What is Empirical Research? Definition, Methods, Examples
Appinio Research · 09.02.2024 · 36min read
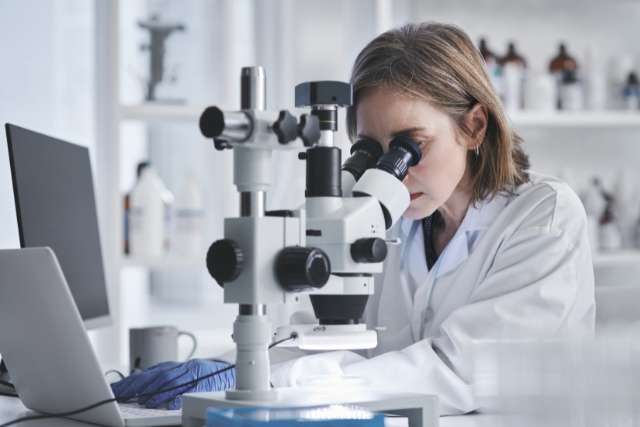
Ever wondered how we gather the facts, unveil hidden truths, and make informed decisions in a world filled with questions? Empirical research holds the key.
In this guide, we'll delve deep into the art and science of empirical research, unraveling its methods, mysteries, and manifold applications. From defining the core principles to mastering data analysis and reporting findings, we're here to equip you with the knowledge and tools to navigate the empirical landscape.
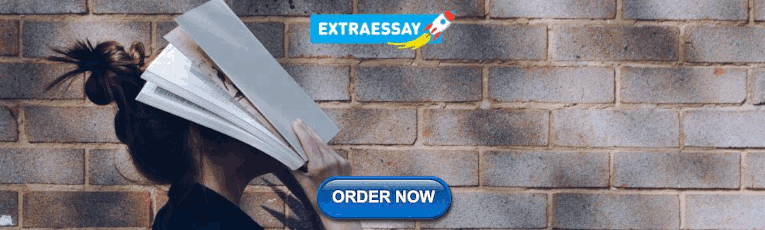
What is Empirical Research?
Empirical research is the cornerstone of scientific inquiry, providing a systematic and structured approach to investigating the world around us. It is the process of gathering and analyzing empirical or observable data to test hypotheses, answer research questions, or gain insights into various phenomena. This form of research relies on evidence derived from direct observation or experimentation, allowing researchers to draw conclusions based on real-world data rather than purely theoretical or speculative reasoning.
Characteristics of Empirical Research
Empirical research is characterized by several key features:
- Observation and Measurement : It involves the systematic observation or measurement of variables, events, or behaviors.
- Data Collection : Researchers collect data through various methods, such as surveys, experiments, observations, or interviews.
- Testable Hypotheses : Empirical research often starts with testable hypotheses that are evaluated using collected data.
- Quantitative or Qualitative Data : Data can be quantitative (numerical) or qualitative (non-numerical), depending on the research design.
- Statistical Analysis : Quantitative data often undergo statistical analysis to determine patterns , relationships, or significance.
- Objectivity and Replicability : Empirical research strives for objectivity, minimizing researcher bias . It should be replicable, allowing other researchers to conduct the same study to verify results.
- Conclusions and Generalizations : Empirical research generates findings based on data and aims to make generalizations about larger populations or phenomena.
Importance of Empirical Research
Empirical research plays a pivotal role in advancing knowledge across various disciplines. Its importance extends to academia, industry, and society as a whole. Here are several reasons why empirical research is essential:
- Evidence-Based Knowledge : Empirical research provides a solid foundation of evidence-based knowledge. It enables us to test hypotheses, confirm or refute theories, and build a robust understanding of the world.
- Scientific Progress : In the scientific community, empirical research fuels progress by expanding the boundaries of existing knowledge. It contributes to the development of theories and the formulation of new research questions.
- Problem Solving : Empirical research is instrumental in addressing real-world problems and challenges. It offers insights and data-driven solutions to complex issues in fields like healthcare, economics, and environmental science.
- Informed Decision-Making : In policymaking, business, and healthcare, empirical research informs decision-makers by providing data-driven insights. It guides strategies, investments, and policies for optimal outcomes.
- Quality Assurance : Empirical research is essential for quality assurance and validation in various industries, including pharmaceuticals, manufacturing, and technology. It ensures that products and processes meet established standards.
- Continuous Improvement : Businesses and organizations use empirical research to evaluate performance, customer satisfaction, and product effectiveness. This data-driven approach fosters continuous improvement and innovation.
- Human Advancement : Empirical research in fields like medicine and psychology contributes to the betterment of human health and well-being. It leads to medical breakthroughs, improved therapies, and enhanced psychological interventions.
- Critical Thinking and Problem Solving : Engaging in empirical research fosters critical thinking skills, problem-solving abilities, and a deep appreciation for evidence-based decision-making.
Empirical research empowers us to explore, understand, and improve the world around us. It forms the bedrock of scientific inquiry and drives progress in countless domains, shaping our understanding of both the natural and social sciences.
How to Conduct Empirical Research?
So, you've decided to dive into the world of empirical research. Let's begin by exploring the crucial steps involved in getting started with your research project.
1. Select a Research Topic
Selecting the right research topic is the cornerstone of a successful empirical study. It's essential to choose a topic that not only piques your interest but also aligns with your research goals and objectives. Here's how to go about it:
- Identify Your Interests : Start by reflecting on your passions and interests. What topics fascinate you the most? Your enthusiasm will be your driving force throughout the research process.
- Brainstorm Ideas : Engage in brainstorming sessions to generate potential research topics. Consider the questions you've always wanted to answer or the issues that intrigue you.
- Relevance and Significance : Assess the relevance and significance of your chosen topic. Does it contribute to existing knowledge? Is it a pressing issue in your field of study or the broader community?
- Feasibility : Evaluate the feasibility of your research topic. Do you have access to the necessary resources, data, and participants (if applicable)?
2. Formulate Research Questions
Once you've narrowed down your research topic, the next step is to formulate clear and precise research questions . These questions will guide your entire research process and shape your study's direction. To create effective research questions:
- Specificity : Ensure that your research questions are specific and focused. Vague or overly broad questions can lead to inconclusive results.
- Relevance : Your research questions should directly relate to your chosen topic. They should address gaps in knowledge or contribute to solving a particular problem.
- Testability : Ensure that your questions are testable through empirical methods. You should be able to gather data and analyze it to answer these questions.
- Avoid Bias : Craft your questions in a way that avoids leading or biased language. Maintain neutrality to uphold the integrity of your research.
3. Review Existing Literature
Before you embark on your empirical research journey, it's essential to immerse yourself in the existing body of literature related to your chosen topic. This step, often referred to as a literature review, serves several purposes:
- Contextualization : Understand the historical context and current state of research in your field. What have previous studies found, and what questions remain unanswered?
- Identifying Gaps : Identify gaps or areas where existing research falls short. These gaps will help you formulate meaningful research questions and hypotheses.
- Theory Development : If your study is theoretical, consider how existing theories apply to your topic. If it's empirical, understand how previous studies have approached data collection and analysis.
- Methodological Insights : Learn from the methodologies employed in previous research. What methods were successful, and what challenges did researchers face?
4. Define Variables
Variables are fundamental components of empirical research. They are the factors or characteristics that can change or be manipulated during your study. Properly defining and categorizing variables is crucial for the clarity and validity of your research. Here's what you need to know:
- Independent Variables : These are the variables that you, as the researcher, manipulate or control. They are the "cause" in cause-and-effect relationships.
- Dependent Variables : Dependent variables are the outcomes or responses that you measure or observe. They are the "effect" influenced by changes in independent variables.
- Operational Definitions : To ensure consistency and clarity, provide operational definitions for your variables. Specify how you will measure or manipulate each variable.
- Control Variables : In some studies, controlling for other variables that may influence your dependent variable is essential. These are known as control variables.
Understanding these foundational aspects of empirical research will set a solid foundation for the rest of your journey. Now that you've grasped the essentials of getting started, let's delve deeper into the intricacies of research design.
Empirical Research Design
Now that you've selected your research topic, formulated research questions, and defined your variables, it's time to delve into the heart of your empirical research journey – research design . This pivotal step determines how you will collect data and what methods you'll employ to answer your research questions. Let's explore the various facets of research design in detail.
Types of Empirical Research
Empirical research can take on several forms, each with its own unique approach and methodologies. Understanding the different types of empirical research will help you choose the most suitable design for your study. Here are some common types:
- Experimental Research : In this type, researchers manipulate one or more independent variables to observe their impact on dependent variables. It's highly controlled and often conducted in a laboratory setting.
- Observational Research : Observational research involves the systematic observation of subjects or phenomena without intervention. Researchers are passive observers, documenting behaviors, events, or patterns.
- Survey Research : Surveys are used to collect data through structured questionnaires or interviews. This method is efficient for gathering information from a large number of participants.
- Case Study Research : Case studies focus on in-depth exploration of one or a few cases. Researchers gather detailed information through various sources such as interviews, documents, and observations.
- Qualitative Research : Qualitative research aims to understand behaviors, experiences, and opinions in depth. It often involves open-ended questions, interviews, and thematic analysis.
- Quantitative Research : Quantitative research collects numerical data and relies on statistical analysis to draw conclusions. It involves structured questionnaires, experiments, and surveys.
Your choice of research type should align with your research questions and objectives. Experimental research, for example, is ideal for testing cause-and-effect relationships, while qualitative research is more suitable for exploring complex phenomena.
Experimental Design
Experimental research is a systematic approach to studying causal relationships. It's characterized by the manipulation of one or more independent variables while controlling for other factors. Here are some key aspects of experimental design:
- Control and Experimental Groups : Participants are randomly assigned to either a control group or an experimental group. The independent variable is manipulated for the experimental group but not for the control group.
- Randomization : Randomization is crucial to eliminate bias in group assignment. It ensures that each participant has an equal chance of being in either group.
- Hypothesis Testing : Experimental research often involves hypothesis testing. Researchers formulate hypotheses about the expected effects of the independent variable and use statistical analysis to test these hypotheses.
Observational Design
Observational research entails careful and systematic observation of subjects or phenomena. It's advantageous when you want to understand natural behaviors or events. Key aspects of observational design include:
- Participant Observation : Researchers immerse themselves in the environment they are studying. They become part of the group being observed, allowing for a deep understanding of behaviors.
- Non-Participant Observation : In non-participant observation, researchers remain separate from the subjects. They observe and document behaviors without direct involvement.
- Data Collection Methods : Observational research can involve various data collection methods, such as field notes, video recordings, photographs, or coding of observed behaviors.
Survey Design
Surveys are a popular choice for collecting data from a large number of participants. Effective survey design is essential to ensure the validity and reliability of your data. Consider the following:
- Questionnaire Design : Create clear and concise questions that are easy for participants to understand. Avoid leading or biased questions.
- Sampling Methods : Decide on the appropriate sampling method for your study, whether it's random, stratified, or convenience sampling.
- Data Collection Tools : Choose the right tools for data collection, whether it's paper surveys, online questionnaires, or face-to-face interviews.
Case Study Design
Case studies are an in-depth exploration of one or a few cases to gain a deep understanding of a particular phenomenon. Key aspects of case study design include:
- Single Case vs. Multiple Case Studies : Decide whether you'll focus on a single case or multiple cases. Single case studies are intensive and allow for detailed examination, while multiple case studies provide comparative insights.
- Data Collection Methods : Gather data through interviews, observations, document analysis, or a combination of these methods.
Qualitative vs. Quantitative Research
In empirical research, you'll often encounter the distinction between qualitative and quantitative research . Here's a closer look at these two approaches:
- Qualitative Research : Qualitative research seeks an in-depth understanding of human behavior, experiences, and perspectives. It involves open-ended questions, interviews, and the analysis of textual or narrative data. Qualitative research is exploratory and often used when the research question is complex and requires a nuanced understanding.
- Quantitative Research : Quantitative research collects numerical data and employs statistical analysis to draw conclusions. It involves structured questionnaires, experiments, and surveys. Quantitative research is ideal for testing hypotheses and establishing cause-and-effect relationships.
Understanding the various research design options is crucial in determining the most appropriate approach for your study. Your choice should align with your research questions, objectives, and the nature of the phenomenon you're investigating.
Data Collection for Empirical Research
Now that you've established your research design, it's time to roll up your sleeves and collect the data that will fuel your empirical research. Effective data collection is essential for obtaining accurate and reliable results.
Sampling Methods
Sampling methods are critical in empirical research, as they determine the subset of individuals or elements from your target population that you will study. Here are some standard sampling methods:
- Random Sampling : Random sampling ensures that every member of the population has an equal chance of being selected. It minimizes bias and is often used in quantitative research.
- Stratified Sampling : Stratified sampling involves dividing the population into subgroups or strata based on specific characteristics (e.g., age, gender, location). Samples are then randomly selected from each stratum, ensuring representation of all subgroups.
- Convenience Sampling : Convenience sampling involves selecting participants who are readily available or easily accessible. While it's convenient, it may introduce bias and limit the generalizability of results.
- Snowball Sampling : Snowball sampling is instrumental when studying hard-to-reach or hidden populations. One participant leads you to another, creating a "snowball" effect. This method is common in qualitative research.
- Purposive Sampling : In purposive sampling, researchers deliberately select participants who meet specific criteria relevant to their research questions. It's often used in qualitative studies to gather in-depth information.
The choice of sampling method depends on the nature of your research, available resources, and the degree of precision required. It's crucial to carefully consider your sampling strategy to ensure that your sample accurately represents your target population.
Data Collection Instruments
Data collection instruments are the tools you use to gather information from your participants or sources. These instruments should be designed to capture the data you need accurately. Here are some popular data collection instruments:
- Questionnaires : Questionnaires consist of structured questions with predefined response options. When designing questionnaires, consider the clarity of questions, the order of questions, and the response format (e.g., Likert scale , multiple-choice).
- Interviews : Interviews involve direct communication between the researcher and participants. They can be structured (with predetermined questions) or unstructured (open-ended). Effective interviews require active listening and probing for deeper insights.
- Observations : Observations entail systematically and objectively recording behaviors, events, or phenomena. Researchers must establish clear criteria for what to observe, how to record observations, and when to observe.
- Surveys : Surveys are a common data collection instrument for quantitative research. They can be administered through various means, including online surveys, paper surveys, and telephone surveys.
- Documents and Archives : In some cases, data may be collected from existing documents, records, or archives. Ensure that the sources are reliable, relevant, and properly documented.
To streamline your process and gather insights with precision and efficiency, consider leveraging innovative tools like Appinio . With Appinio's intuitive platform, you can harness the power of real-time consumer data to inform your research decisions effectively. Whether you're conducting surveys, interviews, or observations, Appinio empowers you to define your target audience, collect data from diverse demographics, and analyze results seamlessly.
By incorporating Appinio into your data collection toolkit, you can unlock a world of possibilities and elevate the impact of your empirical research. Ready to revolutionize your approach to data collection?
Book a Demo
Data Collection Procedures
Data collection procedures outline the step-by-step process for gathering data. These procedures should be meticulously planned and executed to maintain the integrity of your research.
- Training : If you have a research team, ensure that they are trained in data collection methods and protocols. Consistency in data collection is crucial.
- Pilot Testing : Before launching your data collection, conduct a pilot test with a small group to identify any potential problems with your instruments or procedures. Make necessary adjustments based on feedback.
- Data Recording : Establish a systematic method for recording data. This may include timestamps, codes, or identifiers for each data point.
- Data Security : Safeguard the confidentiality and security of collected data. Ensure that only authorized individuals have access to the data.
- Data Storage : Properly organize and store your data in a secure location, whether in physical or digital form. Back up data to prevent loss.
Ethical Considerations
Ethical considerations are paramount in empirical research, as they ensure the well-being and rights of participants are protected.
- Informed Consent : Obtain informed consent from participants, providing clear information about the research purpose, procedures, risks, and their right to withdraw at any time.
- Privacy and Confidentiality : Protect the privacy and confidentiality of participants. Ensure that data is anonymized and sensitive information is kept confidential.
- Beneficence : Ensure that your research benefits participants and society while minimizing harm. Consider the potential risks and benefits of your study.
- Honesty and Integrity : Conduct research with honesty and integrity. Report findings accurately and transparently, even if they are not what you expected.
- Respect for Participants : Treat participants with respect, dignity, and sensitivity to cultural differences. Avoid any form of coercion or manipulation.
- Institutional Review Board (IRB) : If required, seek approval from an IRB or ethics committee before conducting your research, particularly when working with human participants.
Adhering to ethical guidelines is not only essential for the ethical conduct of research but also crucial for the credibility and validity of your study. Ethical research practices build trust between researchers and participants and contribute to the advancement of knowledge with integrity.
With a solid understanding of data collection, including sampling methods, instruments, procedures, and ethical considerations, you are now well-equipped to gather the data needed to answer your research questions.
Empirical Research Data Analysis
Now comes the exciting phase of data analysis, where the raw data you've diligently collected starts to yield insights and answers to your research questions. We will explore the various aspects of data analysis, from preparing your data to drawing meaningful conclusions through statistics and visualization.
Data Preparation
Data preparation is the crucial first step in data analysis. It involves cleaning, organizing, and transforming your raw data into a format that is ready for analysis. Effective data preparation ensures the accuracy and reliability of your results.
- Data Cleaning : Identify and rectify errors, missing values, and inconsistencies in your dataset. This may involve correcting typos, removing outliers, and imputing missing data.
- Data Coding : Assign numerical values or codes to categorical variables to make them suitable for statistical analysis. For example, converting "Yes" and "No" to 1 and 0.
- Data Transformation : Transform variables as needed to meet the assumptions of the statistical tests you plan to use. Common transformations include logarithmic or square root transformations.
- Data Integration : If your data comes from multiple sources, integrate it into a unified dataset, ensuring that variables match and align.
- Data Documentation : Maintain clear documentation of all data preparation steps, as well as the rationale behind each decision. This transparency is essential for replicability.
Effective data preparation lays the foundation for accurate and meaningful analysis. It allows you to trust the results that will follow in the subsequent stages.
Descriptive Statistics
Descriptive statistics help you summarize and make sense of your data by providing a clear overview of its key characteristics. These statistics are essential for understanding the central tendencies, variability, and distribution of your variables. Descriptive statistics include:
- Measures of Central Tendency : These include the mean (average), median (middle value), and mode (most frequent value). They help you understand the typical or central value of your data.
- Measures of Dispersion : Measures like the range, variance, and standard deviation provide insights into the spread or variability of your data points.
- Frequency Distributions : Creating frequency distributions or histograms allows you to visualize the distribution of your data across different values or categories.
Descriptive statistics provide the initial insights needed to understand your data's basic characteristics, which can inform further analysis.
Inferential Statistics
Inferential statistics take your analysis to the next level by allowing you to make inferences or predictions about a larger population based on your sample data. These methods help you test hypotheses and draw meaningful conclusions. Key concepts in inferential statistics include:
- Hypothesis Testing : Hypothesis tests (e.g., t-tests, chi-squared tests) help you determine whether observed differences or associations in your data are statistically significant or occurred by chance.
- Confidence Intervals : Confidence intervals provide a range within which population parameters (e.g., population mean) are likely to fall based on your sample data.
- Regression Analysis : Regression models (linear, logistic, etc.) help you explore relationships between variables and make predictions.
- Analysis of Variance (ANOVA) : ANOVA tests are used to compare means between multiple groups, allowing you to assess whether differences are statistically significant.
Inferential statistics are powerful tools for drawing conclusions from your data and assessing the generalizability of your findings to the broader population.
Qualitative Data Analysis
Qualitative data analysis is employed when working with non-numerical data, such as text, interviews, or open-ended survey responses. It focuses on understanding the underlying themes, patterns, and meanings within qualitative data. Qualitative analysis techniques include:
- Thematic Analysis : Identifying and analyzing recurring themes or patterns within textual data.
- Content Analysis : Categorizing and coding qualitative data to extract meaningful insights.
- Grounded Theory : Developing theories or frameworks based on emergent themes from the data.
- Narrative Analysis : Examining the structure and content of narratives to uncover meaning.
Qualitative data analysis provides a rich and nuanced understanding of complex phenomena and human experiences.
Data Visualization
Data visualization is the art of representing data graphically to make complex information more understandable and accessible. Effective data visualization can reveal patterns, trends, and outliers in your data. Common types of data visualization include:
- Bar Charts and Histograms : Used to display the distribution of categorical data or discrete data .
- Line Charts : Ideal for showing trends and changes in data over time.
- Scatter Plots : Visualize relationships and correlations between two variables.
- Pie Charts : Display the composition of a whole in terms of its parts.
- Heatmaps : Depict patterns and relationships in multidimensional data through color-coding.
- Box Plots : Provide a summary of the data distribution, including outliers.
- Interactive Dashboards : Create dynamic visualizations that allow users to explore data interactively.
Data visualization not only enhances your understanding of the data but also serves as a powerful communication tool to convey your findings to others.
As you embark on the data analysis phase of your empirical research, remember that the specific methods and techniques you choose will depend on your research questions, data type, and objectives. Effective data analysis transforms raw data into valuable insights, bringing you closer to the answers you seek.
How to Report Empirical Research Results?
At this stage, you get to share your empirical research findings with the world. Effective reporting and presentation of your results are crucial for communicating your research's impact and insights.
1. Write the Research Paper
Writing a research paper is the culmination of your empirical research journey. It's where you synthesize your findings, provide context, and contribute to the body of knowledge in your field.
- Title and Abstract : Craft a clear and concise title that reflects your research's essence. The abstract should provide a brief summary of your research objectives, methods, findings, and implications.
- Introduction : In the introduction, introduce your research topic, state your research questions or hypotheses, and explain the significance of your study. Provide context by discussing relevant literature.
- Methods : Describe your research design, data collection methods, and sampling procedures. Be precise and transparent, allowing readers to understand how you conducted your study.
- Results : Present your findings in a clear and organized manner. Use tables, graphs, and statistical analyses to support your results. Avoid interpreting your findings in this section; focus on the presentation of raw data.
- Discussion : Interpret your findings and discuss their implications. Relate your results to your research questions and the existing literature. Address any limitations of your study and suggest avenues for future research.
- Conclusion : Summarize the key points of your research and its significance. Restate your main findings and their implications.
- References : Cite all sources used in your research following a specific citation style (e.g., APA, MLA, Chicago). Ensure accuracy and consistency in your citations.
- Appendices : Include any supplementary material, such as questionnaires, data coding sheets, or additional analyses, in the appendices.
Writing a research paper is a skill that improves with practice. Ensure clarity, coherence, and conciseness in your writing to make your research accessible to a broader audience.
2. Create Visuals and Tables
Visuals and tables are powerful tools for presenting complex data in an accessible and understandable manner.
- Clarity : Ensure that your visuals and tables are clear and easy to interpret. Use descriptive titles and labels.
- Consistency : Maintain consistency in formatting, such as font size and style, across all visuals and tables.
- Appropriateness : Choose the most suitable visual representation for your data. Bar charts, line graphs, and scatter plots work well for different types of data.
- Simplicity : Avoid clutter and unnecessary details. Focus on conveying the main points.
- Accessibility : Make sure your visuals and tables are accessible to a broad audience, including those with visual impairments.
- Captions : Include informative captions that explain the significance of each visual or table.
Compelling visuals and tables enhance the reader's understanding of your research and can be the key to conveying complex information efficiently.
3. Interpret Findings
Interpreting your findings is where you bridge the gap between data and meaning. It's your opportunity to provide context, discuss implications, and offer insights. When interpreting your findings:
- Relate to Research Questions : Discuss how your findings directly address your research questions or hypotheses.
- Compare with Literature : Analyze how your results align with or deviate from previous research in your field. What insights can you draw from these comparisons?
- Discuss Limitations : Be transparent about the limitations of your study. Address any constraints, biases, or potential sources of error.
- Practical Implications : Explore the real-world implications of your findings. How can they be applied or inform decision-making?
- Future Research Directions : Suggest areas for future research based on the gaps or unanswered questions that emerged from your study.
Interpreting findings goes beyond simply presenting data; it's about weaving a narrative that helps readers grasp the significance of your research in the broader context.
With your research paper written, structured, and enriched with visuals, and your findings expertly interpreted, you are now prepared to communicate your research effectively. Sharing your insights and contributing to the body of knowledge in your field is a significant accomplishment in empirical research.
Examples of Empirical Research
To solidify your understanding of empirical research, let's delve into some real-world examples across different fields. These examples will illustrate how empirical research is applied to gather data, analyze findings, and draw conclusions.
Social Sciences
In the realm of social sciences, consider a sociological study exploring the impact of socioeconomic status on educational attainment. Researchers gather data from a diverse group of individuals, including their family backgrounds, income levels, and academic achievements.
Through statistical analysis, they can identify correlations and trends, revealing whether individuals from lower socioeconomic backgrounds are less likely to attain higher levels of education. This empirical research helps shed light on societal inequalities and informs policymakers on potential interventions to address disparities in educational access.
Environmental Science
Environmental scientists often employ empirical research to assess the effects of environmental changes. For instance, researchers studying the impact of climate change on wildlife might collect data on animal populations, weather patterns, and habitat conditions over an extended period.
By analyzing this empirical data, they can identify correlations between climate fluctuations and changes in wildlife behavior, migration patterns, or population sizes. This empirical research is crucial for understanding the ecological consequences of climate change and informing conservation efforts.
Business and Economics
In the business world, empirical research is essential for making data-driven decisions. Consider a market research study conducted by a business seeking to launch a new product. They collect data through surveys , focus groups , and consumer behavior analysis.
By examining this empirical data, the company can gauge consumer preferences, demand, and potential market size. Empirical research in business helps guide product development, pricing strategies, and marketing campaigns, increasing the likelihood of a successful product launch.
Psychological studies frequently rely on empirical research to understand human behavior and cognition. For instance, a psychologist interested in examining the impact of stress on memory might design an experiment. Participants are exposed to stress-inducing situations, and their memory performance is assessed through various tasks.
By analyzing the data collected, the psychologist can determine whether stress has a significant effect on memory recall. This empirical research contributes to our understanding of the complex interplay between psychological factors and cognitive processes.
These examples highlight the versatility and applicability of empirical research across diverse fields. Whether in medicine, social sciences, environmental science, business, or psychology, empirical research serves as a fundamental tool for gaining insights, testing hypotheses, and driving advancements in knowledge and practice.
Conclusion for Empirical Research
Empirical research is a powerful tool for gaining insights, testing hypotheses, and making informed decisions. By following the steps outlined in this guide, you've learned how to select research topics, collect data, analyze findings, and effectively communicate your research to the world. Remember, empirical research is a journey of discovery, and each step you take brings you closer to a deeper understanding of the world around you. Whether you're a scientist, a student, or someone curious about the process, the principles of empirical research empower you to explore, learn, and contribute to the ever-expanding realm of knowledge.
How to Collect Data for Empirical Research?
Introducing Appinio , the real-time market research platform revolutionizing how companies gather consumer insights for their empirical research endeavors. With Appinio, you can conduct your own market research in minutes, gaining valuable data to fuel your data-driven decisions.
Appinio is more than just a market research platform; it's a catalyst for transforming the way you approach empirical research, making it exciting, intuitive, and seamlessly integrated into your decision-making process.
Here's why Appinio is the go-to solution for empirical research:
- From Questions to Insights in Minutes : With Appinio's streamlined process, you can go from formulating your research questions to obtaining actionable insights in a matter of minutes, saving you time and effort.
- Intuitive Platform for Everyone : No need for a PhD in research; Appinio's platform is designed to be intuitive and user-friendly, ensuring that anyone can navigate and utilize it effectively.
- Rapid Response Times : With an average field time of under 23 minutes for 1,000 respondents, Appinio delivers rapid results, allowing you to gather data swiftly and efficiently.
- Global Reach with Targeted Precision : With access to over 90 countries and the ability to define target groups based on 1200+ characteristics, Appinio empowers you to reach your desired audience with precision and ease.
