- Open access
- Published: 08 June 2022
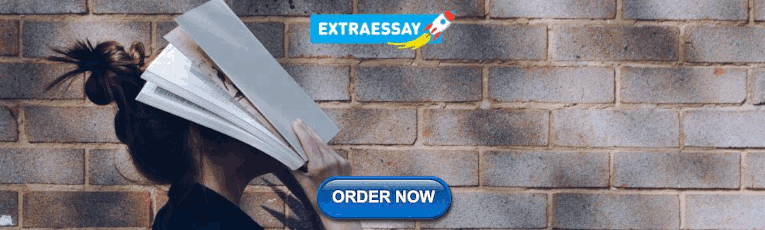
A systematic review on digital literacy
- Hasan Tinmaz ORCID: orcid.org/0000-0003-4310-0848 1 ,
- Yoo-Taek Lee ORCID: orcid.org/0000-0002-1913-9059 2 ,
- Mina Fanea-Ivanovici ORCID: orcid.org/0000-0003-2921-2990 3 &
- Hasnan Baber ORCID: orcid.org/0000-0002-8951-3501 4
Smart Learning Environments volume 9 , Article number: 21 ( 2022 ) Cite this article
49k Accesses
39 Citations
10 Altmetric
Metrics details
The purpose of this study is to discover the main themes and categories of the research studies regarding digital literacy. To serve this purpose, the databases of WoS/Clarivate Analytics, Proquest Central, Emerald Management Journals, Jstor Business College Collections and Scopus/Elsevier were searched with four keyword-combinations and final forty-three articles were included in the dataset. The researchers applied a systematic literature review method to the dataset. The preliminary findings demonstrated that there is a growing prevalence of digital literacy articles starting from the year 2013. The dominant research methodology of the reviewed articles is qualitative. The four major themes revealed from the qualitative content analysis are: digital literacy, digital competencies, digital skills and digital thinking. Under each theme, the categories and their frequencies are analysed. Recommendations for further research and for real life implementations are generated.
Introduction
The extant literature on digital literacy, skills and competencies is rich in definitions and classifications, but there is still no consensus on the larger themes and subsumed themes categories. (Heitin, 2016 ). To exemplify, existing inventories of Internet skills suffer from ‘incompleteness and over-simplification, conceptual ambiguity’ (van Deursen et al., 2015 ), and Internet skills are only a part of digital skills. While there is already a plethora of research in this field, this research paper hereby aims to provide a general framework of digital areas and themes that can best describe digital (cap)abilities in the novel context of Industry 4.0 and the accelerated pandemic-triggered digitalisation. The areas and themes can represent the starting point for drafting a contemporary digital literacy framework.
Sousa and Rocha ( 2019 ) explained that there is a stake of digital skills for disruptive digital business, and they connect it to the latest developments, such as the Internet of Things (IoT), cloud technology, big data, artificial intelligence, and robotics. The topic is even more important given the large disparities in digital literacy across regions (Tinmaz et al., 2022 ). More precisely, digital inequalities encompass skills, along with access, usage and self-perceptions. These inequalities need to be addressed, as they are credited with a ‘potential to shape life chances in multiple ways’ (Robinson et al., 2015 ), e.g., academic performance, labour market competitiveness, health, civic and political participation. Steps have been successfully taken to address physical access gaps, but skills gaps are still looming (Van Deursen & Van Dijk, 2010a ). Moreover, digital inequalities have grown larger due to the COVID-19 pandemic, and they influenced the very state of health of the most vulnerable categories of population or their employability in a time when digital skills are required (Baber et al., 2022 ; Beaunoyer, Dupéré & Guitton, 2020 ).
The systematic review the researchers propose is a useful updated instrument of classification and inventory for digital literacy. Considering the latest developments in the economy and in line with current digitalisation needs, digitally literate population may assist policymakers in various fields, e.g., education, administration, healthcare system, and managers of companies and other concerned organisations that need to stay competitive and to employ competitive workforce. Therefore, it is indispensably vital to comprehend the big picture of digital literacy related research.
Literature review
Since the advent of Digital Literacy, scholars have been concerned with identifying and classifying the various (cap)abilities related to its operation. Using the most cited academic papers in this stream of research, several classifications of digital-related literacies, competencies, and skills emerged.
Digital literacies
Digital literacy, which is one of the challenges of integration of technology in academic courses (Blau, Shamir-Inbal & Avdiel, 2020 ), has been defined in the current literature as the competencies and skills required for navigating a fragmented and complex information ecosystem (Eshet, 2004 ). A ‘Digital Literacy Framework’ was designed by Eshet-Alkalai ( 2012 ), comprising six categories: (a) photo-visual thinking (understanding and using visual information); (b) real-time thinking (simultaneously processing a variety of stimuli); (c) information thinking (evaluating and combining information from multiple digital sources); (d) branching thinking (navigating in non-linear hyper-media environments); (e) reproduction thinking (creating outcomes using technological tools by designing new content or remixing existing digital content); (f) social-emotional thinking (understanding and applying cyberspace rules). According to Heitin ( 2016 ), digital literacy groups the following clusters: (a) finding and consuming digital content; (b) creating digital content; (c) communicating or sharing digital content. Hence, the literature describes the digital literacy in many ways by associating a set of various technical and non-technical elements.
- Digital competencies
The Digital Competence Framework for Citizens (DigComp 2.1.), the most recent framework proposed by the European Union, which is currently under review and undergoing an updating process, contains five competency areas: (a) information and data literacy, (b) communication and collaboration, (c) digital content creation, (d) safety, and (e) problem solving (Carretero, Vuorikari & Punie, 2017 ). Digital competency had previously been described in a technical fashion by Ferrari ( 2012 ) as a set comprising information skills, communication skills, content creation skills, safety skills, and problem-solving skills, which later outlined the areas of competence in DigComp 2.1, too.
- Digital skills
Ng ( 2012 ) pointed out the following three categories of digital skills: (a) technological (using technological tools); (b) cognitive (thinking critically when managing information); (c) social (communicating and socialising). A set of Internet skill was suggested by Van Deursen and Van Dijk ( 2009 , 2010b ), which contains: (a) operational skills (basic skills in using internet technology), (b) formal Internet skills (navigation and orientation skills); (c) information Internet skills (fulfilling information needs), and (d) strategic Internet skills (using the internet to reach goals). In 2014, the same authors added communication and content creation skills to the initial framework (van Dijk & van Deursen). Similarly, Helsper and Eynon ( 2013 ) put forward a set of four digital skills: technical, social, critical, and creative skills. Furthermore, van Deursen et al. ( 2015 ) built a set of items and factors to measure Internet skills: operational, information navigation, social, creative, mobile. More recent literature (vaan Laar et al., 2017 ) divides digital skills into seven core categories: technical, information management, communication, collaboration, creativity, critical thinking, and problem solving.
It is worth mentioning that the various methodologies used to classify digital literacy are overlapping or non-exhaustive, which confirms the conceptual ambiguity mentioned by van Deursen et al. ( 2015 ).
- Digital thinking
Thinking skills (along with digital skills) have been acknowledged to be a significant element of digital literacy in the educational process context (Ferrari, 2012 ). In fact, critical thinking, creativity, and innovation are at the very core of DigComp. Information and Communication Technology as a support for thinking is a learning objective in any school curriculum. In the same vein, analytical thinking and interdisciplinary thinking, which help solve problems, are yet other concerns of educators in the Industry 4.0 (Ozkan-Ozen & Kazancoglu, 2021 ).
However, we have recently witnessed a shift of focus from learning how to use information and communication technologies to using it while staying safe in the cyber-environment and being aware of alternative facts. Digital thinking would encompass identifying fake news, misinformation, and echo chambers (Sulzer, 2018 ). Not least important, concern about cybersecurity has grown especially in times of political, social or economic turmoil, such as the elections or the Covid-19 crisis (Sulzer, 2018 ; Puig, Blanco-Anaya & Perez-Maceira, 2021 ).
Ultimately, this systematic review paper focuses on the following major research questions as follows:
Research question 1: What is the yearly distribution of digital literacy related papers?
Research question 2: What are the research methods for digital literacy related papers?
Research question 3: What are the main themes in digital literacy related papers?
Research question 4: What are the concentrated categories (under revealed main themes) in digital literacy related papers?
This study employed the systematic review method where the authors scrutinized the existing literature around the major research question of digital literacy. As Uman ( 2011 ) pointed, in systematic literature review, the findings of the earlier research are examined for the identification of consistent and repetitive themes. The systematic review method differs from literature review with its well managed and highly organized qualitative scrutiny processes where researchers tend to cover less materials from fewer number of databases to write their literature review (Kowalczyk & Truluck, 2013 ; Robinson & Lowe, 2015 ).
Data collection
To address major research objectives, the following five important databases are selected due to their digital literacy focused research dominance: 1. WoS/Clarivate Analytics, 2. Proquest Central; 3. Emerald Management Journals; 4. Jstor Business College Collections; 5. Scopus/Elsevier.
The search was made in the second half of June 2021, in abstract and key words written in English language. We only kept research articles and book chapters (herein referred to as papers). Our purpose was to identify a set of digital literacy areas, or an inventory of such areas and topics. To serve that purpose, systematic review was utilized with the following synonym key words for the search: ‘digital literacy’, ‘digital skills’, ‘digital competence’ and ‘digital fluency’, to find the mainstream literature dealing with the topic. These key words were unfolded as a result of the consultation with the subject matter experts (two board members from Korean Digital Literacy Association and two professors from technology studies department). Below are the four key word combinations used in the search: “Digital literacy AND systematic review”, “Digital skills AND systematic review”, “Digital competence AND systematic review”, and “Digital fluency AND systematic review”.
A sequential systematic search was made in the five databases mentioned above. Thus, from one database to another, duplicate papers were manually excluded in a cascade manner to extract only unique results and to make the research smoother to conduct. At this stage, we kept 47 papers. Further exclusion criteria were applied. Thus, only full-text items written in English were selected, and in doing so, three papers were excluded (no full text available), and one other paper was excluded because it was not written in English, but in Spanish. Therefore, we investigated a total number of 43 papers, as shown in Table 1 . “ Appendix A ” shows the list of these papers with full references.
Data analysis
The 43 papers selected after the application of the inclusion and exclusion criteria, respectively, were reviewed the materials independently by two researchers who were from two different countries. The researchers identified all topics pertaining to digital literacy, as they appeared in the papers. Next, a third researcher independently analysed these findings by excluded duplicates A qualitative content analysis was manually performed by calculating the frequency of major themes in all papers, where the raw data was compared and contrasted (Fraenkel et al., 2012 ). All three reviewers independently list the words and how the context in which they appeared and then the three reviewers collectively decided for how it should be categorized. Lastly, it is vital to remind that literature review of this article was written after the identification of the themes appeared as a result of our qualitative analyses. Therefore, the authors decided to shape the literature review structure based on the themes.
As an answer to the first research question (the yearly distribution of digital literacy related papers), Fig. 1 demonstrates the yearly distribution of digital literacy related papers. It is seen that there is an increasing trend about the digital literacy papers.
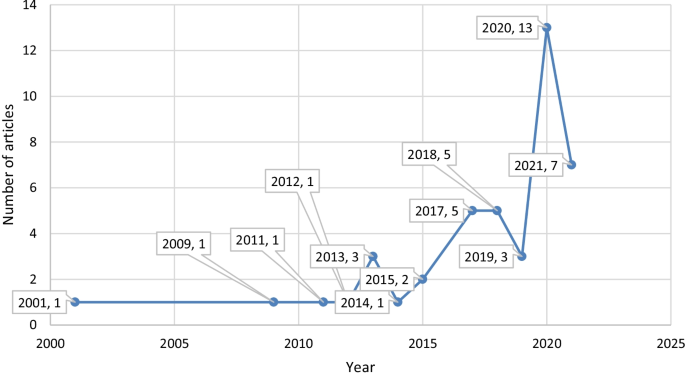
Yearly distribution of digital literacy related papers
Research question number two (The research methods for digital literacy related papers) concentrates on what research methods are employed for these digital literacy related papers. As Fig. 2 shows, most of the papers were using the qualitative method. Not stated refers to book chapters.
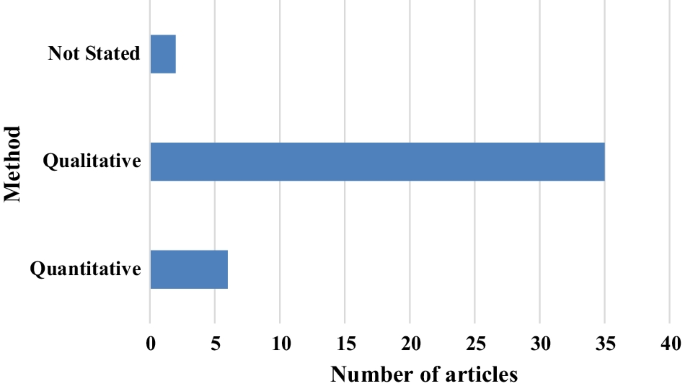
Research methods used in the reviewed articles
When forty-three articles were analysed for the main themes as in research question number three (The main themes in digital literacy related papers), the overall findings were categorized around four major themes: (i) literacies, (ii) competencies, (iii) skills, and (iv) thinking. Under every major theme, the categories were listed and explained as in research question number four (The concentrated categories (under revealed main themes) in digital literacy related papers).
The authors utilized an overt categorization for the depiction of these major themes. For example, when the ‘creativity’ was labelled as a skill, the authors also categorized it under the ‘skills’ theme. Similarly, when ‘creativity’ was mentioned as a competency, the authors listed it under the ‘competencies’ theme. Therefore, it is possible to recognize the same finding under different major themes.
Major theme 1: literacies
Digital literacy being the major concern of this paper was observed to be blatantly mentioned in five papers out forty-three. One of these articles described digital literacy as the human proficiencies to live, learn and work in the current digital society. In addition to these five articles, two additional papers used the same term as ‘critical digital literacy’ by describing it as a person’s or a society’s accessibility and assessment level interaction with digital technologies to utilize and/or create information. Table 2 summarizes the major categories under ‘Literacies’ major theme.
Computer literacy, media literacy and cultural literacy were the second most common literacy (n = 5). One of the article branches computer literacy as tool (detailing with software and hardware uses) and resource (focusing on information processing capacity of a computer) literacies. Cultural literacy was emphasized as a vital element for functioning in an intercultural team on a digital project.
Disciplinary literacy (n = 4) was referring to utilizing different computer programs (n = 2) or technical gadgets (n = 2) with a specific emphasis on required cognitive, affective and psychomotor skills to be able to work in any digital context (n = 3), serving for the using (n = 2), creating and applying (n = 2) digital literacy in real life.
Data literacy, technology literacy and multiliteracy were the third frequent categories (n = 3). The ‘multiliteracy’ was referring to the innate nature of digital technologies, which have been infused into many aspects of human lives.
Last but not least, Internet literacy, mobile literacy, web literacy, new literacy, personal literacy and research literacy were discussed in forty-three article findings. Web literacy was focusing on being able to connect with people on the web (n = 2), discover the web content (especially the navigation on a hyper-textual platform), and learn web related skills through practical web experiences. Personal literacy was highlighting digital identity management. Research literacy was not only concentrating on conducting scientific research ability but also finding available scholarship online.
Twenty-four other categories are unfolded from the results sections of forty-three articles. Table 3 presents the list of these other literacies where the authors sorted the categories in an ascending alphabetical order without any other sorting criterion. Primarily, search, tagging, filtering and attention literacies were mainly underlining their roles in information processing. Furthermore, social-structural literacy was indicated as the recognition of the social circumstances and generation of information. Another information-related literacy was pointed as publishing literacy, which is the ability to disseminate information via different digital channels.
While above listed personal literacy was referring to digital identity management, network literacy was explained as someone’s social networking ability to manage the digital relationship with other people. Additionally, participatory literacy was defined as the necessary abilities to join an online team working on online content production.
Emerging technology literacy was stipulated as an essential ability to recognize and appreciate the most recent and innovative technologies in along with smart choices related to these technologies. Additionally, the critical literacy was added as an ability to make smart judgements on the cost benefit analysis of these recent technologies.
Last of all, basic, intermediate, and advanced digital assessment literacies were specified for educational institutions that are planning to integrate various digital tools to conduct instructional assessments in their bodies.
Major theme 2: competencies
The second major theme was revealed as competencies. The authors directly categorized the findings that are specified with the word of competency. Table 4 summarizes the entire category set for the competencies major theme.
The most common category was the ‘digital competence’ (n = 14) where one of the articles points to that category as ‘generic digital competence’ referring to someone’s creativity for multimedia development (video editing was emphasized). Under this broad category, the following sub-categories were associated:
Problem solving (n = 10)
Safety (n = 7)
Information processing (n = 5)
Content creation (n = 5)
Communication (n = 2)
Digital rights (n = 1)
Digital emotional intelligence (n = 1)
Digital teamwork (n = 1)
Big data utilization (n = 1)
Artificial Intelligence utilization (n = 1)
Virtual leadership (n = 1)
Self-disruption (in along with the pace of digitalization) (n = 1)
Like ‘digital competency’, five additional articles especially coined the term as ‘digital competence as a life skill’. Deeper analysis demonstrated the following points: social competences (n = 4), communication in mother tongue (n = 3) and foreign language (n = 2), entrepreneurship (n = 3), civic competence (n = 2), fundamental science (n = 1), technology (n = 1) and mathematics (n = 1) competences, learning to learn (n = 1) and self-initiative (n = 1).
Moreover, competencies were linked to workplace digital competencies in three articles and highlighted as significant for employability (n = 3) and ‘economic engagement’ (n = 3). Digital competencies were also detailed for leisure (n = 2) and communication (n = 2). Furthermore, two articles pointed digital competencies as an inter-cultural competency and one as a cross-cultural competency. Lastly, the ‘digital nativity’ (n = 1) was clarified as someone’s innate competency of being able to feel contented and satisfied with digital technologies.
Major theme 3: skills
The third major observed theme was ‘skills’, which was dominantly gathered around information literacy skills (n = 19) and information and communication technologies skills (n = 18). Table 5 demonstrates the categories with more than one occurrence.
Table 6 summarizes the sub-categories of the two most frequent categories of ‘skills’ major theme. The information literacy skills noticeably concentrate on the steps of information processing; evaluation (n = 6), utilization (n = 4), finding (n = 3), locating (n = 2) information. Moreover, the importance of trial/error process, being a lifelong learner, feeling a need for information and so forth were evidently listed under this sub-category. On the other hand, ICT skills were grouped around cognitive and affective domains. For instance, while technical skills in general and use of social media, coding, multimedia, chat or emailing in specific were reported in cognitive domain, attitude, intention, and belief towards ICT were mentioned as the elements of affective domain.
Communication skills (n = 9) were multi-dimensional for different societies, cultures, and globalized contexts, requiring linguistic skills. Collaboration skills (n = 9) are also recurrently cited with an explicit emphasis for virtual platforms.
‘Ethics for digital environment’ encapsulated ethical use of information (n = 4) and different technologies (n = 2), knowing digital laws (n = 2) and responsibilities (n = 2) in along with digital rights and obligations (n = 1), having digital awareness (n = 1), following digital etiquettes (n = 1), treating other people with respect (n = 1) including no cyber-bullying (n = 1) and no stealing or damaging other people (n = 1).
‘Digital fluency’ involved digital access (n = 2) by using different software and hardware (n = 2) in online platforms (n = 1) or communication tools (n = 1) or within programming environments (n = 1). Digital fluency also underlined following recent technological advancements (n = 1) and knowledge (n = 1) including digital health and wellness (n = 1) dimension.
‘Social intelligence’ related to understanding digital culture (n = 1), the concept of digital exclusion (n = 1) and digital divide (n = 3). ‘Research skills’ were detailed with searching academic information (n = 3) on databases such as Web of Science and Scopus (n = 2) and their citation, summarization, and quotation (n = 2).
‘Digital teaching’ was described as a skill (n = 2) in Table 4 whereas it was also labelled as a competence (n = 1) as shown in Table 3 . Similarly, while learning to learn (n = 1) was coined under competencies in Table 3 , digital learning (n = 2, Table 4 ) and life-long learning (n = 1, Table 5 ) were stated as learning related skills. Moreover, learning was used with the following three terms: learning readiness (n = 1), self-paced learning (n = 1) and learning flexibility (n = 1).
Table 7 shows other categories listed below the ‘skills’ major theme. The list covers not only the software such as GIS, text mining, mapping, or bibliometric analysis programs but also the conceptual skills such as the fourth industrial revolution and information management.
Major theme 4: thinking
The last identified major theme was the different types of ‘thinking’. As Table 8 shows, ‘critical thinking’ was the most frequent thinking category (n = 4). Except computational thinking, the other categories were not detailed.
Computational thinking (n = 3) was associated with the general logic of how a computer works and sub-categorized into the following steps; construction of the problem (n = 3), abstraction (n = 1), disintegration of the problem (n = 2), data collection, (n = 2), data analysis (n = 2), algorithmic design (n = 2), parallelization & iteration (n = 1), automation (n = 1), generalization (n = 1), and evaluation (n = 2).
A transversal analysis of digital literacy categories reveals the following fields of digital literacy application:
Technological advancement (IT, ICT, Industry 4.0, IoT, text mining, GIS, bibliometric analysis, mapping data, technology, AI, big data)
Networking (Internet, web, connectivity, network, safety)
Information (media, news, communication)
Creative-cultural industries (culture, publishing, film, TV, leisure, content creation)
Academia (research, documentation, library)
Citizenship (participation, society, social intelligence, awareness, politics, rights, legal use, ethics)
Education (life skills, problem solving, teaching, learning, education, lifelong learning)
Professional life (work, teamwork, collaboration, economy, commerce, leadership, decision making)
Personal level (critical thinking, evaluation, analytical thinking, innovative thinking)
This systematic review on digital literacy concentrated on forty-three articles from the databases of WoS/Clarivate Analytics, Proquest Central, Emerald Management Journals, Jstor Business College Collections and Scopus/Elsevier. The initial results revealed that there is an increasing trend on digital literacy focused academic papers. Research work in digital literacy is critical in a context of disruptive digital business, and more recently, the pandemic-triggered accelerated digitalisation (Beaunoyer, Dupéré & Guitton, 2020 ; Sousa & Rocha 2019 ). Moreover, most of these papers were employing qualitative research methods. The raw data of these articles were analysed qualitatively using systematic literature review to reveal major themes and categories. Four major themes that appeared are: digital literacy, digital competencies, digital skills and thinking.
Whereas the mainstream literature describes digital literacy as a set of photo-visual, real-time, information, branching, reproduction and social-emotional thinking (Eshet-Alkalai, 2012 ) or as a set of precise specific operations, i.e., finding, consuming, creating, communicating and sharing digital content (Heitin, 2016 ), this study reveals that digital literacy revolves around and is in connection with the concepts of computer literacy, media literacy, cultural literacy or disciplinary literacy. In other words, the present systematic review indicates that digital literacy is far broader than specific tasks, englobing the entire sphere of computer operation and media use in a cultural context.
The digital competence yardstick, DigComp (Carretero, Vuorikari & Punie, 2017 ) suggests that the main digital competencies cover information and data literacy, communication and collaboration, digital content creation, safety, and problem solving. Similarly, the findings of this research place digital competencies in relation to problem solving, safety, information processing, content creation and communication. Therefore, the findings of the systematic literature review are, to a large extent, in line with the existing framework used in the European Union.
The investigation of the main keywords associated with digital skills has revealed that information literacy, ICT, communication, collaboration, digital content creation, research and decision-making skill are the most representative. In a structured way, the existing literature groups these skills in technological, cognitive, and social (Ng, 2012 ) or, more extensively, into operational, formal, information Internet, strategic, communication and content creation (van Dijk & van Deursen, 2014 ). In time, the literature has become richer in frameworks, and prolific authors have improved their results. As such, more recent research (vaan Laar et al., 2017 ) use the following categories: technical, information management, communication, collaboration, creativity, critical thinking, and problem solving.
Whereas digital thinking was observed to be mostly related with critical thinking and computational thinking, DigComp connects it with critical thinking, creativity, and innovation, on the one hand, and researchers highlight fake news, misinformation, cybersecurity, and echo chambers as exponents of digital thinking, on the other hand (Sulzer, 2018 ; Puig, Blanco-Anaya & Perez-Maceira, 2021 ).
This systematic review research study looks ahead to offer an initial step and guideline for the development of a more contemporary digital literacy framework including digital literacy major themes and factors. The researchers provide the following recommendations for both researchers and practitioners.
Recommendations for prospective research
By considering the major qualitative research trend, it seems apparent that more quantitative research-oriented studies are needed. Although it requires more effort and time, mixed method studies will help understand digital literacy holistically.
As digital literacy is an umbrella term for many different technologies, specific case studies need be designed, such as digital literacy for artificial intelligence or digital literacy for drones’ usage.
Digital literacy affects different areas of human lives, such as education, business, health, governance, and so forth. Therefore, different case studies could be carried out for each of these unique dimensions of our lives. For instance, it is worth investigating the role of digital literacy on lifelong learning in particular, and on education in general, as well as the digital upskilling effects on the labour market flexibility.
Further experimental studies on digital literacy are necessary to realize how certain variables (for instance, age, gender, socioeconomic status, cognitive abilities, etc.) affect this concept overtly or covertly. Moreover, the digital divide issue needs to be analysed through the lens of its main determinants.
New bibliometric analysis method can be implemented on digital literacy documents to reveal more information on how these works are related or centred on what major topic. This visual approach will assist to realize the big picture within the digital literacy framework.
Recommendations for practitioners
The digital literacy stakeholders, policymakers in education and managers in private organizations, need to be aware that there are many dimensions and variables regarding the implementation of digital literacy. In that case, stakeholders must comprehend their beneficiaries or the participants more deeply to increase the effect of digital literacy related activities. For example, critical thinking and problem-solving skills and abilities are mentioned to affect digital literacy. Hence, stakeholders have to initially understand whether the participants have enough entry level critical thinking and problem solving.
Development of digital literacy for different groups of people requires more energy, since each group might require a different set of skills, abilities, or competencies. Hence, different subject matter experts, such as technologists, instructional designers, content experts, should join the team.
It is indispensably vital to develop different digital frameworks for different technologies (basic or advanced) or different contexts (different levels of schooling or various industries).
These frameworks should be updated regularly as digital fields are evolving rapidly. Every year, committees should gather around to understand new technological trends and decide whether they should address the changes into their frameworks.
Understanding digital literacy in a thorough manner can enable decision makers to correctly implement and apply policies addressing the digital divide that is reflected onto various aspects of life, e.g., health, employment, education, especially in turbulent times such as the COVID-19 pandemic is.
Lastly, it is also essential to state the study limitations. This study is limited to the analysis of a certain number of papers, obtained from using the selected keywords and databases. Therefore, an extension can be made by adding other keywords and searching other databases.
Availability of data and materials
The authors present the articles used for the study in “ Appendix A ”.
Baber, H., Fanea-Ivanovici, M., Lee, Y. T., & Tinmaz, H. (2022). A bibliometric analysis of digital literacy research and emerging themes pre-during COVID-19 pandemic. Information and Learning Sciences . https://doi.org/10.1108/ILS-10-2021-0090 .
Article Google Scholar
Beaunoyer, E., Dupéré, S., & Guitton, M. J. (2020). COVID-19 and digital inequalities: Reciprocal impacts and mitigation strategies. Computers in Human Behavior, 111 , 10642. https://doi.org/10.1016/j.chb.2020.106424
Blau, I., Shamir-Inbal, T., & Avdiel, O. (2020). How does the pedagogical design of a technology-enhanced collaborative academic course promote digital literacies, self-regulation, and perceived learning of students? The Internet and Higher Education, 45 , 100722. https://doi.org/10.1016/j.iheduc.2019.100722
Carretero, S., Vuorikari, R., & Punie, Y. (2017). DigComp 2.1: The Digital Competence Framework for Citizens with eight proficiency levels and examples of use (No. JRC106281). Joint Research Centre, https://publications.jrc.ec.europa.eu/repository/handle/JRC106281
Eshet, Y. (2004). Digital literacy: A conceptual framework for survival skills in the digital era. Journal of Educational Multimedia and Hypermedia , 13 (1), 93–106, https://www.learntechlib.org/primary/p/4793/
Eshet-Alkalai, Y. (2012). Thinking in the digital era: A revised model for digital literacy. Issues in Informing Science and Information Technology, 9 (2), 267–276. https://doi.org/10.28945/1621
Ferrari, A. (2012). Digital competence in practice: An analysis of frameworks. JCR IPTS, Sevilla. https://ifap.ru/library/book522.pdf
Fraenkel, J. R., Wallen, N. E., & Hyun, H. H. (2012). How to design and evaluate research in education (8th ed.). Mc Graw Hill.
Google Scholar
Heitin, L. (2016). What is digital literacy? Education Week, https://www.edweek.org/teaching-learning/what-is-digital-literacy/2016/11
Helsper, E. J., & Eynon, R. (2013). Distinct skill pathways to digital engagement. European Journal of Communication, 28 (6), 696–713. https://doi.org/10.1177/0267323113499113
Kowalczyk, N., & Truluck, C. (2013). Literature reviews and systematic reviews: What is the difference ? . Radiologic Technology, 85 (2), 219–222.
Ng, W. (2012). Can we teach digital natives digital literacy? Computers & Education, 59 (3), 1065–1078. https://doi.org/10.1016/j.compedu.2012.04.016
Ozkan-Ozen, Y. D., & Kazancoglu, Y. (2021). Analysing workforce development challenges in the Industry 4.0. International Journal of Manpower . https://doi.org/10.1108/IJM-03-2021-0167
Puig, B., Blanco-Anaya, P., & Perez-Maceira, J. J. (2021). “Fake News” or Real Science? Critical thinking to assess information on COVID-19. Frontiers in Education, 6 , 646909. https://doi.org/10.3389/feduc.2021.646909
Robinson, L., Cotten, S. R., Ono, H., Quan-Haase, A., Mesch, G., Chen, W., Schulz, J., Hale, T. M., & Stern, M. J. (2015). Digital inequalities and why they matter. Information, Communication & Society, 18 (5), 569–582. https://doi.org/10.1080/1369118X.2015.1012532
Robinson, P., & Lowe, J. (2015). Literature reviews vs systematic reviews. Australian and New Zealand Journal of Public Health, 39 (2), 103. https://doi.org/10.1111/1753-6405.12393
Sousa, M. J., & Rocha, A. (2019). Skills for disruptive digital business. Journal of Business Research, 94 , 257–263. https://doi.org/10.1016/j.jbusres.2017.12.051
Sulzer, A. (2018). (Re)conceptualizing digital literacies before and after the election of Trump. English Teaching: Practice & Critique, 17 (2), 58–71. https://doi.org/10.1108/ETPC-06-2017-0098
Tinmaz, H., Fanea-Ivanovici, M., & Baber, H. (2022). A snapshot of digital literacy. Library Hi Tech News , (ahead-of-print).
Uman, L. S. (2011). Systematic reviews and meta-analyses. Journal of the Canadian Academy of Child and Adolescent Psychiatry, 20 (1), 57–59.
Van Deursen, A. J. A. M., Helsper, E. J., & Eynon, R. (2015). Development and validation of the Internet Skills Scale (ISS). Information, Communication & Society, 19 (6), 804–823. https://doi.org/10.1080/1369118X.2015.1078834
Van Deursen, A. J. A. M., & van Dijk, J. A. G. M. (2009). Using the internet: Skills related problems in users’ online behaviour. Interacting with Computers, 21 , 393–402. https://doi.org/10.1016/j.intcom.2009.06.005
Van Deursen, A. J. A. M., & van Dijk, J. A. G. M. (2010a). Measuring internet skills. International Journal of Human-Computer Interaction, 26 (10), 891–916. https://doi.org/10.1080/10447318.2010.496338
Van Deursen, A. J. A. M., & van Dijk, J. A. G. M. (2010b). Internet skills and the digital divide. New Media & Society, 13 (6), 893–911. https://doi.org/10.1177/1461444810386774
van Dijk, J. A. G. M., & Van Deursen, A. J. A. M. (2014). Digital skills, unlocking the information society . Palgrave MacMillan.
van Laar, E., van Deursen, A. J. A. M., van Dijk, J. A. G. M., & de Haan, J. (2017). The relation between 21st-century skills and digital skills: A systematic literature review. Computer in Human Behavior, 72 , 577–588. https://doi.org/10.1016/j.chb.2017.03.010
Download references
This research is funded by Woosong University Academic Research in 2022.
Author information
Authors and affiliations.
AI & Big Data Department, Endicott College of International Studies, Woosong University, Daejeon, South Korea
Hasan Tinmaz
Endicott College of International Studies, Woosong University, Daejeon, South Korea
Yoo-Taek Lee
Department of Economics and Economic Policies, Bucharest University of Economic Studies, Bucharest, Romania
Mina Fanea-Ivanovici
Abu Dhabi School of Management, Abu Dhabi, United Arab Emirates
Hasnan Baber
You can also search for this author in PubMed Google Scholar
Contributions
The authors worked together on the manuscript equally. All authors have read and approved the final manuscript.
Corresponding author
Correspondence to Hasnan Baber .
Ethics declarations
Competing of interests.
The authors declare that they have no competing interests.
Additional information
Publisher's note.
Springer Nature remains neutral with regard to jurisdictional claims in published maps and institutional affiliations.
Rights and permissions
Open Access This article is licensed under a Creative Commons Attribution 4.0 International License, which permits use, sharing, adaptation, distribution and reproduction in any medium or format, as long as you give appropriate credit to the original author(s) and the source, provide a link to the Creative Commons licence, and indicate if changes were made. The images or other third party material in this article are included in the article's Creative Commons licence, unless indicated otherwise in a credit line to the material. If material is not included in the article's Creative Commons licence and your intended use is not permitted by statutory regulation or exceeds the permitted use, you will need to obtain permission directly from the copyright holder. To view a copy of this licence, visit http://creativecommons.org/licenses/by/4.0/ .
Reprints and permissions
About this article
Cite this article.
Tinmaz, H., Lee, YT., Fanea-Ivanovici, M. et al. A systematic review on digital literacy. Smart Learn. Environ. 9 , 21 (2022). https://doi.org/10.1186/s40561-022-00204-y
Download citation
Received : 23 February 2022
Accepted : 01 June 2022
Published : 08 June 2022
DOI : https://doi.org/10.1186/s40561-022-00204-y
Share this article
Anyone you share the following link with will be able to read this content:
Sorry, a shareable link is not currently available for this article.
Provided by the Springer Nature SharedIt content-sharing initiative
- Digital literacy
- Systematic review
- Qualitative research
Students’ Computer Literacy and Academic Performance
This study determined the level of computer literacy and its influence on the academic performance of junior high school students. Specifically, it probed into the students’ attitude toward computers and sought answers to the following: the extent of students’ computer literacy in terms of Word Processing, Spreadsheet, Presentation, and General Computing; their academic performance based on the mean percentage scores during the first and second quarters of the school year 2019-2020; issues and problems encountered by them relative to the extent of their computer literacy; and the solutions that may be suggested by themselves to address the constraints they encountered relative to the extent of their computer literacy. Also, by employing descriptive-correlational analysis, the study examined the significant differences in the extent of students’ computer literacy in said areas when paired according to their attitude toward computers and the significant relationship between their academic performance and the extent of their computer literacy in terms of the identified areas. Generally, the findings of the study revealed that the students needed to enhance the extent of their computer literacy in the areas of word processing, spreadsheet, presentation, and general computing. The results also signified that the greater the extent of their computer literacy in said areas, the higher their academic performance. This implied that classroom intervention activities are imperative to enhance the extent of the students' computer literacy. Thus, teachers should support them by implementing an intervention program that improves students’ level of computer literacy in the specific areas mentioned.
- Related Documents
High school students’ academic performance associated with psychological aspects, body practices and physical activity
The objective of this study was to verify the association of psychological factors and body practices with the academic performance of high school students. A sample of 330 students participated, made up of 167 girls and 163 boys. Likert scale instruments were used for collecting information on self-concept, and on general and academic self-efficacy. Time spent on moderate to vigorous physical activity was recorded, and so was participation in several types of body practices, such as sports, dances, martial arts, performing arts, and systematic physical exercises. Academic achievement was referred to from the students' grades on regular subjects. Linear regression analysis was used for verifying the association of independent variables with academic performance. The adjusted regression model explains between 7% and 36% of academic performance variance, whereas Self-Concept explains academic performance on six of the twelve subjects, and the mean of the grades, with Beta values between 0.13 (p = 0.02) for Sociology and 0.28 (p <0.01) for Mathematics. Academic self-efficacy explained performance on eleven subjects and the mean of the grades, with Beta values between 0.21 (p <0.01) for Physical Education and Philosophy, and 0.44 (p <0.01) for Biology. Participation in extracurricular activities involving body practices explained academic performance on six subjects and the mean of the grades, with Beta values between 0.14 (p = 0.02) for Sociology and 0.31 (p <0.01) for Arts. The studied psychological variables and participation in projects concerning body practices during extracurricular activities correlated with academic achievement as to several school subjects, and with the mean of the grades.
THE EFFECT OF ACADEMIC PERFORMANCE ON CLASSROOM ENVIRONMENT FOR JUNIOR HIGH SCHOOL STUDENTS
Evaluating the influence of anxiety and depression on academic performance in high school students.
The purpose of this study was to evaluate whether anxiety and depression have a negative effect on academic performance. This small-scale study assessed the relationship between these factors. A cross-sectional questionnaire survey was carried out. A randomized sample of 52 high school students based in various schools across Lahore participated in the survey. Of the participants, 32 were males and 20 were females. As a measure of anxiety and depression 14 questions from the DASS-21 were utilized (The 7 questions pertaining to the measure of stress were not included in this study). Based on the DASS-21 score obtained by the respondents they were classified under categories of normal, mild, moderate, severe and extremely severe levels of anxiety and depression. As a measure of academic performance, the Grade Point Average (GPA) of the students was obtained via the survey. The mean average GPA was calculated for all the students falling under the aforementioned categories pertaining to anxiety and depression separately and these averages were compared. It was found that the mean average GPA was highest in students falling under the categories of normal and mild levels of anxiety and depression. Whereas, mean average GPA was lower in students suffering from severe levels of anxiety and depression. These findings suggest the need to come up with methods to combat anxiety and depression in high school students as these factors impede academic performance.
Improved Academic Performance in Junior High School Students
Gender dysphoria in adolescent population: a 5-year replication study.
The aim of this study was to explore whether there has been an increase in prevalence and changes in sex ratio in feelings of gender dysphoria (GD) in an adolescent population in Northern Europe, and to study the impact of invalid responding on this topic. We replicated an earlier survey among junior high school students in Tampere, Finland. All first and second year students, aged 16–18, in the participating schools were invited to respond to an anonymous classroom survey on gender experience during the 2012–2013 school year and in the spring and autumn terms of 2017. Gender identity/GD was measured using the GIDYQ-A. A total of 318 male and 401 female youth participated in 2012–2013, and 326 male and 701 female youth in 2017. In the earlier survey, the GIDYQ-A scores, both among males and females, were strongly skewed toward a cis-gender experience with very narrow interquartile ranges. Of males, 2.2%, and of females, 0.5% nevertheless reported possibly clinically significant GD. The 2017 GIDYQ-A distribution was similarly skewed. The proportion of those reporting potentially clinically significant GD was 3.6% among males and 2.3% among females. Validity screening proved to have a considerable impact on conclusions. GD seems to have increased in prevalence in the adolescent population.
Seeing Is Believing: Experimental Evidence on the Impact of Eyeglasses on Academic Performance, Aspirations, and Dropout among Junior High School Students in Rural China
Computer literacy objectives for junior high school students, visual impairment, spectacle wear and academic performance among junior high school students in western china.
AbstractIn September 2013, 2248 students from 36 junior high schools in Shaanxi Province underwent assessment of visual acuity (VA), completed a questionnaire about their spectacle use and were administered a standardized mathematics examination. Among 2,248 students (mean age 13.6 years, 52% male), visual impairment was present in 699 (31%, 95% Confidence Interval 29-33%). Spectacle wear was observed in 360 of 966 children needing glasses (37%). Ownership of spectacles among children needing glasses was associated with poorer uncorrected vision in the better-seeing eye (P <0.001) and paternal educational (p=0.001), but not age, sex, boarding at school, both parents having out-migrated for work or maternal education. Spectacle ownership among children with visual impairment was associated with better test performance (P=0.035). Therefore, visual impairment and non-wear of spectacle were common. Wearing spectacles was associated with better academic performance in this cross-sectional analysis, consistent with recent trial results among younger children.
Comparative Study on Teaching Reading Using Know, Want, Learnt (KWL) and Direct Instruction Method (DIM)
<p>The aims of this research are to find out: (1) whether there is a significant difference in reading skill between the students taught by using KWL method and those taught by using DIM; and (2) whether KWL method is more effective than DIM to teach reading. This experimental study used 22 students for the experimental group and 22 students for the control group. The data were collected by using reading test and then analyzed by using t-test formula. The computation of the data shows that the t-observation (t<sub>o</sub>) is 1.988, which is higher than the t-table <sub>(42,</sub> <sub>0.05)</sub>: 1.960. Therefore, it can be concluded that there is a significant difference in reading skill between the students taught by using KWL method and those taught by using DIM. The mean of the group of students taught by using KWL method is 75.14, while the mean of the group of students taught by using DIM is 69.13. Therefore, it can be concluded that KWL method is more effective to teach reading than DIM for junior high school students.</p>
Rancang Bangun Sistem Informasi Analisis Kepuasan dalam Proses Belajar Mengajar di SMA Negeri 3 Mataram Menggunakan MetodeQuality Function Deployment
A senior high school is responsible to deliver competitive human resources. A high school that has good facilities are more popular and attractive for junior high school students. To evaluate the school quality of service, we build a system to analyze the student's satisfaction with the learning process and services. To conduct the analysis, an information system was built to identify what components of the learning process needed to be improved. The method used is Quality Function Deployment (QFD) that clearly defines the needs and the expectations of students. The evaluation process was done by asking students to fill out a satisfaction questionnaire through the system. To get the analysis continuously this evaluation process can be carried out annually at the end of each school year. By using this computerized system, the evaluation results are obtained faster and more accurate.
Export Citation Format
Share document.
Digital Competences: Computer and Information Literacy and Computational Thinking
- Reference work entry
- First Online: 22 September 2022
- pp 1273–1297
- Cite this reference work entry
- Wolfram Schulz 4 ,
- Julian Fraillon 4 ,
- John Ainley 4 &
- Daniel Duckworth 4
Part of the book series: Springer International Handbooks of Education ((SIHE))
786 Accesses
This chapter will describe how the cross-curricular learning area of digital competence has been conceptualized and assessed as part of national and international large-scale assessments. Digital competence can be conceived as an overarching concept that includes ICT literacy (or computer and information literacy) and computational thinking. This chapter will outline the conceptual background as well as the history of developing studies assessing students’ ICT-related knowledge, skills, and understanding, both in selected national assessments and in cross-national studies. It will discuss the challenges faced by assessments in this area with regard to new developments in digital technologies that impact on the aim of obtaining comparable measures of digital competence such as computer and information literacy and computational thinking across national contexts and over time.
Taking the example of the IEA International Computer and Information Literacy Study (ICILS) as the only study dedicated to comprehensively assessing this learning area from a cross-national perspective, this chapter outlines the development of its conceptual framework, the assessment strategy, and the broad range of contextual information gathered as part of this survey as well as the main outcomes from the second assessment cycle conducted in 2018. This chapter also includes a description of the assessment of computational thinking as part of ICILS 2018 and describes the relationship of this new construct with young people’s computer and information literacy. While not indicating a unidimensional construct, results from ICILS 2018 show that both computer information literacy and computational thinking are positively correlated and have highly similar associations with contextual factors. However, there are some interesting differences, in particular, in their respective relationships with students’ gender.
This is a preview of subscription content, log in via an institution to check access.
Access this chapter
- Available as PDF
- Read on any device
- Instant download
- Own it forever
- Available as EPUB and PDF
- Durable hardcover edition
- Dispatched in 3 to 5 business days
- Free shipping worldwide - see info
Tax calculation will be finalised at checkout
Purchases are for personal use only
Institutional subscriptions
Aesaert, K., van Braak, J., van Nijlen, D., & Vanderlinde, R. (2015). Primary school pupils’ ICT competences: Extensive model and scale development. Computers & Education, 81 , 326–344. https://doi.org/10.1016/j.compedu.2014.10.021
Article Google Scholar
Aesaert, K., van Nijlen, D., Vanderlinde, R., & van Braak, J. (2014). Direct measures of digital information processing and communication skills in primary education: Using item response theory for the development and validation of an ICT competence scale. Computers & Education, 76 , 168–181. https://doi.org/10.1016/j.compedu.2014.03.013
Ainley, J. (2018). Students and their computer literacy: Evidence and curriculum implications. In J. Voogt, G. Knezek, R. Christensen, & K. W. Lai (Eds.), Second handbook of information technology in primary and secondary education (pp. 69–88). Springer International Handbooks of Education. Springer. https://doi.org/10.1007/978-3-319-53803-7_4-1
Chapter Google Scholar
Australian Curriculum and Reporting Authority [ACARA]. (2018). National assessment program – ICT literacy years 6 & 10 2017 report. Australian Curriculum and Reporting Authority (ACARA).
Google Scholar
Australian Curriculum and Reporting Authority [ACARA]. (2020). General capabilities . . Australian Curriculum . https://acara.edu.au/curriculum/foundation-year-10/general-capabilities
Bienkowski, M., Snow, E., Rutstein, D. W., & Grover, S. (2015). Assessment design patterns for computational thinking practices in secondary computer science: A first look (SRI technical report). SRI International. Retrieved from http://pact.sri.com/resources.html
Bocconi, S., Chioccariello, A., Detori, G., Ferrari, A., & Engelhardt, K. (2016). Developing computational thinking in compulsory education. Implications for policy and practice . Joint research centre report EUR 28295 EN. Publications Office of the European Union. Retrieved from https://doi.org/10.2791/792158 .
Brese, F., & Mirazchiyski, P. (2013). Measuring students’ family background in large-scale education studies (IERI monograph series). Issues and methodologies in large-scale assessments (special issue 2).
Chen, G., Shen, J., Barth-Cohen, L., Jiang, S., Huang, X., & Eltoukhy, M. (2017). Assessing elementary students’ computational thinking in everyday reasoning and robotics programming. Computers & Education, 109 , 162–175. Retrieved from https://doi.org/10.1016/j.compedu.2017.03.001
Claro, M., Preiss, D., San Martin, E., Jara, I., Hinostraoza, J. E., Valenzuela, S., Cortes, F., & Nussbaum, M. (2012). Assessment of 21st century ICT skills in Chile: Test design and results from high school level students. Computers & Education, 59 , 1042–1053. https://doi.org/10.1016/j.compedu.2012.04.004
Ebbs, D., & Friedman, T. (2015). Translation and verification of ICILS 2013 instruments. In J. Fraillon, W. Schulz, T. Friedman, J. Ainley, & E. Gebhardt (Eds.), ICILS 2013 technical report (pp. 55–66). International Association for the Evaluation of Educational Achievement (IEA).
Educational Testing Service [ETS]. (2002). Digital transformation: A framework for ICT literacy . Educational Testing Service. http://www.ets.org/Media/Tests/Information_and_Communication_Technology_Literacy/ictreport.pdf
Fraillon, J. (2018). International studies on information technology literacy in education. In J. Voogt, G. Knezek, R. Christensen, & K. W. Lai (Eds.), Second handbook of information technology in primary and secondary education . Springer International Handbooks of Education.
Fraillon, J. (2020). ICILS 2018 test development. In J. Fraillon, J. Ainley, W. Schulz, T. Friedman, & D. Duckworth (Eds.), ICILS 2018 technical report (pp. 11–30). International Association for the Evaluation of Educational Achievement (IEA).
Fraillon, J., Ainley, J., Schulz, W., Duckworth, D., & Friedman, T. (2019). IEA international computer and information literacy study 2018 assessment framework . Springer. Retrieved from https://www.springer.com/gp/book/9783030193881
Book Google Scholar
Fraillon, J., Ainley, J., Schulz, W., Friedman, T., & Duckworth, D. (2020). Preparing for life in a digital world: IEA international computer and information literacy study, 2018 international report . International Association for the Evaluation of Educational Achievement.
Fraillon, J., Carstens, R., & Meyer, S. (2020). Computer-based assessment systems. In J. Fraillon, J. Ainley, W. Schulz, T. Friedman, & D. Duckworth (Eds.), ICILS 2018 technical report (pp. 31–38). International Association for the Evaluation of educational achievement (IEA).
Fraillon, J., Schulz, W., & Ainley, J. (2013). International computer and information literacy study: Assessment framework . International Association for the Evaluation of Educational Achievement.
Fraillon, J., Schulz, W., & Ainley, J. (2014). Preparing for life in a digital age: The international computer and information literacy study international report . International Association for the Evaluation of Educational Achievement.
Griffin, P., & Care, E. (2015). Assessment and teaching of 21st century skills: Methods and approach . Springer.
Grover, S., & Pea, R. (2013). Computational thinking in K–12: A review of the state of the field. Educational Researcher, 42 (1), 38–43.
Hatlevik, O. (2009). How to identify and understand digital literacy among 9th grade Norwegian students: Examining the influences from school and home on students’ digital literacy. Nordic Journal of Digital Literacy, 4 , 159–175.
Kaarakainen, M., Kivinen, O., & Vainio, T. (2018). Performance-based testing for ICT skills assessing: A case study of students and teachers’ ICT skills in Finnish schools. Universal Access in the Information Society, 17 (2), 349–360.
Kim, H. S., Kil, H. J., & Shin, A. (2014). An analysis of variables affecting the ICT literacy level of Korean elementary school students. Computers & Education, 77 , 29–38. Retrieved from https://doi.org/10.1016/j.compedu.2014.04.009 .
Kim, J., & Lee, W. (2013). Meanings of criteria and norms: Analyses and comparisons of ICT literacy competencies of middle school students. Computers & Education, 64 , 81–94. Retrieved from https://doi.org/10.1016/j.compedu.2012.12.018 .
Lähdemäki, J. (2019). Case study: The Finnish national curriculum 2016 – A co-created national education policy. In J. Cook (Ed.), Sustainability, human Well-being, and the future of education . Palgrave Macmillan. https://doi.org/10.1007/978-3-319-78580-6_13
Lockheed, M., & Mandinach, E. B. (1986). Trends in educational computing: Decreasing interest and the changing focus of instruction. Educational Researcher, 15 (5), 21–26.
Lye, S. Y., & Koh, J. H. L. (2014). Review on teaching and learning of computational thinking through programming: What is next for K-12? Computers in Human Behavior, 41 , 51–61. Retrieved from https://doi.org/10.1016/j.chb.2014.09.012 .
Mullis, I. V. S., Martin, M. O., Foy, P., & Hooper, M. (2017). ePIRLS 2016 international results in online informational reading . Retrieved from Boston College, TIMSS & PIRLS International Study Center website: http://timssandpirls.bc.edu/pirls2016/international-results/
National Assessment Governing Board [NAGB]. (2013). 2014 abridged technology and engineering literacy framework for the 2014 national assessment of educational progress . National Assessment Governing Board.
National Center for Educational Statistics [NCES]. (2016). 2014 nations report card: Technology & Engineering Literacy (TEL) . https://www.nationsreportcard.gov/tel_2014/
National Center for Educational Statistics [NCES]. (2020). NAEP Technology & Engineering Literacy (TEL) report card . https://www.nationsreportcard.gov/tel/
Ockwell, L., Draganov, A., & Schulz, W. (2020). Scaling procedures for ICILS 2018 test items. In J. Fraillon, J. Ainley, W. Schulz, T. Friedman, & D. Duckworth (Eds.), ICILS 2018 technical report (pp. 133–158).
Organisation for Economic Cooperation and Development [OECD]. (2011). PISA 2009 results: Students on-line digital technologies and performance . OECD.
Organisation for Economic Cooperation and Development [OECD]. (2013). OECD skills outlook 2013: First results from the survey of adult skills . OECD. https://doi.org/10.1787/9789264204256-en .
Organisation for Economic Cooperation and Development [OECD]. (2016). PISA 2015 results volume V. Collaborative problem solving . OECD. https://doi.org/10.1787/9789264285521-en .
Orti-Colon, A. M., & Marato Romo, J. L. (2016). Teaching with scratch in compulsory secondary education. International Journal of Emerging Technologies in Learning, 11 (2), 67–70. Retrieved from https://doi.org/10.3991/ijet.v11i02.5094 .
Pelgrum, W. J., & Plomp, T. J. (1993). What do students know, learn and think about computers? In W. J. Pelgrum, I. A. J. Rienen, & T. J. Plomp (Eds.), Schools, teachers, students and computers: A cross-national perspective (IEA-Comped study stage 2) (pp. 49–70). IEA ERIC number: ED372734.
Rasch, G. (1960). Probabilistic models for some intelligence and attainment tests . Copenhagen, Denmark: Nielsen & Lydiche.
Rohatgi, A., Scherer, R., & Hatlevik, O. (2016). The role of ICT self-efficacy for students’ ICT use and their achievement in a computer and information literacy test. Computers & Education, 102 , 103–116.
Rutstein, D. W., Snow, E. B., & Bienkowski, M. (2014). Computational thinking practices: Analyzing and modelling a critical domain in computer science education . Paper presented at the annual meeting of the American Educational Research Association. Retrieved from https://www.aera.net/PublicationsOnline-Paper-Repository/AERA-Online-Paper-Repository
Scheerens, J. (Ed.) (2017). Opportunity to learn, curriculum alignment and test preparation: A research review . Springer, Cham. https://doi.org/10.1007/978-3-319-43110-9
Schulz, W. (2020). The reporting of ICILS 2018 results. In J. Fraillon, W. Schulz, T. Friedman, & S. Meyer (Eds.), ICILS 2018 technical report (pp. 221–233). International Association for the Evaluation of Educational Achievement (IEA).
Schulz, W., & Friedman, T. (2020). Scaling procedures for ICILS 2018 questionnaire items. In J. Fraillon, J. Ainley, W. Schulz, T. Friedman, & D. Duckworth (Eds.), ICILS 2018 technical report (pp. 159–219). International Association for the Evaluation of Educational Achievement (IEA).
Shute, V. J., Sun, C., & Asbell-Clarke, J. (2017). Demystifying computational thinking. Educational Research Review, 22 , 142–158. Retrieved from 10.1016/j.edurev.2017.09.003.
Siddiq, F., Hatlevik, O. E., Olsen, R. V., Throndsen, I., & Scherer, R. (2016). Taking a future perspective by learning from the past: A systematic review of assessment instruments that aim to measure primary and secondary school students’ ICT literacy. Educational Research Review, 19 , 58–84.
Tatnall, A., & Davey, B. (Eds.). (2014). Reflections on the history of computers in education: Early use of computers and teaching about computing in schools (IFIP Advances in Information and Communication Technology). Springer.
Uerz, D., Volman, M., & Kral, M. (2018). Teacher educators’ competences in fostering student teachers’ proficiency in teaching and learning with technology: An overview of relevant research literature. Teaching and Teacher Education, 70 , 12–23. https://doi.org/10.1016/j.tate.2017.11.005
United Nations. (2016). Global indicator framework for the sustainable development goals and targets for the 2030 agenda for sustainable development. Annex: A/RES/21/313. Retrieved from: https://unstats.un.org/sdgs/indicators/Global%20Indicator%20Framework_A.RES.71.313%20Annex.pdf
von Davier, M., Gonzalez, E., & Mislevy, R. (2009). What are plausible values and why are they useful? IERI Monograph Series: Issues and Methodologies in Large-Scale Assessments, 2 , 9–36.
Voogt, J., Fisser, P., Good, J., Mishra, P., & Yadav, A. (2015). Computational thinking in compulsory education: Towards an agenda for research and practice. Education and Information Technologies, 20 (4), 715–728.
Wang, J. (2005). Relationship between mathematics and science achievement at the 8th grade. International Online Journal of Science and Mathematics Education, 5 , 1–17.
Wing, J. M. (2006). Computational thinking. Communications of the ACM, 49 , 33–35. Retrieved from https://doi.org/10.1145/1118178.111821
Zhong, B., Wang, Q., Chen, J., & Li, Y. (2016). An exploration of three-dimensional integrated assessment for computational thinking. Journal of Educational Computing Research, 53 (4), 562–590. Retrieved from https://journals.sagepub.com/doi/pdf/10.1177/0735633115608444
Download references
Author information
Authors and affiliations.
Australian Council for Educational Research, Camberwell, VIC, Australia
Wolfram Schulz, Julian Fraillon, John Ainley & Daniel Duckworth
You can also search for this author in PubMed Google Scholar
Corresponding author
Correspondence to Wolfram Schulz .
Editor information
Editors and affiliations.
Department of Teacher Education and School Research, Faculty of Educational Sciences, University of Oslo, Oslo, Norway
Trude Nilsen
IEA Hamburg, Hamburg, Hamburg, Germany
Agnes Stancel-Piątak
University of Gothenburg, Gothenburg, Sweden
Jan-Eric Gustafsson
Section Editor information
CEMO, University of Oslo, Oslo, Norway
Ronny Scherer
Rights and permissions
Reprints and permissions
Copyright information
© 2022 Springer Nature Switzerland AG
About this entry
Cite this entry.
Schulz, W., Fraillon, J., Ainley, J., Duckworth, D. (2022). Digital Competences: Computer and Information Literacy and Computational Thinking. In: Nilsen, T., Stancel-Piątak, A., Gustafsson, JE. (eds) International Handbook of Comparative Large-Scale Studies in Education. Springer International Handbooks of Education. Springer, Cham. https://doi.org/10.1007/978-3-030-88178-8_43
Download citation
DOI : https://doi.org/10.1007/978-3-030-88178-8_43
Published : 22 September 2022
Publisher Name : Springer, Cham
Print ISBN : 978-3-030-88177-1
Online ISBN : 978-3-030-88178-8
eBook Packages : Education Reference Module Humanities and Social Sciences Reference Module Education
Share this entry
Anyone you share the following link with will be able to read this content:
Sorry, a shareable link is not currently available for this article.
Provided by the Springer Nature SharedIt content-sharing initiative
- Publish with us
Policies and ethics
- Find a journal
- Track your research
- Review article
- Open access
- Published: 02 October 2017
Computer-based technology and student engagement: a critical review of the literature
- Laura A. Schindler ORCID: orcid.org/0000-0001-8730-5189 1 ,
- Gary J. Burkholder 2 , 3 ,
- Osama A. Morad 1 &
- Craig Marsh 4
International Journal of Educational Technology in Higher Education volume 14 , Article number: 25 ( 2017 ) Cite this article
390k Accesses
138 Citations
39 Altmetric
Metrics details
Computer-based technology has infiltrated many aspects of life and industry, yet there is little understanding of how it can be used to promote student engagement, a concept receiving strong attention in higher education due to its association with a number of positive academic outcomes. The purpose of this article is to present a critical review of the literature from the past 5 years related to how web-conferencing software, blogs, wikis, social networking sites ( Facebook and Twitter ), and digital games influence student engagement. We prefaced the findings with a substantive overview of student engagement definitions and indicators, which revealed three types of engagement (behavioral, emotional, and cognitive) that informed how we classified articles. Our findings suggest that digital games provide the most far-reaching influence across different types of student engagement, followed by web-conferencing and Facebook . Findings regarding wikis, blogs, and Twitter are less conclusive and significantly limited in number of studies conducted within the past 5 years. Overall, the findings provide preliminary support that computer-based technology influences student engagement, however, additional research is needed to confirm and build on these findings. We conclude the article by providing a list of recommendations for practice, with the intent of increasing understanding of how computer-based technology may be purposefully implemented to achieve the greatest gains in student engagement.
Introduction
The digital revolution has profoundly affected daily living, evident in the ubiquity of mobile devices and the seamless integration of technology into common tasks such as shopping, reading, and finding directions (Anderson, 2016 ; Smith & Anderson, 2016 ; Zickuhr & Raine, 2014 ). The use of computers, mobile devices, and the Internet is at its highest level to date and expected to continue to increase as technology becomes more accessible, particularly for users in developing countries (Poushter, 2016 ). In addition, there is a growing number of people who are smartphone dependent, relying solely on smartphones for Internet access (Anderson & Horrigan, 2016 ) rather than more expensive devices such as laptops and tablets. Greater access to and demand for technology has presented unique opportunities and challenges for many industries, some of which have thrived by effectively digitizing their operations and services (e.g., finance, media) and others that have struggled to keep up with the pace of technological innovation (e.g., education, healthcare) (Gandhi, Khanna, & Ramaswamy, 2016 ).
Integrating technology into teaching and learning is not a new challenge for universities. Since the 1900s, administrators and faculty have grappled with how to effectively use technical innovations such as video and audio recordings, email, and teleconferencing to augment or replace traditional instructional delivery methods (Kaware & Sain, 2015 ; Westera, 2015 ). Within the past two decades, however, this challenge has been much more difficult due to the sheer volume of new technologies on the market. For example, in the span of 7 years (from 2008 to 2015), the number of active apps in Apple’s App Store increased from 5000 to 1.75 million. Over the next 4 years, the number of apps is projected to rise by 73%, totaling over 5 million (Nelson, 2016 ). Further compounding this challenge is the limited shelf life of new devices and software combined with significant internal organizational barriers that hinder universities from efficiently and effectively integrating new technologies (Amirault, 2012 ; Kinchin, 2012 ; Linder-VanBerschot & Summers 2015 ; Westera, 2015 ).
Many organizational barriers to technology integration arise from competing tensions between institutional policy and practice and faculty beliefs and abilities. For example, university administrators may view technology as a tool to attract and retain students, whereas faculty may struggle to determine how technology coincides with existing pedagogy (Lawrence & Lentle-Keenan, 2013 ; Lin, Singer, & Ha, 2010 ). In addition, some faculty may be hesitant to use technology due to lack of technical knowledge and/or skepticism about the efficacy of technology to improve student learning outcomes (Ashrafzadeh & Sayadian, 2015 ; Buchanan, Sainter, & Saunders, 2013 ; Hauptman, 2015 ; Johnson, 2013 ; Kidd, Davis, & Larke, 2016 ; Kopcha, Rieber, & Walker, 2016 ; Lawrence & Lentle-Keenan, 2013 ; Lewis, Fretwell, Ryan, & Parham, 2013 ; Reid, 2014 ). Organizational barriers to technology adoption are particularly problematic given the growing demands and perceived benefits among students about using technology to learn (Amirault, 2012 ; Cassidy et al., 2014 ; Gikas & Grant, 2013 ; Paul & Cochran, 2013 ). Surveys suggest that two-thirds of students use mobile devices for learning and believe that technology can help them achieve learning outcomes and better prepare them for a workforce that is increasingly dependent on technology (Chen, Seilhamer, Bennett, & Bauer, 2015 ; Dahlstrom, 2012 ). Universities that fail to effectively integrate technology into the learning experience miss opportunities to improve student outcomes and meet the expectations of a student body that has grown accustomed to the integration of technology into every facet of life (Amirault, 2012 ; Cook & Sonnenberg, 2014 ; Revere & Kovach, 2011 ; Sun & Chen, 2016 ; Westera, 2015 ).
