Home Blog Design Understanding Data Presentations (Guide + Examples)
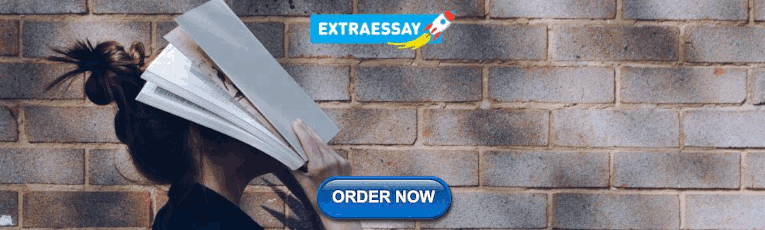
Understanding Data Presentations (Guide + Examples)
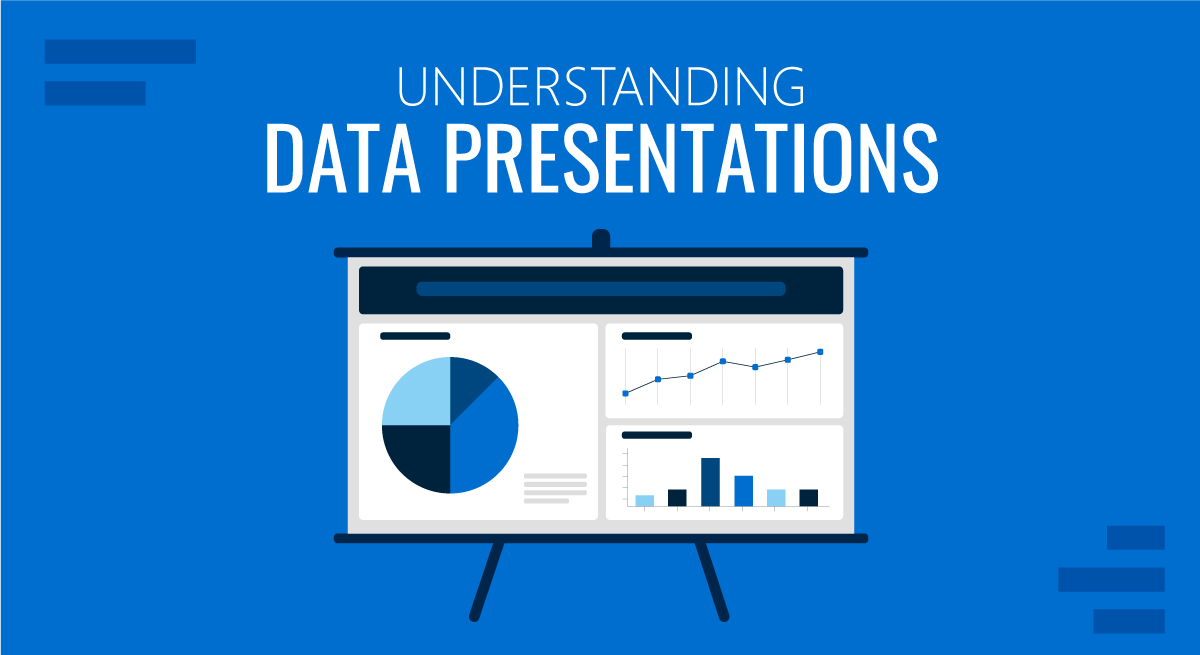
In this age of overwhelming information, the skill to effectively convey data has become extremely valuable. Initiating a discussion on data presentation types involves thoughtful consideration of the nature of your data and the message you aim to convey. Different types of visualizations serve distinct purposes. Whether you’re dealing with how to develop a report or simply trying to communicate complex information, how you present data influences how well your audience understands and engages with it. This extensive guide leads you through the different ways of data presentation.
Table of Contents
What is a Data Presentation?
What should a data presentation include, line graphs, treemap chart, scatter plot, how to choose a data presentation type, recommended data presentation templates, common mistakes done in data presentation.
A data presentation is a slide deck that aims to disclose quantitative information to an audience through the use of visual formats and narrative techniques derived from data analysis, making complex data understandable and actionable. This process requires a series of tools, such as charts, graphs, tables, infographics, dashboards, and so on, supported by concise textual explanations to improve understanding and boost retention rate.
Data presentations require us to cull data in a format that allows the presenter to highlight trends, patterns, and insights so that the audience can act upon the shared information. In a few words, the goal of data presentations is to enable viewers to grasp complicated concepts or trends quickly, facilitating informed decision-making or deeper analysis.
Data presentations go beyond the mere usage of graphical elements. Seasoned presenters encompass visuals with the art of data storytelling , so the speech skillfully connects the points through a narrative that resonates with the audience. Depending on the purpose – inspire, persuade, inform, support decision-making processes, etc. – is the data presentation format that is better suited to help us in this journey.
To nail your upcoming data presentation, ensure to count with the following elements:
- Clear Objectives: Understand the intent of your presentation before selecting the graphical layout and metaphors to make content easier to grasp.
- Engaging introduction: Use a powerful hook from the get-go. For instance, you can ask a big question or present a problem that your data will answer. Take a look at our guide on how to start a presentation for tips & insights.
- Structured Narrative: Your data presentation must tell a coherent story. This means a beginning where you present the context, a middle section in which you present the data, and an ending that uses a call-to-action. Check our guide on presentation structure for further information.
- Visual Elements: These are the charts, graphs, and other elements of visual communication we ought to use to present data. This article will cover one by one the different types of data representation methods we can use, and provide further guidance on choosing between them.
- Insights and Analysis: This is not just showcasing a graph and letting people get an idea about it. A proper data presentation includes the interpretation of that data, the reason why it’s included, and why it matters to your research.
- Conclusion & CTA: Ending your presentation with a call to action is necessary. Whether you intend to wow your audience into acquiring your services, inspire them to change the world, or whatever the purpose of your presentation, there must be a stage in which you convey all that you shared and show the path to staying in touch. Plan ahead whether you want to use a thank-you slide, a video presentation, or which method is apt and tailored to the kind of presentation you deliver.
- Q&A Session: After your speech is concluded, allocate 3-5 minutes for the audience to raise any questions about the information you disclosed. This is an extra chance to establish your authority on the topic. Check our guide on questions and answer sessions in presentations here.
Bar charts are a graphical representation of data using rectangular bars to show quantities or frequencies in an established category. They make it easy for readers to spot patterns or trends. Bar charts can be horizontal or vertical, although the vertical format is commonly known as a column chart. They display categorical, discrete, or continuous variables grouped in class intervals [1] . They include an axis and a set of labeled bars horizontally or vertically. These bars represent the frequencies of variable values or the values themselves. Numbers on the y-axis of a vertical bar chart or the x-axis of a horizontal bar chart are called the scale.
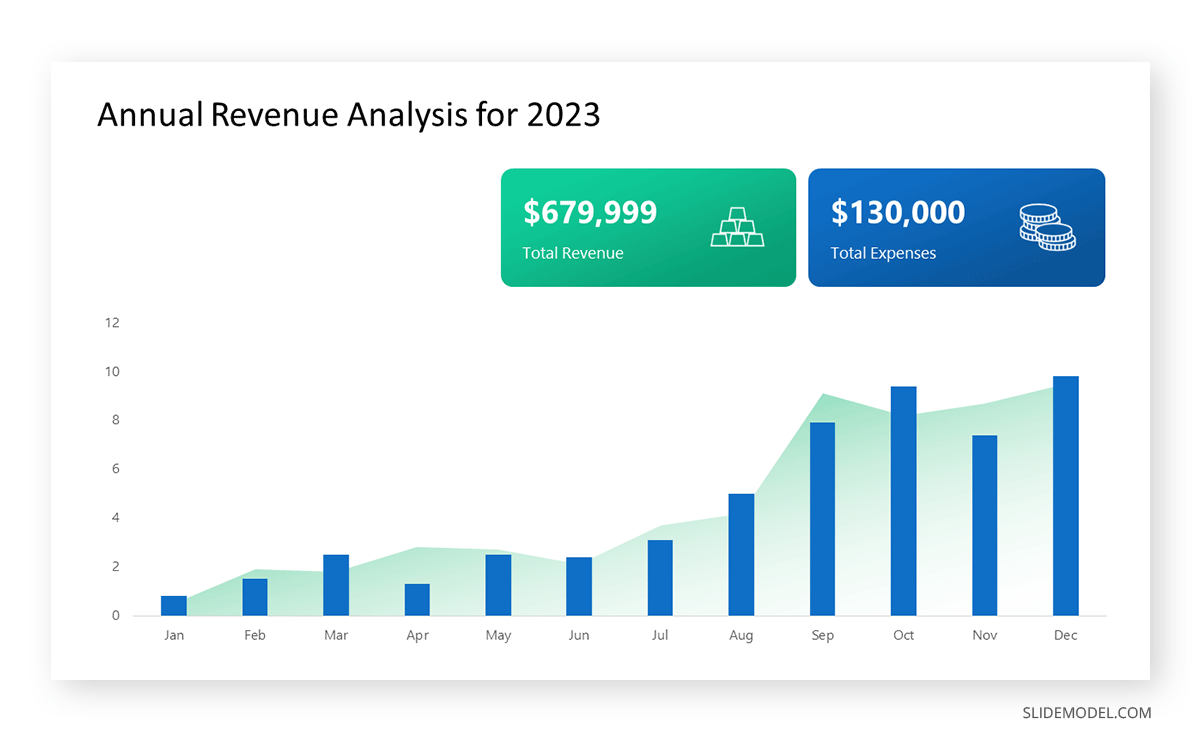
Real-Life Application of Bar Charts
Let’s say a sales manager is presenting sales to their audience. Using a bar chart, he follows these steps.
Step 1: Selecting Data
The first step is to identify the specific data you will present to your audience.
The sales manager has highlighted these products for the presentation.
- Product A: Men’s Shoes
- Product B: Women’s Apparel
- Product C: Electronics
- Product D: Home Decor
Step 2: Choosing Orientation
Opt for a vertical layout for simplicity. Vertical bar charts help compare different categories in case there are not too many categories [1] . They can also help show different trends. A vertical bar chart is used where each bar represents one of the four chosen products. After plotting the data, it is seen that the height of each bar directly represents the sales performance of the respective product.
It is visible that the tallest bar (Electronics – Product C) is showing the highest sales. However, the shorter bars (Women’s Apparel – Product B and Home Decor – Product D) need attention. It indicates areas that require further analysis or strategies for improvement.
Step 3: Colorful Insights
Different colors are used to differentiate each product. It is essential to show a color-coded chart where the audience can distinguish between products.
- Men’s Shoes (Product A): Yellow
- Women’s Apparel (Product B): Orange
- Electronics (Product C): Violet
- Home Decor (Product D): Blue
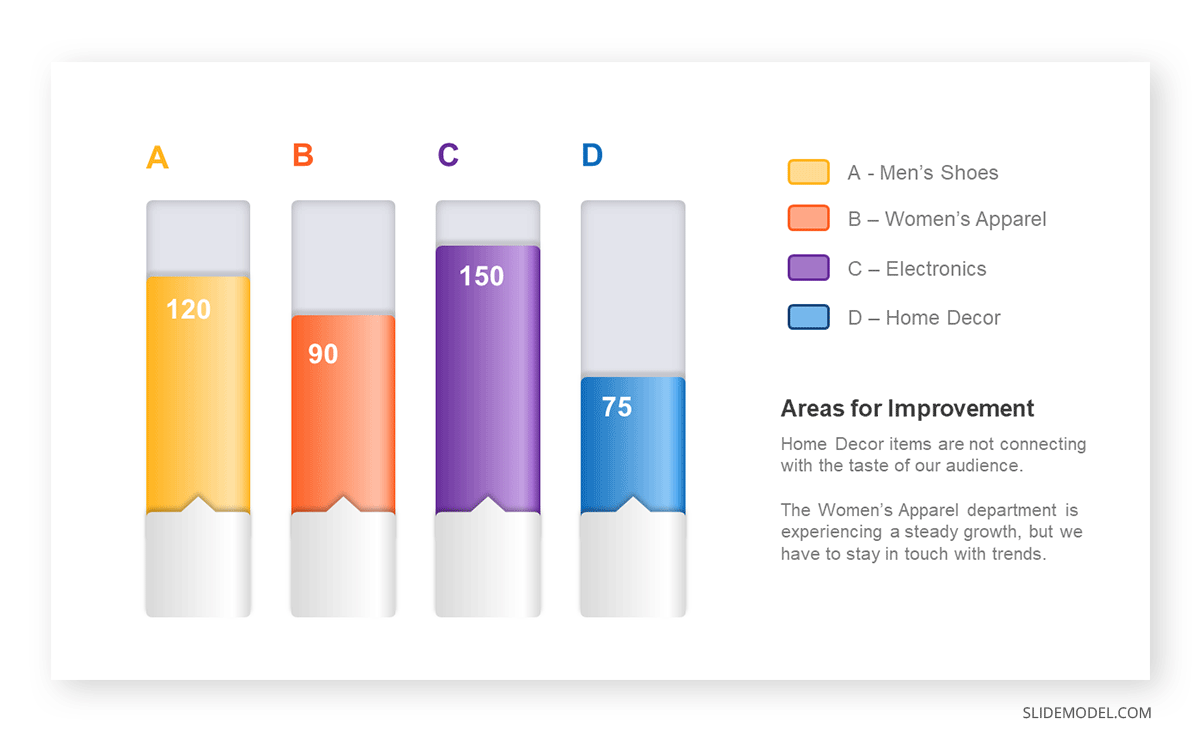
Bar charts are straightforward and easily understandable for presenting data. They are versatile when comparing products or any categorical data [2] . Bar charts adapt seamlessly to retail scenarios. Despite that, bar charts have a few shortcomings. They cannot illustrate data trends over time. Besides, overloading the chart with numerous products can lead to visual clutter, diminishing its effectiveness.
For more information, check our collection of bar chart templates for PowerPoint .
Line graphs help illustrate data trends, progressions, or fluctuations by connecting a series of data points called ‘markers’ with straight line segments. This provides a straightforward representation of how values change [5] . Their versatility makes them invaluable for scenarios requiring a visual understanding of continuous data. In addition, line graphs are also useful for comparing multiple datasets over the same timeline. Using multiple line graphs allows us to compare more than one data set. They simplify complex information so the audience can quickly grasp the ups and downs of values. From tracking stock prices to analyzing experimental results, you can use line graphs to show how data changes over a continuous timeline. They show trends with simplicity and clarity.
Real-life Application of Line Graphs
To understand line graphs thoroughly, we will use a real case. Imagine you’re a financial analyst presenting a tech company’s monthly sales for a licensed product over the past year. Investors want insights into sales behavior by month, how market trends may have influenced sales performance and reception to the new pricing strategy. To present data via a line graph, you will complete these steps.
First, you need to gather the data. In this case, your data will be the sales numbers. For example:
- January: $45,000
- February: $55,000
- March: $45,000
- April: $60,000
- May: $ 70,000
- June: $65,000
- July: $62,000
- August: $68,000
- September: $81,000
- October: $76,000
- November: $87,000
- December: $91,000
After choosing the data, the next step is to select the orientation. Like bar charts, you can use vertical or horizontal line graphs. However, we want to keep this simple, so we will keep the timeline (x-axis) horizontal while the sales numbers (y-axis) vertical.
Step 3: Connecting Trends
After adding the data to your preferred software, you will plot a line graph. In the graph, each month’s sales are represented by data points connected by a line.
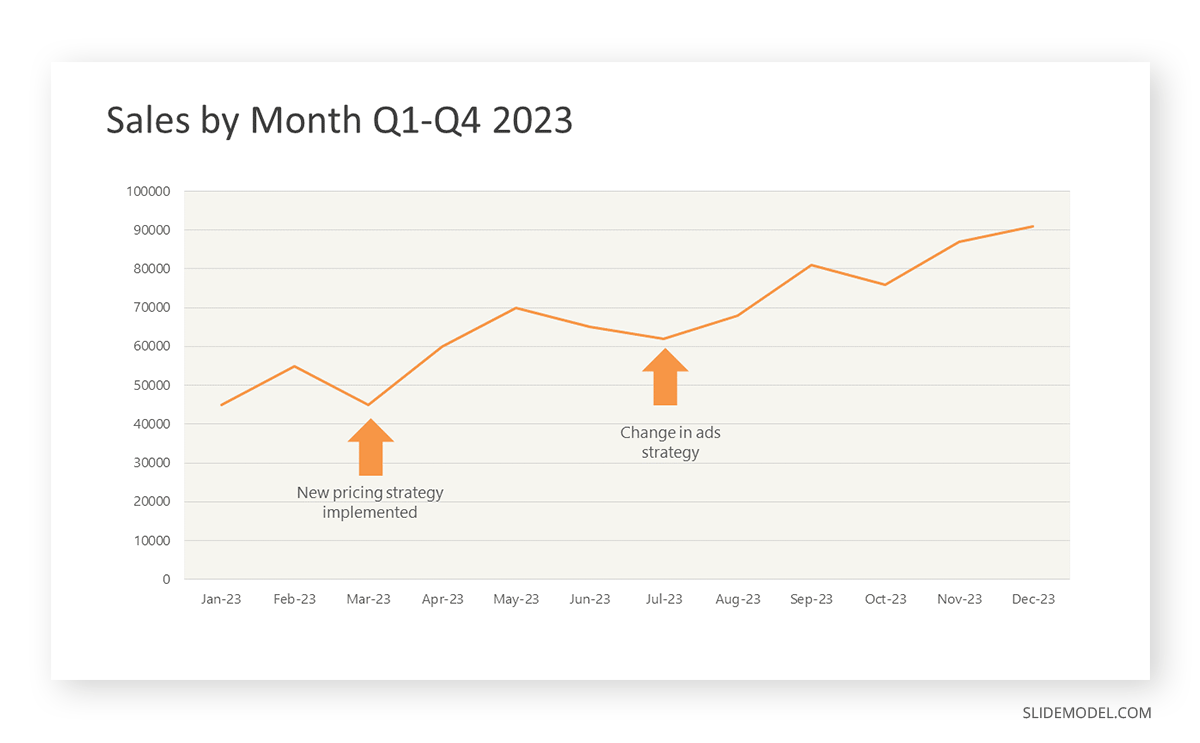
Step 4: Adding Clarity with Color
If there are multiple lines, you can also add colors to highlight each one, making it easier to follow.
Line graphs excel at visually presenting trends over time. These presentation aids identify patterns, like upward or downward trends. However, too many data points can clutter the graph, making it harder to interpret. Line graphs work best with continuous data but are not suitable for categories.
For more information, check our collection of line chart templates for PowerPoint and our article about how to make a presentation graph .
A data dashboard is a visual tool for analyzing information. Different graphs, charts, and tables are consolidated in a layout to showcase the information required to achieve one or more objectives. Dashboards help quickly see Key Performance Indicators (KPIs). You don’t make new visuals in the dashboard; instead, you use it to display visuals you’ve already made in worksheets [3] .
Keeping the number of visuals on a dashboard to three or four is recommended. Adding too many can make it hard to see the main points [4]. Dashboards can be used for business analytics to analyze sales, revenue, and marketing metrics at a time. They are also used in the manufacturing industry, as they allow users to grasp the entire production scenario at the moment while tracking the core KPIs for each line.
Real-Life Application of a Dashboard
Consider a project manager presenting a software development project’s progress to a tech company’s leadership team. He follows the following steps.
Step 1: Defining Key Metrics
To effectively communicate the project’s status, identify key metrics such as completion status, budget, and bug resolution rates. Then, choose measurable metrics aligned with project objectives.
Step 2: Choosing Visualization Widgets
After finalizing the data, presentation aids that align with each metric are selected. For this project, the project manager chooses a progress bar for the completion status and uses bar charts for budget allocation. Likewise, he implements line charts for bug resolution rates.
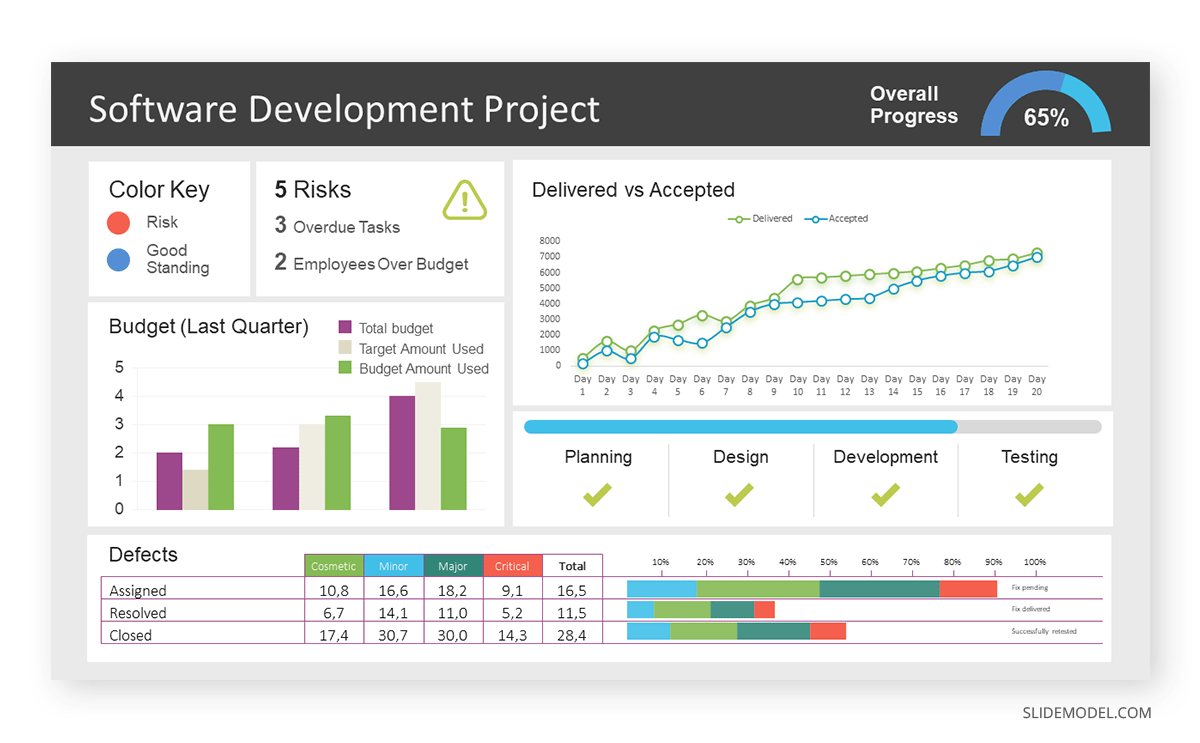
Step 3: Dashboard Layout
Key metrics are prominently placed in the dashboard for easy visibility, and the manager ensures that it appears clean and organized.
Dashboards provide a comprehensive view of key project metrics. Users can interact with data, customize views, and drill down for detailed analysis. However, creating an effective dashboard requires careful planning to avoid clutter. Besides, dashboards rely on the availability and accuracy of underlying data sources.
For more information, check our article on how to design a dashboard presentation , and discover our collection of dashboard PowerPoint templates .
Treemap charts represent hierarchical data structured in a series of nested rectangles [6] . As each branch of the ‘tree’ is given a rectangle, smaller tiles can be seen representing sub-branches, meaning elements on a lower hierarchical level than the parent rectangle. Each one of those rectangular nodes is built by representing an area proportional to the specified data dimension.
Treemaps are useful for visualizing large datasets in compact space. It is easy to identify patterns, such as which categories are dominant. Common applications of the treemap chart are seen in the IT industry, such as resource allocation, disk space management, website analytics, etc. Also, they can be used in multiple industries like healthcare data analysis, market share across different product categories, or even in finance to visualize portfolios.
Real-Life Application of a Treemap Chart
Let’s consider a financial scenario where a financial team wants to represent the budget allocation of a company. There is a hierarchy in the process, so it is helpful to use a treemap chart. In the chart, the top-level rectangle could represent the total budget, and it would be subdivided into smaller rectangles, each denoting a specific department. Further subdivisions within these smaller rectangles might represent individual projects or cost categories.
Step 1: Define Your Data Hierarchy
While presenting data on the budget allocation, start by outlining the hierarchical structure. The sequence will be like the overall budget at the top, followed by departments, projects within each department, and finally, individual cost categories for each project.
- Top-level rectangle: Total Budget
- Second-level rectangles: Departments (Engineering, Marketing, Sales)
- Third-level rectangles: Projects within each department
- Fourth-level rectangles: Cost categories for each project (Personnel, Marketing Expenses, Equipment)
Step 2: Choose a Suitable Tool
It’s time to select a data visualization tool supporting Treemaps. Popular choices include Tableau, Microsoft Power BI, PowerPoint, or even coding with libraries like D3.js. It is vital to ensure that the chosen tool provides customization options for colors, labels, and hierarchical structures.
Here, the team uses PowerPoint for this guide because of its user-friendly interface and robust Treemap capabilities.
Step 3: Make a Treemap Chart with PowerPoint
After opening the PowerPoint presentation, they chose “SmartArt” to form the chart. The SmartArt Graphic window has a “Hierarchy” category on the left. Here, you will see multiple options. You can choose any layout that resembles a Treemap. The “Table Hierarchy” or “Organization Chart” options can be adapted. The team selects the Table Hierarchy as it looks close to a Treemap.
Step 5: Input Your Data
After that, a new window will open with a basic structure. They add the data one by one by clicking on the text boxes. They start with the top-level rectangle, representing the total budget.
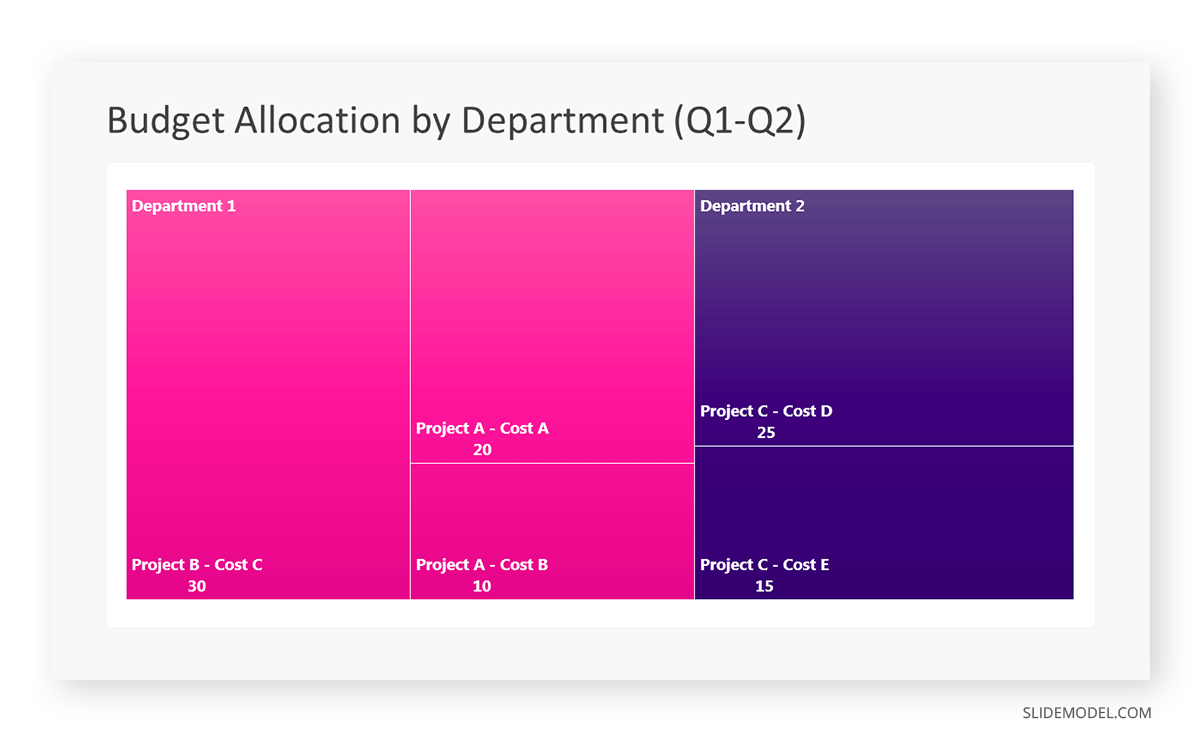
Step 6: Customize the Treemap
By clicking on each shape, they customize its color, size, and label. At the same time, they can adjust the font size, style, and color of labels by using the options in the “Format” tab in PowerPoint. Using different colors for each level enhances the visual difference.
Treemaps excel at illustrating hierarchical structures. These charts make it easy to understand relationships and dependencies. They efficiently use space, compactly displaying a large amount of data, reducing the need for excessive scrolling or navigation. Additionally, using colors enhances the understanding of data by representing different variables or categories.
In some cases, treemaps might become complex, especially with deep hierarchies. It becomes challenging for some users to interpret the chart. At the same time, displaying detailed information within each rectangle might be constrained by space. It potentially limits the amount of data that can be shown clearly. Without proper labeling and color coding, there’s a risk of misinterpretation.
A heatmap is a data visualization tool that uses color coding to represent values across a two-dimensional surface. In these, colors replace numbers to indicate the magnitude of each cell. This color-shaded matrix display is valuable for summarizing and understanding data sets with a glance [7] . The intensity of the color corresponds to the value it represents, making it easy to identify patterns, trends, and variations in the data.
As a tool, heatmaps help businesses analyze website interactions, revealing user behavior patterns and preferences to enhance overall user experience. In addition, companies use heatmaps to assess content engagement, identifying popular sections and areas of improvement for more effective communication. They excel at highlighting patterns and trends in large datasets, making it easy to identify areas of interest.
We can implement heatmaps to express multiple data types, such as numerical values, percentages, or even categorical data. Heatmaps help us easily spot areas with lots of activity, making them helpful in figuring out clusters [8] . When making these maps, it is important to pick colors carefully. The colors need to show the differences between groups or levels of something. And it is good to use colors that people with colorblindness can easily see.
Check our detailed guide on how to create a heatmap here. Also discover our collection of heatmap PowerPoint templates .
Pie charts are circular statistical graphics divided into slices to illustrate numerical proportions. Each slice represents a proportionate part of the whole, making it easy to visualize the contribution of each component to the total.
The size of the pie charts is influenced by the value of data points within each pie. The total of all data points in a pie determines its size. The pie with the highest data points appears as the largest, whereas the others are proportionally smaller. However, you can present all pies of the same size if proportional representation is not required [9] . Sometimes, pie charts are difficult to read, or additional information is required. A variation of this tool can be used instead, known as the donut chart , which has the same structure but a blank center, creating a ring shape. Presenters can add extra information, and the ring shape helps to declutter the graph.