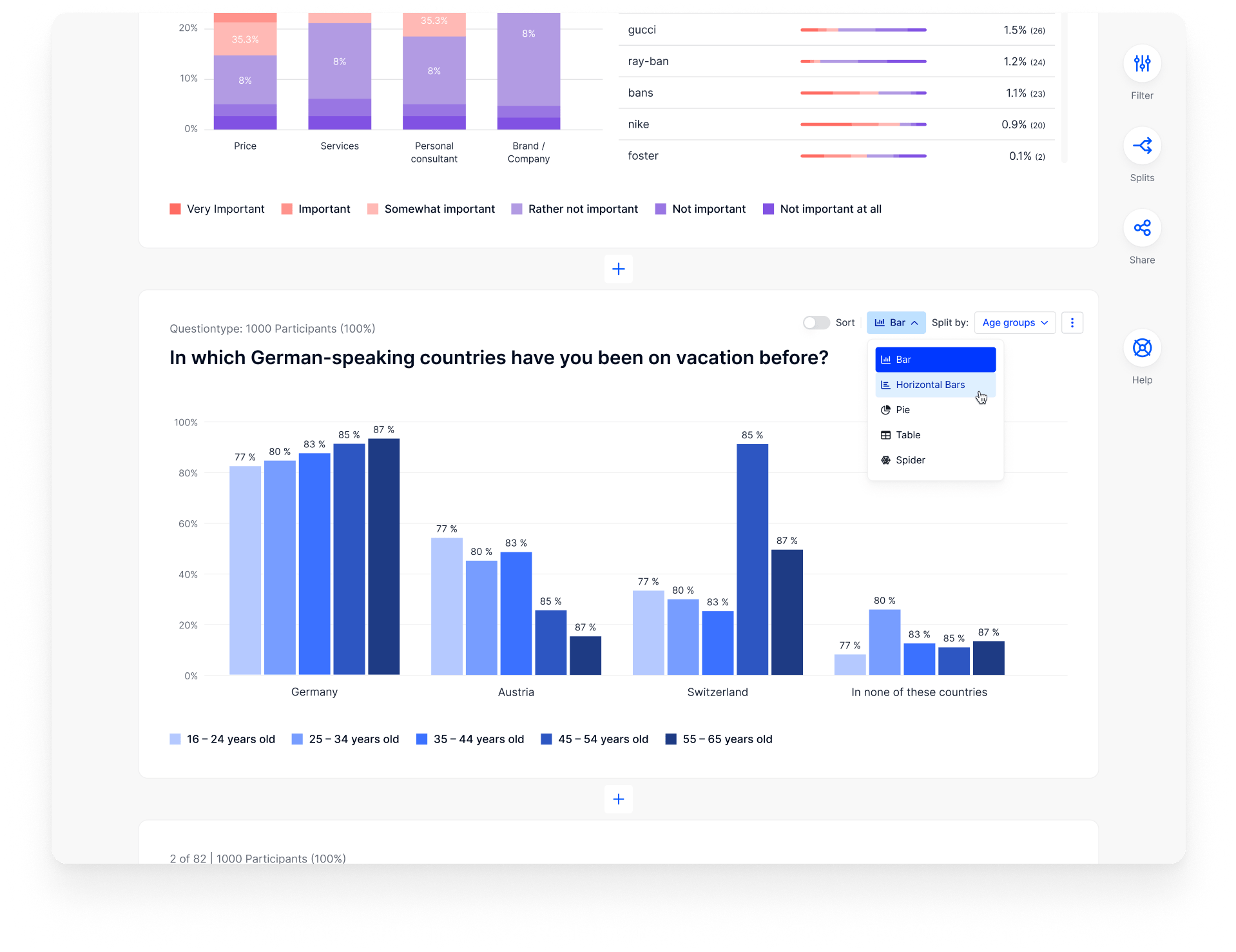
Get free access to the platform!
Join the loop 💌
Be the first to hear about new updates, product news, and data insights. We'll send it all straight to your inbox.
Get the latest market research news straight to your inbox! 💌
Wait, there's more
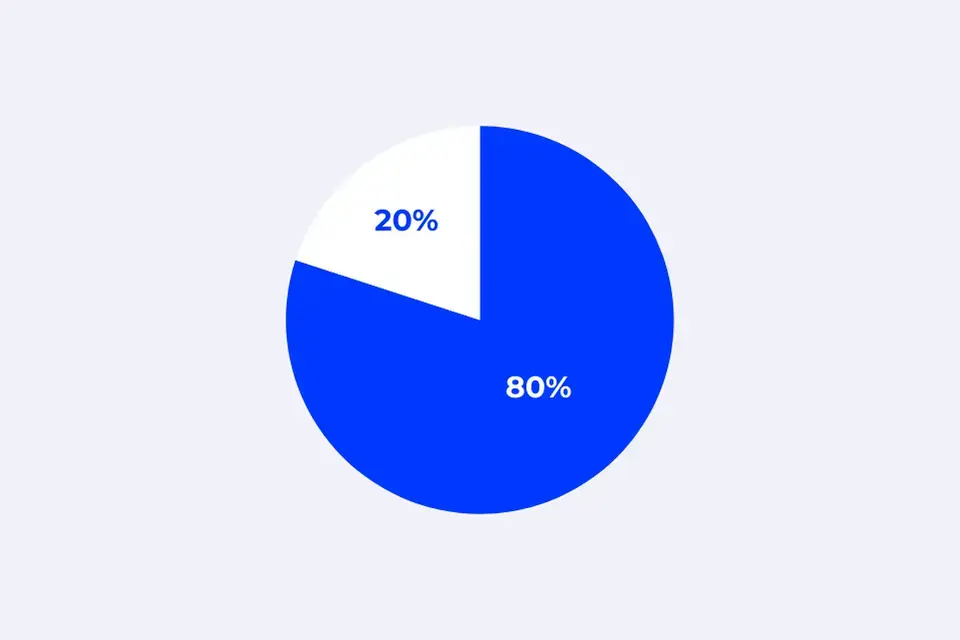
30.05.2024 | 29min read
Pareto Analysis: Definition, Pareto Chart, Examples
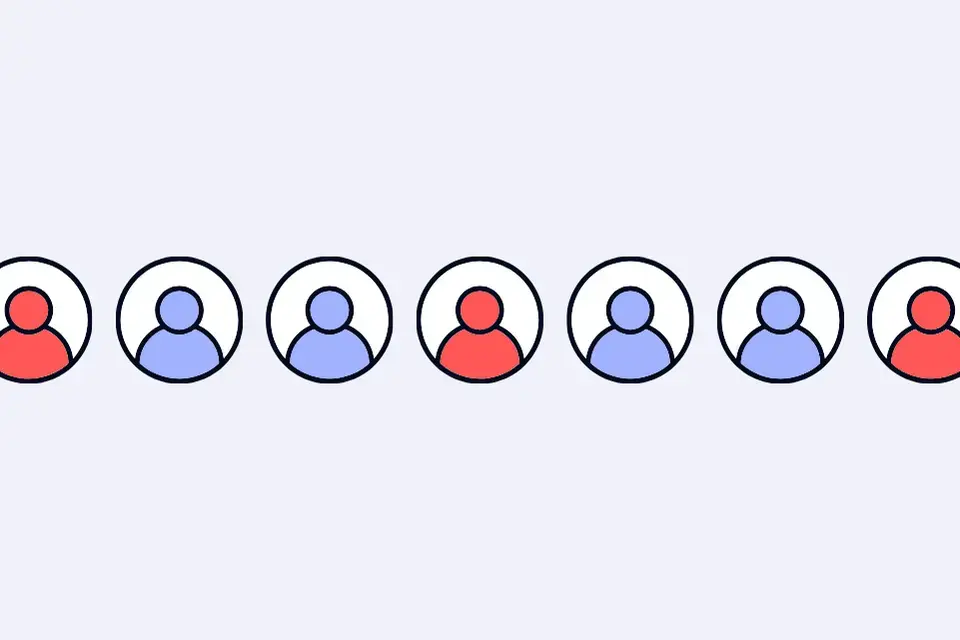
28.05.2024 | 32min read
What is Systematic Sampling? Definition, Types, Examples
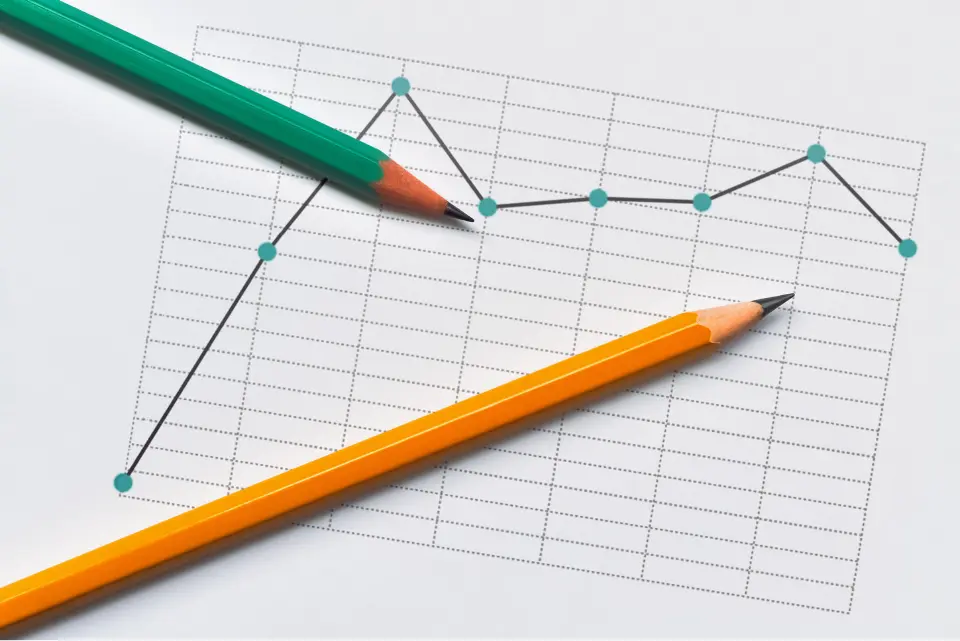
16.05.2024 | 30min read
Time Series Analysis: Definition, Types, Techniques, Examples
Penn State University Libraries
Empirical research in the social sciences and education.
- What is Empirical Research and How to Read It
- Finding Empirical Research in Library Databases
- Designing Empirical Research
- Ethics, Cultural Responsiveness, and Anti-Racism in Research
- Citing, Writing, and Presenting Your Work
Contact the Librarian at your campus for more help!

Introduction: What is Empirical Research?
Empirical research is based on observed and measured phenomena and derives knowledge from actual experience rather than from theory or belief.
How do you know if a study is empirical? Read the subheadings within the article, book, or report and look for a description of the research "methodology." Ask yourself: Could I recreate this study and test these results?
Key characteristics to look for:
- Specific research questions to be answered
- Definition of the population, behavior, or phenomena being studied
- Description of the process used to study this population or phenomena, including selection criteria, controls, and testing instruments (such as surveys)
Another hint: some scholarly journals use a specific layout, called the "IMRaD" format, to communicate empirical research findings. Such articles typically have 4 components:
- Introduction : sometimes called "literature review" -- what is currently known about the topic -- usually includes a theoretical framework and/or discussion of previous studies
- Methodology: sometimes called "research design" -- how to recreate the study -- usually describes the population, research process, and analytical tools used in the present study
- Results : sometimes called "findings" -- what was learned through the study -- usually appears as statistical data or as substantial quotations from research participants
- Discussion : sometimes called "conclusion" or "implications" -- why the study is important -- usually describes how the research results influence professional practices or future studies
Reading and Evaluating Scholarly Materials
Reading research can be a challenge. However, the tutorials and videos below can help. They explain what scholarly articles look like, how to read them, and how to evaluate them:
- CRAAP Checklist A frequently-used checklist that helps you examine the currency, relevance, authority, accuracy, and purpose of an information source.
- IF I APPLY A newer model of evaluating sources which encourages you to think about your own biases as a reader, as well as concerns about the item you are reading.
- Credo Video: How to Read Scholarly Materials (4 min.)
- Credo Tutorial: How to Read Scholarly Materials
- Credo Tutorial: Evaluating Information
- Credo Video: Evaluating Statistics (4 min.)
- Next: Finding Empirical Research in Library Databases >>
- Last Updated: Feb 18, 2024 8:33 PM
- URL: https://guides.libraries.psu.edu/emp
Thank you for visiting nature.com. You are using a browser version with limited support for CSS. To obtain the best experience, we recommend you use a more up to date browser (or turn off compatibility mode in Internet Explorer). In the meantime, to ensure continued support, we are displaying the site without styles and JavaScript.
- View all journals
- My Account Login
- Explore content
- About the journal
- Publish with us
- Sign up for alerts
- Open access
- Published: 07 August 2019
A national experiment reveals where a growth mindset improves achievement
- David S. Yeager 1 ,
- Paul Hanselman 2 ,
- Gregory M. Walton 3 ,
- Jared S. Murray 1 ,
- Robert Crosnoe 1 ,
- Chandra Muller 1 ,
- Elizabeth Tipton 4 ,
- Barbara Schneider 5 ,
- Chris S. Hulleman 6 ,
- Cintia P. Hinojosa 7 ,
- David Paunesku 8 ,
- Carissa Romero 9 ,
- Kate Flint 10 ,
- Alice Roberts 10 ,
- Jill Trott 10 ,
- Ronaldo Iachan 10 ,
- Jenny Buontempo 1 ,
- Sophia Man Yang 1 ,
- Carlos M. Carvalho 1 ,
- P. Richard Hahn 11 ,
- Maithreyi Gopalan 12 ,
- Pratik Mhatre 1 ,
- Ronald Ferguson 13 ,
- Angela L. Duckworth 14 &
- Carol S. Dweck 3
Nature volume 573 , pages 364–369 ( 2019 ) Cite this article
284k Accesses
625 Citations
1161 Altmetric
Metrics details
- Human behaviour
- Risk factors
A global priority for the behavioural sciences is to develop cost-effective, scalable interventions that could improve the academic outcomes of adolescents at a population level, but no such interventions have so far been evaluated in a population-generalizable sample. Here we show that a short (less than one hour), online growth mindset intervention—which teaches that intellectual abilities can be developed—improved grades among lower-achieving students and increased overall enrolment to advanced mathematics courses in a nationally representative sample of students in secondary education in the United States. Notably, the study identified school contexts that sustained the effects of the growth mindset intervention: the intervention changed grades when peer norms aligned with the messages of the intervention. Confidence in the conclusions of this study comes from independent data collection and processing, pre-registration of analyses, and corroboration of results by a blinded Bayesian analysis.
Similar content being viewed by others
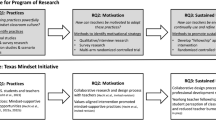
Shifting the mindset culture to address global educational disparities
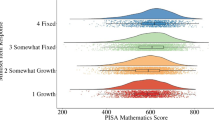
Growth mindset and academic outcomes: a comparison of US and Chinese students
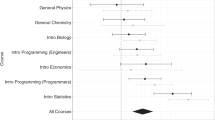
Real-world effectiveness of a social-psychological intervention translated from controlled trials to classrooms
About 20% of students in the United States will not finish high school on time 1 . These students are at a high risk of poverty, poor health and early mortality in the current global economy 2 , 3 , 4 . Indeed, a Lancet commission concluded that improving secondary education outcomes for adolescents “presents the single best investment for health and wellbeing” 5 .
The transition to secondary school represents an important period of flexibility in the educational trajectories of adolescents 6 . In the United States, the grades of students tend to decrease during the transition to the ninth grade (age 14–15 years, UK year 10), and often do not recover 7 . When such students underperform in or opt out of rigorous coursework, they are far less likely to leave secondary school prepared for college or university or for advanced courses in college or university 8 , 9 . In this way, early problems in the transition to secondary school can compound over time into large differences in human capital in adulthood.
One way to improve academic success across the transition to secondary school is through social–psychological interventions, which change how adolescents think or feel about themselves and their schoolwork and thereby encourage students to take advantage of learning opportunities in school 10 , 11 . The specific intervention evaluated here—a growth mindset of intelligence intervention—addresses the beliefs of adolescents about the nature of intelligence, leading students to see intellectual abilities not as fixed but as capable of growth in response to dedicated effort, trying new strategies and seeking help when appropriate 12 , 13 , 14 , 15 , 16 . This can be especially important in a society that conveys a fixed mindset (a view that intelligence is fixed), which can imply that feeling challenged and having to put in effort means that one is not naturally talented and is unlikely to succeed 12 .
The growth mindset intervention communicates a memorable metaphor: that the brain is like a muscle that grows stronger and smarter when it undergoes rigorous learning experiences 14 . Adolescents hear the metaphor in the context of the neuroscience of learning, they reflect on ways to strengthen their brains through schoolwork, and they internalize the message by teaching it to a future first-year ninth grade student who is struggling at the start of the year. The intervention can lead to sustained academic improvement through self-reinforcing cycles of motivation and learning-oriented behaviour. For example, a growth mindset can motivate students to take on more rigorous learning experiences and to persist when encountering difficulties. Their behaviour may then be reinforced by the school context, such as more positive and learning-oriented responses from peers or instructors 10 , 17 .
Initial intervention studies with adolescents taught a growth mindset in multi-session (for example, eight classroom sessions 15 ), interactive workshops delivered by highly trained adults; however, these were not readily scalable. Subsequent growth mindset interventions were briefer and self-administered online, although lower effect sizes were, of course, expected. Nonetheless, previous randomized evaluations, including a pre-registered replication, found that online growth mindset interventions improved grades for the targeted group of students in secondary education who previously showed lower achievement 13 , 16 , 18 . These findings are important because previously low-achieving students are the group that shows the steepest decline in grades during the transition to secondary school 19 , and these findings are consistent with theory because a growth mindset should be most beneficial for students confronting challenges 20 .
Here we report the results of the National Study of Learning Mindsets, which examined the effects of a short, online growth mindset intervention in a nationally representative sample of high schools in the United States (Fig. 1 ). With this unique dataset we tested the hypotheses that the intervention would improve grades among lower-achieving students and overall uptake of advanced courses in this national sample.
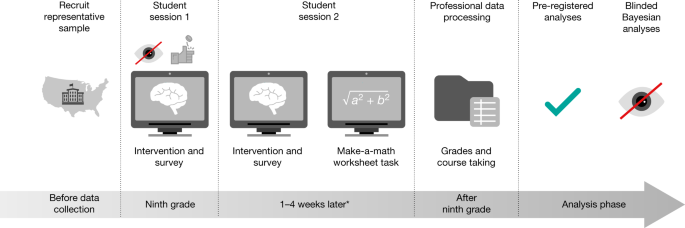
Between August and November 2015, 82% of schools delivered the intervention; the remaining 18% delivered the intervention in January or February of 2016. Asterisk indicates that the median number of days between sessions 1 and 2 among schools implementing the intervention in the autumn was 21 days; for spring-implementing schools it was 27 days. The coin-tossing symbol indicates that random assignment was made during session 1. The tick symbol indicates that a comprehensive analysis plan was pre-registered at https://osf.io/tn6g4 . The blind-eye symbol indicates that, first, teachers and researchers were kept blinded to students’ random assignment to condition, and, second, the Bayesian, machine-learning robustness tests were conducted by analysts who at the time were blinded to study hypotheses and to the identities of the variables.
A focus on heterogeneity
The study was also designed with the purpose of understanding for whom and under what conditions the growth mindset intervention improves grades. That is, it examined potential sources of cross-site treatment effect heterogeneity. One reason why understanding heterogeneity of effects is important is because most interventions that are effective in initial efficacy trials go on to show weaker or no effects when they are scaled up in effectiveness trials that deliver treatments under everyday conditions to more heterogeneous samples 21 , 22 , 23 . Without clear evidence about why average effect sizes differ in later-conducted studies—evidence that could be acquired from a systematic investigation of effect heterogeneity—researchers may prematurely discard interventions that yield low average effects but could provide meaningful and replicable benefits at scale for targeted groups 21 , 23 .
Further, analyses of treatment effect heterogeneity can reveal critical evidence about contextual mechanisms that sustain intervention effects. If school contexts differ in the availability of the resources or experiences needed to sustain the offered belief change and enhanced motivation following an intervention, then the effects of the intervention should differ across these school contexts as well 10 , 11 .
Sociological theory highlights two broad dimensions of school contexts that might sustain or impede belief change and enhanced motivation among students treated by a growth mindset intervention 6 . First, schools with the least ‘formal’ resources, such as high-quality curricula and instruction, may not offer the learning opportunities for students to be able to capitalize on the intervention, while those with the most resources may not need the intervention. Second, some schools may not have the ‘informal’ resources needed to sustain the intervention effect, such as peer norms that support students when they take on challenges and persist in the face of intellectual difficulty. We hypothesized that both of these dimensions would significantly moderate growth mindset intervention effects.
Historically, the scientific methods used to answer questions about the heterogeneity of intervention effects have been underdeveloped and underused 21 , 24 , 25 . Common problems in the literature are: (1) imprecise site-level impact estimates (because of cluster-level random assignment); (2) inconsistent fidelity to intervention protocols across sites (which can obscure the workings of the cross-site moderators of interest); (3) non-representative sampling of sites (which causes site selection bias 22 , 26 ); and (4) multiple post hoc tests for the sources of treatment effect size heterogeneity (which increases the probability of false discoveries 24 ).
We overcame all of these problems in a single study. We randomized students to condition within schools and consistently had high fidelity of implementation across sites (see Supplementary Information section 5 ). We addressed site selection bias by contracting a professional research company, which recruited a sample of schools that generalized to the entire population of ninth-grade students attending regular US public schools 27 (that is, schools that run on government funds; see Supplementary Information section 3 ). Next, the study used analysis methods that avoided false conclusions about subgroup effects, by generating a limited number of moderation hypotheses (two), pre-registering a limited number of statistical tests and conducting a blinded Bayesian analysis that can provide rigorous confirmation of the results (Fig. 1 ).
Expected effect sizes
In this kind of study, it is important to ask what size of effect would be meaningful. As a leading educational economist concluded, “in real-world settings, a fifth of a standard deviation [0.20 s.d.] is a large effect” 28 . This statement is justified by the ‘best evidence synthesis’ movement 29 , which recommends the use of empirical benchmarks, not from laboratory studies, but from the highest-quality field research on factors affecting objective educational outcomes 30 , 31 . A standardized mean difference effect size of 0.20 s.d. is considered ‘large’ because it is: (1) roughly how much improvement results from a year of classroom learning for ninth-grade students, as shown by standardized tests 30 ; (2) at the high end of estimates for the effect of having a very high-quality teacher (versus an average teacher) for one year 32 ; and (3) at the uppermost end of empirical distributions of real-world effect sizes from diverse randomized trials that target adolescents 31 . Notably, the highly-cited ‘nudges’ studied by behavioural economists and others, when aimed at influencing real-world outcomes that unfold over time (such as college enrolment or energy conservation 33 ) rather than one-time choices, rarely, if ever, exceed 0.20 s.d. and typically have much smaller effect sizes.
Returning to educational benchmarks, 0.20 s.d. and 0.23 s.d. were the two largest effects observed in a recent cohort analysis of the results of all of the pre-registered, randomized trials that evaluated promising interventions for secondary schools funded as part of the US federal government’s i3 initiative 34 (the median effect for these promising interventions was 0.03 s.d.; see Supplementary Information section 11 ). The interventions in the i3 initiative typically targeted lower-achieving students or schools, involved training teachers or changing curricula, consumed considerable classroom time, and cost several thousand US dollars per student. Moreover, they were all conducted in non-representative samples of convenience that can overestimate effects. Therefore, it would be noteworthy if a short, low-cost, scalable growth mindset intervention, conducted in a nationally representative sample, could achieve a meaningful proportion of the largest effects seen for past traditional interventions, within the targeted, pre-registered group of lower-achieving students.
Defining the primary outcome and student subgroup
The primary outcome was the post-intervention grade point average (GPA) in core ninth-grade classes (mathematics, science, English or language arts, and social studies), obtained from administrative data sources of the schools (as described in the pre-analysis plan found in the Supplementary Information section 13 and at https://osf.io 35 ). Following the pre-registered analysis plan, we report results for the targeted group of n = 6,320 students who were lower-achieving relative to peers in the same school. This group is typically targeted by comprehensive programmes evaluated in randomized trials in education, as there is an urgent need to improve their educational trajectories. The justification for predicting effects in the lower-achieving group is that (1) this group benefitted in previous growth mindset trials; (2) lower-achieving students may be undergoing more academic difficulties and therefore may benefit more from a growth mindset that alters the interpretation of these difficulties; and (3) students who already have a high GPA may have less room to improve their GPAs. We defined students as relatively lower-achieving if they were earning GPAs at or below the school-specific median in the term before random assignment or, if they were missing prior GPA data, if they were below the school-specific median on academic variables used to impute prior GPA (as described in the analysis plan). Supplementary analyses for the sample overall can be found in Extended Data Table 1 , and robustness analyses for the definition of lower-achieving students are included in Extended Data Fig. 1 ( Supplementary Information section 7 ).
Average effects on mindset
Among lower-achieving adolescents, the growth mindset intervention reduced the prevalence of fixed mindset beliefs relative to the control condition, reported at the end of the second treatment session, unstandardized B = −0.38 (95% confidence interval = −0.31, −0.46), standard error of the regression coefficient (s.e.) = 0.04, n = 5,650 students, k = 65 schools, t = −10.14, P < 0.001, standardized mean difference effect size of 0.33.
Average effects on core course GPAs
In line with our first major prediction, lower-achieving adolescents earned higher GPAs in core classes at the end of the ninth grade when assigned to the growth mindset intervention, B = 0.10 grade points (95% confidence interval = 0.04, 0.16), s.e. = 0.03, n = 6,320, k = 65, t = 3.51, P = 0.001, standardized mean difference effect size of 0.11, relative to comparable students in the control condition. This conclusion is robust to alternative model specifications that deviate from the pre-registered model (Extended Data Fig. 1 ).
To map the growth mindset intervention effect onto a policy-relevant indicator of high school success, we analysed poor performance rates, defined as the percentage of adolescents who earned a GPA below 2.0 on a four-point scale (that is, a ‘D’ or an ‘F’; as described in the pre-analysis plan). Poor performance rates are relevant because recent changes in US federal laws (the Every Student Succeeds Act 36 ), have led many states to adopt reductions in the poor performance rates in the ninth grade as a key metric for school accountability. More than three million ninth-grade students attend regular US public schools each year, and half are lower-achieving according to our definition. The model estimates that 5.3% (95% confidence interval = −1.7, −9.0), s.e. = 1.8, t = 2.95, P = 0.005 of 1.5 million students in the United States per year would be prevented from being ‘off track’ for graduation by the brief and low-cost growth mindset intervention, representing a reduction from 46% to 41%, which is a relative risk reduction of 11% (that is, 0.05/0.46).
Average effects on mathematics and science GPAs
A secondary analysis focused on the outcome of GPAs in only mathematics and science (as described in the analysis plan). Mathematics and science are relevant because a popular belief in the United States links mathematics and science learning to ‘raw’ or ‘innate’ abilities 37 —a view that the growth mindset intervention seeks to correct. In addition, success in mathematics and science strongly predicts long-term economic welfare and well-being 38 . Analyses of outcomes for mathematics and science supported the same conclusions ( B = 0.10 for mathematics and science GPAs compared to B = 0.10 for core GPAs; Extended Data Tables 1 – 3 ).
Quantifying heterogeneity
The intervention was expected to homogeneously change the mindsets of students across schools—as this would indicate high fidelity of implementation—however, it was expected to heterogeneously change lower-achieving students’ GPAs, as this would indicate potential school differences in the contextual mechanisms that sustain an initial treatment effect. As predicted, a mixed-effects model found no significant variability in the treatment effect on self-reported mindsets across schools (unstandardized \(\hat{\tau }=0.08\) , Q 64 = 57.2, P = 0.714), whereas significant variability was found in the effect on GPAs among lower-achieving students across schools (unstandardized \(\hat{\tau }=0.09\) , Q 64 = 85.5, P = 0.038) 39 (Extended Data Fig. 2 ).
