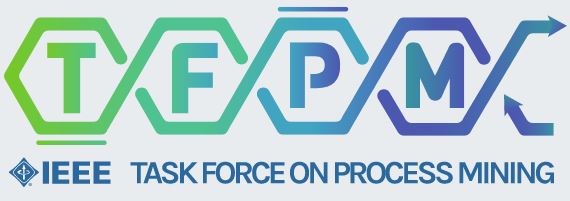
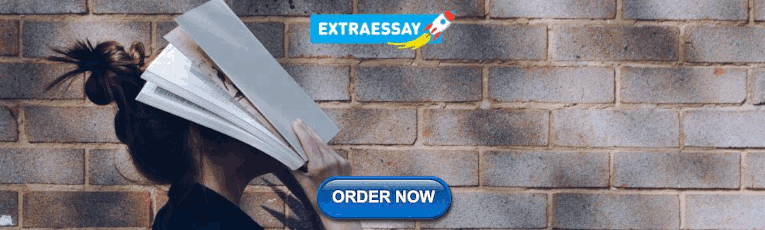
Process Mining Dissertation Award
The IEEE Task Force for Process Mining is happy to announce the 2020 edition of the Best Process Mining PhD Dissertation Award.
The award will be delivered during the 2nd International Conference on Process Mining (ICPM2020), 5-8 October 2020.
Eligibility
Eligible candidates are those who officially obtained a PhD degree in 2017, 2018, or 2019, with a dissertation focused on process mining. We welcome theses that contributed to advance the state of the art in the foundations, engineering, and on-field application of process mining techniques. In this context, the term “process mining” has to be understood in a broad sense: using event data produced during the execution of business, software, or system processes, in order to extract fact-based knowledge and insights on such processes.
For a thesis to be eligible, we also require that thesis-related results have been published in at least one flagship conference/journal for process mining, such as for example ICPM, BPM, CAiSE, EDOC, Petri Nets, ICDM, Information Systems, IEEE TKDE, DKE, ACM TOSEM, IEEE TSC, IEEE TSE, ToPNoC, ...
We remark that applications to other dissertation award initiatives are permitted.
The winner will receive the award at the ICPM’2020 Conference (see https://icpmconference.org/2020/ ).
The award comes with the option of publishing the thesis with Springer, in the LNBIP series.
Nomination and Submission
Candidates are nominated by their primary supervisor via a nomination letter. Each supervisor is only allowed to nominate one candidate. The candidate is responsible for submitting the application via Easychair: https://easychair.org/conferences/?conf=icpm2020 (selecting the “Best Process Mining PhD Dissertation Award” track).
The application consists of the following parts, which have all to be concatenated in a single PDF file (respecting the order):
- An extended abstract of the thesis, positioning the work in the state of the art and highlighting its main results, novelty, and (potential) impact [3-5 pages]
- A nomination letter by the supervisor [1-2 pages]
- The PhD evaluation report, including the reviews of the dissertation
- Full CV of the candidate, including the list of publications
- The dissertation itself
Questions and enquiries can be directed to the chair of the jury: Marco Montali, http://www.inf.unibz.it/~montali
Selection Process
The selection process consists of two steps:
- The jury evaluates the applications and identifies a shortlist of three candidates.
- The three candidates are invited to give a presentation to the jury in an online session. The jury then selects the winner.
The selection is based on the following criteria:
- originality and depth of contribution;
- methodological soundness;
- form and quality of presentation,
- significance and potential impact for the research field.
Important Dates
- Application Deadline : 10 June 2020
- Notification of Short-listing : 31 July 2020
- Online Presentations by Short-listed Candidates : End of August 2020 (Exact day will be negotiated with the short-listed candidates)
- Edition 2022
- Edition 2021
- Edition 2020
- Edition 2019
- Edition 2015
- Edition 2014
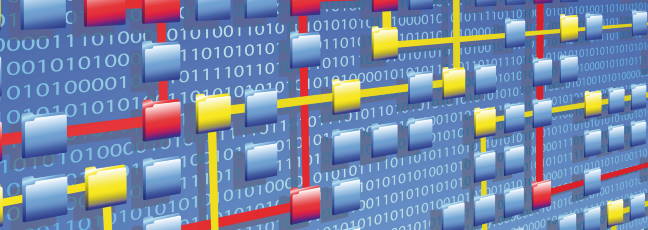
What is your profile?
In this menu you can find the most relevant resources based on the typical visitor profiles:
- Case studies
- Dissertation award
- BPI Challenge
Professional
- XES Standard
- Educational Programs
General public
- P.M. Manifesto
- skip navigation
- Privacy Policy
- search search
- Campusplan Campus Map

- Thesis Projects
- ECTS Approval
Process Mining in Manufacturing: A Causal AI Approach
- Subject: Process Mining in Manufacturing: A Causal AI Approach
- Type: Master's thesis
- Date: Vergeben
Joshua Holstein
Background:
The landscape of modern manufacturing industries is witnessing an exponential increase in the volume of data generated by machinery. This data, albeit a rich resource, is underutilized when it comes to refining production processes. Traditional data analysis often zeroes in on singular features and, in the process, overlooks the complex relationships and correlations among these features. This oversight leads to missed opportunities for enhancing efficiency and more accurate defect detection. The introduction of sophisticated methodologies such as process mining and causal AI aims to decipher these hidden correlations and provide actionable insights.
Research Goal:
In association with Bayer AG, the objective of this thesis project is to utilize process mining techniques and causal AI for an in-depth analysis of machine-generated data in the manufacturing context. The aim is not merely to identify correlations between data features but to interpret and articulate these relationships in a way that is beneficial to domain experts. To achieve this, you will engage with industry professionals through interviews to understand their perspectives and needs concerning this kind of analysis. The insights gained will then be implemented in a Python environment for further evaluation and testing.
In the course of this thesis, you will:
- Apply your theoretical understanding to address real-world manufacturing problems
- Explore advanced techniques such as process mining and causal AI
- Collaborate closely with domain experts in the effort to enhance the efficiency and environmental sustainability of manufacturing processes
We invite applications from those who:
- Have a strong interest in machine learning and data analysis
- Are self-driven and goal-oriented, eager to address contemporary real-world problems, and motivated to contribute original ideas
- Are comfortable engaging with professionals, for instance, through interview studies
- Possess excellent English language skills, as the thesis will be written in English
Start: Immediately
Duration: 6 months
Location: Bayer AG, Remote or nearby Grenzach-Whylen
We promise a challenging research topic, attentive mentorship, and an environment that fosters both practical and theoretical skills. Interested candidates should send their CV, transcript of records, and a brief letter of motivation to [email protected].
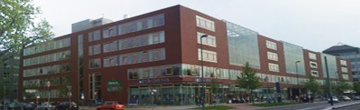
- Contact/Imprint
Humboldt-Universität zu Berlin - Faculty of Mathematics and Natural Sciences - Process Management and Information Systems
Bachelor and master thesis.
General Information
Our team offers bachelor and master thesis topics as well as student projects to be written in English.
There are two application windows in a year in which new topics are available. The first window is open from February 1st until April 1st. The second window is open from July 1st until October 1st. During these windows students can express their interest in a topic by sending an email Dr. Saimir Bala (firstname[dot]lastname[at]hu-berlin.de).
There are biannually info sessions where we explain the process of writing a thesis with our team. The next info session is scheduled for Februray 21 st , 2024 [zoom link we be available soon].
The last info session took place on September 28th, 2023. Here you can find the slides ( part I , part II ) and recordings ( part I , part II ) of previous info sessions .
Furthermore, find below a summary of guidelines for working on your thesis with us.
Process Overview
- There are two main time windows in which the team proposes new topics: Feb 1st – Apr 1st and Jul 1st – Oct 1st
- Within these windows students can apply for an open topic (see list of open topics below)
- Application is done by sending an email to Dr. Saimir Bala (firstname[dot]lastname[at]hu-berlin.de).
- We collect your applications and make a topic-student assignment in two rounds. First round on March, second round after the deadline.
- Once a student has been matched to a supervisor, a meeting is scheduled to scope the topic.
- Then, students must submit a research proposal to the supervisor within a month.
- If the proposal is graded as passed, the supervision is officially registered
- Once the thesis work is concluded, the thesis defense is scheduled within a dedicated defense slot.
Important Dates
09.02.2024: New topics released. Students can express their interest.
21.02.2024: Info session at 12:00 [Zoom link here ]
01.03.2024: Topic assignment (1st round)
01.04.2024: Expression of interest deadline
08.04.2024: Topic assignment (2nd round)
08.05.2024: Research proposal submission
15.05.2024: Official start (if proposal sufficient)
Please consider the following hints and guidelines for working on your thesis:
- Templates for thesis and proposal: https://www.informatik.hu-berlin.de/de/studium/formulare/vorlagen
- Page limits are as follows
- page limit is for Bachelor Informatik 40 pages and for Kombibachelor Lehramt Informatik 30 pages
- page limit is for Master Informatik 80 pages and for Master Information Systems 60 pages
- The limits do not include cover, table of content, references, and appendices.
Prerequisites
The candidate is expected to be familiar with the general rules of writing a scientific paper. Some general references are helpful for framing any thesis, no matter which topic:
- Wil van der Aalst: How to Write Beautiful Process and Data Science Papers? Archive Report (2022).
