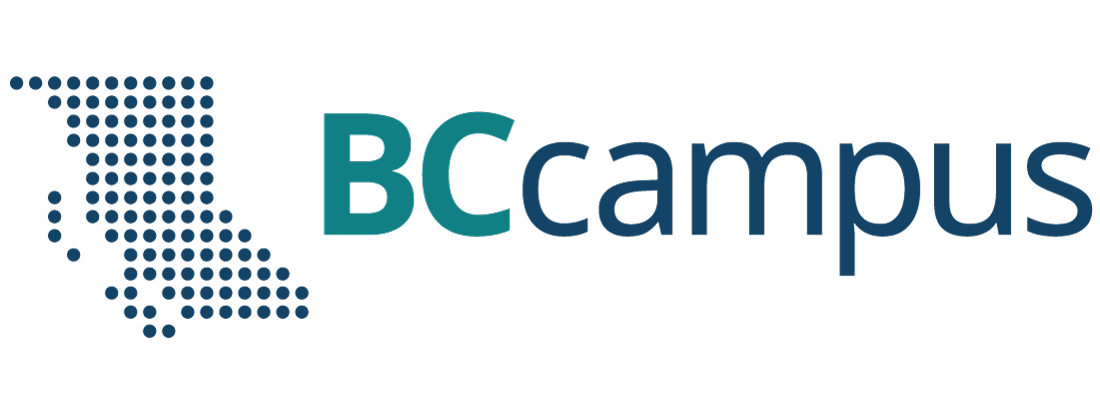
Want to create or adapt books like this? Learn more about how Pressbooks supports open publishing practices.
Chapter 1: Introduction to Research Methods
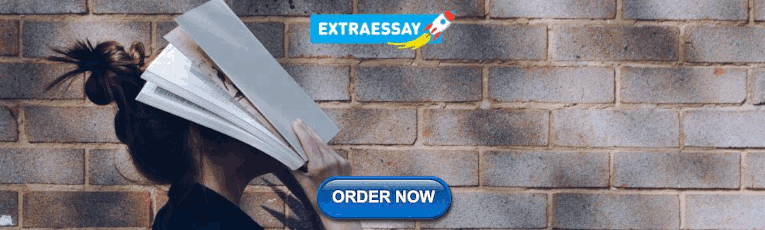
1.4 Understanding Key Research Concepts and Terms
In this textbook you will be exposed to many terms and concepts associated with research methods, particularly as they relate to the research planning decisions you must make along the way. Figure 1.1 will help you contextualize many of these terms and understand the research process. This general chart begins with two key concepts: ontology and epistemology, advances through other concepts, and concludes with three research methodological approaches: qualitative, quantitative and mixed methods.
Research does not end with making decisions about the type of methods you will use; we could argue that the work is just beginning at this point. Figure 1.3 does not represent an all-encompassing list of concepts and terms related to research methods. Keep in mind that each strategy has its own data collection and analysis approaches associated with the various methodological approaches you choose. Figure 1.1 is intentioned to provide a general overview of the research concept. You may want to keep this figure handy as you read through the various chapters.
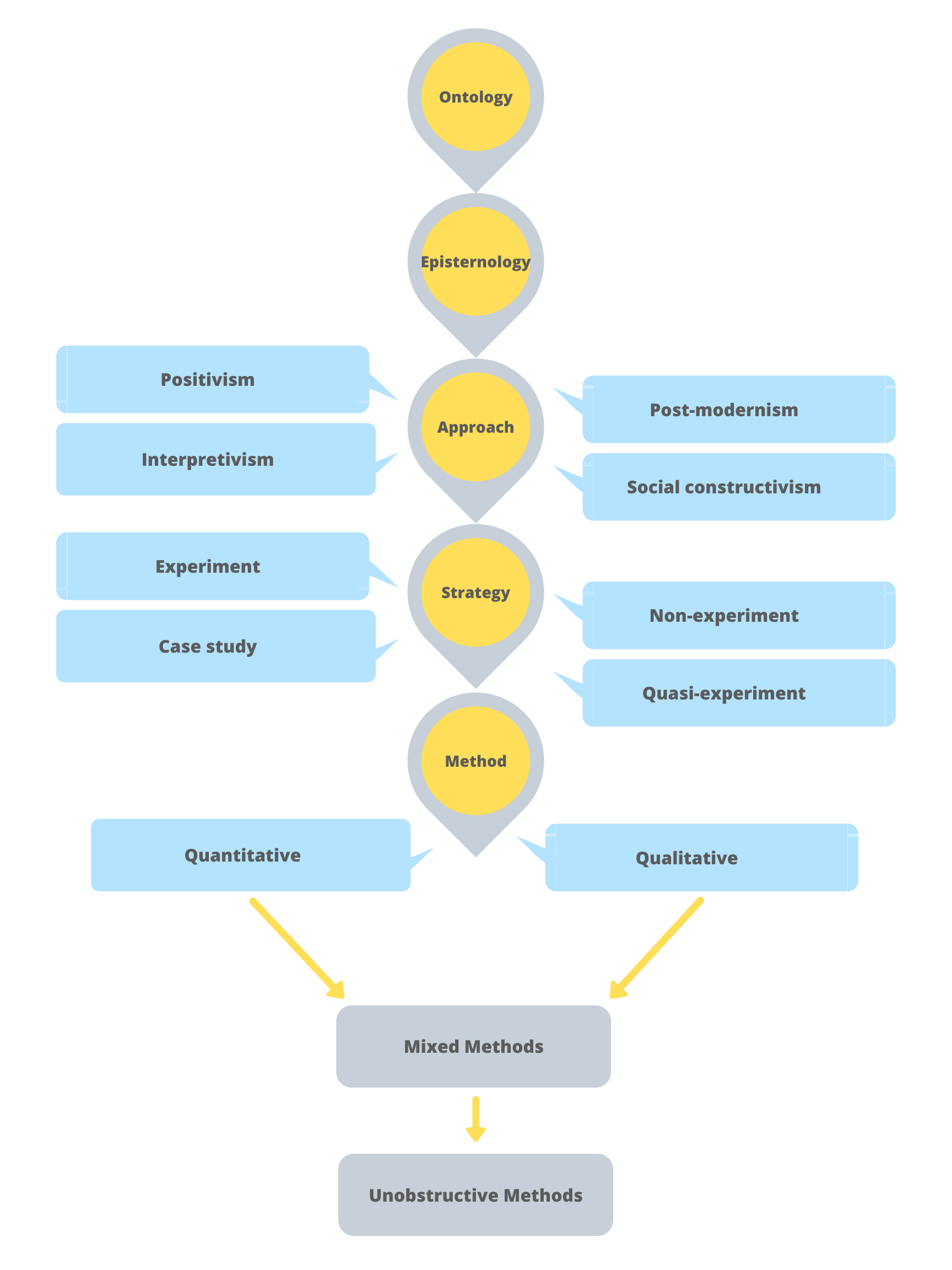
Ontology & Epistemology
Thinking about what you know and how you know what you know involves questions of ontology and epistemology. Perhaps you have heard these concepts before in a philosophy class? These concepts are relevant to the work of sociologists as well. As sociologists (those who undertake socially-focused research), we want to understand some aspect of our social world. Usually, we are not starting with zero knowledge. In fact, we usually start with some understanding of three concepts: 1) what is; 2) what can be known about what is; and, 3) what the best mechanism happens to be for learning about what is (Saylor Academy, 2012). In the following sections, we will define these concepts and provide an example of the terms, ontology and epistemology.
Ontology is a Greek word that means the study, theory, or science of being. Ontology is concerned with the what is or the nature of reality (Saunders, Lewis, & Thornhill, 2009). It can involve some very large and difficult to answer questions, such as:
- What is the purpose of life?
- What, if anything, exists beyond our universe?
- What categories does it belong to?
- Is there such a thing as objective reality?
- What does the verb “to be” mean?
Ontology is comprised of two aspects: objectivism and subjectivism. Objectivism means that social entities exist externally to the social actors who are concerned with their existence. Subjectivism means that social phenomena are created from the perceptions and actions of the social actors who are concerned with their existence (Saunders, et al., 2009). Figure 1.2 provides an example of a similar research project to be undertaken by two different students. While the projects being proposed by the students are similar, they each have different research questions. Read the scenario and then answer the questions that follow.
Subjectivist and objectivist approaches (adapted from Saunders et al., 2009)
Ana is an Emergency & Security Management Studies (ESMS) student at a local college. She is just beginning her capstone research project and she plans to do research at the City of Vancouver. Her research question is: What is the role of City of Vancouver managers in the Emergency Management Department (EMD) in enabling positive community relationships? She will be collecting data related to the roles and duties of managers in enabling positive community relationships.
Robert is also an ESMS student at the same college. He, too, will be undertaking his research at the City of Vancouver. His research question is: What is the effect of the City of Vancouver’s corporate culture in enabling EMD managers to develop a positive relationship with the local community? He will be collecting data related to perceptions of corporate culture and its effect on enabling positive community-emergency management department relationships.
Before the students begin collecting data, they learn that six months ago, the long-time emergency department manager and assistance manager both retired. They have been replaced by two senior staff managers who have Bachelor’s degrees in Emergency Services Management. These new managers are considered more up-to-date and knowledgeable on emergency services management, given their specialized academic training and practical on-the-job work experience in this department. The new managers have essentially the same job duties and operate under the same procedures as the managers they replaced. When Ana and Robert approach the managers to ask them to participate in their separate studies, the new managers state that they are just new on the job and probably cannot answer the research questions; they decline to participate. Ana and Robert are worried that they will need to start all over again with a new research project. They return to their supervisors to get their opinions on what they should do.
Before reading about their supervisors’ responses, answer the following questions:
- Is Ana’s research question indicative of an objectivist or a subjectivist approach?
- Is Robert’s research question indicative of an objectivist or a subjectivist approach?
- Given your answer in question 1, which managers could Ana interview (new, old, or both) for her research study? Why?
- Given your answer in question 2, which managers could Robert interview (new, old, or both) for his research study? Why?
Ana’s supervisor tells her that her research question is set up for an objectivist approach. Her supervisor tells her that in her study the social entity (the City) exists in reality external to the social actors (the managers), i.e., there is a formal management structure at the City that has largely remained unchanged since the old managers left and the new ones started. The procedures remain the same regardless of whoever occupies those positions. As such, Ana, using an objectivist approach, could state that the new managers have job descriptions which describe their duties and that they are a part of a formal structure with a hierarchy of people reporting to them and to whom they report. She could further state that this hierarchy, which is unique to this organization, also resembles hierarchies found in other similar organizations. As such, she can argue that the new managers will be able to speak about the role they play in enabling positive community relationships. Their answers would likely be no different than those of the old managers, because the management structure and the procedures remain the same. Therefore, she could go back to the new managers and ask them to participate in her research study.
Robert’s supervisor tells him that his research is set up for a subjectivist approach. In his study, the social phenomena (the effect of corporate culture on the relationship with the community) is created from the perceptions and consequent actions of the social actors (the managers); i.e., the corporate culture at the City continually influences the process of social interaction, and these interactions influence perceptions of the relationship with the community. The relationship is in a constant state of revision. As such, Robert, using a subjectivist approach, could state that the new managers may have had few interactions with the community members to date and therefore may not be fully cognizant of how the corporate culture affects the department’s relationship with the community. While it would be important to get the new managers’ perceptions, he would also need to speak with the previous managers to get their perceptions from the time they were employed in their positions. This is because the community-department relationship is in a state of constant revision, which is influenced by the various managers’ perceptions of the corporate culture and its effect on their ability to form positive community relationships. Therefore, he could go back to the current managers and ask them to participate in his study, and also ask that the department please contact the previous managers to see if they would be willing to participate in his study.
As you can see the research question of each study guides the decision as to whether the researcher should take a subjective or an objective ontological approach. This decision, in turn, guides their approach to the research study, including whom they should interview.
Epistemology
Epistemology has to do with knowledge. Rather than dealing with questions about what is, epistemology deals with questions of how we know what is. In sociology, there are many ways to uncover knowledge. We might interview people to understand public opinion about a topic, or perhaps observe them in their natural environment. We could avoid face-to-face interaction altogether by mailing people surveys to complete on their own or by reading people’s opinions in newspaper editorials. Each method of data collection comes with its own set of epistemological assumptions about how to find things out (Saylor Academy, 2012). There are two main subsections of epistemology: positivist and interpretivist philosophies. We will examine these philosophies or paradigms in the following sections.
Research Methods for the Social Sciences: An Introduction Copyright © 2020 by Valerie Sheppard is licensed under a Creative Commons Attribution-NonCommercial-ShareAlike 4.0 International License , except where otherwise noted.
Share This Book
- Skip to main content
- Skip to primary sidebar
- Skip to footer
- QuestionPro

- Solutions Industries Gaming Automotive Sports and events Education Government Travel & Hospitality Financial Services Healthcare Cannabis Technology Use Case NPS+ Communities Audience Contactless surveys Mobile LivePolls Member Experience GDPR Positive People Science 360 Feedback Surveys
- Resources Blog eBooks Survey Templates Case Studies Training Help center

Home Market Research
What is Research: Definition, Methods, Types & Examples
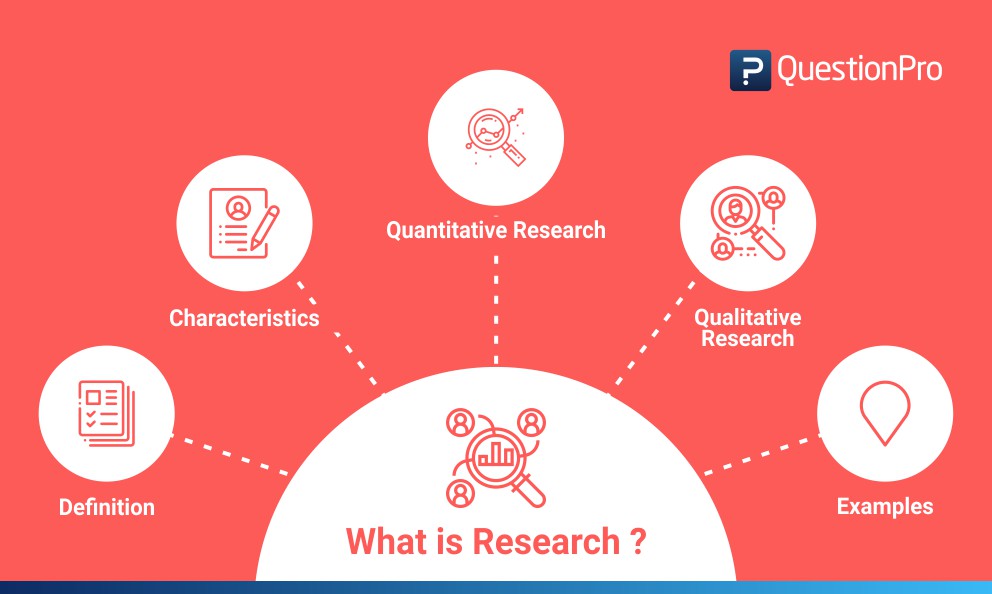
The search for knowledge is closely linked to the object of study; that is, to the reconstruction of the facts that will provide an explanation to an observed event and that at first sight can be considered as a problem. It is very human to seek answers and satisfy our curiosity. Let’s talk about research.
Content Index
What is Research?
What are the characteristics of research.
- Comparative analysis chart
Qualitative methods
Quantitative methods, 8 tips for conducting accurate research.
Research is the careful consideration of study regarding a particular concern or research problem using scientific methods. According to the American sociologist Earl Robert Babbie, “research is a systematic inquiry to describe, explain, predict, and control the observed phenomenon. It involves inductive and deductive methods.”
Inductive methods analyze an observed event, while deductive methods verify the observed event. Inductive approaches are associated with qualitative research , and deductive methods are more commonly associated with quantitative analysis .
Research is conducted with a purpose to:
- Identify potential and new customers
- Understand existing customers
- Set pragmatic goals
- Develop productive market strategies
- Address business challenges
- Put together a business expansion plan
- Identify new business opportunities
- Good research follows a systematic approach to capture accurate data. Researchers need to practice ethics and a code of conduct while making observations or drawing conclusions.
- The analysis is based on logical reasoning and involves both inductive and deductive methods.
- Real-time data and knowledge is derived from actual observations in natural settings.
- There is an in-depth analysis of all data collected so that there are no anomalies associated with it.
- It creates a path for generating new questions. Existing data helps create more research opportunities.
- It is analytical and uses all the available data so that there is no ambiguity in inference.
- Accuracy is one of the most critical aspects of research. The information must be accurate and correct. For example, laboratories provide a controlled environment to collect data. Accuracy is measured in the instruments used, the calibrations of instruments or tools, and the experiment’s final result.
What is the purpose of research?
There are three main purposes:
- Exploratory: As the name suggests, researchers conduct exploratory studies to explore a group of questions. The answers and analytics may not offer a conclusion to the perceived problem. It is undertaken to handle new problem areas that haven’t been explored before. This exploratory data analysis process lays the foundation for more conclusive data collection and analysis.
LEARN ABOUT: Descriptive Analysis
- Descriptive: It focuses on expanding knowledge on current issues through a process of data collection. Descriptive research describe the behavior of a sample population. Only one variable is required to conduct the study. The three primary purposes of descriptive studies are describing, explaining, and validating the findings. For example, a study conducted to know if top-level management leaders in the 21st century possess the moral right to receive a considerable sum of money from the company profit.
LEARN ABOUT: Best Data Collection Tools
- Explanatory: Causal research or explanatory research is conducted to understand the impact of specific changes in existing standard procedures. Running experiments is the most popular form. For example, a study that is conducted to understand the effect of rebranding on customer loyalty.
Here is a comparative analysis chart for a better understanding:
It begins by asking the right questions and choosing an appropriate method to investigate the problem. After collecting answers to your questions, you can analyze the findings or observations to draw reasonable conclusions.
When it comes to customers and market studies, the more thorough your questions, the better the analysis. You get essential insights into brand perception and product needs by thoroughly collecting customer data through surveys and questionnaires . You can use this data to make smart decisions about your marketing strategies to position your business effectively.
To make sense of your study and get insights faster, it helps to use a research repository as a single source of truth in your organization and manage your research data in one centralized data repository .
Types of research methods and Examples
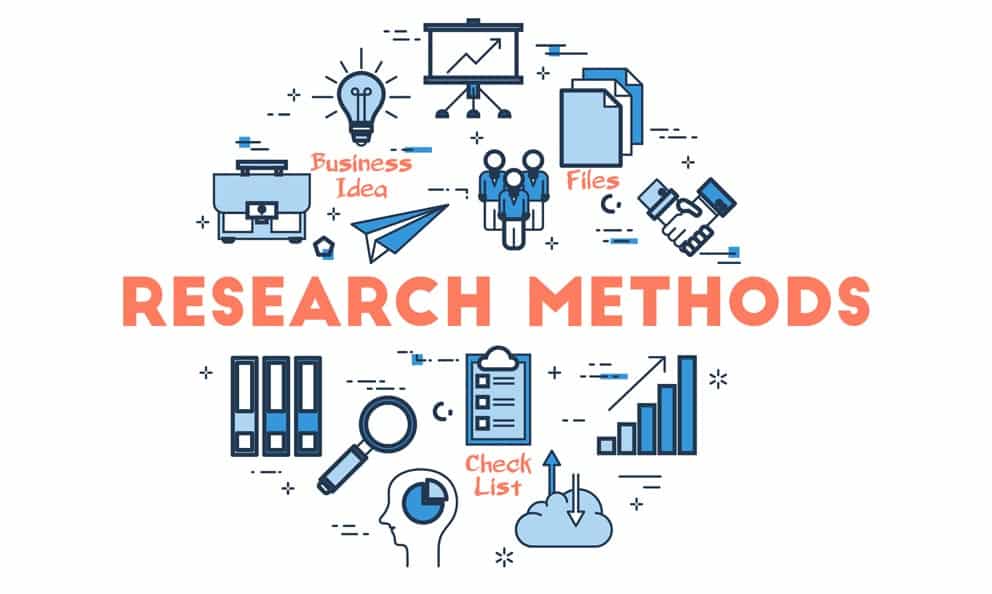
Research methods are broadly classified as Qualitative and Quantitative .
Both methods have distinctive properties and data collection methods .
Qualitative research is a method that collects data using conversational methods, usually open-ended questions . The responses collected are essentially non-numerical. This method helps a researcher understand what participants think and why they think in a particular way.
Types of qualitative methods include:
- One-to-one Interview
- Focus Groups
- Ethnographic studies
- Text Analysis
Quantitative methods deal with numbers and measurable forms . It uses a systematic way of investigating events or data. It answers questions to justify relationships with measurable variables to either explain, predict, or control a phenomenon.
Types of quantitative methods include:
- Survey research
- Descriptive research
- Correlational research
LEARN MORE: Descriptive Research vs Correlational Research
Remember, it is only valuable and useful when it is valid, accurate, and reliable. Incorrect results can lead to customer churn and a decrease in sales.
It is essential to ensure that your data is:
- Valid – founded, logical, rigorous, and impartial.
- Accurate – free of errors and including required details.
- Reliable – other people who investigate in the same way can produce similar results.
- Timely – current and collected within an appropriate time frame.
- Complete – includes all the data you need to support your business decisions.
Gather insights
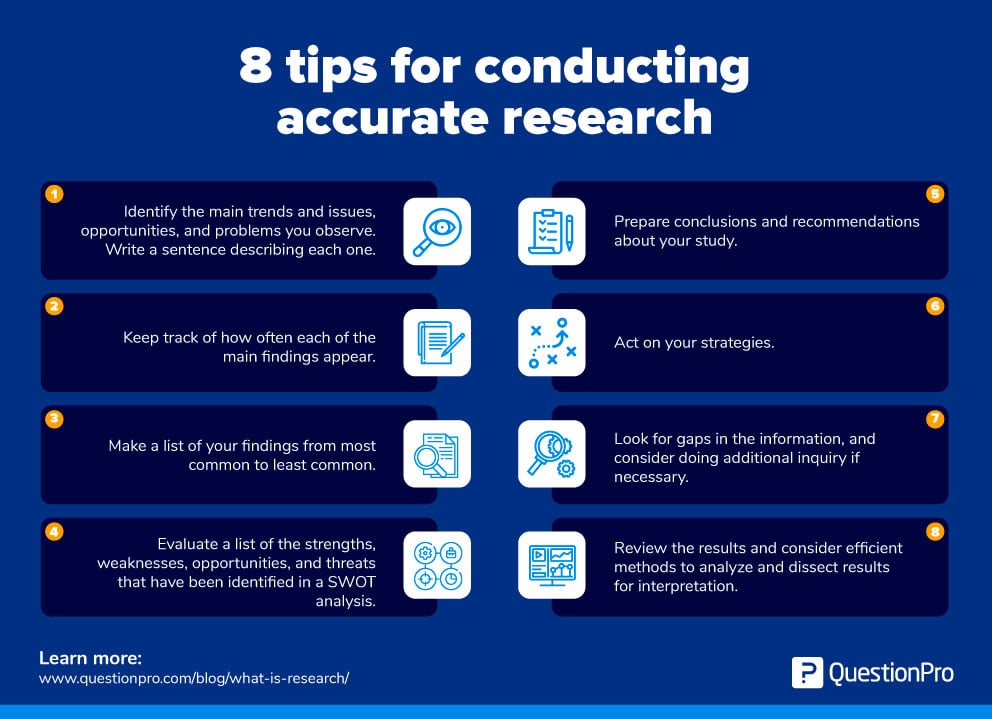
- Identify the main trends and issues, opportunities, and problems you observe. Write a sentence describing each one.
- Keep track of the frequency with which each of the main findings appears.
- Make a list of your findings from the most common to the least common.
- Evaluate a list of the strengths, weaknesses, opportunities, and threats identified in a SWOT analysis .
- Prepare conclusions and recommendations about your study.
- Act on your strategies
- Look for gaps in the information, and consider doing additional inquiry if necessary
- Plan to review the results and consider efficient methods to analyze and interpret results.
Review your goals before making any conclusions about your study. Remember how the process you have completed and the data you have gathered help answer your questions. Ask yourself if what your analysis revealed facilitates the identification of your conclusions and recommendations.
LEARN MORE ABOUT OUR SOFTWARE FREE TRIAL
MORE LIKE THIS
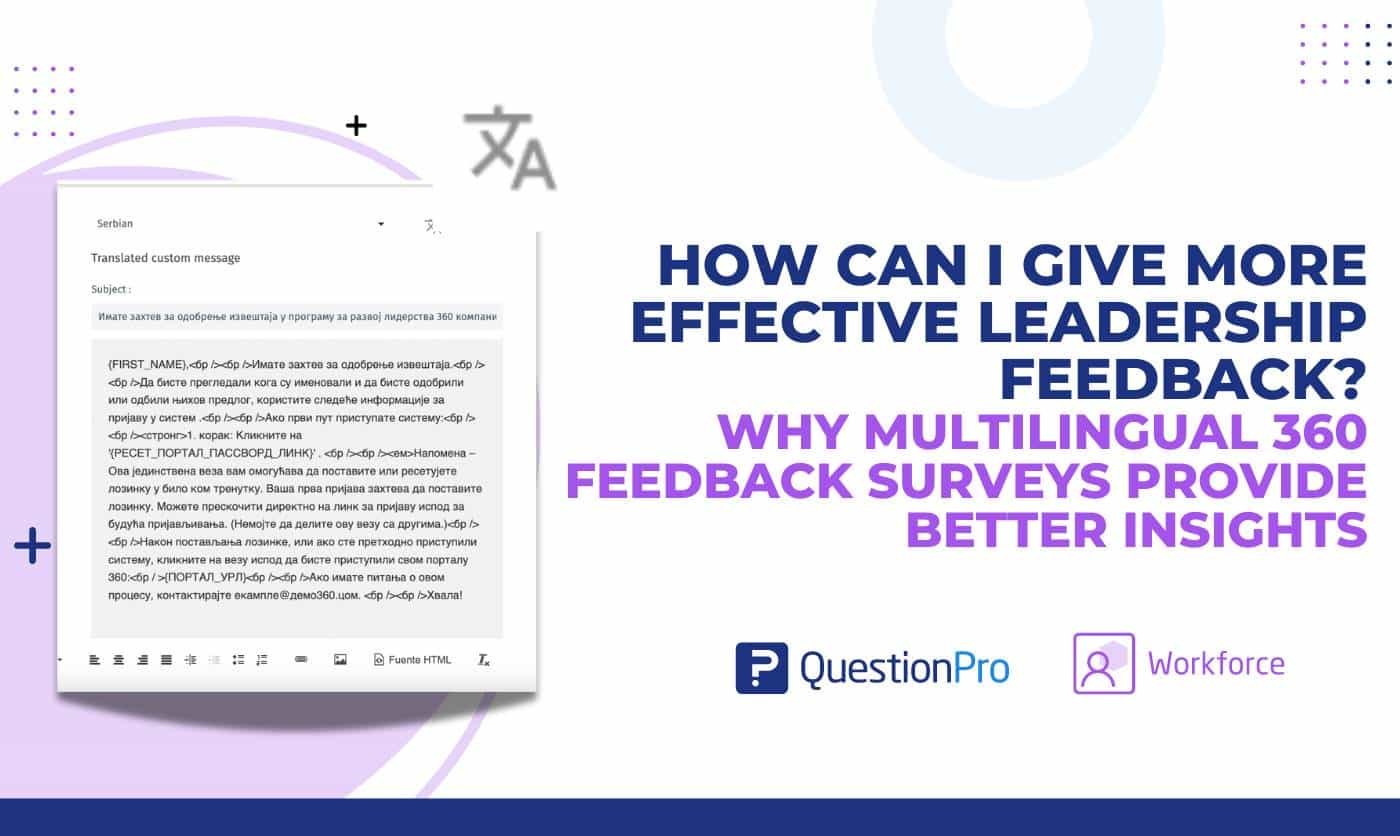
Why Multilingual 360 Feedback Surveys Provide Better Insights
Jun 3, 2024
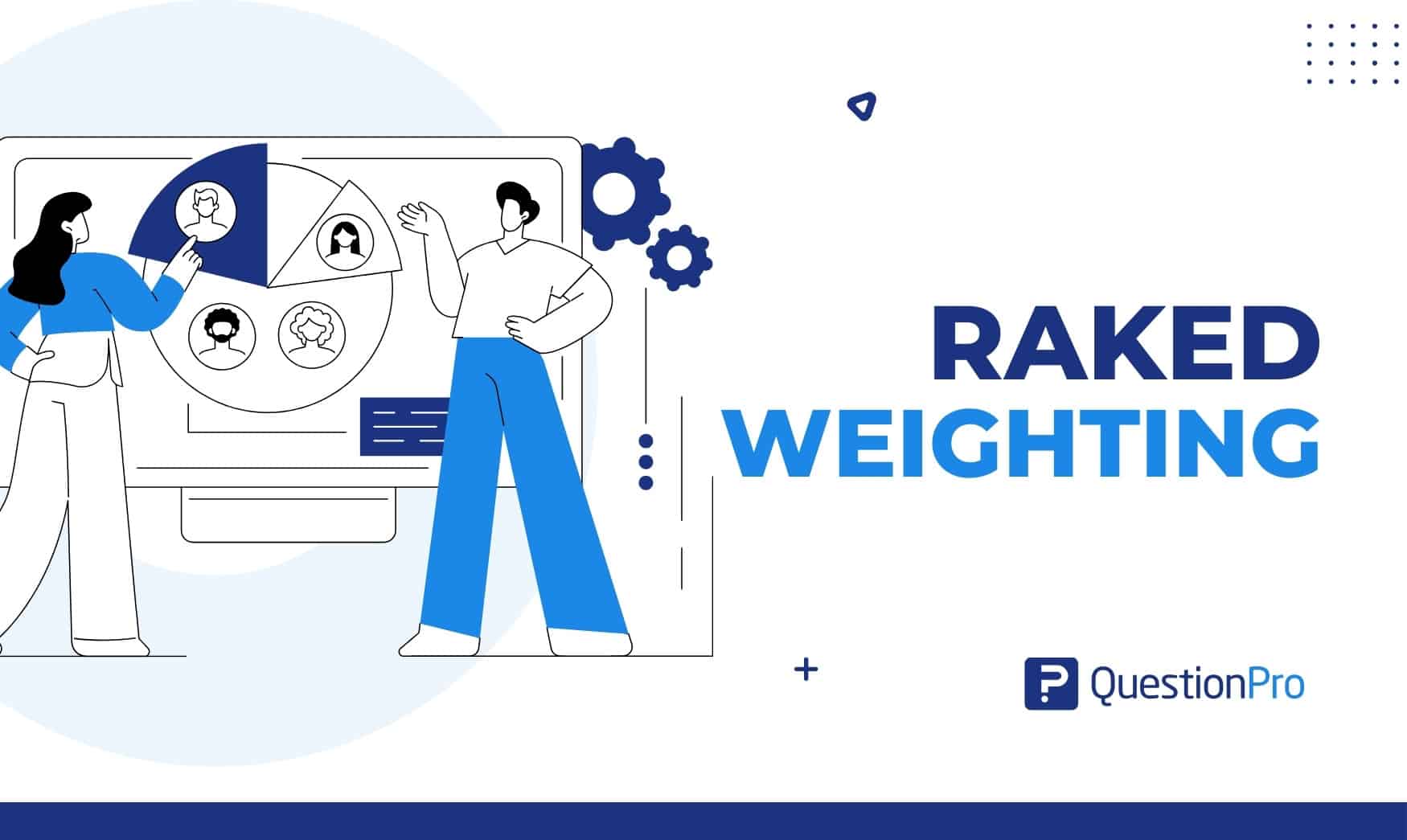
Raked Weighting: A Key Tool for Accurate Survey Results
May 31, 2024
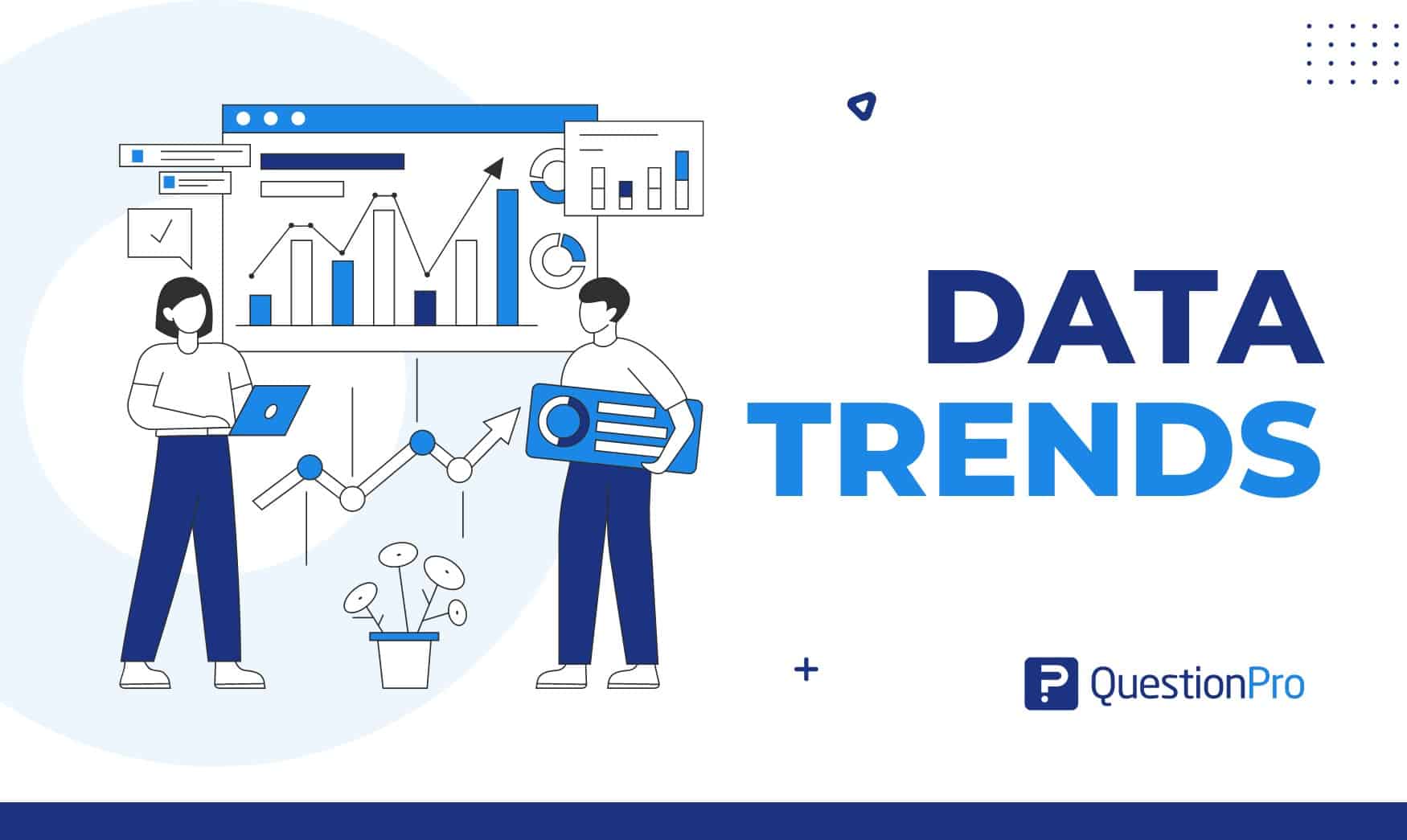
Top 8 Data Trends to Understand the Future of Data
May 30, 2024
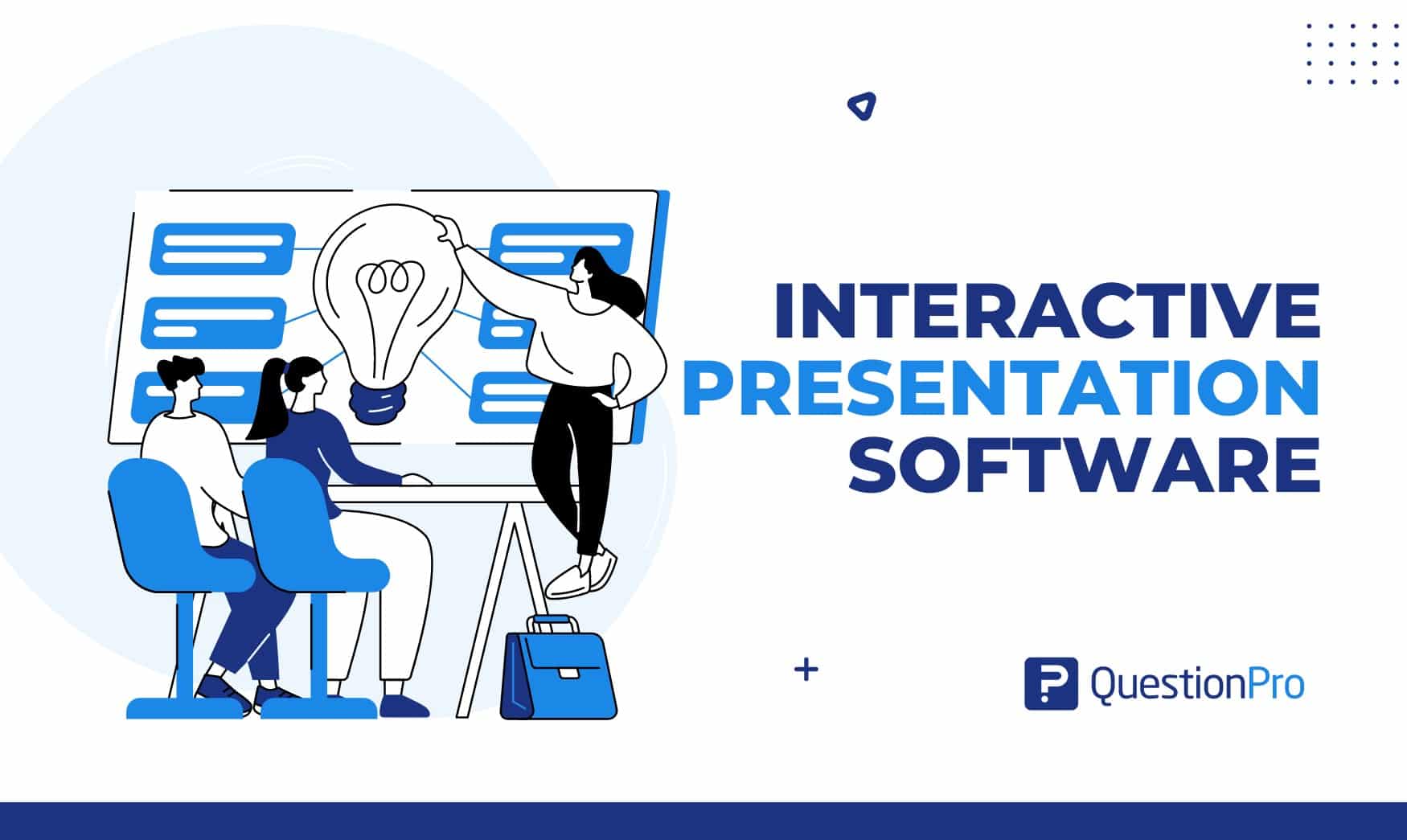
Top 12 Interactive Presentation Software to Engage Your User
May 29, 2024
Other categories
- Academic Research
- Artificial Intelligence
- Assessments
- Brand Awareness
- Case Studies
- Communities
- Consumer Insights
- Customer effort score
- Customer Engagement
- Customer Experience
- Customer Loyalty
- Customer Research
- Customer Satisfaction
- Employee Benefits
- Employee Engagement
- Employee Retention
- Friday Five
- General Data Protection Regulation
- Insights Hub
- Life@QuestionPro
- Market Research
- Mobile diaries
- Mobile Surveys
- New Features
- Online Communities
- Question Types
- Questionnaire
- QuestionPro Products
- Release Notes
- Research Tools and Apps
- Revenue at Risk
- Survey Templates
- Training Tips
- Uncategorized
- Video Learning Series
- What’s Coming Up
- Workforce Intelligence

