Does Homework Improve Academic Achievement?
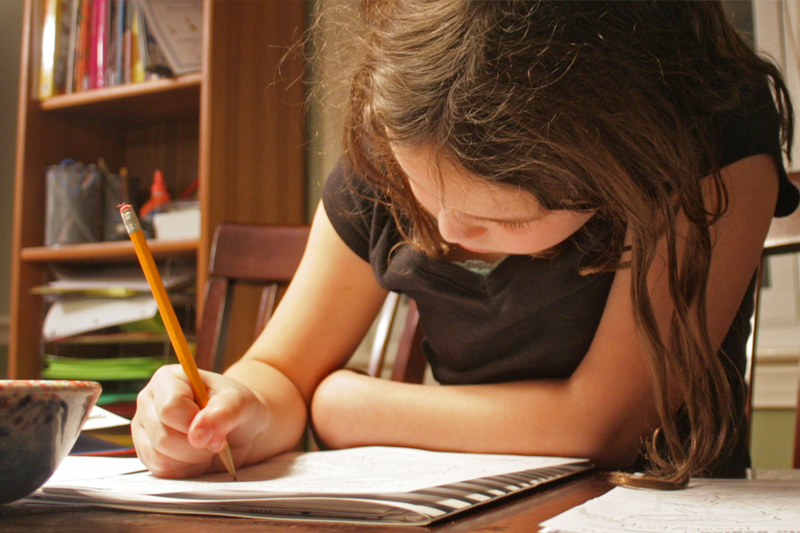
- Share this story on facebook
- Share this story on twitter
- Share this story on reddit
- Share this story on linkedin
- Get this story's permalink
- Print this story

Educators should be thrilled by these numbers. Pleasing a majority of parents regarding homework and having equal numbers of dissenters shouting "too much!" and "too little!" is about as good as they can hope for.
But opinions cannot tell us whether homework works; only research can, which is why my colleagues and I have conducted a combined analysis of dozens of homework studies to examine whether homework is beneficial and what amount of homework is appropriate for our children.
The homework question is best answered by comparing students who are assigned homework with students assigned no homework but who are similar in other ways. The results of such studies suggest that homework can improve students' scores on the class tests that come at the end of a topic. Students assigned homework in 2nd grade did better on math, 3rd and 4th graders did better on English skills and vocabulary, 5th graders on social studies, 9th through 12th graders on American history, and 12th graders on Shakespeare.
Less authoritative are 12 studies that link the amount of homework to achievement, but control for lots of other factors that might influence this connection. These types of studies, often based on national samples of students, also find a positive link between time on homework and achievement.
Yet other studies simply correlate homework and achievement with no attempt to control for student differences. In 35 such studies, about 77 percent find the link between homework and achievement is positive. Most interesting, though, is these results suggest little or no relationship between homework and achievement for elementary school students.
Why might that be? Younger children have less developed study habits and are less able to tune out distractions at home. Studies also suggest that young students who are struggling in school take more time to complete homework assignments simply because these assignments are more difficult for them.

These recommendations are consistent with the conclusions reached by our analysis. Practice assignments do improve scores on class tests at all grade levels. A little amount of homework may help elementary school students build study habits. Homework for junior high students appears to reach the point of diminishing returns after about 90 minutes a night. For high school students, the positive line continues to climb until between 90 minutes and 2½ hours of homework a night, after which returns diminish.
Beyond achievement, proponents of homework argue that it can have many other beneficial effects. They claim it can help students develop good study habits so they are ready to grow as their cognitive capacities mature. It can help students recognize that learning can occur at home as well as at school. Homework can foster independent learning and responsible character traits. And it can give parents an opportunity to see what's going on at school and let them express positive attitudes toward achievement.
Opponents of homework counter that it can also have negative effects. They argue it can lead to boredom with schoolwork, since all activities remain interesting only for so long. Homework can deny students access to leisure activities that also teach important life skills. Parents can get too involved in homework -- pressuring their child and confusing him by using different instructional techniques than the teacher.
My feeling is that homework policies should prescribe amounts of homework consistent with the research evidence, but which also give individual schools and teachers some flexibility to take into account the unique needs and circumstances of their students and families. In general, teachers should avoid either extreme.
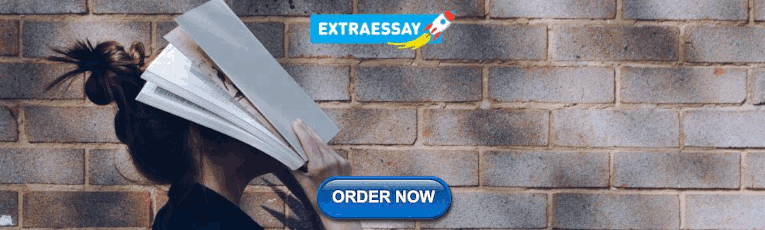
Link to this page
Copy and paste the URL below to share this page.
Does Homework Really Help Students Learn?
A conversation with a Wheelock researcher, a BU student, and a fourth-grade teacher
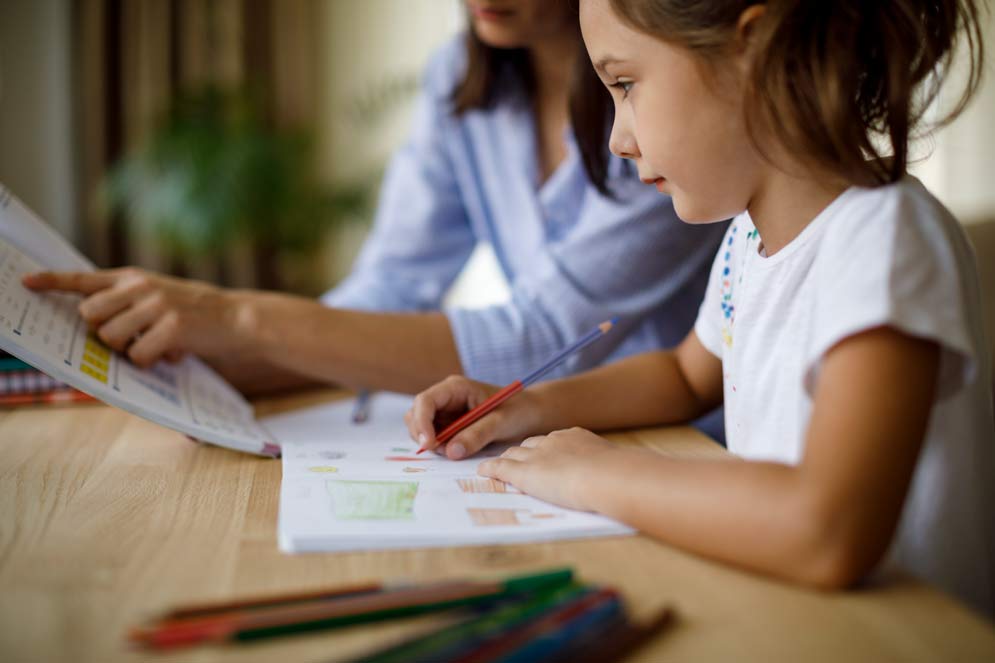
“Quality homework is engaging and relevant to kids’ lives,” says Wheelock’s Janine Bempechat. “It gives them autonomy and engages them in the community and with their families. In some subjects, like math, worksheets can be very helpful. It has to do with the value of practicing over and over.” Photo by iStock/Glenn Cook Photography
Do your homework.
If only it were that simple.
Educators have debated the merits of homework since the late 19th century. In recent years, amid concerns of some parents and teachers that children are being stressed out by too much homework, things have only gotten more fraught.
“Homework is complicated,” says developmental psychologist Janine Bempechat, a Wheelock College of Education & Human Development clinical professor. The author of the essay “ The Case for (Quality) Homework—Why It Improves Learning and How Parents Can Help ” in the winter 2019 issue of Education Next , Bempechat has studied how the debate about homework is influencing teacher preparation, parent and student beliefs about learning, and school policies.
She worries especially about socioeconomically disadvantaged students from low-performing schools who, according to research by Bempechat and others, get little or no homework.
BU Today sat down with Bempechat and Erin Bruce (Wheelock’17,’18), a new fourth-grade teacher at a suburban Boston school, and future teacher freshman Emma Ardizzone (Wheelock) to talk about what quality homework looks like, how it can help children learn, and how schools can equip teachers to design it, evaluate it, and facilitate parents’ role in it.
BU Today: Parents and educators who are against homework in elementary school say there is no research definitively linking it to academic performance for kids in the early grades. You’ve said that they’re missing the point.
Bempechat : I think teachers assign homework in elementary school as a way to help kids develop skills they’ll need when they’re older—to begin to instill a sense of responsibility and to learn planning and organizational skills. That’s what I think is the greatest value of homework—in cultivating beliefs about learning and skills associated with academic success. If we greatly reduce or eliminate homework in elementary school, we deprive kids and parents of opportunities to instill these important learning habits and skills.
We do know that beginning in late middle school, and continuing through high school, there is a strong and positive correlation between homework completion and academic success.
That’s what I think is the greatest value of homework—in cultivating beliefs about learning and skills associated with academic success.
You talk about the importance of quality homework. What is that?
Quality homework is engaging and relevant to kids’ lives. It gives them autonomy and engages them in the community and with their families. In some subjects, like math, worksheets can be very helpful. It has to do with the value of practicing over and over.
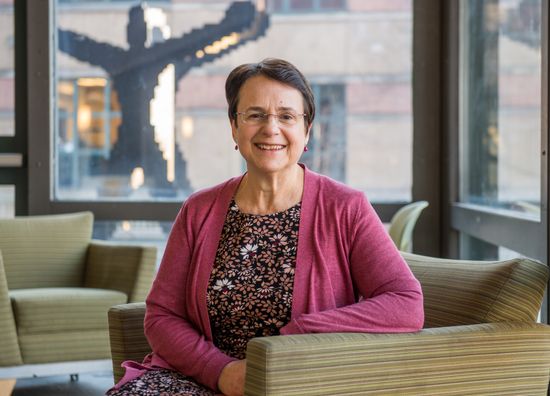
What are your concerns about homework and low-income children?
The argument that some people make—that homework “punishes the poor” because lower-income parents may not be as well-equipped as affluent parents to help their children with homework—is very troubling to me. There are no parents who don’t care about their children’s learning. Parents don’t actually have to help with homework completion in order for kids to do well. They can help in other ways—by helping children organize a study space, providing snacks, being there as a support, helping children work in groups with siblings or friends.
Isn’t the discussion about getting rid of homework happening mostly in affluent communities?
Yes, and the stories we hear of kids being stressed out from too much homework—four or five hours of homework a night—are real. That’s problematic for physical and mental health and overall well-being. But the research shows that higher-income students get a lot more homework than lower-income kids.
Teachers may not have as high expectations for lower-income children. Schools should bear responsibility for providing supports for kids to be able to get their homework done—after-school clubs, community support, peer group support. It does kids a disservice when our expectations are lower for them.
The conversation around homework is to some extent a social class and social justice issue. If we eliminate homework for all children because affluent children have too much, we’re really doing a disservice to low-income children. They need the challenge, and every student can rise to the challenge with enough supports in place.
What did you learn by studying how education schools are preparing future teachers to handle homework?
My colleague, Margarita Jimenez-Silva, at the University of California, Davis, School of Education, and I interviewed faculty members at education schools, as well as supervising teachers, to find out how students are being prepared. And it seemed that they weren’t. There didn’t seem to be any readings on the research, or conversations on what high-quality homework is and how to design it.
Erin, what kind of training did you get in handling homework?
Bruce : I had phenomenal professors at Wheelock, but homework just didn’t come up. I did lots of student teaching. I’ve been in classrooms where the teachers didn’t assign any homework, and I’ve been in rooms where they assigned hours of homework a night. But I never even considered homework as something that was my decision. I just thought it was something I’d pull out of a book and it’d be done.
I started giving homework on the first night of school this year. My first assignment was to go home and draw a picture of the room where you do your homework. I want to know if it’s at a table and if there are chairs around it and if mom’s cooking dinner while you’re doing homework.
The second night I asked them to talk to a grown-up about how are you going to be able to get your homework done during the week. The kids really enjoyed it. There’s a running joke that I’m teaching life skills.
Friday nights, I read all my kids’ responses to me on their homework from the week and it’s wonderful. They pour their hearts out. It’s like we’re having a conversation on my couch Friday night.
It matters to know that the teacher cares about you and that what you think matters to the teacher. Homework is a vehicle to connect home and school…for parents to know teachers are welcoming to them and their families.
Bempechat : I can’t imagine that most new teachers would have the intuition Erin had in designing homework the way she did.
Ardizzone : Conversations with kids about homework, feeling you’re being listened to—that’s such a big part of wanting to do homework….I grew up in Westchester County. It was a pretty demanding school district. My junior year English teacher—I loved her—she would give us feedback, have meetings with all of us. She’d say, “If you have any questions, if you have anything you want to talk about, you can talk to me, here are my office hours.” It felt like she actually cared.
Bempechat : It matters to know that the teacher cares about you and that what you think matters to the teacher. Homework is a vehicle to connect home and school…for parents to know teachers are welcoming to them and their families.
Ardizzone : But can’t it lead to parents being overbearing and too involved in their children’s lives as students?
Bempechat : There’s good help and there’s bad help. The bad help is what you’re describing—when parents hover inappropriately, when they micromanage, when they see their children confused and struggling and tell them what to do.
Good help is when parents recognize there’s a struggle going on and instead ask informative questions: “Where do you think you went wrong?” They give hints, or pointers, rather than saying, “You missed this,” or “You didn’t read that.”
Bruce : I hope something comes of this. I hope BU or Wheelock can think of some way to make this a more pressing issue. As a first-year teacher, it was not something I even thought about on the first day of school—until a kid raised his hand and said, “Do we have homework?” It would have been wonderful if I’d had a plan from day one.
Explore Related Topics:
- Share this story
Senior Contributing Editor

Sara Rimer A journalist for more than three decades, Sara Rimer worked at the Miami Herald , Washington Post and, for 26 years, the New York Times , where she was the New England bureau chief, and a national reporter covering education, aging, immigration, and other social justice issues. Her stories on the death penalty’s inequities were nominated for a Pulitzer Prize and cited in the U.S. Supreme Court’s decision outlawing the execution of people with intellectual disabilities. Her journalism honors include Columbia University’s Meyer Berger award for in-depth human interest reporting. She holds a BA degree in American Studies from the University of Michigan. Profile
She can be reached at [email protected] .
Comments & Discussion
Boston University moderates comments to facilitate an informed, substantive, civil conversation. Abusive, profane, self-promotional, misleading, incoherent or off-topic comments will be rejected. Moderators are staffed during regular business hours (EST) and can only accept comments written in English. Statistics or facts must include a citation or a link to the citation.
There are 81 comments on Does Homework Really Help Students Learn?
Insightful! The values about homework in elementary schools are well aligned with my intuition as a parent.
when i finish my work i do my homework and i sometimes forget what to do because i did not get enough sleep
same omg it does not help me it is stressful and if I have it in more than one class I hate it.
Same I think my parent wants to help me but, she doesn’t care if I get bad grades so I just try my best and my grades are great.
I think that last question about Good help from parents is not know to all parents, we do as our parents did or how we best think it can be done, so maybe coaching parents or giving them resources on how to help with homework would be very beneficial for the parent on how to help and for the teacher to have consistency and improve homework results, and of course for the child. I do see how homework helps reaffirm the knowledge obtained in the classroom, I also have the ability to see progress and it is a time I share with my kids
The answer to the headline question is a no-brainer – a more pressing problem is why there is a difference in how students from different cultures succeed. Perfect example is the student population at BU – why is there a majority population of Asian students and only about 3% black students at BU? In fact at some universities there are law suits by Asians to stop discrimination and quotas against admitting Asian students because the real truth is that as a group they are demonstrating better qualifications for admittance, while at the same time there are quotas and reduced requirements for black students to boost their portion of the student population because as a group they do more poorly in meeting admissions standards – and it is not about the Benjamins. The real problem is that in our PC society no one has the gazuntas to explore this issue as it may reveal that all people are not created equal after all. Or is it just environmental cultural differences??????
I get you have a concern about the issue but that is not even what the point of this article is about. If you have an issue please take this to the site we have and only post your opinion about the actual topic
This is not at all what the article is talking about.
This literally has nothing to do with the article brought up. You should really take your opinions somewhere else before you speak about something that doesn’t make sense.
we have the same name
so they have the same name what of it?
lol you tell her
totally agree
What does that have to do with homework, that is not what the article talks about AT ALL.
Yes, I think homework plays an important role in the development of student life. Through homework, students have to face challenges on a daily basis and they try to solve them quickly.I am an intense online tutor at 24x7homeworkhelp and I give homework to my students at that level in which they handle it easily.
More than two-thirds of students said they used alcohol and drugs, primarily marijuana, to cope with stress.
You know what’s funny? I got this assignment to write an argument for homework about homework and this article was really helpful and understandable, and I also agree with this article’s point of view.
I also got the same task as you! I was looking for some good resources and I found this! I really found this article useful and easy to understand, just like you! ^^
i think that homework is the best thing that a child can have on the school because it help them with their thinking and memory.
I am a child myself and i think homework is a terrific pass time because i can’t play video games during the week. It also helps me set goals.
Homework is not harmful ,but it will if there is too much
I feel like, from a minors point of view that we shouldn’t get homework. Not only is the homework stressful, but it takes us away from relaxing and being social. For example, me and my friends was supposed to hang at the mall last week but we had to postpone it since we all had some sort of work to do. Our minds shouldn’t be focused on finishing an assignment that in realty, doesn’t matter. I completely understand that we should have homework. I have to write a paper on the unimportance of homework so thanks.
homework isn’t that bad
Are you a student? if not then i don’t really think you know how much and how severe todays homework really is
i am a student and i do not enjoy homework because i practice my sport 4 out of the five days we have school for 4 hours and that’s not even counting the commute time or the fact i still have to shower and eat dinner when i get home. its draining!
i totally agree with you. these people are such boomers
why just why
they do make a really good point, i think that there should be a limit though. hours and hours of homework can be really stressful, and the extra work isn’t making a difference to our learning, but i do believe homework should be optional and extra credit. that would make it for students to not have the leaning stress of a assignment and if you have a low grade you you can catch up.
Studies show that homework improves student achievement in terms of improved grades, test results, and the likelihood to attend college. Research published in the High School Journal indicates that students who spent between 31 and 90 minutes each day on homework “scored about 40 points higher on the SAT-Mathematics subtest than their peers, who reported spending no time on homework each day, on average.” On both standardized tests and grades, students in classes that were assigned homework outperformed 69% of students who didn’t have homework. A majority of studies on homework’s impact – 64% in one meta-study and 72% in another – showed that take home assignments were effective at improving academic achievement. Research by the Institute for the Study of Labor (IZA) concluded that increased homework led to better GPAs and higher probability of college attendance for high school boys. In fact, boys who attended college did more than three hours of additional homework per week in high school.
So how are your measuring student achievement? That’s the real question. The argument that doing homework is simply a tool for teaching responsibility isn’t enough for me. We can teach responsibility in a number of ways. Also the poor argument that parents don’t need to help with homework, and that students can do it on their own, is wishful thinking at best. It completely ignores neurodiverse students. Students in poverty aren’t magically going to find a space to do homework, a friend’s or siblings to help them do it, and snacks to eat. I feel like the author of this piece has never set foot in a classroom of students.
THIS. This article is pathetic coming from a university. So intellectually dishonest, refusing to address the havoc of capitalism and poverty plays on academic success in life. How can they in one sentence use poor kids in an argument and never once address that poor children have access to damn near 0 of the resources affluent kids have? Draw me a picture and let’s talk about feelings lmao what a joke is that gonna put food in their belly so they can have the calories to burn in order to use their brain to study? What about quiet their 7 other siblings that they share a single bedroom with for hours? Is it gonna force the single mom to magically be at home and at work at the same time to cook food while you study and be there to throw an encouraging word?
