News alert: UC Berkeley has announced its next university librarian
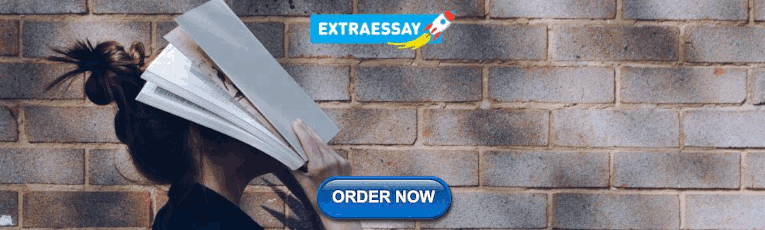
Secondary menu
- Log in to your Library account
- Hours and Maps
- Connect from Off Campus
- UC Berkeley Home
Search form
Research methods--quantitative, qualitative, and more: overview.
- Quantitative Research
- Qualitative Research
- Data Science Methods (Machine Learning, AI, Big Data)
- Text Mining and Computational Text Analysis
- Evidence Synthesis/Systematic Reviews
- Get Data, Get Help!
About Research Methods
This guide provides an overview of research methods, how to choose and use them, and supports and resources at UC Berkeley.
As Patten and Newhart note in the book Understanding Research Methods , "Research methods are the building blocks of the scientific enterprise. They are the "how" for building systematic knowledge. The accumulation of knowledge through research is by its nature a collective endeavor. Each well-designed study provides evidence that may support, amend, refute, or deepen the understanding of existing knowledge...Decisions are important throughout the practice of research and are designed to help researchers collect evidence that includes the full spectrum of the phenomenon under study, to maintain logical rules, and to mitigate or account for possible sources of bias. In many ways, learning research methods is learning how to see and make these decisions."
The choice of methods varies by discipline, by the kind of phenomenon being studied and the data being used to study it, by the technology available, and more. This guide is an introduction, but if you don't see what you need here, always contact your subject librarian, and/or take a look to see if there's a library research guide that will answer your question.
Suggestions for changes and additions to this guide are welcome!
START HERE: SAGE Research Methods
Without question, the most comprehensive resource available from the library is SAGE Research Methods. HERE IS THE ONLINE GUIDE to this one-stop shopping collection, and some helpful links are below:
- SAGE Research Methods
- Little Green Books (Quantitative Methods)
- Little Blue Books (Qualitative Methods)
- Dictionaries and Encyclopedias
- Case studies of real research projects
- Sample datasets for hands-on practice
- Streaming video--see methods come to life
- Methodspace- -a community for researchers
- SAGE Research Methods Course Mapping
Library Data Services at UC Berkeley
Library Data Services Program and Digital Scholarship Services
The LDSP offers a variety of services and tools ! From this link, check out pages for each of the following topics: discovering data, managing data, collecting data, GIS data, text data mining, publishing data, digital scholarship, open science, and the Research Data Management Program.
Be sure also to check out the visual guide to where to seek assistance on campus with any research question you may have!
Library GIS Services
Other Data Services at Berkeley
D-Lab Supports Berkeley faculty, staff, and graduate students with research in data intensive social science, including a wide range of training and workshop offerings Dryad Dryad is a simple self-service tool for researchers to use in publishing their datasets. It provides tools for the effective publication of and access to research data. Geospatial Innovation Facility (GIF) Provides leadership and training across a broad array of integrated mapping technologies on campu Research Data Management A UC Berkeley guide and consulting service for research data management issues
General Research Methods Resources
Here are some general resources for assistance:
- Assistance from ICPSR (must create an account to access): Getting Help with Data , and Resources for Students
- Wiley Stats Ref for background information on statistics topics
- Survey Documentation and Analysis (SDA) . Program for easy web-based analysis of survey data.
Consultants
- D-Lab/Data Science Discovery Consultants Request help with your research project from peer consultants.
