Thank you for visiting nature.com. You are using a browser version with limited support for CSS. To obtain the best experience, we recommend you use a more up to date browser (or turn off compatibility mode in Internet Explorer). In the meantime, to ensure continued support, we are displaying the site without styles and JavaScript.
- View all journals
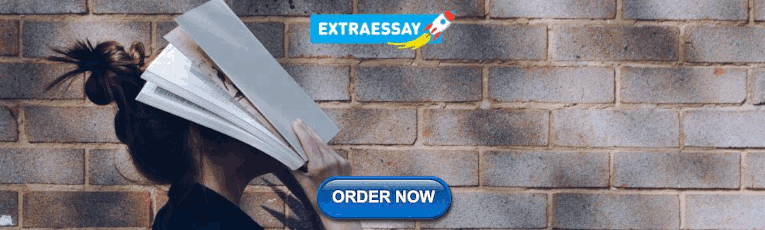
Language and linguistics articles from across Nature Portfolio
Latest research and reviews.
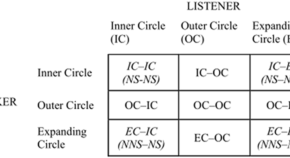
International intelligibility of English spoken by college students in the Bashu dialect area of China
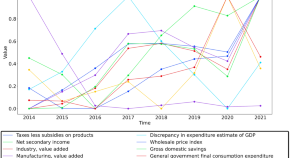
Social, economic, and demographic factors drive the emergence of Hinglish code-mixing on social media
- Ayan Sengupta
- Tanmoy Chakraborty
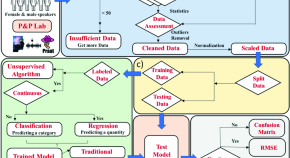
Multi-class identification of tonal contrasts in Chokri using supervised machine learning algorithms
- Amalesh Gope
- Anusuya Pal
- Dinkur Borah
Looking back to move forward: comparison of instructors’ and undergraduates’ retrospection on the effectiveness of online learning using the nine-outcome influencing factors
- Shanshan Hao
Volunteer translators in non-governmental organizations: exploring their identity and power through discourse analysis
- María del Mar Sánchez Ramos
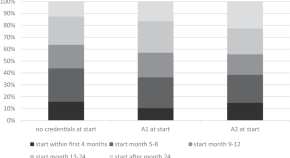
Does timing matter? Language course participation and language outcomes amongst new immigrants
News and Comment
Time to revise the terminology we use to regulate water management practices.
- Paul Jeffrey
- Heather Smith
- Francis Hassard
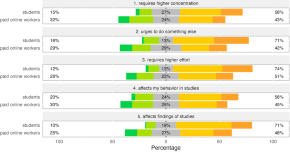
Is boredom a source of noise and/or a confound in behavioral science research?
Behavioral researchers tend to study behavior in highly controlled laboratory settings to minimize the effects of potential confounders. Yet, while doing so, the artificial setup itself might unintentionally introduce noise or confounders, such as boredom. In this perspective, we draw upon theoretical and empirical evidence to make the case that (a) some experimental setups are likely to induce boredom in participants, (b) the degree of boredom induced might differ between individuals as a function of differences in trait boredom, (c) boredom can impair participants’ attention, can make study participation more effortful, and can increase the urge to do something else (i.e., to disengage from the study). Most importantly, we argue that some participants might adjust their behavior because they are bored. Considering boredom’s potential for adding noise to data, or for being an unwanted confound, we discuss a set of recommendations on how to control for and deal with the occurrence and effects of boredom in behavioral science research.
- Maria Meier
- Corinna S. Martarelli
- Wanja Wolff
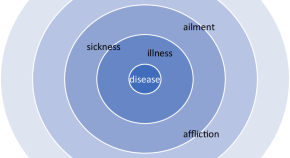
Exploration of the social and philosophical underpinning of ‘the patient’—what this means for people with a long-term condition
Should healthcare professionals use the term ‘patient’? A patient is a social construct, in a biomedical model, in which each actor has their role to play. This model has been criticised as belonging to an era of medical hegemony and (mis)represents an individual seeking healthcare as one who is simply a passive participant and recipient of care. The ‘Language Matters’ campaign, for people living with diabetes, has sought to address the role of language in interactions between healthcare providers. A key point raised in the campaign is whether someone who feels well, but has ongoing healthcare input, should be referred to as a patient? In this article, we address the concept of a patient and how its use can belie a particular mindset (or ‘discourse’) in which power is established in a relationship and can lead to individuals being defined by their condition. However, for some linguistic communities (such as nurses and doctors), a patient may be considered less as one over whom they have dominion, but rather someone for whom they have specific responsibilities and duty of care. Drawing upon the philosophical theories of language—that the meaning and inference of a word is dependent on its use—we argue that the context in which use of the term patient occurs is crucial. Without more fundamental cultural disruption of the biomedical model, word substitution, in itself, will not change perception.
- M. B. Whyte
Approaching the neuroscience of language
- Marika Gobbo
Neural evidence of word prediction
- Jane Aristia
The usefulness of ChatGPT for psychotherapists and patients
ChatGPT is a chatbot based on a large language model. Its application possibilities are extensive, and it is freely accessible to all people, including psychotherapists and individuals with mental illnesses. Some blog posts about the possible use of ChatGPT as a psychotherapist or as a supplement to psychotherapy already exist. Based on three detailed chats, the author analyzed the chatbot’s responses to psychotherapists seeking assistance, to patients looking for support between psychotherapy sessions, during their psychotherapists’ vacations, and to people suffering from mental illnesses who are not yet in psychotherapy. The results suggest that ChatGPT offers an interesting complement to psychotherapy and an easily accessible, good (and currently free) place to go for people with mental-health problems who have not yet sought professional help and have no psychotherapeutic experience. The information is, however, one-sided, and in any future regulation of AI it must also be made clear that the proposals are not only insufficient as a psychotherapy substitute, but also have a bias that favors certain methods while not even mentioning other approaches that may be more helpful for some people.
- Paolo Raile
Quick links
- Explore articles by subject
- Guide to authors
- Editorial policies

- Architecture and Design
- Asian and Pacific Studies
- Business and Economics
- Classical and Ancient Near Eastern Studies
- Computer Sciences
- Cultural Studies
- Engineering
- General Interest
- Geosciences
- Industrial Chemistry
- Islamic and Middle Eastern Studies
- Jewish Studies
- Library and Information Science, Book Studies
- Life Sciences
- Linguistics and Semiotics
- Literary Studies
- Materials Sciences
- Mathematics
- Social Sciences
- Sports and Recreation
- Theology and Religion
- Publish your article
- The role of authors
- Promoting your article
- Abstracting & indexing
- Publishing Ethics
- Why publish with De Gruyter
- How to publish with De Gruyter
- Our book series
- Our subject areas
- Your digital product at De Gruyter
- Contribute to our reference works
- Product information
- Tools & resources
- Product Information
- Promotional Materials
- Orders and Inquiries
- FAQ for Library Suppliers and Book Sellers
- Repository Policy
- Free access policy
- Open Access agreements
- Database portals
- For Authors
- Customer service
- People + Culture
- Journal Management
- How to join us
- Working at De Gruyter
- Mission & Vision
- De Gruyter Foundation
- De Gruyter Ebound
- Our Responsibility
- Partner publishers

