Systematic Reviews and Meta Analysis
- Getting Started
- Guides and Standards
- Review Protocols
- Databases and Sources
- Randomized Controlled Trials
- Controlled Clinical Trials
- Observational Designs
- Tests of Diagnostic Accuracy
- Software and Tools
- Where do I get all those articles?
- Collaborations
- EPI 233/528
- Countway Mediated Search
- Risk of Bias (RoB)
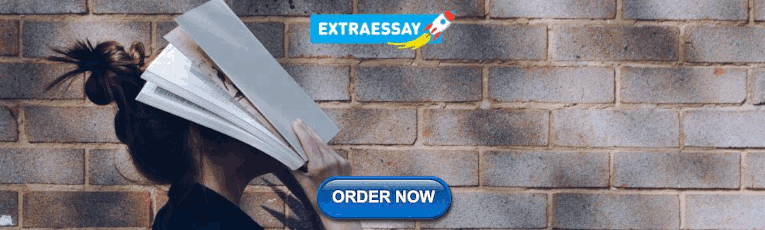
Systematic review Q & A
What is a systematic review.
A systematic review is guided filtering and synthesis of all available evidence addressing a specific, focused research question, generally about a specific intervention or exposure. The use of standardized, systematic methods and pre-selected eligibility criteria reduce the risk of bias in identifying, selecting and analyzing relevant studies. A well-designed systematic review includes clear objectives, pre-selected criteria for identifying eligible studies, an explicit methodology, a thorough and reproducible search of the literature, an assessment of the validity or risk of bias of each included study, and a systematic synthesis, analysis and presentation of the findings of the included studies. A systematic review may include a meta-analysis.
For details about carrying out systematic reviews, see the Guides and Standards section of this guide.
Is my research topic appropriate for systematic review methods?
A systematic review is best deployed to test a specific hypothesis about a healthcare or public health intervention or exposure. By focusing on a single intervention or a few specific interventions for a particular condition, the investigator can ensure a manageable results set. Moreover, examining a single or small set of related interventions, exposures, or outcomes, will simplify the assessment of studies and the synthesis of the findings.
Systematic reviews are poor tools for hypothesis generation: for instance, to determine what interventions have been used to increase the awareness and acceptability of a vaccine or to investigate the ways that predictive analytics have been used in health care management. In the first case, we don't know what interventions to search for and so have to screen all the articles about awareness and acceptability. In the second, there is no agreed on set of methods that make up predictive analytics, and health care management is far too broad. The search will necessarily be incomplete, vague and very large all at the same time. In most cases, reviews without clearly and exactly specified populations, interventions, exposures, and outcomes will produce results sets that quickly outstrip the resources of a small team and offer no consistent way to assess and synthesize findings from the studies that are identified.
If not a systematic review, then what?
You might consider performing a scoping review . This framework allows iterative searching over a reduced number of data sources and no requirement to assess individual studies for risk of bias. The framework includes built-in mechanisms to adjust the analysis as the work progresses and more is learned about the topic. A scoping review won't help you limit the number of records you'll need to screen (broad questions lead to large results sets) but may give you means of dealing with a large set of results.
This tool can help you decide what kind of review is right for your question.
Can my student complete a systematic review during her summer project?
Probably not. Systematic reviews are a lot of work. Including creating the protocol, building and running a quality search, collecting all the papers, evaluating the studies that meet the inclusion criteria and extracting and analyzing the summary data, a well done review can require dozens to hundreds of hours of work that can span several months. Moreover, a systematic review requires subject expertise, statistical support and a librarian to help design and run the search. Be aware that librarians sometimes have queues for their search time. It may take several weeks to complete and run a search. Moreover, all guidelines for carrying out systematic reviews recommend that at least two subject experts screen the studies identified in the search. The first round of screening can consume 1 hour per screener for every 100-200 records. A systematic review is a labor-intensive team effort.
How can I know if my topic has been been reviewed already?
Before starting out on a systematic review, check to see if someone has done it already. In PubMed you can use the systematic review subset to limit to a broad group of papers that is enriched for systematic reviews. You can invoke the subset by selecting if from the Article Types filters to the left of your PubMed results, or you can append AND systematic[sb] to your search. For example:
"neoadjuvant chemotherapy" AND systematic[sb]
The systematic review subset is very noisy, however. To quickly focus on systematic reviews (knowing that you may be missing some), simply search for the word systematic in the title:
"neoadjuvant chemotherapy" AND systematic[ti]
Any PRISMA-compliant systematic review will be captured by this method since including the words "systematic review" in the title is a requirement of the PRISMA checklist. Cochrane systematic reviews do not include 'systematic' in the title, however. It's worth checking the Cochrane Database of Systematic Reviews independently.
You can also search for protocols that will indicate that another group has set out on a similar project. Many investigators will register their protocols in PROSPERO , a registry of review protocols. Other published protocols as well as Cochrane Review protocols appear in the Cochrane Methodology Register, a part of the Cochrane Library .
- Next: Guides and Standards >>
- Last Updated: Feb 26, 2024 3:17 PM
- URL: https://guides.library.harvard.edu/meta-analysis
How to Do a Systematic Review: A Best Practice Guide for Conducting and Reporting Narrative Reviews, Meta-Analyses, and Meta-Syntheses
Affiliations.
- 1 Behavioural Science Centre, Stirling Management School, University of Stirling, Stirling FK9 4LA, United Kingdom; email: [email protected].
- 2 Department of Psychological and Behavioural Science, London School of Economics and Political Science, London WC2A 2AE, United Kingdom.
- 3 Department of Statistics, Northwestern University, Evanston, Illinois 60208, USA; email: [email protected].
- PMID: 30089228
- DOI: 10.1146/annurev-psych-010418-102803
Systematic reviews are characterized by a methodical and replicable methodology and presentation. They involve a comprehensive search to locate all relevant published and unpublished work on a subject; a systematic integration of search results; and a critique of the extent, nature, and quality of evidence in relation to a particular research question. The best reviews synthesize studies to draw broad theoretical conclusions about what a literature means, linking theory to evidence and evidence to theory. This guide describes how to plan, conduct, organize, and present a systematic review of quantitative (meta-analysis) or qualitative (narrative review, meta-synthesis) information. We outline core standards and principles and describe commonly encountered problems. Although this guide targets psychological scientists, its high level of abstraction makes it potentially relevant to any subject area or discipline. We argue that systematic reviews are a key methodology for clarifying whether and how research findings replicate and for explaining possible inconsistencies, and we call for researchers to conduct systematic reviews to help elucidate whether there is a replication crisis.
Keywords: evidence; guide; meta-analysis; meta-synthesis; narrative; systematic review; theory.
- Guidelines as Topic
- Meta-Analysis as Topic*
- Publication Bias
- Review Literature as Topic
- Systematic Reviews as Topic*
- - Google Chrome
Intended for healthcare professionals
- Access provided by Google Indexer
- My email alerts
- BMA member login
- Username * Password * Forgot your log in details? Need to activate BMA Member Log In Log in via OpenAthens Log in via your institution

Search form
- Advanced search
- Search responses
- Search blogs
- The PRISMA 2020...
The PRISMA 2020 statement: an updated guideline for reporting systematic reviews
PRISMA 2020 explanation and elaboration: updated guidance and exemplars for reporting systematic reviews
- Related content
- Peer review
- Matthew J Page , senior research fellow 1 ,
- Joanne E McKenzie , associate professor 1 ,
- Patrick M Bossuyt , professor 2 ,
- Isabelle Boutron , professor 3 ,
- Tammy C Hoffmann , professor 4 ,
- Cynthia D Mulrow , professor 5 ,
- Larissa Shamseer , doctoral student 6 ,
- Jennifer M Tetzlaff , research product specialist 7 ,
- Elie A Akl , professor 8 ,
- Sue E Brennan , senior research fellow 1 ,
- Roger Chou , professor 9 ,
- Julie Glanville , associate director 10 ,
- Jeremy M Grimshaw , professor 11 ,
- Asbjørn Hróbjartsson , professor 12 ,
- Manoj M Lalu , associate scientist and assistant professor 13 ,
- Tianjing Li , associate professor 14 ,
- Elizabeth W Loder , professor 15 ,
- Evan Mayo-Wilson , associate professor 16 ,
- Steve McDonald , senior research fellow 1 ,
- Luke A McGuinness , research associate 17 ,
- Lesley A Stewart , professor and director 18 ,
- James Thomas , professor 19 ,
- Andrea C Tricco , scientist and associate professor 20 ,
- Vivian A Welch , associate professor 21 ,
- Penny Whiting , associate professor 17 ,
- David Moher , director and professor 22
- 1 School of Public Health and Preventive Medicine, Monash University, Melbourne, Australia
- 2 Department of Clinical Epidemiology, Biostatistics and Bioinformatics, Amsterdam University Medical Centres, University of Amsterdam, Amsterdam, Netherlands
- 3 Université de Paris, Centre of Epidemiology and Statistics (CRESS), Inserm, F 75004 Paris, France
- 4 Institute for Evidence-Based Healthcare, Faculty of Health Sciences and Medicine, Bond University, Gold Coast, Australia
- 5 University of Texas Health Science Center at San Antonio, San Antonio, Texas, USA; Annals of Internal Medicine
- 6 Knowledge Translation Program, Li Ka Shing Knowledge Institute, Toronto, Canada; School of Epidemiology and Public Health, Faculty of Medicine, University of Ottawa, Ottawa, Canada
- 7 Evidence Partners, Ottawa, Canada
- 8 Clinical Research Institute, American University of Beirut, Beirut, Lebanon; Department of Health Research Methods, Evidence, and Impact, McMaster University, Hamilton, Ontario, Canada
- 9 Department of Medical Informatics and Clinical Epidemiology, Oregon Health & Science University, Portland, Oregon, USA
- 10 York Health Economics Consortium (YHEC Ltd), University of York, York, UK
- 11 Clinical Epidemiology Program, Ottawa Hospital Research Institute, Ottawa, Canada; School of Epidemiology and Public Health, University of Ottawa, Ottawa, Canada; Department of Medicine, University of Ottawa, Ottawa, Canada
- 12 Centre for Evidence-Based Medicine Odense (CEBMO) and Cochrane Denmark, Department of Clinical Research, University of Southern Denmark, Odense, Denmark; Open Patient data Exploratory Network (OPEN), Odense University Hospital, Odense, Denmark
- 13 Department of Anesthesiology and Pain Medicine, The Ottawa Hospital, Ottawa, Canada; Clinical Epidemiology Program, Blueprint Translational Research Group, Ottawa Hospital Research Institute, Ottawa, Canada; Regenerative Medicine Program, Ottawa Hospital Research Institute, Ottawa, Canada
- 14 Department of Ophthalmology, School of Medicine, University of Colorado Denver, Denver, Colorado, United States; Department of Epidemiology, Johns Hopkins Bloomberg School of Public Health, Baltimore, Maryland, USA
- 15 Division of Headache, Department of Neurology, Brigham and Women's Hospital, Harvard Medical School, Boston, Massachusetts, USA; Head of Research, The BMJ , London, UK
- 16 Department of Epidemiology and Biostatistics, Indiana University School of Public Health-Bloomington, Bloomington, Indiana, USA
- 17 Population Health Sciences, Bristol Medical School, University of Bristol, Bristol, UK
- 18 Centre for Reviews and Dissemination, University of York, York, UK
- 19 EPPI-Centre, UCL Social Research Institute, University College London, London, UK
- 20 Li Ka Shing Knowledge Institute of St. Michael's Hospital, Unity Health Toronto, Toronto, Canada; Epidemiology Division of the Dalla Lana School of Public Health and the Institute of Health Management, Policy, and Evaluation, University of Toronto, Toronto, Canada; Queen's Collaboration for Health Care Quality Joanna Briggs Institute Centre of Excellence, Queen's University, Kingston, Canada
- 21 Methods Centre, Bruyère Research Institute, Ottawa, Ontario, Canada; School of Epidemiology and Public Health, Faculty of Medicine, University of Ottawa, Ottawa, Canada
- 22 Centre for Journalology, Clinical Epidemiology Program, Ottawa Hospital Research Institute, Ottawa, Canada; School of Epidemiology and Public Health, Faculty of Medicine, University of Ottawa, Ottawa, Canada
- Correspondence to: M J Page matthew.page{at}monash.edu
- Accepted 4 January 2021
The Preferred Reporting Items for Systematic reviews and Meta-Analyses (PRISMA) statement, published in 2009, was designed to help systematic reviewers transparently report why the review was done, what the authors did, and what they found. Over the past decade, advances in systematic review methodology and terminology have necessitated an update to the guideline. The PRISMA 2020 statement replaces the 2009 statement and includes new reporting guidance that reflects advances in methods to identify, select, appraise, and synthesise studies. The structure and presentation of the items have been modified to facilitate implementation. In this article, we present the PRISMA 2020 27-item checklist, an expanded checklist that details reporting recommendations for each item, the PRISMA 2020 abstract checklist, and the revised flow diagrams for original and updated reviews.
Systematic reviews serve many critical roles. They can provide syntheses of the state of knowledge in a field, from which future research priorities can be identified; they can address questions that otherwise could not be answered by individual studies; they can identify problems in primary research that should be rectified in future studies; and they can generate or evaluate theories about how or why phenomena occur. Systematic reviews therefore generate various types of knowledge for different users of reviews (such as patients, healthcare providers, researchers, and policy makers). 1 2 To ensure a systematic review is valuable to users, authors should prepare a transparent, complete, and accurate account of why the review was done, what they did (such as how studies were identified and selected) and what they found (such as characteristics of contributing studies and results of meta-analyses). Up-to-date reporting guidance facilitates authors achieving this. 3
The Preferred Reporting Items for Systematic reviews and Meta-Analyses (PRISMA) statement published in 2009 (hereafter referred to as PRISMA 2009) 4 5 6 7 8 9 10 is a reporting guideline designed to address poor reporting of systematic reviews. 11 The PRISMA 2009 statement comprised a checklist of 27 items recommended for reporting in systematic reviews and an “explanation and elaboration” paper 12 13 14 15 16 providing additional reporting guidance for each item, along with exemplars of reporting. The recommendations have been widely endorsed and adopted, as evidenced by its co-publication in multiple journals, citation in over 60 000 reports (Scopus, August 2020), endorsement from almost 200 journals and systematic review organisations, and adoption in various disciplines. Evidence from observational studies suggests that use of the PRISMA 2009 statement is associated with more complete reporting of systematic reviews, 17 18 19 20 although more could be done to improve adherence to the guideline. 21
Many innovations in the conduct of systematic reviews have occurred since publication of the PRISMA 2009 statement. For example, technological advances have enabled the use of natural language processing and machine learning to identify relevant evidence, 22 23 24 methods have been proposed to synthesise and present findings when meta-analysis is not possible or appropriate, 25 26 27 and new methods have been developed to assess the risk of bias in results of included studies. 28 29 Evidence on sources of bias in systematic reviews has accrued, culminating in the development of new tools to appraise the conduct of systematic reviews. 30 31 Terminology used to describe particular review processes has also evolved, as in the shift from assessing “quality” to assessing “certainty” in the body of evidence. 32 In addition, the publishing landscape has transformed, with multiple avenues now available for registering and disseminating systematic review protocols, 33 34 disseminating reports of systematic reviews, and sharing data and materials, such as preprint servers and publicly accessible repositories. To capture these advances in the reporting of systematic reviews necessitated an update to the PRISMA 2009 statement.
Summary points
To ensure a systematic review is valuable to users, authors should prepare a transparent, complete, and accurate account of why the review was done, what they did, and what they found
The PRISMA 2020 statement provides updated reporting guidance for systematic reviews that reflects advances in methods to identify, select, appraise, and synthesise studies
The PRISMA 2020 statement consists of a 27-item checklist, an expanded checklist that details reporting recommendations for each item, the PRISMA 2020 abstract checklist, and revised flow diagrams for original and updated reviews
We anticipate that the PRISMA 2020 statement will benefit authors, editors, and peer reviewers of systematic reviews, and different users of reviews, including guideline developers, policy makers, healthcare providers, patients, and other stakeholders
Development of PRISMA 2020
A complete description of the methods used to develop PRISMA 2020 is available elsewhere. 35 We identified PRISMA 2009 items that were often reported incompletely by examining the results of studies investigating the transparency of reporting of published reviews. 17 21 36 37 We identified possible modifications to the PRISMA 2009 statement by reviewing 60 documents providing reporting guidance for systematic reviews (including reporting guidelines, handbooks, tools, and meta-research studies). 38 These reviews of the literature were used to inform the content of a survey with suggested possible modifications to the 27 items in PRISMA 2009 and possible additional items. Respondents were asked whether they believed we should keep each PRISMA 2009 item as is, modify it, or remove it, and whether we should add each additional item. Systematic review methodologists and journal editors were invited to complete the online survey (110 of 220 invited responded). We discussed proposed content and wording of the PRISMA 2020 statement, as informed by the review and survey results, at a 21-member, two-day, in-person meeting in September 2018 in Edinburgh, Scotland. Throughout 2019 and 2020, we circulated an initial draft and five revisions of the checklist and explanation and elaboration paper to co-authors for feedback. In April 2020, we invited 22 systematic reviewers who had expressed interest in providing feedback on the PRISMA 2020 checklist to share their views (via an online survey) on the layout and terminology used in a preliminary version of the checklist. Feedback was received from 15 individuals and considered by the first author, and any revisions deemed necessary were incorporated before the final version was approved and endorsed by all co-authors.
The PRISMA 2020 statement
Scope of the guideline.
The PRISMA 2020 statement has been designed primarily for systematic reviews of studies that evaluate the effects of health interventions, irrespective of the design of the included studies. However, the checklist items are applicable to reports of systematic reviews evaluating other interventions (such as social or educational interventions), and many items are applicable to systematic reviews with objectives other than evaluating interventions (such as evaluating aetiology, prevalence, or prognosis). PRISMA 2020 is intended for use in systematic reviews that include synthesis (such as pairwise meta-analysis or other statistical synthesis methods) or do not include synthesis (for example, because only one eligible study is identified). The PRISMA 2020 items are relevant for mixed-methods systematic reviews (which include quantitative and qualitative studies), but reporting guidelines addressing the presentation and synthesis of qualitative data should also be consulted. 39 40 PRISMA 2020 can be used for original systematic reviews, updated systematic reviews, or continually updated (“living”) systematic reviews. However, for updated and living systematic reviews, there may be some additional considerations that need to be addressed. Where there is relevant content from other reporting guidelines, we reference these guidelines within the items in the explanation and elaboration paper 41 (such as PRISMA-Search 42 in items 6 and 7, Synthesis without meta-analysis (SWiM) reporting guideline 27 in item 13d). Box 1 includes a glossary of terms used throughout the PRISMA 2020 statement.
Glossary of terms
Systematic review —A review that uses explicit, systematic methods to collate and synthesise findings of studies that address a clearly formulated question 43
Statistical synthesis —The combination of quantitative results of two or more studies. This encompasses meta-analysis of effect estimates (described below) and other methods, such as combining P values, calculating the range and distribution of observed effects, and vote counting based on the direction of effect (see McKenzie and Brennan 25 for a description of each method)
Meta-analysis of effect estimates —A statistical technique used to synthesise results when study effect estimates and their variances are available, yielding a quantitative summary of results 25
Outcome —An event or measurement collected for participants in a study (such as quality of life, mortality)
Result —The combination of a point estimate (such as a mean difference, risk ratio, or proportion) and a measure of its precision (such as a confidence/credible interval) for a particular outcome
Report —A document (paper or electronic) supplying information about a particular study. It could be a journal article, preprint, conference abstract, study register entry, clinical study report, dissertation, unpublished manuscript, government report, or any other document providing relevant information
Record —The title or abstract (or both) of a report indexed in a database or website (such as a title or abstract for an article indexed in Medline). Records that refer to the same report (such as the same journal article) are “duplicates”; however, records that refer to reports that are merely similar (such as a similar abstract submitted to two different conferences) should be considered unique.
Study —An investigation, such as a clinical trial, that includes a defined group of participants and one or more interventions and outcomes. A “study” might have multiple reports. For example, reports could include the protocol, statistical analysis plan, baseline characteristics, results for the primary outcome, results for harms, results for secondary outcomes, and results for additional mediator and moderator analyses
PRISMA 2020 is not intended to guide systematic review conduct, for which comprehensive resources are available. 43 44 45 46 However, familiarity with PRISMA 2020 is useful when planning and conducting systematic reviews to ensure that all recommended information is captured. PRISMA 2020 should not be used to assess the conduct or methodological quality of systematic reviews; other tools exist for this purpose. 30 31 Furthermore, PRISMA 2020 is not intended to inform the reporting of systematic review protocols, for which a separate statement is available (PRISMA for Protocols (PRISMA-P) 2015 statement 47 48 ). Finally, extensions to the PRISMA 2009 statement have been developed to guide reporting of network meta-analyses, 49 meta-analyses of individual participant data, 50 systematic reviews of harms, 51 systematic reviews of diagnostic test accuracy studies, 52 and scoping reviews 53 ; for these types of reviews we recommend authors report their review in accordance with the recommendations in PRISMA 2020 along with the guidance specific to the extension.
How to use PRISMA 2020
The PRISMA 2020 statement (including the checklists, explanation and elaboration, and flow diagram) replaces the PRISMA 2009 statement, which should no longer be used. Box 2 summarises noteworthy changes from the PRISMA 2009 statement. The PRISMA 2020 checklist includes seven sections with 27 items, some of which include sub-items ( table 1 ). A checklist for journal and conference abstracts for systematic reviews is included in PRISMA 2020. This abstract checklist is an update of the 2013 PRISMA for Abstracts statement, 54 reflecting new and modified content in PRISMA 2020 ( table 2 ). A template PRISMA flow diagram is provided, which can be modified depending on whether the systematic review is original or updated ( fig 1 ).
Noteworthy changes to the PRISMA 2009 statement
Inclusion of the abstract reporting checklist within PRISMA 2020 (see item #2 and table 2 ).
Movement of the ‘Protocol and registration’ item from the start of the Methods section of the checklist to a new Other section, with addition of a sub-item recommending authors describe amendments to information provided at registration or in the protocol (see item #24a-24c).
Modification of the ‘Search’ item to recommend authors present full search strategies for all databases, registers and websites searched, not just at least one database (see item #7).
Modification of the ‘Study selection’ item in the Methods section to emphasise the reporting of how many reviewers screened each record and each report retrieved, whether they worked independently, and if applicable, details of automation tools used in the process (see item #8).
Addition of a sub-item to the ‘Data items’ item recommending authors report how outcomes were defined, which results were sought, and methods for selecting a subset of results from included studies (see item #10a).
Splitting of the ‘Synthesis of results’ item in the Methods section into six sub-items recommending authors describe: the processes used to decide which studies were eligible for each synthesis; any methods required to prepare the data for synthesis; any methods used to tabulate or visually display results of individual studies and syntheses; any methods used to synthesise results; any methods used to explore possible causes of heterogeneity among study results (such as subgroup analysis, meta-regression); and any sensitivity analyses used to assess robustness of the synthesised results (see item #13a-13f).
Addition of a sub-item to the ‘Study selection’ item in the Results section recommending authors cite studies that might appear to meet the inclusion criteria, but which were excluded, and explain why they were excluded (see item #16b).
Splitting of the ‘Synthesis of results’ item in the Results section into four sub-items recommending authors: briefly summarise the characteristics and risk of bias among studies contributing to the synthesis; present results of all statistical syntheses conducted; present results of any investigations of possible causes of heterogeneity among study results; and present results of any sensitivity analyses (see item #20a-20d).
Addition of new items recommending authors report methods for and results of an assessment of certainty (or confidence) in the body of evidence for an outcome (see items #15 and #22).
Addition of a new item recommending authors declare any competing interests (see item #26).
Addition of a new item recommending authors indicate whether data, analytic code and other materials used in the review are publicly available and if so, where they can be found (see item #27).
PRISMA 2020 item checklist
- View inline
PRISMA 2020 for Abstracts checklist*

PRISMA 2020 flow diagram template for systematic reviews. The new design is adapted from flow diagrams proposed by Boers, 55 Mayo-Wilson et al. 56 and Stovold et al. 57 The boxes in grey should only be completed if applicable; otherwise they should be removed from the flow diagram. Note that a “report” could be a journal article, preprint, conference abstract, study register entry, clinical study report, dissertation, unpublished manuscript, government report or any other document providing relevant information.
- Download figure
- Open in new tab
- Download powerpoint
We recommend authors refer to PRISMA 2020 early in the writing process, because prospective consideration of the items may help to ensure that all the items are addressed. To help keep track of which items have been reported, the PRISMA statement website ( http://www.prisma-statement.org/ ) includes fillable templates of the checklists to download and complete (also available in the data supplement on bmj.com). We have also created a web application that allows users to complete the checklist via a user-friendly interface 58 (available at https://prisma.shinyapps.io/checklist/ and adapted from the Transparency Checklist app 59 ). The completed checklist can be exported to Word or PDF. Editable templates of the flow diagram can also be downloaded from the PRISMA statement website.
We have prepared an updated explanation and elaboration paper, in which we explain why reporting of each item is recommended and present bullet points that detail the reporting recommendations (which we refer to as elements). 41 The bullet-point structure is new to PRISMA 2020 and has been adopted to facilitate implementation of the guidance. 60 61 An expanded checklist, which comprises an abridged version of the elements presented in the explanation and elaboration paper, with references and some examples removed, is available in the data supplement on bmj.com. Consulting the explanation and elaboration paper is recommended if further clarity or information is required.
Journals and publishers might impose word and section limits, and limits on the number of tables and figures allowed in the main report. In such cases, if the relevant information for some items already appears in a publicly accessible review protocol, referring to the protocol may suffice. Alternatively, placing detailed descriptions of the methods used or additional results (such as for less critical outcomes) in supplementary files is recommended. Ideally, supplementary files should be deposited to a general-purpose or institutional open-access repository that provides free and permanent access to the material (such as Open Science Framework, Dryad, figshare). A reference or link to the additional information should be included in the main report. Finally, although PRISMA 2020 provides a template for where information might be located, the suggested location should not be seen as prescriptive; the guiding principle is to ensure the information is reported.
Use of PRISMA 2020 has the potential to benefit many stakeholders. Complete reporting allows readers to assess the appropriateness of the methods, and therefore the trustworthiness of the findings. Presenting and summarising characteristics of studies contributing to a synthesis allows healthcare providers and policy makers to evaluate the applicability of the findings to their setting. Describing the certainty in the body of evidence for an outcome and the implications of findings should help policy makers, managers, and other decision makers formulate appropriate recommendations for practice or policy. Complete reporting of all PRISMA 2020 items also facilitates replication and review updates, as well as inclusion of systematic reviews in overviews (of systematic reviews) and guidelines, so teams can leverage work that is already done and decrease research waste. 36 62 63
We updated the PRISMA 2009 statement by adapting the EQUATOR Network’s guidance for developing health research reporting guidelines. 64 We evaluated the reporting completeness of published systematic reviews, 17 21 36 37 reviewed the items included in other documents providing guidance for systematic reviews, 38 surveyed systematic review methodologists and journal editors for their views on how to revise the original PRISMA statement, 35 discussed the findings at an in-person meeting, and prepared this document through an iterative process. Our recommendations are informed by the reviews and survey conducted before the in-person meeting, theoretical considerations about which items facilitate replication and help users assess the risk of bias and applicability of systematic reviews, and co-authors’ experience with authoring and using systematic reviews.
Various strategies to increase the use of reporting guidelines and improve reporting have been proposed. They include educators introducing reporting guidelines into graduate curricula to promote good reporting habits of early career scientists 65 ; journal editors and regulators endorsing use of reporting guidelines 18 ; peer reviewers evaluating adherence to reporting guidelines 61 66 ; journals requiring authors to indicate where in their manuscript they have adhered to each reporting item 67 ; and authors using online writing tools that prompt complete reporting at the writing stage. 60 Multi-pronged interventions, where more than one of these strategies are combined, may be more effective (such as completion of checklists coupled with editorial checks). 68 However, of 31 interventions proposed to increase adherence to reporting guidelines, the effects of only 11 have been evaluated, mostly in observational studies at high risk of bias due to confounding. 69 It is therefore unclear which strategies should be used. Future research might explore barriers and facilitators to the use of PRISMA 2020 by authors, editors, and peer reviewers, designing interventions that address the identified barriers, and evaluating those interventions using randomised trials. To inform possible revisions to the guideline, it would also be valuable to conduct think-aloud studies 70 to understand how systematic reviewers interpret the items, and reliability studies to identify items where there is varied interpretation of the items.
We encourage readers to submit evidence that informs any of the recommendations in PRISMA 2020 (via the PRISMA statement website: http://www.prisma-statement.org/ ). To enhance accessibility of PRISMA 2020, several translations of the guideline are under way (see available translations at the PRISMA statement website). We encourage journal editors and publishers to raise awareness of PRISMA 2020 (for example, by referring to it in journal “Instructions to authors”), endorsing its use, advising editors and peer reviewers to evaluate submitted systematic reviews against the PRISMA 2020 checklists, and making changes to journal policies to accommodate the new reporting recommendations. We recommend existing PRISMA extensions 47 49 50 51 52 53 71 72 be updated to reflect PRISMA 2020 and advise developers of new PRISMA extensions to use PRISMA 2020 as the foundation document.
We anticipate that the PRISMA 2020 statement will benefit authors, editors, and peer reviewers of systematic reviews, and different users of reviews, including guideline developers, policy makers, healthcare providers, patients, and other stakeholders. Ultimately, we hope that uptake of the guideline will lead to more transparent, complete, and accurate reporting of systematic reviews, thus facilitating evidence based decision making.
Acknowledgments
We dedicate this paper to the late Douglas G Altman and Alessandro Liberati, whose contributions were fundamental to the development and implementation of the original PRISMA statement.
We thank the following contributors who completed the survey to inform discussions at the development meeting: Xavier Armoiry, Edoardo Aromataris, Ana Patricia Ayala, Ethan M Balk, Virginia Barbour, Elaine Beller, Jesse A Berlin, Lisa Bero, Zhao-Xiang Bian, Jean Joel Bigna, Ferrán Catalá-López, Anna Chaimani, Mike Clarke, Tammy Clifford, Ioana A Cristea, Miranda Cumpston, Sofia Dias, Corinna Dressler, Ivan D Florez, Joel J Gagnier, Chantelle Garritty, Long Ge, Davina Ghersi, Sean Grant, Gordon Guyatt, Neal R Haddaway, Julian PT Higgins, Sally Hopewell, Brian Hutton, Jamie J Kirkham, Jos Kleijnen, Julia Koricheva, Joey SW Kwong, Toby J Lasserson, Julia H Littell, Yoon K Loke, Malcolm R Macleod, Chris G Maher, Ana Marušic, Dimitris Mavridis, Jessie McGowan, Matthew DF McInnes, Philippa Middleton, Karel G Moons, Zachary Munn, Jane Noyes, Barbara Nußbaumer-Streit, Donald L Patrick, Tatiana Pereira-Cenci, Ba’ Pham, Bob Phillips, Dawid Pieper, Michelle Pollock, Daniel S Quintana, Drummond Rennie, Melissa L Rethlefsen, Hannah R Rothstein, Maroeska M Rovers, Rebecca Ryan, Georgia Salanti, Ian J Saldanha, Margaret Sampson, Nancy Santesso, Rafael Sarkis-Onofre, Jelena Savović, Christopher H Schmid, Kenneth F Schulz, Guido Schwarzer, Beverley J Shea, Paul G Shekelle, Farhad Shokraneh, Mark Simmonds, Nicole Skoetz, Sharon E Straus, Anneliese Synnot, Emily E Tanner-Smith, Brett D Thombs, Hilary Thomson, Alexander Tsertsvadze, Peter Tugwell, Tari Turner, Lesley Uttley, Jeffrey C Valentine, Matt Vassar, Areti Angeliki Veroniki, Meera Viswanathan, Cole Wayant, Paul Whaley, and Kehu Yang. We thank the following contributors who provided feedback on a preliminary version of the PRISMA 2020 checklist: Jo Abbott, Fionn Büttner, Patricia Correia-Santos, Victoria Freeman, Emily A Hennessy, Rakibul Islam, Amalia (Emily) Karahalios, Kasper Krommes, Andreas Lundh, Dafne Port Nascimento, Davina Robson, Catherine Schenck-Yglesias, Mary M Scott, Sarah Tanveer and Pavel Zhelnov. We thank Abigail H Goben, Melissa L Rethlefsen, Tanja Rombey, Anna Scott, and Farhad Shokraneh for their helpful comments on the preprints of the PRISMA 2020 papers. We thank Edoardo Aromataris, Stephanie Chang, Toby Lasserson and David Schriger for their helpful peer review comments on the PRISMA 2020 papers.
Contributors: JEM and DM are joint senior authors. MJP, JEM, PMB, IB, TCH, CDM, LS, and DM conceived this paper and designed the literature review and survey conducted to inform the guideline content. MJP conducted the literature review, administered the survey and analysed the data for both. MJP prepared all materials for the development meeting. MJP and JEM presented proposals at the development meeting. All authors except for TCH, JMT, EAA, SEB, and LAM attended the development meeting. MJP and JEM took and consolidated notes from the development meeting. MJP and JEM led the drafting and editing of the article. JEM, PMB, IB, TCH, LS, JMT, EAA, SEB, RC, JG, AH, TL, EMW, SM, LAM, LAS, JT, ACT, PW, and DM drafted particular sections of the article. All authors were involved in revising the article critically for important intellectual content. All authors approved the final version of the article. MJP is the guarantor of this work. The corresponding author attests that all listed authors meet authorship criteria and that no others meeting the criteria have been omitted.