- Research data (RDM) consulting Meet with RDM consultants before designing the data security, storage, and sharing aspects of your qualitative project.
- Statistics Department Consulting Services A service in which advanced graduate students, under faculty supervision, are available to consult during specified hours in the Fall and Spring semesters.
Related Resourcex
- IRB / CPHS Qualitative research projects with human subjects often require that you go through an ethics review.
- OURS (Office of Undergraduate Research and Scholarships) OURS supports undergraduates who want to embark on research projects and assistantships. In particular, check out their "Getting Started in Research" workshops
- Sponsored Projects Sponsored projects works with researchers applying for major external grants.
- Next: Quantitative Research >>
- Last Updated: Apr 25, 2024 11:09 AM
- URL: https://guides.lib.berkeley.edu/researchmethods
Module 2: Research Methods in Learning and Behavior
Module Overview
Module 2 will cover the critical issue of how research is conducted in the experimental analysis of behavior. To do this, we will discuss the scientific method, research designs, the apparatus we use, how we collect data, and dependent measures used to show that learning has occurred. We also will break down the structure of a research article and make a case for the use of both humans and animals in learning and behavior research.
Module Outline
2.1. The Scientific Method
2.2. research designs used in the experimental analysis of behavior, 2.3. dependent measures, 2.4. animal and human research.
Module Learning Outcomes
- Describe the steps in the scientific method and how this process is utilized in the experimental analysis of behavior.
- Describe specific research designs, data collection methods, and apparatus used in the experimental analysis of behavior.
- Understand the basic structure of a research article.
- List and describe dependent measures used in learning experiments.
- Explain why animals are used in learning research.
- Describe safeguards to protect human beings in scientific research.
Section Learning Objectives
- Define scientific method.
- Outline and describe the steps of the scientific method, defining all key terms.
- Define functional relationship and explain how it produces a contingency.
- Explain the concept of a behavioral definition.
- Distinguish between stimuli and responses and define related concepts.
- Distinguish types of contiguity, and the term from contingency.
- Describe the typical phases in learning research.
2.1.1. The Steps of The Scientific Method
In Module 1, we learned that psychology was the scientific study of behavior and mental processes. We will spend quite a lot of time on the behavior and mental processes part, but before we proceed, it is prudent to elaborate more on what makes psychology scientific. It is safe to say that most people not within our discipline or a sister science would be surprised to learn that psychology utilizes the scientific method at all.
So what is the scientific method? Simply, the scientific method is a systematic method for gathering knowledge about the world around us. The key word here is that it is systematic, meaning there is a set way to use it. What is that way? Well, depending on what source you look at it can include a varying number of steps. For our purposes, the following will be used:
Table 2.1: The Steps of the Scientific Method
2.1.2. Making Cause and Effect Statements in the Experimental Analysis of Behavior
As you have seen, scientists seek to make causal statements about what they are studying. In the study of learning and behavior, we call this a functional relationship. This occurs when we can say a target behavior has changed due to the use of a procedure/treatment/strategy and this relationship has been replicated at least one other time. A contingency is when one thing occurs due to another. Think of it as an if-then statement. If I do X then Y will happen. We can also say that when we experience Y that X preceded it. Concerning a functional relationship, if I introduce a treatment, then the animal responds as such or if that animal pushes the lever, then she receives a food pellet.
To help arrive at a functional relationship, we have to understand what we are studying. In science, we say we operationally define our variables. In the realm of learning, we call this a behavioral definition, or a precise, objective, unambiguous description of the behavior. The key is that we must state our behavioral definition with enough precision that anyone can read it and be able to accurately measure the behavior when it occurs.
2.1.3. Frequently Used Terms in the Experimental Analysis of Behavior
In the experimental analysis of behavior, we frequently talk about an animal or person experiencing a trial. Simply, a trial is one instance or attempt at learning. Each time a rat is placed in a maze this is considered one trial. We can then determine if learning is occurring using different dependent measures described in Section 2.3. If a child is asked to complete a math problem and then a second is introduced, and then a third, each practice problem represents a trial.