Also the “parents don’t need to be a parent and be able to guide their kid at all academically they just need to exist in the next room” is wild. Its one thing if a parent straight up is not equipped but to say kids can just figured it out is…. wow coming from an educator What’s next the teacher doesn’t need to teach cause the kid can just follow the packet and figure it out?
Well then get a tutor right? Oh wait you are poor only affluent kids can afford a tutor for their hours of homework a day were they on average have none of the worries a poor child does. Does this address that poor children are more likely to also suffer abuse and mental illness? Like mentioned what about kids that can’t learn or comprehend the forced standardized way? Just let em fail? These children regularly are not in “special education”(some of those are a joke in their own and full of neglect and abuse) programs cause most aren’t even acknowledged as having disabilities or disorders.
But yes all and all those pesky poor kids just aren’t being worked hard enough lol pretty sure poor children’s existence just in childhood is more work, stress, and responsibility alone than an affluent child’s entire life cycle. Love they never once talked about the quality of education in the classroom being so bad between the poor and affluent it can qualify as segregation, just basically blamed poor people for being lazy, good job capitalism for failing us once again!
why the hell?
you should feel bad for saying this, this article can be helpful for people who has to write a essay about it
This is more of a political rant than it is about homework
I know a teacher who has told his students their homework is to find something they are interested in, pursue it and then come share what they learn. The student responses are quite compelling. One girl taught herself German so she could talk to her grandfather. One boy did a research project on Nelson Mandela because the teacher had mentioned him in class. Another boy, a both on the autism spectrum, fixed his family’s computer. The list goes on. This is fourth grade. I think students are highly motivated to learn, when we step aside and encourage them.
The whole point of homework is to give the students a chance to use the material that they have been presented with in class. If they never have the opportunity to use that information, and discover that it is actually useful, it will be in one ear and out the other. As a science teacher, it is critical that the students are challenged to use the material they have been presented with, which gives them the opportunity to actually think about it rather than regurgitate “facts”. Well designed homework forces the student to think conceptually, as opposed to regurgitation, which is never a pretty sight
Wonderful discussion. and yes, homework helps in learning and building skills in students.
not true it just causes kids to stress
Homework can be both beneficial and unuseful, if you will. There are students who are gifted in all subjects in school and ones with disabilities. Why should the students who are gifted get the lucky break, whereas the people who have disabilities suffer? The people who were born with this “gift” go through school with ease whereas people with disabilities struggle with the work given to them. I speak from experience because I am one of those students: the ones with disabilities. Homework doesn’t benefit “us”, it only tears us down and put us in an abyss of confusion and stress and hopelessness because we can’t learn as fast as others. Or we can’t handle the amount of work given whereas the gifted students go through it with ease. It just brings us down and makes us feel lost; because no mater what, it feels like we are destined to fail. It feels like we weren’t “cut out” for success.
homework does help
here is the thing though, if a child is shoved in the face with a whole ton of homework that isn’t really even considered homework it is assignments, it’s not helpful. the teacher should make homework more of a fun learning experience rather than something that is dreaded
This article was wonderful, I am going to ask my teachers about extra, or at all giving homework.
I agree. Especially when you have homework before an exam. Which is distasteful as you’ll need that time to study. It doesn’t make any sense, nor does us doing homework really matters as It’s just facts thrown at us.
Homework is too severe and is just too much for students, schools need to decrease the amount of homework. When teachers assign homework they forget that the students have other classes that give them the same amount of homework each day. Students need to work on social skills and life skills.
I disagree.
Beyond achievement, proponents of homework argue that it can have many other beneficial effects. They claim it can help students develop good study habits so they are ready to grow as their cognitive capacities mature. It can help students recognize that learning can occur at home as well as at school. Homework can foster independent learning and responsible character traits. And it can give parents an opportunity to see what’s going on at school and let them express positive attitudes toward achievement.
Homework is helpful because homework helps us by teaching us how to learn a specific topic.
As a student myself, I can say that I have almost never gotten the full 9 hours of recommended sleep time, because of homework. (Now I’m writing an essay on it in the middle of the night D=)
I am a 10 year old kid doing a report about “Is homework good or bad” for homework before i was going to do homework is bad but the sources from this site changed my mind!
Homeowkr is god for stusenrs
I agree with hunter because homework can be so stressful especially with this whole covid thing no one has time for homework and every one just wants to get back to there normal lives it is especially stressful when you go on a 2 week vaca 3 weeks into the new school year and and then less then a week after you come back from the vaca you are out for over a month because of covid and you have no way to get the assignment done and turned in
As great as homework is said to be in the is article, I feel like the viewpoint of the students was left out. Every where I go on the internet researching about this topic it almost always has interviews from teachers, professors, and the like. However isn’t that a little biased? Of course teachers are going to be for homework, they’re not the ones that have to stay up past midnight completing the homework from not just one class, but all of them. I just feel like this site is one-sided and you should include what the students of today think of spending four hours every night completing 6-8 classes worth of work.
Are we talking about homework or practice? Those are two very different things and can result in different outcomes.
Homework is a graded assignment. I do not know of research showing the benefits of graded assignments going home.
Practice; however, can be extremely beneficial, especially if there is some sort of feedback (not a grade but feedback). That feedback can come from the teacher, another student or even an automated grading program.
As a former band director, I assigned daily practice. I never once thought it would be appropriate for me to require the students to turn in a recording of their practice for me to grade. Instead, I had in-class assignments/assessments that were graded and directly related to the practice assigned.
I would really like to read articles on “homework” that truly distinguish between the two.
oof i feel bad good luck!
thank you guys for the artical because I have to finish an assingment. yes i did cite it but just thanks
thx for the article guys.
Homework is good
I think homework is helpful AND harmful. Sometimes u can’t get sleep bc of homework but it helps u practice for school too so idk.
I agree with this Article. And does anyone know when this was published. I would like to know.
It was published FEb 19, 2019.
Studies have shown that homework improved student achievement in terms of improved grades, test results, and the likelihood to attend college.
i think homework can help kids but at the same time not help kids
This article is so out of touch with majority of homes it would be laughable if it wasn’t so incredibly sad.
There is no value to homework all it does is add stress to already stressed homes. Parents or adults magically having the time or energy to shepherd kids through homework is dome sort of 1950’s fantasy.
What lala land do these teachers live in?
Homework gives noting to the kid
Homework is Bad
homework is bad.
why do kids even have homework?
Comments are closed.
Latest from Bostonia
Opening doors: michele courton brown (cas’83), six bu alums to remember this memorial day, american academy of arts & sciences welcomes five bu members, com’s newest journalism grad took her time, could boston be the next city to impose congestion pricing, alum has traveled the world to witness total solar eclipses, opening doors: rhonda harrison (eng’98,’04, grs’04), campus reacts and responds to israel-hamas war, reading list: what the pandemic revealed, remembering com’s david anable, cas’ john stone, “intellectual brilliance and brilliant kindness”, one good deed: christine kannler (cas’96, sph’00, camed’00), william fairfield warren society inducts new members, spreading art appreciation, restoring the “black angels” to medical history, in the kitchen with jacques pépin, feedback: readers weigh in on bu’s new president, com’s new expert on misinformation, and what’s really dividing the nation, the gifts of great teaching, sth’s walter fluker honored by roosevelt institute, alum’s debut book is a ramadan story for children.

An official website of the United States government
The .gov means it’s official. Federal government websites often end in .gov or .mil. Before sharing sensitive information, make sure you’re on a federal government site.
The site is secure. The https:// ensures that you are connecting to the official website and that any information you provide is encrypted and transmitted securely.
- Publications
- Account settings
Preview improvements coming to the PMC website in October 2024. Learn More or Try it out now .
- Advanced Search
- Journal List
- Front Psychol
Students' Achievement and Homework Assignment Strategies
Rubén fernández-alonso.
1 Department of Education Sciences, University of Oviedo, Oviedo, Spain
2 Department of Education, Principality of Asturias Government, Oviedo, Spain
Marcos Álvarez-Díaz
Javier suárez-Álvarez.
3 Department of Psychology, University of Oviedo, Oviedo, Spain
José Muñiz
The optimum time students should spend on homework has been widely researched although the results are far from unanimous. The main objective of this research is to analyze how homework assignment strategies in schools affect students' academic performance and the differences in students' time spent on homework. Participants were a representative sample of Spanish adolescents ( N = 26,543) with a mean age of 14.4 (±0.75), 49.7% girls. A test battery was used to measure academic performance in four subjects: Spanish, Mathematics, Science, and Citizenship. A questionnaire allowed the measurement of the indicators used for the description of homework and control variables. Two three-level hierarchical-linear models (student, school, autonomous community) were produced for each subject being evaluated. The relationship between academic results and homework time is negative at the individual level but positive at school level. An increase in the amount of homework a school assigns is associated with an increase in the differences in student time spent on homework. An optimum amount of homework is proposed which schools should assign to maximize gains in achievement for students overall.
The role of homework in academic achievement is an age-old debate (Walberg et al., 1985 ) that has swung between times when it was thought to be a tool for improving a country's competitiveness and times when it was almost outlawed. So Cooper ( 2001 ) talks about the battle over homework and the debates and rows continue (Walberg et al., 1985 , 1986 ; Barber, 1986 ). It is considered a complicated subject (Corno, 1996 ), mysterious (Trautwein and Köller, 2003 ), a chameleon (Trautwein et al., 2009b ), or Janus-faced (Flunger et al., 2015 ). One must agree with Cooper et al. ( 2006 ) that homework is a practice full of contradictions, where positive and negative effects coincide. As such, depending on our preferences, it is possible to find data which support the argument that homework benefits all students (Cooper, 1989 ), or that it does not matter and should be abolished (Barber, 1986 ). Equally, one might argue a compensatory effect as it favors students with more difficulties (Epstein and Van Voorhis, 2001 ), or on the contrary, that it is a source of inequality as it specifically benefits those better placed on the social ladder (Rømming, 2011 ). Furthermore, this issue has jumped over the school wall and entered the home, contributing to the polemic by becoming a common topic about which it is possible to have an opinion without being well informed, something that Goldstein ( 1960 ) warned of decades ago after reviewing almost 300 pieces of writing on the topic in Education Index and finding that only 6% were empirical studies.
The relationship between homework time and educational outcomes has traditionally been the most researched aspect (Cooper, 1989 ; Cooper et al., 2006 ; Fan et al., 2017 ), although conclusions have evolved over time. The first experimental studies (Paschal et al., 1984 ) worked from the hypothesis that time spent on homework was a reflection of an individual student's commitment and diligence and as such the relationship between time spent on homework and achievement should be positive. This was roughly the idea at the end of the twentieth century, when more positive effects had been found than negative (Cooper, 1989 ), although it was also known that the relationship was not strictly linear (Cooper and Valentine, 2001 ), and that its strength depended on the student's age- stronger in post-compulsory secondary education than in compulsory education and almost zero in primary education (Cooper et al., 2012 ). With the turn of the century, hierarchical-linear models ran counter to this idea by showing that homework was a multilevel situation and the effect of homework on outcomes depended on classroom factors (e.g., frequency or amount of assigned homework) more than on an individual's attitude (Trautwein and Köller, 2003 ). Research with a multilevel approach indicated that individual variations in time spent had little effect on academic results (Farrow et al., 1999 ; De Jong et al., 2000 ; Dettmers et al., 2010 ; Murillo and Martínez-Garrido, 2013 ; Fernández-Alonso et al., 2014 ; Núñez et al., 2014 ; Servicio de Evaluación Educativa del Principado de Asturias, 2016 ) and that when statistically significant results were found, the effect was negative (Trautwein, 2007 ; Trautwein et al., 2009b ; Lubbers et al., 2010 ; Chang et al., 2014 ). The reasons for this null or negative relationship lie in the fact that those variables which are positively associated with homework time are antagonistic when predicting academic performance. For example, some students may not need to spend much time on homework because they learn quickly and have good cognitive skills and previous knowledge (Trautwein, 2007 ; Dettmers et al., 2010 ), or maybe because they are not very persistent in their work and do not finish homework tasks (Flunger et al., 2015 ). Similarly, students may spend more time on homework because they have difficulties learning and concentrating, low expectations and motivation or because they need more direct help (Trautwein et al., 2006 ), or maybe because they put in a lot of effort and take a lot of care with their work (Flunger et al., 2015 ). Something similar happens with sociological variables such as gender: Girls spend more time on homework (Gershenson and Holt, 2015 ) but, compared to boys, in standardized tests they have better results in reading and worse results in Science and Mathematics (OECD, 2013a ).
On the other hand, thanks to multilevel studies, systematic effects on performance have been found when homework time is considered at the class or school level. De Jong et al. ( 2000 ) found that the number of assigned homework tasks in a year was positively and significantly related to results in mathematics. Equally, the volume or amount of homework (mean homework time for the group) and the frequency of homework assignment have positive effects on achievement. The data suggests that when frequency and volume are considered together, the former has more impact on results than the latter (Trautwein et al., 2002 ; Trautwein, 2007 ). In fact, it has been estimated that in classrooms where homework is always assigned there are gains in mathematics and science of 20% of a standard deviation over those classrooms which sometimes assign homework (Fernández-Alonso et al., 2015 ). Significant results have also been found in research which considered only homework volume at the classroom or school level. Dettmers et al. ( 2009 ) concluded that the school-level effect of homework is positive in the majority of participating countries in PISA 2003, and the OECD ( 2013b ), with data from PISA 2012, confirms that schools in which students have more weekly homework demonstrate better results once certain school and student-background variables are discounted. To put it briefly, homework has a multilevel nature (Trautwein and Köller, 2003 ) in which the variables have different significance and effects according to the level of analysis, in this case a positive effect at class level, and a negative or null effect in most cases at the level of the individual. Furthermore, the fact that the clearest effects are seen at the classroom and school level highlights the role of homework policy in schools and teaching, over and above the time individual students spend on homework.
From this complex context, this current study aims to explore the relationships between the strategies schools use to assign homework and the consequences that has on students' academic performance and on the students' own homework strategies. There are two specific objectives, firstly, to systematically analyze the differential effect of time spent on homework on educational performance, both at school and individual level. We hypothesize a positive effect for homework time at school level, and a negative effect at the individual level. Secondly, the influence of homework quantity assigned by schools on the distribution of time spent by students on homework will be investigated. This will test the previously unexplored hypothesis that an increase in the amount of homework assigned by each school will create an increase in differences, both in time spent on homework by the students, and in academic results. Confirming this hypothesis would mean that an excessive amount of homework assigned by schools would penalize those students who for various reasons (pace of work, gaps in learning, difficulties concentrating, overexertion) need to spend more time completing their homework than their peers. In order to resolve this apparent paradox we will calculate the optimum volume of homework that schools should assign in order to benefit the largest number of students without contributing to an increase in differences, that is, without harming educational equity.
Participants
The population was defined as those students in year 8 of compulsory education in the academic year 2009/10 in Spain. In order to provide a representative sample, a stratified random sampling was carried out from the 19 autonomous regions in Spain. The sample was selected from each stratum according to a two-stage cluster design (OECD, 2009 , 2011 , 2014a ; Ministerio de Educación, 2011 ). In the first stage, the primary units of the sample were the schools, which were selected with a probability proportional to the number of students in the 8th grade. The more 8th grade students in a given school, the higher the likelihood of the school being selected. In the second stage, 35 students were selected from each school through simple, systematic sampling. A detailed, step-by-step description of the sampling procedure may be found in OECD ( 2011 ). The subsequent sample numbered 29,153 students from 933 schools. Some students were excluded due to lack of information (absences on the test day), or for having special educational needs. The baseline sample was finally made up of 26,543 students. The mean student age was 14.4 with a standard deviation of 0.75, rank of age from 13 to 16. Some 66.2% attended a state school; 49.7% were girls; 87.8% were Spanish nationals; 73.5% were in the school year appropriate to their age, the remaining 26.5% were at least 1 year behind in terms of their age.
Test application, marking, and data recording were contracted out via public tendering, and were carried out by qualified personnel unconnected to the schools. The evaluation, was performed on two consecutive days, each day having two 50 min sessions separated by a break. At the end of the second day the students completed a context questionnaire which included questions related to homework. The evaluation was carried out in compliance with current ethical standards in Spain. Families of the students selected to participate in the evaluation were informed about the study by the school administrations, and were able to choose whether those students would participate in the study or not.
Instruments
Tests of academic performance.
The performance test battery consisted of 342 items evaluating four subjects: Spanish (106 items), mathematics (73 items), science (78), and citizenship (85). The items, completed on paper, were in various formats and were subject to binary scoring, except 21 items which were coded on a polytomous scale, between 0 and 2 points (Ministerio de Educación, 2011 ). As a single student is not capable of answering the complete item pool in the time given, the items were distributed across various booklets following a matrix design (Fernández-Alonso and Muñiz, 2011 ). The mean Cronbach α for the booklets ranged from 0.72 (mathematics) to 0.89 (Spanish). Student scores were calculated adjusting the bank of items to Rasch's IRT model using the ConQuest 2.0 program (Wu et al., 2007 ) and were expressed in a scale with mean and standard deviation of 500 and 100 points respectively. The student's scores were divided into five categories, estimated using the plausible values method. In large scale assessments this method is better at recovering the true population parameters (e.g., mean, standard deviation) than estimates of scores using methods of maximum likelihood or expected a-posteriori estimations (Mislevy et al., 1992 ; OECD, 2009 ; von Davier et al., 2009 ).
Homework variables
A questionnaire was made up of a mix of items which allowed the calculation of the indicators used for the description of homework variables. Daily minutes spent on homework was calculated from a multiple choice question with the following options: (a) Generally I don't have homework; (b) 1 h or less; (c) Between 1 and 2 h; (d) Between 2 and 3 h; (e) More than 3 h. The options were recoded as follows: (a) = 0 min.; (b) = 45 min.; (c) = 90 min.; (d) = 150 min.; (e) = 210 min. According to Trautwein and Köller ( 2003 ) the average homework time of the students in a school could be regarded as a good proxy for the amount of homework assigned by the teacher. So the mean of this variable for each school was used as an estimator of Amount or volume of homework assigned .
Control variables
Four variables were included to describe sociological factors about the students, three were binary: Gender (1 = female ); Nationality (1 = Spanish; 0 = other ); School type (1 = state school; 0 = private ). The fourth variable was Socioeconomic and cultural index (SECI), which is constructed with information about family qualifications and professions, along with the availability of various material and cultural resources at home. It is expressed in standardized points, N(0,1) . Three variables were used to gather educational history: Appropriate School Year (1 = being in the school year appropriate to their age ; 0 = repeated a school year) . The other two adjustment variables were Academic Expectations and Motivation which were included for two reasons: they are both closely connected to academic achievement (Suárez-Álvarez et al., 2014 ). Their position as adjustment factors is justified because, in an ex-post facto descriptive design such as this, both expectations and motivation may be thought of as background variables that the student brings with them on the day of the test. Academic expectations for finishing education was measured with a multiple-choice item where the score corresponds to the years spent in education in order to reach that level of qualification: compulsory secondary education (10 points); further secondary education (12 points); non-university higher education (14 points); University qualification (16 points). Motivation was constructed from the answers to six four-point Likert items, where 1 means strongly disagree with the sentence and 4 means strongly agree. Students scoring highly in this variable are agreeing with statements such as “at school I learn useful and interesting things.” A Confirmatory Factor Analysis was performed using a Maximum Likelihood robust estimation method (MLMV) and the items fit an essentially unidimensional scale: CFI = 0.954; TLI = 0.915; SRMR = 0.037; RMSEA = 0.087 (90% CI = 0.084–0.091).
As this was an official evaluation, the tests used were created by experts in the various fields, contracted by the Spanish Ministry of Education in collaboration with the regional education authorities.