Moderation by school achievement level
First, we tested competing hypotheses about whether the formal resources of the school explained the heterogeneity of effects. Before analysing the data, we expected that in schools that are unable to provide high-quality learning opportunities (the lowest-achieving schools), treated students might not sustain a desire to learn. But we also expected that other schools (the highest-achieving schools) might have such ample resources to prevent failure such that a growth mindset intervention would not add much.
The heterogeneity analyses found support for the latter expectation, but not the former. Treatment effects on ninth-grade GPAs among lower-achieving students were smaller in schools with higher achievement levels, intervention × school achievement level (continuous) interaction, unstandardized B = −0.07 (95% confidence interval = 0.02, 0.13), s.e. = 0.03, z = −2.76, n = 6,320, k = 65, P = 0.006, standardized β = −0.25. In follow-up analyses with categorical indicators for school achievement, medium-achieving schools (middle 50%) showed larger effects than higher-achieving schools (top 25%). Low-achieving schools (bottom 25%) did not significantly differ from medium-achieving schools (Extended Data Table 2 ); however, this non-significant difference should be interpreted cautiously, owing to wide confidence intervals for the subgroup of lowest-achieving schools.
Moderation by peer norms
Second, we examined whether students might be discouraged from acting on their enhanced growth mindset when they attend schools in which peer norms were unsupportive of challenge-seeking, whereas peer norms that support challenge-seeking might function to sustain the effects of the intervention over time. We measured peer norms by administering a behavioural challenge-seeking task (the ‘make-a-math-worksheet’ task) at the end of the second intervention session (Fig. 1 ) and aggregating the values of the control group to the school level.
The pre-registered mixed-effects model yielded a positive and significant intervention × behavioural challenge-seeking norms interaction for GPA among the targeted group of lower-achieving adolescents, such that the intervention produced a greater difference in end-of-year GPAs relative to the control group when the behavioural norm that surrounded students was supportive of the growth mindset belief system, B = 0.11 (95% confidence interval = 0.01, 0.21), s.e. = 0.05, z = 2.18, n = 6,320, k = 65, P = 0.029, β = 0.23. The same conclusion was supported in a secondary analysis of only mathematics and science GPAs (Extended Data Table 2 ).
Subgroup effect sizes
Putting together the two pre-registered moderators (school achievement level and school norms), the conditional average treatment effect (CATEs) on core GPAs within low- and medium-achieving schools (combined) was 0.14 grade points when the school was in the third quartile of behavioural norms and 0.18 grade points when the school was in the fourth and highest quartile of behavioural norms, as shown in Fig. 2 . For mathematics and science grades, the CATEs ranged from 0.16 to 0.25 grade points in the same subgroups of low- and medium-achieving schools with more supportive behavioural norms (for results separating low- and medium-achieving schools, see Fig. 2c, d and Extended Data Table 3 ). We also found that even the high-achieving schools showed meaningful treatment effects among their lower achievers on mathematics and science GPAs when they had norms that supported challenge seeking—0.08 and 0.11 grade points for the third and fourth quartiles of school norms, respectively, in the high-achieving schools ( P = 0.002; Extended Data Table 3 ).
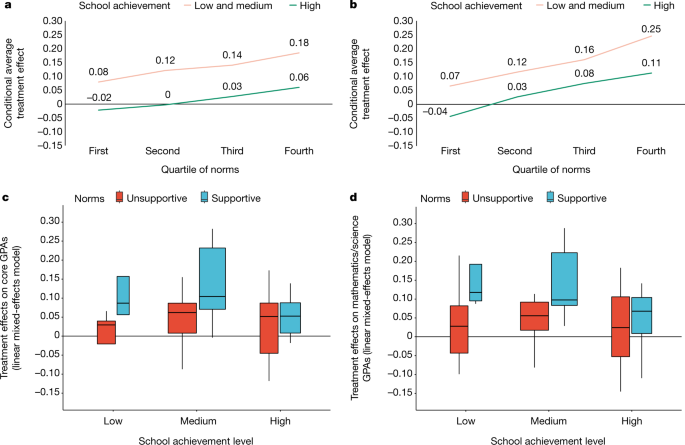
a , c , Treatment effects on core course grade point averages (GPAs). b , d , Treatment effects on GPAs of only mathematics and science. a , b , The CATEs represent the estimated subgroup treatment effects from the pre-registered linear mixed-effects model, with survey weights, when fixing the racial/ethnic composition of the schools to the population median to remove any potential confounding effect of that variable on moderation hypothesis tests. Achievement levels: low, 25th percentile or lower; middle, 25th–75th percentile; high, 75th percentile or higher, which follows the categories set in the sampling plan and in the pre-registration. Norms indicate the behavioural challenge-seeking norms, as measured by the responses of the control group to the make-a-math-worksheet task after session 2. c , d , Box plots represent unconditional treatment effects (one for each school) estimated in the pre-registered linear mixed-effects regression model with no school-level moderators, as specified for research question 3 in the pre-analysis plan and described in the Supplementary Information section 7.4 . The distribution of the school-level treatment effects was re-scaled to the cross-site standard deviation, in accordance with standard practice. Dark lines correspond to the median school in a subgroup and the boxes correspond to the middle 75% of the distribution (the interquartile range). Supportive schools are defined as above the population median (third and fourth quartiles); unsupportive schools are defined as those below the population median (first and second quartiles). n = 6,320 students in k = 65 schools.
Source data
Bayesian robustness analysis.
A team of statisticians, at the time blind to study hypotheses, re-analysed the dataset using a conservative Bayesian machine-learning algorithm, called Bayesian causal forest (BCF). BCF has been shown by both its creators and other leading statisticians in open head-to-head competitions to be the most effective of the state-of-the-art methods for identifying systematic sources of treatment effect heterogeneity, while avoiding false positives 40 , 41 .
The BCF analysis assigned a near-certain posterior probability that the population-average treatment effect (PATE) among lower-achieving students was positive and greater than zero, P PATE > 0 ≥ 0.999, providing strong evidence of positive average treatment effects. BCF also found stronger CATEs in schools with positive challenge-seeking norms, and weaker effects in the highest-achieving schools (Extended Data Fig. 3 and Supplementary Information section 8 ), providing strong correspondence with the primary analyses.
Advanced mathematics course enrolment in tenth grade
The intervention showed weaker benefits on ninth-grade GPAs in high-achieving schools. However, students in these schools may benefit in other ways. An analysis of enrolment in rigorous mathematics courses in the year after the intervention examined this possibility. The enrolment data were gathered with these analyses in mind but since the analyses were not pre-registered, they are exploratory.
Course enrolment decisions are potentially relevant to all students, both lower- and higher-achieving, so we explored them in the full cohort. We found that the growth mindset intervention increased the likelihood of students taking advanced mathematics (algebra II or higher) in tenth grade by 3 percentage points (95% confidence interval = 0.01, 0.04), s.e. = 0.01, n = 6,690, k = 41, t = 3.18, P = 0.001, from a rate of 33% in the control condition to a rate of 36% in the intervention condition, corresponding to a 9% relative increase. Notably, we discovered a positive intervention × school achievement level (continuous) interaction, ( B = 0.04 (95% confidence interval = 0.00, 0.08), s.e. = 0.02, z = 2.26, P = 0.024, the opposite of what we found for core course GPAs. Within the highest-achieving 25% of schools, the intervention increased the rate at which students took advanced mathematics in tenth grade by 4 percentage points ( t = 2.37, P = 0.018). In the lower 75% of schools—where we found stronger effects on GPA—the increase in the rate at which students took advanced mathematics courses was smaller: 2 percentage points ( t = 2.00, P = 0.045). Thus an exclusive focus on GPA would have obscured intervention benefits among students attending higher-achieving schools.
The National Study of Learning Mindsets showed that a low-cost treatment, delivered in less than an hour, attained a substantial proportion of the effects on grades of the most effective rigorously evaluated adolescent interventions of any cost or duration in the literature within the pre-registered group of lower-achieving students. Moreover, the intervention produced gains in the consequential outcome of advanced mathematics course-taking for students overall, which is meaningful because the rigor of mathematics courses taken in high school strongly predicts later educational attainment 8 , 9 , and educational attainment is one of the leading predictors of longevity and health 38 , 42 . The finding that the growth mindset intervention could redirect critical academic outcomes to such an extent—with no training of teachers; in an effectiveness trial conducted in a population-generalizable sample; with data collected by an independent research company using repeatable procedures; with data processed by a second independent research company; and while adhering to a comprehensive pre-registered analysis plan—is a major advance.
Furthermore, the evidence about the kinds of schools where the growth mindset treatment effect on grades was sustained, and where it was not, has important implications for future interventions. We might have expected that the intervention would compensate for unsupportive school norms, and that students who already had supportive peer norms would not need the intervention as much. Instead, it was when the peer norm supported the adoption of intellectual challenges that the intervention promoted sustained benefits in the form of higher grades.
Perhaps students in unsupportive peer climates risked paying a social price for taking on intellectual challenges in front of peers who thought it undesirable to do so. Sustained change may therefore require both a high-quality seed (an adaptive belief system conveyed by a compelling intervention) and conductive soil in which that seed can grow (a context congruent with the proffered belief system). A limitation of our moderation results, of course, is that we cannot draw causal conclusions about the effects of the school norm, as the norms were measured, not manipulated. It is encouraging that a Bayesian analysis, reported in the Supplementary Information section 8, yielded evidence consistent with a causal interpretation of the school norms variable. The present research therefore sets the stage for a new era of experimental research that seeks to enhance both students’ mindsets and the school environments that support student learning.
We emphasize that not all forms of growth mindset interventions can be expected to increase grades or advanced course-taking, even in the targeted subgroups 11 , 12 . New growth mindset interventions that go beyond the module and population tested here will need to be subjected to rigorous development and validation processes, as the current programme was 13 .
Finally, this study offers lessons for the science of adolescent behaviour change. Beliefs—and particularly beliefs that affect how students make sense of ongoing challenges—are important during high-stakes developmental turning points such as pubertal maturation 43 , 44 or the transition to secondary school 6 . Indeed, new interventions in the future should address the interpretation of other challenges that adolescents experience, including social and interpersonal difficulties, to affect outcomes (such as depression) that thus far have proven difficult to address 43 . And the combined importance of belief change and school environments in our study underscores the need for interdisciplinary research to understand the numerous influences on adolescents’ developmental trajectories.
Ethics approval
Approval for this study was obtained from the Institutional Review Board at Stanford University (30387), ICF (FWA00000845), and the University of Texas at Austin (#2016-03-0042). In most schools this experiment was conducted as a programme evaluation carried out at the request of the participating school district 45 . When required by school districts, parents were informed of the programme evaluation in advance and given the opportunity to withdraw their children from the study. Informed student assent was obtained from all participants.
Participants
Data came from the National Study of Learning Mindsets 45 , which is a stratified random sample of 65 regular public schools in the United States that included 12,490 ninth-grade adolescents who were individually randomized to condition. The number of schools invited to participate was determined by a power analysis to detect reasonable estimates of cross-site heterogeneity; as many of the invited schools as possible were recruited into the study. Grades were obtained from the schools of the students, and analyses focused on the lower-achieving subgroup of students (those below the within-school median). The sample reflected the diversity of young people in the United States: 11% self-reported being black/African-American, 4% Asian-American, 24% Latino/Latina, 43% white and 18% another race or ethnicity; 29% reported that their mother had a bachelor’s degree or higher. To prevent deductive disclosure for potentially-small subgroups of students, and consistent with best practices for other public-use datasets, the policies for the National Study of Learning Mindsets require analysts to round all sample sizes to the nearest 10, so this was done here.
Data collection
To ensure that the study procedures were repeatable by third parties and therefore scalable, and to increase the independence of the results, two different professional research companies, who were not involved in developing the materials or study hypotheses, were contracted. One company (ICF) drew the sample, recruited schools, arranged for treatment delivery, supervised and implemented the data collection protocol, obtained administrative data, and cleaned and merged data. They did this work blind to the treatment conditions of the students. This company worked in concert with a technology vendor (PERTS), which delivered the intervention, executed random assignment, tracked student response rates, scheduled make-up sessions and kept all parties blind to condition assignment. A second professional research company (MDRC) processed the data merged by ICF and produced an analytic grades file, blind to the consequences of their decisions for the estimated treatment effects, as described in Supplementary Information section 12 . Those data were shared with the authors of this paper, who analysed the data following a pre-registered analysis plan (see Supplementary Information section 13 ; MDRC will later produce its own independent report using its processed data, and retained the right to deviate from our pre-analysis plan).
Selection of schools was stratified by school achievement and minority composition. A simple random sample would not have yielded sufficient numbers of rare types of schools, such as high-minority schools with medium or high levels of achievement. This was because school achievement level—one of the two candidate moderators—was strongly associated with school racial/ethnic composition 46 (percentage of Black/African-American or Hispanic/Latino/Latina students, r = −0.66).
A total of 139 schools were selected without replacement from a sampling frame of roughly 12,000 regular US public high schools, which serve the vast majority of students in the United States. Regular US public schools exclude charter or private schools, schools serving speciality populations such as students with physical disabilities, alternative schools, schools that have fewer than 25 ninth-grade students enrolled and schools in which ninth grade is not the lowest grade in the school.
Of the 139 schools, 65 schools agreed, participated and provided student records. Another 11 schools agreed and participated but did not provide student grades or course-taking records; therefore, the data of their students are not analysed here. School nonresponse did not appear to compromise representativeness. We calculated the Tipton generalizability index 47 , a measure of similarity between an analytic sample and the overall sampling frame, along eight student demographic and school achievement benchmarks obtained from official government sources 27 . The index ranges from 0 to 1, with a value of 0.90 corresponding to essentially a random sample. The National Study of Learning Mindsets showed a Tipton generalizability index of 0.98, which is very high (see Supplementary Information section 3 ).
Within schools, the average student response rate for eligible students was 92% and the median school had a response rate of 98% (see definitions in Supplementary Information section 5 ). This response rate was obtained by extensive efforts to recruit students into make-up sessions if students were absent and it was aided by a software system, developed by the technology vendor (PERTS), that kept track of student participation. A high within-school response rate was important because lower-achieving students, our target group, are typically more likely to be absent.
Growth mindset intervention content
In preparing the intervention to be scalable, we revised past growth mindset interventions to focus on the perspectives, concerns and reading levels of ninth-grade students in the United States, through an intensive research and development process that involved interviews, focus groups and randomized pilot experiments with thousands of adolescents 13 .
The control condition, focusing on brain functions, was similar to the growth mindset intervention, but did not address beliefs about intelligence. Screenshots from both interventions can be found in Supplementary Information section 4 , and a detailed description of the general intervention content has previously been published 13 . The intervention consisted of two self-administered online sessions that lasted approximately 25 min each and occurred roughly 20 days apart during regular school hours (Fig. 1 ).
The growth mindset intervention aimed to reduce the negative effort beliefs of students (the belief that having to try hard or ask for help means you lack ability), fixed-trait attributions (the attribution that failure stems from low ability) and performance avoidance goals (the goal of never looking stupid). These are the documented mediators of the negative effect of a fixed mindset on grades 12 , 15 , 48 and the growth mindset intervention aims to reduce them. The intervention did not only contradict these beliefs but also used a series of interesting and guided exercises to reduce their credibility.
The first session of the intervention covered the basic idea of a growth mindset—that an individual’s intellectual abilities can be developed in response to effort, taking on challenging work, improving one’s learning strategies, and asking for appropriate help. The second session invited students to deepen their understanding of this idea and its application in their lives. Notably, students were not told outright that they should work hard or employ particular study or learning strategies. Rather, effort and strategy revision were described as general behaviours through which students could develop their abilities and thereby achieve their goals.
The materials presented here sought to make the ideas compelling and help adolescents to put them into practice. It therefore featured stories from both older students and admired adults about a growth mindset, and interactive sections in which students reflected on their own learning in school and how a growth mindset could help a struggling ninth-grade student next year. The intervention style is described in greater detail in a paper reporting the pilot study for the present research 13 and in a recent review article 12 .
Among these features, our intervention mentioned effort as one means to develop intellectual ability. Although we cannot isolate the effect of the growth mindset message from a message about effort alone, it is unlikely that the mere mention of effort to high school students would be sufficient to increase grades and challenge seeking. In part this is because adolescents often already receive a great deal of pressure from adults to try hard in school.
Intervention delivery and fidelity
The intervention and control sessions were delivered as early in the school year as possible, to increase the opportunity to set in motion a positive self-reinforcing cycle. In total 82% of students received the intervention in the autumn semester before the Thanksgiving holiday in the United States (that is, before late November) and the rest received the intervention in January or February; see Supplementary Information section 5 for more detail. The computer software of the technology vendor randomly assigned adolescents to intervention or control materials. Students also answered various survey questions. All parties were blind to condition assignment, and students and teachers were not told the purpose of the study to prevent expectancy effects.
The data collection procedures yielded high implementation fidelity across the participating schools, according to metrics listed in the pre-registered analysis plan. In the median school, treated students viewed 97% of screens and wrote a response for 96% of open-ended questions. In addition, in the median school 91% students reported that most or all of their peers worked carefully and quietly on the materials. Fidelity statistics are reported in full in Supplementary Information section 5.6 ; Extended Data Table 2 shows that the treatment effect heterogeneity conclusions were unchanged when controlling for the interaction of treatment and school-level fidelity as intended.
Self-reported fixed mindset
Students indicated how much they agreed with three statements such as “You have a certain amount of intelligence, and you really can’t do much to change it” (1, strongly disagree; 6, strongly agree). Higher values corresponded to a more fixed mindset; the pre-analysis plan predicted that the intervention would reduce these self-reports.
GPAs . Schools provided the grades of each student in each course for the eight and ninth grade. Decisions about which courses counted for which content area were made independently by a research company (MDRC; see Supplementary Information section 12 ). The GPAs are a theoretically relevant outcome because grades are commonly understood to reflect sustained motivation, rather than only prior knowledge. It is also a practically relevant outcome because, as noted, GPA is a strong predictor of adult educational attainment, health and well-being, even when controlling for high school test scores 38 .
School achievement level
The school achievement level moderator was a latent variable that was derived from publicly available indicators of the performance of the school on state and national tests and related factors 45 , 46 , standardized to have mean = 0 and s.d. = 1 in the population of the more than 12,000 US public schools.
Behavioural challenge-seeking norms of the schools
The challenge-seeking norm of each school was assessed through a behavioural measure called the make-a-math-worksheet task 13 . Students completed the task towards the end of the second session, after having completed the intervention or control content. They chose from mathematical problems that were described either as challenging and offering the chance to learn a lot or as easy and not leading to much learning. Students were told that they could complete the problems at the end of the session if there was time. The school norm was estimated by taking the average number of challenging mathematical problems that adolescents in the control condition attending a given school chose to work on. Evidence for the validity of the challenge-seeking norm is presented in the Supplementary Information section 10 .
Norms of self-reported mindset of the schools
A parallel analysis focused on norms for self-reported mindsets in each school, defined as the average fixed mindset self-reports (described above) of students before random assignment. The private beliefs of peers were thought to be less likely to be visible and therefore less likely to induce conformity and moderate treatment effects, relative to peer behaviours 49 ; hence self-reported beliefs were not expected to be significant moderators. Self-reported mindset norms did not yield significant moderation (see Extended Data Table 2 ).
Course enrolment to advanced mathematics
We analysed data from 41 schools who provided data that allowed us to calculate rates at which students took an advanced mathematics course (that is, algebra II or higher) in tenth grade, the school year after the intervention. Six additional schools provided tenth grade course-taking data but did not differentiate among mathematics courses. We expected average effects of the treatment on challenging course taking in tenth grade to be small because not all students were eligible for advanced mathematics and not all schools allow students to change course pathways. However, some students might have made their way into more advanced mathematics classes or remained in an advanced pathway rather than dropping to an easier pathway. These challenge-seeking decisions are potentially relevant to both lower- and higher-achieving students, so we explored them in the full sample of students in the 41 included schools.
Analysis methods
We used intention-to-treat analyses; this means that data were analysed for all students who were randomized to an experimental condition and whose outcome data could be linked. A complier average causal effects analysis yielded the same conclusions but had slightly larger effect sizes (see Supplementary Information section 9 ). Here we report only the more conservative intention-to-treat effect sizes. Standardized effect sizes reported here were standardized mean difference effect sizes and were calculated by dividing the treatment effect coefficients by the raw standard deviation of the control group for the outcome, which is the typical effect size estimate in education evaluation experiments. Frequentist P values reported throughout are always from two-tailed hypothesis tests.
Model for average treatment effects
Analyses to estimate average treatment effects for an individual person used a cluster-robust fixed-effects linear regression model with school as fixed effect that incorporated weights provided by statisticians from ICF, with cluster defined as the primary sampling unit. Coefficients were therefore generalizable to the population of inference, which is students attending regular public schools in the United States. For the t distribution, the degrees of freedom is 46, which is equal to the number of clusters (or primary sampling units, which was 51) minus the number of sampling strata (which was 5) 45 .
Model for the heterogeneity of effects
To examine cross-school heterogeneity in the treatment effect among lower-achieving students, we estimated multilevel mixed effects models (level 1, students; level 2, schools) with fixed intercepts for schools and a random slope that varied across schools, following current recommended practices 39 . The model included school-centred student-level covariates (prior performance and demographics; see the Supplementary Information section 7 ) to make site-level estimates as precise as possible. This analysis controlled for school-level average student racial/ethnic composition and its interaction with the treatment status variable to account for confounding of student body racial/ethnic composition with school achievement levels. Student body racial/ethnic composition interactions were never significant at P < 0.05 and so we do not discuss them further (but they were always included in the models, as pre-registered).
A final pre-registered robustness analysis was conducted to reduce the influence of two possible sources of bias: awareness of study hypotheses when conducting analyses and misspecification of the regression model (see the Supplemental Information, section 13, p. 12 ). Statisticians who were not involved in the study design and unaware of the moderation hypotheses re-analysed a blinded dataset that masked the identities of the variables. They did so using an algorithm that has emerged as a leading approach for understanding moderators of treatments: BCF 40 . The BCF algorithm uses machine learning tools to discover (or rule out) higher-order interactions and nonlinear relations among covariates and moderators. It is conservative because it uses regularization and strong prior distributions to prevent false discoveries. Evidence for the robustness of the moderation analysis in our pre-registered model comes from correspondence with the estimated moderator effects of BCF in the part of the distribution where there are the most schools (that is, in the middle of the distribution), because this is where the BCF algorithm is designed to have confidence in its estimates (Extended Data Fig. 3 ).
Reporting summary
Further information on research design is available in the Nature Research Reporting Summary linked to this paper.
Data availability
Technical documentation for the National Study of Learning Mindsets is available from ICPSR at the University of Michigan ( https://doi.org/10.3886/ICPSR37353.v1 ). Aggregate data are available at https://osf.io/r82dw/ . Student-level data are protected by data sharing agreements with the participating districts; de-identified data can be accessed by researchers who agree to terms of data use, including required training and approvals from the University of Texas Institutional Review Board and analysis on a secure server. To request access to data, researchers should contact [email protected]. The pre-registered analysis plan can be found at https://osf.io/tn6g4 . The intervention module will not be commercialized and will be available at no cost to all secondary schools in the United States or Canada that wish to use it via https://www.perts.net/ . Selections from the intervention materials are included in the Supplementary Information. Researchers wishing to access full intervention materials should contact [email protected] and must agree to terms of use, including non-commercialization of the intervention.
Code availability
Syntax can be found at https://osf.io/r82dw/ or by contacting [email protected].
McFarland, J., Stark, P. & Cui, J. Trends in High School Dropout and Completion Rates in the United States: 2013 (US Department of Education, 2016).
Autor, D. H. Skills, education, and the rise of earnings inequality among the “other 99 percent”. Science 344 , 843–851 (2014).
Article CAS ADS Google Scholar
Fischer, C. S. & Hout, M. Century of Difference (Russell Sage Foundation, 2006).
Rose, H. & Betts, J. R. The effect of high school courses on earnings. Rev. Econ. Stat . 86 , 497–513 (2004).
Article Google Scholar
Patton, G. C. et al. Our future: a Lancet commission on adolescent health and wellbeing. Lancet 387 , 2423–2478 (2016).
Crosnoe, R. Fitting In, Standing Out: Navigating the Social Challenges of high School to Get an Education (Cambridge Univ. Press, 2011).
Sutton, A., Langenkamp, A. G., Muller, C. & Schiller, K. S. Who gets ahead and who falls behind during the transition to high school? Academic performance at the intersection of race/ethnicity and gender. Soc. Probl . 65 , 154–173 (2018).
Adelman, C. The Toolbox Revisited: Paths to Degree Completion from High School through College (US Department of Education, 2006).
Schiller, K. S., Schmidt, W. H., Muller, C. & Houang, R. Hidden disparities: how courses and curricula shape opportunities in mathematics during high school. Equity Excell. Educ . 43 , 414–433 (2010).
Walton, G. M. & Wilson, T. D. Wise interventions: psychological remedies for social and personal problems. Psychol. Rev . 125 , 617–655 (2018).
Yeager, D. S. & Walton, G. M. Social–psychological interventions in education: they’re not magic. Rev. Educ. Res . 81 , 267–301 (2011).
Dweck, C. S. & Yeager, D. S. Mindsets: a view from two eras. Perspect. Psychol. Sci . 14 , 481–496 (2019).
Yeager, D. S. et al. Using design thinking to improve psychological interventions: the case of the growth mindset during the transition to high school. J. Educ. Psychol . 108 , 374–391 (2016).
Aronson, J. M., Fried, C. B. & Good, C. Reducing the effects of stereotype threat on African American college students by shaping theories of intelligence. J. Exp. Soc. Psychol . 38 , 113–125 (2002).
Blackwell, L. S., Trzesniewski, K. H. & Dweck, C. S. Implicit theories of intelligence predict achievement across an adolescent transition: a longitudinal study and an intervention. Child Dev . 78 , 246–263 (2007).
Paunesku, D. et al. Mind-set interventions are a scalable treatment for academic underachievement. Psychol. Sci . 26 , 784–793 (2015).
Cohen, G. L., Garcia, J., Purdie-Vaughns, V., Apfel, N. & Brzustoski, P. Recursive processes in self-affirmation: intervening to close the minority achievement gap. Science 324 , 400–403 (2009).
Good, C., Aronson, J. & Inzlicht, M. Improving adolescents’ standardized test performance: an intervention to reduce the effects of stereotype threat. J. Appl. Dev. Psychol . 24 , 645–662 (2003).
Benner, A. D. The transition to high school: current knowledge, future directions. Educ. Psychol. Rev . 23 , 299–328 (2011).
Burnette, J. L., O’Boyle, E. H., VanEpps, E. M., Pollack, J. M. & Finkel, E. J. Mind-sets matter: a meta-analytic review of implicit theories and self-regulation. Psychol. Bull . 139 , 655–701 (2013).
Greenberg, M. T. & Abenavoli, R. Universal interventions: fully exploring their impacts and potential to produce population-level impacts. J. Res. Educ. Eff . 10 , 40–67 (2017).
Google Scholar
Allcott, H. Site selection bias in program evaluation. Q. J. Econ . 130 , 1117–1165 (2015).
Singal, A. G., Higgins, P. D. R. & Waljee, A. K. A primer on effectiveness and efficacy trials. Clin. Transl. Gastroenterol . 5 , e45 (2014).
Article CAS Google Scholar
Bloom, H. S. & Michalopoulos, C. When is the story in the subgroups? Strategies for interpreting and reporting intervention effects for subgroups. Prev. Sci . 14 , 179–188 (2013).
Reardon, S. F. & Stuart, E. A. Editors’ introduction: theme issue on variation in treatment effects. J. Res. Educ. Eff . 10 , 671–674 (2017).