As you saw in Module 1, behaviorism is the science of stimuli and responses. What do these terms indicate? Stimuli are the environmental events that have the potential to trigger behavior, called a response . If your significant other does something nice for you and you say, ‘Thank you,’ the kind act is the stimulus which leads to your response of thanking him/her. Stimuli have to be sensed to bring about a response. This occurs through the five senses — vision, hearing, touch, smell, and taste. Stimuli can take on two forms. Appetitive stimuli are those that an organism desires and seeks out while aversive stimuli are readily avoided. An example of the former would be food or water and the latter is exemplified by extremes of temperature, shock, or a spanking by a parent.
As you will come to see in Module 6, we can make a stimulus more desirable or undesirable, called an establishing operation , or make it less desirable or undesirable, called an abolishing operation . Such techniques are called motivating operations . Food may be seen as more attractive, desirable, or pleasant if we are hungry but less desirable (or more undesirable) if we are full. A punishment such as taking away video games is more undesirable if the child likes to play games such as Call of Duty or Madden but is less undesirable (or maybe even has no impact) if they do not enjoy video games. Linked to the discussion above, food is an appetitive stimulus and could be an establishing operation if we are hungry. A valued video game also represents an establishing operation if we threaten its removal, and we will want to avoid such punishment, which makes the threat an aversive stimulus.
As noted earlier, the response is simply the behavior that is made and can take on many different forms. A dog may learn to salivate (response) to the sound of a bell (stimulus). A person may begin going to the gym if he or she seeks to gain tokens to purchase back up reinforcers (more on this in Module 7). A person may work harder in the future if they received a compliment from their boss today (either through email and visual or spoken or through hearing).
Another important concept is contiguity and occurs when two events are associated with one another because they occur together closely, whether in time called temporal contiguity or in space called spatial contiguity . In the case of time, we may come to associate thanking someone for saying ‘good job’ if we hear others doing this and the two verbal behaviors occur very close in time. Usually, the ‘Thank you’ (or other response) follows the praise within seconds. In the case of space, we may learn to use a spatula to flip our hamburgers on the grill if the spatula is placed next to the stove and not in another room. Do not confuse contiguity with contingency. Though the terms look the same they have very different meanings.
Finally, in learning research, we often distinguish two phases — baseline and treatment. Baseline Phase occurs before any strategy or strategies are put into effect. This phase will essentially be used to compare against the treatment phase. We are also trying to find out exactly how much of the target behavior the person or animal is engaging in. Treatment Phase occurs when the strategy or strategies are used, or you might say when the manipulation is implemented. Note that in behavior modification we also talk about what is called the maintenance phase. More on this in Module 7.
- List the five main research methods used in psychology.
- Describe observational research, listing its advantages and disadvantages.
- Describe the case study approach to research, listing its advantages and disadvantages.
- Describe survey research, listing its advantages and disadvantages.
- Describe correlational research, listing its advantages and disadvantages.
- Describe experimental research, listing its advantages and disadvantages.
- Define key terms related to experiments.
- Describe specific types of experimental designs used in learning research.
- Describe the ways we gather data in learning research (or applied behavior analysis).
- Outline the types of apparatus used in learning experiments.
- Outline the parts of a research article and describe their function.
Step 3 called on the scientist to test his or her hypothesis. Psychology as a discipline uses five main research designs to do just that. These include observational research, case studies, surveys, correlational designs, and experiments.
2.2.1. Observational Research
In terms of naturalistic observation , the scientist studies human or animal behavior in its natural environment which could include the home, school, or a forest. The researcher counts, measures, and rates behavior in a systematic way and at times uses multiple judges to ensure accuracy in how the behavior is being measured. This is called inter-rater reliability . The advantage of this method is that you witness behavior as it occurs and it is not tainted by the experimenter. The disadvantage is that it could take a long time for the behavior to occur and if the researcher is detected then this may influence the behavior of those being observed. In the case of the latter, the behavior of the observed becomes artificial .