- Jan Recker: Scientific Research in Information Systems: A Beginner's Guide . Springer, Heidelberg, Germany (2021).
- Jan Mendling, Benoit Depaire, Henrik Leopold: Methodology of Algorithm Engineering . Archive Report (2023).
- Claes Wohlin, Pär Runeson, Martin Höst, Magnus Ohlsson, Björn Regnell, Anders Wesslén Experimentation in software engineering . Springer Science & Business Media (2012).
- Ken Peffers, Tuure Tuunanen, Marcus A. Rothenberger, Samir Chatterjee: A Design Science Research Methodology for Information Systems Research . J of Management Information Systems 24(3): 45-77 (2008).
- Barbara Kitchenham, Rialette Pretorius, David Budgen, Pearl Brereton, Mark Turner, Mahmood Niazi, Stephen G. Linkman: Systematic literature reviews in software engineering - A tertiary study . Information & Software Technology 52(8): 792-805 (2010).
- Lagendijk, Ad. Survival Guide for Scientists: Writing, Presentation, Email . Amsterdam University Press (2008).
- Adam LeBrocq: Journal of the Association for Information Systems Style Guide. https://aisel.aisnet.org/cais/cais_style_guide.pdf
In agreement with the supervisor an individual list of expected readings should be studied by the student in preparation of the actual work on the thesis.
The grading of the thesis takes various criteria into account, relating both to the thesis as a product and the process of establishing its content. These include, but are not limited to:
- Correctness of spelling and grammar
- Aesthetic appeal of documents and figures
- Compliance with formal rules
- Appropriateness of thesis structure
- Coverage of relevant literature
- Appropriateness of research question and method
- Diligence of own research work
- Significance of research results
- Punctuality of work progress
- Proactiveness of handling research progress
Recent Topics
If you are interested in one of the following topics, please send an email expressing your interest to Dr. Saimir Bala (firstname[dot]lastname[at]hu-berlin.de). Please explain why this topic is interesting for you and how it fits your prior studies. Also explain what are your strengths in your studies and in which semester of your studies you are.
Topic 1: Actionable Recommendation for Learner in Learning Management System based on Process Mining (Bachelor/Master)
This study explores the advantages of process mining in learning management systems to provide actionable recommendations to learners. By leveraging data-driven insights, it aims to enhance the learning experience by offering personalized guidance and suggestions to learners, ultimately improving their educational outcomes. This research delves into the potential benefits of process mining in the educational context, highlighting its capacity to empower learners on their educational journeys.
Initial References :
- Wambsganss, Thiemo; Schmitt, Anuschka; Mahnig, Thomas; Ott, Anja; Soellner, Sigitai; Ngo, Ngoc Anh; and Geyer-Klingeberg, Jerome, "The Potential of Technology-Mediated Learning Processes: A Taxonomy and Research Agenda for Educational Process Mining" (2021). ICIS 2021 Proceedings. 1. https://aisel.aisnet.org/icis2021/diglearn_curricula/diglearn_curricula/1
- AlQaheri, H.; Panda, M. An Education Process Mining Framework: Unveiling Meaningful Information for Understanding Students’ Learning Behavior and Improving Teaching Quality. Information 2022, 13, 29. https://doi.org/10.3390/info13010029
- Bala, S., Revoredo, K., Mendling, J. (2023). Process Mining for Analyzing Open Questions Computer-Aided Examinations. In: Montali, M., Senderovich, A., Weidlich, M. (eds) Process Mining Workshops. ICPM 2022. Lecture Notes in Business Information Processing, vol 468. Springer, Cham. https://doi.org/10.1007/978-3-031-27815-0_41
Supervisor: Rachmadita Andre Swari
Topic 2: Process discovery based on undesirable traces (Bachelor/Master)
Background : Process discovery techniques have been used to automatically learn a process model using observed traces (i.e., event logs). The traces are assumed correct and the final process model is expected to explain all the traces (i.e., desirable fitness of 1). However, the final model may also explain behaviors that are known by the specialist to be undesirable.
Research problem : The core research problem addressed is: How to learn a process model considering undesirable traces?
The aim is to propose a method to process discovery that learns using desirable and undesirable trace data.
Requirements : The candidate must have previous knowledge of process mining and software development. Further desirable requirements are pro-activity and self-organization. Initial Reference
- Revoredo, K.: On the use of domain knowledge for process model repair. Softw. Syst. Model. (2022)
Supervisor: Kate Cerqueira Revoredo
Topic 3: Context-aware process monitoring (Bachelor/Master)
Background : Business process monitoring is one of the phases of the BPM cycle concerned with extracting insights from the execution of a process. The digitization of the processes of an organisation has made available a vast amount of trace data about the execution of these processes, which allows for the use of data-driven process monitoring techniques. Given that, in many situations, it is not enough to just directly use the activities information present in the trace data of the process to achieve an accurate output, recent approaches have considered other sources of information combined with activities information, such as sensors data, or domain knowledge. However,, in most situations, additional data is used in a non-systematic way.
Research problem : The core research problem addressed is: How can contextual data be used for process monitoring? The aim is to propose a method to process monitoring using contextual data.
Requirements : The candidate must have previous knowledge of process mining and software development. Further desirable requirements are pro-activity and self-organization. Initial References:
- da Cunha Mattos, T., Santoro, F.M., Revoredo, K., Nunes, V.T.: A formal representation for context-aware business processes. Computers in Industry 65(8) (2014) 1193–1214
- Chamorro, A.E.M., Revoredo, K., Resinas, M., del-R ́ıo-Ortega, A., Santoro, F.M., Ruiz-Cort ́es, A.: Context-aware process performance indicator prediction. IEEE Access 8 (2020) 222050–222063
- Bayomie, D., Revoredo, K., Mendling, J.: Multi-perspective process analysis: Mining the association between control flow and data objects. In: CAiSE. Volume 13295 of Lecture Notes in Computer Science., Springer (2022) 72–89
Topics 4: Uses of Models in Agile Software Development (Bachelor/Master)
Motivation & problem : Modeling is a key topic in software engineering. In software development projects, among other aspects, modeling supports the developer in understanding the design by providing an overview and a tool for communication with fellow developers and other stakeholders. The benefits of models for supporting system analysis and design activities have been highlighted regarding their cognitive effectiveness, often in the context of traditional methodologies. However, these benefits have also been discussed in the agile scene, but it is still not clear to what extent models are used in agile software development projects.
Objectives : conduct a systematic review of the literature, identify the uses of models in agile software development, categorize and prioritize them, and propose a framework to support agile software development based on these findings. The findings shall be evaluated according to the perspective of practitioners.
Prerequisites : (1) Basic knowledge of agile software development methodologies; (2) Intermediate knowledge of models used in software development; (3) Pro-activity, self-organization, attention to detail (desirable).
Initial References:
- Ambler, Scott W. The object primer: Agile model-driven development with UML 2.0. Cambridge University Press, 2004.
- Alfraihi, Hessa Abdulrahman A., and Kevin Charles Lano. "The integration of agile development and model driven development: A systematic literature review." The 5th International Confrence on Model-Driven Engineeing and Software Development (2017).
- Wagner, Stefan, Daniel Méndez Fernández, Michael Felderer, Antonio Vetrò, Marcos Kalinowski, Roel Wieringa, Dietmar Pfahl et al. "Status quo in requirements engineering: A theory and a global family of surveys." ACM Transactions on Software Engineering and Methodology (TOSEM) 28, no. 2 (2019): 1-48.
- Petre, Marian. "UML in practice." In 2013 35th international conference on software engineering (icse), pp. 722-731. IEEE, 2013.
Supervisor: Cielo González Moyano
Topic 5: Artificial Intelligence in Project Management for Software Development Projects (Master)
Motivation & problem : Artificial intelligence is applied in software engineering management for taking decisions, estimating, managing technical debt, and planning, just to provide some examples. These applications have been widely studied by researchers. However, there is no study that presents a deep overview of how artificial intelligence is used for management activities in software development projects. Given the rising interest in artificial intelligence and the need of optimizing management in software projects, having a holistic overview can potentially be beneficial for practitioners and researchers.
Objectives : conduct a systematic review of the literature to identify the status quo on the topic. The findings shall be evaluated from the perspective of practitioners. The results shall be used to provide a framework that supports project managers of software development projects.
Prerequisites : (1) Basic knowledge of project management for software development projects; (2) Intermediate knowledge of artificial intelligence; (3) Pro-activity, self-organization, attention to detail (desirable).
Initial references:
- Perkusich, Mirko, et al. "Intelligent software engineering in the context of agile software development: A systematic literature review." Information and Software Technology 119 (2020): 106241.
- Kotti, Z., Galanopoulou, R., & Spinellis, D. (2023). Machine learning for software engineering: A tertiary study. ACM Computing Surveys, 55(12), 1-39.
- Fridgeirsson, Thordur Vikingur, et al. "An authoritative study on the near future effect of artificial intelligence on project management knowledge areas." Sustainability 13.4 (2021): 2345.