Getting Started: Library Research Strategy
- Choosing Your Topic
- Gathering Background Information
- Defining Key Terms
- Crafting a Research Question
- Gathering Relevant Information
- Evaluating Sources This link opens in a new window
- Formulating a Thesis Statement
- Avoiding Plagiarism This link opens in a new window
- Citation Styles This link opens in a new window
If you have chosen a topic, you may break the topic down into a few main concepts and then list and/or define key terms related to that concept. If you have performed some background searching, you can include some of the words that were used to describe your topic.
For example, if your topic deals with the relationship between teenage smoking and advertising in the United States, the following key terms may apply:
smoking -- tobacco -- nicotine -- cigarettes
teenage -- adolescents -- children -- teens -- youth
advertising -- marketing -- media -- commercials -- TV -- billboards
When listing the key terms or concepts of your topic, be sure to consider synonyms for these terms as well. Since research is an iterative process, you will also find additional key terms to utilize through the resources you encounter throughout your research process.
- << Previous: Gathering Background Information
- Next: Crafting a Research Question >>
- Last Updated: Mar 11, 2024 4:57 PM
- URL: https://libguides.chapman.edu/strategy
Key concepts in research
Key concepts, action research.
In education, action research typically refers to a cycle of reflective inquiry to understand and improve practice. The cycle typically involves the steps of identifying the problem, developing a research plan, collecting and analysing data, incorporating findings into planning, implementing actions, and monitoring and evaluation.
An approach is the term AERO uses to refer to a practice, program or policy.
association (or correlation)
An association is when there is a relationship between two elements, factors or events, but the association cannot be proved or explained. Associations can be positive (for example, higher socioeconomic status is associated with higher student achievement) or negative (for example, higher student absenteeism is associated with lower student achievement).
A baseline is information from an initial point in time, often used for comparison to see how things change over time.
causation (or cause(s)/causal/causal evidence)
Causation is when one element, factor or event is known to cause another (for example, a particular teaching practice is known to lead to improvements in student test scores). To prove causation between two things (let’s call them A and B ), researchers need to show: 1. that there is an association between A and B ; 2. that A happens before B ; and 3. that B is not caused by a third thing (that is, C or D ). In education settings, proving causation is often challenging because of the many influences on teacher and student outcomes.
comparison groups (or control groups)
A comparison group is a group of people in a research study whose responses or outcomes function as a comparison against which the effect of the approach being tested can be measured. Comparison groups receive a different treatment to the group receiving the treatment or approach being tested. There can be any number of comparison groups in a study. A comparison group is called a ‘control group’ when it receives no treatment at all.
context (or contextual factors)
Context is the social, cultural and environmental factors found in research settings. Taking context into account in research studies is important because context can affect the outcomes of research (i.e. evidence generated in one context may not necessarily apply to a different context). Evidence is most relevant when it has been generated in a context similar to the context in which it will be applied. Examples of ‘context’ may include location, demographics of research participants, or the level of organisational support for the particular approach being researched.
Data is information that is collected and analysed in order to produce findings and/or to inform decision-making. Data can be qualitative (for example, teacher observations or quotes from students) or quantitative (for example, student test scores or attendance data).
effective/ness
An educational approach is effective if it causes (see causation above) a desired change in a particular outcome. This desired change can be an increase in an outcome (for example, increases in student achievement) or it can be a decrease in an outcome (for example, reduction in student absenteeism).
empirical research
Empirical research presents observable data to substantiate its claims. This data may be primary data (observation and measurement of phenomena or events directly experienced by the researcher) or secondary data (data that has already been collected by other researchers).
Evaluation is the systematic and objective assessment of an approach. Evaluation provides evidence of what has been done well, what could be done better, the extent to which objectives have been achieved and/or the impact of the approach. This evidence can then be used to inform ongoing decision-making regarding the approach.
evidence (or education evidence)
Evidence is any type of information that supports an assertion, hypothesis or claim. There are many types of evidence in education, including insights drawn from child or student assessments, classroom observations, recommendations from popular education books and findings from research studies and syntheses. AERO refers to two types of evidence in its work:
- research evidence: This is academic research, such as causal research or synthesis research, which uses rigorous methods to provide insights into educational practice.
- practitioner-generated evidence: This is evidence generated through practitioners in their daily practice (for example, teacher observations, information gained from formative assessments or insights from student feedback on teacher practice).
evidence-based practice
Evidence-based practices are educational approaches that are backed up by research evidence. This means there is broad consensus from rigorously conducted evaluations that they work.
evidence-informed practice
Evidence-informed practice is an educational approach that is applied using evidence from research together with a practitioner’s professional expertise and judgement. The expertise and judgement used by practitioners can be based on knowledge or understanding of their children and students, or the environment in which they work.
experimental design
Experimental design is the process of planning an experiment that can establish a ‘cause and effect’ relationship (that is, an experiment to determine the specific factors that influence an outcome). Experimental designs account for all other factors that could influence an outcome, so the cause of an effect can be isolated.
generalisable
Findings from a piece of research are generalisable if they are:
- a fair representation of trends in the wider population from which the study participants were sampled and/or
- applicable to settings or contexts other than those in which the study was conducted.
hierarchy of evidence
Hierarchies of evidence are sometimes used to rank evidence according to rigour, helping people to compare and evaluate the quality of different types of research evidence. The higher up on the hierarchy, the more rigorous the methodology. Instead of a hierarchy, AERO uses Standards of evidence – a continuum of four levels of confidence along which rigour and relevance increase.
history effect
A history effect is the descriptive term for influences that occur at the same time as an approach is being evaluated and/or influences that occur between the approach being implemented and the outcomes being measured. For example, researchers may want to know the effect of a particular teacher’s writing program on student writing test scores. However, to do this they need to separate the effects of any influences that occur simultaneously (for example, other teachers using different writing strategies with these students) and/or those that occur in the two weeks between the implementation of the writing program and the writing test (for example, a whole-school writing celebration).
hypothesise
To hypothesise is to put forward an assumption or idea so that it can be tested to see whether it might be true.
intervention (or treatment)
An approach that is applied to address a problem is sometimes referred to as an intervention ; for example, a teacher may implement a certain early literacy intervention to support struggling readers. In a research study, an intervention is the approach that is being investigated, tested or evaluated. An intervention is sometimes called a ‘treatment’ in a research study.
literature review
A literature review identifies, evaluates and synthesises the relevant literature within a particular field of research. It usually discusses common and emerging approaches, notable patterns and trends, areas of conflict and controversies, and gaps within the relevant literature. Literature reviews do not usually explicitly state the methods used to identify, evaluate or synthesise the relevant literature.
maturation effect
Maturation effects are the effects in a setting where an approach is applied that occur naturally (that would have occurred anyway), as opposed to the effects that occur as a result of the approach. For example, researchers may want to know the effect of a particular educational program on student social and emotional skills. However, social and emotional skills develop over time as children mature, and so researchers need to distinguish between the effect of the educational program and the effects of natural development as a result of students getting older.
meta-analysis
A meta-analysis uses statistical methods to summarise the results of individual studies. It is designed to assess the behaviours that lead to a particular approach working and/or to provide an estimate of how much more likely one approach is to work over another. It is the quantitative version of a literature review or systematic review.
mixed-methods research
Mixed-methods research is research that uses both qualitative (non-numerical data) and quantitative (numerical data) research methods.
A monograph is an academic piece of writing on a single subject or aspect of a subject that presents the findings of primary research and/or original scholarship. It is usually written by one person.
outcome measure
An outcome measure is an observation that can be used to measure the effect of a particular approach. Outcome measures can be qualitative (such as quotes or observations) or quantitative (such as test scores). For example, when examining whether a particular approach helps students understand a concept, a teacher could set an assessment. The student assessment score could then be used as an outcome measure of student understanding.
peer review
Peer review is the assessment of research by others working in the same or a related field. The assessment is based on the expertise and experience of the researcher undertaking the review and should be impartial and independent.
A pilot study, pilot project or pilot experiment is a small-scale trial that is conducted in order to test the effects of an approach before implementing it on a larger scale. A pilot project can also help to determine feasibility, cost, adverse events and necessary improvements to the approach.
positive effect
If a study shows that an approach leads to the desired outcome, it is said to have a positive effect . Conversely, if a study shows that an approach has the opposite of the desired outcome, it is said to have a negative effect.
primary study
A primary study is an individual study which reports on data collected and analysed by the researchers themselves. Primary studies are designed according to the type of research question being answered - for example, they may use qualitative methods, quantitative methods, or be mixed-methods research. The findings from a number of primary studies may be synthesised in meta-analyses, systematic reviews, rapid reviews or literature reviews.
qualitative methods
Qualitative methods involve collecting and analysing non-numerical data, and may include observations, interviews, questionnaires, focus groups, and documents and artifact analysis. Qualitative methods can be used to understand concepts, opinions or experiences as well as to gather in-depth insights into a problem or generate new ideas.
quantitative methods
Quantitative methods involve collecting and analysing numerical data. Quantitative methods are generally used to find patterns and averages, make predictions, test causal relationships and generalise results to wider populations.
quasi-experimental design
A quasi-experimental design is a research methodology that aims to establish a ‘cause and effect’ relationship (that is, to determine the specific factors that influence an outcome), but it cannot completely eliminate all factors that could influence an outcome (that is, there may still be an element of subjectiveness in the findings).
randomised controlled trial
A randomised controlled trial is a trial of a particular approach that is set up in such as a way that allows researchers to test its effects. In a randomised controlled trial, subjects are randomly assigned to one of two groups: one receiving the approach) that is being tested (the experimental group), and the other receiving an alternative approach or no approach (the comparison group or control). After the trial period, differences between the groups can be attributed to the approach being tested. Researchers and teachers who use randomisation must take into account ethical concerns, such as whether it is ethical to withhold treatment from subjects in the comparison group.
rapid review
A rapid review is an evidence-based (or ‘objective’) approach to searching and synthesising research evidence. It uses similar steps to a systematic review, but simplifies or skips some steps so that findings can be reached more quickly (making them more current). Rapid reviews answer a precise, clearly defined question and explicitly outline: the methods for data collection, the methods for data extraction, the number of papers included in the review and the methods for data analysis.
relevant evidence
Relevant evidence is evidence produced in contexts that are similar to one’s own context. Evidence can also be considered relevant when it is derived from a large number of studies conducted over a wide range of contexts.
research (types)
Research is ‘the creation of new knowledge and/or the use of existing knowledge in a new and creative way so as to generate new concepts, methodologies, inventions and understandings’ (Australian Research Council, 2015). There are many types of research. For example:
- exploratory research involves investigating an issue or problem. It aims to better understand this problem and sometimes leads to the formation of hypotheses or theories about the problem.
- descriptive research describes a population, situation or event that is being studied. It focuses on developing knowledge about what exists and what is happening.
- causal research (also known as ‘ evaluative research’ ) uses experimentation to determine whether a cause-and-effect relationship exists between two or more elements, features or factors.
- synthesis research combines, compares and links existing information to provide a summary and/or new insights or information about a given topic.
research methods
Research methods are the methods used to conduct research. Research methods are generally classified as ‘qualitative’ or ‘quantitative’. When both methods are used, it is referred to as ‘mixed methods’ research. Qualitative methods involve collecting and analysing non-numerical data (such as observations, interviews, questionnaires, focus groups, documents and artifacts). Qualitative methods can be used to understand concepts, opinions or experiences as well as to gather in-depth insights into a problem or generate new ideas. Quantitative methods involve collecting and analysing numerical data. Quantitative methods are generally used to find patterns and averages, make predictions, test causal relationships and generalise results to wider populations.
rigour (rigorous evidence)
Evidence is considered rigorous when it proves that a particular approach causes a particular outcome. Rigorous evidence is produced by using specialised research methods that can identify the impact of one particular influence. The most common research method used to produce rigorous evidence is the randomised controlled trial. However, there are many other methods that can produce rigorous evidence, whether qualitative, quantitative or mixed methods. What is important in producing rigorous evidence is that the research method can rule out the effects of as many other influences as possible.
A rubric is a set of criteria that can be used to make consistent judgements. In education settings, a rubric is usually used to help assess learning or development in a particular area.
sample size
When studying a large population, it is not possible to include every individual. Research studies usually include a certain number of individuals to represent the population. Those that are included in the study are referred to as a sample of the population. Sample size refers to the number of people in a sample. Generally, the larger the sample size, the more accurate the research findings. If a sample is too small, it will not provide a fair picture of the whole population.
selection bias
Selection bias is when the sample in a study does not represent the general population. Selection bias can occur in two ways: 1. when individuals selected in a research study have characteristics that make them different to the general population; or 2. when individuals opt into a research study and have characteristics different to the general population. Selection bias can affect the outcome of a study, as it is possible that any effect detected by the research is due to the specific characteristics of the sample, rather than the approach itself.
seminal research
Seminal research is a term used to describe studies that are recognised within a particular discipline as presenting an idea of significant and enduring importance or influence.
statistical significance
A data analysis result is statistically significant when it is likely to be true, rather than by chance. Researchers often use statistical significance to describe the confidence in their results.
systematic review
A systematic review is an evidence-based (or ‘objective’) approach to a literature review. Systematic reviews answer a precise, clearly defined question to produce evidence to underpin a piece of research. A systematic review must explicitly outline: the methods for data collection, the methods for data extraction, the number of papers included in the review, and the methods for data analysis.
Validation is the process of determining whether the way you are measuring something is appropriate given the research aims and conclusions of the study. There are many considerations when determining whether the way you measure is ‘appropriate’. These include but are not limited to:
- whether the way you measure is reliable (for example, will different researchers score a teacher in the same way when using this observation framework?)
- whether it provides data that accurately represents the outcome (for example, is a student’s score on this twenty-question reading comprehension test an accurate reflection of their reading ability?)
- whether the way you measure should be used given the consequences (for example, should we rely on this data when deciding whether to ask a student to repeat a year?).
- Evidence - use & generation
- Actionable insights into Australian education
- Assessing research evidence
- Searching for research: Worksheet
- Interactive evidence decision-making tool
- Assessing whether evidence is relevant to your context
Australia's national education evidence body
Qualitative vs Quantitative Research Methods & Data Analysis
Saul Mcleod, PhD
Editor-in-Chief for Simply Psychology
BSc (Hons) Psychology, MRes, PhD, University of Manchester
Saul Mcleod, PhD., is a qualified psychology teacher with over 18 years of experience in further and higher education. He has been published in peer-reviewed journals, including the Journal of Clinical Psychology.
Learn about our Editorial Process
Olivia Guy-Evans, MSc
Associate Editor for Simply Psychology
BSc (Hons) Psychology, MSc Psychology of Education
Olivia Guy-Evans is a writer and associate editor for Simply Psychology. She has previously worked in healthcare and educational sectors.
On This Page:
What is the difference between quantitative and qualitative?
The main difference between quantitative and qualitative research is the type of data they collect and analyze.
Quantitative research collects numerical data and analyzes it using statistical methods. The aim is to produce objective, empirical data that can be measured and expressed in numerical terms. Quantitative research is often used to test hypotheses, identify patterns, and make predictions.
Qualitative research , on the other hand, collects non-numerical data such as words, images, and sounds. The focus is on exploring subjective experiences, opinions, and attitudes, often through observation and interviews.
Qualitative research aims to produce rich and detailed descriptions of the phenomenon being studied, and to uncover new insights and meanings.
Quantitative data is information about quantities, and therefore numbers, and qualitative data is descriptive, and regards phenomenon which can be observed but not measured, such as language.
What Is Qualitative Research?
Qualitative research is the process of collecting, analyzing, and interpreting non-numerical data, such as language. Qualitative research can be used to understand how an individual subjectively perceives and gives meaning to their social reality.
Qualitative data is non-numerical data, such as text, video, photographs, or audio recordings. This type of data can be collected using diary accounts or in-depth interviews and analyzed using grounded theory or thematic analysis.
Qualitative research is multimethod in focus, involving an interpretive, naturalistic approach to its subject matter. This means that qualitative researchers study things in their natural settings, attempting to make sense of, or interpret, phenomena in terms of the meanings people bring to them. Denzin and Lincoln (1994, p. 2)
Interest in qualitative data came about as the result of the dissatisfaction of some psychologists (e.g., Carl Rogers) with the scientific study of psychologists such as behaviorists (e.g., Skinner ).
Since psychologists study people, the traditional approach to science is not seen as an appropriate way of carrying out research since it fails to capture the totality of human experience and the essence of being human. Exploring participants’ experiences is known as a phenomenological approach (re: Humanism ).
Qualitative research is primarily concerned with meaning, subjectivity, and lived experience. The goal is to understand the quality and texture of people’s experiences, how they make sense of them, and the implications for their lives.
Qualitative research aims to understand the social reality of individuals, groups, and cultures as nearly as possible as participants feel or live it. Thus, people and groups are studied in their natural setting.
Some examples of qualitative research questions are provided, such as what an experience feels like, how people talk about something, how they make sense of an experience, and how events unfold for people.
Research following a qualitative approach is exploratory and seeks to explain ‘how’ and ‘why’ a particular phenomenon, or behavior, operates as it does in a particular context. It can be used to generate hypotheses and theories from the data.
Qualitative Methods
There are different types of qualitative research methods, including diary accounts, in-depth interviews , documents, focus groups , case study research , and ethnography.
The results of qualitative methods provide a deep understanding of how people perceive their social realities and in consequence, how they act within the social world.
The researcher has several methods for collecting empirical materials, ranging from the interview to direct observation, to the analysis of artifacts, documents, and cultural records, to the use of visual materials or personal experience. Denzin and Lincoln (1994, p. 14)
Here are some examples of qualitative data:
Interview transcripts : Verbatim records of what participants said during an interview or focus group. They allow researchers to identify common themes and patterns, and draw conclusions based on the data. Interview transcripts can also be useful in providing direct quotes and examples to support research findings.
Observations : The researcher typically takes detailed notes on what they observe, including any contextual information, nonverbal cues, or other relevant details. The resulting observational data can be analyzed to gain insights into social phenomena, such as human behavior, social interactions, and cultural practices.
Unstructured interviews : generate qualitative data through the use of open questions. This allows the respondent to talk in some depth, choosing their own words. This helps the researcher develop a real sense of a person’s understanding of a situation.
Diaries or journals : Written accounts of personal experiences or reflections.
Notice that qualitative data could be much more than just words or text. Photographs, videos, sound recordings, and so on, can be considered qualitative data. Visual data can be used to understand behaviors, environments, and social interactions.
Qualitative Data Analysis
Qualitative research is endlessly creative and interpretive. The researcher does not just leave the field with mountains of empirical data and then easily write up his or her findings.
Qualitative interpretations are constructed, and various techniques can be used to make sense of the data, such as content analysis, grounded theory (Glaser & Strauss, 1967), thematic analysis (Braun & Clarke, 2006), or discourse analysis.
For example, thematic analysis is a qualitative approach that involves identifying implicit or explicit ideas within the data. Themes will often emerge once the data has been coded .
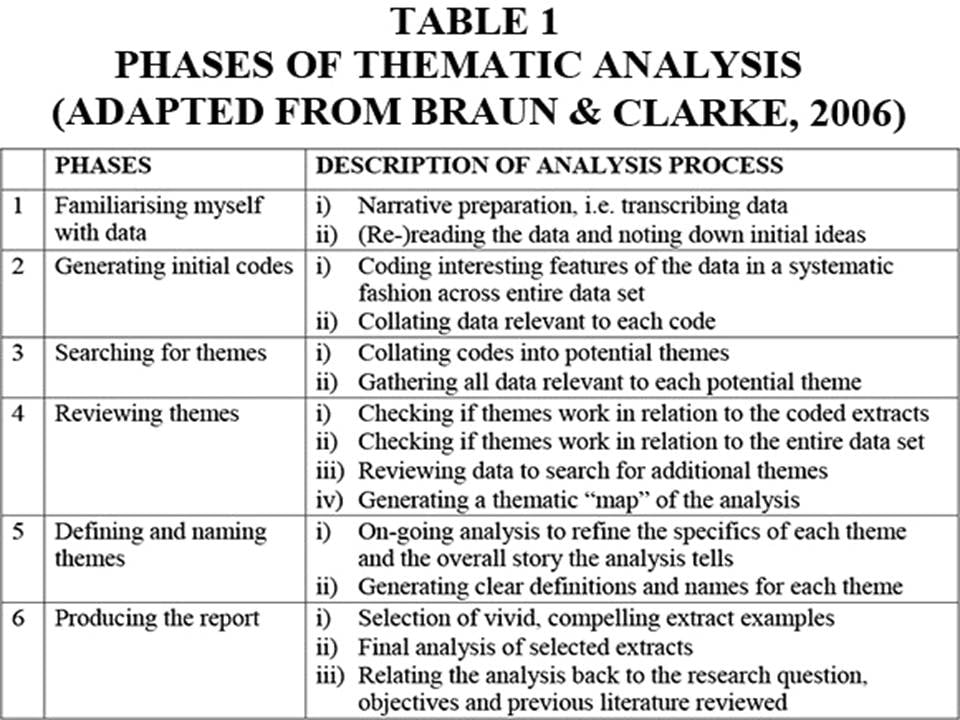
Key Features
- Events can be understood adequately only if they are seen in context. Therefore, a qualitative researcher immerses her/himself in the field, in natural surroundings. The contexts of inquiry are not contrived; they are natural. Nothing is predefined or taken for granted.
- Qualitative researchers want those who are studied to speak for themselves, to provide their perspectives in words and other actions. Therefore, qualitative research is an interactive process in which the persons studied teach the researcher about their lives.
- The qualitative researcher is an integral part of the data; without the active participation of the researcher, no data exists.
- The study’s design evolves during the research and can be adjusted or changed as it progresses. For the qualitative researcher, there is no single reality. It is subjective and exists only in reference to the observer.
- The theory is data-driven and emerges as part of the research process, evolving from the data as they are collected.
Limitations of Qualitative Research
- Because of the time and costs involved, qualitative designs do not generally draw samples from large-scale data sets.
- The problem of adequate validity or reliability is a major criticism. Because of the subjective nature of qualitative data and its origin in single contexts, it is difficult to apply conventional standards of reliability and validity. For example, because of the central role played by the researcher in the generation of data, it is not possible to replicate qualitative studies.
- Also, contexts, situations, events, conditions, and interactions cannot be replicated to any extent, nor can generalizations be made to a wider context than the one studied with confidence.
- The time required for data collection, analysis, and interpretation is lengthy. Analysis of qualitative data is difficult, and expert knowledge of an area is necessary to interpret qualitative data. Great care must be taken when doing so, for example, looking for mental illness symptoms.
Advantages of Qualitative Research
- Because of close researcher involvement, the researcher gains an insider’s view of the field. This allows the researcher to find issues that are often missed (such as subtleties and complexities) by the scientific, more positivistic inquiries.
- Qualitative descriptions can be important in suggesting possible relationships, causes, effects, and dynamic processes.
- Qualitative analysis allows for ambiguities/contradictions in the data, which reflect social reality (Denscombe, 2010).
- Qualitative research uses a descriptive, narrative style; this research might be of particular benefit to the practitioner as she or he could turn to qualitative reports to examine forms of knowledge that might otherwise be unavailable, thereby gaining new insight.
What Is Quantitative Research?
Quantitative research involves the process of objectively collecting and analyzing numerical data to describe, predict, or control variables of interest.
The goals of quantitative research are to test causal relationships between variables , make predictions, and generalize results to wider populations.
Quantitative researchers aim to establish general laws of behavior and phenomenon across different settings/contexts. Research is used to test a theory and ultimately support or reject it.
Quantitative Methods
Experiments typically yield quantitative data, as they are concerned with measuring things. However, other research methods, such as controlled observations and questionnaires , can produce both quantitative information.
For example, a rating scale or closed questions on a questionnaire would generate quantitative data as these produce either numerical data or data that can be put into categories (e.g., “yes,” “no” answers).
Experimental methods limit how research participants react to and express appropriate social behavior.
Findings are, therefore, likely to be context-bound and simply a reflection of the assumptions that the researcher brings to the investigation.
There are numerous examples of quantitative data in psychological research, including mental health. Here are a few examples:
Another example is the Experience in Close Relationships Scale (ECR), a self-report questionnaire widely used to assess adult attachment styles .
The ECR provides quantitative data that can be used to assess attachment styles and predict relationship outcomes.
Neuroimaging data : Neuroimaging techniques, such as MRI and fMRI, provide quantitative data on brain structure and function.
This data can be analyzed to identify brain regions involved in specific mental processes or disorders.
For example, the Beck Depression Inventory (BDI) is a clinician-administered questionnaire widely used to assess the severity of depressive symptoms in individuals.
The BDI consists of 21 questions, each scored on a scale of 0 to 3, with higher scores indicating more severe depressive symptoms.
Quantitative Data Analysis
Statistics help us turn quantitative data into useful information to help with decision-making. We can use statistics to summarize our data, describing patterns, relationships, and connections. Statistics can be descriptive or inferential.
Descriptive statistics help us to summarize our data. In contrast, inferential statistics are used to identify statistically significant differences between groups of data (such as intervention and control groups in a randomized control study).
- Quantitative researchers try to control extraneous variables by conducting their studies in the lab.
- The research aims for objectivity (i.e., without bias) and is separated from the data.
- The design of the study is determined before it begins.
- For the quantitative researcher, the reality is objective, exists separately from the researcher, and can be seen by anyone.
- Research is used to test a theory and ultimately support or reject it.
Limitations of Quantitative Research
- Context: Quantitative experiments do not take place in natural settings. In addition, they do not allow participants to explain their choices or the meaning of the questions they may have for those participants (Carr, 1994).
- Researcher expertise: Poor knowledge of the application of statistical analysis may negatively affect analysis and subsequent interpretation (Black, 1999).
- Variability of data quantity: Large sample sizes are needed for more accurate analysis. Small-scale quantitative studies may be less reliable because of the low quantity of data (Denscombe, 2010). This also affects the ability to generalize study findings to wider populations.
- Confirmation bias: The researcher might miss observing phenomena because of focus on theory or hypothesis testing rather than on the theory of hypothesis generation.
Advantages of Quantitative Research
- Scientific objectivity: Quantitative data can be interpreted with statistical analysis, and since statistics are based on the principles of mathematics, the quantitative approach is viewed as scientifically objective and rational (Carr, 1994; Denscombe, 2010).
- Useful for testing and validating already constructed theories.
- Rapid analysis: Sophisticated software removes much of the need for prolonged data analysis, especially with large volumes of data involved (Antonius, 2003).
- Replication: Quantitative data is based on measured values and can be checked by others because numerical data is less open to ambiguities of interpretation.
- Hypotheses can also be tested because of statistical analysis (Antonius, 2003).
Antonius, R. (2003). Interpreting quantitative data with SPSS . Sage.
Black, T. R. (1999). Doing quantitative research in the social sciences: An integrated approach to research design, measurement and statistics . Sage.
Braun, V. & Clarke, V. (2006). Using thematic analysis in psychology . Qualitative Research in Psychology , 3, 77–101.
Carr, L. T. (1994). The strengths and weaknesses of quantitative and qualitative research : what method for nursing? Journal of advanced nursing, 20(4) , 716-721.
Denscombe, M. (2010). The Good Research Guide: for small-scale social research. McGraw Hill.
Denzin, N., & Lincoln. Y. (1994). Handbook of Qualitative Research. Thousand Oaks, CA, US: Sage Publications Inc.
Glaser, B. G., Strauss, A. L., & Strutzel, E. (1968). The discovery of grounded theory; strategies for qualitative research. Nursing research, 17(4) , 364.
Minichiello, V. (1990). In-Depth Interviewing: Researching People. Longman Cheshire.
Punch, K. (1998). Introduction to Social Research: Quantitative and Qualitative Approaches. London: Sage
Further Information
- Designing qualitative research
- Methods of data collection and analysis
- Introduction to quantitative and qualitative research
- Checklists for improving rigour in qualitative research: a case of the tail wagging the dog?
- Qualitative research in health care: Analysing qualitative data
- Qualitative data analysis: the framework approach
- Using the framework method for the analysis of
- Qualitative data in multi-disciplinary health research
- Content Analysis
- Grounded Theory
- Thematic Analysis