Data analyses
Firstly the descriptive statistics and Pearson correlations between the variables were calculated. Then, using the HLM 6.03 program (Raudenbush et al., 2004 ), two three-level hierarchical-linear models (student, school, autonomous community) were produced for each subject being evaluated: a null model (without predictor variables) and a random intercept model in which adjustment variables and homework variables were introduced at the same time. Given that HLM does not return standardized coefficients, all of the variables were standardized around the general mean, which allows the interpretation of the results as classical standardized regression analysis coefficients. Levels 2 and 3 variables were constructed from means of standardized level 1 variables and were not re-standardized. Level 1 variables were introduced without centering except for four cases: study time, motivation, expectation, and socioeconomic and cultural level which were centered on the school mean to control composition effects (Xu and Wu, 2013 ) and estimate the effect of differences in homework time among the students within the same school. The range of missing variable cases was very small, between 1 and 3%. Recovery was carried out using the procedure described in Fernández-Alonso et al. ( 2012 ).
The results are presented in two ways: the tables show standardized coefficients while in the figures the data are presented in a real scale, taking advantage of the fact that a scale with a 100 point standard deviation allows the expression of the effect of the variables and the differences between groups as percentage increases in standardized points.
Table Table1 1 shows the descriptive statistics and the matrix of correlations between the study variables. As can be seen in the table, the relationship between the variables turned out to be in the expected direction, with the closest correlations between the different academic performance scores and socioeconomic level, appropriate school year, and student expectations. The nationality variable gave the highest asymmetry and kurtosis, which was to be expected as the majority of the sample are Spanish.
Descriptive statistics and Pearson correlation matrix between the variables .
Table Table2 2 shows the distribution of variance in the null model. In the four subjects taken together, 85% of the variance was found at the student level, 10% was variance between schools, and 5% variance between regions. Although the 10% of variance between schools could seem modest, underlying that there were large differences. For example, in Spanish the 95% plausible value range for the school means ranged between 577 and 439 points, practically 1.5 standard deviations, which shows that schools have a significant impact on student results.
Distribution of the variance in the null model .
Table Table3 3 gives the standardized coefficients of the independent variables of the four multilevel models, as well as the percentage of variance explained by each level.
Multilevel models for prediction of achievement in four subjects .
β, Standardized weight; SE, Standard Error; SECI, Socioeconomic and cultural index; AC, Autonomous Communities .
The results indicated that the adjustment variables behaved satisfactorily, with enough control to analyze the net effects of the homework variables. This was backed up by two results, firstly, the two variables with highest standardized coefficients were those related to educational history: academic expectations at the time of the test, and being in the school year corresponding to age. Motivation demonstrated a smaller effect but one which was significant in all cases. Secondly, the adjustment variables explained the majority of the variance in the results. The percentages of total explained variance in Table Table2 2 were calculated with all variables. However, if the strategy had been to introduce the adjustment variables first and then add in the homework variables, the explanatory gain in the second model would have been about 2% in each subject.
The amount of homework turned out to be positively and significantly associated with the results in the four subjects. In a 100 point scale of standard deviation, controlling for other variables, it was estimated that for each 10 min added to the daily volume of homework, schools would achieve between 4.1 and 4.8 points more in each subject, with the exception of mathematics where the increase would be around 2.5 points. In other words, an increase of between 15 and 29 points in the school mean is predicted for each additional hour of homework volume of the school as a whole. This school level gain, however, would only occur if the students spent exactly the same time on homework as their school mean. As the regression coefficient of student homework time is negative and the variable is centered on the level of the school, the model predicts deterioration in results for those students who spend more time than their class mean on homework, and an improvement for those who finish their homework more quickly than the mean of their classmates.
Furthermore, the results demonstrated a positive association between the amount of homework assigned in a school and the differences in time needed by the students to complete their homework. Figure Figure1 1 shows the relationship between volume of homework (expressed as mean daily minutes of homework by school) and the differences in time spent by students (expressed as the standard deviation from the mean school daily minutes). The correlation between the variables was 0.69 and the regression gradient indicates that schools which assigned 60 min of homework per day had a standard deviation in time spent by students on homework of approximately 25 min, whereas in those schools assigning 120 min of homework, the standard deviation was twice as long, and was over 50 min. So schools which assigned more homework also tended to demonstrate greater differences in the time students need to spend on that homework.

Relationship between school homework volume and differences in time needed by students to complete homework .
Figure Figure2 2 shows the effect on results in mathematics of the combination of homework time, homework amount, and the variance of homework time associated with the amount of homework assigned in two types of schools: in type 1 schools the amount of homework assigned is 1 h, and in type 2 schools the amount of homework 2 h. The result in mathematics was used as a dependent variable because, as previously noted, it was the subject where the effect was smallest and as such is the most conservative prediction. With other subjects the results might be even clearer.

Prediction of results for quick and slow students according to school homework size .
Looking at the first standard deviation of student homework time shown in the first graph, it was estimated that in type 1 schools, which assign 1 h of daily homework, a quick student (one who finishes their homework before 85% of their classmates) would spend a little over half an hour (35 min), whereas the slower student, who spends more time than 85% of classmates, would need almost an hour and a half of work each day (85 min). In type 2 schools, where the homework amount is 2 h a day, the differences increase from just over an hour (65 min for a quick student) to almost 3 h (175 min for a slow student). Figure Figure2 2 shows how the differences in performance would vary within a school between the more and lesser able students according to amount of homework assigned. In type 1 schools, with 1 h of homework per day, the difference in achievement between quick and slow students would be around 5% of a standard deviation, while in schools assigning 2 h per day the difference would be 12%. On the other hand, the slow student in a type 2 school would score 6 points more than the quick student in a type 1 school. However, to achieve this, the slow student in a type 2 school would need to spend five times as much time on homework in a week (20.4 weekly hours rather than 4.1). It seems like a lot of work for such a small gain.
Discussion and conclusions
The data in this study reaffirm the multilevel nature of homework (Trautwein and Köller, 2003 ) and support this study's first hypothesis: the amount of homework (mean daily minutes the student spends on homework) is positively associated with academic results, whereas the time students spent on homework considered individually is negatively associated with academic results. These findings are in line with previous research, which indicate that school-level variables, such as amount of homework assigned, have more explanatory power than individual variables such as time spent (De Jong et al., 2000 ; Dettmers et al., 2010 ; Scheerens et al., 2013 ; Fernández-Alonso et al., 2015 ). In this case it was found that for each additional hour of homework assigned by a school, a gain of 25% of a standard deviation is expected in all subjects except mathematics, where the gain is around 15%. On the basis of this evidence, common sense would dictate the conclusion that frequent and abundant homework assignment may be one way to improve school efficiency.
However, as noted previously, the relationship between homework and achievement is paradoxical- appearances are deceptive and first conclusions are not always confirmed. Analysis demonstrates another two complementary pieces of data which, read together, raise questions about the previous conclusion. In the first place, time spent on homework at the individual level was found to have a negative effect on achievement, which confirms the findings of other multilevel-approach research (Trautwein, 2007 ; Trautwein et al., 2009b ; Chang et al., 2014 ; Fernández-Alonso et al., 2016 ). Furthermore, it was found that an increase in assigned homework volume is associated with an increase in the differences in time students need to complete it. Taken together, the conclusion is that, schools with more homework tend to exhibit more variation in student achievement. These results seem to confirm our second hypothesis, as a positive covariation was found between the amount of homework in a school (the mean homework time by school) and the increase in differences within the school, both in student homework time and in the academic results themselves. The data seem to be in line with those who argue that homework is a source of inequity because it affects those less academically-advantaged students and students with greater limitations in their home environments (Kohn, 2006 ; Rømming, 2011 ; OECD, 2013b ).
This new data has clear implications for educational action and school homework policies, especially in compulsory education. If quality compulsory education is that which offers the best results for the largest number (Barber and Mourshed, 2007 ; Mourshed et al., 2010 ), then assigning an excessive volume of homework at those school levels could accentuate differences, affecting students who are slower, have more gaps in their knowledge, or are less privileged, and can make them feel overwhelmed by the amount of homework assigned to them (Martinez, 2011 ; OECD, 2014b ; Suárez et al., 2016 ). The data show that in a school with 60 min of assigned homework, a quick student will need just 4 h a week to finish their homework, whereas a slow student will spend 10 h a week, 2.5 times longer, with the additional aggravation of scoring one twentieth of a standard deviation below their quicker classmates. And in a school assigning 120 min of homework per day, a quick student will need 7.5 h per week whereas a slow student will have to triple this time (20 h per week) to achieve a result one eighth worse, that is, more time for a relatively worse result.
It might be argued that the differences are not very large, as between 1 and 2 h of assigned homework, the level of inequality increases 7% on a standardized scale. But this percentage increase has been estimated after statistically, or artificially, accounting for sociological and psychological student factors and other variables at school and region level. The adjustment variables influence both achievement and time spent on homework, so it is likely that in a real classroom situation the differences estimated here might be even larger. This is especially important in comprehensive education systems, like the Spanish (Eurydice, 2015 ), in which the classroom groups are extremely heterogeneous, with a variety of students in the same class in terms of ability, interest, and motivation, in which the aforementioned variables may operate more strongly.
The results of this research must be interpreted bearing in mind a number of limitations. The most significant limitation in the research design is the lack of a measure of previous achievement, whether an ad hoc test (Murillo and Martínez-Garrido, 2013 ) or school grades (Núñez et al., 2014 ), which would allow adjustment of the data. In an attempt to alleviate this, our research has placed special emphasis on the construction of variables which would work to exclude academic history from the model. The use of the repetition of school year variable was unavoidable because Spain has one of the highest levels of repetition in the European Union (Eurydice, 2011 ) and repeating students achieve worse academic results (Ministerio de Educación, 2011 ). Similarly, the expectation and motivation variables were included in the group of adjustment factors assuming that in this research they could be considered background variables. In this way, once the background factors are discounted, the homework variables explain 2% of the total variance, which is similar to estimations from other multilevel studies (De Jong et al., 2000 ; Trautwein, 2007 ; Dettmers et al., 2009 ; Fernández-Alonso et al., 2016 ). On the other hand, the statistical models used to analyze the data are correlational, and as such, one can only speak of an association between variables and not of directionality or causality in the analysis. As Trautwein and Lüdtke ( 2009 ) noted, the word “effect” must be understood as “predictive effect.” In other words, it is possible to say that the amount of homework is connected to performance; however, it is not possible to say in which direction the association runs. Another aspect to be borne in mind is that the homework time measures are generic -not segregated by subject- when it its understood that time spent and homework behavior are not consistent across all subjects (Trautwein et al., 2006 ; Trautwein and Lüdtke, 2007 ). Nonetheless, when the dependent variable is academic results it has been found that the relationship between homework time and achievement is relatively stable across all subjects (Lubbers et al., 2010 ; Chang et al., 2014 ) which leads us to believe that the results given here would have changed very little even if the homework-related variables had been separated by subject.
Future lines of research should be aimed toward the creation of comprehensive models which incorporate a holistic vision of homework. It must be recognized that not all of the time spent on homework by a student is time well spent (Valle et al., 2015 ). In addition, research has demonstrated the importance of other variables related to student behavior such as rate of completion, the homework environment, organization, and task management, autonomy, parenting styles, effort, and the use of study techniques (Zimmerman and Kitsantas, 2005 ; Xu, 2008 , 2013 ; Kitsantas and Zimmerman, 2009 ; Kitsantas et al., 2011 ; Ramdass and Zimmerman, 2011 ; Bembenutty and White, 2013 ; Xu and Wu, 2013 ; Xu et al., 2014 ; Rosário et al., 2015a ; Osorio and González-Cámara, 2016 ; Valle et al., 2016 ), as well as the role of expectation, value given to the task, and personality traits (Lubbers et al., 2010 ; Goetz et al., 2012 ; Pedrosa et al., 2016 ). Along the same lines, research has also indicated other important variables related to teacher homework policies, such as reasons for assignment, control and feedback, assignment characteristics, and the adaptation of tasks to the students' level of learning (Trautwein et al., 2009a ; Dettmers et al., 2010 ; Patall et al., 2010 ; Buijs and Admiraal, 2013 ; Murillo and Martínez-Garrido, 2013 ; Rosário et al., 2015b ). All of these should be considered in a comprehensive model of homework.
In short, the data seem to indicate that in year 8 of compulsory education, 60–70 min of homework a day is a recommendation that, slightly more optimistically than Cooper's ( 2001 ) “10 min rule,” gives a reasonable gain for the whole school, without exaggerating differences or harming students with greater learning difficulties or who work more slowly, and is in line with other available evidence (Fernández-Alonso et al., 2015 ). These results have significant implications when it comes to setting educational policy in schools, sending a clear message to head teachers, teachers and those responsible for education. The results of this research show that assigning large volumes of homework increases inequality between students in pursuit of minimal gains in achievement for those who least need it. Therefore, in terms of school efficiency, and with the aim of improving equity in schools it is recommended that educational policies be established which optimize all students' achievement.
Ethics statement
This study was carried out in accordance with the recommendations of the University of Oviedo with written informed consent from all subjects. All subjects gave written informed consent in accordance with the Declaration of Helsinki. The protocol was approved by the University of Oviedo.
Author contributions
RF and JM have designed the research; RF and JS have analyzed the data; MA and JM have interpreted the data; RF, MA, and JS have drafted the paper; JM has revised it critically; all authors have provided final approval of the version to be published and have ensured the accuracy and integrity of the work.
This research was funded by the Ministerio de Economía y Competitividad del Gobierno de España. References: PSI2014-56114-P, BES2012-053488. We would like to express our utmost gratitude to the Ministerio de Educación Cultura y Deporte del Gobierno de España and to the Consejería de Educación y Cultura del Gobierno del Principado de Asturias, without whose collaboration this research would not have been possible.
Conflict of interest statement
The authors declare that the research was conducted in the absence of any commercial or financial relationships that could be construed as a potential conflict of interest.
- Barber B. (1986). Homework does not belong on the agenda for educational reform . Educ. Leadersh. 43 , 55–57. [ Google Scholar ]
- Barber M., Mourshed M. (2007). How the World's Best-Performing School Systems Come Out on Top. McKinsey and Company . Available online at: http://mckinseyonsociety.com/downloads/reports/Education/Worlds_School_Systems_Final.pdf (Accessed January 25, 2016).
- Bembenutty H., White M. C. (2013). Academic performance and satisfaction with homework completion among college students . Learn. Individ. Differ. 24 , 83–88. 10.1016/j.lindif.2012.10.013 [ CrossRef ] [ Google Scholar ]
- Buijs M., Admiraal W. (2013). Homework assignments to enhance student engagement in secondary education . Eur. J. Psychol. Educ. 28 , 767–779. 10.1007/s10212-012-0139-0 [ CrossRef ] [ Google Scholar ]
- Chang C. B., Wall D., Tare M., Golonka E., Vatz K. (2014). Relations of attitudes toward homework and time spent on homework to course outcomes: the case of foreign language learning . J. Educ. Psychol. 106 , 1049–1065. 10.1037/a0036497 [ CrossRef ] [ Google Scholar ]
- Cooper H. (1989). Synthesis of research on homework . Educ. Leadersh. 47 , 85–91. [ Google Scholar ]
- Cooper H. (2001). The Battle Over Homework: Common Ground for Administrators, Teachers, and Parents . Thousand Oaks, CA: Sage. [ Google Scholar ]
- Cooper H., Robinson J. C., Patall E. A. (2006). Does homework improve academic achievement? A synthesis of research, 1987-2003 . Rev. Educ. Res. 76 , 1–62. 10.3102/00346543076001001 [ CrossRef ] [ Google Scholar ]
- Cooper H., Steenbergen-Hu S., Dent A. L. (2012). Homework , in APA Educational Psychology Handbook , Vol. 3 : Application to Learning and Teaching , eds Harris K. R., Graham S., Urdan T. (Washington, DC: American Psychological Association; ), 475–495. [ Google Scholar ]
- Cooper H., Valentine J. C. (2001). Using research to answer practical questions about homework . Educ. Psychol. 36 , 143–153. 10.1207/S15326985EP3603_1 [ CrossRef ] [ Google Scholar ]
- Corno L. (1996). Homework is a complicated thing . Educ. Res. 25 , 27–30. 10.3102/0013189X025008027 [ CrossRef ] [ Google Scholar ]
- De Jong R., Westerhof K. J., Creemers B. P. M. (2000). Homework and student math achievement in junior high schools . Educ. Res. Eval. 6 , 130–157. 10.1076/1380-3611(200006)6:2;1-E;F130 [ CrossRef ] [ Google Scholar ]
- Dettmers S., Trautwein U., Lüdtke M., Kunter M., Baumert J. (2010). Homework works if homework quality is high: using multilevel modeling to predict the development of achievement in mathematics . J. Educ. Psychol. 102 , 467–482. 10.1037/a0018453 [ CrossRef ] [ Google Scholar ]
- Dettmers S., Trautwein U., Lüdtke O. (2009). The relationship between homework time and achievement is not universal: evidence from multilevel analyses in 40 countries . Sch. Eff. Sch. Improv. 20 , 375–405. 10.1080/09243450902904601 [ CrossRef ] [ Google Scholar ]
- Epstein J. L., Van Voorhis F. L. (2001). More than minutes: teachers' roles in designing homework . Educ. Psychol. 36 , 181–193. 10.1207/S15326985EP3603_4 [ CrossRef ] [ Google Scholar ]
- Eurydice (2015). The Structure of the European Education Systems 2015/16: Schematic Diagrams. Luxembourg: Publications Office of the European Union . Available online at: https://webgate.ec.europa.eu/fpfis/mwikis/eurydice/index.php/Publications:The_Structure_of_the_European_Education_Systems_2015/16:_Schematic_Diagrams (Accessed January 25, 2016).
- Eurydice (2011). Grade Retention during Compulsory Education in Europe: Regulations and Statistics . Luxembourg: Publications Office of the European Union. [ Google Scholar ]
- Fan H., Xu J., Cai Z., He J., Fan X. (2017). Homework and students' achievement in math and science: a 30-year meta-analysis, 1986-2015 . Educ. Res. Rev. 20 , 35–54. 10.1016/j.edurev.2016.11.003 [ CrossRef ] [ Google Scholar ]
- Farrow S., Tymms P., Henderson B. (1999). Homework and attainment in primary schools . Br. Educ. Res. J. 25 , 323–341. 10.1080/0141192990250304 [ CrossRef ] [ Google Scholar ]
- Fernández-Alonso R., Muñiz J. (2011). Diseños de cuadernillos para la evaluación de competencias b1sicas . Aula Abierta 39 , 3–34. [ Google Scholar ]
- Fernández-Alonso R., Suárez-Álvarez J., Muñiz J. (2012). Imputación de datos perdidos en las evaluaciones diagnósticas educativas. [Imputation methods for missing data in educational diagnostic evaluation]. Psicothema 24 , 167–175. [ PubMed ] [ Google Scholar ]
- Fernández-Alonso R., Suárez-Álvarez J., Muñiz J. (2014). Tareas escolares en el hogar y rendimiento en matemáticas: una aproximación multinivel con estudiantes de enseñanza primaria. [Homework and academic performance in mathematics: A multilevel approach with primary school student]. Rev. Psicol. Educ. 9 , 15–30. [ Google Scholar ]
- Fernández-Alonso R., Suárez-Álvarez J., Muñiz J. (2015). Adolescents' homework performance in mathematics and science: personal factors and teaching practices . J. Educ. Psychol. 107 , 1075–1085. 10.1037/edu0000032 [ CrossRef ] [ Google Scholar ]
- Fernández-Alonso R., Suárez-Álvarez J., Muñiz J. (2016). Homework and performance in mathematics: the role of the teacher, the family and the student's background . Rev. Psicod. 21 , 5–23. 10.1387/RevPsicodidact.13939 [ CrossRef ] [ Google Scholar ]
- Flunger B., Trautwein U., Nagengast B., Lüdtke O., Niggli A., Schnyder I. (2015). The Janus-faced nature of time spent on homework: using latent profile analyses to predict academic achievement over a school year . Lear. Instr. 39 , 97–106. 10.1016/j.learninstruc.2015.05.008 [ CrossRef ] [ Google Scholar ]
- Gershenson S., Holt S. B. (2015). Gender gaps in high school students' homework time . Educ. Res. 44 , 432–441. 10.3102/0013189X15616123 [ CrossRef ] [ Google Scholar ]
- Goetz T., Nett U. E., Martiny S. E., Hall N. C., Pekrun R., Dettmers S., et al. (2012). Students' emotions during homework: structures, self-concept antecedents, and achievement outcomes . Learn. Individ. Differ. 22 , 225–234. 10.1016/j.lindif.2011.04.006 [ CrossRef ] [ Google Scholar ]
- Goldstein A. (1960). Does homework help? A review of research . Elementary Sch. J. 60 , 212–224. 10.1086/459804 [ CrossRef ] [ Google Scholar ]
- Kitsantas A., Cheema J., Ware H. (2011). The role of homework support resources, time spent on homework, and self-efficacy beliefs in mathematics achievement . J. Adv. Acad. 22 , 312–341. 10.1177/1932202X1102200206 [ CrossRef ] [ Google Scholar ]
- Kitsantas A., Zimmerman B. J. (2009). College students homework and academic achievement: the mediating role of self-regulatory beliefs . Metacognition Learn. 4 , 1556–1623. 10.1007/s11409-008-9028-y [ CrossRef ] [ Google Scholar ]
- Kohn A. (2006). Abusing research: the study of homework and other examples . Phi Delta Kappan 88 , 9–22. 10.1177/003172170608800105 [ CrossRef ] [ Google Scholar ]
- Lubbers M. J., Van Der Werf M. P. C., Kuyper H., Hendriks A. A. J. (2010). Does homework behavior mediate the relation between personality and academic performance? Learn. Individ. Differ. 20 , 203–208. 10.1016/j.lindif.2010.01.005 [ CrossRef ] [ Google Scholar ]
- Martinez S. (2011). An examination of Latino students' homework routines . J. Latinos Educ. 10 , 354–368. 10.1080/15348431.2011.605688 [ CrossRef ] [ Google Scholar ]
- Mislevy R. J., Beaton A. E., Kaplan B., Sheehan K. M. (1992). Estimating population characteristics from sparse matrix samples of item responses . J. Educ. Meas. 29 , 133–161. 10.1111/j.1745-3984.1992.tb00371.x [ CrossRef ] [ Google Scholar ]
- Ministerio de Educación (2011). Evaluación General de Diagnóstico 2010. Educación Secundaria Obligatoria. Informe de Resultados . Madrid: Instituto de Evaluación; Available online at: http://www.mecd.gob.es/dctm/ievaluacion/informe-egd-2010.pdf?documentId=0901e72b80d5ad3e (Accessed January 25, 2016). [ Google Scholar ]
- Mourshed M., Chijioke C., Barber M. (2010). How the World's Most Improved School Systems Keep Getting Better. McKinsey and Company . Available online at: http://mckinseyonsociety.com/downloads/reports/Education/How-the-Worlds-Most-Improved-School-Systems-Keep-Getting-Better_Download-version_Final.pdf (Accessed January 25, 2016).