Topic 6: Literature Review on Business Intelligence and Human Factors (Bachelor or Master in Information Systems)
Motivation & problem : New types of analytical tools fundamentally change the way how process analysts do their work, with the expectation to drastically impact various professional services including auditing or business process management. Recent years have seen an increasing uptake of process mining tools by corporations and by professional services companies, where they are used to support the analysis of business processes. Recent research on the organisational impact of process mining highlights benefits for process awareness and overall value creation, but potential negative effects are hardly understood.
Primary objective : Review the literature on business intelligence and big data analytics and investigate where negative effects as discussed by Sutton et al (2023) and Parasuraman et al (2000) are discussed.
Prerequisites : (1) Basic knowledge of process mining; (2) Basic knowledge of business process management; (3) Interest in human-computer interaction and engineering psychology.
- Sutton, S. G., Arnold, V., & Holt, M. (2023). An extension of the theory of technology dominance: Capturing the underlying causal complexity. International Journal of Accounting Information Systems, 50, 100626.
- Grover, V., Chiang, R. H., Liang, T. P., & Zhang, D. (2018). Creating strategic business value from big data analytics: A research framework. Journal of management information systems, 35(2), 388-423.
- Parasuraman, R., Sheridan, T. B., & Wickens, C. D. (2000). A model for types and levels of human interaction with automation. IEEE Transactions on systems, man, and cybernetics-Part A: Systems and Humans, 30(3), 286-297.
- Zimmermann, L., Zebra, F., & Weber, B. (2023). What makes life for process mining analysts difficult? A reflection of challenges. Software and Systems Modeling, 1-29.
Supervisor: Jan Mendling
Topic 7: Visualizing Cyclic Time Arrangements in Process Graphs (Bachelor/Master)
Time is essential to understanding processes, yet most process mining approaches are limited to depicting time within a process graph as textual cues or color schemes. Adapting the visual appearance of process graphs to various time arrangements may enhance the accessibility for finding bottlenecks or delays. An example is aligning process graphs along a linear timeline [1]. In cases where processes involve repetitive patterns, such as in chronic health care or crop management, a cyclic arrangement may be useful. However, for the latter, an adequate solution in process mining is needed.
This thesis aims to develop and exemplify a design method for a visual solution in process mining that allows for exploring a cyclic time arrangement in a process graph. We will adapt the research objectives to align with the experience and study goals of the student.
- H. Kaur, J. Mendling, C. Rubensson, and T. Kampik, “Timeline-based Process Discovery,” CoRR, abs/2401.04114, 2024. Available: https://doi.org/10.48550/arXiv.2401.04114
- A. Yeshchenko and J. Mendling, “A Survey of Approaches for Event Sequence Analysis and Visualization using the ESeVis Framework.,” CoRR, abs/2202.07941, 2022. Available: https://arxiv.org/abs/2202.07941
- W. Aigner, S. Miksch, H. Schumann, and C. Tominski, Visualization of Time-Oriented Data. in Human-Computer Interaction Series. London: Springer London, 2011. Available: https://doi.org/10.1007/978-0-85729-079-3.
Supervisor: Christoffer Rubensson
Topic 8: Advanced Resource Analysis in Process Mining (Bachelor/Master)
In the last decade, process mining techniques have been developed to study human behavior in event data, such as the strength of collaboration between co-workers or even stress levels at a workplace. Since measuring human behavior is complex, this is a welcoming alternative to more labor-intensive methods like surveys. Still, most techniques are relatively simple but could be improved by applying theoretical frameworks from social science.
This thesis aims to develop a resource analysis approach (e.g., a metric, a concept, or a framework) in process mining grounded in an existing theory from social science. We will adapt the research objectives to align with the experience and study goals of the student.
- J. Nakatumba and W. M. P. van der Aalst, “Analyzing Resource Behavior Using Process Mining,” in Business Process Management Workshops. BPM 2009. Lecture Notes in Business Information Processing, S. Rinderle-Ma, S. Sadiq, and F. Leymann, Eds., Berlin, Heidelberg: Springer Berlin Heidelberg, 2010. Available: https://doi.org/10.1007/978-3-642-12186-9_8.
- A. Pika, M. Leyer, M. T. Wynn, C. J. Fidge, A. H. M. Ter Hofstede, and W. M. P. Van der Aalst, “Mining Resource Profiles from Event Logs,” in ACM Transactions on Management Information Systems, vol. 8, no. 1, 1:1-30, 2017. Available: https://doi.org/10.1145/3041218.
- Z. Huang, X. Lu, and H. Duan, “Resource behavior measure and application in business process management,” in Expert Systems with Applications, vol. 39, no. 7, 6458–6468, 2012. Available: https://doi.org/10.1016/j.eswa.2011.12.061.
Topic 9: Anthropomorphic Perceptions of Large Language Models: what is the gender of ChatGPT and its Counterparts? (Bachelor/Master)
Description : In today's digital era, Large Language Models (LLMs) like ChatGPT are transforming the way we interact with technology, often blurring the boundaries between machine and human cognition. This thesis delves into the intriguing realm of anthropomorphism, the human tendency to attribute human-like qualities to non-human entities. Specifically, this research aims to uncover laypeople's underlying beliefs and implicit conceptions about ChatGPT and similar models concerning an implicit gender attribution. By designing and conducting a survey, the thesis will gain insights into individuals' perception of these cutting-edge technologies. The findings can potentially illuminate not only our relationship with LLMs but also the broader implications of human-machine interactions in an increasingly AI-driven world.
- Deshpande, A., Rajpurohit, T., Narasimhan, K., & Kalyan, A. (2023). Anthropomorphization of AI: Opportunities and Risks (arXiv:2305.14784). arXiv. https://doi.org/10.48550/arXiv.2305.14784
- Farina, M., & Lavazza, A. (2023). ChatGPT in society: Emerging issues. Frontiers in Artificial Intelligence, 6. https://www.frontiersin.org/articles/10.3389/frai.2023.1130913
- Aşkın, G., Saltık, İ., Boz, T. E., & Urgen, B. A. (2023). Gendered Actions with a Genderless Robot: Gender Attribution to Humanoid Robots in Action. International Journal of Social Robotics, 15(11), 1915–1931. https://doi.org/10.1007/s12369-022-00964-0
Supervisor: Jennifer Haase
Topic 10: Process prediction using object-centric event log (Bachelor/Master)
Business process prediction involves forecasting specific details, such as the next activity to be performed, the time remaining for the completion of a process instance, or key process indicators, for an ongoing process instance. Currently, the techniques rely on XES event logs as input data. However, the field of process mining is shifting towards utilizing object-centric event logs, which offer a comprehensive multidimensional view of the data. Despite this advancement, object-centric event logs have been underutilized as input for process prediction.
Research problem: The core research problem addressed is: How can process prediction benefit from an object-centric event log? The aim is to propose a method to process prediction using object-centric event log.
Requirements: The candidate must have previous knowledge of process mining and software development. Further desirable requirements are pro-activity and self-organization.
Initial references
- An Empirical Investigation of Different Classifiers, Encoding, and Ensemble Schemes for Next Event Prediction Using Business Process Event Logs. ACM Trans. Intell. Syst. Technol. 11(6): 68:1-68:34 (2020)
- Uncovering Object-Centric Data in Classical Event Logs for the Automated Transformation from XES to OCEL. BPM 2022: 379-396
- Benedikt Knopp, Wil M. P. van der Aalst:Order Management Object-centric Event Log in OCEL 2.0 Standard. Zenodo, 2023
Supervisor: Kate Revoredo
Topic 11: Causation discovery for process prediction (Bachelor/Master)
Business process prediction involves forecasting specific details, such as the next activity to be performed, the time remaining for the completion of a process instance, or key process indicators, for an ongoing process instance. Currently, most techniques rely on the order in which the events happened without considering the cause-effect relation among them.
Research problem : The core research problem addressed is: How can process prediction benefit from the cause-effect relation among the events? The aim is to propose a method to discover the cause relation among events and use this information for process prediction.
Requirements: The candidate must have previous knowledge of process mining, statistics, and software development. Further desirable requirements are pro-activity and self-organization.
- An Empirical Investigation of Different Classifiers, Encoding, and Ensemble Schemes for Next Event Prediction Using Business Process Event Logs. ACM Trans. Intell. Syst. Technol. 11(6): 68:1-68:34 (2020)
- Jens Brunk, Matthias Stierle, Leon Papke, Kate Revoredo, Martin Matzner, Jörg Becker: Cause vs. effect in context-sensitive prediction of business process instances. Inf. Syst. 95: 101635 (2021)
- Pearl,J.(2011).Bayesiannetworks.
Topic 12: Literature review on quality characteristics in dashboards, business intelligence systems, balanced scorecards, and other reporting solutions: a study of visualization methods (Bachelor)
This bachelor thesis aims to conduct a comprehensive literature review on quality characteristics in dashboards, business intelligence systems, balanced scorecards, and other reporting solutions. The focus will be on comparing various visualization methods employed in these systems. The study intends to provide insights into the key features that contribute to the effectiveness and user satisfaction of visual reporting tools, helping to guide the selection and implementation of suitable solutions in diverse organizational contexts. Initial references :
- Burstein, F., & Holsapple, C. W. (2008). Handbook on Decision Support Systems 2. https://www.academia.edu/83497312/Handbook_on_Decision_Support_Systems_2
- Trieu, V.-H. (2023). Towards an understanding of actual business intelligence technology use: An individual user perspective. Information Technology & People, 36(1), 409–432.
