservoirs based on machine learning. Geol. J. (Chichester, England). 58 (6), 2321–2341 (2023).
Fan, J., Shi, J., Wan, X., Xie, Q. & Wang, C. Classification evaluation method for Chang 7 oil group of Yanchang formation in Ordos Basin. J. Pet. Explor. Prod. Te. 12 , 825–834 (2021).
Niu, D. et al. Multi-scale classification and evaluation of shale reservoirs and “sweet spot” prediction of the second and third members of the Qingshankou Formation in the Songliao Basin based on machine learning. J. Petrol Sci. Eng. 216 , 110678 (2022).
Li, C. et al. Oil charging pore throat threshold and accumulation effectiveness of tight sandstone reservoir using the physical simulation experiments combined with NMR. J. Petrol. Sci. Eng. 208 , 109–338 (2022).
Li, S. et al. The dissolution characteristics of the Chang 8 tight reservoir and its quantitative influence on porosity in the Jiyuan area, Ordos Basin, China. J. Nat. Gas Geosci. 3 (2), 95–108 (2018).
Song, X. et al. Analysis of the influence of micro-pore structure on oil occurrence using nano-CT scanning and nuclear magnetic resonance technology: An example from Chang 8 tight sandstone reservoir, Jiyuan, Ordos Basin. Processes 11 , 11274 (2023).
Tong, Q. et al. Research on sand body architecture at the intersection of a bidirectional sedimentary system in the Jiyuan area of Ordos Basin. Sci. Rep. 13 , 12261 (2023).
Fu, S. et al. Accurate characterization of full pore size distribution of tight sandstones by low-temperature nitrogen gas adsorption and high-pressure mercury intrusion combination method. Energy Sci. Eng. 9 (1), 80–100 (2021).
Li, P. et al. Occurrence characteristics and main controlling factors of movable fluids in Chang 81 reservoir, Maling Oilfield, Ordos Basin, China. J. Petrol. Explor. Prod. Technol. 9 (1), 17–29 (2018).
Li, C., Chen, G., Li, X., Zhou, Q. & Sun, Z. The occurrence of tight oil in the Chang 8 lacustrine sandstone of the Huaqing area, Ordos Basin, China: Insights into the content of adsorbed oil and its controlling factors. J. Nat. Gas Geosci. 7 (1), 27–37 (2022).
Gong, Y. & Liu, K. Pore throat size distribution and oiliness of tight sands-A case study of the Southern Songliao Basin, China. J. Petrol. Sci. Eng. 184 , 106508 (2020).
Liu, Y. et al. A novel experimental investigation on the occurrence state of fluids in microscale pores of tight reservoirs. J. Petrol. Sci. Eng. 196 , 107656 (2021).
Sandhya, N. & Baviskar, A. A quick & automated method for measuring. Am. Biol. Teach. 73 (9), 554–556 (2011).
Curtis, M. E., Cardott, B. J. & Sondergeld, C. H. Development to for organic porosity in the Woodford shale with increasing thermal maturity. Int. J. Coal Geol. 26 (31), 26–30 (2012).
Keller, L. M., Schuetz, P. & Erni, R. Characterization of multi-scale micro-structural features in opalinus clay. Microporous Mesoporous Mater. 83 , 84–90 (2013).
Jin, L. et al. Evolution of porosity and geochemistry in Mar cell us formation black shale during weathering. Chem. Geol. 50 , 51–56 (2013).
Rine, J. M. et al. Comparison of porosity distribution with in selected north American shale units by SEM examination of argon-ion-milled samples. Electron Microsc. Shale Hydrocarbon Reserv. AAPG Memoir. 102 , 137–152 (2013).
Zhao, J. Y. et al. A quantitative evaluation for well pattern adaptability in ultra-low permeability oil reservoirs: A case study of Triassic Chang 6 and Chang 8 reservoirs in Ordos Basin. Pet. Explor. Dev. 45 (3), 482–488 (2018).
Dong, Q., Dai Yin, Y. & Ya Zhou, Z. Fine classification of ultra-low permeability reservoirs around the Placanticline of Da Qing oilfield (PR of China). J. Petrol. Sci. Eng. 174 , 1042–1052 (2019).
Gao, Y. et al. Application of an analytic hierarchy process to hydro-carbon accumulation coefficient estimation. Petrol. Sci. 7 (3), 337–346 (2010).
Liu, Y. et al. A reservoir quality evaluation approach for tight sandstone reservoirs based on the gray correlation algorithm: A case study of the Chang 6 layer in the W area of the as oilfield, Ordos Basin. Energy Explor. Exploit. 39 (4), 1027–1056 (2021).
Shi, B., Chang, X., Yin, W., Li, Y. & Mao, L. Quantitative evaluation model for tight sandstone reservoirs based on statistical methods—A case study of the Triassic Chang 8 tight sandstones, Zhenjing area, Ordos Basin, China. J. Petrol. Sci. Eng. 173 , 601–616 (2019).
Liu, B. The analytic hierarchy process for the reservoir evaluation in Chaoyanggou oilfield. Adv. Petrol. Explor. Dev. 6 , 46–50 (2014).
Shang, Y. Z. Application of analytical hierarchy process in the low-grade oil reservoirs evaluation. Daqing Petrol. Geol. Oilfield Dev. 33 , 55–59 (2014).
Xi, Y. et al. Application of analytic hierarchy process in mineral prospecting prediction based on an integrated geology—aerogeophysics—geochemistry model. Minerals 13 (7), 978 (2023).
Lai, F. et al. Crushability evaluation of shale gas reservoir based on analytic hierarchy process. Spec. Oil Gas Reserv. 25 (3), 154–159 (2018).
Elhaj, M.A., Imtiaz, S. A., Naterer, G. F. & Zendehboudi, S. Production optimization of hydrocarbon reservoirs by entropy generation minimization. J. Nat. Gas Sci. Eng . 83 , 103538 (2020).
Szabo, N. P. et al. Cluster analysis of core measurements using heterogeneous data sources: An application to complex Miocene reservoirs. J. Petrol. Sci. Eng. 178 , 575–585 (2019).
Oliveira, G. P., Santos, M. D. & Roque, W. L. Constrained clustering approaches to identify hydraulic flow units in petroleum reservoirs. J. Petrol. Sci. Eng. 186 , 106732 (2020).
Jia, A., Wei, Y. & Jin, Y. Progress in key technologies for evaluating marine shale gas development in China. Petrol. Explor. Dev. 43 (6), 1035–1042 (2016).
Xiao, L., Bi, L., Yi, T., Lei, Y. & Wei, Q. Pore structure characteristics and influencing factors of tight reservoirs controlled by different provenance systems: A case study of the Chang 7 members in Heshui and Xin’anbian of the Ordos Basin. Energies 16 , 34108 (2023).
Dong, J. et al. Pore structure and fractal characteristics of tight sandstone: A case study for Huagang Formation in the Xihu Sag, East China Sea Basin, China. Energies 16 , 20134 (2023).
Gao, J. et al. Study on the coupling law between pore-scale fluid flow capacity and pore-throat configuration in tight sandstone reservoirs. Geofluids 2023 (1), 1693773 (2023).
Zhang, R. et al. Microscopic pore structures and their controlling factors of the lower carboniferous Luzhai Shale in Guizhong depression, China. Geofluids 2023 , 8890709 (2023).
Du, M. et al. Study on the quantitative characterization and heterogeneity of pore structure in deep ultra-high pressure tight glutenite reservoirs. Minerals 13 , 6015 (2023).
Wu, B. H. et al. Integrated classification method of tight sandstone reservoir based on principal component analysis-simulated annealing genetic algorithm-fuzzy cluster means. Petrol. Sci. 20 (5), 2747–2758 (2023).
Lu, X., Xing, X., Hu, K. & Zhou, B. Classification and evaluation of tight sandstone reservoirs based on MK-SVM. Processes. 11 (9), 2678 (2023).
Qiu, X. et al. Quantitative evaluation of reservoir quality of tight oil sandstones in Chang 7 member of Ordos Basin. Front. Earth Sci. 10 , 1046489 (2023).
Download references
Acknowledgements
This research was sponsored by Natural Science Basic Research Plan in Shaanxi Province of China (Grant No. 2017JM4013; Grant No. 2020JQ-798).