Funding: There was no direct funding for this research. MJP is supported by an Australian Research Council Discovery Early Career Researcher Award (DE200101618) and was previously supported by an Australian National Health and Medical Research Council (NHMRC) Early Career Fellowship (1088535) during the conduct of this research. JEM is supported by an Australian NHMRC Career Development Fellowship (1143429). TCH is supported by an Australian NHMRC Senior Research Fellowship (1154607). JMT is supported by Evidence Partners Inc. JMG is supported by a Tier 1 Canada Research Chair in Health Knowledge Transfer and Uptake. MML is supported by The Ottawa Hospital Anaesthesia Alternate Funds Association and a Faculty of Medicine Junior Research Chair. TL is supported by funding from the National Eye Institute (UG1EY020522), National Institutes of Health, United States. LAM is supported by a National Institute for Health Research Doctoral Research Fellowship (DRF-2018-11-ST2-048). ACT is supported by a Tier 2 Canada Research Chair in Knowledge Synthesis. DM is supported in part by a University Research Chair, University of Ottawa. The funders had no role in considering the study design or in the collection, analysis, interpretation of data, writing of the report, or decision to submit the article for publication.
Competing interests: All authors have completed the ICMJE uniform disclosure form at http://www.icmje.org/conflicts-of-interest/ and declare: EL is head of research for the BMJ ; MJP is an editorial board member for PLOS Medicine ; ACT is an associate editor and MJP, TL, EMW, and DM are editorial board members for the Journal of Clinical Epidemiology ; DM and LAS were editors in chief, LS, JMT, and ACT are associate editors, and JG is an editorial board member for Systematic Reviews . None of these authors were involved in the peer review process or decision to publish. TCH has received personal fees from Elsevier outside the submitted work. EMW has received personal fees from the American Journal for Public Health , for which he is the editor for systematic reviews. VW is editor in chief of the Campbell Collaboration, which produces systematic reviews, and co-convenor of the Campbell and Cochrane equity methods group. DM is chair of the EQUATOR Network, IB is adjunct director of the French EQUATOR Centre and TCH is co-director of the Australasian EQUATOR Centre, which advocates for the use of reporting guidelines to improve the quality of reporting in research articles. JMT received salary from Evidence Partners, creator of DistillerSR software for systematic reviews; Evidence Partners was not involved in the design or outcomes of the statement, and the views expressed solely represent those of the author.
Provenance and peer review: Not commissioned; externally peer reviewed.
Patient and public involvement: Patients and the public were not involved in this methodological research. We plan to disseminate the research widely, including to community participants in evidence synthesis organisations.
This is an Open Access article distributed in accordance with the terms of the Creative Commons Attribution (CC BY 4.0) license, which permits others to distribute, remix, adapt and build upon this work, for commercial use, provided the original work is properly cited. See: http://creativecommons.org/licenses/by/4.0/ .
- Gurevitch J ,
- Koricheva J ,
- Nakagawa S ,
- Liberati A ,
- Tetzlaff J ,
- Altman DG ,
- PRISMA Group
- Tricco AC ,
- Sampson M ,
- Shamseer L ,
- Leoncini E ,
- de Belvis G ,
- Ricciardi W ,
- Fowler AJ ,
- Leclercq V ,
- Beaudart C ,
- Ajamieh S ,
- Rabenda V ,
- Tirelli E ,
- O’Mara-Eves A ,
- McNaught J ,
- Ananiadou S
- Marshall IJ ,
- Noel-Storr A ,
- Higgins JPT ,
- Chandler J ,
- McKenzie JE ,
- López-López JA ,
- Becker BJ ,
- Campbell M ,
- Sterne JAC ,
- Savović J ,
- Sterne JA ,
- Hernán MA ,
- Reeves BC ,
- Whiting P ,
- Higgins JP ,
- ROBIS group
- Hultcrantz M ,
- Stewart L ,
- Bossuyt PM ,
- Flemming K ,
- McInnes E ,
- France EF ,
- Cunningham M ,
- Rethlefsen ML ,
- Kirtley S ,
- Waffenschmidt S ,
- PRISMA-S Group
- ↵ Higgins JPT, Thomas J, Chandler J, et al, eds. Cochrane Handbook for Systematic Reviews of Interventions : Version 6.0. Cochrane, 2019. Available from https://training.cochrane.org/handbook .
- Dekkers OM ,
- Vandenbroucke JP ,
- Cevallos M ,
- Renehan AG ,
- ↵ Cooper H, Hedges LV, Valentine JV, eds. The Handbook of Research Synthesis and Meta-Analysis. Russell Sage Foundation, 2019.
- IOM (Institute of Medicine)
- PRISMA-P Group
- Salanti G ,
- Caldwell DM ,
- Stewart LA ,
- PRISMA-IPD Development Group
- Zorzela L ,
- Ioannidis JP ,
- PRISMAHarms Group
- McInnes MDF ,
- Thombs BD ,
- and the PRISMA-DTA Group
- Beller EM ,
- Glasziou PP ,
- PRISMA for Abstracts Group
- Mayo-Wilson E ,
- Dickersin K ,
- MUDS investigators
- Stovold E ,
- Beecher D ,
- Noel-Storr A
- McGuinness LA
- Sarafoglou A ,
- Boutron I ,
- Giraudeau B ,
- Porcher R ,
- Chauvin A ,
- Schulz KF ,
- Schroter S ,
- Stevens A ,
- Weinstein E ,
- Macleod MR ,
- IICARus Collaboration
- Kirkham JJ ,
- Petticrew M ,
- Tugwell P ,
- PRISMA-Equity Bellagio group
- Locations and Hours
- UCLA Library
- Research Guides
- Biomedical Library Guides
Systematic Reviews
- Types of Literature Reviews
What Makes a Systematic Review Different from Other Types of Reviews?
- Planning Your Systematic Review
- Database Searching
- Creating the Search
- Search Filters and Hedges
- Grey Literature
- Managing and Appraising Results
- Further Resources
Reproduced from Grant, M. J. and Booth, A. (2009), A typology of reviews: an analysis of 14 review types and associated methodologies. Health Information & Libraries Journal, 26: 91–108. doi:10.1111/j.1471-1842.2009.00848.x
- << Previous: Home
- Next: Planning Your Systematic Review >>
- Last Updated: Apr 17, 2024 2:02 PM
- URL: https://guides.library.ucla.edu/systematicreviews
Systematic reviews in sentiment analysis: a tertiary study
- Open access
- Published: 03 March 2021
- Volume 54 , pages 4997–5053, ( 2021 )
Cite this article
You have full access to this open access article
- Alexander Ligthart 1 ,
- Cagatay Catal ORCID: orcid.org/0000-0003-0959-2930 2 &
- Bedir Tekinerdogan 1
31k Accesses
122 Citations
1 Altmetric
Explore all metrics
With advanced digitalisation, we can observe a massive increase of user-generated content on the web that provides opinions of people on different subjects. Sentiment analysis is the computational study of analysing people's feelings and opinions for an entity. The field of sentiment analysis has been the topic of extensive research in the past decades. In this paper, we present the results of a tertiary study, which aims to investigate the current state of the research in this field by synthesizing the results of published secondary studies (i.e., systematic literature review and systematic mapping study) on sentiment analysis. This tertiary study follows the guidelines of systematic literature reviews (SLR) and covers only secondary studies. The outcome of this tertiary study provides a comprehensive overview of the key topics and the different approaches for a variety of tasks in sentiment analysis. Different features, algorithms, and datasets used in sentiment analysis models are mapped. Challenges and open problems are identified that can help to identify points that require research efforts in sentiment analysis. In addition to the tertiary study, we also identified recent 112 deep learning-based sentiment analysis papers and categorized them based on the applied deep learning algorithms. According to this analysis, LSTM and CNN algorithms are the most used deep learning algorithms for sentiment analysis.
Similar content being viewed by others
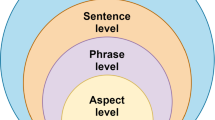
A survey on sentiment analysis methods, applications, and challenges
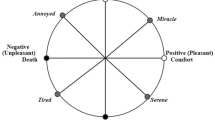
A review on sentiment analysis and emotion detection from text
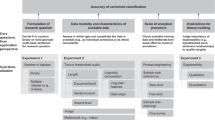
Sentiment Analysis in the Age of Generative AI
Avoid common mistakes on your manuscript.
1 Introduction
Sentiment analysis or opinion mining is the computational study of people's opinions, sentiments, emotions, and attitudes towards entities such as products, services, issues, events, topics, and their attributes (Liu 2015). As such, sentiment analysis can allow tracking the mood of the public about a particular entity to create actionable knowledge. Also, this type of knowledge can be used to understand, explain, and predict social phenomena (Pozzi et al. 2017 ). For the business domain, sentiment analysis plays a vital role in enabling businesses to improve strategy and gain insight into customers' feedback about their products. In today's customer-oriented business culture, understanding the customer is increasingly important (Chagas et al. 2018 ).
The explosive growth of discussion platforms, product review websites, e-commerce, and social media facilitates a continuous stream of thoughts and opinions. This growth makes it challenging for companies to get a better understanding of customers' aggregate opinions and attitudes towards products. The explosion of internet-generated content coupled with techniques like sentiment analysis provides opportunities for marketers to gain intelligence on consumers' attitudes towards their products (Rambocas and Pacheco 2018 ). Extracting sentiments from product reviews helps marketers to reach out to customers who need extra care, which will improve customer satisfaction, sales, and ultimately benefits businesses (Vyas and Uma 2019 ).
Sentiment analysis is a multidisciplinary field, including psychology, sociology, natural language processing, and machine learning. Recently, the exponentially growing amounts of data and computing power enabled more advanced forms of analytics. Machine learning, therefore, became a dominant tool for sentiment analysis. There is an abundance of scientific literature available on sentiment analysis, and there are also several secondary studies conducted on the topic.
A secondary study can be considered as a review of primary studies that empirically analyze one or more research questions (Nurdiani et al. 2016 ). The use of secondary studies (i.e., systematic reviews) in software engineering was suggested in 2004, and the term “Evidence-based Software Engineering” (EBSE) was coined by Kitchenham et al. ( 2004 ). Nowadays, secondary studies are widely used as a well-established tool in software engineering research (Budgen et al. 2018 ). The following two kinds of secondary studies can be conducted within the scope of EBSE:
Systematic Literature Review (SLR): An SLR study aims to identify relevant primary studies, extract the required information regarding the research questions (RQs), and synthesize the information to respond to these RQs. It follows a well-defined methodology and assesses the literature in an unbiased and repeatable way (Kitchenham and Charters 2007 ).
Systematic Mapping Study (SMS): An SMS study presents an overview of a particular research area by categorizing and mapping the studies based on several dimensions (i.e., facets) (Petersen et al. 2008 ).
SLR and SMS studies are different than traditional review papers (a.k.a., survey articles) because we systematically search in electronic databases and follow a well-defined protocol to identify the articles. There are also several differences between SLR and SMS studies (Catal and Mishra 2013 ; Kitchenham et al. 2010b ). For instance, while RQs of the SLR studies are very specific, RQs of SMS are general. The search process of the SLR is driven by research questions, but the search process of the SMS is based on the research topic. For the SLR, all relevant papers must be retrieved, and quality assessments of identified articles must be performed; however, requirements for the SMS are less stringent.
When there is a sufficient number of secondary studies on a research topic, a tertiary study can be performed (Kitchenham et al. 2010a ; Nurdiani et al. 2016 ). A tertiary study synthesizes data from secondary studies and provides a comprehensive review of research in a research area (Rios et al. 2018 ). They are used to summarize the existing secondary studies and can be considered as a special form of review that uses other secondary studies as primary studies (Raatikainen et al. 2019 ).
Although sentiment analysis has been the topic of some SLR studies, a tertiary study characterizing these systematic reviews has not been performed yet. As such, the aim of our study is to identify and characterize systematic reviews in sentiment analysis and present a consolidated view of the published literature to better understand the limitations and challenges of sentiment analysis. We follow the research methodology guidelines suggested for the tertiary studies (Kitchenham et al. 2010a ).
The objective of this study is thus to better understand the sentiment analysis research area by synthesizing results of these secondary studies, namely SLR and SMS, and providing a thorough overview of the topic. The methodology that we followed applies a systematic literature review to a sample of systematic reviews, and therefore, this type of tertiary study is valuable to determine the potential research areas for further research.
As part of this tertiary study, different models, tasks, features, datasets, and approaches in sentiment analysis have been mapped and also, challenges and open problems in this field are identified. Although tertiary studies have been performed for other topics in several fields such as software engineering and software testing (Raatikainen et al. 2019 ; Nurdiani et al. 2016 ; Verner et al. 2014 ; Cruzes and Dybå, 2011 ; Cadavid et al. 2020 ), this is the first study that performs a tertiary study on sentiment analysis.
The main contributions of this article are three-fold:
We present the results of the first tertiary study in the literature on sentiment analysis.
We identify systematic review studies of sentiment analysis systematically and explain the consolidated view of these systematic studies.
We support our study with recent survey papers that review deep learning-based sentiment analysis papers and explain the popular lexicons in this field.
The rest of the paper is organized as follows: Sect. 2 provides the background and related work. Section 3 explains the methodology, which was followed in this study. Section 4 presents the results in detail. Section 5 provides the discussion, and Sect. 6 explains the conclusions.
2 Background and related work
Sentiment analysis and opinion mining are often used interchangeably. Some researchers indicate a subtle difference between sentiments and opinions, namely that opinions are more concrete thoughts, whereas sentiments are feelings (Pozzi et al. 2017 ). However, sentiment and opinion are related constructs, and both sentiment and opinion are included when referring to either one. This research adopts sentiment analysis as a general term for both opinion mining and sentiment analysis.
Sentiment analysis is a broad concept that consists of many different tasks, approaches, and types of analysis, which are explained in this section. In addition, an overview of sentiment analysis is represented in Fig. 1 , which is adapted from (Hemmatian and Sohrabi 2017 ; Kumar and Jaiswal 2020 ; Mite-Baidal et al. 2018 ; Pozzi et al. 2017 ; Ravi and Ravi 2015 ). Cambria et al. ( 2017 ) stated that a holistic approach to sentiment analysis is required, and only categorization or classification is not sufficient. They presented the problem as a three-layer structure that includes 15 Natural Language Processing (NLP) problems as follows:
Syntactics layer: Microtext normalization, sentence boundary disambiguation, POS tagging, text chunking, and lemmatization
Semantics layer: Word sense disambiguation, concept extraction, named entity recognition, anaphora resolution, and subjectivity detection
Pragmatics layer: Personality recognition, sarcasm detection, metaphor understanding, aspect extraction, and polarity detection
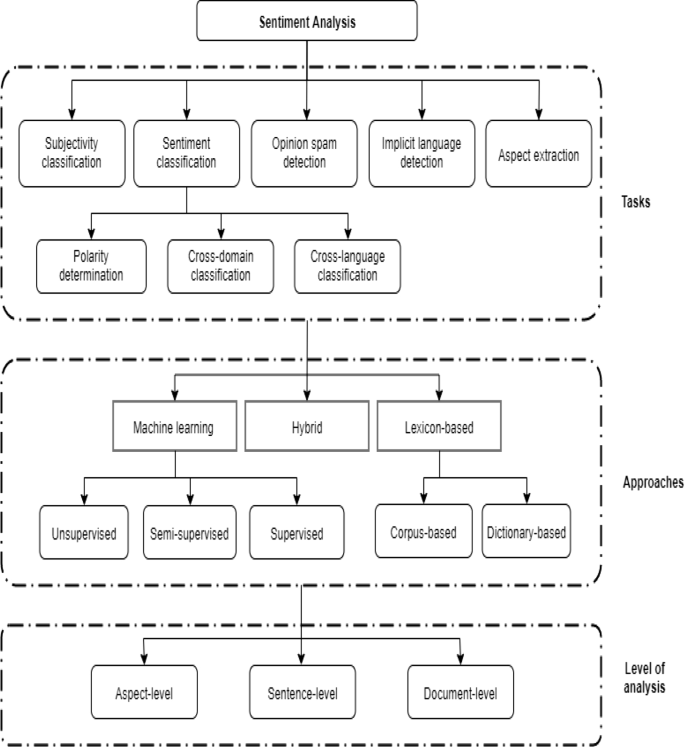
Sentiment analysis concept overview
Cambria ( 2016 ) state that approaches for sentiment analysis and affective computing can be divided into the following three categories: knowledge-based techniques, statistical approaches (e.g., machine learning and deep learning approaches), and hybrid techniques that combine the knowledge-based and statistical techniques.
Sentiment analysis models can adopt different pre-processing methods and apply a variety of feature selection methods. While pre-processing means transforming the text into normalized tokens (e.g., removing article words and applying the stemming or lemmatization techniques), feature selection means determining what features will be used as inputs. In the following subsections, related tasks, approaches, and levels of analysis are presented in detail.
2.1.1 Sentiment classification
One of the most widely known and researched tasks in sentiment analysis is sentiment classification. Polarity determination is a subtask of sentiment classification and is often improperly used when referring to sentiment analysis. However, it is merely a subtask aimed at identifying sentiment polarity in each text document. Traditionally, polarity is classified as either positive or negative (Wang et al. 2014 ). Some studies include a third class called neutral . Cross-domain and cross-language classification are subtasks of sentiment classification that aim to transfer knowledge from a data-rich source domain to a target domain where data and labels are limited. The cross-domain analysis predicts the sentiment of a target domain, with a model (partly) trained on a more data-rich source domain. A popular method is to extract domain invariant features whose distribution in the source domain is close to that of the target domain (Peng et al. 2018 ). The model can be extended with target domain-specific information. The cross-language analysis is practiced in a similar way by training a model on a source language dataset and testing it on a different language where data is limited, for example by translating the target language to the source language before processing (Can et al. 2018 ). Xia et al. ( 2015 ) stated that opinion-level context is beneficial to solve polarity ambiguity of sentiment words and applied the Bayesian model. Word polarity ambiguity is one of the challenges that need to be addressed for sentiment analysis. Vechtomova ( 2017 ) showed that the information retrieval-based model is an alternative to machine learning-based approaches for word polarity disambiguation.
2.1.2 Subjectivity classification
Subjectivity classification is a task to determine the existence of subjectivity in the text (Kasmuri and Basiron 2017 ). The goal of subjectivity classification is to restrict unwanted objective data objects for further processing (Kamal 2013 ). It is often considered the first step in sentiment analysis. Subjectivity classification detects subjective clues , words that carry emotion or subjective notions like ‘expensive’, ‘easy’, and ‘better’ (Kasmuri and Basiron 2017 ). These clues are used to classify text objects as subjective or objective.
2.1.3 Opinion spam detection
The growing popularity of e-commerce websites and review websites caused opinion spam detection to be a prominent issue in sentiment analysis. Opinion spams also referred to as false or fake reviews are intelligently written comments that either promote or discredit a product. Opinion spam detection aims to identify three types of features that relate to a fake review: review content, metadata of review, and real-life knowledge about the product (Ravi and Ravi 2015 ). Review content is often analyzed with machine learning techniques to uncover deception. Metadata includes the star rating, IP address, geo-location, user-id, etc.; however, in many cases, it is not accessible for analysis. The third method includes real-life knowledge. For instance, if a product has a good reputation, and suddenly the inferior product is rated superior in some period, reviews of that period might be suspected.
2.1.4 Implicit language detection
Implicit language refers to humor, sarcasm, and irony. There are vagueness and ambiguity in this form of speech, which is sometimes hard to detect even for humans. However, an implicit meaning to a sentence can completely flip the polarity of a sentence. Implicit language detection often aims at understanding facts related to an event. For example, in the phrase “I love pain”, pain is a factual word with a negative polarity load. The contradiction of the factual word ‘pain’ and subjective word ‘love’ can indicate sarcasm, irony, and humor. More traditional methods for implicit language detection include exploring clues such as emoticons, expressions for laughter, and heavy punctuation mark usage (Filatova 2012 ).
2.1.5 Aspect extraction
Aspect extraction refers to retrieving the target entity and aspects of the target entity in the document. The target entity can be a product, person, event, organization, etc. (Akshi Kumar and Sebastian 2012 ). People's opinions on various parts of a product need to be identified for fine-grained sentiment analysis (Ravi and Ravi 2015 ). Aspect extraction is especially important in sentiment analysis of social media and blogs that often do not have predefined topics.
Multiple methods exist for aspect extraction. The first and most traditional method is frequency-based analysis. This method finds frequently used nouns or compound nouns (POS tags), which are likely to be aspects. A rule of thumb that is often used is that if the (compound) noun occurs in at least 1% of the sentences, it is considered an aspect. This straightforward method turns out to be quite powerful (Schouten and Frasincar 2016 ). However, there are some drawbacks to this method (e.g., not all nouns are referring to aspects).
Syntax-based methods find aspects by means of syntactic relations they are in. A simple example is identifying aspects that are preceded by a modifying adjective that is a sentiment word. This method allows for low-frequency aspects to be identified. The drawback of this method is that many relations need to be found for complete coverage, which requires knowledge of sentiment words. Extra aspects can be found if more sentiment words that serve as adjectives can be identified. Qiu et al. ( 2009 ) propose a syntax-based algorithm that identifies aspects as well as sentiment words that works both ways. The algorithm identifies sentiment words for known aspects and aspects for known sentiment words.
2.2 Approaches
2.2.1 machine learning-based approaches.
Machine learning approaches for sentiment analysis tasks can be divided into three categories: unsupervised learning, semi-supervised learning, and supervised learning.
The unsupervised learning methods group unlabelled data into clusters that are similar to each other. For example, the algorithm can consider data as similar based on common words or word pairs in the document (Li and Liu 2014 ).
Semi-supervised learning uses both labeled and unlabelled data in the training process (da Silva et al. 2016a , b ). A set of unlabelled data is complemented with some examples of labeled data (often limited) included building a classifier. This technique can yield decent accuracy and requires less human effort compared to supervised learning. In cross-domain and cross-language classification, domain, or language invariant features can be extracted with the help of unlabelled data, while fine-tuning the classifier with labeled target data (Peng et al. 2018 ). Semi-supervised learning is especially popular for Twitter sentiment analysis, where large sets of unlabelled data are available (da Silva et al. 2016a , b ). Hussain and Cambria ( 2018 ) compared the computational complexity of several semi-supervised learning methods and presented a new semi-supervised model based on biased SVM (bSVM) and biased Regularized Least Squares (bRLS). Wu et al. ( 2019 ) developed a semi-supervised Dimensional Sentiment Analysis (DSA) model using the variational autoencoder algorithm. DSA calculates the sentiment score of texts based on several dimensions, such as dominance, valence, and arousal. Xu and Tan ( 2019 ) proposed the target-oriented semi-supervised sequential generative model (TSSGM) for target-oriented aspect-based sentiment analysis and showed that this approach outperforms two semi-supervised learning methods. Han et al. (2019) developed a semi-supervised model using dynamic thresholding and multiple classifiers for sentiment analysis. They evaluated their model on the Large Movie Review dataset and showed that it provides higher performance than the other models. Duan et al. ( 2020 ) proposed the Generative Emotion Model with Categorized Words (GEM-CW) model for stock message sentiment classification and demonstrated that this model is effective. Gupta et al. ( 2018 ) investigated the semi-supervised approaches for low resource sentiment classification and showed that their proposed methods improve the model performance against supervised learning models.
The most widely known machine learning method is supervised learning. This approach trains a model with labeled source data. The trained model can subsequently make predictions for an output considering new unlabelled input data. In most cases, supervised learning often outperforms unsupervised and semi-supervised learning approaches, but the dependency on labeled training data can require lots of human effort and is therefore sometimes inefficient (Hemmatian and Sohrabi 2017 ).
Machine learning methods are increasingly popular for aspect extraction. The most commonly used approach for aspect extraction is topic modeling , an unsupervised method that assumes any document contains a certain amount of hidden topics (Hemmatian and Sohrabi 2017 ). Latent Dirichlet Allocation (LDA) algorithm, which has many different variations, is a popular topic modeling algorithm (Nguyen and Shirai 2015 ) that allows observations to be explained by unsupervised grouping of similar data. LDA outputs some topics of a text document and attributes each word in the document to one of the identified topics. The drawback of machine learning methods is that they require lots of labeled data.
2.2.2 Deep learning-based approaches
Deep learning is a sub-branch of machine learning that uses deep neural networks. Recently, deep learning algorithms have been widely applied for sentiment analysis. In this section, first, we discuss the articles that present an overview of papers that applied deep learning for sentiment analysis. These articles are neither SLR nor SMS papers. Instead, they are either traditional review (a.k.a., survey) articles or comparative assessment papers that explain the existing deep learning-based approaches in addition to the experimental analysis. Later, we also present some of the deep learning-based models used in sentiment analysis papers.
In Table 1 , we present the survey papers that analyzed deep learning-based sentiment analysis papers. In this table, we also show the number of papers investigated in these survey papers.
Dang et al. ( 2020 ) presented a summary of 32 deep learning-based sentiment analysis papers and analyzed the performance of Deep Neural Networks (DNN), Convolutional Neural Networks (CNN), and Recurrent Neural Networks (RNN) on eight datasets. They selected these deep learning algorithms because they are the most widely used deep learning algorithms according to their analysis of 32 deep learning-based sentiment analysis papers. They used both word embedding and term frequency-inverse document frequency (TF-IDF) to prepare inputs for classification algorithms and reported that the RNN-based model using word embedding achieved the best performance among other algorithms. However, the processing time of the RNN-based model is ten times larger than the CNN-based one. In addition, they reported that the following deep learning algorithms were used in the 32 deep learning-based sentiment analysis papers: CNN, Long-Short Term Memory (LSTM) (tree-LSTM, discourse-LSTM, coattention-LSTM, bi-LSTM), Gated Recurrent Units (GRU), RNN, Coattention-MemNet, Latent Rating Neural Network (LRNN), Simple Recurrent Networks (SRN), and Recurrent Neural Tensor Network (RNTN)).
Yadav and Vishwakarma (2019) reviewed 130 research papers that apply deep learning techniques in sentiment analysis. They identified the following deep learning methods used for sentiment analysis: CNN, Recursive Neural Network (Rec NN), RNN (LSTM and GRU), Deep Belief Networks (DBN), Attention-based Network, Bi-RNN, and Capsule Network. They reported that LSTM provides better results, and the use of deep learning approaches for sentiment analysis is promising. However, they stated that they require a huge amount of data, and there is a lack of training datasets.
Zhang et al. ( 2018 ) published a survey article on the application of deep learning methods for sentiment analysis. They explained several papers that address one of the following levels: document level, sentence level, and the aspect level sentiment classification. The applied algorithms per analysis level are listed as follows:
Document-level sentiment classification: Artificial Neural Networks (ANN), Stacked Denoising Autoencoder (DSA), Denoising Autoencoder, CNN, LSTM, GRU, Memory Network, and GRU-based Encoder
Sentence-level sentiment classification: CNN, RNN, Semi-supervised Recursive Autoencoders Network (RAE), Recursive Neural Network, Recursive Neural Tensor Network, Dynamic CNN, LSTM, CNN-LSTM, Bi-LSTM, and Recurrent Random Walk Network
Aspect-level sentiment classification: Adaptive Recursive Neural Network, LSTM, Bi-LSTM, Attention-based LSTM, Memory Network, Interactive Attention Network, Recurrent Attention Network, and Dyadic Memory Network
Rojas‐Barahona (2016) presented an overview of deep learning approaches used for sentiment analysis and divided the techniques into the following categories:
Non-Recursive Neural Networks: RNN (variant: Bi-RNN), LSTM (variant: Bi-LSTM), and CNN (variants: CNN-Multichannel, CNN-non-static, Dynamic CNN)
Recursive Neural Networks: Recursive Autoencoders and Constituency Tree Recursive Neural Networks
Combination of Non-Recursive and Recursive Methods: Tree-Long Short-Term Memory (Tree-LSTM) and Deep Recursive Neural Networks (Deep RsNN)
For the movie reviews dataset, Rojas‐Barahona (2016) showed that the Dynamic CNN model provides the best performance. For the Sentiment TreeBank dataset, the Constituency Tree‐LSTM that is a Recursive Neural Network outperforms all the other algorithms.
Habimana et al. ( 2020a ) reviewed papers that applied deep learning algorithms for sentiment analysis and also performed several experiments with the specified algorithms on different datasets. They reported that dynamic sentiment analysis, sentiment analysis for heterogeneous information, and language structure are the main challenges for the sentiment analysis research field. They categorized the techniques used in the papers based on several analysis levels that are listed as follows:
Document-level Sentiment Analysis: CNN-based models, RNN with attention-based models, RNN with the user and product attention-based models, Adversarial Network Models, and Hybrid Models
Sentence-Level Sentiment Classification: Unsupervised Pre-Trained Networks (UPN), CNN, Recurrent Neural Networks, Deep Reinforcement Learning (DRL), RNN, RNN with cognition attention-based models
Aspect-based Sentiment Analysis: Attention-based models with aspect information, attention-based models with the aspect context, RNN with attention memory model, RNN with commonsense knowledge model, CNN-based model, and Hybrid model
Do et al. ( 2019 ) presented an overview of over 40 deep learning approaches used for aspect-based sentiment analysis. They categorized papers based on the following categories: CNN, RNN, Recursive Neural Network, and Hybrid methods. Also, they presented the advantages, disadvantages, and implications for aspect-based sentiment analysis (ABSA). They concluded that deep learning and ABSA are still in the early stages, and there are four main challenges in this field, namely domain adaptation, multi-lingual application, technical requirements (labeled data and computational resources and time), and linguistic complications.
Minaee et al. ( 2020 ) reviewed more than 150 deep learning-based text classification studies and presented their strengths and contributions. 22 of these studies proposed approaches for sentiment analysis. They provided more than 40 popular text classification datasets and showed the performance of some deep learning models on popular datasets. Since they did not only focus on sentiment analysis problems, they explained other kinds of models used for other tasks such as news categorization, topic analysis, question answering (QA), and natural language inference. They explained the following deep learning models in their paper: Feed-forward neural networks, RNN-based models, CNN-based models, Capsule Neural Networks, Models with attention mechanism, Memory augmented networks, Transformers, Graph Neural Networks, Siamese Neural Networks, Hybrid models, Autoencoders, Adversarial training, and Reinforcement learning. The challenges reported in this study are new datasets for multi-lingual text classification, interpretable deep learning models, and memory-efficient models. They concluded that the use of deep learning in text classification improves the performance of the models.
Some of the highly cited deep learning-based sentiment analysis papers are shown in Table 2 .
Kim ( 2014 ) performed several experiments with the CNN algorithm for sentence classification and showed that even with little parameter tuning, the CNN model that includes only one convolutional layer provides better performance than the state-of-the-art models of sentiment analysis.
Wang et al. ( 2016 ) developed an attention-based LSTM approach that can learn aspect embeddings. These aspects are used to compute the attention weights. Their models provided a state-of-the-art performance on SemEval 2014 dataset. Similarly, Pergola et al. ( 2019 ) proposed a topic-dependent attention model for sentiment classification and showed that the use of recurrent unit and multi-task learning provides better representations for accurate sentiment analysis.
Chen et al. ( 2017 ) developed the Recurrent Attention on Memory (RAM) model and showed that their model outperforms other state-of-the-art techniques on four datasets, namely SemEval 2014 (two datasets), Twitter dataset, and Chinese news comment dataset. Multiple attentions were combined with a Recurrent Neural Network in this study.
Ma et al. ( 2018 ) incorporated a hierarchical attention mechanism to the LSTM network and also extended the LSTM cell to incorporate commonsense knowledge. They demonstrated that the combination of this new LSTM model called Sentic LSTM and the attention architecture outperforms the other models for targeted aspect-based sentiment analysis.
Chen et al. ( 2016 ) developed a hierarchical LSTM model that incorporates user and product information via different levels of attention. They showed that their model achieves significant improvements over models without user and product information on IMDB, Yelp2013, and Yelp2014 datasets.
Wehrmann et al. ( 2017 ) proposed a language-agnostic sentiment analysis model based on the CNN algorithm, and the model does not require any translation. They demonstrated that their model outperforms other models on a dataset, including tweets from four languages, namely English, German, Spanish, and Portuguese. The dataset consists of 1.6 million annotated tweets (i.e., positive, negative, and neutral) from 13 European languages.
Ebrahimi et al. ( 2017 ) presented the challenges of building a sentiment analysis platform and focused on the 2016 US presidential election. They reported that they reached the best accuracy using the CNN algorithm, and the content-related challenges were hashtags, links, and sarcasm.
Poria et al. ( 2018 ) investigated three deep learning-based architectures for multimodal sentiment analysis and created a baseline based on state-of-the-art models.
Xu et al. ( 2019 ) developed an improved word representation approach, used the weighted word vectors as input into the Bi-LSTM model, obtained the comment text representation, and applied the feedforward neural network classifier to predict the comment sentiment tendency.
Majumder et al. ( 2019 ) proposed a GRU-based Neural Network that can be trained on sarcasm or sentiment datasets. They demonstrated that multitask learning-based approaches provide better performance than standalone classifiers developed on sarcasm and sentiment datasets.