The purpose of this paper is to provide a literature review on how computer-based technology influences student engagement within higher education settings. We focused on computer-based technology given the specific types of technologies (i.e., web-conferencing software, blogs, wikis, social networking sites, and digital games) that emerged from a broad search of the literature, which is described in more detail below. Computer-based technology (hereafter referred to as technology) requires the use of specific hardware, software, and micro processing features available on a computer or mobile device. We also focused on student engagement as the dependent variable of interest because it encompasses many different aspects of the teaching and learning process (Bryson & Hand, 2007 ; Fredricks, Blumenfeld, & Parks, 1994; Wimpenny & Savin-Baden, 2013 ), compared narrower variables in the literature such as final grades or exam scores. Furthermore, student engagement has received significant attention over the past several decades due to shifts towards student-centered, constructivist instructional methods (Haggis, 2009 ; Wright, 2011 ), mounting pressures to improve teaching and learning outcomes (Axelson & Flick, 2011 ; Kuh, 2009 ), and promising studies suggesting relationships between student engagement and positive academic outcomes (Carini, Kuh, & Klein, 2006 ; Center for Postsecondary Research, 2016 ; Hu & McCormick, 2012 ). Despite the interest in student engagement and the demand for more technology in higher education, there are no articles offering a comprehensive review of how these two variables intersect. Similarly, while many existing student engagement conceptual models have expanded to include factors that influence student engagement, none highlight the overt role of technology in the engagement process (Kahu, 2013 ; Lam, Wong, Yang, & Yi, 2012 ; Nora, Barlow, & Crisp, 2005 ; Wimpenny & Savin-Baden, 2013 ; Zepke & Leach, 2010 ).
Our review aims to address existing gaps in the student engagement literature and seeks to determine whether student engagement models should be expanded to include technology. The review also addresses some of the organizational barriers to technology integration (e.g., faculty uncertainty and skepticism about technology) by providing a comprehensive account of the research evidence regarding how technology influences student engagement. One limitation of the literature, however, is the lack of detail regarding how teaching and learning practices were used to select and integrate technology into learning. For example, the methodology section of many studies does not include a pedagogical justification for why a particular technology was used or details about the design of the learning activity itself. Therefore, it often is unclear how teaching and learning practices may have affected student engagement levels. We revisit this issue in more detail at the end of this paper in our discussions of areas for future research and recommendations for practice. We initiated our literature review by conducting a broad search for articles published within the past 5 years, using the key words technology and higher education , in Google Scholar and the following research databases: Academic Search Complete, Communication & Mass Media Complete, Computers & Applied Sciences Complete, Education Research Complete, ERIC, PsycARTICLES, and PsycINFO . Our initial search revealed themes regarding which technologies were most prevalent in the literature (e.g., social networking, digital games), which then lead to several, more targeted searches of the same databases using specific keywords such as Facebook and student engagement. After both broad and targeted searches, we identified five technologies (web-conferencing software, blogs, wikis, social networking sites, and digital games) to include in our review.
We chose to focus on technologies for which there were multiple studies published, allowing us to identify areas of convergence and divergence in the literature and draw conclusions about positive and negative effects on student engagement. In total, we identified 69 articles relevant to our review, with 36 pertaining to social networking sites (21 for Facebook and 15 for Twitter ), 14 pertaining to digital games, seven pertaining to wikis, and six pertaining to blogs and web-conferencing software respectively. Articles were categorized according to their influence on specific types of student engagement, which will be described in more detail below. In some instances, one article pertained to multiple types of engagement. In the sections that follow, we will provide an overview of student engagement, including an explanation of common definitions and indicators of engagement, followed by a synthesis of how each type of technology influences student engagement. Finally, we will discuss areas for future research and make recommendations for practice.
- Student engagement
Interest in student engagement began over 70 years ago with Ralph Tyler’s research on the relationship between time spent on coursework and learning (Axelson & Flick, 2011 ; Kuh, 2009 ). Since then, the study of student engagement has evolved and expanded considerably, through the seminal works of Pace ( 1980 ; 1984 ) and Astin ( 1984 ) about how quantity and quality of student effort affect learning and many more recent studies on the environmental conditions and individual dispositions that contribute to student engagement (Bakker, Vergel, & Kuntze, 2015 ; Gilboy, Heinerichs, & Pazzaglia, 2015 ; Martin, Goldwasser, & Galentino, 2017 ; Pellas, 2014 ). Perhaps the most well-known resource on student engagement is the National Survey of Student Engagement (NSSE), an instrument designed to assess student participation in various educational activities (Kuh, 2009 ). The NSSE and other engagement instruments like it have been used in many studies that link student engagement to positive student outcomes such as higher grades, retention, persistence, and completion (Leach, 2016 ; McClenney, Marti, & Adkins, 2012 ; Trowler & Trowler, 2010 ), further convincing universities that student engagement is an important factor in the teaching and learning process. However, despite the increased interest in student engagement, its meaning is generally not well understood or agreed upon.
Student engagement is a broad and complex phenomenon for which there are many definitions grounded in psychological, social, and/or cultural perspectives (Fredricks et al., 1994; Wimpenny & Savin-Baden, 2013 ; Zepke & Leach, 2010 ). Review of definitions revealed that student engagement is defined in two ways. One set of definitions refer to student engagement as a desired outcome reflective of a student’s thoughts, feelings, and behaviors about learning. For example, Kahu ( 2013 ) defines student engagement as an “individual psychological state” that includes a student’s affect, cognition, and behavior (p. 764). Other definitions focus primarily on student behavior, suggesting that engagement is the “extent to which students are engaging in activities that higher education research has shown to be linked with high-quality learning outcomes” (Krause & Coates, 2008 , p. 493) or the “quality of effort and involvement in productive learning activities” (Kuh, 2009 , p. 6). Another set of definitions refer to student engagement as a process involving both the student and the university. For example, Trowler ( 2010 ) defined student engagement as “the interaction between the time, effort and other relevant resources invested by both students and their institutions intended to optimize the student experience and enhance the learning outcomes and development of students and the performance, and reputation of the institution” (p. 2). Similarly, the NSSE website indicates that student engagement is “the amount of time and effort students put into their studies and other educationally purposeful activities” as well as “how the institution deploys its resources and organizes the curriculum and other learning opportunities to get students to participate in activities that decades of research studies show are linked to student learning” (Center for Postsecondary Research, 2017 , para. 1).
Many existing models of student engagement reflect the latter set of definitions, depicting engagement as a complex, psychosocial process involving both student and university characteristics. Such models organize the engagement process into three areas: factors that influence student engagement (e.g., institutional culture, curriculum, and teaching practices), indicators of student engagement (e.g., interest in learning, interaction with instructors and peers, and meaningful processing of information), and outcomes of student engagement (e.g., academic achievement, retention, and personal growth) (Kahu, 2013 ; Lam et al., 2012 ; Nora et al., 2005 ). In this review, we examine the literature to determine whether technology influences student engagement. In addition, we will use Fredricks et al. ( 2004 ) typology of student engagement to organize and present research findings, which suggests that there are three types of engagement (behavioral, emotional, and cognitive). The typology is useful because it is broad in scope, encompassing different types of engagement that capture a range of student experiences, rather than narrower typologies that offer specific or prescriptive conceptualizations of student engagement. In addition, this typology is student-centered, focusing exclusively on student-focused indicators rather than combining student indicators with confounding variables, such as faculty behavior, curriculum design, and campus environment (Coates, 2008 ; Kuh, 2009 ). While such variables are important in the discussion of student engagement, perhaps as factors that may influence engagement, they are not true indicators of student engagement. Using the typology as a guide, we examined recent student engagement research, models, and measures to gain a better understanding of how behavioral, emotional, and cognitive student engagement are conceptualized and to identify specific indicators that correspond with each type of engagement, as shown in Fig. 1 .
Conceptual framework of types and indicators of student engagement
Behavioral engagement is the degree to which students are actively involved in learning activities (Fredricks et al., 2004 ; Kahu, 2013 ; Zepke, 2014 ). Indicators of behavioral engagement include time and effort spent participating in learning activities (Coates, 2008 ; Fredricks et al., 2004 ; Kahu, 2013 ; Kuh, 2009 ; Lam et al., 2012 ; Lester, 2013 ; Trowler, 2010 ) and interaction with peers, faculty, and staff (Coates, 2008 ; Kahu, 2013 ; Kuh, 2009 ; Bryson & Hand, 2007 ; Wimpenny & Savin-Baden, 2013 : Zepke & Leach, 2010 ). Indicators of behavioral engagement reflect observable student actions and most closely align with Pace ( 1980 ) and Astin’s ( 1984 ) original conceptualizations of student engagement as quantity and quality of effort towards learning. Emotional engagement is students’ affective reactions to learning (Fredricks et al., 2004 ; Lester, 2013 ; Trowler, 2010 ). Indicators of emotional engagement include attitudes, interests, and values towards learning (Fredricks et al., 2004 ; Kahu, 2013 ; Lester, 2013 ; Trowler, 2010 ; Wimpenny & Savin-Baden, 2013 ; Witkowski & Cornell, 2015 ) and a perceived sense of belonging within a learning community (Fredricks et al., 2004 ; Kahu, 2013 ; Lester, 2013 ; Trowler, 2010 ; Wimpenny & Savin-Baden, 2013 ). Emotional engagement often is assessed using self-report measures (Fredricks et al., 2004 ) and provides insight into how students feel about a particular topic, delivery method, or instructor. Finally, cognitive engagement is the degree to which students invest in learning and expend mental effort to comprehend and master content (Fredricks et al., 2004 ; Lester, 2013 ). Indicators of cognitive engagement include: motivation to learn (Lester, 2013 ; Richardson & Newby, 2006 ; Zepke & Leach, 2010 ); persistence to overcome academic challenges and meet/exceed requirements (Fredricks et al., 2004 ; Kuh, 2009 ; Trowler, 2010 ); and deep processing of information (Fredricks et al., 2004 ; Kahu, 2013 ; Lam et al., 2012 ; Richardson & Newby, 2006 ) through critical thinking (Coates, 2008 ; Witkowski & Cornell, 2015 ), self-regulation (e.g., set goals, plan, organize study effort, and monitor learning; Fredricks et al., 2004 ; Lester, 2013 ), and the active construction of knowledge (Coates, 2008 ; Kuh, 2009 ). While cognitive engagement includes motivational aspects, much of the literature focuses on how students use active learning and higher-order thinking, in some form, to achieve content mastery. For example, there is significant emphasis on the importance of deep learning, which involves analyzing new learning in relation previous knowledge, compared to surface learning, which is limited to memorization, recall, and rehearsal (Fredricks et al., 2004 ; Kahu, 2013 ; Lam et al., 2012 ).
While each type of engagement has distinct features, there is some overlap across cognitive, behavioral, and emotional domains. In instances where an indicator could correspond with more than one type of engagement, we chose to match the indicator to the type of engagement that most closely aligned, based on our review of the engagement literature and our interpretation of the indicators. Similarly, there is also some overlap among indicators. As a result, we combined and subsumed similar indicators found in the literature, where appropriate, to avoid redundancy. Achieving an in-depth understanding of student engagement and associated indicators was an important pre-cursor to our review of the technology literature. Very few articles used the term student engagement as a dependent variable given the concept is so broad and multidimensional. We found that specific indicators (e.g., interaction, sense of belonging, and knowledge construction) of student engagement were more common in the literature as dependent variables. Next, we will provide a synthesis of the findings regarding how different types of technology influence behavioral, emotional, and cognitive student engagement and associated indicators.
Influence of technology on student engagement
We identified five technologies post-literature search (i.e., web-conferencing, blogs, wikis, social networking sites , and digital games) to include in our review, based on frequency in which they appeared in the literature over the past 5 years. One commonality among these technologies is their potential value in supporting a constructivist approach to learning, characterized by the active discovery of knowledge through reflection of experiences with one’s environment, the connection of new knowledge to prior knowledge, and interaction with others (Boghossian, 2006 ; Clements, 2015 ). Another commonality is that most of the technologies, except perhaps for digital games, are designed primarily to promote interaction and collaboration with others. Our search yielded very few studies on how informational technologies, such as video lectures and podcasts, influence student engagement. Therefore, these technologies are notably absent from our review. Unlike the technologies we identified earlier, informational technologies reflect a behaviorist approach to learning in which students are passive recipients of knowledge that is transmitted from an expert (Boghossian, 2006 ). The lack of recent research on how informational technologies affect student engagement may be due to the increasing shift from instructor-centered, behaviorist approaches to student-centered, constructivist approaches within higher education (Haggis, 2009 ; Wright, 2011 ) along with the ubiquity of web 2.0 technologies.
- Web-conferencing
Web-conferencing software provides a virtual meeting space where users login simultaneously and communicate about a given topic. While each software application is unique, many share similar features such as audio, video, or instant messaging options for real-time communication; screen sharing, whiteboards, and digital pens for presentations and demonstrations; polls and quizzes for gauging comprehension or eliciting feedback; and breakout rooms for small group work (Bower, 2011 ; Hudson, Knight, & Collins, 2012 ; Martin, Parker, & Deale, 2012 ; McBrien, Jones, & Cheng, 2009 ). Of the technologies included in this literature review, web-conferencing software most closely mimics the face-to-face classroom environment, providing a space where instructors and students can hear and see each other in real-time as typical classroom activities (i.e., delivering lectures, discussing course content, asking/answering questions) are carried out (Francescucci & Foster, 2013 ; Hudson et al., 2012 ). Studies on web-conferencing software deployed Adobe Connect, Cisco WebEx, Horizon Wimba, or Blackboard Collaborate and made use of multiple features, such as screen sharing, instant messaging, polling, and break out rooms. In addition, most of the studies integrated web-conferencing software into courses on a voluntary basis to supplement traditional instructional methods (Andrew, Maslin-Prothero, & Ewens, 2015 ; Armstrong & Thornton, 2012 ; Francescucci & Foster, 2013 ; Hudson et al., 2012 ; Martin et al., 2012 ; Wdowik, 2014 ). Existing studies on web-conferencing pertain to all three types of student engagement.
Studies on web-conferencing and behavioral engagement reveal mixed findings. For example, voluntary attendance in web-conferencing sessions ranged from 54 to 57% (Andrew et al., 2015 ; Armstrong & Thornton, 2012 ) and, in a comparison between a blended course with regular web-conferencing sessions and a traditional, face-to-face course, researchers found no significant difference in student attendance in courses. However, students in the blended course reported higher levels of class participation compared to students in the face-to-face course (Francescucci & Foster, 2013 ). These findings suggest while web-conferencing may not boost attendance, especially if voluntary, it may offer more opportunities for class participation, perhaps through the use of communication channels typically not available in a traditional, face-to-face course (e.g., instant messaging, anonymous polling). Studies on web-conferencing and interaction, another behavioral indicator, support this assertion. For example, researchers found that students use various features of web-conferencing software (e.g., polling, instant message, break-out rooms) to interact with peers and the instructor by asking questions, expressing opinions and ideas, sharing resources, and discussing academic content (Andrew et al., 2015 ; Armstrong & Thornton, 2012 ; Hudson et al., 2012 ; Martin et al., 2012 ; Wdowik, 2014 ).
Studies on web-conferencing and cognitive engagement are more conclusive than those for behavioral engagement, although are fewer in number. Findings suggest that students who participated in web-conferencing demonstrated critical reflection and enhanced learning through interactions with others (Armstrong & Thornton, 2012 ), higher-order thinking (e.g., problem-solving, synthesis, evaluation) in response to challenging assignments (Wdowik, 2014 ), and motivation to learn, particularly when using polling features (Hudson et al., 2012 ). There is only one study examining how web-conferencing affects emotional engagement, although it is positive suggesting that students who participated in web-conferences had higher levels of interest in course content than those who did not (Francescucci & Foster, 2013 ). One possible reason for the positive cognitive and emotional engagement findings may be that web-conferencing software provides many features that promote active learning. For example, whiteboards and breakout rooms provide opportunities for real-time, collaborative problem-solving activities and discussions. However, additional studies are needed to isolate and compare specific web-conferencing features to determine which have the greatest effect on student engagement.
A blog, which is short for Weblog, is a collection of personal journal entries, published online and presented chronologically, to which readers (or subscribers) may respond by providing additional commentary or feedback. In order to create a blog, one must compose content for an entry, which may include text, hyperlinks, graphics, audio, or video, publish the content online using a blogging application, and alert subscribers that new content is posted. Blogs may be informal and personal in nature or may serve as formal commentary in a specific genre, such as in politics or education (Coghlan et al., 2007 ). Fortunately, many blog applications are free, and many learning management systems (LMSs) offer a blogging feature that is seamlessly integrated into the online classroom. The ease of blogging has attracted attention from educators, who currently use blogs as an instructional tool for the expression of ideas, opinions, and experiences and for promoting dialogue on a wide range of academic topics (Garrity, Jones, VanderZwan, de la Rocha, & Epstein, 2014 ; Wang, 2008 ).
Studies on blogs show consistently positive findings for many of the behavioral and emotional engagement indicators. For example, students reported that blogs promoted interaction with others, through greater communication and information sharing with peers (Chu, Chan, & Tiwari, 2012 ; Ivala & Gachago, 2012 ; Mansouri & Piki, 2016 ), and analyses of blog posts show evidence of students elaborating on one another’s ideas and sharing experiences and conceptions of course content (Sharma & Tietjen, 2016 ). Blogs also contribute to emotional engagement by providing students with opportunities to express their feelings about learning and by encouraging positive attitudes about learning (Dos & Demir, 2013 ; Chu et al., 2012 ; Yang & Chang, 2012 ). For example, Dos and Demir ( 2013 ) found that students expressed prejudices and fears about specific course topics in their blog posts. In addition, Yang and Chang ( 2012 ) found that interactive blogging, where comment features were enabled, lead to more positive attitudes about course content and peers compared to solitary blogging, where comment features were disabled.
The literature on blogs and cognitive engagement is less consistent. Some studies suggest that blogs may help students engage in active learning, problem-solving, and reflection (Chawinga, 2017 ; Chu et al., 2012 ; Ivala & Gachago, 2012 ; Mansouri & Piki, 2016 ), while other studies suggest that students’ blog posts show very little evidence of higher-order thinking (Dos & Demir, 2013 ; Sharma & Tietjen, 2016 ). The inconsistency in findings may be due to the wording of blog instructions. Students may not necessarily demonstrate or engage in deep processing of information unless explicitly instructed to do so. Unfortunately, it is difficult to determine whether the wording of blog assignments contributed to the mixed results because many of the studies did not provide assignment details. However, studies pertaining to other technologies suggest that assignment wording that lacks specificity or requires low-level thinking can have detrimental effects on student engagement outcomes (Hou, Wang, Lin, & Chang, 2015 ; Prestridge, 2014 ). Therefore, blog assignments that are vague or require only low-level thinking may have adverse effects on cognitive engagement.
A wiki is a web page that can be edited by multiple users at once (Nakamaru, 2012 ). Wikis have gained popularity in educational settings as a viable tool for group projects where group members can work collaboratively to develop content (i.e., writings, hyperlinks, images, graphics, media) and keep track of revisions through an extensive versioning system (Roussinos & Jimoyiannis, 2013 ). Most studies on wikis pertain to behavioral engagement, with far fewer studies on cognitive engagement and none on emotional engagement. Studies pertaining to behavioral engagement reveal mixed results, with some showing very little enduring participation in wikis beyond the first few weeks of the course (Nakamaru, 2012 ; Salaber, 2014 ) and another showing active participation, as seen in high numbers of posts and edits (Roussinos & Jimoyiannis, 2013 ). The most notable difference between these studies is the presence of grading, which may account for the inconsistencies in findings. For example, in studies where participation was low, wikis were ungraded, suggesting that students may need extra motivation and encouragement to use wikis (Nakamaru, 2012 ; Salaber, 2014 ). Findings regarding the use of wikis for promoting interaction are also inconsistent. In some studies, students reported that wikis were useful for interaction, teamwork, collaboration, and group networking (Camacho, Carrión, Chayah, & Campos, 2016 ; Martínez, Medina, Albalat, & Rubió, 2013 ; Morely, 2012 ; Calabretto & Rao, 2011 ) and researchers found evidence of substantial collaboration among students (e.g., sharing ideas, opinions, and points of view) in wiki activity (Hewege & Perera, 2013 ); however, Miller, Norris, and Bookstaver ( 2012 ) found that only 58% of students reported that wikis promoted collegiality among peers. The findings in the latter study were unexpected and may be due to design flaws in the wiki assignments. For example, the authors noted that wiki assignments were not explicitly referred to in face-to-face classes; therefore, this disconnect may have prevented students from building on interactive momentum achieved during out-of-class wiki assignments (Miller et al., 2012 ).
Studies regarding cognitive engagement are limited in number but more consistent than those concerning behavioral engagement, suggesting that wikis promote high levels of knowledge construction (i.e., evaluation of arguments, the integration of multiple viewpoints, new understanding of course topics; Hewege & Perera, 2013 ), and are useful for reflection, reinforcing course content, and applying academic skills (Miller et al., 2012 ). Overall, there is mixed support for the use of wikis to promote behavioral engagement, although making wiki assignments mandatory and explicitly referring to wikis in class may help bolster participation and interaction. In addition, there is some support for using wikis to promote cognitive engagement, but additional studies are needed to confirm and expand on findings as well as explore the effect of wikis on emotional engagement.
Social networking sites
Social networking is “the practice of expanding knowledge by making connections with individuals of similar interests” (Gunawardena et al., 2009 , p. 4). Social networking sites, such as Facebook, Twitter, Instagram, and LinkedIn, allow users to create and share digital content publicly or with others to whom they are connected and communicate privately through messaging features. Two of the most popular social networking sites in the educational literature are Facebook and Twitter (Camus, Hurt, Larson, & Prevost, 2016 ; Manca & Ranieri, 2013 ), which is consistent with recent statistics suggesting that both sites also are exceedingly popular among the general population (Greenwood, Perrin, & Duggan, 2016 ). In the sections that follow, we examine how both Facebook and Twitter influence different types of student engagement.
Facebook is a web-based service that allows users to create a public or private profile and invite others to connect. Users may build social, academic, and professional connections by posting messages in various media formats (i.e., text, pictures, videos) and commenting on, liking, and reacting to others’ messages (Bowman & Akcaoglu, 2014 ; Maben, Edwards, & Malone, 2014 ; Hou et al., 2015 ). Within an educational context, Facebook has often been used as a supplementary instructional tool to lectures or LMSs to support class discussions or develop, deliver, and share academic content and resources. Many instructors have opted to create private Facebook groups, offering an added layer of security and privacy because groups are not accessible to strangers (Bahati, 2015 ; Bowman & Akcaoglu, 2014 ; Clements, 2015 ; Dougherty & Andercheck, 2014 ; Esteves, 2012 ; Shraim, 2014 ; Maben et al., 2014 ; Manca & Ranieri, 2013 ; Naghdipour & Eldridge, 2016 ; Rambe, 2012 ). The majority of studies on Facebook address behavioral indicators of student engagement, with far fewer focusing on emotional or cognitive engagement.
Studies that examine the influence of Facebook on behavioral engagement focus both on participation in learning activities and interaction with peers and instructors. In most studies, Facebook activities were voluntary and participation rates ranged from 16 to 95%, with an average of rate of 47% (Bahati, 2015 ; Bowman & Akcaoglu, 2014 ; Dougherty & Andercheck, 2014 ; Fagioli, Rios-Aguilar, & Deil-Amen, 2015 ; Rambe, 2012 ; Staines & Lauchs, 2013 ). Participation was assessed by tracking how many students joined course- or university-specific Facebook groups (Bahati, 2015 ; Bowman & Akcaoglu, 2014 ; Fagioli et al., 2015 ), visited or followed course-specific Facebook pages (DiVall & Kirwin, 2012 ; Staines & Lauchs, 2013 ), or posted at least once in a course-specific Facebook page (Rambe, 2012 ). The lowest levels of participation (16%) arose from a study where community college students were invited to use the Schools App, a free application that connects students to their university’s private Facebook community. While the authors acknowledged that building an online community of college students is difficult (Fagioli et al., 2015 ), downloading the Schools App may have been a deterrent to widespread participation. In addition, use of the app was not tied to any specific courses or assignments; therefore, students may have lacked adequate incentive to use it. The highest level of participation (95%) in the literature arose from a study in which the instructor created a Facebook page where students could find or post study tips or ask questions. Followership to the page was highest around exams, when students likely had stronger motivations to access study tips and ask the instructor questions (DiVall & Kirwin, 2012 ). The wide range of participation in Facebook activities suggests that some students may be intrinsically motivated to participate, while other students may need some external encouragement. For example, Bahati ( 2015 ) found that when students assumed that a course-specific Facebook was voluntary, only 23% participated, but when the instructor confirmed that the Facebook group was, in fact, mandatory, the level of participation rose to 94%.
While voluntary participation in Facebook activities may be lower than desired or expected (Dyson, Vickers, Turtle, Cowan, & Tassone, 2015 ; Fagioli et al., 2015 ; Naghdipour & Eldridge, 2016 ; Rambe, 2012 ), students seem to have a clear preference for Facebook compared to other instructional tools (Clements, 2015 ; DiVall & Kirwin, 2012 ; Hurt et al., 2012 ; Hou et al., 2015 ; Kent, 2013 ). For example, in one study where an instructor shared course-related information in a Facebook group, in the LMS, and through email, the level of participation in the Facebook group was ten times higher than in email or the LMS (Clements, 2015 ). In other studies, class discussions held in Facebook resulted in greater levels of participation and dialogue than class discussions held in LMS discussion forums (Camus et al., 2016 ; Hurt et al., 2012 ; Kent, 2013 ). Researchers found that preference for Facebook over the university’s LMS is due to perceptions that the LMS is outdated and unorganized and reports that Facebook is more familiar, convenient, and accessible given that many students already visit the social networking site multiple times per day (Clements, 2015 ; Dougherty & Andercheck, 2014 ; Hurt et al., 2012 ; Kent, 2013 ). In addition, students report that Facebook helps them stay engaged in learning through collaboration and interaction with both peers and instructors (Bahati, 2015 ; Shraim, 2014 ), which is evident in Facebook posts where students collaborated to study for exams, consulted on technical and theoretical problem solving, discussed course content, exchanged learning resources, and expressed opinions as well as academic successes and challenges (Bowman & Akcaoglu, 2014 ; Dougherty & Andercheck, 2014 ; Esteves, 2012 Ivala & Gachago, 2012 ; Maben et al., 2014 ; Rambe, 2012 ; van Beynen & Swenson, 2016 ).
There is far less evidence in the literature about the use of Facebook for emotional and cognitive engagement. In terms of emotional engagement, studies suggest that students feel positively about being part of a course-specific Facebook group and that Facebook is useful for expressing feelings about learning and concerns for peers, through features such as the “like” button and emoticons (Bowman & Akcaoglu, 2014 ; Dougherty & Andercheck, 2014 ; Naghdipour & Eldridge, 2016 ). In addition, being involved in a course-specific Facebook group was positively related to students’ sense of belonging in the course (Dougherty & Andercheck, 2014 ). The research on cognitive engagement is less conclusive, with some studies suggesting that Facebook participation is related to academic persistence (Fagioli et al., 2015 ) and self-regulation (Dougherty & Andercheck, 2014 ) while other studies show low levels of knowledge construction in Facebook posts (Hou et al., 2015 ), particularly when compared to discussions held in the LMS. One possible reason may be because the LMS is associated with formal, academic interactions while Facebook is associated with informal, social interactions (Camus et al., 2016 ). While additional research is needed to confirm the efficacy of Facebook for promoting cognitive engagement, studies suggest that Facebook may be a viable tool for increasing specific behavioral and emotional engagement indicators, such as interactions with others and a sense of belonging within a learning community.
Twitter is a web-based service where subscribers can post short messages, called tweets, in real-time that are no longer than 140 characters in length. Tweets may contain hyperlinks to other websites, images, graphics, and/or videos and may be tagged by topic using the hashtag symbol before the designated label (e.g., #elearning). Twitter subscribers may “follow” other users and gain access to their tweets and also may “retweet” messages that have already been posted (Hennessy, Kirkpatrick, Smith, & Border, 2016 ; Osgerby & Rush, 2015 ; Prestridge, 2014 ; West, Moore, & Barry, 2015 ; Tiernan, 2014 ;). Instructors may use Twitter to post updates about the course, clarify expectations, direct students to additional learning materials, and encourage students to discuss course content (Bista, 2015 ; Williams & Whiting, 2016 ). Several of the studies on the use of Twitter included broad, all-encompassing measures of student engagement and produced mixed findings. For example, some studies suggest that Twitter increases student engagement (Evans, 2014 ; Gagnon, 2015 ; Junco, Heibergert, & Loken, 2011 ) while other studies suggest that Twitter has little to no influence on student engagement (Junco, Elavsky, & Heiberger, 2013 ; McKay, Sanko, Shekhter, & Birnbach, 2014 ). In both studies suggesting little to no influence on student engagement, Twitter use was voluntary and in one of the studies faculty involvement in Twitter was low, which may account for the negative findings (Junco et al., 2013 ; McKay et al., 2014 ). Conversely, in the studies that show positive findings, Twitter use was mandatory and often directly integrated with required assignments (Evans, 2014 ; Gagnon, 2015 ; Junco et al., 2011 ). Therefore, making Twitter use mandatory, increasing faculty involvement in Twitter, and integrating Twitter into assignments may help to increase student engagement.
Studies pertaining to specific behavioral student engagement indicators also reveal mixed findings. For example, in studies where course-related Twitter use was voluntary, 45-91% of students reported using Twitter during the term (Hennessy et al., 2016 ; Junco et al., 2013 ; Ross, Banow, & Yu, 2015 ; Tiernan, 2014 ; Williams & Whiting, 2016 ), but only 30-36% reported making contributions to the course-specific Twitter page (Hennessy et al., 2016 ; Tiernan, 2014 ; Ross et al., 2015 ; Williams & Whiting, 2016 ). The study that reported a 91% participation rate was unique because the course-specific Twitter page was accessible via a public link. Therefore, students who chose only to view the content (58%), rather than contribute to the page, did not have to create a Twitter account (Hennessy et al., 2016 ). The convenience of not having to create an account may be one reason for much higher participation rates. In terms of low participation rates, a lack of literacy, familiarity, and interest in Twitter , as well as a preference for Facebook , are cited as contributing factors (Bista, 2015 ; McKay et al., 2014 ; Mysko & Delgaty, 2015 ; Osgerby & Rush, 2015 ; Tiernan, 2014 ). However, when the use of Twitter was required and integrated into class discussions, the participation rate was 100% (Gagnon, 2015 ). Similarly, 46% of students in one study indicated that they would have been more motivated to participate in Twitter activities if they were graded (Osgerby & Rush, 2015 ), again confirming the power of extrinsic motivating factors.
Studies also show mixed results for the use of Twitter to promote interactions with peers and instructors. Researchers found that when instructors used Twitter to post updates about the course, ask and answer questions, and encourage students to tweet about course content, there was evidence of student-student and student-instructor interactions in tweets (Hennessy et al., 2016 ; Tiernan, 2014 ). Some students echoed these findings, suggesting that Twitter is useful for sharing ideas and resources, discussing course content, asking the instructor questions, and networking (Chawinga, 2017 ; Evans, 2014 ; Gagnon, 2015 ; Hennessy et al., 2016 ; Mysko & Delgaty, 2015 ; West et al., 2015 ) and is preferable over speaking aloud in class because it is more comfortable, less threatening, and more concise due to the 140 character limit (Gagnon, 2015 ; Mysko & Delgaty, 2015 ; Tiernan, 2014 ). Conversely, other students reported that Twitter was not useful for improving interaction because they viewed it predominately for social, rather than academic, interactions and they found the 140 character limit to be frustrating and restrictive. A theme among the latter studies was that a large proportion of the sample had never used Twitter before (Bista, 2015 ; McKay et al., 2014 ; Osgerby & Rush, 2015 ), which may have contributed to negative perceptions.
The literature on the use of Twitter for cognitive and emotional engagement is minimal but nonetheless promising in terms of promoting knowledge gains, the practical application of content, and a sense of belonging among users. For example, using Twitter to respond to questions that arose in lectures and tweet about course content throughout the term is associated with increased understanding of course content and application of knowledge (Kim et al., 2015 ; Tiernan, 2014 ; West et al., 2015 ). While the underlying mechanisms pertaining to why Twitter promotes an understanding of content and application of knowledge are not entirely clear, Tiernan ( 2014 ) suggests that one possible reason may be that Twitter helps to break down communication barriers, encouraging shy or timid students to participate in discussions that ultimately are richer in dialogue and debate. In terms of emotional engagement, students who participated in a large, class-specific Twitter page were more likely to feel a sense of community and belonging compared to those who did not participate because they could more easily find support from and share resources with other Twitter users (Ross et al., 2015 ). Despite the positive findings about the use of Twitter for cognitive and emotional engagement, more studies are needed to confirm existing results regarding behavioral engagement and target additional engagement indicators such as motivation, persistence, and attitudes, interests, and values about learning. In addition, given the strong negative perceptions of Twitter that still exist, additional studies are needed to confirm Twitter ’s efficacy for promoting different types of behavioral engagement among both novice and experienced Twitter users, particularly when compared to more familiar tools such as Facebook or LMS discussion forums.
- Digital games
Digital games are “applications using the characteristics of video and computer games to create engaging and immersive learning experiences for delivery of specified learning goals, outcomes and experiences” (de Freitas, 2006 , p. 9). Digital games often serve the dual purpose of promoting the achievement of learning outcomes while making learning fun by providing simulations of real-world scenarios as well as role play, problem-solving, and drill and repeat activities (Boyle et al., 2016 ; Connolly, Boyle, MacArthur, Hainey, & Boyle, 2012 ; Scarlet & Ampolos, 2013 ; Whitton, 2011 ). In addition, gamified elements, such as digital badges and leaderboards, may be integrated into instruction to provide additional motivation for completing assigned readings and other learning activities (Armier, Shepherd, & Skrabut, 2016 ; Hew, Huang, Chu, & Chiu, 2016 ). The pedagogical benefits of digital games are somewhat distinct from the other technologies addressed in this review, which are designed primarily for social interaction. While digital games may be played in teams or allow one player to compete against another, the focus of their design often is on providing opportunities for students to interact with academic content in a virtual environment through decision-making, problem-solving, and reward mechanisms. For example, a digital game may require students to adopt a role as CEO in a computer-simulated business environment, make decisions about a series of organizational issues, and respond to the consequences of those decisions. In this example and others, digital games use adaptive learning principles, where the learning environment is re-configured or modified in response to the actions and needs of students (Bower, 2016 ). Most of the studies on digital games focused on cognitive and emotional indicators of student engagement, in contrast to the previous technologies addressed in this review which primarily focused on behavioral indicators of engagement.
Existing studies provide support for the influence of digital games on cognitive engagement, through achieving a greater understanding of course content and demonstrating higher-order thinking skills (Beckem & Watkins, 2012 ; Farley, 2013 ; Ke, Xie, & Xie, 2016 ; Marriott, Tan, & Marriott, 2015 ), particularly when compared to traditional instructional methods, such as giving lectures or assigning textbook readings (Lu, Hallinger, & Showanasai, 2014 ; Siddique, Ling, Roberson, Xu, & Geng, 2013 ; Zimmermann, 2013 ). For example, in a study comparing courses that offered computer simulations of business challenges (e.g, implementing a new information technology system, managing a startup company, and managing a brand of medicine in a simulated market environment) and courses that did not, students in simulation-based courses reported higher levels of action-directed learning (i.e., connecting theory to practice in a business context) than students in traditional, non-simulation-based courses (Lu et al., 2014 ). Similarly, engineering students who participated in a car simulator game, which was designed to help students apply and reinforce the knowledge gained from lectures, demonstrated higher levels of critical thinking (i.e., analysis, evaluation) on a quiz than students who only attended lectures (Siddique et al., 2013 ).
Motivation is another cognitive engagement indicator that is linked to digital games (Armier et al., 2016 ; Chang & Wei, 2016 ; Dichev & Dicheva, 2017 ; Grimley, Green, Nilsen, & Thompson, 2012 ; Hew et al., 2016 ; Ibáñez, Di-Serio, & Delgado-Kloos, 2014 ; Ke et al., 2016 ; Liu, Cheng, & Huang, 2011 ; Nadolny & Halabi, 2016 ). Researchers found that incorporating gamified elements into courses, such as giving students digital rewards (e.g., redeemable points, trophies, and badges) for participating in learning activities or creating competition through the use of leaderboards where students can see how they rank against other students positively affects student motivation to complete learning tasks (Armier et al., 2016 ; Chang & Wei, 2016 ; Hew et al., 2016 ; Nadolny & Halabi, 2016 ). In addition, students who participated in gamified elements, such as trying to earn digital badges, were more motivated to complete particularly difficult learning activities (Hew et al., 2016 ) and showed persistence in exceeding learning requirements (Ibáñez et al., 2014 ). Research on emotional engagement may help to explain these findings. Studies suggest that digital games positively affect student attitudes about learning, evident in student reports that games are fun, interesting, and enjoyable (Beckem & Watkins, 2012 ; Farley, 2013 ; Grimley et al., 2012 ; Hew et al., 2016 ; Liu et al., 2011 ; Zimmermann, 2013 ), which may account for higher levels of student motivation in courses that offered digital games.
Research on digital games and behavioral engagement is more limited, with only one study suggesting that games lead to greater participation in educational activities (Hew et al., 2016 ). Therefore, more research is needed to explore how digital games may influence behavioral engagement. In addition, research is needed to determine whether the underlying technology associated with digital games (e.g., computer-based simulations and virtual realities) produce positive engagement outcomes or whether common mechanisms associated with both digital and non-digital games (e.g., role play, rewards, and competition) account for those outcomes. For example, studies in which non-digital, face-to-face games were used also showed positive effects on student engagement (Antunes, Pacheco, & Giovanela, 2012 ; Auman, 2011 ; Coffey, Miller, & Feuerstein, 2011 ; Crocco, Offenholley, & Hernandez, 2016 ; Poole, Kemp, Williams, & Patterson, 2014 ; Scarlet & Ampolos, 2013 ); therefore, it is unclear if and how digitizing games contributes to student engagement.
Discussion and implications
Student engagement is linked to a number of academic outcomes, such as retention, grade point average, and graduation rates (Carini et al., 2006 ; Center for Postsecondary Research, 2016 ; Hu & McCormick, 2012 ). As a result, universities have shown a strong interest in how to increase student engagement, particularly given rising external pressures to improve learning outcomes and prepare students for academic success (Axelson & Flick, 2011 ; Kuh, 2009 ). There are various models of student engagement that identify factors that influence student engagement (Kahu, 2013 ; Lam et al., 2012 ; Nora et al., 2005 ; Wimpenny & Savin-Baden, 2013 ; Zepke & Leach, 2010 ); however, none include the overt role of technology despite the growing trend and student demands to integrate technology into the learning experience (Amirault, 2012 ; Cook & Sonnenberg, 2014 ; Revere & Kovach, 2011 ; Sun & Chen, 2016 ; Westera, 2015 ). Therefore, the primary purpose of our literature review was to explore whether technology influences student engagement. The secondary purpose was to address skepticism and uncertainty about pedagogical benefits of technology (Ashrafzadeh & Sayadian, 2015 ; Kopcha et al., 2016 ; Reid, 2014 ) by reviewing the literature regarding the efficacy of specific technologies (i.e., web-conferencing software, blogs, wikis, social networking sites, and digital games) for promoting student engagement and offering recommendations for effective implementation, which are included at the end of this paper. In the sections that follow, we provide an overview of the findings, an explanation of existing methodological limitations and areas for future research, and a list of best practices for integrating the technologies we reviewed into the teaching and learning process.
Summary of findings
Findings from our literature review provide preliminary support for including technology as a factor that influences student engagement in existing models (Table 1 ). One overarching theme is that most of the technologies we reviewed had a positive influence on multiple indicators of student engagement, which may lead to a larger return on investment in terms of learning outcomes. For example, digital games influence all three types of student engagement and six of the seven indicators we identified, surpassing the other technologies in this review. There were several key differences in the design and pedagogical use between digital games and other technologies that may explain these findings. First, digital games were designed to provide authentic learning contexts in which students could practice skills and apply learning (Beckem & Watkins, 2012 ; Farley, 2013 ; Grimley et al., 2012 ; Ke et al., 2016 ; Liu et al., 2011 ; Lu et al., 2014 ; Marriott et al., 2015 ; Siddique et al., 2013 ), which is consistent with experiential learning and adult learning theories. Experiential learning theory suggests that learning occurs through interaction with one’s environment (Kolb, 2014 ) while adult learning theory suggests that adult learners want to be actively involved in the learning process and be able apply learning to real life situations and problems (Cercone, 2008 ). Second, students reported that digital games (and gamified elements) are fun, enjoyable, and interesting (Beckem & Watkins, 2012 ; Farley, 2013 ; Grimley et al., 2012 ; Hew et al., 2016 ; Liu et al., 2011 ; Zimmermann, 2013 ), feelings that are associated with a flow-like state where one is completely immersed in and engaged with the activity (Csikszentmihalyi, 1988 ; Weibel, Wissmath, Habegger, Steiner, & Groner, 2008 ). Third, digital games were closely integrated into the curriculum as required activities (Farley, 2013 ; Grimley et al., 2012 , Ke et al., 2016 ; Liu et al., 2011 ; Marriott et al., 2015 ; Siddique et al., 2013 ) as opposed to wikis, Facebook , and Twitter , which were often voluntary and used to supplement lectures (Dougherty & Andercheck, 2014 Nakamaru, 2012 ; Prestridge, 2014 ; Rambe, 2012 ).
Web-conferencing software and Facebook also yielded the most positive findings, influencing four of the seven indicators of student engagement, compared to other collaborative technologies, such as blogs, wikis, and Twitter . Web-conferencing software was unique due to the sheer number of collaborative features it offers, providing multiple ways for students to actively engage with course content (screen sharing, whiteboards, digital pens) and interact with peers and the instructor (audio, video, text chats, breakout rooms) (Bower, 2011 ; Hudson et al., 2012 ; Martin et al., 2012 ; McBrien et al., 2009 ); this may account for the effects on multiple indicators of student engagement. Positive findings regarding Facebook ’s influence on student engagement could be explained by a strong familiarity and preference for the social networking site (Clements, 2015 ; DiVall & Kirwin, 2012 ; Hurt et al., 2012 ; Hou et al., 2015 ; Kent, 2013 ; Manca & Ranieri, 2013 ), compared to Twitter which was less familiar or interesting to students (Bista, 2015 ; McKay et al., 2014 ; Mysko & Delgaty, 2015 ; Osgerby & Rush, 2015 ; Tiernan, 2014 ). Wikis had the lowest influence on student engagement, with mixed findings regarding behavioral engagement, limited, but conclusive findings, regarding one indicator of cognitive engagement (deep processing of information), and no studies pertaining to other indicators of cognitive engagement (motivation, persistence) or emotional engagement.
Another theme that arose was the prevalence of mixed findings across multiple technologies regarding behavioral engagement. Overall, the vast majority of studies addressed behavioral engagement, and we expected that technologies designed specifically for social interaction, such as web-conferencing, wikis, and social networking sites, would yield more conclusive findings. However, one possible reason for the mixed findings may be that the technologies were voluntary in many studies, resulting in lower than desired participation rates and missed opportunities for interaction (Armstrong & Thornton, 2012 ; Fagioli et al., 2015 ; Nakamaru, 2012 ; Rambe, 2012 ; Ross et al., 2015 ; Williams & Whiting, 2016 ), and mandatory in a few studies, yielding higher levels of participation and interaction (Bahati, 2015 ; Gagnon, 2015 ; Roussinos & Jimoyiannis, 2013 ). Another possible reason for the mixed findings is that measures of variables differed across studies. For example, in some studies participation meant that a student signed up for a Twitter account (Tiernan, 2014 ), used the Twitter account for class (Williams & Whiting, 2016 ), or viewed the course-specific Twitter page (Hennessy et al., 2016 ). The pedagogical uses of the technologies also varied considerably across studies, making it difficult to make comparisons. For example, Facebook was used in studies to share learning materials (Clements, 2015 ; Dyson et al., 2015 ), answer student questions about academic content or administrative issues (Rambe, 2012 ), prepare for upcoming exams and share study tips (Bowman & Akcaoglu, 2014 ; DiVall & Kirwin, 2012 ), complete group work (Hou et al., 2015 ; Staines & Lauchs, 2013 ), and discuss course content (Camus et al., 2016 ; Kent, 2013 ; Hurt et al., 2012 ). Finally, cognitive indicators (motivation and persistence) drew the fewest amount of studies, which suggests that research is needed to determine whether technologies affect these indicators.
Methodological limitations
While there appears to be preliminary support for the use of many of the technologies to promote student engagement, there are significant methodological limitations in the literature and, as a result, findings should be interpreted with caution. First, many studies used small sample sizes and were limited to one course, one degree level, and one university. Therefore, generalizability is limited. Second, very few studies used experimental or quasi-experimental designs; therefore, very little evidence exists to substantiate a cause and effect relationship between technologies and student engagement indicators. In addition, in many studies that did use experimental or quasi-experimental designs, participants were not randomized; rather, participants who volunteered to use a specific technology were compared to those who chose not to use the technology. As a result, there is a possibility that fundamental differences between users and non-users could have affected the engagement results. Furthermore, many of the studies did not isolate specific technological features (e.g, using only the breakout rooms for group work in web-conferencing software, rather than using the chat feature, screen sharing, and breakout rooms for group work). Using multiple features at once could have conflated student engagement results. Third, many studies relied on one source to measure technological and engagement variables (single source bias), such as self-report data (i.e., reported usage of technology and perceptions of student engagement), which may have affected the validity of the results. Fourth, many studies were conducted during a very brief timeframe, such as one academic term. As a result, positive student engagement findings may be attributed to a “novelty effect” (Dichev & Dicheva, 2017 ) associated with using a new technology. Finally, many studies lack adequate details about learning activities, raising questions about whether poor instructional design may have adversely affected results. For example, an instructor may intend to elicit higher-order thinking from students, but if learning activity instructions are written using low-level verbs, such as identify, describe, and summarize, students will be less likely to engage in higher-order thinking.
Areas for future research
The findings of our literature review suggest that the influence of technology on student engagement is still a developing area of knowledge that requires additional research to build on promising, but limited, evidence, clarify mixed findings, and address several gaps in the literature. As such, our recommendations for future areas of research are as follows:
Examine the effect of collaborative technologies (i.e., web-conferencing, blogs, wikis, social networking sites ) on emotional and cognitive student engagement. There are significant gaps in the literature regarding whether these technologies affect attitudes, interests, and values about learning; a sense of belonging within a learning community; motivation to learn; and persistence to overcome academic challenges and meet or exceed requirements.
Clarify mixed findings, particularly regarding how web-conferencing software, wikis, and Facebook and Twitter affect participation in learning activities. Researchers should make considerable efforts to gain consensus or increase consistency on how participation is measured (e.g., visited Facebook group or contributed one post a week) in order to make meaningful comparisons and draw conclusions about the efficacy of various technologies for promoting behavioral engagement. In addition, further research is needed to clarify findings regarding how wikis and Twitter influence interaction and how blogs and Facebook influence deep processing of information. Future research studies should include justifications for the pedagogical use of specific technologies and detailed instructions for learning activities to minimize adverse findings from poor instructional design and to encourage replication.
Conduct longitudinal studies over several academic terms and across multiple academic disciplines, degree levels, and institutions to determine long-term effects of specific technologies on student engagement and to increase generalizability of findings. Also, future studies should take individual factors into account, such as gender, age, and prior experience with the technology. Studies suggest that a lack of prior experience or familiarity with Twitter was a barrier to Twitter use in educational settings (Bista, 2015 , Mysko & Delgaty, 2015 , Tiernan, 2014 ); therefore, future studies should take prior experience into account.
Compare student engagement outcomes between and among different technologies and non-technologies. For example, studies suggest that students prefer Facebook over Twitter (Bista, 2015 ; Osgerby & Rush, 2015 ), but there were no studies that compared these technologies for promoting student engagement. Also, studies are needed to isolate and compare different features within the same technology to determine which might be most effective for increasing engagement. Finally, studies on digital games (Beckem & Watkins, 2012 ; Grimley et al., 2012 ; Ke et al., 2016 ; Lu et al., 2014 ; Marriott et al., 2015 ; Siddique et al., 2013 ) and face-to-face games (Antunes et al., 2012 ; Auman, 2011 ; Coffey et al., 2011 ; Crocco et al., 2016 ; Poole et al., 2014 ; Scarlet & Ampolos, 2013 ) show similar, positive effects on student engagement, therefore, additional research is needed to determine the degree to which the delivery method (i.e.., digital versus face-to-face) accounts for positive gains in student engagement.
Determine whether other technologies not included in this review influence student engagement. Facebook and Twitter regularly appear in the literature regarding social networking, but it is unclear how other popular social networking sites, such as LinkedIn, Instagram, and Flickr, influence student engagement. Future research should focus on the efficacy of these and other popular social networking sites for promoting student engagement. In addition, there were very few studies about whether informational technologies, which involve the one-way transmission of information to students, affect different types of student engagement. Future research should examine whether informational technologies, such as video lectures, podcasts, and pre-recorded narrated Power Point presentations or screen casts, affect student engagement. Finally, studies should examine the influence of mobile software and technologies, such as educational apps or smartphones, on student engagement.
Achieve greater consensus on the meaning of student engagement and its distinction from similar concepts in the literature, such as social and cognitive presence (Garrison & Arbaugh, 2007 )
Recommendations for practice
Despite the existing gaps and mixed findings in the literature, we were able to compile a list of recommendations for when and how to use technology to increase the likelihood of promoting student engagement. What follows is not an exhaustive list; rather, it is a synthesis of both research findings and lessons learned from the studies we reviewed. There may be other recommendations to add to this list; however, our intent is to provide some useful information to help address barriers to technology integration among faculty who feel uncertain or unprepared to use technology (Ashrafzadeh & Sayadian, 2015 ; Hauptman, 2015 ; Kidd et al., 2016 ; Reid, 2014 ) and to add to the body of practical knowledge in instructional design and delivery. Our recommendations for practice are as follows:
Consider context before selecting technologies. Contextual factors such as existing technological infrastructure and requirements, program and course characteristics, and the intended audience will help determine which technologies, if any, are most appropriate (Bullen & Morgan, 2011 ; Bullen, Morgan, & Qayyum, 2011 ). For example, requiring students to use a blog that is not well integrated with the existing LMS may prove too frustrating for both the instructor and students. Similarly, integrating Facebook- and Twitter- based learning activities throughout a marketing program may be more appropriate, given the subject matter, compared to doing so in an engineering or accounting program where social media is less integral to the profession. Finally, do not assume that students appreciate or are familiar with all technologies. For example, students who did not already have Facebook or Twitter accounts were less likely to use either for learning purposes and perceived setting up an account to be an increase in workload (Bista, 2015 , Clements, 2015 ; DiVall & Kirwin, 2012 ; Hennessy et al., 2016 ; Mysko & Delgaty, 2015 , Tiernan, 2014 ). Therefore, prior to using any technology, instructors may want to determine how many students already have accounts and/or are familiar with the technology.
Carefully select technologies based on their strengths and limitations and the intended learning outcome. For example, Twitter is limited to 140 characters, making it a viable tool for learning activities that require brevity. In one study, an instructor used Twitter for short pop quizzes during lectures, where the first few students to tweet the correct answer received additional points (Kim et al., 2015 ), which helped students practice applying knowledge. In addition, studies show that students perceive Twitter and Facebook to be primarily for social interactions (Camus et al., 2016 ; Ross et al., 2015 ), which may make these technologies viable tools for sharing resources, giving brief opinions about news stories pertaining to course content, or having casual conversations with classmates rather than full-fledged scholarly discourse.
Incentivize students to use technology, either by assigning regular grades or giving extra credit. The average participation rates in voluntary web-conferencing, Facebook , and Twitter learning activities in studies we reviewed was 52% (Andrew et al., 2015 ; Armstrong & Thornton, 2012 ; Bahati, 2015 ; Bowman & Akcaoglu, 2014 ; Divall & Kirwin, 2012 ; Dougherty & Andercheck, 2014 ; Fagioli et al., 2015 ; Hennessy et al., 2016 ; Junco et al., 2013 ; Rambe, 2012 ; Ross et al., 2015 ; Staines & Lauchs, 2013 ; Tiernan, 2014 ; Williams & Whiting, 2016 ). While there were far fewer studies on the use of technology for graded or mandatory learning activities, the average participation rate reported in those studies was 97% (Bahati2015; Gagnon, 2015 ), suggesting that grading may be a key factor in ensuring students participate.
Communicate clear guidelines for technology use. Prior to the implementation of technology in a course, students may benefit from an overview the technology, including its navigational features, privacy settings, and security (Andrew et al., 2015 ; Hurt et al., 2012 ; Martin et al., 2012 ) and a set of guidelines for how to use the technology effectively and professionally within an educational setting (Miller et al., 2012 ; Prestridge, 2014 ; Staines & Lauchs, 2013 ; West et al., 2015 ). In addition, giving students examples of exemplary and poor entries and posts may also help to clarify how they are expected to use the technology (Shraim, 2014 ; Roussinos & Jimoyiannis, 2013 ). Also, if instructors expect students to use technology to demonstrate higher-order thinking or to interact with peers, there should be explicit instructions to do so. For example, Prestridge ( 2014 ) found that students used Twitter to ask the instructor questions but very few interacted with peers because they were not explicitly asked to do so. Similarly, Hou et al., 2015 reported low levels of knowledge construction in Facebook , admitting that the wording of the learning activity (e.g., explore and present applications of computer networking) and the lack of probing questions in the instructions may have been to blame.
Use technology to provide authentic and integrated learning experiences. In many studies, instructors used digital games to simulate authentic environments in which students could apply new knowledge and skills, which ultimately lead to a greater understanding of content and evidence of higher-order thinking (Beckem & Watkins, 2012 ; Liu et al., 2011 ; Lu et al., 2014 ; Marriott et al., 2015 ; Siddique et al., 2013 ). For example, in one study, students were required to play the role of a stock trader in a simulated trading environment and they reported that the simulation helped them engage in critical reflection, enabling them to identify their mistakes and weaknesses in their trading approaches and strategies (Marriott et al., 2015 ). In addition, integrating technology into regularly-scheduled classroom activities, such as lectures, may help to promote student engagement. For example, in one study, the instructor posed a question in class, asked students to respond aloud or tweet their response, and projected the Twitter page so that everyone could see the tweets in class, which lead to favorable comments about the usefulness of Twitter to promote engagement (Tiernan, 2014 ).
Actively participate in using the technologies assigned to students during the first few weeks of the course to generate interest (Dougherty & Andercheck, 2014 ; West et al., 2015 ) and, preferably, throughout the course to answer questions, encourage dialogue, correct misconceptions, and address inappropriate behavior (Bowman & Akcaoglu, 2014 ; Hennessy et al., 2016 ; Junco et al., 2013 ; Roussinos & Jimoyiannis, 2013 ). Miller et al. ( 2012 ) found that faculty encouragement and prompting was associated with increases in students’ expression of ideas and the degree to which they edited and elaborated on their peers’ work in a course-specific wiki.
Be mindful of privacy, security, and accessibility issues. In many studies, instructors took necessary steps to help ensure privacy and security by creating closed Facebook groups and private Twitter pages, accessible only to students in the course (Bahati, 2015 ; Bista, 2015 ; Bowman & Akcaoglu, 2014 ; Esteves, 2012 ; Rambe, 2012 ; Tiernan, 2014 ; Williams & Whiting, 2016 ) and by offering training to students on how to use privacy and security settings (Hurt et al., 2012 ). Instructors also made efforts to increase accessibility of web-conferencing software by including a phone number for students unable to access audio or video through their computer and by recording and archiving sessions for students unable to attend due to pre-existing conflicts (Andrew et al., 2015 ; Martin et al., 2012 ). In the future, instructors should also keep in mind that some technologies, like Facebook and Twitter , are not accessible to students living in China; therefore, alternative arrangements may need to be made.
In 1985, Steve Jobs predicted that computers and software would revolutionize the way we learn. Over 30 years later, his prediction has yet to be fully confirmed in the student engagement literature; however, our findings offer preliminary evidence that the potential is there. Of the technologies we reviewed, digital games, web-conferencing software, and Facebook had the most far-reaching effects across multiple types and indicators of student engagement, suggesting that technology should be considered a factor that influences student engagement in existing models. Findings regarding blogs, wikis, and Twitter, however, are less convincing, given a lack of studies in relation to engagement indicators or mixed findings. Significant methodological limitations may account for the wide range of findings in the literature. For example, small sample sizes, inconsistent measurement of variables, lack of comparison groups, and missing details about specific, pedagogical uses of technologies threaten the validity and reliability of findings. Therefore, more rigorous and robust research is needed to confirm and build upon limited but positive findings, clarify mixed findings, and address gaps particularly regarding how different technologies influence emotional and cognitive indicators of engagement.
Abbreviations
Learning management system
Amirault, R. J. (2012). Distance learning in the 21 st century university. Quarterly Review of Distance Education, 13 (4), 253–265.
Google Scholar
Anderson, M. (2016). More Americans using smartphones for getting directions, streaming TV . Washington, D.C.: Pew Research Center Retrieved from http://www.pewresearch.org/fact-tank/2016/01/29/us-smartphone-use/ .
Anderson, M., & Horrigan, J. B. (2016). Smartphones help those without broadband get online, but don’t necessary bridge the digital divide . Washington, D.C.: Pew Research Center Retrieved from http://www.pewresearch.org/fact-tank/2016/10/03/smartphones-help-those-without-broadband-get-online-but-dont-necessarily-bridge-the-digital-divide/ .
Andrew, L., Maslin-Prothero, S., & Ewens, B. (2015). Enhancing the online learning experience using virtual interactive classrooms. Australian Journal of Advanced Nursing, 32 (4), 22–31.
Antunes, M., Pacheco, M. R., & Giovanela, M. (2012). Design and implementation of an educational game for teaching chemistry in higher education. Journal of Chemical Education, 89 (4), 517–521. doi: 10.1021/ed2003077 .
Article Google Scholar
Armier, D. J., Shepherd, C. E., & Skrabut, S. (2016). Using game elements to increase student engagement in course assignments. College Teaching, 64 (2), 64–72 https://doi.org/10.1080/87567555.2015.1094439 .
Armstrong, A., & Thornton, N. (2012). Incorporating Brookfield’s discussion techniques synchronously into asynchronous online courses. Quarterly Review of Distance Education, 13 (1), 1–9.
Ashrafzadeh, A., & Sayadian, S. (2015). University instructors’ concerns and perceptions of technology integration. Computers in Human Behavior, 49 , 62–73. doi: 10.1016/j.chb.2015.01.071 .
Astin, A. W. (1984). Student involvement: A developmental theory for higher education. Journal of College Student Personnel, 25 (4), 297–308.
Auman, C. (2011). Using simulation games to increase student and instructor engagement. College Teaching, 59 (4), 154–161. doi: 10.1080/87567555 .
Axelson, R. D., & Flick, A. (2011). Defining student engagement. Change: The magazine of higher learning, 43 (1), 38–43.
Bahati, B. (2015). Extending student discussions beyond lecture room walls via Facebook. Journal of Education and Practice, 6 (15), 160–171.
Bakker, A. B., Vergel, A. I. S., & Kuntze, J. (2015). Student engagement and performance: A weekly diary study on the role of openness. Motivation and Emotion, 39 (1), 49–62. doi: 10.1007/s11031-014-9422-5 .
Beckem, J. I., & Watkins, M. (2012). Bringing life to learning: Immersive experiential learning simulations for online and blended courses. Journal if Asynchronous Learning Networks, 16 (5), 61–70 https://doi.org/10.24059/olj.v16i5.287 .
Bista, K. (2015). Is Twitter an effective pedagogical tool in higher education? Perspectives of education graduate students. Journal of the Scholarship Of Teaching And Learning, 15 (2), 83–102 https://doi.org/10.14434/josotl.v15i2.12825 .
Boghossian, P. (2006). Behaviorism, constructivism, and Socratic pedagogy. Educational Philosophy and Theory, 38 (6), 713–722 https://doi.org/10.1111/j.1469-5812.2006.00226.x .
Bower, M. (2011). Redesigning a web-conferencing environment to scaffold computing students’ creative design processes. Journal of Educational Technology & Society, 14 (1), 27–42.
MathSciNet Google Scholar
Bower, M. (2016). A framework for adaptive learning design in a Web-conferencing environment. Journal of Interactive Media in Education, 2016 (1), 11 http://doi.org/10.5334/jime.406 .
Article MathSciNet Google Scholar
Bowman, N. D., & Akcaoglu, M. (2014). “I see smart people!”: Using Facebook to supplement cognitive and affective learning in the university mass lecture. The Internet and Higher Education, 23 , 1–8. doi: 10.1016/j.iheduc.2014.05.003 .