Stuart, E. A., Bell, S. H., Ebnesajjad, C., Olsen, R. B. & Orr, L. L. Characteristics of school districts that participate in rigorous national educational evaluations. J. Res. Educ. Eff . 10 , 168–206 (2017).
PubMed Google Scholar
Gopalan, M. & Tipton, E. Is the National Study of Learning Mindsets nationally-representative? https://psyarxiv.com/dvmr7/ (2018).
Dynarski, S. M. For Better Learning in College Lectures, Lay Down The Laptop and Pick Up a Pen (The Brookings Institution, 2017).
Slavin, R. E. Best-evidence synthesis: an alternative to meta-analytic and traditional reviews. Educ. Res . 15 , 5–11 (1986).
Hill, C. J., Bloom, H. S., Black, A. R. & Lipsey, M. W. Empirical benchmarks for interpreting effect sizes in research. Child Dev. Perspect . 2 , 172–177 (2008).
Kraft, M. Interpreting Effect Sizes of Education Interventions https://scholar.harvard.edu/files/mkraft/files/kraft_2018_interpreting_effect_sizes.pdf (Brown University, 2018).
Hanushek, E. Valuing teachers: how much is a good teacher worth? Educ. Next 11 , 40–45 (2011).
Benartzi, S. et al. Should governments invest more in nudging? Psychol. Sci . 28 , 1041–1055 (2017).
Boulay, B. et al. The Investing in Innovation Fund: Summary of 67 Evaluations (US Department of Education, 2018).
Yeager, D. S. National Study of Learning Mindsets - One Year Impact Analysis. https://osf.io/tn6g4 (2017).
Alexander, L. Every Student Succeeds Act. 114th Congress Public Law No. 114-95 https://www.congress.gov/bill/114th-congress/senate-bill/1177/text (US Congress, 2015).
Leslie, S.-J., Cimpian, A., Meyer, M. & Freeland, E. Expectations of brilliance underlie gender distributions across academic disciplines. Science 347 , 262–265 (2015).
Carroll, J. M., Muller, C., Grodsky, E. & Warren, J. R. Tracking health inequalities from high school to midlife. Soc. Forces 96 , 591–628 (2017).
Bloom, H. S., Raudenbush, S. W., Weiss, M. J. & Porter, K. Using multisite experiments to study cross-site variation in treatment effects: a hybrid approach with fixed intercepts and a random treatment coefficient. J. Res. Educ. Eff . 10 , 817–842 (2017).
Hahn, P. R., Murray, J. S. & Carvalho, C. Bayesian regression tree models for causal inference: regularization, confounding, and heterogeneous effects. Preprint at https://arxiv.org/abs/1706.09523 (2017).
Dorie, V., Hill, J., Shalit, U., Scott, M. & Cervone, D. Automated versus do-it-yourself methods for causal inference: lessons learned from a data analysis competition. Statist. Sci. 34 , 43–68 (2019).
Kaplan, R. M. More Than Medicine: The Broken Promise of American Health (Harvard Univ. Press, 2019).
Yeager, D. S., Dahl, R. E. & Dweck, C. S. Why interventions to influence adolescent behavior often fail but could succeed. Perspect. Psychol. Sci . 13 , 101–122 (2018).
Dahl, R. E., Allen, N. B., Wilbrecht, L. & Suleiman, A. B. Importance of investing in adolescence from a developmental science perspective. Nature 554 , 441–450 (2018).
Yeager, D. S. The National Study of Learning Mindsets, [United States], 2015–2016 (ICPSR 37353). 10.3886/ICPSR37353.v1 (2019).
Tipton, E., Yeager, D. S., Iachan, R. & Schneider, B. in Experimental Methods in Survey Research: Techniques that Combine Random Sampling with Random Assignment (ed. Lavrakas, P. J.) (Wiley, 2019).
Tipton, E. How generalizable is your experiment? An index for comparing experimental samples and populations. J. Educ. Behav. Stat . 39 , 478–501 (2014).
Robins, R. W. & Pals, J. L. Implicit self-theories in the academic domain: implications for goal orientation, attributions, affect, and self-esteem change. Self. Identity 1 , 313–336 (2002).
Paluck, E. L. Reducing intergroup prejudice and conflict using the media: a field experiment in Rwanda. J. Pers. Soc. Psychol . 96 , 574–587 (2009).
Download references
Acknowledgements
This manuscript uses data from the National Study of Learning Mindsets (principal investigator, D.Y.; co-investigators: R.C., C.S.D., C.M., B.S. and G.M.W.; https://doi.org/10.3886/ICPSR37353.v1 ). The programme and surveys were administered using systems and processes developed by the Project for Education Research That Scales (PERTS ( https://www.perts.net/ ); principal investigator, D.P.). Data collection was carried out by an independent contractor, ICF (project directors, K.F. and A.R.). Planning meetings were hosted by the Mindset Scholars Network at the Center for Advanced Study in the Behavioral Sciences (CASBS) with support from a grant from Raikes Foundation to CASBS (principal investigator, M. Levi), and the study received assistance or advice from M. Shankar, T. Brock, C. Bryan, C. Macrander, T. Wilson, E. Konar, E. Horng, J. Axt, T. Rogers, A. Gelman, H. Bloom and M. Weiss. We are grateful for feedback on a preprint from L. Quay, D. Bailey, J. Harackiewicz, R. Dahl, A. Suleiman and M. Greenberg. Funding was provided by the Raikes Foundation, the William T. Grant Foundation, the Spencer Foundation, the Bezos Family Foundation, the Character Laboratory, the Houston Endowment, the Yidan Prize for Education Research, the National Science Foundation under grant number HRD 1761179, a personal gift from A. Duckworth and the President and Dean of Humanities and Social Sciences at Stanford University. Preparation of the manuscript and the development of the analytical approach were supported by National Institute of Child Health and Human Development (10.13039/100000071 R01HD084772), P2C-HD042849 (to the Population Research Center (PRC) at The University of Texas at Austin). The content is solely the responsibility of the authors and does not necessarily represent the official views of the National Institutes of Health and the National Science Foundation.
Author information
Authors and affiliations.
University of Texas at Austin, Austin, TX, USA
David S. Yeager, Jared S. Murray, Robert Crosnoe, Chandra Muller, Jenny Buontempo, Sophia Man Yang, Carlos M. Carvalho & Pratik Mhatre
University of California, Irvine, Irvine, CA, USA
Paul Hanselman
Stanford University, Stanford, CA, USA
Gregory M. Walton & Carol S. Dweck
Northwestern University, Evanston, IL, USA
Elizabeth Tipton
Michigan State University, East Lansing, MI, USA
Barbara Schneider
University of Virginia, Charlottesville, VA, USA
Chris S. Hulleman
University of Chicago, Chicago, IL, USA
Cintia P. Hinojosa
Project for Education Research that Scales, San Francisco, CA, USA
David Paunesku
Paradigm Strategy Inc., San Francisco, CA, USA
Carissa Romero
ICF, Fairfax, VA, USA
Kate Flint, Alice Roberts, Jill Trott & Ronaldo Iachan
Arizona State University, Tempe, AZ, USA
P. Richard Hahn
The Pennsylvania State University, University Park, PA, USA
Maithreyi Gopalan
Harvard University, Cambridge, MA, USA
Ronald Ferguson
University of Pennsylvania, Philadelphia, PA, USA
Angela L. Duckworth
You can also search for this author in PubMed Google Scholar
Contributions
D.S.Y. conceived the study and led the design, analysis and writing; C.S.D. was involved in every phase of the study, particularly the conception of the study, the study design, the preparation of intervention materials, the interpretation of analyses and the writing of the manuscript; G.M.W. co-conceived the study, contributed to intervention material design and assisted with the interpretation of analyses and writing of the manuscript. P.H. contributed to the design of the study and the conceptualization of the moderators, co-developed the analysis plan, carried out statistical analyses, developed the Supplementary Information and assisted with the writing of the manuscript; R.C. and C.M. contributed to study design, analysis and writing of the paper, especially with respect to sociological theory about context effects; E.T., R.I., D.S.Y. and B.S. developed the school sampling plan; C.R. co-developed the intervention content; D.P. and PERTS developed the intervention delivery and survey data collection software; K.F., A.R., J.T., and R.I. led data collection and independently cleaned and merged raw data prior to access by the analysts; J.S.M., C.M.C. and P.R.H. executed a blinded analysis of the data and contributed to the writing of the paper; C.S.H., A.L.D. and D.S.Y. co-developed the behavioural norms measure; M.G., P.M., J.B. and S.Y.H. contributed to data analysis; R.F. contributed to study design.
Corresponding authors
Correspondence to David S. Yeager or Paul Hanselman .
Ethics declarations
Competing interests.
The authors declare no competing interests for this study. Several authors have disseminated growth mindset research to public audiences and have complied with their institutional financial interest disclosure requirements; currently no financial conflicts of interest have been identified. Specifically, D.P. is the co-founder and executive director at PERTS, an institute at Stanford University that offers free growth mindset interventions and measures to schools, and authors D.S.Y, C.S.D., G.W., A.L.D., D.P., and C.H. have disseminated findings from research to K-12 schools, universities, non-profit entities, or private entities via paid or unpaid speaking appearances or consulting. None of the authors has a financial relationship with any entity that sells growth mindset products or services.
Additional information
Publisher’s note: Springer Nature remains neutral with regard to jurisdictional claims in published maps and institutional affiliations.
Peer review information Nature thanks Eric Grodsky, Luke Miratrix and the other, anonymous, reviewer(s) for their contribution to the peer review of this work.
Extended data figures and tables
Extended data fig. 1 the finding that the growth mindset effect on gpa is positive among lower-achieving students is robust to deviations from the pre-registered statistical model..
a , b , Each estimate represents an unstandardized treatment effect on GPA (on a 0 to 4.3 scale) estimated in separate fixed-effects regression models with school as a fixed effect. Most of the alternative specifications were known to produce less-valid tests of the hypothesis, but some of them required fewer subjective judgments and so it was informative to show that the main conclusion of a positive treatment effect was supported even with a suboptimal model specification. Examples include revising the core GPA outcome to include non-core classes such as speech, debate or electives (because this does not involve coding of core classes; see ‘Includes Non-Core Courses’), or revising the post-treatment marking period to include pre-treatment data in cases in which schools implemented the intervention in the Spring (because this does not involve coding pre- and post-treatment making periods; see ‘Includes Some Pre-Treatment GPA’). a , The effects of changing just one or two model specifications at a time while leaving the rest of the pre-registered model specifications the same. Open circles represent the pre-registered definition of lower-achieving students (below the school-specific median), and filled dots represent the alternative definition of lower-achieving students (below the school-specific median and below a 3.0 GPA out of 4.3). b , A histogram of all possible combinations of the alternative model specifications that shows that effects are uniformly positive. Note that the treatment effect estimates on the far left of b are from clearly less-valid models; for example, they insufficiently control for prior achievement, they drop participants with missing data, they do not use survey weights (so results are not representative and therefore do not answer our research questions). Panels a and b both show that even exercising all of these degrees of freedom in a way that could obscure true treatment effects still yields positive point estimates. Further explanations of why the alternatives were not selected for the pre-registration are included in Supplementary Information section 7.3 .
Extended Data Fig. 2 The growth mindset intervention effect in a given school is almost always positive, although there is significant heterogeneity across schools.
a , b , Mindset treatment effects on for core course GPAs ( a ) and mathematics/science GPAs ( b ). Estimates were generated using the pre-registered linear mixed-effects model (see Supplementary Information section 7 , RQ3). Note that the treatment effect at any individual school is likely to have a very wide confidence interval even when there is a true positive effect, owing to small sample sizes for each school on its own. Therefore, as with any multi-site trial, effects of individual schools are not expected to be significantly different from zero even though the average treatment effect is significantly different from zero. The plotted treatment effects were estimated in an unconditional model with no cross-level interactions (that is, without consideration of the potential moderators) and so the points are shrunken towards the sample mean. Thus, these plotted estimates do not correspond to the estimated CATEs reported in the paper or in Extended Data Table 3 .
Extended Data Fig. 3 A BCF analysis reproduces the same pattern of moderation by norms as the pre-registered linear mixed-effects model
The BCF analysis uses a nonparametric Bayesian model designed to shrink effect sizes to see if any effect can update a relatively strong prior centered on null effects and biased toward low degrees of treatment effect moderation. a , b , Data points correspond to school-level treatment effects estimated by the pre-registered linear mixed-effects model ( a ) or the BCF model ( b ). Treatment effects refers to the difference between the treatment and control groups in terms of mathematics/science GPAs at the end of ninth grade in a school, adjusting for pre-random-assignment covariates and including survey weights. The models included three school-level moderators of the student-level randomized treatment: the achievement level (categorical, dummies for low and high, medium group as the reference category in the linear model), the behavioural growth mindset norms (continuous) and the percentage of racial or ethnic minority students (continuous) of the schools. School-level treatment effects include the fitted values plus the model-estimated, school-specific random effect. Challenge-seeking behavioural norm refers to the average number of challenging mathematics problems (out of 8) chosen by students in the control group in a given school. N , the number of lower-achieving students in a school. Percent minority, the percentage of students who identify as black, African-American, Hispanic, Latino or indigenous American, split at the school-level population median (26% of the student body of the school). The dashed lines represent the estimated intercept and slope for the linear trend of the estimated treatment effects in norms. b , The coloured lines represent LOESS smoothing curves for the trend in norms of the estimated treatment effects, fitted to the estimated school-level treatment effects within achievement groups and weighted by school sample size. The area between thevertical lines is the interquartile range (IQR) of norms, where neither model is extrapolating. The two models agree broadly about average effects, particularly within the IQR of norms, while BCF estimated somewhat lower degrees of heterogeneity and extrapolates in a fundamentally different fashion at the extremes of norms (since it is a nonlinear model).Recall BCF is designed to shrink toward an overall effect size of zero, and to shrink CATEs of similar schools towards one another, in order to avoid over-fitting the data. Unlike the preregistered linear models, BCF was specified with no prior hypotheses about the functional form of moderation (nonlinearities and/or interactions between multiple moderators and treatment) so this shrinkage is necessary to obtain stable estimates of treatment effects. However, it does lead to smaller estimates of effect sizes and a lower estimated degree of moderation relative to the preregistered linear mixed effects model.
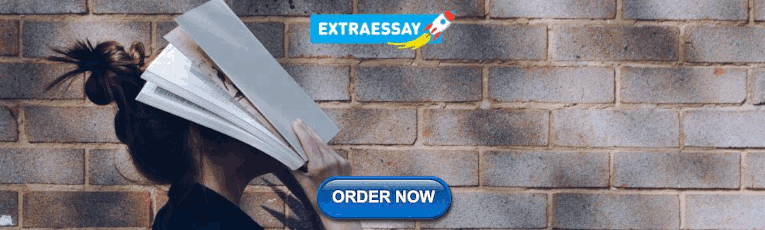
Supplementary information
Supplementary information.
This file contains Supplementary Methods and Information – see the Supplementary Contents page for full details.
Reporting Summary
Source data fig. 2, source data extended data fig. 1, source data extended data fig. 2, source data extended data fig. 3, rights and permissions.
Open Access This article is licensed under a Creative Commons Attribution 4.0 International License, which permits use, sharing, adaptation, distribution and reproduction in any medium or format, as long as you give appropriate credit to the original author(s) and the source, provide a link to the Creative Commons license, and indicate if changes were made. The images or other third party material in this article are included in the article’s Creative Commons license, unless indicated otherwise in a credit line to the material. If material is not included in the article’s Creative Commons license and your intended use is not permitted by statutory regulation or exceeds the permitted use, you will need to obtain permission directly from the copyright holder. To view a copy of this license, visit http://creativecommons.org/licenses/by/4.0/ .
Reprints and permissions
About this article
Cite this article.
Yeager, D.S., Hanselman, P., Walton, G.M. et al. A national experiment reveals where a growth mindset improves achievement. Nature 573 , 364–369 (2019). https://doi.org/10.1038/s41586-019-1466-y
Download citation
Received : 11 May 2018
Accepted : 07 July 2019
Published : 07 August 2019
Issue Date : 19 September 2019
DOI : https://doi.org/10.1038/s41586-019-1466-y
Share this article
Anyone you share the following link with will be able to read this content:
Sorry, a shareable link is not currently available for this article.
Provided by the Springer Nature SharedIt content-sharing initiative
By submitting a comment you agree to abide by our Terms and Community Guidelines . If you find something abusive or that does not comply with our terms or guidelines please flag it as inappropriate.
Quick links
- Explore articles by subject
- Guide to authors
- Editorial policies
Sign up for the Nature Briefing newsletter — what matters in science, free to your inbox daily.


- Getting Started
- Topic Overviews
- Other Databases
- Tests and Measures
What is an Empirical Study?
Literature review.
- Organize Your Sources
- NEW: LibKey Nomad
An empirical article reports the findings of a study conducted by the authors and uses data gathered from an experiment or observation. An empirical study is verifiable and "based on facts, systematic observation, or experiment, rather than theory or general philosophical principle" ( APA Databases Methodology Field Values ). In other words, it tells the story of a research conducted, doing it in great detail. The study may utilize quantitative research methods to produce numerical data and seek to find a causal relationship between two or more variables. Conversely, it may use qualitative research methods, which involves collecting non-numerical data to analyze concepts, opinions, or experiences.
Key parts of an empirical article:
- Abstract - Provides a brief overview of the research.
- Introduction - The introduction provides a review of previous research on the topic and states the hypothesis.
- Methods - The methods area describes how the research was conducted, identifies the design of the study, the participants, and any measurements that were taken during the study.
- Results - The results section describes the outcome of the study.
- Discussion (or conclusion) - The discussion section addresses the researchers' interpretations of their study and any future implications from their findings.
- References - A list of works that were cited in the study.
- What is a Lit. Review?
- Purpose of a Lit. Review
- Limitations
- Non-Empirical Research
- Useful Links/Additional Info
A review of the published resources related to a specific issue, area of research, or specific theory. It provides a summary, description, and critical evaluation of each resource.
A literature review:
- Synthesizes and places into context the research and scholarly literature relevant to the topic.
- Maps the different approaches to a given question and reveals patterns.
- Forms the foundation for subsequent research
- Justifies the significance of the new investigation.
- Contains the most pertinent studies and points to important past and current research and practices.
A Lit. Review provides background and context; it shows how your research will contribute to the field.
There are generally five parts to a literature review:
- Introduction
- Bibliography
A literature review should:
- Provide a comprehensive and updated review of the literature
- Explain why this review has taken place
- Articulate a position or hypothesis
- Acknowledge and account for conflicting and corroborating points of view
Add / Reorder
A lit. review's purpose is to offer an overview of the significant works published on a topic. It can be written as an introduction to a study in order to:
- Demonstrate how a study fills a gap in research
- Compare a study with other research that's been done
It could be a separate work (a research article on its own) that:
- Organizes or describes a topic
- Describes variables within a particular issue/problem
Some limitations of a literature review include:
- It's a snapshot in time. Unlike other reviews, this one has beginning, a middle and an end. Future developments could make your work less relevant.
- It may be too focused. Some niche studies may miss the bigger picture.
- It can be difficult to be comprehensive. There is no way to ensure that all the literature on a topic was considered.
- It is easy to be biased if you stick to top tier journals. There may be other places where people are publishing exemplary research. Look to open access publications and conferences to reflect a more inclusive collection. Also, make sure to include opposing views (and not just supporting evidence).
Non-Empirical Research articles focus more on theories, methods and their implications for research. Non-Empirical Research can include comprehensive reviews and articles that focus on methodology. They rely on empirical research literature as well but does not need to be essentially data-driven.
Write a Literature Review (UCSC)
- Literature Review (Purdue)
- Overview: Lit Reviews (UNC)
- Review of Literature (UW-Madison)
- << Previous: Tests and Measures
- Next: Organize Your Sources >>
- Last Updated: Apr 9, 2024 10:58 AM
- URL: https://libguides.macalester.edu/psyc
The Empirical Research: Context, Data, and Methods
- First Online: 01 September 2023
Cite this chapter
We’re sorry, something doesn't seem to be working properly.
Please try refreshing the page. If that doesn't work, please contact support so we can address the problem.
- Eleonora Rossero 2
55 Accesses
This chapter constitutes a reflexive account, necessary to clarify the theoretical assumptions, the researcher’s characteristics, and the methods employed. First of all, I will begin by introducing the seminal work of Erving Goffman on asylums, as well as more recent ethnographic contributions on acute mental healthcare. Then, I provide a description of the context in which the empirical study took place, the research design, and the techniques employed. Lastly, I will reflect on the personal implications of being on the field.
This is a preview of subscription content, log in via an institution to check access.
Access this chapter
- Available as PDF
- Read on any device
- Instant download
- Own it forever
- Available as EPUB and PDF
- Durable hardcover edition
- Dispatched in 3 to 5 business days
- Free shipping worldwide - see info
Tax calculation will be finalised at checkout
Purchases are for personal use only
Institutional subscriptions
His name was Andrea Soldi, he was 45 years old, and he suffered from schizophrenia. He died on August 5, 2015, in Turin, after the violent intervention of municipal police officers who were there to execute a mandatory medical treatment (“ Trattamento Sanitario Obbligatorio ”, TSO). The police officers and the psychiatrist have been convicted for his death in October 2020, with a sentence ( Corte d’Appello ) of one year and six months.
The not-so-frequently deep and prolonged crisis this boy was undergoing put the Violet Centre’s professionals under much pressure. On one hand, this allowed the raising of many crucial issues I had the opportunity to discuss with them as they unfolded in front of my eyes. On the other hand, the time they dedicated to me and my questions despite the challenging situation counts double. For this, I feel deeply grateful to them.
I also had the impression that for some nurses the constant presence of strangers was disturbing. In particular, I remember hearing the following exclamation as I was introduced to a nurse I met for the first time: “oh, so we also have sociologists now?”. I understand the problem here was not about me personally, but nevertheless I did not perceive it as a pleasant welcoming.
Åkerström, M. (2002). Slaps, punches, pinches—But not violence: Boundary-work in nursing homes for the elderly. Symbolic Interaction, 25 (4), 515–536.
Article Google Scholar
Allen, D. (2001). Narrating nursing jurisdiction: “Atrocity stories” and “boundary-work”. Symbolic Interaction, 24 (1), 75–103.
Babini, V. (2009). Liberi tutti: manicomi e psichiatri in Italia: una storia del Novecento . il Mulino.
Google Scholar
Bonner, G., Lowe, T., Rawcliffe, D., & Wellman, N. (2002). Trauma for all: A pilot study of the subjective experience of physical restraint for mental health inpatients and staff in the UK. Journal of Psychiatric and Mental Health Nursing, 9 (4), 465–473.
Article PubMed Google Scholar
Brown, B., Crawford, P., Gilbert, P., Gilbert, J., & Gale, C. (2014). Practical compassions: Repertoires of practice and compassion talk in acute mental healthcare. Sociology of Health & Illness, 36 (3), 383–399.
Buus, N. (2008). Negotiating clinical knowledge: A field study of psychiatric nurses’ everyday communication. Nursing Inquiry, 15 (3), 189–198.
Cardano, M. (2011). La ricerca qualitativa . il Mulino.
Cardano, M. (2020). Defending qualitative research: Design, analysis and textualization . Routledge.
Book Google Scholar
Cetina, K. K., Schatzki, T. R., & Von Savigny, E. (Eds.). (2005). The practice turn in contemporary theory . Routledge.
Cussins, C. M. (1996). Ontological choreography: Agency through objectification in infertility clinics. Social Studies of Science, 26 (3), 575–610.
Dell’Acqua, G., Norcio, B., de Girolamo, G., Barbato, A., Bracco, R., Gaddini, A., Miglio, R., Morosini, P., Picardi, A., Rossi, E., Rucci, P., & Santone, G. (2007). Caratteristiche e attività delle strutture di ricovero per pazienti psichiatrici acuti: i risultati dell’indagine nazionale “Progress Acuti”. Giornale Italiano di Psicopatologia, 13 , 26–39.
Denzin, N. K., & Lincoln, Y. S. (Eds.). (2017). The Sage handbook of qualitative research . Sage.
Di Lorenzo, R., Baraldi, S., Ferrara, M., Mimmi, S., & Rigatelli, M. (2012). Physical restraints in an Italian psychiatric ward: Clinical reasons and staff organization problems. Perspectives in Psychiatric Care, 48 (2), 95–107.
Di Napoli, W., & Andreatta, O. (2014). A “no-restraint” psychiatric department: Operative protocols and outcome data from the “opened-doors experience” in Trento. Psychiatria Danubina, 26 (1), 138–141.
PubMed Google Scholar
Ferioli, V. (2013). Contenzione: aspetti clinici, giuridici e psico-dinamici. Psichiatria e Psicoterapia, 32 (1), 29–44.
Foucault, M. (2006a). History of madness . Routledge.
Foucault, M. (2006b). Psychiatric power: Lectures at the Collège de France, 1973–1974 . Palgrave Macmillan.
Gieryn, T. F. (1983). Boundary-work and the demarcation of science from non-science: Strains and interests in professional ideologies of scientists. American Sociological Review, 48 , 781–795.
Goffman, E. (1961). Asylums: Essays on the social situations of mental patients and other inmates . Anchor Books.
Gomm, R., Hammersley, M., & Foster, P. (Eds.). (2000). Case study method: Key issues, key texts . Sage.
Goodwin, C. (1994). Professional vision. American Anthropologist, 96 (3), 606–633.
Griffin, C., & Bengry-Howell, A. (2017). Ethnography. In C. Willig & W. Sainton Rogers (Eds.), The SAGE handbook of qualitative research in psychology (pp. 38–54). SAGE Publications Ltd.
Chapter Google Scholar
Hamilton, B. E., & Manias, E. (2007). Rethinking nurses’ observations: Psychiatric nursing skills and invisibility in an acute inpatient setting. Social Science & Medicine, 65 (2), 331–343.
Jacob, J. D., Holmes, D., Rioux, D., & Corneau, P. (2018). Patients’ perspective on mechanical restraints in acute and emergency psychiatric settings: A poststructural feminist analysis. In J. M. Kilty & E. Dej (Eds.), Containing madness. Gender and ‘psy’ in institutional contexts (pp. 93–117). Palgrave Macmillan.
Johansson, I. M., Skärsäter, I., & Danielson, E. (2006). The health-care environment on a locked psychiatric ward: An ethnographic study. International Journal of Mental Health Nursing, 15 (4), 242–250.
Johnston, M. S., & Kilty, J. M. (2014). Power, control and coercion: Exploring hyper-masculine performativity by private guards in a psychiatric ward setting. In D. Holmes, A. Perron, & J. D. Jacob (Eds.), Power and the psychiatric apparatus: Repression, transformation and assistance (pp. 61–90). Ashgate Publishing, Ltd.
Katz, P., & Kirkland, F. R. (1990). Violence and social structure on mental hospital wards. Psychiatry, 53 (3), 262–277.
Kersting, X. A. K., Hirsch, S., & Steinert, T. (2019). Physical harm and death in the context of coercive measures in psychiatric patients: A systematic review. Frontiers in Psychiatry, 10 , 400.
Article PubMed PubMed Central Google Scholar
Lamont, M., & Molnár, V. (2002). The study of boundaries in the social sciences. Annual Review of Sociology, 28 (1), 167–195.
Lave, J., & Wenger, E. (1991). Situated learning: Legitimate peripheral participation . Cambridge University Press.
Law, J. (2010). Care and killing: Tensions in veterinary practice. In A. Mol, I. Moser, & J. Pols (Eds.), Care in practice: On tinkering in clinics, homes and farms (Vol. 8, pp. 57–72). transcript Verlag.
Mason, J. (2002). Qualitative Researching , 2nd Edition. London: Sage Publications.
Mauceri, S. (Ed.). (2017). “Contenere” la contenzione meccanica in Italia. Primo rapporto sui diritti negati dalla pratica di legare coercitivamente i pazienti psichiatrici nei SPDC. A Buon Diritto—Quaderni .