- Murillo F. J., Martínez-Garrido C. (2013). Homework influence on academic performance. A study of iberoamerican students of primary education . J. Psychodidactics 18 , 157–171. 10.1387/RevPsicodidact.6156 [ CrossRef ] [ Google Scholar ]
- Núñez J. C., Vallejo G., Rosário P., Tuero E., Valle A. (2014). Student, teacher, and school context variables predicting academic achievement in biology: analysis from a multilevel perspective . J. Psychodidactics 19 , 145–171. 10.1387/RevPsicodidact.7127 [ CrossRef ] [ Google Scholar ]
- OECD (2009). PISA Data Analysis Manual: SPSS, 2nd Edn . Paris: OECD Publishing. [ Google Scholar ]
- OECD (2011). School Sampling Preparation Manual. PISA 2012 Main Survey. Paris: OECD Publishing; Available online at: https://www.oecd.org/pisa/pisaproducts/PISA2012MS-SamplingGuidelines-.pdf (Accessed January 6, 2017). [ Google Scholar ]
- OECD (2013a). PISA 2012 Results: What Students Know and Can Do. Student Performance in Mathematics, Reading and Science (Volume I) . Paris: OECD Publishing. [ Google Scholar ]
- OECD (2013b). PISA 2012 Results: What Makes Schools Successful? Resources, Policies and Practices (Volume IV). Paris: OECD Publishing. [ Google Scholar ]
- OECD (2014a). PISA 2012 Technical Report. Paris: OECD Publishing; Available online at: http://www.oecd.org/pisa/pisaproducts/PISA-2012-technical-report-final.pdf (Accessed January 25, 2016). [ Google Scholar ]
- OECD (2014b). Does Homework Perpetuate Inequities in Education? PISA in Focus . Paris: OECD Publishing. [ Google Scholar ]
- Osorio A., González-Cámara M. (2016). Testing the alleged superiority of the indulgent parenting style among Spanish adolescents . Psicothema 28 , 414–420. 10.7334/psicothema2015.314 [ PubMed ] [ CrossRef ] [ Google Scholar ]
- Paschal R. A., Weinstein T., Walberg H. J. (1984). The effects of homework on learning: a quantitative synthesis . J. Educ. Res. 78 , 97–104. 10.1080/00220671.1984.10885581 [ CrossRef ] [ Google Scholar ]
- Patall E. A., Cooper H., Wynn S. R. (2010). The effectiveness and relative importance of providing choices in the classroom . J. Educ. Psychol. 102 , 896–915. 10.1037/a0019545 [ CrossRef ] [ Google Scholar ]
- Pedrosa I., Suárez-Álvarez J., García-Cueto E., Muñiz J. (2016). A computerized adaptive test for enterprising personality assessment in youth . Psicothema 28 , 471–478. 10.7334/psicothema2016.68 [ PubMed ] [ CrossRef ] [ Google Scholar ]
- Ramdass D., Zimmerman B. J. (2011). Developing self-regulation skills: the important role of homework . J. Adv. Acad. 22 , 194–218. 10.1177/1932202X1102200202 [ CrossRef ] [ Google Scholar ]
- Raudenbush S. W., Bryk A. S., Cheong Y. F., Congdon R. T. (2004). HLM6: Hierarchical Linear and Nonlinear Modeling . Chicago: Scientific Software International. [ Google Scholar ]
- Rømming M. (2011). Who benefits from homework assignments? Econ. Educ. Rev. 30 , 55–64. 10.1016/j.econedurev.2010.07.001 [ CrossRef ] [ Google Scholar ]
- Rosário P., Núñez J. C., Vallejo G., Cunha J., Nunes T., Mourão R., et al. (2015a). Does homework design matter? The role of homework's purpose in student mathematics achievement . Contemp. Educ. Psychol. 43 , 10–24. 10.1016/j.cedpsych.2015.08.001 [ CrossRef ] [ Google Scholar ]
- Rosário P., Núñez J. C., Vallejo G., Cunha J., Nunes T., Suárez N., et al.. (2015b). The effects of teachers' homework follow-up practices on students' EFL performance: a randomized-group design . Front. Psychol. 6 :1528. 10.3389/fpsyg.2015.01528 [ PMC free article ] [ PubMed ] [ CrossRef ] [ Google Scholar ]
- Servicio de Evaluación Educativa del Principado de Asturias (2016). La relación entre el tiempo de deberes y los resultados académicos [The Relationship between Homework Time and Academic Performance]. Informes de Evaluación, 1 . Oviedo: Consejería de Educación y Cultura del Gobierno del Principado de Asturias. [ Google Scholar ]
- Scheerens J., Hendriks M., Luyten H., Sleegers P., Cees G. (2013). Productive Time in Education. A Review of the Effectiveness of Teaching Time at School, Homework and Extended Time Outside School Hours. Enschede: University of Twente . Available online at: http://doc.utwente.nl/86371/ (Accessed January 25, 2016).
- Suárez-Álvarez J., Fernández-Alonso R., Muñiz J. (2014). Self-concept, motivation, expectations and socioeconomic level as predictors of academic performance in mathematics . Learn. Indiv. Diff. 30 , 118–123. 10.1016/j.lindif.2013.10.019 [ CrossRef ] [ Google Scholar ]
- Suárez N., Regueiro B., Epstein J. L., Piñeiro I., Díaz S. M., Valle A. (2016). Homework involvement and academic achievement of native and immigrant students . Front. Psychol. 7 :1517. 10.3389/fpsyg.2016.01517 [ PMC free article ] [ PubMed ] [ CrossRef ] [ Google Scholar ]
- Trautwein U. (2007). The homework–achievement relation reconsidered: differentiating homework time, homework frequency, and homework effort . Learn. Instr. 17 , 372–388. 10.1016/j.learninstruc.2007.02.009 [ CrossRef ] [ Google Scholar ]
- Trautwein U., Köller O. (2003). The relationship between homework and achievement: still much of a mystery . Educ. Psychol. Rev. 15 , 115–145. 10.1023/A:1023460414243 [ CrossRef ] [ Google Scholar ]
- Trautwein U., Köller O., Schmitz B., Baumert J. (2002). Do homework assignments enhance achievement? A multilevel analysis in 7th grade mathematics . Contemp. Educ. Psychol. 27 , 26–50. 10.1006/ceps.2001.1084 [ CrossRef ] [ Google Scholar ]
- Trautwein U., Lüdtke O., Schnyder I., Niggli A. (2006). Predicting homework effort: support for a domain-specific, multilevel homework model . J. Educ. Psychol. 98 , 438–456. 10.1037/0022-0663.98.2.438 [ CrossRef ] [ Google Scholar ]
- Trautwein U., Lüdtke O. (2007). Students' self-reported effort and time on homework in six school subjects: between-student differences and within-student variation . J. Educ. Psychol. 99 , 432–444. 10.1037/0022-0663.99.2.432 [ CrossRef ] [ Google Scholar ]
- Trautwein U., Lüdtke O. (2009). Predicting homework motivation and homework effort in six school subjects: the role of person and family characteristics, classroom factors, and school track . Learn. Instr. 19 , 243–258. 10.1016/j.learninstruc.2008.05.001 [ CrossRef ] [ Google Scholar ]
- Trautwein U., Niggli A., Schnyder I., Lüdtke O. (2009a). Between-teacher differences in homework assignments and the development of students' homework effort, homework emotions, and achievement . J. Educ. Psychol. 101 , 176–189. 10.1037/0022-0663.101.1.176 [ CrossRef ] [ Google Scholar ]
- Trautwein U., Schnyder I., Niggli A., Neumann M., Lüdtke O. (2009b). Chameleon effects in homework research: the homework–achievement association depends on the measures used and the level of analysis chosen . Contemp. Educ. Psychol. 34 , 77–88. 10.1016/j.cedpsych.2008.09.001 [ CrossRef ] [ Google Scholar ]
- Valle A., Pan I., Regueiro B., Suárez N., Tuero E., Nunes A. R. (2015). Predicting approach to homework in primary school students . Psicothema 27 , 334–340. 10.7334/psicothema2015.118 [ PubMed ] [ CrossRef ] [ Google Scholar ]
- Valle A., Regueiro B., Núñez J. C., Rodríguez S., Piñero I., Rosário P. (2016). Academic goals, student homework engagement, and academic achievement in elementary school . Front. Psychol. 7 :463. 10.3389/fpsyg.2016.00463 [ PMC free article ] [ PubMed ] [ CrossRef ] [ Google Scholar ]
- von Davier M., Gonzalez E., Mislevy R. J. (2009). What are Plausible Values and Why are They Useful?. IERI Monograph Series. Issues and Methodologies in Large-Scale Assessments. Available online at: http://www.ierinstitute.org/fileadmin/Documents/IERI_Monograph/IERI_Monograph_Volume_02.pdf (Accessed January 15, 2017).
- Walberg H. J., Paschal R. A., Weinstein T. (1985). Homework's powerful effects on learning . Educ. Leadersh. 42 , 76–79. [ Google Scholar ]
- Walberg H. J., Paschal R. A., Weinstein T. (1986). Walberg and colleagues reply: effective schools use homework effectively . Educ. Leadersh. 43 , 58. [ Google Scholar ]
- Wu M. L., Adams R. J., Wilson M. R., Haldane S. A. (2007). ACER ConQuest 2.0: Generalised Item Response Modelling Software . Camberwell, VIC: Australian Council for Educational Research. [ Google Scholar ]
- Xu J. (2008). Models of secondary school students' interest in homework: a multilevel analysis . Am. Educ. Res. J. 45 , 1180–1205. 10.3102/0002831208323276 [ CrossRef ] [ Google Scholar ]
- Xu J. (2013). Why do students have difficulties completing homework? The need for homework management . J. Educ. Train. Stud. 1 , 98–105. 10.11114/jets.v1i1.78 [ CrossRef ] [ Google Scholar ]
- Xu J., Wu H. (2013). Self-regulation of homework behavior: homework management at the secondary school level . J. Educ. Res. 106 , 1–13. 10.1080/00220671.2012.658457 [ CrossRef ] [ Google Scholar ]
- Xu J., Yuan R., Xu B., Xu M. (2014). Modeling students' time management in math homework . Learn. Individ. Differ. 34 , 33–42. 10.1016/j.lindif.2014.05.011 [ CrossRef ] [ Google Scholar ]
- Zimmerman B. J., Kitsantas A. (2005). Homework practices and academic achievement: the mediating role of self-efficacy and perceived responsibility beliefs . Contemp. Educ. Psychol. 30 , 397–417. 10.1016/j.cedpsych.2005.05.003 [ CrossRef ] [ Google Scholar ]
Does really educational robotics improve secondary school students’ course motivation, achievement and attitude?
- Open access
- Published: 31 May 2024
Cite this article
You have full access to this open access article
- Nuray Akman Selcuk ORCID: orcid.org/0009-0009-5745-7937 1 ,
- Sevda Kucuk ORCID: orcid.org/0000-0002-2679-5177 2 &
- Burak Sisman ORCID: orcid.org/0000-0002-7472-279X 3
This study examines the impact of educational robotics applications in Information Technologies and Software courses on the motivation, attitude towards robotics, and academic achievement of 6th-grade secondary school students. The research employed pre-experimental method encompassing 112 students. Students got training in robotics using the Arduino education kit over the course of eight weeks consisted of programming and electronic concepts. Findings indicate that while students' motivation levels were moderate both before and after the implementation, there was a relative decrease in course motivation scores after the implementation. Students exhibited positive attitudes towards robotics and achieved a good level of success. Additionally, a significant effect of motivation on attitudes towards robotics was observed. Gender was found to have no effects on motivation, attitude, or achievement. It was determined that students held positive attitudes towards robotics and developed favorable views of their robotics skills. The implications are discussed in terms of theoretical insights, practices and directions for further research.
Avoid common mistakes on your manuscript.
1 Introduction
Today, technology has been rapidly developing in all areas of life, and the production of functional technologies in various sectors has become increasingly important. In the 21st-century, when technology is at the heart of life, it is emphasized that students, from early childhood, need an education that enables them to use computer sciences effectively and also develop their production-oriented and collaborative skills (Bers, 2010 , 2019 ; El-Hamamsy et al., 2021a ; Kazakoff et al., 2013 ; Settle & Perkovic, 2010 ). Fundamental skills such as problem-solving, creativity, algorithmic and computational thinking, necessary for computer experts and people of all ages, professions, and fields, are emphasized under 21st-century skills (Resnick, 2013 ; Shin et al., 2013 ; Wing, 2006 ). These skills can be imparted through teaching programming and computer science (El-Hamamsy et al., 2021b ; Kert et al., 2020 ; Shin et al., 2013 ). Accordingly, for the new generation of global citizens to creatively use technology according to their needs, adapt to changing demands and production styles, and remain an active member of a productive technology world, knowledge of programming languages is crucial (Aytekin et al., 2018 ; Cross et al., 2016 ).
Various platforms (such as Alice, Blockly, Code Org, Scratch, Mblock, and KoduLab) exist to facilitate teaching programming skills to young students. Most of these tools, which are often free, enable individuals to easily develop programs using drag-and-drop or puzzle-like techniques. Such platforms are also referred to as block-based programming environments. These block-based platforms, unlike text-based programming tools with complex code structures, facilitate the learning of algorithms and programming for young students due to their drag-and-drop nature (Bers, 2019 ; Kert et al., 2020 ; Strawhacker & Bers, 2015 ; Todorovska & Bogdanova, 2020 ). In recent years, studies on educational robotics (ER) applications have also begun to appear in the literature alongside block-based platforms for programming instruction. In ER, robotics education kits containing microcontrollers, various sensors, gears, and motors, such as Lego, Robotis, and VEX, as well as cost-effective, open-source electronic boards like Arduino, different types of motors, and sensors are used. This diversity enriches ER applications with a wide range of tools.
ER provides students with an interactive learning environment that offers hands-on and experiential learning opportunities (Yolcu & Demirer, 2023 ). The robot-building process is a comprehensive and collaborative endeavor, integrating various disciplines such as mechanics, electronics, and programming. Robotics education encompasses all these processes. Typically, this education covers mechanical components and basic design principles from a mechanical perspective, circuit components and sensors from an electronics viewpoint, and for programming, algorithms, and fundamentals of programming. Students design their robots by following step-by-step guidebooks, in which they familiarize themselves with materials and apply design principles. While working with electronic components, they gain knowledge of basic electronics through microcontrollers and sensors.
Robot programming is a process where developers or end-users create a robot program to implement specific behaviors. In the context of ER, robots are programmed by students. Students can give instructions to make robots behave in a certain way through a user interface like graphical (block-based) or natural language (text-based). The advantage of robot programming is that it provides immediate feedback to students on whether the robot behaves as intended. This prompts students to wonder why the robot behaves in a certain way, ask questions, seek solutions, and solve problems (Bravo et al., 2017 ). The ability of ER to show the output of developed codes not only on a computer screen but also on a physical robot helps students concretize abstract concepts and code structures. It positively affects their motivation by allowing them to immediately see the real-life impact of their programmed applications (Kert et al., 2020 ; Ntourou et al., 2021 ; Sisman et al., 2021 ; Stewart et al., 2021 ; Wu & Chen, 2021 ).
In ER activities, students experience design and implementation processes similar to those of an engineer (Carro et al., 2021 ; Jackson et al., 2021 ). ER processes closely mirror the engineering steps followed in a real robot development process. The engineering design process involves developing a system, component, or process to meet specific needs (ABET, 2015 ). Students who experience these processes can gain engineering design skills. Although the engineering design process is described in the literature with various names and stages, it fundamentally includes similar phases. According to the Next Generation Science Standards [NGSS] ( 2013 ), these stages are defining the problem, doing research, identifying needs, generating alternative solutions, selecting and developing the best solutions, creating a model, testing, and making necessary corrections. The engineering design process, considering younger age groups, is built on a six-stage cycle: (1) ask, (2) imagine, (3) plan, (4) create (5) test & improve (6) share (Fig. 1 ).
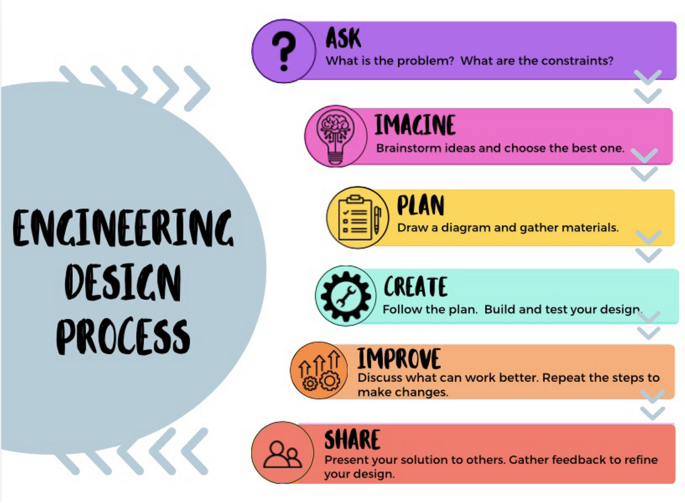
Engineering design process
Engineering activities and goals are valuable and inherently motivating because individuals are naturally driven to make things and benefit from curiosity about how things work (Brophy et al., 2008 ). Rogers and Portsmore ( 2004 ) state that reducing engineering to elementary school age is based on constructivist philosophy; according to this philosophy, students learn better while working with materials that allow them to design and construct meaningful artifacts for people. In this study, lesson plans based on the engineering design process provided in Fig. 1 above were used. Thus, it aimed to ensure that students adopt engineering design processes as a lasting learning experience.
