Human Factors in Phishing Attacks: A Systematic Literature Review

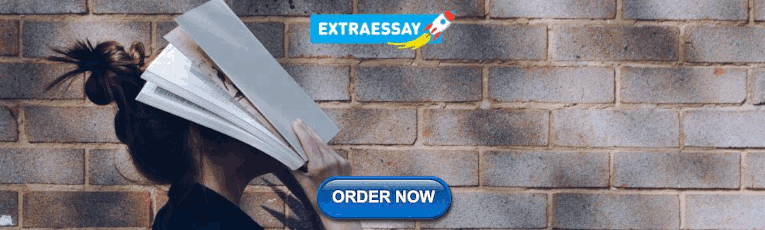
New Citation Alert added!
This alert has been successfully added and will be sent to:
You will be notified whenever a record that you have chosen has been cited.
To manage your alert preferences, click on the button below.
New Citation Alert!
Please log in to your account
Information & Contributors
Bibliometrics & citations.
- Yasin A Fatima R Wen L JiangBin Z Niazi M (2025) What goes wrong during phishing education? A probe into a game-based assessment with unfavorable results Entertainment Computing 10.1016/j.entcom.2024.100815 52 (100815) Online publication date: Jan-2025 https://doi.org/10.1016/j.entcom.2024.100815
- Fan Z Li W Laskey K Chang K (2024) Investigation of Phishing Susceptibility with Explainable Artificial Intelligence Future Internet 10.3390/fi16010031 16 :1 (31) Online publication date: 17-Jan-2024 https://doi.org/10.3390/fi16010031
- Katsarakes E Edwards M Still J (2024) Where Do Users Look When Deciding If a Text Message is Safe or Malicious? Proceedings of the Human Factors and Ergonomics Society Annual Meeting 10.1177/10711813241264204 Online publication date: 12-Aug-2024 https://doi.org/10.1177/10711813241264204
- Show More Cited By
Index Terms
Human-centered computing
Human computer interaction (HCI)
Security and privacy
Human and societal aspects of security and privacy
Intrusion/anomaly detection and malware mitigation
Social engineering attacks
Recommendations
Mitigating phishing attacks: an overview.
Social engineering is the process of getting a person to provide a service or complete a task that may give away private or confidential information. Phishing is the most common type of social engineering. In phishing, an attacker poses as a trustworthy ...
Defending against phishing attacks: taxonomy of methods, current issues and future directions
Internet technology is so pervasive today, for example, from online social networking to online banking, it has made people's lives more comfortable. Due the growth of Internet technology, security threats to systems and networks are relentlessly ...
Fighting against phishing attacks: state of the art and future challenges
In the last few years, phishing scams have rapidly grown posing huge threat to global Internet security. Today, phishing attack is one of the most common and serious threats over Internet where cyber attackers try to steal user's personal or financial ...
Information
Published in.

