Have a language expert improve your writing
Run a free plagiarism check in 10 minutes, generate accurate citations for free.
- Knowledge Base
Methodology
- Descriptive Research | Definition, Types, Methods & Examples
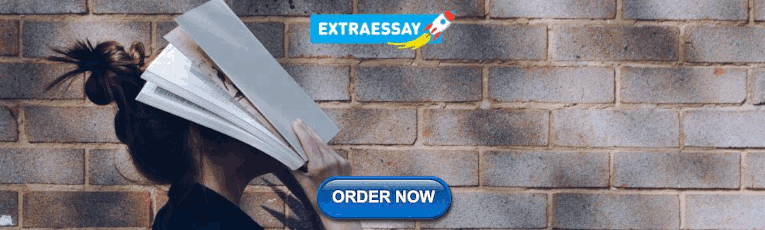
Descriptive Research | Definition, Types, Methods & Examples
Published on May 15, 2019 by Shona McCombes . Revised on June 22, 2023.
Descriptive research aims to accurately and systematically describe a population, situation or phenomenon. It can answer what , where , when and how questions , but not why questions.
A descriptive research design can use a wide variety of research methods to investigate one or more variables . Unlike in experimental research , the researcher does not control or manipulate any of the variables, but only observes and measures them.
Table of contents
When to use a descriptive research design, descriptive research methods, other interesting articles.
Descriptive research is an appropriate choice when the research aim is to identify characteristics, frequencies, trends, and categories.
It is useful when not much is known yet about the topic or problem. Before you can research why something happens, you need to understand how, when and where it happens.
Descriptive research question examples
- How has the Amsterdam housing market changed over the past 20 years?
- Do customers of company X prefer product X or product Y?
- What are the main genetic, behavioural and morphological differences between European wildcats and domestic cats?
- What are the most popular online news sources among under-18s?
- How prevalent is disease A in population B?
Receive feedback on language, structure, and formatting
Professional editors proofread and edit your paper by focusing on:
- Academic style
- Vague sentences
- Style consistency
See an example
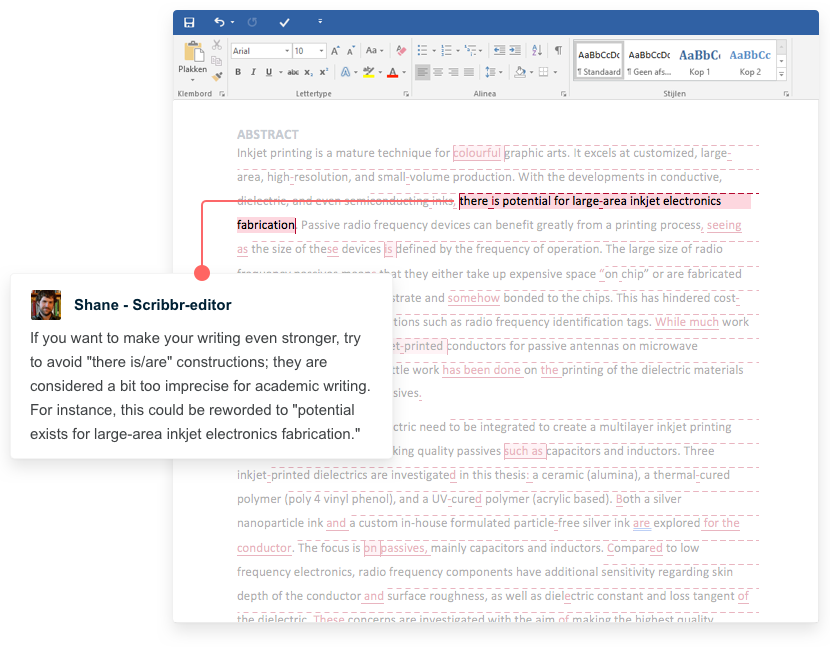
Descriptive research is usually defined as a type of quantitative research , though qualitative research can also be used for descriptive purposes. The research design should be carefully developed to ensure that the results are valid and reliable .
Survey research allows you to gather large volumes of data that can be analyzed for frequencies, averages and patterns. Common uses of surveys include:
- Describing the demographics of a country or region
- Gauging public opinion on political and social topics
- Evaluating satisfaction with a company’s products or an organization’s services
Observations
Observations allow you to gather data on behaviours and phenomena without having to rely on the honesty and accuracy of respondents. This method is often used by psychological, social and market researchers to understand how people act in real-life situations.
Observation of physical entities and phenomena is also an important part of research in the natural sciences. Before you can develop testable hypotheses , models or theories, it’s necessary to observe and systematically describe the subject under investigation.
Case studies
A case study can be used to describe the characteristics of a specific subject (such as a person, group, event or organization). Instead of gathering a large volume of data to identify patterns across time or location, case studies gather detailed data to identify the characteristics of a narrowly defined subject.
Rather than aiming to describe generalizable facts, case studies often focus on unusual or interesting cases that challenge assumptions, add complexity, or reveal something new about a research problem .
If you want to know more about statistics , methodology , or research bias , make sure to check out some of our other articles with explanations and examples.
- Normal distribution
- Degrees of freedom
- Null hypothesis
- Discourse analysis
- Control groups
- Mixed methods research
- Non-probability sampling
- Quantitative research
- Ecological validity
Research bias
- Rosenthal effect
- Implicit bias
- Cognitive bias
- Selection bias
- Negativity bias
- Status quo bias
Cite this Scribbr article
If you want to cite this source, you can copy and paste the citation or click the “Cite this Scribbr article” button to automatically add the citation to our free Citation Generator.
McCombes, S. (2023, June 22). Descriptive Research | Definition, Types, Methods & Examples. Scribbr. Retrieved June 22, 2024, from https://www.scribbr.com/methodology/descriptive-research/
Is this article helpful?
Shona McCombes
Other students also liked, what is quantitative research | definition, uses & methods, correlational research | when & how to use, descriptive statistics | definitions, types, examples, "i thought ai proofreading was useless but..".
I've been using Scribbr for years now and I know it's a service that won't disappoint. It does a good job spotting mistakes”
Chapter 2: Psychological Research
Descriptive research.
Psychologists use descriptive, experimental, and correlational methods to conduct research. Descriptive, or qualitative, methods include the case study, naturalistic observation, surveys, archival research, longitudinal research, and cross-sectional research.
https://assessments.lumenlearning.com/assessments/2706
There are many research methods available to psychologists in their efforts to understand, describe, and explain behavior and the cognitive and biological processes that underlie it. Some methods rely on observational techniques. Other approaches involve interactions between the researcher and the individuals who are being studied—ranging from a series of simple questions to extensive, in-depth interviews—to well-controlled experiments.
The three main categories of psychological research are descriptive, correlational, and experimental research. Research studies that do not test specific relationships between variables are called descriptive, or qualitative, studies . These studies are used to describe general or specific behaviors and attributes that are observed and measured. In the early stages of research it might be difficult to form a hypothesis, especially when there is not any existing literature in the area. In these situations designing an experiment would be premature, as the question of interest is not yet clearly defined as a hypothesis. Often a researcher will begin with a non-experimental approach, such as a descriptive study, to gather more information about the topic before designing an experiment or correlational study to address a specific hypothesis.
Video 1. Descriptive Research Design provides explanation and examples for quantitative descriptive research. A closed-captioned version of this video is available here .
Descriptive research is distinct from correlational research , in which psychologists formally test whether a relationship exists between two or more variables. Experimental research goes a step further beyond descriptive and correlational research and randomly assigns people to different conditions, using hypothesis testing to make inferences about how these conditions affect behavior. It aims to determine if one variable directly impacts and causes another. Correlational and experimental research both typically use hypothesis testing, whereas descriptive research does not.
Each of these research methods has unique strengths and weaknesses, and each method may only be appropriate for certain types of research questions. For example, studies that rely primarily on observation produce incredible amounts of information, but the ability to apply this information to the larger population is somewhat limited because of small sample sizes. Survey research, on the other hand, allows researchers to easily collect data from relatively large samples. While this allows for results to be generalized to the larger population more easily, the information that can be collected on any given survey is somewhat limited and subject to problems associated with any type of self-reported data. Some researchers conduct archival research by using existing records. While this can be a fairly inexpensive way to collect data that can provide insight into a number of research questions, researchers using this approach have no control on how or what kind of data was collected.
Correlational research can find a relationship between two variables, but the only way a researcher can claim that the relationship between the variables is cause and effect is to perform an experiment. In experimental research, which will be discussed later in the text, there is a tremendous amount of control over variables of interest. While this is a powerful approach, experiments are often conducted in very artificial settings. This calls into question the validity of experimental findings with regard to how they would apply in real-world settings. In addition, many of the questions that psychologists would like to answer cannot be pursued through experimental research because of ethical concerns.
Data Collection
Regardless of the method of research, data collection will be necessary. The method of data collection selected will primarily depend on the type of information the researcher needs for their study; however, other factors, such as time, resources, and even ethical considerations can influence the selection of a data collection method. All of these factors need to be considered when selecting a data collection method because each method has unique strengths and weaknesses. We will discuss the uses and assessment of the most common data collection methods: observation, surveys, archival data, and tests.
Observation
If you want to understand how behavior occurs, one of the best ways to gain information is to simply observe the behavior in its natural context. However, people might change their behavior in unexpected ways if they know they are being observed. How do researchers obtain accurate information when people tend to hide their natural behavior? As an example, imagine that your professor asks everyone in your class to raise their hand if they always wash their hands after using the restroom. Chances are that almost everyone in the classroom will raise their hand, but do you think hand washing after every trip to the restroom is really that universal?
This is very similar to the phenomenon mentioned earlier in this module: many individuals do not feel comfortable answering a question honestly. But if we are committed to finding out the facts about handwashing, we have other options available to us.
Suppose we send a classmate into the restroom to actually watch whether everyone washes their hands after using the restroom. Will our observer blend into the restroom environment by wearing a white lab coat, sitting with a clipboard, and staring at the sinks? We want our researcher to be inconspicuous—perhaps standing at one of the sinks pretending to put in contact lenses while secretly recording the relevant information. This type of observational study is called naturalistic observation : observing behavior in its natural setting. To better understand peer exclusion, Suzanne Fanger collaborated with colleagues at the University of Texas to observe the behavior of preschool children on a playground. How did the observers remain inconspicuous over the duration of the study? They equipped a few of the children with wireless microphones (which the children quickly forgot about) and observed while taking notes from a distance. Also, the children in that particular preschool (a “laboratory preschool”) were accustomed to having observers on the playground (Fanger, Frankel, & Hazen, 2012).
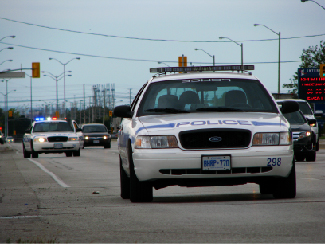
Figure 1 . Seeing a police car behind you would probably affect your driving behavior. (credit: Michael Gil)
It is critical that the observer be as unobtrusive and as inconspicuous as possible: when people know they are being watched, they are less likely to behave naturally. If you have any doubt about this, ask yourself how your driving behavior might differ in two situations: In the first situation, you are driving down a deserted highway during the middle of the day; in the second situation, you are being followed by a police car down the same deserted highway (Figure 1).
It should be pointed out that naturalistic observation is not limited to research involving humans. Indeed, some of the best-known examples of naturalistic observation involve researchers going into the field to observe various kinds of animals in their own environments. As with human studies, the researchers maintain their distance and avoid interfering with the animal subjects so as not to influence their natural behaviors. Scientists have used this technique to study social hierarchies and interactions among animals ranging from ground squirrels to gorillas. The information provided by these studies is invaluable in understanding how those animals organize socially and communicate with one another. The anthropologist Jane Goodall, for example, spent nearly five decades observing the behavior of chimpanzees in Africa (Figure 2). As an illustration of the types of concerns that a researcher might encounter in naturalistic observation, some scientists criticized Goodall for giving the chimps names instead of referring to them by numbers—using names was thought to undermine the emotional detachment required for the objectivity of the study (McKie, 2010).
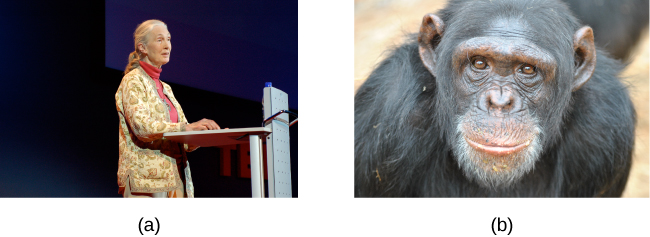
Figure 2 . (a) Jane Goodall made a career of conducting naturalistic observations of (b) chimpanzee behavior. (credit “Jane Goodall”: modification of work by Erik Hersman; “chimpanzee”: modification of work by “Afrika Force”/Flickr.com)
The greatest benefit of naturalistic observation is the validity, or accuracy, of information collected unobtrusively in a natural setting. Having individuals behave as they normally would in a given situation means that we have a higher degree of ecological validity, or realism, than we might achieve with other research approaches. Therefore, our ability to generalize the findings of the research to real-world situations is enhanced. If done correctly, we need not worry about people or animals modifying their behavior simply because they are being observed. Sometimes, people may assume that reality programs give us a glimpse into authentic human behavior. However, the principle of inconspicuous observation is violated as reality stars are followed by camera crews and are interviewed on camera for personal confessionals. Given that environment, we must doubt how natural and realistic their behaviors are.
The major downside of naturalistic observation is that they are often difficult to set up and control. In our restroom study, what if you stood in the restroom all day prepared to record people’s handwashing behavior and no one came in? Or, what if you have been closely observing a troop of gorillas for weeks only to find that they migrated to a new place while you were sleeping in your tent? The benefit of realistic data comes at a cost. As a researcher, you have no control of when (or if) you have behavior to observe. In addition, this type of observational research often requires significant investments of time, money, and a good dose of luck.
Sometimes studies involve structured observation. In these cases, people are observed while engaging in set, specific tasks. An excellent example of structured observation comes from Strange Situation by Mary Ainsworth (you will read more about this in the module on lifespan development). The Strange Situation is a procedure used to evaluate attachment styles that exist between an infant and caregiver. In this scenario, caregivers bring their infants into a room filled with toys. The Strange Situation involves a number of phases, including a stranger coming into the room, the caregiver leaving the room, and the caregiver’s return to the room. The infant’s behavior is closely monitored at each phase, but it is the behavior of the infant upon being reunited with the caregiver that is most telling in terms of characterizing the infant’s attachment style with the caregiver.
Another potential problem in observational research is observer bias . Generally, people who act as observers are closely involved in the research project and may unconsciously skew their observations to fit their research goals or expectations. To protect against this type of bias, researchers should have clear criteria established for the types of behaviors recorded and how those behaviors should be classified. In addition, researchers often compare observations of the same event by multiple observers, in order to test inter-rater reliability : a measure of reliability that assesses the consistency of observations by different observers.
Often, psychologists develop surveys as a means of gathering data. Surveys are lists of questions to be answered by research participants, and can be delivered as paper-and-pencil questionnaires, administered electronically, or conducted verbally (Figure 3). Generally, the survey itself can be completed in a short time, and the ease of administering a survey makes it easy to collect data from a large number of people.
Surveys allow researchers to gather data from larger samples than may be afforded by other research methods . A sample is a subset of individuals selected from a population , which is the overall group of individuals that the researchers are interested in. Researchers study the sample and seek to generalize their findings to the population.
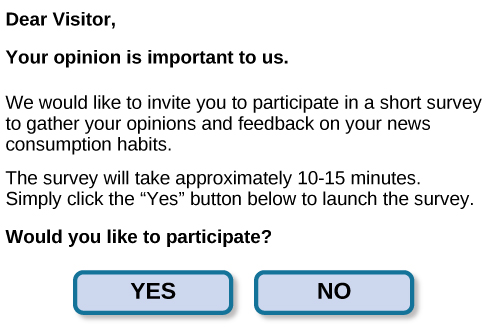
Figure 3 . Surveys can be administered in a number of ways, including electronically administered research, like the survey shown here. (credit: Robert Nyman)
There is both strength and weakness of the survey in comparison to case studies. By using surveys, we can collect information from a larger sample of people. A larger sample is better able to reflect the actual diversity of the population, thus allowing better generalizability. Therefore, if our sample is sufficiently large and diverse, we can assume that the data we collect from the survey can be generalized to the larger population with more certainty than the information collected through a case study. However, given the greater number of people involved, we are not able to collect the same depth of information on each person that would be collected in a case study.
Another potential weakness of surveys is something we touched on earlier in this module: people don’t always give accurate responses. They may lie, misremember, or answer questions in a way that they think makes them look good. For example, people may report drinking less alcohol than is actually the case.
Any number of research questions can be answered through the use of surveys. One real-world example is the research conducted by Jenkins, Ruppel, Kizer, Yehl, and Griffin (2012) about the backlash against the US Arab-American community following the terrorist attacks of September 11, 2001. Jenkins and colleagues wanted to determine to what extent these negative attitudes toward Arab-Americans still existed nearly a decade after the attacks occurred. In one study, 140 research participants filled out a survey with 10 questions, including questions asking directly about the participant’s overt prejudicial attitudes toward people of various ethnicities. The survey also asked indirect questions about how likely the participant would be to interact with a person of a given ethnicity in a variety of settings (such as, “How likely do you think it is that you would introduce yourself to a person of Arab-American descent?”). The results of the research suggested that participants were unwilling to report prejudicial attitudes toward any ethnic group. However, there were significant differences between their pattern of responses to questions about social interaction with Arab-Americans compared to other ethnic groups: they indicated less willingness for social interaction with Arab-Americans compared to the other ethnic groups. This suggested that the participants harbored subtle forms of prejudice against Arab-Americans, despite their assertions that this was not the case (Jenkins et al., 2012).
Archival Data and Case Studies
Some researchers gain access to large amounts of data without interacting with a single research participant. Instead, they use existing records to answer various research questions. This type of research approach is known as archival research. Archival research relies on looking at past records or data sets to look for interesting patterns or relationships.
For example, a researcher might access the academic records of all individuals who enrolled in college within the past ten years and calculate how long it took them to complete their degrees, as well as course loads, grades, and extracurricular involvement. Archival research could provide important information about who is most likely to complete their education, and it could help identify important risk factors for struggling students (Figure 4).
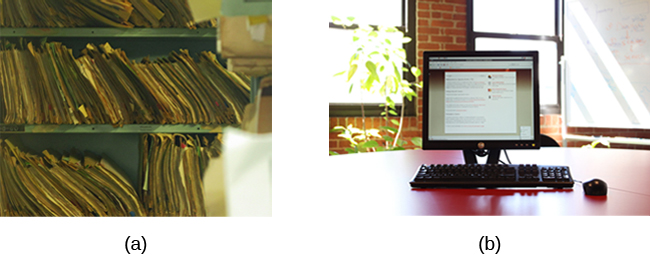
Figure 4 . A researcher doing archival research examines records, whether archived as a (a) hardcopy or (b) electronically. (credit “paper files”: modification of work by “Newtown graffiti”/Flickr; “computer”: modification of work by INPIVIC Family/Flickr)
In comparing archival research to other research methods, there are several important distinctions. For one, the researcher employing archival research never directly interacts with research participants. Therefore, the investment of time and money to collect data is considerably less with archival research. Additionally, researchers have no control over what information was originally collected. Therefore, research questions have to be tailored so they can be answered within the structure of the existing data sets. There is also no guarantee of consistency between the records from one source to another, which might make comparing and contrasting different data sets problematic.
https://assessments.lumenlearning.com/assessments/2712
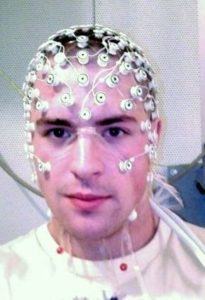
A good test will aid researchers in assessing a particular psychological construct. What is a good test? Researchers want a test that is standardized, reliable, and valid. A standardized test is one that is administered, scored, and analyzed in the same way for each participant. This minimizes differences in test scores due to confounding factors, such as variability in the testing environment or scoring process, and assures that scores are comparable. Reliability refers to the consistency of a measure. Researchers consider three types of consistency: over time (test-retest reliability), across items (internal consistency), and across different researchers (interrater reliability). Validity is the extent to which the scores from a measure represent the variable they are intended to. When a measure has good test-retest reliability and internal consistency, researchers should be more confident that the scores represent what they are supposed to.
There are various types of tests used in psychological research. Self-report measures are those in which participants report on their own thoughts, feelings, and actions, such as the Rosenberg Self-Esteem Scale or the Big Five Personality Test. Some tests measure performance, ability, aptitude, or skill, like the Stanford-Binet Intelligence Scale or the SATs.There are also tests that measure physiological states, including electrical activity or blood flow in the brain.
Video 2. Methods of Data Collection explains various means for gathering data for quantitative and qualitative research. A closed-captioned version of this video is available here .
Studying Changes over Time
Sometimes, especially in developmental research, the researcher is interested in examining changes over time and will need to consider a research design that will capture these changes. Remember, research methods are tools that are used to collect information, while r esearch design is the strategy or blueprint for deciding how to collect and analyze information. Research design dictates which methods are used and how. There are three types of developmental research designs: cross-sectional, longitudinal, and sequential.
Video 3. Developmental Research Designs
Cross-Sectional Design
The majority of developmental studies use cross-sectional designs because they are less time-consuming and less expensive than other developmental designs. Cross-sectional research designs are used to examine behavior in participants of different ages who are tested at the same point in time. Let’s suppose that researchers are interested in the relationship between intelligence and aging. They might have a hypothesis that intelligence declines as people get older. The researchers might choose to give a particular intelligence test to individuals who are 20 years old, individuals who are 50 years old, and individuals who are 80 years old at the same time and compare the data from each age group. This research is cross-sectional in design because the researchers plan to examine the intelligence scores of individuals of different ages within the same study at the same time; they are taking a “cross-section” of people at one point in time. Let’s say that the comparisons find that the 80-year-old adults score lower on the intelligence test than the 50-year-old adults, and the 50-year-old adults score lower on the intelligence test than the 20-year-old adults. Based on these data, the researchers might conclude that individuals become less intelligent as they get older. Would that be a valid (accurate) interpretation of the results?
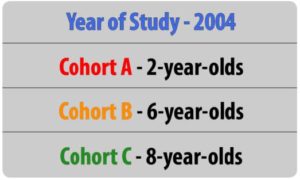
Figure 5. Example of cross-sectional research design
No, that would not be a valid conclusion because the researchers did not follow individuals as they aged from 20 to 50 to 80 years old. One of the primary limitations of cross-sectional research is that the results yield information about age differences not necessarily changes over time. That is, although the study described above can show that the 80-year-olds scored lower on the intelligence test than the 50-year-olds, and the 50-year-olds scored lower than the 20-year-olds, the data used for this conclusion were collected from different individuals (or groups). It could be, for instance, that when these 20-year-olds get older, they will still score just as high on the intelligence test as they did at age 20. Similarly, maybe the 80-year-olds would have scored relatively low on the intelligence test when they were young; the researchers don’t know for certain because they did not follow the same individuals as they got older.
With each cohort being members of a different generation, it is also possible that the differences found between the groups are not due to age, per se, but due to cohort effects. Differences between these cohorts’ IQ results could be due to differences in life experiences specific to their generation, such as differences in education, economic conditions, advances in technology, or changes in health and nutrition standards, and not due to age-related changes.
Another disadvantage of cross-sectional research is that it is limited to one time of measurement. Data are collected at one point in time, and it’s possible that something could have happened in that year in history that affected all of the participants, although possibly each cohort may have been affected differently.
Longitudinal Research Design
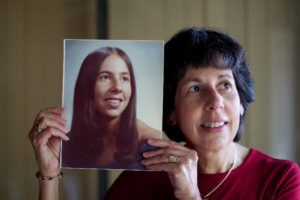
Longitudinal research designs are used to examine behavior in the same individuals over time. For instance, with our example of studying intelligence and aging, a researcher might conduct a longitudinal study to examine whether 20-year-olds become less intelligent with age over time. To this end, a researcher might give an intelligence test to individuals when they are 20 years old, again when they are 50 years old, and then again when they are 80 years old. This study is longitudinal in nature because the researcher plans to study the same individuals as they age. Based on these data, the pattern of intelligence and age might look different than from the cross-sectional research; it might be found that participants’ intelligence scores are higher at age 50 than at age 20 and then remain stable or decline a little by age 80. How can that be when cross-sectional research revealed declines in intelligence with age?

Figure 6. Example of a longitudinal research design
Since longitudinal research happens over a period of time (which could be short term, as in months, but is often longer, as in years), there is a risk of attrition. Attrition occurs when participants fail to complete all portions of a study. Participants may move, change their phone numbers, die, or simply become disinterested in participating over time. Researchers should account for the possibility of attrition by enrolling a larger sample into their study initially, as some participants will likely drop out over time. There is also something known as selective attrition— this means that certain groups of individuals may tend to drop out. It is often the least healthy, least educated, and lower socioeconomic participants who tend to drop out over time. That means that the remaining participants may no longer be representative of the whole population, as they are, in general, healthier, better educated, and have more money. This could be a factor in why our hypothetical research found a more optimistic picture of intelligence and aging as the years went by. What can researchers do about selective attrition? At each time of testing, they could randomly recruit more participants from the same cohort as the original members to replace those who have dropped out.
The results from longitudinal studies may also be impacted by repeated assessments. Consider how well you would do on a math test if you were given the exact same exam every day for a week. Your performance would likely improve over time, not necessarily because you developed better math abilities, but because you were continuously practicing the same math problems. This phenomenon is known as a practice effect. Practice effects occur when participants become better at a task over time because they have done it again and again (not due to natural psychological development). So our participants may have become familiar with the intelligence test each time (and with the computerized testing administration).
Another limitation of longitudinal research is that the data are limited to only one cohort. As an example, think about how comfortable the participants in the 2010 cohort of 20-year-olds are with computers. Since only one cohort is being studied, there is no way to know if findings would be different from other cohorts. In addition, changes that are found as individuals age over time could be due to age or to time of measurement effects. That is, the participants are tested at different periods in history, so the variables of age and time of measurement could be confounded (mixed up). For example, what if there is a major shift in workplace training and education between 2020 and 2040, and many of the participants experience a lot more formal education in adulthood, which positively impacts their intelligence scores in 2040? Researchers wouldn’t know if the intelligence scores increased due to growing older or due to a more educated workforce over time between measurements.
Sequential Research Design
Sequential research designs include elements of both longitudinal and cross-sectional research designs. Similar to longitudinal designs, sequential research features participants who are followed over time; similar to cross-sectional designs, sequential research includes participants of different ages. This research design is also distinct from those that have been discussed previously in that individuals of different ages are enrolled into a study at various points in time to examine age-related changes, development within the same individuals as they age, and to account for the possibility of cohort and/or time of measurement effects
Consider, once again, our example of intelligence and aging. In a study with a sequential design, a researcher might recruit three separate groups of participants (Groups A, B, and C). Group A would be recruited when they are 20 years old in 2010 and would be tested again when they are 50 and 80 years old in 2040 and 2070, respectively (similar in design to the longitudinal study described previously). Group B would be recruited when they are 20 years old in 2040 and would be tested again when they are 50 years old in 2070. Group C would be recruited when they are 20 years old in 2070, and so on.
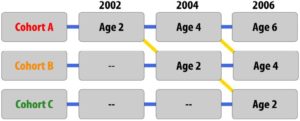
Figure 7. Example of sequential research design
Studies with sequential designs are powerful because they allow for both longitudinal and cross-sectional comparisons—changes and/or stability with age over time can be measured and compared with differences between age and cohort groups. This research design also allows for the examination of cohort and time of measurement effects. For example, the researcher could examine the intelligence scores of 20-year-olds at different times in history and different cohorts (follow the yellow diagonal lines in figure 3). This might be examined by researchers who are interested in sociocultural and historical changes (because we know that lifespan development is multidisciplinary). One way of looking at the usefulness of the various developmental research designs was described by Schaie and Baltes (1975): cross-sectional and longitudinal designs might reveal change patterns while sequential designs might identify developmental origins for the observed change patterns.
Since they include elements of longitudinal and cross-sectional designs, sequential research has many of the same strengths and limitations as these other approaches. For example, sequential work may require less time and effort than longitudinal research (if data are collected more frequently than over the 30-year spans in our example) but more time and effort than cross-sectional research. Although practice effects may be an issue if participants are asked to complete the same tasks or assessments over time, attrition may be less problematic than what is commonly experienced in longitudinal research since participants may not have to remain involved in the study for such a long period of time.
Comparing Developmental Research Designs
When considering the best research design to use in their research, scientists think about their main research question and the best way to come up with an answer. A table of advantages and disadvantages for each of the described research designs is provided here to help you as you consider what sorts of studies would be best conducted using each of these different approaches.
Table 1. Advantages and disadvantages of different research designs
Cross-Sectional | ||
Longitudinal | ||
Sequential |
- Introductory content. Provided by : Lumen Learning. License : CC BY-NC-SA: Attribution-NonCommercial-ShareAlike
- Modification, adaptation, and original content. Provided by : Lumen Learning. License : CC BY-SA: Attribution-ShareAlike
- Paragraph on correlation. Authored by : Christie Napa Scollon. Provided by : Singapore Management University. Located at : http://nobaproject.com/modules/research-designs?r=MTc0ODYsMjMzNjQ%3D . Project : The Noba Project. License : CC BY-NC-SA: Attribution-NonCommercial-ShareAlike
- Psychology, Approaches to Research. Authored by : OpenStax College. Located at : http://cnx.org/contents/[email protected]:mfArybye@7/Analyzing-Findings . License : CC BY: Attribution . License Terms : Download for free at http://cnx.org/contents/[email protected]
- Lec 2 | MIT 9.00SC Introduction to Psychology, Spring 2011. Authored by : John Gabrieli. Provided by : MIT OpenCourseWare. Located at : https://www.youtube.com/watch?v=syXplPKQb_o . License : CC BY-NC-SA: Attribution-NonCommercial-ShareAlike
- Descriptive Research. Provided by : Boundless. Located at : https://courses.lumenlearning.com/boundless-psychology/ . License : CC BY-SA: Attribution-ShareAlike
- Researchers review documents. Authored by : National Cancer Institute. Provided by : Wikimedia. Located at : https://commons.wikimedia.org/wiki/File:Researchers_review_documents.jpg . License : Public Domain: No Known Copyright
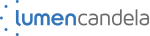
Privacy Policy
Research Methods In Psychology
Saul Mcleod, PhD
Editor-in-Chief for Simply Psychology
BSc (Hons) Psychology, MRes, PhD, University of Manchester
Saul Mcleod, PhD., is a qualified psychology teacher with over 18 years of experience in further and higher education. He has been published in peer-reviewed journals, including the Journal of Clinical Psychology.
Learn about our Editorial Process
Olivia Guy-Evans, MSc
Associate Editor for Simply Psychology
BSc (Hons) Psychology, MSc Psychology of Education
Olivia Guy-Evans is a writer and associate editor for Simply Psychology. She has previously worked in healthcare and educational sectors.
Research methods in psychology are systematic procedures used to observe, describe, predict, and explain behavior and mental processes. They include experiments, surveys, case studies, and naturalistic observations, ensuring data collection is objective and reliable to understand and explain psychological phenomena.
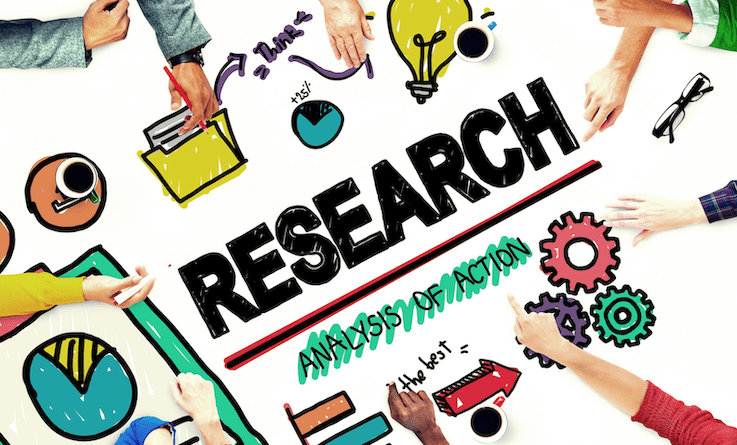
Hypotheses are statements about the prediction of the results, that can be verified or disproved by some investigation.
There are four types of hypotheses :
- Null Hypotheses (H0 ) – these predict that no difference will be found in the results between the conditions. Typically these are written ‘There will be no difference…’
- Alternative Hypotheses (Ha or H1) – these predict that there will be a significant difference in the results between the two conditions. This is also known as the experimental hypothesis.
- One-tailed (directional) hypotheses – these state the specific direction the researcher expects the results to move in, e.g. higher, lower, more, less. In a correlation study, the predicted direction of the correlation can be either positive or negative.
- Two-tailed (non-directional) hypotheses – these state that a difference will be found between the conditions of the independent variable but does not state the direction of a difference or relationship. Typically these are always written ‘There will be a difference ….’
All research has an alternative hypothesis (either a one-tailed or two-tailed) and a corresponding null hypothesis.
Once the research is conducted and results are found, psychologists must accept one hypothesis and reject the other.
So, if a difference is found, the Psychologist would accept the alternative hypothesis and reject the null. The opposite applies if no difference is found.
Sampling techniques
Sampling is the process of selecting a representative group from the population under study.
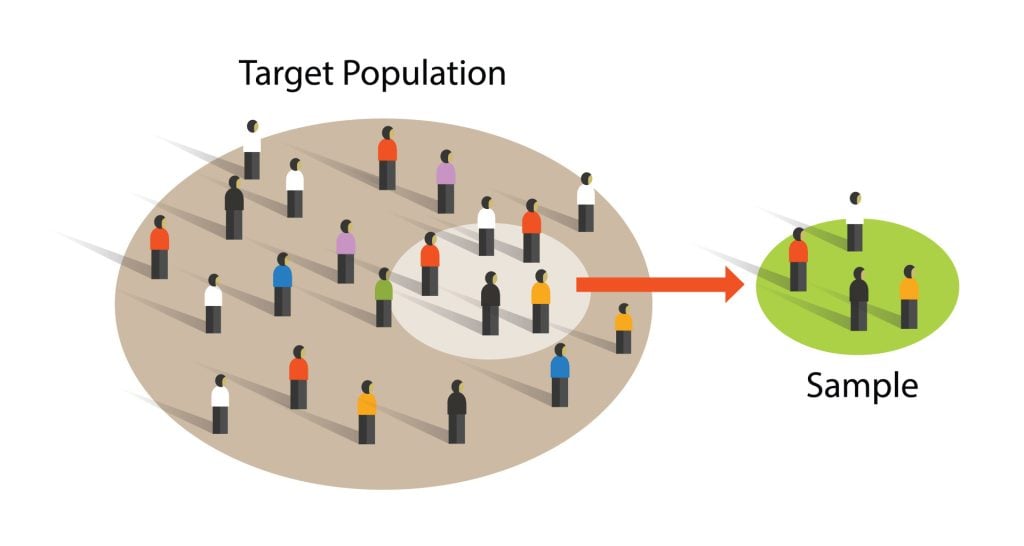
A sample is the participants you select from a target population (the group you are interested in) to make generalizations about.
Representative means the extent to which a sample mirrors a researcher’s target population and reflects its characteristics.
Generalisability means the extent to which their findings can be applied to the larger population of which their sample was a part.
- Volunteer sample : where participants pick themselves through newspaper adverts, noticeboards or online.
- Opportunity sampling : also known as convenience sampling , uses people who are available at the time the study is carried out and willing to take part. It is based on convenience.
- Random sampling : when every person in the target population has an equal chance of being selected. An example of random sampling would be picking names out of a hat.
- Systematic sampling : when a system is used to select participants. Picking every Nth person from all possible participants. N = the number of people in the research population / the number of people needed for the sample.
- Stratified sampling : when you identify the subgroups and select participants in proportion to their occurrences.
- Snowball sampling : when researchers find a few participants, and then ask them to find participants themselves and so on.
- Quota sampling : when researchers will be told to ensure the sample fits certain quotas, for example they might be told to find 90 participants, with 30 of them being unemployed.
Experiments always have an independent and dependent variable .
- The independent variable is the one the experimenter manipulates (the thing that changes between the conditions the participants are placed into). It is assumed to have a direct effect on the dependent variable.
- The dependent variable is the thing being measured, or the results of the experiment.
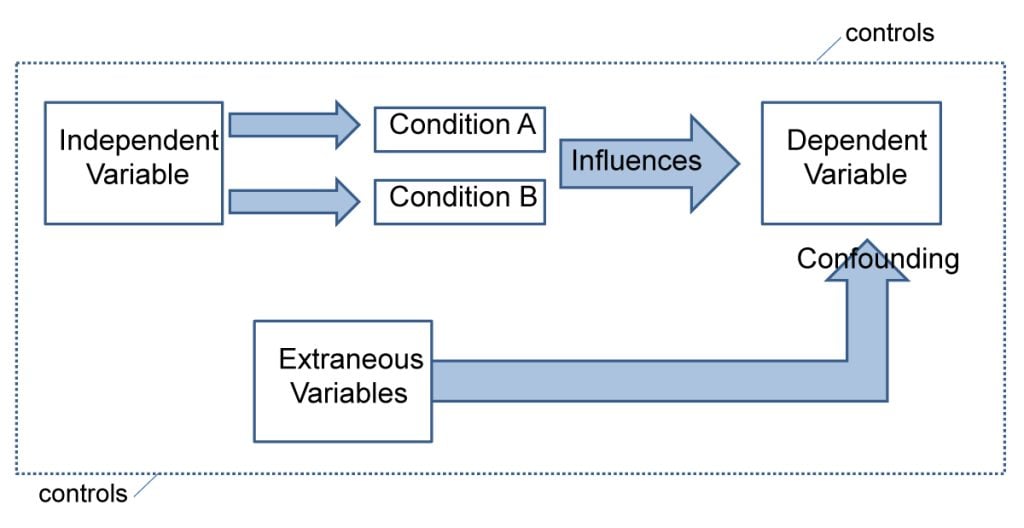
Operationalization of variables means making them measurable/quantifiable. We must use operationalization to ensure that variables are in a form that can be easily tested.
For instance, we can’t really measure ‘happiness’, but we can measure how many times a person smiles within a two-hour period.
By operationalizing variables, we make it easy for someone else to replicate our research. Remember, this is important because we can check if our findings are reliable.
Extraneous variables are all variables which are not independent variable but could affect the results of the experiment.
It can be a natural characteristic of the participant, such as intelligence levels, gender, or age for example, or it could be a situational feature of the environment such as lighting or noise.
Demand characteristics are a type of extraneous variable that occurs if the participants work out the aims of the research study, they may begin to behave in a certain way.
For example, in Milgram’s research , critics argued that participants worked out that the shocks were not real and they administered them as they thought this was what was required of them.
Extraneous variables must be controlled so that they do not affect (confound) the results.
Randomly allocating participants to their conditions or using a matched pairs experimental design can help to reduce participant variables.
Situational variables are controlled by using standardized procedures, ensuring every participant in a given condition is treated in the same way
Experimental Design
Experimental design refers to how participants are allocated to each condition of the independent variable, such as a control or experimental group.
- Independent design ( between-groups design ): each participant is selected for only one group. With the independent design, the most common way of deciding which participants go into which group is by means of randomization.
- Matched participants design : each participant is selected for only one group, but the participants in the two groups are matched for some relevant factor or factors (e.g. ability; sex; age).
- Repeated measures design ( within groups) : each participant appears in both groups, so that there are exactly the same participants in each group.
- The main problem with the repeated measures design is that there may well be order effects. Their experiences during the experiment may change the participants in various ways.
- They may perform better when they appear in the second group because they have gained useful information about the experiment or about the task. On the other hand, they may perform less well on the second occasion because of tiredness or boredom.
- Counterbalancing is the best way of preventing order effects from disrupting the findings of an experiment, and involves ensuring that each condition is equally likely to be used first and second by the participants.
If we wish to compare two groups with respect to a given independent variable, it is essential to make sure that the two groups do not differ in any other important way.
Experimental Methods
All experimental methods involve an iv (independent variable) and dv (dependent variable)..
- Field experiments are conducted in the everyday (natural) environment of the participants. The experimenter still manipulates the IV, but in a real-life setting. It may be possible to control extraneous variables, though such control is more difficult than in a lab experiment.
- Natural experiments are when a naturally occurring IV is investigated that isn’t deliberately manipulated, it exists anyway. Participants are not randomly allocated, and the natural event may only occur rarely.
Case studies are in-depth investigations of a person, group, event, or community. It uses information from a range of sources, such as from the person concerned and also from their family and friends.
Many techniques may be used such as interviews, psychological tests, observations and experiments. Case studies are generally longitudinal: in other words, they follow the individual or group over an extended period of time.
Case studies are widely used in psychology and among the best-known ones carried out were by Sigmund Freud . He conducted very detailed investigations into the private lives of his patients in an attempt to both understand and help them overcome their illnesses.
Case studies provide rich qualitative data and have high levels of ecological validity. However, it is difficult to generalize from individual cases as each one has unique characteristics.
Correlational Studies
Correlation means association; it is a measure of the extent to which two variables are related. One of the variables can be regarded as the predictor variable with the other one as the outcome variable.
Correlational studies typically involve obtaining two different measures from a group of participants, and then assessing the degree of association between the measures.
The predictor variable can be seen as occurring before the outcome variable in some sense. It is called the predictor variable, because it forms the basis for predicting the value of the outcome variable.
Relationships between variables can be displayed on a graph or as a numerical score called a correlation coefficient.
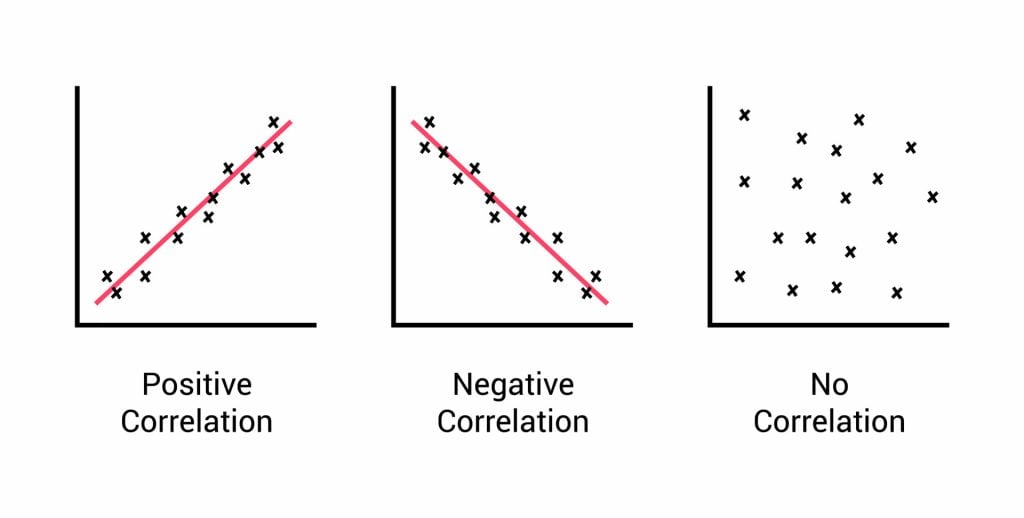
- If an increase in one variable tends to be associated with an increase in the other, then this is known as a positive correlation .
- If an increase in one variable tends to be associated with a decrease in the other, then this is known as a negative correlation .
- A zero correlation occurs when there is no relationship between variables.
After looking at the scattergraph, if we want to be sure that a significant relationship does exist between the two variables, a statistical test of correlation can be conducted, such as Spearman’s rho.
The test will give us a score, called a correlation coefficient . This is a value between 0 and 1, and the closer to 1 the score is, the stronger the relationship between the variables. This value can be both positive e.g. 0.63, or negative -0.63.
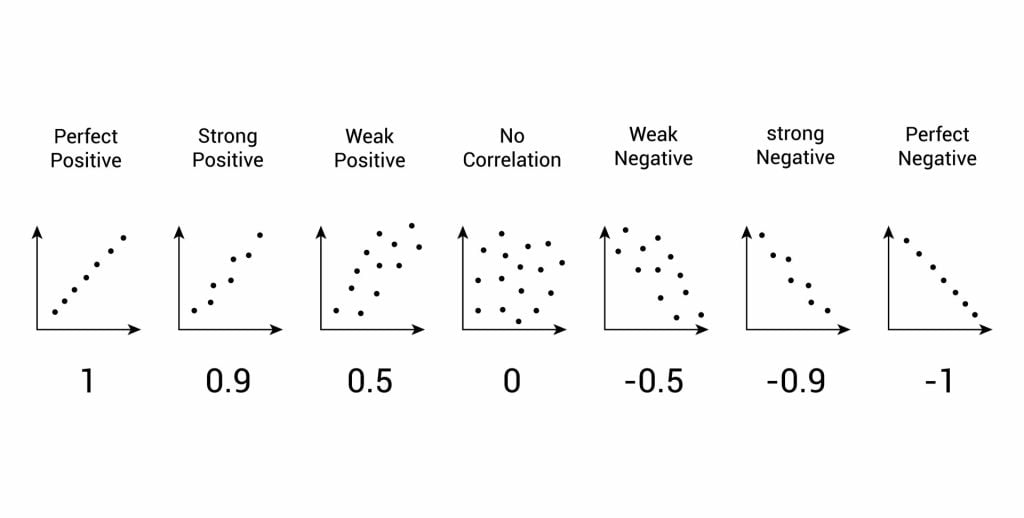
A correlation between variables, however, does not automatically mean that the change in one variable is the cause of the change in the values of the other variable. A correlation only shows if there is a relationship between variables.
Correlation does not always prove causation, as a third variable may be involved.
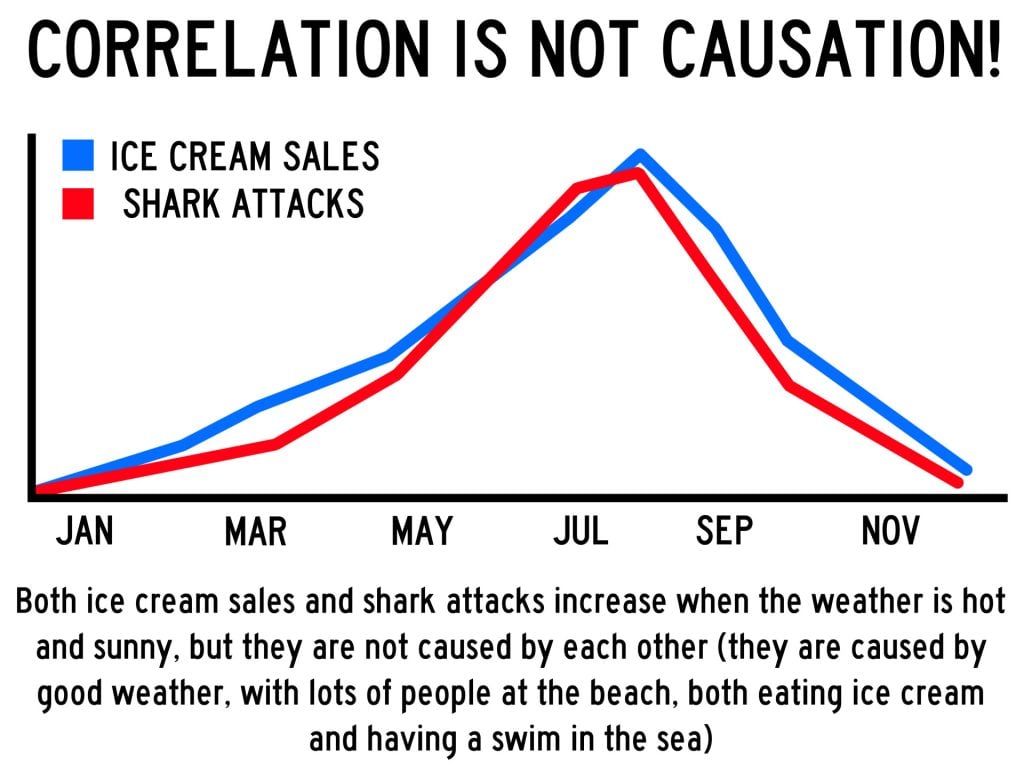
Interview Methods
Interviews are commonly divided into two types: structured and unstructured.
A fixed, predetermined set of questions is put to every participant in the same order and in the same way.
Responses are recorded on a questionnaire, and the researcher presets the order and wording of questions, and sometimes the range of alternative answers.
The interviewer stays within their role and maintains social distance from the interviewee.
There are no set questions, and the participant can raise whatever topics he/she feels are relevant and ask them in their own way. Questions are posed about participants’ answers to the subject
Unstructured interviews are most useful in qualitative research to analyze attitudes and values.
Though they rarely provide a valid basis for generalization, their main advantage is that they enable the researcher to probe social actors’ subjective point of view.
Questionnaire Method
Questionnaires can be thought of as a kind of written interview. They can be carried out face to face, by telephone, or post.
The choice of questions is important because of the need to avoid bias or ambiguity in the questions, ‘leading’ the respondent or causing offense.
- Open questions are designed to encourage a full, meaningful answer using the subject’s own knowledge and feelings. They provide insights into feelings, opinions, and understanding. Example: “How do you feel about that situation?”
- Closed questions can be answered with a simple “yes” or “no” or specific information, limiting the depth of response. They are useful for gathering specific facts or confirming details. Example: “Do you feel anxious in crowds?”
Its other practical advantages are that it is cheaper than face-to-face interviews and can be used to contact many respondents scattered over a wide area relatively quickly.
Observations
There are different types of observation methods :
- Covert observation is where the researcher doesn’t tell the participants they are being observed until after the study is complete. There could be ethical problems or deception and consent with this particular observation method.
- Overt observation is where a researcher tells the participants they are being observed and what they are being observed for.
- Controlled : behavior is observed under controlled laboratory conditions (e.g., Bandura’s Bobo doll study).
- Natural : Here, spontaneous behavior is recorded in a natural setting.
- Participant : Here, the observer has direct contact with the group of people they are observing. The researcher becomes a member of the group they are researching.
- Non-participant (aka “fly on the wall): The researcher does not have direct contact with the people being observed. The observation of participants’ behavior is from a distance
Pilot Study
A pilot study is a small scale preliminary study conducted in order to evaluate the feasibility of the key s teps in a future, full-scale project.
A pilot study is an initial run-through of the procedures to be used in an investigation; it involves selecting a few people and trying out the study on them. It is possible to save time, and in some cases, money, by identifying any flaws in the procedures designed by the researcher.
A pilot study can help the researcher spot any ambiguities (i.e. unusual things) or confusion in the information given to participants or problems with the task devised.
Sometimes the task is too hard, and the researcher may get a floor effect, because none of the participants can score at all or can complete the task – all performances are low.
The opposite effect is a ceiling effect, when the task is so easy that all achieve virtually full marks or top performances and are “hitting the ceiling”.
Research Design
In cross-sectional research , a researcher compares multiple segments of the population at the same time
Sometimes, we want to see how people change over time, as in studies of human development and lifespan. Longitudinal research is a research design in which data-gathering is administered repeatedly over an extended period of time.
In cohort studies , the participants must share a common factor or characteristic such as age, demographic, or occupation. A cohort study is a type of longitudinal study in which researchers monitor and observe a chosen population over an extended period.
Triangulation means using more than one research method to improve the study’s validity.
Reliability
Reliability is a measure of consistency, if a particular measurement is repeated and the same result is obtained then it is described as being reliable.
- Test-retest reliability : assessing the same person on two different occasions which shows the extent to which the test produces the same answers.
- Inter-observer reliability : the extent to which there is an agreement between two or more observers.
Meta-Analysis
A meta-analysis is a systematic review that involves identifying an aim and then searching for research studies that have addressed similar aims/hypotheses.
This is done by looking through various databases, and then decisions are made about what studies are to be included/excluded.
Strengths: Increases the conclusions’ validity as they’re based on a wider range.
Weaknesses: Research designs in studies can vary, so they are not truly comparable.
Peer Review
A researcher submits an article to a journal. The choice of the journal may be determined by the journal’s audience or prestige.
The journal selects two or more appropriate experts (psychologists working in a similar field) to peer review the article without payment. The peer reviewers assess: the methods and designs used, originality of the findings, the validity of the original research findings and its content, structure and language.
Feedback from the reviewer determines whether the article is accepted. The article may be: Accepted as it is, accepted with revisions, sent back to the author to revise and re-submit or rejected without the possibility of submission.
The editor makes the final decision whether to accept or reject the research report based on the reviewers comments/ recommendations.
Peer review is important because it prevent faulty data from entering the public domain, it provides a way of checking the validity of findings and the quality of the methodology and is used to assess the research rating of university departments.
Peer reviews may be an ideal, whereas in practice there are lots of problems. For example, it slows publication down and may prevent unusual, new work being published. Some reviewers might use it as an opportunity to prevent competing researchers from publishing work.
Some people doubt whether peer review can really prevent the publication of fraudulent research.
The advent of the internet means that a lot of research and academic comment is being published without official peer reviews than before, though systems are evolving on the internet where everyone really has a chance to offer their opinions and police the quality of research.
Types of Data
- Quantitative data is numerical data e.g. reaction time or number of mistakes. It represents how much or how long, how many there are of something. A tally of behavioral categories and closed questions in a questionnaire collect quantitative data.
- Qualitative data is virtually any type of information that can be observed and recorded that is not numerical in nature and can be in the form of written or verbal communication. Open questions in questionnaires and accounts from observational studies collect qualitative data.
- Primary data is first-hand data collected for the purpose of the investigation.
- Secondary data is information that has been collected by someone other than the person who is conducting the research e.g. taken from journals, books or articles.
Validity means how well a piece of research actually measures what it sets out to, or how well it reflects the reality it claims to represent.
Validity is whether the observed effect is genuine and represents what is actually out there in the world.
- Concurrent validity is the extent to which a psychological measure relates to an existing similar measure and obtains close results. For example, a new intelligence test compared to an established test.
- Face validity : does the test measure what it’s supposed to measure ‘on the face of it’. This is done by ‘eyeballing’ the measuring or by passing it to an expert to check.
- Ecological validit y is the extent to which findings from a research study can be generalized to other settings / real life.
- Temporal validity is the extent to which findings from a research study can be generalized to other historical times.
Features of Science
- Paradigm – A set of shared assumptions and agreed methods within a scientific discipline.
- Paradigm shift – The result of the scientific revolution: a significant change in the dominant unifying theory within a scientific discipline.
- Objectivity – When all sources of personal bias are minimised so not to distort or influence the research process.
- Empirical method – Scientific approaches that are based on the gathering of evidence through direct observation and experience.
- Replicability – The extent to which scientific procedures and findings can be repeated by other researchers.
- Falsifiability – The principle that a theory cannot be considered scientific unless it admits the possibility of being proved untrue.
Statistical Testing
A significant result is one where there is a low probability that chance factors were responsible for any observed difference, correlation, or association in the variables tested.
If our test is significant, we can reject our null hypothesis and accept our alternative hypothesis.
If our test is not significant, we can accept our null hypothesis and reject our alternative hypothesis. A null hypothesis is a statement of no effect.
In Psychology, we use p < 0.05 (as it strikes a balance between making a type I and II error) but p < 0.01 is used in tests that could cause harm like introducing a new drug.
A type I error is when the null hypothesis is rejected when it should have been accepted (happens when a lenient significance level is used, an error of optimism).
A type II error is when the null hypothesis is accepted when it should have been rejected (happens when a stringent significance level is used, an error of pessimism).
Ethical Issues
- Informed consent is when participants are able to make an informed judgment about whether to take part. It causes them to guess the aims of the study and change their behavior.
- To deal with it, we can gain presumptive consent or ask them to formally indicate their agreement to participate but it may invalidate the purpose of the study and it is not guaranteed that the participants would understand.
- Deception should only be used when it is approved by an ethics committee, as it involves deliberately misleading or withholding information. Participants should be fully debriefed after the study but debriefing can’t turn the clock back.
- All participants should be informed at the beginning that they have the right to withdraw if they ever feel distressed or uncomfortable.
- It causes bias as the ones that stayed are obedient and some may not withdraw as they may have been given incentives or feel like they’re spoiling the study. Researchers can offer the right to withdraw data after participation.
- Participants should all have protection from harm . The researcher should avoid risks greater than those experienced in everyday life and they should stop the study if any harm is suspected. However, the harm may not be apparent at the time of the study.
- Confidentiality concerns the communication of personal information. The researchers should not record any names but use numbers or false names though it may not be possible as it is sometimes possible to work out who the researchers were.

