- Hispanoamérica
- Work at ArchDaily
- Terms of Use
- Privacy Policy
- Cookie Policy
- Dining Hall
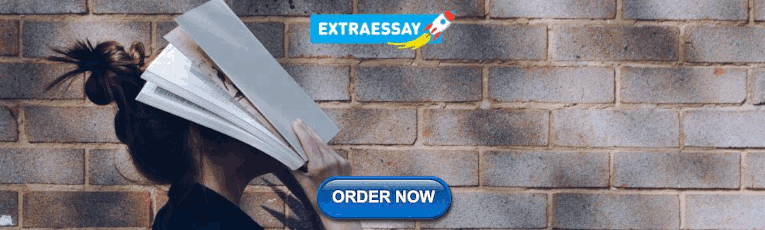
University Canteen and Restaurant / LGLS Architects
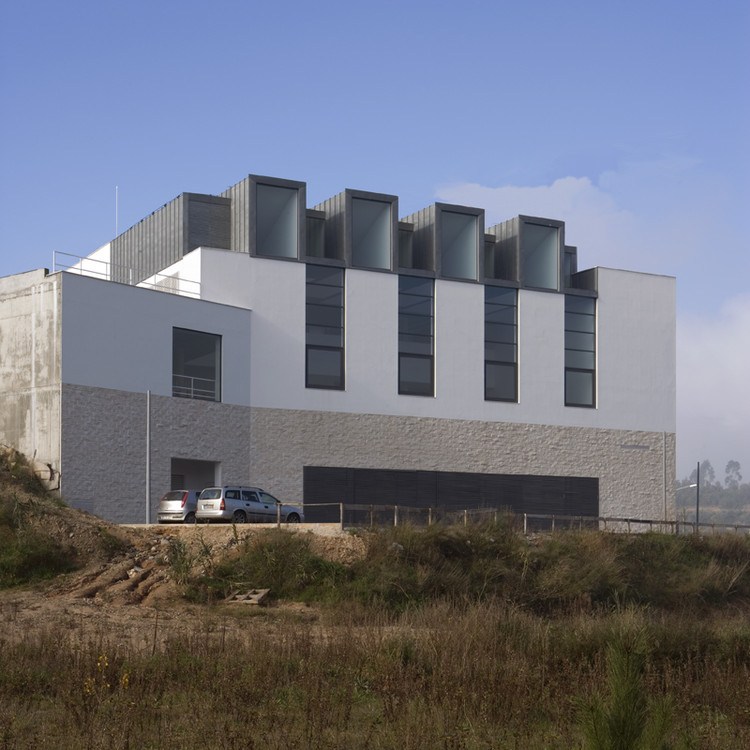
- Curated by ArchDaily
- Architects: LGLS Architects : LGLS Arquitectos
- Year Completion year of this architecture project Year: 2011
- Photographs Photographs: Luis Ferreira Alves
Text description provided by the architects. A canteen is a natural meeting place on a University Campus. The lunch break, usually understood as the moment one escapes from classes, is usually welcomed by one and all and this should be reflected in the architecture. Though sometimes neglected, even in a country like Portugal, which is so proud of its culinary achievements, here in Coimbra it was accorded due attention. The new canteen by LGLS aims to celebrate the importance of that moment of the day.
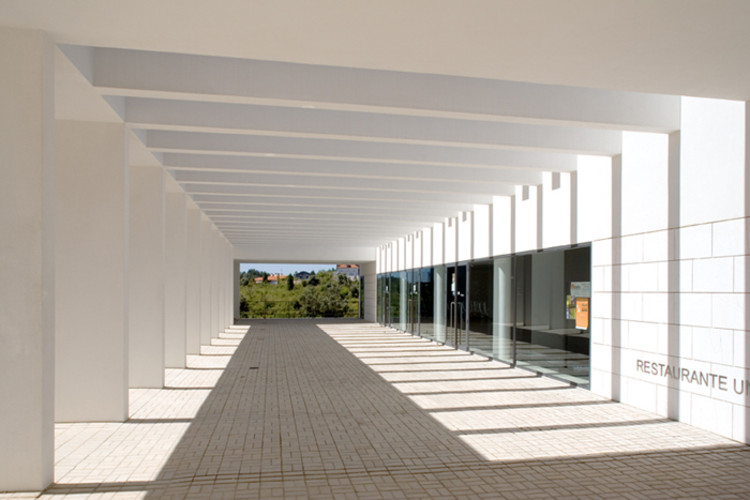
The canteen is situated on the new Campus III of the University of Coimbra . Given that this is the oldest University in Portugal, established over 700 years ago, it naturally carries a significant amount of symbolism and tradition. Its historic buildings are the symbol of the city itself, and the two new campuses bear an architectural responsibility to support these values.
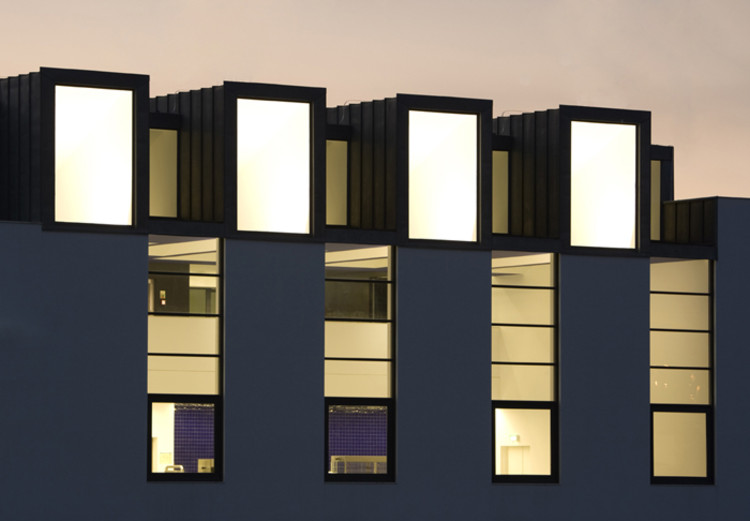
Campus II (engineering) is nearing completion while Campus III (medical sciences) is still under construction. Both display a lively architectural ensemble, as nearly all the buildings are the result of national competitions which gave younger architects an opportunity to emerge from amongst the work of already established offices.
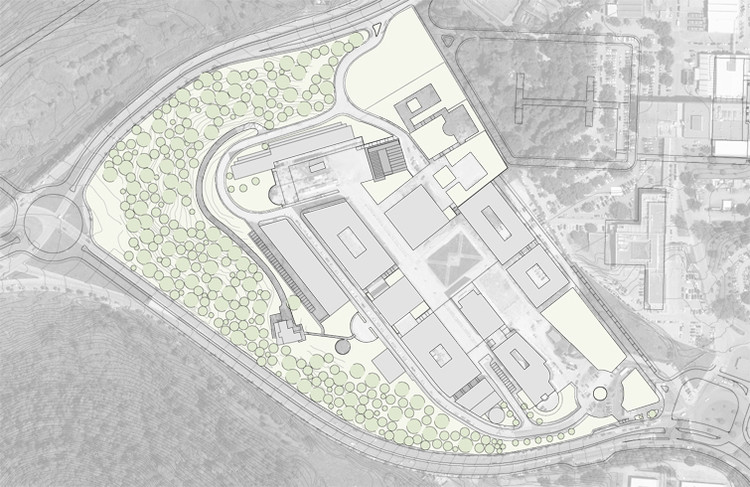
Appropriately, given its social importance, the canteen is one of the first buildings to be finished on Campus III. Situated on the edge of the campus, looking away from the city and facing its main road ring, the canteen is the built result of a competition won back in 2001 by the Lisbon-based architectural practice, LGLS.
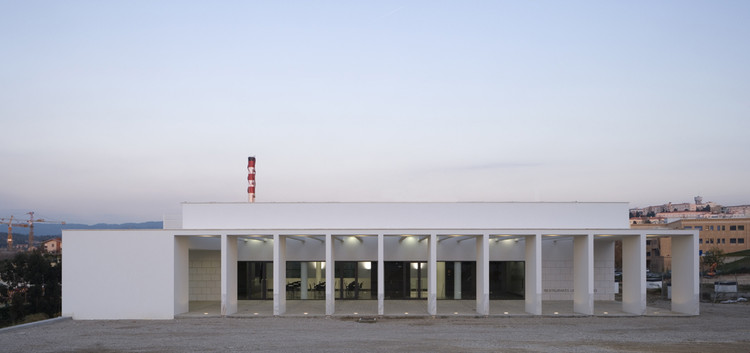
Conditioned by a sharply sloping site and a limited budget, the project quickly evolved into a play of scale, space, light and color that offers us a surprisingly large and luminous interior wrapped in what at first sight seems to be a fairly compact external package.
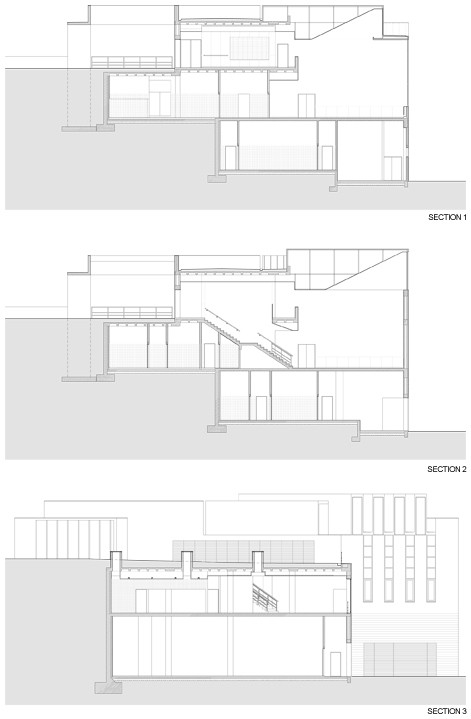
Public access is located on the upper level. The building then develops downwards, generating its strongest external moment at the opposite end from the entry point, thereby twisting the meaning of 'main facade' and allowing for an internal shift in scale that makes the light-flooded refectory so impressive.
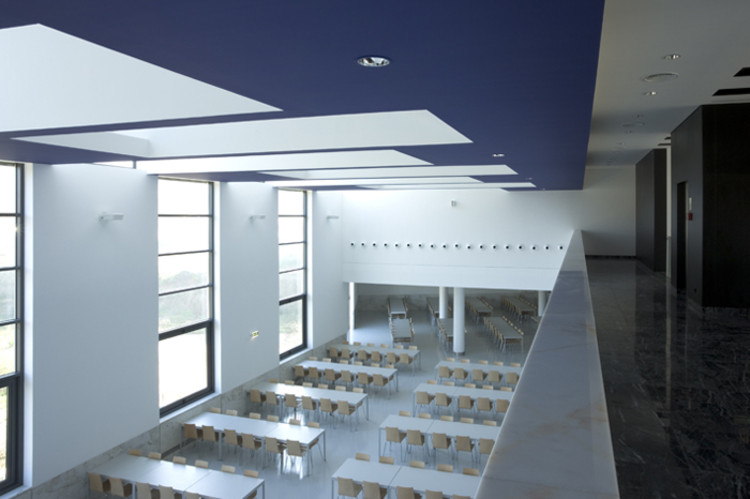
The composure displayed by the building when approached from within the Campus, with its low-profile main elevation hidden behind a sun-filtering pergola, is a key moment in the architectural promenade that runs through the building, down to the main refectory. You end up following the light in search of the space where everybody congregates.
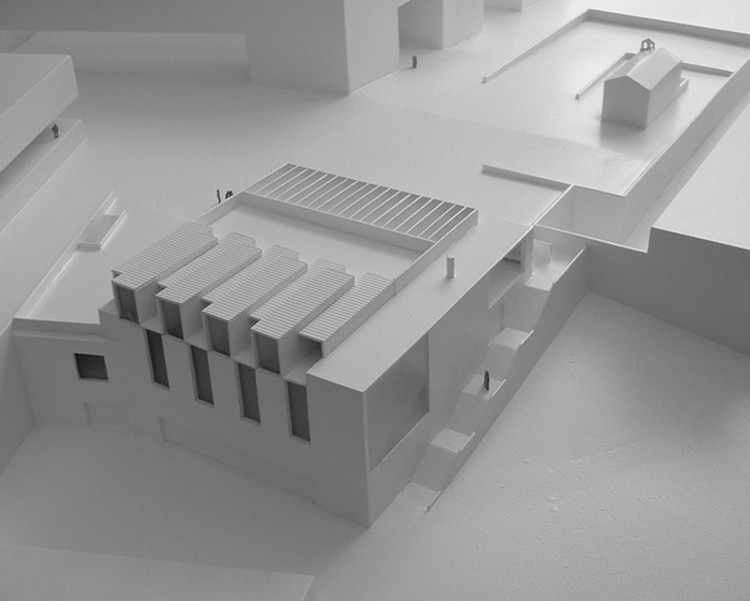
The internal colour scheme, tied to distinct ceiling heights, clearly the internal functions and instinctively organizes the users. The upper floor contains the wash rooms and the cafeteria (both painted in black) which ends in a full-length balcony above the main refectory below. This translates into two differentiated canteen systems for the students (one tiled in blue with a high ceiling and one tiled in red with a low ceiling) and a restaurant for teachers and university guests (panelled in wood). The bottom level is technical, including storage spaces, staff changing rooms and so on.
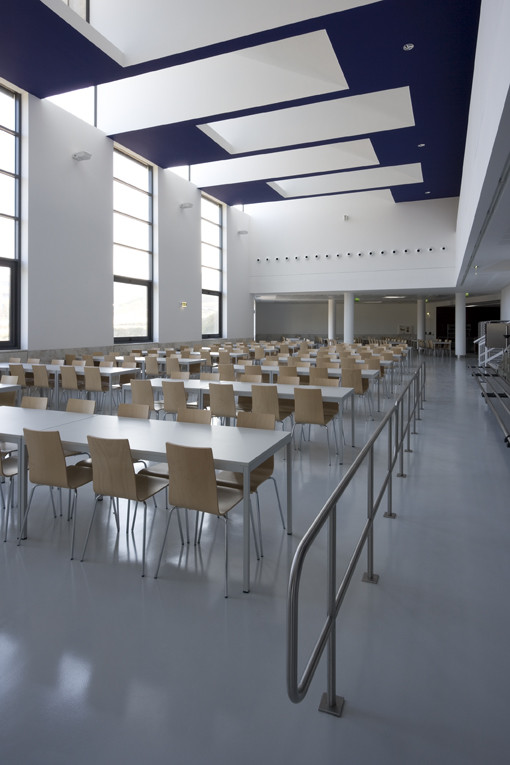
An artful juxtaposition of simple architectural elements turns the smallest building on the campus into a well-proportioned environment for socializing in the middle of the academic day.
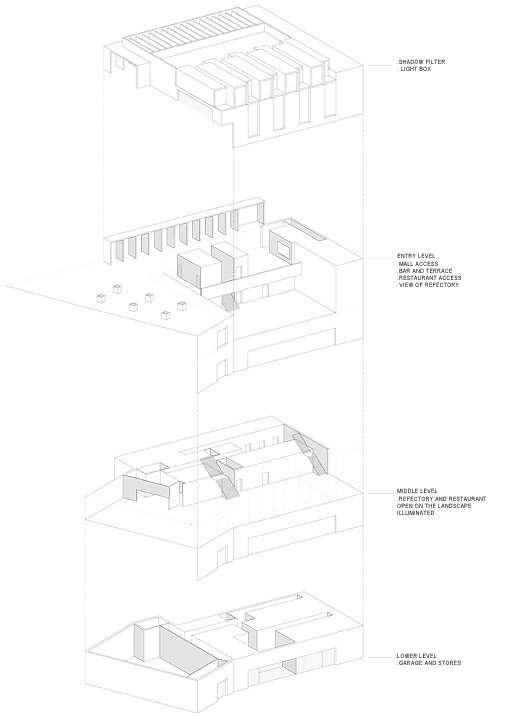
Project gallery
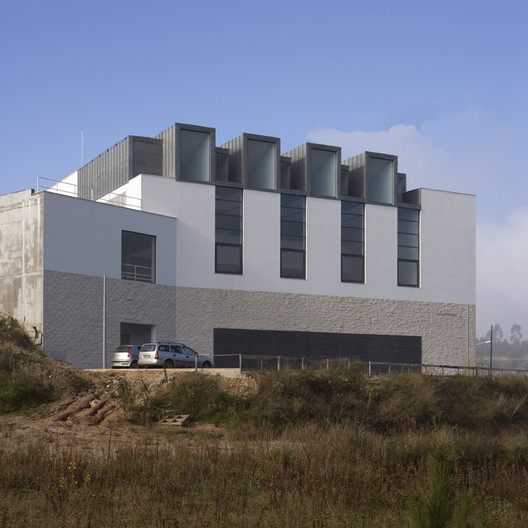
Project location
Address: coimbra, portugal.
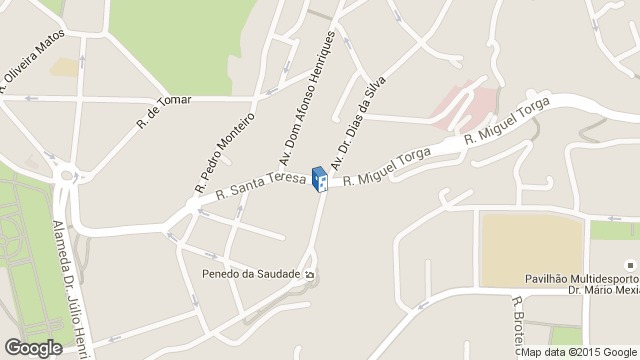
- Sustainability
世界上最受欢迎的建筑网站现已推出你的母语版本!
想浏览archdaily中国吗, you've started following your first account, did you know.
You'll now receive updates based on what you follow! Personalize your stream and start following your favorite authors, offices and users.
Check the latest Desks Privacy Furniture
Check the latest Desks Bureaus
Click through the PLOS taxonomy to find articles in your field.
For more information about PLOS Subject Areas, click here .
Loading metrics
Open Access
Peer-reviewed
Research Article
Analysis of college students’ canteen consumption by broad learning clustering: A case study in Guangdong Province, China
Roles Data curation, Methodology, Writing – original draft
Affiliation School of Accounting, Guangzhou Huashang College, Guangzhou, China
Roles Formal analysis, Methodology, Software
Roles Investigation, Validation, Writing – review & editing
Affiliation School of Data Science, Guangzhou Huashang College, Guangzhou, China
Roles Software, Validation, Visualization
Roles Conceptualization, Funding acquisition, Methodology, Resources, Writing – review & editing
* E-mail: [email protected]