University of Sydney, Australia
Association for Computing Machinery
New York, NY, United States
Publication History
Permissions, check for updates, author tags.
- human factors
- cybersecurity
Funding Sources
- Italian Ministry of University and Research (MUR)
- PON projects LIFT, TALIsMAn, and SIMPLe
- “Dipartimento di Eccellenza”
- DATACLOUD, DESTINI, and FIRST
- RoMA—Resilience of Metropolitan Areas
Contributors
Other metrics, bibliometrics, article metrics.
- 35 Total Citations View Citations
- 3,604 Total Downloads
- Downloads (Last 12 months) 1,158
- Downloads (Last 6 weeks) 99
- Guo S Fan Y (2024) X-Phishing-Writer: A Framework for Cross-lingual Phishing E-mail Generation ACM Transactions on Asian and Low-Resource Language Information Processing 10.1145/3670402 23 :7 (1-34) Online publication date: 26-Jun-2024 https://dl.acm.org/doi/10.1145/3670402
- Kanaoka A Isohara T (2024) Enhancing Smishing Detection in AR Environments: Cross-Device Solutions for Seamless Reality 2024 IEEE Conference on Virtual Reality and 3D User Interfaces Abstracts and Workshops (VRW) 10.1109/VRW62533.2024.00108 (565-572) Online publication date: 16-Mar-2024 https://doi.org/10.1109/VRW62533.2024.00108
- Sarker O Jayatilaka A Haggag S Liu C Babar M (2024) A Multi-vocal Literature Review on challenges and critical success factors of phishing education, training and awareness Journal of Systems and Software 10.1016/j.jss.2023.111899 208 :C Online publication date: 4-Mar-2024 https://dl.acm.org/doi/10.1016/j.jss.2023.111899
- Varshney G Kumawat R Varadharajan V Tupakula U Gupta C (2024) Anti-phishing Expert Systems with Applications: An International Journal 10.1016/j.eswa.2023.122199 238 :PF Online publication date: 27-Feb-2024 https://dl.acm.org/doi/10.1016/j.eswa.2023.122199
- Baltuttis D Teubner T (2024) Effects of visual risk indicators on phishing detection behavior: An eye-tracking experiment Computers & Security 10.1016/j.cose.2024.103940 144 (103940) Online publication date: Sep-2024 https://doi.org/10.1016/j.cose.2024.103940
- Marshall N Sturman D Auton J (2024) Exploring the evidence for email phishing training Computers and Security 10.1016/j.cose.2023.103695 139 :C Online publication date: 16-May-2024 https://dl.acm.org/doi/10.1016/j.cose.2023.103695
- Chen R Li Z Han W Zhang J (2024) A Survey of Attack Techniques Based on MITRE ATT&CK Enterprise Matrix Network Simulation and Evaluation 10.1007/978-981-97-4522-7_13 (188-199) Online publication date: 2-Aug-2024 https://doi.org/10.1007/978-981-97-4522-7_13
View Options
Login options.
Check if you have access through your login credentials or your institution to get full access on this article.
Full Access
View options.
View or Download as a PDF file.
View online with eReader .
HTML Format
View this article in HTML Format.
Share this Publication link
Copying failed.
Share on social media
Affiliations, export citations.
- Please download or close your previous search result export first before starting a new bulk export. Preview is not available. By clicking download, a status dialog will open to start the export process. The process may take a few minutes but once it finishes a file will be downloadable from your browser. You may continue to browse the DL while the export process is in progress. Download
- Download citation
- Copy citation
We are preparing your search results for download ...
We will inform you here when the file is ready.
Your file of search results citations is now ready.
Your search export query has expired. Please try again.
A comprehensive survey of AI-enabled phishing attacks detection techniques
- Published: 23 October 2020
- Volume 76 , pages 139–154, ( 2021 )
Cite this article
- Abdul Basit 1 ,
- Maham Zafar 1 ,
- Xuan Liu ORCID: orcid.org/0000-0002-7966-4488 2 ,
- Abdul Rehman Javed 3 ,
- Zunera Jalil 3 &
- Kashif Kifayat 3
48k Accesses
167 Citations
4 Altmetric
Explore all metrics
In recent times, a phishing attack has become one of the most prominent attacks faced by internet users, governments, and service-providing organizations. In a phishing attack, the attacker(s) collects the client’s sensitive data (i.e., user account login details, credit/debit card numbers, etc.) by using spoofed emails or fake websites. Phishing websites are common entry points of online social engineering attacks, including numerous frauds on the websites. In such types of attacks, the attacker(s) create website pages by copying the behavior of legitimate websites and sends URL(s) to the targeted victims through spam messages, texts, or social networking. To provide a thorough understanding of phishing attack(s), this paper provides a literature review of Artificial Intelligence (AI) techniques: Machine Learning, Deep Learning, Hybrid Learning, and Scenario-based techniques for phishing attack detection. This paper also presents the comparison of different studies detecting the phishing attack for each AI technique and examines the qualities and shortcomings of these methodologies. Furthermore, this paper provides a comprehensive set of current challenges of phishing attacks and future research direction in this domain.
Similar content being viewed by others
Classification of Phishing Attack Solutions by Employing Deep Learning Techniques: A Systematic Literature Review
A Survey on Phishing Website Detection Using Deep Neural Networks
An Exploratory Study of Automated Anti-phishing System
Explore related subjects.
- Artificial Intelligence
Avoid common mistakes on your manuscript.
1 Introduction
The process of protecting cyberspace from attacks has come to be known as Cyber Security [ 16 , 32 , 37 ]. Cyber Security is all about protecting, preventing, and recovering all the resources that use the internet from cyber-attacks [ 20 , 38 , 47 ]. The complexity in the cybersecurity domain increases daily, which makes identifying, analyzing, and controlling the relevant risk events significant challenges. Cyberattacks are digital malicious attempts to steal, damage, or intrude into the personal or organizational confidential data [ 2 ]. Phishing attack uses fake websites to take sensitive client data, for example, account login credentials, credit card numbers, etc. In the year of 2018, the Anti-Phishing Working Group (APWG) detailed above 51,401 special phishing websites. Another report by RSA assessed that worldwide associations endured losses adding up to $9 billion just due to phishing attack happenings in the year 2016 [ 26 ]. These stats have demonstrated that the current anti-phishing techniques and endeavors are not effective. Figure 1 shows how a typical phishing attack activity happens.
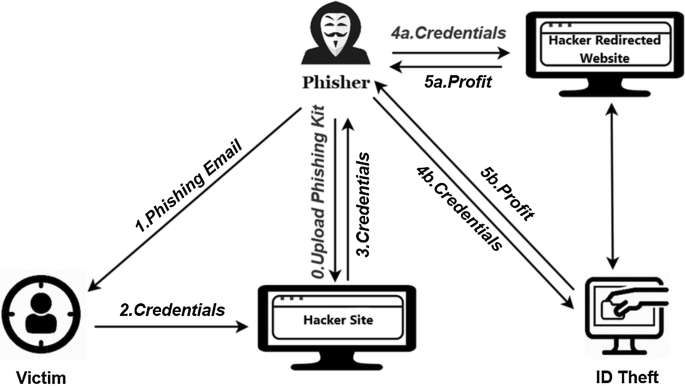
Phishing attack diagram [ 26 ]
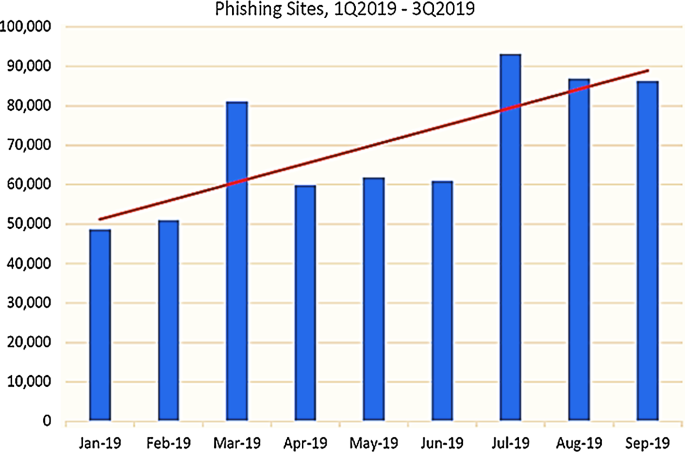
Phishing report for third quarter of the year 2019 [ 1 ]
Personal computer clients are victims of phishing attack because of the five primary reasons [ 60 ]: (1) Users do not have brief information about Uniform Resource Locator (URLs), (2) the exact idea about which pages can be trusted, (3) entire location of the page because of the redirection or hidden URLs, (4) the URL possess many possible options, or some pages accidentally entered, (5) Users cannot differentiate a phishing website page from the legitimate ones.
Phishing websites are common entry points of online social engineering attacks, including numerous ongoing web scams [ 30 ]. In such type of attacks, the attackers create website pages by copying genuine websites and send suspicious URLs to the targeted victims through spam messages, texts, or online social networking. An attacker scatters a fake variant of an original website, through email, phone, or content messages [ 5 ], with the expectation that the targeted victims would accept the cases in the email made. They will likely target the victim to include their personal or highly sensitive data (e.g., bank details, government savings number, etc.). A phishing attack brings about an attacker acquiring bank card information and login data. In any case, there are a few methods to battle phishing [ 27 ]. The expanded utilization of Artificial Intelligence (AI) has affected essentially every industry, including cyber-security. On account of email security, AI has brought speed, accuracy, and the capacity to do a detailed investigation. AI can detect spam, phishing, skewers phishing, and different sorts of attacks utilizing previous knowledge in the form of datasets. These type of attacks likely creates a negative impact on clients’ trust toward social services such as web services. According to the APWG report, 1,220,523 phishing attacks have been reported in 2016, which is 65% more expansion than 2015 [ 1 ]. Figure 2 shows the Phishing Report for the third quarter of 2019.
As per Parekh et al. [ 51 ], a generic phishing attack has four stages. First, the phisher makes and sets up a fake website that looks like an authentic website. Secondly, the person sends a URL connection of the website to a targeted victim pretending like a genuine organization, user, or association. Thirdly, the person in question will be tempted to visit the injected fake website. Fourth, the unfortunate targeted victim will click on the fake source link and give his/her valuable data as input. By utilizing the individual data of the person in question, impersonation activities will be performed by the phisher. APWG contributes individual reports on phishing URLs and analyzes the regularly evolving nature and procedures of cybercrimes. The Anti-Phishing Working Group (APWG) tracks the number of interesting phishing websites, an essential proportion of phishing over the globe. Phishing locales dictate the interesting base URLs. The absolute number of phishing websites recognized by APWG in the 3rd quarter-2019 was 266,387 [ 3 ]. This was 46% from the 182,465 seen in Q2 and in Q4-2018 practically twofold 138,328 was seen.
Figure 3 shows the most targeted industries in 2019. Attacks on distributed storage and record facilitating websites, financial institutions stayed more frequent, and attacks on the gaming, protection, vitality, government, and human services areas were less prominent during the 3rd quarter [ 3 ].
MarkMonitor is an online brand insurance association, verifying licensed innovation. In the 3rd quarter of 2019, the greatest focus of phishing remained Software as a service (SaaS) and webmail websites. Phishers keep on collecting credentials to these sorts of websites, using them to execute business email compromises (BEC) and to enter corporate SaaS accounts.
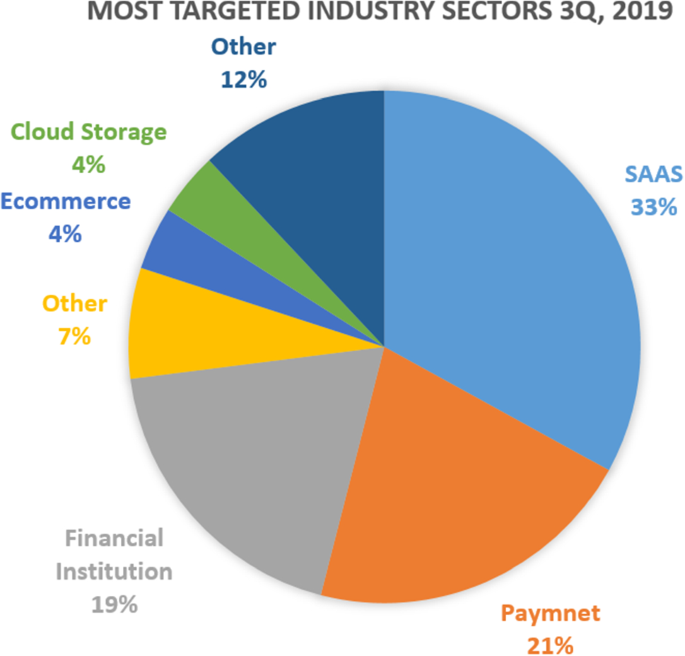
Most targeted industry sectors—3rd quarter 2019 [ 3 ]
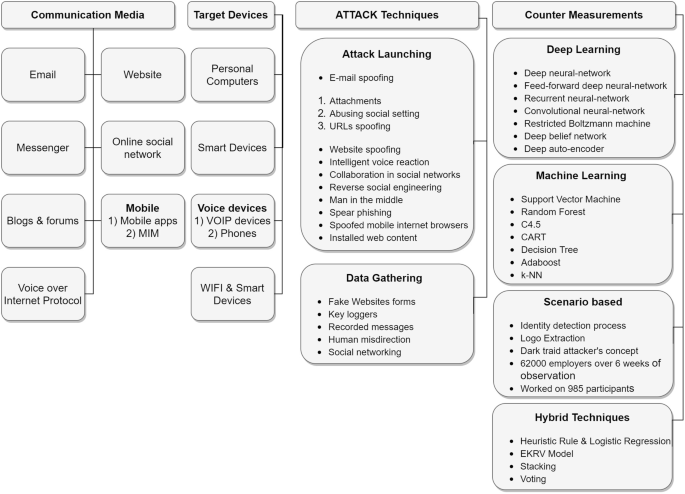
Taxonomy of this survey focusing on phishing attack detection studies
This survey covers the four aspects of a phishing attack: communication media, target devices, attack technique, and counter-measures as shown in Fig. 4 . Human collaboration is a communication media with an application targeted by the attack. Seven types of communication media which include Email, Messenger, Blog & Forum, Voice over internet protocol, Website, Online Social Network (OSN), and Mobile platform are identified from the literature. For the selection of attack strategies, our devices play a significant role as victims interact online through physical devices. Phishing attack may target personal computers, smart devices, voices devices, and/or WiFi-smart devices which includes VOIP devices as well as mobile phone device.
Attack techniques are grouped into two categories: attack launching and data collection. For attack launching, several techniques are identified such as email spoofing, attachments, abusing social settings, URLs spoofing, website spoofing, intelligent voice reaction, collaboration in a social network, reserve social engineering, man in the middle attack, spear phishing, spoofed mobile internet browser and installed web content. Meanwhile, for data collection during and after the victim’s interaction with attacks, various data collection techniques are used [ 49 ]. There are two types of data collection techniques, one is automated data collection techniques (such as fake websites forms, key loggers, and recorded messages) and the other is manual data collection techniques (such as human misdirection and social networking). Then, there are counter-measures for victim’s data collected or used before and after the attack. These counter-measures are used to detect and prevent attacks. We categorized counter-measurement into four groups (1) Deep learning-based Techniques, (2) Machine learning Techniques, (3) Scenario-based Techniques, and (4) Hybrid Techniques.
To the best of our knowledge, existing literature [ 11 , 18 , 28 , 40 , 62 ] include a limited number of surveys focusing more on providing an overview of attack detection techniques. These surveys do not include details about all deep learning, machine learning, hybrid, and scenario based techniques. Besides, these surveys lack in providing an extensive discussion about current and future challenges for phishing attack detection.
Keeping in sight the above limitations, this article makes the following contributions:
Provide a comprehensive and easy-to-follow survey focusing on deep learning, machine learning, hybrid learning, and scenario-based techniques for phishing attack detection.
Provide an extensive discussion on various phishing attack techniques and comparison of results reported by various studies.
Provide an overview of current practices, challenges, and future research directions for phishing attack detection.
The study is divided into the following sections: Sect. 1 present the introduction of phishing attacks. Section 2 presents the literature survey focusing on deep learning, machine learning, hybrid learning, and scenario-based phishing attack detection techniques and presents the comparison of these techniques. Section 3 presents a discussion on various approaches used in literature. Section 4 present the current and future challenges. Section 5 concludes the paper with recommendations for future research.
2 Literature survey
This paper explores detailed literature available in prominent journals, conferences, and chapters. This paper explores relevant articles from Springer, IEEE, Elsevier, Wiley, Taylor & Francis, and other well-known publishers. This literature review is formulated after an exhaustive search on the existing literature published in the last 10 years.
A phishing attack is one of the most serious threats for any organization and in this section, we present the work done on phishing attacks in more depth along with its different types. Initially, the phishing attacks were performed on telephone networks also known as Phone Phreaking which is the reason the term “fishing” was replaced with the term “Phishing”, ph replaced f in fishing. From the reports of the anti-phishing working group (APWG) [ 1 ], it can be confirmed that phishing was discovered in 1996 when America-on-line (AOL) accounts were attacked by social engineering. Phishing turns into a danger to numerous people, especially individuals who are unaware of the dangers while being in the internet world. In light of a report created by the Federal Bureau of Investigation (FBI) [ 4 ], from October-2013 to February-2016, a phishing attack caused severe damage of 2.3 billion dollars. In general, users tend to overlook the URL of a website. At times, phishing tricks connected through phishing websites can be effectively prevented by seeing whether a URL is of phishing or an authentic website. For the situation where a website is suspected as a targeted phish, a client can escape from the criminal’s trap.
The conventional approaches for phishing attack detection give low accuracy and can recognize only about 20% of phishing attacks. Machine learning approaches give good outcomes for phishing detection but are time-consuming even on the small-sized datasets and not scale-able. Phishing recognition by heuristics techniques gives high false-positive rates. Client mindfulness is a significant issue, for resistance against phishing attacks. Fake URLs are utilized by phisher, to catch confidential private data of the targeted victim like bank account data, personal data, username, secret password, etc.
Previous work on phishing attack detection has focused on one or more techniques to improve accuracy however, accuracy can be further improved by feature reduction and by using an ensemble model. Existing work done for phishing attack detection can be placed in four categories:
Deep learning for phishing attack detection
Machine learning for phishing attack detection
Scenario-based phishing attack detection
Hybrid learning based Phishing attack detection
2.1 Deep learning (DL) for phishing attack detection
This section describes the DL approaches-based intrusion detection systems. Recent advancements in DL approaches suggested that the classification of phishing websites using deep NN should outperform the traditional Machine Learning (ML) algorithms. However, the results of utilizing deep NN heavily depend on the setting of different learning parameters [ 61 ]. There exist multiple DL approaches used for cybersecurity intrusion detection [ 25 ], namely, (1) deep neural-network, (2) feed-forward deep neural-network, (3) recurrent neural-network, (4) convolutional neural-network, (5) restricted Boltzmann machine, (6) deep belief network, (7) deep auto-encoder. Figure 5 shows the working of deep learning models. A batch of input data is fed to the neurons and assigned some weights to predict the phishing attack or legitimate traffic.
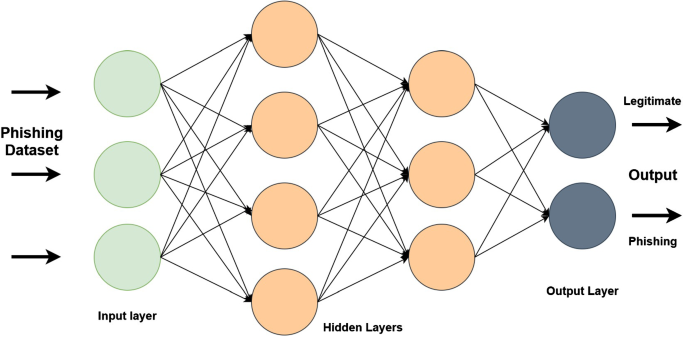
Authors in Benavides et al. [ 15 ] work to incorporate a combination of each chosen work and the classification. They characterize the DL calculations chosen in every arrangement, which yielded that the most regularly utilized are the Deep Neural Network (DNN) and Convolutional Neural Network (CNN) among all. Diverse DL approaches have been presented and analyzed, but there exists a research gap in the use of DL calculations in recognition of cyber-attacks.
Authors in Shie [ 55 ] worked on the examination of different techniques and talked about different strategies for precisely recognizing phishing attacks. Of the evaluated strategies, DL procedures that used feature extraction shows good performance because of high accuracy, while being robust. Classifications models also depict good performance. Authors in Maurya and Jain [ 46 ] proposed an anti-phishing structure that depends on utilizing a phishing identification model dependent on DL, at the ISP’s level to guarantee security at a vertical scale as opposed to even execution. This methodology includes a transitional security layer at ISPs and is set between various workers and end-clients. The proficiency of executing this structure lies in the way that a solitary purpose of blocking can guarantee a large number of clients being protected from a specific phishing attack. The calculation overhead for phishing discovery models is restricted distinctly to ISPs and end users are granted secure assistance independent of their framework designs without highly efficient processing machines.
Authors in Subasi et al. [ 57 ] proposed a comparison of Adaboost and multi boosting for detecting the phishing website. They used the UCI machine learning repository dataset having 11,055 instances, and 30 features. AdaBoost and multi boost are the proposed ensemble learners in this research to upgrade the presentation of phishing attack calculations. Ensemble models improve the exhibition of the classifiers in terms of precision, F-measure, and ROC region. Experimental results reveal that by utilizing ensemble models, it is possible to recognize phishing pages with a precision of 97.61%. Authors in Abdelhamid et al. [ 9 ] proposed a comparison based on model content and features. They used a dataset from PhishTank, containing around 11,000 examples. They used an approach named enhanced dynamic rule induction (eDRI) and claimed that dynamic rule induction (eDRI) is the first algorithm of machine learning and DL which has been applied to an anti-phishing tool. This algorithm passes datasets with two main threshold frequencies and rules strength. The training dataset only stores “strong” features and these features become part of the rule while others are removed.
Authors in Mao et al. [ 44 ] proposed a learning-based system to choose page design comparability used to distinguish phishing attack pages. for effective page layout features, they characterized the guidelines and build up a phishing page classifier with two conventional learning-algorithms, SVM and DT. They tested the methodology on real website page tests from phishtank.com and alexa.com. Authors in Jain and Gupta [ 34 ] proposed techniques and have performed experiments on more than two datasets. First from Phishtank containing 1528 phishing websites, second from Openphish: which contains 613 phishing websites, third from Alexa: which contains 1600 legitimate websites, fourth from payment gateway: which contains 66 legitimate websites, and fifth from top banking website: which contains 252 legitimate websites. By applying machine-learning algorithms, they improved accuracy for phishing detection. They used RF, SVM, Neural-Networks (NN), LR, and NB. They used a feature extraction approach on the client-side.
Authors in Li et al. [ 42 ] proposed a novel approach in which the URL is sent as input and the URL, as well as HTML related features, are extracted. After feature extraction, a stacking model is used to combine classifiers. They performed experiments on different datasets: The first one was obtained from Phishtank, with 2000 web pages (1000 legitimate and 1000 phishing). The second dataset is a larger one with 49,947 web pages (30,873 legitimate, and 19,074 phishing) and was taken from Alexa. They used a support vector machine, NN, DT, RF, and combined these through stacking to achieve better accuracy. This research achieves good accuracy using different classifiers.
Some studies are limited to few classifiers and some used many classifiers, but their techniques were not efficient or accurate. Two datasets have been commonly used by researchers in past and these are publicly accessible from Phishtank and UCI machine learning repository. ML techniques have been used but without feature reduction, and some studies used only a few classifiers to compare their results.
2.2 Machine learning (ML) for phishing attack detection
ML approaches are popular for phishing websites detection and it becomes a simple classification problem. To train a machine learning model for a learning-based detection system, the data at hand must-have features that are related to phishing and legitimate website classes. Different classifiers are used to detect a phishing attack. Previous studies show that detection accuracy is high as robust ML techniques are used. Several feature selection techniques are used to reduce features. Figure 6 shows the working of the machine learning model. A batch of input data is given as input for training to the machine learning model to predict the phishing attack or legitimate traffic.
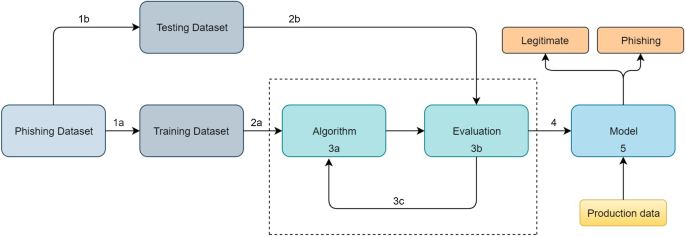
By reducing features, dataset visualization becomes more efficient and understandable. The most significant classifiers that were used in various studies and are found to give good phishing attack detection accuracy are C4.5, k-NN, and SVM. These classifiers are based on DTs such as C4.5, so it gives the maximum accuracy and efficiency to detect a phishing attack. To further explore the detection of phishing attacks, researchers have mentioned the limitations of their work. Many highlighted a common limitation that ensemble learning techniques are not used, and in some studies, feature reduction was not done. Authors in James et al. [ 36 ] used different classifiers such as C4.5, IBK, NB, and SVM. Similarly, authors in Liew et al. [ 43 ] used RF to distinguish phishing attacks from original web pages. Authors in Adebowale et al. [ 10 ] used the Adaptive Neuro-Fuzzy Inference System based robust scheme using the integrated features for phishing attack detection and protection.
Authors in Zamir et al. [ 65 ] presented an examination of supervised learning and stacking models to recognize phishing websites. The rationale behind these experiments was to improve the classification precision through proposed features with PCA and the stacking of the most efficient classifiers. Stacking (RF, NN, stowing) outperformed other classifiers with proposed features N1 and N2. The experiments were performed on the phishing websites datasets. The data-set contained 32 pre-processed features with 11,055 websites. Authors in Alsariera et al. [ 13 ] used four meta-student models: AdaBoost-Extra Tree (ABET), Bagging-Extra tree (BET), Rotation Forest-Extra Tree (RoFBET), and LogitBoost-Extra Tree (LBET), using the extra-tree base classifier. The proposed meta-algorithms were fitted for phishing website datasets, and their performance was tested. Furthermore, the proposed models beat existing ML-based models in phishing attack recognition. Thus, they suggest the appropriation of meta-algorithms when building phishing attack identification models.
Authors in El Aassal et al. [ 22 ] proposed a benchmarking structure called PhishBench, which enables us to assess and analyze the existing features for phishing detection and completely understand indistinguishable test conditions, i.e., unified framework specification, datasets, classifiers, and performance measurements. The examinations indicated that the classification execution dropped when the proportion among phishing and authentic decreases towards 1 to 10. The decrease in execution extended from 5.9 to 42% in F1-score. Furthermore, PhishBench was likewise used to test past techniques on new and diverse datasets.
Authors in Subasi and Kremic [ 56 ] proposed an intelligent phishing website identification system. They utilized unique ML models to classify websites as genuine or phishing. A few classification methods were used to implement an accurate and smart phishing website detecting structure. ROC area, F-measure, and AUC were used to assess the performance of ML techniques. Results demonstrated that Adaboost with SVM performed best among all other classification techniques achieving the highest accuracy of 97.61%. Authors in Ali and Malebary [ 12 ] proposed a phishing website detection technique utilizing Particle Swarm Optimization (PSO) based component weighting to improve the detection of phishing websites. Their proposed approach recommends using PSO to weigh different websites, effectively accomplishing higher accuracy when distinguishing phishing websites. In particular, the proposed PSO based website features weighting is utilized to separate different features in websites, given how significantly these contribute towards distinguishing the phishing from real websites. Results showed that the ML models improved with the proposed PSO-based component weighting to effectively distinguish, and monitor both phishing and real websites separately.
Authors in James et al. [ 36 ] used datasets from Alexa and Phishtank. Their proposed approach read the URL one by one and analyze the host-name URL and path to classify into an attack or legitimate activity using four classifiers: NB, DT, KNN, and Support Vector Machine (SVM). Authors in Subasi et al. [ 57 ] used Artificial Neural Network (ANN), KNN, SVM, RF, Rotation Forest, and C4.5. They discussed in detail how these classifiers are very accurate in detecting a phishing attack. They claim that the accuracy of the RF is not more than 97.26%. All other classifiers got the same accuracy as given in the study. Authors in Hutchinson et al. [ 31 ] proposed a study on phishing website detection focusing on features selection. They used the dataset of the UCI machine learning repository that contains 11,055 URLs and 30 features and divided these features into six groups. They selected three groups and concluded that these groups are suitable options for accurate phishing attack detection.
Authors in Abdelhamid et al. [ 9 ] creates a method called Enhanced Dynamic Rule Induction (eDRI) to detect phishing attacks. They used feature extraction, Remove replace feature selection technique (RRFST), and ANOVA to reduce features. The results show that they have the highest accuracies of 93.5% in comparison with other studies. The research [ 29 ] proposed a feature selection technique named as Remove Replace Feature Selection Technique (RRFST). They claim that they got the phishing email dataset from the khoonji’s anti-phishing website containing 47 features. The DT was used to predict the performance measures.
Authors in Tyagi et al. [ 58 ] used a dataset from the UCI machine learning repository that contains unique 2456 URL instances, and 11,055 total number of URLs that have 6157 phishing websites and 4898 legitimate websites. They extracted 30 features of URLs and used these features to predict the phishing attack. There were two possible outcomes whether the user has to be notified that the website is a phishing or aware user that the website is safe. They used ML algorithms such as DT, RF, Gradient Boosting (GBM), Generalized Linear Model (GLM), and PCA. The authors in Chen and Chen [ 17 ] used the SMOTE method which improves the detection coverage of the model. They trained machine learning models including bagging, RF, and XGboost. Their proposed method achieved the highest accuracy through the XGboost method. They used the dataset of Phishtank which has 24,471 phishing websites and 3850 legitimate websites.
Authors in Joshi et al. [ 39 ] used a RF algorithm as a binary classifier and reliefF algorithm for feature selection algorithm. They used the dataset from the Mendeley website which is given as input to the feature selection algorithm to select efficient features. Next, they trained a RF algorithm over the selected features to predict the phishing attack. Authors in Ubing et al. [ 59 ] proposed their work on ensemble Learning. They used ensemble learning through three techniques that were bagging, boosting, stacking. Their dataset contains 30 features with a result column of 5126 records. The dataset is taken from UCI, which is publicly accessible. They had combined their classifiers to acquire the maximum accuracy which they got from a DT. Authors in Mao et al. [ 45 ] used different machine learning classifiers that include SVM, DT, AdaBoost, and RF to predict the phishing attack. Authors in Sahingoz et al. [ 54 ] created their dataset. The dataset contains 73,575 URLs, and out of this 36,400 legitimate URLs and 37,175 phishing URLs. As they mentioned that Phishtank doesn’t give a free dataset on the web page therefore they created their dataset. They used seven classification-algorithms and natural-language-processing (NLP) based features for phishing attack detection.
Table 1 presents the summary of ML approaches for phishing websites detection. Table shows that some studies provide highly efficient results for phishing attack detection.
2.3 Scenario-based phishing attack detection
In this section, we provide a comparison of scenario-based phishing attack detection used by various researchers. The comparison of scenario-based techniques to detect a phishing attack is shown in Table 2 . Studies show that different scenarios worked with various methods and provides different outcomes.
Authors in Begum and Badugu [ 14 ] discussed some approaches which are useful to detect a phishing attack. They performed a detailed survey of existing techniques such as Machine Learning (ML) based approaches, Non-machine Learning-based approaches, Neural Network-based approaches, and Behavior-based detection approaches for phishing attack detection. Authors in Yasin et al. [ 64 ] consolidated various studies that researchers have used to clarify different exercises of social specialists. Moreover, they proposed that a higher comprehension of the social engineering attack scenarios would be possible utilizing topical and game-based investigation techniques. The proposed strategy for interpreting social engineering attack scenario is one such endeavor to empower people to comprehend general attack scenarios. Even though the underlying outcomes have demonstrated neutral outcomes, the hypothetically predictable system of this strategy despite everything, merits future augmentation and re-performance.
Authors in Fatima et al. [ 23 ] presented PhishI as a precise way to deal with structure genuine games for security training. They characterize a game structure system that incorporates the group of information on social networking, that needs authoritative players. They used stick phishing as a guide to show how the proposed approach functions, and afterward assessed the learning impacts of the produced game dependent on observational information gathered from the student’s movement. In the PhishI game, members are needed to trade phishing messages and have the option to remark on the viability of the attack scenario. Results demonstrated that student’s attention to spear-phishing chances is improved and that the protection from the first potential attack is upgraded. Moreover, the game demonstrated a beneficial outcome on members’ comprehension of extreme online data and information disclosure.
Authors in Chiew et al. [ 18 ] concentrated phishing attacks in detail through their features of the medium and vector which they live in and their specialized methodologies. Besides, they accept this information will assist the overall population by taking preparatory and preventive activities against these phishing attacks and the policies to execute approaches to check any further misuse by the phishers. Relying just on client instruction as a preventive measure in a phishing attack is not sufficient. Their survey shows that the improvement of clever frameworks to counter these specialized methodologies is required, as such countermeasures will have the option to recognize and disable both existing attacks and new phishing dangers.