Author information
Authors and affiliations.
State Key Laboratory of Continental Dynamics, Northwest University, Xi’an, 710069, China
Xinglei Song, Congjun Feng, Xinhui Pan & Yanlong Ge
Department of Geology, Northwest University, No. 229, Taibai North Road, Xi’an, 710069, Shaanxi, China
School of Petroleum Engineering, Xi’an Shiyou University, Xi’an, 710065, China
Engineering Research Center of Development and Management for Low to Ultra-Low Permeability Oil & Gas Reservoirs in West China, Ministry of Education, Xi’an, 710065, China
Xi’an Key Laboratory of Tight Oil (Shale Oil) Development, Xi’an, 710065, China
PetroChina Research Institute of Petroleum Exploration & Development, Beijing, 100083, People’s Republic of China
School of Petroleum Engineering and Environmental Engineering, Yan’an University, Yan’an, 716000, China
You can also search for this author in PubMed Google Scholar
Contributions
Xinglei Song: Investigation, Formal analysis, Conceptualization, Data Curation, Writing-Original Draft; Congjun Feng: Writing-Review & Editing, Supervision, Funding acquisition,Methodology; Teng Li: Investigation, Resources, Data Curation, Writing-Review & Editing; Qin Zhang: Investigation, Resources, Data Curation; Xinhui Pan: Supervision, Project administration; Mengsi Sun: Supervision, Writing-Review & Editing, Project administration; Yanlong Ge: Investigation, Resources, Data Curation. All authors have read and agreed to the published version of the manuscript.
Corresponding author
Correspondence to Congjun Feng .
Ethics declarations
Competing interests.
The authors declare no competing interests.
Additional information
Publisher's note.
Springer Nature remains neutral with regard to jurisdictional claims in published maps and institutional affiliations.
Rights and permissions
Open Access This article is licensed under a Creative Commons Attribution-NonCommercial-NoDerivatives 4.0 International License, which permits any non-commercial use, sharing, distribution and reproduction in any medium or format, as long as you give appropriate credit to the original author(s) and the source, provide a link to the Creative Commons licence, and indicate if you modified the licensed material. You do not have permission under this licence to share adapted material derived from this article or parts of it. The images or other third party material in this article are included in the article’s Creative Commons licence, unless indicated otherwise in a credit line to the material. If material is not included in the article’s Creative Commons licence and your intended use is not permitted by statutory regulation or exceeds the permitted use, you will need to obtain permission directly from the copyright holder. To view a copy of this licence, visit http://creativecommons.org/licenses/by-nc-nd/4.0/ .
Reprints and permissions
About this article
Cite this article.
Song, X., Feng, C., Li, T. et al. Quantitative classification evaluation model for tight sandstone reservoirs based on machine learning. Sci Rep 14 , 20712 (2024). https://doi.org/10.1038/s41598-024-71351-0
Download citation
Received : 22 April 2024
Accepted : 27 August 2024
Published : 05 September 2024
DOI : https://doi.org/10.1038/s41598-024-71351-0
Share this article
Anyone you share the following link with will be able to read this content:
Sorry, a shareable link is not currently available for this article.
Provided by the Springer Nature SharedIt content-sharing initiative
- Tight sandstone
- Pore structure
- Quantitative evaluation
- High-pressure mercury injection
- Image recognition
- Machine learning
By submitting a comment you agree to abide by our Terms and Community Guidelines . If you find something abusive or that does not comply with our terms or guidelines please flag it as inappropriate.
Quick links
- Explore articles by subject
- Guide to authors
- Editorial policies
Sign up for the Nature Briefing newsletter — what matters in science, free to your inbox daily.


An official website of the United States government.
Here’s how you know
The .gov means it’s official. Federal government websites often end in .gov or .mil. Before sharing sensitive information, make sure you’re on a federal government site.
The site is secure. The https:// ensures that you are connecting to the official website and that any information you provide is encrypted and transmitted securely.
- American Job Centers
- Apprenticeship
- Demonstration Grants
- Farmworkers
- Federal Bonding Program
- Foreign Labor Certification
- Indians and Native Americans
- Job Seekers
- Layoffs and Rapid Response
- National Dislocated Worker Grants
- Older Workers
- Skills Training Grants
- Trade Adjustment Assistance
- Unemployment Insurance
- Workforce Innovation and Opportunity Act (WIOA)
- WIOA Adult Program
- Advisories and Directives
- Regulations
- Labor Surplus Area
- Performance
- Recovery-Ready Workplace Resource Hub
- Research and Evaluation
- ETA News Releases
- Regional Offices
- Freedom of Information Act
- Office of Apprenticeship
- Office of Foreign Labor Certification
- Office of Grants Management
- Office of Job Corps
- Office of Unemployment Insurance (1-877-S-2JOBS)
Workforce Development in Rural Areas
Publication info, country, state or territory, description.
This report examines the provision of workforce development services in rural areas and the challenges rural One-Stop systems face in providing those services. Key research questions included understanding: rural One-Stop service networks of access points and their challenges, other means of providing workforce development services to rural residents, rural One-Stop partnerships and the role of different partners, cost and support for One-Stop centers, competition for workforce development services and availability of workforce development providers, and changes over time to workforce service delivery systems and services. -->
To explore the key research topics, Social Policy Research Associates designed a mixed-methods study that involved the following four principal tasks that were carried out from August 2004 to May 2005: (1) study design and literature review, (2) a quantitative and geospatial analysis of local office and One-Stop center distribution, (3) qualitative site visits to five rural local workforce investment areas in five states, and (4) analysis and reporting.
The findings of this report are primarily based on the five qualitative site visits conducted in five states and rural areas. These states and the local areas were chosen in consultation with the U.S. Department of Labor’s Employment and Training Administration ( --> ETA ), and were aimed at including a diverse group of sites in terms of degree of rurality, ETA regions, and number of workforce development access points. Site visitors spent four days at each of these sites, interviewing a variety of local respondents and visiting different types of access points.
- Open access
- Published: 04 September 2024
Detection and molecular characterization of major enteric pathogens in calves in central Ethiopia
- Julia Bergholm 1 ,
- Tesfaye Sisay Tessema 2 ,
- Anne-Lie Blomström 1 &
- Mikael Berg 1
BMC Veterinary Research volume 20 , Article number: 389 ( 2024 ) Cite this article
Metrics details
Calf diarrhea is a major cause of morbidity and mortality in the livestock sector worldwide and it can be caused by multiple infectious agents. In Ethiopia, cattle are the most economically important species within the livestock sector, but at the same time the young animals suffer from high rates of morbidity and mortality due to calf diarrhea. However, studies including both screening and molecular characterization of bovine enteric pathogens are lacking. Therefore, we aimed to both detect and molecularly characterize four of the major enteric pathogens in calf diarrhea, Enterotoxigenic Escherichia coli (E. coli K99 +), Cryptosporidium spp . , rotavirus A (RVA), and bovine coronavirus (BCoV) in calves from central Ethiopia. Diarrheic and non-diarrheic calves were included in the study and fecal samples were analyzed with antigen-ELISA and quantitative real-time PCR (qPCR). Positive samples were further characterized by genotyping PCRs.
All four pathogens were detected in both diarrheic and non-diarrheic calves using qPCR and further characterization showed the presence of three Cryptosporidium species, C. andersoni , C. bovis and C. ryanae . Furthermore, genotyping of RVA-positive samples found a common bovine genotype G10P[11], as well as a more unusual G-type, G24. To our knowledge this is the first detection of the G24 RVA genotype in Ethiopia as well as in Africa. Lastly, investigation of the spike gene revealed two distinct BCoV strains, one classical BCoV strain and one bovine-like CoV strain.
Conclusions
Our results show that Cryptosporidium spp . , E. coli K99 + , RVA and BCoV circulate in calves from central Ethiopia. Furthermore, our findings of the rare RVA G-type G24 and a bovine-like CoV demonstrates the importance of genetic characterization.