Your purchase has been completed. Your documents are now available to view.
Linguistics
An interdisciplinary journal of the language sciences.
- Print ISSN: 0024-3949
- Type: Journal
- Language: English
- Publisher: De Gruyter Mouton
- First published: January 1, 1963
- Publication Frequency: 6 Issues per Year
- Audience: linguists, scholars and students in the field of linguistics
Linguistics and Language: A Research Guide: Journal Articles & Dissertations
- Library Support for Linguistics
- Reference Sources
- Journal Articles & Dissertations
- World Languages
- About English
- Catalogs and Bibliographies
- Corpora / Text Analysis
- Data Management
- Linguistics @ Cornell
- Linguistics Associations and Resources
- Citation and Style Manuals
- Off Campus Access
Finding Journal Articles and Dissertations
Primary online indexes and databases for linguistics.
- LLBA Language, Linguistics and Behavior Abstracts Covers all aspects of the study of language including phonetics, phonology, morphology, syntax and semantics. Documents indexed include journal articles, book reviews, books, book chapters, dissertations and working papers.
- Linguistic Bibliography Online "Contains over 440,000 detailed bibliographical descriptions of linguistic publications on general and language-specific theoretical linguistics. While the bibliography aims to cover all languages of the world, particular attention is given to the inclusion of publications on endangered and lesser-studied languages. Publications in any language are collected, analyzed and annotated (using a state-of-the-art system of subject and language keywords) by an international team of linguists and bibliographers from all over the world." [Introduction]
- MLA International Bibliography An international index and database providing references to scholarly articles from over 4000 journals in literature, folklore, literary theory, semiotics, and linguistics.
- ProQuest Dissertations and Theses Global 1731 to the present. Many are full-text online. Others (citation-only sources) are available through interlibrary loan.
Print Indexes for Linguistics
- Bibliographie linguistischer Literatur (BLL) Call Number: Uris Library Stacks Oversize Z 7003 .B58 ++ Cancelled after 2018. BLL covers articles in periodicals and essays in collective works, including conference and congress proceedings and festschriften. The number of periodicals it covers has gradually increased from 123 in Band 1 to about 770 titles in Band 12 (1986). Coverage is international in scope with a one- or two-year time lag. Besides a division on general linguistics it also includes divisions on English, German, and Romance linguistics. Each of these divisions is subdivided into a form section, a systematic section, and a language section (the latter missing of course in the general linguistics division). The systematic section of each division contains all the entries for that division classified under appropriate subject categories. These entries may also qualify for listing again in the form and/or language sections. This whole classification scheme is fully explained in the introduction which, beginning with Band 7, appears in both German and English, as do the headings. A cumulative author index and subject and name index complete each annual volume. This index and the Bibliographie Linguistique/Linguistic Bibliography are international in scope. The BLL, however, is more current and has the advantage of a subject index. On the other hand it does not begin to cover the variety of languages that the Bibliographie Linguistique does. (De Miller)
- Bibliographie linguistique Call Number: Uris Library Stacks Z 7001 .P451 Library has 1984-2004. See also: Online edition, above.
Related Indexes and Databases
- Language Teaching [journal] Available online via Cambridge Core. The Cornell Library also has the print edition from 1982 - 2006. " ... offers critical survey articles of recent research on specific topics, second and foreign languages and countries, and invites original research articles reporting on replication studies and meta-analyses. The journal also includes regional surveys of outstanding doctoral dissertations, topic-based research timelines, theme-based research agendas, recent plenary conference speeches, and research-in-progress reports." [publisher]
- PsycINFO Access to the international literature in psychology and related behavioral and social sciences, including psychiatry, sociology, anthropology, education, pharmacology, and linguistics.
- Sociology Source Ultimate Offers coverage from all sub-disciplines of sociology.
- Web of Science Web of Science indexes journal articles in the sciences, social sciences, and humanities. It is also a citation database that allows cited- reference searching to identify articles that have cited a particular article or author.
Online Repositories
Increasingly scholars are submitting their papers to open access archives. These digital repositories capture, store, index, preserve, and redistribute digital research material. Many materials archived in digital repositories are searchable by search engines such as Google, as opposed to being sequestered in proprietary databases such as JSTOR or ProQuest.
- LingBuzz LingBuzz is an openly accessible repository of scholarly papers, discussions and other documents for linguistics.
- semanticsarchive.net For exchanging papers of interest to natural language semanticists and philosophers of language. Maintained by the Linguistic Society of America.
- ROA: Rutgers Optimality Archive "The Rutgers Optimality Archive is a distribution point for research in Optimality Theory and its conceptual affiliates." -- home page
- << Previous: Reference Sources
- Next: World Languages >>
- Last Updated: Apr 9, 2024 9:49 AM
- URL: https://guides.library.cornell.edu/linguistics
Articles on Linguistics
Displaying 1 - 20 of 359 articles.
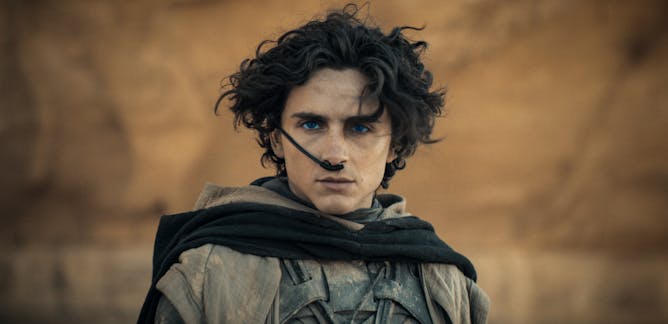
Diplomacy and resistance: how Dune shows us the power of language – including sign language
Gemma King , Australian National University
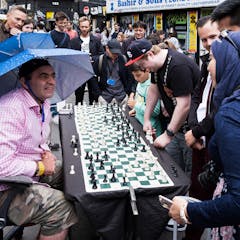
‘Bengali Cockney, Black Cockney, East End Cockney, Essex Cockney, Jewish Cockney, Sylheti Cockney’: why community languages matter
Christopher Strelluf , University of Warwick
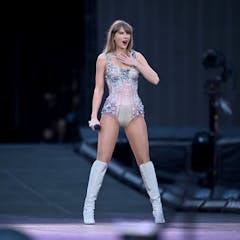
Are you ready for it? ‘ Yeah-nah ’ comes back stronger – with a little help from Taylor Swift
Kate Burridge , Monash University and Isabelle Burke , Monash University
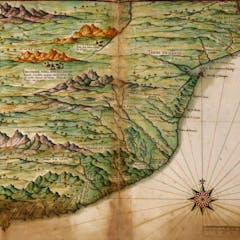
What are Sabaki languages? How people formed ethnic groups along the coast of east Africa
Daren Ray , Brigham Young University
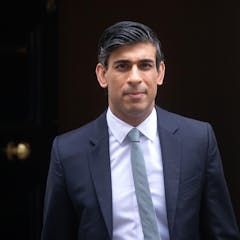
Don’t blame parents for wanting their children to speak differently – blame society
Rob Drummond , Manchester Metropolitan University
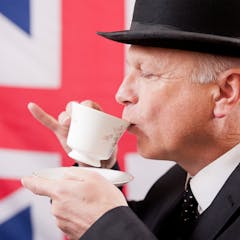
Tea, weather and being on time: analysis of 100 million words reveals what Brits talk about most
Vaclav Brezina , Lancaster University
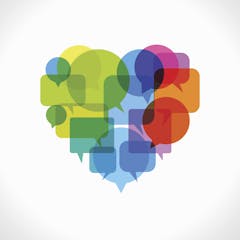
Are you really in love? How expanding your love lexicon can change your relationships and how you see yourself
Georgi Gardiner , University of Tennessee
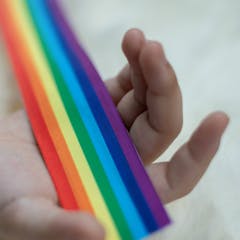
How the tide turned on transgender support charity Mermaids
Aimee Bailey , De Montfort University and Jai Mackenzie , Newman University
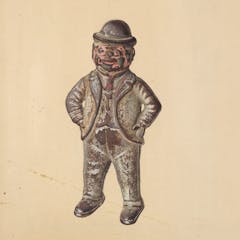
Sellout! How political corruption shaped an American insult
Ian Afflerbach , University of North Georgia
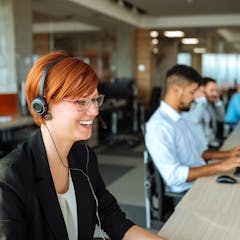
Why AI software ‘softening’ accents is problematic
Grégory Miras , Université de Lorraine
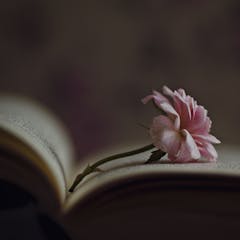
‘Thirst trap’ and ‘edgelord’ were recently added to the dictionary – so why hasn’t ‘nibling’ made the cut?
Roger J. Kreuz , University of Memphis
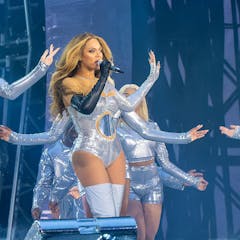
They’re serving what?! How the c-word went from camp to internet mainstream
Christian Ilbury , The University of Edinburgh
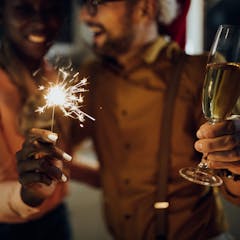
I’m an expert in slang – here are my picks for word of the year
Tony Thorne , King's College London
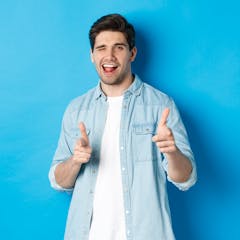
Rizz: I study the history of charisma – here’s why the word of the year is misunderstood
Tom F. Wright , University of Sussex
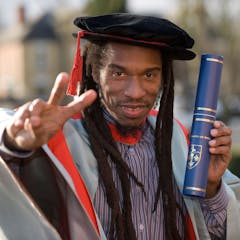
Benjamin Zephaniah: how the poet’s linguistic anarchy and abolitionist politics impacted education – and me
Ian Cushing , Manchester Metropolitan University
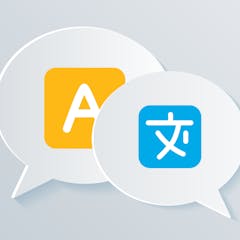
When research study materials don’t speak their participants’ language, data can get lost in translation
Sonia Colina , University of Arizona
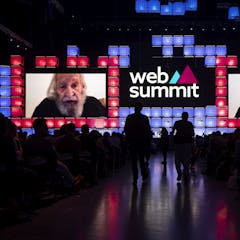
Noam Chomsky turns 95: the social justice advocate paved the way for AI. Does it keep him up at night?
Cameron Shackell , Queensland University of Technology
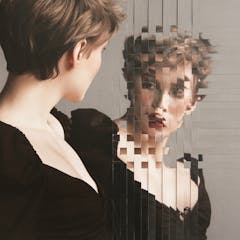
Merriam-Webster ’s word of the year – authentic – reflects growing concerns over AI’s ability to deceive and dehumanize
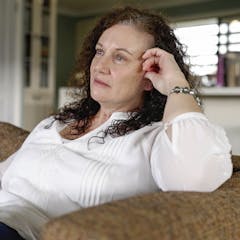
The words that helped wrongly convict Kathleen Folbigg
Kate Burridge , Monash University and Stephen Cordner , Victorian Institute of Forensic Medicine
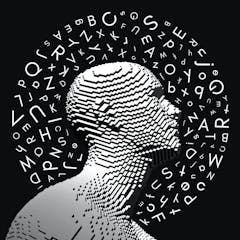
Your mental dictionary is part of what makes you unique − here’s how your brain stores and retrieves words
Nichol Castro , University at Buffalo
Related Topics
- Donald Trump
- English language
- Sociolinguistics
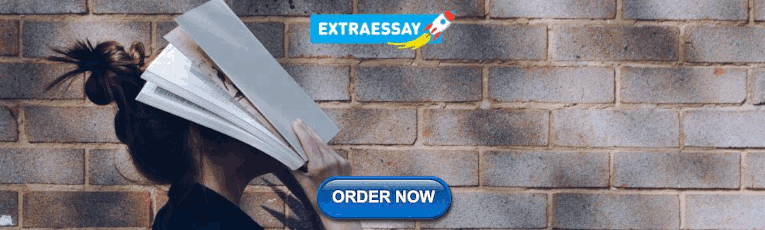
Top contributors
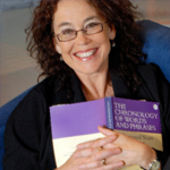
Professor of Linguistics, Monash University
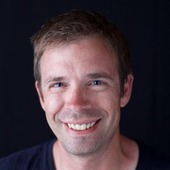
Senior Lecturer in Linguistics, Monash University
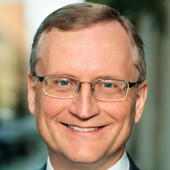
Associate Dean and Professor of Psychology, University of Memphis
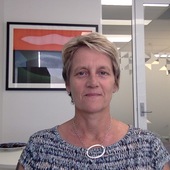
Associate Professor in Linguistics, Macquarie University
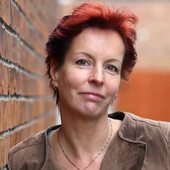
Professor of Linguistics, University of York
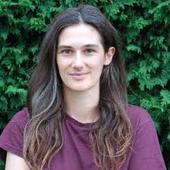
Lecturer in Department of Language and Linguistics, University of Essex
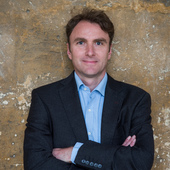
Professor of English Language and Literature, University of Oxford
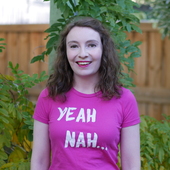
Research fellow in Linguistics, Monash University
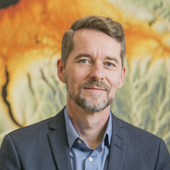
Professor of Psychology, Cornell University
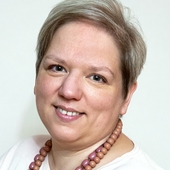
Reader in Discourse Studies, Lancaster University
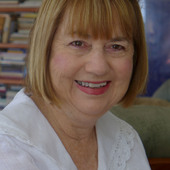
Course coordinator, The University of Queensland
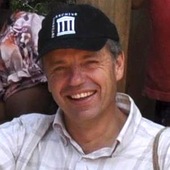
Professor, Charles Darwin University
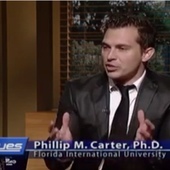
Professor of Linguistics and English, Florida International University
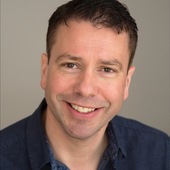
Professor of Linguistics
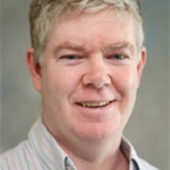
Professor Emeritus of Linguistics, University of Essex
- X (Twitter)
- Unfollow topic Follow topic
- Search Menu
- Advance articles
- Author Guidelines
- Submission Site
- Open Access
- Why Submit?
- About Applied Linguistics
- Editorial Board
- Advertising and Corporate Services
- Journals Career Network
- Self-Archiving Policy
- Dispatch Dates
- Terms and Conditions
- Journals on Oxford Academic
- Books on Oxford Academic
Article Contents
Introduction, literature review, methodology, analysis and results, audience design for chatbots: a way forward, acknowledgments, making the case for audience design in conversational ai: users’ pragmatic strategies and rapport expectations in interaction with a task-oriented chatbot.