- Webster, J., & Watson, R. T. (2002). Analyzing the past to prepare for the future: Writing a literature review. MIS Quarterly, xiii–xxiii.
Supervisor: Kristina Schneider
Topic 13: Analysis of theoretical explanations and scientific theories on transitioning from dashboards to decision making in organizational contexts (Bachelor)
This bachelor thesis seeks to analyze theoretical explanations and scientific theories concerning the transition from dashboards to decision-making processes. Dashboards are widely used tools in organizational contexts for decision-making. The study aims to examine the levels of management where dashboards are employed and how they contribute to the decision-making process within organizations. Initial references :
- Maynard, S., Burstein, F., & Arnott, D. (2001). A multi-faceted decision support system evaluation approach. Journal of Decision Systems, 10(3–4), 395–428.
- Mintzberg, H., Raisinghani, D., & Theoret, A. (1976). The Structure of “Unstructured” Decision Processes. Administrative Science Quarterly, 21(2), 246. https://doi.org/10.2307/2392045
Topic 14: Enhancing Student Engagement in Online Learning Environments through Process Mining (Bachelor/Master)
This study investigates how process mining techniques can be leveraged to enhance student engagement within online learning environments. It explores the utilization of data-driven insights to optimize learning pathways, identify patterns of student interaction, and design personalized interventions to foster greater engagement and participation in digital educational platforms.
- Rohani, N., Gal, K., Gallagher, M., Manataki, A. (2023). Discovering Students’ Learning Strategies in a Visual Programming MOOC Through Process Mining Techniques. In: Montali, M., Senderovich, A., Weidlich, M. (eds) Process Mining Workshops. ICPM 2022. Lecture Notes in Business Information Processing, vol 468. Springer, Cham. https://doi.org/10.1007/978-3-031-27815-0_39
- Umer, R., Susnjak, T., Mathrani, A. and Suriadi, S. (2017), "On predicting academic performance with process mining in learning analytics", Journal of Research in Innovative Teaching & Learning, Vol. 10 No. 2, pp. 160-176. https://doi.org/10.1108/JRIT-09-2017-0022
- Nkomo, L.M., Nat, M. Student Engagement Patterns in a Blended Learning Environment: an Educational Data Mining Approach. TechTrends 65, 808–817 (2021). https://doi.org/10.1007/s11528-021-00638-0
Topic 15: Runtime Prediction of Alignment Construction Algorithms (Bachelor/Master)
Conformance Checking relates a process model to recorded instances of the execution of the process, typically stored in event logs, to determine where expected and actual behaviour deviate from each other. In this context alignment algorithms are regarded as the de facto standard method, due to their interpretability and accuracy in highlighting precise problem areas in the process. Yet, typically run times for alignment construction are prohibitively large, typically caused by a handful of traces in the log, for which the construction of an alignment is especially complex. One possible solution to this problem could lie in predicting the expected runtime of aligning single traces in the log, for instance using regression-based methods and then ignoring traces, that are expected to take long.
In this thesis, the student will:
- derive a methodology for predicting the runtime of alignment construction between event logs and process models
- evaluate the accuracy of the predictor
- assess the factors that influence the runtime of alignments
The student is expected to have knowledge of process mining, conformance checking, and basic knowledge of regression analysis, or willingness to dive deep into these topics.
- Carmona, J., van Dongen, B., Solti, A., & Weidlich, M. (2018). Conformance checking. Switzerland: Springer.
- Backhaus, K., Erichson, B., Weiber, R., Plinke, W. (2016). Regressionsanalyse. In: Multivariate Analysemethoden. Springer Gabler, Berlin, Heidelberg.
Supervisor: Martin Kabierski
Topic 16: Biodiversity-based Saturation for Grounded Theory (Master)
Grounded theory is a research methodology usually applied in qualitative analysis. It involves the collection of data (usually through interviews, surveys, ...), and the deduction of concepts, categories, and ultimately theories that emerge from the collected data. A central question to this iterative data collection-evaluation process is when to stop collecting data. Usually one stops when the categories are saturated, i.e. when no new insights are obtained. Determining when exactly this point has been reached is a topic of discussion and research. Species richness estimators, that estimate the completeness of samples, could be utilized to give saturation estimates that are data-driven and grounded in statistics.
- assess the applicability of species richness estimation for determining saturation in grounded theory
- implement and apply the estimator to qualitative interview data
- evaluate the feasibility of the approach and discuss potential limitations
The student is expected to have a solid understanding of statistics and ideally preliminary experience in the analysis of qualitative data. Note, that the student is not expected to collect data for the thesis. These will be provided by us.
- Strauss, A., & Corbin, J. (1994). Grounded theory methodology: An overview. In N. K. Denzin & Y. S. Lincoln (Eds.), Handbook of qualitative research (pp. 273–285). Sage Publications, Inc.
- Saunders, Benjamin, et al. (2018). Saturation in qualitative research: exploring its conceptualization and operationalization. In: Qual Quant 52 (pp. 1893-1907). Springer
- Colwell, Robert K., et al. "Models and estimators linking individual-based and sample-based rarefaction, extrapolation and comparison of assemblages." _Journal of plant ecology_ 5.1 (2012): 3-21
HU on the internet
- Humboldt University on Facebook
- Die Humboldt-Universität bei BlueSky
- Humboldt University on Instagram
- Humboldt University on YouTube
- Humboldt University on LinkedIn
- RSS-Feeds of the Humboldt University
- Humboldt University on Twitter
- Help & FAQ
Multi-perspective process mining
- Process Analytics
Research output : Thesis › Phd Thesis 1 (Research TU/e / Graduation TU/e)
Bibliographical note
Promotion : time and place.
- 16:00, Auditorium, Senaatszaal
Access to Document
- 20180207_Mannhardt Final published version, 8.08 MB
Celonis - Thesis Award (PhD)
Mannhardt, Felix (Recipient), 2019
Prize : Other › Career, activity or publication related prizes (lifetime, best paper, poster etc.) › Scientific
Process Mining Dissertation Award 2019
Mannhardt, Felix (Recipient), Sept 2019
- Doctor of Philosophy 100%
T1 - Multi-perspective process mining
AU - Mannhardt, F.
N1 - Proefschrift
PY - 2018/2/7
Y1 - 2018/2/7
M3 - Phd Thesis 1 (Research TU/e / Graduation TU/e)
SN - 978-90-386-4438-7
T3 - SIKS dissertation series
PB - Technische Universiteit Eindhoven
CY - Eindhoven
Process Mining in Healthcare: Data Challenges When Answering Frequently Posed Questions
- Conference paper
- Cite this conference paper
- Ronny S. Mans 25 ,
- Wil M. P. van der Aalst 25 ,
- Rob J. B. Vanwersch 25 &
- Arnold J. Moleman 26
Part of the book series: Lecture Notes in Computer Science ((LNAI,volume 7738))
Included in the following conference series:
- International Workshop on Process-oriented Information Systems in Healthcare
- International Workshop on Knowledge Representation for Health Care
2156 Accesses
64 Citations
In hospitals, huge amounts of data are recorded concerning the diagnosis and treatments of patients. Process mining can exploit such data and provide an accurate view on healthcare processes and show how they are really executed. In this paper, we describe the different types of event data found in current Hospital Information Systems (HISs). Based on this classification of available data, open problems and challenges are discussed that need to be solved in order to increase the uptake of process mining in healthcare.
This is a preview of subscription content, log in via an institution to check access.
Access this chapter
- Available as PDF
- Read on any device
- Instant download
- Own it forever
- Compact, lightweight edition
- Dispatched in 3 to 5 business days
- Free shipping worldwide - see info
Tax calculation will be finalised at checkout
Purchases are for personal use only
Institutional subscriptions
Unable to display preview. Download preview PDF.