Pie charts are used in business to show percentage distribution, compare relative sizes of categories, or present straightforward data sets where visualizing ratios is essential.
Real-Life Application of Pie Charts
Consider a scenario where you want to represent the distribution of the data. Each slice of the pie chart would represent a different category, and the size of each slice would indicate the percentage of the total portion allocated to that category.
Step 1: Define Your Data Structure
Imagine you are presenting the distribution of a project budget among different expense categories.
- Column A: Expense Categories (Personnel, Equipment, Marketing, Miscellaneous)
- Column B: Budget Amounts ($40,000, $30,000, $20,000, $10,000) Column B represents the values of your categories in Column A.
Step 2: Insert a Pie Chart
Using any of the accessible tools, you can create a pie chart. The most convenient tools for forming a pie chart in a presentation are presentation tools such as PowerPoint or Google Slides. You will notice that the pie chart assigns each expense category a percentage of the total budget by dividing it by the total budget.
For instance:
- Personnel: $40,000 / ($40,000 + $30,000 + $20,000 + $10,000) = 40%
- Equipment: $30,000 / ($40,000 + $30,000 + $20,000 + $10,000) = 30%
- Marketing: $20,000 / ($40,000 + $30,000 + $20,000 + $10,000) = 20%
- Miscellaneous: $10,000 / ($40,000 + $30,000 + $20,000 + $10,000) = 10%
You can make a chart out of this or just pull out the pie chart from the data.
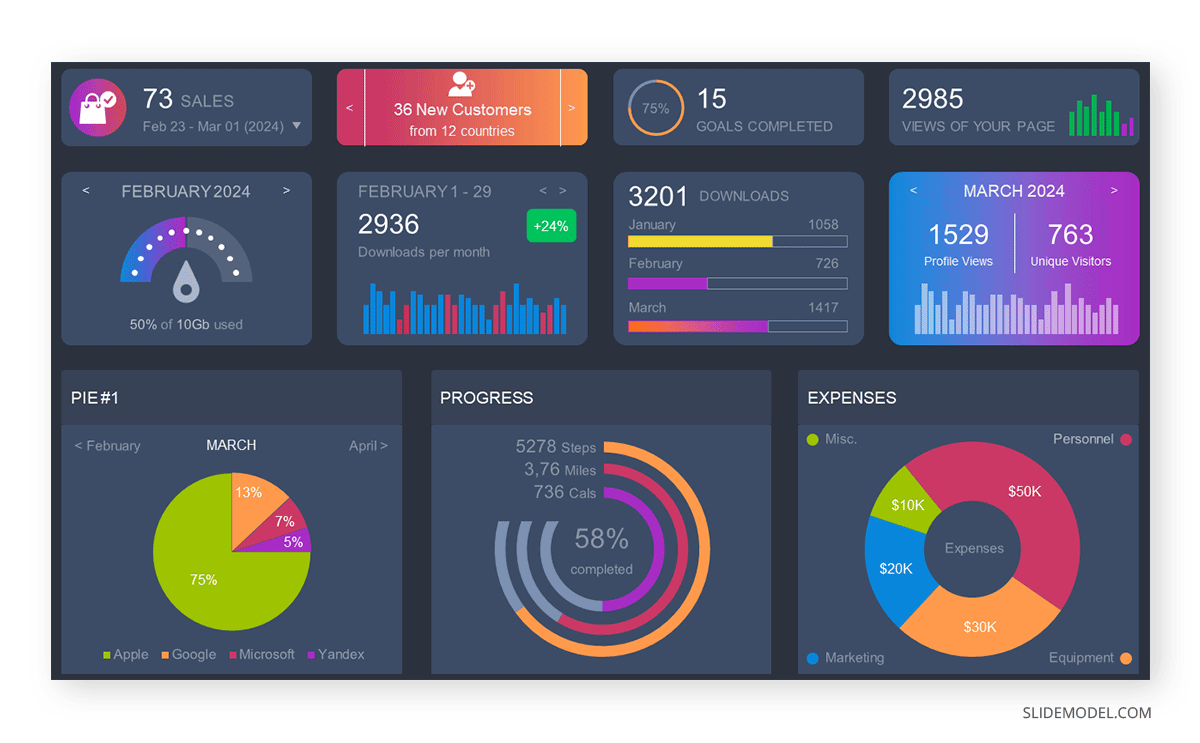
3D pie charts and 3D donut charts are quite popular among the audience. They stand out as visual elements in any presentation slide, so let’s take a look at how our pie chart example would look in 3D pie chart format.
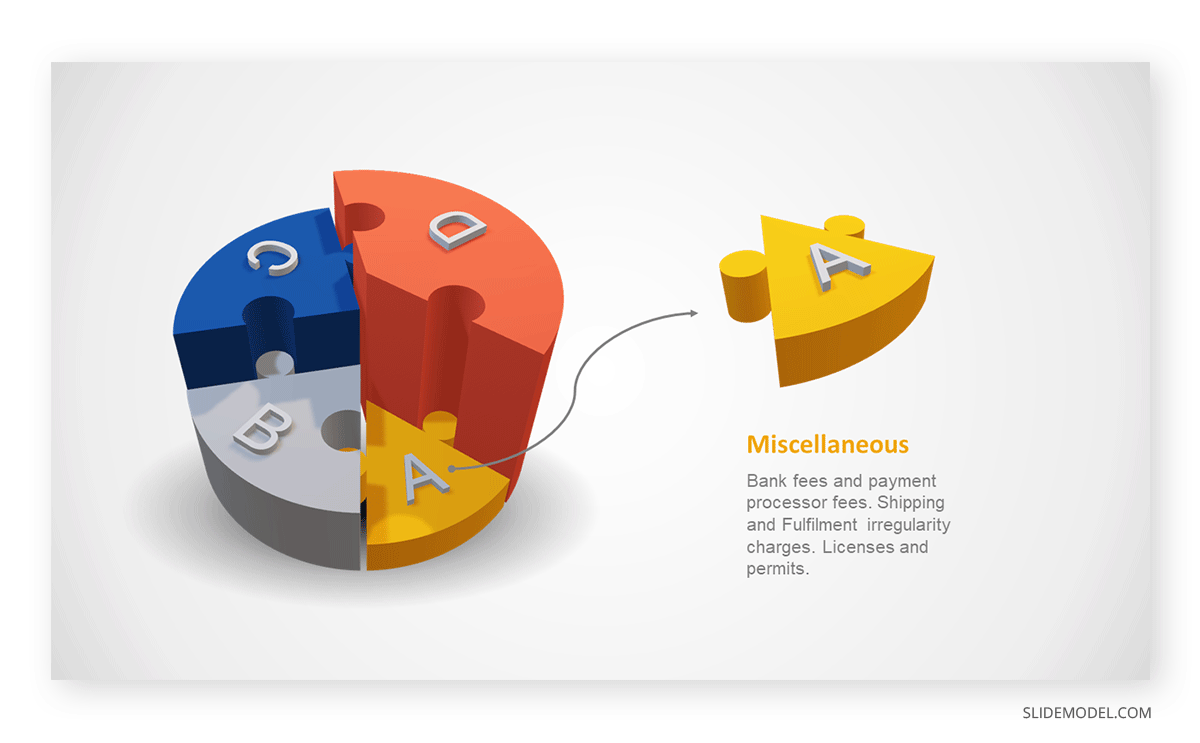
Step 03: Results Interpretation
The pie chart visually illustrates the distribution of the project budget among different expense categories. Personnel constitutes the largest portion at 40%, followed by equipment at 30%, marketing at 20%, and miscellaneous at 10%. This breakdown provides a clear overview of where the project funds are allocated, which helps in informed decision-making and resource management. It is evident that personnel are a significant investment, emphasizing their importance in the overall project budget.
Pie charts provide a straightforward way to represent proportions and percentages. They are easy to understand, even for individuals with limited data analysis experience. These charts work well for small datasets with a limited number of categories.
However, a pie chart can become cluttered and less effective in situations with many categories. Accurate interpretation may be challenging, especially when dealing with slight differences in slice sizes. In addition, these charts are static and do not effectively convey trends over time.
For more information, check our collection of pie chart templates for PowerPoint .
Histograms present the distribution of numerical variables. Unlike a bar chart that records each unique response separately, histograms organize numeric responses into bins and show the frequency of reactions within each bin [10] . The x-axis of a histogram shows the range of values for a numeric variable. At the same time, the y-axis indicates the relative frequencies (percentage of the total counts) for that range of values.
Whenever you want to understand the distribution of your data, check which values are more common, or identify outliers, histograms are your go-to. Think of them as a spotlight on the story your data is telling. A histogram can provide a quick and insightful overview if you’re curious about exam scores, sales figures, or any numerical data distribution.
Real-Life Application of a Histogram
In the histogram data analysis presentation example, imagine an instructor analyzing a class’s grades to identify the most common score range. A histogram could effectively display the distribution. It will show whether most students scored in the average range or if there are significant outliers.
Step 1: Gather Data
He begins by gathering the data. The scores of each student in class are gathered to analyze exam scores.
Names | Score |
---|---|
Alice | 78 |
Bob | 85 |
Clara | 92 |
David | 65 |
Emma | 72 |
Frank | 88 |
Grace | 76 |
Henry | 95 |
Isabel | 81 |
Jack | 70 |
Kate | 60 |
Liam | 89 |
Mia | 75 |
Noah | 84 |
Olivia | 92 |
After arranging the scores in ascending order, bin ranges are set.
Step 2: Define Bins
Bins are like categories that group similar values. Think of them as buckets that organize your data. The presenter decides how wide each bin should be based on the range of the values. For instance, the instructor sets the bin ranges based on score intervals: 60-69, 70-79, 80-89, and 90-100.
Step 3: Count Frequency
Now, he counts how many data points fall into each bin. This step is crucial because it tells you how often specific ranges of values occur. The result is the frequency distribution, showing the occurrences of each group.
Here, the instructor counts the number of students in each category.
- 60-69: 1 student (Kate)
- 70-79: 4 students (David, Emma, Grace, Jack)
- 80-89: 7 students (Alice, Bob, Frank, Isabel, Liam, Mia, Noah)
- 90-100: 3 students (Clara, Henry, Olivia)
Step 4: Create the Histogram
It’s time to turn the data into a visual representation. Draw a bar for each bin on a graph. The width of the bar should correspond to the range of the bin, and the height should correspond to the frequency. To make your histogram understandable, label the X and Y axes.
In this case, the X-axis should represent the bins (e.g., test score ranges), and the Y-axis represents the frequency.
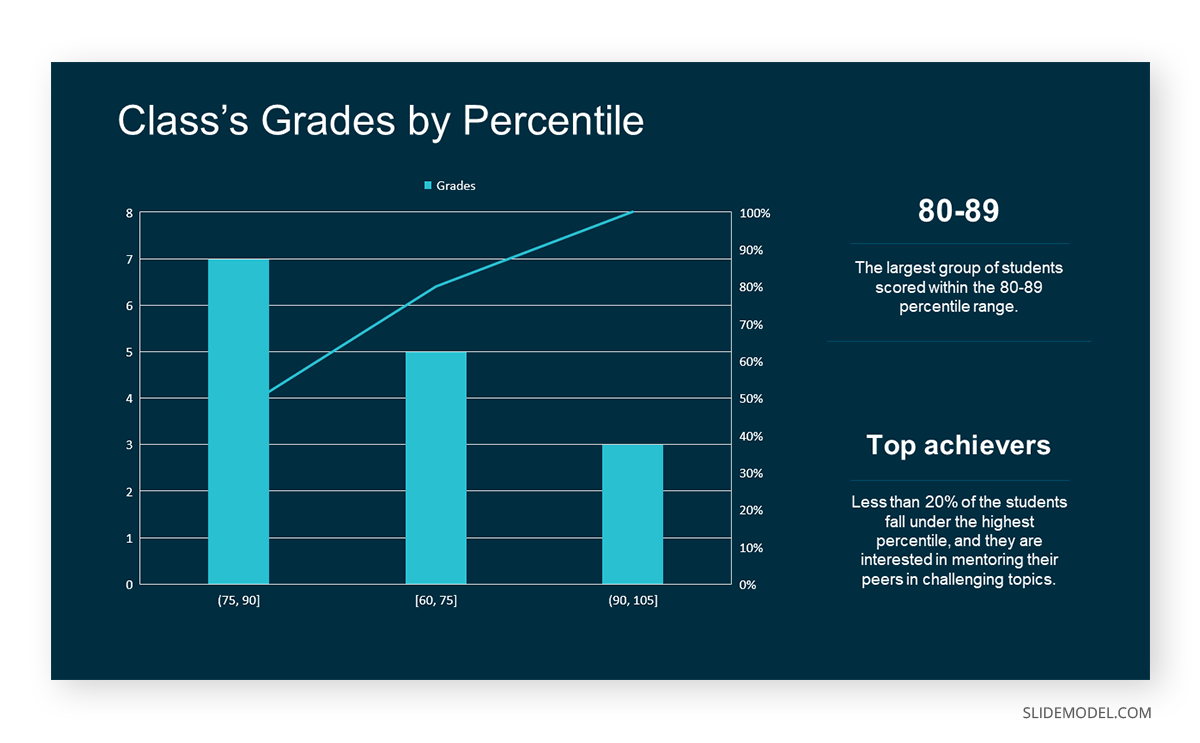
The histogram of the class grades reveals insightful patterns in the distribution. Most students, with seven students, fall within the 80-89 score range. The histogram provides a clear visualization of the class’s performance. It showcases a concentration of grades in the upper-middle range with few outliers at both ends. This analysis helps in understanding the overall academic standing of the class. It also identifies the areas for potential improvement or recognition.
Thus, histograms provide a clear visual representation of data distribution. They are easy to interpret, even for those without a statistical background. They apply to various types of data, including continuous and discrete variables. One weak point is that histograms do not capture detailed patterns in students’ data, with seven compared to other visualization methods.
A scatter plot is a graphical representation of the relationship between two variables. It consists of individual data points on a two-dimensional plane. This plane plots one variable on the x-axis and the other on the y-axis. Each point represents a unique observation. It visualizes patterns, trends, or correlations between the two variables.
Scatter plots are also effective in revealing the strength and direction of relationships. They identify outliers and assess the overall distribution of data points. The points’ dispersion and clustering reflect the relationship’s nature, whether it is positive, negative, or lacks a discernible pattern. In business, scatter plots assess relationships between variables such as marketing cost and sales revenue. They help present data correlations and decision-making.
Real-Life Application of Scatter Plot
A group of scientists is conducting a study on the relationship between daily hours of screen time and sleep quality. After reviewing the data, they managed to create this table to help them build a scatter plot graph:
Participant ID | Daily Hours of Screen Time | Sleep Quality Rating |
---|---|---|
1 | 9 | 3 |
2 | 2 | 8 |
3 | 1 | 9 |
4 | 0 | 10 |
5 | 1 | 9 |
6 | 3 | 7 |
7 | 4 | 7 |
8 | 5 | 6 |
9 | 5 | 6 |
10 | 7 | 3 |
11 | 10 | 1 |
12 | 6 | 5 |
13 | 7 | 3 |
14 | 8 | 2 |
15 | 9 | 2 |
16 | 4 | 7 |
17 | 5 | 6 |
18 | 4 | 7 |
19 | 9 | 2 |
20 | 6 | 4 |
21 | 3 | 7 |
22 | 10 | 1 |
23 | 2 | 8 |
24 | 5 | 6 |
25 | 3 | 7 |
26 | 1 | 9 |
27 | 8 | 2 |
28 | 4 | 6 |
29 | 7 | 3 |
30 | 2 | 8 |
31 | 7 | 4 |
32 | 9 | 2 |
33 | 10 | 1 |
34 | 10 | 1 |
35 | 10 | 1 |
In the provided example, the x-axis represents Daily Hours of Screen Time, and the y-axis represents the Sleep Quality Rating.
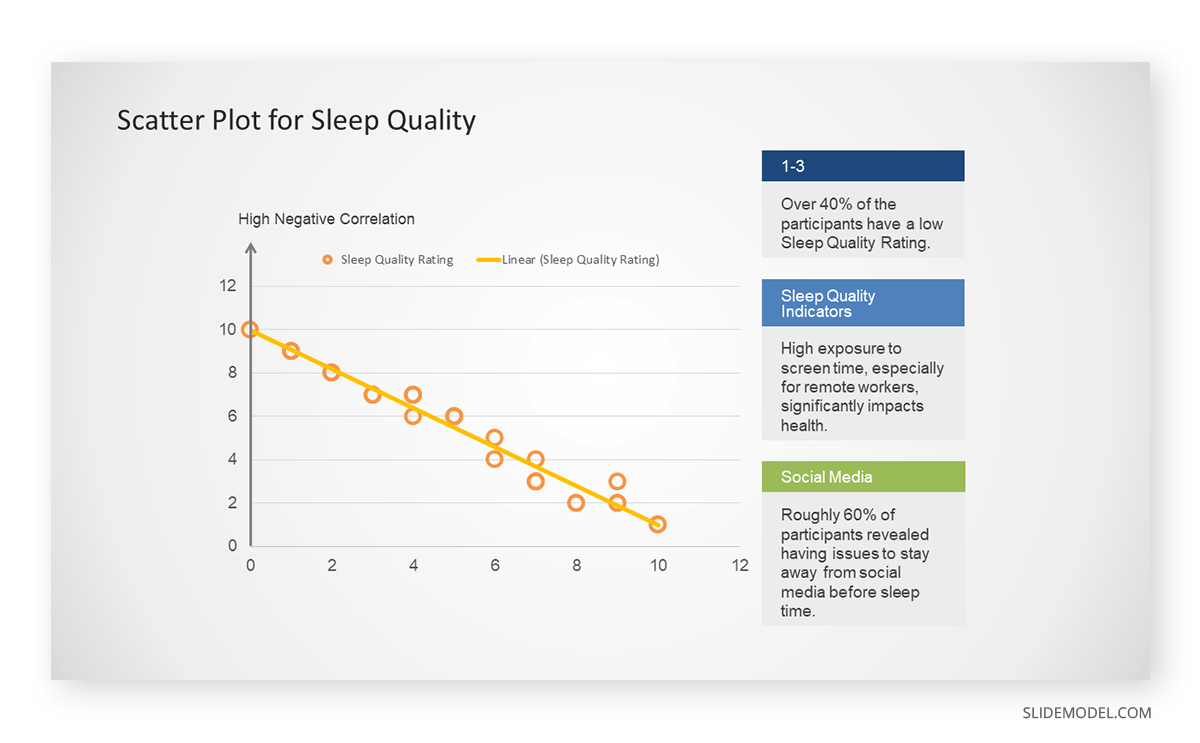
The scientists observe a negative correlation between the amount of screen time and the quality of sleep. This is consistent with their hypothesis that blue light, especially before bedtime, has a significant impact on sleep quality and metabolic processes.
There are a few things to remember when using a scatter plot. Even when a scatter diagram indicates a relationship, it doesn’t mean one variable affects the other. A third factor can influence both variables. The more the plot resembles a straight line, the stronger the relationship is perceived [11] . If it suggests no ties, the observed pattern might be due to random fluctuations in data. When the scatter diagram depicts no correlation, whether the data might be stratified is worth considering.
Choosing the appropriate data presentation type is crucial when making a presentation . Understanding the nature of your data and the message you intend to convey will guide this selection process. For instance, when showcasing quantitative relationships, scatter plots become instrumental in revealing correlations between variables. If the focus is on emphasizing parts of a whole, pie charts offer a concise display of proportions. Histograms, on the other hand, prove valuable for illustrating distributions and frequency patterns.
Bar charts provide a clear visual comparison of different categories. Likewise, line charts excel in showcasing trends over time, while tables are ideal for detailed data examination. Starting a presentation on data presentation types involves evaluating the specific information you want to communicate and selecting the format that aligns with your message. This ensures clarity and resonance with your audience from the beginning of your presentation.
1. Fact Sheet Dashboard for Data Presentation
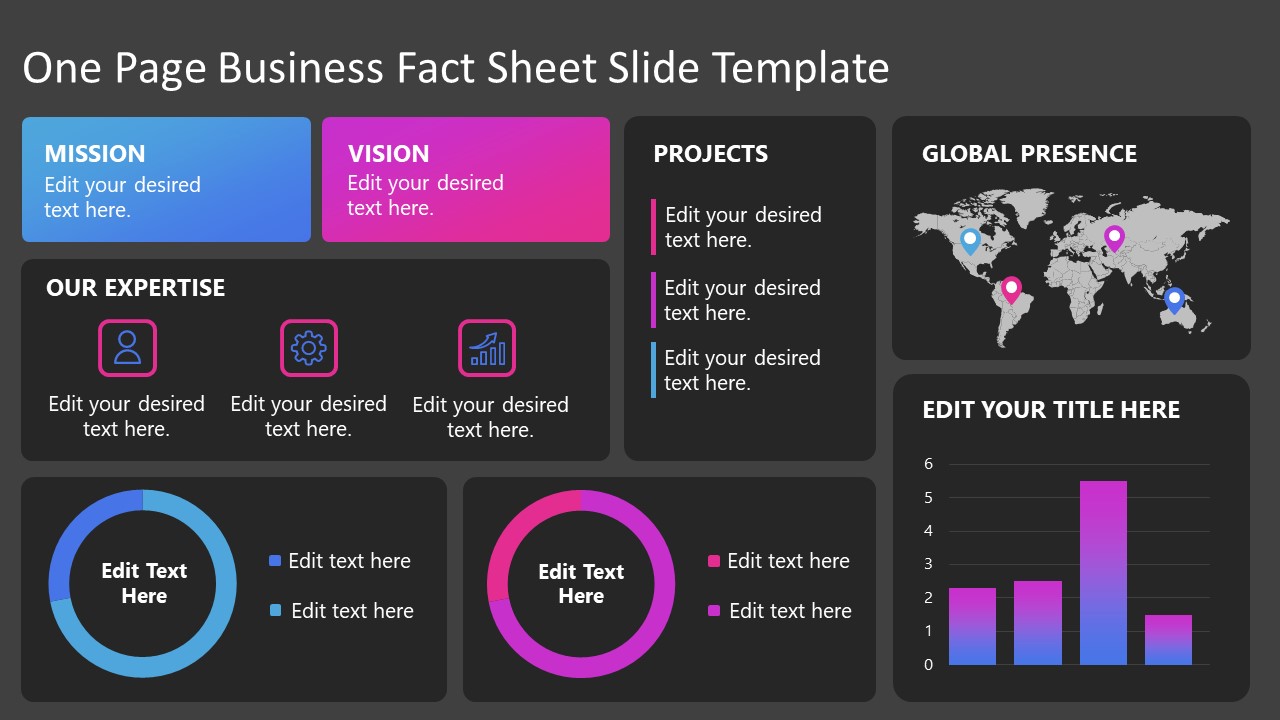
Convey all the data you need to present in this one-pager format, an ideal solution tailored for users looking for presentation aids. Global maps, donut chats, column graphs, and text neatly arranged in a clean layout presented in light and dark themes.
Use This Template
2. 3D Column Chart Infographic PPT Template
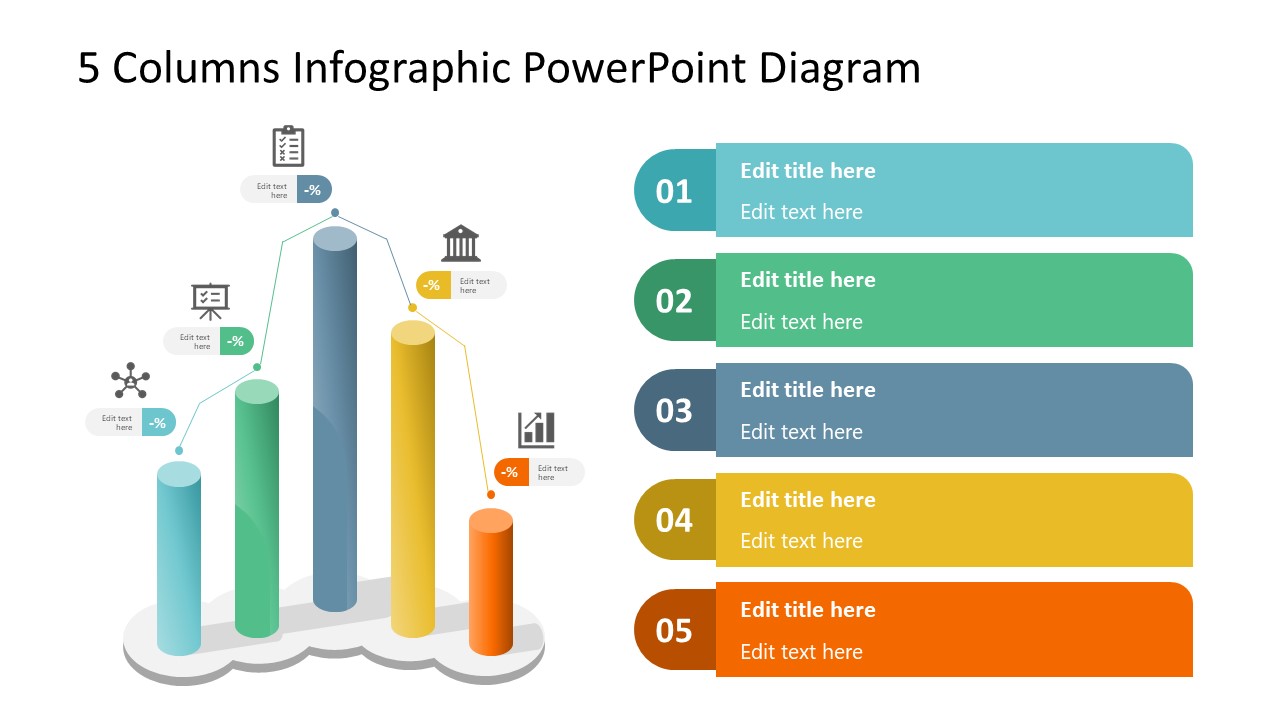
Represent column charts in a highly visual 3D format with this PPT template. A creative way to present data, this template is entirely editable, and we can craft either a one-page infographic or a series of slides explaining what we intend to disclose point by point.
3. Data Circles Infographic PowerPoint Template
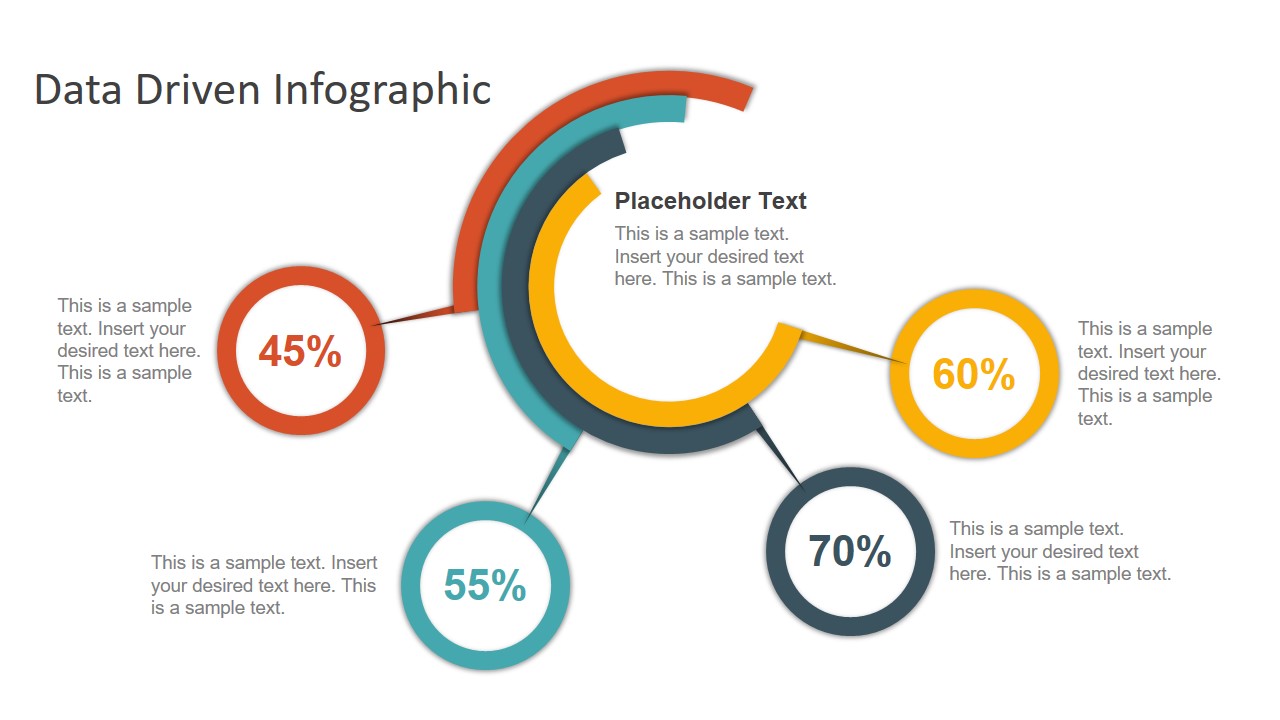
An alternative to the pie chart and donut chart diagrams, this template features a series of curved shapes with bubble callouts as ways of presenting data. Expand the information for each arch in the text placeholder areas.
4. Colorful Metrics Dashboard for Data Presentation
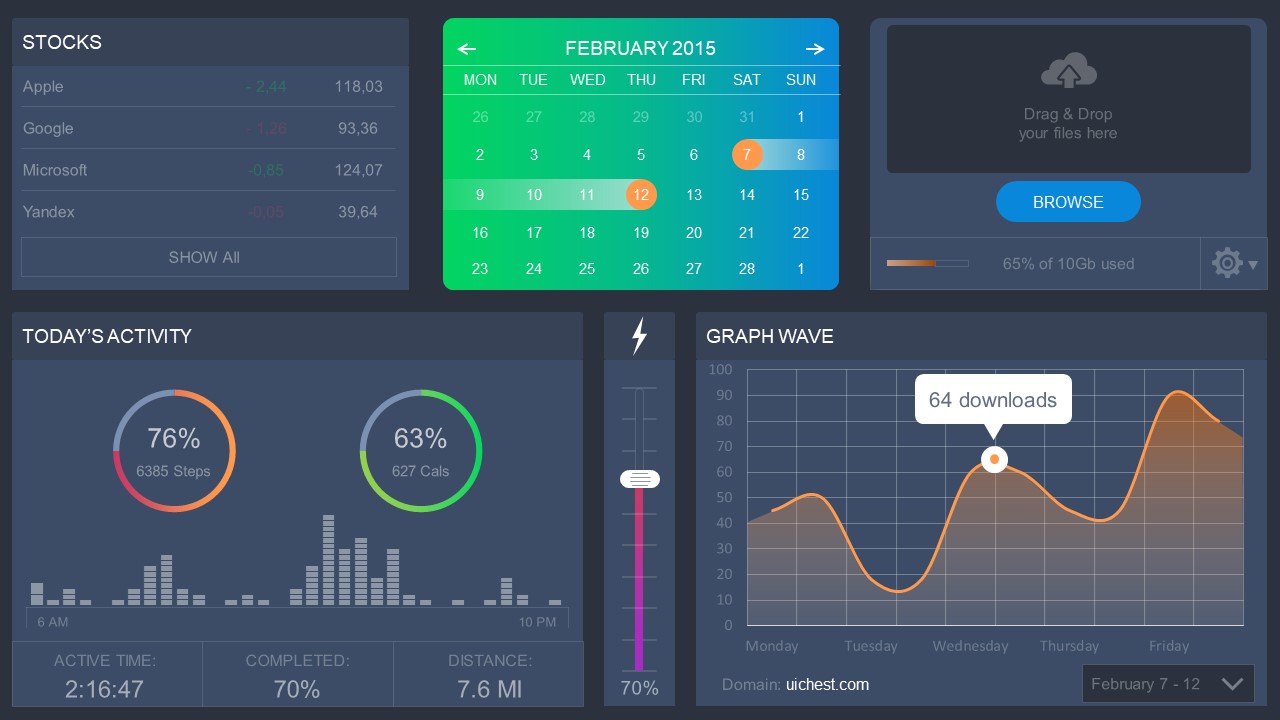
This versatile dashboard template helps us in the presentation of the data by offering several graphs and methods to convert numbers into graphics. Implement it for e-commerce projects, financial projections, project development, and more.
5. Animated Data Presentation Tools for PowerPoint & Google Slides
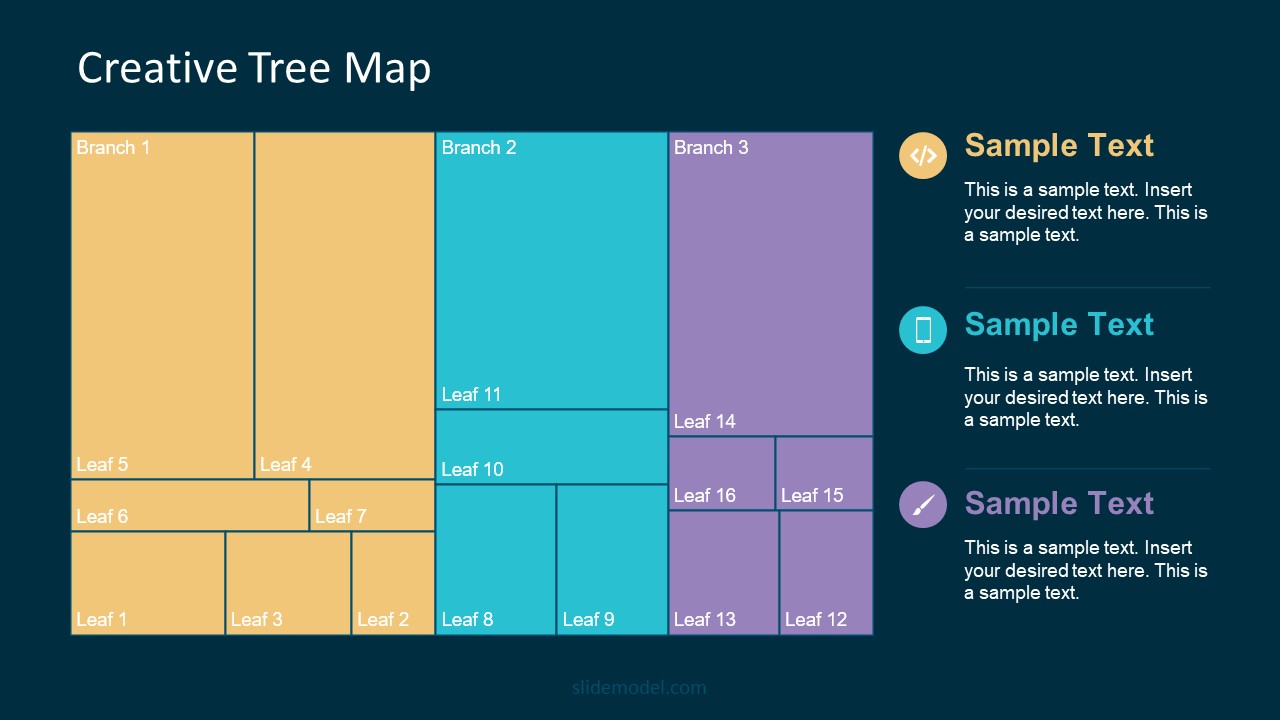
A slide deck filled with most of the tools mentioned in this article, from bar charts, column charts, treemap graphs, pie charts, histogram, etc. Animated effects make each slide look dynamic when sharing data with stakeholders.
6. Statistics Waffle Charts PPT Template for Data Presentations
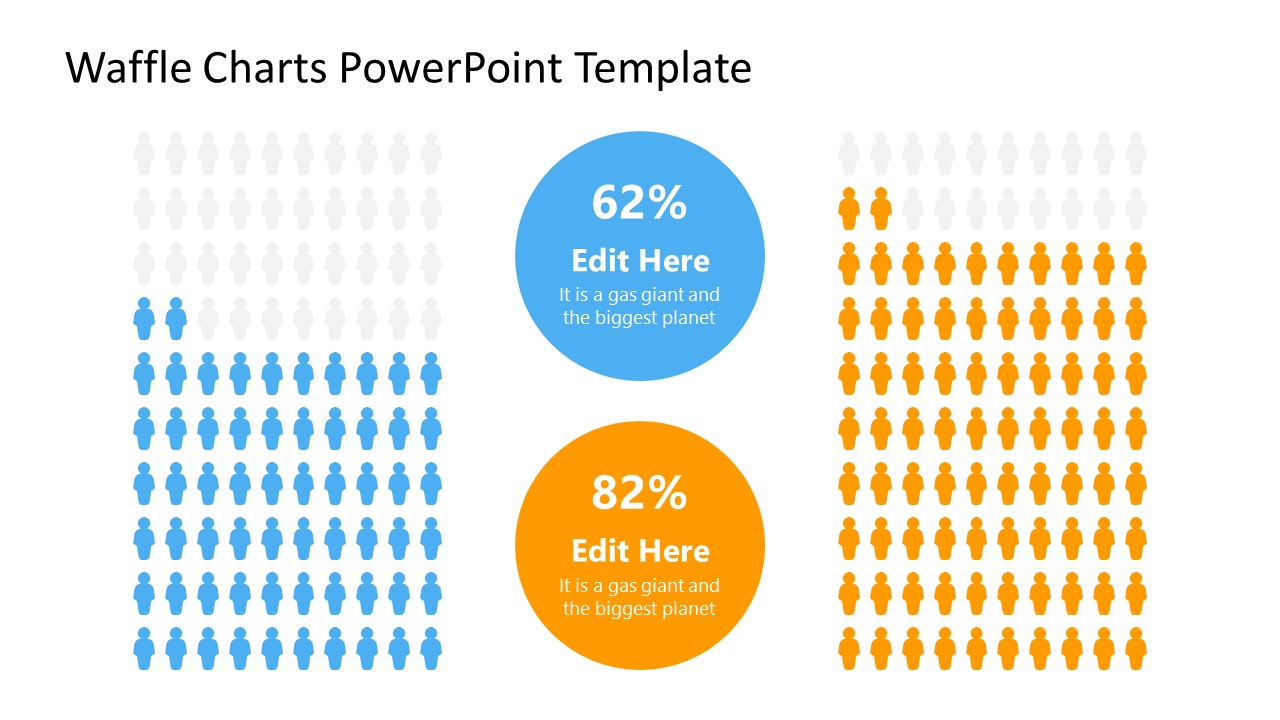
This PPT template helps us how to present data beyond the typical pie chart representation. It is widely used for demographics, so it’s a great fit for marketing teams, data science professionals, HR personnel, and more.
7. Data Presentation Dashboard Template for Google Slides
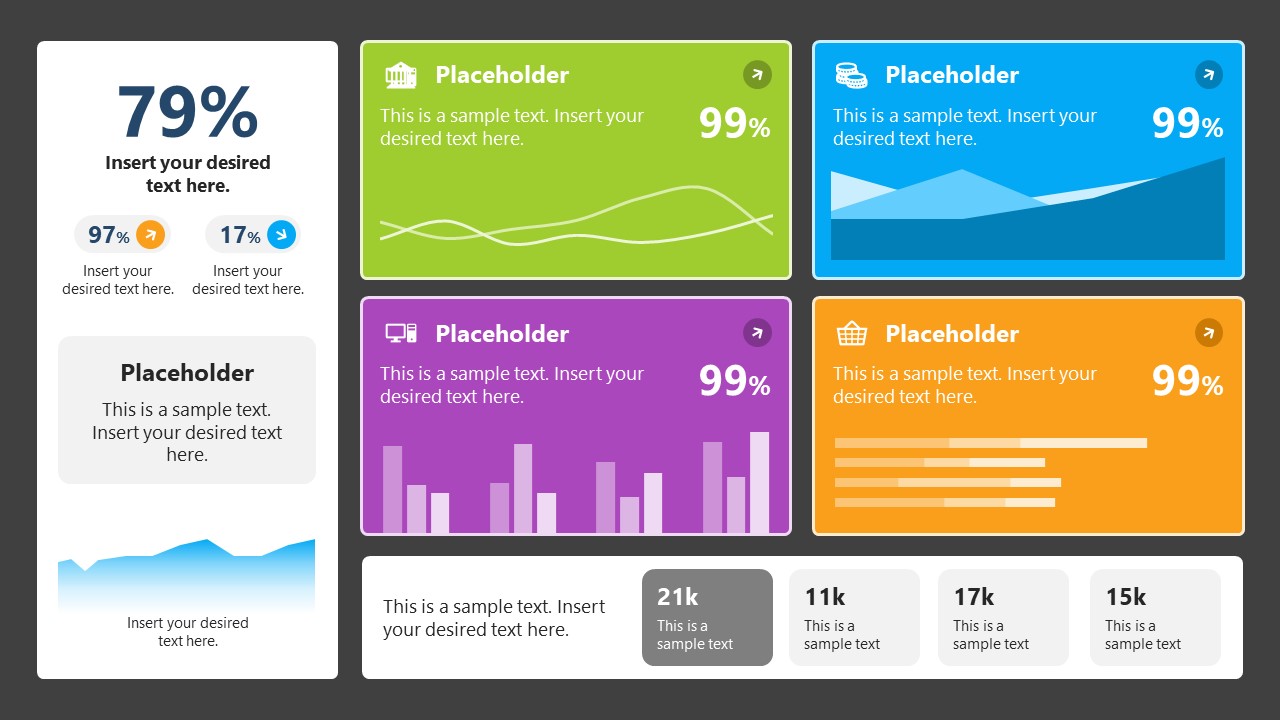
A compendium of tools in dashboard format featuring line graphs, bar charts, column charts, and neatly arranged placeholder text areas.
8. Weather Dashboard for Data Presentation
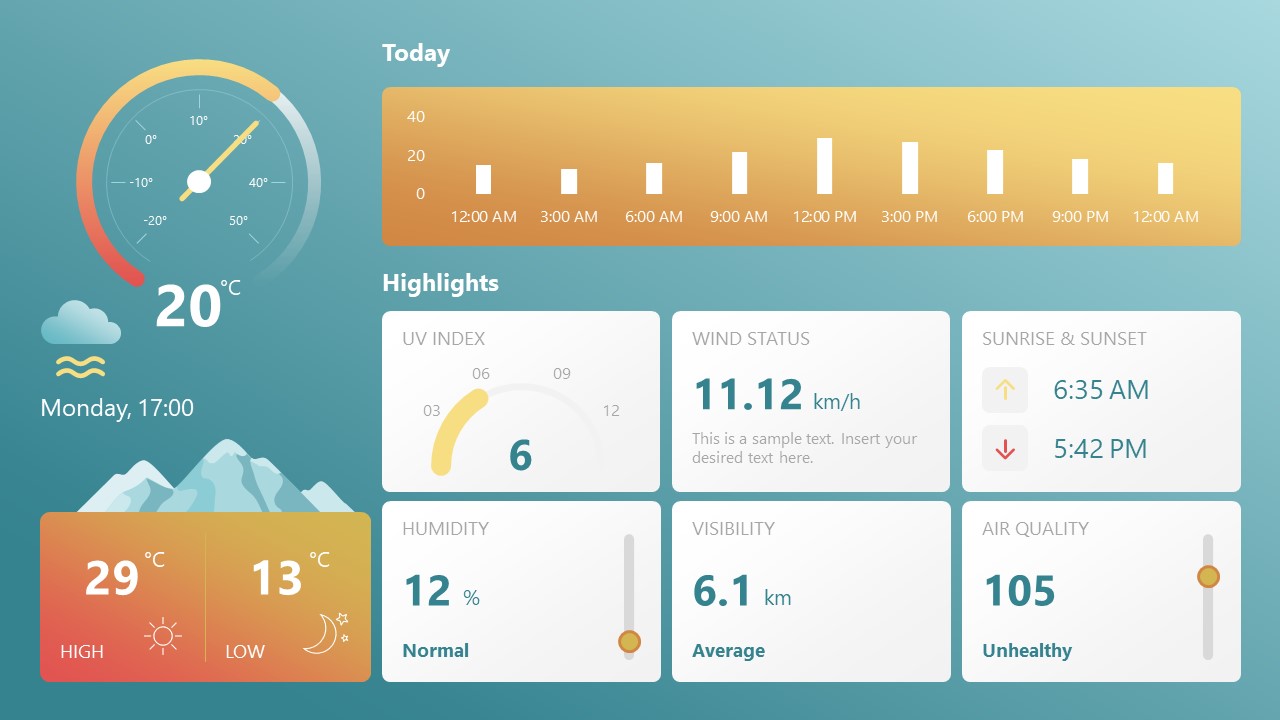
Share weather data for agricultural presentation topics, environmental studies, or any kind of presentation that requires a highly visual layout for weather forecasting on a single day. Two color themes are available.
9. Social Media Marketing Dashboard Data Presentation Template
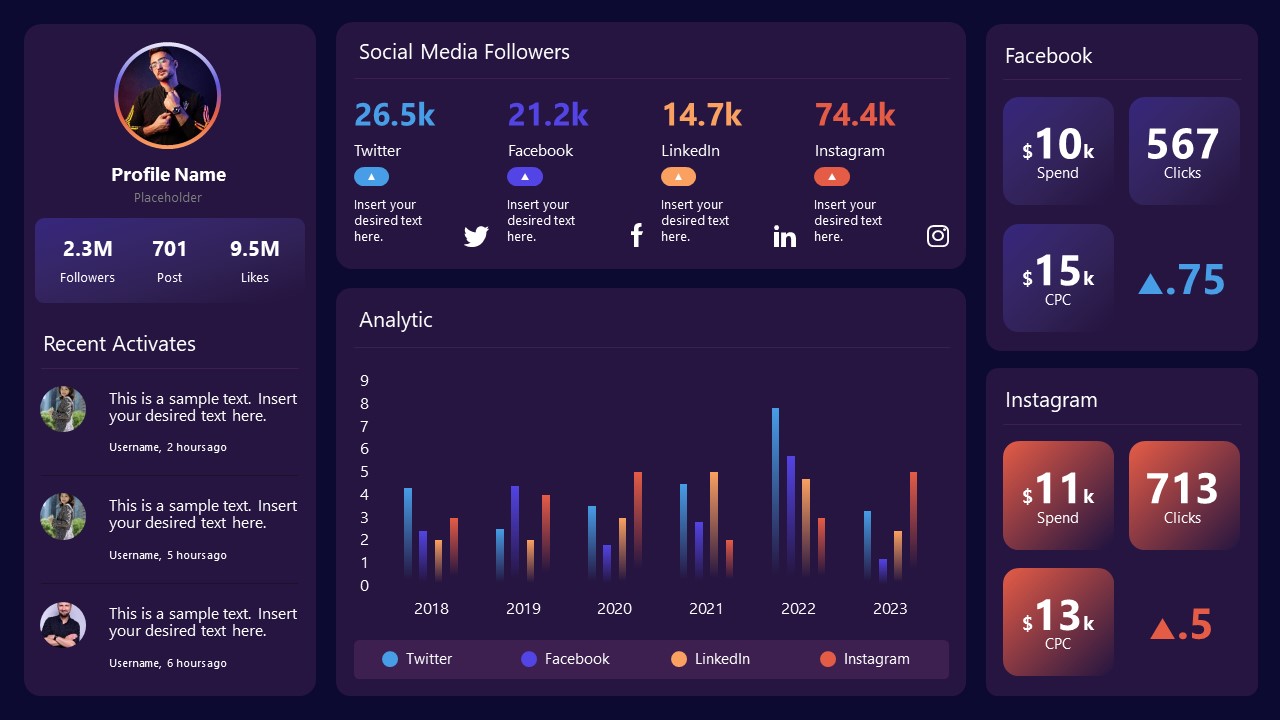
Intended for marketing professionals, this dashboard template for data presentation is a tool for presenting data analytics from social media channels. Two slide layouts featuring line graphs and column charts.
10. Project Management Summary Dashboard Template
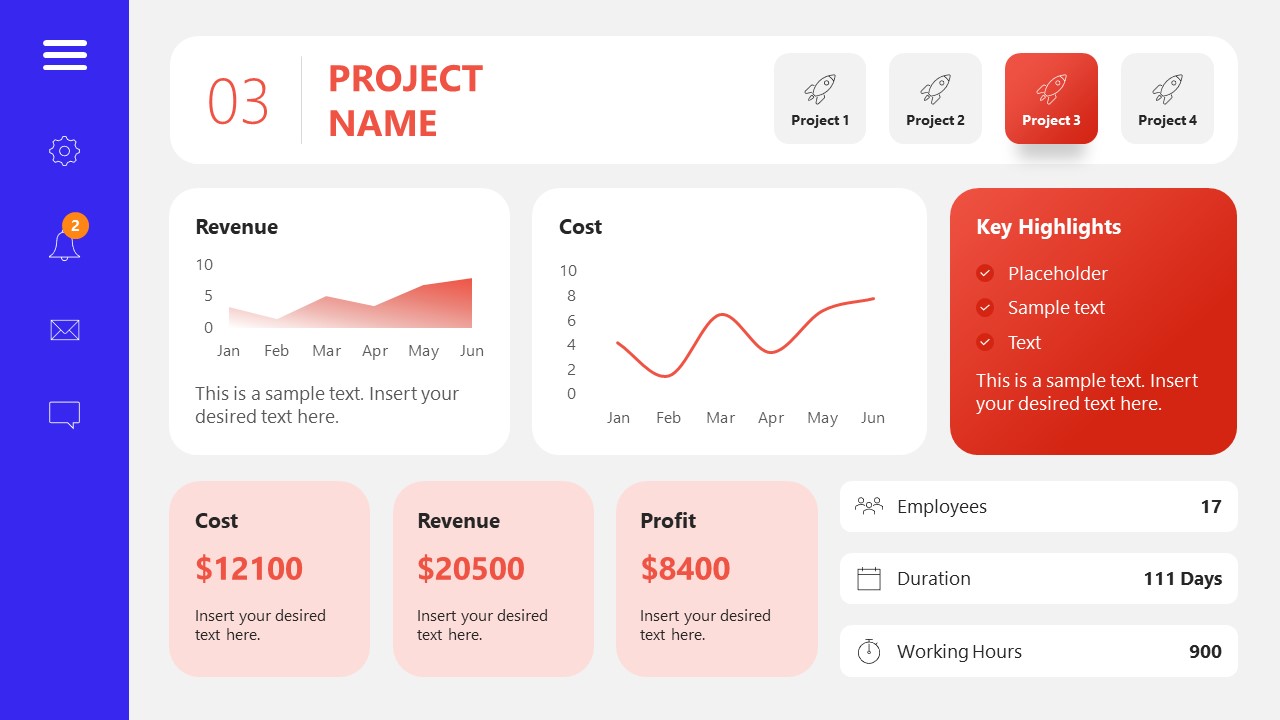
A tool crafted for project managers to deliver highly visual reports on a project’s completion, the profits it delivered for the company, and expenses/time required to execute it. 4 different color layouts are available.
11. Profit & Loss Dashboard for PowerPoint and Google Slides
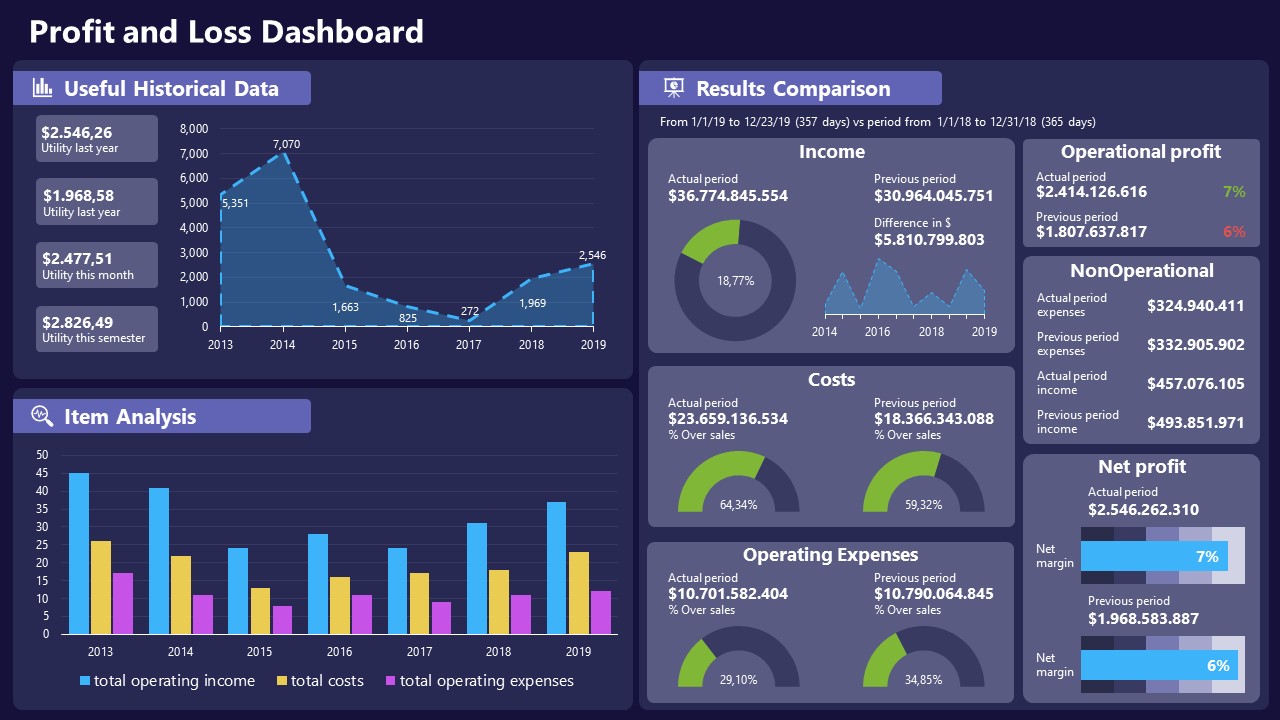
A must-have for finance professionals. This typical profit & loss dashboard includes progress bars, donut charts, column charts, line graphs, and everything that’s required to deliver a comprehensive report about a company’s financial situation.
Overwhelming visuals
One of the mistakes related to using data-presenting methods is including too much data or using overly complex visualizations. They can confuse the audience and dilute the key message.
Inappropriate chart types
Choosing the wrong type of chart for the data at hand can lead to misinterpretation. For example, using a pie chart for data that doesn’t represent parts of a whole is not right.
Lack of context
Failing to provide context or sufficient labeling can make it challenging for the audience to understand the significance of the presented data.
Inconsistency in design
Using inconsistent design elements and color schemes across different visualizations can create confusion and visual disarray.
Failure to provide details
Simply presenting raw data without offering clear insights or takeaways can leave the audience without a meaningful conclusion.
Lack of focus
Not having a clear focus on the key message or main takeaway can result in a presentation that lacks a central theme.
Visual accessibility issues
Overlooking the visual accessibility of charts and graphs can exclude certain audience members who may have difficulty interpreting visual information.
In order to avoid these mistakes in data presentation, presenters can benefit from using presentation templates . These templates provide a structured framework. They ensure consistency, clarity, and an aesthetically pleasing design, enhancing data communication’s overall impact.
Understanding and choosing data presentation types are pivotal in effective communication. Each method serves a unique purpose, so selecting the appropriate one depends on the nature of the data and the message to be conveyed. The diverse array of presentation types offers versatility in visually representing information, from bar charts showing values to pie charts illustrating proportions.
Using the proper method enhances clarity, engages the audience, and ensures that data sets are not just presented but comprehensively understood. By appreciating the strengths and limitations of different presentation types, communicators can tailor their approach to convey information accurately, developing a deeper connection between data and audience understanding.
[1] Government of Canada, S.C. (2021) 5 Data Visualization 5.2 Bar Chart , 5.2 Bar chart . https://www150.statcan.gc.ca/n1/edu/power-pouvoir/ch9/bargraph-diagrammeabarres/5214818-eng.htm
[2] Kosslyn, S.M., 1989. Understanding charts and graphs. Applied cognitive psychology, 3(3), pp.185-225. https://apps.dtic.mil/sti/pdfs/ADA183409.pdf
[3] Creating a Dashboard . https://it.tufts.edu/book/export/html/1870
[4] https://www.goldenwestcollege.edu/research/data-and-more/data-dashboards/index.html
[5] https://www.mit.edu/course/21/21.guide/grf-line.htm
[6] Jadeja, M. and Shah, K., 2015, January. Tree-Map: A Visualization Tool for Large Data. In GSB@ SIGIR (pp. 9-13). https://ceur-ws.org/Vol-1393/gsb15proceedings.pdf#page=15
[7] Heat Maps and Quilt Plots. https://www.publichealth.columbia.edu/research/population-health-methods/heat-maps-and-quilt-plots
[8] EIU QGIS WORKSHOP. https://www.eiu.edu/qgisworkshop/heatmaps.php
[9] About Pie Charts. https://www.mit.edu/~mbarker/formula1/f1help/11-ch-c8.htm
[10] Histograms. https://sites.utexas.edu/sos/guided/descriptive/numericaldd/descriptiven2/histogram/ [11] https://asq.org/quality-resources/scatter-diagram
Like this article? Please share
Data Analysis, Data Science, Data Visualization Filed under Design
Related Articles
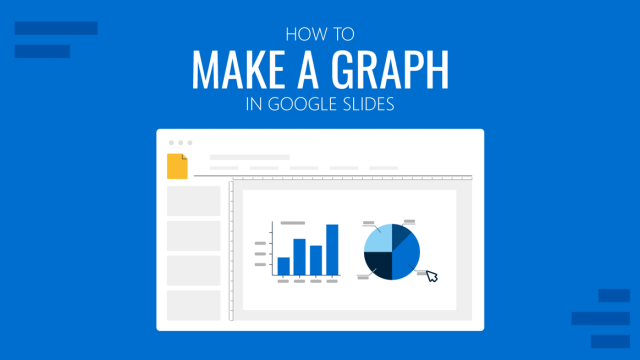
Filed under Google Slides Tutorials • June 3rd, 2024
How To Make a Graph on Google Slides
Creating quality graphics is an essential aspect of designing data presentations. Learn how to make a graph in Google Slides with this guide.
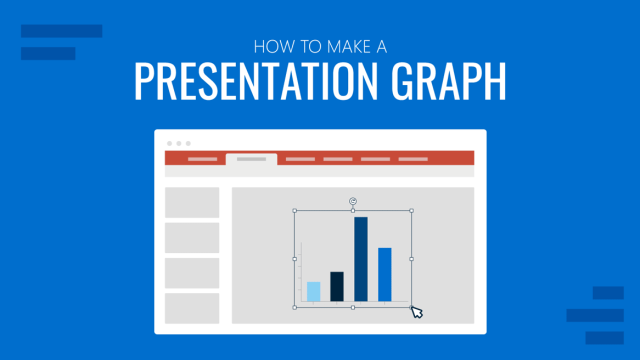
Filed under Design • March 27th, 2024
How to Make a Presentation Graph
Detailed step-by-step instructions to master the art of how to make a presentation graph in PowerPoint and Google Slides. Check it out!
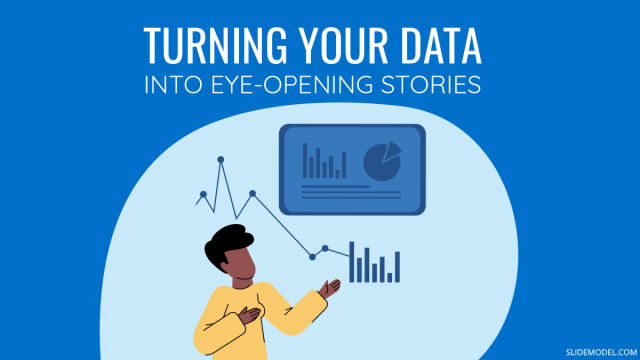
Filed under Presentation Ideas • February 12th, 2024
Turning Your Data into Eye-opening Stories
What is Data Storytelling is a question that people are constantly asking now. If you seek to understand how to create a data storytelling ppt that will complete the information for your audience, you should read this blog post.
Leave a Reply
- Privacy Policy