- Chun Yang,
- Hongwei Wen,
- Darui Jiang,
- Lijuan Xu,
- Shaoyong Hong
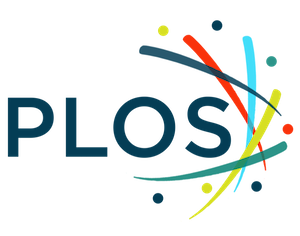
- Published: October 13, 2022
- https://doi.org/10.1371/journal.pone.0276006
- Reader Comments
Investigation on college students’ consumption ability help classify them as from rich or relative poor family, thus to distinguish the students who are in urgent need for government’s economic support. As canteen consumption is the main part of the expenses of the college students, we proposed the adjusted K-means clustering methods for discrimination of the college students at different economic levels. To improve the discrimination accuracy, a broad learning network architecture was built up for extracting informative features from the students’ canteen consumption records. A fuzzy transformed technique was combined in the network architecture to extend the candidate range for identifying implicit informative variables from the single type of consumption data. Then, the broad learning network model is fully trained. We specially designed to train the network parameters in an iterative tuning mode, in order to find the precise properties that reflect the consumption characteristics. The selected feature variables are further delivered to establish the adjusted K-means clustering model. For the case study, the framework of combining the broad learning network with the adjusted K-means method was applied for the discrimination of the canteen consumption data of the college students in Guangdong province, China. Results show that the most optimal broad learning architecture is structured with 14 hidden nodes, the model training and testing results are appreciating. The results indicated that the framework was feasible to classify the students into different economic levels by analyzing their canteen consumption data, so that we are able to distinguish the students who are in need for financial aid.
Citation: Yang C, Wen H, Jiang D, Xu L, Hong S (2022) Analysis of college students’ canteen consumption by broad learning clustering: A case study in Guangdong Province, China. PLoS ONE 17(10): e0276006. https://doi.org/10.1371/journal.pone.0276006
Editor: Brij Bhooshan Gupta, Asia University, TAIWAN
Received: July 19, 2022; Accepted: September 27, 2022; Published: October 13, 2022
Copyright: © 2022 Yang et al. This is an open access article distributed under the terms of the Creative Commons Attribution License , which permits unrestricted use, distribution, and reproduction in any medium, provided the original author and source are credited.
Data Availability: All relevant data are within the manuscript and its Supporting information files.
Funding: This work is funded by Innovation Team Project of Humanities and Social Sciences in Colleges and Universities of Guangdong Province (2020WCXTD008), The Project of Philosophy and Social Sciences in Guangdong Province (GD17XGL19), The Scientific Project of Guangzhou Huashang College (Grant: 2020HSDS03, 2021HSKT01). The funders had no role in study design, data collection and analysis, decision to publish, or preparation of the manuscript.
Competing interests: The authors have declared that no competing interests exist.
1. Introduction
Consumption is a special behavior that reflects the people’s possession of objects. The possession of objective ability is a necessary condition for the implementation of behavior [ 1 ]. Consumption ability is a concept accumulated by the rapid development of the social economic system. For the consumption behaviors, in addition to the knowledge and skills of consumer action strategies, the identification, comparison and selection of products will take extra time and energy, while the price the products account for most of the causal ratio [ 2 ]. Awareness and attention about the well-being of people’s consumption ability and consuming behaviors have increased in many countries, including China. Consumers with more abundant disposable time and energy and sufficient economic conditions have stronger consumption power [ 3 ].
University is a microcosm of a society. College students are participating in the transition to independence on attention to a highlighted set of features from the social character [ 4 ]. College students’ consumption ability is the basis of social consumption behavior. When individuals do not have or have insufficient consumption ability, even if they have consumption intentions, they will eventually lack corresponding consumption behaviors. According to the results of the interviews, it is found that the individual’s lack of consumption connotation knowledge and action knowledge, economic ability constraints, individual inherent habits and preferences such as cynicism, lazy and troublesome personality barriers hinder consumer behavior [ 5 ]. Therefore, investigation on college students’ consumption property help classify them as from rich or relative poor family, thus to distinguish the students who are in urgent need for government’s economic support.
The habitual consumption behavior of the young adults in universities can reflect the economic level and the unbalanced development of the society in the next few years. College students’ consumption behavior is on concern. Eating consumption is recognized as universal consumption among many consumption methods [ 6 , 7 ]. Thus the eating habits of the college students are the key factors that can directly reflect the consumption ability of the students’ families. Generally, the students’ eating habits in colleges and universities are mainly reflected in their canteen consumption records [ 8 ]. The characteristics of canteen consumption will release some prominent marker to tell the economic difference of the students’ families. Consequently, we can use the consumption characteristics of college students spending in the college canteen to classify and discriminate the students’ family economic situation.
In recent years, research on college students’ consumption ability has been actively carried out. For example, Kim et al. discussed the students’ behavior difference about clothing consumption in comparison between the clothing majors and non-majors [ 9 ]. Bruce et al. used conventional statistical methods (such as the p-value and some simple descriptive indicators) to study the male first-year college students’ consumption change on in sugar-sweetened beverage [ 10 ]. Gao et al. performed an empirical study on the mechanism of college students’ green consumption in China [ 11 ]. They used questionnaires and make statistical analysis, mainly accomplished by using the SPSS software and lack of novel design in methodologies. There are relatively few studies concerning on data mining by investigating machine learning methods for qualitative or quantitative analysis [ 12 , 13 ]. A review article discussed the possibility of artificial intelligence technologies be analyzed for enhancing the educational systems for semantic web-based education [ 14 ]. Cloud computing technology was used to analyze the requirements of academic and administrative affairs, education affairs, and affiliated institutions in the colleges and universities [ 15 ]. However, in the era of big data, the consumption data is presented as large volume, low value density, diverse forms, and fast growth [ 16 , 17 ]; machine learning strategy plays an important role in data analysis of the trends in college student consumption. More specifically, the students’ canteen data simply records the consumption amount at each time the students spend. The data is fragmented and dynamically increasing, which is bond to raise some challenging issues that probably hinder the computational model optimization [ 18 ]. Then some multi-variate statistical methods should be introduced into the design of the machine learning algorithmic flow.
Clustering is one of the important machine learning methods in many communities for data classification, discrimination, and pattern recognition [ 19 ]. Basically, it aims to group data points into different clusters based on their similarity or density [ 20 ]. In the past decades, many clustering algorithms have been proposed such as the K-means clustering [ 21 ], spectral clustering [ 22 ], min-max cut [ 23 ], and multi-view clustering [ 24 ]. A parallel fractional lion algorithm is recently reported for data analysis, the algorithm is tested on six standard databases [ 25 ], but it is not examined by practical collected data. The fact that it operates only based on the MapReduce framework limits its further application.
Among the existing clustering methods which are suitable for flexible application, the most popular one is the K-means clustering algorithm because its algorithmic flow is simple and efficient, and its operation only needs to learn a limited number of centroids to minimize the interclass data distances. However, the clustering performance of K-means is much related to its random initialization of the centroids [ 26 ]. Spectral clustering is able to prevent the uncertain interference of the randomness. It characterizes the data connection with an appropriate graph whose vertices represent the data points and the weights represent the connection between data pairs, and tries to partition the vertices into different clusters by minimizing the cut information [ 27 ]. For this consideration, we jointly perform spectral clustering in the K-means process, in the way of adjusting the spectral rotation to derive the underlying data connection. The adjusting process is detailly to perform eigen-decomposition on the graph Laplacian matrix transformed from the original data. The graph Laplacian matrix help to amplify the property of the data, and the eigen-decomposition is functional to extract the features [ 28 ]. After the adjusted operation, the normal K-means clustering is applied. The newly proposed methods is defined as adjusted K-means clustering.
Although the adjusted K-means method is advanced for classifying the students’ canteen consumption data, the data is raw and the feature information of the data is ambiguous. To this end, we design a broad learning neural network architecture to extract the feature variables that are rich in data information. Broad learning architecture is built up based on the broad learning system (BLS) established on the idea of a fully connected neural network (FCNN) accompanied with random vector functional link [ 29 , 30 ]. Specifically, the input data is transformed into numbers of feature nodes by proper mapping functions, and then these features are concatenated together to generate a series of enhancement nodes randomly, which will be used to expand the network architecture in a broad sense. All the features nodes and the outputs of the enhancement nodes are connected together to feed into the output layer. The desired output weights are to be determined by a fast ridge regression of the pseudo-inverse of the system equation [ 31 ]. The weights and biases in BLS are all randomly generated, and further to be trained in data-driven manner. The incremental learning algorithm is incorporated into the BLS which makes the network can be remodeled fast in the broad expansion without a retraining process.
Based on the principle of BLS, the broad learning neural network (BLNN) architecture is constructed as a black box model in our study for the analysis of the college student’s canteen consumption. We designed the architecture to be trained in data iteration for optimal observation of the linkage weights. On the other hand, the BLNN training bias is not considered in the model training process, we designed a fuzzy-transformed strategy to catch the feature information of the data under the condition of a fuzzy interval range. What the fuzzy-transformed strategy does is to make a fault-tolerant mode to ensure a relative large amount of candidate feature variables for BLNN training. The fuzzy-transformation is in fusion design with the principal component analysis (PCA) method. Specifically, the data features were extracted by PCA algorithm before the data was input to the BLNN architecture, and the PCA procedure is improved by using the fuzzy rules of the triangular membership function. The fuzzy transformed PCA technique is able to inhibit the original noise interference and make the explicit characteristics of the principal components much remarkable [ 32 ].
We proposed an adjusted K-means clustering method for the discrimination of the college students based on their canteen consumption data, thus to classify the students into different economic levels. Also, a broad learning neural network is designed to extract the exact data features to improve the discriminant accuracy, and a fuzzy transformed PCA technique is employed to reduce the model uncertainty and thus to help reduce the classification errors. The remainder of this article is organized as follows. Section 2 introduced the adjusted clustering method, the BLNN architecture and the fuzzy transformed PCA technique as well as the specific algorithmic modification. Section 3 describes the data collection and present the descriptive statistical properties. Section 4 applies the fusion of the proposed methods to establish the combined discrimination models in a data-driven manner, to fulfil the model optimization, validation and evaluation. Section 5 makes conclusions.
2. Methodologies
2.1. the adjusted clustering algorithm.
For unsupervised data analysis models, clustering analysis is the simplest classification method. Among the existing clustering methods, the most popular one is K-means clustering due to its good adaptiveness and high efficiency. It is used to tune the cluster centroids by each newly-added testing sample, to minimize the within cluster data distances [ 33 ].
However, the data available for clustering in this work is some designated statistical data which points to some specific properties of the raw data. In this point of view, we proposed an adjusted K-means algorithm as the improvement of the traditional K-means method, so that the cluster operation is much suitable to deal with the comprehensive statistic data. Given a built graph, we employ a two-step spectral clustering procedure. The first step is to perform eigen-decomposition on the graph Laplacian matrix to obtain the scaled cluster indicator matrix; The second step aims to make discretization based on the matrix, to get the final cluster assignment [ 28 ].
Since Y T Y = ( A T A ) −1/2 A T A ( A T A ) −1/2 = I , the embedding Y can be obtained by stacking the eigenvectors of L corresponding to its k smallest eigen values. However, Y is a real-valued matrix by default, then spatial rotation is necessary to perform discretization for the adjusted K-means clustering [ 34 ].
2.2. The broad learning architecture
- PPT PowerPoint slide
- PNG larger image
- TIFF original image
https://doi.org/10.1371/journal.pone.0276006.g001
BLNN is an adaptive learning architecture designed based on the FCNN structure. It has similar data training function as a deep learning framework. BLNN has a widened pseudo input layer that can help to take one pre-clustering feature screening in one network structure. But in contrast to deep learning, broad learning is able to skip the process of long-time consuming on training abundant parameters in different filters and layers [ 29 , 37 ]. The parameters in BLNN could be determined by random projection or by generating pseudo neural nodes to form a wide enough layer for fast tuning. Moreover, the training process can be changed to an incremental learning mode when the network structure is extended in the width direction [ 38 ]. Specific BLNN training procedure is showed as follows,
Considering the general supervised learning task, we are given the training dataset X p × d containing p sample data of d properties, and the target Y p × k marking the p samples as k discriminant classes. Each row data in X and Y denotes the original sample dimensional information and target clustered makers, respectively. The training samples are taken as the original input data with d properties.
Also, the input variables are applied to generate and connect the layer of enhancement nodes. To speed up the training process, the enhancement nodes are obtained in N a groups. Any feasible algorithmic flow can be designed to observe the outputs of the groups of enhancement nodes.
The BLNN architecture is shown in Fig 2 . Practically, the BLNN architecture enables to test different number of hidden nodes ( m ), and adaptively tune the linkage weights ( w 1 and w 2 ) for searching the optimal broad learning network structure. Before model training, we need to select suitable activation functions performing as f 1 and f 2 in advance. Concerning on the BLNN feature extraction by obeying the equations of ( 6 ), ( 7 ) and ( 8 ). The BLNN architecture runs in the complexity of O[ N a 2 × d × p 2 ].
https://doi.org/10.1371/journal.pone.0276006.g002
2.3. Fuzzy PCA transform technique
Fuzzy-transformed design is carried out under the condition of interval range of information data to be considered. It is used as the preprocessing algorithm for preventing the impact of the single type data [ 39 ]. It means to ensure that the fuzzy transform is functional to find the features from the consumption data that are not merely telling the consumption properties, then the model can be improved as conceiving a diversity of data characteristics. The fuzzy transform design of the classical PCA is able to emphasize the explicit informative features of the principal components so that the unnecessary noise can be suppressed.
The algorithm is proceeded by the fuzzy partition of the universe fuzzification. This depends on the feature contribution of the measured data. There are several membership functions can be used or modified to fit a specific target, such as the monotonic, triangular, trapezoidal and bell-shaped functions [ 40 ]. Specifically, the triangular function (see Fig 3 ) can much reduce the computational overhead of fuzzy transform due to simpler operation. The triangular fuzzy transform is briefly outlined as follows,
https://doi.org/10.1371/journal.pone.0276006.g003
The fuzzy PCA transform algorithm is evident to generate a group of enhancement features in machine learning procedures [ 41 ]. Thus we designed to apply fuzzy PCA into the BLNN model to search for brand new network nodes, so that the data features are increasingly emphasized, but the computational complexity is increased by O[ N × k × p ].
3. Data collection and descriptive statistics
Experimental data was from colleges and universities located in Guangdong province, China. We collected a total of 307,968 students’ records from the year 2019 to 2021. The records include students’ personal information such as his/her age, majority and hometown. The properties of students’ consumption behaviors include card recharges, canteen expenses, utility bills, library borrowing, expenses for training and certification, etc. Commonly, every college student goes for meals in the college canteen, their expense on canteen meals is the key factor to tell their family backgrounds and economic status. Thus we targeted on the record of canteen expense data. There are some missing data and abnormal data in the 307,968 sample records. we removed them as they account for a small proportion in the total; then we have 265,972 samples for modeling analysis.
In details, the canteen expense data is large and complex. It shows a long list of students’ consuming records in time orders. There appear heavy repeats of students’ personal information, the meal menus and the price. To reduce the influence of overlapping information, we design to use five common descriptive statistics to summarize the data features. They are the annual-averaged consumption cash amount (yCA), the month-averaged consumption cash amount (mCA), the averaged cash amount by consumption frequency (fCA), the month-averaged consumption frequency (mCF) and the day-averaged consumption frequency (dCF). There indicators are socially meaningful. A large value of yCA, mCA and fCA represents it is economically supported that the student is capable of enjoying good meals in canteen level. On the contrary, a small value of mCF and dCF means that the student is seldom expense on canteen meals, which indicates that he/she is economically supported to have high quality meals in good restaurants other than the college canteen. Fig 4 shows the distribution of the five quantified descriptive statistics.
https://doi.org/10.1371/journal.pone.0276006.g004
Additionally, some students’ personal information inherently influenced their consuming behaviors. On one hand, we surveyed the students’ majorities and summarized them into the subject category. The distribution by subjects is shown in Fig 5 . There are relatively more students involved in the subjects of Engineering, Science, Economics, Medicine and Management, and relatively less students are in Agriculture, Art, Education and Literature. On the other hand, we also performed a statistical analysis of their hometown information. The 21 cities in Guangdong province are grouped into 7 regional segmentations according to their geographical locations. The numbers of students coming from each city group are listed in Table 1 .
https://doi.org/10.1371/journal.pone.0276006.g005
https://doi.org/10.1371/journal.pone.0276006.t001
4. Model designs and result discussions
4.1. adjusted k-means modeling for discriminant metics.
For all of the 265,972 targeting samples, we have the five important descriptive statistic indicators for modeling, i.e. yCA, mCA, fCA, mCF and dCF. The former three represent the consuming cash amount while the latter two are the consuming frequency. They are of different types and the data range vary a lot, thus we have to make a 0–1 normalization before modelling. Besides, the students’ consuming ability is certified by a large consuming cash amount reflected in yCA, mCA and fCA, and also by a small consuming frequency represented in mCF and dCF. As for modeling simplicity, we defined the consuming property vector as v = [yCA, mCA, fCA, 1/mCF, 1/dCF] T for each target student sample, so that the sorting discrimination is totally uniform as from the smallest to the largest on each element. Then we applied the adjusted K-means method to the data for classification of the students’ consuming abilities.
We used the elbow method to select the best number of clusters k . The elbow method defines a distortion quantified index on the objective function. a small value of distortion represents the samples clustered tightly, while a large value means the samples are loose [ 42 ]. We test several candidate k values for different mode of clustering, and find the objective function min Tr for each k . the comparative plot is shown in Fig 6 . The curve indicated that k = 4 is the best choice before the clustering becoming loose. Therefore, we decide to classify the samples into 4 classes according to their consuming property indicators. The 4 classes are generated by the second norm value of the vector v (i.e. ‖ v ‖). The total of 265,972 samples are sorted in the ascending order of ‖ v ‖. To some extent, the sorting list is used to estimate the students’ consumption level. Then we can easily observe 4 piece-wise segments by dividing the minimum-to-maximum range by the points of Q1 (the 25% quantile), Q3 (the 75% quantile) and the median values. The segment from Minima to Q1 is marked as the cluster C 1 , the segment from Q1 to the Median is marked as the cluster C 2 , the segment from the Median to Q3 is marked as the cluster C 3 , and the segment from Q3 to the Maxima is marked as the cluster C 4 (see Table 2 ). Thus we have the number of samples partitioned in each cluster set. The students classified in C 1 , C 2 , C 3 and C 4 are regarded as poor, frugal, normal and affluent, respectively, in the analysis of college canteen consuming behavior. The classification markers are further used to train and evaluate the discrimination models established by BLNN architecture.
https://doi.org/10.1371/journal.pone.0276006.g006
https://doi.org/10.1371/journal.pone.0276006.t002
4.2. Data extended by fuzzy PCA technique
Of the total of 265,972 samples, 186,180 samples (~70%) are used to train the model and 79,792 samples (~30%) used for model test. The test samples are not involved in the model training process. The training sample data X is used as the raw input data for BLNN training. The raw data is extended to produce one enhancement layer by using the fuzzy transform PCA technique, and then the enhancement neural nodes and the raw input data nodes are flattened as a novel extended input layer for broad network learning.
Concerning the raw data, the network architecture generates 5 neural nodes for receiving the 5-dimension normalized property variables [yCA, mCA, fCA, 1/mCF, 1/dCF] T . The 5-dimension property variables were globally converted into common principal components (PCs) sorted in the order of descending contribution rate. Then the PCs were further carried out for the fuzzy transform strategy, in which we used the triangular membership function. There we tuning the number of PCs from 1 to 5, to determine the best number of PCs for fuzzy optimization. The generated variables acquired by fuzzy transformed PCA was used to train the FCNN model. With adaptively searching the network linkage weights, appreciate discriminant results were obtained based on the186,180 training samples. There we observed the best FCNN model with aided transformation by the fuzzy-transform PCA technique. The optimal discrimination model was obtained by using 5 PCs, and the confusion matrix of the training samples was showed in Table 3 . We have the discrimination accuracy as 89.9% from the confusion matrix. Accordingly, we set 5 extended enhancement neural nodes to build up the BLNN network architecture.
https://doi.org/10.1371/journal.pone.0276006.t003
4.3. Data training by BLNN architecture
The refreshed input layer is await for the data input of X ext . Then X ext was delivered to the hidden layer by the algorithmic flow introduced in Section 2.2, generating a series of hidden nodes by activation function mapping of the summation of the input variables. The ReLU function [ 43 ] was applied for the hidden layer activation. The linkage weights ( w 1 ) was adaptively trained for iterative optimization, while the number of hidden nodes ( m ) is preset for tuning in the integer range of m ∈ [1, 2 … 16] ⊂ Z + .
Next, the hidden variables are delivered to the output layer by the similar operation of variable summation and activation function mapping, where we used the Sigmoid function [ 44 ] for output activation. The linkage weights ( w 2 ) was also set for adaptively iterative training. Afterwards, the output variables were involved in the softmax unit, and we used the adjusted K-means method to deduce the discriminant results. As for parameter tuning, different number of hidden nodes released different BLNN training results. We compared observations of model discrimination accuracy for using a changing value of m (see Fig 7 ). It is seen from the figure that we observed the best discrimination accuracy as 93.5% when m = 14. This indicated that the BLNN structure is best optimized by using 14 hidden nodes. Besides, the network structures built up with the numbers of from 11 to 17 hidden nodes are able to reach nearly appreciating discrimination results (see the green points in Fig 7 ). Their discrimination accuracies are also over 92%. For the comparison to the FCNN model, the discriminant confusion matrix of the optimal BLNN model was showed in Table 4 . Although the BLNN model is able to improve the total accuracy, we can see in details that the better results are noticeable for the C 1 class and the C 2 class, while the C 3 and C 4 classes are not so obvious improved. The reasons are probably instinct to the segments for dividing the minimum-to-maximum range by the Q1, Q3 and the median values. The C 1 and C 2 classes are regarded as the poor and frugal level; It means that the students who consumes less in college canteen are relatively more easy to discriminated by the BLNN model. In contrast, the normal and the affluent level students (i.e. the C 3 and C 4 classes) can be classified to an equal extend by using the BLNN or using the FCNN model.
https://doi.org/10.1371/journal.pone.0276006.g007
https://doi.org/10.1371/journal.pone.0276006.t004
4.4. Model evaluation
There are 79,792 samples (~30% of the total) reserved before model training, which are excluded and totally independent from the model training process. The test samples were utilized to evaluate the best BLNN training model, as well as the FCNN model for comparison.
The optimized BLNN is built up with 5 original input nodes and 5 pseudo input nodes for broad learning, thus the extended input layer contains 10 neurons, for accepting the raw data variables (yCA, mCA, fCA, 1/mCF, dCF) and the generated comprehensive variables (PC 1 , PC 2 , PC 3 , PC 4 , PC 5 ) transformed by the fuzzy PCA technique. The optimal network was identified as constructed with 14 hidden nodes by the abovementioned training fulfillment. The linkage weights were automatically tuning by the network adaptive training mechanism. The ReLU function was used as the activation functions for each neural perceptron. The softmax unit was designed to launch the adjusted K-means clustering algorithm for data classification.
The data of the 79,792 test samples were input to test the optimized BLNN model. As the model training process is only to identify the optimal network structure, thus the model evaluation needs to re-train the network linkage weights for any new input. We recorded 20 of the changing classification accuracies during the adaptive iteration on the network linkage weights (showed in Fig 8 ), and the iterative discrimination results of the FCNN model were also noted in the figure for comparison. Fig 8 shows that the BLNN model observed the best discrimination accuracy of 91.4% for the test set samples. In contrast, the FCNN model was not able to reach up to 90%, its highest data only reached 88.2%.
(Note that the label AdjKmeans represents the adjusted K–means method).
https://doi.org/10.1371/journal.pone.0276006.g008
As for comparison, the BLNN and FCNN extracted data features were delivered to establish the common K-means classification model. results were also illustrated in Fig 8 . The comparative results indicated that the proposed BLNN architecture combined with the adjusted K-means clustering is functional to improve the model discrimination accuracy in data analysis for the classification of college student’s canteen consumption levels.
5. Conclusions
A broad learning clustering architecture was built up in this paper for the qualitative analysis of college students’ canteen consumption data. The fuzzy transformed PCA technique was applied to find extended data features before the data was input to the network. the adjusted K-means clustering method was fused in the network softmax unit for optimizing the classification model. Confronting the data of Guangdong college students, the BLNN structure is constructed by an extended input layer, a node-number-tuning hidden layer and an adaptive clustering softmax. The extended input layer was formed by a set of pseudo neural nodes for fast tuning. There identified 10 neural nodes, of which the former 5 are to receive the 5 descriptive statistical features of the raw data, and the latter 5 pseudo nodes are available for taking the 5 fuzzy PCs generated by fuzzy PCA transform technique, in which a triangle membership function was applied. Then the extended input data was trained through the hidden layer, and delivered to a softmax unit for establishing the adjusted K-means clustering models, thus to analyze the students’ canteen consumption data. The set-up BLNN modeling architecture is applied to classify the college students into 5 different economic level, by analyzing their canteen consumption data.
As to find the most optimal BLNN model for the discrimination, the hidden layer of the BLNN architecture was to tune the number of hidden nodes, and to test the model discrimination accuracy. The most optimal BLNN training model is structured as having 14 hidden nodes, the corresponding best training discrimination accuracy is high up to 93.5%. except for the best model, the BLNN models built up with from 11 to 17 hidden nodes were also able to get appreciating discrimination results over 92%. After that, the optimal training model was evaluated by using the test set samples. By 20 iteration model tuning, we observed the best testing discrimination accuracy as 91.4% by the BLNN model, which is better than the conventional FCNN model.
These modeling and comparing results indicated that the designed BLNN architecture is reasonable to get a more accurate discrimination results for the statistical evaluation of college students’ economic level, by analyzing the students’ canteen consumption recorded data. The design of BLNN framework in fusion use of fuzzy transformed PCA technique and the adjusted K-means method is an advanced machine learning mode. It is feasible to enhance the model effects for classifying college students into different economic levels, and thus we are able to make more accurate distinguishment of the students who are in need for financial aid. Further, the framework is prospectively expected to be applied in solving some challenging data mining issues in other fields.
Supporting information
S1 appendix..
https://doi.org/10.1371/journal.pone.0276006.s001
- View Article
- Google Scholar
- PubMed/NCBI
Academia.edu no longer supports Internet Explorer.
To browse Academia.edu and the wider internet faster and more securely, please take a few seconds to upgrade your browser .
Enter the email address you signed up with and we'll email you a reset link.
- We're Hiring!
- Help Center
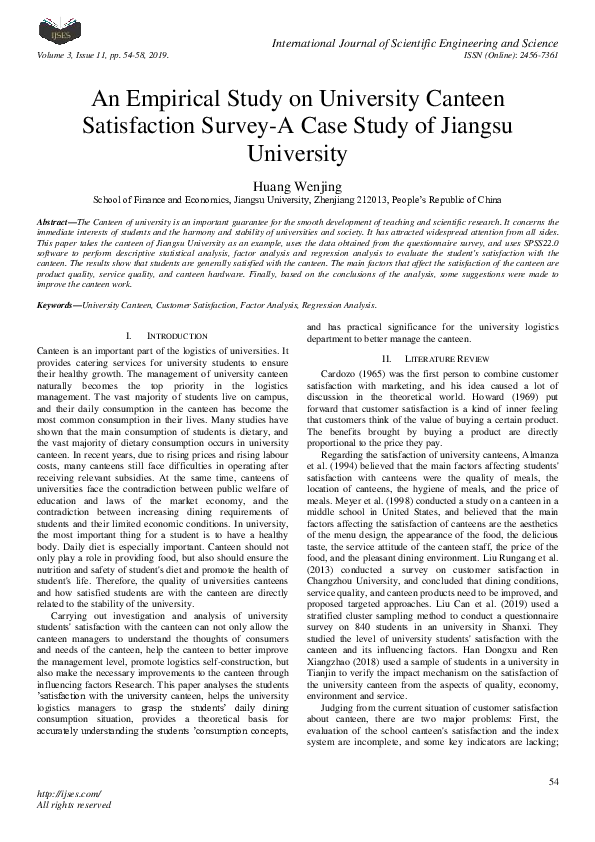
An Empirical Study on University Canteen Satisfaction Survey-A Case Study of Jiangsu University