van der Aalst, W.M.P.: Process Mining: Discovery, Conformance and Enhancement of Business Processes. Springer, Berlin (2011)
MATH Google Scholar
Blum, T., Padoy, N., Feußner, H., Navab, N.: Workflow Mining for Visualization and Analysis of Surgeries. International Journal of Computer Assisted Radiology and Surgery 3, 379–386 (2008)
Article Google Scholar
Alves de Medeiros, A.K., van der Aalst, W.M.P.: Process Mining towards Semantics. In: Dillon, T.S., Chang, E., Meersman, R., Sycara, K. (eds.) Advances in Web Semantics I. LNCS, vol. 4891, pp. 35–80. Springer, Heidelberg (2008)
Google Scholar
Ghionna, L., Greco, G., Guzzo, A., Pontieri, L.: Outlier Detection Techniques for Process Mining Applications. In: An, A., Matwin, S., Raś, Z.W., Ślęzak, D. (eds.) ISMIS 2008. LNCS (LNAI), vol. 4994, pp. 150–159. Springer, Heidelberg (2008)
Günther, C.W., Rozinat, A., van der Aalst, W.M.P., van Uden, K.: Monitoring Deployed Application Usage with Process Mining. BPM Center Report BPM-08-11, BPMcenter.org (2008)
Gupta, S.: Workflow and Process Mining in Healthcare. Master’s thesis. Eindhoven University of Technology, Eindhoven (2007)
Han, B., Jiang, L., Cai, H.: Abnormal Process Instances Identification Method in Healthcare Environment. In: Proceedings of IEEE TrustCom 2011, pp. 1387–1392 (2011)
Haux, R., Winter, A., Ammenwerth, E., Brigl, B.: Strategic Information Management in Hospitals: An Introduction to Hospital Information Systems. Springer, Berlin (2004)
Book Google Scholar
Lang, M., Bürkle, T., Laumann, S., Prokosch, H.-U.: Process Mining for Clinical Workflows: Challenges and Current Limitations. In: Proceedings of MIE 2008. Studies in Health Technology and Informatics, vol. 136, pp. 229–234. IOS Press (2008)
Lenz, R., Reichert, M.: IT Support for Healthcare Processes - Premises, Challenges, Perspectives. Data and Knowledge Engineering 61, 49–58 (2007)
Mans, R.S., Schonenberg, M.H., Leonardi, G., Panzarasa, S., Quaglini, S., van der Aalst, W.M.P.: Process Mining Techniques: An Application to Stroke Care. In: Proceedings of MIE 2008. Studies in Health Technology and Informatics, vol. 136, pp. 573–578. IOS Press (2008)
Mans, R.S., Schonenberg, M.H., Song, M.S., van der Aalst, W.M.P., Bakker, P.J.M.: Application of Process Mining in Healthcare: a Case Study in a Dutch Hospital. In: Fred, A., Filipe, J., Gamboa, H. (eds.) BIOSTEC 2008. CCIS, vol. 25, pp. 425–438. Springer, Heidelberg (2009)
Perimal-Lewis, L., Qin, S., Thompson, C., Hakendorf, P.: Gaining Insight from Patient Journey Data using a Process-Oriented Analysis Approach. In: HIKM 2012. Conferences in Research and Practice in Information Technology, vol. 129, pp. 59–66. Australian Computer Society, Inc. (2012)
Poelmans, J., Dedene, G., Verheyden, G., Van der Mussele, H., Viaene, S., Peters, E.: Combining Business Process and Data Discovery Techniques for Analyzing and Improving Integrated Care Pathways. In: Perner, P. (ed.) ICDM 2010. LNCS, vol. 6171, pp. 505–517. Springer, Heidelberg (2010)
Chapter Google Scholar
Rada, R.: Information Systems and Healthcare Enterprises. IGI Global (2008)
Torres Ramos, L.: Healthcare Process Analysis: Validation and Improvements of a Data-based Method using Process Mining and Visual Analytics. Master’s thesis. Eindhoven University of Technology, Eindhoven (2009)
Rebuge, A., Ferreira, D.R.: Business Process Analysis in Healthcare Environments: A Methodology Based on Process Mining. Information Systems 37(2) (2012)
Riemers, P.: Process Improvement in Healthcare: a Data-based Method using a Combination of Process Mining and Visual Analytics. Master’s thesis. Eindhoven University of Technology, Eindhoven (2009)
Treekoverleg. TR-039, Notitie Streefnormstelling Wachttijden Curatieve Sector (2000) (in Dutch)
van der Werf, J.M.E.M., Verbeek, H.M.W., van der Aalst, W.M.P.: Context-Aware Compliance Checking. In: Barros, A., Gal, A., Kindler, E. (eds.) BPM 2012. LNCS, vol. 7481, pp. 98–113. Springer, Heidelberg (2012)
Zhou, J.Y.: Process mining: Acquiring Objective Process Information for Healthcare Process Management with the CRISP-DM Framework. Master’s thesis. Eindhoven University of Technology, Eindhoven (2009)
Download references
Author information
Authors and affiliations.
Department of Information Systems, Eindhoven University of Technology, P.O. Box 513, NL-5600 MB, Eindhoven, The Netherlands
Ronny S. Mans, Wil M. P. van der Aalst & Rob J. B. Vanwersch
Department of Quality Assurance and Process Innovation, Academic Medical Center, University of Amsterdam, Amsterdam, The Netherlands
Arnold J. Moleman
You can also search for this author in PubMed Google Scholar
Editor information
Editors and affiliations.
Dept. of Computer Science, Data Management, University of Erlangen and Nuremberg, Martensstr. 3, 91058, Erlangen, Germany
Richard Lenz
Institute of Software Technology and Interactive Systems, Information and Software Engineering Gruop, Vienna University of Technology, Favoritenstr. 9-11, 1040, Vienna, Austria
Silvia Miksch
Faculty of Social Sciences, Dept. of Information Systems, University of Haifa, Rabin Bldg., room 7049, 31905, Haifa, Israel
Institute of Databases and Information Systems, Ulm University, Building 027 # room 524, James-Tranck-Ring, 89069, Ulm, Germany
Manfred Reichert
Dept. d’Enginyeria Informàtica i Matimàtiques, Research Group on Artificial Intelligence, Universitat Rovira i Virgili, Av. Països Catalans 26, 43007, Tarragona, Spain
David Riaño
Dept. of AI, Knowledge Representation and Reasoning Gruop, Vrije Universiteit Amsterdam, De Boelelaan 1081A, 1081HV, Amsterdam, The Netherlands
Annette ten Teije
Rights and permissions
Reprints and permissions
Copyright information
© 2013 Springer-Verlag Berlin Heidelberg
About this paper
Cite this paper.
Mans, R.S., van der Aalst, W.M.P., Vanwersch, R.J.B., Moleman, A.J. (2013). Process Mining in Healthcare: Data Challenges When Answering Frequently Posed Questions. In: Lenz, R., Miksch, S., Peleg, M., Reichert, M., Riaño, D., ten Teije, A. (eds) Process Support and Knowledge Representation in Health Care. ProHealth KR4HC 2012 2012. Lecture Notes in Computer Science(), vol 7738. Springer, Berlin, Heidelberg. https://doi.org/10.1007/978-3-642-36438-9_10
Download citation
DOI : https://doi.org/10.1007/978-3-642-36438-9_10
Publisher Name : Springer, Berlin, Heidelberg
Print ISBN : 978-3-642-36437-2
Online ISBN : 978-3-642-36438-9
eBook Packages : Computer Science Computer Science (R0)
Share this paper
Anyone you share the following link with will be able to read this content:
Sorry, a shareable link is not currently available for this article.
Provided by the Springer Nature SharedIt content-sharing initiative
- Publish with us
Policies and ethics
- Find a journal
- Track your research
- Utrecht University Student Theses Repository Home
- UU Theses Repository
Care Mining: An application of Process Mining in Value-Based Healthcare
Publication date
Collections.
Javascript is required
You must have JavaScript enabled in order to access the Celonis website. The web browser you're using either doesn't support JavaScript, or it's being blocked. Please check your browser's help resources to learn whether your browser supports JavaScript and how to allow JavaScript.
Celonis Student Thesis and Research (STaR) Program
We highly welcome bachelor or master students, or doctoral candidates interested in writing their thesis, project study, developer study or research seminar papers on process mining and related aspects. It is our mission to empower the next generation of process miners, and this means that we aim to support you and your research as well as we can.
We believe that process mining can make an impact anywhere in the world, and in almost any discipline. Therefore, we explicitly encourage students of all nationalities and backgrounds to join our Celonis STaR Program!
