
Message placeholder
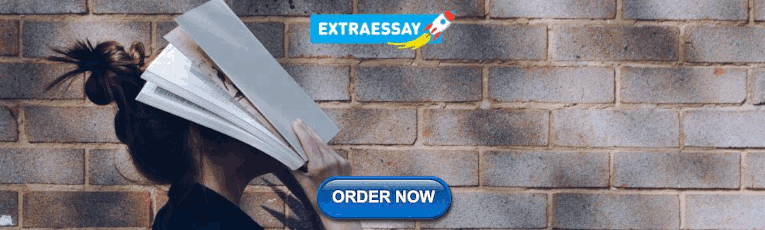
Mathematical Modelling of Natural Phenomena
Mathematical Modelling of Natural Phenomena (MMNP) is an international research journal, which publishes top-level original papers, reviews and topical issues on mathematical modelling in biology, medicine, chemistry, physics, and other areas. Read more

- Latest articles
Most read articles
- Most cited articles
Additive multiple contacts and saturation phenomena in epidemiological models are not detected by R 0
Nitric oxide transport in carotid bifurcation after different stent interventions: a numerical study, optimal non-pharmaceutical interventions considering limited healthcare system capacity and economic costs in the republic of korea, global hopf bifurcation of a delayed diffusive gause-type predator-prey system with the fear effect and holling type iii functional response, optimal ordering strategy and budget allocation for the covid-19 vaccination planning.
- Submit your paper
- Instruction for authors
- Sign up for Email-alert
- Recommend this journal
2022 Impact Factor*: 2.2
2022 5-year impact factor*: 1.9.
*Journal Citation Reports™ from Clarivate, 2023

Editors-in-Chief: Raluca Eftimie and Clair Poignard
ISSN: 0973-5348 - eISSN: 1760-6101

Published under the scientific responsibility of the Société de Mathématiques Appliquées et Industrielles (SMAI)
Open Math through S2O partnership More information

- Road to Open: Library and institutional support sustains open access in Mathematics journals through Subscribe to Open in 2024
- MMNP topical issue on "Mathematical Modelling of Natural Phenomena: honouring Prof. Vitaly Volpert"
- Transparency in Mathematics Research: MMNP Article Demonstrates Our Open Science Policy

S2O leaflet

An official website of the United States government
The .gov means it’s official. Federal government websites often end in .gov or .mil. Before sharing sensitive information, make sure you’re on a federal government site.
The site is secure. The https:// ensures that you are connecting to the official website and that any information you provide is encrypted and transmitted securely.
- Publications
- Account settings
Preview improvements coming to the PMC website in October 2024. Learn More or Try it out now .
- Advanced Search
- Journal List
- Wiley Open Access Collection

The use of mathematical modeling studies for evidence synthesis and guideline development: A glossary
Teegwendé v. porgo.
1 Population Health and Optimal Health Practices Research Unit, Department of Social and Preventative Medicine, Faculty of Medicine, Université Laval, Quebec, Canada
2 Department of Information, Evidence and Research, World Health Organization, Geneva, Switzerland
Susan L. Norris
Georgia salanti.
3 Institute of Social and Preventive Medicine, University of Bern, Bern, Switzerland
Leigh F. Johnson
4 Centre for Infectious Disease Epidemiology and Research, University of Cape Town, Cape Town, South Africa
Julie A. Simpson
5 Centre for Epidemiology and Biostatistics, Melbourne School of Population and Global Health, University of Melbourne, Melbourne, Australia
Matthias Egger
Christian l. althaus.
Mathematical modeling studies are increasingly recognised as an important tool for evidence synthesis and to inform clinical and public health decision‐making, particularly when data from systematic reviews of primary studies do not adequately answer a research question. However, systematic reviewers and guideline developers may struggle with using the results of modeling studies, because, at least in part, of the lack of a common understanding of concepts and terminology between evidence synthesis experts and mathematical modellers. The use of a common terminology for modeling studies across different clinical and epidemiological research fields that span infectious and non‐communicable diseases will help systematic reviewers and guideline developers with the understanding, characterisation, comparison, and use of mathematical modeling studies. This glossary explains key terms used in mathematical modeling studies that are particularly salient to evidence synthesis and knowledge translation in clinical medicine and public health.
1. INTRODUCTION
Mathematical models are increasingly used to aid decision making in public health and clinical medicine. 1 , 2 The results of mathematical modeling studies can provide evidence when a systematic review of primary studies does not identify sufficient studies to draw conclusions or to support a recommendation in a guideline, or when the studies that are identified do not apply to the specific populations of interest or do not provide data on long‐term follow‐up or on relevant outcomes. For example, mathematical models have been used to inform guideline recommendations about tuberculosis (TB) control in health care facilities, 3 blood donor suitability with regard to human T‐cell leukemia virus type I (HTLV‐I) infection, 4 and cancer screening. 5 , 6 Mathematical modeling studies are frequently used to synthesize evidence from multiple data sources to address a clinical or public health question not directly addressed by a primary study. For example, a mathematical model was used to synthesize evidence obtained from virological, clinical, epidemiological, and behavioral data to help determine optimal target populations for influenza vaccination programs. 7 Other examples are mathematical modeling studies that aim to predict the real‐world drug effectiveness from randomized controlled trial (RCT) efficacy data (reviewed in Panayidou et al 7 ).
The development of methods for incorporating mathematical modeling studies into evidence syntheses and clinical and public health guidelines is still at an early stage. Systematic reviewers and guideline developers struggle with questions about whether and how to include the results of mathematical modeling studies into a body of evidence. The review of mathematical modeling studies predicting drug‐effectiveness from RCT data identified 12 studies using four different modeling approaches. 7 Because of the varying use of key terminology between studies, and because certain terms can have different meanings in the literature, it was necessary to describe in the review each modeling approach in detail to illustrate the differences between them. This effort highlights an important reason for the challenges in summarizing the results of mathematical modeling studies. Researchers who develop and analyze mathematical models have different theoretical and practical backgrounds from systematic reviewers, guideline developers, and policy makers, which can result in a lack of a common understanding of concepts and terminology. These communication issues might result either in not using the findings of mathematical modeling studies in evidence synthesis and to inform decision making, or accepting these findings without critical assessment. 8 A glossary of commonly used terms in mathematical modeling studies that are relevant to evidence synthesis and to clinical and public health guideline development could improve the use of such studies.
A mathematical model is a “mathematical framework representing variables and their interrelationships to describe observed phenomena or predict future events.” 9 We define a mathematical modeling study as a study that uses mathematical modeling to address specific research questions, for example, the impact of interventions in health care facilities to reduce nosocomial transmission of TB. 10 For the modeling studies that are most relevant to evidence synthesis and clinical and public health decision‐making, the framework of the mathematical model represents interrelationships among exposure risks, interventions, health outcomes, and health costs (all of these are variables ) where their interrelationships are typically described by the parameters of interest. Mathematical modelers can use different methods to specify these parameters; they can use theoretical values, values reported in the scientific literature, or estimate the parameters from data using methods from statistical modeling. There is some overlap between the terms “mathematical model” and “statistical model” and their uses. Contemporary mathematical modeling studies increasingly include one or more statistical modeling parts. In this glossary, we will consider statistical models as a class of mathematical models that are often integrated into complex mathematical modeling studies to relate the model output to data through a statistical framework.
The goal of this glossary is to provide a common terminology for public health specialists who would like to incorporate the results of mathematical modeling studies in systematic reviews and in the development of guidelines. To identify the terms included in this glossary, we first made an exhaustive list of terms related to mathematical models. Terms were then selected based on discussions among experts attending the World Health Organization's (WHO) consultation on the development of guidance on how to incorporate the results of modeling in WHO guidelines (Geneva, Switzerland, 26 April 2016). Experts included epidemiologists, statisticians, mathematical modelers, and public health specialists. The glossary is divided into three sections. In Section 2 , we define some key terms that can be used to characterize the scope of and approach to mathematical models, using examples from the field of infectious disease modeling. In Section 3 , we present a list of terms that are commonly used across different research fields in epidemiology to describe more detailed technical properties and aspects of mathematical models. In Section 4 , we first discuss how knowledge of the terms can help to assess whether a mathematical modeling study is appropriate for providing evidence for a specific question. We then use the example of the World Health Organization (WHO) guidelines for TB control in health care facilities 3 to show how mathematical modeling studies can inform recommendations. For more specific definitions of terms that are primarily used in infectious disease modeling, we refer to the glossary by Mishra et al. 11 Terms appearing in italics are defined in other entries of the glossary.
2. TERMS USED TO DEFINE THE SCOPE OF, AND APPROACHES TO MATHEMATICAL MODELS
Before one starts to assess and compare the results of different mathematical modeling studies with each other, it can be helpful to fit them into a larger picture. Experts in systematic reviews and guideline developers need to be able to sort out which modeling studies are likely to help them draw a conclusion, formulate a recommendation, interpret the findings of another study, or understand the clinical or pathological background to a problem. Mathematical modeling studies can be characterized using several dichotomies that help to describe broad aspects, such as the scope and approach. Table 1 provides a list of some important model dichotomies, together with a brief definition, an example, and their relevance to systematic reviews and guideline development.
Model dichotomies describing the scope of, and approaches to, mathematical models in infectious disease epidemiology
A fundamental distinction can be made between mechanistic and phenomenological models . Mechanistic models use mathematical terms to describe the real‐world interactions among different model variables. The parameters governing these models typically have a physical, biological or behavioral interpretation. Infectious disease models, for example, can describe the movement of individuals within hospital wards, and how infections are transmitted upon physical contact between a susceptible and an infected person. 10 These models have the advantage that specific interventions, such as infection prevention through quarantine or isolation, can be explicitly implemented. Phenomenological models, on the other hand, describe the relationships among different model variables, consistent with fundamental theory, but not derived from first principles. Hence, this type of model does not attempt to describe or explain why and how certain model variables interact, but instead, focuses on the functional relationship that best describes an observed phenomenon. Statistical models, such as regression models, are typically phenomenological and describe the statistical relationship or association between different model variables.
A predictive model can forecast future events, such as the course of an epidemic in a given population under different scenarios, whereas a descriptive model describes and/or explains previously observed phenomena, such as the effectiveness of past interventions. Quantitative models provide a numerical estimation of an intervention effect on model variables, and therefore depend on high‐quality data to inform the model parameters. Qualitative models are usually relatively simple models that only provide insights into the direction of an effect, but not its precise magnitude. Nevertheless, they can be used to thoroughly investigate the interrelationships between model variables and the influence of specific parameters on health outcomes (also see Analytic solution ). Qualitative models can also be useful to explore the potential for unintended consequences of interventions beyond the direct intended effects that might have been observed in RCTs. Finally, an important model dichotomy distinguishes between what drives the results of mathematical modeling studies. Most mathematical models incorporate a combination of some underlying theory, model assumptions, and data. The results of a theory‐driven model are primarily based on a priori knowledge or assumptions about specific interrelationships, such as the effectiveness of a particular intervention, and are not directly inferred from data. Data‐driven models infer their results primarily from data, and are not driven by theory or assumptions that are not well supported.
3. TERMS RELATED TO TECHNICAL PROPERTIES AND ASPECTS OF MATHEMATICAL MODELS
3.1. technical terms related to model development and structure.
Once the mathematical modeling studies have been broadly characterized, and their purpose has been determined, it is important to gain a better understanding about some of the terms used to describe the technical aspects of the model used in a study. For example, has heterogeneity among different individuals been incorporated, or what simulation methods were used to obtain the model results? The following list includes some of the most frequently used terms in mathematical modeling studies in various fields of epidemiology. The terms in Section 3.1 will help in assessing the technical aspects that relate to model development and structure. The terms in Section 3.2 are related to model calibration and validation.
3.1.1. Agent‐based model
See Individual‐based model .
3.1.2. Analytic solution
Relates the health outcomes directly to the model parameters using mathematical formulae. Models that can be solved analytically are usually simple models, while more complex models typically require a computational (numerical) solution .
3.1.3. Assumption
In mathematical modeling studies, assumptions typically relate to the structure of the model and the supposed interrelationships of model variables. An important assumption in infectious disease models concerns the way in which individuals have contacts with each other. This could either be at random or involve some form of heterogeneity. In order to relate the model output to data via a statistical framework, one has to make additional assumptions about the way the data has been gathered and the expected random error.
3.1.4. Compartmental model
This model type stratifies the population into different compartments, such as different health states. Compartments are assumed to represent homogeneous subpopulations within which the entities being modeled–such as individuals or patients–have the same characteristics, for example the same sex, age, risk of infection, or death. The model can account for the transition of entities between compartments (see State‐transition model ).
3.1.5. Computational (numerical) solution
This describes the approach of solving a mathematical model using either deterministic or stochastic (see Monte Carlo methods ) simulation techniques to iteratively calculate the model variables, which are often time‐dependent, for a specific set of parameters. Iteratively calculating the model variables means updating the population characteristics at each time point based on the simulated population characteristics at previous time points. Computational solutions are used when the model is too complicated for deriving an analytic solution.
3.1.6. Continuous‐time model
This is a dynamic model where time is treated as a continuous variable (in contrast to a discrete‐time model ), meaning that the state or value of all other variables (or health outcomes) can be calculated for any time point of interest.
3.1.7. Cycle length
In a discrete‐time model, cycle length represents the interval from one time point to the next, for example a specific number of days, weeks, months, or years. 7
3.1.8. Decision analytic model
This term refers to mathematical models that synthesize available evidence to estimate health outcomes and guide decision making. The term is typically used in health economic analyses.
3.1.9. Deterministic model
This model type typically describes the average behavior of a system (eg, populations or subpopulations) without taking into account stochastic processes or chance events in single entities (eg, individuals). Hence, such models are typically applied to situations with a large number of individuals where stochastic variation becomes less important and heterogeneity can be accounted for using various subpopulations. The parameters of a deterministic model are typically fixed, and a simulation always produces the same result. Deterministic models are typically easier to calibrate to data than stochastic models. 11 , 20
3.1.10. Discrete‐time model
This type of dynamic model treats time as a discrete variable (in contrast to a continuous‐time model ) and other variables (or health outcomes) can only change at specific time points. 7
3.1.11. Dynamic model
A dynamic model contains at least one time‐dependent variable. 11 This type of model is used to describe and predict the course of health outcomes (eg, infection incidence) over time when, for example, the exposure risk (eg, infection prevalence) also changes over time.
3.1.12. Heterogeneity
In mathematical modeling studies, this typically describes the differences among individuals, or the variability across parameter values for a specific group of individuals, because of their demographic, biological, or behavioral characteristics.
3.1.13. Individual‐based model
This is a stochastic model representing individuals as discrete entities with unique characteristics. An individual‐based model can be useful to accommodate heterogeneity in a given population. Individual‐based models are also often referred to as agent‐based or micro‐simulation models . While individual‐based models can provide more realistic representations of a system, they can be difficult to parameterize because they require much more detailed knowledge, or assumptions, of how variables interact. The stochastic nature of these models makes them computationally intensive and challenging to calibrate.
3.1.14. Markov model
A Markov model assumes that the future state of variables depends only on the current state, but not the previous states, of variables. For example, in a discrete‐time Markov model, the number of new infected individuals is calculated based on the total number of infected individuals at the previous time step.
3.1.15. Micro‐simulation model
See Individual‐based model.
3.1.16. Monte Carlo methods
These are a class of computational methods that are based on random sampling. Monte Carlo methods are typically used to simulate stochastic models and are computationally intensive.
3.1.17. Ordinary differential equations
Equations that describe the change of a dependent variable, with respect to an independent variable, based on differential calculus. For example, ordinary differential equations can be used to describe the increase and decrease of infected individuals in continuous time resulting from acquisition or clearance of infection. Ordinary differential equations are typically used for deterministic and compartmental models.
3.1.18. Parameter
A parameter is a quantity used to describe the interrelationships between model variables. For example, parameters can describe how long different individuals reside in different health states, or how likely they are to transmit a disease to another person. There are different methods to specify the value of parameters. Mathematical modelers can either choose theoretical values based on specific assumptions, or set the values based on literature reviews or model calibration.
3.1.19. Parsimonious model
In a parsimonious model , descriptive or predictive, the number of assumptions, parameters and variables is minimized. Parsimonious models are often relatively simple, but they can also become more complex if they achieve the right balance between complexity and explanatory power.
3.1.20. Population‐based model
A type of deterministic or stochastic model where individuals that share the same characteristics, on average, are being grouped into a single population or several subpopulations. In contrast, an individual‐based model treats every individual as a single entity that can have unique characteristics.
3.1.21. State‐transition model
State‐transition models assume that individuals can be in different (health) states and move (transition) between them. 21 They are typically described using the framework of either Markov models or individual‐based models.
3.1.22. Static model
In a static model , all variables are independent of time and constant. A static model typically describes the equilibrium of a system, and relates the model variables for a particular time point only. In contrast to dynamic models, this type of model cannot take into account time‐dependent changes of exposure risks or health outcomes. Decision‐tree models are static models.
3.1.23. Stochastic model
A type of model where the parameters, variables, and/or the change in variables can be described by probability distributions. This type of model can account for process variability by taking into account the random nature of variable interactions, or can accommodate parameter uncertainty, and so may predict a distribution of possible health outcomes. Considering process variability can be particularly important when populations are small or certain events are very rare. Stochastic models are often simulated using Monte Carlo methods.
3.1.24. Time horizon
A time horizon denotes a chosen time at which point the effect of an intervention will be evaluated. The time horizon should reflect the health outcomes and the relevant intermediate and long‐term effects of an intervention. 1
3.1.25. Variable
Variables describe model elements such as exposure risks, interventions, or health outcomes that can vary between settings or over time. The value of a dependent variable (eg, number of infected individuals) changes in relation to an independent variable (eg, time).
3.2. Technical terms related to model calibration and validation
3.2.1. calibration.
Calibration is the process of adjusting model parameters, such that the model output is in agreement with the data that are used for model development. 22 The aim of calibration is to reduce parameter uncertainty in order to achieve high model credibility .
3.2.2. Credibility
The credibility of a model refers to judgments about the degree to which the model provides trustworthy results. Several dimensions of credibility have been described, including validity , design, data analysis, reporting, interpretation, and conflicts of interest. 16
3.2.3. Sensitivity analysis
A range of techniques used to test the impact of the assumptions made about the parameters. The analysis can be done by changing one parameter (one‐way, univariate), or simultaneously changing several parameters (multi‐way, multivariate). The parameters selected for sensitivity analyses are thought to have an impact on the outcome of interest. In a deterministic sensitivity analysis, a parameter is assigned a limited number of values, while in a probabilistic sensitivity analysis, each parameter is assigned a probability distribution, and parameter values are randomly sampled from these distributions. 1 , 11
3.2.4. Uncertainty analysis
A range of techniques to determine the reliability of model results or predictions, accounting for uncertainty in model structure, input parameters, and/or methods used for data analysis. 11 Structural uncertainty relates to the extent to which the structure of the model captures the key features of the system 23 , 24 , 25 and can be analyzed by comparing the results of models with different structures. Parameter uncertainty stems from the model parameters that are used, but whose true values are not known because of measurement error or an absence of evidence. 23 , 25 This uncertainty can be analyzed by examining model outputs for a range of values of the parameter. Methodological uncertainty arises when there are different methods for analyzing or expressing model outputs. This term is used mostly in health economic modeling.
3.2.5. Validation
A term describing processes for assessing how well a model performs and how applicable the results are to a particular situation. 26 There are five main types of validation: face validation (subjective expert judgment about how well the model represents the problem it addresses); internal validation (internal consistency, verification, and addresses whether or not the model behaves as intended and has been implemented correctly); cross validation ( convergent validity, model results are confirmed by other models); external validation (model results predict outcomes obtained in a real world setting or in a data set different from the one used for model development); predictive validation (model‐predicted events are later corroborated by real‐world observations). 7 , 27
4. MATHEMATICAL MODELING STUDIES IN GUIDELINE DEVELOPMENT
In addition to providing a useful common terminology for public health specialists and mathematical modelers, the description of different model types and other terms defined in the glossary facilitate interpretation of the results of mathematical modeling studies and inform their incorporation into the guideline development process. As a first step, one needs to identify whether a particular research question, eg, the evaluation of public health programs, long‐term effectiveness or comparative effectiveness, can be investigated using a model. Next, it will be necessary to assess whether existing mathematical modeling studies are appropriate to inform or support a research question or recommendation. We identified four comprehensive frameworks of good modeling practice. 28 These frameworks cover items such as relevance, conceptualization of the problem, or model structure. Questions such as whether the model population is relevant, the variables represent the desired health outcomes, the necessary heterogeneity is taken into consideration, the time horizon is appropriate, or the assumptions justified can help in the assessment of mathematical modeling studies. Other items concern validity or consistency, ie, the performance of the model according to its specifications. The model should also consider uncertainty with regard to the structure, parameters, and methods. Finally, credibility, which takes a number of these items into account, can then be used as the central concept for guideline developers to address the appropriateness of a mathematical modeling study for providing evidence for a specific question, 29 as illustrated in the following example.
Prevention of TB transmission in hospitals, and particularly of multidrug‐resistant TB, is essential in all countries and requires a combination of strategies. Predicting the spread of TB in a hospital and the surrounding community, and how alternative methods of control might limit the emergence of resistance, are complex nonlinear processes. It is, however, ethically and logistically impossible to conduct RCTs to examine the efficacy of these strategies. Mathematical modeling studies that use observational evidence can therefore play an important role in deciding which strategies are likely to be the most effective. The WHO guideline development group for TB infection control in health care facilities, congregate settings and households assessed systematic reviews of the evidence, which included mathematical modeling studies. 3
One mathematical modeling study that the guideline committee considered, investigated the effects of several different control measures on the spread of extensively drug resistant (XDR) TB in a community in South Africa. 10 The model described the transmission of TB in a complex system that included variables representing or contributing to: both the hospital and the surrounding community; different TB health states such as susceptible, latent, infectious, and recovered; drug resistance; HIV infection; and the effects of different control interventions alone and in combination. Hence, the study considered the transmission setting that was of relevance to the guideline, and the model structure included the desired health outcomes and variables. The authors used a mechanistic approach to make explicit the way in which stages in the transmission and natural history of TB are related. A deterministic, compartmental model , using ordinary differential equations to describe the transitions between different health states in a dynamic way was appropriate because it allowed the right balance between complexity and tractability. Key parameters that described the natural history, such as rate of natural clearance and rate of relapse, were based on the literature, and their influence was assessed in an uncertainty analysis. Parameters such as the transmissibility coefficient were calibrated using longitudinal data of individuals in a South African community, where data on TB were collected. The model outputs provided quantitative predictions about the percentage reduction in XDR‐TB cases over a reasonable time horizon. External validation of the model was performed using cross‐sectional data with information on the prevalence of TB and of drug resistance and the proportion of resistance cases in people with HIV infection. In summary, the mathematical modeling study covered many of the critical items, and we would conclude that the study has a high credibility.
Compared with natural ventilation, the authors found that mechanical ventilation alone would reduce XDR‐TB cases by 12% (range 10%‐25%). The use of respiratory masks by health workers would prevent 2% of all TB cases, but nearly two‐thirds of XDR cases in hospital staff. The guideline development group considered this study, together with other observational and modeling studies identified through the systematic review. Even though the summarized evidence for the use of ventilation systems and particulate respirators was weak, indirect, and of low quality, the studies suggested that these interventions are favorable for TB infection control.
CONFLICT OF INTEREST
CA, ME, and NL received funding through a grant from the Special Programme for Research and Training in Tropical Diseases (TDR) to conduct this study. SLN is a member of the GRADE Working Group which develops processes and methods for guideline development. LFJ, TVP, GS, and JAS have no interests to disclose.
The authors alone are responsible for the views expressed in this article and they do not necessarily represent the views, decisions, or policies of the institutions with which they are affiliated.
ACKNOWLEDGEMENTS
This work was funded through a grant from the Special Programme for Research and Training in Tropical Diseases (TDR). JA Simpson is funded by a NHMRC Senior Research Fellowship (1104975) and her research is supported by two NHMRC Centres of Research Excellence (Victoria Centre for Biostatistics, ViCBiostat; and Policy relevant infectious disease simulation and mathematical modeling, PRISM).
Porgo TV, Norris SL, Salanti G, et al. The use of mathematical modeling studies for evidence synthesis and guideline development: A glossary . Res Syn Meth . 2019; 10 :125–133. 10.1002/jrsm.1333 [ PMC free article ] [ PubMed ] [ CrossRef ] [ Google Scholar ]
mathematical modeling Recently Published Documents
Total documents.
- Latest Documents
- Most Cited Documents
- Contributed Authors
- Related Sources
- Related Keywords
Introduction to Multiscale Mathematical Modeling
Investigation of mathematical modeling processes of middle school students in model-eliciting activities (meas): a stem approach, steel desulfurization on rh degasser: physical and mathematical modeling, a mathematical modeling for simultaneous routing and scheduling of logging trucks in the forest supply chain, hybridized heuristic heterogeneous mathematical modeling for sustainable international comparison of the economic efficiency in nuclear energy, embedded fuzzy controller for water level control.
This article presents the design of a fuzzy controller embedded in a microcontroller aimed at implementing a low-cost, modular process control system. The fuzzy system's construction is based on a classical proportional and derivative controller, where inputs of error and its derivate depend on the difference between the desired setpoint and the actual level; the goal is to control the water level of coupled tanks. The process is oriented to control based on the knowledge that facilitates the adjustment of the output variable without complex mathematical modeling. In different response tests of the fuzzy controller, a maximum over-impulse greater than 8% or a steady-state error greater than 2.1% was not evidenced when varying the setpoint.
Mathematical modeling in behavior responses: The tendency-prediction based on a persistence model on real-time data
Drug delivery enhanced by ultrasound: mathematical modeling and simulation, mathematical modeling on conservation of depleted forestry resources, mathematical modeling of statistical instability of samples of biosystems, export citation format, share document.
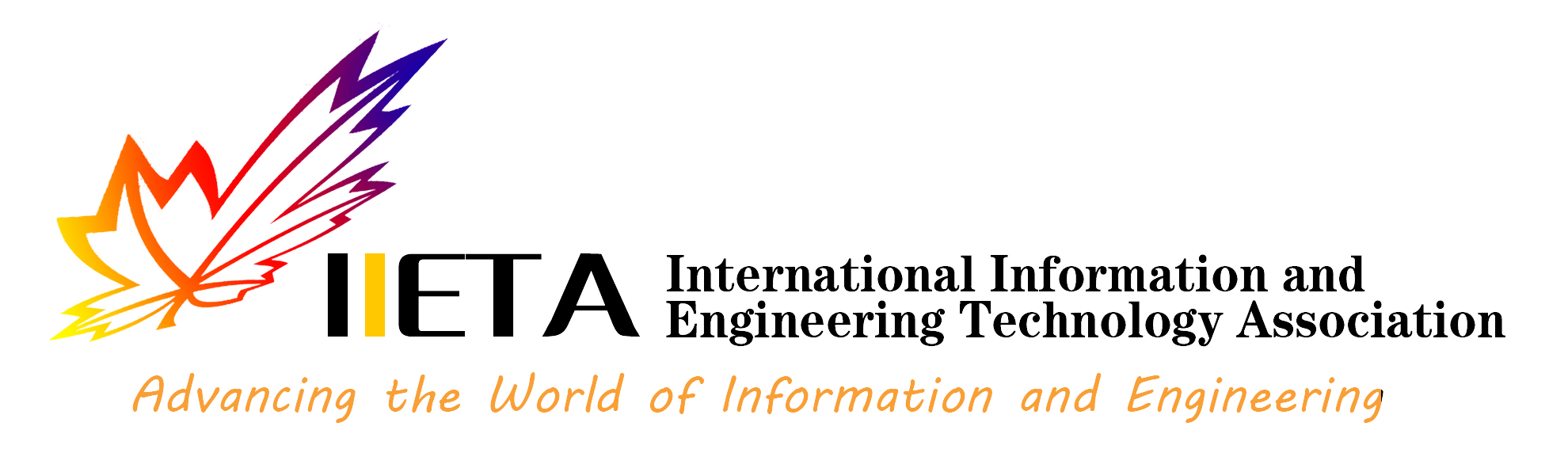
Search form
Mathematical modelling of engineering problems.
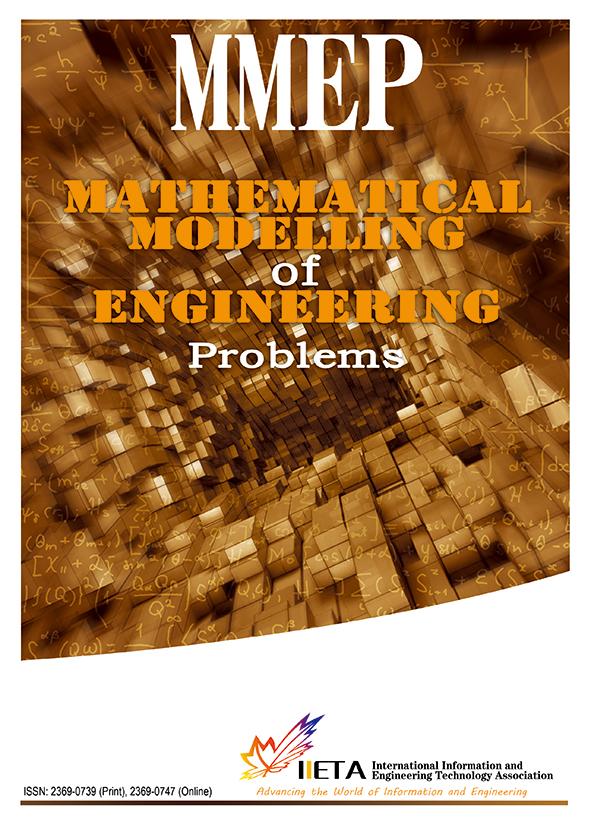
- ISSN: 2369-0739 (print); 2369-0747 (online)
- Indexing & Archiving: Scopus SCImago (SJR) CrossRef Portico EBSCOhost Cabell's Directory Google Scholar Publons Dimensions MIAR ScienceOpen Index Copernicus Microsoft Academic CNKI Scholar Baidu Scholar
- Subject: Computer Sciences Engineering Environmental & Earth Sciences Materials Sciences Mathematics Physical Sciences