Home » Data Interpretation – Process, Methods and Questions
Data Interpretation – Process, Methods and Questions
Table of Contents

Data Interpretation
Definition :
Data interpretation refers to the process of making sense of data by analyzing and drawing conclusions from it. It involves examining data in order to identify patterns, relationships, and trends that can help explain the underlying phenomena being studied. Data interpretation can be used to make informed decisions and solve problems across a wide range of fields, including business, science, and social sciences.
Data Interpretation Process
Here are the steps involved in the data interpretation process:
- Define the research question : The first step in data interpretation is to clearly define the research question. This will help you to focus your analysis and ensure that you are interpreting the data in a way that is relevant to your research objectives.
- Collect the data: The next step is to collect the data. This can be done through a variety of methods such as surveys, interviews, observation, or secondary data sources.
- Clean and organize the data : Once the data has been collected, it is important to clean and organize it. This involves checking for errors, inconsistencies, and missing data. Data cleaning can be a time-consuming process, but it is essential to ensure that the data is accurate and reliable.
- Analyze the data: The next step is to analyze the data. This can involve using statistical software or other tools to calculate summary statistics, create graphs and charts, and identify patterns in the data.
- Interpret the results: Once the data has been analyzed, it is important to interpret the results. This involves looking for patterns, trends, and relationships in the data. It also involves drawing conclusions based on the results of the analysis.
- Communicate the findings : The final step is to communicate the findings. This can involve creating reports, presentations, or visualizations that summarize the key findings of the analysis. It is important to communicate the findings in a way that is clear and concise, and that is tailored to the audience’s needs.
Types of Data Interpretation
There are various types of data interpretation techniques used for analyzing and making sense of data. Here are some of the most common types:
Descriptive Interpretation
This type of interpretation involves summarizing and describing the key features of the data. This can involve calculating measures of central tendency (such as mean, median, and mode), measures of dispersion (such as range, variance, and standard deviation), and creating visualizations such as histograms, box plots, and scatterplots.
Inferential Interpretation
This type of interpretation involves making inferences about a larger population based on a sample of the data. This can involve hypothesis testing, where you test a hypothesis about a population parameter using sample data, or confidence interval estimation, where you estimate a range of values for a population parameter based on sample data.
Predictive Interpretation
This type of interpretation involves using data to make predictions about future outcomes. This can involve building predictive models using statistical techniques such as regression analysis, time-series analysis, or machine learning algorithms.
Exploratory Interpretation
This type of interpretation involves exploring the data to identify patterns and relationships that were not previously known. This can involve data mining techniques such as clustering analysis, principal component analysis, or association rule mining.
Causal Interpretation
This type of interpretation involves identifying causal relationships between variables in the data. This can involve experimental designs, such as randomized controlled trials, or observational studies, such as regression analysis or propensity score matching.
Data Interpretation Methods
There are various methods for data interpretation that can be used to analyze and make sense of data. Here are some of the most common methods:
Statistical Analysis
This method involves using statistical techniques to analyze the data. Statistical analysis can involve descriptive statistics (such as measures of central tendency and dispersion), inferential statistics (such as hypothesis testing and confidence interval estimation), and predictive modeling (such as regression analysis and time-series analysis).
Data Visualization
This method involves using visual representations of the data to identify patterns and trends. Data visualization can involve creating charts, graphs, and other visualizations, such as heat maps or scatterplots.
Text Analysis
This method involves analyzing text data, such as survey responses or social media posts, to identify patterns and themes. Text analysis can involve techniques such as sentiment analysis, topic modeling, and natural language processing.
Machine Learning
This method involves using algorithms to identify patterns in the data and make predictions or classifications. Machine learning can involve techniques such as decision trees, neural networks, and random forests.
Qualitative Analysis
This method involves analyzing non-numeric data, such as interviews or focus group discussions, to identify themes and patterns. Qualitative analysis can involve techniques such as content analysis, grounded theory, and narrative analysis.
Geospatial Analysis
This method involves analyzing spatial data, such as maps or GPS coordinates, to identify patterns and relationships. Geospatial analysis can involve techniques such as spatial autocorrelation, hot spot analysis, and clustering.
Applications of Data Interpretation
Data interpretation has a wide range of applications across different fields, including business, healthcare, education, social sciences, and more. Here are some examples of how data interpretation is used in different applications:
- Business : Data interpretation is widely used in business to inform decision-making, identify market trends, and optimize operations. For example, businesses may analyze sales data to identify the most popular products or customer demographics, or use predictive modeling to forecast demand and adjust pricing accordingly.
- Healthcare : Data interpretation is critical in healthcare for identifying disease patterns, evaluating treatment effectiveness, and improving patient outcomes. For example, healthcare providers may use electronic health records to analyze patient data and identify risk factors for certain diseases or conditions.
- Education : Data interpretation is used in education to assess student performance, identify areas for improvement, and evaluate the effectiveness of instructional methods. For example, schools may analyze test scores to identify students who are struggling and provide targeted interventions to improve their performance.
- Social sciences : Data interpretation is used in social sciences to understand human behavior, attitudes, and perceptions. For example, researchers may analyze survey data to identify patterns in public opinion or use qualitative analysis to understand the experiences of marginalized communities.
- Sports : Data interpretation is increasingly used in sports to inform strategy and improve performance. For example, coaches may analyze performance data to identify areas for improvement or use predictive modeling to assess the likelihood of injuries or other risks.
When to use Data Interpretation
Data interpretation is used to make sense of complex data and to draw conclusions from it. It is particularly useful when working with large datasets or when trying to identify patterns or trends in the data. Data interpretation can be used in a variety of settings, including scientific research, business analysis, and public policy.
In scientific research, data interpretation is often used to draw conclusions from experiments or studies. Researchers use statistical analysis and data visualization techniques to interpret their data and to identify patterns or relationships between variables. This can help them to understand the underlying mechanisms of their research and to develop new hypotheses.
In business analysis, data interpretation is used to analyze market trends and consumer behavior. Companies can use data interpretation to identify patterns in customer buying habits, to understand market trends, and to develop marketing strategies that target specific customer segments.
In public policy, data interpretation is used to inform decision-making and to evaluate the effectiveness of policies and programs. Governments and other organizations use data interpretation to track the impact of policies and programs over time, to identify areas where improvements are needed, and to develop evidence-based policy recommendations.
In general, data interpretation is useful whenever large amounts of data need to be analyzed and understood in order to make informed decisions.
Data Interpretation Examples
Here are some real-time examples of data interpretation:
- Social media analytics : Social media platforms generate vast amounts of data every second, and businesses can use this data to analyze customer behavior, track sentiment, and identify trends. Data interpretation in social media analytics involves analyzing data in real-time to identify patterns and trends that can help businesses make informed decisions about marketing strategies and customer engagement.
- Healthcare analytics: Healthcare organizations use data interpretation to analyze patient data, track outcomes, and identify areas where improvements are needed. Real-time data interpretation can help healthcare providers make quick decisions about patient care, such as identifying patients who are at risk of developing complications or adverse events.
- Financial analysis: Real-time data interpretation is essential for financial analysis, where traders and analysts need to make quick decisions based on changing market conditions. Financial analysts use data interpretation to track market trends, identify opportunities for investment, and develop trading strategies.
- Environmental monitoring : Real-time data interpretation is important for environmental monitoring, where data is collected from various sources such as satellites, sensors, and weather stations. Data interpretation helps to identify patterns and trends that can help predict natural disasters, track changes in the environment, and inform decision-making about environmental policies.
- Traffic management: Real-time data interpretation is used for traffic management, where traffic sensors collect data on traffic flow, congestion, and accidents. Data interpretation helps to identify areas where traffic congestion is high, and helps traffic management authorities make decisions about road maintenance, traffic signal timing, and other strategies to improve traffic flow.
Data Interpretation Questions
Data Interpretation Questions samples:
- Medical : What is the correlation between a patient’s age and their risk of developing a certain disease?
- Environmental Science: What is the trend in the concentration of a certain pollutant in a particular body of water over the past 10 years?
- Finance : What is the correlation between a company’s stock price and its quarterly revenue?
- Education : What is the trend in graduation rates for a particular high school over the past 5 years?
- Marketing : What is the correlation between a company’s advertising budget and its sales revenue?
- Sports : What is the trend in the number of home runs hit by a particular baseball player over the past 3 seasons?
- Social Science: What is the correlation between a person’s level of education and their income level?
In order to answer these questions, you would need to analyze and interpret the data using statistical methods, graphs, and other visualization tools.
Purpose of Data Interpretation
The purpose of data interpretation is to make sense of complex data by analyzing and drawing insights from it. The process of data interpretation involves identifying patterns and trends, making comparisons, and drawing conclusions based on the data. The ultimate goal of data interpretation is to use the insights gained from the analysis to inform decision-making.
Data interpretation is important because it allows individuals and organizations to:
- Understand complex data : Data interpretation helps individuals and organizations to make sense of complex data sets that would otherwise be difficult to understand.
- Identify patterns and trends : Data interpretation helps to identify patterns and trends in data, which can reveal important insights about the underlying processes and relationships.
- Make informed decisions: Data interpretation provides individuals and organizations with the information they need to make informed decisions based on the insights gained from the data analysis.
- Evaluate performance : Data interpretation helps individuals and organizations to evaluate their performance over time and to identify areas where improvements can be made.
- Communicate findings: Data interpretation allows individuals and organizations to communicate their findings to others in a clear and concise manner, which is essential for informing stakeholders and making changes based on the insights gained from the analysis.
Characteristics of Data Interpretation
Here are some characteristics of data interpretation:
- Contextual : Data interpretation is always contextual, meaning that the interpretation of data is dependent on the context in which it is analyzed. The same data may have different meanings depending on the context in which it is analyzed.
- Iterative : Data interpretation is an iterative process, meaning that it often involves multiple rounds of analysis and refinement as more data becomes available or as new insights are gained from the analysis.
- Subjective : Data interpretation is often subjective, as it involves the interpretation of data by individuals who may have different perspectives and biases. It is important to acknowledge and address these biases when interpreting data.
- Analytical : Data interpretation involves the use of analytical tools and techniques to analyze and draw insights from data. These may include statistical analysis, data visualization, and other data analysis methods.
- Evidence-based : Data interpretation is evidence-based, meaning that it is based on the data and the insights gained from the analysis. It is important to ensure that the data used in the analysis is accurate, relevant, and reliable.
- Actionable : Data interpretation is actionable, meaning that it provides insights that can be used to inform decision-making and to drive action. The ultimate goal of data interpretation is to use the insights gained from the analysis to improve performance or to achieve specific goals.
Advantages of Data Interpretation
Data interpretation has several advantages, including:
- Improved decision-making: Data interpretation provides insights that can be used to inform decision-making. By analyzing data and drawing insights from it, individuals and organizations can make informed decisions based on evidence rather than intuition.
- Identification of patterns and trends: Data interpretation helps to identify patterns and trends in data, which can reveal important insights about the underlying processes and relationships. This information can be used to improve performance or to achieve specific goals.
- Evaluation of performance: Data interpretation helps individuals and organizations to evaluate their performance over time and to identify areas where improvements can be made. By analyzing data, organizations can identify strengths and weaknesses and make changes to improve their performance.
- Communication of findings: Data interpretation allows individuals and organizations to communicate their findings to others in a clear and concise manner, which is essential for informing stakeholders and making changes based on the insights gained from the analysis.
- Better resource allocation: Data interpretation can help organizations allocate resources more efficiently by identifying areas where resources are needed most. By analyzing data, organizations can identify areas where resources are being underutilized or where additional resources are needed to improve performance.
- Improved competitiveness : Data interpretation can give organizations a competitive advantage by providing insights that help to improve performance, reduce costs, or identify new opportunities for growth.
Limitations of Data Interpretation
Data interpretation has some limitations, including:
- Limited by the quality of data: The quality of data used in data interpretation can greatly impact the accuracy of the insights gained from the analysis. Poor quality data can lead to incorrect conclusions and decisions.
- Subjectivity: Data interpretation can be subjective, as it involves the interpretation of data by individuals who may have different perspectives and biases. This can lead to different interpretations of the same data.
- Limited by analytical tools: The analytical tools and techniques used in data interpretation can also limit the accuracy of the insights gained from the analysis. Different analytical tools may yield different results, and some tools may not be suitable for certain types of data.
- Time-consuming: Data interpretation can be a time-consuming process, particularly for large and complex data sets. This can make it difficult to quickly make decisions based on the insights gained from the analysis.
- Incomplete data: Data interpretation can be limited by incomplete data sets, which may not provide a complete picture of the situation being analyzed. Incomplete data can lead to incorrect conclusions and decisions.
- Limited by context: Data interpretation is always contextual, meaning that the interpretation of data is dependent on the context in which it is analyzed. The same data may have different meanings depending on the context in which it is analyzed.
Difference between Data Interpretation and Data Analysis
Data interpretation and data analysis are two different but closely related processes in data-driven decision-making.
Data analysis refers to the process of examining and examining data using statistical and computational methods to derive insights and conclusions from it. It involves cleaning, transforming, and modeling the data to uncover patterns, relationships, and trends that can help in understanding the underlying phenomena.
Data interpretation, on the other hand, refers to the process of making sense of the findings from the data analysis by contextualizing them within the larger problem domain. It involves identifying the key takeaways from the data analysis, assessing their relevance and significance to the problem at hand, and communicating the insights in a clear and actionable manner.
In short, data analysis is about uncovering insights from the data, while data interpretation is about making sense of those insights and translating them into actionable recommendations.
About the author
Muhammad Hassan
Researcher, Academic Writer, Web developer
You may also like

Scope of the Research – Writing Guide and...

Research Topics – Ideas and Examples

Research Recommendations – Examples and Writing...

What is a Hypothesis – Types, Examples and...

Future Research – Thesis Guide

Background of The Study – Examples and Writing...
Data Collection, Presentation and Analysis
- First Online: 25 May 2023
Cite this chapter
- Uche M. Mbanaso 4 ,
- Lucienne Abrahams 5 &
- Kennedy Chinedu Okafor 6
760 Accesses
This chapter covers the topics of data collection, data presentation and data analysis. It gives attention to data collection for studies based on experiments, on data derived from existing published or unpublished data sets, on observation, on simulation and digital twins, on surveys, on interviews and on focus group discussions. One of the interesting features of this chapter is the section dealing with using measurement scales in quantitative research, including nominal scales, ordinal scales, interval scales and ratio scales. It explains key facets of qualitative research including ethical clearance requirements. The chapter discusses the importance of data visualization as key to effective presentation of data, including tabular forms, graphical forms and visual charts such as those generated by Atlas.ti analytical software.
This is a preview of subscription content, log in via an institution to check access.
Access this chapter
Subscribe and save.
- Get 10 units per month
- Download Article/Chapter or eBook
- 1 Unit = 1 Article or 1 Chapter
- Cancel anytime
- Available as PDF
- Read on any device
- Instant download
- Own it forever
- Available as EPUB and PDF
- Compact, lightweight edition
- Dispatched in 3 to 5 business days
- Free shipping worldwide - see info
- Durable hardcover edition
Tax calculation will be finalised at checkout
Purchases are for personal use only
Institutional subscriptions
Bibliography
Abdullah, M. F., & Ahmad, K. (2013). The mapping process of unstructured data to structured data. Proceedings of the 2013 International Conference on Research and Innovation in Information Systems (ICRIIS) , Malaysia , 151–155. https://doi.org/10.1109/ICRIIS.2013.6716700
Adnan, K., & Akbar, R. (2019). An analytical study of information extraction from unstructured and multidimensional big data. Journal of Big Data, 6 , 91. https://doi.org/10.1186/s40537-019-0254-8
Article Google Scholar
Alsheref, F. K., & Fattoh, I. E. (2020). Medical text annotation tool based on IBM Watson Platform. Proceedings of the 2020 6th international conference on advanced computing and communication systems (ICACCS) , India , 1312–1316. https://doi.org/10.1109/ICACCS48705.2020.9074309
Cinque, M., Cotroneo, D., Della Corte, R., & Pecchia, A. (2014). What logs should you look at when an application fails? Insights from an industrial case study. Proceedings of the 2014 44th Annual IEEE/IFIP International Conference on Dependable Systems and Networks , USA , 690–695. https://doi.org/10.1109/DSN.2014.69
Gideon, L. (Ed.). (2012). Handbook of survey methodology for the social sciences . Springer.
Google Scholar
Leedy, P., & Ormrod, J. (2015). Practical research planning and design (12th ed.). Pearson Education.
Madaan, A., Wang, X., Hall, W., & Tiropanis, T. (2018). Observing data in IoT worlds: What and how to observe? In Living in the Internet of Things: Cybersecurity of the IoT – 2018 (pp. 1–7). https://doi.org/10.1049/cp.2018.0032
Chapter Google Scholar
Mahajan, P., & Naik, C. (2019). Development of integrated IoT and machine learning based data collection and analysis system for the effective prediction of agricultural residue/biomass availability to regenerate clean energy. Proceedings of the 2019 9th International Conference on Emerging Trends in Engineering and Technology – Signal and Information Processing (ICETET-SIP-19) , India , 1–5. https://doi.org/10.1109/ICETET-SIP-1946815.2019.9092156 .
Mahmud, M. S., Huang, J. Z., Salloum, S., Emara, T. Z., & Sadatdiynov, K. (2020). A survey of data partitioning and sampling methods to support big data analysis. Big Data Mining and Analytics, 3 (2), 85–101. https://doi.org/10.26599/BDMA.2019.9020015
Miswar, S., & Kurniawan, N. B. (2018). A systematic literature review on survey data collection system. Proceedings of the 2018 International Conference on Information Technology Systems and Innovation (ICITSI) , Indonesia , 177–181. https://doi.org/10.1109/ICITSI.2018.8696036
Mosina, C. (2020). Understanding the diffusion of the internet: Redesigning the global diffusion of the internet framework (Research report, Master of Arts in ICT Policy and Regulation). LINK Centre, University of the Witwatersrand. https://hdl.handle.net/10539/30723
Nkamisa, S. (2021). Investigating the integration of drone management systems to create an enabling remote piloted aircraft regulatory environment in South Africa (Research report, Master of Arts in ICT Policy and Regulation). LINK Centre, University of the Witwatersrand. https://hdl.handle.net/10539/33883
QuestionPro. (2020). Survey research: Definition, examples and methods . https://www.questionpro.com/article/survey-research.html
Rajanikanth, J. & Kanth, T. V. R. (2017). An explorative data analysis on Bangalore City Weather with hybrid data mining techniques using R. Proceedings of the 2017 International Conference on Current Trends in Computer, Electrical, Electronics and Communication (CTCEEC) , India , 1121-1125. https://doi/10.1109/CTCEEC.2017.8455008
Rao, R. (2003). From unstructured data to actionable intelligence. IT Professional, 5 , 29–35. https://www.researchgate.net/publication/3426648_From_Unstructured_Data_to_Actionable_Intelligence
Schulze, P. (2009). Design of the research instrument. In P. Schulze (Ed.), Balancing exploitation and exploration: Organizational antecedents and performance effects of innovation strategies (pp. 116–141). Gabler. https://doi.org/10.1007/978-3-8349-8397-8_6
Usanov, A. (2015). Assessing cybersecurity: A meta-analysis of threats, trends and responses to cyber attacks . The Hague Centre for Strategic Studies. https://www.researchgate.net/publication/319677972_Assessing_Cyber_Security_A_Meta-analysis_of_Threats_Trends_and_Responses_to_Cyber_Attacks
Van de Kaa, G., De Vries, H. J., van Heck, E., & van den Ende, J. (2007). The emergence of standards: A meta-analysis. Proceedings of the 2007 40th Annual Hawaii International Conference on Systems Science (HICSS’07) , USA , 173a–173a. https://doi.org/10.1109/HICSS.2007.529
Download references
Author information
Authors and affiliations.
Centre for Cybersecurity Studies, Nasarawa State University, Keffi, Nigeria
Uche M. Mbanaso
LINK Centre, University of the Witwatersrand, Johannesburg, South Africa
Lucienne Abrahams
Department of Mechatronics Engineering, Federal University of Technology, Owerri, Nigeria
Kennedy Chinedu Okafor
You can also search for this author in PubMed Google Scholar
Rights and permissions
Reprints and permissions
Copyright information
© 2023 The Author(s), under exclusive license to Springer Nature Switzerland AG
About this chapter
Mbanaso, U.M., Abrahams, L., Okafor, K.C. (2023). Data Collection, Presentation and Analysis. In: Research Techniques for Computer Science, Information Systems and Cybersecurity. Springer, Cham. https://doi.org/10.1007/978-3-031-30031-8_7
Download citation
DOI : https://doi.org/10.1007/978-3-031-30031-8_7
Published : 25 May 2023
Publisher Name : Springer, Cham
Print ISBN : 978-3-031-30030-1
Online ISBN : 978-3-031-30031-8
eBook Packages : Engineering Engineering (R0)
Share this chapter
Anyone you share the following link with will be able to read this content:
Sorry, a shareable link is not currently available for this article.
Provided by the Springer Nature SharedIt content-sharing initiative
- Publish with us
Policies and ethics
- Find a journal
- Track your research