The Canteen of university is an important guarantee for the smooth development of teaching and scientific research. It concerns the immediate interests of students and the harmony and stability of universities and society. It has attracted widespread attention from all sides. This paper takes the canteen of Jiangsu University as an example, uses the data obtained from the questionnaire survey, and uses SPSS22.0 software to perform descriptive statistical analysis, factor analysis and regression analysis to evaluate the student's satisfaction with the canteen. The results show that students are generally satisfied with the canteen. The main factors that affect the satisfaction of the canteen are product quality, service quality, and canteen hardware. Finally, based on the conclusions of the analysis, some suggestions were made to improve the canteen work.
Related Papers
Asian Social Science
Norazah Mohd Suki
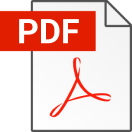
Intan Nurul Azni
The canteen of Sahid University - Jakarta is a canteen which is located at Prof. Dr. Soepomo, SH No. 84, Tebet South Jakarta. The counters were sheltered by the Employee Union of Sahid University - Jakarta. The canteen of Sahid University - Jakarta is able to accommodate 15 counters for catering business. Currently the food and beverage products sold in the canteen are still in conventional method so that consumers are limited to the academic community of Sahid University - Jakarta and the community around the campus. This study aimed to assess the services offered by the canteen. This study also to determine the level of satisfaction of canteen customers on the canteen services, and to identify the problems encountered on the services. Most of customer of this canteen are 16-25 years old (84%) and half number of visitors are senior high school educational background (50%). More than half number of visitors come to Sahid University - Jakarta ocassionally (58%). At the quality of pro...
Asian Journal of Empirical Research
Bakul Sarkar
arvic ricafort
International Journal of Engineering & Technology
dyana chang
This research investigated the effect of food quality, price fairness, staff performance, and ambience on students’ satisfaction of cafeteria food services by comparing responses from Universiti Malaysia Sabah (UMS), Malaysia with Mercu Buana University (MBU), Indonesia. Data was analysed using the Partial Least Squares (PLS) application in SmartPLS computer software version 2.0. Path coefficients from PLS results revealed ambience as the most significant predictor of students’ satisfaction of food services at the university cafeteria in both UMS and MBU settings, followed by aspects of staff performance and food quality. It is imperative for university cafeteria operators at both sites to uplift students’ satisfaction of food services at their cafeterias by enhancing ambiance such as installing decorations that project a warm and cosy environment. The result of these findings could be utilised as guidelines for the respective university management to incorporate elements that could...
Psychology and Education: A Multidisciplinary Journal
Psychology and Education , Rosa Madonna L. Licudan , Dennis Caballes
This study aimed to determine the students' satisfaction on the school canteen operation of Pililla National High School during School Year 2022-2023. The researcher used stratified technique-a probability sampling procedure in which each member of the population has an equal chance of being selected, providing a representative sample that accurately reflects the characteristics of the population being studied. The respondents of the study were 279 Junior High School students and were chosen using stratified sampling technique. This study utilized the mix method of research in which the researcher combines the elements of qualitative and quantitative approaches. The study used the researchers made questionnaire, which includes the data about profile such gender and socioeconomic status as Part I, the level of satisfaction of junior high school students on school canteen operation in Part II and the typical experiences that influence the level of satisfaction of students in the school canteen operations in Part III. The researcher utilized different data gathering techniques such as questionnaire checklist (hard copy and online) and survey form in order to the conduct of this capstone project and will employ different analytical tools to help and justify the conduct of the study. It was discovered that female respondents' outnumbered male and majority of the students belong to socioeconomic status of less than 10, 000, while on the level of satisfaction on school canteen operations, it was found out that cleanliness of the school canteen obtained the highest computed mean and has been identified with the most consistent response among the respondents as revealed by the standard deviation., however, it was also revealed that there is no significant difference in the students' satisfaction on school canteen operations when assessed by the respondents categorized in terms of gender except for the area on nutritional value (p<0.05), and there is no significant difference in the students' satisfaction on school canteen operations based on socioeconomic status reflective of the sig. values all greater than α = 0.05. On the qualitative data, the researchers found out that the experiences that influence student satisfaction in school canteen operations can be complex and multifaceted, and may vary depending on factors such as student demographics, cultural backgrounds, and personal preferences. It is important for canteen operators to take these factors into account when designing and implementing strategies to improve student satisfaction and promote healthy eating habits.
Noor Zainol
The food and beverage industry has been a prominent industry in the world.University food service has also become one of the services that need to be considered in order to gratify students by providing food in universities.University foodservice is one of the largest sectors of the food service industry and moreover, college student consumer group is getting larger.The main objective of this paper is to examine the relationship of food quality and food price on international students‘ satisfaction in UUM cafeterias.A mixed-method approach was applied in this study which includes both qualitative and quantitative methods.In order to identify the current research problem, an initial pre-data collection was being done by distributing comment cards to the international students to gain feedback regarding their satisfaction towards food quality and food price while patronizing in UUM cafeterias. Based on the findings, majority of international students were dissatisfied with the taste, ...
International Journal of Environmental Research and Public Health
Hanna Górska-Warsewicz
The purpose of this study was to identify and analyze consumer choices and service quality in university canteens in Warsaw. Our study consists of two parts. The first part of our research was conducted using a sample of 1250 adult respondents in 25 university canteens located at five higher education institutions. The reasons and frequency for using canteens, types of selected dishes and opinions on a given catering facility management system were analyzed. The second part of the study was conducted as an inspection to assess internal control and reliability of information. The respondents’ opinions are not in line with inspection assessments. This may be due to the fact that students do not pay attention to the quality of services in university canteens or have little knowledge about service, quality of services or hygiene aspects. For a detailed analysis of consumer choices and service quality assessment, we used Analysis of Variance (ANOVA) test and multi-dimensional cluster ana...
Abdallah Mohamed , Eslam Fathy
Food and beverage quality Service quality Reasonable prices and overall student satisfaction In this study, perceptions of different service attributes that have an impact on university students' overall satisfaction were examined. Students using different university cafeterias were selected through convenience samples of different college students at the university. A total number of 543 valid questionnaires were used for statistical analysis. Results indicated that student satisfaction with different service attributes was below average. Moreover, all service attributes were found to have a significant and positive impact on the overall student satisfaction. Based on the results of the current study, several recommendations were derived for university management to increase student satisfaction with food and beverage services provided at different university cafeterias.
European Journal of Tourism, Hospitality and Recreation
Anshul Garg
This study investigates the link between the different service characteristics that have an influence on customer satisfaction in university cafeteria food services. A conceptual model comprising of different variables like food and beverage quality, service quality, food choice, price and value equality, and ambience were studied for customer satisfaction to explore the relationship among them. This study examined how dining experiences influence the satisfaction and loyalty of both non-mature (students) and mature (staff) customers, in order to find their similarities and differences within the context of university cafeteria services. The study was conducted at Taylor's University in Malaysia by using a quantitative research approach, and 231 students and 63 staff members of the university participated in the study. The findings reveal that the various quality factors considered in the study are positively correlated with customer satisfaction and have a significant impact on the satisfaction level of both students and staff who dined at the university cafeteria. It could be concluded from the results that the influence of price offered at the university cafeteria had a significant effect on respondents' loyalty. The result of this study offers a direction towards better the services and facilities, which lead to an increase of the competitive influence of the restaurant business in the marketplace.
Loading Preview
Sorry, preview is currently unavailable. You can download the paper by clicking the button above.
RELATED TOPICS
- We're Hiring!
- Help Center
- Find new research papers in:
- Health Sciences
- Earth Sciences
- Cognitive Science
- Mathematics
- Computer Science
- Academia ©2024
To read this content please select one of the options below:
Please note you do not have access to teaching notes, what influences students’ food waste behaviour in campus canteens.
British Food Journal
ISSN : 0007-070X
Article publication date: 25 May 2022
Issue publication date: 16 January 2023
Reducing food waste is one of the critical challenges of campus canteens. This study aims to test an extended theory of planned behaviour that incorporates moral norm, food taste and campus food-saving climate to understand the influence factors of food waste behaviour on campuses.
Design/methodology/approach
To evaluate the proposed model, an online survey was utilized to collect 513 valid questionnaires.
The findings suggest that (1) attitudes towards food waste, subjective norm and perceived behavioural control have a significant influence on intention to reduce food waste; (2) Moral norm and food taste are shown to have a favourable effect on the intention to reduce food waste; (3) Food waste behaviour is significantly determined by intention to reduce food waste; (4) Campus food-saving climate negatively moderates the relationship between intention to reduce food waste and food waste behaviour.
Originality/value
This study paves the way to enhance the understanding of the factors of food waste among college students. In addition, this study develops practical strategies to prevent large amounts of food from being wasted on campus.
- Theory of planned behaviour
Acknowledgements
This study was supported by China Postdoctoral Science Foundation (Grant No. 2020M670170), National Natural Science Foundation of China (Grant No. 71672008; 91746210; 72050410354; 71772012).
Wang, H. , Ma, B. , Cudjoe, D. , Farrukh, M. and Bai, R. (2023), "What influences students’ food waste behaviour in campus canteens?", British Food Journal , Vol. 125 No. 2, pp. 381-395. https://doi.org/10.1108/BFJ-10-2021-1103
Emerald Publishing Limited
Copyright © 2022, Emerald Publishing Limited
Related articles
We’re listening — tell us what you think, something didn’t work….
Report bugs here
All feedback is valuable
Please share your general feedback
Join us on our journey
Platform update page.
Visit emeraldpublishing.com/platformupdate to discover the latest news and updates
Questions & More Information
Answers to the most commonly asked questions here

An official website of the United States government
The .gov means it’s official. Federal government websites often end in .gov or .mil. Before sharing sensitive information, make sure you’re on a federal government site.
The site is secure. The https:// ensures that you are connecting to the official website and that any information you provide is encrypted and transmitted securely.
- Publications
- Account settings
Preview improvements coming to the PMC website in October 2024. Learn More or Try it out now .
- Advanced Search
- Journal List