Related Articles
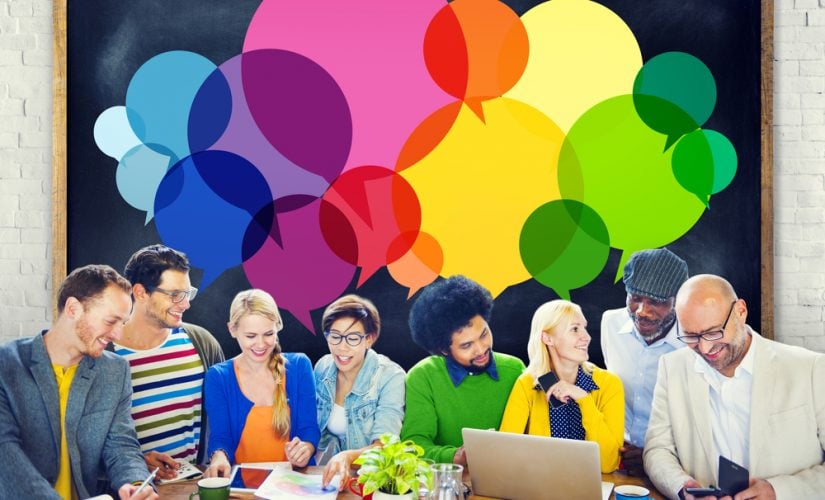
Research Methodology
Conversation Analysis
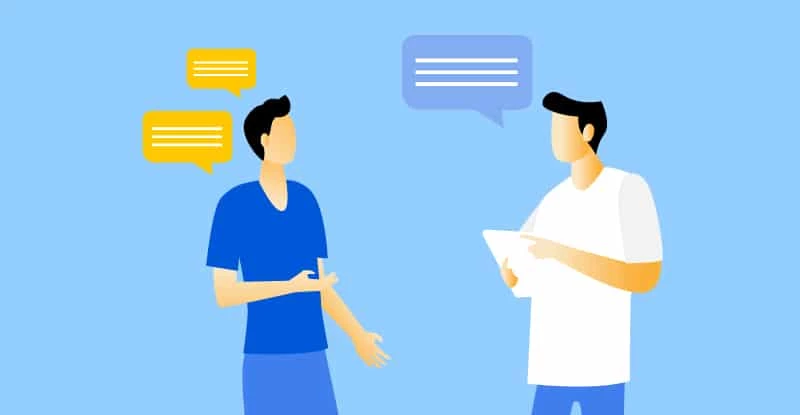
Discourse Analysis
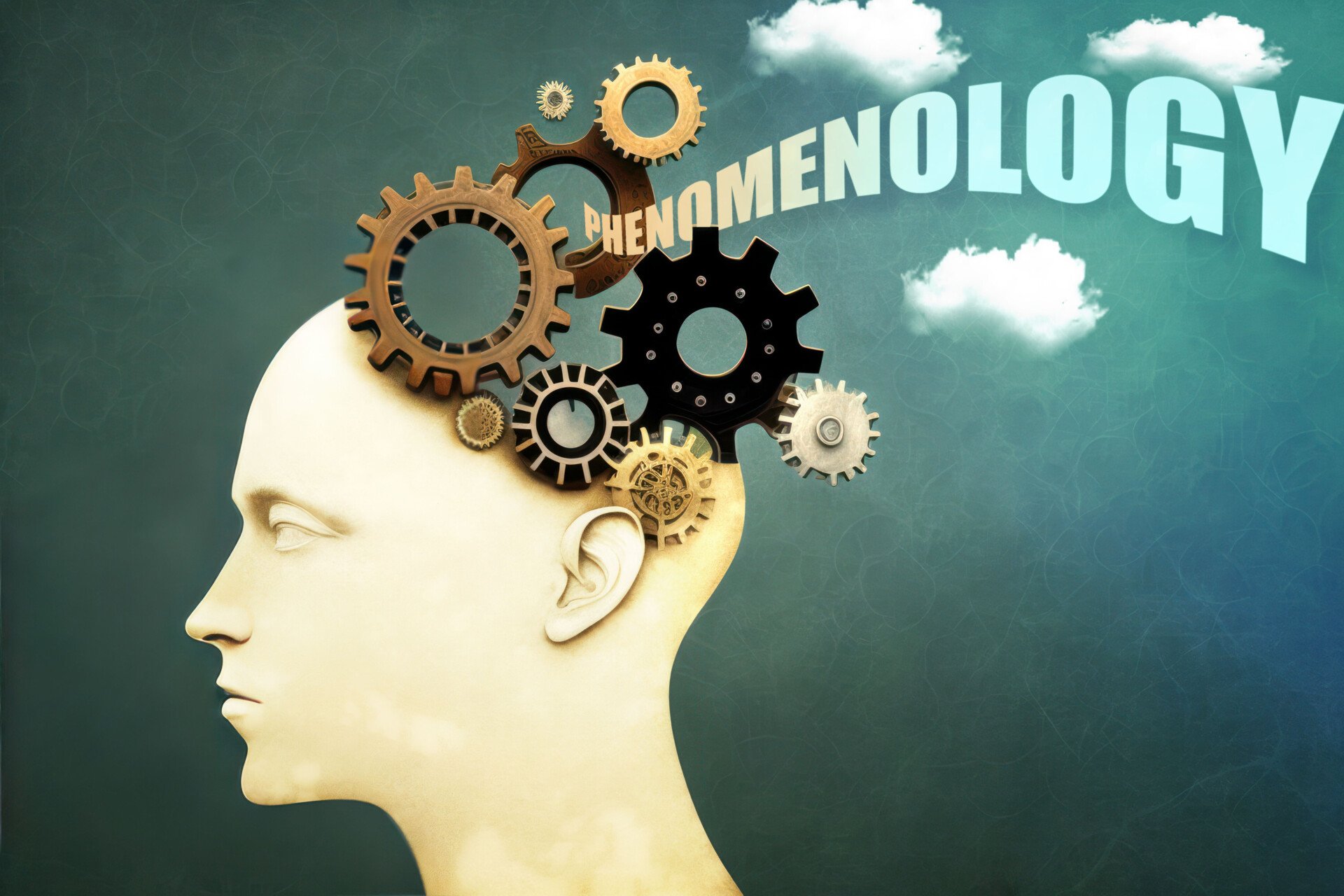
Phenomenology In Qualitative Research
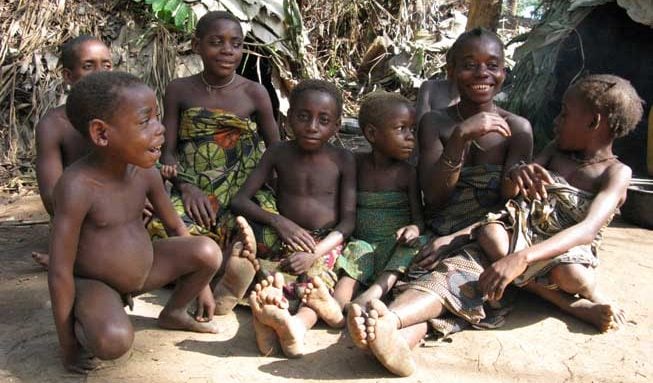
Ethnography In Qualitative Research
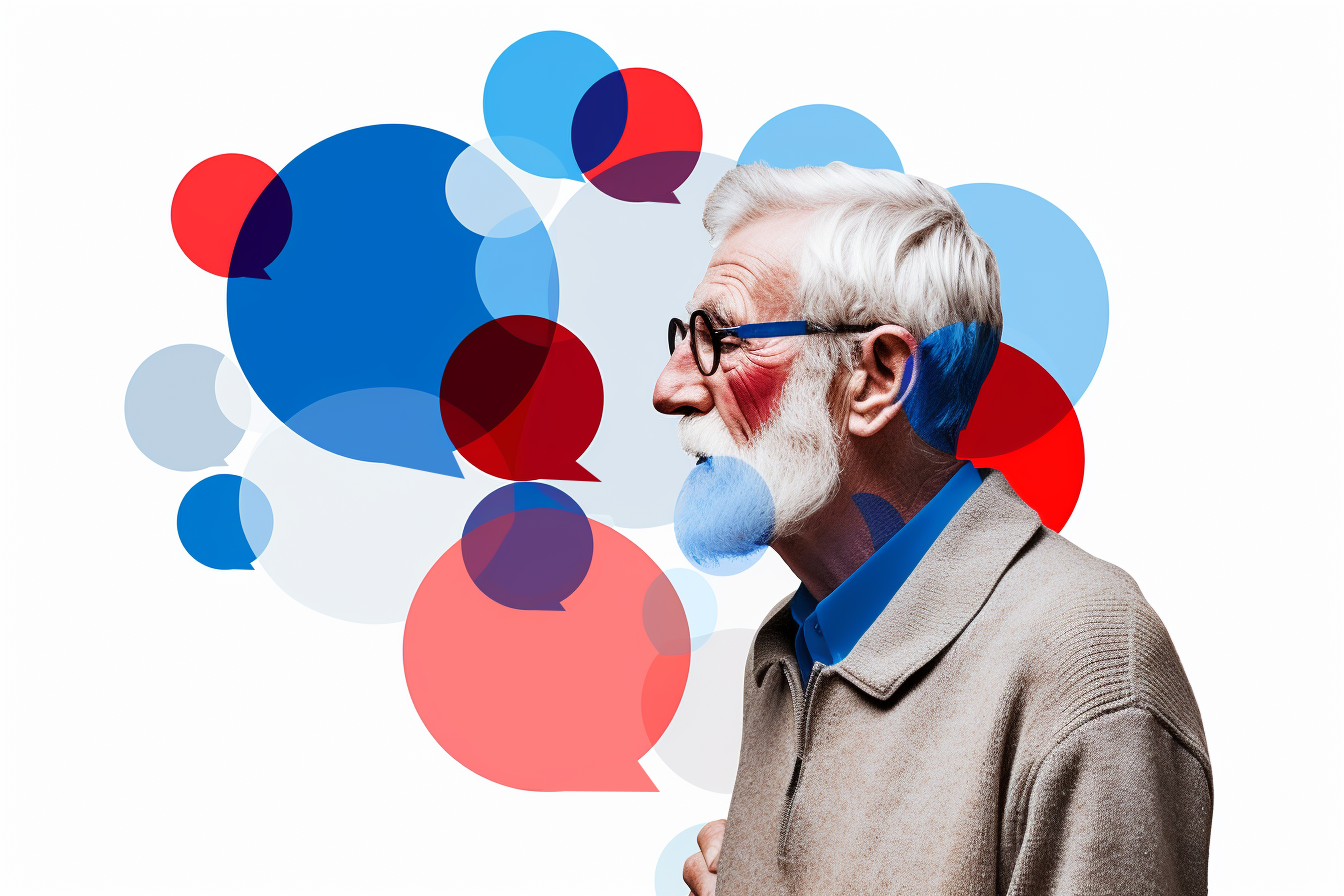
Narrative Analysis In Qualitative Research
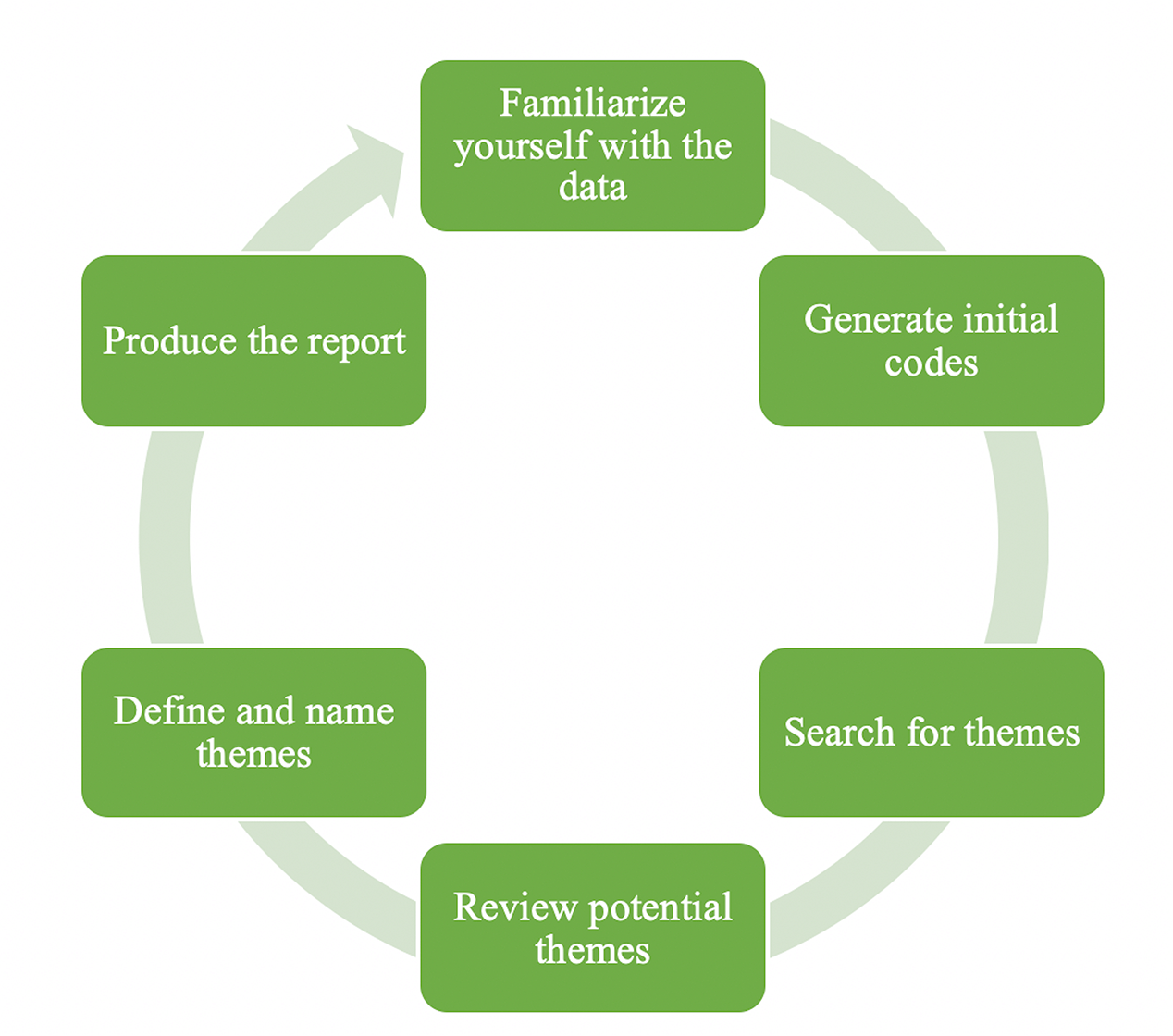
Thematic Analysis: A Step by Step Guide

Want to create or adapt books like this? Learn more about how Pressbooks supports open publishing practices.
2.2 Psychologists Use Descriptive, Correlational, and Experimental Research Designs to Understand Behavior
Learning objectives.
- Differentiate the goals of descriptive, correlational, and experimental research designs and explain the advantages and disadvantages of each.
- Explain the goals of descriptive research and the statistical techniques used to interpret it.
- Summarize the uses of correlational research and describe why correlational research cannot be used to infer causality.
- Review the procedures of experimental research and explain how it can be used to draw causal inferences.
Psychologists agree that if their ideas and theories about human behavior are to be taken seriously, they must be backed up by data. However, the research of different psychologists is designed with different goals in mind, and the different goals require different approaches. These varying approaches, summarized in Table 2.2 “Characteristics of the Three Research Designs” , are known as research designs . A research design is the specific method a researcher uses to collect, analyze, and interpret data . Psychologists use three major types of research designs in their research, and each provides an essential avenue for scientific investigation. Descriptive research is research designed to provide a snapshot of the current state of affairs . Correlational research is research designed to discover relationships among variables and to allow the prediction of future events from present knowledge . Experimental research is research in which initial equivalence among research participants in more than one group is created, followed by a manipulation of a given experience for these groups and a measurement of the influence of the manipulation . Each of the three research designs varies according to its strengths and limitations, and it is important to understand how each differs.
Table 2.2 Characteristics of the Three Research Designs
Research design | Goal | Advantages | Disadvantages |
---|---|---|---|
Descriptive | To create a snapshot of the current state of affairs | Provides a relatively complete picture of what is occurring at a given time. Allows the development of questions for further study. | Does not assess relationships among variables. May be unethical if participants do not know they are being observed. |
Correlational | To assess the relationships between and among two or more variables | Allows testing of expected relationships between and among variables and the making of predictions. Can assess these relationships in everyday life events. | Cannot be used to draw inferences about the causal relationships between and among the variables. |
Experimental | To assess the causal impact of one or more experimental manipulations on a dependent variable | Allows drawing of conclusions about the causal relationships among variables. | Cannot experimentally manipulate many important variables. May be expensive and time consuming. |
There are three major research designs used by psychologists, and each has its own advantages and disadvantages. |
Stangor, C. (2011). Research methods for the behavioral sciences (4th ed.). Mountain View, CA: Cengage.
Descriptive Research: Assessing the Current State of Affairs
Descriptive research is designed to create a snapshot of the current thoughts, feelings, or behavior of individuals. This section reviews three types of descriptive research: case studies , surveys , and naturalistic observation .
Sometimes the data in a descriptive research project are based on only a small set of individuals, often only one person or a single small group. These research designs are known as case studies — descriptive records of one or more individual’s experiences and behavior . Sometimes case studies involve ordinary individuals, as when developmental psychologist Jean Piaget used his observation of his own children to develop his stage theory of cognitive development. More frequently, case studies are conducted on individuals who have unusual or abnormal experiences or characteristics or who find themselves in particularly difficult or stressful situations. The assumption is that by carefully studying individuals who are socially marginal, who are experiencing unusual situations, or who are going through a difficult phase in their lives, we can learn something about human nature.
Sigmund Freud was a master of using the psychological difficulties of individuals to draw conclusions about basic psychological processes. Freud wrote case studies of some of his most interesting patients and used these careful examinations to develop his important theories of personality. One classic example is Freud’s description of “Little Hans,” a child whose fear of horses the psychoanalyst interpreted in terms of repressed sexual impulses and the Oedipus complex (Freud (1909/1964).

Political polls reported in newspapers and on the Internet are descriptive research designs that provide snapshots of the likely voting behavior of a population.
Another well-known case study is Phineas Gage, a man whose thoughts and emotions were extensively studied by cognitive psychologists after a railroad spike was blasted through his skull in an accident. Although there is question about the interpretation of this case study (Kotowicz, 2007), it did provide early evidence that the brain’s frontal lobe is involved in emotion and morality (Damasio et al., 2005). An interesting example of a case study in clinical psychology is described by Rokeach (1964), who investigated in detail the beliefs and interactions among three patients with schizophrenia, all of whom were convinced they were Jesus Christ.
In other cases the data from descriptive research projects come in the form of a survey — a measure administered through either an interview or a written questionnaire to get a picture of the beliefs or behaviors of a sample of people of interest . The people chosen to participate in the research (known as the sample ) are selected to be representative of all the people that the researcher wishes to know about (the population ). In election polls, for instance, a sample is taken from the population of all “likely voters” in the upcoming elections.
The results of surveys may sometimes be rather mundane, such as “Nine out of ten doctors prefer Tymenocin,” or “The median income in Montgomery County is $36,712.” Yet other times (particularly in discussions of social behavior), the results can be shocking: “More than 40,000 people are killed by gunfire in the United States every year,” or “More than 60% of women between the ages of 50 and 60 suffer from depression.” Descriptive research is frequently used by psychologists to get an estimate of the prevalence (or incidence ) of psychological disorders.
A final type of descriptive research—known as naturalistic observation —is research based on the observation of everyday events . For instance, a developmental psychologist who watches children on a playground and describes what they say to each other while they play is conducting descriptive research, as is a biopsychologist who observes animals in their natural habitats. One example of observational research involves a systematic procedure known as the strange situation , used to get a picture of how adults and young children interact. The data that are collected in the strange situation are systematically coded in a coding sheet such as that shown in Table 2.3 “Sample Coding Form Used to Assess Child’s and Mother’s Behavior in the Strange Situation” .
Table 2.3 Sample Coding Form Used to Assess Child’s and Mother’s Behavior in the Strange Situation
Coder name: | ||||
---|---|---|---|---|
Mother and baby play alone | ||||
Mother puts baby down | ||||
Stranger enters room | ||||
Mother leaves room; stranger plays with baby | ||||
Mother reenters, greets and may comfort baby, then leaves again | ||||
Stranger tries to play with baby | ||||
Mother reenters and picks up baby | ||||
The baby moves toward, grasps, or climbs on the adult. | ||||
The baby resists being put down by the adult by crying or trying to climb back up. | ||||
The baby pushes, hits, or squirms to be put down from the adult’s arms. | ||||
The baby turns away or moves away from the adult. | ||||
This table represents a sample coding sheet from an episode of the “strange situation,” in which an infant (usually about 1 year old) is observed playing in a room with two adults—the child’s mother and a stranger. Each of the four coding categories is scored by the coder from 1 (the baby makes no effort to engage in the behavior) to 7 (the baby makes a significant effort to engage in the behavior). More information about the meaning of the coding can be found in Ainsworth, Blehar, Waters, and Wall (1978). |
The results of descriptive research projects are analyzed using descriptive statistics — numbers that summarize the distribution of scores on a measured variable . Most variables have distributions similar to that shown in Figure 2.5 “Height Distribution” , where most of the scores are located near the center of the distribution, and the distribution is symmetrical and bell-shaped. A data distribution that is shaped like a bell is known as a normal distribution .
Table 2.4 Height and Family Income for 25 Students
Student name | Height in inches | Family income in dollars |
---|---|---|
Lauren | 62 | 48,000 |
Courtnie | 62 | 57,000 |
Leslie | 63 | 93,000 |
Renee | 64 | 107,000 |
Katherine | 64 | 110,000 |
Jordan | 65 | 93,000 |
Rabiah | 66 | 46,000 |
Alina | 66 | 84,000 |
Young Su | 67 | 68,000 |
Martin | 67 | 49,000 |
Hanzhu | 67 | 73,000 |
Caitlin | 67 | 3,800,000 |
Steven | 67 | 107,000 |
Emily | 67 | 64,000 |
Amy | 68 | 67,000 |
Jonathan | 68 | 51,000 |
Julian | 68 | 48,000 |
Alissa | 68 | 93,000 |
Christine | 69 | 93,000 |
Candace | 69 | 111,000 |
Xiaohua | 69 | 56,000 |
Charlie | 70 | 94,000 |
Timothy | 71 | 73,000 |
Ariane | 72 | 70,000 |
Logan | 72 | 44,000 |
Figure 2.5 Height Distribution
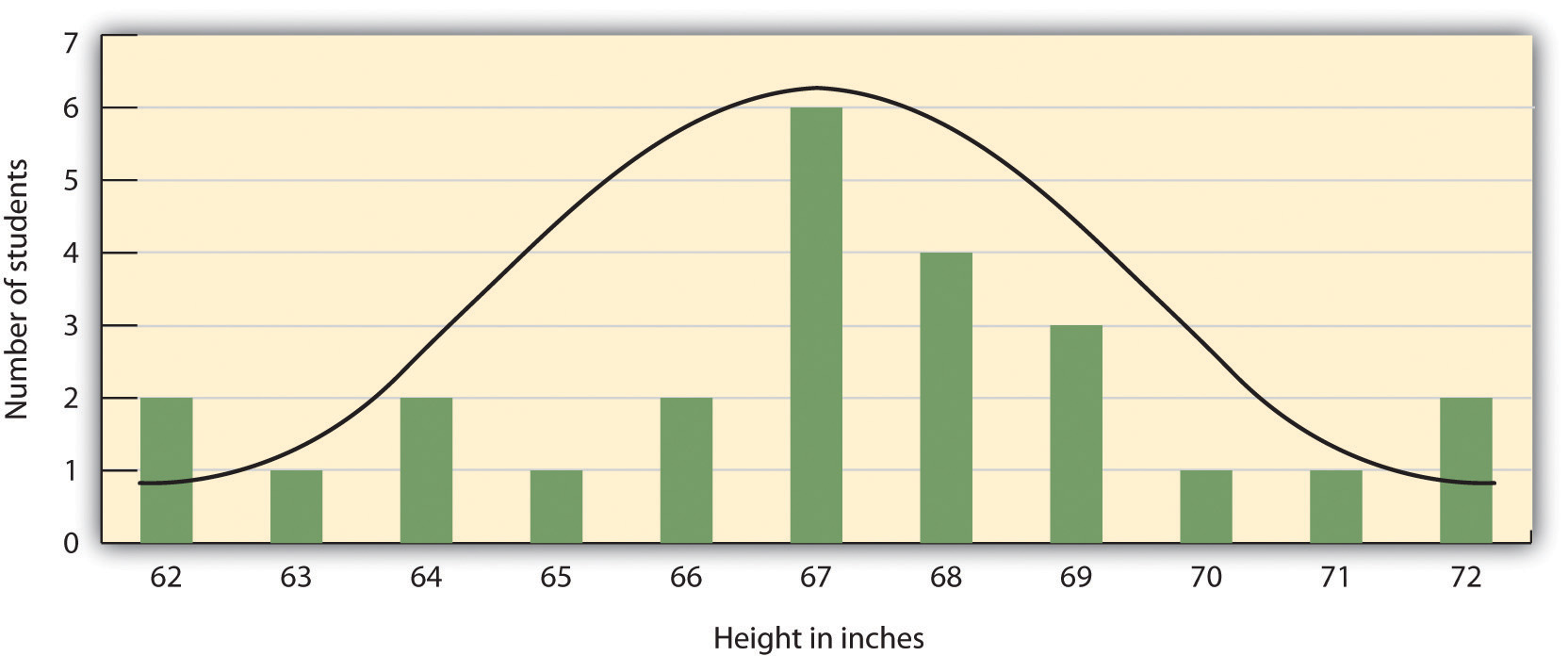
The distribution of the heights of the students in a class will form a normal distribution. In this sample the mean ( M ) = 67.12 and the standard deviation ( s ) = 2.74.
A distribution can be described in terms of its central tendency —that is, the point in the distribution around which the data are centered—and its dispersion , or spread. The arithmetic average, or arithmetic mean , is the most commonly used measure of central tendency . It is computed by calculating the sum of all the scores of the variable and dividing this sum by the number of participants in the distribution (denoted by the letter N ). In the data presented in Figure 2.5 “Height Distribution” , the mean height of the students is 67.12 inches. The sample mean is usually indicated by the letter M .
In some cases, however, the data distribution is not symmetrical. This occurs when there are one or more extreme scores (known as outliers ) at one end of the distribution. Consider, for instance, the variable of family income (see Figure 2.6 “Family Income Distribution” ), which includes an outlier (a value of $3,800,000). In this case the mean is not a good measure of central tendency. Although it appears from Figure 2.6 “Family Income Distribution” that the central tendency of the family income variable should be around $70,000, the mean family income is actually $223,960. The single very extreme income has a disproportionate impact on the mean, resulting in a value that does not well represent the central tendency.
The median is used as an alternative measure of central tendency when distributions are not symmetrical. The median is the score in the center of the distribution, meaning that 50% of the scores are greater than the median and 50% of the scores are less than the median . In our case, the median household income ($73,000) is a much better indication of central tendency than is the mean household income ($223,960).
Figure 2.6 Family Income Distribution
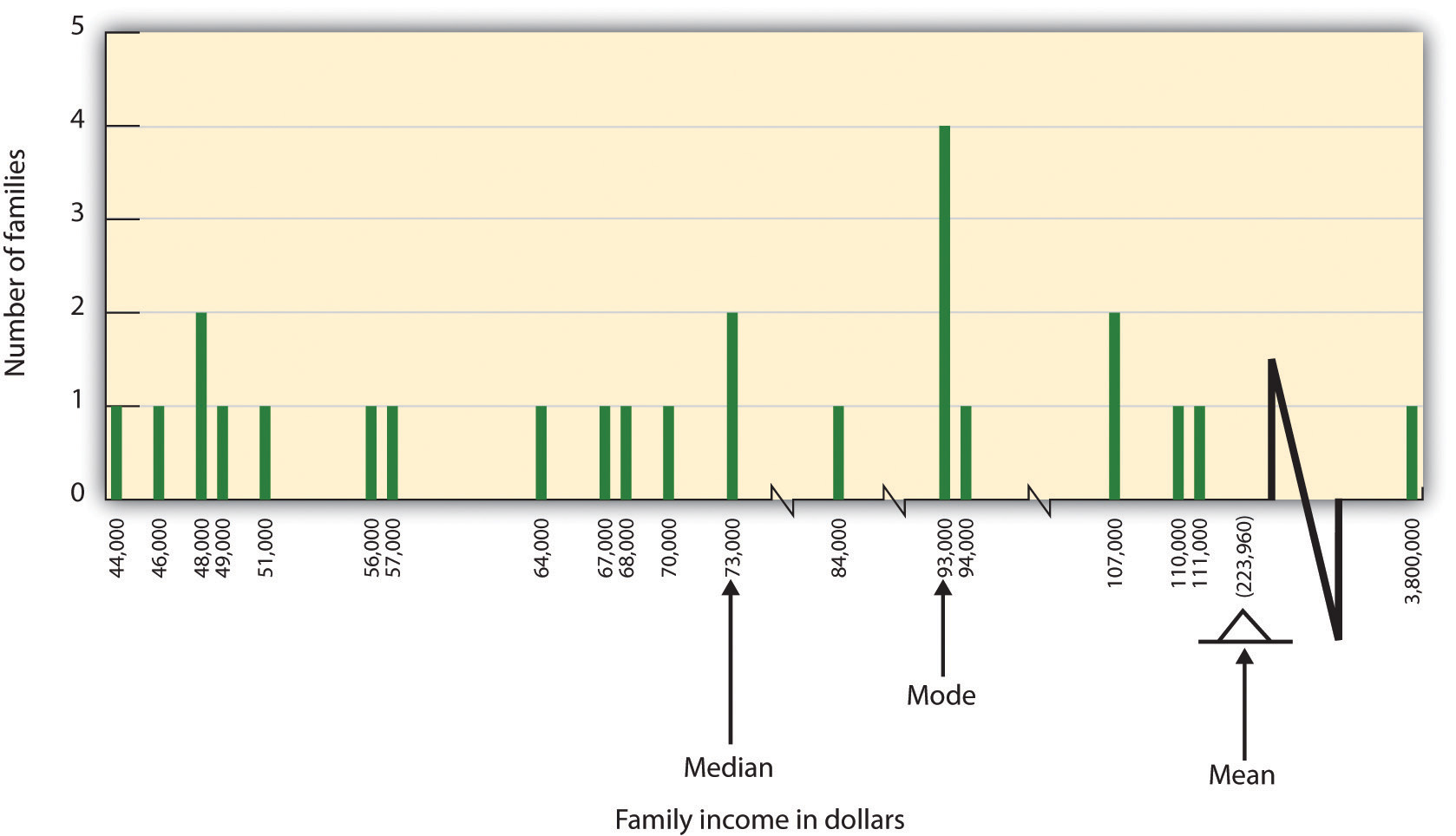
The distribution of family incomes is likely to be nonsymmetrical because some incomes can be very large in comparison to most incomes. In this case the median or the mode is a better indicator of central tendency than is the mean.
A final measure of central tendency, known as the mode , represents the value that occurs most frequently in the distribution . You can see from Figure 2.6 “Family Income Distribution” that the mode for the family income variable is $93,000 (it occurs four times).
In addition to summarizing the central tendency of a distribution, descriptive statistics convey information about how the scores of the variable are spread around the central tendency. Dispersion refers to the extent to which the scores are all tightly clustered around the central tendency, like this:
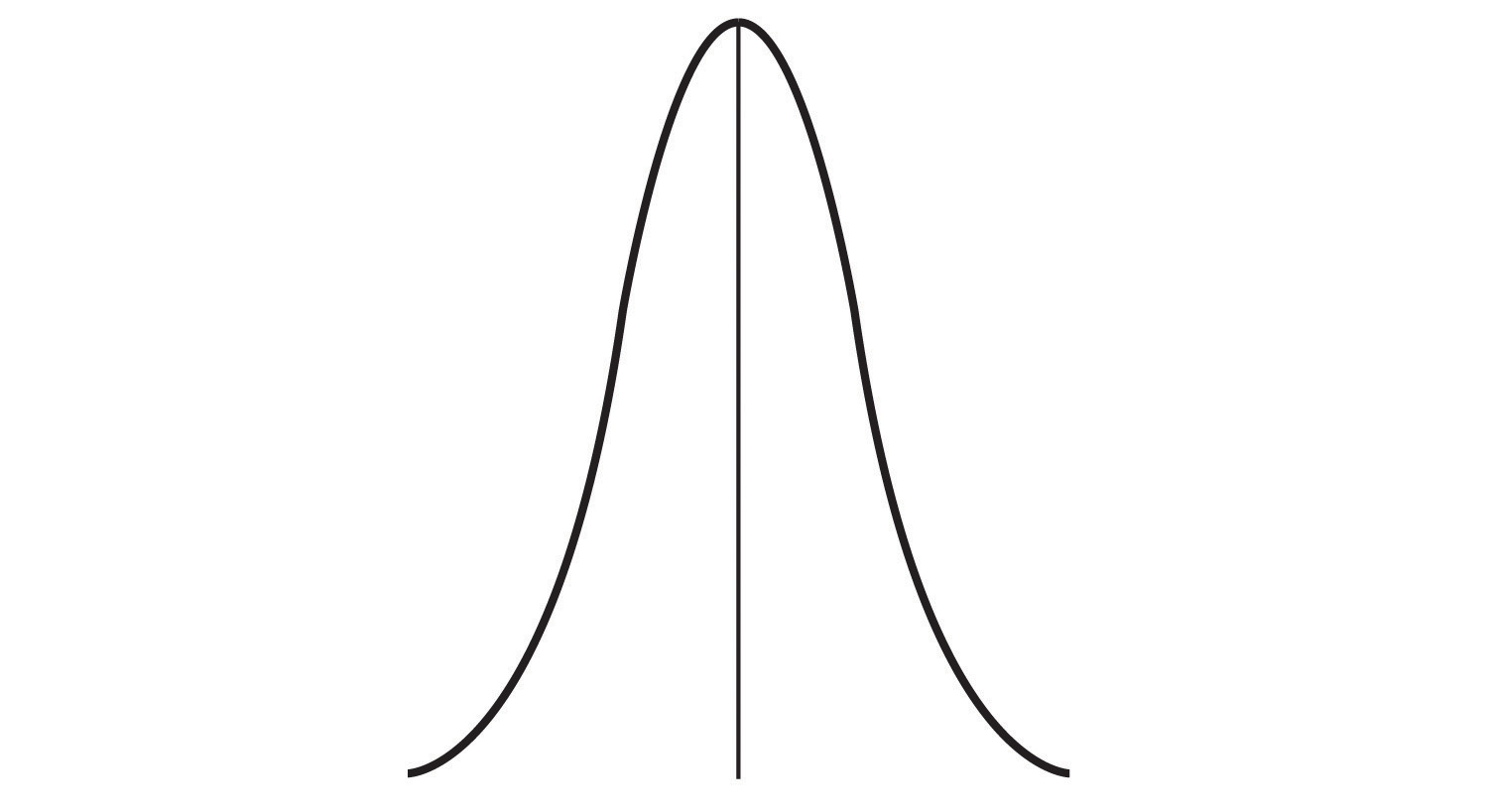
Or they may be more spread out away from it, like this:
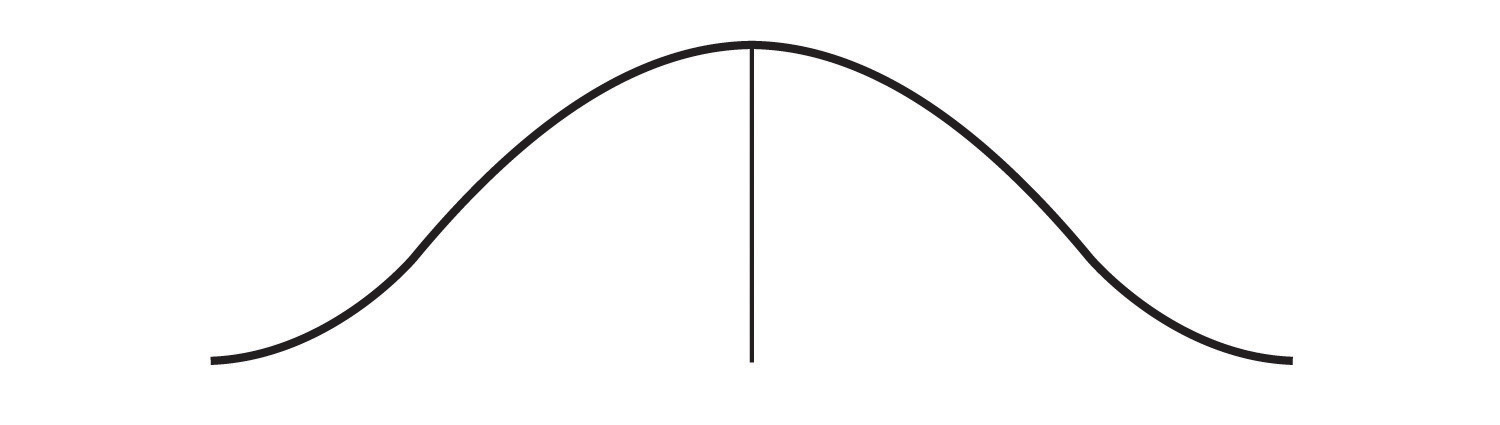
One simple measure of dispersion is to find the largest (the maximum ) and the smallest (the minimum ) observed values of the variable and to compute the range of the variable as the maximum observed score minus the minimum observed score. You can check that the range of the height variable in Figure 2.5 “Height Distribution” is 72 – 62 = 10. The standard deviation , symbolized as s , is the most commonly used measure of dispersion . Distributions with a larger standard deviation have more spread. The standard deviation of the height variable is s = 2.74, and the standard deviation of the family income variable is s = $745,337.
An advantage of descriptive research is that it attempts to capture the complexity of everyday behavior. Case studies provide detailed information about a single person or a small group of people, surveys capture the thoughts or reported behaviors of a large population of people, and naturalistic observation objectively records the behavior of people or animals as it occurs naturally. Thus descriptive research is used to provide a relatively complete understanding of what is currently happening.
Despite these advantages, descriptive research has a distinct disadvantage in that, although it allows us to get an idea of what is currently happening, it is usually limited to static pictures. Although descriptions of particular experiences may be interesting, they are not always transferable to other individuals in other situations, nor do they tell us exactly why specific behaviors or events occurred. For instance, descriptions of individuals who have suffered a stressful event, such as a war or an earthquake, can be used to understand the individuals’ reactions to the event but cannot tell us anything about the long-term effects of the stress. And because there is no comparison group that did not experience the stressful situation, we cannot know what these individuals would be like if they hadn’t had the stressful experience.
Correlational Research: Seeking Relationships Among Variables
In contrast to descriptive research, which is designed primarily to provide static pictures, correlational research involves the measurement of two or more relevant variables and an assessment of the relationship between or among those variables. For instance, the variables of height and weight are systematically related (correlated) because taller people generally weigh more than shorter people. In the same way, study time and memory errors are also related, because the more time a person is given to study a list of words, the fewer errors he or she will make. When there are two variables in the research design, one of them is called the predictor variable and the other the outcome variable . The research design can be visualized like this, where the curved arrow represents the expected correlation between the two variables:
Figure 2.2.2

One way of organizing the data from a correlational study with two variables is to graph the values of each of the measured variables using a scatter plot . As you can see in Figure 2.10 “Examples of Scatter Plots” , a scatter plot is a visual image of the relationship between two variables . A point is plotted for each individual at the intersection of his or her scores for the two variables. When the association between the variables on the scatter plot can be easily approximated with a straight line, as in parts (a) and (b) of Figure 2.10 “Examples of Scatter Plots” , the variables are said to have a linear relationship .
When the straight line indicates that individuals who have above-average values for one variable also tend to have above-average values for the other variable, as in part (a), the relationship is said to be positive linear . Examples of positive linear relationships include those between height and weight, between education and income, and between age and mathematical abilities in children. In each case people who score higher on one of the variables also tend to score higher on the other variable. Negative linear relationships , in contrast, as shown in part (b), occur when above-average values for one variable tend to be associated with below-average values for the other variable. Examples of negative linear relationships include those between the age of a child and the number of diapers the child uses, and between practice on and errors made on a learning task. In these cases people who score higher on one of the variables tend to score lower on the other variable.
Relationships between variables that cannot be described with a straight line are known as nonlinear relationships . Part (c) of Figure 2.10 “Examples of Scatter Plots” shows a common pattern in which the distribution of the points is essentially random. In this case there is no relationship at all between the two variables, and they are said to be independent . Parts (d) and (e) of Figure 2.10 “Examples of Scatter Plots” show patterns of association in which, although there is an association, the points are not well described by a single straight line. For instance, part (d) shows the type of relationship that frequently occurs between anxiety and performance. Increases in anxiety from low to moderate levels are associated with performance increases, whereas increases in anxiety from moderate to high levels are associated with decreases in performance. Relationships that change in direction and thus are not described by a single straight line are called curvilinear relationships .
Figure 2.10 Examples of Scatter Plots
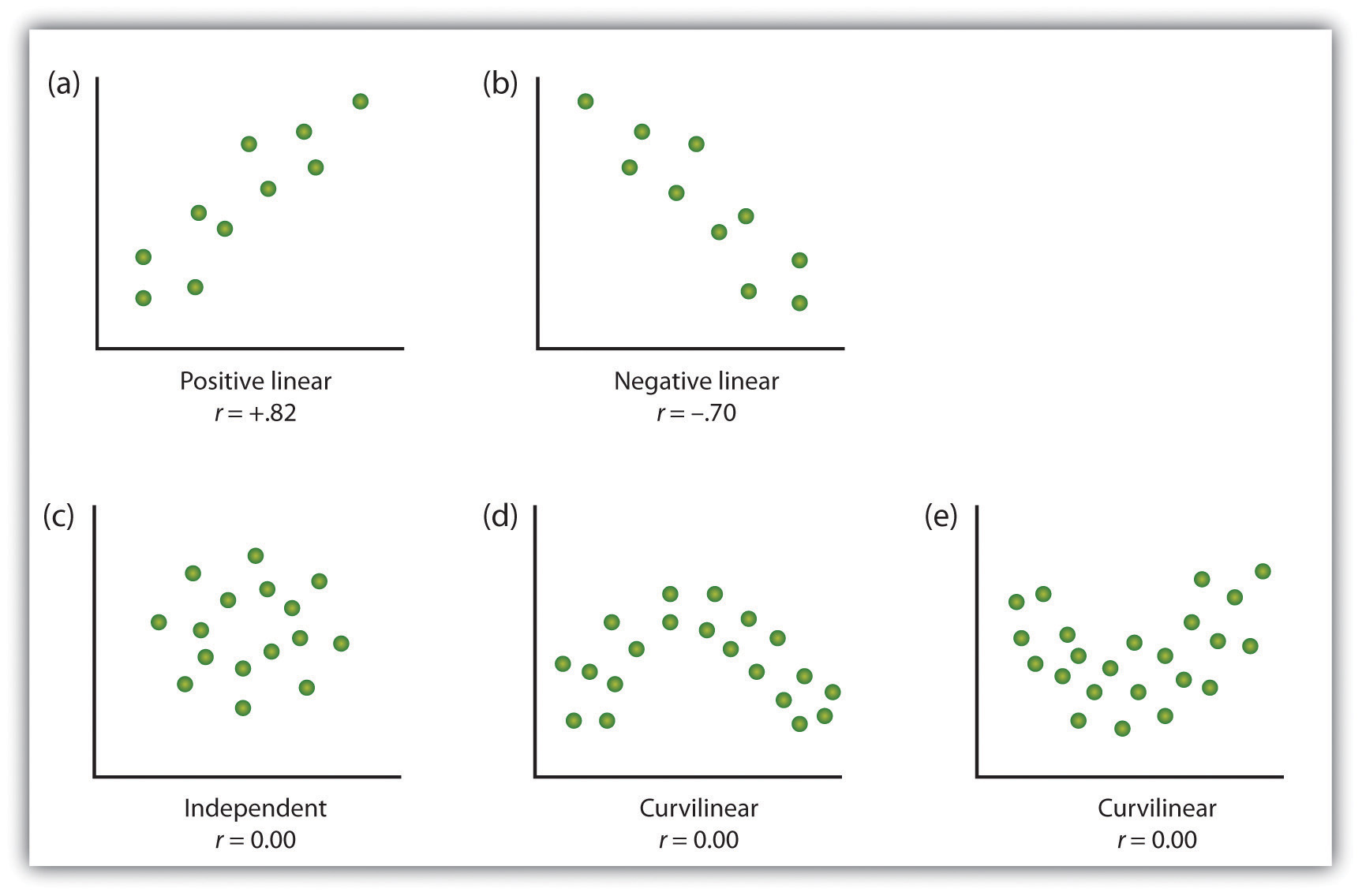
Some examples of relationships between two variables as shown in scatter plots. Note that the Pearson correlation coefficient ( r ) between variables that have curvilinear relationships will likely be close to zero.
Adapted from Stangor, C. (2011). Research methods for the behavioral sciences (4th ed.). Mountain View, CA: Cengage.
The most common statistical measure of the strength of linear relationships among variables is the Pearson correlation coefficient , which is symbolized by the letter r . The value of the correlation coefficient ranges from r = –1.00 to r = +1.00. The direction of the linear relationship is indicated by the sign of the correlation coefficient. Positive values of r (such as r = .54 or r = .67) indicate that the relationship is positive linear (i.e., the pattern of the dots on the scatter plot runs from the lower left to the upper right), whereas negative values of r (such as r = –.30 or r = –.72) indicate negative linear relationships (i.e., the dots run from the upper left to the lower right). The strength of the linear relationship is indexed by the distance of the correlation coefficient from zero (its absolute value). For instance, r = –.54 is a stronger relationship than r = .30, and r = .72 is a stronger relationship than r = –.57. Because the Pearson correlation coefficient only measures linear relationships, variables that have curvilinear relationships are not well described by r , and the observed correlation will be close to zero.
It is also possible to study relationships among more than two measures at the same time. A research design in which more than one predictor variable is used to predict a single outcome variable is analyzed through multiple regression (Aiken & West, 1991). Multiple regression is a statistical technique, based on correlation coefficients among variables, that allows predicting a single outcome variable from more than one predictor variable . For instance, Figure 2.11 “Prediction of Job Performance From Three Predictor Variables” shows a multiple regression analysis in which three predictor variables are used to predict a single outcome. The use of multiple regression analysis shows an important advantage of correlational research designs—they can be used to make predictions about a person’s likely score on an outcome variable (e.g., job performance) based on knowledge of other variables.
Figure 2.11 Prediction of Job Performance From Three Predictor Variables
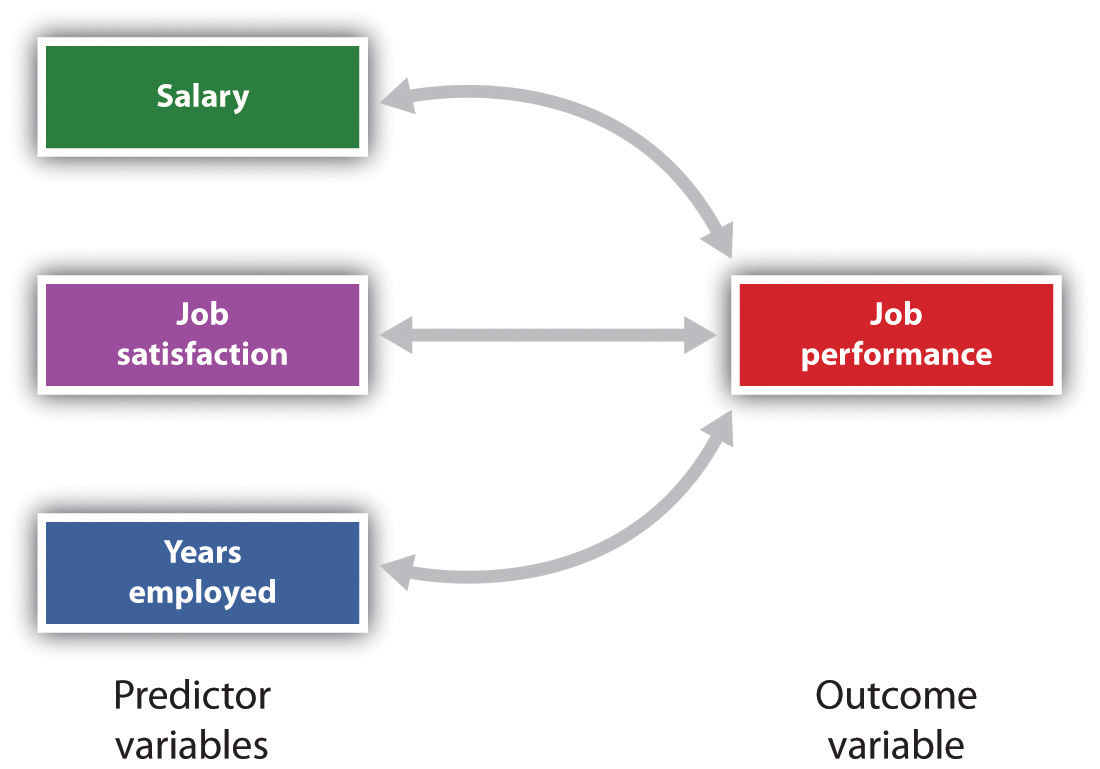
Multiple regression allows scientists to predict the scores on a single outcome variable using more than one predictor variable.
An important limitation of correlational research designs is that they cannot be used to draw conclusions about the causal relationships among the measured variables. Consider, for instance, a researcher who has hypothesized that viewing violent behavior will cause increased aggressive play in children. He has collected, from a sample of fourth-grade children, a measure of how many violent television shows each child views during the week, as well as a measure of how aggressively each child plays on the school playground. From his collected data, the researcher discovers a positive correlation between the two measured variables.
Although this positive correlation appears to support the researcher’s hypothesis, it cannot be taken to indicate that viewing violent television causes aggressive behavior. Although the researcher is tempted to assume that viewing violent television causes aggressive play,

there are other possibilities. One alternate possibility is that the causal direction is exactly opposite from what has been hypothesized. Perhaps children who have behaved aggressively at school develop residual excitement that leads them to want to watch violent television shows at home:

Although this possibility may seem less likely, there is no way to rule out the possibility of such reverse causation on the basis of this observed correlation. It is also possible that both causal directions are operating and that the two variables cause each other:

Still another possible explanation for the observed correlation is that it has been produced by the presence of a common-causal variable (also known as a third variable ). A common-causal variable is a variable that is not part of the research hypothesis but that causes both the predictor and the outcome variable and thus produces the observed correlation between them . In our example a potential common-causal variable is the discipline style of the children’s parents. Parents who use a harsh and punitive discipline style may produce children who both like to watch violent television and who behave aggressively in comparison to children whose parents use less harsh discipline:

In this case, television viewing and aggressive play would be positively correlated (as indicated by the curved arrow between them), even though neither one caused the other but they were both caused by the discipline style of the parents (the straight arrows). When the predictor and outcome variables are both caused by a common-causal variable, the observed relationship between them is said to be spurious . A spurious relationship is a relationship between two variables in which a common-causal variable produces and “explains away” the relationship . If effects of the common-causal variable were taken away, or controlled for, the relationship between the predictor and outcome variables would disappear. In the example the relationship between aggression and television viewing might be spurious because by controlling for the effect of the parents’ disciplining style, the relationship between television viewing and aggressive behavior might go away.
Common-causal variables in correlational research designs can be thought of as “mystery” variables because, as they have not been measured, their presence and identity are usually unknown to the researcher. Since it is not possible to measure every variable that could cause both the predictor and outcome variables, the existence of an unknown common-causal variable is always a possibility. For this reason, we are left with the basic limitation of correlational research: Correlation does not demonstrate causation. It is important that when you read about correlational research projects, you keep in mind the possibility of spurious relationships, and be sure to interpret the findings appropriately. Although correlational research is sometimes reported as demonstrating causality without any mention being made of the possibility of reverse causation or common-causal variables, informed consumers of research, like you, are aware of these interpretational problems.
In sum, correlational research designs have both strengths and limitations. One strength is that they can be used when experimental research is not possible because the predictor variables cannot be manipulated. Correlational designs also have the advantage of allowing the researcher to study behavior as it occurs in everyday life. And we can also use correlational designs to make predictions—for instance, to predict from the scores on their battery of tests the success of job trainees during a training session. But we cannot use such correlational information to determine whether the training caused better job performance. For that, researchers rely on experiments.
Experimental Research: Understanding the Causes of Behavior
The goal of experimental research design is to provide more definitive conclusions about the causal relationships among the variables in the research hypothesis than is available from correlational designs. In an experimental research design, the variables of interest are called the independent variable (or variables ) and the dependent variable . The independent variable in an experiment is the causing variable that is created (manipulated) by the experimenter . The dependent variable in an experiment is a measured variable that is expected to be influenced by the experimental manipulation . The research hypothesis suggests that the manipulated independent variable or variables will cause changes in the measured dependent variables. We can diagram the research hypothesis by using an arrow that points in one direction. This demonstrates the expected direction of causality:
Figure 2.2.3