Authors in Yao et al. [ 63 ] used the logo extraction method by using the identity detection process to detect phishing. Two non-overlapping datasets were made from a sum of 726 pages. Phishing pages are from the PhishTank website, and the legitimate website pages are from the Alexa website as they limited their work by not using the DL technique. The authors gave the concept of dark triad attackers. Phishing exertion and execution, and end-users’ arrangement of emails are the theoretical approach of the dark triad method. They had limited their work as end-client members may have been hyper-mindful of potential duplicity and in this way progressively careful in their ratings of each email than they would be in their normal workplace. Authors in Williams et al. [ 62 ] uses a mixed approach to detect a phishing attack. They used ensemble learning to investigate 62, 000 instances over a six-week time frame to detect phishing messages, called spear phishing. As they had a drawback of just taking information from two organizations, employee observations and encounters are probably going to be affected by a scope of components that might be explicit to the association considered.
Authors in Parsons et al. [ 52 ] used the method of ANOVA. In a scenario-based phishing study, they took a total of 985 participants completed to play a role. Two-way repeated-measures analysis of variance (ANOVA) was led to survey the impact of email authenticity and that impact was focused on the study. This investigation included only one phishing and one certifiable email with one of the standards and did not test the impact of numerous standards inside an email. Following are the comparison of specific classifier known as RF which is the most used algorithm by the researchers.
Table 3 provides a comparison of RF classifiers with different datasets and different approaches. Some studies reduced features without creating a lot of impact on accuracy and the remaining studies focused on accuracy. Authors in Subasi et al. [ 57 ] used different classifiers to detect phishing attacks and they achieved an accuracy of 97.36% by RF algorithm.
Authors in Tyagi et al. [ 58 ] used 30 features to detect the attack by RF. They used other classifiers as well but their result on RF was better than other classifiers. Similarly, authors in Mao et al. [ 45 ] collected the dataset of 49 phishing websites from PhishinTank.com . They used four learning classifiers to detect phishing attacks and concluded that the RF classifiers are much better than others. Authors in Jagadeesan et al. [ 33 ] used two datasets one from UCI Machine Learning Repository having 30 features and one target class, containing 2456 instances of phishing and non-phishing URLs. The second dataset comprises of 1353 URLs with 10 features, grouped into 3 classifications: phishing, non-phishing and suspicious. They concluded that RF provides better accuracy than that of support vector machine. Authors in Joshi et al. [ 39 ] used the dataset from Mendeley website which is publicly accessible. The dataset contains 5000 legitimate and 5000 phishing records. Authors in Sahingoz et al. [ 54 ] used Ebbu2017 Phishing Dataset containing 73,575 URLs in which 36,400 are legitimate URLs and 37,175 are phishing URLs. They proposed seven different classification algorithms including Natural Language Processing (NLP) based features. They actually used a dataset which is not used commonly for detecting phishing attack.
Authors in Williams et al. [ 62 ] conducted two studies considering different aspects of emails. The email that is received, the person who received that email, and the context of the email all the theoretical approaches were studied in this paper. They believe that the current study will provide a way to theoretical development in this field. They considered 62,000 employers over 6 weeks and observed the individuals and targeted phishing emails known as spear phishing. Authors in Parsons et al. [ 52 ] proposed and worked on 985 participants who completed a role in a scenario-based phishing study. They used two-way repeated-measures analysis of variance which was named (ANOVA) to assess the effect of email legitimacy and email influence. The email which was used in their research indicates that the recipient has previously donated to some charity.
Authors in Yao et al. [ 63 ] proposed a methodology which mainly includes two processes: logon extraction and identity detection. The proposed methodology describes that the logon extraction extracted the logo from the image from the two-dimensional code after performing image processing. Next, the identity detection process assessed the relationship between the actual identity of the website and it’s described identity. If the identity is actual then the website is legitimate, if it is not then this is a phishing website. They created two datasets which are non-overlapping datasets from 726 web pages. The dataset contains phishing web pages and legitimate web pages. The legitimate pages are taken from Alexa, whereas the phishing pages are taken from Phishtank. They believe that logo extraction can be improved in the future. Authors in Curtis et al. [ 21 ] introduced the dark triad attacker’s concepts. They used a dark triad score to complete the 27 items short dark triad with both attackers. The end-users were asked to participate in the scenario to assign scores based on psychopathy, narcissism, and Machiavellianism.
2.4 Hybrid learning (HL) based phishing attack detection
In this section, we present the comparison of HL models which are used by state-of-the-art studies as shown in Tables 4 and 5 The studies show how the accuracies got improved by ensemble and HL techniques.
Authors in Kumar et al. [ 41 ] separated some irrelevant features from the content and pictures and applied SVM as a binary classifier. They group the real and phished messages with strategies like Text parsing, word tokenization, and stop word evacuation. The authors in Jain et al. [ 35 ] utilized TF-IDF to locate the most significant features of the website to be used in the search question, yet it has been well adjusted to improve execution. The proposed approach has been discovered to be more accurate for their methodology against existing techniques utilizing the traditional TF-IDF approach.
Authors in Adebowale et al. [ 10 ] proposed a hybrid approach comprising Search and Heuristic Rule and Logistic Regression (SHLR) for efficient phishing attack detection. Authors proposed three steps approach: (1) the most of website shown in the result of a search query is legal if the web page domain matches the domain name of the websites retrieved in results against the query, (2) the heuristic rules defined by the character features (3) an ML model to predict the web page to be either a legal web page or a phishing attack. Authors in Patil et al. [ 53 ] used LR, DT, and RF techniques to detect a phishing attack, and they believe the RF is a much-improved way to detect the attack. The drawback of this system is detecting some minimal false-positive and false-negative results. Authors in Niranjan et al. [ 48 ] used the UCI dataset on phishing containing 6157 legitimate and 4898 phishing instances out of a total of 11,055 instances. The EKRV model was used that involves a combination of KNN and random committee techniques. Authors in Chiew et al. [ 19 ] used two datasets one from 5000 phishing web-pages based on URLs from PhishTank and second OpenPhish. Another 5000 legitimate web-pages were based on URLs from Alexa and the Common Crawl5 archive. They used Hybrid Ensemble Strategy. Authors in Pandey et al. [ 50 ] used a dataset from the Website phishing dataset, available online in a repository of the University of California. This dataset has 10 features and 1353 instances. They trained an RF-SVM hybrid model that achieved an accuracy of 94%.
Authors in Niranjan et al. [ 48 ] proposed an ensemble technique through the voting and stacking method. They selected the UCI ML phishing dataset and take only 23 features out of 30 features for further attack detection. Out of a total of 11,055 instances, the dataset has 6157 legitimate and 4898 phishing instances. They used the EKRV model to predict the phishing attack. Authors in Patil et al. [ 53 ] proposed a hybrid solution that uses three approaches: blacklist and whitelist, heuristics, and visual similarity. The proposed methodology monitors all traffic on the end-user system and compares each URL with the white list of trusted domains. The website analyzes various details for features. The three outcomes are suspicious websites, phishing websites, and legitimate websites. The ML classifier is used to collect data and to generate a score. If the score is greater than the threshold, then they marked the URL as a phishing attack and immediately blocked it. They used LR, DT, and RF to predict the accuracy of their test websites.
Authors in Jagadeesan et al. [ 33 ] utilized RF and SVM to detect phishing attacks. They used two types of datasets the first one is from the UCI machine learning repository which has 30 features. This dataset consists of 2456 entries of phishing and non-phishing URLs. The second dataset consists of 1353 URLs which has 10 features and three categories: Phishing, non-Phishing, and suspicious. Authors in Pandey et al. [ 50 ] used the dataset of a repository of the University of California. The dataset has 10 features and 1353 instances. They trained a hybrid model comprising RF and SVM which they utilize to predict the accuracy.
3 Discussion
Phishing is a deceitful attempt to obtain sensitive data using social networking approaches, for example, usernames and passwords in an endeavor to deceive website users and getting their sensitive credentials [ 24 ]. Phishers prey on human emotion and the urge to follow instructions in a flow. Phishing is so omnipresent in the internet world that it has become a constant threat. In phishing, the biggest challenge is that the attackers are continuously devising new approaches to deceive clients such that they fall prey to their phishing traps.
A comparative study of previous works using different approaches is discussed in the above section with details. Machine learning based approaches, deep learning based approaches, scenario-based approaches, and hybrid techniques are deployed in past to tackle this problem. A detailed comparative analysis revealed that machine learning methods are the most frequently used and effective methods to detect a phishing attack. Different classification methods such as SVM, RF, ANN, C4.5, k-NN, DT have been used. Techniques with feature reduction give better performance. Classification is done through ELM, SVM, LR, C4.5, LC-ELM, kNN, XGB, and feature selection with ANOVA detected phishing attack with 99.2% accuracy, which is highest among all methods proposed so far but with trade-offs in terms of computational cost.
The RF method gives the best performance with the highest accuracy among any other classification methods on different datasets. Several studies proved that more than 95% attack detection accuracy can be achieved using a RF classification method. UCI machine learning dataset is the common dataset that has been used by researchers for phishing attack detection in past.
In various studies, the researchers also created a scenario-based environment to detect phishing attacks but these solutions are only applicable for a particular environment. Individual users in each organization exhibit different behaviors and individuals in the organization are sometimes aware of the scenarios. The hybrid learning approach is another way to detect phishing attacks as it occasionally gave better accuracy than that of a RF. Researchers are of the view that some ensemble models can further improve performance.
Nowadays phishing attacks defense is probably considered a hard job by system security experts. With low false positives, a feasible detection system should be there to identify phishing attacks. The defense approaches talked about so far are based on machine learning and deep learning algorithms. Besides having high computational costs, these methods have high false-positive rates; however, better at distinguishing phishing attacks. The machine learning techniques provide the best results when compared with other different approaches. The most effective defense for phishing attacks is an educated and well aware employee. But still, people are people with their built features of curiosity. They have a thirst to explore and know more. To mitigate the risks of falling victim to phishing tricks, organizations should try to keep employees away from their inherent core processes and make them develop a mindset that will abstain from clicking suspicious links and webpages.
4 Current practices and future challenges
A phishing attack is still considered a fascinating form of attack to lure a novice internet user to pass his/her private confidential data to the attackers. There are different measures available, yet at whatever point a solution is proposed to overcome these attacks, attackers consider the vulnerabilities of that solution to continue with their attacks. Several solutions to control phishing attacks have been proposed in past. A recent increase in the number of phishing attacks linked to COVID-19 performed between March 1 and March 23, 2020, and attacks performed on online collaboration tools (ZOOM, Microsoft Teams, etc.) has led researchers to pay more attention in this research domain. Most of the working be it at government or the corporate level, educational activities, businesses, as well as non-commercial activities, have switched online from the traditional on-premises approach. More users are relying on the web to perform their routine work. This has increased the importance of having a comprehensive phishing attack detection solution with better accuracy and better response time [ 6 , 7 , 8 ].
The conventional approaches for phishing attack detection are not accurate and can recognize only about 20% of phishing attacks. ML approaches give better results but with scalability trade-off and time-consuming even on the small-sized datasets. Phishing detection by heuristics techniques gives high false-positive rates. User cautiousness is a key requirement to prevent phishing attacks. Besides educating the client regarding safe browsing, some changes can be done in the user interfaces such as giving dynamic warnings and consequently identifying malicious emails. As the classified resources are accessible to the IoT gadgets, but their security architectures and features are not mature so far which makes them an exceptionally obvious target for the attackers.
Phishing is a door for all kinds of malware and ransomware. Malware attacks on organizations use ransomware and ransomware operators demand heavy amount as ransom in exchange for not disclosing stolen data which is a recent trend in 2020. Phishing scams in 2020 are deliberately impersonating COVID-19 and healthcare-related organizations and individuals by exploiting the unprepared users. It is better to safeguard doors at our ends and be proactive in defense rather than thinking about reactive strategies to combat once a phishing attack has happened.
Fake websites with phishing appear to be original but it is hard to identify as attackers imitate the appearance and functionality of real websites. Prevention is better than cure so there is a need for anti-phishing frameworks or plug-ins with web browsers. These plug-ins or frameworks may perform content filtering and identify as well as block suspected phishing websites to proceed further. An automated reporting feature can be added that can report phishing attacks to the organization from the user’s end such as a bank, government organization, etc. The time lost on remediation after a phishing attack can have a damaging impact on the productivity and profitability of businesses. In the current scenario, organizations need to provide their employees with awareness and feasible solutions to detect and report phishing attacks proactively and promptly before it causes any harm.
In the future, an all-inclusive phishing attack detection solution can be designed to identify, report, and block malicious web websites without the user’s involvement. If a website is asking for login credentials or sensitive information, a framework or smart web plug-in solution should be responsible to ensure the website is legitimate and inform the owner (organization, business, etc.) beforehand. Web pages health checking during user browsing has become a need of the time and a scalable, as well as a robust solution, is needed.
5 Conclusion
This survey enables researchers to comprehend the various methods, challenges, and trends for phishing attack detection. Nowadays, prevention from phishing attacks is considered a tough job in the system security domain. An efficient detection system ought to have the option to identify phishing attacks with low false positives. The protection strategies talked about in this paper are data mining and heuristics, ML, and deep learning algorithms. With high computational expenses, heuristic and data mining methods have high FP rates, however better at distinguishing phishing attacks. The ML procedures give the best outcomes when contrasted with different strategies. A portion of the ML procedures can identify TP up to 99%. As malicious URLs are created every other day and the attackers are using techniques to fool users and modify the URLs to attack. Nowadays deep learning and machine learning methods are used to detect a phishing attack. classification methods such as RF, SVM, C4.5, DT, PCA, k-NN are also common. These methods are most useful and effective for detecting the phishing attack. Future research can be done for a more scalable and robust method including the smart plugin solutions to tag/label if the website is legitimate or leading towards a phishing attack.
Abbreviations
Support vector machine
Random forest
Instant base learner
Artificial neural network
Rotation forest
Decision forest
Enhanced dynamic rule induction
Linear regression
Classification and regression tree
Extreme gradient boost
Gradient boosting decision tree
Neural-networks
Gradient boosting machine
Generalized linear model
Navies Bayes
K-nearest neighbor
Combination extreme learning machine
Extreme learning machine
Random committee
Principle component analysis
(2016). Apwg trend report. http://docs.apwg.org/reports/apwg_trends_report_q4_2016.pdf . Accessed from 20 July 2020
(2018) Phishing activity trends report. http://docs.apwg.org/reports/apwg_trends_report_q2_2018.pdf . Accessed from 20 July 2020
(2019) Apwg trend report. https://docs.apwg.org/reports/apwg_trends_report_q3_2019.pdf . Accessed from 20 July 2020
(2019) Fbi warns of dramatic increase in business e-mail compromise (bec) schemes—fbi. https://www.fbi.gov/contact-us/field-offices/memphis/news/press-releases/fbi-warns-of-dramatic-increase-in-business-e-mail-compromise-bec-schemes . Accessed from 20 July 2020
(2019) What is phishing? https://www.phishing.org/what-is-phishing . Accessed from 20 July 2020
(2020) Coronavirus-related spear phishing attacks see 667% increase. https://www.securitymagazine.com/articles/92157-coronavirus-related-spear-phishing-attacks-see-667-increase-in-march-2020 . Accessed from 20 July 2020
(2020) Cost of black market phishing kits soars 149% in 2019. https://www.infosecurity-magazine.com/news/black-phishing-kits/ . Accessed from 20 July 2020
(2020) Recent phishing attacks. https://www.infosec.gov.hk/english/anti/recent.html . Accessed from 20 July 2020
Abdelhamid, N., Thabtah, F., Abdel-jaber, H. (2017). Phishing detection: A recent intelligent machine learning comparison based on models content and features. In 2017 IEEE international conference on intelligence and security informatics (ISI) (pp. 72–77). IEEE.
Adebowale, M. A., Lwin, K. T., Sanchez, E., & Hossain, M. A. (2019). Intelligent web-phishing detection and protection scheme using integrated features of images, frames and text. Expert Systems with Applications , 115 , 300–313.
Article Google Scholar
Aleroud, A., & Zhou, L. (2017). Phishing environments, techniques, and countermeasures: A survey. Computers and Security , 68 , 160–196.
Ali, W., & Malebary, S. (2020). Particle swarm optimization-based feature weighting for improving intelligent phishing website detection. IEEE Access , 8 , 116766–116780.
Alsariera, Y. A., Adeyemo, V. E., Balogun, A. O., & Alazzawi, A. K. (2020). Ai meta-learners and extra-trees algorithm for the detection of phishing websites. IEEE Access , 8 , 142532–142542.
Begum, A., & Badugu, S. (2020). A study of malicious url detection using machine learning and heuristic approaches. In Advances in decision sciences, security and computer vision, image processing (pp. 587–597). Berlin: Springer.
Benavides, E., Fuertes, W., Sanchez, S., & Sanchez, M. (2020). Classification of phishing attack solutions by employing deep learning techniques: A systematic literature review. In Developments and advances in defense and security (pp. 51–64). Springer.
Cabaj, K., Domingos, D., Kotulski, Z., & Respício, A. (2018). Cybersecurity education: Evolution of the discipline and analysis of master programs. Computers and Security , 75 , 24–35.
Chen, Y. H., & Chen, J. L. (2019). Ai@ ntiphish—machine learning mechanisms for cyber-phishing attack. IEICE Transactions on Information and Systems , 102 (5), 878–887.
Chiew, K. L., Yong, K. S. C., & Tan, C. L. (2018). A survey of phishing attacks: Their types, vectors and technical approaches. Expert Systems with Applications , 106 , 1–20.
Chiew, K. L., Tan, C. L., Wong, K., Yong, K. S., & Tiong, W. K. (2019). A new hybrid ensemble feature selection framework for machine learning-based phishing detection system. Information Sciences , 484 , 153–166.
Conklin, W. A., Cline, R. E., & Roosa, T. (2014). Re-engineering cybersecurity education in the us: An analysis of the critical factors. In 2014 47th Hawaii international conference on system sciences (pp. 2006–2014). IEEE.
Curtis, S. R., Rajivan, P., Jones, D. N., & Gonzalez, C. (2018). Phishing attempts among the dark triad: Patterns of attack and vulnerability. Computers in Human Behavior , 87 , 174–182.
El Aassal, A., Baki, S., Das, A., & Verma, R. M. (2020). An in-depth benchmarking and evaluation of phishing detection research for security needs. IEEE Access , 8 , 22170–22192.
Fatima, R., Yasin, A., Liu, L., & Wang, J. (2019). How persuasive is a phishing email? A phishing game for phishing awareness. Journal of Computer Security , 27 (6), 581–612.
Feng, Q., Tseng, K. K., Pan, J. S., Cheng, P., & Chen, C. (2011). New anti-phishing method with two types of passwords in openid system. In 2011 Fifth international conference on genetic and evolutionary computing (pp. 69–72). IEEE.
Ferrag, M. A., Maglaras, L., Moschoyiannis, S., & Janicke, H. (2020). Deep learning for cyber security intrusion detection: Approaches, datasets, and comparative study. Journal of Information Security and Applications , 50 , 102419.
Forecast. (2017). Global fraud and cybercrime forecast. https://www.rsa.com/en-us/blog/2016-12/2017-global-fraud-cybercrime-forecast . Accessed from 20 July 2020
Gupta, B. B., Tewari, A., Jain, A. K., & Agrawal, D. P. (2017). Fighting against phishing attacks: State of the art and future challenges. Neural Computing and Applications , 28 (12), 3629–3654.
Gupta, B. B., Arachchilage, N. A., & Psannis, K. E. (2018). Defending against phishing attacks: Taxonomy of methods, current issues and future directions. Telecommunication Systems , 67 (2), 247–267.
Hota, H., Shrivas, A., & Hota, R. (2018). An ensemble model for detecting phishing attack with proposed remove-replace feature selection technique. Procedia Computer Science , 132 , 900–907.
Hulten, G. J., Rehfuss, P. S., Rounthwaite, R., Goodman, J. T., Seshadrinathan, G., Penta, A. P., Mishra, M., Deyo, R. C., Haber, E. J., & Snelling, D. A. W. et al. (2014). Finding phishing sites . US Patent 8,839,418.
Hutchinson, S., Zhang, Z., & Liu, Q. (2018). Detecting phishing websites with random forest. In International conference on machine learning and intelligent communications (pp. 470–479). Springer.
Iwendi, C., Jalil, Z., Javed, A. R., Reddy, T., Kaluri, R., Srivastava, G., et al. (2020). Keysplitwatermark: Zero watermarking algorithm for software protection against cyber-attacks. IEEE Access , 8 , 72650–72660.
Jagadeesan, S., Chaturvedi, A., & Kumar, S. (2018). Url phishing analysis using random forest. International Journal of Pure and Applied Mathematics , 118 (20), 4159–4163.
Google Scholar
Jain, A. K., & Gupta, B. B. (2018). Towards detection of phishing websites on client-side using machine learning based approach. Telecommunication Systems , 68 (4), 687–700.
Jain, A. K., Parashar, S., Katare, P., & Sharma, I. (2020). Phishskape: A content based approach to escape phishing attacks. Procedia Computer Science , 171 , 1102–1109.
James, J., Sandhya, L., & Thomas, C. (2013). Detection of phishing urls using machine learning techniques. In 2013 International conference on control communication and computing (ICCC) (pp. 304–309). IEEE.
Javed, A. R., Jalil, Z., Moqurrab, S. A., Abbas, S., & Liu, X. (2020). Ensemble adaboost classifier for accurate and fast detection of botnet attacks in connected vehicles. Transactions on Emerging Telecommunications Technologies .
Javed, A. R., Usman, M., Rehman, S. U., Khan, M. U., & Haghighi, M. S. (2020). Anomaly detection in automated vehicles using multistage attention-based convolutional neural network. IEEE Transactions on Intelligent Transportation Systems , pp. 1–10.
Joshi, A., Pattanshetti, P., & Tanuja, R. (2019). Phishing attack detection using feature selection techniques. In International conference on communication and information processing (ICCIP), Nutan College of Engineering and Research .
Khonji, M., Iraqi, Y., & Jones, A. (2013). Phishing detection: A literature survey. IEEE Communications Surveys and Tutorials , 15 (4), 2091–2121.
Kumar, A., Chatterjee, J. M., & Díaz, V. G. (2020). A novel hybrid approach of svm combined with nlp and probabilistic neural network for email phishing. International Journal of Electrical and Computer Engineering , 10 (1), 486.
Li, Y., Yang, Z., Chen, X., Yuan, H., & Liu, W. (2019). A stacking model using url and html features for phishing webpage detection. Future Generation Computer Systems , 94 , 27–39.
Liew, S. W., Sani, N. F. M., Abdullah, M. T., Yaakob, R., & Sharum, M. Y. (2019). An effective security alert mechanism for real-time phishing tweet detection on twitter. Computers and Security , 83 , 201–207.
Mao, J., Bian, J., Tian, W., Zhu, S., Wei, T., Li, A., et al. (2018). Detecting phishing websites via aggregation analysis of page layouts. Procedia Computer Science , 129 , 224–230.
Mao, J., Bian, J., Tian, W., Zhu, S., Wei, T., Li, A., et al. (2019). Phishing page detection via learning classifiers from page layout feature. EURASIP Journal on Wireless Communications and Networking , 2019 (1), 43.
Maurya, S., & Jain, A. (2020). Deep learning to combat phishing. Journal of Statistics and Management Systems , pp. 1–13.
Mittal, M., Iwendi, C., Khan, S., & Rehman Javed, A. (2020). Analysis of security and energy efficiency for shortest route discovery in low-energy adaptive clustering hierarchy protocol using Levenberg–Marquardt neural network and gated recurrent unit for intrusion detection system. Transactions on Emerging Telecommunications Technologies , p. e3997.
Niranjan, A., Haripriya, D., Pooja, R., Sarah, S., Shenoy, P. D., & Venugopal, K. (2019). Ekrv: Ensemble of knn and random committee using voting for efficient classification of phishing. In Progress in advanced computing and intelligent engineering (pp. 403–414). Springer.
Ollmann, G. (2004). The phishing guide understanding and preventing phishing attacks . NGS Software Insight Security Research.
Pandey, A., Gill, N., Nadendla, K. S. P., & Thaseen, I. S. (2018). Identification of phishing attack in websites using random forest-svm hybrid model. In International conference on intelligent systems design and applications (pp. 120–128). Springer.
Parekh, S., Parikh, D., Kotak, S., & Sankhe, S. (2018). A new method for detection of phishing websites: Url detection. In 2018 Second international conference on inventive communication and computational technologies (ICICCT) (pp. 949–952). IEEE.
Parsons, K., Butavicius, M., Delfabbro, P., & Lillie, M. (2019). Predicting susceptibility to social influence in phishing emails. International Journal of Human-Computer Studies , 128 , 17–26.
Patil, V., Thakkar, P., Shah, C., Bhat, T., & Godse, S. (2018). Detection and prevention of phishing websites using machine learning approach. In 2018 Fourth international conference on computing communication control and automation (ICCUBEA) (pp. 1–5). IEEE.
Sahingoz, O. K., Buber, E., Demir, O., & Diri, B. (2019). Machine learning based phishing detection from urls. Expert Systems with Applications , 117 , 345–357.
Shie, E. W. S. (2020). Critical analysis of current research aimed at improving detection of phishing attacks . Selected computing research papers, p. 45.
Subasi, A., & Kremic, E. (2020). Comparison of adaboost with multiboosting for phishing website detection. Procedia Computer Science , 168 , 272–278.
Subasi, A., Molah, E., Almkallawi, F., & Chaudhery, T. J. (2017). Intelligent phishing website detection using random forest classifier. In 2017 International conference on electrical and computing technologies and applications (ICECTA) (pp. 1–5). IEEE.
Tyagi, I., Shad, J., Sharma, S., Gaur, S., & Kaur, G. (2018). A novel machine learning approach to detect phishing websites. In 2018 5th International conference on signal processing and integrated networks (SPIN) (pp. 425–430). IEEE.
Ubing, A. A., Jasmi, S. K. B., Abdullah, A., Jhanjhi, N., & Supramaniam, M. (2019). Phishing website detection: An improved accuracy through feature selection and ensemble learning. International Journal of Advanced Computer Science and Applications , 10 (1), 252–257.
Volkamer, M., Renaud, K., Reinheimer, B., & Kunz, A. (2017). User experiences of torpedo: Tooltip-powered phishing email detection. Computers and Security , 71 , 100–113.
Vrbančič, G., Fister Jr, I., & Podgorelec, V. (2018). Swarm intelligence approaches for parameter setting of deep learning neural network: Case study on phishing websites classification. In Proceedings of the 8th international conference on web intelligence, mining and semantics (pp. 1–8).
Williams, E. J., Hinds, J., & Joinson, A. N. (2018). Exploring susceptibility to phishing in the workplace. International Journal of Human-Computer Studies , 120 , 1–13.
Yao, W., Ding Y., & Li, X. (2018). Logophish: A new two-dimensional code phishing attack detection method. In 2018 IEEE international conference on parallel and distributed processing with applications, ubiquitous computing and communications, big data and cloud computing, social computing and networking, sustainable computing and communications (ISPA/IUCC/BDCloud/SocialCom/SustainCom) (pp. 231–236). IEEE.
Yasin, A., Fatima, R., Liu, L., Yasin, A., & Wang, J. (2019). Contemplating social engineering studies and attack scenarios: A review study. Security and Privacy , 2 (4), e73.
Zamir, A., Khan, H. U., Iqbal, T., Yousaf, N., Aslam, F., Anjum, A., et al. (2020). Phishing web site detection using diverse machine learning algorithms. The Electronic Library .
Download references
Author information
Authors and affiliations.
Department of Computer Science, Air University, E-9, Islamabad, Pakistan
Abdul Basit & Maham Zafar
School of Information Engineering, Yangzhou University, Yangzhou, China
Department of Cyber Security, Air University, E-9, Islamabad, Pakistan
Abdul Rehman Javed, Zunera Jalil & Kashif Kifayat
You can also search for this author in PubMed Google Scholar
Corresponding author
Correspondence to Xuan Liu .
Additional information
Publisher's note.
Springer Nature remains neutral with regard to jurisdictional claims in published maps and institutional affiliations.
Rights and permissions
Reprints and permissions
About this article
Basit, A., Zafar, M., Liu, X. et al. A comprehensive survey of AI-enabled phishing attacks detection techniques. Telecommun Syst 76 , 139–154 (2021). https://doi.org/10.1007/s11235-020-00733-2
Download citation
Accepted : 09 October 2020
Published : 23 October 2020
Issue Date : January 2021
DOI : https://doi.org/10.1007/s11235-020-00733-2
Share this article
Anyone you share the following link with will be able to read this content:
Sorry, a shareable link is not currently available for this article.
Provided by the Springer Nature SharedIt content-sharing initiative
- Phishing attack
- Security threats
- Advanced phishing techniques
- Cyberattack
- Internet security
- Machine learning
- Deep learning
- Hybrid learning
- Find a journal
- Publish with us
- Track your research
Information
- Author Services
Initiatives
You are accessing a machine-readable page. In order to be human-readable, please install an RSS reader.
All articles published by MDPI are made immediately available worldwide under an open access license. No special permission is required to reuse all or part of the article published by MDPI, including figures and tables. For articles published under an open access Creative Common CC BY license, any part of the article may be reused without permission provided that the original article is clearly cited. For more information, please refer to https://www.mdpi.com/openaccess .
Feature papers represent the most advanced research with significant potential for high impact in the field. A Feature Paper should be a substantial original Article that involves several techniques or approaches, provides an outlook for future research directions and describes possible research applications.
Feature papers are submitted upon individual invitation or recommendation by the scientific editors and must receive positive feedback from the reviewers.
Editor’s Choice articles are based on recommendations by the scientific editors of MDPI journals from around the world. Editors select a small number of articles recently published in the journal that they believe will be particularly interesting to readers, or important in the respective research area. The aim is to provide a snapshot of some of the most exciting work published in the various research areas of the journal.
Original Submission Date Received: .
- Active Journals
- Find a Journal
- Proceedings Series
- For Authors
- For Reviewers
- For Editors
- For Librarians
- For Publishers
- For Societies
- For Conference Organizers
- Open Access Policy
- Institutional Open Access Program
- Special Issues Guidelines
- Editorial Process
- Research and Publication Ethics
- Article Processing Charges
- Testimonials
- Preprints.org
- SciProfiles
- Encyclopedia
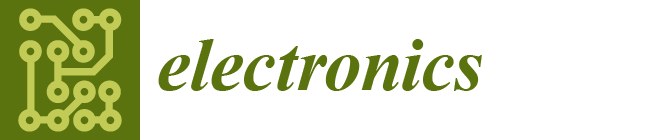
Article Menu