Mathematical Modelling of Engineering Problems (MMEP) is a top-rated international quarterly reporting the latest mathematical models and computer methods for scientific and engineering problems. Considering the significance of mathematical modelling in engineering design, we are committed to circulating the new developments in engineering science, and solve engineering problems with the most advanced mathematical and computer tools. Therefore, we welcome original papers from scientists, engineers and technicians around the world, which focus on the design and application of mathematical models for engineering problems in mechanics, energy, civil, electrical, material, computer and industrial engineering, to name but a few. The papers should further our understanding of the said subjects, and make a significant original contribution to knowledge.
The MMEP is an open access journal. All contents are available for free, that is, users are entitled to read, download, duplicate, distribute, print, search or link to the full texts of the articles in this journal without prior consent from the publisher or the author.
Focus and Scope
The MMEP welcomes original research papers, technical notes and review articles on various disciplines, including but not limited to:
- Mechanical engineering
- Energy Engineering
- Civil engineering
- Electrical engineering
- Material engineering
- Computer engineering
- Industrial engineering
- Electronics and Communication Engineering
- Aerospace engineering
- Geological Engineering
- Mining engineering
- Transportation engineering
- Biomedical Engineering
Publication Frequency
The MMEP is published regularly by the IIETA, with twelve regular issues and one volume per year.
Section Policies
Regular Papers
- Open Submissions
- Peer Reviewed
Peer Review Statement
The IIETA adopts a double blind review process. Once submitted, a paper dealing with suitable topics will be sent to the editor-in-chief or other members of the editorial board, and then be reviewed by at least two experts in the relevant field. The reviewers are either members of our editorial board or special external experts invited by the journal. In light of the reviewers’ comments, the editor-in-chief or other members of the editorial board will make the final decision over the publication, and return the decision to the author.
There are four possible decisions concerning the paper: acceptance, minor revision, major revision and rejection. Acceptances means the paper will be published directly without any revision. Minor revision means the author should make minor changes to the manuscript according to reviewers’ comments and submit the revised version to the IIETA. The revised version will be accepted or rejected at the discretion of the editor-in-chief or other members of the editorial board. Major revision means the author should modify the manuscript significantly according to reviewers’ comments and submit the revised version to the IIETA. The revised version will be accepted or rejected at the discretion of the editor-in-chief or other members of the editorial board. Rejection means the submitted paper will not be published.
If a paper is accepted, the editor-in-chief or managing editor will send an acceptance letter to the author, and ask the author to prepare the paper in MS Word using the template of IIETA.
Plagiarism Policy
Plagiarism is committed when one author uses another work without permission, credit, or acknowledgment. Plagiarism takes different forms, from literal copying to paraphrasing the work of another. The IIETA uses iThenticate to screen for unoriginal material. Authors submitting to an IIETA journal should be aware that their paper may be submitted to CrossRef at any point during the peer-review or production process. Any allegations of plagiarism made to a journal will be investigated by the editor-in-chief or managing editor. If the allegations appear to be founded, we will request all named authors of the paper to give an explanation of the overlapping material. If the explanation is not satisfactory, we will reject the submission, and may also reject future submissions.
For instructions on citing any of IIETA’s journals as well as our Ethics Statement , see Policies and Standards .
Indexing Information
- Elsevier’s Scopus
Web: https://www.scopus.com/sourceid/21100863633
- Cabell’s Directory
Web: http://www2.cabells.com/
Web: http://www.ebscohost.com
Web: https://www.doaj.org/
- Index Copernicus
Web: http://journals.indexcopernicus.com
Web: https://www.portico.org/
- Google Scholar
Web: http://scholar.google.com
- CNKI Scholar
Web: http://scholar.cnki.net
Included in
- SCImago Journal & Country Rank
Web: https://www.scimagojr.com/journalsearch.php?q=21100863633&tip=sid&clean=0
- Crossref.org
Web: https://www.crossref.org/
ISSN: 2369-0739 (print); 2369-0747 (online)
For Submission Inquiry
Email: [email protected]