Research Focus: Video Games and Aggression
Consider an experiment conducted by Anderson and Dill (2000). The study was designed to test the hypothesis that viewing violent video games would increase aggressive behavior. In this research, male and female undergraduates from Iowa State University were given a chance to play with either a violent video game (Wolfenstein 3D) or a nonviolent video game (Myst). During the experimental session, the participants played their assigned video games for 15 minutes. Then, after the play, each participant played a competitive game with an opponent in which the participant could deliver blasts of white noise through the earphones of the opponent. The operational definition of the dependent variable (aggressive behavior) was the level and duration of noise delivered to the opponent. The design of the experiment is shown in Figure 2.17 “An Experimental Research Design” .
Figure 2.17 An Experimental Research Design
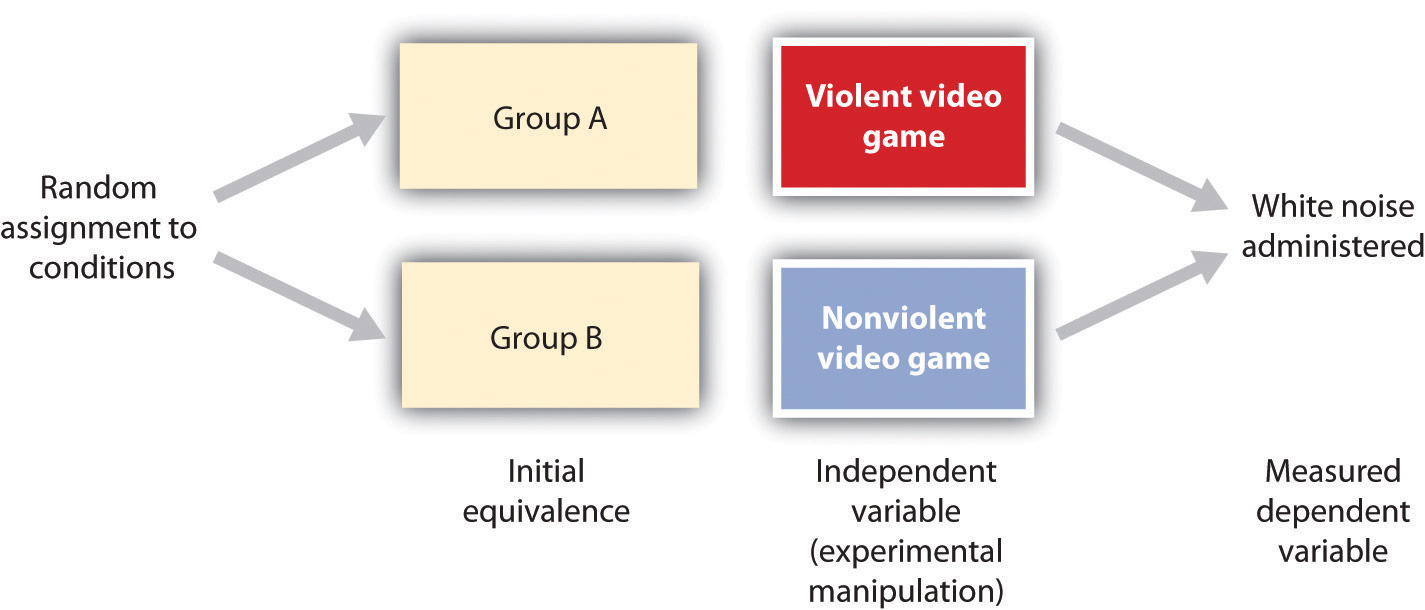
Two advantages of the experimental research design are (1) the assurance that the independent variable (also known as the experimental manipulation) occurs prior to the measured dependent variable, and (2) the creation of initial equivalence between the conditions of the experiment (in this case by using random assignment to conditions).
Experimental designs have two very nice features. For one, they guarantee that the independent variable occurs prior to the measurement of the dependent variable. This eliminates the possibility of reverse causation. Second, the influence of common-causal variables is controlled, and thus eliminated, by creating initial equivalence among the participants in each of the experimental conditions before the manipulation occurs.
The most common method of creating equivalence among the experimental conditions is through random assignment to conditions , a procedure in which the condition that each participant is assigned to is determined through a random process, such as drawing numbers out of an envelope or using a random number table . Anderson and Dill first randomly assigned about 100 participants to each of their two groups (Group A and Group B). Because they used random assignment to conditions, they could be confident that, before the experimental manipulation occurred, the students in Group A were, on average, equivalent to the students in Group B on every possible variable, including variables that are likely to be related to aggression, such as parental discipline style, peer relationships, hormone levels, diet—and in fact everything else.
Then, after they had created initial equivalence, Anderson and Dill created the experimental manipulation—they had the participants in Group A play the violent game and the participants in Group B play the nonviolent game. Then they compared the dependent variable (the white noise blasts) between the two groups, finding that the students who had viewed the violent video game gave significantly longer noise blasts than did the students who had played the nonviolent game.
Anderson and Dill had from the outset created initial equivalence between the groups. This initial equivalence allowed them to observe differences in the white noise levels between the two groups after the experimental manipulation, leading to the conclusion that it was the independent variable (and not some other variable) that caused these differences. The idea is that the only thing that was different between the students in the two groups was the video game they had played.
Despite the advantage of determining causation, experiments do have limitations. One is that they are often conducted in laboratory situations rather than in the everyday lives of people. Therefore, we do not know whether results that we find in a laboratory setting will necessarily hold up in everyday life. Second, and more important, is that some of the most interesting and key social variables cannot be experimentally manipulated. If we want to study the influence of the size of a mob on the destructiveness of its behavior, or to compare the personality characteristics of people who join suicide cults with those of people who do not join such cults, these relationships must be assessed using correlational designs, because it is simply not possible to experimentally manipulate these variables.
Key Takeaways
- Descriptive, correlational, and experimental research designs are used to collect and analyze data.
- Descriptive designs include case studies, surveys, and naturalistic observation. The goal of these designs is to get a picture of the current thoughts, feelings, or behaviors in a given group of people. Descriptive research is summarized using descriptive statistics.
- Correlational research designs measure two or more relevant variables and assess a relationship between or among them. The variables may be presented on a scatter plot to visually show the relationships. The Pearson Correlation Coefficient ( r ) is a measure of the strength of linear relationship between two variables.
- Common-causal variables may cause both the predictor and outcome variable in a correlational design, producing a spurious relationship. The possibility of common-causal variables makes it impossible to draw causal conclusions from correlational research designs.
- Experimental research involves the manipulation of an independent variable and the measurement of a dependent variable. Random assignment to conditions is normally used to create initial equivalence between the groups, allowing researchers to draw causal conclusions.
Exercises and Critical Thinking
- There is a negative correlation between the row that a student sits in in a large class (when the rows are numbered from front to back) and his or her final grade in the class. Do you think this represents a causal relationship or a spurious relationship, and why?
- Think of two variables (other than those mentioned in this book) that are likely to be correlated, but in which the correlation is probably spurious. What is the likely common-causal variable that is producing the relationship?
- Imagine a researcher wants to test the hypothesis that participating in psychotherapy will cause a decrease in reported anxiety. Describe the type of research design the investigator might use to draw this conclusion. What would be the independent and dependent variables in the research?
Aiken, L., & West, S. (1991). Multiple regression: Testing and interpreting interactions . Newbury Park, CA: Sage.
Ainsworth, M. S., Blehar, M. C., Waters, E., & Wall, S. (1978). Patterns of attachment: A psychological study of the strange situation . Hillsdale, NJ: Lawrence Erlbaum Associates.
Anderson, C. A., & Dill, K. E. (2000). Video games and aggressive thoughts, feelings, and behavior in the laboratory and in life. Journal of Personality and Social Psychology, 78 (4), 772–790.
Damasio, H., Grabowski, T., Frank, R., Galaburda, A. M., Damasio, A. R., Cacioppo, J. T., & Berntson, G. G. (2005). The return of Phineas Gage: Clues about the brain from the skull of a famous patient. In Social neuroscience: Key readings. (pp. 21–28). New York, NY: Psychology Press.
Freud, S. (1964). Analysis of phobia in a five-year-old boy. In E. A. Southwell & M. Merbaum (Eds.), Personality: Readings in theory and research (pp. 3–32). Belmont, CA: Wadsworth. (Original work published 1909)
Kotowicz, Z. (2007). The strange case of Phineas Gage. History of the Human Sciences, 20 (1), 115–131.
Rokeach, M. (1964). The three Christs of Ypsilanti: A psychological study . New York, NY: Knopf.
Introduction to Psychology Copyright © 2015 by University of Minnesota is licensed under a Creative Commons Attribution-NonCommercial-ShareAlike 4.0 International License , except where otherwise noted.
- Bipolar Disorder
- Therapy Center
- When To See a Therapist
- Types of Therapy
- Best Online Therapy
- Best Couples Therapy
- Best Family Therapy
- Managing Stress
- Sleep and Dreaming
- Understanding Emotions
- Self-Improvement
- Healthy Relationships
- Student Resources
- Personality Types
- Guided Meditations
- Verywell Mind Insights
- 2024 Verywell Mind 25
- Mental Health in the Classroom
- Editorial Process
- Meet Our Review Board
- Crisis Support
Understanding Methods for Research in Psychology
A Psychology Research Methods Study Guide
Kendra Cherry, MS, is a psychosocial rehabilitation specialist, psychology educator, and author of the "Everything Psychology Book."
:max_bytes(150000):strip_icc():format(webp)/IMG_9791-89504ab694d54b66bbd72cb84ffb860e.jpg)
Emily is a board-certified science editor who has worked with top digital publishing brands like Voices for Biodiversity, Study.com, GoodTherapy, Vox, and Verywell.
:max_bytes(150000):strip_icc():format(webp)/Emily-Swaim-1000-0f3197de18f74329aeffb690a177160c.jpg)
Types of Research in Psychology
- Cross-Sectional vs. Longitudinal Research
- Reliability and Validity
Glossary of Terms
Research in psychology focuses on a variety of topics , ranging from the development of infants to the behavior of social groups. Psychologists use the scientific method to investigate questions both systematically and empirically.
Research in psychology is important because it provides us with valuable information that helps to improve human lives. By learning more about the brain, cognition, behavior, and mental health conditions, researchers are able to solve real-world problems that affect our day-to-day lives.
At a Glance
Knowing more about how research in psychology is conducted can give you a better understanding of what those findings might mean to you. Psychology experiments can range from simple to complex, but there are some basic terms and concepts that all psychology students should understand.
Start your studies by learning more about the different types of research, the basics of experimental design, and the relationships between variables.
Research in Psychology: The Basics
The first step in your review should include a basic introduction to psychology research methods . Psychology research can have a variety of goals. What researchers learn can be used to describe, explain, predict, or change human behavior.
Psychologists use the scientific method to conduct studies and research in psychology. The basic process of conducting psychology research involves asking a question, designing a study, collecting data, analyzing results, reaching conclusions, and sharing the findings.
The Scientific Method in Psychology Research
The steps of the scientific method in psychology research are:
- Make an observation
- Ask a research question and make predictions about what you expect to find
- Test your hypothesis and gather data
- Examine the results and form conclusions
- Report your findings
Research in psychology can take several different forms. It can describe a phenomenon, explore the causes of a phenomenon, or look at relationships between one or more variables. Three of the main types of psychological research focus on:
Descriptive Studies
This type of research can tell us more about what is happening in a specific population. It relies on techniques such as observation, surveys, and case studies.
Correlational Studies
Correlational research is frequently used in psychology to look for relationships between variables. While research look at how variables are related, they do not manipulate any of the variables.
While correlational studies can suggest a relationship between two variables, finding a correlation does not prove that one variable causes a change in another. In other words, correlation does not equal causation.
Experimental Research Methods
Experiments are a research method that can look at whether changes in one variable cause changes in another. The simple experiment is one of the most basic methods of determining if there is a cause-and-effect relationship between two variables.
A simple experiment utilizes a control group of participants who receive no treatment and an experimental group of participants who receive the treatment.
Experimenters then compare the results of the two groups to determine if the treatment had an effect.
Cross-Sectional vs. Longitudinal Research in Psychology
Research in psychology can also involve collecting data at a single point in time, or gathering information at several points over a period of time.
Cross-Sectional Research
In a cross-sectional study , researchers collect data from participants at a single point in time. These are descriptive type of research and cannot be used to determine cause and effect because researchers do not manipulate the independent variables.
However, cross-sectional research does allow researchers to look at the characteristics of the population and explore relationships between different variables at a single point in time.
Longitudinal Research
A longitudinal study is a type of research in psychology that involves looking at the same group of participants over a period of time. Researchers start by collecting initial data that serves as a baseline, and then collect follow-up data at certain intervals. These studies can last days, months, or years.
The longest longitudinal study in psychology was started in 1921 and the study is planned to continue until the last participant dies or withdraws. As of 2003, more than 200 of the partipants were still alive.
The Reliability and Validity of Research in Psychology
Reliability and validity are two concepts that are also critical in psychology research. In order to trust the results, we need to know if the findings are consistent (reliability) and that we are actually measuring what we think we are measuring (validity).
Reliability
Reliability is a vital component of a valid psychological test. What is reliability? How do we measure it? Simply put, reliability refers to the consistency of a measure. A test is considered reliable if we get the same result repeatedly.
When determining the merits of a psychological test, validity is one of the most important factors to consider. What exactly is validity? One of the greatest concerns when creating a psychological test is whether or not it actually measures what we think it is measuring.
For example, a test might be designed to measure a stable personality trait but instead measures transitory emotions generated by situational or environmental conditions. A valid test ensures that the results accurately reflect the dimension undergoing assessment.
Review some of the key terms that you should know and understand about psychology research methods. Spend some time studying these terms and definitions before your exam. Some key terms that you should know include:
- Correlation
- Demand characteristic
- Dependent variable
- Hawthorne effect
- Independent variable
- Naturalistic observation
- Placebo effect
- Random assignment
- Replication
- Selective attrition
Erol A. How to conduct scientific research ? Noro Psikiyatr Ars . 2017;54(2):97-98. doi:10.5152/npa.2017.0120102
Aggarwal R, Ranganathan P. Study designs: Part 2 - Descriptive studies . Perspect Clin Res . 2019;10(1):34-36. doi:10.4103/picr.PICR_154_18
Curtis EA, Comiskey C, Dempsey O. Importance and use of correlational research . Nurse Res . 2016;23(6):20-25. doi:10.7748/nr.2016.e1382
Wang X, Cheng Z. Cross-sectional studies: Strengths, weaknesses, and recommendations . Chest . 2020;158(1S):S65-S71. doi:10.1016/j.chest.2020.03.012
Caruana EJ, Roman M, Hernández-Sánchez J, Solli P. Longitudinal studies . J Thorac Dis . 2015;7(11):E537-E540. doi:10.3978/j.issn.2072-1439.2015.10.63
Stanford Magazine. The vexing legacy of Lewis Terman .
By Kendra Cherry, MSEd Kendra Cherry, MS, is a psychosocial rehabilitation specialist, psychology educator, and author of the "Everything Psychology Book."
Want to create or adapt books like this? Learn more about how Pressbooks supports open publishing practices.
Ch 2: Psychological Research Methods
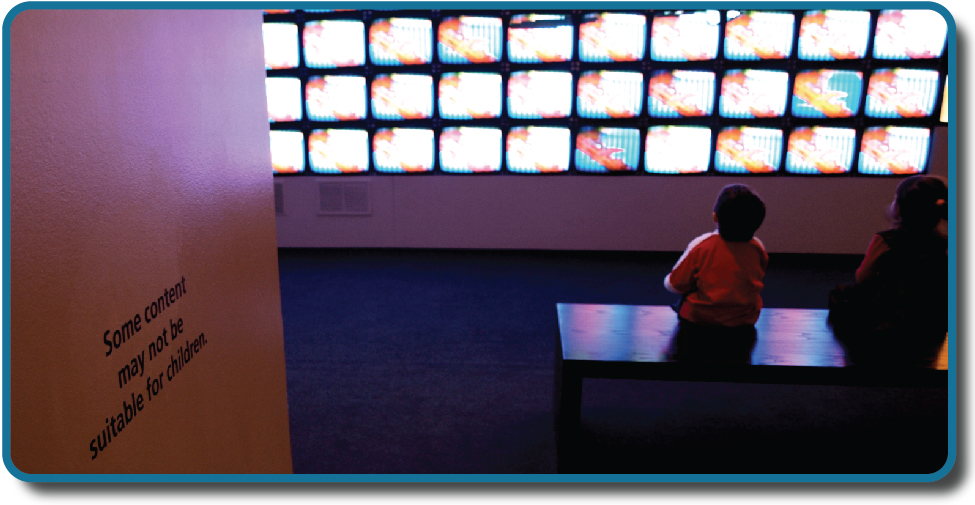
Have you ever wondered whether the violence you see on television affects your behavior? Are you more likely to behave aggressively in real life after watching people behave violently in dramatic situations on the screen? Or, could seeing fictional violence actually get aggression out of your system, causing you to be more peaceful? How are children influenced by the media they are exposed to? A psychologist interested in the relationship between behavior and exposure to violent images might ask these very questions.
The topic of violence in the media today is contentious. Since ancient times, humans have been concerned about the effects of new technologies on our behaviors and thinking processes. The Greek philosopher Socrates, for example, worried that writing—a new technology at that time—would diminish people’s ability to remember because they could rely on written records rather than committing information to memory. In our world of quickly changing technologies, questions about the effects of media continue to emerge. Is it okay to talk on a cell phone while driving? Are headphones good to use in a car? What impact does text messaging have on reaction time while driving? These are types of questions that psychologist David Strayer asks in his lab.
Watch this short video to see how Strayer utilizes the scientific method to reach important conclusions regarding technology and driving safety.
You can view the transcript for “Understanding driver distraction” here (opens in new window) .
How can we go about finding answers that are supported not by mere opinion, but by evidence that we can all agree on? The findings of psychological research can help us navigate issues like this.
Introduction to the Scientific Method
Learning objectives.
- Explain the steps of the scientific method
- Describe why the scientific method is important to psychology
- Summarize the processes of informed consent and debriefing
- Explain how research involving humans or animals is regulated
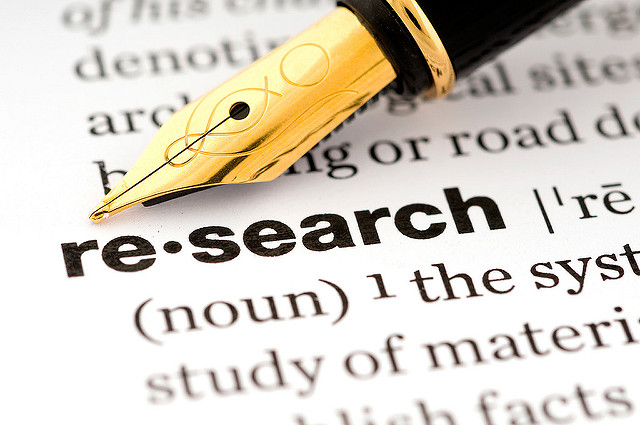
Scientists are engaged in explaining and understanding how the world around them works, and they are able to do so by coming up with theories that generate hypotheses that are testable and falsifiable. Theories that stand up to their tests are retained and refined, while those that do not are discarded or modified. In this way, research enables scientists to separate fact from simple opinion. Having good information generated from research aids in making wise decisions both in public policy and in our personal lives. In this section, you’ll see how psychologists use the scientific method to study and understand behavior.
The Scientific Process
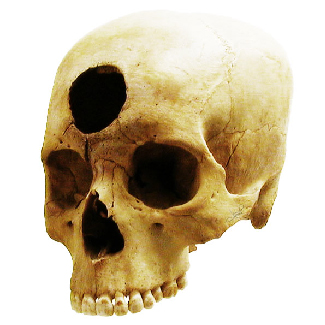
The goal of all scientists is to better understand the world around them. Psychologists focus their attention on understanding behavior, as well as the cognitive (mental) and physiological (body) processes that underlie behavior. In contrast to other methods that people use to understand the behavior of others, such as intuition and personal experience, the hallmark of scientific research is that there is evidence to support a claim. Scientific knowledge is empirical : It is grounded in objective, tangible evidence that can be observed time and time again, regardless of who is observing.
While behavior is observable, the mind is not. If someone is crying, we can see the behavior. However, the reason for the behavior is more difficult to determine. Is the person crying due to being sad, in pain, or happy? Sometimes we can learn the reason for someone’s behavior by simply asking a question, like “Why are you crying?” However, there are situations in which an individual is either uncomfortable or unwilling to answer the question honestly, or is incapable of answering. For example, infants would not be able to explain why they are crying. In such circumstances, the psychologist must be creative in finding ways to better understand behavior. This module explores how scientific knowledge is generated, and how important that knowledge is in forming decisions in our personal lives and in the public domain.
Process of Scientific Research
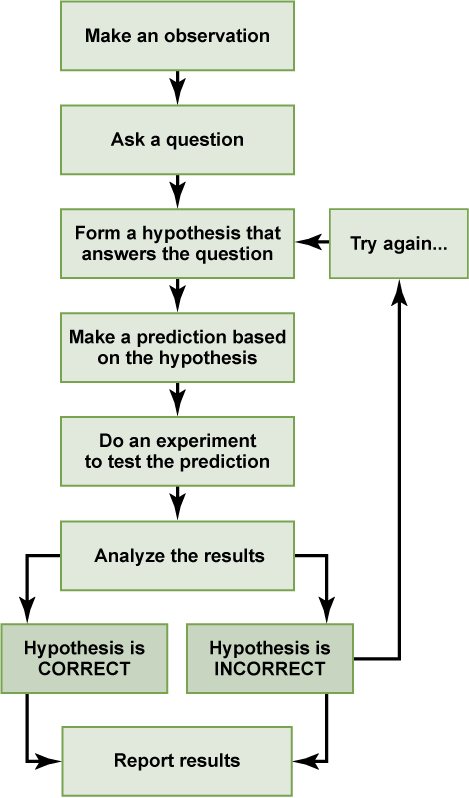
Scientific knowledge is advanced through a process known as the scientific method. Basically, ideas (in the form of theories and hypotheses) are tested against the real world (in the form of empirical observations), and those empirical observations lead to more ideas that are tested against the real world, and so on.
The basic steps in the scientific method are:
- Observe a natural phenomenon and define a question about it
- Make a hypothesis, or potential solution to the question
- Test the hypothesis
- If the hypothesis is true, find more evidence or find counter-evidence
- If the hypothesis is false, create a new hypothesis or try again
- Draw conclusions and repeat–the scientific method is never-ending, and no result is ever considered perfect
In order to ask an important question that may improve our understanding of the world, a researcher must first observe natural phenomena. By making observations, a researcher can define a useful question. After finding a question to answer, the researcher can then make a prediction (a hypothesis) about what he or she thinks the answer will be. This prediction is usually a statement about the relationship between two or more variables. After making a hypothesis, the researcher will then design an experiment to test his or her hypothesis and evaluate the data gathered. These data will either support or refute the hypothesis. Based on the conclusions drawn from the data, the researcher will then find more evidence to support the hypothesis, look for counter-evidence to further strengthen the hypothesis, revise the hypothesis and create a new experiment, or continue to incorporate the information gathered to answer the research question.
Basic Principles of the Scientific Method
Two key concepts in the scientific approach are theory and hypothesis. A theory is a well-developed set of ideas that propose an explanation for observed phenomena that can be used to make predictions about future observations. A hypothesis is a testable prediction that is arrived at logically from a theory. It is often worded as an if-then statement (e.g., if I study all night, I will get a passing grade on the test). The hypothesis is extremely important because it bridges the gap between the realm of ideas and the real world. As specific hypotheses are tested, theories are modified and refined to reflect and incorporate the result of these tests.
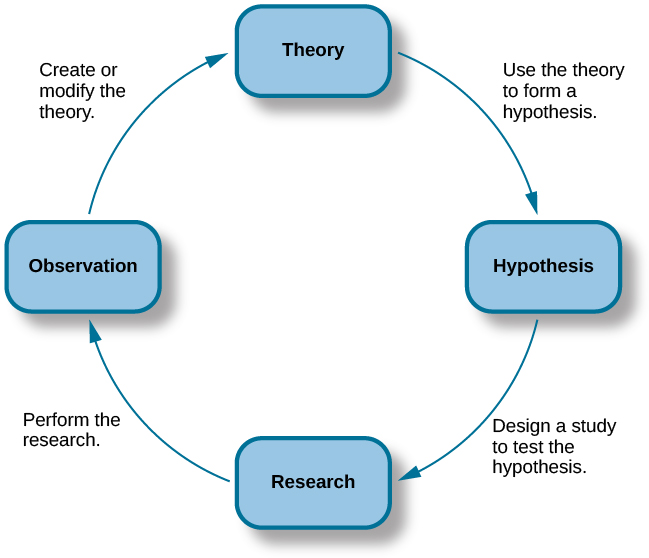
Other key components in following the scientific method include verifiability, predictability, falsifiability, and fairness. Verifiability means that an experiment must be replicable by another researcher. To achieve verifiability, researchers must make sure to document their methods and clearly explain how their experiment is structured and why it produces certain results.
Predictability in a scientific theory implies that the theory should enable us to make predictions about future events. The precision of these predictions is a measure of the strength of the theory.
Falsifiability refers to whether a hypothesis can be disproved. For a hypothesis to be falsifiable, it must be logically possible to make an observation or do a physical experiment that would show that there is no support for the hypothesis. Even when a hypothesis cannot be shown to be false, that does not necessarily mean it is not valid. Future testing may disprove the hypothesis. This does not mean that a hypothesis has to be shown to be false, just that it can be tested.
To determine whether a hypothesis is supported or not supported, psychological researchers must conduct hypothesis testing using statistics. Hypothesis testing is a type of statistics that determines the probability of a hypothesis being true or false. If hypothesis testing reveals that results were “statistically significant,” this means that there was support for the hypothesis and that the researchers can be reasonably confident that their result was not due to random chance. If the results are not statistically significant, this means that the researchers’ hypothesis was not supported.
Fairness implies that all data must be considered when evaluating a hypothesis. A researcher cannot pick and choose what data to keep and what to discard or focus specifically on data that support or do not support a particular hypothesis. All data must be accounted for, even if they invalidate the hypothesis.
Applying the Scientific Method
To see how this process works, let’s consider a specific theory and a hypothesis that might be generated from that theory. As you’ll learn in a later module, the James-Lange theory of emotion asserts that emotional experience relies on the physiological arousal associated with the emotional state. If you walked out of your home and discovered a very aggressive snake waiting on your doorstep, your heart would begin to race and your stomach churn. According to the James-Lange theory, these physiological changes would result in your feeling of fear. A hypothesis that could be derived from this theory might be that a person who is unaware of the physiological arousal that the sight of the snake elicits will not feel fear.
Remember that a good scientific hypothesis is falsifiable, or capable of being shown to be incorrect. Recall from the introductory module that Sigmund Freud had lots of interesting ideas to explain various human behaviors (Figure 5). However, a major criticism of Freud’s theories is that many of his ideas are not falsifiable; for example, it is impossible to imagine empirical observations that would disprove the existence of the id, the ego, and the superego—the three elements of personality described in Freud’s theories. Despite this, Freud’s theories are widely taught in introductory psychology texts because of their historical significance for personality psychology and psychotherapy, and these remain the root of all modern forms of therapy.
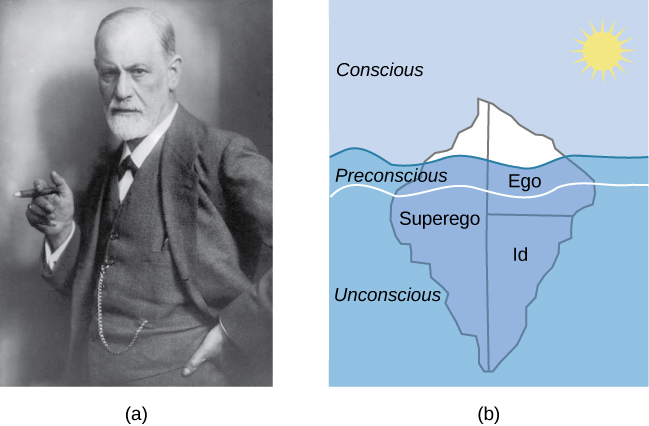
In contrast, the James-Lange theory does generate falsifiable hypotheses, such as the one described above. Some individuals who suffer significant injuries to their spinal columns are unable to feel the bodily changes that often accompany emotional experiences. Therefore, we could test the hypothesis by determining how emotional experiences differ between individuals who have the ability to detect these changes in their physiological arousal and those who do not. In fact, this research has been conducted and while the emotional experiences of people deprived of an awareness of their physiological arousal may be less intense, they still experience emotion (Chwalisz, Diener, & Gallagher, 1988).
Link to Learning
Why the scientific method is important for psychology.
The use of the scientific method is one of the main features that separates modern psychology from earlier philosophical inquiries about the mind. Compared to chemistry, physics, and other “natural sciences,” psychology has long been considered one of the “social sciences” because of the subjective nature of the things it seeks to study. Many of the concepts that psychologists are interested in—such as aspects of the human mind, behavior, and emotions—are subjective and cannot be directly measured. Psychologists often rely instead on behavioral observations and self-reported data, which are considered by some to be illegitimate or lacking in methodological rigor. Applying the scientific method to psychology, therefore, helps to standardize the approach to understanding its very different types of information.
The scientific method allows psychological data to be replicated and confirmed in many instances, under different circumstances, and by a variety of researchers. Through replication of experiments, new generations of psychologists can reduce errors and broaden the applicability of theories. It also allows theories to be tested and validated instead of simply being conjectures that could never be verified or falsified. All of this allows psychologists to gain a stronger understanding of how the human mind works.
Scientific articles published in journals and psychology papers written in the style of the American Psychological Association (i.e., in “APA style”) are structured around the scientific method. These papers include an Introduction, which introduces the background information and outlines the hypotheses; a Methods section, which outlines the specifics of how the experiment was conducted to test the hypothesis; a Results section, which includes the statistics that tested the hypothesis and state whether it was supported or not supported, and a Discussion and Conclusion, which state the implications of finding support for, or no support for, the hypothesis. Writing articles and papers that adhere to the scientific method makes it easy for future researchers to repeat the study and attempt to replicate the results.
Ethics in Research
Today, scientists agree that good research is ethical in nature and is guided by a basic respect for human dignity and safety. However, as you will read in the Tuskegee Syphilis Study, this has not always been the case. Modern researchers must demonstrate that the research they perform is ethically sound. This section presents how ethical considerations affect the design and implementation of research conducted today.
Research Involving Human Participants
Any experiment involving the participation of human subjects is governed by extensive, strict guidelines designed to ensure that the experiment does not result in harm. Any research institution that receives federal support for research involving human participants must have access to an institutional review board (IRB) . The IRB is a committee of individuals often made up of members of the institution’s administration, scientists, and community members (Figure 6). The purpose of the IRB is to review proposals for research that involves human participants. The IRB reviews these proposals with the principles mentioned above in mind, and generally, approval from the IRB is required in order for the experiment to proceed.
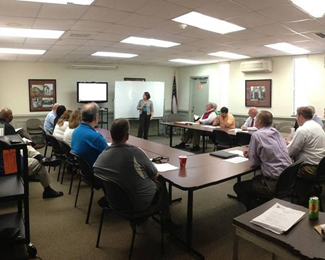
An institution’s IRB requires several components in any experiment it approves. For one, each participant must sign an informed consent form before they can participate in the experiment. An informed consent form provides a written description of what participants can expect during the experiment, including potential risks and implications of the research. It also lets participants know that their involvement is completely voluntary and can be discontinued without penalty at any time. Furthermore, the informed consent guarantees that any data collected in the experiment will remain completely confidential. In cases where research participants are under the age of 18, the parents or legal guardians are required to sign the informed consent form.
While the informed consent form should be as honest as possible in describing exactly what participants will be doing, sometimes deception is necessary to prevent participants’ knowledge of the exact research question from affecting the results of the study. Deception involves purposely misleading experiment participants in order to maintain the integrity of the experiment, but not to the point where the deception could be considered harmful. For example, if we are interested in how our opinion of someone is affected by their attire, we might use deception in describing the experiment to prevent that knowledge from affecting participants’ responses. In cases where deception is involved, participants must receive a full debriefing upon conclusion of the study—complete, honest information about the purpose of the experiment, how the data collected will be used, the reasons why deception was necessary, and information about how to obtain additional information about the study.
Dig Deeper: Ethics and the Tuskegee Syphilis Study
Unfortunately, the ethical guidelines that exist for research today were not always applied in the past. In 1932, poor, rural, black, male sharecroppers from Tuskegee, Alabama, were recruited to participate in an experiment conducted by the U.S. Public Health Service, with the aim of studying syphilis in black men (Figure 7). In exchange for free medical care, meals, and burial insurance, 600 men agreed to participate in the study. A little more than half of the men tested positive for syphilis, and they served as the experimental group (given that the researchers could not randomly assign participants to groups, this represents a quasi-experiment). The remaining syphilis-free individuals served as the control group. However, those individuals that tested positive for syphilis were never informed that they had the disease.
While there was no treatment for syphilis when the study began, by 1947 penicillin was recognized as an effective treatment for the disease. Despite this, no penicillin was administered to the participants in this study, and the participants were not allowed to seek treatment at any other facilities if they continued in the study. Over the course of 40 years, many of the participants unknowingly spread syphilis to their wives (and subsequently their children born from their wives) and eventually died because they never received treatment for the disease. This study was discontinued in 1972 when the experiment was discovered by the national press (Tuskegee University, n.d.). The resulting outrage over the experiment led directly to the National Research Act of 1974 and the strict ethical guidelines for research on humans described in this chapter. Why is this study unethical? How were the men who participated and their families harmed as a function of this research?
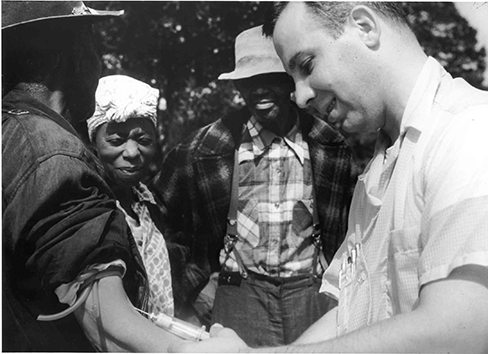
Learn more about the Tuskegee Syphilis Study on the CDC website .
Research Involving Animal Subjects
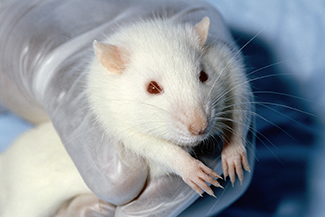
This does not mean that animal researchers are immune to ethical concerns. Indeed, the humane and ethical treatment of animal research subjects is a critical aspect of this type of research. Researchers must design their experiments to minimize any pain or distress experienced by animals serving as research subjects.
Whereas IRBs review research proposals that involve human participants, animal experimental proposals are reviewed by an Institutional Animal Care and Use Committee (IACUC) . An IACUC consists of institutional administrators, scientists, veterinarians, and community members. This committee is charged with ensuring that all experimental proposals require the humane treatment of animal research subjects. It also conducts semi-annual inspections of all animal facilities to ensure that the research protocols are being followed. No animal research project can proceed without the committee’s approval.
Introduction to Approaches to Research
- Differentiate between descriptive, correlational, and experimental research
- Explain the strengths and weaknesses of case studies, naturalistic observation, and surveys
- Describe the strength and weaknesses of archival research
- Compare longitudinal and cross-sectional approaches to research
- Explain what a correlation coefficient tells us about the relationship between variables
- Describe why correlation does not mean causation
- Describe the experimental process, including ways to control for bias
- Identify and differentiate between independent and dependent variables
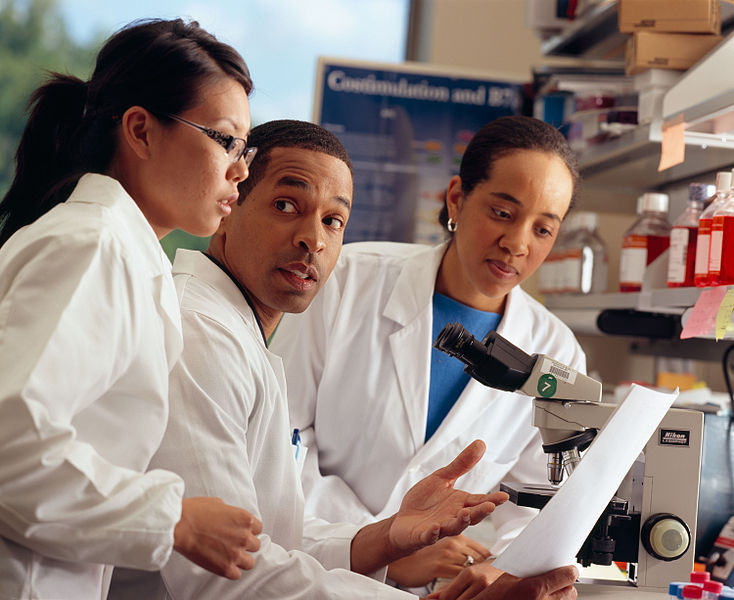
Psychologists use descriptive, experimental, and correlational methods to conduct research. Descriptive, or qualitative, methods include the case study, naturalistic observation, surveys, archival research, longitudinal research, and cross-sectional research.
Experiments are conducted in order to determine cause-and-effect relationships. In ideal experimental design, the only difference between the experimental and control groups is whether participants are exposed to the experimental manipulation. Each group goes through all phases of the experiment, but each group will experience a different level of the independent variable: the experimental group is exposed to the experimental manipulation, and the control group is not exposed to the experimental manipulation. The researcher then measures the changes that are produced in the dependent variable in each group. Once data is collected from both groups, it is analyzed statistically to determine if there are meaningful differences between the groups.
When scientists passively observe and measure phenomena it is called correlational research. Here, psychologists do not intervene and change behavior, as they do in experiments. In correlational research, they identify patterns of relationships, but usually cannot infer what causes what. Importantly, with correlational research, you can examine only two variables at a time, no more and no less.
Watch It: More on Research
If you enjoy learning through lectures and want an interesting and comprehensive summary of this section, then click on the Youtube link to watch a lecture given by MIT Professor John Gabrieli . Start at the 30:45 minute mark and watch through the end to hear examples of actual psychological studies and how they were analyzed. Listen for references to independent and dependent variables, experimenter bias, and double-blind studies. In the lecture, you’ll learn about breaking social norms, “WEIRD” research, why expectations matter, how a warm cup of coffee might make you nicer, why you should change your answer on a multiple choice test, and why praise for intelligence won’t make you any smarter.
You can view the transcript for “Lec 2 | MIT 9.00SC Introduction to Psychology, Spring 2011” here (opens in new window) .
Descriptive Research
There are many research methods available to psychologists in their efforts to understand, describe, and explain behavior and the cognitive and biological processes that underlie it. Some methods rely on observational techniques. Other approaches involve interactions between the researcher and the individuals who are being studied—ranging from a series of simple questions to extensive, in-depth interviews—to well-controlled experiments.
The three main categories of psychological research are descriptive, correlational, and experimental research. Research studies that do not test specific relationships between variables are called descriptive, or qualitative, studies . These studies are used to describe general or specific behaviors and attributes that are observed and measured. In the early stages of research it might be difficult to form a hypothesis, especially when there is not any existing literature in the area. In these situations designing an experiment would be premature, as the question of interest is not yet clearly defined as a hypothesis. Often a researcher will begin with a non-experimental approach, such as a descriptive study, to gather more information about the topic before designing an experiment or correlational study to address a specific hypothesis. Descriptive research is distinct from correlational research , in which psychologists formally test whether a relationship exists between two or more variables. Experimental research goes a step further beyond descriptive and correlational research and randomly assigns people to different conditions, using hypothesis testing to make inferences about how these conditions affect behavior. It aims to determine if one variable directly impacts and causes another. Correlational and experimental research both typically use hypothesis testing, whereas descriptive research does not.
Each of these research methods has unique strengths and weaknesses, and each method may only be appropriate for certain types of research questions. For example, studies that rely primarily on observation produce incredible amounts of information, but the ability to apply this information to the larger population is somewhat limited because of small sample sizes. Survey research, on the other hand, allows researchers to easily collect data from relatively large samples. While this allows for results to be generalized to the larger population more easily, the information that can be collected on any given survey is somewhat limited and subject to problems associated with any type of self-reported data. Some researchers conduct archival research by using existing records. While this can be a fairly inexpensive way to collect data that can provide insight into a number of research questions, researchers using this approach have no control on how or what kind of data was collected.
Correlational research can find a relationship between two variables, but the only way a researcher can claim that the relationship between the variables is cause and effect is to perform an experiment. In experimental research, which will be discussed later in the text, there is a tremendous amount of control over variables of interest. While this is a powerful approach, experiments are often conducted in very artificial settings. This calls into question the validity of experimental findings with regard to how they would apply in real-world settings. In addition, many of the questions that psychologists would like to answer cannot be pursued through experimental research because of ethical concerns.
The three main types of descriptive studies are, naturalistic observation, case studies, and surveys.
Naturalistic Observation
If you want to understand how behavior occurs, one of the best ways to gain information is to simply observe the behavior in its natural context. However, people might change their behavior in unexpected ways if they know they are being observed. How do researchers obtain accurate information when people tend to hide their natural behavior? As an example, imagine that your professor asks everyone in your class to raise their hand if they always wash their hands after using the restroom. Chances are that almost everyone in the classroom will raise their hand, but do you think hand washing after every trip to the restroom is really that universal?
This is very similar to the phenomenon mentioned earlier in this module: many individuals do not feel comfortable answering a question honestly. But if we are committed to finding out the facts about hand washing, we have other options available to us.
Suppose we send a classmate into the restroom to actually watch whether everyone washes their hands after using the restroom. Will our observer blend into the restroom environment by wearing a white lab coat, sitting with a clipboard, and staring at the sinks? We want our researcher to be inconspicuous—perhaps standing at one of the sinks pretending to put in contact lenses while secretly recording the relevant information. This type of observational study is called naturalistic observation : observing behavior in its natural setting. To better understand peer exclusion, Suzanne Fanger collaborated with colleagues at the University of Texas to observe the behavior of preschool children on a playground. How did the observers remain inconspicuous over the duration of the study? They equipped a few of the children with wireless microphones (which the children quickly forgot about) and observed while taking notes from a distance. Also, the children in that particular preschool (a “laboratory preschool”) were accustomed to having observers on the playground (Fanger, Frankel, & Hazen, 2012).
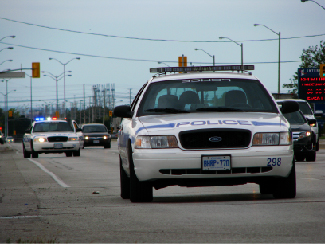
It is critical that the observer be as unobtrusive and as inconspicuous as possible: when people know they are being watched, they are less likely to behave naturally. If you have any doubt about this, ask yourself how your driving behavior might differ in two situations: In the first situation, you are driving down a deserted highway during the middle of the day; in the second situation, you are being followed by a police car down the same deserted highway (Figure 9).
It should be pointed out that naturalistic observation is not limited to research involving humans. Indeed, some of the best-known examples of naturalistic observation involve researchers going into the field to observe various kinds of animals in their own environments. As with human studies, the researchers maintain their distance and avoid interfering with the animal subjects so as not to influence their natural behaviors. Scientists have used this technique to study social hierarchies and interactions among animals ranging from ground squirrels to gorillas. The information provided by these studies is invaluable in understanding how those animals organize socially and communicate with one another. The anthropologist Jane Goodall, for example, spent nearly five decades observing the behavior of chimpanzees in Africa (Figure 10). As an illustration of the types of concerns that a researcher might encounter in naturalistic observation, some scientists criticized Goodall for giving the chimps names instead of referring to them by numbers—using names was thought to undermine the emotional detachment required for the objectivity of the study (McKie, 2010).
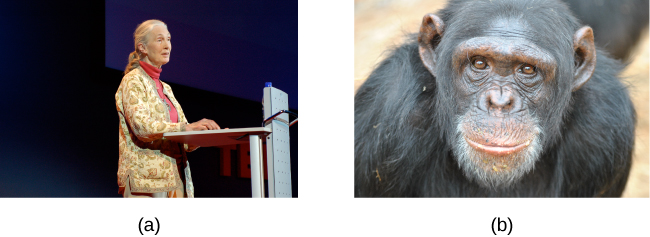
The greatest benefit of naturalistic observation is the validity, or accuracy, of information collected unobtrusively in a natural setting. Having individuals behave as they normally would in a given situation means that we have a higher degree of ecological validity, or realism, than we might achieve with other research approaches. Therefore, our ability to generalize the findings of the research to real-world situations is enhanced. If done correctly, we need not worry about people or animals modifying their behavior simply because they are being observed. Sometimes, people may assume that reality programs give us a glimpse into authentic human behavior. However, the principle of inconspicuous observation is violated as reality stars are followed by camera crews and are interviewed on camera for personal confessionals. Given that environment, we must doubt how natural and realistic their behaviors are.
The major downside of naturalistic observation is that they are often difficult to set up and control. In our restroom study, what if you stood in the restroom all day prepared to record people’s hand washing behavior and no one came in? Or, what if you have been closely observing a troop of gorillas for weeks only to find that they migrated to a new place while you were sleeping in your tent? The benefit of realistic data comes at a cost. As a researcher you have no control of when (or if) you have behavior to observe. In addition, this type of observational research often requires significant investments of time, money, and a good dose of luck.
Sometimes studies involve structured observation. In these cases, people are observed while engaging in set, specific tasks. An excellent example of structured observation comes from Strange Situation by Mary Ainsworth (you will read more about this in the module on lifespan development). The Strange Situation is a procedure used to evaluate attachment styles that exist between an infant and caregiver. In this scenario, caregivers bring their infants into a room filled with toys. The Strange Situation involves a number of phases, including a stranger coming into the room, the caregiver leaving the room, and the caregiver’s return to the room. The infant’s behavior is closely monitored at each phase, but it is the behavior of the infant upon being reunited with the caregiver that is most telling in terms of characterizing the infant’s attachment style with the caregiver.
Another potential problem in observational research is observer bias . Generally, people who act as observers are closely involved in the research project and may unconsciously skew their observations to fit their research goals or expectations. To protect against this type of bias, researchers should have clear criteria established for the types of behaviors recorded and how those behaviors should be classified. In addition, researchers often compare observations of the same event by multiple observers, in order to test inter-rater reliability : a measure of reliability that assesses the consistency of observations by different observers.
Case Studies
In 2011, the New York Times published a feature story on Krista and Tatiana Hogan, Canadian twin girls. These particular twins are unique because Krista and Tatiana are conjoined twins, connected at the head. There is evidence that the two girls are connected in a part of the brain called the thalamus, which is a major sensory relay center. Most incoming sensory information is sent through the thalamus before reaching higher regions of the cerebral cortex for processing.
The implications of this potential connection mean that it might be possible for one twin to experience the sensations of the other twin. For instance, if Krista is watching a particularly funny television program, Tatiana might smile or laugh even if she is not watching the program. This particular possibility has piqued the interest of many neuroscientists who seek to understand how the brain uses sensory information.
These twins represent an enormous resource in the study of the brain, and since their condition is very rare, it is likely that as long as their family agrees, scientists will follow these girls very closely throughout their lives to gain as much information as possible (Dominus, 2011).
In observational research, scientists are conducting a clinical or case study when they focus on one person or just a few individuals. Indeed, some scientists spend their entire careers studying just 10–20 individuals. Why would they do this? Obviously, when they focus their attention on a very small number of people, they can gain a tremendous amount of insight into those cases. The richness of information that is collected in clinical or case studies is unmatched by any other single research method. This allows the researcher to have a very deep understanding of the individuals and the particular phenomenon being studied.
If clinical or case studies provide so much information, why are they not more frequent among researchers? As it turns out, the major benefit of this particular approach is also a weakness. As mentioned earlier, this approach is often used when studying individuals who are interesting to researchers because they have a rare characteristic. Therefore, the individuals who serve as the focus of case studies are not like most other people. If scientists ultimately want to explain all behavior, focusing attention on such a special group of people can make it difficult to generalize any observations to the larger population as a whole. Generalizing refers to the ability to apply the findings of a particular research project to larger segments of society. Again, case studies provide enormous amounts of information, but since the cases are so specific, the potential to apply what’s learned to the average person may be very limited.
Often, psychologists develop surveys as a means of gathering data. Surveys are lists of questions to be answered by research participants, and can be delivered as paper-and-pencil questionnaires, administered electronically, or conducted verbally (Figure 11). Generally, the survey itself can be completed in a short time, and the ease of administering a survey makes it easy to collect data from a large number of people.
Surveys allow researchers to gather data from larger samples than may be afforded by other research methods . A sample is a subset of individuals selected from a population , which is the overall group of individuals that the researchers are interested in. Researchers study the sample and seek to generalize their findings to the population.
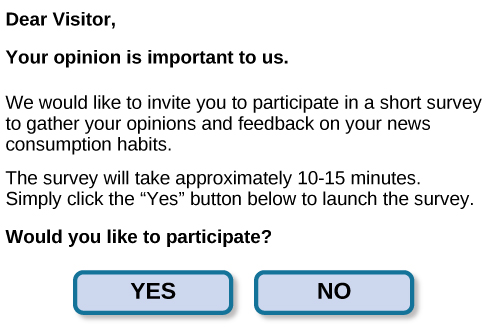
There is both strength and weakness of the survey in comparison to case studies. By using surveys, we can collect information from a larger sample of people. A larger sample is better able to reflect the actual diversity of the population, thus allowing better generalizability. Therefore, if our sample is sufficiently large and diverse, we can assume that the data we collect from the survey can be generalized to the larger population with more certainty than the information collected through a case study. However, given the greater number of people involved, we are not able to collect the same depth of information on each person that would be collected in a case study.
Another potential weakness of surveys is something we touched on earlier in this chapter: people don’t always give accurate responses. They may lie, misremember, or answer questions in a way that they think makes them look good. For example, people may report drinking less alcohol than is actually the case.
Any number of research questions can be answered through the use of surveys. One real-world example is the research conducted by Jenkins, Ruppel, Kizer, Yehl, and Griffin (2012) about the backlash against the US Arab-American community following the terrorist attacks of September 11, 2001. Jenkins and colleagues wanted to determine to what extent these negative attitudes toward Arab-Americans still existed nearly a decade after the attacks occurred. In one study, 140 research participants filled out a survey with 10 questions, including questions asking directly about the participant’s overt prejudicial attitudes toward people of various ethnicities. The survey also asked indirect questions about how likely the participant would be to interact with a person of a given ethnicity in a variety of settings (such as, “How likely do you think it is that you would introduce yourself to a person of Arab-American descent?”). The results of the research suggested that participants were unwilling to report prejudicial attitudes toward any ethnic group. However, there were significant differences between their pattern of responses to questions about social interaction with Arab-Americans compared to other ethnic groups: they indicated less willingness for social interaction with Arab-Americans compared to the other ethnic groups. This suggested that the participants harbored subtle forms of prejudice against Arab-Americans, despite their assertions that this was not the case (Jenkins et al., 2012).
Think It Over
Archival research.
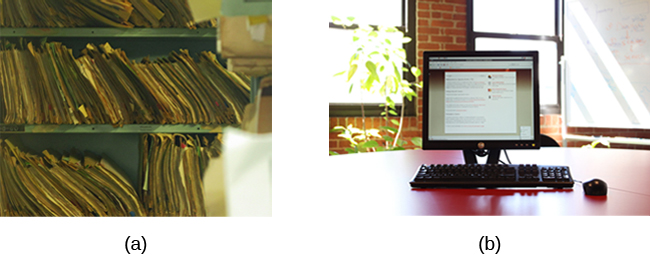
In comparing archival research to other research methods, there are several important distinctions. For one, the researcher employing archival research never directly interacts with research participants. Therefore, the investment of time and money to collect data is considerably less with archival research. Additionally, researchers have no control over what information was originally collected. Therefore, research questions have to be tailored so they can be answered within the structure of the existing data sets. There is also no guarantee of consistency between the records from one source to another, which might make comparing and contrasting different data sets problematic.
Longitudinal and Cross-Sectional Research
Sometimes we want to see how people change over time, as in studies of human development and lifespan. When we test the same group of individuals repeatedly over an extended period of time, we are conducting longitudinal research. Longitudinal research is a research design in which data-gathering is administered repeatedly over an extended period of time. For example, we may survey a group of individuals about their dietary habits at age 20, retest them a decade later at age 30, and then again at age 40.
Another approach is cross-sectional research . In cross-sectional research, a researcher compares multiple segments of the population at the same time. Using the dietary habits example above, the researcher might directly compare different groups of people by age. Instead of observing a group of people for 20 years to see how their dietary habits changed from decade to decade, the researcher would study a group of 20-year-old individuals and compare them to a group of 30-year-old individuals and a group of 40-year-old individuals. While cross-sectional research requires a shorter-term investment, it is also limited by differences that exist between the different generations (or cohorts) that have nothing to do with age per se, but rather reflect the social and cultural experiences of different generations of individuals make them different from one another.
To illustrate this concept, consider the following survey findings. In recent years there has been significant growth in the popular support of same-sex marriage. Many studies on this topic break down survey participants into different age groups. In general, younger people are more supportive of same-sex marriage than are those who are older (Jones, 2013). Does this mean that as we age we become less open to the idea of same-sex marriage, or does this mean that older individuals have different perspectives because of the social climates in which they grew up? Longitudinal research is a powerful approach because the same individuals are involved in the research project over time, which means that the researchers need to be less concerned with differences among cohorts affecting the results of their study.
Often longitudinal studies are employed when researching various diseases in an effort to understand particular risk factors. Such studies often involve tens of thousands of individuals who are followed for several decades. Given the enormous number of people involved in these studies, researchers can feel confident that their findings can be generalized to the larger population. The Cancer Prevention Study-3 (CPS-3) is one of a series of longitudinal studies sponsored by the American Cancer Society aimed at determining predictive risk factors associated with cancer. When participants enter the study, they complete a survey about their lives and family histories, providing information on factors that might cause or prevent the development of cancer. Then every few years the participants receive additional surveys to complete. In the end, hundreds of thousands of participants will be tracked over 20 years to determine which of them develop cancer and which do not.
Clearly, this type of research is important and potentially very informative. For instance, earlier longitudinal studies sponsored by the American Cancer Society provided some of the first scientific demonstrations of the now well-established links between increased rates of cancer and smoking (American Cancer Society, n.d.) (Figure 13).
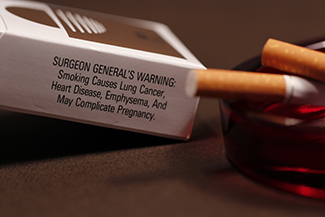
As with any research strategy, longitudinal research is not without limitations. For one, these studies require an incredible time investment by the researcher and research participants. Given that some longitudinal studies take years, if not decades, to complete, the results will not be known for a considerable period of time. In addition to the time demands, these studies also require a substantial financial investment. Many researchers are unable to commit the resources necessary to see a longitudinal project through to the end.
Research participants must also be willing to continue their participation for an extended period of time, and this can be problematic. People move, get married and take new names, get ill, and eventually die. Even without significant life changes, some people may simply choose to discontinue their participation in the project. As a result, the attrition rates, or reduction in the number of research participants due to dropouts, in longitudinal studies are quite high and increases over the course of a project. For this reason, researchers using this approach typically recruit many participants fully expecting that a substantial number will drop out before the end. As the study progresses, they continually check whether the sample still represents the larger population, and make adjustments as necessary.
Correlational Research
Did you know that as sales in ice cream increase, so does the overall rate of crime? Is it possible that indulging in your favorite flavor of ice cream could send you on a crime spree? Or, after committing crime do you think you might decide to treat yourself to a cone? There is no question that a relationship exists between ice cream and crime (e.g., Harper, 2013), but it would be pretty foolish to decide that one thing actually caused the other to occur.
It is much more likely that both ice cream sales and crime rates are related to the temperature outside. When the temperature is warm, there are lots of people out of their houses, interacting with each other, getting annoyed with one another, and sometimes committing crimes. Also, when it is warm outside, we are more likely to seek a cool treat like ice cream. How do we determine if there is indeed a relationship between two things? And when there is a relationship, how can we discern whether it is attributable to coincidence or causation?
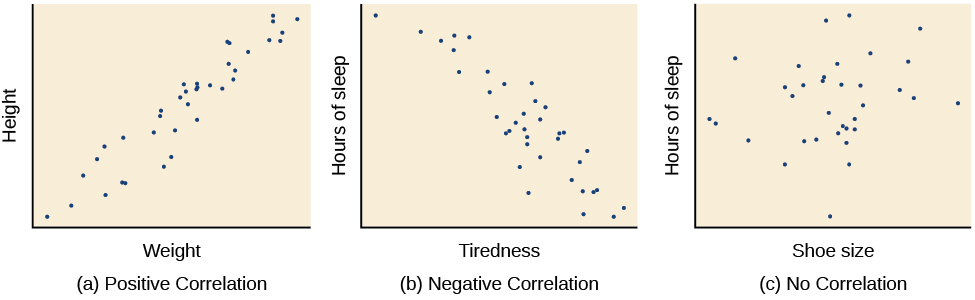
Correlation Does Not Indicate Causation
Correlational research is useful because it allows us to discover the strength and direction of relationships that exist between two variables. However, correlation is limited because establishing the existence of a relationship tells us little about cause and effect . While variables are sometimes correlated because one does cause the other, it could also be that some other factor, a confounding variable , is actually causing the systematic movement in our variables of interest. In the ice cream/crime rate example mentioned earlier, temperature is a confounding variable that could account for the relationship between the two variables.
Even when we cannot point to clear confounding variables, we should not assume that a correlation between two variables implies that one variable causes changes in another. This can be frustrating when a cause-and-effect relationship seems clear and intuitive. Think back to our discussion of the research done by the American Cancer Society and how their research projects were some of the first demonstrations of the link between smoking and cancer. It seems reasonable to assume that smoking causes cancer, but if we were limited to correlational research , we would be overstepping our bounds by making this assumption.
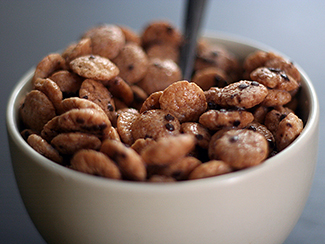
Unfortunately, people mistakenly make claims of causation as a function of correlations all the time. Such claims are especially common in advertisements and news stories. For example, recent research found that people who eat cereal on a regular basis achieve healthier weights than those who rarely eat cereal (Frantzen, Treviño, Echon, Garcia-Dominic, & DiMarco, 2013; Barton et al., 2005). Guess how the cereal companies report this finding. Does eating cereal really cause an individual to maintain a healthy weight, or are there other possible explanations, such as, someone at a healthy weight is more likely to regularly eat a healthy breakfast than someone who is obese or someone who avoids meals in an attempt to diet (Figure 15)? While correlational research is invaluable in identifying relationships among variables, a major limitation is the inability to establish causality. Psychologists want to make statements about cause and effect, but the only way to do that is to conduct an experiment to answer a research question. The next section describes how scientific experiments incorporate methods that eliminate, or control for, alternative explanations, which allow researchers to explore how changes in one variable cause changes in another variable.
Watch this clip from Freakonomics for an example of how correlation does not indicate causation.
You can view the transcript for “Correlation vs. Causality: Freakonomics Movie” here (opens in new window) .
Illusory Correlations
The temptation to make erroneous cause-and-effect statements based on correlational research is not the only way we tend to misinterpret data. We also tend to make the mistake of illusory correlations, especially with unsystematic observations. Illusory correlations , or false correlations, occur when people believe that relationships exist between two things when no such relationship exists. One well-known illusory correlation is the supposed effect that the moon’s phases have on human behavior. Many people passionately assert that human behavior is affected by the phase of the moon, and specifically, that people act strangely when the moon is full (Figure 16).
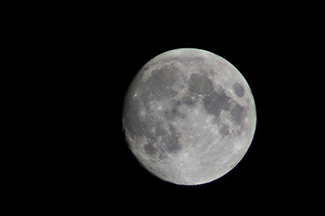
There is no denying that the moon exerts a powerful influence on our planet. The ebb and flow of the ocean’s tides are tightly tied to the gravitational forces of the moon. Many people believe, therefore, that it is logical that we are affected by the moon as well. After all, our bodies are largely made up of water. A meta-analysis of nearly 40 studies consistently demonstrated, however, that the relationship between the moon and our behavior does not exist (Rotton & Kelly, 1985). While we may pay more attention to odd behavior during the full phase of the moon, the rates of odd behavior remain constant throughout the lunar cycle.
Why are we so apt to believe in illusory correlations like this? Often we read or hear about them and simply accept the information as valid. Or, we have a hunch about how something works and then look for evidence to support that hunch, ignoring evidence that would tell us our hunch is false; this is known as confirmation bias . Other times, we find illusory correlations based on the information that comes most easily to mind, even if that information is severely limited. And while we may feel confident that we can use these relationships to better understand and predict the world around us, illusory correlations can have significant drawbacks. For example, research suggests that illusory correlations—in which certain behaviors are inaccurately attributed to certain groups—are involved in the formation of prejudicial attitudes that can ultimately lead to discriminatory behavior (Fiedler, 2004).
We all have a tendency to make illusory correlations from time to time. Try to think of an illusory correlation that is held by you, a family member, or a close friend. How do you think this illusory correlation came about and what can be done in the future to combat them?
Experiments
Causality: conducting experiments and using the data, experimental hypothesis.
In order to conduct an experiment, a researcher must have a specific hypothesis to be tested. As you’ve learned, hypotheses can be formulated either through direct observation of the real world or after careful review of previous research. For example, if you think that children should not be allowed to watch violent programming on television because doing so would cause them to behave more violently, then you have basically formulated a hypothesis—namely, that watching violent television programs causes children to behave more violently. How might you have arrived at this particular hypothesis? You may have younger relatives who watch cartoons featuring characters using martial arts to save the world from evildoers, with an impressive array of punching, kicking, and defensive postures. You notice that after watching these programs for a while, your young relatives mimic the fighting behavior of the characters portrayed in the cartoon (Figure 17).
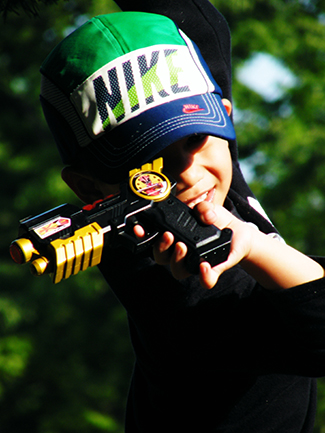
These sorts of personal observations are what often lead us to formulate a specific hypothesis, but we cannot use limited personal observations and anecdotal evidence to rigorously test our hypothesis. Instead, to find out if real-world data supports our hypothesis, we have to conduct an experiment.
Designing an Experiment
The most basic experimental design involves two groups: the experimental group and the control group. The two groups are designed to be the same except for one difference— experimental manipulation. The experimental group gets the experimental manipulation—that is, the treatment or variable being tested (in this case, violent TV images)—and the control group does not. Since experimental manipulation is the only difference between the experimental and control groups, we can be sure that any differences between the two are due to experimental manipulation rather than chance.
In our example of how violent television programming might affect violent behavior in children, we have the experimental group view violent television programming for a specified time and then measure their violent behavior. We measure the violent behavior in our control group after they watch nonviolent television programming for the same amount of time. It is important for the control group to be treated similarly to the experimental group, with the exception that the control group does not receive the experimental manipulation. Therefore, we have the control group watch non-violent television programming for the same amount of time as the experimental group.
We also need to precisely define, or operationalize, what is considered violent and nonviolent. An operational definition is a description of how we will measure our variables, and it is important in allowing others understand exactly how and what a researcher measures in a particular experiment. In operationalizing violent behavior, we might choose to count only physical acts like kicking or punching as instances of this behavior, or we also may choose to include angry verbal exchanges. Whatever we determine, it is important that we operationalize violent behavior in such a way that anyone who hears about our study for the first time knows exactly what we mean by violence. This aids peoples’ ability to interpret our data as well as their capacity to repeat our experiment should they choose to do so.
Once we have operationalized what is considered violent television programming and what is considered violent behavior from our experiment participants, we need to establish how we will run our experiment. In this case, we might have participants watch a 30-minute television program (either violent or nonviolent, depending on their group membership) before sending them out to a playground for an hour where their behavior is observed and the number and type of violent acts is recorded.
Ideally, the people who observe and record the children’s behavior are unaware of who was assigned to the experimental or control group, in order to control for experimenter bias. Experimenter bias refers to the possibility that a researcher’s expectations might skew the results of the study. Remember, conducting an experiment requires a lot of planning, and the people involved in the research project have a vested interest in supporting their hypotheses. If the observers knew which child was in which group, it might influence how much attention they paid to each child’s behavior as well as how they interpreted that behavior. By being blind to which child is in which group, we protect against those biases. This situation is a single-blind study , meaning that one of the groups (participants) are unaware as to which group they are in (experiment or control group) while the researcher who developed the experiment knows which participants are in each group.
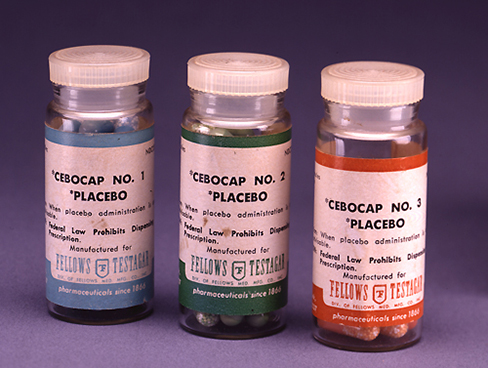
In a double-blind study , both the researchers and the participants are blind to group assignments. Why would a researcher want to run a study where no one knows who is in which group? Because by doing so, we can control for both experimenter and participant expectations. If you are familiar with the phrase placebo effect, you already have some idea as to why this is an important consideration. The placebo effect occurs when people’s expectations or beliefs influence or determine their experience in a given situation. In other words, simply expecting something to happen can actually make it happen.
The placebo effect is commonly described in terms of testing the effectiveness of a new medication. Imagine that you work in a pharmaceutical company, and you think you have a new drug that is effective in treating depression. To demonstrate that your medication is effective, you run an experiment with two groups: The experimental group receives the medication, and the control group does not. But you don’t want participants to know whether they received the drug or not.
Why is that? Imagine that you are a participant in this study, and you have just taken a pill that you think will improve your mood. Because you expect the pill to have an effect, you might feel better simply because you took the pill and not because of any drug actually contained in the pill—this is the placebo effect.
To make sure that any effects on mood are due to the drug and not due to expectations, the control group receives a placebo (in this case a sugar pill). Now everyone gets a pill, and once again neither the researcher nor the experimental participants know who got the drug and who got the sugar pill. Any differences in mood between the experimental and control groups can now be attributed to the drug itself rather than to experimenter bias or participant expectations (Figure 18).
Independent and Dependent Variables
In a research experiment, we strive to study whether changes in one thing cause changes in another. To achieve this, we must pay attention to two important variables, or things that can be changed, in any experimental study: the independent variable and the dependent variable. An independent variable is manipulated or controlled by the experimenter. In a well-designed experimental study, the independent variable is the only important difference between the experimental and control groups. In our example of how violent television programs affect children’s display of violent behavior, the independent variable is the type of program—violent or nonviolent—viewed by participants in the study (Figure 19). A dependent variable is what the researcher measures to see how much effect the independent variable had. In our example, the dependent variable is the number of violent acts displayed by the experimental participants.
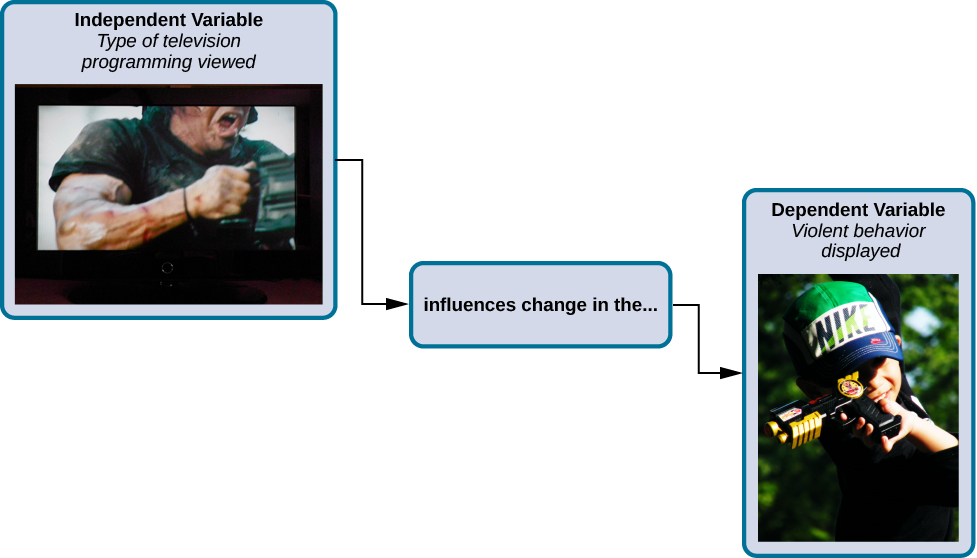
We expect that the dependent variable will change as a function of the independent variable. In other words, the dependent variable depends on the independent variable. A good way to think about the relationship between the independent and dependent variables is with this question: What effect does the independent variable have on the dependent variable? Returning to our example, what effect does watching a half hour of violent television programming or nonviolent television programming have on the number of incidents of physical aggression displayed on the playground?
Selecting and Assigning Experimental Participants
Now that our study is designed, we need to obtain a sample of individuals to include in our experiment. Our study involves human participants so we need to determine who to include. Participants are the subjects of psychological research, and as the name implies, individuals who are involved in psychological research actively participate in the process. Often, psychological research projects rely on college students to serve as participants. In fact, the vast majority of research in psychology subfields has historically involved students as research participants (Sears, 1986; Arnett, 2008). But are college students truly representative of the general population? College students tend to be younger, more educated, more liberal, and less diverse than the general population. Although using students as test subjects is an accepted practice, relying on such a limited pool of research participants can be problematic because it is difficult to generalize findings to the larger population.
Our hypothetical experiment involves children, and we must first generate a sample of child participants. Samples are used because populations are usually too large to reasonably involve every member in our particular experiment (Figure 20). If possible, we should use a random sample (there are other types of samples, but for the purposes of this section, we will focus on random samples). A random sample is a subset of a larger population in which every member of the population has an equal chance of being selected. Random samples are preferred because if the sample is large enough we can be reasonably sure that the participating individuals are representative of the larger population. This means that the percentages of characteristics in the sample—sex, ethnicity, socioeconomic level, and any other characteristics that might affect the results—are close to those percentages in the larger population.
In our example, let’s say we decide our population of interest is fourth graders. But all fourth graders is a very large population, so we need to be more specific; instead we might say our population of interest is all fourth graders in a particular city. We should include students from various income brackets, family situations, races, ethnicities, religions, and geographic areas of town. With this more manageable population, we can work with the local schools in selecting a random sample of around 200 fourth graders who we want to participate in our experiment.
In summary, because we cannot test all of the fourth graders in a city, we want to find a group of about 200 that reflects the composition of that city. With a representative group, we can generalize our findings to the larger population without fear of our sample being biased in some way.
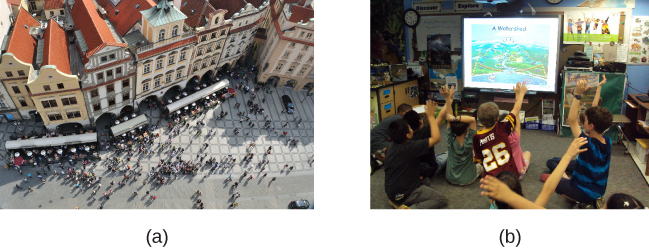
Now that we have a sample, the next step of the experimental process is to split the participants into experimental and control groups through random assignment. With random assignment , all participants have an equal chance of being assigned to either group. There is statistical software that will randomly assign each of the fourth graders in the sample to either the experimental or the control group.
Random assignment is critical for sound experimental design. With sufficiently large samples, random assignment makes it unlikely that there are systematic differences between the groups. So, for instance, it would be very unlikely that we would get one group composed entirely of males, a given ethnic identity, or a given religious ideology. This is important because if the groups were systematically different before the experiment began, we would not know the origin of any differences we find between the groups: Were the differences preexisting, or were they caused by manipulation of the independent variable? Random assignment allows us to assume that any differences observed between experimental and control groups result from the manipulation of the independent variable.
Issues to Consider
While experiments allow scientists to make cause-and-effect claims, they are not without problems. True experiments require the experimenter to manipulate an independent variable, and that can complicate many questions that psychologists might want to address. For instance, imagine that you want to know what effect sex (the independent variable) has on spatial memory (the dependent variable). Although you can certainly look for differences between males and females on a task that taps into spatial memory, you cannot directly control a person’s sex. We categorize this type of research approach as quasi-experimental and recognize that we cannot make cause-and-effect claims in these circumstances.
Experimenters are also limited by ethical constraints. For instance, you would not be able to conduct an experiment designed to determine if experiencing abuse as a child leads to lower levels of self-esteem among adults. To conduct such an experiment, you would need to randomly assign some experimental participants to a group that receives abuse, and that experiment would be unethical.
Introduction to Statistical Thinking
Psychologists use statistics to assist them in analyzing data, and also to give more precise measurements to describe whether something is statistically significant. Analyzing data using statistics enables researchers to find patterns, make claims, and share their results with others. In this section, you’ll learn about some of the tools that psychologists use in statistical analysis.
- Define reliability and validity
- Describe the importance of distributional thinking and the role of p-values in statistical inference
- Describe the role of random sampling and random assignment in drawing cause-and-effect conclusions
- Describe the basic structure of a psychological research article
Interpreting Experimental Findings
Once data is collected from both the experimental and the control groups, a statistical analysis is conducted to find out if there are meaningful differences between the two groups. A statistical analysis determines how likely any difference found is due to chance (and thus not meaningful). In psychology, group differences are considered meaningful, or significant, if the odds that these differences occurred by chance alone are 5 percent or less. Stated another way, if we repeated this experiment 100 times, we would expect to find the same results at least 95 times out of 100.
The greatest strength of experiments is the ability to assert that any significant differences in the findings are caused by the independent variable. This occurs because random selection, random assignment, and a design that limits the effects of both experimenter bias and participant expectancy should create groups that are similar in composition and treatment. Therefore, any difference between the groups is attributable to the independent variable, and now we can finally make a causal statement. If we find that watching a violent television program results in more violent behavior than watching a nonviolent program, we can safely say that watching violent television programs causes an increase in the display of violent behavior.
Reporting Research
When psychologists complete a research project, they generally want to share their findings with other scientists. The American Psychological Association (APA) publishes a manual detailing how to write a paper for submission to scientific journals. Unlike an article that might be published in a magazine like Psychology Today, which targets a general audience with an interest in psychology, scientific journals generally publish peer-reviewed journal articles aimed at an audience of professionals and scholars who are actively involved in research themselves.
A peer-reviewed journal article is read by several other scientists (generally anonymously) with expertise in the subject matter. These peer reviewers provide feedback—to both the author and the journal editor—regarding the quality of the draft. Peer reviewers look for a strong rationale for the research being described, a clear description of how the research was conducted, and evidence that the research was conducted in an ethical manner. They also look for flaws in the study’s design, methods, and statistical analyses. They check that the conclusions drawn by the authors seem reasonable given the observations made during the research. Peer reviewers also comment on how valuable the research is in advancing the discipline’s knowledge. This helps prevent unnecessary duplication of research findings in the scientific literature and, to some extent, ensures that each research article provides new information. Ultimately, the journal editor will compile all of the peer reviewer feedback and determine whether the article will be published in its current state (a rare occurrence), published with revisions, or not accepted for publication.
Peer review provides some degree of quality control for psychological research. Poorly conceived or executed studies can be weeded out, and even well-designed research can be improved by the revisions suggested. Peer review also ensures that the research is described clearly enough to allow other scientists to replicate it, meaning they can repeat the experiment using different samples to determine reliability. Sometimes replications involve additional measures that expand on the original finding. In any case, each replication serves to provide more evidence to support the original research findings. Successful replications of published research make scientists more apt to adopt those findings, while repeated failures tend to cast doubt on the legitimacy of the original article and lead scientists to look elsewhere. For example, it would be a major advancement in the medical field if a published study indicated that taking a new drug helped individuals achieve a healthy weight without changing their diet. But if other scientists could not replicate the results, the original study’s claims would be questioned.
Dig Deeper: The Vaccine-Autism Myth and the Retraction of Published Studies
Some scientists have claimed that routine childhood vaccines cause some children to develop autism, and, in fact, several peer-reviewed publications published research making these claims. Since the initial reports, large-scale epidemiological research has suggested that vaccinations are not responsible for causing autism and that it is much safer to have your child vaccinated than not. Furthermore, several of the original studies making this claim have since been retracted.
A published piece of work can be rescinded when data is called into question because of falsification, fabrication, or serious research design problems. Once rescinded, the scientific community is informed that there are serious problems with the original publication. Retractions can be initiated by the researcher who led the study, by research collaborators, by the institution that employed the researcher, or by the editorial board of the journal in which the article was originally published. In the vaccine-autism case, the retraction was made because of a significant conflict of interest in which the leading researcher had a financial interest in establishing a link between childhood vaccines and autism (Offit, 2008). Unfortunately, the initial studies received so much media attention that many parents around the world became hesitant to have their children vaccinated (Figure 21). For more information about how the vaccine/autism story unfolded, as well as the repercussions of this story, take a look at Paul Offit’s book, Autism’s False Prophets: Bad Science, Risky Medicine, and the Search for a Cure.
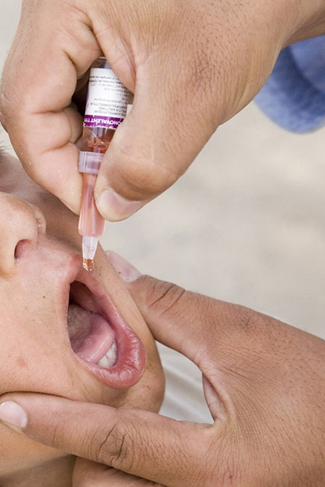
Reliability and Validity
Dig deeper: everyday connection: how valid is the sat.
Standardized tests like the SAT are supposed to measure an individual’s aptitude for a college education, but how reliable and valid are such tests? Research conducted by the College Board suggests that scores on the SAT have high predictive validity for first-year college students’ GPA (Kobrin, Patterson, Shaw, Mattern, & Barbuti, 2008). In this context, predictive validity refers to the test’s ability to effectively predict the GPA of college freshmen. Given that many institutions of higher education require the SAT for admission, this high degree of predictive validity might be comforting.
However, the emphasis placed on SAT scores in college admissions has generated some controversy on a number of fronts. For one, some researchers assert that the SAT is a biased test that places minority students at a disadvantage and unfairly reduces the likelihood of being admitted into a college (Santelices & Wilson, 2010). Additionally, some research has suggested that the predictive validity of the SAT is grossly exaggerated in how well it is able to predict the GPA of first-year college students. In fact, it has been suggested that the SAT’s predictive validity may be overestimated by as much as 150% (Rothstein, 2004). Many institutions of higher education are beginning to consider de-emphasizing the significance of SAT scores in making admission decisions (Rimer, 2008).
In 2014, College Board president David Coleman expressed his awareness of these problems, recognizing that college success is more accurately predicted by high school grades than by SAT scores. To address these concerns, he has called for significant changes to the SAT exam (Lewin, 2014).
Statistical Significance
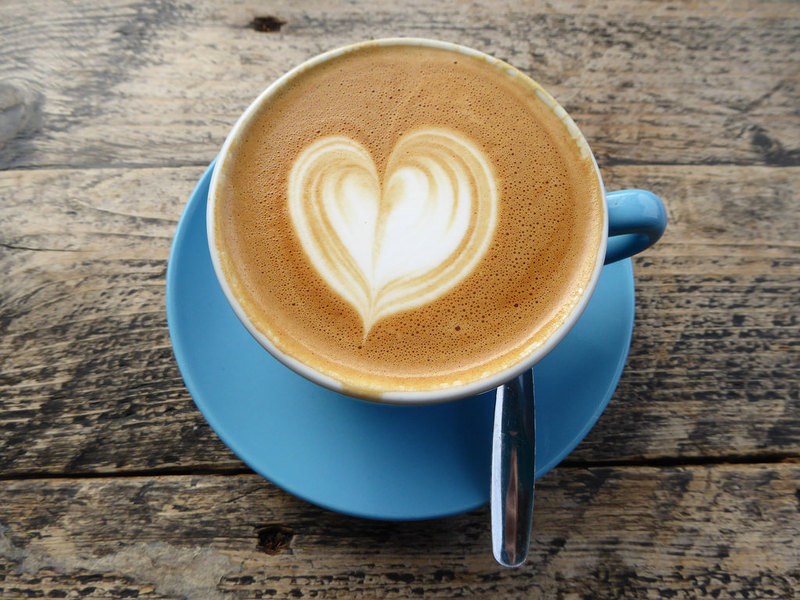
Does drinking coffee actually increase your life expectancy? A recent study (Freedman, Park, Abnet, Hollenbeck, & Sinha, 2012) found that men who drank at least six cups of coffee a day also had a 10% lower chance of dying (women’s chances were 15% lower) than those who drank none. Does this mean you should pick up or increase your own coffee habit? We will explore these results in more depth in the next section about drawing conclusions from statistics. Modern society has become awash in studies such as this; you can read about several such studies in the news every day.
Conducting such a study well, and interpreting the results of such studies requires understanding basic ideas of statistics , the science of gaining insight from data. Key components to a statistical investigation are:
- Planning the study: Start by asking a testable research question and deciding how to collect data. For example, how long was the study period of the coffee study? How many people were recruited for the study, how were they recruited, and from where? How old were they? What other variables were recorded about the individuals? Were changes made to the participants’ coffee habits during the course of the study?
- Examining the data: What are appropriate ways to examine the data? What graphs are relevant, and what do they reveal? What descriptive statistics can be calculated to summarize relevant aspects of the data, and what do they reveal? What patterns do you see in the data? Are there any individual observations that deviate from the overall pattern, and what do they reveal? For example, in the coffee study, did the proportions differ when we compared the smokers to the non-smokers?
- Inferring from the data: What are valid statistical methods for drawing inferences “beyond” the data you collected? In the coffee study, is the 10%–15% reduction in risk of death something that could have happened just by chance?
- Drawing conclusions: Based on what you learned from your data, what conclusions can you draw? Who do you think these conclusions apply to? (Were the people in the coffee study older? Healthy? Living in cities?) Can you draw a cause-and-effect conclusion about your treatments? (Are scientists now saying that the coffee drinking is the cause of the decreased risk of death?)
Notice that the numerical analysis (“crunching numbers” on the computer) comprises only a small part of overall statistical investigation. In this section, you will see how we can answer some of these questions and what questions you should be asking about any statistical investigation you read about.
Distributional Thinking
When data are collected to address a particular question, an important first step is to think of meaningful ways to organize and examine the data. Let’s take a look at an example.
Example 1 : Researchers investigated whether cancer pamphlets are written at an appropriate level to be read and understood by cancer patients (Short, Moriarty, & Cooley, 1995). Tests of reading ability were given to 63 patients. In addition, readability level was determined for a sample of 30 pamphlets, based on characteristics such as the lengths of words and sentences in the pamphlet. The results, reported in terms of grade levels, are displayed in Figure 23.