After investigating these above-mentioned survey and highly cited articles, we searched in Google Scholar by using our search criteria (i.e., “deep learning” and “sentiment analysis”) to reach the recent state-of-the-art deep learning-based studies published in 2020. We retrieved 112 deep learning-based sentiment analysis papers published in 2020 and extracted the applied deep learning algorithms from these papers. In Appendix (Table 16 ), we present these recent deep learning-based sentiment analysis papers. In Table 3 , we show the distribution of applied deep learning algorithms used in these 112 recent papers.
According to this table, the most applied algorithm is the LSTM algorithm (i.e., 35.53%) and the second most used algorithm is CNN (i.e., 33.33%). The other widely used algorithms are GUR (i.e., 8.77%) and RNN (i.e., 7.89%) algorithms. However, the other well-known deep learning algorithms such as DNN, Recursive Neural Network (ReNN), Capsule Network (CapN), Generative Adversarial Network (GAN), Deep Q-Network, and Autoencoder have not been preferred much and used only in a few studies. Most of the hybrid approaches also combined the CNN and LSTM algorithms and therefore, they were represented under these categories. As this analysis indicates, most of the recent deep learning-based studies followed the supervised learning machine learning approach.
2.2.3 Lexicon-based approaches
The traditional approach for sentiment analysis is the lexicon-based approach (Hemmatian and Sohrabi 2017 ). Lexicon-based methods scan through the documents for words that express positive or negative feelings to humans. Negatives words would be ‘bad’, ‘ugly’, ‘scary’ while positive words are, for example, ‘good’ or ‘beautiful’. The values of these words are documented in a lexicon. Words with high positive or negative values are mostly adjectives and adverbs. Sentiment analysis shows to be extremely dependent on the domain of interest (Vinodhini 2012 ). For example, analyzing movie reviews can yield very different results compared to analyzing Twitter data due to different forms of language used. Therefore, the lexicon used for sentiment analysis needs to be adjusted according to the domain of interest. This can be a time-consuming process. However, lexicon-based methods do not require training data, which is a big advantage (Shayaa et al. 2018 ).
There are two main approaches to creating sentiment lexicons: dictionary-based and corpus-based. The dictionary-based approach starts with a small set of sentiment words, and iteratively expands the lexicon with synonyms and antonyms from existing dictionaries. In most cases, the dictionary-based approach works best for general purposes. Corpus-based lexicons can be tailored to specific domains. The approach starts with a list of general-purpose sentiment words and discovers other sentiment words from a domain corpus based on co-occurring word patterns (Mite-Baidal et al. 2018 ).
2.2.4 Hybrid approaches
There are different hybrid approaches in the literature. Some of them aim to extend machine learning models with lexicon-based knowledge (Behera et al. 2016 ). The goal is to combine both methods to yield optimal results using an effective feature set of both lexicon and machine learning-based techniques (Munir Ahmad et al. 2017 ). This way, the deficiencies and limitations of both approaches can be overcome.
Recently, researchers focused on the integration of symbolic and subsymbolic Artificial Intelligence (AI) for sentiment analysis (Cambria et al. 2020 ). Machine learning (also, deep learning) is considered to be a bottom-up approach and applies subsymbolic AI. This is extremely useful for exploring a huge amount of data and discovering interesting patterns in the data. Although this type of bottom-up approach works quite well for image classification tasks, they are not very effective for natural language processing tasks. For effective communication, we learn many issues such as cultural awareness and commonsense in a top-down manner instead of a bottom-up manner (Cambria et al. 2020 ). Therefore, these researchers applied subsymbolic AI (i.e., deep learning) to recognize patterns in text and represented them in a knowledge base using symbolic AI (i.e., logic and semantic networks). They built a new commonsense knowledge base called SenticNet for the sentiment analysis problem and concluded that coupling symbolic AI and subsymbolic AI is crucial to passing to the natural language understanding stage from natural language processing.
Minaee et al. ( 2019 ) developed an ensemble model using LSTM and CNN algorithm and demonstrated that this ensemble model provides better performance than the individual models.
2.2.5 Milestones of sentiment analysis research
Recently, Poria et al. ( 2020 ) investigated the challenges and new research directions in sentiment analysis research. Also, they presented the key milestones of sentiment analysis for the last two decades. We adapted their timeline figure for the last decade. In Fig. 2 , we present the most promising works of sentiment analysis research. For a more detailed illustration of milestones, we refer the readers to the article of Poria et al. ( 2020 ).
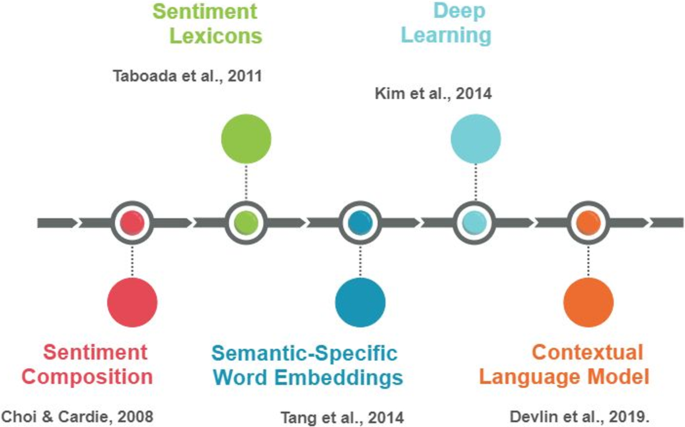
Milestones of sentiment analysis research for the last decade
2.3 Levels of analysis
Sentiment analysis can be implemented at the following three levels: document, sentence, and aspect level. We elaborate on these in the next paragraphs.
2.3.1 Document-level
Document-level analysis considers the whole text document as a unit of analysis (Wang et al. 2014 ). It is a simplified task that presumes that the entire document originates from a single opinion holder. Document analysis comes with some issues, namely that there could be multiple and mixed opinions in a document expressed in many different ways, sometimes with implicit language (Akshi Kumar and Sebastian 2012 ). Typically, documents are revised on a sentence or aspect level before determining the polarity of the entire text document.
2.3.2 Sentence-level:
Sentence-level analysis considers specific sentences in a text and is especially used for subjectivity classification. Text documents typically consist of sentences that either contain opinion or not. Subjectivity classification analyses individual sentences in a document to detect whether the sentence contains facts or emotions and opinions. The main goal of subjectivity classification is to exclude sentences that do not contain sentiment or opinion (Akshi Kumar and Sebastian 2012 ). This analysis often includes subjectivity classification as a step to either include or exclude sentences for analysis.
2.3.3 Aspect-level
Aspect-level analysis is a challenging topic in sentiment analysis. It refers to analyzing sentiments about specific entities and their aspects in a text document, not merely the overall sentiment of the document (Tun Thura Thet et al. 2010 ). It is also known as entity-level or feature-level analysis. Even though the general sentiment of a document may be classified as positive or negative, the opinion holder can have a divergent opinion about specific aspects of an entity (Akshi Kumar and Sebastian 2012 ). In order to measure aspect-level opinion, aspects of the entity need to be identified. Valdivia et al. ( 2017 ) stated that aspect-based sentiment analysis is beneficial to the business manager because customer opinions are extracted in a transparent way. Also, they reported that ironic expression detection in TripAdvisor is still an open problem and also, labeling of reviews should not only focus on user ratings because some users write positive sentences on negative user ratings and vice versa. Poria et al. ( 2016 ) proposed a new algorithm called Sentic LDA (Latent Dirichlet Allocation) and improved the LDA algorithm with semantic similarity for aspect-based sentiment analysis. They concluded that this new algorithm helps researchers to pass to the semantics analysis from the syntactical analysis in aspect-based sentiment analysis by using the common-sense computing (Cambria et al. 2009 ) and improves the clustering process (Poria et al. 2016 ).
2.4 Popular lexicons
Several survey articles discussed the popular lexicons used in sentiment analysis. Dang et al. ( 2020 ) reported the following popular sentiment analysis lexicons in their article: Sentiment 140, Tweets Airline, Tweets Semeval, IMDB Movie Reviews (1), IMDB Movie Reviews (2), Cornell Movie Reviews, Book Reviews, and Music Reviews datasets. Habimana et al. ( 2020a ) explained the following popular lexicons in their survey article: IMDB, IMDB2, SST-5, SST-2, Amazon, SemEval 2014-D1, SemEval 2014-D2, SemEval 2017, STS, STS-Gold, Yelp, HR (Chinese), MR, Sanders, Deutsche Bahn (Deutsch), ASTD (Arabic), YouTube, CMU-MOSI, and CMU-MOSEI. Do et al. ( 2019 ) reported the following datasets widely used in sentiment analysis papers: Customer review data, SemEval 2014, SemEval 2015, SemEval 2016, ICWSM 2010 JDPA Sentiment Corpus, Darmstadt Service Review Corpus, FiQA ABSA, and target-dependent Twitter sentiment classification dataset. Minaee et al. ( 2020 ) explained the following datasets used for sentiment analysis: Yelp, IMDB, Movie Review, SST, MPQA, Amazon, and aspect-based sentiment analysis datasets (SemEval 2014 Task-4, Twitter, and SentiHood). Researchers who would like to perform a new study are suggested to look at these articles because links and other details per lexicon are presented in detail in these articles.
2.5 Advantages, disadvantages, and performance of the models
Several studies have been performed to compare the performance of existing models for sentiment analysis. Each model has its own advantages and weaknesses. For the aspect-based sentiment analysis, Do et al. ( 2019 ) divided models based on the following three categories: CNN, RNN, and Recurrent Neural Networks. The advantages of CNN-based models are fast computation, the ability to extract local patterns and represent non-linear dynamics. The disadvantage of the CNN-based model is the high demand for data. The advantages of RNN-based models are that they do not require a huge amount of data, they have a distributed hidden state that stores previous computations, and they require fewer parameters. The disadvantages are that they cannot capture long-term dependencies, and they select the last hidden state to represent the sentence. The advantages of Recurrent Neural Networks are their simple architectures and their ability to learn tree structures. The disadvantages are that they require parsers that might be slow, and they are still at early stages. It was reported that RNN-based models provide better performance than CNN-based models, and more research is required for Recurrent Neural Networks.
Yadav and Vishwakarma (2019) reported that deep learning-based models are gaining popularity for different sentiment analysis tasks. They stated that CNN followed by LSTM (an RNN algorithm) provides the highest accuracy for document-level sentiment classification, researchers focused on RNN algorithms (particularly, LSTM) for sentence-level sentiment classification and aspect-level sentiment classification, and RNN models the best-performing ones for multi-domain sentiment classification. They also discussed the merits and demerits of CNN, Recursive Neural Networks (RecNN), RNN, LSTM, GRU, DBN models.
The advantage of DBN is the ability to learn the dimension of vocabulary using different layers. The disadvantages of DBN are that they are computationally expensive and unable to remember the previous task.
The advantage of GRU is that it is computationally less expensive, it has a less complex structure, and it can capture interdependencies between sentences. The disadvantage of GRU is that it does not have a memory unit, and its performance is lower than the LSTM model on larger datasets.
The advantage of LSTM is that they perform better than CNN, they can extract sequential information, and they can forget/remember things selectively. The disadvantage of LSTM is that it is considerably slower, each output should be reconciled to a sentence, and it is computationally expensive.
The advantage of RNN models is that they provide better performance than CNN models, have fewer parameters, and capture long-distance dependency features. The disadvantage of RNN models is that they cannot process long sequences.
The advantage of CNN models is that they are less expensive in terms of computational complexity and faster compared to RNN, LSTM, and GRU algorithms. Also, they can discover relevant features from different parts of the word. The disadvantage of LSTM models is that they cannot preserve long-term dependency and ignores this type of long-distance features.
The advantage of RecNN is that they are good at learning hierarchical structure and therefore, they provide better performance for NLP tasks. The disadvantage of RecNN models is that their efficiency is dramatically affected in the case of informal data that do not have grammatical rules and training can be difficult because structure changes for every sample.
Despite the excellent performance of deep learning models, there are some drawbacks. The following drawbacks are discussed by Yadav and Vishwakarma (2019):
A huge amount of data is required to train the models and finding these large datasets is not easy in many cases
They work like a black box, it is hard to understand how they predict the sentiment of the text
The performance of the models is affected by the hyperparameters and the selection of these hyperparameters is very challenging
Training time is very long and most of the time they require GPU support and large RAM
Yadav and Vishwakarma (2019) performed experiments to compare the execution time and accuracy of several deep learning algorithms. They reported that the LSTM algorithm and its variations such as Bi-LSTM and GRU require long training and execution time compared to other deep learning models. However, these LSTM-based algorithms better performance. Therefore, there is a trade-off between time and accuracy parameters when selecting the deep learning model.
3 Methodology
In this section, the methodology of our tertiary study is presented. This study can be considered as a systematic review study that targets secondary studies on sentiment analysis, which is a widely researched topic. There are several reviews and mapping studies available on sentiment analysis in the literature. In this section, we focus on synthesizing the results of these secondary studies. Hence, we conduct a tertiary study. The study design is based on the systematic literature review (SLR) protocol suggested by Kitchenham and Charters ( 2007 ) and the format followed by the tertiary study papers of Curcio et al. ( 2019 ); Raatikainen et al. ( 2019 ). This study reviews two types of secondary studies:
SLR: These studies are performed to aggregate results related to specific research questions.
SMS: These studies aim to find and classify primary studies in a specific research topic. This method is more explorative compared to the SLR and is used to identify available literature prior to undertaking an SLR.
Both are considered secondary studies as they review primary studies. A pragmatic comparison between SLR and SMS is discussed by Kitchenham et al. ( 2011 ). Three main phases for conducting this research are planning, conducting, and reporting the review (Kitchenham 2004 ). Planning refers to identifying the need for the review and developing the review protocol. The goal of this tertiary study is to gather a broad overview of the current state of the art in sentiment analysis and to identify open problems and challenges in the field.
3.1 Research questions
The following research questions have been defined for this study:
RQ1 What are the adopted features (input/output) in sentiment analysis?
RQ2 What are the adopted approaches in sentiment analysis?
RQ3 What domains have been addressed in the adopted data sets?
RQ4 What are the challenges and open problems with respect to sentiment analysis?
3.2 Search process
This section provides insight into the process of determining secondary studies to include. Not all databases are equally relevant to this research topic. Databases that are used to identify secondary studies are adopted from the search strategy of secondary studies on sentiment analysis (Genc-Nayebi and Abran 2017 ; Hemmatian and Sohrabi 2017 ; Kumar and Jaiswal 2020 ; Sharma and Dutta 2018 ). The following databases are included in this study: IEEE, Science Direct, ACM, Springer, Wiley, and Scopus . To find the relevant literature, databases are searched for the title, abstract, and keywords based on the following query:
(“sentiment analysis” OR “sentiment classification” OR “opinion mining”) AND (“SLR” OR “systematic literature review” OR “systematic mapping” OR “mapping study”)
This query results in 43 hits. As stated before, this study only considers systematic literature reviews and systematic mapping studies since they are considered of higher quality and more in-depth compared to survey articles. Inclusion and exclusion criteria are formulated, as shown in Table 4 .
All secondary studies are analyzed and classified according to the inclusion and exclusion criteria in Table 4 . After this process, 16 secondary studies are selected.
3.3 Quality assessment
The confidence placed in the secondary studies is on the quality assessment of the articles. For a tertiary study, the quality assessment is especially important (Goulão et al. 2016 ). The DARE criteria proposed by York University Centre for Reviews and Dissemination (CDR) and adopted in this study are often used in the context of software engineering (Goulão et al. 2016 ; Rios et al. 2018 ; Curcio et al. 2019 ; Goulão et al. 2016 ; Kitchenham et al. 2010a ). The criteria are based on four questions (CQs), as shown in Table 5 . For each selected article, the criteria are scored based on a three-point scale, as described in Table 6 , adopted from (Kitchenham et al. 2010a , b ).
The scoring procedure is Yes = 1, Partial = 0.5, and No = 0. The assessment is conducted by the researchers. The results of the quality assessment are shown in Table 7 . Two studies are excluded based on the results, leaving a total amount of 14 studies remaining for analysis.
3.4 Additional data
In order to provide an overview of the selected secondary studies, Table 8 shows the following data extracted from the articles: Research focus, number of primary studies included in the review, year of publication, paper type (conference/journal/book chapter), and source. In addition, an overview of the research questions of the secondary studies is provided, as shown in Table 9 . The reference numbers in Table 8 are used throughout the rest of this paper.
This section addresses the results of the research questions derived from 14 secondary studies. For each research question, tables with aggregate results and in-depth descriptions and interpretations are presented. The selected secondary studies discuss specific sentiment analysis tasks. It is important to note that different tasks in sentiment analysis require different features and approaches. Therefore, a brief overview of each paper is presented. Note that in-depth analysis and synthesis of the articles are presented later in this section.
Genc-Nayebi and Abran ( 2017 ) identify mobile app store opinion mining techniques. Their paper is mainly focused on statistical data mining techniques based on manual classification and correlation analysis. Some machine learning algorithms are discussed in the context of cross-domain analysis and app aspect-extraction. Some interesting challenges in sentiment analysis are proposed.
Al-Moslmi et al. ( 2017 ) review the cross-domain sentiment analysis. Specific algorithms for cross-domain sentiment analysis are described.
Qazi et al. ( 2017 ) research the opinion types and sentiment analysis. Opinion types are classified into the following three categories: regular, comparative, and suggestive. Several supervised machine learning techniques are used. Sentiment classification algorithms are mapped.
Ahmed Ibrahim and Salim ( 2013 ) perform sentiment analysis of Arabic tweets. Their study is focused on mapping features and techniques used for general sentiment analysis.
Shayaa et al. ( 2018 ) research the big data approach to sentiment analysis. A solid overview of machine learning methods and challenges is presented.
A. Kumar and Sharma ( 2017 ) research sentiment analysis for government intelligence. Techniques and datasets are mapped.
M. Ahmad et al. ( 2018 ) focus their research on SVM classification. SVM is the most used machine learning technique in sentiment classification.
A. Kumar and Jaiswal ( 2020 ) discuss soft computing techniques for sentiment analysis on Twitter. Soft computing techniques include machine learning techniques. Deep learning (CNN in particular) is mentioned as upcoming in recent articles. KPIs are described thoroughly.
A. Kumar and Garg ( 2019 ) research context-based sentiment analysis. They stress the importance of subjectivity in sentiment analysis and show that deep learning offers opportunities for context-based sentiment analysis.
Kasmuri and Basiron ( 2017 ) research the subjectivity analysis. Its purpose is to determine whether the text is subjective or objective with objective clues. Subjectivity analysis is a classification problem, and thus, machine learning algorithms are widely used.
Madhala et al. ( 2018 ) research customer emotion analysis. They review articles that classify emotions from 4 to 51 different classes.
Mite-Baidal et al. ( 2018 ) research sentiment analysis in the education domain. E-learning is upcoming, and due to the online nature, lots of review data is generated on forums of MOOCS and social media.
Salah et al. ( 2019 ) research the social media sentiment analysis. Mainly twitter data is used because of the high dimensionality (e. g., retweets, location, user followers no.) and structure.
De Oliveira Lima et al. ( 2018 ) research opinion mining of hotel reviews specifically aimed at sustainability practices aspects. Limited information on used features is available. The following sections dive into the different models that are used in sentiment analysis, including adopted features, approaches, and datasets.
4.1 RQ1 “What are the adopted features in sentiment analysis?”
Table 10 depicts the common input and output features that articles present for the sentiment analysis approach. Checkmarks indicate that the features are explicitly discussed in the referred article. Traditional approaches commonly use Bag-Of-Words (BOW) method. BOW counts the words, referred to as n-grams , in the text and creates a sparse vector with 1 s for present words and 0 s for absent words. These vectors are used as input to machine learning models. N-grams are sets of words that occur next to each other that are combined into one feature. This way, the order of words will be maintained when the text is vectorized. Part-Of-Speech (POS) tags provide feature tags for similar words with a different part of speech in the context. The term frequency-inverse document frequency (TF-IDF) method highlights words or word pairs that often occur in one document but are low in frequency in the entire text corpus. Negation is an important feature to include in lexicon-based approaches. Negation means contradicting or denying something, which can flip the polarity of an opinion or sentiment.
Word embeddings are often used as feature learning techniques in deep learning models. Word embeddings are dense vectors with real numbers for a word or sentence in the text based on the context of the word in the text corpus. This approach, although considered promising, is only discussed to a limited extent in the selected articles.
Output variables differ per sentiment analysis task. Output classes of the identified secondary studies are polarity, subjectivity, emotions classes, or spam identification. Polarity indicates the extent to which the input is considered positive or negative in sentiment. In most cases, the output is classified in a binary way, either positive or negative. Some models include a neutral class as well. Multiple classes of polarity are shown to drastically reduce performance (Al-Moslmi et al. 2017 ) and are, therefore, not frequently used. One study (Madhala et al. 2018 ) focuses specifically on emotion classification, with up to 51 different classes of emotions. Some studies (Ahmed Ibrahim and Salim 2013 ; Kasmuri and Basiron 2017 ) include subjectivity analysis as part of sentiment analysis. Finally, spam detection is an important task in sentiment analysis, referring to extracting illegitimate means for a review. Examples of spam are untruthful opinions, reviews on the brand instead of on the product, and non-reviews like advertisements and random questions or text (Jindal and Liu 2008).
A clear pattern exists in the use of input and output features. Traditional machine learning models commonly use unigrams and n-grams as input. Variable features are TF-IDF values and POS-tags. Not every feature extraction method is as effective in differing domains. Combinations of input features are often made to reach better performance. Word embeddings are upcoming input features. The most recent articles (Kumar and Garg 2019 ; Kumar and Jaiswal 2020 ) explicitly discuss them. Text classification with word embeddings as input is considered a promising technique that is often combined with deep learning methods like recurrent neural networks. The output shows a similar pattern with common and variable features. The common feature is polarity, and variable output features include emotions, subjectivity, and spam type.
4.2 RQ2 “What are the adopted approaches in sentiment analysis?”
Different tasks in sentiment analysis require different approaches. Therefore, it is important to note which task requires which approach. Table 11 shows the categories that are used throughout different sentiment analysis tasks.
Table 12 depicts the commonly used approaches for sentiment analysis per selected paper. Machine learning algorithms, including deep learning (DL), unsupervised learning, and ensemble learning, are widely used for sentiment analysis tasks, as well as lexicon-based and hybrid methods. Checkmarks indicate that approaches are explicitly discussed in the referred article. Results are divided into five categories with specific subcategories. Each category with corresponding subcategories are described as follows:
4.2.1 Deep learning
Deep learning models are complex architectures with multiple layers of neural networks to progressively extract high-level features from input. CNN uses convolutional filters to recognize patterns in data. CNN is widely used in image recognition and, to a lesser extent, in the field of NLP. RNN is designed for recognizing sequential patterns. RNN is especially powerful in cases where context is critical. For this reason, RNN is very promising in sentiment analysis. LSTM networks are a special kind of RNN, that is capable of learning long-term context and dependencies. LSTM is especially powerful in NLP, where long-term dependencies are often important. The discussed deep learning algorithms are considered promising techniques and able to boost the performance of NLP tasks (Socher et al. 2013 ).
4.2.2 Traditional machine learning
Traditional ML algorithms are still widely used in all kinds of sentiment analysis tasks, including sentiment classification. While deep learning is a promising field, in many cases, traditional ML performs sufficiently well or even better for a specific task compared to deep learning methods, usually on smaller datasets. The traditional supervised machine learning algorithms are Support Vector Machines (SVM), Naive Bayes (NB), Neural Networks (NN), Logistic Regression (LogR), Maximum Entropy (ME), k-Nearest Neighbor (kNN), Random Forest (RF), and Decision Trees (DT).
4.2.3 Lexicon-based
Lexicon-based learning is a traditional approach to sentiment analysis. Lexicon-based methods scan through the documents for words that express positive or negative feelings to humans. Words are defined in a lexicon beforehand, so no learning data is required for this approach.
4.2.4 Hybrid models
In the context of sentiment classification, hybrid models combine the lexicon-based approach with machine learning techniques (Behera et al. 2016 ) to create a lexicon-enhanced classifier. Lexicons are used for defining domain-related features that are used as input for a machine learning classifier.
4.2.5 Ensemble classification
Ensemble classifiers approach adopts multiple learning algorithms to obtain better performance (Behera et al. 2016 ). Three main types of ensemble classification methods are bagging (bootstrap aggregating), boosting, and stacking. The bagging method independently learns homogeneous algorithms with data points randomly picked from the training set, following a deterministic averaging process. Boosting learns homogeneous algorithms in a sequential and adaptive way before following an averaging process. Stacking learns heterogeneous classifiers in parallel and combines them to predict an output. An overview of ensemble classifiers is shown in Table 13 .
Support Vector Machines (SVM) is the dominant algorithm in the field of sentiment classification. All selected papers include SVM for classification purposes, and in most cases, this technique yields the best performance. Naive Bayes is the second most used algorithm and is praised for its high performance despite the simplicity of the technique. Besides these two dominant algorithms, methods like NN, LogR, ME, kNN, RF, and DT are used throughout different tasks of sentiment analysis. A popular unsupervised approach for aspect extraction is LDA. Hybrid approaches to sentiment classification have been effective by using domain-specific knowledge to create extra features that enhance the performance of the model. Ensemble and hybrid methods often improve the performance and reliability of predictions.
Deep learning algorithms are rising techniques in sentiment analysis. Especially, RNNs and the more complex RNN architecture, LSTM, are increasing in popularity. Even though deep learning is promising for increasing the performance of NLP and sentiment analysis models (Al-Moslmi et al. 2017 ; Kumar and Garg 2019 ; Kumar and Jaiswal 2020 ; Socher et al. 2013 ), the selected papers only discuss deep learning to a limited extent. The papers that discuss deep learning algorithms are recent papers published in 2018 and 2019, which stresses that sentiment analysis is a timely research subject and that the state-of-the-art is evolving rapidly. Figure 3 shows the year-wise distribution of selected articles. Except for one study from 2013, all selected studies are published in 2017, 2018, and 2019.
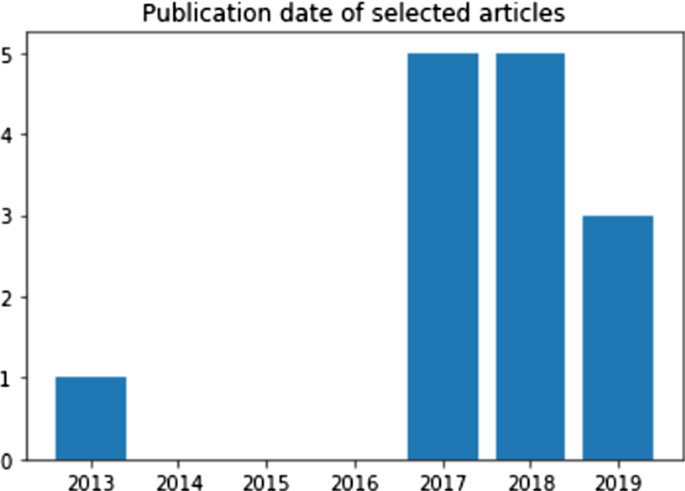
Publication dates of the selected articles
4.3 RQ3 “What domains have been addressed in the adopted data sets?”
Datasets for sentiment analysis are typically user-generated textual content. The text differs a lot depending on the domain and platform that the content is derived from. For example, social media data is usually very subjective and full of informal speech, whereas news article websites are mostly objective and formally written. Twitter data is limited to a certain number of characters and contains hashtags and references, whereas product review websites take a specific product into account and describe this in-depth. ML models trained on a specific domain provide poor performance when tested on a dataset from a different domain. Different domains have different language use and, therefore, require different methods for analysis. Table 14 depicts the domains of the adopted datasets per study. Checkmarks indicate that datasets from the domain are explicitly mentioned in the referred article.
Social media data is the most widely used source of data. This data is usually easy to obtain through APIs. Especially, tweets are popular because they are relatively similar in format (e.g., a limited number of characters). Twitter has an API where tweets can be scraped on specific subjects, time range, hashtags, etc. Tweets contain worldwide real-time information on entities. Furthermore, scraped tweets contain information about the location, number of retweets, number of likes, and much more. Some reviewed articles focus specifically on Twitter data (Ahmed Ibrahim and Salim 2013 ; Kumar and Jaiswal 2020 ). Other social media platforms like Facebook and Tumblr are also used for sentiment analysis.
Reviews of products, hotels, and movies are also commonly used for text classification models. Reviews are usually combined with a star rating (i.e., label), which makes them suitable for machine learning models. Star ratings indicate polarity. This way, no labor-intensive manual labeling process or predefined lexicon is required.
4.4 RQ4 “What are the challenges and open problems with respect to sentiment analysis?”
All of the 14 selected papers include challenges and open problems in sentiment analysis. Table 15 shows the challenges that are explicitly described in the papers. These challenges are categorized and sorted by the number of selected papers that explicitly mention the challenge.
Domain dependency is a well-known challenge in sentiment analysis; most of the models that we build are dependent on the domain it was built in. Linguistic dependency is the second most stated and well-known challenge that originates from the same deeper problem. Specific text corpora per domain or language need to be available for the optimal performance of the ML model. Some studies investigate multi-lingual or multi-domain models.
Most papers use English text corpora. Spanish and Chinese are the second most used languages in sentiment analysis. Limited literature is available in other languages. Some studies attempted to create a multi-language model (Al-Moslmi et al. 2017 ), but this is still a challenging task (Kumar and Garg 2019 ; Qazi et al. 2017 ). Multi-lingual systems are an interesting topic for further research.
Deep learning is a promising but complex technique where syntactic structures and word order can be retained. Deep learning still poses some challenges and is not widely researched in the selected articles. Opinion spam or fake review detection is a prominent issue in sentiment analysis where the internet has become an integral part of life, and false information spreads just as fast as accurate information on the web (Vosoughi et al. 2018 ). Another major challenge is the multi-class classification. In general, more output classes in a classifier reduce the performance (Al-Moslmi et al. 2017 ). Multiple polarity classes and multiple classes of emotions (Madhala et al. 2018 ) have been shown to dramatically reduce the performance of the model.
Further challenges are incomplete information, implicit language, typos, slang, and all other kinds of inconsistencies in language use. Combining text with corresponding pictures, audio, and video is also challenging.
5 Discussion
The goal of this study is to present an overview of the current application of machine learning models and corresponding challenges in sentiment analysis. This is done by critically analyzing the selected secondary studies and extracting the relevant data considering the predefined research questions. This tertiary study follows the guidelines proposed by Kitchenham and Charters ( 2007 ) for conducting systematic literature reviews. The study initially selected 16 secondary studies. After the quality assessment, 14 secondary papers remained for data extraction. The research methodology is transparent and designed in such a way that it can be reproduced by other researchers. Like any secondary study, there are also some limitations to this tertiary study.
The SLRs included in this study have their specific research focus on sentiment analysis. Even though the methodology of the 14 secondary studies is similar, the documentation of techniques and methods differs a lot. Besides that, some SLR papers are more comprehensive than others. This made the data extraction process harder and prone to mistakes. Another limitation concerns the selection process. The criteria for inclusion are restricted to SLR and SMS papers. Some other studies chose to include non-systematic literature reviews as well to complement results, but we did not include traditional survey papers because they do not systematically synthesize the papers in a field.
The first threat to validity is related to the inclusion criteria for methods in research questions. Checkmarks in the tables of RQ2, RQ3, and RQ4 are placed when something is explicitly mentioned in the referred paper. The included secondary studies have their specific research focus with different sentiment analysis tasks and corresponding machine learning approaches. For instance, Kasmuri and Basiron ( 2017 ) discuss subjectivity classification, which typically uses different approaches compared to other sentiment analysis tasks. This variation in research focus influences the checkmarks placed in the tables.
Another threat related to inclusion criteria is that some secondary studies have more included papers than others. For example, Kumar and Sharma ( 2017 ) included 194 primary studies, where Mite-Baidal et al. ( 2018 ) only included eight primary studies. It is likely that papers with a higher number of included primary articles mention more different techniques and challenges, and thus, more checkmarks are placed in the tables compared to papers with a lower number of primary articles included.
Lastly, this tertiary study only considers the selected secondary papers and does not consult the primary papers selected by the secondary papers. If any mistakes are made in the documentation of results in the secondary articles, these mistakes will be reflected in this study as well.
6 Conclusion and future work
This study provides the results of a tertiary study on sentiment analysis methods whereby we aimed to highlight the adopted features (input/output), adopted approaches, the adopted data sets, and the challenges with respect to sentiment analysis. The answers to the research questions were derived based on in-depth secondary studies.
A different number of input and output features could be identified. Interestingly, some features appeared to be described in all the secondary studies, while other features were more specific to a selected set of secondary studies. The results further indicate that sentiment analysis has been applied in various domains, among which social media is the most popular. Also, the study showed that different domains require the use of different techniques.
There also seems a trend towards using more complex deep learning techniques, since they can detect more complex patterns in text and perform particularly well with larger datasets. In some use cases like, for example, advertisement, slight improvements in performance that can be obtained through deep learning can have a great impact. However, it should be noted that traditional machine learning models are less computationally expensive and perform sufficiently well for sentiment analysis tasks. They are widely praised for their performance and efficiency.
This study showed that the most prominent challenges in sentiment analysis are domain and language dependency. Specific text corpora are required for differing languages and domains of interest. Attempts for cross-domain and multi-lingual sentiment analysis models have been made, but this challenging task should be explored further. Other prominent challenges are opinion spam detection and the application of deep learning for sentiment analysis tasks. Overall, the study shows that sentiment analysis is a timely and important research topic. The adoption of a tertiary study showed the additional value that could not be derived from each of the secondary studies.