- Article contents
- Figures & tables
- Supplementary Data
Doris Dippold, Making the Case for Audience Design in Conversational AI: Users’ Pragmatic Strategies and Rapport Expectations in Interaction with a Task-Oriented Chatbot, Applied Linguistics , 2024;, amae033, https://doi.org/10.1093/applin/amae033
- Permissions Icon Permissions
With chatbots becoming more and more prevalent in commercial and service contexts, they need to be designed to provide equitable access to services for all user groups. This paper argues that insights into users’ pragmatic strategies and rapport expectations can inform the audience design of chatbots and ensure that all users can equally benefit from the services they facilitate. The argument is underpinned by the analysis of simulated user interactions with a chatbot facilitating health appointment bookings, users’ introspective comments on their interactions, and users’ qualitative survey comments. The study shows that users’ pragmatic strategies show considerable variation. It also shows the negative impact of user experiences when the chatbot’s language and interaction patterns do not align with users’ rapport expectations. In closing, the paper uses these findings to define audience design for chatbots and discuss how audience design can be realized and supported by research.
Chatbots are becoming ever more common in daily life, taking over customer service functions, offering advice, and facilitating access to public services, for example, through appointment booking. In this paper, I aim to show that improved audience design—the adaptive design of bot dialogue in line with users’ pragmatic practices and expectations—will better align services with principles of equality (giving everyone equal opportunities), diversity (valuing difference), and inclusion (ensuring access to services).
The cornerstone of my approach is an investigation of chatbot users’ pragmatic strategies. Whilst the analytical focus is primarily on particular politeness strategies ( Brown and Levinson 1987 ), the paper will also look at users’ deployment of other pragmatic strategies. These include greetings and partings which serve as access rituals ( Goffman 1971 : 79) and as face-saving strategies ( Laver 1981 ), self-disclosure ( Haugh and Carbaugh 2015 ), discourse markers ( Fraser 1999 ), expressions of thanks as an indication of alignment ( Aston 1995 ), and the use of pronouns and names as forms of address in interaction with an appointment booking chatbot. These are triangulated with user comments, made in surveys and interviews, on how these pragmatic strategies connect to their expectations for rapport ( Spencer-Oatey 2008 ). In chatbot research, investigations of users’ pragmatic strategies are still relatively scarce, in particular those using ethnographic and qualitative approaches (see literature review). This study investigates these strategies in a semi-naturalistic setting and thus addresses a gap in human–machine interaction research, underpinning a discussion on how cultural sensitivity within chatbot interactions can be enhanced in the future.
To introduce my argument regarding the need for audience design, a look at two frequently cited studies of language use in interethnic and intercultural contexts. We start with Gumperz’ (1982) case study relating to events at Heathrow Airport in the late 1970s. Pakistani and Indian staff there were hired to serve food at a staff canteen but were perceived as rude and uncooperative by British supervisors and customers. After recording service interactions in the cafeteria, Gumperz found that the Indian and Pakistani staff tended to use falling intonation when making service-related requests. Interpreting these intonation patterns against their background expectations of phonetic patterns, British speakers of English interpreted these requests as unnecessary statements rather than offers and judged the Indian and Pakistani staff to be rude.
More than 20 years later, Spencer-Oatey and Xing (2003) and Spencer-Oatey (2005) investigated participants’ pragmatic strategies and rapport expectations in a Chinese-British business meeting. Drawing on transcriptions of video recordings, retrospective comments by participants and researcher observations, the authors showed that rapport between participants is centred around and can be threatened by individuals’ perceptions of different aspects of language and discourse, for example, stylistic choices, turn management, topic choice, sequencing of information, and so on, as well as differences in their assessment of contextual factors.
These classic case studies have one central aspect in common: they all emphasize how specific language strategies, dialogue patterns, and conversational expectations drive individuals’ perceptions of other social actors. In this paper, I will take these ideas forward to propose that artificial social actors such as chatbots need to be subjected to audience design to mimic the way that human social actors adapt to different audiences, no matter whether these differences are defined by users’ linguistic or cultural backgrounds or other factors, such as age.
The paper starts with a literature review in which I will introduce the notion of audience design, review previous research on users’ expectations of chatbot dialogue and describe the main theoretical approaches framing this study, politeness theory ( Brown and Levinson 1987 ), and rapport management ( Spencer-Oatey 2008 ). In the methodology section, I will discuss the triangulated research design consisting of an analysis of user interactions with a chatbot, surveys, and user interviews, participant recruitment and the analytical approach. I present the results in the form of the case studies of two users’ interactions with the chatbot, followed by a discussion of users’ pragmatic strategies and rapport expectations against the backdrop of the three rapport management dimensions of face, sociality rights, and interactional goals. I close with a definition of audience design for chatbots, its importance and a research agenda for audience design in human–machine interaction.
Introducing audience design
The concept of audience design derives from Bell (1984 , 2001 ). The key take-home-message is that ‘speakers design their style primarily for and in response to their audience’ (143). Bell (1984) roots the concept explicitly in sociolinguistic theory, defining the factors influencing language variation in the design of speech for different audiences as both linguistic (e.g. phonology, syntax, etc.) and extralinguistic, including interspeaker (class, age, etc.) and intraspeaker (addressee, topic, etc.) variation. In his ‘reworked’ audience design concept, Bell (2001) further stresses that style shift also includes encoding the speaker’s own identity in relation to their audience. Audience design shows reflections of Hymes’ (1974) ‘SPEAKING’ mnemonic which purports that linguistic competence requires an acknowledgement of context in speaking. It also integrates Gumperz’s (1982) notion of contextualization cues that describes how listeners interpret verbal and nonverbal features in line with their expectations of social interaction.
Previous research on users’ expectations of chatbot dialogue
The majority of the research on people’s perceptions of interactions with bots and other forms of conversational AI so far uses experimental methods rather than data gathered ‘in the wild’. Their insights underline not only the importance of building conversational agents that have the ability to build and sustain rapport with their users but also the importance of aligning the language strategies of the bot with the expectations of their users. For example, Srinivasan (2016) systematically manipulated the politeness levels of requests (e.g. positive politeness, negative politeness, etc.) used by chatbots in interaction with users. They found that the use of positive politeness was more likely to entice people to help the robots complete tasks, such as to open a door. Salem et al. (2014) investigated the effects of politeness and culture on robot acceptance and anthropomorphization. They found that the more polite robot was perceived to exhibit greater warmth than the control robot. Moreover, they also found that native speakers of English and of Arabic evaluated the robot differently, suggesting that differences in language and sociocultural background between users may be guiding users’ perceptions. Finally, the importance of conversational agents building rapport with users was shown in a study by Bickmore and Pickard (2005) who found that an artificial agent supporting users with a new fitness regime attracted more respect, was liked more, and generated more trust than a non-relational agent and made users more likely to want to continue working with the agent.
The importance of alignment of conversational AI systems with user preferences and expectations has been confirmed in research on text-based chatbots and voice–user interfaces. Cowan et al. (2016) operationalized dialectal variation as a factor for users’ perceptions of the credibility of a navigation system. They found that a navigation system speaking with an Irish voice was considered more credible by its Irish users, no matter how accurate it was. Li and Mao (2015) confirmed the importance of user alignment with their study on a virtual health advisory system. They showed that, when the system’s communication style aligned with a user’s style, it was perceived to be more enjoyable, credible, informative, and transparent. Moreover, Følstad et al. (2020), in a questionnaire-based study on user experiences with chatbots, found that user experience, user needs, and user expectations varied with age. Younger users expected more elements that allowed for the engagement with the bot to be evocative or stimulating, whereas older users preferred elements that were oriented towards the task and general productivity. Van der Goot (2021) described customers’ ‘communication journeys’ with chatbots through the help of interviews with respondents of different genders, ages, and educational levels. They found that customer journeys were strongly influenced by customers’ prior expectations of the interaction, their experiences during the conversations, the effort required, and the extent to which the bot appeared to be human-like. Finally, the study also revealed that there were differences in users’ expectations of language style, for example, of the use of formal vs. informal style.
Another tranche of studies describes how users interact with conversational AI by drawing on the micro-analytical techniques provided by conversation analysis. For example, Hill et al. (2015) investigated differences between human–human interaction via instant messenger and human–chatbot interaction. They found that users’ pragmatic strategies were linked to their perceptions of how human-like their conversational partner was. When interacting with chatbots, users used more profanity and less rich vocabulary than when they were interacting with humans via instant messenger. Braunger et al ’.s (2017) study on user interactions with an in-car spoken dialogue system revealed that speakers were influenced by their beliefs about the system, leading them to adapt to the system, for example, through the use of short concise phrases. Finally, Pelikan (2015) and Pelikan and Broth (2016) investigated how humans adapt to embodied robots, revealing that users adapted their turn design and turn-taking strategies to be in line with their expectations of the conversations.
So far, there is a scarcity of studies that explicitly investigate users’ pragmatic strategies in interaction with chatbots. In one study which is based on the same dataset than the one for this paper ( Dippold 2023 ). I revealed that some users’ strategies that are more akin to human–human interaction are less likely to be successful than those that accommodate the chatbot’s strategies. The only cross-cultural study of users’ pragmatic strategies in interaction with chatbots known to me is Timpe-Laughlin and Dombi’s (2020) comparison of L2 Hungarian and L1 Japanese learners’ English requests to an automated agent. The study not only showed distinct differences in both groups’ strategies to learners’ requests elicited in other interlanguage studies of requests, such as more direct strategies and more internal rather than external modifiers. In addition, it also showed differences in the realization of requests between the two groups. This study is significant as it is perhaps the first attempt to systematically describe the request strategies of different linguistic and cultural groups, insights from which are important to inform audience design through a window into users’ practices.
In summary, existing studies suggest that users have more positive perceptions of conversational systems that are perceived to be creating rapport with users and whose spoken or written output strategies align with user characteristics and expectations. Studies also found that users adapt their pragmatic strategies to their perceptions and expectations of the conversational system. This suggests that a more user-centred design of chatbot language and interaction patterns needs to consider users’ expectations and preferences as well as their pragmatic strategies in interaction with chatbots. This study thus seeks to address this research gap by combining an investigation into users’ rapport expectations—as supported by the chatbot’s dialogue patterns—with an exploration of users’ own pragmatic strategies.
Research questions
This paper supports the research agenda of human–machine interaction by investigating two central questions:
What pragmatic strategies do people use when interacting with chatbots?
In what way do users’ rapport expectations influence their enjoyment of interacting with the chatbot?
The insights from both of these research questions will allow for ethnographically grounded and qualitative insights into users’ authentic pragmatic strategies and rapport expectations and the relationship between these strategies and expectations. Given the experimental focus of most prior studies and a lack of investigation into pragmatic strategies in human–machine contexts, this study thus addresses an important research need. This empirically informed discussion on these issues will later feed into a more extensive discussion of the need for audience design for AI and how audience design can be practically realized and supported through research.
Theoretical frameworks
This paper will answer these questions by drawing on two main theoretical approaches. For the analysis of users’ pragmatic strategies (research question 1), politeness theory ( Brown and Levinson 1987 ) is the guiding theoretical framework. This seminal work on politeness distinguishes positive politeness strategies (addressing human needs for connection and approval), negative politeness strategies (addressing human needs for distance and avoiding imposition), off-record politeness (indirect speech acts), and on-record strategies which are delivered without any mitigation. In addition, the consideration of users’ pragmatic strategies also involves other indicators, such as greetings and partings, self-disclosure, discourse markers, expressions of thanks and forms of address.