Related Articles
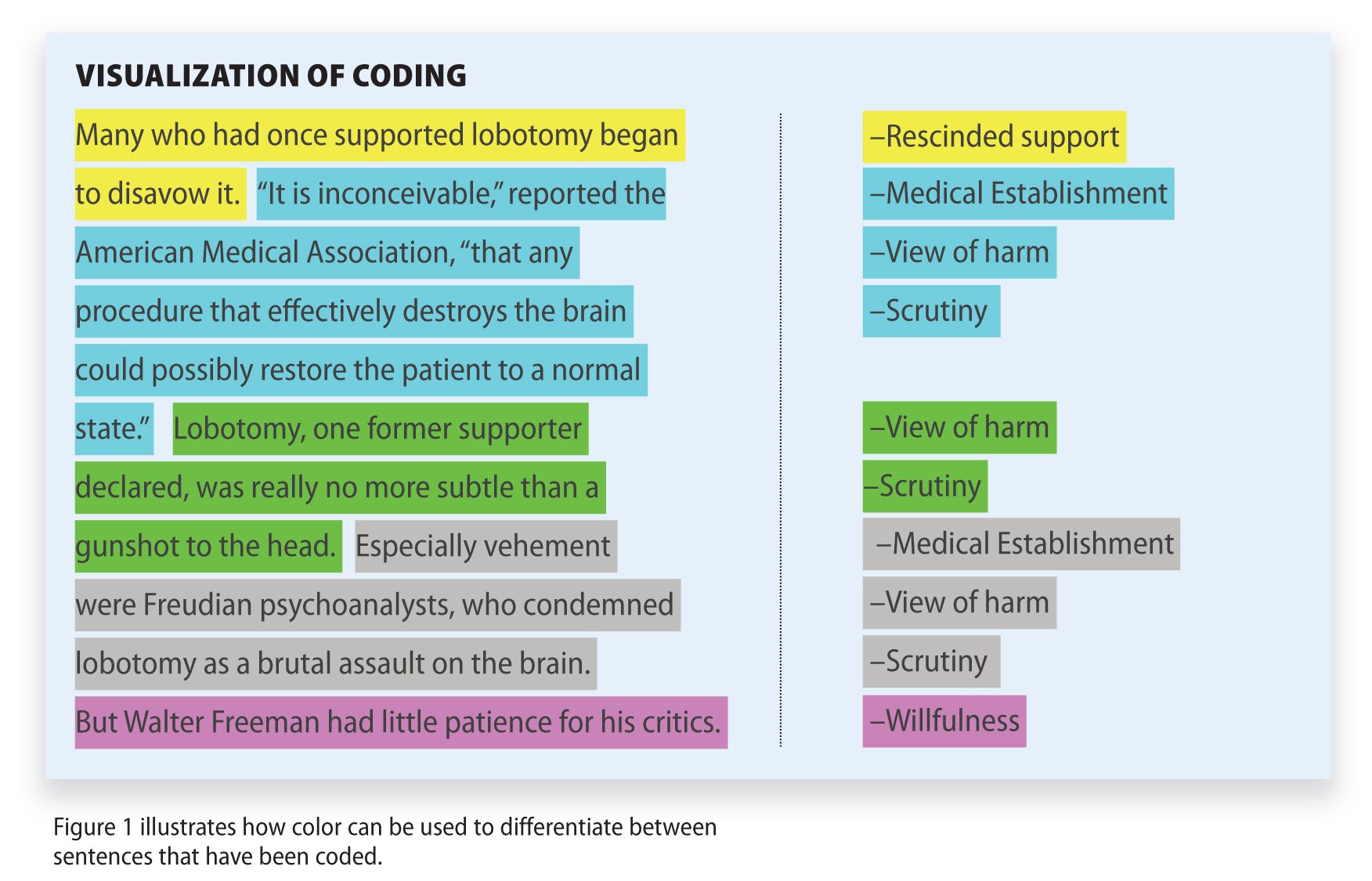
Research Methodology
Qualitative Data Coding
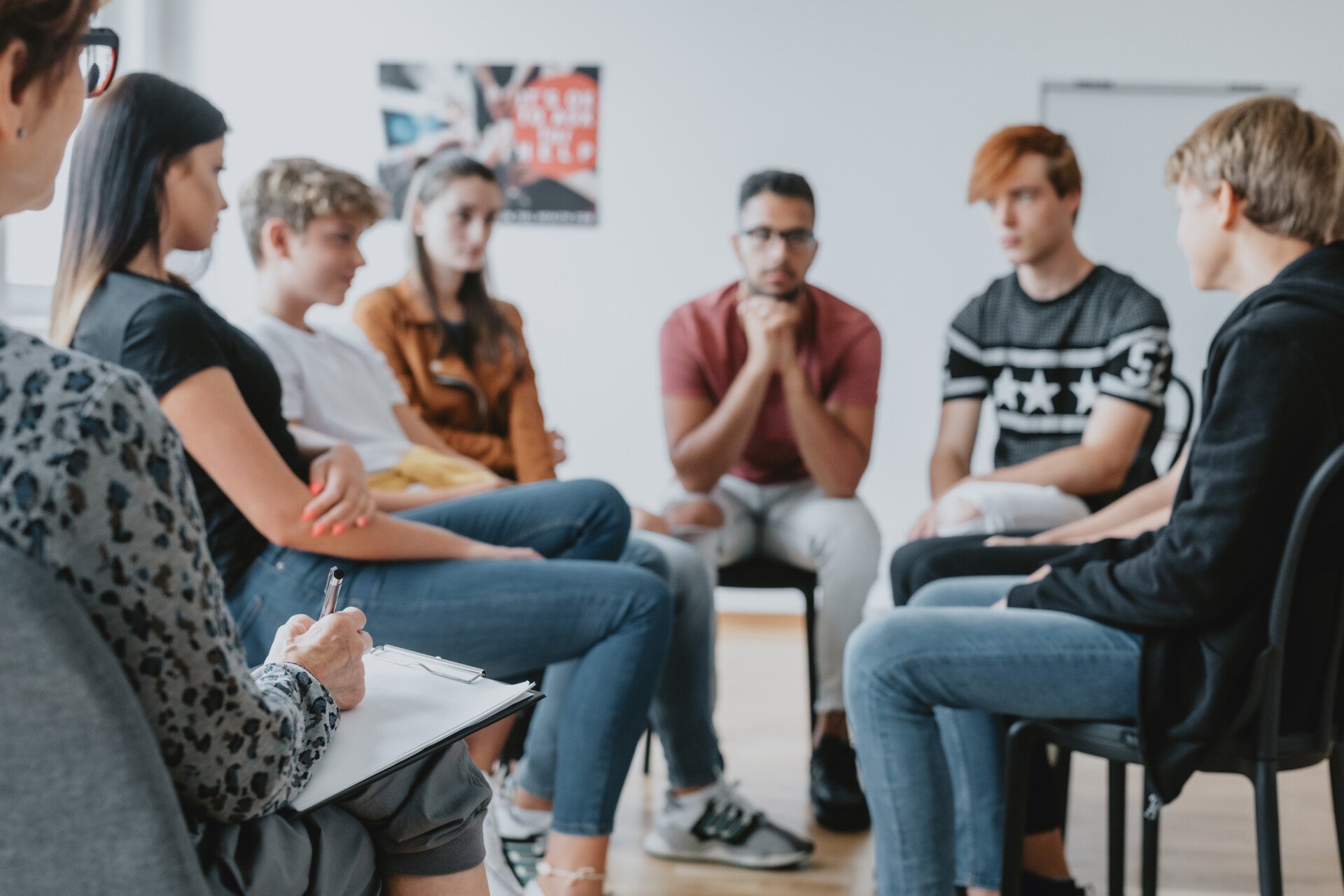
What Is a Focus Group?
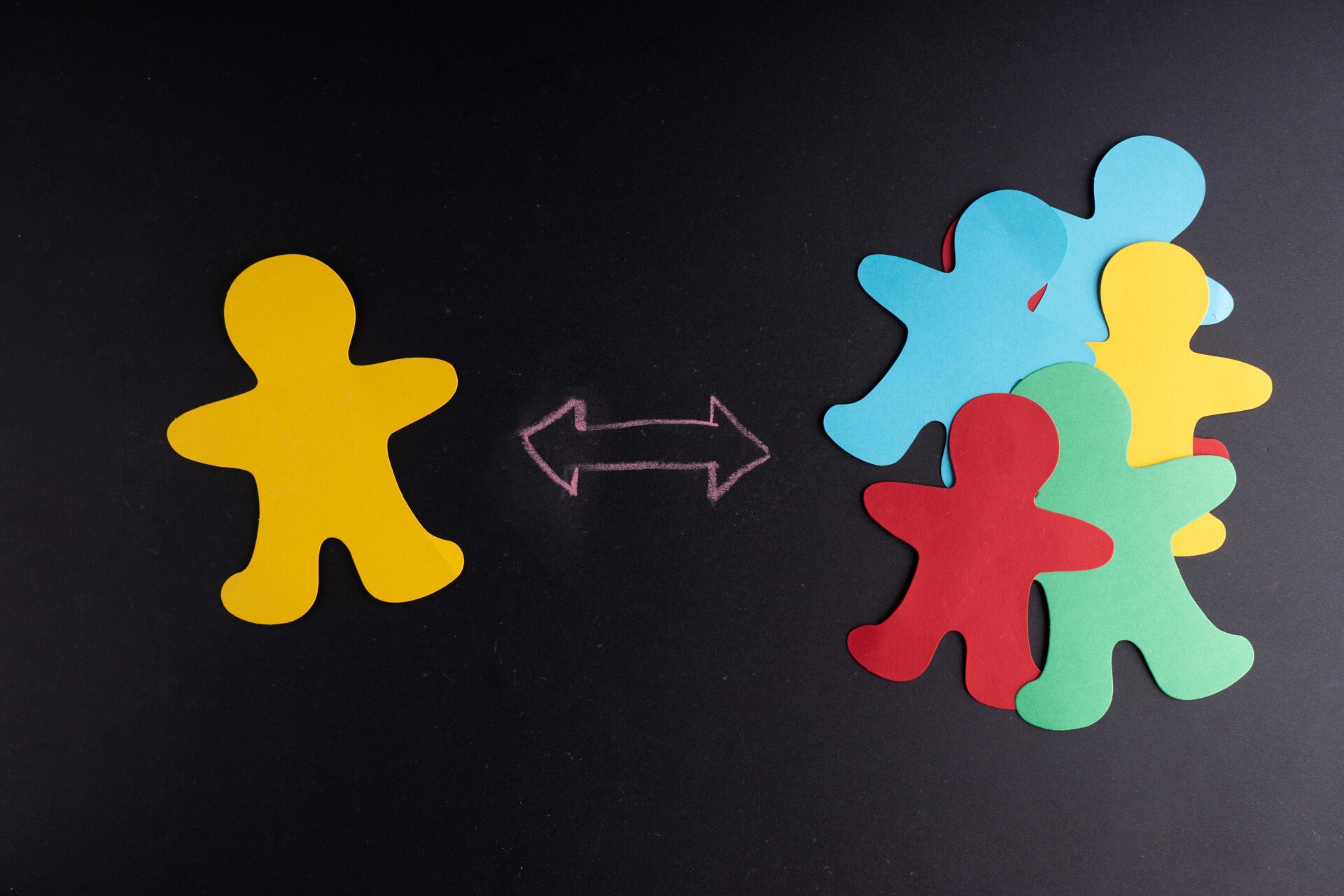
Cross-Cultural Research Methodology In Psychology
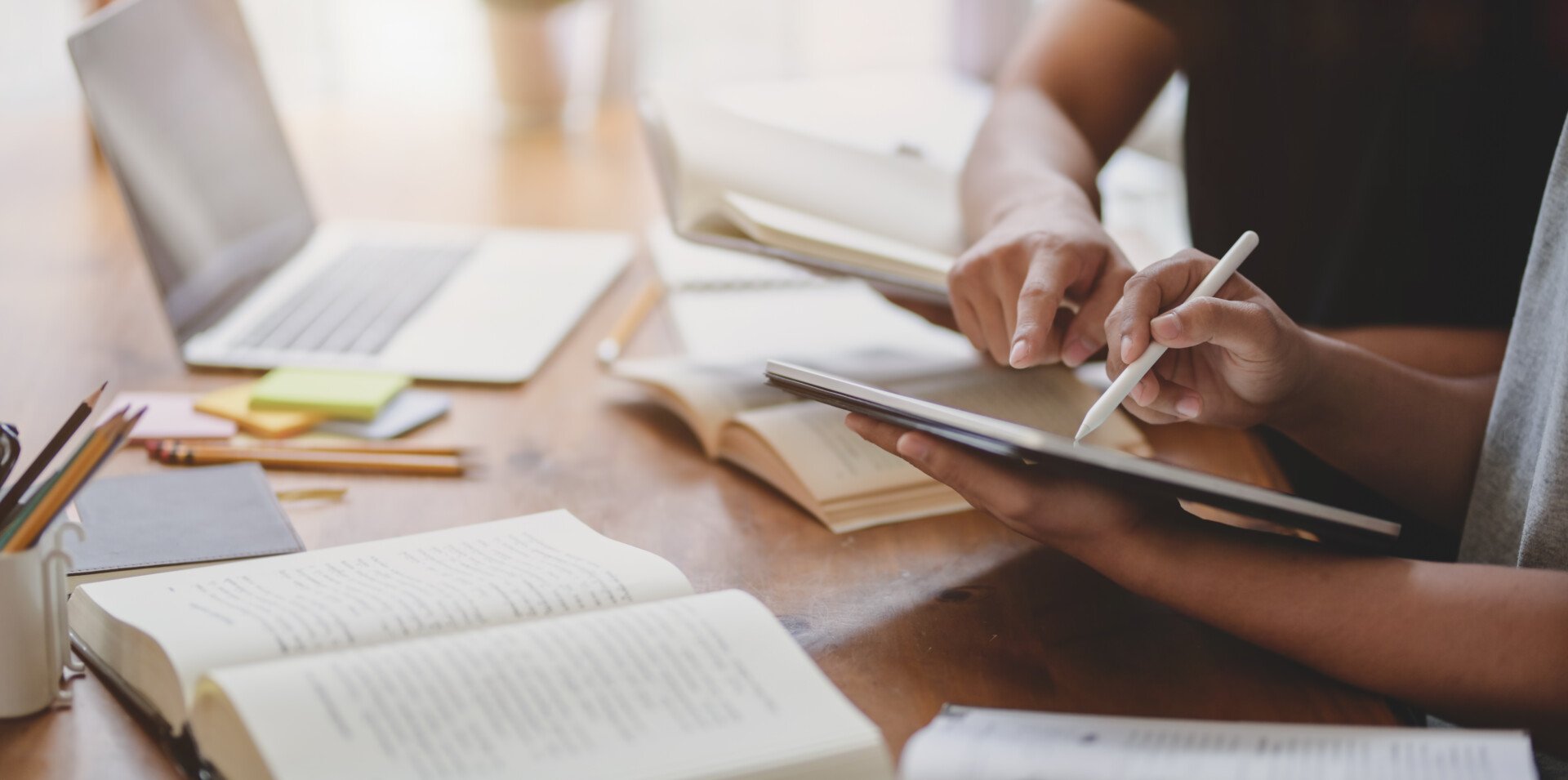
What Is Internal Validity In Research?
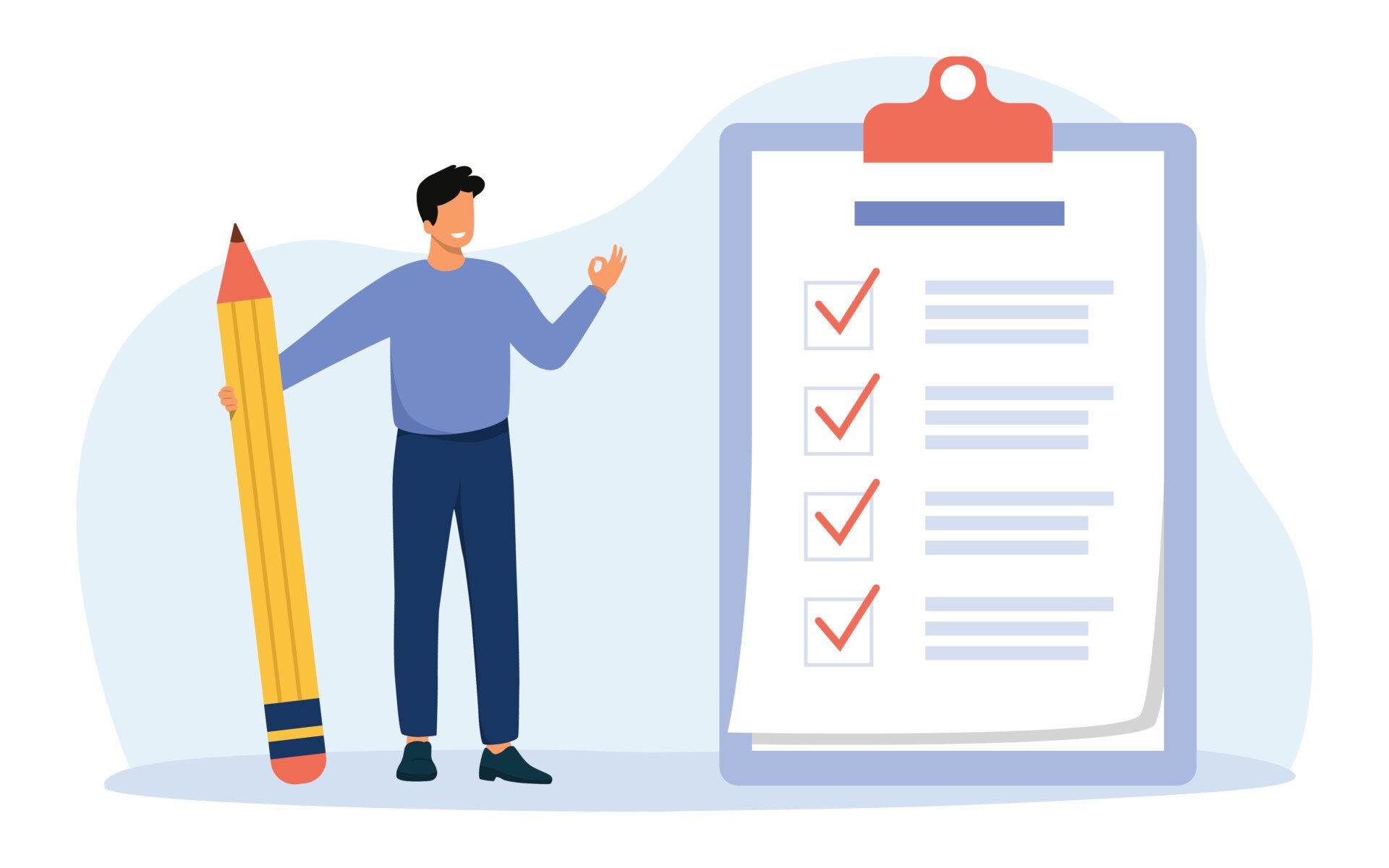
Research Methodology , Statistics
What Is Face Validity In Research? Importance & How To Measure
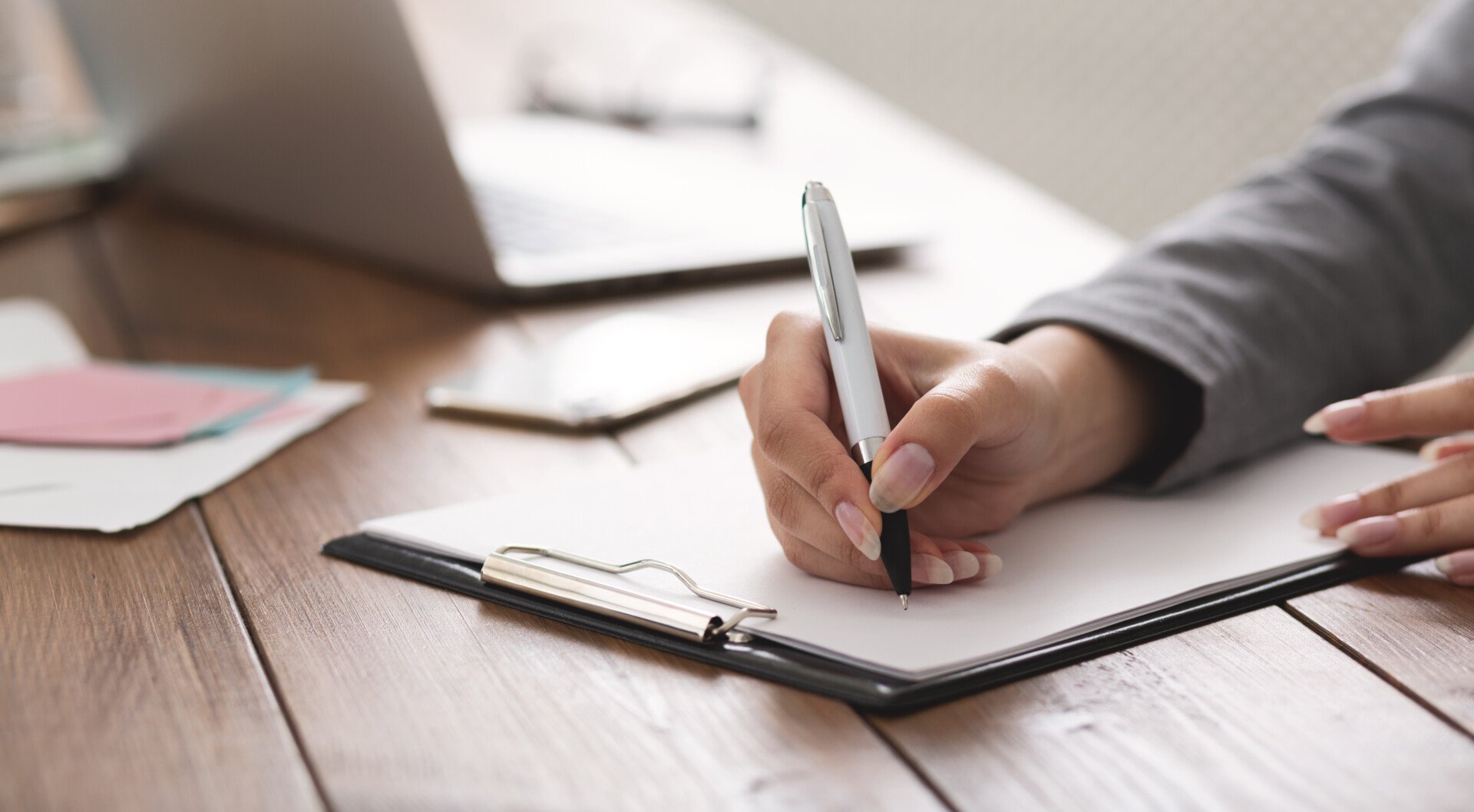
Criterion Validity: Definition & Examples
Fastest Nurse Insight Engine
- MEDICAL ASSISSTANT
- Abdominal Key
- Anesthesia Key
- Basicmedical Key
- Otolaryngology & Ophthalmology
- Musculoskeletal Key
- Obstetric, Gynecology and Pediatric
- Oncology & Hematology
- Plastic Surgery & Dermatology
- Clinical Dentistry
- Radiology Key
- Thoracic Key
- Veterinary Medicine
- Gold Membership
Key concepts in research
2. Key concepts in research Key points • Research is a lot easier to appreciate through an understanding of some of the concepts covered in this chapter. • Quantitative and qualitative approaches to research relate to the different research designs, and are based on philosophical beliefs about the nature of empirical evidence, that is, evidence collected in the real world through the senses. Quantitative research is based on the belief that the truth of a situation exists in an objective state outside the personal views or perceptions of the individual. It emphasises accuracy, and produces numerical data. Qualitative researchers believe that the truth of a situation is produced by our subjective experience, and that we need to look at things from an individual’s point of view. Midwifery is concerned with issues that draw on both beliefs. • Research questions can relate to three levels of exploration. Level-one questions relate to describing one variable, usually about which little is known, or that has rarely been the subject of research. Level-two questions look for relationships between variables but where little theory exists. Level-three questions relate to questions where theory exists and the aim is to test hypotheses based on the theory. • Variables are the elements in which the researcher is interested. In level-three questions, there will be a dependent variable that is the outcome or effect, and one or more independent variables that are presumed to influence or cause the dependent variable. • Concept definitions relate to how the researcher defines the topic in which they are interested. This can be thought of as a dictionary definition or alternative word for the topic of interest. • Operational definitions refer to the way in which a concept is measured. It reduces the vagueness of such words as comfort, pain, and benefit by producing a clear specification of how the researcher will make them visible in a specific study. • Theoretical and conceptual frameworks provide the context and meaning for the ideas and concepts contained in a study. • Reliability, validity, bias and rigour relate first to the extent to which the tool of data collection is accurate and consistent between different measurements, or different researchers. Validity relates to whether the method does measure what the researcher intends it to measure. Bias is the extent to which the findings are distorted either by the choice of subjects or the method of measurement. Rigour is the extent to which the researcher has attempted to conduct the study to ensure accuracy and high-quality research. This chapter will examine some of the important concepts used by researchers and simplify the language by helping you to understand its meaning. The language of research can appear to be composed of ‘jargon’, that is, unhelpful and meaningless words. This can form a barrier to understanding research, as people resent the use of words they do not understand, particularly if they feel they are just being used for effect. However, in reality, the words are a shorthand for complex ideas, and once the most commonly used words are understood, research can take on a completely different level of understanding. The chapter will also cover some of the important issues that researchers face when demonstrating that their research is accurate and carried out to a high standard. These are called ‘methodological issues’. An important starting point is to recognise that research takes many different forms; in this book we will focus specifically on research examining midwifery issues, carried out on the whole by midwives. In Chapter 1 research was defined as the systematic collection of information using carefully designed and controlled methods that answer a specific question objectively and as accurately as possible. This definition can look similar to audit and so lead to some confusion between these two sources of information. The basic difference between the two, however, is that the key role of research is to extend knowledge and understanding of a particular topic or issue through the systematic collection of information that leads to generalisations about the topic examined. Research conclusions are usually placed within a context of existing knowledge. That is, they are usually compared to previous research that has examined the same topic in order to confirm existing knowledge or help to clarify or extend it. The purpose is always to enrich our understanding of the topic so that we can better use, or control its features. Audit, on the other hand, is usually interested in the performance level of a part of a service, and a comparison of results against an agreed standard (or previous audit results) that may allow action to be taken. Watson and Keady (2008) suggest that we can think of audit as management activity concerned with measuring the extent to which agreed standards for clinical practice or procedures are being met or are reaching a sufficient level. Gerrish and Lacey (2010) agree, saying it is a process of measuring care against predetermined standards. This is very different from the way research is designed to increasing our overall understanding of a topic and which can be applied generally, rather than the very specific location to which audit data can apply. One problem in trying to define research is that it is similar to words such as ‘care’, ‘birth’, or ‘midwifery’; it is used as though it consisted of a single entity when, in fact, in can take many different alternative forms. This means that once we decide to study it, we have to learn something about the many forms it can take. At this stage it is useful to think of research as a process that will follow a number of principles or guidelines that will change depending on the type or category of research considered. In this book we will focus on midwifery research, that is, research that explores the problems and issues of direct concern to the midwife and that has implications for the work of the midwife more than any other discipline. Quantitative and qualitative research These two concepts are an ideal starting point for learning about research as they categorise very different approaches to thinking about the role of research and the beliefs or philosophies underpinning its production. This is important as it explains why some studies look very different from others. If we know why they differ we can make the best use of both types. Although Chapter 4 on quantitative and qualitative research explores the differences in more detail in, here we need outline ideas associated with them, and the implications these have for midwifery research and knowledge. Historically, research has been synonymous with the word ‘scientific’, often associated with words like ‘objective’ or ‘accurate’, as these are two key characteristics that ‘good’ research is presumed to posses. Gerrish and Lacey (2010: 8) see a scientific approach to research as indicating ‘a rigorous approach to a systematic form of enquiry’. The philosophy or belief on which this approach is based is that the natural or ‘real’ world does not depend on an individual’s experience of it to exist and that it is open to study and quantification. In other words, it can be measured in some way independent of the person doing the measuring. This type of research can be characterised as ‘ quantitative’ research as it attempts to quantify concepts, such as blood pressure, family size and even pain, in the form of a numeric value. These numbers can be summarised and allow the use of a range of statistical techniques to give the results greater usefulness and meaning ( Chapter 13 ). The purpose of quantitative research is seen as the search for relationships between things in the world so that we can understand the way they act and relate together. The ultimate aim of this understanding is to be able to control the elements in our world that impact on human existence. Our understanding of gravity and how we are influenced by its ‘laws’ is a good example of this measurement and developing of relationships leading to theories about ‘how things are’. In midwifery, an example may be the search for a relationship between physical skin-to-skin contact with the baby at birth and parental feelings of emotional attachment so this pattern can be measured and demonstrated to be advantageous. This scientific view is one ‘ paradigm’ or total way of looking at things (world view) in research. It is the one embraced by medical research as the ‘right’ and ‘proper’ approach for a profession that is concerned with clinical outcomes. These words have been put in inverted commas to show that there may not be total agreement on this statement, and it is open to debate whether the belief applies in all circumstances. We must remember that this is only one approach to research and, without suggesting that it is not an indispensable approach in midwifery, that there are other, just as legitimate ways of conducting a study in addition to counting or measuring something that can also extend midwifery knowledge and practice. Qualitative research (sometimes referred to as representing a naturalistic paradigm as it avoids controlling situations) is the second in the pair of concepts that go to make up the two largest research approaches in midwifery. This has a different view of the characteristics of knowledge and the best way of conducting research to discover, extend or confirm that knowledge. It is believed that the real world can only be understood through our personal experience of it, and everything depends on how we experience and interpret that experience. This explains why some people are afraid of spiders or going to the dentist. It is a product of how people experience them, or the associations they hold for the individual. It does not mean that spiders or dentists themselves are frightening. Naturalistic or qualitative researchers believe that if we are to understand a topic we need to look at it through the eyes of those who experience it and try to understand it from their point of view. This way of thinking creates a different understanding of reality and the type of research we need to capture it accurately. This kind of research produces qualitative data in the form of verbal or written statements and dialogue, or extensive descriptions of observed human activity and behaviour. It uses methods such as interviews or observations, and information taken from documents such as diaries or health records that capture perceptions, interpretations, experiences or understanding. One of the guiding principles of qualitative research is that it tries to capture people’s thoughts and feelings in their own words. So, questionnaires with fixed-choice options would not be classed as qualitative research even though they may have tried to see things from the individual’s point of view, as the list of alternative answers has been developed by the researcher. This format does not allow individuals to express ideas and answers in their own words, only in those of the researchers who have designed the alternatives and selected what they think are relevant alternatives. An important visual distinction between quantitative and qualitative research is the presentation of data. Quantitative research will use numerical or visual forms of data presentation such as tables, bar charts and histograms (more of these in Chapter 13 on statistics). This form of data presentation is not a main feature of qualitative research, although some studies may present a table showing details of the sample, such as age, number of children, etc. It is more usual for qualitative results to avoid numbers and simply present broad theme headings and discuss the type of comments made, often with examples of direct quotations or dialogue. As will be seen in Chapter 4 , these two forms of research are so different they are almost two different entities. The importance of this is that we must avoid criticising qualitative research using the criteria of a quantitative approach. Which of these two approaches is best suited to midwifery research? The answer is, the one that is most appropriate to the question posed. If the midwifery question is one of quantity, or frequency, particularly in regard to clinical outcomes, then a quantitative approach will be appropriate; if the question is one of perceptions, understanding and interpretations, then the best approach will be qualitative. Levels of questions in research There is no shortage of questions that need to be answered through midwifery research. From the research point of view, it is the question posed by the researcher that results in the aim of the research. The aim usually begins with the word ‘to’ as in: … this study aims to examine how a certain group of midwives (the participants) conceptualise the phenomenon of the ‘good’ midwife and the ‘good’ leader. Byrom and Downe (2010: 127) Research questions will differ in their complexity and this will have implications for the way a study is designed. Wood and Ross-Kerr (2006) make a useful distinction between what they call the three levels of research question. These levels are influenced by how much is known about a particular subject, or how much theory exists in relation to it ( Table 2.1 ). The advantage of this system is that it allows you to predict the way a study should be structured to answer a question at each of the levels. Table 2.1 Levels of research questions Level of question Description Type of research Level 1 Examines one variable (or a series of variables) but without looking for patterns between variables. Exploratory situation where little is known about the topic. Quantitative descriptive, e.g. survey Qualitative study: all types are level 1. Level 2
Share this:
- Click to share on Twitter (Opens in new window)
- Click to share on Facebook (Opens in new window)
Related posts:
- The basic framework of research
- The research question
- The challenge of the future
- Sampling methods
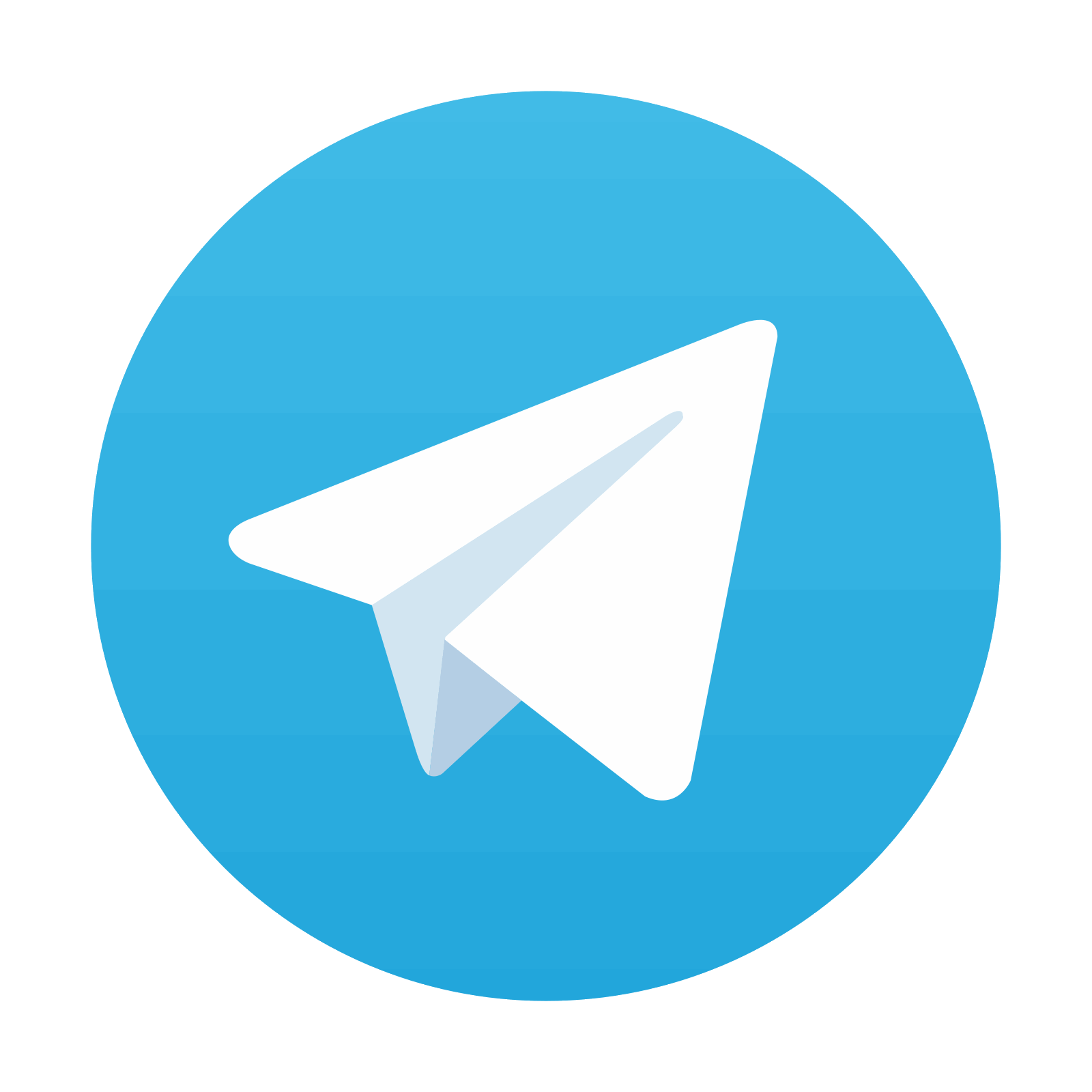
Stay updated, free articles. Join our Telegram channel
Comments are closed for this page.