Analysis of college students’ canteen consumption by broad learning clustering: A case study in Guangdong Province, China
1 School of Accounting, Guangzhou Huashang College, Guangzhou, China
Hongwei Wen
Darui jiang.
2 School of Data Science, Guangzhou Huashang College, Guangzhou, China
Shaoyong Hong
Associated data.
All relevant data are within the manuscript and its Supporting information files.
Investigation on college students’ consumption ability help classify them as from rich or relative poor family, thus to distinguish the students who are in urgent need for government’s economic support. As canteen consumption is the main part of the expenses of the college students, we proposed the adjusted K-means clustering methods for discrimination of the college students at different economic levels. To improve the discrimination accuracy, a broad learning network architecture was built up for extracting informative features from the students’ canteen consumption records. A fuzzy transformed technique was combined in the network architecture to extend the candidate range for identifying implicit informative variables from the single type of consumption data. Then, the broad learning network model is fully trained. We specially designed to train the network parameters in an iterative tuning mode, in order to find the precise properties that reflect the consumption characteristics. The selected feature variables are further delivered to establish the adjusted K-means clustering model. For the case study, the framework of combining the broad learning network with the adjusted K-means method was applied for the discrimination of the canteen consumption data of the college students in Guangdong province, China. Results show that the most optimal broad learning architecture is structured with 14 hidden nodes, the model training and testing results are appreciating. The results indicated that the framework was feasible to classify the students into different economic levels by analyzing their canteen consumption data, so that we are able to distinguish the students who are in need for financial aid.
1. Introduction
Consumption is a special behavior that reflects the people’s possession of objects. The possession of objective ability is a necessary condition for the implementation of behavior [ 1 ]. Consumption ability is a concept accumulated by the rapid development of the social economic system. For the consumption behaviors, in addition to the knowledge and skills of consumer action strategies, the identification, comparison and selection of products will take extra time and energy, while the price the products account for most of the causal ratio [ 2 ]. Awareness and attention about the well-being of people’s consumption ability and consuming behaviors have increased in many countries, including China. Consumers with more abundant disposable time and energy and sufficient economic conditions have stronger consumption power [ 3 ].
University is a microcosm of a society. College students are participating in the transition to independence on attention to a highlighted set of features from the social character [ 4 ]. College students’ consumption ability is the basis of social consumption behavior. When individuals do not have or have insufficient consumption ability, even if they have consumption intentions, they will eventually lack corresponding consumption behaviors. According to the results of the interviews, it is found that the individual’s lack of consumption connotation knowledge and action knowledge, economic ability constraints, individual inherent habits and preferences such as cynicism, lazy and troublesome personality barriers hinder consumer behavior [ 5 ]. Therefore, investigation on college students’ consumption property help classify them as from rich or relative poor family, thus to distinguish the students who are in urgent need for government’s economic support.
The habitual consumption behavior of the young adults in universities can reflect the economic level and the unbalanced development of the society in the next few years. College students’ consumption behavior is on concern. Eating consumption is recognized as universal consumption among many consumption methods [ 6 , 7 ]. Thus the eating habits of the college students are the key factors that can directly reflect the consumption ability of the students’ families. Generally, the students’ eating habits in colleges and universities are mainly reflected in their canteen consumption records [ 8 ]. The characteristics of canteen consumption will release some prominent marker to tell the economic difference of the students’ families. Consequently, we can use the consumption characteristics of college students spending in the college canteen to classify and discriminate the students’ family economic situation.
In recent years, research on college students’ consumption ability has been actively carried out. For example, Kim et al. discussed the students’ behavior difference about clothing consumption in comparison between the clothing majors and non-majors [ 9 ]. Bruce et al. used conventional statistical methods (such as the p-value and some simple descriptive indicators) to study the male first-year college students’ consumption change on in sugar-sweetened beverage [ 10 ]. Gao et al. performed an empirical study on the mechanism of college students’ green consumption in China [ 11 ]. They used questionnaires and make statistical analysis, mainly accomplished by using the SPSS software and lack of novel design in methodologies. There are relatively few studies concerning on data mining by investigating machine learning methods for qualitative or quantitative analysis [ 12 , 13 ]. A review article discussed the possibility of artificial intelligence technologies be analyzed for enhancing the educational systems for semantic web-based education [ 14 ]. Cloud computing technology was used to analyze the requirements of academic and administrative affairs, education affairs, and affiliated institutions in the colleges and universities [ 15 ]. However, in the era of big data, the consumption data is presented as large volume, low value density, diverse forms, and fast growth [ 16 , 17 ]; machine learning strategy plays an important role in data analysis of the trends in college student consumption. More specifically, the students’ canteen data simply records the consumption amount at each time the students spend. The data is fragmented and dynamically increasing, which is bond to raise some challenging issues that probably hinder the computational model optimization [ 18 ]. Then some multi-variate statistical methods should be introduced into the design of the machine learning algorithmic flow.
Clustering is one of the important machine learning methods in many communities for data classification, discrimination, and pattern recognition [ 19 ]. Basically, it aims to group data points into different clusters based on their similarity or density [ 20 ]. In the past decades, many clustering algorithms have been proposed such as the K-means clustering [ 21 ], spectral clustering [ 22 ], min-max cut [ 23 ], and multi-view clustering [ 24 ]. A parallel fractional lion algorithm is recently reported for data analysis, the algorithm is tested on six standard databases [ 25 ], but it is not examined by practical collected data. The fact that it operates only based on the MapReduce framework limits its further application.
Among the existing clustering methods which are suitable for flexible application, the most popular one is the K-means clustering algorithm because its algorithmic flow is simple and efficient, and its operation only needs to learn a limited number of centroids to minimize the interclass data distances. However, the clustering performance of K-means is much related to its random initialization of the centroids [ 26 ]. Spectral clustering is able to prevent the uncertain interference of the randomness. It characterizes the data connection with an appropriate graph whose vertices represent the data points and the weights represent the connection between data pairs, and tries to partition the vertices into different clusters by minimizing the cut information [ 27 ]. For this consideration, we jointly perform spectral clustering in the K-means process, in the way of adjusting the spectral rotation to derive the underlying data connection. The adjusting process is detailly to perform eigen-decomposition on the graph Laplacian matrix transformed from the original data. The graph Laplacian matrix help to amplify the property of the data, and the eigen-decomposition is functional to extract the features [ 28 ]. After the adjusted operation, the normal K-means clustering is applied. The newly proposed methods is defined as adjusted K-means clustering.
Although the adjusted K-means method is advanced for classifying the students’ canteen consumption data, the data is raw and the feature information of the data is ambiguous. To this end, we design a broad learning neural network architecture to extract the feature variables that are rich in data information. Broad learning architecture is built up based on the broad learning system (BLS) established on the idea of a fully connected neural network (FCNN) accompanied with random vector functional link [ 29 , 30 ]. Specifically, the input data is transformed into numbers of feature nodes by proper mapping functions, and then these features are concatenated together to generate a series of enhancement nodes randomly, which will be used to expand the network architecture in a broad sense. All the features nodes and the outputs of the enhancement nodes are connected together to feed into the output layer. The desired output weights are to be determined by a fast ridge regression of the pseudo-inverse of the system equation [ 31 ]. The weights and biases in BLS are all randomly generated, and further to be trained in data-driven manner. The incremental learning algorithm is incorporated into the BLS which makes the network can be remodeled fast in the broad expansion without a retraining process.
Based on the principle of BLS, the broad learning neural network (BLNN) architecture is constructed as a black box model in our study for the analysis of the college student’s canteen consumption. We designed the architecture to be trained in data iteration for optimal observation of the linkage weights. On the other hand, the BLNN training bias is not considered in the model training process, we designed a fuzzy-transformed strategy to catch the feature information of the data under the condition of a fuzzy interval range. What the fuzzy-transformed strategy does is to make a fault-tolerant mode to ensure a relative large amount of candidate feature variables for BLNN training. The fuzzy-transformation is in fusion design with the principal component analysis (PCA) method. Specifically, the data features were extracted by PCA algorithm before the data was input to the BLNN architecture, and the PCA procedure is improved by using the fuzzy rules of the triangular membership function. The fuzzy transformed PCA technique is able to inhibit the original noise interference and make the explicit characteristics of the principal components much remarkable [ 32 ].
We proposed an adjusted K-means clustering method for the discrimination of the college students based on their canteen consumption data, thus to classify the students into different economic levels. Also, a broad learning neural network is designed to extract the exact data features to improve the discriminant accuracy, and a fuzzy transformed PCA technique is employed to reduce the model uncertainty and thus to help reduce the classification errors. The remainder of this article is organized as follows. Section 2 introduced the adjusted clustering method, the BLNN architecture and the fuzzy transformed PCA technique as well as the specific algorithmic modification. Section 3 describes the data collection and present the descriptive statistical properties. Section 4 applies the fusion of the proposed methods to establish the combined discrimination models in a data-driven manner, to fulfil the model optimization, validation and evaluation. Section 5 makes conclusions.
2. Methodologies
2.1. the adjusted clustering algorithm.
For unsupervised data analysis models, clustering analysis is the simplest classification method. Among the existing clustering methods, the most popular one is K-means clustering due to its good adaptiveness and high efficiency. It is used to tune the cluster centroids by each newly-added testing sample, to minimize the within cluster data distances [ 33 ].
The principle of K-means is understandable. Given a data matrix X = [ x 1 , x 2 ,…, x p ] ∈ R d × p , the K-means clustering aims to partition X into k clusters ({ C s | s = 1, 2, …, k }, 1 ≤ k ≤ p ), to achieve that the within-cluster sum of squared distances can be minimized and the sum of squared distances between different clusters can be maximized. The objective function of K-means clustering is
where ∥·∥ applies the Euclidean norm operation, x i is the i -th sample in the C s cluster set, μ s is the centroid of C s . To optimize the K-means results, the membership of each data point and the centroid of each cluster are alternately updated.
However, the data available for clustering in this work is some designated statistical data which points to some specific properties of the raw data. In this point of view, we proposed an adjusted K-means algorithm as the improvement of the traditional K-means method, so that the cluster operation is much suitable to deal with the comprehensive statistic data. Given a built graph, we employ a two-step spectral clustering procedure. The first step is to perform eigen-decomposition on the graph Laplacian matrix to obtain the scaled cluster indicator matrix; The second step aims to make discretization based on the matrix, to get the final cluster assignment [ 28 ].
In the adjusted K-means details, we firstly construct a graph affinity matrix A p × p according to certain similarity measures to depict the relationship in the data. Let a j be the j -th vector of the matrix A = { a j | j = 1, 2 … p }, which corresponds to the cluster indicator vector for x i . Each element of vector a j is defined as 0–1 binary values, 1 means that x i ∈ C s , and 0 means otherwise. By defining the scaled cluster indicator matrix as Y = A ( A T A ) −1/2 whose column vectors are given by
where p s is the number of data in the s -th cluster. Then, the objective function of adjusted clustering can be formulated as
where L is a kind of Laplacian matrix defined as L = D − A , in which D is a diagonal matrix with its j -th diagonal element calculated by the numerical summation of the vector a j . The operator Tr(∙) is to calculate the trace of the matrix.
Since Y T Y = ( A T A ) −1/2 A T A ( A T A ) −1/2 = I , the embedding Y can be obtained by stacking the eigenvectors of L corresponding to its k smallest eigen values. However, Y is a real-valued matrix by default, then spatial rotation is necessary to perform discretization for the adjusted K-means clustering [ 34 ].
Suppose R is an arbitrary orthogonal matrix, for any solution Y , the matrix product YR is a new generated solution from Y by applying the corresponding spatial rotation. Therefore, spatial rotation aims at finding a proper orthogonal and normalized R such that the resultant YR is closer to the discrete indicator than Y in K-means. Mathematically, it aims to minimize the distance of YR and Y , namely,
where 1 k and 1 p are the all-one column vectors with sizes of k × 1 and p × 1, respectively. We can easily solve the function by using the alternative optimization method, therefore the final cluster assignment can be obtained. By the computation of formulae ( 2 ), ( 3 ) and ( 4 ), we can estimate that the adjusted K-means procedure is designed to run within an effort of O[ d 6 × p 2 ].
2.2. The broad learning architecture
The broad learning neural network architecture is built up on the basis of a fully connected neural network (FCNN). An FCNN model is for training an adaptive machine learning model by increase input of data information [ 35 ]. For instance, a basic single-hidden-layered FCNN structure is formed as shown in Fig 1 . The original data x = ( x 1 , …, x d ) T is taken as the input, delivered to the hidden layer with activation mapping on the linear summation of x . Further the generated hidden variables are moved into a softmax unit, fitting a calibration model with multi-variate methods, to generated a discriminant/predictive results as the output data. The FCNN computation is formulated as
where f ν ( ν = 1,2) is the activation functions, w ν ( ν = 1,2) is the linkage weights, and b ν ( ν = 1,2) is the constant thresholds. Subscript ν = 1 points to the calculation from the input layer to the hidden layer, ν = 2 means that from the hidden layer to the softmax unit, and finally to obtain the discriminant/predictive results in the output layer. On some loose definition, the softmax unit is taken as part of the output layer [ 36 ].

BLNN is an adaptive learning architecture designed based on the FCNN structure. It has similar data training function as a deep learning framework. BLNN has a widened pseudo input layer that can help to take one pre-clustering feature screening in one network structure. But in contrast to deep learning, broad learning is able to skip the process of long-time consuming on training abundant parameters in different filters and layers [ 29 , 37 ]. The parameters in BLNN could be determined by random projection or by generating pseudo neural nodes to form a wide enough layer for fast tuning. Moreover, the training process can be changed to an incremental learning mode when the network structure is extended in the width direction [ 38 ]. Specific BLNN training procedure is showed as follows,
Considering the general supervised learning task, we are given the training dataset X p × d containing p sample data of d properties, and the target Y p × k marking the p samples as k discriminant classes. Each row data in X and Y denotes the original sample dimensional information and target clustered makers, respectively. The training samples are taken as the original input data with d properties.
Also, the input variables are applied to generate and connect the layer of enhancement nodes. To speed up the training process, the enhancement nodes are obtained in N a groups. Any feasible algorithmic flow can be designed to observe the outputs of the groups of enhancement nodes.
Furthermore, the neurons in different enhancement layers are taken as added input nodes to widen the range of the input layer. The BLNN mechanism is to gather all of the enhancement nodes as well as the original input nodes, and flatten them as a brand new extended input layer.
where T i represents the i -th group of enhancement nodes obtained by a same transformation rule.
Thus the BLNN architecture is built up by re-constructing the FCNN with the new input data X ext , and the hidden variables are obtained in vector as
where H = H p × m . The hidden variables are moved through the softmax unit and give discriminant/predictive results in the output layer, i.e.
The BLNN architecture is shown in Fig 2 . Practically, the BLNN architecture enables to test different number of hidden nodes ( m ), and adaptively tune the linkage weights ( w 1 and w 2 ) for searching the optimal broad learning network structure. Before model training, we need to select suitable activation functions performing as f 1 and f 2 in advance. Concerning on the BLNN feature extraction by obeying the equations of ( 6 ), ( 7 ) and ( 8 ). The BLNN architecture runs in the complexity of O[ N a 2 × d × p 2 ].

2.3. Fuzzy PCA transform technique
Fuzzy-transformed design is carried out under the condition of interval range of information data to be considered. It is used as the preprocessing algorithm for preventing the impact of the single type data [ 39 ]. It means to ensure that the fuzzy transform is functional to find the features from the consumption data that are not merely telling the consumption properties, then the model can be improved as conceiving a diversity of data characteristics. The fuzzy transform design of the classical PCA is able to emphasize the explicit informative features of the principal components so that the unnecessary noise can be suppressed.
The algorithm is proceeded by the fuzzy partition of the universe fuzzification. This depends on the feature contribution of the measured data. There are several membership functions can be used or modified to fit a specific target, such as the monotonic, triangular, trapezoidal and bell-shaped functions [ 40 ]. Specifically, the triangular function (see Fig 3 ) can much reduce the computational overhead of fuzzy transform due to simpler operation. The triangular fuzzy transform is briefly outlined as follows,

First to calculate the membership function M n ,
where the operator [·] is to get the floor integer; ∥·∥ applies the Euclidean norm operation; x 2 k −1 = { x 1 , x 3 , x 5 …} and x 2 k = { x 2 , x 4 , x 6 …} are the original dataset; c 2 k −1 = { c 1 , c 3 , c 5 …} and c 2 k = { c 2 , c 4 , c 6 …} are the principal components extracted by PCA.
Next to perform the fuzzy transform F n of the n -th principal component.
where the integral interval [ a , b ] piece-wise equals to [‖ x 2 k −1 ‖, ‖ x 2 k ‖].
The fuzzy PCA transform algorithm is evident to generate a group of enhancement features in machine learning procedures [ 41 ]. Thus we designed to apply fuzzy PCA into the BLNN model to search for brand new network nodes, so that the data features are increasingly emphasized, but the computational complexity is increased by O[ N × k × p ].
3. Data collection and descriptive statistics
Experimental data was from colleges and universities located in Guangdong province, China. We collected a total of 307,968 students’ records from the year 2019 to 2021. The records include students’ personal information such as his/her age, majority and hometown. The properties of students’ consumption behaviors include card recharges, canteen expenses, utility bills, library borrowing, expenses for training and certification, etc. Commonly, every college student goes for meals in the college canteen, their expense on canteen meals is the key factor to tell their family backgrounds and economic status. Thus we targeted on the record of canteen expense data. There are some missing data and abnormal data in the 307,968 sample records. we removed them as they account for a small proportion in the total; then we have 265,972 samples for modeling analysis.
In details, the canteen expense data is large and complex. It shows a long list of students’ consuming records in time orders. There appear heavy repeats of students’ personal information, the meal menus and the price. To reduce the influence of overlapping information, we design to use five common descriptive statistics to summarize the data features. They are the annual-averaged consumption cash amount (yCA), the month-averaged consumption cash amount (mCA), the averaged cash amount by consumption frequency (fCA), the month-averaged consumption frequency (mCF) and the day-averaged consumption frequency (dCF). There indicators are socially meaningful. A large value of yCA, mCA and fCA represents it is economically supported that the student is capable of enjoying good meals in canteen level. On the contrary, a small value of mCF and dCF means that the student is seldom expense on canteen meals, which indicates that he/she is economically supported to have high quality meals in good restaurants other than the college canteen. Fig 4 shows the distribution of the five quantified descriptive statistics.

Additionally, some students’ personal information inherently influenced their consuming behaviors. On one hand, we surveyed the students’ majorities and summarized them into the subject category. The distribution by subjects is shown in Fig 5 . There are relatively more students involved in the subjects of Engineering, Science, Economics, Medicine and Management, and relatively less students are in Agriculture, Art, Education and Literature. On the other hand, we also performed a statistical analysis of their hometown information. The 21 cities in Guangdong province are grouped into 7 regional segmentations according to their geographical locations. The numbers of students coming from each city group are listed in Table 1 .