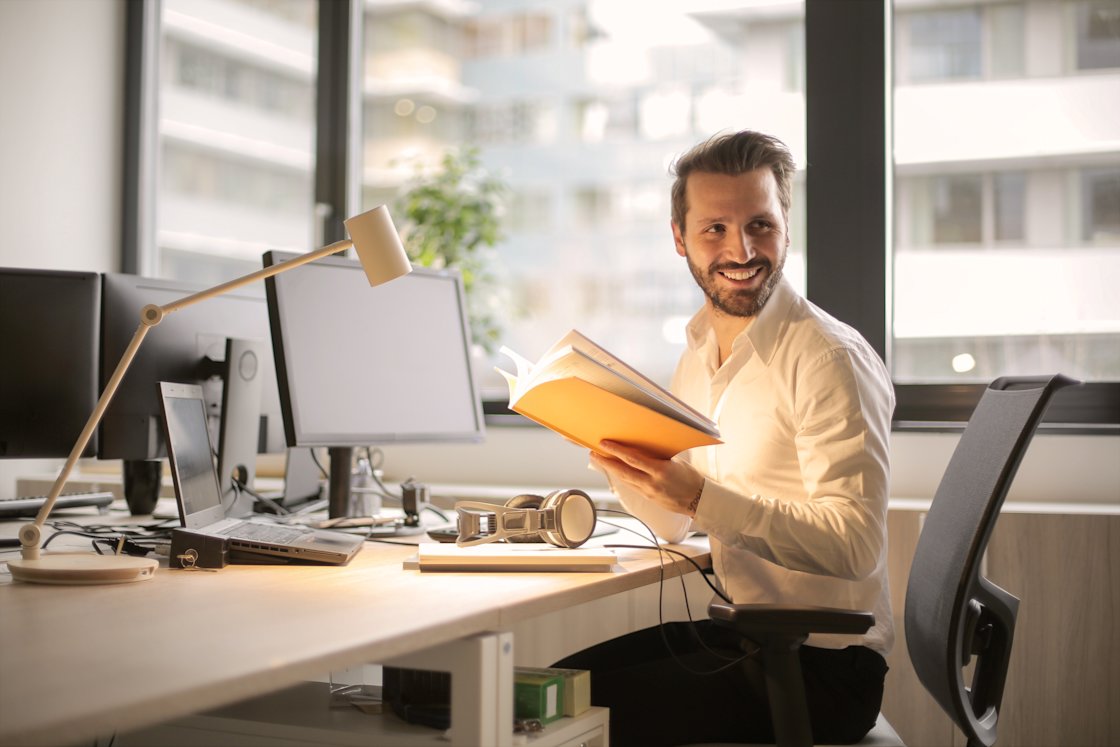
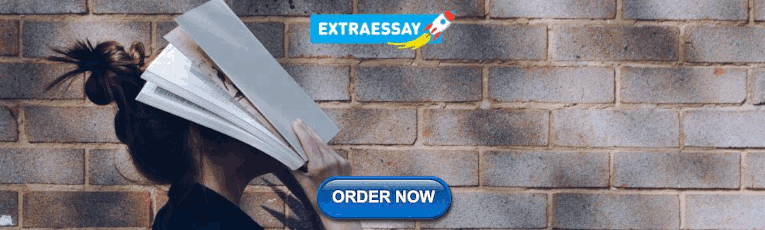
Join the Celonis STaR Program
Sign up for the Celonis STaR Program by completing the sign-up form below and receive all the information you may need to successfully write your thesis, project or developer study or research seminar papers:
Directions on how to get your hands on the academic version of the Celonis Software for free
Training and material on how to use, modify, work and upload data within our Celonis Software in the Celonis Academy
Access to our vibrant community of process mining enthusiasts on our Celopeers Forum
Help from our Academic Research & Innovation Coordinator
Moreover, if you need additional resources from our side, such as access to further experts, interviews, or if you are interested in starting a project with another academic partner or one of our customers, we could help you. In this case, we need a One-Page pitch from you, explaining what you need from our side, the scientific excellence of the topic, and its relevance for Celonis (and/or other involved stakeholders). You will receive a template for this pitch after signing up for Celonis STaR Program. The pitches are evaluated internally at least twice a month, and you will hear from us shortly.
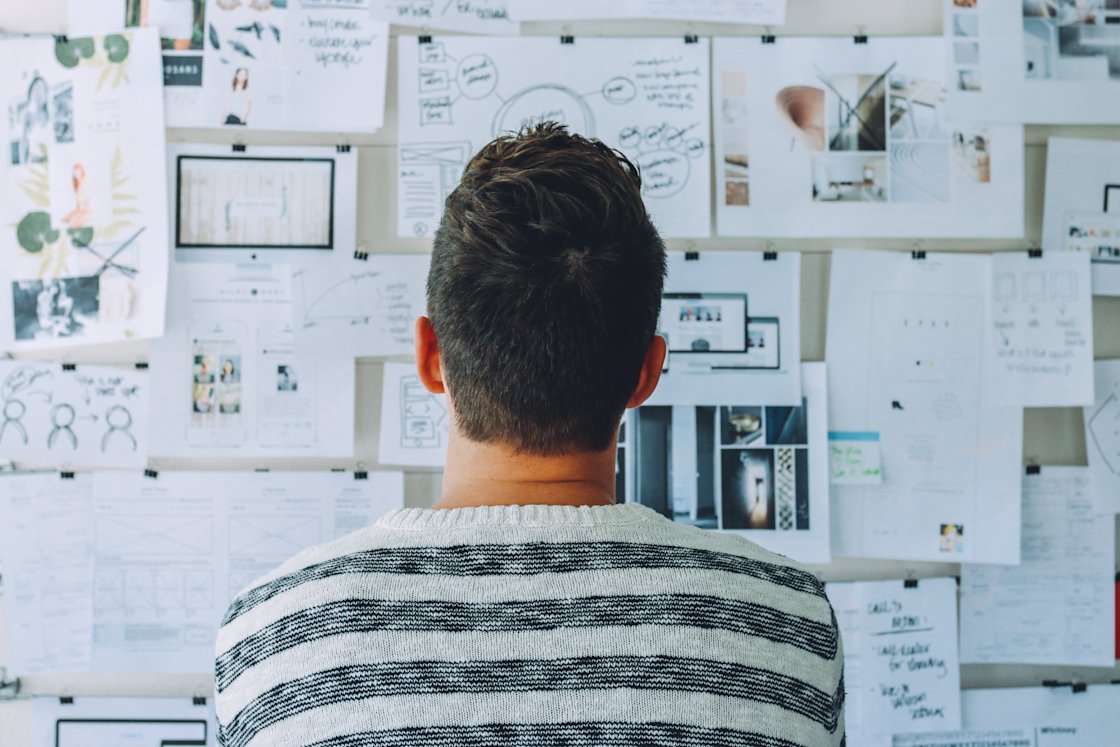
Sign up here for the Celonis STaR Program!
Sign up here to receive instant access to our resources.
Frequently Asked Questions
Are there any limitations as to which countries students can come from or which universities they are working with.
We especially encourage students from outside of Europe to take part in the Celonis STaR Program. All our materials are available in English.
However, Celonis is legally unable to transact (directly or indirectly) with the following countries: Cuba, Iran, North Korea, Sudan, and Syria. In addition, Celonis does not do business in Russia. If the institution you are studying/working at or with is located in/belongs to one of these countries, then you will need to wait until the situation changes to apply for Celonis STaR Program.
Can I schedule a personal meeting with the Research & Innovation Coordinator?
Due to capacity reasons, we do not schedule calls with students. For every question you have regarding your project with Celonis, please first read the FAQs and the information and instructions we have given you in our welcome package (you get this after signing up for our STaR program).
If you have questions that are not answered by the FAQs, please check our Celonis Community (you will get the information how to engage here in your welcome letter after signing up)
If you have still not received the information you are looking for, you can write an email to [email protected] and state as a Subject “STaR Program + (topic of your question)”.
- Please tell us specifically what your question is and where you need support. Our team will then get back to you. Because we get many requests, following up on your request can take up some days.
Can you help me find an academic supervisor?
If you already know your topic but do not have an academic supervisor yet, we can check within our network whether we know a suitable researcher who can support you. We cannot promise you that we will find somebody, but in most cases this is feasible. It depends on your university whether external supervisors are accepted.
Please note that all communication and the checking of all regulations is in your responsibility. We can only support in making the first introduction.
Do I need to sign any documents to work with you on my project? What about Data Declarations and Non-Disclosure?
If you need to sign a Non Disclosure Agreement (NDA) depends on your topic. If you only use our standard material and don’t need anything further you don’t need to sign any documents.
If you need access to internal information at Celonis and your thesis contains confidential information (technical data, customer data), you have to sign a Non-Disclosure-Agreement (NDA).
If your project will contain this internal information, your academic supervisor will have to sign an NDA as well, and the project must not be published.
Please also check the Thesis Guidelines in your Welcome Letter for specific information on Non-Disclosure Agreements and Data Security.
How can Celonis support my project?
Celonis can provide researchers and thesis students free access to the academic version of our software and help you analyze your own data. We can provide our expertise and help you get started by giving you access to our digital training platform. We will also connect you with fellow students and researchers through the Celonis community platform. This support is entirely provided online by signing up to our thesis program.
Please do not contact us directly, but first follow the steps of this program. In some cases, we can also provide further support such as contact to experts or interviews. In these cases, we perform a pre-selection of relevant topics through a pitch, for which you can access a template after registration.
How do I find a suitable topic?
You as a student are responsible for finding a topic you want to research on. As a company we have no resources to guide you in finding a research topic. Please frame your topic in agreement with your academic supervisor. If you are writing your thesis with a company, please also make sure that the research topic is in line with your company supervisor.
How closely are you working with external students on their projects?
External students (those not working at Celonis) need to be mainly supervised by their academic and/or company supervisors. We do not have the capacity to support every project extensively.
I am a working student at a company and I would like to use the Celonis Academic Intelligent Business Cloud for my project, which is at the same time a commercial project at the company I work for. Is this possible?
Yes, but only if you use the academic version of our software just for the scope of the project, and only you personally have access to the Celonis account. Any primarily commercial use of our academic version is prohibited, as stated in the terms of use.
Is it also possible to write a doctoral/PhD thesis with support by Celonis?
Yes! If you want to write your PhD Thesis using Celonis materials, please sign up for our STaR programme. You may book an appointment with our Research & Innovation Coordinator, who will be able to discuss the scope of our involvement. However, we do not give out any PhD scholarships or working positions only with the purpose of writing a PhD thesis.
Is there a possibility to work with Celonis as a working student while I write my thesis?
At the moment, we do not offer working student positions only for the purpose of writing a thesis. However, you can check our career page for open internship or working student positions and then contact us to find out if a combination with your thesis topic is possible.
What does the Celonis STaR Program cover and what not?
What we provide after you have signed up for our Thesis Program:
- We provide students and researchers (after signing up for our STaR program) with free access to our Celonis Software the Execution Management System (EMS).
- We provide free access to our Online Academy and train you to become a process mining expert.