The following future directions and challenges have also been mainly discussed in deep learning-based survey papers: New datasets are required for more challenging tasks, common sense knowledge must be modeled, interpretable deep learning-based models must be developed, and memory-efficient models are required (Minaee et al. 2020 ). Domain adaptation techniques are needed, multi-lingual applications should be addressed, technical requirements such as a huge amount of labeled data requirement must be considered, and linguistic complications must be investigated (Do et al. 2019 ). Popular deep learning techniques such as deep reinforcement learning and generative adversarial networks can be evaluated to solve some challenging tasks, advantages of the BERT algorithm can be considered, language structures (e.g., slangs) can be investigated in detail, dynamic sentiment analysis can be studied, and sentiment analysis for heterogeneous data can be implemented (Habimana et al. 2020a ). Dependency trees in recursive neural networks can be investigated, domain adaptation can be analyzed in detail, and linguistic-subjective phenomena (e.g., irony and sarcasm) can be studied (Rojas-Barahona 2016 ). Different applications of sentiment analysis (e.g., medical domain and security screening of employees) can be implemented, and transfer learning approaches can be analyzed for sentiment classification (Yadav and Vishwakarma 2019). Comparative studies should be extended with new approaches and new datasets, and also hybrid approaches to reduce computational cost and improve performance must be developed (Dang et al. 2020 ).
Abid F, Li C, Alam M (2020) Multi-source social media data sentiment analysis using bidirectional recurrent convolutional neural networks. Comput Commun 157:102–115
Article Google Scholar
Ahmad M, Aftab S, Ali I, Hameed N (2017) Hybrid tools and techniques for sentiment analysis: a review 8(4):7
Ahmad M, Aftab S, Bashir MS, Hameed N (2018) Sentiment analysis using SVM: a systematic literature review. Int J Adv Comput Sci Appl 9(2):182–188 ( Scopus )
Google Scholar
Ahmed Ibrahim M, Salim N (2013) Opinion analysis for twitter and Arabic tweets: a systematic literature review. J Theor Appl Inf Technol 56(3):338–348 ( Scopus )
Alam M, Abid F, Guangpei C, Yunrong LV (2020) Social media sentiment analysis through parallel dilated convolutional neural network for smart city applications. Comput Commun 154:129–137
Alarifi A, Tolba A, Al-Makhadmeh Z, Said W (2020) A big data approach to sentiment analysis using greedy feature selection with cat swarm optimization-based long short-term memory neural networks. J Supercomput 76(6):4414–4429
Alexandridis G, Michalakis K, Aliprantis J, Polydoras P, Tsantilas P, Caridakis G (2020) A deep learning approach to aspect-based sentiment prediction. In: IFIP International conference on artificial ıntelligence applications and ınnovations. Springer, Cham, pp 397–408
Al-Moslmi T, Omar N, Abdullah S, Albared M (2017) Approaches to cross-domain sentiment analysis: a systematic literature review. IEEE Access 5:16173–16192 ( Scopus )
Almotairi, M. (2009) A framework for successful CRM implementation. In: European and mediterranean conference on information systems. pp 1–14
Aslam A, Qamar U, Saqib P, Ayesha R, Qadeer A (2020) A novel framework for sentiment analysis using deep learning. In: 2020 22nd International conference on advanced communication technology (ICACT). IEEE, pp 525–529
Basiri ME, Abdar M, Cifci MA, Nemati S, Acharya UR (2020) A novel method for sentiment classification of drug reviews using fusion of deep and machine learning techniques. Knowl-Based Syst 198:1–19
Becker JU, Greve G, Albers S (2009) The impact of technological and organizational implementation of CRM on customer acquisition, maintenance, and retention. Int J Res Mark 26(3):207–215
Behera RN, Manan R, Dash S (2016) Ensemble based hybrid machine learning approach for sentiment classification-a review. Int J Comput Appl 146(6):31–36
Beseiso M, Elmousalami H (2020) Subword attentive model for arabic sentiment analysis: a deep learning approach. ACM Trans Asian Low-Resour Lang Inf Process (TALLIP) 19(2):1–17
Blum A, Mitchell T (1998) Combining labeled and unlabeled data with co-training. Proc Eleventh Ann Conf Comput Learn Theory COLT’ 98:92–100
Article MathSciNet Google Scholar
Bondielli A, Marcelloni F (2019) A survey on fake news and rumour detection techniques. Inf Sci 497:38–55
Budgen D, Brereton P, Drummond S, Williams N (2018) Reporting systematic reviews: some lessons from a tertiary study. Inf Softw Technol 95:62–74
Cadavid H, Andrikopoulos V, Avgeriou P (2020) Architecting systems of systems: a tertiary study. Inf Softw Technol 118(106202):1–18
Cai Y, Huang Q, Lin Z, Xu J, Chen Z, Li Q (2020) Recurrent neural network with pooling operation and attention mechanism for sentiment analysis: a multi-task learning approach. Knowl-Based Syst 203(105856):1–12
Cambria E (2016) Affective computing and sentiment analysis. IEEE Intell Syst 31(2):102–107
Cambria E, Hussain A, Havasi C, Eckl C (2009) Common sense computing: from the society of mind to digital intuition and beyond. In: European workshop on biometrics and ıdentity management. Springer, Berlin, Heidelberg, pp 252–259
Cambria E, Poria S, Gelbukh A, Thelwall M (2017) Sentiment analysis is a big suitcase. IEEE Intell Syst 32(6):74–80
Cambria E, Li Y, Xing FZ, Poria S, Kwok K (2020) SenticNet 6: ensemble application of symbolic and subsymbolic AI for sentiment analysis. In: Proceedings of the 29th ACM International conference on ınformation & knowledge management. pp 105–114
Can EF, Ezen-Can A., & Can, F. (2018). Multi-lingual sentiment analysis: an RNN-based framework for limited data. In: Proceedings of ACM SIGIR 2018 workshop on learning from limited or noisy data, Ann Arbor
Catal C, Mishra D (2013) Test case prioritization: a systematic mapping study. Softw Qual J 21(3):445–478
Chandra Y, Jana A (2020) Sentiment analysis using machine learning and deep learning. In: 2020 7th International conference on computing for sustainable global development (INDIACom). IEEE, pp. 1–4
Chapelle O, Zien A (2005) Semi-supervised classification by low density separation. In: AISTATS vol 2005, pp 57–64
Che S, Li X (2020) HCI with DEEP learning for sentiment analysis of corporate social responsibility report. Curr Psychol. https://doi.org/10.1007/s12144-020-00789-y
Chen IJ, Popovich K (2003) Understanding customer relationship management (CRM). Bus Process Manag J 9(5):672–688. https://doi.org/10.1108/14637150310496758
Chen L, Chen G, Wang F (2015) Recommender systems based on user reviews: the state of the art. User Model User-Adap Inter 25(2):99–154. https://doi.org/10.1007/s11257-015-9155-5
Chen H, Sun M, Tu C, Lin Y, Liu Z (2016) Neural sentiment classification with user and product attention. In: Proceedings of the 2016 conference on empirical methods in natural language processing. pp 1650–1659
Chen P, Sun Z, Bing L, Yang W (2017) Recurrent attention network on memory for aspect sentiment analysis. In: Proceedings of the 2017 conference on empirical methods in natural language processing. pp 452–461
Chen H, Liu J, Lv Y, Li MH, Liu M, Zheng Q (2018) Semi-supervised clue fusion for spammer detection in Sina Weibo. Inf Fusion 44:22–32. https://doi.org/10.1016/j.inffus.2017.11.002
Cheng Y, Yao L, Xiang G, Zhang G, Tang T, Zhong L (2020) Text sentiment orientation analysis based on multi-channel CNN and bidirectional GRU with attention mechanism. IEEE Access 8:134964–134975
Choi Y, Cardie C (2008) Learning with compositional semantics as structural inference for subsentential sentiment analysis. In: Proceedings of the 2008 conference on empirical methods in natural language processing. pp 793–801
Colón-Ruiz C, Segura-Bedmar I (2020) Comparing deep learning architectures for sentiment analysis on drug reviews. J Biomed Inform 110(103539):1–11
Crawford M, Khoshgoftaar TM, Prusa JD, Richter AN, Al Najada H (2015) Survey of review spam detection using machine learning techniques. J Big Data 2(1):23. https://doi.org/10.1186/s40537-015-0029-9
Cruzes DS, Dybå T (2011) Research synthesis in software engineering: a tertiary study. Inf Softw Technol 53(5):440–455
Curcio K, Santana R, Reinehr S, Malucelli A (2019) Usability in agile software development: a tertiary study. Comput Stand Interfaces 64:61–77. https://doi.org/10.1016/j.csi.2018.12.003
Da Silva NFF, Coletta LFS, Hruschka ER, Hruschka ER Jr (2016a) Using unsupervised information to improve semi-supervised tweet sentiment classification. Inf Sci 355:348–365. https://doi.org/10.1016/j.ins.2016.02.002
Dang NC, Moreno-García MN, De la Prieta F (2020) Sentiment analysis based on deep learning: a comparative study. Electronics 9(3):483
Dashtipour K, Gogate M, Li J, Jiang F, Kong B, Hussain A (2020) A hybrid persian sentiment analysis framework: Integrating dependency grammar based rules and deep neural networks. Neurocomputing 380:1–10
Da’u A, Salim N, Rabiu I, Osman A (2020a) Recommendation system exploiting aspect-based opinion mining with deep learning method. Inf Sci 512:1279–1292
Da’u A, Salim N, Rabiu I, Osman A (2020b) Weighted aspect-based opinion mining using deep learning for recommender system. Expert Syst Appl 140(112871):1–12
De Oliveira Lima T, Colaco Junior M, Nunes MASN (2018) Mining on line general opinions about sustainability of hotels: a systematic literature mapping. In: Gervasi O, Murgante B, Misra S, Stankova E, Torre CM, Rocha AMAC, Taniar D, Apduhan BO, Tarantino E, Ryu Y (eds) Computational science and ıts applications–ICCSA 2018. Springer, New York, pp 558–574
Chapter Google Scholar
Dessí D, Dragoni M, Fenu G, Marras M, Recupero DR (2020) Deep learning adaptation with word embeddings for sentiment analysis on online course reviews. deep learning-based approaches for sentiment analysis. Springer, Singapore, pp 57–83
Devlin J, Chang MW, Lee K, Toutanova K (2019) BERT: Pre-training of deep bidirectional transformers for language understanding. In: Proceedings of the 2019 conference of the north american chapter of the association for computational linguistics: human language technologies vol 1 (Long and Short Papers). pp 4171–4186
Dietterich TG (2002) Machine learning for sequential data: a review. In: Caelli T, Amin A, Duin RPW, de Ridder D, Kamel M (eds) Structural, syntactic, and statistical pattern recognition. Springer, Berlin Heidelberg, pp 15–30
Do HH, Prasad PWC, Maag A, Alsadoon A (2019) Deep learning for aspect-based sentiment analysis: a comparative review. Expert Syst Appl 118:272–299
Dong M, Li Y, Tang X, Xu J, Bi S, Cai Y (2020a) Variable convolution and pooling convolutional neural network for text sentiment classification. IEEE Access 8:16174–16186
Dong Y, Fu Y, Wang L, Chen Y, Dong Y, Li J (2020b) A sentiment analysis method of capsule network based on BiLSTM. IEEE Access 8:37014–37020
Duan J, Luo B, Zeng J (2020) Semi-supervised Learning with generative model for sentiment classification of stock messages. Expert Syst Appl 158(113540):1–9
Ebrahimi M, Yazdavar AH, Sheth A (2017) Challenges of sentiment analysis for dynamic events. IEEE Intell Syst 32(5):70–75
Elmuti D, Jia H, Gray D (2009) Customer relationship management strategic application and organizational effectiveness: An empirical investigation. J Strateg Mark 17(1):75–96. https://doi.org/10.1080/09652540802619301
Filatova, E. (2012). Irony and sarcasm: corpus generation and analysis using crowdsourcing. In: Lrec, pp 392–398
Gan C, Wang L, Zhang Z, Wang Z (2020a) Sparse attention based separable dilated convolutional neural network for targeted sentiment analysis. Knowl-Based Syst 188(104827):1–10
Gan C, Wang L, Zhang Z (2020b) Multi-entity sentiment analysis using self-attention based hierarchical dilated convolutional neural network. Future Gener Comput Syst 112:116–125
Genc-Nayebi N, Abran A (2017) A systematic literature review: opinion mining studies from mobile app store user reviews. J Syst Softw 125:207–219. https://doi.org/10.1016/j.jss.2016.11.027
Ghorbani M, Bahaghighat M, Xin Q, Özen F (2020) ConvLSTMConv network: a deep learning approach for sentiment analysis in cloud computing. J Cloud Comput 9(1):1–12
Gieseke F, Airola A, pahikkala T, Oliver K (2012) Sparse quasi-newton optimization for semi-supervised support vector machines. Proceedings of the 1st ınternational conference on pattern recognition applications and methods 45–54. https://doi.org/ https://doi.org/10.5220/0003755300450054
Giménez M, Palanca J, Botti V (2020) Semantic-based padding in convolutional neural networks for improving the performance in natural language processing. a case of study in sentiment analysis. Neurocomputing 378:315–323
Gneiser MS (2010) Value-Based CRM. Bus Inf Syst Eng 2(2):95–103. https://doi.org/10.1007/s12599-010-0095-7
Goldberg AB, Zhu X (2006) Seeing stars when there aren’t many stars: graph-based semi-supervised learning for sentiment categorization. In: Proceedings of textgraphs: the first workshop on graph based methods for natural language processing. pp 45–52
Goulão M, Amaral V, Mernik M (2016) Quality in model-driven engineering: a tertiary study. Softw Qual J 24(3):601–633. https://doi.org/10.1007/s11219-016-9324-8
Gu T, Xu G, Luo J (2020) Sentiment analysis via deep multichannel neural networks with variational information bottleneck. IEEE Access 8:121014–121021
Gupta R, Sahu S, Espy-Wilson C, Narayanan S (2018) Semi-supervised and transfer learning approaches for low resource sentiment classification. In: 2018 IEEE International conference on acoustics, speech and signal processing (ICASSP). IEEE, pp 5109–5113
Habimana O, Li Y, Li R, Gu X, Yu G (2020a) Sentiment analysis using deep learning approaches: an overview. Sci China Inf Sci 63(1):1–36
Habimana O, Li Y, Li R, Gu X, Yan W (2020b) Attentive convolutional gated recurrent network: a contextual model to sentiment analysis. Int J Mach Learn Cybern 11:2637–2651
Hameed Z, Garcia-Zapirain B (2020) Sentiment classification using a single-layered BiLSTM model. IEEE Access 8:73992–74001
Han Y, Liu Y, Jin Z (2020a) Sentiment analysis via semi-supervised learning: a model based on dynamic threshold and multi-classifiers. Neural Comput Appl 32(9):5117–5129
Han Y, Liu M, Jing W (2020b) Aspect-level drug reviews sentiment analysis based on double BiGRU and knowledge transfer. IEEE Access 8:21314–21325
Haralabopoulos G, Anagnostopoulos I, McAuley D (2020) Ensemble deep learning for multilabel binary classification of user-generated content. Algorithms 13(4):83
Hassan R, Islam MR (2019) Detection of fake online reviews using semi-supervised and supervised learning. In: 2019 International conference on electrical, computer and communication engineering (ECCE). pp 1–5. https://doi.org/ https://doi.org/10.1109/ECACE.2019.8679186
Hemmatian F, Sohrabi MK (2017) A survey on classification techniques for opinion mining and sentiment analysis. Artif Intell Rev 52(3):1495–1545. https://doi.org/10.1007/s10462-017-9599-6
Huang M, Xie H, Rao Y, Feng J, Wang FL (2020b) Sentiment strength detection with a context-dependent lexicon-based convolutional neural network. Inf Sci 520:389–399
Huang F, Wei K, Weng J, Li Z (2020a) Attention-based modality-gated networks for image-text sentiment analysis. ACM Trans Multimed Comput Commun Appl (TOMM) 16(3):1–19
Huang M, Xie H, Rao Y, Liu Y, Poon LK, Wang FL (2020c) Lexicon-based sentiment convolutional neural networks for online review analysis. IEEE Trans Affect Comput (Early Access), 1–1
Hung BT (2020) Domain-specific versus general-purpose word representations in sentiment analysis for deep learning models. Frontiers in ıntelligent computing: theory and applications. Springer, Singapore, pp 252–264
Hung BT (2020) Integrating sentiment analysis in recommender systems. Reliability and statistical computing. Springer, Cham, pp 127–137
Hussain A, Cambria E (2018) Semi-supervised learning for big social data analysis. Neurocomputing 275:1662–1673
Ishaya T, Folarin M (2012) A service oriented approach to business intelligence in telecoms industry. Telemat Inform 29(3):273–285. https://doi.org/10.1016/j.tele.2012.01.004
Ji C, Wu H (2020) Cascade architecture with rhetoric long short-term memory for complex sentence sentiment analysis. Neurocomputing 405:161–172
Jia Z, Bai X, Pang S (2020) Hierarchical gated deep memory network with position-aware for aspect-based sentiment analysis. IEEE Access 8:136340–136347
Jiang T, Wang J, Liu Z, Ling Y (2020) Fusion-extraction network for multimodal sentiment analysis. Pacific-Asia conference on knowledge discovery and data mining. Springer, Cham, pp 785–797
Jin N, Wu J, Ma X, Yan K, Mo Y (2020) Multi-task learning model based on multi-scale CNN and LSTM for sentiment classification. IEEE Access 8:77060–77072
Josiassen A, Assaf AG, Cvelbar LK (2014) CRM and the bottom line: do all CRM dimensions affect firm performance? Int J Hosp Manag 36:130–136. https://doi.org/10.1016/j.ijhm.2013.08.005
Kabra A, Shrawne S (2020) Location-wise news headlines classification and sentiment analysis: a deep learning approach. International conference on ıntelligent computing and smart communication 2019. Springer, Singapore, pp 383–391
Kamal A (2013) Subjectivity classification using machine learning techniques for mining feature-opinion pairs from web opinion sources 10(5):191–200
Kamal N, Andrew M, Tom M (2006) Semi-supervised text classification using EM. In: Chapelle O, Scholkopf B, Zien A (eds) Semi-supervised learning. The MIT Press, Cambridge, pp 32–55. https://doi.org/10.7551/mitpress/9780262033589.003.0003
Kansara D, Sawant V (2020) Comparison of traditional machine learning and deep learning approaches for sentiment analysis. Advanced computing technologies and applications. Springer, Singapore, pp 365–377
Karimpour J, Noroozi AA, Alizadeh S (2012) Web spam detection by learning from small labeled samples. Int J Comput Appl 50(21):1–5. https://doi.org/10.5120/7924-0993
Kasmuri E, Basiron H (2017) Subjectivity analysis in opinion mining—a systematic literature review. Int J Adv Soft Comput Appl 9(3):132–159 ( Scopus )
Khan M, Malviya A (2020) Big data approach for sentiment analysis of twitter data using Hadoop framework and deep learning. In: 2020 International conference on emerging trends in ınformation technology and engineering (ic-ETITE). IEEE, pp 1–5
Khedkar S, Shinde S (2020) Deep learning and ensemble approach for praise or complaint classification. Procedia Comput Sci 167:449–458
Khedkar S, Shinde S (2020a) Deep learning-based approach to classify praises or complaints. In: Proceeding of ınternational conference on computational science and applications: ICCSA 2019. Springer, New York, p 391
Kim Y (2014) Convolutional neural networks for sentence classification. In: Proceedings of the 2014 conference on empirical methods in natural language processing (EMNLP). pp 1746–1751
Kim H-S, Kim Y-G (2009) A CRM performance measurement framework: Its development process and application. Ind Mark Manag 38(4):477–489. https://doi.org/10.1016/j.indmarman.2008.04.008
Kiran R, Kumar P, Bhasker B (2020) OSLCFit (organic simultaneous LSTM and CNN fit): a novel deep learning based solution for sentiment polarity classification of reviews. Expert Syst Appl 157(113488):1–12
Kitchenham B (2004) Procedures for performing systematic reviews, vol 33. Keele University, Keele, UK, pp 1–26
Kitchenham B, Charters S (2007) Guidelines for performing systematic literature reviews in software engineering version 2.3. Engineering, 45(4ve), p 1051
Kitchenham BA, Dyba T, Jorgensen M (2004) Evidence-based software engineering. In: Proceedings 26th ınternational conference on software engineering. IEEE, pp 273–281
Kitchenham B, Pretorius R, Budgen D, Pearl Brereton O, Turner M, Niazi M, Linkman S (2010) Systematic literature reviews in software engineering–a tertiary study. Inf Softw Technol 52(8):792–805. https://doi.org/10.1016/j.infsof.2010.03.006
Kitchenham BA, Budgen D, Brereton OP (2010b) The value of mapping studies–a participant-observer case study. In: 14th international conference on evaluation and assessment in software engineering (ease). pp 1–9
Kitchenham BA, Budgen D, Pearl Brereton O (2011) Using mapping studies as the basis for further research–a participant-observer case study. Inf Softw Technol 53(6):638–651. https://doi.org/10.1016/j.infsof.2010.12.011
Koksal O, Tekinerdogan B (2017) Feature-driven domain analysis of session layer protocols of internet of things. IEEE Int Congr Internet Things (ICIOT) 2017:105–112. https://doi.org/10.1109/IEEE.ICIOT.2017.19
Krouska A, Troussas C, Virvou M (2020) Deep learning for twitter sentiment analysis: the effect of pre-trained word embedding. Machine learning paradigms. Springer, Cham, pp 111–124
Kula S, Choraś M, Kozik R, Ksieniewicz P, Woźniak M (2020) Sentiment analysis for fake news detection by means of neural networks. International conference on computational science. Springer, Cham, pp 653–666
Kumar V (2010) Customer relationship management. In: Wiley ınternational encyclopedia of marketing. American Cancer Society, Georgia. https://onlinelibrary.wiley.com/doi/abs/ https://doi.org/10.1002/9781444316568.wiem01015
Kumar A, Garg G (2019) Systematic literature review on context-based sentiment analysis in social multimedia. Multimed Tools Appl 79:15349–15380
Kumar R, Garg S (2020) Aspect-based sentiment analysis using deep learning convolutional neural network. Information and communication technology for sustainable development. Springer, Singapore, pp 43–52
Kumar A, Jaiswal A (2020) Systematic literature review of sentiment analysis on Twitter using soft computing techniques. Concurr Comput Pract Exp 32(1):e5107
Kumar NS, Malarvizhi N (2020) Bi-directional LSTM–CNN combined method for sentiment analysis in part of speech tagging (PoS). Int J Speech Technol 23:373–380
Kumar V, Reinartz W (2016) Creating enduring customer value. J Mark 80(6):36–68. https://doi.org/10.1509/jm.15.0414
Kumar A, Sebastian TM (2012) Sentiment analysis: a perspective on its past, present and future. Int J Intell Syst Appl 4(10):1–14. https://doi.org/10.5815/ijisa.2012.10.01
Kumar A, Sharan A (2020) Deep learning-based frameworks for aspect-based sentiment analysis. Deep learning-based approaches for sentiment analysis. Springer, Singapore, pp 139–158
Kumar A, Sharma A (2017) Systematic literature review on opinion mining of big data for government intelligence. Webology 14(2):6–47 ( Scopus )
Kumar R, Pannu HS, Malhi AK (2020) Aspect-based sentiment analysis using deep networks and stochastic optimization. Neural Comput Appl 32(8):3221–3235
Kumar A, Srinivasan K, Cheng WH, Zomaya AY (2020) Hybrid context enriched deep learning model for fine-grained sentiment analysis in textual and visual semiotic modality social data. Inf Process Manag 57(1):102141
Ładyżyński P, Żbikowski K, Gawrysiak P (2019) Direct marketing campaigns in retail banking with the use of deep learning and random forests. Expert Syst Appl 134:28–35. https://doi.org/10.1016/j.eswa.2019.05.020
Lai Y, Zhang L, Han D, Zhou R, Wang G (2020) Fine-grained emotion classification of Chinese microblogs based on graph convolution networks. World Wide Web 23(5):2771–2787
Li G, Liu F (2014) Sentiment analysis based on clustering: a framework in improving accuracy and recognizing neutral opinions. Appl Intell 40(3):441–452. https://doi.org/10.1007/s10489-013-0463-3
Li F, Huang M, Yang Y, Zhu X (2011) Learning to identify review spam. In: Proceedings of the twenty-second international joint conference on artificial ıntelligence-volume Vol 3. pp 2488–2493
Li W, Zhu L, Shi Y, Guo K, Zheng Y (2020) User reviews: Sentiment analysis using lexicon integrated two-channel CNN-LSTM family models. Appl Soft Comput 94(106435):1–11
Li L, Goh TT, Jin D (2020) How textual quality of online reviews affect classification performance: a case of deep learning sentiment analysis. Neural Comput Appl 32(9):4387–4415
Li D, Rzepka R, Ptaszynski M, Araki K (2020) HEMOS: a novel deep learning-based fine-grained humor detecting method for sentiment analysis of social media. Inf Process Manag 57(6):102290
Lim WL, Ho CC, Ting CY (2020) Tweet sentiment analysis using deep learning with nearby locations as features. Computational science and technology. Springer, Singapore, pp 291–299
Lin Y, Li J, Yang L, Xu K, Lin H (2020) Sentiment analysis with comparison enhanced deep neural network. IEEE Access 8:78378–78384
Ling M, Chen Q, Sun Q, Jia Y (2020) Hybrid neural network for sina weibo sentiment analysis. IEEE Trans Comput Soc Syst 7(4):983–990
Liu B (2020) Text sentiment analysis based on CBOW model and deep learning in big data environment. J Ambient Intell Humaniz Comput 11(2):451–458
Liu Q, Mukaidani H (2020) Effective-target representation via LSTM with attention for aspect-level sentiment analysis. In: 2020 ınternational conference on artificial ıntelligence in ınformation and communication (ICAIIC). IEEE, pp 336–340
Liu N, Shen B (2020) Aspect-based sentiment analysis with gated alternate neural network. Knowl-Based Syst 188(105010):1–14
Liu N, Shen B (2020) ReMemNN: a novel memory neural network for powerful interaction in aspect-based sentiment analysis. Neurocomputing 395:66–77
Lou Y, Zhang Y, Li F, Qian T, Ji D (2020) Emoji-based sentiment analysis using attention networks. ACM Trans Asian Low-Resour Lang Inf Process (TALLIP) 19(5):1–13
Lu Q, Zhu Z, Zhang D, Wu W, Guo Q (2020) Interactive rule attention network for aspect-level sentiment analysis. IEEE Access 8:52505–52516
Lu G, Zhao X, Yin J, Yang W, Li B (2020) Multi-task learning using variational auto-encoder for sentiment classification. Pattern Recogn Lett 132:115–122
Luo J, Huang S, Wang R (2020) A fine-grained sentiment analysis of online guest reviews of economy hotels in China. J Hosp Mark Manag 1–25
Ma Y, Peng H, Cambria E (2018) Targeted aspect-based sentiment analysis via embedding commonsense knowledge into an attentive LSTM. In; Proceedings of the AAAI conference on artificial ıntelligence vol 32. pp 5876–5883
Madhala P, Jussila J, Aramo-Immonen H, Suominen A (2018) Systematic literature review on customer emotions in social media. In: ECSM 2018 5th European conference on social media. Academic Conferences and publishing limited, South Oxfordshire, pp 154–162
Maglogiannis IG (ed) (2007) Emerging artificial intelligence applications in computer engineering: real word ai systems with applications in ehealth, hci, information retrieval and pervasive technologies, vol 160. Ios Press, Amsterdam
Mahmood Z, Safder I, Nawab RMA, Bukhari F, Nawaz R, Alfakeeh AS, Hassan SU (2020) Deep sentiments in Roman Urdu text using recurrent convolutional neural network model. Inf Process Manag 57(4):102233
Majumder N, Poria S, Peng H, Chhaya N, Cambria E, Gelbukh A (2019) Sentiment and sarcasm classification with multitask learning. IEEE Intell Syst 34(3):38–43
Meškelė D, Frasincar F (2020) ALDONAr: a hybrid solution for sentence-level aspect-based sentiment analysis using a lexicalized domain ontology and a regularized neural attention model. Inf Process Manag 57(3):102211
Minaee S, Azimi E, Abdolrashidi A (2019) Deep-sentiment: sentiment analysis using ensemble of cnn and bi-lstm models. http://arxiv.org/abs/arXiv:1904.04206
Minaee S, Kalchbrenner N, Cambria E, Nikzad N, Chenaghlu M, Gao J (2020) Deep learning based text classification: a comprehensive review 1(1):1–43. http://arxiv.org/abs/arXiv:2004.03705
Mite-Baidal K, Delgado-Vera C, Solís-Avilés E, Espinoza AH, Ortiz-Zambrano J, Varela-Tapia E (2018) Sentiment analysis in education domain: a systematic literature review. Commun Comput Inf Sci 883:285–297. https://doi.org/10.1007/978-3-030-00940-3_21 ( Scopus )
Naseem U, Razzak I, Musial K, Imran M (2020) Transformer based deep intelligent contextual embedding for twitter sentiment analysis. Future Gener Comput Syst 113:58–69
Nguyen TH, Shirai K (2015) Topic modeling based sentiment analysis on social media for stock market prediction. In: Proceedings of the 53rd annual meeting of the association for computational linguistics and the 7th ınternational joint conference on natural language processing vol 1. pp 1354–1364. https://doi.org/ https://doi.org/10.3115/v1/P15-1131
Nigam K, Ghani R (2000) Analyzing the effectiveness and applicability of co-training. In: Proceedings of the ninth ınternational conference on ınformation and knowledge management—CIKM '00. pp 86–93. https://doi.org/ https://doi.org/10.1145/354756.354805
Nurdiani I, Börstler J, Fricker SA (2016) The impacts of agile and lean practices on project constraints: a tertiary study. J Syst Softw 119:162–183
Ombabi AH, Ouarda W, Alimi AM (2020) Deep learning CNN–LSTM framework for Arabic sentiment analysis using textual information shared in social networks. Soc Netw Anal Min 10(1):1–13
Onan A (2020) Mining opinions from instructor evaluation reviews: a deep learning approach. Comput Appl Eng Edu 28(1):117–138
Onan A (2020a) Sentiment analysis on massive open online course evaluations: A text mining and deep learning approach. Comput Appl Eng Edu 1–18
Onan A (2020b) Sentiment analysis on product reviews based on weighted word embeddings and deep neural networks. Concurr Comput Pract Exp e5909
Ott M, Choi Y, Cardie C, Hancock JT (2011) Finding deceptive opinion spam by any stretch of the imagination. In: Proceedings of the 49th annual meeting of the association for computational linguistics: human language technologies vol 1. pp 309–319
Pan Y, Liang M (2020) Chinese text sentiment analysis based on BI-GRU and self-attention. In: 2020 IEEE 4th ınformation technology, networking, electronic and automation control conference (ITNEC) vol. 1. IEEE, pp 1983–1988
Parimala M, Swarna Priya RM, Praveen Kumar Reddy M, Lal Chowdhary C, Kumar Poluru R, Khan S (2020) Spatiotemporal‐based sentiment analysis on tweets for risk assessment of event using deep learning approach. Softw Pract Exp 1–21
Park HJ, Song M, Shin KS (2020) Deep learning models and datasets for aspect term sentiment classification: implementing holistic recurrent attention on target-dependent memories. Knowl-Based Syst 187(104825):1–15
Patel P, Patel D, Naik C (2020) Sentiment analysis on movie review using deep learning RNN method. Intelligent data engineering and analytics. Springer, Singapore, pp 155–163
Pavlinek M, Podgorelec V (2017) Text classification method based on self-training and LDA topic models. Expert Syst Appl 80:83–93. https://doi.org/10.1016/j.eswa.2017.03.020
Payne A, Frow P (2005) A strategic framework for customer relationship management. J Mark 69(4):167–176. https://doi.org/10.1509/jmkg.2005.69.4.167
Peng M, Zhang Q, Jiang Y, Huang X (2018) Cross-domain sentiment classification with target domain specific ınformation. In: Proceedings of the 56th Annual Meeting of the Association for Computational Linguistics vol 1. pp 2505–2513. https://doi.org/ https://doi.org/10.18653/v1/P18-1233
Peng H, Xu L, Bing L, Huang F, Lu W, Si L (2020) Knowing what, how and why: a near complete solution for aspect-based sentiment analysis. In AAAI. pp 8600–8607
Pergola G, Gui L, He Y (2019) TDAM: a topic-dependent attention model for sentiment analysis. Inf Process Manag 56(6):102084
Petersen K, Feldt R, Mujtaba S, Mattsson M (2008) Systematic mapping studies in software engineering. In: 12th international conference on evaluation and assessment in software engineering (EASE) 12. pp 1–10
Phillips-Wren G, Hoskisson A (2015) An analytical journey towards big data. J Decis Syst 24(1):87–102. https://doi.org/10.1080/12460125.2015.994333
Poria S, Chaturvedi I, Cambria E, Bisio F (2016) Sentic LDA: ımproving on LDA with semantic similarity for aspect-based sentiment analysis. In: 2016 international joint conference on neural networks (IJCNN). IEEE, pp 4465–4473
Poria S, Majumder N, Hazarika D, Cambria E, Gelbukh A, Hussain A (2018) Multimodal sentiment analysis: addressing key issues and setting up the baselines. IEEE Intell Syst 33(6):17–25
Poria S, Hazarika D, Majumder N, Mihalcea R (2020) Beneath the tip of the ıceberg: current challenges and new directions in sentiment analysis research. http://arxiv.org/abs/arXiv:2005.00357
Portugal I, Alencar P, Cowan D (2018) The use of machine learning algorithms in recommender systems: a systematic review. Expert Syst Appl 97:205–227. https://doi.org/10.1016/j.eswa.2017.12.020
Pozzi FA, Fersini E, Messina E, Liu B (2017) Challenges of sentiment analysis in social networks: an overview. In: Pozzi FA, Fersini E, Messina E, Liu B (eds) Sentiment analysis in social networks. Morgan Kaufmann, Burlington, pp 1–11
Pröllochs N, Feuerriegel S, Lutz B, Neumann D (2020) Negation scope detection for sentiment analysis: a reinforcement learning framework for replicating human interpretations. Inf Sci 536:205–221
Qazi A, Raj RG, Hardaker G, Standing C (2017) A systematic literature review on opinion types and sentiment analysis techniques: tasks and challenges. Internet Res 27(3):608–630. https://doi.org/10.1108/IntR-04-2016-0086 ( Scopus )
Qiu G, Liu B, Bu J, Chen C (2009) Expanding domain sentiment lexicon through double propagation. In: IJCAI vol 9. pp 1199–1204
Raatikainen M, Tiihonen J, Männistö T (2019) Software product lines and variability modeling: a tertiary study. J Syst Softw 149:485–510. https://doi.org/10.1016/j.jss.2018.12.027