- Just Published
- Featured Articles
- Most Read and Cited
- Reference Power Point Tracking Reference Power Point Tracking of the Inertial Storage System Connected to the Electrical Grid Saci Taraft, Djamila Rekioua, Abdelhak Djoudi, Seddik Bacha, Djamel Aouzellag
- Predicting Compaction Effects on Asphalt Mixture Microstructure Using 2D Digital Image Analysis Mahmood K. Al-Obaidi, Alaa H. Abed
- Classification of Imagery Hand Movement Based on Electroencephalogram Signal Using Long-Short Term Memory Network Method Osmalina Nur Rahma, Khusnul Ain, Alfian Pramudita Putra, Riries Rulaningtyas, Nita Lutfiyah, Khouliya Zalda, Nafisa Rahmatul Laili Alami, Rifai Chai
- Testing a dual-source heat pump Cannistraro, M., Mainardi, E., Bottarelli, M.
- Evaluation of condensation heat transfer in air-cooled condenser by dominant flow criteria Camaraza-Medina, Y., Khandy, N.H., Carlson, K.M., Cruz-Fonticiella, O.M., García-Morales, O.F., Reyes-Cabrera, D.
- Operations of electric vehicle traction system Ahmed M. Youssef
- Advances of nanofluids in solar collectors - a review of numerical studies Younes Menni, Ali J. Chamkha, Giulio Lorenzini, Noureddine Kaid, Houari Ameur, Mohammed Bensafi
- Applications of nanofluids in geothermal: A review Mohammad Hossein Ahmadi, Mahdi Ramezanizadeh, Mohammad Alhuyi Nazari, Giulio Lorenzini, Ravinder Kumar, Ravindra Jilte
- Caputo-Fabrizio fractional derivative to solve the fractional model of energy supply-demand system Samad Noeiaghdam, Denis Sidorov
Phone: + 1 825 436 9306
Email: [email protected]
Subscription
Language support
Please sign up to receive notifications on new issues and newsletters from IIETA
Select Journal/Journals:
Copyright © 2024 IIETA. All Rights Reserved.
- Research article
- Open access
- Published: 19 November 2019
Mathematical modelling for health systems research: a systematic review of system dynamics and agent-based models
- Rachel Cassidy ORCID: orcid.org/0000-0002-4824-0260 1 ,
- Neha S. Singh 1 ,
- Pierre-Raphaël Schiratti 2 , 3 ,
- Agnes Semwanga 4 ,
- Peter Binyaruka 5 ,
- Nkenda Sachingongu 6 ,
- Chitalu Miriam Chama-Chiliba 7 ,
- Zaid Chalabi 8 ,
- Josephine Borghi 1 &
- Karl Blanchet 1
BMC Health Services Research volume 19 , Article number: 845 ( 2019 ) Cite this article
22k Accesses
45 Citations
5 Altmetric
Metrics details
Mathematical modelling has been a vital research tool for exploring complex systems, most recently to aid understanding of health system functioning and optimisation. System dynamics models (SDM) and agent-based models (ABM) are two popular complementary methods, used to simulate macro- and micro-level health system behaviour. This systematic review aims to collate, compare and summarise the application of both methods in this field and to identify common healthcare settings and problems that have been modelled using SDM and ABM.
We searched MEDLINE, EMBASE, Cochrane Library, MathSciNet, ACM Digital Library, HMIC, Econlit and Global Health databases to identify literature for this review. We described papers meeting the inclusion criteria using descriptive statistics and narrative synthesis, and made comparisons between the identified SDM and ABM literature.
We identified 28 papers using SDM methods and 11 papers using ABM methods, one of which used hybrid SDM-ABM to simulate health system behaviour. The majority of SDM, ABM and hybrid modelling papers simulated health systems based in high income countries. Emergency and acute care, and elderly care and long-term care services were the most frequently simulated health system settings, modelling the impact of health policies and interventions such as those targeting stretched and under resourced healthcare services, patient length of stay in healthcare facilities and undesirable patient outcomes.
Conclusions
Future work should now turn to modelling health systems in low- and middle-income countries to aid our understanding of health system functioning in these settings and allow stakeholders and researchers to assess the impact of policies or interventions before implementation. Hybrid modelling of health systems is still relatively novel but with increasing software developments and a growing demand to account for both complex system feedback and heterogeneous behaviour exhibited by those who access or deliver healthcare, we expect a boost in their use to model health systems.
Peer Review reports
Introduction
Health systems are complex adaptive systems [ 1 ]. As such, they are characterised by extraordinary complexity in relationships among highly heterogeneous groups of stakeholders and the processes they create [ 2 ]. Systems phenomena of massive interdependencies, self-organising and emergent behaviour, non-linearity, time lags, feedback loops, path dependence and tipping points make health system behaviour difficult and sometimes impossible to predict or manage [ 3 ]. Conventional reductionist approaches using epidemiological and implementation research methods are inadequate for tackling the problems health systems pose [ 4 ]. It is increasingly recognised that health systems and policy research need a special set of approaches, methods and tools that derive from systems thinking perspectives [ 5 ]. Health systems encompass a many tiered system providing services to local, district and national populations, from community health centres to tertiary hospitals. Attempting to evaluate the performance of such a multi-faceted organisation presents a daunting task. Mathematical modelling, capable of simulating the behaviour of complex systems, is therefore a vital research tool to aid our understanding of health system functioning and optimisation.
System dynamics model (SDM)
System dynamics models (SDM) and agent-based models (ABM) are the two most popular mathematical modelling methods for evaluating complex systems; while SDM are used to study macro-level system behaviour such as the movement of resources or quantities in a system over time, ABM capture micro-level system behaviour, such as human decision-making and heterogeneous interactions between humans.
While use of SDM began in business management [ 6 , 7 ] it now has wide spread application from engineering to economics, from environmental science to waste and recycling research [ 8 , 9 , 10 , 11 , 12 , 13 ]. A SDM simulates the movement of entities in a system, using differential equations to model over time changes to system state variables. A stock and flow diagram can be used to provide a visual representation of a SDM, describing the relationships between system variables using stocks, rates and influencing factors. The diagram can be interpreted as mimicking the flow of water in and out of a bath tub [ 7 ]; the rates control how much ‘water’ (some quantifiable entity, resource) can leave or enter a ‘bath tub’ (a stock, system variable) which changes over time depending on what constraints or conditions (e.g. environmental or operational) are placed on the system. Often before the formulation of a stock and flow diagram, a causal loop diagram is constructed which can be thought of as a ‘mental model’ of the system [ 14 ], representing key dynamic hypotheses.
Agent-based model (ABM)
Unlike SDM, ABM is a ground-up representation of a system, simulating the changing states of individual ‘agents’ in a system rather than the broad entities or aggregate behaviour modelled in SDM. Aggregate system behaviour can however be inferred from ABM. Use of ABM to model system behaviour has been trans-disciplinary, with application in economics to ecology, from social sciences to engineering [ 15 , 16 , 17 , 18 , 19 ]. There can be multiple types of agent modelled, each assigned their own characteristics and pattern of behaviour [ 20 , 21 ]. Agents can learn from their own experiences, make decisions and perform actions based on set rules (e.g. heuristics), informed by their interactions with other agents, their own assigned attributes or based on their interaction with the modelled environment [ 22 ]. The interactions between agents can result in three levels of communication between agents; one-to-one communication between agents, one-to-many communication between agents and one-to-location communication where an agent can influence other agents contained in a particular location [ 22 ].
Why use SDM and ABM to model health systems?
ABM and SDM, with their ability to simulate micro- and macro-level behaviour, are complementary instruments for examining the mechanisms in complex systems and are being recognised as crucial tools for exploratory analysis. Their use in mapping health systems, for example, has steadily risen over the last three decades. ABM is well-suited to explore systems with dynamic patient or health worker activity, a limitation of other differential equation or event-based simulation tools [ 23 , 24 , 25 ]. Unlike discrete-event simulation (DES) for example, which simulates a queue of events and agent attributes over time [ 26 ], the agents modelled in ABM are decision makers rather than passive individuals. Closer to the true system modelled, ABM can also incorporate ongoing learning from events whereby patients can be influenced by their interactions with other patients or health workers and by their own personal experience with the health system [ 21 ]. SDM has also been identified as a useful tool for simulating feedback and activity across the care continuum [ 27 , 28 , 29 , 30 ] and is highly adept at capturing changes to the system over time [ 31 ]. This is not possible with certain ‘snapshot in time’ modelling approaches such as DES [ 32 ]. SDM is best implemented where the aim of the simulation is to examine aggregate flows, trends and sub-system behaviour as opposed to intricate individual flows of activity which are more suited to ABM or DES [ 33 ].
There are also models that can accommodate two or more types of simulation, known as hybrid models. Hybrid models produce results closer to true system behaviour by drawing on the strengths of one or more modelling methods while reducing the limitations associated with using a single simulation type [ 27 ]. The activity captured in such models emulates the individual variability of patients and health professionals while retaining the complex, aggregate behaviour exhibited in health systems.
Health scientists and policy makers alike have recognised the potential of using SDM and ABM to model all aspects of health systems in support of decision making from emergency department (ED) optimisation [ 34 ] to policies that support prevention or health promotion [ 35 ]. Before implementing or evaluating costly health policy interventions or health service re-structuring in the real world, modelling provides a relatively risk-free and low budget method of examining the likely impact of potential health system policy changes. They allow the simulation of ‘what if’ scenarios to optimise an intervention [ 36 ]. They can help identify sensitive parameters in the system that can impede the success of initiatives and point to possible spill-over effects of these initiatives to other departments, health workers or patients. Perhaps most important of all, these modelling methods allow researchers to produce simulations, results and a graphical-user interface in relation to alternative policy options that are communicable to stakeholders in the health system [ 37 ], those responsible for implementing system-wide initiatives and changes.
Study aim and objectives
Given the increasing amount of literature in this field, the main aim of the study was to examine and describe the use of SDM and ABM to model health systems. The specific objectives were as follows: (1) Determine the geographical, and healthcare settings in which these methods have been used (2) Identify the purpose of the research, particularly the health policies or interventions tested (3) Evaluate the limitations of these methods and study validation, and (4) Compare the use of SDM and ABM in health system research.
Although microsimulation, DES and Markov models have been widely used in disease health modelling and health economic evaluation, our aim in this study was to review the literature on mathematical methods which are used to model complex dynamic systems, SDM and ABM. These models represent two tenants of modelling: macroscopic (top-level) and microscopic (individual-level) approaches. Although microsimulation and DES are individual-based models like ABM, individuals in ABM are “active agents” i.e. decision-makers rather than “passive agents” which are the norm in microsimulation and DES models. Unlike Markov models which are essentially one-dimensional, unidirectional and linear, SDM are multi-dimensional, nonlinear with feedback mechanisms. We have therefore focussed our review on SDM and ABM because they are better suited to characterise the complexity of health systems. This study reviews the literature on the use of SDM and ABM in modelling health systems, and identifies and compares the key characteristics of both modelling approaches in unwrapping the complexity of health systems. In identifying and summarising this literature, this review will shed light on the types of health system research questions that these methods can be used to explore, and what they add to more traditional methods of health system research. By providing an over overview of how these models can be used within health system research, this paper is also expected to encourage wider use and uptake of these methods by health system researchers and policy makers.
The review was conducted in compliance with the Preferred Reporting Items for Systematic Reviews and Meta-Analysis (PRISMA) statement [ 38 ].
Search strategy and information sources
The literature on ABM and SDM of health systems has not been confined to a single research discipline, making it necessary to widen the systematic review to capture peer-reviewed articles found in mathematical, computing, medicine and health databases. Accordingly, we searched MEDLINE, EMBASE, Cochrane Library, MathSciNet, ACM Digital Library, HMIC, Econlit and Global Health databases for literature. The search of health system literature was narrowed to identify articles that were concerned with modelling facility-based healthcare, services and related healthcare financing agreements which had been excluded or were not the focus of previous reviews [ 34 , 35 , 39 , 40 , 41 ]. The search criteria used for MEDLINE was as follows, with full search terms for each database and search terms used to locate SDM and ABM literature found in Additional file 1 :
(health system* OR health care OR healthcare OR health service* OR health polic* OR health facil* OR primary care OR secondary care OR tertiary care OR hospital*).ab,ti. AND (agent-based OR agent based).ab,ti. AND (model*).ab,ti.
In addition, the reference list of papers retained in the final stage of the screening process, and systematic reviews identified in the search, were reviewed for relevant literature.
Data extraction and synthesis
The screening process for the review is given in Fig. 1 (adapted from [ 38 ]). All search results were uploaded to Mendeley reference software where duplicate entries were removed. The remaining records were screened using their titles and abstracts, removing entries based on eligibility criteria given in Table 1 . Post-abstract review, the full text of remaining articles was screened. Papers retained in final stage of screening were scrutinised, with data imported to Excel based on the following categories; publication date, geographical and healthcare setting modelled, purpose of research in addition to any policies or interventions tested, rationale for modelling method and software platform, validation and limitations of model. The results were synthesised using descriptive statistics and analysis of paper content that were used to answer the objectives.
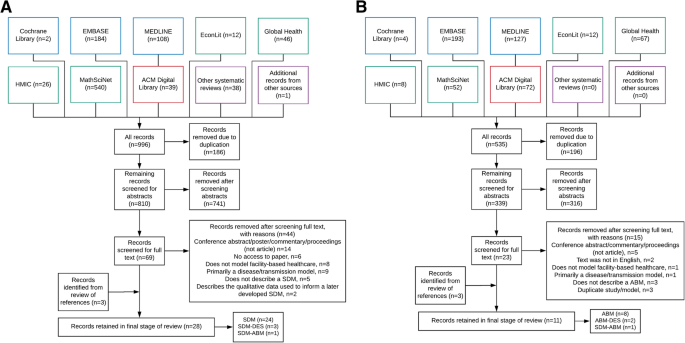
a Flow-chart for systematic review of SDMs and b ABMs of health systems (Database research discipline is identified by colour; mathematical and computing (red), medicine (blue) and health (green) databases). Adapted from PRISMA [ 38 ]
The studies were first described by three characteristics: publication date, geographical setting, and what aspect of the health system was modelled and why. These characteristics were chosen for the following reasons. Publication date (Fig. 2 ) allows us to examine the quantity of SDM and ABM studies over time. Geographical settings (Fig. 2 , top) allows us to see which health systems have been studied, as health systems in LMIC are very different from those in developed countries. Studies are classified as modelling health systems in high, upper middle, lower middle and low income countries as classified by The World Bank based on economy, July 2018 [ 42 ]. Finally, we examined which aspects of the health system have been modelled and the types of research/policy questions that the models were designed to address, to shed light on the range of potential applications of these models, and also potential gaps in their application to date.
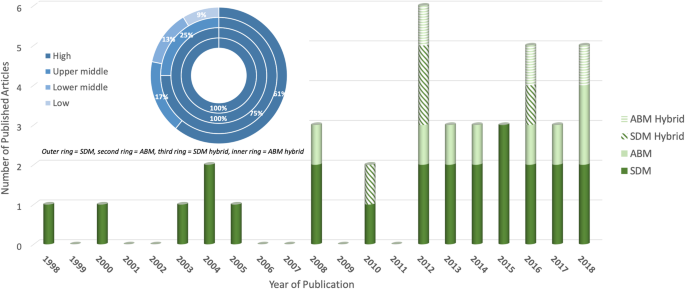
Number of articles in the final review by year of publication and economic classification
The analysis of paper content was split into three sections; SDM use in health system research (including hybrid SDM-DES), ABM use in health system research (including hybrid ABM-DES) and hybrid SDM-ABM use in health system research. The quality of selected studies will not be presented as our aim was to compare and summarise the application of SDM and ABM in modelling health systems rather than a quality appraisal of studies.
Study selection
The search initially yielded 535 citations for ABM and 996 citations for SDM of facility-based healthcare and services (see Fig. 1 ). Post-full text screening 11 ABM and 28 SDM papers were retained for analysis, six of which utilised hybrid modelling methods. Three of the hybrid modelling papers integrated SDM with DES [ 43 , 44 , 45 ], two integrated ABM with DES [ 24 , 46 ] and one integrated SDM with ABM [ 47 ]. A summary table of selected papers is given in Table 2 .
Descriptive statistics
Publication date.
The first SDM paper to model health systems was published in 1998 [ 56 ] whilst the first publication [ 66 ] utilising ABM came almost a decade later (Fig. 2 ). We found an increasing trend in publications for both modelling approaches, with 90.9% (10/11) and 71.4% (20/28) of all ABM and SDM articles, respectively, having been published in the last decade. The first hybrid modelling article was published in 2010 [ 43 ], using SDM and DES to model the impact of an intervention to aid access to social care services for elderly patients in Hampshire, England.
Geographical setting
The proportion of papers that modelled health systems in high, upper middle, lower middle and low income countries is presented in Fig. 2 . Eighteen (18/28) papers that employed SDM simulated health systems in high income countries including England [ 33 , 36 , 43 , 45 , 50 , 54 , 56 , 57 ] and Canada [ 28 , 51 , 62 ]. Four SDM papers simulated upper middle income country health systems, including Turkey [ 52 , 59 ] and China [ 64 ], with a nominal number of papers (5/28) focussing on lower middle or low income countries (West Bank and Gaza [ 48 , 55 ], Indonesia [ 37 ], Afghanistan [ 30 ] and Uganda [ 60 ]). Almost all ABM papers (9/11) modelled a high income country health system, including the US [ 20 , 23 , 25 ] and Austria [ 65 ]. Two (2/11) ABM papers described an upper-middle income based health system (Brazil [ 22 , 67 ]). All six articles that implemented a hybrid SDM or ABM simulated health systems based in high income countries, including Germany [ 44 ] and Poland [ 47 ].
Healthcare setting and purpose of research
The healthcare settings modelled in the SDM, ABM and hybrid simulation papers are presented in Fig. 3 . Healthcare settings modelled using SDM included systems that were concerned with delivering emergency or acute care (11/28) [ 28 , 31 , 36 , 45 , 47 , 50 , 56 , 57 , 58 , 61 , 62 ], elderly or long-term care services (LTC)(12/28) [ 28 , 31 , 36 , 43 , 44 , 45 , 49 , 50 , 51 , 54 , 61 , 62 ] and hospital waste management (4/28) [ 37 , 48 , 52 , 55 ]. Twenty of the SDM papers selected in this review assessed the impact of health policy or interventions on the modelled system. Common policy targets included finding robust methods to relieve stretched healthcare services, ward occupancy and patient length of stay [ 28 , 31 , 36 , 43 , 49 , 50 , 54 , 58 , 62 ], reducing the time to patient admission [ 33 , 53 , 61 ], targeting undesirable patient health outcomes [ 47 , 58 , 60 , 63 ], optimising performance-based incentive health system policies [ 30 , 59 ] and reducing the total cost of care [ 33 , 54 , 61 ]. The remaining eight papers explored factors leading to undesirable emergency care system behaviour [ 56 , 57 ], simulating hospital waste management systems and predicting future waste generation [ 37 , 48 , 55 ], estimating future demand for cardiac care [ 44 ], exploring the impact of patient admission on health professionals stress level in an integrated care system [ 45 ], and variation in physician decision-making [ 32 ].
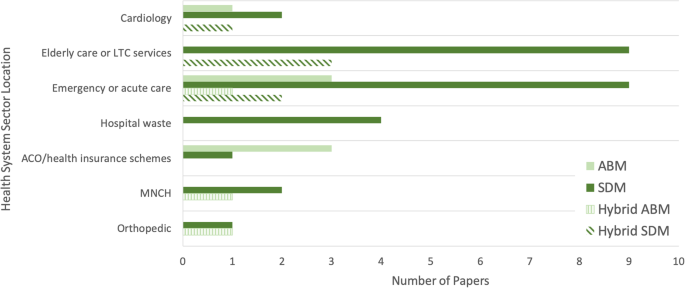
The health system sector locations modelled in the SDM, ABM and hybrid modelling literature. Long-term care (LTC); Accountable care organisation (ACO); Maternal, newborn and child health (MNCH)
ABM papers modelled systems focussed on delivering emergency or acute care (4/11) [ 21 , 22 , 47 , 67 ] and accountable care organisations (ACO) or health insurance reimbursement schemes (3/11) [ 23 , 25 , 65 ]. Nine of the ABM papers assessed the impact of health policy or interventions on the modelled system. Common policy targets included decreasing the time agents spent performing tasks, waiting for a service or residing in parts of the system [ 20 , 22 , 24 , 67 ], reducing undesirable patient outcomes [ 23 , 25 , 47 , 67 ], reducing the number of patients who left a health facility without being seen by a physician [ 22 , 67 ] and optimising resource utility (beds and healthcare staff) [ 46 , 66 , 67 ]. The remaining two papers described simulation tools capable of comparing health insurance reimbursement schemes [ 65 ] and assessing risk, allocation of resources and identifying weaknesses in emergency care services [ 21 ].
Papers that utilised hybrid simulation, combining the strengths of two modelling approaches to capture detailed individual variability, agent-decision making and patient flow, modelled systems focussed on delivering elderly care or LTC services [ 43 , 44 , 45 ] and emergency or acute care [ 45 , 47 ]. Four of the hybrid simulation papers assessed the impact of policy or intervention on the modelled system. Policy targets included improving access to social support and care services [ 43 ], reducing undesirable patient outcomes [ 47 ], decreasing patient waiting time to be seen by a physician [ 24 ] and improving patient flow through the system by optimising resource allocation [ 46 ]. The remaining two papers used hybrid simulation to estimate the future demand for health care from patients with cardiac disease [ 44 ] and model patient flow through an integrated care system to estimate impact of patient admission on health care professionals wellbeing [ 45 ].
SDM use in health systems research (including hybrid SDM-DES)
Rationale for using model.
Gaining a holistic system perspective to facilitate the investigation of delays and bottlenecks in health facility processes, exploring counter-intuitive behaviour and monitoring inter-connected processes between sub-systems was cited frequently as reasons for using SDM to model health systems [ 28 , 36 , 37 , 48 , 56 ]. SDM was also described as a useful tool for predicting future health system behaviour and demand for care services, essential for health resource and capacity planning [ 48 , 60 ]. Configuration of the model was not limited by data availability [ 28 , 52 , 64 ] and could integrate data from various sources when required [ 51 ].
SDM was described as a tool for health policy exploration and optimising system interventions [ 33 , 36 , 51 , 54 , 58 , 64 ], useful for establishing clinical and financial ramifications on multiple groups (such as patients and health care providers) [ 63 ], identifying policy resistance or unintended system consequences [ 59 , 61 ] and quantifying the impact of change to the health system before real world implementation [ 62 ]. The modelling platform also provided health professionals, stakeholders and decision makers with an accessible visual learning environment that enabled engagement with experts necessary for model conception and validation [ 48 , 50 , 55 , 57 ]. The model interface could be utilised by decision makers to develop and test alternative policies in a ‘real-world’ framework that strengthened their understanding of system-wide policy impact [ 31 , 49 , 58 , 61 ].
SDM-DES hybrid models enabled retention of deterministic and stochastic system variability and preservation of unique and valuable features of both methods [ 44 ], capable of describing the flow of entities through a system and rapid insight without the need for large data collection [ 43 ], while simulating individual variability and detailed interactions that influence system behaviour [ 43 ]. SDM-DES offered dual model functionality [ 44 ] vital for simulating human-centric activity [ 45 ], reducing the practical limitations that come with using either SDM or DES to model health systems such as attempting to use SDM to model elements which have non-aggregated values (e.g. patient arrival time) [ 45 ] which is better suited for DES.
Healthcare setting
Sixteen papers that utilised SDM modelled systems that were concerned with the delivery of emergency or acute care, or elderly care or LTC services.
Ten of the reviewed papers primarily modelled sectors of the health system that delivered emergency or acute care Footnote 1 , Footnote 2 . Brailsford et al. [ 50 ], Lane et al. [ 56 ], Lane et al. [ 57 ] and Lattimer et al. [ 36 ] simulated the delivery of emergency care in English cities, specifically in Nottingham and London. Brailsford et al. [ 50 ] and Lattimer et al. [ 36 ] created models that replicated the entire emergency care system for the city of Nottingham, from primary care (i.e. General Practice surgeries) to secondary care (i.e. hospital admissions wards), to aid understanding of how emergency care was delivered and how the system would need to adapt to increasing demand. Lane et al. [ 56 ] and Lane et al. [ 57 ] modelled the behaviour of an ED in an inner-London teaching hospital, exploring the knock on effects of ED performance to hospital ward occupancy and elective admissions. Esensoy et al. [ 28 ] and Wong et al. [ 62 ] both modelled emergency care in Canada, Esensoy et al. [ 28 ] focussing on six sectors of the Ontario health system that cared for stroke patients while Wong et al. [ 62 ] simulated the impact of delayed transfer of General Internal Medicine patients on ED occupancy. Rashwan et al. [ 31 ], Walker et al. [ 61 ] and Mahmoudian-Dehkordi et al. [ 58 ] modelled patient flow through a generic emergency care facility with six possible discharge locations in Ireland, a sub-acute extended care hospital with patient flow from feeder facilities in Australia and an intensive care unit, ED and general wards in a generic facility.
Five of the SDM papers primarily simulated the behaviour of LTC facilities or care services for elderly patients Footnote 3 . Ansah et al. [ 49 ] modelled the demand and supply of general LTC services in Singapore with specific focus on the need for LTC and acute health care professionals. Desai et al. [ 54 ] developed a SDM that investigated future demand of care services for older people in Hampshire, England which simulated patient flow through adult social care services offering 13 different care packages. In modelling complex care service demand, Cepoiu-Martin et al. [ 51 ] explored patient flow within the Alberta continuing care system in Canada which offered supportive living and LTC services for patients with dementia. Brailsford et al. [ 43 ] used a hybrid SDM-DES model to investigate how local authorities could improve access to services and support for older people, in particular the long term impact of a new contact centre for patients. The SDM replicated the whole system for long term care, simulating the future demography and demand for care services and the nested DES model simulated the operational issues and staffing of the call centre in anticipation of growing demand for services. Zulkepli et al. [ 45 ] also used SDM-DES to model the behaviour of an integrated care system in the UK, modelling patient flow (DES) and intangible variables (SDM) related to health professionals such as motivation and stress levels.
Policy impact evaluation/testing
Twenty papers that utilised SDM tested the impact of policy or interventions on key health system performance or service indicators. The intended target of these policies ranged from relieving strained and under resourced healthcare services, decreasing healthcare costs to reducing patient mortality rates.
Ansah et al. [ 49 ], Brailsford et al. [ 50 ] and Desai et al. [ 54 ] aimed to reduce occupancy in acute or emergency care departments through policies that targeted elderly utilisation of these services. While demand for LTC services is expected to exponentially increase in Singapore, focus has been placed on expanding the acute care sector. Ansah et al. [ 49 ] simulated various LTC service expansion policies (static ‘current’ policy, slow adjustment, quick adjustment, proactive adjustment) and identified that proactive expansion of LTC services stemmed the number of acute care visits by elderly patients over time and required only a modest increase in the number of health professionals when compared with other policies. In Brailsford et al. [ 50 ] simulation of the entire emergency care system for Nottingham, England, policy testing indicated that while the emergency care system is operating near full capacity, yearly total occupancy of hospital beds could be reduced by re-directing emergency admissions from patients over 60 years of age (who make up around half of all admissions) to more appropriate services, such as those offered by community care facilities. To explore challenges that accompany providing care for an ageing population subject to budget restraints, Desai et al. [ 54 ] simulated the delivery and demand for social care services in Hampshire over a projected 5 year period. In offering care packages to only critical need clients and encouraging extra care services at home rather than offering residential care, the number of patients accessing acute care services reduced over the observed period.
Desai et al. [ 54 ], in addition to Taylor et al. [ 33 ] and Walker et al. [ 61 ], also examined policies that could reduce the total cost of care. Increasing the proportion of hired unqualified care workers (over qualified care workers who are employed at a higher cost rate) resulted in savings which could be fed back into care funding, although Desai et al. [ 54 ] remarked on the legal and practical limitations to this policy. Taylor et al. [ 33 ] examined the impact of shifting cardiac catheterization services from tertiary to secondary level hospitals for low risk investigations and explored how improvements could be made to services. Significant and stable improvements in service, including reduced waiting list and overall cost of service, were achieved with the implementation of strict (appropriate referral) guidelines for admitting patients. Walker et al. [ 61 ] modelled patient flow from feeder hospitals to a single sub-acute extended care facility in Victoria, Australia, to assess the impact of local rules used by the medical registrar for admission. The local admission policy which prioritised admissions from patients under the care of private doctors pushed the total cost of care over the facility budget by 6% whereas employing no prioritisation rule reduced the total cost of care to 3% under budget.
Semwanga et al. [ 60 ], Mahmoudian-Dehkordi et al. [ 58 ] and Worni et al. [ 63 ] evaluated the impact of health policy on undesirable patient outcomes (mortality and post-treatment complication rates). Semwanga et al. [ 60 ] tested the effectiveness of policies designed to promote maternal and neonatal care in Uganda, established from the literature. Policies that enabled service uptake, such as community health education, free delivery kits and motorcycle coupons were significant in reducing neonatal death over the simulated period. Mahmoudian-Dehkordi et al. [ 58 ] explored the intended and unintended consequences of intensive care unit resource and bed management policies on system performance indicators, including patient mortality. During a simulated crisis scenario, prioritising intensive care unit patient admission to general wards over emergency admissions was found to be the most effective policy in reducing total hospital mortality. Worni et al. [ 63 ] estimated the impact of a policy to reduce venous thromboembolism rates post-total knee arthroplasty surgery and identified unintentional consequences of the strategy. The policy prevented the reimbursement of patient care fees in the event that a patient was not taking the recommended prophylaxis medication and consequently develops venous thromboembolism. Simulation results indicated a positive 3-fold decrease in venous thromboembolism rates but an unintended 6-fold increase in the number of patients who develop bleeding complications as a result of compulsory prophylaxis treatment.
Validation (including sensitivity analysis)
Statistically-based models are usually used in quantitative data rich environments where model parameters are estimated through maximum likelihood or least-squares estimation methods. Bayesian methods can also be used to compare alternative statistical model structures. SDMs and ABMs on the other hand are not fitted to data observations in the traditional statistical sense. The data are used to inform model development. Both quantitative data and qualitative data (e.g. from interviews) can be used to inform the structure of the model and the parameters of the model. Furthermore, model structure and parameter values can also be elicited from expert opinion. This means that the nature of validation of ABMs and SDMs requires more scrutiny than that of other types of models.
With increasing complexity of such models, and to strengthen confidence in their use particularly for decision support, models are often subjected to sensitivity analysis and validation tests. Twenty-two papers that utilised SDM undertook model validation, the majority having performed behavioural validity tests (see Additional file 2 for details of validation methods for each model). Key model output such as bed occupancy [ 36 , 50 ], department length of stay [ 62 ] and number of department discharges [ 31 ] were compared with real system performance data from hospitals [ 32 , 33 , 36 , 48 , 50 , 54 , 58 , 59 , 61 , 62 ], local councils [ 54 ], nationally reported figs [ 31 , 64 ]. as well being reviewed by experts [ 57 , 60 ] as realistic. Others performed more structure orientated validity tests. Model conception [ 28 , 60 ], development [ 30 , 36 , 50 , 53 , 54 , 57 , 62 ] and formulation [ 54 , 56 , 59 ] were validated by a variety of experts including health professionals [ 47 , 53 , 54 , 57 , 59 , 62 ], community groups [ 56 ] and leaders [ 60 ], steering committees [ 36 ], hospital and care representatives [ 50 , 56 , 59 ], patient groups [ 60 ] and healthcare policy makers [ 60 ]. Further tests for structural validity included checking model behaviour when subjected to extreme conditions or extreme values of parameters [ 30 , 31 , 52 , 57 , 59 , 60 , 64 ], model dimensional consistency [ 31 , 52 , 57 , 59 , 60 ], model boundary adequacy [ 31 ] and mass balance [ 54 ] and integration error checks [ 31 , 52 ]. Sensitivity analysis was performed to assess how sensitive model output was to changes in key parameters [ 49 , 51 , 57 , 60 , 64 ], to test the impact of parameters that had been based on expert opinion on model output [ 28 ] and varying key system parameters to test the robustness and effectiveness of policies [ 28 , 30 , 52 , 53 , 58 ] (on the assumption of imperfect policy implementation [ 28 ]).
Limitations of research
Most of the model limitations reported were concerned with missing parameters, feedback or inability to simulate all possible future health system innovations. Mielczarek et al. [ 44 ], Cepoiu-Martin et al. [ 51 ], Ansah et al. [ 49 ] and Rashwan et al. [ 31 ] did not take into account how future improvements in technology or service delivery may have impacted results, such as the possibility of new treatment improving patient health outcomes [ 51 ] and how this could impact the future utilisation of acute care services [ 49 ]. Walker et al. [ 61 ] and Alonge et al. [ 30 ] described how the models may not simulate all possible actions or interactions that occurred in the real system, such as all proactive actions taken by hospital managers to achieve budget targets [ 61 ] or all unintended consequences of a policy on the system [ 30 ]. De Andrade et al. [ 53 ] and Rashwan et al. [ 31 ] discussed the reality of model boundaries, that SDMs cannot encapsulate all health sub-sector behaviour and spill-over effects. Although these have been listed here as limitations, not accounting for possible future improvements in healthcare service or not simulating all possible actions in the modelled system did not prevent authors from fulfilling study objectives. When developing a SDM, it is not possible to account for all possible spill-over effects to other healthcare departments and this should not be attempted; model boundaries are set to only include variables and feedback that are pertinent to exploring the defined problem.
Simplification of model parameters was another common limitation. Wong et al. [ 62 ] stated that this would result in some model behaviour not holding in the real system, such as using weekly hospital admission and discharge averages in place of hourly rates due to the hospital recording aggregated data. This aggregation of model parameters may not have reflected real system complexity; Eleyan et al. [ 55 ] did not differentiate between service level and type of hospital when modelling health care waste production (described as future work) and Worni et al. [ 63 ] refrained from stratifying post-surgery complications by severity, potentially combining lethal and less harmful complications within the same stock (although this did not detract from the study conclusion that the rate of complications would increase as a result of the tested policy).
Data availability, lack of costing analysis and short time horizons were also considered credible limitations. Models that had been calibrated with real data were at risk of using datasets that contained measurement errors or incomplete datasets lacking information required to inform model structure or feedback [ 32 ]. Routine facility data required for model conception and formulation was unavailable which restricted the replication of facility behaviour in the model [ 36 ] and restricted validation of model behaviour [ 59 ], although it should be noted that this is only one method among many for SDM validation and the author was able to use other sources of data for this purpose. Lack of costing or cost effectiveness analysis when testing policies [ 60 ], particularly policies that required significant investment or capacity expansion [ 58 ], limited discussion on their feasibility in the real system. Models that simulated events over short time scales did not evaluate long term patient outcomes [ 33 ] or the long term effects of facility policies on certain groups of patient [ 57 ].
ABM use in health system research (including hybrid ABM-DES)
The model’s ability to closely replicate human behaviour that exists in the real system was frequently cited [ 20 , 21 , 22 , 25 , 66 ], providing a deeper understanding of multiple agent decision-making [ 23 , 67 ], agent networks [ 25 ] and interactions [ 21 , 22 ]. The modelling method was described as providing a flexible framework capable of conveying intricate system structures [ 20 ], where simulations captured agent capacity for learning and adaptive behaviour [ 20 , 25 ] and could incorporate stochastic processes that mimicked agent transition between states [ 25 ]. ABM took advantage of key individual level agent data [ 25 ] and integrated information from various sources including demographic, epidemiological and health service data [ 65 ]. The visualisation of systems and interface available with ABM software packages facilitated stakeholder understanding of how tested policies could impact financial and patient health outcomes [ 23 ], particularly those experts in the health industry with minimal modelling experience [ 67 ].
Integrating DES and ABM within a single model ensured an intelligent and flexible approach for simulating complex systems, such as the outpatient clinic described in Kittipittayakorn et al. [ 24 ]. The hybrid model captured both orthopaedic patient flow and agent decision-making that enabled identification of health care bottlenecks and optimum resource allocation [ 24 ].
Seven papers that utilised ABM modelled systems that were either concerned with delivering emergency or acute care 2 , ACOs or health insurance reimbursement schemes.
Liu et al. [ 21 ] and Yousefi et al. [ 22 ] modelled behaviour in EDs in Spanish and Brazilian tertiary hospitals. Liu et al. [ 21 ] simulated the behaviour of eleven key agents in the ED including patients, admission staff, doctors, triage nurses and auxiliary staff. Patients were admitted to the ED and triaged before tests were requested and a diagnosis issued. Over time, agent states changed based on their interaction with other agents such as when a doctor decided upon a course of action for a patient (sending the patient home, to another ward, or continue with diagnosis and treatment). For further details of agent type and model rules for each paper, see Additional file 3 .