1.1 Theoretical background
Although ER has only recently become widespread, its origins date back much further. ER applications are based on constructivism and constructionism theories. The views of Piaget ( 1973 ) and Papert ( 1980 ), suggesting that children in the learning process are not passive recipients but actively construct their knowledge, have been influential in the field of ER. Active thinking and practical activities during the learning process enable learners to create concepts and rules (Ackermann, 2001 ; Alimisis, 2013 ; Harel & Papert, 1991 ). Constructionism emphasizes student-centered learning through the exploration of concrete objects and the importance of connecting prior knowledge and new information in the real world (Alimisis & Kynigos, 2009 ). Based on this perspective, students are introduced to programming education and engineering practices from an early age through ER (Arís & Orcos, 2019 ; Berland & Wilensky, 2015 ; Bers, 2010 ; Bers & Portsmore, 2005 ; El-Hamamsy et al., 2021b ; Elkin et al., 2016 ; Kazakoff et al., 2013 ; Sullivan & Bers, 2018 ). Current studies in the field of ER are of significant importance. This study investigates the effects of ER on secondary school students' motivation, attitudes, and achievements.
In many studies in the field of education, students' success and failure are often explained through the concept of motivation (Fryer & Bovee, 2016 ; Gabrielle, 2003 ; Guilloteaux & Dörnyei, 2008 ). Learners' motivation is crucial in the learning and teaching process. Accordingly, focusing on the use of technology has been a key approach in research aimed at increasing motivation in the classroom and assisting students (Di Serio et al., 2013 ; Huitt, 2001 ). Compared to those lacking motivation, these studies have revealed that students willing to learn tend to participate more, show perseverance, and complete tasks. On the other hand, a lack of motivation in students has been identified as leading to lower their academic performance and even to increase their academic failure (Molaee & Dortaj, 2015 ). Learners' motivation occupies a pivotal role in the learning and teaching process (Keller, 1987 , 2009 ). Although many researchers have suggested that ER can enhance learners' motivation for learning, entertainment and interaction alone, if only emerging from the technology itself without integrated teaching strategies, cannot sustain learning motivation, which may rapidly decline over time. Numerous studies have identified a close relationship between the sustainability of learning motivation and teaching strategies. In this context, learners should be well directed with suitable teaching strategies to increase and maintain their learning motivation. In other words, introducing ER to learners may boost learning motivation at the start of learning activities, but appropriate teaching strategies are essential (Hung et al., 2013 ).
Numerous studies have been conducted to date explaining how motivation can be provided, developing various theories in the process. However, most of these theories describe how motivation occurs and on what it depends. Distinctively, the ARCS (Attention- Relevance- Confidence- Satisfaction) motivation model, proposed by John Keller ( 1987 ), is a model that facilitates both the provision and sustainability of motivation. The ARCS motivation model offers the opportunity to design the motivation process as a whole by synthesizing motivational theories and concepts. The "Attention, Relevance, Confidence, and Satisfaction" (ARCS) motivation model, a teaching strategy developed to motivate students, focuses on both intrinsic and extrinsic motivations to increase students' motivation and interest (Keller, 1987 ). According to research, each component of the ARCS model plays a critical role in enhancing students' motivation during the learning process (Di Serio et al., 2013 ). Yıldız et al. ( 2019 ) in their study, indicate that there is a substantial body of work on this model in technology-related fields (computer-web based training, mobile learning, virtual worlds, etc.), and accordingly, instructional designs based on technological areas improve students' academic achievement, motivation levels, and motivational characteristics. This study has been conducted by applying the ARCS model to investigate the impact of ER activities on secondary school students' course motivation, thereby contributing to the limited literature on the use of ER within the framework of the ARCS model.
Student attitudes are also one of the key factors affecting the learning process. Factors such as students' level of maturity and readiness, interests, needs, attitudes, and values can affect their willingness to learn (Tay & Akyürek Tay, 2004 ). Attitudes express an individual's positive or negative learned predispositions towards an object or concept. Studies have shown that there is a significant relationship between students' academic achievements and their attitudes (Mohd et al., 2011 ; Nicolaidou & Philippou, 2003 ). In skill development processes across various sectors, students' attitudes and interests play a critical role. Attitudes often encompass a wide range of emotional behaviors including preference, acceptance, appreciation, and commitment, and are frequently used to express students' intrinsic values or areas of interest (Welch, 2010 ). In course design, it is important to identify students' attitudes towards the course and the factors affecting these attitudes.
1.2 Literature review
The interdisciplinary nature of ER enables students to find links between different subjects and have a more comprehensive understanding of these subjects as a whole. In this way, ER offers a more extensive and integrated approach in education (Sun & Zhou, 2023 ). Studies on ER have seen a significant increase in recent years. Many of these studies focus on the instruction of robotics and its inclusion in STEM (Science, Technology, Engineering, Mathematics) processes (Anwar et al., 2019 ; Atman Uslu et al., 2022 ; Jung & Won, 2018 ; Karim et al., 2015 ; Zhang et al., 2021 ). The use of educational robots in teaching processes provides significant positive effects. These effects include the development of computational thinking skills (Sapounidis et al., 2023 ; Wu & Su, 2021 ; Zhang et al., 2021 ), enhancement of problem-solving and creative thinking abilities (Arís & Orcos, 2019 ; Karim et al., 2015 ; Zhang & Zhu, 2022 ), promotion of positive attitudes towards courses (Erol et al., 2023 ; Hussain et al., 2006 ; T.T. Wu & Chen, 2021 ), and strengthening of motivation (Arís & Orcos, 2019 ; Carro et al., 2021 ; Erol et al., 2023 ; Ribeiro et al., 2008 ; Sáez López et al., 2020 ; T.T. Wu & Chen, 2021 ). The integration of ER into STEM education plays a significant role in enhancing students' problem-solving abilities and teamwork skills (Arís & Orcos, 2019 ; Erol et al., 2023 ; Kucuk & Sisman, 2020 ; Ouyang & Xu, 2024 ; Stewart et al., 2021 ). Moreover, ER increases interest and participation in the STEM field, positively affecting students' attitudes towards STEM disciplines (Atman Uslu et al., 2022 ; Chen & Chang, 2018 ; Erol et al., 2023 ; Karim et al., 2015 ; Kim & Lee., 2016 ; Ouyang & Xu, 2024 ; Stewart et al., 2021 ). The focal points of studies that examine ER from different angles and offer various perspectives are summarized in Table 1 .
Furthermore, ER is used to facilitate a faster understanding of abstract concepts and to allow the visual and physical application of computer science concepts, thereby enhancing programming and IT skills (Angeli & Valanides, 2020 ; Atmatzidou & Demetriadis, 2016 ; Noh & Lee, 2020 ). Erol et al. ( 2023 ), in their study investigating the impact of robotic activities with Arduino on students' attitudes towards ICT (Information and Communication Technology) courses and STEM, revealed that robot design activities conducted with Arduino enhance students' attitudes towards engineering and technology within the context of STEM and ICT courses. Furthermore, student feedback indicates that educational robotic activities are fun, engaging, interesting, and hands-on.
However, it is noted that using ER is a more effective method for developing students' algorithm solving, data processing, and basic programming skills, as well as increasing self-confidence, but not necessarily effective for problem-solving skills (Kert et al., 2020 ). In contrast, students may find robotic activities diverse, challenging, complex, and time-consuming (Erol et al., 2023 ). Technical issues, limitations of the robot sets used, limited availability of robots outside of class, and restricted time periods throughout the term have also been found to not positively impact class motivation (McWhorter, 2008 ).
Some studies, carried out in the past years, have shown that boys generally perform at a higher level than girls in terms of computer experience (Papastergiou, 2009 ), participation in programming and robotics activities (Rusk et al., 2008 ), and attitude (Baser, 2013 ). Additionally, there are findings indicating that boys have more confidence and higher achievement in STEM fields (American Association of University Women & Greenberg Lake the Analysis Group, 1994 ; Comber et al., 1997 ; Pajares & Schunk, 2001 ; Wang & Degol, 2017 ). However, in recent years, this gender gap has been narrowing. ER activities have become a positive and encouraging tool for women, helping to remove societal biases and bridge the gender gap in computer sciences and STEM fields (Atmatzidou & Demetriadis, 2016 ; Jackson et al., 2021 ; Sullivan & Bers, 2018 ). Most recent research in computer sciences suggests that the difference between men and women is not significant (Atmatzidou & Demetriadis, 2016 ; Noh & Lee, 2020 ). Studies on gender in ER and STEM show that gender does not affect STEM attitudes (Kucuk & Sisman, 2020 ), and both genders can have a successful and beneficial experience in ER activities as well as in STEM and computer sciences, without gender being a limitation (Beisser, 2005 ; Cheng et al., 2013 ; Hussain et al., 2006 ; Kaloti-Hallak et al., 2015 ; Master et al., 2017 ; Ribeiro et al., 2008 ).
1.3 Rationale and importance of the study
Software programming influences all aspects of human production and life in the data age, thereby increasingly emphasizing the value of programming education (Sun & Zhou, 2023 ). Moreover, programming has been redefined as part of the new K-12 computer curriculum reform worldwide. The OECD ( 2016 ) states that programming ability is no longer just essential for computer professionals but also a fundamental skill for children living in the intelligent era of the twenty-first century. This situation highlights the importance of providing students with a broader capacity for thinking and the ability to interact with technology.
In programming instruction, the challenge of concretizing often abstract concepts encountered by students can impact their attitudes towards programming and their learning motivation (Anwar et al., 2019 ; Erol et al., 2023 ; Hodges et al., 2020 ; Jdeed et al., 2020 ; Jung & Won, 2018 ; Kert et al., 2020 ; Kim & Lee, 2016 ; Sisman et al., 2021 ). Indeed, attitude and motivation are among the most important factors affecting learning (Bixler, 2006 ; Malone, 1981 ; Tay & Akyürek Tay, 2004 ;).
Research indicates that ER activities play a positive role in learning, foster creative thinking, and enhance problem-solving skills (Zhang & Zhu, 2022 ). Interaction with robots also increases motivation, engagement, and attitudes towards education (Erol et al., 2023 ; Ouyang & Xu, 2024 ; Yolcu & Demirer, 2023 ). Moreover, the simplification of robot design and assembly processes, the inclusion of visual drag-and-drop programming, and the decreasing cost of educational robot platforms are ushering in a new era for educational technologies (Karim et al., 2015 ).
The benefits and advantages provided by ER applications, such as their interdisciplinary relationship, the various skills gained during the learning process, assistance in concretizing abstract concepts, and the immediate observation of programming outputs on robots, make creating effective learning environments with robotic activities appealing. Ouyang and Xu ( 2024 ) emphasize in their study that to fully realize the potential of educational robotics, it's necessary to go beyond focusing solely on robotics itself. The study highlights the importance of integrating interdisciplinary approaches and diverse educational strategies in the development and design of ER applications, in a manner that can maximize learning effects technologically. However, studies on a curriculum integrated with motivation-based ER are quite limited in the literature and on the agendas of many countries (El-Hamamsy et al., 2021a ). Within this study, a teaching program has been developed using the ARCS motivation model, which emphasizes the motivational aspect of instruction and highlights the importance of the motivation factor at every stage of teaching. Important cognitive-affective variables such as motivation, achievement, and attitude have been examined. The study sought answers to the following research questions in a 6th-grade Information Technologies and Software course in which educational robotics applications were implemented:
RQ 1. What are the levels of students' course motivation, robotics attitudes, and achievements?
RQ 2. What is the effect of educational robotics applications on students' course motivation?
RQ 2.a. Is there a significant difference between pre-test and post-test motivation scores according to students' initial motivation levels for the course?
RQ 3. Does students' course motivation show any significant differences according to gender?
RQ 4. Do students' achievements and robotics attitudes show any significant differences according to their course motivation levels and gender?
In this study examining the impact of educational robotics applications on secondary school students' course motivation, achievements, and attitudes towards robotics, the pre-test-post-test single-group experimental design model, which is a pre-experimental method of quantitative approaches, has been utilized. In this design, the effect of the experimental procedure is tested on a single group. Measurements related to the dependent variable for the subjects are obtained before the procedure as a pre-test, and afterward as a post-test, using the same subjects and the same measurement tools (Creswell, 2014 ; Fraenkel et al., 2018 ). The study investigates students' course motivation before and after the experimental application. Additionally, students' attitudes towards educational robotics and learning performance have been revealed following the experimental application.
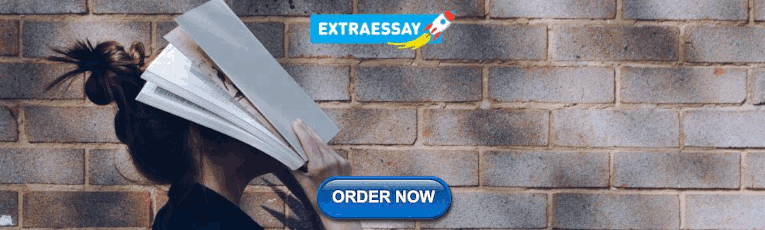
2.1 Participants and the research process
The study group consisted of 112 sixth-grade students (49 girls, 63 boys, aged 11–12) from four different classes of a state school in a socio-economically disadvantaged area. These sixth-grade students had previously attended a two-hour weekly Information Technologies and Software course in the fifth grade, where they were introduced to basic information technologies and computer literacy skills. In the first term of the sixth grade, they were taught coding using the block-based Scratch program. In the second term, ER applications were conducted for 12 weeks as a part of this study. Parental consent was obtained from the students' parents before starting the study, and ethical committee approvals were secured from the relevant institutions.
In the implementation, daily plans and class activities, prepared within the framework of "ARCS Motivation Strategies" and the "Engineering Design Process”, included both programming concepts such as loops, variables, and robotics learning outcomes. The lesson content and plan flow were implemented after receiving expert opinion. For the implementation, an Arduino education kit capable of carrying out the activities in the lesson plans was prepared. Robotics materials used during class activities, including Arduino Uno R3, breadboard, LED, resistor, RGB LED, buzzer, LDR sensor, ultrasonic distance sensor, potentiometer, and jumper cables, were placed in a hobby box, with one set provided for every two students. The pairs were attentively formed to create heterogeneous groups in terms of ability, gender, achievement, and personal characteristics, to integrate students with low skills and learning difficulties into the learning process and also to develop higher learning skills. This approach aimed to increase efficiency by promoting collaboration in groups while maintaining students' motivation and attention in classes. The bi-weekly two-hour lesson plans prepared by the researchers, based on ARCS principles and strategies and steps of the engineering design process, were finalized after being reviewed by two field experts. Table 2 displays an example two-hour weekly plan. The implementation of this plan, including the "Let's Light an LED Activity" circuit diagram and codes, is shown in Fig. 2 . Images from the implementation process are presented in Fig. 3 .
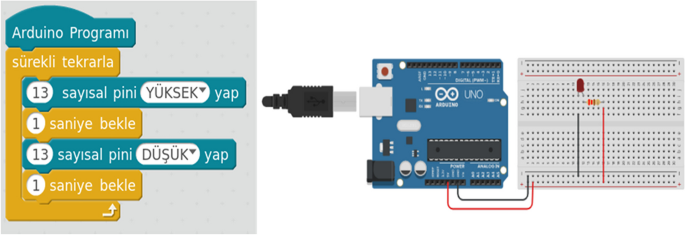
LED lighting activity Mblock codes and circuit diagram
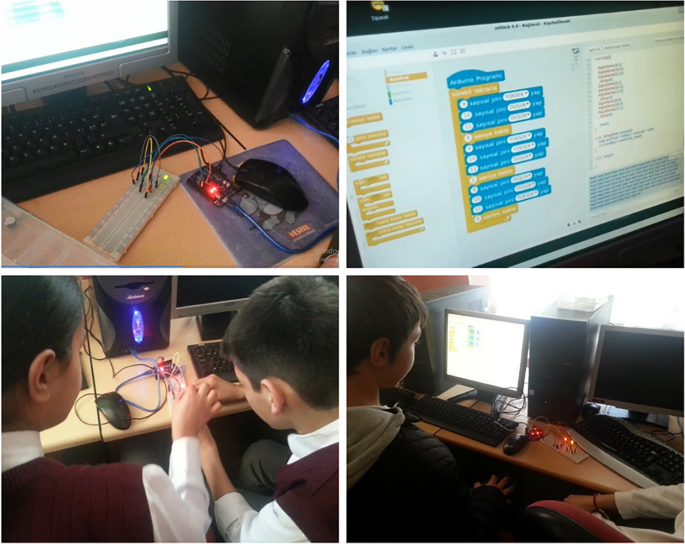
Images from the application process
2.1.1 Data collection instruments
In the research, the Course Interest Scale, Robotics Attitude Scale, and Achievement Test were used as data collection tools. Details of each data collection instrument are provided below.
2.1.1.1 Course Interest Scale (CIS)
The Course Interest Survey (CIS), originally published by Keller ( 2009 ), has been adapted into Turkish by Dincer ( 2015 ). Based on the ARCS Model, the original version of the CIS is a 34-item Likert-type scale (very true, true, moderately true, slightly true, not true) developed to encompass the components of attention, relevance, confidence, and satisfaction, which are the four sub-dimensions named identically to the components of the ARCS Model. In the ARCS motivation model, the attention category involves arousing and sustaining curiosity and interest (Sample Item 4: "There were many things in this course that caught my interest"), the relevance category addresses situations related to learners' needs, interests, and motivations (Sample Item 18: "The content of this course was relevant to my expectations and goals"), the confidence category is about creating a positive expectation of success among learners (Sample Item 3: "I was confident I would succeed in this course"), and the satisfaction category includes providing intrinsic and extrinsic supports to encourage student effort (Sample Item 10: "Learning the topics of this course was satisfying"). The original scale's Cronbach Alpha internal consistency coefficient is 0.95, while the adapted scale's Cronbach Alpha internal consistency coefficient is 0.91. The data obtained indicate that the adapted scale is a valid and reliable instrument for application among middle school students. The scale, which has a midpoint of 96, determines the lowest possible score as 32 and the highest as 160. Based on this information, it is recommended that the scoring and interpretation of the CIS and its sub-dimensions should be conducted according to the principles of normal distribution, within these specified lower and upper limits. Interpretations of the CIS scores and its sub-dimensions are shown in Table 3 .
2.1.1.2 Robotics Attitude Scale (RAS)
In the study, to determine students' attitudes towards ER activities, the Robotics Attitude Scale (RAS), developed by Cross et al. ( 2016 ) and adapted to Turkish by Sisman and Kucuk ( 2018 ), was used. The scale consists of four sub-dimensions: learning desire, self-confidence, computational thinking, and teamwork, and includes 24 items in a 5-point Likert format. The Cronbach Alpha value of the scale has been calculated as 0.932.
The scale includes a dimension for Learning Desire, which aims to uncover students' curiosity and interest in robotics with items such as "I want to learn more about robotics" and "robotics interests me." In the Confidence dimension, items like "I believe I can become an expert in the field of robotics" and "I can build a robot" are designed to assess students' belief in their ability to build and program robots. The Computational Thinking dimension includes items such as "I am good at logical thinking" and "I solve problems in a logical way" to reveal students' approaches to solving problems they encounter. Lastly, the Teamwork dimension contains items like "I can communicate my ideas to my group" and "I enjoy working in a group" to determine students' views on working in group settings.
2.1.1.3 Achievement test
To measure students' achievements in educational robotics applications, the researcher developed an achievement test and a practical exam consisting of multiple-choice and fill-in-the-blank questions. The achievement test encompasses the outcomes of "Problem Solving, Programming, and Original Product Development" units in the Information Technologies and Software Course, as well as the outcomes associated with robotics applications aligned with these goals. These outcomes were selected based on a review of existing literature, consultations with subject matter experts, and findings from preliminary studies to comprehensively understand the effects of ER applications on student achievement. The outcomes were structured around four main components: loops, conditions, variables in programming, and robotics achievements. These outcomes were included in the achievement test after necessary adjustments based on the feedback of three field experts. A pool of items, mostly at the comprehension and application levels, was created for the achievement test, which included multiple-choice and fill-in-the-blank questions. In addition to the achievement test, the researchers prepared a practical exam for each class, consisting of two questions per class, eight questions in total. These practical questions, related to everyday life, were posed to students as real-life problems, with the expectation that they would approach them solution-focused and solve them using their learned robotics knowledge. The practical exam was assessed through a practice control form prepared based on the defined outcomes. Students' achievement scores were calculated by averaging the scores obtained from the achievement test and the practical exam.