Full access? Get Clinical Tree
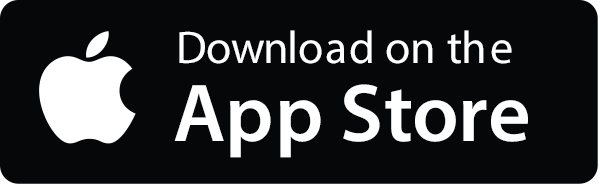
- Do Not Sell My Personal Info
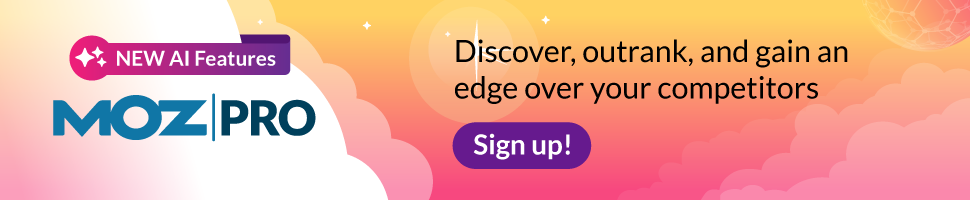
- ⋅
Keyword Research: An In-Depth Beginner’s Guide
Keyword research is the foundation of search engine optimization. This guide covers what it is and how you should do keyword research.
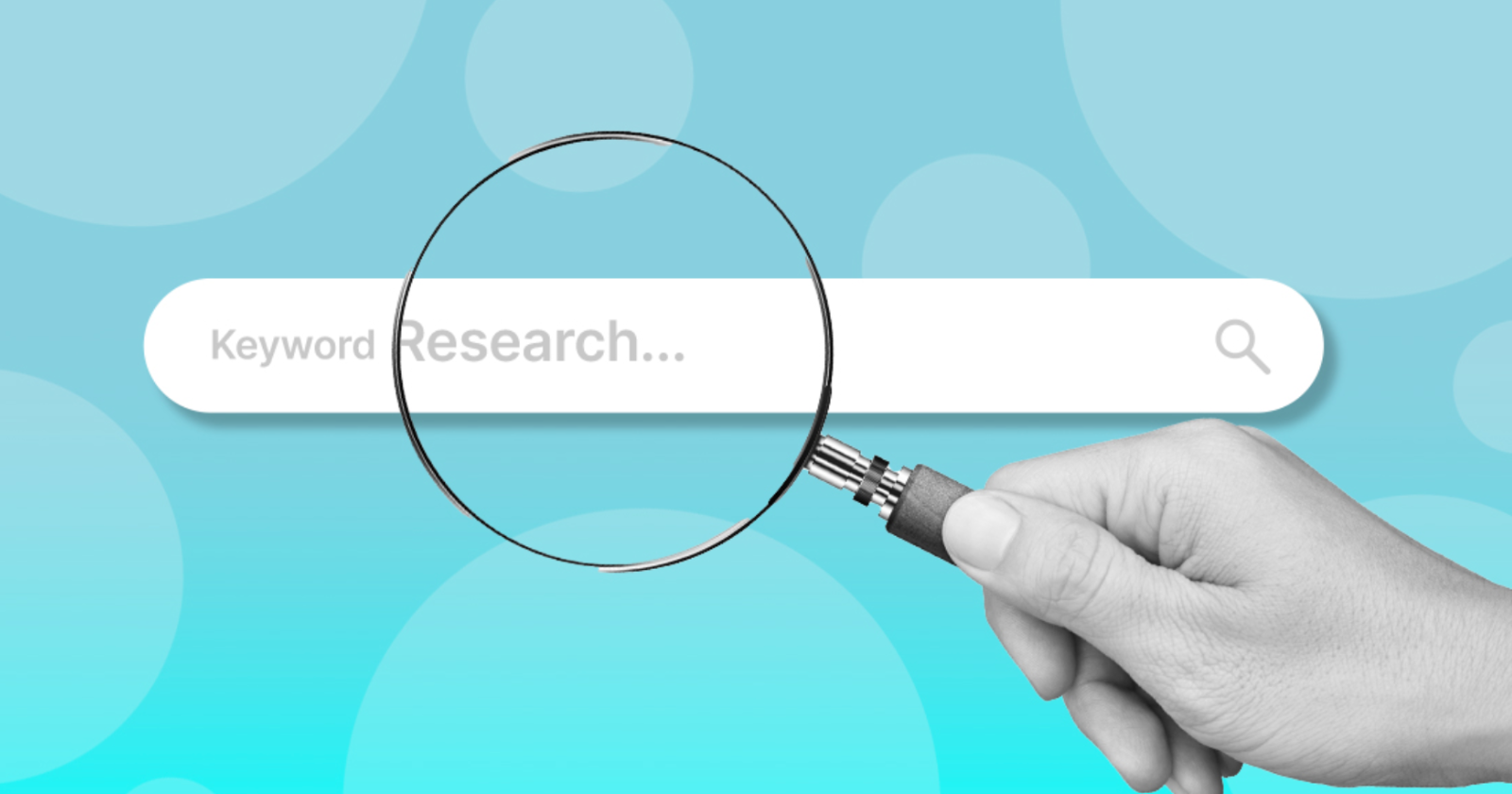
Keyword research is the foundation of search engine optimization, and without it, you cannot expect to create sustainable and repeatable visibility.
Today, SEO is a much wider discipline than in the early days of the industry and has been segmented into many verticals.
But, at the core, SEO is about finding opportunities online and capturing relevant traffic to a website through visibility in search engines. Keyword research is at the heart of that SEO strategy.
This guide explains what keyword research is, why it’s important, and how you can make a start for a successful SEO strategy.
What Is Keyword Research?
Keyword research is a process of finding words, queries, and phrases that users are searching for, which means a keyword that has search volume.
Research involves connecting the relevance of keywords to a website and its individual pages so that the user can find the best page to answer their query, known as search intent.
Keyword research also involves categorizing search queries into the different stages of a user journey and different categories of search, such as transactional, navigational, and informational.
Good keyword research enables users to find what they need:
- Shoppers who want to buy something can find the right product page.
- A user that wants to know ‘how to’ can find a page that explains a process in-depth.
- Users who want to research a person or brand can find out about that entity.
Keyword research should also carefully consider if ranking on a keyword is worth the effort it would take to rank highly and get visitors. Not all traffic is equal.
Download the ebook, How To Do Keyword Research For SEO .
Why Keyword Research Is Important For SEO
A search engine is an information retrieval system built around the queries that a user inputs to find an answer or relevant information to their search query.
The predominant focus of Google is to connect a user with the best answer to their query and the best website page so that a user is satisfied. Understanding this underlines SEO.
Good keyword research is the foundation of how a business can connect with its potential customers and audience. Understanding this helps to understand a good SEO strategy.
A business strategy starts with understanding its audience and their needs.
- What do they want?
- What do they need?
- What keeps them awake at night?
- What could solve their problem?
Keyword research is an extension of understanding your audience by first considering their needs and then the phrases, keywords, or queries they use to find solutions.
Keyword research is also important for SEO because it can show you where the opportunities are by knowing what your audience is searching for.
This will help you to find new areas of business and to prioritize where to focus attention and resources.
Keyword research will also help you to calculate where you can expect a return on investment to justify your efforts:
- Can a keyword deliver relevant traffic that has the possibility to convert to an end goal?
- What is an estimation of that traffic, and how much is each visitor worth to your business?
Basically, keyword research is the ultimate business research tool.
Read more: Why Keywords Are Still So Very Important For SEO
Keyword Research Basics
Monthly search volume.
Monthly Search Volume (MSV) is a predominant measure of keyword value. It’s a useful metric as a starting point to consider if anyone is searching for that keyword, but it shouldn’t be used in isolation or as the only measure of value.
Just because a keyword has a high MSV doesn’t mean it is the right keyword for you to rank on.
High-volume keywords generally deliver ‘browsing’ traffic at the top of the funnel. They are useful for brand awareness but not for direct conversion.
Low-volume keywords can be much more valuable because they can deliver users who are ready to buy a product.
Read more: A Complete Guide To Keyword Search Volume For SEO
User Intent
User intent refers to what type of result they want to see when they search for a query – the intention of their search.
You will hear user intent talked about a lot in keyword research, as it is one of the most important factors in the process.
User intent is important in two ways, firstly because your primary aim in creating content and pages on a website are to provide a user with information that they want to know.
There’s no point in creating a page about what you care about – your user only cares about their problems and needs.
You can have the best page in the world about the history of cupcakes, but if a user searching for [cupcake] wants a recipe for cupcakes, then they will not click on your link.
Secondly, Google considers relevance when serving results pages (as we said above, they want to deliver the best result for a query). So, the better your page fits user intent, the better it might rank.
Read more: How People Search: Understanding User Intent
When Google considers which pages it will show in search results, the algorithm will look at other pages that users are clicking on for that query.
If we have a query such as [cupcake], Google has to consider if a user wants to know what a cupcake is, how to make a cupcake, or wants to buy a cupcake.
By looking at a search result page, you can get a good idea of user intent from the other results.
Reviewing the search results page of a query should be part of your research process for every keyword you want to consider.
Keyword relevance and user intent are much the same things. It’s about knowing what the user really means when they search. This is more ambiguous for head keywords and less so for long-tail queries.
Long-Tail keywords
Long-tail keywords are called long-tail because they fall to the right of the search demand curve – where the graph looks like a long tail stretching to the right.
The search demand curve is a graph that shows keywords with high volume to the left and lower search volumes to the right.
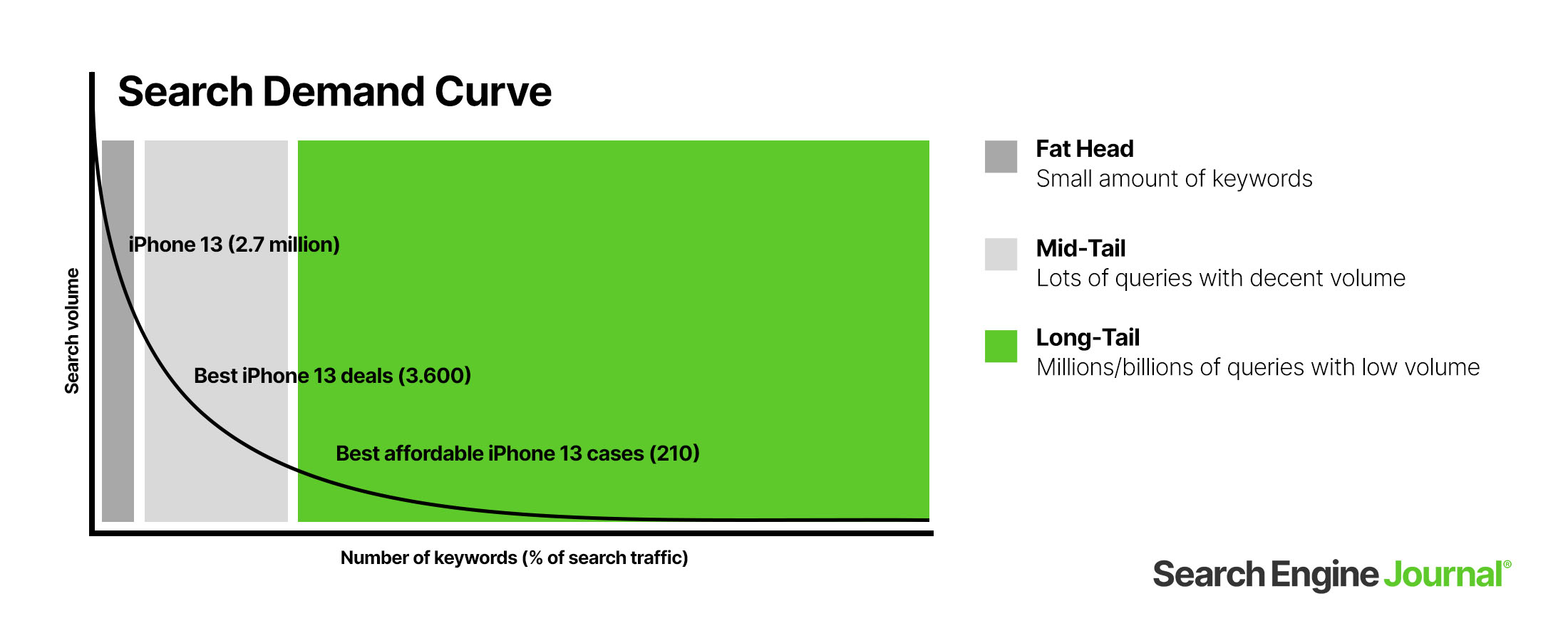
Longer queries that are more focused fall to the right. Head terms with broad meaning and high volume fall to the left.
The value of long-tail keywords is that they are usually highly focused terms that convert well, as users are actively looking for something very specific.
For example, [iPhone 13] is a head term with high volume (2.7 million MSV), and [Best affordable iPhone 13 cases] (210 MSV) is a long-tail keyword with far fewer searches but would have a high conversion rate.
Long-tail keywords are useful to include in a keyword strategy because they are usually much easier to rank for and achievable for a new website, and the cumulative volume of many long-tail keywords adds up to considerable targeted traffic.
This is a much more stable strategy than focusing on one high-volume ambiguous keyword.
Read more: Long-Tail Keyword Strategy: Why & How To Target Intent For SEO
Types Of Search Query
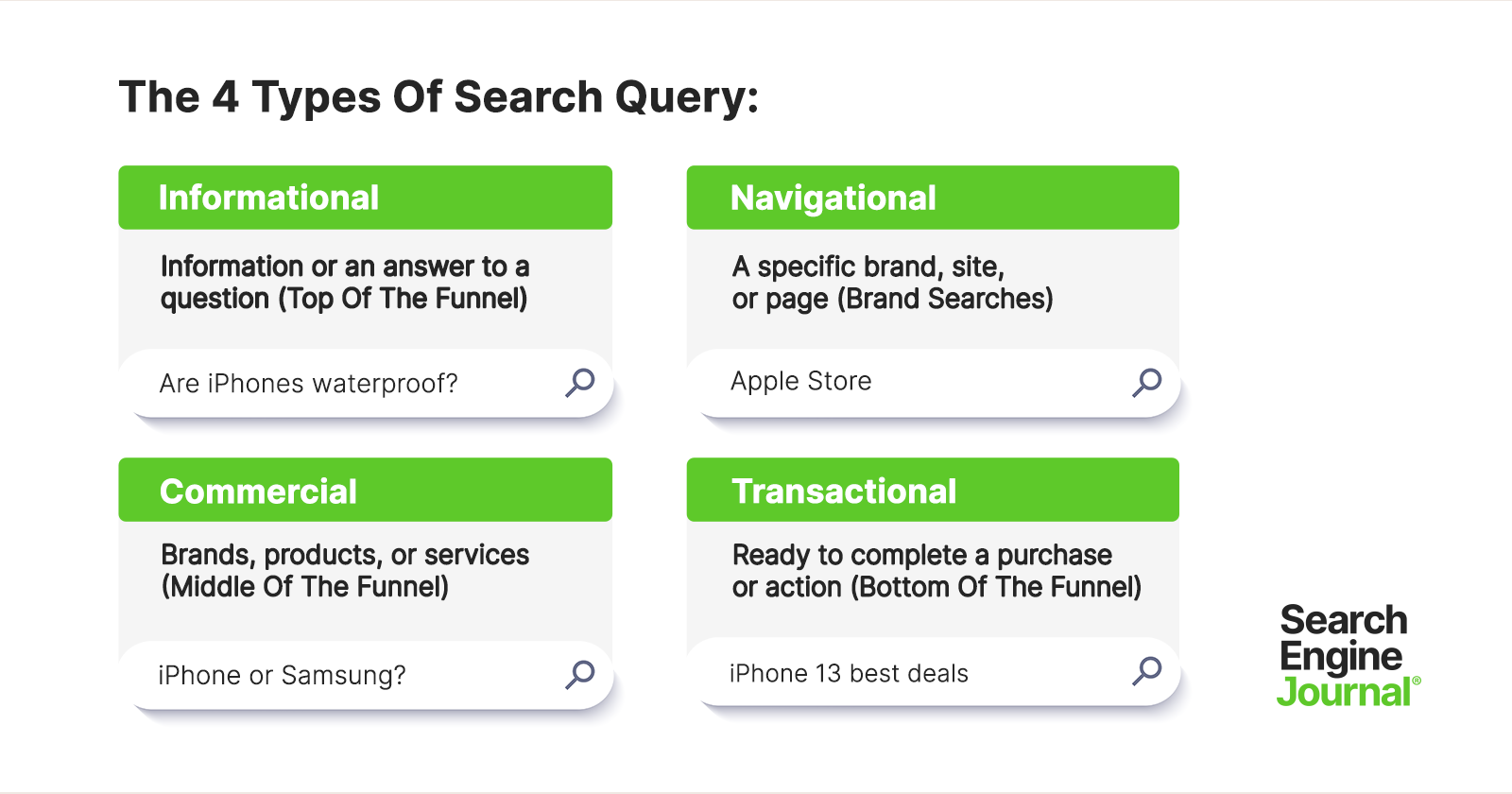
There are four types of keywords that are useful to understand as they categorize the different user intents and can help when planning a keyword strategy.
- Informational – users looking for information or an answer to a question (top of the funnel).
- Navigational – users wanting to find a specific brand, site, or page (brand searches).
- Commercial – users researching brands, products, or services (middle of the funnel).
- Transactional – users ready to complete a purchase or action (bottom of the funnel).
Local keywords can also be considered another category.
How To Do Keyword Research
Now that you have a better understanding of the basics of keyword research, we can look at where you would start with your research and keyword strategy.
1. How To Find Keyword Ideas
The first stage of keyword research is to brainstorm ideas for seed keywords, and there are several ways to do this.
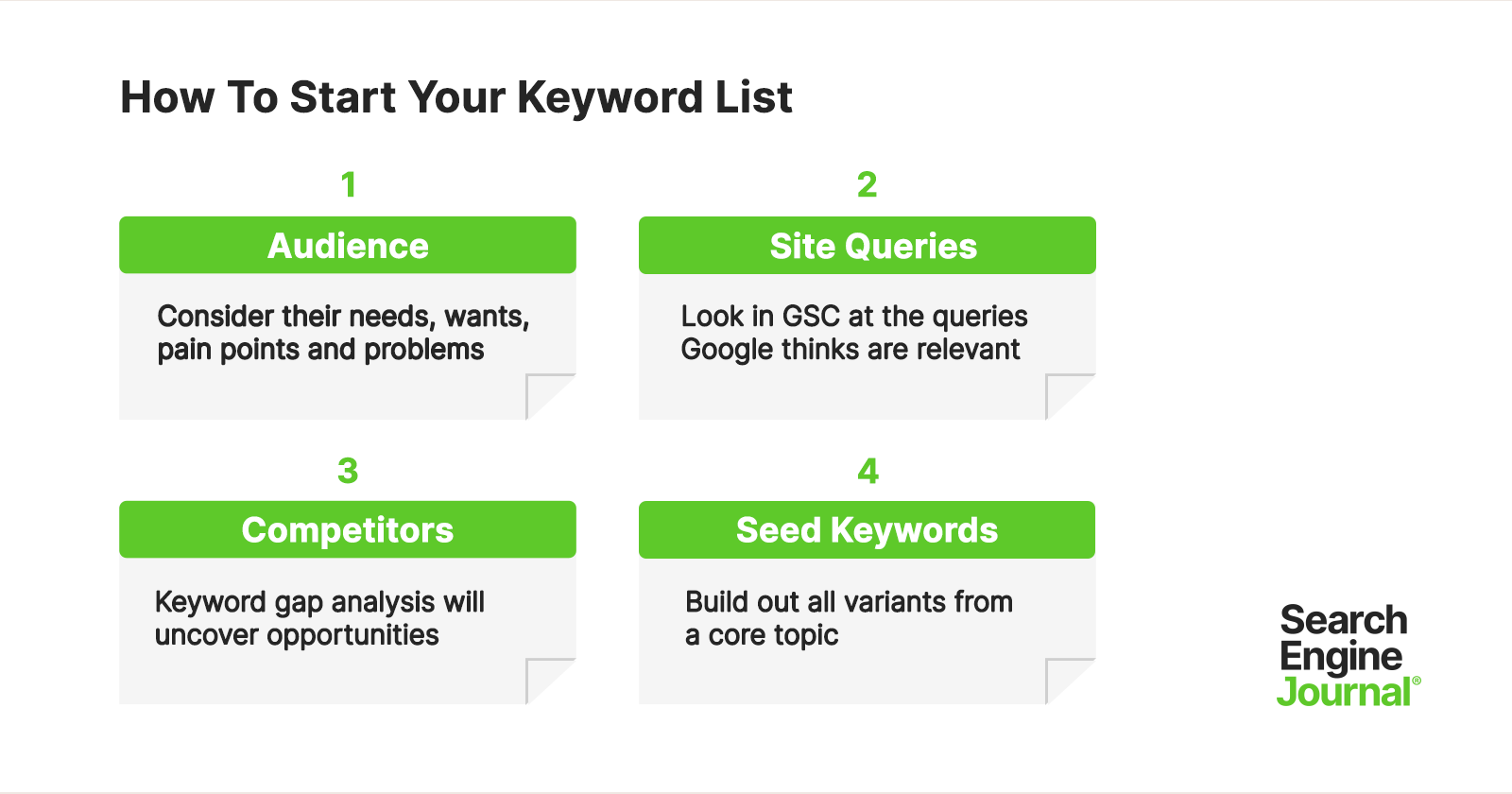
Your Target Audience
Everything starts with your audience and what they want. Think about their needs, wants, and especially their pain points and problems.
Start to compile your wide list of words, ideas, and topics that surround your niche or business.
Think About Questions
Question-based keyword queries are valuable as they can help you to capture featured snippets and can be a way to jump rank on highly competitive keywords.
Ask your sales team and review CRM data to find the questions that your audience is asking.
Also, think of question modifiers that will start to build out your list:
- What [is a road bike].
- How to [ride a road bike].
- When is [the best time to service a road bike].
- What is [the best road bike].
- Where is [road bike shop].
Current Site Queries
If your site already has some online history, then Google Search Console can tell you what Google thinks your website is relevant for. This is insightful to tell you if your site is conveying the right message and to find opportunities.
If Google is showing a lot of queries that have nothing to do with your product or brand, then you need to apply more keyword focus to your pages.
Look for queries that are position 10 or greater, have reasonable impressions, and you think are relevant to your business.
These are potential quick-win opportunities that you can capture by making improvements and optimizing pages for the query.
Read more: A Complete Google Search Console Guide For SEO Pros
Competitors’ Keywords
Your competitors are a gold mine of information because they might already have invested in extensive research.
A business should be constantly monitoring its competitors anyway, so keep an eye on what content they are producing and the terms they target.
A keyword gap analysis will help you find opportunities your competitors are targeting that you might not have considered.
Read more: Competitor Keyword Analysis: 5 Ways To Fill The Gaps In Your Organic Strategy & Get More Traffic
Seed Keywords
Start with high-level ‘seed’ keywords that you can use as a starting point to then open up variants and related queries.
Seed keywords are ‘big’ head terms such as [iPhone], [trainers], [road bike], or [cupcake].
For each seed keyword, start to think of topics that are related:
- Road bike maintenance.
- Road bike training.
- Road bike clothing.
- Road bike lights.
Also, use question modifiers and buying modifiers such as:
- Best [road bike].
- Buy [road bike].
- Price [for women’s road bike].
- [road bikes for hire] near me.
- Reviews [of road bikes under $1,000].
When you have finished this process, you should have a raw list of potential keywords grouped in topics – such as maintenance, clothing, training, etc.
2. How To Analyze Keywords
Once you have your raw list, it’s time to start to analyze and sort by value and opportunity.
Search Volume
Search volume will tell you if anyone is actively searching for this term.
For a first-stage strategy, you should aim for keywords with a mid-range and long-tail volume for quick wins and then build up to approach more competitive terms with higher volumes.
Head keywords with super high volumes (like ‘iPhone’) are not the best keywords to focus on as they can be too ambiguous and rarely have a specific intent.
Also, the amount of work that is needed to rank can be too high a barrier for entry unless you have an established domain of significant authority.
If a tool shows a keyword with zero search volume, this doesn’t always mean you should discard the term.
If the keyword is shown in the tool, then there can be value to consider targeting it in your strategy. However, make sure you know your audience and what is relevant to them before you invest resources in a zero-volume keyword.
Read more: Why You Should Target Zero Search Volume Keywords
Search Intent
After you have sorted your raw lists by search volumes, it’s then time to review the intent for each keyword that you would like to target.
The SERP will tell you everything that you need to know, and you should always review SERPs for clues on how to construct content and rank.
Look at the other listing that rank highly:
- Are they ‘how-to’ guides that indicate it’s informational?
- Do the titles say ‘buy,’ ‘best,’ or include product names?
- Is there a shopping carousel that indicates a buying keyword?
- Is there a location map that indicates it’s a local search?
Tag each keyword type and then consider which are the strongest keywords from each group.
You can also use a research tool that will tell you the type of keyword.
Topic Clusters
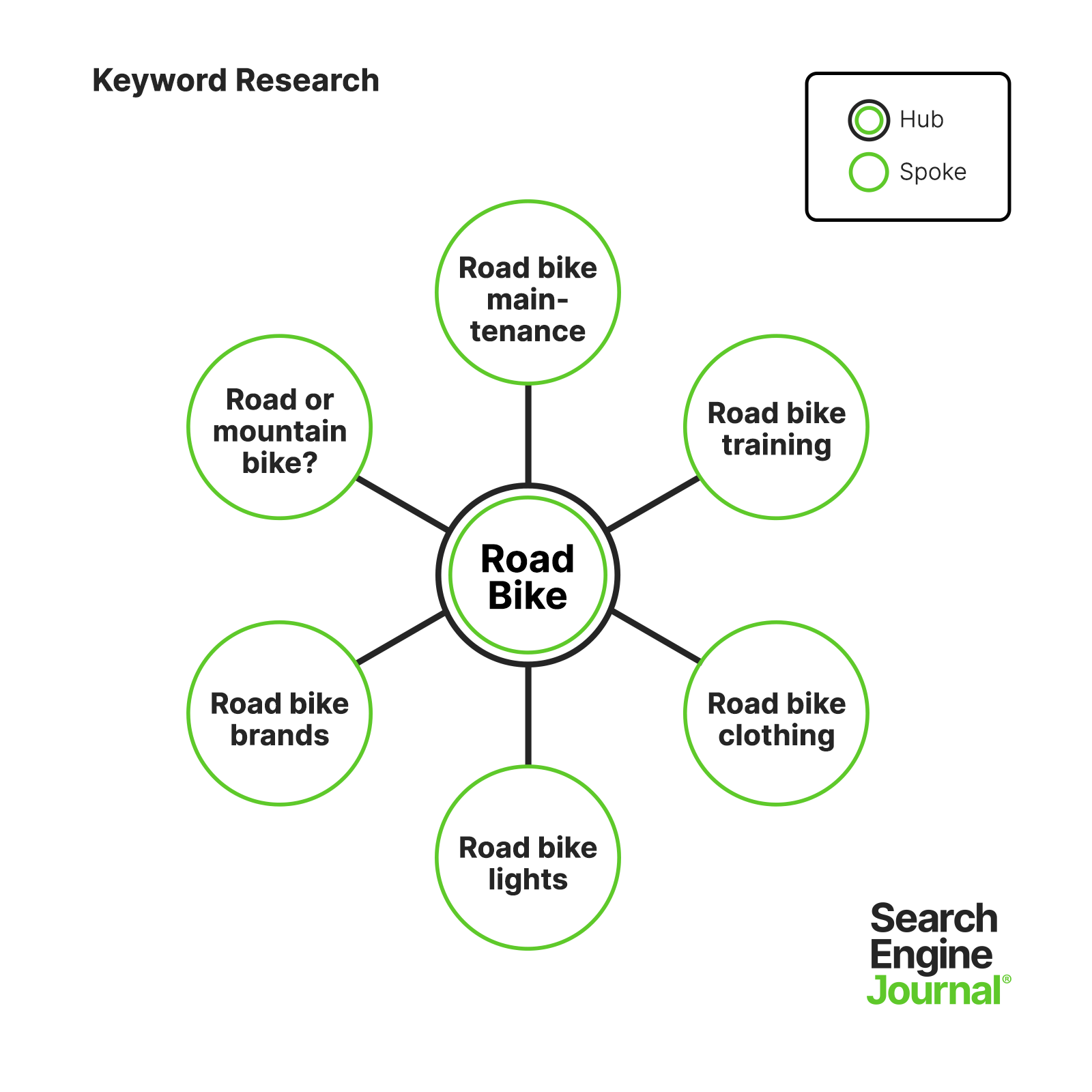
Grouping keywords into topic clusters is an advanced keyword strategy that can help to strengthen the topic authority of a site.
To do this, you would start with a high-volume head keyword and then research a series of keywords that supports that head term.
After creating pages of content that target each keyword, you use internal linking to connect pages with the same topic.
Read more: Keyword Clusters: How To Level Up Your SEO Content Strategy
3. How To Choose Organic Keywords
After sorting the volumes, intent, and topics, you will need to decide if you have a chance of ranking on a term by looking at how much competition there is for each keyword.
Keyword Difficulty
Keyword difficulty is one of the most important keyword metrics when doing your research.
If a keyword is so competitive that you need hundreds of thousands of dollars to rank, then you need to get strategic.
The easiest way to calculate keyword difficulty is to use a research tool that gives a score for each keyword.
Or, you can refer to Google Keyword Planner Tool and look at the CPC and level of difficulty. The higher the CPC bid, the higher the competition.
If you are starting out, first approach the lower competition keywords that are achievable and then build your way up to more competitive terms.
Read more: Why Keyword Research Is Useful For SEO & How To Rank
Connecting To Your Objectives And Goals
Unless a keyword can actually deliver a result for you – do you want to target it?
As we said above, targeting head terms is not the best strategy as they will, at best, deliver browsing or drive-by visitors. Unless you are a big brand with a big budget that is aiming for brand awareness, this is not the best application of your resources and budget.
Choosing your keyword priority should start with what can give you the best return in the shortest time frame.
Good keyword research is not just about trying to target a high-volume popular keyword. A good keyword strategy is about finding the right keywords for your needs and outcome. Always keep that front and center.
Read more: How To Calculate ROI For SEO When Targeting A Set Of Keywords
Watch John Mueller talk about ranking for head keywords in this video from the 38:55 minute mark.
Using Keyword Research Tools
Doing your research without a tool is limited; for the most in-depth keyword research, you need help to find keyword opportunities you had not thought of.
The following keyword research tools are all free versions that you can start out with.
Google Keyword Planner
The original keyword tool has evolved over the years, but it still remains one of the best free keyword tools and a good starting point to find seed keywords and keyword ideas.
The Google tool is aligned with Google Ads, so the data is skewed towards paid ads but is still valuable for research.
You need a Google Ads account to access the tool. Google will try to force you to set up an active campaign, but you can access the account by setting up an account without a campaign.
Without a campaign running, you will only get limited search volumes displayed in ranges, but the tool is still useful for its suggestions of keyword ideas. If you have an active campaign, Google will show you the monthly search volume.
You can add up to 10 seed keywords and get a list of suggested keywords, and run competitor URLs in the tool to find keywords they are targeting. Doing this is a great place to start building out raw lists of keywords to work from.
Read more: How To Use Google Keyword Planner
Read more: 9 Creative Ways To Use Google’s Keyword Planner Tool
Google Trends
Google Trends offers data based on actual search query data. It doesn’t provide search volumes, but the data in Google trends can be compared with actual search volumes from other tools so you can get a comparative feel of what the actual volumes are.
Where Trends excels is to identify trending topics and subtopics in a niche and to find geographic search trends in a local area. Trends will recommend related keywords that are currently growing in popularity.
As part of a keyword strategy, this can show you where to focus resources and when to stop investing in terms.
Read more: How To Use Google Trends For SEO
Google Autocomplete
Previously known as Suggest, Autocomplete is integrated into the Google search box to help users complete their search with what Google calls ‘predictions.’
Google takes its predictions from common searches and trending searches.
As the suggestions are all variations around the topic you are typing, the results shown give you an insight into other related terms that users could be looking for.
Checking the predictions that Google provides in Autocomplete can help you find more variations and keywords to consider.
Read more: Google Autocomplete: A Complete SEO Guide
Answer The Public
Answer The Public is a powerful tool that scrapes data from Google Autocomplete and connects a seed keyword with a variety of modifiers to produce a list of variants.
Answer The Public will quickly provide a list of suggestions, especially based on questions that you can use as a raw list to then review.
Read more: More Free Keyword Research Tools
Paid Keyword Research Tools
Free keyword tools are great to get you started and to create raw lists of keywords that you can then drill into.
However, for the best results, you might want to invest in a paid competitive analysis tool that can help you get monthly search volume and keyword difficulty data.
Some tools will also help with assigning topics and clustering for more advanced keyword strategies.
Anyone who does keyword research in-depth or to an advanced level will have their own process. They will also use a variety of tools and a combination of paid and free resources to get the best results.
Read more: Best Keyword Research Tools
Advanced Keyword Strategies
Now that you have an understanding of how to get started with keyword research, experiment with a few different websites and niches. Doing the work yourself is the best way to learn.
Once you have a better understanding in practice, move into more advanced methods and strategies to take your keyword research to the next level.
Advanced Keyword Research
- B2B Keyword Research Done Right With Practical Examples
- Keyword Clusters: How To Level Up Your SEO Content Strategy
- Building A Keyword Strategy For Comparison Content
Featured Image: Paulo Bobita/Search Engine Journal
Shelley Walsh is the SEO Content Strategist at SEJ & produces the Pioneers, a series about the history of SEO ...
Subscribe To Our Newsletter.
Conquer your day with daily search marketing news.
Research Tips and Tricks
- Narrowing Topics
- Research Questions and Key Terms
- Combining Search Terms
- Finding Resources: Databases
- The Rhetorical Situation
Creating Key Terms
What do you even search for once you've got your topic and research question solidified, or at least started? Well, you take the most important words in your research statement/question and use them as key terms. Use those key terms in conjunction with each other (see the section on "Combining Key Terms" for advice about how to do so). Also, use synonyms of your key terms.
Example Key Terms
Research question:.
- What effect would paying college athletes have on collegiate sports?
- college athletes
- college sports
- << Previous: Narrowing Topics
- Next: Combining Search Terms >>
- Last Updated: Jan 26, 2024 10:10 AM
- URL: https://guides.library.unk.edu/research-tips
- University of Nebraska Kearney
- Library Policies
- Accessibility
- Research Guides
- Citation Guides
- A to Z Databases
- Open Nebraska
- Interlibrary Loan
2508 11th Avenue, Kearney, NE 68849-2240
Circulation Desk: 308-865-8599 Main Office: 308-865-8535
Ask A Librarian
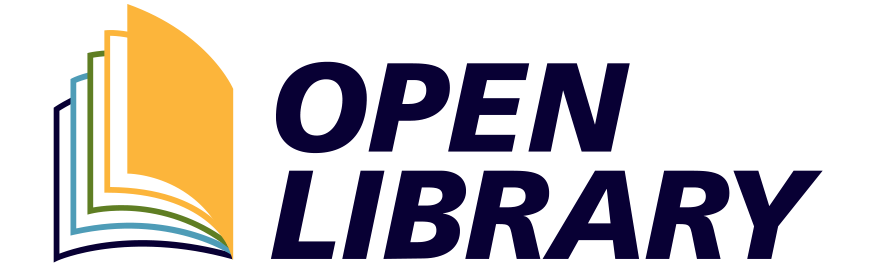
Want to create or adapt books like this? Learn more about how Pressbooks supports open publishing practices.
In this textbook you will be exposed to many terms and concepts associated with research methods, particularly as they relate to the research planning decisions you must make along the way. Figure 1.1 will help you contextualize many of these terms and understand the research process. This general chart begins with two key concepts: ontology and epistemology, advances through other concepts, and concludes with three research methodological approaches: qualitative, quantitative and mixed methods.
Research does not end with making decisions about the type of methods you will use; we could argue that the work is just beginning at this point. Figure 1.3 does not represent an all-encompassing list of concepts and terms related to research methods. Keep in mind that each strategy has its own data collection and analysis approaches associated with the various methodological approaches you choose. Figure 1.1 is intentioned to provide a general overview of the research concept. You may want to keep this figure handy as you read through the various chapters.
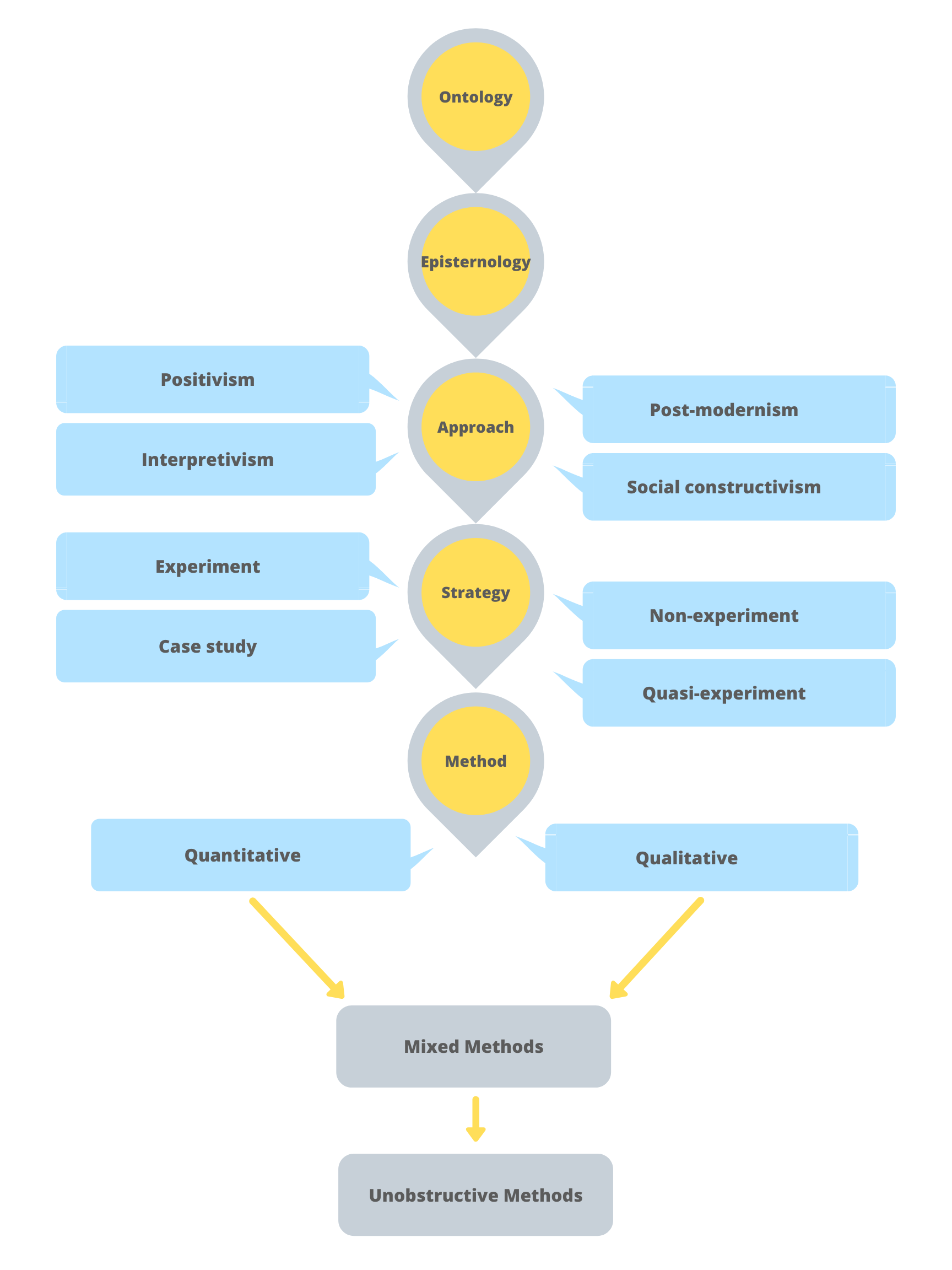
Figure 1.3: Shows the research paradigms and research process © JIBC 2019
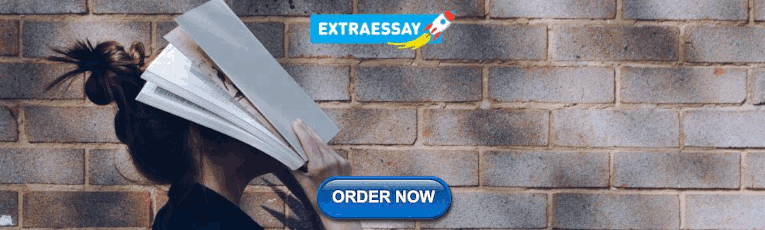
Ontology & Epistemology
Thinking about what you know and how you know what you know involves questions of ontology and epistemology. Perhaps you have heard these concepts before in a philosophy class? These concepts are relevant to the work of sociologists as well. As sociologists (those who undertake socially-focused research), we want to understand some aspect of our social world. Usually, we are not starting with zero knowledge. In fact, we usually start with some understanding of three concepts: 1) what is; 2) what can be known about what is; and, 3) what the best mechanism happens to be for learning about what is (Saylor Academy, 2012). In the following sections, we will define these concepts and provide an example of the terms, ontology and epistemology.
Ontology is a Greek word that means the study, theory, or science of being. Ontology is concerned with the what is or the nature of reality (Saunders, Lewis, & Thornhill, 2009). It can involve some very large and difficult to answer questions, such as:
- What is the purpose of life?
- What, if anything, exists beyond our universe?
- What categories does it belong to?
- Is there such a thing as objective reality?
- What does the verb “to be” mean?
Ontology is comprised of two aspects: objectivism and subjectivism. Objectivism means that social entities exist externally to the social actors who are concerned with their existence. Subjectivism means that social phenomena are created from the perceptions and actions of the social actors who are concerned with their existence (Saunders, et al., 2009). Figure 1.2 provides an example of a similar research project to be undertaken by two different students. While the projects being proposed by the students are similar, they each have different research questions. Read the scenario and then answer the questions that follow.
Subjectivist and objectivist approaches (adapted from Saunders et al., 2009)
Ana is an Emergency & Security Management Studies (ESMS) student at a local college. She is just beginning her capstone research project and she plans to do research at the City of Vancouver. Her research question is: What is the role of City of Vancouver managers in the Emergency Management Department (EMD) in enabling positive community relationships? She will be collecting data related to the roles and duties of managers in enabling positive community relationships.
Robert is also an ESMS student at the same college. He, too, will be undertaking his research at the City of Vancouver. His research question is: What is the effect of the City of Vancouver’s corporate culture in enabling EMD managers to develop a positive relationship with the local community? He will be collecting data related to perceptions of corporate culture and its effect on enabling positive community-emergency management department relationships.
Before the students begin collecting data, they learn that six months ago, the long-time emergency department manager and assistance manager both retired. They have been replaced by two senior staff managers who have Bachelor’s degrees in Emergency Services Management. These new managers are considered more up-to-date and knowledgeable on emergency services management, given their specialized academic training and practical on-the-job work experience in this department. The new managers have essentially the same job duties and operate under the same procedures as the managers they replaced. When Ana and Robert approach the managers to ask them to participate in their separate studies, the new managers state that they are just new on the job and probably cannot answer the research questions; they decline to participate. Ana and Robert are worried that they will need to start all over again with a new research project. They return to their supervisors to get their opinions on what they should do.
Before reading about their supervisors’ responses, answer the following questions:
- Is Ana’s research question indicative of an objectivist or a subjectivist approach?
- Is Robert’s research question indicative of an objectivist or a subjectivist approach?
- Given your answer in question 1, which managers could Ana interview (new, old, or both) for her research study? Why?
- Given your answer in question 2, which managers could Robert interview (new, old, or both) for his research study? Why?
Ana’s supervisor tells her that her research question is set up for an objectivist approach. Her supervisor tells her that in her study the social entity (the City) exists in reality external to the social actors (the managers), i.e., there is a formal management structure at the City that has largely remained unchanged since the old managers left and the new ones started. The procedures remain the same regardless of whoever occupies those positions. As such, Ana, using an objectivist approach, could state that the new managers have job descriptions which describe their duties and that they are a part of a formal structure with a hierarchy of people reporting to them and to whom they report. She could further state that this hierarchy, which is unique to this organization, also resembles hierarchies found in other similar organizations. As such, she can argue that the new managers will be able to speak about the role they play in enabling positive community relationships. Their answers would likely be no different than those of the old managers, because the management structure and the procedures remain the same. Therefore, she could go back to the new managers and ask them to participate in her research study.
Robert’s supervisor tells him that his research is set up for a subjectivist approach. In his study, the social phenomena (the effect of corporate culture on the relationship with the community) is created from the perceptions and consequent actions of the social actors (the managers); i.e., the corporate culture at the City continually influences the process of social interaction, and these interactions influence perceptions of the relationship with the community. The relationship is in a constant state of revision. As such, Robert, using a subjectivist approach, could state that the new managers may have had few interactions with the community members to date and therefore may not be fully cognizant of how the corporate culture affects the department’s relationship with the community. While it would be important to get the new managers’ perceptions, he would also need to speak with the previous managers to get their perceptions from the time they were employed in their positions. This is because the community-department relationship is in a state of constant revision, which is influenced by the various managers’ perceptions of the corporate culture and its effect on their ability to form positive community relationships. Therefore, he could go back to the current managers and ask them to participate in his study, and also ask that the department please contact the previous managers to see if they would be willing to participate in his study.
As you can see the research question of each study guides the decision as to whether the researcher should take a subjective or an objective ontological approach. This decision, in turn, guides their approach to the research study, including whom they should interview.
Epistemology
Epistemology has to do with knowledge. Rather than dealing with questions about what is, epistemology deals with questions of how we know what is. In sociology, there are many ways to uncover knowledge. We might interview people to understand public opinion about a topic, or perhaps observe them in their natural environment. We could avoid face-to-face interaction altogether by mailing people surveys to complete on their own or by reading people’s opinions in newspaper editorials. Each method of data collection comes with its own set of epistemological assumptions about how to find things out (Saylor Academy, 2012). There are two main subsections of epistemology: positivist and interpretivist philosophies. We will examine these philosophies or paradigms in the following sections.
Research Methods, Data Collection and Ethics Copyright © 2020 by Valerie Sheppard is licensed under a Creative Commons Attribution-NonCommercial-ShareAlike 4.0 International License , except where otherwise noted.
Share This Book
Live revision! Join us for our free exam revision livestreams Watch now →
Reference Library
Collections
- See what's new
- All Resources
- Student Resources
- Assessment Resources
- Teaching Resources
- CPD Courses
- Livestreams
Study notes, videos, interactive activities and more!
Psychology news, insights and enrichment
Currated collections of free resources
Browse resources by topic
- All Psychology Resources
Resource Selections
Currated lists of resources
Study Notes
Research Methods Key Term Glossary
Last updated 22 Mar 2021
- Share on Facebook
- Share on Twitter
- Share by Email
This key term glossary provides brief definitions for the core terms and concepts covered in Research Methods for A Level Psychology.
Don't forget to also make full use of our research methods study notes and revision quizzes to support your studies and exam revision.
The researcher’s area of interest – what they are looking at (e.g. to investigate helping behaviour).
A graph that shows the data in the form of categories (e.g. behaviours observed) that the researcher wishes to compare.
Behavioural categories
Key behaviours or, collections of behaviour, that the researcher conducting the observation will pay attention to and record
In-depth investigation of a single person, group or event, where data are gathered from a variety of sources and by using several different methods (e.g. observations & interviews).
Closed questions
Questions where there are fixed choices of responses e.g. yes/no. They generate quantitative data
Co-variables
The variables investigated in a correlation
Concurrent validity
Comparing a new test with another test of the same thing to see if they produce similar results. If they do then the new test has concurrent validity
Confidentiality
Unless agreed beforehand, participants have the right to expect that all data collected during a research study will remain confidential and anonymous.
Confounding variable
An extraneous variable that varies systematically with the IV so we cannot be sure of the true source of the change to the DV
Content analysis
Technique used to analyse qualitative data which involves coding the written data into categories – converting qualitative data into quantitative data.
Control group
A group that is treated normally and gives us a measure of how people behave when they are not exposed to the experimental treatment (e.g. allowed to sleep normally).
Controlled observation
An observation study where the researchers control some variables - often takes place in laboratory setting
Correlational analysis
A mathematical technique where the researcher looks to see whether scores for two covariables are related
Counterbalancing
A way of trying to control for order effects in a repeated measures design, e.g. half the participants do condition A followed by B and the other half do B followed by A
Covert observation
Also known as an undisclosed observation as the participants do not know their behaviour is being observed
Critical value
The value that a test statistic must reach in order for the hypothesis to be accepted.
After completing the research, the true aim is revealed to the participant. Aim of debriefing = to return the person to the state s/he was in before they took part.
Involves misleading participants about the purpose of s study.
Demand characteristics
Occur when participants try to make sense of the research situation they are in and try to guess the purpose of the research or try to present themselves in a good way.
Dependent variable
The variable that is measured to tell you the outcome.
Descriptive statistics
Analysis of data that helps describe, show or summarize data in a meaningful way
Directional hypothesis
A one-tailed hypothesis that states the direction of the difference or relationship (e.g. boys are more helpful than girls).
Dispersion measure
A dispersion measure shows how a set of data is spread out, examples are the range and the standard deviation
Double blind control
Participants are not told the true purpose of the research and the experimenter is also blind to at least some aspects of the research design.
Ecological validity
The extent to which the findings of a research study are able to be generalized to real-life settings
Ethical guidelines
These are provided by the BPS - they are the ‘rules’ by which all psychologists should operate, including those carrying out research.
Ethical issues
There are 3 main ethical issues that occur in psychological research – deception, lack of informed consent and lack of protection of participants.
Evaluation apprehension
Participants’ behaviour is distorted as they fear being judged by observers
Event sampling
A target behaviour is identified and the observer records it every time it occurs
Experimental group
The group that received the experimental treatment (e.g. sleep deprivation)
External validity
Whether it is possible to generalise the results beyond the experimental setting.
Extraneous variable
Variables that if not controlled may affect the DV and provide a false impression than an IV has produced changes when it hasn’t.
Face validity
Simple way of assessing whether a test measures what it claims to measure which is concerned with face value – e.g. does an IQ test look like it tests intelligence.
Field experiment
An experiment that takes place in a natural setting where the experimenter manipulates the IV and measures the DV
A graph that is used for continuous data (e.g. test scores). There should be no space between the bars, because the data is continuous.
This is a formal statement or prediction of what the researcher expects to find. It needs to be testable.
Independent groups design
An experimental design where each participants only takes part in one condition of the IV
Independent variable
The variable that the experimenter manipulates (changes).
Inferential statistics
Inferential statistics are ways of analyzing data using statistical tests that allow the researcher to make conclusions about whether a hypothesis was supported by the results.
Informed consent
Psychologists should ensure that all participants are helped to understand fully all aspects of the research before they agree (give consent) to take part
Inter-observer reliability
The extent to which two or more observers are observing and recording behaviour in the same way
Internal validity
In relation to experiments, whether the results were due to the manipulation of the IV rather than other factors such as extraneous variables or demand characteristics.
Interval level data
Data measured in fixed units with equal distance between points on the scale
Investigator effects
These result from the effects of a researcher’s behaviour and characteristics on an investigation.
Laboratory experiment
An experiment that takes place in a controlled environment where the experimenter manipulates the IV and measures the DV
Matched pairs design
An experimental design where pairs of participants are matched on important characteristics and one member allocated to each condition of the IV
Measure of central tendency calculated by adding all the scores in a set of data together and dividing by the total number of scores
Measures of central tendency
A measurement of data that indicates where the middle of the information lies e.g. mean, median or mode
Measure of central tendency calculated by arranging scores in a set of data from lowest to highest and finding the middle score
Meta-analysis
A technique where rather than conducting new research with participants, the researchers examine the results of several studies that have already been conducted
Measure of central tendency which is the most frequently occurring score in a set of data
Natural experiment
An experiment where the change in the IV already exists rather than being manipulated by the experimenter
Naturalistic observation
An observation study conducted in the environment where the behaviour would normally occur
Negative correlation
A relationship exists between two covariables where as one increases, the other decreases
Nominal level data
Frequency count data that consists of the number of participants falling into categories. (e.g. 7 people passed their driving test first time, 6 didn’t).
Non-directional hypothesis
A two-tailed hypothesis that does not predict the direction of the difference or relationship (e.g. girls and boys are different in terms of helpfulness).
Normal distribution
An arrangement of a data that is symmetrical and forms a bell shaped pattern where the mean, median and mode all fall in the centre at the highest peak
Observed value
The value that you have obtained from conducting your statistical test
Observer bias
Occurs when the observers know the aims of the study study or the hypotheses and allow this knowledge to influence their observations
Open questions
Questions where there is no fixed response and participants can give any answer they like. They generate qualitative data.
Operationalising variables
This means clearly describing the variables (IV and DV) in terms of how they will be manipulated (IV) or measured (DV).
Opportunity sample
A sampling technique where participants are chosen because they are easily available
Order effects
Order effects can occur in a repeated measures design and refers to how the positioning of tasks influences the outcome e.g. practice effect or boredom effect on second task
Ordinal level data
Data that is capable of being out into rank order (e.g. places in a beauty contest, or ratings for attractiveness).
Overt observation
Also known as a disclosed observation as the participants given their permission for their behaviour to be observed
Participant observation
Observation study where the researcher actually joins the group or takes part in the situation they are observing.
Peer review
Before going to publication, a research report is sent other psychologists who are knowledgeable in the research topic for them to review the study, and check for any problems
Pilot study
A small scale study conducted to ensure the method will work according to plan. If it doesn’t then amendments can be made.
Positive correlation
A relationship exists between two covariables where as one increases, so does the other
Presumptive consent
Asking a group of people from the same target population as the sample whether they would agree to take part in such a study, if yes then presume the sample would
Primary data
Information that the researcher has collected him/herself for a specific purpose e.g. data from an experiment or observation
Prior general consent
Before participants are recruited they are asked whether they are prepared to take part in research where they might be deceived about the true purpose
Probability
How likely something is to happen – can be expressed as a number (0.5) or a percentage (50% change of tossing coin and getting a head)
Protection of participants
Participants should be protected from physical or mental health, including stress - risk of harm must be no greater than that to which they are exposed in everyday life
Qualitative data
Descriptive information that is expressed in words
Quantitative data
Information that can be measured and written down with numbers.
Quasi experiment
An experiment often conducted in controlled conditions where the IV simply exists so there can be no random allocation to the conditions
Questionnaire
A set of written questions that participants fill in themselves
Random sampling
A sampling technique where everyone in the target population has an equal chance of being selected
Randomisation
Refers to the practice of using chance methods (e.g. flipping a coin' to allocate participants to the conditions of an investigation
The distance between the lowest and the highest value in a set of scores.
A measure of dispersion which involves subtracting the lowest score from the highest score in a set of data
Reliability
Whether something is consistent. In the case of a study, whether it is replicable.
Repeated measures design
An experimental design where each participants takes part in both/all conditions of the IV
Representative sample
A sample that that closely matched the target population as a whole in terms of key variables and characteristics
Retrospective consent
Once the true nature of the research has been revealed, participants should be given the right to withdraw their data if they are not happy.
Right to withdraw
Participants should be aware that they can leave the study at any time, even if they have been paid to take part.
A group of people that are drawn from the target population to take part in a research investigation
Scattergram
Used to plot correlations where each pair of values is plotted against each other to see if there is a relationship between them.
Secondary data
Information that someone else has collected e.g. the work of other psychologists or government statistics
Semi-structured interview
Interview that has some pre-determined questions, but the interviewer can develop others in response to answers given by the participant
A statistical test used to analyse the direction of differences of scores between the same or matched pairs of subjects under two experimental conditions
Significance
If the result of a statistical test is significant it is highly unlikely to have occurred by chance
Single-blind control
Participants are not told the true purpose of the research
Skewed distribution
An arrangement of data that is not symmetrical as data is clustered ro one end of the distribution
Social desirability bias
Participants’ behaviour is distorted as they modify this in order to be seen in a positive light.
Standard deviation
A measure of the average spread of scores around the mean. The greater the standard deviation the more spread out the scores are. .
Standardised instructions
The instructions given to each participant are kept identical – to help prevent experimenter bias.
Standardised procedures
In every step of the research all the participants are treated in exactly the same way and so all have the same experience.
Stratified sample
A sampling technique where groups of participants are selected in proportion to their frequency in the target population
Structured interview
Interview where the questions are fixed and the interviewer reads them out and records the responses
Structured observation
An observation study using predetermined coding scheme to record the participants' behaviour
Systematic sample
A sampling technique where every nth person in a list of the target population is selected
Target population
The group that the researchers draws the sample from and wants to be able to generalise the findings to
Temporal validity
Refers to how likely it is that the time period when a study was conducted has influenced the findings and whether they can be generalised to other periods in time
Test-retest reliability
Involves presenting the same participants with the same test or questionnaire on two separate occasions and seeing whether there is a positive correlation between the two
Thematic analysis
A method for analysing qualitative data which involves identifying, analysing and reporting patterns within the data
Time sampling
A way of sampling the behaviour that is being observed by recording what happens in a series of fixed time intervals.
Type 1 error
Is a false positive. It is where you accept the alternative/experimental hypothesis when it is false
Type 2 error
Is a false negative. It is where you accept the null hypothesis when it is false
Unstructured interview
Also know as a clinical interview, there are no fixed questions just general aims and it is more like a conversation
Unstructured observation
Observation where there is no checklist so every behaviour seen is written down in an much detail as possible
Whether something is true – measures what it sets out to measure.
Volunteer sample
A sampling technique where participants put themselves forward to take part in research, often by answering an advertisement
You might also like
Explanations for conformity, cultural variations in attachment, emergence of psychology as a science: the laboratory experiment, scoville and milner (1957), kohlberg (1968), schizophrenia: what is schizophrenia, biopsychology: the pns – somatic and autonomic nervous systems, relationships: duck's phase model of relationship breakdown, our subjects.
- › Criminology
- › Economics
- › Geography
- › Health & Social Care
- › Psychology
- › Sociology
- › Teaching & learning resources
- › Student revision workshops
- › Online student courses
- › CPD for teachers
- › Livestreams
- › Teaching jobs
Boston House, 214 High Street, Boston Spa, West Yorkshire, LS23 6AD Tel: 01937 848885
- › Contact us
- › Terms of use
- › Privacy & cookies
© 2002-2024 Tutor2u Limited. Company Reg no: 04489574. VAT reg no 816865400.