- Data vary . More specifically, values of a variable (such as reading level of a cancer patient or readability level of a cancer pamphlet) vary.
- Analyzing the pattern of variation, called the distribution of the variable, often reveals insights.
Addressing the research question of whether the cancer pamphlets are written at appropriate levels for the cancer patients requires comparing the two distributions. A naïve comparison might focus only on the centers of the distributions. Both medians turn out to be ninth grade, but considering only medians ignores the variability and the overall distributions of these data. A more illuminating approach is to compare the entire distributions, for example with a graph, as in Figure 24.
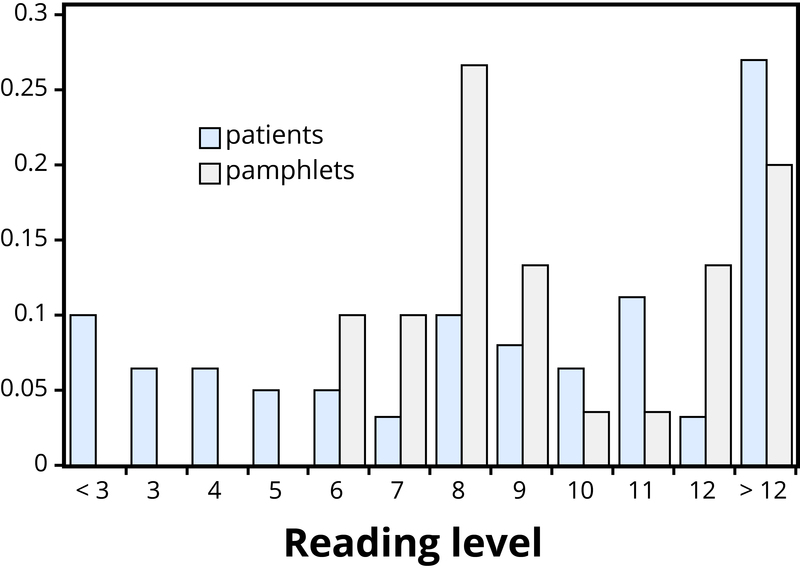
Figure 24 makes clear that the two distributions are not well aligned at all. The most glaring discrepancy is that many patients (17/63, or 27%, to be precise) have a reading level below that of the most readable pamphlet. These patients will need help to understand the information provided in the cancer pamphlets. Notice that this conclusion follows from considering the distributions as a whole, not simply measures of center or variability, and that the graph contrasts those distributions more immediately than the frequency tables.
Finding Significance in Data
Even when we find patterns in data, often there is still uncertainty in various aspects of the data. For example, there may be potential for measurement errors (even your own body temperature can fluctuate by almost 1°F over the course of the day). Or we may only have a “snapshot” of observations from a more long-term process or only a small subset of individuals from the population of interest. In such cases, how can we determine whether patterns we see in our small set of data is convincing evidence of a systematic phenomenon in the larger process or population? Let’s take a look at another example.
Example 2 : In a study reported in the November 2007 issue of Nature , researchers investigated whether pre-verbal infants take into account an individual’s actions toward others in evaluating that individual as appealing or aversive (Hamlin, Wynn, & Bloom, 2007). In one component of the study, 10-month-old infants were shown a “climber” character (a piece of wood with “googly” eyes glued onto it) that could not make it up a hill in two tries. Then the infants were shown two scenarios for the climber’s next try, one where the climber was pushed to the top of the hill by another character (“helper”), and one where the climber was pushed back down the hill by another character (“hinderer”). The infant was alternately shown these two scenarios several times. Then the infant was presented with two pieces of wood (representing the helper and the hinderer characters) and asked to pick one to play with.
The researchers found that of the 16 infants who made a clear choice, 14 chose to play with the helper toy. One possible explanation for this clear majority result is that the helping behavior of the one toy increases the infants’ likelihood of choosing that toy. But are there other possible explanations? What about the color of the toy? Well, prior to collecting the data, the researchers arranged so that each color and shape (red square and blue circle) would be seen by the same number of infants. Or maybe the infants had right-handed tendencies and so picked whichever toy was closer to their right hand?
Well, prior to collecting the data, the researchers arranged it so half the infants saw the helper toy on the right and half on the left. Or, maybe the shapes of these wooden characters (square, triangle, circle) had an effect? Perhaps, but again, the researchers controlled for this by rotating which shape was the helper toy, the hinderer toy, and the climber. When designing experiments, it is important to control for as many variables as might affect the responses as possible. It is beginning to appear that the researchers accounted for all the other plausible explanations. But there is one more important consideration that cannot be controlled—if we did the study again with these 16 infants, they might not make the same choices. In other words, there is some randomness inherent in their selection process.
Maybe each infant had no genuine preference at all, and it was simply “random luck” that led to 14 infants picking the helper toy. Although this random component cannot be controlled, we can apply a probability model to investigate the pattern of results that would occur in the long run if random chance were the only factor.
If the infants were equally likely to pick between the two toys, then each infant had a 50% chance of picking the helper toy. It’s like each infant tossed a coin, and if it landed heads, the infant picked the helper toy. So if we tossed a coin 16 times, could it land heads 14 times? Sure, it’s possible, but it turns out to be very unlikely. Getting 14 (or more) heads in 16 tosses is about as likely as tossing a coin and getting 9 heads in a row. This probability is referred to as a p-value . The p-value represents the likelihood that experimental results happened by chance. Within psychology, the most common standard for p-values is “p < .05”. What this means is that there is less than a 5% probability that the results happened just by random chance, and therefore a 95% probability that the results reflect a meaningful pattern in human psychology. We call this statistical significance .
So, in the study above, if we assume that each infant was choosing equally, then the probability that 14 or more out of 16 infants would choose the helper toy is found to be 0.0021. We have only two logical possibilities: either the infants have a genuine preference for the helper toy, or the infants have no preference (50/50) and an outcome that would occur only 2 times in 1,000 iterations happened in this study. Because this p-value of 0.0021 is quite small, we conclude that the study provides very strong evidence that these infants have a genuine preference for the helper toy.
If we compare the p-value to some cut-off value, like 0.05, we see that the p=value is smaller. Because the p-value is smaller than that cut-off value, then we reject the hypothesis that only random chance was at play here. In this case, these researchers would conclude that significantly more than half of the infants in the study chose the helper toy, giving strong evidence of a genuine preference for the toy with the helping behavior.
Drawing Conclusions from Statistics
Generalizability.
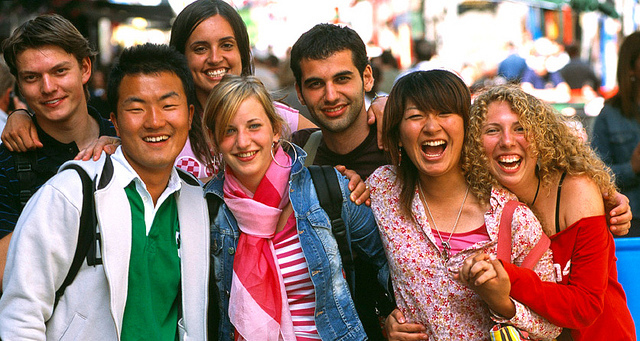
One limitation to the study mentioned previously about the babies choosing the “helper” toy is that the conclusion only applies to the 16 infants in the study. We don’t know much about how those 16 infants were selected. Suppose we want to select a subset of individuals (a sample ) from a much larger group of individuals (the population ) in such a way that conclusions from the sample can be generalized to the larger population. This is the question faced by pollsters every day.
Example 3 : The General Social Survey (GSS) is a survey on societal trends conducted every other year in the United States. Based on a sample of about 2,000 adult Americans, researchers make claims about what percentage of the U.S. population consider themselves to be “liberal,” what percentage consider themselves “happy,” what percentage feel “rushed” in their daily lives, and many other issues. The key to making these claims about the larger population of all American adults lies in how the sample is selected. The goal is to select a sample that is representative of the population, and a common way to achieve this goal is to select a r andom sample that gives every member of the population an equal chance of being selected for the sample. In its simplest form, random sampling involves numbering every member of the population and then using a computer to randomly select the subset to be surveyed. Most polls don’t operate exactly like this, but they do use probability-based sampling methods to select individuals from nationally representative panels.
In 2004, the GSS reported that 817 of 977 respondents (or 83.6%) indicated that they always or sometimes feel rushed. This is a clear majority, but we again need to consider variation due to random sampling . Fortunately, we can use the same probability model we did in the previous example to investigate the probable size of this error. (Note, we can use the coin-tossing model when the actual population size is much, much larger than the sample size, as then we can still consider the probability to be the same for every individual in the sample.) This probability model predicts that the sample result will be within 3 percentage points of the population value (roughly 1 over the square root of the sample size, the margin of error. A statistician would conclude, with 95% confidence, that between 80.6% and 86.6% of all adult Americans in 2004 would have responded that they sometimes or always feel rushed.
The key to the margin of error is that when we use a probability sampling method, we can make claims about how often (in the long run, with repeated random sampling) the sample result would fall within a certain distance from the unknown population value by chance (meaning by random sampling variation) alone. Conversely, non-random samples are often suspect to bias, meaning the sampling method systematically over-represents some segments of the population and under-represents others. We also still need to consider other sources of bias, such as individuals not responding honestly. These sources of error are not measured by the margin of error.
Cause and Effect
In many research studies, the primary question of interest concerns differences between groups. Then the question becomes how were the groups formed (e.g., selecting people who already drink coffee vs. those who don’t). In some studies, the researchers actively form the groups themselves. But then we have a similar question—could any differences we observe in the groups be an artifact of that group-formation process? Or maybe the difference we observe in the groups is so large that we can discount a “fluke” in the group-formation process as a reasonable explanation for what we find?
Example 4 : A psychology study investigated whether people tend to display more creativity when they are thinking about intrinsic (internal) or extrinsic (external) motivations (Ramsey & Schafer, 2002, based on a study by Amabile, 1985). The subjects were 47 people with extensive experience with creative writing. Subjects began by answering survey questions about either intrinsic motivations for writing (such as the pleasure of self-expression) or extrinsic motivations (such as public recognition). Then all subjects were instructed to write a haiku, and those poems were evaluated for creativity by a panel of judges. The researchers conjectured beforehand that subjects who were thinking about intrinsic motivations would display more creativity than subjects who were thinking about extrinsic motivations. The creativity scores from the 47 subjects in this study are displayed in Figure 26, where higher scores indicate more creativity.
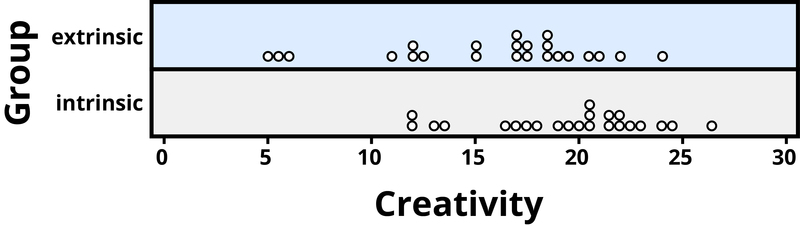
In this example, the key question is whether the type of motivation affects creativity scores. In particular, do subjects who were asked about intrinsic motivations tend to have higher creativity scores than subjects who were asked about extrinsic motivations?
Figure 26 reveals that both motivation groups saw considerable variability in creativity scores, and these scores have considerable overlap between the groups. In other words, it’s certainly not always the case that those with extrinsic motivations have higher creativity than those with intrinsic motivations, but there may still be a statistical tendency in this direction. (Psychologist Keith Stanovich (2013) refers to people’s difficulties with thinking about such probabilistic tendencies as “the Achilles heel of human cognition.”)
The mean creativity score is 19.88 for the intrinsic group, compared to 15.74 for the extrinsic group, which supports the researchers’ conjecture. Yet comparing only the means of the two groups fails to consider the variability of creativity scores in the groups. We can measure variability with statistics using, for instance, the standard deviation: 5.25 for the extrinsic group and 4.40 for the intrinsic group. The standard deviations tell us that most of the creativity scores are within about 5 points of the mean score in each group. We see that the mean score for the intrinsic group lies within one standard deviation of the mean score for extrinsic group. So, although there is a tendency for the creativity scores to be higher in the intrinsic group, on average, the difference is not extremely large.
We again want to consider possible explanations for this difference. The study only involved individuals with extensive creative writing experience. Although this limits the population to which we can generalize, it does not explain why the mean creativity score was a bit larger for the intrinsic group than for the extrinsic group. Maybe women tend to receive higher creativity scores? Here is where we need to focus on how the individuals were assigned to the motivation groups. If only women were in the intrinsic motivation group and only men in the extrinsic group, then this would present a problem because we wouldn’t know if the intrinsic group did better because of the different type of motivation or because they were women. However, the researchers guarded against such a problem by randomly assigning the individuals to the motivation groups. Like flipping a coin, each individual was just as likely to be assigned to either type of motivation. Why is this helpful? Because this random assignment tends to balance out all the variables related to creativity we can think of, and even those we don’t think of in advance, between the two groups. So we should have a similar male/female split between the two groups; we should have a similar age distribution between the two groups; we should have a similar distribution of educational background between the two groups; and so on. Random assignment should produce groups that are as similar as possible except for the type of motivation, which presumably eliminates all those other variables as possible explanations for the observed tendency for higher scores in the intrinsic group.
But does this always work? No, so by “luck of the draw” the groups may be a little different prior to answering the motivation survey. So then the question is, is it possible that an unlucky random assignment is responsible for the observed difference in creativity scores between the groups? In other words, suppose each individual’s poem was going to get the same creativity score no matter which group they were assigned to, that the type of motivation in no way impacted their score. Then how often would the random-assignment process alone lead to a difference in mean creativity scores as large (or larger) than 19.88 – 15.74 = 4.14 points?
We again want to apply to a probability model to approximate a p-value , but this time the model will be a bit different. Think of writing everyone’s creativity scores on an index card, shuffling up the index cards, and then dealing out 23 to the extrinsic motivation group and 24 to the intrinsic motivation group, and finding the difference in the group means. We (better yet, the computer) can repeat this process over and over to see how often, when the scores don’t change, random assignment leads to a difference in means at least as large as 4.41. Figure 27 shows the results from 1,000 such hypothetical random assignments for these scores.
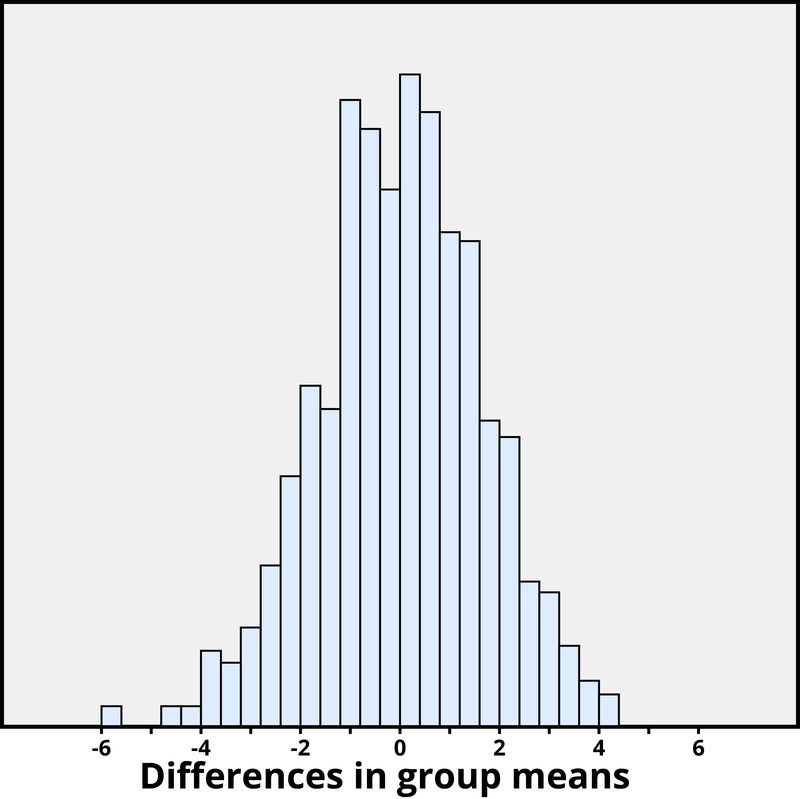
Only 2 of the 1,000 simulated random assignments produced a difference in group means of 4.41 or larger. In other words, the approximate p-value is 2/1000 = 0.002. This small p-value indicates that it would be very surprising for the random assignment process alone to produce such a large difference in group means. Therefore, as with Example 2, we have strong evidence that focusing on intrinsic motivations tends to increase creativity scores, as compared to thinking about extrinsic motivations.
Notice that the previous statement implies a cause-and-effect relationship between motivation and creativity score; is such a strong conclusion justified? Yes, because of the random assignment used in the study. That should have balanced out any other variables between the two groups, so now that the small p-value convinces us that the higher mean in the intrinsic group wasn’t just a coincidence, the only reasonable explanation left is the difference in the type of motivation. Can we generalize this conclusion to everyone? Not necessarily—we could cautiously generalize this conclusion to individuals with extensive experience in creative writing similar the individuals in this study, but we would still want to know more about how these individuals were selected to participate.
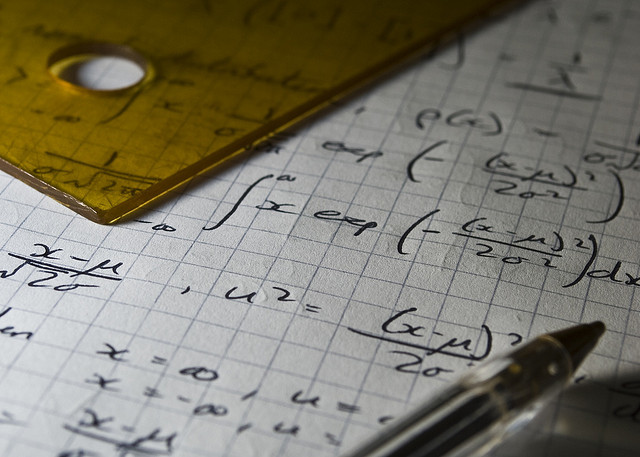
Statistical thinking involves the careful design of a study to collect meaningful data to answer a focused research question, detailed analysis of patterns in the data, and drawing conclusions that go beyond the observed data. Random sampling is paramount to generalizing results from our sample to a larger population, and random assignment is key to drawing cause-and-effect conclusions. With both kinds of randomness, probability models help us assess how much random variation we can expect in our results, in order to determine whether our results could happen by chance alone and to estimate a margin of error.
So where does this leave us with regard to the coffee study mentioned previously (the Freedman, Park, Abnet, Hollenbeck, & Sinha, 2012 found that men who drank at least six cups of coffee a day had a 10% lower chance of dying (women 15% lower) than those who drank none)? We can answer many of the questions:
- This was a 14-year study conducted by researchers at the National Cancer Institute.
- The results were published in the June issue of the New England Journal of Medicine , a respected, peer-reviewed journal.
- The study reviewed coffee habits of more than 402,000 people ages 50 to 71 from six states and two metropolitan areas. Those with cancer, heart disease, and stroke were excluded at the start of the study. Coffee consumption was assessed once at the start of the study.
- About 52,000 people died during the course of the study.
- People who drank between two and five cups of coffee daily showed a lower risk as well, but the amount of reduction increased for those drinking six or more cups.
- The sample sizes were fairly large and so the p-values are quite small, even though percent reduction in risk was not extremely large (dropping from a 12% chance to about 10%–11%).
- Whether coffee was caffeinated or decaffeinated did not appear to affect the results.
- This was an observational study, so no cause-and-effect conclusions can be drawn between coffee drinking and increased longevity, contrary to the impression conveyed by many news headlines about this study. In particular, it’s possible that those with chronic diseases don’t tend to drink coffee.
This study needs to be reviewed in the larger context of similar studies and consistency of results across studies, with the constant caution that this was not a randomized experiment. Whereas a statistical analysis can still “adjust” for other potential confounding variables, we are not yet convinced that researchers have identified them all or completely isolated why this decrease in death risk is evident. Researchers can now take the findings of this study and develop more focused studies that address new questions.
Explore these outside resources to learn more about applied statistics:
- Video about p-values: P-Value Extravaganza
- Interactive web applets for teaching and learning statistics
- Inter-university Consortium for Political and Social Research where you can find and analyze data.
- The Consortium for the Advancement of Undergraduate Statistics
- Find a recent research article in your field and answer the following: What was the primary research question? How were individuals selected to participate in the study? Were summary results provided? How strong is the evidence presented in favor or against the research question? Was random assignment used? Summarize the main conclusions from the study, addressing the issues of statistical significance, statistical confidence, generalizability, and cause and effect. Do you agree with the conclusions drawn from this study, based on the study design and the results presented?
- Is it reasonable to use a random sample of 1,000 individuals to draw conclusions about all U.S. adults? Explain why or why not.
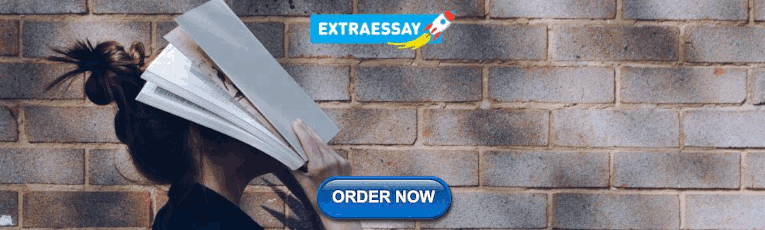
How to Read Research
In this course and throughout your academic career, you’ll be reading journal articles (meaning they were published by experts in a peer-reviewed journal) and reports that explain psychological research. It’s important to understand the format of these articles so that you can read them strategically and understand the information presented. Scientific articles vary in content or structure, depending on the type of journal to which they will be submitted. Psychological articles and many papers in the social sciences follow the writing guidelines and format dictated by the American Psychological Association (APA). In general, the structure follows: abstract, introduction, methods, results, discussion, and references.
- Abstract : the abstract is the concise summary of the article. It summarizes the most important features of the manuscript, providing the reader with a global first impression on the article. It is generally just one paragraph that explains the experiment as well as a short synopsis of the results.
- Introduction : this section provides background information about the origin and purpose of performing the experiment or study. It reviews previous research and presents existing theories on the topic.
- Method : this section covers the methodologies used to investigate the research question, including the identification of participants , procedures , and materials as well as a description of the actual procedure . It should be sufficiently detailed to allow for replication.
- Results : the results section presents key findings of the research, including reference to indicators of statistical significance.
- Discussion : this section provides an interpretation of the findings, states their significance for current research, and derives implications for theory and practice. Alternative interpretations for findings are also provided, particularly when it is not possible to conclude for the directionality of the effects. In the discussion, authors also acknowledge the strengths and limitations/weaknesses of the study and offer concrete directions about for future research.
Watch this 3-minute video for an explanation on how to read scholarly articles. Look closely at the example article shared just before the two minute mark.
https://digitalcommons.coastal.edu/kimbel-library-instructional-videos/9/
Practice identifying these key components in the following experiment: Food-Induced Emotional Resonance Improves Emotion Recognition.
In this chapter, you learned to
- define and apply the scientific method to psychology
- describe the strengths and weaknesses of descriptive, experimental, and correlational research
- define the basic elements of a statistical investigation
Putting It Together: Psychological Research
Psychologists use the scientific method to examine human behavior and mental processes. Some of the methods you learned about include descriptive, experimental, and correlational research designs.
Watch the CrashCourse video to review the material you learned, then read through the following examples and see if you can come up with your own design for each type of study.
You can view the transcript for “Psychological Research: Crash Course Psychology #2” here (opens in new window).
Case Study: a detailed analysis of a particular person, group, business, event, etc. This approach is commonly used to to learn more about rare examples with the goal of describing that particular thing.
- Ted Bundy was one of America’s most notorious serial killers who murdered at least 30 women and was executed in 1989. Dr. Al Carlisle evaluated Bundy when he was first arrested and conducted a psychological analysis of Bundy’s development of his sexual fantasies merging into reality (Ramsland, 2012). Carlisle believes that there was a gradual evolution of three processes that guided his actions: fantasy, dissociation, and compartmentalization (Ramsland, 2012). Read Imagining Ted Bundy (http://goo.gl/rGqcUv) for more information on this case study.
Naturalistic Observation : a researcher unobtrusively collects information without the participant’s awareness.
- Drain and Engelhardt (2013) observed six nonverbal children with autism’s evoked and spontaneous communicative acts. Each of the children attended a school for children with autism and were in different classes. They were observed for 30 minutes of each school day. By observing these children without them knowing, they were able to see true communicative acts without any external influences.
Survey : participants are asked to provide information or responses to questions on a survey or structure assessment.
- Educational psychologists can ask students to report their grade point average and what, if anything, they eat for breakfast on an average day. A healthy breakfast has been associated with better academic performance (Digangi’s 1999).
- Anderson (1987) tried to find the relationship between uncomfortably hot temperatures and aggressive behavior, which was then looked at with two studies done on violent and nonviolent crime. Based on previous research that had been done by Anderson and Anderson (1984), it was predicted that violent crimes would be more prevalent during the hotter time of year and the years in which it was hotter weather in general. The study confirmed this prediction.
Longitudinal Study: researchers recruit a sample of participants and track them for an extended period of time.
- In a study of a representative sample of 856 children Eron and his colleagues (1972) found that a boy’s exposure to media violence at age eight was significantly related to his aggressive behavior ten years later, after he graduated from high school.
Cross-Sectional Study: researchers gather participants from different groups (commonly different ages) and look for differences between the groups.
- In 1996, Russell surveyed people of varying age groups and found that people in their 20s tend to report being more lonely than people in their 70s.
Correlational Design: two different variables are measured to determine whether there is a relationship between them.
- Thornhill et al. (2003) had people rate how physically attractive they found other people to be. They then had them separately smell t-shirts those people had worn (without knowing which clothes belonged to whom) and rate how good or bad their body oder was. They found that the more attractive someone was the more pleasant their body order was rated to be.
- Clinical psychologists can test a new pharmaceutical treatment for depression by giving some patients the new pill and others an already-tested one to see which is the more effective treatment.
American Cancer Society. (n.d.). History of the cancer prevention studies. Retrieved from http://www.cancer.org/research/researchtopreventcancer/history-cancer-prevention-study
American Psychological Association. (2009). Publication Manual of the American Psychological Association (6th ed.). Washington, DC: Author.
American Psychological Association. (n.d.). Research with animals in psychology. Retrieved from https://www.apa.org/research/responsible/research-animals.pdf
Arnett, J. (2008). The neglected 95%: Why American psychology needs to become less American. American Psychologist, 63(7), 602–614.
Barton, B. A., Eldridge, A. L., Thompson, D., Affenito, S. G., Striegel-Moore, R. H., Franko, D. L., . . . Crockett, S. J. (2005). The relationship of breakfast and cereal consumption to nutrient intake and body mass index: The national heart, lung, and blood institute growth and health study. Journal of the American Dietetic Association, 105(9), 1383–1389. Retrieved from http://dx.doi.org/10.1016/j.jada.2005.06.003
Chwalisz, K., Diener, E., & Gallagher, D. (1988). Autonomic arousal feedback and emotional experience: Evidence from the spinal cord injured. Journal of Personality and Social Psychology, 54, 820–828.
Dominus, S. (2011, May 25). Could conjoined twins share a mind? New York Times Sunday Magazine. Retrieved from http://www.nytimes.com/2011/05/29/magazine/could-conjoined-twins-share-a-mind.html?_r=5&hp&
Fanger, S. M., Frankel, L. A., & Hazen, N. (2012). Peer exclusion in preschool children’s play: Naturalistic observations in a playground setting. Merrill-Palmer Quarterly, 58, 224–254.
Fiedler, K. (2004). Illusory correlation. In R. F. Pohl (Ed.), Cognitive illusions: A handbook on fallacies and biases in thinking, judgment and memory (pp. 97–114). New York, NY: Psychology Press.
Frantzen, L. B., Treviño, R. P., Echon, R. M., Garcia-Dominic, O., & DiMarco, N. (2013). Association between frequency of ready-to-eat cereal consumption, nutrient intakes, and body mass index in fourth- to sixth-grade low-income minority children. Journal of the Academy of Nutrition and Dietetics, 113(4), 511–519.
Harper, J. (2013, July 5). Ice cream and crime: Where cold cuisine and hot disputes intersect. The Times-Picaune. Retrieved from http://www.nola.com/crime/index.ssf/2013/07/ice_cream_and_crime_where_hot.html
Jenkins, W. J., Ruppel, S. E., Kizer, J. B., Yehl, J. L., & Griffin, J. L. (2012). An examination of post 9-11 attitudes towards Arab Americans. North American Journal of Psychology, 14, 77–84.
Jones, J. M. (2013, May 13). Same-sex marriage support solidifies above 50% in U.S. Gallup Politics. Retrieved from http://www.gallup.com/poll/162398/sex-marriage-support-solidifies-above.aspx
Kobrin, J. L., Patterson, B. F., Shaw, E. J., Mattern, K. D., & Barbuti, S. M. (2008). Validity of the SAT for predicting first-year college grade point average (Research Report No. 2008-5). Retrieved from https://research.collegeboard.org/sites/default/files/publications/2012/7/researchreport-2008-5-validity-sat-predicting-first-year-college-grade-point-average.pdf
Lewin, T. (2014, March 5). A new SAT aims to realign with schoolwork. New York Times. Retreived from http://www.nytimes.com/2014/03/06/education/major-changes-in-sat-announced-by-college-board.html.
Lowry, M., Dean, K., & Manders, K. (2010). The link between sleep quantity and academic performance for the college student. Sentience: The University of Minnesota Undergraduate Journal of Psychology, 3(Spring), 16–19. Retrieved from http://www.psych.umn.edu/sentience/files/SENTIENCE_Vol3.pdf
McKie, R. (2010, June 26). Chimps with everything: Jane Goodall’s 50 years in the jungle. The Guardian. Retrieved from http://www.theguardian.com/science/2010/jun/27/jane-goodall-chimps-africa-interview
Offit, P. (2008). Autism’s false prophets: Bad science, risky medicine, and the search for a cure. New York: Columbia University Press.
Perkins, H. W., Haines, M. P., & Rice, R. (2005). Misperceiving the college drinking norm and related problems: A nationwide study of exposure to prevention information, perceived norms and student alcohol misuse. J. Stud. Alcohol, 66(4), 470–478.
Rimer, S. (2008, September 21). College panel calls for less focus on SATs. The New York Times. Retrieved from http://www.nytimes.com/2008/09/22/education/22admissions.html?_r=0
Rothstein, J. M. (2004). College performance predictions and the SAT. Journal of Econometrics, 121, 297–317.
Rotton, J., & Kelly, I. W. (1985). Much ado about the full moon: A meta-analysis of lunar-lunacy research. Psychological Bulletin, 97(2), 286–306. doi:10.1037/0033-2909.97.2.286
Santelices, M. V., & Wilson, M. (2010). Unfair treatment? The case of Freedle, the SAT, and the standardization approach to differential item functioning. Harvard Education Review, 80, 106–134.
Sears, D. O. (1986). College sophomores in the laboratory: Influences of a narrow data base on social psychology’s view of human nature. Journal of Personality and Social Psychology, 51, 515–530.
Tuskegee University. (n.d.). About the USPHS Syphilis Study. Retrieved from http://www.tuskegee.edu/about_us/centers_of_excellence/bioethics_center/about_the_usphs_syphilis_study.aspx.
CC licensed content, Original
- Psychological Research Methods. Provided by : Karenna Malavanti. License : CC BY-SA: Attribution ShareAlike
CC licensed content, Shared previously
- Psychological Research. Provided by : OpenStax College. License : CC BY: Attribution . License Terms : Download for free at https://openstax.org/books/psychology-2e/pages/1-introduction. Located at : https://openstax.org/books/psychology-2e/pages/2-introduction .
- Why It Matters: Psychological Research. Provided by : Lumen Learning. License : CC BY: Attribution Located at: https://pressbooks.online.ucf.edu/lumenpsychology/chapter/introduction-15/
- Introduction to The Scientific Method. Provided by : Lumen Learning. License : CC BY: Attribution Located at: https://pressbooks.online.ucf.edu/lumenpsychology/chapter/outcome-the-scientific-method/
- Research picture. Authored by : Mediterranean Center of Medical Sciences. Provided by : Flickr. License : CC BY: Attribution Located at : https://www.flickr.com/photos/mcmscience/17664002728 .
- The Scientific Process. Provided by : Lumen Learning. License : CC BY-SA: Attribution ShareAlike Located at: https://pressbooks.online.ucf.edu/lumenpsychology/chapter/reading-the-scientific-process/
- Ethics in Research. Provided by : Lumen Learning. License : CC BY: Attribution Located at: https://pressbooks.online.ucf.edu/lumenpsychology/chapter/ethics/
- Ethics. Authored by : OpenStax College. Located at : https://openstax.org/books/psychology-2e/pages/2-4-ethics . License : CC BY: Attribution . License Terms : Download for free at https://openstax.org/books/psychology-2e/pages/1-introduction .
- Introduction to Approaches to Research. Provided by : Lumen Learning. License : CC BY-NC-SA: Attribution NonCommercial ShareAlike Located at: https://pressbooks.online.ucf.edu/lumenpsychology/chapter/outcome-approaches-to-research/
- Lec 2 | MIT 9.00SC Introduction to Psychology, Spring 2011. Authored by : John Gabrieli. Provided by : MIT OpenCourseWare. License : CC BY-NC-SA: Attribution-NonCommercial-ShareAlike Located at : https://www.youtube.com/watch?v=syXplPKQb_o .
- Paragraph on correlation. Authored by : Christie Napa Scollon. Provided by : Singapore Management University. License : CC BY-NC-SA: Attribution-NonCommercial-ShareAlike Located at : http://nobaproject.com/modules/research-designs?r=MTc0ODYsMjMzNjQ%3D . Project : The Noba Project.
- Descriptive Research. Provided by : Lumen Learning. License : CC BY-SA: Attribution ShareAlike Located at: https://pressbooks.online.ucf.edu/lumenpsychology/chapter/reading-clinical-or-case-studies/
- Approaches to Research. Authored by : OpenStax College. License : CC BY: Attribution . License Terms : Download for free at https://openstax.org/books/psychology-2e/pages/1-introduction. Located at : https://openstax.org/books/psychology-2e/pages/2-2-approaches-to-research
- Analyzing Findings. Authored by : OpenStax College. Located at : https://openstax.org/books/psychology-2e/pages/2-3-analyzing-findings . License : CC BY: Attribution . License Terms : Download for free at https://openstax.org/books/psychology-2e/pages/1-introduction.
- Experiments. Provided by : Lumen Learning. License : CC BY: Attribution Located at: https://pressbooks.online.ucf.edu/lumenpsychology/chapter/reading-conducting-experiments/
- Research Review. Authored by : Jessica Traylor for Lumen Learning. License : CC BY: Attribution Located at: https://pressbooks.online.ucf.edu/lumenpsychology/chapter/reading-conducting-experiments/
- Introduction to Statistics. Provided by : Lumen Learning. License : CC BY: Attribution Located at: https://pressbooks.online.ucf.edu/lumenpsychology/chapter/outcome-statistical-thinking/
- histogram. Authored by : Fisher’s Iris flower data set. Provided by : Wikipedia.
- License : CC BY-SA: Attribution-ShareAlike Located at : https://en.wikipedia.org/wiki/Wikipedia:Meetup/DC/Statistics_Edit-a-thon#/media/File:Fisher_iris_versicolor_sepalwidth.svg .
- Statistical Thinking. Authored by : Beth Chance and Allan Rossman . Provided by : California Polytechnic State University, San Luis Obispo.
- License : CC BY-NC-SA: Attribution-NonCommerci al-S hareAlike . License Terms : http://nobaproject.com/license-agreement Located at : http://nobaproject.com/modules/statistical-thinking . Project : The Noba Project.
- Drawing Conclusions from Statistics. Authored by: Pat Carroll and Lumen Learning. Provided by : Lumen Learning. License : CC BY: Attribution Located at: https://pressbooks.online.ucf.edu/lumenpsychology/chapter/reading-drawing-conclusions-from-statistics/
- Statistical Thinking. Authored by : Beth Chance and Allan Rossman, California Polytechnic State University, San Luis Obispo. Provided by : Noba. License: CC BY-NC-SA: Attribution-NonCommercial-ShareAlike Located at : http://nobaproject.com/modules/statistical-thinking .
- The Replication Crisis. Authored by : Colin Thomas William. Provided by : Ivy Tech Community College. License: CC BY: Attribution
- How to Read Research. Provided by : Lumen Learning. License : CC BY: Attribution Located at: https://pressbooks.online.ucf.edu/lumenpsychology/chapter/how-to-read-research/
- What is a Scholarly Article? Kimbel Library First Year Experience Instructional Videos. 9. Authored by: Joshua Vossler, John Watts, and Tim Hodge. Provided by : Coastal Carolina University License : CC BY NC ND: Attribution-NonCommercial-NoDerivatives Located at : https://digitalcommons.coastal.edu/kimbel-library-instructional-videos/9/
- Putting It Together: Psychological Research. Provided by : Lumen Learning. License : CC BY: Attribution Located at: https://pressbooks.online.ucf.edu/lumenpsychology/chapter/putting-it-together-psychological-research/
- Research. Provided by : Lumen Learning. License : CC BY: Attribution Located at:
All rights reserved content
- Understanding Driver Distraction. Provided by : American Psychological Association. License : Other. License Terms: Standard YouTube License Located at : https://www.youtube.com/watch?v=XToWVxS_9lA&list=PLxf85IzktYWJ9MrXwt5GGX3W-16XgrwPW&index=9 .
- Correlation vs. Causality: Freakonomics Movie. License : Other. License Terms : Standard YouTube License Located at : https://www.youtube.com/watch?v=lbODqslc4Tg.
- Psychological Research – Crash Course Psychology #2. Authored by : Hank Green. Provided by : Crash Course. License : Other. License Terms : Standard YouTube License Located at : https://www.youtube.com/watch?v=hFV71QPvX2I .
Public domain content
- Researchers review documents. Authored by : National Cancer Institute. Provided by : Wikimedia. Located at : https://commons.wikimedia.org/wiki/File:Researchers_review_documents.jpg . License : Public Domain: No Known Copyright
grounded in objective, tangible evidence that can be observed time and time again, regardless of who is observing
well-developed set of ideas that propose an explanation for observed phenomena
(plural: hypotheses) tentative and testable statement about the relationship between two or more variables
an experiment must be replicable by another researcher
implies that a theory should enable us to make predictions about future events
able to be disproven by experimental results
implies that all data must be considered when evaluating a hypothesis
committee of administrators, scientists, and community members that reviews proposals for research involving human participants
process of informing a research participant about what to expect during an experiment, any risks involved, and the implications of the research, and then obtaining the person’s consent to participate
purposely misleading experiment participants in order to maintain the integrity of the experiment
when an experiment involved deception, participants are told complete and truthful information about the experiment at its conclusion
committee of administrators, scientists, veterinarians, and community members that reviews proposals for research involving non-human animals
research studies that do not test specific relationships between variables
research investigating the relationship between two or more variables
research method that uses hypothesis testing to make inferences about how one variable impacts and causes another
observation of behavior in its natural setting
inferring that the results for a sample apply to the larger population
when observations may be skewed to align with observer expectations
measure of agreement among observers on how they record and classify a particular event
observational research study focusing on one or a few people
list of questions to be answered by research participants—given as paper-and-pencil questionnaires, administered electronically, or conducted verbally—allowing researchers to collect data from a large number of people
subset of individuals selected from the larger population
overall group of individuals that the researchers are interested in
method of research using past records or data sets to answer various research questions, or to search for interesting patterns or relationships
studies in which the same group of individuals is surveyed or measured repeatedly over an extended period of time
compares multiple segments of a population at a single time
reduction in number of research participants as some drop out of the study over time
relationship between two or more variables; when two variables are correlated, one variable changes as the other does
number from -1 to +1, indicating the strength and direction of the relationship between variables, and usually represented by r
two variables change in the same direction, both becoming either larger or smaller
two variables change in different directions, with one becoming larger as the other becomes smaller; a negative correlation is not the same thing as no correlation
changes in one variable cause the changes in the other variable; can be determined only through an experimental research design
unanticipated outside factor that affects both variables of interest, often giving the false impression that changes in one variable causes changes in the other variable, when, in actuality, the outside factor causes changes in both variables
seeing relationships between two things when in reality no such relationship exists
tendency to ignore evidence that disproves ideas or beliefs
group designed to answer the research question; experimental manipulation is the only difference between the experimental and control groups, so any differences between the two are due to experimental manipulation rather than chance
serves as a basis for comparison and controls for chance factors that might influence the results of the study—by holding such factors constant across groups so that the experimental manipulation is the only difference between groups
description of what actions and operations will be used to measure the dependent variables and manipulate the independent variables
researcher expectations skew the results of the study
experiment in which the researcher knows which participants are in the experimental group and which are in the control group
experiment in which both the researchers and the participants are blind to group assignments
people's expectations or beliefs influencing or determining their experience in a given situation
variable that is influenced or controlled by the experimenter; in a sound experimental study, the independent variable is the only important difference between the experimental and control group
variable that the researcher measures to see how much effect the independent variable had
subjects of psychological research
subset of a larger population in which every member of the population has an equal chance of being selected
method of experimental group assignment in which all participants have an equal chance of being assigned to either group
consistency and reproducibility of a given result
accuracy of a given result in measuring what it is designed to measure
determines how likely any difference between experimental groups is due to chance
statistical probability that represents the likelihood that experimental results happened by chance
Psychological Science is the scientific study of mind, brain, and behavior. We will explore what it means to be human in this class. It has never been more important for us to understand what makes people tick, how to evaluate information critically, and the importance of history. Psychology can also help you in your future career; indeed, there are very little jobs out there with no human interaction!
Because psychology is a science, we analyze human behavior through the scientific method. There are several ways to investigate human phenomena, such as observation, experiments, and more. We will discuss the basics, pros and cons of each! We will also dig deeper into the important ethical guidelines that psychologists must follow in order to do research. Lastly, we will briefly introduce ourselves to statistics, the language of scientific research. While reading the content in these chapters, try to find examples of material that can fit with the themes of the course.
To get us started:
- The study of the mind moved away Introspection to reaction time studies as we learned more about empiricism
- Psychologists work in careers outside of the typical "clinician" role. We advise in human factors, education, policy, and more!
- While completing an observation study, psychologists will work to aggregate common themes to explain the behavior of the group (sample) as a whole. In doing so, we still allow for normal variation from the group!
- The IRB and IACUC are important in ensuring ethics are maintained for both human and animal subjects
Psychological Science: Understanding Human Behavior Copyright © by Karenna Malavanti is licensed under a Creative Commons Attribution-NonCommercial-ShareAlike 4.0 International License , except where otherwise noted.
Share This Book