Number of students | Percentage | In-group cities | |
---|---|---|---|
Region 1 | 57,449 | 21.6% | Foshan, Guangzhou |
Region 2 | 48,672 | 18.3% | Dongguan, Huizhou, Shenzhen |
Region 3 | 40,961 | 15.4% | Jiangmen, Zhongshan, Zhuhai |
Region 4 | 32,183 | 12.1% | Maoming, Yangjiang, Zhanjiang |
Region 5 | 27,129 | 10.2% | Qingyuan, Yunfu, Zhaoqing |
Region 6 | 28,993 | 10.9% | Heyuan, Meizhou, Shaoguan |
Region 7 | 30,585 | 11.5% | Chaozhou, Jieyang, Shantou, Shanwei |
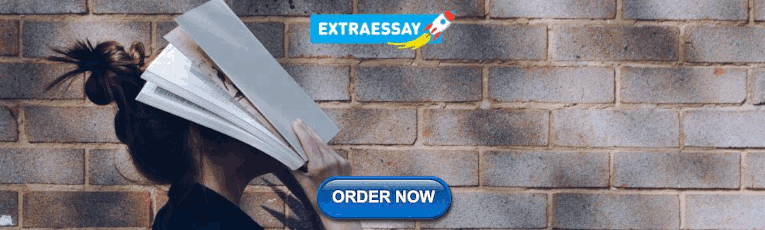
4. Model designs and result discussions
4.1. adjusted k-means modeling for discriminant metics.
For all of the 265,972 targeting samples, we have the five important descriptive statistic indicators for modeling, i.e. yCA, mCA, fCA, mCF and dCF. The former three represent the consuming cash amount while the latter two are the consuming frequency. They are of different types and the data range vary a lot, thus we have to make a 0–1 normalization before modelling. Besides, the students’ consuming ability is certified by a large consuming cash amount reflected in yCA, mCA and fCA, and also by a small consuming frequency represented in mCF and dCF. As for modeling simplicity, we defined the consuming property vector as v = [yCA, mCA, fCA, 1/mCF, 1/dCF] T for each target student sample, so that the sorting discrimination is totally uniform as from the smallest to the largest on each element. Then we applied the adjusted K-means method to the data for classification of the students’ consuming abilities.
We used the elbow method to select the best number of clusters k . The elbow method defines a distortion quantified index on the objective function. a small value of distortion represents the samples clustered tightly, while a large value means the samples are loose [ 42 ]. We test several candidate k values for different mode of clustering, and find the objective function min Tr for each k . the comparative plot is shown in Fig 6 . The curve indicated that k = 4 is the best choice before the clustering becoming loose. Therefore, we decide to classify the samples into 4 classes according to their consuming property indicators. The 4 classes are generated by the second norm value of the vector v (i.e. ‖ v ‖). The total of 265,972 samples are sorted in the ascending order of ‖ v ‖. To some extent, the sorting list is used to estimate the students’ consumption level. Then we can easily observe 4 piece-wise segments by dividing the minimum-to-maximum range by the points of Q1 (the 25% quantile), Q3 (the 75% quantile) and the median values. The segment from Minima to Q1 is marked as the cluster C 1 , the segment from Q1 to the Median is marked as the cluster C 2 , the segment from the Median to Q3 is marked as the cluster C 3 , and the segment from Q3 to the Maxima is marked as the cluster C 4 (see Table 2 ). Thus we have the number of samples partitioned in each cluster set. The students classified in C 1 , C 2 , C 3 and C 4 are regarded as poor, frugal, normal and affluent, respectively, in the analysis of college canteen consuming behavior. The classification markers are further used to train and evaluate the discrimination models established by BLNN architecture.

Segment 1 | Segment 2 | Segment 3 | Segment 4 | |
---|---|---|---|---|
∥ ∥ | ∈ [Minima, Q1] | ∈ [Q1, Median] | ∈ [Median, Q3] | ∈ [Q3, Maxima] |
Clustering class | ||||
No. of samples | 49,562 | 75,519 | 83,757 | 57,134 |
Regarded as | Poor | Frugal | Normal | Affluent |
4.2. Data extended by fuzzy PCA technique
Of the total of 265,972 samples, 186,180 samples (~70%) are used to train the model and 79,792 samples (~30%) used for model test. The test samples are not involved in the model training process. The training sample data X is used as the raw input data for BLNN training. The raw data is extended to produce one enhancement layer by using the fuzzy transform PCA technique, and then the enhancement neural nodes and the raw input data nodes are flattened as a novel extended input layer for broad network learning.
Concerning the raw data, the network architecture generates 5 neural nodes for receiving the 5-dimension normalized property variables [yCA, mCA, fCA, 1/mCF, 1/dCF] T . The 5-dimension property variables were globally converted into common principal components (PCs) sorted in the order of descending contribution rate. Then the PCs were further carried out for the fuzzy transform strategy, in which we used the triangular membership function. There we tuning the number of PCs from 1 to 5, to determine the best number of PCs for fuzzy optimization. The generated variables acquired by fuzzy transformed PCA was used to train the FCNN model. With adaptively searching the network linkage weights, appreciate discriminant results were obtained based on the186,180 training samples. There we observed the best FCNN model with aided transformation by the fuzzy-transform PCA technique. The optimal discrimination model was obtained by using 5 PCs, and the confusion matrix of the training samples was showed in Table 3 . We have the discrimination accuracy as 89.9% from the confusion matrix. Accordingly, we set 5 extended enhancement neural nodes to build up the BLNN network architecture.
Classification by FCNN model with fuzzy PCA transform | Summation by available marker | |||||
Available marker | 1,027 | 859 | 2,094 | 34,693 | ||
1,733 | 1,962 | 1,517 | 52,863 | |||
1,532 | 1,794 | 1,431 | 58,630 | |||
1,274 | 1,596 | 1,977 | 39,994 | |||
Summation by classification model | 35,252 | 52,068 | 58,671 | 40,189 | ||
4.3. Data training by BLNN architecture
The BLNN training model is established by using the above-introduced BLNN architecture. We construct the raw input layer as having 5 neural nodes, for correspondence to receive the training sample data X which have 5 different properties. By testing different number of fuzzy PCs in Section 4.2, we finally decide to use 5 different transformed variables as the enhancement neural nodes for broad learning. The newly generated 5 PCs were included in one group denoted as T 1 = {PC 1 , PC 2 , PC 3 , PC 4 , PC 5 }. Then the BLNN model is driven by flattening the original 5 input neurons ( X ) and the extended 5 enhancement neurons ( T 1 ) into a novel broadened layer. The broaden layer is taken as the refreshed input layer for network training. Accordingly, the original data X are extended to be X ext , which have a total of 10 different variables as follows,
The refreshed input layer is await for the data input of X ext . Then X ext was delivered to the hidden layer by the algorithmic flow introduced in Section 2.2, generating a series of hidden nodes by activation function mapping of the summation of the input variables. The ReLU function [ 43 ] was applied for the hidden layer activation. The linkage weights ( w 1 ) was adaptively trained for iterative optimization, while the number of hidden nodes ( m ) is preset for tuning in the integer range of m ∈ [1, 2 … 16] ⊂ Z + .
Next, the hidden variables are delivered to the output layer by the similar operation of variable summation and activation function mapping, where we used the Sigmoid function [ 44 ] for output activation. The linkage weights ( w 2 ) was also set for adaptively iterative training. Afterwards, the output variables were involved in the softmax unit, and we used the adjusted K-means method to deduce the discriminant results. As for parameter tuning, different number of hidden nodes released different BLNN training results. We compared observations of model discrimination accuracy for using a changing value of m (see Fig 7 ). It is seen from the figure that we observed the best discrimination accuracy as 93.5% when m = 14. This indicated that the BLNN structure is best optimized by using 14 hidden nodes. Besides, the network structures built up with the numbers of from 11 to 17 hidden nodes are able to reach nearly appreciating discrimination results (see the green points in Fig 7 ). Their discrimination accuracies are also over 92%. For the comparison to the FCNN model, the discriminant confusion matrix of the optimal BLNN model was showed in Table 4 . Although the BLNN model is able to improve the total accuracy, we can see in details that the better results are noticeable for the C 1 class and the C 2 class, while the C 3 and C 4 classes are not so obvious improved. The reasons are probably instinct to the segments for dividing the minimum-to-maximum range by the Q1, Q3 and the median values. The C 1 and C 2 classes are regarded as the poor and frugal level; It means that the students who consumes less in college canteen are relatively more easy to discriminated by the BLNN model. In contrast, the normal and the affluent level students (i.e. the C 3 and C 4 classes) can be classified to an equal extend by using the BLNN or using the FCNN model.

Classification optimized BLNN model with 14 hidden neurons | Summation by available marker | |||||
Available marker | 726 | 621 | 1,172 | 34,693 | ||
1,134 | 1,486 | 819 | 52,863 | |||
988 | 1,209 | 1,087 | 58,630 | |||
669 | 951 | 1,229 | 39,994 | |||
Summation by classification model | 34,965 | 52,310 | 58,682 | 40,223 | ||
4.4. Model evaluation
There are 79,792 samples (~30% of the total) reserved before model training, which are excluded and totally independent from the model training process. The test samples were utilized to evaluate the best BLNN training model, as well as the FCNN model for comparison.
The optimized BLNN is built up with 5 original input nodes and 5 pseudo input nodes for broad learning, thus the extended input layer contains 10 neurons, for accepting the raw data variables (yCA, mCA, fCA, 1/mCF, dCF) and the generated comprehensive variables (PC 1 , PC 2 , PC 3 , PC 4 , PC 5 ) transformed by the fuzzy PCA technique. The optimal network was identified as constructed with 14 hidden nodes by the abovementioned training fulfillment. The linkage weights were automatically tuning by the network adaptive training mechanism. The ReLU function was used as the activation functions for each neural perceptron. The softmax unit was designed to launch the adjusted K-means clustering algorithm for data classification.
The data of the 79,792 test samples were input to test the optimized BLNN model. As the model training process is only to identify the optimal network structure, thus the model evaluation needs to re-train the network linkage weights for any new input. We recorded 20 of the changing classification accuracies during the adaptive iteration on the network linkage weights (showed in Fig 8 ), and the iterative discrimination results of the FCNN model were also noted in the figure for comparison. Fig 8 shows that the BLNN model observed the best discrimination accuracy of 91.4% for the test set samples. In contrast, the FCNN model was not able to reach up to 90%, its highest data only reached 88.2%.

(Note that the label AdjKmeans represents the adjusted K–means method).
As for comparison, the BLNN and FCNN extracted data features were delivered to establish the common K-means classification model. results were also illustrated in Fig 8 . The comparative results indicated that the proposed BLNN architecture combined with the adjusted K-means clustering is functional to improve the model discrimination accuracy in data analysis for the classification of college student’s canteen consumption levels.
5. Conclusions
A broad learning clustering architecture was built up in this paper for the qualitative analysis of college students’ canteen consumption data. The fuzzy transformed PCA technique was applied to find extended data features before the data was input to the network. the adjusted K-means clustering method was fused in the network softmax unit for optimizing the classification model. Confronting the data of Guangdong college students, the BLNN structure is constructed by an extended input layer, a node-number-tuning hidden layer and an adaptive clustering softmax. The extended input layer was formed by a set of pseudo neural nodes for fast tuning. There identified 10 neural nodes, of which the former 5 are to receive the 5 descriptive statistical features of the raw data, and the latter 5 pseudo nodes are available for taking the 5 fuzzy PCs generated by fuzzy PCA transform technique, in which a triangle membership function was applied. Then the extended input data was trained through the hidden layer, and delivered to a softmax unit for establishing the adjusted K-means clustering models, thus to analyze the students’ canteen consumption data. The set-up BLNN modeling architecture is applied to classify the college students into 5 different economic level, by analyzing their canteen consumption data.
As to find the most optimal BLNN model for the discrimination, the hidden layer of the BLNN architecture was to tune the number of hidden nodes, and to test the model discrimination accuracy. The most optimal BLNN training model is structured as having 14 hidden nodes, the corresponding best training discrimination accuracy is high up to 93.5%. except for the best model, the BLNN models built up with from 11 to 17 hidden nodes were also able to get appreciating discrimination results over 92%. After that, the optimal training model was evaluated by using the test set samples. By 20 iteration model tuning, we observed the best testing discrimination accuracy as 91.4% by the BLNN model, which is better than the conventional FCNN model.
These modeling and comparing results indicated that the designed BLNN architecture is reasonable to get a more accurate discrimination results for the statistical evaluation of college students’ economic level, by analyzing the students’ canteen consumption recorded data. The design of BLNN framework in fusion use of fuzzy transformed PCA technique and the adjusted K-means method is an advanced machine learning mode. It is feasible to enhance the model effects for classifying college students into different economic levels, and thus we are able to make more accurate distinguishment of the students who are in need for financial aid. Further, the framework is prospectively expected to be applied in solving some challenging data mining issues in other fields.
Supporting information
S1 appendix, funding statement.
This work is funded by Innovation Team Project of Humanities and Social Sciences in Colleges and Universities of Guangdong Province (2020WCXTD008), The Project of Philosophy and Social Sciences in Guangdong Province (GD17XGL19), The Scientific Project of Guangzhou Huashang College (Grant: 2020HSDS03, 2021HSKT01). The funders had no role in study design, data collection and analysis, decision to publish, or preparation of the manuscript.
Data Availability
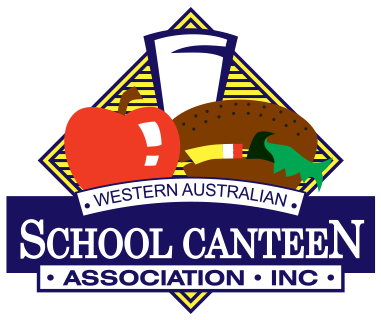
Case Studies
Search for Recipes, Resources and More Search
- More Information
WASCA Wednesday
- Canteens in the News
- WASCA YouTube
- Promotion Material and Resources
- Theme Days and Marketing Messages
- Advocacy in Action
Learning from others is human nature and we know that canteens have great stories they love to share. See the Harvest case studies for practical examples of how Parent bodies, Canteen staff and Volunteers have connected with their school communities to run successful food services. Plus see the WASCA Wednesday Facebook posts for more short sharp examples.

‘HARVEST: Bright ideas from the field’ aims to motivate and inspire schools and their canteens to provide and promote healthy choices.

As a valued WASCA member we would love to help you share your small milestones, ideas or wins via ‘WASCA Wednesday’.

- Star Choice™ Program
- Canteen Operations
- Canteen Promotion
- School Community
- Preparing Food Safely
- Recipes & Healthy Eating
WASCA is proud to acknowledge Aboriginal and Torres Strait Islander wisdom and concepts of traditional and holistic health and wellbeing. WASCA pays respect to the Traditional Custodians of Country throughout Australia and acknowledges Elders past and present and their ongoing connection to the lands and waterways.
Find what you’re looking for
Search terms, recipe categories.
- Sweets Misc (11)
- Salads (22)
- Savoury Meals (47)
- Small Savoury Bites (32)
- Savoury Snacks (12)
- Muffins (21)
- Biscuits and Slices (22)
- Bliss Balls (8)
- Breads, Scones and Pikelets (23)
- Dips and Spreads (15)
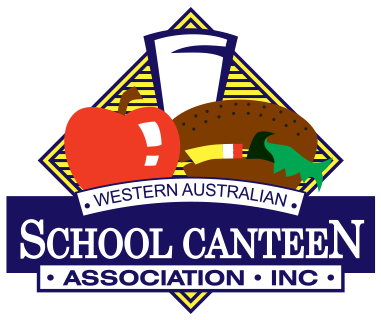
- Search Menu
- Sign in through your institution
- Advance Articles
- Author Guidelines
- Open Access Options
- Self-Archiving Policy
- About International Journal of Low-Carbon Technologies
- Editorial Board
- Advertising and Corporate Services
- Journals Career Network
- Dispatch Dates
- Journals on Oxford Academic
- Books on Oxford Academic

Article Contents
1 introduction, 2 case study: shunde polytechnic, 3 methodology, 4 results and discussion, 5 conclusions, acknowledgements, author contributions.
- < Previous
Study on the effects of low-carbon education on the carbon emissions of college students: a case study in Guangdong Province