What is Data Interpretation? Tools, Techniques, Examples
By Hady ElHady
July 14, 2023
Get Started With a Prebuilt Model
Start with a free template and upgrade when needed.
In today’s data-driven world, the ability to interpret and extract valuable insights from data is crucial for making informed decisions. Data interpretation involves analyzing and making sense of data to uncover patterns, relationships, and trends that can guide strategic actions.
Whether you’re a business professional, researcher, or data enthusiast, this guide will equip you with the knowledge and techniques to master the art of data interpretation.
What is Data Interpretation?
Data interpretation is the process of analyzing and making sense of data to extract valuable insights and draw meaningful conclusions. It involves examining patterns, relationships, and trends within the data to uncover actionable information. Data interpretation goes beyond merely collecting and organizing data; it is about extracting knowledge and deriving meaningful implications from the data at hand.
Why is Data Interpretation Important?
In today’s data-driven world, data interpretation holds immense importance across various industries and domains. Here are some key reasons why data interpretation is crucial:
- Informed Decision-Making: Data interpretation enables informed decision-making by providing evidence-based insights. It helps individuals and organizations make choices supported by data-driven evidence, rather than relying on intuition or assumptions .
- Identifying Opportunities and Risks: Effective data interpretation helps identify opportunities for growth and innovation. By analyzing patterns and trends within the data, organizations can uncover new market segments, consumer preferences, and emerging trends. Simultaneously, data interpretation also helps identify potential risks and challenges that need to be addressed proactively.
- Optimizing Performance: By analyzing data and extracting insights, organizations can identify areas for improvement and optimize their performance. Data interpretation allows for identifying bottlenecks, inefficiencies, and areas of optimization across various processes, such as supply chain management, production, and customer service.
- Enhancing Customer Experience: Data interpretation plays a vital role in understanding customer behavior and preferences. By analyzing customer data, organizations can personalize their offerings, improve customer experience, and tailor marketing strategies to target specific customer segments effectively.
- Predictive Analytics and Forecasting: Data interpretation enables predictive analytics and forecasting, allowing organizations to anticipate future trends and make strategic plans accordingly. By analyzing historical data patterns, organizations can make predictions and forecast future outcomes, facilitating proactive decision-making and risk mitigation.
- Evidence-Based Research and Policy Making: In fields such as healthcare, social sciences, and public policy, data interpretation plays a crucial role in conducting evidence-based research and policy-making. By analyzing relevant data, researchers and policymakers can identify trends, assess the effectiveness of interventions, and make informed decisions that impact society positively.
- Competitive Advantage: Organizations that excel in data interpretation gain a competitive edge. By leveraging data insights, organizations can make informed strategic decisions, innovate faster, and respond promptly to market changes. This enables them to stay ahead of their competitors in today’s fast-paced business environment.
In summary, data interpretation is essential for leveraging the power of data and transforming it into actionable insights. It enables organizations and individuals to make informed decisions, identify opportunities and risks, optimize performance, enhance customer experience, predict future trends, and gain a competitive advantage in their respective domains.
The Role of Data Interpretation in Decision-Making Processes
Data interpretation plays a crucial role in decision-making processes across organizations and industries. It empowers decision-makers with valuable insights and helps guide their actions. Here are some key roles that data interpretation fulfills in decision-making:
- Informing Strategic Planning : Data interpretation provides decision-makers with a comprehensive understanding of the current state of affairs and the factors influencing their organization or industry. By analyzing relevant data, decision-makers can assess market trends, customer preferences, and competitive landscapes. These insights inform the strategic planning process, guiding the formulation of goals, objectives, and action plans.
- Identifying Problem Areas and Opportunities: Effective data interpretation helps identify problem areas and opportunities for improvement. By analyzing data patterns and trends, decision-makers can identify bottlenecks, inefficiencies, or underutilized resources. This enables them to address challenges and capitalize on opportunities, enhancing overall performance and competitiveness.
- Risk Assessment and Mitigation: Data interpretation allows decision-makers to assess and mitigate risks. By analyzing historical data, market trends, and external factors, decision-makers can identify potential risks and vulnerabilities. This understanding helps in developing risk management strategies and contingency plans to mitigate the impact of risks and uncertainties.
- Facilitating Evidence-Based Decision-Making: Data interpretation enables evidence-based decision-making by providing objective insights and factual evidence. Instead of relying solely on intuition or subjective opinions, decision-makers can base their choices on concrete data-driven evidence. This leads to more accurate and reliable decision-making, reducing the likelihood of biases or errors.
- Measuring and Evaluating Performance: Data interpretation helps decision-makers measure and evaluate the performance of various aspects of their organization. By analyzing key performance indicators (KPIs) and relevant metrics, decision-makers can track progress towards goals, assess the effectiveness of strategies and initiatives, and identify areas for improvement. This data-driven evaluation enables evidence-based adjustments and ensures that resources are allocated optimally.
- Enabling Predictive Analytics and Forecasting: Data interpretation plays a critical role in predictive analytics and forecasting. Decision-makers can analyze historical data patterns to make predictions and forecast future trends. This capability empowers organizations to anticipate market changes, customer behavior, and emerging opportunities. By making informed decisions based on predictive insights, decision-makers can stay ahead of the curve and proactively respond to future developments.
- Supporting Continuous Improvement: Data interpretation facilitates a culture of continuous improvement within organizations. By regularly analyzing data, decision-makers can monitor performance, identify areas for enhancement, and implement data-driven improvements. This iterative process of analyzing data, making adjustments, and measuring outcomes enables organizations to continuously refine their strategies and operations.
In summary, data interpretation is integral to effective decision-making. It informs strategic planning, identifies problem areas and opportunities, assesses and mitigates risks, facilitates evidence-based decision-making, measures performance, enables predictive analytics, and supports continuous improvement. By harnessing the power of data interpretation, decision-makers can make well-informed, data-driven decisions that lead to improved outcomes and success in their endeavors.
Understanding Data
Before delving into data interpretation, it’s essential to understand the fundamentals of data. Data can be categorized into qualitative and quantitative types, each requiring different analysis methods. Qualitative data represents non-numerical information, such as opinions or descriptions, while quantitative data consists of measurable quantities.
Types of Data
- Qualitative data: Includes observations, interviews, survey responses, and other subjective information.
- Quantitative data: Comprises numerical data collected through measurements, counts, or ratings.
Data Collection Methods
To perform effective data interpretation, you need to be aware of the various methods used to collect data. These methods can include surveys, experiments, observations, interviews, and more. Proper data collection techniques ensure the accuracy and reliability of the data.
Data Sources and Reliability
When working with data, it’s important to consider the source and reliability of the data. Reliable sources include official statistics, reputable research studies, and well-designed surveys. Assessing the credibility of the data source helps you determine its accuracy and validity.
Data Preprocessing and Cleaning
Before diving into data interpretation, it’s crucial to preprocess and clean the data to remove any inconsistencies or errors. This step involves identifying missing values, outliers, and data inconsistencies, as well as handling them appropriately. Data preprocessing ensures that the data is in a suitable format for analysis.
Exploratory Data Analysis: Unveiling Insights from Data
Exploratory Data Analysis (EDA) is a vital step in data interpretation, helping you understand the data’s characteristics and uncover initial insights. By employing various graphical and statistical techniques, you can gain a deeper understanding of the data patterns and relationships.
Univariate Analysis
Univariate analysis focuses on examining individual variables in isolation, revealing their distribution and basic characteristics. Here are some common techniques used in univariate analysis:
- Histograms: Graphical representations of the frequency distribution of a variable. Histograms display data in bins or intervals, providing a visual depiction of the data’s distribution.
- Box plots: Box plots summarize the distribution of a variable by displaying its quartiles, median, and any potential outliers. They offer a concise overview of the data’s central tendency and spread.
- Frequency distributions: Tabular representations that show the number of occurrences or frequencies of different values or ranges of a variable.
Bivariate Analysis
Bivariate analysis explores the relationship between two variables, examining how they interact and influence each other. By visualizing and analyzing the connections between variables, you can identify correlations and patterns. Some common techniques for bivariate analysis include:
- Scatter plots: Graphical representations that display the relationship between two continuous variables. Scatter plots help identify potential linear or nonlinear associations between the variables.
- Correlation analysis: Statistical measure of the strength and direction of the relationship between two variables. Correlation coefficients, such as Pearson’s correlation coefficient, range from -1 to 1, with higher absolute values indicating stronger correlations.
- Heatmaps: Visual representations that use color intensity to show the strength of relationships between two categorical variables. Heatmaps help identify patterns and associations between variables.
Multivariate Analysis
Multivariate analysis involves the examination of three or more variables simultaneously. This analysis technique provides a deeper understanding of complex relationships and interactions among multiple variables. Some common methods used in multivariate analysis include:
- Dimensionality reduction techniques: Approaches like Principal Component Analysis (PCA) or t-Distributed Stochastic Neighbor Embedding (t-SNE) reduce high-dimensional data into lower dimensions, simplifying analysis and visualization.
- Cluster analysis: Grouping data points based on similarities or dissimilarities. Cluster analysis helps identify patterns or subgroups within the data.
Descriptive Statistics: Understanding Data’s Central Tendency and Variability
Descriptive statistics provides a summary of the main features of a dataset, focusing on measures of central tendency and variability. These statistics offer a comprehensive overview of the data’s characteristics and aid in understanding its distribution and spread.
Measures of Central Tendency
Measures of central tendency describe the central or average value around which the data tends to cluster. Here are some commonly used measures of central tendency:
- Mean: The arithmetic average of a dataset, calculated by summing all values and dividing by the total number of observations.
- Median: The middle value in a dataset when arranged in ascending or descending order. The median is less sensitive to extreme values than the mean.
- Mode: The most frequently occurring value in a dataset.
Measures of Dispersion
Measures of dispersion quantify the spread or variability of the data points. Understanding variability is essential for assessing the data’s reliability and drawing meaningful conclusions. Common measures of dispersion include:
- Range: The difference between the maximum and minimum values in a dataset, providing a simple measure of spread.
- Variance: The average squared deviation from the mean, measuring the dispersion of data points around the mean.
- Standard Deviation: The square root of the variance, representing the average distance between each data point and the mean.
Percentiles and Quartiles
Percentiles and quartiles divide the dataset into equal parts, allowing you to understand the distribution of values within specific ranges. They provide insights into the relative position of individual data points in comparison to the entire dataset.
- Percentiles: Divisions of data into 100 equal parts, indicating the percentage of values that fall below a given value. The median corresponds to the 50th percentile.
- Quartiles: Divisions of data into four equal parts, denoted as the first quartile (Q1), median (Q2), and third quartile (Q3). The interquartile range (IQR) measures the spread between Q1 and Q3.
Skewness and Kurtosis
Skewness and kurtosis measure the shape and distribution of data. They provide insights into the symmetry, tail heaviness, and peakness of the distribution.
- Skewness: Measures the asymmetry of the data distribution. Positive skewness indicates a longer tail on the right side, while negative skewness suggests a longer tail on the left side.
- Kurtosis: Measures the peakedness or flatness of the data distribution. Positive kurtosis indicates a sharper peak and heavier tails, while negative kurtosis suggests a flatter peak and lighter tails.
Inferential Statistics: Drawing Inferences and Making Hypotheses
Inferential statistics involves making inferences and drawing conclusions about a population based on a sample of data. It allows you to generalize findings beyond the observed data and make predictions or test hypotheses. This section covers key techniques and concepts in inferential statistics.
Hypothesis Testing
Hypothesis testing involves making statistical inferences about population parameters based on sample data. It helps determine the validity of a claim or hypothesis by examining the evidence provided by the data. The hypothesis testing process typically involves the following steps:
- Formulate hypotheses: Define the null hypothesis (H0) and alternative hypothesis (Ha) based on the research question or claim.
- Select a significance level: Determine the acceptable level of error (alpha) to guide the decision-making process.
- Collect and analyze data: Gather and analyze the sample data using appropriate statistical tests.
- Calculate the test statistic: Compute the test statistic based on the selected test and the sample data.
- Determine the critical region: Identify the critical region based on the significance level and the test statistic’s distribution.
- Make a decision: Compare the test statistic with the critical region and either reject or fail to reject the null hypothesis.
- Draw conclusions: Interpret the results and make conclusions based on the decision made in the previous step.
Confidence Intervals
Confidence intervals provide a range of values within which the population parameter is likely to fall. They quantify the uncertainty associated with estimating population parameters based on sample data. The construction of a confidence interval involves:
- Select a confidence level: Choose the desired level of confidence, typically expressed as a percentage (e.g., 95% confidence level).
- Compute the sample statistic: Calculate the sample statistic (e.g., sample mean) from the sample data.
- Determine the margin of error: Determine the margin of error, which represents the maximum likely distance between the sample statistic and the population parameter.
- Construct the confidence interval: Establish the upper and lower bounds of the confidence interval using the sample statistic and the margin of error.
- Interpret the confidence interval: Interpret the confidence interval in the context of the problem, acknowledging the level of confidence and the potential range of population values.
Parametric and Non-parametric Tests
In inferential statistics, different tests are used based on the nature of the data and the assumptions made about the population distribution. Parametric tests assume specific population distributions, such as the normal distribution, while non-parametric tests make fewer assumptions. Some commonly used parametric and non-parametric tests include:
- t-tests: Compare means between two groups or assess differences in paired observations.
- Analysis of Variance (ANOVA): Compare means among multiple groups.
- Chi-square test: Assess the association between categorical variables.
- Mann-Whitney U test: Compare medians between two independent groups.
- Kruskal-Wallis test: Compare medians among multiple independent groups.
- Spearman’s rank correlation: Measure the strength and direction of monotonic relationships between variables.
Correlation and Regression Analysis
Correlation and regression analysis explore the relationship between variables, helping understand how changes in one variable affect another. These analyses are particularly useful in predicting and modeling outcomes based on explanatory variables.
- Correlation analysis: Determines the strength and direction of the linear relationship between two continuous variables using correlation coefficients, such as Pearson’s correlation coefficient.
- Regression analysis: Models the relationship between a dependent variable and one or more independent variables, allowing you to estimate the impact of the independent variables on the dependent variable. It provides insights into the direction, magnitude, and significance of these relationships.
Data Interpretation Techniques: Unlocking Insights for Informed Decisions
Data interpretation techniques enable you to extract actionable insights from your data, empowering you to make informed decisions. We’ll explore key techniques that facilitate pattern recognition, trend analysis , comparative analysis , predictive modeling, and causal inference.
Pattern Recognition and Trend Analysis
Identifying patterns and trends in data helps uncover valuable insights that can guide decision-making. Several techniques aid in recognizing patterns and analyzing trends:
- Time series analysis: Analyzes data points collected over time to identify recurring patterns and trends.
- Moving averages: Smooths out fluctuations in data, highlighting underlying trends and patterns.
- Seasonal decomposition: Separates a time series into its seasonal, trend, and residual components.
- Cluster analysis: Groups similar data points together, identifying patterns or segments within the data.
- Association rule mining: Discovers relationships and dependencies between variables, uncovering valuable patterns and trends.
Comparative Analysis
Comparative analysis involves comparing different subsets of data or variables to identify similarities, differences, or relationships. This analysis helps uncover insights into the factors that contribute to variations in the data.
- Cross-tabulation: Compares two or more categorical variables to understand the relationships and dependencies between them.
- ANOVA (Analysis of Variance): Assesses differences in means among multiple groups to identify significant variations.
- Comparative visualizations: Graphical representations, such as bar charts or box plots, help compare data across categories or groups.
Predictive Modeling and Forecasting
Predictive modeling uses historical data to build mathematical models that can predict future outcomes. This technique leverages machine learning algorithms to uncover patterns and relationships in data, enabling accurate predictions.
- Regression models: Build mathematical equations to predict the value of a dependent variable based on independent variables.
- Time series forecasting: Utilizes historical time series data to predict future values, considering factors like trend, seasonality, and cyclical patterns.
- Machine learning algorithms: Employ advanced algorithms, such as decision trees, random forests, or neural networks, to generate accurate predictions based on complex data patterns.
Causal Inference and Experimentation
Causal inference aims to establish cause-and-effect relationships between variables, helping determine the impact of certain factors on an outcome. Experimental design and controlled studies are essential for establishing causal relationships.
- Randomized controlled trials (RCTs): Divide participants into treatment and control groups to assess the causal effects of an intervention.
- Quasi-experimental designs: Apply treatment to specific groups, allowing for some level of control but not full randomization.
- Difference-in-differences analysis: Compares changes in outcomes between treatment and control groups before and after an intervention or treatment.
Data Visualization Techniques: Communicating Insights Effectively
Data visualization is a powerful tool for presenting data in a visually appealing and informative manner. Visual representations help simplify complex information, enabling effective communication and understanding.
Importance of Data Visualization
Data visualization serves multiple purposes in data interpretation and analysis. It allows you to:
- Simplify complex data: Visual representations simplify complex information, making it easier to understand and interpret.
- Spot patterns and trends: Visualizations help identify patterns, trends, and anomalies that may not be apparent in raw data.
- Communicate insights: Visualizations are effective in conveying insights to different stakeholders and audiences.
- Support decision-making: Well-designed visualizations facilitate informed decision-making by providing a clear understanding of the data.
Choosing the Right Visualization Method
Selecting the appropriate visualization method is crucial to effectively communicate your data. Different types of data and insights are best represented using specific visualization techniques. Consider the following factors when choosing a visualization method:
- Data type: Determine whether the data is categorical, ordinal, or numerical.
- Insights to convey: Identify the key messages or patterns you want to communicate.
- Audience and context: Consider the knowledge level and preferences of the audience, as well as the context in which the visualization will be presented.
Common Data Visualization Tools and Software
Several tools and software applications simplify the process of creating visually appealing and interactive data visualizations. Some widely used tools include:
- Tableau: A powerful business intelligence and data visualization tool that allows you to create interactive dashboards, charts, and maps.
- Power BI: Microsoft’s business analytics tool that enables data visualization, exploration, and collaboration.
- Python libraries: Matplotlib, Seaborn, and Plotly are popular Python libraries for creating static and interactive visualizations.
- R programming: R offers a wide range of packages, such as ggplot2 and Shiny, for creating visually appealing data visualizations.
Best Practices for Creating Effective Visualizations
Creating effective visualizations requires attention to design principles and best practices. By following these guidelines, you can ensure that your visualizations effectively communicate insights:
- Simplify and declutter: Eliminate unnecessary elements, labels, or decorations that may distract from the main message.
- Use appropriate chart types: Select chart types that best represent your data and the relationships you want to convey.
- Highlight important information: Use color, size, or annotations to draw attention to key insights or trends in your data.
- Ensure readability and accessibility: Use clear labels, appropriate font sizes, and sufficient contrast to make your visualizations easily readable.
- Tell a story: Organize your visualizations in a logical order and guide the viewer’s attention to the most important aspects of the data.
- Iterate and refine: Continuously refine and improve your visualizations based on feedback and testing.
Data Interpretation in Specific Domains: Unlocking Domain-Specific Insights
Data interpretation plays a vital role across various industries and domains. Let’s explore how data interpretation is applied in specific fields, providing real-world examples and applications.
Marketing and Consumer Behavior
In the marketing field, data interpretation helps businesses understand consumer behavior, market trends, and the effectiveness of marketing campaigns. Key applications include:
- Customer segmentation: Identifying distinct customer groups based on demographics, preferences, or buying patterns.
- Market research : Analyzing survey data or social media sentiment to gain insights into consumer opinions and preferences.
- Campaign analysis: Assessing the impact and ROI of marketing campaigns through data analysis and interpretation.
Financial Analysis and Investment Decisions
Data interpretation is crucial in financial analysis and investment decision-making. It enables the identification of market trends, risk assessment , and portfolio optimization. Key applications include:
- Financial statement analysis: Interpreting financial statements to assess a company’s financial health , profitability , and growth potential.
- Risk analysis: Evaluating investment risks by analyzing historical data, market trends, and financial indicators.
- Portfolio management: Utilizing data analysis to optimize investment portfolios based on risk-return trade-offs and diversification.
Healthcare and Medical Research
Data interpretation plays a significant role in healthcare and medical research, aiding in understanding patient outcomes, disease patterns, and treatment effectiveness. Key applications include:
- Clinical trials: Analyzing clinical trial data to assess the safety and efficacy of new treatments or interventions.
- Epidemiological studies: Interpreting population-level data to identify disease risk factors and patterns.
- Healthcare analytics: Leveraging patient data to improve healthcare delivery, optimize resource allocation, and enhance patient outcomes.
Social Sciences and Public Policy
Data interpretation is integral to social sciences and public policy, informing evidence-based decision-making and policy formulation. Key applications include:
- Survey analysis: Interpreting survey data to understand public opinion, social attitudes, and behavior patterns.
- Policy evaluation: Analyzing data to assess the effectiveness and impact of public policies or interventions.
- Crime analysis: Utilizing data interpretation techniques to identify crime patterns, hotspots, and trends, aiding law enforcement and policy formulation.
Data Interpretation Tools and Software: Empowering Your Analysis
Several software tools facilitate data interpretation, analysis, and visualization, providing a range of features and functionalities. Understanding and leveraging these tools can enhance your data interpretation capabilities.
Spreadsheet Software
Spreadsheet software like Excel and Google Sheets offer a wide range of data analysis and interpretation functionalities. These tools allow you to:
- Perform calculations: Use formulas and functions to compute descriptive statistics, create pivot tables, or analyze data.
- Visualize data: Create charts, graphs, and tables to visualize and summarize data effectively.
- Manipulate and clean data: Utilize built-in functions and features to clean, transform, and preprocess data.
Statistical Software
Statistical software packages, such as R and Python, provide a more comprehensive and powerful environment for data interpretation. These tools offer advanced statistical analysis capabilities, including:
- Data manipulation: Perform data transformations, filtering, and merging to prepare data for analysis.
- Statistical modeling: Build regression models, conduct hypothesis tests, and perform advanced statistical analyses.
- Visualization: Generate high-quality visualizations and interactive plots to explore and present data effectively.
Business Intelligence Tools
Business intelligence (BI) tools, such as Tableau and Power BI, enable interactive data exploration, analysis, and visualization. These tools provide:
- Drag-and-drop functionality: Easily create interactive dashboards, reports, and visualizations without extensive coding.
- Data integration: Connect to multiple data sources and perform data blending for comprehensive analysis.
- Real-time data analysis: Analyze and visualize live data streams for up-to-date insights and decision-making.
Data Mining and Machine Learning Tools
Data mining and machine learning tools offer advanced algorithms and techniques for extracting insights from complex datasets. Some popular tools include:
- Python libraries: Scikit-learn, TensorFlow, and PyTorch provide comprehensive machine learning and data mining functionalities.
- R packages: Packages like caret, randomForest, and xgboost offer a wide range of algorithms for predictive modeling and data mining.
- Big data tools: Apache Spark, Hadoop, and Apache Flink provide distributed computing frameworks for processing and analyzing large-scale datasets.
Common Challenges and Pitfalls in Data Interpretation: Navigating the Data Maze
Data interpretation comes with its own set of challenges and potential pitfalls. Being aware of these challenges can help you avoid common errors and ensure the accuracy and validity of your interpretations.
Sampling Bias and Data Quality Issues
Sampling bias occurs when the sample data is not representative of the population, leading to biased interpretations. Common types of sampling bias include selection bias, non-response bias, and volunteer bias. To mitigate these issues, consider:
- Random sampling: Implement random sampling techniques to ensure representativeness.
- Sample size: Use appropriate sample sizes to reduce sampling errors and increase the accuracy of interpretations.
- Data quality checks: Scrutinize data for completeness, accuracy, and consistency before analysis.
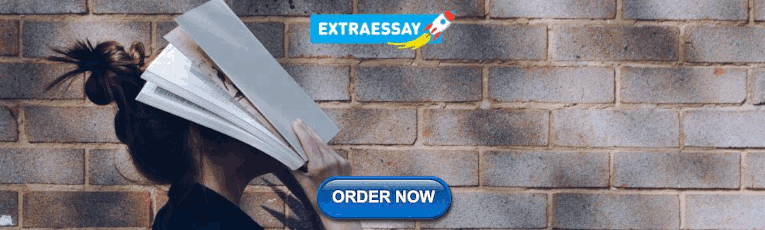
Overfitting and Spurious Correlations
Overfitting occurs when a model fits the noise or random variations in the data instead of the underlying patterns. Spurious correlations, on the other hand, arise when variables appear to be related but are not causally connected. To avoid these issues:
- Use appropriate model complexity: Avoid overcomplicating models and select the level of complexity that best fits the data.
- Validate models: Test the model’s performance on unseen data to ensure generalizability.
- Consider causal relationships: Be cautious in interpreting correlations and explore causal mechanisms before inferring causation.
Misinterpretation of Statistical Results
Misinterpretation of statistical results can lead to inaccurate conclusions and misguided actions. Common pitfalls include misreading p-values, misinterpreting confidence intervals, and misattributing causality. To prevent misinterpretation:
- Understand statistical concepts: Familiarize yourself with key statistical concepts, such as p-values, confidence intervals, and effect sizes.
- Provide context: Consider the broader context, study design, and limitations when interpreting statistical results.
- Consult experts: Seek guidance from statisticians or domain experts to ensure accurate interpretation.
Simpson’s Paradox and Confounding Variables
Simpson’s paradox occurs when a trend or relationship observed within subgroups of data reverses when the groups are combined. Confounding variables, or lurking variables, can distort or confound the interpretation of relationships between variables. To address these challenges:
- Account for confounding variables: Identify and account for potential confounders when analyzing relationships between variables.
- Analyze subgroups: Analyze data within subgroups to identify patterns and trends, ensuring the validity of interpretations.
- Contextualize interpretations: Consider the potential impact of confounding variables and provide nuanced interpretations.
Best Practices for Effective Data Interpretation: Making Informed Decisions
Effective data interpretation relies on following best practices throughout the entire process, from data collection to drawing conclusions. By adhering to these best practices, you can enhance the accuracy and validity of your interpretations.
Clearly Define Research Questions and Objectives
Before embarking on data interpretation, clearly define your research questions and objectives. This clarity will guide your analysis, ensuring you focus on the most relevant aspects of the data.
Use Appropriate Statistical Methods for the Data Type
Select the appropriate statistical methods based on the nature of your data. Different data types require different analysis techniques, so choose the methods that best align with your data characteristics.
Conduct Sensitivity Analysis and Robustness Checks
Perform sensitivity analysis and robustness checks to assess the stability and reliability of your results. Varying assumptions, sample sizes, or methodologies can help validate the robustness of your interpretations.
Communicate Findings Accurately and Effectively
When communicating your data interpretations, consider your audience and their level of understanding. Present your findings in a clear, concise, and visually appealing manner to effectively convey the insights derived from your analysis.
Data Interpretation Examples: Applying Techniques to Real-World Scenarios
To gain a better understanding of how data interpretation techniques can be applied in practice, let’s explore some real-world examples. These examples demonstrate how different industries and domains leverage data interpretation to extract meaningful insights and drive decision-making.
Example 1: Retail Sales Analysis
A retail company wants to analyze its sales data to uncover patterns and optimize its marketing strategies. By applying data interpretation techniques, they can:
- Perform sales trend analysis : Analyze sales data over time to identify seasonal patterns, peak sales periods, and fluctuations in customer demand.
- Conduct customer segmentation: Segment customers based on purchase behavior, demographics, or preferences to personalize marketing campaigns and offers.
- Analyze product performance: Examine sales data for each product category to identify top-selling items, underperforming products, and opportunities for cross-selling or upselling.
- Evaluate marketing campaigns: Analyze the impact of marketing initiatives on sales by comparing promotional periods, advertising channels, or customer responses.
- Forecast future sales: Utilize historical sales data and predictive models to forecast future sales trends, helping the company optimize inventory management and resource allocation.
Example 2: Healthcare Outcome Analysis
A healthcare organization aims to improve patient outcomes and optimize resource allocation. Through data interpretation, they can:
- Analyze patient data: Extract insights from electronic health records, medical history, and treatment outcomes to identify factors impacting patient outcomes.
- Identify risk factors: Analyze patient populations to identify common risk factors associated with specific medical conditions or adverse events.
- Conduct comparative effectiveness research: Compare different treatment methods or interventions to assess their impact on patient outcomes and inform evidence-based treatment decisions.
- Optimize resource allocation: Analyze healthcare utilization patterns to allocate resources effectively, optimize staffing levels, and improve operational efficiency.
- Evaluate intervention effectiveness: Analyze intervention programs to assess their effectiveness in improving patient outcomes, such as reducing readmission rates or hospital-acquired infections.
Example 3: Financial Investment Analysis
An investment firm wants to make data-driven investment decisions and assess portfolio performance. By applying data interpretation techniques, they can:
- Perform market trend analysis : Analyze historical market data, economic indicators, and sector performance to identify investment opportunities and predict market trends.
- Conduct risk analysis: Assess the risk associated with different investment options by analyzing historical returns, volatility, and correlations with market indices.
- Perform portfolio optimization: Utilize quantitative models and optimization techniques to construct diversified portfolios that maximize returns while managing risk.
- Monitor portfolio performance: Analyze portfolio returns, compare them against benchmarks, and conduct attribution analysis to identify the sources of portfolio performance.
- Perform scenario analysis : Assess the impact of potential market scenarios, economic changes, or geopolitical events on investment portfolios to inform risk management strategies.
These examples illustrate how data interpretation techniques can be applied across various industries and domains. By leveraging data effectively, organizations can unlock valuable insights, optimize strategies, and make informed decisions that drive success.
Data interpretation is a fundamental skill for unlocking the power of data and making informed decisions. By understanding the various techniques, best practices, and challenges in data interpretation, you can confidently navigate the complex landscape of data analysis and uncover valuable insights.
As you embark on your data interpretation journey, remember to embrace curiosity, rigor, and a continuous learning mindset. The ability to extract meaningful insights from data will empower you to drive positive change in your organization or field.
Get Started With a Prebuilt Template!
Looking to streamline your business financial modeling process with a prebuilt customizable template? Say goodbye to the hassle of building a financial model from scratch and get started right away with one of our premium templates.
- Save time with no need to create a financial model from scratch.
- Reduce errors with prebuilt formulas and calculations.
- Customize to your needs by adding/deleting sections and adjusting formulas.
- Automatically calculate key metrics for valuable insights.
- Make informed decisions about your strategy and goals with a clear picture of your business performance and financial health .
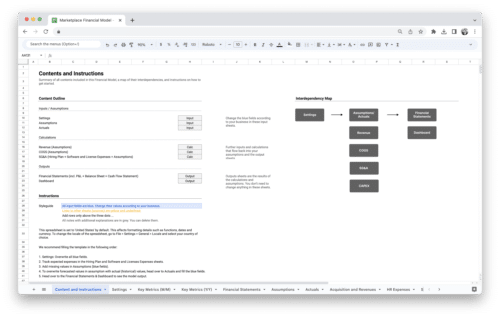
Marketplace Financial Model Template
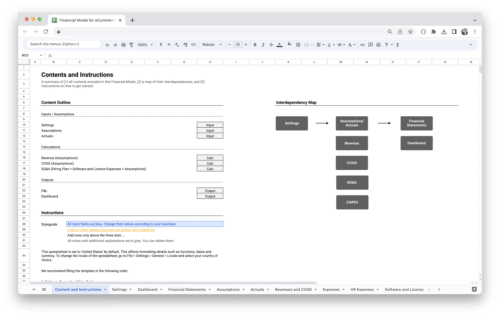
E-Commerce Financial Model Template
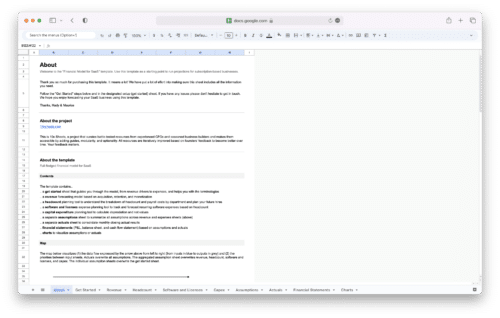
SaaS Financial Model Template
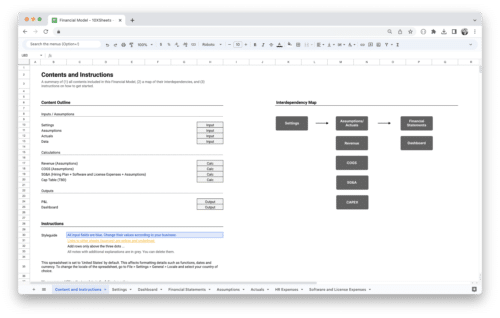
Standard Financial Model Template
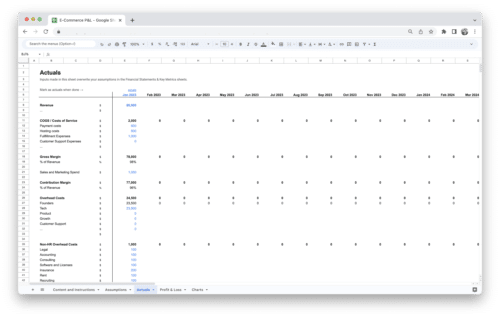
E-Commerce Profit and Loss Statement
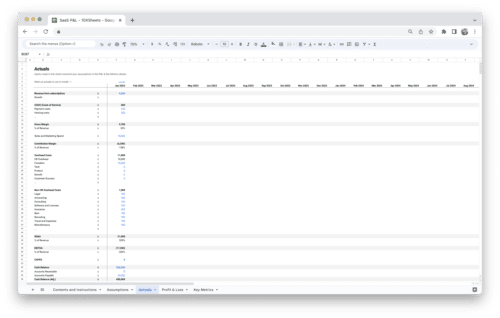
SaaS Profit and Loss Statement
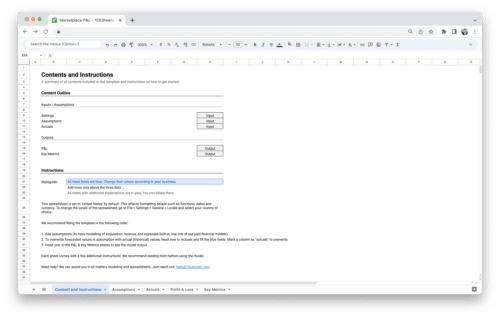
Marketplace Profit and Loss Statement
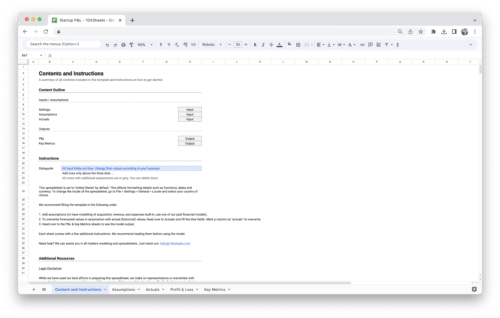
Startup Profit and Loss Statement
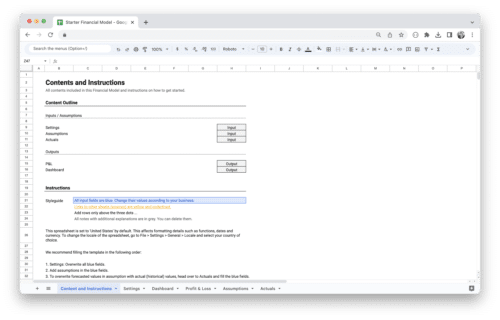
Startup Financial Model Template
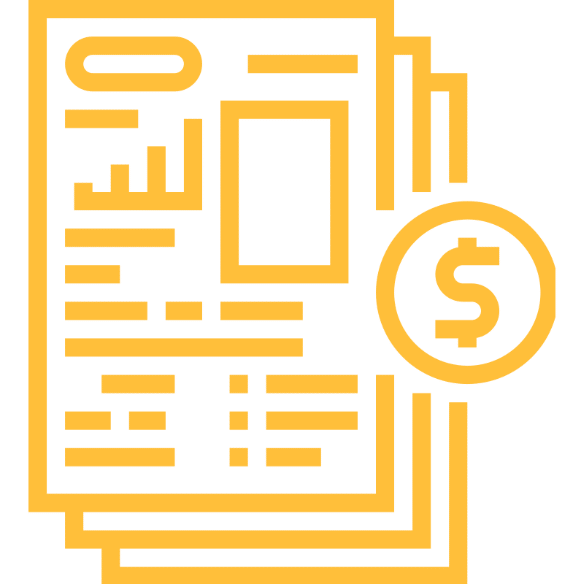
Expert Templates For You
Don’t settle for mediocre templates. Get started with premium spreadsheets and financial models customizable to your unique business needs to help you save time and streamline your processes.
Receive Exclusive Updates
Get notified of new templates and business resources to help grow your business. Join our community of forward-thinking entrepreneurs and stay ahead of the game!
[email protected]
© Copyright 2024 | 10XSheets | All Rights Reserved
Your email *
Your message
Send a copy to your email
Call Us Today! +91 99907 48956 | [email protected]

It is the simplest form of data Presentation often used in schools or universities to provide a clearer picture to students, who are better able to capture the concepts effectively through a pictorial Presentation of simple data.
2. Column chart

It is a simplified version of the pictorial Presentation which involves the management of a larger amount of data being shared during the presentations and providing suitable clarity to the insights of the data.
3. Pie Charts

Pie charts provide a very descriptive & a 2D depiction of the data pertaining to comparisons or resemblance of data in two separate fields.
4. Bar charts

A bar chart that shows the accumulation of data with cuboid bars with different dimensions & lengths which are directly proportionate to the values they represent. The bars can be placed either vertically or horizontally depending on the data being represented.
5. Histograms
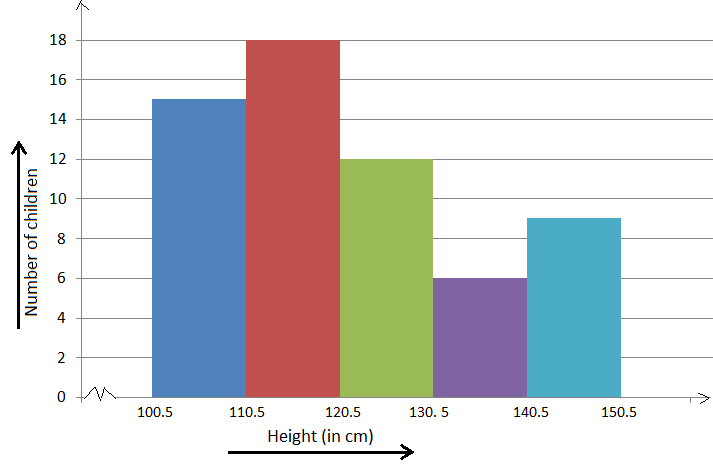
It is a perfect Presentation of the spread of numerical data. The main differentiation that separates data graphs and histograms are the gaps in the data graphs.
6. Box plots

Box plot or Box-plot is a way of representing groups of numerical data through quartiles. Data Presentation is easier with this style of graph dealing with the extraction of data to the minutes of difference.

Map Data graphs help you with data Presentation over an area to display the areas of concern. Map graphs are useful to make an exact depiction of data over a vast case scenario.
All these visual presentations share a common goal of creating meaningful insights and a platform to understand and manage the data in relation to the growth and expansion of one’s in-depth understanding of data & details to plan or execute future decisions or actions.
Importance of Data Presentation
Data Presentation could be both can be a deal maker or deal breaker based on the delivery of the content in the context of visual depiction.
Data Presentation tools are powerful communication tools that can simplify the data by making it easily understandable & readable at the same time while attracting & keeping the interest of its readers and effectively showcase large amounts of complex data in a simplified manner.
If the user can create an insightful presentation of the data in hand with the same sets of facts and figures, then the results promise to be impressive.
There have been situations where the user has had a great amount of data and vision for expansion but the presentation drowned his/her vision.
To impress the higher management and top brass of a firm, effective presentation of data is needed.
Data Presentation helps the clients or the audience to not spend time grasping the concept and the future alternatives of the business and to convince them to invest in the company & turn it profitable both for the investors & the company.
Although data presentation has a lot to offer, the following are some of the major reason behind the essence of an effective presentation:-
- Many consumers or higher authorities are interested in the interpretation of data, not the raw data itself. Therefore, after the analysis of the data, users should represent the data with a visual aspect for better understanding and knowledge.
- The user should not overwhelm the audience with a number of slides of the presentation and inject an ample amount of texts as pictures that will speak for themselves.
- Data presentation often happens in a nutshell with each department showcasing their achievements towards company growth through a graph or a histogram.
- Providing a brief description would help the user to attain attention in a small amount of time while informing the audience about the context of the presentation
- The inclusion of pictures, charts, graphs and tables in the presentation help for better understanding the potential outcomes.
- An effective presentation would allow the organization to determine the difference with the fellow organization and acknowledge its flaws. Comparison of data would assist them in decision making.
Recommended Courses

Data Visualization
Using powerbi &tableau.

