- Corpus ID: 5940305
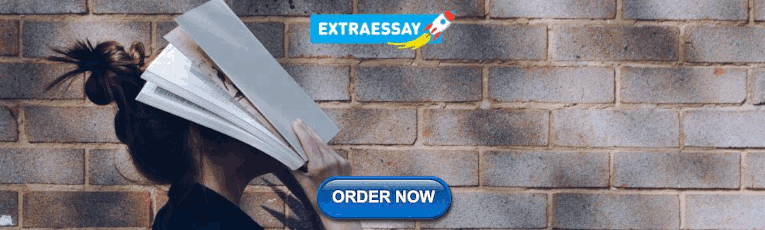
The Gender Similarities Hypothesis
Janet Shibley
- Published 2005
Figures and Tables from this paper
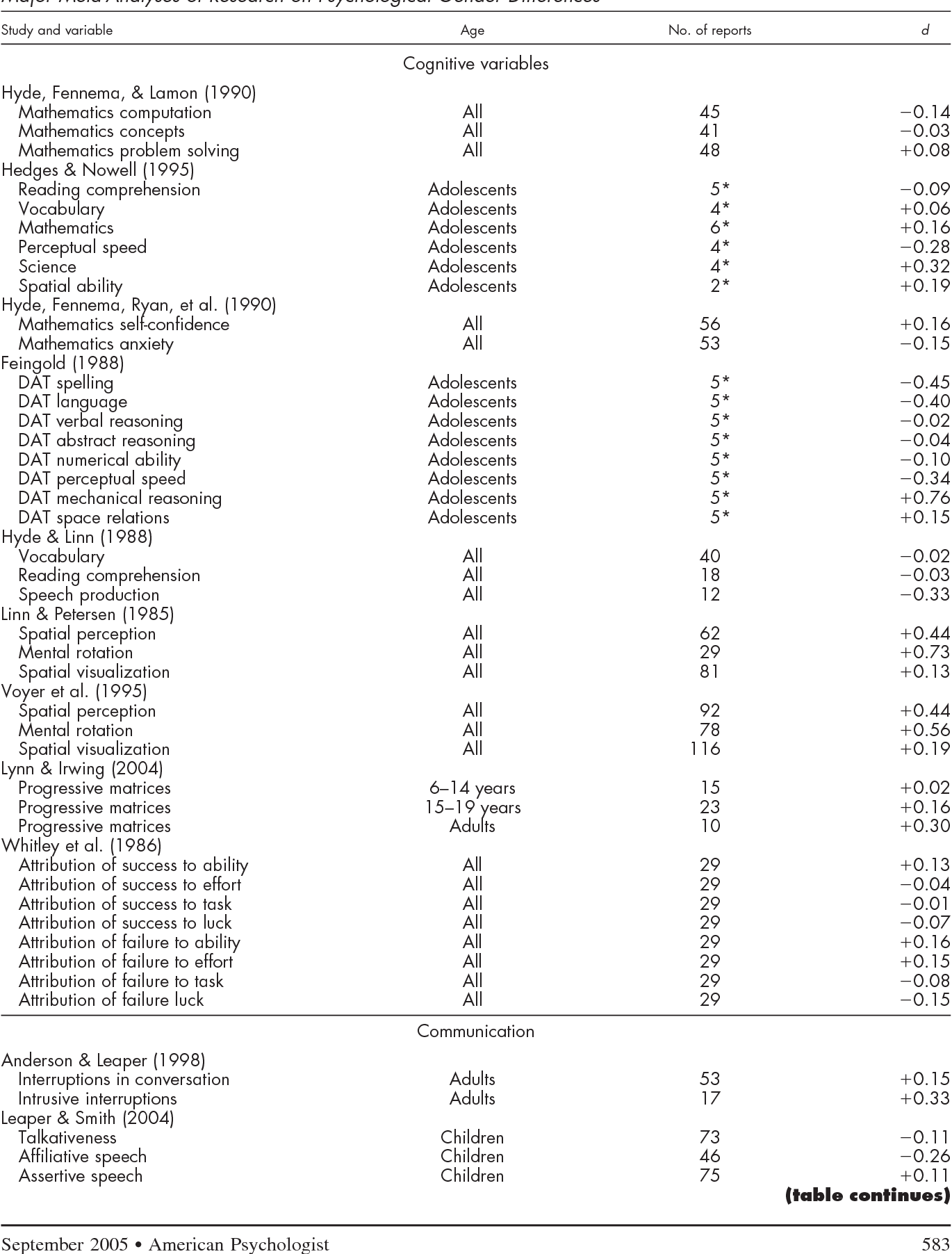
2,516 Citations
New directions in the study of gender similarities and differences.
- Highly Influenced
New Directions in the Study of Gender Similarities and
Gender differences in personality and social behavior, gender and media: content, uses, and impact, gender differences in personality and interests: when, where, and why, the intersection of gender, social class, and cultural context: a meta-analysis, lay beliefs about competition: scale development and gender differences, a feminist review of behavioral economic research on gender differences, what do true gender ratios and stereotype norms really tell us, understanding magnitude of sex/gender differences 1 understanding the magnitude of psychological differences between women and men requires seeing the forest and the trees, 97 references, magnitude of psychological gender differences. another side to the story..
- Highly Influential
Sex differences and similarities in job attribute preferences: a meta-analysis.
Gender differences in sexuality: a meta-analysis., estimating gender differences in the comprehension and preference of moral issues., gender comparisons of mathematics attitudes and affect: a meta-analysis, gender differences in moral orientation: a meta-analysis., gender differences in personality: a meta-analysis., gender differences in computer-related attitudes and behavior: a meta-analysis, emotional arousal and gender differences in aggression: a meta‐analysis, gender and the evaluation of leaders: a meta-analysis., related papers.
Showing 1 through 3 of 0 Related Papers
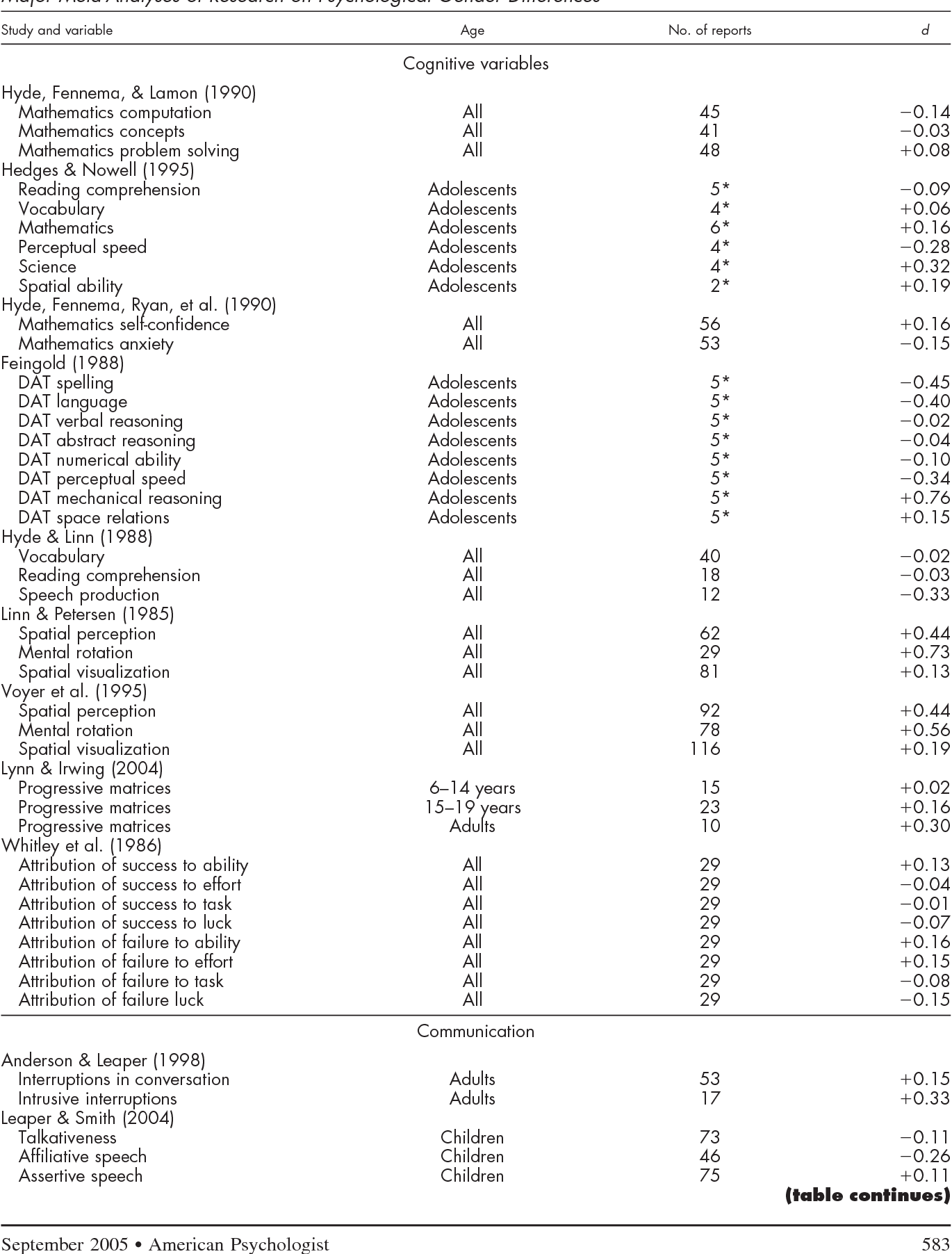
Published in 2005
- A-Z Publications
Annual Review of Psychology
Volume 65, 2014, review article, gender similarities and differences.
- Janet Shibley Hyde 1
- View Affiliations Hide Affiliations Affiliations: Department of Psychology, University of Wisconsin, Madison, Wisconsin 53706; email: [email protected]
- Vol. 65:373-398 (Volume publication date January 2014) https://doi.org/10.1146/annurev-psych-010213-115057
- First published as a Review in Advance on June 26, 2013
- © Annual Reviews
Whether men and women are fundamentally different or similar has been debated for more than a century. This review summarizes major theories designed to explain gender differences: evolutionary theories, cognitive social learning theory, sociocultural theory, and expectancy-value theory. The gender similarities hypothesis raises the possibility of theorizing gender similarities. Statistical methods for the analysis of gender differences and similarities are reviewed, including effect sizes, meta-analysis, taxometric analysis, and equivalence testing. Then, relying mainly on evidence from meta-analyses, gender differences are reviewed in cognitive performance (e.g., math performance), personality and social behaviors (e.g., temperament, emotions, aggression, and leadership), and psychological well-being. The evidence on gender differences in variance is summarized. The final sections explore applications of intersectionality and directions for future research.
Article metrics loading...
Full text loading...
Data & Media loading...
- Article Type: Review Article
Most Read This Month
Most cited most cited rss feed, job burnout, executive functions, social cognitive theory: an agentic perspective, on happiness and human potentials: a review of research on hedonic and eudaimonic well-being, sources of method bias in social science research and recommendations on how to control it, mediation analysis, missing data analysis: making it work in the real world, grounded cognition, personality structure: emergence of the five-factor model, motivational beliefs, values, and goals.
Publication Date: 03 Jan 2014
Online Option
Sign in to access your institutional or personal subscription or get immediate access to your online copy - available in PDF and ePub formats
The Gender Similarities Hypothesis: Insights From A Multilevel Analysis of High-Stakes Examination Results in Mathematics
- Original Article
- Open access
- Published: 12 July 2021
- Volume 85 , pages 481–496, ( 2021 )
Cite this article
You have full access to this open access article
- Ian Cantley ORCID: orcid.org/0000-0002-8995-6281 1 &
- James McAllister 1
4393 Accesses
7 Citations
10 Altmetric
Explore all metrics
A Publisher Correction to this article was published on 16 August 2021
This article has been updated
The current study involved multilevel analysis of high-stakes examination results (i.e., GCSE) in Northern Ireland to investigate gender differentials in mathematical achievement, whereas most previous research in the area used results from low-stakes tests (i.e., PISA, TIMSS). The analysis supported the gender similarities hypothesis with respect to both overall and content domain-specific mathematical attainment. Similar conclusions were drawn from the current study as have been reported in studies into gender differentials using data from low-stakes assessments in the respective jurisdiction. This suggests that previously expressed concerns in the literature about the viability of using data derived from low-stakes assessments to accurately assess gender differentials in achievement may be unfounded. Furthermore, the context for the current study permitted an investigation into the effects of school type (grammar versus non-grammar) and gender on overall and domain-specific mathematical achievement, an area that has received scant attention in the literature. School type was not found to have an effect on the applicability of the gender similarities hypothesis with respect to mathematical achievement. The study findings are likely to prove useful to researchers and policymakers who are interested in gender equity issues in mathematics.
Similar content being viewed by others
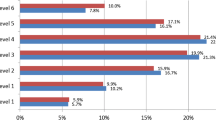
Gender equity in mathematical achievement: the case of China
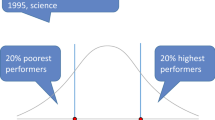
Trends in gender gaps: using 20 years of evidence from TIMSS
Gender Differences in School Achievement
Avoid common mistakes on your manuscript.
Mathematical proficiency is considered an important skill to acquire as part of a good education. This is because of its pivotal role in everyday life and in many vocations (Leder & Forgasz, 2018 ; Valero, 2017 ). The issue of gender differences in mathematical performance is an important and contested one, particularly against the backdrop of the stereotypical view that women lack mathematical ability (Franceschini et al., 2014 ).
The analysis reported upon in this paper is used to test the gender similarities hypothesis (Hyde, 2005 , 2014 , 2016 ) in relation to student achievement in high-stakes mathematics examinations in Northern Ireland. Based on a review of 46 meta-analyses, Hyde ( 2005 ) concluded that, “males and females are similar on most, but not all, psychological variables” (p. 581). In her work, Hyde ( 2005 ) applied Cohen’s d standardised measure of effect size to assess gender differences in psychological variables, with positive values of d favouring boys and men and negative values favouring girls and women. The following ranges were used to evaluate the magnitudes of effects: \(\left|d\right|\le .10\) is close-to-zero; \(.10<\left|d\right|\le .35\) is small; \(.35<\left|d\right|\le .65\) is moderate; \(.65<\left|d\right|\le 1.00\) is large; and \(\left|d\right|>1.00\) is very large. Hyde’s ( 2005 ) research revealed close-to-zero or small gender differences in mathematics-related constructs, with the exception of spatial ability, which had moderate to large effect sizes in favour of boys. The small, or close-to-zero, gender differences in mathematical performance have been supported by more recent meta-analytic studies (e.g., Lindberg et al., 2010 ), but national gender differentials have been shown to exhibit substantial variability (Else-Quest et al., 2010 ; Nollenberger et al., 2016 ; Rodríguez-Planas & Nollenberger, 2018 ). It is important to note that the research described here was almost exclusively conducted in the United States, either as a nation or in specific states or school districts. By contrast, the use of Northern Ireland as a site for the current study offers a distinct context to investigate gender differentials in mathematical achievement.
A large proportion of the recent research on gender differentials in mathematical achievement has been based on analyses of student performance in large-scale international assessments such as the Programme for International Student Assessment (PISA) and the Trends in International Mathematics and Science Study (TIMSS) (e.g., Ayalon & Livneh, 2013 ; Brunner et al., 2013 ; Demir et al., 2010 ; Else-Quest et al., 2010 ; Innabi & Dodeen, 2018 ; Liu & Wilson, 2009 ; Louis & Mistele, 2012 ; Meggiolaro, 2018 ). Given that international large-scale assessments have no direct consequences for participants, it has been suggested that their low-stakes nature can negatively impinge upon the amount of effort that students invest in their completion. This may compromise the validity and reliability of the associated ability measurements (Eklöf, 2010 ), which has the potential to negatively impact the reliability of gender differentials in achievement derived from large-scale international assessments.
Gender differentials in mathematical achievement have been shown to vary according to mathematical content domains such as number work, algebra, geometry and measures, and statistics and probability (e.g., Li et al., 2018 ; McGraw et al., 2006 ; Taylor & Lee, 2012 ). For example, a large male advantage in geometry has been reported by a number of scholars (e.g., Leahey & Guo, 2001 ; McGraw et al., 2006 ; Taylor & Lee, 2012 ). However, less attention has been paid to this issue than overall mathematical performance. The current article addresses this gap in the literature. This is an important area for investigation because some existing gender-focused research has demonstrated that women’s study of, and achievement in, different content domains influence their uptake of the physical sciences, engineering, mathematics, and computer science in tertiary level education (Nix & Perez-Felkner, 2019 ; Perez-Felkner et al., 2012 ; Watt et al., 2012 ).
Background and Study Context
To further illuminate the gaps in existing work, this article reports on an analysis of a sample of high-stakes public examination results in mathematics from General Certificate of Secondary Education (GCSE) mathematics examinations, which are taken at the end of compulsory post-primary education in Northern Ireland, and have a pivotal role in determining students’ future educational and vocational pathways. The analysis has been undertaken for both lower-ability and higher-ability students, who sit GCSE mathematics at foundation tier and higher tier respectively. Foundation tier examinations tend to be taken by lower-ability students who do not pursue STEM-related courses after GCSE, while higher tier examinations are taken by higher-ability students who may pursue STEM-related courses beyond GCSE. The analysis of gender differences in mathematical achievement by content domain (number, algebra, geometry and measures, or statistics and probability), in addition to overall mathematical achievement, is an important feature of the current study. Limited attention has been paid to variation in gendered achievement by mathematical content domain (i.e., in number work, algebra, geometry and measures, and statistics and probability) in the existing literature, which places considerable emphasis on overall mathematical achievement. This is an important area for investigation since mathematical performance has a tendency to be viewed as a monolith (Miner, 2019 ).
Northern Ireland provides an appropriate context for the current study since it is a jurisdiction that has attracted less attention in the global literature and also exhibits negligible gender differentials in mathematical achievement in large-scale international comparative studies such as PISA and TIMSS (Jerrim & Shure, 2016 ; Mullis et al., 2016 ; Wheater et al., 2013 ). Furthermore, Northern Ireland operates a system of academic selection, whereby children are selected for different types of post-primary schools, either grammar or non-grammar, on the basis of academic ability at 10 or 11 years of age. Grammar schools offer a more academically-oriented curriculum than non-grammar schools, which place a greater emphasis on the development of practical and vocational skills. However, it is noteworthy that students from lower socioeconomic backgrounds are less likely to attend grammar schools than their counterparts from more privileged backgrounds. For example, during the 2015–2016 school year, 13.9% of grammar school students were entitled to free school meals (which is considered to be a measure of socioeconomic advantage/disadvantage in Northern Ireland, with lower percentages indicating that students come from more affluent backgrounds), compared to 39.9% of non-grammar school students (Department of Education, 2016 ). Whilst grammar school students have been shown to significantly outperform their non-grammar school counterparts in international comparative studies of mathematical achievement (Jerrim & Shure, 2016 ), there is limited evidence in the literature pertaining to how gender differences in mathematical achievement vary by school type. The current study also addresses this gap in the current evidence base.
In the current research, multilevel models were used to analyse gender differences in overall mathematical achievement, and achievement in the various content domains of mathematics, based on results obtained in Northern Irish GCSE mathematics examinations. A multilevel regression analysis was also used to test for the effects of interactions of gender with type of post-primary school attended on overall mathematical achievement, and achievement in the different mathematical content domains. Given the dearth of studies that directly analyse high-stakes examination results in mathematics from a gender equity perspective using multilevel models, the current study makes a timely contribution to the literature pertaining to gender effects in mathematical achievement.