- Article contents
- Figures & tables
- Supplementary Data
Zhang Junting, Lyu Shun, Chen Zefeng, Study on the effects of low-carbon education on the carbon emissions of college students: a case study in Guangdong Province, International Journal of Low-Carbon Technologies , Volume 19, 2024, Pages 1425–1431, https://doi.org/10.1093/ijlct/ctae097
- Permissions Icon Permissions
College students are the main force in low-carbon society and social governance, and therefore, their awareness of low-carbon is of vital importance. This article indicates a case study about the effects of low-carbon education on the carbon emissions of college students. In 2019, low-carbon education was promoted on the campus of Shunde Polytechnic and questionnaire survey and interview method were used to study the carbon emissions and low-carbon behaviors of college students after 3 years. Based on the results, the carbon emissions of college students in the aspects of food and daily use accounted for 53.8% and 40.8%, respectively, of the total carbon emissions. In the aspect of food, wine and staple foods accounted for a higher proportion. Their per capital carbon emissions were 291.536 kg and 454.651 kg, respectively. The main contributor to daily use was laptops, which emitted 530.351 kg of carbon per capital. The carbon emissions of clothing, housing, and transportation were relatively low. It is found that low-carbon facilities and measures and low-carbon living environments in colleges have a very positive effect on the reduction of carbon emissions of college students. But the disunity between knowing and doing is still common among college students. Therefore, further measures must be taken to improve the situation.
Over the past decades, China’s economy has achieved rapid development, accompanied by the increasing consumption of fossil energy such as oil and coal. In this process, the ecological environment has suffered serious damage, such as global warming, sea level rise, and many other hazards [ 1 ]. These problems are contrary to the direction of realizing sustainable economic and social development.
According to the latest analysis data from the International Energy Agency (IEA), China has become the largest carbon emitter. In 2021, China’s carbon dioxide emissions exceeded 12.9 billion tons, accounting for 33% of the global total and twice that of the USA. According to the China Energy Big Data Report (2022), China’s coal consumption in 2021 was 4.87 billion tons, an increase of 4% year-on-year, and coal accounted for 47.7% of the total energy consumption, which is related to the coal-dominated energy consumption structure in China [ 2 ]. In the long run, the traditional energy production and consumption structure is not sustainable, and the damage caused to the ecological environment is irreversible. In this case, many countries begin to attach importance to low-carbon economies and use various fiscal and tax policies to promote the development of low-carbon economies. The development of low-carbon economy has become a new direction for China’s economic transformation [ 3 ].
In light of its economic development, the Chinese government has proposed to build and improve a green, low-carbon, and circular economic system and achieve the commitment of “carbon peak” by 2030 and “carbon neutrality” by 2060 at an early date. In October 2021, the Chinese government proposed to establish a green and low carbon development economic system and promote the transformation of economic and social development to green and low carbon, which is the long-term strategy to realize sustainable development [ 4 ].
College students are important driving forces for environmental protection and sustainable development. Their understanding of the environment will determine the development trend of social environment and ecology in the future. Improving their environmental literacy has become a strategic task for the whole society. As a special group, college students have strong plasticity and influence [ 5 ]. On the one hand, there are still high carbon behaviors in college students’ living habits, but based on their plasticity. It is feasible to study and analyze college students’ behaviors and give them correct guidance. On the other hand, the behavioral concept of college students can produce a demonstration effect, which has a strong social radiation effect. Therefore, improving the low-carbon literacy of college students is an important aspect of accelerating the formation of low-carbon environmental protection lifestyle and consumption mode in the public, which is conducive to the formation of a low energy, low consumption, and low-expenditure lifestyle in the whole society and promoting the construction of ecological civilization [ 6 ].
Low-carbon work has gradually shifted from the macro perspective to individual lifestyle carbon emissions. Shui and Dowlatabadi [ 7 ] propose the consumer lifestyle model law to explore the impact of consumer lifestyle on the environment in 2005. In 2006, Britain’s environment minister proposed a personal carbon cap [ 8 ]. Rahman et al. [ 9 ] introduced the Carbon Footprint Calculator app in 2009 as a basis for dynamically tracking individual carbon footprints. In 2009, Riddell et al . [ 10 ] estimated that college students each produced nearly 4 t carbon emissions per year. In 2013, Larsen et al. [ 11 ] calculated the carbon footprint of the Norwegian University of Science and Technology by applying the environment extended I/O model, showing that the average student contribution to carbon emissions was 4.6 t/a.
The current research on college students’ carbon emissions mainly focuses on four aspects: (i) The analysis of college students’ carbon footprint and the research on emission reduction measures. Yixin et al. ’s research [ 12 ] shows that the carbon emissions of college students in Beijing have different proportions in different aspects of clothing, food, housing, and transportation; (ii) Research on low carbon lifestyle of college students. Zeguang et al . [ 13 ] studied the cognition and practice of low carbon consumption among college students and pointed out that college students should establish a correct consumption concept, put forward a series of measures to practice low carbon lifestyle and (iii) explore the relevant influencing factors of college students’ low-carbon consumption behavior. Ma Xiaoxu’s research [ 14 ] shows that environmental responsibility awareness, environmental impact concern, and low carbon concern have a significant impact on college students’ low carbon consumption intention; (iv) Research on college students’ low-carbon life education. Xin and Zhendong’s research [ 15 ] shows that low-carbon life education aims to cultivate low-carbon values, develop ecological morality, establish a correct view of nature, and disseminate low-carbon ideas, popularize low-carbon knowledge, develop low-carbon behavior content, and cultivate group and individual autonomy and consciousness of low-carbon life. In a word, the current research on college students’ carbon emissions shows multiangle and multilevel development, which has guiding significance for the research in this article.
In this article, a college in Guangdong, China was taken as a case study, which lasted for 3 years (from 2019 to 2022). In this study, the students’ carbon emission behavior was firstly investigated and suggestions for carbon reduction at campus were given, and the college adopted several measures of low-carbon education to improve the students’ carbon emission behavior, then an investigation was conducted again to know the students carbon footprints. By comparing the results before and after college’s activity, the effects of low-carbon education could be fully understood, and effective suggestion could also be provided. The investigation and research in this article not only grasp an important aspect of college students’ culture, moral quality, and sense of mission but also have important academic value and extensive social significance.
Shunde Polytechnic is a public college in Guangdong Province which is located in the south China. According to the statistical data for the year 2022, the campus covers an area of 1.2 million m 2 , with a construction area of more than 600 000 m 2 . It has 11 secondary colleges and 53 majors, with a total of 16 000 full-time students, 2781 adult education students, and 1086 faculty and staff members.
Figure 1 shows the CO 2 emission of university students from different countries compared to other countries. The Chinese students have much smaller CO 2 emission. But there is still space for CO 2 emission reduction among the Chinese university students. In order to better understand the students’ carbon footprints, a survey has been conducted in Shunde Polytechnic to investigate the students’ energy behavior in 2019. Figure 2 shows the composition of students’ energy consumption. It can be seen that students consume more electricity in dorms and research building, and the electricity in these two places can easily be affected by students’ behavior.
![college canteen case study The CO2 emission of university students from different countries [16].](https://oup.silverchair-cdn.com/oup/backfile/Content_public/Journal/ijlct/19/10.1093_ijlct_ctae097/2/m_ctae097f1.jpeg?Expires=1721046948&Signature=xykNEYaJpQFXkdmMV3-x9LkT-T6pe0cETW3rNX9fNnQBM1HOrVmNoo3ipgfSI8MfBcCSPugUTbtgTpxbNRfwRaXgoueNOEFanQS4LMTDlE29Vbcjl8EXnoyNLI49IioqrOeDAv7dSsoSxloFMWyz-DdAnKAOQ~GDc-zXFFy2otQcLNf28npXxBn~4GNAUAFe~Wznqc0g7QncaOmVVjUZX9cBmdEfkfdahewN1zf9AG5PoB4BRBKkPhGOhzL5w9Xa1CedufGMOznIMcWJykCXFjOXlCFB3m-9qJww4vepH~IVilOQhropW0NUx9M7qlJdVbmlMHzGuukSgOh-O55DQA__&Key-Pair-Id=APKAIE5G5CRDK6RD3PGA)
The CO 2 emission of university students from different countries [ 16 ].

The composition of students’ energy consumption in 2019.
To further reduce the CO 2 emission of students at Shunde Polytechnic, the college proposed several low-carbon education measures, e.g. low-carbon seminar, poster, low-carbon behavior evaluation, etc. After 3 years of adjustment, a carbon footprint investigation was conducted again to obtain an in-depth understanding on the effects of low-carbon education on the carbon footprint of college students, and corresponding measures for further carbon reduction were proposed based on the research.
3.1 Carbon emission calculation
At present, there are two authoritative methods for the calculation of carbon emissions, one is from a macro perspective, and the other is from a micro perspective. For the calculation from the macro perspective, the guidance document is mainly based on the ‘National Greenhouse Gas Inventory’ guidelines issued by the United Nations. In the calculation of carbon emissions, it is necessary to first classify carbon emission sources and establish a subset until all carbon emission sources are covered. For the calculation from the micro perspective, the most critical step is to calculate the carbon emission factor of different products, obtain the unit carbon emission of the product, and obtain the total carbon emissions. In this article, the calculation method from the bottom to the top microlevel is used to determine the carbon emission factors and calculate the total carbon emission. The expression is as follows:
where CE is the carbon emissions of a certain lifestyle; A x means the activity level of one detail in the lifestyle; and EF x refers to the carbon emission factor of one detail in the lifestyle. The carbon emission factors of different items are shown in Table 1 .
Carbon emission factors [ 17 ] .
. | . | . | . |
---|---|---|---|
Housing | Water | 0.93 | kgCO /m |
Electricity | 0.612 | kgCO /kWh | |
Clothing | Cloths | 5.7 | kgCO /piece |
Washing powder | 0.72 | kgCO /kg | |
Transportation | Buses | 0.0045 | kgCO /min |
Taxis | 0.15 | kgCO /min | |
Electric motor | 0.0535 | kgCO /min | |
Bicycle | 0.0113 | kgCO /min | |
Food | Rice | 2.7 | kgCO /kg |
Wheaten food | 1.25 | kgCO /kg | |
Beef and mutton | 1.3657 | kgCO /kg | |
Pork | 1.1892 | kgCO /kg | |
Fruits | 0.0498 | kgCO /kg | |
Vegetables | 0.0274 | kgCO /kg | |
Milk | 0.4256 | kgCO /kg | |
Eggs | 0.9026 | kgCO /kg | |
Alcohol | 1.1293 | kgCO /kg | |
Cigarette | 0.96 | kgCO /package | |
Daily use | Plastic bags | 0.0001 | kgCO /each |
Disposable cutlery | 0.023 | kgCO /set | |
Paper products | 3.5 | kgCO /kg | |
Laptops | 482 | kgCO /each |
. | . | . | . |
---|---|---|---|
Housing | Water | 0.93 | kgCO /m |
Electricity | 0.612 | kgCO /kWh | |
Clothing | Cloths | 5.7 | kgCO /piece |
Washing powder | 0.72 | kgCO /kg | |
Transportation | Buses | 0.0045 | kgCO /min |
Taxis | 0.15 | kgCO /min | |
Electric motor | 0.0535 | kgCO /min | |
Bicycle | 0.0113 | kgCO /min | |
Food | Rice | 2.7 | kgCO /kg |
Wheaten food | 1.25 | kgCO /kg | |
Beef and mutton | 1.3657 | kgCO /kg | |
Pork | 1.1892 | kgCO /kg | |
Fruits | 0.0498 | kgCO /kg | |
Vegetables | 0.0274 | kgCO /kg | |
Milk | 0.4256 | kgCO /kg | |
Eggs | 0.9026 | kgCO /kg | |
Alcohol | 1.1293 | kgCO /kg | |
Cigarette | 0.96 | kgCO /package | |
Daily use | Plastic bags | 0.0001 | kgCO /each |
Disposable cutlery | 0.023 | kgCO /set | |
Paper products | 3.5 | kgCO /kg | |
Laptops | 482 | kgCO /each |
3.2 Data collection
This research follows three basic principles: the combination of theoretical analysis and practice, the combination of qualitative analysis and quantitative research, and interdisciplinary research. In terms of specific methods of data collection, it mainly adopts the literature analysis method, questionnaire survey method, and interview method.
(1) Literature analysis
The literature analysis method is a theoretical method of modern science and technology research, that deeply consults, analyzes, and sorts out the scientific data of the literature of related things and uses it to find the physical nature and scientific attributes of related things. In terms of this research, literature related to carbon emission assessment, low carbon environmental protection education for college students, and low carbon environmental protection social governance was collected and sorted out, and some relevant literature was reviewed.
(2) Questionnaire survey
Questionnaire survey is a research method that collects relevant information by asking questions in written form. Students from a college in Guangdong, China were selected as key respondents and totally 1200 students were investigated randomly by questionnaires and 1097 valid questionnaires were collected. Considering the impact of age and gender on the research results, the age and gender of the respondents are both generally equally distributed.
The purpose of the questionnaire survey was to understand the low-carbon environmental awareness and behavior of college students, and the survey object was mainly college students. The students who participated in the survey were freshman, sophomore, junior, and senior, accounting for 22.17%, 23.22%, 33.48%, and 21.13%, respectively. Among the students who participated in this questionnaire survey, male students accounted for 49.5% of the total number, while female students accounted for 50.5%. From this data, it can be seen that the students participating in the survey come from different genders and grades, and the questionnaire had certain rationality.
The questionnaire mainly consisted of two parts. The first part was about carbon emissions of college students. From the aspects of clothing, food, housing, transportation, daily use, and environmental protection behavior, the differences in carbon emission behavior of college students were analyzed from four aspects: consumption characteristics, individual characteristics, consciousness characteristics and behavior characteristics. The main influencing factors of carbon emission were found. The detailed contents information of this part of questionnaire is shown in Table 2 . The second part of the questionnaire was about environmental protection awareness, attitude to low-carbon living, low-carbon education in college, and importance of low-carbon education for social governance. The main aim of this part was to obtain an in-depth understanding of students’ environmental protection behavior.
(3) Interview
Detailed contents of different aspects in the questionnaire .
. | . |
---|---|
Clothing | The purchase of clothing, washing powder, and washing liquid in each school year |
Food | Staple food, complementary food, fruit, milk, alcohol, and tobacco |
Housing | Daily water and electricity use |
Transportation | The time and means of transportation used in daily trip |
Daily use | Daily use of electronic equipment, disposable chopsticks, express delivery, paper products, and plastic bags |
. | . |
---|---|
Clothing | The purchase of clothing, washing powder, and washing liquid in each school year |
Food | Staple food, complementary food, fruit, milk, alcohol, and tobacco |
Housing | Daily water and electricity use |
Transportation | The time and means of transportation used in daily trip |
Daily use | Daily use of electronic equipment, disposable chopsticks, express delivery, paper products, and plastic bags |
The interview method mainly refers to a method of collecting other relevant resources and literature in a purposeful, reasonable, and systematic way, in which the investigator conducts oral conversations with other respondents and asks them about their feelings or views about the phenomenon in a specific time or situation. In this actual research, the authors first prepared an interview outline on the basis of fully understanding the research content, and then conducted in-depth interviews with college students, college teachers, and social personnel. The interview could help the authors to gain a well understanding of the status of low-carbon education among college students from a more comprehensive perspective and help us further understand the importance of low carbon education for the development of students and social governance. Finally, the interview results are helpful to provide reasonable suggestions for further improvement of low-carbon education for college students.
4.1 Preliminary statistics of questionnaire survey
Data observed from the questionnaire were firstly collected and analyzed. It can be observed from the data that from the aspect of water usage, the number of college students taking a shower less than 100 minutes per week accounted for 66.5%, 100–200 minutes accounted for 26.8%, and more than 200 minutes accounted for 6.7%. Most students used water for a reasonable time, but there were some students who used water for a long time, resulting in a waste of resources. The electricity in the dormitory was limited by the objective conditions of the dormitory, which were basically limited to lighting electricity and low-power electrical appliances, and the most important high-power electrical facilities were air conditioners. In this respect, carbon emissions have not changed significantly from before.
According to public statistics, the carbon emissions of private transportation in China show an increasing trend year by year. Among public transportation, taxis account for the largest proportion of carbon emissions. The survey found that more than 86.2% of college students chose low-carbon emission transportation modes, such as buses and bicycles, when traveling. Due to the reasons of COVID-19, most college students only had activities near the college, and even if they need to travel, the travel time was short. In terms of the choice of travelling tools, most college students were more inclined to choose public transportation, so the carbon emissions caused by traveling are relatively small.
In terms of the use of disposable tableware, 55.6% of the students used disposable tableware between 0 and 20 times per week, and the carbon emissions generated by disposable tableware accounted for 48.3% of the survey group. More than 42.2% of students used disposable tableware more than 20 times a week, accounting for a high proportion, indicating that there was a large space for improving students’ low-carbon awareness.
4.2 Carbon emission structure analysis
According to the data obtained from the survey, the selection of main activity data in the analysis is introduced: The quantity of clothing purchased and the laundry behavior will have a great impact on the carbon emissions of clothing. In the aspect of clothing, the excessive carbon emission was obvious, so the quantity of clothing and the amount of laundry detergent were selected as the main factors affecting clothing. The amount of food has an important impact on carbon emissions. In terms of food, staple food, auxiliary food, and daily consumption of fruit, milk, wine, and tobacco were selected as the measurement criteria. Water resources are widely used in the life of college students, and the resulting carbon emissions cannot be underestimated. Therefore, the water that college students frequently use in daily life was selected for consideration. For daily consumption products, express delivery, plastic bags, disposable chopsticks, laptops, and paper products that had a greater impact on carbon emissions were selected for analysis. In terms of transportation, the variables of subway, bus, taxi, electric vehicle, and bicycle were selected for analysis. Through the above analysis, the numerical characteristics of carbon emissions from students’ life in Shunde Polytechnic can be found, and the distribution of carbon emissions from the investigated objects can be better reflected.
According to the analysis results for each variable, it can be seen that the distribution basically conforms to the sampling requirements. The total carbon emission of college students at Shunde Polytechnic was 18201.72 kg, of which the per capital carbon emission of clothing, food, housing, transportation, and daily use were 819.08, 9592.31, 91.01, 418.64, and 7280.69 kg, respectively.
The proportions of carbon emissions from different aspects of college students are shown in Fig. 3 . It can be seen that the life carbon emissions of college students at Shunde Polytechnic are mainly concentrated in the aspects of food and daily use, with the total proportion of about 94%. Carbon emissions from food occupy the first place in all aspects of life. The shares of carbon emissions from different food types in colleges are shown in Fig. 4 , among them, vegetables, eggs, fruits, milk, meat, tobacco, drinks, staple food, and other carbon emissions accounted for 0.19%, 2.16%, 0.32%, 1.86%, 21.44%, 1.07%, 28.49%, and 44.47% of the food carbon emissions, respectively. Alcohol and staple foods accounted for 72.96% of food’s total carbon emissions. It can be seen that the unreasonable diet structure of students in Shunde Polytechnic was very serious, and there were generally bad habits such as picky eating and drinking drinks. In this aspect, there is a large space for carbon emission reduction. From the perspective of per capital carbon emissions of specific behaviors, carbon emissions from clothing purchased during the school year accounted for a relatively large proportion in the aspect of clothing, whose per capital carbon emissions are 40.597 kg. In terms of food, drinks and staple foods occupied a relatively large proportion of carbon emissions (291.536 kg and 454.651 kg, respectively). In the daily use aspect, which accounted for a relatively large of carbon emissions was the laptop with a per capital carbon emissions as high as 530.351 kg, plastic bags released less carbon emissions with a per capital carbon emissions of only 0.0002 kg. It can be seen that most of the college students have a healthy and good living habits. In terms of transportation, the carbon emission is relatively small due to the influence of COVID-19 in recent years. The proportion of carbon emissions from food and daily use is closely related to the higher education environment received by college students, but there is still a large part of the room for emission reduction.

Carbon emission of college students from different aspects.