- Within our StaR program package you also get access to some literature and our Celosphere Conference contents.
- If you need further support e.g. interviews, use cases etc. you can apply for this by handing in a pitch for your project. All information on this is coming with our welcome package after signing up for the program.
- We connect you to other students via our Celonis Community.
- We provide you with all information you need to do your project using the Celonis Software with our welcome package and the FAQs that are regularly updated.
- If questions are not covered by the FAQs you can check out our Celonis Community (you will get the information how to access with your welcome letter after you signed up for the Thesis Program).
- If your question is still open after those steps, email us via [email protected] and state as a Subject “STaR Program + (topic of your question).
What we do not provide. Due to capacity reasons we can not…
- support you if you have not signed up for our STaR Program.
- help you find a topic.
- help you frame your project - unless you are working at Celonis (please reach out to your academic supervisor for topic collection and framing).
- provide personal calls (does not apply for people working at Celonis).
- provide you with interviews or data (unless you apply for support via a thesis pitch - only the best students with a high impact for Celonis will be accepted).
- proofread your paper.
- answer any question that is already answered by the FAQs.
Subscribe to our newsletter
Insights to inbox - Monthly newsletter
Thank you for your subscription
Introduction to celonis process mining demo.
Learn the basics of Celonis Process Mining in just 20 minutes.
- Artificial Intelligence
- Generative AI
- Business Operations
- Cloud Computing
- Data Center
- Data Management
- Emerging Technology
- Enterprise Applications
- IT Leadership
- Digital Transformation
- IT Strategy
- IT Management
- Diversity and Inclusion
- IT Operations
- Project Management
- Software Development
- Vendors and Providers
- Enterprise Buyer’s Guides
- United States
- Middle East
- España (Spain)
- Italia (Italy)
- Netherlands
- United Kingdom
- New Zealand
- Newsletters
- Foundry Careers
- Terms of Service
- Privacy Policy
- Cookie Policy
- Copyright Notice
- Member Preferences
- About AdChoices
- Your California Privacy Rights
Our Network
- Computerworld
- Network World

Process mining helps IT leaders modernize business operations
With a blend of data science and process improvement, cios are mining data to transform business processes for efficiency, improved resource allocation, and better results..

Following a legislative review of state purchases in fiscal year 2022, the state of Oklahoma discovered that its agencies had procured more than $3 billion worth of goods and services outside the oversight of its Office of Management and Enterprise Services (OMES) Central Purchasing division.
“Understandably, state leaders were concerned,” says CIO Joe McIntosh. “The legislative review identified issues in the procurement process that created gaps in transparency and accountability. As a result, taxpayer dollars were being spent without appropriate checks and balances.”
To address the problem, the Legislative Office of Fiscal Transparency and the state governor tasked OMES with increasing oversight of state purchasing to maintain proper compliance with state laws and regulations.
“One of our key directives was to audit procurement for all state agencies by the end of the calendar year 2023,” McIntosh says. “To achieve that, we needed a cost-effective solution that could streamline workflows, provide a robust view of procurement processes in real-time, identify rogue spending, and enable us to audit all agencies by the Dec. 31 deadline.”
To do that, McIntosh and OMES turned to process mining, a technique for analyzing event data to better understand and improve operational processes. Process mining encompasses both data science and process management, providing a way to analyze digital footprints and event logs so that organizations can identify bottlenecks and other issues in core business processes.
Process mining on the rise
Operational efficiency remains a top CIO concern, and with the rise of digitization, redesigning business processes is increasingly becoming a key strategic initiatives for CIOs. One in three respondents to the 2024 State of the CIO survey said they anticipated spending more time revamping business processes compared to other IT activities within the next three years, up from 25% today. That figure polled No. 2 behind driving business innovation as CIOs’ most anticipated focus beginning next year.
To tackle that issue, some early movers, like Oklahoma’s McIntosh, are turning to process mining platforms to help achieve their goals.
Process mining provides the potential to enable organizations make quicker, more informed decisions when overhauling business processes by leveraging data for insights. By using the information gleaned from process mining, companies can better streamline workflows, enhance resource allocation, and automate repetitive tasks.
And the market for process mining tools is growing. According to a November 2023 report by global market research and management consulting company Global Market Insights, the process mining market is estimated to expand at a compound annual growth rate of more than 40% over the next decade, reaching $31.52 billion by 2032.
Among the key growth factors are increased demand for process efficiency and the integration of artificial intelligence into process mining solutions. AI enhances process mining by automating complex data analysis, uncovering intricate patterns, and predicting process behavior, according to the report.
“The push for efficiency, automation, and insight are driving leading companies to process mining,” says Ray Wang, principal analyst and founder of Constellation Research. “The demand is coming from companies [that] have been told by strategy firms that they must cut costs to preserve margins. Once these projects are in place, there is a realization that process mining is the foundation for AI-driven process orchestration and automation.”
Organizations, like OMES, that have deployed process mining are seeing significant gains.
Modernizing with purpose
In June 2023, Oklahoma began using process mining tools from Celonis to analyze procurement processes based on purchasing laws and massive amounts of financial data.
“As a government agency, it’s our responsibility to identify where things are slipping through the cracks and correct them as quickly and effectively as possible,” McIntosh says.
Among the goals were to gain greater insight into procurement, slash maverick spending, cut audit times, and reduce legal and financial risks to the state.
“With these tools, we’ve gained real-time insights into how taxpayer dollars are being spent, specifically through reviewing purchase orders and purchase card transactions,” McIntosh says. “This approach offers a comprehensive view of purchasing activities and notifies us of deviations, critical to ensuring compliance.”
Process mining has helped the state address decades-old problems that many government agencies are familiar with, including outdated approaches with data scattered across various systems, emails, spreadsheets, and written documents; limited resources for reviewing purchase orders; and complex processes, with 122 agencies using various purchasing procedures and approvals.
With the Celonis process mining platform in place, OMES has a consolidated dashboard of financial and procurement data that can map the trajectory of purchases to ensure everything is on track and in compliance, McIntosh says. “OMES was able to review more than 16,000 purchase orders valued at over $2.26 billion in a matter of weeks,” he says.
Previously, processing this amount of data would be tedious and require the state to contract about 45 people. “Now, OMES can do much more in a significantly shorter timeframe, saving us thousands of hours in manual labor and tens of thousands of tax dollars in wages,” McIntosh says. “Plus, this allows us to redirect our workforce to other areas where they’re needed.”
In less than 12 weeks, the platform enabled OMES to oversee 100% of spending, reviewing more than 24,000 purchase orders worth $4.58 billion.
“Process mining has been a great asset to the state, helping to drive efficiencies and — perhaps more importantly — transparency, which is crucial for accountability and compliance,” McIntosh says. “The state now has the tools to make data-informed decisions that ultimately benefit taxpayers, who want to see their money put toward improving their lives, like schools, parks, libraries, and police and fire departments. That isn’t possible without a comprehensive view of the state’s procurement activities.”
Streamlining freight operations
International freight forwarding and logistics company Albini & Pitigliani S.p.A. (ALPI) is also benefiting from process mining.
The company began using a platform from Apromore in late 2022, with the goals of identifying steps in processes that were taking the longest or that were causing the most errors; measuring the effectiveness of process changes implemented; gaining a competitive advantage by improving processes; and improving customer satisfaction by reducing process time and errors.
“Our initial approach to process mining focused on analyzing the end-to-end process within our main Transport Management System [TMS],” says Antonio Mortello, technology manager at ALPI. “The goal was to identify where our colleagues were encountering issues and delays, even if they were not consciously aware of them.”
The analysis posed several challenges. For one thing, there was more than one flow across many stages of the process. In addition, it was necessary to understand the unique characteristics of each specific route. And some operational staff were hesitant to adopt changes.
“To tackle these issues, we began by examining the TMS logs to map and categorize all the stored information, aiming to add a process mining layer to our activities,” Mortello says. “We analyzed traffic patterns to identify areas with a more linear process flow, which helped us focus our efforts.”
After these initial steps, the IT team designed a flow and a dashboard for compliance analysis across three traffic types. “We used these dashboards to track key performance indicators [KPIs] relevant to our area managers,” Mortello says. “This process provided valuable insights into our operations, allowing us to pinpoint the most time-consuming steps, whether due to waiting times from third parties or reworks.”
The next focus is on streamlining the process flow with external offices and identifying opportunities to automate steps in information exchange. “By addressing these areas, we hope to achieve a smoother operational flow and improve overall efficiency,” Mortello says.
Using the Apromore platform, ALPI’s IT team effectively identified the root causes of problems, created operational process digital twins to model and test simulations, and worked with subject matter experts to implement changes that resulted in a 75% reduction in errors in fulfillment by improving communication between different departments, a 15% increase in sales through reducing the number of steps in the sales process, and a 20% increase in customer satisfaction, among other benefits.
Process mining tips
Successful deployment and maintenance of process mining requires a clear vision from the management team and board, Mortello says, as well as commitment and persistence.
“Process mining doesn’t usually yield immediate, tangible results, but it can offer unique insights into how a company operates,” he says. “A leadership team with a long-term vision is crucial to ensure the technology is utilized to its full potential.”