- Subscribe SciFeed
- Google Scholar
- on Google Scholar
- Table of Contents
Find support for a specific problem in the support section of our website.
Please let us know what you think of our products and services.
Visit our dedicated information section to learn more about MDPI.
JSmol Viewer
A systematic review on deep-learning-based phishing email detection.
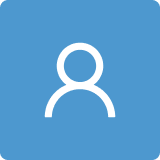
1. Introduction
1.1. our contribution, 1.2. organization of the document, 2. methodology, 2.1. research question and search strategy, 2.2. study selection, 2.3. data extraction and analysis, 2.4. quality assessment, 2.5. inclusion and exclusion criteria, 2.5.1. inclusion criteria.
- The paper must contain empirical results on deep-learning-based phishing detection.
- The paper must be published in the English language.
2.5.2. Exclusion Criteria
- The paper is not available in full-text format.
- The paper is not related to the research question.
- The paper is a duplicate publication. The paper is a review article or a meta-analysis.
- The paper is a conference abstract or poster presentation.
- The paper is a book, book chapter, or thesis.
- The paper is of low quality, as determined by the QATQS.
3. Literature Survey and Findings
3.1. research papers published in 2017 and before, 3.2. research papers published in 2018, 3.3. research papers published in 2019, 3.4. research papers published in 2020, 3.5. research papers published in 2021, 3.6. research papers published in 2022, 3.7. research papers published in 2023, 4. results and analysis, 4.1. findings of data analysis, 4.2. limitations found, 4.3. future direction, 4.3.1. privacy preservation, 4.3.2. increasing dataset size and optimizing feature selection, 4.3.3. broader email content analysis, 4.3.4. handling modern phishing techniques, 4.3.5. handling concept drift, 4.3.6. consideration of additional factors, 4.3.7. comparison with state-of-the-art techniques, 4.3.8. hyperparameter optimization and more deep learning architectures, 4.3.9. real-time dataset and processing, 4.3.10. exploration of other machine learning techniques, 4.3.11. incorporating additional data sources, 4.3.12. enriching the dataset, 4.3.13. exploring attackers’ behavior and modus operandi, 4.3.14. testing on other domains, 5. conclusions, author contributions, data availability statement, conflicts of interest.
- Alshingiti, Z.; Alaqel, R.; Al-Muhtadi, J.; Haq, Q.E.U.; Saleem, K.; Faheem, M.H. A Deep Learning-Based Phishing Detection System Using CNN, LSTM, and LSTM-CNN. Electronics 2023 , 12 , 232. [ Google Scholar ] [ CrossRef ]
- Tsohou, A.; Diamantopoulou, V.; Gritzalis, S.; Lambrinoudakis, C. Cyber insurance: State of the art, trends and future directions. Int. J. Inf. Secur. 2023 , 22 , 737–748. [ Google Scholar ] [ CrossRef ]
- Sheng, S.; Wardman, B.; Warner, G.; Cranor, L.; Hong, J.; Zhang, C. An Empirical Analysis of Phishing Blacklists. In Proceedings of the Sixth Conference on Email and Anti-Spam, Mountain View, CA, USA, 16–17 July 2009. [ Google Scholar ]
- Edge, M.E.; Sampaio, P.R.F. A survey of signature based methods for financial fraud detection. Comput. Secur. 2009 , 28 , 381–394. [ Google Scholar ] [ CrossRef ]
- Safi, A.; Singh, S. A systematic literature review on phishing website detection techniques. J. King Saud Univ. Comput. Inf. Sci. 2023 , 35 , 590–611. [ Google Scholar ] [ CrossRef ]
- Aldawood, H.; Skinner, G. An Advanced Taxonomy for Social Engineering Attacks. Int. J. Comput. Appl. 2020 , 177 , 1–11. [ Google Scholar ] [ CrossRef ]
- Aleroud, A.; Zhou, L. Phishing environments, techniques, and countermeasures: A survey. Comput. Secur. 2017 , 68 , 160–196. [ Google Scholar ] [ CrossRef ]
- Kocher, G.; Kumar, G. Machine learning and deep learning methods for intrusion detection systems: Recent developments and challenges. Soft Comput. 2021 , 25 , 9731–9763. [ Google Scholar ] [ CrossRef ]
- Chen, D.; Wawrzynski, P.; Lv, Z. Cyber security in smart cities: A review of deep learning-based applications and case studies. Sustain. Cities Soc. 2021 , 66 , 102655. [ Google Scholar ] [ CrossRef ]
- Adebowale, M.A.; Lwin, K.T.; Hossain, M.A. Deep learning with convolutional neural network and long short-term memory for phishing detection. In Proceedings of the 2019 13th International Conference on Software, Knowledge, Information Management and Applications (SKIMA), Island of Ulkulhas, Maldives, 26–28 August 2019; pp. 1–8. [ Google Scholar ]
- Thomas, B.; Ciliska, D.; Dobbins, M.; Micucci, S. A Process for Systematically Reviewing the Literature: Providing the Research Evidence for Public Health Nursing Interventions. Worldviews Evid.-Based Nurs. 2004 , 1 , 176–184. [ Google Scholar ] [ CrossRef ]
- Nosseir, A.; Nagati, K.; Taj-Eddin, I. Intelligent word-based spam filter detection using multi-neural networks. Int. J. Comput. Sci. Issues (IJCSI) 2013 , 10 Pt 1 , 17. [ Google Scholar ]
- Almomani, A.; Gupta, B.B.; Wan, T.C.; Altaher, A.; Manickam, S. Phishing dynamic evolving neural fuzzy framework for online detection zero-day phishing email. Indian J. Sci. Technol. 2013 , 6 , 3960–3964. [ Google Scholar ] [ CrossRef ]
- Hamid, I.R.A.; Abawajy, J.; Kim, T.H. Using feature selection and classification scheme for automating phishing email detection. Stud. Inform. Control. 2013 , 22 , 61–70. [ Google Scholar ] [ CrossRef ]
- Jameel, N.G.M.; George, L.E. Detection of phishing emails using feed forward neural network. Int. J. Comput. Appl. 2013 , 77 , 10–15. [ Google Scholar ]
- Soni, A.N. Spam-e-mail-detection-using-advanced-deep-convolution-neuralnetwork-algorithms. J. Innov. Dev. Pharm. Tech. Sci. 2019 , 2 , 74–80. [ Google Scholar ]
- Zhang, N.; Yuan, Y. Phishing Detection Using Neural Network. Available online: http://cs229.stanford.edu/proj2012/ZhangYuan-PhishingDetectionUsingNeuralNetwork.pdf (accessed on 1 October 2023).
- Kufandirimbwa, O.; Gotora, R. Spam detection using artificial neural networks (perceptron learning rule). Online J. Phys. Environ. Sci. Res. 2012 , 1 , 22–29. [ Google Scholar ]
- Abu-Nimeh, S.; Nappa, D.; Wang, X.; Nair, S. A comparison of machine learning techniques for phishing detection. In Proceedings of the Anti-Phishing Working Groups 2nd Annual eCrime Researchers Summit, Pittsburgh, PA, USA, 4–5 October 2007; pp. 60–69. [ Google Scholar ]
- Chandan, C.J.; Chheda, H.P.; Gosar, D.M.; Shah, H.R.; Bhave, P.U. A Machine learning approach for detection of phished websites using neural networks. Int. J. Recent Innov. Trends Comput. Commun. 2014 , 2 , 42054209. [ Google Scholar ]
- Alkaht, I.J.; Al Khatib, B. Filtering SPAM Using Several Stages Neural Networks. Int. Rev. Comput. Softw. (IRECOS) 2016 , 11 , 123–132. [ Google Scholar ] [ CrossRef ]
- Coyotes, C.; Mohan, V.S.; Naveen, J.; Vinayakumar, R.; Soman, K.P.; Verma, A.D.R. ARES: Automatic rogue email spotter. In Proceedings of the 1st AntiPhishing Shared Pilot at 4th ACM International Workshop on Security and Privacy Analytics (IWSPA), Tempe, AZ, USA, 1–11 March 2018. [ Google Scholar ]
- Smadi, S.; Aslam, N.; Zhang, L. Detection of online phishing email using dynamic evolving neural network based on reinforcement learning. Decis. Support Syst. 2018 , 107 , 88–102. [ Google Scholar ] [ CrossRef ]
- Hiransha, M.; Unnithan, N.A.; Vinayakumar, R.; Soman, K.; Verma, A.D.R. Deep learning based phishing e-mail detection. In Proceedings of the 1st AntiPhishing Shared Pilot at 4th ACM International Workshop Security Privacy Analytics (IWSPA), Tempe, AZ, USA, 1–11 March 2018; pp. 1–5. [ Google Scholar ]
- Barushka, A.; Hajek, P. Spam filtering using integrated distribution-based balancing approach and regularized deep neural networks. Appl. Intell. 2018 , 48 , 3538–3556. [ Google Scholar ] [ CrossRef ]
- Fang, Y.; Zhang, C.; Huang, C.; Liu, L.; Yang, Y. Phishing Email Detection Using Improved RCNN Model With Multilevel Vectors and Attention Mechanism. IEEE Access 2019 , 7 , 56329–56340. [ Google Scholar ] [ CrossRef ]
- Harikrishnan, N.B.; Vinayakumar, R.; Soman, K.P.; Poornachandran, P. Time split based pre-processing with a data-driven approach for malicious url detection. Cybersecur. Secur. Inf. Syst. Chall. Solut. Smart Environ. 2019 , 43–65. [ Google Scholar ] [ CrossRef ]
- Ali, W.; Ahmed, A.A. Hybrid intelligent phishing website prediction using deep neural networks with genetic algorithm-based feature selection and weighting. IET Inf. Secur. 2019 , 13 , 659–669. [ Google Scholar ] [ CrossRef ]
- Oña, D.; Zapata, L.; Fuertes, W.; Rodríguez, G.; Benavides, E.; Toulkeridis, T. Phishing attacks: Detecting and preventing infected e-mails using machine learning methods. In Proceedings of the 2019 3rd Cyber Security in Networking Conference (CSNet), IEEE, Quito, Ecuador, 23–25 October 2019; pp. 161–163. [ Google Scholar ]
- Nguyen, M.; Nguyen, T.; Nguyen, T.H. A deep learning model with hierarchical lstms and supervised attention for anti-phishing. CEUR Workshop Proc. 2018 , 2124 , 29–38. [ Google Scholar ]
- Wei, B.; Hamad, R.A.; Yang, L.; He, X.; Wang, H.; Gao, B.; Woo, W.L. A deep-learning-driven light-weight phishing detection sensor. Sensors 2019 , 19 , 4258. [ Google Scholar ] [ CrossRef ] [ PubMed ]
- Vinayakumar, R.; Soman, K.P.; Poornachandran, P.; Akarsh, S.; Elhoseny, M. Deep learning framework for cyber threat situational awareness based on email and url data analysis. In Cybersecurity and Secure Information Systems: Challenges and Solutions in Smart Environments ; Springer: Berlin/Heidelberg, Germany, 2019; pp. 87–124. [ Google Scholar ]
- Yang, P.; Zhao, G.; Zeng, P. Phishing Website Detection Based on Multidimensional Features Driven by Deep Learning. IEEE Access 2019 , 7 , 15196–15209. [ Google Scholar ] [ CrossRef ]
- Saha, I.; Sarma, D.; Chakma, R.J.; Alam, M.N.; Sultana, A.; Hossain, S. Phishing attacks detection using deep learning approach. In Proceedings of the 2020 Third International Conference on Smart Systems and Inventive Technology (ICSSIT), IEEE, Tirunelveli, India, 20–22 August 2020; pp. 1180–1185. [ Google Scholar ]
- Thapa, C.; Tang, J.W.; Abuadbba, A.; Gao, Y.; Camtepe, S.; Nepal, S.; Almashor, M.; Zheng, Y. Evaluation of Federated Learning in Phishing Email Detection. Sensors 2023 , 23 , 4346. [ Google Scholar ] [ CrossRef ] [ PubMed ]
- Adebowale, M.A.; Lwin, K.T.; Hossain, M.A. Intelligent phishing detection scheme using deep learning algorithms. J. Enterp. Inf. Manag. 2020 , 36 , 747–766. [ Google Scholar ] [ CrossRef ]
- Alotaibi, R.; Al-Turaiki, I.; Alakeel, F. Mitigating email phishing attacks using convolutional neural networks. In Proceedings of the 2020 3rd International Conference on Computer Applications & Information Security (ICCAIS), IEEE, Riyadh, Saudi Arabia, 19–21 March 2020; pp. 1–6. [ Google Scholar ]
- Baccouche, A.; Ahmed, S.; Sierra-Sosa, D.; Elmaghraby, A. Malicious text identification: Deep learning from public comments and emails. Information 2020 , 11 , 312. [ Google Scholar ] [ CrossRef ]
- Soon, G.K.; On, C.K.; Rusli, N.M.; Fun, T.S.; Alfred, R.; Guan, T.T. March. Comparison of simple feedforward neural network, recurrent neural network and ensemble neural networks in phishing detection. J. Phys. Conf. Ser. 2020 , 1502 , 012033. [ Google Scholar ] [ CrossRef ]
- Alauthman, M. Botnet Spam E-Mail Detection Using Deep Recurrent Neural Network. Int. J. Emerg. Trends Eng. Res. 2020 , 8 , 1979–1986. [ Google Scholar ] [ CrossRef ]
- Eryılmaz, E.E.; Şahin, D.Ö.; Kılıç, E. Filtering turkish spam using LSTM from deep learning techniques. In Proceedings of the 2020 8th International Symposium on Digital Forensics and Security, ISDFS, IEEE, Beirut, Lebanon, 1–2 June 2020; pp. 1–6. [ Google Scholar ]
- Halgaš, L.; Agrafiotis, I.; Nurse, J.R. Catching the Phish: Detecting phishing attacks using recurrent neural networks (RNNs). In Proceedings of the Information Security Applications: 20th International Conference, WISA 2019, Jeju Island, Republic of Korea, 21–24 August 2019; pp. 219–233. [ Google Scholar ]
- Isik, S.; Kurt, Z.; Anagun, Y.; Ozkan, K. Spam E-mail Classification Recurrent Neural Networks for Spam E-mail Classification on an Agglutinative Language. Int. J. Intell. Syst. Appl. Eng. 2020 , 8 , 221–227. [ Google Scholar ] [ CrossRef ]
- AlEroud, A.; Karabatis, G. Bypassing detection of URL-based phishing attacks using generative adversarial deep neural networks. In Proceedings of the Sixth International Workshop on Security and Privacy Analytics, New Orleans, LA, USA, 18 March 2020; pp. 53–60. [ Google Scholar ]
- Castillo, E.; Dhaduvai, S.; Liu, P.; Thakur, K.S.; Dalton, A.; Strzalkowski, T. Email threat detection using distinct neural network approaches. In Proceedings of the First International Workshop on Social Threats in Online Conversations: Understanding and Management, Marseille, France, 11–16 May 2020; pp. 48–55. [ Google Scholar ]
- Kumar, A.; Chatterjee, J.M.; Díaz, V.G. A novel hybrid approach of SVM combined with NLP and probabilistic neural network for email phishing. Int. J. Electr. Comput. Eng. (IJECE) 2020 , 10 , 486–493. [ Google Scholar ] [ CrossRef ]
- Opara, C.; Wei, B.; Chen, Y. HTMLPhish: Enabling phishing web page detection by applying deep learning techniques on HTML analysis. In Proceedings of the 2020 International Joint Conference on Neural Networks (IJCNN), Glasgow, UK, 19–24 July 2020; pp. 1–8. [ Google Scholar ]
- AbdulNabi, I.; Yaseen, Q. Spam Email Detection Using Deep Learning Techniques. Procedia Comput. Sci. 2021 , 184 , 853–858. [ Google Scholar ] [ CrossRef ]
- Otter, D.W.; Medina, J.R.; Kalita, J.K. A Survey of the Usages of Deep Learning for Natural Language Processing. IEEE Trans. Neural Networks Learn. Syst. 2020 , 32 , 604–624. [ Google Scholar ] [ CrossRef ]
- Alhogail, A.; Alsabih, A. Applying machine learning and natural language processing to detect phishing email. Comput. Secur. 2021 , 110 , 102414. [ Google Scholar ] [ CrossRef ]
- Bagui, S.; Nandi, D.; Bagui, S.; White, R.J. Machine learning and deep learning for phishing email classification using one-hot encoding. J. Comput. Sci. 2021 , 17 , 610–623. [ Google Scholar ] [ CrossRef ]
- Lee, J.; Tang, F.; Ye, P.; Abbasi, F.; Hay, P.; Divakaran, D.M. D-Fence: A flexible, efficient, and comprehensive phishing email detection system. In Proceedings of the 2021 IEEE European Symposium on Security and Privacy (EuroS&P), IEEE, Vienna, Austria, 7–11 September 2021; pp. 578–597. [ Google Scholar ]
- Manaswini, M.; Srinivasu, D.N. Phishing Email Detection Model using Improved Recurrent Convolutional Neural Networks and Multilevel Vectors. Ann. Rom. Soc. Cell Biol. 2021 , 25 , 16674–16681. [ Google Scholar ]
- Ghaleb, S.A.A.; Mohamad, M.; Fadzli, S.A.; Ghanem, W.A.H.M. Training Neural Networks by Enhance Grasshopper Optimization Algorithm for Spam Detection System. IEEE Access 2021 , 9 , 116768–116813. [ Google Scholar ] [ CrossRef ]
- Eckhardt, R.; Bagui, S. Convolutional Neural Networks and Long Short Term Memory for Phishing Email Classification. Int. J. Comput. Sci. Inf. Secur. 2021 , 19 , 27–35. [ Google Scholar ]
- Sheneamer, A. Comparison of Deep and Traditional Learning Methods for Email Spam Filtering. Int. J. Adv. Comput. Sci. Appl. 2021 , 12 , 560–565. [ Google Scholar ] [ CrossRef ]
- Dubey, K.A.; Ganesh, K.B.; Gowtham, V.; Balakrishnan, M.D. Phishing email detection. Int. J. Emerg. Technol. Comput. Sci. Electron. (IJETCSE) 2021 , 28 , 1–4. [ Google Scholar ]
- Samarthrao, K.V.; Rohokale, V.M. Enhancement of email spam detection using improved deep learning algorithms for cyber security. J. Comput. Secur. 2022 , 30 , 231–264. [ Google Scholar ] [ CrossRef ]
- Dewis, M.; Viana, T. Phish Responder: A Hybrid Machine Learning Approach to Detect Phishing and Spam Emails. Appl. Syst. Innov. 2022 , 5 , 73. [ Google Scholar ] [ CrossRef ]
- Khan, S.A.; Iqbal, K.; Mohammad, N.; Akbar, R.; Ali, S.S.A.; Siddiqui, A.A. A Novel Fuzzy-Logic-Based Multi-Criteria Metric for Performance Evaluation of Spam Email Detection Algorithms. Appl. Sci. 2022 , 12 , 7043. [ Google Scholar ] [ CrossRef ]
- Malhotra, P.; Malik, S. Spam Email Detection Using Machine Learning and Deep Learning Techniques. In Proceedings of the International Conference on Innovative Computing & Communication (ICICC), Delhi, India, 24 June 2022. [ Google Scholar ] [ CrossRef ]
- Korkmaz, M.; Koçyiğit, E.; Şahingöz, Ö.; Diri, B. A Hybrid Phishing Detection System by Using Deep Learning-Based URL and Content Analysis. Elektron. Ir Elektrotechnika 2022 , 28 , 80–89. [ Google Scholar ] [ CrossRef ]
- Zhu, E.; Yuan, Q.; Chen, Z.; Li, X.; Fang, X. CCBLA: A Lightweight Phishing Detection Model Based on CNN, BiLSTM, and Attention Mechanism. Cogn. Comput. 2022 , 15 , 1320–1333. [ Google Scholar ] [ CrossRef ]
- Nooraee, M.; Ghaffari, H. Optimization and Improvement of Spam Email Detection Using Deep Learning Approaches. J. Comput. Robot. 2022 , 15 , 61–70. [ Google Scholar ]
- Prosun, P.R.K.; Alam, K.S.; Bhowmik, S. Improved Spam Email Filtering Architecture Using Several Feature Extraction Techniques. In Proceedings of the International Conference on Big Data, IoT, and Machine Learning: BIM 2021, Cox’s Bazar, Bangladesh, 23–25 September 2021; Springer: Singapore, 2021; pp. 665–675. [ Google Scholar ]
- Jafar, M.T.; Al-Fawa’reh, M.; Barhoush, M.; Alshira’H, M.H. Enhanced Analysis Approach to Detect Phishing Attacks During COVID-19 Crisis. Cybern. Inf. Technol. 2022 , 22 , 60–76. [ Google Scholar ] [ CrossRef ]
- Do, N.Q.; Selamat, A.; Krejcar, O.; Herrera-Viedma, E.; Fujita, H. Deep Learning for Phishing Detection: Taxonomy, Current Challenges and Future Directions. IEEE Access 2022 , 10 , 36429–36463. [ Google Scholar ] [ CrossRef ]
- Zhou, M.-G.; Liu, Z.-P.; Yin, H.-L.; Li, C.-L.; Xu, T.-K.; Chen, Z.-B. Quantum Neural Network for Quantum Neural Computing. Research 2023 , 6 , 0134. [ Google Scholar ] [ CrossRef ] [ PubMed ]
- Rafat, K.F.; Xin, Q.; Javed, A.R.; Jalil, Z.; Ahmad, R.Z. Evading obscure communication from spam emails. Math. Biosci. Eng. 2021 , 19 , 1926–1943. [ Google Scholar ] [ CrossRef ] [ PubMed ]
- Rathee, D.; Mann, S. Detection of E-Mail Phishing Attacks – using Machine Learning and Deep Learning. Int. J. Comput. Appl. 2022 , 183 , 1–7. [ Google Scholar ] [ CrossRef ]
- Mughaid, A.; AlZu’bi, S.; Hnaif, A.; Taamneh, S.; Alnajjar, A.; Abu Elsoud, E. An intelligent cyber security phishing detection system using deep learning techniques. Clust. Comput. 2022 , 25 , 3819–3828. [ Google Scholar ] [ CrossRef ] [ PubMed ]
- Butt, U.A.; Amin, R.; Aldabbas, H.; Mohan, S.; Alouffi, B.; Ahmadian, A. Cloud-based email phishing attack using machine and deep learning algorithm. Complex Intell. Syst. 2022 , 9 , 3043–3070. [ Google Scholar ] [ CrossRef ]
- Logavarshini, G.; Yogalakshmi, S. E-Mail Spam Classification Via Deep Learning and Natural Language Processing. Int. J. Res. Publ. Rev. 2022 , 2582 , 7421. [ Google Scholar ]
- Ghaleb, S.A.A.; Mohamad, M.; Ghanem, W.A.H.M.; Nasser, A.B.; Ghetas, M.; Abdullahi, A.M.; Saleh, S.A.M.; Arshad, H.; Omolara, A.E.; Abiodun, O.I. Feature Selection by Multiobjective Optimization: Application to Spam Detection System by Neural Networks and Grasshopper Optimization Algorithm. IEEE Access 2022 , 10 , 98475–98489. [ Google Scholar ] [ CrossRef ]
- Babu, D.K. Phishing Detection in Emails Using Multi-Convolutional Neural Network Fusion. Ph.D. Thesis, National College of Ireland, Dublin, Ireland, 2022. [ Google Scholar ]
- Shmalko, M.; Abuadbba, A.; Gaire, R.; Wu, T.; Paik, H.Y.; Nepal, S. Profiler: Profile-Based Model to Detect Phishing Emails. arXiv 2022 , arXiv:2208.08745. [ Google Scholar ]
- Muralidharan, T.; Nissim, N. Improving malicious email detection through novel designated deep-learning architectures utilizing entire email. Neural Networks 2023 , 157 , 257–279. [ Google Scholar ] [ CrossRef ]
- Bountakas, P.; Xenakis, C. HELPHED: Hybrid Ensemble Learning PHishing Email Detection. J. Netw. Comput. Appl. 2023 , 210 , 103545. [ Google Scholar ] [ CrossRef ]
- Wen, T.; Xiao, Y.; Wang, A.; Wang, H. A novel hybrid feature fusion model for detecting phishing scam on Ethereum using deep neural network. Expert Syst. Appl. 2023 , 211 , 118463. [ Google Scholar ] [ CrossRef ]
- Liu, Z.-P.; Zhou, M.-G.; Liu, W.-B.; Li, C.-L.; Gu, J.; Yin, H.-L.; Chen, Z.-B. Automated machine learning for secure key rate in discrete-modulated continuous-variable quantum key distribution. Opt. Express 2022 , 30 , 15024–15036. [ Google Scholar ] [ CrossRef ] [ PubMed ]
Click here to enlarge figure
Ref | Method | Data | Result | Innovations | Limitations |
---|---|---|---|---|---|
[ ] | CNN, MLP, RNN | Self-generated emails dataset | Accuracy: 93.1% | Highlighted issues related to imbalance data | Highly imbalanced nature of the dataset |
[ ] | NN | SpanAssian | Accuracy: 99.07% | Provided guidelines to improve offline data | Needed to enrich the offline dataset to enhance model performance |
[ ] | CEN-Deepspam | Self-generated emails dataset | Accuracy: 95.5% | Larger dataset could improve accuracy | Additional dataset required to validate the result |
[ ] | DBB-RDNN-ReL | Enron, SpamAssassin, SMS Spam Colection | Accuracy: 96.1% | DBBRDNN-ReL model outperformed compared to other models | Slow processing |
Ref | Method | Data | Result | Innovations | Limitations |
---|---|---|---|---|---|
[ ] | THEMIS | Enron and SpamAssassin | Accuracy: 99.85% | Utilized unbalanced dataset | Limited to detecting phishing emails with header |
[ ] | NB, DT, AB, RF, DNN, RNN, CNN | PhishTank | Accuracy: 88.5% | Tf-idf presentation is better than feature hashing and embedding | Limited real-time dataset |
[ ] | DNN | UCI phishing websites | Accuracy: 95% | Hybrid model performs better for classification | Feature selection requires longer time |
[ ] | NN | Debian and PhishTank | Accuracy: 93.9% | Better accuracy | Limited use of deep learning |
[ ] | LSTM | Data-no-header and data-full-header | Accuracy: 89.34% | - | Low effectiveness |
[ ] | Multi-spatial CNN | Self-generated emails dataset | Accuracy: 86.63% | 30% reduction in the execution time | Did not compare model’s performance with other state-of-the-art methods |
[ ] | CNN, RNN, CNN-RNN, CNN-LSTM | Spam dataset. URL dataset | Recall: 99% | Better performance in detecting malware | Performance could be improved by adding sub-modules |
[ ] | CNN, RNN, LSTM, CNN-RNN | Self-generated emails dataset | Accuracy: 98.99% | High accuracy and low FPR | Focused on a single type of phishing attack |
Ref | Method | Data | Result | Innovations | Limitations |
---|---|---|---|---|---|
[ ] | IPDS | URLs | Accuracy: 93.28% | Novel approach to differentiate phishing and legitimate URLs | Ensuring the availability of the dataset would be challenging |
[ ] | CNN | PhishingCorpus and SpamAssasin | Accuracy: 99.42% | Used a huge dataset to detect phishing emails | Used a smaller dataset |
[ ] | Multi-label LSTM | Self-generated emails dataset | Accuracy: 92.7% | Used combined dataset | No comparison of the results |
[ ] | GRU-RNN+SVM | Spambase dataset | Accuracy: 98.7% | Claimed higher accuracy | Limited to one dataset |
[ ] | LSTM+Keras | 800 Turkish emails dataset | Accuracy: 100% | Proposed hybrid model | Limited dataset |
[ ] | RNNs | SA-JN and En-JN datasets | Accuracy: 98.91% and 96.74% | Outperformed state-of-the-art systems | Unrealistically hard |
[ ] | ANN, LSTM, and BILSTM | Self-generated Turkish emails dataset | Accuracy: 100% | Highest accuracy | Focused on the Turkish language only |
[ ] | GAN-based | PhishTank and MillerSmiles | TPR: 97% | Has used actual phishing dataset | Controlled environment |
[ ] | ML, DL, NLP | Rnron, APWG | Accuracy: 93% | - | Limited dataset |
[ ] | SVM combined with NLP and PNN | Self-generated emails dataset | Accuracy: 89% | Probabilistic NN would be more accurate in phishing detection | Only works on a small phishing dataset |
[ ] | CNN | HTML documents | Accuracy: 93% | Automatic phishing web page detection | Limited to HTML document analysis |
Ref | Method | Data | Result | Innovations | Limitations |
---|---|---|---|---|---|
[ ] | GCN+NLP | Self-generated email body text dataset | Accuracy: 98.2% | Enhance phishing detection on the email body text | Tested only English corpus |
[ ] | CNN and LSTM | Self-generated emails dataset | Accuracy: 96.34% | CNN with word embedding is most accurate | Tested only English corpus |
[ ] | D-Fence | Self-generated emails dataset | Accuracy: 99% | D-Fence maintained a high detection rate | Relied on multiple modules |
[ ] | Themis | Self-generated emails dataset | Accuracy: 99.87% | Combined email head and body | Focused only on analyzing the email structure |
[ ] | MLP | SpamBase, SpamAssassin, UK-2011 Webspam | Accuracy: 98.1% | Used several dataset and features | Spam detection study is inadequate |
[ ] | CNN and LSTM | Two datasets | Accuracy: 98.3% | Adam optimizer outperformed the SGD optimizer | Comparison limited to textual data classification |
[ ] | CNN | Self-generated emails dataset | Accuracy: 96.52% | Automated features extraction | Limited datasets |
Ref | Method | Data | Result | Innovations | Limitations |
---|---|---|---|---|---|
[ ] | Fitness-oriented, Levy improvement-based Dragonfly | N/A | Accuracy: 14.93% | Better performance than DT, KNN, and SVM | Misclassification existed |
[ ] | DL+NLP | Text-based and numerical-based datasets | Accuracy: 99% (text-based) and 94% (numerical-based) | Phish Responder better than other models | Limited data used; no explanation on the dataset employed |
[ ] | ML and DL | N/A | Accuracy: 98.5% | BiLSTM classifier performed better | Dataset did not contain variety of spam emails |
[ ] | TshPhish | PhishTank | Accuracy: 98.37% | Improved feature selection through evolutionary algorithms | Low recall rate |
[ ] | CCBLA | Two datasets | Accuracy: 99.85% | Combined CNN, bi-directional LSTM, and attention mechanism | Huge time consumption |
[ ] | LSTM and Glove word embedding | Two datasets | Accuracy: 98.39% and 99.49% | Used multiple datasets | Limited to one language |
[ ] | ML-based voting model | N/A | Accuracy: 98% | Used various feature retrieval algorithms | Lack of benchmark datasets |
[ ] | GRU-based Phishing URL detection | Phishing URLs | Accuracy: 98.30% | Highly accurate classifier | Limited detection of phishing attacks during COVID-19 |
[ ] | Deep learning | N/A | Accuracy: 92% | Incorporated less explored DL techniques | No details of empirical analysis |
[ ] | ML and DL | Spamassassin | Precision: 95.26%, recall: 97.18%, F1-score: 96% | Focused on the limitations of ML and DL algorithms | Broader email content analysis |
[ ] | DL | Email text | Accuracy: 88–100% | - | Cannot effectively handle modern phishing techniques |
[ ] | RCNN | Email Structure | N/A | Examined emails at multiple levels, including the header, body, character, and words | Limited to detecting phishing emails with header |
[ ] | Multiobjective optimization | SpamBase, SpamAssassin, and UK-2011 datasets | Accuracy: 97.5%, 98.3%, and 96.4% | - | Limited to detecting spam |
Ref | Method | Data | Result | Innovations | Limitations |
---|---|---|---|---|---|
[ ] | Deep ensemble learning | Email segments | AUC of 0.993 and TPR of 5% | Higher AUC result | Focus on privacy preservation in future work. |
[ ] | HELPHED | Imbalanced | F1-score: 99.42% | Superior result in the imbalance dataset | Focused on the detection and did not address prevention or mitigation of attacks. The dataset was imbalanced. |
[ ] | LBPS | Ethereum data | F1-score: 97.86% | Phishing scam account detection model | Tested the LBPS model only on Ethereum data. |
The statements, opinions and data contained in all publications are solely those of the individual author(s) and contributor(s) and not of MDPI and/or the editor(s). MDPI and/or the editor(s) disclaim responsibility for any injury to people or property resulting from any ideas, methods, instructions or products referred to in the content. |
Share and Cite
Thakur, K.; Ali, M.L.; Obaidat, M.A.; Kamruzzaman, A. A Systematic Review on Deep-Learning-Based Phishing Email Detection. Electronics 2023 , 12 , 4545. https://doi.org/10.3390/electronics12214545
Thakur K, Ali ML, Obaidat MA, Kamruzzaman A. A Systematic Review on Deep-Learning-Based Phishing Email Detection. Electronics . 2023; 12(21):4545. https://doi.org/10.3390/electronics12214545
Thakur, Kutub, Md Liakat Ali, Muath A. Obaidat, and Abu Kamruzzaman. 2023. "A Systematic Review on Deep-Learning-Based Phishing Email Detection" Electronics 12, no. 21: 4545. https://doi.org/10.3390/electronics12214545
Article Metrics
Article access statistics, further information, mdpi initiatives, follow mdpi.
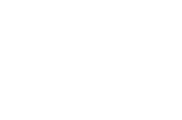
Subscribe to receive issue release notifications and newsletters from MDPI journals
Thank you for visiting nature.com. You are using a browser version with limited support for CSS. To obtain the best experience, we recommend you use a more up to date browser (or turn off compatibility mode in Internet Explorer). In the meantime, to ensure continued support, we are displaying the site without styles and JavaScript.
- View all journals
- Explore content
- About the journal
- Publish with us
- Sign up for alerts
- Open access
- Published: 25 May 2022
An effective detection approach for phishing websites using URL and HTML features
- Ali Aljofey 1 , 2 ,
- Qingshan Jiang 1 ,
- Abdur Rasool 1 , 2 ,
- Hui Chen 1 , 2 ,
- Wenyin Liu 3 ,
- Qiang Qu 1 &
- Yang Wang 4
Scientific Reports volume 12 , Article number: 8842 ( 2022 ) Cite this article
24k Accesses
37 Citations
Metrics details
- Computer science
- Information technology
- Scientific data
Today's growing phishing websites pose significant threats due to their extremely undetectable risk. They anticipate internet users to mistake them as genuine ones in order to reveal user information and privacy, such as login ids, pass-words, credit card numbers, etc. without notice. This paper proposes a new approach to solve the anti-phishing problem. The new features of this approach can be represented by URL character sequence without phishing prior knowledge, various hyperlink information, and textual content of the webpage, which are combined and fed to train the XGBoost classifier. One of the major contributions of this paper is the selection of different new features, which are capable enough to detect 0-h attacks, and these features do not depend on any third-party services. In particular, we extract character level Term Frequency-Inverse Document Frequency (TF-IDF) features from noisy parts of HTML and plaintext of the given webpage. Moreover, our proposed hyperlink features determine the relationship between the content and the URL of a webpage. Due to the absence of publicly available large phishing data sets, we needed to create our own data set with 60,252 webpages to validate the proposed solution. This data contains 32,972 benign webpages and 27,280 phishing webpages. For evaluations, the performance of each category of the proposed feature set is evaluated, and various classification algorithms are employed. From the empirical results, it was observed that the proposed individual features are valuable for phishing detection. However, the integration of all the features improves the detection of phishing sites with significant accuracy. The proposed approach achieved an accuracy of 96.76% with only 1.39% false-positive rate on our dataset, and an accuracy of 98.48% with 2.09% false-positive rate on benchmark dataset, which outperforms the existing baseline approaches.
Similar content being viewed by others
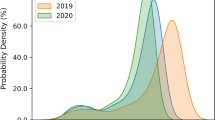
Life-long phishing attack detection using continual learning
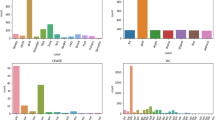
Machine learning-based guilt detection in text
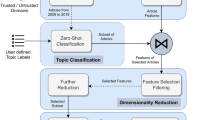
A Multiple change-point detection framework on linguistic characteristics of real versus fake news articles
Introduction.
Phishing offenses are increasing, resulting in billions of dollars in loss 1 . In these attacks, users enter their critical (i.e., credit card details, passwords, etc.) to the forged website which appears to be legitimate. The Software-as-a-Service (SaaS) and webmail sites are the most common targets of phishing 2 . The phisher makes websites that look very similar to the benign websites. The phishing website link is then sent to millions of internet users via emails and other communication media. These types of cyber-attacks are usually activated by emails, instant messages, or phone calls 3 . The aim of the phishing attack is not only to steal the victims' personality, but it can also be performed to spread other types of malware such as ransomware, to exploit approach weaknesses, or to receive monetary profits 4 . According to the Anti-Phishing Working Group (APWG) report in the 3rd Quarter of 2020, the number of phishing attacks has grown since March, and 28,093 unique phishing sites have been detected between July to September 2 . The average amount demanded during wire transfer Business E-mail Compromise (BEC) attacks was $48,000 in the third quarter, down from $80,000 in the second quarter and $54,000 in the first.
Detecting and preventing phishing offenses is a significant challenge for researchers due to the way phishers carry out the attack to bypass the existing anti-phishing techniques. Moreover, the phisher can even target some educated and experienced users by using new phishing scams. Thus, software-based phishing detection techniques are preferred for fighting against the phishing attack. Mostly available methods for detecting phishing attacks are blacklists/whitelists 5 , natural language processing 6 , visual similarity 7 , rules 8 , machine learning techniques 9 , 10 , etc. Techniques based on blacklists/whitelists fail to detect unlisted phishing sites (i.e. 0-h attacks) as well as these methods fail when blacklisted URL is encountered with minor changes. In the machine learning based techniques, a classification model is trained using various heuristic features (i.e., URL, webpage content, website traffic, search engine, WHOIS record, and Page Rank) in order to improve detection efficiency. However, these heuristic features are not warranted to present in all phishing websites and might also present in the benign websites, which may cause a classification error. Moreover, some of the heuristic features are hard to access and third-party dependent. Some third-party services (i.e., page rank, search engine indexing, WHOIS etc.) may not be sufficient to identify phishing websites that are hosted on hacked servers and these websites are inaccurately identified as benign websites because they are contained in search results. Websites hosted on compromised servers are usually more than a day old unlike other phishing websites which only take a few hours. Also, these services inaccurately identify the new benign website as a phishing site due to the lack of domain age. The visual similarity-based heuristic techniques compare the new website with the pre-stored signature of the website. The website’s visual signature includes screenshots, font styles, images, page layouts, logos, etc. Thus, these techniques cannot identify the fresh phishing websites and generate a high false-negative rate (phishing to benign). The URL based technique does not consider the HTML of the webpage and may misjudge some of the malicious websites hosted on free or compromised servers. Many existing approaches 11 , 12 , 13 extract hand-crafted URL based features, e.g., number of dots, presence of special “@”, “#”, “–” symbol, URL length, brand names in URL, position of Top-Level domain, check hostname for IP address, presence of multiple TLDs, etc. However, there are still hurdles to extracting manual URL features due to the fact that human effort requires time and extra maintenance labor costs. Detecting and preventing phishing offense is a major defiance for researchers because the scammer carries out these offenses in a way that can avoid current anti-phishing methods. Hence, the use of hybrid methods rather than a single approach is highly recommended by the networks security manager.
This paper provides an efficient solution for phishing detection that extracts the features from website's URL and HTML source code. Specifically, we proposed a hybrid feature set including URL character sequence features without expert’s knowledge, various hyperlink information, plaintext and noisy HTML data-based features within the HTML source code. These features are then used to create feature vector required for training the proposed approach by XGBoost classifier. Extensive experiments show that the proposed anti-phishing approach has attained competitive performance on real dataset in terms of different evaluation statistics.
Our anti-phishing approach has been designed to meet the following requirements.
High detection efficiency: To provide high detection efficiency, incorrect classification of benign sites as phishing (false-positive) should be minimal and correct classification of phishing sites (true-positive) should be high.
Real-time detection: The prediction of the phishing detection approach must be provided before exposing the user's personal information on the phishing website.
Target independent: Due to the features extracted from both URL and HTML the proposed approach can detect new phishing websites targeting any benign website (zero-day attack).
Third-party independent: The feature set defined in our work are lightweight and client-side adaptable, which do not rely on third-party services such as blacklist/whitelist, Domain Name System (DNS) records, WHOIS record (domain age), search engine indexing, network traffic measures, etc. Though third-party services may raise the effectiveness of the detection approach, they might misclassify benign websites if a benign website is newly registered. Furthermore, the DNS database and domain age record may be poisoned and lead to false negative results (phishing to benign).
Hence, a light-weight technique is needed for phishing websites detection adaptable at client side. The major contributions in this paper are itemized as follows.
We propose a phishing detection approach, which extracts efficient features from the URL and HTML of the given webpage without relying on third-party services. Thus, it can be adaptable at the client side and specify better privacy.
We proposed eight novel features including URL character sequence features (F1), textual content character level (F2), various hyperlink features (F3, F4, F5, F6, F7, and F14) along with seven existing features adopted from the literature.
We conducted extensive experiments using various machine learning algorithms to measure the efficiency of the proposed features. Evaluation results manifest that the proposed approach precisely identifies the legitimate websites as it has a high true negative rate and very less false positive rate.
We release a real phishing webpage detection dataset to be used by other researchers on this topic.
The rest of this paper is structured as follows: The " Related work " section first reviews the related works about phishing detection. Then the " Proposed approach " section presents an overview of our proposed solution and describes the proposed features set to train the machine learning algorithms. The " Experiments and result analysis ” section introduces extensive experiments including the experimental dataset and results evaluations. Furthermore, the " Discussion and limitation " section contains a discussion and limitations of the proposed approach. Finally, the " Conclusion " section concludes the paper and discusses future work.
Related work
This section provides an overview of the proposed phishing detection techniques in the literature. Phishing methods are divided into two categories; expanding the user awareness to distinguish the characteristics of phishing and benign webpages 14 , and using some extra software. Software-based techniques are further categorized into list-based detection, and machine learning-based detection. However, the problem of phishing is so sophisticated that there is no definitive solution to efficiently bypass all threats; thus, multiple techniques are often dedicated to restrain particular phishing offenses.
List-based detection
List-based phishing detection methods use either whitelist or blacklist-based technique. A blacklist contains a list of suspicious domains, URLs, and IP addresses, which are used to validate if a URL is fraudulent. Simultaneously, the whitelist is a list of legitimate domains, URLs, and IP addresses used to validate a suspected URL. Wang et al. 15 , Jain and Gupta 5 and Han et al. 16 use white list-based method for the detection of suspected URL. Blacklist-based methods are widely used in openly available anti-phishing toolbars, such as Google safe browsing, which maintains a blacklist of URLs and provides warnings to users once a URL is considered as phishing. Prakash et al. 17 proposed a technique to predict phishing URLs called Phishnet. In this technique, phishing URLs are identified from the existing blacklisted URLs using the directory structure, equivalent IP address, and brand name. Felegyhazi et al. 18 developed a method that compares the domain name and name server information of new suspicious URLs to the information of blacklisted URLs for the classification process. Sheng et al. 19 demonstrated that a forged domain was added to the blacklist after a considerable amount of time, and approximately 50–80% of the forged domains were appended after the attack was carried out. Since thousands of deceptive websites are launched every day, the blacklist requires to be updated periodically from its source. Thus, machine learning-based detection techniques are more efficient in dealing with phishing offenses.
Machine learning-based detection
Data mining techniques have provided outstanding performance in many applications, e.g., data security and privacy 20 , game theory 21 , blockchain systems 22 , healthcare 23 , etc. Due to the recent development of phishing detection methods, various machine learning-based techniques have also been employed 6 , 9 , 10 , 13 to investigate the legality of websites. The effectiveness of these methods relies on feature collection, training data, and classification algorithm. The feature collection is extracted from different sources, e.g., URL, webpage content, third party services, etc. However, some of the heuristic features are hard to access and time-consuming, which makes some machine learning approaches demand high computations to extract these features.
Jain and Gupta 24 proposed an anti-phishing approach that extracts the features from the URL and source code of the webpage and does not rely on any third-party services. Although the proposed approach attained high accuracy in detecting phishing webpages, it used a limited dataset (2141 phishing and 1918 legitimate webpages). The same authors 9 present a phishing detection method that can identify phishing attacks by analyzing the hyperlinks extracted from the HTML of the webpage. The proposed method is a client-side and language-independent solution. However, it entirely depends on the HTML of the webpage and may incorrectly classify the phishing webpages if the attacker changes all webpage resource references (i.e., Javascript, CSS, images, etc.). Rao and Pais 25 proposed a two-level anti-phishing technique called BlackPhish. At first level, a blacklist of signatures is created using visual similarity based features (i.e., file names, paths, and screenshots) rather than using blacklist of URLs. At second level, heuristic features are extracted from URL and HTML to identify the phishing websites which override the first level filter. In spite of that, the legitimate websites always undergo two-level filtering. In some researches 26 authors used search engine-based mechanism to authenticate the webpage as first-level authentication. In the second level authentication, various hyperlinks within the HTML of the website are processed for the phishing websites detection. Although the use of search engine-based techniques increases the number of legitimate websites correctly identified as legitimate, it also increases the number of legitimate websites incorrectly identified as phishing when newly created authentic websites are not found in the top results of search engine. Search based approaches assume that genuine website appears in the top search results.
In a recent study, Rao et al. 27 proposed a new phishing websites detection method with word embedding extracted from plain text and domain specific text of the html source code. They implemented different word embedding to evaluate their model using ensemble and multimodal techniques. However, the proposed method is entirely dependent on plain text and domain specific text, and may fail when the text is replaced with images. Some researchers have tried to identify phishing attacks by extracting different hyperlink relationships from webpages. Guo et al. 28 proposed a phishing webpages detection approach which they called HinPhish. The approach establishes a heterogeneous information network (HIN) based on domain nodes and loading resources nodes and establishes three relationships between the four hyperlinks: external link, empty link, internal link and relative link. Then, they applied an authority ranking algorithm to calculate the effect of different relationships and obtain a quantitative score for each node.
In Sahingoz et al. 6 work, the distributed representation of words is adopted within a specific URL, and then seven various machine learning classifiers are employed to identify whether a suspicious URL is a phishing website. Rao et al. 13 proposed an anti-phishing technique called CatchPhish. They extracted hand-crafted and Term Frequency-Inverse Document Frequency (TF-IDF) features from URLs, then trained a classifier on the features using random forest algorithm. Although the above methods have shown satisfactory performance, they suffer from the following restrictions: (1) inability to handle unobserved characters because the URLs usually contain meaningless and unknown words that are not in the training set; (2) they do not consider the content of the website. Accordingly, some URLs, which are distinctive to others but imitate the legitimate sites, may not be identified based on URL string. As their work is only based on URL features, which is not enough to detect the phishing websites. However, we have provided an effective solution by proposing our approach to this domain by utilizing three different types of features to detect the phishing website more efficiently. Specifically, we proposed a hybrid feature set consisting of URL character sequence, various hyperlinks information, and textual content-based features.
Deep learning methods have been used for phishing detection e.g., Convolutional Neural Network (CNN), Deep Neural Network (DNN), Recurrent Neural Network (RNN), and Recurrent Convolutional Neural Networks (RCNN) due to the success of the Natural Language Processing (NLP) attained by these techniques. However, deep learning methods are not employed much in phishing detection due to the inclusive training time. Aljofey et al. 3 proposed a phishing detection approach with a character level convolutional neural network based on URL. The proposed approach was compared by using various machine and deep learning algorithms, and different types of features such as TF-IDF characters, count vectors, and manually-crafted features. Le et al. 29 provided a URLNet method to detect phishing webpage from URL. They extract character-level and word-level features from URL strings and employ CNN networks for training and testing. Chatterjee and Namin 30 introduced a phishing detection technique based on deep reinforcement learning to identify phishing URLs. They used their model on a balanced, labeled dataset of benign and phishing URLs, extracting 14 hand-crafted features from the given URLs to train the proposed model. In recent studies, Xiao et al. 31 proposed phishing website detection approach named CNN–MHSA. CNN network is applied to extract characters features from URLs. In the meanwhile, multi-head self-attention (MHSA) mechanism is employed to calculate the corresponding weights for the CNN learned features. Zheng et al. 32 proposed a new Highway Deep Pyramid Neural Network (HDP-CNN) which is a deep convolutional network that integrates both character-level and word-level embedding representation to identify whether a given URL is phishing or legitimate. Albeit the above approaches have shown valuable performances, they might misclassify phishing websites hosted on compromised servers since the features are extracted only from the URL of the website.
The features extracted in some previous studies are based on manual work and require additional effort since these features need to be reset according to the dataset, which may lead to overfitting of anti-phishing solutions. We got the motivation from the above-mentioned studies and proposed our approach. In which, the current work extract character sequences feature from URL without manual intervention. Moreover, our approach employs noisy data of HTML, plaintext, and hyperlinks information of the website with the benefit of identifying new phishing websites. Table 1 presents the detailed comparison of existing machine learning based phishing detection approaches.
Proposed approach
Our approach extracts and analyzes different features of suspected webpages for effective identification of large-scale phishing offenses. The main contribution of this paper is the combined uses of these feature set. For improving the detection accuracy of phishing webpages, we have proposed eight new features. Our proposed features determine the relationship between the URL of the webpage and the webpage content.
System architecture
The overall architecture of the proposed approach is divided into three phases. In the first phase, all the essential features are extracted and HTML source code will be crawled. The second phase applies feature vectorization to generate a particular feature vector for each webpage. The third phase identifies if the given webpage is phishing. Figure 1 shows the system structure of the proposed approach. Details of each phase are described as follows.
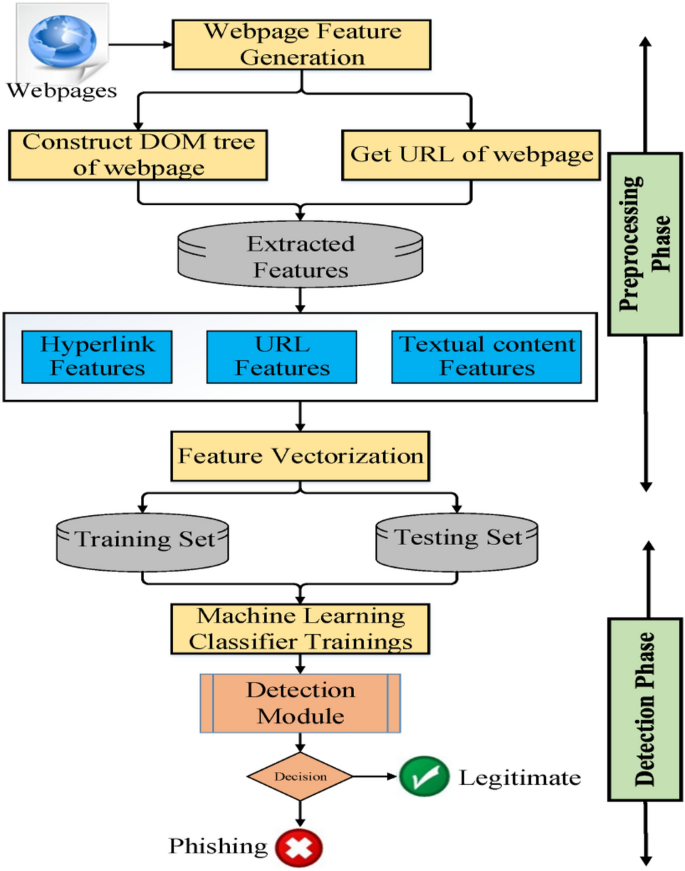
General architecture of the proposed approach.
Feature generation
The features are generated in this component. Our features are based on the URL and HTML source code of the webpage. A Document Object Model (DOM) tree of the webpage is used to extract the hyperlink and textual content features using a web crawler automatically. The features of our approach are categorized into four groups as depicted in Table 2 . In particular, features F1–F7, and F14 are new and proposed by us; Features F8–F13, and F15 are taken from other approaches 9 , 11 , 12 , 24 , 33 but we adjusted them for better results. Moreover, the observational method and strategy regarding the interpretation of these features are applied differently in our approach. A detailed explanation of the proposed features is provided in the feature extraction section of this paper.
Feature vectorization
After the features are extracted, we apply feature vectorization to generate a particular feature vector for each webpage to create a labeled dataset. We integrate URL character sequences features with textual content TF-IDF features and hyperlink information features to create feature vector required for training the proposed approach. The hyperlink features combination outputs 13-dimensional feature vector as \(F_{H} = \left\langle {f_{3} ,f_{4} ,f_{5} , \ldots ,f_{{15}} } \right\rangle\) , and the URL character sequence features combination outputs 200-dimensional feature vector as \(F_{U} = \left\langle {c_{1} ,c_{2} ,c_{3} , \ldots ,c_{{200}} } \right\rangle\) , we set a fixed URL length to 200. If the URL length is greater than 200, the additional part will be ignored. Otherwise, we put a 0 in the remainder of the URL string. The setting of this value depends on the distribution of URL lengths within our dataset. We have noticed that most of the URL lengths are less than 200 which means that when a vector is long, it may contain useless information, in contrast when the feature vector is too short, it may contain insufficient features. TF-IDF character level combination outputs \(D\) -dimensional feature vector as \(F_{T} = \left\langle {t_{1} ,t_{2} ,t_{3} , \ldots ,t_{D} } \right\rangle\) where \(D\) is the size of dictionary computed from the textual content corpus. It is observed from the experimental analysis that the size of dictionary \(D\) = 20,332 and the size increases with an increase in number of corpus. The above three feature vectors are combined to generate final feature vector \(F_{V} = F_{T} \cup F_{U} \cup F_{H} = \left\langle {t_{1} ,t_{2} , \ldots ,t_{D} ,c_{1} ,c_{2} \ldots ,c_{{200}} ,f_{3} ,f_{4} ,f_{5} , \ldots ,f_{{15}} } \right\rangle\) that is fed as input to machine learning algorithms to classify the website.
Detection module
The Detection phase includes building a strong classifier by using the boosting method, XGBoost classifier. Boosting integrates many weak and relatively accurate classifiers to build a strong and therefore robust classifier for detecting phishing offences. Boosting also helps to combine diverse features resulting in improved classification performance 34 . Here, XGBoost classifier is employed on integrated feature sets of URL character sequence \({F}_{U}\) , various hyperlinks information \({F}_{H}\) , login form features \({F}_{L}\) , and textual content-based features \({F}_{T}\) to build a strong classifier for phishing detection. In the training phase, XGBoost classifier is trained using the feature vector \(({F}_{U}\cup {F}_{H} \cup {F}_{L} \cup {F}_{T})\) collected from each record in the training dataset. At the testing phase, the classifier detects whether a particular website is a malicious website or not. The detailed description is shown in Fig. 2 .
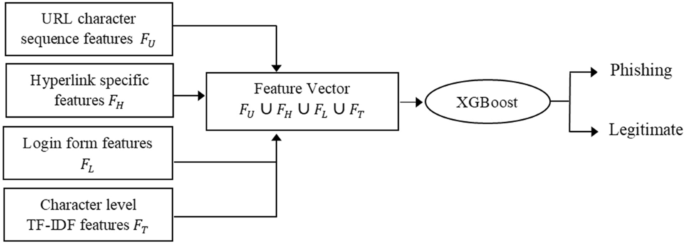
Phishing detection algorithm.
Features extraction
Due to the limited search engine and third-party methods discussed in the literature, we extract the particular features from the client side in our approach. We have introduced eleven hyperlink features (F3–F13), two login form features (F14 and F15), character level TF-IDF features (F2), and URL character sequence features (F1). All these features are discussed in the following subsections.
URL character sequence features (F1)
The URL stands for Uniform Resource Locator. It is used for providing the location of the resources on the web such as images, files, hypertext, video, etc. URL. Each URL starts with a protocol (http, https, and ftp) used to access the resource requested. In this part, we extract character sequence features from URL. We employ the method used in 35 to process the URL at the character level. More information is contained at the character level. Phishers also imitate the URLs of legitimate websites by changing many unnoticeable characters, e.g., “ www.icbc.com ” as “ www.1cbc.com ”. Character level URL processing is a solution to the out of vocabulary problem. Character level sequences identify substantial information from specific groups of characters that appear together which could be a symptom of phishing. In general, a URL is a string of characters or words where some words have little semantic meanings. Character sequences help find this sensitive information and improve the efficiency of phishing URL detection. During the learning task, machine learning techniques can be applied directly using the extracted character sequence features without the expert intervention. The main processes of character sequences generating include: preparing the character vocabulary, creating a tokenizer object using Keras preprocessing package ( https://Keras.io ) to process URLs in char level and add a “UNK” token to the vocabulary after the max value of chars dictionary, transforming text of URLs to sequence of tokens, and padding the sequence of URLs to ensure equal length vectors. The description of URL features extraction is shown in Algorithm 1.
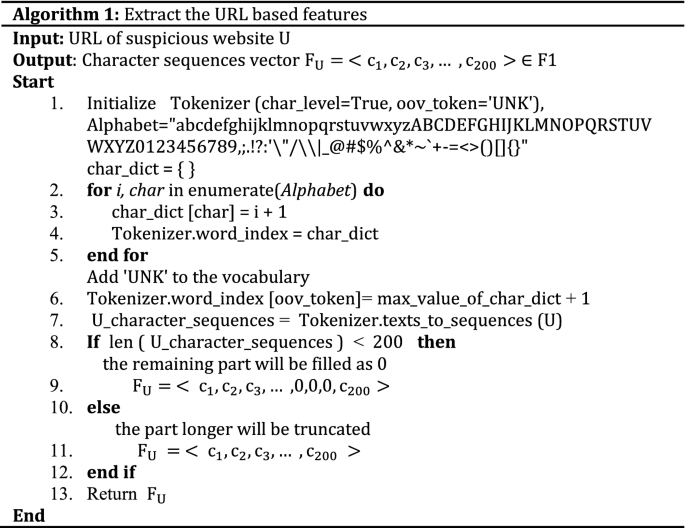
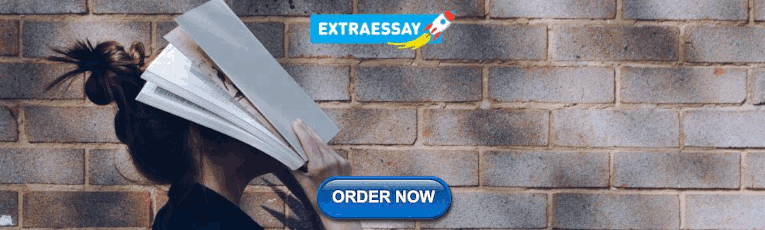
HTML features
The webpage source code is the programming behind any webpage, or software. In case of websites, this code can be viewed by anyone using various tools, even in the web browser itself. In this section, we extract the textual and hyperlink features existing in the HTML source code of the webpage.
Textual content-based features (F2)
TF-IDF stands for Term Frequency-Inverse Document Frequency. TF-IDF weight is a statistical measure that tells us the importance of a term in a corpus of documents 36 . TF-IDF vectors can be created at various levels of input tokens (words, characters, n-grams) 37 . It is observed that TF-IDF technique has been implemented in many approaches to catch phish of webpages by inspecting URLs 13 , obtain the indirect associated links 38 , target website 11 , and validity of suspected website 39 . In spite of TF-IDF technique extracts outstanding keywords from the text content of the webpage, it has some limitations. One of the limitations is that TF-IDF technique fails when the extracted keywords are meaningless, misspelled, skipped or replaced with images. Since plaintext and noisy data (i.e., attribute values for div, h1, h2, body and form tags) are extracted in our approach from the given webpage using BeautifulSoup parser, TF-IDF character level technique is applied with max features as 25,000. To obtain valid textual information, extra portions (i.e., JavaScript code, CSS code, punctuation symbols, and numbers) of the webpage are removed through regular expressions, including Natural Language Processing packages ( http://www.nltk.org/nltk_data/ ) such as sentence segmentation, word tokenization, text lemmatization and stemming as shown in Fig. 3 .