Getting Started with Research
- Introduction: Information Literacy is Integral to Academic Research
- Scholarship as Conversation /Research IS Conversation
- The Research Process
- Background Research Tips
- Developing a Research Question
- Identifying Key Words
From Research Question(s) to Keywords
One thing to keep in mind is that our focus is on using keywords in research databases rather than in a Web search such as in Google. Database searching requires a more structured approach to search them effectively. Once you have selected a topic for your research, the next step is identifying the keywords critical to describing your topic and generating more before you start searching one of the research databases
Let's say that your research question is: Does media have an impact on the self image of teenagers?
The next step is to pull out the key concepts. This may seem simple but identifying the main concepts in the research question is important for a successful search. This question has three major concepts: Media, Self-image and Teenagers. These keywords will be the building blocks of our search. It’s best to search by keywords instead of phrases or sentences because the more words you add to a search box, the fewer results you’ll get back. You are more likely to find useful articles if you start your search with a minimal number of keywords .
Note: You probably noticed the absence of “impact” as one of the keywords. “Impact” is certainly an important concept but starting out it will be of more interest to us to see what kind of publications are available on self-image, teenagers and media in total. Let’s hold “impact” in reserve in case we need to refine our search later.
We can’t stop here with only those three keywords! There may be synonyms for your keywords that can help broaden your search or better describe your topic, not only to make your search comprehensive but to also improve your search results. Since this step can require more creativity it can be useful to brainstorm synonyms with friends or classmates. What other words can describe your main concepts? Start making a list of your alternative keywords and synonyms and have them ready when you start searching. You may need them!
It may be helpful to use a table, such as the one below to brainstorm alternative keywords/synonyms:

- << Previous: Developing a Research Question
- Last Updated: Oct 17, 2023 8:42 AM
- URL: https://library.defiance.edu/InfoLit-GettingStarted
Pilgrim Library :
419-783-2481 , library@ defiance.edu , click the purple "ask us" side tab above.
- Privacy Policy

Home » Variables in Research – Definition, Types and Examples
Variables in Research – Definition, Types and Examples
Table of Contents

Variables in Research
Definition:
In Research, Variables refer to characteristics or attributes that can be measured, manipulated, or controlled. They are the factors that researchers observe or manipulate to understand the relationship between them and the outcomes of interest.
Types of Variables in Research
Types of Variables in Research are as follows:
Independent Variable
This is the variable that is manipulated by the researcher. It is also known as the predictor variable, as it is used to predict changes in the dependent variable. Examples of independent variables include age, gender, dosage, and treatment type.
Dependent Variable
This is the variable that is measured or observed to determine the effects of the independent variable. It is also known as the outcome variable, as it is the variable that is affected by the independent variable. Examples of dependent variables include blood pressure, test scores, and reaction time.
Confounding Variable
This is a variable that can affect the relationship between the independent variable and the dependent variable. It is a variable that is not being studied but could impact the results of the study. For example, in a study on the effects of a new drug on a disease, a confounding variable could be the patient’s age, as older patients may have more severe symptoms.
Mediating Variable
This is a variable that explains the relationship between the independent variable and the dependent variable. It is a variable that comes in between the independent and dependent variables and is affected by the independent variable, which then affects the dependent variable. For example, in a study on the relationship between exercise and weight loss, the mediating variable could be metabolism, as exercise can increase metabolism, which can then lead to weight loss.
Moderator Variable
This is a variable that affects the strength or direction of the relationship between the independent variable and the dependent variable. It is a variable that influences the effect of the independent variable on the dependent variable. For example, in a study on the effects of caffeine on cognitive performance, the moderator variable could be age, as older adults may be more sensitive to the effects of caffeine than younger adults.
Control Variable
This is a variable that is held constant or controlled by the researcher to ensure that it does not affect the relationship between the independent variable and the dependent variable. Control variables are important to ensure that any observed effects are due to the independent variable and not to other factors. For example, in a study on the effects of a new teaching method on student performance, the control variables could include class size, teacher experience, and student demographics.
Continuous Variable
This is a variable that can take on any value within a certain range. Continuous variables can be measured on a scale and are often used in statistical analyses. Examples of continuous variables include height, weight, and temperature.
Categorical Variable
This is a variable that can take on a limited number of values or categories. Categorical variables can be nominal or ordinal. Nominal variables have no inherent order, while ordinal variables have a natural order. Examples of categorical variables include gender, race, and educational level.
Discrete Variable
This is a variable that can only take on specific values. Discrete variables are often used in counting or frequency analyses. Examples of discrete variables include the number of siblings a person has, the number of times a person exercises in a week, and the number of students in a classroom.
Dummy Variable
This is a variable that takes on only two values, typically 0 and 1, and is used to represent categorical variables in statistical analyses. Dummy variables are often used when a categorical variable cannot be used directly in an analysis. For example, in a study on the effects of gender on income, a dummy variable could be created, with 0 representing female and 1 representing male.
Extraneous Variable
This is a variable that has no relationship with the independent or dependent variable but can affect the outcome of the study. Extraneous variables can lead to erroneous conclusions and can be controlled through random assignment or statistical techniques.
Latent Variable
This is a variable that cannot be directly observed or measured, but is inferred from other variables. Latent variables are often used in psychological or social research to represent constructs such as personality traits, attitudes, or beliefs.
Moderator-mediator Variable
This is a variable that acts both as a moderator and a mediator. It can moderate the relationship between the independent and dependent variables and also mediate the relationship between the independent and dependent variables. Moderator-mediator variables are often used in complex statistical analyses.
Variables Analysis Methods
There are different methods to analyze variables in research, including:
- Descriptive statistics: This involves analyzing and summarizing data using measures such as mean, median, mode, range, standard deviation, and frequency distribution. Descriptive statistics are useful for understanding the basic characteristics of a data set.
- Inferential statistics : This involves making inferences about a population based on sample data. Inferential statistics use techniques such as hypothesis testing, confidence intervals, and regression analysis to draw conclusions from data.
- Correlation analysis: This involves examining the relationship between two or more variables. Correlation analysis can determine the strength and direction of the relationship between variables, and can be used to make predictions about future outcomes.
- Regression analysis: This involves examining the relationship between an independent variable and a dependent variable. Regression analysis can be used to predict the value of the dependent variable based on the value of the independent variable, and can also determine the significance of the relationship between the two variables.
- Factor analysis: This involves identifying patterns and relationships among a large number of variables. Factor analysis can be used to reduce the complexity of a data set and identify underlying factors or dimensions.
- Cluster analysis: This involves grouping data into clusters based on similarities between variables. Cluster analysis can be used to identify patterns or segments within a data set, and can be useful for market segmentation or customer profiling.
- Multivariate analysis : This involves analyzing multiple variables simultaneously. Multivariate analysis can be used to understand complex relationships between variables, and can be useful in fields such as social science, finance, and marketing.
Examples of Variables
- Age : This is a continuous variable that represents the age of an individual in years.
- Gender : This is a categorical variable that represents the biological sex of an individual and can take on values such as male and female.
- Education level: This is a categorical variable that represents the level of education completed by an individual and can take on values such as high school, college, and graduate school.
- Income : This is a continuous variable that represents the amount of money earned by an individual in a year.
- Weight : This is a continuous variable that represents the weight of an individual in kilograms or pounds.
- Ethnicity : This is a categorical variable that represents the ethnic background of an individual and can take on values such as Hispanic, African American, and Asian.
- Time spent on social media : This is a continuous variable that represents the amount of time an individual spends on social media in minutes or hours per day.
- Marital status: This is a categorical variable that represents the marital status of an individual and can take on values such as married, divorced, and single.
- Blood pressure : This is a continuous variable that represents the force of blood against the walls of arteries in millimeters of mercury.
- Job satisfaction : This is a continuous variable that represents an individual’s level of satisfaction with their job and can be measured using a Likert scale.
Applications of Variables
Variables are used in many different applications across various fields. Here are some examples:
- Scientific research: Variables are used in scientific research to understand the relationships between different factors and to make predictions about future outcomes. For example, scientists may study the effects of different variables on plant growth or the impact of environmental factors on animal behavior.
- Business and marketing: Variables are used in business and marketing to understand customer behavior and to make decisions about product development and marketing strategies. For example, businesses may study variables such as consumer preferences, spending habits, and market trends to identify opportunities for growth.
- Healthcare : Variables are used in healthcare to monitor patient health and to make treatment decisions. For example, doctors may use variables such as blood pressure, heart rate, and cholesterol levels to diagnose and treat cardiovascular disease.
- Education : Variables are used in education to measure student performance and to evaluate the effectiveness of teaching strategies. For example, teachers may use variables such as test scores, attendance, and class participation to assess student learning.
- Social sciences : Variables are used in social sciences to study human behavior and to understand the factors that influence social interactions. For example, sociologists may study variables such as income, education level, and family structure to examine patterns of social inequality.
Purpose of Variables
Variables serve several purposes in research, including:
- To provide a way of measuring and quantifying concepts: Variables help researchers measure and quantify abstract concepts such as attitudes, behaviors, and perceptions. By assigning numerical values to these concepts, researchers can analyze and compare data to draw meaningful conclusions.
- To help explain relationships between different factors: Variables help researchers identify and explain relationships between different factors. By analyzing how changes in one variable affect another variable, researchers can gain insight into the complex interplay between different factors.
- To make predictions about future outcomes : Variables help researchers make predictions about future outcomes based on past observations. By analyzing patterns and relationships between different variables, researchers can make informed predictions about how different factors may affect future outcomes.
- To test hypotheses: Variables help researchers test hypotheses and theories. By collecting and analyzing data on different variables, researchers can test whether their predictions are accurate and whether their hypotheses are supported by the evidence.
Characteristics of Variables
Characteristics of Variables are as follows:
- Measurement : Variables can be measured using different scales, such as nominal, ordinal, interval, or ratio scales. The scale used to measure a variable can affect the type of statistical analysis that can be applied.
- Range : Variables have a range of values that they can take on. The range can be finite, such as the number of students in a class, or infinite, such as the range of possible values for a continuous variable like temperature.
- Variability : Variables can have different levels of variability, which refers to the degree to which the values of the variable differ from each other. Highly variable variables have a wide range of values, while low variability variables have values that are more similar to each other.
- Validity and reliability : Variables should be both valid and reliable to ensure accurate and consistent measurement. Validity refers to the extent to which a variable measures what it is intended to measure, while reliability refers to the consistency of the measurement over time.
- Directionality: Some variables have directionality, meaning that the relationship between the variables is not symmetrical. For example, in a study of the relationship between smoking and lung cancer, smoking is the independent variable and lung cancer is the dependent variable.
Advantages of Variables
Here are some of the advantages of using variables in research:
- Control : Variables allow researchers to control the effects of external factors that could influence the outcome of the study. By manipulating and controlling variables, researchers can isolate the effects of specific factors and measure their impact on the outcome.
- Replicability : Variables make it possible for other researchers to replicate the study and test its findings. By defining and measuring variables consistently, other researchers can conduct similar studies to validate the original findings.
- Accuracy : Variables make it possible to measure phenomena accurately and objectively. By defining and measuring variables precisely, researchers can reduce bias and increase the accuracy of their findings.
- Generalizability : Variables allow researchers to generalize their findings to larger populations. By selecting variables that are representative of the population, researchers can draw conclusions that are applicable to a broader range of individuals.
- Clarity : Variables help researchers to communicate their findings more clearly and effectively. By defining and categorizing variables, researchers can organize and present their findings in a way that is easily understandable to others.
Disadvantages of Variables
Here are some of the main disadvantages of using variables in research:
- Simplification : Variables may oversimplify the complexity of real-world phenomena. By breaking down a phenomenon into variables, researchers may lose important information and context, which can affect the accuracy and generalizability of their findings.
- Measurement error : Variables rely on accurate and precise measurement, and measurement error can affect the reliability and validity of research findings. The use of subjective or poorly defined variables can also introduce measurement error into the study.
- Confounding variables : Confounding variables are factors that are not measured but that affect the relationship between the variables of interest. If confounding variables are not accounted for, they can distort or obscure the relationship between the variables of interest.
- Limited scope: Variables are defined by the researcher, and the scope of the study is therefore limited by the researcher’s choice of variables. This can lead to a narrow focus that overlooks important aspects of the phenomenon being studied.
- Ethical concerns: The selection and measurement of variables may raise ethical concerns, especially in studies involving human subjects. For example, using variables that are related to sensitive topics, such as race or sexuality, may raise concerns about privacy and discrimination.
About the author
Muhammad Hassan
Researcher, Academic Writer, Web developer
You may also like

Quantitative Variable – Definition, Types and...

Categorical Variable – Definition, Types and...

Dependent Variable – Definition, Types and...

Continuous Variable – Definition, Types and...