Peer Review reports
Ethiopia is situated in eastern Africa and is home to the largest livestock population on the continent. Livestock plays a key role in Ethiopia’s agricultural production and cattle are considered the most economically important species, with over 12 million of the 14 million livestock-holding households owning at least one cattle [ 1 , 2 ]. However, high morbidity and mortality, especially among young animals, results in large losses in the livestock sector [ 3 ]. This impacts both animal health and the people depending on livestock for their livelihood. Diarrheic disease has been reported to be a leading cause of mortality and morbidity in neonatal calves all over Ethiopia [ 4 , 5 , 6 , 7 ].
Neonatal calf diarrhea is a multifactorial disease and calves are especially susceptible to diarrheic disease during their first weeks of life, when the immune system is not yet fully developed. Diarrheic disease in calves can be caused by multiple bacterial, protozoan, and viral agents, but some are considered major enteric pathogens. Four of the major enteric agents include Enterotoxigenic Escherichia coli (E. coli K99 +), Cryptosporidium spp . , rotavirus, and coronavirus [ 8 ]. E. coli K99 + is a gram-negative bacterium that primarily causes diarrhea within the first days of the neonatal calf’s life. It attaches and colonizes the intestinal epithelium and secretes heat-labile enterotoxin, causing diarrhea [ 9 , 10 ]. Cryptosporidium is a unicellular protozoan that causes enteric disease in a variety of mammals, including humans. Four species of Cryptosporidium are commonly found in cattle: C. parvum , C. bovis , C. ryanae and C. andersoni. However, mainly C. parvum is associated with clinical disease in neonatal calves [ 11 ]. Rotaviruses are double-stranded RNA viruses that cause enteric disease in both humans and animals. In calves, rotavirus type A (RVA) is the most common subtype, primarily causing diarrhea within the first 14 days of life [ 8 ]. RVAs can be classified based on the two outer capsid proteins, the surface glycoprotein encoded by the VP7 gene (G-type), and the protease-sensitive attachment protein encoded by the VP4 gene (P-type) [ 12 ]. Bovine coronavirus (BCoV) is a single-stranded RNA virus that belongs to the species Betacoronavirus 1 . BCoV is associated with diarrhea in neonatal calves and winter dysentery in adult cattle. Furthermore, BCoV can infect the respiratory tract, causing respiratory disease in cattle of all ages [ 13 ]. The S1 subunit of the spike gene is variable and has been utilized for molecular epidemiology of BCoV strains [ 14 , 15 ].
All four enteric pathogens have been detected at different prevalence rates in calves in Ethiopia [ 16 , 17 , 18 , 19 ]. However, studies including molecular characterization of the pathogens are lacking. Therefore, the aim of this study was to investigate and characterize the genotypes of Cryptosporidium spp . , E. coli K99 + , RVA and BCoV circulating in calves in central Ethiopia.
Detection of enteric pathogens with antigen-ELISA and qPCR
A total of 47 calves (< 2 months of age) were sampled in four different towns in central Ethiopia. Antigen-ELISA (Ag-ELISA) was used to screen 44 of the fecal samples for E. coli K99 + , Cryptosporidium spp . , RVA and BCoV. Following analysis with Ag-ELISA, all 47 samples were screened for the same enteric pathogens using quantitative real-time (qPCR) (Table 1 ). When using qPCR, a higher proportion of positive samples was observed for all four tested pathogens in comparison to Ag-ELISA. Cryptosporidium spp. was the most common pathogen identified in all calves followed by RVA when analyzed with qPCR, with 72.3% and 19.1% of samples being positive for each pathogen, respectively. When using Ag-ELISA the positivity rate was 4.5% for Cryptosporidium spp. and 2.3% for RVA. qPCR and Ag-ELISA detected E. coli K99 + at the rates of 10.6% and 4.5%, respectively. Lastly, 8.5% of the calves were positive for BCoV with qPCR and no calves tested positive for BCoV with the Ag-ELISA kit.
E. coli K99 + , Cryptosporidium spp . , RVA and BCoV were found in both diarrheic and non-diarrheic calves. Eight of the calves tested negative for all four enteric pathogens when screened with qPCR. Of the calves that tested negative five were diarrheic and three non-diarrheic (Fig. 1 A). Of the positive calves, the majority tested positive for one pathogen (60%, 28/47), followed by two pathogens (19%, 9/47), and lastly three pathogens (4%, 2/47) (Fig. 1 B). Both diarrheic and non-diarrheic calves tested positive for one and two pathogens. The two calves that tested positive for three pathogens were both diarrheic (data not shown).
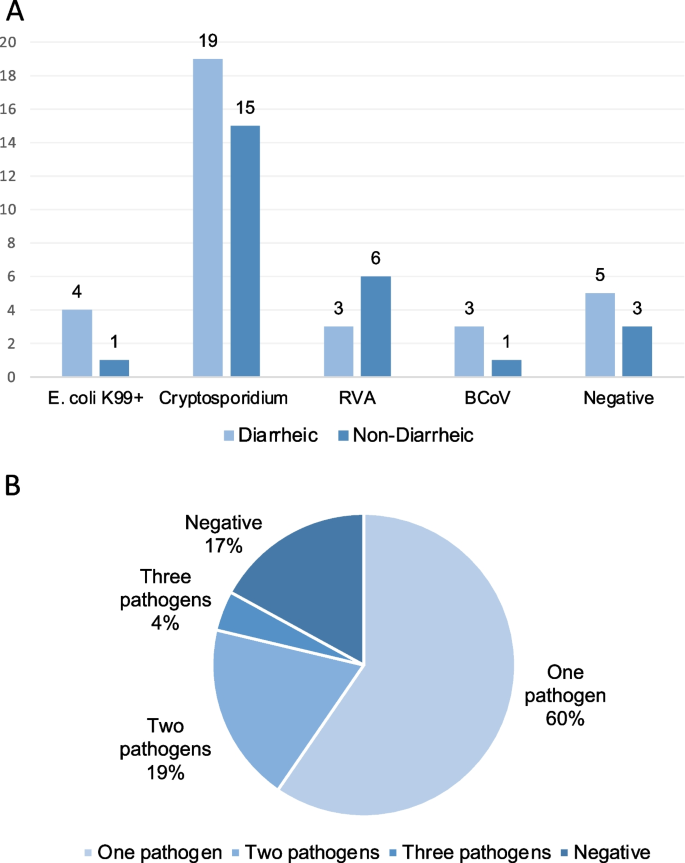
qPCR based detection rates of each pathogen based on diarrheal status. Numbers above bars indicate number of qPCR positive animals ( A ). Percentage of calves testing positive for one, two, three or no pathogens with qPCR ( B )
Cryptosporidium species
All 34 qPCR Cryptosporidium -positive samples generated the expected 18S rRNA gene products in nested PCR. Three species of Cryptosporidium were identified, C. andersoni , C. bovis and C. ryanae . C. andersoni was the most common species (62%, 21/34), followed by C. bovis (21%, 7/34) and C. ryanae (15%, 5/34). One sample that was positive using qPCR was not possible to genotype. Interestingly, no C. parvum was detected in any of the positive samples. Furthermore, the two specimens that tested positive for Cryptosporidium with Ag-ELISA were molecularly characterized as C. andersoni.
G- and P-typing of RVA
To determine G- and P-genotypes of RVA-positive samples partial fragments of the VP7 and VP4 gene were amplified. G-type was determined for nine RVA-positive samples, generating the expected VP7 gene products after nested PCR. Two different G-types were detected, G10 in eight samples, and G24 in one sample. Characterization of the VP4 gene found one P-type, P[11]. However, P-typing was not possible for eight of the RVA-positive samples. In total, three genotypes were found, G10P[11] (1/9), G24P[x] (1/9) and G10P[x] (7/9). The G24P[x] strain was isolated from a calf on a farm in Sebeta and the remaining samples genotyped as G10P[11] and G10P[x] originated from three different farms in Holeta.
Phylogenetic analysis was performed on the VP7 gene of the Ethiopian G10P[11] and G24P[x] strains. For VP4 analysis only G10P[11] was included. Phylogenetic analysis revealed that the VP7 genes of G10P[11] and G24P[x] grouped with sequences from the respective genotypes, G10 and G24 (Fig. 2 A). A similar tree structure was observed for the VP4 gene of G10P[11], clustering with other P[11] isolates (Fig. 2 B).