In addition, Spencer-Oatey’s (2008) rapport management framework provides the backdrop to the analysis for research question 2. The framework describes rapport as ‘people’s subjective perceptions of (dis)harmony, smoothness-turbulence and warmth-antagonism in interpersonal relations’ ( Spencer-Oatey and Franklin 2009 : 102) and as managed across three dimensions ( Spencer-Oatey 2008 ). The first one of these is face (the need to have personal qualities and social roles upheld) and sociality rights and obligations, consisting of equity rights (rights to personal consideration from others) and association rights (rights to an association with others that is in keeping with the relationship we have with them). The third dimension is that of interactional goals , distinguishing whether speakers focus primarily on completing the task (transactional goals) or on establishing a relationship (relational goals).
Data collection instruments
The data were collected as part of a research project conducted conjointly with start-up company spryt.com. SPRYT have developed an intelligent patient scheduling system that allows patients—currently only using English—to schedule medical appointments via text-based interactions on WhatsApp. Patients interact with a digital receptionist—the chatbot—called ‘Asa’ to schedule appointments, amend or cancel appointments, or respond to a medical screening questionnaire.
As a result of the main author’s post on a UK National Health System (NHS) AI discussion board, SPRYT asked for their support in supporting Asa’s development through her academic expertise. This research project represented the first time the scheduling system was tested with users in a simulated setting and the methodology was developed in collaboration with SPRYT. After the project, the main author gave feedback to SPRYT on aspects of Asa’s dialogue design. The author received no monetary compensation for this work but has benefited from producing publications and evidence of research impact.
Data were gathered through three research instruments:
User experience interviews (see supplementary data A ): Ten interviews were planned in collaboration with an experienced UX researcher. Interviews initially centred on users’ routines and everyday lives and then their general booking experiences for booking both health-related and other appointments. After this phase of the interview, the researcher then triggered Asa to contact the user via WhatsApp on their phone. Users were given a range of tasks to complete with Asa—for example, scheduling an appointment, cancelling an appointment, answering the patient invitation questionnaire. With every bot turn, they were asked what they liked about Asa’s response, what they disliked, what they thought they should be doing next, and what they would actually do next.
Survey (see supplementary data B ): After the user experience interviews, spryt.com made some changes to the wording of some turns and to the interaction structure of Asa, based on the researchers’ recommendations. Further data were then gathered through a survey which again asked for users’ experiences of booking, scheduling, and cancelling appointments and responding to the medical screening questionnaire. The survey included a mixture of closed and open-ended questions. Participants completed the survey soon after interacting with Asa. 32 participants were recruited for this stage.
User–bot interactions: Across the interview and the survey stage, 36 users interacted with Asa and made their interactions available for analysis. In the case of the interactions gathered as part of the interview stage, it is possible to directly crossmatch these to participant interviews that allows for insights into specific moments in the interactions. As the surveys were anonymous, answers within the survey and the interactions of respondents with Asa cannot be cross-matched.
At the time when data were collected, Asa generated templated responses from a pre-programmed response pool which were designed by the company CEO and the wider technical team. For the first 10 of the user interactions with Asa (interview stage), the system was not set up to greet users by their own name, which resulted in a greeting of ‘Hi, Test’. In the survey stage, Asa greeted users by their own names, which have been pseudonymized to maintain anonymity.
In the transcripts presented in this paper, see example 3 below Asa’s turns are represented in italics, whereas users’ responses are represented in regular typeface. Words framed by an asterisk * on each side (see, e.g. 3, 1, 4–5) would have appeared as bold on the users’ mobile phone. The purpose of this was to indicate users that using these words in a response would start a specific conversational path. However, this was not made explicit to users. Statements introduced by a dot • (e.g. lines 7–10) would have appeared as a line-separated bullet point to users.
1 What do I need to do before the appointment?
2 You can find us at, Stag Hill, University Campus, Guildford, GU2 7XH, UK
3 Can I eat before an mri
4 Are you trying to say? *general information* *schedule* appointment *prepare* for
5 appointment *None* of these
6 Prepare for appointment
7 The scan will be around 30 min • *Unless we let you know otherwise*, you can eat and drink
8 as normal and take any prescribed medication • You’re welcome to bring a friend or a relative
9 with you • The radiography team with explain the process once you arrive Do you have any
10 other questions or concerns about what to expect on the day?
11 Will it hurt
12 Sorry, I didn’t understand that. Can you say that in a different way?
13 Can I cancel the appointment
14 Okay,. Please let me know the number of the appointment you would like to cancel: 1.
15 Screening on Tue 22 Mar ‘22 at 16:00
Participant recruitment and demographics
Participants were recruited through the researchers’ social media channels as well as the university’s experimental platform. In the interviews, the majority of participants were undergraduate and postgraduate university students, in addition to two professionals who took part in the research due to professional interest in chatbot development. In the survey, the majority of participants (45 per cent) were between 18 and 24 years old and more than 70 per cent described their ethnicity as ‘White’. Due to the relatively low participant number, I will use the data only to derive theoretical insights rather than attempting to provide verifiable insights into different user group’s pragmatic strategies and rapport expectations.
Participants in both stages of the project were provided with an information sheet. Interview participants signed a written consent form, and participants in the survey stage indicated their consent electronically before starting the survey.
Data analysis
User–bot interactions..
User–bot interactions were downloaded from SPRYT’s dashboard for the trial clinic that was specifically created for the purpose of this project. The transcript files were uploaded into the qualitative analysis programme NVivo. NVivo was then used to code the data with respect to users’ pragmatic strategies, including
directly addressing Asa, for example, Hi, 2 nd person pronouns (‘you’)
discourse markers (e.g. actually , sorry to be a pain)
greetings and partings (e.g. Goodbye)
self-disclosure (e.g. the user telling Asa their name)
expressions of thanks (e.g. Okay, thank you )
expressions of positive politeness, negative politeness, off-record and on-record politeness strategies in users’ requests for information, requests for scheduling, cancellation or rescheduling and their confirmations of attendance or compliance.
The paper will discuss to what extent these pragmatic strategies betray users’ orientations to Asa to be relationship-focussed and anthropomorphic—that is, users orient to the agent as humanlike—or task-focused, orientating to Asa as a machine ( Waytz et al. 2010 ).
Interviews and surveys.
The interviews were recorded on MS Teams. The automatically generated transcripts were downloaded and edited into a word-level transcript by a research assistant, after which they were uploaded onto Nvivo. Users’ responses to the open questions in the survey were also extracted and uploaded onto Nvivo.
The qualitative data from both the interviews and the survey were analysed using reflexive thematic analysis ( Braun and Clarke 2019 ) describes themes as ‘creative and interpretive stories about the data, produced at the intersection of the researcher’s theoretical assumptions, their analytic resources and skill, and the data themselves’ (594). Whilst the initial data approach was ‘bottom-up’, inductive, and data driven, I soon realized that one of the main stories users were telling was about their needs when booking appointments more generally, and their experiences and needs when booking through Asa (e.g. efficiency, confidentiality, being listened to). Consequently, I started using a deductive approach, drawing on a combination of semantic coding—using what a respondent has said or written—and latent coding—interpreting underlying meaning and ideas ( Byrne 2022 )—to code the entire dataset against this theme and these sub-themes. Given the analytical focus on issues of rapport, instances in which participants talked about ‘incidents’ within the interaction with Asa which has a negative effect (e.g. feeling annoyed, insulted, etc.) or a positive effect (feeling happy, proud, a sense of achievement) on them (see Spencer-Oatey 2002 ), were particularly helpful in this phase of latent coding.
In the subsequent section, I will draw on the analysis of the users’ pragmatic strategies in interaction with Asa as well as the analysis of interview and survey data in relation to the rapport management framework. The presentation of the results starts with two case studies. Subsequently, I will discuss users’ comments from the surveys and the interviews in relation to issues of rapport, linking these whenever possible to the interactional segments they relate to.
Case study 1: a relationship-focused and polite user
1 Hi Miriam, I’m Asa your virtual receptionist from University Of Surrey. Here is how I can
2 help. 3 I can *schedule* appointments, help you *prepare* for your appointment or answer a *question* you
5 Hello, I was wondering if I could book an MRI scan?
Miriam (a pseudonym) was a participant in the second stage of the project. Example (1) is the start of the interaction with Asa, the bot (l. 1–3).
1 Sure, what date works for you?
2 I can do any day next week other than Wednesday
3 Please pick a time 08:00 09:00 10:00 11:00 12:00 13:00 14:00 15:00 16:00 17:00 18:00 19:00
5 What day?
6 Did you mean? clinic’s *opening times* *hello* *prepare* for appointment *None* of these
7 None of these
8 Sorry, I didn’t quite get that. I’m still learning. Can you please rephrase the date/time you
9 provided? Thanks, Asa
10 Tuesday would be best
11 Sorry, I didn’t understand that. Can you say that in a different way?
12 Can I have the scan on Tuesday
Miriam reacts to Asa’s introduction by greeting Asa (‘hello’) and with a request to book an MRI scan. The request is made as a conventionally indirect request, with ‘I am wondering’ forestalling the actual request (l. 4).
Example (2) is taken from Miriam’s booking interaction with Asa. After Asa’s prompt to provide a date (l. 1), Miriam indicates her availability, though the only ‘named’ day within her turn is the day when she is not available (l. 2). This causes Asa to move the conversation on to the next question about preferred appointment times (l. 3). Given the lack of clarity with regard to the day of the appointment, Miriam reacts by asking a question to confirm what day the appointment will take place (l. 5). Asa does not understand and requests repair (l. 6), to which Miriam reacts by using one of Asa’s prompts from the previous turn (l. 7). Miriam subsequently reacts to Asa’s second and third repair request (l. 8-9, l. 11) by attempting again to confirm a day of the appointment. Both requests include negative politeness marker, for example, ‘would be’ (l. 10) and ‘can I have’ (l. 12).
14 Okay. Please let me know the number of the appointment you would like to cancel: 1.
Example (3) represents a point in the interaction at which Miriam is trying to ask questions about the procedure. After her question about actions to take ahead of the procedure (l. 1) is not understood by Asa (l. 2), she provides a ‘repaired’ turn twice (l. 3, 6). Asa does not understand Miriam’s new information request (l. 11) prompting Miriam to move out from the information-seeking sequence into a cancellation sequence. When making the cancellation request she does so again in a conventionally indirect manner (‘can I cancel’, l. 13).
Case study 2: a task-focused user
John (also a pseudonym) also interacted with Asa during the survey stage of the project. Example (4) showcases John’s complete booking interaction with Asa.
1 Hi John, I’m Asa your virtual receptionist from University Of Surrey. Here is how I can help. I
can 2 *schedule* appointments, help you *prepare* for your appointment or answer a
*question* you may 3 have
5 Sure, what date works for you?
7 Okay. you can choose the time of day you want for your appointment: • Morning (8am-12pm)
• 8 Afternoon (12pm-5pm) • Evening (5pm-8pm) • Any (8am-8pm)
10 Please put in your preferred time 08:00 09:00 10:00 11:00 None
12 I can offer you these appointment times 08:00 09:00 10:00 11:00 None
14 Hi John. I’m Asa, your virtual receptionist. Happy to confirm your Screening appointment is
now15 booked for Wed 23 Mar ‘22 at 10:00. Is this ok?
After Asa’s standard introduction (l. 1–3), John’s first turn consists of only one word, indicating his need to schedule an appointment (l. 4). As the interaction progresses, John’s subsequent answers are equally short. For example, his requests include no additional politeness markers (e.g. l. 6), and he also uses Asa’s own prompts from the previous turn to progress the interaction (e.g. ‘morning’, l. 9). This is particularly evident when his original date input is not understood by Asa (l. 11), and he subsequently reverts to a time format that is more acceptable to Asa (l. 13). This leads to successful confirmation of the appointment (l. 14–15).
2 •You’re welcome to bring a friend or a relative with you • The radiography team with explain
3 the process once you arrive • Please tell us about any special circumstances (for example, if
4 you need wheel-chair access, if you are hard of hearing, if you have poor eyesight or if you are
5 claustrophobic) Do you have any other questions or concerns about what to expect on the
7 Directions
8 Here you go...
2 Okay. Please let me know the number of the appointment you would like to cancel: 1.
3 Screening on Wed 23 Mar ‘22 at 10:00
5 Could you tell us why you have cancelled your appointment? Was it related to: 1. Work
6 commitments 2. Transport issues 3. Concerns regarding the appointment 4. Appointment no