Rababah K, Mohd H, Ibrahim H (2011) A unified definition of CRM towards the successful adoption and implementation. Acad Res Int 1(1):220–228
Rambocas M, Pacheco BG (2018) Online sentiment analysis in marketing research: a review. J Res Interact Mark 12(2):146–163. https://doi.org/10.1108/JRIM-05-2017-0030
Rao AVSR, Ranjana P (2020) Deep learning method to ıdentify the demographic attribute to enhance effectiveness of sentiment analysis. Innovations in computer science and engineering. Springer, Singapore, pp 275–285
Ravi K, Ravi V (2015) A survey on opinion mining and sentiment analysis: tasks, approaches and applications. Knowl-Based Syst 89:14–46. https://doi.org/10.1016/j.knosys.2015.06.015
Ray P, Chakrabarti A (2020) A mixed approach of deep learning method and rule-based method to improve aspect level sentiment analysis. Appl Comput Inform. https://doi.org/10.1016/j.aci.2019.02.002
Reddy YCAP, Viswanath P, Eswara Reddy B (2018) Semi-supervised learning: a brief review. Int J Eng Technol 7(18):81
Reichheld FF, Schefter P (2000) E-loyalty: your secret weapon on the web. Harv Bus Rev 78(4):105–113
Reinartz W, Krafft M, Hoyer WD (2004) The customer relationship management process: its measurement and impact on performance. J Mark Res 41(3):293–305. https://doi.org/10.1509/jmkr.41.3.293.35991
Ren Z, Zeng G, Chen L, Zhang Q, Zhang C, Pan D (2020) A lexicon-enhanced attention network for aspect-level sentiment analysis. IEEE Access 8:93464–93471
Ren F, Feng L, Xiao D, Cai M, Cheng S (2020) DNet: a lightweight and efficient model for aspect based sentiment analysis. Expert Syst Appl 151(113393):1–10
Ren L, Xu B, Lin H, Liu X, Yang L (2020) Sarcasm detection with sentiment semantics enhanced multi-level memory network. Neurocomputing 401:320–326
Rios N, de Mendonça Neto MG, Spínola RO (2018) A tertiary study on technical debt: types, management strategies, research trends, and base information for practitioners. Inf Softw Technol 102:117–145. https://doi.org/10.1016/j.infsof.2018.05.010
Rodrigues Chagas BN, Nogueira Viana JA, Reinhold O, Lobato F, Jacob AFL, Alt R (2018) Current applications of machine learning techniques in CRM: a literature review and practical implications. IEEE/WIC/ACM Int Conf Web Intell (WI) 2018:452–458. https://doi.org/10.1109/WI.2018.00-53
Rojas-Barahona LM (2016) Deep learning for sentiment analysis. Lang Linguist Compass 10(12):701–719
Rout JK, Dalmia A, Choo K-KR, Bakshi S, Jena SK (2017) Revisiting semi-supervised learning for online deceptive review detection. IEEE Access 5:1319–1327. https://doi.org/10.1109/ACCESS.2017.2655032
Rygielski C, Wang J-C, Yen DC (2002) Data mining techniques for customer relationship management. Technol Soc 24(4):483–502. https://doi.org/10.1016/S0160-791X(02)00038-6
Sabbeh SF (2018) Machine-learning techniques for customer retention: A comparative study. Int J Adv Comput Sci Appl 9(2):273–281
Sadr H, Pedram MM, Teshnehlab M (2020) Multi-view deep network: a deep model based on learning features from heterogeneous neural networks for sentiment analysis. IEEE Access 8:86984–86997
Salah Z, Al-Ghuwairi A-RF, Baarah A, Aloqaily A, Qadoumi B, Alhayek M, Alhijawi B (2019) A systematic review on opinion mining and sentiment analysis in social media. Int J Bus Inf Syst 31(4):530–554. https://doi.org/10.1504/IJBIS.2019.101585 ( Scopus )
Salur MU, Aydin I (2020) A novel hybrid deep learning model for sentiment classification. IEEE Access 8:58080–58093
Sangeetha K, Prabha D (2020) Sentiment analysis of student feedback using multi-head attention fusion model of word and context embedding for LSTM. J Ambient Intell Humaniz Comput. https://doi.org/10.1007/s12652-020-01791-9
Sankar H, Subramaniyaswamy V, Vijayakumar V, Arun Kumar S, Logesh R, Umamakeswari AJSP (2020) Intelligent sentiment analysis approach using edge computing-based deep learning technique. Softw Pract Exp 50(5):645–657
Sawant SS, Prabukumar M (2018) A review on graph-based semi-supervised learning methods for hyperspectral image classification. Egypt J Remote Sens Space Sci 23(2):243–248. https://doi.org/10.1016/j.ejrs.2018.11.001
Schouten K, Frasincar F (2016) Survey on aspect-level sentiment analysis. IEEE Trans Knowl Data Eng 28(3):813–830. https://doi.org/10.1109/TKDE.2015.2485209
Seo S, Kim C, Kim H, Mo K, Kang P (2020) Comparative study of deep learning-based sentiment classification. IEEE Access 8:6861–6875
Shakeel MH, Karim A (2020) Adapting deep learning for sentiment classification of code-switched informal short text. In: Proceedings of the 35th annual ACM symposium on applied computing. pp 903–906
Sharma SS, Dutta G (2018) Polarity determination of movie reviews: a systematic literature review. Int J of Innov Knowl Concepts 6:12
Shayaa S, Jaafar NI, Bahri S, Sulaiman A, Seuk Wai P, Wai Chung Y, Piprani AZ, Al-Garadi MA (2018) Sentiment analysis of big data: Methods, applications, and open challenges. IEEE Access 6:37807–37827. https://doi.org/10.1109/ACCESS.2018.2851311 ( Scopus )
Shirani-Mehr H (2014) Applications of deep learning to sentiment analysis of movie reviews. Tech Report 1–8
Shuang K, Yang Q, Loo J, Li R, Gu M (2020) Feature distillation network for aspect-based sentiment analysis. Inf Fusion 61:13–23
Silva NFFD, Coletta LFS, Hruschka ER (2016) A survey and comparative study of tweet sentiment analysis via semi-supervised learning. ACM Comput Surv 49(1):1–26. https://doi.org/10.1145/2932708
Singh PK, Sharma S, Paul S (2020) Identifying hidden sentiment in text using deep neural network. In 2nd ınternational conference on data, engineering and applications (IDEA). IEEE, pp 1–5
Socher R, Perelygin A, Wu J, Chuang J, Manning CD, Ng AY, Potts C (2013) Recursive deep models for semantic compositionality over a sentiment treebank. In: Proceedings of the 2013 conference on empirical methods in natural language processing. pp 1631–1642
Studiawan H, Sohel F, Payne C (2020) Sentiment analysis in a forensic timeline with deep learning. IEEE Access 8:60664–60675
Su YJ, Hu WC, Jiang JH, Su RY (2020) A novel LMAEB-CNN model for Chinese microblog sentiment analysis. J Supercomput 76:9127–9141
Sun X, He J (2020) A novel approach to generate a large scale of supervised data for short text sentiment analysis. Multimed Tools Appl 79(9):5439–5459
Taboada M, Brooke J, Tofiloski M, Voll K, Stede M (2011) Lexicon-based methods for sentiment analysis. Comput Linguist 37(2):267–307
Tang, D., Wei, F., Yang, N., Zhou, M., Liu, T., & Qin, B. (2014, June). Learning sentiment-specific word embedding for twitter sentiment classification. In Proceedings of the 52nd Annual Meeting of the Association for Computational Linguistics vol 1. pp 1555–1565
Tao J, Fang X (2020) Toward multi-label sentiment analysis: a transfer learning based approach. J Big Data 7(1):1–26
Thet TT, Na J-C, Khoo CSG (2010) Aspect-based sentiment analysis of movie reviews on discussion boards. J Inf Sci 36(6):823–848. https://doi.org/10.1177/0165551510388123
Tran TU, Hoang HTT, Huynh HX (2020) Bidirectional ındependently long short-term memory and conditional random field ıntegrated model for aspect extraction in sentiment analysis. Frontiers in ıntelligent computing: theory and applications. Springer, Singapore, pp 131–140
Tsai C, Hu Y, Hung C, Hsu Y (2013) A comparative study of hybrid machine learning techniques for customer lifetime value prediction. Kybernetes 42(3):357–370. https://doi.org/10.1108/03684921311323626
Ullah MA, Marium SM, Begum SA, Dipa NS (2020) An algorithm and method for sentiment analysis using the text and emoticon. ICT Express 6(4):357–360
Usama M, Ahmad B, Song E, Hossain MS, Alrashoud M, Muhammad G (2020) Attention-based sentiment analysis using convolutional and recurrent neural network. Future Gener Comput Syst 113:571–578
Valdivia A, Luzón MV, Herrera F (2017) Sentiment analysis in tripadvisor. IEEE Intell Syst 32(4):72–77
Valdivia A, Martínez-Cámara E, Chaturvedi I, Luzón MV, Cambria E, Ong YS, Herrera F (2020) What do people think about this monument? understanding negative reviews via deep learning, clustering and descriptive rules. J Ambient Intell Humaniz Comput 11(1):39–52
Vechtomova O (2017) Disambiguating context-dependent polarity of words: an information retrieval approach. Inf Process Manag 53(5):1062–1079
Venkatakrishnan S, Kaushik A, Verma JK (2020) Sentiment analysis on google play store data using deep learning. Applications of machine learning. Springer, Singapore, pp 15–30
Verhoef PC, Venkatesan R, McAlister L, Malthouse EC, Krafft M, Ganesan S (2010) CRM in data-rich multichannel retailing environments: a review and future research directions. J Interact Mark 24(2):121–137. https://doi.org/10.1016/j.intmar.2010.02.009
Verner JM, Brereton OP, Kitchenham BA, Turner M, Niazi M (2014) Risks and risk mitigation in global software development: a tertiary study. Inf Softw Technol 56(1):54–78
Vinodhini G, Chandrasekaran RM (2012) Sentiment analysis and opinion mining: a survey. Int J 2(6):282–292
Vosoughi S, Roy D, Aral S (2018) The spread of true and false news online. Science 359(6380):1146–1151
Vyas V, Uma V (2019) Approaches to sentiment analysis on product reviews. Sentiment analysis and knowledge discovery in contemporary business. IGI Global, Pennsylvania, pp 15–30
Wadawadagi R, Pagi V (2020) Sentiment analysis with deep neural networks: comparative study and performance assessment. Artif Intell Rev 53:6155–6195
Wang G, Sun J, Ma J, Xu K, Gu J (2014) Sentiment classification: the contribution of ensemble learning. Decis Support Syst 57:77–93. https://doi.org/10.1016/j.dss.2013.08.002
Wang Y, Huang M, Zhu X, Zhao L (2016) Attention-based LSTM for aspect-level sentiment classification. In: Proceedings of the 2016 conference on empirical methods in natural language processing. pp 606–615
Wang S, Zhu Y, Gao W, Cao M, Li M (2020) Emotion-semantic-enhanced bidirectional LSTM with multi-head attention mechanism for microblog sentiment analysis. Information 11(5):280
Wehrmann J, Becker W, Cagnini HE, Barros RC (2017) A character-based convolutional neural network for language-agnostic Twitter sentiment analysis. In: 2017 International joint conference on neural networks (IJCNN). IEEE, pp 2384–2391
Wilcox PA, Gurău C (2003) Business modelling with UML: the implementation of CRM systems for online retailing. J Retail Consum Serv 10(3):181–191. https://doi.org/10.1016/S0969-6989(03)00004-3
Winer RS (2001) A framework for customer relationship management. Calif Manag Rev 43(4):89–105. https://doi.org/10.2307/41166102
Wu C, Wu F, Wu S, Yuan Z, Liu J, Huang Y (2019) Semi-supervised dimensional sentiment analysis with variational autoencoder. Knowl-Based Syst 165:30–39
Xi D, Zhuang F, Zhou G, Cheng X, Lin F, He Q (2020) Domain adaptation with category attention network for deep sentiment analysis. In: Proceedings of the web conference 2020. pp 3133–3139
Xia Y, Cambria E, Hussain A, Zhao H (2015) Word polarity disambiguation using bayesian model and opinion-level features. Cognit Comput 7(3):369–380
Xu W, Tan Y (2019) Semi-supervised target-oriented sentiment classification. Neurocomputing 337:120–128
Xu G, Meng Y, Qiu X, Yu Z, Wu X (2019) Sentiment analysis of comment texts based on BiLSTM. IEEE Access 7:51522–51532
Yadav A, Vishwakarma DK (2020) Sentiment analysis using deep learning architectures: a review. Artif Intell Rev 53(6):4335–4385
Yadav A, Vishwakarma DK (2020) A deep learning architecture of RA-DLNet for visual sentiment analysis. Multimed Syst 26:431–451
Yang L, Li Y, Wang J, Sherratt RS (2020) Sentiment analysis for E-commerce product reviews in chinese based on sentiment lexicon and deep learning. IEEE Access 8:23522–23530
Yao F, Wang Y (2020) Domain-specific sentiment analysis for tweets during hurricanes (DSSA-H): a domain-adversarial neural-network-based approach. Comput Environ Urban Syst 83(101522):1–14
Yarowsky D (1995) Unsupervised word sense disambiguation rivaling supervised methods. In: Proceedings of the 33rd annual meeting on association for computational linguistics. pp189–196. https://doi.org/ https://doi.org/10.3115/981658.981684
Yildirim S (2020) Comparing deep neural networks to traditional models for sentiment analysis in Turkish language. Deep learning-based approaches for sentiment analysis. Springer, Singapore, pp 311–319
Zerbino P, Aloini D, Dulmin R, Mininno V (2018) Big Data-enabled customer relationship management: a holistic approach. Inf Process Manag 54(5):818–846. https://doi.org/10.1016/j.ipm.2017.10.005
Zhang L, Wang S, Liu B (2018) Deep learning for sentiment analysis: a survey. Wiley Interdiscip Rev Data Min Knowl Discov 8(4):e1253
Zhang B, Li X, Xu X, Leung KC, Chen Z, Ye Y (2020) Knowledge guided capsule attention network for aspect-based sentiment analysis. IEEE/ACM Trans Audio Speech Lang Process 28:2538–2551
Zhang S, Xu X, Pang Y, Han J (2020) Multi-layer attention based CNN for target-dependent sentiment classification. Neural Process Lett 51(3):2089–2103
Zhao P, Hou L, Wu O (2020) Modeling sentiment dependencies with graph convolutional networks for aspect-level sentiment classification. Knowl-Based Syst 193(105443):1–10
Zhou J, Huang JX, Hu QV, He L (2020) Is position important? deep multi-task learning for aspect-based sentiment analysis. Appl Intell 50:3367–3378
Zhu X, Ghahramani Z (2002) Learning from labeled and unlabeled data with label propagation. Technical report CMU-CALD-02–107, Carnegie Mellon University. 8
Zhu X, Yin S, Chen Z (2020) Attention based BiLSTM-MCNN for sentiment analysis. In: 2020 IEEE 5th international conference on cloud computing and big data analytics (ICCCBDA). IEEE, pp 170–174
Zuo E, Zhao H, Chen B, Chen Q (2020) Context-specific heterogeneous graph convolutional network for implicit sentiment analysis. IEEE Access 8:37967–37975
Download references
Open Access funding provided by the Qatar National Library.
Author information
Authors and affiliations.
Information Technology Group, Wageningen University & Research, Wageningen, The Netherlands
Alexander Ligthart & Bedir Tekinerdogan
Department of Computer Science & Engineering, Qatar University, Doha, Qatar
Cagatay Catal
You can also search for this author in PubMed Google Scholar
Corresponding author
Correspondence to Cagatay Catal .
Ethics declarations
Conflict of interest.
The authors have no conflicts of interest to declare that are relevant to the content of this article.
Additional information
Publisher's note.
Springer Nature remains neutral with regard to jurisdictional claims in published maps and institutional affiliations.
Rights and permissions
Open Access This article is licensed under a Creative Commons Attribution 4.0 International License, which permits use, sharing, adaptation, distribution and reproduction in any medium or format, as long as you give appropriate credit to the original author(s) and the source, provide a link to the Creative Commons licence, and indicate if changes were made. The images or other third party material in this article are included in the article's Creative Commons licence, unless indicated otherwise in a credit line to the material. If material is not included in the article's Creative Commons licence and your intended use is not permitted by statutory regulation or exceeds the permitted use, you will need to obtain permission directly from the copyright holder. To view a copy of this licence, visit http://creativecommons.org/licenses/by/4.0/ .
Reprints and permissions
About this article
Ligthart, A., Catal, C. & Tekinerdogan, B. Systematic reviews in sentiment analysis: a tertiary study. Artif Intell Rev 54 , 4997–5053 (2021). https://doi.org/10.1007/s10462-021-09973-3
Download citation
Accepted : 08 February 2021
Published : 03 March 2021
Issue Date : October 2021
DOI : https://doi.org/10.1007/s10462-021-09973-3
Share this article
Anyone you share the following link with will be able to read this content:
Sorry, a shareable link is not currently available for this article.
Provided by the Springer Nature SharedIt content-sharing initiative
- Sentiment analysis
- Tertiary study
- Systematic literature review
- Sentiment classification
- Find a journal
- Publish with us
- Track your research

Systematic Reviews & Meta-Analysis
- Identifying Your Research Question
- Developing Your Protocol
- Conducting Your Search
- Screening & Selection
- Data Extraction & Appraisal
- Meta-Analyses
- Writing the Systematic Review
- Suggested Readings
What is a Systematic Review?
A systematic review attempts to identify, appraise and synthesize all available relevant evidence that to answer a specific, focus research question. Researchers conducting systematic reviews use standardized, systematic methods and pre-selected eligibility criteria to reduce the risk of bias in identifying, selecting and analyzing relevant studies.
Prepared by the Cochrane Consumers and Communication Group, La Trobe University and generously support by Cochrane Australia. Written by Jack Nunn and Sophie Hill.
What to Consider Before Starting a Systematic Review
Traditional literature reviews differ from systematic reviews in many ways, and especially on how they are conducted and time commitment required.
When systematic reviews SHOULD be done :
- If you have a clearly defined research question with established inclusion and exclusion criteria
- To test a specific hypothesis to ensure a manageable results set
- When there is a large body of primary research on your specific research question
- When you have team of at least three people assembled to help conduct the systematic review
- When a transparent search methodology and replicability are needed
- When an existing systematic review is outdated (consider updating the existing review)
- When no ongoing or existing systematic review addresses your research question
When Systematic reviews SHOULD NOT be done:
- Systematic reviews without a clear and specified research question with details such as populations, interventions, exposures, and outcomes, will produce large and inconsistent search results to screen, and offer no consistent way to assess and synthesize findings from the studies that are identified.
- Systematic reviews are a lot of work. Including creating the protocol, building and running a quality search, collecting all the papers, evaluating the studies that meet the inclusion criteria and extracting and analyzing the summary data, a well done review can require dozens to hundreds of hours of work that can span several months.
- All systematic review guidelines recommend that at least two subject experts screen the studies identified in the search. The first round of screening can consume 1 hour per screener for every 100-200 records. A systematic review is a team effort.
Systematic Review Steps & Timeline
Systematic reviews require time and effort to complete. It should not be expected to be complete a systematic review in a matter of months. An average time to complete a systematic review is between 12-18 months. The Cochrane Handbook for Systematic Reviews of Interventions suggests the following timeline to complete a review:
Cochrane Handbook for Systematic Reviews of Interventions Version 5.1.0 [updated March 2011]. The Cochrane Collaboration, 2011. Available from https://training.cochrane.org/handbook/current .
Assembling Your Team
A systematic review can't be done alone. You should carefully consider all of the expertise you will need to define your research question, search for evidence, appraise/grade the evidence, and potentially complete a statistical meta-analysis of the data. A recommended systematic review team would consist of the following:
- 2 or more subject experts on the topic of the study. These experts will screen and appraised the evidence, and a third may be necessary to settle any disagreements.
- A library or expert skillful enough to perform complex literature search.
- A statistician if a meta-analysis is to be performed.
- Next: Identifying Your Research Question >>
- Last Updated: Jun 5, 2024 8:45 AM
- URL: https://libguides.chapman.edu/systematic_reviews
Loading metrics
Open Access
Peer-reviewed
Research Article
Functional connectivity changes in the brain of adolescents with internet addiction: A systematic literature review of imaging studies
Roles Conceptualization, Data curation, Formal analysis, Investigation, Methodology, Project administration, Software, Validation, Visualization, Writing – original draft, Writing – review & editing
Affiliation Child and Adolescent Mental Health, Department of Brain Sciences, Great Ormond Street Institute of Child Health, University College London, London, United Kingdom
Roles Conceptualization, Supervision, Validation, Writing – review & editing
* E-mail: [email protected]
Affiliation Behavioural Brain Sciences Unit, Population Policy Practice Programme, Great Ormond Street Institute of Child Health, University College London, London, United Kingdom

- Max L. Y. Chang,
- Irene O. Lee
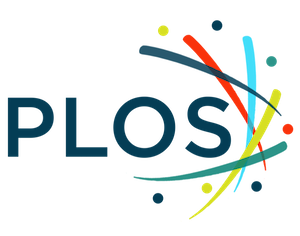
- Published: June 4, 2024
- https://doi.org/10.1371/journal.pmen.0000022
- Peer Review
- Reader Comments
Internet usage has seen a stark global rise over the last few decades, particularly among adolescents and young people, who have also been diagnosed increasingly with internet addiction (IA). IA impacts several neural networks that influence an adolescent’s behaviour and development. This article issued a literature review on the resting-state and task-based functional magnetic resonance imaging (fMRI) studies to inspect the consequences of IA on the functional connectivity (FC) in the adolescent brain and its subsequent effects on their behaviour and development. A systematic search was conducted from two databases, PubMed and PsycINFO, to select eligible articles according to the inclusion and exclusion criteria. Eligibility criteria was especially stringent regarding the adolescent age range (10–19) and formal diagnosis of IA. Bias and quality of individual studies were evaluated. The fMRI results from 12 articles demonstrated that the effects of IA were seen throughout multiple neural networks: a mix of increases/decreases in FC in the default mode network; an overall decrease in FC in the executive control network; and no clear increase or decrease in FC within the salience network and reward pathway. The FC changes led to addictive behaviour and tendencies in adolescents. The subsequent behavioural changes are associated with the mechanisms relating to the areas of cognitive control, reward valuation, motor coordination, and the developing adolescent brain. Our results presented the FC alterations in numerous brain regions of adolescents with IA leading to the behavioural and developmental changes. Research on this topic had a low frequency with adolescent samples and were primarily produced in Asian countries. Future research studies of comparing results from Western adolescent samples provide more insight on therapeutic intervention.
Citation: Chang MLY, Lee IO (2024) Functional connectivity changes in the brain of adolescents with internet addiction: A systematic literature review of imaging studies. PLOS Ment Health 1(1): e0000022. https://doi.org/10.1371/journal.pmen.0000022
Editor: Kizito Omona, Uganda Martyrs University, UGANDA
Received: December 29, 2023; Accepted: March 18, 2024; Published: June 4, 2024
Copyright: © 2024 Chang, Lee. This is an open access article distributed under the terms of the Creative Commons Attribution License , which permits unrestricted use, distribution, and reproduction in any medium, provided the original author and source are credited.
Data Availability: All relevant data are within the paper and its Supporting information files.
Funding: The authors received no specific funding for this work.
Competing interests: The authors have declared that no competing interests exist.
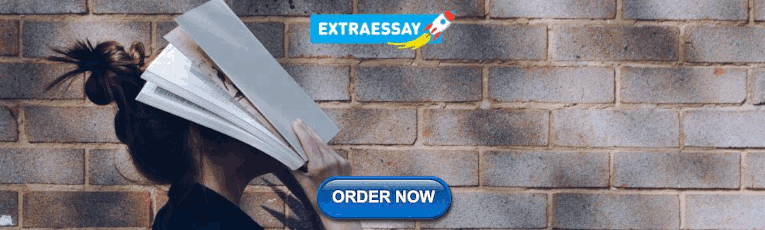
Introduction
The behavioural addiction brought on by excessive internet use has become a rising source of concern [ 1 ] since the last decade. According to clinical studies, individuals with Internet Addiction (IA) or Internet Gaming Disorder (IGD) may have a range of biopsychosocial effects and is classified as an impulse-control disorder owing to its resemblance to pathological gambling and substance addiction [ 2 , 3 ]. IA has been defined by researchers as a person’s inability to resist the urge to use the internet, which has negative effects on their psychological well-being as well as their social, academic, and professional lives [ 4 ]. The symptoms can have serious physical and interpersonal repercussions and are linked to mood modification, salience, tolerance, impulsivity, and conflict [ 5 ]. In severe circumstances, people may experience severe pain in their bodies or health issues like carpal tunnel syndrome, dry eyes, irregular eating and disrupted sleep [ 6 ]. Additionally, IA is significantly linked to comorbidities with other psychiatric disorders [ 7 ].
Stevens et al (2021) reviewed 53 studies including 17 countries and reported the global prevalence of IA was 3.05% [ 8 ]. Asian countries had a higher prevalence (5.1%) than European countries (2.7%) [ 8 ]. Strikingly, adolescents and young adults had a global IGD prevalence rate of 9.9% which matches previous literature that reported historically higher prevalence among adolescent populations compared to adults [ 8 , 9 ]. Over 80% of adolescent population in the UK, the USA, and Asia have direct access to the internet [ 10 ]. Children and adolescents frequently spend more time on media (possibly 7 hours and 22 minutes per day) than at school or sleeping [ 11 ]. Developing nations have also shown a sharp rise in teenage internet usage despite having lower internet penetration rates [ 10 ]. Concerns regarding the possible harms that overt internet use could do to adolescents and their development have arisen because of this surge, especially the significant impacts by the COVID-19 pandemic [ 12 ]. The growing prevalence and neurocognitive consequences of IA among adolescents makes this population a vital area of study [ 13 ].
Adolescence is a crucial developmental stage during which people go through significant changes in their biology, cognition, and personalities [ 14 ]. Adolescents’ emotional-behavioural functioning is hyperactivated, which creates risk of psychopathological vulnerability [ 15 ]. In accordance with clinical study results [ 16 ], this emotional hyperactivity is supported by a high level of neuronal plasticity. This plasticity enables teenagers to adapt to the numerous physical and emotional changes that occur during puberty as well as develop communication techniques and gain independence [ 16 ]. However, the strong neuronal plasticity is also associated with risk-taking and sensation seeking [ 17 ] which may lead to IA.
Despite the fact that the precise neuronal mechanisms underlying IA are still largely unclear, functional magnetic resonance imaging (fMRI) method has been used by scientists as an important framework to examine the neuropathological changes occurring in IA, particularly in the form of functional connectivity (FC) [ 18 ]. fMRI research study has shown that IA alters both the functional and structural makeup of the brain [ 3 ].
We hypothesise that IA has widespread neurological alteration effects rather than being limited to a few specific brain regions. Further hypothesis holds that according to these alterations of FC between the brain regions or certain neural networks, adolescents with IA would experience behavioural changes. An investigation of these domains could be useful for creating better procedures and standards as well as minimising the negative effects of overt internet use. This literature review aims to summarise and analyse the evidence of various imaging studies that have investigated the effects of IA on the FC in adolescents. This will be addressed through two research questions:
- How does internet addiction affect the functional connectivity in the adolescent brain?
- How is adolescent behaviour and development impacted by functional connectivity changes due to internet addiction?
The review protocol was conducted in line with the Preferred Reporting Items for Systematic Reviews and Meta-Analyses (PRISMA) guidelines (see S1 Checklist ).
Search strategy and selection process
A systematic search was conducted up until April 2023 from two sources of database, PubMed and PsycINFO, using a range of terms relevant to the title and research questions (see full list of search terms in S1 Appendix ). All the searched articles can be accessed in the S1 Data . The eligible articles were selected according to the inclusion and exclusion criteria. Inclusion criteria used for the present review were: (i) participants in the studies with clinical diagnosis of IA; (ii) participants between the ages of 10 and 19; (iii) imaging research investigations; (iv) works published between January 2013 and April 2023; (v) written in English language; (vi) peer-reviewed papers and (vii) full text. The numbers of articles excluded due to not meeting the inclusion criteria are shown in Fig 1 . Each study’s title and abstract were screened for eligibility.
- PPT PowerPoint slide
- PNG larger image
- TIFF original image
https://doi.org/10.1371/journal.pmen.0000022.g001
Quality appraisal
Full texts of all potentially relevant studies were then retrieved and further appraised for eligibility. Furthermore, articles were critically appraised based on the GRADE (Grading of Recommendations, Assessment, Development, and Evaluations) framework to evaluate the individual study for both quality and bias. The subsequent quality levels were then appraised to each article and listed as either low, moderate, or high.
Data collection process
Data that satisfied the inclusion requirements was entered into an excel sheet for data extraction and further selection. An article’s author, publication year, country, age range, participant sample size, sex, area of interest, measures, outcome and article quality were all included in the data extraction spreadsheet. Studies looking at FC, for instance, were grouped, while studies looking at FC in specific area were further divided into sub-groups.
Data synthesis and analysis
Articles were classified according to their location in the brain as well as the network or pathway they were a part of to create a coherent narrative between the selected studies. Conclusions concerning various research trends relevant to particular groupings were drawn from these groupings and subgroupings. To maintain the offered information in a prominent manner, these assertions were entered into the data extraction excel spreadsheet.
With the search performed on the selected databases, 238 articles in total were identified (see Fig 1 ). 15 duplicated articles were eliminated, and another 6 items were removed for various other reasons. Title and abstract screening eliminated 184 articles because they were not in English (number of article, n, = 7), did not include imaging components (n = 47), had adult participants (n = 53), did not have a clinical diagnosis of IA (n = 19), did not address FC in the brain (n = 20), and were published outside the desired timeframe (n = 38). A further 21 papers were eliminated for failing to meet inclusion requirements after the remaining 33 articles underwent full-text eligibility screening. A total of 12 papers were deemed eligible for this review analysis.
Characteristics of the included studies, as depicted in the data extraction sheet in Table 1 provide information of the author(s), publication year, sample size, study location, age range, gender, area of interest, outcome, measures used and quality appraisal. Most of the studies in this review utilised resting state functional magnetic resonance imaging techniques (n = 7), with several studies demonstrating task-based fMRI procedures (n = 3), and the remaining studies utilising whole-brain imaging measures (n = 2). The studies were all conducted in Asiatic countries, specifically coming from China (8), Korea (3), and Indonesia (1). Sample sizes ranged from 12 to 31 participants with most of the imaging studies having comparable sample sizes. Majority of the studies included a mix of male and female participants (n = 8) with several studies having a male only participant pool (n = 3). All except one of the mixed gender studies had a majority male participant pool. One study did not disclose their data on the gender demographics of their experiment. Study years ranged from 2013–2022, with 2 studies in 2013, 3 studies in 2014, 3 studies in 2015, 1 study in 2017, 1 study in 2020, 1 study in 2021, and 1 study in 2022.
https://doi.org/10.1371/journal.pmen.0000022.t001
(1) How does internet addiction affect the functional connectivity in the adolescent brain?
The included studies were organised according to the brain region or network that they were observing. The specific networks affected by IA were the default mode network, executive control system, salience network and reward pathway. These networks are vital components of adolescent behaviour and development [ 31 ]. The studies in each section were then grouped into subsections according to their specific brain regions within their network.
Default mode network (DMN)/reward network.