Boyle, E. A., Hainey, T., Connolly, T. M., Gray, G., Earp, J., Ott, M., et al. (2016). An update to the systematic literature review of empirical evidence of the impacts and outcomes of computer games and serious games. Computers & Education, 94 , 178–192. doi: 10.1016/j.compedu.2015.11.003 .
Bryson, C., & Hand, L. (2007). The role of engagement in inspiring teaching and learning. Innovations in Education and Teaching International, 44 (4), 349–362. doi: 10.1080/14703290701602748 .
Buchanan, T., Sainter, P., & Saunders, G. (2013). Factors affecting faculty use of learning technologies: Implications for models of technology adoption. Journal of Computer in Higher Education, 25 (1), 1–11.
Bullen, M., & Morgan, T. (2011). Digital learners not digital natives. La Cuestión Universitaria, 7 , 60–68.
Bullen, M., Morgan, T., & Qayyum, A. (2011). Digital learners in higher education: Generation is not the issue. Canadian Journal of Learning and Technology, 37 (1), 1–24.
Calabretto, J., & Rao, D. (2011). Wikis to support collaboration of pharmacy students in medication management workshops -- a pilot project. International Journal of Pharmacy Education & Practice, 8 (2), 1–12.
Camacho, M. E., Carrión, M. D., Chayah, M., & Campos, J. M. (2016). The use of wiki to promote students’ learning in higher education (Degree in Pharmacy). International Journal of Educational Technology in Higher Education, 13 (1), 1–8 https://doi.org/10.1186/s41239-016-0025-y .
Camus, M., Hurt, N. E., Larson, L. R., & Prevost, L. (2016). Facebook as an online teaching tool: Effects on student participation, learning, and overall course performance. College Teaching, 64 (2), 84–94 https://doi.org/10.1080/87567555.2015.1099093 .
Carini, R. M., Kuh, G. D., & Klein, S. P. (2006). Student engagement and student learning: Testing the linkages. Research in Higher Education, 47 (1), 1–32. doi: 10.1007/s11162-005-8150-9 .
Cassidy, E. D., Colmenares, A., Jones, G., Manolovitz, T., Shen, L., & Vieira, S. (2014). Higher Education and Emerging Technologies: Shifting Trends in Student Usage. The Journal of Academic Librarianship, 40 , 124–133. doi: 10.1016/j.acalib.2014.02.003 .
Center for Postsecondary Research (2016). Engagement insights: Survey findings on the quality of undergraduate education . Retrieved from http://nsse.indiana.edu/NSSE_2016_Results/pdf/NSSE_2016_Annual_Results.pdf .
Center for Postsecondary Research (2017). About NSSE. Retrieved on February 15, 2017 from http://nsse.indiana.edu/html/about.cfm
Cercone, K. (2008). Characteristics of adult learners with implications for online learning design. AACE Journal, 16 (2), 137–159.
Chang, J. W., & Wei, H. Y. (2016). Exploring Engaging Gamification Mechanics in Massive Online Open Courses. Educational Technology & Society, 19 (2), 177–203.
Chawinga, W. D. (2017). Taking social media to a university classroom: teaching and learning using Twitter and blogs. International Journal of Educational Technology in Higher Education, 14 (1), 3 https://doi.org/10.1186/s41239-017-0041-6 .
Chen, B., Seilhamer, R., Bennett, L., & Bauer, S. (2015). Students’ mobile learning practices in higher education: A multi-year study. In EDUCAUSE Review Retrieved from http://er.educause.edu/articles/2015/6/students-mobile-learning-practices-in-higher-education-a-multiyear-study .
Chu, S. K., Chan, C. K., & Tiwari, A. F. (2012). Using blogs to support learning during internship. Computers & Education, 58 (3), 989–1000. doi: 10.1016/j.compedu.2011.08.027 .
Clements, J. C. (2015). Using Facebook to enhance independent student engagement: A case study of first-year undergraduates. Higher Education Studies, 5 (4), 131–146 https://doi.org/10.5539/hes.v5n4p131 .
Coates, H. (2008). Attracting, engaging and retaining: New conversations about learning . Camberwell: Australian Council for Educational Research Retrieved from http://research.acer.edu.au/cgi/viewcontent.cgi?article=1015&context=ausse .
Coffey, D. J., Miller, W. J., & Feuerstein, D. (2011). Classroom as reality: Demonstrating campaign effects through live simulation. Journal of Political Science Education, 7 (1), 14–33.
Coghlan, E., Crawford, J. Little, J., Lomas, C., Lombardi, M., Oblinger, D., & Windham, C. (2007). ELI Discovery Tool: Guide to Blogging . Retrieved from https://net.educause.edu/ir/library/pdf/ELI8006.pdf .
Connolly, T. M., Boyle, E. A., MacArthur, E., Hainey, T., & Boyle, J. M. (2012). A systematic literature review of empirical evidence on computer games and serious games. Computers & Education, 59 , 661–686. doi: 10.1016/j.compedu.2012.03.004 .
Cook, C. W., & Sonnenberg, C. (2014). Technology and online education: Models for change. ASBBS E-Journal, 10 (1), 43–59.
Crocco, F., Offenholley, K., & Hernandez, C. (2016). A proof-of-concept study of game-based learning in higher education. Simulation & Gaming, 47 (4), 403–422. doi: 10.1177/1046878116632484 .
Csikszentmihalyi, M. (1988). The flow experience and its significance for human psychology. In M. Csikszentmihalyi & I. Csikszentmihalyi (Eds.), Optimal experience: Psychological studies of flow in consciousness (pp. 15–13). Cambridge, UK: Cambridge University Press.
Chapter Google Scholar
Dahlstrom, E. (2012). ECAR study of undergraduate students and information technology, 2012 (Research Report). Retrieved from http://net.educause.edu/ir/library/pdf/ERS1208/ERS1208.pdf
de Freitas, S. (2006). Learning in immersive worlds: A review of game-based learning . Retrieved from https://curve.coventry.ac.uk/open/file/aeedcd86-bc4c-40fe-bfdf-df22ee53a495/1/learning%20in%20immersive%20worlds.pdf .
Dichev, C., & Dicheva, D. (2017). Gamifying education: What is known, what is believed and what remains uncertain: A critical review. International Journal of Educational Technology in Higher Education, 14 (9), 1–36. doi: 10.1186/s41239-017-0042-5 .
DiVall, M. V., & Kirwin, J. L. (2012). Using Facebook to facilitate course-related discussion between students and faculty members. American Journal of Pharmaceutical Education, 76 (2), 1–5 https://doi.org/10.5688/ajpe76232 .
Dos, B., & Demir, S. (2013). The analysis of the blogs created in a blended course through the reflective thinking perspective. Educational Sciences: Theory & Practice, 13 (2), 1335–1344.
Dougherty, K., & Andercheck, B. (2014). Using Facebook to engage learners in a large introductory course. Teaching Sociology, 42 (2), 95–104 https://doi.org/10.1177/0092055x14521022 .
Dyson, B., Vickers, K., Turtle, J., Cowan, S., & Tassone, A. (2015). Evaluating the use of Facebook to increase student engagement and understanding in lecture-based classes. Higher Education: The International Journal of Higher Education and Educational Planning, 69 (2), 303–313 https://doi.org/10.1007/s10734-014-9776-3.
Esteves, K. K. (2012). Exploring Facebook to enhance learning and student engagement: A case from the University of Philippines (UP) Open University. Malaysian Journal of Distance Education, 14 (1), 1–15.
Evans, C. (2014). Twitter for teaching: Can social media be used to enhance the process of learning? British Journal of Educational Technology, 45 (5), 902–915 https://doi.org/10.1111/bjet.12099 .
Fagioli, L., Rios-Aguilar, C., & Deil-Amen, R. (2015). Changing the context of student engagement: Using Facebook to increase community college student persistence and success. Teachers College Record, 17 , 1–42.
Farley, P. C. (2013). Using the computer game “FoldIt” to entice students to explore external representations of protein structure in a biochemistry course for nonmajors. Biochemistry and Molecular Biology Education, 41 (1), 56–57 https://doi.org/10.1002/bmb.20655 .
Francescucci, A., & Foster, M. (2013). The VIRI classroom: The impact of blended synchronous online courses on student performance, engagement, and satisfaction. Canadian Journal of Higher Education, 43 (3), 78–91.
Fredricks, J., Blumenfeld, P., & Paris, A. (2004). School engagement: Potential of the concept, state of the evidence. Review of Educational Research, 74 (1), 59–109. doi: 10.3102/00346543074001059 .
Gagnon, K. (2015). Using twitter in health professional education: A case study. Journal of Allied Health, 44 (1), 25–33.
Gandhi, P., Khanna, S., & Ramaswamy, S. (2016). Which industries are the most digital (and why?) . Retrieved from https://hbr.org/2016/04/a-chart-that-shows-which-industries-are-the-most-digital-and-why .
Garrison, D. R., & Arbaugh, J. B. (2007). Researching the community of inquiry framework: Review, issues, and future directions. The Internet and Higher Education, 10 (3), 157–172 http://dx.doi.org/10.1016/j.iheduc.2007.04.001 .
Garrity, M. K., Jones, K., VanderZwan, K. J., de la Rocha, A. R., & Epstein, I. (2014). Integrative review of blogging: Implications for nursing education. Journal of Nursing Education, 53 (7), 395–401. doi: 10.3928/01484834-20140620-01 .
Gikas, J., & Grant, M. M. (2013). Mobile computing devices in higher education: Student perspectives on learning with cellphones, smartphones & social media. The Internet and Higher Education, 19 , 18–26 http://dx.doi.org/10.1016/j.iheduc.2013.06.002 .
Gilboy, M. B., Heinerichs, S., & Pazzaglia, G. (2015). Enhancing student engagement using the flipped classroom. Journal of Nutrition Education and Behavior, 47 (1), 109–114 http://dx.doi.org/10.1016/j.jneb.2014.08.008 .
Greenwood, S., Perrin, A., & Duggan, M. (2016). Social media update 2016 . Washington.: Pew Research Center Retrieved from http://www.pewinternet.org/2016/11/11/social-media-update-2016/ .
Grimley, M., Green, R., Nilsen, T., & Thompson, D. (2012). Comparing computer game and traditional lecture using experience ratings from high and low achieving students. Australasian Journal of Educational Technology, 28 (4), 619–638 https://doi.org/10.14742/ajet.831 .
Gunawardena, C. N., Hermans, M. B., Sanchez, D., Richmond, C., Bohley, M., & Tuttle, R. (2009). A theoretical framework for building online communities of practice with social networking tools. Educational Media International, 46 (1), 3–16 https://doi.org/10.1080/09523980802588626 .
Haggis, T. (2009). What have we been thinking of? A critical overview of 40 years of student learning research in higher education. Studies in Higher Education, 34 (4), 377–390. doi: 10.1080/03075070902771903 .
Hauptman, P.H. (2015). Mobile technology in college instruction. Faculty perceptions and barriers to adoption (Doctoral dissertation). Retrieved from ProQuest. (AAI3712404).
Hennessy, C. M., Kirkpatrick, E., Smith, C. F., & Border, S. (2016). Social media and anatomy education: Using twitter to enhance the student learning experience in anatomy. Anatomical Sciences Education, 9 (6), 505–515 https://doi.org/10.1002/ase.1610 .
Hew, K. F., Huang, B., Chu, K. S., & Chiu, D. K. (2016). Engaging Asian students through game mechanics: Findings from two experiment studies. Computers & Education, 93 , 221–236. doi: 10.1016/j.compedu.2015.10.010 .
Hewege, C. R., & Perera, L. R. (2013). Pedagogical significance of wikis: Towards gaining effective learning outcomes. Journal of International Education in Business, 6 (1), 51–70 https://doi.org/10.1108/18363261311314953 .
Hou, H., Wang, S., Lin, P., & Chang, K. (2015). Exploring the learner’s knowledge construction and cognitive patterns of different asynchronous platforms: comparison of an online discussion forum and Facebook. Innovations in Education and Teaching International, 52 (6), 610–620. doi: 10.1080/14703297.2013.847381 .
Hu, S., & McCormick, A. C. (2012). An engagement-based student typology and its relationship to college outcomes. Research in Higher Education, 53 , 738–754. doi: 10.1007/s11162-012-9254-7 .
Hudson, T. M., Knight, V., & Collins, B. C. (2012). Perceived effectiveness of web conferencing software in the digital environment to deliver a graduate course in applied behavior analysis. Rural Special Education Quarterly, 31 (2), 27–39.
Hurt, N. E., Moss, G. S., Bradley, C. L., Larson, L. R., Lovelace, M. D., & Prevost, L. B. (2012). The ‘Facebook’ effect: College students’ perceptions of online discussions in the age of social networking. International Journal for the Scholarship of Teaching & Learning, 6 (2), 1–24 https://doi.org/10.20429/ijsotl.2012.060210 .
Ibáñez, M. B., Di-Serio, A., & Delgado-Kloos, C. (2014). Gamification for engaging computer science students in learning activities: A case study. IEEE Transactions on Learning Technologies, 7 (3), 291–301 https://doi.org/10.1109/tlt.2014.2329293 .
Ivala, E., & Gachago, D. (2012). Social media for enhancing student engagement: The use of facebook and blogs at a university of technology. South African Journal of Higher Education, 26 (1), 152–167.
Johnson, D. R. (2013). Technological change and professional control in the professoriate. Science, Technology & Human Values, 38 (1), 126–149. doi: 10.1177/0162243911430236 .
Junco, R., Elavsky, C. M., & Heiberger, G. (2013). Putting Twitter to the test: Assessing outcomes for student collaboration, engagement and success. British Journal of Educational Technology, 44 (2), 273–287. doi: 10.1111/j.1467-8535.2012.01284.x .
Junco, R., Heibergert, G., & Loken, E. (2011). The effect of Twitter on college student engagement and grades. Journal of Computer Assisted Learning, 27 (2), 119–132. doi: 10.1111/j.1365-2729.2010.00387.x .
Kahu, E. R. (2013). Framing student engagement in higher education. Studies in Higher Education, 38 (5), 758–773. doi: 10.1080/03075079.2011.598505 .
Kaware, S. S., & Sain, S. K. (2015). ICT Application in Education: An Overview. International Journal of Multidisciplinary Approach & Studies, 2 (1), 25–32.
Ke, F., Xie, K., & Xie, Y. (2016). Game-based learning engagement: A theory- and data-driven exploration. British Journal of Educational Technology, 47 (6), 1183–1201 https://doi.org/10.1111/bjet.12314 .
Kent, M. (2013). Changing the conversation: Facebook as a venue for online class discussion in higher education. Journal of Online Learning & Teaching, 9 (4), 546–565 https://doi.org/10.1353/rhe.2015.0000 .
Kidd, T., Davis, T., & Larke, P. (2016). Experience, adoption, and technology: Exploring the phenomenological experiences of faculty involved in online teaching at once school of public health. International Journal of E-Learning, 15 (1), 71–99.
Kim, Y., Jeong, S., Ji, Y., Lee, S., Kwon, K. H., & Jeon, J. W. (2015). Smartphone response system using twitter to enable effective interaction and improve engagement in large classrooms. IEEE Transactions on Education, 58 (2), 98–103 https://doi.org/10.1109/te.2014.2329651 .
Kinchin. (2012). Avoiding technology-enhanced non-learning. British Journal of Educational Technology, 43 (2), E43–E48.
Kolb, D. A. (2014). Experiential learning: Experience as the source of learning and development (2nd ed.). Upper Saddle River: Pearson Education, Inc..
Kopcha, T. J., Rieber, L. P., & Walker, B. B. (2016). Understanding university faculty perceptions about innovation in teaching and technology. British Journal of Educational Technology, 47 (5), 945–957. doi: 10.1111/bjet.12361 .
Krause, K., & Coates, H. (2008). Students’ engagement in first-year university. Assessment and Evaluation in Higher Education, 33 (5), 493–505. doi: 10.1080/02602930701698892 .
Kuh, G. D. (2009). The National Survey of Student Engagement: Conceptual and empirical foundations. New Directions for Institutional Research, 141 , 5–20.
Lam, S., Wong, B., Yang, H., & Yi, L. (2012). Understanding student engagement with a contextual model. In S. L. Christenson, A. L. Reschly, & C. Wylie (Eds.), Handbook of Research on Student Engagement (pp. 403–419). New York: Springer.
Lawrence, B., & Lentle-Keenan, S. (2013). Teaching beliefs and practice, institutional context, and the uptake of Web-based technology. Distance Education, 34 (1), 4–20.
Leach, L. (2016). Enhancing student engagement in one institution. Journal of Further and Higher Education, 40 (1), 23–47.
Lester, D. (2013). A review of the student engagement literature. Focus on Colleges, Universities, and Schools, 7 (1), 1–8.
Lewis, C. C., Fretwell, C. E., Ryan, J., & Parham, J. B. (2013). Faculty use of established and emerging technologies in higher education: A unified theory of acceptance and use of technology perspective. International Journal of Higher Education, 2 (2), 22–34 http://dx.doi.org/10.5430/ijhe.v2n2p22 .
Lin, C., Singer, R., & Ha, L. (2010). Why university members use and resist technology? A structure enactment perspective. Journal of Computing in Higher Education, 22 (1), 38–59. doi: 10.1007/s12528-010-9028-1 .
Linder-VanBerschot, J. A., & Summers, L. L. (2015). Designing instruction in the face of technology transience. Quarterly Review of Distance Education, 16 (2), 107–118.
Liu, C., Cheng, Y., & Huang, C. (2011). The effect of simulation games on the learning of computational problem solving. Computers & Education, 57 (3), 1907–1918 https://doi.org/10.1016/j.compedu.2011.04.002 .
Lu, J., Hallinger, P., & Showanasai, P. (2014). Simulation-based learning in management education: A longitudinal quasi-experimental evaluation of instructional effectiveness. Journal of Management Development, 33 (3), 218–244. doi: 10.1108/JMD-11-2011-0115 .
Maben, S., Edwards, J., & Malone, D. (2014). Online engagement through Facebook groups in face-to-face undergraduate communication courses: A case study. Southwestern Mass Communication Journal, 29 (2), 1–27.
Manca, S., & Ranieri, M. (2013). Is it a tool suitable for learning? A critical review of the literature on Facebook as a technology-enhanced learning environment. Journal of Computer Assisted Learning, 29 (6), 487–504. doi: 10.1111/jcal.12007 .
Mansouri, S. A., & Piki, A. (2016). An exploration into the impact of blogs on students’ learning: Case studies in postgraduate business education. Innovations in Education And Teaching International, 53 (3), 260–273 http://dx.doi.org/10.1080/14703297.2014.997777 .
Marriott, P., Tan, S. W., & Marriot, N. (2015). Experiential learning – A case study of the use of computerized stock market trading simulation in finance education. Accounting Education, 24 (6), 480–497 http://dx.doi.org/10.1080/09639284.2015.1072728 .
Martin, F., Parker, M. A., & Deale, D. F. (2012). Examining interactivity in synchronous virtual classrooms. International Review of Research in Open and Distance Learning, 13 (3), 227–261.
Martin, K., Goldwasser, M., & Galentino, R. (2017). Impact of Cohort Bonds on Student Satisfaction and Engagement. Current Issues in Education, 19 (3), 1–14.
Martínez, A. A., Medina, F. X., Albalat, J. A. P., & Rubió, F. S. (2013). Challenges and opportunities of 2.0 tools for the interdisciplinary study of nutrition: The case of the Mediterranean Diet wiki. International Journal of Educational Technology in Higher Education, 10 (1), 210–225 https://doi.org/10.7238/rusc.v10i1.1341 .
McBrien, J. L., Jones, P., & Cheng, R. (2009). Virtual spaces: Employing a synchronous online classroom to facilitate student engagement in online learning. International Review of Research in Open and Distance Learning, 10 (3), 1–17 https://doi.org/10.19173/irrodl.v10i3.605 .
McClenney, K., Marti, C. N., & Adkins, C. (2012). Student engagement and student outcomes: Key findings from “CCSSE” validation research . Austin: Community College Survey of Student Engagement.
McKay, M., Sanko, J., Shekhter, I., & Birnbach, D. (2014). Twitter as a tool to enhance student engagement during an interprofessional patient safety course. Journal of Interprofessional Care, 28 (6), 565–567 https://doi.org/10.3109/13561820.2014.912618 .
Miller, A. D., Norris, L. B., & Bookstaver, P. B. (2012). Use of wikis in pharmacy hybrid elective courses. Currents in Pharmacy Teaching & Learning, 4 (4), 256–261. doi: 10.1016/j.cptl.2012.05.004 .
Morley, D. A. (2012). Enhancing networking and proactive learning skills in the first year university experience through the use of wikis. Nurse Education Today, 32 (3), 261–266.
Mysko, C., & Delgaty, L. (2015). How and why are students using Twitter for #meded? Integrating Twitter into undergraduate medical education to promote active learning. Annual Review of Education, Communication & Language Sciences, 12 , 24–52.
Nadolny, L., & Halabi, A. (2016). Student participation and achievement in a large lecture course with game-based learning. Simulation and Gaming, 47 (1), 51–72. doi: 10.1177/1046878115620388 .
Naghdipour, B., & Eldridge, N. H. (2016). Incorporating social networking sites into traditional pedagogy: A case of facebook. TechTrends, 60 (6), 591–597 http://dx.doi.org/10.1007/s11528-016-0118-4 .
Nakamaru, S. (2012). Investment and return: Wiki engagement in a “remedial” ESL writing course. Journal of Research on Technology in Education, 44 (4), 273–291.
Nelson, R. (2016). Apple’s app store will hit 5 million apps by 2020, more than doubling its current size . Retrieved from https://sensortower.com/blog/app-store-growth-forecast-2020 .
Nora, A., Barlow, E., & Crisp, G. (2005). Student persistence and degree attainment beyond the first year in college. In A. Seidman (Ed.), College Student Retention (pp. 129–154). Westport: Praeger Publishers.
Osgerby, J., & Rush, D. (2015). An exploratory case study examining undergraduate accounting students’ perceptions of using Twitter as a learning support tool. International Journal of Management Education, 13 (3), 337–348. doi: 10.1016/j.ijme.2015.10.002 .
Pace, C. R. (1980). Measuring the quality of student effort. Current Issues in Higher Education, 2 , 10–16.
Pace, C. R. (1984). Student effort: A new key to assessing quality . Los Angeles: University of California, Higher Education Research Institute.
Paul, J. A., & Cochran, J. D. (2013). Key interactions for online programs between faculty, students, technologies, and educational institutions: A holistic framework. Quarterly Review of Distance Education, 14 (1), 49–62.
Pellas, N. (2014). The influence of computer self-efficacy, metacognitive self-regulation, and self-esteem on student engagement in online learning programs: Evidence from the virtual world of Second Life. Computers in Human Behavior, 35 , 157–170. doi: 10.1016/j.chb.2014.02.048 .
Poole, S. M., Kemp, E., Williams, K. H., & Patterson, L. (2014). Get your head in the game: Using gamification in business education to connect with Generation Y. Journal for Excellence in Business Education, 3 (2), 1–9.
Poushter, J. (2016). Smartphone ownership and internet usage continues to climb in emerging economies . Washington, D.C.: Pew Research Center Retrieved from http://www.pewglobal.org/2016/02/22/smartphone-ownership-and-internet-usage-continues-to-climb-in-emerging-economies/ .
Prestridge, S. (2014). A focus on students’ use of Twitter - their interactions with each other, content and interface. Active Learning in Higher Education, 15 (2), 101–115.
Rambe, P. (2012). Activity theory and technology mediated interaction: Cognitive scaffolding using question-based consultation on “Facebook”. Australasian Journal of Educational Technology, 28 (8), 1333–1361 https://doi.org/10.14742/ajet.775 .
Reid, P. (2014). Categories for barriers to adoption of instructional technologies. Education and Information Technologies, 19 (2), 383–407.
Revere, L., & Kovach, J. V. (2011). Online technologies for engagement learning: A meaningful synthesis for educators. Quarterly Review of Distance Education, 12 (2), 113–124.
Richardson, J. C., & Newby, T. (2006). The role of students’ cognitive engagement in online learning. American Journal of Distance Education, 20 (1), 23–37 http://dx.doi.org/10.1207/s15389286ajde2001_3 .
Ross, H. M., Banow, R., & Yu, S. (2015). The use of Twitter in large lecture courses: Do the students see a benefit? Contemporary Educational Technology, 6 (2), 126–139.
Roussinos, D., & Jimoyiannis, A. (2013). Analysis of students’ participation patterns and learning presence in a wiki-based project. Educational Media International, 50 (4), 306–324 https://doi.org/10.1080/09523987.2013.863471 .
Salaber, J. (2014). Facilitating student engagement and collaboration in a large postgraduate course using wiki-based activities. International Journal of Management Education, 12 (2), 115–126. doi: 10.1016/j.ijme.2014.03.006 .
Scarlet, J., & Ampolos, L. (2013). Using game-based learning to teach psychopharmacology. Psychology Learning and Teaching, 12 (1), 64–70 https://doi.org/10.2304/plat.2013.12.1.64 .
Sharma, P., & Tietjen, P. (2016). Examining patterns of participation and meaning making in student blogs: A case study in higher education. American Journal of Distance Education, 30 (1), 2–13 http://dx.doi.org/10.1080/08923647.2016.1119605 .
Shraim, K. Y. (2014). Pedagogical innovation within Facebook: A case study in tertiary education in Palestine. International Journal of Emerging Technologies in Learning, 9 (8), 25–31. doi: 10.3991/ijet.v9i8.3805 .
Siddique, Z., Ling, C., Roberson, P., Xu, Y., & Geng, X. (2013). Facilitating higher-order learning through computer games. Journal of Mechanical Design, 135 (12), 121004–121010.
Smith, A., & Anderson, M. (2016). Online Shopping and E-Commerce . Washington, D.C.: Pew Research Center Retrieved from http://www.pewinternet.org/2016/12/19/online-shopping-and-e-commerce/ .
Staines, Z., & Lauchs, M. (2013). Students’ engagement with Facebook in a university undergraduate policing unit. Australasian Journal of Educational Technology, 29 (6), 792–805 https://doi.org/10.14742/ajet.270 .
Sun, A., & Chen, X. (2016). Online education and its effective practice: A research review. Journal of Information Technology Education: Research, 15 , 157–190.
Tiernan, P. (2014). A study of the use of Twitter by students for lecture engagement and discussion. Education and Information Technologies, 19 (4), 673–690 https://doi.org/10.1007/s10639-012-9246-4 .
Trowler, V. (2010). Student engagement literature review . Lancaster: Lancaster University Retrieved from http://www.lancaster.ac.uk/staff/trowler/StudentEngagementLiteratureReview.pdf .
Trowler, V., & Trowler, P. (2010). Student engagement evidence summary . Lancaster: Lancaster University Retrieved from http://eprints.lancs.ac.uk/61680/1/Deliverable_2._Evidence_Summary._Nov_2010.pdf .
van Beynen, K., & Swenson, C. (2016). Exploring peer-to-peer library content and engagement on a student-run Facebook group. College & Research Libraries, 77 (1), 34–50 https://doi.org/10.5860/crl.77.1.34 .
Wang, S. (2008). Blogs in education. In M. Pagani (Ed.), Encyclopedia of Multimedia Technology and Networking (2nd ed., pp. 134–139). Hershey: Information Sciences Reference.
Wdowik, S. (2014). Using a synchronous online learning environment to promote and enhance transactional engagement beyond the classroom. Campus — Wide Information Systems, 31 (4), 264–275. doi: 10.1108/CWIS-10-2013-0057 .
Weibel, D., Wissmath, B., Habegger, S., Steiner, Y., & Groner, R. (2008). Playing online games against computer-vs. human-controlled opponents: Effects on presence, flow, and enjoyment. Computers in Human Behavior, 24 (5), 2274–2291 https://doi.org/10.1016/j.chb.2007.11.002 .
West, B., Moore, H., & Barry, B. (2015). Beyond the tweet: Using Twitter to enhance engagement, learning, and success among first-year students. Journal of Marketing Education, 37 (3), 160–170. doi: 10.1177/0273475315586061 .
Westera, W. (2015). Reframing the role of educational media technologies. Quarterly Review of Distance Education, 16 (2), 19–32.
Whitton, N. (2011). Game engagement theory and adult learning. Simulation & Gaming, 42 (5), 596–609.
Williams, D., & Whiting, A. (2016). Exploring the relationship between student engagement, Twitter, and a learning management system: A study of undergraduate marketing students. International Journal of Teaching & Learning in Higher Education, 28 (3), 302–313.
Wimpenny, K., & Savin-Baden, M. (2013). Alienation, agency, and authenticity: A synthesis of the literature on student engagement. Teaching in Higher Education, 18 (3), 311–326. doi: 10.1080/13562517.2012.725223 .
Witkowski, P., & Cornell, T. (2015). An Investigation into Student Engagement in Higher Education Classrooms. InSight: A Journal of Scholarly Teaching, 10 , 56–67.
Wright, G. B. (2011). Student-centered learning in higher education. International Journal of Teaching and Learning in Higher Education, 23 (3), 92–97.
Yang, C., & Chang, Y. (2012). Assessing the effects of interactive blogging on student attitudes towards peer interaction, learning motivation, and academic achievements. Journal of Computer Assisted Learning, 28 (2), 126–135 https://doi.org/10.1111/j.1365-2729.2011.00423.x .
Zepke, N. (2014). Student engagement research in higher education: questioning an academic orthodoxy. Teaching in Higher Education, 19 (6), 697–708 http://dx.doi.org/10.1080/13562517.2014.901956 .
Zepke, N., & Leach, L. (2010). Improving student engagement: Ten proposals for action. Active Learning in Higher Education, 11 (3), 167–177. doi: 10.1177/1469787410379680 .
Zickuhr, K., & Raine, L. (2014). E-reading rises as device ownership jumps . Washington, D.C.: Pew Research Center Retrieved from http://www.pewinternet.org/2014/01/16/e-reading-rises-as-device-ownership-jumps/ .
Zimmermann, L. K. (2013). Using a virtual simulation program to teach child development. College Teaching, 61 (4), 138–142. doi: 10.1080/87567555.2013.817377 .
Download references
Acknowledgements
Not applicable.
This research was supported in part by a Laureate Education, Incl. David A. Wilson research grant study awarded to the second author, “A Comparative Analysis of Student Engagement and Critical Thinking in Two Approaches to the Online Classroom”.
Availability of data and materials
Authors’ contributions.
The first and second authors contributed significantly to the writing, review, and conceptual thinking of the manuscript. The third author provided a first detailed outline of what the paper could address, and the fourth offer provided input and feedback through critical review. All authors read and approved the final manuscript.
Competing interests
The authors declare that they have no competing interests.
Consent for publication
Ethics approval and consent to participate.
The parent study was approved by the University of Liverpool Online International Online Ethics Review Committee, approval number 04-24-2015-01.
Publisher’s Note
Springer Nature remains neutral with regard to jurisdictional claims in published maps and institutional affiliations.
Author information
Authors and affiliations.
University of Liverpool Online, Liverpool, UK
Laura A. Schindler & Osama A. Morad
Laureate Education, Inc., Baltimore, USA
Gary J. Burkholder
Walden University, Minneapolis, USA
University of Lincoln, Lincoln, UK
Craig Marsh
You can also search for this author in PubMed Google Scholar
Corresponding author
Correspondence to Laura A. Schindler .
Rights and permissions
Open Access This article is distributed under the terms of the Creative Commons Attribution 4.0 International License ( http://creativecommons.org/licenses/by/4.0/ ), which permits unrestricted use, distribution, and reproduction in any medium, provided you give appropriate credit to the original author(s) and the source, provide a link to the Creative Commons license, and indicate if changes were made.
Reprints and permissions
About this article
Cite this article.
Schindler, L.A., Burkholder, G.J., Morad, O.A. et al. Computer-based technology and student engagement: a critical review of the literature. Int J Educ Technol High Educ 14 , 25 (2017). https://doi.org/10.1186/s41239-017-0063-0
Download citation
Received : 31 March 2017
Accepted : 06 June 2017
Published : 02 October 2017
DOI : https://doi.org/10.1186/s41239-017-0063-0
Share this article
Anyone you share the following link with will be able to read this content:
Sorry, a shareable link is not currently available for this article.
Provided by the Springer Nature SharedIt content-sharing initiative
- Social networking
- Research article
- Open access
- Published: 19 June 2006
Computer literacy and attitudes towards e-learning among first year medical students
- Thomas Michael Link 1 &
- Richard Marz 1
BMC Medical Education volume 6 , Article number: 34 ( 2006 ) Cite this article
62k Accesses
87 Citations
Metrics details
At the Medical University of Vienna, most information for students is available only online. In 2005, an e-learning project was initiated and there are plans to introduce a learning management system. In this study, we estimate the level of students' computer skills, the number of students having difficulty with e-learning, and the number of students opposed to e-learning.
The study was conducted in an introductory course on computer-based and web-based training (CBT/WBT). Students were asked to fill out a questionnaire online that covered a wide range of relevant attitudes and experiences.
While the great majority of students possess sufficient computer skills and acknowledge the advantages of interactive and multimedia-enhanced learning material, a small percentage lacks basic computer skills and/or is very skeptical about e-learning. There is also a consistently significant albeit weak gender difference in available computer infrastructure and Internet access. As for student attitudes toward e-learning, we found that age, computer use, and previous exposure to computers are more important than gender. A sizable number of students, 12% of the total, make little or no use of existing e-learning offerings.
Many students would benefit from a basic introduction to computers and to the relevant computer-based resources of the university. Given to the wide range of computer skills among students, a single computer course for all students would not be useful nor would it be accepted. Special measures should be taken to prevent students who lack computer skills from being disadvantaged or from developing computer-hostile attitudes.
Peer Review reports
Computer literacy has been a subject of educational research ever since personal computers were introduced to the classroom, either as teaching aids or as tools for self-study. In the 1980s, research on computer literacy focused on the question whether medical students were ready for the foreseeable omnipresence of computers in the future doctors' professional environments [ 1 – 4 ], i.e., whether they possessed the necessary computer skills [ 2 , 5 – 9 ]. The vision of a knowledge-based society saw future economic wealth dependent on people's abilities to deal with the growing information load and to adapt to an ever-changing working environment [ 10 – 13 ]. It was assumed that computers would become ubiquitous tools for managing medical knowledge [ 14 ]. In some medical schools, a privately owned computer was made a requirement for medical students [ 15 , 16 ].
E-Learning, in particular the use of learning management systems (LMSs), introduced a new aspect. Researchers [ 17 ] suggested that some students may lack the necessary skills to use web-based learning platforms effectively and are therefore handicapped. This issue is often discussed in the context of gender differences. The main concern is that female students are at a disadvantage due to different patterns of computer usage, e.g. a less dominant style of discussion in web-based communication [ 18 , 19 ]. These gender differences can be observed in students' computer-related behaviors but also in their attitudes towards computer-based and web-based training (CBT/WBT). In a Danish study, Dørup [ 9 ] reported that among first-year students, 46% of the men were in favor of replacing "traditional teaching with use of computers if possible" while only 22% women agreed with this statement.
In 2004, 80% of Austria's 20–29 year olds had Internet access and 75% of university and high school students used a computer daily [ 20 ]. We can thus assume that, in general, students entering university have good basic computer skills. Studies nevertheless demonstrate that there is a considerable difference in computer use according to students' disciplines. Middendorff [ 21 ] reports that German medical students spend an average of 8 hours per week at the computer (including private activities). This is the lowest value of all disciplines, what makes it difficult to draw conclusions about medical students' computer use from general surveys. Often the degree of "informational fluency" remains at a basic level and students tend to over-estimate their computer skills [ 22 ].
This study examines the level of computer literacy and patterns of computer usage of first-year medical students at the Medical University of Vienna. It was conducted in an introductory course for first-year students on CBT/WBT. The goal of the study was to determine the need for such introductory courses and to provide information that could be used to improve them. A secondary aim was to identify difficulties that may be encountered in implementing a university-wide LMS due to students' lack of computer literacy or low acceptance of e-learning. While multimedia learning programs have been praised for their educational superiority, actual use of these programs has sometimes failed to meet our expectations.
Since autumn 2003, we have required students to take an introductory course on CBT/WBT as a single 90-minute class session. This course is held for first-year students (about 1500 students took it in 2004 and 2005) and second-year students (about 600 students from 2003 to 2005) [ 23 ]. The course serves two main purposes:
To ensure a certain level of computer and information literacy, including online communication skills.
To acquaint students with computer and web-based learning materials.
In 2003 and 2004, students had to review web-based learning programs (e.g. [ 24 ]) and post their statements in a dedicated online forum. In the course for first-year students we used a student-developed platform [ 25 ]. In the course for second-year students, we used Manila [ 26 ] in 2003 and TikiWiki [ 27 ] in 2004 as a collaboration tool. In 2005, we switched to tools that were partly self-developed and less demanding with respect to the server load.
This paper reports on data from an online survey for the 2004 course for first-year students. Participation in the survey was voluntary and anonymous (though students were asked to give their student ID if they wanted to). The tutors were not able to determine who has or has not filled out the questionnaire. Using class time for students to fill out the questionnaire nevertheless ensured a high response rate of 79%.
A total of 1232 questionnaires were completed, 1160 of which remained in the data set after applying some filtering rules in order to eliminate records of uncertain origin. The gender breakdown of respondents was 61% female and 39% male. This corresponds exactly to the gender breakdown of the 1560 students entering the study module (61% female and 39% male). We thus conclude that our sample was representative of the 2004 cohort. Missing values due to non-responses are not included in tables or figures. Differences between the reported counts and the sample size (n = 1160) are thus due to missing responses.
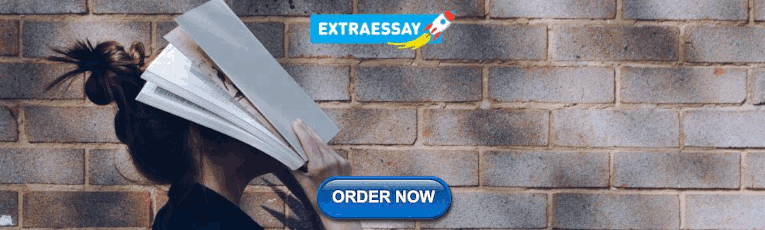
Questionnaire
The questionnaire [ 28 ] (see Additional file 1 ) was designed to collect the following information:
Overall evaluation of the course
Attitudes towards e-learning as well as previous experiences and expectations about the use of CBT/WBT
Computer and Internet usage
Extent of students' private computer infrastructure
Basic demographic data.
In the following, we will focus on students' computer usage and private computer infrastructure as well as their attitudes toward e-learning.
Attitudes towards e-learning (understood as an umbrella concept for learning methods supported by information- and communication technologies (ICT) in general) were determined by the students' agreement or disagreement with several statements about the importance of ICT in medical education. These statements contained items like "Web-based learning programs are able to replace lectures" or "In medical teaching, there is no need for the use of Web-based programs." The students rated their agreement or disagreement on a bi-polar eight-point Likert scale. For the purpose of comparability with Dørup [ 9 ], we recoded their answers into dichotomous variables. As computer use and attitudes towards e-learning were measured on an ordinal scale, we accordingly used Spearman rho to describe the statistical relationship of these variables with other items. For other metric variables Pearson r was used.
Computer infrastructure
Almost all students (94%) have access to a privately owned PC they can use for their studies, which is either owned by the students themselves (74%) or shared with family members or roommates (20%). Only 5% rely primarily on public computer facilities (Table 1 ).
Student-owned PCs are on average 2.3 years old; 92% are newer than 5 years, 87% newer than 4 years. This corresponds to the life span of computers in companies or public administration offices. Only 3.2% of the students have a computer older than 6 years. Male students' PCs (mean ± SD: 2 ± 1.42 years) are newer than those owned by women (2.5 ± 2.05 years). The 95% confidence interval for the difference is 0.33..0.79 years.
Internet access
The great majority of students also have access to the Internet, though the quality of connectivity varies widely; 60% have access via ADSL, cable TV, or LAN (which, however, usually signifies the use of public facilities at the university or elsewhere); 37% have access using a telephone connection (modem or ISDN) (Table 2 ). The type of Internet access differs according to gender (Cramer V = 0.28, p = 0.001). Male students tend to have faster Internet access while older technologies (e.g. modem) are more common among women. The proportion of modem users is twice as high among women (33%) than among men (15%).
Computer use
Types of computer use.
Students are familiar with e-mail and the use of the Internet for information research; 94% of the students communicate via e-mail and 97% use the Internet for information research at least several times per month. While the use of word processors is very common (82% use such a program several times a month), students are less familiar with other program types (Table 3 ).
Very few medical students have experience in Web design or the creation of HTML documents (5% at least weekly) and thus make no use of the Internet for publishing or more sophisticated collaboration purposes. The frequencies of using communication technologies other than e-mail, e.g., chats (21%), forums and bulletin boards (13%), are also low.
One noteworthy detail is the proportion of students who use computers for organizing appointments, to do lists, or making notes: 28% use such a personal organizer software several times per week, which may point to the use of personal digital assistants (PDA) or smart cell phones.
Except for the categories "Word Processor" and "E-mail," male students use the computer significantly more often than women. The strength of this statistical relationship is weak. Spearman rho is highest for the categories "Web-design" (r s = 0.25, p = 0.001), "Games" (r s = 0.23, p = 0.001), "Forums" (r s = 0.21, p = 0.001), and "Spreadsheets" (r s = 0.20, p = 0.001).
Age when using a computer for the first time
Half of all students (50%) used a computer for the first time by the age of 11 (mean 11.2 ± 3.77 SD). By the time they entered university, i.e., before the age of 18, fully 96% of all students had begun to use computers. The average age when students began using computers for the first time is slightly lower for men (10.7 ± 3.40 years) than women (11.5 ± 3.96 years). The 95% confidence interval for this difference is 0.33..1.24 years.
Prior experiences and expectations
Half of the students (49%) report using a computer or Web-based learning program at least once per month. In order to determine how many students have little or no experience with e-learning, we consolidated answers to questions about four different kinds of e-learning programs (information retrieval, downloading scripts, LMS, and CBT/WBT) into one index. Because of the high response rates for "downloading learning material," we defined inexperienced users as those who answered "less often" or "never" to questions about at least three of these kinds of programs. Following this typology, 12% of the students are inexperienced, having used at most one kind of e-learning program at least once per term (Table 4 ).
The majority of students (66%) have already used a computer or Web-based dictionary like the Pschyrembel medical dictionary, which is one of the standard references used by Vienna medical students. Half of them (50%) have used an online image repository at least once and 42% have used some kind of online quiz to test their knowledge (Table 5 ). Other kinds of learning programs, such as those associated with a constructivist approach, are less well known among first-year Vienna students. The results given in Tables 4 and 5 relating to students' use of LMS are inconsistent. This inconsistency arises most likely from the students' lack of understanding of what a LMS is since very few lecturers use this kind of software to support their courses.
About 10% of the students have never used any of the above-mentioned kinds of e-learning programs and 4.4% do not regard any of them as helpful. Those who regard only two or fewer as helpful tend to prefer learning programs that have no "built-in" educational theory, such as encyclopedias (38%), image collections (23%), and quizzes (23%). The number of different kinds of programs that students have experience with and that they consider helpful correlates with Pearson r = 0.32 (p = 0.001) – the more kinds of programs they know, the more kinds they consider useful.
A majority of the students agree (median = 2, interquartile range = 3) that CBT/WBT should be offered as a supplement to lectures and seminars (Figure 1 ). On the other hand, most students disagree with the statement that e-learning should replace these traditional forms of teaching (median = 7, IQR = 4).
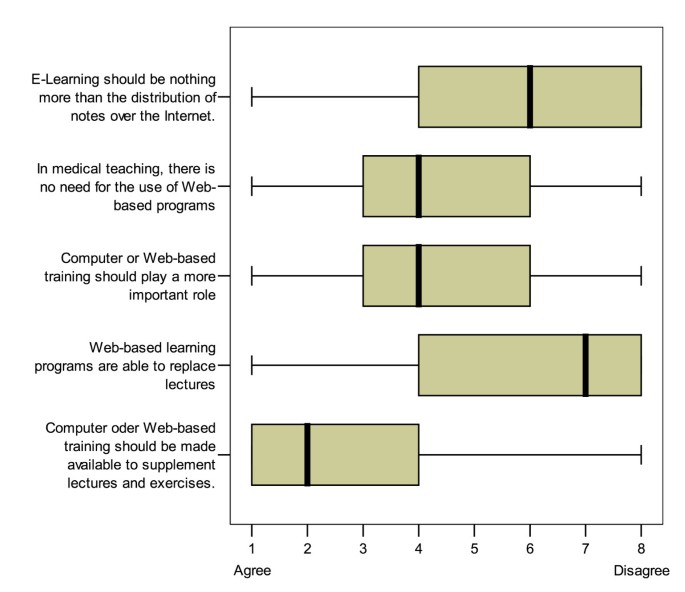
Students' agreement or disagreement with statements on the usefulness of e-learning . The x-axis represents the values of an 8-point bi-polar rating scale: 1 = strong agreement, 8 = strong disagreement. The boxes show the quartiles (25% of the distribution) and the median (50% cut).
Men (median = 6) tend to be slightly more in favor of replacing traditional lectures with CBT/WBT than women (median = 7). The strength of this effect is negligible (r s = 0.06, p = 0.041). After recoding to a dichotomous scale (1..4 = pro, 5..8 = contra), 28% of male and 25% of female students can be considered favoring the replacement of traditional teaching methods with e-learning. The gender difference is slightly bigger for the item "Computer or Web-based training should play a more important role" but still hardly noteworthy (r s = 0.16, p = 0.001). In general, the following variables have bigger effects on e-learning-related attitudes than gender per se:
Lack of experience with CBT/WBT
Productive computer and Internet use (e.g. spreadsheets, organizer, word processor, graphics, e-mail, Web design, and information research).
We consolidated statements 2 to 4 in Figure 1 into one index (Cronbach alpha = 0.65; inclusion of the items 1 and 5 leads to a slight decrease in reliability). In a regression model (Table 6 ) that includes the above 3 variables and gender (R 2 adj = 0.15, p = 0.001, SEE = 1.54), gender is not statistically significant (p = 0.41). When the stepwise regression method is used, gender is excluded from the final model.
Computer infrastructure and internet access
A sizable number of students still have Internet access only via dial-up connections using a modem. This mode of Internet access is slow and impedes the use of synchronous communication tools that require one to stay online for a long period of time. Even if the majority of students do have broadband access to the Internet, mandatory e-learning solutions cannot rely on synchronous online communication tools like chats and on extensive video material, e.g. recordings from lectures. Instead, preference should be given to asynchronous online communication tools and textual information along with videos. Asynchronous communication tools also have the advantage that teachers and students do not have to be online at the same time.
Computer use patterns
Only a small number of students have experience with Internet publishing and asynchronous communication tools like BBS or forums. Thus, most of our students are rather passive Internet users and miss out on numerous possibilities of virtual communities and Web-based publishing. The lack of experience with synchronous and asynchronous online communication, with the exception of e-mail, may cause problems when using the collaboration tools included in an LMS [ 29 ].
Attitudes towards e-learning
Most students agree that e-learning could serve as a supplement for lectures and seminars. However, about as many students disagree with the statement that e-learning could replace traditional ways of teaching. In the Danish context, Dørup [ 9 ] reported a slightly greater proportion of first-year medical students in favor of replacing traditional lectures with e-learning (47% men, 22% women). These higher levels of agreement could be explained by the different response scales used but also by the fact that Danish people in general are reported [ 30 ] to be more "digital literate" than Austrians – although this difference cannot be claimed for persons under 24 years of age [ 30 ].
The intensity of computer use and previous experience with CBT/WBT have the greatest effect on students' attitudes towards e-learning. The explanation for this could be a general discomfort with the technology that makes students who lack experience with ICT express themselves cautiously about its use in education [ 31 ]. It could also be explained by the relative novelty of e-learning and students' difficulties in integrating CBT/WBT into their way of learning [ 32 ].
Most students seem to acknowledge the range of possibilities of new media to enhance their learning experience although they consider CBT/WBT a supplement to rather than a replacement of other learning materials. However, there is also a group of students who are strictly opposed to CBT/WBT (4.4% of the first-year students do not value any of the kinds of programs mentioned above). More disturbing, 24% strongly agree (values 1 and 2 on an 8-point rating scale) with the statement that the Medical University of Vienna could do well without CBT/WBT. When introducing an online LMS or Web-based learning program, special care should be taken not to lose these students because of the choice of a certain learning technology.
In December 2005, we also held a few focus groups with teachers and students on a similar subject. In the course of these discussions it became clear how some characteristics of the new curriculum, especially the emphasis on the MCQ-based year-end examinations, impeded the use of CBT/WBT. In these discussions the students had doubts about the usability and efficiency of e-learning (with regard to costs, handling of ICT, but also learning efficiency) while they still acknowledged the possibilities of ICT support with respect to visualization, simulation, self-quizzing, and fast information retrieval from several sources such as encyclopedias or Web pages.
Gender differences
We were able to identify gender differences for all computer-related variables. In sum, men make more frequent use of computers and have access to better computer infrastructure and faster Internet connections. While this difference is quite consistent over several variables, the strength of the statistical relationship is weak and, with respect to students' attitudes towards e-learning, overshadowed by other variables (e.g. previous exposure to CBT/WBT) that are more important for predicting students' attitudes.
With respect to the implementation of an LMS, the most important difference between men and women is the relatively high number of women still using a slow dial-up connection to the Internet, which could impede the use of synchronous communication tools or multimedia-rich Web applications. Well planned use of e-learning and supportive measures should help to neutralize this difference. Although women have less experience with forums, Gunn [ 19 ] showed that these differences in online communication behavior do not necessarily result in worse examination outcomes.
E-Learning must be appropriate to students' level of computer expertise in order not to become a source of frustration. Courses to develop students' computer skills can improve this situation by influencing students' attitudes and capabilities. Our conclusions with respect to such introductory courses are twofold. Students certainly need some kind of formal introduction to the new ICT for learning purposes. But due to the wide range of previous experience and computer skills, there is no one-size-fits-all course design available. Such a course should either be split into several tracks according to students' different levels of computer literacy [ 33 ], or it should be held only for students with little or no computer experience.
There is, however, the danger that precisely those students who need this course the most will hesitate to attend it voluntarily. It is difficult to say how these students could be persuaded to take such a course despite their skepticism towards ICT and e-learning. One strategy would be to emphasize the practical value for solving everyday problems and obtaining useful information. Once they have learned how computers help them solve recurring problems, they will perhaps develop more computer-friendly attitudes. Another solution could be to make the course compulsory but to make the impact negligible for students with good ICT knowledge. This could be achieved with a Web-based entry test. Students who pass the test would be exempted from having to take the course.
When introducing a campus-wide LMS, one has to take into consideration that some students lack the necessary computer skills or infrastructure to participate effectively in online courses, and that others are strictly opposed to e-learning. Introducing a campus-wide e-learning solution thus poses not only technical and organizational challenges but also calls for a promotional strategy. In the future, we can expect more students to think of computers as standard tools for learning as schools make more use ICT in their classrooms. For example, an "avant-garde" of Vienna medical students already created an online forum [ 34 – 36 ] for informally exchanging information about courses as well as students authored learning materials.
Bresnitz EA, Stettin GD, Gabrielson IW: A survey of computer literacy among medical students. J Med Educ. 1986, 61: 410-2.
Google Scholar
Osman LM, Muir AL: Computer skills and attitudes to computer-aided learning among medical students. Med Educ. 1994, 28: 381-5.
Article Google Scholar
Koschmann T: Medical education and computer literacy: learning about, through, and with computers. Acad Med. 1995, 70: 818-21. 10.1097/00001888-199509000-00018.
McGowan JJ, Berner ES: Computer in medical education. International handbook of research in medical education. Edited by: Norman GR, van der Vleuten CPM, Newble DI. 2002, Dordrecht (Netherlands): Kluwer, 537-79.
Chapter Google Scholar
SEUSISS Project. Survey of European universities skills in ICT of students and staff. Final report. 2003, Edinburgh (United Kingdom): University of Edinburgh, [ http://www.intermedia.uib.no/seusiss/seusissreport.pdf ]
Asgari-Jirhandeh N, Haywood J: Computer awareness among medical students: a survey. Med Educ. 1997, 31 (3): 225-9.
Seago BL, Schlesinger JB, Hampton CL: Using a decade of data on medical student computer literacy for strategic planning. J Med Libr Assoc. 2002, 90 (2): 202-209. [ http://www.pubmedcentral.gov/articlerender.fcgi?tool=pubmed&pubmedid=11999178 ]
Hollander S: Assessing and enhancing medical students' computer skills: a two-year experience. Bull Med Libr Assoc. 1999, 87 (1): 67-73. [ http://www.pubmedcentral.gov/articlerender.fcgi?tool=pubmed&pubmedid=9934531 ]
Dørup J: Experience and attitudes towards information technology among first-year medical students in Denmark: longitudinal questionnaire survey. J Med Internet Res. 2004, 6 (1): e10-10.2196/jmir.6.1.e10. [ http://www.jmir.org/2004/1/e10/ ]
Bell D: The coming of the post-industrial society. 1999, New York: Basic Books, [1973]
Lyotard JF: The postmodern condition: a report on knowledge. 1994, Manchester: Manchester University Press, [1979]
Book Google Scholar
Virkus S: Information literacy in Europe: a literature review. Information Research. 2003, 8 (4): paper no. 159, [ http://informationr.net/ir/8-4/paper159.html ]
OECD: The knowledge-based economy. 1996, Paris (France): OECD, [ http://www.oecd.org/dataoecd/51/8/1913021.pdf ]
Faughnan JG, Elson R: Information technology and the clinical curriculum: some predictions and their implications for the class of 2003. Acad Med. 1998, 73 (7): 766-769. 10.1097/00001888-199807000-00013.
Mavis BE, Smith JM: Mandatory microcomputers: potential effects on medical school recruitment and admissions. Medical Education Online. 1997, 2: 5-[ http://www.med-ed-online.org/volume2.htm ]
Blue AV, Elam CL, Rubeck R, Nora LM: Implementing a requirement for computer ownership: One medical school's experience. Medical Education Online. 1997, 2-[ http://www.med-ed-online.org/t0000002.htm ]
Brüchner K: A longitudinal analysis of the development of learner-prerequisites for e-learning using a typology of computer use [in German]. Proceedings "med:u – e-learning in medical education", 7.-8.11.03 [in German]. 2003, Kiel (Germany): University of Kiel, 15-32.
Richter T, Naumann J, Horz H: Computer literacy, computer-related attitudes, and computer use among male and female students [in German]. People and computer. 1st interdisciplinary conference [in German]. Edited by: Oberquelle H, Oppermann R, Krause J Stuttgart. 2001, (Germany): B.G. Teubner, 71-80. [ http://mc.informatik.uni-hamburg.de/konferenzbaende/mc2001/V23.pdf ]
Gunn C: Dominant or different? Gender issues in computer supported learning. Journal of Asynchronous Learning Networks. 2003, 7 (1): 14-30. [ http://www.aln.org/publications/jaln/v7n1/pdf/v7n1_gunn.pdf ]
Integral. Austrian Internet Monitor – Internet development [in German]. Vienna (Austria): Integral. 2005
Middendorff E: Computer use and new media in higher education. Results from the 16th social survey of the German Students Association conducted by the HIS Higher Education-Information-System [in German]. 2002, Bonn (Germany): Bundesministerium für Bildung und Forschung
Gavriilidis K: No leap in quality ahead – empiric results concerning the integration and use of electronic information in higher education [in German]. Virtual campus: scenarios, strategies, studies [in German]. Edited by: Wagner E, Kindt M. 2001, Münster: Waxmann, 266-274.
Link TM, Marz R: Introduction to computer-based learning. Science and medicine. A textbook for the special studies module (SSM1) [in German]. Edited by: Trappl R. 2005, Vienna: Veritas, 147-162.
HemoSurf. [ http://www.aum.iawf.unibe.ch/vlz/BWL/HemoSurf/ ]
eMed. [ http://emed.mitsch.cc ]
Manila. [ http://manila.userland.com ]
TikiWiki. [ http://tikiwiki.org ]
1 st year students 2004 questionnaire. the orginal version in German http://www.meduniwien.ac.at/user/thomas.link/b2/index.php?review=04w , [ http://www.meduniwien.ac.at/user/thomas.link/b2_en/index.php?review=04w ]
Stokes CW, Cannavina C, Cannavina G: The state of readiness of student health professionals for web-based learning environments. Health Informatics Journal. 2004, 10 (3): 195-204. 10.1177/1460458204045434.
SIBIS – Statistical Indicators Benchmarking the Information Society: SIBIS pocket book 2002/03. Measuring the information society in the EU, the EU accession countries, Switzerland and the US. 2003, Bonn (Germany) empirica, [ http://www.sibis-eu.org/publications/pocketbook.htm ]
SPOT+: Students' perspective on technology. Survey report. 2004, [ http://www.spotplus.odl.org/downloads/Survey_report_final.pdf ]
Schanze S: The use of concept mapping in the med:u project – e-learning in medical education. A tool for structuring complex information and for testing learning performance [in German]. Evaluation of e-learning. Goals, methodological aspects, future [in German]. Edited by: Meister DM, Tergan S, Zentel P. 2004, Münster (Germany): Waxmann, 171-187.
Gibson KE, Silverberg M: A two-year experience teaching computer literacy to first-year medical students using skill-based cohorts. Bull Med Libr Assoc. 2000, 88 (2): 157-164.
MCW Portal. [ http://www.mcw-portal.com ]
Med-Forum. [ http://www.med-forum.at/ ]
Medizinstudium.at. [ http://www.medizinstudium.at ]
Pre-publication history
The pre-publication history for this paper can be accessed here: http://www.biomedcentral.com/1472-6920/6/34/prepub
Download references
Acknowledgements
We thank Thomas Benesch for statistical advice. We would also like to thank Jens Dørup, William Fulton, and Sean Marz for critically reading the manuscript and their helpful suggestions.
Author information
Authors and affiliations.
Core Unit for Medical Education, Medical University of Vienna, Vienna, Austria
Thomas Michael Link & Richard Marz
You can also search for this author in PubMed Google Scholar
Corresponding author
Correspondence to Thomas Michael Link .
Additional information
Competing interests.
The author(s) declare that they have no competing interests.
Authors' contributions
RM and TML planned and organized courses [ 23 ] to promote computer literacy among medical students.
TML was responsible for designing the study, implementing the online questionnaire, analyzing the data, writing the first draft, and proofreading the final draft.
RM was responsible for designing the course content, recruiting and training the tutors and supervising all aspects of the course. He revised the article extensively.
Both authors read and approved the final version.
Thomas Michael Link and Richard Marz contributed equally to this work.
Electronic supplementary material
12909_2006_133_moesm1_esm.htm.
Additional File 1: This is the HTML output of the English translation of the questionnaire we used. The HTML was originally generated by a PHP script. As this is only the HTML representation of the questionnaire, clicking on the "Submit" button has no effect. The file can be viewed in any Internet browser. (HTM 53 KB)
Authors’ original submitted files for images
Below are the links to the authors’ original submitted files for images.
Authors’ original file for figure 1
Authors’ original file for figure 2, authors’ original file for figure 3, rights and permissions.
This article is published under license to BioMed Central Ltd. This is an Open Access article distributed under the terms of the Creative Commons Attribution License ( http://creativecommons.org/licenses/by/2.0 ), which permits unrestricted use, distribution, and reproduction in any medium, provided the original work is properly cited.
Reprints and permissions
About this article
Cite this article.
Link, T.M., Marz, R. Computer literacy and attitudes towards e-learning among first year medical students. BMC Med Educ 6 , 34 (2006). https://doi.org/10.1186/1472-6920-6-34
Download citation
Received : 02 March 2006
Accepted : 19 June 2006
Published : 19 June 2006
DOI : https://doi.org/10.1186/1472-6920-6-34
Share this article
Anyone you share the following link with will be able to read this content:
Sorry, a shareable link is not currently available for this article.
Provided by the Springer Nature SharedIt content-sharing initiative
- Internet Access
- Computer Literacy
- Computer Skill
- Computer Infrastructure
- Basic Computer Skill
BMC Medical Education
ISSN: 1472-6920
- Submission enquiries: [email protected]
- General enquiries: [email protected]
Numbers, Facts and Trends Shaping Your World
Read our research on:
Full Topic List
Regions & Countries
- Publications
- Our Methods
- Short Reads
- Tools & Resources
Read Our Research On:
- Digital Readiness Gaps
Americans fall along a spectrum of preparedness when it comes to using tech tools to pursue learning online, and many are not eager or ready to take the plunge
Table of contents.
- 1. The meaning of digital readiness
- 2. The spectrum of digital readiness for e-learning
- 3. Greater digital readiness translates to higher level of use of technology in learning
- Appendix: Detail on digital readiness and other metrics across groups
- Acknowledgments
- Methodology
For many years concerns about “digital divides” centered primarily on whether people had access to digital technologies. Now, those worried about these issues also focus on the degree to which people succeed or struggle when they use technology to try to navigate their environments, solve problems, and make decisions. A recent Pew Research Center report showed that adoption of technology for adult learning in both personal and job-related activities varies by people’s socio-economic status, their race and ethnicity, and their level of access to home broadband and smartphones. Another report showed that some users are unable to make the internet and mobile devices function adequately for key activities such as looking for jobs .
In this report, we use newly released Pew Research Center survey findings to address a related issue: digital readiness. The new analysis explores the attitudes and behaviors that underpin people’s preparedness and comfort in using digital tools for learning as we measured it in a survey about people’s activities for personal learning .
Specifically, we assess American adults according to five main factors: their confidence in using computers, their facility with getting new technology to work, their use of digital tools for learning, their ability to determine the trustworthiness of online information, and their familiarity with contemporary “education tech” terms. It is important to note that the findings here just cover people’s learning activities in digital spaces and do not address the full range of important things that people can do online or their “readiness” to perform them.
To better understand the way in which different groups of Americans line up when it comes to digital readiness, researchers used a statistical technique called cluster analysis that places people into groups based on similarities in their answers to key questions.
The analysis shows there are several distinct groups of Americans who fall along a spectrum of digital readiness from relatively more prepared to relatively hesitant. Those who tend to be hesitant about embracing technology in learning are below average on the measures of readiness, such as needing help with new electronic gadgets or having difficulty determining whether online information is trustworthy. Those whose profiles indicate a higher level of preparedness for using tech in learning are collectively above average on measures of digital readiness.
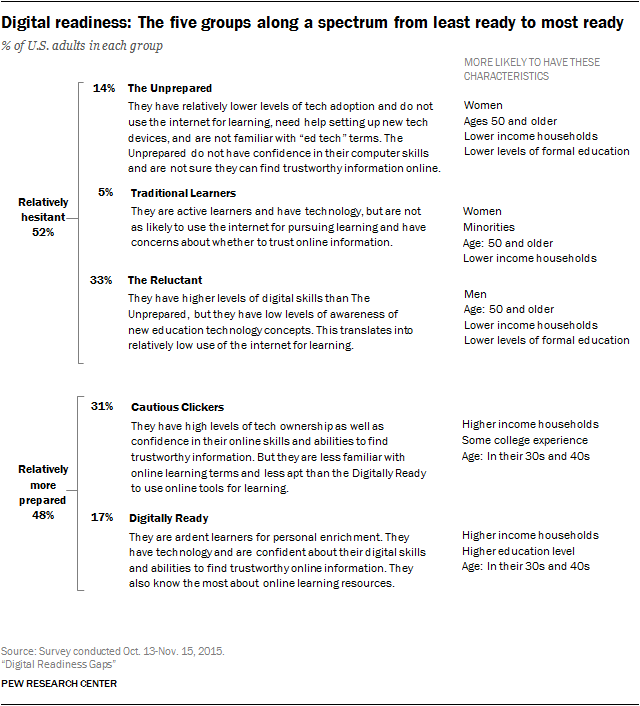
Relatively Hesitant – 52% of adults in three distinct groups. This overall cohort is made up of three different clusters of people who are less likely to use digital tools in their learning. This has to do, in part, with the fact that these groups have generally lower levels of involvement with personal learning activities. It is also tied to their professed lower level of digital skills and trust in the online environment.
- A group of 14% of adults make up The Unprepared . This group has both low levels of digital skills and limited trust in online information. The Unprepared rank at the bottom of those who use the internet to pursue learning, and they are the least digitally ready of all the groups.
- We call one small group Traditional Learners, and they make up of 5% of Americans. They are active learners, but use traditional means to pursue their interests. They are less likely to fully engage with digital tools, because they have concerns about the trustworthiness of online information.
- A larger group, The Reluctant, make up 33% of all adults. They have higher levels of digital skills than The Unprepared, but very low levels of awareness of new “education tech” concepts and relatively lower levels of performing personal learning activities of any kind. This is correlated with their general lack of use of the internet in learning.
Relatively more prepared – 48% of adults in two distinct groups. This cohort is made up of two groups who are above average in their likeliness to use online tools for learning.
- A group we call Cautious Clickers comprises 31% of adults. They have tech resources at their disposal, trust and confidence in using the internet, and the educational underpinnings to put digital resources to use for their learning pursuits. But they have not waded into e-learning to the extent the Digitally Ready have and are not as likely to have used the internet for some or all of their learning.
- Finally, there are the Digitally Ready . They make up 17% of adults, and they are active learners and confident in their ability to use digital tools to pursue learning. They are aware of the latest “ed tech” tools and are, relative to others, more likely to use them in the course of their personal learning. The Digitally Ready, in other words, have high demand for learning and use a range of tools to pursue it – including, to an extent significantly greater than the rest of the population, digital outlets such as online courses or extensive online research.
There are several important qualifying notes to sound about this analysis. First, the research focuses on a particular activity – online learning. The findings are not necessarily projectable to people’s capacity (or lack of capacity) to perform health-related web searches, use mobile apps for civic activities, or use smartphones to apply for a job.
Second, while there are numerical descriptions of the groups, there is some fluidity in the boundaries of the groups. Unlike many other statistical techniques, cluster analysis does not require a single “correct” result. Instead, researchers run numerous versions of it (e.g., asking it to produce different numbers of clusters) and judge each result by how analytically practical and substantively meaningful it is. Fortunately, nearly every version produced had a great deal in common with the others, giving us confidence that the pattern of divisions were genuine and that the comparative shares of those who were relatively ready and not ready each constituted about half of Americans.
Third, it is important to note that the findings represent a snapshot of where adults are today in a fairly nascent stage of e-learning in society. The groupings reported here may well change in the coming years as people’s understanding of e-tools grows and as the creators of technology related to e-learning evolve it and attempt to make it more user friendly.
Even allowing for those caveats, the findings add additional context to insights about those who pursue personal learning activities. Although factors such as educational attainment or age might influence whether people use digital tools in learning, other things such as people’s digital skills and their trust in online information may also loom large. These “readiness” factors, separate and apart from demographic ones, are the focus in this report.
The results are also significant in light of Americans’ expressed interest in learning and personal growth. Most Americans said in the Center survey that they like to look for opportunities to grow as people: 58% said this applies to them “very well” and another 31% said it applies to them “somewhat well.” Additionally, as they age, many Americans say they hope to stay active and engaged with the world .
Sign up for our weekly newsletter
Fresh data delivery Saturday mornings
Sign up for The Briefing
Weekly updates on the world of news & information
- Digital Divide
- Education & Learning Online
- Emerging Technology
- Platforms & Services
- Technology Adoption
Americans’ Use of Mobile Technology and Home Broadband
Teens and internet, device access fact sheet, what americans know about ai, cybersecurity and big tech, how teens navigate school during covid-19, what we know about online learning and the homework gap amid the pandemic, most popular, report materials.
- Oct. 13-Nov. 15, 2015 – Educational Ecosystem
1615 L St. NW, Suite 800 Washington, DC 20036 USA (+1) 202-419-4300 | Main (+1) 202-857-8562 | Fax (+1) 202-419-4372 | Media Inquiries
Research Topics
- Age & Generations
- Coronavirus (COVID-19)
- Economy & Work
- Family & Relationships
- Gender & LGBTQ
- Immigration & Migration
- International Affairs
- Internet & Technology
- Methodological Research
- News Habits & Media
- Non-U.S. Governments
- Other Topics
- Politics & Policy
- Race & Ethnicity
- Email Newsletters
ABOUT PEW RESEARCH CENTER Pew Research Center is a nonpartisan fact tank that informs the public about the issues, attitudes and trends shaping the world. It conducts public opinion polling, demographic research, media content analysis and other empirical social science research. Pew Research Center does not take policy positions. It is a subsidiary of The Pew Charitable Trusts .
Copyright 2024 Pew Research Center