Historical Overview of Research on Gender and Mathematical Achievement
Traditionally, mathematics has been viewed as a discipline that is more accessible to men than women (Henrion, 1997 ). Early researchers in the field reported that there were no significant differences between boys’ and girls’ mathematical achievements in early primary school, but that differences in favour of boys began to emerge as children progressed in their education (e.g., Fennema, 1974 ). However, some inconsistencies were noted in the gender differentials, and the gender favoured, in different mathematical content domains and/or different countries (Hanna et al., 1990 ; Smith & Walker, 1988 ). Interestingly, the greatest differentials in favour of boys’ mathematical achievements usually occurred amongst high-achieving students (Benbow & Stanley, 1980 ).
More recent research on gender effects in mathematical achievement, conducted during the early part of the twenty-first century, has broadly supported the findings reported by the pioneering researchers in the area. For example, in a study designed to investigate gender differences in the mathematical achievement of children aged nine, 12 or 15 years in Northern Taiwan, Chen and colleagues ( 2013 ) reported that gender had small to moderate effects on mathematical achievement, and that gender differences in favour of boys increased with age. These findings are corroborated by the work of Contini et al. ( 2017 ), who found that, after controlling for a variety of other background variables in an Italian context, boys perform better in mathematics than girls, and that the difference increases with age, with the greatest differentials for the highest-attaining students. These findings are consistent with those reported by Matteucci and Mignani ( 2011 ), who also investigated gender differences in mathematics for Italian students. Gender differentials in favour of boys’ mathematical achievement have been highlighted by numerous researchers in relation to other national contexts (e.g., Dickerson et al., 2015 ; Leahey & Guo, 2001 ; Pargulski & Reynolds, 2017 ; Rodríguez-Planas & Nollenberger, 2018 ). However, it is important to observe that boys do not have a universal advantage in terms of mathematical achievement. This is exemplified by the fact Innabi and Dodeen ( 2018 ) concluded that 8 th grade girls in Jordan outperformed boys in mathematics, but with the caveat that boys had a greater likelihood of correctly answering more demanding problems set in unfamiliar contexts, while girls were more likely to correctly answer routine problems. Gender differences in favour of boys have been reported in many studies involving PISA (Organisation for Economic Co-operation and Development (OECD, 2015 , 2016 ), but the findings are more subtle in the case of TIMSS. This is illustrated by the fact no significant differences between boys’ and girls’ mathematics scores were recorded in 26 of the 39 countries participating in the TIMSS 2015 8th grade study (Mullis et al., 2016 ).
It is important to highlight that a very high proportion of previous research into gender differentials, including all studies cited thus far in the current section, was conducted using the results of low-stakes assessments of mathematical achievement, which had no direct consequences for study participants. There is less evidence pertaining to gender differences in relation to test results garnered from high-stakes assessments. Notable exceptions include Cox et al.’s ( 2004 ) study in an Australian context, and Zawistowska’s ( 2017 ) study in a Polish context. Cox et al. ( 2004 ) reported that, on average, girls outperformed boys in almost all mathematical subjects for the majority of the years considered in the study, while Zawistowska ( 2017 ) concluded there were marginal gender differences in mathematical attainment, although boys demonstrated a pronounced advantage at the highest levels of performance.
Gender Differentials in International Large-Scale Assessments: Limitations of Low-Stakes Testing
The mathematical achievement of students from different countries is currently assessed in two major international comparative studies: the quadrennial TIMSS, which was inaugurated by the International Association for the Evaluation of Educational Achievement (IEA) in 1995, and the triennial PISA, which was introduced by the OECD in 2000. Both tests are deemed to yield valid international comparisons of students’ mathematical achievements, and to have the potential to inform the decision-making of mathematics education policymakers in participating jurisdictions (Dossey & Wu, 2013 ), although the robustness of their underpinning measurement model has been challenged by some researchers (e.g., Cantley, 2015 , 2017 , 2019 ; Dohn, 2007 ; Goldstein, 2004 ). TIMSS test items are focused on assessing samples of 4th grade and 8th grade students’ mastery of the content of the school mathematics curriculum, while PISA items attempt to assess the mathematical problem-solving skills of a sample of 15 year-olds in the context of real life scenarios. According to Dossey and Wu ( 2013 ), “although PISA’s results provide a picture of students’ capabilities, they provide less direct relationships to the schooling students have received” (p. 1013). However, they acknowledge that, despite the dubious link between schooling and PISA outcomes, the results “may provide a better picture of the future capabilities of nations’ students to cope with everyday applications of mathematics” (p. 1013).
From a gender equity perspective, it is therefore concerning that the PISA 2012 study, which focused on mathematics, revealed that boys’ mean mathematics score exceeded that of girls by 11 points. Boys outperformed girls in 38 of the 65 participating jurisdictions, whereas girls performed better than boys in just five countries (OECD, 2014 , 2015 ). However, although the gender difference was significant at the 5% level, it is noteworthy that the male advantage was small ( \(d=.109\) ), and likely to be of limited practical significance. Furthermore, it is noteworthy that gender differences in PISA mathematical achievement vary considerably by country (Else-Quest et al., 2010 ). Indeed, in a number of jurisdictions, such as Northern Ireland, there were very small differences between boys’ and girls’ mathematical achievement levels in PISA 2012, and the associated effect size of \(d=.118\) confirms that boys’ scores only exceeded those for girls by a small margin (OECD, 2015 ; Wheater et al., 2013 ).
DeMars et al. ( 2013 ) noted that girls tend to expend greater effort than boys when confronted with low-stakes tests to the extent that “it is possible to observe sizeable gender differences in performance on low-stakes assessments partly or fully due to gender differences in test-taking motivation” (p. 79). Furthermore, Barry and colleagues ( 2010 ) posit that students are likely to invest greater effort in high-stakes testing situations where the test result has tangible personal consequences which, according to DeMars et al.’s ( 2013 ) argument, may influence the observed gender differentials in mathematical achievement for studies such as TIMSS and PISA. This stance is also advocated by Guez et al. ( 2020 ), who argue that gender differences in academic achievement are modulated by the stakes associated with the assessment used. The high-stakes test results that feature in the current research permitted an investigation into the import of these concerns.
Country-Specific Factors That may Potentially Influence Gender Differentials in Mathematical Achievement
In addition to differential performance by content domain, a number of other factors have been linked to gender differences in mathematical achievement. For example, gender social norms in a particular country have been shown to influence gender differentials in mathematical achievement (Rodríguez-Planas & Nollenberger, 2018 ). Ayalon and Livneh’s ( 2013 ) research revealed that countries with greater degrees of standardisation in terms of their curriculum, and where the curriculum is governed via the use of national examinations, have lower gender differences in mathematical achievement, although no evidence was found of a relationship between such standardisation measures and a country’s average mathematical achievement. However, Leder and Forgasz ( 2018 ) stressed that the design of assessment instruments, including the response format employed (e.g., free response or multiple choice), is an important contributory factor to gender effects in mathematical achievement.
The jurisdiction where the current research was conducted (Northern Ireland) operates a bipartite, selective education system, whereby some students complete their post-primary education in more academically-oriented grammar schools, with the remainder attending non-selective post-primary schools, which cater for students of all abilities. The use of academic selection is a highly contentious issue in Northern Ireland. Although students from lower socioeconomic backgrounds are less likely to gain admission to grammar schools (Gardner, 2016 ), these schools remain popular with many parents. This is particularly worrying since there are high levels of social disadvantage through poverty in many areas of Northern Ireland, thus meaning that academic selection perpetuates social class divisions (Gardner, 2016 ). Although the available data in the current study did not permit an analysis of the interaction of individual students’ socioeconomic statuses and genders on mathematical achievement, it did facilitate an analysis of gender differentials in achievement by school type (grammar versus non-grammar), which addresses a gap in the research evidence in its own right.
Aims of Current Study and Research Questions
The aim of the current study was to examine gender differentials in both overall mathematical achievement, and achievement in various content domains (number, algebra, geometry and measures, and statistics and probability). This aim was addressed by examining a sample of Northern Irish students’ total scores and domain-specific scores derived from item-level scores in GCSE mathematics. Furthermore, the data were analysed to test for the effects of interactions of gender with school type on both overall and domain-specific achievement.
The specific research questions addressed in the current study are as follows:
What is the magnitude of the gender difference for students’ overall achievement in a high-stakes Northern Irish mathematics examination?
What are the magnitudes of the gender differences for students’ achievement in the different content domains of a high-stakes Northern Irish mathematics examination?
How do the magnitudes of gender differences in mathematical achievement vary by school type for a high-stakes Northern Irish mathematics examination?
Methodology
The current study.
The current study involved analysis of the results obtained by a sample of students in the summer 2016 Northern Ireland Council for the Curriculum, Examinations and Assessment (NICCEA) GCSE mathematics examinations. The NICCEA GCSE mathematics specification at that time was unitised and had two tiers of entry: foundation (usually entered by lower attaining students) and higher tier (usually entered by higher attaining students). A choice of units, with different levels of challenge, was available at each tier to cater for the wide range of mathematical abilities of candidates taking the tier. Scores from two units were used to determine the GCSE mathematics grade at certification stage. Each unit contained questions designed to assess students’ knowledge, understanding, and problem-solving skills in relation to the four content domains: number, algebra, geometry and measures, statistics and probability. Unit assessments were marked in raw marks but, since candidates could take unit assessments in different examination series, raw marks were adjusted to uniform marks to compensate for any variation in difficulty of the assessments between series. This ensured that candidates who demonstrated the same level of achievement in a unit taken in different examination series finished up with the same uniform mark. Uniform marks from two assessment units were added to obtain a total uniform mark which determined the final GCSE mathematics grade.
NICCEA supplied the authors with anonymised item level raw marks, together with the total uniform mark, gender, and type of school attended (grammar or non-grammar) for each candidate who took a GCSE mathematics assessment unit in the summer 2016 examination series. Recall that grammar schools provide an academically-oriented curriculum, whereas non-grammar schools provide a practically/vocationally-oriented curriculum. Unfortunately, additional sociodemographic information pertaining to candidates was not available in the dataset supplied by NICCEA. The research entailed analysis of the results obtained by candidates taking the most popular combination of assessment units at foundation tier for whom certification of the qualification was also requested in summer 2016 (units T2 and T5), and likewise at higher tier (units T4 and T6). Further details pertaining to unit content are available from NICCEA ( 2017 ).
Initially, each question, at both foundation and higher tiers, was independently classified into one of the four content domains (number, algebra, geometry and measures, or statistics and probability) by both authors. Table 1 presents one sample question for each content domain at both foundation and higher tiers. Initial coding of questions resulted in 80% agreement between the authors at foundation tier, and 87.5% agreement at higher tier, regarding the distribution of the 200 raw marks available at each tier across the four content domains. Differences were resolved through discussion and agreement. Based on the supplied item level raw marks, the raw percentage scores obtained by candidates in each of the four content domains were then calculated.
The research was conducted in line with the research governance regulations of Queen’s University Belfast, and the current study was approved by the research ethics committee of the university.
Participants and Sampling
The participants consisted of those NICCEA GCSE mathematics candidates for whom certification was requested in summer 2016, but who also took both assessment units that contributed to the certification (at foundation or higher tier, as appropriate) in the summer 2016 examination series. This was to allow for direct comparability of student achievement in each of the four mathematics content domains since the candidates concerned would have been tasked with answering exactly the same questions. Although certificates were issued for 16,351 candidates in summer 2016, the requirement for candidates to have taken both contributory assessment units in that series meant the sample consisted of 1,118 candidates from 113 schools at foundation tier (51.8% boys, 48.2% girls), and 2,762 candidates from 91 schools at higher tier (50.5% boys, 49.5% girls).
The variables included in the analysis are detailed below. The same variables featured in the analysis of both foundation and higher tier results.
Standardised total uniform score ( \({\boldsymbol{z}}_{\boldsymbol{t}\boldsymbol{o}\boldsymbol{t}\boldsymbol{a}\boldsymbol{l}}\) ). The total uniform score used to determine GCSE grade at certification, standardised ( M = 0, SD = 1) for the sampled participants at either foundation or higher tier, as appropriate.
Gender. Girls [Women] (0) or Boys [Men] (1).
School type ( SchType ). Non-grammar (0) or Grammar (1).
Standardised number score ( \({\boldsymbol{z}}_{\boldsymbol{n}\boldsymbol{u}\boldsymbol{m}}\) ). Score in the number content domain, standardised ( M = 0, SD = 1) for the sampled participants at either foundation or higher tier, as appropriate.
Standardised algebra score ( \({\boldsymbol{z}}_{\boldsymbol{a}\boldsymbol{l}\boldsymbol{g}}\) ). Score in the algebra content domain, standardised ( M = 0, SD = 1) for the sampled participants at either foundation or higher tier, as appropriate.
Standardised geometry and measures score ( \({\boldsymbol{z}}_{\boldsymbol{g}\boldsymbol{m}}\) ). Score in the geometry and measures content domain, standardised ( M = 0, SD = 1) for the sampled participants at either foundation or higher tier, as appropriate.
Standardised statistics and probability score ( \({\boldsymbol{z}}_{\boldsymbol{s}\boldsymbol{p}}\) ). Score in the statistics and probability content domain, standardised ( M = 0, SD = 1) for the sampled participants at either foundation or higher tier, as appropriate.
Statistical Analysis
Descriptive statistics were used to provide an overview of the foundation and higher tier samples, including the means and standard deviations of boys’ and girls’ standardised total uniform scores, and standardised content domain-specific scores, for all school types, and also for grammar and non-grammar schools. Since the samples were clustered within schools, scores for students within the same school were likely to have more in common than scores of students from other schools (Cohen et al., 2011 ). Therefore, two-level multilevel regression models, with student at level one and school at level two, were used to address the three research questions. Multilevel modelling takes cognisance of the clustering of students within schools, and facilitates estimation of the variance in the dependent variable that is due to differences both within and between different schools (Field, 2013 ; Robson & Pevalin, 2016 ). Such an approach ensured that the standard errors of the regression coefficients were not underestimated (Woltman et al., 2012 ).
The first two research questions were addressed by estimating, for each tier (foundation and higher), the following two-level random intercept models:
where \({z}_{ij}\) denotes the standardised score ( \({z}_{total}\) for research question one, and \({z}_{num}\) , \({z}_{alg}\) , \({z}_{gm}\) or \({z}_{sp}\) for research question two) for student \(i\) within school \(j\) ; \({\beta }_{0}\) is the overall mean of the standardised score across all schools; \({u}_{0j}\) is the random error at level two; \({\varepsilon }_{ij}\) is the level one residual; and \({\beta }_{1}\) is the \({Gender}_{ij}\) slope coefficient. A significant gender differential in mathematical achievement is indicated by a non-zero (significant) \({\beta }_{1}\) coefficient. Since the outcome scores are standardised, Lorah ( 2018 ) suggests that the \({\beta }_{1}\) coefficients provide an appropriate measure of the effect size after controlling for nesting within schools. It has been proposed by some researchers that Cohen’s d , which measures the standardised mean difference between the two groups defined by a dichotomous variable (such as \(Gender\) ) is an appropriate measure of effect size for a dichotomous covariate in a multilevel regression model (Snijders & Bosker, 2012 ). However, Cohen’s d simply quantifies the relationship between the dependent variable and the dichotomous covariate without controlling for clustering effects at level two of the model. Cohen’s d is thus an inappropriate measure of effect size for data with a hierarchical structure, such as those in the current study. Instead, the \({\beta }_{1}\) coefficient in the model indicates the expected number of standard deviation increases in mathematical achievement associated with being male, but controlling for school effects, and therefore represents a more robust measure of effect size.
The third research question was addressed by estimating, for each tier (foundation and higher), the following two-level random intercept models:
where \({z}_{ij}\) denotes the standardised score ( \({z}_{total}\) , \({z}_{num}\) , \({z}_{alg}\) , \({z}_{gm}\) or \({z}_{sp}\) as appropriate) for student \(i\) within school \(j\) ; \({\beta }_{0}\) is the overall mean of the standardised score across all schools; \({u}_{0j}\) is the random error at level two; \({\varepsilon }_{ij}\) is the level one residual; \({\beta }_{1}\) is the \({Gender}_{ij}\) slope coefficient; \({\beta }_{2}\) is the \({SchType}_{j}\) slope coefficient; and \({\beta }_{3}\) is the slope coefficient for the interaction of \(Gender\) and \(SchType\) .
All statistical calculations were performed using Stata version 14.
Descriptive Statistics
Tables 2 and 3 summarise, for foundation and higher tiers respectively, means and standard deviations for girls’ and boys’ overall mathematical achievement and achievement in each of the four content domains (number, algebra, geometry and measures, statistics and probability). However, because of the hierarchical structure of the dataset, the magnitudes of the gender differentials in achievement are more accurately assessed in the subsequent sections, which present the results of multilevel regression analyses that control for school effects.
Multilevel Assessment of Gender Effects in Overall and Domain-Specific Mathematical Achievement
A two-level multilevel analysis (with student at level one and school at level two) was performed to assess gender differentials in overall and content domain-specific achievement at both foundation and higher tiers, while controlling for clustering effects within schools. Initially, for each tier, a null model with no covariates, represented by Eq. ( 1 ), was estimated for each of the five achievement-related variables. The null model permitted investigation of whether there was evidence to justify the existence of random intercepts for the grouping variable at level two (school), and therefore whether multilevel modelling was necessary. Gender was then introduced as a level one explanatory variable to estimate the multilevel model represented by Eq. ( 2 ) for each of the five achievement variables. The estimated regression coefficients (with standard errors in parentheses) are presented in Table 4 for foundation tier and Table 5 for higher tier. Level one and level two variances, together with the intraclass correlation coefficient (ICC) and log likelihood, are also included for each model in Tables 4 and 5 .
The null models for overall mathematical achievement, at both foundation and higher tiers, indicated that substantial proportions of the variation in achievement were explained by differences between schools, 25.1% at foundation tier and 28.0% at higher tier, as revealed by the ICCs of .251 and .280 respectively. This confirmed that multilevel modelling was appropriate when assessing the influence of gender on overall achievement at both tiers. However, when gender was added as a level one explanatory variable to both the foundation and higher tier models, there was no appreciable change in either the ICCs or the log likelihood functions, which suggested that gender had minimal influence in explaining differences in overall achievement. This is further reinforced by the fact the gender coefficients of .027 and .057 for foundation and higher tiers respectively were not significant, even at the 5% level. The small, positive magnitudes of these effect sizes indicate that, for both tiers, boys scored very marginally higher than girls on average, but the differentials are negligible for practical purposes. On average, boys scored .027 of a standard deviation higher than girls at foundation tier, and .057 of a standard deviation at higher tier.
At foundation tier, only algebra and geometry and measures, had significant gender coefficients (at the 1% level) of -.173 and .170 respectively. These values showed that, at foundation tier, boys on average scored .173 of a standard deviation lower than girls in algebra, but .170 of a standard deviation higher in geometry and measures. Although these differentials are greater in magnitude than those for overall achievement, both are relatively small. At higher tier, only number and geometry and measures revealed significant gender differentials, with small effect sizes of .098 and .108 respectively. These values indicate that, at higher tier, boys on average scored .098 of a standard deviation higher than girls in the number domain and .108 of a standard deviation higher in geometry and measures.
Effects of School Type and Gender on Overall and Domain-Specific Mathematical Achievement
To investigate the effects of school type and gender on overall and domain-specific mathematical achievement at each tier (foundation and higher), two-level random intercept models (again with student at level one and school at level two) were fitted with the standardised score for mathematical achievement as the dependent variable. Initially, gender and school type were used as covariates, but the interaction term between gender and school type was then introduced. The results of these multilevel analyses are presented in Tables 6 and 7 .