Feedback for the achievement test was sought from different field experts such as a measurement and evaluation expert for the content and face validity, four Information Technology teachers for content and content and structure, and two language teachers and two subject matter experts for language review. The expert group reviewed the prepared achievement test in terms of content, learning outcomes, and language. Based on their feedback, some questions in the item pool were revised, and changes were made to the options and stems of the items. As a result, the achievement test included 12 multiple-choice and 3 fill-in-the-blank questions. The test was scored out of 100 points, and the grading was done in accordance with the Ministry of National Education's directive: “85–100—Excellent,” “70–84—Good,” “60–69—Medium,” “50–59—Pass,” and “0–49—Fail.”
2.1.2 Data analysis
In the analysis of the collected data, the SPSS.21 software was used to initially conduct descriptive statistics such as frequency, percentage, mean, and standard deviation. In the study, dependent t-tests, independent t-tests, and multivariate analysis of variance (MANOVA) were performed upon meeting the assumptions for each test (Field, 2009 ). A significance level of 0.05 was used for all statistical analyses. The distributions of the data, examined through skewness and kurtosis coefficients, indicated that all variables fell within the + 1 to -1 range, suggesting a normal distribution of the data (Tabachnick & Fidell, 2007 ). Effect size values in the study were calculated and presented using Cohen’s d and η 2 . Cohen's effect size values are interpreted as Small: 0.20, Medium: 0.50, and Large: 0.80. In MANOVA tests, partial η 2 values of 0.01 indicate a low effect size, 0.06 a medium effect size, and 0.14 and above a large effect size (Cohen, 1988 ).
3.1 Students' motivation, achievement, and attitudes towards robotics
The descriptive data related to students' course motivation, achievement, and attitudes towards robotics at the end of the application are presented in Table 4 . As seen in Table 4 , it has been found that students' motivation towards the course (M = 120.47, SD = 22.6) and their achievements (M = 69.35, SD = 10.17) are at a medium level. Students' attitudes towards robotics have been determined to be at a good level (M = 3.54, SD = 0.74).
3.2 Comparison of pre-test and post-test scores for students' motivation towards the course
A dependent t-test was conducted to determine whether there was a significant difference in the pre-test and post-test average scores of students' motivation towards the course. The results revealed a significant decrease in the students' course motivation scores ( t = 4.36; p < 0.05). The effect size value was found to be 0.39, indicating a moderate level of impact (Table 5 ).
Additionally, the dependent t-test results, conducted to determine whether there was a significant difference between pre-test and post-test scores in students' initial motivation levels towards the course, are presented in Table 6 . The students' motivation levels towards the course were identified based on their responses to the motivation scale administered at the beginning of the term. In this regard, it was determined that 26 students had high motivation towards the course before the implementation, while 86 students had moderate motivation levels.
For the 86 students who initially had a moderate level of motivation towards the course, it was observed that their average motivation scores significantly decreased after the implementation of educational robotics applications (pre-test = 125.23, SD = 18.37, post-test = 112.34, SD post-test = 19.29). The effect size emerged as high at 0.68.
For the 26 students who initially had a high level of motivation towards the course, it was observed that their average motivation scores increased following the implementation (pre-test = 139.23, SD pre-test = 13.34, post-test = 147.38, SD post-test = 4.57). The effect size was high at 0.82.
3.3 Students' motivation towards the course by gender
The results of the independent t-test conducted to determine whether there was a significant difference in students' motivation towards the course based on gender are presented in Table 7 . It was found that both male and female students had a moderate level of motivation towards the course (Mean (male) = 121.40, SD (male) = 21.23, Mean (female) = 119.29, SD (female) = 24.63), and that there was no significant difference in course-related motivation by gender (p > 0.05.
3.4 The impact of students' motivation levels and gender on their achievement and attitudes towards robotics
A Multivariate Analysis of Variance (MANOVA) was conducted to determine if there was a significant effect of students' motivation levels towards the course and their gender on their achievements and attitudes towards robotics. The results are presented in Table 8 .
Upon examining Table 8 , it is evident that the motivation level towards the course has a significant effect on achievement scores and attitudes towards robotics (Wilks' Λ = 0.704, F(2,107) = 3.92, p < 0.05). The effect size is determined to be high at 0.14. It has been found that neither gender nor the interaction of gender with motivation level significantly affects students' achievement scores and attitudes towards robotics.
Table 9 displays the effects of the dependent variables, robotics attitude and achievement scores, in relation to the independent variables of gender and motivation level towards the course. According to the findings from the MANOVA test, while there is no significant effect of motivation level towards the course and gender on achievement scores (p > 0.05), the motivation level towards the course significantly affects attitudes towards robotics (p < 0.05).
Within the scope of this research question, a second MANOVA test was conducted to investigate which sub-dimensions the significant effect of motivation level towards the course had on attitudes towards robotics. As seen in Table 10 , it was found that the motivation level towards the course created a significant difference in the sub-dimensions of attitudes towards robotics (Wilks' Λ = 0.760, F(4,107) = 3.92, p < 0.05). The effect size was determined to be 0.24, indicating a high level.
While the motivation level towards the course has a significant effect on the sub-dimensions of attitudes towards robotics, such as desire to learn, confidence, and computational thinking (p < 0.05), it does not have a significant impact on the teamwork sub-dimension (p > 0.05). When examining the magnitude of the significant effect of motivation level towards the course on the sub-dimensions of attitudes towards robotics, it was found that desire to learn (η2 = 0.190) has a large effect size, whereas confidence (η2 = 0.136) and computational thinking (η2 = 0.123) have a medium level of effect size.
4 Discussion and conclusion
This research investigated the effects of an educational robotics program developed with the ARCS motivation model on students' motivation towards the course, their achievements, attitudes towards robotics, and whether gender had any influences on these factors. The implementation conducted for the study revealed that students had a good level of attitude towards robotics and their achievements were also close to being good. However, a more detailed examination of the results showed that despite students' motivation towards the course being moderate both before and after the application, there was a relative decrease in the average motivation scores after the application. The higher level of interest towards the course initially might be due to the block-based Scratch programming studied by the students in the first term of the Information Technologies and Software course and perceived as easier and more enjoyable, thereby motivating and engaging students. However, this study also suggests that creating electronic circuits, encountering new concepts like resistance, sensors, grounding, and resolving wiring complexities could have increased cognitive load and thus, students' motivation decreased. Indeed, studies indicate that students perform better and exhibit higher motivation when using block-based Scratch in programming instruction, as it offers a more engaging learning environment by visualizing program structures (Kert et al., 2020 ; Bers, 2019 ; Kazakoff et al., 2013 ; Resnick et al., 2003 ; Ruf et al., 2014 ; Strawhacker & Bers, 2015). Tlili et al. ( 2017 ), in their study, implemented a "learning by doing" strategy for undergraduate computer science students and applied Keller's ARCS motivational model. These approaches were observed to increase student motivation, keep them actively engaged, and assist them in acquiring the necessary technical skills to develop their educational games. Keller's ARCS motivational model is designed to increase students' interest in the learning process, strengthen the relevance of learning materials to their personal and professional goals, support their confidence towards success, and maximize their satisfaction from the learning experiences (Keller, 1987 ). Therefore, although some students found educational robotics activities difficult, there was no significant decrease in their motivation for the course.
Contrarily, Przybylla and Romeike ( 2014 ) have noted that while physical programming is seen as an exciting phenomenon by many teachers, the technical complexity of activities like breadboarding and soldering often makes them unsuitable for classroom use. In this study, it was proposed that teachers could solve these problems using ready-made robot sets like MyIG, TinkerKit, Hummingbird, etc. However, it has been observed that the cost of educational robotic activities conducted with these ready-made sets is significantly higher compared to the materials used in this study. Additionally, factors such as students only being able to work during laboratory hours, lack of resources to revise topics outside of class hours, insufficient weekly lesson hours, and large class sizes may have contributed to a relative decrease in students' motivation towards the course. Parallel to this conclusion, similar findings are reported in the literature (Beug, 2012 ; Fagin & Merkle, 2002 ; McWhorter, 2008 ; Reich-Stiebert & Eyssel, 2015 ). Beug ( 2012 ) in his study concluded that Arduino is not suitable for teaching programming concepts at a beginner level, noting that some students in the Arduino group got bored during activities, possibly due to technical difficulties related to the Arduino board. Ouyang and Xu ( 2024 ) in their research, showed that ER is more effective at high school and higher education levels compared to other educational levels (e.g., elementary, middle school). Due to the complex functions of ER, it is considered that most educational robotics might be more suitable for older students (e.g., students in higher education) rather than younger ones (e.g., elementary school students).
Fagin and Merkle ( 2002 ) associated the negative results of their research with the lack of equipment for robotic students to work with outside class hours. They suggested allowing students to take robots home after class or providing more out-of-class lab time. McWhorter ( 2008 ) identified potential reasons for the lack of positive quantitative outcomes in course motivation from robotic activities, including technical problems, limitations of the robot set used, limited availability of robots outside of class, and the restricted time allocated for robotic activities throughout the term.
On the other hand, Saleiro et al. ( 2013 ) indicated in their study that robot systems which do not require additional software installation (based on PIC microcontroller, Arduino, or Raspberry Pi and programmed with Blockly) were successfully used even by 3rd and 4th-grade students. Moreover, the use of robotics in education has been found effective in increasing students' willingness to collaborate with each other and in enhancing their desire and motivation for learning activities, as evidenced in various studies (Arís & Orcos, 2019 ; Atmatzidou & Demetriadis, 2016 ; Bargagna et al., 2019 ; Benitti, 2012 ; Erol et al., 2023 ; Gupta et al., 2012 ; Highfield, 2010 ; Jdeed et al., 2020 ; Kucuk & Sisman, 2017 ; Ouyang & Xu, 2024 ; Ribeiro et al., 2008 ; Rubio et al., 2013 ; Sáez López et al., 2020 ; Stewart et al., 2021 ; Wei et al., 2011 ).
When examining the initial motivation levels of students towards the course, it was found that the 86 students with a moderate level of initial interest experienced a decrease in their interest after the implementation, while the 26 students with a high level of initial interest showed an increase in their interest. In other words, for students already highly interested in the course, robotic activities were engaging and enhanced their motivation. Literature review also indicates that many studies on motivation report similar positive outcomes (Arís & Orcos, 2019 ; Erol et al., 2023 ; Kaloti-Hallak et al., 2015 ; Kert et al., 2020 ; Kucuk & Sisman, 2017 ; Ribeiro et al., 2008 ; Rubio et al., 2013 ; Sáez López et al., 2020 ; Saleiro et al., 2013 ).
According to the findings from the robotics attitude scale, students developed positive attitudes towards robotics and their attitudes were found to be at a good level after the application. Consistently, literature also indicates that robotic activities positively influence students' attitudes towards computer sciences and STEM (Science, Technology, Engineering, and Mathematics) fields (Anwar et al., 2019 ; Arís & Orcos, 2019 ; Chen & Chang, 2018 ; Erol et al., 2023 ; Hussain et al., 2006 ; Jung & Won, 2018 ; Kaloti-Hallak et al., 2015 ; Kandlhofer & Steinbauer, 2016 ; Kucuk & Sisman, 2020 ; Liu, 2010 ; Ouyang & Xu, 2024 ; Ribeiro et al., 2008 ; Rubio et al., 2013 ; Somyurek, 2015 ; Zhang et al., 2021 ).
The average achievement scores of students, calculated by combining the results of the success test and the practical exam conducted at the end of the educational robotics activities, are quite close to a good level. The fact that students had learned block-based coding in the first term of this course may have contributed to this outcome. Additionally, students who did not perform well in written exams may have been able to demonstrate their robotic skills more comfortably in the practical exam, which could have had a positive effect on the results.
Another result of the study is the significant effect of motivation level towards the course on attitudes towards robotics. It can be said that students with a high level of motivation towards the course are more interested in the field of computing and its applications. This may have led to the development of positive attitudes towards robotics. Additionally, it was determined that the motivation level towards the course has a significant effect on the sub-dimensions of attitudes towards robotics, including desire to learn, confidence, and computational thinking. However, no significant effects were determined on the teamwork sub-dimension of the robotics attitude. In the ARCS motivation model used in the study, the attention category, by arousing and maintaining curiosity and interest, may have increased the desire to learn. The relevance category, by helping students perceive teaching needs as consistent with their goals, compatible with their learning styles, and related to their past experiences, may have positively influenced their computational thinking. The confidence category, by creating a positive expectation for success, may have positively impacted students' self-confidence. The grouping of students in pairs may not have provided an adequate team working environment, which could be why no effect was observed in the teamwork dimension.
The study also revealed that gender as an independent variable did not have any effects on students' motivation towards the course, their attitudes towards robotics, or their achievement. This finding aligns with previous research in science, mathematics, technology, and engineering activities, where gender differences have been a notable point of discussion. Historically, many studies have highlighted gender differences, showing that males often had more confidence and higher achievement in fields related to science, technology, engineering, and mathematics (STEM) (American Association of University Women & Greenberg Lake-the Analysis Group, 1994 ; Comber et al., 1997 ; Pajares & Schunk, 2001 ; Papastergiou, 2009 ; Wang & Degol, 2017 ). Research has also indicated that males generally exhibited higher levels of computer experience (Papastergiou, 2009 ), participation in programming and robotics activities (Rusk et al., 2008 ), and attitudes towards these fields (Baser, 2013 ) than females.
However, in the last decade, this gender gap has been gradually decreasing. Recent literature suggests that robotics activities attract equal interest from both genders, and gender does not delimitate it. Studies have shown that there are no significant differences in learning outcomes based on gender in the context of robotics (Cheng et al., 2013 ; Hussain et al., 2006 ; Jackson et al., 2021 ; Kaloti-Hallak et al., 2015 ; Ribeiro et al., 2008 ; Sullivan & Bers, 2018 ). This shift may be attributed to various factors, including changes in societal attitudes, more inclusive educational practices, and a broader recognition of the importance of engaging all students, regardless of gender, in STEM fields.
5 Limitations and implications
The limitations of this study include its implementation with a limited number of students over a short period, in a single-group pre-test post-test design at a school with a low socio-economic status. Another limitation is that students' motivation towards the course and their attitudes towards robotics were determined through self-reports. The fact that the educational robotics (ER) activities were conducted only with one type of robotics set might also be considered a limitation. Additionally, the limited classroom hours for students to access ER sets and the lack of such equipment at their homes constrain the further study.
For future research, teaching activities could be designed using different robotics sets. More comprehensive collaborative activities could be planned to foster a team-working spirit among students. Some opportunities could be provided for students to practice more what they learn in class during their out-of-class time. Future studies could examine students' course motivation, achievements, and attitudes towards robotics using larger sample groups and longer-term implementations, including experimental and control group designs. Investigating how students' cognitive and affective states are influenced in environments using different ER sets and various teaching designs would be valuable. Additionally, students' experiences related to the process could be explored more in-depth through qualitative studies, providing a more comprehensive understanding of the impact and dynamics of educational robotics in learning environments.
Data availability
The datasets analyzed during the current study are available from the corresponding author on reasonable request.
Accreditation Board for Engineering and Technology, Inc., ABET. (2015). Criteria for accrediting engineering programs. Retrieved from https://www.abet.org/wp-content/uploads/2015/10/E001-16-17-EAC-Criteria-10-20-15.pdf . Accessed 11.08.2023.
Ackermann, E. (2001). Piaget’s constructivism, Papert’s constructionism : What’s the difference ? 1–11.
Alimisis, D. (2013). Educational robotics: Open questions and new challenges. Themes in Science and Technology Education, 6 (1), 63–71.
Google Scholar
Alimisis, A. D., & Kynigos, C. (2009). Constructionism and robotics in education. Teacher Education on Robotics-Enhanced Costructivist Pedagogical Methods , 11–26.
American Association of University Women & Greenberg Lake the Analysis Group. (1994). Shortchanging Girls, Shortchanging America: Executive Summary: A Nationwide Poll that Assesses Self-esteem, Educational Experiences, Interest in Math and Science, and Career Aspirations of Girls and Boys Ages 9–15 . https://www.aauwbakersfield.com/uploads/9/6/2/5/96257912/shortchanging_girls_shortchanging_america.pdf/ . Accessed 20 Oct 2018.
Angeli, C., & Valanides, N. (2020). Developing young children’s computational thinking with educational robotics: An interaction effect between gender and scaffolding strategy. Computers in Human Behavior, 105 . https://doi.org/10.1016/j.chb.2019.106225
Anwar, S., Bascou, N. A., Menekse, M., & Kardgar, A. (2019). A systematic review of studies on educational robotics. Journal of Pre-College Engineering Education Research (J-PEER) , 9(2). https://doi.org/10.7771/2157-9288.1223 .
Arís, N., & Orcos, L. (2019). Educational robotics in the stage of secondary education: Empirical study on motivation and STEM skills. Education Sciences, 9 (2), 73. https://doi.org/10.3390/educsci9020073
Article Google Scholar
Atman Uslu, N., Yavuz, G. O., & Koçak Usluel, Y. (2022). A systematic review study on educational robotics and robots. Interactive Learning Environments, 31 (9), 5874–5898. https://doi.org/10.1080/10494820.2021.2023890
Atmatzidou, S., & Demetriadis, S. (2016). Advancing students’ computational thinking skills through educational robotics : A study on age and gender relevant differences. Robotics and Autonomous Systems, 75 , 661–670. https://doi.org/10.1016/j.robot.2015.10.008
Atmatzidou, S., Demetriadis, S., & Nika, P. (2018). How does the degree of guidance support students’ metacognitive and problem-solving skills in educational robotics? Journal of Science Education and Technology, 27 (1), 70–85. https://doi.org/10.1007/s10956-017-9709-x
Aytekin, A., Sonmez Cakir, F., Yucel, Y. B., & Kulaoz, İ. (2018). Coding science directed to future and some methods to be available and coding learned. Eurasian Journal of Researches in Social and Economics (EJRSE) , 24–41.
Bargagna, S., Castro, E., Cecchi, F., Cioni, G., Dario, P., Dell’Omo, M., Di Lieto, M. C., Inguaggiato, E., Martinelli, A., Pecini, C., & Sgandurra, G. (2019). Educational robotics in down syndrome: A feasibility study. Technology, Knowledge and Learning, 24 (2), 315–323. https://doi.org/10.1007/s10758-018-9366-z
Baser, M. (2013). Attitude, gender and achievement in computer programming. Online Submission, 14 (2), 248–255. https://doi.org/10.5829/idosi.mejsr.2013.14.2.2007
Beisser, S. R. (2005). An examination of gender differences in elementary constructionist classrooms using Lego/Logo instruction. Computers in the Schools, 22 (3–4), 7–19. https://doi.org/10.1300/j025v22n03_02
Benitti, F. B. V. (2012). Exploring the educational potential of robotics in schools: A systematic review. Computers & Education, 58 (3), 978–988. https://doi.org/10.1016/j.compedu.2011.10.006
Berland, M., & Wilensky, U. (2015). Comparing virtual and physical robotics environments for supporting complex systems and computational thinking. Journal of Science Education and Technology, 24 (5), 628–647. https://doi.org/10.1007/s10956-015-9552-x
Bers, M. U. (2019). Coding as another language: A pedagogical approach for teaching computer science in early childhood. Journal of Computers in Education, 6 (4), 499–528. https://doi.org/10.1007/s40692-019-00147-3
Bers, M. U. (2010). The TangibleK robotics program: Applied computational thinking for young children. Early Childhood Research and Practice, 12 (2), 1–20.