The process of generating text features.
Phishers usually mimic the textual content of the target website to trick the user. Moreover, phishers may mistake or override some texts (i.e., title, copyright, metadata, etc.) and tags in phishing webpages to bypass revealing the actual identification of the webpage. However, tag attributes stay the same to preserve the visual similarity between phishing and targeted site using the same style and theme as that of the benign webpage. Therefore, it is needful to extract the text features (plaintext and noisy part of HTML) of the webpage. The basic of this step is to extract the vectored representation of the text and the effective webpage content. A TF-IDF object is employed to vectorize text of the webpage. The detailed process of the text vector generation algorithm as follows.
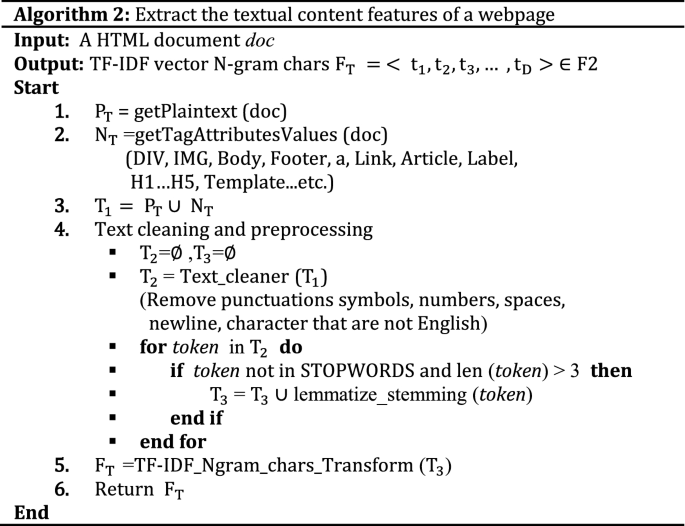
Script, CSS, img, and anchor files (F3, F4, F5, and F6)
External JavaScript or external Cascading Style Sheets (CSS) files are separate files that can be accessed by creating a link within the head section of a webpage. JavaScript, CSS, images, etc. files may contain malicious code while loading a webpage or clicking on a specific link. Moreover, phishing websites have fragile and unprofessional content as the number of hyperlinks referring to a different domain name increases. We can use <img> and <script> tags that have the "src" attribute to extract images and external JavaScript files in the website. Similarly, CSS and anchor files are within "href" attribute in <link> and <a> tags. In Eqs. ( 1 – 4 ), basically we calculated the rate of img and script tags that have the “src” attribute, link and anchor tags that have “href” attribute to the total hyperlinks available in a webpage, these tags usually link to image, Javascript, anchor, and CSS files required for a website
where \({\text{F}}_{\text{Script}\_\text{files}}\) , \({\text{F}}_{\text{CSS}\_\text{files}}\) , \({\text{F}}_{\text{Img}\_\text{files}}\) , \({\text{F}}_{\text{a}\_\text{files}}\) are the numbers of Javascript, CSS, image, anchor files existing in a webpage, and \({\text{F}}_{\text{Total}}\) is the total hyperlinks available in a webpage.
Empty hyperlinks (F7 and F8)
In the empty hyperlink, the “href” or “src” attributes of anchor, link, script, or img tags do not contain any URL. The empty link returns on the same webpage again when the user clicks on it. A benign website contains many webpages; thus, the scammer does not place any values in hyperlinks to make a phishing website behave like the benign website, and the hyperlinks look active on the phishing website. For example, <a href = “#”>, <a href = “#content”> and <a href = “javascript:void(0);”> HTML coding are used to design null hyperlinks 24 . To establish the empty hyperlink features, we define the rate of empty hyperlinks to the total number of hyperlinks available in a webpage, and the rate of anchor tag without “href” attribute to the total number of hyperlinks in a webpage. Following formulas are used to compute empty hyperlink features
where \({\text{F}}_{\text{a}\_\text{null}}\) and \({\text{F}}_{\text{null}}\) are the numbers of anchor tags without href attribute, and null hyperlinks in a webpage.
Total hyperlinks feature (F9)
Phishing websites usually contain minimal pages as compared to benign websites. Furthermore, sometimes the phishing webpage does not contain any hyperlink because the phishers usually only create a login page. Equation ( 7 ) computes the number of hyperlinks in a webpage by extracting the hyperlinks from an anchor, link, script, and img tags in the HTML source code.
Internal and external hyperlinks (F10, F11, and F12)
The base domain name in the external hyperlink is different from the website domain name, unlike the internal hyperlink; the base domain name is the same as the website domain name. The phishing websites may contain many external hyperlinks that indicate to the target websites due to the cybercriminals commonly copy the HTML code from the targeted authorized websites to create their phishing websites. Most of hyperlinks in a benign website contain the similar base domain name, whereas many hyperlinks in a phishing site may include the corresponding benign website domain. In our approach, the internal and external hyperlinks are extracted from the “src” attribute of img, script, frame tags, “action” attribute of form tag, and “href” attribute of the anchor and link tags. We compute the rate of internal hyperlinks to the total links available in a webpage (Eq. 8 ) to establish the internal hyperlink feature, and the rate of external hyperlinks to the total links (Eq. 9 ) to set the external hyperlink feature. Moreover, to set the external/internal hyperlink feature, we compute the rate of external hyperlinks to the internal hyperlinks (Eq. 10 ). A specified number has been used as a way of detecting the suspected websites in some previous studies 5 , 9 , 24 that these features used for classification. For example, if the rate of external hyperlinks to the total links is greater than 0.5, it will indicate that the website is phishing. However, determining a specific number as a parametric detection may cause errors in classification.
where \({\text{F}}_{\text{Internal}}\) , \({\text{F}}_{\text{External}}\) , and \({\text{F}}_{\text{Total}}\) are the number of external, internal, and total hyperlinks in a website.
Error in hyperlinks (F13)
Phishers sometimes add some hyperlinks in the fake website which are dead or broken links. In the hyperlink error feature, we check whether the hyperlink is a valid URL in the website. We do not consider the 403 and 404 error response code of hyperlinks due to the time consumed of the internet access to get the response code of each link. Hyperlink error is defined by dividing the total number of invalid links to the total links as represented in Eq. ( 11 )
where \({\text{F}}_{\text{Error}}\) is the total invalid hyperlinks.
Login form features (F14 and F15)
In the fraudulent website, the common trick to acquire the user's personal information is to include a login form. In the benign webpage, the action attribute of login form commonly includes a hyperlink that has the similar base domain as appear in in the browser address bar 24 . However, in the phishing websites, the form action attribute includes a URL that has a different base domain (external link), empty link, or not valid URL (Eq. 13 ). The suspicious form feature (Eq. 14 ) is defined by dividing the total number of suspicious forms S to the total forms available in a webpage (Eq. 12 )
where \({\text{F}}_{\text{S}}\) and \({\text{L}}_{\text{Total}}\) are the number of suspicious forms and total forms present in a webpage.
Figure 4 shows a comparison between benign and fishing hyperlink features based on the average occurrence rate per feature within each website in our dataset. From the figure, we noticed that the ratios of the external hyperlinks to the internal hyperlinks, and null hyperlinks in the phishing websites are higher than that in benign websites. Whereas, benign sites contain more anchor files, internal hyperlinks, and total hyperlinks.
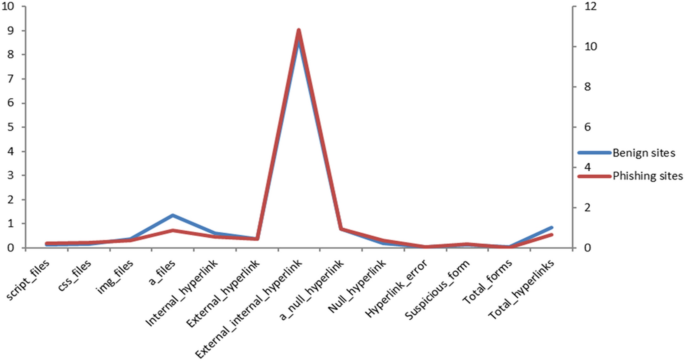
Distribution of hyperlink-based features in our data.
Classification algorithms
To measure the effectiveness of the proposed features, we have used various machine learning classifiers such as eXtreme Gradient Boosting (XGBoost), Random Forest, Logistic Regression, Naïve Bayes, and Ensemble of Random Forest and Adaboost classifiers to train our proposed approach. The major aim of comparing different classifiers is to expose the best classifier fit for our feature set. To apply different machine learning classifiers, Scikit-learn.org package is used, and Python is employed for feature extraction. From the empirical results, we noticed that XGBoost outperformed other classifiers. XGBoost algorithm is a type of ensemble classifiers, that transform weak learners to robust ones and convenient for our proposed feature set, thus it has high performance.
XGBoost (extreme gradient boosting) is a scalable machine learning system for tree boosting proposed by Chen and Guestrin 40 . Suppose there are \(N\) websites in the dataset \(\left\{ {\left( {x_{i} ,y_{i} } \right)|i = 1,2,...,N} \right\}\) , where \(x_{i} \in R^{d}\) is the extracted features associated with the \(i - th\) website, \(y_{i} \in \left\{ {0,\left. 1 \right\}} \right.\) is the class label, such that \(y_{i} = 1\) if and only if the website is a labelled phishing website. The final output \(f_{K} \left( x \right)\) of model is as follows 41 , 46 :
where l is the training loss function and \(\Omega \left( {G_{k}} \right) = \gamma T + \frac{1}{2}\lambda \sum\limits_{t = 1}^{T} {\omega_{t}^{2} }\) is the regulation term, since XGBoost introduces additive training and all previous k-1 base learners are fixed, here we assumed that we are in step k that optimizes our function \(f_{k} \left( x \right)\) , T is the number of leaves nodes in the base learner G k , γ is the complexity of each leaf, λ is a parameter to scale the penalty, and ω t is the output value at each final leaf node. If we apply the Taylor expansion to expand the Loss function at f k-1 ( x ) we will have 41 :
where \(g_{i} = \frac{{\partial l\left( {y_{i} ,f_{k - 1} \left( {x_{i} } \right)} \right)}}{{\partial f_{k - 1} \left( x \right)}},h_{i} = \frac{{\partial l\left( {y_{i} ,f_{k - 1} \left( {x_{i} } \right)} \right)}}{{\partial f_{k - 1}^{2} \left( x \right)}}\) are respectively first and second derivative of the Loss function.
XGBoost classifier is a type of ensemble classifiers, that transform weak learners to robust ones and convenient for our proposed feature set for the prediction of phishing websites, thus it has high performance. Moreover, XGBoost provides a number of advantages, some of which include: (i) The strength to handle missing values existing within the training set, (ii) handling huge datasets that do not fit into memory and (iii) For faster computing, XGBoost can make use of multiple cores on the CPU. The websites are classified into two possible categories: phishing and benign using a binary classifier. When a user requests a new site, the trained XGBoost classifier determines the validity of a particular webpage from the created feature vector.
Experiments and result analysis
In this section we describe the training and testing dataset, performance metrics, implementation details, and outcomes of our approach. The proposed features described in “ Features extraction ” section are used to build a binary classifier, which classify phishing and benign websites accurately.
We collected the dataset from two sources for our experimental implementation. The benign webpages are collected in February 2020 from Stuff Gate 42 , whereas the phishing webpages are collected from PhishTank 43 , which have been validated from August 2016 to April 2020. Our dataset consists of 60,252 webpages and their HTML source codes, wherein 27,280 ones are phishing and 32,972 ones are benign. Table 3 provides the distribution of the benign and phishing instances. We have divided the dataset into two groups where D1 is our dataset, and D2 is dataset used in existing literature 6 . The database management system (i.e., pgAdmin) has been employed with python to import and pre-process the data. The data sets were randomly split in 80:20 ratios for training and testing, respectively.
Performance metrics
To measure the performance of proposed anti-phishing approach, we used different statistical metrics such true-positive rate (TPR), true-negative rate (TNR), false-positive rate (FPR), false-negative rate (FNR), sensitivity or recall, accuracy (Acc), precision (Pre), F-Score, AUC, and they are presented in Table 4 . \({N}_{B}\) and \({N}_{P}\) indicate the total number of benign and phishing websites, respectively. \({N}_{B\to B}\) are the benign websites are correctly marked as benign, \({N}_{B\to P}\) are the benign websites are incorrectly marked as phishing, \({N}_{P\to P}\) are the phishing websites are correctly marked as phishing, and \({N}_{P\to B}\) are the phishing websites are incorrectly marked as benign. The receiver operating characteristic (ROC) arch and AUC are commonly used to evaluate the measures of a binary classifier. The horizontal coordinate of the ROC arch is FPR, which indicates the probability that the benign website is misclassified as a phishing; the ordinate is TPR, which indicates the probability that the phishing website is identified as a phishing.
Evaluation of features
In this section, we evaluated the performance of our proposed features (URL and HTML). We have implemented different Machine Learning (ML) classifiers for feature evaluation used in our approach. In Table 5 , we extracted various text features such as TF-IDF word level, TF-IDF N-gram level (the length of n-gram between 2 and 3), TF-IDF character level, count vectors (bag-of-words), word sequences vectors, global to vector (GloVe) pre-trained word embedding, trained word embedding, character sequences vectors and implemented various classifiers such as XGBoost, Random forest, logistic regression, Naïve Bayes, Deep Neural Networks (DNN), Convolutional Neural Networks (CNN), and Long Short-Term Memory (LSTM) network. The main intention of this experiment was to reveal the best textual content features convenient for our data. From the experimental results, it is noticed that TF-IDF character level features outperformed other features with significant accuracy, precision, F-Score, Recall, and AUC using XGBoost and DNN classifiers. Hence, we implemented TF-IDF character level technique to generate text features (F2) of the webpage. Figure 5 presents the performance of textual content-based features. As shown in the figure, text features can correctly filter a high amount of phishing websites and achieved an accuracy of 88.82%.
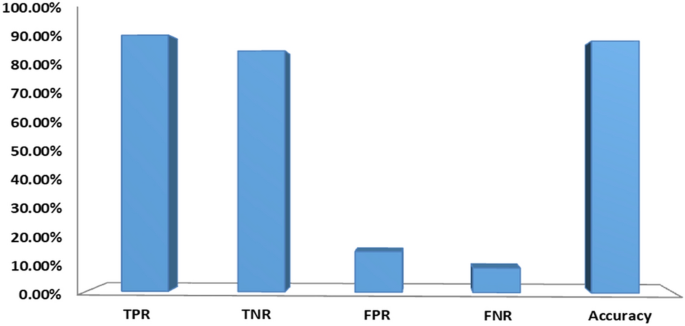
Performance of textual content features.
Table 6 shows the experiment results with hyperlinks features. From the empirical results, it is noticed that Random Forest classifier superior to the other classifiers with an accuracy of 82.27%, precision of 77.59%, F_Measure of 81.63%, recall of 86.10%, and AUC of 82.57%. It is also noticed that ensemble and XGBoost classifiers attained good accuracy of 82.18% and 80.49%, respectively. Figure 6 presents the classification results of hyperlink based features (F3–F15). As shown in the figure, hyperlink based features can accurately clarify 79.04% of benign websites and 86.10% of phishing websites.
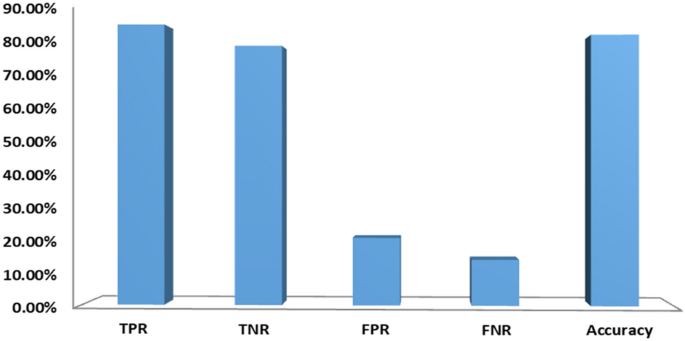
Performance of hyperlink based features.
In Table 7 , we integrated features of URL and HTML (hyperlink and text) using various classifiers to verify complementary behavior in phishing websites detection. From the empirical results, it is noticed that LR classifier has sufficient accuracy, precision, F-Score, AUC, and recall in terms of the HTML features. In contrast, NB classifier has good accuracy, precision, F-Score, AUC, and recall with respect to combining all the features. RF and ensemble classifiers achieved high accuracy, recall, F-Score, and AUC with respect to URL based features. XGBoost classifier outperformed the others with an accuracy of 96.76%, F-Score of 96.38%, AUC of 96.58% and recall of 94.56% with respect to combining all the features. It is observed that URL and HTML features are valuable in phishing detection. However, one type of feature is not suitable to identify all kinds of phishing webpages and does not result in high accuracy. Thus, we have combined all features to get more comprehensive features. The results on various classifiers of combined feature set are also shown in Fig. 7 . In Fig. 8 we compare the three feature sets in terms of accuracy, TNR, FPR, FNR, and TPR.
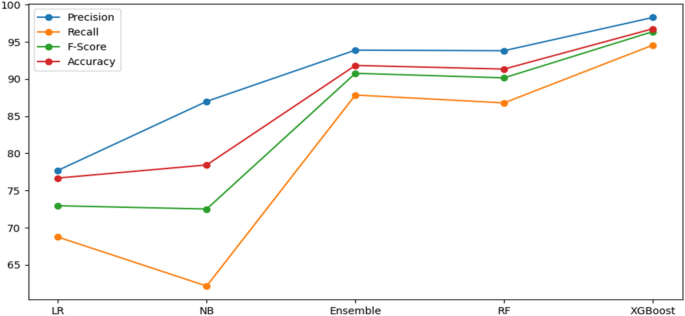
Test results of various classifiers with respect to combined features.
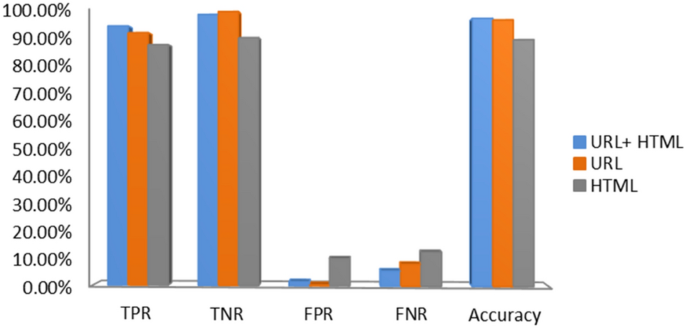
Performance of different feature combinations using XGBoost on dataset D1.
The confusion matrix is used to measure results where each row of the matrix represents the instances in a predicted class, while each column represents the instances in an actual class (or vice versa). The confusion matrix of the proposed approach is created as represented in Table 8 . From the results, combining all kind of features together as an entity correctly identified 5212 out of 5512 phishing webpages and 6448 out of 6539 benign webpages and attained an accuracy of 96.76%. Our approach results in low false positive rate (i.e., less than 1.39% of benign webpages incorrectly classified as phishing), and high true positive rate (i.e., more than 94.56% of phishing webpages accurately classified). We have also tested our feature sets (URL and HTML) on the existing dataset D2. Since dataset D2 only contains legitimate and malicious URLs, we needed to extract the HTML source code features for these URLs. The results are given in Table 9 and Fig. 9 . From the results, it is noticed that combining all kinds of features had outperformed other feature sets with a significant accuracy of 98.48%, TPR of 99.04%, and FPR of 2.09%.
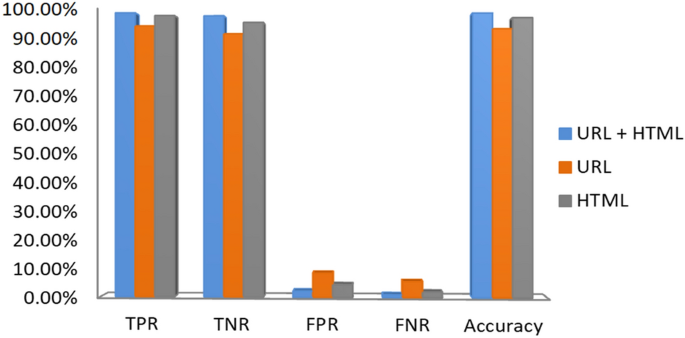
Performance of the proposed approach on dataset D2.
Comparison with existing approaches
In this experiment, we compare our approach with existing anti-phishing approaches. Notice that we have applied Le et al. 29 and Aljofey et al. 3 works on dataset D1 to evaluate the efficiency of the proposed approach. While for comparison of the proposed approach with Sahingoz et al. 6 , Rao et al. 13 , Chatterjee and Namin 30 works, we evaluated our approach on benchmark dataset D2 6 , 13 , 30 based on the four-statistics metrics used in the papers. The comparison results are shown in Table 10 . From the results, it is observed that our approach gives better performance than other approaches discussed in the literature, which shows the efficiency of detecting phishing websites over the existing approaches.
In Table 11 , we implemented Le et al. 29 and Aljofey et al. 3 methods to our dataset D1 and our approach outperformed the others with an accuracy of 96.76%, precision of 98.28%, and F-Score of 96.38%. It should also be mentioned that Aljofey et al. method achieved 97.86% recall, which is 3.3% greater than our method, whereas our approach gives TNR that is higher by 4.97%, and FPR that is lesser by 4.96%. Our approach accurately identifies the legitimate websites with a high TNR and low FPR. Some phishing detection methods achieve high recall, however inaccurate classification of the legitimate websites is more serious compared to inaccurate classification of the phishing sites.
Discussion and limitations
The phishing website seems similar to its benign official website, and the defiance is how to distinguish between them. This paper proposed a novel anti-phishing approach, which involves different features (URL, hyperlink, and text) that have never been taken into consideration. The proposed approach is a completely client-side solution. We applied these features on various machine learning algorithms and found that XGBoost attained the best performance. Our major aim is to design a real-time approach, which has a high true-negative rate and low false-positive rate. The results show that our approach correctly filtered the benign webpages with a low amount of benign webpages incorrectly classified as phishing. In the process of phishing webpage classification, we construct the dataset by extracting the relevant and useful features from benign and phishing webpages.
A desktop machine having a core™ i7 processor with 3.4 GHz clock speed and 16 GB RAM is used to executed the proposed anti-phishing approach. Since Python provides excellent support of its libraries and has sensible compile-time, the proposed approach is implemented using Python programming language. BeautifulSoup library is employed to parse the HTML of the specified URL. The detection time is the time between entering URL to generating outputs. When the URL is entered as a parameter, the approach attempts to fetch all specific features from the URL and HTML code of the webpage as debated in feature extraction section. This is followed by current URL classification in form of benign or phishing based on the value of the extracted feature. The total execution time of our approach in phishing webpage detection is around 2–3 s, which is quite low and acceptable in a real-time environment. Response time depends on different factors, such as input size, internet speed, and server configuration. Using our data D1, we also attempted to compute the time taken for training, testing and detecting of proposed approach (all feature combinations) for the webpage classification. The results are given in Table 12 .
In pursuit of a further understanding of the learning capabilities, we also present the classification error as well as log loss regarding the number of iterations implemented by XGBoost. Log loss, short for logarithmic loss is a loss function for classification that indicates the price paid for the inaccuracy of predictions in classification problems. Figure 10 show the logarithmic loss and the classification error of the XGBoost approach for each epoch on the training and test dataset D1. From reviewing the figure, we might note that the learning algorithm is converging after approximately 100 iterations.
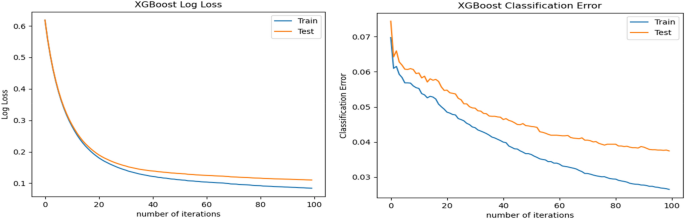
XGBoost learning curve of logarithmic loss and classification error on dataset D1.
Limitations
Although our proposed approach has attained outstanding accuracy, it has some limitations. First limitation is that the textual features of our phishing detection approach depend on the English language. This may cause an error in generating efficient classification results when the suspicious webpage includes language other than English. About half (60.5%) of the websites use English as a text language 44 . However, our approach employs URL, noisy part of HTML, and hyperlink based features, which are language-independent features. The second limitation is that despite the proposed approach uses URL based features, our approach may fail to identify the phishing websites in case when the phishers use the embedded objects (i.e., Javascript, images, Flash, etc.) to obscure the textual content and HTML coding from the anti-phishing solutions. Many attackers use single server-side scripting to hide the HTML source code. Based on our experiments, we noticed that legitimate pages usually contain rich textual content features, and high amount of hyperlinks (At least one hyperlink in the HTML source code). At present, some phishing webpages include malware, for example, a Trojan horse that installs on user’s system when the user opens the website. Hence, the next limitation of this approach is that it is not sufficiently capable of detecting attached malware because our approach does not read and process content from the web page's external files, whether they are cross-domain or not. Finally, our approach's training time is relatively long due to the high dimensional vector generated by textual content features. However, the trained approach is much better than the existing baseline methods in terms of accuracy.
Conclusion and future work
Phishing website attacks are a massive challenge for researchers, and they continue to show a rising trend in recent years. Blacklist/whitelist techniques are the traditional way to alleviate such threats. However, these methods fail to detect non-blacklisted phishing websites (i.e., 0-day attacks). As an improvement, machine learning techniques are being used to increase detection efficiency and reduce the misclassification ratio. However, some of them extract features from third-party services, search engines, website traffic, etc., which are complicated and difficult to access. In this paper, we propose a machine learning-based approach which can speedily and precisely detect phishing websites using URL and HTML features of the given webpage. The proposed approach is a completely client-side solution, and does not rely on any third-party services. It uses URL character sequence features without expert intervention, and hyperlink specific features that determine the relationship between the content and the URL of a webpage. Moreover, our approach extracts TF-IDF character level features from the plaintext and noisy part of the given webpage's HTML.
A new dataset is constructed to measure the performance of the phishing detection approach, and various classification algorithms are employed. Furthermore, the performance of each category of the proposed feature set is also evaluated. According to the empirical and comparison results from the implemented classification algorithms, the XGBoost classifier with integration of all kinds of features provides the best performance. It acquired 1.39% false-positive rate and 96.76% of overall detection accuracy on our dataset. An accuracy of 98.48% with a 2.09% false-positive rate on a benchmark dataset.
In future work, we plane to include some new features to detect the phishing websites that contain malware. As we said in “ Limitations ” section, our approach could not detect the attached malware with phishing webpage. Nowadays, blockchain technology is more popular and seems to be a perfect target for phishing attacks like phishing scams on the blockchain. Blockchain is an open and distributed ledger that can effectively register transactions between receiving and sending parties, demonstrably and constantly, making it common among investors 45 . Thus, detecting phishing scams in the blockchain environment is a defiance for more research and evolution. Moreover, detecting phishing attacks in mobile devices is another important topic in this area due to the popularity of smart phones 47 , which has made them a common target of phishing offenses.
Data availability
The dataset generated during the current study are available in the Google Drive repository: https://drive.google.com/file/d/18ZZHsCeMmF9HKTaL_yd41oJ_3Fgk0gWE/view?usp=sharing .
RSA. Rsa fraud report. https://go.rsa.com/l/797543/2020-07-08/3njln/797543/48525/RSA_Fraud_Report_Q1_2020.pdf (2020) (Accessed 14 January 2021).
APWG. Phishing Attack Trends Reports, 24, November 2020. https://docs.apwg.org/reports/apwg_trends_report_q3_2020.pdf (2020) (Accessed 14 January 2021).
Aljofey, A., Jiang, Q., Qu, Q., Huang, M. & Niyigena, J.-P. An effective phishing detection model based on character level convolutional neural network from URL. Electronics 9 , 1514 (2020).
Article Google Scholar
Dhamija, R., Tygar, J.D., & Hearst, M. Why phishing works. in Proceedings of the SIGCHI Conference on Human Factors in Computing Systems, Montreal, QC, Canada, 22–27 April 2006 , 581–590 (2006).
Jain, A. K. & Gupta, B. B. A novel approach to protect against phishing attacks at client side using auto-updated white-list. EURASIP J. on Info. Security. 9 , 1–11. https://doi.org/10.1186/s13635-016-0034-3 (2016).
Sahingoz, O. K., Buber, E., Demir, O. & Diri, B. Machine learning based phishing detection from URLs. Expert Syst. Appl. 2019 (117), 345–357 (2019).
Haruta, S. , Asahina, H., & Sasase, I. Visual Similarity-based Phishing Detection Scheme using Image and CSS with Target Website Finder. 978-1-5090-5019-2/17/$31.00 ©2017 IEEE (2017).
Cook, D. L., Gurbani, V. K., & Daniluk, M. Phishwish: A stateless phishing filter using minimal rules. in Financial Cryptography and Data Security , (ed. Gene Tsudik) 324, (Berlin, Heidelberg, Springer-Verlag, 2008).
Jain, A. K. & Gupta, B. B. A machine learning based approach for phishing detection using hyperlinks information. J. Ambient. Intell. Humaniz. Comput. https://doi.org/10.1007/s12652-018-0798-z (2018).
Li, Y., Yang, Z., Chen, X., Yuan, H. & Liu, W. A stacking model using URL and HTML features for phishing webpage detection. Futur. Gener. Comput. Syst. 94 , 27–39 (2019).
Article ADS Google Scholar
Xiang, G., Hong, J., Rose, C. P. & Cranor, L. CANTINA+: a feature rich machine learning framework for detecting phishing web sites. ACM Trans. Inf. Syst. Secur. 14 (2), 1–28. https://doi.org/10.1145/2019599.2019606 (2011).
Zhang, W., Jiang, Q., Chen, L. & Li, C. Two-stage ELM for phishing Web pages detection using hybrid features. World Wide Web 20 (4), 797–813 (2017).
Rao, R. S., Vaishnavi, T. & Pais, A. R. CatchPhish: Detection of phishing websites by inspecting URLs. J. Ambient. Intell. Humanized Comput. 11 , 813–825 (2019).
Arachchilage, N. A. G., Love, S. & Beznosov, K. Phishing threat avoidance behaviour: An empirical investigation. Comput. Hum. Behav. 60 , 185–197 (2016).
Wang, Y., Agrawal, R., & Choi, B.Y. Light weight anti-phishing with user whitelisting in a web browser. in Region 5 conference, 2008 IEEE, IEEE , 1–4 (2008).
Han, W., Cao, Y., Bertino, E. & Yong, J. Using automated individual white-list to protect web digital identities. Expert Syst. Appl. 39 (15), 11861–11869 (2012).
Prakash, P., Kumar, M., Kompella, R.R., Gupta, M. Phishnet: Predictive blacklisting to detect phishing attacks. in INFOCOM, 2010 Proceedings IEEE, IEEE , 1–5. https://doi.org/10.1109/INFCOM.2010.5462216 (2010)
Felegyhazi, M., Kreibich, C. & Paxson, V. On the potential of proactive domain blacklisting. LEET 10 , 6–6 (2010).
Google Scholar
Sheng, S., Wardman, B., Warner, G., Cranor, L.F., Hong, J., & Zhang, C. An empirical analysis of phishing blacklists. in Proceedings of the 6th Conference on Email and Anti-Spam (CEAS’09) (2010).
Qi, L. et al. Privacy-aware data fusion and prediction with spatial-temporal context for smart city industrial environment. IEEE Trans. Ind. Inform. 17 (6), 4159–4167. https://doi.org/10.1109/TII.2020.3012157 (2021).
Liu, Y. et al. A label noise filtering and label missing supplement framework based on game theory. Digital Commun. Netw. https://doi.org/10.1016/j.dcan.2021.12.008 (2022).
Muzammal, M., Qu, Q. & Nasrulin B. Renovating blockchain with distributed databases: An open source system. Future Gener. Comput. Syst. 90 , 105–117. https://doi.org/10.1016/j.future.2018.07.042 (2019).
Liu, Y. et al. Bidirectional GRU networks-based next POI category prediction for healthcare. Int. J. Intell. Syst. https://doi.org/10.1002/int.22710 (2021).
Jain, A. K. & Gupta, B. B. Towards detection of phishing websites on client-side using machine learning based approach. Telecommun. Syst. https://doi.org/10.1007/s11235-017-0414-0 (2017).
Rao, R. S. & Pais, A. R. Two level filtering mechanism to detect phishing sites using lightweight visual similarity approach. J. Ambient. Intell. Humaniz. Comput. https://doi.org/10.1007/s12652-019-01637-z (2019).
Jain, A. K. & Gupta, B. B. Two-level authentication approach to protect from phishing attacks in real time. J. Ambient. Intell. Human Comput. https://doi.org/10.1007/s12652-017-0616-z (2017).
Rao, R. S., Umarekar, A. & Pais, A. R. Application of word embedding and machine learning in detecting phishing websites. Telecommun. Syst. 79 , 33–45. https://doi.org/10.1007/s11235-021-00850-6 (2022).
Guo, B. et al. HinPhish: An effective phishing detection approach based on heterogeneous information networks. Appl. Sci. 11 (20), 9733. https://doi.org/10.3390/app11209733 (2021).
Le, H., Pham, Q., Sahoo, D., & Hoi, S.C.H. Urlnet: Learning a URL representation with deep learning for malicious URL detection. arXiv 2018, arXiv: 1802.03162 (2018).
Chatterjee, M., & Namin, A.S. Detecting phishing websites through deep reinforcement learning. in 2019 IEEE 43rd Annual Computer Software and Applications Conference (COMPSAC) . 978-1-7281-2607-4/19/$31.00 ©2019 IEEE. (IEE Computer Society, 2019). https://doi.org/10.1109/COMPSAC.2019.10211 .
Xiao, X., Zhang, D., Hu, G., Jiang, Y. & Xia, S. CNN-MHSA: A convolutional neural network and multi-head self- attention combined approach for detecting phishing websites. Neural Netw. 125 , 303–312. https://doi.org/10.1016/j.neunet.2020.02.013 (2020).
Article PubMed Google Scholar
Zheng, F., Yan Q., Victor C.M. Leung, F. Richard Yu, Ming Z. HDP-CNN: Highway deep pyramid convolution neural network combining word-level and character-level representations for phishing website detection, computers & security. https://doi.org/10.1016/j.cose.2021.102584 (2021)
Mohammad, R. M., Thabtah, F. & McCluskey, L. Predicting phishing websites based on self-structuring neural network. Neural Comput. Appl. 25 (2), 443–458 (2014).
Ramanathan, V. & Wechsler, H. Phishing detection and impersonated entity discovery using Conditional Random Field and Latent Dirichlet Allocation. Comput. Security. 34 , 123–139 (2013).
Zhang, X., Zhao, J., & LeCun, Y. Character-level convolutional networks for text classification. in Proceedings of the Advances in Neural Information Processing Systems 28 (NIPS 2015), Montreal, QC, Canada, 7–12 December 2015 (2015).
Stecanella, B. What is TF-IDF? https://monkeylearn.com/blog/what-is-tf-idf/ . (2019) (Accessed 20 December 2020).
Bansal, S.A. Comprehensive guide to understand and implement text classification in python. https://www.analyticsvidhya.com/blog/2018/04/a-comprehensive-guide-to-understand-andimplement-text-classification-in-python/ (2018) (Accessed 1 July 2020).
Ramesh, G., Krishnamurthi, I. & Kumar, K. S. S. An efficacious method for detecting phishing webpages through target domain identification. Decis. Support Syst. 2014 (61), 12–22 (2014).
Zhang, Y., Hong, J.I., & Cranor, L.F. Cantina: A content- based approach to detecting phishing websites. in Proceedings of the 16th International Conference on World Wide Web, Banff, AB, Canada, 8–12 May 2007 , 639–648 (2007).
Chen, T., & Guestrin, C.: Xgboost: A scalable tree boosting system. in Proceedings of the 22nd ACM Sigkdd International Conference on Knowledge Discovery and Data Mining. ACM , 785–794 (2016)
Aljofey, A., Jiang, Q. & Qu, Q. A supervised learning model for detecting Ponzi contracts in Ethereum Blockchain. In Big Data and Security. ICBDS 2021. Communications in Computer and Information Science Vol. 1563 (eds Tian, Y. et al. ) (Springer, 2022). https://doi.org/10.1007/978-981-19-0852-1_52 .
Chapter Google Scholar
http://stuffgate.com/stuff/website/ . (Accessed February 2020).
http://www.phishtank.com . (Accessed April 2020).
Usage of content languages for websites. https://w3techs.com/technologies/overview/content_language/all . (2021) (Accessed 19 January 2021).
Iansiti, M. & Lakhani, K. R. The truth about blockchain. Harvard Bus. Rev. 95 (1), 118–127 (2017).
https://github.com/YC-Coder-Chen/Tree-Math/blob/master/XGboost.md . (Accessed September 2021).
Qu, Q., Liu, S., Yang, B. & Jensen, C. S. Efficient top-k spatial locality search for co-located spatial web objects. 2014 IEEE 15th International Conference on Mobile Data Management. 1 , 269–278 (2014).
Download references
Acknowledgements
This research work is supported by the National Key Research and Development Program of China Grant nos. 2021YFF1200104 and 2021YFF1200100.
Author information
Authors and affiliations.
Shenzhen Key Laboratory for High Performance Data Mining, Shenzhen Institute of Advanced Technology, Chinese Academy of Sciences, Shenzhen, 518055, China
Ali Aljofey, Qingshan Jiang, Abdur Rasool, Hui Chen & Qiang Qu
Shenzhen College of Advanced Technology, University of Chinese Academy of Sciences, Beijing, 100049, China
Ali Aljofey, Abdur Rasool & Hui Chen
Department of Computer Science, Guangdong University of Technology, Guangzhou, China
Cloud Computing Center, Shenzhen Institute of Advanced Technology, Chinese Academy of Sciences, Shenzhen, 518055, China
You can also search for this author in PubMed Google Scholar
Contributions
Data curation, A.A. and Q.J.; Funding acquisition, Q.J. and Q.Q.; Investigation, Q.J. and Q.Q.; Methodology, A.A. and Q.J.; Project administration, Q.J.; Software, A.A.; Supervision, Q.J.; Validation, A.R. and H.C.; Writing—original draft, A.A.; Writing—review & editing, Q.J., W.L, Y.W, and Q.Q; All authors reviewed the manuscript.
Corresponding author
Correspondence to Qingshan Jiang .
Ethics declarations
Competing interests.
The authors declare no competing interests.
Additional information
Publisher's note.
Springer Nature remains neutral with regard to jurisdictional claims in published maps and institutional affiliations.
Rights and permissions
Open Access This article is licensed under a Creative Commons Attribution 4.0 International License, which permits use, sharing, adaptation, distribution and reproduction in any medium or format, as long as you give appropriate credit to the original author(s) and the source, provide a link to the Creative Commons licence, and indicate if changes were made. The images or other third party material in this article are included in the article's Creative Commons licence, unless indicated otherwise in a credit line to the material. If material is not included in the article's Creative Commons licence and your intended use is not permitted by statutory regulation or exceeds the permitted use, you will need to obtain permission directly from the copyright holder. To view a copy of this licence, visit http://creativecommons.org/licenses/by/4.0/ .
Reprints and permissions
About this article
Cite this article.
Aljofey, A., Jiang, Q., Rasool, A. et al. An effective detection approach for phishing websites using URL and HTML features. Sci Rep 12 , 8842 (2022). https://doi.org/10.1038/s41598-022-10841-5
Download citation
Received : 17 December 2021
Accepted : 06 April 2022
Published : 25 May 2022
DOI : https://doi.org/10.1038/s41598-022-10841-5
Share this article
Anyone you share the following link with will be able to read this content:
Sorry, a shareable link is not currently available for this article.
Provided by the Springer Nature SharedIt content-sharing initiative
This article is cited by
Spark-based multi-verse optimizer as wrapper features selection algorithm for phishing attack challenge.
- Jamil Al-Sawwa
- Mohammad Almseidin
- Remah Younisse
Cluster Computing (2024)
Detection of phishing URLs with deep learning based on GAN-CNN-LSTM network and swarm intelligence algorithms
- Abbas Jabr Saleh Albahadili
- Ayhan Akbas
- Javad Rahebi
Signal, Image and Video Processing (2024)
A CNN-Based SIA Screenshot Method to Visually Identify Phishing Websites
- Dong-Jie Liu
- Jong-Hyouk Lee
Journal of Network and Systems Management (2024)
- Adnan Noor Mian
- Sanaullah Manzoor
Scientific Reports (2023)
By submitting a comment you agree to abide by our Terms and Community Guidelines . If you find something abusive or that does not comply with our terms or guidelines please flag it as inappropriate.
Quick links
- Explore articles by subject
- Guide to authors
- Editorial policies
Sign up for the Nature Briefing: AI and Robotics newsletter — what matters in AI and robotics research, free to your inbox weekly.