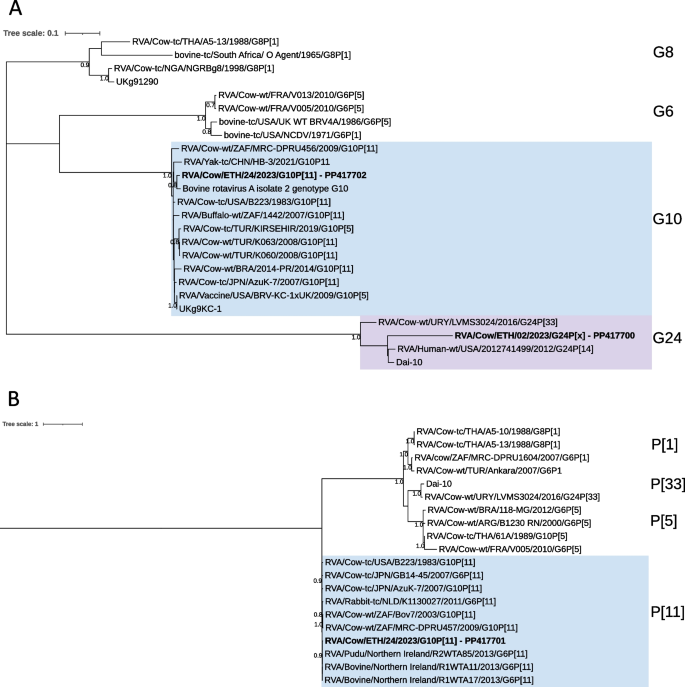
Maximum likelihood trees of the VP7 gene ( A ) and partial VP4 gene (nt 11–887, VP8* segment) ( B ) rooted at the midpoint. G10 and P[11] genotypes are highlighted with a blue background and G24 genotypes with a purple background. Strains identified in the current study are indicated by bold text. Bootstrap values > 0.7 are displayed
Molecular characterization of the S1 subunit of BCoV
Four samples tested positive for BCoV in qPCR and were successfully genotyped by amplification and sequencing of the hypervariable region of the S1 subunit. BCoV/Cow/ETH/2023/25 and BCoV/Cow/ETH/2023/26 were isolated from the same farm in Holeta. BLASTn search identified BCoV strains isolated from cattle in France as closest relatives, with > 99% nucleotide identity. The other two samples, BCoV/Cow/ETH/2023/40 and BCoV/Cow/ETH/2023/42 were isolated from the same farm in Sululta. Interestingly, the most similar sequence identified with BLASTn with 96.9% identical nucleotides, was a bovine-like coronavirus (CoV) isolated from a white oryx ( Oryx leucoryx ) in Israel. Phylogenetic analysis of the S1 hypervariable region revealed that samples BCoV/Cow/ETH/2023/25 and BCoV/Cow/ETH/2023/26 clustered with classical BCoV strains isolated from cattle in different countries. As indicated by the BLASTn search, BCoV/Cow/ETH/2023/40 and BCoV/Cow/ETH/2023/42 grouped with bovine-like CoVs isolated from different animal species instead of classical BCoV (Fig. 3 ).
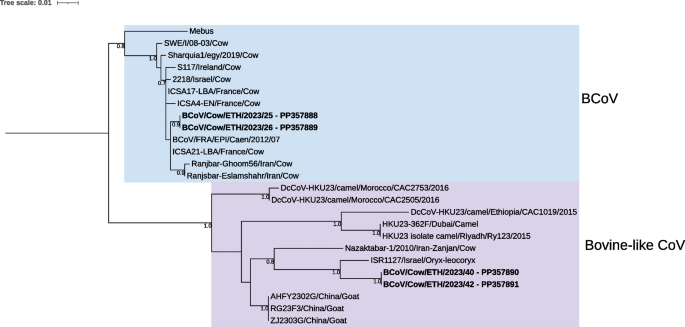
Maximum likelihood tree of the hypervariable region of the S1 subunit (nt: 1332–1778 of Mebus, U00735) rooted at the midpoint. Classical BCoV strains are highlighted with a blue background and bovine-like CoV strains with a purple background. Strains identified in the current study are indicated by bold text. Bootstrap values > 0.7 are displayed
The present study identified and molecularly characterized Cryptosporidium , E. coli K99 + , RVA and BCoV in calves from central Ethiopia and all four pathogens were detected using qPCR. Furthermore, the four major enteric pathogens were found in fecal samples from both diarrheic and non-diarrheic calves. Similar findings have been observed in a previous study conducted in Ethiopia [ 20 ], as well as in reports from other countries [ 21 , 22 ]. All four pathogens are causative agents of neonatal calf diarrhea; however, the clinical picture can differ depending on immune status of the animal and at what stage of the infection the sample is taken. A majority of the screened calves tested positive for one or more pathogens, however, eight calves tested negative for both Cryptosporidium , E. coli K99 + , RVA and BCoV. Five of the calves testing negative had active diarrhea during sampling, indicating that other pathogens, not tested for in this study, could be the causative agents of the disease. Screening for additional enteric pathogens or metagenomics-based analysis of the samples could provide insight on other microbes possibly involved in the disease.
When screening the samples with the commercial Ag-ELISA kit fewer samples tested positive compared to qPCR and no BCoV could be identified using the Ag-ELISA. Three out of the four samples that were positive in both qPCR and Ag-ELISA had lower ct values (ct < 30). Together, this indicates a lower sensitivity of the commercial Ag-ELISA or difference in surface antigenicity between the pathogens detected by the kit and the pathogens circulating in the country. Ag-ELISAs are often less sensitive than qPCR [ 8 , 23 ] but can provide a preliminary overview of what pathogens are circulating in animals when access to laboratory facilities with molecular techniques is limited.
qPCR detected E. coli K99 + at the rate of 10.6% (5/47) in calves from Holeta and Bishoftu. Being a small sample size, no conclusions can be drawn on the true prevalence rate of E. coli K99 + in the calf population. However, similar detection rates have been seen for E. coli K99 + in previous studies on calves in Ethiopia, reporting E. coli K99 + in 11.1–22.1% of sampled calves [ 17 , 19 ].
Cryptosporidium spp . was the most commonly detected enteric pathogen using qPCR. Genotyping using the partial 18S rRNA gene showed three different species among the qPCR positive calves, C. andersoni , C. bovis and C. ryanae. One sample was un-typeable even after repeated trials of sequencing as well as cloning. Most likely there was an insufficient amount of genetic material available for molecular characterization as the sample had a high ct-value in the qPCR (data not shown). Interestingly, no samples were genotyped as C. parvum, which is believed to be the most predominant Cryptosporidium species among neonatal calves, while infection with C. andersoni , C. bovis and C. ryanae is mostly associated with older calves [ 11 ]. However, our finding correlates with another study from central Ethiopia which screened over 400 calves below five months of age. The authors detected C. andersoni , C. bovis and C. ryanae but no C. parvum , suggesting that C. parvum could have a low prevalence in calves in the central region of Ethiopia [ 24 ]. Moreover, a study from 2019 in Sweden investigated the infection dynamics of C. bovis and C. ryanae in dairy herds free from C. parvum infection. The study found that C. bovis and C. ryanae infections were most prevalent in calves aged 4–5 weeks, which is earlier than reported in other studies, indicating that the absence of C. parvum affects the infection dynamics of C. bovis and C. ryanae [ 25 ]. This provides a possible explanation to the previous and current findings of C. andersoni , C. bovis and C. ryanae in young calves in the central region of Ethiopia.
Genotyping of the nine RVA-positive samples found two G-types, G10 and G24 and one P-type, P[11]. To our knowledge this is the first genotyping of bovine RVAs in Ethiopia. However, a majority of the RVA positive samples could not be P-typed, which is most likely explained by few viral RNA copies being available in these samples, all having ct values > 30 in the qPCR (data not shown). G10[P11] is an RVA genotype commonly found in cattle [ 26 ] and the genotype identified in the current study clustered with other G10 and P[11] sequences isolated from different countries. More unusual is the G24P[x] strain identified in a calf from Sebeta, a G-type which has only been detected at three occasions prior to this study, twice in cattle from Japan and Uruguay (G24P[33]) [ 27 , 28 ], and once in a human in USA (G24P[14] genotype) [ 29 ]. Phylogenetic analysis revealed that the G24 sequence from the current study grouped with the isolated G24P[33] strains from Japan and Uruguay as well as the human G24P[14] strain. To our knowledge, this is the first detection of the G24 G-type in Ethiopia, as well as in Africa. Being segmented viruses, RVAs are capable of reassortment and interspecies transmission, and the G24P[33] and G24P[14] strains are hypothesized to have arisen from reassortment between RVAs from different species. Further characterization of the remaining segments by high-throughput sequencing could reveal information on the origin and possible reassortment events of the identified G24P[x] strain.