An official website of the United States government
The .gov means it’s official. Federal government websites often end in .gov or .mil. Before sharing sensitive information, make sure you’re on a federal government site.
The site is secure. The https:// ensures that you are connecting to the official website and that any information you provide is encrypted and transmitted securely.
- Publications
- Account settings
Preview improvements coming to the PMC website in October 2024. Learn More or Try it out now .
- Advanced Search
- Journal List
- v.8(3); 2022 Mar

Influence of computers in students’ academic achievement
Associated data.
Data will be made available on request.
With fast-growing technology, schools have to adapt and use technology constantly as a tool to grow. This study aims to understand the influence of computer factors on students' academic achievement. We propose a model on the influence of computer attitudes, computer learning environments, computer learning motivations, computer confidence, computer use, computer self-efficacy, loneliness, mothers' education, parents' marital status and family size on academic achievement (AA). To validate the conceptual model, 286 students aged 16–18 years old answered an online questionnaire. The most important drivers that positively affect AA are computer use, employment motivations, and mothers' education. While enjoyment attitudes, school environment, interest motivations, and loneliness influence AA negatively. Also, family size and computer self-efficacy work as moderators, and computer use works as a mediator between computer learning environments and academic achievement.
Academic achievement; Computers; Family; Learning; Students.
1. Introduction
Countries are constantly facing everchanging economic challenges and social transformations due to globalisation and technology development. Education helps overcome these challenges by developing knowledge and high skills, allowing better opportunities and faster economic progression ( OECD, 2019 ). Computers and information technology have become key to educational institutions worldwide ( Hsu and Huang, 2006 ). With the advantages of the digital era through digital markets, advanced scientific and social networks, there is a growth in innovation, development and employment ( OECD, 2015 ). Education needs to adapt to social changes, students' needs, and technology growth ( OECD, 2019 ), the perfect example of this adaptation is during the recent pandemic. The COVID-19 pandemic (meaning "CO" - corona; "VI" – virus; "D" – disease; "19" - "2019″) started in December 2019 in Wuhan, a province of China. It is caused by a highly contagious virus that has already claimed millions of lives worldwide ( Roy et al., 2020 ). The virus forced schools to close, and since classes had to continue, teachers and students had to adapt, resorting to virtual classes ( Ng and Peggy, 2020 ). However, it impacted academic life in yet unknown dimensions ( Rajkumar, 2020 ).
Digital technology provides access to high-quality learning and consequently allows schools to develop their teaching and learning methods ( Ertmer et al., 2012 ). Nonetheless, access to computers at home or the internet is not equal in every dwelling, and some students have the disadvantage of not having parental support or engagement to learn by themselves online. For these reasons, the pandemic can bestow tremendous advantages in digital education and academic achievement or significant disadvantages, mostly in developing countries. Therefore, access to technology is not enough; fostering a close relationship between families and teachers is essential ( OECD, 2020 ). Technology has been an invaluable tool, and it is being taken under consideration in students' academic achievement, including not only in access to the internet but also the way students use it ( Levine and Donitsa-Schmidt, 1998 ; Torres-Díaz et al., 2016 ; Voogt et al., 2013 ). Schools are expected to have a particular concern regarding integrating computers in classroom teaching ( Schmid and Petko, 2019 ), and technical devices such as computers, laptops, tablets and mobile phones should be included wisely in adolescent education. Through the information gathered, this study was motivated mainly by the atual pandemic context and the important role technology has on the academic achievement.
Over the years, researchers have tried to identify the variables that contribute to academic excellence in an attempt to understand which factors lead to better students' performance ( Valli Jayanthi et al., 2014 ). A vast number of studies have been conducted to identify predictors of academic achievement ( Gonzalez-pienda et al., 2002 ; J. Lee, Shute and Lee, 2010 ; Suárez-álvarez et al., 2014 ) although few have studied computer influences on the prediction of students' academic achievement.
Since there is a need to extend innovations in education ( Admiraal et al., 2017 ), we identified a need to investigate how students' relationships with computers impact their academic performance to understand the real impact of computers on schooling. To the best of our knowledge, some studies address computers' impact on academic achievement, but the data available is not totally enlightening. With the actual context of the pandemic, this subject gains additional importance, comparing technology use and academic achievement (AA) in such a tumultuous time for the world. This study presents three contributions. Firstly , it identifies which the best computer-related determinants to understand AA are through a research model that combines computer-related variables to students' grades. In this way, we identify the factors that lead to better academic achievement, helping schools and parents use them as a strategic advantage. Secondly , it investigates the moderation effect of family size and computer self-efficacy and the mediation effect of computer use between the factors identified and AA. Finally , to understand how the COVID-19 pandemic is influencing students' AA, using the variable loneliness, we explore how forced social isolation affected the use of computers and students' academic achievement in the pandemic period.
A literature review is presented in the next section. Section 3 introduces a theoretical model explaining academic achievement. Section 4 elucidates on the data-collection methods, followed by the results in Section 5 . The results are discussed in Section 6 , and conclusions are outlined in the final section.
2. Literature review and hypotheses
2.1. computer attitudes.
Attitudes and perceptions play a pivotal role in learning behaviours. Some researchers tested a model based on the concept of the attitude-behaviour theory, which argues that beliefs lead to attitudes, and attitudes are an essential factor to predict behaviour ( Levine and Donitsa-Schmidt, 1998 ). They predicted that computer use leads to more computer confidence and positive attitudes towards computers, and these elements influence each other. The computer attitudes refer to the opinion of students about: the stereotypes of those who use the computer the most – stereotypes; the use of computers for education purposes – educational; and about the use of the computer for fun – enjoyment. In their view, student achievement is a reflection of their behaviour in school. Even with the change of technology over time, recent studies support their theory that positive computer attitudes and positive computer confidence continue to lead to better outcomes ( Lee et al., 2019 ). Stereotypes associated with computers are usually on gender, proving the idea that women have less computer knowledge than men ( Punter et al., 2017 ). However, there are no results on how other stereotypes, such as the lack of computer use by athletes', or even if the concept of people who use computers are considered nerds, negatively affects the confidence of those who use computers.
Regarding the attitudes of enjoyment and educational use of computers, there is no consensus in the literature. Some researchers found a positive association between students' academic achievement and computer use for interactive social media and video gaming, as well as for educational purposes ( Bowers and Berland, 2013 ; Tang and Patrick, 2018 ), although other researchers have found that students who play more videogames have worse results in school ( Bae and Wickrama, 2015 ), some previous studies suggest that the technology intervention has a positive effect on students' attitudes toward the use of computers for educational purposes ( Gibson et al., 2014 ). Others show concerns on the effects of technology and social media use on students' outcomes and confirm that students who have lower grades spend more time using computers for fun ( Bae and Wickrama, 2015 ; Tang and Patrick, 2018 ), others find no evidence that using computers for fun causes higher or lower achievement ( Hamiyet, 2015 ). Milani et al. (2019) demonstrated that using computers with moderate levels of video gaming may improve student achievement because it increases visual-spatial skills ( Milani et al., 2019 ) when complemented with educational use such as homework, extracurricular activities, and reading ( Bowers and Berland, 2013 ). Regarding the effect on computer confidence, we expect students to feel confident about using computers when using them for school ( Claro et al., 2012 ) and even more when using them for recreational purposes. Taking this background into account, we propose the following hypotheses.
Educational attitudes have a positive effect on computer confidence.
Educational attitudes have a positive effect on academic achievement.
Stereotype attitudes have a negative effect on computer confidence.
Enjoyment attitudes have a positive effect on computer confidence.
Enjoyment attitudes have a negative effect on academic achievement.
2.2. Learning environments and motivations
The environment where students learn can affect their attitudes ( Hsu and Huang, 2006 ). Studies have found that students achieve higher grades when they have a computer at home ( Fairlie, 2012 ; Fairlie et al., 2010 ) and use it daily to facilitate their school work ( Gu and Xu, 2019 ), suggesting that home computers improve educational outcomes and computer skills, leading to more efficient use of computers ( Fairlie and London, 2012 ). Many researchers pointed to a positive impact of computer use in schools on students' educational outcomes ( Bayrak and Bayram, 2010 ; Murillo-Zamorano et al., 2019 ; Xiao and Sun, 2021 ). The integration of computers in the classroom positively influences the interaction between students and increases learning and teaching ( Murillo-Zamorano et al., 2019 ). Experimental class manipulations using a computer in class were tested over the years, with positive results: students' academic achievement increases when a computer assists them in learning ( Bayrak and Bayram, 2010 ). However, most students show dissatisfaction with the learning environment of schools ( Hsu and Huang, 2006 ). So, we propose that home and school environments positively influence computer use in general and student achievement particularly, as hypothesised below.
Home environments have a positive effect on computer use.
Home environments have a positive effect on academic achievement.
Computer use mediates the effect of home environment on academic achievement
School environments have a positive effect on computer use.
School environments have a positive effect on academic achievement
Computer use mediates the effect of school environment on academic achievement
Regarding motivations, several types of motivations have already been studied to predict academic achievement, and the best predictor so far is associated with interest. If the student is interested, he will engage in the activity independently, and there is also evidence that interest motivations directly affect reading achievements ( Habók et al., 2020 ). When analysing students' motivations for using computers, studies show that using computers at school and for schoolwork results in higher motivation when studying and positively impacts academic achievement ( Partovi and Razavi, 2019 ). Likewise, when the students' perceptions of learning motivations are improved, there is an increasing computer use by the students and, as a result, it enhances their computer self-efficacy - perceived skill on the use ( Rohatgi et al., 2016 ) - indirectly ( Hsu and Huang, 2006 ). Therefore, in order to increase computer self-efficacy, students need to use computers more frequently. Previous results indicate that interest motivations positively affect computer use and computer self-efficacy, predicting that when student interests in computers are higher, student computer self-efficacy increases. Students are also motivated by employment and recognise that computer abilities can help them get a good job ( Hsu and Huang, 2006 ). This factor can be predicted by self-efficacy because it defines the confidence and ability on achieving success ( Serge et al., 2018 ). A study showed that learners who are more engaged and motivated use more technology for their learning purposes, most likely for individual learning than for collaborative tasks ( Lee et al., 2019 ). Regarding the use of technology, students who use it more are more motivated to do it and have better grades ( Higgins, Huscroft-D’Angelo and Crawford, 2019 ), and students who are motivated by attaining better grades tend to use e-learning more ( Dunn and Kennedy, 2019 ). In line with the literature, we expect the confirmation of the presented hypotheses.
Interest motivations have a positive effect on computer use.
Interest motivations have a positive effect on academic achievement.
Interest motivations have a positive effect on computer self-efficacy.
Employment motivations have a positive effect on computer self-efficacy.
Employment motivations have a positive effect on academic achievement.
2.3. Computer confidence, computer use & computer self-efficacy
Hands-on experience with technology is the most important factor in increasing students' confidence while using it and consequently increasing their perceived computer self-efficacy ( Hatlevik and Bjarnø, 2021 ). Students with access to a computer are more involved and interested in their classwork ( Gibson et al., 2014 ). Higher commitment to school, curiosity, and positivism can help students develop motivation and interest in school subjects, leading to higher self-efficacy and consequently better academic achievement ( Stajkovic et al., 2018 ).
Computer use has a positive effect on computer confidence.
Computer confidence has a positive effect on computer self-efficacy.
Computer confidence has a positive effect on academic achievement.
Computer use has a positive effect on academic achievement.
We know from previous literature that employment motivations positively influence academic achievement, and computer self-efficacy is also a significant influence factor on employment ( Serge et al., 2018 ) to explain academic achievement, so we believe that computer self-efficacy can moderate this relation by proposing H14 .
Computer self-efficacy moderates the effect of employment motivations on academic achievement.
2.4. Loneliness
Due to the coronavirus pandemic, schools were closed to slow down the virus transmission as a control measure, affecting half of the students globally ( Viner et al., 2020 ). Schools were forced to adapt during coronavirus outbreaks since campus classes were suspended, and online platforms have been exploited to conduct virtual classes ( Ng and Peggy, 2020 ). Ng and Peggy (2020) states that virtual classes can improve students' learning outcomes if all students are self-disciplined. However, self-isolation may affect people's mental health ( Roy et al., 2020 ), primarily impacting adolescents, influencing their behaviours and achievement in academic pursuits. Interaction with others is a pivotal factor for academic performance since students who engage with colleagues and teachers tend to have more academic success than those who study by themselves ( Torres-Díaz et al., 2016 ). Loneliness or social isolation is linked to anxiety and self-esteem ( Helm et al., 2020 ), leading to unhealthy smartphone use ( Shen and Wang, 2019 ) and sedentary behaviours ( Werneck et al., 2019 ), motivating us to posit the following.
Loneliness has a negative effect on academic achievement.
2.5. Family and students' factors
Technology use is linked to additional factors that influence adolescents' academic outcomes such as family socioeconomic factors – in particular, parents' occupation, marital status ( Abosede and Akintola, 2016 ; Asendorpf and Conner, 2012 ), parents' educational level ( Chesters and Daly, 2017 ) and family size - and student socio-emotional factors - such as relationship with colleagues, student motivation and anxiety ( Balogun et al., 2017 ). Family involvement and closeness to younger progeny have positive impacts on their achievements ( Fang, 2020 ), so we believe that the relation between using computers in a school environment on academic achievement, verified above, may change depending on the family size. Also, we know from the previous results that computer use has increased with the pandemic due to online classes, and family context has a significant impact on home computer use, so we predict a moderation effect on the relation between computer use and academic achievement. The psychological status of parents, mostly their marital status and economic status, has a powerful association with the family environment and consequently on their child's educational attainments ( Poon, 2020 ). We predict there is a positive impact of mothers' education on academic achievement since the maternal figure is the most relevant for children ( Abosede and Akintola, 2016 ). Expecting that the higher the level of education of mothers, the better the students result at school, also, we predict that parents being married have a positive influence on students' results, H15 and H16 .
Family size moderates the school environment on academic achievement.
Family size moderates computer use on academic achievement.
Parents marital status has a positive effect on academic achievement.
Mothers' education has a positive effect on academic achievement.
According to their age and gender, students' grades can differ independently of their family characteristics: female students tend to achieve higher scores than male students ( Valli Jayanthi et al., 2014 ) and older students showed lower grades compared to younger students ( Chowa et al., 2015 ). Some of these factors are not of primary interest for this study. Nevertheless, it is crucial to include them in the research to control for bias since they influence the association between the use of technology and adolescents' outcomes ( Tang and Patrick, 2018 ). We have therefore used age and gender as a control variable on our research model.
2.6. Conceptual model
Figure 1 illustrates our proposed model. We focus our research on computers and their influence on academic achievement. The drivers shown in the research model emerged from the literature above. We first gathered information and identified the main factors that influence academic achievement through computer use, and from the most significant constructs relating to computers and academic achievement, we examined and analysed their viability on the study. From the computers' context, the most significant constructs found were computer attitudes (educational attitudes, enjoyable attitudes, stereotypes attitudes), computer use, computer confidence ( Levine and Donitsa-Schmidt, 1998 ), computer self-efficacy, learning environments (home environment, school environment) and learning motivations (interest motivations, employment motivations) ( Hsu and Huang, 2006 ). We identified loneliness as the most relevant construct from the pandemic context considering its impact on academic achievement ( Helm et al., 2020 ). We identified mothers' education, marital status, and family size as the most relevant influencers from the family context. Finally, with our central construct, academic achievement, we are trying to understand how it is impacted by computers, the pandemic and family factors from students' points of view. So, the proposed model tries to predict AA through students' computer attitudes, learning environments, learning motivations, computer confidence, computer use, computer self-efficacy and loneliness, adding sociodemographic data related to students and their families - parents' marital status, mothers' education and family size, where the latter only works as a moderator, including two additional control variables, age and gender. This model integrates several constructs on the literature relevant to the study of computers influence on academic achievement since is essential to fortify and unify the knowledge in this investigation field. As explained above, the model merges two existing models ( Hsu and Huang, 2006 ; Levine and Donitsa-Schmidt, 1998 ), allowing us to update the previous results and test new hypothesis. Additionally, the integration of the covid pandemic context brings a different and important analysis of today's reality.