An official website of the United States government
The .gov means it’s official. Federal government websites often end in .gov or .mil. Before sharing sensitive information, make sure you’re on a federal government site.
The site is secure. The https:// ensures that you are connecting to the official website and that any information you provide is encrypted and transmitted securely.
- Publications
- Account settings
Preview improvements coming to the PMC website in October 2024. Learn More or Try it out now .
- Advanced Search
- Journal List
- Elsevier - PMC COVID-19 Collection

The development of phishing during the COVID-19 pandemic: An analysis of over 1100 targeted domains
Raphael hoheisel.
a Department of High-tech Business and Entrepreneurship (IEBIS), University of Twente, The Netherlands
Guido van Capelleveen
b Faculty of Economics and Business, Section Business Analytics, University of Amsterdam, The Netherlands
Dipti K. Sarmah
c Faculty of Electrical Engineering, Mathematics and Computer Science (SCS), University of Twente, The Netherlands
Marianne Junger
Associated data.
The data that has been used is confidential.
To design preventive policy measures for email phishing, it is helpful to be aware of the phishing schemes and trends that are currently applied. How phishing schemes and patterns emerge and adapt is an ongoing field of study. Existing phishing works already reveal a rich set of phishing schemes, patterns, and trends that provide insight into the mechanisms used. However, there seems to be limited knowledge about how email phishing is affected in periods of social disturbance, such as COVID-19 in which phishing numbers have quadrupled. Therefore, we investigate how the COVID-19 pandemic influences the phishing emails sent during the first year of the pandemic. The email content (header data and html body, excl. attachments) is evaluated to assess how the pandemic influences the topics of phishing emails over time (peaks and trends), whether email campaigns correlate with momentous events and trends of the COVID-19 pandemic, and what hidden content revealed. This is studied through an in-depth analysis of the body of 500.000 phishing emails addressed to Dutch registered top-level domains collected during the start of the pandemic. The study reveals that most COVID-19 related phishing emails follow known patterns indicating that perpetrators are more likely to adapt than to reinvent their schemes.
1. Introduction
The crisis resulting from the COVID-19 pandemic has had profound implications worldwide, on, among others, global health and health systems ( Walker et al., 2020 ), the global social and economic situation, and almost every other aspect of daily life ( Atkeson, 2020 , Nicola, Alsafi, Sohrabi, Kerwan, Al-Jabir, Iosifidis, Agha, Agha, 2020 ). In particular, lockdown measures and social distancing have caused a great change in the routine activities of many people. For instance, in countries around the world, the pandemic had a dramatic impact on travel patterns, such as the number of trips, distances travelled, purpose of travel, and choice of travel mode ( Cats and Hoogendoorn, 2020 ). There was a decrease in the use of cars and public transport, as well as an increase in walking and cycling, which involved more recreational trips. Other changes in activity patterns occur more in online shopping. Dutch data showed a shift in movements in time and space, but not necessarily in the number of trips that people have been making. For example, the pedestrian data show more walks in parks on the weekends while far fewer people walk on the streets ( Cats and Hoogendoorn, 2020 ). Overall, the Dutch went out less often to buy groceries, shop, exercise, and visit people ( de Haas et al., 2020 ). A lot of research shows that opportunities for crime and people’s routine activities are relatively strongly related to crime. The amount of time individuals spent outdoors and the activities they are involved in are in strong correlation with their likelihood of becoming a victim of a broad variety of crime types, including property crime ( Kennedy, Forde, 1990 , van Kesteren et al., 2013 ), violence ( Sherman, Gartin, Buerger, 1989 , Tilley, Sidebottom, 2015 ), and fraud ( Holtfreter et al., 2008 ). As COVID-19 changed opportunities for crime, it is plausible that the lockdown affected crime rates. This also suggests that the societal changes because of COVID-19 would also impact trends in crime-related activities.
In the US ( Ashby, 2020 , Boman, Gallupe, 2020 , Bullinger, Carr, Packham, 2020 , Felson, Jiang, Xu, 2020 , Mohler et al., 2020 ) and in Canada ( Hodgkinson and Andresen, 2020 ), countries with a more non-committal approach to covid restrictions (e.g., lockdowns), declines in physical crime were indeed found during the pandemic, but overall results seemed to be relatively inconsistent. The studies show that there were usually no significant changes in the frequency of serious assaults in public or in the frequency of serious assaults in residences. In some US cities, there were reductions in residential burglary but little change in non-residential burglary ( Ashby, 2020 , Boman, Gallupe, 2020 , Bullinger, Carr, Packham, 2020 , Felson, Jiang, Xu, 2020 , Mohler et al., 2020 ). European studies seemed to find a stronger impact of the measures taken to fight the virus. In France, almost all crimes and the associated measures for about every type of crime showed a very strong decline during the lockdown. More specifically, fraud overall declined as well ( InterStats, 2020 ). Similarly, in the UK victim surveys found a decline in crime of 32% (excluding fraud and cybercrime) and a similar decline of 31% in police-recorded crime. Fraud and computer misuse also fell by 16% ( Office for National Statistics UK, August, 2020 ). These findings illustrate the extent to which offenders are responsive to the context and respond quickly and flexibly to changed circumstances. It seems plausible that the impact of crime is proportional to the extent to which stringent measures were taken by governments and followed up by citizens in each country. Stringency index numbers during the first lockdown (March 2020) provide an indication of this difference ( Mathieu et al., 2020 ). This might explain the difference between the USA and Canada and Europe and suggest the impact of the lockdown on routine activities and opportunities in Europe and in North America.
Fewer studies investigated the impact of the COVID-19 crisis on cybercrime. While there was a decrease in physical-related crime activities ( Europol, 2020 ), e.g., property crime, during the first COVID-19 outbreak in Europe, a noticeable shift and surge took place towards online fraudulent activities ( Buil-Gil et al., 2020 ). A significant increase in particular was observed in phishing, which has quadrupled during the outbreak ( APWG, 2020a ) and has increased eightfold since then ( APWG, 2022 ). In the Netherlands and other countries, phishing is considered a criminal activity and is actively prosecuted ( Rechtsraak, 2022 ). Fraudsters have often “benefited” from disasters ( Aguirre and Lane, 2019 ). To illustrate, attackers have made extensive use of the COVID-19 crisis to design phishing emails. Typical examples reported in the media are zoom phishing emails, fraudulent CEO emails, and phishing emails aimed at healthcare institutions ( APWG, 2020b ). This sudden rise of COVID-19 phishing fraud as a global problem may be explained by the COVID-19 outbreak. That is, because the social disturbance resulting from a disaster makes society typically more vulnerable to fraudulent activities, hence, more susceptible to phishing attacks ( Aguirre and Lane, 2019 ). We should be aware of the magnitude of impact these COVID-19 related fraudulent activities may cause. In particular, because this impact is often underestimated ( Lastdrager, 2018 ). Phishing, apart from its effectivity to gain direct financial gain ( Laan, 2021 ), is also the typical starting point for leads to successful cyber attacks and resulting data breaches, of course, associated with all sorts of financial losses ( CNBC , Lastdrager, 2018 ). All such organizational as well as societal costs ask for preventive measures to increase resilience against cyber-attacks, such as awareness campaigns, and the ability to timely scale customer support when novel phishing schemes or adaptions are noticed or expected.
Based on these observations, we must recognize the importance of analyzing new phishing behavior that appeared during the pandemic. Therefore, this study focuses on COVID-19 related phishing emails to understand better how attackers adapt to new societal conditions.
This analysis produced two types of contributions. On the one hand, this paper provides insights that lead to revealing patterns of fraudulent characteristics that were applied in the phishing schemes, which may be used in support of societal awareness campaigns of phishing that reduce the societal costs of cybercrime. On the other hand, this paper shows that phishing scheme adoption is commonly observed, and seems preferable to novel scheme development. This paper further reflects on this adaption choice by attackers from multiple theory perspectives to explain this behavior.
The rest of this paper is organized as follows. Section 2 reviews the related work. Section 3 explains the research method. Section 4 presents the results of the COVID-19 phishing email analysis. Finally, Section 5 discusses all results followed by conclusions in Section 6 .
2. Related work
The increase in cyber attacks during the pandemic has triggered several studies researching how cybercriminals develop new attacking strategies and fraudulent operations. These new studies roughly focused on two types of cybercrime, i.e., cyber-dependent crime ( Furnell et al., 2015 ) and cyber-enabled crime ( Lallie et al., 2021 ); where cyber-dependent crime covers hacking, malware, and denial of service, cyber-enabled crimes covers financial fraud, phishing, pharming, and extortion. This related work section provides a review of the literature related to cybercrime during the COVID-19 pandemic, with a focus on phishing.
A number of studies are conducted that aim to prevent cybercrime during the COVID-19 pandemic. In one case study by Groenendaal and Helsloot (2021) , cyber strength during COVID-19 is analyzed and possible approaches for improvement are discussed. The authors followed a well-accepted theory in cyber resilience, i.e., the resilience analysis grid proposed by Hollnagel (2017) . The grid allows organisations to measure the performance on four potentials: (i) anticipate, (ii) monitor, (iii) respond, and (iv) learn, as is suggested that the potentials are dependent and alignment would create better cyber resilience. In addition, several studies address the need for more awareness about cyber attacks given the increase in cyber attacks during the crisis. Alzubaidi (2021) contributed to this direction by surveying the level of cyber awareness in Saudi Arabia. The study discusses the most common security tools used by internet users and their cyber security habits. The authors recognized security awareness training is a must for different organizations, especially in the field of phishing due to the increase in cyber-attacks. Since cyber-criminals frequently use different mechanisms for online scamming, awareness about possible strategies contributes to protecting them from such crimes. This pandemic offered them a chance to exploit these attacking mechanisms and apply them to those already worried. Chawki (2021) performed case studies in the USA and the European Union and proposes plausible ways to safeguard online users from such attacks. The results from the cyber-criminal forums indicate that healthcare agencies were prime targets for such fraudulent activities where attackers gain visibility on confidential documents about patients ( Alghamdi, 2022 , Chawki, 2021 , Gafni, Pavel, 2021 ).
Other researchers compared the criminal patterns during the COVID-19 outbreak to other pandemic outbreaks. Levi and Smith (2021) compared COVID-19 with the Spanish flu pandemic of 2018 by analyzing the common features that lead to different crimes impacting society. Along with the Spanish flu, they focused their interest on other different flues that occurred in the world, such as the Asian flu (1957–58), the Hong Kong flu (1968), and the Swine flu (2009–10). The comparative approach resulted also in the identification of new types of attacks during this pandemic and a proposal of best practices to avoid such attacks.
Furthermore, phishing emails have been analysed in several studies with the aim to detect time patterns during the COVID-19 pandemic. To understand diverse attacks during the pandemic, Lallie et al. (2021) proposed a world timeline analysis of COVID-19 (from 2019 to 2020). They searched for patterns occurring along with COVID-19 related events in different countries (e.g., China, the UK, Spain, the USA, Italy, and the Philippines). Events included, among others, government announcements and articles and reports published by the media. The study concluded that 86% of cyber-attacks out of 43 involved phishing and/or smishing. The researchers further identified new malicious website domain registrations with Corona-related keywords and proposed a few solutions to diminish the cyber-attack rate. The study on cybercrime and its trends are further analyzed by Kemp et al. (2021) based on the reported crime in the UK. They considered the timeline analysis which further can be deviated based on the number of crimes reported during the moment. Also, Venkatesha et al. (2021) performed a similar study by identifying the cause of social engineering attacks during the COVID-19 pandemic and proposed a few techniques to avoid such attacks. The work of Sood et al. (2021) detect trends in the total number of malware and phishing-related messages blocked by Google during April 2020 in both emails and communication tools such as Google Meet ( Kumaran and Lugani, 2020 ).
Another group of studies focuses on identifying and aggregating the modus operandi used in phishing emails during the COVID-19 pandemic. A survey of Al-Qahtani and Cresci, 2022 reviewed 54 studies about phishing attacks and analysed the modus operandi and the proposed techniques for detecting COVID-19 phishing, smishing, and vishing attacks. As indicated in the Microsoft Digital Defense Report, phishing attacks consist of almost 70% of all cyber attacks ( Kaliňák, 2021 ). The work of Akdemir and Yenal (2021) analysed 208 COVID-19 phishing emails in April 2020 and identified 9 subjects that were used to target organizations and individual users, being fear appeals, urgency cues, source credibility, authority, liking, social proof, consistency commitment, scarcity, reciprocity (many of Cialdini’s principles Cialdini and Sagarin, 2005 ). The study by Sharevski et al. (2022) explored, in a laboratory setting, the susceptibility of people to phishing via QR codes, a technology often used during the COVID-19 pandemic.
There is also attention to the automated classification of COVID-19 related phishing emails. Since phishing has grown to substantial sizes, multiple researchers ( Alsmadi, Alhami, 2015 , Hamid, Abawajy, 2013 , Karim, Azam, Shanmugam, Kannoorpatti, 2020 ) considered machine-learning algorithms such as K -means, OPTICS, K -modes, etc. for email clustering ( Zubair et al., 2021 ) and classifying the email contents according to similarity of features to quickly gain insights into the malicious activities performed by the attackers, i.e., the modus operandi. There are behaviour-based classification methods ( Hamid, Abawajy, 2011 , Toolan, Carthy, 2010 ) investigated along with the content analysis of the emails ( Basnet, Sung, 2010 , Fette, Sadeh, Tomasic, 2007 ), and email profiling methods studied to detect patterns in, for example, important email features such as hyperlinks, email subject, ( Gansterer, Pölz, 2009 , Hamid, Abawajy, 2013 , Yearwood, Mammadov, Webb, 2012 ) header and domain features ( Karim et al., 2020 ), and URLs in the message content ( Afandi, Hamid, 2021 , Ispahany, Islam, 2021 ). The literature further reports on systems and case studies of automatic phishing classification (i.e., to determine if emails are phishing or not). In that regard, Karim et al. (2020) proposed an automated framework for anti-spam detection that exploited unsupervised methodologies. Ispahany and Islam (2021) proposed a machine-learning classification technique for detecting malicious URLs. In the proposed framework of Xia et al. (2021) , COVID-19 related keywords were identified to detect malicious domains. In addition, Afandi and Hamid (2021) exploited the KNN algorithm to detect phishing hyperlinks by considering the four datasets PhishTank, Kaggle, SpyCloud, and DomainTool. However, their study was limited to five features of the hyperlinks which have more room to analyze the fact in detail. Further, Kawaoka et al. (2021) ; Pletinckx et al. (2021) worked in a related path to analyze early COVID-19 related domain name registrations. Patgiri et al. (2019) ; Patil and Patil (2018) ; Rameem Zahra et al. (2021) use machine learning methods, such as decision trees and fuzzy logic, to learn the malicious URLs. Also, natural language processing techniques ( Sahingoz et al., 2019 ) and Shannon’s entropy ( Verma and Das, 2017 ) are used to determine the maliciousness of a URL. At last, a case study on Twitter data explores the malicious and inconsistent URLs during COVID-19 to identify link-sharing patterns ( Horawalavithana et al., 2021 ). The authors suggest improving topic moderation techniques on Twitter data that mitigate the intent of poor players in promoting malicious activities. Besides, one can further investigate the quality of these poor players and how they can effectively plan their road map during the crisis.
Finally, studies have been conducted that concentrate on the crime patterns and the shifts to online crime in general ( Hardyns et al., 2021 ), and victimization during COVID-19. Hardyns et al. (2021) , for example, studied common crime patterns such as burglary, violence, vehicle theft during the pandemic in Belgium. They found that, for example, cases of domestic violence and the general crime rate reported during the Corona period were similar from 2015 to 2019 but growth was observed for cybercrimes, particularly phishing and online scams. Therefore, victimization should also be taken into account to understand and analyze the activities performed by attackers, as the fraud committed during COVID-19 affects the victims socially and mentally. Such a study was conducted by Kennedy et al. (2021) by surveying 2200 Americans during COVID-19. Although the paper discusses the facts of the victimization and proposes solutions to mitigate cybercrimes at a particular time of COVID-19, the authors also point out that proposed solutions are consistent with studies conducted in other periods before the pandemic.
As discussed in the literature ( Aleroud and Zhou, 2017 ), many studies have been conducted to understand and analyze the behavior of cybercriminals. Furthermore, various methods have been proposed to mitigate malicious activities. However, a thorough analysis on understanding the characteristics of phishing emails during COVID-19 is lacking. In the present study, we analyze the behaviour of cyber criminals concerning phishing emails received at firm domains in the Netherlands. In addition, the research examines the impact of COVID-19 on phishing emails by considering various trends and events announced by the government.
3. Methodology
With the importance stressed for analyzing new phishing behavior that appeared during the pandemic, the present study focuses on COVID-19 related phishing emails including an analysis of the contents and a trend analysis, to understand better how attackers adapted to new societal conditions. The goal of the analysis is to create insights into applied patterns abusing the COVID-19 pandemic to deceive people with their phishing schemes. This leads to the following key research question: which effects did COVID-19 have on patterns in phishing emails?
The key research question is concerned with creating explanations for applied practices (behavior) of cyber criminals in a time of crisis. This is a typical interpretive question since it aims to gain in-depth knowledge of actor behavior in their natural context while developing an empathetic understanding of their actions ( Goldkuhl, 2012 ). As phishing is an illegal activity in most countries it is difficult to directly interact with actors on a large scale to study the patterns of behavior. Also, in the Netherlands, email phishing is a criminal activity that is actively monitored and prosecuted ( Fraudehelpdesk , Rechtsraak ). The data trail phishers create, however, prevails as a rich source to gain a large-scale overview and create insights into COVID-19 related phishing. Therefore, we adopt a quantitative research approach. The data collection is based on a document (email) analysis and the empirical method selected is content analysis. We intentionally do not differentiate between perpetrators’ motivations and specific types of criminals such as nation-state actors, hacktivists, or people motivated by the thrill of criminal activities. Although it would be interesting to determine the motivation of the sender of the phishing emails studied, our data does not allow us to identify the perpetrator, nor do we consider it to be the scope of this study. The study aims to understand the emergence and adaption of content related to COVID-19.
3.1. Dataset description
The dataset used in this research contains COVID-19 related phishing emails. This data was collected by Tesorion. 1 The emails are collected via 1105 top-level domains 2 that were previously managed by Tesorion, but are taken out of use. The data was collected between Jan 17th 2020 and 8th of March 2021. The selection of this data is based on the initial start of collection by Tesorion just before the European pandemic outbreak until about one year after the first COVID-19 restrictions were announced in Europe. The inclusion criteria for emails to be classified as COVID-19 related emails was based on a list of COVID-19 related keywords such as Covid-19, corona, or Pandemic (for the full list see Appendix A ). The list of keywords has been derived from several other papers and online sources discussing corona-related phishing and corona-related spam ( Chen, Lerman, Ferrara, 2020 , Cinelli, Quattrociocchi, Galeazzi, Valensise, Brugnoli, Schmidt, Zola, Zollo, Scala, 2020 , Kouzy, Abi Jaoude, Kraitem, El Alam, Karam, Adib, Zarka, Traboulsi, Akl, Baddour, 2020 , Kousha, Thelwall , Mimecast, 2020 ). The total number of corona related emails received to these domains is 1.076.541. The emails contain the following key features that are used for the analysis, mail_id, received_date, from_address, subject, filename, hash, plain_body, html_body to_domain_id, and attachment
3.2. Pre-processing data
To prepare the data, we follow the guideline for pre-processing as described in Gibert et al. (2016) . This resulted in the following filtering and pre-processing steps for this study (see Fig. 1 for process flow):
- 1. First, we divide the initial data set (1.076.541 emails) into emails having attachments (148.295) and those without (928.246). This study focuses solely on the analysis of emails without attachments to gain insights as we are highly interested in the body content of emails that can be further analyzed by NLP techniques. Attachment analysis is much more software injection oriented, and falls beyond the scoping of this paper, but is addressed later as future work.
- 2. On the COVID-19 related email data (without attachments) we apply a number of filters to the html body content with the aim to retrieve the textual content only so that it can be used for topic modelling. The following functions are applied in order: (i) use of the beautifulsoup python package ( Crummy, 2021 ) to get textual contents of emails, (ii) remove email addresses, (iii) remove all non- ascii characters, (iv) lowercase all words, (v) remove urls, (vi) remove html special characters, (vii) remove all types of brackets, (vii) remove unnecessary white spaces, tabs and newlines, (viii) remove (e) numerations, (iX) remove punctuation.
- 3. The initial dataset contains emails in different languages such as English, Dutch, French, and German. Therefore, we determined the language of each email using the Python package CLD3 ( Google, 2020 ) and only applied further pre-processing on English emails. By targeting only English language-based emails we reduce the challenges of analyzing emails in other languages while doing topic clustering. That further helped to achieve the research goals effectively. This step reduces the dataset to 594.895 emails.
- 4. In addition, we removed emails with duplicate email body which further reduced the data set to 104.228 unique emails.
- 5. In the next step, we determined for the emails which of them are phishing emails. This was achieved with the help of the VirusTotal ( VirusTotal, 2020 ) API, resulting in the identification of 29.171 phishing emails. VirusTotal is to date considered one the top performing tool for classifying phishing emails ( Choo et al., 2022a ). Current studies evaluate the accuracy of the VirusTotal phishing classification to be at 81.72% ( Choo et al., 2022a ). To determine whether a URL is regarded as phishing, VirusTotal queries over 70 antivirus scanners and services to return whether and how many services flagged a submitted URL as malicious ( VirusTotal, 2022 ). The topic model analysis, further described in Section 3.3 , is based on those phishing emails. The motivation for taking all phishing emails (including similar emails) as the basis for the topic model analysis is to consider all possibly relevant topics.
- 6. To prepare for topic analysis, we removed common words from the email body to derive a more refined set of words determining topic clusters. Firstly, we remove all common words and stop words based in the NLTK corpus ( NLTK, 2021 ). Secondly, we filter the 35.000 least significant words according to Term Frequency Inverse Document Frequency (TFIDF) ( Luhn, 1957 , Spark Jones, 1972 ). This number was determined through human experimentation. Finally, we remove keywords that had too much overlap with other clusters when indicated by at least 2 authors of this paper, with the goal to make topic clusters more distinct from each other. The keywords removed are: “view”, “email”, “click”, “offer”, “shop”, “free”, “com”, “open”, “sale”, “house”, “health”, “detail”, “unsubscribe”, “company”, “store”, “app”, “address”, “buy”, “receive”, “day”, “delay”, “business”, “south”, “said”, “product”, “delay”, “game”, “week”, “new”, “test”, “covid”, “coronavirus”, “trade”, “united”, “best”, “service”, “time”, “change”, “online”.
- 7. In the next step, we removed phishing emails, which do not have an identical but very similar email body, using the discrete cosine similarity measure ( Manning et al., 2008 ). The goal of this step is to reduce noise to better identify existing trends. We used a similarity value of 0.95. This number is determined through human inspection ( Akhtar et al., 2017 ) about the effectiveness of duplicates removal (reviewing small samples of emails over the similarity value and whether these concern near duplicates or not). This refers to searching for an optimal True Positive/False Positive rate based on the parameter setting (here similarity value), but on a small sample rather than the full dataset since the data is not annotated for similar items and doing so would require severe efforts. The removal reduced the phishing emails to 11.765, and formed the basis for the trend and timeline analysis (see Sections 4.2 and 4.2.2 ).
- 8. After having identified meaningful patterns, a set of emails remained in which potentially more patterns could be found. Therefore, we removed identified patterns as well as emails that can be grouped together but do not describe a technical or semantic pattern used by criminals (see Section 4.5 ). As an example, we identified around 100 Google Alert emails, 3 which haven been possibly falsely classified as phishing. This step reduced the dataset size to 7.397 emails.

Overview of the methodology.
3.3. Analysis approach
In order to investigate how attackers use COVID-19 keywords in their phishing schemes, we searched for a model that can represent texts of different sizes in a feature space that clustering algorithms can work with (see Fig. 1 , topic modelling). We decided on using a Doc2Vec ( Le and Mikolov, 2014 ) method in combination with clustering algorithm k -means ( Lloyd, 1982 ), similar to the approaches by Budiarto et al. (2021) or Wang and Kwok (2021) . The choice for selecting Doc2Vec over other methods, such as bag-of-words ( Harris, 1954 ) to represent textual data, was its ability to incorporate the semantics of a text in its model ( Le and Mikolov, 2014 ). In the course of the analysis, we realized that this method does not work well with our data. The used clustering method ( k -means) could not find meaningful clusters. We did not investigate in detail why Doc2Vec did not work on the data used in this study however, we suspect that the quality of the data in terms of large differences in lengths of emails, semantically incorrect emails (seemingly randomly combined text blocks) and emails having multiple topics, was not good enough to create satisfying results. As a result we tried a popular statistical model, Latent dirichlet allocation (LDA) ( Blei et al., 2003 ), to find clusters (topics) in the dataset. The standard Gensim LDA model ( Řehuřek, 2021 ) is used in combination with the pyLDAvis ( Mabey, 2021 ) library to visualize the topic clusters. In order to get the ideal number of clusters, we tried several values to see with which number of clusters we get a reasonable outcome (see Section 4.1 ).
The second and third analysis concern trends and timelines. We tried to understand whether phishing emails follow any trends or relate to specific events. To get insights into the general timeline of phishing emails, we used standard python visualization libraries such as matplotlib ( Matplotlib.org, 2022 ) to create time plots. When we observed spikes or other interesting points in the graphs, we investigated manually what types of emails are part of that spike. This research further investigates whether phishing campaigns made use of current events related to corona. As a reference, we used the timelines of COVID-19 measures and other related events of the Dutch government ( Ministerie van Volksgezondheid, 2023 ) and the WHO ( World Health Organization, 2022 ). For the verification, we inspected the days where high number of emails were received and manually checked emails whether they mention any events around that day that are listed in the timelines. The fourth analysis searches for date patterns. The fifth analysis is concerned with hidden content. During the topic model analysis, we observed that emails contained hidden text, e.g., white letters on white background. We then used regular expressions to find more of this type of emails to get a better insight into this pattern (see Section 4.3 ). Finally, we assessed if dominant patterns or trends would have distorted data that would impair our view on existing patterns. We subtracted the identified patterns to assess if the remainder contained interesting patterns. For the verification process, we formed two assumptions with which we could verify whether our findings are proven to be correct.
COVID-19 Phishing Patterns.
Pattern name | Pattern description | Pattern type | Relation to COVID-19 | Attacker motivation | Revealed in | Literature |
---|---|---|---|---|---|---|
Hidden pixel | A pattern frequently observed to add hidden textual content to an email in a small image usually with the intend to mislead spam filters to classify the email as genuine | Recurring | Predominant use of COVID-19 related news articles | To disguise | , | Web bugs ( , ) tracking pixel ( ) |
White color font | A pattern frequently observed to add hidden textual content to an email at the background usually with the intend to mislead spam filters to classify the email as genuine | Recurring | Predominant use of COVID-19 related news articles | To disguise | , | Hidden salting ( , , ) |
HTML Email Preheader Text | A pattern frequently observed to add textual content to an email usually with the intend to mislead spam filters to classify the email as genuine | Recurring | Predominant use of news articles; both COVID-19 and non COVID-19 related | To disguise | HTML tag ( ) | |
Unsubscribe button | Fictive clickable link stating “unsubscribe”. Variants display “Manage subscriptions” | Recurring | No | To disguise | Unsubscribe” spam attack ( ) | |
Encoded HTML | Emails contain base64 encoded instructions (e.g., POST requests). Often the emails ask to enter credentials into a field in the email (e.g., fake login to see document) | Recurring | No | To disguise | Unicode ( ) encoding ( ) | |
Bit.ly links | Emails start with malicious bit.ly link, accompanied by news headlines. | Recurring | No | To disguise | Verification | URL features url shortener / tracker ( , , , ) |
E-moji in subject | An emoji is added to the subject line (various emojis observed) | Recurring | No | To disguise | Verification | Obfuscated words ( ) |
Clickable image | Emails frequently include a clickable image (via i.imgur.com) that links towards a phishing website. | Recurring | No | To disguise | Image features ( ) | |
News headlines | The email subject header is disguised by a real news headline to gain your interest and forwarding you to a fake store to purchase and extort and swindle your data | Recurring | Predominant use of corona-related news articles | To gain interest | Obfuscated words ( ) fake news headlines ( ) | |
Face-mask | Emails are trying to scare you into buying face masks with the purpose to collect personal information | Recurring | Yes | To gain interest | , , | Profiled purchasing ( ) compulsive buying ( ) deals too good to be true ( ) |
Home warranty | Emails that are trying to scare you into take out an home insurance policy with the purpose to collect personal information | Recurring | No | To gain interest | Verification | Profiled purchasing ( ) compulsive buying ( ) deals too good to be true ( ) |
Topic shift to Medical | Phishing emails concerned with the topic clusters “medical” & “information” increased substantially higher than other topics | Trend shift | Phishers targeting medical products and services | Likely higher victimization rate | Topic analysis | |
Phishing campaign spikes | Many of the peaks in the analysis are caused by phishing campaigns. Phishing emails conform an email template (same email format, but slightly differentiated content, e.g., different company name), domain sender, etc., hence, are assumed to originate from the same attacker. Phishing campaigns, however, do not correlate to specific events. | Recurring | Likely higher victimization rate | Spikes e.g., ( , ) | ||
Dutch phishing rise march 2020 | The rise of phishing emails in the Netherlands corresponds in a broad sense to the announced measures taken by the Dutch government, and highly relates to the announcement classifying COVID-19 as a pandemic | Trend shift | Dutch COVID-19 restriction announcements | Likely higher victimization rate | ||
Day and Time-depend susceptibility to phishing | It is apparent that the day of the week, and the time of the day play an influential role in the assumptions of attackers when people are most susceptible to phishing. Patterns follow work-week patterns of employees (mon-fri), with specific hours of breaktime, and email reading patterns | Recurring | Likely higher victimization rate | Weekend pattern ( , ) peak pattern ( ) |
- 2. If we remove the dominant pattern, there are no other spikes appearing in the data. That means, it is likely that we have caught the largest campaigns that are event/date specific.
4. Analysis: patterns and potential explanations
This section presents and interprets the results of this study. First, the results of the topic model are presented in Section 4.1 , followed by an overview of the number of received phishing emails during the time frame of the dataset ( Section 4.2 ). Subsequent sections discuss correlations between phishing emails and COVID-19 related events, as well as findings in domains, time and date of received emails ( Sections 4.2.1 – 4.2.3 ). Following this, Section 4.3 highlights identified trends and patterns. Then, we conduct the verification ( Section 4.4 ). The chapter ends with a summary of the identified patterns ( Section 4.5 ).
4.1. Topic analysis
The topic analysis clustered all emails classified as phishing emails in 22 topics, then further merged to 17 clusters to finally derive to 6 unique high-level topics (see Fig. 2 ). The LDA algorithm was used to identify the most satisfying number of topics. In order to assess the coherence of the formed topics in a technical way, we relied on metrics such as C_V metric, UMASS and normalized pointwise mutual information (NPMI) ( Röder et al., 2015 ), with values 0.582, − 2.799 , and − 0.00376 respectively. Röder et al. (2015) suggest that NPMI is in this regard the best topic coherence metric for optimization. Obtaining a score close to zero is a good result, but should be seen in the context of the data source. To determine the ‘goodness’ of topic independence, we relied on the visual inspection of 50 randomly selected emails from each topic/cluster by maintaining the balance between the efforts and the number of emails in each topic. If there was an explanatory pattern among the emails, e.g., the majority concerns Nigerian prince scams, we accepted a topic cluster as reasonably coherent. Even though the topic clusters were initially perceived as sufficiently coherent, certain topics were so closely related that they could be merged together and in a following step associated with a more general topic as seen in Fig. 2 . The process of merging these topics was carried out in two steps: (i) from 22 to 17 to find sufficiently distinct topics, and (ii) from 17 to 6 to group more refined topics in a more general way. The colored numbers in Fig. 2 refer to the size of the topic in relation to the cumulative size of all 6 topics. The blue is based on the phishing dataset and the red one on the reduced dataset where similar emails have been removed (see Fig. 1 ).