BCoV was identified in four calves from two different farms, one in Holeta and one in Sululta. Molecular characterization of the spike gene revealed that the two BCoV isolates from Holeta (BCoV/Cow/ETH/2023/25 and BCoV/Cow/ETH/2023/26) belonged to classical BcoV, clustering with other BCoV isolated from cattle from different countries. However, the two isolates from the farm in Sululta (BCoV/Cow/ETH/2023/40 and BCoV/Cow/ETH/2023/42), differed from the classical BCoV strains and grouped with bovine-like CoVs isolated from different animals. Furthermore, a Dromedary camel CoV-HKU23 (DcCoV-HKU23) isolated from a dromedary camel in Ethiopia in 2015 [ 30 ] clustered with BCoV/Cow/ETH/2023/40 and BCoV/Cow/ETH/2023/42, demonstrating that the strain identified in the two calves from Sululta is more similar to the Ethiopian DcCoV-HKU23 than to the classical BCoV strain isolated from the two calves in the nearby town, Holeta. BCoV and bovine-like CoVs are genetically similar and belong to the same species, Betacoronavirus 1 , and are known to be able to cross the species barrier [ 13 ]. This suggests that either the calves in Sululta have been infected with a bovine-like CoV, either from contact with dromedary camels or other ruminants, or that a BCoV originating from bovine-like CoVs circulates among calves together with classical BCoV strains. Sequencing of the full BCoV and bovine-like CoV genomes could provide a better picture of the evolution and common ancestors, as well as potential recombination events between different strains.
This study has some limitations that need to be addressed. First is the small sample size, which resulted from a limited study time and restrictions in the field. Secondly, both the geographical area and study time of two months prevent any conclusions from being drawn on the seasonality of the pathogens or their geographical spread in Ethiopia. However, with molecular characterization of pathogens in calf diarrhea lacking in Ethiopia, this study provides important insight into circulating species and genotypes. The results presented here can hopefully aid and promote future studies on the subject in Ethiopia, involving more animals and additional regions.
In summary, we detected Cryptosporidium spp . , E. coli K99 + , RVA and BCoV in calves from central Ethiopia. Cryptosporidium , RVA and BCoV were further characterized to determine species and genotypes and to our knowledge this is the first molecular characterization of bovine RVAs and BCoV in Ethiopia. Our findings of the rare RVA G-type G24P[x] and a bovine-like CoV demonstrates the importance of genetic characterization in combination with conventional screening. It enables the discovery of novel or uncommon variants of different pathogens that would most likely remain undetected with normal screening methods. This knowledge is key to detect emerging strains that can affect the animal health or have possible zoonotic potential, as well as providing better vaccination strategies and treatment options.
Study area and sample collection
The study was conducted in four small towns, Sebeta, Holeta, Sululta and Bishoftu, outside the capital Addis Ababa in central Ethiopia during January and February 2023 (Fig. 4 ). A total of 47 calves (diarrheic = 26, non-diarrheic = 21) up to two months of age from 16 different farms were included in the study using purposive sampling. A trained veterinarian sampled all calves included in the study and determined if they were diarrheic or non-diarrheic. Fecal samples were collected directly from the rectum in sterile stool cups and transported under cold chain from the field to the Institute of Biotechnology, AAU, Addis Ababa, where the samples were stored at -20 °C until further processing. Information on health status and age of each sampled calf was retrieved from the animal owner using a questionnaire form (Supplementary data).
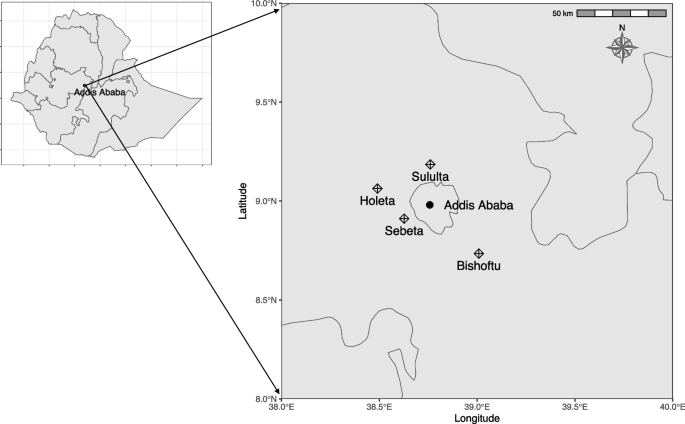
Map showing the location of the towns in Ethiopia where sampling was conducted. The towns Sebeta, Holeta, Sululta and Bishoftu are represented by diamonds. The capital Addis Ababa is represented by a black circle. Maps were generated using R software (version 4.2.2)
Sample preparation
All fecal samples were divided into three aliquots. For DNA and RNA extraction two fecal aliquots were suspended in DNA/RNA Shield™ (10% w/v) and homogenized using ZR BashingBead™ Lysis Tubes (Zymo research, Irvine, CA, USA) and a Vortex-Genie in combination with a horizontal tube holder (Thermo Fisher Scientific, Waltham, MA, USA). The homogenization was done according to manufacturer’s instructions for lower speed bead beating devices. The homogenates were centrifuged at 12,000 × g for 1 min and the supernatant was collected for future analysis. Homogenates to be used for RNA extraction were further processed by filtering with ultracentrifuge membrane filters (0.45 μM; Utrafree®-MC centrifugal Filter, Darmstadt, Germany) at 12,000 × g for 4 min. Lastly, one aliquot was kept untreated for analysis with Ag-ELISA.
Antigen-ELISA
The untreated fecal samples were screened for the presence of E. coli K99 + , Cryptosporidium spp . , RVA and BCoV using the commercial Ag-ELISA kit BIO K 151 (Bio-X Diagnostics, Belgium) following manufacturer’s instructions. After adding stop solution, the optical densities were measured at 450 nm using a Multiskan™ FC Microplate Photometer (Thermo Fisher Scientific, Waltham, MA, USA). Due to limited access to Ag-ELISA kits three non-diarrheic samples were excluded from the analysis.
Nucleic acid extraction and cDNA synthesis
DNA was extracted using the Quick-DNA™ Fecal/Soil Microbe Miniprep Kit (Zymo research, Irvine, CA, USA) according to manufacturer´s instructions. The DNA was eluted in 100 µL of DNA elution buffer and stored at -80 °C until further use. Total RNA was extracted using a combination of TRIzol (Invitrogen, Carlsbad, CA, USA) and GeneJet RNA extraction columns (Thermo Fisher Scientific, Waltham, MA, USA). In short, an equal volume of TRIzol was added to the filtered samples and mixed. Following addition of chloroform, the aqueous phase was diluted with an equal volume of 70% ethanol and purified with GeneJet columns. The RNA was eluted in 40 µL of nuclease-free water and stored at -80 °C until further use. cDNA synthesis was performed on the extracted RNA using SuperScript III reverse transcriptase (Invitrogen, Carlsbad, CA, USA) with 5 µL of RNA template, according to manufacturer’s instructions.
qPCR for the detection of E. coli K99 + , Cryptosporidium spp., RVA and BCoV was performed using a 20 µL iTaq Universal Probes Supermix reaction with 300–600 nM primers, 100–200 nM probe and 2 µL of template. Each reaction was run as singleplex with pathogen-specific primers (Table 2 ) on a C1000 Touch Thermal Cycler™ (Bio-Rad, Hercules, CA, USA). Positive and negative controls were included in all qPCR runs. Number of cycles and cut-off values for each qPCR is provided in supplementary table S1.