7 longer needed 5. Other
Example (5) is from a sequence in which John asks questions about the preparation for the appointment. He does so without any further politeness markers in a one-word turn (‘prepare’, l. 1). Similarly, he makes his request for directions in only one word, too (l. 7).
When cancelling his appointment (example 6), John makes the same stylistic choices as observed previously. He makes a request for cancellation of one single word (‘cancel’, l. 1), and subsequently uses Asa’s own prompts, in this case, the numeric indicators of preference from the previous turn to proceed with the cancellation sequence (e.g. l. 4, l. 8).
These examples from John’s interaction with Asa show that Miriam and John use different linguistic means to fulfil the same tasks. Miriam greets Asa as if ‘she’ is a human and makes the vast majority of her requests with negative politeness markers. These mitigate the force of the request, indicating that the requests might constitute an imposition on the addressee. Hence, Miriam uses similar strategies to those she might use with a human addressee to whom a request might indeed constitute a face-threatening act that limits their freedom of action.
In contrast, John makes his requests with minimal turns, using just a simple keyword. He is also very adept at using Asa’s own prompts when they are provided, a strategy which can be described as ‘upward convergence’ or ‘accommodation’ to the bot ( Giles and Ogay 2007 : 295). Hence, John uses strategies that are different from what one might expect in face-to-face social interaction. The differences between Miriam’s and John’s strategies may mean different things. One could see them as an indication that Miriam positions Asa as a human, while John perceives Asa as dehumanized and thus not worthy of the social conventions that are the norm when speaking to a human addressee. However, as discussed previously ( Dippold 2023 ), these differences could also be due to the fact that Miriam is a less experienced bot user than John and thus less aware of the sociolinguistic environment human–chatbot interaction represents.
Rapport expectations, rapport threat, and beliefs about language
As discussed in the literature review, Spencer-Oatey’s rapport management framework consists of three interrelated dimensions: face, sociality rights, and interactional goals. I will now use the insights from the thematic analysis to discuss each of these aspects in turn.
Face: Users’ rapport expectations can be threatened when language and interaction patterns do not support users’ face needs or wants. This is shown in example (7) and the respective user comment on the example:
1 We want to ask a few simple, medical questions before the scan. Do you have 2–3 min now to
2 a nswer some questions? This is for your safety. Please reply with yes/no
4 Thanks. These safety questions take just 3 min, and help us ensure everything is set up
5 correctly
6 1.00/0. Do you have a cardiac (heart) pacemaker? Yes/No
User comment (1)—relating to example (7):
I feel this question is pretty like out of the blue is very like cardiac output peacemaker. I think it’s quite like it has nothing to do with for why I wanted the MRI. I feel like it’s just really random and kind of it doesn’t make sense this has nothing to do with your why I contacted them in the 1st place so I don’t know why they. It just makes me feel like I don’t know why they want me to tell them about this.
Example 7 is from the first part of the medical screening questionnaire, with the first question inquiring whether the user has a cardiac pacemaker. The user (see user comment 1) perceives this as a threat to rapport because of the immediacy of the question and the lack of explanation of its relevance, leading to threat to negative face due to the perceived imposition caused.
Also relating to the medical screening questionnaire was a user comment from the survey:
User comment (2):
It was easy to do but seemed quite robotic and impersonal. When I answered yes to questions I was expecting Asa to ask me for further information or details but she didn’t. It also didn’t give me the opportunity to provide further details e.g., what if my answer wasn’t just Yes or No. The answers left no space for in between answers/grey areas.
This user reports a perceived threat to rapport on the dimension of a positive face because of Asa’s perceived ‘impersonal’ style, which does not allow for the user’s want for connection to be satisfied. This is linked specifically to the lack of follow-up questions by Asa on affirmative answers to the medical screening questions, and the fact that the questions and answers did not leave any room for vagueness.
Sociality rights.
In addition to rapport threat based on ‘face’, user comment (2) can also be read as threatening rapport on accounts of equity rights as the lack of room for elaboration shows a lack of consideration for the users’ needs. Indeed, many of the comments in both surveys and interviews discuss rapport threat based on perceived equity rights violations, such as when a question is not answered (user comment 3) or Asa displays a lack of understanding (user comment 4):
User comment (3):
They ignored my question completely and it made me agitated.
User comment (4):
Too robotic, only understands key words from my phrases, I had to send at least 3 messages for her to understand what I meant.
Cost–benefit considerations come into play in some user comments:
1 What date works for you?
2 4/02/2022
3 Our clinic is open Monday through Friday only. Please try specifying a date that does not
4 fall on weekends.
6 Please choose a convenient time of day - any - Morning - Afternoon - Evening
8 Please choose a time slot 09:00 10:00 11:00 None
User comment (5)—relating to example (8):
For example, I think I don’t like the idea for it. I I am just giving a day Tuesday, for example, but I still can’t. No exactly which date is available can be the 8th of Tuesday the 8th. It can be 11. Choose day, so, uh, it’s a bit overwhelming because I can be available on that day, but I may not be available. May have other responsibilities other days, so I cannot speak exactly at 8. I think I am looking for more freedom to choose the time the date exactly.
This user (user comment 5) bemoans the fact that the booking dialogue does not afford sufficient opportunity for consideration of their needs. Whilst Asa offers a date, there is no certainty that the user is available at the specific time(s) offered by Asa. The user thus felt that their personal freedom to make choices was restricted—an issue also relating to negative face—making the dialogue quite ‘costly’ in terms of the effort required to achieve a successful booking.
There are also user comments that indicate rapport being threatened based on association rights:
User comment (6):
Quick and easy. Although I selected option 3 ‘concerns regarding the appointment’ and it didn’t prompt me to discuss my concerns or call anyone. That might leave me up in the air as a worried potential patient not sure who to turn to for advice.
This user describes Asa’s lack of response to their indicating that they had concerns about the appointment. These concerns were neither addressed by Asa nor did a human respond to them in a follow-up call. This goes against this user’s perceived rights to an association with a medical service provider who responds to patient concerns.
Interactional goals.
This rapport dimension is concerned with whether users have a mainly relational or a mainly transactional orientation to the interactions with Asa. In the analysis of interviews ‘efficiency’ emerged as an important need reported by users, betraying a transactional orientation focussed primarily on achieving task goals quickly and efficiently. This is in opposition to another user need described through the theme of ‘personalization’. User comments 7 and 8 and their associated interaction transcripts illustrate these contrasting user needs and goals:
User comment (7)—relating to example (9):
I think because they just went straight like oh hi and they just meet and they didn’t really introduce themselves. I kind of thought like OK this is kind of looked like a robotic response and also kind of like when they said please choose a convenient time for you. Please choose. It’s kind of like no one’s there, it’s just the automatic responses. Please choose like I think if it was someone behind the text, I think they’d be more like oh can you or I don’t know, it’s just something a bit more humanely kind of, not just kind of that direct.
1 Hi Test, I’m Asa from—Asa Clinic, your clinic assistant. Here is how I can help. Just type:
2 *Schedule/ Reschedule/ Cancel* - Book New/Manage existing appointment(s) *Prep* -
3 For info on how to prepare for your appointment *FAQ* - For our frequently answered
4 questions page *General* - For general info (opening times, phone no., directions/maps etc.)
5 *Admin* - To speak with someone
7 Hi Test, Is there anything I can help you with?
8 I would like to book an MRI appointment please
9 What date works for you?
10 Can I book it for the 28th January
In relation to example (9), this user discusses their need for a more personalized experience to fulfil core interpersonal/social goals of the encounter, perceiving the dialogue overall as rather ‘robotic’ and expressing a wish for it to be less ‘direct’ and involving more direct forms of address, for example, ‘can you’. It is interesting to note that the user here also betrays an overall anthropomorphic orientation to the dialogue, as evidenced by them greeting Asa (l. 6) and their use of politeness strategies in their booking request (l. 8).
Reflecting on their own interaction with Asa, another user displays a more transactional orientation:
User comment (8):
In theory this would be great because all I know is that if it was working then I’m not waiting around. I can ask and see what my options are boom and then I have my appointment over and done with without having to wait through a waiting time. And I’ve also got proof as well over the text message to show that even though they’re hopefully will send me a confirmation message. There’s also proof via the text that they have texted me saying these are my options and that’s the option that I’ve chosen.
This user emphasizes primarily the speed and efficiency with which appointments are made by Asa compared to booking appointments with the help of a human receptionist. Part of that appreciation for efficiency is also the fact that, given the interaction is recorded in written form, there is a record of the booking.
In addition, the data also revealed instances in which users orient in parallel to social and transactional goals and value both the anthropomorphic and the robotic features of social interaction.
User comment (9):
Yeah, because I did this kind of like interaction before. I know like the procedure and I know like this is not a real person so the responding speed is it’s good. You know they’re not like holding back to process the information I type in. So maybe the AI smart. It’s smart enough to go through the process. And second, you know the languages for me is. Understandable, it’s clear. but however I like, I said before like they don’t have like this small talk like they don’t have like opening talk that to to make the people who want to book appointment feel welcome, I feel like. but this is it’s pretty good. It’s pretty good. Yeah, because it’s super convenient.
In comment (9), the user, on one hand, expresses appreciation for the effectiveness and speed by which bookings can be made. On the other hand, they also comment on the fact that, in the earlier stages of the interaction, Asa the bot offers hardly any small talk to make the user feel welcome. This shows that conflicting rapport orientations and language beliefs can co-exist within individuals. These may in turn be reflected within their ‘practiced’ orientations.
This paper aimed to answer the following two central research questions:
What pragmatic strategies people use when interacting with chatbots?
The data presented above show that, even though most users taking part in this project are from a similar demographic group, their pragmatic strategies show considerable variation. They also show that, at times, these strategies contravene expectations. For example, users may talk to Asa as if they were a human—using politeness features as if trying to protect Asa’s face, referring to the Asa by name—even when Asa does not understand their input and is ineffective in completing the task. This suggests that some users are finding it difficult to detach themselves from ‘learned’ human–human interaction behaviours. This project did not investigate whether users’ pragmatic strategies change depending on whether Asa is more or less anthropomorphized, but this is a possible topic for further investigation.
Moreover, the data show that, if Asa’s language and interaction patterns fail to align with users’ rapport expectations, users are likely to have more negative experiences of their interactions. These of course have the potential to disengage users from interacting with bots. It is highly likely that, in a more diverse user group, differences in users’ expectations and practices would likely be even more pronounced. Moreover, in a real-life context with more at stake, users’ negative experiences and perceptions of their expectations being unmet would have been exacerbated and led to disengagement and dissatisfaction with the service.
What is audience design for chatbots and why is it needed?
Interactional sociolinguistics research in professional contexts has revealed that variation in language practices and in perceptions of appropriateness can lead to inequalities, for example in terms of access of linguistic minorities to the job market ( Roberts 2021 ). Research by the King’s Fund (2020) also warned that inequalities in access to and experience of health services can arise because ‘information is not communicated in an easily understandable or culturally sensitive way’. Given the growing importance of chatbots in commercial as well as public service contexts including the health services, it is vital that this situation is not mirrored when services—such as, in this simulated example, medical appointment booking—are delivered through and accessed through chatbots.
This paper has shown that, whilst users’ pragmatic practices and preferences vary, Asa’s contributions to the dialogue remain stable, however, users interact with them. I argue that to provide all user groups with a good user experience, and thus equal access to services delivered by chatbots, chatbot design needs to take account of a diversity of expectations through audience design, focussing in particular on meeting users’ rapport expectations. I define audience design for chatbots as follows:
Audience design for chatbots is the adaptive design of chatbot language and interaction patterns in response to the chatbot user.
Examples of adaptive design might include the following (in a non-exhaustive list):