Overview of topics and how they were merged. Advertisement : COVID-19 related emails that advertise various types of products to the recipient. News : COVID-19 related emails containing news to the reader on all sorts of topics. Information : COVID-19 related emails with the goal to inform the reader about various business topics/situations/regulations etc. Government : COVID-19 related emails that concern political or governmental affairs. Medical : COVID-19 related emails that are focused on health or healthcare in a broader sense. Other : COVID-19 related emails to which no general topic was found.
Figure 3 shows the size of the each of these clusters over time, based on the same dataset as the topic model. It becomes clear that the number of COVID-19 related phishing email is the highest at the beginning of the pandemic in the Netherlands. Especially emails with health related topics (medical) show a high increase decrease during this period. This might indicate that phishers were particularly framing emails around medical services or goods at the beginning of the pandemic when those products where in high demand. Figure 3 shows an increase of the topics: 1) information, 2) news, 3) advertisement, and 4) medical, related to the two lock-downs in the Netherlands (start ‘intelligent lockdown’ 23rd March-31 May 2020, and ‘full lockdown’ 15th Dec 2020-23rd Jan 2021), while the : 5) government, and 6) other, remain relatively constant.

Size of abstract topic clusters per month, based on the phishing dataset.
4.2. Timeline overview
Figure 4 shows the number of COVID-19 phishing emails over the time period in which the phishing emails were collected. The figure shows some spikes on days or short periods in which the number of COVID-19 phishing emails are substantially higher than during other periods.

Timeline of filtered COVID-19 phishing emails.
4.2.1. Timeline correlated COVID-19 events
We analyse correlations between events concerning measures or other events regarding the COVID-19 pandemic and the contents of phishing emails. The initial assumption was that some spikes and trends (in Fig. 4 ) would correlate with specific events. However, none of the spikes could be traced back to corona-related events using the approach explained in Section 3.3 . It seems, therefore, that most large phishing campaigns are not event-related, last a few weeks, and the launching of new campaigns follow a steady pacing pattern resulting in continuous steady amount of COVID-19 phishing emails. All the spikes (in Fig. 4 ) could be explained by phishing campaigns that were sent out with a slightly altered content but in a similar structured format. We did find trend alternations associated with corona-related events.
- - On the 12th March a press conference was held in The Netherlands in which the first nation-wide strict measures were announced (e.g., canceling events and closing higher education).
- - On the 16th March followed a TV speech of the Dutch prime minister (Mark Rutte) in which he addressed the nation about the notion of the COVID-19 virus (last address to the Nation was in 1973 oil crisis).
- • From April 2020 the trend is slowly decreasing until August 2020 after which it stabilizes.
- • The low amount of COVID-19 related emails in the summer months could be caused by the ease of restrictions during that time (email is less read and people are less scared of COVID-19 hence fall for phishing schemes).
4.2.2. Date patterns
Working patterns of cyber-criminals are considered when dates, time (in hour), and/or number of emails seem to correlate.
As showcased in Fig. 5 , there is a growth pattern in COVID-19 phishing emails between 9:00 (strongest increase) and 17:00 (strongest decrease after the plateau), reflecting the “9:00 to 17:00” workweek, the common working pattern (before COVID-19) of many organizations (i.e., the start for Dutch organizations is generally at 8:30 and ends at 17:00 with a half an hour break at 12:30–13:00). Remarkable is the highest peak at 11:00 (a little after the second coffee break, on average starting 10:30 lasting some 10 to 15 min). The peak is followed by a ‘lunch time’ dip from 12:00–13:00-, followed by a plateau from 14:00 to 16:00. The second slight peak at 16:00 may be explained by phishers aiming for the ‘getting to home early to pick up my kid rush’. This way it may be easier to deceive people and secondly may provide more time for phishing to be discovered since the employee went home, leaving work tasks and taking off their work minds. The distribution on the weekend is more varied and coincides with the 11 h peak during working days. In addition, it shows that also on the weekend most emails are received during working hours. There are two hypotheses to explain the highlighted patterns between 9:00 and 17:00 of Fig. 5 . On the one hand, it could be that criminals believe that by following a usual working day makes their phishing more effective, but the emails are sent automatically. On the other hand, it could reflect the working hours of criminals showing that they also follow a ‘9:00–17:00’ job and send emails during their working hours.

No. of COVID-19 phishing emails by hour of day (time zone AMS (UTC + 01:00)) during the week and weekend.
In line with Ramzan and Wüest (2007) and Lastdrager (2018) , we notice a sharp drop of nearly 50% in received emails during the weekend as reflected in Fig. 6 .

COVID-19phishing emails by day of week.
4.2.3. Phishing domains
Figure 7 shows the number of phishing emails containing URLs with the domains listed in the legend. For this analysis, the 5 most occurring phishing domains are selected. The lifetime of the domains varies greatly. For example, all emails with phishing URLs from ‘kiolyduke.casa’ and ‘unfortunatedeadly.icu’ are received in a span of less than 4 h. Phishing campaigns lasting for several hours to a few days is in line with the findings of other researchers, such as McGrath and Gupta (2008) ; Moore and Clayton (2007) ; Oest et al. (2020) . In contrast, emails containing phishing URLs from the ‘covidvirus.guru’ domain appeared over several months. The extended use of the domains ‘edmcn.cn’ and ‘app1.ftrans01.com’ is likely due to those being domains of content sharing providers.

Temporal overview of domain URLs used in COVID-19 related phishing emails.
4.3. Hidden text analysis
The motivation of identifying hidden text is to recognize new phishing schemes adopted by attackers during the pandemic.
The first phishing scheme was found consisting of the pattern that text was obfuscated by coloring text white on a white background as shown in Box Box1 , 1 , making it invisible for the reader.

Hidden text.
Some emails contain the small font-size (usually 1 or 2 pixels, with the exceptional case of 0.001 px) as well as the white color trick. The hidden texts are either a collection of nonsense words (refer to Example 1 ) varying form a few words to a paragraph of text, or short texts taken from online sources, e.g., news websites such as BBC.com.
In many phishing emails, we observe the appearance of these small samples of non-sense text repeatedly within a single email. It is likely that these fragments are used to circumvent spam-filters by adding seemingly reliable data into the mail to disguise real phishing intentions.
The emails that adopted the hidden text are frequently about face mask offers (see Fig. 9 (d)). Those emails are sent from different addresses and have different contents and subjects, which could indicate that those are created by different adversaries. However, there is no easily observable relationship that explains the coherence between the use of the phishing pattern (hidden text) and selling of masks.

Examples of different phishing emails and how they mention COVID-19. The references cited in this figure are [ Andrew and Yeung (2020) ; ... Ferreira and Teles (2019) ; ... Lin et al. (2019) ; ... WHO (2020) .]
Another remarkable observation is that a substantial part of the mails (although from different senders and relate to different subjects), make use of the trick to include an clickable image (via i.imgur.com, see Fig. 8 ) that forwards you to the intended malicious website, which is positioned at the bottom section of the mail and displays a fictive clickable link stating “Unsubscribe Here”. Variants display “Manage subscriptions” or “if you do not wish to continue receiving email newsletters click here”.

Example unsubscribe image hosted on i.imgur.com.
4.4. Verification
By inspecting the resulting dataset (dominant patterns removed), we observe that the trends discussed in Section 4.2.2 (emails are received mostly during working hours, large decrease of emails on the weekends) are also present, which supports assumption 1. For assumption 2, we examined whether there are any spikes appearing in the timeline of received phishing emails (similarly as in Section 4.2.1 ). We could identify multiple peaks, however, we could not find any major patterns or relations in these emails to any specific event, hence, conclude there is support for our second assumption. However, we did find emails sharing a characteristic. For example, we identified emails containing base64 encoded instructions, or emails with bit.ly links and news headlines. Table 1 lists all our findings in that regard.
4.5. Summary of patterns
We first provide an overview of different identified COVID-19 related patterns in Table 1 . Then, we show how the results of 4.2.1 , 4.2.2 and 4.3 were verified and checked for incompleteness.
The different identified ways in which COVID-19 related keywords have been used to frame phishing emails can be classified in three types. Fig. 9 (a)–(d) presents these different ways.
The three different existing relations (See Table 2 ), show that criminals made use of the COVID-19 pandemic to persuade recipients into clicking a malicious link out of curiosity/need (Example (Example9(b) 9 (b) and (d)) or understanding for disruptions/errors (example (example9(c)). 9 (c)). Besides, criminals use such keywords to pass spam filters either intentionally (actively use COVID-19 related fragments of news articles etc.) or unintentionally since new articles during that time were often related to COVID-19.
Description of relation types of COVID-19 to phishing emails.
Relationship | Relationship description |
---|---|
Direct relation to COVID-19 | The email relates to COVID-19 directly as the main topic of the email ( (b)). |
Indirect relation to COVID-19 | The email mentions something about COVID-19, however, the main topic is not directly related to it ( (c)). |
Hidden relation to COVID-19 | The email shows no sign of a relation to COVID-19. However, the HTML code of the email contains text which is related to COVID-19 ( (a), HTML content not shown). |
No relation | The email shows no relation to COVID-19. |
5. Discussion of research contributions
First, we revisit the research questions, and then summarize the contributing findings and cover the limitations and implications.
5.1. Revisiting research questions
Crime changes and adapts to new circumstances such as those resulting from the COVID-19 pandemic. Different studies already have highlighted these changes in crime such as computer misuse ( Office for National Statistics UK, August, 2020 ) or fraud ( InterStats, 2020 ). This study is concerned with phishing, and the question: which effects did COVID-19 had on patterns in phishing emails? The initial expectation was that phishing would increase and the criminals would try to exploit uncertainties around the virus and introduced measures in their phishing emails. This study shows that there was a high increase of COVID-19 related phishing emails after the first restrictions had been introduced in the Netherlands. It also shows that criminals did make use of COVID-19 related content in their phishing emails.
The findings in Sections 4.2 and 4.2.1 show that in the beginning of the pandemic in the Netherlands phishing emails increased in numbers and healthcare related content such as selling masks formed prominent topics. Research from ( Aguirre and Lane, 2019 ) indicates that fraud occurs at the beginning of disasters, which may explain this high increase in the first two months.
In general, we identified three different ways COVID-19 related content has been used in phishing emails (see Section 4.5 ). First is direct use, which gives the impression of providing help such as applying for monetary help or access to goods protecting against the virus. The second way was to make use of the pandemic in a more passive way by mentioning the pandemic but the main topic is about something else. The third way of using content regarding COVID-19 in phishing emails was the use of text, e.g., parts of news articles, which was included in the HTML code of the email but not visible to the reader.
Regarding all our findings, it could be that only a very small number of criminals caused a large number of emails and thus our findings reflect the behaviour of a small number of criminals. We tried to assess this with our trend verification process but it still could be that this finding cannot be generalized. It is possible that the first three identified ways of how COVID-19 related content is embedded in phishing emails do not reflect the approach the criminals pursued. For example, it is possible that hidden content in emails is not about the Coronavirus on purpose but due to the increased number of news articles about this topic. This would still make this approach a relevant finding, however, it would be unrelated to the COVID-19 pandemic. The finding that some phishing emails contain hidden (invisible) text is a known deceptive technique used in phishing emails as mentioned by Bergholz et al. (2010b) . However, the paper showed that COVID-19 related content is also used for this approach. Findings on working days of criminals are in line with research presented by Ramzan and Wüest (2007) and Lastdrager (2018) .
Section 4.2.1 revealed the finding that the volume of phishing emails followed the development of the pandemic in the Netherlands, showing a high increase after the fist measures were introduced. However, we could not find emails which directly relate to specific COVID-19 events such as introduced counter measures and restrictions. One can argue that phishing emails offering financial support such as shown in Fig. 9 (b), are related to the introduction of such relief funds and thus co-occur with COVID-19 related events. However, in this research the focus was to find out whether phishing emails referenced specific COVID-19 related restrictions, measures and developments shortly after they have been introduced or observed.
It is not possible to rule out that criminals did not reference specific events in any of their phishing emails. However, this research shows that this has not been done on a large scale. Researchers, for example Bitaab et al. (2020) , also identified phishing emails impersonating a COVID-19 relief fund.
Table 1 lists identified patterns and if applicable references to researchers who identified similar patterns. The table highlights that phishing emails often contained adaptations of known patterns. For example, adding COVID-19 related text in white color (invisible) to an email is a pattern has been adapted but previously described more generally as hidden salting by Bergholz et al. (2008) . In contrast, this study identified very few novel patterns, suggesting that attackers favor adaption over innovation for the vast majority of phishing emails. The rational choice theory on crime of Cornish and Clarke (2016) can explain this behaviour as it argues that decision-making during crime scripts are majorly cost dependent. More specifically, Kirton’s Adaption-Innovation Theory, supports this cost difference by showing that adaptions are generally associated with lesser resource investments, than innovation ( Kirton, 1976 ). The adversarial can alter it schemes most cost effectively, by assessing the cost-risk (i.e., low risk - low reward is preferred over high risk - high reward) ( Junger et al., 2020 ) of component alterations based on both the perspective-based view ( Hunton, 2009 ) and the process-based view ( Maymí et al., 2017 ). In the perspective-based view, the phishing scheme would be evaluated for adaption based on seven distinct components, from the globalized environment, criminal or illicit intent, to data objectives, to exploitation tactics, attack methods, networked technology, or evasion and concealment ( Hunton, 2009 ). On the other hand, the process-based views reviews using a pre-known set procedures and techniques as alternative elements to alter schemes quickly. MITRE ATT&CK, which is based on the Cyber Kill Chain, is an example of such framework ( Maymí et al., 2017 ).
5.2. Limitations
The study’s limitations are as follows: Firstly, there is no comparison to data (long) before the COVID-19 pandemic or after to detect differences in trends and patterns. Furthermore, the study relied on an unsupervised classification algorithm used to classify phishing emails, but such method has its imperfections (Virustotal inaccuracy Choo et al., 2022b ). In addition, the type of emails in the dataset adds to the limitations of our study. The data does not include all emails sent to specific domains, but only those classified as spam by Tesorion and containing a COVID-19 related keyword (see Table A.3 ). As a result, we could not analyse phishing emails with characteristics and patterns that could circumvent Tesorion’s spam filter (proprietary, not known to the researchers). This may have affected our observation that phishing patterns were mostly adaptations of existing ones. Furthermore, the data is limited to Dutch firms, while observing slightly other COVID-19 restrictions in other continents, this might affect the generalization to social conditions ( Ashby, 2020 , Boman, Gallupe, 2020 , Bullinger, Carr, Packham, 2020 , Felson, Jiang, Xu, 2020 , Hodgkinson, Andresen, 2020 , Mohler et al., 2020 ). Another limitation is that data has been restricted by English emails. Finally, there is no comparison to non-COVID-19 related phishing emails.
5.3. Implications
There are a number of implications of this study:
- • The general rise of phishing and COVID-19 related phishing indicates that phishing is considered a lucrative business for adversaries and requires increased attention for policy makers to counteract preventive measurements, e.g., increased resource allocation, more or adapted awareness campaigns and altering phishing scheme detection in algorithms.
- • The technique of topic clustering helps to detect shifts in phishing schemes operated, which is useful information for awareness campaign designers to recognize what are the topics or schemes that need to be explained to the wider public to prevent victimization.
- • The confirmation of misuse of the chaos induced by COVID-19 for developing phishing schemes implies that we should be extra careful to expect shifts in crime, fraudulent and phishing patterns. This could lead to the thought to predict or relate to other disturbing societal changes and to prepare for such foreseen impactful changes.
6. Conclusion and future work
In this paper, we studied the surge and shift of phishing patterns during the COVID-19 pandemic. We observed a large increase of COVID-19 related phishing emails in the beginning of the pandemic in the Netherlands. Although we could relate COVID-19 content frequently to the schemes, we did not see a direct relation in phishing emails to specific events or measures against the spread of the virus. Additionally, we confirm existing knowledge on time patterns, such as that most phishing emails were received during working hours during the week.
The contributions of the research are in two-fold: i) methodology to identify topics in COVID-19 phishing emails, and ii) an analysis of phishing patterns, its adaptions and innovations. For the first part, we observed the following:
- • The LDA model worked more effective than the combination of Doc2Vec and k -means for our dataset as it allows to focus on more contextual information in comparison to the Doc2Vec model. Furthermore, it is not affected by unrealistic clustering outcomes resulting from k -means that has a characteristic of hard clustering. Further, it is important for such studies to consider various aspects of the dataset and analysis such as the number of emails, email lenght, email content, size of clusters, distance between clusters, and the choice of the model and its optimization.
- • The TF-IDF is effective in identifying irrelevant terms resulting in more coherent topics and a less complex model.
Regarding the analysis of phishing patterns we found that
- • The overwhelming presence of COVID-19 in people's lives, for example through lockdowns, contributed to an increased use of COVID-19 related content in phishing emails.
- • Offender schemes are modified to COVID-19 topics (e.g., face masks), but the modi operandi are adapted to its context (exaptation).
- • This adaptive behavior by offenders can be understood by Cornish’s rational choice theory on crime and Kirton’s Adaption-Innovation Theory.
This paper’s findings contribute to institutes who develop awareness campaigns or phishing detection systems. Furthermore, this work can be interesting to academicians who work on phishing patterns developments and are curious to do further study on the challenges/limitations of the research as highlighted in the future work.
Future work could concentrate on our data limitations of COVID-19 related phishing emails during the pandemic, missing data before and after the pandemic as well as data on ’normal’ phishing during the same time. Moreover, future work could focus on a larger scale comparative study that could reveal changes in the behavior of criminals or principles of persuasion used. Such study could include time frames before and after the pandemic as well a broader scope, such as including non COVID-19 related phishing emails and attachments, that could improve the insights on how and if criminals adapt their phishing schemes to the COVID-19 pandemic. Another aspect would be to enhance the data pre-processing and the classification methods. The pre-processing could be improved in terms of complexity and regarding the selection of words to exclude for the LDA model. The classification algorithm requires optimization to reduce false positives. To obtain insight on the notion of phishing emails it could be beneficial to perform a sentiment analysis. In addition, the analysis of phishing emails written in different languages, including Dutch, on the targeted Dutch domains could give insights on differences in the design of phishing emails in different languages.
CRediT authorship contribution statement
Raphael Hoheisel: Conceptualization, Data curation, Formal analysis, Investigation, Writing – original draft, Writing – review & editing. Guido van Capelleveen: Conceptualization, Formal analysis, Funding acquisition, Methodology, Project administration, Writing – original draft, Writing – review & editing. Dipti K. Sarmah: Conceptualization, Funding acquisition, Project administration, Methodology, Writing – original draft, Writing – review & editing. Marianne Junger: Conceptualization, Supervision, Writing – original draft, Writing – review & editing.
Declaration of Competing Interest
The authors declare that they have no known competing financial interests or personal relationships that could have appeared to influence the work reported in this paper.
Acknowledgment
This research has received funding from the University of Twente, BMS COVID-19 Fund. We thank Tesorion Technology B.V., and in particular Dr. Wouter de Vries, for providing the phishing email data.
Biographies

Raphael Hoheisel is a master’s student at the University of Twente with a specialization in cyber security. For his master’s thesis he worked together with a private security company to study ransomware cases in more detail. His research interest expands towards ransomware into the general area of cybercrime. You can reach him at [email protected] .

Guido van Capelleveen is an assistant professor at the Department of Business Analytics, University of Amsterdam. He received his Ph.D. from the University of Twente on the topic of Industrial Symbiosis Recommender Systems. His research interests are in the area of data analytics and data science with applications to real world practice, currently focused on applications for sustainability. His work has been accepted in venues as Decision Support Systems, the International Journal of Accounting Information Systems, Environmental Modelling & Software, the Journal of Environmental Management, and Expert systems with applications, among others. You can reach Guido at [email protected] .

Dipti K. Sarmah is a Lecturer in the group Services and Cyber Security (SCS) at the University of Twente. During her Ph.D., she worked on developing a high-capacity and robust image steganography method. Her research interests are not only limited to the field of steganography, and cryptography, and its applications, but also the study and analysis of human behavior for Cyber security. Her research work is published in the Journal of Information Security and Applications, Information Sciences, etc. She is also the author of a book published in the Intelligent Systems Reference Library, Springer. Dipti can be reached at [email protected] .