Mezzina, R. (2014). Community mental health care in Trieste and beyond: An “Open Door–No Restraint” system of care for recovery and citizenship. The Journal of Nervous and Mental Disease, 202 (6), 440–445.
Morrison, E. F. (1990). The tradition of toughness: A study of nonprofessional nursing care in psychiatric settings. Image: The Journal of Nursing Scholarship, 22 (1), 32–38.
Okin, R. (2020). The Trieste model. In T. Burns & J. Foot (Eds.), Basaglia’s international legacy: From asylum to community (pp. 317–331). Oxford University Press.
Oliveira, T. T. S. D. S., Fabrici, E. P., & Santos, M. A. D. (2018). Structure and functioning of a mental health team of Trieste in its members’ perspective: A qualitative study. Psicologia em Pesquisa, 12 (2), 24–35.
Pilgrim, D. (2002). The biopsychosocial model in Anglo-American psychiatry: Past, present and future? Journal of Mental Health, 11 (6), 585–594.
Pols, J. (2003). Enforcing patient rights or improving care? The interference of two modes of doing good in mental health care. Sociology of Health & Illness, 25 (4), 320–347.
Prior, P. M. (1995). Surviving psychiatric institutionalisation: A case study. Sociology of Health & Illness, 17 (5), 651–667.
Quirk, A., & Lelliott, P. (2001). What do we know about life on acute psychiatric wards in the UK? A review of the research evidence. Social Science & Medicine, 53 (12), 1565–1574.
Quirk, A., & Lelliott, P. (2004). Users’ experiences of inpatient services. In P. Campling, S. Davies, & G. Farquharson (Eds.), From toxic institutions to therapeutic environments . Gaskell.
Quirk, A., Lelliott, P., & Seale, C. (2006). The permeable institution: An ethnographic study of three acute psychiatric wards in London. Social Science & Medicine, 63 (8), 2105–2117.
Ragin, C. C., & Becker, H. S. (Eds.). (1992). What is a case?: Exploring the foundations of social inquiry . Cambridge University Press.
Rhodes, L. A. (1991). Emptying beds: The work of an emergency psychiatric unit . University of California Press.
Rogers, A., & Pilgrim, D. (2014). A sociology of mental health and illness . McGraw-Hill Education UK.
Toresini, L. (2007). SPDC no restraint. La sfida della cura. In AA.VV., I Servizi Psichiatrici di Diagnosi e Cura. L’utopia della cura in ospedale . Edizioni Co.Pro.S, Caltagirone.
Working Party on Psychiatry and Human Rights. (2000). White Paper on the protection of the human rights and dignity of people suffering from mental disorder, especially those placed as involuntary patients in a psychiatric establishment . Strasbourg.
Download references
Author information
Authors and affiliations.
University of Turin, Turin, Italy
Eleonora Rossero
You can also search for this author in PubMed Google Scholar
Rights and permissions
Reprints and permissions
Copyright information
© 2023 The Author(s), under exclusive license to Springer Nature Switzerland AG
About this chapter
Rossero, E. (2023). The Empirical Research: Context, Data, and Methods. In: Care in a Time of Crisis. Palgrave Macmillan, Cham. https://doi.org/10.1007/978-3-031-34418-3_4
Download citation
DOI : https://doi.org/10.1007/978-3-031-34418-3_4
Published : 01 September 2023
Publisher Name : Palgrave Macmillan, Cham
Print ISBN : 978-3-031-34417-6
Online ISBN : 978-3-031-34418-3
eBook Packages : Behavioral Science and Psychology Behavioral Science and Psychology (R0)
Share this chapter
Anyone you share the following link with will be able to read this content:
Sorry, a shareable link is not currently available for this article.
Provided by the Springer Nature SharedIt content-sharing initiative
- Publish with us
Policies and ethics
- Find a journal
- Track your research

Understanding the Empirical Method in Research Methodology

Table of Contents
Have you ever wondered how scientists gather evidence to support their theories? Or what steps researchers take to ensure that their findings are reliable and not just based on speculation? The answer lies in a cornerstone of scientific investigation known as the empirical method . This approach to research is all about collecting data and observing the world to form solid, evidence-based conclusions. Let’s dive into the empirical method’s fascinating world and understand why it’s so critical in research methodology.
What is the empirical method?
The empirical method is a way of gaining knowledge by means of direct and indirect observation or experience. It’s fundamentally based on the idea that knowledge comes from sensory experience and can be acquired through observation and experimentation. This method stands in contrast to approaches that rely solely on theoretical or logical means.
The role of observation in the empirical method
Observation is at the heart of the empirical method. It involves using your senses to gather information about the world. This could be as simple as noting the color of a flower or as complex as using advanced technology to observe the behavior of microscopic organisms. The key is that the observations must be systematic and replicable, providing reliable data that can be used to draw conclusions.
Data collection: qualitative and quantitative
Different types of data can be collected using the empirical method:
- Qualitative data – This data type is descriptive and conceptual, often collected through interviews, observations, and case studies.
- Quantitative data – This involves numerical data collected through methods like surveys, experiments, and statistical analysis.
Empirical vs. experimental methods
While the empirical method is often associated with experimentation, it’s important to distinguish between the two. Experimental methods involve controlled tests where the researcher manipulates one variable to observe the effect on another. In contrast, the empirical method doesn’t necessarily involve manipulation. Instead, it focuses on observing and collecting data in natural settings, offering a broader understanding of phenomena as they occur in real life.
Why the distinction matters
Understanding the difference between empirical and experimental methods is crucial because it affects how research is conducted and how results are interpreted. Empirical research can provide a more naturalistic view of the subject matter, whereas experimental research can offer more control over variables and potentially more precise outcomes.
The significance of experiential learning
The empirical method has deep roots in experiential learning, which emphasizes learning through experience. This connection is vital because it underlines the importance of engaging with the subject matter at a practical level, rather than just theoretically. It’s a hands-on approach to knowledge that has been valued since the time of Aristotle.
Developing theories from empirical research
One of the most significant aspects of the empirical method is its role in theory development . Researchers collect and analyze data, and from these findings, they can formulate or refine theories. Theories that are supported by empirical evidence tend to be more robust and widely accepted in the scientific community.
Applying the empirical method in various fields
The empirical method is not limited to the natural sciences. It’s used across a range of disciplines, from social sciences to humanities, to understand different aspects of the world. For instance:
- In psychology , researchers might use the empirical method to observe and record behaviors to understand the underlying mental processes.
- In sociology , it could involve studying social interactions to draw conclusions about societal structures.
- In economics , empirical data might be used to test the validity of economic theories or to measure market trends.
Challenges and limitations
Despite its importance, the empirical method has its challenges and limitations. One major challenge is ensuring that observations and data collection are unbiased. Additionally, not all phenomena are easily observable, and some may require more complex or abstract approaches.
The empirical method is a fundamental aspect of research methodology that has stood the test of time. By relying on observation and data collection, it allows researchers to ground their theories in reality, providing a solid foundation for knowledge. Whether it’s used in the hard sciences, social sciences, or humanities, the empirical method continues to be a critical tool for understanding our complex world.
How do you think the empirical method affects the credibility of research findings? And can you think of a situation where empirical methods might be difficult to apply but still necessary for advancing knowledge? Let’s discuss these thought-provoking questions and consider the breadth of the empirical method’s impact on the pursuit of understanding.
How useful was this post?
Click on a star to rate it!
Average rating / 5. Vote count:
No votes so far! Be the first to rate this post.
We are sorry that this post was not useful for you!
Let us improve this post!
Tell us how we can improve this post?
Submit a Comment Cancel reply
Your email address will not be published. Required fields are marked *
Save my name, email, and website in this browser for the next time I comment.
Submit Comment
Research Methodology
1 Introduction to Research in General
- Research in General
- Research Circle
- Tools of Research
- Methods: Quantitative or Qualitative
- The Product: Research Report or Papers
2 Original Unity of Philosophy and Science
- Myth Philosophy and Science: Original Unity
- The Myth: A Spiritual Metaphor
- Myth Philosophy and Science
- The Greek Quest for Unity
- The Ionian School
- Towards a Grand Unification Theory or Theory of Everything
- Einstein’s Perennial Quest for Unity
3 Evolution of the Distinct Methods of Science
- Definition of Scientific Method
- The Evolution of Scientific Methods
- Theory-Dependence of Observation
- Scope of Science and Scientific Methods
- Prevalent Mistakes in Applying the Scientific Method
4 Relation of Scientific and Philosophical Methods
- Definitions of Scientific and Philosophical method
- Philosophical method
- Scientific method
- The relation
- The Importance of Philosophical and scientific methods
5 Dialectical Method
- Introduction and a Brief Survey of the Method
- Types of Dialectics
- Dialectics in Classical Philosophy
- Dialectics in Modern Philosophy
- Critique of Dialectical Method
6 Rational Method
- Understanding Rationalism
- Rational Method of Investigation
- Descartes’ Rational Method
- Leibniz’ Aim of Philosophy
- Spinoza’ Aim of Philosophy
7 Empirical Method
- Common Features of Philosophical Method
- Empirical Method
- Exposition of Empiricism
- Locke’s Empirical Method
- Berkeley’s Empirical Method
- David Hume’s Empirical Method
8 Critical Method
- Basic Features of Critical Theory
- On Instrumental Reason
- Conception of Society
- Human History as Dialectic of Enlightenment
- Substantive Reason
- Habermasian Critical Theory
- Habermas’ Theory of Society
- Habermas’ Critique of Scientism
- Theory of Communicative Action
- Discourse Ethics of Habermas
9 Phenomenological Method (Western and Indian)
- Phenomenology in Philosophy
- Phenomenology as a Method
- Phenomenological Analysis of Knowledge
- Phenomenological Reduction
- Husserl’s Triad: Ego Cogito Cogitata
- Intentionality
- Understanding ‘Consciousness’
- Phenomenological Method in Indian Tradition
- Phenomenological Method in Religion
10 Analytical Method (Western and Indian)
- Analysis in History of Philosophy
- Conceptual Analysis
- Analysis as a Method
- Analysis in Logical Atomism and Logical Positivism
- Analytic Method in Ethics
- Language Analysis
- Quine’s Analytical Method
- Analysis in Indian Traditions
11 Hermeneutical Method (Western and Indian)
- The Power (Sakti) to Convey Meaning
- Three Meanings
- Pre-understanding
- The Semantic Autonomy of the Text
- Towards a Fusion of Horizons
- The Hermeneutical Circle
- The True Scandal of the Text
- Literary Forms
12 Deconstructive Method
- The Seminal Idea of Deconstruction in Heidegger
- Deconstruction in Derrida
- Structuralism and Post-structuralism
- Sign Signifier and Signified
- Writing and Trace
- Deconstruction as a Strategic Reading
- The Logic of Supplement
- No Outside-text
13 Method of Bibliography
- Preparing to Write
- Writing a Paper
- The Main Divisions of a Paper
- Writing Bibliography in Turabian and APA
- Sample Bibliography
14 Method of Footnotes
- Citations and Notes
- General Hints for Footnotes
- Writing Footnotes
- Examples of Footnote or Endnote
- Example of a Research Article
15 Method of Notes Taking
- Methods of Note-taking
- Note Book Style
- Note taking in a Computer
- Types of Note-taking
- Notes from Field Research
- Errors to be Avoided
16 Method of Thesis Proposal and Presentation
- Preliminary Section
- Presenting the Problem of the Thesis
- Design of the Study
- Main Body of the Thesis
- Conclusion Summary and Recommendations
- Reference Material
Share on Mastodon
- Skip to main content
- Skip to primary sidebar
- Skip to footer
- QuestionPro

- Solutions Industries Gaming Automotive Sports and events Education Government Travel & Hospitality Financial Services Healthcare Cannabis Technology Use Case NPS+ Communities Audience Contactless surveys Mobile LivePolls Member Experience GDPR Positive People Science 360 Feedback Surveys
- Resources Blog eBooks Survey Templates Case Studies Training Help center

Home Market Research
Empirical Research: Definition, Methods, Types and Examples
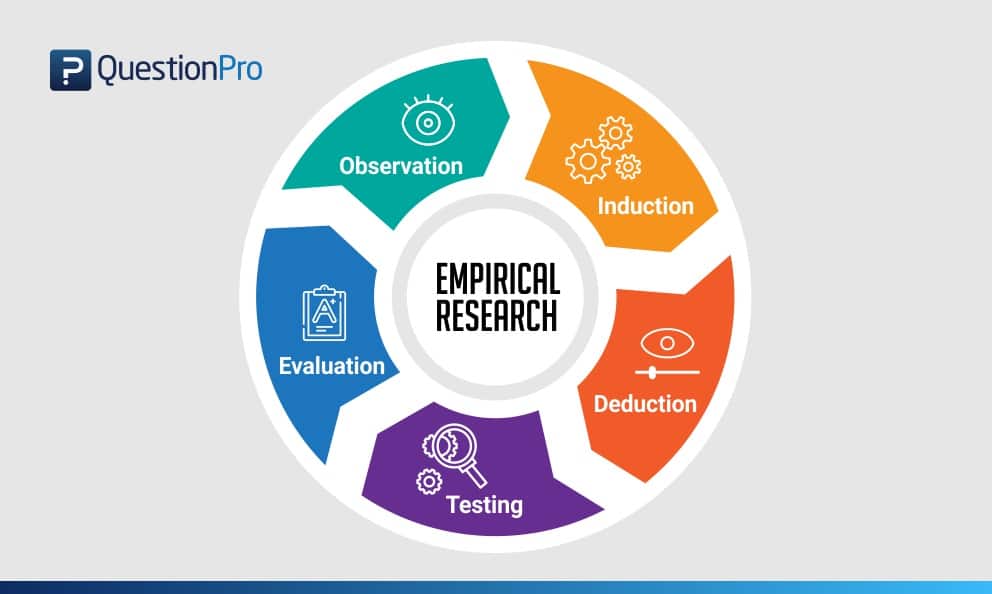
Content Index
Empirical research: Definition
Empirical research: origin, quantitative research methods, qualitative research methods, steps for conducting empirical research, empirical research methodology cycle, advantages of empirical research, disadvantages of empirical research, why is there a need for empirical research.
Empirical research is defined as any research where conclusions of the study is strictly drawn from concretely empirical evidence, and therefore “verifiable” evidence.
This empirical evidence can be gathered using quantitative market research and qualitative market research methods.
For example: A research is being conducted to find out if listening to happy music in the workplace while working may promote creativity? An experiment is conducted by using a music website survey on a set of audience who are exposed to happy music and another set who are not listening to music at all, and the subjects are then observed. The results derived from such a research will give empirical evidence if it does promote creativity or not.
LEARN ABOUT: Behavioral Research
You must have heard the quote” I will not believe it unless I see it”. This came from the ancient empiricists, a fundamental understanding that powered the emergence of medieval science during the renaissance period and laid the foundation of modern science, as we know it today. The word itself has its roots in greek. It is derived from the greek word empeirikos which means “experienced”.
In today’s world, the word empirical refers to collection of data using evidence that is collected through observation or experience or by using calibrated scientific instruments. All of the above origins have one thing in common which is dependence of observation and experiments to collect data and test them to come up with conclusions.
LEARN ABOUT: Causal Research
Types and methodologies of empirical research
Empirical research can be conducted and analysed using qualitative or quantitative methods.
- Quantitative research : Quantitative research methods are used to gather information through numerical data. It is used to quantify opinions, behaviors or other defined variables . These are predetermined and are in a more structured format. Some of the commonly used methods are survey, longitudinal studies, polls, etc
- Qualitative research: Qualitative research methods are used to gather non numerical data. It is used to find meanings, opinions, or the underlying reasons from its subjects. These methods are unstructured or semi structured. The sample size for such a research is usually small and it is a conversational type of method to provide more insight or in-depth information about the problem Some of the most popular forms of methods are focus groups, experiments, interviews, etc.
Data collected from these will need to be analysed. Empirical evidence can also be analysed either quantitatively and qualitatively. Using this, the researcher can answer empirical questions which have to be clearly defined and answerable with the findings he has got. The type of research design used will vary depending on the field in which it is going to be used. Many of them might choose to do a collective research involving quantitative and qualitative method to better answer questions which cannot be studied in a laboratory setting.
LEARN ABOUT: Qualitative Research Questions and Questionnaires
Quantitative research methods aid in analyzing the empirical evidence gathered. By using these a researcher can find out if his hypothesis is supported or not.
- Survey research: Survey research generally involves a large audience to collect a large amount of data. This is a quantitative method having a predetermined set of closed questions which are pretty easy to answer. Because of the simplicity of such a method, high responses are achieved. It is one of the most commonly used methods for all kinds of research in today’s world.
Previously, surveys were taken face to face only with maybe a recorder. However, with advancement in technology and for ease, new mediums such as emails , or social media have emerged.
For example: Depletion of energy resources is a growing concern and hence there is a need for awareness about renewable energy. According to recent studies, fossil fuels still account for around 80% of energy consumption in the United States. Even though there is a rise in the use of green energy every year, there are certain parameters because of which the general population is still not opting for green energy. In order to understand why, a survey can be conducted to gather opinions of the general population about green energy and the factors that influence their choice of switching to renewable energy. Such a survey can help institutions or governing bodies to promote appropriate awareness and incentive schemes to push the use of greener energy.
Learn more: Renewable Energy Survey Template Descriptive Research vs Correlational Research
- Experimental research: In experimental research , an experiment is set up and a hypothesis is tested by creating a situation in which one of the variable is manipulated. This is also used to check cause and effect. It is tested to see what happens to the independent variable if the other one is removed or altered. The process for such a method is usually proposing a hypothesis, experimenting on it, analyzing the findings and reporting the findings to understand if it supports the theory or not.
For example: A particular product company is trying to find what is the reason for them to not be able to capture the market. So the organisation makes changes in each one of the processes like manufacturing, marketing, sales and operations. Through the experiment they understand that sales training directly impacts the market coverage for their product. If the person is trained well, then the product will have better coverage.
- Correlational research: Correlational research is used to find relation between two set of variables . Regression analysis is generally used to predict outcomes of such a method. It can be positive, negative or neutral correlation.
LEARN ABOUT: Level of Analysis
For example: Higher educated individuals will get higher paying jobs. This means higher education enables the individual to high paying job and less education will lead to lower paying jobs.
- Longitudinal study: Longitudinal study is used to understand the traits or behavior of a subject under observation after repeatedly testing the subject over a period of time. Data collected from such a method can be qualitative or quantitative in nature.
For example: A research to find out benefits of exercise. The target is asked to exercise everyday for a particular period of time and the results show higher endurance, stamina, and muscle growth. This supports the fact that exercise benefits an individual body.
- Cross sectional: Cross sectional study is an observational type of method, in which a set of audience is observed at a given point in time. In this type, the set of people are chosen in a fashion which depicts similarity in all the variables except the one which is being researched. This type does not enable the researcher to establish a cause and effect relationship as it is not observed for a continuous time period. It is majorly used by healthcare sector or the retail industry.
For example: A medical study to find the prevalence of under-nutrition disorders in kids of a given population. This will involve looking at a wide range of parameters like age, ethnicity, location, incomes and social backgrounds. If a significant number of kids coming from poor families show under-nutrition disorders, the researcher can further investigate into it. Usually a cross sectional study is followed by a longitudinal study to find out the exact reason.
- Causal-Comparative research : This method is based on comparison. It is mainly used to find out cause-effect relationship between two variables or even multiple variables.
For example: A researcher measured the productivity of employees in a company which gave breaks to the employees during work and compared that to the employees of the company which did not give breaks at all.
LEARN ABOUT: Action Research
Some research questions need to be analysed qualitatively, as quantitative methods are not applicable there. In many cases, in-depth information is needed or a researcher may need to observe a target audience behavior, hence the results needed are in a descriptive analysis form. Qualitative research results will be descriptive rather than predictive. It enables the researcher to build or support theories for future potential quantitative research. In such a situation qualitative research methods are used to derive a conclusion to support the theory or hypothesis being studied.
LEARN ABOUT: Qualitative Interview
- Case study: Case study method is used to find more information through carefully analyzing existing cases. It is very often used for business research or to gather empirical evidence for investigation purpose. It is a method to investigate a problem within its real life context through existing cases. The researcher has to carefully analyse making sure the parameter and variables in the existing case are the same as to the case that is being investigated. Using the findings from the case study, conclusions can be drawn regarding the topic that is being studied.
For example: A report mentioning the solution provided by a company to its client. The challenges they faced during initiation and deployment, the findings of the case and solutions they offered for the problems. Such case studies are used by most companies as it forms an empirical evidence for the company to promote in order to get more business.
- Observational method: Observational method is a process to observe and gather data from its target. Since it is a qualitative method it is time consuming and very personal. It can be said that observational research method is a part of ethnographic research which is also used to gather empirical evidence. This is usually a qualitative form of research, however in some cases it can be quantitative as well depending on what is being studied.
For example: setting up a research to observe a particular animal in the rain-forests of amazon. Such a research usually take a lot of time as observation has to be done for a set amount of time to study patterns or behavior of the subject. Another example used widely nowadays is to observe people shopping in a mall to figure out buying behavior of consumers.
- One-on-one interview: Such a method is purely qualitative and one of the most widely used. The reason being it enables a researcher get precise meaningful data if the right questions are asked. It is a conversational method where in-depth data can be gathered depending on where the conversation leads.
For example: A one-on-one interview with the finance minister to gather data on financial policies of the country and its implications on the public.
- Focus groups: Focus groups are used when a researcher wants to find answers to why, what and how questions. A small group is generally chosen for such a method and it is not necessary to interact with the group in person. A moderator is generally needed in case the group is being addressed in person. This is widely used by product companies to collect data about their brands and the product.
For example: A mobile phone manufacturer wanting to have a feedback on the dimensions of one of their models which is yet to be launched. Such studies help the company meet the demand of the customer and position their model appropriately in the market.
- Text analysis: Text analysis method is a little new compared to the other types. Such a method is used to analyse social life by going through images or words used by the individual. In today’s world, with social media playing a major part of everyone’s life, such a method enables the research to follow the pattern that relates to his study.
For example: A lot of companies ask for feedback from the customer in detail mentioning how satisfied are they with their customer support team. Such data enables the researcher to take appropriate decisions to make their support team better.
Sometimes a combination of the methods is also needed for some questions that cannot be answered using only one type of method especially when a researcher needs to gain a complete understanding of complex subject matter.
We recently published a blog that talks about examples of qualitative data in education ; why don’t you check it out for more ideas?
Since empirical research is based on observation and capturing experiences, it is important to plan the steps to conduct the experiment and how to analyse it. This will enable the researcher to resolve problems or obstacles which can occur during the experiment.
Step #1: Define the purpose of the research
This is the step where the researcher has to answer questions like what exactly do I want to find out? What is the problem statement? Are there any issues in terms of the availability of knowledge, data, time or resources. Will this research be more beneficial than what it will cost.
Before going ahead, a researcher has to clearly define his purpose for the research and set up a plan to carry out further tasks.
Step #2 : Supporting theories and relevant literature
The researcher needs to find out if there are theories which can be linked to his research problem . He has to figure out if any theory can help him support his findings. All kind of relevant literature will help the researcher to find if there are others who have researched this before, or what are the problems faced during this research. The researcher will also have to set up assumptions and also find out if there is any history regarding his research problem
Step #3: Creation of Hypothesis and measurement
Before beginning the actual research he needs to provide himself a working hypothesis or guess what will be the probable result. Researcher has to set up variables, decide the environment for the research and find out how can he relate between the variables.
Researcher will also need to define the units of measurements, tolerable degree for errors, and find out if the measurement chosen will be acceptable by others.
Step #4: Methodology, research design and data collection
In this step, the researcher has to define a strategy for conducting his research. He has to set up experiments to collect data which will enable him to propose the hypothesis. The researcher will decide whether he will need experimental or non experimental method for conducting the research. The type of research design will vary depending on the field in which the research is being conducted. Last but not the least, the researcher will have to find out parameters that will affect the validity of the research design. Data collection will need to be done by choosing appropriate samples depending on the research question. To carry out the research, he can use one of the many sampling techniques. Once data collection is complete, researcher will have empirical data which needs to be analysed.
LEARN ABOUT: Best Data Collection Tools
Step #5: Data Analysis and result
Data analysis can be done in two ways, qualitatively and quantitatively. Researcher will need to find out what qualitative method or quantitative method will be needed or will he need a combination of both. Depending on the unit of analysis of his data, he will know if his hypothesis is supported or rejected. Analyzing this data is the most important part to support his hypothesis.
Step #6: Conclusion
A report will need to be made with the findings of the research. The researcher can give the theories and literature that support his research. He can make suggestions or recommendations for further research on his topic.
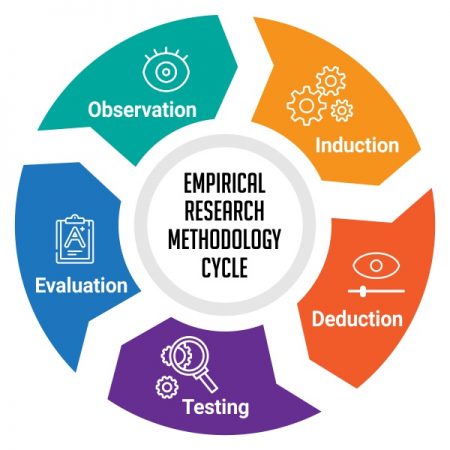
A.D. de Groot, a famous dutch psychologist and a chess expert conducted some of the most notable experiments using chess in the 1940’s. During his study, he came up with a cycle which is consistent and now widely used to conduct empirical research. It consists of 5 phases with each phase being as important as the next one. The empirical cycle captures the process of coming up with hypothesis about how certain subjects work or behave and then testing these hypothesis against empirical data in a systematic and rigorous approach. It can be said that it characterizes the deductive approach to science. Following is the empirical cycle.
- Observation: At this phase an idea is sparked for proposing a hypothesis. During this phase empirical data is gathered using observation. For example: a particular species of flower bloom in a different color only during a specific season.
- Induction: Inductive reasoning is then carried out to form a general conclusion from the data gathered through observation. For example: As stated above it is observed that the species of flower blooms in a different color during a specific season. A researcher may ask a question “does the temperature in the season cause the color change in the flower?” He can assume that is the case, however it is a mere conjecture and hence an experiment needs to be set up to support this hypothesis. So he tags a few set of flowers kept at a different temperature and observes if they still change the color?
- Deduction: This phase helps the researcher to deduce a conclusion out of his experiment. This has to be based on logic and rationality to come up with specific unbiased results.For example: In the experiment, if the tagged flowers in a different temperature environment do not change the color then it can be concluded that temperature plays a role in changing the color of the bloom.
- Testing: This phase involves the researcher to return to empirical methods to put his hypothesis to the test. The researcher now needs to make sense of his data and hence needs to use statistical analysis plans to determine the temperature and bloom color relationship. If the researcher finds out that most flowers bloom a different color when exposed to the certain temperature and the others do not when the temperature is different, he has found support to his hypothesis. Please note this not proof but just a support to his hypothesis.
- Evaluation: This phase is generally forgotten by most but is an important one to keep gaining knowledge. During this phase the researcher puts forth the data he has collected, the support argument and his conclusion. The researcher also states the limitations for the experiment and his hypothesis and suggests tips for others to pick it up and continue a more in-depth research for others in the future. LEARN MORE: Population vs Sample
LEARN MORE: Population vs Sample
There is a reason why empirical research is one of the most widely used method. There are a few advantages associated with it. Following are a few of them.
- It is used to authenticate traditional research through various experiments and observations.
- This research methodology makes the research being conducted more competent and authentic.
- It enables a researcher understand the dynamic changes that can happen and change his strategy accordingly.
- The level of control in such a research is high so the researcher can control multiple variables.
- It plays a vital role in increasing internal validity .
Even though empirical research makes the research more competent and authentic, it does have a few disadvantages. Following are a few of them.