Politeness strategies used by the chatbot, e.g. when making requests to the user or apologizing to the users asking for an apology. Research on cross-cultural pragmatics in human–human interaction (see e.g. House and Kadar 2021 ) has demonstrated clearly that there is cross-cultural variation in the way politeness strategies are produced and perceived. Therefore, there is every reason to assume that there is variation in chatbot users’ preferences across user groups.
Phatic elements, such as greetings, partings, backchannels are used in conversation to establish social contact between participants. Kulkarni (2013) , exploring phatic elements in instant messaging interactions, argues that ‘it is possible that data sets belonging to different cultures will suggest different requirements of contact’ (134). This suggests again that adaptive design of phatic elements in chatbot dialogue might be able to better engage specific user groups.
Adaptive user support: As the two examples from the case studies have shown, some users use pragmatic strategies that are conducive to effective interaction with chatbots, whereas other users apply strategies that are less effective (see also Dippold 2023 ). Adaptive dialogue design for chatbots would include the bot recognizing which user will need more assistance, and consequently provide this assistance, for example by making the ‘rules of the game’ more explicit, in particular on what strategies to use to ensure the bot understands etc.
Engaging in audience design goes some way toward making chatbots user- rather than technology-centred ( Kopp and Krämer 2021 ). Microsoft’s (2019) guidelines for human-AI interaction design, already include references to audience design, for example, ‘Match relevant social norms: Ensure that experience is delivered in a way that users would expect, given their social and cultural context’ and ‘Mitigate social biases: Ensure the AI system’s language and behaviours do not reinforce undesirable and unfair stereotypes and biases’.
This view is supported by Sutton et al .’s ( 2019 ) research on sociophonetic design strategies in the development of voice–user interfaces such as Siri and Amazon Alexa. The authors argue that the VUI voices need to be ‘designed for diversification’ (11), considering users’ voice-based preferences. They also argue that there is a need ‘to focus on developing tools and techniques that allow the mining of and inference of voice-based preferences, while also dynamically respond to users’ (12). I will use the next section of this paper to propose that the same considerations also apply to text-based conversational AI and to describe a research agenda that supports this purpose.
A research agenda for audience design
When the conversational pathways of chatbots are scripted and defined, this is usually done with the support of conversation designers. Based on the data analysed in this paper, I purport that audience design should be seen as an aim as well as an outcome of conversation design. As an aim , audience design is able to support principles of inclusive design, defined as ‘design that considers the full range of human diversity with respect to ability, language, culture, gender, age and other forms of human difference’ ( Deibel and Evanhoe 2021 : 270). In the UK, the Equality Act ( National Archives 2010 ) stipulates that public bodies, including the NHS, have a duty to eliminate discrimination and advance equality of opportunity. Whilst the Equality Act does not include language as a protected characteristic, it does include age and race, which, as the literature review has shown (e.g. Gumperz 1982 ; Følstad et al. 2020 ; Van der Goot 2021 ) has links to language-based preferences.
As an outcome , effective audience design needs to be underpinned by methods and approaches that allow for true user-centredness. To achieve this, ethnographic approaches that investigate user interactions with AI, and investigations that focus on user perceptions need to move out of simulated and experimental environments (see literature review) into real-life contexts in order to truly reflect the user experiences when higher stakes area attached to a task. In addition, co-design methods ( Steen 2013 ) need to be adapted to exploring the influence of wider social factors on users’ pragmatic strategies and preferences. In the case of chatbot dialogue, users would be involved with writing how they imagine the optimal dialogue to be. Finally, as I have argued previously ( Dippold et al. 2020 ), any research that has the purpose of underpinning audience design for chatbots will additionally benefit from analysis of user–bot interactions to provide differentiated insights into users’ rapport expectations and pragmatic practices. Previous studies suggest that the success of chatbot dialogue is influenced by how users interact with the system ( Kvale et al. 2019 ) and by users’ degrees of experience in interacting with chatbots effectively ( Dippold 2023 )
There are, of course, possible limitations to audience design in relation to the dynamic recognition of user preferences. As research on human–human interaction has shown, service user identities should not be seen as stable, but as constantly evolving ( Feller 2014 ; Flinkfeldt et al. 2021 ). Adaptive audience design would thus need to use natural language processing to dynamically feed analyses of users’ own pragmatic strategies and preferences into a decision-making module, with the goal of creating chatbot dialogue that dynamically adapts to these strategies and preferences.
Limitations
This study has a number of limitations. It is based on a small dataset of user–bot interactions that were gathered in a simulated rather than a real-life environment. However, the study was not designed to provide empirical proof of how targeted audience design can improve audience engagement and trust. Instead, this study has shown, in a qualitative way, the need for audience design and pathways toward its implementation. In the future, larger empirical studies are necessary to gather data on user–chatbot interactions, to implement audience design, and to then evaluate its success through empirical methods (e.g. O’Brien et al. 2018 ; Gulati et al. 2019 ; Chaves et al. 2022 ).
In addition, Bell’s concept of audience design ( Bell 1984 , 2001 ), which underpins this study, was of course never developed for contexts in which one of the ‘speakers’ is an artificial rather than a human agent. In contrast to humans, chatbots do not have any inherent ability to assess who the audience is and what their practices and preferences may be. The ability to recognize users’ rapport expectations thus needs to be ‘taught’ to bots through a machine-learning approach that extracts codes and infers these based on users’ pragmatic strategies. Braunger et al. (2017) , Cruz-Benito et al. (2017) demonstrate this concept by using machine-learning algorithms to build predictive models of user preferencing and behaviour, allowing a complex survey to be adapted to different users. Whilst this process will of course be subject to its own shortcomings, flaws, and errors, it has the potential to positively support efforts for more equitable participation in commercial and public services delivered by chatbots.
Finally, this study also did not gather data ‘in the wild’, investigating authentic user interactions with a chatbot with a real-life transactional goal (such as booking a medical appointment), but drew on simulated data Whilst this approach puts some limits on the ecologic validity of the results—their generalizability to real-life settings ( Andrade 2018 )—the triangulated, qualitative approach, both in terms of data collection points and analytical perspective used in this study, still constitutes a significant step-change in human–machine interaction research.
This paper had a combined theoretical and practical aim: it has developed the concept of ‘audience design’ for chatbots and other forms of conversational AI, arguing that careful audience design allows AI-based social interaction to consider the needs of specific user groups, moving away from a ‘one size fits all’ approach. Through excerpts from user interactions with AI, user interviews, and surveys it has also shown that insights users’ pragmatic practices and their rapport expectations can provide an ideal underpinning for audience design. With a commitment to audience design, conversational AI can be developed in a people-centric way, increasing user engagement with and enjoyment of bots and related technologies and providing equitable access to services.
Notes on Contributor
Doris Dippold is a senior lecturer in Intercultural Communication at the University of Surrey. She is a fellow of the Surrey Institute for People-Centred AI and co-leads Working Group 8 (Language Variation, Pragmatics, and Interaction) of the Research Network LITHME (Language in the Human Machine Era). She has published extensively on conversational AI, chatbots, intercultural pragmatics, English as a lingua franca, internationalization of higher education, and classroom interaction. Her collaboration with amo.ai led to improvements in dialogue design of a business-to-business chatbot. Currently, she is supporting spryt.com with research on audience design for their medical appointment booking chatbot. Address for correspondence: School of Literature and Languages, University of Surrey, School of Literature and Languages, Guildford GU27XH, UK < [email protected] >
The author wishes to thank Adeeba Ahmad for her support with data analysis and Marion Heron for her feedback on the first draft of the paper.
This work was supported by the University of Surrey ESRC Impact Acceleration Account.
Andrade , C. 2018 . ‘Internal, external, and ecological validity in research design, conduct, and evaluation,’ Indian Journal of Psychological Medicine 40 / 5 : 498 – 9 .
Google Scholar
Aston , G. 1995 . ‘Say “Thank You”: Some pragmatic constraints in conversational closings,’ Applied Linguistics 16 / 1 : 57 – 86 .
Bell , A. 2001 . ‘Back in style: Reworking audience design’ in Eckert , P. and Rickfort , J. (eds.), Style and Sociolinguistic Variation . Cambridge University Press ; 139 – 169 .
Bell , A. 1984 . ‘Language style as audience design,’ Language in Society 13 / 2 : 145 – 204 .
Bickmore , T. and R. Picard . 2005 . ‘Establishing and maintaining long-term human-computer relationships,’ ACM Transactions on Human-Computer Interaction 12 / 2 : 293 – 327 .
Braun , V. and V. Clarke . 2019 . ‘Reflecting on reflexive thematic analysis,’ Quality Research in Sport, Exercise and Health 11 / 4 : 589 – 97 .
Braunger , P. , Maier , W. , Wessling , J. and Werner , S. 2017 . ‘Natural language input for in-car spoken dialog systems: How natural is natural’ , Proceedings of the SIGDIAL 2017 Conference. Saarbrücken , Association for Computational Linguistics , 137 – 46 .
Brown , S. and Levinson , P. 1987 . Politeness. Some Universals in Language Usage . Cambridge University Press .
Google Preview
Byrne , D. 2022 . ‘A worked example of Braun and Clarke’s approach to reflexive thematic analysis,’ Quality & Quantity 56 : 1391 – 412 .
Chaves , A. et al. . 2022 . ‘Chatbots language design: The influence of language variation on user experience with tourist assistant chatbots,’ ACM Transactions on Computer-Human Interaction 29 / 2 : 1 – 38 .
Cowan , B.R. et al. . 2016 . ‘Towards understanding how speech output affects navigation system credibility’ , Proceedings of CHI 2016, San Jose , Association for Computing Machinery , 2805 – 12 .
Cruz-Benito , J. et al. . 2017 . ‘Enabling adaptability in web forms based on user characteristics detection through A/B testing and machine learning,’ IEEE Access 6 / 2251 : 2251 – 2265 .
Deibel , D. and Evenhoe , R. 2021 . Conversations With Things: UX Design for Chat and Voice . Rosenfeld Media .
Dippold , D. 2023 . ‘“Can I have the scan on Tuesday?” User repair in interaction with a task-oriented chatbot and the question of communication skills for AI,’ Journal of Pragmatics 204 : 21 – 32 .
Dippold , D. et al. . 2020 . ‘A turn to language: How interactional sociolinguistics informs the redesign of prompt:response chatbot turns,’ Discourse, Context and Media 37 : 100432 .
Feller , S. 2014 . ‘The good, the bad, and the ugly: The co-construction of identities in dialog,’ Language and Dialogue 4 / 3 : 341 – 56 .
Flinkfeldt , M. , S. Parslow , and E. Stokoe . 2021 . ‘How categorization impacts the design of requests: Asking for email addresses in call-centre interaction,’ Language in Society 51 : 693 – 716 .
Følstad , A. and P. Brandtzæg . 2020 . ‘Users’ experiences with chatbots: Findings from a questionnaire study,’ Quality and User Experience 5 / 3 .
Fraser , B. 1999 . ‘What are discourse markers?,’ Journal of Pragmatics 31 / 7 : 931 – 52 .
Giles , H. and Ogay , H. 2007 . ‘Communication accommodation theory’ in Whaley , B. and Samter , W. (eds), Explaining Communication: Contemporary Theories and Exemplars . Lawrence Erlbaum ; 293 – 310 .
Goffman , E. 1971 . Relations in Public: Microstudies of the Public Order . Basic Books .
Gulati , S. , S. Sousa , and D. Lamas . 2019 . ‘Design, development and evaluation of a human-computer trust scale,’ Behaviour & Information Technology 38 / 10 : 1004 – 15 .
Gumperz , J. 1982 . Discourse Strategies . Cambridge University Press .
Haugh , M. and D. Carbaugh . 2015 . ‘Self-disclosure in initial interactions amongst speakers of American and Australian English,’ Multilingua 34 / 4 : 461 – 93 .
Hill , J. , W. Ford , and I. Farreras . 2015 . ‘Real conversations with artificial intelligence: A comparison between human-human online conversations and human-chatbot conversations,’ Computers in Human Behavior 49 : 245 – 50 .
House , J. and Kadar , D. 2021 . Cross-Cultural Pragmatics . Cambridge University Press .
Hymes , D. 1974 . Foundations in Sociolinguistics: An Ethnographic Approach . Routledge .
King’s Fund . 2020 . ‘ What are Health Inequalities?,’ available at https://www.kingsfund.org.uk/publications/what-are-health-inequalities . Accessed 21 February 2024 .
Kopp , S. and N. Krämer . 2021 . ‘Revisiting human-agent communication: The importance of joint co-co-construction and understanding mental states,’ Frontiers in Psychology 12 : 580955 .
Kulkarni , D. 2013 . ‘Exploring Jacobson’s “phatic function” in instant messaging interactions,’ Discourse & Communication 8 / 2 : 117 – 36 .
Kvale , K. , Sell , O. , Hodnebrog , S. , and Følstad , A. 2019 . ‘Improving conversations: Lessons learnt from manual analysis of chatbot dialogues’ in: Følstad , A . et al. (eds.), International workshop on chatbot research and design. Springer International Publishing ; 187 – 200 .
Laver , J. 1981 . ‘Linguistic routines and politeness in greeting and parting’ in: Coulmas , F . (ed.), Explorations in Standardized Communication Situations and Prepatterned Speech. Volume 2: Conversational Routine . De Gruyter Mouton ; 289 – 304 .
Li , M. and J. Mao . 2015 . ‘Hedonic or utilitarian? Exploring the impact of communication style alignment on user’s perception of virtual health advisory services,’ International Journal of Information Management 35 / 2 : 229 – 43 .