Marianne Junger received the Ph.D. degree in law from the Free University of Amsterdam, Amsterdam, the Netherlands, in 1990. She is the Emeritus Professor of Cyber Security and Business Continuity with the University of Twente, Enschede, the Netherlands. Her research investigates the human factors of fraud and cybercrime. More specifically, she investigates victimization, disclosure, and privacy issues. She founded the Crime Science journal together with Pieter Hartel and was an Associate Editor for 6 years. Her research was sponsored by, among others, the Dutch Police, NWO, ZonMw (for health research), and the European Union.
1 Tesorion is a Dutch cybersecurity firm located in Enschede and Leusden providing managed cybersecurity services to 500 firms.
2 Further details regarding these domains have not been shared with us.
3 Emails about alerts that can be created via https://www.google.com/alerts
Appendix A. Full list of keywords
Keywords used to filter the emails.
Email filter keywords | ||
---|---|---|
Covid-19 | 2019_ncov | Corona |
COVID-19 | COVID19 | COVID19 |
covid-19 | 2019nCoV | corona |
coronavirusupdates | COVID19 | NCOV19 |
SARS-CoV-2 | Pandemic | Coronapocalypse |
2019-nCoV | CDC | Wuhan |
Kungflu | N95 | epidemic |
Panic Shopping | stayhomechallenge | Chinese virus |
safeathome | stayathome | covididiot |
Coronavirus | COVID19 | coronavirus |
SARS-CoV-2 | outbreak | Wuhanlockdown |
mondmasker | NCOV2019 | lockdown |
covid | Panic Buying | Koronavirus |
Novel coronavirus | Wuhanvirus | coronaviruses |
Facemask | Coronavirus disease 2019 | PPE shortage |
mondkap | flatten the curve | SocialDistancing |
face mask | COVID 19 | coronavirusoutbreak |
Wuhancoronavirus | Corona virus |
Data availability
- Adebowale M., Lwin K., Sánchez E., Hossain M. Intelligent web-phishing detection and protection scheme using integrated features of images, frames and text. Expert Syst. Appl. 2019; 115 :300–313. doi: 10.1016/j.eswa.2018.07.067. [ CrossRef ] [ Google Scholar ]
- Afandi N.A., Hamid I.R.A. COVID-19 phishing detection based on hyperlink using k -nearest neighbor (KNN) algorithm. Appl. Inf. Technol. Comput. Sci. 2021; 2 (2):287–301. [ Google Scholar ] https://publisher.uthm.edu.my/periodicals/index.php/aitcs/article/view/2317
- Aguirre B., Lane D. Fraud in disaster: rethinking the phases. Int. J. Disaster Risk Reduct. 2019; 39 :101232. doi: 10.1016/j.ijdrr.2019.101232. [ CrossRef ] [ Google Scholar ]
- Akdemir N., Yenal S. How phishers exploit the coronavirus pandemic: acontent analysis of COVID-19 themed phishing emails. SAGE Open. 2021; 11 (3) doi: 10.1177/21582440211031879. [ CrossRef ] [ Google Scholar ] 21582440211031879
- Akhtar, M., Kumar, A., Ghosal, D., Ekbal, A., Bhattacharyya, P., 2017. A multilayer perceptron based ensemble technique for fine-grained financial sentiment analysis. pp. 540–546. 10.18653/v1/D17-1057
- Aleroud A., Zhou L. Phishing environments, techniques, and countermeasures: a survey. Comput. Secur. 2017; 68 :160–196. doi: 10.1016/j.cose.2017.04.006. [ CrossRef ] [ Google Scholar ]
- Alghamdi A. 2022 2nd International Conference on Computing and Information Technology (ICCIT) 2022. Cybersecurity threats to healthcare sectors during COVID-19; pp. 87–92. [ CrossRef ] [ Google Scholar ]
- Al-Qahtani A.F., Cresci S. The COVID-19 scamdemic: a survey of phishing attacks and their countermeasures during COVID-19. IET Inf. Secur. 2022; 16 :324–345. [ PMC free article ] [ PubMed ] [ Google Scholar ]
- Alsmadi I., Alhami I. Clustering and classification of email contents. J. King Saud Univ. - Comput. Inf. Sci. 2015; 27 (1):46–57. doi: 10.1016/j.jksuci.2014.03.014. [ CrossRef ] [ Google Scholar ]
- Alzubaidi A. Measuring the level of cyber-security awareness for cybercrime in Saudi Arabia. Heliyon, Natl. Lib. Med. 2021; 7 (1) doi: 10.1016/j.heliyon.2021.e06016. [ PMC free article ] [ PubMed ] [ CrossRef ] [ Google Scholar ]
- APWG . Technical Report. APWG; 2020. Phishing Activity Trend Reports. 3rd Quarter 2020. [ Google Scholar ] Accessed: 2020-11-26
- APWG . Technical Report. APWG; 2020. Trend Reports. 1st Quarter 2020 Plus COVID-19 Coverage. [ Google Scholar ] Accessed: 2020-11-23
- APWG . Technical Report. APWG; 2022. Trend Reports. 1st Quarter 2022. [ Google Scholar ] Accessed: 2022-06-30
- Ashby M.P. Initial evidence on the relationship between the coronavirus pandemic and crime in the United States. Crime Sci. 2020; 9 :1–16. [ PMC free article ] [ PubMed ] [ Google Scholar ]
- Atkeson A. Report. National Bureau of Economic Research; 2020. What Will be the Economic Impact of COVID-19 in the US? Rough Estimates of Disease Scenarios. [ Google Scholar ]
- Basnet R.B., Sung A.H. International conference on information security and artificial intelligence (ISAI) Citeseer; 2010. Classifying phishing emails using confidence-weighted linear classifiers; pp. 108–112. [ Google Scholar ]
- Bergholz A., De Beer J., Glahn S., Moens M.-F., Paaß G., Strobel S. New filtering approaches for phishing email. J. Comput. Secur. 2010; 18 (1):7–35. [ Google Scholar ]
- Bergholz A., Paass G., Reichartz F., Strobel S., Moens M.-F., Witten B. CEAS. vol. 9. 2008. Detecting known and new salting tricks in unwanted emails. [ Google Scholar ]
- Bhardwaj A., Sapra V., Kumar A., Kumar N., Arthi S. Why is phishing still successful? Comput. Fraud Secur. 2020; 2020 (9):15–19. [ Google Scholar ]
- Bitaab M., Cho H., Oest A., Zhang P., Sun Z., Pourmohamad R., Kim D., Bao T., Wang R., Shoshitaishvili Y., Doupé A., Ahn G.-J. 2020 APWG Symposium on Electronic Crime Research (eCrime) 2020. Scam pandemic: how attackers exploit public fear through phishing; pp. 1–10. [ CrossRef ] [ Google Scholar ]
- Blancaflor E.B., Alfonso A.B., Banganay K., et al. Proceedings of the International Conference on Industrial Engineering and Operations Management. 2021. Let’s go phishing: a phishing awareness campaign using smishing, email phishing, and social media phishing tools. [ Google Scholar ]
- Blei D.M., Ng A.Y., Jordan M.I. Latent dirichlet allocation. J. Mach. Learn. Res. 2003; 3 (Jan):993–1022. [ Google Scholar ]
- Boman J.H., Gallupe O. Has COVID-19 changed crime? Crime rates in the United States during the pandemic. Am. J. Crim. Justice. 2020; 45 (4):537–545. [ PMC free article ] [ PubMed ] [ Google Scholar ]
- Budiarto A., Rahutomo R., Putra H.N., Cenggoro T.W., Kacamarga M.F., Pardamean B. Unsupervised news topic modelling with Doc2Vec and spherical clustering. Procedia Comput. Sci. 2021; 179 :40–46. doi: 10.1016/j.procs.2020.12.007. [ CrossRef ] [ Google Scholar ] 5th International Conference on Computer Science and Computational Intelligence 2020
- Buil-Gil D., Miró-Llinares F., Moneva A., Kemp S., Díaz-Castaño N. Cybercrime and shifts in opportunities during COVID-19: a preliminary analysis in the uk. Eur. Soc. 2020; 0 (0):1–13. doi: 10.1080/14616696.2020.1804973. [ CrossRef ] [ Google Scholar ]
- Bullinger L.R., Carr J.B., Packham A. Report. National Bureau of Economic Research; 2020. COVID-19 and Crime: Effects of Stay-at-Home Orders on Domestic Violence (Pre-Print) [ Google Scholar ] https://www.nber.org/papers/w27667
- Andrew, S., Yeung, J., 2020. Masks can’t stop the coronavirus in the US, but hysteria has led to bulk-buying, price-gouging and serious fear for the future. Accessed: 2023-01-14. https://edition.cnn.com/2020/02/29/health/coronavirus-mask-hysteria-us-trnd/index.html .
- Chawki M. In: Intelligent Computing. Arai K., editor. Springer International Publishing; Cham: 2021. Cybercrime in the context of COVID-19; pp. 986–1002. [ CrossRef ] [ Google Scholar ]
- Chen E., Lerman K., Ferrara E. Tracking social media discourse about the COVID-19pandemic: development of a public coronavirus twitter data set. JMIR Public Health Surveill. 2020; 6 (2):e19273. doi: 10.2196/19273. [ PMC free article ] [ PubMed ] [ CrossRef ] [ Google Scholar ]
- Cats, O., Hoogendoorn, S., 2020. Accessed: 2023-02-27. https://www.tudelft.nl/en/covid/exit-strategies/the-role-of-and-impact-on-mobility-on-the-course-of-the-virus/ .
- Choo, E., Nabeel, M., De Silva, R., Yu, T., Khalil, I., 2022a. A large scale study and classification of virustotal reports on phishing and malware urls. 10.48550/ARXIV.2205.13155
- Choo, E., Nabeel, M., De Silva, R., Yu, T., Khalil, I., 2022b. A large scale study and classification of virustotal reports on phishing and malware urls. arXiv preprint arXiv:2205.13155
- Cialdini, R. B., Sagarin, B. J., 2005. Principles of interpersonal influence.
- Cinelli M., Quattrociocchi W., Galeazzi A., Valensise C.M., Brugnoli E., Schmidt A.L., Zola P., Zollo F., Scala A. The COVID-19 social media infodemic. Sci. Rep. 2020; 10 (1):16598. doi: 10.1038/s41598-020-73510-5. [ PMC free article ] [ PubMed ] [ CrossRef ] [ Google Scholar ]
- CNBC, 2020. Cybercrime ramps up amid coronavirus chaos, costing companies billions. Accessed: 2020-11-23, https://www.cnbc.com/2020/07/29/cybercrime-ramps-up-amid-coronavirus-chaos-costing-companies-billions.html .
- Cornish D.B., Clarke R.V. Environmental Criminology and Crime analysis. Routledge; 2016. The rational choice perspective; pp. 48–80. [ Google Scholar ]
- Crummy, 2021. Beautiful soup. Accessed: 2021-12-15, https://www.crummy.com/software/BeautifulSoup/ .
- Cucinotta D., Vanelli M. Who declares COVID-19 a pandemic. Acta Bio Medica. 2020; 91 (1):157. [ PMC free article ] [ PubMed ] [ Google Scholar ]
- de Haas M., Faber R., Hamersma M. How COVID-19 and the Dutch ‘intelligent lockdown’ change activities, work and travel behaviour: evidence from longitudinal data in the netherlands. Transp. Res. Interdiscip. Perspect. 2020; 6 (100150) [ PMC free article ] [ PubMed ] [ Google Scholar ]
- Drury V., Lux L., Meyer U. Proceedings of the 17th International Conference on Availability, Reliability and Security. Association for Computing Machinery; New York, NY, USA: 2022. Dating phish: An analysis of the life cycles of phishing attacks and campaigns. [ CrossRef ] [ Google Scholar ]
- Europol . Technical Report. Europol; 2020. Pandemic Profiteering how Criminals Exploit the COVID-19 Crisis. [ Google Scholar ] Accessed: 2020-11-23
- Felson M., Jiang S., Xu Y. Routine activity effects of the COVID-19 pandemic on burglary in detroit, March, 2020. Crime Sci. 2020; 9 (1):1–7. [ PMC free article ] [ PubMed ] [ Google Scholar ]
- Ferreira A., Teles S. Persuasion: how phishing emails can influence users and bypass security measures. Int. J. Human-Computer Stud. 2019; 125 :19–31. doi: 10.1016/j.ijhcs.2018.12.004. [ CrossRef ] [ Google Scholar ]
- Fette I., Sadeh N., Tomasic A. Proceedings of the 16th International Conference on World Wide Web. Association for Computing Machinery; New York, NY, USA: 2007. Learning to detect phishing emails; pp. 649–656. [ CrossRef ] [ Google Scholar ]
- Fraudehelpdesk, 2023. About fraud help desk. Accessed: 2023-14-01, https://www.fraudehelpdesk.nl/fraudhelpdesk-the-dutch-national-anti-fraud-hotline/ .
- Furnell S., Emm D., Papadaki M. The challenge of measuring cyber-dependent crimes. Comput. Fraud Secur. 2015; 2015 (10):5–12. doi: 10.1016/S1361-3723(15)30093-2. [ CrossRef ] [ Google Scholar ]
- Gafni R., Pavel T. Cyberattacks against the health-care sectors during the COVID-19 pandemic. Inf. Comput. Secur. 2021; 30 (1):137–150. doi: 10.1108/ICS-05-2021-0059. [ CrossRef ] [ Google Scholar ]
- Gansterer W.N., Pölz D. European Conference on Information Retrieval. Springer; 2009. E-mail classification for phishing defense; pp. 449–460. [ Google Scholar ]
- Gibert K., Sànchez-Marrè M., Izquierdo J. A survey on pre-processing techniques: relevant issues in the context of environmental data mining. AI Commun. 2016; 29 (6):627–663. [ Google Scholar ]
- Goldkuhl G. Pragmatism vs. interpretivism in qualitative information systems research. Eur. J. Inf. Syst. 2012; 21 (2):135–146. [ Google Scholar ]
- Google, 2020. Compact language detector v3 (CLD3). Accessed: 2021-06-21, https://github.com/google/cld3 .
- Groenendaal J., Helsloot I. Cyber resilience during the COVID-19 pandemic crisis: a case study. J. Conting. Crisis Manag. 2021; 29 (4):439–444. doi: 10.1111/1468-5973.12360. [ CrossRef ] [ Google Scholar ]
- Halevi, T., Memon, N., Nov, O., 2015. Spear-Phishing in the Wild: A Real-World Study of Personality, Phishing Self-Efficacy and Vulnerability to Spear-Phishing Attacks (January 2, 2015).
- Hamid I.R.A., Abawajy J. 2011, Algorithms and Architectures for Parallel Processing. ICA3PP 2011. Lecture Notes in Computer Science. vol. 7017. 2011. Hybrid feature selection for phishing email detection; pp. 266–275. [ CrossRef ] [ Google Scholar ]
- Hamid I.R.A., Abawajy J.H. 2013 12th IEEE International Conference on Trust, Security and Privacy in Computing and Communications. IEEE; 2013. Profiling phishing email based on clustering approach; pp. 628–635. [ Google Scholar ]
- Hardyns W., Schokkenbroek J.M., Schapansky E., Keygnaert I., Ponnet K., Vandeviver C. Technical Report. Ghent University; 2021. Patterns of Crime During the COVID-19 Pandemic in Belgium. [ Google Scholar ] http://doi.org/10.31235/osf.io/r34x8
- Harris Z.S. Distributional structure. Word. 1954; 10 (2–3):146–162. [ Google Scholar ]
- Hodgkinson T., Andresen M.A. Show me a man or a woman alone and i’ll show you a saint: changes in the frequency of criminal incidents during the COVID-19pandemic. J. Crim. Justice. 2020; 69 :101706. doi: 10.1016/j.jcrimjus.2020.101706. [ PMC free article ] [ PubMed ] [ CrossRef ] [ Google Scholar ]
- Hollnagel E. Resilience Engineering in Practice. CRC Press; 2017. Epilogue: rag–the resilience analysis grid; pp. 275–296. [ Google Scholar ]
- Holtfreter K., Reisig M.D., Pratt T.C. Low self-control, routine activities, and fraud victimization. Criminology. 2008; 46 (1):189–220. doi: 10.1111/j.1745-9125.2008.00101.x. [ CrossRef ] [ Google Scholar ]
- Horawalavithana S., De Silva R., Nabeel M., Elvitigala C., Wijesekara P., Iamnitchi A. In: Social, Cultural, and Behavioral Modeling. Thomson R., Hussain M.N., Dancy C., Pyke A., editors. Springer International Publishing; Cham: 2021. Malicious and low credibility urls on twitter during the astrazeneca COVID-19 vaccine development; pp. 3–12. [ Google Scholar ]
- Hu H., Peng P., Wang G. 2019 IEEE Symposium on Security and Privacy (SP) 2019. Characterizing pixel tracking through the lens of disposable email services; pp. 365–379. [ CrossRef ] [ Google Scholar ]
- Hunton P. The growing phenomenon of crime and the internet: acybercrime execution and analysis model. Comput. Law Secur. Rev. 2009; 25 (6):528–535. doi: 10.1016/j.clsr.2009.09.005. [ CrossRef ] [ Google Scholar ]
- Ispahany J., Islam R. 2021 IEEE International Conference on Pervasive Computing and Communications Workshops and Other Affiliated Events (PerCom Workshops) 2021. Detecting malicious COVID-19 urls using machine learning techniques; pp. 718–723. [ CrossRef ] [ Google Scholar ]
- Jáñez-Martino F., Alaiz-Rodríguez R., González-Castro V., Fidalgo E., Alegre E. A review of spam email detection: analysis of spammer strategies and the dataset shift problem. Artif. Intell. 2022; 56 :1–29. [ Google Scholar ]
- Junger M., Wang V., Schlömer M. Fraud against businesses both online and offline: crime scripts, business characteristics, efforts, and benefits. Crime Sci. 2020; 9 (1):1–15. [ Google Scholar ]
- Kaliňák, V., 2021. Psychology of phishing attacks during crises: the case of COVID-19 pandemic.
- Karim A., Azam S., Shanmugam B., Kannoorpatti K. Efficient clustering of emails into spam and ham: the foundational study of a comprehensive unsupervised framework. IEEE Access. 2020; 8 :154759–154788. doi: 10.1109/ACCESS.2020.3017082. [ CrossRef ] [ Google Scholar ]
- Kawaoka R., Chiba D., Watanabe T., Akiyama M., Mori T. International Conference on Passive and Active Network Measurement. Springer; 2021. A first look at COVID-19 domain names: origin and implications; pp. 39–53. [ Google Scholar ]
- Kemp S., Buil-Gil D., Moneva A., Miró-Llinares F., Díaz-Castaño N. Empty streets, busy internet: a time-series analysis of cybercrime and fraud trends during COVID-19. J. Contemp. Crim. Justice. 2021; 37 (4):480–501. doi: 10.1177/10439862211027986. [ CrossRef ] [ Google Scholar ]
- Kennedy J.P., Rorie M., Benson M.L. COVID-19 frauds: an exploratory study of victimization during a global crisis. Criminol. Public Policy. 2021; 20 (3):493–543. doi: 10.1111/1745-9133.12554. [ PMC free article ] [ PubMed ] [ CrossRef ] [ Google Scholar ]
- Kennedy L.W., Forde D.R. Routine activities and crime: an analysis of victimization in canada. Criminology. 1990; 28 (1):137–152. [ Google Scholar ]
- Kirlappos I., Sasse M.A. Security education against phishing: a modest proposal for a major rethink. IEEE Secur. Privacy. 2011; 10 (2):24–32. [ Google Scholar ]
- Kirton M. Adaptors and innovators - description and measure. J. Appl. Psychol. 1976; 61 (5):622–629. doi: 10.1037/0021-9010.61.5.622. [ CrossRef ] [ Google Scholar ]
- Kousha, K., Thelwall, M., 2020. COVID-19 publications: database coverage, citations, readers, tweets, news, facebook walls, reddit posts. arXiv:2004.10400
- Kouzy R., Abi Jaoude J., Kraitem A., El Alam M.B., Karam B., Adib E., Zarka J., Traboulsi C., Akl E.W., Baddour K. Coronavirus goes viral: quantifying the COVID-19misinformation epidemic on twitter. Cureus. 2020; 12 (3):e7255. doi: 10.7759/cureus.7255. [ PMC free article ] [ PubMed ] [ CrossRef ] [ Google Scholar ]
- InterStats, 2020. Analyse conjoncturelle des crimes et délits enregistrés par la police et la gendarmerie à la fin du mois d'août 2020 . Paris, France: Service statistique ministériel de la sécurité intérieure. Retrieved from: https://www.interieur.gouv.fr/Interstats/Actualites/Interstats-Conjoncture-N-60-Septembre-2020
- Kumaran, N., Lugani, S., 2020. Protecting businesses against cyber threats during COVID-19 and beyond. Google Cloud. Accessed: 2023-02-27. https://cloud.google.com/blog/products/identity-security/protecting-against-cyber-threats-during-covid-19-and-beyond .
- Laan, J., 2021. The impact of the corona-pandemic on the business model of cybercrime. http://essay.utwente.nl/87830/ .
- Lallie H.S., Shepherd L.A., Nurse J.R., Erola A., Epiphaniou G., Maple C., Bellekens X. Cyber security in the age of COVID-19: a timeline and analysis of cyber-crime and cyber-attacks during the pandemic. Comput. Secur. 2021; 105 :102248. doi: 10.1016/j.cose.2021.102248. [ PMC free article ] [ PubMed ] [ CrossRef ] [ Google Scholar ]
- Lastdrager E. From Fishing to Phishing. University of Twente, Netherlands; 2018. [ Google Scholar ]
- Le, Q. V., Mikolov, T., 2014. Distributed representations of sentences and documents. CoRR abs/1405.4053 . http://arxiv.org/abs/1405.4053 .
- Legg P., Blackman T. 2019 International Conference on Cyber Situational Awareness, Data Analytics And Assessment (Cyber SA) 2019. Tools and techniques for improving cyber situational awareness of targeted phishing attacks; pp. 1–4. [ CrossRef ] [ Google Scholar ]
- Levi M., Smith R.G. Technical Report. Australian Institute of Criminology; 2021. Fraud and its Relationship to Pandemics and Economic Crises: From Spanish flu to COVID-19. [ Google Scholar ]
- Lin, T., Capecci, D. E., Ellis, D. M., Rocha, H. A., Dommaraju, S., Oliveira, D. S., Ebner, N. C., 2019. Susceptibility to spear-phishing emails: effects of internet user demographics and email content 26(5). 10.1145/3336141 [ PMC free article ] [ PubMed ]
- Liu C., Stamm S. Proceedings of the Anti-Phishing Working Groups 2nd Annual eCrime Researchers Summit. 2007. Fighting unicode-obfuscated spam; pp. 45–59. [ Google Scholar ]
- Lloyd S. Least squares quantization in PCM. IEEE Trans. Inf. Theory. 1982; 28 (2):129–137. [ Google Scholar ]
- Luhn H.P. A statistical approach to mechanized encoding and searching of literary information. IBM J. Res. Dev. 1957; 1 (4):309–317. doi: 10.1147/rd.14.0309. [ CrossRef ] [ Google Scholar ]
- Mabey, B., 2021. pyldavis 3.1. Accessed 2021-12-10. https://pypi.org/project/pyLDAvis/ .
- Manning C.D., Raghavan P., Schütze H., et al. vol. 1. Cambridge University Press Cambridge; 2008. Introduction to Information Retrieval. [ Google Scholar ]
- Martin D., Wu H., Alsaid A. Hidden surveillance by web sites: web bugs in contemporary use. Commun. ACM. 2003; 46 (12):258–264. [ Google Scholar ]
- Mathieu E., Ritchie H., Rodés-Guirao L., Appel C., Giattino C., Hasell J., Macdonald B., Dattani S., Beltekian D., Ortiz-Ospina E., Roser M. Our World in Data. 2020. Coronavirus pandemic (COVID-19) [ Google Scholar ] https://ourworldindata.org/coronavirus
- Mailtrap, 2022.   and html space challenges and tricks. Accessed 2022-01-07. https://mailtrap.io/blog/nbsp/ .
- Matplotlib.org, 2022. Matplotlib - Visualization with Python. Accessed: 2022-06-30. https://matplotlib.org/ .
- Maymí F., Bixler R., Jones R., Lathrop S. 2017 IEEE International Conference on Big Data (Big Data) IEEE; 2017. Towards a definition of cyberspace tactics, techniques and procedures; pp. 4674–4679. [ Google Scholar ]
- McGrath, D. K., Gupta, M., 2008. Behind phishing: an examination of phisher modi operandi https://www.usenix.org/legacy/event/leet08/tech/full_papers/mcgrath/mcgrath_html/ .
- McRae C.M., Vaughn R.B. 2007 40th Annual Hawaii International Conference on System Sciences (HICSS’07) 2007. Phighting the phisher: using web bugs and honeytokens to investigate the source of phishing attacks; p. 270c. [ CrossRef ] [ Google Scholar ]
- Mimecast, 2020. Coronavirus phishing attacks speed up across the globe | mimecast blog. Accessed: 2020-08-10. https://www.mimecast.com/blog/coronavirus-phishing-attacks-speed-up-globally/ .
- Mohler G., Bertozzi A.L., Carter J., Short M.B., Sledge D., Tita G.E., Uchida C.D., Brantingham P.J. Impact of social distancing during COVID-19 pandemic on crime in los angeles and Indianapolis. J. Crim. Just. 2020; 68 :101692. [ PMC free article ] [ PubMed ] [ Google Scholar ]
- Moore T., Clayton R. Proceedings of the Anti-Phishing Working Groups 2nd Annual ECrime Researchers Summit. Association for Computing Machinery; New York, NY, USA: 2007. Examining the impact of website take-down on phishing; pp. 1–13. [ CrossRef ] [ Google Scholar ]
- Nicola M., Alsafi Z., Sohrabi C., Kerwan A., Al-Jabir A., Iosifidis C., Agha M., Agha R. The socio-economic implications of the coronavirus pandemic (COVID-19): a review. Int. J. Surg. 2020; 78 :185. [ PMC free article ] [ PubMed ] [ Google Scholar ]
- Niu W., Zhang X., Yang G., Ma Z., Zhuo Z. 2017 IEEE International Symposium on Parallel and Distributed Processing with Applications and 2017 IEEE International Conference on Ubiquitous Computing and Communications (ISPA/IUCC) IEEE; 2017. Phishing emails detection using CS-SVM; pp. 1054–1059. [ Google Scholar ]
- Ministerie van Volksgezondheid W. e. S., 2023. Confirmed cases | Coronavirus Dashboard | Government.nl. Accessed: 2022-03-27. https://coronadashboard.government.nl .
- NLTK, 2021. Natural language toolkit (NLTK). Accessed: 2021-12-15, https://github.com/nltk/nltk .
- Oest A., Zhang P., Wardman B., Nunes E., Burgis J., Zand A., Thomas K., Doupé A., Ahn G.-J. Proceedings of the 29th USENIX Conference on Security Symposium. USENIX Association; USA: 2020. Sunrise to sunset: analyzing the end-to-end life cycle and effectiveness of phishing attacks at scale. [ Google Scholar ]
- Office for National Statistics UK, August, 2020. https://www.gov.uk/government/statistics/coronavirus-and-crime-in-england-and-wales-august-2020 .
- Patgiri R., Katari H., Kumar R., Sharma D. International Conference on Distributed Computing and Internet Technology. Springer; 2019. Empirical study on malicious url detection using machine learning; pp. 380–388. [ Google Scholar ]
- Patil D.R., Patil J.B. Malicious urls detection using decision tree classifiers and majority voting technique. Cybern. Inf. Technol. 2018; 18 (1):11–29. [ Google Scholar ]
- Petelka J., Zou Y., Schaub F. Proceedings of the 2019 CHI Conference on Human Factors in Computing Systems. 2019. Put your warning where your link is: Improving and evaluating email phishing warnings; pp. 1–15. [ Google Scholar ]
- Pletinckx S., Jansen G.H., Brussen A., van Wegberg R. 2021 12th International Conference on Information and Communication Systems (ICICS) 2021. Cash for the register? Capturing rationales of early COVID-19 domain registrations at internet-scale; pp. 41–48. [ CrossRef ] [ Google Scholar ]
- Rameem Zahra S., Ahsan Chishti M., Iqbal Baba A., Wu F. Detecting COVID-19 chaos driven phishing/malicious url attacks by a fuzzy logic and data mining based intelligence system. Egyptian Inform. J. 2021 doi: 10.1016/j.eij.2021.12.003. [ CrossRef ] [ Google Scholar ]
- Ramzan Z., Wüest C. CEAS. Citeseer; 2007. Phishing attacks: analyzing trends in 2006. [ Google Scholar ]
- Rechtsraak, D., 2022. Uitspraak, afdeling strafrecht. Accessed: 2023-14-01, https://uitspraken.rechtspraak.nl/#!/details?id=ECLI:NL:GHARL:2022:10845 .
- Řehuřek, R., 2021. Gensim: topic modelling for humans. Accessed: 2021-12-15, https://radimrehurek.com/gensim/models/ldamodel.html .
- Röder M., Both A., Hinneburg A. Proceedings of the Eighth ACM International Conference on Web Search and Data Mining. Association for Computing Machinery; New York, NY, USA: 2015. Exploring the space of topic coherence measures; pp. 399–408. [ CrossRef ] [ Google Scholar ]
- Sahingoz O.K., Buber E., Demir O., Diri B. Machine learning based phishing detection from urls. Expert Syst. Appl. 2019; 117 :345–357. [ Google Scholar ]
- Sarno D.M., Black J., Harris K., Harris M., Koontz P., Paradise E. Proceedings of the Human Factors and Ergonomics Society Annual Meeting. vol. 66. SAGE Publications Sage CA: Los Angeles, CA; 2022. Fall for one, fall for all: understanding deception detection in phishing emails, scam texts messages, and fake news headlines; p. 1115. [ Google Scholar ]
- Sharevski F., Devine A., Pieroni E., Jachim P. Proceedings of the 2022 European Symposium on Usable Security. Association for Computing Machinery; New York, NY, USA: 2022. Phishing with malicious QR codes; pp. 160–171. [ CrossRef ] [ Google Scholar ]
- Sherman L.W., Gartin P.R., Buerger M.E. Hot spots of predatory crime: routine activities and the criminology of place*. Criminology. 1989; 27 (1):27–56. [ Google Scholar ]
- Sood A.K., Talluri S., Nagal A., SL R.R., Chaturvedi R. The COVID-19 threat landscape. Comput. Fraud Secur. 2021; 2021 (9):10–15. [ Google Scholar ]
- Spark Jones K. A statistical interpretation of term specificity and its application in retrieval. J. Doc. 1972; 28 (1):11–21. doi: 10.1108/eb026526. [ CrossRef ] [ Google Scholar ]
- Tilley N., Sidebottom A. Wiley; Oxford, UK: 2015. Routine Activities and Opportunity Theory; pp. 331–348. [ Google Scholar ] book section 21
- Toolan F., Carthy J. 2010 eCrime Researchers Summit, 2010. vol. 7017. 2010. Feature selection for spam and phishing detection; pp. 1–12. [ CrossRef ] [ Google Scholar ]
- Tsow A., Jakobsson M. Indiana University; 2007. Deceit and Deception: A Large User Study of Phishing; p. 2007. [ Google Scholar ] Retrieved September 9
- Van Der Heijden A., Allodi L. 28th USENIX Security Symposium (USENIX Security 19) 2019. Cognitive triaging of phishing attacks; pp. 1309–1326. [ Google Scholar ]
- van Kesteren J., van Dijk J., Mayhew P. The international crime victims surveys: aretrospective. Int. Rev. Vict. 2013; 20 (1):49–69. [ Google Scholar ]
- Venkatesha S., Reddy K.R., Chandavarkar B. Social engineering attacks during the COVID-19pandemic. SN Comput. Sci. 2021; 2 (2):1–9. doi: 10.1007/s42979-020-00443-1. [ PMC free article ] [ PubMed ] [ CrossRef ] [ Google Scholar ]
- Verma R., Das A. Proceedings of the 3rd ACM on International Workshop on Security and Privacy Analytics. 2017. What’s in a url: fast feature extraction and malicious url detection; pp. 55–63. [ Google Scholar ]
- VirusTotal, 2020. Virustotal api: getting started with v2. Accessed: 2020-11-23, https://developers.virustotal.com/reference/overview .
- VirusTotal, 2022. How it works. Accessed: 2022-01-08, https://support.virustotal.com/hc/en-us/articles/115002126889-How-it-works .
- Walker P., Whittaker C., Watson O., Baguelin M., Ainslie K., Bhatia S., Bhatt S., Boonyasiri A., Boyd O., Cattarino L. Journal Article. Imperial College London; 2020. Report 12: The Global Impact of COVID-19 and Strategies for Mitigation and Suppression. [ CrossRef ] [ Google Scholar ]
- Wang G., Kwok S.W.H. 2021 IEEE EMBS International Conference on Biomedical and Health Informatics (BHI) 2021. Using k -means clustering method with Doc2Vec to understand the twitter users’ opinions on COVID-19 vaccination; pp. 1–4. [ CrossRef ] [ Google Scholar ]
- Xia P., Nabeel M., Khalil I., Wang H., Yu T. Proceedings of the Eleventh ACM Conference on Data and Application Security and Privacy. Association for Computing Machinery; New York, NY, USA: 2021. Identifying and characterizing COVID-19 themed malicious domain campaigns; pp. 209–220. [ CrossRef ] [ Google Scholar ]
- Yearwood J., Mammadov M., Webb D. Profiling phishing activity based on hyperlinks extracted from phishing emails. Soc. Netw. Anal. Min. 2012; 2 (1):5–16. [ Google Scholar ]
- Zubair M., Asif Iqbal M., Shil A., Haque E., Moshiul Hoque M., Sarker I.H. In: Hybrid Intelligent Systems. Abraham A., Hanne T., Castillo O., Gandhi N., Nogueira Rios T., Hong T.-P., editors. Springer International Publishing; Cham: 2021. An efficient k -means clustering algorithm for analysing COVID-19; pp. 422–432. [ Google Scholar ]
- WHO, 2020. Shortage of personal protective equipment endangering health workers worldwide. Accessed: 2023-01-14. https://www.who.int/news/item/03-03-2020-shortage-of-personal-protective-equipment-endangering-health-workers-worldwide .
- World Health Organization, 2022. Timeline: WHO’s COVID-19 response. Accessed: 2022-03-07. https://www.who.int/emergencies/diseases/novel-coronavirus-2019/interactive-timeline .
IEEE Account
- Change Username/Password
- Update Address
Purchase Details
- Payment Options
- Order History
- View Purchased Documents
Profile Information
- Communications Preferences
- Profession and Education
- Technical Interests
- US & Canada: +1 800 678 4333
- Worldwide: +1 732 981 0060
- Contact & Support
- About IEEE Xplore
- Accessibility
- Terms of Use
- Nondiscrimination Policy
- Privacy & Opting Out of Cookies
A not-for-profit organization, IEEE is the world's largest technical professional organization dedicated to advancing technology for the benefit of humanity. © Copyright 2024 IEEE - All rights reserved. Use of this web site signifies your agreement to the terms and conditions.
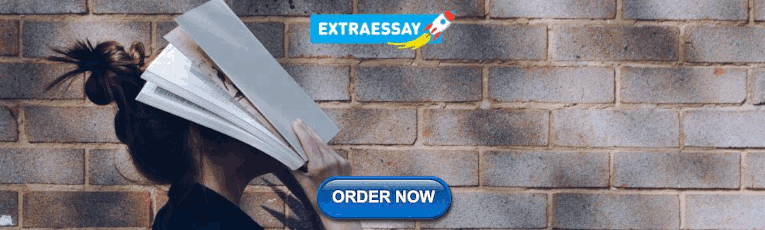
IMAGES
VIDEO
COMMENTS
However, info-security professionals reported a higher frequency of all types of social engineering attacks year-on-year according to a report presented by Proofpoint. Spear phishing increased to 64% in 2018 from 53% in 2017, Vishing and/or SMishing increased to 49% from 45%, and USB attacks increased to 4% from 3%.
This article proposes a new detailed. anatomy of phishing which involves attack phases, attacker 's types, vulnerabilities, threats, targets, attack mediums, and attacking techniques. Moreover ...
Phishing is a social engineering attack (Paliath et al., 2020, Nakamura and Dobashi, 2019, Zabihimayvan and Doran, 2019) identified as the most common method used by cybercriminals to get access to an internet user's personal information such as credit card information, usernames, and passwords (Ramana et al., 2021, Faris and Yazid, 2021).Sometimes, attackers perform phishing attacks to ...
The paper presents the outcomes of SLR conducted while focusing on four research questions. The paper advocates that technology-only solutions are never going to be enough to protect against attacks targeted toward human users, therefore, there is a need to consider the role and abilities of human users in the development of anti-phishing ...
Phishing attacks have been the most common crime from 2020, with phishing incidents nearly doubled in regularity . Business Email Compromise attacks are the most common and amount to huge losses. These phishing attacks come in the form of a request, urgent, important, seeking attention and often requiring some form of payment .
anti-phishing toolbars, machine learning, and artificial intelligence are among t he technolo gies. deployed to detect and prevent phishing attacks. Continuous research and innovation, along with ...
Still within the large body of initial research on phishing attacks and COVID‐19, other papers investigated a number of more specific issues. For example, the work in [ 20 ] focussed on challenges of the heathcare sector, by outlining why cyber‐attacks have been particularly problematic during COVID‐19 and by defining the ways in which ...
Abstract. Phishing is the fraudulent attempt to obtain sensitive information by disguising oneself as a trustworthy entity in digital communication. It is a type of cyber attack often successful because users are not aware of their vulnerabilities or are unable to understand the risks. This article presents a systematic literature review ...
The evolution of phishing, its life cycle, and attacker motivation are also covered. A detailed taxonomy of phishing attacks on desktops as well as mobile devices is presented. Various open research challenges or research gaps have been identified and discussed. Characteristics of phishing attacks during the COVID-19 pandemic are studied in ...
This research paper presents a comprehensive study of phishing attacks and their countermeasures. Phishing attacks are a major threat to individuals and organizations worldwide, and understanding ...
Phishing attacks are on the increase. The fact that our ways of living, studying and working have drastically changed as a result of the COVID pandemic (i.e., almost everything being done online) has created many new cyber security concerns. In particular, with the move to remote working, the number of phishing emails threatening employees has increased. The 2020 Phishing Attack Landscape ...
Therefore, this paper presents a detailed analysis of phishing attack methods and defense techniques. This survey is presented in five folds. First, we discuss in detail the lifecycle of phishing attack, its history, and motivation behind this attack. Second, we present various distribution methods that are used to spread phishing attacks.
This paper explores continual learning (CL) techniques for sustained phishing detection performance over time. To demonstrate this behavior, we collect phishing and benign samples for three ...
This paper also presents the comparison of different studies detecting the phishing attack for each AI technique and examines the qualities and shortcomings of these methodologies. Furthermore, this paper provides a comprehensive set of current challenges of phishing attacks and future research direction in this domain.
Phishing attacks are a growing concern for individuals and organizations alike, with the potential to cause significant financial and reputational damage. Traditional methods for detecting phishing attacks, such as blacklists and signature-based techniques, have limitations that have led to developing more advanced techniques. In recent years, machine learning and deep learning techniques have ...
Phishing Attacks: A Recent Comprehensive Study and a New Anatomy. Zainab Alkhalil, Chaminda Hewage*, Liqaa Nawaf and Imtiaz Khan. Cardiff School of Technologies, Cardiff Metropolitan University, Cardiff, United Kingdom. With the significant growth of internet usage, people increasingly share their personal information online.
To summarize, this paper makes the following contributions: 1) Extensive measurement study on human factors of phish-ing and phishing prevention in large organizations. 2) Supportive results for several previous research findings with improved ecological validity. 3) Contradicting findings that challenge the conclusions of
Phishing offenses are increasing, resulting in billions of dollars in loss 1.In these attacks, users enter their critical (i.e., credit card details, passwords, etc.) to the forged website which ...
A survey of Al-Qahtani and Cresci, 2022 reviewed 54 studies about phishing attacks and analysed the modus operandi and the proposed techniques for detecting COVID-19 phishing, smishing, and vishing attacks. As indicated in the Microsoft Digital Defense Report, phishing attacks consist of almost 70% of all cyber attacks (Kaliňák, 2021).
these attacks use social engineering techniques to deceive endusers, indicating the importance of user- -focused studies to help prevent future attacks. We provide a detailed overview of phishing research that has focused on users by conducting a systematic literature review of peer-reviewed academic papers published in ACM Digital Library.
h to find out different types of phishing and anti-phishing techniques. Research study evaluated that spear phishing, Email Spoofing, Email Manipul. tion and phone phishing are the most commonly used phishing techniques. On the other hand, according to the SLR, machine learning approaches have the highest accuracy of preventing.
The persistent and evolving threat of phishing attacks demands effective and adaptive detection techniques. This research paper presents a comprehensive evaluation and comparison of various machine learning approaches to detect phishing attacks. We investigated five prominent algorithms: Logistic Regression, Support Vector Machine (SVM), K-Nearest Neighbors (K-NN), Naive Bayes, and Extreme ...
Phishing is. one such type of methodologies which are used to acquire the. information. Phishing is a cyber crime in which emails, telephone, text messages, personally identifiable information ...