- Such a research needs patience as it can be very time consuming. The researcher has to collect data from multiple sources and the parameters involved are quite a few, which will lead to a time consuming research.
- Most of the time, a researcher will need to conduct research at different locations or in different environments, this can lead to an expensive affair.
- There are a few rules in which experiments can be performed and hence permissions are needed. Many a times, it is very difficult to get certain permissions to carry out different methods of this research.
- Collection of data can be a problem sometimes, as it has to be collected from a variety of sources through different methods.
LEARN ABOUT: Social Communication Questionnaire
Empirical research is important in today’s world because most people believe in something only that they can see, hear or experience. It is used to validate multiple hypothesis and increase human knowledge and continue doing it to keep advancing in various fields.
For example: Pharmaceutical companies use empirical research to try out a specific drug on controlled groups or random groups to study the effect and cause. This way, they prove certain theories they had proposed for the specific drug. Such research is very important as sometimes it can lead to finding a cure for a disease that has existed for many years. It is useful in science and many other fields like history, social sciences, business, etc.
LEARN ABOUT: 12 Best Tools for Researchers
With the advancement in today’s world, empirical research has become critical and a norm in many fields to support their hypothesis and gain more knowledge. The methods mentioned above are very useful for carrying out such research. However, a number of new methods will keep coming up as the nature of new investigative questions keeps getting unique or changing.
Create a single source of real data with a built-for-insights platform. Store past data, add nuggets of insights, and import research data from various sources into a CRM for insights. Build on ever-growing research with a real-time dashboard in a unified research management platform to turn insights into knowledge.
LEARN MORE FREE TRIAL
MORE LIKE THIS
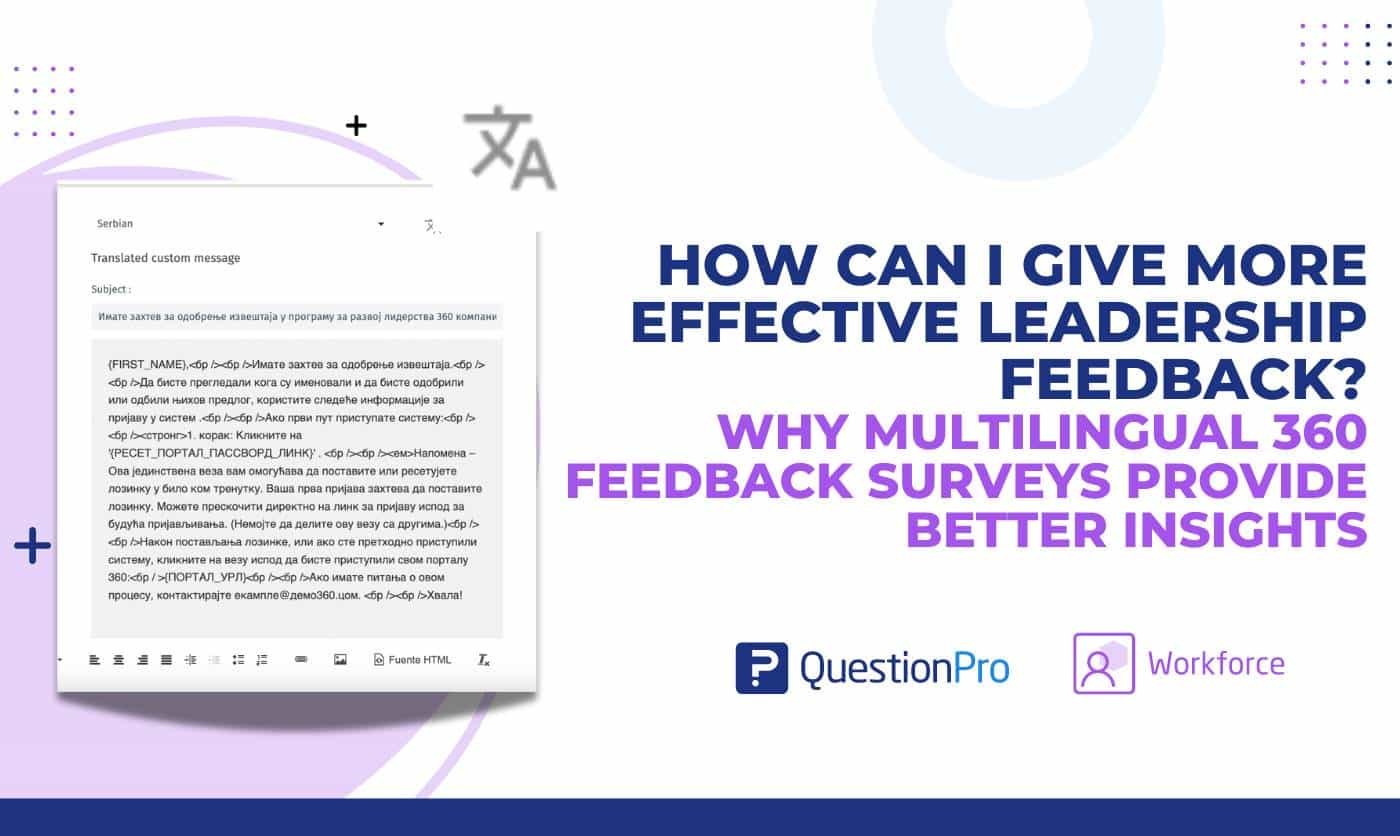
Why Multilingual 360 Feedback Surveys Provide Better Insights
Jun 3, 2024
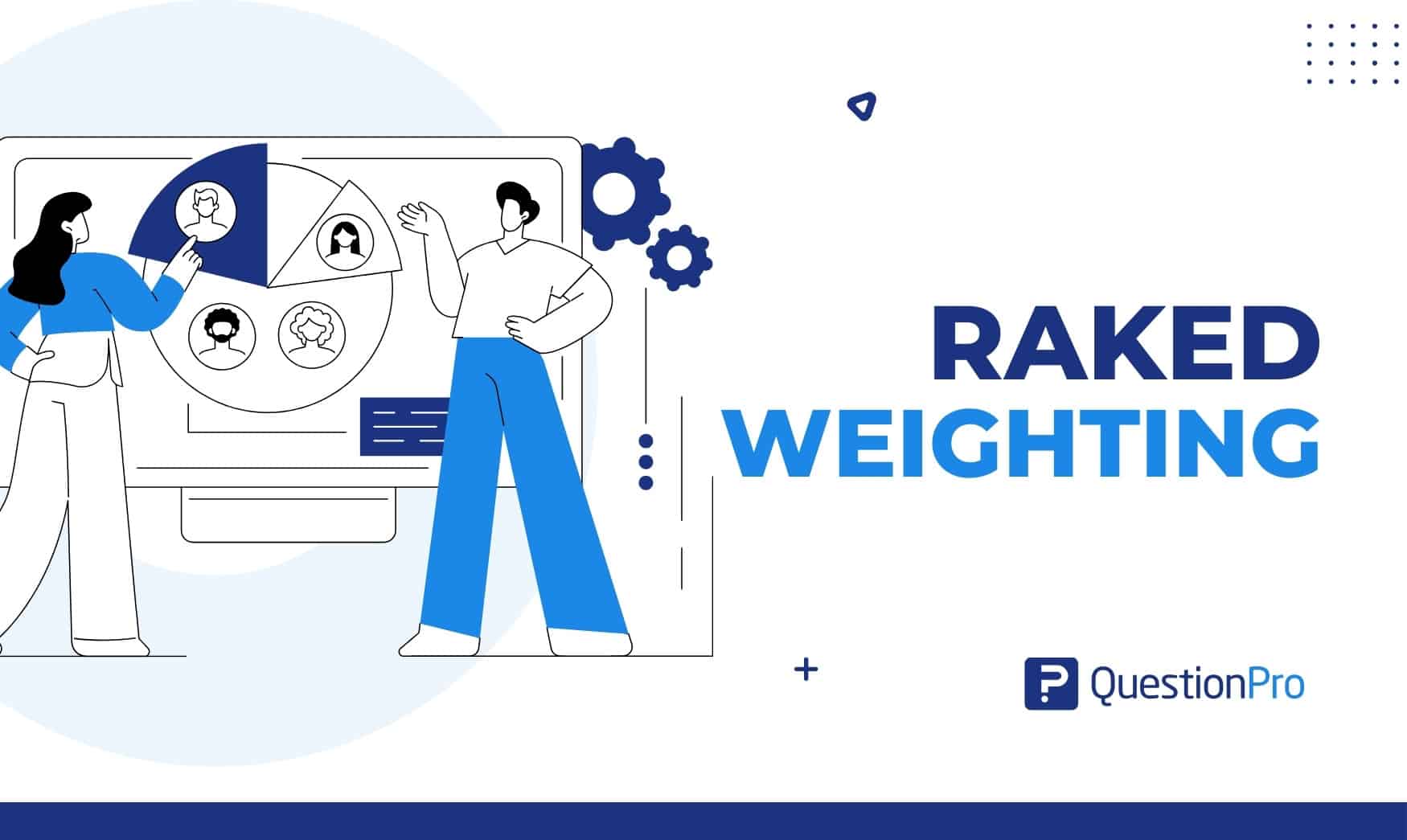
Raked Weighting: A Key Tool for Accurate Survey Results
May 31, 2024
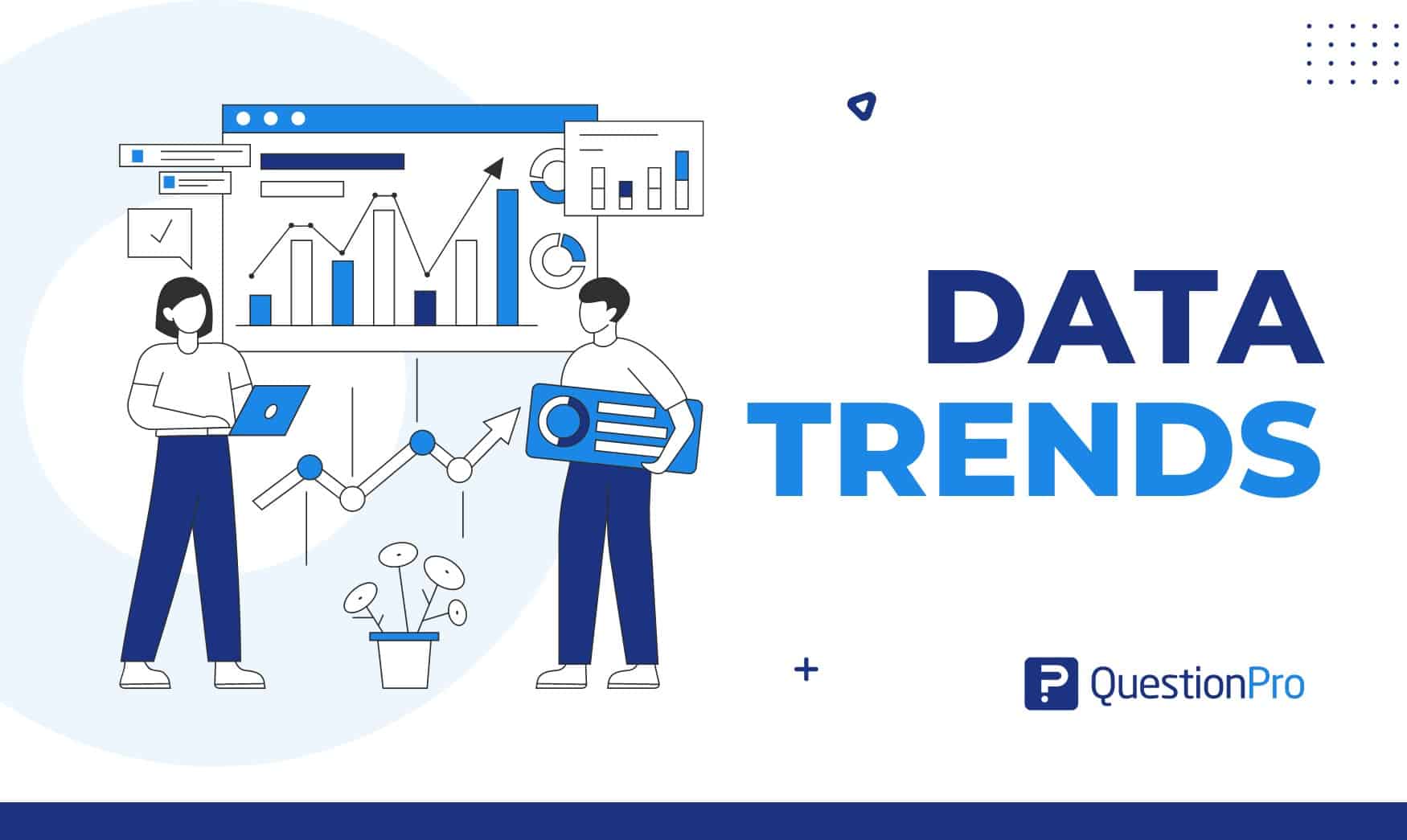
Top 8 Data Trends to Understand the Future of Data
May 30, 2024
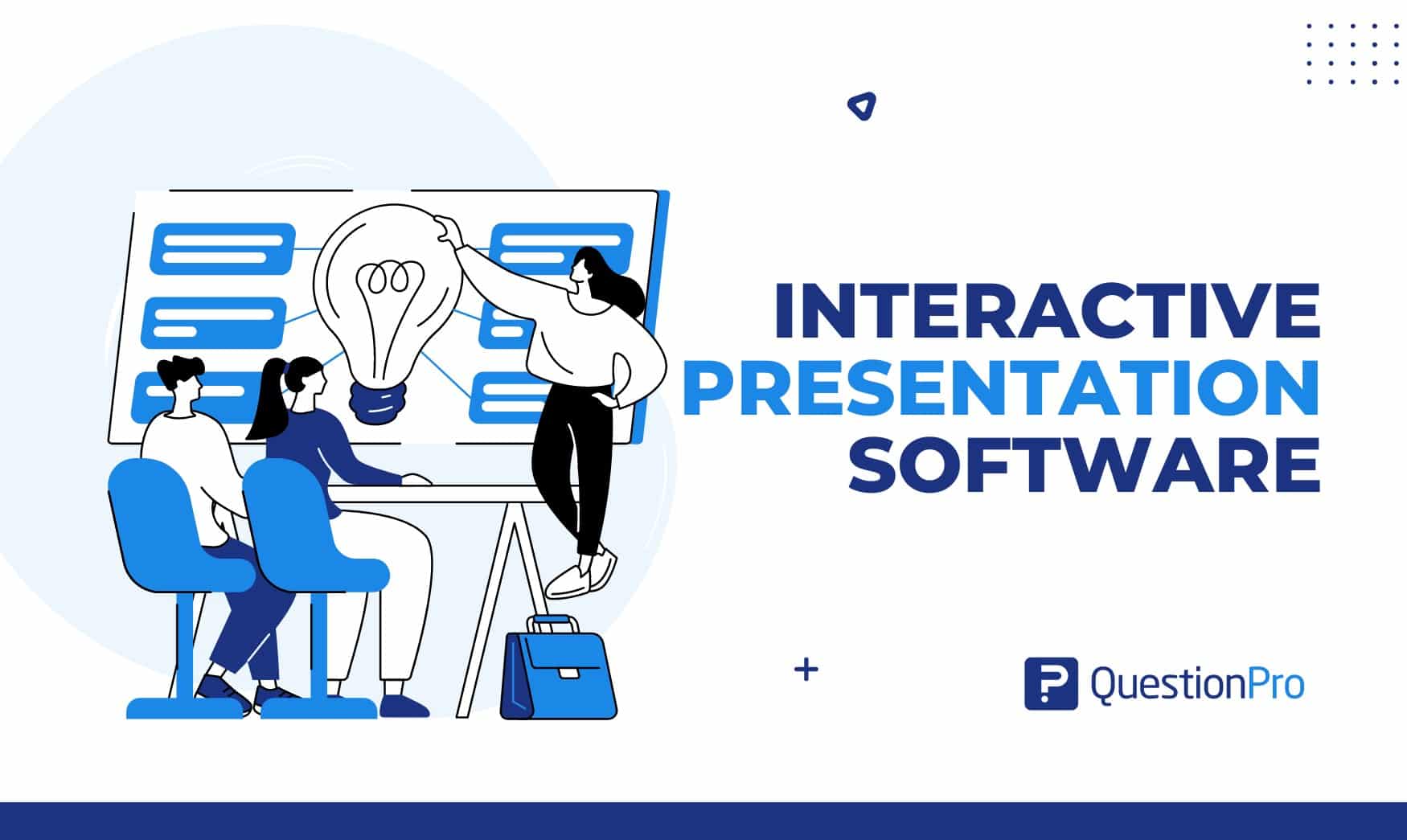
Top 12 Interactive Presentation Software to Engage Your User
May 29, 2024
Other categories
- Academic Research
- Artificial Intelligence
- Assessments
- Brand Awareness
- Case Studies
- Communities
- Consumer Insights
- Customer effort score
- Customer Engagement
- Customer Experience
- Customer Loyalty
- Customer Research
- Customer Satisfaction
- Employee Benefits
- Employee Engagement
- Employee Retention
- Friday Five
- General Data Protection Regulation
- Insights Hub
- Life@QuestionPro
- Market Research
- Mobile diaries
- Mobile Surveys
- New Features
- Online Communities
- Question Types
- Questionnaire
- QuestionPro Products
- Release Notes
- Research Tools and Apps
- Revenue at Risk
- Survey Templates
- Training Tips
- Uncategorized
- Video Learning Series
- What’s Coming Up
- Workforce Intelligence

An official website of the United States government
The .gov means it's official. Federal government websites often end in .gov or .mil. Before sharing sensitive information, make sure you're on a federal government site.
The site is secure. The https:// ensures that you are connecting to the official website and that any information you provide is encrypted and transmitted securely.
- Publications
- Account settings
- Browse Titles
NCBI Bookshelf. A service of the National Library of Medicine, National Institutes of Health.

Observational Studies: Empirical Evidence of Their Contributions to Comparative Effectiveness Reviews
Research White Papers
Investigators: Jennifer C Seida , MPH, Donna M Dryden , PhD, and Lisa Hartling , PhD.
Affiliations
- Copyright and Permissions
Structured Abstract
Introduction:.
Although observational studies are increasingly being used to address gaps in the evidence from randomized controlled trials, the effect they have on the results and conclusions of systematic reviews is unclear. Our objectives were to evaluate: (1) how often observational studies are searched for and included in comparative effectiveness reviews (CERs); (2) the rationale for including or excluding observational studies; (3) how data from observational studies are appraised, analyzed, and graded; and (4) the impact of observational studies on the strength of evidence (SOE) and overall conclusions.
In June 2013 we searched the Effective Health Care Program Web site for final reports of CERs. One reviewer screened titles, abstracts, and Key Questions for CERs that examined a therapeutic or preventive intervention provided at an individual patient level. We selected a 25 percent sample of the most recent eligible CERs. Data were extracted by one reviewer and verified by a second reviewer. We extracted the number and type of study designs included and the approaches to quality assessment, presentation of results, and grading the SOE. We identified all comparisons for which both trials and observational studies provided data, and evaluated whether observational studies had an impact on the SOE and conclusions. We applied an RCT filter to the searches to determine the impact on search yield.
From 129 records we identified 88 eligible CERs. Our final sample included 23 CERs published since November 2012. EPCs searched for observational studies in 20 CERs, of which 18 included a median of 11 (interquartile range: 2, 31) studies. Sixteen CERs incorporated the observational studies in their SOE assessments. We identified 78 comparisons from 12 CERs for which both trials and observational studies provided evidence; observational studies had an impact on SOE and conclusions for 19 (24 percent) of the comparisons. There was considerable diversity across the CERs regarding decisions to include or exclude observational studies, the study designs considered, and the approaches used to appraise, synthesize, and grade the SOE. Applying an RCT filter reduced the search yield by 65 percent (range 39 to 92 percent).
Discussion:
Reporting guidelines and methods guidance relating to observational studies are needed in order to ensure clarity and consistency across Evidence-based Practice Centers. It was not always clear that the inclusion of observational studies added value in light of the additional resources needed to search for, select, appraise, and analyze such studies.
- Collapse All
- Introduction
- Limitations
- Summary and Conclusion
- Appendix A Data Extraction Form
- Appendix B Included Comparative Effectiveness Reviews
- Appendix C Search Yields With and Without an RCT Filter
Prepared for: Agency for Healthcare Research and Quality, U.S. Department of Health and Human Services 1 , Contract No. 290-2013-00013-I. Prepared by: Alberta Evidence-based Practice Center, Edmonton, Alberta, Canada
Suggested citation:
Seida JC, Dryden DM, Hartling L. Observational Studies: Empirical Evidence of Their Contributions to Comparative Effectiveness Reviews. Methods White Paper (Prepared by the University of Alberta Evidence-based Practice Center under Contract No. 290-2013-00013-I.) AHRQ Publication No. 14-EHC002-EF. Rockville, MD: Agency for Healthcare Research and Quality. December 2013. www.effectivehealthcare.ahrq.gov/reports/final.cfm .
This report is based on research conducted by the Alberta Evidence-based Practice Center (EPC) under contract to the Agency for Healthcare Research and Quality (AHRQ), Rockville, MD (Contract No. 290-2013-00013-I). The findings and conclusions in this document are those of the authors, who are responsible for its contents; the findings and conclusions do not necessarily represent the views of AHRQ. Therefore, no statement in this report should be construed as an official position of AHRQ or of the U.S. Department of Health and Human Services.
The information in this report is intended to help health care decisionmakers—patients and clinicians, health system leaders, and policymakers, among others—make well-informed decisions and thereby improve the quality of health care services. This report is not intended to be a substitute for the application of clinical judgment. Anyone who makes decisions concerning the provision of clinical care should consider this report in the same way as any medical reference and in conjunction with all other pertinent information, i.e., in the context of available resources and circumstances presented by individual patients.
This report may be used, in whole or in part, as the basis for development of clinical practice guidelines and other quality enhancement tools, or as a basis for reimbursement and coverage policies. AHRQ or U.S. Department of Health and Human Services endorsement of such derivative products may not be stated or implied.
None of the investigators have any affiliations or financial involvement that conflicts with the material presented in this report.
540 Gaither Road, Rockville, MD 20850; www .ahrq.gov
- Cite this Page Seida JC, Dryden DM, Hartling L. Observational Studies: Empirical Evidence of Their Contributions to Comparative Effectiveness Reviews [Internet]. Rockville (MD): Agency for Healthcare Research and Quality (US); 2013 Dec.
- PDF version of this title (264K)
Other titles in these collections
- AHRQ Methods for Effective Health Care
- Health Services/Technology Assessment Texts (HSTAT)
Related information
- NLM Catalog Related NLM Catalog Entries
Similar articles in PubMed
- The value of including observational studies in systematic reviews was unclear: a descriptive study. [J Clin Epidemiol. 2014] The value of including observational studies in systematic reviews was unclear: a descriptive study. Seida J, Dryden DM, Hartling L. J Clin Epidemiol. 2014 Dec; 67(12):1343-52. Epub 2014 Sep 22.
- Review Reliability Testing of the AHRQ EPC Approach to Grading the Strength of Evidence in Comparative Effectiveness Reviews [ 2012] Review Reliability Testing of the AHRQ EPC Approach to Grading the Strength of Evidence in Comparative Effectiveness Reviews Berkman ND, Lohr KN, Morgan LC, Richmond E, Kuo TM, Morton S, Viswanathan M, Kamerow D, West S, Tant E. 2012 May
- Review Lipid Screening in Childhood for Detection of Multifactorial Dyslipidemia: A Systematic Evidence Review for the U.S. Preventive Services Task Force [ 2016] Review Lipid Screening in Childhood for Detection of Multifactorial Dyslipidemia: A Systematic Evidence Review for the U.S. Preventive Services Task Force Lozano P, Henrikson NB, Morrison CC, Dunn J, Nguyen M, Blasi P, Whitlock EP. 2016 Aug
- Review Lipid Screening in Childhood and Adolescence for Detection of Familial Hypercholesterolemia: A Systematic Evidence Review for the U.S. Preventive Services Task Force [ 2016] Review Lipid Screening in Childhood and Adolescence for Detection of Familial Hypercholesterolemia: A Systematic Evidence Review for the U.S. Preventive Services Task Force Lozano P, Henrikson NB, Dunn J, Morrison CC, Nguyen M, Blasi PR, Anderson ML, Whitlock E. 2016 Aug
- Review Low-Dose Aspirin for the Prevention of Morbidity and Mortality From Preeclampsia: A Systematic Evidence Review for the U.S. Preventive Services Task Force [ 2014] Review Low-Dose Aspirin for the Prevention of Morbidity and Mortality From Preeclampsia: A Systematic Evidence Review for the U.S. Preventive Services Task Force Henderson JT, Whitlock EP, O'Conner E, Senger CA, Thompson JH, Rowland MG. 2014 Apr
Recent Activity
- Observational Studies: Empirical Evidence of Their Contributions to Comparative ... Observational Studies: Empirical Evidence of Their Contributions to Comparative Effectiveness Reviews
Your browsing activity is empty.
Activity recording is turned off.
Turn recording back on
Connect with NLM
National Library of Medicine 8600 Rockville Pike Bethesda, MD 20894
Web Policies FOIA HHS Vulnerability Disclosure
Help Accessibility Careers

- Global (EN)
- Albania (en)
- Algeria (fr)
- Argentina (es)
- Armenia (en)
- Australia (en)
- Austria (de)
- Austria (en)
- Azerbaijan (en)
- Bahamas (en)
- Bahrain (en)
- Bangladesh (en)
- Barbados (en)
- Belgium (en)
- Belgium (nl)
- Bermuda (en)
- Bosnia and Herzegovina (en)
- Brasil (pt)
- Brazil (en)
- British Virgin Islands (en)
- Bulgaria (en)
- Cambodia (en)
- Cameroon (fr)
- Canada (en)
- Canada (fr)
- Cayman Islands (en)
- Channel Islands (en)
- Colombia (es)
- Costa Rica (es)
- Croatia (en)
- Cyprus (en)
- Czech Republic (cs)
- Czech Republic (en)
- DR Congo (fr)
- Denmark (da)
- Denmark (en)
- Ecuador (es)
- Estonia (en)
- Estonia (et)
- Finland (fi)
- France (fr)
- Georgia (en)
- Germany (de)
- Germany (en)
- Gibraltar (en)
- Greece (el)
- Greece (en)
- Hong Kong SAR (en)
- Hungary (en)
- Hungary (hu)
- Iceland (is)
- Indonesia (en)
- Ireland (en)
- Isle of Man (en)
- Israel (en)
- Ivory Coast (fr)
- Jamaica (en)
- Jordan (en)
- Kazakhstan (en)
- Kazakhstan (kk)
- Kazakhstan (ru)
- Kuwait (en)
- Latvia (en)
- Latvia (lv)
- Lebanon (en)
- Lithuania (en)
- Lithuania (lt)
- Luxembourg (en)
- Macau SAR (en)
- Malaysia (en)
- Mauritius (en)
- Mexico (es)
- Moldova (en)
- Monaco (en)
- Monaco (fr)
- Mongolia (en)
- Montenegro (en)
- Mozambique (en)
- Myanmar (en)
- Namibia (en)
- Netherlands (en)
- Netherlands (nl)
- New Zealand (en)
- Nigeria (en)
- North Macedonia (en)
- Norway (nb)
- Pakistan (en)
- Panama (es)
- Philippines (en)
- Poland (en)
- Poland (pl)
- Portugal (en)
- Portugal (pt)
- Romania (en)
- Romania (ro)
- Saudi Arabia (en)
- Serbia (en)
- Singapore (en)
- Slovakia (en)
- Slovakia (sk)
- Slovenia (en)
- South Africa (en)
- Sri Lanka (en)
- Sweden (sv)
- Switzerland (de)
- Switzerland (en)
- Switzerland (fr)
- Taiwan (en)
- Taiwan (zh)
- Thailand (en)
- Trinidad and Tobago (en)
- Tunisia (en)
- Tunisia (fr)
- Turkey (en)
- Turkey (tr)
- Ukraine (en)
- Ukraine (ru)
- Ukraine (uk)
- United Arab Emirates (en)
- United Kingdom (en)
- United States (en)
- Uruguay (es)
- Uzbekistan (en)
- Uzbekistan (ru)
- Venezuela (es)
- Vietnam (en)
- Vietnam (vi)
- Zambia (en)
- Zimbabwe (en)
- Financial Reporting View
- Women's Leadership
- Corporate Finance
- Board Leadership
- Executive Education
Fresh thinking and actionable insights that address critical issues your organization faces.