Qualitative Variable – Types and Examples

Ratio Variable – Definition, Purpose and Examples
4 surprising ways to find happiness, according to studies
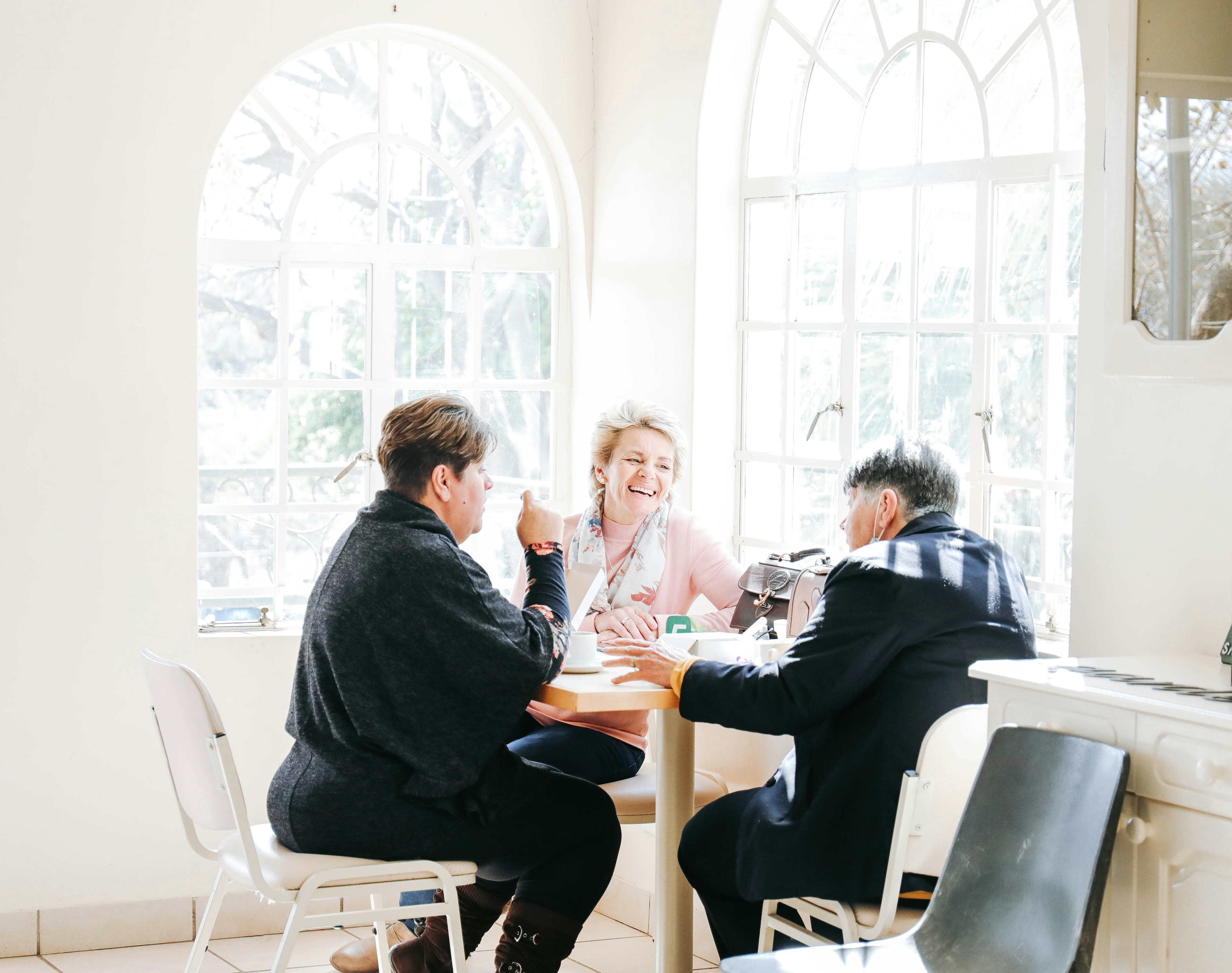
In an ageing world, social connections are crucial. Image: Unsplash/Melanie Stander
.chakra .wef-1c7l3mo{-webkit-transition:all 0.15s ease-out;transition:all 0.15s ease-out;cursor:pointer;-webkit-text-decoration:none;text-decoration:none;outline:none;color:inherit;}.chakra .wef-1c7l3mo:hover,.chakra .wef-1c7l3mo[data-hover]{-webkit-text-decoration:underline;text-decoration:underline;}.chakra .wef-1c7l3mo:focus,.chakra .wef-1c7l3mo[data-focus]{box-shadow:0 0 0 3px rgba(168,203,251,0.5);} Michael Purton
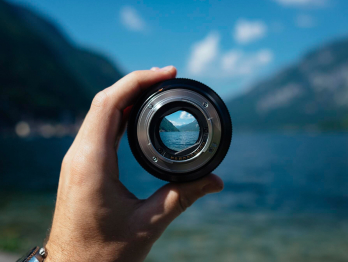
.chakra .wef-1nk5u5d{margin-top:16px;margin-bottom:16px;line-height:1.388;color:#2846F8;font-size:1.25rem;}@media screen and (min-width:56.5rem){.chakra .wef-1nk5u5d{font-size:1.125rem;}} Get involved .chakra .wef-9dduvl{margin-top:16px;margin-bottom:16px;line-height:1.388;font-size:1.25rem;}@media screen and (min-width:56.5rem){.chakra .wef-9dduvl{font-size:1.125rem;}} with our crowdsourced digital platform to deliver impact at scale
- There are ‘hacks’ to happiness, a university study has found.
- Having a sense of meaning is more important than pleasure, another study found.
- Social connections that prevent loneliness are crucial for happiness in our ageing world, according to a World Economic Forum initiative.
We all want to be happy, but achieving happiness can feel far from straightforward.
Could science help us?
Yes, according to studies. One research programme found that there are seven ‘hacks’ to being happy, another discovered that purpose is more important than pleasure, while a third revealed that spending time among birdlife is the answer.
As the World Happiness Report 2024 reveals the countries with the happiest citizens , here are four facts about happiness which may surprise you.
Have you read?
A generation adrift: why young people are less happy and what we can do about it, charted: the happiest countries in the world, why wellbeing and happiness hold the key to a healthy ageing society in japan, 1. hack your way to happiness.
There are seven “happiness hacks” , according to a study by the University of Bristol, in the UK.
The Science of Happiness programme questioned 228 psychology undergraduates who had taken a positive psychology course.
Immediately after taking the course, the students reported a 10-15% improvement in their wellbeing, while researchers found that, by continuing to practise the activities they had been taught, more than half the group maintained their positive outlook one to two years afterwards .
Dr Bruce Hood, senior author of the study, has identified seven “happiness hacks”. In case you want to try them out for yourself, they are:
- Performing acts of kindness
- Increasing social connections, including initiating conversations with people you don’t know
- Savouring experiences
- Deliberately drawing attention to the positive events and aspects of the day
- Practising feeling grateful, and endeavouring to thank people
- Being physically active
- Exploring mindfulness and other meditation techniques.
One in four people will experience mental illness in their lives, costing the global economy an estimated $6 trillion by 2030.
Mental ill-health is the leading cause of disability and poor life outcomes in young people aged 10–24 years, contributing up to 45% of the overall burden of disease in this age-group. Yet globally, young people have the worst access to youth mental health care within the lifespan and across all the stages of illness (particularly during the early stages).
In response, the Forum has launched a global dialogue series to discuss the ideas, tools and architecture in which public and private stakeholders can build an ecosystem for health promotion and disease management on mental health.
One of the current key priorities is to support global efforts toward mental health outcomes - promoting key recommendations toward achieving the global targets on mental health, such as the WHO Knowledge-Action-Portal and the Countdown Global Mental Health
Read more about the work of our Platform for Shaping the Future of Health and Healthcare , and contact us to get involved.
2. Purpose rather than pleasure is the key to happiness
When it comes to being happy, having a sense of meaning in life is more important than pleasure , according to a study by the ESCP Business School.
The research investigated how pleasure, meaning and spirituality affected life satisfaction levels in 2,615 people spread across six continents and representing different cultural contexts.
And they discovered that meaning is a stronger predictor of life satisfaction than pleasure and spirituality.
The study’s authors, writing in a World Economic Forum piece, said the findings could be valuable for bosses looking for new ways to enhance staff wellbeing. Managers “could benefit from widening their focus on pleasure-based benefits such as financial rewards, to include giving employees a sense of meaning , by volunteering their time, for example,” they said.
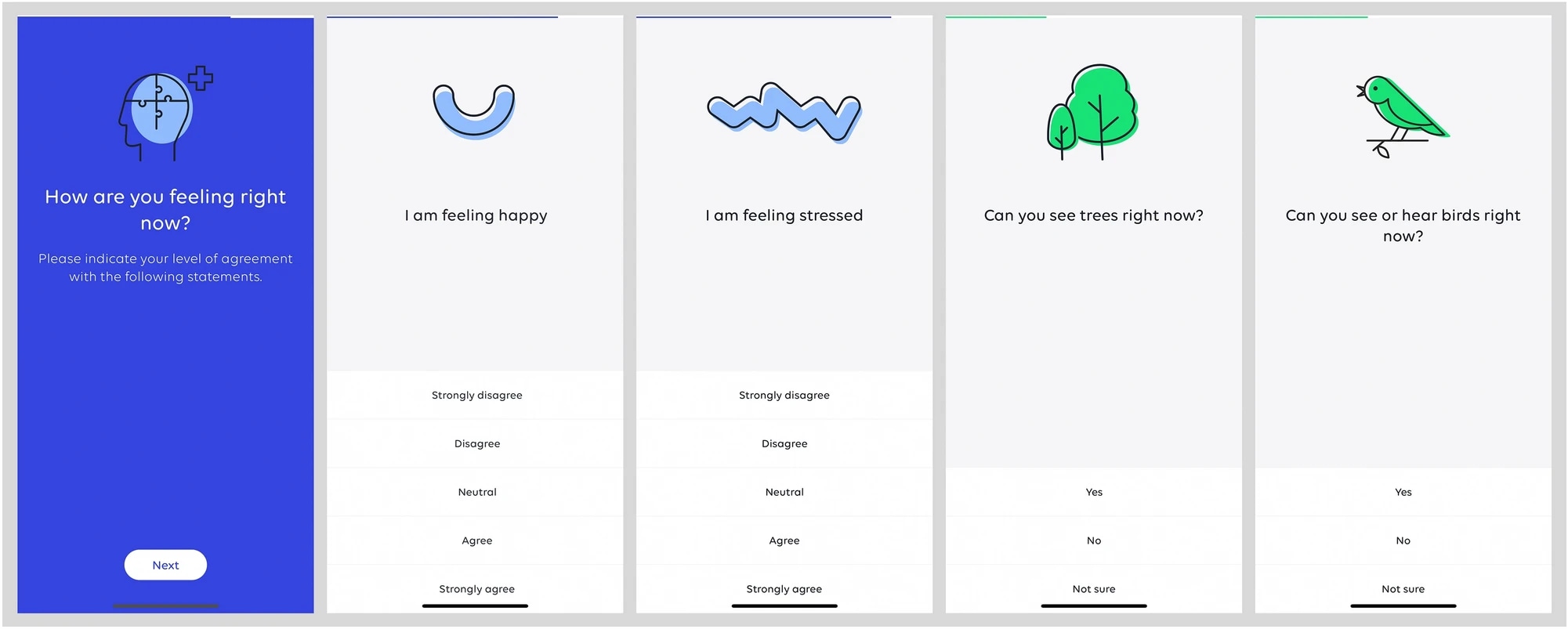
3. Just seeing or hearing birds can make you happier
Achieving happiness, or at least an improvement in your sense of well-being, doesn’t have to be complicated, it turns out.
A King’s College London study discovered “ significant positive associations between seeing or hearing birds and mental wellbeing ”.
Using an app, more than 1,200 people from around the world “were prompted at random intervals to record how they were feeling, including whether they were happy or stressed , whether they could see trees, and whether they could see or hear birds,” reports The Guardian.
And it turns out that everyday encounters with birdlife can be linked to “time-lasting improvements in mental well-being”. What’s more, say the study’s authors, “these improvements were evident not only in healthy people but also in those with a diagnosis of depression, the most common mental illness across the world”.
4. Good relationships make us happy – and live longer
Harvard University researchers have spent 85 years trying to scientifically prove what makes us happy. And they think they’ve found the answer – being sociable.
The study focused on 724 participants, from adolescence to old age. Regardless of material wealth, physical health or job status, what was striking over the course of the project was that people with strong, supportive relationships were found to be happier , healthier and live longer than those without.
The study also found that “social fitness” – the ability to build and maintain strong relationships – was more important to a long and happy life than genes, social class, or IQ.
“Personal connection creates mental and emotional stimulation, which are automatic mood boosters ,” said the project’s director, Dr Waldinger, “while isolation is a mood buster.”
Isolation is one of the challenges facing our ageing population. To that end, the Forum has developed six ‘longevity economy’ principles .
Principle five is to ‘ Design systems and environments for social connection and purpose ’. “Social connection is integral to healthy longevity,” says the report. “Socially isolated older adults have a higher risk of poor health and earlier death. Intentional design of systems and environments for social connection can mitigate these impacts,” it says.
Don't miss any update on this topic
Create a free account and access your personalized content collection with our latest publications and analyses.
License and Republishing
World Economic Forum articles may be republished in accordance with the Creative Commons Attribution-NonCommercial-NoDerivatives 4.0 International Public License, and in accordance with our Terms of Use.
The views expressed in this article are those of the author alone and not the World Economic Forum.
The Agenda .chakra .wef-n7bacu{margin-top:16px;margin-bottom:16px;line-height:1.388;font-weight:400;} Weekly
A weekly update of the most important issues driving the global agenda
.chakra .wef-1dtnjt5{display:-webkit-box;display:-webkit-flex;display:-ms-flexbox;display:flex;-webkit-align-items:center;-webkit-box-align:center;-ms-flex-align:center;align-items:center;-webkit-flex-wrap:wrap;-ms-flex-wrap:wrap;flex-wrap:wrap;} More on Wellbeing and Mental Health .chakra .wef-17xejub{-webkit-flex:1;-ms-flex:1;flex:1;justify-self:stretch;-webkit-align-self:stretch;-ms-flex-item-align:stretch;align-self:stretch;} .chakra .wef-nr1rr4{display:-webkit-inline-box;display:-webkit-inline-flex;display:-ms-inline-flexbox;display:inline-flex;white-space:normal;vertical-align:middle;text-transform:uppercase;font-size:0.75rem;border-radius:0.25rem;font-weight:700;-webkit-align-items:center;-webkit-box-align:center;-ms-flex-align:center;align-items:center;line-height:1.2;-webkit-letter-spacing:1.25px;-moz-letter-spacing:1.25px;-ms-letter-spacing:1.25px;letter-spacing:1.25px;background:none;padding:0px;color:#B3B3B3;-webkit-box-decoration-break:clone;box-decoration-break:clone;-webkit-box-decoration-break:clone;}@media screen and (min-width:37.5rem){.chakra .wef-nr1rr4{font-size:0.875rem;}}@media screen and (min-width:56.5rem){.chakra .wef-nr1rr4{font-size:1rem;}} See all
Olena Zelenska: War is harming global mental health — even for people living in safety
Olena Zelenska
May 31, 2024
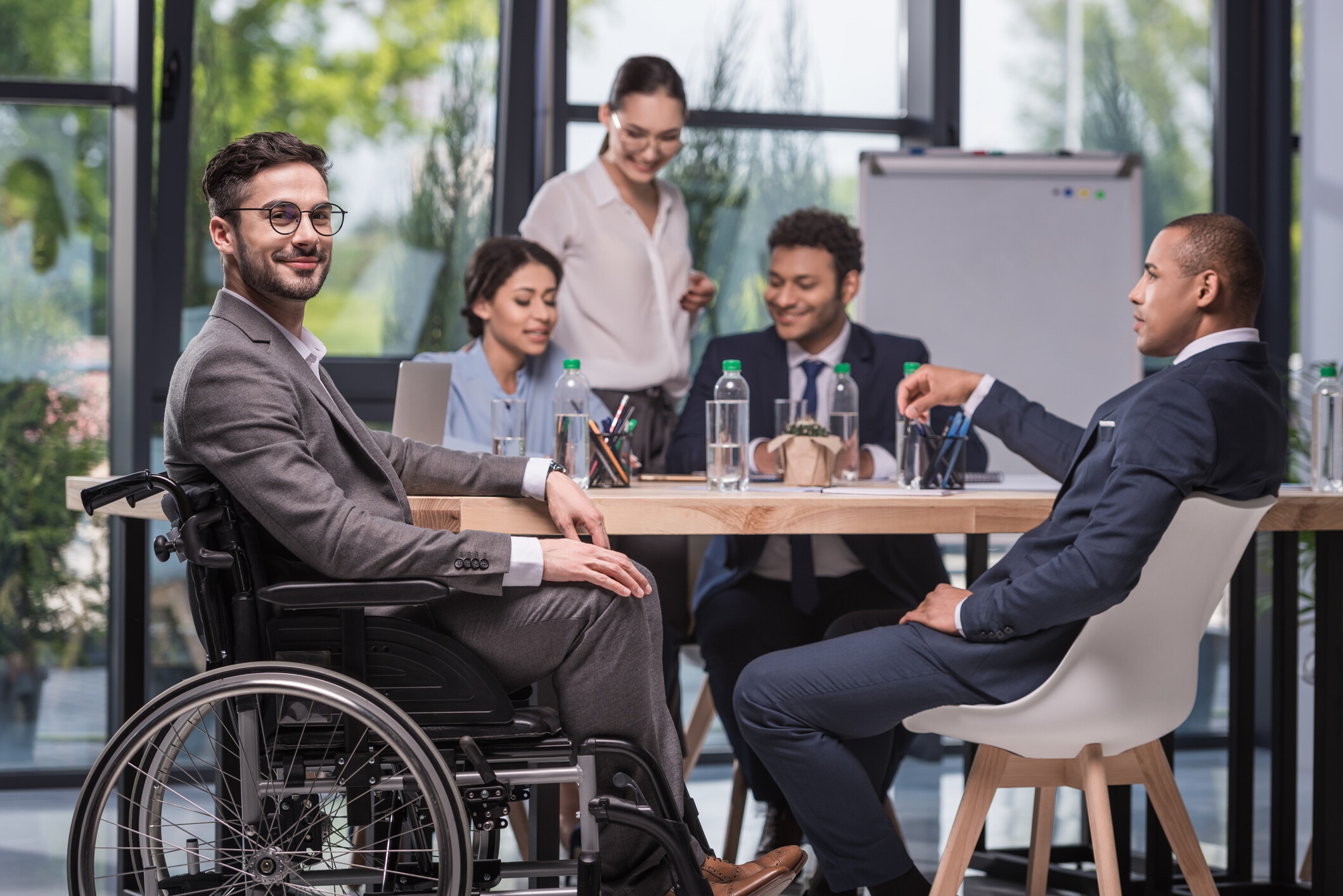
Improving workplace productivity requires a holistic approach to employee health and well-being
Susan Garfield, Ruma Bhargava and Eric Kostegan
May 30, 2024
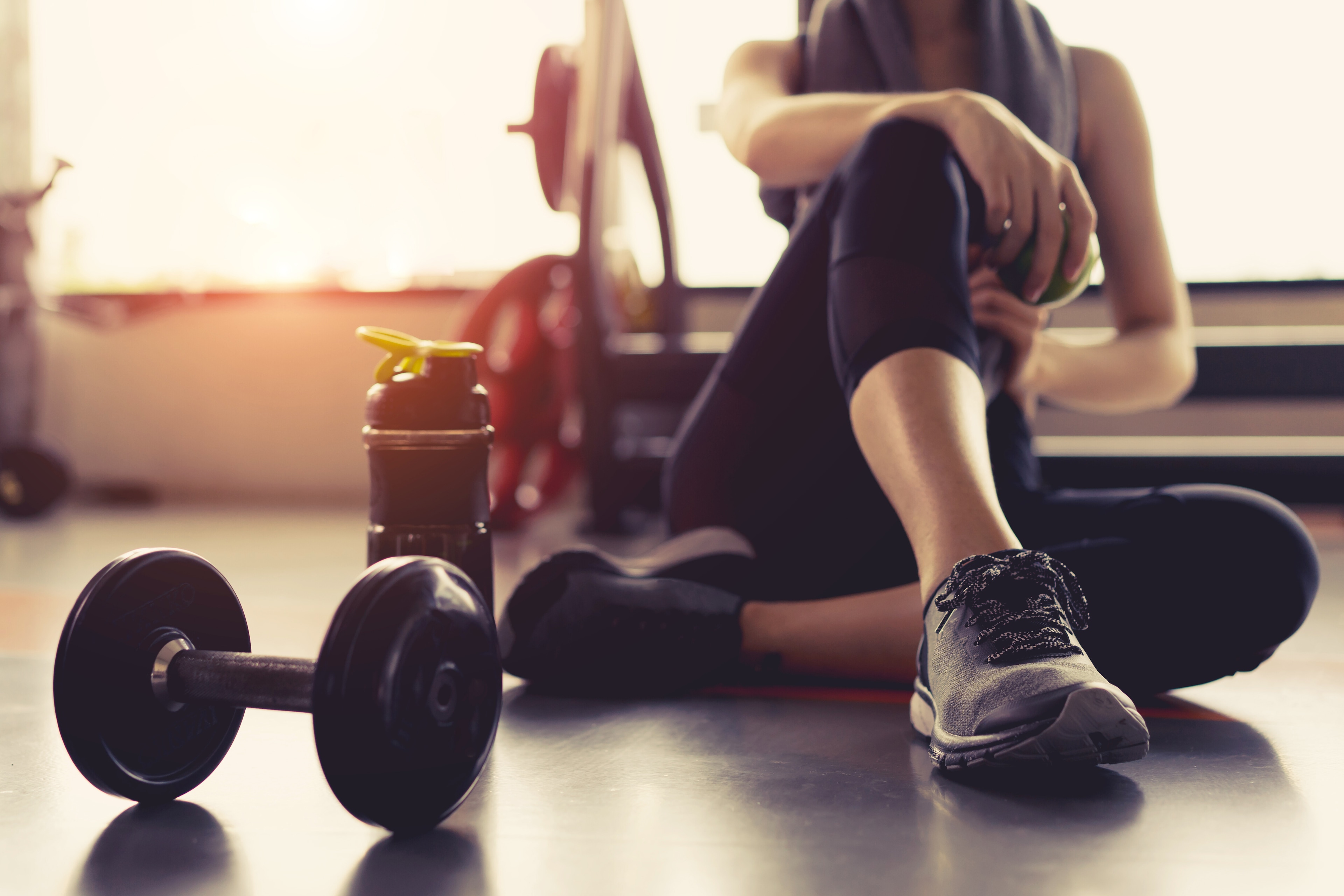
Why movement is the best prescription for a healthy workforce
Emma Mason Zwiebler
May 28, 2024
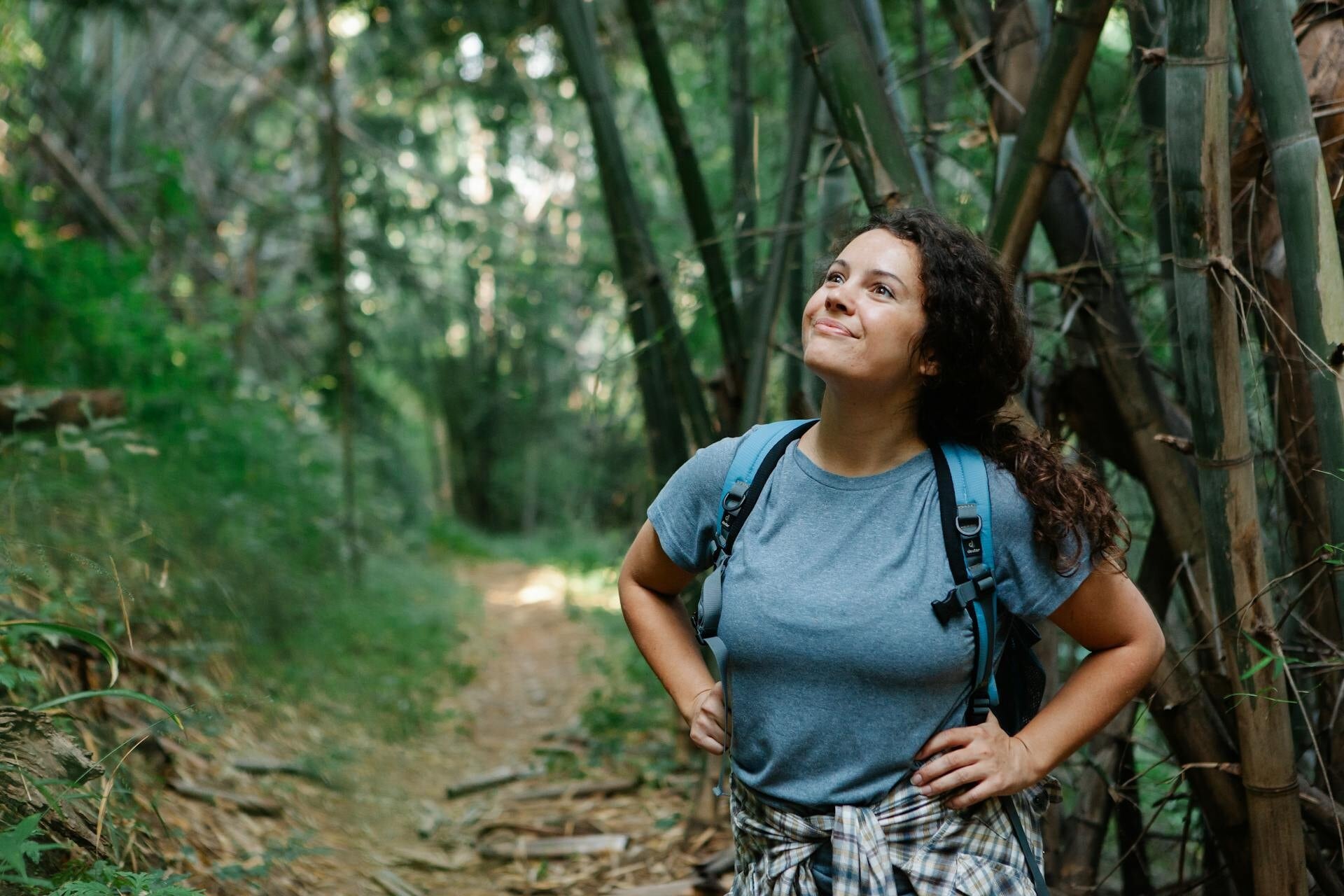
The fascinating link between biodiversity and mental wellbeing
Andrea Mechelli
May 15, 2024
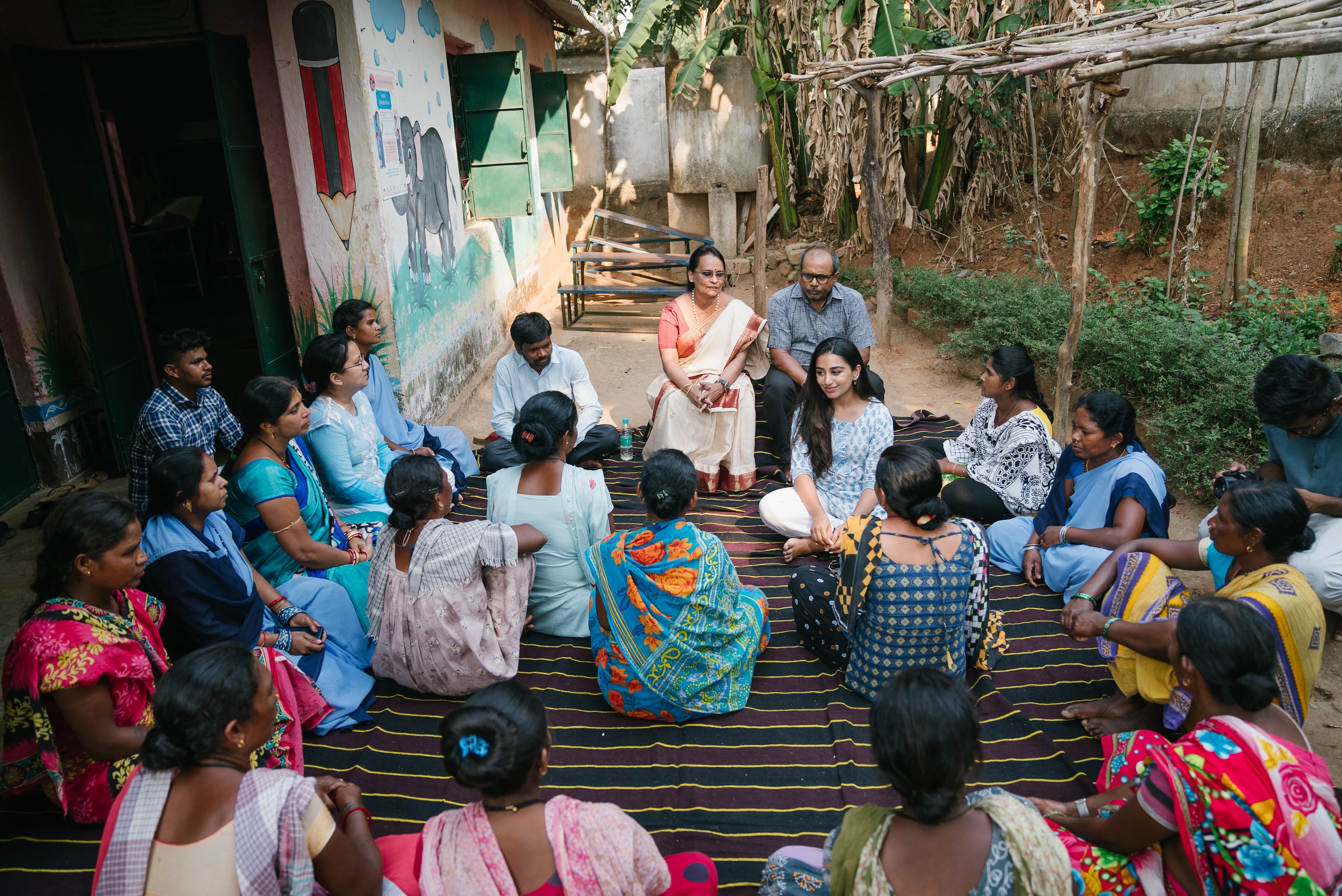
How philanthropy is empowering India's mental health sector
Kiran Mazumdar-Shaw
May 2, 2024
From 'Quit-Tok' to proximity bias, here are 11 buzzwords from the world of hybrid work
Kate Whiting
April 17, 2024
The four building blocks of change
Large-scale organizational change has always been difficult, and there’s no shortage of research showing that a majority of transformations continue to fail. Today’s dynamic environment adds an extra level of urgency and complexity. Companies must increasingly react to sudden shifts in the marketplace, to other external shocks, and to the imperatives of new business models. The stakes are higher than ever.
So what’s to be done? In both research and practice, we find that transformations stand the best chance of success when they focus on four key actions to change mind-sets and behavior: fostering understanding and conviction, reinforcing changes through formal mechanisms, developing talent and skills, and role modeling. Collectively labeled the “influence model,” these ideas were introduced more than a dozen years ago in a McKinsey Quarterly article, “ The psychology of change management .” They were based on academic research and practical experience—what we saw worked and what didn’t.
Digital technologies and the changing nature of the workforce have created new opportunities and challenges for the influence model (for more on the relationship between those trends and the model, see this article’s companion, “ Winning hearts and minds in the 21st century ”). But it still works overall, a decade and a half later (exhibit). In a recent McKinsey Global Survey, we examined successful transformations and found that they were nearly eight times more likely to use all four actions as opposed to just one. 1 1. See “ The science of organizational transformations ,” September 2015. Building both on classic and new academic research, the present article supplies a primer on the model and its four building blocks: what they are, how they work, and why they matter.
Fostering understanding and conviction
We know from research that human beings strive for congruence between their beliefs and their actions and experience dissonance when these are misaligned. Believing in the “why” behind a change can therefore inspire people to change their behavior. In practice, however, we find that many transformation leaders falsely assume that the “why” is clear to the broader organization and consequently fail to spend enough time communicating the rationale behind change efforts.
This common pitfall is predictable. Research shows that people frequently overestimate the extent to which others share their own attitudes, beliefs, and opinions—a tendency known as the false-consensus effect. Studies also highlight another contributing phenomenon, the “curse of knowledge”: people find it difficult to imagine that others don’t know something that they themselves do know. To illustrate this tendency, a Stanford study asked participants to tap out the rhythms of well-known songs and predict the likelihood that others would guess what they were. The tappers predicted that the listeners would identify half of the songs correctly; in reality, they did so less than 5 percent of the time. 2 2. Chip Heath and Dan Heath, “The curse of knowledge,” Harvard Business Review , December 2006, Volume 8, Number 6, hbr.org.
Therefore, in times of transformation, we recommend that leaders develop a change story that helps all stakeholders understand where the company is headed, why it is changing, and why this change is important. Building in a feedback loop to sense how the story is being received is also useful. These change stories not only help get out the message but also, recent research finds, serve as an effective influencing tool. Stories are particularly effective in selling brands. 3 3. Harrison Monarth, “The irresistible power of storytelling as a strategic business tool,” Harvard Business Review , March 11, 2014, hbr.org.
Even 15 years ago, at the time of the original article, digital advances were starting to make employees feel involved in transformations, allowing them to participate in shaping the direction of their companies. In 2006, for example, IBM used its intranet to conduct two 72-hour “jam sessions” to engage employees, clients, and other stakeholders in an online debate about business opportunities. No fewer than 150,000 visitors attended from 104 countries and 67 different companies, and there were 46,000 posts. 4 4. Icons of Progress , “A global innovation jam,” ibm.com. As we explain in “Winning hearts and minds in the 21st century,” social and mobile technologies have since created a wide range of new opportunities to build the commitment of employees to change.
Reinforcing with formal mechanisms
Psychologists have long known that behavior often stems from direct association and reinforcement. Back in the 1920s, Ivan Pavlov’s classical conditioning research showed how the repeated association between two stimuli—the sound of a bell and the delivery of food—eventually led dogs to salivate upon hearing the bell alone. Researchers later extended this work on conditioning to humans, demonstrating how children could learn to fear a rat when it was associated with a loud noise. 5 5. John B. Watson and Rosalie Rayner, “Conditioned emotional reactions,” Journal of Experimental Psychology , 1920, Volume 3, Number 1, pp. 1–14. Of course, this conditioning isn’t limited to negative associations or to animals. The perfume industry recognizes how the mere scent of someone you love can induce feelings of love and longing.
Reinforcement can also be conscious, shaped by the expected rewards and punishments associated with specific forms of behavior. B. F. Skinner’s work on operant conditioning showed how pairing positive reinforcements such as food with desired behavior could be used, for example, to teach pigeons to play Ping-Pong. This concept, which isn’t hard to grasp, is deeply embedded in organizations. Many people who have had commissions-based sales jobs will understand the point—being paid more for working harder can sometimes be a strong incentive.
Despite the importance of reinforcement, organizations often fail to use it correctly. In a seminal paper “On the folly of rewarding A, while hoping for B,” management scholar Steven Kerr described numerous examples of organizational-reward systems that are misaligned with the desired behavior, which is therefore neglected. 6 6. Steven Kerr, “On the folly of rewarding A, while hoping for B,” Academy of Management Journal , 1975, Volume 18, Number 4, pp. 769–83. Some of the paper’s examples—such as the way university professors are rewarded for their research publications, while society expects them to be good teachers—are still relevant today. We ourselves have witnessed this phenomenon in a global refining organization facing market pressure. By squeezing maintenance expenditures and rewarding employees who cut them, the company in effect treated that part of the budget as a “super KPI.” Yet at the same time, its stated objective was reliable maintenance.
Even when organizations use money as a reinforcement correctly, they often delude themselves into thinking that it alone will suffice. Research examining the relationship between money and experienced happiness—moods and general well-being—suggests a law of diminishing returns. The relationship may disappear altogether after around $75,000, a much lower ceiling than most executives assume. 7 7. Belinda Luscombe, “Do we need $75,000 a year to be happy?” Time , September 6, 2010, time.com.
Would you like to learn more about our People & Organizational Performance Practice ?
Money isn’t the only motivator, of course. Victor Vroom’s classic research on expectancy theory explained how the tendency to behave in certain ways depends on the expectation that the effort will result in the desired kind of performance, that this performance will be rewarded, and that the reward will be desirable. 8 8. Victor Vroom, Work and motivation , New York: John Wiley, 1964. When a Middle Eastern telecommunications company recently examined performance drivers, it found that collaboration and purpose were more important than compensation (see “Ahead of the curve: The future of performance management,” forthcoming on McKinsey.com). The company therefore moved from awarding minor individual bonuses for performance to celebrating how specific teams made a real difference in the lives of their customers. This move increased motivation while also saving the organization millions.
How these reinforcements are delivered also matters. It has long been clear that predictability makes them less effective; intermittent reinforcement provides a more powerful hook, as slot-machine operators have learned to their advantage. Further, people react negatively if they feel that reinforcements aren’t distributed fairly. Research on equity theory describes how employees compare their job inputs and outcomes with reference-comparison targets, such as coworkers who have been promoted ahead of them or their own experiences at past jobs. 9 9. J. S. Adams, “Inequity in social exchanges,” Advances in Experimental Social Psychology , 1965, Volume 2, pp. 267–300. We therefore recommend that organizations neutralize compensation as a source of anxiety and instead focus on what really drives performance—such as collaboration and purpose, in the case of the Middle Eastern telecom company previously mentioned.
Developing talent and skills
Thankfully, you can teach an old dog new tricks. Human brains are not fixed; neuroscience research shows that they remain plastic well into adulthood. Illustrating this concept, scientific investigation has found that the brains of London taxi drivers, who spend years memorizing thousands of streets and local attractions, showed unique gray-matter volume differences in the hippocampus compared with the brains of other people. Research linked these differences to the taxi drivers’ extraordinary special knowledge. 10 10. Eleanor Maguire, Katherine Woollett, and Hugo Spires, “London taxi drivers and bus drivers: A structural MRI and neuropsychological analysis,” Hippocampus , 2006, Volume 16, pp. 1091–1101.
Despite an amazing ability to learn new things, human beings all too often lack insight into what they need to know but don’t. Biases, for example, can lead people to overlook their limitations and be overconfident of their abilities. Highlighting this point, studies have found that over 90 percent of US drivers rate themselves above average, nearly 70 percent of professors consider themselves in the top 25 percent for teaching ability, and 84 percent of Frenchmen believe they are above-average lovers. 11 11. The art of thinking clearly, “The overconfidence effect: Why you systematically overestimate your knowledge and abilities,” blog entry by Rolf Dobelli, June 11, 2013, psychologytoday.com. This self-serving bias can lead to blind spots, making people too confident about some of their abilities and unaware of what they need to learn. In the workplace, the “mum effect”—a proclivity to keep quiet about unpleasant, unfavorable messages—often compounds these self-serving tendencies. 12 12. Eliezer Yariv, “‘Mum effect’: Principals’ reluctance to submit negative feedback,” Journal of Managerial Psychology , 2006, Volume 21, Number 6, pp. 533–46.
Even when people overcome such biases and actually want to improve, they can handicap themselves by doubting their ability to change. Classic psychological research by Martin Seligman and his colleagues explained how animals and people can fall into a state of learned helplessness—passive acceptance and resignation that develops as a result of repeated exposure to negative events perceived as unavoidable. The researchers found that dogs exposed to unavoidable shocks gave up trying to escape and, when later given an opportunity to do so, stayed put and accepted the shocks as inevitable. 13 13. Martin Seligman and Steven Maier, “Failure to escape traumatic shock,” Journal of Experimental Psychology , 1967, Volume 74, Number 1, pp. 1–9. Like animals, people who believe that developing new skills won’t change a situation are more likely to be passive. You see this all around the economy—from employees who stop offering new ideas after earlier ones have been challenged to unemployed job seekers who give up looking for work after multiple rejections.
Instilling a sense of control and competence can promote an active effort to improve. As expectancy theory holds, people are more motivated to achieve their goals when they believe that greater individual effort will increase performance. 14 14. Victor Vroom, Work and motivation , New York: John Wiley, 1964. Fortunately, new technologies now give organizations more creative opportunities than ever to showcase examples of how that can actually happen.
Role modeling
Research tells us that role modeling occurs both unconsciously and consciously. Unconsciously, people often find themselves mimicking the emotions, behavior, speech patterns, expressions, and moods of others without even realizing that they are doing so. They also consciously align their own thinking and behavior with those of other people—to learn, to determine what’s right, and sometimes just to fit in.
While role modeling is commonly associated with high-power leaders such as Abraham Lincoln and Bill Gates, it isn’t limited to people in formal positions of authority. Smart organizations seeking to win their employees’ support for major transformation efforts recognize that key opinion leaders may exert more influence than CEOs. Nor is role modeling limited to individuals. Everyone has the power to model roles, and groups of people may exert the most powerful influence of all. Robert Cialdini, a well-respected professor of psychology and marketing, examined the power of “social proof”—a mental shortcut people use to judge what is correct by determining what others think is correct. No wonder TV shows have been using canned laughter for decades; believing that other people find a show funny makes us more likely to find it funny too.
Today’s increasingly connected digital world provides more opportunities than ever to share information about how others think and behave. Ever found yourself swayed by the number of positive reviews on Yelp? Or perceiving a Twitter user with a million followers as more reputable than one with only a dozen? You’re not imagining this. Users can now “buy followers” to help those users or their brands seem popular or even start trending.
The endurance of the influence model shouldn’t be surprising: powerful forces of human nature underlie it. More surprising, perhaps, is how often leaders still embark on large-scale change efforts without seriously focusing on building conviction or reinforcing it through formal mechanisms, the development of skills, and role modeling. While these priorities sound like common sense, it’s easy to miss one or more of them amid the maelstrom of activity that often accompanies significant changes in organizational direction. Leaders should address these building blocks systematically because, as research and experience demonstrate, all four together make a bigger impact.
Tessa Basford is a consultant in McKinsey’s Washington, DC, office; Bill Schaninger is a director in the Philadelphia office.
Explore a career with us
Related articles.
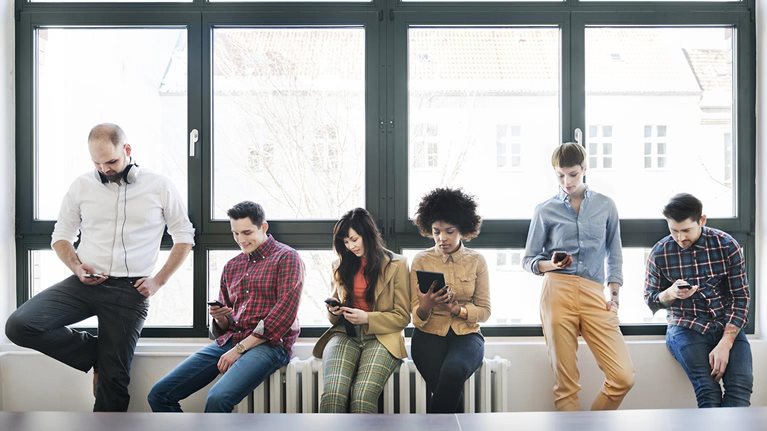
Winning hearts and minds in the 21st century