At both foundation and higher tiers, school type is a highly significant predictor (at the .1% level) of overall and domain-specific mathematical achievement, with grammar school students performing significantly better than their non-grammar school counterparts. This corroborates Jerrim and Shure’s ( 2016 ) evidence of achievement differentials between grammar and non-grammar school students in Northern Ireland. It is also noteworthy that the majority of students at foundation tier attended non-grammar schools, while the majority at higher tier attended grammar schools.
At foundation tier, the ICCs and log likelihoods in Table 6 indicate that the addition of school type as a level two explanatory variable in the model for overall mathematical achievement further reduced the variance between schools and also improved upon the fit of the model, relative to the null plus gender model presented in Table 4 . However, the gender coefficient was still not significant, nor was the coefficient of the gender x school type interaction term, thus implying that school type did not have a bearing on the gender differential in overall achievement at foundation tier. Gender differentials for the four content domains at foundation tier were also in line with those presented in Table 4 . It is notable that the coefficients of the gender x school type interaction terms were not significant, thus confirming that school type did not significantly influence gender differentials within the four content domains. At higher tier, the addition of school type as a level two explanatory variable in the models yielded similar conclusions about the statistical significance of the gender coefficients to those obtained for the null plus gender models presented in Table 5 . As for foundation tier, none of the coefficients for the gender x school type interaction terms were significant, thus suggesting that school type did not have an impact on gender differentials at higher tier.
Discussion and Conclusions
Summary of findings.
What is the magnitude of the gender difference for students’ overall achievement in a high-stakes Northern Irish mathematics examination? Findings from the current study provide evidence in support of the gender similarities hypothesis (Hyde, 2005 , 2014 , 2016 ). Specifically, the results of the multilevel analysis confirmed that the differences between boys’ and girls’ overall mathematical achievement in the high-stakes examination were close-to-zero for both foundation and higher tiers, with effect sizes of .027 and .057 respectively. This is consistent with other research focusing on gender differentials in overall mathematical achievement within a Northern Irish context, albeit research that was not undertaken using the results obtained in high-stakes examinations (Jerrim & Shure, 2016 ; Mullis et al., 2016 ; Wheater et al., 2013 ).
What are the magnitudes of the gender differences for students’ achievement in the different content domains of a high-stakes Northern Irish mathematics examination? The multilevel analysis indicated that gender differentials in the foundation tier examination were significant at the 1% level for both algebra and geometry and measures, with relatively small effect sizes of -.173 and .170 respectively. These demonstrated a small female advantage in algebra and a similar small male advantage in relation to geometry and measures. Interestingly, the multilevel analysis also highlighted a very small differential in favour of girls for algebra at higher tier, although it did not attain statistical significance, even at the 5% level. At higher tier, only the number and geometry and measures content domains exhibited significant gender differentials in favour of boys, with small effect sizes of .098 and .108 respectively. However, it is important to note that all of the effect sizes are small and offer evidence to support the gender similarities hypothesis in respect of domain-specific mathematical achievement (Hyde, 2005 , 2014 , 2016 ).
The existence of significant, but small, gender effects in relation to content domain-specific achievement contradicts some existing research that was conducted using international large-scale assessments of mathematical achievement in Northern Ireland (e.g., Wheater et al., 2013 ). However, the findings resonate with the conclusions reported by some other scholars internationally. For example, Leahey and Guo ( 2001 ) and Liu and Wilson ( 2009 ) both concluded that boys demonstrated significantly higher achievement than girls in geometry. Furthermore, the female advantage in algebraic achievement identified in the current study aligns with the findings of Louis and Mistele ( 2012 ), who reported that, in their US-focused research, algebra was the only mathematical content domain where girls outperformed boys. Therefore, there are slight disparities between the conclusions drawn from recent research into Northern Irish gender differentials in content domain-specific mathematical achievement that was conducted using comparative international assessment data (e.g., from PISA) and the findings of the current study. The disparity is particularly apparent in relation to the gender differential in favour of males for achievement in geometry and measures that featured at both tiers in the current study, but it is noteworthy that all of the effect sizes are small.
How do the magnitudes of gender differences in mathematical achievement vary by school type for a high-stakes Northern Irish mathematics examination? Results of the multilevel analysis highlight that, while grammar school students consistently outperformed their non-grammar school counterparts at both tiers of entry (with large effect sizes), school type did not have a bearing on gender differentials for either overall or domain-specific mathematical achievement. However, it is important to note that the majority of students at foundation tier attended non-grammar schools, while the majority at higher tier attended grammar schools, thus suggesting a potential equity issue pertaining to tier of entry.
Limitations and Future Research Directions
Although the current study involved multilevel analysis of high-stakes mathematics examination results of 3,880 students (1,118 of whom took foundation tier, and 2,762 of whom took higher tier examinations), this represented just 23.7% of the 16,351 candidates who received a NICCEA GCSE mathematics certificate in summer 2016. The sample was chosen to include only those candidates who took the most popular combination of assessment units, at both foundation and higher tiers, for whom certification of the qualification was requested in summer 2016, and where both contributory assessment units were also taken during that examination series. Although this ensured that identical questions were attempted by all candidates within a particular tier, and thus permitted domain-specific achievement to be assessed more reliably, it does restrict the generalisability of the findings.
The current study also attempted to offer some insights into the similarities and differences in the conclusions that can be drawn from investigations into gender differentials in achievement using different data sources, namely the results of high-stakes examinations versus performance in low-stakes assessments. The credence that can be accorded to these insights is restricted by the use of different samples of students, although some reassurance can be taken from the relatively large sample used in the current study. Clearly, it would be preferable to undertake a study involving the analysis of high-stakes examination results for a sample of students who also participated in low stakes assessments such as PISA or TIMSS, so that more robust comparisons between the different approaches to assessing gender differentials could be established.
The current study focused on overall and content domain-specific achievement. However, there is scope to classify the various items on the assessment units into cognitive domains (e.g., knowledge, application, reasoning, problem-solving) rather than content domains, to facilitate an analysis of gender differentials in cognitive domain-specific achievement. In addition, a more fine-grained analysis of domain-specific achievement, coupled with scrutiny of the actual assessment items, may offer useful insights into assessment practices that inadvertently lead to gender differentials in achievement. For example, it is conceivable that some assessment practices could be experienced differently by boys [men] and girls [women], and that these different experiences may actually precipitate gender differentials in achievement.
Finally, the lack of student sociodemographic information in the dataset supplied by NICCEA significantly limited the analysis that could be performed. Nevertheless, the analysis that was possible has contributed new knowledge to the field of gender differences in mathematical achievement.
Practice Implications
On the basis of the findings of the current research, coupled with the overview of previous studies outlined in the literature review, some recommendations can be proffered for mathematics education policymakers in Northern Ireland, which are also likely to have considerable import for policymakers in other international contexts. The results of the multilevel analysis of high-stakes mathematics examination scores suggest that there is gender equity in overall mathematical achievement in Northern Ireland. Given that this resonates with the findings of other recent studies, it is conceivable that the Northern Ireland education system, together with its mathematics curriculum and assessment arrangements are conducive to promoting gender equity in overall mathematical achievement. Therefore, consideration of Northern Ireland as a case study may serve as a useful starting point for other countries with an interest in promoting more gender-equitable outcomes in mathematical achievement.
Whilst there is gender equity in overall achievement, small but significant differences are apparent for achievement in some of the content domains. In particular, the gender equity in overall achievement masks a consistent, small male advantage in geometry and measures. This contradicts other recent findings on gender differentials for content domain-specific achievement in Northern Ireland (e.g., Wheater et al., 2013 ), but corroborates the conclusions reported by scholars working in other international contexts (e.g., Leahey & Guo, 2001 ; Liu & Wilson, 2009 ). This suggests that it is appropriate, both in Northern Ireland, and in other countries, to investigate interventions aimed at improving girls’ achievement in geometry. For example, Meggiolaro ( 2018 ) demonstrated that the use of some ICT applications can lead to achievement gains in geometry for girls, and it is suggested that the potential of such applications should be explored as a vehicle for promoting higher levels of gender equity in mathematical learning outcomes, both in Northern Ireland and elsewhere. To this end, the dynamic geometry capabilities of software such as GeoGebra are likely to have beneficial roles to play in enhancing pedagogy.
The current study offers some useful insights into the appropriateness of using data from low-stakes assessments, such as PISA and TIMSS, rather than high-stakes assessments (with real consequences for students), to assess gender differentials in mathematical achievement. Both approaches led to similar conclusions about gender differentials in overall mathematical achievement in Northern Ireland, but a more subtle picture emerged in relation to content domain-specific achievement. Small, but significant, gender differentials in content domain achievement were apparent in the research conducted using high-stakes examination results, but it is important to note that the observed effect sizes are small. This indicates that studies using PISA data do offer useful insights into gender equity issues in mathematical achievement, despite concerns some researchers have raised about the utility of low-stakes tests for accurately assessing gender differentials in achievement. It has been suggested that the low-stakes nature of tests such as those used in PISA and TIMSS may potentially lead to differential gender effects in test-taking motivation, which could in turn precipitate gender differentials in achievement (Barry et al., 2010 ; DeMars et al., 2013 ; Eklöf, 2010 ; Guez et al., 2020 ). However, evidence from the current study, which entailed a multilevel analysis of high-stakes mathematics examination results from a jurisdiction where no significant gender differentials in mathematical achievement were apparent in PISA, suggests that these concerns are unfounded.
Conclusions
Gender stereotyping is prevalent in mathematics, which is often viewed as an academic discipline that is more appropriate for men than women due to inherent differences between the genders (Franceschini et al., 2014 ). The findings of the current research, using results from high-stakes summative assessments in mathematics, have demonstrated that there are negligible or small gender differentials in mathematical achievement. These findings support the gender similarities hypothesis, which asserts that males and females are similar on most, but not all, psychological variables (Hyde, 2005 , 2014 , 2016 ). No significant differences between boys and girls were found in relation to overall mathematical achievement, but small differences in favour of males were apparent in relation to achievement in the geometry and measures content domain. However, the magnitudes of the associated effect sizes mean that the differences are likely to be of limited practical significance. Therefore, it is imperative that damaging gender stereotyping in mathematics is dispensed with as a matter of urgency, and that improvements are made to mathematics pedagogy to allow girls to realise their mathematical potential. To this end, the current article has offered some suggestions regarding potential improvements to girls’ learning experiences in geometry.
The current study also provided some insights into the viability of using data from low-stakes assessments such as PISA or TIMSS to accurately investigate gender differentials in achievement. Similar conclusions were drawn from the analysis of both these low-stakes assessments and high-stakes public examination results, thus indicating that research based on low-stakes assessments (such as PISA or TIMSS) does offer useful insights into gender differentials in achievement, thus contradicting the findings of Guez et al. ( 2020 ). Finally, findings from this study augment the evidence base pertaining to gender effects in mathematical achievement since they confirmed that school type, selective versus non-selective, did not have an effect on gender differences, an area where there was scant evidence in the existing literature.
The current study therefore offers useful insights for researchers and policymakers with an interest in enhancing gender equity in mathematical achievement, which is a highly desirable characteristic of any high-performing education system.
Data Availability
Data is available from the first author upon request.
Change history
16 august 2021.
A Correction to this paper has been published: https://doi.org/10.1007/s11199-021-01241-6
Ayalon, H., & Livneh, I. (2013). Educational standardization and gender differences in mathematics achievement: A comparative study. Social Science Research, 42 (2), 432–445. https://doi.org/10.1016/j.ssresearch.2012.10.001
Article PubMed Google Scholar
Barry, C. L., Horst, S. J., Finney, S. J., Brown, A. R., & Kopp, J. P. (2010). Do examinees have similar test-taking effort? A high-stakes question for low-stakes testing. International Journal of Testing, 10 (4), 342–363. https://doi.org/10.1080/15305058.2010.508569
Article Google Scholar
Benbow, C. P., & Stanley, J. (1980). Sex differences in mathematical ability: Facto or artefact? Science, 210 (4475), 1262–1264. https://doi.org/10.1126/science.7434028
Brunner, M., Gogol, K. M., Sonnleitner, P., Keller, U., Krauss, S., & Preckel, F. (2013). Gender differences in the mean level, variability, and profile shape of student achievement: Results from 41 countries. Intelligence, 41 (5), 378–395. https://doi.org/10.1016/j.intell.2013.05.009
Cantley, I. (2015). How secure is a Newtonian paradigm for psychological and educational measurement? Theory & Psychology, 25 (1), 117–138. https://doi.org/10.1177/0959354314561141
Cantley, I. (2017). A quantum measurement paradigm for educational predicates: Implications for validity in educational measurement. Educational Philosophy and Theory, 49 (4), 405–421. https://doi.org/10.1080/00131857.2015.1048668
Cantley, I. (2019). PISA and policy-borrowing: A philosophical perspective on their interplay in mathematics education. Educational Philosophy and Theory, 51 (12), 1200–1215. https://doi.org/10.1080/00131857.2018.1523005
Chen, H.-Y., Chen, M.-F., Lee, Y.-S., Chen, H.-P., & Keith, T. Z. (2013). Gender reality regarding mathematic outcomes of students aged 9 to 15 years in Taiwan. Learning and Individual Differences, 26 , 55–63. https://doi.org/10.1016/j.lindif.2013.04.009
Cohen, L., Manion, L., & Morrison, K. (2011). Research methods in education (7th ed.). Routledge.
Contini, D., Di Tommaso, M. L., & Mendolia, S. (2017). The gender gap in mathematics achievement: Evidence from Italian data. Economics of Education Review, 58 , 32–42. https://doi.org/10.1016/j.econedurev.2017.03.001
Cox, P. J., Leder, G. C., & Forgasz, H. J. (2004). Victorian Certificate of Education: Mathematics, science and gender. Australian Journal of Education, 48 (1), 27–46. https://doi.org/10.1177/000494410404800103
DeMars, C. E., Bashko, B. M., & Socha, A. B. (2013). The role of gender in test-taking motivation under low-stakes conditions. Research & Practice in Assessment , 8 (1), 69–82. https://files.eric.ed.gov/fulltext/EJ1062839.pdf .
Demir, I., Kiliç, S., & Ünal, H. (2010). Effects of students’ and schools’ characteristics on mathematics achievement: Findings from PISA 2006. Procedia Social and Behavioral Sciences, 2 (2), 3099–3103. https://doi.org/10.1016/j.sbspro.2010.03.472
Department of Education. (2016). Statistical bulletin 3/2016: Annual enrolments at schools and in funded pre-school education in Northern Ireland, 2015/16 . Retrieved from https://www.education-ni.gov.uk/sites/default/files/publications/education/Statistical%20Bulletin%201516%20-%20March%20%2820.07.16%20update%29.PDF .
Dickerson, A., McIntosh, S., & Valente, C. (2015). Do the maths: An analysis of the gender gap in mathematics in Africa. Economics of Education Review, 46 , 1–22. https://doi.org/10.1016/j.econedurev.2015.02.005
Dohn, N. B. (2007). Knowledge and skills for PISA – assessing the assessment. Journal of Philosophy of Education, 41 (1), 1–16. https://doi.org/10.1111/j.1467-9752.2007.00542.x
Dossey, J. A., & Wu, M. L. (2013). Implications of international studies for national and local policy in mathematics education. In M. A. (Ken) Clements, A. Bishop, C. Keitel-Kreidt, J. Kilpatrick, & F. K. S. Leung (Eds.), Third international handbook of mathematics education (pp. 1009–1042). Springer.
Eklöf, H. (2010). Skill and will: Test-taking motivation and assessment quality. Assessment in Education: Principles, Policy & Practice, 17 (4), 345–356. https://doi.org/10.1080/0969594x.2010.516569
Else-Quest, N. M., Hyde, J. S., & Linn, M. C. (2010). Cross-national patterns of gender differences in mathematics: A meta-analysis. Psychological Bulletin, 136 (1), 103–127. https://doi.org/10.1037/a0018053
Fennema, E. (1974). Mathematics learning and the sexes: A review. Journal for Research in Mathematics Education, 5 (3), 126–139. https://doi.org/10.2307/748949
Field, A. (2013). Discovering statistics using IBM SPSS statistics (4th ed.). SAGE Publishing.
Franceschini, G., Galli, S., Chiesi, F., & Primi, C. (2014). Implicit gender-math stereotype and women’s susceptibility to stereotype threat and stereotype lift. Learning and Individual Differences, 32 , 273–277. https://doi.org/10.1016/j.lindif.2014.03.020
Gardner, J. (2016). Education in Northern Ireland since the Good Friday Agreement: Kabuki theatre meets danse macabre. Oxford Review of Education, 42 (3), 346–361. https://doi.org/10.1080/03054985.2016.1184869
Goldstein, H. (2004). International comparisons of student attainment: Some issues arising from the PISA study. Assessment in Education: Principles, Policy & Practice, 11 (3), 319–330. https://doi.org/10.1080/0969594042000304618
Guez, A., Peyre, H., & Ramus, F. (2020). Sex differences in academic achievement are modulated by evaluation type. Learning and Individual Differences, 83–84 , 101935. https://doi.org/10.1016/j.lindif.2020.101935
Hanna, G., Kündiger, E., & Larouche, C. (1990). Mathematical achievement of grade 12 girls in fifteen countries. In L. Burton (Ed.), Gender and mathematics: An international perspective (pp. 87–97). Cassell.
Henrion, C. (1997). Women in mathematics: The addition of difference . Indiana University Press.
Hyde, J. S. (2005). The gender similarities hypothesis. American Psychologist, 60 (6), 581–592. https://doi.org/10.1037/0003-066x.60.6.581
Hyde, J. S. (2014). Gender similarities and differences. Annual Review of Psychology, 65 (1), 373–398. https://doi.org/10.1146/annurev-psych-010213-115057
Hyde, J. S. (2016). Sex and cognition: Gender and cognitive functions. Current Opinion in Neurobiology, 38 , 53–56. https://doi.org/10.1016/j.conb.2016.02.007
Innabi, H., & Dodeen, H. (2018). Gender differences in mathematics achievement in Jordan: A differential item functioning analysis of the 2015 TIMSS. School Science and Mathematics, 118 (3–4), 127–137. https://doi.org/10.1111/ssm.12269
Jerrim, J., & Shure, N. (2016). Achievement of 15-year-olds in Northern Ireland: PISA 2015 national report . UCL Institute of Education. https://www.education-ni.gov.uk/sites/default/files/publications/education/ACHIEVEMENT%20OF%2015%20YEAR-OLDS%20IN%20NORTHERN%20IRELAND%20PISA%202015%20NATIONAL%20REPORT..PDF .