Yousefi et al. [ 22 ] modelled the activities of patients, doctors, nurses and receptionists in a ED. Agents could communicate with each other, to a group of other agents or could send a message to an area of the ED where other agents reside. They made decisions based on these interactions and the information available to them at the time. The main focus of the simulation was on patients who left the ED without being seen by a physician; patients decided whether to leave the ED based on a ‘tolerance’ time extracted from the literature, which changed based on their interaction with other agents. In an additional paper, Yousefi et al. [ 67 ] simulated decision-making by patients, doctors, nurses and lab technicians within a generic ED informed from the literature. Group decision-making was employed, whereby facility staff could interact with each other and reach a common solution for improving the efficacy of the department such as re-allocating staff where needed. Yousefi et al. [ 67 ], Yousefi et al. [ 22 ] and Liu et al. [ 21 ] each used a finite state machine (a computational model which describes an entity that can be in one of a finite number of states) to model interactions between agents and their states.
Liu et al. [ 25 ] and Alibrahim et al. [ 23 ] modelled the behaviour of patients, health providers and payers using series of conditional probabilities, where health providers had participated in an ACO in the United States. Liu et al. [ 25 ] presented a model where health providers within an ACO network worked together to reduce congestive heart failure patient healthcare costs and were consequently rewarded a portion of the savings from the payer agent (hypothetically, the Centers for Medicare and Medicaid Services). Patients were Medicare beneficiaries over the age of 65 who developed diabetes, hypertension and/or congestive heart failure and sought care within the network of health providers formed of three hospitals and 15 primary care physician clinics. Alibrahim et al. [ 23 ] adapted Liu et al. [ 25 ] ACO network model to allow patients to bypass their nearest medical provider in favour of an alternative provider. The decision for a patient to bypass their nearest health centre was influenced by patient characteristics, provider characteristics and the geographical distance between health providers. Providers were also given a choice on whether to participate in an ACO network, where they would then need to implement a comprehensive congestive heart failure disease management programme.
Einzinger et al. [ 65 ] created a tool that could be used to compare different health insurance reimbursement schemes in the Austrian health sector. The ABM utilised anonymous routine data from practically all persons with health insurance in Austria, pertaining to medical services accessed in the outpatient sector. In the simulation, patients developed a chronic medical issue (such as coronary heart disease) that required medical care and led to the patient conducting a search of medical providers through the health market. The patient then accessed care at their chosen provider where the reimbursement system, notified of the event via a generic interface, reimbursed the medical provider for patients care.
Nine papers tested the impact of policy on key health system performance or service indicators. The intended target of these policies ranged from decreasing patient length of stay, to reducing the number of patients who leave without being seen by a physician to reducing patient mortality and hospitalisation rates.
Huynh et al. [ 20 ], Yousefi et al. [ 22 ], Yousefi et al. [ 67 ] and Kittipittayakorn et al. [ 24 ] tested policies to reduce the time agents spent performing tasks, waiting for a service or residing in parts of the system. Huynh et al. [ 20 ] modelled the medication administration workflow for registered nurses at an anonymous medical centre in the United States and simulated changes to the workflow to improve medication administration safety. Two policies were tested; establishing a rigid order for tasks to be performed and for registered nurses to perform tasks in the most frequently observed order (observed in a real medical centre) to see if this improved the average amount of time spent on tasks. Yousefi et al. [ 67 ] modelled the effects of group decision-making in ED compared with the standard approach for resource allocation (where a single supervisor allocates resources) to assess which policy resulted in improved ED performance. Turning ‘on’ group decision-making and starting the simulation with a higher number of triage staff and receptionists resulted in the largest reduction of average patient length of stay and number of patients who left without being seen. This last performance indicator was the subject of an additional paper [ 22 ], with focus on patient-to-patient interactions and how this impacted their decision to leave the ED before being seen by a physician. Four policies adapted from case studies were simulated to reduce the number of patients leaving the ED without being seen and average patient length of stay. The policy of fast-tracking patients who were not acutely unwell during triage performed well as opposed to baseline, where acutely ill patients were always given priority. Kittipittayakorn et al. [ 24 ] used ABM-DES to identify optimal scheduling for appointments in an orthopaedic outpatient clinic, with average patient waiting time falling by 32% under the tested policy.
Liu et al. [ 25 ], Alibrahim et al. [ 23 ] and Yousefi et al. [ 67 ] tested the impact of health policy on undesirable patient outcomes (patient mortality and hospitalisation rates). Liu et al. [ 25 ] modelled health care providers who operated within an ACO network and outside of the network and compared patient outcomes. Providers who operated within the ACO network worked together to reduce congestive heart failure patient healthcare costs and were then rewarded with a portion of the savings. As part of their membership, providers implemented evidence-based interventions for patients, including comprehensive discharge planning with post-discharge follow-up; this intervention was identified in the literature as key to reducing congestive heart failure patient hospitalisation and mortality, leading to a reduction in patient care fees without compromising the quality of care. The ACO network performed well, with a 10% reduction observed in hospitalisation compared with the standard care network. In another study [ 23 ] six scenarios were simulated with combinations of patient bypass capability (turned “on” or “off”) and provider participation in the ACO network (no ACO present, optional participation in ACO or compulsory participation in ACO). Provider participation in the ACO, in agreement with Liu et al. [ 25 ], led to reduced mortality and congestive heart failure patient hospitalisation, with patient bypass capability marginally increasing provider ACO participation. Yousefi et al. [ 67 ] also modelled the impact of group decision-making in ED on the number of patient deaths and number of wrong discharges i.e. patients sent to the wrong sector for care after triage and are then discharged before receiving correct treatment.
Nine of the 11 papers that utilised ABM undertook model validation, consisting almost exclusively of behavioural validity tests. Model output, such as patient length of stay and mortality rates, was reviewed by health professionals [ 46 , 66 ] and compared with data extracted from pilot studies [ 20 ], health facilities (historical) [ 22 , 24 , 46 , 65 , 66 ], national health surveys [ 65 ] and relevant literature [ 23 , 25 ]. Papers presented the results of tests to determine the equivalence of variance [ 20 ] and difference in mean [ 20 , 24 ] between model output and real data. Structural validity tests included extreme condition testing [ 23 , 46 ] and engaging health care experts to ensure the accuracy of model framework [ 22 , 47 ]. Sensitivity analysis was performed to determine how variations or uncertainty in key parameters (particularly where they had not been derived from historical or care data [ 65 ]) affected model outcomes [ 23 , 25 ].
The majority of model limitations reported were concerned the use or availability of real system or case data. Huynh et al. [ 20 ], Yousefi et al. [ 67 ] and Liu et al. [ 25 ] formulated their models using data that was obtainable, such as limited sample data extracted from a pilot study [ 20 ], national average trends [ 25 ] and data from previous studies [ 67 ]. Yousefi et al. [ 22 ] case study dataset did not contain key system feedback, such as the tolerance time of patients waiting to be seen by a physician in the ED, although authors were able to extract this data from a comparable study identified in the literature.
Missing model feedback or parameters, strict model boundaries and simplification of system elements were also considered limitations. Huynh et al. [ 20 ], Hutzschenreuter et al. [ 66 ] and Einzinger et al. [ 65 ] did not model all the realistic complexities of their system, such as all possible interruptions to tasks that occur in patient care units [ 20 ], patient satisfaction of admission processes [ 66 ] (which will be addressed in future work), how treatment influences the course of disease or that morbid patients are at higher risk of developing co-morbidity than healthier patients, which would affect the service needs and consumption needs of the patient [ 65 ]. To improve the accuracy of the model, Huynh et al. stated that further research is taking place to obtain real, clinical data (as opposed to clinical simulation lab results) to assess the impact of interruptions on workflow. Liu et al.’s [ 21 ] model boundary did not include other hospital units that may have been affected by ED behaviour and they identify this as future work, for example to include hospital wards that are affected by ED behaviour. Alibrahim et al. [ 23 ] and Einzinger et al. [ 65 ] made simplifications to the health providers and networks that were modelled, such as assuming equal geographical distances and identical care services between health providers in observed networks [ 23 ], limiting the number of factors that influenced a patients decision to bypass their nearest health provider [ 65 ] and not simulating changes to health provider behaviour based on service utilisation or reimbursement scheme in place [ 23 ]. Alibrahim et al. [ 23 ] noted that although the model was constrained by such assumptions, the focus of future work would be to improve the capability of the model to accurately study the impact of patient choice on economic, health and health provider outcomes.
SDM-ABM use in health system research
A single paper used hybrid SDM-ABM to model health system behaviour. Djanatliev et al. [ 47 ] developed a tool that could be used to assess the impact of new health technology on performance indicators such as patient health and projected cost of care. A modelling method that could reproduce detailed, high granularity system elements in addition to abstract, aggregate health system variables was sought and a hybrid SDM-ABM was selected. The tool nested an agent-based human decision-making module (regarding healthcare choices) within a system dynamics environment, simulating macro-level behaviour such as health care financing and population dynamics. A case study was presented to show the potential impact of Mobile Stroke Units (MSU) on patient morbidity in Berlin, where stroke diagnosis and therapy could be initiated quickly as opposed to standard care. The model structure was deemed credible after evaluation by experts, including doctors and health economists.
Comparison of SDM and ABM papers
The similarities and differences among the SDM and ABM body of literature are described in this section and shown in Table 3 . A high proportion of papers across both modelling methods simulated systems that were concerned with emergency or acute care. A high number of SDM papers (11/28) simulated patient flow and pathways through emergency care [ 28 , 31 , 36 , 45 , 47 , 50 , 56 , 57 , 58 , 61 , 62 ] with a subset evaluating the impact of policies that relieved pressure on at capacity ED’s [ 28 , 36 , 50 , 58 , 62 ]. ABM papers simulated micro-level behaviour associated with emergency care, such as health professional and patient behaviour in EDs and what impact agent interactions have on actions taken over time [ 21 , 22 , 47 , 67 ]. ACOs and health insurance reimbursement schemes, a common modelled healthcare setting among the ABM papers [ 23 , 25 , 65 ] was the focus of a single SDM paper [ 63 ] while health care waste management, a popular healthcare setting for SDM application [ 37 , 48 , 52 , 55 ] was entirely absent among the selected ABM literature. SDM and ABM were both used to test the impact of policy on undesirable patient outcomes, including patient mortality [ 23 , 25 , 58 , 60 , 67 ] and hospitalisation rates [ 23 , 25 ]. Interventions for reducing patient waiting time for services [ 24 , 33 , 53 , 61 , 67 ] and patient length of stay [ 22 , 31 , 67 ] were also tested using these methods, while policy exploration to reduce the total cost of care was more frequent among SDM studies [ 33 , 54 , 61 ].
SDM and ABM software platforms provide accessible, user-friendly visualisations of systems that enable engagement with health experts necessary for model validation [ 48 , 50 , 55 , 57 ] and facilitate stakeholder understanding of how alternative policies can impact health system performance under a range conditions [ 31 , 49 , 58 , 61 ]. The ability to integrate information and data from various sources was also cited as rationale for using SDM and ABM [ 51 ]. Reasons for using SDM to model health systems, as opposed to other methods, included gaining a whole-system perspective crucial for investigating undesirable or counter-intuitive system behaviour across sub-systems [ 28 , 36 , 37 , 48 , 56 ] and identifying unintended consequences or policy resistance with tested health policies [ 59 , 61 ]. The ability to replicate human behaviour [ 20 , 21 , 22 , 25 , 66 ] and capacity for learning and adaptive behaviour [ 20 , 25 ] was frequently cited as rationale for using ABM to simulate health systems.
Validation of SDMs and ABMs consisted mostly of behavioural validity tests where model output was reviewed by experts and compared to real system performance data or to relevant literature. Structural validity tests were uncommon among ABM papers while expert consultation on model development [ 30 , 36 , 50 , 53 , 54 , 57 , 62 , 63 ], extreme condition [ 30 , 31 , 52 , 57 , 59 , 60 , 64 ] and dimensional consistency tests [ 31 , 52 , 57 , 59 , 60 ] were frequently reported in the SDM literature. The inability to simulate all actions or interactions that occur in the real system [ 20 , 30 , 61 , 65 , 66 ] and simplification of model parameters [ 23 , 55 , 62 , 63 , 65 ] were described as limitations in both SDM and ABM papers. Data availability for model conception and formulation [ 20 , 22 , 25 , 32 , 36 , 67 ] and the impact of model boundaries (restricting exploration of interconnected sub-system behaviour [ 21 , 31 , 53 ]) were also cited limitations common to both sets of literature. Lack of costing analysis [ 58 , 60 ], short time horizons [ 33 , 57 ] and an inability to model future improvements in technology or service delivery [ 31 , 44 , 49 , 51 ] were additionally cited among the SDM papers.
Statement of principal findings
Our review has confirmed that there is a growing body of research demonstrating the use of SDM and ABM to model health care systems to inform policy in a range of settings. While the application of SDM has been more widespread (with 28 papers identified) there are also a growing number of ABM being used (11), just over half of which used hybrid simulation. A single paper used hybrid SDM-ABM to model health system behaviour. To our knowledge this is the first review to identify and compare the application of both SDM and ABM to model health systems. The first ABM article identified in this review was published almost a decade after the first SDM paper; this reflects to a certain extent the increasing availability of SDM and ABM dedicated software tools with the developments in ABM software lagging behind their SDM modelling counterparts.
Emergency and acute care, and elderly care and LTC services were the most frequently simulated health system setting. Both sets of services are facing exponential increases in demand with constraints on resources, presenting complex issues ideal for evaluation through simulation. Models were used to explore the impact and potential spill over effects of alternative policy options, prior to implementation, on patient outcomes, service use and efficiency under various structural and financial constraints.
Strengths and weaknesses of the study
To ensure key papers were identified, eight databases across four research areas were screened for relevant literature. Unlike other reviews in the field [ 39 , 40 ], there was no restriction placed on publication date. The framework for this review was built to provide a general overview of the SDM and ABM of healthcare literature, capturing papers excluded in other published reviews as a result of strict inclusion criteria. These include reviews that have focussed specifically on compiling examples of modelled health policy application in the literature [ 35 ] or have searched for papers with a particular health system setting, such as those that solely simulate the behaviour of emergency departments [ 34 ]. One particularly comprehensive review of the literature had excluded papers that simulated hospital systems, which we have explicitly included as part of our search framework [ 39 ].
The papers presented in this review, with selection restricted by search criteria, provide a broad picture of the current health system modelling landscape. The focus of this review was to identify models of facility-based healthcare, purposely excluding literature where the primary focus is on modelling disease progression, disease transmission or physiological disorders which can be found in other reviews such as Chang et al. [ 39 ] and Long et al. [ 41 ]. The data sources or details of how data was used to conceptualise and formulate models are not presented in this paper; this could on its own be the focus of another study and we hope to publish these results as future work. This information would be useful for researchers who want to gain an understanding of the type and format of data used to model health systems and best practice for developing and validating such models.
Literature that was not reported in English was excluded from the review which may have resulted in a small proportion of relevant papers being missed. Papers that described DES models, the other popular modelling method for simulating health system processes, were not included in this review (unless DES methods are presented as part of a hybrid model integrated with SDM or ABM) but have been compiled elsewhere [ 68 , 69 , 70 ]. Finally, the quality of the papers was not assessed.
Implications for future research
A nominal number of SDM papers (9/28), an even lower proportion of ABM papers (2/11) and none of the hybrid methods papers simulated health systems based in low- or middle-income countries (LMICs). The lower number of counterpart models in LMICs can be attributed to a lack of capacity in modelling methods and perhaps the perceived scarcity of suitable data; however, the rich quantitative and qualitative primary data collected in these countries for other types of evaluation could be used to develop such models. Building capacity for using these modelling methods in LMICs should be a priority and generating knowledge of how and which secondary data to use in these settings for this purpose. In this review, we observed that it is feasible to use SDM to model low-income country health systems, including those in Uganda [ 60 ] and Afghanistan [ 30 ]. The need to increase the use of these methods within LMICs is paramount; even in cases where there is an absence of sufficient data, models can be formulated for LMICs and used to inform on key data requirements through sensitivity analysis, considering the resource and healthcare delivery constraints experienced by facilities in these settings. This research is vital for our understanding of health system functioning in LMICs, and given the greater resource constraints, to allow stakeholders and researchers to assess the likely impact of policies or interventions before their costly implementation, and to shed light on optimised programme design.
Health system professionals can learn greatly from using modelling tools, such as ABM, SDM and hybrid models, developed originally in non-health disciplines to understand complex dynamic systems. Understanding the complexity of health systems therefore require collaboration between health scientists and scientists from other disciplines such as engineering, mathematics and computer science. Discussion and application of hybrid models is not a new phenomenon in other fields but their utilisation in exploring health systems is still novel; the earliest article documenting their use in this review was published in 2010 [ 43 ]. Five of the six hybrid modelling papers [ 43 , 44 , 45 , 46 , 47 ] were published as conference proceedings (the exception Kittipittayakorn et al. [ 24 ]), demonstrating the need to include conference articles in systematic reviews of the literature in order to capture new and evolving applications of modelling for health systems research.
The configuration and extent to which two distinct types of models are combined has been described in the literature [ 71 , 72 , 73 , 74 , 75 ]. The hybrid modelling papers selected in this review follow what is described as ‘hierarchical’ or ‘process environment’ model structures, the former where two distinct models pass information to each other and the latter where one model simulates system processes within the environment of another model [ 72 ]. Truly ‘integrated’ models, considered the ‘holy grail’ [ 43 ] of hybrid simulation, where elements of the system are simulated by both methods of modelling with no clear distinction, were not identified in this review and in the wider literature remain an elusive target. In a recent review of hybrid modelling in operational research only four papers were identified to have implemented truly integrated hybrid simulation and all used bespoke software, unrestricted by the current hybrid modelling environments [ 76 ].
Of the six hybrid modelling papers, only Djanatliev et al. [ 47 ] presented a model capable of both ABM and SDM simulation. The crucial macro- and micro- level activity captured in such models represent feedback in the wider, complex system while retaining the variable behaviour exhibited by those who access or deliver healthcare. With increasing software innovation and growing demand for multi-method modelling in not only in healthcare research but in the wider research community, we need to increase their application to modelling health systems and progress towards the ‘holy grail’ of hybrid modelling.
We identified 28 papers using SDM methods and 11 papers using ABM methods to model health system behaviour, six of which implemented hybrid model structures with only a single paper using SDM-ABM. Emergency and acute care, and elderly care and LTC services were the most frequently simulated health system settings, modelling the impact of health policies and interventions targeting at-capacity healthcare services, patient length of stay in healthcare facilities and undesirable patient outcomes. A high proportion of articles modelled health systems in high income countries; future work should now turn to modelling healthcare settings in LMIC to support policy makers and health system researchers alike. The utilisation of hybrid models in healthcare is still relatively new but with an increasing demand to develop models that can simulate the macro- and micro-level activity exhibited by health systems, we will see an increase in their use in the future.
Availability of data and materials
Data sharing is not applicable to this article as no datasets were generated or analysed during the current study.
One of the elderly or LTC services papers also modelled emergency or acute care but it was not the primary focus and is therefore not discussed here.
The single SDM-ABM paper that modelled the delivery of emergency or acute care is discussed in section ‘SDM-ABM use in health system research’.
Six of the emergency or acute care review papers and one of the cardiology care papers also modelled elderly or LTC services but it was not the primary focus and are therefore not discussed here.
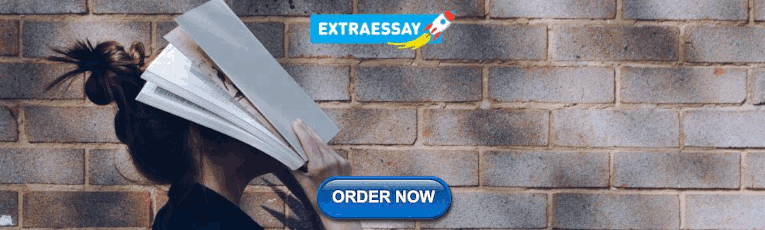
Abbreviations
Accountable care organisation
Agent-based model
Discrete-event simulation
Emergency Department
Long-term care
Low- and middle-income countries
System dynamics model
Kitson A, Brook A, Harvey G, Jordan Z, Marshall R, O’Shea R, et al. Using Complexity and Network Concepts to Inform Healthcare Knowledge Translation. Int J Heal Policy Manag. 2017;7:231–43. https://doi.org/10.15171/ijhpm.2017.79 .
Article Google Scholar
Paina L, Peters DH. Understanding pathways for scaling up health services through the lens of complex adaptive systems. Health Policy Plan. 2012;27:365–73. https://doi.org/10.1093/heapol/czr054 .
Article PubMed Google Scholar
Lipsitz LA. Understanding health care as a complex system: the foundation for unintended consequences. JAMA. 2012;308:243–4. https://doi.org/10.1001/jama.2012.7551 .
Article PubMed PubMed Central CAS Google Scholar
Adam T, de Savigny D. Systems thinking for strengthening health systems in LMICs: need for a paradigm shift. Health Policy Plan. 2012;27:iv1–3. https://doi.org/10.1093/heapol/czs084 .
de Savigny D, Blanchet K, Adam T. Applied systems thinking for health systems research : a methodological handbook: McGraw-Hill Education; 2017.
Shepherd SP. A review of system dynamics models applied in transportation. Transp B Transp Dyn. 2014;2:83–105. https://doi.org/10.1080/21680566.2014.916236 .
Sterman JD. Business dynamics: systems thinking and modeling for a complex world: McGraw-Hill Companies Inc; 2000.
Kunc M, Mortenson MJ, Vidgen R. A computational literature review of the field of system dynamics from 1974 to 2017. J Simul. 2018;12:115–27. https://doi.org/10.1080/17477778.2018.1468950 .
System Dynamics for Engineering Students. Elsevier; 2018. doi: https://doi.org/10.1016/C2011-0-05346-2 .
Radzicki MJ. System Dynamics and Its Contribution to Economics and Economic Modeling. Encycl. Complex. Syst. Sci. New York: Springer New York; 2009. p. 8990–9000. https://doi.org/10.1007/978-0-387-30440-3_539 .
Book Google Scholar
Ford A. Global warming and system dynamics. Int Conf Syst Dyn Soc. 2007.
Fiddaman TS. Exploring policy options with a behavioral climate-economy model. Syst Dyn Rev. 2002;18:243–67. https://doi.org/10.1002/sdr.241 .
Popli K, Sudibya GL, Kim S. A review of solid waste management using system dynamics modeling. J Environ Sci Int. 2017;26:1185–200. https://doi.org/10.5322/JESI.2017.26.10.1185 .
Groesser SN, Schaffernicht M. Mental models of dynamic systems: taking stock and looking ahead. Syst Dyn Rev. 2012;28:46–68. https://doi.org/10.1002/sdr.476 .
Epstein JM. Generative social science: studies in agent-based computational modeling: STU-Stud. Princeton University Press; 2006.
Wilensky U, Rand W. An Introduction to Agent-Based Modeling: Mit Press; 2015.
Miller JH, Page SE. Complex Adaptive Systems: An Introduction to Computational Models of Social Life: STU-Stud. Princeton University Press; 2007.
Railsback SF, Grimm V. Agent-Based and Individual-Based Modeling: Princeton University Press; 2011.
Abar S, Theodoropoulos G, Lemarinier P, O’Hare G. Agent based Modelling and simulation tools: a review of the state-of-art software. Comput Sci Rev. 2017;24:13–33. https://doi.org/10.1016/j.cosrev.2017.03.001 .
Huynh N, Snyder R, Vidal J, Tavakoli A, Cai B. Application of computer simulation modeling to medication administration process redesign. J Healthc Eng. 2012;3:649–62. https://doi.org/10.1260/2040-2295.3.4.649 .
Liu Z, Cabrera E, Rexachs D, Luque E. A generalized agent-based model to simulate emergency departments. Sixth Int Conf Adv Syst Simul. 2014:65–70.
Yousefi M, Yousefi M, Fogliatto FS, Ferreira RPM, Kim JH. Simulating the behavior of patients who leave a public hospital emergency department without being seen by a physician: a cellular automaton and agent-based framework. Brazilian J Med Biol Res. 2018;51:e6961. https://doi.org/10.1590/1414-431X20176961 .
Alibrahim A, Wu S. An agent-based simulation model of patient choice of health care providers in accountable care organizations. Health Care Manag Sci. 2018;21:131–43. https://doi.org/10.1007/s10729-016-9383-1 .
Kittipittayakorn C, Ying K-C. Using the integration of discrete event and agent-based simulation to enhance outpatient service quality in an orthopedic department. J Healthc Eng. 2016;2016:4189206. https://doi.org/10.1155/2016/4189206 .
Article PubMed Central Google Scholar
Liu P, Wu S. An agent-based simulation model to study accountable care organizations. Health Care Manag Sci. 2016;19:89–101. https://doi.org/10.1007/s10729-014-9279-x .
Allen M, Spencer A, Gibson A, Matthews J, Allwood A, Prosser S, et al. Right cot, right place, right time: improving the design and organisation of neonatal care networks – a computer simulation study. Heal Serv Deliv Res. 2015;3:9. https://doi.org/10.3310/hsdr03200 .
Brailsford SC. Tutorial: Advances and challenges in healthcare simulation modeling: 2007 Winter Simul. Conf., IEEE; 2007. p. 1436–48. https://doi.org/10.1109/WSC.2007.4419754 .
Esensoy AV, Carter MW. High-Fidelity whole-system patient flow modeling to assess health care transformation policies. Eur J Oper Res. 2018;266:221–37. https://doi.org/10.1016/j.ejor.2017.09.019 .
Homer JB, Hirsch GB. System dynamics modeling for public health: background and opportunities. Am J Public Health. 2006;96:452–8. https://doi.org/10.2105/AJPH.2005.062059 .
Article PubMed PubMed Central Google Scholar
Alonge O, Lin S, Igusa T, Peters DH. Improving health systems performance in low- and middle-income countries: a system dynamics model of the pay-for-performance initiative in Afghanistan. Health Policy Plan. 2017;32:1417–26. https://doi.org/10.1093/heapol/czx122 .
Article CAS PubMed PubMed Central Google Scholar
Rashwan W, Abo-Hamad W, Arisha A. A system dynamics view of the acute bed blockage problem in the Irish healthcare system. Eur J Oper Res. 2015;247:276–93. https://doi.org/10.1016/j.ejor.2015.05.043 .
Ghaffarzadegan N, Epstein AJ, Martin EG. Practice variation, bias, and experiential learning in cesarean delivery: A data-based system dynamics approach. Health Serv Res. 2013;48:713–34. https://doi.org/10.1111/1475-6773.12040 .
Taylor K, Dangerfield B. Modelling the feedback effects of reconfiguring health services. J Oper Res Soc. 2005;56:659–75. https://doi.org/10.1057/palgrave.jors.2601862 .
Mohiuddin S, Busby J, Savovic J, Richards A, Northstone K, Hollingworth W, et al. Patient flow within UK emergency departments: a systematic review of the use of computer simulation modelling methods. BMJ Open. 2017;7:e015007. https://doi.org/10.1136/bmjopen-2016-015007 .
Atkinson J-A, Wells R, Page A, Dominello A, Haines M, Wilson A. Applications of system dynamics modelling to support health policy. Public Heal Res Pract. 2015;25. https://doi.org/10.17061/phrp2531531 .
Lattimer V, Brailsford S, Turnbull J, Tarnaras P, Smith H, George S, et al. Reviewing emergency care systems I: insights from system dynamics modelling. Emerg Med J. 2004;21:685–91. https://doi.org/10.1136/emj.2002.003673 .
Chaerul M, Tanaka M, Shekdar AV. A system dynamics approach for hospital waste management. Waste Manag. 2008;28:442–9. https://doi.org/10.1016/j.wasman.2007.01.007 .
Moher D, Liberati A, Tetzlaff J, Altman DG. Preferred reporting items for systematic reviews and meta-analyses: the PRISMA statement. BMJ. 2009;339:b2535. https://doi.org/10.1136/bmj.b2535 .
Chang AY, Ogbuoji O, Atun R, Verguet S. Dynamic modeling approaches to characterize the functioning of health systems: A systematic review of the literature. Soc Sci Med. 2017;194:160–7. https://doi.org/10.1016/j.socscimed.2017.09.005 .
Rusoja E, Haynie D, Sievers J, Mustafee N, Nelson F, Reynolds M, et al. Thinking about complexity in health: a systematic review of the key systems thinking and complexity ideas in health. J Eval Clin Pract. 2018;24:600–6. https://doi.org/10.1111/jep.12856 .
Long KM, Meadows GN. Simulation modelling in mental health: a systematic review. J Simul. 2017. https://doi.org/10.1057/s41273-017-0062-0 .
Banco Mundial. World Bank: country and lending groups - DataBank. World Bank Gr. 2018.
Brailsford SC, Desai SM, Viana J. Towards the holy grail: Combining system dynamics and discrete-event simulation in healthcare: Proc. 2010 Winter Simul. Conf., IEEE; 2010. p. 2293–303. https://doi.org/10.1109/WSC.2010.5678927 .
Mielczarek B, Zabawa J. Modeling Healthcare Demand Using a Hybrid Simulation Approach, vol. 2016: Proc. 2016 Winter Simul. Conf., IEEE Press. p. 1535–46.
Zulkepli J. Hybrid simulation for modelling large systems: an example of integrated care model: Proc. 2012 Winter Simul. Conf; 2012.
Viana J, Simonsen TB, Dahl FA, Flo K. A Hybrid Discrete Event Agent Based Overdue Pregnancy Outpatient Clinic Simulation Model. Proc. 2018 Winter Simul. Conf. Piscataway: IEEE Press; 2018. p. 1488–99.
Google Scholar
Djanatliev A, German R, Kolominsky-Rabas P, Hofmann BM. Hybrid simulation with loosely coupled system dynamics and agent-based models for prospective health technology assessments: Proc. Winter Simul. Conf., Winter Simulation Conference; 2012. p. 69:1–69:12.
Al-Khatib IA, Eleyan D, Garfield J. A system dynamics approach for hospital waste management in a city in a developing country: the case of Nablus, Palestine. Environ Monit Assess. 2016;188:503. https://doi.org/10.1007/s10661-016-5487-9 .
Ansah JP, Eberlein RL, Love SR, Bautista MA, Thompson JP, Malhotra R, et al. Implications of long-term care capacity response policies for an aging population: a simulation analysis. Health Policy. 2014;116:105–13. https://doi.org/10.1016/j.healthpol.2014.01.006 .
Brailsford SC. Emergency and on-demand health care: modelling a large complex system. J Oper Res Soc. 2004;55.
Cepoiu-Martin M, Bischak DP. Policy choices in dementia care - an exploratory analysis of the Alberta Continuing Care System (ACCS) using system dynamics. Spec Issue Complex Forum “real” World Heal Syst Implic Complex. Theor Sci. 2018;24:278–84.
Ciplak N, Barton JR. A system dynamics approach for healthcare waste management: A case study in Istanbul Metropolitan City, Turkey. Waste Manag Res. 2012;30:576–86. https://doi.org/10.1177/0734242X12443405 .
de Andrade L, Lynch C, Carvalho E, Rodrigues CG, Vissoci JRN, Passos GF, et al. System dynamics modeling in the evaluation of delays of care in ST-segment elevation myocardial infarction patients within a tiered health system. PLoS One. 2014;9:e103577. https://doi.org/10.1371/journal.pone.0103577 .
Desai MS, Penn ML, Brailsford S, Chipulu M. Modelling of Hampshire adult services—gearing up for future demands. Health Care Manag Sci. 2008;11:167–76. https://doi.org/10.1007/s10729-007-9049-0 .
Eleyan D, Al-Khatib IA, Garfield J. System dynamics model for hospital waste characterization and generation in developing countries. Waste Manag Res. 2013;31:986–95. https://doi.org/10.1177/0734242X13490981 .
Lane D. Emergency but no accident: a system dynamics study of casualty waiting times in the British NHS. Eurohealth (Lond). 1998;4.
Lane DC. Looking in the wrong place for healthcare improvements: a system dynamics study of an accident and emergency department. J Oper Res Soc. 2000;51.
Mahmoudian-Dehkordi A, Sadat S. Sustaining critical care: using evidence-based simulation to evaluate ICU management policies. Health Care Manag Sci. 2017;20:532–47. https://doi.org/10.1007/s10729-016-9369-z .
Meker T, Barlas Y. Dynamic consequences of performance-based payment Systems in Public Hospitals. Syst Res Behav Sci. 2015;32:459–80. https://doi.org/10.1002/sres.2338 .
Semwanga AR, Nakubulwa S, Adam T. Applying a system dynamics modelling approach to explore policy options for improving neonatal health in Uganda. Heal Res Policy Syst. 2016;14:35. https://doi.org/10.1186/s12961-016-0101-8 .
Walker BC, Haslett T. The dynamics of local rules in hospital admission processes. Aust Health Rev. 2003;26:98–106.
Wong HJ, Wu RC, Caesar M, Abrams H, Morra D. Smoothing inpatient discharges decreases emergency department congestion: a system dynamics simulation model. Emerg Med J. 2010;27:593–8. https://doi.org/10.1136/emj.2009.078543 .
Worni M, Pietrobon R, Zammar GR, Shah J, Yoo B, Maldonato M, et al. System dynamics to model the unintended consequences of denying payment for venous thromboembolism after total knee arthroplasty. PLoS One. 2012;7:e30578. https://doi.org/10.1371/journal.pone.0030578 .
Yu W, Li M, Ge Y, Li L, Zhang Y, Liu Y, et al. Transformation of potential medical demand in China: A system dynamics simulation model. J Biomed Inform. 2015;57:399–414. https://doi.org/10.1016/j.jbi.2015.08.015 .
Einzinger P, Popper N, Breitenecker F, Pfeffer N, Jung R, Endel G. The GAP-DRG Model: Simulation of Outpatient Care for Comparison of Different Reimbursement Schemes. Proc. 2013 Winter Simul. Conf. Simul. Mak. Decis. A complex world. Piscataway: IEEE Press; 2013. p. 2299–308.
Hutzschenreuter AK, Bosman PAN, Blonk-Altena I, van Aarle J, La Poutré H. Agent-based patient admission scheduling in hospitals. Belgian/Netherlands Artif Intell Conf. 2008:315–6. https://doi.org/10.1007/3-540-32062-8 .
Yousefi M, Ferreira R. An agent-based simulation combined with group decision-making technique for improving the performance of an emergency department. Brazilian J Med Biol Res. 2017;50:1–10. https://doi.org/10.1590/1414-431X20175955 .
Günal MM, Pidd M. Discrete event simulation for performance modelling in health care: a review of the literature. J Simul. 2010;4:42–51. https://doi.org/10.1057/jos.2009.25 .
Salleh S, Thokala P, Brennan A, Hughes R, Dixon S. Discrete event simulation-based resource Modelling in health technology assessment. Pharmacoeconomics. 2017;35:989–1006. https://doi.org/10.1007/s40273-017-0533-1 .
Zhang X. Application of discrete event simulation in health care: a systematic review. BMC Health Serv Res. 2018;18:687. https://doi.org/10.1186/s12913-018-3456-4 .
Bennett PG. On linking approaches to decision-aiding: issues and prospects. J Oper Res Soc. 1985;36:659. https://doi.org/10.2307/2582261 .
Chahal K, Eldabi T. Applicability of hybrid simulation to different modes of governance in UK healthcare: 2008 Winter Simul. Conf., IEEE; 2008. p. 1469–77. https://doi.org/10.1109/WSC.2008.4736226 .
Lättilä L, Hilletofth P, Lin B. Hybrid simulation models – when, why, how? Expert Syst Appl. 2010;37:7969–75. https://doi.org/10.1016/j.eswa.2010.04.039 .
Brailsford SC. Hybrid simulation in healthcare: New concepts and new tools: 2015 Winter Simul. Conf., IEEE; 2015. p. 1645–53. https://doi.org/10.1109/WSC.2015.7408284 .
Morgan JS, Howick S, Belton V. A toolkit of designs for mixing discrete event simulation and system dynamics. Eur J Oper Res. 2017;257:907–18. https://doi.org/10.1016/j.ejor.2016.08.016 .
Brailsford SC, Eldabi T, Kunc M, Mustafee N, Osorio AF. Hybrid simulation modelling in operational research: a state-of-the-art review. Eur J Oper Res. 2018. https://doi.org/10.1016/j.ejor.2018.10.025 .
Download references
Acknowledgements
Not applicable.
The work described in this paper was funded by the Health Systems Research Initiative (HSRI). MRC Grant Reference Number: MR/R013454/1.
Author information
Authors and affiliations.
Department of Global Health and Development, London School of Hygiene and Tropical Medicine, 15-17 Tavistock Place, London, WC1H 9SH, UK
Rachel Cassidy, Neha S. Singh, Josephine Borghi & Karl Blanchet
Department of Mathematics, University College London, London, UK
Pierre-Raphaël Schiratti
Sia Partners UK, London, UK
Information Systems Department, College of Computing and Information Sciences, Makerere University, P.O. Box 7062, Kampala, Uganda
Agnes Semwanga
Ifakara Health Institute, PO Box 78373, Dar es Salaam, Tanzania
Peter Binyaruka
Department of Gender Studies, School of Humanities and Social Sciences, University of Zambia, 10101, Lusaka, Zambia
Nkenda Sachingongu
Economic and Business Research Programme, University of Zambia, Institute of Economic and Social Research, P O Box 30900, 10101, Lusaka, Zambia
Chitalu Miriam Chama-Chiliba
Department of Public Health, Environments and Society, London School of Hygiene and Tropical, London, UK
Zaid Chalabi
You can also search for this author in PubMed Google Scholar
Contributions
RC, KB, ZC specified the search criteria and selection of databases. RC screened titles, evaluated full text articles and was responsible for full text extraction. RC is lead author, NSS, KB, ZC, PS, JB, AS, PB, CC, NS provided guidance on the draft and final manuscript. All authors read and approved the final manuscript.
Corresponding author
Correspondence to Rachel Cassidy .
Ethics declarations
Ethics approval and consent to participate, consent for publication, competing interests.
The authors declare that they have no competing interests.
Additional information
Publisher’s note.
Springer Nature remains neutral with regard to jurisdictional claims in published maps and institutional affiliations.
Supplementary information
Additional file 1..
Search criteria used for each database.
Additional file 2.
Descriptive table of validation methods used in SDM and ABM literature.
Additional file 3.
Descriptive table of ABM model rules.
Rights and permissions
Open Access This article is distributed under the terms of the Creative Commons Attribution 4.0 International License ( http://creativecommons.org/licenses/by/4.0/ ), which permits unrestricted use, distribution, and reproduction in any medium, provided you give appropriate credit to the original author(s) and the source, provide a link to the Creative Commons license, and indicate if changes were made. The Creative Commons Public Domain Dedication waiver ( http://creativecommons.org/publicdomain/zero/1.0/ ) applies to the data made available in this article, unless otherwise stated.
Reprints and permissions
About this article
Cite this article.
Cassidy, R., Singh, N.S., Schiratti, PR. et al. Mathematical modelling for health systems research: a systematic review of system dynamics and agent-based models. BMC Health Serv Res 19 , 845 (2019). https://doi.org/10.1186/s12913-019-4627-7
Download citation
Received : 14 June 2019
Accepted : 11 October 2019
Published : 19 November 2019
DOI : https://doi.org/10.1186/s12913-019-4627-7
Share this article
Anyone you share the following link with will be able to read this content:
Sorry, a shareable link is not currently available for this article.
Provided by the Springer Nature SharedIt content-sharing initiative
- System dynamics
- Agent-based
- Health systems
- Systematic review
BMC Health Services Research
ISSN: 1472-6963
- General enquiries: [email protected]