- Insights by Industry
- Insights by Topic
KPMG's multi-disciplinary approach and deep, practical industry knowledge help clients meet challenges and respond to opportunities.
- Advisory Services
- Audit Services
- Tax Services
Services to meet your business goals
Technology Alliances
KPMG has market-leading alliances with many of the world's leading software and services vendors.
Helping clients meet their business challenges begins with an in-depth understanding of the industries in which they work. That’s why KPMG LLP established its industry-driven structure. In fact, KPMG LLP was the first of the Big Four firms to organize itself along the same industry lines as clients.
- Our Industries
How We Work
We bring together passionate problem-solvers, innovative technologies, and full-service capabilities to create opportunity with every insight.
- What sets us apart
Careers & Culture
What is culture? Culture is how we do things around here. It is the combination of a predominant mindset, actions (both big and small) that we all commit to every day, and the underlying processes, programs and systems supporting how work gets done.
Relevant Results
Sorry, there are no results matching your search..
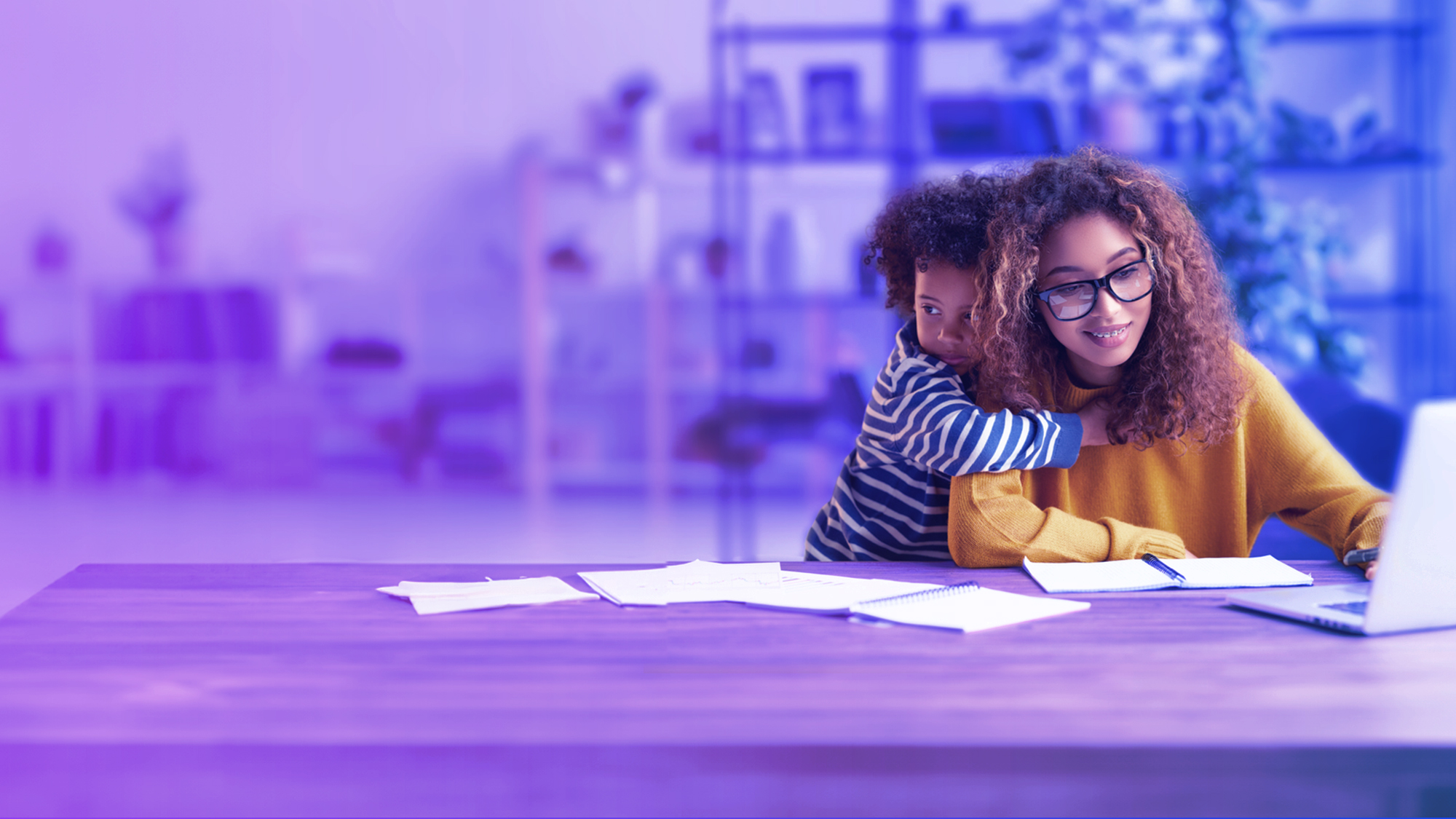
Crisis in childcare and the state of work in America
May 28, 2024
Introduction
The childcare crisis, which was simmering prior to the pandemic, has come to a boil. Pandemic-era aid has lapsed or is about to lapse. Work from home (WFH) and more flexible work schedules helped some working parents, but most workers still need to work in person. Millennials are in the thick of it. They make up the largest generation of thirty-somethings we have ever seen and are now forming households and having families.
Women are bearing more of the costs, but all of us are paying the price. Absences due to childcare problems remain elevated, hours parents can work are dropping, coworkers are picking up the slack and children are suffering. Those shifts are hurting bottom lines and eroding our potential to grow as an economy.
This report provides a deep dive into the childcare crisis, its breadth, who it is hitting the hardest and how it is undermining potential business and overall economic growth. Recent economic gains are only papering over a much more deep-seated problem. There are solutions, with companies and states stepping up to the plate. The challenge is to get more on board and catch up with peers around the world to unlock the potential of our workers, employers and economy.
Assessing the crisis: runaway costs and a lack of access
Chart 1 shows the yawning gap between the costs of childcare versus the overall consumer price index (CPI). Between 1991 and 2024, the costs for daycare and preschool rose at nearly twice the pace of overall inflation.
CPI, all items vs. daycare & preschool (January 1991 - April 2024)
Index, dec 1990=100, SA
A baseline estimate of childcare affordability is when the cost comprises up to 7% of family household income. Today, childcare costs are often in the 10-20% range. 1
Childcare is not available for many Americans where they live. As of 2018, some 51% of Americans lived in what is known as a “childcare desert.” 2 Those are areas where access to childcare facilities is either non-existent or the slots available at licensed daycare facilities are three times the number of children.
The shortage of workers in the childcare sector remains acute. Workers in the sector earn less than $15 per hour, well below the wages in other low-wage sectors. That raises the question about the quality of workers childcare centers can attract in an already tight labor market.
Many states have made significant investments in childcare services; it is one of the few bipartisan issues. The most proactive states view it as an advantage when competing for business and workers. Access to universal pre-school, known as pre-K, is a factor on which states are ranked for determining the state attractiveness for businesses.
The problem is that those programs are still ramping up and large gaps remain. Many states have yet to adopt them, and those that do only offer services for children three or four years of age. Care for infants and toddlers is still lacking. These initial years are when parents make key decisions about employment.
There are also consequences for children’s education. Research by the American Enterprise Institute shows that absences from school have increased and become chronic, especially in low-income households. 3 One way that low-income parents are dealing with the need to work and the gap in childcare is having older children watch their younger siblings. That adds insult to injury to the learning lost during the pandemic and is yet another hurdle to social mobility.
Headwinds to labor force participation
The participation rate of prime-age workers, defined as adults aged 25-54, hit its pre-recession peak in 2023 and remained above its February 2020 level in early 2024.
Sadly, those gains have done little to recoup what was lost in the 2000s. Prime-age labor force participation was 83.5% in April 2024, still more than a percentage point below the peak it hit during the boom of the late 1990s. That translates to roughly 1.2 million fewer 25–54-year-old workers in the labor force, all else equal.
Those figures are even more discouraging given the context of the tightest labor market conditions we have seen in decades. In April, the unemployment rate was below 4% for the 27 th consecutive month, tying the record last hit in the 1960s.
Why does that matter? Tight labor markets force employers to cast a larger net and lure people who might have otherwise stayed on the periphery into the labor force. They also enable workers to find better matches and escape under-employment, which boosts productivity.
Women doing better, but still lagging
Chart 2 breaks down the labor force participation rate by gender. Two takeaways are:
- Women’s participation in the labor market hit a record high in 2023 and again in April 2024, following a 23-year hiatus.
- Men’s participation in the labor market has basically recovered what it lost to the recession for the first time since the 1950s but remains on a multi-decade downtrend.
The gains made by women were all that more impressive given the employment shock of the COVID-recession. The recession was dubbed a “she-cession” for the disproportionate hit it dealt to low-wage jobs in the service sector, which tend to be dominated by women. (See Chart 2.)
Prime-age (25-54 years) workers labor force participation rate (January 1948 - April 2024)
Percent, SA
A Brookings study found that women with children under the age of 5, especially highly educated, married or foreign-born, accounted for the bulk of that rise. 4 Women in the workforce still face a penalty for having children, though WFH has muted that penalty. 5 The challenge is that the share of workers who can work fully or even partially remotely is still low. Nearly 60% of all workers still need to work fully in-person. 6
Immigration also picked up after the pandemic; foreign-born workers participate at higher rates than native-born workers.
Women with a high school degree or less had a harder time recovering. Work done by the ADP Research Institute showed that women are now working one hour less on average per week than they were in 2019. 7
The hours worked gap between men and women has also grown by an hour, which is a setback to weekly earnings. Women aged 16-24 and 25-35 were hit hardest in the wake of the pandemic. Men in those age groups saw no change in the hours they worked from 2019 to 2023.
Women with young children have a much lower labor force participation rate as compared to other groups. Women with children under six had a participation rate of only 69% in 2023, while men in the same group had a rate of 95%. Participation among women with children between six and 17 was 78%, while that for men was 92%.
No one expects the labor force participation rate of women to necessarily equal that of men. Parents should be able to choose to work or stay at home if they can afford it. However, the US has gone from leading its peers in women’s workforce participation rates in the 1990s to lagging them dramatically today. That suggests a more systemic problem.
US moves from a leader to a laggard
Women’s prime-age participation rate in the US now lags its peers, including Japan, as well as many developing countries. In 2022, Germany, France, Canada, Austria and Sweden, among others, all had participation rates at least seven percentage points higher than the US.
According to a study by the US Department of Labor, if the US had the same participation rate as Canada and Germany, around five million more women would be in the workforce today. 8 That translates to approximately $775 billion (about $2,400 per person in the US) in additional economic activity each year in addition to better outcomes for children.
Men are not immune to disruptions from childcare problems. Their participation in the labor force has largely recovered since the pandemic but remains on a long-term downward trend. There is a whole field of research studying the causes of this. As men take more active roles in childcare, they are also facing the challenges of being full-time workers and full-time parents.
The Federal Reserve’s new survey about US households found that 29% of prime-age women were not working for pay. 9 Nearly two-fifths of this group cited childcare responsibilities as a reason why. This raises the question about how much of a choice mothers with children have to work or not to work.
Voluntary work not truly “voluntary”
The Bureau of Labor Statistics (BLS) definitions separate part-time work into economic reasons, or involuntary part-time work , and noneconomic reasons, or voluntary part-time work . 10 Involuntary part-time work includes reasons such as “slack work; unfavorable business conditions; inability to find full-time work; and seasonal declines in demand.”
Voluntary part-time work includes the following reasons: “illness or other health or medical limitations; childcare problems ; family or personal obligations ; in school or training; retirement or Social Security limits on earnings; having a job where full-time work is less than 35 hours” (emphasis added).
A rise in voluntary part-time work is typically seen as a sign that economic conditions for workers are improving. However, the surge in voluntary part-time work we have seen in recent years may not all be by choice.
Someone who must devote a significant amount of time to caregiving responsibilities due to high costs and the lack of public and private benefits would still respond to a surveyor of the Household Survey that they prefer part-time work. That might conceal their true preferences and obscure both the current state of work and the crisis in care in the US.
The number of voluntary part-time workers has spiked in recent years. It reached a new series high in March 2024 at 22.9 million and remains elevated at 22.3 million (seasonally adjusted) as of April 2024.
Chart 3 shows the large uptick in part-time work due to childcare problems. Childcare problems as a share of total voluntary part-time work have also risen from an average of 3.8% pre-2010 to 4.4% in the 2010s to 4.9% since the pandemic. Historically, women, teenagers and older individuals are overrepresented among voluntary part-time workers. 11
Working part-time due to childcare problems (January 1994 - April 2024)
Thousands, 3 month moving average, NSA
Full-time workers are not immune to the struggles due to childcare. The number of full-time workers who had to cut work hours to fewer than 35 per week due to childcare problems spiked in response to initial lockdowns and the pivot online. The ranks of those workers have abated slightly but remain well above pre-pandemic levels. (See Chart 4.)
Parents are settling in and scraping by after the initial chaos of the pandemic. Some work is better than no work, but the elevated levels of both data series point to the underlying stress in the labor market due to the childcare crisis and its negative impact on employment and output.
Full-time workers who worked part-time due to childcare problems (January 2003 - April 2024)
Moreover, the data does not fully capture the degree to which childcare problems are affecting working parents, and therefore employers. In recent years, many parents have had to take care of a child who is home due to illness, not in school or a lapse in coverage by a care provider.
They may be able to take a sick day or vacation time but that is not always possible. Doing work and childcare at the same time leads to inevitably lower quality work and more stress. These unpredictable absences and distractions are costly for employers and increase the burden to coworkers, which is accelerating burnout and undermining productivity.
Flexible work arrangements help
In the wake of the pandemic, more flexible working arrangements have become the norm, including full-time remote, or WFH, and different hybrid models. This sudden increase in flexibility, especially WFH, was met with a backlash. Debates immediately arose about WFH’s effect on employee satisfaction and its impact on productivity and profits.
Research has yielded a range of findings, from lower productivity to no effect to higher productivity. Research by Stanford University Professor Nick Bloom and colleagues found that WFH resulted in higher productivity and lower wage growth. 12 Firms save money on wages and office space with fully remote and hybrid options.
Mothers with young children (especially those who are college-educated) have benefitted from WFH. 13
Indeed Hiring Lab data show that women are more likely to say that remote work is a reason for looking for new employment. 14
Hybrid work tends to boost productivity, as it allows for more mentoring, collaboration and engagement. 15 The peak days in the office are Tuesday through Thursday. Younger and older workers want to be in offices more than millennials in their thirties.
Millennials moved the furthest from their offices, seeking space and schools for their burgeoning families. 16 That shift in commutes has made it harder for companies to bring the largest share of the labor force back into offices.
When it comes to return-to-office (RTO) mandates, the evidence is clear. Research found that RTO mandates do not increase firm performance but do decrease employee satisfaction. 17 An MIT Sloan article found that such mandates decrease employee engagement and increase turnover, especially for high performers and caregivers.
The KPMG U.S. CEO Outlook Pulse Survey , a survey of 100 CEOs of large companies, found that CEOs are increasingly predicting that hybrid work is here to stay. Only 34% stated employees would be back in the office within three years, down from 62% last year.
The problem is that flexible work arrangements tend to accrue to white collar workers. However, there are options that in-person establishments are exploring, including four-day work weeks. One study conducted in the UK on 61 companies that experimented with a 4-day workweek found that workers were more efficient, happier and had lower turnover rates. 18
These debates will continue, but more flexible work arrangements are here to stay. Companies should pay attention to their employees’ different preferences. Instead of a strict company-wide policy, different business units, functions or teams could experiment with what their ideal arrangements might be.
Productivity growth still suppressed
Productivity slowed dramatically in the wake of the 2008-09 recession and remains below its pre-pandemic trend, despite substantial gains in 2023. The challenge is how to change that.
Generative AI (Gen AI) has extraordinary potential. Work done by the Census suggests that the number of firms investing in GenAI is low but moving up rapidly – it has increased from about 3.7% of all businesses in September 2023 to 5.4% in February 2024. 19 Adoption is following a U-shape, with the largest and smallest firms investing the most.
The KPMG U.S. CEO Outlook Pulse Survey also found that nearly all CEOs will continue investing in GenAI next year with 41% responding they will increase their investments. Nearly 70% of CEOs stated that their companies are using GenAI to fill gaps in the workforce.
The costs of GenAI shift the dynamics of use cases that are theoretically versus economically feasible, at least for now. It has variable costs (e.g., costs increase as use increases). Add the time it takes to adopt a new technology, and GenAI alone cannot solve our current productivity challenges; it typically takes at least a decade to see the benefits of a technological innovation.
Even if adoption is much more rapid than historical norms, that means we are not likely to see the economy-wide effects of GenAI until the late 2020s and early 2030s. That raises the question: how can firms boost productivity growth more today?
This is where childcare policies can play a larger role. A growing body of research shows the benefits that companies and the economy at large can gain from childcare support and benefits.
Childcare issues are leading to absenteeism and its associated costs for employers. Chart 5 shows that American workers are increasingly taking time off work due to childcare problems.
Full-time workers who missed work due to childcare problems (January 2003 - April 2024)
Companies also benefit via improvements in employee satisfaction and engagement, retention and productivity. One study found that companies offering caregiving benefits were rewarded with higher retention and employee productivity. 20 Company case studies found that every dollar spent on caregiving benefits resulted in gains of $18.93.
As firms benefit, so does the economy. Research has shown the clear link between childcare and better employment outcomes, especially for women. A meta-analysis of the empirical literature about childcare costs and employment found that “a 10% reduction in the price of childcare would lead to a 0.25-11% increase in maternal employment, likely near 0.5-2.5%.” 21
An increase in labor force participation leads to an increase in economic growth. An Economist Impact study found that increased access to childcare could result in millions of women entering the workforce, increasing GDP growth between 0.19% to 1.09% per country per year. 22
Childcare as a strategic investment
American workers’ access to childcare from employers is low. According to the BLS, as of March 2023 only 13% of full-time and 6% of part-time private industry workers have access to employer-provided childcare benefits. 23 These figures vary by income – higher-earners are more than three times as likely to have access than lower-earners. 24 Those who are least able to afford childcare are the least likely to have access, disproportionately affecting people of color.
Childcare-related benefits are increasingly on the agenda in corporate America. A recent care.com survey found that 56% of employers responded that childcare benefits will be prioritized in 2024. 25 An analysis by Revelio Labs found that job postings mentioning childcare benefits have increased since 2021. 26 They remain very low overall at only 2% of postings.
Working parents are increasingly looking for these benefits. A survey of more than 2,000 parents with children conducted by KinderCare and Harris Poll found that 72% of respondents “say that if they knew they would always have quality childcare coverage, they would be able to focus better on their work.” 27
That shows the potential gains in engagement and productivity that could come from childcare policies. Up to 57% said they “would [even] take a pay cut to work for a company that provided childcare benefits.” Moreover, 46% of the respondents in the Modern Family Index say they “want help paying for childcare.” 28
Companies face many constraints and competing demands. They cannot provide everything to everyone. However, many companies are expanding and publicly disclosing their childcare-related benefits. They are meeting demands by top talent, especially millennials, and they have reaped the business benefits by doing so. Companies disclosing these policies are dispersed across industries and geographies with different types of workforces.
Employer-provided childcare-related benefits include subsidized childcare or childcare discounts; on-site childcare; backup childcare; dependent care flexible savings account (FSA); childcare referral services; or flexible working hours.
Childcare-related policies should be understood as strategic investments. Though there is no one-size-fits-all childcare-related policy, companies can identify the right policies for their workforce by actively engaging with employees.
Frontline workers may prefer on-site childcare. White collar workers may instead seek flexible work options and subsidized center-based daycare. These are not zero sum. Listening to employees will help avoid losing top talent.
After instituting a new policy, companies can make sure employees are aware of it, encourage employees to use it and evaluate its effects on employee engagement, satisfaction and productivity. This data-driven approach will ensure the policies are achieving the best ROI.
In addition to childcare-related benefits, another policy that leads to positive outcomes is paid parental leave, which can help parents navigate the dizzying initial period of a child’s life.
Parental leave expanded
The United States is one of just seven countries in the world without paid maternity leave and one of 83 without paid paternity leave. 29 For those countries with mandatory paid parental leave, the average time is 29 weeks for maternity leave and 16 weeks for paternity leave.
In the US, the Family and Medical Leave Act (FMLA) guarantees that eligible workers have access to up to 12 weeks of unpaid leave. 30 Only 56% of American workers are eligible. The share is lower for people of color and low-income workers.
With the absence of federal policy, state and local governments have started to step in. As of January 2024, 13 states and Washington DC offer paid parental leave. 31 This means that 37 states do not. Among those that do, not all offer 12 weeks, whereas the evidence-based minimum best practice for paid parental leave is 26 weeks. 32
Data show that American workers are increasingly taking time off from work for parental leave. (See Chart 6). The number reached record highs in the data series two separate times in 2023. It is on a general upward trend despite recent declines.
Though usage has grown across the board, one estimate found that fewer than 5% of working fathers take more than two weeks of paid parental leave (and three-fifths of low-income fathers take no time). 33 This reflects access and economic necessities, but it also reveals the staying power of traditional gender norms and the social stigma around working men taking leave to care for their babies.
Full-time workers who missed work due to maternity or paternity leave (January 2003 - April 2024)
Increased usage of parental leave is due to state government policies and the fact that large companies are increasingly offering paid parental leave as an employee benefit. Public disclosure of paid parental leave among the Russell 1000 universe is growing but is still relatively low. 34 To ensure equity, the best practice is to provide parity in paid parental leave, but three-quarters of R1000 companies do not offer parity.
Company policies include 26 weeks of paid leave for all parents, including after birth, adoption, surrogacy or foster care placement. Many companies enhanced their paid parental leave in 2023, increasing the number of weeks offered for both maternity and paternity leave. Not all companies are moving in this direction; some have announced cuts to their policy.
Research shows the benefits of paid parental leave. It increases employee commitment and cooperation, reduces absenteeism and helps companies attract and retain top talent and thus lower turnover and hiring costs. 35
Moreover, paid parental leave results in better health outcomes for mothers and children. 36 When men take paternity leave, research shows it leads to lower divorce rates, closer relationships between fathers and children and lower rates of postpartum depression and other maternal health issues. 37
One study found that maternal earnings increase 6.7% each month that fathers stay on paternity leave. 38 Paid parental leave also saves lives. 39 California’s eight-week partial paid family leave law reduced infant mortality rates and prevented the deaths of 339 infants.
As many small and mid-sized companies may not be able to provide paid leave, states can play a key role to help employers boost retention and productivity with these benefits. California’s paid leave program is 100% funded via employee contributions to the state disability insurance fund and provides partial-wage replacement for up to eight weeks to eligible workers.
Bottom Line
The crisis in childcare in the US is a headwind for women’s labor force participation and parents more broadly and is disrupting the lives of children, extended families and coworkers. It is exacerbating income, gender and racial inequalities.
Conversely, efficient and relatively inexpensive childcare benefits can boost participation in the labor force and productivity growth. The benefits tend to accrue to white collar workers but can also lift the fortunes of low-income households and their children. Many states are actively trying to level the playing field and enact policies that benefit a larger swath of constituents. Large gaps remain.
Leading companies understand this. They are competing via the expansion and disclosure of their childcare policies. It is rare to see so many incentives align as they do with childcare policies. The benefit versus the cost proposition is clear – it is a win-win.
1 “ Childcare Prices as a Share of Median Family Income by Age of Children and Care Setting .” Women’s Bureau, US Department of Labor.
2 “ Do You Live in a Child Care Desert? ” Center for American Progress. 2020.
3 Malkus, Nat. “ Long COVID for Public Schools: Chronic Absenteeism Before and After the Pandemic .” American Enterprise Institute. January 2024.
4 Bauer, Lauren and Sarah Yu Wang. “ Prime-age women are going above and beyond in the labor market recovery .” Brookings. August 30, 2023.
5 Kleven, Henrik, Camille Landais and Gabriel Leite-Mariante. “ The Child Penalty Atlas .” NBER Working Paper 31649. February 2024 and Harrington, Emma and Matthew E. Kahn. “ Has the Rise of Work-from-Home Reduced the Motherhood Penalty in the Labor Market? ” October 31, 2023.
6 Barrero, Jose Maria, Nicholas Bloom and Steven J. Davis. “ The Evolution of Working from Home .” WFH Research. July 2023.
7 “ People are working less .” Today at Work. ADP Research Institute. Issue 5. March 2024.
8 Glynn, Sarah Jane. “ The Cost of Doing Nothing, 2023 Update: The Price We STILL Pay without Policies to Support Working Families .” US Department of Labor. November 2023.
9 “ Economic Well-Being of U.S. Households in 2023 .” Board of Governors of the Federal Reserve System. May 2024.
10 “ Labor Force Statistics from the Current Population Survey, Concepts and Definitions (CPS) .” US Bureau of Labor Statistics. November 28, 2023.
11 Dunn, Megan. “ Who chooses part-time work and why? ” Monthly Labor Review. US Bureau of Labor Statistics. March 2018.
12 Shah, Krishan et. al. “ Managers say working from home is here to stay .” VoxEU, CEPR. February 18, 2024.
13 Casselman, Ben, Emma Goldberg and Ella Koeze. “ Who Still Works from Home? ” The New York Times. March 8, 2024.
14 Bunker, Nick. “ Remote Work Holds More Appeal for Female Job Seekers .” Indeed Hiring Lab. March 27, 2024.
15 Bloom, Nicholas, Ruobing Han and James Liang. “ How Hybrid Working from Home Works Out .” NBER Working Paper 30292. January 2023.
16 Akan, Mert et. al. “ Americans Now Live Farther From Their Employers .” February 2024.
17 Ding, Yuye and Mark (Shuai) Ma. “ Return-to-Office Mandates .” SSRN. January 26, 2024.
18 “ Making it Stick: The UK Four Day Week Pilot One Year On .” Autonomy. February 2024.
19 “ Business Trends and Outlook Survey .” United States Census Bureau. May 9, 2024.
20 “ The R.O.I. of Caregiving Benefits .” VIVVI and The Fifth Trimester. February 2024.
21 Morrissey, Taryn W. “ Child care and parent labor force participation: a review of the research literature .” Review of Economics of the Household. Springer. Volume 1. March 2017.
22 Ballesteros, Monica, Shreya Mukarji and Lavanya Sayal. “ The childcare dividend initiative: bridging the access gap: quantifying the economic returns of public investment in childcare .” Economist Impact. 2023.
23 “ Percentage of private industry workers with access to employer-provided benefits by work status, March 2023 .” US Bureau of Labor Statistics.
24 “ Percentage of civilian workers with access to quality-of-life benefits by worker characteristic, March 2023 .” US Bureau of Labor Statistics.
25 “ 2024 Future of Benefits Report .” Care.com. March 2024.
26 Abdelwahed, Loujaina and Beyza Arslan. “ Are Current Child Care Benefits Enough to Keep Mothers at Work? ” Revelio Labs. October 19, 2023.
27 “ KinderCare Confidence Index .” KinderCare Learning Companies. 2024.
28 “ 10th Annual Modern Family Index: The New Working Parent .” Bright Horizons. 2024.
29 Cain Miller, Claire. “ The World ‘Has Found a Way to Do This’: The U.S. Lags on Paid Leave .” The New York Times. October 25, 2021.
30 “ Family and Medical Leave Act .” US Department of Labor.