Leahey, E., & Guo, G. (2001). Gender differences in mathematical trajectories. Social Forces, 80 (2), 713–732. https://doi.org/10.1353/sof.2001.0102
Leder, G. C., & Forgasz, H. J. (2018). Measuring who counts: Gender and mathematics assessment. ZDM, 50 (4), 687–697. https://doi.org/10.1007/s11858-018-0939-z
Li, M., Zhang, Y., Liu, H., & Hao, Y. (2018). Gender differences in mathematics achievement in Beijing: A meta-analysis. British Journal of Educational Psychology, 88 (4), 566–583. https://doi.org/10.1111/bjep.12203
Lindberg, S. M., Hyde, J. S., Peterson, J. L., & Linn, M. C. (2010). New trends in gender and mathematics performance: A meta-analysis. Psychological Bulletin, 136 (6), 1123–1135. https://doi.org/10.1037/a0021276
Article PubMed PubMed Central Google Scholar
Liu, O. L., & Wilson, M. (2009). Gender differences in large-scale math assessments: PISA trend 2000 and 2003. Applied Measurement in Education, 22 (2), 164–184. https://doi.org/10.1080/08957340902754635
Lorah, J. (2018). Effect size measures for multilevel models: Definition, interpretation, and TIMSS example. Large-Scale Assessments in Education, 6 (1), 1–11. https://doi.org/10.1186/s40536-018-0061-2
Louis, R. A., & Mistele, J. M. (2012). The differences in scores and self-efficacy by student gender in mathematics and science. International Journal of Science and Mathematics Education, 10 (5), 1163–1190. https://doi.org/10.1007/s10763-011-9325-9
Matteucci, M., & Mignani, S. (2011). Gender differences in performance in mathematics at the end of lower secondary school in Italy. Learning and Individual Differences, 21 (5), 543–548. https://doi.org/10.1016/j.lindif.2011.03.001
McGraw, R., Lubienski, S. T., & Strutchens, M. E. (2006). A closer look at gender in NAEP mathematics achievement and affect data: Intersections with achievement, race/ethnicity, and socioeconomic status. Journal for Research in Mathematics Education , 37 (2), 129–150. https://www.jstor.org/stable/30034845 .
Meggiolaro, S. (2018). Information and communication technologies use, gender and mathematics achievement: Evidence from Italy. Social Psychology of Education: An International Journal, 21 (2), 497–516. https://doi.org/10.1007/s11218-017-9425-7
Miner, M. A. (2019). Unpacking the monolith: Intersecting gender and citizenship status in STEM graduate education. International Journal of Sociology and Social Policy, 39 (9–10), 661–679. https://doi.org/10.1108/ijssp-05-2019-0101
Mullis, I. V. S., Martin, M. O., Foy, P., & Hooper, M. (2016). TIMSS 2015 international results in mathematics . Boston College, Trends in International Mathematics and Science Study (TIMSS) & Progress in International Reading Literacy Study (PIRLS) International Study Center. http://timssandpirls.bc.edu/timss2015/international-results/ .
Nix, S., & Perez-Felkner, L. (2019). Difficulty orientations, gender, and race/ethnicity: An intersectional analysis of pathways to STEM degrees. Social Sciences, 8 (2), 43. https://doi.org/10.3390/socsci8020043
Nollenberger, N., Rodríguez-Planas, N., & Sevilla, A. (2016). The math gender gap: The role of culture. American Economic Review, 106 (5), 257–261. https://doi.org/10.1257/aer.p20161121
Northern Ireland Council for the Curriculum, Examinations and Assessment (2017). GCSE Mathematics (2017) . https://ccea.org.uk/key-stage-4/gcse/subjects/gcse-mathematics-2017
Organisation for Economic Co-operation and Development (2014). PISA 2012 results: What students know and can do – Student performance in mathematics, reading and science (volume I, revised edition, February 2014) . OECD. https://www.oecd.org/pisa/keyfindings/pisa-2012-results-volume-I.pdf .
Organisation for Economic Co-operation and Development (2015). The ABC of gender equality in education: Aptitude, behaviour, confidence . OECD. https://www.oecd.org/pisa/keyfindings/pisa-2012-results-gender-eng.pdf .
Organisation for Economic Co-operation and Development (2016). PISA 2015 results (volume I): Excellence and equity in education . OECD. https://www.oecd-ilibrary.org/docserver/9789264266490-en.pdf?expires=1625054287&id=id&accname=guest&checksum=90800465380342A8017AA703D2C4803F .
Pargulski, J. R., & Reynolds, M. R. (2017). Sex differences in achievement: Distributions matter. Personality and Individual Differences, 104 , 272–278. https://doi.org/10.1016/j.paid.2016.08.016
Perez-Felkner, L., McDonald, S.-K., Schneider, B., & Grogan, E. (2012). Female and male adolescents’ subjective orientations to mathematics and the influence of those orientations on postsecondary majors. Developmental Psychology, 48 (6), 1658–1673. https://doi.org/10.1037/a0027020
Robson, K., & Pevalin, D. (2016). Multilevel modelling in plain language . SAGE Publishing.
Rodríguez-Planas, N., & Nollenberger, N. (2018). Let the girls learn! It is not only about math … it’s about gender social norms. Economics of Education Review, 62 , 230–253. https://doi.org/10.1016/j.econedurev.2017.11.006
Smith, S. E., & Walker, W. J. (1988). Sex differences on New York state Regents examinations: Support for the differential course-taking hypothesis. Journal for Research in Mathematics Education, 19 (1), 81–85. https://doi.org/10.5951/jresematheduc.19.1.0081
Snijders, T. A. B., & Bosker, R. J. (2012). Multilevel analysis: An introduction to basic and advanced multilevel modeling (2 nd ed.). SAGE Publishing.
Taylor, C. S., & Lee, Y. (2012). Gender DIF in reading and mathematics tests with mixed item formats. Applied Measurement in Education, 25 (3), 246–280. https://doi.org/10.1080/08957347.2012.687650
Valero, P. (2017). Mathematics for all, economic growth, and the making of the citizen-worker. In T. S. Popkewitz, J. Diaz, & C. Kirchgasler (Eds.), Political sociology and transnational educational studies: The styles of reason governing teaching, curriculum and teacher education (pp. 117–132). Routledge.
Watt, H. M. G., Shapka, J. D., Morris, Z. A., Durik, A. M., Keating, D. P., & Eccles, J. S. (2012). Gendered motivational processes affecting high school mathematics participation, educational aspirations, and career plans: A comparison of samples from Australia, Canada, and the United States. Developmental Psychology, 48 (6), 1594–1611. https://doi.org/10.1037/a0027838
Wheater, R., Ager, R., Burge, B., & Sizmur, J. (2013). Student achievement in Northern Ireland: Results in mathematics, science, and reading among 15-year-olds from the OECD PISA 2012 study . National Foundation for Educational Research. https://www.nfer.ac.uk/publications/pquk03/pquk03.pdf .
Woltman, H., Feldstain, A., Mackay, J. C., & Rocchi, M. (2012). An introduction to hierarchical linear modeling. Tutorials in Quantitative Methods for Psychology , 8 (1), 52–69. https://doi.org/10.20982/tqmp.08.1.p052 .
Zawistowska, A. (2017). Gender differences in high-stakes maths testing: Findings from Poland. Studies in Logic, Grammar and Rhetoric, 50 (1), 205–226. https://doi.org/10.1515/slgr-2017-0025
Download references
No external funding was received for this project.
Author information
Authors and affiliations.
School of Social Sciences, Education and Social Work, Queen’s University Belfast, 69-71 University Street, Belfast, BT7 1HL, UK
Ian Cantley & James McAllister
You can also search for this author in PubMed Google Scholar
Corresponding author
Correspondence to Ian Cantley .
Ethics declarations
Ethical approval.
The study was approved by the research ethics committee of the university.
Research Involving Human and Animal Participants
The research involved the analysis of anonymised data and was conducted in line with the research governance regulations of Queen’s University Belfast.
Conflict of Interest
There are no conflicts of interest.
Additional information
Publisher's note.
Springer Nature remains neutral with regard to jurisdictional claims in published maps and institutional affiliations.
The original version of this article was revised: Several words that are needed for adequate interpretation of the text in the second sentence of the third paragraph under the Limitations and Future Directions section is missing.
Rights and permissions
Open Access This article is licensed under a Creative Commons Attribution 4.0 International License, which permits use, sharing, adaptation, distribution and reproduction in any medium or format, as long as you give appropriate credit to the original author(s) and the source, provide a link to the Creative Commons licence, and indicate if changes were made. The images or other third party material in this article are included in the article's Creative Commons licence, unless indicated otherwise in a credit line to the material. If material is not included in the article's Creative Commons licence and your intended use is not permitted by statutory regulation or exceeds the permitted use, you will need to obtain permission directly from the copyright holder. To view a copy of this licence, visit http://creativecommons.org/licenses/by/4.0/ .
Reprints and permissions
About this article
Cantley, I., McAllister, J. The Gender Similarities Hypothesis: Insights From A Multilevel Analysis of High-Stakes Examination Results in Mathematics. Sex Roles 85 , 481–496 (2021). https://doi.org/10.1007/s11199-021-01234-5
Download citation
Accepted : 30 June 2021
Published : 12 July 2021
Issue Date : October 2021
DOI : https://doi.org/10.1007/s11199-021-01234-5
Share this article
Anyone you share the following link with will be able to read this content:
Sorry, a shareable link is not currently available for this article.
Provided by the Springer Nature SharedIt content-sharing initiative
- Gender equity
- Mathematics
- High-stakes assessments
- Domain-specific achievement
- Find a journal
- Publish with us
- Track your research

An official website of the United States government
The .gov means it’s official. Federal government websites often end in .gov or .mil. Before sharing sensitive information, make sure you’re on a federal government site.
The site is secure. The https:// ensures that you are connecting to the official website and that any information you provide is encrypted and transmitted securely.
- Publications
- Account settings
- My Bibliography
- Collections
- Citation manager
Save citation to file
Email citation, add to collections.
- Create a new collection
- Add to an existing collection
Add to My Bibliography
Your saved search, create a file for external citation management software, your rss feed.
- Search in PubMed
- Search in NLM Catalog
- Add to Search
Gender similarities and differences
Affiliation.
- 1 Department of Psychology, University of Wisconsin, Madison, Wisconsin 53706; email: [email protected].
- PMID: 23808917
- DOI: 10.1146/annurev-psych-010213-115057
Whether men and women are fundamentally different or similar has been debated for more than a century. This review summarizes major theories designed to explain gender differences: evolutionary theories, cognitive social learning theory, sociocultural theory, and expectancy-value theory. The gender similarities hypothesis raises the possibility of theorizing gender similarities. Statistical methods for the analysis of gender differences and similarities are reviewed, including effect sizes, meta-analysis, taxometric analysis, and equivalence testing. Then, relying mainly on evidence from meta-analyses, gender differences are reviewed in cognitive performance (e.g., math performance), personality and social behaviors (e.g., temperament, emotions, aggression, and leadership), and psychological well-being. The evidence on gender differences in variance is summarized. The final sections explore applications of intersectionality and directions for future research.
PubMed Disclaimer
Similar articles
- Evaluating gender similarities and differences using metasynthesis. Zell E, Krizan Z, Teeter SR. Zell E, et al. Am Psychol. 2015 Jan;70(1):10-20. doi: 10.1037/a0038208. Am Psychol. 2015. PMID: 25581005
- Sex differences in cooperation: a meta-analytic review of social dilemmas. Balliet D, Li NP, Macfarlan SJ, Van Vugt M. Balliet D, et al. Psychol Bull. 2011 Nov;137(6):881-909. doi: 10.1037/a0025354. Psychol Bull. 2011. PMID: 21910518 Review.
- Sex differences in aggression among children of low and high gender inequality backgrounds: a comparison of gender role and sexual selection theories. Nivette AE, Eisner M, Malti T, Ribeaud D. Nivette AE, et al. Aggress Behav. 2014 Sep-Oct;40(5):451-64. doi: 10.1002/ab.21530. Epub 2014 Feb 13. Aggress Behav. 2014. PMID: 24526324
- Gender similarities and differences in children's social behavior: finding personality in contextualized patterns of adaptation. Zakriski AL, Wright JC, Underwood MK. Zakriski AL, et al. J Pers Soc Psychol. 2005 May;88(5):844-55. doi: 10.1037/0022-3514.88.5.844. J Pers Soc Psychol. 2005. PMID: 15898879
- The gender similarities hypothesis. Hyde JS. Hyde JS. Am Psychol. 2005 Sep;60(6):581-592. doi: 10.1037/0003-066X.60.6.581. Am Psychol. 2005. PMID: 16173891 Review.
- Do Sex and Gender Have Separate Identities? Quintana GR, Pfaus JG. Quintana GR, et al. Arch Sex Behav. 2024 Aug 6. doi: 10.1007/s10508-024-02933-2. Online ahead of print. Arch Sex Behav. 2024. PMID: 39105983
- Disentangling the within- and between-person effects of personality on income for men and women. Kemp S, Yogeeswaran K, Stronge S, Yaghoubi M, Sibley CG. Kemp S, et al. R Soc Open Sci. 2024 May 22;11(5):231750. doi: 10.1098/rsos.231750. eCollection 2024 May. R Soc Open Sci. 2024. PMID: 39076795 Free PMC article.
- Perceptions of Skills Needed for STEM Jobs: Links to Academic Self-Concepts, Job Interests, Job Gender Stereotypes, and Spatial Ability in Young Adults. Signorella ML, Liben LS. Signorella ML, et al. J Intell. 2024 Jun 27;12(7):63. doi: 10.3390/jintelligence12070063. J Intell. 2024. PMID: 39057183 Free PMC article.
- Unveiling gender differences in psychophysiological dynamics: support for a two-dimensional autonomic space approach. Menashri Sinai Y, Ma YXJ, Abba Daleski M, Gannot S, Bartsch RP, Gordon I. Menashri Sinai Y, et al. Front Hum Neurosci. 2024 Mar 13;18:1363891. doi: 10.3389/fnhum.2024.1363891. eCollection 2024. Front Hum Neurosci. 2024. PMID: 38545517 Free PMC article.
- Are many sex/gender differences really power differences? Galinsky AD, Turek A, Agarwal G, Anicich EM, Rucker DD, Bowles HR, Liberman N, Levin C, Magee JC. Galinsky AD, et al. PNAS Nexus. 2024 Feb 27;3(2):pgae025. doi: 10.1093/pnasnexus/pgae025. eCollection 2024 Feb. PNAS Nexus. 2024. PMID: 38415218 Free PMC article.
Publication types
- Search in MeSH
LinkOut - more resources
Full text sources.
- Ingenta plc
- Ovid Technologies, Inc.
Other Literature Sources
- scite Smart Citations
- Citation Manager
NCBI Literature Resources
MeSH PMC Bookshelf Disclaimer
The PubMed wordmark and PubMed logo are registered trademarks of the U.S. Department of Health and Human Services (HHS). Unauthorized use of these marks is strictly prohibited.

Men and Women: No Big Difference
The truth about gender "differences".
Mars-Venus sex differences appear to be as mythical as the Man in the Moon. A 2005 analysis of 46 meta-analyses that were conducted during the last two decades of the 20th century underscores that men and women are basically alike in terms of personality, cognitive ability and leadership. Psychologist Janet Shibley Hyde, PhD, of the University of Wisconsin in Madison, discovered that males and females from childhood to adulthood are more alike than different on most psychological variables, resulting in what she calls a gender similarities hypothesis . Using meta-analytical techniques that revolutionized the study of gender differences starting in the 1980s, she analyzed how prior research assessed the impact of gender on many psychological traits and abilities, including cognitive abilities, verbal and nonverbal communication, aggression, leadership, self-esteem, moral reasoning and motor behaviors.
Hyde observed that across the dozens of studies, consistent with the gender similarities hypothesis, gender differences had either no or a very small effect on most of the psychological variables examined. Only a few main differences appeared: Compared with women, men could throw farther, were more physically aggressive, masturbated more, and held more positive attitudes about sex in uncommitted relationships.
Furthermore, Hyde found that gender differences seem to depend on the context in which they were measured. In studies designed to eliminate gender norms, researchers demonstrated that gender roles and social context strongly determined a person's actions. For example, after participants in one experiment were told that they would not be identified as male or female, nor did they wear any identification, none conformed to stereotypes about their sex when given the chance to be aggressive. In fact, they did the opposite of what would be expected - women were more aggressive and men were more passive.
Finally, Hyde's 2005 report looked into the developmental course of possible gender differences - how any apparent gap may open or close over time. The analysis presented evidence that gender differences fluctuate with age, growing smaller or larger at different times in the life span. This fluctuation indicates again that any differences are not stable.
Learning Gender-Difference Myths
Media depictions of men and women as fundamentally "different" appear to perpetuate misconceptions - despite the lack of evidence. The resulting "urban legends" of gender difference can affect men and women at work and at home, as parents and as partners. As an example, workplace studies show that women who go against the caring, nurturing feminine stereotype may pay dearly for it when being hired or evaluated. And when it comes to personal relationships, best-selling books and popular magazines often claim that women and men don't get along because they communicate too differently. Hyde suggests instead that men and women stop talking prematurely because they have been led to believe that they can't change supposedly "innate" sex-based traits.
Hyde has observed that children also suffer the consequences of exaggerated claims of gender difference -- for example, the widespread belief that boys are better than girls in math. However, according to her meta-analysis, boys and girls perform equally well in math until high school, at which point boys do gain a small advantage. That may not reflect biology as much as social expectations, many psychologists believe. For example, the original Teen Talk Barbie ™, before she was pulled from the market after consumer protest, said, "Math class is tough."
As a result of stereotyped thinking, mathematically talented elementary-school girls may be overlooked by parents who have lower expectations for a daughter's success in math. Hyde cites prior research showing that parents' expectations of their children's success in math relate strongly to the children's self-confidence and performance.
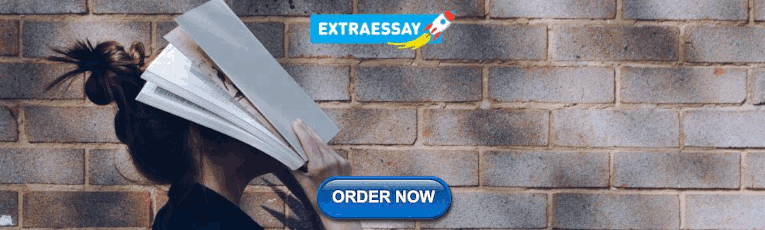
Moving Past Myth
Hyde and her colleagues hope that people use the consistent evidence that males and females are basically alike to alleviate misunderstanding and correct unequal treatment. Hyde is far from alone in her observation that the clear misrepresentation of sex differences, given the lack of evidence, harms men and women of all ages. In a September 2005 press release on her research issued by the American Psychological Association (APA), she said, "The claims [of gender difference] can hurt women's opportunities in the workplace, dissuade couples from trying to resolve conflict and communication problems and cause unnecessary obstacles that hurt children and adolescents' self-esteem."
Psychologist Diane Halpern, PhD, a professor at Claremont College and past-president (2005) of the American Psychological Association, points out that even where there are patterns of cognitive differences between males and females, "differences are not deficiencies." She continues, "Even when differences are found, we cannot conclude that they are immutable because the continuous interplay of biological and environmental influences can change the size and direction of the effects some time in the future."
The differences that are supported by the evidence cause concern, she believes, because they are sometimes used to support prejudicial beliefs and discriminatory actions against girls and women. She suggests that anyone reading about gender differences consider whether the size of the differences are large enough to be meaningful, recognize that biological and environmental variables interact and influence one other, and remember that the conclusions that we accept today could change in the future.
Cited Research
Archer, J. (2004). Sex differences in aggression in real-world settings: A meta-analytic review. Review of General Psychology, 8 , 291-322.
Barnett, R. & Rivers, C. (2004). Same difference: How gender myths are hurting our relationships, our children, and our jobs. New York: Basic Books.
Eaton, W. O., & Enns, L. R. (1986). Sex differences in human motor activity level. Psychological Bulletin, 100 , 19-28.
Feingold, A. (1994). Gender differences in personality: A meta-analysis. Psychological Bulletin, 116 , 429-456.
Halpern, D. F. (2000). Sex Differences in Cognitive Abilities (3rd Edition). Mahwah, NJ: Lawrence Erlbaum, Associates, Inc. Publishers.
Halpern, D. F. (2004). A cognitive-process taxonomy for sex differences in cognitive abilities. Current Directions in Psychological Science, 13 (4), 135-139.