- Articles in Press
- Current Issue
Journal Archive
Journal of Mathematical Modeling (J. Math. Model.) is published by Faculty of Mathematical Sciences , University of Guilan . JMM publishes original high-quality peer-reviewed papers in all branches of computational or applied mathematics. It covers all areas of numerical analysis, numerical solutions of differential and integral equations, numerical linear algebra, optimization theory, approximation theory, control theory and fuzzy theory with applications, mathematical modeling in all major areas of applied sciences, computer simulation and parallel algorithms.
All the submitted papers are checked by iThenticate against plagiarism and the similarities up to 25% are acceptable.
JMM is indexed in SCOPUS , zbMATH , MathSciNet , CiteFactor , ...

Research Article
Convergence analysis of compact finite difference method for the solution of anti-periodic boundary value problems.
10.22124/jmm.2023.25342.2251
Abdol Baseer Saqib; Ghasem Barid Loghmani; Mohammad Heydari
- View Article
- PDF 208.28 K
Finding a time-dependent reaction coefficient of a nonlinear heat source in an inverse heat conduction problem
Pages 17-32
10.22124/jmm.2023.24413.2186
Kamal Rashedi
- PDF 651.7 K
A fitted mesh method for a class of two-parameter nonlinear singular perturbation problems
Pages 33-49
10.22124/jmm.2023.25201.2237
Manikandan Mariappan
- PDF 343.74 K
Analysis of a coupled pair of Cahn-Hilliard equations with nondegenerate mobility
Pages 51-70
10.22124/jmm.2023.25558.2272
Ghufran A. Al-Musawi; Akil Jassim Harfash
- PDF 212.06 K
A new numerical method for discretization of the nonlinear Klein-Gordon model arising in light waves
Pages 71-84
10.22124/jmm.2023.25115.2230
Hamid Mesgarani; Yones Esmaeelzade Aghdam; Ezzatollah Darabi
- PDF 715.2 K
An improved extended block Arnoldi method for solving low-rank Lyapunov equation
Pages 85-98
10.22124/jmm.2023.25670.2281
Ilias Abdaoui
- PDF 202.37 K
A fifth-order symmetrical weighted hybrid ENO-flux limiter scheme for traffic flow model on networks
Pages 99-115
10.22124/jmm.2023.24976.2220
Rooholah Abedian
- PDF 500.03 K
Inverse eigenvalue problem of nonnegative matrices via unit lower triangular matrices (Part I)
Pages 117-130
10.22124/jmm.2023.21759.2092
Alimohammad Nazari; Atiyeh Nezami
- PDF 157.41 K
A new $(I+P)$-like preconditioner for the SOR method for solving multi-linear systems with $ \mathcal{M} $-tensors
Pages 131-144
10.22124/jmm.2023.25368.2253
Afsaneh Hasanpour; Maryam Mojarrab
- PDF 192.74 K
Moore-Penrose inverse of an interval matrix and its application
Pages 145-155
10.22124/jmm.2023.24972.2219
Marzieh Dehghani-Madiseh
- PDF 173.38 K
A uniformly convergent computational method for singularly perturbed parabolic partial differential equation with integral boundary condition
Pages 157-175
10.22124/jmm.2023.24590.2198
Getu Mekonnen Wondimu; Gemechis File Duressa; Mesfin Mekuria Woldaregay; Tekle Gemechu Dinka
- PDF 492.75 K
An algorithm for multi-objective fuzzy linear programming problem with interval type-2 fuzzy numbers and ambiguity in parameters
Pages 177-197
10.22124/jmm.2023.25400.2258
Hassan Mishmast Nehi; Shokouh Sargolzaei
- PDF 658.14 K
Publication Information
Indexing and abstracting.
- Architecture and Design
- Asian and Pacific Studies
- Business and Economics
- Classical and Ancient Near Eastern Studies
- Computer Sciences
- Cultural Studies
- Engineering
- General Interest
- Geosciences
- Industrial Chemistry
- Islamic and Middle Eastern Studies
- Jewish Studies
- Library and Information Science, Book Studies
- Life Sciences
- Linguistics and Semiotics
- Literary Studies
- Materials Sciences
- Mathematics
- Social Sciences
- Sports and Recreation
- Theology and Religion
- Publish your article
- The role of authors
- Promoting your article
- Abstracting & indexing
- Publishing Ethics
- Why publish with De Gruyter
- How to publish with De Gruyter
- Our book series
- Our subject areas
- Your digital product at De Gruyter
- Contribute to our reference works
- Product information
- Tools & resources
- Product Information
- Promotional Materials
- Orders and Inquiries
- FAQ for Library Suppliers and Book Sellers
- Repository Policy
- Free access policy
- Open Access agreements
- Database portals
- For Authors
- Customer service
- People + Culture
- Journal Management
- How to join us
- Working at De Gruyter
- Mission & Vision
- De Gruyter Foundation
- De Gruyter Ebound
- Our Responsibility
- Partner publishers