Genotyping PCRs and sequencing
To determine Cryptosporidium species, a nested PCR was utilized to amplify an 830 bp fragment of the 18S rRNA gene, using primers 5′-TTC TAG AGC TAA TAC ATG CG-3′ and 5′-CCC ATT TCC TTC GAA ACA GGA-′3 in the primary PCR and 5´-GGA AGG GTT GTA TTT ATT AGA TAA AG-3′ and 5´-AAG GAG TAA GGA ACA ACC TCC A-3′ in the secondary PCR [ 36 , 37 ].
All samples that tested positive for RVA in the qPCR were subjected to nested PCRs to determine G-type (VP7) and P-type (VP4). For VP7, primers Gra-5 and Gra-3 were used [ 38 ] together with primers VP7-up2 and VP7-down3 [ 39 ] generating a 956 bp product. If no product was visible a second PCR designed for RVA samples with a lower viral load was applied. Primers N-VP7F1 and N-VP7R1 were used in the primary reaction, followed by a secondary reaction with primers N-VP7F2 and N-VP7R2, amplifying a 193 bp product [ 40 ]. For partial amplification of the VP4 gene, primers Con-3 and Con-2 were used [ 41 ], generating an 850 bp product. If amplification was unsuccessful with primers Con-3 and Con-2, the protocol by Mijatovic-Rustempasic et. al with primers N-VP4F1 and N-VP4R1 in the primary PCR and primers N-VP4F2 and N-VP4R2 in the secondary PCR was used [ 40 ].
Molecular characterization of BCoV was performed by amplifying the hypervariable region of S1 subunit of the spike gene. Primers S1HS and S1HA were used in the first reaction and primers S1NS and S1NA in the second reaction, amplifying a 488 bp product [ 15 ]. Modified primers were used on BCoV-positive samples that did not generate any product using the original nested PCR. In the new reaction primer S1HS together with S1HA - mod (5´-C A GA T ACACGACCACTAT-3´) was used, followed by primers S1NS - mod (5´-GTTTCTGTTAGCAG A TT A AA-3´) and S1NA - mod (5´-ATATTACACCT G TC T CCTTG-3´). Modified bases in the primer sequences are indicated by bold text.
All PCR reactions were run for 35 cycles in a 25 µL reaction with Invitrogen™ Platinum Superfi DNA Polymerase (Invitrogen, Carlsbad, CA, USA), 0.5 µM of each primer and 2 µL of template according to manufacturer’s instructions. PCR products were visualized on 1–2% agarose gels, depending on product size, stained with GelRed. Gels were run at 100 V for 60 min and purified using the GeneJET™ gel extraction kit (Thermo Fisher Scientific, Waltham, MA, USA). Purified products were sent for sequencing at Macrogen Europe. If sequencing failed or was of poor quality the PCR product was cloned into the pJET1.2/blunt vector using the CloneJET PCR Cloning Kit (Thermo Fisher Scientific, Waltham, MA, USA) and transformed into Dh5α competent cells. Plasmid DNA was subsequently purified using the GeneJET™ Plasmid Miniprep Kit (Thermo Fisher Scientific, Waltham, MA, USA) and sent for re-sequencing.
Sequence analysis and phylogenetics
Forward and reverse sequences were assembled and manually curated in Geneious prime (version 2023.2.1). Consensus sequences were compared to nucleotide reference sequences using BLASTn (Basic Local Alignment Search Tool, NCBI, http://www.ncbi.nlm.nih.gov/blast/Blast.cgi ) to determine genotypes. Representative sequences were deposited into NCBI GenBank under accession numbers PP358250-52 ( Cryptosporidium ), PP417700-02 (RVA) and PP357888-91 (BCoV).
Reference sequences (supplementary tables S2-S4) were downloaded from GenBank and aligned with sequences from the study in Geneious prime (version 2023.2.1) using MUSCLE [ 42 ]. Aligned sequences were imported into MEGA X [ 43 ] and phylogenetic trees were constructed using the maximum likelihood method with 1,000 bootstrap replicates. The best fitting substitution model was determined for each dataset by lowest BIC score. The phylogenetic trees were visualized in Interactive Tree Of Life (iTOL) version 6.8.1 [ 44 ].
Availability of data and materials
Representative nucleotide sequences are available at NCBI GenBank under accession numbers PP358250-52 ( Cryptosporidium ), PP417700-02 (RVA) and PP357888-91 (BCoV). The remaining datasets used and analyzed during the current study are available from the corresponding author on reasonable request.
FAO. The future of livestock in Ethiopia. Opportunitues and challenges in the face of uncertainty. 2019.
Google Scholar
Mekuriaw Z, Harris-Coble L. Ethiopia´s Livestock Systems: Overview and Areas of Inquiry. Gainesville: Feed the Future Innovation Lab for Livestock Systems; 2021.
Shapiro BI, Gebru G, Desta S, Negassa A, Nigussie K, Aboset G, et al. Ethiopia livestock sector analysis. ILRI Project Report. Nairobi: International Livestock Research Institute (ILRI); 2017.
Abebe R, Dema T, Libiyos Y, Teherku W, Regassa A, Fekadu A, et al. Longitudinal study of calf morbidity and mortality and the associated risk factors on urban and peri-urban dairy farms in southern Ethiopia. BMC Vet Res. 2023;19(1):15. https://doi.org/10.1186/s12917-023-03574-8 .
Article PubMed PubMed Central Google Scholar
Hadgu A, Lemma A, Yilma T, Fesseha H. Major Causes of Calf and Lamb Mortality and Morbidity and Associated Risk Factors in the Mixed Crop-Livestock Production System in Jamma District, South Wollo. Ethiopia Veterinary Medicine International. 2021;2021:6689154. https://doi.org/10.1155/2021/6689154 .
Article PubMed Google Scholar
Hordofa D, Abunna F, Megersa B, Abebe R. Incidence of morbidity and mortality in calves from birth to six months of age and associated risk factors on dairy farms in Hawassa city, southern Ethiopia. Heliyon. 2021;7(12): e08546. https://doi.org/10.1016/j.heliyon.2021.e08546 .
Article CAS PubMed PubMed Central Google Scholar
Fentie T, Guta S, Mekonen G, Temesgen W, Melaku A, Asefa G, et al. Assessment of Major Causes of Calf Mortality in Urban and Periurban Dairy Production System of Ethiopia. Vet Med Int. 2020;2020:3075429. https://doi.org/10.1155/2020/3075429 .
Cho YI, Yoon KJ. An overview of calf diarrhea - infectious etiology, diagnosis, and intervention. J Vet Sci. 2014;15(1):1–17. https://doi.org/10.4142/jvs.2014.15.1.1 .
Dubreuil JD, Isaacson RE, Schifferli DM. Animal Enterotoxigenic Escherichia coli. EcoSal Plus. 2016;7(1). https://doi.org/10.1128/ecosalplus.ESP-0006-2016 .
Nagy B, Fekete PZ. Enterotoxigenic Escherichia coli in veterinary medicine. Int J Med Microbiol. 2005;295(6–7):443–54. https://doi.org/10.1016/j.ijmm.2005.07.003 .
Article CAS PubMed Google Scholar
Thomson S, Hamilton CA, Hope JC, Katzer F, Mabbott NA, Morrison LJ, et al. Bovine cryptosporidiosis: impact, host-parasite interaction and control strategies. Vet Res. 2017;48(1):42. https://doi.org/10.1186/s13567-017-0447-0 .
Desselberger U. Rotaviruses. Virus Res. 2014;190:75–96. https://doi.org/10.1016/j.virusres.2014.06.016 .
Vlasova AN, Saif LJ. Bovine Coronavirus and the Associated Diseases. Front Vet Sci. 2021;8: 643220. https://doi.org/10.3389/fvets.2021.643220 .
Hasoksuz M, Sreevatsan S, Cho K-O, Hoet AE, Saif LJ. Molecular analysis of the S1 subunit of the spike glycoprotein of respiratory and enteric bovine coronavirus isolates. Virus Res. 2002;84(1-2):101-9.
Brandao PE, Gregori F, Richtzenhain LJ, Rosales CA, Villarreal LY, Jerez JA. Molecular analysis of Brazilian strains of bovine coronavirus (BCoV) reveals a deletion within the hypervariable region of the S1 subunit of the spike glycoprotein also found in human coronavirus OC43. Arch Virol. 2006;151(9):1735–48. https://doi.org/10.1007/s00705-006-0752-9 .