31 “ State Paid Family Leave Laws Across the U.S. ” Bipartisan Policy Center. January 16, 2024.
32 “ Paid Family Leave: How Much Time Is Enough? ” New America.
33 Turner, Terry. “ Average Paid Maternity Leave by State: 2024 Statistics .” Annuity.org. January 10, 2024.
34 Avila, Jordyn and Matthew Nestler. “ Only 9% of America’s Largest Companies Provide Parity in Paid Parental Leave of 12+ Weeks for Primary and Secondary Caregivers .” JUST Capital. April 27, 2023.
35 Bartel et. al. “ The impact of paid family leave on employers: evidence from New York .” Community, Work & Family. January 29, 2023; Shaak, Natalie. “ Making the Case for Paid Family Leave .” Policy Brief. Drexel University Dornsife School of Public Health. March 2023; and Davison, H. Kristl and Adam Scott Blackburn. “ The Case for Offering Paid Leave: Benefits to the Employer, Employee, and Society .” Compens Benefits Rev. 55 (1). January 2023.
36 Van Niel, Maureen Sayres et. al. “ The Impact of Paid Maternity Leave on the Mental and Physical Health of Mothers and Children: A Review of the Literature and Policy Implications .” Harvard Review of Psychiatry. 28 (2). 2020.
37 Saxbe, Darby and Sofia Cardenas. “ What Paternity Leave Does for a Father’s Brain .” The New York Times. November 8, 2021.
38 Johansson, Elly-Ann. “ The effect of own and spousal parental leave on earnings .” The Institute for Labour Market Policy Evaluation. Working Paper. March 22, 2010.
39 Chen, Feng. “ Does paid family leave save infant lives? Evidence from California’s paid family leave program .” Contemporary Economic Policy. 41 (2). October 5, 2022.
Explore more
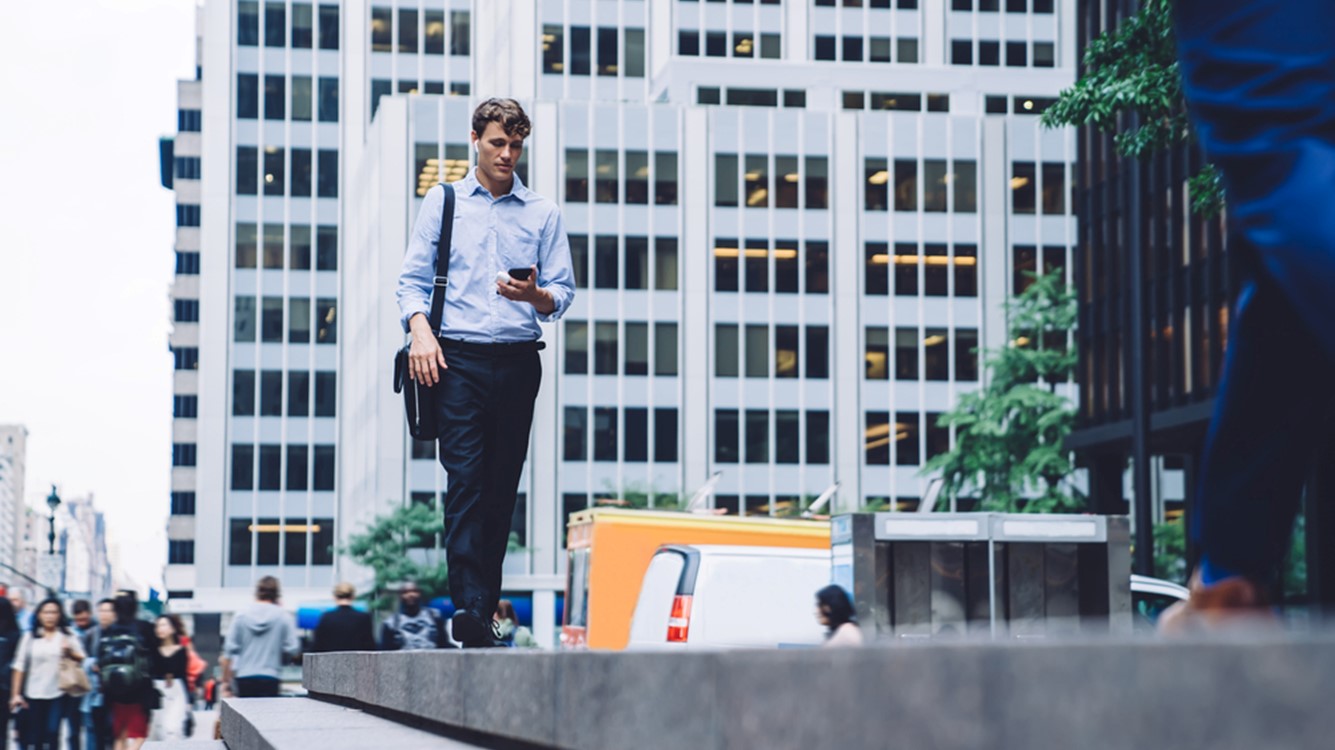
April showers
The first rate cut is still expected in December.
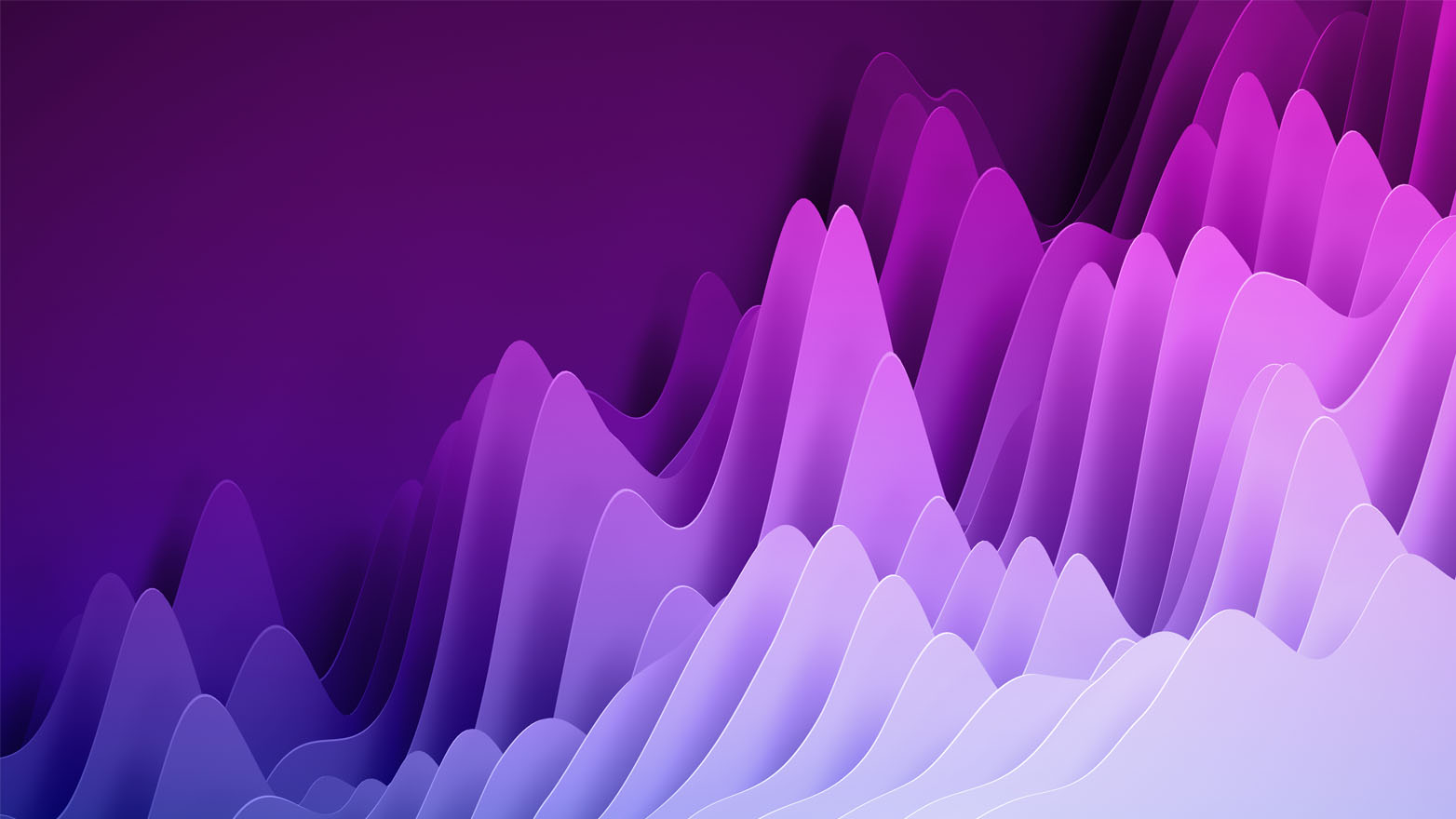
KPMG Economics
A source for unbiased economic intelligence to help improve strategic decision-making.
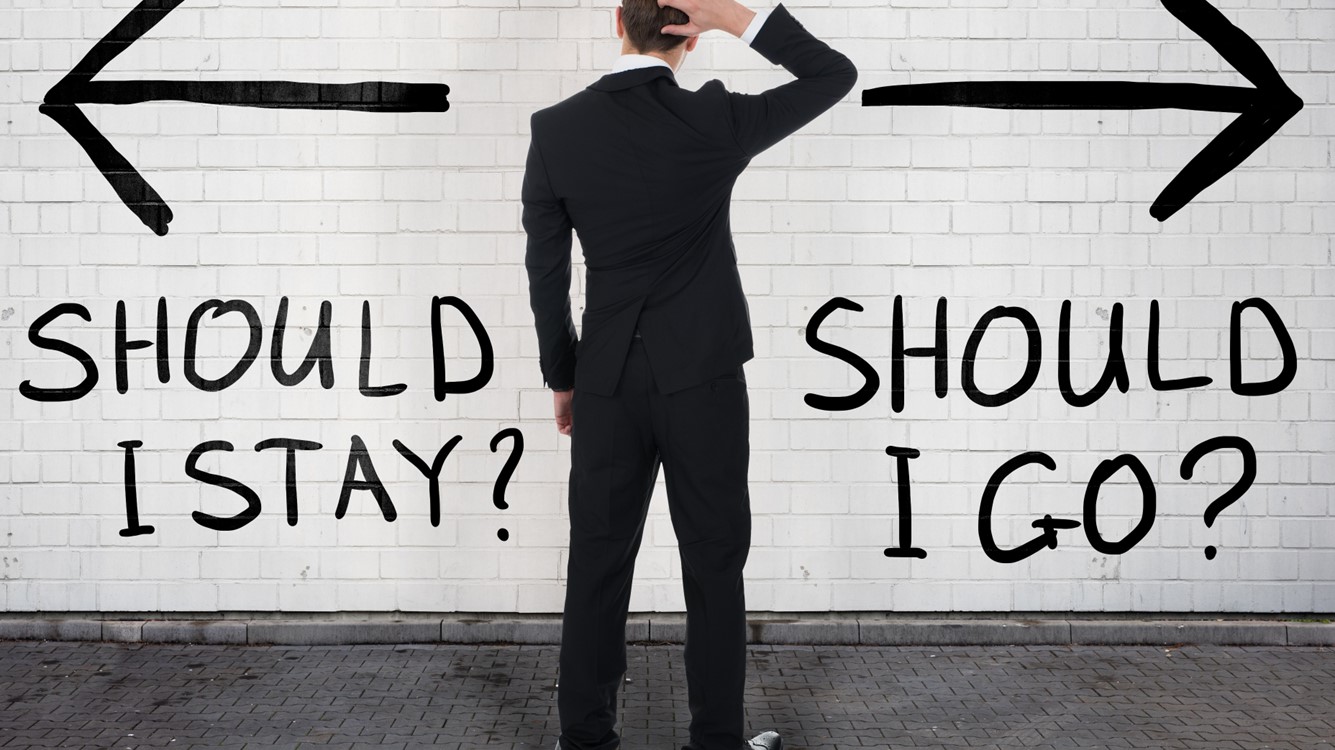
Navigating policy purgatory: Inflation and the challenge for the Fed
The central banker’s worst nightmare is to cut rates, then have to raise them.
Subscribe to insights from KPMG Economics
KPMG Economics distributes a wide selection of insight and analysis to help businesses make informed decisions.
Meet our team
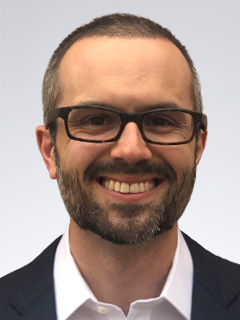
By submitting, you agree that KPMG LLP may process any personal information you provide pursuant to KPMG LLP's Privacy Statement .
Thank you for contacting KPMG. We will respond to you as soon as possible.
Contact KPMG
Job seekers
Visit our careers section or search our jobs database.
Use the RFP submission form to detail the services KPMG can help assist you with.
Office locations
International hotline
You can confidentially report concerns to the KPMG International hotline
Press contacts
Do you need to speak with our Press Office? Here's how to get in touch.
Million Dollar Sense
15 Signs You're Financially Better off Than the Average American, Backed by Research
Posted: June 2, 2024 | Last updated: June 2, 2024
#1 You Have a Sizeable Emergency Fund
If you’ve established an emergency fund capable of covering at least three to six months of living expenses, you’re better off than many Americans who struggle to save. Having a sizable emergency fund provides a financial safety net, allowing you to weather unexpected setbacks such as job loss or medical emergencies without resorting to high-interest debt or depleting your retirement savings.
Source: Wellsfargo
#2 Not Living Paycheck to Paycheck
According to a recent study, nearly 80% of Americans live paycheck to paycheck. So, if you’re not living paycheck to paycheck, you’re in a more secure financial position than many Americans who struggle to cover basic expenses between paydays. Living paycheck to paycheck can lead to financial stress and limited opportunities for savings or investments.
Source: Forbes
Like our content? Be sure to follow us .
#3 Minimal Debt
If you’ve managed to keep your debt levels significantly lower than the average American, you’re in a better financial position. The average American household debt load is $101,915 (including mortgage). High debt burdens can weigh heavily on individuals, leading to stress and financial instability. By prioritizing debt repayment and avoiding unnecessary borrowing, you’re able to allocate more of your income towards savings and investments, setting yourself up for long-term financial success.
Source: Debt.org
#4 Investment Portfolio
If you’ve built a diversified investment portfolio aligned with your financial goals and risk tolerance, you’re ahead of the curve compared to many Americans who may not invest or have a poorly diversified portfolio. Investing allows your money to grow over time, outpacing inflation and increasing your net worth. By regularly reviewing and adjusting your investments, you’re able to capitalize on market opportunities and minimize potential losses.
Source: Gallup
#5 High Credit Score
If you have an excellent credit score, you have access to better credit terms and lower interest rates, saving you money over time. A high credit score reflects responsible financial behavior such as paying bills on time, keeping credit card balances low, and avoiding excessive borrowing, making you a lower credit risk in the eyes of lenders. The average American credit score is around 715, so having a score significantly above that puts you in a strong position.
Source: Experian
#6 Retirement Savings
If you’re actively saving for retirement and have a well-defined plan in place, you’re ahead of many Americans who may not prioritize retirement savings or lack a clear strategy. In fact, a recent survey revealed that nearly 50% of Americans don’t have a dedicated retirement savings account. Saving early and regularly for retirement allows your investments to compound over time, maximizing your retirement nest egg.
Source: Yahoo Finance
#7 Homeownership
If you own a home with manageable mortgage payments, you’re in a better financial position than renters who may face rising rents and lack the opportunity to build equity. Homeownership provides stability and the potential for appreciation, allowing you to build wealth over time. Additionally, mortgage interest and property tax deductions can provide valuable tax benefits, further enhancing your financial situation.
Source: First Home Mortgage
#8 Educational Investments
If you’ve invested in your education or skills development, you’re better positioned to increase your earning potential and advance your career. Higher levels of education are associated with higher lifetime earnings and lower unemployment rates, providing greater financial security and opportunities for advancement.
Source: Faster Capital
#9 Frugal Lifestyle Choices
Did you know that an average American spends $18,000 per year on non-essential things? If you’re someone who prioritizes value over extravagance, you may be better off than an average American. Frugal living allows you to stretch your dollars further, avoid unnecessary expenses, and build wealth over time, setting you on a path toward financial independence and security.
#10 Financial Literacy
If you possess a strong understanding of personal finance concepts and strategies, you’re better equipped to make informed financial decisions and avoid common pitfalls. Financial literacy empowers you to manage your money effectively, plan for the future, and navigate complex financial situations with confidence. However, according to a recent survey, only 57% of American adults are considered financially literate.
Source: Market Watch
#11 Regular Budgeting
According to an NFCC survey, 61% of Americans don't have a budget. If you adhere to a strict budget and financial plan, you’re more likely to achieve your financial goals and avoid common pitfalls such as overspending or living beyond your means. Budgeting helps you track your income and expenses, identify areas where you can cut costs, and prioritize savings and investments, setting you on a path toward financial success.
Source: National Foundation for Credit Counseling
#12 Multiple Income Streams
If you’ve diversified your income sources to include passive income streams such as investments, rental properties, or side businesses, you’re better positioned for financial stability than those reliant solely on a single source of income. Multiple income streams provide resilience against job loss or economic downturns, ensuring a more secure financial future.
#13 Ownership of Appreciating Assets
If you possess appreciating assets such as valuable collectibles, art, or real estate investments, you’re in a better financial position than the average American, who may primarily rely on depreciating assets like cars or consumer goods. Appreciating assets not only preserves wealth but also has the potential to generate passive income or significant returns over time.
#14 Employment Stability
Did you know that there are more than 6 million unemployed people in America? If you’re fortunate enough to have a stable job with consistent income and prospects for career advancement, you’re in a more secure financial position than many. This stability not only ensures a regular paycheck to cover expenses but also opens doors for professional growth and increased earning potential over time.
Source: US Bureau of Labor Statistics
#15 Regularly Maxing Out Retirement Contributions
If you consistently contribute the maximum allowed amount to your retirement accounts, such as your 401(k) or IRA, you’re ahead financially. Many Americans contribute less than the maximum or don’t contribute at all, missing out on valuable tax benefits and potential employer matches. By maximizing your contributions, you’re supercharging your retirement savings and setting yourself up for a more comfortable future.
Disclaimer – This list is solely the author's opinion based on research and publicly available information.
15 Everyday Things Baby Boomers Had 40 Years Ago That Are Luxuries Now
As we ride the waves of technological progress, manufacturing efficiencies and cheap supply chains, some everyday aspects that were once standard now seem like relics of a bygone era. Let us take a nostalgic trip down memory lane as social media users reminisce about luxuries that were once ordinary.
11 Things You're Too Old For After Age 50
Reaching your 50s brings about a shift in priorities and perspectives. It’s a time to reevaluate habits and behaviors that might no longer serve you as you embrace the wisdom that comes with age. In this article, we’ll explore 11 aspects of life that you might want to leave behind once you hit the big 5-0. From focusing on health and relationships to letting go of unnecessary stressors, this guide offers practical advice for navigating the later stages of adulthood with clarity and purpose.
11 Things You're Too Old For After Age 50
More for You
California Democrat Calls Out Colleagues in Impassioned Speech: 'I'm Done'
"The First Time I Visited The US I Thought This Was A Restaurant Scam": Non-Americans Are Sharing The Things That Are Totally Common In The US But Bizarre In Other Countries
Ukraine-Russia war – live: Kyiv fires US weapon inside Russia as Zelensky accuses China of ‘sabotage’
This Is What a TSA Agent First Notices About You
This 3,000-Year-Old Sword Was So Well Preserved That It 'Almost Still Shines'
Are Retirees Ready for a 21% Cut to Social Security Benefits? Here's Exactly When It Could Happen.
Mom Shares Tip That Helped Her Drop 160 Pounds Healthily—Without Fad Diets
Alan Dershowitz advises Trump's attorney to 'move immediately' for sentencing: 'Timing is everything'
Do you get a jolt when you're falling asleep? Here's the reason behind that and other strange bodily reactions.
Fact Check: We Looked into Actor Terrence Howard's Claim That He Holds Patent on Augmented/Virtual Reality Technology
The best work-life balance advice came from my 24-year-old boss. Here are the 5 lessons I carry with me at every job.
Vitamins to prevent hair loss and gray hair
Plane Lands at O'Hare Airport — CDC Called Over What's Inside Passenger's Bag
WNBA Veteran Brittney Griner Delivers Four-Word Take on Caitlin Clark, Angel Reese
Map reveals best places to live in the US if nuclear war breaks out
‘Wheel of Fortune’ Host Pat Sajak Loses it While Contestants Celebrate Incorrect Guess
I moved from the US to Ireland. Here are 11 things that surprised me most.
12 Prediabetes Foods to Avoid—and Plenty More to Enjoy
This Small Town in Tennessee Was Just Named the Best Place to Retire in the U.S. South
Older People Are Holding Nothing Back About What It's Really Like To Get Old
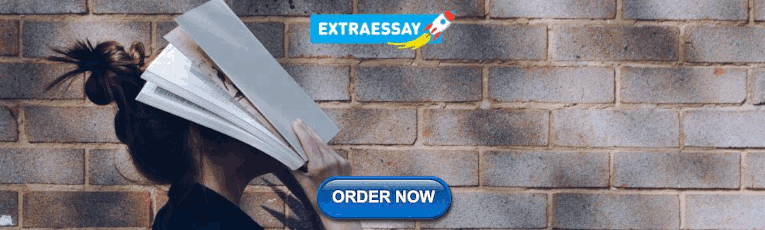
IMAGES
VIDEO
COMMENTS
Race plays an important role in how people think, develop, and behave. In the current article, we queried more than 26,000 empirical articles published between 1974 and 2018 in top-tier cognitive, developmental, and social psychology journals to document how often psychological research acknowledges this reality and to examine whether people who edit, write, and participate in the research are ...
Empirical research is characterized by several key features: Observation and Measurement: It involves the systematic observation or measurement of variables, events, or behaviors. Data Collection: Researchers collect data through various methods, such as surveys, experiments, observations, or interviews.
Another hint: some scholarly journals use a specific layout, called the "IMRaD" format, to communicate empirical research findings. Such articles typically have 4 components: Introduction : sometimes called "literature review" -- what is currently known about the topic -- usually includes a theoretical framework and/or discussion of previous ...
A US national experiment showed that a short, online, self-administered growth mindset intervention can increase adolescents' grades and advanced course-taking, and identified the ...
Share this content. Empirical research is research that is based on observation and measurement of phenomena, as directly experienced by the researcher. The data thus gathered may be compared against a theory or hypothesis, but the results are still based on real life experience. The data gathered is all primary data, although secondary data ...
An empirical article reports the findings of a study conducted by the authors and uses data gathered from an experiment or observation. An empirical study is verifiable and "based on facts, systematic observation, or experiment, rather than theory or general philosophical principle" (APA Databases Methodology Field Values).In other words, it tells the story of a research conducted, doing it in ...
different mediums since 1992 that juxtaposes the contemporary field of reading. digitally against the long-established and deep-rooted research on reading in print. Our goal of understanding print ...
The concrete empirical contexts that hosted the research, identified as information-rich cases that could function as best examples of the studied phenomenon, are the following. The first case, representing the "restraint" model, comprises an acute psychiatric ward, which I will call the Pine Ward, and a Mental Health Centre I call the ...
The empirical method is a fundamental aspect of research methodology that has stood the test of time. By relying on observation and data collection, it allows researchers to ground their theories in reality, providing a solid foundation for knowledge. Whether it's used in the hard sciences, social sciences, or humanities, the empirical method ...
cognitive theory of multimedia learning (CTML) has been built on decades of. empirical research addressing processing demands of texts that incorporate both linguistic and visuographic content. The CTML model outlines 12 prin- ciples of text and visual integration, such as the coherence principle or the.
By increasing the inferential value of our empirical research in these ways, the conclusions we draw from it can reveal interesting insights that can better prepare individual entrepreneurs (rather than the "average" entrepreneur) to more effectively address the important problems they face.
Steps for conducting empirical research. Since empirical research is based on observation and capturing experiences, it is important to plan the steps to conduct the experiment and how to analyse it. This will enable the researcher to resolve problems or obstacles which can occur during the experiment. Step #1: Define the purpose of the research
Research problems and hypotheses are important means for attaining valuable knowledge. They are pointers or guides to such knowledge, or as formulated by Kerlinger ( 1986, p. 19): " … they direct investigation.". There are many kinds of problems and hypotheses, and they may play various roles in knowledge construction.
Empirical research reveals that on average, 2%-4% of the population spend about ____ of their time daydreaming. 10%. About us. About Quizlet; How Quizlet works; Careers; Advertise with us; Get the app; For students. Flashcards; Test; Learn; Solutions; Q-Chat: AI Tutor; Spaced Repetition; Modern Learning Lab; Quizlet Plus; For teachers. Live;
This systematic literature review was undertaken primarily to examine the role that print and digitally mediums play in text comprehension. Overall, results suggest that medium plays an influential role under certain text or task conditions or for certain readers. Additional goals were to identify how researchers defined and measured comprehension, and the various trends that have emerged over ...
Although observational studies are increasingly being used to address gaps in the evidence from randomized controlled trials, the effect they have on the results and conclusions of systematic reviews is unclear. Our objectives were to evaluate: (1) how often observational studies are searched for and included in comparative effectiveness reviews (CERs); (2) the rationale for including or ...
readers. I outline four reporting templates for quantitative empirical studies that are suited to differing reader needs. Keywords: reporting; epistemology; uncertainty; model selection. Science is a social process. Most of what we think we know we have learned from others, and the impact of our own research depends on how well others can make ...
As indicated in Fig. 1, empirical research reveals that these types of shareholder activism are associated with small or negligible changes in target firm value. At the other extreme, corporate takeovers typically involve the formation of large blockholdings and create large changes in firm valuation that average 15.3%.
The purpose of this article is to argue that quantitative research could be more defensible if contested assumptions about measurement were abandoned, and to illustrate this thesis with the example of the better-than-average-effect (BTAE). If research on the BTAE is conceptualized as an interpretive endeavor, one can provide arguments that do ...
Empirical research reveals that on average people spend about 10% of their time daydreaming. In addition, daydreaming is a short period dispassion from one people in the instant environment throughout which the contact of the person with reality is blurry and partially replaced by a visionary fantasy which is most particularly one of happy pleasant opinions, ambitions, imaginary as coming to ...
Based on empirical research, it has been revealed that an average person, spends approximately 50 percent of their time daydreaming. Yet, some of these people experience leading their daydreams to negative outcomes, while others think positively of their daydreams.
As indicated in the Academic Research Capsule 4-1, RBV theory may be helpful in identifying diversification targets. D. Empirical research by Neffke reveals that it is the nature of a firm's human capital, more than any other variable in the firm's value chain, that impacts that firm's choice of diversification targets.
A meta-analysis of the empirical literature about childcare costs and employment found that "a 10% reduction in the price of childcare would lead to a .25-11% increase in maternal employment, likely near 0.5-2.5%." 21. An increase in labor force participation leads to an increase in economic growth.
D.Empirical research by Neffke reveals that it is the nature of a firm's human capital, more than any other variable in the firm's value chain, that impacts that firm's choice of diversification targets. ... D.Departures from industry average financial ratios do not always indicate that a firm is doing especially well or badly.
The research also found the average adult claims to smile at a stranger five times a week - but 16 per cent admit they rarely or never return the gesture. ... Study reveals the top 30 simple ...
Discover the key indicators that reveal you're doing better than most Americans. From savings habits to debt management, these 15 signs will help you gauge your financial health. Let's explore ...
Tax policy is critical for business operations and export decisions. However, the relationship between tax sharing and export has been less frequently discussed. This paper explicitly examines the underexplored tax-sharing system's effect on manufacturing exporters' export quality and develops four hypotheses. We use data on Chinese manufacturing exporters and prefecture-level tax-sharing ...