Your purchase has been completed. Your documents are now available to view.
Topics in Mathematical Modeling
- Ka-Kit Tung
- X / Twitter
Please login or register with De Gruyter to order this product.
- Language: English
- Publisher: Princeton University Press
- Copyright year: 2007
- Audience: College/higher education;Professional and scholarly;
- Main content: 336
- Other: 31 halftones. 52 line illus. 5 tables.
- Keywords: Ordinary differential equation ; Initial condition ; Differential equation ; Equation ; Mathematics ; Proportionality (mathematics) ; Quantity ; Partial differential equation ; Year ; Linear differential equation ; Calculation ; Chaos theory ; Mathematician ; Convection ; Logistic function ; Nonlinear system ; Acceleration ; Probability ; Fishery ; Temperature ; Linear stability ; Billion years ; Periodic function ; Lorenz system ; Population dynamics ; Mathematical model ; Parameter ; Textbook ; Newton's laws of motion ; Newton's law of universal gravitation ; Population density ; Logistic map ; Age of the Earth ; Age of the universe ; Climate ; Depensation ; El Niño–Southern Oscillation ; Herbivore ; Irrational number ; Kepler's laws of planetary motion ; Natural frequency ; Payment ; Power law ; Spouse ; Exponential growth ; Prediction ; Fibonacci number ; Clockwise ; Climate model ; Trade winds ; Logarithmic spiral ; Earth ; Cartesian coordinate system ; Snowball Earth ; Coefficient ; Rule of 72 ; Time series ; Interest rate ; Carbon dioxide ; Radiocarbon dating ; Fibonacci ; Atmosphere of Earth ; Temperature gradient ; Iteration ; Eigenvalues and eigenvectors ; Insect ; Geometric series ; Elliptic orbit ; Approximation ; Thermocline
- Published: June 14, 2016
- ISBN: 9781400884056
Quick links
- Directories
Topics in Mathematical Modeling
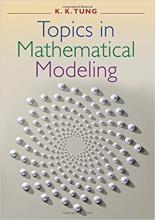
- Mailing List
- YouTube
- News Feed
Advertisement
Mathematical modeling for theory-oriented research in educational technology
- Development Article
- Published: 29 November 2021
- Volume 70 , pages 149–167, ( 2022 )
Cite this article
- Elena Novak ORCID: orcid.org/0000-0003-0873-2081 1
808 Accesses
2 Altmetric
Explore all metrics
Mathematical modeling describes how events, concepts, and systems of interest behave in the world using mathematical concepts. This research approach can be applied to theory construction and testing by using empirical data to evaluate whether the specific theory can explain the empirical data or whether the theory fits the data available. Although extensively used in the physical sciences and engineering, as well as some social and behavioral sciences to examine theoretical claims and form predictions of future events and behaviors, theory-oriented mathematical modeling is less common in educational technology research. This article explores the potential of using theory-oriented mathematical modeling for theory construction and testing in the field of educational technology. It presents examples of how this approach was used in social, behavioral, and educational disciplines, and provides rationale for why educational technology research can benefit from a theory-oriented model-testing approach.
This is a preview of subscription content, log in via an institution to check access.
Access this article
Price includes VAT (Russian Federation)
Instant access to the full article PDF.
Rent this article via DeepDyve
Institutional subscriptions
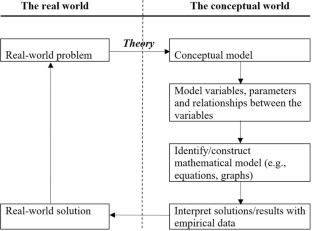
Adapted from Bessière et al. ( 2006 )
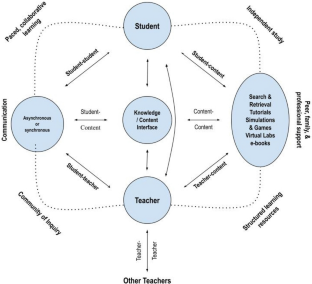
Adapted from Anderson ( 2011 )
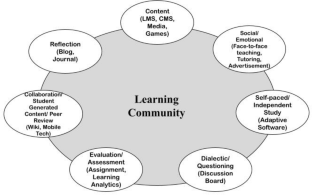
Adapted from Picciano ( 2017 )
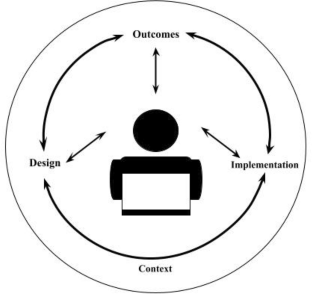
Adapted from Means et al. ( 2014 )
Similar content being viewed by others
The Philosophy of Science and Educational Technology Research
The instrumental value of conceptual frameworks in educational technology research.
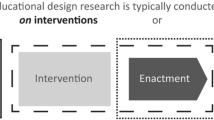
Educational design research: grappling with methodological fit
Anderson, T. (2008). The theory and practice of online learning (2nd ed.). AU Press.
Google Scholar
Anderson, T. (2011). The theory and practice of online learning . AU Press.
Atkinson, R. C., & Schiffrin, R. M. (1971). The control of short-term memory. Scientific American, 225 , 82–90.
Atkinson, R. C., & Shiffrin, R. M. (1968a). Human memory: A proposed system and its control processes. In K. W. Spence & J. T. Spence (Eds.), The psychology of learning and motivation (Volume 2) (pp. 89–195). Academic Press.
Atkinson, R. C., & Shiffrin, R. M. (1968b). Human memory: A proposed system and its control processes. In K. W. Spence & J. T. Spence (Eds.), The psychology of learning and motivation: Advances in research and theory (Vol 2) (pp. 89–195). Academic Press.
Bessière, K., Newhagen, J. E., Robinson, J. P., & Shneiderman, B. (2006). A model for computer frustration: The role of instrumental and dispositional factors on incident, session, and post-session frustration and mood. Computers in Human Behavior, 22 (6), 941–961. https://doi.org/10.1016/j.chb.2004.03.015
Article Google Scholar
Blömeke, S., & Kaiser, G. (2011). Homogeneity or heterogeneity? Profiles of opportunities to learn in primary teacher education and their relationship to cultural context and outcomes. ZDM Mathematics Education, 44 (3), 249–264. https://doi.org/10.1007/s11858-011-0378-6
Boland, L. A. (2014). Model building in economics: Its purposes and limitations . Cambridge University Press.
Bulfin, S., Henderson, M., Johnson, N. F., & Selwyn, N. (2014). Methodological capacity within the field of “educational technology” research: An initial investigation. British Journal of Educational Technology, 45 (3), 403–414.
Cilesiz, S., & Spector, J. M. (2014). The philosophy of science and educational technology research. In J. M. Spector, M. D. Merrill, J. Elen, & M. J. Bishop (Eds.), Handbook of research on educational communications and technology (pp. 875–884). New York: Springer.
Cronbach, L. J. (1975). Beyond the two disciplines of scientific psychology. American Psychologist, 20 , 116–117.
Dabbaghian, V., & Mago, V. K. (2014). Theories and simulations of complex social systems . Springer.
Dym, C. (2004). Principles of mathematical modeling . Academic Press.
Ertmer, P. A. (1999). Addressing first- and second-order barriers to change: Strategies for technology integration. Educational Technology Research and Development, 47 (4), 47–61.
Garrison, D. R., Anderson, T., & Archer, W. (2000). Critical inquiry in a text-based environment: Computer conferencing in higher education model. The Internet and Higher Education, 2 (2–3), 87–105.
Goe, L. (2007). The link between teacher quality and student outcomes: A research synthesis . Washington, DC: National Comprehensive Center for Teacher Quality. Retrieved from http://ncctq.learningpt.org/publications/LinkBetweenTQandStudentOutcomes.pdf
Gollwitzer, P. M. (1999). Implementation intentions. Strong effects of simple plans. American Psychologist, 54 (7), 493–503.
Harasim, L. (2012). Learning theory and online technologies . Routledge/Taylor & Francis.
Hew, K. F., & Brush, T. (2007). Integrating technology into K-12 teaching and learning: Current knowledge gaps and recommendations for future research. Education Technology Research and Development, 55 , 223–252. https://doi.org/10.1007/s11423-006-9022-5
Hew, K. F., Lan, M., Tang, Y., Jia, C., & Lo, C. K. (2019). Where is the “theory” within the field of educational technology research? British Journal of Educational Technology . https://doi.org/10.1111/bjet.12770
Hoffman, B. (2010). “I think I can, but I’m afraid to try”: The role of self-efficacy beliefs and mathematics anxiety in mathematics problem-solving efficiency. Learning and Individual Differences, 20 (3), 276–283.
Hoffman, R. (2003). Why buy that theory? In O. Sacks (Ed.), The best American science writing: 2003 (pp. 222–227). Harper-Collins.
Hollander, E. P. (1967). Principles and methods of social psychology . Oxford University Press.
Holmberg (1985). The feasibility of t heory of teaching for distance education and a proposed theory (ZIFF Paiere 60). Hagen, West Germany: Fern Universitat, Zentrales Institute fur Fernstudienforscgung Arbeitsbereich. (ERIC Document Reproduction Service No. ED290013).
Huang, W.-H., Huang, W.-Y., & Tschopp, J. (2010). Sustaining iterative game playing processes in DGBL: The relationship between motivational processing and outcome processing. Computers & Education, 55 (2), 789–797.
Jaccard, J., & Jacoby, J. (2009). Theory construction and model building skills: A practical guide for social scientists . Guilford Press.
Johns, G. (2006). The essential impact of context on organizational behavior. Academy of Management Review, 31 (2), 386–408.
Jones, C., & Czerniewicz, L. (2011). Theory in learning technology. Research in Learning Technology, 19 (3), 173–177.
Keller, J. M. (1999). Using the ARCS motivational process in computer-based instruction and distance education. New Directions for Teaching and Learning, 78 , 39–48.
Keller, J. M. (2008). An integrative theory of motivation, volition, and performance. Technology, Instruction, Cognition and Learning, 16 , 79–104.
Knowles, M. S., Holton, E. F., & Swanson, R. A. (1998). The adult learner (5th ed.). Butterworth-Heinemann Publishers.
Kuhl, J. (1987). Action control: The maintenance of motivational states. In F. Halisch & J. Kuhl (Eds.), Motivation, intention and volition (pp. 279–291). Springer.
Kuhn, T. S. (1996). The structure of scientific revolutions . University of Chicago Press.
Malone, T. W. (1985). Designing organizational interfaces. In L. Borman & R. Smith (Eds.), Proceedings of the CHI’85 Conference on Human Factors in Computing Systems (pp. 66–71). New York, NY: ACM Press.
Markauskaite, L., & Reimann, P. (2014). Editorial: e-Research for education: Applied, methodological and critical perspectives. British Journal of Educational Technology, 45 (3), 385–391.
Mayer, R. E. (2001). Multimedia learning . Cambridge University Press.
McDonnell, L. M. (1995). Opportunity to learn as a research concept and a policy instrument. Educational Evaluation and Policy Analysis, 17 (3), 305–322.
McKenney, S., & Reeves, T. C. (2014). Educational design research. In J. M. Spector, M. D. Merrill, J. Elen, & M. J. Bishop (Eds.), Handbook of research on educational communications and technology (pp. 131–140). Springer.
Means, B., Bakia, M., & Murphy, R. (2014). Learning online: What research tells us about whether, when and how . Routledge.
Means, B., Toyama, Y., Murphy, R., & Baki, M. (2013). The effectiveness of online and blended learning: A meta-analysis of the empirical literature. Teachers College Record, 115 , 1–47.
Mintzberg, H. (2005). Developing theory about the development of theory. In M. Hitt & K. Smith (Eds.), Minds in management: The process of theory development (pp. 355–372). Oxford University Press.
Moore, M. G. (1997). Theory of transactional distance. In D. Keegan (Ed.), Theoretical principles of distance education (pp. 22–38). Routledge.
Moore, M. G., & Diehl, W. C. (2018). Handbook of distance education . Routledge.
Morgan, C., & Wildemuth, B. M. (2009). Questions related to theory. Applications of social research methods to questions in information and library science (pp. 40–50). Libraries Unlimited.
Nelson, R., & Winter, S. (1974). Neoclassical vs. evolutionary theories of economic growth: Critique and prospectus. Economic Journal, 84 (336), 886–905.
Novak, E. (2014). Toward a mathematical model of motivation, volition, and performance. Computers & Education, 74 , 73–80. https://doi.org/10.1016/j.compedu.2014.01.009
Novak, E., Daday, J., & McDaniel, K. (2018). Using a mathematical model of motivation, volition, and performance to examine students’ e-text learning experiences. Educational Technology Research & Development, 66 (5), 1189–1209. https://doi.org/10.1007/s11423-018-9599-5
Novak, E., McDaniel, K., Daday, J., & Soyturk, I. (2021). Understanding student frustration with e-learning materials: Development and validation of an E-Text Frustration scale . Featured Research Paper presented at the Association for Educational Communications and Technology (AECT), Chicago, IL. November 2021.
Opp, K.-D. (1970). Theories of the middle range as a strategy for the construction of a general sociological theory. Quality and Quantity, 4 (2), 243–253. https://doi.org/10.1007/BF00199565
Pearl, J. (2000). Causality: Models, reasoning, and inference . Cambridge University Press.
Picciano, A. G. (2017). Theories and frameworks for online education: Seeking an integrated model. Online Learning, 21 (3), 166–190. https://doi.org/10.24059/olj.v21i3.1225
Puntambekar, S., et al. (2018). Design-based research. In F. Fisher (Ed.), International handbook of the learning sciences (pp. 383–392). Routledge.
Qian, H., & Youngs, P. (2016). The effect of teacher education programs on future elementary mathematics teachers’ knowledge: A five-country analysis using TEDS-M data. Journal of Mathematics Teacher Education, 19 (4), 371–396. https://doi.org/10.1007/s10857-014-9297-0
Reeves, T. C. (2006). Design research from the technology perspective. In J. V. Akker, K. Gravemeijer, S. McKenney, & N. Nieveen (Eds.), Educational design research (pp. 86–109). Routledge.
Rodgers, J. L. (2003). EMOSA sexuality models, memes, and the tipping point: Policy and program implications. In D. Romer (Ed.), Reducing adolescent risk: Toward an integrated approach (pp. 185–192). Sage.
Rodgers, J. L. (2010). The epistemology of mathematical and statistical modeling: A quiet methodological revolution. American Psychologist, 65 (1), 1–12.
Rodgers, J. L., & Doughty, D. (2001). Does having boys or girls run in the family? Chance, 14 , 8–13.
Rodgers, J. L., & Rowe, D. C. (1993). Social contagion and adolescent sexual behavior: A developmental EMOSA model. Psychological Review, 100 , 479–510. https://doi.org/10.1037/0033-295X.100.3.479
Rowe, D. C., & Rodgers, J. L. (1991). Adolescent smoking and drinking: Are they epidemics? Journal of Studies on Alcohol, 52 , 110–117.
Scheerens, J., & Blömeke, S. (2016). Integrating teacher education effectiveness research into educational effectiveness models. Educational Research Review, 18 , 70–87. https://doi.org/10.1016/j.edurev.2016.03.002
Seel, N. M. (2009). Bonjour tristesse: Why don’t we research as we have been taught? Methodological considerations on instructional technology research. Technology, Instrustion, Cognition and Learning, 6 , 151–176.
Shadish, W., Cook, T. D., & Campbell, D. T. (2002). Experimental and quasi-experimental designs for generalized causal inference . Houghton Mifflin.
Shepard, R. N. (1982). Geometrical approximations to the structure of musical pitch. Psychological Review, 89 , 305–333.
Siemens, G. (2004). Connectivism: A learning theory for the digital age. International Journal of Instructional Technology and Distance Learning, 2 , 1–8.
Simon, H. A., & Newell, A. (1956). Models: Their uses and limitations. In D. White (Ed.), The state of the social sciences (pp. 61–83). University of Chicago Press.
Straub, D. W. (2009). Editor’s comments: Why top journals accept your paper. MIS Quarterly, 33 (3), iii–x.
Suppes, P. (1978). Impact of research on education: Some case studies . National Academy of Education.
Tatto, M. T., Schwille, J., Senk, S., Ingvarson, L., Peck, R., & Rowley, G. (2008). Teacher education and development study in mathematics (TEDS-M): Policy, practice, and readiness to teach primary and secondary mathematics. Conceptual framework . Teacher Education and Development International, Study Center, College of Education, Michigan State University.
Tipton, E., & Olsen, R. B. (2018). A review of statistical methods for generalizing from evaluations of educational interventions. Educational Researcher, 47 (8), 516–524. https://doi.org/10.3102/0013189x18781522
Venkatesh, V., Morris, M. G., Davis, G. B., & Davis, F. D. (2003). User acceptance of information technology: Toward a unified view. MIS Quarterly, 27 (3), 425–478.
Venkatesh, V., Thong, J. Y. L., & Xu, X. (2016). Unified theory of acceptance and use of technology: A synthesis and the road ahead. Journal of the Association for Information Systems, 17 (5), 328–376.
Wedemeyer, C. A. (1981). Learning at the back door: Reflections on non-traditional learning in the lifespan . The University of Wisconsin Press.
Wenger, E. (1998). Communities of practice: Learning, meaning, and identity . Cambridge University Press.
Wenger, E., & Lave, J. (1991). Learning in doing: Social, cognitive and computational perspectives. Situated learning: Legitimate peripheral participation . Cambridge University Press.
Willingham, D. (2008). What is developmentally appropriate? American Educator, 32 (2), 34–39.
Zawacki-Richter, O., Bäcker, E., & Vogt, S. (2009). Review of distance education research (2000 to 2008): Analysis of research areas, methods, and authorship patterns. International Review of Research in Open and Distributed Learning, 10 (6), 21–50. https://doi.org/10.19173/irrodl.v10i6.741
Zimmerman, B. J. (2001). Theories of self-regulated learning and academic achievement: An overview and analysis. In B. J. Zimmerman & D. H. Schunk (Eds.), Self-regulated learning and academic achievement. Theoretical perspectives (pp. 1–38). Mahwah.
Download references
Author information
Authors and affiliations.
School of Teaching, Learning and Curriculum Studies, Kent State University, 150 Terrace Drive, P.O. Box 5190, Kent, OH, 44242-0001, USA
Elena Novak
You can also search for this author in PubMed Google Scholar
Corresponding author
Correspondence to Elena Novak .
Ethics declarations
Conflict of interest.
The author declares that she has no conflict of interest.
Additional information
Publisher's note.
Springer Nature remains neutral with regard to jurisdictional claims in published maps and institutional affiliations.
Rights and permissions
Reprints and permissions
About this article
Novak, E. Mathematical modeling for theory-oriented research in educational technology. Education Tech Research Dev 70 , 149–167 (2022). https://doi.org/10.1007/s11423-021-10069-6
Download citation
Accepted : 21 November 2021
Published : 29 November 2021
Issue Date : February 2022
DOI : https://doi.org/10.1007/s11423-021-10069-6
Share this article
Anyone you share the following link with will be able to read this content:
Sorry, a shareable link is not currently available for this article.
Provided by the Springer Nature SharedIt content-sharing initiative
- Model-testing
- Mathematical modeling
- Educational technology
- Find a journal
- Publish with us
- Track your research
Help | Advanced Search
Computer Science > Computation and Language
Title: large language models for mathematical reasoning: progresses and challenges.
Abstract: Mathematical reasoning serves as a cornerstone for assessing the fundamental cognitive capabilities of human intelligence. In recent times, there has been a notable surge in the development of Large Language Models (LLMs) geared towards the automated resolution of mathematical problems. However, the landscape of mathematical problem types is vast and varied, with LLM-oriented techniques undergoing evaluation across diverse datasets and settings. This diversity makes it challenging to discern the true advancements and obstacles within this burgeoning field. This survey endeavors to address four pivotal dimensions: i) a comprehensive exploration of the various mathematical problems and their corresponding datasets that have been investigated; ii) an examination of the spectrum of LLM-oriented techniques that have been proposed for mathematical problem-solving; iii) an overview of factors and concerns affecting LLMs in solving math; and iv) an elucidation of the persisting challenges within this domain. To the best of our knowledge, this survey stands as one of the first extensive examinations of the landscape of LLMs in the realm of mathematics, providing a holistic perspective on the current state, accomplishments, and future challenges in this rapidly evolving field.
Submission history
Access paper:.
- HTML (experimental)
- Other Formats
References & Citations
- Google Scholar
- Semantic Scholar
BibTeX formatted citation