It’s also important to thoroughly analyze processes prior to “fixing” them. “Make sure you have a good handle on the process you think you have and the ones you really have,” Constellation Research’s Wang says. “What we see across the board is a quick realization that what’s assumed and what’s done is very different.”
Another good practice is to create a bridge between the technical team implementing process mining and the operational team being analyzed, Mortello says. “This ‘middleware’ role helps ensure that both sides speak the same language and understand the purpose of process mining,” he says. “It’s essential to communicate to the operational team that process mining is a tool designed to help them improve processes, not to monitor their daily activities.”
It’s also important to foster a culture of continuous improvement. “This means encouraging the operational teams to provide feedback and suggest enhancements,” Mortello says. “By creating an environment where the target team feels empowered to contribute ideas, you can turn process mining from a purely analytical tool into a collaborative solution.”
This continuous feedback loop helps ensure that the technology evolves to meet the needs of the people using it, leading to more effective and sustainable outcomes, Mortello says.
One of the most important factors for success is to work closely with the process mining vendor, even after the platform has been deployed, OMES’s McIntosh says.
“In general, I believe it’s very important to work with a process mining supplier that doesn’t just sell you software,” he adds. Organizations need to work with technology partners that are invested in their success, understand their goals, and are willing to work with them to make corrections as needed, he says.
“Also, when deploying process mining, public sector agencies and companies should have a clear vision of what they want to achieve,” McIntosh says. “Our goal was to increase transparency and save taxpayer dollars wherever possible. From there, we had our priorities mapped out: get better insight into procurement, reduce wasteful spending, and accelerate audit times.”
Process mining is like many other technology tools in that organizations can get more out of it if they are more strategic about how they use it, McIntosh says. “It isn’t a problem-solving silver bullet, so to speak, for all things,” he says. “However, it’s a great tool that helps to simplify things in ways that couldn’t be done otherwise.”
When selecting a process mining platform, look for one that offers flexibility in end-to-end process mining discovery, from preparing the data to visualizing it, Mortello says. “This flexibility was crucial for us, as it allowed our team to tailor the process according to our unique needs and challenges.”
ALPI was able to customize and control every step of the process. “We wanted a tool that provided us with the licenses and rights to manage the process independently, without being restricted by external constraints or additional costs for customization,” Mortello says.
Related content
It leaders’ ai talent needs hinge on reskilling, skills the irish government cio uses to advance digital transformation, 3 reasons you should adopt cloud monitoring, faultless with serverless: cloud best practices for optimized returns, from our editors straight to your inbox.

Bob Violino is a freelance writer who covers a variety of technology and business topics.
More from this author
Oracle makes its pitch for the enterprise cloud. should cios listen, the early returns on gen ai for software development, 6 best practices for better vendor management, most popular authors.

Show me more

How Italian CIOs produce value with gen AI

CIO Bhavani Amirthalingam on driving change in the AI era

Rimini Street’s Eric Helmer and the top questions to ask about vendor support

CIO Leadership Live India with Partha Protim Mondal, CIO, Berger Paints

CIO Leadership Live Middle East with Nani M. Butti, Bahrain Supreme Council for Youth & Sports

Learn the top challenges cybersecurity leaders face in 2024

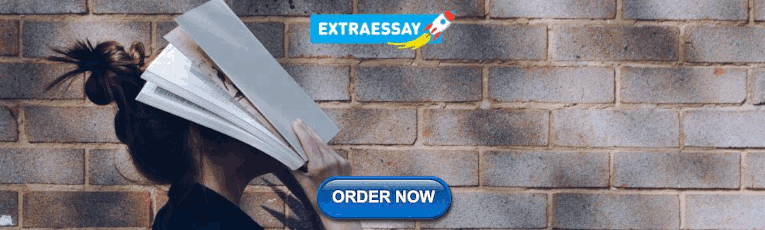
IMAGES
VIDEO
COMMENTS
The thesis studies process mining and investigate existing applications to understand process mining capabilities and industry best practices to implement process mining. The thesis aims to identify how a company can initiate process mining and scale it on enterprise-level and what elements are needed to operate it successfully. ...
This thesis will introduce the most important process mining techniques and apply them to uses cases that are based on real life event data. Three process mining tools, ProM, Disco and Celonis, will be introduced and used to apply process mining techniques. 1. Introduction.
implementation of Industry 4.0 and study how Process Mining technologies can help to achieve it. The purpose of this study is to open a debate and discuss why companies that look forward to become Industry 4.0 standards might not be benefiting from technologies such as Process Mining, which can facilitate their journey.
This Master thesis is the result of 8 months of research on process mining, process performance, and the intriguing world where business and IT meet. I would not have been able to complete my master thesis project without the help and guidance of a lot of people, and therefore I would like to take the time to thank them (again).
Purpose - The purpose of this paper is to present a comparison of a number of business process mining tools currently available in the UK market. An outline of the practice of business process ...
Abstract ii Abstract Context: Process mining is an approach that exploits event logs to discover real processes executed in organizations, enabling them to (re)design and improve process models. Goal modelling, on the other hand, is a requirements engineering (RE) approach mainly used to
Through process mining it is possible to (i) discover the process that better describes the user behavior, (ii) nd useful insights, (iii) discover and compare the processes of di erent behavi-oural clusters of users. Moreover, this thesis aims to make a second contribution by bridging the gap between process mining and recommender systems worlds.
lenges to the problem of process mining. Using the Design Science Research Methodology, this thesis introduces a new method to study, discover, and analyze cloud-based application processes using process mining tech-niques. It explores the applications and known challenges related to process mining in
assignment is to use process mining as the replacement tool. In this thesis, a new methodology for benchmarking business processes by means of process mining is proposed. Towards designing the new methodology, two high-level methodologies, one for benchmarking projects and one for process mining projects, were combined into one.
Katri Tuunanen: Supporting implementation and use of process mining Master's Thesis Tampere University Master's Programme in Information and Knowledge Management November 2021 In competitive and continuously changing business environments, companies have a constant
Currently, the process mining aims at an automatic extraction of process knowledge from the event logs recorded by information systems, and, therefore, by using these techniques, it becomes possible to grasp the complex nature of industrial processes. In fact, most of the industrial processes change over time, and through the process mining ...
Chapter 1 Introduction This thesis is the result of a graduation project carried out within Fluxicon1 and the Architecture of Infor- mation System (AIS) group2 of the Mathematics and Computer Science Department of TU/e. The goal of the project was the evaluation of commercial process mining systems based on a defined set of criteria.
Process Mining Dissertation Award. The IEEE Task Force for Process Mining is happy to announce the 2020 edition of the Best Process Mining PhD Dissertation Award. The award will be delivered during the 2nd International Conference on Process Mining (ICPM2020), 5-8 October 2020.
This thesis analyses problems related to the applicability, in business en-vironments, of Process Mining tools and techniques. The first contribution reported in this thesis consists in a presentation of the state of the art of Process Mining and a characterization of compa-nies, in terms of their "process awareness". The work continues ...
Another methodological contribution of this thesis is our analysis of the problems typically faced when applying process mining to flexible environments. When event logs from flexible processes are analyzed by traditional process mining algorithms, they typically yield large, highly unstructured, and essentially useless "spaghetti" models.
This oversight leads to missed opportunities for enhancing efficiency and more accurate defect detection. The introduction of sophisticated methodologies such as process mining and causal AI aims to decipher these hidden correlations and provide actionable insights. Research Goal: In association with Bayer AG, the objective of this thesis ...
However, for the latter, an adequate solution in process mining is needed. This thesis aims to develop and exemplify a design method for a visual solution in process mining that allows for exploring a cyclic time arrangement in a process graph. We will adapt the research objectives to align with the experience and study goals of the student ...
In this case, the algorithms would run much faster than the time required to transfer the data over the network. 4.4 Parallel computing for process mining. Parallel computing has been applied in many areas, such as text mining [52,53], data visualization [54], document clustering [55], pattern mining [56] and many others.
uni-stuttgart.de
Process Mining Dissertation Award 2019 . Mannhardt, Felix (Recipient), Sept 2019. ... T1 - Multi-perspective process mining. AU - Mannhardt, F. N1 - Proefschrift. PY - 2018/2/7. Y1 - 2018/2/7. M3 - Phd Thesis 1 (Research TU/e / Graduation TU/e) SN - 978-90-386-4438-7.
Rebuge, A., Ferreira, D.R.: Business Process Analysis in Healthcare Environments: A Methodology Based on Process Mining. Information Systems 37(2) (2012) Google Scholar Riemers, P.: Process Improvement in Healthcare: a Data-based Method using a Combination of Process Mining and Visual Analytics. Master's thesis.
This thesis is an analysis of the care processes, with the following research question: "How can Process Mining strengthen Value-Based Health Care?" The costs in this thesis refer to performance times of care activities. A well-known concept in health care provides this research with a base and structure to investigate care processes: Value ...
Celonis Student Thesis and Research (STaR) Program. We highly welcome bachelor or master students, or doctoral candidates interested in writing their thesis, project study, developer study or research seminar papers on process mining and related aspects. It is our mission to empower the next generation of process miners, and this means that we ...
Process mining provides the potential to enable organizations make quicker, more informed decisions when overhauling business processes by leveraging data for insights. By using the information ...