Out of the 12 studies, 3 have specifically studied the default mode network (DMN), and 3 observed whole-brain FC that partially included components of the DMN. The effect of IA on the various centres of the DMN was not unilaterally the same. The findings illustrate a complex mix of increases and decreases in FC depending on the specific region in the DMN (see Table 2 and Fig 2 ). The alteration of FC in posterior cingulate cortex (PCC) in the DMN was the most frequently reported area in adolescents with IA, which involved in attentional processes [ 32 ], but Lee et al. (2020) additionally found alterations of FC in other brain regions, such as anterior insula cortex, a node in the DMN that controls the integration of motivational and cognitive processes [ 20 ].
https://doi.org/10.1371/journal.pmen.0000022.g002
The overall changes of functional connectivity in the brain network including default mode network (DMN), executive control network (ECN), salience network (SN) and reward network. IA = Internet Addiction, FC = Functional Connectivity.
https://doi.org/10.1371/journal.pmen.0000022.t002
Ding et al. (2013) revealed altered FC in the cerebellum, the middle temporal gyrus, and the medial prefrontal cortex (mPFC) [ 22 ]. They found that the bilateral inferior parietal lobule, left superior parietal lobule, and right inferior temporal gyrus had decreased FC, while the bilateral posterior lobe of the cerebellum and the medial temporal gyrus had increased FC [ 22 ]. The right middle temporal gyrus was found to have 111 cluster voxels (t = 3.52, p<0.05) and the right inferior parietal lobule was found to have 324 cluster voxels (t = -4.07, p<0.05) with an extent threshold of 54 voxels (figures above this threshold are deemed significant) [ 22 ]. Additionally, there was a negative correlation, with 95 cluster voxels (p<0.05) between the FC of the left superior parietal lobule and the PCC with the Chen Internet Addiction Scores (CIAS) which are used to determine the severity of IA [ 22 ]. On the other hand, in regions of the reward system, connection with the PCC was positively connected with CIAS scores [ 22 ]. The most significant was the right praecuneus with 219 cluster voxels (p<0.05) [ 22 ]. Wang et al. (2017) also discovered that adolescents with IA had 33% less FC in the left inferior parietal lobule and 20% less FC in the dorsal mPFC [ 24 ]. A potential connection between the effects of substance use and overt internet use is revealed by the generally decreased FC in these areas of the DMN of teenagers with drug addiction and IA [ 35 ].
The putamen was one of the main regions of reduced FC in adolescents with IA [ 19 ]. The putamen and the insula-operculum demonstrated significant group differences regarding functional connectivity with a cluster size of 251 and an extent threshold of 250 (Z = 3.40, p<0.05) [ 19 ]. The molecular mechanisms behind addiction disorders have been intimately connected to decreased striatal dopaminergic function [ 19 ], making this function crucial.
Executive Control Network (ECN).
5 studies out of 12 have specifically viewed parts of the executive control network (ECN) and 3 studies observed whole-brain FC. The effects of IA on the ECN’s constituent parts were consistent across all the studies examined for this analysis (see Table 2 and Fig 3 ). The results showed a notable decline in all the ECN’s major centres. Li et al. (2014) used fMRI imaging and a behavioural task to study response inhibition in adolescents with IA [ 25 ] and found decreased activation at the striatum and frontal gyrus, particularly a reduction in FC at inferior frontal gyrus, in the IA group compared to controls [ 25 ]. The inferior frontal gyrus showed a reduction in FC in comparison to the controls with a cluster size of 71 (t = 4.18, p<0.05) [ 25 ]. In addition, the frontal-basal ganglia pathways in the adolescents with IA showed little effective connection between areas and increased degrees of response inhibition [ 25 ].
https://doi.org/10.1371/journal.pmen.0000022.g003
Lin et al. (2015) found that adolescents with IA demonstrated disrupted corticostriatal FC compared to controls [ 33 ]. The corticostriatal circuitry experienced decreased connectivity with the caudate, bilateral anterior cingulate cortex (ACC), as well as the striatum and frontal gyrus [ 33 ]. The inferior ventral striatum showed significantly reduced FC with the subcallosal ACC and caudate head with cluster size of 101 (t = -4.64, p<0.05) [ 33 ]. Decreased FC in the caudate implies dysfunction of the corticostriatal-limbic circuitry involved in cognitive and emotional control [ 36 ]. The decrease in FC in both the striatum and frontal gyrus is related to inhibitory control, a common deficit seen with disruptions with the ECN [ 33 ].
The dorsolateral prefrontal cortex (DLPFC), ACC, and right supplementary motor area (SMA) of the prefrontal cortex were all found to have significantly decreased grey matter volume [ 29 ]. In addition, the DLPFC, insula, temporal cortices, as well as significant subcortical regions like the striatum and thalamus, showed decreased FC [ 29 ]. According to Tremblay (2009), the striatum plays a significant role in the processing of rewards, decision-making, and motivation [ 37 ]. Chen et al. (2020) reported that the IA group demonstrated increased impulsivity as well as decreased reaction inhibition using a Stroop colour-word task [ 26 ]. Furthermore, Chen et al. (2020) observed that the left DLPFC and dorsal striatum experienced a negative connection efficiency value, specifically demonstrating that the dorsal striatum activity suppressed the left DLPFC [ 27 ].
Salience network (SN).
Out of the 12 chosen studies, 3 studies specifically looked at the salience network (SN) and 3 studies have observed whole-brain FC. Relative to the DMN and ECN, the findings on the SN were slightly sparser. Despite this, adolescents with IA demonstrated a moderate decrease in FC, as well as other measures like fibre connectivity and cognitive control, when compared to healthy control (see Table 2 and Fig 4 ).
https://doi.org/10.1371/journal.pmen.0000022.g004
Xing et al. (2014) used both dorsal anterior cingulate cortex (dACC) and insula to test FC changes in the SN of adolescents with IA and found decreased structural connectivity in the SN as well as decreased fractional anisotropy (FA) that correlated to behaviour performance in the Stroop colour word-task [ 21 ]. They examined the dACC and insula to determine whether the SN’s disrupted connectivity may be linked to the SN’s disruption of regulation, which would explain the impaired cognitive control seen in adolescents with IA. However, researchers did not find significant FC differences in the SN when compared to the controls [ 21 ]. These results provided evidence for the structural changes in the interconnectivity within SN in adolescents with IA.
Wang et al. (2017) investigated network interactions between the DMN, ECN, SN and reward pathway in IA subjects [ 24 ] (see Fig 5 ), and found 40% reduction of FC between the DMN and specific regions of the SN, such as the insula, in comparison to the controls (p = 0.008) [ 24 ]. The anterior insula and dACC are two areas that are impacted by this altered FC [ 24 ]. This finding supports the idea that IA has similar neurobiological abnormalities with other addictive illnesses, which is in line with a study that discovered disruptive changes in the SN and DMN’s interaction in cocaine addiction [ 38 ]. The insula has also been linked to the intensity of symptoms and has been implicated in the development of IA [ 39 ].
“+” indicates an increase in behaivour; “-”indicates a decrease in behaviour; solid arrows indicate a direct network interaction; and the dotted arrows indicates a reduction in network interaction. This diagram depicts network interactions juxtaposed with engaging in internet related behaviours. Through the neural interactions, the diagram illustrates how the networks inhibit or amplify internet usage and vice versa. Furthermore, it demonstrates how the SN mediates both the DMN and ECN.
https://doi.org/10.1371/journal.pmen.0000022.g005
(2) How is adolescent behaviour and development impacted by functional connectivity changes due to internet addiction?
The findings that IA individuals demonstrate an overall decrease in FC in the DMN is supported by numerous research [ 24 ]. Drug addict populations also exhibited similar decline in FC in the DMN [ 40 ]. The disruption of attentional orientation and self-referential processing for both substance and behavioural addiction was then hypothesised to be caused by DMN anomalies in FC [ 41 ].
In adolescents with IA, decline of FC in the parietal lobule affects visuospatial task-related behaviour [ 22 ], short-term memory [ 42 ], and the ability of controlling attention or restraining motor responses during response inhibition tests [ 42 ]. Cue-induced gaming cravings are influenced by the DMN [ 43 ]. A visual processing area called the praecuneus links gaming cues to internal information [ 22 ]. A meta-analysis found that the posterior cingulate cortex activity of individuals with IA during cue-reactivity tasks was connected with their gaming time [ 44 ], suggesting that excessive gaming may impair DMN function and that individuals with IA exert more cognitive effort to control it. Findings for the behavioural consequences of FC changes in the DMN illustrate its underlying role in regulating impulsivity, self-monitoring, and cognitive control.
Furthermore, Ding et al. (2013) reported an activation of components of the reward pathway, including areas like the nucleus accumbens, praecuneus, SMA, caudate, and thalamus, in connection to the DMN [ 22 ]. The increased FC of the limbic and reward networks have been confirmed to be a major biomarker for IA [ 45 , 46 ]. The increased reinforcement in these networks increases the strength of reward stimuli and makes it more difficult for other networks, namely the ECN, to down-regulate the increased attention [ 29 ] (See Fig 5 ).
Executive control network (ECN).
The numerous IA-affected components in the ECN have a role in a variety of behaviours that are connected to both response inhibition and emotional regulation [ 47 ]. For instance, brain regions like the striatum, which are linked to impulsivity and the reward system, are heavily involved in the act of playing online games [ 47 ]. Online game play activates the striatum, which suppresses the left DLPFC in ECN [ 48 ]. As a result, people with IA may find it difficult to control their want to play online games [ 48 ]. This system thus causes impulsive and protracted gaming conduct, lack of inhibitory control leading to the continued use of internet in an overt manner despite a variety of negative effects, personal distress, and signs of psychological dependence [ 33 ] (See Fig 5 ).
Wang et al. (2017) report that disruptions in cognitive control networks within the ECN are frequently linked to characteristics of substance addiction [ 24 ]. With samples that were addicted to heroin and cocaine, previous studies discovered abnormal FC in the ECN and the PFC [ 49 ]. Electronic gaming is known to promote striatal dopamine release, similar to drug addiction [ 50 ]. According to Drgonova and Walther (2016), it is hypothesised that dopamine could stimulate the reward system of the striatum in the brain, leading to a loss of impulse control and a failure of prefrontal lobe executive inhibitory control [ 51 ]. In the end, IA’s resemblance to drug use disorders may point to vital biomarkers or underlying mechanisms that explain how cognitive control and impulsive behaviour are related.
A task-related fMRI study found that the decrease in FC between the left DLPFC and dorsal striatum was congruent with an increase in impulsivity in adolescents with IA [ 26 ]. The lack of response inhibition from the ECN results in a loss of control over internet usage and a reduced capacity to display goal-directed behaviour [ 33 ]. Previous studies have linked the alteration of the ECN in IA with higher cue reactivity and impaired ability to self-regulate internet specific stimuli [ 52 ].
Salience network (SN)/ other networks.
Xing et al. (2014) investigated the significance of the SN regarding cognitive control in teenagers with IA [ 21 ]. The SN, which is composed of the ACC and insula, has been demonstrated to control dynamic changes in other networks to modify cognitive performance [ 21 ]. The ACC is engaged in conflict monitoring and cognitive control, according to previous neuroimaging research [ 53 ]. The insula is a region that integrates interoceptive states into conscious feelings [ 54 ]. The results from Xing et al. (2014) showed declines in the SN regarding its structural connectivity and fractional anisotropy, even though they did not observe any appreciable change in FC in the IA participants [ 21 ]. Due to the small sample size, the results may have indicated that FC methods are not sensitive enough to detect the significant functional changes [ 21 ]. However, task performance behaviours associated with impaired cognitive control in adolescents with IA were correlated with these findings [ 21 ]. Our comprehension of the SN’s broader function in IA can be enhanced by this relationship.
Research study supports the idea that different psychological issues are caused by the functional reorganisation of expansive brain networks, such that strong association between SN and DMN may provide neurological underpinnings at the system level for the uncontrollable character of internet-using behaviours [ 24 ]. In the study by Wang et al. (2017), the decreased interconnectivity between the SN and DMN, comprising regions such the DLPFC and the insula, suggests that adolescents with IA may struggle to effectively inhibit DMN activity during internally focused processing, leading to poorly managed desires or preoccupations to use the internet [ 24 ] (See Fig 5 ). Subsequently, this may cause a failure to inhibit DMN activity as well as a restriction of ECN functionality [ 55 ]. As a result, the adolescent experiences an increased salience and sensitivity towards internet addicting cues making it difficult to avoid these triggers [ 56 ].
The primary aim of this review was to present a summary of how internet addiction impacts on the functional connectivity of adolescent brain. Subsequently, the influence of IA on the adolescent brain was compartmentalised into three sections: alterations of FC at various brain regions, specific FC relationships, and behavioural/developmental changes. Overall, the specific effects of IA on the adolescent brain were not completely clear, given the variety of FC changes. However, there were overarching behavioural, network and developmental trends that were supported that provided insight on adolescent development.
The first hypothesis that was held about this question was that IA was widespread and would be regionally similar to substance-use and gambling addiction. After conducting a review of the information in the chosen articles, the hypothesis was predictably supported. The regions of the brain affected by IA are widespread and influence multiple networks, mainly DMN, ECN, SN and reward pathway. In the DMN, there was a complex mix of increases and decreases within the network. However, in the ECN, the alterations of FC were more unilaterally decreased, but the findings of SN and reward pathway were not quite clear. Overall, the FC changes within adolescents with IA are very much network specific and lay a solid foundation from which to understand the subsequent behaviour changes that arise from the disorder.
The second hypothesis placed emphasis on the importance of between network interactions and within network interactions in the continuation of IA and the development of its behavioural symptoms. The results from the findings involving the networks, DMN, SN, ECN and reward system, support this hypothesis (see Fig 5 ). Studies confirm the influence of all these neural networks on reward valuation, impulsivity, salience to stimuli, cue reactivity and other changes that alter behaviour towards the internet use. Many of these changes are connected to the inherent nature of the adolescent brain.
There are multiple explanations that underlie the vulnerability of the adolescent brain towards IA related urges. Several of them have to do with the inherent nature and underlying mechanisms of the adolescent brain. Children’s emotional, social, and cognitive capacities grow exponentially during childhood and adolescence [ 57 ]. Early teenagers go through a process called “social reorientation” that is characterised by heightened sensitivity to social cues and peer connections [ 58 ]. Adolescents’ improvements in their social skills coincide with changes in their brains’ anatomical and functional organisation [ 59 ]. Functional hubs exhibit growing connectivity strength [ 60 ], suggesting increased functional integration during development. During this time, the brain’s functional networks change from an anatomically dominant structure to a scattered architecture [ 60 ].
The adolescent brain is very responsive to synaptic reorganisation and experience cues [ 61 ]. As a result, one of the distinguishing traits of the maturation of adolescent brains is the variation in neural network trajectory [ 62 ]. Important weaknesses of the adolescent brain that may explain the neurobiological change brought on by external stimuli are illustrated by features like the functional gaps between networks and the inadequate segregation of networks [ 62 ].
The implications of these findings towards adolescent behaviour are significant. Although the exact changes and mechanisms are not fully clear, the observed changes in functional connectivity have the capacity of influencing several aspects of adolescent development. For example, functional connectivity has been utilised to investigate attachment styles in adolescents [ 63 ]. It was observed that adolescent attachment styles were negatively associated with caudate-prefrontal connectivity, but positively with the putamen-visual area connectivity [ 63 ]. Both named areas were also influenced by the onset of internet addiction, possibly providing a connection between the two. Another study associated neighbourhood/socioeconomic disadvantage with functional connectivity alterations in the DMN and dorsal attention network [ 64 ]. The study also found multivariate brain behaviour relationships between the altered/disadvantaged functional connectivity and mental health and cognition [ 64 ]. This conclusion supports the notion that the functional connectivity alterations observed in IA are associated with specific adolescent behaviours as well as the fact that functional connectivity can be utilised as a platform onto which to compare various neurologic conditions.
Limitations/strengths
There were several limitations that were related to the conduction of the review as well as the data extracted from the articles. Firstly, the study followed a systematic literature review design when analysing the fMRI studies. The data pulled from these imaging studies were namely qualitative and were subject to bias contrasting the quantitative nature of statistical analysis. Components of the study, such as sample sizes, effect sizes, and demographics were not weighted or controlled. The second limitation brought up by a similar review was the lack of a universal consensus of terminology given IA [ 47 ]. Globally, authors writing about this topic use an array of terminology including online gaming addiction, internet addiction, internet gaming disorder, and problematic internet use. Often, authors use multiple terms interchangeably which makes it difficult to depict the subtle similarities and differences between the terms.
Reviewing the explicit limitations in each of the included studies, two major limitations were brought up in many of the articles. One was relating to the cross-sectional nature of the included studies. Due to the inherent qualities of a cross-sectional study, the studies did not provide clear evidence that IA played a causal role towards the development of the adolescent brain. While several biopsychosocial factors mediate these interactions, task-based measures that combine executive functions with imaging results reinforce the assumed connection between the two that is utilised by the papers studying IA. Another limitation regarded the small sample size of the included studies, which averaged to around 20 participants. The small sample size can influence the generalisation of the results as well as the effectiveness of statistical analyses. Ultimately, both included study specific limitations illustrate the need for future studies to clarify the causal relationship between the alterations of FC and the development of IA.
Another vital limitation was the limited number of studies applying imaging techniques for investigations on IA in adolescents were a uniformly Far East collection of studies. The reason for this was because the studies included in this review were the only fMRI studies that were found that adhered to the strict adolescent age restriction. The adolescent age range given by the WHO (10–19 years old) [ 65 ] was strictly followed. It is important to note that a multitude of studies found in the initial search utilised an older adolescent demographic that was slightly higher than the WHO age range and had a mean age that was outside of the limitations. As a result, the results of this review are biased and based on the 12 studies that met the inclusion and exclusion criteria.
Regarding the global nature of the research, although the journals that the studies were published in were all established western journals, the collection of studies were found to all originate from Asian countries, namely China and Korea. Subsequently, it pulls into question if the results and measures from these studies are generalisable towards a western population. As stated previously, Asian countries have a higher prevalence of IA, which may be the reasoning to why the majority of studies are from there [ 8 ]. However, in an additional search including other age groups, it was found that a high majority of all FC studies on IA were done in Asian countries. Interestingly, western papers studying fMRI FC were primarily focused on gambling and substance-use addiction disorders. The western papers on IA were less focused on fMRI FC but more on other components of IA such as sleep, game-genre, and other non-imaging related factors. This demonstrated an overall lack of western fMRI studies on IA. It is important to note that both western and eastern fMRI studies on IA presented an overall lack on children and adolescents in general.
Despite the several limitations, this review provided a clear reflection on the state of the data. The strengths of the review include the strict inclusion/exclusion criteria that filtered through studies and only included ones that contained a purely adolescent sample. As a result, the information presented in this review was specific to the review’s aims. Given the sparse nature of adolescent specific fMRI studies on the FC changes in IA, this review successfully provided a much-needed niche representation of adolescent specific results. Furthermore, the review provided a thorough functional explanation of the DMN, ECN, SN and reward pathway making it accessible to readers new to the topic.
Future directions and implications
Through the search process of the review, there were more imaging studies focused on older adolescence and adulthood. Furthermore, finding a review that covered a strictly adolescent population, focused on FC changes, and was specifically depicting IA, was proven difficult. Many related reviews, such as Tereshchenko and Kasparov (2019), looked at risk factors related to the biopsychosocial model, but did not tackle specific alterations in specific structural or functional changes in the brain [ 66 ]. Weinstein (2017) found similar structural and functional results as well as the role IA has in altering response inhibition and reward valuation in adolescents with IA [ 47 ]. Overall, the accumulated findings only paint an emerging pattern which aligns with similar substance-use and gambling disorders. Future studies require more specificity in depicting the interactions between neural networks, as well as more literature on adolescent and comorbid populations. One future field of interest is the incorporation of more task-based fMRI data. Advances in resting-state fMRI methods have yet to be reflected or confirmed in task-based fMRI methods [ 62 ]. Due to the fact that network connectivity is shaped by different tasks, it is critical to confirm that the findings of the resting state fMRI studies also apply to the task based ones [ 62 ]. Subsequently, work in this area will confirm if intrinsic connectivity networks function in resting state will function similarly during goal directed behaviour [ 62 ]. An elevated focus on adolescent populations as well as task-based fMRI methodology will help uncover to what extent adolescent network connectivity maturation facilitates behavioural and cognitive development [ 62 ].
A treatment implication is the potential usage of bupropion for the treatment of IA. Bupropion has been previously used to treat patients with gambling disorder and has been effective in decreasing overall gambling behaviour as well as money spent while gambling [ 67 ]. Bae et al. (2018) found a decrease in clinical symptoms of IA in line with a 12-week bupropion treatment [ 31 ]. The study found that bupropion altered the FC of both the DMN and ECN which in turn decreased impulsivity and attentional deficits for the individuals with IA [ 31 ]. Interventions like bupropion illustrate the importance of understanding the fundamental mechanisms that underlie disorders like IA.
The goal for this review was to summarise the current literature on functional connectivity changes in adolescents with internet addiction. The findings answered the primary research questions that were directed at FC alterations within several networks of the adolescent brain and how that influenced their behaviour and development. Overall, the research demonstrated several wide-ranging effects that influenced the DMN, SN, ECN, and reward centres. Additionally, the findings gave ground to important details such as the maturation of the adolescent brain, the high prevalence of Asian originated studies, and the importance of task-based studies in this field. The process of making this review allowed for a thorough understanding IA and adolescent brain interactions.
Given the influx of technology and media in the lives and education of children and adolescents, an increase in prevalence and focus on internet related behavioural changes is imperative towards future children/adolescent mental health. Events such as COVID-19 act to expose the consequences of extended internet usage on the development and lifestyle of specifically young people. While it is important for parents and older generations to be wary of these changes, it is important for them to develop a base understanding of the issue and not dismiss it as an all-bad or all-good scenario. Future research on IA will aim to better understand the causal relationship between IA and psychological symptoms that coincide with it. The current literature regarding functional connectivity changes in adolescents is limited and requires future studies to test with larger sample sizes, comorbid populations, and populations outside Far East Asia.
This review aimed to demonstrate the inner workings of how IA alters the connection between the primary behavioural networks in the adolescent brain. Predictably, the present answers merely paint an unfinished picture that does not necessarily depict internet usage as overwhelmingly positive or negative. Alternatively, the research points towards emerging patterns that can direct individuals on the consequences of certain variables or risk factors. A clearer depiction of the mechanisms of IA would allow physicians to screen and treat the onset of IA more effectively. Clinically, this could be in the form of more streamlined and accurate sessions of CBT or family therapy, targeting key symptoms of IA. Alternatively clinicians could potentially prescribe treatment such as bupropion to target FC in certain regions of the brain. Furthermore, parental education on IA is another possible avenue of prevention from a public health standpoint. Parents who are aware of the early signs and onset of IA will more effectively handle screen time, impulsivity, and minimize the risk factors surrounding IA.
Additionally, an increased attention towards internet related fMRI research is needed in the West, as mentioned previously. Despite cultural differences, Western countries may hold similarities to the eastern countries with a high prevalence of IA, like China and Korea, regarding the implications of the internet and IA. The increasing influence of the internet on the world may contribute to an overall increase in the global prevalence of IA. Nonetheless, the high saturation of eastern studies in this field should be replicated with a Western sample to determine if the same FC alterations occur. A growing interest in internet related research and education within the West will hopefully lead to the knowledge of healthier internet habits and coping strategies among parents with children and adolescents. Furthermore, IA research has the potential to become a crucial proxy for which to study adolescent brain maturation and development.
Supporting information
S1 checklist. prisma checklist..
https://doi.org/10.1371/journal.pmen.0000022.s001
S1 Appendix. Search strategies with all the terms.
https://doi.org/10.1371/journal.pmen.0000022.s002
S1 Data. Article screening records with details of categorized content.
https://doi.org/10.1371/journal.pmen.0000022.s003
Acknowledgments
The authors thank https://www.stockio.com/free-clipart/brain-01 (with attribution to Stockio.com); and https://www.rawpixel.com/image/6442258/png-sticker-vintage for the free images used to create Figs 2 – 4 .
- View Article
- PubMed/NCBI
- Google Scholar
- 2. Association AP. Diagnostic and statistical manual of mental disorders: DSM-5. 5 ed. Washington, D.C.: American Psychiatric Publishing; 2013.
- 10. Stats IW. World Internet Users Statistics and World Population Stats 2013 [ http://www.internetworldstats.com/stats.htm .
- 11. Rideout VJR M. B. The common sense census: media use by tweens and teens. San Francisco, CA: Common Sense Media; 2019.
- 37. Tremblay L. The Ventral Striatum. Handbook of Reward and Decision Making: Academic Press; 2009.
- 57. Bhana A. Middle childhood and pre-adolescence. Promoting mental health in scarce-resource contexts: emerging evidence and practice. Cape Town: HSRC Press; 2010. p. 124–42.
- 65. Organization WH. Adolescent Health 2023 [ https://www.who.int/health-topics/adolescent-health#tab=tab_1 .
Thank you for visiting nature.com. You are using a browser version with limited support for CSS. To obtain the best experience, we recommend you use a more up to date browser (or turn off compatibility mode in Internet Explorer). In the meantime, to ensure continued support, we are displaying the site without styles and JavaScript.
- View all journals
- My Account Login
- Explore content
- About the journal
- Publish with us
- Sign up for alerts
- Review Article
- Open access
- Published: 03 June 2024
The effectiveness of digital twins in promoting precision health across the entire population: a systematic review
- Mei-di Shen 1 ,
- Si-bing Chen 2 &
- Xiang-dong Ding ORCID: orcid.org/0009-0001-1925-0654 2
npj Digital Medicine volume 7 , Article number: 145 ( 2024 ) Cite this article
38 Accesses
2 Altmetric
Metrics details
- Public health
- Risk factors
- Signs and symptoms
Digital twins represent a promising technology within the domain of precision healthcare, offering significant prospects for individualized medical interventions. Existing systematic reviews, however, mainly focus on the technological dimensions of digital twins, with a limited exploration of their impact on health-related outcomes. Therefore, this systematic review aims to explore the efficacy of digital twins in improving precision healthcare at the population level. The literature search for this study encompassed PubMed, Embase, Web of Science, Cochrane Library, CINAHL, SinoMed, CNKI, and Wanfang Database to retrieve potentially relevant records. Patient health-related outcomes were synthesized employing quantitative content analysis, whereas the Joanna Briggs Institute (JBI) scales were used to evaluate the quality and potential bias inherent in each selected study. Following established inclusion and exclusion criteria, 12 studies were screened from an initial 1321 records for further analysis. These studies included patients with various conditions, including cancers, type 2 diabetes, multiple sclerosis, heart failure, qi deficiency, post-hepatectomy liver failure, and dental issues. The review coded three types of interventions: personalized health management, precision individual therapy effects, and predicting individual risk, leading to a total of 45 outcomes being measured. The collective effectiveness of these outcomes at the population level was calculated at 80% (36 out of 45). No studies exhibited unacceptable differences in quality. Overall, employing digital twins in precision health demonstrates practical advantages, warranting its expanded use to facilitate the transition from the development phase to broad application.
PROSPERO registry: CRD42024507256.
Similar content being viewed by others
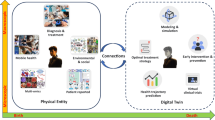
Digital twins for health: a scoping review
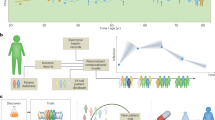
Digital twins in medicine
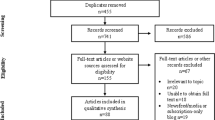
The health digital twin to tackle cardiovascular disease—a review of an emerging interdisciplinary field
Introduction.
Precision health represents a paradigm shift from the conventional “one size fits all” medical approach, focusing on specific diagnosis, treatment, and health management by incorporating individualized factors such as omics data, clinical information, and health outcomes 1 , 2 . This approach significantly impacts various diseases, potentially improving overall health while reducing healthcare costs 3 , 4 . Within this context, digital twins emerged as a promising technology 5 , creating digital replicas of the human body through two key steps: building mappings and enabling dynamic evolution 6 . Unlike traditional data mining methods, digital twins consider individual variability, providing continuous, dynamic recommendations for clinical practice 7 . This approach has gained significant attention among researchers, highlighting its potential applications in advancing precision health.
Several systematic reviews have explored the advancement of digital twins within the healthcare sector. One rapid review 8 identified four core functionalities of digital twins in healthcare management: safety management, information management, health management/well-being promotion, and operational control. Another systematic review 9 , through an analysis of 22 selected publications, summarized the diverse application scenarios of digital twins in healthcare, confirming their potential in continuous monitoring, personalized therapy, and hospital management. Furthermore, a quantitative review 10 assessed 94 high-quality articles published from 2018 to 2022, revealing a primary focus on technological advancements (such as artificial intelligence and the Internet of Things) and application scenarios (including personalized, precise, and real-time healthcare solutions), thus highlighting the pivotal role of digital twins technology in the field of precision health. Another systematic review 11 , incorporating 18 framework papers or reviews, underscored the need for ongoing research into digital twins’ healthcare applications, especially during the COVID-19 pandemic. Moreover, a systematic review 12 on the application of digital twins in cardiovascular diseases presented proof-of-concept and data-driven approaches, offering valuable insights for implementing digital twins in this specific medical area.
While the existing literature offers valuable insights into the technological aspects of digital twins in healthcare, these systematic reviews failed to thoroughly examine the actual impacts on population health. Despite the increasing interest and expanding body of research on digital twins in healthcare, the direct effects on patient health-related outcomes remain unclear. This knowledge gap highlights the need to investigate how digital twins promote and restore patient health, which is vital for advancing precision health technologies. Therefore, the objective of our systematic review is to assess the effectiveness of digital twins in improving health-related outcomes at the population level, providing a clearer understanding of their practical benefits in the context of precision health.
Search results
The selection process for the systematic review is outlined in the PRISMA flow chart (Fig. 1 ). Initially, 1321 records were identified. Of these, 446 duplicates (446/1321, 33.76%) were removed, leaving 875 records (875/1321, 66.24%) for title and abstract screening. Applying the pre-defined inclusion and exclusion criteria led to the exclusion of 858 records (858/875, 98.06%), leaving 17 records (17/875, 1.94%) for full-text review. Further scrutiny resulted in the exclusion of one study (1/17, 5.88%) lacking health-related outcomes and four studies (4/17, 23.53%) with overlapping data. Ultimately, 12 (12/17, 70.59%) original studies 13 , 14 , 15 , 16 , 17 , 18 , 19 , 20 , 21 , 22 , 23 , 24 were included in the systematic review. Supplementary Table 1 provides a summary of the reasons for exclusion at the full-text reading phase.
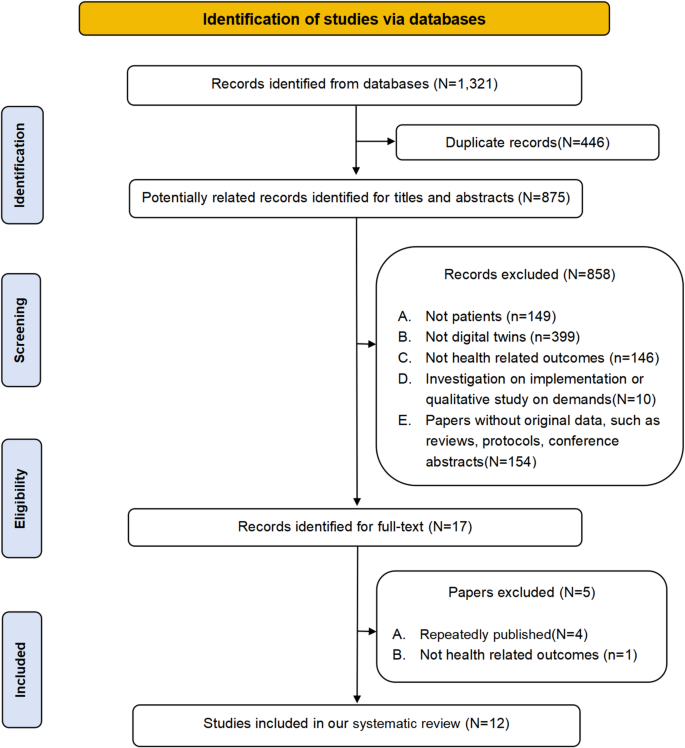
Flow chart of included studies in the systematic review.
Study characteristics
The studies included in this systematic review were published between 2021 (2/12, 16.67%) 23 , 24 and 2023 (8/12, 66.67%) 13 , 14 , 15 , 16 , 17 , 18 , 19 , 20 . Originating from diverse regions, 4/12 studies (33.33%) were from Asia 13 , 14 , 21 , 24 , 5/12 (41.67%) from America 15 , 17 , 19 , 20 , 22 , and 3/12 (25.00%) from Europe 16 , 18 , 23 . The review encompassed various study designs, including randomized controlled trials (1/12, 8.33%) 14 , quasi-experiments (6/12, 50.00%) 13 , 15 , 16 , 18 , 19 , 21 , and cohort studies (5/12, 41.67%) 17 , 20 , 22 , 23 , 24 . The sample sizes ranged from 15 13 to 3500 patients 19 . Five studies assessed the impact of digital twins on virtual patients 15 , 16 , 18 , 19 , 20 , while seven examined their effect on real-world patients 13 , 14 , 17 , 21 , 22 , 23 , 24 . These patients included had various diseases, including cancer (4/12, 33.33%) 15 , 16 , 19 , 22 , type 2 diabetes (2/12, 16.66%) 13 , 14 , multiple sclerosis (2/12, 16.66%) 17 , 18 , qi deficiency (1/12, 8.33%) 21 , heart failure (1/12, 8.33%) 20 , post-hepatectomy liver failure (1/12, 8.33%) 23 , and dental issues (1/12, 8.33%) 24 . This review coded interventions into three types: personalized health management (3/12, 25.00%) 13 , 14 , 21 , precision individual therapy effects (3/12, 25.00%) 15 , 16 , 18 , 19 , 20 , 22 , and predicting individual risk (3/12, 25.00%) 17 , 23 , 24 , with a total of 45 measured outcomes. Characteristics of the included studies are detailed in Table 1 .