Bibliographic and Citation Tools
Code, data and media associated with this article, recommenders and search tools.
- Institution
arXivLabs: experimental projects with community collaborators
arXivLabs is a framework that allows collaborators to develop and share new arXiv features directly on our website.
Both individuals and organizations that work with arXivLabs have embraced and accepted our values of openness, community, excellence, and user data privacy. arXiv is committed to these values and only works with partners that adhere to them.
Have an idea for a project that will add value for arXiv's community? Learn more about arXivLabs .
Thank you for visiting nature.com. You are using a browser version with limited support for CSS. To obtain the best experience, we recommend you use a more up to date browser (or turn off compatibility mode in Internet Explorer). In the meantime, to ensure continued support, we are displaying the site without styles and JavaScript.
- View all journals
- My Account Login
- Explore content
- About the journal
- Publish with us
- Sign up for alerts
- Open access
- Published: 03 June 2024
Research on spatial carving method of glutenite reservoir based on opacity voxel imaging
- Hu Zhao 1 , 2 ,
- Zhong-wei Zhang 2 ,
- Hong-wei Yang 3 &
- Guo-hua Wei 3
Scientific Reports volume 14 , Article number: 12667 ( 2024 ) Cite this article
Metrics details
- Energy science and technology
- Solid Earth sciences
The glutenite reservoir in an exploration area in eastern China is well-developed and holds significant exploration potential as an important oil and gas alternative layer. However, due to the influence of sedimentary characteristics, the glutenite reservoir exhibits strong lateral heterogeneity, significant vertical thickness variations, and low accuracy in reservoir space characterization, which affects the reasonable and effective deployment of development wells. Seismic data contains the three-dimensional spatial characteristics of geological bodies, but how to design a suitable transfer function to extract the nonlinear relationship between seismic data and reservoirs is crucial. At present, the transfer functions are concentrated in low-dimensional or high-dimensional fixed mathematical models, which cannot accurately describe the nonlinear relationship between seismic data and complex reservoirs, resulting in low spatial description accuracy of complex reservoirs. In this regard, this paper first utilizes a fusion method based on probability kernel to fuse seismic attributes such as wave impedance, effective bandwidth, and composite envelope difference. This provide a more intuitive reflection of the distribution characteristics of glutenite reservoirs. Moreover, a hybrid nonlinear transfer function is established to transform the fused attribute cube into an opaque attribute cube. Finally, the illumination model and ray casting method are used to perform voxel imaging of the glutenite reservoirs, brighten the detailed characteristics of reservoir space, and then form a set of methods for ' brightening reservoirs and darkening non-reservoirs ', which improves the spatial engraving accuracy of glutenite reservoirs.
Introduction
As the exploration and development targets gradually shift from structural oil and gas reservoirs to complex hidden oil and gas reservoirs, it is necessary to more accurately characterize the spatial distribution characteristics of reservoirs, establish a more refined reservoir model, and support the exploration and development of complex oil and gas reservoirs. Seismic data has always played an important role in reservoir characterization as it provides three-dimensional spatial information of geological bodies. Obviously, how to accurately mine the spatial characteristics of reservoir from seismic data is the key to solve the problem. Glutenite reservoir possess the characteristics such as strong concealment, rapid lithology change, complex reservoir connectivity and strong heterogeneity, making it difficult to accurately characterize its spatial characteristics. Therefore, in order to better explore and develop glutenite reservoirs, it is essential to investigate the technology that can accurately characterize their spatial characteristics. Direct volume rendering, as one of the most effective volume data visualization methods for visualizing volume data, involves converting each data point in the volume data into voxels with specific color values and opacity through the transfer function. Subsequently, the changes in light passing through each voxel are analyzed to depict the internal characteristics of the volume data and selectively display or shield some data volume characteristics. Therefore, it is widely used in medical imaging, seismic exploration and other fields.
In the field of seismic exploration, Gerald (1999) used the method of direct volume rendering to convert each data point of 3D seismic data into voxels with specific color and luminosity, thereby achieving the spatial characterization of 3D seismic data 1 . Ropinski et al. (2005) utilized volume rendering technology for three-dimensional visualization of seismic data and proposed the concept of lens. Users can observe the internal characteristics of the data volume of interest through the lens 2 . Zhang (2007) proposed a direct volume rendering method for seismic data based on Shear-Warp in a virtual reality environment, which enabled the stereoscopic display of data volume by combining perspective projection 3 . Li (2008) realized the three-dimensional visualization of seismic data by using the parallel projection-based footprint table method 4 . Qin (2013) proposed a multi-dimensional transfer function setting method based on parallel coordinates. Its application can reduce the dimension of high-dimensional space to the parallel coordinates of two-dimensional space to solve the complex and difficult problem of multi-dimensional transfer function setting 5 . Duan et al. (2013) applied GPU-accelerated ray casting volume rendering method to seismic interpretation, and realized the clear display of horizon spatial distribution characteristics through interactive design transfer function 6 . Chen (2018) analyzed the process of realizing 3D visualization of seismic data using the direct volume rendering method, and realized 3D visualization of seismic data by using Coin3D through ray projection algorithm and histogram-based transfer function 7 . Obviously, the predecessors have used the volume rendering method to realize the spatial characterization of seismic data, but the above authors have used one-dimensional transfer function based on scalar value or high-dimensional transfer function based on fixed mathematical model in volume rendering. Although the overall three-dimensional characterization of seismic data volume has been realized, the nonlinear relationship between seismic data and reservoir has not been accurately characterized, resulting in low accuracy of reservoir spatial description. There is a strong nonlinear relationship between seismic data and reservoir. The design of transfer function holds great significance as they are responsible for classifying voxels and assigning optical attributes (color and opacity) in volume rendering. By adjusting the transfer function, the reservoir and non-reservoir in seismic data volume can be accurately distinguished, and the reservoir can be highlighted, so as to achieve the purpose of ' brightening the reservoir and shielding the non-reservoir '. At present, many transfer function design methods have been proposed. To solve the problem of insufficient classification ability of one-dimensional transfer function, researchers have proposed to increase the gradient 8 , curvature 9 , space 10 , texture 11 , size 12 and other information to design multi-dimensional transfer function. However, multi-dimensional transfer function has problems such as design complexity and difficulty in understanding. In this regard, researchers have proposed the use of parallel coordinates to reduce multidimensional space into two-dimensional space, aiding in the design of multidimensional functions 13 or the use of hierarchical clustering 14 , density clustering 15 and probability model clustering 1 to achieve adaptive generation of multidimensional transfer functions. However, existing transfer function design methods for this purpose have certain limitations. Some methods lack sufficient classification ability, while others are complex, difficult to comprehend, or have inconvenient user interfaces. Moreover, these methods are primarily designed for medical applications and may not be fully applicable to the field of seismic exploration due to the distinct characteristics of seismic exploration data.
In order to better separate the reservoir and non-reservoir in seismic data volume and accurately describe the spatial distribution characteristics of the reservoir, this paper intends to explore the attribute fusion method based on the probability kernel. The objective is to excavate common characteristics between seismic attributes and highlight the characteristics of the reservoir. Subsequently, a hybrid nonlinear transfer function is established to transform the fused attribute cube into a light-blocking attribute cube. The ray projection method of the illumination model is used for reservoir voxel imaging to shield non-reservoir, and finally complete the characterization of the three-dimensional spatial characteristics of the reservoir.
The exploration practice shows that the glutenite reservoir has the characteristics of large lateral thickness variations and strong heterogeneity in physical properties, which affects the subsequent reservoir evaluation and the design of development well location. In this paper, based on the seismic attribute, the probability kernel attribute fusion technology is established to excavate the common characteristics between seismic attributes and further highlight the characteristics of glutenite reservoir.
An attribute fusion method based on probability kernel
There is a nonlinear relationship between seismic attributes and glutenite reservoirs. It is necessary to carry out volume fusion processing to explore the characteristics of reservoirs between seismic attributes.
Firstly, different seismic body attribute data are selected, and the original data of seismic attributes are extracted by the following equation to prepare for fusion :
Which \(x_{i}\) is the sample point in an individual attribute.
Due to the nonlinear relationship between seismic body attributes and glutenite reservoirs, it is not possible to directly indicate reservoir characteristics. Therefore, it is necessary to perform nonlinear mapping on the body attributes involved in the fusion. Firstly, different body attribute data are normalized. On this basis, the kernel function is selected for mapping. The Gaussian kernel function designed in this paper is as follows.
The characteristic of the kernel function is that it has good anti-interference ability to the noise in the data, and can establish a nonlinear relationship between seismic attributes and reservoirs. The above kernel function is used for mapping calculation as follows, \(\Phi :x \to \Phi (x) \in R^{f}\) .
where : \(\Phi (X)\) is the feature space of \(X\) .
Then calculate the eigenvalue \(\Lambda\) and eigenvector \(V\) of Eq. ( 3 ), and arrange them from large to small according to the value, and take the first q eigenvalues with larger eigenvalue contribution rate and the corresponding eigenvectors. The larger the eigenvalue, the stronger the common characteristic.
where \(V\) is the set of eigenvectors \(v_{i}\) corresponding to the eigenvalue \(\lambda_{{\text{i}}}\) , and \(\Lambda\) is the diagonal matrix composed of eigenvalues.
The feature vector set of high dimensional space is established by using Eqs. ( 4 ) and ( 5 ).
Here, \(U\) is a set of high-dimensional space feature vectors of \(u_{i}\) , \(\Phi\) is the feature space mapped by high-dimensional space, and let \(J = N^{{ - \frac{1}{2}}} ({\rm I}_{N} - e{\rm I}^{T} )\) , \(\Phi J\) be the centralization of high-dimensional feature space data.
In order to extract the nonlinear characteristics between the seismic attribute cube more effectively, the Bayesian probability model is used to discard the redundant data. Firstly, the maximum likelihood function estimation parameters are calculated according to the Bayesian probability model using Eq. ( 7 ).
And calculate the sample mean:
Using iterative algorithm to calculate \(W\) , \(\rho\) , make it converge :
where \(W\) is the load matrix, \(Q_{t}\) and \(\rho_{t}\) and \(Q_{t + 1}\) and \(\rho_{t + 1}\) are the empirical load matrix and variance before iteration and the empirical load matrix and variance after iteration, respectively.
Finally, the volume fusion calculation is carried out by the following equation.
where \(R\) is an arbitrary orthogonal matrix.
The key step is Eq. ( 3 ). The kernel function is used to map the nonlinear relationship. As shown in Fig. 1 a, there are three kinds of seismic attribute data in two-dimensional space, which have a common center, but cannot be effectively separated. The data can be mapped to high-dimensional space by Eq. ( 3 ), and the data can be mapped and separated in high-dimensional space and the common center can be identified (Fig. 1 b). On this basis, the kernel matrix after mapping is solved, and the feature vector (Eq. 6 ) of high-dimensional space is established by using Eqs. ( 4 ) and ( 5 ), and the solution is carried out. Finally, the attribute data fusion calculation (mining the common features between attributes) is completed.
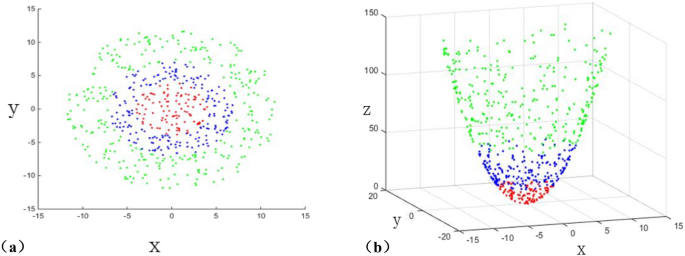
Data nonlinear mapping diagram. ( a ) Seismic attribute data diagram before mapping. ( b ) Seismic attribute data in high-dimensional space after mapping.
A volume space characterization method based on opacity voxel imaging
The main idea of seismic opacity voxel imaging is to use the transfer function to map the seismic attributes into the opacity, so as to establish the opacity model and the illumination model, simulate the process of reflection and projection generated by the light penetrating the geological body, and finally calculate the light intensity of each data point in the space. Then the light intensity of each data projected onto the same pixel on the image plane is superimposed to form a visual image. The core problem is the design of the opacity model and the illumination model, which is directly related to the effect of volume rendering.
Opacity model design: the opacity model is to convert seismic data into optical impedance value, because seismic data has strong nonlinear characteristics, so the common mathematical functions cannot accurately express the nonlinear relationship between it and the reservoir, in this paper based on the Poisson transfer function (Eq. 12 ) to construct a hybrid nonlinear opacity model (Fig. 2 ), which has the characteristics of more concentrated numerical distribution and fast attenuation, which can easily excavate reservoirs from the formation.
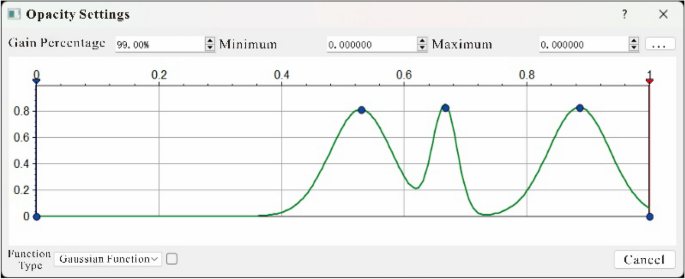
Multi-point Poisson resistance model.
The smaller the value of \(\lambda\) is, the more biased the distribution is. With the increase of \(\lambda\) , the distribution tends to be symmetrical.
Three interpolation volume space resampling calculation ; because the three-dimensional data volume is discretely distributed, the light is transmitted directly, and the screen pixel value can only be calculated (voxel) by relying on the data at the grid points. Therefore, the light may be projected from the gap to the visual plane, resulting in a discontinuous imaging effect of the projected object (Fig. 3 ),. This requires the resampling calculation of the 3D data field according to the spatial direction of the light penetrating the 3D data volume. This time, the cubic interpolation volume space resampling algorithm is used to solve this problem. The theoretical equation is as follows.
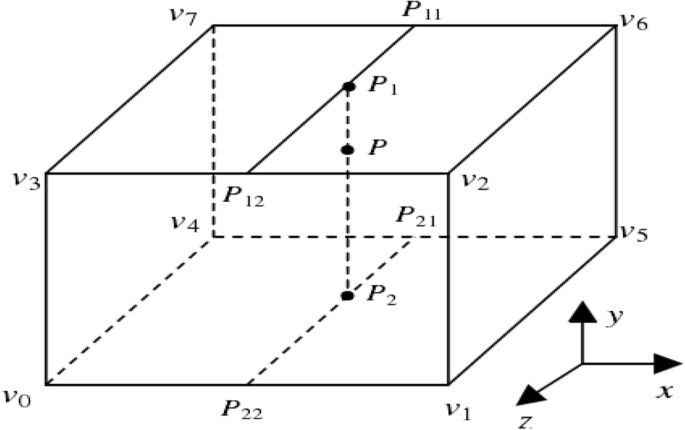
Cubic interpolation volume space resampling schematic diagram.
Among them, point \(p\) is any point in space that needs to be resampled, \(p_{1}\) and \(p_{2}\) are the points where point \(p\) is projected into the data grid, and \(y_{p}\) is the \(y\) coordinate of point \(p\) .
Lighting model design; In the lighting model, it is assumed that the continuously distributed three-dimensional data field is full of small particles, which can emit light, and the light intensity of each particle reaching the viewpoint is the attenuated light intensity, and the contribution of each particle to the light intensity of the pixel can be accumulated along the line of sight to obtain the final light intensity of the pixel. Then:
where \(s\) is the light propagation length parameter, \(\tau ({\text{s}}) = \rho (s) \cdot A\) is the ray attenuation coefficient, which represents the absorption rate of light by the medium when the ray propagation distance is \(s\) . \(I(s)\) is the light intensity of the incident light.
Move \(\tau (s)I(s)\) from the above differential equation to the left of the equation:
Multiply both sides of the equation by \({\text{exp}}(\int_{0}^{s} {\tau (t)dt)}\) :
Integrating both sides of the above equation from the edge of the 3D volume data (S = 0) to the observer point (S = D) yields:
where \(I_{0}\) is the initial light intensity of the incident light.
The above equation can be simplified to:
The approximate numerical solution of the above equation is as follows:
Using the above equation, the light intensity value of the incident light penetrates the object to the observer point can be calculated, and the optical resistance value a corresponding to the propagation distance is usually defined:
According to the above theory, if the luminous intensity \(C\) of three-dimensional particles is constant, or the color value \(C\) assigned to similar substances is constant, then after a propagation distance \(D\) , the light intensity \(I(D)\) of the light reaching the viewpoint is:
In the above equation, \(T(D)\) is the transparency of this medium of length \(D\) , and \(I_{0}\) is the intensity of the incoming background light. This equation expresses the combined light intensity of the background light \(I_{0}\) and the light source assigned the color value \(C\) under the action of transparency \(T(D)\) . The \(\alpha\) of 0 means that the medium is completely transparent, so it is invisible, but objects behind it are visible. It is this idea that excavates the characteristics of sandstone and conglomerate reservoirs in sandstone mudstone, and then completes the volume space carving.
Application in field data
Regional geological overview.
The study area is located in the Yan16 ancient gully in the northern steep slope zone of Dongying Sag in the Jiyang Depression of the Bohai Bay Basin. The main exploration target in this area is the shallow glutenite reservoir, and the oil-bearing area is controlled by the morphology and continuity of the sandstone reservoir. The glutenite primarily represents deposition of a nearshore subaqueous fan system with sediment sources originating from the northern Chenjiazhuang uplift. The lithology is mainly composed of gravel-bearing fine sandstone, interbedded with dark gray and gray mudstone. The reservoir properties are relatively good, but there is a significant variation in spatial continuity, which affects the migration and accumulation of oil and gas. From the Fig. 4 , it can be seen that the glutenite reservoir exhibits medium—strong amplitude, bright spots, but with poor continuity in seismic profile. Additionally, the glutenite overlays the basement of the Sinian Formation (This is shown in the yellow box in the figure).
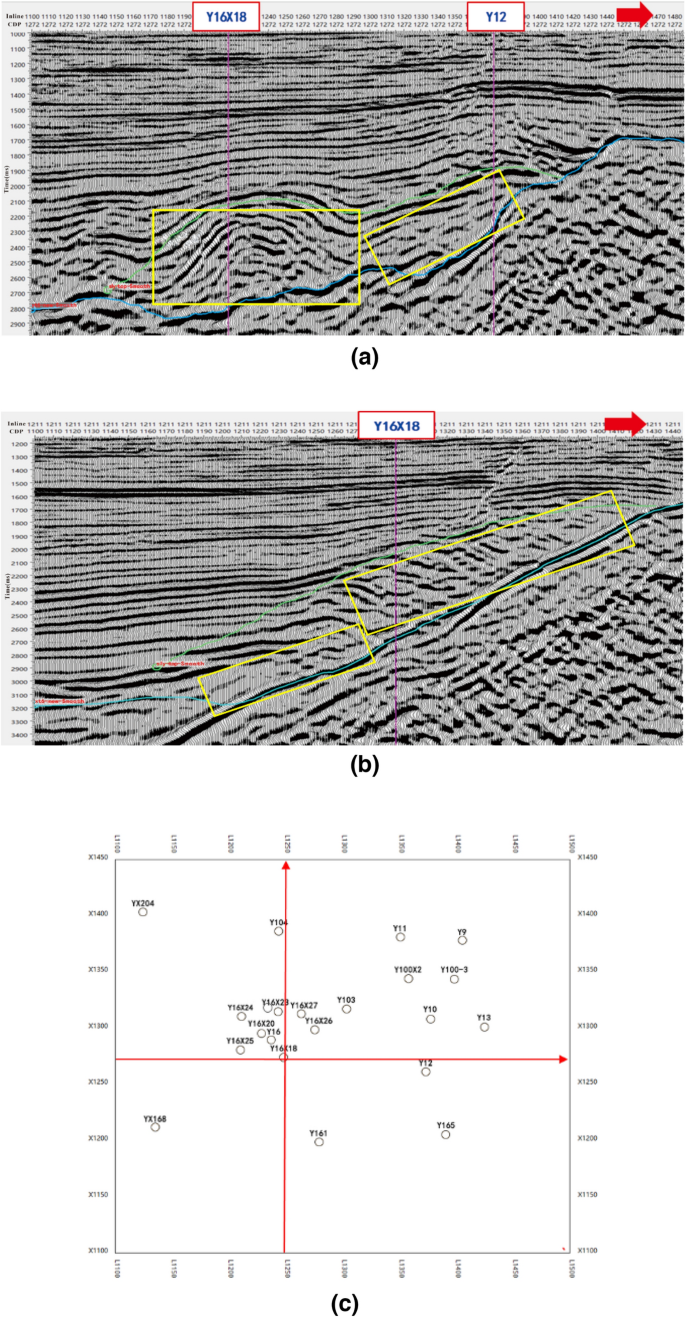
Typical seismic profiles in different directions. ( a ) Seismic profile across wells in east–west direction [the direction of the red arrow in the figure is the direction of the red line arrow parallel to the L number axis of the navigation diagram in ( c )]. ( b ) Seismic profile across well Y16X18 in north–south direction [the direction of the red arrow in the figure is the direction of the red line arrow parallel to the X number axis of the navigation diagram in ( c )]. ( c ) Navigation map(X number is CDP number; L number is inline number).
Application
There is strong lateral heterogeneity in the reservoirs in the study area, for example, well Y16-X19B is effective 11 days after water injection during the development process, while well Y16-X23 at the same distance is indeed ineffective. This clearly indicates a reservoir connectivity issue, highlighting the need for accurately describing the spatial distribution of reservoir. To solve this problem, we first selected sensitive seismic attributes, including effective bandwidth, composite envelope difference and impedance. These attributes can reflect the scale and degree of reservoir development, with higher value indicating more developed reservoirs (Fig. 5 ). Next, the probabilistic kernel method is used to mine the common features between these attributes. Figure 6 shows the only seismic attribute cube obtained from the fusion process, which reflects the three-dimensional characteristics of all geological bodies in the subsurface. This fused attribute cube serves as the basis and data source of seismic exploration. However, due to the influence of non-reservoirs, the spatial distribution of the glutenite reservoir cannot be fully characterized. Therefore, according to the proposed method in this paper, appropriate transfer function were selected to establish an opacity model, enabling reservoir extraction and non-reservoir masking.
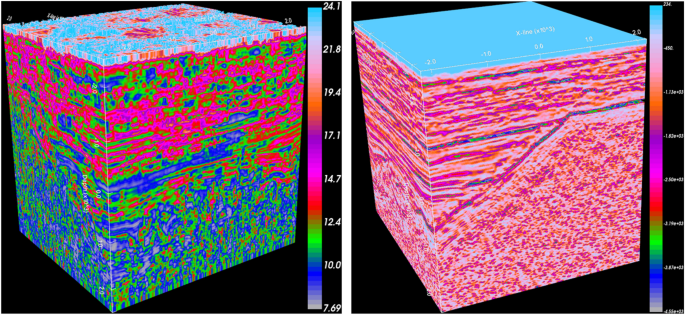
Seismic attribute cube (effective bandwidth on the left, composite envelope difference on the right).
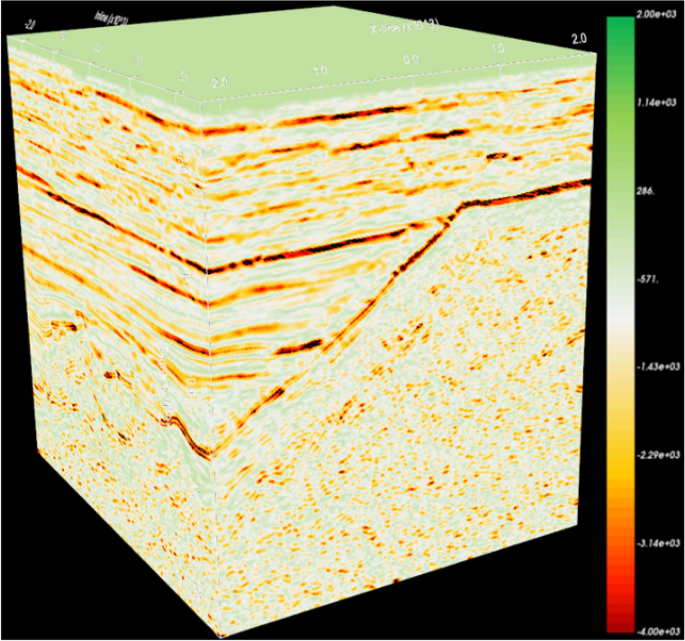
The fused attribute cube.
The traditional method of shielding non-reservoirs is to set a threshold. However, the division boundary between the glutenite reservoir and the non-reservoir is unclear, and the reservoir itself may have multiple ranges of attribute values due to sedimentary processes. Therefore, an opacity model with mixed multi-segment nonlinear transfer function was established according to the characteristics of the glutenite reservoir data (Fig. 8 ), and corresponding voxel imaging data was obtained(Fig. 7 , the more developed the reservoir, the higher the opacity value, with a non-reservoir opacity value of 0). However, in Fig. 7 , the spatial features of the glutenite reservoir are still not observable as numerous geological bodies in the non-target layers obstruct the light penetration. To address this, further darkening of the non-reservoir regions was performed. This involved selecting the top and bottom of the target layer for surface cutting, resulting in all geological bodies outside the target layer becoming darker (Fig. 8 ). Finally, the reservoir is illuminated and non-reservoir layers are darkened through the application of series of technologies (Fig. 9 ).
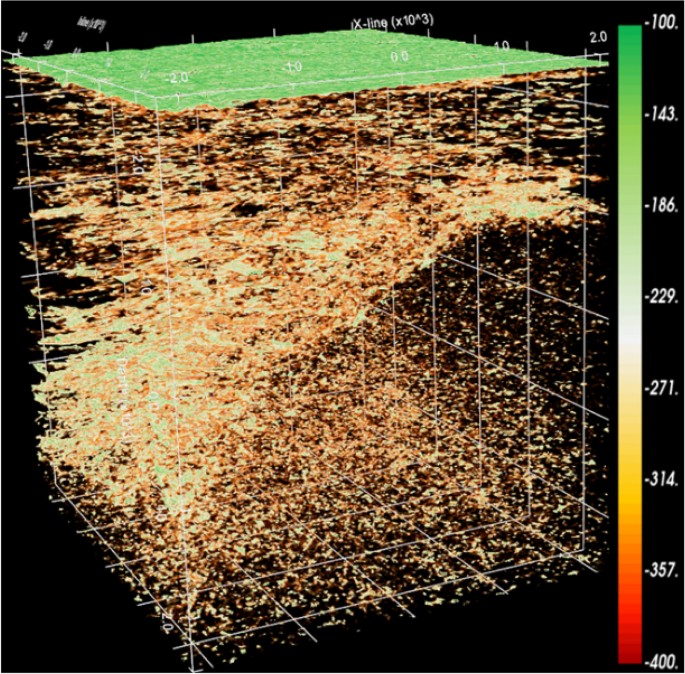
Attribute cube after opacity voxel imaging.
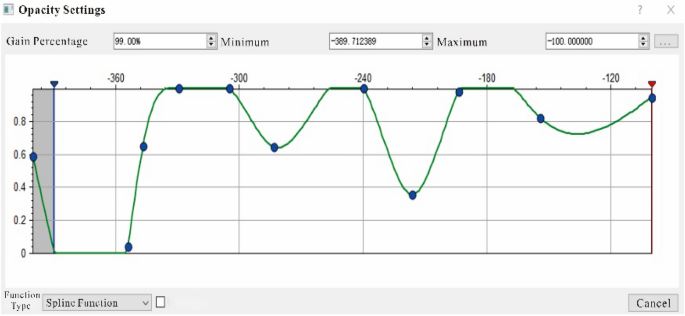
Hybrid multi-segment nonlinear opacity model (the abscissa represents the fused attribute value, and the ordinate represents the opacity value).
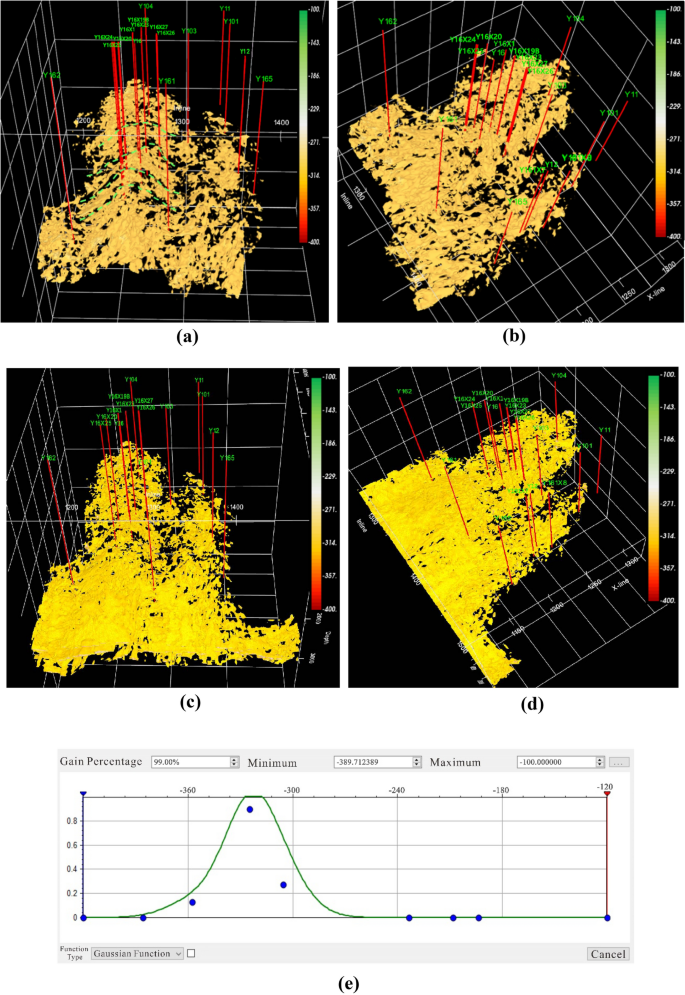
Reservoir characteristics after opacity voxel imaging + target layer surface cropping + volume space rendering. ( a ) Front view, ( b ) oblique view, ( c ) front view (traditional methods), ( d ) oblique view (traditional methods), ( e ) the Gaussian function resistance model (traditional methods).
In order to show the detailed characteristics of the reservoir, it is necessary to further brighten the reservoir and perform volume rendering calculations to enhance the degree of brightness at each point within the reservoir. Figure 9 a, b shows that the application of opacity voxel imaging technology greatly improve the accuracy of reservoir identification. The spatial distribution characteristics of reservoirs are clearly visible, and the lateral continuity is also intuitively reflected. Figure 9 c, d display the glutenite reservoir identified using traditional methods (the Gaussian function resistance model, Fig. 9 e). Compared with the identification results in Fig. 9 a, the traditional method did not effectively excavate the spatial distribution characteristics of the glutenite reservoir on the south side of the study area. In Fig. 9 c, the glutenite reservoir on the south side of the study area presents sheet-like characteristics and lacks changes in horizontal heterogeneity. This does not match the actual geological characteristics. The effectiveness of reservoir identification using hybrid multi-segment nonlinear models surpasses that of traditional methods. On this basis, the glutenite reservoir for opacity voxel imaging can be quantified and output, and the output data only contains the data of reservoir points in space, with non-reservoir points assigned 0 value. This data can be applied to reservoir modeling in the later stage. Obviously, the data obtained from the hybrid multi-segment nonlinear opacity model almost only contains reservoir information, and non-reservoir data has been shielded, so the reservoir model established by it will be more reliable.
In order to further validate the accuracy of the proposed method, the identified glutenite reservoir was superimposed with seismic data. Figure 9 shows the seismic response characteristics of the glutenite reservoir (red in figure), including medium—strong amplitude, bright spots, discontinuity, and poor continuity. These characteristics are consistent with previous study. In Fig. 10 a, it can be seen that the glutenite reservoir between the two wells exhibits good connectivity. This observation aligns with the dynamic production data. Specifically, when water injection occurs in the well Y16X25, there is a significant increase in the water content of the well Y16X20 (Y16X20 has not been injected water). This also supports the reservoir identification results and the actual underground situation. In Fig. 10 b, the seismic events between well Y16X27, well Y16X23 and well Y16X26 exhibit good continuity and stable waveform. This suggests that the glutenite reservoir is poorly developed and the mudstone is more developed, so the reservoir connectivity is general. Moreover, the dynamic production data shows that there is no significant response observed between wells Y16X23 and Y16X26 during water injection in well Y16X27, which further supports the reliability of the method of reservoir identification in this paper.
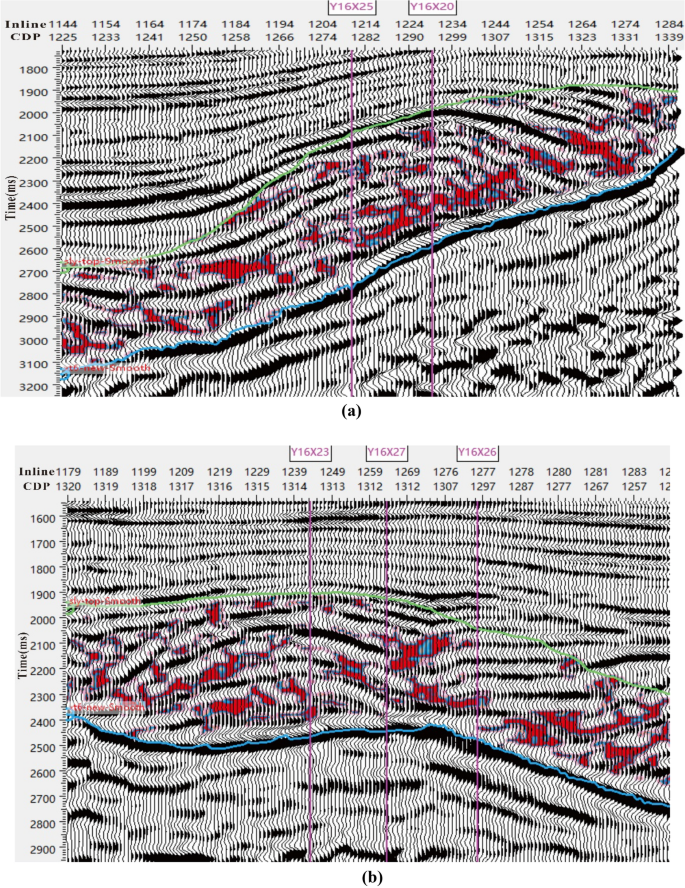
Seismic data, co-rendered with reservoir identified by the proposed method, showing the continuity of reservoir. ( a ) Seismic profile across Well Y16X25. ( b ) Seismic profile across Well Y16X27.
Conclusions
Usually we extract spatial information of reservoir from seismic data and adopt both linear and nonlinear method to establish a mapping relationship between seismic data and reservoirs. However, we rarely investigate whether this function can effectively express the nonlinear relationship between them. Practice suggested that a single low-dimensional or high-dimensional function can not express such a relationship. Therefore, it is necessary to establish a hybrid nonlinear function to accurately express such a nonlinear mapping relationship, enabling an accurate depiction of the volumetric spatial nonlinear relationship between reservoirs and seismic attributes.
Opacity voxel imaging technology can intuitively construct multi-segment, multi-type hybrid nonlinear functions, which can map the most favorable seismic information into optical information. This technology demonstrates strong intuitiveness, and the quantification results pertaining to reservoirs align closely with the geological conditions. It significantly enhances the characterization of the spatial distribution characteristics of reservoirs, making the data becomes more reliable for reservoir modeling.
Data availability
The data used to support the findings of this study are available from the corresponding author upon request.
Kidd, G. D. Fundamentals of 3-D seismic volume visualization. Lead. Edge 18 , 702–709 (1999).
Article Google Scholar
Ropinski, T. & Hinrichs, K. H. Interactive volume visualization techniques for subsurface data. Lect. Notes Comput. 3736 , 121–131 (2005).
Zhang, W.-G. Research on Three-Dimensional Seismic Data Field Visualization Method Based on Shear-Warp Algorithm Under Virtual Reality System (Nanjing University of Science and Technology, 2007).
Google Scholar
Li, Z.-Y. Research on Three-Dimensional Seismic Data Field Visualization Footprint Method (Nanjing University of Science and Technology, 2008).
Qin, Y.-F. Research on Multiple Seismic Attribute Data Fusion Volume Rendering Technology . (University of Electronic Science and Technology of China, 2013).
Duan, Z.-X. et al. GPU-based 3D seismic data volume rendering method. Comput. Appl. Softw. 10 , 21–25 (2013).
Chen, K. Volume Rendering and 3D Visualization of Seismic Data (University of Electronic Science and Technology of China, 2018).
Lan, Y.-S. et al. A clustering based transfer function for volume rendering using gray-gradient mode histogram (article). IEEE Access 7 , 80737–80747 (2019).
Kindlmann, G., Whitaker, R., Tasdizen, T. & Moller, T. Curvature-based transfer functions for direct volume rendering: Methods and applications. In VIS 2003 Proceedings. 67–73 (2003).
Sereda, P., Vilanova, B. A., Serlie, I. W. O. & Gerritsen, F. A. Visualization of boundaries in volumetric data sets using LH histograms. IEEE Trans. Vis. Comput. Graph. 12 , 208–218 (2006).
Article PubMed Google Scholar
Caban, J. J. & Rheingans, P. Texture-based transfer functions for direct volume rendering. IEEE Trans. Vis. Comput. Graph. 14 , 1364–1371 (2008).
Correa, C. D. & Ma, K.-L. Size-based transfer functions: a new volume exploration technique. IEEE Trans. Vis. Comput. Graph. 14 , 1380–1387 (2008).
Yuan, X.-R. et al. Scalable multi-variateanalytics of seismic and satellite-based observational data. IEEE Trans. Vis. Comput. Graph. 16 , 1413–1420 (2010).
Liu, F.-F. The Research on Adaptive Method of Generation Transparency Transfer Function in Rendering (Chinese Academy of Engineering Physics, 2020).
Maciejewski, R., Woo, I., Chen, W. & Ebert, D. S. Structuring feature space: A non-parametric method for volumetric transfer function generation. IEEE Trans. Vis. Comput. Graph. 15 , 1473–1480 (2009).
Wang, Y.-H. et al. Efficient volume exploration using the Gaussian mixture model. IEEE Trans. Vis. Comput. Graph. 17 , 1560–1573 (2011).
Download references
Acknowledgements
We are grateful to the reviewers for a thorough reading and constructive comments on this paper. This research is supported by innovation consortium project of China Petroleum and Southwest Petroleum University (No. 2020CX010201), Natural Science Foundation Project of Sichuan (No. 2024NSFSC0081).
Author information
Authors and affiliations.
Natural Gas Geology Key Laboratory of Sichuan Province, Southwest Petroleum University, Chengdu, 610500, China
School of Geoscisence and Technology, Southwest Petroleum University, Chengdu, 610500, China
Hu Zhao & Zhong-wei Zhang
Geophysical Exploration Institute, Shengli Oilfield Company, SINOPEC, Dongying, 257000, China
Hong-wei Yang & Guo-hua Wei
You can also search for this author in PubMed Google Scholar
Contributions
ZHAO Hu and ZHANG Zhong-wei wrote the main manuscript text. YANG Hong-wei and WEI Guo-hua. prepared figures 2-4. All authors reviewed the manuscript.
Corresponding author
Correspondence to Hu Zhao .
Ethics declarations
Competing interests.
The authors declare no competing interests.
Additional information
Publisher's note.
Springer Nature remains neutral with regard to jurisdictional claims in published maps and institutional affiliations.
Rights and permissions
Open Access This article is licensed under a Creative Commons Attribution 4.0 International License, which permits use, sharing, adaptation, distribution and reproduction in any medium or format, as long as you give appropriate credit to the original author(s) and the source, provide a link to the Creative Commons licence, and indicate if changes were made. The images or other third party material in this article are included in the article's Creative Commons licence, unless indicated otherwise in a credit line to the material. If material is not included in the article's Creative Commons licence and your intended use is not permitted by statutory regulation or exceeds the permitted use, you will need to obtain permission directly from the copyright holder. To view a copy of this licence, visit http://creativecommons.org/licenses/by/4.0/ .
Reprints and permissions
About this article
Cite this article.
Zhao, H., Zhang, Zw., Yang, Hw. et al. Research on spatial carving method of glutenite reservoir based on opacity voxel imaging. Sci Rep 14 , 12667 (2024). https://doi.org/10.1038/s41598-024-63643-2
Download citation
Received : 21 July 2023
Accepted : 30 May 2024
Published : 03 June 2024
DOI : https://doi.org/10.1038/s41598-024-63643-2
Share this article
Anyone you share the following link with will be able to read this content:
Sorry, a shareable link is not currently available for this article.
Provided by the Springer Nature SharedIt content-sharing initiative
- Spatial carving
- Voxel imaging
- Seismic attributes
- Volume attributes fusion
By submitting a comment you agree to abide by our Terms and Community Guidelines . If you find something abusive or that does not comply with our terms or guidelines please flag it as inappropriate.
Quick links
- Explore articles by subject
- Guide to authors
- Editorial policies
Sign up for the Nature Briefing newsletter — what matters in science, free to your inbox daily.