Hyde, J. S., Fennema, E., & Lamon, S. (1990). Gender differences in mathematics performance: A meta-analysis. Psychological Bulletin, 107 , 139-155.
Hyde, J. S. (2005). The Gender Similarities Hypothesis. American Psychologist , Vol. 60, No. 6.
Leaper, C. & Smith, T. E. (2004). A meta-analytic review of gender variations in children's language use: Talkativeness, affiliative speech, and assertive speech. Developmental Psychology, 40 , 993-1027.
Oliver, M. B. & Hyde, J. S. (1993). Gender differences in sexuality: A meta-analysis. Psychological Bulletin, 114 , 29-51.
Spencer, S. J., Steele, C. M. & Quinn, D. M. (1999). Stereotype threat and women's math performance. Journal of Experimental Social Psychology, 35 , 4-28.
Voyer, D., Voyer, S., & Bryden, M. P., (1995). Magnitude of sex differences in spatial abilities: A meta-analysis and consideration of critical variables. Psychological Bulletin, 117 , 250-270.
American Psychological Association, October 20, 2005
Thank you for visiting nature.com. You are using a browser version with limited support for CSS. To obtain the best experience, we recommend you use a more up to date browser (or turn off compatibility mode in Internet Explorer). In the meantime, to ensure continued support, we are displaying the site without styles and JavaScript.
- View all journals
- Explore content
- About the journal
- Publish with us
- Sign up for alerts
- Open access
- Published: 08 November 2019
Gender similarities in the brain during mathematics development
- Alyssa J. Kersey ORCID: orcid.org/0000-0001-6685-5982 1 , 2 ,
- Kelsey D. Csumitta ORCID: orcid.org/0000-0001-9488-7981 1 &
- Jessica F. Cantlon ORCID: orcid.org/0000-0003-0207-5615 1 , 3
npj Science of Learning volume 4 , Article number: 19 ( 2019 ) Cite this article
38k Accesses
26 Citations
565 Altmetric
Metrics details
- Human behaviour
- Intelligence
Some scientists and public figures have hypothesized that women and men differ in their pursuit of careers in science, technology, engineering, and mathematics (STEM) owing to biological differences in mathematics aptitude. However, little evidence supports such claims. Some studies of children and adults show gender differences in mathematics performance but in those studies it is impossible to disentangle intrinsic, biological differences from sociocultural influences. To investigate the early biology of mathematics and gender, we tested for gender differences in the neural processes of mathematics in young children. We measured 3–10-year-old children’s neural development with functional magnetic resonance imaging (fMRI) during naturalistic viewing of mathematics education videos. We implemented both frequentist and Bayesian analyses that quantify gender similarities and differences in neural processes. Across all analyses girls and boys showed significant gender similarities in neural functioning, indicating that boys and girls engage the same neural system during mathematics development.
Similar content being viewed by others
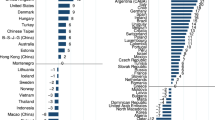
The effect of language on performance: do gendered languages fail women in maths?
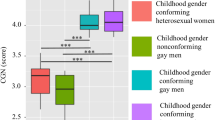
Male sexual orientation, gender nonconformity, and neural activity during mental rotations: an fMRI study
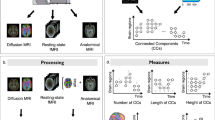
Differences in spatiotemporal brain network dynamics of Montessori and traditionally schooled students
Introduction.
Limited evidence for intrinsic, biological gender differences in mathematics ability has fueled debate about the underrepresentation of girls and women in STEM fields (science, technology, engineering, and mathematics). Some have suggested that girls and women are underrepresented in careers in STEM owing to biological differences. 1 Biological sex differences manifest in aspects of brain function, particularly those related to neuroendocrinology, 2 , 3 , 4 but many measures indicate that neural variability is a continuum wherein the brains of males and females reflect one heterogenous population rather than two distinct groups. 4 In the domain of mathematics, the evidence for biologically based gender differences is weak because when gender differences are observed, the studies fail to differentiate intrinsic biological factors from sociocultural ones. 5 , 6 , 7 , 8 , 9 Moreover, behavioral studies often find no gender differences in mathematical cognition in early childhood, and there are no prior functional neuroimaging studies of biological gender differences in mathematical cognition during early childhood. 5 , 8 , 9 , 10 , 11 , 12 , 13 In order to understand the origins of mathematics ability, and whether there are any gender differences, it is important to ask whether boys and girls begin development with biological differences in mathematical processing. Here, we combine frequentist and Bayesian statistical approaches to test for gender similarities and differences in the neural processing of mathematics during early childhood. We use “gender” instead of “sex” throughout this manuscript, which accords with the relevant literature, 12 , 13 , 14 and because we collected parental report of children’s gender and did not measure their chromosomes.
Although evidence for behavioral gender differences in mathematics is weak in older children, adolescents, and adults, it is important to consider when and how any differences might emerge. One possibility is that despite established gender similarities on behavioral tasks in early childhood, 5 , 10 , 12 , 13 the underlying biological or neural processes could differ between boys and girls. For example, boys’ and girls’ incorrect responses could result from different neural processes (e.g., inefficient recruitment of math processing regions vs inefficient response selection mechanisms). Boys’ and girls’ error rates could yield the same levels on behavioral tests of mathematics but the biology that underlies the errors in each gender group could differ. Alternatively, boys and girls may show significant, widespread biological similarities in the neural processes of mathematics during early childhood. This outcome would be consistent with yet untested claims that boys and girls share a core biology for mathematical cognition.
To compare the neural processes underlying mathematics development, we used functional magnetic resonance imaging (fMRI) to measure neural activity in 3–10-year-old children while they watched video clips that targeted early childhood mathematics skills (e.g., counting, addition; see Methods for more details). In total, 104 children (55 girls) participated in one of three natural viewing tasks (2 published studies 15 , 16 + 1 unpublished study, under review). Data were combined across natural viewing tasks by normalizing each subject to a within-task adult baseline using intersubject correlations. 17 This approach yields 80% power to achieve a medium-effect size of d = 0.55, p < 0.05 for independent samples t tests.
Intersubject correlations were conducted by comparing each child with every other child and comparing each child with every adult within a comparison group (63 total adults, 25 women, who watched one set of video clips). This resulted in an index of ‘neural maturity’ (child-to-adult intersubject correlations) and an index of ‘neural similarity’ (child-to-child intersubject correlations). The majority of analyses focus on similarities and differences in ‘neural maturity’, which indicates how well-developed and adult-like each child’s brain is during mathematical processing. 15 , 16 Neural maturity was calculated by conducting intersubject correlations 17 of the neural timecourses across the entire video between children and adults in every voxel of the brain (see Methods for details). Thus, it assesses the degree to which children’s neural activity resembled that of adults who watched the same video and allows the data to be combined across studies in a meaningful way. Within-child comparisons of neural maturity calculated to women vs men revealed that children’s neural maturity did not statistically differ based on the gender of the adult comparison group (see Methods for details). Therefore, for each child, their measure of neural maturity is averaged across all adults who watched the same video.
Girls’ and boys’ neural maturity were statistically compared across five whole-brain analyses which test for differences in mean neural maturity, similarities in mean neural maturity, differences in variance of neural maturity, and differences in the rate of mathematics development. First, we conducted frequentist statistical tests of differences (two-sided, independent samples t tests) and similarities in neural maturity. Similarities in mean neural maturity were assessed using statistical equivalence statistics. Testing for statistical equivalence is critical for evaluating gender similarities because a null result from a t test only suggests that there is not enough evidence to conclude that a difference exists—to address this we conducted a statistical test of similarity, Schuirmann’s Test of Equivalence. 18 This test uses two one-sided t tests to determine the likelihood that the mean difference between two groups falls within a specified similarity range (consistent with previous work that tested for similarities and differences in SAT scores, we used a similarity range of 2/3 of a standard deviation 19 ). Complementary to this approach, we conducted a Bayes Factor analysis, which also allows for the interpretation of both significant differences and significant similarities. The Bayes Factor analysis weighs the evidence for an alternative hypothesis against the evidence for the null hypothesis by taking the ratio of the posterior probabilities for the two hypotheses (the Bayes Factor). Bayes Factors > 3 indicate substantial, interpretable evidence for the corresponding hypothesis, and Bayes Factors < 3 suggest that the evidence is only anecdotal. Following previous work, 13 the prior for the alternative hypothesis of gender differences was the default Cauchy distribution centered on the prior for the null hypothesis with a width of 0.707. The prior for the null hypothesis was 0. To test for differences in neural variance between boys and girls across the whole brain, we used Levene’s Test of Variance. Some have claimed that differences in the upper and lower tails of the distributions drive gender differences. 20 Variance thus is an important measure because previous work shows that gender differences in variance can exist even when mean performance is the same. 10 , 21 Following the whole-brain analyses, we present more-detailed region-of-interest analyses on regions of the number processing network (bilateral intraparietal sulcus, bilateral inferior frontal gyrus, and anterior cingulate cortex), which were defined from an independent functional localizer scan. In a final analysis, we calculated intersubject correlations across children to obtain measures of within-gender and between-gender neural similarity. We then directly compared children’s neural similarity as calculated with children of the same and of the different gender. If gender differences in neural activity have a biological categorical origin rooted in childhood, these categorical differences should be evident in the brain. In contrast, if gender differences in neural activity do not originate from categorical differences in early childhood, there should instead be widespread similarities.
Frequentist and Bayesian comparisons of neural maturity
We used intersubject correlations to compare girls’ and boys’ temporal patterns of neural activity across the whole brain during the educational videos. First, we compared girls’ and boys’ overall neural maturity using frequentist statistics. A whole-brain t test revealed no differences in neural maturity between groups (threshold = t (102) ≥ 2.36, voxel-wise p ≤ 0.01, cluster corrected to p < 0.05, cluster threshold = 29 voxels). Next, gender similarities were assessed using Schuirmann’s Test of Equivalence. 18 Findings of statistical equivalence would suggest that children’s neural processing of mathematics comprises one heterogenous group rather than two distinct gender groups. In fact, girls and boys showed statistically equivalent levels of neural maturity throughout the brain (Fig. 1a , light purple; minimum t(102) ≥ 2.36, maximum one-sided p ≤ 0.01), suggesting that the neural processing of mathematics develops at similar rates in boys and girls. In terms of differences in neural variance, Levene’s Test of Variance revealed one small region of right posterior parietal cortex where groups differed in variance but not in mean activation, with girls showing greater variance than boys (TAL peak: 18, −64, 43; F(1,102) ≥ 6.89, voxel-wise p ≤ 0.01, cluster corrected to p < 0.05, cluster threshold = 11 voxels, Supplementary Fig. 1 ). This variance cluster was small (15 voxels) and girls exhibited more variance than boys at equal neural amplitudes to boys, which did not result in a mean difference between groups in this region.
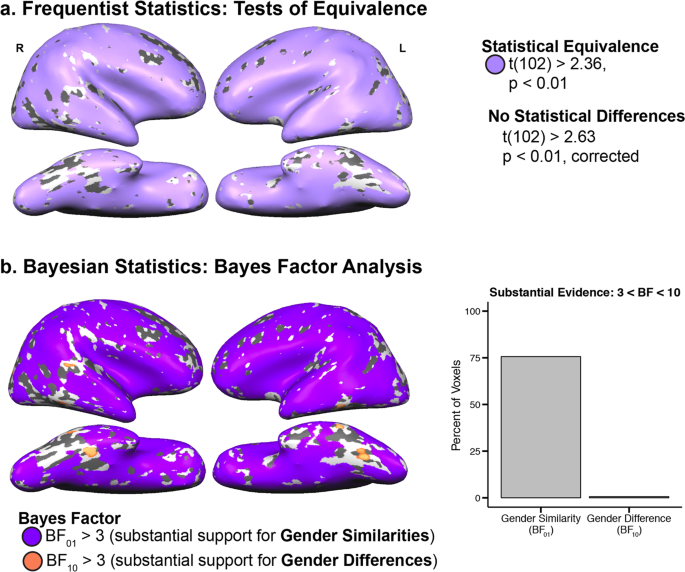
Whole-brain analyses. a Frequentist statistical tests of gender differences and similarities (light purple) of neural maturity. Using frequentist analyses ( t tests), there are no regions showing significant gender differences at the standard threshold. b Whole-brain Bayes Factor analysis showing substantial evidence of gender differences (orange) and gender similarities (dark purple) of neural maturity. The plot to the right shows the percent of voxels across the brain that show substantial support for gender similarities and differences. The regions that show evidence of gender similarities are consistent across frequentist and Bayesian approaches
The pattern of large-scale statistical similarities between boys and girls from the frequentist analyses was replicated in the Bayesian analysis. In each voxel of the brain, the weight of evidence for the null and alternative hypotheses were indexed by the Bayes factor (B 01 for the null hypothesis of gender similarities, B 10 for the alternative hypothesis of gender differences). Bayes factors suggesting that the data provide substantial support of the hypotheses are displayed in Fig. 1b (B 01 > 3, in purple indicating gender similarities, B 10 > 3 in orange indicating greater neural maturity in girls; no cortical regions showed substantial support for greater neural maturity in boys). Less than 1% of voxels (0.8%) showed substantial or strong (B 10 > 10) evidence of gender differences (Fig. 1b ; 23.6% voxels did not substantially support either similarities or differences in the Bayesian analysis).
Similarities in math processing networks
Importantly, across all three natural viewing tasks, children engaged numerical processes in the brain. Children showed number-selective neural activation in the intraparietal sulci (IPS) during the mathematics content in the educational videos (Fig. 2 ) consistent with previous fMRI research on numerical cognition in children and adults. 15 , 16 Boys and girls showed equivalent mathematics-related neural responses (see Fig. 2 ). This is evidence that children engaged mathematical neural processes during the educational videos, and that boys and girls did so equally.

Number and math selectivity in the intraparietal sulci (IPS). Individual data for boys are shown in blue and for girls are shown in red. Error bars represent ±1 standard error of the mean. a Percent signal change for number clips vs non-number clips in Study 1—redrawn data 15 (RIPS: t(25) = 4.01, p = 0.0005, 95% CI = 0.19–0.60, Cohen’s d = 0.79; LIPS: t(25) = 1.51, p = 0.26, 95% CI = −0.09–0.33, Cohen’s d = 0.23). b Preference for math clips vs non-math clips in Study 2—redrawn data 16 (RIPS: t(34) = 6.86, p < 0.0001, 95% CI = 0.12–0.23, Cohen’s d = 1.16; LIPS: t(34) = 4.12, p = 0.0002, 95% CI = 0.05–0.15, Cohen’s d = 0.70). c Slope of % signal change across increasing counting vs alphabet sequences in Study 3 (higher slope = greater sensitivity to sequence; RIPS: t(42) = 3.21, p = 0.003, 95% CI = 0.35–1.56, Cohen’s d = 0.49; LIPS: t(42) = 2.55, p = 0.014, 95% CI = 0.14–1.17, Cohen’s d = 0.39). Independent t tests suggest no differences between girls and boys (max t value = 0.84, min p value = 0.41) and Bayes Factor analyses suggest anecdotal to substantial evidence for gender similarities (BF 10 for gender differences: 0.30–0.47; BF 01 for gender similarities: 2.14–3.25). Although, samples for each individual study are small, effects are consistent with the larger patterns reported in the following analyses
Next, we compared the rate of mathematics development in boys and girls. Ninety-seven children completed the Test of Early Mathematics Ability to evaluate their mathematics skills (TEMA-3 22 ; n = 50 girls, 3.12–8.96 years; 47 boys, 3.33–9.08 years). Math ability was statistically equivalent across children and did not show gender differences in mean ability or variance (Fig. 3a ; t test: t(95) = 0.57, p = 0.57, girls’ mean = 33.62, boys’ mean = 35.96, 95% CI = −10.42–5.74; Tests of Equivalence: t 1 (95) = 3.84, p < 0.001, t 2 (95) = −2.70, p = 0.004; Test of Variance: F(1,95) = 0.29, p = 0.59, girls’ sd = 20.19, boys’ sd = 19.87; descriptively there were more girls than boys in the upper tail of the distribution: 14 girls and 10 boys). Nor did the relation between gender and math ability change across age (Fig. 3a ; Regression of TEMA-3 on gender and age: R 2 = 0.79, F(3,93) = 118.5, p < 0.0001, Gender: b = 1.22, t(93) = 0.163, p = 0.871; Age: b = 11.74, t = 13.97, p < 0.0001; Gender × Age Interaction: b = 0.26, t = 0.21, p = 0.84). These behavioral data were previously included as part of a larger behavioral study that showed no differences and statistical equivalence in math ability in this age group. 12
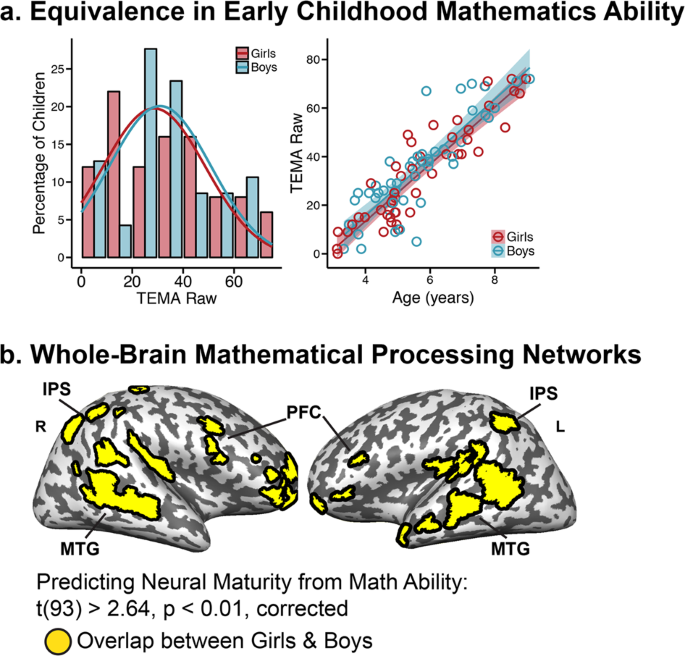
Gender similarities in math ability. a Left: distributions of TEMA-3 scores for girls (red) and boys (blue). Right: TEMA-3 scores increase with age for girls (red) and boys (blue), shaded regions around the line represent ±1 standard error of the mean. b Regions where boys and girls showed a relation between neural maturity and math ability. Importantly, no cortical regions showed an interaction between math ability and gender. Abbreviations: IPS = intraparietal sulcus, PFC = prefrontal cortex, MTG = middle temporal gyrus, R = right, L = left
We then identified mathematical processing networks by testing for regions that showed higher neural maturity in children with stronger math skills. Math ability, gender, and the interaction between math ability and gender were entered as predictors of neural maturity in a whole-brain regression. This regression revealed that math ability predicted neural maturity in both gender groups in the IPS, prefrontal cortex, and middle temporal gyrus (Fig. 3b ; t(93) ≥ 2.63 voxel-wise p ≤ 0.01, cluster corrected to p < 0.05, girls: r(48) ≥ 0.36 with cluster threshold of 109 voxels, boys: r(45) ≥ 0.37 with cluster threshold of 69 voxels, see Supplementary Fig. 2 for separate maps). These regions are consistent with those that show a correlation between neural maturity and math ability when collapsed across gender (r(95) ≥ 0.26, p ≤ 0.01, corrected, Supplementary Fig. 2 ). No cortical regions showed significant interactions between math ability and gender (t(93) ≤ 2.63, threshold: voxel-wise p ≤ 0.01, cluster corrected to p < 0.05, threshold = 110 voxels), indicating that the relation between math ability and neural maturity does not depend on gender. In other words, mathematical processing networks develop at the same rate for girls and boys.