Tableau for Data Analysis

MySQL Certification Program

The PowerBI Masterclass
Need help call our support team 7:00 am to 10:00 pm (ist) at (+91 999-074-8956 | 9650-308-956), keep in touch, email: [email protected].
WhatsApp us
A Guide to Effective Data Presentation
Key objectives of data presentation, charts and graphs for great visuals, storytelling with data, visuals, and text, audiences and data presentation, the main idea in data presentation, storyboarding and data presentation, additional resources, data presentation.
Tools for effective data presentation
Financial analysts are required to present their findings in a neat, clear, and straightforward manner. They spend most of their time working with spreadsheets in MS Excel, building financial models , and crunching numbers. These models and calculations can be pretty extensive and complex and may only be understood by the analyst who created them. Effective data presentation skills are critical for being a world-class financial analyst .
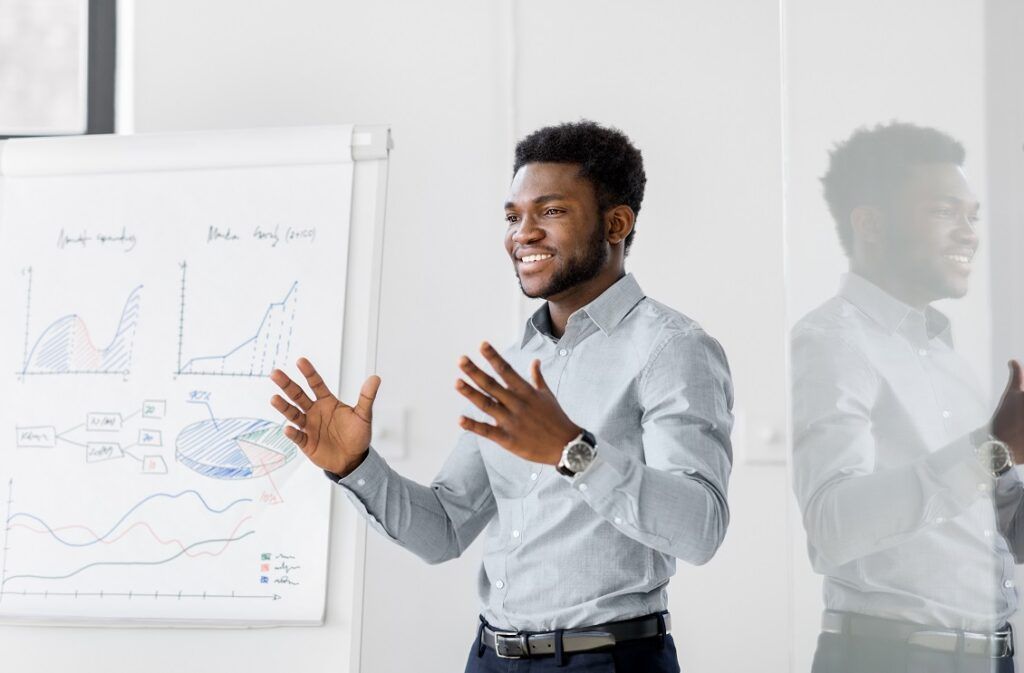
It is the analyst’s job to effectively communicate the output to the target audience, such as the management team or a company’s external investors. This requires focusing on the main points, facts, insights, and recommendations that will prompt the necessary action from the audience.
One challenge is making intricate and elaborate work easy to comprehend through great visuals and dashboards. For example, tables, graphs, and charts are tools that an analyst can use to their advantage to give deeper meaning to a company’s financial information. These tools organize relevant numbers that are rather dull and give life and story to them.
Here are some key objectives to think about when presenting financial analysis:
- Visual communication
- Audience and context
- Charts, graphs, and images
- Focus on important points
- Design principles
- Storytelling
- Persuasiveness
For a breakdown of these objectives, check out Excel Dashboards & Data Visualization course to help you become a world-class financial analyst.
Charts and graphs make any financial analysis readable, easy to follow, and provide great data presentation. They are often included in the financial model’s output, which is essential for the key decision-makers in a company.
The decision-makers comprise executives and managers who usually won’t have enough time to synthesize and interpret data on their own to make sound business decisions. Therefore, it is the job of the analyst to enhance the decision-making process and help guide the executives and managers to create value for the company.
When an analyst uses charts, it is necessary to be aware of what good charts and bad charts look like and how to avoid the latter when telling a story with data.
Examples of Good Charts
As for great visuals, you can quickly see what’s going on with the data presentation, saving you time for deciphering their actual meaning. More importantly, great visuals facilitate business decision-making because their goal is to provide persuasive, clear, and unambiguous numeric communication.
For reference, take a look at the example below that shows a dashboard, which includes a gauge chart for growth rates, a bar chart for the number of orders, an area chart for company revenues, and a line chart for EBITDA margins.
To learn the step-by-step process of creating these essential tools in MS Excel, watch our video course titled “ Excel Dashboard & Data Visualization .” Aside from what is given in the example below, our course will also teach how you can use other tables and charts to make your financial analysis stand out professionally.
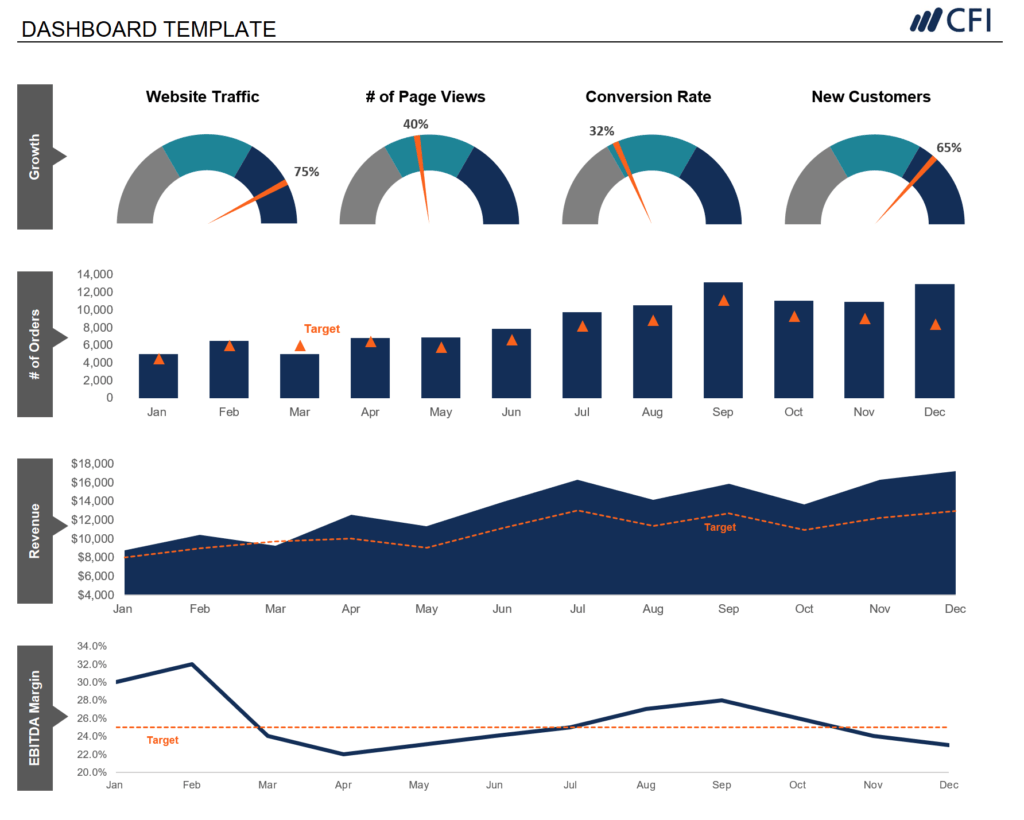
Learn how to build the graph above in our Dashboards Course !
Example of Poorly Crafted Charts
A bad chart, as seen below, will give the reader a difficult time to find the main takeaway of a report or presentation, because it contains too many colors, labels, and legends, and thus, will often look too busy. It also doesn’t help much if a chart, such as a pie chart, is displayed in 3D, as it skews the size and perceived value of the underlying data. A bad chart will be hard to follow and understand.
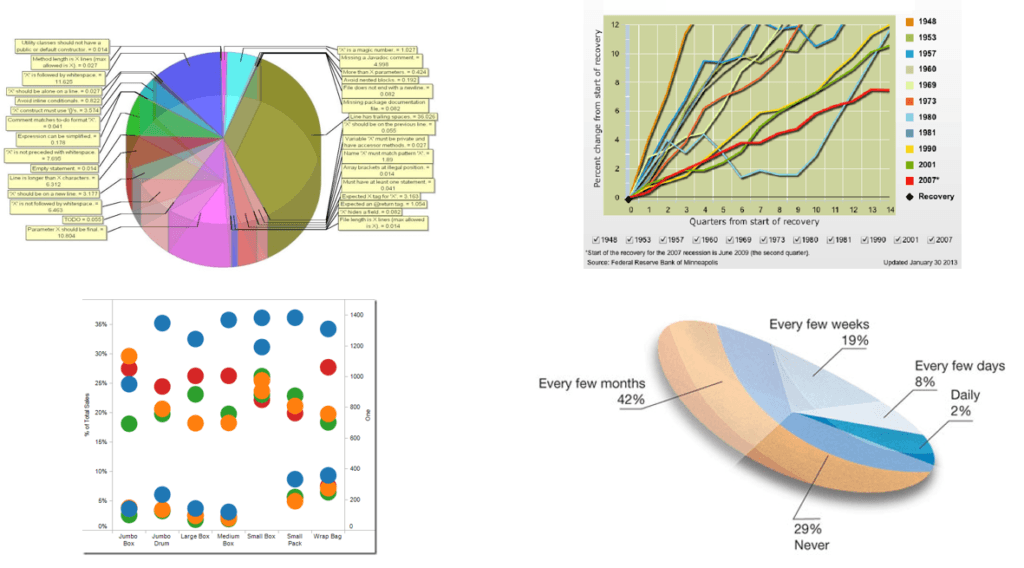
Aside from understanding the meaning of the numbers, a financial analyst must learn to combine numbers and language to craft an effective story. Relying only on data for a presentation may leave your audience finding it difficult to read, interpret, and analyze your data. You must do the work for them, and a good story will be easier to follow. It will help you arrive at the main points faster, rather than just solely presenting your report or live presentation with numbers.
The data can be in the form of revenues, expenses, profits, and cash flow. Simply adding notes, comments, and opinions to each line item will add an extra layer of insight, angle, and a new perspective to the report.
Furthermore, by combining data, visuals, and text, your audience will get a clear understanding of the current situation, past events, and possible conclusions and recommendations that can be made for the future.
The simple diagram below shows the different categories of your audience.
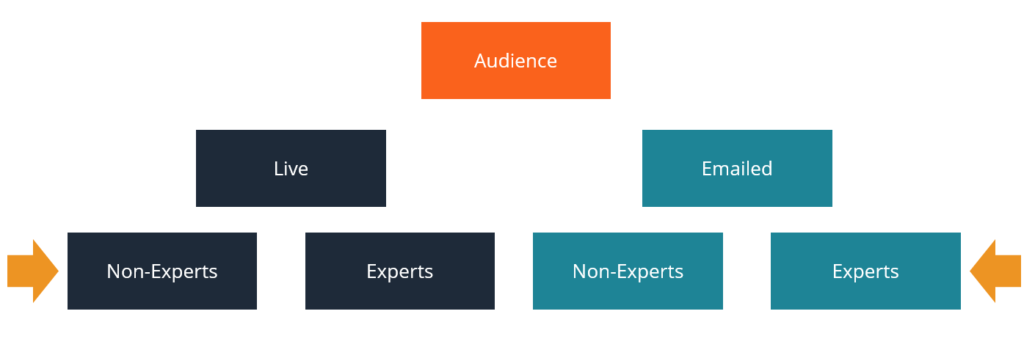
This chart is taken from our course on how to present data .
Internal Audience
An internal audience can either be the executives of the company or any employee who works in that company. For executives, the purpose of communicating a data-filled presentation is to give an update about a certain business activity such as a project or an initiative.
Another important purpose is to facilitate decision-making on managing the company’s operations, growing its core business, acquiring new markets and customers, investing in R&D, and other considerations. Knowing the relevant data and information beforehand will guide the decision-makers in making the right choices that will best position the company toward more success.
External Audience
An external audience can either be the company’s existing clients, where there are projects in progress, or new clients that the company wants to build a relationship with and win new business from. The other external audience is the general public, such as the company’s external shareholders and prospective investors of the company.
When it comes to winning new business, the analyst’s presentation will be more promotional and sales-oriented, whereas a project update will contain more specific information for the client, usually with lots of industry jargon.
Audiences for Live and Emailed Presentation
A live presentation contains more visuals and storytelling to connect more with the audience. It must be more precise and should get to the point faster and avoid long-winded speech or text because of limited time.
In contrast, an emailed presentation is expected to be read, so it will include more text. Just like a document or a book, it will include more detailed information, because its context will not be explained with a voice-over as in a live presentation.
When it comes to details, acronyms, and jargon in the presentation, these things depend on whether your audience are experts or not.
Every great presentation requires a clear “main idea”. It is the core purpose of the presentation and should be addressed clearly. Its significance should be highlighted and should cause the targeted audience to take some action on the matter.
An example of a serious and profound idea is given below.
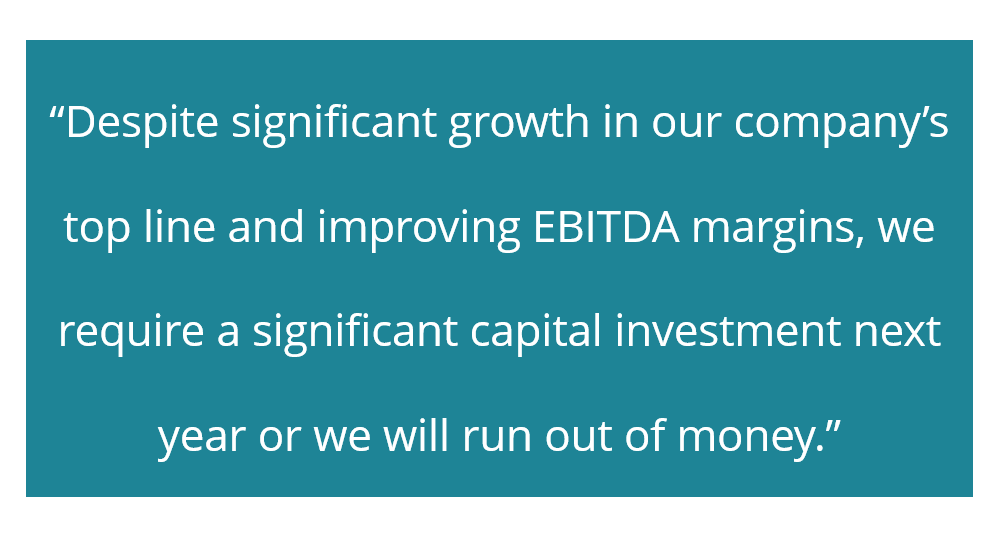
To communicate this big idea, we have to come up with appropriate and effective visual displays to show both the good and bad things surrounding the idea. It should put emphasis and attention on the most important part, which is the critical cash balance and capital investment situation for next year. This is an important component of data presentation.
The storyboarding below is how an analyst would build the presentation based on the big idea. Once the issue or the main idea has been introduced, it will be followed by a demonstration of the positive aspects of the company’s performance, as well as the negative aspects, which are more important and will likely require more attention.
Various ideas will then be suggested to solve the negative issues. However, before choosing the best option, a comparison of the different outcomes of the suggested ideas will be performed. Finally, a recommendation will be made that centers around the optimal choice to address the imminent problem highlighted in the big idea.
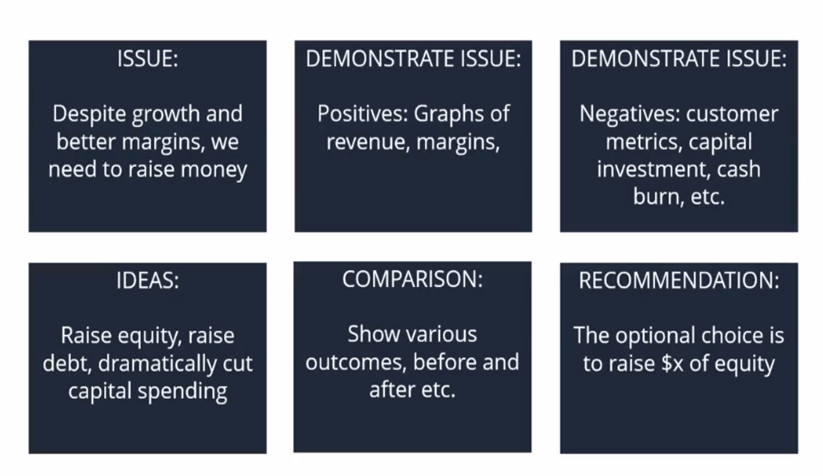
This storyboard is taken from our course on how to present data .
To get to the final point (recommendation), a great deal of analysis has been performed, which includes the charts and graphs discussed earlier, to make the whole presentation easy to follow, convincing, and compelling for your audience.
CFI offers the Business Intelligence & Data Analyst (BIDA)® certification program for those looking to take their careers to the next level. To keep learning and developing your knowledge base, please explore the additional relevant resources below:
- Investment Banking Pitch Books
- Excel Dashboards
- Financial Modeling Guide
- Startup Pitch Book
- See all business intelligence resources
- Share this article
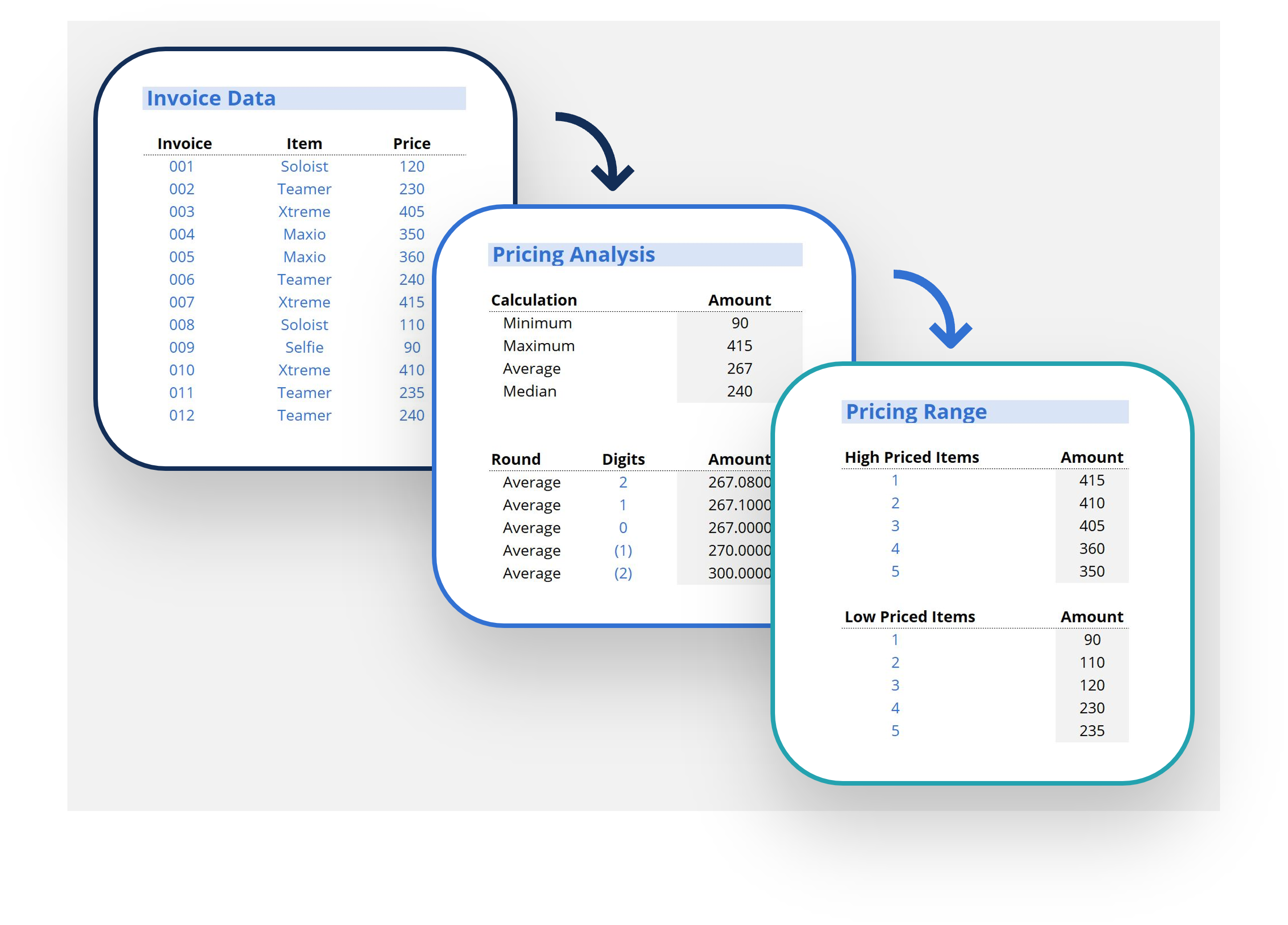
Create a free account to unlock this Template
Access and download collection of free Templates to help power your productivity and performance.
Already have an account? Log in
Supercharge your skills with Premium Templates
Take your learning and productivity to the next level with our Premium Templates.
Upgrading to a paid membership gives you access to our extensive collection of plug-and-play Templates designed to power your performance—as well as CFI's full course catalog and accredited Certification Programs.
Already have a Self-Study or Full-Immersion membership? Log in
Access Exclusive Templates
Gain unlimited access to more than 250 productivity Templates, CFI's full course catalog and accredited Certification Programs, hundreds of resources, expert reviews and support, the chance to work with real-world finance and research tools, and more.
Already have a Full-Immersion membership? Log in
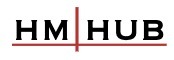
Data Analysis, Interpretation, and Presentation Techniques: A Guide to Making Sense of Your Research Data
by Prince Kumar
Last updated: 27 February 2023
Table of Contents
Data analysis, interpretation, and presentation are crucial aspects of conducting high-quality research. Data analysis involves processing and analyzing the data to derive meaningful insights, while data interpretation involves making sense of the insights and drawing conclusions. Data presentation involves presenting the data in a clear and concise way to communicate the research findings. In this article, we will discuss the techniques for data analysis, interpretation, and presentation.
1. Data Analysis Techniques
Data analysis techniques involve processing and analyzing the data to derive meaningful insights. The choice of data analysis technique depends on the research question and objectives. Some common data analysis techniques are:
a. Descriptive Statistics
Descriptive statistics involves summarizing and describing the data using measures such as mean, median, and standard deviation.
b. Inferential Statistics
Inferential statistics involves making inferences about the population based on the sample data. This technique involves hypothesis testing, confidence intervals, and regression analysis.
c. Content Analysis
Content analysis involves analyzing the text, images, or videos to identify patterns and themes.
d. Data Mining
Data mining involves using statistical and machine learning techniques to analyze large datasets and identify patterns.
2. Data Interpretation Techniques
Data interpretation involves making sense of the insights derived from the data analysis. The choice of data interpretation technique depends on the research question and objectives. Some common data interpretation techniques are:
a. Data Visualization
Data visualization involves presenting the data in a visual format, such as charts, graphs, or tables, to communicate the insights effectively.
b. Storytelling
Storytelling involves presenting the data in a narrative format, such as a story, to make the insights more relatable and memorable.
c. Comparative Analysis
Comparative analysis involves comparing the research findings with the existing literature or benchmarks to draw conclusions.
3. Data Presentation Techniques
Data presentation involves presenting the data in a clear and concise way to communicate the research findings. The choice of data presentation technique depends on the research question and objectives. Some common data presentation techniques are:
a. Tables and Graphs
Tables and graphs are effective data presentation techniques for presenting numerical data.
b. Infographics
Infographics are effective data presentation techniques for presenting complex data in a visual and easy-to-understand format.
c. Data Storytelling
Data storytelling involves presenting the data in a narrative format to communicate the research findings effectively.
In conclusion, data analysis, interpretation, and presentation are crucial aspects of conducting high-quality research. By using the appropriate data analysis, interpretation, and presentation techniques, researchers can derive meaningful insights, make sense of the insights, and communicate the research findings effectively. By conducting high-quality data analysis, interpretation, and presentation in research, researchers can provide valuable insights into the research question and objectives.
How useful was this post?
5 star mean very useful & 1 star means not useful at all.
Average rating / 5. Vote count:
No votes so far! Be the first to rate this post.
We are sorry that this post was not useful for you! 😔
Let us improve this post!
Tell us how we can improve this post?
Syllabus – Research Methodology
01 Introduction To Research Methodology
- Meaning and objectives of Research
- Types of Research
- Research Approaches
- Significance of Research
- Research methods vs Methodology
- Research Process
- Criteria of Good Research
- Problems faced by Researchers
- Techniques Involved in defining a problem
02 Research Design
- Meaning and Need for Research Design
- Features and important concepts relating to research design
- Different Research design
- Important Experimental Designs
03 Sample Design
- Introduction to Sample design
- Censure and sample survey
- Implications of Sample design
- Steps in sampling design
- Criteria for selecting a sampling procedure
- Characteristics of a good sample design
- Different types of Sample design
- Measurement Scales
- Important scaling Techniques
04 Methods of Data Collection
- Introduction
- Collection of Primary Data
- Collection through Questionnaire and schedule collection of secondary data
- Differences in Questionnaire and schedule
- Different methods to collect secondary data
05 Data Analysis Interpretation and Presentation Techniques
- Hypothesis Testing
- Basic concepts concerning Hypothesis Testing
- Procedure and flow diagram for Hypothesis Testing
- Test of Significance
- Chi-Square Analysis
- Report Presentation Techniques
- Skip to main content
- Skip to "About this site"
Language selection
- Français
- Search and menus
Publications
Statistics canada quality guidelines.
- Introduction
- Survey steps
- More information
Data analysis and presentation
Archived content.
Information identified as archived is provided for reference, research or recordkeeping purposes. It is not subject to the Government of Canada Web Standards and has not been altered or updated since it was archived. Please " contact us " to request a format other than those available.
This page has been archived on the Web.
Scope and purpose Principles Guidelines Quality indicators References
Scope and purpose
Data analysis is the process of developing answers to questions through the examination and interpretation of data. The basic steps in the analytic process consist of identifying issues, determining the availability of suitable data, deciding on which methods are appropriate for answering the questions of interest, applying the methods and evaluating, summarizing and communicating the results.
Analytical results underscore the usefulness of data sources by shedding light on relevant issues. Some Statistics Canada programs depend on analytical output as a major data product because, for confidentiality reasons, it is not possible to release the microdata to the public. Data analysis also plays a key role in data quality assessment by pointing to data quality problems in a given survey. Analysis can thus influence future improvements to the survey process.
Data analysis is essential for understanding results from surveys, administrative sources and pilot studies; for providing information on data gaps; for designing and redesigning surveys; for planning new statistical activities; and for formulating quality objectives.
Results of data analysis are often published or summarized in official Statistics Canada releases.
A statistical agency is concerned with the relevance and usefulness to users of the information contained in its data. Analysis is the principal tool for obtaining information from the data.
Data from a survey can be used for descriptive or analytic studies. Descriptive studies are directed at the estimation of summary measures of a target population, for example, the average profits of owner-operated businesses in 2005 or the proportion of 2007 high school graduates who went on to higher education in the next twelve months. Analytical studies may be used to explain the behaviour of and relationships among characteristics; for example, a study of risk factors for obesity in children would be analytic.
To be effective, the analyst needs to understand the relevant issues both current and those likely to emerge in the future and how to present the results to the audience. The study of background information allows the analyst to choose suitable data sources and appropriate statistical methods. Any conclusions presented in an analysis, including those that can impact public policy, must be supported by the data being analyzed.
Initial preparation
Prior to conducting an analytical study the following questions should be addressed:
Objectives. What are the objectives of this analysis? What issue am I addressing? What question(s) will I answer?
Justification. Why is this issue interesting? How will these answers contribute to existing knowledge? How is this study relevant?
Data. What data am I using? Why it is the best source for this analysis? Are there any limitations?
Analytical methods. What statistical techniques are appropriate? Will they satisfy the objectives?
Audience. Who is interested in this issue and why?
Suitable data
Ensure that the data are appropriate for the analysis to be carried out. This requires investigation of a wide range of details such as whether the target population of the data source is sufficiently related to the target population of the analysis, whether the source variables and their concepts and definitions are relevant to the study, whether the longitudinal or cross-sectional nature of the data source is appropriate for the analysis, whether the sample size in the study domain is sufficient to obtain meaningful results and whether the quality of the data, as outlined in the survey documentation or assessed through analysis is sufficient.
If more than one data source is being used for the analysis, investigate whether the sources are consistent and how they may be appropriately integrated into the analysis.
Appropriate methods and tools
Choose an analytical approach that is appropriate for the question being investigated and the data to be analyzed.
When analyzing data from a probability sample, analytical methods that ignore the survey design can be appropriate, provided that sufficient model conditions for analysis are met. (See Binder and Roberts, 2003.) However, methods that incorporate the sample design information will generally be effective even when some aspects of the model are incorrectly specified.
Assess whether the survey design information can be incorporated into the analysis and if so how this should be done such as using design-based methods. See Binder and Roberts (2009) and Thompson (1997) for discussion of approaches to inferences on data from a probability sample.
See Chambers and Skinner (2003), Korn and Graubard (1999), Lehtonen and Pahkinen (1995), Lohr (1999), and Skinner, Holt and Smith (1989) for a number of examples illustrating design-based analytical methods.
For a design-based analysis consult the survey documentation about the recommended approach for variance estimation for the survey. If the data from more than one survey are included in the same analysis, determine whether or not the different samples were independently selected and how this would impact the appropriate approach to variance estimation.
The data files for probability surveys frequently contain more than one weight variable, particularly if the survey is longitudinal or if it has both cross-sectional and longitudinal purposes. Consult the survey documentation and survey experts if it is not obvious as to which might be the best weight to be used in any particular design-based analysis.
When analyzing data from a probability survey, there may be insufficient design information available to carry out analyses using a full design-based approach. Assess the alternatives.
Consult with experts on the subject matter, on the data source and on the statistical methods if any of these is unfamiliar to you.
Having determined the appropriate analytical method for the data, investigate the software choices that are available to apply the method. If analyzing data from a probability sample by design-based methods, use software specifically for survey data since standard analytical software packages that can produce weighted point estimates do not correctly calculate variances for survey-weighted estimates.
It is advisable to use commercial software, if suitable, for implementing the chosen analyses, since these software packages have usually undergone more testing than non-commercial software.
Determine whether it is necessary to reformat your data in order to use the selected software.
Include a variety of diagnostics among your analytical methods if you are fitting any models to your data.
Refer to the documentation about the data source to determine the degree and types of missing data and the processing of missing data that has been performed. This information will be a starting point for what further work may be required.
Consider how unit and/or item nonresponse could be handled in the analysis, taking into consideration the degree and types of missing data in the data sources being used.
Consider whether imputed values should be included in the analysis and if so, how they should be handled. If imputed values are not used, consideration must be given to what other methods may be used to properly account for the effect of nonresponse in the analysis.
If the analysis includes modelling, it could be appropriate to include some aspects of nonresponse in the analytical model.
Report any caveats about how the approaches used to handle missing data could have impact on results
Interpretation of results
Since most analyses are based on observational studies rather than on the results of a controlled experiment, avoid drawing conclusions concerning causality.
When studying changes over time, beware of focusing on short-term trends without inspecting them in light of medium-and long-term trends. Frequently, short-term trends are merely minor fluctuations around a more important medium- and/or long-term trend.
Where possible, avoid arbitrary time reference points. Instead, use meaningful points of reference, such as the last major turning point for economic data, generation-to-generation differences for demographic statistics, and legislative changes for social statistics.
Presentation of results
Focus the article on the important variables and topics. Trying to be too comprehensive will often interfere with a strong story line.
Arrange ideas in a logical order and in order of relevance or importance. Use headings, subheadings and sidebars to strengthen the organization of the article.
Keep the language as simple as the subject permits. Depending on the targeted audience for the article, some loss of precision may sometimes be an acceptable trade-off for more readable text.
Use graphs in addition to text and tables to communicate the message. Use headings that capture the meaning ( e.g. "Women's earnings still trail men's") in preference to traditional chart titles ( e.g. "Income by age and sex"). Always help readers understand the information in the tables and charts by discussing it in the text.
When tables are used, take care that the overall format contributes to the clarity of the data in the tables and prevents misinterpretation. This includes spacing; the wording, placement and appearance of titles; row and column headings and other labeling.
Explain rounding practices or procedures. In the presentation of rounded data, do not use more significant digits than are consistent with the accuracy of the data.
Satisfy any confidentiality requirements ( e.g. minimum cell sizes) imposed by the surveys or administrative sources whose data are being analysed.
Include information about the data sources used and any shortcomings in the data that may have affected the analysis. Either have a section in the paper about the data or a reference to where the reader can get the details.
Include information about the analytical methods and tools used. Either have a section on methods or a reference to where the reader can get the details.
Include information regarding the quality of the results. Standard errors, confidence intervals and/or coefficients of variation provide the reader important information about data quality. The choice of indicator may vary depending on where the article is published.
Ensure that all references are accurate, consistent and are referenced in the text.
Check for errors in the article. Check details such as the consistency of figures used in the text, tables and charts, the accuracy of external data, and simple arithmetic.
Ensure that the intentions stated in the introduction are fulfilled by the rest of the article. Make sure that the conclusions are consistent with the evidence.
Have the article reviewed by others for relevance, accuracy and comprehensibility, regardless of where it is to be disseminated. As a good practice, ask someone from the data providing division to review how the data were used. If the article is to be disseminated outside of Statistics Canada, it must undergo institutional and peer review as specified in the Policy on the Review of Information Products (Statistics Canada, 2003).
If the article is to be disseminated in a Statistics Canada publication make sure that it complies with the current Statistics Canada Publishing Standards. These standards affect graphs, tables and style, among other things.
As a good practice, consider presenting the results to peers prior to finalizing the text. This is another kind of peer review that can help improve the article. Always do a dry run of presentations involving external audiences.
Refer to available documents that could provide further guidance for improvement of your article, such as Guidelines on Writing Analytical Articles (Statistics Canada 2008 ) and the Style Guide (Statistics Canada 2004)
Quality indicators
Main quality elements: relevance, interpretability, accuracy, accessibility
An analytical product is relevant if there is an audience who is (or will be) interested in the results of the study.
For the interpretability of an analytical article to be high, the style of writing must suit the intended audience. As well, sufficient details must be provided that another person, if allowed access to the data, could replicate the results.
For an analytical product to be accurate, appropriate methods and tools need to be used to produce the results.
For an analytical product to be accessible, it must be available to people for whom the research results would be useful.
Binder, D.A. and G.R. Roberts. 2003. "Design-based methods for estimating model parameters." In Analysis of Survey Data. R.L. Chambers and C.J. Skinner ( eds. ) Chichester. Wiley. p. 29-48.
Binder, D.A. and G. Roberts. 2009. "Design and Model Based Inference for Model Parameters." In Handbook of Statistics 29B: Sample Surveys: Inference and Analysis. Pfeffermann, D. and Rao, C.R. ( eds. ) Vol. 29B. Chapter 24. Amsterdam.Elsevier. 666 p.
Chambers, R.L. and C.J. Skinner ( eds. ) 2003. Analysis of Survey Data. Chichester. Wiley. 398 p.
Korn, E.L. and B.I. Graubard. 1999. Analysis of Health Surveys. New York. Wiley. 408 p.
Lehtonen, R. and E.J. Pahkinen. 2004. Practical Methods for Design and Analysis of Complex Surveys.Second edition. Chichester. Wiley.
Lohr, S.L. 1999. Sampling: Design and Analysis. Duxbury Press. 512 p.
Skinner, C.K., D.Holt and T.M.F. Smith. 1989. Analysis of Complex Surveys. Chichester. Wiley. 328 p.
Thompson, M.E. 1997. Theory of Sample Surveys. London. Chapman and Hall. 312 p.
Statistics Canada. 2003. "Policy on the Review of Information Products." Statistics Canada Policy Manual. Section 2.5. Last updated March 4, 2009.
Statistics Canada. 2004. Style Guide. Last updated October 6, 2004.
Statistics Canada. 2008. Guidelines on Writing Analytical Articles. Last updated September 16, 2008.
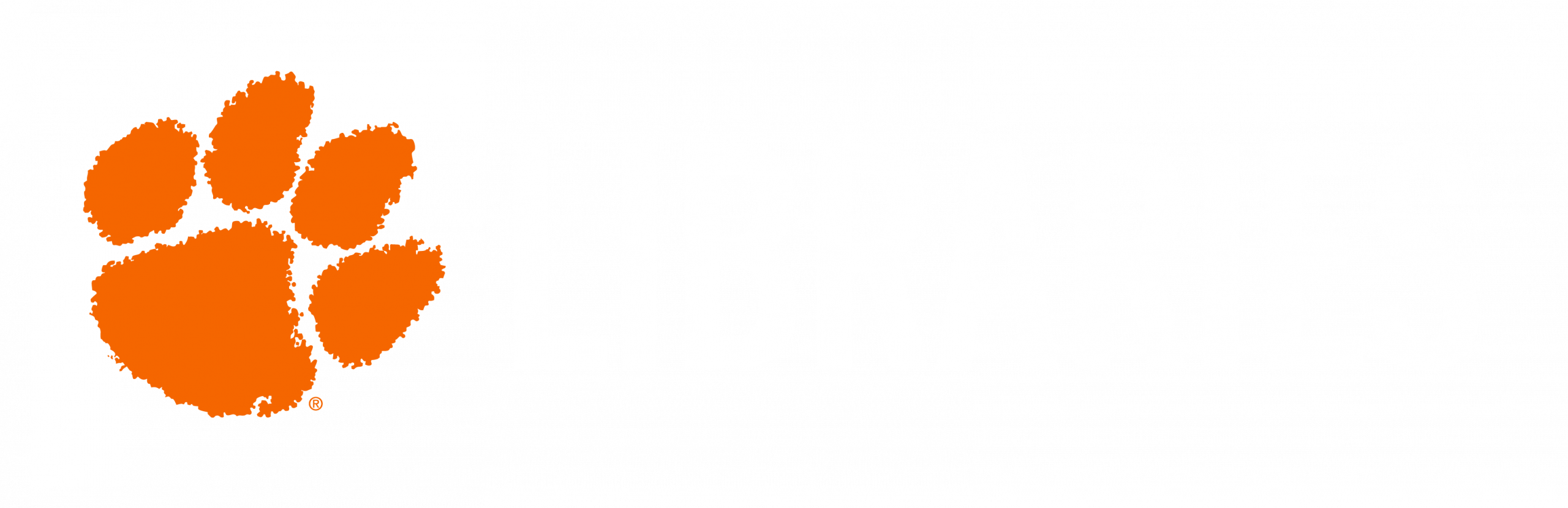
- Books, Articles & More
- Course Reserves
- Research & Course Guides
- Interlibrary Loan
- PASCAL Delivers
- Scan and Deliver
- Off-Campus Delivery Service
- Explore - State Park Passes
- Borrowing Privileges
- Textbook Lending
- Borrow from Area Libraries
- CU-ICAR Library
- Gunnin Architecture Library
- Education Media Center & Digital Media Learning Lab
- Historic Properties
- Special Collections and Archives
- Oral Histories
- Digital Collections
- Clemson Open
- Services for Community Members
- Finding Library Materials
- Borrowing Help
- Offsite Shelving
- Circulation Policies
- Library Fines and Fees
- Proxy Card Service
- Ask a Librarian
- Frequently Asked Questions
- Citation Management
- Subject Librarians
- Undergraduate Research Award
- Learning Commons
- Course Instruction
- Open Educational Resources (OER)
- Data Services
- Digital Projects Support
- Publishing & Copyright
- Clemson University Press
- Getting Started with Research
- Purchase Request
- Space Booking
- Library Locations
- Adobe Studio and Makerspace
- Digital Media Learning Lab
- Geospatial Center
- Data Visualization Lab
- Social Media Listening Center
- Starbucks - Cooper Library
- POD - Cooper Library
- Reflection Room
- Find Printers & Scanners
- Academic Success Center
- CCIT Support Center
- Michelin Career Center
- Writing Lab
- Visit the Libraries
- Locations & Parking
- Event Spaces
- Exhibit Spaces
- Welcome from the Dean
- Staff Directory
- Organizational Chart
- Employment Opportunities
- Annual Report 2023
- Giving Home
- Honor with Books Fund
- Friends of the Libraries
- Records Management
- Make a Suggestion
- Accessibility
- Cooper 2.0: A Vision for the Future
- LibQUAL+ 2023
- Clemson Libraries Advisory Committee
- Clemson Libraries Student Advisory Group
- Open Access Task Force
- R.M. Cooper Library
- Library Depot
- Facilities Projects and Updates
- Report a Facilities Issue
- Toggle Dropdown --> R.M. Cooper Library 8:00 AM - 10:00 PM ▾ CU-ICAR Library: 10:00 AM - 2:00 PM Cheezem Education Center (OLLI at Patrick Square): 9:00 AM - 4:00 PM Special Collections and Archives: 9:00 AM - 4:00 PM Gunnin Architecture Library: 8:30 AM - 4:30 PM Education Media Center: 8:00 AM - 4:30 PM -->
Data Visualization Lab offers assistance with data analysis and presentation
--> Posted on August 20, 2024
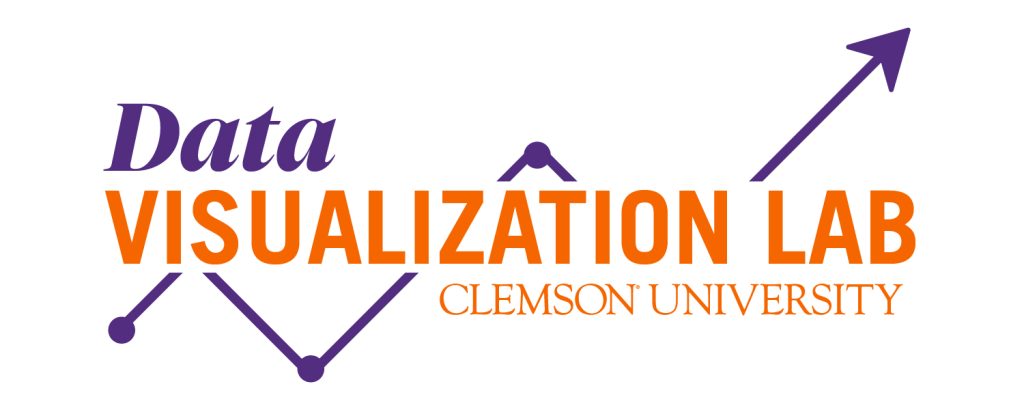
For the Fall of 2024, the lab will be open from 9 a.m. to 5 p.m. Monday through Friday. The lab will also host workshops and lunch-and-learn sessions throughout the semester.
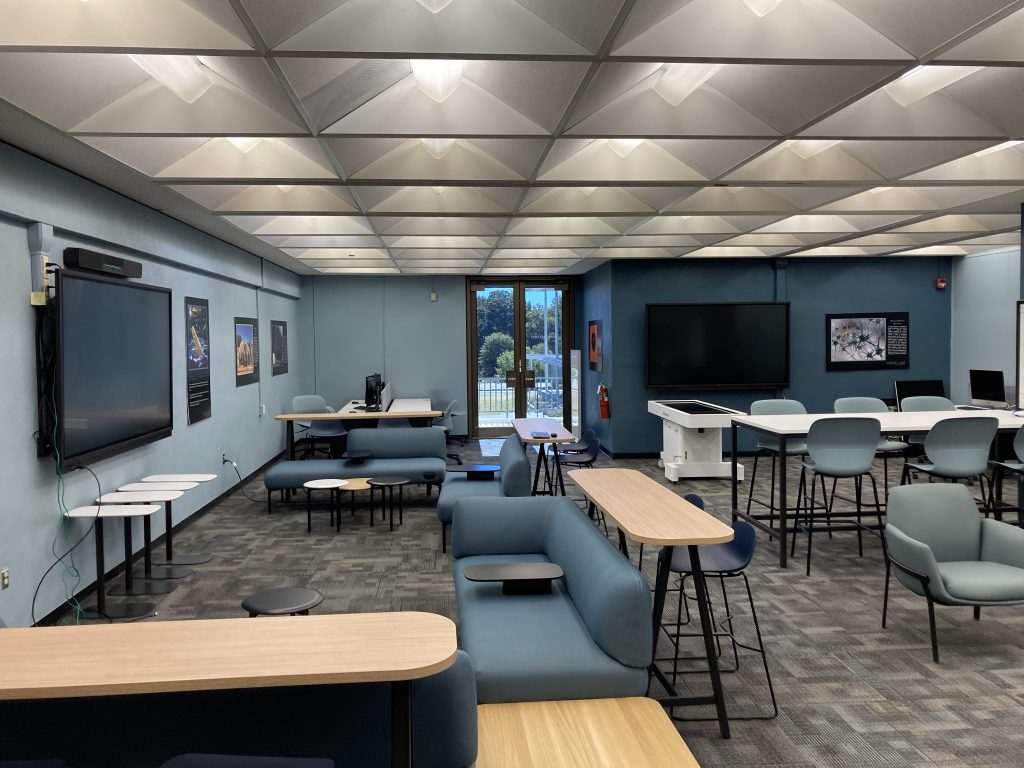
“The lab is here to support anyone on campus with their data — with sharing it, making visualizations with it, making it viewable and accessible,” said Data Services Librarian Stacie Powell. “We not only offer training on how to use data visualization software, but we also offer assistance to more advanced users who might need help with coding or other issues.”
Walk-ins during open hours are welcome, and appointments for assistance can be made online .