Microsoft . 2019 . ‘ Guidelines for human-AI interaction design ,’ available at https://www.microsoft.com/en-us/research/blog/guidelines-for-human-ai-interaction-design/ . Accessed 6 March 2023 .
National Archives . 2010 . ‘ Equality act ,’ available at https://www.legislation.gov.uk/ukpga/2010/15/section/149 . Accessed 6 March 2023 .
O’Brien , H. and CairnsHall , M. 2018 . ‘A practical approach to measuring user engagement with the refined user engagement scale (UES) and new UES short form,’ International Journal of Human-Computer Studies 112 : 28 – 39 .
Pelikan , H. 2015 . ‘ How humans adapt to a robot recipient: An interaction analysis perspective on human-robot interaction’, Bachelor thesis, University of Osnabrück .
Pelikan , H. and Broth , M. , 2016 . ‘Why that Nao? How humans adapt to a conventional humanoid robot in taking turns-at-talk’ , CHI ‘16: Proceedings of the 2016 CHI Conference on Human Factors in Computing Systems, 4921 – 32 .
Roberts , C. 2021 . Linguistic Penalties and the Job Interview . Equinox .
Salem , M. , Yiadee , M. , and Sakr , M. 2014 . ‘Marhaba, how can I help you? Effects of politeness and culture on robot acceptance and anthropomorphization’ , 9th ACM/IEEE International Conference on Human-Robot Interaction (HRI), 74 – 81 .
Spencer-Oatey , H. 2005 . ‘(Im)politeness, face and perceptions of rapport: Unpackaging their bases and interrelationships,’ Journal of Politeness Research 1 : 95 – 119 .
Spencer-Oatey , H. 2008 . Culturally Speaking: Culture, Communication and Politeness Theory . Bloomsbury Publishing .
Spencer-Oatey , H. 2002 . ‘Managing rapport in talk. Using rapport sensitive incidents to explore the motivational concerns underlying the management of relations,’ Journal of Pragmatics 34 / 5 : 529 – 45 .
Spencer-Oatey , H. and Franklin , P. 2009 . Intercultural Interaction: A Multidisciplinary Approach to Intercultural Communication . Palgrave Macmillan .
Spencer-Oatey , H. and J. Xing . 2003 . ‘Managing rapport in intercultural business interactions: A comparison of two Chinese-British welcome meetings,’ Journal of Intercultural Studies 24 / 1 : 33 – 46 .
Srinivasan , V. 2016 . ‘Help me please: Robot politeness strategies for soliciting help from people’ , CHI ‘16: Proceedings of the 2016 CHI Conference on Human Factors in Computing Systems, 4945 – 55 .
Steen , M. 2013 . ‘Co-design as a process of joint inquiry and imagination,’ Design Issues 29 / 2 : 16 – 28 .
Sutton , S. , Faulkes , P. , Kirk , D. and Lawson , S. 2019 . ‘Voice as a design Material: Sociophonetic inspired design strategies in human-computer interaction’ , CHI ‘19: Proceedings of the 2019 CHI Conference on Human Factors in Computing Systems, 1 – 14 .
Timpe-Laughlin , V. and J. Dombi . 2020 . ‘Exploring L2 learners’ request behavior in a multi-turn conversation with a fully automated agent,’ Intercultural Pragmatics 17 / 2 : 221 – 57 .
Van der Goot , M. , Hafkamp , L. , and Dankfort , Z. 2021 . ‘Customer service chatbots: A qualitative interview study into the communication journey of customers’ in Følstad , A. et al. (eds.), Chatbot Research and Design. 6th International Workshop: Conversations 2020 . Springer ; 190 – 204 .
Waytz , A. , N. Eplez , and J. Cacioppo . 2010 . ‘Social cognition unbound: Insights into anthropomorphism and dehumanization,’ Current Research in Psychological Science 19 / 1 : 58 – 62 .
Supplementary data
Email alerts, citing articles via, looking for your next opportunity.
- Recommend to your Library
Affiliations
- Online ISSN 1477-450X
- Print ISSN 0142-6001
- Copyright © 2024 Oxford University Press
- About Oxford Academic
- Publish journals with us
- University press partners
- What we publish
- New features
- Open access
- Institutional account management
- Rights and permissions
- Get help with access
- Accessibility
- Advertising
- Media enquiries
- Oxford University Press
- Oxford Languages
- University of Oxford
Oxford University Press is a department of the University of Oxford. It furthers the University's objective of excellence in research, scholarship, and education by publishing worldwide
- Copyright © 2024 Oxford University Press
- Cookie settings
- Cookie policy
- Privacy policy
- Legal notice
This Feature Is Available To Subscribers Only
Sign In or Create an Account
This PDF is available to Subscribers Only
For full access to this pdf, sign in to an existing account, or purchase an annual subscription.
Social Sciences
© 2024 Inquiries Journal/Student Pulse LLC . All rights reserved. ISSN: 2153-5760.
Disclaimer: content on this website is for informational purposes only. It is not intended to provide medical or other professional advice. Moreover, the views expressed here do not necessarily represent the views of Inquiries Journal or Student Pulse, its owners, staff, contributors, or affiliates.
Home | Current Issue | Blog | Archives | About The Journal | Submissions Terms of Use :: Privacy Policy :: Contact
Need an Account?
Forgot password? Reset your password »
Interdisciplinary Graduate Program in Neuroscience
Ashby martin featured in graduate college news article about his neurolinguistic research for betterment of multilingual communities.
At Iowa, Martin studies developmental neurolinguistics, particularly in young children who are bilingual in Spanish and English. His focus is on “numbers as language”, and he examines the neurological impact and visual representation of shifting between the individual’s multiple linguistic repertories through neurological imaging.
Read the entire article here .
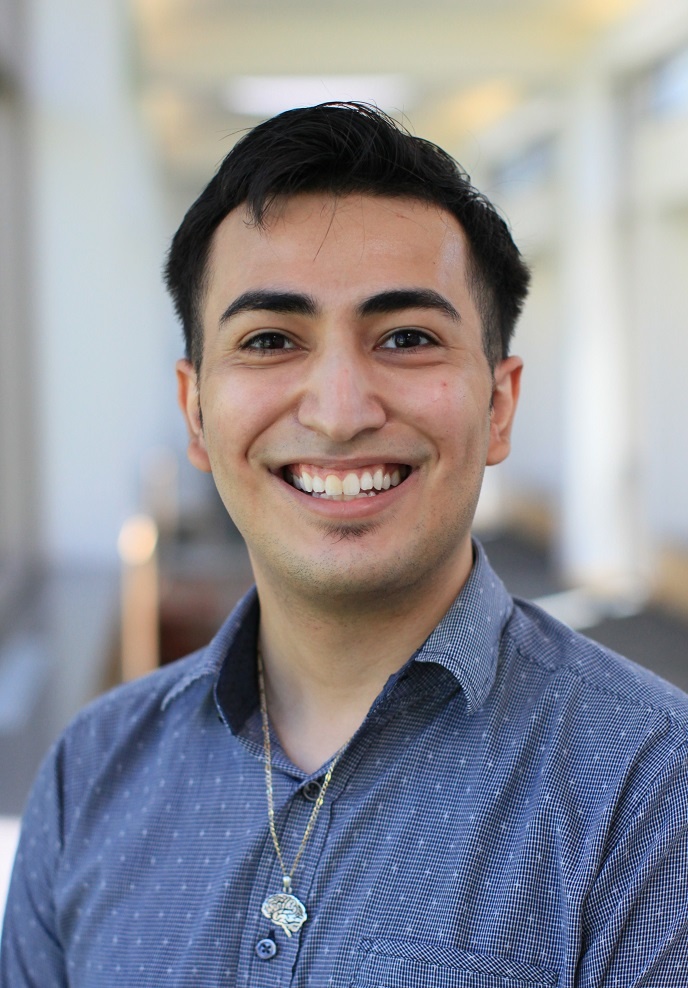
Are Microsoft and OpenAI becoming full-on frenemies?
Hello and welcome to Eye on AI!
Microsoft and OpenAI have long had a complex, codependent relationship that always makes me want to sing Avril Lavigne (“Why’d ya have to go and make things so complicated?”).
It all began with Microsoft’s $1 billion investment in OpenAI in 2019 and ramped up with its $10 billion commitment in 2023, much of which is in the form of cloud compute purchases instead of cash. In exchange, Microsoft got the right to reuse OpenAI’s models and became a minority holder specifically in the profit-making part of OpenAI’s business which, in turn, is “ legally bound to pursue the Nonprofit’s mission.”
That arrangement gives OpenAI some unusual powers. According to OpenAI, its nonprofit board will determine when the company has “ attained AGI ,” or artificial general intelligence—the point at which AI finally outperforms humans at “economically valuable work.” Once the board decides AGI has been reached, such a system will be “excluded from IP licenses and other commercial terms with Microsoft.” Translation: Once OpenAI achieves its stated mission of reaching AGI, Microsoft will presumably not have access to or be able to profit from OpenAI’s AGI-level technology.
Issues around OpenAI’s nonprofit board came to a head when it suddenly fired CEO Sam Altman—and then quickly reinstated him—in November 2023. Before his ouster, Altman said he and Microsoft CEO Satya Nadella were “nowhere near the frenemy territory,” saying the relationship was “really good.”
But recent developments leave me wondering whether the Big Tech behemoth and the $80 billion startup are becoming full-on frenemies. After all, Nadella was blindsided by Altman’s ouster, learning of it just minutes before the rest of the world. And on a joint episode of the Pivot and On with Kara Swisher podcasts during the Altman drama, he said, “One thing, I’ll be very, very clear, is we’re never going to get back into a situation where we get surprised like this, ever again.… That’s done.”
These days, Microsoft is clearly hedging its bets: Less than two months ago, Microsoft announced it was forming a new organization, called Microsoft AI, with Mustafa Suleyman, founder of DeepMind and cofounder of Inflection AI, as its CEO. Microsoft also paid $650 million for the rights to Inflection’s intellectual property. And yesterday, The Information reported that Microsoft is readying a new, massive, state-of-the-art AI model to compete with OpenAI (as well as Google and Anthropic). The new model, internally referred to as MAI-1, is being overseen by Suleyman.
Meanwhile, OpenAI’s Altman appears less focused on the part of the business that benefits Microsoft, and more on the company’s relentless pursuit to reach AGI. Addressing students at Stanford University last week, he honed in on his belief that any cost in support of that mission is justified.
“There is probably some more business-minded person than me at OpenAI somewhere worried about how much we’re spending, but I kinda don’t,” he said . “Whether we burn $500 million a year or $5 billion—or $50 billion a year—I don’t care, I genuinely don’t,” he continued. “As long as we can figure out a way to pay the bills, we’re making AGI. It’s going to be expensive.”
Whether Microsoft will keep investing in OpenAI towards that mega-cost, ill-defined mission remains to be seen. After all, according to an internal email released last week as part of the ongoing U.S. Justice Department antitrust case against Google, Microsoft initially invested in OpenAI in 2019 because it was “very worried” that Google was years ahead in scaling up its AI efforts.
“We are multiple years behind the competition in terms of machine learning scale,” Microsoft’s CTO Kevin Scott wrote in the 2019 email to Nadella and Microsoft founder Bill Gates.
Now, of course, Microsoft is seen as a clear AI leader. Nadella, for his part, appears to be shrewd at playing a multidimensional chess game meant to make sure the company does not fall behind again in AI. At the same time, the OpenAI drama continues: OpenAI is said to be planning the release of an AI-powered search product similar to Perplexity and competitive with Google. But just this morning, The Information reported that OpenAI may postpone an event where its leaders were expected to share updates and show product demonstrations—though it is unclear what the event was about.
So, are Microsoft and OpenAI full-on frenemies at this point? Or will the Nadella-Altman bromance continue? As recently as January, the two showed a united front in a discussion at Davos about their partnership. But either way, it looks like Microsoft isn’t taking any chances.
With that, here’s the AI news.
Sharon Goldman [email protected] @sharongoldman
AI IN THE NEWS
Microsoft-backed Wayve raises $1 billion for its AI-powered self-driving technology. London-based self-driving startup Wayve announced a massive $1.05 billion Series C investment round today, led by SoftBank and including new investor Nvidia and existing investor Microsoft. Founded in 2017, Wayve was the first to develop and test a fully autonomous driving system powered by deep learning on public roads. Today, the company said that it's building foundation models for autonomous driving, similar to a 'GPT for driving.’
Met Gala taps custom OpenAI chatbot. Chatbots are certainly showing up everywhere—but do they really need to be at the Met Gala? Still, the Wall Street Journal reported yesterday that this year’s exhibition featured a custom chatbot installation powered by GPT-4 associated with a wedding gown from the 1930s once worn by New York socialite Natalie Potter. The chatbot answered visitor questions about her life and dress, in the persona of Potter.
Apple is reportedly getting into the AI chip business. According to the Wall Street Journal , Apple has been working on developing its own chip designed to run AI software in data centers. Humorously called Project ACDC (AI Chip Data Center), the effort has apparently been in the works for several years—building on Apple’s longtime efforts to build chips for iPhones, iPads, Apple Watch, and Mac computers. There is no word whether the new chip will be part of Apple’s promised AI product announcements at its Worldwide Developer Conference in June.
Employers are getting hundreds of identical AI-written cover letters from Gen Z applicants. It’s likely no surprise that Gen Z, as digital natives and young job-seekers, are particularly keen to use generative AI like ChatGPT to help with their search. But it might be more shocking to hear that the cohort is less than savvy about how to use the tools so that they help, not harm, their prospects. CNBC interviewed a career consultant who said “employers are getting hundreds of the exact same cover letters word for word,” or answers to job application questions that are the same. Young people “need to educate themselves” on how to use ChatGPT properly and not just to copy answers, the career consultant added.
FORTUNE ON AI
OpenAI’s Sam Altman doesn’t care how much AGI will cost: Even if he spends $50 billion a year, some breakthroughs for mankind are priceless —by Christiaan Hetzner
Runway’s second-ever AI Film Festival walks the line between movie business’ past and its future —by Allie Garflinkle
A 2019 email from Microsoft’s CTO to CEO Satya Nadella and Bill Gates shows how spooked the company was by AI rivals Google and OpenAI — by Paolo Confino
I’m paid $14 an hour to rate AI-generated Google search results. Subcontractors like me do key work but don’t get fair wages or benefits —by Toni Allen
‘Splintered’ AI regulations could harm pursuit of advancements, understanding of where the guardrails lie —by John Kell
Tech leaders crave ‘peace of mind’ with AI in the cloud —by John Kell
AI CALENDAR
May 21-23 : Microsoft Build in Seattle
June 5: FedScoop’s FedTalks 2024 in Washington, D.C.
June 25-27: 2024 IEEE Conference on Artificial Intelligence in Singapore
July 15-17 : Fortune Brainstorm Tech in Park City, Utah (register here )
July 30-31: Fortune Brainstorm AI Singapore (register here )
Aug. 12-14: Ai4 2024 in Las Vegas
AI RESEARCH
Meta researchers say predicting multiple 'tokens' is key to improving LLM speed and accuracy. Large language models may seem amazingly smart, but in fact, they are simply predicting what the next word should be based on the billions of words they have been trained on. To get even more specific, they are trained on pieces of words, called 'tokens.' Typically, LLMs predict one word at a time—but in a recent study , Meta researchers suggest improving the accuracy and speed of AI large language models (LLMs) by making them predict multiple tokens at once. The study found that the technique could become a powerful tool for some LLM applications—making them faster and more accurate, at a lower cost.
An AI deepfake from the Met Gala fooled Katy Perry’s mom. Is this a sign of what’s to come?
The Metropolitan Museum of Art’s annual Gala last night showcased glittering stars and even more sparkling high fashion. But while pop stars Katy Perry and Rhianna did not attend the event, AI images made it seem like they did—and the deepfakes, which were right on theme for the Met’s "Sleeping Beauties: Reawakening Fashion" theme, even fooled Katy Perry’s own mother. The AI image of Katy Perry in a long gown adorned with flowers went viral on social media, while Perry finally posted it on Instagram with the caption “couldn’t make it to the MET, had to work.” It left many wondering: Is this just the tip of the AI deepfake iceberg? The answer is clearly a resounding yes .
Latest in Newsletters