Changing change management
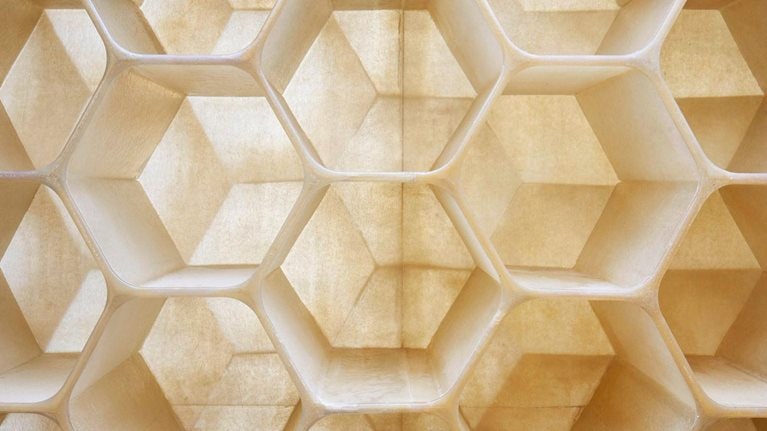
Digital hives: Creating a surge around change
- Research article
- Open access
- Published: 31 May 2024
Deep learning-based automatic measurement system for patellar height: a multicenter retrospective study
- Zeyu Liu 1 ,
- Jiangjiang Wu 2 ,
- Zhipeng Qin 4 ,
- Run Tian 1 na1 &
- Chunsheng Wang 1 na1
Journal of Orthopaedic Surgery and Research volume 19 , Article number: 324 ( 2024 ) Cite this article
52 Accesses
Metrics details
The patellar height index is important; however, the measurement procedures are time-consuming and prone to significant variability among and within observers. We developed a deep learning-based automatic measurement system for the patellar height and evaluated its performance and generalization ability to accurately measure the patellar height index.
We developed a dataset containing 3,923 lateral knee X-ray images. Notably, all X-ray images were from three tertiary level A hospitals, and 2,341 cases were included in the analysis after screening. By manually labeling key points, the model was trained using the residual network (ResNet) and high-resolution network (HRNet) for human pose estimation architectures to measure the patellar height index. Various data enhancement techniques were used to enhance the robustness of the model. The root mean square error (RMSE), object keypoint similarity (OKS), and percentage of correct keypoint (PCK) metrics were used to evaluate the training results. In addition, we used the intraclass correlation coefficient (ICC) to assess the consistency between manual and automatic measurements.
The HRNet model performed excellently in keypoint detection tasks by comparing different deep learning models. Furthermore, the pose_hrnet_w48 model was particularly outstanding in the RMSE, OKS, and PCK metrics, and the Insall–Salvati index (ISI) automatically calculated by this model was also highly consistent with the manual measurements (intraclass correlation coefficient [ICC], 0.809–0.885). This evidence demonstrates the accuracy and generalizability of this deep learning system in practical applications.
We successfully developed a deep learning-based automatic measurement system for the patellar height. The system demonstrated accuracy comparable to that of experienced radiologists and a strong generalizability across different datasets. It provides an essential tool for assessing and treating knee diseases early and monitoring and rehabilitation after knee surgery. Due to the potential bias in the selection of datasets in this study, different datasets should be examined in the future to optimize the model so that it can be reliably applied in clinical practice.
Trial registration
The study was registered at the Medical Research Registration and Filing Information System (medicalresearch.org.cn) MR-61-23-013065. Date of registration: May 04, 2023 (retrospectively registered).
The patellar height is important in the anatomy and biomechanics of the patellofemoral joint. Recently, several studies have shown that an abnormal patellar height is associated with various knee diseases. These diseases include patellar dislocation [ 1 , 2 ], patellar instability [ 3 ], Osgood–Schlatter disease [ 4 , 5 ], anterior knee pain [ 6 ], chondromalacia patella [ 7 ], and anterior cruciate ligament (ACL) injuries [ 8 , 9 ]. Moreover, abnormalities in the patellar height are closely linked to complications and poor recovery after total knee arthroplasty (TKA) [ 10 , 11 , 12 ], tibial osteotomy [ 13 ], and ACL reconstruction [ 14 ]. Therefore, early assessment and treatment of abnormal patellar height are vital to effectively control symptoms, prevent and alleviate related diseases, and improve patients’ quality of life.
The patellar height is typically measured directly or indirectly using radiological or magnetic resonance imaging (MRI) methods [ 15 ]. However, these standard procedures are lengthy, time-consuming, repetitive, and require additional computational support. They are prone to significant variability among and within observers, which may affect the accuracy of the measurements [ 16 , 17 ].
Recently, deep-learning algorithms have been increasingly applied across various aspects of the medical field, particularly in orthopedics. Kim et al. [ 18 ] automated the detection and segmentation of lumbar vertebrae from radiographs to assess compressive fractures. Similarly, Krogue et al. [ 19 ] implemented the automatic identification and classification of hip fractures. Regarding surgical planning, Qu et al. [ 20 ] utilized MRI for the precise segmentation of pelvic bone tumors, aiding the development of effective surgical plans for tumor excision and reconstruction. Von Schacky et al. [ 21 ] segmented and classified primary bone tumors, whereas Consalvo et al. [ 22 ] have detected and differentiated Ewing’s sarcoma and acute osteomyelitis. Leung et al. [ 23 ] have predicted the risks of TKA in patients with osteoarthritis for risk assessment. Ye et al. [ 24 ] developed a deep learning-based automatic measurement algorithm using a convolutional neural network (CNN) with VGG16 as the encoder. Kwolek et al. [ 25 ] employed the YOLO neural network and U-Net for detecting and segmenting the patellofemoral joint, enabling automatic measurements of the Caton-Deschamps index (CDI) and Blackburne-Peel index.
Deep-learning algorithms are particularly effective at navigating the complex anatomical structures and variations between normal and pathological states in humans. They excel at identifying and learning intricate patterns from extensive datasets, which is crucial for accurately measuring patellar height in various radiographic images [ 26 ]. Furthermore, the automation capabilities of deep learning significantly streamline the measurement process, reducing both the variability and the time required for manual assessments [ 27 ].
Therefore, our objective was to develop a novel deep learning-based algorithm to automatically measure patellar height, to enable high-precision and rapid analysis of medical images.
Study aim, design, and setting
This multicenter retrospective study aimed to develop a deep learning-based algorithm to automatically measure patellar height parameters in lateral knee radiographs and evaluate its performance and generalization ability. We utilized a dataset containing X-ray images from three tertiary level A hospitals.
The images used in this study’s dataset were obtained from three tertiary A-grade comprehensive hospitals: The Second Affiliated Hospital of Xi’an Jiaotong University, Xi’an Honghui Hospital, and The Second Affiliated Hospital of Shanxi Medical University. This retrospective study was approved by the ethics committees of the three hospitals. The ethics committees waived the requirement for informed consent due to the study’s retrospective nature. We continuously collected imaging and clinical data between April 2022 and December 2023 through the imaging and hospital information system. Patients aged ≥ 20 years (with mature bones) undergoing a lateral knee radiograph were eligible for inclusion. The exclusion criteria were as follows: (1) knee osteoarthritis (Kellgren–Lawrence grade > 2); (2) poor quality radiographs with insufficient rotation (the distance between the edge of the femoral condyles ˃5 mm) or knee flexion ˂30°; (3) overlapping knees; and (4) unclear superior or inferior poles of the patellar or patellar ligament endpoints. The osteoarthritis criteria were used to include radiographs with clear patellar height markers. We retrospectively analyzed 3,923 knee joints, of which 2,341 met the inclusion criteria. A random selection of 90% of the cases from The Second Affiliated Hospital of Xi’an Jiaotong University (2,017 X-ray images) was used for training, and 10% (224 X-ray images) were used as the internal test set. For external validation, we further evaluated datasets from the Xi’an Honghui Hospital (60 X-ray images as external test set 1) and The Second Affiliated Hospital of Shanxi Medical University (40 X-ray images as external test set 2).
The dataset required labeling before training the neural network. The LabelMe software was used to manually annotate the keypoints in the lateral knee radiographs, generating JSON format annotation files. Three keypoints were labeled from the 2,341 images. Images from each of the three hospitals were annotated by radiologists (R1, R2, and R3) with over 10 years of experience from their respective institutions. Figures 1 , 2 and 3 illustrate the data collection process, keypoint annotation demonstration, and overall model architecture, respectively.
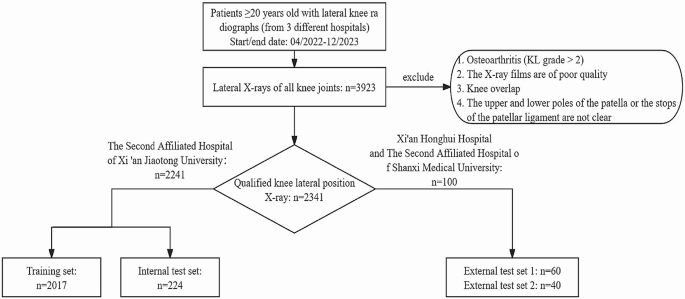
Data collection process
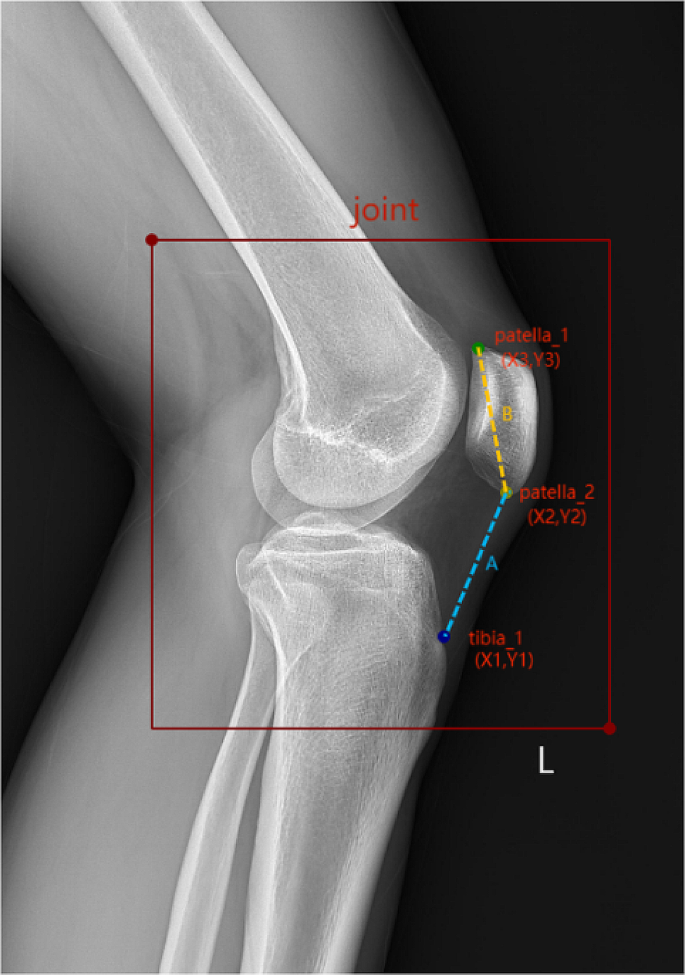
Keypoint marking and calculation method for the ISI of PH. Patella_1, upper pole of patella; patella_2, inferior pole of patella; tibia_1, insertion of the patellar ligament. The ISI is the ratio of A to B; \({\rm{A}}\, = \,\sqrt {{\rm{X}}1 - {\rm{X}}2{^2} + {\rm{Y}}1 - {\rm{Y}}2{^2}}\) , \({\rm{B}}\, = \,\sqrt {{\rm{X}}2 - {\rm{X}}3{^2} + {\rm{Y}}2 - {\rm{Y}}3{^2}}\) ISI, Insall–Salvati Index
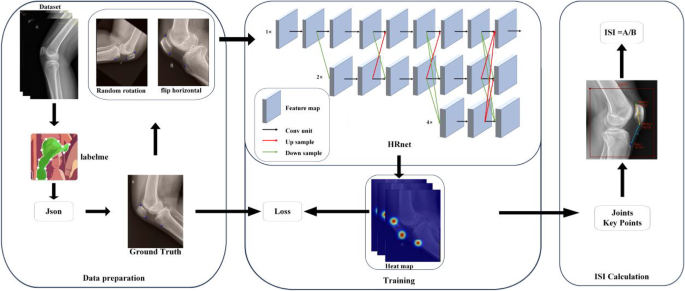
Overall model architecture diagram
Dataset preparation
An experiment was conducted using the PyTorch 1.10 framework in Python 3.8 (Python Software Foundation, Wilmington, DE, USA) and Cuda 11.3 (Nvidia Corp., Santa Clara, CA, USA) environments to demonstrate the performance and effectiveness of the constructed model. The experimental setup was based on the Ubuntu 18.04 operating system (Canonical Ltd., London, England) with an Intel i7-10700 F processor (Intel, Santa Clara, CA, USA) and was equipped with an Nvidia GeForce RTX 4070 GPU (Nvidia Corp.) and 32 GB RAM. We optimized several parameters of our deep learning models to balance computational efficiency and training effectiveness based on the capabilities of our NVIDIA GeForce RTX 4070 GPU, which has 12 GB of VRAM. We chose a batch size of 8 after extensive testing, which maximized VRAM usage and model training speed, particularly when using the computationally intensive pose_hrnet_w48 model with an input size of 384 × 288. For consistency, this batch size was applied across all models. The Adam optimizer was selected for its robust performance and rapid convergence. To manage computational load while preserving image quality, we experimented with input sizes of 256 × 192 and 384 × 288 pixels. Through preliminary testing, this approach not only reduced the volume of data processed by the model but also enhanced training and inference efficiency without significant feature loss. Therefore, we uniformly adjusted the image size to 256 × 192 or 384 × 288 pixels and employed data augmentation techniques such as random rotation, scaling, and flipping to enhance the model’s robustness and generalization ability.
Model selection
The residual network for human pose estimation (Pose_ResNet) is an advanced human pose estimation method that leverages the robustness of deep residual networks (ResNets) to effectively locate human body keypoints [ 28 , 29 ]. The network initially extracts image features through convolutional layers, which can recognize complex patterns in the images and provide the necessary visual information for detecting human-body keypoints. The output is a set of heat maps, each corresponding to a specific body keypoint, representing the probabilistic distribution of the keypoint’s possible locations within the image. During training, the loss function typically compared the predicted heat maps with the true heat maps to optimize the network parameters. The final keypoint locations are determined through post-processing steps such as finding the local maxima in the heatmaps. This architecture was built on the fundamental principles of ResNet by integrating deep convolutional layers with residual connections. These connections mitigate the issue of vanishing gradients, thereby enabling the training of deeper networks to enhance feature extraction without losing crucial information during the process.
The High-Resolution Network for Human Pose Estimation (Pose_HRNet) is an efficient network for human pose estimation designed to improve keypoint detection accuracy by maintaining high-resolution feature maps throughout the process [ 30 , 31 , 32 , 33 ]. Starting with a high-resolution subnetwork, it progressively adds high-to-low-resolution subnetworks in more stages. Then, it connects multiresolution subnetworks in parallel to learn features on different scales. This multiscale approach enhances the robustness of the model to various challenges in pose estimation, such as occlusions and varying sizes. The global context and local detail information are effectively fused throughout the process by exchanging information across parallel multiresolution subnetworks. Finally, the keypoints are estimated on the network’s output high-resolution feature maps. This approach benefits from semantically richer and spatially precise result representations.
Performance and generalization of keypoint detection
We assessed the landmark detection performance of five models, pose_resnet_50, pose_resnet_101, pose_resnet_152, pose_hrnet_w32, and pose_hrnet_w48, using root mean square error (RMSE), object keypoint similarity (OKS), and percentage of correct keypoints (PCK). Furthermore, we compared the parameter counts and computational complexities of the models. Subsequently, an appropriate model was selected to evaluate the performance of the external test dataset. This was performed to assess the accuracy of knee joint radiographs obtained from different devices across various algorithms.
RMSE measures the model accuracy using the square difference between the average predicted and actual values, indicating the error magnitude.
OKS evaluates keypoint detection accuracy by considering object scale and keypoint distance, offering a normalized accuracy measure that penalizes larger errors more.
PCK calculates the share of keypoints accurately detected within a set distance from true positions, reflecting the model’s precision in keypoint localization.
Performance and generalization of insall–Salvati index (ISI) measurement
The analyses compared the average ISI test results across the three test sets. In addition, we calculated the intraclass correlation coefficient (ICC) to evaluate the reliability and consistency of the manual and automatic measurements. The ICC reflects abstract consistency, with values ≥ 0.75 considered sufficiently reliable [ 34 ]. This approach was used to assess the performance and generalizability of ISI measurements.
Statistical analysis
Statistical analyses were performed using PASW Statistics v18 (IBM Corp., Armonk, NY, USA) and Microsoft Excel 2019 (Microsoft Corp., Redmond, WA, USA). We employed ANOVA to compare the mean ages of different groups to identify any statistically significant differences in age distributions. For categorical variables such as sex, side, osteoarthritis grade, and surgery status, chi-square tests were utilized to assess the homogeneity of these variables across the groups. All statistical tests were two-tailed, and a p-value of less than 0.05 was considered to indicate statistical significance.
General data distributions
In this study of 2,241 knee radiographs, the average ages of the patients forming the training, internal, first external, and second external sets, were 50.10 ± 17.77 years (range, 20–87 years), 50.76 ± 16.96 years (range, 21–83 years), 48.88 ± 15.01 years (range, 23–86 years), and 47.43 ± 13.01 years (range, 20–81 years), respectively. Table 1 presents the overall patient characteristics.
Quantitative analysis
Experiments were conducted on keypoint-detection tasks using different deep-learning models. We primarily compared five models: pose_resnet_50, pose_resnet_101, pose_resnet_152, pose_hrnet_w32, and pose_hrnet_w48. We examined the impact of the input sizes of 256 × 192 and 384 × 288 pixels on model performance. In the comparative analysis, we focused on the number of model parameters (#Params), computational complexity (GFLOPs), and three performance metrics: RMSE, OKS, and PCK. The results are shown in Table 2 ; Figs. 4 , 5 and 6 .
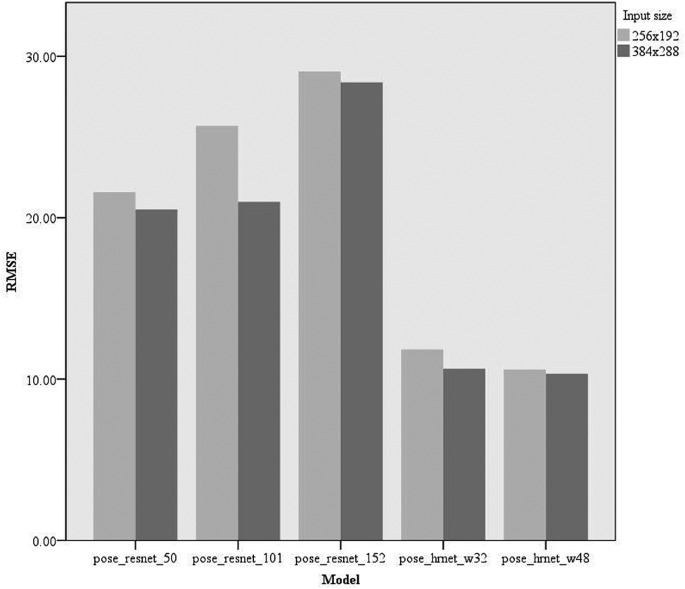
Comparison of RMSE of different models RMSE, root mean square error
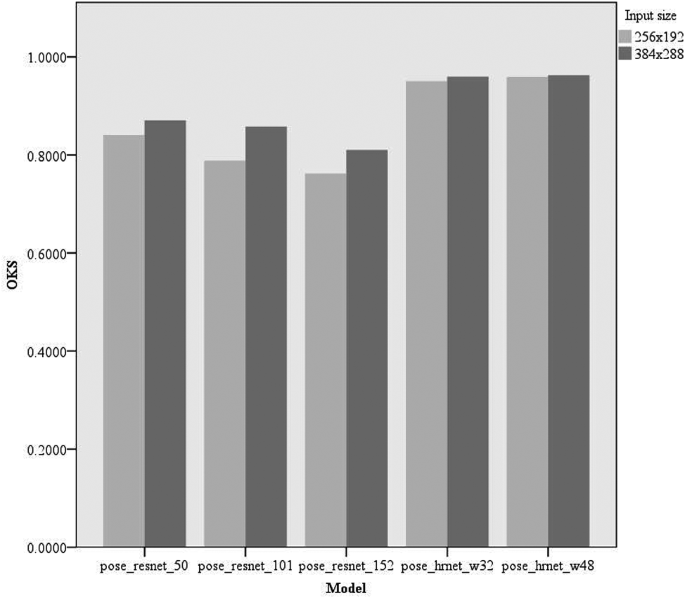
Comparison of OKS of different models OKS, object keypoint similarity
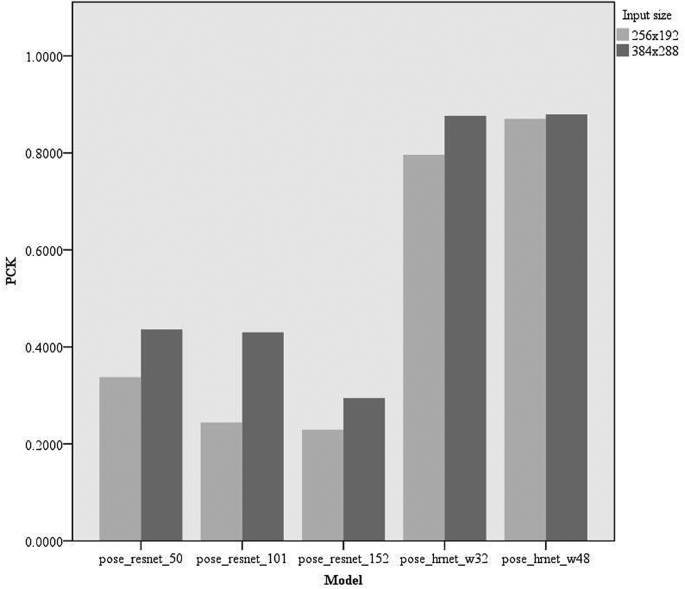
Comparison of PCK of different models PCK, percentage of correct keypoints
Model Parameters and Computational Complexity: The parameter counts for pose_resnet_50, pose_resnet_101, and pose_resnet_152 were 34.0 M, 53.0 M, and 68.6 M, respectively, with computational complexities of 8.9, 12.4, and 15.7 GFLOPs, respectively. In contrast, pose_hrnet_w32 and pose_hrnet_w48 had parameter counts of 28.5 M and 63.6 M, respectively, with computational complexities of 7.1 and 14.6 GFLOPs, respectively. A positive correlation was observed between the model parameter count and computational complexity. The results of the performance analysis are as follows:
RMSE: The pose_hrnet_w48 model scored 10.60 and the pose_hrnet_w32 score was 11.84. However, the pose_resnet series had higher RMSE values with pose_resnet_152 having the highest value of 29.06.
OKS: The pose_hrnet_w48 model scored the highest at 0.9592, while pose_hrnet_w32 scored 0.9505. In the pose_resnet series, pose_resnet_50 scored 0.8408.
PCK: The pose_hrnet_w48 model had the highest score of 0.8705. The pose_hrnet_w32 model scored 0.7961. The Pose_ResNet series generally exhibited lower PCK scores.
Qualitative analysis
From the image analysis perspective, our patellar height measurement system can accurately identify the patella’s upper and lower poles and the patellar tendon’s endpoints. The precision of this identification is high, with virtually no deviation discernible to the human eye (Fig. 7 ). Therefore, it is evident from the effectiveness diagrams of patellar keypoint detection after total knee arthroplasty that the patellar height measurement system can still effectively identify and measure keypoints even with the implantation of knee prostheses (Fig. 8 ).
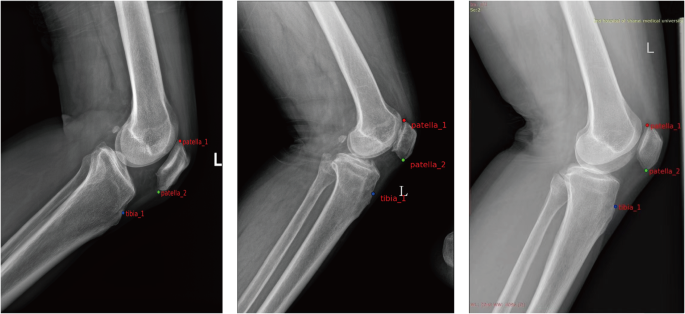
Visualized results of keypoint detection on three datasets. From left to right are the internal test set, external test set 1, and external test set 2

Rendering of keypoint detection after total knee replacement
Generalized analysis
Our study also included an analysis of the model’s generalizability. Tables 3 and 4 show the model’s performance on external test sets from other institutions. In the external test set 1 from Xi’an Honghui Hospital, as presented in Table 3 , the HRNet model exhibited an RMSE value between 2 and 3, an OKS > 0.96, and a PCK > 0.99. Similarly, on external test set 2 from the Second Affiliated Hospital of Shanxi Medical University, as presented in Table 4 , HRNet’s RMSE ranged between 7 and 9, with an OKS > 0.94 and a PCK > 0.88.
Performance and generalization of the ISI measurement
Radiologists with over 10 years of experience calculated the ISI index values with averages of 1.0444 ± 0.1453, 1.0587 ± 0.1727, and 1.0826 ± 0.1448 for the internal test set, external test set 1, and external test set 2, respectively. The deep learning algorithm based on pose_hrnet_w48 automatically calculated ISI index values with averages of 1.0377 ± 0.1323, 1.0637 ± 0.1900, and 1.0492 ± 0.1363 for the internal test set, external test set 1, and external test set 2, respectively (Table 5 ). ICC was used to evaluate the consistency between manual and automatic measurements. For R1 vs. Model, the ICC = 0.809; R2 vs. Model: ICC = 0.885; and R3 vs. Model: ICC = 0.830 (Table 6 ).
Currently, insufficient attention has been given to the measurement of patellar height indices, primarily for the following reasons. First, there is a lack of comprehensive understanding among physicians regarding the role of this index in the diagnosis and assessment of knee joint diseases. However, the patellar height index is crucial to diagnosing abnormal patellar positions and related diseases. Balcarek et al. [ 2 ] confirmed that patella alta significantly correlated with increased incidences of patellar dislocations, identifying it as a key risk factor for instability. Dejour et al. [ 3 ] found that 24% of patients with patellar instability exhibited patella alta, which impaired knee mechanics and heightened dislocation risks. Similarly, Visuri et al. [ 5 ] linked patella alta with Osgood–Schlatter disease, emphasizing its typical presentation of a higher patellar position. Luyckx et al. [ 6 ] explored how patella alta affected anterior knee pain by altering joint forces and pressures. Lu et al. [ 7 ] reported that abnormal patellar heights disrupted knee biomechanics, potentially increasing cartilage damage. Furthermore, Degnan et al. [ 9 ] discovered that a high Insall–Salvati ratio was a significant indicator of ACL damage risks in children, while Nishizawa et al. [ 12 ] noted that patella elevation in posterior-stabilized TKA led to increased joint space by reducing flexion restrictions.
Second, the manual measurement of patellar height indices involves subjectivity and variability, as doctors or technicians rely on personal experience and judgment, leading to potentially different results among various people taking measurements [ 16 , 17 ]. Finally, manual measurements are time-consuming and inefficient, particularly when dealing with large datasets. Therefore, there is an urgent need to develop an automated, objective, and rapid tool to measure patellar height indices.
With the widespread application of deep learning algorithms in the medical field, researchers have begun to explore using this advanced technology to automatically measure the patellar height index. Ye et al. [ 24 ] developed an automatic measurement algorithm based on deep learning, employing a CNN framework for landmark detection, with the VGG16 network serving as the core encoder. This method demonstrated high accuracy for the ISI, CDI, and a new method proposed by the Keerati Index (KI). However, it was slightly less accurate for the modified CDI. Kwolek et al. [ 25 ] used the “You Only Look Once” neural network to detect the patellofemoral joint area. They employed U-Net for bone segmentation, enabling the automatic measurement of the CDI and Blackburne–Peel index, thereby facilitating the calculation of the patellar height.
This study introduces a novel measurement system of patella height based on deep learning that integrates an HRNet for two-dimensional keypoint detection and mathematical formulae for analyzing joint coordinates, requiring only a single image input to obtain results. To ensure the reliability of the labeled data for the patellar height measurement system, several steps were taken to address inter-observer variability and to enhance consistency during the annotation process. First, the key points on the lateral knee radiographs were manually annotated by experienced radiologists, each with over ten years of experience at their respective institutions. This level of expertise ensured high standards of annotation accuracy. Additionally, the annotations underwent multiple review rounds to ensure no significant discrepancies between observers. Second, the ICC was used to evaluate the consistency between manual and automatic measurements, with ICC values ranging from 0.809 to 0.885. The close ICC values indirectly indicate a high reliability in annotations among different observers, as the model’s automatic measurements were trained using the manual annotations from one hospital’s radiologists. Thirdly, the LabelMe software was used to label the dataset, ensuring standardized annotations across all images. These measures collectively helped to maintain the reliability of the annotations, enabling the effective training and validation of the deep learning models for measuring patellar height.
All hardware selections were made to facilitate the training and inference of our models. We first considered software environment compatibility; PyTorch 1.10 and Python 3.8 are compatible with the reproduction code of our models. The choice of CUDA version 11.3 was dictated by its compatibility with the selected RTX 4070 GPU and the PyTorch framework. Ubuntu 18.04, a popular and stable Linux distribution, was chosen for its reliability and security, although the version of the operating system does not impact experimental results. In terms of hardware performance, the choice of GPU is crucial. Ideally, the more advanced the GPU, the better; however, given our current experimental conditions, we used the Nvidia GeForce RTX 4070 GPU. It has 12 GB of VRAM and 5888 CUDA cores, offering 29 TFLOPS of computational power, more than sufficient for training HRNet. The Intel i7-10700 F processor, with 8 cores and 16 threads, a base frequency of 2.9 GHz, and a turbo frequency of 4.8 GHz, provides robust multi-core and high-frequency capabilities suitable for CPU-intensive tasks such as data preprocessing and model evaluation. The 32 GB of memory ensures that training is not bottlenecked by memory limitations. Overall, these choices were made based on a comprehensive consideration of experimental conditions, compatibility, and performance.
The differences in the distribution of osteoarthritis severity across the datasets ( p < 0.05) may be linked to the smaller sample sizes in the external test sets. However, due to the large sample size of the training set, it may not affect the effectiveness of key point identification in the test set.
Lower RMSE values correlate with higher model accuracy in predicting patellar height, crucial for ensuring reliable measurements in clinical environments. Higher OKS scores signify that the model’s predictions are both precise and consistent across various knee sizes and positions, enhancing its utility in diverse clinical scenarios. Additionally, a high PCK score demonstrates the model’s reliability in identifying keypoints on the patella and related structures, essential for diagnosing conditions such as patellar instability or dislocation. Pose_hrnet_w48 and pose_hrnet_w32 demonstrated higher accuracy and efficiency than other models in this keypoint detection task, especially pose_hrnet_w48, which performed the best across all performance metrics (Figs. 4 , 5 and 6 ). Although the pose_hrnet series models had higher parameter counts and computational complexities, their performance improvements were significant. Conversely, although the pose_resnet series models had relatively lower computational complexities, their accuracy and efficiency in keypoint detection need to be enhanced. Therefore, selecting an appropriate model for keypoint detection tasks requires a comprehensive consideration of the availability of computational resources and the demand for detection performance. The speed of the various models discussed in this study is sufficient to meet the needs of clinical real-time image processing. Given the critical importance of accuracy in patellar height measurement in clinical practice, we ultimately selected the pose_hrnet_w48 model from HRNet as the benchmark model for our measurement system. Despite its larger parameter size and relatively high computational demand (measured in GFLOPS), it excelled in key performance metrics, achieving an OKS of 0.9625, a RMSE of 10.33, and a PCK of 0.8795. Compared with models based on ResNet, this represents a significant improvement in these metrics. Furthermore, the pose_hrnet_w48 model processes images at 31.80 frames per second (FPS), indicating its capacity to handle 31.80 images per second.
The model’s performance on external test sets further showcased the strong generalizability of the HRNet model for detecting knee joint keypoints across various datasets. In addition, a comparison of the average ISI across the three test sets shows that the difference between the predicted and actual ISI values is negligible. A comparison of the ISI values obtained by radiologists from different hospitals (R1, R2, R3) with those calculated by our deep learning model based on pose_hrnet_w48 for patellar height measurement showed excellent reliability in the results obtained by the artificial intelligence calculation compared with those by the radiologists, indicating that the model has produced results similar to those of radiologists with extensive experience (R1 vs. Model: ICC = 0.809; R2 vs. Model: ICC = 0.885; R3 vs. Model: ICC = 0.830). These results demonstrate the precision and reliability of the patellar height measurement system based on pose_hrnet_w48 across various datasets.
This system’s primary advantages and improvements are mainly reflected in the following aspects. First, unlike the serial convolution method from high to low resolution adopted by existing networks such as ResNet and vanishing gradient (VGGNet), HRNet employs a parallel connection strategy. This maintains a high-resolution expression throughout the inference process and achieves multiscale fusion, allowing for a richer and more accurate estimation of keypoints on high-resolution feature maps [ 30 ]. Second, considering that abnormalities in patellar height are closely associated with complications or poor recovery after TKA [ 10 , 11 , 12 ] and ACL reconstruction [ 14 ], we specifically included the lateral knee radiographs of patients after these surgeries in our custom dataset. Finally, the training dataset for this study was exclusively derived from the Second Affiliated Hospital of Xi’an Jiaotong University, while datasets from the Second Affiliated Hospital of Shanxi Medical University and the Xi’an Honghui Cross Hospital were used solely for testing to validate the generalization capabilities of the deep learning model. Differences in imaging protocols and parameter settings across hospitals are real, but were substantially mitigated during the image preprocessing stage of the deep learning model through adjustments in resolution and the application of data augmentation techniques such as random flipping and rotation. These methods significantly enhanced the model’s robustness. The rationale behind this setup was to test the applicability of the model trained on data from the Second Affiliated Hospital of Xi’an Jiaotong University in broader contexts. The successful performance of the model on datasets from various hospitals indicates its strong potential for clinical application.
In the future, the application of automated patellar height measurement will reduce human errors and variability associated with manual methods, which is critical for diagnosing diseases related to abnormal patellar heights. Accurate patellar height indices will also aid orthopedic surgeons in devising treatment strategies, including surgical planning such as alignment and balance of the patellofemoral joint in TKA. Additionally, this technology can effectively monitor disease progression and recovery post-surgery, ensuring timely and appropriate interventions that can shorten recovery times and improve overall patient outcomes.
Compared to previously developed models for automatically measuring patellar height using deep learning, this study utilizes a large, multicenter dataset and the advanced HRNet architecture, achieving notably low RMSE and high OKS and PCK scores. Despite the outstanding results achieved in this study, there were some limitations. First, training and testing the model on specific hospital datasets may not fully represent the global diversity of knee joint anatomy. Additionally, county and township hospitals may employ different imaging protocols for knee X-rays, which could impact the model’s ability to accurately detect keypoints. Second, the dataset primarily included images with clear patellar height markers, which may have affected the outcomes in patients with abnormal patellar morphology. Third, as a purely linear measurement method, the ISI only considers the patellar height parameter and fails to comprehensively evaluate other anatomical patella structures, such as the patellar type and posterior tibial slope. To mitigate these limitations, future research should train the model with datasets from different races, regions, populations, and devices and focus on optimizing specific patient groups to enhance the model’s applicability across different patient types. By integrating more closely with clinical practice, the model could be guided towards improvements and optimizations for patients with abnormal patellar morphology. Furthermore, consideration should be given to introducing more anatomical structure indicators to comprehensively assess patellar anatomy and the use of three-dimensional imaging techniques (such as computed tomography and MRI) for a comprehensive assessment of patellar anatomy and function. By implementing these measures, we anticipate effectively improving the limitations of the existing model, thereby enabling its true application in clinical settings.
Conclusions
This study successfully developed and validated a deep learning-based automatic patellar height measurement system. This system can accurately measure the patellar height index, performing on par with experienced radiologists. Extensive dataset testing demonstrated the system’s excellent generalization ability and reliability, particularly for processing radiographs from different hospitals and equipment. This measurement system is expected to help in the assessment, treatment, and postoperative monitoring of knee joint diseases, thereby providing a powerful tool for enhancing patients’ quality of life. Due to the potential bias in the selection of datasets in this study, the model still has some shortcomings. In the future, further optimizing and incorporating more anatomical structure indicators will significantly improve the application scope and accuracy of the system, offering a more precise and comprehensive assessment tool for clinical use.
Data availability
The datasets used and/or analyzed in the current study are available from the corresponding author upon reasonable request.
Abbreviations
Root mean square error
Object keypoint similarity
Percentage of correct keypoints
Intraclass correlation coefficient
Residual network for human pose estimation
High-resolution network for human pose estimation
Magnetic resonance imaging
Total knee arthroplasty
Anterior cruciate ligament
Insall–Salvati index
Convolutional neural network
Visual geometry group 16
Caton–Deschamps index
Keerati index
Jaquith BP, Parikh SN. Predictors of recurrent patellar instability in children and adolescents after first-time dislocation. J Pediatr Orthop. 2017;37:484–90. https://doi.org/10.1097/BPO.0000000000000674 .
Article PubMed Google Scholar
Balcarek P, Ammon J, Frosch S, Walde TA, Schüttrumpf JP, Ferlemann KG, et al. Magnetic resonance imaging characteristics of the medial patellofemoral ligament lesion in acute lateral patellar dislocations considering trochlear dysplasia, Patella alta, and tibial tuberosity-trochlear groove distance. Arthroscopy. 2010;26:926–35. https://doi.org/10.1016/j.arthro.2009.11.004 .
Dejour H, Walch G, Nove-Josserand L, Guier C. Factors of patellar instability: an anatomic radiographic study. Knee Surg Sports Traumatol Arthrosc. 1994;2:19–26. https://doi.org/10.1007/BF01552649 .
Article CAS PubMed Google Scholar
Aparicio G, Abril JC, Calvo E, Alvarez L. Radiologic study of patellar height in Osgood-Schlatter disease. J Pediatr Orthop. 1997;17:63–6. https://doi.org/10.1097/01241398-199701000-00015 .
Visuri T, Pihlajamäki HK, Mattila VM, Kiuru M. Elongated patellae at the final stage of Osgood-Schlatter disease: a radiographic study. Knee. 2007;14:198–203. https://doi.org/10.1016/j.knee.2007.03.003 .
Luyckx T, Didden K, Vandenneucker H, Labey L, Innocenti B, Bellemans J. Is there a biomechanical explanation for anterior knee pain in patients with Patella alta? Influence of patellar height on patellofemoral contact force, contact area and contact pressure. J Bone Joint Surg Br. 2009;91:344–50. https://doi.org/10.1302/0301-620X.91B3.21592 .
Lu W, Yang J, Chen S, Zhu Y, Zhu C. Abnormal patella height based on Insall-Salvati ratio and its correlation with patellar cartilage lesions: an extremity-dedicated low-field magnetic resonance imaging analysis of 1703 Chinese cases. Scand J Surg. 2016;105:197–203. https://doi.org/10.1177/1457496915607409 .
Akgün AS, Agirman M. Associations between anterior cruciate ligament injuries and Patella alta and trochlear dysplasia in adults using magnetic resonance imaging. J Knee Surg. 2021;34:1220–6. https://doi.org/10.1055/s-0040-1702198 .
Degnan AJ, Maldjian C, Adam RJ, Fu FH, Di Domenica M. Comparison of Insall-Salvati ratios in children with an acute anterior cruciate ligament tear and a matched control population. AJR Am J Roentgenol. 2015;204:161–6. https://doi.org/10.2214/AJR.13.12435 .
Lum ZC, Saiz AM, Pereira GC, Meehan JP. Patella baja in total knee arthroplasty. J Am Acad Orthop Surg. 2020;28:316–23. https://doi.org/10.5435/JAAOS-D-19-00422 .
Tischer T, Geier A, Lutter C, Enz A, Bader R, Kebbach M. Patella height influences patellofemoral contact and kinematics following cruciate-retaining total knee replacement. J Orthop Res. 2023;41:793–802. https://doi.org/10.1002/jor.25425 .
Nishizawa Y, Matsumoto T, Kubo S, Muratsu H, Matsushita T, Oka S, et al. The influence of patella height on soft tissue balance in cruciate-retaining and posterior-stabilised total knee arthroplasty. Int Orthop. 2013;37:421–5. https://doi.org/10.1007/s00264-012-1749-5 .
Portner O. High tibial valgus osteotomy: closing, opening or combined? Patellar height as a determining factor. Clin Orthop Relat Res. 2014;472:3432–40. https://doi.org/10.1007/s11999-014-3821-5 .
Article PubMed PubMed Central Google Scholar
Aglietti P, Buzzi R, D’andria S, Zaccherotti G. Patellofemoral problems after intraarticular anterior cruciate ligament reconstruction. Clin Orthop Relat Res. 1993;288195–204. https://doi.org/10.1097/00003086-199303000-00025 .
Igoumenou VG, Dimopoulos L, Mavrogenis AF. Patellar height assessment methods: an update. JBJS Rev. 2019;7:e4. https://doi.org/10.2106/JBJS.RVW.18.00038 .
Smith TO, Davies L, Toms AP, Hing CB, Donell ST. The reliability and validity of radiological assessment for patellar instability. A systematic review and meta-analysis. Skelet Radiol. 2011;40:399–414. https://doi.org/10.1007/s00256-010-0961-x .
Article Google Scholar
Chen HC, Lin CJ, Wu CH, Wang CK, Sun YN. Automatic Insall-Salvati ratio measurement on lateral knee x-ray images using model-guided landmark localization. Phys Med Biol. 2010;55:6785–800. https://doi.org/10.1088/0031-9155/55/22/012 .
Kim KC, Cho HC, Jang TJ, Choi JM, Seo JK. Automatic detection and segmentation of lumbar vertebrae from X-ray images for compression fracture evaluation. Comput Methods Programs Biomed. 2021;200:105833. https://doi.org/10.1016/j.cmpb.2020.105833 .
Krogue JD, Cheng KV, Hwang KM, Toogood P, Meinberg EG, Geiger EJ, et al. Automatic hip fracture identification and functional subclassification with deep learning. Radiol Artif Intell. 2020;2:e190023. https://doi.org/10.1148/ryai.2020190023 .
Qu Y, Li X, Yan Z, Zhao L, Zhang L, Liu C, et al. Surgical planning of pelvic tumor using multi-view CNN with relation-context representation learning. Med Image Anal. 2021;69:101954. https://doi.org/10.1016/j.media.2020.101954 .
von Schacky CE, Wilhelm NJ, Schäfer VS, Leonhardt Y, Gassert FG, Foreman SC, et al. Multitask deep learning for segmentation and classification of primary bone tumors on radiographs. Radiology. 2021;301:398–406. https://doi.org/10.1148/radiol.2021204531 .
Consalvo S, Hinterwimmer F, Neumann J, Steinborn M, Salzmann M, Seidl F, et al. Two-phase deep learning algorithm for detection and differentiation of ewing sarcoma and acute osteomyelitis in paediatric radiographs. Anticancer Res. 2022;42:4371–80. https://doi.org/10.21873/anticanres.15937 .
Leung K, Zhang B, Tan J, Shen Y, Geras KJ, Babb JS, et al. Prediction of total knee replacement and diagnosis of osteoarthritis by using deep learning on knee radiographs: data from the osteoarthritis initiative. Radiology. 2020;296:584–93. https://doi.org/10.1148/radiol.2020192091 .
Ye Q, Shen Q, Yang W, Huang S, Jiang Z, He L, et al. Development of automatic measurement for patellar height based on deep learning and knee radiographs. Eur Radiol. 2020;30:4974–84. https://doi.org/10.1007/s00330-020-06856-z .
Kwolek K, Grzelecki D, Kwolek K, Marczak D, Kowalczewski J, Tyrakowski M. Automated patellar height assessment on high-resolution radiographs with a novel deep learning-based approach. World J Orthop. 2023;14:387–98. https://doi.org/10.5312/wjo.v14.i6.387 .
Bonaldi L, Pretto A, Pirri C, Uccheddu F, Fontanella CG, Stecco C. Deep learning-based medical images segmentation of Musculoskeletal anatomical structures: a survey of bottlenecks and strategies. Bioengineering. 2023;10:137. https://doi.org/10.3390/bioengineering10020137 .
Sharbatdaran A, Romano D, Teichman K, Dev H, Raza SI, Goel A, et al. Deep learning automation of kidney, liver, and spleen segmentation for Organ volume measurements in autosomal Dominant polycystic kidney disease. Tomography. 2022;8:1804–19. https://doi.org/10.21873/anticanres.15937 .
Papandreou G, Zhu T, Kanazawa N, Toshev A, Tompson J, Bregler C et al. Towards Accurate Multi-person Pose Estimation in the Wild; 2017. arXiv.org. Ithaca: Cornell University. Library. https://doi.org/10.1109/CVPR.2017.395 .
Lee J, Kim T, Beak S, Moon Y, Jeong J. Real-time pose estimation based on ResNet-50 for rapid safety prevention and accident detection for field workers. Electronics. 2023;12:3513. https://doi.org/10.3390/electronics12163513 .
Li Y, Jia S, Li Q, BalanceHRNet. An effective network for bottom-up human pose estimation. Neural Netw. 2023;161:297–305. https://doi.org/10.1016/j.neunet.2023.01.036 .
Li Q, Zhang Z, Xiao F, Zhang F, Bhanu B. Dite-HRNet: Dynamic Lightweight High-Resolution Network for Human Pose Estimation; 2022. arXiv.org. Ithaca: Cornell University. Library. https://doi.org/10.24963/ijcai.2022/153 .
Cheng B, Xiao B, Wang J, Shi H, Huang TS, Zhang L, HigherHRNet. Scale-Aware Representation Learning for Bottom-Up Human Pose Estimation. In: Proceedings of the IEEE/CVF conference on computer vision and pattern recognition 2020 (pp. 5386–5395). https://doi.org/10.48550/arXiv.1908.10357 .
Wang J, Sun K, Cheng T, Jiang B, Deng C, Zhao Y, et al. Deep high-resolution representation learning for visual recognition. IEEE Trans Pattern Anal Mach Intell. 2021;43:3349–64. https://doi.org/10.1109/TPAMI.2020.2983686 .
Zou GY. Sample size formulas for estimating intraclass correlation coefficients with precision and assurance. Stat Med. 2012;31:3972–81. https://doi.org/10.1002/sim.5466 .
Download references
Acknowledgements
Not applicable.
This study was supported by the Key Program of Shaanxi Province (2023-YBSF-102) and the National Key Research and Development Program of China (2021YFC2401435).
Author information
Chunsheng Wang and Run Tian contributed equally to this work.
Authors and Affiliations
Department of Bone and Joint Surgery, The Second Affiliated Hospital of Xi’an Jiaotong University, Xi’an, Shaanxi, China
Zeyu Liu, Run Tian & Chunsheng Wang
College of Information and Communication Engineering, Harbin Engineering University, Heilongjiang, Harbin, China
Jiangjiang Wu
Department of Orthopedics, Xi’an Honghui Hospital, Xi’an, Shaanxi, China
Department of Orthopedics, The Second Affiliated Hospital of Shanxi Medical University, Taiyuan, Shanxi, China
Zhipeng Qin
You can also search for this author in PubMed Google Scholar
Contributions
All the authors contributed substantially to the conception and design of this study. Zeyu Liu conceived and designed the work, collected original data, and wrote and edited the manuscript. Jiangjiang Wu analyzed and interpreted the collected data and provided the technical support required for the work. Xu Gao and Zhipeng Qin collected original data. Professor Run Tian and Chunsheng Wang directed the conception and design of the work and revised the manuscript.
Corresponding authors
Correspondence to Run Tian or Chunsheng Wang .
Ethics declarations
Ethics approval and consent to participate.
We have obtained the approval of the Medical Ethics Committee of the Second Affiliated Hospital of Xi ‘an Jiaotong University (reference number 2023-025). The Medical Ethics Committee waived the requirement for informed consent based on the study’s retrospective nature.
Consent for publication
All authors have reviewed and approved the final version of the manuscript and provided consent for publication.
Competing interests
The authors declare that they have no competing interests.
Additional information
Publisher’s note.
Springer Nature remains neutral with regard to jurisdictional claims in published maps and institutional affiliations.
Rights and permissions
Open Access This article is licensed under a Creative Commons Attribution 4.0 International License, which permits use, sharing, adaptation, distribution and reproduction in any medium or format, as long as you give appropriate credit to the original author(s) and the source, provide a link to the Creative Commons licence, and indicate if changes were made. The images or other third party material in this article are included in the article’s Creative Commons licence, unless indicated otherwise in a credit line to the material. If material is not included in the article’s Creative Commons licence and your intended use is not permitted by statutory regulation or exceeds the permitted use, you will need to obtain permission directly from the copyright holder. To view a copy of this licence, visit http://creativecommons.org/licenses/by/4.0/ . The Creative Commons Public Domain Dedication waiver ( http://creativecommons.org/publicdomain/zero/1.0/ ) applies to the data made available in this article, unless otherwise stated in a credit line to the data.
Reprints and permissions
About this article
Cite this article.
Liu, Z., Wu, J., Gao, X. et al. Deep learning-based automatic measurement system for patellar height: a multicenter retrospective study. J Orthop Surg Res 19 , 324 (2024). https://doi.org/10.1186/s13018-024-04809-6
Download citation
Received : 23 March 2024
Accepted : 22 May 2024
Published : 31 May 2024
DOI : https://doi.org/10.1186/s13018-024-04809-6
Share this article
Anyone you share the following link with will be able to read this content:
Sorry, a shareable link is not currently available for this article.
Provided by the Springer Nature SharedIt content-sharing initiative
- Deep learning
- Patellar height index
- Radiographic imaging
- Keypoint detection
Journal of Orthopaedic Surgery and Research
ISSN: 1749-799X
- Submission enquiries: [email protected]
Money blog: Emirates boss says Heathrow is like a 'Second World War airport'
The president of Emirates airlines has said Heathrow Airport is "not good enough" and looks like a Second World War airport. Read this and the rest of today's consumer and personal finance news below - and leave your thoughts in the comments box.
Tuesday 4 June 2024 11:26, UK
- Heathrow is like a Second World War airport, says Emirates boss
- Gas prices hit highest level since December
- Earnings of M&S and Sainsbury's bosses revealed
- Glitch that delayed 500,000 benefit payments 'fixed', HMRC says
Essential reads
- The five different types of shopping addict
- How much are student loans, when do you start paying back and what is the interest?
- Your rights when deliveries or returns don't arrive - and why leaving instructions could jeopardise them
- Think twice before buying your holiday clothes from Zara
- Where is all the money going? Here's who is really responsible for concert tickets going crazy
- Would tourist tax put you off visiting Scotland?
- Best of the Money blog - an archive
Ask a question or make a comment
Company records have revealed the bosses of M&S and Sainsbury's were paid about £5m each last year.
M&S chief executive Stuart Machin received a record £4.73m payout - a 75% increase on his earnings the previous year.
Of this, £908,000 was fixed pay while the rest was performance-related.
Co-chief executive Katie Bickerstaffe, who has a four-day working pattern, took home a £4.41m payout.
Her earnings were 85% higher than last year.
The pair have overseen a successful turnaround for the retailer, which saw its profits surge by 58% in the 52 weeks to 30 March.
Meanwhile, Sainsbury's chief executive Simon Roberts took home £4.91m in the last year - a dip of 5.8% on last year's payout.
Mr Roberts's base salary increased by 3.8% to £933,000, but his bonus decreased.
Sainsbury's saw its pre-tax profit rise by 1.6%.
By Sarah Taaffe-Maguire , business reporter
Good news for motorists - the oil price has fallen again today to a low not seen since the start of February.
The international oil price benchmark, a barrel of Brent crude, costs $77.25 - down from $92 in April. It's lowered amid speculation that major oil producing countries in the OPEC+ (the Organisation of the Petroleum Exporting Countries) group will increase exports later this year.
A pound still buys roughly the same amount of euro as it has over the past week (€1.1743) and more dollars ($1.2776) than it has in the past two months.
The index of most valuable companies on the London Stock Exchange - the FTSE 100 - is down 0.63% this morning. One of the biggest fallers is the bank Standard and Chartered. Its share price is down more than 3% after reports that it's being accused in a New York court of funding terrorism, an accusation it denies.
The president of Emirates airlines has said Heathrow Airport is "not good enough" and looks like a Second World War airport.
Sir Tim Clark said the airport was putting its shareholders before running a world-class business.
"I was at Heathrow the other day and walking out of our lounge the ceiling height is awful," he said.
"It looks like a utilitarian structure, post-Second World War. It is just not good enough."
He argued Terminal 3, where Emirates is based, should be redesigned to make it better for passengers.
Heathrow is "seriously lagging behind" in its customer experience, Mr Clark said.
"It's an old airport. I'm afraid it's very difficult. You need to open up the whole terminal. Where we are based, new airports are being built employing the latest technologies to streamline the process of all the customer-facing elements. That is not the case at Heathrow."
Heathrow told The Telegraph: "Every pound we want to spend on improving airport facilities needs approval from our regulator. Despite having our proposals cut back in the current regulatory settlement, we will still invest £3.6bn upgrading our infrastructure over the next three years.
"We will continue to invest and to work with our airline partners to build an airport fit for the future."
Wholesale costs for natural gas have hit their highest levels across Europe since December last year - threatening a future spike in energy bills.
The cause is a key Norwegian export operation being shut down due to a cracked pipe.
The damage, discovered aboard the Sleipner Riser platform, prompted wider energy infrastructure to be halted including the Nyhamna processing plant which exports gas to the UK, pipeline operator Gassco said.
Alfred Hansen, the company's head of pipeline system operations, told the Reuters news agency: "This has big consequences from a supply perspective."
Read more on this, and how there's better new on oil prices, below ...
Yesterday we talked about the dopamine hit you get when shopping - and spoke to a psychologist about how you might learn to control it.
But what if it's gone too far? That's what we're discussing in the second of this four-part series this week.
A piece by the Royal College of Psychiatrists , published by Cambridge University Press in 2012, said shopping addiction, often referred to as compulsive buying disorder (CBD), "was first described by the German psychiatrist Emil Kraepelin almost a century ago".
He called the disorder "oniomania" (from the Greek onios, meaning "for sale", and mania, meaning "insanity").
A hundred years on, the World Health Organisation doesn't classify shopping addiction as a mental illness, unlike gambling, video game addiction, pyromania and kleptomania - but psychologists are taking note of the subject.
According to a 2021 paper in the Journal of Behavioral Addictions, potential symptoms of a compulsive shopping disorder include:
- Preoccupation with shopping (an irresistible urge to buy a product);
- Reduced control over buying behaviours;
- Buying products but not using them for the purposes they were intended to serve;
- Using shopping to regulate mood;
- Negative consequences afterwards such as guilt, shame, debt, relationship problems;
- Negative mood and cognitive symptoms if attempting to stop.
Donald W Black, a prominent American psychiatrist, has written extensively on the subject. He says the "disorder has a lifetime prevalence of 5.8% in the US general population".
There has been much debate about whether CBD is a valid mental illness - amid concerns of over medicalising. However, a growing number of rehab clinics are offering treatment.
The Priory's website says: "If you are addicted to spending money, and are finding that it is affecting your finances, relationships, health and quality of life, this is just as serious as any other addiction."
The Abbey Care Foundation says signs you may have a shopping addiction include juggling multiple credit cards, hiding extravagant spending from your family, hoarding things you don't use and getting angry at anyone who tries to get in the way of your spending.
The foundation even breaks down different types of shopping addict:
- Bargain-seekers: These people have a shopping habit of actively seeking items on sale. When they spot items for less than their perceived value, they purchase them. This behaviour makes them feel like they are winning and relieves shopping addiction.
- Collectors: This shopping addiction entails seeking out different versions of a particular item. The desire to collect or complete a set of similar items drives this addiction.
- Show-offs: The compulsive behaviour is driven by the desire to buy high-value items. In some cases, the individual's self-worth or self-esteem is attached to making such purchases.
- Trophy-hunters: The shopping addiction is for rare, expensive items. The individual intentionally looks for the most expensive or rarest items and gains satisfaction in buying them.
- Shopping bulimics: This shopping addiction is like the eating disorder known as bulimia nervosa. Individuals categorised as shopping bulimics make large, frequent purchases only to request later refunds. They do so to cushion themselves from the financial consequences of making such large purchases.
Join us tomorrow as we speak to a woman for who this used to be all too real - leading her into £40,000 of debt.
Passengers will soon be able to book British Airways flights in a few clicks as part of a major revamp by the airline.
The company told The Telegraph it wanted to style its website on the "three clicks and you can check out" approach of Amazon.
British Airways said it was spending £7bn on a revamp, which would see the company's app and website relaunched.
Other changes will include new planes, revamped seats and refurbished airport lounges.
Customers will also be able to rebook, claim a refund and cancel flights online.
The new website is currently being trialled by people flying from London Gatwick to Montpellier in France, Antalya in Turkey and Bari, Cagliari and Catania in Italy.
Basically, student finance is a government-financed loan that covers university students' tuition fees and living costs for the duration of their study.
There are two main types of loan, tuition and maintenance - we'll take each in turn.
Tuition fees
Undergraduate courses in England generally cost students about £9,250 a year.
That's a lot for a young person (or their family) to cover, so the government offers to pay that outright, direct to the university, on their behalf.
This is known as your tuition loan - we'll come to how this is repaid later.
Maintenance loans
These help students cover day-to-day costs, such as rent and food, while studying.
For the 2024-25 academic year, students can borrow anywhere between £4,327 and £13,348 for each year of study - depending on where you live, where you're going to study and your family's financial situation.
See how much you could be entitled to by clicking here .
The various plans
Here's where it gets more complicated. What plan you may be on is listed below...
Why no Plan 3? The repayment plan for postgraduate loans in England and Wales is actually Plan 3.
In the UK, you pay nothing up front, and the amount you pay back each month is determined by how much you earn.
You'll repay a percentage of your income over the threshold for your type of loan, depending on how often you get paid - see the table below for the thresholds.
With those thresholds in mind, you'll repay either:
- 9% of your income over the threshold if you're on Plan 1, 2, 4 or 5
- 6% of your income over the threshold if you're on a postgraduate loan (Plan 3)
If you're on multiple plans, the rules are slightly different.
If you don't have a postgraduate loan, you'll repay 9% of your income over the lowest threshold out of the plan types you have.
In this scenario, you'll only have a single repayment taken each time you get paid, even if you're on more than one plan type.
But if you do have a postgraduate loan, you'll repay 6% of your income over the postgraduate loan threshold and 9% of your income over the lowest threshold for any other plan types you have.
You don't need to worry about paying it off each month yourself if you're employed - the money will be deducted from your earnings before it hits your account, like income tax.
Interest rates
Like any loan, you'll be paying back what you owe plus a little bit on the top - known as interest.
With student loans, that extra on the top isn't so little right now, as it is linked to retail price rises.
- 6.25% if you're on Plan 1
- 7.8% if you're on Plan 2
- 6.25% if you're on Plan 4
- 7.8% if you're on Plan 5
- 7.8% if you're on a postgraduate loan plan (Plan 3)
Read other entries in our Basically... series...
Royal Mail's incoming owner has refused to rule out stamp price hikes under his leadership.
In fact, Czech billionaire Daniel Kretinsky seemed to suggest there might be more increases to come.
"I can't make unconditional commitments," he told The Times when questioned on the topic.
"[If] your circulation is 50% of what it was … you either need to go home, or you need to increase the unit price and hope that people will pay for it. Because if not, you are making losses.
"You can be loss-making for a year or two, but you can't be in a loss for 20 years. It's simple maths. There's no mystery to it."
First class stamp prices have more than doubled since 2018 from 67p to £1.35.
The businessman, nicknamed the Czech Sphinx, had his £3.6bn offer accepted by the postal service's parent company, International Distribution Services, last week.
It said the agreement included a series of "contractual commitments" to protect public service aspects of the Royal Mail - such as its universal service obligation to "one-price-goes-anywhere" first-class post six days a week.
Many were shocked by the deal, with Royal Mail reporting losses of £1m a day in recent years.
You can read more about the Czech Sphinx below...
A major error that meant 500,000 families did not receive their scheduled child benefit today has been "fixed", HMRC has said.
In a post on X, HMRC said affected families would get the money on Wednesday morning, two days after the payments were due...
Multiple readers have got in touch to say they had been affected by the problem, which meant almost a third of payments scheduled for today were not made.
Reader Susan1984 said: "When should we expect to receive the missing payment? This has left not just me but so many more families with kids completely stuck for food and fuel this morning."
Earlier, HMRC apologised and said it was working urgently to resolve the issue, which would not affected payments scheduled for tomorrow (see post at 14.41).
Child benefit is usually paid every four weeks on a Monday or a Tuesday at a rate of £25.60 for an eldest or only child and £16.95 for each additional child.
The bank has today announced wholesale rate hikes across its residential and buy-to-let mortgage product ranges.
The new rates, which come into effect tomorrow, will be applied largely across its two, three and five-year fixed rates for purchase and remortgage.
However, a number of rates available to existing HSBC customers looking to switch will also see increases.
Brokers say more lenders could increase rates this week.
This is thanks to an uptick in swap rates due to hopes fading for a cut to the base rate set by the Bank of England in June.
Here is what some industry insiders told Newspage...
The dreaded 'higher for longer' scenario is no longer a mere notion: it's the harsh reality for many. It looks like these elevated rates are here to stay for the foreseeable future. Ranald Mitchell, director at Charwin Private Clients
We can now expect more awkward conversations with clients who have read that rates are coming down and inflation is under control. HSBC have been fairly competitive recently so the hope is that they just need to turn the tap off a little to catch up and this isn't an upward trend that will continue into the summer period. David Stirling, independent financial advisor at Mint Mortgages and Protection
HSBC is one of several lenders to already have announced changes this week. Even with the higher rates on offer, I would not suggest waiting in the hope of a drop any time soon. My advice to borrowers is take control of the situation and start the process of arranging a new deal as early as possible, secure a rate and, if a better one materialises, change to it. Simon Bridgland, broker/director at Release Freedom
Be the first to get Breaking News
Install the Sky News app for free