- SUGGESTED TOPICS
- The Magazine
- Newsletters
- Managing Yourself
- Managing Teams
- Work-life Balance
- The Big Idea
- Data & Visuals
- Reading Lists
- Case Selections
- HBR Learning
- Topic Feeds
- Account Settings
- Email Preferences
Where Data-Driven Decision-Making Can Go Wrong
- Michael Luca
- Amy C. Edmondson

When considering internal data or the results of a study, often business leaders either take the evidence presented as gospel or dismiss it altogether. Both approaches are misguided. What leaders need to do instead is conduct rigorous discussions that assess any findings and whether they apply to the situation in question.
Such conversations should explore the internal validity of any analysis (whether it accurately answers the question) as well as its external validity (the extent to which results can be generalized from one context to another). To avoid missteps, you need to separate causation from correlation and control for confounding factors. You should examine the sample size and setting of the research and the period over which it was conducted. You must ensure that you’re measuring an outcome that really matters instead of one that is simply easy to measure. And you need to look for—or undertake—other research that might confirm or contradict the evidence.
By employing a systematic approach to the collection and interpretation of information, you can more effectively reap the benefits of the ever-increasing mountain of external and internal data and make better decisions.
Five pitfalls to avoid
Idea in Brief
The problem.
When managers are presented with internal data or an external study, all too often they either automatically accept its accuracy and relevance to their business or dismiss it out of hand.
Why It Happens
Leaders mistakenly conflate causation with correlation, underestimate the importance of sample size, focus on the wrong outcomes, misjudge generalizability, or overweight a specific result.
The Right Approach
Leaders should ask probing questions about the evidence in a rigorous discussion about its usefulness. They should create a psychologically safe environment so that participants will feel comfortable offering diverse points of view.
Let’s say you’re leading a meeting about the hourly pay of your company’s warehouse employees. For several years it has automatically been increased by small amounts to keep up with inflation. Citing a study of a large company that found that higher pay improved productivity so much that it boosted profits, someone on your team advocates for a different approach: a substantial raise of $2 an hour for all workers in the warehouse. What would you do?
- Michael Luca is a professor of business administration and the director of the Technology and Society Initiative at Johns Hopkins University, Carey Business School.
- Amy C. Edmondson is the Novartis Professor of Leadership and Management at Harvard Business School. Her latest book is Right Kind of Wrong: The Science of Failing Well (Atria Books, 2023).

Partner Center
Academia.edu no longer supports Internet Explorer.
To browse Academia.edu and the wider internet faster and more securely, please take a few seconds to upgrade your browser .
Enter the email address you signed up with and we'll email you a reset link.
- We're Hiring!
- Help Center
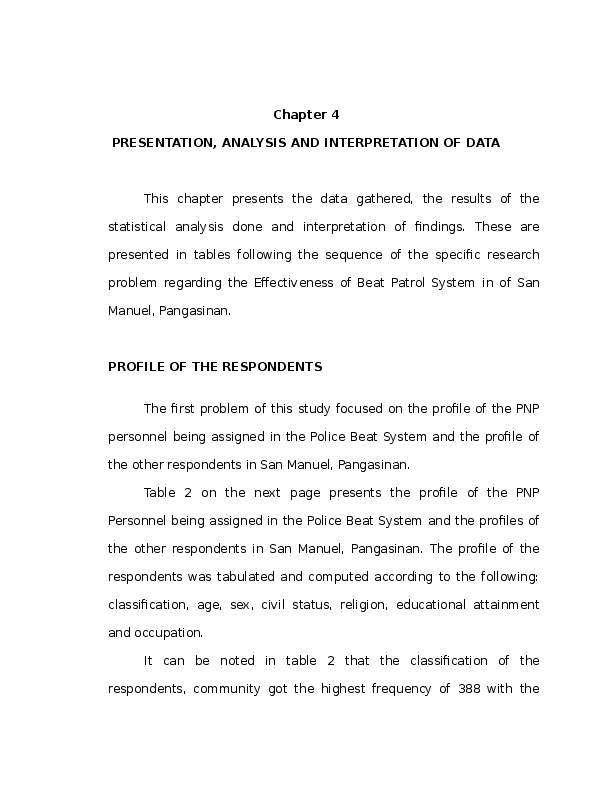
Chapter 4 PRESENTATION, ANALYSIS AND INTERPRETATION OF DATA

Related Papers
SMCC Higher Education Research Journal
Gian Venci Alonzo
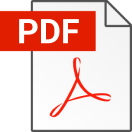
International Journal of Research -GRANTHAALAYAH
Sherill A . Gilbas
This paper highlights the trust, respect, safety and security ratings of the community to the Philippine National Police (PNP) in the Province of Albay. It presents the sectoral ratings to PNP programs. The survey utilized a structured interview with 200 sample respondents from Albay coming from different sectors. Male respondents outnumbered female respondents. The majority of the respondents are 41-50 years old, at least high school graduates and are married. The respondents gave the highest net rating on respect, followed by net rating on trust and the lowest net rating on safety and security on the performance of the PNP. Moreover, a high net rating on commitment of support to the identified programs of the PNP was also attained from the respondents. The highest net rating of support is given to the PNP’s anti-illegal drugs program, followed by anti-terrorism, anti-riding in tandem and anti-illegal gambling programs. The ratings of the PNP obtained from the different sectors of...
Charlie Rosales
IOER International Multidisciplinary Research Journal
IOER International Multidisciplinary Research Journal ( IIMRJ)
Police organizations have conducted operational activities to reduce the opportunity for would-be criminals to commit crimes. This operational activity includes patrol, traffic management, and investigation. In this study, the extent of police operational activities in Pagadian City, Zamboanga del Sur, Philippines, was evaluated to determine the extent of police operational activities and to test the association between the crime rate and the extent of police operation activities. This study utilized a quantitative descriptive research method. The respondents were 142 active police officers who were chosen purposively by employing total enumeration. The gathering of data was done using a self-made questionnaire, which underwent validation and reliability testing. The statistical tools used were frequency count, mean computation, percentage, and regression analysis. The results revealed that more respondents were 31-35 years old and above. Most of them were male, bachelor's degree holders, attended training and seminars for 50 hours, and served the police force for 15 years and below. Patrolling and investigation were found to be much observable while traffic management was observable. As for index crime, there were more crimes against the person committed than crimes against property. As for non-index crimes, there were more other non-index crimes compared to the violation of special laws. Patrolling has a positive influence on the commission and non-commission of both index and non-index crimes. This study also recommends intensive patrolling on hot-spot areas for criminal presence and activity, strengthening traffic management practices, procurement of traffic lights, improving traffic signs, and intensive implementation of traffic laws and regulations.
International Journal of Social Sciences and Humanities Research
Bro. Jose Arnold L . Alferez, OCDS
Effective law enforcement service demands that the law enforcement officers are diligent and effective in their duties and responsibilities. They should be punctual and alert while on their respective beats. They should respect the human rights of the people of the community they serve. They should even patrol their beats on foot so that their visibility would be more evident thus curtailing the criminal impulses of the criminally inclined, instead of whisking through the vicinity on the " flying visit" to their assigned places without even giving the people a glimpse of their presence. Transparency is the call of effective law enforcement service. However, effective law enforcement necessitates that the police command should be provided police equipment like two-way radios so that they could readily call for assistance whenever necessary, in order to improve the delivery of services and the maintenance of peace and order. Furthermore, a strong partnership between the police and the community will help ensure the success of the Philippine National Police in its drive against criminality. The findings of this study showed that the police force of the municipality of Pinamungajan, Cebu did their best under the circumstances they had to work in, but their efforts were not equally recognized by the people of the community. Hence, the need for support from the local officials and the people in the community are important factors that would facilitate the effectiveness of the law enforcement service.
Filius Populi
The Philippine National Police has implemented the new rank classification and abbreviation that shall be used in all manner of organization communications. Interview method was used to gather the information from the PNP RCADD respondents and selected community residents. Focus Group Discussion was conducted among the Barangay Officials to validate the data gathered. The findings of the study as follows: The respondents were not fully aware yet on the modified new rank classification applied in the PNP organization today; They shared diverse insights both positive and negative about the PNP modified new rank classification and it can offer a positive outcome in the long-run . Respondents were satisfied with the implementation of the PNP community relations program under the new rank classification. However, the modified new rank classification of the PNP would have the following positive implications: The new rank would mean higher people’s expectations; bring new image of the PNP;...
josefina B A L U C A N A G bitonio
DISSERTATION ABSTRACT Title : THE WOMEN AND CHILDREN PROTECTION SERVICES OF THE PHILIPPINE NATIONAL POLICE-CORDILLERA ADMINISTRATIVE REGION Researcher : MELY RITA D. ANAMONG-DAVIS Institution : Lyceum-Northwestern University, Dagupan City Degree : DOCTOR IN PUBLIC ADMINISTRATION Date : April 5, 2013 Abstract : This research sought to evaluate the provision of services provided by the members of the Women and Children Protection Desk of the Philippine National Police (PNP) Cordillera Administrative Region . The descriptive-evaluative research design was used in this study with the questionnaire, interviews as the data- gathering tool in the evaluation of the WCPD of the PNP services rendered to the victims-survivors of violence in the Cordillera Region. The types and statistics of cases investigated by the members of the WCPD of the PNP in the Cordillera were provided by the different offices of the WCPD of the PNP particularly the Regional and Provincial Offices. On the other hand, the acquired data from the respondents describes the capability of the WCPD office and personnel, relative to the organizational structure, financial resources, human resources, equipment and facilities; the extent of the mandated services provided for the victims-survivors of violence, level of satisfaction of the WCPD clientele and the problems encountered by the members of the WCPD of the PNP in providing the services to its clientele. Based on the findings, a proposal were formulated to enhance the quality or quantity of the services rendered to the victims of abuses and violence. Two hundred thirty (230) respondents were employed to answer the questionnaire to get the needed data, 160 from the police officers and 70 from the clientele of the WCPD. In the treatment of the data, SPSS version 20 was used in the analysis of data, Paired t-test for the determination of the significant difference in the perceptions of the two groups of respondents on the extent of provision of the mandated services by the WCPD of the PNP in the Cordillera and Spearman rank correlation for determining the level of satisfaction of the victims-survivors related to their perception on the extent of services provided by the Women and Children Protection Desk of the PNP. The findings of the study were the following: 1) Cases handled by the members of the WCPD of the PNP are physical injuries, violation of RA 9262, Rape and Acts of lasciviousness are the myriad cases committed against women; for crimes against children, rape, physical injuries, other forms of RA 7610 and acts of lasciviousness ; and for the crimes committed by the Children in Conflict with the law theft and robbery for intent to gain and material gain, physical injuries, rape and acts of lasciviousness are the majority they committed. The fact of this case is that 16 children were involved in the commission of rape where the youngest perpetrator is 7 years old. 2. On the capability of the members of the WCPD of the PNP, police officers believed that WCPD investigators are capable in providing the services to the victims of violence while the clientele respondents states otherwise that on some point along capability on human resources states that the number of police women assigned with the WCPD of the PNP is not sufficient to provide the services to its clientele. 3. On the extent of the mandated services provided to the victims-survivors of violence by the members of the WCPD of the PNP, perceptions of the police officers that to a great extent the members of the WCPD provide the services while the perceptions of the clientele is just on average extent on the services provided to them. 3.1. On the significant difference in the perceptions of the two groups of respondent on the extent of provision of the mandated services by the WCPD of the PNP in the Cordillera, there is a significant difference in the perception of the two groups of respondents on the extent of mandated services provided by the WCPD of the PNP in the Cordillera. The result indicates that the performance of the WCPD in rendering service is inadequate in the perception of its clients. 4) The satisfaction level of the clientele on the extent of services provided by the WCPD of the PNP is just moderate. This validates the result of the extent of the mandated services provided to the victims-survivors of violence by the WCPD investigators to be just on average. 5. On the level of satisfaction of the victims-survivors related to their perception on the extent of services provided by the Women and Children Protection Desk of the PNP revealed that WCPD clients is higher with greater extent of services being rendered by the WCPD. It indicates that the WCPD of the PNP in Cordillera should strive more to really fulfill the needed services to be provided with its clients. Likewise, on the level of satisfaction of the victims-survivors related to the capability of the WCPD of the PNP Cordillera in providing their mandated services disclosed that the more capable of the WCPD of the PNP in Cordillera will definitely provide an intense delivery of services to its clients. 7) Lastly, for the problems encountered by the WCPD of the PNP in providing services the following are considered a) no imagery tool kit purposely for the children’s victim to illicit information regarding the incident; b) the insufficient number of female police officers to investigate cases of women and children; c) lack of training of WCPD officers in handling VAWC cases and other gender-based crimes and d) service vehicle purposely for WCPD use only. Based on the findings and conclusion, the following recommendations are offered. 1. The propose strategies to enhance the services provided to the victims-survivors by the WCPD investigators must be intensely implemented: 1.a. There should be budgetary allocations for WCPD to enhance their capability to provide services and to fulfill the satisfaction of their clientele. 1.b. Increase the number of the female police officers assigned with the WCPD to sustain the 24/7 availability of investigators. 1.c. There should be a continuous conduct of specialized training on the Investigation of Crimes involving Women and children to all WCPD officers to include policemen for conclusive delivery of services for the victims of violence. 1.d. Purchase of the imagery tool kit purposely for the children’s victim of sexual abuse to illicit information regarding the incident.1.e. Issuance of service vehicle purposely for the Women and Children Protection Desk.1.f. Provide computer sets for WCPD.1.g. Provide communication equipment to be issued with the WCPD. 1.h. To improve the quality and consistency of WCPD services, a constant monitoring scheme and or clientele feedback should be implemented to understand the ways that service can be improved. 1.i. Develop and sustain the collaborative effort of the multidisciplinary team to meet the specific protocol designed to meet the needs of the victims of violence.1.j. To prevent new victims of violence, there should be a persistent campaign through advocacy and the education of the community in every barangay in coordination with the different member agencies. 2. A follow-up study should be conducted to cover other areas particularly target respondents on the level of satisfaction on the services provided for the victims of violence which is the main purpose of the establishment of the Women and Children Protection Desk.
Maita P Guadamor
Loading Preview
Sorry, preview is currently unavailable. You can download the paper by clicking the button above.
RELATED PAPERS
Joanna Tolentino
Neiza Valerio
Texas State PA Applied Research Projects
International Journal of Advanced Research in Management and Social Sciences
Melody Utang
International Review of Humanities Studies
bayu suseno
Research on humanities and social sciences
melkisedek neolaka
Proceedings of the International Conference on Social Science 2019 (ICSS 2019)
Elvis Lumingkewas
MARK PATALINGHUG
johnmarkoy johnmarkoy
Carlos Barrachina
januaryn Jose Aydinan
The International Journal of Social Sciences and Humanities Invention
Mohammad Mujaheed Hassan
Mark Joseph Oñate
East Asian Journal of Multidisciplinary Research
Russel Aporbo
eugene loria
Peter Kreuzer
Eula De Luna
La Ode Husen
Judge Eliza B. Yu
Publisher ijmra.us UGC Approved
- We're Hiring!
- Help Center
- Find new research papers in:
- Health Sciences
- Earth Sciences
- Cognitive Science
- Mathematics
- Computer Science
- Academia ©2024
More From Forbes
How to upskill family office employees. five steps to get started..
- Share to Facebook
- Share to Twitter
- Share to Linkedin
As family offices continue to evolve with technological advancements, the need to upskill employees ... [+] across a wide array of remits has become crucial.
Among technological progress and societal shifts, there is a global trend to upskill and re-skill employees; the World Economic Forum's Future of Jobs Report indicated in 2023 that 44% of workers' skills will be disrupted in the next five years thanks to automation, AI, and other new developments that will significantly change our expectation of, and approach to, work.
Similarly, as family offices continue to evolve with technological advancements, the need to upskill employees has become crucial; the integration of digitisation, artificial intelligence (AI), and new software tools (including the transition from Excel ) is reshaping the family office landscape - creating a demand for employees who are adept at using these technologies.
The Importance of Upskilling
In the fast-paced environment of family offices, the ability to adapt to technological changes is vital. Upskilling employees ensures that they can navigate the complexities of new software and AI tools, ultimately enhancing operational efficiency and decision-making.
With the added risk of poor cybersecurity, upskilling family office employees can be a positive step in mitigating risks and ensuring that family office operations remain secure.
Additionally, investing in employee development fosters a culture of continuous learning. Given that many family offices struggle to attract top talent, this is a great approach to garner the attention of new candidates and cultivate higher employee satisfaction and retention.
‘Inside Out 2’ Debuts On Digital Streaming This Week
Nyt ‘strands’ hints, spangram and answers for sunday, august 18th, acclaimed horror thriller oddity debuts on digital streaming this week, technology-focused upskilling areas.
While family offices are many and varied, there are generally five key areas that - given their sophistication and nuance - are essential for staff to upskill in and understand.
Digital Literacy
Understanding basic digital tools and platforms is foundational.
Employees should be comfortable using cloud-based software, data management systems, and communication tools to perform their roles effectively. Many family offices in generational transition may have principals or staff more familiar with analogue or dated tool sets.
For family offices to cater to newer generations, understanding digital tooling and both its advantages and limitations is a great starting place.
Data Analysis and Interpretation
With the increasing reliance on data-driven decision-making, employees must be skilled in analysing and interpreting data.
Training in data visualisation tools and statistical analysis can empower employees to extract meaningful insights from complex datasets.
This can be made more pressing by either a shift from Excel to new tools, or by simply adopting one or several new software platforms. Ensuring integrity of data and information in this transition is critical - and this focus area can help navigate what can typically be a complex change.
AI and Machine Learning
While AI has been a buzzword for many years, the topic has reached fever pitch in recent times thanks to the proliferation of LLMs.
While few family office employees need to be AI experts, familiarity with AI and machine learning concepts can help employees understand how these technologies can be applied to streamline operations and improve services.
Offering workshops or courses on AI basics can demystify these technologies and encourage innovative thinking. Similarly, familiarity with these working areas can also bolster a foundational understanding of digital security and information retention.
Cybersecurity Awareness
On that topic, cybersecurity is paramount
Employees should be trained in best practices for protecting data, recognising phishing attempts, and maintaining secure communication channels.
In a recent poll, 54% of family office respondents indicated that they had dedicated external resources towards preventing cybersecurity breaches - a positive start. However, what remains crucial is to involve operational staff in educational and operational training to ensure that this awareness and skillset reaches all hands at once.
Financial Technology (FinTech)
The integration of FinTech solutions is transforming financial services.
While deep expertise need not be needed, employees should be knowledgeable about emerging financial technologies, such as blockchain and digital currencies, to stay ahead of industry trends.
The task of managing complex financial instruments in a family office is only set to become more challenging as financial instruments similarly become more complex. An appreciation of newer trends, and an investigation where new technologies become salient, should be key for staff.
Practical Upskilling Strategies
To enrich their staff’s skills, family offices can develop customised training programmes that address the specific needs and goals of the family office. This can include workshops, seminars, and online courses that focus on relevant skills and technologies.
Similarly, encouraging a culture of mentorship where experienced employees share their knowledge with colleagues can provide beneficial; Peer learning groups can also facilitate knowledge exchange and foster collaboration.
Where generational attitudes may differ, involving employees in cross-functional projects that require them to apply new skills and collaborate with different teams can reinforce learning and promote a deeper understanding of how different functions interconnect.
The Role of Leadership in Upskilling
Family office principals and leaders must demonstrate a commitment to employee development by allocating resources and prioritising upskilling initiatives. Leaders should actively participate in training sessions and communicate the value of upskilling to the entire team. in order to cultivate a culture that encourages curiosity and experimentation.
Similarly, acknowledging and rewarding employees who actively engage in upskilling efforts could come in the form of promotions, bonuses, or public acknowledgment of achievements.
5 Short Steps To Get Started
Identify skill gaps: Conduct a thorough analysis of current and future skill needs.
Create personalised learning plans: Develop tailored development paths for each employee.
Allocate dedicated learning time: Schedule regular training sessions and provide flexible learning options.
Foster a learning culture: Encourage knowledge sharing and peer-to-peer learning.
Measure and evaluate: Track training outcomes and adjust your program accordingly.
By focusing on key areas of education and implementing practical strategies, family offices can ensure their teams are well-equipped to thrive in a rapidly changing landscape.
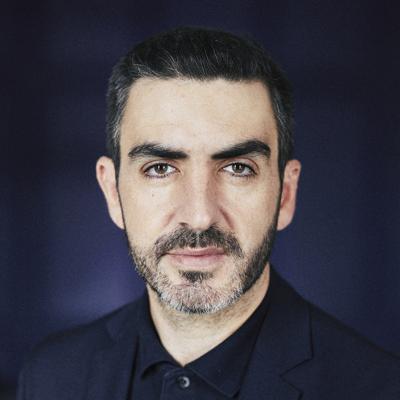
- Editorial Standards
- Reprints & Permissions
Join The Conversation
One Community. Many Voices. Create a free account to share your thoughts.
Forbes Community Guidelines
Our community is about connecting people through open and thoughtful conversations. We want our readers to share their views and exchange ideas and facts in a safe space.
In order to do so, please follow the posting rules in our site's Terms of Service. We've summarized some of those key rules below. Simply put, keep it civil.
Your post will be rejected if we notice that it seems to contain:
- False or intentionally out-of-context or misleading information
- Insults, profanity, incoherent, obscene or inflammatory language or threats of any kind
- Attacks on the identity of other commenters or the article's author
- Content that otherwise violates our site's terms.
User accounts will be blocked if we notice or believe that users are engaged in:
- Continuous attempts to re-post comments that have been previously moderated/rejected
- Racist, sexist, homophobic or other discriminatory comments
- Attempts or tactics that put the site security at risk
- Actions that otherwise violate our site's terms.
So, how can you be a power user?
- Stay on topic and share your insights
- Feel free to be clear and thoughtful to get your point across
- ‘Like’ or ‘Dislike’ to show your point of view.
- Protect your community.
- Use the report tool to alert us when someone breaks the rules.
Thanks for reading our community guidelines. Please read the full list of posting rules found in our site's Terms of Service.

An official website of the United States government
The .gov means it’s official. Federal government websites often end in .gov or .mil. Before sharing sensitive information, make sure you’re on a federal government site.
The site is secure. The https:// ensures that you are connecting to the official website and that any information you provide is encrypted and transmitted securely.
- Publications
- Account settings
Preview improvements coming to the PMC website in October 2024. Learn More or Try it out now .
- Advanced Search
- Journal List
- HHS Author Manuscripts
- PMC10370480

Distributional Cost-Effectiveness of Equity-Enhancing Gene Therapy in Sickle Cell Disease in the United States
Author Contributions : Conception and design: G. Goshua, L. Krishnamurti, A. Pandya.
Drafting of the article: G. Goshua, C. Calhoun, L.P. James, A. Luviano, A. Pandya.
Critical revision for important intellectual content: G. Goshua, C. Calhoun, S. Ito, L.P. James, L. Krishnamurti, A. Pandya.
Final approval of the article: G. Goshua, C. Calhoun, S. Ito, L.P. James, A. Luviano, L. Krishnamurti, A. Pandya.
Statistical expertise: G. Goshua, L.P. James, A. Pandya.
Administrative, technical, or logistic support: G. Goshua.
Collection and assembly of data: G. Goshua.
Associated Data
Background:.
Gene therapy is a potential cure for sickle cell disease (SCD). Conventional cost-effectiveness analysis (CEA) does not capture the effects of treatments on disparities in SCD, but distributional CEA (DCEA) uses equity weights to incorporate these considerations.
To compare gene therapy versus standard of care (SOC) in patients with SCD by using conventional CEA and DCEA.
Markov model.
Data Sources:
Claims data and other published sources.
Target Population:
Birth cohort of patients with SCD.
Time Horizon:
Perspective:.
U.S. health system.
Intervention:
Gene therapy at age 12 years versus SOC.
Outcome Measures:
Incremental cost-effectiveness ratio (ICER) (in dollars per quality-adjusted life-years [QALYs] gained) and threshold inequality aversion parameter (equity weight).
Results of Base-Case Analysis:
Gene therapy versus SOC for females yielded 25.5 versus 15.7 (males: 24.4 vs. 15.5) discounted lifetime QALYs at costs of $2.8 million and $1.0 million (males: $2.8 million and $1.2 million), respectively, with an ICER of $176 000 per QALY (full SCD population). The inequality aversion parameter would need to be 0.90 for the full SCD population for gene therapy to be preferred per DCEA standards.
Results of Sensitivity Analysis:
SOC was favored in 100.0% (females) and 87.1% (males) of 10 000 probabilistic iterations at a willingness-to-pay threshold of $100 000 per QALY. Gene therapy would need to cost less than $1.79 million to meet conventional CEA standards.
Limitation:
Benchmark equity weights (as opposed to SCD-specific weights) were used to interpret DCEA results.
Conclusion:
Gene therapy is cost-ineffective per conventional CEA standards but can be an equitable therapeutic strategy for persons living with SCD in the United States per DCEA standards.
Patients with sickle cell disease (SCD) face substantial mortality risks and decreased quality of life for every year they live with the disease ( 1 ). Once approved in the United States, gene therapy treatment for eligible patients living with SCD would allow the possibility of lifelong disease remission without the concomitant risks associated with allo-transplantation, such as graft-versus-host disease.
Based on previously approved indications, gene therapy administered 1 time for patients with SCD may cost more than $2 million per treated patient ( 2 , 3 ). Although gene therapy is cost-ineffective when it is theoretically administered at birth using a willingness-to-pay threshold of $100 000 per quality-adjusted life-year and conventional cost-effectiveness criteria in the United States ( 4 ), these estimates do not quantitatively account for health inequities among patients with SCD. Specifically, conventional cost-effectiveness analysis (CEA) weighs all outcomes equally across the population, regardless of what subpopulations receive the health benefits or incur the costs of additional health care spending. Although the First Panel on Cost-Effectiveness in Health and Medicine recommended in 1996 that researchers highlight the distributive implications of CEA results, the authors did not recommend explicitly weighting outcomes to provide a quantitative recommendation directly informed by distributive equity ( 5 ). Since then, newer methods, such as distributional CEA (DCEA), have emerged and were endorsed by the Second Panel on Cost-Effectiveness in Health and Medicine in 2016 to quantitatively weigh potential tradeoffs between conventional cost-effectiveness results and their effects on existing health disparities ( 6 ). However, such equity-informed CEAs have rarely been conducted for North American settings ( 7 ). We sought to begin to address this knowledge gap by conducting a DCEA for gene therapy in SCD to weigh the tradeoffs between cost-effectiveness and the effect of this treatment on the health disparity between persons with and without SCD.
Overview of DCEA
Per conventional CEA theory, total population health decreases when any part of a limited budget is spent on cost-ineffective care instead of cost-effective options. However, this notion of opportunity cost does not account for the effect of health care spending on salient health disparities. Distributional CEA, a newer method, uses equity weights to combine these effects on disparities with conventional CEA outcomes. If the societal weight placed on a more equal distribution of health in the population is high enough, DCEA and conventional CEA can result in different recommendations for conventionally cost-effective interventions that exacerbate existing disparities or conventionally cost-ineffective interventions that successfully reduce health disparities, as would be the case for a gene therapy cure for SCD at sufficiently high prices. The total cost of a gene therapy cure for SCD would be paid by commercially insured persons with SCD (through co-insurance and health insurance premium payments) and without SCD (only through premiums) and by taxpayers (for example, for patients with SCD who are covered by Medicaid). When equity receives no weight (that is, when the inequality aversion parameter is equal to zero in the commonly used Atkinson Index–based DCEA formulation), the opportunity costs of this spending are weighed against the health gains among patients with SCD who are receiving the gene therapy cure. When the inequality aversion parameter is greater than zero, DCEA gives additional credit to the gene therapy cure for creating a more equal distribution of health and cost outcomes between persons with and without SCD. We describe DCEA methods in more detail in the Supplement (available at Annals.org ).
Study Cohort
We built a Markov simulation model of patients with a diagnosis of mild, moderate, or severe SCD. Disease severity was based on the annual number of vaso-occlusive crises requiring hospitalization, which has been used in clinical trials and has previously been shown to affect patient quality of life ( 8 – 11 ). Specifically, mild disease is classified as zero vaso-occlusive crises requiring hospitalization per year, moderate disease is classified as 1 vaso-occlusive crisis requiring hospitalization per year, and severe disease is classified as 2 or more vaso-occlusive crises requiring hospitalization per year. We used a prior published analysis of commercial claims data from the OptumRx database to inform cost and natural history of disease inputs ( 4 ). Patients with SCD in this data set were propensity score matched to patients without SCD based on the following covariates: race, sex, geographic division, year of birth, index year, plan characteristics, and education.
Comparators
We compared 2 treatment strategies for treating females and males with SCD: gene therapy versus the standard of care (SOC) ( Figure 1 ). Standard of care for patients with SCD includes hydroxyurea-anchored treatment, attention to recommended vaccine maintenance, and early and prompt treatment of pain and infection with opioid and antibiotic therapy, respectively. It also includes judicious blood transfusion support that provides necessary transfusions while balancing lifelong risks for allo-immunization. Since 2017, the U.S. Food and Drug Administration (FDA) has approved 3 additional therapeutic options for patients with SCD: L-glutamine, crizanlizumab, and voxelotor. L-glutamine and crizanlizumab effectively decrease the burden of vaso-occlusive crises for patients with SCD, and voxelotor increases hemoglobin levels ( 8 , 9 , 11 ). We assumed an age of 12 years at the start of treatment, corresponding to the earliest age in current prospective clinical trial data in gene modification and gene addition therapy ( 12 , 13 ).