Conceptual model.
3.1. Participants and procedure
For this study, we developed a questionnaire for students enrolled in public high schools. The survey, with an estimated completion time of 8 min was sent by e-mail to several schools in Portugal to achieve more diversity within the collected answers. The participants consented to the use of their information as long as it was anonymous and confidential. The questionnaire was answered online and comprised 26 closed questions (please, see Appendix A ) inquiring about computer attitudes, motivations, use at home and school, frequency of use, students' grade average from 0 to 20 marks, and sociodemographic information. With this data, we can compare and analyse the impact of their type of use and opinion about computers on their achievement in school. The study's target population were 16 to 18-year-old adolescents in the 10 th , 11 th and 12 th grades at secondary schools. This range of students allowed us to surround a group of people with similar maturity and identical needs in digital use. We chose to study public school students because teaching methods in private schools are quite different, as are the type of students and families who choose private schools. Also, most students in Portugal study at public schools, and it seems more coherent to study only public education since it is more accessible to address. According to the Ethics Committee of NOVA IMS and MagIC Research Center regulations, this project was considered to meet the requirements, being considered approved.
A pilot test with 30 answers allowed us to comprehend the viability of some survey questions and their order, and afterwards, when evaluating the model, the strength of constructs led us to drop a few items due to the lack of importance and correlations within them. The pilot test allowed us to improve the questionnaire to facilitate answering and adapt the research model initially built. After the complete collection of data, we considered only student responses 100% completed, amounting to 286 valid responses, from a total of 465 answers. We had 98 boys and 188 girls among the respondents, with an average age of 17 years old, with an average global grade of 15 points (on a scale from 0 to 20). Students' academic achievement was measured through students' average grades - on reading, mathematics and global average grade. Computer use was measured through a scale range from 1 (never) to 5 (every day) to measure the frequency of use. A 3-item loneliness scale was used to assess the loneliness construct ( Hughes et al., 2004 ) based on the UCLA Loneliness Scale ( Russel, 1996 ). This scale has been used in several studies recently ( Helm et al., 2020 ; Liu et al., 2020 ; Shen and Wang, 2019 ) to study loneliness as a consequence of the coronavirus. The remaining items, apart from the demographic variables (age, gender, marital status, mothers' education, family size), were measured through a scale range from 1 (strongly disagree) to 5 (strongly agree).
4. Analysis and results
We used structural equation modelling (SEM) to test the relations estimated in our theoretical model and its effects ( Marsh et al., 2004 ). Consequently, we applied partial least squares (PLS), a method used to develop theories in explanatory research. The use of PLS-SEM is to maximise the explained variance in the dependent constructs and evaluate data quality, knowing that it is a method that works better on bigger sample sizes and larger complexity with less restrictive assumptions on data (Joe F Hair et al., 2014 ). We used the partial least squares method as the recommended two-step approach that first tests the reliability and validity of the measurement model and then assesses the structural model ( Anderson and Gerbing, 1988 ).
4.1. Measurement model
Measurement models measure the relation between the latent variables and their indicators for both reflective and formative constructs. In this study, all constructs are reflective except computer use, which is formative.
The internal consistency, convergent validity and discriminatory validity must be verified to assess the reflective measurement model. The composite reliability (CR), shown in Appendix B, is higher than 0.7 in all constructs, reflecting internal consistency ( Mcintosh et al., 2014 ). Also, by analysing the loadings of the items, which are all higher than 0,6, we can conclude there is indicator reliability. To demonstrate convergent validity, we verify the average variance extracted (AVE) values of constructs, and they are all higher than 0.5 (please see Appendix B), confirming there is convergent validity ( Sarstedt et al., 2017 ). To analyse discriminant validity, we implemented three methods - the Fornell-Larcker criterion, the loadings and cross-loadings analysis, and the heterotrait-monotrait ratio (HTMT) methodology. The Fornell-Larcker criterion supports that the AVE square root of each construct should be higher than the correlation between constructs ( Fornell and Larcker, 1981 ), which Appendix B can confirm. The second criteria support that the loadings should be higher than the respective cross-loadings (Joseph F Hair et al., 2014 ), which is observed in Appendix C. The HTMT method sustains that the HTMT values should be lower than 0.9 (Joseph F Hair et al., 2017 ; Sarstedt et al., 2017 ), confirmed by Appendix D. Thus, all the constructs have discriminant validity.
In order to assess the validity of the formative construct computer use, we assessed the model for multicollinearity using (variance inflation factor) VIF. Table 1 shows the VIF values are all under 5 (Joseph F Hair et al., 2017 ), as the threshold indicates it should be, so the model does not have multicollinearity problems. In terms of significance, the three items are statistically significant (p < 0.05), as Table 1 confirms, concluding that the formative construct is reliable.
Table 1
Formative measurement model evaluation.
Note: ∗p < 0.05, ∗∗p < 0.01, ∗∗∗p < 0.001.
We can conclude that both reflective and formative constructs present a good measurement model. For this reason, we can move to the structural model.
4.2. Structural model
To estimate the structural model, first, we assessed the VIF to check the model for multicollinearity issues. The VIF values are below the threshold of 5 ( Sarstedt et al., 2017 ), so the model does not have multicollinearity problems. To evaluate the statistical significance of the path coefficients, we did a bootstrap with 5000 resamples. Results from the model are presented in Figure 2 .