Debelo M, Abdela H, Tesfaye A, Tiruneh A, Mekonnen G, Asefa Z, et al. Prevalence of Bovine Rotavirus and Coronavirus in Neonatal Calves in Dairy Farms of Addis Ababa, Ethiopia: Preliminary Study. Biomed Res Int. 2021;2021:5778455. https://doi.org/10.1155/2021/5778455 .
Abraham G, Roeder PL, Zewdu R. Agents associated with neonatal diarrhoea in Ethiopian dairy calves. Trop Anim Health Prod. 1992;24(2):74-80.
Seid U, Dawo F, Tesfaye A, Ahmednur M. Isolation and Characterization of Coronavirus and Rotavirus Associated with Calves in Central Part of Oromia. Ethiopia Vet Med Int. 2020;2020:8869970. https://doi.org/10.1155/2020/8869970 .
Wong JT, Lane JK, Allan FK, Vidal G, Vance C, Donadeu M, et al. Reducing Calf Mortality in Ethiopia. Animals (Basel). 2022;12(16). https://doi.org/10.3390/ani12162126 .
Lane J, Vidal G, Smith W, Jackson W, Fentie T, Kebede N. LSIL Ethiopia Young Stock Mortality Animal Health and Disease Surveillance Data. Harvard Dataverse, V1. 2022. https://doi.org/10.7910/DVN/E6EV2K .
Caffarena RD, Casaux ML, Schild CO, Fraga M, Castells M, Colina R, et al. Causes of neonatal calf diarrhea and mortality in pasture-based dairy herds in Uruguay: a farm-matched case-control study. Braz J Microbiol. 2021;52(2):977–88. https://doi.org/10.1007/s42770-021-00440-3 .
Bartels CJ, Holzhauer M, Jorritsma R, Swart WA, Lam TJ. Prevalence, prediction and risk factors of enteropathogens in normal and non-normal faeces of young Dutch dairy calves. Prev Vet Med. 2010;93(2–3):162–9. https://doi.org/10.1016/j.prevetmed.2009.09.020 .
Izzo MM, Kirkland PD, Gu X, Lele Y, Gunn AA, House JK. Comparison of three diagnostic techniques for detection of rotavirus and coronavirus in calf faeces in Australia. Aust Vet J. 2012;90(4):122–9. https://doi.org/10.1111/j.1751-0813.2011.00891.x .
Wegayehu T, Karim R, Anberber M, Adamu H, Erko B, Zhang L, et al. Prevalence and Genetic Characterization of Cryptosporidium Species in Dairy Calves in Central Ethiopia. PLoS ONE. 2016;11(5): e0154647. https://doi.org/10.1371/journal.pone.0154647 .
Åberg M, Emanuelson U, Troell K, Björkman C. Infection dynamics of Cryptosporidium bovis and Cryptosporidium ryanae in a Swedish dairy herd. Veterinary Parasitology. 2019;276. https://doi.org/10.1016/j.vpoa.2019.100010 .
Geletu US, Usmael MA, Bari FD. Rotavirus in Calves and Its Zoonotic Importance. Vet Med Int. 2021;2021:6639701. https://doi.org/10.1155/2021/6639701 .
Abe M, Ito N, Masatani T, Nakagawa K, Yamaoka S, Kanamaru Y, et al. Whole genome characterization of new bovine rotavirus G21P[29] and G24P[33] strains provides evidence for interspecies transmission. J Gen Virol. 2011;92(Pt 4):952–60. https://doi.org/10.1099/vir.0.028175-0 .
Castells M, Caffarena RD, Casaux ML, Schild C, Mino S, Castells F, et al. Phylogenetic Analyses of Rotavirus A from Cattle in Uruguay Reveal the Circulation of Common and Uncommon Genotypes and Suggest Interspecies Transmission. Pathogens. 2020;9(7). https://doi.org/10.3390/pathogens9070570 .
Ward ML, Mijatovic-Rustempasic S, Roy S, Rungsrisuriyachai K, Boom JA, Sahni LC, et al. Molecular characterization of the first G24P[14] rotavirus strain detected in humans. Infect Genet Evol. 2016;43:338–42. https://doi.org/10.1016/j.meegid.2016.05.033 .
So RTY, Chu DKW, Miguel E, Perera R, Oladipo JO, Fassi-Fihri O, et al. Diversity of Dromedary Camel Coronavirus HKU23 in African Camels Revealed Multiple Recombination Events among Closely Related Betacoronaviruses of the Subgenus Embecovirus. J Virol. 2019;93(23). https://doi.org/10.1128/JVI.01236-19 .
West DM, Sprigings KA, Cassar C, Wakeley PR, Sawyer J, Davies RH. Rapid detection of Escherichia coli virulence factor genes using multiplex real-time TaqMan PCR assays. Vet Microbiol. 2007;122(3–4):323–31. https://doi.org/10.1016/j.vetmic.2007.01.026 .
Cho YI, Han JI, Wang C, Cooper V, Schwartz K, Engelken T, et al. Case-control study of microbiological etiology associated with calf diarrhea. Vet Microbiol. 2013;166(3–4):375–85. https://doi.org/10.1016/j.vetmic.2013.07.001 .
Stroup S, Tongjai S, Swai N, Maro A, Kibiki G, Houpt ER. Dual probe DNA capture for sensitive real-time PCR detection of Cryptosporidium and Giardia. Mol Cell Probes. 2012;26(2):104–6. https://doi.org/10.1016/j.mcp.2011.12.003 .
Bernandes NTCG. Development PCR Real-Time Taqman® system for rotavirus detection in clinical samples of cattle. Thesis [Doctorate in Science]. São Paulo: University of São Paulo; 2016.
Decaro N, Elia G, Campolo M, Desario C, Mari V, Radogna A, et al. Detection of bovine coronavirus using a TaqMan-based real-time RT-PCR assay. J Virol Methods. 2008;151(2):167–71. https://doi.org/10.1016/j.jviromet.2008.05.016 .
Xiao L, Escalante L, Yang C, Sulaiman I, Escalante AA, Montali RJ, et al. Phylogenetic analysis of Cryptosporidium parasites based on the small-subunit rRNA gene locus. Appl Environ Microbiol. 1999. https://doi.org/10.1128/AEM.65.4.1578-1583 .
Santin M, Trout JM, Xiao L, Zhou L, Greiner E, Fayer R. Prevalence and age-related variation of Cryptosporidium species and genotypes in dairy calves. Vet Parasitol. 2004;122(2):103–17. https://doi.org/10.1016/j.vetpar.2004.03.020 .
Badaracco A, Garaicoechea L, Rodriguez D, Uriarte EL, Odeon A, Bilbao G, et al. Bovine rotavirus strains circulating in beef and dairy herds in Argentina from 2004 to 2010. Vet Microbiol. 2012;158(3–4):394–9. https://doi.org/10.1016/j.vetmic.2011.12.011 .
Abe M, Ito N, Morikawa S, Takasu M, Murase T, Kawashima T, et al. Molecular epidemiology of rotaviruses among healthy calves in Japan: isolation of a novel bovine rotavirus bearing new P and G genotypes. Virus Res. 2009;144(1–2):250–7. https://doi.org/10.1016/j.virusres.2009.05.005 .
Mijatovic-Rustempasic S, Esona MD, Williams AL, Bowen MD. Sensitive and specific nested PCR assay for detection of rotavirus A in samples with a low viral load. J Virol Methods. 2016;236:41–6. https://doi.org/10.1016/j.jviromet.2016.07.007 .
Gouvea V, Santos N, Timenetsky MC. VP4 Typing of Bovine and Porcine Group A Rotaviruses by PCR. J Clin Microbiol. 1994. https://doi.org/10.1128/jcm.32.5.1333-1337 .
Edgar RC. MUSCLE: multiple sequence alignment with high accuracy and high throughput. Nucleic Acids Res. 2004;32(5):1792–7. https://doi.org/10.1093/nar/gkh340 .
Kumar S, Stecher G, Li M, Knyaz C, Tamura K. MEGA X: Molecular Evolutionary Genetics Analysis across Computing Platforms. Mol Biol Evol. 2018;35(6):1547–9. https://doi.org/10.1093/molbev/msy096 .