The shares of carbon emissions from different food types in colleges.
In terms of environmental behavior, students at Shunde Polytechnic are analyzed in four aspects: waste clothing disposal method, daily traveling method, meal surplus, and disposable container use. Among them, 15.4% of students chose to discard their old clothing, 23.4% chose to donate them, 5.6% chose others, and 55.6% chose to leave them idle. Among the daily travel modes, 82.4% of college students choose walking, 7.1% choose electric vehicles, 6.8% choose bicycles, 1.4% choose buses, and nearly 1.0% choose taxis, rail transit, and motor vehicles. 57.9% of students have little surplus, 37.9% have little surplus, and 4.2% have a lot of surplus. In terms of takeaway containers, 61.2% of college students used plastic bags, 27.9% used disposable plastic cutlery, 0.9% used paper bags, and 10.0% used self-catering cutlery.
According to the results of relevant analysis, 68.1% of college students in Foshan choose to discard or idle old clothing, indicating that college students still have not completed the effective use of clothing in the disposal of old clothing. In terms of daily travel, 89.2% of college students chose walking and cycling to travel, indicating that the students possess low-carbon behavior in traveling. In terms of meal leftovers, 95.8% of college students have little leftovers, and their overall behavior is good. In terms of disposable container, 89.1% of college students choose to use plastic bags and disposable plastic tableware. The main reason is that most students give priority to the convenience of take-out food, resulting in the lack of environmental protection behavior.
4.3 Low-carbon awareness analysis
In the following research, the authors investigated the low-carbon awareness of college students by questionnaires, and the main results are presented and discussed in this part.
In the respondents, 42% of the students think that individuals play a very large role in low-carbon process, while 48% of the students think individuals play a relatively large role. However, there are still 10% of the students think that individuals play no role in low-carbon process. As to whether they pay attention to the news about environmental protection and carbon reduction, 26% of the students answered often, 62% chose occasionally, and 12% never pay attention to the news about environmental protection. This shows that college students’ attention to low-carbon issues needs to be strengthened.
About the understanding level of the concept of low carbon and environmental protection, 58.60% of the students knew roughly the meaning and content, 25.15% of the students were familiar with the meaning and content, 12.12% of the students had heard but did not know the meaning, and 4.13% of the students had never heard. Only nearly 25% of students are familiar with the content and meaning of ‘low-carbon and environmental protection’, which is worth our deep thought. The college, government, and relevant groups should strengthen the publicity of the concept of ‘low-carbon environmental protection’ in colleges. Only by letting more people know this knowledge, we can achieve low-carbon environmental protection better.
What are the main channels through which college students learn about the concept of low carbon and environmental protection? According to the survey data, it is mainly through the Internet, accounting for 79.8%. In fact, this channel also reflects the contemporary college students contact with the Internet for lots of time. Most of the time is with computers and mobile phones. 55.6% of college students learn about it through TV and radio. The rest of the students learn mainly through newspapers, magazines, and other channels. Therefore, if colleges and relevant government departments want to increase the spread of the concept of low carbon and environmental protection among college students, releasing this knowledge on the Internet can be a good means of publicity.
In terms of students’ attitude toward low-carbon and environmental protection, we designed ‘Do you think the promotion and practice of low carbon and environmental protection are closely related to college students?’. According to the statistics, 66.70% of the students think it is relevant, college students are the main force in environmental protection; 9.09% of the students think it is irrelevant and think it is the government’s business; 24.21% of the students are not clear. It can be seen from this set of data that most students hold a positive attitude toward it, believing that the promotion of ‘low carbon and environmental protection’ is related to themselves. For those students whose attitude is not clear or negative, they should be guided positively and improve their understanding.
4.4 Interview analysis
To understand the status of low-carbon education in colleges, several college students and tutors were interviewed. The main aim of the interview was to collect the low-carbon activities and measures in colleges and suggestions from college students and tutors.
In terms of low-carbon activities and measures in colleges, about 55% interviewees thought it was not enough. The colleges put more attention on professional education, but less on low-carbon education as the main job of colleges is to solve the employment problem of students. Based on the interview of tutors, they were not forced to add low-carbon education to their courses because they had heavy teaching tasks. Besides, only little college associations are about low-carbon environmental protections, thus the opportunities for tutors and students to participate in low-carbon environmental activities are very limited, resulting in limited low-carbon propagandizing and popularizing.
Low-carbon living environment in colleges should be further built. For example, several students mentioned that they had limited methods to dispose waste cloths, based on previous questionnaire. 68.1% of college students choose to discard or idle old clothing. Based on the interview, the interviewees indicated that the handling facilities were not enough, and they had no way to sell or donate their clothes even they wished to. In the canteens, disposable plastic food container, cutlery, and plastic bags usually severed to students due to the severe take-out demand, but unfortunately, most of the students would not bring their own food containers. Furthermore, the canteens usually interfere with students’ meal choices very little, even the students waste much food, they do not need to take responsibility for your actions.
Last but not least, the disunity of knowing and doing is very common among college students. For example, nearly all the students know that the disposable cutlery has negative effects on the low-carbon process and environmental protection, 61.2% of college students will use plastic bags and 27.9% use disposable plastic cutlery because of the convenience and low cost of disposable cutlery. When questioned ‘would you support the ban on using disposable cutlery and plastic bags in the college canteen’, only 56% of the interviewees support it, which showed that there is still a distance between having environmental awareness and actually taking action to protect the environment.
4.5 Suggestions
Based on the research, several suggestions are proposed for the promotion of low-carbon education in colleges.
Firstly, we will promote the improvement of college students’ environmental literacy and normalize environmental science popularization. Specifically, measures can be taken from the following two aspects. Firstly, national environmental protection education, the annual ‘World Environment Day’, ‘National Tree Planting Week’, and other special activity days are used to organize various forms of activities to promote environmental protection. Secondly, it is necessary to create an environment protection atmosphere in the whole society and to guide young people to take an active part in environmental protection activities such as planting trees. Besides, it is urgent to improve and strengthen the organization of large-scale voluntary activities, as well as the training, incentive, guarantee, feedback, and supervision mechanism of volunteers.
Secondly, various activities should be taken to enhance public environmental awareness. First, public courses on environmental protection should be offered. Colleges and universities should set up courses related to ecological and environmental protection to improve the cognitive ability of environmental protection of college students and actively guide them to establish scientific ecological and environmental awareness. Second, various forms of environmental protection activities should be organized. By organizing environmental protection activities, college students can understand the importance of keeping the environment clean and maintaining the balance of the ecological environment and develop the good habit of protecting the environment. Thirdly, research on environmental protection should be encouraged. Colleges and universities can organize students to carry out research on environmental protection topics, so that students can ask questions, decide the direction of research, collect and sort out data, draw conclusions, and take social actions in the process of implementing project plans. By doing environmental protection research, the students’ environmental awareness could be improved.
Thirdly, students themselves should learn more about low-carbon life and eliminate the behavior of comparison and waste. From the perspective of the school, it is necessary to create a good low-carbon environment, provide students with low-carbon life as far as possible, and integrate low-carbon into their daily life.
Based on the collection and collation of 1097 valid questionnaires, this article conducted an in-depth exploration of different aspects of college students’ carbon emissions in Shunde Polytechnic. Based on the research, the following conclusions can be drawn:
(1) The carbon emissions of college students in the aspects of food and daily use accounted for 53.8% and 40.8%, relatively in the total carbon emissions. In the aspect of food, wine and staple food accounted for a higher proportion. Their per capital carbon emissions are 291. 536 kg and 454.651 kg, respectively. The main contributor to daily use is laptops, which emit 530.351 kg of carbon per capital.
(2) The carbon emissions of clothing, housing, and transportation are relatively low, respectively, accounting for 3.3%, 0.3%, and 1.8% of the total carbon emissions.
(3) It is found that the low-carbon facilities and measures and low-carbon living environments in colleges have a very positive effect on the reduction of carbon footprint of college students. But the disunity of knowing and doing is still common among college students. Therefore, further measures must be taken to improve the situation.
The authors would appreciate The Plan of Philosophy and Social Science of Guangdong Province 2023 Annual Discipline Co-Constructive Project (Project No. GD23XGL121), 2023 Young Innovative Talent Category of Colleges and Universities in Guangdong Province (Project No. 2023WQNCX255), Special Funds for the Cultivation of 2023 Guangdong College Students’ Scientific and Technological Innovation. (‘Climbing Program’ Special Funds Project No.pdjh2023b1077).
Junting Zhang (Formal analysis [equal], Investigation [equal], Methodology [equal]), Shun Lyu (CRediT contribution not specified), Shun Lyu (Conceptualization [lead], Funding acquisition [lead], Resources [lead], Supervision [lead]), Chen Zefeng (Data curation [equal], Software [equal], Validation [equal]).
None declared.
Xiang Y . Evaluating parallelized support vector regression and nearest neighbor regression with different input variations for estimating daily global solar radiation of the humid subtropical region in China . Int J Low-Carbon Technol 2023 ; 18 : 95 – 110 . https://doi.org/10.1093/ijlct/ctad005 .
Google Scholar
Yuekun L . Fiscal and taxation policy research on promoting low carbon economy development in Liaoning Province . Shenyang, China : Shenyang University , 2022 .
Google Preview
Shi H , Chai J , Quanying L . et al. The impact of China's low-carbon transition on economy, society and energy in 2030 based on CO2 emissions drivers . Energy 2022 ; 239 :122336. https://doi.org/10.1016/j.energy.2021.122336 .
Jin C , Lv Z , Li Z . et al. Green finance, renewable energy and carbon neutrality in OECD countries . Renew Energy 2023 ; 211 : 279 – 84 . https://doi.org/10.1016/j.renene.2023.04.105 .
Liu J , Tian J , Lyu W . et al. The impact of COVID-19 on reducing carbon emissions: from the angle of international student mobility . Appl Energy 2022 ; 317 :119136. https://doi.org/10.1016/j.apenergy.2022.119136 .
Si H , Li N , Duan X . et al. Understanding the public's willingness to participate in the carbon generalized system of preferences (CGSP): an innovative mechanism to drive low-carbon behavior in China . Sustain Prod Consump 2023 ; 38 : 1 – 12 . https://doi.org/10.1016/j.spc.2023.03.016 .
Shui B , Dowlatabadi H . Consumer lifestyle approach to US energy use and the related CO emissions . Energy Policy 2005 ; 33 : 197 – 208 . https://doi.org/10.1016/S0301-4215(03)00210-6 .
Paterson M , Stripple J . My space: governing individuals’carbon emissions . Soc Space 2010 ; 28 : 341 – 62 . https://doi.org/10.1068/d4109 .
Rahman F , Ahamed SI , Hoque E . et al. UCFC-ubiquitous personal carbon footprint calculation platform. Chicago, IL, USA . In: International Conference on Green Computing IEEE Computer Society 2010 , pp. 425 – 32 .
Riddell W , Bhatia KK , Parisi M . et al. Assessing carbon dioxide emissions from energy use at a university . Int J Sustain High Educ 2009 ; 10 : 266 – 78 . https://doi.org/10.1108/14676370910972576 .
Larsen HN , Pettersen J , Solli C . et al. Investigating the carbon footprint of a university-the case of NTNU . J Clean Prod 2013 ; 48 : 39 – 47 . https://doi.org/10.1016/j.jclepro.2011.10.007 .
Liu Y , Wang J , Fu B . et al. Influence of college students’ life style on carbon emission in Beijing . J Arid Land Resour Environ 2019 ; 32 : 31 – 6 .
Zeguang Z , Xiumei D . Study on low-carbon consumption strategy of college students . China Adult Educ 2013 ; 13 : 60 – 2 .
Xiaoxu M . Influencing factors of low-carbon consumption behavior of college students based on structural equation model-a case study in Yangzhou University . Consum Econ 2015 ; 31 : 87 – 91 .
Xin Z , Zhendong D . Analysis on the connotation and content of low-carbon life education for college students . Heilongjiang Res Higher Educ 2014 ; 5 : 26 – 8 .
Jiao P , Xiaoming A , Xin L . et al. Structural characteristics of college student’ carbon emission in Hebei Province and the path of carbon emission reduction . J Arid Land Res Environ 2023 ; 37 : 8 – 14 .
Li X , Tan H , Rackes A . Carbon footprint analysis of student behavior for a sustainable university campus in China . J Clean Prod 2015 ; 106 : 97 – 108 . https://doi.org/10.1016/j.jclepro.2014.11.084 .
Month: | Total Views: |
---|---|
June 2024 | 50 |
Email alerts
Citing articles via, affiliations.
- Online ISSN 1748-1325
- Print ISSN 1748-1317
- Copyright © 2024 Oxford University Press
- About Oxford Academic
- Publish journals with us
- University press partners
- What we publish
- New features
- Open access
- Institutional account management
- Rights and permissions
- Get help with access
- Accessibility
- Advertising
- Media enquiries
- Oxford University Press
- Oxford Languages
- University of Oxford
Oxford University Press is a department of the University of Oxford. It furthers the University's objective of excellence in research, scholarship, and education by publishing worldwide
- Copyright © 2024 Oxford University Press
- Cookie settings
- Cookie policy
- Privacy policy
- Legal notice
This Feature Is Available To Subscribers Only
Sign In or Create an Account
This PDF is available to Subscribers Only
For full access to this pdf, sign in to an existing account, or purchase an annual subscription.