Conceptual model results.
The model explains 30.5% of computer confidence. Educational attitudes (β = 0.307, p < 0.001), stereotype attitudes (β = - 0.160, p < 0.01), enjoyment attitudes (β = 0.236, p < 0.001) and computer use (β = 0.136, p < 0.05) are statistically significant in explaining computer confidence, confirming hypotheses H1 a, H2 , H3 a and H8 . The explained variation of computer use is 42,5%. The results show that home environment (β = 0.421, p < 0.001), school environment (β = 0.317, p < 0.05) and interest motivations (β = 0.124, p < 0.05) are statistically significant and have a positive influence on computer use, thus hypotheses H4 a, H5 a and H6 a are supported. The model explains 35.8% of computer self-efficacy. The home environment construct (β = 0.200, p < 0.01), interest motivations (β = - 0.156, p < 0.05), and employment motivations (β = 0.217, p < 0.01) are statistically significant however, home environment and employment motivation show a positive influence on computer self-efficacy, supporting hypotheses H4 c, H7 a and interest motivations show a negative influence on computer self-efficacy where we expected a positive influence, rejecting H6 c.
The model explains 31.1% of students' academic achievement. Enjoyment attitudes (β = - 0.162, p < 0.05), employment motivations (β = 0.183, p < 0.05), computer use (β = 0.257, p < 0.05), loneliness (β = - 0.150, p < 0.05) and mother's education (β = 0.135, p < 0.05) are statistically significant in explaining academic achievement, supporting the hypotheses, H3 b, H7 b, H11 , H13 and H16 . We reject respective hypotheses H5 b and H6 b respectively, despite school environment (β = - 0.246, p < 0.001) and interest motivations (β = - 0.159, p < 0.05), being statistically significant, because we suggested that school environment and interest motivations would positively influence academic achievement, and the results observe a negative influence. Educational attitudes (β = -0.003, p > 0.05), home environment (β = 0.100, p > 0.05), computer confidence (0.105, p > 0.05) and parental marital status (β = 0.067, p > 0.05) show a non-significant effect on explaining academic achievement, rejecting H1 b, H4 b, H10 and H15 . The moderation effect of computer self-efficacy in employment motivations (β = 0.108, p < 0.05) is statistically significant, supporting H12 . The moderation effect of family size on school environment (β = 0.141, p < 0.05) and on computer use (β = - 0.233, p < 0.01) is statistically significant, supporting H14 a and H14 b.
Table 2 summarises the research hypotheses results. We can conclude that 17 of the 25 proposed hypotheses were supported.
Table 2
Research hypotheses results.
Notes: n.a. - not applicable; ∗ significant at p < 0.05; ∗∗ significant at p < 0.01; ∗∗∗ significant at p < 0.001.
5. Discussion
This research model contributes to and extends the literature review on computers and academic achievement. This study relates academic achievement with loneliness, family and computer-related variables such as computer confidence, computer self-efficacy, computer attitudes, computer learning motivations and computer learning environments.
The results show that educational and enjoyment computer attitudes positively influence computer confidence, while stereotype attitudes negatively influence it. We expected this negative relation regarding stereotypes since there are the same results regarding stereotypes on gender and age ( Punter et al., 2017 ), although similar results concerning stereotypes on computer users have not yet been found. As for the influence of attitudes on academic achievement, educational computer attitudes do not have a statistically significant relationship with academic achievement. On the other hand, enjoyable computer attitudes have a significant negative impact on academic achievement, which leads us to conclude that there is no relation between computers as an educational tool and academic achievement. In fact, apart from some specific high school vocational courses oriented to computing skills, most classes happen in a classic lecture setting and rely mostly on textbook manuals as learning tools, which can help explain the results regarding educational computer attitude. However, using computers for recreational purposes negatively influences students' academic achievement, as similar results have already been observed - students who play more video games have a lower achievement ( Tang and Patrick, 2018 ). Two possible reasons can explain this phenomenon. First, because young adults are so engaged and skilled with technology use for game playing and social media that they do not make the best use of these skills for academic purposes, for instance ( Gurung and Rutledge, 2014 ) and second, because excessive use and multitasking can lead to distractions and lack of time to study ( Rashid and Asghar, 2016 ).
The construct computer use, measured as the frequency of use, positively impacts computer confidence and academic achievement. Thus, the greater the use of computers, the more confident students are while using them, and so the more use of the computer, the better the performance achieved. Several other studies contradict the negative influence verified between school environment and academic achievement ( Bayrak and Bayram, 2010 ; Carle et al., 2009 ; Murillo-Zamorano et al., 2019 ). However, this can be explained by the rapid development of computer technology and the massive use of computers at home compared to the lack of use at school due to schools' technology being obsolete, and students preferring the home environment.
The results demonstrate that computer use works as a full mediator for home environment and academic achievement since there is no relation between home environment and academic achievement, contrary to another study ( Fairlie et al., 2010 ). However, with computer use as a mediator, we suggest that the home environment influences academic achievement when computer use increases since there is a positive relation between home environment and computer use ( Hsu and Huang, 2006 ), i.e., students who use a computer at home have better results. Also, computer use works as a partial mediator for the school environment and academic achievement. Hence, we suggest that, although the use of computers at school already directly (but negatively) influences students' performance, computer use mediates this relation positively. This effect is likely due to the fact that even though there is an effort to implement digital transformation in the education sector, there is still a lack of computers at schools: most students do not have easy access to computers in school (high schools in Portugal have an average 4.2 students per computer), but those who use them benefit on their grades. These results allow us to confirm our second contribution, the investigation of the mediation effect of computer use between the factors identified and academic achievement. The mediation results are shown in Table 3 .
Table 3
Hypotheses testing on mediation.
Note: ∗ |t|> 1.96 and p-value = 0.05.; ∗∗ |t| > 2.57 and p-value = 0.01; ∗∗∗ |t| > 3.291 and p-value = 0.001.
Regarding motivations, interest motivation impacts computer use positively, as concluded by other similar findings ( Rohatgi et al., 2016 ), i.e. the more interested students are in computers, the more they use them. Nonetheless, it negatively influences academic achievement and computer self-efficacy, concluding that the bigger the interest motivation, the more the use of computers but the lower the achievement and the computer self-efficacy. These two negative relations are quite controversial compared to the literature. However, it may mean that the more interest in computers, the more use for recreational purposes, negatively impacting academic achievement ( Rashid and Asghar, 2016 ). The more interest students have in computers, the more knowledge of using the devices, and the perceived efficacy starts to decrease. Thus further research is needed to draw any conclusions on this.
Computer confidence has a strong positive effect on computer self-efficacy, meaning that the perceived computer self-efficacy increases when the confidence in the device is higher, as stated in similar findings ( Hatlevik and Bjarnø, 2021 ). Although, we cannot conclude there is a relation between computer confidence and academic achievement. All the previous results allow us to reflect on the influence that the computer-related variables studied have on the student performance, contributing with data for future research and confirming our first contribution of the study.
The loneliness construct, used as a measure of coronavirus effects, negatively influenced academic achievement, as expected. While students were in lockdown having remote classes, without any presential contact with their school, teachers, and colleagues, the feeling of loneliness and isolation negatively impacted their performance indeed, as observed in our results. These results confirm our contribution to understanding how the COVID-19 pandemic influences students’ academic achievement. Recent studies found negative impacts of loneliness ( Roy et al., 2020 ) on students, demonstrating the importance of cooperating with colleagues ( Torres-Díaz et al., 2016 ). However, there are yet no results of the direct impact of loneliness deriving from the pandemic on academic achievement.
There are three moderation hypotheses using family size and computer self-efficacy. From the family size moderator, we can conclude that family size influences the relation between school environment and academic achievement. In Figure 3 , we can see that when the family size decreases, the negative impact the school environment has on academic achievement increases, suggesting that the smaller the family, the students tend to have worse grades when studying in a school environment. Regarding family size in the relation between computer use and academic achievement, shown in Figure 4 , when the family size decreases, computer use is more important to explain academic achievement because when the family is small, students need to use the computer more to achieve better results. Relating to the computer self-efficacy moderator, in Figure 5 , it impacts the relationship between employment motivations and academic achievement positively, meaning that the better students perceive their computer self-efficacy, the stronger positive impact employment motivation has on academic achievement. This effect can be explained due to the increase of technological jobs: students who feel more capable in their computer skills (with a higher computer self-efficacy) and are more motivated to pursue a technological career have higher academic achievement. These results allow us to confirm our second contribution, the investigation of the moderation effect family size and computer self-efficacy.