APA Handbook of Research Methods in Psychology
Available formats.
- Table of contents
- Contributor bios
- Book details
With significant new and updated content across dozens of chapters, this second edition presents the most exhaustive treatment available of the techniques psychologists and others have developed to help them pursue a shared understanding of why humans think, feel, and behave the way they do.
The initial chapters in this indispensable three-volume handbook address broad, crosscutting issues faced by researchers: the philosophical, ethical, and societal underpinnings of psychological research. Next, chapters detail the research planning process, describe the range of measurement techniques that psychologists most often use to collect data, consider how to determine the best measurement techniques for a particular purpose, and examine ways to assess the trustworthiness of measures.
Additional chapters cover various aspects of quantitative, qualitative, neuropsychological, and biological research designs, presenting an array of options and their nuanced distinctions. Chapters on techniques for data analysis follow, and important issues in writing up research to share with the community of psychologists are discussed in the handbook’s concluding chapters.
Among the newly written chapters in the second edition, the handbook’s stellar roster of authors cover literature searching, workflow and reproducibility, research funding, neuroimaging, various facets of a wide range of research designs and data analysis methods, and updated information on the publication process, including research data management and sharing, questionable practices in statistical analysis, and ethical issues in manuscript preparation and authorship.
Volume 1. Foundations, Planning, Measures, and Psychometrics
Editorial Board
About the Editors
Contributors
A Note from the Publisher
Introduction: Objectives of Psychological Research and Their Relations to Research Methods
Part I. Philosophical, Ethical, and Societal Underpinnings of Psychological Research
- Chapter 1. Perspectives on the Epistemological Bases for Qualitative Research Carla Willig
- Chapter 2. Frameworks for Causal Inference in Psychological Science Peter M. Steiner, William R. Shadish, and Kristynn J. Sullivan
- Chapter 3. Ethics in Psychological Research: Guidelines and Regulations Adam L. Fried and Kate L. Jansen
- Chapter 4. Ethics and Regulation of Research With Nonhuman Animals Sangeeta Panicker, Chana K. Akins, and Beth Ann Rice
- Chapter 5. Cross-Cultural Research Methods David Masumoto and Fons J. R. van de Vijver
- Chapter 6.Research With Populations that Experience Marginalization George P. Knight, Rebecca M. B. White, Stefanie Martinez-Fuentes, Mark W. Roosa, and Adriana J. Umaña-Taylor
Part II. Planning Research
- Chapter 7. Developing Testable and Important Research Questions Frederick T. L. Leong, Neal Schmitt, and Brent J. Lyons
- Chapter 8. Searching With a Purpose: How to Use Literature Searching to Support Your Research Diana Ramirez and Margaret J. Foster
- Chapter 9. Psychological Measurement: Scaling and Analysis Heather Hayes and Susan E. Embretson
- Chapter 10. Sample Size Planning Ken Kelley, Samantha F. Anderson, and Scott E. Maxwell
- Chapter 11. Workflow and Reproducibility Oliver Kirchkamp
- Chapter 12. Obtaining and Evaluating Research Funding Jonathan S. Comer and Amanda L. Sanchez
Part III. Measurement Methods
- Chapter 13. Behavioral Observation Roger Bakeman and Vicenç Quera
- Chapter 14. Question Order Effects Lisa Lee, Parvati Krishnamurty, and Struther Van Horn
- Chapter 15. Interviews and Interviewing Techniques Anna Madill
- Chapter 16. Using Intensive Longitudinal Methods in Psychological Research Masumi Iida, Patrick E. Shrout, Jean-Philippe Laurenceau, and Niall Bolger
- Chapter 17. Automated Analyses of Natural Language in Psychological Research Laura K. Allen, Arthur C. Graesser, and Danielle S. McNamara
- Chapter 18. Objective Tests as Instruments of Psychological Theory and Research David Watson
- Chapter 19. Norm- and Criterion-Referenced Testing Kurt F. Geisinger
- Chapter 20. The Current Status of "Projective" "Tests" Robert E. McGrath, Alec Twibell, and Elizabeth J. Carroll
- Chapter 21. Brief Instruments and Short Forms Emily A. Atkinson, Carolyn M. Pearson Carter, Jessica L. Combs Rohr, and Gregory T. Smith
- Chapter 22. Eye Movements, Pupillometry, and Cognitive Processes Simon P. Liversedge, Sara V. Milledge, and Hazel I. Blythe
- Chapter 23. Response Times Roger Ratcliff
- Chapter 24. Psychophysics: Concepts, Methods, and Frontiers Allie C. Hexley, Takuma Morimoto, and Manuel Spitschan
- Chapter 25. The Perimetric Physiological Measurement of Psychological Constructs Louis G. Tassinary, Ursula Hess, Luis M. Carcoba, and Joseph M. Orr
- Chapter 26. Salivary Hormone Assays Linda Becker, Nicholas Rohleder, and Oliver C. Schultheiss
- Chapter 27. Electro- and Magnetoencephalographic Methods in Psychology Eddie Harmon-Jones, David M. Amodio, Philip A. Gable, and Suzanne Dikker
- Chapter 28. Event-Related Potentials Steven J. Luck
- Chapter 29. Functional Neuroimaging Megan T. deBettencourt, Wilma A. Bainbridge, Monica D. Rosenberg
- Chapter 30. Noninvasive Stimulation of the Cerebral Cortex Dennis J. L. G. Schutter
- Chapter 31. Combined Neuroimaging Methods Marius Moisa and Christian C. Ruff
- Chapter 32. Neuroimaging Analysis Methods Yanyu Xiong and Sharlene D. Newman
Part IV. Psychometrics
- Chapter 33. Reliability Sean P. Lane, Elizabeth N. Aslinger, and Patrick E. Shrout
- Chapter 34. Generalizability Theory Xiaohong Gao and Deborah J. Harris
- Chapter 35. Construct Validity Kevin J. Grimm and Keith F. Widaman
- Chapter 36. Item-Level Factor Nisha C. Gottfredson, Brian D. Stucky, and A. T. Panter
- Chapter 37. Item Response Theory Steven P. Reise and Tyler M. Moore
- Chapter 38. Measuring Test Performance With Signal Detection Theory Techniques Teresa A. Treat and Richard J. Viken
Volume 2. Research Designs: Quantitative, Qualitative, Neuropsychological, and Biological
Part I. Qualitative Research Methods
- Chapter 1. Developments in Qualitative Inquiry Sarah Riley and Andrea LaMarre
- Chapter 2. Metasynthesis of Qualitative Research Sally Thorne
- Chapter 3. Grounded Theory and Psychological Research Robert Thornberg, Elaine Keane, and Malgorzata Wójcik
- Chapter 4. Thematic Analysis Virginia Braun and Victoria Clarke
- Chapter 5. Phenomenological Methodology, Methods, and Procedures for Research in Psychology Frederick J. Wertz
- Chapter 6. Narrative Analysis Javier Monforte and Brett Smith
- Chapter 7. Ethnomethodology and Conversation Analysis Paul ten Have
- Chapter 8. Discourse Analysis and Discursive Psychology Chris McVittie and Andy McKinlay
- Chapter 9. Ethnography in Psychological Research Elizabeth Fein and Jonathan Yahalom
- Chapter 10. Visual Research in Psychology Paula Reavey, Jon Prosser, and Steven D. Brown
- Chapter 11. Researching the Temporal Karen Henwood and Fiona Shirani
Part II. Working Across Epistemologies, Methodologies, and Methods
- Chapter 12. Mixed Methods Research in Psychology Timothy C. Guetterman and Analay Perez
- Chapter 13. The "Cases Within Trials" (CWT) Method: An Example of a Mixed-Methods Research Design Daniel B. Fishman
- Chapter 14. Researching With American Indian and Alaska Native Communities: Pursuing Partnerships for Psychological Inquiry in Service to Indigenous Futurity Joseph P. Gone
- Chapter 15. Participatory Action Research as Movement Toward Radical Relationality, Epistemic Justice, and Transformative Intervention: A Multivocal Reflection Urmitapa Dutta, Jesica Siham Fernández, Anne Galletta, and Regina Day Langhout
Part III. Sampling Across People and Time
- Chapter 16. Introduction to Survey Sampling Roger Tourangeau and Ting Yan
- Chapter 17. Epidemiology Rumi Kato Price and Heidi H. Tastet
- Chapter 18. Collecting Longitudinal Data: Present Issues and Future Challenges Simran K. Johal, Rohit Batra, and Emilio Ferrer
- Chapter 19. Using the Internet to Collect Data Ulf-Dietrich Reips
Part IV. Building and Testing Models
- Chapter 20. Statistical Mediation Analysis David P. MacKinnon, Jeewon Cheong, Angela G. Pirlott, and Heather L. Smyth
- Chapter 21. Structural Equation Modeling with Latent Variables Rick H. Hoyle and Nisha C. Gottfredson
- Chapter 22. Mathematical Psychology Parker Smith, Yanjun Liu, James T. Townsend, and Trisha Van Zandt
- Chapter 23. Computational Modeling Adele Diederich
- Chapter 24. Fundamentals of Bootstrapping and Monte Carlo Methods William Howard Beasley, Patrick O'Keefe, and Joseph Lee Rodgers
- Chapter 25. Designing Simulation Studies Xitao Fan
- Chapter 26. Bayesian Modeling for Psychologists: An Applied Approach Fred M. Feinberg and Richard Gonzalez
Part V. Designs Involving Experimental Manipulations
- Chapter 27. Randomized Designs in Psychological Research Larry Christensen, Lisa A. Turner, and R. Burke Johnson
- Chapter 28. Nonequivalent Comparison Group Designs Henry May and Zachary K. Collier
- Chapter 29. Regression Discontinuity Designs Charles S. Reichardt and Gary T. Henry
- Chapter 30. Treatment Validity for Intervention Studies Dianne L. Chambless and Steven D. Hollon
- Chapter 31. Translational Research Michael T. Bardo, Christopher Cappelli, and Mary Ann Pentz
- Chapter 32. Program Evaluation: Outcomes and Costs of Putting Psychology to Work Brian T. Yates
Part VI. Quantitative Research Designs Involving Single Participants or Units
- Chapter 33. Single-Case Experimental Design John M. Ferron, Megan Kirby, and Lodi Lipien
- Chapter 34. Time Series Designs Bradley J. Bartos, Richard McCleary, and David McDowall
Part VII. Designs in Neuropsychology and Biological Psychology
- Chapter 35. Case Studies in Neuropsychology Randi C. Martin, Simon Fischer-Baum, and Corinne M. Pettigrew
- Chapter 36. Group Studies in Experimental Neuropsychology Avinash R Vaidya, Maia Pujara, and Lesley K. Fellows
- Chapter 37. Genetic Methods in Psychology Terrell A. Hicks, Daniel Bustamante, Karestan C. Koenen, Nicole R. Nugent, and Ananda B. Amstadter
- Chapter 38. Human Genetic Epidemiology Floris Huider, Lannie Ligthart, Yuri Milaneschi, Brenda W. J. H. Penninx, and Dorret I. Boomsma
Volume 3. Data Analysis and Research Publication
Part I. Quantitative Data Analysis
- Chapter 1. Methods for Dealing With Bad Data and Inadequate Models: Distributions, Linear Models, and Beyond Rand R. Wilcox and Guillaume A. Rousselet
- Chapter 2. Maximum Likelihood and Multiple Imputation Missing Data Handling: How They Work, and How to Make Them Work in Practice Timothy Hayes and Craig K. Enders
- Chapter 3. Exploratory Data Analysis Paul F. Velleman and David C. Hoaglin
- Chapter 4. Graphic Displays of Data Leland Wilkinson
- Chapter 5. Estimating and Visualizing Interactions in Moderated Multiple Regression Connor J. McCabe and Kevin M. King
- Chapter 6. Effect Size Estimation Michael Borenstein
- Chapter 7. Measures of Clinically Significant Change Russell J. Bailey, Benjamin M. Ogles, and Michael J. Lambert
- Chapter 8. Analysis of Variance and the General Linear Model James Jaccard and Ai Bo
- Chapter 9. Generalized Linear Models David Rindskopf
- Chapter 10. Multilevel Modeling for Psychologists John B. Nezlek
- Chapter 11. Longitudinal Data Analysis Andrew K. Littlefield
- Chapter 12. Event History Analysis Fetene B. Tekle and Jeroen K. Vermunt
- Chapter 13. Latent State-Trait Models Rolf Steyer, Christian Geiser, and Christiane Loßnitzer
- Chapter 14. Latent Variable Modeling of Continuous Growth David A. Cole, Jeffrey A. Ciesla, and Qimin Liu
- Chapter 15. Dynamical Systems and Differential Equation Models of Change Steven M. Boker and Robert G. Moulder
- Chapter 16. A Multivariate Growth Curve Model for Three-Level Data Patrick J. Curran, Chris L. Strauss, Ethan M. McCormick, and James S. McGinley
- Chapter 17. Exploratory Factor Analysis and Confirmatory Factor Analysis Keith F. Widaman and Jonathan Lee Helm
- Chapter 18. Latent Class and Latent Profile Models Brian P. Flaherty, Liying Wang, and Cara J. Kiff
- Chapter 19. Decision Trees and Ensemble Methods in the Behavioral Sciences Kevin J. Grimm, Ross Jacobucci, and John J. McArdle
- Chapter 20. Using the Social Relations Model to Understand Interpersonal Perception and Behavior P. Niels Christensen, Deborah A. Kashy, and Katelin E. Leahy
- Chapter 21. Dyadic Data Analysis Richard Gonzalez and Dale Griffin
- Chapter 22. The Data of Others: New and Old Faces of Archival Research Sophie Pychlau and David T. Wagner
- Chapter 23. Social Network Analysis in Psychology: Recent Breakthroughs in Methods and Theories Wei Wang, Tobias Stark, James D. Westaby, Adam K. Parr, and Daniel A. Newman
- Chapter 24. Meta-Analysis Jeffrey C. Valentine, Therese D. Pigott, and Joseph Morris
Part II. Publishing and the Publication Process
- Chapter 25. Research Data Management and Sharing Katherine G. Akers and John A. Borghi
- Chapter 26. Questionable Practices in Statistical Analysis Rex B. Kline
- Chapter 27. Ethical Issues in Manuscript Preparation and Authorship Jennifer Crocker
Harris Cooper, PhD, is the Hugo L. Blomquist professor, emeritus, in the Department of Psychology and Neuroscience at Duke University. His research interests concern research synthesis and research methodology, and he also studies the application of social and developmental psychology to education policy. His book Research Synthesis and Meta-Analysis: A Step-by-Step Approach (2017) is in its fifth edition. He is the coeditor of the Handbook of Research Synthesis and Meta-Analysis (3 rd ed. 2019).
In 2007, Dr. Cooper was the recipient of the Frederick Mosteller Award for Contributions to Research Synthesis Methodology, and in 2008 he received the Ingram Olkin Award for Distinguished Lifetime Contribution to Research Synthesis from the Society for Research Synthesis Methodology.
He served as the chair of the Department of Psychology and Neuroscience at Duke University from 2009 to 2014, and from 2017 to 2018 he served as the dean of social science at Duke. Dr. Cooper chaired the first APA committee that developed guidelines for information about research that should be included in manuscripts submitted to APA journals. He currently serves as the editor of American Psychologist, the flagship journal of APA.
Marc N. Coutanche, PhD, is an associate professor of psychology and research scientist in the Learning Research and Development Center at the University of Pittsburgh. Dr. Coutanche directs a program of cognitive neuroscience research and develops and tests new computational techniques to identify and understand the neural information present within neuroimaging data.
His work has been funded by the National Institutes of Health, National Science Foundation, American Psychological Foundation, and other organizations, and he has published in a variety of journals.
Dr. Coutanche received his PhD from the University of Pennsylvania, and conducted postdoctoral training at Yale University. He received a Howard Hughes Medical Institute International Student Research Fellowship and Ruth L. Kirschstein Postdoctoral National Research Service Award, and was named a 2019 Rising Star by the Association for Psychological Science.
Linda M. McMullen, PhD, is professor emerita of psychology at the University of Saskatchewan, Canada. Over her career, she has contributed to the development of qualitative inquiry in psychology through teaching, curriculum development, and pedagogical scholarship; original research; and service to the qualitative research community.
Dr. McMullen introduced qualitative inquiry into both the graduate and undergraduate curriculum in her home department, taught courses at both levels for many years, and has published articles, coedited special issues, and written a book ( Essentials of Discursive Psychology ) that is part of APA’s series on qualitative methodologies, among other works. She has been engaged with building the Society for Qualitative Inquiry in Psychology (SQIP; a section of Division 5 of the APA) into a vibrant scholarly society since its earliest days, and took on many leadership roles while working as a university professor.
Dr. McMullen’s contributions have been recognized by Division 5 of the APA, the Canadian Psychological Association, and the Saskatchewan Psychological Association.
Abigail Panter, PhD, is the senior associate dean for undergraduate education and a professor of psychology in the L. L. Thurstone Psychometric Laboratory at University of North Carolina at Chapel Hill. She is past president of APA’s Division 5, Quantitative and Qualitative Methods.
As a quantitative psychologist, she develops instruments, research designs and data-analytic strategies for applied research questions in higher education, personality, and health. She serves as a program evaluator for UNC’s Chancellor’s Science Scholars Program, and was also principal investigator for The Finish Line Project, a $3 million grant from the U.S. Department of Education that systematically investigated new supports and academic initiatives, especially for first-generation college students.
Her books include the APA Dictionary of Statistics and Research Methods (2014), the APA Handbook of Research Methods in Psychology (first edition; 2012), the Handbook of Ethics in Quantitative Methodology (2011), and the SAGE Handbook of Methods in Social Psychology (2004), among others.
David Rindskopf, PhD, is distinguished professor at the City University of New York Graduate Center, specializing in research methodology and statistics. His main interests are in Bayesian statistics, causal inference, categorical data analysis, meta-analysis, and latent variable models.
He is a fellow of the American Statistical Association and the American Educational Research Association, and is past president of the Society of Multivariate Experimental Psychology and the New York Chapter of the American Statistical Association.
Kenneth J. Sher, PhD, is chancellor’s professor and curators’ distinguished professor of psychological sciences, emeritus, at the University of Missouri. He received his PhD in clinical psychology from Indiana University (1980) and his clinical internship training at Brown University (1981).
His primary areas of research focus on etiological processes in the development of alcohol dependence, factors that affect the course of drinking and alcohol use disorders throughout adulthood, longitudinal research methodology, psychiatric comorbidity, and nosology. At the University of Missouri he directed the predoctoral and postdoctoral training program in alcohol studies, and his research has been continually funded by the National Institute on Alcohol Abuse and Alcoholism for more than 35 years.
Dr. Sher’s research contributions have been recognized by professional societies including the Research Society on Alcoholism and APA, and throughout his career, he has been heavily involved in service to professional societies and scholarly publications.
You may also like
How to Mix Methods
Using Science to Inform Educational Practices
Descriptive Research
There are many research methods available to psychologists in their efforts to understand, describe, and explain behavior. Some methods rely on observational techniques. Other approaches involve interactions between the researcher and the individuals who are being studied—ranging from a series of simple questions to extensive, in-depth interviews—to well-controlled experiments. The main categories of psychological research are descriptive, correlational, and experimental research. Each of these research methods has unique strengths and weaknesses, and each method may only be appropriate for certain types of research questions.
Research studies that do not test specific relationships between variables are called descriptive studies . For this method, the research question or hypothesis can be about a single variable (e.g., How accurate are people’s first impressions?) or can be a broad and exploratory question (e.g., What is it like to be a working mother diagnosed with depression?). The variable of the study is measured and reported without any further relationship analysis. A researcher might choose this method if they only needed to report information, such as a tally, an average, or a list of responses. Descriptive research can answer interesting and important questions, but what it cannot do is answer questions about relationships between variables.
Video 2.4.1. Descriptive Research Design provides explanation and examples for quantitative descriptive research. A closed-captioned version of this video is available here .
Descriptive research is distinct from correlational research , in which researchers formally test whether a relationship exists between two or more variables. Experimental research goes a step further beyond descriptive and correlational research and randomly assigns people to different conditions, using hypothesis testing to make inferences about causal relationships between variables. We will discuss each of these methods more in-depth later.
Table 2.4.1. Comparison of research design methods
Research design | Goal | Advantages | Disadvantages |
---|---|---|---|
Descriptive | To create a snapshot of the current state of affairs | Provides a relatively complete picture of what is occurring at a given time. Allows the development of questions for further study. | Does not assess relationships among variables. Maybe unethical if participants do not know they are being observed. |
Correlational | To assess the relationships between and among two or more variables | Allows testing of expected relationships between and among variables and the making of predictions. Can assess these relationships in everyday life events. | Cannot be used to draw inferences about the causal relationships between and among the variables. |
Experimental | To assess the causal impact of one or more experimental manipulations on a dependent variable | Allows drawing conclusions about the causal relationships among variables. | Cannot experimentally manipulate many important variables. May be expensive and time-consuming. |
Stangor, 2011. |
Candela Citations
- Descriptive Research. Authored by : Nicole Arduini-Van Hoose. Provided by : Hudson Valley Community College. Retrieved from : https://courses.lumenlearning.com/edpsy/chapter/descriptive-research/. License : CC BY-NC-SA: Attribution-NonCommercial-ShareAlike
- Descriptive Research. Authored by : Nicole Arduini-Van Hoose. Provided by : Hudson Valley Community College. Retrieved from : https://courses.lumenlearning.com/adolescent/chapter/descriptive-research/. License : CC BY-NC-SA: Attribution-NonCommercial-ShareAlike
Educational Psychology Copyright © 2020 by Nicole Arduini-Van Hoose is licensed under a Creative Commons Attribution-NonCommercial-ShareAlike 4.0 International License , except where otherwise noted.
Share This Book
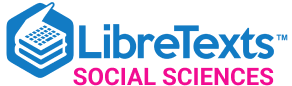
- school Campus Bookshelves
- menu_book Bookshelves
- perm_media Learning Objects
- login Login
- how_to_reg Request Instructor Account
- hub Instructor Commons
Margin Size
- Download Page (PDF)
- Download Full Book (PDF)
- Periodic Table
- Physics Constants
- Scientific Calculator
- Reference & Cite
- Tools expand_more
- Readability
selected template will load here
This action is not available.
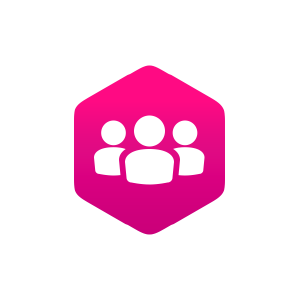
1.4: Psychologists Use Descriptive, Correlational, and Experimental Research Designs to Understand Behavior
- Last updated
- Save as PDF
- Page ID 107148

\( \newcommand{\vecs}[1]{\overset { \scriptstyle \rightharpoonup} {\mathbf{#1}} } \)
\( \newcommand{\vecd}[1]{\overset{-\!-\!\rightharpoonup}{\vphantom{a}\smash {#1}}} \)
\( \newcommand{\id}{\mathrm{id}}\) \( \newcommand{\Span}{\mathrm{span}}\)
( \newcommand{\kernel}{\mathrm{null}\,}\) \( \newcommand{\range}{\mathrm{range}\,}\)
\( \newcommand{\RealPart}{\mathrm{Re}}\) \( \newcommand{\ImaginaryPart}{\mathrm{Im}}\)
\( \newcommand{\Argument}{\mathrm{Arg}}\) \( \newcommand{\norm}[1]{\| #1 \|}\)
\( \newcommand{\inner}[2]{\langle #1, #2 \rangle}\)
\( \newcommand{\Span}{\mathrm{span}}\)
\( \newcommand{\id}{\mathrm{id}}\)
\( \newcommand{\kernel}{\mathrm{null}\,}\)
\( \newcommand{\range}{\mathrm{range}\,}\)
\( \newcommand{\RealPart}{\mathrm{Re}}\)
\( \newcommand{\ImaginaryPart}{\mathrm{Im}}\)
\( \newcommand{\Argument}{\mathrm{Arg}}\)
\( \newcommand{\norm}[1]{\| #1 \|}\)
\( \newcommand{\Span}{\mathrm{span}}\) \( \newcommand{\AA}{\unicode[.8,0]{x212B}}\)
\( \newcommand{\vectorA}[1]{\vec{#1}} % arrow\)
\( \newcommand{\vectorAt}[1]{\vec{\text{#1}}} % arrow\)
\( \newcommand{\vectorB}[1]{\overset { \scriptstyle \rightharpoonup} {\mathbf{#1}} } \)
\( \newcommand{\vectorC}[1]{\textbf{#1}} \)
\( \newcommand{\vectorD}[1]{\overrightarrow{#1}} \)
\( \newcommand{\vectorDt}[1]{\overrightarrow{\text{#1}}} \)
\( \newcommand{\vectE}[1]{\overset{-\!-\!\rightharpoonup}{\vphantom{a}\smash{\mathbf {#1}}}} \)
Learning Objectives
- Differentiate the goals of descriptive, correlational, and experimental research designs and explain the advantages and disadvantages of each.
- Explain the goals of descriptive research and the statistical techniques used to interpret it.
- Summarize the uses of correlational research and describe why correlational research cannot be used to infer causality.
- Review the procedures of experimental research and explain how it can be used to draw causal inferences.
Psychologists agree that if their ideas and theories about human behavior are to be taken seriously, they must be backed up by data. However, the research of different psychologists is designed with different goals in mind, and the different goals require different approaches. These varying approaches, summarized in Table \(\PageIndex{2}\), are known as research designs . A research design is the specific method a researcher uses to collect, analyze, and interpret data . Psychologists use three major types of research designs in their research, and each provides an essential avenue for scientific investigation. Descriptive research is research designed to provide a snapshot of the current state of affairs . Correlational research is research designed to discover relationships among variables and to allow the prediction of future events from present knowledge . Experimental research is research in which initial comparability among research participants in more than one group is created, followed by a manipulation of a given experience for these groups and a measurement of the influence of the manipulation . Each of the three research designs varies according to its strengths and limitations, and it is important to understand how each differs.
Research design | Goal | Advantages | Disadvantages |
---|---|---|---|
Descriptive | To create a snapshot of the current state of affairs | Provides a relatively complete picture of what is occurring at a given time. Allows the development of questions for further study. | Does not assess relationships among variables. May be unethical if participants do not know they are being observed. |
Correlational | To assess the relationships between and among two or more variables | Allows observation of expected relationships between and among variables and the making of predictions. Can assess these relationships in everyday life events. | Cannot be used to draw inferences about the causal relationships between and among the variables. Data are descriptive - no manipulation is introduced. |
Experimental | To assess the causal impact of one or more experimental manipulations on a dependent variable | Allows drawing of conclusions about the causal relationships among variables. | Cannot experimentally manipulate many important variables. May be expensive and time consuming. |
There are three major research designs used by psychologists, and each has its own advantages and disadvantages. Stangor, C. (2011). (4th ed.). Mountain View, CA: Cengage. |
Descriptive Research: Assessing the Current State of Affairs
Descriptive research is designed to create a snapshot of the current thoughts, feelings, or behavior of individuals. This section reviews three types of descriptive research: case studies , surveys , and naturalistic observation .
Sometimes the data in a descriptive research project are based on only a small set of individuals, often only one person or a single small group. These research designs are known as case studies— descriptive records of one or more individual’s experiences and behavior . Sometimes case studies involve ordinary individuals, as when developmental psychologist Jean Piaget used his observation of his own children to develop his stage theory of cognitive development. More frequently, case studies are conducted on individuals who have unusual or abnormal experiences or characteristics or who find themselves in particularly difficult or stressful situations. The assumption is that by carefully studying individuals who are socially marginal, who are experiencing unusual situations, or who are going through a difficult phase in their lives, we can learn something about human nature.
Sigmund Freud was a master of using the psychological difficulties of individuals to draw conclusions about basic psychological processes. Freud wrote case studies of some of his most interesting patients and used these careful examinations to develop his important theories of personality. One classic example is Freud’s description of “Little Hans,” a child whose fear of horses the psychoanalyst interpreted in terms of repressed sexual impulses and the Oedipus complex (Freud (1909/1964).
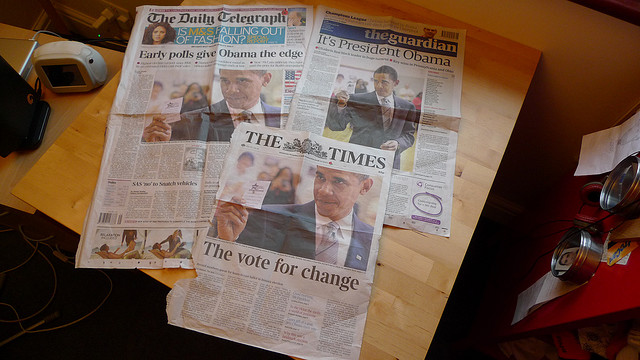
Another well-known case study is Phineas Gage, a man whose thoughts and emotions were extensively studied by cognitive psychologists after a railroad spike was blasted through his skull in an accident. Although there is question about the interpretation of this case study (Kotowicz, 2007), it did provide early evidence that the brain’s frontal lobe is involved in emotion and morality (Damasio et al., 2005). An interesting example of a case study in clinical psychology is described by Rokeach (1964), who investigated in detail the beliefs and interactions among three patients with schizophrenia, all of whom were convinced they were Jesus Christ.
In other cases the data from descriptive research projects come in the form of a survey— a measure administered through either an interview or a written questionnaire to get a picture of the beliefs or behaviors of a sample of people of interest . The people chosen to participate in the research (known as the sample) are selected to be representative of all the people that the researcher wishes to know about (the population). In election polls, for instance, a sample is taken from the population of all “likely voters” in the upcoming elections.
The results of surveys may sometimes be rather mundane, such as “Nine out of ten doctors prefer Tymenocin,” or “The median income in Montgomery County is $36,712.” Yet other times (particularly in discussions of social behavior), the results can be shocking: “More than 40,000 people are killed by gunfire in the United States every year,” or “More than 60% of women between the ages of 50 and 60 suffer from depression.” Descriptive research is frequently used by psychologists to get an estimate of the prevalence (or incidence ) of psychological disorders.
A final type of descriptive research—known as naturalistic observation—is research based on the observation of everyday events . For instance, a developmental psychologist who watches children on a playground and describes what they say to each other while they play is conducting descriptive research, as is a biopsychologist who observes animals in their natural habitats. One example of observational research involves a systematic procedure known as the strange situation , used to get a picture of how adults and young children interact. The data that are collected in the strange situation are systematically coded in a coding sheet such as that shown in Table \(\PageIndex{3}\).
Coder name: | ||||
---|---|---|---|---|
Mother and baby play alone | ||||
Mother puts baby down | ||||
Stranger enters room | ||||
Mother leaves room; stranger plays with baby | ||||
Mother reenters, greets and may comfort baby, then leaves again | ||||
Stranger tries to play with baby | ||||
Mother reenters and picks up baby | ||||
The baby moves toward, grasps, or climbs on the adult. | ||||
The baby resists being put down by the adult by crying or trying to climb back up. | ||||
The baby pushes, hits, or squirms to be put down from the adult’s arms. | ||||
The baby turns away or moves away from the adult. | ||||
This table represents a sample coding sheet from an episode of the “strange situation,” in which an infant (usually about 1 year old) is observed playing in a room with two adults—the child’s mother and a stranger. Each of the four coding categories is scored by the coder from 1 (the baby makes no effort to engage in the behavior) to 7 (the baby makes a significant effort to engage in the behavior). More information about the meaning of the coding can be found in Ainsworth, Blehar, Waters, and Wall (1978). Stangor, C. (2011). (4th ed.). Mountain View, CA: Cengage. |
The results of descriptive research projects are analyzed using descriptive statistics— numbers that summarize the distribution of scores on a measured variable . Most variables have distributions similar to that shown in Figure \(\PageIndex{5}\), where most of the scores are located near the center of the distribution, and the distribution is symmetrical and bell-shaped. A data distribution that is shaped like a bell is known as a normal distribution.
Student name | Height in inches | Family income in dollars |
---|---|---|
Lauren | 62 | 48,000 |
Courtnie | 62 | 57,000 |
Leslie | 63 | 93,000 |
Renee | 64 | 107,000 |
Katherine | 64 | 110,000 |
Jordan | 65 | 93,000 |
Rabiah | 66 | 46,000 |
Alina | 66 | 84,000 |
Young Su | 67 | 68,000 |
Martin | 67 | 49,000 |
Hanzhu | 67 | 73,000 |
Caitlin | 67 | 3,800,000 |
Steven | 67 | 107,000 |
Emily | 67 | 64,000 |
Amy | 68 | 67,000 |
Jonathan | 68 | 51,000 |
Julian | 68 | 48,000 |
Alissa | 68 | 93,000 |
Christine | 69 | 93,000 |
Candace | 69 | 111,000 |
Xiaohua | 69 | 56,000 |
Charlie | 70 | 94,000 |
Timothy | 71 | 73,000 |
Ariane | 72 | 70,000 |
Logan | 72 | 44,000 |
A distribution can be described in terms of its central tendency —that is, the point in the distribution around which the data are centered—and its dispersion , or spread. The arithmetic average, or arithmetic mean, is the most commonly used measure of central tendency . It is computed by calculating the sum of all the scores of the variable and dividing this sum by the number of participants in the distribution (denoted by the letter N ). In the data presented in Figure \(\PageIndex{5}\), the mean height of the students is 67.12 inches. The sample mean is usually indicated by the letter M .
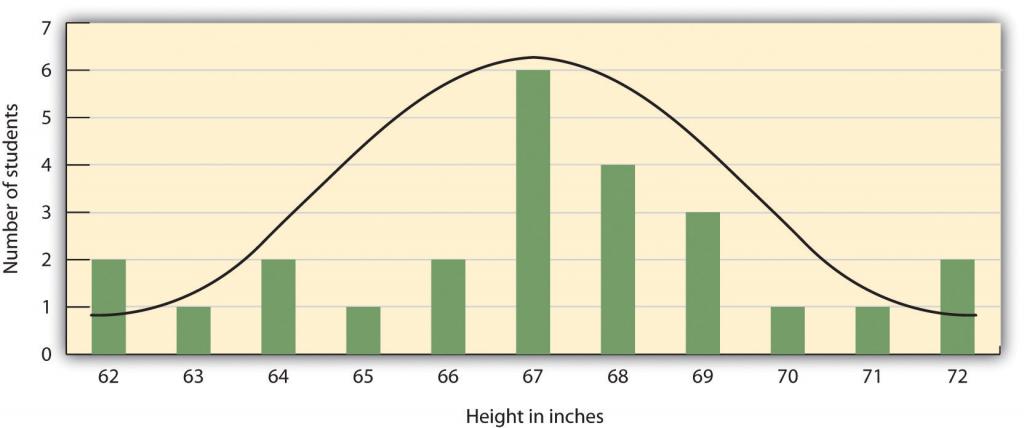
In some cases, however, the data distribution is not symmetrical. This occurs when there are one or more extreme scores (known as outliers ) at one end of the distribution. Consider, for instance, the variable of family income (Figure \(\PageIndex{6}\)), which includes an outlier (a value of $3,800,000). In this case the mean is not a good measure of central tendency. Although it appears from Figure \(\PageIndex{6}\) that the central tendency of the family income variable should be around $70,000, the mean family income is actually $223,960. The single very extreme income has a disproportionate impact on the mean, resulting in a value that does not well represent the central tendency.
The median is used as an alternative measure of central tendency when distributions are not symmetrical. The median is the score in the center of the distribution, meaning that 50% of the scores are greater than the median and 50% of the scores are less than the median . In our case, the median household income ($73,000) is a much better indication of central tendency than is the mean household income ($223,960).
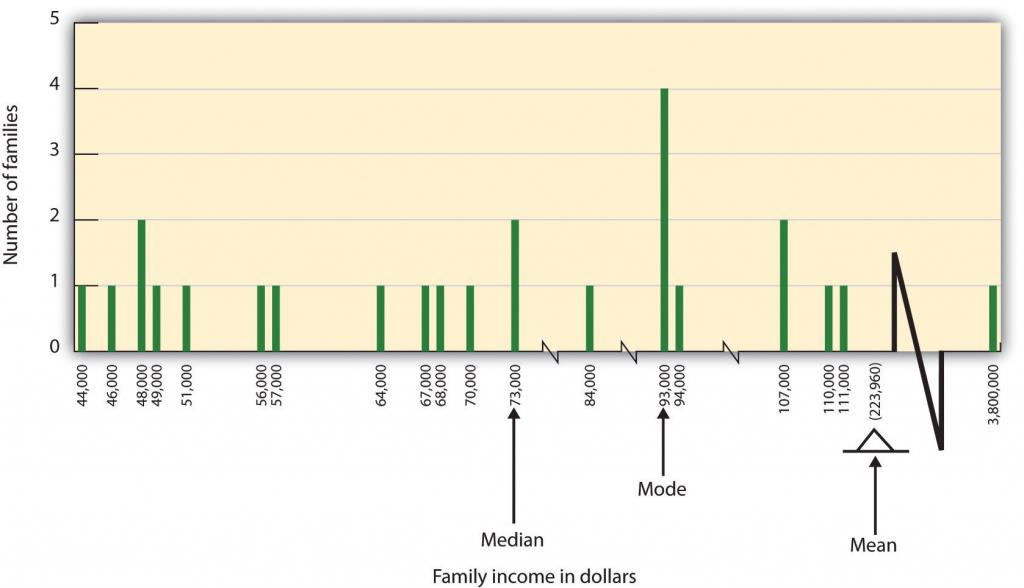
A final measure of central tendency, known as the mode, represents the value that occurs most frequently in the distribution . You can see from Figure \(\PageIndex{6}\) that the mode for the family income variable is $93,000 (it occurs four times).
In addition to summarizing the central tendency of a distribution, descriptive statistics convey information about how the scores of the variable are spread around the central tendency. Dispersion refers to the extent to which the scores are all tightly clustered around the central tendency, like this:
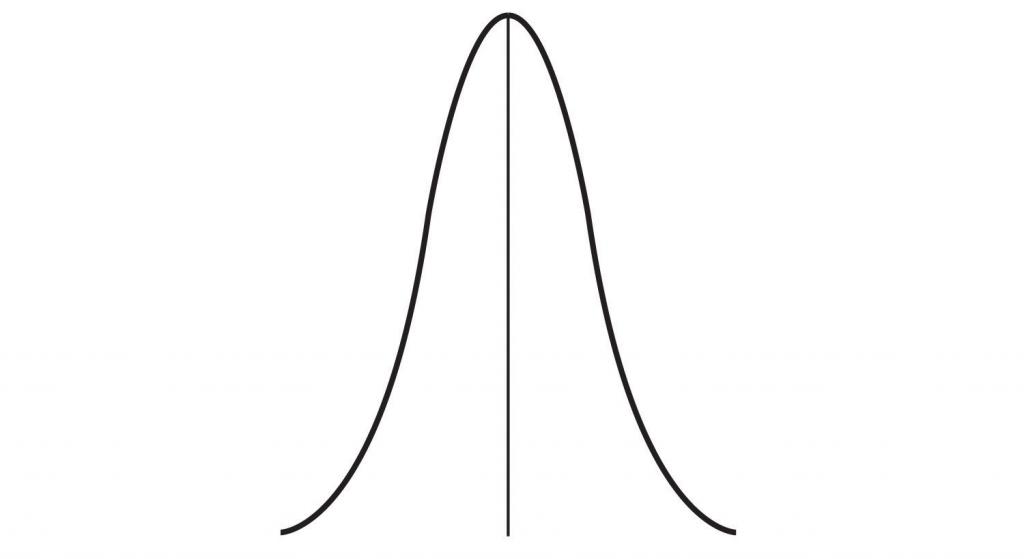
Or they may be more spread out away from it, like this:
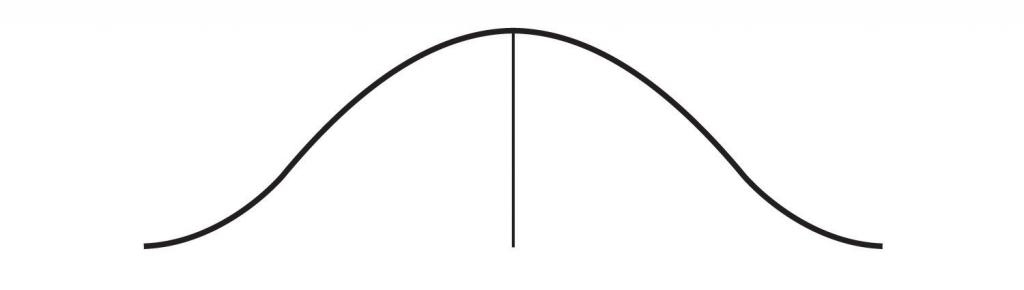
One simple measure of dispersion is to find the largest (the maximum ) and the smallest (the minimum ) observed values of the variable and to compute the range of the variable as the maximum observed score minus the minimum observed score. You can check that the range of the height variable in Figure \(\PageIndex{5}\) is 72 – 62 = 10. The standard deviation, symbolized as s , is the most commonly used measure of dispersion . Distributions with a larger standard deviation have more spread. The standard deviation of the height variable is s = 2.74, and the standard deviation of the family income variable is s = $745,337.
An advantage of descriptive research is that it attempts to capture the complexity of everyday behavior. Case studies provide detailed information about a single person or a small group of people, surveys capture the thoughts or reported behaviors of a large population of people, and naturalistic observation objectively records the behavior of people or animals as it occurs naturally. Thus descriptive research is used to provide a relatively complete understanding of what is currently happening.
Despite these advantages, descriptive research has a distinct disadvantage in that, although it allows us to get an idea of what is currently happening, it is usually limited to static pictures. Although descriptions of particular experiences may be interesting, they are not always transferable to other individuals in other situations, nor do they tell us exactly why specific behaviors or events occurred. For instance, descriptions of individuals who have suffered a stressful event, such as a war or an earthquake, can be used to understand the individuals’ reactions to the event but cannot tell us anything about the long-term effects of the stress. And because there is no comparison group that did not experience the stressful situation, we cannot know what these individuals would be like if they hadn’t had the stressful experience.
Correlational Research: Seeking Relationships Among Variables
In contrast to descriptive research, which is designed primarily to provide static pictures, correlational research involves the measurement of two or more relevant variables and an assessment of the relationship between or among those variables. For instance, the variables of height and weight are systematically related (correlated) because taller people generally weigh more than shorter people. In the same way, study time and memory errors are also related, because the more time a person is given to study a list of words, the fewer errors he or she will make.
One way of organizing the data from a correlational study with two variables is to graph the values of each of the measured variables using a scatter plot . As you can see in Figure \(\PageIndex{10}\), a scatter plot is a visual image of the relationship between two variables . A point is plotted for each individual at the intersection of his or her scores for the two variables. When the association between the variables on the scatter plot can be easily approximated with a straight line, as in parts (a) and (b) of Figure \(\PageIndex{10}\), the variables are said to have a linear relationship .
When the straight line indicates that individuals who have above-average values for one variable also tend to have above-average values for the other variable, as in part (a), the relationship is said to be positive linear . Examples of positive linear relationships include those between height and weight, between education and income, and between age and mathematical abilities in children. In each case people who score higher on one of the variables also tend to score higher on the other variable. Negative linear relationships , in contrast, as shown in part (b), occur when above-average values for one variable tend to be associated with below-average values for the other variable. Examples of negative linear relationships include those between the age of a child and the number of diapers the child uses, and between practice on and errors made on a learning task. In these cases people who score higher on one of the variables tend to score lower on the other variable.
Relationships between variables that cannot be described with a straight line are known as nonlinear relationships . Part (c) of Figure \(\PageIndex{10}\) shows a common pattern in which the distribution of the points is essentially random. In this case there is no relationship at all between the two variables, and they are said to be independent . Parts (d) and (e) of Figure \(\PageIndex{10}\) show patterns of association in which, although there is an association, the points are not well described by a single straight line. For instance, part (d) shows the type of relationship that frequently occurs between anxiety and performance. Increases in anxiety from low to moderate levels are associated with performance increases, whereas increases in anxiety from moderate to high levels are associated with decreases in performance. Relationships that change in direction and thus are not described by a single straight line are called curvilinear relationships .
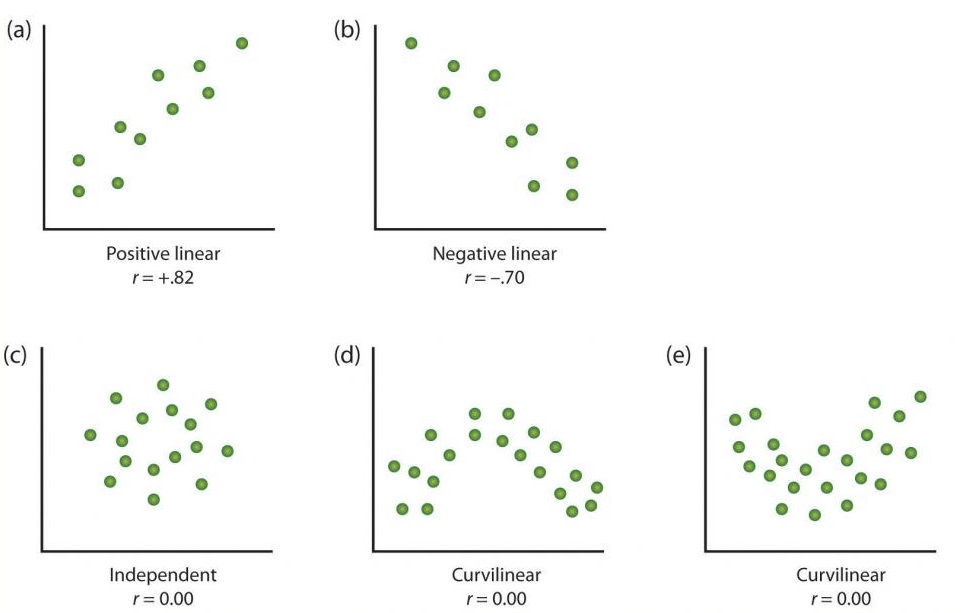
The most common statistical measure of the strength of linear relationships among variables is the Pearson correlation coefficient , which is symbolized by the letter r . The value of the correlation coefficient ranges from r = –1.00 to r = +1.00. The direction of the linear relationship is indicated by the sign of the correlation coefficient. Positive values of r (such as r = 0.54 or r = 0.67) indicate that the relationship is positive linear (i.e., the pattern of the dots on the scatter plot runs from the lower left to the upper right), whereas negative values of r (such as r = –0.30 or r = –0.72) indicate negative linear relationships (i.e., the dots run from the upper left to the lower right). The strength of the linear relationship is indexed by the distance of the correlation coefficient from zero (its absolute value). For instance, r = -0.54 is a stronger relationship than r = 0.30, and r = 0.72 is a stronger relationship than r = –0.57. Because the Pearson correlation coefficient only measures linear relationships, variables that have curvilinear relationships are not well described by r , and the observed correlation will be close to zero.
It is also possible to study relationships among more than two measures at the same time. A research design in which more than one variable is used to predict a single outcome variable is analyzed through multiple regression (Aiken & West, 1991). Multiple regression is a statistical technique, based on correlation coefficients among variables, that allows predicting a single outcome variable from more than one predictor variable . For instance, Figure \(\PageIndex{11}\) shows a multiple regression analysis in which three predictor variables are used to predict a single outcome. The use of multiple regression analysis shows an important advantage of correlational research designs—they can be used to make predictions about a person’s likely score on an outcome variable (e.g., job performance) based on knowledge of other variables. It is important, however, to keep in mind that using data to make a prediction is not the same thing as being able to say that one thing causes another. Height and weight, for example, are correlated - but you can't state that one "causes" the other.
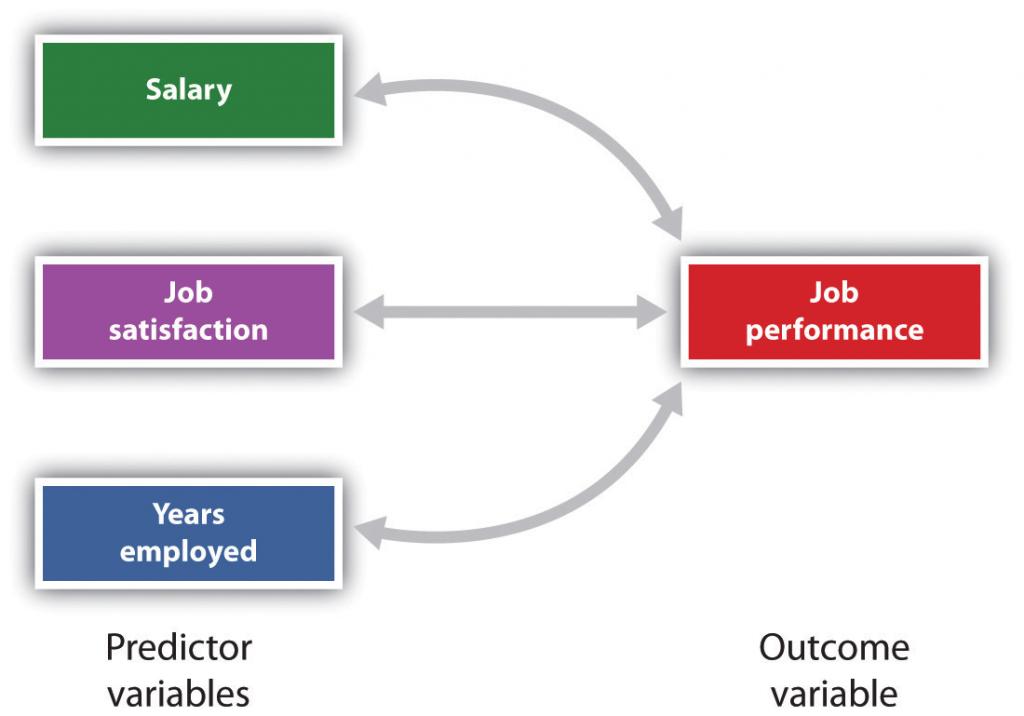
An important limitation of correlational research designs is that they cannot be used to draw conclusions about the causal relationships among the measured variables. Consider, for instance, a researcher who has hypothesized that viewing violent behavior will cause increased aggressive play in children. He has collected, from a sample of fourth-grade children, a measure of how many violent television shows each child views during the week, as well as a measure of how aggressively each child plays on the school playground. From his collected data, the researcher discovers a positive correlation between the two measured variables.
Although this positive correlation appears to support the researcher’s hypothesis, it cannot be taken to indicate that viewing violent television causes aggressive behavior. Although the researcher is tempted to assume that viewing violent television causes aggressive play,

there are other possibilities. One alternate possibility is that the causal direction is exactly opposite from what has been hypothesized. Perhaps children who have behaved aggressively at school develop residual excitement that leads them to want to watch violent television shows at home:

Although this possibility may seem less likely, there is no way to rule out the possibility of such reverse causation on the basis of this observed correlation. It is also possible that both causal directions are operating and that the two variables cause each other:

Still another possible explanation for the observed correlation is that it has been produced by the presence of a common-causal variable (also known as a third variable ). A common-causal variable is a variable that is not part of the research hypothesis but that impacts both of the measured variables and thus produces the observed correlation between them . In our example a potential common-causal variable is the discipline style of the children’s parents. Parents who use a harsh and punitive discipline style may produce children who both like to watch violent television and who behave aggressively in comparison to children whose parents use less harsh discipline:
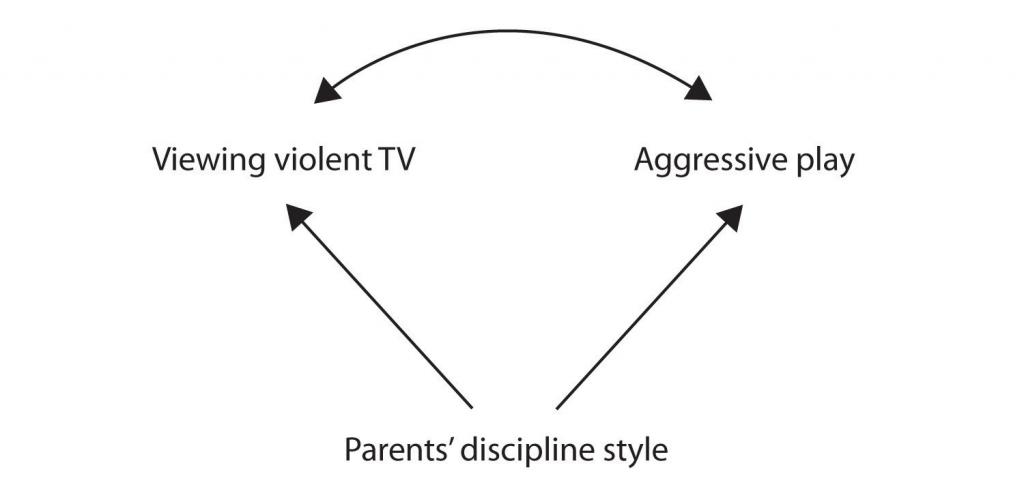
In this case, television viewing and aggressive play would be positively correlated (as indicated by the curved arrow between them), even though neither one caused the other but they were both caused by the discipline style of the parents (the straight arrows). When both of the measured variables are both impacted by a common-causal variable, the observed relationship between them is said to be spurious . A spurious relationship is a relationship between two variables in which a common-causal variable produces and “explains away” the relationship . If effects of the common-causal variable were taken away, or controlled for, the relationship between the predictor and outcome variables would disappear. In the example the relationship between aggression and television viewing might be spurious because by controlling for the effect of the parents’ disciplining style, the relationship between television viewing and aggressive behavior might go away.
Common-causal variables in correlational research designs can be thought of as “mystery” variables because, as they have not been measured, their presence and identity are usually unknown to the researcher. Since it is not possible to measure every variable that could impact your results, the existence of an unknown common-causal variable is always a possibility. For this reason, we are left with the basic limitation of correlational research: Correlation does not demonstrate causation. It is important that when you read about correlational research projects, you keep in mind the possibility of spurious relationships, and be sure to interpret the findings appropriately. Although correlational research is sometimes reported as demonstrating causality without any mention being made of the possibility of reverse causation or common-causal variables, informed consumers of research, like you, are aware of these interpretational problems. It should be noted that one is also unable to control - or measure - all variables that could impact your results in an experiment - but experiments can lead to conclusions about cause.
In sum, correlational research designs have both strengths and limitations. One strength is that they can be used when experimental research is not possible because the variables of interest cannot be manipulated. Correlational designs also have the advantage of allowing the researcher to study behavior as it occurs in everyday life. And we can also use correlational designs to make predictions—for instance, to predict from the scores on their battery of tests the success of job trainees during a training session. But we cannot use such correlational information to determine whether the training caused better job performance. For that, researchers rely on experiments.
Experimental Research: Understanding the Causes of Behavior
The goal of experimental research design is to provide more definitive conclusions about the causal relationships among the variables in the research hypothesis than is available from correlational designs. In an experimental research design, the variables of interest are called the independent variable (or variables ) and the dependent variable . The independent variable in an experiment is the causing variable that is created (manipulated) by the experimenter . The dependent variable in an experiment is a measured variable that is expected to be influenced by the experimental manipulation . The research hypothesis suggests that the manipulated independent variable or variables will cause changes in the measured dependent variables. We can diagram the research hypothesis by using an arrow that points in one direction. This demonstrates the expected direction of causality:

Research Focus: Video Games and Aggression
Consider an experiment conducted by Anderson and Dill (2000). The study was designed to test the hypothesis that viewing violent video games would increase aggressive behavior. In this research, male and female undergraduates from Iowa State University were given a chance to play with either a violent video game (Wolfenstein 3D) or a nonviolent video game (Myst). During the experimental session, the participants played their assigned video games for 15 minutes. Then, after the play, each participant played a competitive game with an opponent in which the participant could deliver blasts of white noise through the earphones of the opponent. The operational definition of the dependent variable (aggressive behavior) was the level and duration of noise delivered to the opponent. The design of the experiment is shown in Figure \(\PageIndex{17}\).
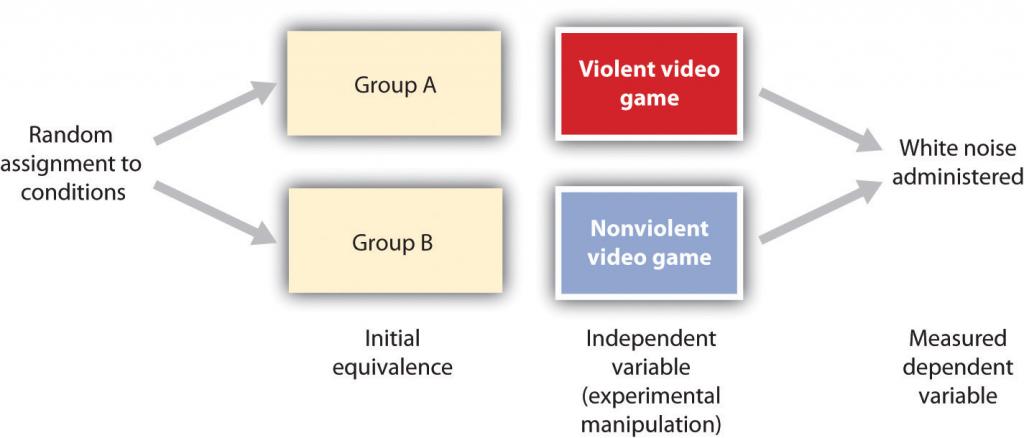
Two advantages of the experimental research design are (1) the assurance that the independent variable (also known as the experimental manipulation) occurs prior to the measured dependent variable, and (2) the creation of initial equivalence between the conditions of the experiment (in this case by using random assignment to conditions).
Experimental designs have two very nice features. For one, they guarantee that the independent variable occurs prior to the measurement of the dependent variable. This eliminates the possibility of reverse causation. Second, the influence of common-causal variables is controlled, and thus eliminated, by creating initial equivalence among the participants in each of the experimental conditions before the manipulation occurs.
The most common method of creating equivalence among the experimental conditions is through random assignment to conditions, a procedure in which the condition that each participant is assigned to is determined through a random process, such as drawing numbers out of an envelope or using a random number table . Anderson and Dill first randomly assigned about 100 participants to each of their two groups (Group A and Group B). Because they used random assignment to conditions, they could be confident that, before the experimental manipulation occurred, the students in Group A were, on average, equivalent to the students in Group B on every possible variable, including variables that are likely to be related to aggression, such as parental discipline style, peer relationships, hormone levels, diet—and in fact everything else.
Then, after they had created initial equivalence, Anderson and Dill created the experimental manipulation—they had the participants in Group A play the violent game and the participants in Group B play the nonviolent game. Then they compared the dependent variable (the white noise blasts) between the two groups, finding that the students who had viewed the violent video game gave significantly longer noise blasts than did the students who had played the nonviolent game.
Anderson and Dill had from the outset created initial equivalence between the groups. This initial equivalence allowed them to observe differences in the white noise levels between the two groups after the experimental manipulation, leading to the conclusion that it was the independent variable (and not some other variable) that caused these differences. The idea is that the only thing that was different between the students in the two groups was the video game they had played.
Despite the advantage of determining causation, experiments do have limitations. One is that they are often conducted in laboratory situations rather than in the everyday lives of people. Therefore, we do not know whether results that we find in a laboratory setting will necessarily hold up in everyday life. Second, and more important, is that some of the most interesting and key social variables cannot be experimentally manipulated. If we want to study the influence of the size of a mob on the destructiveness of its behavior, or to compare the personality characteristics of people who join suicide cults with those of people who do not join such cults, these relationships must be assessed using correlational designs, because it is simply not possible to experimentally manipulate these variables.
Key Takeaways
- Descriptive, correlational, and experimental research designs are used to collect and analyze data.
- Descriptive designs include case studies, surveys, and naturalistic observation. The goal of these designs is to get a picture of the current thoughts, feelings, or behaviors in a given group of people. Descriptive research is summarized using descriptive statistics.
- Correlational research designs measure two or more relevant variables and assess a relationship between or among them. The variables may be presented on a scatter plot to visually show the relationships. The Pearson Correlation Coefficient ( r ) is a measure of the strength of linear relationship between two variables.
- Common-causal variables may impact the measured variables in a correlational design, producing a spurious relationship. The possibility of common-causal variables makes it impossible to draw causal conclusions from correlational research designs.
- Experimental research involves the manipulation of an independent variable and the measurement of a dependent variable. Random assignment to conditions is normally used to create initial equivalence (comparability) between the groups, allowing researchers to draw causal conclusions.
Exercises and Critical Thinking
- There is a negative correlation between the row that a student sits in in a large class (when the rows are numbered from front to back) and his or her final grade in the class. Do you think this represents a causal relationship or a spurious relationship, and why?
- Think of two variables (other than those mentioned in this book) that are likely to be correlated, but in which the correlation is probably spurious. What is the likely common-causal variable that is producing the relationship?
- Imagine a researcher wants to test the hypothesis that participating in psychotherapy will cause a decrease in reported anxiety. Describe the type of research design the investigator might use to draw this conclusion. What would be the independent and dependent variables in the research?
Updates and Edits
This page was forked and modified on January 14, 2021. Slight language modifications were made and references to "predictor" and "outcome" variables with respect to correlational data were modified. While correlational data can be be used to make predictions, referring to the variables in a correlational study as predictors and outcomes may inappropriately imply a causal relationship.
Aiken, L., & West, S. (1991). Multiple regression: Testing and interpreting interactions . Newbury Park, CA: Sage.
Ainsworth, M. S., Blehar, M. C., Waters, E., & Wall, S. (1978). Patterns of attachment: A psychological study of the strange situation . Hillsdale, NJ: Lawrence Erlbaum Associates.
Anderson, C. A., & Dill, K. E. (2000). Video games and aggressive thoughts, feelings, and behavior in the laboratory and in life. Journal of Personality and Social Psychology, 78 (4), 772–790.
Damasio, H., Grabowski, T., Frank, R., Galaburda, A. M., Damasio, A. R., Cacioppo, J. T., & Berntson, G. G. (2005). The return of Phineas Gage: Clues about the brain from the skull of a famous patient. In Social neuroscience: Key readings. (pp. 21–28). New York, NY: Psychology Press.
Freud, S. (1964). Analysis of phobia in a five-year-old boy. In E. A. Southwell & M. Merbaum (Eds.), Personality: Readings in theory and research (pp. 3–32). Belmont, CA: Wadsworth. (Original work published 1909)
Kotowicz, Z. (2007). The strange case of Phineas Gage. History of the Human Sciences, 20 (1), 115–131.
Rokeach, M. (1964). The three Christs of Ypsilanti: A psychological study . New York, NY: Knopf.
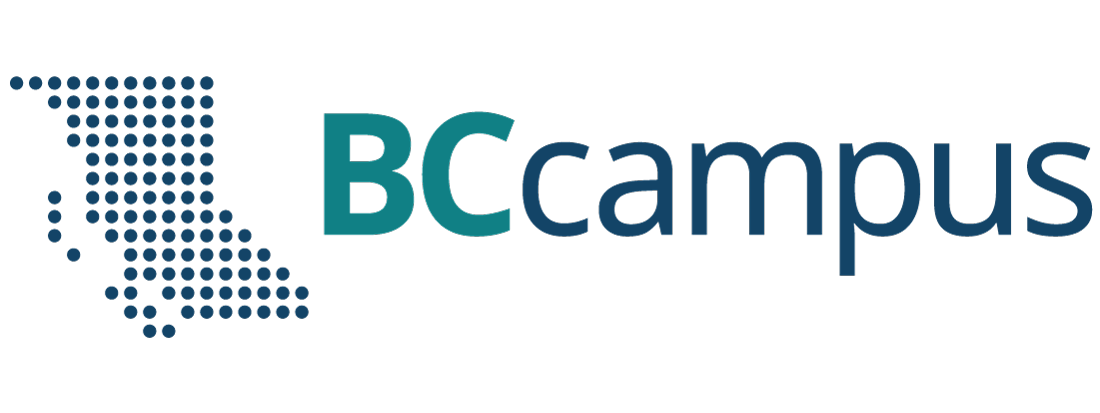
Want to create or adapt books like this? Learn more about how Pressbooks supports open publishing practices.
Chapter 3. Psychological Science
3.2 Psychologists Use Descriptive, Correlational, and Experimental Research Designs to Understand Behaviour
Learning objectives.
- Differentiate the goals of descriptive, correlational, and experimental research designs and explain the advantages and disadvantages of each.
- Explain the goals of descriptive research and the statistical techniques used to interpret it.
- Summarize the uses of correlational research and describe why correlational research cannot be used to infer causality.
- Review the procedures of experimental research and explain how it can be used to draw causal inferences.
Psychologists agree that if their ideas and theories about human behaviour are to be taken seriously, they must be backed up by data. However, the research of different psychologists is designed with different goals in mind, and the different goals require different approaches. These varying approaches, summarized in Table 3.2, are known as research designs . A research design is the specific method a researcher uses to collect, analyze, and interpret data . Psychologists use three major types of research designs in their research, and each provides an essential avenue for scientific investigation. Descriptive research is research designed to provide a snapshot of the current state of affairs . Correlational research is research designed to discover relationships among variables and to allow the prediction of future events from present knowledge . Experimental research is research in which initial equivalence among research participants in more than one group is created, followed by a manipulation of a given experience for these groups and a measurement of the influence of the manipulation . Each of the three research designs varies according to its strengths and limitations, and it is important to understand how each differs.
Research design | Goal | Advantages | Disadvantages |
---|---|---|---|
Descriptive | To create a snapshot of the current state of affairs | Provides a relatively complete picture of what is occurring at a given time. Allows the development of questions for further study. | Does not assess relationships among variables. May be unethical if participants do not know they are being observed. |
Correlational | To assess the relationships between and among two or more variables | Allows testing of expected relationships between and among variables and the making of predictions. Can assess these relationships in everyday life events. | Cannot be used to draw inferences about the causal relationships between and among the variables. |
Experimental | To assess the causal impact of one or more experimental manipulations on a dependent variable | Allows drawing of conclusions about the causal relationships among variables. | Cannot experimentally manipulate many important variables. May be expensive and time consuming. |
Source: Stangor, 2011. |
Descriptive Research: Assessing the Current State of Affairs
Descriptive research is designed to create a snapshot of the current thoughts, feelings, or behaviour of individuals. This section reviews three types of descriptive research : case studies , surveys , and naturalistic observation (Figure 3.4).
Sometimes the data in a descriptive research project are based on only a small set of individuals, often only one person or a single small group. These research designs are known as case studies — descriptive records of one or more individual’s experiences and behaviour . Sometimes case studies involve ordinary individuals, as when developmental psychologist Jean Piaget used his observation of his own children to develop his stage theory of cognitive development. More frequently, case studies are conducted on individuals who have unusual or abnormal experiences or characteristics or who find themselves in particularly difficult or stressful situations. The assumption is that by carefully studying individuals who are socially marginal, who are experiencing unusual situations, or who are going through a difficult phase in their lives, we can learn something about human nature.
Sigmund Freud was a master of using the psychological difficulties of individuals to draw conclusions about basic psychological processes. Freud wrote case studies of some of his most interesting patients and used these careful examinations to develop his important theories of personality. One classic example is Freud’s description of “Little Hans,” a child whose fear of horses the psychoanalyst interpreted in terms of repressed sexual impulses and the Oedipus complex (Freud, 1909/1964).
Another well-known case study is Phineas Gage, a man whose thoughts and emotions were extensively studied by cognitive psychologists after a railroad spike was blasted through his skull in an accident. Although there are questions about the interpretation of this case study (Kotowicz, 2007), it did provide early evidence that the brain’s frontal lobe is involved in emotion and morality (Damasio et al., 2005). An interesting example of a case study in clinical psychology is described by Rokeach (1964), who investigated in detail the beliefs of and interactions among three patients with schizophrenia, all of whom were convinced they were Jesus Christ.
In other cases the data from descriptive research projects come in the form of a survey — a measure administered through either an interview or a written questionnaire to get a picture of the beliefs or behaviours of a sample of people of interest . The people chosen to participate in the research (known as the sample) are selected to be representative of all the people that the researcher wishes to know about (the population). In election polls, for instance, a sample is taken from the population of all “likely voters” in the upcoming elections.
The results of surveys may sometimes be rather mundane, such as “Nine out of 10 doctors prefer Tymenocin” or “The median income in the city of Hamilton is $46,712.” Yet other times (particularly in discussions of social behaviour), the results can be shocking: “More than 40,000 people are killed by gunfire in the United States every year” or “More than 60% of women between the ages of 50 and 60 suffer from depression.” Descriptive research is frequently used by psychologists to get an estimate of the prevalence (or incidence ) of psychological disorders.
A final type of descriptive research — known as naturalistic observation — is research based on the observation of everyday events . For instance, a developmental psychologist who watches children on a playground and describes what they say to each other while they play is conducting descriptive research, as is a biopsychologist who observes animals in their natural habitats. One example of observational research involves a systematic procedure known as the strange situation , used to get a picture of how adults and young children interact. The data that are collected in the strange situation are systematically coded in a coding sheet such as that shown in Table 3.3.
Coder name: | ||||
This table represents a sample coding sheet from an episode of the “strange situation,” in which an infant (usually about one year old) is observed playing in a room with two adults — the child’s mother and a stranger. Each of the four coding categories is scored by the coder from 1 (the baby makes no effort to engage in the behaviour) to 7 (the baby makes a significant effort to engage in the behaviour). More information about the meaning of the coding can be found in Ainsworth, Blehar, Waters, and Wall (1978). | ||||
Coding categories explained | ||||
Proximity | The baby moves toward, grasps, or climbs on the adult. | |||
Maintaining contact | The baby resists being put down by the adult by crying or trying to climb back up. | |||
Resistance | The baby pushes, hits, or squirms to be put down from the adult’s arms. | |||
Avoidance | The baby turns away or moves away from the adult. | |||
Episode | Coding categories | |||
---|---|---|---|---|
Proximity | Contact | Resistance | Avoidance | |
Mother and baby play alone | 1 | 1 | 1 | 1 |
Mother puts baby down | 4 | 1 | 1 | 1 |
Stranger enters room | 1 | 2 | 3 | 1 |
Mother leaves room; stranger plays with baby | 1 | 3 | 1 | 1 |
Mother re-enters, greets and may comfort baby, then leaves again | 4 | 2 | 1 | 2 |
Stranger tries to play with baby | 1 | 3 | 1 | 1 |
Mother re-enters and picks up baby | 6 | 6 | 1 | 2 |
Source: Stang0r, 2011. |
The results of descriptive research projects are analyzed using descriptive statistics — numbers that summarize the distribution of scores on a measured variable . Most variables have distributions similar to that shown in Figure 3.5 where most of the scores are located near the centre of the distribution, and the distribution is symmetrical and bell-shaped. A data distribution that is shaped like a bell is known as a normal distribution .
A distribution can be described in terms of its central tendency — that is, the point in the distribution around which the data are centred — and its dispersion, or spread . The arithmetic average, or arithmetic mean , symbolized by the letter M , is the most commonly used measure of central tendency . It is computed by calculating the sum of all the scores of the variable and dividing this sum by the number of participants in the distribution (denoted by the letter N ). In the data presented in Figure 3.5 the mean height of the students is 67.12 inches (170.5 cm). The sample mean is usually indicated by the letter M .
In some cases, however, the data distribution is not symmetrical. This occurs when there are one or more extreme scores (known as outliers ) at one end of the distribution. Consider, for instance, the variable of family income (see Figure 3.6), which includes an outlier (a value of $3,800,000). In this case the mean is not a good measure of central tendency. Although it appears from Figure 3.6 that the central tendency of the family income variable should be around $70,000, the mean family income is actually $223,960. The single very extreme income has a disproportionate impact on the mean, resulting in a value that does not well represent the central tendency.
The median is used as an alternative measure of central tendency when distributions are not symmetrical. The median is the score in the center of the distribution, meaning that 50% of the scores are greater than the median and 50% of the scores are less than the median . In our case, the median household income ($73,000) is a much better indication of central tendency than is the mean household income ($223,960).
A final measure of central tendency, known as the mode , represents the value that occurs most frequently in the distribution . You can see from Figure 3.6 that the mode for the family income variable is $93,000 (it occurs four times).
In addition to summarizing the central tendency of a distribution, descriptive statistics convey information about how the scores of the variable are spread around the central tendency. Dispersion refers to the extent to which the scores are all tightly clustered around the central tendency , as seen in Figure 3.7.
Or they may be more spread out away from it, as seen in Figure 3.8.
One simple measure of dispersion is to find the largest (the maximum ) and the smallest (the minimum ) observed values of the variable and to compute the range of the variable as the maximum observed score minus the minimum observed score. You can check that the range of the height variable in Figure 3.5 is 72 – 62 = 10. The standard deviation , symbolized as s , is the most commonly used measure of dispersion . Distributions with a larger standard deviation have more spread. The standard deviation of the height variable is s = 2.74, and the standard deviation of the family income variable is s = $745,337.
An advantage of descriptive research is that it attempts to capture the complexity of everyday behaviour. Case studies provide detailed information about a single person or a small group of people, surveys capture the thoughts or reported behaviours of a large population of people, and naturalistic observation objectively records the behaviour of people or animals as it occurs naturally. Thus descriptive research is used to provide a relatively complete understanding of what is currently happening.
Despite these advantages, descriptive research has a distinct disadvantage in that, although it allows us to get an idea of what is currently happening, it is usually limited to static pictures. Although descriptions of particular experiences may be interesting, they are not always transferable to other individuals in other situations, nor do they tell us exactly why specific behaviours or events occurred. For instance, descriptions of individuals who have suffered a stressful event, such as a war or an earthquake, can be used to understand the individuals’ reactions to the event but cannot tell us anything about the long-term effects of the stress. And because there is no comparison group that did not experience the stressful situation, we cannot know what these individuals would be like if they hadn’t had the stressful experience.
Correlational Research: Seeking Relationships among Variables
In contrast to descriptive research, which is designed primarily to provide static pictures, correlational research involves the measurement of two or more relevant variables and an assessment of the relationship between or among those variables. For instance, the variables of height and weight are systematically related (correlated) because taller people generally weigh more than shorter people. In the same way, study time and memory errors are also related, because the more time a person is given to study a list of words, the fewer errors he or she will make. When there are two variables in the research design, one of them is called the predictor variable and the other the outcome variable . The research design can be visualized as shown in Figure 3.9, where the curved arrow represents the expected correlation between these two variables.
One way of organizing the data from a correlational study with two variables is to graph the values of each of the measured variables using a scatter plot . As you can see in Figure 3.10 a scatter plot is a visual image of the relationship between two variables . A point is plotted for each individual at the intersection of his or her scores for the two variables. When the association between the variables on the scatter plot can be easily approximated with a straight line , as in parts (a) and (b) of Figure 3.10 the variables are said to have a linear relationship .
When the straight line indicates that individuals who have above-average values for one variable also tend to have above-average values for the other variable , as in part (a), the relationship is said to be positive linear . Examples of positive linear relationships include those between height and weight, between education and income, and between age and mathematical abilities in children. In each case, people who score higher on one of the variables also tend to score higher on the other variable. Negative linear relationships , in contrast, as shown in part (b), occur when above-average values for one variable tend to be associated with below-average values for the other variable. Examples of negative linear relationships include those between the age of a child and the number of diapers the child uses, and between practice on and errors made on a learning task. In these cases, people who score higher on one of the variables tend to score lower on the other variable.
Relationships between variables that cannot be described with a straight line are known as nonlinear relationships . Part (c) of Figure 3.10 shows a common pattern in which the distribution of the points is essentially random. In this case there is no relationship at all between the two variables, and they are said to be independent . Parts (d) and (e) of Figure 3.10 show patterns of association in which, although there is an association, the points are not well described by a single straight line. For instance, part (d) shows the type of relationship that frequently occurs between anxiety and performance. Increases in anxiety from low to moderate levels are associated with performance increases, whereas increases in anxiety from moderate to high levels are associated with decreases in performance. Relationships that change in direction and thus are not described by a single straight line are called curvilinear relationships .
The most common statistical measure of the strength of linear relationships among variables is the Pearson correlation coefficient , which is symbolized by the letter r . The value of the correlation coefficient ranges from r = –1.00 to r = +1.00. The direction of the linear relationship is indicated by the sign of the correlation coefficient. Positive values of r (such as r = .54 or r = .67) indicate that the relationship is positive linear (i.e., the pattern of the dots on the scatter plot runs from the lower left to the upper right), whereas negative values of r (such as r = –.30 or r = –.72) indicate negative linear relationships (i.e., the dots run from the upper left to the lower right). The strength of the linear relationship is indexed by the distance of the correlation coefficient from zero (its absolute value). For instance, r = –.54 is a stronger relationship than r = .30, and r = .72 is a stronger relationship than r = –.57. Because the Pearson correlation coefficient only measures linear relationships, variables that have curvilinear relationships are not well described by r , and the observed correlation will be close to zero.
It is also possible to study relationships among more than two measures at the same time. A research design in which more than one predictor variable is used to predict a single outcome variable is analyzed through multiple regression (Aiken & West, 1991). Multiple regression is a statistical technique, based on correlation coefficients among variables, that allows predicting a single outcome variable from more than one predictor variable . For instance, Figure 3.11 shows a multiple regression analysis in which three predictor variables (Salary, job satisfaction, and years employed) are used to predict a single outcome (job performance). The use of multiple regression analysis shows an important advantage of correlational research designs — they can be used to make predictions about a person’s likely score on an outcome variable (e.g., job performance) based on knowledge of other variables.
An important limitation of correlational research designs is that they cannot be used to draw conclusions about the causal relationships among the measured variables. Consider, for instance, a researcher who has hypothesized that viewing violent behaviour will cause increased aggressive play in children. He has collected, from a sample of Grade 4 children, a measure of how many violent television shows each child views during the week, as well as a measure of how aggressively each child plays on the school playground. From his collected data, the researcher discovers a positive correlation between the two measured variables.
Although this positive correlation appears to support the researcher’s hypothesis, it cannot be taken to indicate that viewing violent television causes aggressive behaviour. Although the researcher is tempted to assume that viewing violent television causes aggressive play, there are other possibilities. One alternative possibility is that the causal direction is exactly opposite from what has been hypothesized. Perhaps children who have behaved aggressively at school develop residual excitement that leads them to want to watch violent television shows at home (Figure 3.13):
Although this possibility may seem less likely, there is no way to rule out the possibility of such reverse causation on the basis of this observed correlation. It is also possible that both causal directions are operating and that the two variables cause each other (Figure 3.14).
Still another possible explanation for the observed correlation is that it has been produced by the presence of a common-causal variable (also known as a third variable ). A common-causal variable is a variable that is not part of the research hypothesis but that causes both the predictor and the outcome variable and thus produces the observed correlation between them . In our example, a potential common-causal variable is the discipline style of the children’s parents. Parents who use a harsh and punitive discipline style may produce children who like to watch violent television and who also behave aggressively in comparison to children whose parents use less harsh discipline (Figure 3.15)
In this case, television viewing and aggressive play would be positively correlated (as indicated by the curved arrow between them), even though neither one caused the other but they were both caused by the discipline style of the parents (the straight arrows). When the predictor and outcome variables are both caused by a common-causal variable, the observed relationship between them is said to be spurious . A spurious relationship is a relationship between two variables in which a common-causal variable produces and “explains away” the relationship . If effects of the common-causal variable were taken away, or controlled for, the relationship between the predictor and outcome variables would disappear. In the example, the relationship between aggression and television viewing might be spurious because by controlling for the effect of the parents’ disciplining style, the relationship between television viewing and aggressive behaviour might go away.
Common-causal variables in correlational research designs can be thought of as mystery variables because, as they have not been measured, their presence and identity are usually unknown to the researcher. Since it is not possible to measure every variable that could cause both the predictor and outcome variables, the existence of an unknown common-causal variable is always a possibility. For this reason, we are left with the basic limitation of correlational research: correlation does not demonstrate causation. It is important that when you read about correlational research projects, you keep in mind the possibility of spurious relationships, and be sure to interpret the findings appropriately. Although correlational research is sometimes reported as demonstrating causality without any mention being made of the possibility of reverse causation or common-causal variables, informed consumers of research, like you, are aware of these interpretational problems.
In sum, correlational research designs have both strengths and limitations. One strength is that they can be used when experimental research is not possible because the predictor variables cannot be manipulated. Correlational designs also have the advantage of allowing the researcher to study behaviour as it occurs in everyday life. And we can also use correlational designs to make predictions — for instance, to predict from the scores on their battery of tests the success of job trainees during a training session. But we cannot use such correlational information to determine whether the training caused better job performance. For that, researchers rely on experiments.
Experimental Research: Understanding the Causes of Behaviour
The goal of experimental research design is to provide more definitive conclusions about the causal relationships among the variables in the research hypothesis than is available from correlational designs. In an experimental research design, the variables of interest are called the independent variable (or variables ) and the dependent variable . The independent variable in an experiment is the causing variable that is created (manipulated) by the experimenter . The dependent variable in an experiment is a measured variable that is expected to be influenced by the experimental manipulation . The research hypothesis suggests that the manipulated independent variable or variables will cause changes in the measured dependent variables. We can diagram the research hypothesis by using an arrow that points in one direction. This demonstrates the expected direction of causality (Figure 3.16):
Research Focus: Video Games and Aggression
Consider an experiment conducted by Anderson and Dill (2000). The study was designed to test the hypothesis that viewing violent video games would increase aggressive behaviour. In this research, male and female undergraduates from Iowa State University were given a chance to play with either a violent video game (Wolfenstein 3D) or a nonviolent video game (Myst). During the experimental session, the participants played their assigned video games for 15 minutes. Then, after the play, each participant played a competitive game with an opponent in which the participant could deliver blasts of white noise through the earphones of the opponent. The operational definition of the dependent variable (aggressive behaviour) was the level and duration of noise delivered to the opponent. The design of the experiment is shown in Figure 3.17
Two advantages of the experimental research design are (a) the assurance that the independent variable (also known as the experimental manipulation ) occurs prior to the measured dependent variable, and (b) the creation of initial equivalence between the conditions of the experiment (in this case by using random assignment to conditions).
Experimental designs have two very nice features. For one, they guarantee that the independent variable occurs prior to the measurement of the dependent variable. This eliminates the possibility of reverse causation. Second, the influence of common-causal variables is controlled, and thus eliminated, by creating initial equivalence among the participants in each of the experimental conditions before the manipulation occurs.
The most common method of creating equivalence among the experimental conditions is through random assignment to conditions, a procedure in which the condition that each participant is assigned to is determined through a random process, such as drawing numbers out of an envelope or using a random number table . Anderson and Dill first randomly assigned about 100 participants to each of their two groups (Group A and Group B). Because they used random assignment to conditions, they could be confident that, before the experimental manipulation occurred, the students in Group A were, on average, equivalent to the students in Group B on every possible variable, including variables that are likely to be related to aggression, such as parental discipline style, peer relationships, hormone levels, diet — and in fact everything else.
Then, after they had created initial equivalence, Anderson and Dill created the experimental manipulation — they had the participants in Group A play the violent game and the participants in Group B play the nonviolent game. Then they compared the dependent variable (the white noise blasts) between the two groups, finding that the students who had viewed the violent video game gave significantly longer noise blasts than did the students who had played the nonviolent game.
Anderson and Dill had from the outset created initial equivalence between the groups. This initial equivalence allowed them to observe differences in the white noise levels between the two groups after the experimental manipulation, leading to the conclusion that it was the independent variable (and not some other variable) that caused these differences. The idea is that the only thing that was different between the students in the two groups was the video game they had played.
Despite the advantage of determining causation, experiments do have limitations. One is that they are often conducted in laboratory situations rather than in the everyday lives of people. Therefore, we do not know whether results that we find in a laboratory setting will necessarily hold up in everyday life. Second, and more important, is that some of the most interesting and key social variables cannot be experimentally manipulated. If we want to study the influence of the size of a mob on the destructiveness of its behaviour, or to compare the personality characteristics of people who join suicide cults with those of people who do not join such cults, these relationships must be assessed using correlational designs, because it is simply not possible to experimentally manipulate these variables.
Key Takeaways
- Descriptive, correlational, and experimental research designs are used to collect and analyze data.
- Descriptive designs include case studies, surveys, and naturalistic observation. The goal of these designs is to get a picture of the current thoughts, feelings, or behaviours in a given group of people. Descriptive research is summarized using descriptive statistics.
- Correlational research designs measure two or more relevant variables and assess a relationship between or among them. The variables may be presented on a scatter plot to visually show the relationships. The Pearson Correlation Coefficient ( r ) is a measure of the strength of linear relationship between two variables.
- Common-causal variables may cause both the predictor and outcome variable in a correlational design, producing a spurious relationship. The possibility of common-causal variables makes it impossible to draw causal conclusions from correlational research designs.
- Experimental research involves the manipulation of an independent variable and the measurement of a dependent variable. Random assignment to conditions is normally used to create initial equivalence between the groups, allowing researchers to draw causal conclusions.
Exercises and Critical Thinking
- There is a negative correlation between the row that a student sits in in a large class (when the rows are numbered from front to back) and his or her final grade in the class. Do you think this represents a causal relationship or a spurious relationship, and why?
- Think of two variables (other than those mentioned in this book) that are likely to be correlated, but in which the correlation is probably spurious. What is the likely common-causal variable that is producing the relationship?
- Imagine a researcher wants to test the hypothesis that participating in psychotherapy will cause a decrease in reported anxiety. Describe the type of research design the investigator might use to draw this conclusion. What would be the independent and dependent variables in the research?
Image Attributions
Figure 3.4: “ Reading newspaper ” by Alaskan Dude (http://commons.wikimedia.org/wiki/File:Reading_newspaper.jpg) is licensed under CC BY 2.0
Aiken, L., & West, S. (1991). Multiple regression: Testing and interpreting interactions . Newbury Park, CA: Sage.
Ainsworth, M. S., Blehar, M. C., Waters, E., & Wall, S. (1978). Patterns of attachment: A psychological study of the strange situation . Hillsdale, NJ: Lawrence Erlbaum Associates.
Anderson, C. A., & Dill, K. E. (2000). Video games and aggressive thoughts, feelings, and behavior in the laboratory and in life. Journal of Personality and Social Psychology, 78 (4), 772–790.
Damasio, H., Grabowski, T., Frank, R., Galaburda, A. M., Damasio, A. R., Cacioppo, J. T., & Berntson, G. G. (2005). The return of Phineas Gage: Clues about the brain from the skull of a famous patient. In Social neuroscience: Key readings. (pp. 21–28). New York, NY: Psychology Press.
Freud, S. (1909/1964). Analysis of phobia in a five-year-old boy. In E. A. Southwell & M. Merbaum (Eds.), Personality: Readings in theory and research (pp. 3–32). Belmont, CA: Wadsworth. (Original work published 1909).
Kotowicz, Z. (2007). The strange case of Phineas Gage. History of the Human Sciences, 20 (1), 115–131.
Rokeach, M. (1964). The three Christs of Ypsilanti: A psychological study . New York, NY: Knopf.
Stangor, C. (2011). Research methods for the behavioural sciences (4th ed.). Mountain View, CA: Cengage.
Long Descriptions
Figure 3.6 long description: There are 25 families. 24 families have an income between $44,000 and $111,000 and one family has an income of $3,800,000. The mean income is $223,960 while the median income is $73,000. [Return to Figure 3.6]
Figure 3.10 long description: Types of scatter plots.
- Positive linear, r=positive .82. The plots on the graph form a rough line that runs from lower left to upper right.
- Negative linear, r=negative .70. The plots on the graph form a rough line that runs from upper left to lower right.
- Independent, r=0.00. The plots on the graph are spread out around the centre.
- Curvilinear, r=0.00. The plots of the graph form a rough line that goes up and then down like a hill.
- Curvilinear, r=0.00. The plots on the graph for a rough line that goes down and then up like a ditch.
[Return to Figure 3.10]
Introduction to Psychology - 1st Canadian Edition Copyright © 2014 by Jennifer Walinga and Charles Stangor is licensed under a Creative Commons Attribution-NonCommercial-ShareAlike 4.0 International License , except where otherwise noted.
Share This Book
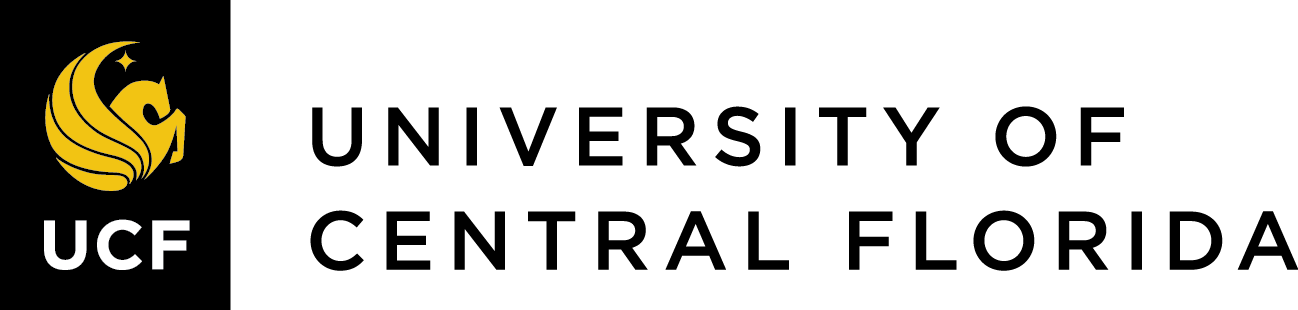
Psychological Research
Descriptive Research
Learning objectives.
- Differentiate between descriptive, experimental, and correlational research
- Explain the strengths and weaknesses of case studies, naturalistic observation, and surveys
There are many research methods available to psychologists in their efforts to understand, describe, and explain behavior and the cognitive and biological processes that underlie it. Some methods rely on observational techniques. Other approaches involve interactions between the researcher and the individuals who are being studied—ranging from a series of simple questions to extensive, in-depth interviews—to well-controlled experiments.
The three main categories of psychological research are descriptive, correlational, and experimental research. Research studies that do not test specific relationships between variables are called descriptive, or qualitative, studies . These studies are used to describe general or specific behaviors and attributes that are observed and measured. In the early stages of research it might be difficult to form a hypothesis, especially when there is not any existing literature in the area. In these situations designing an experiment would be premature, as the question of interest is not yet clearly defined as a hypothesis. Often a researcher will begin with a non-experimental approach, such as a descriptive study, to gather more information about the topic before designing an experiment or correlational study to address a specific hypothesis. Descriptive research is distinct from correlational research , in which psychologists formally test whether a relationship exists between two or more variables. Experimental research goes a step further beyond descriptive and correlational research and randomly assigns people to different conditions, using hypothesis testing to make inferences about how these conditions affect behavior. It aims to determine if one variable directly impacts and causes another. Correlational and experimental research both typically use hypothesis testing, whereas descriptive research does not.
Each of these research methods has unique strengths and weaknesses, and each method may only be appropriate for certain types of research questions. For example, studies that rely primarily on observation produce incredible amounts of information, but the ability to apply this information to the larger population is somewhat limited because of small sample sizes. Survey research, on the other hand, allows researchers to easily collect data from relatively large samples. While this allows for results to be generalized to the larger population more easily, the information that can be collected on any given survey is somewhat limited and subject to problems associated with any type of self-reported data. Some researchers conduct archival research by using existing records. While this can be a fairly inexpensive way to collect data that can provide insight into a number of research questions, researchers using this approach have no control on how or what kind of data was collected.
Correlational research can find a relationship between two variables, but the only way a researcher can claim that the relationship between the variables is cause and effect is to perform an experiment. In experimental research, which will be discussed later in the text, there is a tremendous amount of control over variables of interest. While this is a powerful approach, experiments are often conducted in very artificial settings. This calls into question the validity of experimental findings with regard to how they would apply in real-world settings. In addition, many of the questions that psychologists would like to answer cannot be pursued through experimental research because of ethical concerns.
The three main types of descriptive studies are case studies, naturalistic observation, and surveys.
Case Studies
In 2011, the New York Times published a feature story on Krista and Tatiana Hogan, Canadian twin girls. These particular twins are unique because Krista and Tatiana are conjoined twins, connected at the head. There is evidence that the two girls are connected in a part of the brain called the thalamus, which is a major sensory relay center. Most incoming sensory information is sent through the thalamus before reaching higher regions of the cerebral cortex for processing.
Link to Learning
To learn more about Krista and Tatiana, watch this video about their lives as conjoined twins.
The implications of this potential connection mean that it might be possible for one twin to experience the sensations of the other twin. For instance, if Krista is watching a particularly funny television program, Tatiana might smile or laugh even if she is not watching the program. This particular possibility has piqued the interest of many neuroscientists who seek to understand how the brain uses sensory information.
These twins represent an enormous resource in the study of the brain, and since their condition is very rare, it is likely that as long as their family agrees, scientists will follow these girls very closely throughout their lives to gain as much information as possible (Dominus, 2011).
In observational research, scientists are conducting a clinical or case study when they focus on one person or just a few individuals. Indeed, some scientists spend their entire careers studying just 10–20 individuals. Why would they do this? Obviously, when they focus their attention on a very small number of people, they can gain a tremendous amount of insight into those cases. The richness of information that is collected in clinical or case studies is unmatched by any other single research method. This allows the researcher to have a very deep understanding of the individuals and the particular phenomenon being studied.
If clinical or case studies provide so much information, why are they not more frequent among researchers? As it turns out, the major benefit of this particular approach is also a weakness. As mentioned earlier, this approach is often used when studying individuals who are interesting to researchers because they have a rare characteristic. Therefore, the individuals who serve as the focus of case studies are not like most other people. If scientists ultimately want to explain all behavior, focusing attention on such a special group of people can make it difficult to generalize any observations to the larger population as a whole. Generalizing refers to the ability to apply the findings of a particular research project to larger segments of society. Again, case studies provide enormous amounts of information, but since the cases are so specific, the potential to apply what’s learned to the average person may be very limited.
Naturalistic Observation
If you want to understand how behavior occurs, one of the best ways to gain information is to simply observe the behavior in its natural context. However, people might change their behavior in unexpected ways if they know they are being observed. How do researchers obtain accurate information when people tend to hide their natural behavior? As an example, imagine that your professor asks everyone in your class to raise their hand if they always wash their hands after using the restroom. Chances are that almost everyone in the classroom will raise their hand, but do you think hand washing after every trip to the restroom is really that universal?
This is very similar to the phenomenon mentioned earlier in this module: many individuals do not feel comfortable answering a question honestly. But if we are committed to finding out the facts about hand washing, we have other options available to us.
Suppose we send a classmate into the restroom to actually watch whether everyone washes their hands after using the restroom. Will our observer blend into the restroom environment by wearing a white lab coat, sitting with a clipboard, and staring at the sinks? We want our researcher to be inconspicuous—perhaps standing at one of the sinks pretending to put in contact lenses while secretly recording the relevant information. This type of observational study is called naturalistic observation : observing behavior in its natural setting. To better understand peer exclusion, Suzanne Fanger collaborated with colleagues at the University of Texas to observe the behavior of preschool children on a playground. How did the observers remain inconspicuous over the duration of the study? They equipped a few of the children with wireless microphones (which the children quickly forgot about) and observed while taking notes from a distance. Also, the children in that particular preschool (a “laboratory preschool”) were accustomed to having observers on the playground (Fanger, Frankel, & Hazen, 2012).
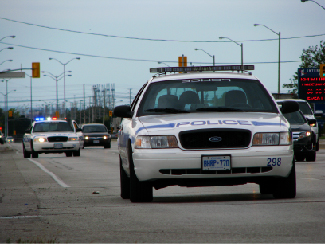
It is critical that the observer be as unobtrusive and as inconspicuous as possible: when people know they are being watched, they are less likely to behave naturally. If you have any doubt about this, ask yourself how your driving behavior might differ in two situations: In the first situation, you are driving down a deserted highway during the middle of the day; in the second situation, you are being followed by a police car down the same deserted highway (Figure 1).
It should be pointed out that naturalistic observation is not limited to research involving humans. Indeed, some of the best-known examples of naturalistic observation involve researchers going into the field to observe various kinds of animals in their own environments. As with human studies, the researchers maintain their distance and avoid interfering with the animal subjects so as not to influence their natural behaviors. Scientists have used this technique to study social hierarchies and interactions among animals ranging from ground squirrels to gorillas. The information provided by these studies is invaluable in understanding how those animals organize socially and communicate with one another. The anthropologist Jane Goodall, for example, spent nearly five decades observing the behavior of chimpanzees in Africa (Figure 2). As an illustration of the types of concerns that a researcher might encounter in naturalistic observation, some scientists criticized Goodall for giving the chimps names instead of referring to them by numbers—using names was thought to undermine the emotional detachment required for the objectivity of the study (McKie, 2010).
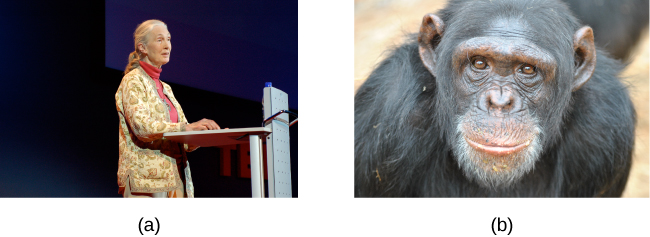
The greatest benefit of naturalistic observation is the validity, or accuracy, of information collected unobtrusively in a natural setting. Having individuals behave as they normally would in a given situation means that we have a higher degree of ecological validity, or realism, than we might achieve with other research approaches. Therefore, our ability to generalize the findings of the research to real-world situations is enhanced. If done correctly, we need not worry about people or animals modifying their behavior simply because they are being observed. Sometimes, people may assume that reality programs give us a glimpse into authentic human behavior. However, the principle of inconspicuous observation is violated as reality stars are followed by camera crews and are interviewed on camera for personal confessionals. Given that environment, we must doubt how natural and realistic their behaviors are.
The major downside of naturalistic observation is that they are often difficult to set up and control. In our restroom study, what if you stood in the restroom all day prepared to record people’s hand washing behavior and no one came in? Or, what if you have been closely observing a troop of gorillas for weeks only to find that they migrated to a new place while you were sleeping in your tent? The benefit of realistic data comes at a cost. As a researcher you have no control of when (or if) you have behavior to observe. In addition, this type of observational research often requires significant investments of time, money, and a good dose of luck.
Sometimes studies involve structured observation. In these cases, people are observed while engaging in set, specific tasks. An excellent example of structured observation comes from Strange Situation by Mary Ainsworth (you will read more about this in the module on lifespan development). The Strange Situation is a procedure used to evaluate attachment styles that exist between an infant and caregiver. In this scenario, caregivers bring their infants into a room filled with toys. The Strange Situation involves a number of phases, including a stranger coming into the room, the caregiver leaving the room, and the caregiver’s return to the room. The infant’s behavior is closely monitored at each phase, but it is the behavior of the infant upon being reunited with the caregiver that is most telling in terms of characterizing the infant’s attachment style with the caregiver.
Another potential problem in observational research is observer bias . Generally, people who act as observers are closely involved in the research project and may unconsciously skew their observations to fit their research goals or expectations. To protect against this type of bias, researchers should have clear criteria established for the types of behaviors recorded and how those behaviors should be classified. In addition, researchers often compare observations of the same event by multiple observers, in order to test inter-rater reliability : a measure of reliability that assesses the consistency of observations by different observers.
Often, psychologists develop surveys as a means of gathering data. Surveys are lists of questions to be answered by research participants, and can be delivered as paper-and-pencil questionnaires, administered electronically, or conducted verbally (Figure 3). Generally, the survey itself can be completed in a short time, and the ease of administering a survey makes it easy to collect data from a large number of people.
Surveys allow researchers to gather data from larger samples than may be afforded by other research methods . A sample is a subset of individuals selected from a population , which is the overall group of individuals that the researchers are interested in. Researchers study the sample and seek to generalize their findings to the population.
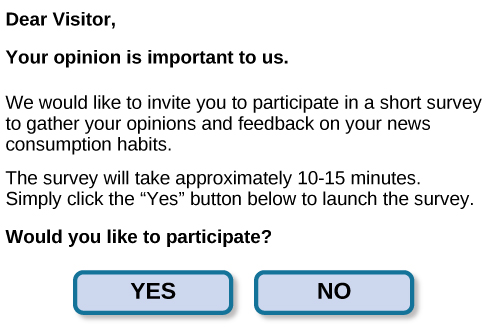
There is both strength and weakness of the survey in comparison to case studies. By using surveys, we can collect information from a larger sample of people. A larger sample is better able to reflect the actual diversity of the population, thus allowing better generalizability. Therefore, if our sample is sufficiently large and diverse, we can assume that the data we collect from the survey can be generalized to the larger population with more certainty than the information collected through a case study. However, given the greater number of people involved, we are not able to collect the same depth of information on each person that would be collected in a case study.
Another potential weakness of surveys is something we touched on earlier in this module: people don’t always give accurate responses. They may lie, misremember, or answer questions in a way that they think makes them look good. For example, people may report drinking less alcohol than is actually the case.
Any number of research questions can be answered through the use of surveys. One real-world example is the research conducted by Jenkins, Ruppel, Kizer, Yehl, and Griffin (2012) about the backlash against the US Arab-American community following the terrorist attacks of September 11, 2001. Jenkins and colleagues wanted to determine to what extent these negative attitudes toward Arab-Americans still existed nearly a decade after the attacks occurred. In one study, 140 research participants filled out a survey with 10 questions, including questions asking directly about the participant’s overt prejudicial attitudes toward people of various ethnicities. The survey also asked indirect questions about how likely the participant would be to interact with a person of a given ethnicity in a variety of settings (such as, “How likely do you think it is that you would introduce yourself to a person of Arab-American descent?”). The results of the research suggested that participants were unwilling to report prejudicial attitudes toward any ethnic group. However, there were significant differences between their pattern of responses to questions about social interaction with Arab-Americans compared to other ethnic groups: they indicated less willingness for social interaction with Arab-Americans compared to the other ethnic groups. This suggested that the participants harbored subtle forms of prejudice against Arab-Americans, despite their assertions that this was not the case (Jenkins et al., 2012).
Think It Over
A friend of yours is working part-time in a local pet store. Your friend has become increasingly interested in how dogs normally communicate and interact with each other, and is thinking of visiting a local veterinary clinic to see how dogs interact in the waiting room. After reading this section, do you think this is the best way to better understand such interactions? Do you have any suggestions that might result in more valid data?
CC licensed content, Original
- Modification and adaptation. Provided by : Lumen Learning. License : CC BY-SA: Attribution-ShareAlike
CC licensed content, Shared previously
- Approaches to Research. Authored by : OpenStax College. Located at : https://openstax.org/books/psychology-2e/pages/2-2-approaches-to-research . License : CC BY: Attribution . License Terms : Download for free at https://openstax.org/books/psychology-2e/pages/1-introduction.
- Descriptive Research. Provided by : Boundless. Located at : https://www.boundless.com/psychology/textbooks/boundless-psychology-textbook/researching-psychology-2/types-of-research-studies-27/descriptive-research-124-12659/ . License : CC BY-SA: Attribution-ShareAlike
research studies that do not test specific relationships between variables; they are used to describe general or specific behaviors and attributes that are observed and measured
tests whether a relationship exists between two or more variables
tests a hypothesis to determine cause and effect relationships
observational research study focusing on one or a few people
observation of behavior in its natural setting
inferring that the results for a sample apply to the larger population
when observations may be skewed to align with observer expectations
measure of agreement among observers on how they record and classify a particular event
list of questions to be answered by research participants—given as paper-and-pencil questionnaires, administered electronically, or conducted verbally—allowing researchers to collect data from a large number of people
the collection of individuals on which we collect data.
a larger collection of individuals that we would like to generalize our results to.
General Psychology Copyright © by OpenStax and Lumen Learning is licensed under a Creative Commons Attribution 4.0 International License , except where otherwise noted.
Share This Book
Psychological Research
Descriptive Research
There are many research methods available to psychologists in their efforts to understand, describe, and explain behavior. Some methods rely on observational techniques. Other approaches involve interactions between the researcher and the individuals who are being studied—ranging from a series of simple questions to extensive, in-depth interviews—to well-controlled experiments. The main categories of psychological research are descriptive, correlational, and experimental research. Each of these research methods has unique strengths and weaknesses, and each method may only be appropriate for certain types of research questions.
Research studies that do not test specific relationships between variables are called descriptive studies . For this method, the research question or hypothesis can be about a single variable (e.g., How accurate are people’s first impressions?) or can be a broad and exploratory question (e.g., What is it like to be a working mother diagnosed with depression?). The variable of the study is measured and reported without any further relationship analysis. A researcher might choose this method if they only needed to report information, such as a tally, an average, or a list of responses. Descriptive research can answer interesting and important questions, but what it cannot do is answer questions about relationships between variables.
Video 2.4.1. Descriptive Research Design provides explanation and examples for quantitative descriptive research. A closed-captioned version of this video is available here .
Descriptive research is distinct from correlational research , in which researchers formally test whether a relationship exists between two or more variables. Experimental research goes a step further beyond descriptive and correlational research and randomly assigns people to different conditions, using hypothesis testing to make inferences about causal relationships between variables. We will discuss each of these methods more in-depth later.
Table 2.4.1. Comparison of research design methods
Research design | Goal | Advantages | Disadvantages |
---|---|---|---|
Descriptive | To create a snapshot of the current state of affairs | Provides a relatively complete picture of what is occurring at a given time. Allows the development of questions for further study. | Does not assess relationships among variables. May be unethical if participants do not know they are being observed. |
Correlational | To assess the relationships between and among two or more variables | Allows testing of expected relationships between and among variables and the making of predictions. Can assess these relationships in everyday life events. | Cannot be used to draw inferences about the causal relationships between and among the variables. |
Experimental | To assess the causal impact of one or more experimental manipulations on a dependent variable | Allows drawing of conclusions about the causal relationships among variables. | Cannot experimentally manipulate many important variables. May be expensive and time-consuming. |
Source: Stangor, 2011. |
Candela Citations
- Descriptive Research. Authored by : Nicole Arduini-Van Hoose. Provided by : Hudson Valley Community College. Retrieved from : https://courses.lumenlearning.com/adolescent/chapter/descriptive-research/. License : CC BY-NC-SA: Attribution-NonCommercial-ShareAlike
- Introduction to Psychology. Authored by : Charles Stangor and Jennifer Walinga. Provided by : BC Campus Open Education. Retrieved from : https://opentextbc.ca/introductiontopsychology/. License : CC BY-NC-SA: Attribution-NonCommercial-ShareAlike
- Descriptive Research Design. Authored by : Nicole Arduini-Van Hoose. Provided by : Hudson Valley Community College. Retrieved from : https://hvcc.techsmithrelay.com/LNvM. License : CC BY-NC-SA: Attribution-NonCommercial-ShareAlike
Adolescent Psychology Copyright © 2020 by Nicole Arduini-Van Hoose is licensed under a Creative Commons Attribution-NonCommercial-ShareAlike 4.0 International License , except where otherwise noted.
Share This Book