Risk of bias assessment
The risk of bias for the studies included in this review is summarized in Fig. 2 . In the single RCT 14 assessed, 10 out of 13 items received positive responses. Limitations were observed due to incomplete reporting of baseline characteristics and issues with blinding. Among the six quasi-experimental studies evaluated, five (83.33%) 13 , 15 , 16 , 18 , 21 achieved at least six positive responses, indicating an acceptable quality, while one study (16.67%) 19 fell slightly below this threshold with five positive responses. The primary challenges in these quasi-experimental studies were due to the lack of control groups, inadequate baseline comparisons, and limited follow-up reporting. Four out of five (80.00%) 17 , 20 , 22 , 23 of the cohort studies met or exceeded the criterion with at least eight positive responses, demonstrating their acceptable quality. However, one study (20.00%) 24 had a lower score due to incomplete data regarding loss to follow-up and the specifics of the interventions applied. Table 1 elaborates on the specific reasons for these assessments. Despite these concerns, the overall quality of the included studies is considered a generally acceptable risk of bias.
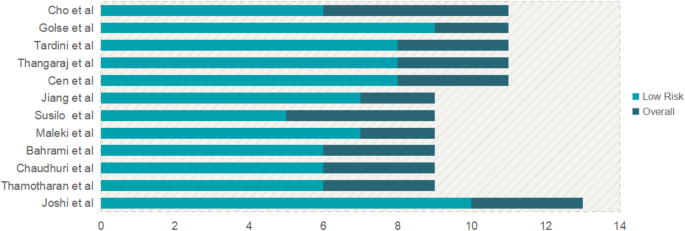
The summary of bias risk via the Joanna Briggs Institute assessment tools.
The impact of digital twins on health-related outcomes among patients
This review includes 12 studies that collectively assessed 45 outcomes, achieving an overall effectiveness rate of 80% (36 out of 45 outcomes), as depicted in Fig. 3a . The digital twins analyzed were coded into three functional categories: personalized health management, precision individual therapy effects, and predicting individual risks. A comprehensive analysis of the effectiveness of digital twins across these categories is provided, detailing the impact and outcomes associated with each function.
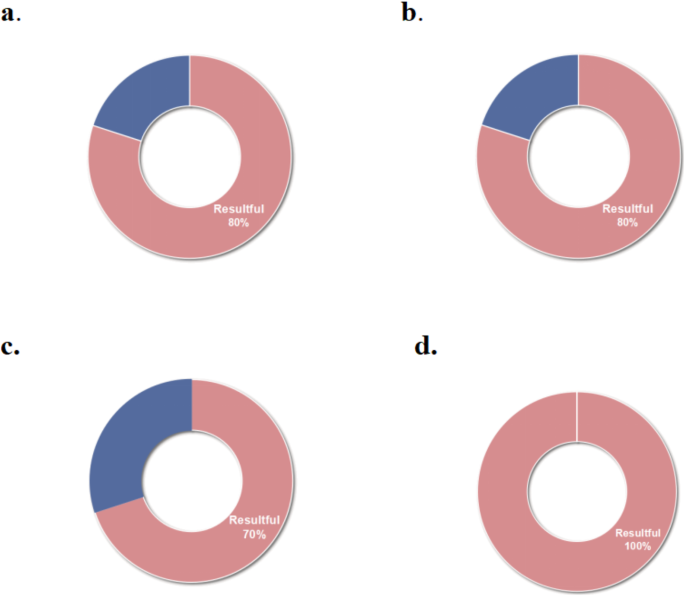
a The overall effectiveness of digital twins; b The effectiveness of personalized health management driven by digital twins; c The effectiveness of precision individualized therapy effects driven by digital twins; d The effectiveness of prediction of individual risk driven by digital twins.
The effectiveness of digital twins in personalized health management
In this review, three studies 13 , 14 , 21 employing digital twins for personalized health management reported an effectiveness of 80% (24 out of 30 outcomes), as shown in Fig. 3b . A self-control study 13 involving 15 elderly patients with diabetes, used virtual patient representations based on health information to guide individualized insulin infusion. Over 14 days, this approach improved the time in range (TIR) from 3–75% to 86–97%, decreased hypoglycemia duration from 0–22% to 0–9%, and reduced hyperglycemia time from 0–98% to 0–12%. A 1-year randomized controlled trial 14 with 319 type 2 diabetes patients, implemented personalized digital twins interventions based on nutrition, activity, and sleep. This trial demonstrated significant improvements in Hemoglobin A1c (HbA1C), Homeostatic Model Assessment 2 of Insulin Resistance (HOMA2-IR), Nonalcoholic Fatty Liver Disease Liver Fat Score (NAFLD-LFS), and Nonalcoholic Fatty Liver Disease Fibrosis Score (NAFLD-NFS), and other primary outcomes (all, P < 0.001; Table 2 ). However, no significant changes were observed in weight, Alanine Aminotransferase (ALT), Fibrosis-4 Score (FIB4), and AST to Platelet Ratio Index (APRI) (all, P > 0.05). A non-randomized controlled trial 21 introduced a digital twin-based Traditional Chinese Medicine (TCM) health management platform for patients with qi deficiency. It was found to significantly improve blood pressure, main and secondary TCM symptoms, total TCM symptom scores, and quality of life (all, P < 0.05). Nonetheless, no significant improvements were observed in heart rate and BMI (all, P > 0.05; Table 2 ).
The effectiveness of digital twins in precision individual therapy effects
Six studies 15 , 16 , 18 , 19 , 20 , 22 focused on the precision of individual therapy effects using digital twins, demonstrating a 70% effectiveness rate (7 out of 10 outcomes), as detailed in Fig. 3c . In a self-control study 15 , a data-driven approach was employed to create digital twins, generating 100 virtual patients to predict the potential tumor biology outcomes of radiotherapy regimens with varying contents and doses. This study showed that personalized radiotherapy plans derived from digital twins could extend the median tumor progression time by approximately six days and reduce radiation doses by 16.7%. Bahrami et al. 16 created 3000 virtual patients experiencing cancer pain to administer precision dosing of fentanyl transdermal patch therapy. The intervention led to a 16% decrease in average pain intensity and an additional median pain-free duration of 23 hours, extending from 72 hours in cancer patients. Another quasi-experimental study 18 created 3000 virtual patients with multiple sclerosis to assess the impact of Ocrelizumab. Findings indicated Ocrelizumab can resulted in a reduction in relapses (0.191 [0.143, 0.239]) and lymphopenic adverse events (83.73% vs . 19.9%) compared to a placebo. American researchers 19 developed a quantitative systems pharmacology model using digital twins to identify the optimal dosing for aggressive non-Hodgkin lymphoma patients. This approach resulted in at least a 50% tumor size reduction by day 42 among 3500 virtual patients. A cohort study 20 assessed the 5-year composite cardiovascular outcomes in 2173 virtual patients who were treated with spironolactone or left untreated and indicated no statistically significant inter-group differences (0.85, [0.69–1.04]). Tardini et al. 22 employed digital twins to optimize multi-step treatment for oropharyngeal squamous cell carcinoma in 134 patients. The optimized treatment selection through digital twins predicted increased survival rates by 3.73 (−0.75, 8.96) and dysphagia rates by 0.75 (−4.48, 6.72) compared to clinician decisions, with no statistical significance.
The effectiveness of digital twins in predicting individual risk
Three studies 17 , 23 , 24 employing digital twins to predict individual patient risks demonstrated a 100% effectiveness rate (5 out of 5 outcomes), as shown in Fig. 3d . A cohort study 17 used digital twins to forecast the onset age for disease-specific brain atrophy in patients with multiple sclerosis. Findings indicated that the onset of progressive brain tissue loss, on average, preceded clinical symptoms by 5-6 years among the 519 patients ( P < 0.01). Another study 23 focused on predicting postoperative liver failure in 47 patients undergoing major hepatectomy through mathematical models of blood circulation. The study highlighted that elevated Postoperative Portal Vein pressure (PPV) and Portocaval Gradient (PCG) values above 17.5 mmHg and 13.5 mmHg, respectively, correlated with the measured values (all, P < 0.0001; Table 2 ). These indicators were effective in predicting post-hepatectomy liver failure, accurately identifying three out of four patients who experienced this complication. Cho et al. 24 created digital twins for 50 adult female patients using facial scans and cone-beam computed tomography images to evaluate the anteroposterior position of the maxillary central incisors and forehead inclination. The analysis demonstrated significant differences in the position of the maxillary central incisors ( P = 0.04) and forehead inclination ( P = 0.02) between the two groups.
This systematic review outlines the effectiveness of digital twins in improving health-related outcomes across various diseases, including cancers, type 2 diabetes, multiple sclerosis, qi deficiency, heart failure, post-hepatectomy liver failure, and dental issues, at the population level. Distinct from prior reviews that focused on the technological dimensions of digital twins, our analysis shows the practical applications of digital twins in healthcare. The applications have been categorized into three main areas: personalized health management, precision individual therapy effects, and predicting individual risks, encompassing a total of 45 outcomes. An overall effectiveness of 80% was observed across these outcomes. This review offers valuable insights into the application of digital twins in precision health and supports the transition of digital twins from construction to population-wide implementation.
Digital twins play a crucial role in achieving precision health 25 . They serve as virtual models of human organs, tissues, cells, or microenvironments, dynamically updating based on real-time data to offer feedback for interventions on their real counterparts 26 , 27 . Digital twins can solve complex problems in personalized health management 28 , 29 and enable comprehensive, proactive, and precise healthcare 30 . In the studies reviewed, researchers implemented digital twins by creating virtual patients based on personal health data and using simulations to generate personalized recommendations and predictions. It is worth noting that while certain indicators have not experienced significant improvement in personalized health management for patients with type 2 diabetes and Qi deficiency, it does not undermine the effectiveness of digital twins. Firstly, these studies have demonstrated significant improvements in primary outcome measures. Secondly, improving health-related outcomes in chronic diseases is an ongoing, complex process heavily influenced by changes in health behaviors 31 , 32 . While digital twins can provide personalized health guidance based on individual health data, their impact on actual behaviors warrants further investigation.
The dual nature of medications, providing benefits yet potentially leading to severe clinical outcomes like morbidity or mortality, must be carefully considered. The impact of therapy is subject to various factors, including the drug attributes and the specific disease characteristics 33 . Achieving accurate medication administration remains a significant challenge for healthcare providers 34 , underscoring the need for innovative methodologies like computational precise drug delivery 35 , 36 , a example highlighted in our review of digital twins. Regarding the prediction of individual therapy effects for conditions such as cancer, multiple sclerosis, and heart failure, six studies within this review have reported partly significant improvements in patient health-related outcomes. These advancements facilitate the tailored selection and dosing of therapy, underscoring the ability of digital twins to optimize patient-specific treatment plans effectively.
Furthermore, digital twins can enhance clinical understanding and personalize disease risk prediction 37 . It enables a quantitative understanding and prediction of individuals by continuously predicting and evaluating patient data in a virtual environment 38 . In patients with multiple sclerosis, digital twins have facilitated predictions regarding the onset of disease-specific brain atrophy, allowing for early intervention strategies. Similarly, digital twins assessed the risk of liver failure after liver resection, aiding healthcare professionals in making timely decisions. Moreover, the application of digital twins in the three-dimensional analysis of patients with dental problems has demonstrated highly effective clinical significance, underscoring its potential across various medical specialties. In summary, the adoption of digital twins has significantly contributed to advancing precision health and restoring patient well-being by creating virtual patients based on personal health data and using simulations to generate personalized recommendations and predictions.
Recent studies have introduced various digital twin systems, covering areas such as hospital management 8 , remote monitoring 9 , and diagnosing and treating various conditions 39 , 40 . Nevertheless, these systems were not included in this review due to the lack of detailed descriptions at the population health level, which constrains the broader application of this emerging technology. Our analysis underscores the reported effectiveness of digital twins, providing unique opportunities for dynamic prevention and precise intervention across different diseases. Multiple research methodologies and outcome measures poses a challenge for quantitative publication detection. This systematic review employed a comprehensive retrieval strategy across various databases for screening articles on the effectiveness of digital twins, to reduce the omission of negative results. And four repeated publications were excluded based on authors, affiliation, population, and other criteria to mitigate the bias of overestimating the digital twins effect due to repeated publication.
However, there are still limitations. Firstly, the limited published research on digital twins’ application at the population level hinders the ability to perform a quantitative meta-analysis, possibly limiting our findings’ interpretability. We encourage reporting additional high-quality randomized controlled trials on the applicability of digital twins to facilitate quantitative analysis of their effectiveness in precision health at the population level. Secondly, this review assessed the effectiveness of digital twins primarily through statistical significance ( P -value or 95% confidence interval). However, there are four quasi-experimental studies did not report statistical significance. One of the limitations of this study is the use of significant changes in author self-reports as a criterion in these four quasi-experimental studies for identifying effectiveness. In clinical practice, the author’s self-reported clinical significance can also provide the effectiveness of digital twins. Thirdly, by focusing solely on studies published in Chinese and English, this review may have omitted relevant research available in other languages, potentially limiting the scope of the analyzed literature. Lastly, our review primarily emphasized reporting statistical differences between groups. Future work should incorporate more application feedback from real patients to expose digital twins to the nuances of actual patient populations.
The application of digital twins is currently limited and primarily focused on precision health for individual patients. Expanding digital twins’ application from individual to group precision health is recommended to signify a more extensive integration in healthcare settings. This expansion involves sharing real-time data and integrating medical information across diverse medical institutions within a region, signifying the development of group precision health. Investigating both personalized medical care and collective health management has significant implications for improving medical diagnosis and treatment approaches, predicting disease risks, optimizing health management strategies, and reducing societal healthcare costs 41 .
Digital twins intervention encompasses various aspects such as health management, decision-making, and prediction, among others 9 . It represents a technological and conceptual innovation in traditional population health intervention. However, the current content design of the digital twins intervention is insufficient and suggests that it should be improved by incorporating more effective content strategies tailored to the characteristics of the target population. Findings from this study indicate that interventions did not differ significantly in our study is from digital twins driven by personalized health management, which means that compared with the other two function-driven digital twins, personalized health management needs to receive more attention to enhance its effect in population-level. For example, within the sphere of chronic disease management, integrating effective behavioral change strategies into digital twins is advisable to positively influence health-related indicators, such as weight and BMI. The effectiveness of such digital behavior change strategies has been reported in previous studies 42 , 43 . The consensus among researchers on the importance of combining effective content strategies with digital intervention technologies underscores the potential for this approach to improve patient health-related outcomes significantly.
The applications of digital twins in precision health are mainly focused on model establishment and prediction description, with limited implementation in multi-center settings. A more robust and detailed data foundation is recommended to improve clinical decision-making and reduce the likelihood of imprecise treatments. This requires continuous updating and capturing of dynamic information by digital twins in the future, as well as the improvement of the data platform that facilitates mapping, interaction, and iterative optimization. Integrating digital twins effectively into clinical workflows can support clinical interventions, assist physicians in making informed decisions, and increase the standard of patient care 6 .
The accessibility of health data is a significant challenge for the clinical implementation of digital twins. Although the internet and information technology have significantly enhanced health data availability, health data, including information systems and electronic health records, remain heterogeneous and are difficult to share 44 . Health data often contains confidential patient information, as well as unreliable information, posing challenges for implementing digital twins in healthcare settings. The primary technology utilized in digital twins, artificial intelligence algorithms, demands high-performance hardware devices and software platforms for data analysis 45 , necessitating healthcare organizations to allocate increased investment and budget for computing infrastructure supporting digital twins’ application. Therefore, future research should be focused on the technical aspects of digital twins to resolve these challenges. The automated processing of health data using a large language model and the rapid conversion of complex natural language texts into comprehensive knowledge texts are encouraged. The development of high-performance computing technology is essential for cost-effective computing requirements, which can facilitate the application of digital twins in clinical practice 46 .
Overall, this systematic review offers a comprehensive overview of digital twins in precision health, examining their impact at the population level. The findings indicate a significant overall effectiveness rate of 80% for the measured outcomes, highlighting digital twins’ pivotal role in advancing precision health. Future research should broaden the application of digital twins across various populations, integrate proven content strategies, and implement these approaches in various healthcare settings. Such efforts will maximize the benefits of digital technologies in healthcare, promoting more precise and efficacious strategies, thereby elevating patient outcomes and improving overall healthcare experiences. While digital twins offer great promise for precision health, their broad adoption and practical implementation are still in the early stages. Development, and application are essential to unlock the full potential of digital twins in revolutionizing healthcare delivery.
This systematic review was performed following the Preferred Reporting Items for Systematic Reviews and Meta-Analysis (PRISMA) guidelines 47 . The protocol for this systematic review was prospectively registered on PROSPERO, which can be accessed via the following link: https://www.crd.york.ac.uk/prospero/display_record.php?ID=CRD42024507256 . The registered protocol underwent an update, which included polishing the title of the article, modifying the limitation of the control group and language in the inclusion/exclusion criteria, and refining the process of data synthesis and analysis to enhance that clarity and readability of this systematic review. These modifications were updated in the revision notes section of the PROSPERO.
Literature search strategy
Literature searches were conducted in PubMed, Embase, Web of Science, Cochrane Library, CINAHL, SinoMed, CNKI, and Wanfang Database, covering publications up to December 24, 2023. A comprehensive search strategy was developed using a combination of Medical Subject Headings terms and free-text terms, as detailed in Supplementary Table 2 . Furthermore, reference lists of articles and reviews meeting the inclusion criteria were reviewed for additional relevant studies.
Inclusion and exclusion criteria
The inclusion criteria for this systematic review included: 1) Population: Patients diagnosed with any diseases or symptoms; 2) Intervention: Any interventions involving digital twins; 3) Controls: Non-digital twin groups, such as standard care or conventional therapy, as well as no control group; 4) Outcomes: Health-related outcomes as the primary outcomes of interest; 5) Study design: All study designs that measured patient health-related outcomes after digital twins were included, including intervention studies and predictive cohort studies.
Initially, duplicates were removed. Exclusion criteria included: 1) Papers lacking original data, such as reviews, protocols, and conference abstracts; 2) Studies not in English or Chinese; 3) Surveys focusing on implementation and qualitative studies related to requirements. In cases of data duplication, the most comprehensive data report was included.
Study selection and Data extraction
Following the automatic removal of duplicates, two independent reviewers (MD.SHEN and SB.CHEN) conducted initial screenings of titles and abstracts against the predefined inclusion and exclusion criteria to identify potentially relevant studies. Afterward, the same reviewers examined the full texts of these shortlisted articles to confirm their suitability for inclusion. This process also involved checking the reference lists of these articles for any additional studies that might meet the criteria. Data from the included studies were systematically extracted using a pre-designed extraction form. Recorded information included the first author’s name, publication year, country of origin, type of study, sample size, study population, intervention, controls, measurements, and an appraisal of each study. Disagreements between the reviewers were resolved by consultation with a third senior reviewer (XD.DING), ensuring consensus.
Quality appraisal
The Joanna Briggs Institute (JBI) scales 48 were used to assess the quality and potential bias of each study included in the review, employing specific tools tailored to the type of study under evaluation. These tools feature response options of “yes,” “no,” “unclear,” or “not applicable” for each assessment item. For randomized controlled trials (RCTs), the JBI scale includes 13 items, with answering “yes” to at least six items indicating a high-quality study. Quasi-experimental studies were evaluated using a nine-item checklist, where five or more positive responses qualify the research as high quality. Cohort studies underwent evaluation through an 11-item checklist, with six or more affirmative responses indicating high quality. The assessment was independently carried out by two reviewers (MD.SHEN and SB.CHEN), and any disagreements were resolved through consultation with a third senior reviewer (XD.DING), ensuring the integrity and accuracy of the quality assessment.
Data synthesis and analysis
Given the heterogeneity in type of study and outcome measures, a meta-analysis was deemed unfeasible. Instead, a quantitative content analysis was employed to analyze all the selected studies 49 , 50 . Key information was extracted using a pre-designed standardized form, including the first author’s name, patient characteristics, intervention functional characteristics, measurements, results, effectiveness, and adverse events. Two reviewers (MD.SHEN and SB.CHEN) independently coded digital twin technology into three categories for descriptive analysis: personalized health management, precision individual therapy effects, and predicting individual risk, based on its functional characteristics. The Kappa statistic was applied to evaluate the inter-rater reliability during the coding process, yielding a value of 0.871, which signifies good agreement between the researchers 51 , 52 . The assessment of digital twins effectiveness was based on statistical significance ( P -value or 95% confidence interval). Outcomes with statistical significance were labeled as “resultful,” whereas those lacking statistical significance were deemed “resultless.” For quasi-experimental studies, significant changes in the authors’ self-reports were used to determine the effectiveness in the absence of reporting of statistical significance. The proportion of effectiveness was calculated as the number of “resultful” indicators divided by the total number of outcomes within each category.
Data availability
Data sharing is not applicable to this article as no datasets were generated or analyzed during the current study.
Code availability
Code sharing is not applicable to this article as no codes were generated or analyzed during the current study.
Fu, M. R. et al. Precision health: A nursing perspective. Int. J. Nurs. Sci. 7 , 5–12 (2020).
PubMed Google Scholar
Naithani, N., Sinha, S., Misra, P., Vasudevan, B. & Sahu, R. Precision medicine: Concept and tools. Med. J., Armed Forces India 77 , 249–257 (2021).
Article PubMed Google Scholar
Payne, K. & Gavan, S. P. Economics and precision medicine. Handb. Exp. Pharmacol. 280 , 263–281 (2023).
Ielapi, N. et al. Precision medicine and precision nursing: the era of biomarkers and precision health. Int. J. Gen. Med. 13 , 1705–1711 (2020).
Article PubMed PubMed Central Google Scholar
Corral-Acero, J. et al. The ‘Digital Twin’ to enable the vision of precision cardiology. Eur. Heart J. 41 , 4556–4564 (2020).
Ferdousi, R., Laamarti, F., Hossain, M. A., Yang, C. S. & Saddik, A. E. Digital twins for well-being: an overview. Digital Twin 1 , 2022 (2022).
Article Google Scholar
Vallée, A. Digital twin for healthcare systems. Front. Digital health 5 , 1253050 (2023).
Elkefi, S. & Asan, O. Digital twins for managing health care systems: rapid literature review. J. Med. Internet Res. 24 , e37641 (2022).
Sun, T., He, X. & Li, Z. Digital twin in healthcare: Recent updates and challenges. Digital Health 9 , 20552076221149651 (2023).
Sheng, B. et al. Detecting latent topics and trends of digital twins in healthcare: A structural topic model-based systematic review. Digital Health 9 , 20552076231203672 (2023).
Khan, A. et al. A scoping review of digital twins in the context of the Covid-19 pandemic. Biomed. Eng. Comput. Biol. 13 , 11795972221102115 (2022).
Coorey, G. et al. The health digital twin to tackle cardiovascular disease-a review of an emerging interdisciplinary field. NPJ Digital Med. 5 , 126 (2022).
Thamotharan, P. et al. Human Digital Twin for Personalized Elderly Type 2 Diabetes Management. J. Clin. Med. 12 , https://doi.org/10.3390/jcm12062094 (2023).
Joshi, S. et al. Digital twin-enabled personalized nutrition improves metabolic dysfunction-associated fatty liver disease in type 2 diabetes: results of a 1-year randomized controlled study. Endocr. Pract. : Off. J. Am. Coll. Endocrinol. Am. Assoc. Clin. Endocrinologists 29 , 960–970 (2023).
Chaudhuri, A. et al. Predictive digital twin for optimizing patient-specific radiotherapy regimens under uncertainty in high-grade gliomas. Front. Artif. Intell. 6 , 1222612–1222612 (2023).
Bahrami, F., Rossi, R. M., De Nys, K. & Defraeye, T. An individualized digital twin of a patient for transdermal fentanyl therapy for chronic pain management. Drug Deliv. Transl. Res. 13 , 2272–2285 (2023).
Article CAS PubMed PubMed Central Google Scholar
Cen, S., Gebregziabher, M., Moazami, S., Azevedo, C. J. & Pelletier, D. Toward precision medicine using a “digital twin” approach: modeling the onset of disease-specific brain atrophy in individuals with multiple sclerosis. Sci. Rep. 13 , 16279 (2023).
Maleki, A. et al. Moving forward through the in silico modeling of multiple sclerosis: Treatment layer implementation and validation. Comput. Struct. Biotechnol. J. 21 , 3081–3090 (2023).
Susilo, M. E. et al. Systems-based digital twins to help characterize clinical dose–response and propose predictive biomarkers in a Phase I study of bispecific antibody, mosunetuzumab, in NHL. Clin. Transl. Sci. 16 , 1134–1148 (2023).
Thangaraj, P. M., Vasisht Shankar, S., Oikonomou, E. K. & Khera, R. RCT-Twin-GAN Generates Digital Twins of Randomized Control Trials Adapted to Real-world Patients to Enhance their Inference and Application. medRxiv : the preprint server for health sciences , https://doi.org/10.1101/2023.12.06.23299464 (2023).
Jiang, J., Li, Q. & Yang, F. TCM Physical Health Management Training and Nursing Effect Evaluation Based on Digital Twin. Sci. Progr. 2022 , https://doi.org/10.1155/2022/3907481 (2022).
Tardini, E. et al. Optimal treatment selection in sequential systemic and locoregional therapy of oropharyngeal squamous carcinomas: deep Q-learning with a patient-physician digital twin dyad. J. Med. Int. Res. 24 , e29455 (2022).
Google Scholar
Golse, N. et al. Predicting the risk of post-hepatectomy portal hypertension using a digital twin: A clinical proof of concept. J. Hepatol. 74 , 661–669 (2021).
Cho, S.-W. et al. Sagittal relationship between the maxillary central incisors and the forehead in digital twins of korean adult females. J. Personal. Med. 11 , https://doi.org/10.3390/jpm11030203 (2021).
Imoto, S., Hasegawa, T. & Yamaguchi, R. Data science and precision health care. Nutr. Rev. 78 , 53–57 (2020).
Drummond, D. & Coulet, A. Technical, ethical, legal, and societal challenges with digital twin systems for the management of chronic diseases in children and young people. J. Med. Internet Res. 24 , e39698 (2022).
Bertezene, S. The digital twin in health: Organizational contributions and epistemological limits in a context of health crisis. Med. Sci. M/S 38 , 663–668 (2022).
Johnson, K. B. et al. Precision Medicine, AI, and the Future of Personalized Health Care. Clin. Transl. Sci. 14 , 86–93 (2021).
Powell, J. & Li, X. Integrated, data-driven health management: A step closer to personalized and predictive healthcare. Cell Syst. 13 , 201–203 (2022).
Article CAS PubMed Google Scholar
Delpierre, C. & Lefèvre, T. Precision and personalized medicine: What their current definition says and silences about the model of health they promote. Implication for the development of personalized health. Front. Sociol. 8 , 1112159 (2023).
Raiff, B. R., Burrows, C. & Dwyer, M. Behavior-analytic approaches to the management of diabetes mellitus: current status and future directions. Behav. Anal. Pract. 14 , 240–252 (2021).
Ahern, D. K. et al. Behavior-based diabetes management: impact on care, hospitalizations, and costs. Am. J. Managed care 27 , 96–102 (2021).
Tyson, R. J. et al. Precision dosing priority criteria: drug, disease, and patient population variables. Front. Pharmacol. 11 , 420 (2020).
Walton, R., Dovey, S., Harvey, E. & Freemantle, N. Computer support for determining drug dose: systematic review and meta-analysis. BMJ (Clin. Res.) 318 , 984–990 (1999).
Article CAS Google Scholar
Friedrichs, M. & Shoshi, A. History and future of KALIS: Towards computer-assisted decision making in prescriptive medicine. J. Integr. Bioinform. 16 , https://doi.org/10.1515/jib-2019-0011 (2019).
Zhao, H. et al. Identifying the serious clinical outcomes of adverse reactions to drugs by a multi-task deep learning framework. Commun. Biol. 6 , 870 (2023).
Thiong’o, G. M. & Rutka, J. T. Digital twin technology: the future of predicting neurological complications of pediatric cancers and their treatment. Front. Oncol. 11 , 781499 (2021).
Sun, T., He, X., Song, X., Shu, L. & Li, Z. The digital twin in medicine: a key to the future of healthcare? Front. Med. 9 , 907066 (2022).
Sarp, S., Kuzlu, M., Zhao, Y. & Gueler, O. Digital twin in healthcare: a study for chronic wound management. IEEE J. Biomed. health Inform. 27 , 5634–5643 (2023).
Chu, Y., Li, S., Tang, J. & Wu, H. The potential of the Medical Digital Twin in diabetes management: a review. Front. Med. 10 , 1178912 (2023).
Barricelli, B. R., Casiraghi, E. & Fogli, D. A survey on digital twin: definitions, characteristics, applications, and design implications. IEEE Access 7 , 167653–167671 (2019).
Keller, R. et al. Digital behavior change interventions for the prevention and management of type 2 diabetes: systematic market analysis. J. Med. Internet Res. 24 , e33348 (2022).
Priesterroth, L., Grammes, J., Holtz, K., Reinwarth, A. & Kubiak, T. Gamification and behavior change techniques in diabetes self-management apps. J. diabetes Sci. Technol. 13 , 954–958 (2019).
Venkatesh, K. P., Raza, M. M. & Kvedar, J. C. Health digital twins as tools for precision medicine: Considerations for computation, implementation, and regulation. NPJ digital Med. 5 , 150 (2022).
Venkatesh, K. P., Brito, G. & Kamel Boulos, M. N. Health digital twins in life science and health care innovation. Annu. Rev. Pharmacol. Toxicol. 64 , 159–170 (2024).
Katsoulakis, E. et al. Digital twins for health: a scoping review. NPJ Digital Med. 7 , 77 (2024).
Page, M. J. et al. The PRISMA 2020 statement: an updated guideline for reporting systematic reviews. BMJ (Clin. Res. ed.) 372 , n71 (2021).
Barker, T. H. et al. Revising the JBI quantitative critical appraisal tools to improve their applicability: an overview of methods and the development process. JBI Evid. Synth. 21 , 478–493 (2023).
Manganello, J. & Blake, N. A study of quantitative content analysis of health messages in U.S. media from 1985 to 2005. Health Commun. 25 , 387–396 (2010).
Giannantonio, C. M. Content Analysis: An Introduction to Its Methodology, 2nd edition. Organ. Res. Methods 13 , 392–394 (2010).
Rigby, A. S. Statistical methods in epidemiology. v. Towards an understanding of the kappa coefficient. Disabil. Rehabilitation 22 , 339–344 (2000).
Lantz, C. A. & Nebenzahl, E. Behavior and interpretation of the kappa statistic: resolution of the two paradoxes. J. Clin. Epidemiol. 49 , 431–434 (1996).
Download references
Acknowledgements
This research did not receive any specific grant from funding agencies in the public, commercial or not-for-profit sectors.
Author information
Authors and affiliations.
School of Nursing, Peking University, Beijing, China
Mei-di Shen
Department of Plastic and Reconstructive Microsurgery, China-Japan Union Hospital, Jilin University, Changchun, Jilin, China
Si-bing Chen & Xiang-dong Ding
You can also search for this author in PubMed Google Scholar
Contributions
MD.SHEN contributed to the data collection, analysis and the manuscript writing. SB.CHEN contributed to the data collection and analysis. XD.DING contributed to the critical revision of the manuscript as well as the initial study conception. All authors read and approved the final manuscript, and jointly take responsibility for the decision to submit this work for publication.
Corresponding author
Correspondence to Xiang-dong Ding .
Ethics declarations
Competing interests.
The authors declare no competing interests.
Additional information
Publisher’s note Springer Nature remains neutral with regard to jurisdictional claims in published maps and institutional affiliations.
Supplementary information
Supplementary file, rights and permissions.
Open Access This article is licensed under a Creative Commons Attribution 4.0 International License, which permits use, sharing, adaptation, distribution and reproduction in any medium or format, as long as you give appropriate credit to the original author(s) and the source, provide a link to the Creative Commons licence, and indicate if changes were made. The images or other third party material in this article are included in the article’s Creative Commons licence, unless indicated otherwise in a credit line to the material. If material is not included in the article’s Creative Commons licence and your intended use is not permitted by statutory regulation or exceeds the permitted use, you will need to obtain permission directly from the copyright holder. To view a copy of this licence, visit http://creativecommons.org/licenses/by/4.0/ .
Reprints and permissions
About this article
Cite this article.
Shen, Md., Chen, Sb. & Ding, Xd. The effectiveness of digital twins in promoting precision health across the entire population: a systematic review. npj Digit. Med. 7 , 145 (2024). https://doi.org/10.1038/s41746-024-01146-0
Download citation
Received : 29 January 2024
Accepted : 22 May 2024
Published : 03 June 2024
DOI : https://doi.org/10.1038/s41746-024-01146-0
Share this article
Anyone you share the following link with will be able to read this content:
Sorry, a shareable link is not currently available for this article.
Provided by the Springer Nature SharedIt content-sharing initiative
Quick links
- Explore articles by subject
- Guide to authors
- Editorial policies
Sign up for the Nature Briefing newsletter — what matters in science, free to your inbox daily.


An official website of the United States government
The .gov means it’s official. Federal government websites often end in .gov or .mil. Before sharing sensitive information, make sure you’re on a federal government site.
The site is secure. The https:// ensures that you are connecting to the official website and that any information you provide is encrypted and transmitted securely.