Structural model (variance-based technique) for academic achievement.

In this study, we found that marital status does not have any effect on academic achievement, but mothers' education has a positive impact on students' achievement, reinforcing the literature ( Abosede and Akintola, 2016 ).
5.1. Practical implications
Academic achievement is a widely topic studied because there is an ongoing concern for understanding the factors that lead to better academic achievements. Since students practically depend on computers for school nowadays, we tried to relate the most studied computer variables in the literature with academic achievement, expecting results that answer the gaps identified in the literature. To our knowledge, no study has yet provided a conclusion on the influence of loneliness provoked by the COVID-19 pandemic on academic achievement, neither of interest and employment motivations on AA. Moreover, there is no consensus in the literature on the influence of the use of computers for fun and academic performance. We can contribute to the literature with the answers to these questions: students who feel lonely have worse academic achievement, students motivated by an interest in computers have worse academic achievement and students motivated by the expectation of having a good job have better grades. Also, enjoyable computer attitudes negatively influence academic achievement, so the students who find the computer a good tool for recreational purposes have worse grades.
Contrary to the literature, we found that computer confidence does not influence academic achievement; apart from this, we concur with the available results published by other researchers. There are clear positive implications on using computers in education, and consequently, in students' outcomes. Therefore, teachers and parents should encourage using computers in adolescents' education to improve their school performance and future.
5.2. Limitations and further research
The present study has some limitations that point to future research directions on the role of students' academic achievement and its predictors. First, the data collected does not have sufficient diversity in country dispersity and gender balance since most participants were girls hailing from Portugal. Also, better results can be obtained with a more significant sample. Secondly, the fact that we are going through a pandemic forced schools and students to attend classes online, which on the one hand, is an advantage because it provides the opportunity to study loneliness deriving from the pandemic. On the other hand, it could bias the students' answers to the questionnaire and the subsequent results because their opinion on computers could have changed during home-schooling compared to the usual previous schooling method since the literature is related to regular presential school attendance.
In further research, other factors regarding loneliness should be studied to understand the impact of coronavirus on students' lives better, comparing pre-pandemic and pandemic daily computer usage. Other factors such as addiction to technology should be analysed.
6. Conclusions
This study proposes a theoretical model on the influence of several computer factors on the academic achievement of high school students. The results, in general, empirically support the literature in similar findings. The proposed conceptual model explains 31.1% of academic achievement. We found that students who use computers for recreational purposes or feel that a computer is a tool to "pass the time" or play games are those who have the worst grades. We can conclude this through the negative relation between enjoyment attitudes and academic achievement. Nevertheless, there is no relation between students who perceive computers as an educational tool and their academic achievement. We believe this conclusion results from how teenagers use their computers and smartphones excessively, not prioritising the use for school, leading to the observed results. Our results also show that there are still stereotypes about who uses computers most. Respondents believe that peers who play sports do not have the same likelihood of using computers excessively, and those that frequently use computers are not sociable. This mindset leads to less confidence in computers.
A significant conclusion was found regarding the computer use environment, though the mediation effect of computer use. When students use the computer at home, they need to use it frequently to influence their academic achievement, but when students use the computer at school, it will influence their academic achievement positively independently of the frequency of use. However, the frequency of computer use itself influences academic achievement. As we expected, the feelings of loneliness associated with the coronavirus negatively influence students' academic achievement, an important new conclusion in the literature. The moderation effect on family size allows us to conclude that students with a smaller family tend to have worse grades when studying in a school environment and need to use computers more to have better school results than those in larger families. Moreover, the moderation effect on computer self-efficacy lets us conclude that students who perceive better computer self-efficacy, have better grades and academic achievement is influenced by employment motivation.
Declarations
Author contribution statement.
Sofia Simões: Conceived and designed the experiments; Performed the experiments; Analyzed and interpreted the data; Contributed reagents, materials, analysis tools or data; Wrote the paper.
Tiago Oliveira: Conceived and designed the experiments; Analyzed and interpreted the data; Contributed reagents, materials, analysis tools or data; Wrote the paper.
Catarina Nunes Analyzed and interpreted the data; Wrote the paper.
Funding statement
This work was supported by FCT (Fundação para a Ciência e a Tecnologia) under project DSAIPA/DS/0032/2018 (DS4AA).
Data availability statement
Declaration of interests statement.
The authors declare no conflict of interest.
Additional information
No additional information is available for this paper.
Appendix A. Constructs table
Notes: 1, 2, 3, 4, 5, 6, 7, 9, 10 Range scale from 1 (Strongly Disagree) to 5 (Strongly Agree); 8 Range scale from 1 (Never) to 5 (Everyday); 11 Ordinal Scale (Hardly ever, some of the time, often); 12 Ratio scale from 0 to 20 (number); 13 Nominal scale (number); 14 Nominal scale (married, divorced, in a domestic partnership, widowed, other); 15 Ordinal scale (less than high school, high school or equivalent, bachelor's degree, master's degree, doctorate, other); 16 Ratio scale (number); 17 Nominal scale (male, female).
Appendix B. Descriptive statistics, correlation, composite reliability (CR), and average variance extracted (AVE)
Note: Values in diagonal (bold) are the AVE square root.
Appendix C. Outer Loadings and Cross-Loadings
Appendix d. heterotrait-monotrait ratio (htmt).
- Abosede S., Akintola O. Mothers ’ employment , marital status and educational level on students ’ academic achievement in business studies. Multidiscip. Res. 2016; 4 (2):159–165. [ Google Scholar ]
- Admiraal W., Louws M., Lockhorst D., Paas T., Buynsters M., Cviko A., Kester L. Teachers in school-based technology innovations: a typology of their beliefs on teaching and technology. Comput. Educ. 2017; 114 :57–68. [ Google Scholar ]
- Anderson J.C., Gerbing D.W. Structural equation modeling in practice : a review and recommended two-step approach. Psychol. Bull. 1988; 103 (3):411–423. [ Google Scholar ]
- Asendorpf J.B., Conner M. Conflict resolution as a dyadic mediator: considering the partner pespective on conflict resolution. Eur. J. Pers. 2012; 119 :108–119. [ Google Scholar ]
- Bae D., Wickrama K.A.S. Family socioeconomic status and academic achievement among Korean adolescents: linking mechanisms of family processes and adolescents’ time use. J. Early Adolesc. 2015; 35 (7):1014–1038. [ Google Scholar ]
- Balogun A.G., Balogun S.K., Onyencho C.V. Test anxiety and academic performance among undergraduates: the moderating role of achievement motivation. Spanish J. Psychol. 2017; 20 :1–8. [ PubMed ] [ Google Scholar ]
- Bayrak B.K., Bayram H. The effect of computer aided teaching method on the student’s academic achievement in the science and technology course. Procedia - Soc. Behav. Sci. 2010; 9 :235–238. [ Google Scholar ]
- Bowers A.J., Berland M. Does recreational computer use affect high school achievement? Educ. Technol. Res. Dev. 2013; 61 (1):51–69. [ Google Scholar ]
- Carle A.C., Jaffee D., Miller D. Engaging college science students and changing academic achievement with technology: a quasi-experimental preliminary investigation. Comput. Educ. 2009; 52 (2):376–380. [ Google Scholar ]
- Chesters J., Daly A. Do peer effects mediate the association between family socio-economic status and educational achievement? Aust. J. Soc. Issues. 2017; 52 (1):65–77. [ Google Scholar ]
- Chowa G.A.N., Masa R.D., Ramos Y., Ansong D. How do student and school characteristics influence youth academic achievement in Ghana? A hierarchical linear modeling of Ghana YouthSave baseline data. Int. J. Educ. Dev. 2015; 45 :129–140. [ Google Scholar ]
- Claro M., Preiss D.D., San Martín E., Jara I., Hinostroza J.E., Valenzuela S., Nussbaum M. Assessment of 21st century ICT skills in Chile: test design and results from high school level students. Comput. Educ. 2012; 59 (3):1042–1053. [ Google Scholar ]
- Dunn T.J., Kennedy M. Technology enhanced learning in higher education; motivations, engagement and academic achievement. Comput. Educ. 2019; 137 (March):104–113. [ Google Scholar ]
- Ertmer P.A., Ottenbreit-Leftwich A.T., Sadik O., Sendurur E., Sendurur P. Teacher beliefs and technology integration practices: a critical relationship. Comput. Educ. 2012; 59 (2):423–435. [ Google Scholar ]
- Fairlie R.W. Academic achievement, technology and race: experimental evidence. Econ. Educ. Rev. 2012; 31 (5):663–679. [ Google Scholar ]
- Fairlie R.W., Beltran D.O., Das K.K. Home computers and educational outcomes: evidence from the NLSY97 and cps. Econ. Inq. 2010; 48 (3):771–792. [ Google Scholar ]
- Fairlie R.W., London R.A. The effects of home computers on educational outcomes: evidence from a field experiment with community college students. Econ. J. 2012; 122 (561):727–753. [ Google Scholar ]
- Fang L. Acculturation and academic achievement of rural to urban migrant youth: the role of school satisfaction and family closeness. Int. J. Intercult. Relat. 2020; 74 (November 2019):149–160. [ Google Scholar ]
- Fornell C., Larcker D. Evaluating structural equation models with unobservable variables and measurement error. J. Market. Res. 1981; 18 (1):39–50. [ Google Scholar ]
- Gibson P.A., Stringer K., Cotten S.R., Simoni Z., O’Neal L.J., Howell-Moroney M. Changing teachers, changing students? the impact of a teacher-focused intervention on students’ computer usage, attitudes, and anxiety. Comput. Educ. 2014; 71 :165–174. [ Google Scholar ]
- Gonzalez-pienda J.A., Nunez J.C., Gonzalez-pumariega S., Alvarez L., Roces C., Garcia M. A structural equation model of parental involvement, motivational and aptitudinal characteristics, and academic achievement. J. Exp. Educ. 2002; 70 (3):257–287. [ Google Scholar ]
- Gu X., Xu H. Missing piece in understanding student learning: out-of-school computer use. J. Educ. Comput. Res. 2019; 57 (2):320–342. [ Google Scholar ]
- Gurung B., Rutledge D. Digital learners and the overlapping of their personal and educational digital engagement. Comput. Educ. 2014; 77 :91–100. [ Google Scholar ]
- Habók A., Magyar A., Németh M.B., Csapó B. Motivation and self-related beliefs as predictors of academic achievement in reading and mathematics: structural equation models of longitudinal data. Int. J. Educ. Res. 2020; 103 (May):101634. [ Google Scholar ]
- Hair Joe F., Ringle C.M., Sarstedt M., Hair J.F., Ringle C.M., Sarstedt M. 2014. PLS-SEM : Indeed a Silver Bullet; pp. 37–41. [ Google Scholar ]
- Hair Joseph F., Hult Gt., Ringle C.M., Sarstedt M. second ed. SAGE Publications, Inc; Los Angeles: 2017. A Primer on Partial Least Squares Structural Equation Modeling (PLS-SEM) [ Google Scholar ]
- Hamiyet S. The effects of computer games on the achievement of basic mathematical skills. Educ. Res. Rev. 2015; 10 (22):2846–2853. [ Google Scholar ]
- Hatlevik O.E., Bjarnø V. Examining the relationship between resilience to digital distractions , ICT self-ef fi cacy , motivation , approaches to studying , and time spent on individual studies. Teach. Teach. Educ. 2021; 102 :103326. [ Google Scholar ]
- Helm P.J., Jimenez T., Bultmann M., Lifshin U., Greenberg J., Arndt J. Existential isolation, loneliness, and attachment in young adults. Pers. Indiv. Differ. 2020; 159 (February):109890. [ Google Scholar ]
- Higgins K., Huscroft-D’Angelo J., Crawford L. Effects of technology in mathematics on achievement, motivation, and attitude: a Meta-Analysis. J. Educ. Comput. Res. 2019; 57 (2):283–319. [ Google Scholar ]
- Hsu W.K.K., Huang S.H.S. Determinants of computer self-efficacy - an examination of learning motivations and learning environments. J. Educ. Comput. Res. 2006; 35 (3):245–265. [ Google Scholar ]
- Hughes M.E., Waite L.J., Hawkley L.C., Cacioppo J.T. A short scale for measuring loneliness in large surveys: results from two population-based studies. Res. Aging. 2004; 26 (6):655–672. [ PMC free article ] [ PubMed ] [ Google Scholar ]
- Lee C., Yeung A.S., Cheung K.W. Learner perceptions versus technology usage: a study of adolescent English learners in Hong Kong secondary schools. Comput. Educ. 2019; 133 (August 2017):13–26. [ Google Scholar ]
- Lee J., Shute V.J., Lee J. 2010. Personal and Social-Contextual Factors in K – 12 Academic Performance : an Integrative Perspective on Student Learning Personal and Social-Contextual Factors in K – 12 Academic Performance : an Integrative Perspective on Student Learning; pp. 37–41. (December 2014) [ Google Scholar ]
- Levine T., Donitsa-Schmidt S. Computer use, confidence, attitudes, and knowledge: a causal analysis. Comput. Hum. Behav. 1998; 14 (1):125–146. [ Google Scholar ]
- Liu C.H., Zhang E., Wong G.T.F., Hyun S., Hahm H. Chris. Factors associated with depression, anxiety, and PTSD symptomatology during the COVID-19 pandemic: clinical implications for U.S. young adult mental health. Psychiatr. Res. 2020; 290 [ PMC free article ] [ PubMed ] [ Google Scholar ]
- Marsh H.W., Wen Z., Hau K.T. Structural equation models of latent interactions: evaluation of alternative estimation strategies and indicator construction. Psychol. Methods. 2004; 9 (3):275–300. [ PubMed ] [ Google Scholar ]
- Mcintosh C.N., Edwards J., Antonakis J. 2014. Reflections on Partial Least Squares Path Modeling. [ Google Scholar ]
- Milani L., Grumi S., Di Blasio P. Positive effects of videogame use on visuospatial competencies: the impact of visualization style in preadolescents and adolescents. Front. Psychol. 2019; 10 (MAY):1–9. [ PMC free article ] [ PubMed ] [ Google Scholar ]
- Murillo-Zamorano L.R., López Sánchez J.Á., Godoy-Caballero A.L. How the flipped classroom affects knowledge, skills, and engagement in higher education: effects on students’ satisfaction. Comput. Educ. 2019; 141 October 2018. [ Google Scholar ]
- Ng Y.-M., Peggy P.L. Coronavirus disease (COVID-19) prevention: virtual classroom education for hand hygiene. Nurse Educ. Pract. 2020:102782. [ PMC free article ] [ PubMed ] [ Google Scholar ]
- OECD . 2015. OECD Digital Economy Outlook 2015. [ Google Scholar ]
- OECD . 2019. Benchmarking Higher Education System Performance: Norway. [ Google Scholar ]
- OECD . 2020. Learning Remotely when Schools Close : How Well Are Students and Schools Prepared ? Insights from PISA. [ Google Scholar ]
- Partovi T., Razavi M.R. The effect of game-based learning on academic achievement motivation of elementary school students. Learn. Motiv. 2019; 68 (August):101592. [ Google Scholar ]
- Poon K. The impact of socioeconomic status on parental factors in promoting academic achievement in Chinese children. Int. J. Educ. Dev. 2020; 75 (December 2019):102175. [ Google Scholar ]
- Punter R.A., Meelissen M.R.M., Glas C.A.W. Gender differences in computer and information literacy: an exploration of the performances of girls and boys in ICILS 2013. Eur. Educ. Res. J. 2017; 16 (6):762–780. [ Google Scholar ]
- Rajkumar R.P. COVID-19 and mental health: a review of the existing literature. Asian J. Psychiatr. 2020; 52 (March):102066. [ PMC free article ] [ PubMed ] [ Google Scholar ]
- Rashid T., Asghar H.M. Technology use, self-directed learning, student engagement and academic performance: examining the interrelations. Comput. Hum. Behav. 2016; 63 :604–612. [ Google Scholar ]
- Rohatgi A., Scherer R., Hatlevik O.E. The role of ICT self-efficacy for students ’ ICT use and their achievement in a computer and information literacy test. Comput. Educ. 2016; 102 :103–116. [ Google Scholar ]
- Roy D., Tripathy S., Kar S.K., Sharma N., Verma S.K., Kaushal V. Study of knowledge, attitude, anxiety & perceived mental healthcare need in Indian population during COVID-19 pandemic. Asian J. Psychiatr. 2020; 51 :102083. [ PMC free article ] [ PubMed ] [ Google Scholar ]
- Russel D. UCLA loneliness scale. J. Pers. Assess. 1996; 66 (1):20–40. [ PubMed ] [ Google Scholar ]
- Sarstedt M., Ringle C.M., Hair J.F. 2017. Partial Least Squares Structural Equation Modeling. [ Google Scholar ]
- Schmid R., Petko D. Does the use of educational technology in personalized learning environments correlate with self-reported digital skills and beliefs of secondary-school students? Comput. Educ. 2019; 136 :75–86. [ Google Scholar ]
- Serge P., Veiga M., Turban D.B. Insight into job search self-regulation : effects of employment self-efficacy and perceived progress on job search intensity. J. Vocat. Behav. 2018; 108 :57–66. [ Google Scholar ]
- Shen X., Wang J.L. Loneliness and excessive smartphone use among Chinese college students: moderated mediation effect of perceived stressed and motivation. Comput. Hum. Behav. 2019; 95 (January):31–36. [ Google Scholar ]
- Stajkovic A.D., Bandura A., Locke E.A., Lee D., Sergent K. Test of three conceptual models of influence of the big five personality traits and self-efficacy on academic performance: a meta-analytic path-analysis. Pers. Indiv. Differ. 2018; 120 (August 2017):238–245. [ Google Scholar ]
- Suárez-álvarez J., Fernández-alonso R., Muñiz J. Self-concept, motivation, expectations, and socioeconomic level as predictors of academic performance in mathematics. Learn. Indiv Differ. 2014; 30 :118–123. [ Google Scholar ]
- Tang S., Patrick M.E. Technology and interactive social media use among 8th and 10th graders in the U.S. and associations with homework and school grades. Comput. Hum. Behav. 2018; 86 :34–44. [ PMC free article ] [ PubMed ] [ Google Scholar ]
- Tesfagiorgis M., Tsegai S., Mengesha T., Craft J., Tessema M. The correlation between parental socioeconomic status (SES) and children’s academic achievement: the case of Eritrea. Child. Youth Serv. Rev. 2020; 116 (July):105242. [ Google Scholar ]
- Torres-Díaz J.C., Duart J.M., Gómez-Alvarado H.F., Marín-Gutiérrez I., Segarra-Faggioni V. Internet use and academic success in university students. Comunicar. 2016; 24 (48):61–70. [ Google Scholar ]
- Valli Jayanthi S., Balakrishnan S., Lim Siok Ching A., Aaqilah Abdul Latiff N., Nasirudeen A.M.A. Factors contributing to academic performance of students in a tertiary institution in Singapore. Am. J. Educ. Res. 2014; 2 (9):752–758. [ Google Scholar ]
- Viner R.M., Russell S.J., Croker H., Packer J., Ward J., Stansfield C., Booy R. School closure and management practices during coronavirus outbreaks including COVID-19: a rapid systematic review. Lancet Child Adolescent Health. 2020; 4 (5):397–404. [ PMC free article ] [ PubMed ] [ Google Scholar ]
- Voogt J., Erstad O., Dede C., Mishra P. Challenges to learning and schooling in the digital networked world of the 21st century. J. Comput. Assist. Learn. 2013; 29 (5):403–413. [ Google Scholar ]
- Werneck A.O., Collings P.J., Barboza L.L., Stubbs B., Silva D.R. Associations of sedentary behaviors and physical activity with social isolation in 100,839 school students: the Brazilian Scholar Health Survey. Gen. Hosp. Psychiatr. 2019; 59 (April):7–13. [ PubMed ] [ Google Scholar ]
- Xiao F., Sun L. Profiles of student ICT use and their relations to background, motivational factors, and academic achievement. J. Res. Technol. Educ. 2021 0(0),1–17. [ Google Scholar ]
- HPCWire - ORNL Researchers Develop an AI-driven Tool for Nea...
- The Mirage - AI Streamlines Cancer Pathology Reporting at Oak R...
- HPC Wire - OLCF’s Summit Supercomputer Lives for Another Year...
- WATE - NASA uses Oak Ridge National Lab’s supercomputers ...
- InsideHPC - Exascale’s New Software Frontier: E3SM-MMF
- Staff Directory
Search the Site