Bers, M. U., & Portsmore, M. (2005). Teaching partnerships: Early childhood and engineering students teaching math and science through robotics. Journal of Science Education and Technology, 14 (1), 59–73. https://doi.org/10.1007/s10956-005-2734-1
Beug, A. (2012). Teaching introductory programming concepts: A comparison of Scratch and Arduino (Master's thesis, California Polytechnic State University, San Luis Obispo). https://doi.org/10.15368/theses.2012.95 .
Bixler, B. (2006). Motivation and its relationship to the design of educational games. NMC Conference, Cleveland, Ohio, 10 (07).
Bravo, F. A., Gonzalez, A. M., & Gonzalez, E. (2017). A review of intuitive robot programming environments for educational purposes. 2017 IEEE 3rd Colombian Conference on Automatic Control, CCAC 2017 - Conference Proceedings , 2018 - Janua (pp. 1–6). https://doi.org/10.1109/CCAC.2017.8276396 .
Brophy, S., Klein, S., Portsmore, M., & Rogers, C. (2008). Advancing engineering education in P-12 classrooms. Journal of Engineering Education, 97 (3), 369–387. https://doi.org/10.1002/j.2168-9830.2008.tb00985.x
Cam, E., & Kıyıcı, M. (2022). The impact of robotics assisted programming education on academic success, problem solving skills and motivation. Journal of Educational Technology and Online Learning, 5 (1), 47–65. https://doi.org/10.31681/jetol.1028825
Carro, G., Sancristobal, E., & Plaza, P. (2021). Robotics as a tool to awaken interest in engineering and computing among children and young people. Revista Iberoamericana De Tecnologias Del Aprendizaje, 16 (2), 204–212. https://doi.org/10.1109/RITA.2021.3089919
Chalmers, C. (2018). Robotics and computational thinking in primary school. International Journal of Child-Computer Interaction, 17 , 93–100. https://doi.org/10.1016/j.ijcci.2018.06.003
Chang, C. C., & Chen, Y. (2020). Cognition, attitude, and interest in cross-disciplinary i-STEM robotics curriculum developed by thematic integration approaches of webbed and threaded models: A concurrent embedded mixed methods study. Journal of Science Education and Technology, 29 (5), 622–634. https://doi.org/10.1007/s10956-020-09822-4
Chen, Y., & Chang, C. C. (2018). The impact of an integrated robotics STEM course with a sailboat topic on high school students’ perceptions of integrative STEM, interest, and career orientation. Eurasia Journal of Mathematics, Science and Technology Education, 14 (12), 1614.
Cheng, C. C., Huang, P. L., & Huang, K. H. (2013). Cooperative learning in Lego robotics projects: Exploring the impacts of group formation on interaction and achievement. Journal of Networks, 8 (7), 1529–1535. https://doi.org/10.4304/jnw.8.7.1529-1535
Cohen, J. (1988). Statistical power analysis for the behavioral sciences. In Routledge Academic . https://doi.org/10.2307/2529115 .
Comber, C., Colley, A., Hargreaves, D. J., & Dorn, L. (1997). The effects of age, gender, and computer experience upon computer attitudes. Educational Research, 39 (2), 123–133. https://doi.org/10.1080/0013188970390201
Creswell, J. W. (2014). Research Design: Qualitative, quantitative, and mixed methods approaches (4nd ed.). Sage. Clark, (4th ed.). SAGE.
Cross, J., Hamner, E., Zito, L., Nourbakhshh, I., & Bernstein, D. (2016). Development of an assessment for measuring middle school student attitudes towards robotics activities. 2016 IEEE Frontiers in Education Conference (FIE) . https://doi.org/10.1109/fie.2016.7757677 .
Di Serio, Á., Ibáñez, M. B., & Kloos, C. D. (2013). Impact of an augmented reality system on students’ motivation for a visual art course. Computers & Education, 68 , 586–596. https://doi.org/10.1016/j.compedu.2012.03.002
Dincer, S. (2015). The effect of computer-aided instruction software prepared by using different pedagogical agents to students' academic achievements, motivations, interests in their courses, judgements about their computer-aided instruction and cognitive loads (Doctoral dissertation, Çukurova University).
Eguchi, A. (2014). Robotics as a learning tool for educational transformation. International Workshop Teaching Robotics, Teaching with Robotics & International Conference Robotics in Education , 27–34. https://doi.org/10.4018/978-1-4666-8363-1.ch002 .
Eguchi, A. (2016). RoboCupJunior for promoting STEM education, 21st century skills, and technological advancement through robotics competition. Robotics and Autonomous Systems, 75 , 692–699. https://doi.org/10.1016/j.robot.2015.05.013
El-Hamamsy, L., Bruno, B., Chessel-Lazzarotto, F., Chevalier, M., Roy, D., Zufferey, J. D., & Mondada, F. (2021). The symbiotic relationship between educational robotics and computer science in formal education. Education and Information Technologies, 26 (5), 5077–5107. https://doi.org/10.1007/s10639-021-10494-3
El-Hamamsy, L., Chessel-Lazzarotto, F., Bruno, B., Roy, D., Cahlikova, T., Chevalier, M., Parriaux, G., Pellet, J. P., Lanarès, J., Zufferey, J. D., & Mondada, F. (2021). A computer science and robotics integration model for primary school: Evaluation of a large-scale in-service K-4 teacher-training program. Education and Information Technologies, 26 (3), 2445–2475. https://doi.org/10.1007/s10639-020-10355-5
Elkin, M., Sullivan, A., & Bers, M. U. (2016). Programming with the Kibo robotics kit in preschool classrooms. Computers in the Schools, 33 (3), 169–186. https://doi.org/10.1080/07380569.2016.1216251
Erol, O., Sevim-Cirak, N., & Baser Gulsoy, V. G. (2023). The effects of educational robotics activities on students’ attitudes towards stem and ict courses. International Journal of Technology in Education, 6 (2), 203–223. https://doi.org/10.46328/ijte.365
Fagin, B. S., & Merkle, L. (2002). Quantitative analysis of the effects of robots on introductory computer science education. Journal on Educational Resources in Computing, 2 (4), 1–17. https://doi.org/10.1145/949257.949259
Field, A. (2009). Discovering statistics using SPSS. In Sage Publication (Vol. 58). https://doi.org/10.1234/12345678 .
Fraenkel, J., Wallen, N., & Hyun, H. (2018). How to design and evaluate research in education (10th ed.). McGraw-Hill.
Fryer, L. K., & Bovee, H. N. (2016). Internet and higher education supporting students ’ motivation for e-learning : Teachers matter on and off line. The Internet and Higher Education, 30 , 21–29. https://doi.org/10.1016/j.iheduc.2016.03.003
Gabrielle, D. (2003). The effects of technology-mediated instructional strategies on motivation, performance, and self-directed learning. In EdMedia+ Innovate Learning (pp. 2568–2575). Association for the Advancement of Computing in Education (AACE).
Gerecke, U., & Wagner, B. (2007). The challenges and benefits of using robots in higher education. Intelligent Automation & Soft Computing, 13 (1), 29–43.
Guilloteaux, M. J., & Dörnyei, Z. (2008). Motivating language learners: A classroom-oriented investigation of the effects of motivational strategies on student motivation. TESOL Quarterly, 42 (1), 55–77.
Gupta, N., Tejovanth, N., & Murthy, P. (2012). Learning by creating: Interactive programming for Indian high schools. Proceedings - 2012 IEEE International Conference on Technology Enhanced Education, ICTEE 2012 , 4–6. https://doi.org/10.1109/ICTEE.2012.6208643 .
Harel, I., & Papert, S. (1991). Situating Constructionism. Constructionism , 1–16.
Highfield, K. (2010). Robotic toys as a catalyst for mathematical problem solving. Australian Primary Mathematics Classroom, 15 (2), 22–28.
Hodges, S., Sentance, S., Finney, J., & Ball, T. (2020). Physical computing: A key element of modern computer science education. Computer, 53 (4), 20–30. https://doi.org/10.1109/MC.2019.2935058
Huitt, W. (2001). Motivation to learn: An overview. Educational Psychology Interactive, 12 (3), 29–36.
Hung, I. C., Chao, K. J., Lee, L., & Chen, N. S. (2013). Designing a robot teaching assistant for enhancing and sustaining learning motivation. Interactive Learning Environments, 21 (2), 156–171. https://doi.org/10.1080/10494820.2012.705855
Hussain, S., Lindh, J., & Shukur, G. (2006). The effect of LEGO training on pupils’ school performance in mathematics, problem solving ability and attitude: Swedish data. Educational Technology and Society, 9 (3), 182–194.
Ioannou, A., & Makridou, E. (2018). Exploring the potentials of educational robotics in the development of computational thinking: A summary of current research and practical proposal for future work. Education and Information Technologies, 23 (6), 2531–2544. https://doi.org/10.1007/s10639-018-9729-z
Jackson, A., Mentzer, N., & Kramer-Bottiglio, R. (2021). Increasing gender diversity in engineering using soft robotics. Journal of Engineering Education, 110 (1), 143–160. https://doi.org/10.1002/jee.20378
Jdeed, M., Schranz, M., & Elmenreich, W. (2020). A study using the low-cost swarm robotics platform spiderino in education. Computers and Education Open, 1 (November), 100017. https://doi.org/10.1016/j.caeo.2020.100017
Jung, S. E., & Won, E. S. (2018). Systematic review of research trends in robotics education for young children. Sustainability (Switzerland), 10 (4), 1–24. https://doi.org/10.3390/su10040905
Kaloti-Hallak, F., Armoni, M., & Ben-Ari, M. (2015, November). Students' attitudes and motivation during robotics activities. In Proceedings of the Workshop in Primary and Secondary Computing Education (pp. 102–110).
Kandlhofer, M., & Steinbauer, G. (2016). Evaluating the impact of educational robotics on pupils ’ technical- and social-skills and science related attitudes. Robotics and Autonomous Systems, 75 , 679–685. https://doi.org/10.1016/j.robot.2015.09.007
Karaman, S., Anders, A., Boulet, M., Connor, J., Gregson, K., Guerra, W., Guldner, O., Mohamoud, M., Plancher, B., Shin, R., & Vivilecchia, J. (2017). Project-based, collaborative, algorithmic robotics for high school students: Programming self-driving race cars at MIT. ISEC 2017 - Proceedings of the 7th IEEE Integrated STEM Education Conference , 195–203. https://doi.org/10.1109/ISECon.2017.7910242 .
Karim, M. E., Lemaignan, S., & Mondada, F. (2015). A review: Can robots reshape K-12 STEM education? 2015 IEEE International Workshop on Advanced Robotics and its Social Impacts (ARSO) . https://doi.org/10.1109/ARSO.2015.7428217 .
Kazakoff, E. R., Sullivan, A., & Bers, M. U. (2013). The effect of a classroom-based intensive robotics and programming workshop on sequencing ability in early childhood. Early Childhood Education Journal, 41 (4), 245–255. https://doi.org/10.1007/s10643-012-0554-5
Keller, J. M. (1987). Development and use of the ARCS model of instructional design. Journal of Instructional Development, 10 (3), 2. https://doi.org/10.1007/BF02905780
Article MathSciNet Google Scholar
Keller, J. M. (2009). Motivational design for learning and performance: The ARCS model approach. Springer Science & Business Media.
Kert, S. B., Erkoç, M. F., & Yeni, S. (2020). The effect of robotics on six graders’ academic achievement, computational thinking skills, and conceptual knowledge levels. Thinking Skills and Creativity, 38 (1), 100714. https://doi.org/10.1016/j.tsc.2020.100714
Kim, S. W., & Lee, Y. (2016). The effect of robot programming education on attitudes towards robots. Indian journal of science and technology, 9 (24), 1–11. https://doi.org/10.17485/ijst/2016/v9i24/96104
Kucuk, S., & Sisman, B. (2017). Behavioral patterns of elementary students and teachers in one-to-one robotics instruction. Computers and Education, 111 , 31–43. https://doi.org/10.1016/j.compedu.2017.04.002
Kucuk, S., & Sisman, B. (2020). Students’ attitudes towards robotics and STEM: Differences based on gender and robotics experience. International Journal of Child-Computer Interaction, 23–24 , 100167. https://doi.org/10.1016/j.ijcci.2020.100167
Lin, C. H., Liu, E. Z. F., Kou, C. H., Virnes, M., Sutinen, E., & Cheng, S. S. (2009). A case analysis of creative spiral instruction model and students’ creative problem solving performance in a Lego® robotics course. In Learning by Playing. Game-based Education System Design and Development: 4th International Conference on E-Learning and Games, Edutainment 2009, Banff, Canada, August 9–11, 2009. Proceedings 4 (pp. 501–505). Springer Berlin Heidelberg.
Liu, E. Z. F. (2010). Early adolescents’ perceptions of educational robots and learning of robotics. British Journal of Educational Technology, 41 (3), 44–48. https://doi.org/10.1111/j.1467-8535.2009.00944.x
Malone, T. W. (1981). Toward a theory of intrinsically motivating instruction. Cognitive Science, 5 (4), 333–369.
Master, A., Cheryan, S., Moscatelli, A., & Meltzoff, A. N. (2017). Programming experience promotes higher STEM motivation among first-grade girls. Journal of Experimental Child Psychology, 160 , 92–106.
McWhorter, W. I. (2008). The effectiveness of using LEGO Mindstorms robotics activities to influence self-regulated learning in a university introductory computer programming course. University of North Texas.
Mohd, N., Mahmood, T. F. P. T., & Ismail, M. N. (2011). Factors that influence students in mathematics achievement. International Journal of Academic Research, 3 (3), 49–54.
Molaee, Z., & Dortaj, F. (2015). Improving L2 learning: An ARCS instructional-motivational approach. Procedia - Social and Behavioral Sciences, 171 , 1214–1222. https://doi.org/10.1016/j.sbspro.2015.01.234
NGSS Lead States. (2013). Next generation science standards: For states, by states. The National Academies Press.
Nicolaidou, M., & Philippou, G. (2003). Attitudes towards mathematics, self-efficacy and achievement in problem solving. European Research in Mathematics Education III , 1 (11).
Noh, J., & Lee, J. (2020). Effects of robotics programming on the computational thinking and creativity of elementary school students. Educational Technology Research and Development, 68 , 463–484.
Ntourou, V., Kalogiannakis, M., & Psycharis, S. (2021). A study of the impact of arduino and visual programming in self-efficacy, motivation, computational thinking and 5th grade students’ perceptions on electricity. Eurasia Journal of Mathematics, Science and Technology Education, 17 (5), 1–11. https://doi.org/10.29333/ejmste/10842
OECD. (2016). Skills for a digital world . Organisation for Economic Co-operation and Development. https://www.oecd.org/employment/emp/Skills-for-a-Digital-World.pdf . Accessed 14 Aug 2023.
Okita, S. Y. (2014). The relative merits of transparency: Investigating situations that support the use of robotics in developing student learning adaptability across virtual and physical computing platforms. British Journal of Educational Technology, 45 (5), 844–862.
Ouyang, F., & Xu, W. (2024). The effects of educational robotics in STEM education: A multilevel meta-analysis. International Journal of STEM Education, 11 (1). https://doi.org/10.1186/s40594-024-00469-4
Pajares, F., & Schunk, D. (2001). The development of academic self-efficacy. In A. Wigfield & J. Eccles (Eds.), Development of Achievement Motivation (pp. 16–32). United States: Vol. 1446, 2004.
Papastergiou, M. (2009). Digital game-based learning in high school computer science education: Impact on educational effectiveness and student motivation. Computers & Education, 52 (1), 1–12. https://doi.org/10.1016/j.compedu.2008.06.004
Papert, S. (1980). Mindstorms: Children, computers and powerful ideas. In New Ideas in Psychology (Vol. 1). https://doi.org/10.1016/0732-118X(83)90034-X .
Piaget, J. (1973). To understand is to invent: the future of education (G. Roberts, Trans.). Grossman Publishers, A Division of the Viking Press 148.
Przybylla, M., & Romeike, R. (2014). Overcoming Issues with Students’ Perceptions of Informatics in Everyday Life and Education with Physical Computing. In Local Proceeding of the 7th International Conference on Informatics in Schools: Situation, Evolution and Perspectives ISSEP 2014 .
Reich-Stiebert, N., & Eyssel, F. (2015). Learning with educational companion robots? Toward attitudes on education robots, predictors of attitudes, and application potentials for education robots. International Journal of Social Robotics, 7 (5), 875–888. https://doi.org/10.1007/s12369-015-0308-9
Resnick, M. (2013). Learn to code, code to learn. EdSurge .
Resnick, M., Kafai, Y., & Maeda, J. (2003). A networked, media-rich programming environment to enhance technological fluency at after-school centers in economically-disadvantaged communities. National Science Foundation (Information Technology Research) , 1–14.
Ribeiro, C., Costa, M. F. M., & Rocha, M. (2008). A study of educational robotics in elementary schools. In Proceedings of the 4th International Conference on Hands-on Science: Development Diversity and Inclusion in Science Education (pp. 580–595).
Rogers, C., & Portsmore, M. (2004). Bringing engineering to elementary school. Journal of STEM Education, 5 (3), 17–29.
Rubio, M., Hierro, C., & Pablo, Á. (2013). Using arduino to enhance computer programming courses in science and engineering. Proceedings of the EDULEARN13 , 72 (July), 5127–5133.
Ruf, A., Mühling, A., & Hubwieser, P. (2014). Scratch vs. Karel - impact on learning outcomes and motivation. ACM International Conference Proceeding Series , 50–59. https://doi.org/10.1145/2670757.2670772 .
Rusk, N., Resnick, M., Berg, R., & Pezalla-Granlund, M. (2008). New pathways into robotics: Strategies for broadening participation. Journal of Science Education and Technology, 17 (1), 59–69. https://doi.org/10.1007/s10956-007-9082-2
Sáez López, J. M., Buceta Otero, R., & De Lara García-Cervigón, S. (2020). Introducing robotics and block programming in elementary education. RIED. Revista Iberoamericana de Educación a Distancia, 24 (1), 95. https://doi.org/10.5944/ried.24.1.27649
Saleiro, M., Carmo, B., Rodrigues, J. M., & du Buf, J. H. (2013). A low-cost classroom-oriented educational robotics system. In Social Robotics: 5th International Conference, ICSR 2013, Bristol, UK, October 27–29, 2013, Proceedings 5 (pp. 74–83). Springer International Publishing.
Sapounidis, T., Tselegkaridis, S., & Stamovlasis, D. (2023). Educational robotics and STEM in primary education: A review and a meta-analysis. Journal of Research on Technology in Education . https://doi.org/10.1080/15391523.2022.2160394
Settle, A., & Perkovic, L. (2010). Computational thinking across the curriculum: A conceptual framework.
Shin, S., Park, P., & Bae, Y. (2013). The effects of an information-technology gifted program on friendship using scratch programming language and clutter. International Journal of Computer and Communication Engineering, 2 (3), 246–249. https://doi.org/10.7763/IJCCE.2013.V2.181
Sisman, B., & Kucuk, S. (2018). A validity and reliability study of the Turkish robotics attitude scale for secondary school students. Ege Eğitim Dergisi, July , 262–283. https://doi.org/10.12984/egeefd.414091 .
Sisman, B., Kucuk, S., & Yaman, Y. (2021). The effects of robotics training on children ’ s spatial ability and attitude toward STEM. International Journal of Social Robotics, 13 (2), 379–389. https://doi.org/10.1007/s12369-020-00646-9
Somyurek, S. (2015). An effective educational tool: Construction kits for fun and meaningful learning. International Journal of Technology and Design Education, 25 (1), 25–41. https://doi.org/10.1007/s10798-014-9272-1
Stewart, W. H., Baek, Y., Kwid, G., & Taylor, K. (2021). Exploring factors that influence computational thinking skills in elementary students’ collaborative robotics. Journal of Educational Computing Research, 59 (6), 1208–1239. https://doi.org/10.1177/0735633121992479
Strawhacker, A., & Bers, M. U. (2015). “I want my robot to look for food”: Comparing kindergartner’s programming comprehension using tangible, graphic, and hybrid user interfaces. International Journal of Technology and Design Education, 25 (3), 293–319. https://doi.org/10.1007/s10798-014-9287-7
Sullivan, A., & Bers, M. U. (2018). Investigating the use of robotics to increase girls ’ interest in engineering during early elementary school. International Journal of Technology and Design Education, 29 (5), 1033–1051. https://doi.org/10.1007/s10798-018-9483-y
Sun, L., & Zhou, D. (2023). Effective instruction conditions for educational robotics to develop programming ability of K-12 students: A meta-analysis. Journal of Computer Assisted Learning, 39 (2), 380–398.