- Publications
- Account settings
Preview improvements coming to the PMC website in October 2024. Learn More or Try it out now .
- Advanced Search
- Journal List
- Wiley Open Access Collection

An overview of methodological approaches in systematic reviews
Prabhakar veginadu.
1 Department of Rural Clinical Sciences, La Trobe Rural Health School, La Trobe University, Bendigo Victoria, Australia
Hanny Calache
2 Lincoln International Institute for Rural Health, University of Lincoln, Brayford Pool, Lincoln UK
Akshaya Pandian
3 Department of Orthodontics, Saveetha Dental College, Chennai Tamil Nadu, India
Mohd Masood
Associated data.
APPENDIX B: List of excluded studies with detailed reasons for exclusion
APPENDIX C: Quality assessment of included reviews using AMSTAR 2
The aim of this overview is to identify and collate evidence from existing published systematic review (SR) articles evaluating various methodological approaches used at each stage of an SR.
The search was conducted in five electronic databases from inception to November 2020 and updated in February 2022: MEDLINE, Embase, Web of Science Core Collection, Cochrane Database of Systematic Reviews, and APA PsycINFO. Title and abstract screening were performed in two stages by one reviewer, supported by a second reviewer. Full‐text screening, data extraction, and quality appraisal were performed by two reviewers independently. The quality of the included SRs was assessed using the AMSTAR 2 checklist.
The search retrieved 41,556 unique citations, of which 9 SRs were deemed eligible for inclusion in final synthesis. Included SRs evaluated 24 unique methodological approaches used for defining the review scope and eligibility, literature search, screening, data extraction, and quality appraisal in the SR process. Limited evidence supports the following (a) searching multiple resources (electronic databases, handsearching, and reference lists) to identify relevant literature; (b) excluding non‐English, gray, and unpublished literature, and (c) use of text‐mining approaches during title and abstract screening.
The overview identified limited SR‐level evidence on various methodological approaches currently employed during five of the seven fundamental steps in the SR process, as well as some methodological modifications currently used in expedited SRs. Overall, findings of this overview highlight the dearth of published SRs focused on SR methodologies and this warrants future work in this area.
1. INTRODUCTION
Evidence synthesis is a prerequisite for knowledge translation. 1 A well conducted systematic review (SR), often in conjunction with meta‐analyses (MA) when appropriate, is considered the “gold standard” of methods for synthesizing evidence related to a topic of interest. 2 The central strength of an SR is the transparency of the methods used to systematically search, appraise, and synthesize the available evidence. 3 Several guidelines, developed by various organizations, are available for the conduct of an SR; 4 , 5 , 6 , 7 among these, Cochrane is considered a pioneer in developing rigorous and highly structured methodology for the conduct of SRs. 8 The guidelines developed by these organizations outline seven fundamental steps required in SR process: defining the scope of the review and eligibility criteria, literature searching and retrieval, selecting eligible studies, extracting relevant data, assessing risk of bias (RoB) in included studies, synthesizing results, and assessing certainty of evidence (CoE) and presenting findings. 4 , 5 , 6 , 7
The methodological rigor involved in an SR can require a significant amount of time and resource, which may not always be available. 9 As a result, there has been a proliferation of modifications made to the traditional SR process, such as refining, shortening, bypassing, or omitting one or more steps, 10 , 11 for example, limits on the number and type of databases searched, limits on publication date, language, and types of studies included, and limiting to one reviewer for screening and selection of studies, as opposed to two or more reviewers. 10 , 11 These methodological modifications are made to accommodate the needs of and resource constraints of the reviewers and stakeholders (e.g., organizations, policymakers, health care professionals, and other knowledge users). While such modifications are considered time and resource efficient, they may introduce bias in the review process reducing their usefulness. 5
Substantial research has been conducted examining various approaches used in the standardized SR methodology and their impact on the validity of SR results. There are a number of published reviews examining the approaches or modifications corresponding to single 12 , 13 or multiple steps 14 involved in an SR. However, there is yet to be a comprehensive summary of the SR‐level evidence for all the seven fundamental steps in an SR. Such a holistic evidence synthesis will provide an empirical basis to confirm the validity of current accepted practices in the conduct of SRs. Furthermore, sometimes there is a balance that needs to be achieved between the resource availability and the need to synthesize the evidence in the best way possible, given the constraints. This evidence base will also inform the choice of modifications to be made to the SR methods, as well as the potential impact of these modifications on the SR results. An overview is considered the choice of approach for summarizing existing evidence on a broad topic, directing the reader to evidence, or highlighting the gaps in evidence, where the evidence is derived exclusively from SRs. 15 Therefore, for this review, an overview approach was used to (a) identify and collate evidence from existing published SR articles evaluating various methodological approaches employed in each of the seven fundamental steps of an SR and (b) highlight both the gaps in the current research and the potential areas for future research on the methods employed in SRs.
An a priori protocol was developed for this overview but was not registered with the International Prospective Register of Systematic Reviews (PROSPERO), as the review was primarily methodological in nature and did not meet PROSPERO eligibility criteria for registration. The protocol is available from the corresponding author upon reasonable request. This overview was conducted based on the guidelines for the conduct of overviews as outlined in The Cochrane Handbook. 15 Reporting followed the Preferred Reporting Items for Systematic reviews and Meta‐analyses (PRISMA) statement. 3
2.1. Eligibility criteria
Only published SRs, with or without associated MA, were included in this overview. We adopted the defining characteristics of SRs from The Cochrane Handbook. 5 According to The Cochrane Handbook, a review was considered systematic if it satisfied the following criteria: (a) clearly states the objectives and eligibility criteria for study inclusion; (b) provides reproducible methodology; (c) includes a systematic search to identify all eligible studies; (d) reports assessment of validity of findings of included studies (e.g., RoB assessment of the included studies); (e) systematically presents all the characteristics or findings of the included studies. 5 Reviews that did not meet all of the above criteria were not considered a SR for this study and were excluded. MA‐only articles were included if it was mentioned that the MA was based on an SR.
SRs and/or MA of primary studies evaluating methodological approaches used in defining review scope and study eligibility, literature search, study selection, data extraction, RoB assessment, data synthesis, and CoE assessment and reporting were included. The methodological approaches examined in these SRs and/or MA can also be related to the substeps or elements of these steps; for example, applying limits on date or type of publication are the elements of literature search. Included SRs examined or compared various aspects of a method or methods, and the associated factors, including but not limited to: precision or effectiveness; accuracy or reliability; impact on the SR and/or MA results; reproducibility of an SR steps or bias occurred; time and/or resource efficiency. SRs assessing the methodological quality of SRs (e.g., adherence to reporting guidelines), evaluating techniques for building search strategies or the use of specific database filters (e.g., use of Boolean operators or search filters for randomized controlled trials), examining various tools used for RoB or CoE assessment (e.g., ROBINS vs. Cochrane RoB tool), or evaluating statistical techniques used in meta‐analyses were excluded. 14
2.2. Search
The search for published SRs was performed on the following scientific databases initially from inception to third week of November 2020 and updated in the last week of February 2022: MEDLINE (via Ovid), Embase (via Ovid), Web of Science Core Collection, Cochrane Database of Systematic Reviews, and American Psychological Association (APA) PsycINFO. Search was restricted to English language publications. Following the objectives of this study, study design filters within databases were used to restrict the search to SRs and MA, where available. The reference lists of included SRs were also searched for potentially relevant publications.
The search terms included keywords, truncations, and subject headings for the key concepts in the review question: SRs and/or MA, methods, and evaluation. Some of the terms were adopted from the search strategy used in a previous review by Robson et al., which reviewed primary studies on methodological approaches used in study selection, data extraction, and quality appraisal steps of SR process. 14 Individual search strategies were developed for respective databases by combining the search terms using appropriate proximity and Boolean operators, along with the related subject headings in order to identify SRs and/or MA. 16 , 17 A senior librarian was consulted in the design of the search terms and strategy. Appendix A presents the detailed search strategies for all five databases.
2.3. Study selection and data extraction
Title and abstract screening of references were performed in three steps. First, one reviewer (PV) screened all the titles and excluded obviously irrelevant citations, for example, articles on topics not related to SRs, non‐SR publications (such as randomized controlled trials, observational studies, scoping reviews, etc.). Next, from the remaining citations, a random sample of 200 titles and abstracts were screened against the predefined eligibility criteria by two reviewers (PV and MM), independently, in duplicate. Discrepancies were discussed and resolved by consensus. This step ensured that the responses of the two reviewers were calibrated for consistency in the application of the eligibility criteria in the screening process. Finally, all the remaining titles and abstracts were reviewed by a single “calibrated” reviewer (PV) to identify potential full‐text records. Full‐text screening was performed by at least two authors independently (PV screened all the records, and duplicate assessment was conducted by MM, HC, or MG), with discrepancies resolved via discussions or by consulting a third reviewer.
Data related to review characteristics, results, key findings, and conclusions were extracted by at least two reviewers independently (PV performed data extraction for all the reviews and duplicate extraction was performed by AP, HC, or MG).
2.4. Quality assessment of included reviews
The quality assessment of the included SRs was performed using the AMSTAR 2 (A MeaSurement Tool to Assess systematic Reviews). The tool consists of a 16‐item checklist addressing critical and noncritical domains. 18 For the purpose of this study, the domain related to MA was reclassified from critical to noncritical, as SRs with and without MA were included. The other six critical domains were used according to the tool guidelines. 18 Two reviewers (PV and AP) independently responded to each of the 16 items in the checklist with either “yes,” “partial yes,” or “no.” Based on the interpretations of the critical and noncritical domains, the overall quality of the review was rated as high, moderate, low, or critically low. 18 Disagreements were resolved through discussion or by consulting a third reviewer.
2.5. Data synthesis
To provide an understandable summary of existing evidence syntheses, characteristics of the methods evaluated in the included SRs were examined and key findings were categorized and presented based on the corresponding step in the SR process. The categories of key elements within each step were discussed and agreed by the authors. Results of the included reviews were tabulated and summarized descriptively, along with a discussion on any overlap in the primary studies. 15 No quantitative analyses of the data were performed.
From 41,556 unique citations identified through literature search, 50 full‐text records were reviewed, and nine systematic reviews 14 , 19 , 20 , 21 , 22 , 23 , 24 , 25 , 26 were deemed eligible for inclusion. The flow of studies through the screening process is presented in Figure 1 . A list of excluded studies with reasons can be found in Appendix B .

Study selection flowchart
3.1. Characteristics of included reviews
Table 1 summarizes the characteristics of included SRs. The majority of the included reviews (six of nine) were published after 2010. 14 , 22 , 23 , 24 , 25 , 26 Four of the nine included SRs were Cochrane reviews. 20 , 21 , 22 , 23 The number of databases searched in the reviews ranged from 2 to 14, 2 reviews searched gray literature sources, 24 , 25 and 7 reviews included a supplementary search strategy to identify relevant literature. 14 , 19 , 20 , 21 , 22 , 23 , 26 Three of the included SRs (all Cochrane reviews) included an integrated MA. 20 , 21 , 23
Characteristics of included studies
SR = systematic review; MA = meta‐analysis; RCT = randomized controlled trial; CCT = controlled clinical trial; N/R = not reported.
The included SRs evaluated 24 unique methodological approaches (26 in total) used across five steps in the SR process; 8 SRs evaluated 6 approaches, 19 , 20 , 21 , 22 , 23 , 24 , 25 , 26 while 1 review evaluated 18 approaches. 14 Exclusion of gray or unpublished literature 21 , 26 and blinding of reviewers for RoB assessment 14 , 23 were evaluated in two reviews each. Included SRs evaluated methods used in five different steps in the SR process, including methods used in defining the scope of review ( n = 3), literature search ( n = 3), study selection ( n = 2), data extraction ( n = 1), and RoB assessment ( n = 2) (Table 2 ).
Summary of findings from review evaluating systematic review methods
There was some overlap in the primary studies evaluated in the included SRs on the same topics: Schmucker et al. 26 and Hopewell et al. 21 ( n = 4), Hopewell et al. 20 and Crumley et al. 19 ( n = 30), and Robson et al. 14 and Morissette et al. 23 ( n = 4). There were no conflicting results between any of the identified SRs on the same topic.
3.2. Methodological quality of included reviews
Overall, the quality of the included reviews was assessed as moderate at best (Table 2 ). The most common critical weakness in the reviews was failure to provide justification for excluding individual studies (four reviews). Detailed quality assessment is provided in Appendix C .
3.3. Evidence on systematic review methods
3.3.1. methods for defining review scope and eligibility.
Two SRs investigated the effect of excluding data obtained from gray or unpublished sources on the pooled effect estimates of MA. 21 , 26 Hopewell et al. 21 reviewed five studies that compared the impact of gray literature on the results of a cohort of MA of RCTs in health care interventions. Gray literature was defined as information published in “print or electronic sources not controlled by commercial or academic publishers.” Findings showed an overall greater treatment effect for published trials than trials reported in gray literature. In a more recent review, Schmucker et al. 26 addressed similar objectives, by investigating gray and unpublished data in medicine. In addition to gray literature, defined similar to the previous review by Hopewell et al., the authors also evaluated unpublished data—defined as “supplemental unpublished data related to published trials, data obtained from the Food and Drug Administration or other regulatory websites or postmarketing analyses hidden from the public.” The review found that in majority of the MA, excluding gray literature had little or no effect on the pooled effect estimates. The evidence was limited to conclude if the data from gray and unpublished literature had an impact on the conclusions of MA. 26
Morrison et al. 24 examined five studies measuring the effect of excluding non‐English language RCTs on the summary treatment effects of SR‐based MA in various fields of conventional medicine. Although none of the included studies reported major difference in the treatment effect estimates between English only and non‐English inclusive MA, the review found inconsistent evidence regarding the methodological and reporting quality of English and non‐English trials. 24 As such, there might be a risk of introducing “language bias” when excluding non‐English language RCTs. The authors also noted that the numbers of non‐English trials vary across medical specialties, as does the impact of these trials on MA results. Based on these findings, Morrison et al. 24 conclude that literature searches must include non‐English studies when resources and time are available to minimize the risk of introducing “language bias.”
3.3.2. Methods for searching studies
Crumley et al. 19 analyzed recall (also referred to as “sensitivity” by some researchers; defined as “percentage of relevant studies identified by the search”) and precision (defined as “percentage of studies identified by the search that were relevant”) when searching a single resource to identify randomized controlled trials and controlled clinical trials, as opposed to searching multiple resources. The studies included in their review frequently compared a MEDLINE only search with the search involving a combination of other resources. The review found low median recall estimates (median values between 24% and 92%) and very low median precisions (median values between 0% and 49%) for most of the electronic databases when searched singularly. 19 A between‐database comparison, based on the type of search strategy used, showed better recall and precision for complex and Cochrane Highly Sensitive search strategies (CHSSS). In conclusion, the authors emphasize that literature searches for trials in SRs must include multiple sources. 19
In an SR comparing handsearching and electronic database searching, Hopewell et al. 20 found that handsearching retrieved more relevant RCTs (retrieval rate of 92%−100%) than searching in a single electronic database (retrieval rates of 67% for PsycINFO/PsycLIT, 55% for MEDLINE, and 49% for Embase). The retrieval rates varied depending on the quality of handsearching, type of electronic search strategy used (e.g., simple, complex or CHSSS), and type of trial reports searched (e.g., full reports, conference abstracts, etc.). The authors concluded that handsearching was particularly important in identifying full trials published in nonindexed journals and in languages other than English, as well as those published as abstracts and letters. 20
The effectiveness of checking reference lists to retrieve additional relevant studies for an SR was investigated by Horsley et al. 22 The review reported that checking reference lists yielded 2.5%–40% more studies depending on the quality and comprehensiveness of the electronic search used. The authors conclude that there is some evidence, although from poor quality studies, to support use of checking reference lists to supplement database searching. 22
3.3.3. Methods for selecting studies
Three approaches relevant to reviewer characteristics, including number, experience, and blinding of reviewers involved in the screening process were highlighted in an SR by Robson et al. 14 Based on the retrieved evidence, the authors recommended that two independent, experienced, and unblinded reviewers be involved in study selection. 14 A modified approach has also been suggested by the review authors, where one reviewer screens and the other reviewer verifies the list of excluded studies, when the resources are limited. It should be noted however this suggestion is likely based on the authors’ opinion, as there was no evidence related to this from the studies included in the review.
Robson et al. 14 also reported two methods describing the use of technology for screening studies: use of Google Translate for translating languages (for example, German language articles to English) to facilitate screening was considered a viable method, while using two computer monitors for screening did not increase the screening efficiency in SR. Title‐first screening was found to be more efficient than simultaneous screening of titles and abstracts, although the gain in time with the former method was lesser than the latter. Therefore, considering that the search results are routinely exported as titles and abstracts, Robson et al. 14 recommend screening titles and abstracts simultaneously. However, the authors note that these conclusions were based on very limited number (in most instances one study per method) of low‐quality studies. 14
3.3.4. Methods for data extraction
Robson et al. 14 examined three approaches for data extraction relevant to reviewer characteristics, including number, experience, and blinding of reviewers (similar to the study selection step). Although based on limited evidence from a small number of studies, the authors recommended use of two experienced and unblinded reviewers for data extraction. The experience of the reviewers was suggested to be especially important when extracting continuous outcomes (or quantitative) data. However, when the resources are limited, data extraction by one reviewer and a verification of the outcomes data by a second reviewer was recommended.
As for the methods involving use of technology, Robson et al. 14 identified limited evidence on the use of two monitors to improve the data extraction efficiency and computer‐assisted programs for graphical data extraction. However, use of Google Translate for data extraction in non‐English articles was not considered to be viable. 14 In the same review, Robson et al. 14 identified evidence supporting contacting authors for obtaining additional relevant data.
3.3.5. Methods for RoB assessment
Two SRs examined the impact of blinding of reviewers for RoB assessments. 14 , 23 Morissette et al. 23 investigated the mean differences between the blinded and unblinded RoB assessment scores and found inconsistent differences among the included studies providing no definitive conclusions. Similar conclusions were drawn in a more recent review by Robson et al., 14 which included four studies on reviewer blinding for RoB assessment that completely overlapped with Morissette et al. 23
Use of experienced reviewers and provision of additional guidance for RoB assessment were examined by Robson et al. 14 The review concluded that providing intensive training and guidance on assessing studies reporting insufficient data to the reviewers improves RoB assessments. 14 Obtaining additional data related to quality assessment by contacting study authors was also found to help the RoB assessments, although based on limited evidence. When assessing the qualitative or mixed method reviews, Robson et al. 14 recommends the use of a structured RoB tool as opposed to an unstructured tool. No SRs were identified on data synthesis and CoE assessment and reporting steps.
4. DISCUSSION
4.1. summary of findings.
Nine SRs examining 24 unique methods used across five steps in the SR process were identified in this overview. The collective evidence supports some current traditional and modified SR practices, while challenging other approaches. However, the quality of the included reviews was assessed to be moderate at best and in the majority of the included SRs, evidence related to the evaluated methods was obtained from very limited numbers of primary studies. As such, the interpretations from these SRs should be made cautiously.
The evidence gathered from the included SRs corroborate a few current SR approaches. 5 For example, it is important to search multiple resources for identifying relevant trials (RCTs and/or CCTs). The resources must include a combination of electronic database searching, handsearching, and reference lists of retrieved articles. 5 However, no SRs have been identified that evaluated the impact of the number of electronic databases searched. A recent study by Halladay et al. 27 found that articles on therapeutic intervention, retrieved by searching databases other than PubMed (including Embase), contributed only a small amount of information to the MA and also had a minimal impact on the MA results. The authors concluded that when the resources are limited and when large number of studies are expected to be retrieved for the SR or MA, PubMed‐only search can yield reliable results. 27
Findings from the included SRs also reiterate some methodological modifications currently employed to “expedite” the SR process. 10 , 11 For example, excluding non‐English language trials and gray/unpublished trials from MA have been shown to have minimal or no impact on the results of MA. 24 , 26 However, the efficiency of these SR methods, in terms of time and the resources used, have not been evaluated in the included SRs. 24 , 26 Of the SRs included, only two have focused on the aspect of efficiency 14 , 25 ; O'Mara‐Eves et al. 25 report some evidence to support the use of text‐mining approaches for title and abstract screening in order to increase the rate of screening. Moreover, only one included SR 14 considered primary studies that evaluated reliability (inter‐ or intra‐reviewer consistency) and accuracy (validity when compared against a “gold standard” method) of the SR methods. This can be attributed to the limited number of primary studies that evaluated these outcomes when evaluating the SR methods. 14 Lack of outcome measures related to reliability, accuracy, and efficiency precludes making definitive recommendations on the use of these methods/modifications. Future research studies must focus on these outcomes.
Some evaluated methods may be relevant to multiple steps; for example, exclusions based on publication status (gray/unpublished literature) and language of publication (non‐English language studies) can be outlined in the a priori eligibility criteria or can be incorporated as search limits in the search strategy. SRs included in this overview focused on the effect of study exclusions on pooled treatment effect estimates or MA conclusions. Excluding studies from the search results, after conducting a comprehensive search, based on different eligibility criteria may yield different results when compared to the results obtained when limiting the search itself. 28 Further studies are required to examine this aspect.
Although we acknowledge the lack of standardized quality assessment tools for methodological study designs, we adhered to the Cochrane criteria for identifying SRs in this overview. This was done to ensure consistency in the quality of the included evidence. As a result, we excluded three reviews that did not provide any form of discussion on the quality of the included studies. The methods investigated in these reviews concern supplementary search, 29 data extraction, 12 and screening. 13 However, methods reported in two of these three reviews, by Mathes et al. 12 and Waffenschmidt et al., 13 have also been examined in the SR by Robson et al., 14 which was included in this overview; in most instances (with the exception of one study included in Mathes et al. 12 and Waffenschmidt et al. 13 each), the studies examined in these excluded reviews overlapped with those in the SR by Robson et al. 14
One of the key gaps in the knowledge observed in this overview was the dearth of SRs on the methods used in the data synthesis component of SR. Narrative and quantitative syntheses are the two most commonly used approaches for synthesizing data in evidence synthesis. 5 There are some published studies on the proposed indications and implications of these two approaches. 30 , 31 These studies found that both data synthesis methods produced comparable results and have their own advantages, suggesting that the choice of the method must be based on the purpose of the review. 31 With increasing number of “expedited” SR approaches (so called “rapid reviews”) avoiding MA, 10 , 11 further research studies are warranted in this area to determine the impact of the type of data synthesis on the results of the SR.
4.2. Implications for future research
The findings of this overview highlight several areas of paucity in primary research and evidence synthesis on SR methods. First, no SRs were identified on methods used in two important components of the SR process, including data synthesis and CoE and reporting. As for the included SRs, a limited number of evaluation studies have been identified for several methods. This indicates that further research is required to corroborate many of the methods recommended in current SR guidelines. 4 , 5 , 6 , 7 Second, some SRs evaluated the impact of methods on the results of quantitative synthesis and MA conclusions. Future research studies must also focus on the interpretations of SR results. 28 , 32 Finally, most of the included SRs were conducted on specific topics related to the field of health care, limiting the generalizability of the findings to other areas. It is important that future research studies evaluating evidence syntheses broaden the objectives and include studies on different topics within the field of health care.
4.3. Strengths and limitations
To our knowledge, this is the first overview summarizing current evidence from SRs and MA on different methodological approaches used in several fundamental steps in SR conduct. The overview methodology followed well established guidelines and strict criteria defined for the inclusion of SRs.
There are several limitations related to the nature of the included reviews. Evidence for most of the methods investigated in the included reviews was derived from a limited number of primary studies. Also, the majority of the included SRs may be considered outdated as they were published (or last updated) more than 5 years ago 33 ; only three of the nine SRs have been published in the last 5 years. 14 , 25 , 26 Therefore, important and recent evidence related to these topics may not have been included. Substantial numbers of included SRs were conducted in the field of health, which may limit the generalizability of the findings. Some method evaluations in the included SRs focused on quantitative analyses components and MA conclusions only. As such, the applicability of these findings to SR more broadly is still unclear. 28 Considering the methodological nature of our overview, limiting the inclusion of SRs according to the Cochrane criteria might have resulted in missing some relevant evidence from those reviews without a quality assessment component. 12 , 13 , 29 Although the included SRs performed some form of quality appraisal of the included studies, most of them did not use a standardized RoB tool, which may impact the confidence in their conclusions. Due to the type of outcome measures used for the method evaluations in the primary studies and the included SRs, some of the identified methods have not been validated against a reference standard.
Some limitations in the overview process must be noted. While our literature search was exhaustive covering five bibliographic databases and supplementary search of reference lists, no gray sources or other evidence resources were searched. Also, the search was primarily conducted in health databases, which might have resulted in missing SRs published in other fields. Moreover, only English language SRs were included for feasibility. As the literature search retrieved large number of citations (i.e., 41,556), the title and abstract screening was performed by a single reviewer, calibrated for consistency in the screening process by another reviewer, owing to time and resource limitations. These might have potentially resulted in some errors when retrieving and selecting relevant SRs. The SR methods were grouped based on key elements of each recommended SR step, as agreed by the authors. This categorization pertains to the identified set of methods and should be considered subjective.
5. CONCLUSIONS
This overview identified limited SR‐level evidence on various methodological approaches currently employed during five of the seven fundamental steps in the SR process. Limited evidence was also identified on some methodological modifications currently used to expedite the SR process. Overall, findings highlight the dearth of SRs on SR methodologies, warranting further work to confirm several current recommendations on conventional and expedited SR processes.
CONFLICT OF INTEREST
The authors declare no conflicts of interest.
Supporting information
APPENDIX A: Detailed search strategies
ACKNOWLEDGMENTS
The first author is supported by a La Trobe University Full Fee Research Scholarship and a Graduate Research Scholarship.
Open Access Funding provided by La Trobe University.
Veginadu P, Calache H, Gussy M, Pandian A, Masood M. An overview of methodological approaches in systematic reviews . J Evid Based Med . 2022; 15 :39–54. 10.1111/jebm.12468 [ PMC free article ] [ PubMed ] [ CrossRef ] [ Google Scholar ]
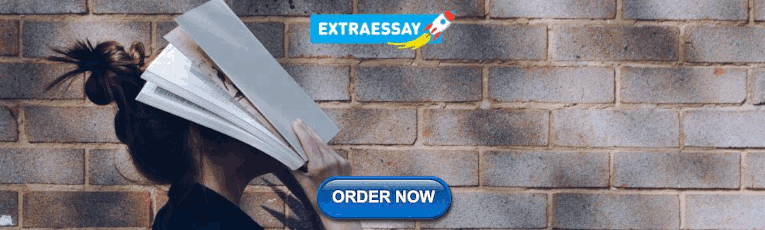
IMAGES
VIDEO
COMMENTS
Introduction. Literature review is an essential feature of academic research. Fundamentally, knowledge advancement must be built on prior existing work. To push the knowledge frontier, we must know where the frontier is. By reviewing relevant literature, we understand the breadth and depth of the existing body of work and identify gaps to explore.
A systematic review collects all possible studies related to a given topic and design, and reviews and analyzes their results [ 1 ]. During the systematic review process, the quality of studies is evaluated, and a statistical meta-analysis of the study results is conducted on the basis of their quality. A meta-analysis is a valid, objective ...
A systematic review is a type of review that uses repeatable methods to find, select, and synthesize all available evidence. It answers a clearly formulated research question and explicitly states the methods used to arrive at the answer. Example: Systematic review. In 2008, Dr. Robert Boyle and his colleagues published a systematic review in ...
Meta-analysis is a statistical tool that provides pooled estimates of effect from the data extracted from individual studies in the systematic review. The graphical output of meta-analysis is a forest plot which provides information on individual studies and the pooled effect. Systematic reviews of literature can be undertaken for all types of ...
A systematic review is guided filtering and synthesis of all available evidence addressing a specific, focused research question, generally about a specific intervention or exposure. The use of standardized, systematic methods and pre-selected eligibility criteria reduce the risk of bias in identifying, selecting and analyzing relevant studies.
Method details Overview. A Systematic Literature Review (SLR) is a research methodology to collect, identify, and critically analyze the available research studies (e.g., articles, conference proceedings, books, dissertations) through a systematic procedure [12].An SLR updates the reader with current literature about a subject [6].The goal is to review critical points of current knowledge on a ...
The best reviews synthesize studies to draw broad theoretical conclusions about what a literature means, linking theory to evidence and evidence to theory. This guide describes how to plan, conduct, organize, and present a systematic review of quantitative (meta-analysis) or qualitative (narrative review, meta-synthesis) information.
A systematic review is a summary of the medical literature that uses explicit and reproducible methods to systematically search, critically appraise, and synthesize on a specific issue. ... Even though systematic review and meta-analysis are considered the best evidence for getting a definitive answer to a research question, there are certain ...
CONCLUSION. Siddaway 16 noted that, "The best reviews synthesize studies to draw broad theoretical conclusions about what the literature means, linking theory to evidence and evidence to theory" (p. 747). To that end, high quality systematic reviews are explicit, rigorous, and reproducible. It is these three criteria that should guide authors seeking to write a systematic review or editors ...
The Preferred Reporting Items for Systematic reviews and Meta-Analyses (PRISMA) statement, published in 2009, was designed to help systematic reviewers transparently report why the review was done, what the authors did, and what they found. Over the past decade, advances in systematic review methodology and terminology have necessitated an update to the guideline. The PRISMA 2020 statement ...
Systematic literature reviews (SRs) are a way of synthesising scientific evidence to answer a particular ... SRs treat the literature review process like a scientific process, and apply concepts of empirical ... Perform sensitivity analysis if possible. If the results of all studies are pooled together in a quantitative analysis, this is called ...
Qualitative, narrative synthesis. Thematic analysis, may include conceptual models. Rapid review. Assessment of what is already known about a policy or practice issue, by using systematic review methods to search and critically appraise existing research. Completeness of searching determined by time constraints.
Review Manager (RevMan) is a web-based software that manages the entire literature review process and meta-analysis. The meta-analyst uploads all studies to RevMan library, where they can be managed and exanimated for inclusion. Like CMA, RevMan enables authors to conduct overall analysis and moderator analysis. 4.4.6.3 Stata
Systematic review and meta-analysis • Synthesizes guidelines for systematic literature reviews • Provides guidelines for conducting a systematic review and meta-analysis in social sciences. Palmatier et al. (2018) Marketing: Review papers and systematic reviews •
Nevertheless, to carry out a systematic literature review /meta-analysis, researchers must deeply understand its methodology. This narrative review aims to act as a learning tool for new ...
This paper presents a method to conduct a systematic literature review (SLR) and meta-analysis studies on environmental science. SLR is a process that allowed to collect relevant evidence on the given topic that fits the pre-specified eligibility criteria and to have an answer for the formulated research questions.
In this section, the methodology of our tertiary study is presented. This study can be considered as a systematic review study that targets secondary studies on sentiment analysis, which is a widely researched topic. There are several reviews and mapping studies available on sentiment analysis in the literature.
What is a Systematic Review? A systematic review attempts to identify, appraise and synthesize all available relevant evidence that to answer a specific, focus research question. Researchers conducting systematic reviews use standardized, systematic methods and pre-selected eligibility criteria to reduce the risk of bias in identifying ...
Other previous attempts at summarising the available evidence refrained from quantification and comprehensive meta-analysis due to methodological difficulties. Today, as a result, no comprehensive systematic review and meta-analysis that aimed to quantify the incidence or severity of antidepressant discontinuation symptoms has been published.
This systematic literature review focuses on assessing the potential utility of bitemark analysis in forensic identification. This review adhered to the PRISMA statement (Preferred Reporting Items for Systematic Reviews and Meta-Analyses) guidelines as outlined by Page et al. (2021) .
A systematic review that incorporates quantitative pooling of similar studies to produce an overall summary of treatment effects is a meta-analysis. A systematic review should have clear, focused clinical objectives containing four elements expressed through the acronym PICO (Patient, group of patients, or problem, an Intervention, a Comparison ...
Internet usage has seen a stark global rise over the last few decades, particularly among adolescents and young people, who have also been diagnosed increasingly with internet addiction (IA). IA impacts several neural networks that influence an adolescent's behaviour and development. This article issued a literature review on the resting-state and task-based functional magnetic resonance ...
This systematic review and meta-analysis were registered prospectively on the International Prospective Register of Systematic Reviews (PROSPERO) database (registration number: CRD42020207504).
Gut microbiome-modulating agents (MMAs), including probiotics, prebiotics, postbiotics, and synbiotics, are shown to ameliorate type 1 diabetes (T1D) by restoring the microbiome from dysbiosis. The objective of this systematic review and meta-analysis was to assess the impact of MMAs on hemoglobin A1c (HbA1c) and biomarkers associated with (T1D). A comprehensive search was conducted in PubMed ...
Work on the "double empathy problem" (DEP) is rapidly growing in academic and applied settings (e.g., clinical practice). It is most popular in research on conditions, like autism, which are characterized by social cognitive difficulties. Drawing from this literature, we propose that, while research on the DEP has the potential to improve understanding of both typical and atypical social ...
Another systematic review 9, through an analysis of 22 selected publications, summarized the diverse application scenarios of digital twins in healthcare, confirming their potential in continuous ...
1. INTRODUCTION. Evidence synthesis is a prerequisite for knowledge translation. 1 A well conducted systematic review (SR), often in conjunction with meta‐analyses (MA) when appropriate, is considered the "gold standard" of methods for synthesizing evidence related to a topic of interest. 2 The central strength of an SR is the transparency of the methods used to systematically search ...