Going Big: World’s Fastest Computer Takes On Large Language Modeling
Frontier could fuel next generation of AI for open science
A team led by researchers at the Department of Energy’s Oak Ridge National Laboratory explored training strategies for one of the largest artificial intelligence models to date with help from the world’s fastest supercomputer.
The findings could help guide training for a new generation of AI models for scientific research.
The study led by ORNL’s Sajal Dash, Feiyi Wang and Prasanna Balaprakash employed Frontier , the world’s first exascale supercomputer, to run the initial stages of training on a large language model similar to OpenAI’s ChatGPT . The research team used a set of test data to project how models with 22 billion, 175 billion, and 1 trillion parameters, or variables, could run across 128 and later 384 of Frontier’s more than 9,400 nodes. The team didn’t attempt to train a full model to completion.
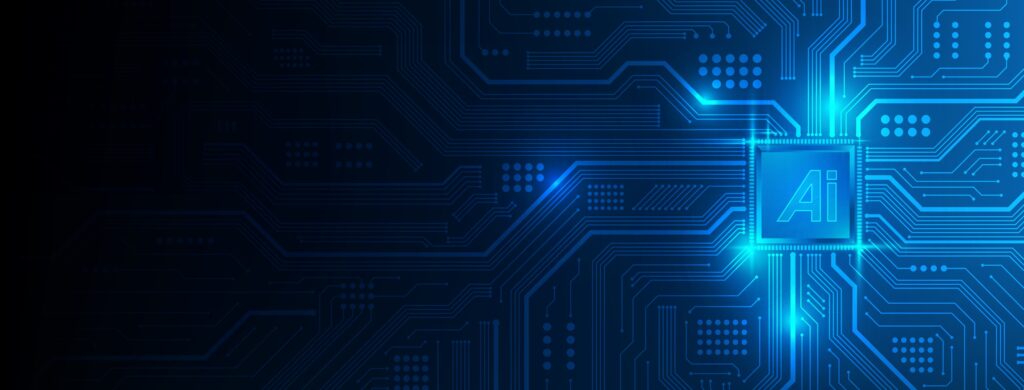
A team led by OLCF researchers used Frontier to explore training strategies for one of the largest artificial intelligence models to date. Credit: Getty Images
“This study and our findings aren’t so much a manual as a potential set of guidelines for users training a large model,” Dash said. “They can draw from our experience to decide how to use Frontier’s resources to train their particular model and make the most effective use of their allotted computing time.”
The team presented the study at the International Supercomputing Conference High Performance 2024 in May in Hamburg, Germany. Fellow scientists Isaac Lyngaas, Junqi Yin, Xiao Wang and Guojing Cong of ORNL and Romaine Egele of Paris-Saclay University also collaborated on the study.
The study focused less on model development than on pinpointing the most efficient ways to exploit the graphics processing units, or GPUs, that power Frontier and similar supercomputers and putting them to work training an AI. Each of Frontier’s nodes relies on four AMD MI250X GPUs for a total of more than 38,000 GPUs.
The training ran for a few hours on about 100 million tokens — basic units of text such as words and characters — of test data. That’s about a ten-thousandth of the necessary data to train a trillion-parameter model to completion and an even smaller fraction of the necessary time.
The research team used the data from those runs to calculate how a trillion-parameter model might perform if trained to completion on Frontier.
“This study was largely an exercise to show we can train this particular size of model on Frontier at this particular scale with this particular level of efficiency,” Wang said. “We didn’t get anywhere near the finish line of a complete large language model.”
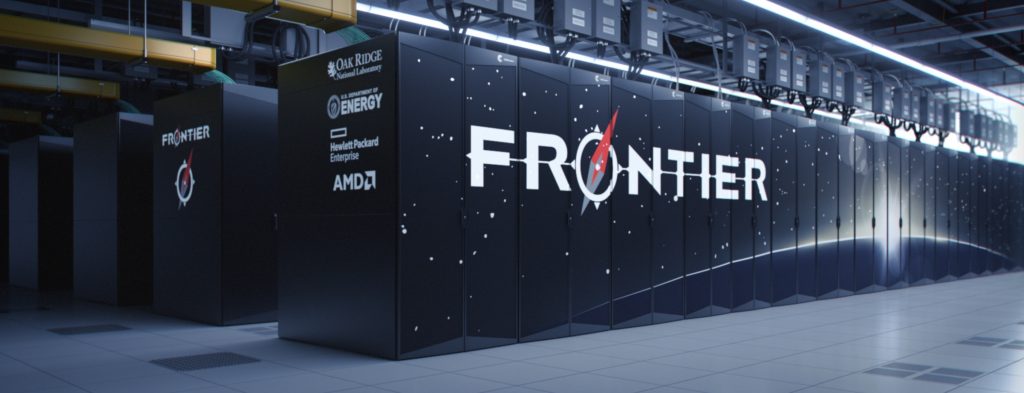
An artist’s rendering of the Frontier supercomputer. Credit: Sibling Rivalry/HPE
Large language models loosely mimic the human brain in their ability to learn and recognize patterns in words and numbers and to improve on that learning over time with additional training. The goal: design a model that can absorb and adjust the lessons learned on training data and apply that knowledge consistently and accurately to new, unfamiliar data and tasks.
The vast datasets and powerful processors needed for such training have remained mostly out of reach of scholars and in the possession of private companies, which tend to guard those resources as proprietary and set strict conditions for use. Those conditions typically limit research opportunities and don’t allow results to be easily verified.
But leadership-class supercomputers like Frontier, which awards computing time to scientific researchers through the DOE’s Innovative and Novel Computational Impact on Theory and Experiment program , could enable a new generation of AI models to be trained more quickly if scientists find the right approach.
“Traditionally, this process has relied on expert knowledge or on trial and error,” said Prasanna Balaprakash, ORNL’s director of AI programs. “One of the highlights of our work in this study is the automation of identifying high-performing strategies among a vast array of options. We leveraged DeepHyper, an open-source scalable tuning software, to automatically determine the optimal settings. We plan to extend this automated approach to fine-tune system-level performance and enhance efficiency at an extreme scale. Furthermore, we have democratized our methodologies and software for the benefit of the scientific community. This strategy ensures that our insights are widely accessible for future research on training large AI foundation models in science.”
The larger the model and its training datasets, the better its performance — but also the higher its demand for computational power. Training a trillion-parameter large language model from the initial stages to completion without optimizations would take months even at Frontier’s world-leading speeds.
The ORNL study examined approaches to data parallelism — a process used by supercomputers like Frontier to break a large problem into smaller problems to reach a solution more quickly — to train AI and how to port that training across proprietary frameworks of GPUs made by competing vendors.
“It’s about finding the best combination of training strategies while getting the best throughput,” Dash said. “Most deep-learning frameworks target the GPUs made by NVIDIA rather than the GPUs made by AMD that power Frontier. We wanted to see if existing models could run on Frontier, how to make the best use of Frontier’s computing power and how to make that level of performance possible across GPU platforms.
“We can’t train a model this size on a single GPU or a single node, for example, and every time we cross the barrier between nodes that requires more communication that consumes more time. How do we slice up the model across GPUs so that we can fit and train the model without losing too much time and energy communicating between nodes?”
The researchers found a blend of parallelism strategies worked best when tailored to the computing platform but said their work’s far from finished.
“The efficiency we achieved on Frontier with this model was decent but not decent enough,” Wang said. “At extreme scale, we achieved 30% efficiency — which means we left about 70% of Frontier’s computing power on the floor. We need much more optimization to make the machine more efficient at this scale.”
The team’s next steps include training a model further with peer-reviewed scientific data across more nodes.
Support for this research came from the DOE Office of Science’s Advanced Scientific Computing Research program and ORNL’s AI Initiative. The OLCF is a DOE Office of Science user facility.
UT-Battelle manages ORNL for DOE’s Office of Science, the single largest supporter of basic research in the physical sciences in the United States. DOE’s Office of Science is working to address some of the most pressing challenges of our time. For more information, visit https://energy.gov/science .
You Might Also Like
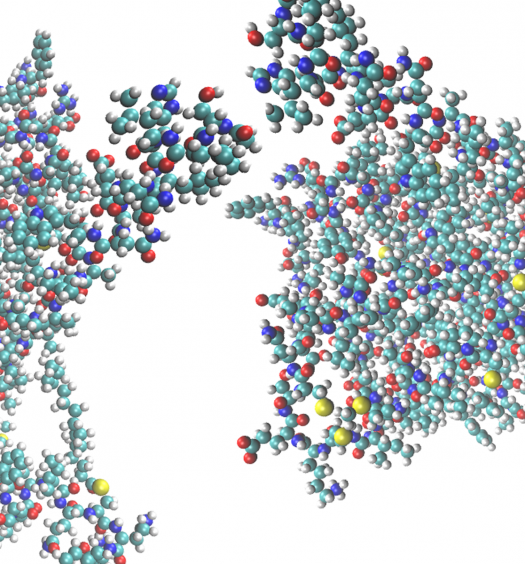
Machine Learning for Better Drug Design

Distilling How Water Turns into Ice

Meet the NCCS and OLCF Director: Arjun Shankar

How Hot Is Too Hot in Fusion?

Frontier Search for Lightweight, Flexible Alloys Wins Gordon Bell Prize

Breaking Benchmarks: Frontier Supercomputer Sets New Standard in Molecular Simulation

Planning for a Smooth Landing on Mars
May 14, 2024
May 13, 2024

Something in the Water Does Not Compute
May 6, 2024

ORNL and SLAC Team Up for Breakthrough Biology Projects

Summit Helps Forge Stronger Flights
April 30, 2024
Oak Ridge Leadership Computing Facility
- Application Software Development
- Data Management
- File and Storage Systems
- Non-Volatile Memory
- Operational Efficiency
- Outreach and Education
- Scientific Research
- System Architecture
Oak Ridge National Laboratory is managed by UT-Battelle for the US Department of Energy.
- DOE Office of Science
- Battelle.org
- Accessibility
Oak Ridge Leadership Computing Facility One Bethel Valley Rd P.O. Box 2008 Oak Ridge, TN 37831
Support Email: [email protected]
Quick Links
- Publications
Connect with OLCF

- Our History
- Director’s Letter
- OLCF Groups
- Industry Partnership Program
- OLCF User Group
- Visiting & Tours
- Compute Systems
- Data & Visualization Resources
- Computer Science
- Earth Science
- Engineering
- Materials Science
- Nuclear Energy
- Getting Started
- System User Guides
- OLCF Policy Guide
- Documents & Forms
- User Assistance
- OLCF in the News
- Center Reports
- Media Assets
Helpful Links
- Acknowledgement Statement
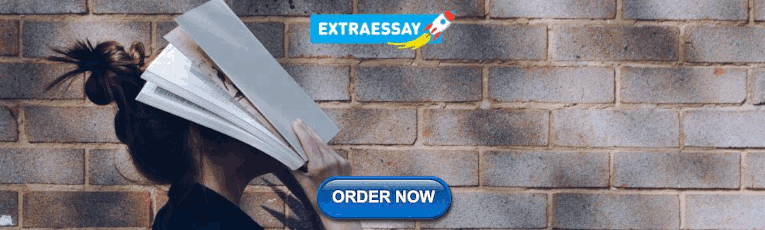
IMAGES
VIDEO
COMMENTS
Abstract. For a number of years, education authorities have responded to the importance of school students developing computer literacy by including it as part of the school curriculum, directly as a cross-curriculum capability, and by assessing the extent to which students are computer literate. Computer literacy and related concepts, such as ...
2019-2020. This study determined t he level of computer literacy and its influence on the academic performance of Grade 10 students in. Silanga National High School under the District of ...
This study aimed to investigate the factors that can affect digital literacy and whether academic achievement in an online course is affected by students' digital literacy. The research questions guiding this study addressed how digital literacy and its different aspects affect and are affected by academic achievement and students ...
Hence, countries around the world have promoted research on students' ICT skills and their inclusion in curricula. Despite these efforts, little is known about what predicts future ICT literacy, and prior research has almost exclusively conducted cross-sectional studies. ... Iea international computer and information literacy study 2018 ...
In J. Fraillon, J. Ainley, W. Schulz, D. Duckworth, & T. Friedman (Eds.), IEA International Computer and Information Literacy Study 2018 Assessment Framework (pp. 13-23). Springer International Publishing. Google Scholar. ... Taiwan. Her research interests center at computer and information literacy, computer programming self-efficacy ...
The present article summarizes past and current empirical studies regarding computer literacy that have implications for educators of students of any age, organized by the developmental domains of childhood, young and middle adulthood, and older adulthood. ... Paralleling the human development literature in general, the research regarding ...
izations of computer literacy that have emerged involve both the use of digital tools and the ideas of information literacy. Studies of computer literacy assume that there is an underlying construct that can be measured in different contexts. This assump-tion is consistent with the view that computer literacy is more than operating
Computer literacy is part of the perception of a new type of literacy that is caused by the use of computers and the application of technology in all aspects of society [1]. Technology changes by ...
The purpose of this study is to discover the main themes and categories of the research studies regarding digital literacy. To serve this purpose, the databases of WoS/Clarivate Analytics, Proquest Central, Emerald Management Journals, Jstor Business College Collections and Scopus/Elsevier were searched with four keyword-combinations and final forty-three articles were included in the dataset ...
The International Computer and Information Literacy Study (ICILS 2013) provides, for the first time, information about students' computer and information literacy (CIL), as well as its acquisition, based on a computer-based test for students and background questionnaires. Among the 21 education systems that participated in ICILS 2013, there are 12 European countries, making secondary ...
This study determined the level of computer literacy and its influence on the academic performance of junior high school students. Specifically, it probed into the students' attitude toward computers and sought answers to the following: the extent of students' computer literacy in terms of Word Processing, Spreadsheet, Presentation, and ...
Digital literacy is defined here as the "capabilities which fit an individual for living, learning and working in a digital society" and as the "integration of computer literacy, information literacy, media literacy, the ability to communicate and collaborate using digital networks, to participate in research and scholarship dependent on ...
Abstract This research examines the influence of digital literacy on preschool children's school readiness and mental health. The analysis dissects psychological resilience's role as a mediator among digitally literate-conscious kids. The underlying theory underpinning the literature is a social learning theory, which provides the paradigm lens for effectively accessing and evaluating ...
This study determined the level of computer literacy and its influence on the academic performance of junior high school students. Specifically, it probed into the
The IEA International Computer and Literacy Study (ICILS) is the only study dedicated to investigating the capabilities of students in secondary education to work with digital technologies, from a comparative international perspective. ... A systematic review of assessment instruments that aim to measure primary and secondary school students ...
Computer-based technology has infiltrated many aspects of life and industry, yet there is little understanding of how it can be used to promote student engagement, a concept receiving strong attention in higher education due to its association with a number of positive academic outcomes. The purpose of this article is to present a critical review of the literature from the past 5 years related ...
Even though there is an abundance of research on computer supported education (CSE), digital literacy (DL), technological literacy (TL), and internet literacy (IL), the correlation between them and their effect on each other have not been analyzed in the literature. ... Noh Y. A study on the effect of digital literacy on information use ...
Computer literacy has been a subject of educational research ever since personal computers were introduced to the classroom, either as teaching aids or as tools for self-study. In the 1980s, research on computer literacy focused on the question whether medical students were ready for the foreseeable omnipresence of computers in the future ...
1. The meaning of digital readiness. 2. The spectrum of digital readiness for e-learning. 3. Greater digital readiness translates to higher level of use of technology in learning. For many years concerns about "digital divides" centered primarily on whether people had access to digital technologies. Now, those worried about these issues ...
The purpose of this study is to discover the main themes and categories of the research studies regarding digital literacy. To serve this purpose, the databases of WoS/Clarivate Analytics, Proquest Central, Emerald Management Journals, Jstor Business College Collections and Scopus/Elsevier were searched with four keyword-combinations and final forty-three articles were included in the dataset.
This research model contributes to and extends the literature review on computers and academic achievement. This study relates academic achievement with loneliness, family and computer-related variables such as computer confidence, computer self-efficacy, computer attitudes, computer learning motivations and computer learning environments.
The study led by ORNL's Sajal Dash, Feiyi Wang and Prasanna Balaprakash employed Frontier, the world's first exascale supercomputer, to run the initial stages of training on a large language model similar to OpenAI's ChatGPT. The research team used a set of test data to project how models with 22 billion, 175 billion, and 1 trillion ...
Following those definitions of computer literacy mentioned by the researchers, computer literacy, in this study, refers to the level of ability and skills regarding the use of computers ...