Case Study: Focused on Accessibility, Chemeketa Community College Makes the Switch to YuJa Panorama Digital Accessibility Platform
Chemeketa Community College is part of the Oregon Community College Distance Learning Association (OCCDLA), which represents the state’s 17 publicly chartered community colleges and their locally elected board members.
Every biennium, the consortium bands together to get licenses for ed-tech tools, apply for grant funding, and more.
As a Digital Accessibility Advocate and Instructional Designer at the institution and a member of the OCCDLA accessibility group, Heather Mariger is actively involved in helping pilot tools, secure licenses, and train others on accessibility best practices. “Some of the OCCDLA institutions are very small and many don’t have an accessibility advocate or a lot of resources,” she explained. “We’re much stronger as a group and we can all help one another.”
Chemeketa was using another accessibility tool, but Mariger shared that her institution and others in the consortium had been experiencing issues with lack of innovation and its customer service.
Read the full case study.
Subscribe to Our Newsletter
Related posts.
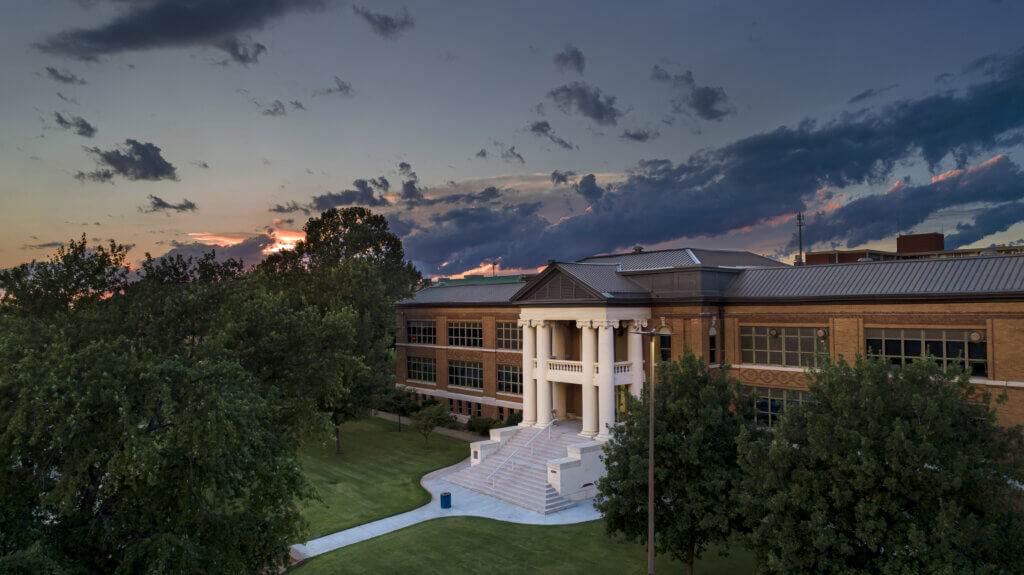
Case Study: Why One of the US’s Leading Regional Universities, Southwestern Oklahoma State University, Made the Switch to the YuJa Panorama Digital Accessibility Platform
They were using another accessibility tool, but “I didn’t feel like it was serving all the needs we needed to have served, and I didn’t feel like their tech support was where it needed to...
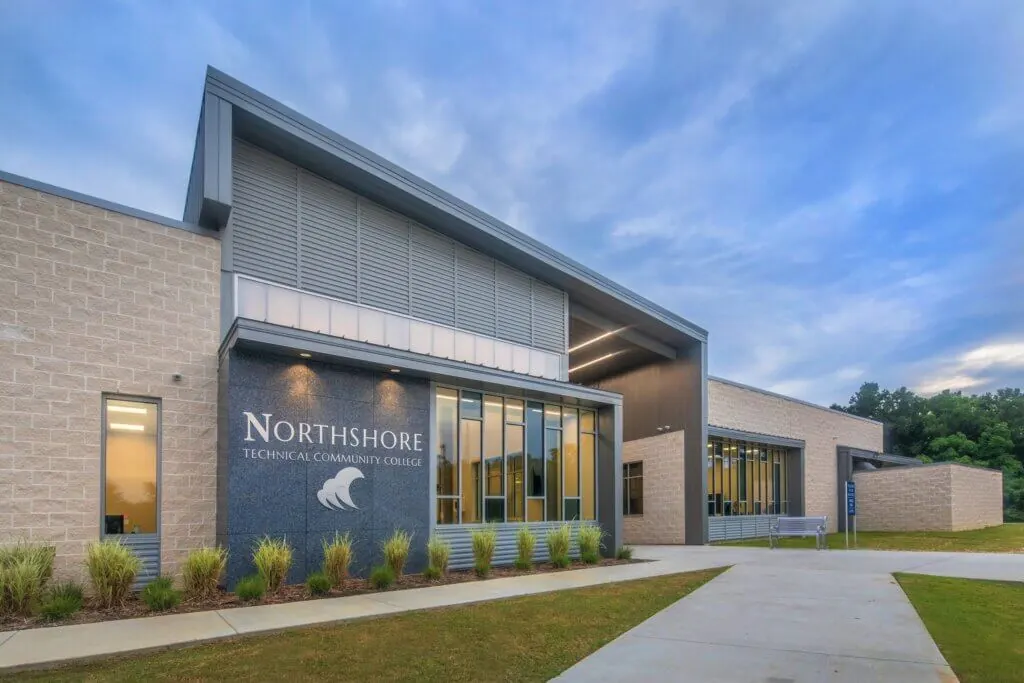
Case Study: How Northshore Technical Community College Increased Accessibility of Course Content by More Than 30 Percent with YuJa Panorama Digital Accessibility Platform
The Louisiana Community and Technical College System (LCTCS) is comprised of 12 institutions that serve more than 150,000 students statewide, including Northshore Technical Community College (NTCC). LCTCS is charged with purchasing technology for all colleges...
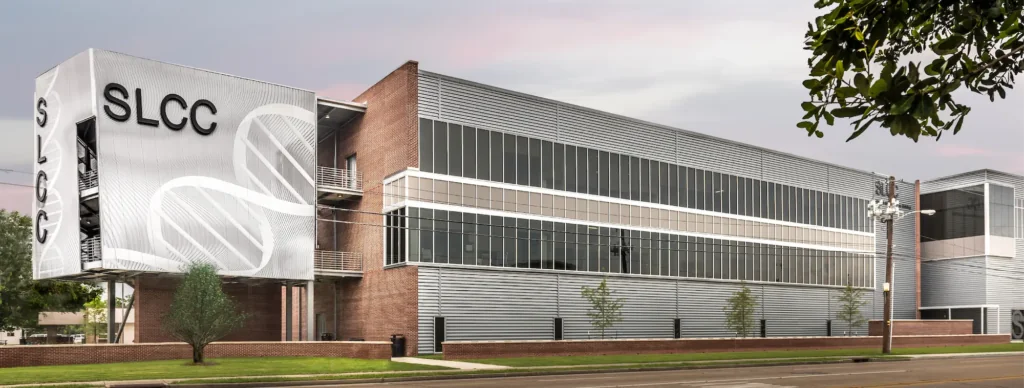
Case Study: How YuJa Panorama Streamlines and Improves Digital Accessibility at South Louisiana Community College
When Dr. Maia Smith, Associate Director of e-Learning at SLCC, began in her role at the college, one of the first tasks she took on was auditing courses against the Quality Matters Higher Ed Course...
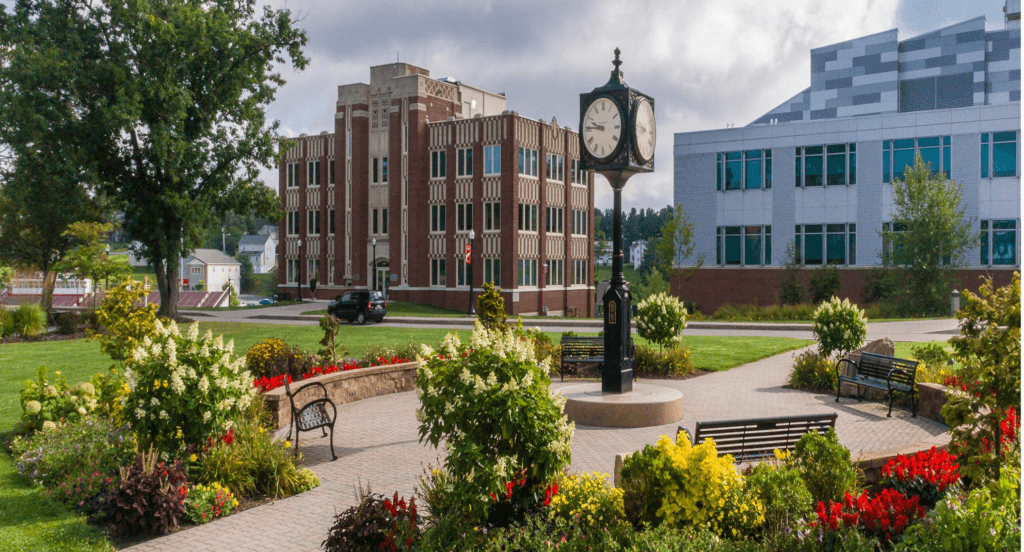
Case Study: YuJa Video Platform and Panorama Digital Accessibility Platform Are a ‘Game Changer’ at Saint Francis University
When the administration at Saint Francis University needed a video solution to be used for teaching and learning, it asked the Center for Excellence in Teaching and Learning (CETL) to help evaluate tools. At that...
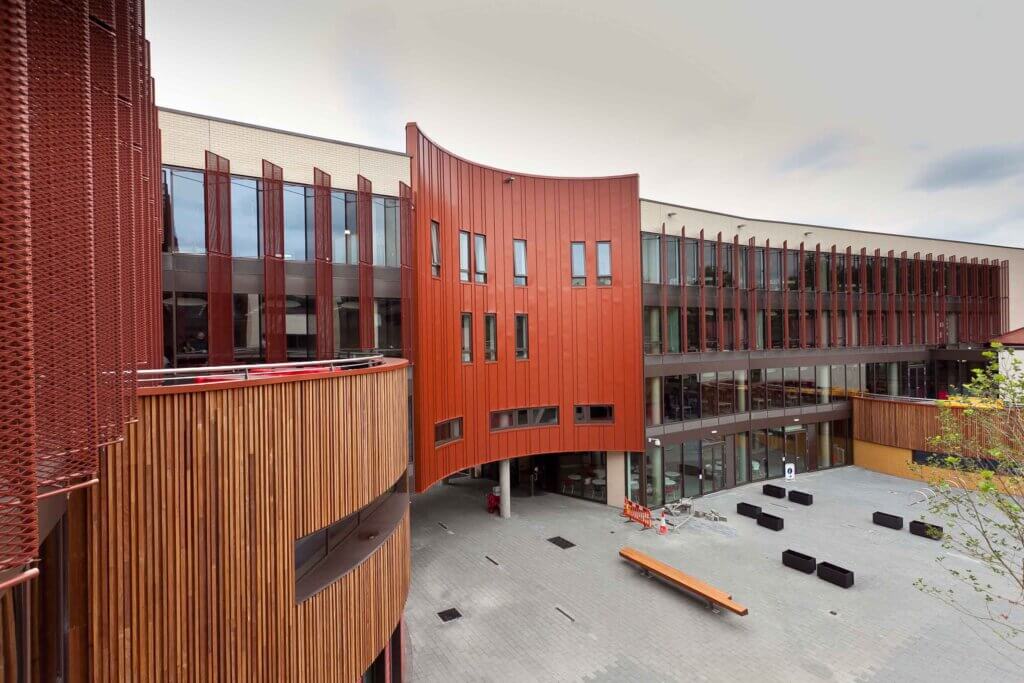
Case Study: How a Top UK University, Anglia Ruskin University, Made the Switch to YuJa to Serve Students Across Five Campuses With a Comprehensive Video Platform
Anglia Ruskin has thousands of instructors at its campuses. The institution wanted an easy-to-use platform that integrates with both the Canvas Learning Management System and Microsoft Teams, and that enables both students and instructors to create,...
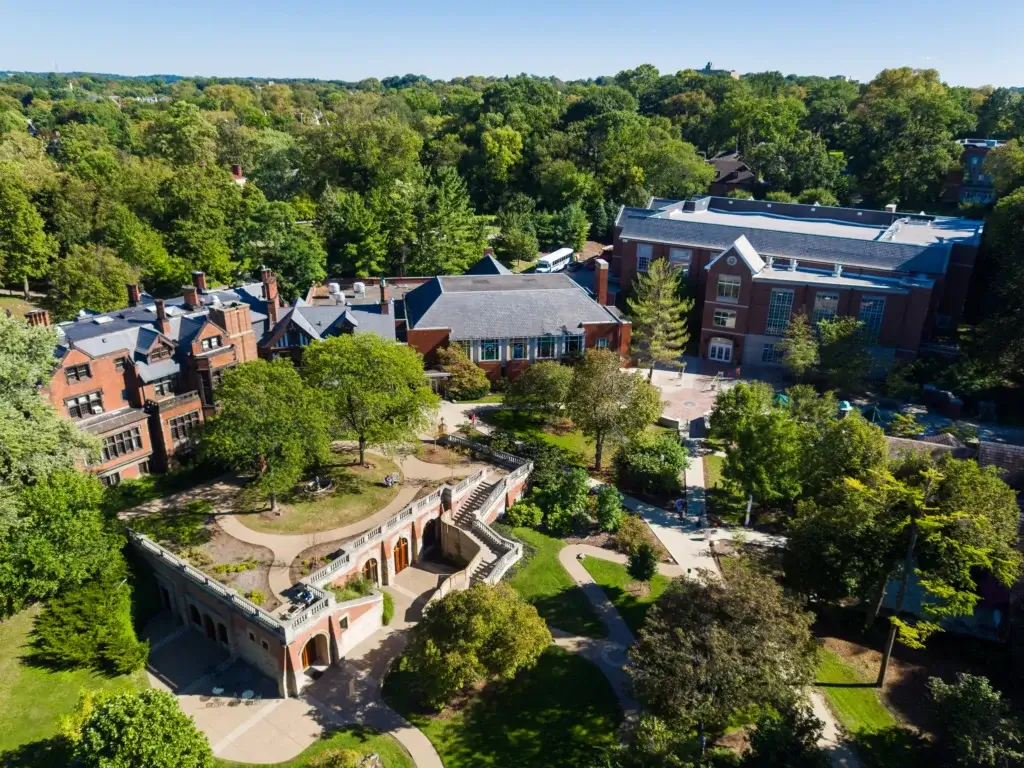
Case Study: Chatham University Makes the Switch to YuJa’s Comprehensive Video Platform for Ease of Use, Robust Capabilities and Ongoing Support
Lauren Panton, Director, Instructional Technology and Teaching Development at Chatham University was at a conference when she learned about YuJa’s suite of ed-tech products. She was particularly interested in the Enterprise Video Platform, an all-in-one,...
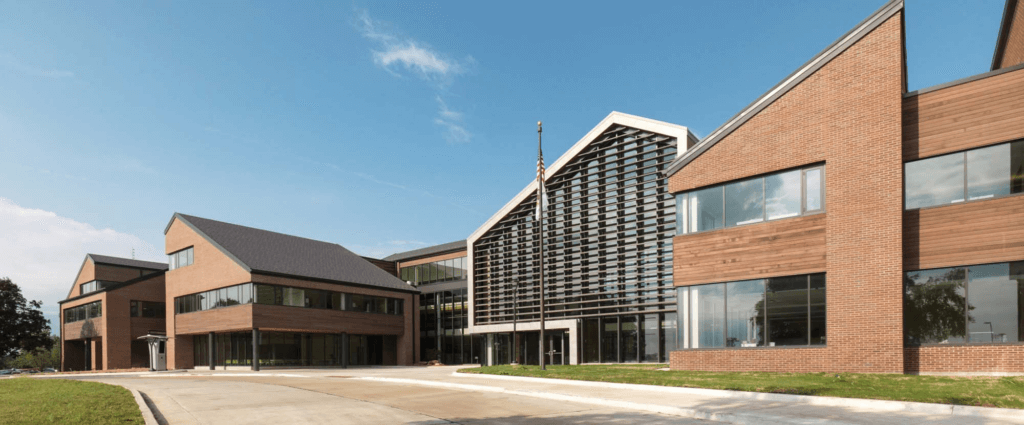
Case Study: How Parkland College Creates a Connected, Engaging Learning Environment for its 20,000 Students With the YuJa Enterprise Video Platform
Lori Wendt, an instructional technology specialist at the institution, led the charge to find a new ed-tech solution. Wendt has a unique perspective both on what instructors want and need, as in addition to her...
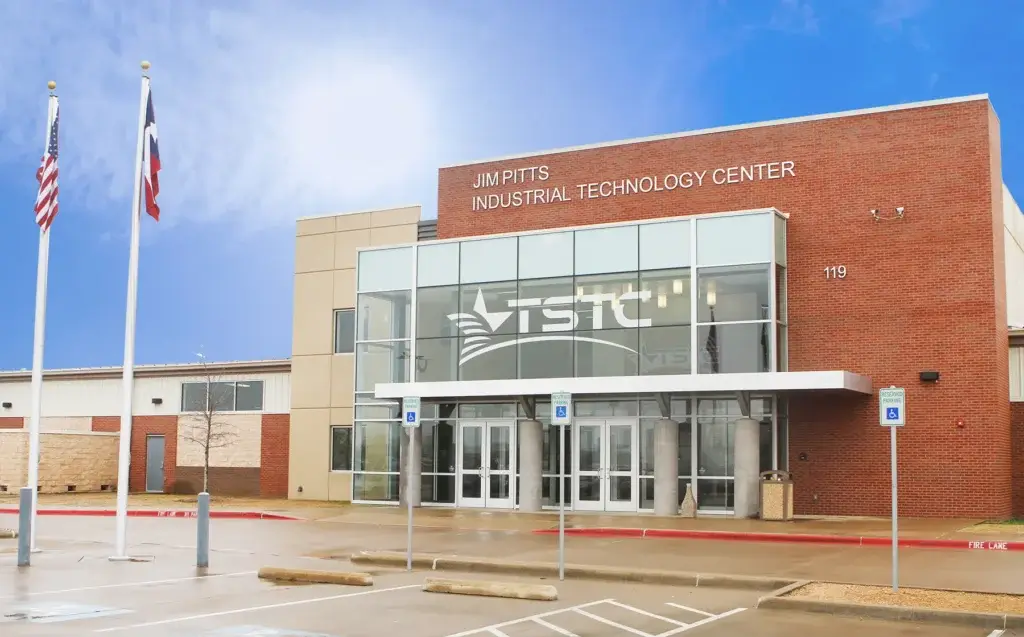
Case Study: How Texas State Technical College Leverages the YuJa Video Platform’s Robust Features to Improve the Educational Experience Campuswide
Texas State Technical College is a coeducational two-year institution of higher education offering courses of study in technical vocational education. Texas State Technical College contributes to the educational and economic development of the state of...
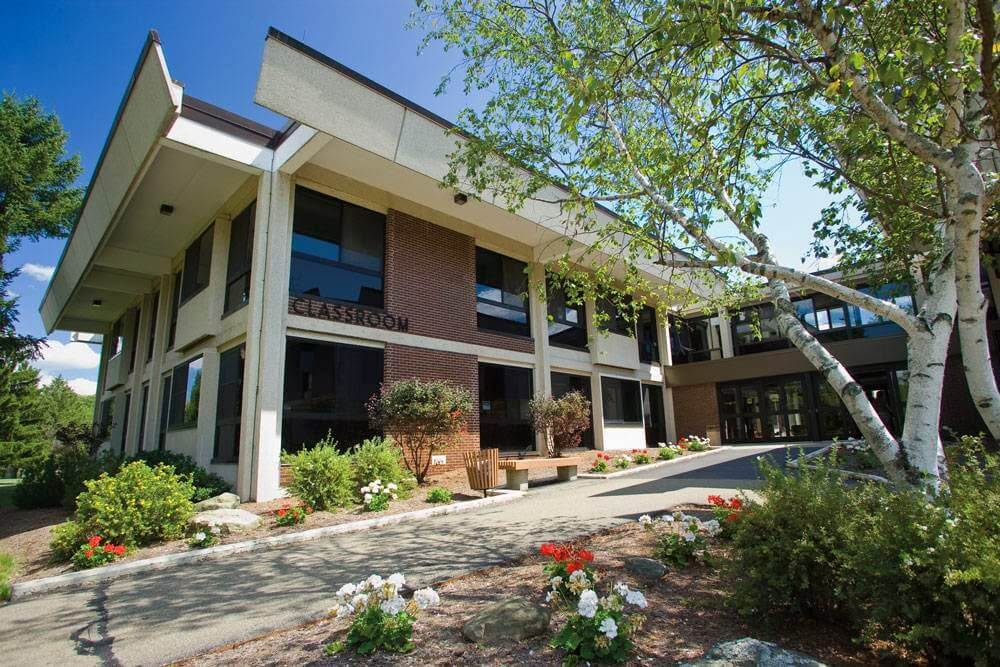
Case Study: SUNY Corning Community College Leverages the YuJa Enterprise Video Platform for Instruction and Other Uses Across its Campus
One of the community colleges in the State University of New York (SUNY) system, Corning Community College is a public community college in Corning, New York. It was initiated in 1957 and moved to its...
Join the Hundreds of Organizations Deploying High-Impact Solutions
Send us an email or message.
Questions or concerns? Please fill out the form below or email [email protected] .
Organization
Additional Info
Send Us an Email or Message
Contact us to learn more, please fill out the below form or email [email protected] .
Privacy Overview
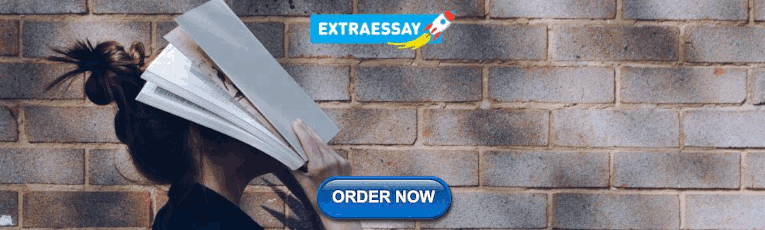
IMAGES
VIDEO
COMMENTS
A canteen serves as a venue for the serving and consumption of food, where a diverse range of snacks and beverages are available for purchase. This purpose of the study was to examine student ...
Oct 31, 2019 •. 10 likes • 9,452 views. AnandKasaudhan. case study of canteen design. 1 of 53. Case study on canteen design - Download as a PDF or view online for free.
Analysis of college students' canteen consumption by broad learning clustering: A case study in Guangdong Province, China Chun Yang1, Hongwei Wen1, Darui Jiang2, Lijuan Xu2, Shaoyong Hong ID 2* 1 School of Accounting, Guangzhou Huashang College, Guangzhou, China, 2 School of Data Science, Guangzhou Huashang College, Guangzhou, China ...
An Empirical Study on University Canteen Satisfaction Survey-A Case Study of Jiangsu University. IJSES Editor. ... If students believe that a college canteen has reduced the quality of the products they receive, they will not accept the foods and facilities. 4. As research reveals, Students are present in the colleges and they would like to ...
Completed in 2011 in Coimbra, Portugal. Images by Luis Ferreira Alves. A canteen is a natural meeting place on a University Campus. The lunch break, usually understood as the moment one escapes ...
The college canteen is an important hub on campus for students, faculty, and staff to grab a quick bite between classes. ... A UI design case study to redesign an example user interface using ...
The university canteen is a queuing system with time dependent arrival patterns and limited resources. It has created the problem of students waiting in the queue during the lunch hour. This study purposively selected a Sri Lankan state university canteen composed of two counters and corresponding queues. The data were collected during the ...
For the case study, the framework of combining the broad learning network with the adjusted K-means method was applied for the discrimination of the canteen consumption data of the college students in Guangdong province, China. Results show that the most optimal broad learning architecture is structured with 14 hidden nodes, the model training ...
Advanced analytics, your own customized portfolio website, and more features to grow your creative career. College canteens is a place where a revolution is much needed to save precious time of students because of heavy crowd during the lunch time, which also results in fraudulent activities and delay in attending classes.During our research ...
ISSN (Online): 2456-7361 An Empirical Study on University Canteen Satisfaction Survey-A Case Study of Jiangsu University Huang Wenjing School of Finance and Economics, Jiangsu University, Zhenjiang 212013, People's Republic of China Abstract—The Canteen of university is an important guarantee for the smooth development of teaching and ...
This research paper examines the impact of canteen services on students' satisfaction, focusing on a case study conducted at a Sri Lankan university. By addressing a gap in the existing knowledge, particularly in the Sri Lankan context regarding the relationship between canteen services and student satisfaction, this research aims to
Financial viability was perceived as the key to sustainability, and in onsite canteen services, the recruitment and retention of a skilled canteen manager was observed as a critical factor. Overall, most school canteen services were currently fulfilling their financial goals and were perceived to be financially viable.
Abstract — The purpose of the study was to determine the relationship between canteen service quality and student satisfaction at Mintal. Comprehensive High School, Mintal, Tugbok, Davao City ...
The findings suggest that (1) attitudes towards food waste, subjective norm and perceived behavioural control have a significant influence on intention to reduce food waste; (2) Moral norm and food taste are shown to have a favourable effect on the intention to reduce food waste; (3) Food waste behaviour is significantly determined by intention ...
PDF | On Dec 27, 2017, M. C. Weerasinghe and others published Service quality of school canteens: a case study from the Western Province, Sri Lanka | Find, read and cite all the research you need ...
UX Case Study - Improve College Canteen Experience. 28. 350
The selected feature variables are further delivered to establish the adjusted K-means clustering model. For the case study, the framework of combining the broad learning network with the adjusted K-means method was applied for the discrimination of the canteen consumption data of the college students in Guangdong province, China.
The school recently had a scratch-cooking canteen embedded within it. Using a case study methodology, this paper investigates whether this type of canteen can lead to the school becoming a more food literate entity by building a scaffolding that can facilitate a greater knowledge of food throughout the school, increased interest in tasting new ...
Thematic analysis of case studies to analyse the interaction between police culture and the handling of sexual misconduct cases; Examine existing design and implementation of training programmes which adhere to the Police (Conduct) Regulations, specifically relating to sexual misconduct within police organisations. References. Casey B. (2023).
This case College Canteen's Decreasing Beverages' Sales: Analysis Dilemmas focus on Raghu's analysis dilemma, the owner-manager of Matrix canteen, which sells various fast food items and beverages. Over the last few months however, he witnessed a steady drop in beverages' sales. Based on his experience, he took all the measures, however, sales did not improve.
For the case study, the framework of combining the broad learning network with the adjusted K-means method was applied for the discrimination of the canteen consumption data of the college ...
Case Studies. Search for Recipes, Resources and More. Learning from others is human nature and we know that canteens have great stories they love to share. See the Harvest case studies for practical examples of how Parent bodies, Canteen staff and Volunteers have connected with their school communities to run successful food services.
This article indicates a case study about the effects of low-carbon education on the carbon emissions of college students. In 2019, low-carbon education was promoted on the campus of Shunde Polytechnic and questionnaire survey and interview method were used to study the carbon emissions and low-carbon behaviors of college students after 3 years.
moderately satisfactory. 2. The level of student satisfaction in the school canteen. of Mintal Comprehensive High School is 3.21 or. moderately satisfied. 3. There was a significant relationship ...
Case Study: How Parkland College Creates a Connected, Engaging Learning Environment for its 20,000 Students With the YuJa Enterprise Video Platform . Lori Wendt, an instructional technology specialist at the institution, led the charge to find a new ed-tech solution. Wendt has a unique perspective both on what instructors want and need, as in ...