Tabachnick, B. G., & Fidell, L. S. (2007). Using multivariate statistics (Allyn & Bacon (Ed.); 7th ed.). Pearson Education.
Tay, B., & Akyürek Tay, B. (2004). The effects of attitude on success in social sciences courses. Türk Eğitim Bilimleri Dergisi, 4 (1), 73–84.
Tlili, A., Essalmi, F., Jemni, M., & Kinshuk. (2017). Towards applying Keller’s ARCS model and learning by doing strategy in classroom courses. In Lecture Notes in Educational Technology (pp. 189–198). https://doi.org/10.1007/978-981-10-2419-1_26 .
Todorovska, K., & Bogdanova, A. M. (2020). Educational robots in preschool education. 17th International Conference on Informatics and Information Technologies - CIIT 2020 , 17–19.
Wang, M. T., & Degol, J. L. (2017). Gender Gap in Science, Technology, Engineering, and Mathematics (STEM): Current Knowledge, Implications for Practice, Policy, and Future Directions. In Educational Psychology Review (Vol. 29, Issue 1, pp. 119–140). Educational Psychology Review. https://doi.org/10.1007/s10648-015-9355-x .
Wei, C. W., Hung, I. C., Lee, L., & Chen, N. S. (2011). A joyful classroom learning system with robot learning companion for children to learn mathematics multiplication. Turkish Online Journal of Educational Technology, 10 (2), 11–23.
Welch, A. G. (2010). Using the TOSRA to assess high school students’ attitudes toward science after competing in the first robotics competition: An exploratory study. Eurasia Journal of Mathematics, Science and Technology Education, 6 (3), 187–197. https://doi.org/10.12973/ejmste/75239
Wing, J. M. (2006). Computational thinking . 49 (3), 1–3. https://doi.org/10.1145/1118178.1118215 .
Wu, T. T., and Chen, J. M. (2021). Combining webduino programming with situated learning to promote computational thinking, motivation, and satisfaction among high school students. Journal of Educational Computing Research, 123 . https://doi.org/10.1177/07356331211039961
Wu, S. Y., & Su, Y. S. (2021). Visual programming environments and computational thinking performance of fifth- and sixth-grade students. Journal of Educational Computing Research, 59 (6), 1075–1092.
Yadagiri, R. G., Krishnamoorthy, S. P., & Kapila, V. (2015). A blocks-based visual environment to teach robot-programming to k-12 students. 122nd ASEE Annual Conference & Exposition , 1–12. https://doi.org/10.18260/p.23358 .
Yıldız, V. A., Baydaş, Ö., & Göktaş, Y. (2019). The ARCS motivation model: A content analysis of applied articles between the years1997–2018. Trakya Education Journal, 9 (4), 723–741. https://doi.org/10.24315/tred.520477
Yolcu, V., & Demirer, V. (2023). The effects of educational robotics in programming education on students’ programming success, computational thinking, and transfer of learning. Computer Applications in Engineering Education, 31 (6), 1633–1647. https://doi.org/10.1002/cae.22664
Zhang, Y., & Zhu, Y. (2022). Effects of educational robotics on the creativity and problem-solving skills of K-12 students: A meta-analysis. Educational Studies, 00 (00), 1–19. https://doi.org/10.1080/03055698.2022.2107873 .
Zhang, Y., Luo, R., Zhu, Y., & Yin, Y. (2021). Educational robots improve K-12 students’ computational thinking and STEM attitudes: Systematic review. Journal of Educational Computing Research, 59 (7), 1450–1481. https://doi.org/10.1177/0735633120982944
Download references
Open access funding provided by the Scientific and Technological Research Council of Türkiye (TÜBİTAK). Scientific Research Project Coordination Unit of Istanbul University-Cerrahpaşa, Grant/Award Number: SDK-2018-27619 and SYL-2018-29217.
Author information
Authors and affiliations.
Behiye Dr. Nevhiz Işıl Anatolian High School, Istanbul, Türkiye
Nuray Akman Selcuk
Department of Instructional Technology, Kazım Karabekir Education Faculty, Ataturk University, Erzurum, Türkiye
Sevda Kucuk
Department of Cognitive Robotics, Delft University of Technology, Delft, Netherlands
Burak Sisman
You can also search for this author in PubMed Google Scholar
Contributions
Dr. Nuray Akman Selcuk is currently working at Behiye Dr. Nevhiz Işıl Anatolian High School as an Information Technologies teacher in Istanbul. She received Phd from Istanbul University Institution of Informatics. Her research interests are information technologies education, technology integration into K-12 education and educational robotics.
Dr. Sevda Kucuk is a professor doctor at the Department of Computer Education & Instructional Technology at Atatürk University. Her research interests are in the augmented reality technology, mobile learning, technology integration, educational robotics, teacher education, distance education, instructional design, instructional strategies, and research methods.
Dr. Burak Sisman is a researcher in the Department of Cognitive Robotics at Delft University of Technology in the Netherlands. His research focuses on educational robotics, STEM education, human-robot interaction, knowledge representation, and artificial intelligence. He has over 18 years of experience in teaching and research. He has conducted various research projects in these fields and has received several patents for his innovative work.
Corresponding author
Correspondence to Sevda Kucuk .
Ethics declarations
Conflict of interest.
The authors declare that they have no conflict of interest.
Additional information
Publisher's note.
Springer Nature remains neutral with regard to jurisdictional claims in published maps and institutional affiliations.
Rights and permissions
Open Access This article is licensed under a Creative Commons Attribution 4.0 International License, which permits use, sharing, adaptation, distribution and reproduction in any medium or format, as long as you give appropriate credit to the original author(s) and the source, provide a link to the Creative Commons licence, and indicate if changes were made. The images or other third party material in this article are included in the article's Creative Commons licence, unless indicated otherwise in a credit line to the material. If material is not included in the article's Creative Commons licence and your intended use is not permitted by statutory regulation or exceeds the permitted use, you will need to obtain permission directly from the copyright holder. To view a copy of this licence, visit http://creativecommons.org/licenses/by/4.0/ .
Reprints and permissions
About this article
Selcuk, N.A., Kucuk, S. & Sisman, B. Does really educational robotics improve secondary school students’ course motivation, achievement and attitude?. Educ Inf Technol (2024). https://doi.org/10.1007/s10639-024-12773-1
Download citation
Received : 10 February 2024
Accepted : 02 May 2024
Published : 31 May 2024
DOI : https://doi.org/10.1007/s10639-024-12773-1
Share this article
Anyone you share the following link with will be able to read this content:
Sorry, a shareable link is not currently available for this article.
Provided by the Springer Nature SharedIt content-sharing initiative
- Secondary education
- Educational robotics
- Gender studies
- Course motivation
- Find a journal
- Publish with us
- Track your research
Academia.edu no longer supports Internet Explorer.
To browse Academia.edu and the wider internet faster and more securely, please take a few seconds to upgrade your browser .
Enter the email address you signed up with and we'll email you a reset link.
- We're Hiring!
- Help Center
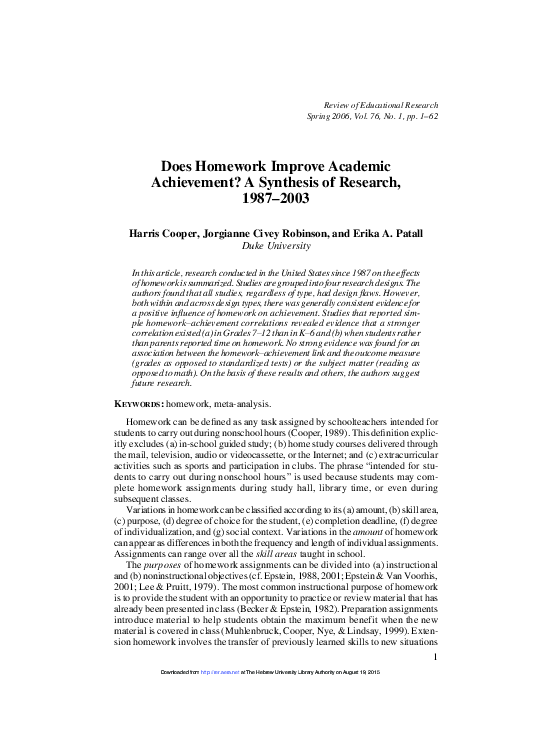
Does Homework Improve Academic Achievement? A Synthesis of Research, 1987–2003

2006, Review of Educational Research
Related Papers
Steven McMullen
In the literature on the impact of homework there is little empirical support for assigning homework to elementary school students. Nevertheless, the practice has become more common, despite popular resistance among many parents and popular media. We examine the effects of both assigning homework and time spent on homework on mathematics and reading achievement using nationally representative longitudinal data on elementary school students. In order to control for important unobserved characteristics and inputs we use empirical specifications that include student fixed effects. We find that this approach consistently indicates that homework has a positive impact on academic achievement, and that less sophisticated empirical approaches will produce misleading results. Additionally, we find that the impact of homework is not uniform across the population, but that some minority groups and low income students get more benefit from homework, indicating that increasing homework assigned could be a valuable policy for decreasing the black-white as well as the high and low-income achievement gap.
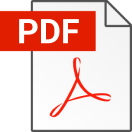
Fatih Mehmet Cigerci
The main purpose of this study was to determine the effect of homework assignments on students' academic achievement. This meta-analysis sought an answer to the research question: "What kind of effect does homework assignment have on students' academic achievement levels?" In this research, meta-analysis was adopted to determine the effect of homework assignments on students' academic achievement. The effect sizes of the studies included in the meta-analysis were compared with regard to their methodological characteristics (research design, sample size, and publication bias) and substantive characteristics (course type, grade level, duration of implementation, instructional level, socioeconomic status, and setting). At the end of the research, it was revealed that homework assignments had a small effect size (d = 0.229) on students' academic achievement levels. Lastly, it was seen that there was not a significant difference with regard to the effect sizes of the studies with respect to all variables, except the course type variable in the research.
Social Psychology of Education
Jim LIndsay
Four explanations were tested for why the correlation between homework and achievement is weaker in elementary school than secondary school. Eighty-two teachers answered questions about their homework practices, and their responses were related to their students' achievement test scores. No evidence was found to suggest the weaker correlation in elementary school associated with a restricted variation in amounts of homework in early grades nor that teachers assigned more homework to poor-performing classes. Evidence did suggest that teachers in early grades assigned homework more often to develop young students' management of time, a skill rarely measured on standardized achievement tests. Also consistent with this hypothesis, elementary school teachers were more likely to use homework to review class material and to go over homework in class, while secondary school teachers more often used homework to prepare for and enrich class lessons. Finally, there was weak evidence ...
Robert Marzano
SSRN Electronic Journal
Charlene Kalenkoski
This study takes advantage of nationally representative panel data on student behavior and academic performance to test two possible policy reforms. First, I examine a policy that increases the amount of homework that students complete. Second, I examine the impact of increasing the amount of homework assigned. Previous studies have not been able to consistently estimate the impact of homework because of important omitted variables and measurement error, which strongly bias the estimated impact of homework time. This paper, however, uses an instrumental variables approach with student fixed effects to account for both time-varying and time-invariant unobserved characteristics and inputs. This approach produces estimates of the impact of homework time on academic achievement that are much larger than those of previous studies. Additionally, these findings suggest that assigning additional homework primarily improves the achievement of low performing students and students in low performing schools. Thus, assigning more homework could help close the gap in achievement between high and low performing students.
Educational Psychology
Marley Watkins
Volume V Issue I
Dr. Anila Fatima Shakil
Homework is the means by which the relationship between home and school is demonstrated and developed, leading to more consistent progress in all aspects of school life. The current research was carried out in Gilgit Baltistan to find out the impact of homework on the academic performance of students at secondary level. The research was observed by teachers of Gilgit Baltistan public schools while 100 teachers were chosen by a random sampling technique as a sample. Questionnaires were as a research instrument. The study found that homework impacts learning for learners, its impact differs with the age of students, and it plays an important role in student achievement. The study proposed that homework should be purposeful, i.e. it should include the introduction of new content, the practise of skills, the creation of any data and the ability for students to explore topics of their own interest.
School Psychology Quarterly
Jodene Fine
Contemporary Educational Psychology
Bernhard Schmitz
RELATED PAPERS
BARIDULA VITO
Geological Quarterly
piotr olchowy
Journal of Molecular Structure
neema ani mangalam
Didier Villemin
Owen Bootman
Manuel Boquete
Genes & Development
Susanne Kirschnek
Turkish Bulletin of Hygiene and Experimental Biology
Journal of Clinical Microbiology
Costi Sifri
Paweł Szymon Towpik
Electronics Letters
Gregory Belenky
Reforma y Democracia
Mauricio Merino Huerta
The Journal of Immunology
Kelly Summers
International Journal of Sustainable Development and Planning
MARIKA GABALLO
Zeyneb Guliyeva
2003 European Control Conference (ECC)
Ahmed Rahmani
American Journal of Physiology-gastrointestinal and Liver Physiology
Terry Machen
The Yearbook of Comparative Literature
Samia Rahimtoola
Economic Paper
Kanayathu Koshy
The journal of allergy and clinical immunology. In practice
Kevin Kennedy
Nature Biotechnology
Richard Vandlen
RELATED TOPICS
- We're Hiring!
- Help Center
- Find new research papers in:
- Health Sciences
- Earth Sciences
- Cognitive Science
- Mathematics
- Computer Science
- Academia ©2024
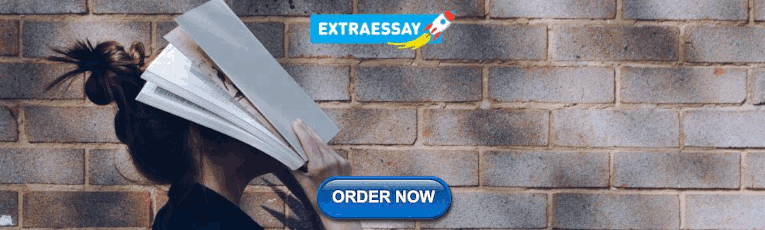
COMMENTS
Student achievement in schools has always been a concern for parents, students, and educators. There have been several theories on the areas of what help students achieve. One of the main factors impacting student achievement has been the use of homework (Collier, 2007). Opinions vary on whether or not homework has positive effects on achievement.
HARRIS COOPER is a Professor of Psychology and Director of the Program in Education, Box 90739, Duke University, Durham, NC 27708-0739; e-mail [email protected] His research interests include how academic activities outside the school day (such as homework, after school programs, and summer school) affect the achievement of children and adolescents; he also studies techniques for improving ...
In this article, research conducted in the United States since 1987 on the effects of homework is summarized. Studies are grouped into four research designs. The authors found that all studies, regardless of type, had design flaws. However, both within and across design types, there was generally consistent evidence for a positive influence of homework on achievement. Studies that reported ...
Many school district policies state that high school students should expect about 30 minutes of homework for each academic course they take, a bit more for honors or advanced placement courses. These recommendations are consistent with the conclusions reached by our analysis. Practice assignments do improve scores on class tests at all grade ...
June 26, 2022 at 12:42 am. Studies have shown that homework improved student achievement in terms of improved grades, test results, and the likelihood to attend college. Link. i think homework can help kids but at the same time not help kids. Link.
Homework assignments are influenced by more factors than any other instruc-tional strategy. Student differences may play a major role because homework allows students considerable discretion about whether, when, and how to complete assign-ments. Teachers may structure and monitor homework in a multitude of ways.
In this article, research conducted in the United States since 1987 on the effects of homework is summarized. Studies are grouped into four research designs. The authors found that all studies, regardless of type, had design flaws. However, both within and across design types, there was generally consistent evidence for a positive influence of homework on achievement. Studies that reported ...
These characteristics encompassed six broad distinctions among studies: (a) the research report; (b) the research design; (c) the homework variable; (d) the sample of students; (e) the measure of achievement, and (f) the estimate of the relationship between homework and achievement.
Most research examines what students do, and whether and how the completion of homework or time spent affects student achievement or success in school (Cooper, 1989; Paschal, Weinstein, & Walberg ...
1. Cooper, Robinson, and Patall (Citation 2006) provide a nice overview of the effects of homework on academic achievement in the education, psychology, and sociology literatures.In general, small positive effects have been found. More recently, using 1990 data from NELS and 2002 data from the Education Longitudinal Study, Maltese, Tai, and Fan (Citation 2012) found no effect of math and ...
Homework can be defined as any task assigned by schoolteachers intended for students to carry out during nonschool hours (Cooper, 1989). This definition explicitly excludes (a) in-school guided study; (b) home study courses delivered through the mail, television, audio or vidéocassette, or the Internet; and (c) extracurricular activities such ...
Does Homework Improve Academic Achievement? A Synthesis of Research, 1987-2003 In this article, the authors summarize research conducted in the United States since 1987 on the effects of homework. Studies are grouped into four research designs. The authors found that all studies, regardless of type, had design flaws.
Does Homework Improve Academic Achievement? A Synthesis of Research, 1987-2003 Harris Cooper, Jorgianne Civey Robinson, and Erika A. Patall Duke University In this article, research conducted in the United States since 1987 on the effects ofhomework is summarized Studies are grouped intofour research designs. The
However, both within and across design types, there was generally consistent evidence for a positive influence of homework on achievement. Studies that reported simple homework-achievement correlations revealed evidence that a stronger correlation existed (a) in Grades 7-12 than in K-6 and (b) when students rather than parents reported ...
The role of homework in academic achievement is an age-old debate (Walberg et al., 1985) that has swung between times when it was thought to be a tool for improving a country's competitiveness and times when it was almost outlawed.So Cooper talks about the battle over homework and the debates and rows continue (Walberg et al., 1985, 1986; Barber, 1986).
Critics have objected that even if homework doesn't increase grades or test scores, it has other benefits, like fostering good study habits and providing parents with a window into what kids are ...
The eligible intervention studies must be clear that the intervention is homework assigned to students to complete during nonschool hours regularly by schoolteachers which aims to improve academic achievement. This does not mean that the intervention must consist of academic activities, but rather that the explicit expectation must be that the ...
At the end of the research, it was revealed that homework assignments had a small effect size (d = 0.229) on students' academic achievement levels. Lastly, it was seen that there was not a significant difference with regard to the effect sizes of the studies with respect to all variables, except the course type variable in the research.
Previous research provides evidence that developing a growth mindset—believing that one's capabilities can improve—promotes academic achievement. Although this phenomenon has undergone prior study in a representative sample of ninth graders in the United States, it has not been studied in representative samples of other grade levels or ...
Past Syntheses of Homework Research. Homework has been an active area of study among American education researchers for the past 70 years. As early as 1927, a study by Hagan (1927) compared the effects. of homework with the effects of in-school supervised study on the achievement of 11- and 12-year-olds.
Analysis and comparison of commitment, homework, extra hours, preliminary grades and testing of students in Mathematics using linear regression model. Arta Aliu S. Rexhepi. Mathematics, Education. 2021. In this paper, a simple and multiple linear regression model has been developed to analyze and compare math test results of two student groups ...
This study examines the impact of educational robotics applications in Information Technologies and Software courses on the motivation, attitude towards robotics, and academic achievement of 6th-grade secondary school students. The research employed pre-experimental method encompassing 112 students. Students got training in robotics using the Arduino education kit over the course of eight ...
The study found that homework impacts learning for learners, its impact differs with the age of students, and it plays an important role in student achievement. The study proposed that homework should be purposeful, i.e. it should include the introduction of new content, the practise of skills, the creation of any data and the ability for ...