- Small Business
- How to Start a Business
How to Start a Business: A Comprehensive Guide and Essential Steps
Building an effective business launch plan
- Search Search Please fill out this field.
Conducting Market Research
Crafting a business plan, reviewing funding options, understanding legal requirements, implementing marketing strategies, the bottom line.
:max_bytes(150000):strip_icc():format(webp)/Headshot-4c571aa3d8044192bcbd7647dd137cf1.jpg)
- How to Start a Business: A Comprehensive Guide and Essential Steps CURRENT ARTICLE
- How to Do Market Research, Types, and Example
- Marketing Strategy: What It Is, How It Works, How To Create One
- Marketing in Business: Strategies and Types Explained
- What Is a Marketing Plan? Types and How to Write One
- Business Development: Definition, Strategies, Steps & Skills
- Business Plan: What It Is, What's Included, and How to Write One
- Small Business Development Center (SBDC): Meaning, Types, Impact
- How to Write a Business Plan for a Loan
- Business Startup Costs: It’s in the Details
- Startup Capital Definition, Types, and Risks
- Bootstrapping Definition, Strategies, and Pros/Cons
- Crowdfunding: What It Is, How It Works, and Popular Websites
- Starting a Business with No Money: How to Begin
- A Comprehensive Guide to Establishing Business Credit
- Equity Financing: What It Is, How It Works, Pros and Cons
- Best Startup Business Loans
- Sole Proprietorship: What It Is, Pros & Cons, and Differences From an LLC
- Partnership: Definition, How It Works, Taxation, and Types
- What is an LLC? Limited Liability Company Structure and Benefits Defined
- Corporation: What It Is and How to Form One
- Starting a Small Business: Your Complete How-to Guide
- Starting an Online Business: A Step-by-Step Guide
- How to Start Your Own Bookkeeping Business: Essential Tips
- How to Start a Successful Dropshipping Business: A Comprehensive Guide
Starting a business in the United States involves a number of different steps spanning legal considerations, market research, creating a business plan, securing funding, and developing a marketing strategy. It also requires decisions about a business’ location, structure, name, taxation, and registration. Here are the key steps involved in starting a business, as well as important aspects of the process for entrepreneurs to consider.
Key Takeaways
- Entrepreneurs should start by conducting market research to understand their industry space, competition, and target customers.
- The next step is to write a comprehensive business plan, outlining the company’s structure, vision, and strategy.
- Securing funding in the form of grants, loans, venture capital, and/or crowdfunded money is crucial if you’re not self-funding.
- When choosing a venue, be aware of local regulations and requirements.
- Design your business structure with an eye to legal aspects, such as taxation and registration.
- Make a strategic marketing plan that addresses the specifics of the business, industry, and target market.
Before starting a business, entrepreneurs should conduct market research to determine their target audience, competition, and market trends. The U.S. Small Business Administration (SBA) breaks down common market considerations as follows:
- Demand : Is there a need for this product or service?
- Market size : How many people might be interested?
- Economic indicators : What are the income, employment rate, and spending habits of potential customers?
- Location : Are the target market and business well situated for each other?
- Competition : What is the market saturation ? Who and how many are you going up against?
- Pricing : What might a customer be willing to pay?
Market research should also include an analysis of market opportunities, barriers to market entry, and industry trends, as well as the competition’s strengths, weaknesses, and market share .
There are various methods for conducting market research, and these will vary depending on the nature of the industry and potential business. Data can come from a variety of places, including statistical agencies, economic and financial institutions, and industry sources, as well as direct consumer research through focus groups, interviews, surveys, and questionnaires.
A comprehensive business plan is like a blueprint. It lays the foundation for business development and affects decision-making, day-to-day operations, and growth. Potential investors or partners may want to review and assess it in advance of agreeing to work together. Financial institutions often request business plans as part of an application for a loan or other forms of capital.
Business plans will differ according to the needs and nature of the company and should only include what makes sense for the business in question. As such, they can vary in length and structure. They can generally be divided into two formats: traditional and lean start-up. The latter is less common and more useful for simple businesses or those that expect to rework their traditional business plan frequently. It provides a vivid snapshot of the company through a small number of elements.
The process of funding a business depends on its needs and the vision and financial situation of its owner. The first step is to calculate the start-up costs . Identify a list of expenses and put a dollar amount to each of them through research and requesting quotes. The SBA has a start-up costs calculator for small businesses that includes common types of business expenses.
The next step is to determine how to get the money. Common methods include:
- Self-funding , also known as “ bootstrapping ”
- Finding investors willing to contribute to your venture capital
- Raising money online by crowdfunding
- Securing a business loan from a bank, an online lender, or a credit union
- Winning a business grant from a donor, usually a government, foundation, charity, or corporation
Different methods suit different businesses, and it’s important to consider the obligations associated with any avenue of funding. For example, investors generally want a degree of control for their money, while self-funding puts business owners fully in charge. Of course, investors also mitigate risk; self-funding does not.
Availability is another consideration. Loans are easier to get than grants, which don’t have to be paid back. Additionally, the federal government doesn’t provide grants for the purposes of starting or growing a business, although private organizations may. However, the SBA does guarantee several categories of loans , accessing capital that may not be available through traditional lenders. No matter the funding method(s), it’s essential to detail how the money will be used and lay out a future financial plan for the business, including sales projections and loan repayments .
Businesses operating in the U.S. are legally subject to regulations at the local, county, state, and federal level involving taxation, business IDs, registrations, and permits.
Choosing a Business Location
Where a business operates will dictate such things as taxes, zoning laws (for brick-and-mortar locations), licenses, and permits. Other considerations when choosing a location might include:
- Human factors : These include target audience and the preferences of business owners and partners regarding convenience, knowledge of the area, and commuting distance.
- Regulations : Government at every level will assert its authority.
- Regionally specific expenses : Examples are average salaries (including required minimum wages), property or rental prices, insurance rates, utilities, and government fees and licensing.
- The tax and financial environment : Tax types include income, sales, corporate, and property, as well as tax credits; available investment incentives and loan programs may also be geographically determined.
Picking a Business Structure
The structure of a business should reflect the desired number of owners, liability characteristics, and tax status. Because these have legal and tax compliance implications , it’s important to understand them fully. If necessary, consult a business counselor, a lawyer, and/or an accountant.
Common business structures include:
- Sole proprietorship : A sole proprietorship is an unincorporated business that has just one owner, who pays personal income tax on its profits.
- Partnership : Partnership options include a limited partnership (LP) and a limited liability partnership (LLP) .
- Limited liability company (LLC) : An LLC protects its owners from personal responsibility for the company’s debts and liabilities.
- Corporation : The different types of corporations include C corp , S corp , B corp , closed corporation , and nonprofit .
Getting a Tax ID Number
A tax ID number is the equivalent of a Social Security number for a business. Whether or not a state and/or federal tax ID number is required will depend on the nature of the business and the location in which it’s registered.
A federal tax ID, also known as an employer identification number (EIN) , is required if a business:
- Operates as a corporation or partnership
- Pays federal taxes
- Has employees
- Files employment, excise, alcohol, tobacco, or firearms tax returns
- Has a Keogh plan
- Withholds taxes on non-wage income to nonresident aliens
- Is involved with certain types of organizations, including trusts, estates, real estate mortgage investment conduits, nonprofits, farmers’ cooperatives, and plan administrators
An EIN can also be useful if you want to open a business bank account, offer an employer-sponsored retirement plan, or apply for federal business licenses and permits. You can get one online from the Internal Revenue Service (IRS) . State websites will do the same for a state tax ID.
Registering a Business
How you register a business will depend on its location, nature, size, and business structure. For example, a small business may not require any steps beyond registering its business name with local and state governments, and business owners whose business name is their own legal name might not need to register at all.
That said, registration can provide personal liability protection, tax-exempt status, and trademark protection, so it can be beneficial even if it’s not strictly required. Overall registration requirements, costs, and documentation will vary depending on the governing jurisdictions and business structure.
Most LLCs, corporations, partnerships, and nonprofits are required to register at the state level and will need a registered agent to file on their behalf. Determining which state to register with can depend on factors such as:
- Whether the business has a physical presence in the state
- If the business often conducts in-person client meetings in the state
- If a large portion of business revenue comes from the state
- Whether the business has employees working in the state
If a business operates in more than one state, it may need to file for foreign qualification in other states in which it conducts business. In this case the business would register in the state in which it was formed (this would be considered the domestic state) and file for foreign qualification in any additional states.
Obtaining Permits
Filing for the applicable government licenses and permits will depend on the industry and nature of the business and might include submitting an application to a federal agency, state, county, and/or city. The SBA lists federally regulated business activities alongside the corresponding license-issuing agency, while state, county, and city regulations can be found on the official government websites for each region.
Every business should have a marketing plan that outlines an overall strategy and the day-to-day tactics used to execute it. A successful marketing plan will lay out tactics for how to connect with customers and convince them to buy what the company is selling.
Marketing plans will vary according to the specifics of the industry, target market, and business, but they should aim to include descriptions of and strategies for the following:
- A target customer : Including market size, demographics, traits, and relevant trends
- Value propositions or business differentiators : An overview of the company’s competitive advantage with regard to employees, certifications, and offerings
- A sales and marketing plan : Including methods, channels, and a customer’s journey through interacting with the business
- Goals : Should cover different aspects of the marketing and sales strategy, such as social media follower growth, public relations opportunities, and sales targets
- An execution plan : Should detail tactics and break down higher-level goals into specific actions
- A budget : Detailing how much different marketing projects and activities will cost
How Much Does It Cost to Start a Business?
Business start-up costs will vary depending on the industry, business activity, and product or service offered. Home-based online businesses will usually cost less than those that require an office setting to meet with customers. The estimated cost can be calculated by first identifying a list of expenses and then researching and requesting quotes for each one. Use the SBA’s start-up costs calculator for common types of expenses associated with starting a small business.
What Should I Do Before Starting a Business?
Entrepreneurs seeking to start their own business should fully research and understand all the legal and funding considerations involved, conduct market research, and create marketing and business plans. They will also need to secure any necessary permits, licenses, funding, and business bank accounts.
What Types of Funding Are Available to Start a Business?
Start-up capital can come in the form of loans, grants, crowdfunding, venture capital, or self-funding. Note that the federal government does not provide grant funding for the purposes of starting a business, although some private sources do.
Do You Need to Write a Business Plan?
Business plans are comprehensive documents that lay out the most important information about a business. They reference its growth, development, and decision-making processes, and financial institutions and potential investors and partners generally request to review them in advance of agreeing to provide funding or to collaborate.
Starting a business is no easy feat, but research and preparation can help smooth the way. Having a firm understanding of your target market, competition, industry, goals, company structure, funding requirements, legal regulations, and marketing strategy, as well as conducting research and consulting experts where necessary, are all things that entrepreneurs can do to set themselves up for success.
U.S. Small Business Administration. “ Market Research and Competitive Analysis .”
U.S. Small Business Administration. “ Write Your Business Plan .”
U.S. Small Business Administration. " Calculate Your Startup Costs ."
U.S. Small Business Administration. “ Fund Your Business .”
U.S. Small Business Administration. “ Grants .”
U.S. Small Business Administration. “ Loans .”
U.S. Small Business Administration. “ Pick Your Business Location .”
U.S. Small Business Administration. “ Choose a Business Structure .”
Internal Revenue Service. “ Do You Need an EIN? ”
U.S. Small Business Administration. “ Get Federal and State Tax ID Numbers .”
U.S. Small Business Administration. “ Register Your Business .”
U.S. Small Business Administration. “ Apply for Licenses and Permits .”
U.S. Small Business Administration. “ Marketing and Sales .”
:max_bytes(150000):strip_icc():format(webp)/GettyImages-1421562920-d5cf7a5a92204c579da450389c6b01a5.jpg)
- Terms of Service
- Editorial Policy
- Privacy Policy
- Your Privacy Choices
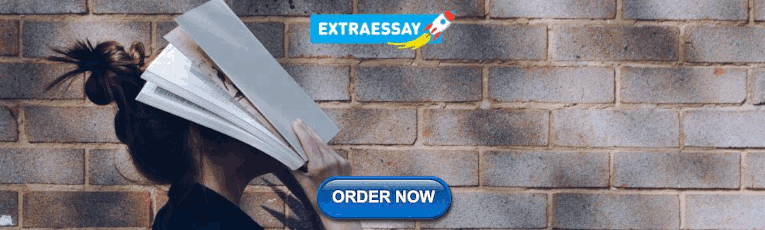
IMAGES
VIDEO
COMMENTS
Figure 1.1 will help you contextualize many of these terms and understand the research process. This general chart begins with two key concepts: ontology and epistemology, advances through other concepts, and concludes with three research methodological approaches: qualitative, quantitative and mixed methods.
hypothesis: a proposition which research sets out to prove or disprove: "experimental" where the hypothesis is a positive statement, or "null" where statement contains a negative. independent variable: a variable that researcher believes precedes, influences or predicts the dependent variable. informed consent: giving potential ...
Research is defined as a meticulous and systematic inquiry process designed to explore and unravel specific subjects or issues with precision. This methodical approach encompasses the thorough collection, rigorous analysis, and insightful interpretation of information, aiming to delve deep into the nuances of a chosen field of study.
Research methods are specific procedures for collecting and analyzing data. Developing your research methods is an integral part of your research design. When planning your methods, there are two key decisions you will make. First, decide how you will collect data. Your methods depend on what type of data you need to answer your research question:
research terminologies in educational research. It provides definitions of many of the terms used in the guidebooks to conducting qualitative, quantitative, and mixed methods of research. The terms are arranged in alphabetical order. Abstract A brief summary of a research project and its findings. A summary of a study that
Research is the careful consideration of study regarding a particular concern or research problem using scientific methods. According to the American sociologist Earl Robert Babbie, "research is a systematic inquiry to describe, explain, predict, and control the observed phenomenon. It involves inductive and deductive methods.".
Defining Key Terms. If you have chosen a topic, you may break the topic down into a few main concepts and then list and/or define key terms related to that concept. If you have performed some background searching, you can include some of the words that were used to describe your topic. For example, if your topic deals with the relationship ...
descriptive research describes a population, situation or event that is being studied. It focuses on developing knowledge about what exists and what is happening. causal research (also known as 'evaluative research') uses experimentation to determine whether a cause-and-effect relationship exists between two or more elements, features or ...
Qualitative research is primarily concerned with meaning, subjectivity, and lived experience. The goal is to understand the quality and texture of people's experiences, how they make sense of them, and the implications for their lives. ... Key Features. Quantitative researchers try to control extraneous variables by conducting their studies ...
Another definition of research is given by John W. Creswell, who states that "research is a process of steps used to collect and analyze information to increase our understanding of a topic or issue". It consists of three steps: pose a question, collect data to answer the question, and present an answer to the question. ...
In educational research the term paradigm is used to describe a researcher's 'worldview' (Mackenzie & Knipe, 2006). This worldview is the perspective, or thinking, or school of thought, or set of shared beliefs, that informs the meaning or interpretation of research data. Or, as Lather (1986) explains, a research paradigm inherently ...
Qualitative research involves collecting and analyzing non-numerical data (e.g., text, video, or audio) to understand concepts, opinions, or experiences. It can be used to gather in-depth insights into a problem or generate new ideas for research. Qualitative research is the opposite of quantitative research, which involves collecting and ...
The first and most important step in the research process is to identify the key concepts of your topic. From these key concepts you will generate the keywords needed to search the library's catalog and article databases. The box to the right explains how to identify key concepts. NOTE: This is not necessarily a thesis, but an exploration of ...
2. Key concepts in research. • Research is a lot easier to appreciate through an understanding of some of the concepts covered in this chapter. • Quantitative and qualitative approaches to research relate to the different research designs, and are based on philosophical beliefs about the nature of empirical evidence, that is, evidence ...
Keyword research is an extension of understanding your audience by first considering their needs and then the phrases, keywords, or queries they use to find solutions. Keyword research is also ...
Well, you take the most important words in your research statement/question and use them as key terms. Use those key terms in conjunction with each other (see the section on "Combining Key Terms" for advice about how to do so). Also, use synonyms of your key terms.
This general chart begins with two key concepts: ontology and epistemology, advances through other concepts, and concludes with three research methodological approaches: qualitative, quantitative and mixed methods. Research does not end with making decisions about the type of methods you will use; we could argue that the work is just beginning ...
Research Methods Key Term Glossary. This key term glossary provides brief definitions for the core terms and concepts covered in Research Methods for A Level Psychology. Don't forget to also make full use of our research methods study notes and revision quizzes to support your studies and exam revision. Aim. The researcher's area of interest ...
The next step is to pull out the key concepts. This may seem simple but identifying the main concepts in the research question is important for a successful search. This question has three major concepts: Media, Self-image and Teenagers. These keywords will be the building blocks of our search. It's best to search by keywords instead of ...
Quantitative research is the process of collecting and analyzing numerical data to describe, predict, or control variables of interest. This type of research helps in testing the causal relationships between variables, making predictions, and generalizing results to wider populations. The purpose of quantitative research is to test a predefined ...
Keyword research starts with a topic, idea, or head keyword, also called a "seed keyword." This seed can come from your industry knowledge or the products and services you provide, from being an active member in related online forums and groups, or through social listening. For example, if you run a bike shop, your seed keywords may be ...
Categorical Variable. This is a variable that can take on a limited number of values or categories. Categorical variables can be nominal or ordinal. Nominal variables have no inherent order, while ordinal variables have a natural order. Examples of categorical variables include gender, race, and educational level.
The research investigated how pleasure, meaning and spirituality affected life satisfaction levels in 2,615 people spread across six continents and representing different cultural contexts. And they discovered that meaning is a stronger predictor of life satisfaction than pleasure and spirituality.
In both research and practice, we find that transformations stand the best chance of success when they focus on four key actions to change mind-sets and behavior: fostering understanding and conviction, reinforcing changes through formal mechanisms, developing talent and skills, and role modeling. Collectively labeled the "influence model ...
The U.S. House of Representatives Committee on Agriculture completed its markup of the Farm, Food and National Security Act of 2024 (Farm Bill) on May 24, 2024, and passed the proposed legislation 33-21, with four Democrats - Reps. Don Davis (D-N.C.), Sanford Bishop (D-Ga.), Eric Sorensen (D-Ill.) and Yadira Caraveo (D-Colo.) - voting in ...
This study explored the positive effects of a six-week Social-Emotional and Ethical Learning® (SEE Learning) program on resilience and social and emotional competences, adapted for elementary students in Daegu, South Korea, a region strongly affected by the first outbreak of COVID-19. A total of 348 third- and fourth-grade students from 15 elementary schools participated, and the curriculum ...
Background The patellar height index is important; however, the measurement procedures are time-consuming and prone to significant variability among and within observers. We developed a deep learning-based automatic measurement system for the patellar height and evaluated its performance and generalization ability to accurately measure the patellar height index. Methods We developed a dataset ...
British Airways to allow passengers to book flights in just a few clicks with Amazon-style app. Passengers will soon be able to book British Airways flights in a few clicks as part of a major ...
Starting a business in the United States involves a number of different steps spanning legal considerations, market research, creating a business plan, securing funding, and developing a marketing ...