To visualize patterns of gender similarities, neural maturity was extracted from an independently-defined number processing network 15 consisting of bilateral IPS, bilateral inferior frontal gyrus, and anterior cingulate cortex (number > face, shape, and word matching; Fig. 4a ; t(17) ≥ 4.04, FDR corrected to p < 0.05). In accord with the whole-brain analyses, these regions showed statistical equivalence, not statistical differences, and no differences in variance (Fig. 4b ; t tests: max t(102) = 1.08, p = 0.28; equivalence-tests: min t 1 (102) = 3.82, p = 0.0001; min absolute value of t 2 (102) = 2.33, p = 0.01; see Supplementary Table 1 for full statistics). Regression analyses revealed that math ability predicted neural maturity throughout the number processing network, particularly in the IPS, but did not interact with gender (interaction predictors: max t(93) = 1.42, p = 0.16, Supplementary Table 1 ). This shows that within key number processing regions of the brain, girls and boys show the same degree of development in mathematical processing.
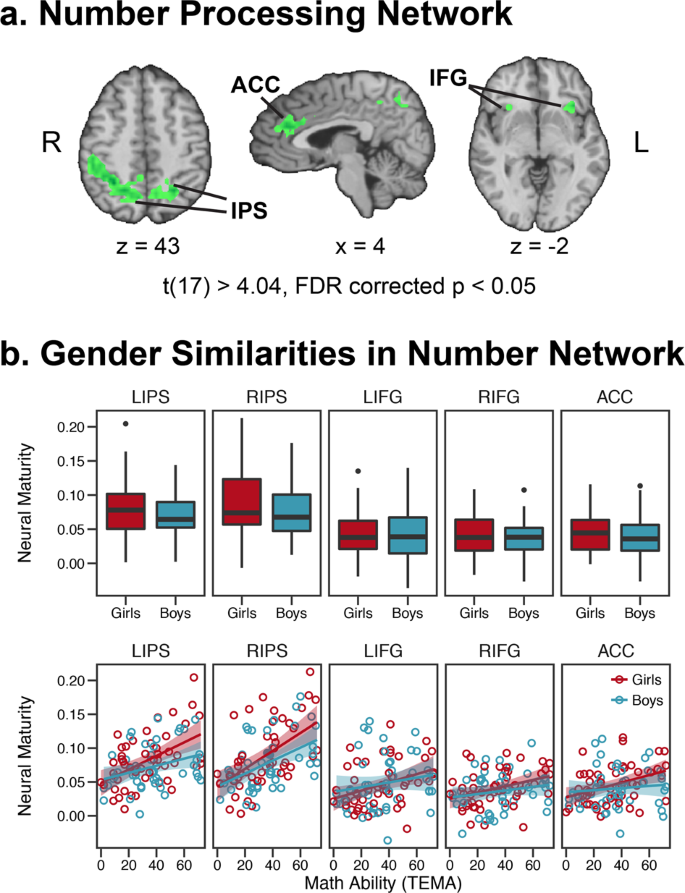
Region-of-interest analyses. a Number processing network identified from an independent localizer (number matching > face, shape, & word matching). b Mean neural maturity in the number network (top) and relation between neural maturity and math ability in the number network (bottom). Boxplot center line identifies the median, the upper whiskers extend from the 75th percentile to the 75th percentile + 1.5 interquartile range, the lower whiskers extend from the 25th percentile to the 25th–1.5 interquartile range. Outliers are those data points beyond the whisker ranges. Shaded regions around the lines in the scatterplots indicate ±1 standard error. Abbreviations: IPS = intraparietal sulcus, ACC = anterior cingulate cortex, IFG = inferior frontal gyrus, R = right, L = left
Whole-brain child-to-child similarity
Finally, we examined neural similarity in children of the same versus different genders. Intersubject correlations were calculated between children, resulting in maps of same-gender neural similarity (comparing girls with girls and boys with boys) and different-gender neural similarity (comparing girls with boys and boys with girls). To determine whether there were differences between same-gender versus different-gender similarity, each child’s whole-brain different-gender similarity map was subtracted from their same-gender similarity map. These difference maps were then subjected to a one-sample t test vs 0. This whole-brain t test revealed no regions that showed a difference in neural activity (threshold: t(103) ≥ 2.62, voxel-wise p ≤ 0.01, corrected to p < 0.05 with a cluster threshold of 19 voxels). Figure 5 shows average neural similarity calculated to children of the same gender (yellow), a different gender (dark green), and the overlap of those maps (light green; r(500) ≥ 0.115, p ≤ 0.01). The regions that showed strong neural similarity between children were identical for statistical comparisons of the same gender and different genders. This again indicates that children’s patterns of neural activity reflect one heterogenous group, rather than two distinct groups based on gender.
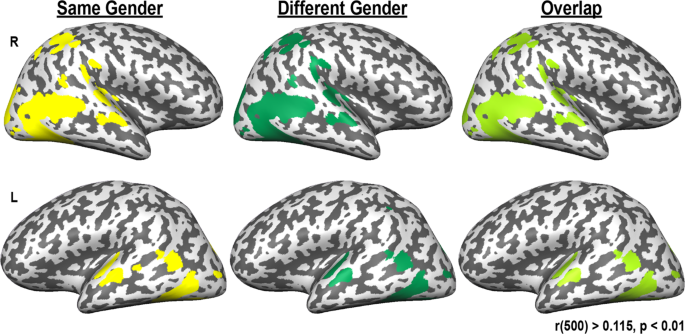
Child-to-child neural saimilarity. Average neural similarity (r(500) ≥ 0.115, p ≤ 0.01) when calculated across children of the same gender (yellow, column 1) and children of different genders (green, column 2). The third column shows the overlap of the first two columns in light green. Importantly, regions are identical across neural similarity calculated to children of the same gender and to children of different genders
Across multiple neural analyses, we show that girls’ and boys’ brains function similarly during mathematical processing. We saw no evidence of gender differences in neural responses to mathematics content, neural responses during educational video viewing, or rates of neural development for mathematical processing in early childhood, and in fact we found statistical equivalence between boys and girls throughout the brain. Tests of statistical equivalence and a Bayes Factor analysis show gender similarities throughout the number processing network. Furthermore, boys’ and girls’ math abilities related to the rate of neural mathematics development in the same brain regions, and neural similarity was consistent across children of the same and of different genders.
Our results are consistent with the ‘Gender Similarities Hypothesis’, which argues that boys and girls function similarly in most areas of cognition. 10 , 23 In particular, gender similarities in early childhood mathematics show, as proposed in the domain of spatial cognition, 14 that gender differences in STEM fields in adults are not derived from intrinsic differences in children’s brains but likely from a complex environmental origin.
Any test of cognitive ability that shows gender differences faces the difficulty of disentangling biological factors from social ones. For instance, 4- to 7-year-old boys show an advantage over girls in tests of spatial skills, but parents also report more-spatial play with their boys compared with their girls, 14 suggesting a possible sociocultural influence on gender differences in spatial cognition. Similarly, in math and science, teachers tend to show differential distributions of time spent encouraging students, praising students, and explaining concepts to students, with boys receiving more time than girls. 24 , 25 , 26 , 27 This is important because teachers’ perceptions of children’s math ability predicts later math achievement scores. 28 Parents’ expectations about their children’s success also correlate with children’s own self-concepts of their abilities and their performance on math tasks. 29 , 30 A strong sociocultural influence on early childhood math achievement makes it difficult to tease apart intrinsic gender differences from sociocultural factors in older children and adults. 8 , 9
Given the broad similarities between boys and girls, gender differences observed in STEM performance during adolescence or adulthood are unlikely to originate from early childhood differences in the brain or cognition. Although gender differences in STEM may emerge later in development or from interactions between STEM training and sexually dimorphic behaviors (e.g., differences in hormone levels following puberty), 6 , 31 , 32 the findings of widespread gender similarities in boys’ and girls’ brains do not support claims of biological gender differences in childhood. Instead, the data show that the neural functions underlying mathematical cognition are similar between genders and represent one heterogeneous population rather than two categorical groups.
Participants
In total, 104 typically-developing 3- to 10-year-old children (55 girls) and 63 adults (35 women) participated in one of three studies. Age was statistically equivalent between girls and boys (t 1 (102) = 3.32, p = 0.0006, t 2 (102) = 3.43, p = 0.0004), and there were no differences in age variability, reflecting an even distribution of age across-gender groups (F(1,102) = 0.07, p = 0.79, girls’ sd = 1.65, boys’ sd = 1.63). Informed written consent was obtained from adult participants and parents of children, and informed written assent was obtained for children 7 years and older. All protocols were approved by the University of Rochester Research Subjects Review Board.
fMRI paradigms
Twenty-six 4- to 10-year-old children (15 girls; girls’ mean age = 6.93 years, boys’ mean age = 7.13 years; range = 4.32–10.80 years) and 20 adults (13 women; women’s mean age = 20.52 years, men’s mean age = 20.98 years; range = 18.9–25.4 years) successfully participated in Study 1. This paradigm consisted of a 20.3-min video containing clips from children’s educational television shows. Clips ranged from 12 to 176 s in length and were edited into a continuous movie. These data have been previously reported 15 as the “natural viewing” task.
Thirty-five 4- to 8-year-old children (17 girls; girls’ mean age = 6.61 years, boys’ mean age = 6.35 years; range = 4.08–8.67 years) and 23 adults (12 women; women’s mean age = 22.13 years, men’s mean age = 22.65 years; range = 18.44–28.09 years) successfully participated in Study 2. The 11.6-min video contained clips from children’s educational television shows. Clips ranged from 12.5 to 32.4 s in length and were edited into a continuous movie. These data have been previously reported 16 as the “natural viewing” task.
Forty-three 3- to 5-year-old children (23 girls; girls’ mean age = 4.54 years, boys’ mean age = 4.71 years; range = 3.12–5.96 years) and 20 adults (10 women; women’s mean age = 23.43 years, men’s mean age = 24.17 years; range = 20.15–31.55 years) successfully participated in Study 3. In this study, participants listened to pre-recorded audio tracks of someone counting or saying the alphabet. Short clips from child-friendly cartoons were presented on the screen during the sequences. Audio tracks were removed from the cartoons and were replaced with quieter, child-friendly instrumental music. Cartoon tracks were matched across sequences. Sequences were presented in 70 s blocks of 60 items presented at a rate of one item every 1.1–1.2 s. Fifteen blocks were presented throughout the experimental paradigm and were separated by 4-s of blackscreen. The scan began and ended with 12 s of blackscreen resulting in a total scan time of 19.2 min.
Number Localizer
Eighteen children from Study 1 (11 girls, 7 boys; girls’ mean age = 6.78 years, boys’ mean age = 7.47 years; range = 4.32–10.8 years) completed a traditional fMRI paradigm in which they compared pairs of stimuli (isolated images of faces, numbers, words or shapes) that were presented on the left and right sides of the screen. Participants reported whether they were the same or different by pressing a button only if the two stimuli matched. Half of the pairs presented were “matches”, whereas the other half were “non-matches”. Number comparisons were made across notation (i.e., dot array compared with Arabic numeral), face comparisons were made across a frontal shot and an oblique view, word comparisons were made across a word in all capital letters and the other in all lowercase letters, and shape comparisons were made across two shape images. Stimuli were presented in a blocked design. Each block consisted of three 2-s trials from the same condition, separated by a 2-s intertrial interval. Three blocks of each condition were semi-randomly presented throughout a run with 8 s of fixation between blocks. These data have been previously reported 15 as the “traditional functional task”.
fMRI session
Prior to the scanning sessions, children participated in a 30-minute training session in a mock scanner to familiarize them with the scanning environment and to practice remaining motionless. Children who completed the Number Localizer paradigm practiced the task prior to the MRI session. Adults received verbal instructions prior to scanning and did not participate in a training session. During the scan, children’s heads were secured with medical tape, headphones, and foam padding, and adults’ heads were secured with headphones and foam padding.
MR parameters
Whole-brain BOLD imaging was conducted on a 3-Tesla Siemens MAGNETOM Trio scanner with a 12-channel head coil at the Rochester Center for Brain Imaging. High-resolution structural T1 contrast images were acquired using a magnetization prepared rapid gradient echo pulse sequence at the start of each session (repetition time (TR) = 2530 ms, echo time (TE) = 3.44 ms, flip angle = 7, field of view (FOV) = 256 mm, matrix = 256 × 256, 192, 176, or 160 slices depending on head size, 1 × 1 × 1 mm sagittal left-to-right slices). An echo-planar imaging pulse sequence with online motion correction was used for T2*contrast (TR = 2000 ms, TE = 30 ms, flip angle = 90 degrees, FOV = 256 mm, matrix 64 × 64, 30 axial oblique slices, parallel to the AC-PC plane, voxel size = 4 × 4 × 4 mm). The primary paradigms from Studies 1, 2, and 3 were 610 volumes, 348 volumes, and 567 volumes, respectively, and the Number Localizer paradigm was distributed over two to four functional runs of 132 volumes each.
Preprocessing
fMRI data were analyzed in BrainVoyager 33 using in-house scripts drawing on the BVQX toolbox. Data from previously published studies were analyzed as originally reported for consistency. 15 , 16 For the Number Localizer and Study 1, which were collected during the same scanning session, the first six TRs of each run were discarded prior to analysis to allow for signal equilibration. For Studies 2 and 3, the first two TRs of each run were discarded. Functional data were registered to high-resolution anatomy images for each participant in native space. Preprocessing consisted of slice scan time corrected (cubic spline interpolation), motion correction with respect to the first volume in the run, and linear trend removal in the temporal domain (cutoff: two cycles within the run). A Gaussian spatial filter with an 8 mm full-width at half-maximum was applied to each volume for Study 1, 15 and a 6 mm full-width at half-maximum was applied to each volume for Studies 2 16 and 3. The functional data from the Number Localizer were not smoothed. Adult and child echo-planar and anatomical volumes were then normalized into Talairach space 34 using piecewise affine transformation based on manual identification of anatomical landmarks. Analyses were performed on processed data in Talairach space. Average framewise displacement 35 , 36 was regressed across the brain for each child to control for sudden changes in volume-to-volume head motion.
fMRI data analyses
Neural maturity: neural data were analyzed using an intersubject correlation approach. 15 , 16 , 17 Between-group intersubject correlations were performed by using the full timecourse of each voxel for each child as a predictor for activation of the corresponding voxel in each adult brain. Functional data from each child were then correlated with that of each adult from the same study to produce paired r-maps. Paired r-maps represent the neural similarity of each child compared with each adult in every voxel of the brain. A single, average brain map was then calculated to represent the neural similarity of each child to all adults. This map is referred to as a map of “neural maturity” because it shows how “adult-like” each child’s neural timecourse appears. To ensure that these similarity maps were not confounded by any adult gender differences prior to averaging, we compared neural maturity calculated to women vs men. For each child, we conducted an Independent Samples t -test to compare neural maturity when calculated to adults of the same vs different gender as the child. This resulted in a map of between-group t values for each child. To determine whether there were any differences that were consistent at the group level, the absolute value of these individual-level between-group t value maps were then subjected to a one-sample t test vs a critical t value of 2.08. The critical value represents the t value at which a difference could be considered significant given the smallest sample of adults ( n = 20 for studies 1 and 3; t(18) = 2.08, p = 0.05). If there were a significant difference between neural maturity calculated to adults of the same vs different gender as the child, the one-sample t test should reveal a positive and significant effect, which would indicate that the absolute values of the individual-level between-group t values are significantly above 2.08. Instead, the whole-brain one-sample t test revealed that at the group level, the absolute values of the individual-level between-group t values were significantly below the critical t value of 2.08 (one-sample t test of absolute value of individual-level between-group t values vs critical t value of 2.08: t(102) ≤ −2.62, p ≤ 0.01). In other words, the majority of individual-level between-group t values were less than the critical t value of 2.08 (range of average absolute value of individual-level between-group t values across voxels: 0–1.90). This indicates that overall, the differences between neural maturity when calculated to adults of the same vs different gender were not different, so neural maturity was not biased by the gender of the adult.
Neural similarity
To compare how similar children were to each other, intersubject correlations were calculated across children following the same procedures as for calculating neural maturity. This resulted in two maps for each child: one representing the average neural similarity of a child to children of the same gender and one representing the average neural similarity of a child to children of the different gender. To compare neural similarity within and across gender, these maps were then subtracted from each other (within-gender similarity−across-gender similarity) and these difference maps were subjected to a one-sample t test vs 0.
Functional data collected during the traditional fMRI paradigm were analyzed using a general linear model (random effects analysis). Experimental events (duration = 10 s) were convolved with a standard dual gamma hemodynamic response function. There were four regressors of interest (corresponding to the four stimulus categories), one regressor for button press, and six regressors of no interest (corresponding to the motion parameters obtained during preprocessing).
Region-of-interest (ROI) analyses
Data were extracted from number network ROIs using MATLAB. Analyses were then conducted using R (version 3.3.1) and R-Studio (version 0.99.902). Independent samples t tests were conducted using the “ t test” function assuming equal variance. Tests of equivalence were conducted using the “TOSTtwo.raw” function from the “TOSTER” package (upper and lower bounds set to ±0.667 × standard deviation of the entire group; alpha = 0.05). This function returns two t values ( t 1 and t 2 ). For statistical equivalence, both t values must be statistically significant. Statistical equivalence is rejected if either t 1 or t 2 does not reach significance. Levene’s Test of Variance was carried out using the “leveneTest” function in the “car” package. Regression analyses were conducted using the built-in “lm” function.
Assessment of math ability
Math skills were evaluated by administering the TEMA-3 22 to participants aged 8 and younger. The TEMA-3 tests a variety of math concepts and is standardized for 3–8-year-old children.
Reporting summary
Further information on research design is available in the Nature Research Reporting Summary linked to this article.
Data availability
These data sets are available from the corresponding author on reasonable request.
Code availability
All code for R and MATLAB are available from the corresponding author on reasonable request.
Levy, J. & Kimura, D. Women, men and the sciences. in The Science on Women and Science (ed. Sommers, C. H.) 202–284 (American Enterprise Institute Washington, 2009).
Sowell, E. R. et al. Sex differences in cortical thickness mapped in 176 healthy individuals between 7 and 87 years of age. Cereb. Cortex 17 , 1550–1560 (2007).
Article Google Scholar
Giedd, J. N., Raznahan, A., Mills, K. L. & Lenroot, R. K. Review: magnetic resonance imaging of male/female differences in human adolescent brain anatomy. Biol. Sex Differ. 3 , 19 (2012).
Joel, D. & Fausto-Sterling, A. Beyond sex differences: New approaches for thinking about variation in brain structure and function. Philos. Trans. R. Soc. B Biol. Sci. 371 , 20150451 (2016).
Spelke, E. S. & Ellison, K. Gender, math and science. in The Science on Women and Science (ed. Sommers, C. H.) 24–53 (American Enterprise Institute Washington, 2009).
Halpern, D. F. et al. The science of sex differences in science and mathematics. Psychol. Sci. Public Interes. 8 , 1–51 (2007).
Hyde, J. S., Fennema, E. & Lamon, S. J. Gender differences in mathematics performance - a metaanalysis. Psychol. Bull. 107 , 139–155 (1990).
Article CAS Google Scholar
Keller, K. & Menon, V. Gender differences in the functional and structural neuroanatomy of mathematical cognition. Neuroimage 47 , 342–352 (2009).
Pletzer, B. Sex differences in number processing: Differential systems for subtraction and multiplication were confirmed in men, but not in women. Sci. Rep. 6 , 36904 (2016).