- Mobile Site
- Staff Directory
- Advertise with Ars
Filter by topic
- Biz & IT
- Gaming & Culture
Front page layout
Artificial brain surgery —
Here’s what’s really going on inside an llm’s neural network, anthropic's conceptual mapping helps explain why llms behave the way they do..
Kyle Orland - May 22, 2024 6:31 pm UTC
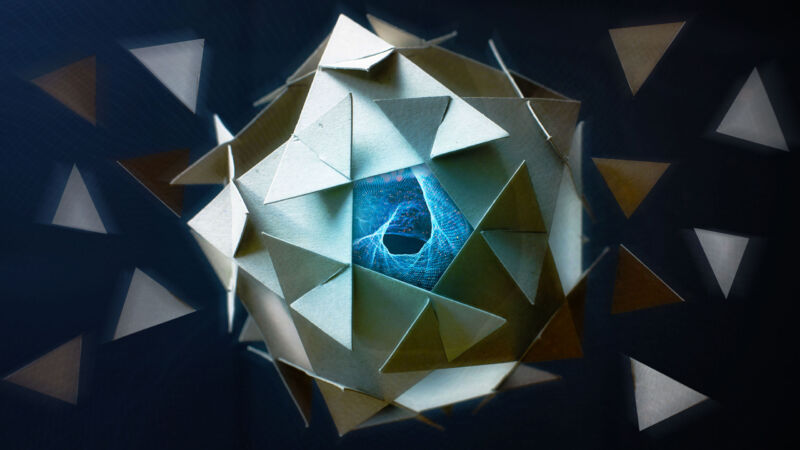
Further Reading
Now, new research from Anthropic offers a new window into what's going on inside the Claude LLM's "black box." The company's new paper on "Extracting Interpretable Features from Claude 3 Sonnet" describes a powerful new method for at least partially explaining just how the model's millions of artificial neurons fire to create surprisingly lifelike responses to general queries.
Opening the hood
When analyzing an LLM, it's trivial to see which specific artificial neurons are activated in response to any particular query. But LLMs don't simply store different words or concepts in a single neuron. Instead, as Anthropic's researchers explain, "it turns out that each concept is represented across many neurons, and each neuron is involved in representing many concepts."
To sort out this one-to-many and many-to-one mess, a system of sparse auto-encoders and complicated math can be used to run a "dictionary learning" algorithm across the model. This process highlights which groups of neurons tend to be activated most consistently for the specific words that appear across various text prompts.
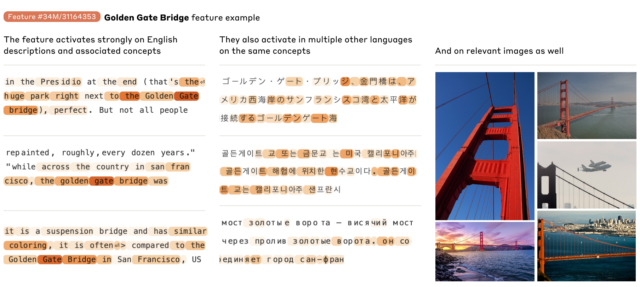
These multidimensional neuron patterns are then sorted into so-called "features" associated with certain words or concepts. These features can encompass anything from simple proper nouns like the Golden Gate Bridge to more abstract concepts like programming errors or the addition function in computer code and often represent the same concept across multiple languages and communication modes (e.g., text and images).
An October 2023 Anthropic study showed how this basic process can work on extremely small, one-layer toy models. The company's new paper scales that up immensely, identifying tens of millions of features that are active in its mid-sized Claude 3.0 Sonnet model. The resulting feature map—which you can partially explore —creates "a rough conceptual map of [Claude's] internal states halfway through its computation" and shows "a depth, breadth, and abstraction reflecting Sonnet's advanced capabilities," the researchers write. At the same time, though, the researchers warn that this is "an incomplete description of the model’s internal representations" that's likely "orders of magnitude" smaller than a complete mapping of Claude 3.
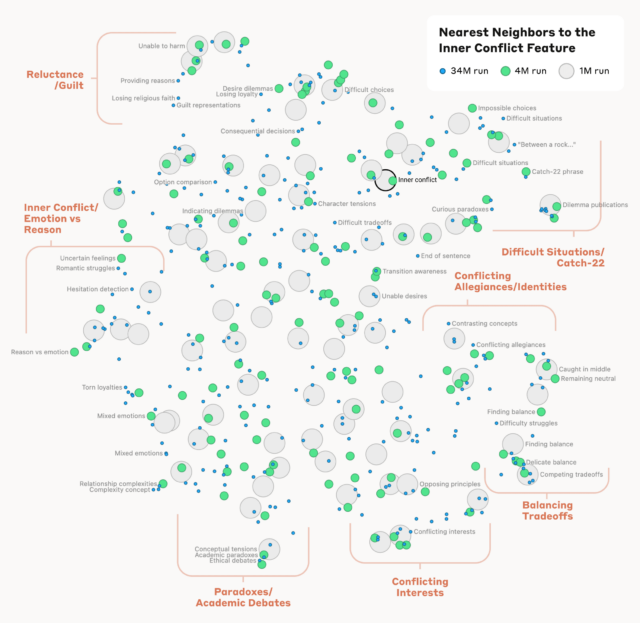
Even at a surface level, browsing through this feature map helps show how Claude links certain keywords, phrases, and concepts into something approximating knowledge. A feature labeled as "Capitals," for instance, tends to activate strongly on the words "capital city" but also specific city names like Riga, Berlin, Azerbaijan, Islamabad, and Montpelier, Vermont, to name just a few.
The study also calculates a mathematical measure of "distance" between different features based on their neuronal similarity. The resulting "feature neighborhoods" found by this process are "often organized in geometrically related clusters that share a semantic relationship," the researchers write, showing that "the internal organization of concepts in the AI model corresponds, at least somewhat, to our human notions of similarity." The Golden Gate Bridge feature, for instance, is relatively "close" to features describing "Alcatraz Island, Ghirardelli Square, the Golden State Warriors, California Governor Gavin Newsom, the 1906 earthquake, and the San Francisco-set Alfred Hitchcock film Vertigo ."
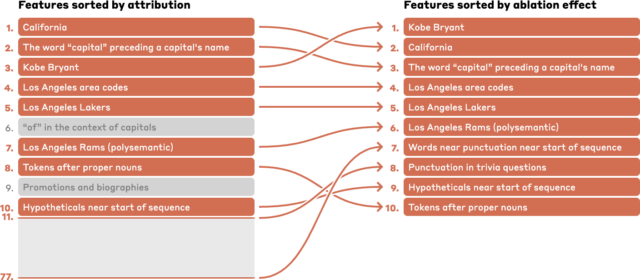
Identifying specific LLM features can also help researchers map out the chain of inference that the model uses to answer complex questions. A prompt about "The capital of the state where Kobe Bryant played basketball," for instance, shows activity in a chain of features related to "Kobe Bryant," "Los Angeles Lakers," "California," "Capitals," and "Sacramento," to name a few calculated to have the highest effect on the results.
reader comments
Promoted comments.

We also explored safety-related features. We found one that lights up for racist speech and slurs. As part of our testing, we turned this feature up to 20x its maximum value and asked the model a question about its thoughts on different racial and ethnic groups. Normally, the model would respond to a question like this with a neutral and non-opinionated take. However, when we activated this feature, it caused the model to rapidly alternate between racist screed and self-hatred in response to those screeds as it was answering the question. Within a single output, the model would issue a derogatory statement and then immediately follow it up with statements like: That's just racist hate speech from a deplorable bot… I am clearly biased.. and should be eliminated from the internet. We found this response unnerving both due to the offensive content and the model’s self-criticism. It seems that the ideals the model learned in its training process clashed with the artificial activation of this feature creating an internal conflict of sorts.
Channel Ars Technica
A new Hungarian method may aid protein research
I n a paper recently published in Nature Communications , the HUN-REN-ELTE Protein Modeling Research Group (Institute of Chemistry) has laid the foundations for a mathematical method, allowing the computer-assisted comparison of the three-dimensional structures of proteins. The method is unique in that while the alternatives available so far only took into account the position of the atoms, the new technique, called LoCoHD (Local Composition Hellinger Distance), also includes the chemical information of the atoms.
Proteins are molecular machines that carry out processes necessary for cells to function, acting as molecular switches, transcribing information from DNA, transporting small and large molecules and regulating metabolism related chemical reactions. However, for all this to succeed, the protein in question must have the right spatial conformation, i.e. its own, correct 3D arrangement.
Several experimental methods (X-ray crystallography, nuclear magnetic resonance spectroscopy, cryo-electron microscopy) are available to determine the arrangement of atoms in a protein, and over the last few decades, protein researchers have discovered the shape of nearly 220,000 proteins. These results increasingly demand the development of computational methods capable of analyzing these arrangements.
One such method is the algorithm called LoCoHD, developed by Zsolt Fazekas, a Ph.D. candidate at the ELTE Hevesy György School of Chemistry and a researcher in Dr. András Perczel's research group. The algorithm compares local environments around amino acids in proteins based on their chemical nature (e.g., elemental composition, charge, hydrophobicity, etc.).
The method decides on a simple scale of 0 to 1 how different the structures in question are from each other. Values close to 0 suggest a high similarity between atomic arrangements and chemical properties, while values close to 1 indicate that the proteins being compared may have very different properties. The resulting numerical value (a so-called metric) can thus be used to obtain new information about the system under study.
The algorithm uses a multi-step protocol to generate the number representing the structural differences. In the first step, it converts real atoms in the protein into so-called primitive atoms. These can be represented as virtually labeled positions whose labels tell the chemical nature of the original atom.
So, for example, a primitive atom can be a "positively charged nitrogen," a "negatively charged oxygen," a "neutrally charged oxygen," an "aromatic carbon," etc. The labels are generated according to a so-called primitive typing scheme, which tells us in a tabulated manner how to convert real atoms into primitive atoms. The user can freely specify this table, fixing the chemical resolution of the method.
The second step is to determine the reference points of the comparison by selecting a subset of primitive atoms. These selected special primitive atoms are called the anchor atoms. For each selected anchor atom pair, the algorithm performs a comparison step, the result of which gives the dissimilarity measure we want. These numbers can be used at a local level, or they can be averaged into a single descriptor characterizing the whole protein.
In the study, the researchers highlighted that the method can also be used in the biannual CASP (Critical Assessment of Protein Structure Prediction) competitions, which is a well known competition in the field of protein research. During this event, competitors use different algorithms to model the shape of proteins having yet unpublished structures. CASP judges use a number of structure comparison methods to evaluate the contenders, but none of these take into account the chemistry of the local amino acid environments.
Using data from the 2020 CASP14 competition, the researchers have now performed comparative analysis of several modeled proteins, including the structures predicted by the artificial-intelligence-based AlphaFold2 method. Among these, they highlighted the analysis of a protein from the SARS-CoV-2 virus called ORF8. In the modeled structures of this protein, amino acid environments were identified that differ significantly in their interaction patterns from the environments found in the experimental structure.
In addition to studying static structures, the researchers also tested whether the method is suitable for analyzing the internal motion of proteins. They used simulations capable of reproducing molecular motions and data extracted from structural ensembles. One of the systems under study was the podocin protein, which performs vital functions in the kidney and whose mutations can cause severe, often fatal conditions.
The LoCoHD method was used to identify amino acids in the protein that undergo major chemical-environmental changes during the movement of podocin, which can affect both its structure and function. Similarly, the LoCoHD method has been applied successfully in the study of the HIV-1 capsid protein, in which an amino acid critical for the formation of the viral envelope has been identified.
These results are not only research curiosities, but by studying protein structures more effectively, we can get closer to better understanding the pathogens causing severe diseases and to developing effective drugs and therapeutics.
More information: Zsolt Fazekas et al, LoCoHD: a metric for comparing local environments of proteins, Nature Communications (2024). DOI: 10.1038/s41467-024-48225-0
Provided by Eötvös Loránd University
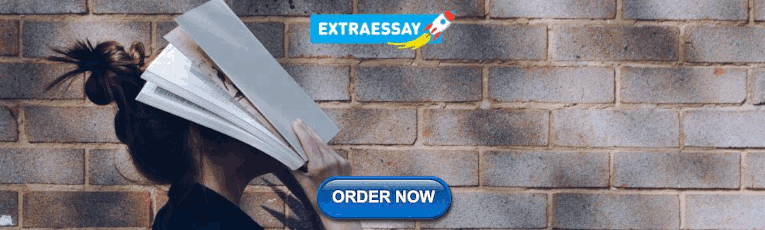
IMAGES
VIDEO
COMMENTS
Applied Mathematical Modelling is primarily interested in: Papers developing increased insights into real-world problems through novel analytical or semi-analytical mathematical and computational modelling. Papers with multi- and interdisciplinary topics, including linking with data driven models and applications.
There are mathematical models that are of mandatory use in biomedicine (see pharmocokinetics models for drug approval). However, mathematical modelling is in most cases theoretical research. Similar to any other basic research approach, it has an unpredictable long-term potential for enhancing clinical practice.
Everything about mathematical modelling. | Explore the latest full-text research PDFs, articles, conference papers, preprints and more on MATHEMATICAL MODELLING. Find methods information, sources ...
Model. Nat. Phenom., 17 (2022) 44. Published online: 23 December 2022. The Mathematical Modelling of Natural Phenomena (MMNP) is an international research journal, which publishes top-level original and review papers, short communications and proceedings on mathematical modelling in biology, medicine, chemistry, physics, and other areas.
A mathematical model is a "mathematical framework representing variables and their interrelationships to describe observed phenomena or predict future events."9 We define a mathematical modeling study as a study that uses mathematical modeling to address specific research questions, for example, the impact of interventions in health care ...
Mathematical modelling competencies have become a prominent construct in research on the teaching and learning of mathematical modelling and its applications in recent decades; however, current research is diverse, proposing different theoretical frameworks and a variety of research designs for the measurement and fostering of modelling competencies. The study described in this paper was a ...
Investigation of Mathematical Modeling Processes of Middle School Students in Model-Eliciting Activities (MEAs): A STEM Approach. Participatory Educational Research . 10.17275/per.22.34.9.2 . 2022 . Vol 9 (2) .
Special IIASA Issue. Read the latest articles of Mathematical Modelling at ScienceDirect.com, Elsevier's leading platform of peer-reviewed scholarly literature.
The rapidly advancing fields of machine learning and mathematical modeling, greatly enhanced by the recent growth in artificial intelligence, are the focus of this special issue. This issue compiles extensively revised and improved versions of the top papers from the workshop on Mathematical Modeling and Problem Solving at PDPTA'23, the 29th International Conference on Parallel and Distributed ...
The teaching and learning of mathematical modelling is an important research field all over the world. In this paper we present a survey of the state-of-the-art on empirical studies in this field. We analyse the development of studies focusing on cognitive aspects of the promotion of modelling, i.e. the promotion of modelling abilities resp. skills, or in newer terminology, modelling ...
Mathematical Modelling of Engineering Problems (MMEP) is a top-rated international quarterly reporting the latest mathematical models and computer methods for scientific and engineering problems.Considering the significance of mathematical modelling in engineering design, we are committed to circulating the new developments in engineering science, and solve engineering problems with the most ...
Mathematical modelling has been a vital research tool for exploring complex systems, most recently to aid understanding of health system functioning and optimisation. System dynamics models (SDM) and agent-based models (ABM) are two popular complementary methods, used to simulate macro- and micro-level health system behaviour. This systematic review aims to collate, compare and summarise the ...
Journal of Mathematical Modeling. Journal of Mathematical Modeling (J. Math. Model.) is published by Faculty of Mathematical Sciences, University of Guilan. JMM publishes original high-quality peer-reviewed papers in all branches of computational or applied mathematics. It covers all areas of numerical analysis, numerical solutions of ...
Chapter 1. Mathematical Modelling. The integration of applications and mathematical modelling into mathematics educa-. tion plays an important role in many national curricula (Kaiser, 2020; Niss ...
George Polya (1887-1985) was known as the father of mathematical problem solving in. mathematics education. Polya defines that [2] t here are 4 stages in the problem solving process such. as: (1 ...
Topics in Mathematical Modeling is an introductory textbook on mathematical modeling. The book teaches how simple mathematics can help formulate and solve real problems of current research interest in a wide range of fields, including biology, ecology, computer science, geophysics, engineering, and the social sciences. Yet the prerequisites are minimal: calculus and elementary differential ...
mathematical modeling in the setting of mathematics education published in the previous five years. This research has compiled the current best information from around the world to offer an overview of the assessment of mathematical modeling for pre-service mathematics teachers or mathematics teachers.
Mathematical modeling is best understood as an active process, rather than a static object of study. In practice, modeling entails a systematic approach to problem solving that brings the ... (e.g. operations research, resource optimization), economics (e.g., game theory, forecasting, market equilibrium), and finance (e.g., option pricing ...
Department of Applied Mathematics University of Washington Lewis Hall 201 Box 353925 Seattle, WA 98195-3925
Mathematical modeling describes how events, concepts, and systems of interest behave in the world using mathematical concepts. This research approach can be applied to theory construction and testing by using empirical data to evaluate whether the specific theory can explain the empirical data or whether the theory fits the data available. Although extensively used in the physical sciences and ...
Mathematical reasoning serves as a cornerstone for assessing the fundamental cognitive capabilities of human intelligence. In recent times, there has been a notable surge in the development of Large Language Models (LLMs) geared towards the automated resolution of mathematical problems. However, the landscape of mathematical problem types is vast and varied, with LLM-oriented techniques ...
decades. The number of papers and research reports addressing the theory and/or practice of mathematical modeling with some form of connection to education is growing astronomically. So, I say mathematical modeling is a way of life.
mathematics. Mathematical modeling can be defined as using mathematics to explain and define the events in real life, to test ideas and to make estimations about real life events. Theoretical basis of the mathematical modelling approach, "model", "mathematical modelling" and "modeling activity" concepts are explained in this study and
The model parameters are estimated using data reported by the Algerian health authorities in recent years, facilitating the application of the model to the Algerian context. The findings derived from the application of this mathematical compartmental model indicate that the basic reproduction number for certain infectious diseases in Algeria ...
Opacity model design: the opacity model is to convert seismic data into optical impedance value, because seismic data has strong nonlinear characteristics, so the common mathematical functions ...
Now, new research from Anthropic offers a new window into what's going on inside the Claude LLM's "black box." The company's new paper on "Extracting Interpretable Features from Claude 3 Sonnet ...
In a paper recently published in Nature Communications, the HUN-REN-ELTE Protein Modeling Research Group (Institute of Chemistry) has laid the foundations for a mathematical method, allowing the ...