State transition diagram.
In the standard of care strategy, patients aged 12 years start in 1 of 3 states of SCD severity (mild, moderate, or severe) and transition every year in a 3×3 matrix to 1 of 3 disease severity states or the dead state due to SCD-specific and background mortality. In the gene therapy strategy, patients aged 12 years start in disease remission, having received gene therapy at age 12 years, and transition to the dead state due to background mortality. SCD = sickle cell disease.
Simulation Model
Transition-state cycles were 12 months in duration with lifetime simulation (lifetime time horizon) to estimate the expected benefits and costs of gene therapy compared with SOC through the end of life, as previously published by Salcedo and colleagues ( 4 ). For the SOC treatment strategy, the patient proceeded from their initial disease severity state (mild, moderate, or severe) and cycled through time-varying disease severity as reflected in 11 years of real-world utilization data in commercially insured patients with SCD (2007 to 2017; OptumRx), with SOC involving treatment with hydroxyurea, opioid therapy, antibiotics, vaccinations, blood transfusions, and stem cell transplantation. For the gene therapy treatment strategy, the patient was assumed to be in lifelong remission from SCD, with data informed by propensity score–matched control patients, as reflected in the same 11 years of real-world data (2007 to 2017; OptumRx).
Annual background mortality probabilities specific to age, sex, race or ethnicity, and disease for patients living with SCD under the SOC strategy were informed by a cohort simulation model of patients in the United States that compared life expectancy in a prevalent SCD cohort with that of a matched cohort of persons without SCD ( 1 ). These were derived from known estimates of SCD-specific birth and mortality rates spanning 2007 to 2016, as reported by the Centers for Disease Control and Prevention ( 14 ). For patients treated successfully with gene therapy, the background mortality probability was assumed to return to the age-, sex-, and race-or ethnicity-specific baseline of the general population living without SCD, which was obtained from the 2018 U.S. Life Tables found in National Vital Statistics Reports ( 15 ). Base-case estimates and ranges for all input parameters used in the model are reported in Table 1 , model verification is summarized in Supplement Figure 1 and Supplement Table 1 (available at Annals.org ), and corresponding clinical estimates are shown in Supplement Table 2 (available at Annals.org ).
Base-Case Input Parameters and Probability Distributions
Input Parameter | Value | Probability Distribution Used in Probabilistic Sensitivity Analysis | Source | |||
---|---|---|---|---|---|---|
2 450 000 | 2100 000 to 2 800 000 | References and | ||||
0.03 | − | Reference | ||||
12 | 12 to 50 | Range from clinical trials ( , ) | ||||
Derived from validation model of 2007–2017 OptumRx data ( ) | ||||||
Females | ||||||
Mild | 56.0 | - | ||||
Moderate | 12.7 | - | ||||
Severe | 29.3 | - | ||||
Dead | 2.0 | − | ||||
Males | ||||||
Mild | 56.4 | - | ||||
Moderate | 14.0 | - | ||||
Severe | 27.3 | - | ||||
Dead | 2.3 | |||||
References , , and | ||||||
Age 1–18 y | 0.69 | -PERT (0.57 to 0.80) | ||||
Age >18 y | 0.68 | -PERT (0.67 to 0.69) | ||||
References , , and | ||||||
Women | ||||||
Age 1–44 y | 0.89 | ( = 870.4, =107.6) | ||||
Age 45–54 y | 0.87 | ( = 983.1, =146.9) | ||||
Age 55–64 y | 0.84 | ( = 1128.1, =214.9) | ||||
Age 65–74 y | 0.84 | ( = 1128.1, =214.9) | ||||
Age ≥ 75 y | 0.82 | ( = 1209.5, = 265.5) | ||||
Men | ||||||
Age 1–44 y | 0.89 | ( = 870.4, =107.6) | ||||
Age 45–54 y | 0.88 | ( = 928.4, =126.6) | ||||
Age 55–64 y | 0.86 | ( = 1034.6, = 168.4) | ||||
Age 65–74 y | 0.87 | ( = 983.1, =146.9) | ||||
Age ≥ 75 y | 0.85 | ( = 1082.9, = 191.1) | ||||
OptumRx, 2007–2017( ) | ||||||
Women | ||||||
Cut point 1 | 1.04 | -PERT (0.76 to 1.32) | ||||
Cut point 2 | 1.86 | -PERT (1.57 to 2.15) | ||||
Moderate SCD | 1.07 | -PERT (0.64 to 1.51) | ||||
Severe SCD | 2.63 | -PERT (2.19 to 3.06) | ||||
Age | −0.03 | -PERT (−0.03 to −0.02) | ||||
Interaction of age and moderate SCD | 0.01 | -PERT (0 to 0.02) | ||||
Interaction of age and severe SCD | 0.02 | -PERT (0.01 to 0.03) | ||||
Men | ||||||
Cut point 1 | 0.79 | -PERT (0.49 to 1.08) | ||||
Cut point 2 | 1.60 | -PERT (1.30 to 1.91) | ||||
Moderate SCD | 0.70 | -PERT (0.23 to 1.17) | ||||
Severe SCD | 1.98 | -PERT (1.53 to 2.42) | ||||
Age | −0.03 | -PERT (−0.04 to −0.02) | ||||
Interaction of age and moderate SCD | 0.01 | -PERT (0 to 0.03) | ||||
Interaction of age and severe SCD | 0.03 | -PERT (0.02 to 0.05) | ||||
OptumRx, 2007–2017( ) | ||||||
Women | ||||||
Intercept | 8.05 | -PERT (7.88 to 8.22) | ||||
Mild SCD | 1.58 | -PERT (1.30 to 1.85) | ||||
Moderate SCD | 1.78 | -PERT (1.48 to 2.09) | ||||
Severe SCD | 3.16 | -PERT (2.88 to 3.45) | ||||
Age | 0.03 | -PERT (0.03 to 0.04) | ||||
Interaction of age and mild SCD | −0.01 | -PERT (−0.02 to −0.01) | ||||
Interaction of age and moderate SCD | −0.01 | -PERT (−0.02 to −0.01) | ||||
Interaction of age and severe SCD | −0.02 | -PERT (−0.03 to −0.02) | ||||
Men | ||||||
Intercept | 8.39 | -PERT (7.51 to 9.28) | ||||
Mild SCD | 2.31 | -PERT (1.16 to 3.09) | ||||
Moderate SCD | 1.14 | -PERT (0.21 to 2.07) | ||||
Severe SCD | 2.36 | -PERT(1.46 to 3.27) | ||||
Age | 0.02 | -PERT (0.01 to 0.04) | ||||
Interaction of age and mild SCD | −0.02 | -PERT (−0.03 to 0) | ||||
Interaction of age and moderate SCD | 0.01 | -PERT (−0.02 to 0.03) | ||||
Interaction of age and severe SCD | −0.01 | -PERT (−0.02 to 0.01) |
PERT = program evaluation and review technique; SCD = sickle cell disease.
We constructed our model using TreeAge Pro Healthcare 2023 (TreeAge Software). The CHEERS (Consolidated Health Economic Evaluation Reporting Standards) reporting guideline was implemented where applicable.
Health and Cost Outcomes
Health outcomes estimated by our model were quantified using quality-adjusted life-years (QALYs), a measure that accounts for both health-related quality of life and length of life. We describe these methods in more detail in the Supplement . All costs were estimated in 2022 U.S. dollars ( 18 ). We used a prior published analysis of commercial claims data from OptumRx to inform annual costs of care under SOC. All costs and transition probabilities by year are provided in the Supplement . The cost of gene therapy ($2 450 000) was estimated as the average of pricing for gene therapy in other approved indications, such as spinal muscular atrophy ($2 100 000) and β -thalassemia ($2 800 000) ( 2 , 3 ). Both cost and health outcomes were discounted by 3% annually ( 19 ). We describe the threshold inequality aversion parameter in more detail in the Supplement .
Conventional CEA and DCEA Outcomes
We performed a conventional CEA from a health care sector perspective, comparing the incremental cost-effectiveness ratio (ICER) of a gene therapy cure versus SOC with a cost-effectiveness threshold of $100 000 per QALY ( 20 , 21 ). For the DCEA, we quantified the threshold equity weight that would set a gene therapy cure intervention equal to the SOC strategy and compared this threshold equity weight with established conventions (the range of commonly used inequality aversion parameters in the United States and Canada is 0.5 to 3.0) ( 22 , 23 ).
Sensitivity Analyses
We performed 1-way sensitivity analyses and scenario analyses to evaluate the effect of specific input parameter values and assumptions on our results. For deterministic sensitivity analyses, we used a 20% multiplicative factor of the base case with 2 exceptions: the range of real-world gene therapy costs for previously approved indications (minimum of $2.1 million in spinal muscular atrophy and maximum of $2.8 million in β -thalassemia), and the gene therapy initiation age range that was compatible with the widest inclusion criteria used in clinical trials (ages 12 to 50 years [ Table 1 ]). The latter was informed for every relevant age by using starting distributions of disease severity in a cohort trace generated from age 0 years under SOC in the model verification. We present a tornado diagram showing the effect of all parameters for which at least a 10% change in the ICER was observed in either direction. We conducted an additional threshold analysis for the cost of gene therapy to identify a cost below which gene therapy would become cost-effective per conventional and distributional CEA criteria. Furthermore, we propagated the sampling uncertainty in the input parameters to the outcomes of our Markov model by performing a probabilistic sensitivity analysis, which is explained further in the Supplement . We also performed scenario analyses for gene therapy duration with effectiveness lasting 10 or 20 years (as opposed to our base-case assumption of lifetime benefit) and analyses that excluded 18.9% of patients with SCD from being eligible for gene therapy because of comorbid pulmonary hypertension or heart failure ( 24 ).
Role of the Funding Source
The funding sources—the Yale Bernard G. Forget Scholars Program, Bunker Endowment, and the National Institute of Allergy and Infectious Diseases—had no role in the study design; collection, analysis, or interpretation of the data; writing of the manuscript; or the decision to submit the manuscript for publication.
Base-Case Conventional CEA
The estimated total cost and QALYs associated with each treatment strategy at a lifetime horizon are reported in Table 2 . For females with SCD, gene therapy versus SOC starting at age 12 years yielded 25.5 versus 15.7 discounted lifetime QALYs at costs of $2.8 million and $1.0 million, respectively. For males with SCD, gene therapy versus SOC starting at age 12 years yielded 24.4 versus 15.5 discounted lifetime QALYs at costs of $2.8 million and $1.2 million, respectively. The ICERs were $178 000 and $174 000 per QALY for females and males, respectively, which exceeded the willingness-to-pay threshold of $100 000 per QALY that is commonly used in conventional CEA in the United States ( 25 ). Gene therapy would need to cost less than $1.69 million for females and less than $1.79 million for males to meet the willingness-to-pay threshold of $100 000 per QALY.
Base-Case Results and Probabilistic Sensitivity Analysis
Variable | Standard of Care | Gene Therapy |
---|---|---|
Cost, $ | 1 120 000 | 2 770 000 |
QALYs | 15.6 | 25.0 |
ICER, | - | 176 000 |
95% credible interval for ICER, | ||
Females | - | 155 000–208 000 |
Males | - | 14 800–243 000 |
Threshold inequality aversion parameter (equity weight) | - | 0.90 |
ICER = incremental cost-effectiveness ratio; QALY = quality-adjusted life-year.
Base-Case DCEA
In the DCEA that weighed the effects of gene therapy on both conventional CEA outcomes and equity considerations, the threshold inequality aversion parameter (equity weight) was 0.90 ( Figure 2 ). This implies that the minimum preference for reducing disparities in SCD care to favor gene therapy over SOC per DCEA standards is in line with prior estimates in the United States. The corresponding threshold price range (per DCEA standards) for a durable SCD gene therapy was $2 100 000 to $7 000 000 across the range of commonly used equity weights in the United States (inequality aversion parameters of 0.5 to 3.0).

Two-way sensitivity analysis on inequality aversion parameter (equity weight) and gene therapy price.
The red and orange areas indicate scenarios in which standard of care is preferred per DCEA standards. The yellow and green areas indicate scenarios in which gene therapy is preferred per DCEA standards. The box indicates the range of commonly used values of equity weights (inequality aversion parameters ranging from 0.5 to 3.0) ( 22 , 23 ) and plausible gene therapy costs. Equity weight values have been estimated to be as high as 10.0 (based on a small U.K. study [26]). The star indicates the threshold equity weight for a gene therapy cure for sickle cell disease (priced at $2450000). DCEA= distributional cost-effectiveness analysis.
In 1-way deterministic sensitivity analyses, the parameters affecting the ICER by at least ±10% from the base-case input values for both sexes included the same 6 parameters with 1 additional parameter for males. The 6 parameters were cost of gene therapy, age at treatment, gamma log-link regression coefficients for the intercept and severe SCD, and QALYs for controls and adults for SCD, and the additional parameter was the cost of mild SCD in males ( Supplement Figure 2 , available at Annals.org ). In probabilistic sensitivity analyses, SOC was favored in 100.0% and 87.1% of 10 000 iterations for females and males ( Figure 3 ). For DCEA, varying the percentage of patients with SCD who are covered by Medicaid up to 70%, as is seen in the pediatric population ( 27 ), resulted in a very small (<0.001) change in the threshold equity weight, which still rounded to 0.90. Threshold equity weights for scenarios with gene therapy durations of 10 and 20 years were 3.0 and 2.1, respectively ( Supplement Table 3 , available at Annals.org ). The threshold equity weight when 18.9% of patients with SCD were excluded due to comorbidities was 0.8 ( Supplement Table 3 ).