An official website of the United States government
The .gov means it’s official. Federal government websites often end in .gov or .mil. Before sharing sensitive information, make sure you’re on a federal government site.
The site is secure. The https:// ensures that you are connecting to the official website and that any information you provide is encrypted and transmitted securely.
- Publications
- Account settings
Preview improvements coming to the PMC website in October 2024. Learn More or Try it out now .
- Advanced Search
- Journal List
- Front Res Metr Anal

The Use of Research Methods in Psychological Research: A Systematised Review
Salomé elizabeth scholtz.
1 Community Psychosocial Research (COMPRES), School of Psychosocial Health, North-West University, Potchefstroom, South Africa
Werner de Klerk
Leon t. de beer.
2 WorkWell Research Institute, North-West University, Potchefstroom, South Africa
Research methods play an imperative role in research quality as well as educating young researchers, however, the application thereof is unclear which can be detrimental to the field of psychology. Therefore, this systematised review aimed to determine what research methods are being used, how these methods are being used and for what topics in the field. Our review of 999 articles from five journals over a period of 5 years indicated that psychology research is conducted in 10 topics via predominantly quantitative research methods. Of these 10 topics, social psychology was the most popular. The remainder of the conducted methodology is described. It was also found that articles lacked rigour and transparency in the used methodology which has implications for replicability. In conclusion this article, provides an overview of all reported methodologies used in a sample of psychology journals. It highlights the popularity and application of methods and designs throughout the article sample as well as an unexpected lack of rigour with regard to most aspects of methodology. Possible sample bias should be considered when interpreting the results of this study. It is recommended that future research should utilise the results of this study to determine the possible impact on the field of psychology as a science and to further investigation into the use of research methods. Results should prompt the following future research into: a lack or rigour and its implication on replication, the use of certain methods above others, publication bias and choice of sampling method.
Introduction
Psychology is an ever-growing and popular field (Gough and Lyons, 2016 ; Clay, 2017 ). Due to this growth and the need for science-based research to base health decisions on (Perestelo-Pérez, 2013 ), the use of research methods in the broad field of psychology is an essential point of investigation (Stangor, 2011 ; Aanstoos, 2014 ). Research methods are therefore viewed as important tools used by researchers to collect data (Nieuwenhuis, 2016 ) and include the following: quantitative, qualitative, mixed method and multi method (Maree, 2016 ). Additionally, researchers also employ various types of literature reviews to address research questions (Grant and Booth, 2009 ). According to literature, what research method is used and why a certain research method is used is complex as it depends on various factors that may include paradigm (O'Neil and Koekemoer, 2016 ), research question (Grix, 2002 ), or the skill and exposure of the researcher (Nind et al., 2015 ). How these research methods are employed is also difficult to discern as research methods are often depicted as having fixed boundaries that are continuously crossed in research (Johnson et al., 2001 ; Sandelowski, 2011 ). Examples of this crossing include adding quantitative aspects to qualitative studies (Sandelowski et al., 2009 ), or stating that a study used a mixed-method design without the study having any characteristics of this design (Truscott et al., 2010 ).
The inappropriate use of research methods affects how students and researchers improve and utilise their research skills (Scott Jones and Goldring, 2015 ), how theories are developed (Ngulube, 2013 ), and the credibility of research results (Levitt et al., 2017 ). This, in turn, can be detrimental to the field (Nind et al., 2015 ), journal publication (Ketchen et al., 2008 ; Ezeh et al., 2010 ), and attempts to address public social issues through psychological research (Dweck, 2017 ). This is especially important given the now well-known replication crisis the field is facing (Earp and Trafimow, 2015 ; Hengartner, 2018 ).
Due to this lack of clarity on method use and the potential impact of inept use of research methods, the aim of this study was to explore the use of research methods in the field of psychology through a review of journal publications. Chaichanasakul et al. ( 2011 ) identify reviewing articles as the opportunity to examine the development, growth and progress of a research area and overall quality of a journal. Studies such as Lee et al. ( 1999 ) as well as Bluhm et al. ( 2011 ) review of qualitative methods has attempted to synthesis the use of research methods and indicated the growth of qualitative research in American and European journals. Research has also focused on the use of research methods in specific sub-disciplines of psychology, for example, in the field of Industrial and Organisational psychology Coetzee and Van Zyl ( 2014 ) found that South African publications tend to consist of cross-sectional quantitative research methods with underrepresented longitudinal studies. Qualitative studies were found to make up 21% of the articles published from 1995 to 2015 in a similar study by O'Neil and Koekemoer ( 2016 ). Other methods in health psychology, such as Mixed methods research have also been reportedly growing in popularity (O'Cathain, 2009 ).
A broad overview of the use of research methods in the field of psychology as a whole is however, not available in the literature. Therefore, our research focused on answering what research methods are being used, how these methods are being used and for what topics in practice (i.e., journal publications) in order to provide a general perspective of method used in psychology publication. We synthesised the collected data into the following format: research topic [areas of scientific discourse in a field or the current needs of a population (Bittermann and Fischer, 2018 )], method [data-gathering tools (Nieuwenhuis, 2016 )], sampling [elements chosen from a population to partake in research (Ritchie et al., 2009 )], data collection [techniques and research strategy (Maree, 2016 )], and data analysis [discovering information by examining bodies of data (Ktepi, 2016 )]. A systematised review of recent articles (2013 to 2017) collected from five different journals in the field of psychological research was conducted.
Grant and Booth ( 2009 ) describe systematised reviews as the review of choice for post-graduate studies, which is employed using some elements of a systematic review and seldom more than one or two databases to catalogue studies after a comprehensive literature search. The aspects used in this systematised review that are similar to that of a systematic review were a full search within the chosen database and data produced in tabular form (Grant and Booth, 2009 ).
Sample sizes and timelines vary in systematised reviews (see Lowe and Moore, 2014 ; Pericall and Taylor, 2014 ; Barr-Walker, 2017 ). With no clear parameters identified in the literature (see Grant and Booth, 2009 ), the sample size of this study was determined by the purpose of the sample (Strydom, 2011 ), and time and cost constraints (Maree and Pietersen, 2016 ). Thus, a non-probability purposive sample (Ritchie et al., 2009 ) of the top five psychology journals from 2013 to 2017 was included in this research study. Per Lee ( 2015 ) American Psychological Association (APA) recommends the use of the most up-to-date sources for data collection with consideration of the context of the research study. As this research study focused on the most recent trends in research methods used in the broad field of psychology, the identified time frame was deemed appropriate.
Psychology journals were only included if they formed part of the top five English journals in the miscellaneous psychology domain of the Scimago Journal and Country Rank (Scimago Journal & Country Rank, 2017 ). The Scimago Journal and Country Rank provides a yearly updated list of publicly accessible journal and country-specific indicators derived from the Scopus® database (Scopus, 2017b ) by means of the Scimago Journal Rank (SJR) indicator developed by Scimago from the algorithm Google PageRank™ (Scimago Journal & Country Rank, 2017 ). Scopus is the largest global database of abstracts and citations from peer-reviewed journals (Scopus, 2017a ). Reasons for the development of the Scimago Journal and Country Rank list was to allow researchers to assess scientific domains, compare country rankings, and compare and analyse journals (Scimago Journal & Country Rank, 2017 ), which supported the aim of this research study. Additionally, the goals of the journals had to focus on topics in psychology in general with no preference to specific research methods and have full-text access to articles.
The following list of top five journals in 2018 fell within the abovementioned inclusion criteria (1) Australian Journal of Psychology, (2) British Journal of Psychology, (3) Europe's Journal of Psychology, (4) International Journal of Psychology and lastly the (5) Journal of Psychology Applied and Interdisciplinary.
Journals were excluded from this systematised review if no full-text versions of their articles were available, if journals explicitly stated a publication preference for certain research methods, or if the journal only published articles in a specific discipline of psychological research (for example, industrial psychology, clinical psychology etc.).
The researchers followed a procedure (see Figure 1 ) adapted from that of Ferreira et al. ( 2016 ) for systematised reviews. Data collection and categorisation commenced on 4 December 2017 and continued until 30 June 2019. All the data was systematically collected and coded manually (Grant and Booth, 2009 ) with an independent person acting as co-coder. Codes of interest included the research topic, method used, the design used, sampling method, and methodology (the method used for data collection and data analysis). These codes were derived from the wording in each article. Themes were created based on the derived codes and checked by the co-coder. Lastly, these themes were catalogued into a table as per the systematised review design.

Systematised review procedure.
According to Johnston et al. ( 2019 ), “literature screening, selection, and data extraction/analyses” (p. 7) are specifically tailored to the aim of a review. Therefore, the steps followed in a systematic review must be reported in a comprehensive and transparent manner. The chosen systematised design adhered to the rigour expected from systematic reviews with regard to full search and data produced in tabular form (Grant and Booth, 2009 ). The rigorous application of the systematic review is, therefore discussed in relation to these two elements.
Firstly, to ensure a comprehensive search, this research study promoted review transparency by following a clear protocol outlined according to each review stage before collecting data (Johnston et al., 2019 ). This protocol was similar to that of Ferreira et al. ( 2016 ) and approved by three research committees/stakeholders and the researchers (Johnston et al., 2019 ). The eligibility criteria for article inclusion was based on the research question and clearly stated, and the process of inclusion was recorded on an electronic spreadsheet to create an evidence trail (Bandara et al., 2015 ; Johnston et al., 2019 ). Microsoft Excel spreadsheets are a popular tool for review studies and can increase the rigour of the review process (Bandara et al., 2015 ). Screening for appropriate articles for inclusion forms an integral part of a systematic review process (Johnston et al., 2019 ). This step was applied to two aspects of this research study: the choice of eligible journals and articles to be included. Suitable journals were selected by the first author and reviewed by the second and third authors. Initially, all articles from the chosen journals were included. Then, by process of elimination, those irrelevant to the research aim, i.e., interview articles or discussions etc., were excluded.
To ensure rigourous data extraction, data was first extracted by one reviewer, and an independent person verified the results for completeness and accuracy (Johnston et al., 2019 ). The research question served as a guide for efficient, organised data extraction (Johnston et al., 2019 ). Data was categorised according to the codes of interest, along with article identifiers for audit trails such as authors, title and aims of articles. The categorised data was based on the aim of the review (Johnston et al., 2019 ) and synthesised in tabular form under methods used, how these methods were used, and for what topics in the field of psychology.
The initial search produced a total of 1,145 articles from the 5 journals identified. Inclusion and exclusion criteria resulted in a final sample of 999 articles ( Figure 2 ). Articles were co-coded into 84 codes, from which 10 themes were derived ( Table 1 ).

Journal article frequency.
Codes used to form themes (research topics).
Social Psychology | 31 | Aggression SP, Attitude SP, Belief SP, Child abuse SP, Conflict SP, Culture SP, Discrimination SP, Economic, Family illness, Family, Group, Help, Immigration, Intergeneration, Judgement, Law, Leadership, Marriage SP, Media, Optimism, Organisational and Social justice, Parenting SP, Politics, Prejudice, Relationships, Religion, Romantic Relationships SP, Sex and attraction, Stereotype, Violence, Work |
Experimental Psychology | 17 | Anxiety, stress and PTSD, Coping, Depression, Emotion, Empathy, Facial research, Fear and threat, Happiness, Humor, Mindfulness, Mortality, Motivation and Achievement, Perception, Rumination, Self, Self-efficacy |
Cognitive Psychology | 12 | Attention, Cognition, Decision making, Impulse, Intelligence, Language, Math, Memory, Mental, Number, Problem solving, Reading |
Health Psychology | 7 | Addiction, Body, Burnout, Health, Illness (Health Psychology), Sleep (Health Psychology), Suicide and Self-harm |
Physiological Psychology | 6 | Gender, Health (Physiological psychology), Illness (Physiological psychology), Mood disorders, Sleep (Physiological psychology), Visual research |
Developmental Psychology | 3 | Attachment, Development, Old age |
Personality | 3 | Machiavellian, Narcissism, Personality |
Psychological Psychology | 3 | Programme, Psychology practice, Theory |
Education and Learning | 1 | Education and Learning |
Psychometrics | 1 | Measure |
Code Total | 84 |
These 10 themes represent the topic section of our research question ( Figure 3 ). All these topics except, for the final one, psychological practice , were found to concur with the research areas in psychology as identified by Weiten ( 2010 ). These research areas were chosen to represent the derived codes as they provided broad definitions that allowed for clear, concise categorisation of the vast amount of data. Article codes were categorised under particular themes/topics if they adhered to the research area definitions created by Weiten ( 2010 ). It is important to note that these areas of research do not refer to specific disciplines in psychology, such as industrial psychology; but to broader fields that may encompass sub-interests of these disciplines.

Topic frequency (international sample).
In the case of developmental psychology , researchers conduct research into human development from childhood to old age. Social psychology includes research on behaviour governed by social drivers. Researchers in the field of educational psychology study how people learn and the best way to teach them. Health psychology aims to determine the effect of psychological factors on physiological health. Physiological psychology , on the other hand, looks at the influence of physiological aspects on behaviour. Experimental psychology is not the only theme that uses experimental research and focuses on the traditional core topics of psychology (for example, sensation). Cognitive psychology studies the higher mental processes. Psychometrics is concerned with measuring capacity or behaviour. Personality research aims to assess and describe consistency in human behaviour (Weiten, 2010 ). The final theme of psychological practice refers to the experiences, techniques, and interventions employed by practitioners, researchers, and academia in the field of psychology.
Articles under these themes were further subdivided into methodologies: method, sampling, design, data collection, and data analysis. The categorisation was based on information stated in the articles and not inferred by the researchers. Data were compiled into two sets of results presented in this article. The first set addresses the aim of this study from the perspective of the topics identified. The second set of results represents a broad overview of the results from the perspective of the methodology employed. The second set of results are discussed in this article, while the first set is presented in table format. The discussion thus provides a broad overview of methods use in psychology (across all themes), while the table format provides readers with in-depth insight into methods used in the individual themes identified. We believe that presenting the data from both perspectives allow readers a broad understanding of the results. Due a large amount of information that made up our results, we followed Cichocka and Jost ( 2014 ) in simplifying our results. Please note that the numbers indicated in the table in terms of methodology differ from the total number of articles. Some articles employed more than one method/sampling technique/design/data collection method/data analysis in their studies.
What follows is the results for what methods are used, how these methods are used, and which topics in psychology they are applied to . Percentages are reported to the second decimal in order to highlight small differences in the occurrence of methodology.
Firstly, with regard to the research methods used, our results show that researchers are more likely to use quantitative research methods (90.22%) compared to all other research methods. Qualitative research was the second most common research method but only made up about 4.79% of the general method usage. Reviews occurred almost as much as qualitative studies (3.91%), as the third most popular method. Mixed-methods research studies (0.98%) occurred across most themes, whereas multi-method research was indicated in only one study and amounted to 0.10% of the methods identified. The specific use of each method in the topics identified is shown in Table 2 and Figure 4 .
Research methods in psychology.
Quantitative | 401 | 162 | 69 | 60 | 52 | 52 | 48 | 28 | 38 | 13 |
Qualitative | 28 | 4 | 1 | 0 | 5 | 2 | 3 | 5 | 0 | 1 |
Review | 11 | 5 | 2 | 0 | 3 | 4 | 1 | 13 | 0 | 1 |
Mixed Methods | 7 | 0 | 0 | 0 | 1 | 0 | 1 | 1 | 0 | 0 |
Multi-method | 0 | 0 | 0 | 0 | 0 | 0 | 0 | 0 | 1 | 0 |
Total | 447 | 171 | 72 | 60 | 61 | 58 | 53 | 47 | 39 | 15 |

Research method frequency in topics.
Secondly, in the case of how these research methods are employed , our study indicated the following.
Sampling −78.34% of the studies in the collected articles did not specify a sampling method. From the remainder of the studies, 13 types of sampling methods were identified. These sampling methods included broad categorisation of a sample as, for example, a probability or non-probability sample. General samples of convenience were the methods most likely to be applied (10.34%), followed by random sampling (3.51%), snowball sampling (2.73%), and purposive (1.37%) and cluster sampling (1.27%). The remainder of the sampling methods occurred to a more limited extent (0–1.0%). See Table 3 and Figure 5 for sampling methods employed in each topic.
Sampling use in the field of psychology.
Not stated | 331 | 153 | 45 | 57 | 49 | 43 | 43 | 38 | 31 | 14 |
Convenience sampling | 55 | 8 | 10 | 1 | 6 | 8 | 9 | 2 | 6 | 1 |
Random sampling | 15 | 3 | 9 | 1 | 2 | 2 | 0 | 2 | 1 | 1 |
Snowball sampling | 14 | 4 | 4 | 1 | 2 | 0 | 0 | 3 | 0 | 0 |
Purposive sampling | 6 | 0 | 2 | 0 | 0 | 2 | 0 | 3 | 1 | 0 |
Cluster sampling | 8 | 1 | 2 | 0 | 0 | 2 | 0 | 0 | 0 | 0 |
Stratified sampling | 4 | 1 | 2 | 0 | 1 | 1 | 0 | 0 | 0 | 0 |
Non-probability sampling | 4 | 0 | 1 | 0 | 0 | 0 | 0 | 0 | 1 | 0 |
Probability sampling | 3 | 1 | 0 | 0 | 0 | 0 | 0 | 0 | 0 | 0 |
Quota sampling | 1 | 0 | 1 | 0 | 0 | 0 | 0 | 0 | 0 | 0 |
Criterion sampling | 1 | 0 | 0 | 0 | 0 | 0 | 0 | 0 | 0 | 0 |
Self-selection sampling | 1 | 0 | 0 | 0 | 0 | 0 | 0 | 0 | 0 | 0 |
Unsystematic sampling | 0 | 1 | 0 | 0 | 0 | 0 | 0 | 0 | 0 | 0 |
Total | 443 | 172 | 76 | 60 | 60 | 58 | 52 | 48 | 40 | 16 |

Sampling method frequency in topics.
Designs were categorised based on the articles' statement thereof. Therefore, it is important to note that, in the case of quantitative studies, non-experimental designs (25.55%) were often indicated due to a lack of experiments and any other indication of design, which, according to Laher ( 2016 ), is a reasonable categorisation. Non-experimental designs should thus be compared with experimental designs only in the description of data, as it could include the use of correlational/cross-sectional designs, which were not overtly stated by the authors. For the remainder of the research methods, “not stated” (7.12%) was assigned to articles without design types indicated.
From the 36 identified designs the most popular designs were cross-sectional (23.17%) and experimental (25.64%), which concurred with the high number of quantitative studies. Longitudinal studies (3.80%), the third most popular design, was used in both quantitative and qualitative studies. Qualitative designs consisted of ethnography (0.38%), interpretative phenomenological designs/phenomenology (0.28%), as well as narrative designs (0.28%). Studies that employed the review method were mostly categorised as “not stated,” with the most often stated review designs being systematic reviews (0.57%). The few mixed method studies employed exploratory, explanatory (0.09%), and concurrent designs (0.19%), with some studies referring to separate designs for the qualitative and quantitative methods. The one study that identified itself as a multi-method study used a longitudinal design. Please see how these designs were employed in each specific topic in Table 4 , Figure 6 .
Design use in the field of psychology.
Experimental design | 82 | 82 | 3 | 60 | 10 | 12 | 8 | 6 | 4 | 3 |
Non-experimental design | 115 | 30 | 51 | 0 | 13 | 17 | 13 | 13 | 14 | 3 |
Cross-sectional design | 123 | 31 | 12 | 1 | 19 | 17 | 21 | 5 | 13 | 2 |
Correlational design | 56 | 12 | 3 | 0 | 10 | 2 | 2 | 0 | 4 | 2 |
Not stated | 37 | 7 | 3 | 0 | 4 | 2 | 4 | 14 | 1 | 3 |
Longitudinal design | 21 | 6 | 2 | 1 | 1 | 2 | 2 | 0 | 2 | 3 |
Quasi-experimental design | 4 | 1 | 0 | 0 | 0 | 0 | 2 | 1 | 0 | 0 |
Systematic review | 3 | 0 | 0 | 0 | 1 | 1 | 0 | 1 | 0 | 0 |
Cross-cultural design | 3 | 0 | 0 | 1 | 0 | 0 | 0 | 1 | 0 | 0 |
Descriptive design | 2 | 0 | 0 | 0 | 0 | 0 | 3 | 0 | 0 | 0 |
Ethnography | 4 | 0 | 0 | 0 | 0 | 0 | 0 | 0 | 0 | 0 |
Literature review | 1 | 1 | 0 | 0 | 1 | 1 | 0 | 0 | 0 | 0 |
Interpretative Phenomenological Analysis (IPA) | 2 | 0 | 0 | 0 | 1 | 0 | 0 | 0 | 0 | 0 |
Narrative design | 1 | 0 | 0 | 0 | 0 | 0 | 1 | 1 | 0 | 0 |
Case-control research design | 0 | 0 | 0 | 0 | 0 | 2 | 0 | 0 | 0 | 0 |
Concurrent data collection design | 1 | 0 | 0 | 0 | 1 | 0 | 0 | 0 | 0 | 0 |
Grounded Theory | 1 | 0 | 0 | 0 | 1 | 0 | 0 | 0 | 0 | 0 |
Narrative review | 0 | 1 | 0 | 0 | 0 | 1 | 0 | 0 | 0 | 0 |
Auto-ethnography | 1 | 0 | 0 | 0 | 0 | 0 | 0 | 0 | 0 | 0 |
Case series evaluation | 0 | 0 | 0 | 0 | 0 | 0 | 0 | 1 | 0 | 0 |
Case study | 1 | 0 | 0 | 0 | 0 | 0 | 0 | 0 | 0 | 0 |
Comprehensive review | 0 | 1 | 0 | 0 | 0 | 0 | 0 | 0 | 0 | 0 |
Descriptive-inferential | 0 | 0 | 0 | 0 | 0 | 0 | 0 | 0 | 1 | 0 |
Explanatory sequential design | 1 | 0 | 0 | 0 | 0 | 0 | 0 | 0 | 0 | 0 |
Exploratory mixed-method | 0 | 0 | 0 | 0 | 1 | 0 | 0 | 1 | 0 | 0 |
Grounded ethnographic design | 0 | 1 | 0 | 0 | 0 | 0 | 0 | 0 | 0 | 0 |
Historical cohort design | 0 | 1 | 0 | 0 | 0 | 0 | 0 | 0 | 0 | 0 |
Historical research | 0 | 0 | 0 | 0 | 0 | 0 | 0 | 1 | 0 | 0 |
interpretivist approach | 0 | 0 | 0 | 0 | 0 | 0 | 0 | 1 | 0 | 0 |
Meta-review | 1 | 0 | 0 | 0 | 0 | 0 | 0 | 1 | 0 | 0 |
Prospective design | 1 | 0 | 0 | 0 | 0 | 0 | 0 | 0 | 0 | 0 |
Qualitative review | 0 | 0 | 0 | 0 | 0 | 0 | 0 | 1 | 0 | 0 |
Qualitative systematic review | 0 | 0 | 0 | 0 | 0 | 1 | 0 | 0 | 0 | 0 |
Short-term prospective design | 0 | 1 | 0 | 0 | 0 | 0 | 0 | 0 | 0 | 0 |
Total | 461 | 175 | 74 | 63 | 63 | 58 | 56 | 48 | 39 | 16 |

Design frequency in topics.
Data collection and analysis —data collection included 30 methods, with the data collection method most often employed being questionnaires (57.84%). The experimental task (16.56%) was the second most preferred collection method, which included established or unique tasks designed by the researchers. Cognitive ability tests (6.84%) were also regularly used along with various forms of interviewing (7.66%). Table 5 and Figure 7 represent data collection use in the various topics. Data analysis consisted of 3,857 occurrences of data analysis categorised into ±188 various data analysis techniques shown in Table 6 and Figures 1 – 7 . Descriptive statistics were the most commonly used (23.49%) along with correlational analysis (17.19%). When using a qualitative method, researchers generally employed thematic analysis (0.52%) or different forms of analysis that led to coding and the creation of themes. Review studies presented few data analysis methods, with most studies categorising their results. Mixed method and multi-method studies followed the analysis methods identified for the qualitative and quantitative studies included.
Data collection in the field of psychology.
Questionnaire | 364 | 113 | 65 | 42 | 40 | 51 | 39 | 24 | 37 | 11 |
Experimental task | 68 | 66 | 3 | 52 | 9 | 5 | 11 | 5 | 5 | 1 |
Cognitive ability test | 9 | 57 | 1 | 12 | 6 | 1 | 5 | 1 | 1 | 0 |
Physiological measure | 3 | 12 | 1 | 6 | 2 | 5 | 3 | 0 | 1 | 0 |
Interview | 19 | 3 | 0 | 1 | 3 | 0 | 2 | 2 | 0 | 1 |
Online scholarly literature | 10 | 4 | 0 | 0 | 3 | 4 | 0 | 10 | 0 | 0 |
Open-ended questions | 15 | 3 | 0 | 1 | 3 | 1 | 2 | 3 | 0 | 0 |
Semi-structured interviews | 10 | 3 | 0 | 0 | 3 | 2 | 1 | 2 | 0 | 1 |
Observation | 10 | 1 | 0 | 0 | 0 | 0 | 0 | 0 | 2 | 0 |
Documents | 5 | 1 | 1 | 0 | 0 | 0 | 0 | 1 | 2 | 0 |
Focus group | 6 | 1 | 2 | 0 | 1 | 0 | 0 | 0 | 0 | 0 |
Not stated | 2 | 1 | 1 | 0 | 0 | 0 | 1 | 4 | 0 | 1 |
Public data | 6 | 1 | 0 | 0 | 0 | 0 | 0 | 2 | 0 | 1 |
Drawing task | 0 | 2 | 0 | 1 | 1 | 1 | 0 | 2 | 0 | 0 |
In-depth interview | 6 | 0 | 0 | 0 | 1 | 0 | 0 | 0 | 0 | 0 |
Structured interview | 0 | 2 | 0 | 0 | 1 | 2 | 0 | 0 | 1 | 0 |
Writing task | 1 | 0 | 0 | 0 | 4 | 0 | 0 | 1 | 0 | 0 |
Questionnaire interviews | 1 | 0 | 1 | 0 | 2 | 0 | 1 | 0 | 0 | 0 |
Non-experimental task | 4 | 0 | 0 | 0 | 0 | 0 | 0 | 0 | 0 | 0 |
Tests | 2 | 2 | 0 | 0 | 0 | 0 | 0 | 0 | 0 | 0 |
Group accounts | 2 | 0 | 0 | 0 | 0 | 0 | 0 | 1 | 0 | 0 |
Open-ended prompts | 1 | 1 | 0 | 0 | 0 | 0 | 0 | 1 | 0 | 0 |
Field notes | 2 | 0 | 0 | 0 | 0 | 0 | 0 | 0 | 0 | 0 |
Open-ended interview | 2 | 0 | 0 | 0 | 0 | 0 | 0 | 0 | 0 | 0 |
Qualitative questions | 0 | 0 | 0 | 0 | 0 | 1 | 0 | 0 | 0 | 1 |
Social media | 1 | 0 | 0 | 0 | 0 | 0 | 0 | 0 | 1 | 0 |
Assessment procedure | 0 | 0 | 0 | 1 | 0 | 0 | 0 | 0 | 0 | 0 |
Closed-ended questions | 0 | 0 | 0 | 0 | 0 | 0 | 0 | 1 | 0 | 0 |
Open discussions | 1 | 0 | 0 | 0 | 0 | 0 | 0 | 0 | 0 | 0 |
Qualitative descriptions | 1 | 0 | 0 | 0 | 0 | 0 | 0 | 0 | 0 | 0 |
Total | 551 | 273 | 75 | 116 | 79 | 73 | 65 | 60 | 50 | 17 |