Hyde, J. S., Lindberg, S. M., Linn, M. C., Ellis, A. B. & Williams, C. C. Gender similarities characterize math performance. Science 321 , 494–495 (2008).
Lindberg, S. M., Hyde, J. S., Petersen, J. L. & Linn, M. C. New trends in gender and mathematics performance: a meta-analysis. Psychol. Bull. 136 , 1123–1135 (2010).
Kersey, A. J., Braham, E. J., Csumitta, K. D., Libertus, M. E. & Cantlon, J. F. No intrinsic gender differences in children’s earliest numerical abilities. npj Sci. Learn. 3 , 12 (2018).
Hutchison, J. E., Lyons, I. M. & Ansari, D. More similar than different: gender differences in children’s basic numerical skills are the exception not the rule. Child Dev. 00 , 1–14 (2018).
Google Scholar
Jirout, J. J. & Newcombe, N. S. Building blocks for developing spatial skills: evidence from a large, representative U.S. sample. Psychol. Sci. 26 , 302–310 (2015).
Cantlon, J. F. & Li, R. Neural activity during natural viewing of Sesame Street statistically predicts test scores in early childhood. PLoS Biol. 11 , e1001462 (2013).
Kersey, A. J., Wakim, K.-M., Li, R. & Cantlon, J. F. Developing, mature, and unique functions of the child’s brain in reading and mathematics. Dev. Cogn. Neurosci. 39 , 100684 (2019).
Hasson, U., Nir, Y., Levy, I., Fuhrmann, G. & Malach, R. Intersubject synchronization of cortical activity during natural vision. Science 303 , 1634–1640 (2004).
Schuirmann, D. J. A comparison of the two one-sided tests procedure and the powers approach for assessing the equivalence of average bioavailability. J. Pharmacokinet. Biopharm. 15 , 657–680 (1987).
Ball, L. C., Cribbie, R. A. & Steele, J. R. Beyond gender differences: using tests of equivalence to evaluate gender similarities. Psychol. Women Q. 37 , 147–154 (2013).
Hedges, L. & Nowell, A. Sex differences in mental test scores, variability, and numbers of high-scoring individuals. Science 269 , 41–45 (1995).
Benbow, C. P. & Stanley, J. C. Sex differences in mathematical reasoning ability: more facts. Science 22 , 1029–1031 (1983).
Ginsburg, H. & Baroody, A. J. Test of Early Mathematics Ability , 3rd Edn (TEMA-3) . (Pro-Ed, 2003).
Hyde, J. S. The gender similarities hypothesis. Am. Psychol. 60 , 581–592 (2005).
American Association of University Women Educational Foundation. How Schools Shortchange Girls. AAUW Rep . 1–13 (1992).
Jones, M. G. & Wheatley, J. Gender differences in teacher-student interactions in science classrooms. J. Res. Sci. Teach. 27 , 861–874 (1990).
Kelly, S. N. Teachers’ useful skills. J. Res. Music Educ. 46 , 374–383 (1998).
Becker, J. R. Differential treatment of females and males in mathematics classes. J. Res. Math. Educ. 12 , 40–53 (1981).
Jussim, L. & Eccles, J. S. Teacher expectations II: constructions and reflection of student achievement. J. Pers. Soc. Psychol. 63 , 947–961 (1992).
Bleeker, M. M. & Jacobs, J. E. Achievement in math and science: do mothers’ beliefs matter 12 years later? J. Educ. Psychol. 96 , 97–109 (2004).
Parsons, J. E., Adler, T. F. & Kaczala, C. M. Socialization of achievement attitudes and beliefs: parental influences. Child Dev. 53 , 310–321 (1982).
Geary, D. C. A model for representing gender differences in the pattern of cognitive abilities. Am. Psychol. 44 , 1155–1156 (1989).
Arnold, A. P. et al. Minireview: sex chromosomes and brain sexual differentiation. Endocrinology 145 , 1057–1062 (2004).
Goebel, R., Esposito, F. & Formisano, E. Analysis of functional image analysis contest (FIAC) data with brainvoyager QX: From single-subject to cortically aligned group general linear model analysis and self-organizing group independent component analysis. Hum. Brain Mapp. 27 , 392–401 (2006).
Talairach, J. & Tournoux, P. Co-Planar Stereotaxic Atlas of the Human Brain . (Thieme, 1988).
Grill-Spector, K., Golarai, G. & Gabrieli, J. Developmental neuroimaging of the human ventral visual cortex. Trends Cogn. Sci. 12 , 152–162 (2008).
Power, J. D., Barnes, K. A., Snyder, A. Z., Schlaggar, B. L. & Petersen, S. E. Spurious but systematic correlations in functional connectivity MRI networks arise from subject motion. Neuroimage 59 , 2142–2154 (2012).
Download references
Acknowledgements
We thank Rosa Li, Courtney Lussier, and Pat Weber for help with data collection and Rosa Li and Kamy Wakim for help with data processing. This work was supported by the National Science Foundation (DRL-1459625 to J.F.C. and DGE- 1419118 to A.J.K.) and the National Institutes of Health (R01 HD064636 to J.F.C).
Author information
Authors and affiliations.
Department of Brain and Cognitive Sciences, University of Rochester, Rochester, NY, 14627, USA
Alyssa J. Kersey, Kelsey D. Csumitta & Jessica F. Cantlon
Department of Psychology, University of Chicago, Chicago, IL, 60637, USA
Alyssa J. Kersey
Department of Psychology, Carnegie Mellon University, Pittsburgh, PA, 15213, USA
Jessica F. Cantlon
You can also search for this author in PubMed Google Scholar
Contributions
All authors designed the research, analyzed the data, and revised and approved the manuscript.
Corresponding author
Correspondence to Alyssa J. Kersey .
Ethics declarations
Competing interests.
The authors declare no competing interests.
Additional information
Publisher’s note Springer Nature remains neutral with regard to jurisdictional claims in published maps and institutional affiliations.
Supplementary information
Kerseycsumittacantlon_supplementalmaterial, reporting summary, rights and permissions.
Open Access This article is licensed under a Creative Commons Attribution 4.0 International License, which permits use, sharing, adaptation, distribution and reproduction in any medium or format, as long as you give appropriate credit to the original author(s) and the source, provide a link to the Creative Commons license, and indicate if changes were made. The images or other third party material in this article are included in the article’s Creative Commons license, unless indicated otherwise in a credit line to the material. If material is not included in the article’s Creative Commons license and your intended use is not permitted by statutory regulation or exceeds the permitted use, you will need to obtain permission directly from the copyright holder. To view a copy of this license, visit http://creativecommons.org/licenses/by/4.0/ .
Reprints and permissions
About this article
Cite this article.
Kersey, A.J., Csumitta, K.D. & Cantlon, J.F. Gender similarities in the brain during mathematics development. npj Sci. Learn. 4 , 19 (2019). https://doi.org/10.1038/s41539-019-0057-x
Download citation
Received : 27 March 2019
Accepted : 11 September 2019
Published : 08 November 2019
DOI : https://doi.org/10.1038/s41539-019-0057-x
Share this article
Anyone you share the following link with will be able to read this content:
Sorry, a shareable link is not currently available for this article.
Provided by the Springer Nature SharedIt content-sharing initiative
This article is cited by
On the association between gender-science stereotypes’ endorsement and gender bias attribution.
- Elena De Gioannis
Quality & Quantity (2024)
The conundrum of gender-science stereotypes: a review and discussion of measurements
Quality & Quantity (2023)
The Representation of Gender Stereotypes in Spanish Mathematics Textbooks for Elementary Education
- Virginia Guichot-Reina
- Ana María De la Torre-Sierra
Sexuality & Culture (2023)
Implicit gender-science stereotypes and college-major intentions of italian adolescents
Social Psychology of Education (2022)
- Tamar Kricheli-Katz
npj Science of Learning (2021)
Quick links
- Explore articles by subject
- Guide to authors
- Editorial policies
Sign up for the Nature Briefing newsletter — what matters in science, free to your inbox daily.


Janet Shibley Hyde Sinks Stereotypes With Data
- Gender Roles
- Mathematical Ability
- Stereotypes
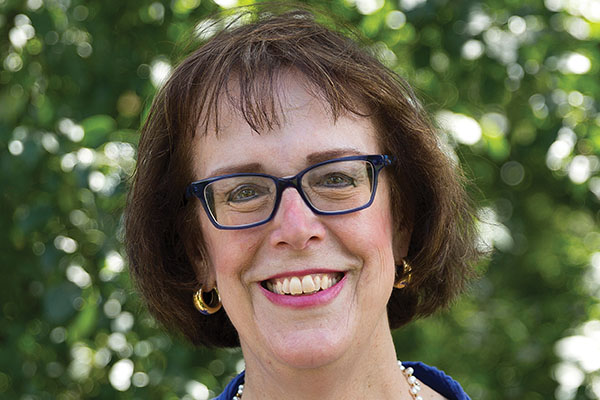
Growing up, one of APS James McKeen Cattell Fellow Janet Shibley Hyde’s favorite activities in school was Around the World, a math game in which students compete to “travel” around their classmates by answering as many problems as possible without making a mistake.
“Nobody told me I was a girl and I wasn’t supposed to be competitive,” Hyde, a professor of psychology and women’s studies at the University of Wisconsin-Madison, said during her Award Address at the 2018 APS Annual Convention in San Francisco. “I just loved the competition, what can I say?”
Even today, the kind of benevolent, and sometimes not so well-meaning, sexism epitomized by John Gray’s 1992 book “Men Are from Mars, Women Are from Venus” remains popular in mass media portrayals of gender. People think they’re being kind when they say women are hardwired by hormones or other “immutable” biological factors to be nurturing and caring, or possess a “nearly psychic” ability to perceive emotions. But none of that corresponds to the data, Hyde explained.
“Unfortunately, it’s all wrong,” she said. “As I’m fond of saying, you’ll never hear a good neuroscientist use the term ‘hardwired’ because the brain is anything but hardwired — it is plastic, so anyone who is trying to sell you on [that notion], you know you have to be suspicious of.”
Hyde’s meta-analyses of the current body of research on gender difference cast aside both the difference and deficit models — the idea that women just aren’t quite as capable as men overall — in support of what she has titled the gender similarities hypothesis: the theory that “men and women are very similar on most (not all) psychological variables.”
Or, to put it even more simply: “women are from Earth and men are from Earth.”
Order and Method
Hyde’s approach to psychological science revolves around quantitative literature reviews, a method of combining the results of numerous studies on a given topic to identify not only whether an effect exists, but how large it is.
Meta-analysis is the “gold standard” for conclusions in medicine, education, and psychological science, Hyde said, and, in the case of gender differences, comes down to computing a weighted average, d, that estimates the standardized difference between male and female scores across a set of studies.
Typically, researchers examining gender differences calculate this number so that it has a positive score if males score higher on a particular measure and a negative score if females score higher. According to psychological scientist Jacob Cohen’s guidelines for interpreting effect sizes, the number can be small (0.2), medium (0.5), or large (0.8) — or, Hyde added, completely trivial (less than 0.1), indicating an almost complete overlap in scores. For example, data indicate that the effect size of the height difference between men and women is about 2.0. This suggests a noticeable and meaningful difference between males and females, but even then, taller-than-average women can still stand head and shoulders above shorter-than-average men. A d of 0.15, on the other hand, would suggest an almost complete overlap in the distributions of scores for males and for females.
One limitation of reviewing research on gender difference, Hyde noted, is that the bulk of studies available for review are based primarily on the gender binary — a social construct facing increasing academic and cultural criticism. As such, the focus tends to be on cisgender men and women to the exclusion of both transgender and non-binary individuals. Hyde’s recent paper, “The future of sex and gender in psychology: Five challenges to the gender binary,” further elaborates on the empirical evidence for a more diverse range of gender experiences.
Running the Numbers on Women in Mathematics
In 2005, former President of Harvard University Lawrence Summers made a lengthy statement to the United States’ National Bureau of Economic Research outlining his belief that women simply don’t have the math ability to compete with men for jobs in science and engineering.
“His speech annoyed me, but I fight not with my fists, but with data, so I went to NSF and got a grant to test whether Larry Summers was right,” Hyde said.
To start, a quick glance at the National Science Foundation’s website nipped the myth that women simply aren’t interested in math in the bud: 47% of undergraduate degrees in mathematics were awarded to women in 2002.
“If women can’t do math, I don’t see how they’re getting half the undergraduate degrees in math, despite what Larry Summers says, so this is our first clue that things are not quite what the stereotypes say,” Hyde quipped.
Next, she held the question of women and girl’s performance in mathematics up to meta-analytic scrutiny using the annual state assessment scores mandated by No Child Left Behind policies in the United States. Just 10 states responded to Hyde’s request for data sorted by gender, grade level, and ethnicity, but even that was enough to provide information on more than 7 million kids.
The result? The difference between boys’ and girls’ scores in grades 2–11 had an effect size that ranged from about 0.01 to 0.06 d, with an average d value for all grades of just 0.0065.
“I don’t know what could be more ‘no gender difference’ than that,” Hyde said.
A previous meta-analysis Hyde had conducted in the 1990s found evidence of a gender performance gap in math, but these new data showed that female students had clearly reached parity with their male peers. Another 1990 study by Max Lummis and Harold W. Stevenson found that boys performed better on word problems than girls in all cultures — but that wasn’t the real takeaway. Lummis and Stevenson also found that American students were outperformed substantially by their peers of both genders in other countries.
“If girls can’t do math, why is it that girls in Taiwan do so much better than American boys?” she asked. “The big effect here is culture and how cultures treat math and how they teach math and so on. The big effect here is not gender differences.”
In fact, a 2008 study by APS Fellow Edward C. Melhuish et al. found that the best predictor of a child’s mathematical ability wasn’t gender, but their mother’s education level, a possible proxy for social class, Hyde said. Other strong predictors included their home learning environment, elementary school quality, and their family’s socioeconomic status, all factors that influence children’s educational outcomes regardless of gender.
Assertions that boys and girls learn differently due to girls’ inherent ability to cooperate and sit still for extended periods of time or higher rates of Attention Deficit/Hyperactivity Disorder (ADHD) in boys simply aren’t supported by the data, Hyde continued, but that hasn’t stopped so-called education “gurus” from promoting single-sex classrooms. In a 2014 meta-analysis of well-controlled studies with random assignment, Erin Pahlke of Whitman College, Hyde, and colleagues found no performance differences in math and other domains between single-sex and co-ed classrooms.
“What you want is a mixing,” Hyde said. “Getting them to work together and minimizing, rather than emphasizing, differences between boys and girls.”
Encouraging existing mathematical talent is important not only on the individual level, where it grants access to prestigious, high-paying jobs, Hyde explained, but on the national level.
“We can’t afford to waste 50% of our talent in the US,” she said. “We’re no longer a manufacturing society. The thing that’s going to save us in the global economic competition is our scientific and technological innovation.”
The Data on Depression
Gender stereotypes aren’t harmful only to women, of course — the depiction of depression as a “female problem” can lead clinicians to overlook boys who are struggling with the condition as well.
Rates of depression in boys and girls were thought to diverge around ages 13 to 15 years old, when rates in girls jump dramatically. But studies on the topic of gender and depression had never been subject to meta-analysis until Hyde and her team took on the challenge in 2017 — possibly because there were over 56,000 studies to wade through on the topic.
Hyde and colleagues narrowed down their search by excluding studies based on convenient samples of college students, focusing instead on representative samples drawing on populations from over 90 countries worldwide. They then split the analysis into two parts: one drawing on diagnostic measure datasets from 66 articles with 1.7 million participants, and the other drawing from 97 articles featuring over 1.9 million participants’ self-reported symptoms of depression.
The diagnosis-based meta-analysis confirmed much of the current thinking on gender and depression. Rather than computing a weighted average, Hyde used a female-to-male odds ratio (OR) to find that, averaged over all ages and nationalities included in the study, roughly 1.95 females per male met the diagnostic criteria for depression — just slightly under the commonly cited 2.0 OR.
Beyond that, there were some surprising findings, Hyde said. To begin with, the OR at age 12 was found to be 2.37, suggesting that gender differences in depression diagnoses may arise even earlier than previously believed.
Furthermore, the OR was highest — at nearly 3.0 — among 13- to 15-year-olds. From there, the OR declined across age groups, reaching the overall average of 2.0 for participants in their 20s, and remaining stable thereafter, a previously unidentified trend in adulthood, Hyde continued.
These findings have significant clinical, methodological, and theoretical implications, Hyde said. An OR of 2.0 suggests that the majority of people with depression are female, but that still means 1/3 of depressed adults are men. Yet the perception of depression as a somehow “feminine” condition can make men reluctant to seek help. The prevalence of studies on depression with single-sex designs that exclude male participants exacerbates these stereotypes, preventing progress on the question of how depression manifests in boys and men, she added.
Theoretically, Hyde continued, conceptualizations of how depression may emerge as a reaction to factors such as pubertal hormones, stress, and sexual harassment victimization in adolescence need to account for the narrowing of the gender gap in the 20s.
When Sexuality Met Gender Empowerment
Educational outcomes and measures of mental health can tell us a lot about the similarities and differences between men and women, but there are few domains of life where traditional stereotypes remain more resilient than when it comes to sexuality. In a 2010 meta-analysis of datasets from 840 articles involving more than 1.4 million participants from 82 countries, Jennifer L. Peterson and Hyde found that although differences between men’s and women’s sexual attitudes (such as supporting gay rights or premarital sex) were generally small or trivial, men were more likely to view pornography, engage in masturbation, and have casual sexual encounters.
The researchers also identified an important moderating factor: gender empowerment. In countries that the United Nations Development Programme rated as having higher gender empowerment — a measure that factors in the ratio of women to men holding parliamentary seats, average estimated income, and the percentage of women working in high-level positions such as senior management, public office, and academic jobs — the behavioral differences between men and women were smaller.
Hyde notes that they did not observe this trend for every behavior they examined, but the overall effect aligns with the social role theory, which posits that men and women behave differently in large part due to societal expectations.
In 2005, when Hyde first put forward the gender similarities hypothesis, the data created a clear pattern in support of her claim: of 124 effect sizes from 46 existing meta-analyses, 78% reported d values that were small or close to zero. The “meta-meta-analysis,” independently replicated in 2015, found evidence of some gender differences, but not nearly enough to suggest that men and women come from different psychological worlds — and studies of very young children suggest that the differences that do exist may take years of socialization to develop.
“What we need to do is think more systematically about where the messages are coming from that create those early differences,” Hyde said. “I don’t for a minute believe they’re hardwired into the brain.”
Hyde, J. S. (2005). The gender similarities hypothesis. American Psychologist , 60(6), 581-592. doi:10.1037/0003-066x.60.6.581
Hyde, J. S., Lindberg, S. M., Linn, M. C., Ellis, A. B., & Williams, C. C. (2008). Gender similarities characterize math performance. Science , 321(5888), 494-495. doi:10.1126/science.1160364
Lummis, M., & Stevenson, H. W. (1990). Gender differences in beliefs and achievement: A cross-cultural study. Developmental Psychology , 26(2), 254-263. doi:10.1037/0012-1649.26.2.254
Melhuish, E. C., Sylva, K., Sammons, P., Siraj-Blatchford, I., Taggart, B., Phan, M. B., & Malin, A. (2008). The early years: Preschool influences on mathematics achievement. Science , 321(5893), 1161-1162. doi:10.1126/science.1158808
Pahlke, E., Hyde, J., & Allison, C. M. (2014). Supplemental material for the effects of single-sex compared with coeducational schooling on students’ performance and attitudes: A meta-analysis. Psychological Bulletin . doi:10.1037/a0035740.supp
Petersen, J. L., & Hyde, J. S. (2010). A meta-analytic review of research on gender differences in sexuality, 1993–2007. Psychological Bulletin , 136(1), 21-38. doi:10.1037/a0017504
I thought that Summers’ point was that although male and female average math scores were much the same, the males showed greater variance. Hence more men at the top and bottom of the scale and thus more men in high-end math discipline. Does Prof. Shibley’s work address this point?