Letunic I, Bork P. Interactive Tree Of Life (iTOL) v5: an online tool for phylogenetic tree display and annotation. Nucleic Acids Res. 2021;49(W1):W293–6. https://doi.org/10.1093/nar/gkab301 .
Download references
Acknowledgements
We would like to acknowledge all the animal owners and calves for participating in this project, without them this study would not have been possible. Secondly, thank you to Asamenew Tesfaye, Mequanent Addisu and all local veterinary staff for assistance during sampling. The authors would also like to acknowledge Anton de Jong, Giulio Grandi, Tomas Jinnerot and Jonas Johansson Wensman at the Swedish Veterinary Agency for providing positive controls for the PCRs and discussions on methodology.
Open access funding provided by Swedish University of Agricultural Sciences. This project was funded from grant 2021–04343 from the Swedish Research Council (Vetenskapsrådet).
Author information
Authors and affiliations.
Department of Animal Biosciences, Swedish University of Agricultural Sciences, Uppsala, Sweden
Julia Bergholm, Anne-Lie Blomström & Mikael Berg
Institute of Biotechnology, Addis Ababa University, Addis Ababa, Ethiopia
Tesfaye Sisay Tessema
You can also search for this author in PubMed Google Scholar
Contributions
JB: Conceptualization, Formal analysis, Investigation, Methodology, Visualization, Writing – original draft, Writing – review and editing. TST: Conceptualization, Funding acquisition, Methodology, Supervision, Resources, Writing – review and editing. A-LB: Conceptualization, Funding acquisition, Methodology, Supervision, Resources, Writing – review and editing. MB: Conceptualization, Funding acquisition, Methodology, Supervision, Resources, Writing – review and editing. All authors have read and approved the final manuscript.
Corresponding author
Correspondence to Julia Bergholm .
Ethics declarations
Ethics approval and consent to participate.
Informed consent was obtained from the animal owners prior to sampling. Ethical statements were received from the Swedish University of Agricultural Sciences (SLU ua 2023.4.1–2475) and from the Institute of Biotechnology, Addis Ababa University (AAU) (IoB/L-4/2016/2024).
Consent for publication
Not applicable.
Competing interests
The authors declare no competing interests.
Additional information
Publisher’s note.
Springer Nature remains neutral with regard to jurisdictional claims in published maps and institutional affiliations.
Supplementary Information
Supplementary material 1., supplementary material 2., rights and permissions.
Open Access This article is licensed under a Creative Commons Attribution 4.0 International License, which permits use, sharing, adaptation, distribution and reproduction in any medium or format, as long as you give appropriate credit to the original author(s) and the source, provide a link to the Creative Commons licence, and indicate if changes were made. The images or other third party material in this article are included in the article's Creative Commons licence, unless indicated otherwise in a credit line to the material. If material is not included in the article's Creative Commons licence and your intended use is not permitted by statutory regulation or exceeds the permitted use, you will need to obtain permission directly from the copyright holder. To view a copy of this licence, visit http://creativecommons.org/licenses/by/4.0/ .
Reprints and permissions
About this article
Cite this article.
Bergholm, J., Tessema, T.S., Blomström, AL. et al. Detection and molecular characterization of major enteric pathogens in calves in central Ethiopia. BMC Vet Res 20 , 389 (2024). https://doi.org/10.1186/s12917-024-04258-7
Download citation
Received : 11 April 2024
Accepted : 29 August 2024
Published : 04 September 2024
DOI : https://doi.org/10.1186/s12917-024-04258-7
Share this article
Anyone you share the following link with will be able to read this content:
Sorry, a shareable link is not currently available for this article.
Provided by the Springer Nature SharedIt content-sharing initiative
- Calf diarrhea
- Cryptosporidium
- E. coli K99 +
- Rotavirus A
- Bovine coronavirus
BMC Veterinary Research
ISSN: 1746-6148
- General enquiries: [email protected]
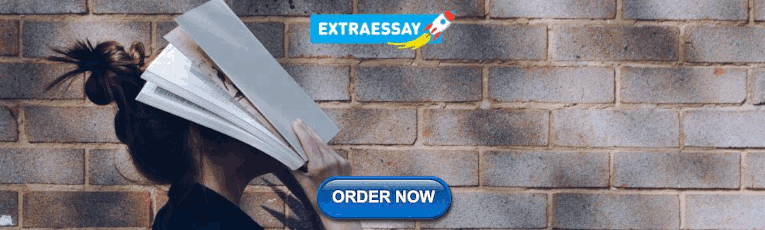
COMMENTS
Quantitative Research. Quantitative research is a type of research that collects and analyzes numerical data to test hypotheses and answer research questions. This research typically involves a large sample size and uses statistical analysis to make inferences about a population based on the data collected. It often involves the use of surveys ...
Types of quantitative research
What Is Quantitative Research? | Definition, Uses & Methods
What is Quantitative Research? Definition, Methods, Types, ...
Quantitative research is a systematic investigation method that relies on numerical data to answer research questions or test hypotheses. Unlike qualitative research, which deals with subjective experiences and meanings, quantitative research aims to quantify relationships, behaviors, or phenomena through statistical analysis .
Chapter Four: Quantitative Methods (Part 1)
Quantitative research
What is Quantitative Research Design? Definition, Types, ...
Quantitative research is a type of research that focuses on collecting and analyzing numerical data to answer research questions. There are two main methods used to conduct quantitative research: 1. Primary Method. There are several methods of primary quantitative research, each with its own strengths and limitations.
What Is Quantitative Research? | Definition & Methods - Scribbr
Quantitative Research Methodologies
Quantitative Research is essentially about numbers and statistical analysis. It's how we turn observations into data that we can measure and analyze in a structured way. This type of research allows us to quantify behaviors, opinions, or phenomena and translate that into figures and statistics that can be used to uncover patterns, test ...
Abstract. In an era of data-driven decision-making, a comprehensive understanding of quantitative research is indispensable. Current guides often provide fragmented insights, failing to offer a holistic view, while more comprehensive sources remain lengthy and less accessible, hindered by physical and proprietary barriers.
Types of Research Designs Compared | Guide & Examples
This is thanks in large part to your strategic research design. As you prepare for your quantitative dissertation research, you'll need to think about structuring your research design. There are several types of quantitative research designs, such as the experimental, comparative or predictive correlational designs.
Different Types of Quantitative Research
Quantitative Research: Types, Characteristics, Methods & ...
(PDF) An Overview of Quantitative Research Methods
Qualitative vs. Quantitative Research | Differences, ...
Quantitative Research: What It Is, Practices & Methods
A Practical Guide to Writing Quantitative and Qualitative ...
Step 2: Gather the Data. Once you've gathered your tools, perform the research and record all relevant results. In the event you have multiple test groups, be sure to keep the results separate for comparison purposes later. As quantitative research is mathematically-based, the majority of data should be based on numbers.
The types of quantitative research results depend on the large sample size. This sample size represents the population. The lager is the sample size; the more valid results will be drawn. Deal with the larger sample data. The types of quantitative research results depend on the large sample size. This sample size represents the population.
In quantitative research, surveys typically include questions that ask respondents to choose a rating from a scale, select one or more items from a list, or other responses that result in numerical data. ... These four types of data are referred to as nominal, ordinal, interval, and ratio. First, let's examine nominal data. With nominal data ...
The case study based on the comprehensive quantitative classification prediction model showed that Q1 ≥ 0.5 and Q2 ≥ 0.5 corresponded to type I reservoirs, while Q1 > 0.7 and Q2 > 0.57 ...
To explore the key research topics, Social Policy Research Associates designed a mixed-methods study that involved the following four principal tasks that were carried out from August 2004 to May 2005: (1) study design and literature review, (2) a quantitative and geospatial analysis of local office and One-Stop center distribution, (3 ...
E. coli K99 + , Cryptosporidium spp., RVA and BCoV were found in both diarrheic and non-diarrheic calves.Eight of the calves tested negative for all four enteric pathogens when screened with qPCR. Of the calves that tested negative five were diarrheic and three non-diarrheic (Fig. 1A). Of the positive calves, the majority tested positive for one pathogen (60%, 28/47), followed by two pathogens ...