Data collection frequency in topics.
Data analysis in the field of psychology.
Not stated | 5 | 1 | 2 | 0 | 0 | 1 | 1 | 5 | 0 | 1 |
Actor-Partner Interdependence Model (APIM) | 4 | 0 | 0 | 0 | 0 | 0 | 0 | 0 | 0 | 0 |
Analysis of Covariance (ANCOVA) | 17 | 8 | 1 | 3 | 4 | 2 | 1 | 0 | 0 | 1 |
Analysis of Variance (ANOVA) | 112 | 60 | 16 | 29 | 15 | 17 | 15 | 6 | 5 | 3 |
Auto-regressive path coefficients | 0 | 0 | 1 | 0 | 0 | 0 | 0 | 0 | 0 | 0 |
Average variance extracted (AVE) | 1 | 0 | 0 | 0 | 0 | 1 | 0 | 0 | 0 | 0 |
Bartholomew's classification system | 1 | 0 | 0 | 0 | 0 | 0 | 0 | 0 | 0 | 0 |
Bayesian analysis | 3 | 0 | 0 | 0 | 1 | 0 | 0 | 0 | 0 | 0 |
Bibliometric analysis | 1 | 1 | 0 | 0 | 0 | 0 | 0 | 1 | 0 | 0 |
Binary logistic regression | 1 | 1 | 0 | 0 | 1 | 4 | 1 | 0 | 0 | 0 |
Binary multilevel regression | 0 | 0 | 0 | 1 | 0 | 0 | 0 | 0 | 0 | 0 |
Binomial and Bernoulli regression models | 2 | 0 | 0 | 0 | 0 | 0 | 0 | 0 | 0 | 0 |
Binomial mixed effects model | 1 | 0 | 0 | 0 | 0 | 0 | 0 | 0 | 0 | 0 |
Bivariate Correlations | 32 | 10 | 3 | 0 | 4 | 3 | 5 | 1 | 1 | 1 |
Bivariate logistic correlations | 1 | 0 | 0 | 0 | 0 | 1 | 0 | 0 | 0 | 0 |
Bootstrapping | 39 | 16 | 2 | 3 | 5 | 1 | 6 | 1 | 2 | 1 |
Canonical correlations | 0 | 0 | 0 | 0 | 0 | 0 | 0 | 0 | 2 | 0 |
Cartesian diagram | 1 | 0 | 0 | 0 | 0 | 0 | 0 | 0 | 0 | 0 |
Case-wise diagnostics | 0 | 1 | 0 | 0 | 0 | 0 | 1 | 0 | 0 | 0 |
Casual network analysis | 0 | 0 | 0 | 1 | 0 | 0 | 0 | 0 | 0 | 0 |
Categorisation | 5 | 2 | 0 | 0 | 1 | 1 | 0 | 4 | 0 | 0 |
Categorisation of responses | 2 | 0 | 0 | 0 | 0 | 0 | 0 | 0 | 0 | 0 |
Category codes | 3 | 1 | 0 | 0 | 0 | 1 | 0 | 0 | 0 | 0 |
Cattell's scree-test | 0 | 0 | 1 | 0 | 0 | 0 | 0 | 0 | 0 | 0 |
Chi-square tests | 52 | 20 | 17 | 5 | 6 | 11 | 8 | 7 | 4 | 3 |
Classic Parallel Analysis (PA) | 0 | 0 | 1 | 0 | 0 | 1 | 0 | 0 | 1 | 0 |
Cluster analysis | 7 | 0 | 0 | 0 | 1 | 1 | 1 | 1 | 0 | 1 |
Coded | 15 | 3 | 1 | 2 | 1 | 1 | 1 | 2 | 1 | 0 |
Cohen d effect size | 14 | 5 | 2 | 1 | 3 | 2 | 3 | 1 | 0 | 1 |
Common method variance (CMV) | 5 | 0 | 1 | 0 | 0 | 0 | 0 | 0 | 0 | 0 |
Comprehensive Meta-Analysis (CMA) | 0 | 0 | 0 | 0 | 0 | 0 | 0 | 0 | 1 | 0 |
Confidence Interval (CI) | 2 | 0 | 0 | 0 | 0 | 1 | 0 | 0 | 0 | 0 |
Confirmatory Factor Analysis (CFA) | 57 | 13 | 40 | 0 | 2 | 4 | 7 | 1 | 3 | 1 |
Content analysis | 9 | 1 | 0 | 0 | 2 | 1 | 0 | 1 | 0 | 0 |
Convergent validity | 1 | 0 | 0 | 0 | 0 | 0 | 0 | 0 | 0 | 0 |
Cook's distance | 0 | 1 | 0 | 0 | 1 | 0 | 0 | 0 | 0 | 0 |
Correlated-trait-correlated-method minus one model | 1 | 0 | 0 | 0 | 0 | 0 | 0 | 0 | 0 | 0 |
Correlational analysis | 259 | 85 | 44 | 18 | 27 | 31 | 34 | 8 | 33 | 8 |
Covariance matrix | 3 | 0 | 1 | 0 | 0 | 0 | 0 | 0 | 0 | 0 |
Covariance modelling | 0 | 1 | 1 | 0 | 0 | 0 | 0 | 0 | 0 | 0 |
Covariance structure analyses | 2 | 0 | 0 | 0 | 0 | 0 | 0 | 0 | 0 | 0 |
Cronbach's alpha | 61 | 14 | 18 | 6 | 5 | 10 | 8 | 3 | 7 | 5 |
Cross-validation | 0 | 0 | 2 | 0 | 0 | 0 | 0 | 0 | 0 | 1 |
Cross-lagged analyses | 1 | 2 | 1 | 0 | 0 | 0 | 1 | 0 | 0 | 0 |
Dependent t-test | 1 | 2 | 0 | 0 | 1 | 1 | 0 | 1 | 0 | 0 |
Descriptive statistics | 324 | 132 | 43 | 49 | 41 | 43 | 36 | 28 | 29 | 10 |
Differentiated analysis | 0 | 0 | 0 | 0 | 0 | 0 | 1 | 0 | 0 | 0 |
Discriminate analysis | 1 | 0 | 2 | 0 | 0 | 0 | 0 | 0 | 0 | 1 |
Discursive psychology | 1 | 0 | 0 | 0 | 0 | 0 | 0 | 0 | 0 | 0 |
Dominance analysis | 1 | 0 | 0 | 0 | 0 | 0 | 0 | 0 | 0 | 0 |
Expectation maximisation | 2 | 1 | 0 | 0 | 0 | 0 | 0 | 1 | 0 | 0 |
Exploratory data Analysis | 1 | 1 | 0 | 0 | 1 | 1 | 0 | 0 | 0 | 0 |
Exploratory Factor Analysis (EFA) | 14 | 5 | 24 | 0 | 1 | 1 | 4 | 0 | 4 | 0 |
Exploratory structural equation modelling (ESEM) | 0 | 0 | 1 | 0 | 0 | 0 | 0 | 0 | 1 | 0 |
Factor analysis | 12 | 4 | 16 | 0 | 2 | 1 | 5 | 0 | 2 | 0 |
Measurement invariance testing | 0 | 0 | 0 | 0 | 0 | 0 | 0 | 0 | 0 | 0 |
Four-way mixed ANOVA | 0 | 1 | 0 | 1 | 0 | 0 | 0 | 0 | 0 | 0 |
Frequency rate | 20 | 1 | 4 | 2 | 1 | 2 | 2 | 2 | 0 | 0 |
Friedman test | 1 | 0 | 0 | 0 | 0 | 0 | 0 | 0 | 0 | 0 |
Games-Howell | 2 | 2 | 0 | 0 | 0 | 1 | 0 | 0 | 0 | 0 |
General linear model analysis | 1 | 2 | 0 | 0 | 0 | 0 | 1 | 1 | 0 | 0 |
Greenhouse-Geisser correction | 2 | 5 | 0 | 0 | 0 | 0 | 1 | 1 | 1 | 1 |
Grounded theory method | 0 | 0 | 0 | 0 | 0 | 0 | 0 | 0 | 0 | 1 |
Grounded theory methodology using open and axial coding | 1 | 0 | 0 | 0 | 0 | 0 | 0 | 0 | 0 | 0 |
Guttman split-half | 0 | 0 | 1 | 0 | 0 | 0 | 0 | 0 | 0 | 0 |
Harman's one-factor test | 13 | 2 | 0 | 0 | 0 | 1 | 2 | 0 | 0 | 0 |
Herman's criteria of experience categorisation | 0 | 0 | 0 | 0 | 0 | 0 | 0 | 1 | 0 | 0 |
Hierarchical CFA (HCFA) | 0 | 0 | 1 | 0 | 0 | 0 | 0 | 0 | 0 | 0 |
Hierarchical cluster analysis | 1 | 0 | 0 | 0 | 0 | 0 | 0 | 0 | 0 | 0 |
Hierarchical Linear Modelling (HLM) | 76 | 22 | 2 | 3 | 7 | 6 | 7 | 4 | 4 | 1 |
Huynh-Felt correction | 1 | 0 | 0 | 0 | 0 | 0 | 0 | 0 | 0 | 0 |
Identified themes | 3 | 0 | 0 | 0 | 1 | 0 | 0 | 0 | 0 | 0 |
Independent samples t-test | 38 | 9 | 4 | 4 | 4 | 8 | 3 | 3 | 1 | 1 |
Inductive open coding | 1 | 0 | 0 | 0 | 0 | 0 | 0 | 0 | 0 | 0 |
Inferential statistics | 2 | 0 | 0 | 0 | 0 | 0 | 1 | 0 | 0 | 0 |
Interclass correlation | 3 | 0 | 1 | 0 | 0 | 0 | 0 | 0 | 0 | 0 |
Internal consistency | 3 | 1 | 2 | 0 | 0 | 0 | 0 | 0 | 0 | 0 |
Interpreted and defined | 0 | 0 | 0 | 0 | 1 | 0 | 0 | 0 | 0 | 0 |
Interpretive Phenomenological Analysis (IPA) | 2 | 1 | 0 | 0 | 1 | 0 | 0 | 0 | 0 | 0 |
Item fit analysis | 1 | 0 | 5 | 0 | 0 | 0 | 0 | 0 | 0 | 0 |
K-means clustering | 0 | 0 | 0 | 0 | 0 | 0 | 0 | 1 | 0 | 0 |
Kaiser-meyer-Olkin measure of sampling adequacy | 2 | 0 | 8 | 0 | 0 | 0 | 2 | 0 | 2 | 0 |
Kendall's coefficients | 3 | 1 | 0 | 0 | 0 | 0 | 0 | 0 | 0 | 0 |
Kolmogorov-Smirnov test | 1 | 2 | 1 | 1 | 2 | 2 | 0 | 0 | 1 | 0 |
Lagged-effects multilevel modelling | 1 | 1 | 0 | 0 | 0 | 0 | 0 | 0 | 0 | 0 |
Latent class differentiation (LCD) | 1 | 0 | 0 | 0 | 0 | 0 | 0 | 0 | 0 | 0 |
Latent cluster analysis | 0 | 0 | 0 | 0 | 0 | 1 | 0 | 0 | 0 | 0 |
Latent growth curve modelling (LGCM) | 1 | 0 | 0 | 0 | 0 | 0 | 0 | 1 | 1 | 0 |
Latent means | 1 | 0 | 0 | 0 | 0 | 0 | 0 | 0 | 0 | 0 |
Latent Profile Analysis (LPA) | 1 | 1 | 0 | 0 | 0 | 0 | 0 | 0 | 0 | 0 |
Linear regressions | 69 | 19 | 4 | 10 | 3 | 12 | 5 | 3 | 13 | 0 |
Linguistic Inquiry and Word Count | 0 | 0 | 0 | 0 | 1 | 0 | 0 | 0 | 0 | 0 |
Listwise deletion method | 0 | 0 | 0 | 0 | 0 | 1 | 0 | 0 | 0 | 0 |
Log-likelihood ratios | 0 | 0 | 0 | 0 | 0 | 1 | 0 | 0 | 0 | 0 |
Logistic mixed-effects model | 1 | 0 | 0 | 0 | 0 | 0 | 0 | 0 | 0 | 0 |
Logistic regression analyses | 17 | 0 | 1 | 0 | 4 | 2 | 1 | 0 | 0 | 1 |
Loglinear Model | 2 | 0 | 0 | 0 | 0 | 0 | 0 | 0 | 0 | 0 |
Mahalanobis distances | 0 | 2 | 0 | 0 | 0 | 1 | 0 | 0 | 0 | 0 |
Mann-Whitney U tests | 6 | 4 | 2 | 1 | 2 | 0 | 2 | 4 | 0 | 0 |
Mauchly's test | 0 | 1 | 0 | 2 | 0 | 0 | 0 | 1 | 0 | 1 |
Maximum likelihood method | 11 | 3 | 9 | 0 | 1 | 3 | 2 | 3 | 1 | 0 |
Maximum-likelihood factor analysis with promax rotation | 0 | 1 | 0 | 0 | 0 | 0 | 0 | 0 | 0 | 0 |
Measurement invariance testing | 4 | 1 | 1 | 0 | 1 | 0 | 0 | 0 | 0 | 0 |
Mediation analysis | 29 | 7 | 1 | 2 | 4 | 3 | 5 | 0 | 3 | 0 |
Meta-analysis | 3 | 0 | 1 | 0 | 0 | 0 | 0 | 1 | 0 | 0 |
Microanalysis | 1 | 0 | 0 | 0 | 0 | 0 | 0 | 0 | 0 | 0 |
Minimum significant difference (MSD) comparison | 0 | 1 | 0 | 0 | 0 | 0 | 0 | 0 | 0 | 0 |
Mixed ANOVAs | 19 | 6 | 0 | 10 | 1 | 2 | 1 | 4 | 1 | 0 |
Mixed linear model | 0 | 0 | 0 | 1 | 0 | 0 | 1 | 0 | 0 | 0 |
Mixed-design ANCOVA | 1 | 1 | 0 | 0 | 0 | 0 | 0 | 0 | 0 | 0 |
Mixed-effects multiple regression models | 1 | 0 | 0 | 0 | 0 | 0 | 0 | 0 | 0 | 0 |
Moderated hierarchical regression model | 1 | 0 | 0 | 0 | 0 | 0 | 0 | 0 | 0 | 0 |
Moderated regression analysis | 8 | 4 | 0 | 0 | 1 | 0 | 1 | 0 | 1 | 0 |
Monte Carlo Markov Chains | 2 | 0 | 1 | 0 | 0 | 0 | 0 | 0 | 0 | 0 |
Multi-group analysis | 3 | 0 | 0 | 0 | 0 | 0 | 0 | 0 | 0 | 0 |
Multidimensional Random Coefficient Multinomial Logit (MRCML) | 0 | 0 | 1 | 0 | 0 | 0 | 0 | 0 | 0 | 0 |
Multidimensional Scaling | 2 | 0 | 0 | 0 | 0 | 0 | 0 | 0 | 0 | 0 |
Multiple-Group Confirmatory Factor Analysis (MGCFA) | 3 | 0 | 0 | 0 | 0 | 2 | 0 | 0 | 0 | 0 |
Multilevel latent class analysis | 1 | 0 | 0 | 0 | 0 | 1 | 0 | 0 | 0 | 0 |
Multilevel modelling | 7 | 2 | 1 | 1 | 1 | 0 | 0 | 1 | 1 | 0 |
Multilevel Structural Equation Modelling (MSEM) | 2 | 0 | 0 | 0 | 0 | 0 | 0 | 0 | 0 | 0 |
Multinominal logistic regression (MLR) | 1 | 0 | 0 | 0 | 0 | 0 | 0 | 0 | 0 | 0 |
Multinominal regression analysis | 1 | 0 | 0 | 0 | 0 | 2 | 0 | 0 | 0 | 0 |
Multiple Indicators Multiple Causes (MIMIC) | 0 | 0 | 0 | 0 | 1 | 1 | 0 | 0 | 0 | 0 |
Multiple mediation analysis | 2 | 6 | 0 | 0 | 2 | 2 | 1 | 0 | 0 | 0 |
Multiple regression | 34 | 15 | 3 | 0 | 3 | 4 | 5 | 0 | 7 | 2 |
Multivariate analysis of co-variance (MANCOVA) | 12 | 2 | 1 | 1 | 0 | 1 | 1 | 0 | 1 | 0 |
Multivariate Analysis of Variance (MANOVA) | 38 | 8 | 4 | 5 | 5 | 6 | 9 | 1 | 1 | 2 |
Multivariate hierarchical linear regression | 1 | 1 | 0 | 0 | 0 | 0 | 0 | 0 | 0 | 0 |
Multivariate linear regression | 0 | 1 | 0 | 0 | 0 | 0 | 1 | 0 | 0 | 0 |
Multivariate logistic regression analyses | 1 | 0 | 0 | 0 | 0 | 0 | 0 | 0 | 0 | 0 |
Multivariate regressions | 2 | 1 | 0 | 0 | 0 | 0 | 1 | 0 | 0 | 0 |
Nagelkerke's R square | 0 | 0 | 0 | 0 | 0 | 1 | 0 | 0 | 0 | 0 |
Narrative analysis | 1 | 0 | 0 | 0 | 0 | 0 | 1 | 0 | 0 | 0 |
Negative binominal regression with log link | 0 | 0 | 0 | 0 | 0 | 1 | 0 | 0 | 0 | 0 |
Newman-Keuls | 0 | 1 | 0 | 0 | 0 | 1 | 0 | 0 | 0 | 0 |
Nomological Validity Analysis | 0 | 0 | 1 | 0 | 0 | 0 | 0 | 0 | 0 | 0 |
One sample t-test | 8 | 10 | 1 | 7 | 4 | 6 | 4 | 0 | 1 | 0 |
Ordinary Least-Square regression (OLS) | 2 | 2 | 0 | 1 | 0 | 0 | 0 | 0 | 0 | 0 |
Pairwise deletion method | 0 | 0 | 0 | 0 | 0 | 1 | 0 | 0 | 0 | 0 |
Pairwise parameter comparison | 4 | 0 | 0 | 0 | 0 | 0 | 2 | 0 | 0 | 0 |
Parametric Analysis | 0 | 0 | 0 | 1 | 0 | 0 | 0 | 0 | 0 | 0 |
Partial Least Squares regression method (PLS) | 1 | 1 | 0 | 0 | 0 | 0 | 0 | 0 | 0 | 0 |
Path analysis | 21 | 9 | 0 | 1 | 2 | 4 | 5 | 1 | 2 | 0 |
Path-analytic model test | 1 | 0 | 0 | 0 | 0 | 0 | 0 | 0 | 0 | 0 |
Phenomenological analysis | 0 | 0 | 1 | 0 | 0 | 0 | 0 | 1 | 0 | 0 |
Polynomial regression analyses | 1 | 0 | 0 | 0 | 0 | 0 | 0 | 0 | 0 | 0 |
Fisher LSD | 0 | 1 | 0 | 0 | 0 | 0 | 0 | 0 | 0 | 0 |
Principal axis factoring | 2 | 1 | 4 | 0 | 0 | 0 | 1 | 0 | 0 | 0 |
Principal component analysis (PCA) | 8 | 1 | 12 | 1 | 1 | 0 | 3 | 2 | 5 | 1 |
Pseudo-panel regression | 1 | 0 | 0 | 0 | 0 | 0 | 0 | 0 | 0 | 0 |
Quantitative content analysis | 0 | 0 | 0 | 0 | 1 | 0 | 0 | 0 | 0 | 0 |
Receiver operating characteristic (ROC) curve analysis | 2 | 0 | 0 | 1 | 0 | 0 | 0 | 0 | 0 | 0 |
Relative weight analysis | 1 | 0 | 0 | 0 | 0 | 0 | 0 | 0 | 0 | 0 |
Repeated measures analyses of variances (rANOVA) | 18 | 22 | 1 | 7 | 5 | 2 | 1 | 1 | 1 | 1 |
Ryan-Einot-Gabriel-Welsch multiple F test | 1 | 0 | 0 | 0 | 0 | 0 | 0 | 0 | 0 | 0 |
Satorra-Bentler scaled chi-square statistic | 0 | 0 | 3 | 0 | 0 | 0 | 0 | 0 | 0 | 0 |
Scheffe's test | 3 | 0 | 0 | 0 | 0 | 1 | 0 | 0 | 0 | 0 |
Sequential multiple mediation analysis | 1 | 0 | 0 | 0 | 0 | 0 | 0 | 0 | 0 | 0 |
Shapiro-Wilk test | 2 | 3 | 0 | 2 | 1 | 0 | 0 | 0 | 0 | 0 |
Sobel Test | 13 | 5 | 0 | 1 | 0 | 2 | 4 | 0 | 0 | 0 |
Squared multiple correlations | 1 | 0 | 0 | 0 | 0 | 0 | 0 | 0 | 0 | 0 |
Squared semi-partial correlations (sr2) | 2 | 0 | 0 | 0 | 0 | 0 | 0 | 0 | 0 | 0 |
Stepwise regression analysis | 3 | 2 | 0 | 0 | 1 | 0 | 0 | 0 | 2 | 0 |
Structural Equation Modelling (SEM) | 56 | 22 | 3 | 3 | 3 | 5 | 5 | 0 | 5 | 3 |
Structure analysis | 0 | 0 | 0 | 0 | 0 | 0 | 1 | 0 | 0 | 0 |
Subsequent t-test | 0 | 0 | 0 | 0 | 1 | 0 | 0 | 0 | 0 | 0 |
Systematic coding- Gemeinschaft-oriented | 1 | 0 | 0 | 0 | 1 | 0 | 0 | 0 | 0 | 0 |
Task analysis | 2 | 0 | 0 | 0 | 0 | 0 | 0 | 0 | 0 | 0 |
Thematic analysis | 11 | 2 | 0 | 0 | 3 | 0 | 2 | 2 | 0 | 0 |
Three (condition)-way ANOVA | 0 | 4 | 0 | 0 | 1 | 0 | 1 | 0 | 0 | 0 |
Three-way hierarchical loglinear analysis | 0 | 2 | 0 | 0 | 0 | 0 | 0 | 0 | 0 | 0 |
Tukey-Kramer corrections | 0 | 0 | 0 | 1 | 0 | 1 | 0 | 0 | 0 | 0 |
Two-paired sample t-test | 7 | 6 | 1 | 1 | 0 | 3 | 1 | 1 | 0 | 1 |
Two-tailed related t-test | 0 | 1 | 1 | 0 | 1 | 0 | 0 | 0 | 0 | 0 |
Unadjusted Logistic regression analysis | 0 | 1 | 0 | 0 | 0 | 0 | 0 | 0 | 0 | 0 |
Univariate generalized linear models (GLM) | 2 | 0 | 0 | 0 | 0 | 0 | 0 | 0 | 0 | 0 |
Variance inflation factor (VIF) | 3 | 1 | 0 | 0 | 0 | 0 | 0 | 0 | 1 | 0 |
Variance-covariance matrix | 1 | 0 | 0 | 0 | 0 | 0 | 0 | 1 | 0 | 0 |
Wald test | 1 | 1 | 0 | 0 | 0 | 0 | 0 | 0 | 0 | 0 |
Ward's hierarchical cluster method | 0 | 0 | 0 | 0 | 0 | 0 | 0 | 0 | 0 | 1 |
Weighted least squares with corrections to means and variances (WLSMV) | 2 | 0 | 0 | 0 | 0 | 0 | 0 | 0 | 0 | 0 |
Welch and Brown-Forsythe F-ratios | 0 | 1 | 0 | 0 | 0 | 1 | 0 | 0 | 0 | 0 |
Wilcoxon signed-rank test | 3 | 3 | 0 | 2 | 0 | 0 | 0 | 2 | 0 | 1 |
Wilks' Lamba | 6 | 0 | 0 | 0 | 0 | 0 | 1 | 0 | 0 | 0 |
Word analysis | 0 | 0 | 0 | 0 | 0 | 0 | 0 | 1 | 0 | 0 |
Word Association Analysis | 1 | 0 | 0 | 0 | 0 | 0 | 0 | 0 | 0 | 0 |
scores | 5 | 6 | 1 | 0 | 1 | 1 | 0 | 1 | 0 | 0 |
Total | 1738 | 635 | 329 | 192 | 198 | 237 | 225 | 117 | 152 | 55 |
Results of the topics researched in psychology can be seen in the tables, as previously stated in this article. It is noteworthy that, of the 10 topics, social psychology accounted for 43.54% of the studies, with cognitive psychology the second most popular research topic at 16.92%. The remainder of the topics only occurred in 4.0–7.0% of the articles considered. A list of the included 999 articles is available under the section “View Articles” on the following website: https://methodgarden.xtrapolate.io/ . This website was created by Scholtz et al. ( 2019 ) to visually present a research framework based on this Article's results.
This systematised review categorised full-length articles from five international journals across the span of 5 years to provide insight into the use of research methods in the field of psychology. Results indicated what methods are used how these methods are being used and for what topics (why) in the included sample of articles. The results should be seen as providing insight into method use and by no means a comprehensive representation of the aforementioned aim due to the limited sample. To our knowledge, this is the first research study to address this topic in this manner. Our discussion attempts to promote a productive way forward in terms of the key results for method use in psychology, especially in the field of academia (Holloway, 2008 ).
With regard to the methods used, our data stayed true to literature, finding only common research methods (Grant and Booth, 2009 ; Maree, 2016 ) that varied in the degree to which they were employed. Quantitative research was found to be the most popular method, as indicated by literature (Breen and Darlaston-Jones, 2010 ; Counsell and Harlow, 2017 ) and previous studies in specific areas of psychology (see Coetzee and Van Zyl, 2014 ). Its long history as the first research method (Leech et al., 2007 ) in the field of psychology as well as researchers' current application of mathematical approaches in their studies (Toomela, 2010 ) might contribute to its popularity today. Whatever the case may be, our results show that, despite the growth in qualitative research (Demuth, 2015 ; Smith and McGannon, 2018 ), quantitative research remains the first choice for article publication in these journals. Despite the included journals indicating openness to articles that apply any research methods. This finding may be due to qualitative research still being seen as a new method (Burman and Whelan, 2011 ) or reviewers' standards being higher for qualitative studies (Bluhm et al., 2011 ). Future research is encouraged into the possible biasness in publication of research methods, additionally further investigation with a different sample into the proclaimed growth of qualitative research may also provide different results.
Review studies were found to surpass that of multi-method and mixed method studies. To this effect Grant and Booth ( 2009 ), state that the increased awareness, journal contribution calls as well as its efficiency in procuring research funds all promote the popularity of reviews. The low frequency of mixed method studies contradicts the view in literature that it's the third most utilised research method (Tashakkori and Teddlie's, 2003 ). Its' low occurrence in this sample could be due to opposing views on mixing methods (Gunasekare, 2015 ) or that authors prefer publishing in mixed method journals, when using this method, or its relative novelty (Ivankova et al., 2016 ). Despite its low occurrence, the application of the mixed methods design in articles was methodologically clear in all cases which were not the case for the remainder of research methods.
Additionally, a substantial number of studies used a combination of methodologies that are not mixed or multi-method studies. Perceived fixed boundaries are according to literature often set aside, as confirmed by this result, in order to investigate the aim of a study, which could create a new and helpful way of understanding the world (Gunasekare, 2015 ). According to Toomela ( 2010 ), this is not unheard of and could be considered a form of “structural systemic science,” as in the case of qualitative methodology (observation) applied in quantitative studies (experimental design) for example. Based on this result, further research into this phenomenon as well as its implications for research methods such as multi and mixed methods is recommended.
Discerning how these research methods were applied, presented some difficulty. In the case of sampling, most studies—regardless of method—did mention some form of inclusion and exclusion criteria, but no definite sampling method. This result, along with the fact that samples often consisted of students from the researchers' own academic institutions, can contribute to literature and debates among academics (Peterson and Merunka, 2014 ; Laher, 2016 ). Samples of convenience and students as participants especially raise questions about the generalisability and applicability of results (Peterson and Merunka, 2014 ). This is because attention to sampling is important as inappropriate sampling can debilitate the legitimacy of interpretations (Onwuegbuzie and Collins, 2017 ). Future investigation into the possible implications of this reported popular use of convenience samples for the field of psychology as well as the reason for this use could provide interesting insight, and is encouraged by this study.
Additionally, and this is indicated in Table 6 , articles seldom report the research designs used, which highlights the pressing aspect of the lack of rigour in the included sample. Rigour with regards to the applied empirical method is imperative in promoting psychology as a science (American Psychological Association, 2020 ). Omitting parts of the research process in publication when it could have been used to inform others' research skills should be questioned, and the influence on the process of replicating results should be considered. Publications are often rejected due to a lack of rigour in the applied method and designs (Fonseca, 2013 ; Laher, 2016 ), calling for increased clarity and knowledge of method application. Replication is a critical part of any field of scientific research and requires the “complete articulation” of the study methods used (Drotar, 2010 , p. 804). The lack of thorough description could be explained by the requirements of certain journals to only report on certain aspects of a research process, especially with regard to the applied design (Laher, 20). However, naming aspects such as sampling and designs, is a requirement according to the APA's Journal Article Reporting Standards (JARS-Quant) (Appelbaum et al., 2018 ). With very little information on how a study was conducted, authors lose a valuable opportunity to enhance research validity, enrich the knowledge of others, and contribute to the growth of psychology and methodology as a whole. In the case of this research study, it also restricted our results to only reported samples and designs, which indicated a preference for certain designs, such as cross-sectional designs for quantitative studies.
Data collection and analysis were for the most part clearly stated. A key result was the versatile use of questionnaires. Researchers would apply a questionnaire in various ways, for example in questionnaire interviews, online surveys, and written questionnaires across most research methods. This may highlight a trend for future research.
With regard to the topics these methods were employed for, our research study found a new field named “psychological practice.” This result may show the growing consciousness of researchers as part of the research process (Denzin and Lincoln, 2003 ), psychological practice, and knowledge generation. The most popular of these topics was social psychology, which is generously covered in journals and by learning societies, as testaments of the institutional support and richness social psychology has in the field of psychology (Chryssochoou, 2015 ). The APA's perspective on 2018 trends in psychology also identifies an increased amount of psychology focus on how social determinants are influencing people's health (Deangelis, 2017 ).
This study was not without limitations and the following should be taken into account. Firstly, this study used a sample of five specific journals to address the aim of the research study, despite general journal aims (as stated on journal websites), this inclusion signified a bias towards the research methods published in these specific journals only and limited generalisability. A broader sample of journals over a different period of time, or a single journal over a longer period of time might provide different results. A second limitation is the use of Excel spreadsheets and an electronic system to log articles, which was a manual process and therefore left room for error (Bandara et al., 2015 ). To address this potential issue, co-coding was performed to reduce error. Lastly, this article categorised data based on the information presented in the article sample; there was no interpretation of what methodology could have been applied or whether the methods stated adhered to the criteria for the methods used. Thus, a large number of articles that did not clearly indicate a research method or design could influence the results of this review. However, this in itself was also a noteworthy result. Future research could review research methods of a broader sample of journals with an interpretive review tool that increases rigour. Additionally, the authors also encourage the future use of systematised review designs as a way to promote a concise procedure in applying this design.
Our research study presented the use of research methods for published articles in the field of psychology as well as recommendations for future research based on these results. Insight into the complex questions identified in literature, regarding what methods are used how these methods are being used and for what topics (why) was gained. This sample preferred quantitative methods, used convenience sampling and presented a lack of rigorous accounts for the remaining methodologies. All methodologies that were clearly indicated in the sample were tabulated to allow researchers insight into the general use of methods and not only the most frequently used methods. The lack of rigorous account of research methods in articles was represented in-depth for each step in the research process and can be of vital importance to address the current replication crisis within the field of psychology. Recommendations for future research aimed to motivate research into the practical implications of the results for psychology, for example, publication bias and the use of convenience samples.
Ethics Statement
This study was cleared by the North-West University Health Research Ethics Committee: NWU-00115-17-S1.
Author Contributions
All authors listed have made a substantial, direct and intellectual contribution to the work, and approved it for publication.
Conflict of Interest
The authors declare that the research was conducted in the absence of any commercial or financial relationships that could be construed as a potential conflict of interest.
- Aanstoos C. M. (2014). Psychology . Available online at: http://eds.a.ebscohost.com.nwulib.nwu.ac.za/eds/detail/detail?sid=18de6c5c-2b03-4eac-94890145eb01bc70%40sessionmgr4006&vid$=$1&hid$=$4113&bdata$=$JnNpdGU9ZWRzL~WxpdmU%3d#AN$=$93871882&db$=$ers
- American Psychological Association (2020). Science of Psychology . Available online at: https://www.apa.org/action/science/
- Appelbaum M., Cooper H., Kline R. B., Mayo-Wilson E., Nezu A. M., Rao S. M. (2018). Journal article reporting standards for quantitative research in psychology: the APA Publications and Communications Board task force report . Am. Psychol. 73 :3. 10.1037/amp0000191 [ PubMed ] [ CrossRef ] [ Google Scholar ]
- Bandara W., Furtmueller E., Gorbacheva E., Miskon S., Beekhuyzen J. (2015). Achieving rigor in literature reviews: insights from qualitative data analysis and tool-support . Commun. Ass. Inform. Syst. 37 , 154–204. 10.17705/1CAIS.03708 [ CrossRef ] [ Google Scholar ]
- Barr-Walker J. (2017). Evidence-based information needs of public health workers: a systematized review . J. Med. Libr. Assoc. 105 , 69–79. 10.5195/JMLA.2017.109 [ PMC free article ] [ PubMed ] [ CrossRef ] [ Google Scholar ]
- Bittermann A., Fischer A. (2018). How to identify hot topics in psychology using topic modeling . Z. Psychol. 226 , 3–13. 10.1027/2151-2604/a000318 [ CrossRef ] [ Google Scholar ]
- Bluhm D. J., Harman W., Lee T. W., Mitchell T. R. (2011). Qualitative research in management: a decade of progress . J. Manage. Stud. 48 , 1866–1891. 10.1111/j.1467-6486.2010.00972.x [ CrossRef ] [ Google Scholar ]
- Breen L. J., Darlaston-Jones D. (2010). Moving beyond the enduring dominance of positivism in psychological research: implications for psychology in Australia . Aust. Psychol. 45 , 67–76. 10.1080/00050060903127481 [ CrossRef ] [ Google Scholar ]
- Burman E., Whelan P. (2011). Problems in / of Qualitative Research . Maidenhead: Open University Press/McGraw Hill. [ Google Scholar ]
- Chaichanasakul A., He Y., Chen H., Allen G. E. K., Khairallah T. S., Ramos K. (2011). Journal of Career Development: a 36-year content analysis (1972–2007) . J. Career. Dev. 38 , 440–455. 10.1177/0894845310380223 [ CrossRef ] [ Google Scholar ]
- Chryssochoou X. (2015). Social Psychology . Inter. Encycl. Soc. Behav. Sci. 22 , 532–537. 10.1016/B978-0-08-097086-8.24095-6 [ CrossRef ] [ Google Scholar ]
- Cichocka A., Jost J. T. (2014). Stripped of illusions? Exploring system justification processes in capitalist and post-Communist societies . Inter. J. Psychol. 49 , 6–29. 10.1002/ijop.12011 [ PubMed ] [ CrossRef ] [ Google Scholar ]
- Clay R. A. (2017). Psychology is More Popular Than Ever. Monitor on Psychology: Trends Report . Available online at: https://www.apa.org/monitor/2017/11/trends-popular
- Coetzee M., Van Zyl L. E. (2014). A review of a decade's scholarly publications (2004–2013) in the South African Journal of Industrial Psychology . SA. J. Psychol . 40 , 1–16. 10.4102/sajip.v40i1.1227 [ CrossRef ] [ Google Scholar ]
- Counsell A., Harlow L. (2017). Reporting practices and use of quantitative methods in Canadian journal articles in psychology . Can. Psychol. 58 , 140–147. 10.1037/cap0000074 [ PMC free article ] [ PubMed ] [ CrossRef ] [ Google Scholar ]
- Deangelis T. (2017). Targeting Social Factors That Undermine Health. Monitor on Psychology: Trends Report . Available online at: https://www.apa.org/monitor/2017/11/trend-social-factors
- Demuth C. (2015). New directions in qualitative research in psychology . Integr. Psychol. Behav. Sci. 49 , 125–133. 10.1007/s12124-015-9303-9 [ PubMed ] [ CrossRef ] [ Google Scholar ]
- Denzin N. K., Lincoln Y. (2003). The Landscape of Qualitative Research: Theories and Issues , 2nd Edn. London: Sage. [ Google Scholar ]
- Drotar D. (2010). A call for replications of research in pediatric psychology and guidance for authors . J. Pediatr. Psychol. 35 , 801–805. 10.1093/jpepsy/jsq049 [ PubMed ] [ CrossRef ] [ Google Scholar ]
- Dweck C. S. (2017). Is psychology headed in the right direction? Yes, no, and maybe . Perspect. Psychol. Sci. 12 , 656–659. 10.1177/1745691616687747 [ PubMed ] [ CrossRef ] [ Google Scholar ]
- Earp B. D., Trafimow D. (2015). Replication, falsification, and the crisis of confidence in social psychology . Front. Psychol. 6 :621. 10.3389/fpsyg.2015.00621 [ PMC free article ] [ PubMed ] [ CrossRef ] [ Google Scholar ]
- Ezeh A. C., Izugbara C. O., Kabiru C. W., Fonn S., Kahn K., Manderson L., et al.. (2010). Building capacity for public and population health research in Africa: the consortium for advanced research training in Africa (CARTA) model . Glob. Health Action 3 :5693. 10.3402/gha.v3i0.5693 [ PMC free article ] [ PubMed ] [ CrossRef ] [ Google Scholar ]
- Ferreira A. L. L., Bessa M. M. M., Drezett J., De Abreu L. C. (2016). Quality of life of the woman carrier of endometriosis: systematized review . Reprod. Clim. 31 , 48–54. 10.1016/j.recli.2015.12.002 [ CrossRef ] [ Google Scholar ]
- Fonseca M. (2013). Most Common Reasons for Journal Rejections . Available online at: http://www.editage.com/insights/most-common-reasons-for-journal-rejections
- Gough B., Lyons A. (2016). The future of qualitative research in psychology: accentuating the positive . Integr. Psychol. Behav. Sci. 50 , 234–243. 10.1007/s12124-015-9320-8 [ PubMed ] [ CrossRef ] [ Google Scholar ]
- Grant M. J., Booth A. (2009). A typology of reviews: an analysis of 14 review types and associated methodologies . Health Info. Libr. J. 26 , 91–108. 10.1111/j.1471-1842.2009.00848.x [ PubMed ] [ CrossRef ] [ Google Scholar ]
- Grix J. (2002). Introducing students to the generic terminology of social research . Politics 22 , 175–186. 10.1111/1467-9256.00173 [ CrossRef ] [ Google Scholar ]
- Gunasekare U. L. T. P. (2015). Mixed research method as the third research paradigm: a literature review . Int. J. Sci. Res. 4 , 361–368. Available online at: https://ssrn.com/abstract=2735996 [ Google Scholar ]
- Hengartner M. P. (2018). Raising awareness for the replication crisis in clinical psychology by focusing on inconsistencies in psychotherapy Research: how much can we rely on published findings from efficacy trials? Front. Psychol. 9 :256. 10.3389/fpsyg.2018.00256 [ PMC free article ] [ PubMed ] [ CrossRef ] [ Google Scholar ]
- Holloway W. (2008). Doing intellectual disagreement differently . Psychoanal. Cult. Soc. 13 , 385–396. 10.1057/pcs.2008.29 [ CrossRef ] [ Google Scholar ]
- Ivankova N. V., Creswell J. W., Plano Clark V. L. (2016). Foundations and Approaches to mixed methods research , in First Steps in Research , 2nd Edn. K. Maree (Pretoria: Van Schaick Publishers; ), 306–335. [ Google Scholar ]
- Johnson M., Long T., White A. (2001). Arguments for British pluralism in qualitative health research . J. Adv. Nurs. 33 , 243–249. 10.1046/j.1365-2648.2001.01659.x [ PubMed ] [ CrossRef ] [ Google Scholar ]
- Johnston A., Kelly S. E., Hsieh S. C., Skidmore B., Wells G. A. (2019). Systematic reviews of clinical practice guidelines: a methodological guide . J. Clin. Epidemiol. 108 , 64–72. 10.1016/j.jclinepi.2018.11.030 [ PubMed ] [ CrossRef ] [ Google Scholar ]
- Ketchen D. J., Jr., Boyd B. K., Bergh D. D. (2008). Research methodology in strategic management: past accomplishments and future challenges . Organ. Res. Methods 11 , 643–658. 10.1177/1094428108319843 [ CrossRef ] [ Google Scholar ]
- Ktepi B. (2016). Data Analytics (DA) . Available online at: https://eds-b-ebscohost-com.nwulib.nwu.ac.za/eds/detail/detail?vid=2&sid=24c978f0-6685-4ed8-ad85-fa5bb04669b9%40sessionmgr101&bdata=JnNpdGU9ZWRzLWxpdmU%3d#AN=113931286&db=ers
- Laher S. (2016). Ostinato rigore: establishing methodological rigour in quantitative research . S. Afr. J. Psychol. 46 , 316–327. 10.1177/0081246316649121 [ CrossRef ] [ Google Scholar ]
- Lee C. (2015). The Myth of the Off-Limits Source . Available online at: http://blog.apastyle.org/apastyle/research/
- Lee T. W., Mitchell T. R., Sablynski C. J. (1999). Qualitative research in organizational and vocational psychology, 1979–1999 . J. Vocat. Behav. 55 , 161–187. 10.1006/jvbe.1999.1707 [ CrossRef ] [ Google Scholar ]
- Leech N. L., Anthony J., Onwuegbuzie A. J. (2007). A typology of mixed methods research designs . Sci. Bus. Media B. V Qual. Quant 43 , 265–275. 10.1007/s11135-007-9105-3 [ CrossRef ] [ Google Scholar ]
- Levitt H. M., Motulsky S. L., Wertz F. J., Morrow S. L., Ponterotto J. G. (2017). Recommendations for designing and reviewing qualitative research in psychology: promoting methodological integrity . Qual. Psychol. 4 , 2–22. 10.1037/qup0000082 [ CrossRef ] [ Google Scholar ]
- Lowe S. M., Moore S. (2014). Social networks and female reproductive choices in the developing world: a systematized review . Rep. Health 11 :85. 10.1186/1742-4755-11-85 [ PMC free article ] [ PubMed ] [ CrossRef ] [ Google Scholar ]
- Maree K. (2016). Planning a research proposal , in First Steps in Research , 2nd Edn, ed Maree K. (Pretoria: Van Schaik Publishers; ), 49–70. [ Google Scholar ]
- Maree K., Pietersen J. (2016). Sampling , in First Steps in Research, 2nd Edn , ed Maree K. (Pretoria: Van Schaik Publishers; ), 191–202. [ Google Scholar ]
- Ngulube P. (2013). Blending qualitative and quantitative research methods in library and information science in sub-Saharan Africa . ESARBICA J. 32 , 10–23. Available online at: http://hdl.handle.net/10500/22397 . [ Google Scholar ]
- Nieuwenhuis J. (2016). Qualitative research designs and data-gathering techniques , in First Steps in Research , 2nd Edn, ed Maree K. (Pretoria: Van Schaik Publishers; ), 71–102. [ Google Scholar ]
- Nind M., Kilburn D., Wiles R. (2015). Using video and dialogue to generate pedagogic knowledge: teachers, learners and researchers reflecting together on the pedagogy of social research methods . Int. J. Soc. Res. Methodol. 18 , 561–576. 10.1080/13645579.2015.1062628 [ CrossRef ] [ Google Scholar ]
- O'Cathain A. (2009). Editorial: mixed methods research in the health sciences—a quiet revolution . J. Mix. Methods 3 , 1–6. 10.1177/1558689808326272 [ CrossRef ] [ Google Scholar ]
- O'Neil S., Koekemoer E. (2016). Two decades of qualitative research in psychology, industrial and organisational psychology and human resource management within South Africa: a critical review . SA J. Indust. Psychol. 42 , 1–16. 10.4102/sajip.v42i1.1350 [ CrossRef ] [ Google Scholar ]
- Onwuegbuzie A. J., Collins K. M. (2017). The role of sampling in mixed methods research enhancing inference quality . Köln Z Soziol. 2 , 133–156. 10.1007/s11577-017-0455-0 [ CrossRef ] [ Google Scholar ]
- Perestelo-Pérez L. (2013). Standards on how to develop and report systematic reviews in psychology and health . Int. J. Clin. Health Psychol. 13 , 49–57. 10.1016/S1697-2600(13)70007-3 [ CrossRef ] [ Google Scholar ]
- Pericall L. M. T., Taylor E. (2014). Family function and its relationship to injury severity and psychiatric outcome in children with acquired brain injury: a systematized review . Dev. Med. Child Neurol. 56 , 19–30. 10.1111/dmcn.12237 [ PubMed ] [ CrossRef ] [ Google Scholar ]
- Peterson R. A., Merunka D. R. (2014). Convenience samples of college students and research reproducibility . J. Bus. Res. 67 , 1035–1041. 10.1016/j.jbusres.2013.08.010 [ CrossRef ] [ Google Scholar ]
- Ritchie J., Lewis J., Elam G. (2009). Designing and selecting samples , in Qualitative Research Practice: A Guide for Social Science Students and Researchers , 2nd Edn, ed Ritchie J., Lewis J. (London: Sage; ), 1–23. [ Google Scholar ]
- Sandelowski M. (2011). When a cigar is not just a cigar: alternative perspectives on data and data analysis . Res. Nurs. Health 34 , 342–352. 10.1002/nur.20437 [ PubMed ] [ CrossRef ] [ Google Scholar ]
- Sandelowski M., Voils C. I., Knafl G. (2009). On quantitizing . J. Mix. Methods Res. 3 , 208–222. 10.1177/1558689809334210 [ PMC free article ] [ PubMed ] [ CrossRef ] [ Google Scholar ]
- Scholtz S. E., De Klerk W., De Beer L. T. (2019). A data generated research framework for conducting research methods in psychological research .
- Scimago Journal & Country Rank (2017). Available online at: http://www.scimagojr.com/journalrank.php?category=3201&year=2015
- Scopus (2017a). About Scopus . Available online at: https://www.scopus.com/home.uri (accessed February 01, 2017).
- Scopus (2017b). Document Search . Available online at: https://www.scopus.com/home.uri (accessed February 01, 2017).
- Scott Jones J., Goldring J. E. (2015). ‘I' m not a quants person'; key strategies in building competence and confidence in staff who teach quantitative research methods . Int. J. Soc. Res. Methodol. 18 , 479–494. 10.1080/13645579.2015.1062623 [ CrossRef ] [ Google Scholar ]
- Smith B., McGannon K. R. (2018). Developing rigor in quantitative research: problems and opportunities within sport and exercise psychology . Int. Rev. Sport Exerc. Psychol. 11 , 101–121. 10.1080/1750984X.2017.1317357 [ CrossRef ] [ Google Scholar ]
- Stangor C. (2011). Introduction to Psychology . Available online at: http://www.saylor.org/books/
- Strydom H. (2011). Sampling in the quantitative paradigm , in Research at Grass Roots; For the Social Sciences and Human Service Professions , 4th Edn, eds de Vos A. S., Strydom H., Fouché C. B., Delport C. S. L. (Pretoria: Van Schaik Publishers; ), 221–234. [ Google Scholar ]
- Tashakkori A., Teddlie C. (2003). Handbook of Mixed Methods in Social & Behavioural Research . Thousand Oaks, CA: SAGE publications. [ Google Scholar ]
- Toomela A. (2010). Quantitative methods in psychology: inevitable and useless . Front. Psychol. 1 :29. 10.3389/fpsyg.2010.00029 [ PMC free article ] [ PubMed ] [ CrossRef ] [ Google Scholar ]
- Truscott D. M., Swars S., Smith S., Thornton-Reid F., Zhao Y., Dooley C., et al.. (2010). A cross-disciplinary examination of the prevalence of mixed methods in educational research: 1995–2005 . Int. J. Soc. Res. Methodol. 13 , 317–328. 10.1080/13645570903097950 [ CrossRef ] [ Google Scholar ]
- Weiten W. (2010). Psychology Themes and Variations . Belmont, CA: Wadsworth. [ Google Scholar ]
Just one more step to your free trial.
.surveysparrow.com
Already using SurveySparrow? Login
By clicking on "Get Started", I agree to the Privacy Policy and Terms of Service .
This site is protected by reCAPTCHA and the Google Privacy Policy and Terms of Service apply.
Enterprise Survey Software
Enterprise Survey Software to thrive in your business ecosystem
NPS® Software
Turn customers into promoters
Offline Survey
Real-time data collection, on the move. Go internet-independent.
360 Assessment
Conduct omnidirectional employee assessments. Increase productivity, grow together.
Reputation Management
Turn your existing customers into raving promoters by monitoring online reviews.
Ticket Management
Build loyalty and advocacy by delivering personalized support experiences that matter.
Chatbot for Website
Collect feedback smartly from your website visitors with the engaging Chatbot for website.
Swift, easy, secure. Scalable for your organization.
Executive Dashboard
Customer journey map, craft beautiful surveys, share surveys, gain rich insights, recurring surveys, white label surveys, embedded surveys, conversational forms, mobile-first surveys, audience management, smart surveys, video surveys, secure surveys, api, webhooks, integrations, survey themes, accept payments, custom workflows, all features, customer experience, employee experience, product experience, marketing experience, sales experience, hospitality & travel, market research, saas startup programs, wall of love, success stories, sparrowcast, nps® benchmarks, learning centre, apps & integrations, testimonials.
Our surveys come with superpowers ⚡
Blog General
Descriptive Research 101: Definition, Methods and Examples
Parvathi vijayamohan.
Last Updated:
5 June 2024
Table Of Contents
- Descriptive Research 101: The Definitive Guide
What is Descriptive Research?
Key characteristics of descriptive research.
- Descriptive Research Methods: The 3 You Need to Know!
Observation
Case studies, 7 types of descriptive research, descriptive research: examples to build your next study, tips to excel at descriptive research.
Imagine you are a detective called to a crime scene. Your job is to study the scene and report whatever you find: whether that’s the half-smoked cigarette on the table or the large “RACHE” written in blood on the wall. That, in a nutshell, is descriptive research .
Researchers often need to do descriptive research on a problem before they attempt to solve it. So in this guide, we’ll take you through:
- What is descriptive research + characteristics
- Descriptive research methods
- Types of descriptive research
- Descriptive research examples
- Tips to excel at the descriptive method
Click to jump to the section that interests you.
Definition: As its name says, descriptive research describes the characteristics of the problem, phenomenon, situation, or group under study.
So the goal of all descriptive studies is to explore the background, details, and existing patterns in the problem to fully understand it. In other words, preliminary research.
However, descriptive research can be both preliminary and conclusive . You can use the data from a descriptive study to make reports and get insights for further planning.
What descriptive research isn’t: Descriptive research finds the what/when/where of a problem, not the why/how .
Because of this, we can’t use the descriptive method to explore cause-and-effect relationships where one variable (like a person’s job role) affects another variable (like their monthly income).
- Answers the “what,” “when,” and “where” of a research problem. For this reason, it is popularly used in market research , awareness surveys , and opinion polls .
- Sets the stage for a research problem. As an early part of the research process, descriptive studies help you dive deeper into the topic.
- Opens the door for further research. You can use descriptive data as the basis for more profound research, analysis and studies.
- Qualitative and quantitative . It is possible to get a balanced mix of numerical responses and open-ended answers from the descriptive method.
- No control or interference with the variables . The researcher simply observes and reports on them. However, specific research software has filters that allow her to zoom in on one variable.
- Done in natural settings . You can get the best results from descriptive research by talking to people, surveying them, or observing them in a suitable environment. For example, suppose you are a website beta testing an app feature. In that case, descriptive research invites users to try the feature, tracking their behavior and then asking their opinions .
- Can be applied to many research methods and areas. Examples include healthcare, SaaS, psychology, political studies, education, and pop culture.
Descriptive Research Methods: The Top Three You Need to Know!
In short, survey research is a brief interview or conversation with a set of prepared questions about a topic.
So you create a questionnaire, share it, and analyze the data you collect for further action. Learn about the differences between surveys and questionnaires here .
You can access free survey templates , over 20+ question types, and pass data to 1,500+ applications with survey software, like SurveySparrow . It enables you to create surveys, share them and capture data with very little effort.
Sign up today to launch stunning surveys for free.
Please enter a valid Email ID.
14-Day Free Trial • No Credit Card Required • No Strings Attached
- Surveys can be hyper-local, regional, or global, depending on your objectives.
- Share surveys in-person, offline, via SMS, email, or QR codes – so many options!
- Easy to automate if you want to conduct many surveys over a period.
The observational method is a type of descriptive research in which you, the researcher, observe ongoing behavior.
Now, there are several (non-creepy) ways you can observe someone. In fact, observational research has three main approaches:
- Covert observation: In true spy fashion, the researcher mixes in with the group undetected or observes from a distance.
- Overt observation : The researcher identifies himself as a researcher – “The name’s Bond. J. Bond.” – and explains the purpose of the study.
- Participatory observation : The researcher participates in what he is observing to understand his topic better.
- Observation is one of the most accurate ways to get data on a subject’s behavior in a natural setting.
- You don’t need to rely on people’s willingness to share information.
- Observation is a universal method that can be applied to any area of research.
In the case study method, you do a detailed study of a specific group, person, or event over a period.
This brings us to a frequently asked question: “What’s the difference between case studies and longitudinal studies?”
A case study will go very in-depth into the subject with one-on-one interviews, observations, and archival research. They are also qualitative, though sometimes they will use numbers and stats.
An example of longitudinal research would be a study of the health of night shift employees vs. general shift employees over a decade. An example of a case study would involve in-depth interviews with Casey, an assistant director of nursing who’s handled the night shift at the hospital for ten years now.
- Due to the focus on a few people, case studies can give you a tremendous amount of information.
- Because of the time and effort involved, a case study engages both researchers and participants.
- Case studies are helpful for ethically investigating unusual, complex, or challenging subjects. An example would be a study of the habits of long-term cocaine users.
Cross-sectional research | Studies a particular group of people or their sections at a given point in time. Example: current social attitudes of Gen Z in the US |
Longitudinal research | Studies a group of people over a long period of time. Example: tracking changes in social attitudes among Gen-Zers from 2022 – 2032. |
Normative research | Compares the results of a study against the existing norms. Example: comparing a verdict in a legal case against similar cases. |
Correlational/relational research | Investigates the type of relationship and patterns between 2 variables. Example: music genres and mental states. |
Comparative research | Compares 2 or more similar people, groups or conditions based on specific traits. Example: job roles of employees in similar positions from two different companies. |
Classification research | Arranges the data into classes according to certain criteria for better analysis. Example: the classification of newly discovered insects into species. |
Archival research | Searching for and extracting information from past records. Example: Tracking US Census data over the decades. |
1. Case Study: Airbnb’s Growth Strategy
In an excellent case study, Tam Al Saad, Principal Consultant, Strategy + Growth at Webprofits, deep dives into how Airbnb attracted and retained 150 million users .
“What Airbnb offers isn’t a cheap place to sleep when you’re on holiday; it’s the opportunity to experience your destination as a local would. It’s the chance to meet the locals, experience the markets, and find non-touristy places.
Sure, you can visit the Louvre, see Buckingham Palace, and climb the Empire State Building, but you can do it as if it were your hometown while staying in a place that has character and feels like a home.” – Tam al Saad, Principal Consultant, Strategy + Growth at Webprofits
2. Observation – Better Tech Experiences for the Elderly
We often think that our elders are so hopeless with technology. But we’re not getting any younger either, and tech is changing at a hair trigger! This article by Annemieke Hendricks shares a wonderful example where researchers compare the levels of technological familiarity between age groups and how that influences usage.
“It is generally assumed that older adults have difficulty using modern electronic devices, such as mobile telephones or computers. Because this age group is growing in most countries, changing products and processes to adapt to their needs is increasingly more important. “ – Annemieke Hendricks, Marketing Communication Specialist, Noldus
3. Surveys – Decoding Sleep with SurveySparrow
SRI International (formerly Stanford Research Institute) – an independent, non-profit research center – wanted to investigate the impact of stress on an adolescent’s sleep. To get those insights, two actions were essential: tracking sleep patterns through wearable devices and sending surveys at a pre-set time – the pre-sleep period.
“With SurveySparrow’s recurring surveys feature, SRI was able to share engaging surveys with their participants exactly at the time they wanted and at the frequency they preferred.”
Read more about this project : How SRI International decoded sleep patterns with SurveySparrow
1: Answer the six Ws –
- Who should we consider?
- What information do we need?
- When should we collect the information?
- Where should we collect the information?
- Why are we obtaining the information?
- Way to collect the information
#2: Introduce and explain your methodological approach
#3: Describe your methods of data collection and/or selection.
#4: Describe your methods of analysis.
#5: Explain the reasoning behind your choices.
#6: Collect data.
#7: Analyze the data. Use software to speed up the process and reduce overthinking and human error.
#8: Report your conclusions and how you drew the results.
Growth Marketer at SurveySparrow
Fledgling growth marketer. Cloud watcher. Aunty to a naughty beagle.
You Might Also Like
10 best enterprise feedback management (efm) software, top 21 lead generation tools in 2024 (cheaper and better), training needs survey questions for employees: examples (+ free template), see it to believe it..
14-Day Free Trial • Cancel Anytime • No Credit Card Required • Need a Demo?
Start your free trial today
No Credit Card Required. 14-Day Free Trial
Request a Demo
Want to learn more about SurveySparrow? We'll be in touch soon!
Scale up your descriptive research with the best survey software
Build surveys that actually work. give surveysparrow a free try today.
14-Day Free Trial • No Credit card required • 40% more completion rate
Hi there, we use cookies to offer you a better browsing experience and to analyze site traffic. By continuing to use our website, you consent to the use of these cookies. Learn More
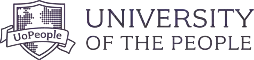
Higher Education News , Tips for Online Students , Tips for Students
A Comprehensive Guide to Different Types of Research

Updated: June 19, 2024
Published: June 15, 2024
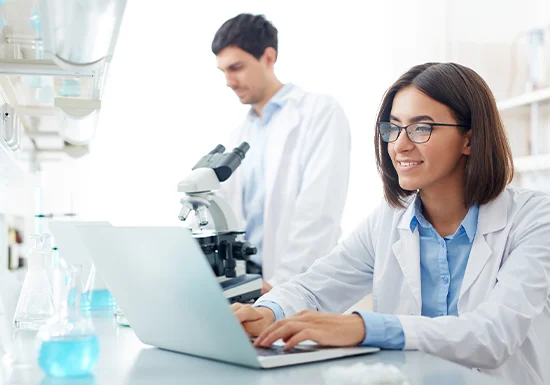
When embarking on a research project, selecting the right methodology can be the difference between success and failure. With various methods available, each suited to different types of research, it’s essential you make an informed choice. This blog post will provide tips on how to choose a research methodology that best fits your research goals .
We’ll start with definitions: Research is the systematic process of exploring, investigating, and discovering new information or validating existing knowledge. It involves defining questions, collecting data, analyzing results, and drawing conclusions.
Meanwhile, a research methodology is a structured plan that outlines how your research is to be conducted. A complete methodology should detail the strategies, processes, and techniques you plan to use for your data collection and analysis.
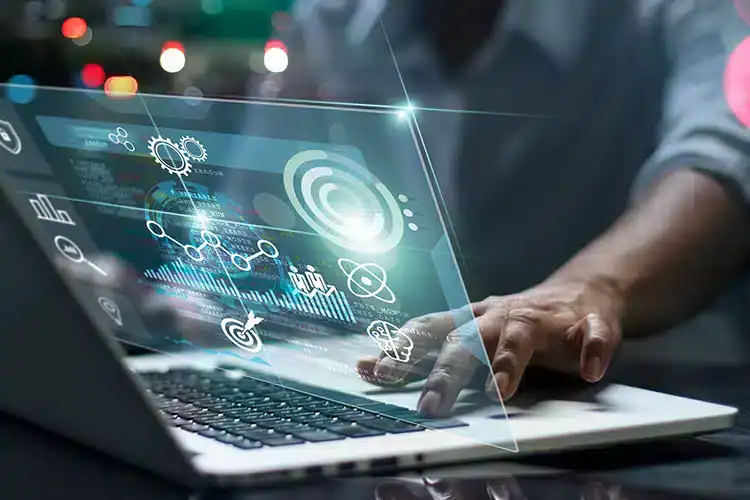
Research Methods
The first step of a research methodology is to identify a focused research topic, which is the question you seek to answer. By setting clear boundaries on the scope of your research, you can concentrate on specific aspects of a problem without being overwhelmed by information. This will produce more accurate findings.
Along with clarifying your research topic, your methodology should also address your research methods. Let’s look at the four main types of research: descriptive, correlational, experimental, and diagnostic.
Descriptive Research
Descriptive research is an approach designed to describe the characteristics of a population systematically and accurately. This method focuses on answering “what” questions by providing detailed observations about the subject. Descriptive research employs surveys, observational studies , and case studies to gather qualitative or quantitative data.
A real-world example of descriptive research is a survey investigating consumer behavior toward a competitor’s product. By analyzing the survey results, the company can gather detailed insights into how consumers perceive a competitor’s product, which can inform their marketing strategies and product development.
Correlational Research
Correlational research examines the statistical relationship between two or more variables to determine whether a relationship exists. Correlational research is particularly useful when ethical or practical constraints prevent experimental manipulation. It is often employed in fields such as psychology, education, and health sciences to provide insights into complex real-world interactions, helping to develop theories and inform further experimental research.
An example of correlational research is the study of the relationship between smoking and lung cancer. Researchers observe and collect data on individuals’ smoking habits and the incidence of lung cancer to determine if there is a correlation between the two variables. This type of research helps identify patterns and relationships, indicating whether increased smoking is associated with higher rates of lung cancer.
Experimental Research
Experimental research is a scientific approach where researchers manipulate one or more independent variables to observe their effect on a dependent variable. This method is designed to establish cause-and-effect relationships. Fields like psychology , medicine, and social sciences frequently employ experimental research to test hypotheses and theories under controlled conditions.
A real-world example of experimental research is Pavlov’s Dog experiment. In this experiment, Ivan Pavlov demonstrated classical conditioning by ringing a bell each time he fed his dogs. After repeating this process multiple times, the dogs began to salivate just by hearing the bell, even when no food was presented. This experiment helped to illustrate how certain stimuli can elicit specific responses through associative learning.
Diagnostic Research
Diagnostic research tries to accurately diagnose a problem by identifying its underlying causes. This type of research is crucial for understanding complex situations where a precise diagnosis is necessary for formulating effective solutions. It involves methods such as case studies and data analysis and often integrates both qualitative and quantitative data to provide a comprehensive view of the issue at hand.
An example of diagnostic research is studying the causes of a specific illness outbreak. During an outbreak of a respiratory virus, researchers might conduct diagnostic research to determine the factors contributing to the spread of the virus. This could involve analyzing patient data, testing environmental samples, and evaluating potential sources of infection. The goal is to identify the root causes and contributing factors to develop effective containment and prevention strategies.
Using an established research method is imperative, no matter if you are researching for marketing , technology , healthcare , engineering, or social science. A methodology lends legitimacy to your research by ensuring your data is both consistent and credible. A well-defined methodology also enhances the reliability and validity of the research findings, which is crucial for drawing accurate and meaningful conclusions.
Additionally, methodologies help researchers stay focused and on track, limiting the scope of the study to relevant questions and objectives. This not only improves the quality of the research but also ensures that the study can be replicated and verified by other researchers, further solidifying its scientific value.
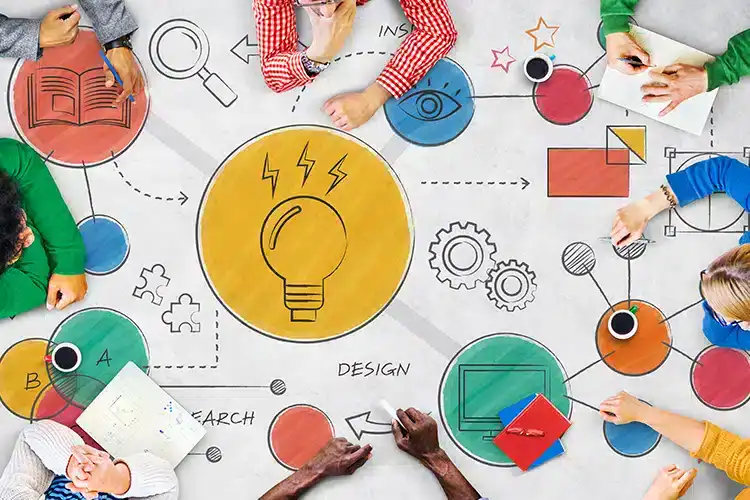
How to Choose a Research Methodology
Choosing the best research methodology for your project involves several key steps to ensure that your approach aligns with your research goals and questions. Here’s a simplified guide to help you make the best choice.
Understand Your Goals
Clearly define the objectives of your research. What do you aim to discover, prove, or understand? Understanding your goals helps in selecting a methodology that aligns with your research purpose.
Consider the Nature of Your Data
Determine whether your research will involve numerical data, textual data, or both. Quantitative methods are best for numerical data, while qualitative methods are suitable for textual or thematic data.
Understand the Purpose of Each Methodology
Becoming familiar with the four types of research – descriptive, correlational, experimental, and diagnostic – will enable you to select the most appropriate method for your research. Many times, you will want to use a combination of methods to gather meaningful data.
Evaluate Resources and Constraints
Consider the resources available to you, including time, budget, and access to data. Some methodologies may require more resources or longer timeframes to implement effectively.
Review Similar Studies
Look at previous research in your field to see which methodologies were successful. This can provide insights and help you choose a proven approach.
By following these steps, you can select a research methodology that best fits your project’s requirements and ensures robust, credible results.
Completing Your Research Project
Upon completing your research, the next critical step is to analyze and interpret the data you’ve collected. This involves summarizing the key findings, identifying patterns, and determining how these results address your initial research questions. By thoroughly examining the data, you can draw meaningful conclusions that contribute to the body of knowledge in your field.
It’s essential that you present these findings clearly and concisely, using charts, graphs, and tables to enhance comprehension. Furthermore, discuss the implications of your results, any limitations encountered during the study, and how your findings align with or challenge existing theories.
Your research project should conclude with a strong statement that encapsulates the essence of your research and its broader impact. This final section should leave readers with a clear understanding of the value of your work and inspire continued exploration and discussion in the field.
Now that you know how to perform quality research , it’s time to get started! Applying the right research methodologies can make a significant difference in the accuracy and reliability of your findings. Remember, the key to successful research is not just in collecting data, but in analyzing it thoughtfully and systematically to draw meaningful conclusions. So, dive in, explore, and contribute to the ever-growing body of knowledge with confidence. Happy researching!
At UoPeople, our blog writers are thinkers, researchers, and experts dedicated to curating articles relevant to our mission: making higher education accessible to everyone.
Related Articles
- Search Menu
Sign in through your institution
- Advance articles
- Virtual Issues
- Author Guidelines
- Open Access
- Self-Archiving Policy
- Why publish with this journal?
- About Journal of Pediatric Psychology
- About the Society of Pediatric Psychology
- Editorial Board
- Student Resources
- Advertising and Corporate Services
- Dispatch Dates
- Journals on Oxford Academic
- Books on Oxford Academic
Feasibility and acceptability of a telehealth intervention for improving peer relationships for adolescents with neurofibromatosis type 1: a single-arm pilot study

- Article contents
- Figures & tables
- Supplementary Data
Danielle M Glad, Sara K Pardej, Ellen Olszewski, Bonita P Klein-Tasman, Feasibility and acceptability of a telehealth intervention for improving peer relationships for adolescents with neurofibromatosis type 1: a single-arm pilot study, Journal of Pediatric Psychology , 2024;, jsae050, https://doi.org/10.1093/jpepsy/jsae050
- Permissions Icon Permissions
Elevated rates of social difficulties are evident for children and adolescents with neurofibromatosis type 1 (NF1) but the effects of social skills interventions have not been investigated for this population. The Program for the Education and Enrichment of Relational Skills (PEERS ® ), a widely established social skills intervention in autism spectrum disorders with expansion to other conditions, was recently modified to be offered virtually. This study examined the feasibility and acceptability of this telehealth intervention.
27 adolescents with NF1 with social skills difficulties and at least 1 caregiver enrolled in the study. 19 of those participants ( M age = 14.21 years, SD = 1.63; 7 females; 79% White) completed PEERS ® via telehealth in a single-arm pilot study. Dropout rates, attendance records, helpfulness of the curriculum topics and caregiver-reported acceptability, including ratings on the Treatment Acceptability Questionnaire, were examined.
Low study drop out (30% of enrolled participants; 14% of participants who began the intervention) and high attendance rates were observed. Caregivers found sessions related to common, everyday interactions most helpful. Adolescents indicated sessions related to having get-togethers and social nuances (e.g., humor) as most helpful. Caregiver ratings indicated acceptability of the intervention.
This investigation supported the feasibility and acceptability of telehealth PEERS ® , a social skills intervention program, among adolescents with NF1 and their caregivers based on attendance patterns as well as appraisal of the curriculum and telehealth modality.
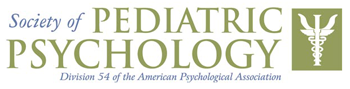
Society of Pediatric Psychology members
Personal account.
- Sign in with email/username & password
- Get email alerts
- Save searches
- Purchase content
- Activate your purchase/trial code
- Add your ORCID iD
Institutional access
Sign in with a library card.
- Sign in with username/password
- Recommend to your librarian
- Institutional account management
- Get help with access
Access to content on Oxford Academic is often provided through institutional subscriptions and purchases. If you are a member of an institution with an active account, you may be able to access content in one of the following ways:
IP based access
Typically, access is provided across an institutional network to a range of IP addresses. This authentication occurs automatically, and it is not possible to sign out of an IP authenticated account.
Choose this option to get remote access when outside your institution. Shibboleth/Open Athens technology is used to provide single sign-on between your institution’s website and Oxford Academic.
- Click Sign in through your institution.
- Select your institution from the list provided, which will take you to your institution's website to sign in.
- When on the institution site, please use the credentials provided by your institution. Do not use an Oxford Academic personal account.
- Following successful sign in, you will be returned to Oxford Academic.
If your institution is not listed or you cannot sign in to your institution’s website, please contact your librarian or administrator.
Enter your library card number to sign in. If you cannot sign in, please contact your librarian.
Society Members
Society member access to a journal is achieved in one of the following ways:
Sign in through society site
Many societies offer single sign-on between the society website and Oxford Academic. If you see ‘Sign in through society site’ in the sign in pane within a journal:
- Click Sign in through society site.
- When on the society site, please use the credentials provided by that society. Do not use an Oxford Academic personal account.
If you do not have a society account or have forgotten your username or password, please contact your society.
Sign in using a personal account
Some societies use Oxford Academic personal accounts to provide access to their members. See below.
A personal account can be used to get email alerts, save searches, purchase content, and activate subscriptions.
Some societies use Oxford Academic personal accounts to provide access to their members.
Viewing your signed in accounts
Click the account icon in the top right to:
- View your signed in personal account and access account management features.
- View the institutional accounts that are providing access.
Signed in but can't access content
Oxford Academic is home to a wide variety of products. The institutional subscription may not cover the content that you are trying to access. If you believe you should have access to that content, please contact your librarian.
For librarians and administrators, your personal account also provides access to institutional account management. Here you will find options to view and activate subscriptions, manage institutional settings and access options, access usage statistics, and more.
Short-term Access
To purchase short-term access, please sign in to your personal account above.
Don't already have a personal account? Register
Email alerts
Citing articles via.
- Recommend to your Library
Affiliations
- Online ISSN 1465-735X
- Print ISSN 0146-8693
- Copyright © 2024 Society of Pediatric Psychology
- About Oxford Academic
- Publish journals with us
- University press partners
- What we publish
- New features
- Open access
- Rights and permissions
- Accessibility
- Advertising
- Media enquiries
- Oxford University Press
- Oxford Languages
- University of Oxford
Oxford University Press is a department of the University of Oxford. It furthers the University's objective of excellence in research, scholarship, and education by publishing worldwide
- Copyright © 2024 Oxford University Press
- Cookie settings
- Cookie policy
- Privacy policy
- Legal notice
This Feature Is Available To Subscribers Only
Sign In or Create an Account
This PDF is available to Subscribers Only
For full access to this pdf, sign in to an existing account, or purchase an annual subscription.
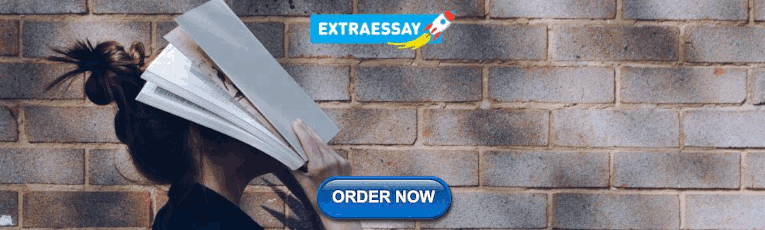
IMAGES
VIDEO
COMMENTS
Learn about the three main methods of descriptive research in psychology: observation, case studies, and surveys. Find out how they are used to define and describe behaviors, patterns, and phenomena in a natural setting.
Descriptive research is one of the key tools needed in any psychology researcher's toolbox in order to create and lead a project that is both equitable and effective. Because psychology, as a field, loves definitions, let's start with one. The University of Minnesota's Introduction to Psychology defines this type of research as one that ...
Learn what descriptive research is and how to use it to describe a population, situation or phenomenon. Find out the common methods, such as surveys, observations and case studies, and see examples of descriptive research questions.
Learn about the types and uses of descriptive, or qualitative, research methods in psychology, such as case study, naturalistic observation, surveys, archival research, longitudinal research, and cross-sectional research. Compare and contrast descriptive research with correlational and experimental research.
Olivia Guy-Evans, MSc. Research methods in psychology are systematic procedures used to observe, describe, predict, and explain behavior and mental processes. They include experiments, surveys, case studies, and naturalistic observations, ensuring data collection is objective and reliable to understand and explain psychological phenomena.
A research design is the specific method a researcher uses to collect, analyze, and interpret data. Psychologists use three major types of research designs in their research, and each provides an essential avenue for scientific investigation. Descriptive research is research designed to provide a snapshot of the current state of affairs.
The three main categories of psychological research are descriptive, correlational, and experimental research. Research studies that do not test specific relationships between variables are called descriptive, or qualitative, studies. These studies are used to describe general or specific behaviors and attributes that are observed and measured.
An example of this type of research in psychology would be changing the length of a specific mental health treatment and measuring the effect on study participants. 2. Descriptive Research . Descriptive research seeks to depict what already exists in a group or population. Three types of psychology research utilizing this method are: Case studies
Title: APA handbook of research methods in psychology / editor-in-Chief Harris Cooper; associate editors Mar c N. Coutanche, Linda M. McMullen, A.T . Panter, David Rindskopf, and Kenneth J. Sher Description: Second Edition. | Washington, DC : American Psychological Association, [2023-] | Series: AP A handbooks in psychology | Revised edition of ...
Research in Psychology: The Basics. The first step in your review should include a basic introduction to psychology research methods. Psychology research can have a variety of goals. What researchers learn can be used to describe, explain, predict, or change human behavior. Psychologists use the scientific method to conduct studies and research ...
Applying the scientific method to psychology, therefore, helps to standardize the approach to understanding its very different types of information. ... Psychologists use descriptive, experimental, and correlational methods to conduct research. Descriptive, or qualitative, methods include the case study, naturalistic observation, surveys ...
Marc N. Coutanche, PhD, is an associate professor of psychology and research scientist in the Learning Research and Development Center at the University of Pittsburgh. Dr. Dr. Coutanche directs a program of cognitive neuroscience research and develops and tests new computational techniques to identify and understand the neural information ...
Video 2.4.1. Descriptive Research Design provides explanation and examples for quantitative descriptive research.A closed-captioned version of this video is available here.. Descriptive research is distinct from correlational research, in which researchers formally test whether a relationship exists between two or more variables. Experimental research goes a step further beyond descriptive and ...
A research design is the specific method a researcher uses to collect, analyze, and interpret data. Psychologists use three major types of research designs in their research, and each provides an essential avenue for scientific investigation. Descriptive research is research designed to provide a snapshot of the current state of affairs.
A research design is the specific method a researcher uses to collect, analyze, and interpret data. Psychologists use three major types of research designs in their research, and each provides an essential avenue for scientific investigation. Descriptive research is research designed to provide a snapshot of the current state of affairs.
Learn about the three main types of descriptive research methods in psychology: case studies, naturalistic observation, and surveys. Compare their strengths and weaknesses, and how they differ from correlational and experimental research.
Experimental research goes a step further beyond descriptive and correlational research and randomly assigns people to different conditions, using hypothesis testing to make inferences about causal relationships between variables. We will discuss each of these methods more in-depth later. Table 2.4.1. Comparison of research design methods
Chapter 2—Psychology's Scientific Methods Learning Goals 1. Explain what makes psychology a science. 2. Discuss the three types of research that are used in psychology. 3. Distinguish between descriptive statistics and inferential statistics. 4. Discuss some research challenges that involve ethics, bias, and information.
Introduction. Psychology is an ever-growing and popular field (Gough and Lyons, 2016; Clay, 2017).Due to this growth and the need for science-based research to base health decisions on (Perestelo-Pérez, 2013), the use of research methods in the broad field of psychology is an essential point of investigation (Stangor, 2011; Aanstoos, 2014).Research methods are therefore viewed as important ...
Descriptive Methods of Research Speaker: Jennifer Hess In this section we're talking about descriptive research methods in psychology. So, starting with the definition of psychology, it is the science of behavior and mental processes. To learn more about the way people think feel and act we have two general types of research.
For example, suppose you are a website beta testing an app feature. In that case, descriptive research invites users to try the feature, tracking their behavior and then asking their opinions. Can be applied to many research methods and areas. Examples include healthcare, SaaS, psychology, political studies, education, and pop culture.
Descriptive research is an approach designed to describe the characteristics of a population systematically and accurately. This method focuses on answering "what" questions by providing detailed observations about the subject. ... It is often employed in fields such as psychology, education, and health sciences to provide insights into ...
research methods that yield descriptions of behavior. What are the types of descriptive research methods? naturalistic and laboratory observation, case study, survey. What is naturalistic observation? descriptive research method in which researchers observe and record behavior in its natural setting without attempting to influence or control ...
Psychologists use descriptive, experimental, and correlational methods to conduct research. Descriptive, or qualitative, methods include the case study, naturalistic observation, surveys, archival research, longitudinal research, and cross-sectional research. Experiments are conducted in order to determine cause-and-effect relationships.
The data were analyzed using IBM SPSS for Windows, version 28. Descriptive statistics were conducted to examine study drop out, treatment attendance, and session content. The percent of participants providing the highest ratings ("very helpful"/"not helpful" ratings) was reported for each item for the curriculum content analysis.