APS regularly opens certain online articles for discussion on our website. Effective February 2021, you must be a logged-in APS member to post comments. By posting a comment, you agree to our Community Guidelines and the display of your profile information, including your name and affiliation. Any opinions, findings, conclusions, or recommendations present in article comments are those of the writers and do not necessarily reflect the views of APS or the article’s author. For more information, please see our Community Guidelines .
Please login with your APS account to comment.

Back Page: Singular Sensation
Clinical psychologist Dominika Ochnik discusses her research into singlehood and well-being, mental health risks among young people, and her plans to study associations between urbanization and mental health.

Up-and-Coming Voices: Artificial Intelligence in Psychological Science
Previews of relevant research by students and early-career scientists.

Encouraging Girls to Roleplay as Successful Female Scientists Could Help Close the Gender Gap in STEM
Girls may persist longer in science activities when they pretend to be successful female scientists. This kind of play-based intervention could help close the gender gap in science, technology, engineering, and math (STEM) fields.
Privacy Overview
Cookie | Duration | Description |
---|---|---|
__cf_bm | 30 minutes | This cookie, set by Cloudflare, is used to support Cloudflare Bot Management. |
Cookie | Duration | Description |
---|---|---|
AWSELBCORS | 5 minutes | This cookie is used by Elastic Load Balancing from Amazon Web Services to effectively balance load on the servers. |
Cookie | Duration | Description |
---|---|---|
at-rand | never | AddThis sets this cookie to track page visits, sources of traffic and share counts. |
CONSENT | 2 years | YouTube sets this cookie via embedded youtube-videos and registers anonymous statistical data. |
uvc | 1 year 27 days | Set by addthis.com to determine the usage of addthis.com service. |
_ga | 2 years | The _ga cookie, installed by Google Analytics, calculates visitor, session and campaign data and also keeps track of site usage for the site's analytics report. The cookie stores information anonymously and assigns a randomly generated number to recognize unique visitors. |
_gat_gtag_UA_3507334_1 | 1 minute | Set by Google to distinguish users. |
_gid | 1 day | Installed by Google Analytics, _gid cookie stores information on how visitors use a website, while also creating an analytics report of the website's performance. Some of the data that are collected include the number of visitors, their source, and the pages they visit anonymously. |
Cookie | Duration | Description |
---|---|---|
loc | 1 year 27 days | AddThis sets this geolocation cookie to help understand the location of users who share the information. |
VISITOR_INFO1_LIVE | 5 months 27 days | A cookie set by YouTube to measure bandwidth that determines whether the user gets the new or old player interface. |
YSC | session | YSC cookie is set by Youtube and is used to track the views of embedded videos on Youtube pages. |
yt-remote-connected-devices | never | YouTube sets this cookie to store the video preferences of the user using embedded YouTube video. |
yt-remote-device-id | never | YouTube sets this cookie to store the video preferences of the user using embedded YouTube video. |
yt.innertube::nextId | never | This cookie, set by YouTube, registers a unique ID to store data on what videos from YouTube the user has seen. |
yt.innertube::requests | never | This cookie, set by YouTube, registers a unique ID to store data on what videos from YouTube the user has seen. |
- Bipolar Disorder
- Therapy Center
- When To See a Therapist
- Types of Therapy
- Best Online Therapy
- Best Couples Therapy
- Managing Stress
- Sleep and Dreaming
- Understanding Emotions
- Self-Improvement
- Healthy Relationships
- Student Resources
- Personality Types
- Sweepstakes
- Guided Meditations
- Verywell Mind Insights
- 2024 Verywell Mind 25
- Mental Health in the Classroom
- Editorial Process
- Meet Our Review Board
- Crisis Support
An Expert-Backed Guide to Body Dysmorphia and Gender Dysphoria
They may sound similar but they're completely different conditions
Getty Images / Verywell Mind
Understanding Dysmorphia
Understanding dysphoria, key differences between dysmorphia and dysphoria, overlapping features and challenges, treatment and support options.
Have you fallen for the dysmorphia and dysphoria homonym trap? I have. These two words might sound similar—and overlap in definitions—but they're two separate conditions.
Body dysmorphia, technically called body dysmorphic disorder, is when one sees flaws in their physical appearance that others cannot perceive whereas gender dysphoria is feeling uncomfortable in one's assigned gender.
At a Glance
Naturally, it's easy to confuse the two but both terms have different treatments and conditions. Treatment for dysphoria often includes gender-affirming surgeries whereas dysmorphia requires psychiatric help and even medication. Despite their different treatment plans, they also have a few similarities such as the barriers to treatment. Ahead you'll find everything you need to know about dysmorphia and dysphoria.
“Body dysmorphia involves perceiving certain physical flaws in your body that are not evident to other people,” explains gender-affirmed therapist Madison McCullough, LCSW . This condition lives under the “ obsessive-compulsive and related disorders ” in the DSM-5 and is driven by anxiety and misperception.
A Quick FYI
Anyone can experience dysmorphia, regardless of their gender. Chances are you have known people who believe their nose is too big or their mouth is oddly shaped. While others may see their body as completely normal in size, the person with dysmorphia perceives it differently.
Characteristics of dysmorphia include:
- Body checking, whether in the mirror alone or posted on social media
- Excessive comparison with other people's bodies
- Consistently seeking validation from others about your appearance
- Compulsive grooming, makeup, exercise, or other lifestyle habits in an attempt to change a body part
- Worrying that your body isn't okay and people will see you as less than accordingly
Some people may keep their dysmorphia to themselves, while others talk about it often. Either way, it can negatively impact one's quality of life. McCullough says dysmorphia can isolate people and lead them to suffer in silence, which can negatively impact their relationships and social support.
Dysmorphia can mistakenly be seen as vanity or self-obsession. It is an obsession in the disordered sense, but it isn't solely about vanity. Rather, it's a mental health condition that can become debilitating.
As big a deal as dysmorphia is, gender dysphoria is even more so, as it can be life-threatening. “Gender dysphoria involves persistent distress around one's embodied experience of gender,” says McCullough. “It can coincide with anxiety and depression, and be detrimental to how people carry themselves in the world.”
While it may seem like a new concept, dysphoria has existed—and researched—for years. It's only recently become more talked about.
Some symptoms of dysphoria include:
- The desire to present physically as a different gender than the one you were assigned at birth
- Feeling that the gender you were assigned is not right for you and is not who you are
- Dislike of sexual or sexualized body parts of yours
- Discomfort with physical or emotional traits you attribute to your assigned gender
- Feeling strongly that a different gender is an accurate representation of you
Just like dysmorphia, a person with dysphoria may discuss their feelings with others or not at all. It might take years for someone to speak about their condition or seek out gender-affirming help.
Did You Know?
While most people with gender dysphoria are transgender , that's not the case for everyone. A non-binary person or agender person can have dysphoria. Really, anyone who feels their body isn't aligned with their gender identity can suffer from dysphoria.
Furthermore, a person experiencing dysphoria can have dysmorphia, particularly around body parts they feel aren't aligned with their gender identity. For example, an assigned female person who identifies as a man may see their hips as very wide, even if they aren't. Conversely, a trans woman might see her shoulders as more broad than others do.
These conditions can and often do overlap, but they are not the same. Dysphoria is about gender identity while dysmorphia is about body image. Specifically, dysmorphia is seeing one's body as flawed in ways other people can't perceive, whereas dysphoria is feeling like your body isn't aligned with the gender you identify as.
A preoccupation with perceived physical flaws, such as thinking you aren't thin enough or your legs are too short.
Anxiety that your body is less than and a compulsive need to “fix” any flaws.
Feeling like the gender you were assigned to is not accurate
Believing your physical features should be of a different gender
Both dysphoria and dysmorphia involve our bodies and our comfort in them. Those who experience either of these conditions feel uncomfortable in their skin and often struggle to verbalize their feelings with others. Moreover, both dysphoria and dysmorphia are often left undiagnosed and untreated, which can impact one's quality of life.
Several boundaries hinder one from getting diagnosed. One of them is the societal stigmas around these conditions. Even after being assessed for these conditions, there's a barrier to treatment. Not all insurances cover the necessary medication or therapies, which makes it difficult for those suffering to seek appropriate help.
Naturally, to move forward from gender dysphoria or body dysmorphia, getting professional help is the right step.
“People experiencing gender dysphoria should seek out a therapist who has extensive experience working with folks across the gender spectrum, and who is knowledgeable about different gender-affirming bodily interventions available to you,” recommends McCullough. This can involve mental health services like talk therapy or gender-affirming physical changes such as surgery.
When it comes to body dysmorphia, treatment usually involves therapy, medication, or both. “People experiencing body dysmorphia often find cognitive behavioral therapy or exposure therapy helpful, as both challenge their critical beliefs about their body and identify more affirming narratives,” says McCullough. Medications like SSRIs are often prescribed for body dysmorphia as they can help lessen anxiety and depression symptoms.
If therapy is unavailable, consider community care instead. LGBT centers often have virtual and in-person support groups that center around gender issues. There's also GLAAD , which has various resources for trans and queer people. Meeting other people experiencing body dysmorphia or gender dysphoria and discussing coping mechanisms are useful tools for recovery.
Gender dysphoria and body dysmorphia are both serious conditions about our perceptions of our body. However, that's where the similarities end. Dysmorphia is an untrue perception of one's physical flaws, whereas dysphoria is a lack of congruity in one's assigned gender and gender identity.
Both conditions can be difficult to discuss with others, impact a person's self-esteem, and lead to a reduced quality of life. And each has available treatments. For gender dysphoria, you'll need gender-affirming care, which includes gender-affirming physical procedures and therapy. For dysmorphia, you'll need psychiatric help and potentially SSRI medication (if your psychiatrist deems it necessary). Even though these conditions can be severe challenges in a person's life, once faced head-on, you can work through them.
Cooper, K., Russell, A., Mandy, W., & Butler, C. (2020). The phenomenology of gender dysphoria in adults: A systematic review and meta-synthesis . Clinical Psychology Review , 80 , 101875. https://doi.org/10.1016/j.cpr.2020.101875
Marques, L., Weingarden, H. M., LeBlanc, N. J., Siev, J., & Wilhelm, S. (2011). The relationship between perceived social support and severity of body dysmorphic disorder symptoms: The role of gender . Revista Brasileira De Psiquiatria (Sao Paulo, Brazil: 1999) , 33 (3), 238–244. https://doi.org/10.1590/s1516-44462011000300006
By Ariane Resnick, CNC Ariane Resnick, CNC is a mental health writer, certified nutritionist, and wellness author who advocates for accessibility and inclusivity.
Advertisement
Supported by
5 Noteworthy Falsehoods Robert F. Kennedy Jr. Has Promoted
A longtime vaccine skeptic, Mr. Kennedy is leaning heavily on misinformation as he mounts a long-shot 2024 campaign.
- Share full article

By Anjali Huynh
He has promoted a conspiracy theory that coronavirus vaccines were developed to control people via microchips . He has endorsed the false notion that antidepressants are linked to school shootings. And he has pushed the decades-old theory that the C.I.A. killed his uncle, former President John F. Kennedy.
Robert F. Kennedy Jr., an environmental lawyer, is a leading vaccine skeptic and purveyor of conspiracy theories who has leaned heavily on misinformation as he mounts his long-shot 2024 campaign for the Democratic nomination.
But as voters express discontentment at a likely rematch between President Biden and former President Donald J. Trump, Mr. Kennedy has garnered as much as 20 percent of the vote in recent Democratic primary polling.
Mr. Biden and the Democratic National Committee have not publicly acknowledged Mr. Kennedy’s candidacy and have declined to comment on his campaign. Nevertheless, the public scrutiny that accompanies a White House bid has highlighted other questionable beliefs and statements Mr. Kennedy has elevated over the years.
Here are five of the many baseless claims Mr. Kennedy has peddled on the campaign trail and beyond.
He has falsely linked vaccines to various medical conditions.
Mr. Kennedy has promoted many false, specious or unproven claims that center on public health and the pharmaceutical industry — most notably, the scientifically discredited belief that childhood vaccines cause autism.
That notion has been rejected by more than a dozen peer-reviewed scientific studies across multiple countries. The National Academy of Medicine reviewed eight vaccines for children and adults and found that with rare exceptions, the vaccines are very safe, according to the Centers for Disease Control and Prevention.
We are having trouble retrieving the article content.
Please enable JavaScript in your browser settings.
Thank you for your patience while we verify access. If you are in Reader mode please exit and log into your Times account, or subscribe for all of The Times.
Thank you for your patience while we verify access.
Already a subscriber? Log in .
Want all of The Times? Subscribe .
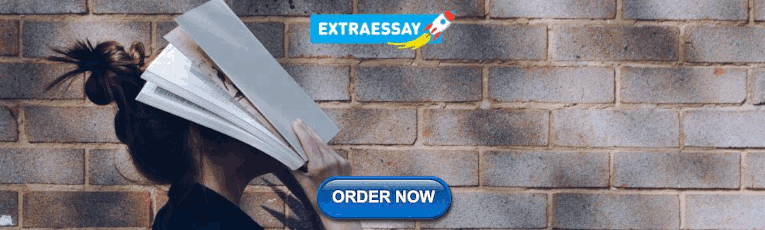
IMAGES
COMMENTS
The gender similarities hypothesis holds that males and females are similar on most, but not all, psychological variables. That is, men and women, as well as boys and girls, are more alike than they are different. In terms of effect sizes, the gender similarities hypothesis states that most psychological gender differences are in the close-to-
The gender similarities hypothesis, proposed by Hyde (2005), states that males and females are similar on most, but not all, psychological variables. Based on a meta-analysis of 46 meta-analyses of psychological gender differences, 30% of effect sizes were trivial in magnitude ( d between 0 and 0.10) and an additional 48% were small (0.10-0.35).
The differences model, which argues that males and females are vastly different psychologically, dominates the popular media. Here, the author advances a very different view, the gender similarities hypothesis, which holds that males and females are similar on most, but not all, psychological variables. Results from a review of 46 meta-analyses ...
Numerous psychological theories lay the foundation for gender similarities in psychological constructs and attempt to explain why gender differences exist. This chapter focuses on three specific theories that are often applied to the theoretical foundation of psychological gender differences. It provides a brief description of evolutionary theory, social structural theory, and cognitive social ...
The gender similarities hypothesis proposed by Hyde ( 2005) argues that women and men are similar on most, but not all, psychological variables. Evidence in favor of this hypothesis as opposed to the gender differences hypothesis is accumulating while acknowledging exceptions and the role of contextual variations.
Results from a review of 46 meta-analyses support the gender similarities hypothesis, which holds that males and females are similar on most, but not all, psychological variables. The differences model, which argues that males and females are vastly different psychologically, dominates the popular media. Here, the author advances a very different view, the gender similarities hypothesis, which ...
The differences model, which argues that males and females are vastly different psychologically, dominates the popular media. Here, the author advances a very different view, the gender similarities hypothesis, which holds that males and females are similar on most, but not all, psychological variables. Results from a review of 46 meta-analyses support the gender similarities hypothesis.
Gender similarities hypothesis: the hypothesis that males and females are similar on most, but not all, psychological variables Meta-analysis: a statistical technique that allows a researcher to combine the results of many research studies on a given topic Effect size: in the study of gender
The Gender Similarities Hypothesis. Janet Shibley. Published 2005. Psychology. TLDR. Results from a review of 46 meta-analyses support the gender similarities hypothesis, which holds that males and females are similar on most, but not all, psychological variables. Expand. gsappweb.rutgers.edu. Save to Library.
The gender similarities hypothesis holds that males. and females are similar on most, but not all, psychological. variables. That is, men and women, as well as boys and. girls, are more alike than ...
Whether men and women are fundamentally different or similar has been debated for more than a century. This review summarizes major theories designed to explain gender differences: evolutionary theories, cognitive social learning theory, sociocultural theory, and expectancy-value theory. The gender similarities hypothesis raises the possibility of theorizing gender similarities.
Whether men and women are fundamentally different or similar has been debated for more than a century. This review summarizes major theories designed to explain gender differences: evolutionary theories, cognitive social learning theory, sociocultural theory, and expectancy-value theory. The gender similarities hypothesis raises the possibility of theorizing gender similarities.
The magnitude of differences between women and men is an unsettled issue in psychological science. One prominent claim, known as the gender-similarities hypothesis, is that women and men are very similar on most psychological variables (Hyde, 2005, 2014).
The analysis reported upon in this paper is used to test the gender similarities hypothesis (Hyde, 2005, 2014, 2016) in relation to student achievement in high-stakes mathematics examinations in Northern Ireland.Based on a review of 46 meta-analyses, Hyde concluded that, "males and females are similar on most, but not all, psychological variables" (p. 581).
Abstract. I review new trends in research on the psychology of gender. The gender similarities hypothesis holds that males and females are similar on most, but not all, psychological variables. Gender is not only an individual-difference or person variable but also a stimulus variable. Emerging approaches to cross-national measurement of ...
The gender similarities hypothesis raises the possibility of theorizing gender similarities. Statistical methods for the analysis of gender differences and similarities are reviewed, including effect sizes, meta-analysis, taxometric analysis, and equivalence testing. Then, relying mainly on evidence from meta-analyses, gender differences are ...
Hyde observed that across the dozens of studies, consistent with the gender similarities hypothesis, gender differences had either no or a very small effect on most of the psychological variables examined. Only a few main differences appeared: Compared with women, men could throw farther, were more physically aggressive, masturbated more, and ...
The gender similarities hypothesis. Am. Psychol. 60, 581-592 (2005). Article Google Scholar American Association of University Women Educational Foundation. How Schools Shortchange Girls.
The Gender Similarities Hypothesis asserts that females and males are more similar than different for most psychological measures. Gender stereotypes hold that males outperform females in mathematics and spatial tests, and females outperform males on verbal tests. According to meta-analyses, however, among both children and adults, females ...
The Gender Similarities Hypothesis (5) provides an alterna-tive view, stating instead that males and females are very similar on most, but not all, psycholog-ical variables. Evidence for Gender Similarities A review of meta-analyses of research on psychological gender differences identified
Conversely, the gender-similarities hypothesis assumes that they're highly similar across many domains. Researchers at the University of North Carolina, Iowa State University and Western Carolina University sought to examine these two hypotheses in a 2015 metastudy, "Evaluating Gender Similarities and Differences Using Metasynthesis.".
In 2005, when Hyde first put forward the gender similarities hypothesis, the data created a clear pattern in support of her claim: of 124 effect sizes from 46 existing meta-analyses, 78% reported d values that were small or close to zero. The "meta-meta-analysis," independently replicated in 2015, found evidence of some gender differences ...
The Gender Similarities Hypothesis provides an alternative view, stating instead that males and females are very similar on most, but not all, ... The article, "Gender Similarities in Mathematics and Science," by J. S. Hyde and M. C. Linn (27 Oct. 2006, p. 599), makes one very important point but obscures another. ...
Learn about the differences (and similarities) between these two conditions. One is about a perception of your body that others can't see, and the other is a misalignment in your gender identity to what you were assigned. Learn about the differences (and similarities) between these two conditions. Menu. Conditions A-Z Addiction; Depression; ADHD;
Robert F. Kennedy Jr. announced in April that he would seek the Democratic presidential nomination. He has garnered an unusual amount of support during a re-election campaign for a sitting president.