Inside two ‘PayPal Mafia’ members’ plans to turn PayPal’s meteoric rise and internal drama into a Hollywood movie

Banks feel FOMO as SEC rules keep them out of crypto custody

A CEO shares his key ingredient for cooling team conflict

Kate Spade confronted the tragic death of its founder head-on. Now its CEO aims to honor the legacy of that loss

Free education benefits are Papa John’s secret sauce to boosting frontline employee retention

Three founders launched HR software unicorn Gusto – then their moms hit it off
Most popular.

‘Housing has hit rock bottom’: Top real estate CEO says high home prices are shutting people out of the market

Bumble’s Whitney Wolfe Herd says your dating ‘AI concierge’ will soon date hundreds of other people’s ‘concierges’ for you

Google employees grill Sundar Pichai and CFO Ruth Porat on why they’re not getting pay rises amid blowout earnings

A 35-year-old junior Bank of America associate suddenly died—and it’s ignited discussions about Wall Street’s intense working conditions

Hedge fund billionaire Ken Griffin says college protests are the result of a ‘cultural revolution’ and Harvard should ’embrace our Western values’

TikTok’s lawsuit against the U.S. reveals billionaire ByteDance founder Zhang Yiming is living in Singapore while keeping Chinese citizenship
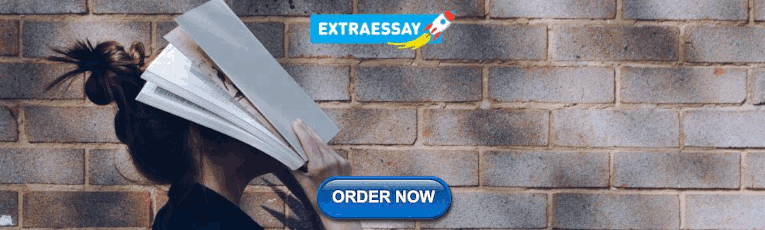
IMAGES
VIDEO
COMMENTS
Drawing upon the philosophical theories of language—that the meaning and inference of a word is dependent on its use—we argue that the context in which use of the term patient occurs is ...
The Journal of Linguistics is an open access journal that is concerned with all branches of theoretical linguistics. Preference is given to original Research Articles which present analyses or generalisations based on sound empirical work, which results in making a clear contribution to current debate in theoretical linguistics.Papers should be accessible to non-specialist linguists with an ...
Journal of Linguistics has as its goal to publish articles that make a clear contribution to current debate in all branches of theoretical linguistics. The journal also provides an excellent survey of recent linguistics publications, with around thirty book reviews in each volume and regular review articles on major works marking important theoretical advances.
Linguistics and Education is a research-oriented journal. Papers may address practical and policy implications for education but must be built on robust research and have a strong conceptual grounding in their analyses and discussions. Linguistics and Education welcomes papers from across disciplinary and interdisciplinary research traditions ...
The Journal of English Linguistics is your premier resource for original linguistic research based on data drawn from the English language, encompassing a broad theoretical and methodological scope. Highlighting theoretically and technologically innovative scholarship, the Journal provides in-depth research and analysis in a variety of areas, including history of English, English grammar ...
Abstract. Our paper examines how selected research articles (RAs) reporting empirical investigations in applied linguistics proceed from first presenting results to eventually offering final conclusions or some other form of closure. After reviewing the literature on relevant aspects of RA structure and its functions, we report the findings of ...
International Association for Applied Linguistics (AILA) AILA (originally founded in 1964 in France) is an international federation of national and regional associations of Applied Linguistics. Find out more. Publishes research into language with relevance to real-world problems. Connections are made between fields, theories, research methods ...
Linguistics publishes articles addressing research questions of current or general relevance that make a significant contribution to our understanding of human language as a system of communication or a cognitive, social and historical phenomenon. This includes aspects of grammatical organization (phonetics, phonology, morphology, syntax) as ...
Language, a journal of the Linguistic Society of America (LSA), has appeared continuously since 1925 (4 issues per year).It publishes scholarly articles that report on original research covering the field of linguistics broadly, thus treating topics that include, among others, linguistic theory (phonology, morphology, syntax, and semantics); language description; language in its social setting ...
Evolution of Applied Linguistics Research Applied linguistics is a relatively youthful field which emerged in the latter of half of the twentieth century; one of the field's flagship journals, Applied Linguistics, published its first issue in 1980 and others are of even more recent vintage. There are common terms that underlie applied ...
The International Journal of Applied Linguistics explores how the knowledge of linguistics is connected to the practical reality of language. This leading linguistics journal is interested in how the particular and the general are inter-related and encourage research which is international in the sense that it shows explicitly how local issues of language use or learning exemplify global concerns.
Besides a division on general linguistics it also includes divisions on English, German, and Romance linguistics. Each of these divisions is subdivided into a form section, a systematic section, and a language section (the latter missing of course in the general linguistics division). ... offers critical survey articles of recent research on ...
Articles on Linguistics. Displaying 1 - 20 of 359 articles. Courtesy Warner Bros. Pictures March 4, 2024 ... Research fellow in Linguistics, Monash University Morten H. Christiansen
The special issue comprises nine substantive research articles together with a comprehensive bibliography dealing with cultural linguistics and world Englishes. ... schemas, categories and metaphors that are central to the cultural-linguistic endeavour. With linguistic data of several text types from West African Englishes, Cameroon English ...
We selected research articles as the academic genre of focus because they are probably the most important type of academic texts that can represent "expert performances" (Bazerman, 1994); and our decision to sample research articles published in Applied Linguistics was driven by its relatively long history (it was established in the 1980s ...
Research on cross-cultural pragmatics in human-human interaction (see e.g. House and Kadar 2021) has demonstrated clearly that there is cross-cultural variation in the way politeness strategies are produced and perceived. Therefore, there is every reason to assume that there is variation in chatbot users' preferences across user groups.
Research articles generate and disseminate disciplinary knowledge in a specific field of study. They are expected to be objective, faceless, and free from expressions of emotion. However, linguistic expressions of confusion that signal authors' affective attitude toward the propositional information are not uncommon in research articles.
High citations most often characterize quality research that reflects the foci of the discipline. This study aims to spotlight the most recent hot topics and the trends looming from the highly cited papers (HCPs) in Web of Science category of linguistics and language & linguistics with bibliometric analysis. The bibliometric information of the 143 HCPs based on Essential Citation Indicators ...
Research - MIT Linguistics
We decided to sample research articles from the journal Applied Linguistics because of its relatively long history (i.e., established in 1980), its broad coverage of topics, and its increasing high impact (e.g., it is ranked the second out of 187 journals in Linguistics and has an IF of 4.286, as shown on its official website on April 23, 2021 ...
Free full-text Linguistics articles. Explore academic scholarship in the Social Sciences and other fields. ... First language attrition (L1) studies are a comparably young and theoretically unspecified field of research in bilingualism. Young, because the first scientifically acclaimed, related article, Andersen's "Determining the ...
The data consisted of single-authored empirical research articles and papers that followed a certain structure (i.e., abstract, introduction, literature review, methodology, results, and conclusion) and were written or published between 2007 and 2015. The four corpora included, in total, 120 applied linguistics research articles and papers.
356 Medical Research Center Iowa City, IA 52242 (319) 335-9968 [email protected]
AI RESEARCH. Meta researchers say predicting multiple 'tokens' is key to improving LLM speed and accuracy. Large language models may seem amazingly smart, but in fact, they are simply predicting ...
Linguistic research on the media has always emphasized the importance of media and their discourses in shaping culture, politics, and social life. It focuses on the macrolevel of discourse structures and on factual genres, particularly news. Most research has been conducted under the umbrella of Critical Discourse Analysis, with an explicit ...
Overall, previous studies have suggested that the distribution of linguistic features in television and film dialog resembles those found in face-to-face conversation. In contrast, research shows that textbook conversations differ considerably from authentic conversations (Le Foll, 2021). Although some textbook conversations can be ...