Cost-effectiveness acceptability curves.
At a willingness-to-pay threshold of $100 000 per QALY, standard of care is favored over gene therapy in 100% and 87.1% of 10 000 iterations for females ( top ) and males ( bottom ) with sickle cell disease, respectively. QALY = quality-adjusted life-year.
We evaluated the conventional and distributional cost-effectiveness of gene therapy for commercially insured persons living with SCD in the United States. Although gene therapy priced across known costs ranging from $2.1 million to $2.8 million did not meet conventional standards for cost-effectiveness, our distributional cost-effectiveness findings suggest that gene therapy could meet distributional cost-effectiveness standards based on commonly used equity weights for the U.S. setting in this price range. If one assumes similar therapeutic efficacy in patients with mild and moderate disease and patients with severe disease, once gene therapy is approved, it could be an equity-enhancing therapeutic strategy for all patients with SCD whose values and preferences align with pursuing this course of therapy.
The value realized in improving the length and quality of life of persons living with SCD after disease-altering gene therapy reflects the high morbidity and mortality averted when disease remission is achieved and the stark disparities in length and quality of life between persons living with SCD and the population at large. Moreover, in the context of the social construct of race, racism has affected the clinical care that patients with SCD receive and has contributed to resource allocation that is discordant with the burden of the lived disease experience in the United States ( 28 ). Although the benefits of lifelong disease remission may not entirely bring this lived experience to the level of that of matched controls living without SCD, the improvement would be marked, and gene therapy may also decrease lifetime out-of-pocket costs for persons living with SCD. For example, a recent analysis of commercially insured persons younger than 65 years who were living with SCD reported SCD-attributable out-of-pocket costs of $42 395 for females and $45 091 for males through age 65 years, constituting an overall 285% increase compared with matched controls ( 29 ). Our DCEA captured the effects of gene therapy on population subgroups (patients with SCD compared with all others in the health care system) with regard to benefits and costs (including cost savings), which is not captured in conventional CEA.
Because clinical trials to date have used a minimum age criterion of 12 years for inclusion, an underlying concern is patient eligibility for gene therapy in real-world practice. Given the importance of robust cardiovascular and pulmonary status in minimizing adverse events from gene therapy, cardiovascular and pulmonary conditions are likely to lead to exclusion of patients from eligibility for gene therapy. For example, if approximately 18.9% of the SCD patient population is ineligible to receive gene therapy due to development of heart failure or pulmonary hypertension by age 12 years, the threshold equity weight is 0.8 for the SCD population ( Supplement Table 3 ). On a population level, this raises the question of optimizing the minimum age for eligibility so that patients are still old enough to tolerate gene therapy and receive its benefits while remaining eligible for treatment. Practically, however, if the eligibility age stays consistent with that studied in initial clinical trials, a coordinated effort across the United States will be required to deliver early and sustained treatment with SOC to bridge as many patients to gene therapy eligibility as possible before the development of exclusionary comorbidities. Another consideration is the durability of gene therapy effectiveness; a shorter duration of gene therapy from 1-time administration would result in a higher ICER and would require correspondingly greater societal weight placed on equity to be recommended in a DCEA ( Supplement Table 3 ).
When faced with the costs of innovative, disease-altering therapies that are administered only once, such as gene therapy and chimeric antigen receptor T-cell therapy (CAR-T), budgetary constraints can and do drive therapy availability for patients. This discord recently led to the withdrawal of gene therapy for β -thalassemia from the European market ( 30 ). Currently and soon-to-be approved indications for gene therapy in the hematology field alone include hemophilia, β -thalassemia, and SCD in addition to CAR-T for a range of malignant diseases, such as acute lymphoblastic leukemia and diffuse large B-cell lymphoma. The costs in the context of the cumulative prevalence of these conditions will continue to put financial pressure on state-funded, federally funded, and commercially funded health plans. The consideration of health inequities, which DCEA explicitly supports, may be an additional helpful metric in this context. However, rather than relying on ranges of commonly used equity weights for the United States, fielding nationally representative surveys would allow the derivation of inequality aversion parameter values that address disease-specific health disparities ( 26 ).
To our knowledge, only 1 other DCEA has been published for the U.S. setting (for COVID-19 treatments), and it did not provide different recommendations using conventional CEA versus DCEA methods ( 31 ). In the United Kingdom, the National Institute for Health and Care Excellence has adopted more lenient cost-effectiveness thresholds for equity-relevant situations, such as end-of-life care ( 32 ), but this decision has been criticized by economists for potentially leading to suboptimal equity-weighted population health outcomes compared with DCEA approaches ( 33 ). Another alternative approach would be to present a visual dashboard of potential tradeoffs between conventional CEA and DCEA results ( 34 ), but unlike DCEA, this approach alone would not provide recommendations to decision makers based on a quantitative framework.
Limitations of this study include model input parameters being informed by real-world data from commercially insured patients rather than patients covered by Medicaid or Medicare, the assumption that 100% of patients receiving gene therapy will benefit from lifelong disease remission, the assumption that lifelong disease remission achieved with gene therapy will successfully bring background mortality to the level among matched controls without SCD, and the fact that all data are from the period through 2017, just before the approval of 3 new disease-modifying FDA-approved agents for SCD. These new and expensive therapies may increase both cost and quality-adjusted life expectancy and could increase the ICER for gene therapy (if the relevant comparator for gene therapy were no longer SOC). Through 2020, use of these agents in commercially insured patients remained below 5% ( 35 ). Furthermore, there is a notable degree of uncertainty in the cost regression coefficient for males living with SCD. We quantified this uncertainty in deterministic and probabilistic sensitivity analysis, which suggests that future studies on the costs of SCD in males could influence conventional and distributional CEAs of gene therapy in this patient group. Finally, the disparity analyzed in our DCEA was that between persons with and without SCD, which does not capture all salient health disparities in the United States. For example, funding an expensive gene therapy for patients with SCD who are covered by Medicaid could displace other spending on persons without SCD covered by Medicaid, who themselves belong to lower-income groups. Health policy decision makers should therefore fully understand the disparities that are and are not addressed in a given DCEA.
In summary, we performed a DCEA evaluating gene therapy versus SOC in persons living with SCD. We found that although gene therapy for persons living with SCD may exceed a cost-effectiveness threshold from a conventional cost-effectiveness perspective, it can be an equitable therapeutic option per DCEA standards with equity weight conventions used in the United States. Studies to determine disease-specific equity weights in addressing this SCD-specific health disparity would further help inform an equitable and value-based price benchmark for gene therapy in the United States.
Supplementary Material
Financial support:.
By the Yale Bernard G. Forget Scholars Program and Bunker Endowment (Dr. Goshua). The project described was supported by grant T32 AI007433 from the National Institute of Allergy and Infectious Diseases. The contents of this article are solely the responsibility of the authors and do not necessarily represent the official views of the National Institutes of Health.
Primary Funding Source:
Yale Bernard G. Forget Scholars Program and Bunker Endowment.
Disclaimer: The content of this article is solely the responsibility of the authors and does not necessarily represent the official views of the funding sources.
Disclosures: Disclosures can be viewed at www.acponline.org/authors/icmje/ConflictOfInterestForms.do?msNum=M22-3272 .
Reproducible Research Statement: Study protocol, statistical code, and data set : Restricted access is available through written agreements with Dr. Goshua (e-mail, [email protected] ).
- Open access
- Published: 12 August 2024
Micropapillary breast carcinoma in comparison with invasive duct carcinoma. Does it have an aggressive clinical presentation and an unfavorable prognosis?
- Yasmine Hany Abdel Moamen Elzohery 1 , 5 ,
- Amira H. Radwan 2 , 5 ,
- Sherihan W. Y. Gareer 2 , 5 ,
- Mona M. Mamdouh 3 , 5 ,
- Inas Moaz 4 , 5 ,
- Abdelrahman Mohammad Khalifa 5 ,
- Osama Abdel Mohen 5 ,
- Mohamed Fathy Abdelfattah Abdelrahman Elithy 5 nAff6 &
- Mahmoud Hassaan 5 nAff7
BMC Cancer volume 24 , Article number: 992 ( 2024 ) Cite this article
336 Accesses
Metrics details
Invasive micropapillary carcinoma (IMPC) was first proposed as an entity by Fisher et al. In the 2003 World Health Organization (WHO) guidelines for histologic classification of the breast tumors. IMPC was recognized as a distinct, rare histological subtype of breast cancer.
IMPC is emerging as a surgical and oncological challenge due to its tendency to manifest as a palpable mass, larger in size and higher in grade than IDC with more rate of lymphovascular invasion (LVI) and lymph node (LN) involvement, which changes the surgical and adjuvant management plans to more aggressive, with comparative prognosis still being a point of ongoing debate.
Aim of the study
In this study, we compared the clinicopathological characteristics, survival and surgical management of breast cancer patients having invasive micropapillary carcinoma pathological subtype in comparison to those having invasive duct carcinoma.
This is a comparative study on female patients presented to Baheya center for early detection and treatment of breast cancer, in the period from 2015 to 2022 diagnosed with breast cancer of IMPC subtype in one group compared with another group of invasive duct carcinoma. we analyzed 138 cases of IMPC and 500 cases of IDC.
The incidence of LVI in the IMPC group was 88.3% in comparison to 47.0% in the IDC group (p < 0.001). IMPC had a higher incidence of lymph node involvement than the IDC group (68.8% and 56% respectively). IMPC had a lower rate of breast conserving surgery (26% vs.37.8%) compared with IDC.
The survival analysis indicated that IMPC patients had no significant difference in overall survival compared with IDC patients and no differences were noted in locoregional recurrence rate and distant metastasis rate comparing IMPCs with IDCs.
The results from our PSM analysis suggested that there was no statistically significant difference in prognosis between IMPC and IDC patients after matching them with similar clinical characteristics. However, IMPC was found to be more aggressive, had larger tumor size, greater lymph node metastasis rate and an advanced tumor stage.
Peer Review reports
Introduction
Breast cancer is the most common cancer in women. In the 2012 World Health Organization (WHO) classification of breast cancer. Breast Cancer is classified into up to 21 different histological types depending on cell growth, morphology and architecture patterns [ 1 ]. The invasive carcinoma of no special type (IBC-NST), which is known as invasive ductal carcinoma (IDC), is the most frequently occurring histological type, which constitutes around 75% of invasive breast carcinoma [ 2 ].
Invasive micropapillary carcinoma (IMPC) was first proposed as an entity by Fisher et al. in 1980 [ 3 ] and first described as the term “invasive micropapillary carcinoma” by Siriaunkgul et al. [ 4 ] in 1993.
In the 2003 World Health Organization (WHO) guidelines for histologic classification of the breast tumors [ 5 ]. IMPC was recognized as a distinct, rare histological subtype of breast cancer. While micropapillary histological architecture is present in 2–8% of breast carcinomas, pure micropapillary carcinoma is uncommon and accounts for 0.9–2% of all breast cancers [ 6 ].
IMPC exhibits more distinct morphologic architecture than the IDC, characterized by pseudopapillary and tubuloalveolar arrangements of tumor cell clusters in clear empty sponge-like spaces that resemble extensive lymphatic invasion [ 7 ]. The neoplastic cell exhibits an “inside-out” pattern, known as the reverse polarity pattern [ 2 ].
Most studies demonstrate that the radiological findings of IMPC are irregular-shaped masses with an angular or spiculated margin on ultrasound, mammography and MRI with heterogeneous enhancement and washout kinetics on MRI [ 8 ].
IMPC had tendency to manifest as a palpable mass, larger in size and higher in grade than IDC with more rate of lymphovascular invasion (LVI) and lymph node (LN) involvement, which changes the surgical and adjuvant management plans to more aggressive, with comparative prognosis still being a point of ongoing debate [ 9 ].
In this study, we compared the clinicopathological characteristics, survival and surgical management of breast cancer patients having invasive micropapillary carcinoma pathological subtype in comparison to those having invasive ductal carcinoma.
Patient and method
This is a comparative study on female patients presented to Baheya center for early detection and treatment of breast cancer, in the period from 2015 to 2022 diagnosed with breast cancer of IMPC subtype in one group compared with another group of invasive duct carcinoma.
This retrospective study analyzed 138 cases of IMPC and 500 cases of IDC. Informed consent was obtained from all patients. Ethical approval is obtained from Baheya center for early detection and treatment of breast cancer and National research center ethics committee. Baheya IRB protocol number:202305150022.
The following clinical-pathological features were analyzed for each case: patient age at diagnosis, clinical presentation, laterality, imaging findings, histopathological examination, treatment plan with either primary surgical intervention or other treatment protocol according to tumor stage and biological subtypes.
A breast pathologist evaluated the tumor size, type, grade, lymphovascular invasion, estrogen receptor (ER), progesterone receptor (PR), human epidermal growth factor receptor 2 (HER2) receptor and the axillary lymph node involvement.
According to the ASCO/CAP guideline update, 2019: Samples with 1% to 100% of tumor nuclei positive for ER or progesterone receptor (PgR) are interpreted as positive. If ER (not PgR), 1% to 10% of tumor cell nuclei are immunoreactive, the sample are reported as ER Low Positive. There are limited data on the overall benefit of endocrine therapies for patients with low level (1%-10%) ER expression, but they currently suggest possible benefit, so patients are considered eligible for endocrine treatment. A sample is considered negative for ER or PgR if < 1% or 0% of tumor cell nuclei are immunoreactive [ 10 ]. An Allred score between 0 and 8. This scoring system looks at what percentage of cells test positive for hormone receptors, along with how well the receptors show up after staining, called intensity: proportion of cells staining (0, no staining; 1, < 1%; 2, between 1 and 10%; 3, between 11 and 33%; 4, between 34 and 66% and 5, between 67%–100% of the cells staining). Intensity of positive tumor cells (0, none; 1, weak, 2, intermediate; and 3, strong) [ 11 ].
HER2 Test Guideline IHC Recommendations, 2018. IHC 0: as defined by no staining observed or membrane staining that is incomplete and is faint/barely perceptible and within < = 10% of the invasive tumor cells. IHC 1 + : as defined by incomplete membrane staining that is faint/barely perceptible and within > 10% of the invasive tumor cells. IHC 2 + : The revised definition of IHC 2 + (equivocal) is weak to moderate complete membrane staining observed in > 10% of tumor cells. IHC 3 + : based on circumferential membrane staining that is complete, intense in > 10% of tumor cells. [ 12 ].
ASCO–CAP HER2 SISH Test Guideline Recommendations,2018 Twenty nuclei (each containing red (Chr17) and black (HER2) signals) should be enumerated. The final results for the HER2 status are reported based on the ratio formed by dividing the sum of HER2 signals for all 20 nuclei divided by the sum of Chromosome 17 signals for all 20 nuclei. The amplification status is defined as Amplified if the HER2/Chromosome 17 ratio > / = 2.0 and the average Her2 gene copy number is > / = 4.0. It is non-Amplified if the HER2/Chromosome 17 ratio < 2.0 with the Her2 gene copy number is < 4.0. If the HER2/Chr17 ratio is < 2 and the Her2 gene copy number is between 4.0 and 6.0, or, HER2/Chr17 ratio is > / = 2 and the Her2 gene copy number is < 4, or HER2/Chr17 ratio is < 2 and the Her2 gene copy number is > / = 6.0, an additional work should be done. [ 12 ].
Follow-up duration was calculated from the date of diagnosis to the date of the last follow-up. Patients still alive at the last follow-up censored or to the date of occurrence of any event or death.
Disease-free survival was defined as the duration (months) from the initial diagnosis of breast cancer to first any type of recurrence (invasive ipsilateral breast tumor recurrence, local invasive recurrence, regional invasive recurrence, invasive contra lateral breast cancer, distant metastasis.
Overall survival (OS) is defined as the time from diagnosis of breast cancer to death from any cause.
Data were statistically analyzed using an IBM-compatible personal computer with Statistical Package for the Social Sciences (SPSS) version 23. Quantitative data were expressed as mean, standard deviation (SD) and range (minimum–maximum). Qualitative data were expressed as Number (N) and percentage (%), while A P value of < 0.05 was statistically significant. For comparison of unmatched data, chi-square tests were used for categorical variables and t-tests or Mann–Whitney tests for continuous variables.
In this study, we analyzed 138 cases of IMPC which presented to our center in the period from 2015 to 2022.We included a total number of 500 cases of IDC as controls with a ratio of controls to cases 4:1.
Propensity score matching (PSM) is a method for filtrating experimental and control cases of similar characteristics, which are called the matching variables, from existing data to make them comparable in a retrospective analysis. PSM reduce the effect of selection bias. So, the comparison of outcomes between two groups can be fair.
The variables for propensity score matching were selected as follows: age (years), tumour size (cm), nodal status, HR status and HER2 status.
To diminish the effects of baseline differences and potential confounds in clinical characteristics and patients across histology subtypes for outcome differences (disease-free survival and overall survival), PSM method was applied with each micropapillary patient matched to one IDC patient who showed similar baseline characteristics in terms of: menopausal status, comorbidities, multiplicity, histologic grade, tumor size, stage, nodal status, ER /PR status. Differences in prognosis were assessed by Kaplan–Meier analysis.
Most of the patients were postmenopausal, the mean age of patients in IMPC group was 57.36 ± 11.321 years while the mean age of the IDC group was 56.63 ± 9.719 years ( p = 0.45) (Table 1 ).
The most common presentation of IMPC on breast mammography was an irregular shaped mass with a non-circumscribed spiculated margin. while, the most common sonographic finding of IMPC was hypoechoic mass with irregular shapes and spiculated margins. Associated microcalcifications were found in 49 patients (35.5%) of IMPC group. Figs. ( 1 , 2 ): Radiological characteristics of IMPC.
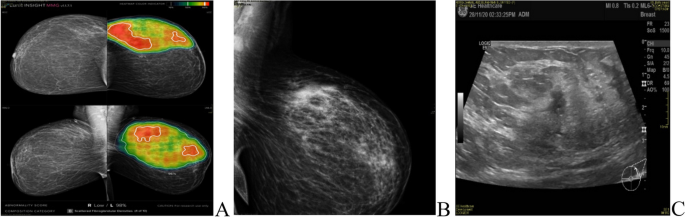
A , B 37-years-old female patient presented with Left breast UOQ extensive fine pleomorphic and amorphous calcifications of segmental distribution, with UOQ multiple indistinct irregular masses. C ultrasound showed left breast UOQ multiple irregular hypoechoic masses with calcific echogenic foci, the largest is seen at 1 o’clock measuring 13 × 15mm. Intraductal echogenic lesions are noted
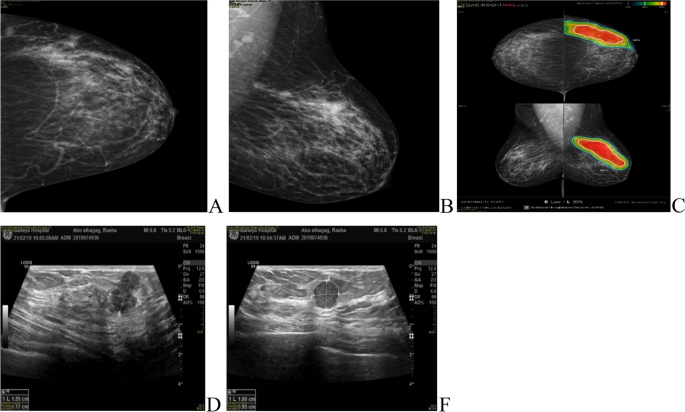
A , B , C 40-years-old female patient presented with left UOQ extensive pleomorphic microcalcifications of segmental distribution reaching the areola, with multiple well-circumscribed small obscured masses. D , E complementary Ultrasound showed left 2 o’clock multiple ill-defined and well-defined hypoechoic masses (BIRADS 5)
All patients underwent axillary sonography where 77 patients (55.8%) of the IMPC group exhibited pathological lymph nodes and 18 patients (13%) had indeterminate lymph nodes demonstrating preserved hila and associated with either a symmetrical increase of their cortical thickness reaching 3mm or with a focal increase in the cortical thickness.
Multiple lesions were detected in 30% of IMPC patients in comparison to 7% of IDC patients. Intra-ductal extension with nipple involvement was found in 44 patients (31.9%) of the IMPC group (Table 2 ).
MRI was done for 5 cases (3.6%), while CESM was performed for 18 cases (13%) of the IMPC group, the commonest presentation of IMPC in contrast study was irregular shaped enhanced mass in 21 patients and non-mass enhancement was found in 5 patients. Figs. ( 3 , 4 ).
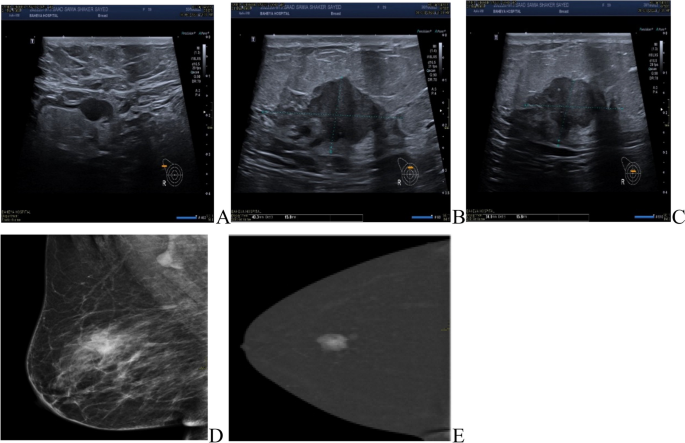
Further imaging modalities. A , B , C 60-years-old female patient had right breast irregular hypoechoic solid mass by ultrasound (BIRADS 5). D , E CESM showed a right breast irregular heterogeneously enhancing solid mass
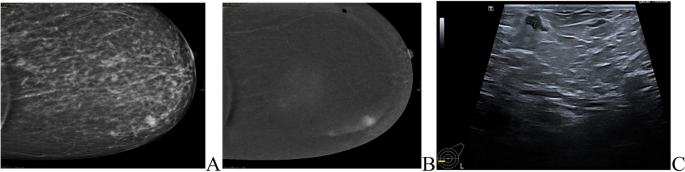
Role of CESM in diagnosis of IMPC patients. A , B 42-years-old patient presented with a left LIQ irregular spiculated mass with suspicious microcalcifications, other similar lesions were seen anterior and posterior at the same line. C Ultrasound showed a heterogeneously hypoechoic irregular mass with a spiculated outline with multiple similar satellite lesions were seen anterior and posterior to the main lesions
The average tumor size in the IMPC and IDC groups was 3.37 ± 2.04 cm and 2.72 ± 1.39 cm, respectively ( P < 0.001).
The percentage of tumors larger than 5cm, was reported 9.5% in IMPC and 7.4% in IDC.
The pure form of IMPC was the most common type and found in 90 cases (65%) and 47 cases (34%) were mixed type where IDC was the commonest associated type.
There are 6 cases in the IMPC group diagnosed as invasive mucinous carcinoma on biopsy, then in the specimen was mixed invasive micropapillary, IBC-NST and invasive mucinous carcinoma.
On core biopsy, 28 cases were diagnosed as IMPC with focal IDC component, but in corresponding specimens 10 cases were only approved to be mixed invasive micropapillary and invasive duct carcinoma, while others diagnosed as pure invasive micropapillary carcinoma without IDC component.
On the other hand, 48 of our cases were diagnosed as IDC on core biopsy, but in the final specimen examination, 17 of these cases were diagnosed as pure invasive micropapillary carcinoma without invasive ductal component.
The explanation of controversy in proper histologic subtyping of carcinoma on core biopsy and the definite subtype on the corresponding specimen was that the ductal component which only represented in the biopsy is a very minor component of the tumor or the limited sampling, tissue fragmentation and architecture distortion in core biopsy may cause diagnostic pitfalls as regard precise subtyping of the tumor.
The incidence of LVI in the IMPC group was 88.3% in comparison to 47.0% in the IDC group ( p < 0.001).
IMPC had a higher incidence of lymph node involvement than the IDC group (68.8% and 56% respectively) with N3 stage reported in 12.4% of IMPC patients.
IMPC had a higher nuclear grade than the IDC group (25.1% and 15.2% respectively).
The percentage of ER-positive patients was 97.8% in the IMPC group and 87.6% in the IDC group ( p < 0.001), while PR-positive cases were 98.6% in the IMPC group and 88.8% in the IDC group ( p < 0.001). HER2 status was positive in 4.3% of IMPCs and 8% of IDCs ( p = 0.23) (Table 3 ) (Figs. 5 , 6 ).
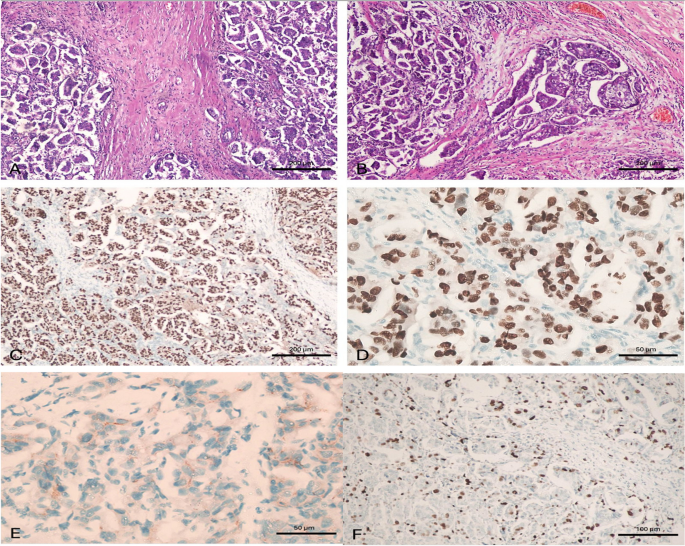
A case of invasive micropapillary carcinoma. A case of invasive micropapillary carcinoma, grade II. A Tissue core biopsy, × 100, B MRM specimen × 100 with Positive metastatic L. nodes 2/15, C ER is positive in > 90% of tumor cells, × 100, D PR is positive in > 90% of tumor cells, × 400, E HER2/neu is negative, × 400 and F) Ki-67 labelling index is high, × 200. This case was considered as luminal type pure invasive micropapillary carcinoma. (100 micron 20__ 50 micron 40)
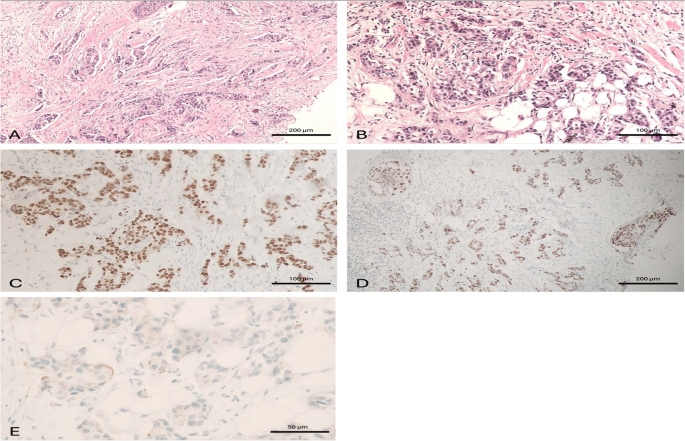
A case of invasive duct carcinoma. A case of invasive duct carcinoma, grade II. A Tissue core biopsy, × 100, B MRM specimen, × 200 with negative L. nodes 0/16, C ER is positive in > 90% of tumor cells, × 200, D PR is positive in > 90% of tumor cells, × 100, E HER2/neu is negative, × 400. This case was considered as luminal type pure invasive duct carcinoma
Regarding definitive surgical management, IMPC had a lower rate of breast conserving surgery (26% vs.37.8%) compared with IDC. While, 49.3% of IMPC patients underwent modified radical mastectomy in comparison to 46% of the IDC patients. Such high incidence of mastectomy was due to the advanced stage at presentation, presence of multiple lesions and presence of intra-ductal extension with nipple involvement.
The incidence of re-surgery in the IMPC group was only in 3 cases, two of them underwent completion mastectomy after the initial conservative breast surgery and axillary clearance. While one patient underwent wider margin excision as positive margin for an invasive residual disease was found.
Two patients in the IMPC group had distant metastasis at the initial diagnosis, they had multiple metastatic lesions and received systemic treatment but one of them underwent palliative mastectomy.
Systemic chemotherapy was administered to 107 patients (77.5%) in the IMPC group and to 207 patients (41%) in the IDC group. Hormonal therapy was administered to all IMPC patients and 76% patients in the IDC group (Table 4 ).
The overall median follow-up duration was 21 months (range 6 – 88 months) with mean follow up duration = 29.8months.
Among the 138 IMPC patients, local recurrence developed in 3 cases, they developed a recurrence at 6,18 and 48 months postoperative. Distant metastasis developed in 5 patients in the form of bone, lung, hepatic and mediastinal lymph node metastasis.
The survival analysis indicated that IMPC patients had no significant difference in overall survival compared with IDC patients and no differences were noted in locoregional recurrence rate comparing IMPCs with IDCs (2.2% and 0.4% respectively). P value for local recurrence = 0.12 (yates corrected chi square).
Distant metastasis rate comparing IMPCs with IDCs was (3.7% and 5.4% respectively). P value for distant metastasis = 0.53 (Table 5 ).
Comparison of OS between IDC and micropapillary cases (Matched by propensity score matching -PSM).
Case Processing Summary
Type | Total N | N of Events | Censored | |
---|---|---|---|---|
N | Percent | |||
IDC | 125 | 7 | 118 | 94.4% |
Micropapillary | 128 | 3 | 125 | 97.7% |
Overall | 253 | 10 | 243 | 96.0% |
Type | Mean survival time | |||
---|---|---|---|---|
Estimate | Std. Error | 95% Confidence Interval | ||
Lower Bound | Upper Bound | |||
IDC | 84.596 | 2.314 | 80.061 | 89.131 |
Micropapillary | 57.530 | .844 | 55.876 | 59.185 |
Overall | 85.807 | 1.633 | 82.606 | 89.008 |
Overall Comparisons
Chi-Square | df | Sig. | |
---|---|---|---|
Log Rank (Mantel-Cox) | .438 | 1 | .508 |
- Test of equality of survival distributions for the different levels type
Disease free survival
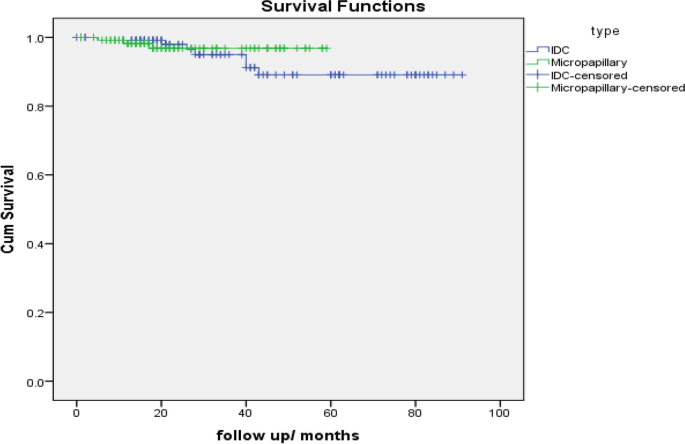
Type | Total N | N of Events | Censored | |
---|---|---|---|---|
N | Percent | |||
IDC | 124 | 11 | 113 | 91.1% |
Micropapillary | 129 | 5 | 124 | 96.1% |
Overall | 253 | 16 | 237 | 93.7% |
Type | Mean | |||
---|---|---|---|---|
Estimate | Std. Error | 95% Confidence Interval | ||
Lower Bound | Upper Bound | |||
IDC | 77.324 | 3.019 | 71.407 | 83.242 |
Micropapillary | 56.062 | 1.355 | 53.407 | 58.718 |
Overall | 78.725 | 2.333 | 74.152 | 83.299 |
Chi-Square | df | Sig. | |
---|---|---|---|
Log Rank (Mantel-Cox) | .380 | 1 | .537 |
- Test of equality of survival distributions for the different levels of type
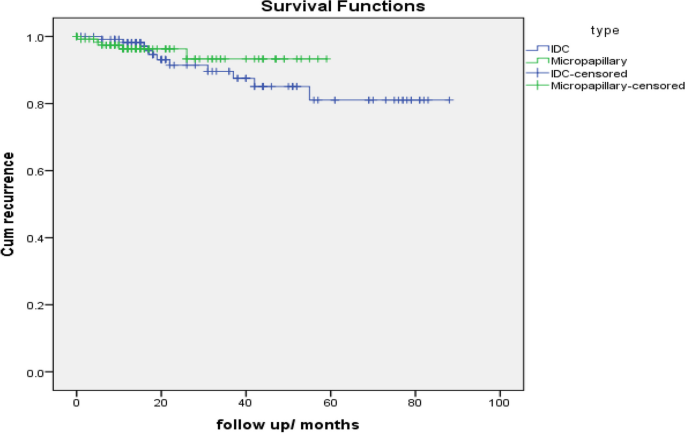
IMPC is a highly invasive type of breast cancer. Hashmi A.A. et al. [ 13 ] found that the incidence of IMPC is very low accounting for 0.76–3.8% of breast carcinomas.
Shi WB et al.; [ 7 ] in a study comparing 188 IMPC cases and 1,289 invasive ductal carcinoma (IDC) cases from China showed that IMPC can occur either alone or mixed with other histological types, such as ductal carcinoma in situ, mucinous carcinoma and IDC. Furthermore, the majority of patients had mixed IMPC.
Fakhry et al. [ 14 ] reported that 64.7% of IMPC patients were pure type. In our study, we found that the pure form of IMPC was the commonest type and presented in 90 patients (65%) and 47 cases (34%) were mixed type which was similar to that reported by Nassar et al. [ 15 ], and Guo et al. [ 16 ] in their studies.
In our study, the commonest finding of IMPC on breast mammography was an irregular shaped mass with a non-circumscribed spiculated margin. While, the commonest sonographic finding of IMPC was hypoechoic mass with irregular shapes and spiculated margins.
These findings were similar to the results demonstrated by Jones et al., [ 17 ] which found that the commonest morphologic finding of IMPC was an irregular high-density lesion (50% of patients) with spiculated margin (42% of patients). However, Günhan-Bilgen et al. [ 18 ] reported that an ovoid or round lesion was found in 53.8% of patients.
Alsharif et al., [ 19 ] reported that the commonest sonographic finding of IMPC was hypoechoic masse (39/41, 95%) with irregular shape (30/41, 73.2%) and angular or spiculated margin (26/41, 63.4%).
In our study, MRI was done for 5 cases (3.6%), while CESM was performed for 18 cases (13%) of the IMPC group, the commonest presentation of IMPC in contrast study was irregular shaped enhanced lesion in 21 cases and non-mass enhancement was presented in 5 cases.
Nangogn et al. [ 20 ] and yoon et al. [ 8 ] recorded that the commonest finding of IMPCs in MRI was spiculated irregular mass with early rapid initial heterogenous enhancement, indicating that the MRI findings correlated with the invasiveness of IMPC.
Fakhry et al. [ 14 ] conducted a study on 68 cases, out of which 17 cases underwent CEM. In all of these cases, the masses showed pathological enhancement, which was either in the form of mass enhancement (12/17 patients, 70.6%) or non-mass enhancement (4/17 patients, 23.5%). The majority of the enhanced masses were irregular in shape (11/12 patients, 91.7%).
All patients underwent axillary sonography and 77 patients (55.8%) of the IMPC group exhibited pathological lymph nodes; this percentage was similar to that recorded by Nangong et al. [ 20 ] which was 54.8% and lower than that recorded by Jones et al. [ 17 ] but higher than that of Günhan et al. [ 18 ] which were 67% and 38% respectively.
Günhan et al. [ 18 ] reported microcalcification in about 66.7% of the cases. In our study, associated microcalcifications were found in 49 patients (35.5%) of the IMPC group. Yun et al. [ 21 ] and Adrada et al. [ 22 ] showed a fine pleomorphic appearance (66.7% and 68%).
Hao et al. [ 23 ] compared the rate of tumors larger than 5cm, reporting 3% in IDC and 4.3% in IMPC. In our study, the rate of tumors larger than 5cm, was reported 7.4% in the IDC patients and 9.5% in the IMPC patients.
Yu et al., et al. [ 24 ] documented in a study comparing 72 cases of IMPC and 144 cases of IDC of the breast that IMPC had a higher nuclear grade than IDC (52.8% vs. 37.5% respectively). In our study, IMPC had a higher nuclear grade than the IDC group (25.1% and 15.2% respectively).
Verras GI et al.; [ 9 ] demonstrated that IMPC was an aggressive breast cancer subtype with a great tendency to lymphovascular invasion and lymph node metastasis. In our study, the incidence of LVI in the IMPC patients was 88.3% in comparison to 47.0% in the IDC patients ( p < 0.001). Tang et al., [ 25 ] also reported that lymphovascular involvement was more common among the IIMPC group than IDC group, with a percentage of 14.7% compared to only 0.1% in the IDC group.
Also, Shi et al. [ 7 ] reported that LVI was detected in 74.5% of cases. Furthermore, the frequency of LVI was found to be greater in IMPC cases when compared to IDC cases. Jones et al., [ 17 ] recorded angiolymphatic invasion in 69% of cases.
Hashmi et al. [ 13 ] reported in his comparative study that nodal involvement was present in 49.5% of IDC patients and N3 stage was only 15.6% in IDC patients compared to 33% in IMPC patients. In our study, the percentage of lymph node involvement of IMPC and IDC patients were 68.8% and 56% respectively with N3 stage reported in 12.4% of IMPC patients.
Guan et al. [ 26 ], Lewis et al., [ 27 ], Pettinato et al., [ 28 ] and De La Cruz et al., [ 29 ] recorded a higher percentage of lymph node metastasis in IMPC patients, reaching 90%, 92.9%,55.2% and 60.9% respectively.
The management of IMPC remains controversial, particularly among breast surgeons. Modified radical mastectomy was the preferred surgical procedure for the majority of IMPC case reports, as found in a study conducted by Yu et al., [ 24 ] where 99% of IMPC cases underwent modified radical mastectomy. Fakhry et al. [ 14 ] reported that 76.5% of the patients underwent modified radical mastectomy. In our study, 49.3% of IMPC patients received modified radical mastectomy.
IMPC patients were also prone to accept BCS rather than mastectomy in the previous series conducted by Lewis GD,et al. [ 27 ] and Vingiani, A. et al. [ 30 ]. However, the precise prognosis value of BCS for patients with IMPC remained unknowable. In our study, IMPC had a lower rate of breast conserving surgery (26% vs.37.8%) compared with IDC.
IMPC was characterized by a high incidence of ER and PR positivity. Our study recorded a high percentage of ER (97.8%) and PR (98.6%) expression. Our findings are similar to those found by Walsh et al., [ 31 ] who reported ER and PR expression of 90% and 70%, respectively. Zekioglu et al. [ 32 ] demonstrated a rate of ER and PR expression of 68% and 61%respectively.
In this study, we reported a relatively lower percentage of HER-2 positivity (4.3%). Also, Nangong et al. [ 20 ] showed HER 2 overexpression in 26.4% of cases.
However, Cui et al. [ 33 ] reported a much higher incidence of HER 2 positivity and Perron et al., [ 34 ] reported that 65% of IMPCs were HER-2 positive.
Chen, A et al. [ 35 ] reported that that the percentage of radiation therapy for IMPC patients was similar to those seen in IDC patients and demonstrates a similar benefit of radiation treatment in both groups. In our study,77.5% patients received radiotherapy in IMPC group in compared to 59.4% patients in IDC group.
Shi et al. [ 7 ] found that patients with IMPC had worse recurrence-free survival (RFS) and overall survival (OS) rates as compared to those with IDC. However, because IMPC is relatively rare, most studies had reported on small sample sizes with limited follow-ups.
Yu et al., [ 24 ] conducted a comparison between IMPC and IDC patients, and the results showed that the IMPC group had a greater tendency for LRR compared to the IDC group ( P = 0.03), but the distant metastasis rate ( P = 0.52) and OS rate ( P = 0.67) of the IMPC showed no statistical differences from the IDC group.
Nevertheless, several recent studies documented that IMPC had better or similar prognosis in comparison to IDC.
Hao et al. [ 23 ] and Vingiani et al. [ 30 ] documented that there was no statistically significant difference in OS and disease-free survival between IMPC patients and IDC patients which was similar to our results. locoregional recurrence rate comparing IMPCs with IDCs was (2.2% and 0.4% respectively). P value for local recurrence = 0.12 (yates corrected chi square). Distant metastasis rate comparing IMPCs with IDCs was (3.7% and 5.4% respectively). P value for distant metastasis = 0.53.
Chen H et al. [ 36 ], compared the overall survival in patient groups with similar nodal involvement and found that IMPC group had better breast cancer–specific survival and overall survival than IDC group.
Availability of data and materials
No datasets were generated or analysed during the current study.
Abbreviations
Invasive micropapillary carcinoma
Invasive duct carcinoma
Modified radical mastectomy
Conserving breast surgery
Estrogen receptor
Progesterone receptor
Lymphovascular invasion
Contrast enhanced spectral mammography
Overall survival
Lakhani SR. International Agency for Research on Cancer Press and World Health Organization. WHO Classification of Tumours of the Breast. Lyon: International Agency for Research on Cancer Press; 2012.
Wu Y, Zhang N, Yang Q. The prognosis of invasive micropapillary carcinoma compared with invasive ductal carcinoma in the breast: a meta-analysis. BMC Cancer. 2017;17:839.
Article PubMed PubMed Central Google Scholar
Fisher ER, Palekar AS, et al. Pathologic findings from the national surgical adjuvant breast project (protocol no. 4). Vi. Invasive papillary cancer. Am J Clin Pathol. 1980;73:313–22.
Article PubMed CAS Google Scholar
Siriaunkgul S, Tavassoli FA. Invasive micropapillary carcinoma of the breast. Mod Pathol. 1993;6:660–2.
PubMed CAS Google Scholar
Hanby AM, walker C, Tavassoli FA, Devilee P. Pathology and Genetics: Tumours of the Breast and Female Genital Organs. WHO Classification of Tumours series. Breast Cancer Res. Lyon: IARC Press; 2004;4(6):133. https://doi.org/10.1186/bcr788 .
Yang YL, Liu BB, Zhang X, Fu L. Invasive micropapillary carcinoma of the breast: an update. Arch Pathol Lab Med. 2016;140(8):799–805. https://doi.org/10.5858/arpa.2016-0040-RA .
Shi WB, Yang LJ, et al. Clinico-pathological features and prognosis of invasive micropapillary carcinoma compared to invasive ductal carcinoma: a population-based study from china. PLoS ONE. 2014;9:e101390.
Yoon GY, Cha JH, Kim HH, Shin HJ, Chae EY, Choi WJ. Comparison of invasive micropapillary and invasive ductal carcinoma of the breast: a matched cohort study. Acta Radiol. 2019;60(11):1405–13.
Article PubMed Google Scholar
Verras GI, et al. Micropapillary breast carcinoma: from molecular pathogenesis to prognosis. Breast Cancer (Dove Med Press). 2022;12(14):41–61.
Google Scholar
Allison KH, Hammond MEH, Dowsett M, McKernin SE, Carey LA, Fitzgibbons PL, et al. Estrogen and Progesterone Receptor Testing in Breast Cancer: ASCO/CAP Guideline Update. J Clin Oncol. 2020;38(12):1346–66. https://doi.org/10.1200/JCO.19.02309 .
Fitzgibbons PL, Dillon DA, Alsabeh R, Berman MA, Hayes DF, Hicks DG, Hughes KS, Nofech-Mozes S. Template for reporting results of biomarker testing of specimens from patients with carcinoma of the breast. Arch Pathol Lab Med. 2014;138(5):595–601.
Ahn S, Woo JW, Lee K, Park SY. HER2 status in breast cancer: changes in guidelines and complicating factors for interpretation. J PatholTransl Med. 2020;54(1):34.
Hashmi AA, et al. Clinicopathologic features of invasive metaplastic and micropapillary breast carcinoma: comparison with invasive ductal carcinoma of breast. BMC Res Notes. 2018;11:1–7.
Article Google Scholar
Fakhry S, et al. Radiological characteristics of invasive micropapillary carcinoma of the breast. Clin Radiol. 2024;79(1):e34–40.
Nassar H, Wallis T, Andea A, et al. Clinicopathologic analysis of invasive micropapillary differentiation in breast carcinoma. Mod Pathol. 2001;14:836e41.
Guo X, Chen L, Lang R, et al. Invasive micropapillary carcinoma of the breast: association of pathologic features with lymph node metastasis. Am J Clin Pathol. 2006;126:740e6.
Jones KN, Guimaraes LS, Reynolds CA, Ghosh K, Degnim AC, Glazebrook KN. Invasive micropapillary carcinoma of the breast: imaging features with clinical and pathologic correlation. AJR Am J Roentgenol. 2013;200:689–95.
Günhan-Bilgen I, et al. Invasive micropapillary carcinoma of the breast: clinical, mammographic, and sonographic findings with histopathologic correlation. AJR Am J Roentgenol. 2002;179:927–31.
Alsharif S, et al. Mammographic, sonographic and MR imaging features of invasive micropapillary breast cancer. Eur J Radiol. 2014;83(8):1375–80.
Nangong J, Cheng Z, Yu L, Zheng X, Ding G. Invasive micropapillary breast carcinoma: a retrospective study on the clinical imaging features and pathologic findings. Front Surg. 2022;23(9):1011773.
Yun SU, Choi BB, Shu KS, et al. Imaging findings of invasive micropapillary carcinoma of the breast. J Breast Cancer. 2012;15:57e64.
Adrada B, Arribas E, Gilcrease M, et al. Invasive micropapillary carcinoma of the breast: mammographic, sonographic, and MRI features. AJR Am J Roentgenol. 2009;193:58e63.
Hao S, Zhao Y, Peng J, et al. Invasive micropapillary carcinoma of the breast had no difference in prognosis compared with invasive ductal carcinoma: a propensity-matched analysis. Sci Rep. 2019;9:1–8.
Yu JI, Choi DH, Huh SJ, et al. Differences in prognostic factors and failure patterns between invasive micropapillary carcinoma and carcinoma with micropapillary component versus invasive ductal carcinoma of the breast: retrospective multicenter case-control study (KROG 13–06). Clin Breast Cancer. 2015;15:353–361.e2.
Tang S-L, Yang J-Q, Du Z-G, et al. Clinicopathologic study of invasive micropapillary carcinoma of the breast. Oncotarget. 2017;8:42455–65.
Guan X, Xu G, Shi A, et al. Comparison of clinicopathological characteristics and prognosis among patients with pure invasive ductal carcinoma, invasive ductal carcinoma coexisted with invasive micropapillary carcinoma, and invasive ductal carcinoma coexisted with ductal carcinoma. Medicine (Baltimore). 2020;99:e23487.
Lewis GD, Xing Y, Haque W, et al. The impact of molecular status on survival outcomes for invasive micropapillary carcinoma of the breast. Breast J. 2019;25:1171e6.
Pettinato G, Pambuccian SE, Di Prisco B, et al. Fine needle aspiration cytology of invasive micropapillary (pseudopapillary) carcinoma of the breast: report of 11 cases with clinicopathologic findings. Acta Cytol. 2002;46:1088e94.
De La Cruz C, et al. Invasive micropapillary carcinoma of the breast: clinicopathological and immunohistochemical study. Pathol Int. 2004;54:90–6.
Vingiani A, et al. The clinical relevance of micropapillary carcinoma of the breast: a case–control study. Histopathology. 2013;63:217–24.
Walsh MM, Bleiweiss IJ. Invasive micropapillary carcinoma of the breast: eighty cases of an underrecognized entity. Hum Pathol. 2001;32:583–9.
Zekioglu O, et al. Invasive micropapillary carcinoma of the breast: high incidence of lymph node metastasis with extranodal extension and its immunohistochemical profile compared with invasive ductal carcinoma. Histopathology. 2004;44:18–23.
Cui ZQ, et al. Clinicopathological features of invasive micropapillary carcinoma of the breast. Oncol Lett. 2015;9:1163–6.
Perron M, Wen HY, Hanna MG, Brogi E, Ross DS. HER2 Immunohistochemistry in invasive micropapillary breast carcinoma: complete assessment of an incomplete pattern. Arch Pathol Lab Med. 2021;145:979–87.
Article PubMed PubMed Central CAS Google Scholar
Chen A, Paulino A, Schwartz M, et al. Population-based comparison of prognostic factors in invasive micropapillary and invasive ductal carcinoma of the breast. Br J Cancer. 2014;111:619–22.
Chen H, Wu K, Wang M, Wang F, Zhang M, Zhang P. Invasive micropapillary carcinoma of the breast has a better long-term survival than invasive ductal carcinoma of the breast in spite of its aggressive clinical presentations: a comparison based on large population database and case–control analysis. Cancer Med. 2017;6:2775–86.
Download references
Acknowledgements
Not applicable.
Open access funding provided by The Science, Technology & Innovation Funding Authority (STDF) in cooperation with The Egyptian Knowledge Bank (EKB).
Author information
Mohamed Fathy Abdelfattah Abdelrahman Elithy
Present address: Department of Surgical Oncology, Faculty of Medicine, Al Azhar University, Cairo, Egypt
Mahmoud Hassaan
Present address: Departement of Surgical Oncology, National Cancer Institute, Cairo University, Giza, Egypt
Authors and Affiliations
Department of General Surgery, Faculty of Medicine, Ain Shams University, Cairo, Egypt
Yasmine Hany Abdel Moamen Elzohery
Department of Radiodiagnosis, NCI, Cairo University, Giza, Egypt
Amira H. Radwan & Sherihan W. Y. Gareer
Department of Pathology, National Cancer Institute, Cairo University, Giza, Egypt
Mona M. Mamdouh
Department of Epidemiology and Preventive Medicine, National Liver Institute, Menoufia, Egypt
Baheya Center for Early Detection and Treatment of Breast Cancer, Giza, Egypt
Yasmine Hany Abdel Moamen Elzohery, Amira H. Radwan, Sherihan W. Y. Gareer, Mona M. Mamdouh, Inas Moaz, Abdelrahman Mohammad Khalifa, Osama Abdel Mohen, Mohamed Fathy Abdelfattah Abdelrahman Elithy & Mahmoud Hassaan
You can also search for this author in PubMed Google Scholar
Contributions
Mohamed fathy participated in the sequence alignment and Yasmine hany drafted the manuscript. Mahmoud Hassan participated in the design of the study. Inas Moaz and Abdelrahman Mohammad performed the statistical analysis. Amira H. Radwan and Sherihan WY Gareer conceived the study. Mona M Mamdouh and Osama abdel Mohen participated in its design and coordination and helped to draft the manuscript. All authors read and approved the final manuscript.
Corresponding author
Correspondence to Yasmine Hany Abdel Moamen Elzohery .
Ethics declarations
Ethics approval and consent to participate.
Available all patients provided informed consent for publication. All patients provided signed written informed consent.
Consent for publication
Ethical approval is obtained from Baheya center for early detection and treatment of breast cancer and National research center ethics committee. Baheya IRB protocol number: 202305150022.
Competing interests
The authors declare no competing interests.
Additional information
Publisher’s note.
Springer Nature remains neutral with regard to jurisdictional claims in published maps and institutional affiliations.
Rights and permissions
Open Access This article is licensed under a Creative Commons Attribution 4.0 International License, which permits use, sharing, adaptation, distribution and reproduction in any medium or format, as long as you give appropriate credit to the original author(s) and the source, provide a link to the Creative Commons licence, and indicate if changes were made. The images or other third party material in this article are included in the article's Creative Commons licence, unless indicated otherwise in a credit line to the material. If material is not included in the article's Creative Commons licence and your intended use is not permitted by statutory regulation or exceeds the permitted use, you will need to obtain permission directly from the copyright holder. To view a copy of this licence, visit http://creativecommons.org/licenses/by/4.0/ . The Creative Commons Public Domain Dedication waiver ( http://creativecommons.org/publicdomain/zero/1.0/ ) applies to the data made available in this article, unless otherwise stated in a credit line to the data.
Reprints and permissions
About this article
Cite this article.
Elzohery, Y.H.A.M., Radwan, A.H., Gareer, S.W.Y. et al. Micropapillary breast carcinoma in comparison with invasive duct carcinoma. Does it have an aggressive clinical presentation and an unfavorable prognosis?. BMC Cancer 24 , 992 (2024). https://doi.org/10.1186/s12885-024-12673-0
Download citation
Received : 05 April 2024
Accepted : 23 July 2024
Published : 12 August 2024
DOI : https://doi.org/10.1186/s12885-024-12673-0
Share this article
Anyone you share the following link with will be able to read this content:
Sorry, a shareable link is not currently available for this article.
Provided by the Springer Nature SharedIt content-sharing initiative
ISSN: 1471-2407
- Submission enquiries: [email protected]
- General enquiries: [email protected]
Grab your spot at the free arXiv Accessibility Forum
Help | Advanced Search
Mathematics > Numerical Analysis
Title: a new interpretation of the weighted pseudoinverse and its applications.
Abstract: Consider the generalized linear least squares (GLS) problem $\min\|Lx\|_2 \ \mathrm{s.t.} \ \|M(Ax-b)\|_2=\min$. The weighted pseudoinverse $A_{ML}^†$ is the matrix that maps $b$ to the minimum 2-norm solution of this GLS problem. By introducing a linear operator induced by $\{A, M, L\}$ between two finite-dimensional Hilbert spaces, we show that the minimum 2-norm solution of the GLS problem is equivalent to the minimum norm solution of a linear least squares problem involving this linear operator, and $A_{ML}^†$ can be expressed as the composition of the Moore-Penrose pseudoinverse of this linear operator and an orthogonal projector. With this new interpretation, we establish the generalized Moore-Penrose equations that completely characterize the weighted pseudoinverse, give a closed-form expression of the weighted pseudoinverse using the generalized singular value decomposition (GSVD), and propose a generalized LSQR (gLSQR) algorithm for iteratively solving the GLS problem. We construct several numerical examples to test the proposed iterative algorithm for solving GLS problems. Our results highlight the close connections between GLS, weighted pseudoinverse, GSVD and gLSQR, providing new tools for both analysis and computations.
Subjects: | Numerical Analysis (math.NA) |
classes: | 15A09, 15A22, 65F10, 65F20 |
Cite as: | [math.NA] |
(or [math.NA] for this version) | |
Focus to learn more arXiv-issued DOI via DataCite |
Submission history
Access paper:.
- HTML (experimental)
- Other Formats

References & Citations
- Google Scholar
- Semantic Scholar
BibTeX formatted citation

Bibliographic and Citation Tools
Code, data and media associated with this article, recommenders and search tools.
- Institution
arXivLabs: experimental projects with community collaborators
arXivLabs is a framework that allows collaborators to develop and share new arXiv features directly on our website.
Both individuals and organizations that work with arXivLabs have embraced and accepted our values of openness, community, excellence, and user data privacy. arXiv is committed to these values and only works with partners that adhere to them.
Have an idea for a project that will add value for arXiv's community? Learn more about arXivLabs .
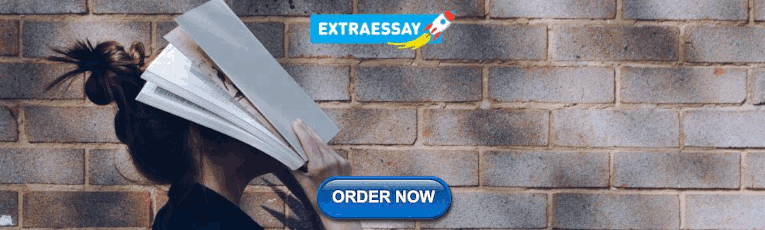
IMAGES
COMMENTS
DATA PRESENTATION, ANALYSIS AND INTERPRETATION. 4.0 Introduction. This chapter is concerned with data pres entation, of the findings obtained through the study. The. findings are presented in ...
A proper data presentation includes the interpretation of that data, the reason why it's included, and why it matters to your research. ... In the histogram data analysis presentation example, imagine an instructor analyzing a class's grades to identify the most common score range. A histogram could effectively display the distribution.
analysis to use on a set of data and the relevant forms of pictorial presentation or data display. The decision is based on the scale of measurement of the data. These scales are nominal, ordinal and numerical. Nominal scale A nominal scale is where: the data can be classified into a non-numerical or named categories, and
TheJoelTruth. While a good presentation has data, data alone doesn't guarantee a good presentation. It's all about how that data is presented. The quickest way to confuse your audience is by ...
The purpose of data interpretation is to make sense of complex data by analyzing and drawing insights from it. The process of data interpretation involves identifying patterns and trends, making comparisons, and drawing conclusions based on the data. The ultimate goal of data interpretation is to use the insights gained from the analysis to ...
By following these guidelines, you can ensure that your data analysis, interpretation, and presentation are thorough, accurate, and compelling, ultimately enhancing the overall quality and impact of your dissertation. ... Data analysis and interpretation are critical stages in your dissertation that transform raw data into meaningful insights ...
There are 4 modules in this course. This course focuses on the analysis and interpretation of data. The focus will be placed on data preparation and description and quantitative and qualitative data analysis. The course commences with a discussion of data preparation, scale internal consistency, appropriate data analysis and the Pearson ...
Abstract. This chapter covers the topics of data collection, data presentation and data analysis. It gives attention to data collection for studies based on experiments, on data derived from existing published or unpublished data sets, on observation, on simulation and digital twins, on surveys, on interviews and on focus group discussions.
Tools, Techniques, Examples - 10XSheets. July 14, 2023. In today's data-driven world, the ability to interpret and extract valuable insights from data is crucial for making informed decisions. Data interpretation involves analyzing and making sense of data to uncover patterns, relationships, and trends that can guide strategic actions.
Data Analysis and Data Presentation have a practical implementation in every possible field. It can range from academic studies, commercial, industrial and marketing activities to professional practices. In its raw form, data can be extremely complicated to decipher and in order to extract meaningful insights from the data, data analysis is an important step towards breaking down data into ...
Key Objectives of Data Presentation. Here are some key objectives to think about when presenting financial analysis: Visual communication. Audience and context. Charts, graphs, and images. Focus on important points. Design principles. Storytelling. Persuasiveness.
Data is interpreted in a descriptive form. This chapter comprises the analysis, presentation and interpretation of the findings resulting from this study. The analysis and interpretation of data is carried out in two phases. The first part, which is based on the results of the questionnaire, deals with a qualitative analysis of data.
View PDF. Presentations, Analysis and Interpretation of Data 125 CHAPTER-4 PRESENTATION, ANALYSIS AND INTERPRETATION OF DATA "Data analysis is the process of bringing order, structure and meaning to the mass of collected data. It is a messy, ambiguous, time consuming, creative, and fascinating process. It does not proceed in a linear fashion ...
Presentations, Analysis and Interpretation of Data 125 CHAPTER-4 PRESENTATION, ANALYSIS AND INTERPRETATION OF DATA "Data analysis is the process of bringing order, structure and meaning to the mass of collected data. It is a messy, ambiguous, time consuming, creative, and fascinating process. It does not proceed in a linear fashion; it is not neat.
Data is the basis of information, reasoning, or calcul ation, it is analysed to obtain. information. Data analysis is a process of inspecting, cleansing, transforming, and data. modeling with the ...
In conclusion, data analysis, interpretation, and presentation are crucial aspects of conducting high-quality research. By using the appropriate data analysis, interpretation, and presentation techniques, researchers can derive meaningful insights, make sense of the insights, and communicate the research findings effectively.
This chapter focuses on data presentation, data analysis and discussion. The data was obtained. by CRDB in budgeting. position (job title) at CRDB in Arusha,T anzania. stage or degree of mental or ...
Data analysis is the process of developing answers to questions through the examination and interpretation of data. The basic steps in the analytic process consist of identifying issues, determining the availability of suitable data, deciding on which methods are appropriate for answering the questions of interest, applying the methods and ...
This document discusses the presentation, analysis, and interpretation of data in research. It outlines three ways of presenting data: textual, tabular, and graphical. Some common graph types are listed. It also describes two approaches to data analysis: qualitative and quantitative. Qualitative analysis does not use precise measurements while quantitative analysis assigns numerical values to ...
The document discusses the presentation, analysis, and interpretation of data. It begins by explaining the differences between these three processes. Presentation of data refers to organizing data in charts, tables or figures. Analysis is the process of inspecting and transforming data to extract useful information. Interpretation refers to reviewing the data to arrive at conclusions. The ...
Chapter-4-Presentation-Analysis-and-Interpretation-of-Data.pdf - Free download as PDF File (.pdf), Text File (.txt) or read online for free. The document provides profiles of research participants in a study on faculty and administrator commitment. It summarizes their characteristics such as gender (59% female), employment status (52% full-time permanent), academic rank (43% assistant ...
The Data Visualization Lab located in room 413, formerly known as the Scholars' Lab, is available to assist students, faculty and staff with a variety of data-related needs, from analysis to visualization to digital research methods. For the Fall of 2024, the lab will be open from 9 a.m. to 5 p.m. Monday through Friday.
By employing a systematic approach to the collection and interpretation of information, you can more effectively reap the benefits of the ever-increasing mountain of external and internal data and ...
Chapter 4 PRESENTATION, ANALYSIS AND INTERPRETATION OF DATA This chapter presents the data gathered, the results of the statistical analysis done and interpretation of findings. These are presented in tables following the sequence of the specific research problem regarding the Effectiveness of Beat Patrol System in of San Manuel, Pangasinan.
Data Analysis and Interpretation. With the increasing reliance on data-driven decision-making, employees must be skilled in analysing and interpreting data.
Furthermore, interval censoring may have made interpretation challenging. The study also did not require patients to have measurable disease, as would be required in clinical trials using RECIST. Finally, although each data provider used patient-level data, aggregate analyses across cohorts were limited to interpretations from summary-level data.
Considering the inherent complexity of DIA metaproteomics data, data analysis strategies specifically designed for interpretation is imperative. From this point of view, we anticipate that deep learning methods and de novo sequencing methods will become more prevalent in the future, potentially improving protein coverage in metaproteomics.
We used a prior published analysis of commercial claims data from OptumRx to inform annual costs of care under SOC. All costs and transition probabilities by year are provided in the Supplement . The cost of gene therapy ($2 450 000) was estimated as the average of pricing for gene therapy in other approved indications, such as spinal muscular ...
Propensity score matching (PSM) is a method for filtrating experimental and control cases of similar characteristics, which are called the matching variables, from existing data to make them comparable in a retrospective analysis. PSM reduce the effect of selection bias. So, the comparison of outcomes between two groups can be fair.
View a PDF of the paper titled A new interpretation of the weighted pseudoinverse and its applications, by Haibo Li ... providing new tools for both analysis and computations. Subjects: Numerical Analysis (math.NA) MSC classes: 15A09, 15A22, 65F10, 65F20: Cite as: arXiv:2408.09412 [math.NA] (or ... Data and Media Associated with this Article ...