Laboratory observation involves observing people or animals in a laboratory setting. The researcher might want to know more about parent-child interactions and so brings a mother and her child into the lab to engage in preplanned tasks such as playing with toys, eating a meal, or the mother leaving the room for a short period of time. The advantage of this method over the naturalistic method is that the experimenter can use sophisticated equipment and videotape the session to examine it later. The problem is that since the subjects know the experimenter is watching them, their behavior could become artificial.
2.2.2. Case Studies
Psychology can also utilize a detailed description of one person or a small group based on careful observation. The advantage of this method is that you arrive at a rich description of the behavior being investigated, but the disadvantage is that what you are learning may be unrepresentative of the larger population and so lacks generalizability . Again, bear in mind that you are studying one person or a very small group. Can you possibly make conclusions about all people from just one or even five or ten? The other issue is that the case study is subject to the bias of the researcher in terms of what is included in the final write up and what is left out. Despite these limitations, case studies can lead us to novel ideas about the cause of a behavior and help us to study unusual conditions that occur too infrequently to study with large sample sizes and in a systematic way.
2.2.3. Surveys/Self-Report Data
A survey is a questionnaire consisting of at least one scale with a number of questions that assess a psychological construct of interest such as parenting style, depression, locus of control, attitudes, or sensation-seeking behavior. It may be administered by paper and pencil or computer. Surveys allow for the collection of large amounts of data quickly, but the actual survey could be tedious for the participant, and social desirability , or when a participant answers questions dishonestly so that he/she is seen in a more favorable light, could be an issue. For instance, if you are asking high school students about their sexual activity, they may not give genuine answers for fear that their parents will find out. Or if you wanted to know about prejudiced attitudes of a group of people, you could use the survey method. You could alternatively gather this information via an interview in a structured, semi-structured, or unstructured fashion. Important to survey research is that you have random sampling, or when everyone in the population has an equal chance of being included in the sample. This helps the survey to be representative of the population, and in terms of key demographic variables such as gender, age, ethnicity, race, education level, and religious orientation. Surveys are not frequently used in the experimental analysis of behavior.
2.2.4. Correlational Research
This research method examines the relationship between two variables or two groups of variables. A numerical measure of the strength of this relationship is derived, called the correlation coefficient , and can range from -1.00, which indicates a perfect inverse relationship meaning that as one variable goes up the other goes down, to 0 or no relationship at all, to +1.00 or a perfect relationship in which as one variable goes up or down so does the other. In terms of a negative correlation we might say that as a parent becomes more rigid, controlling, and cold, the attachment of the child to parent goes down. In contrast, as a parent becomes warmer, more loving, and provides structure, the child becomes more attached. The advantage of correlational research is that you can correlate anything. The disadvantage is also that you can correlate anything. Variables that do not have any relationship to one another could be viewed as related. Yes. This is both an advantage and a disadvantage. For instance, we might correlate instances of making peanut butter and jelly sandwiches with someone we are attracted to sitting near us at lunch. Are the two related? Not likely, unless you make a really good PB&J, but then the person is probably only interested in you for food and not companionship. The main issue here is that correlation does not allow you to make a causal statement.
2.2.5. Experiments
An experiment is a controlled test of a hypothesis in which a researcher manipulates one variable and measures its effect on another. A variable is anything that varies over time or from one situation to the next. Patience could be an example of a variable. Though we may be patient in one situation, we may have less if a second situation occurs close in time. The first could have lowered our ability to cope making an emotional reaction quicker to occur even if the two situations are about the same in terms of impact. Another variable is weight. Anyone who has tried to shed some pounds and weighs in daily knows just how much weight can vary from day to day, or even on the same day. In terms of experiments, the variable that is manipulated is called the independent variable (IV) and the one that is measured is called the dependent variable (DV) .
A common feature of experiments is to have a control group that does not receive the treatment, or is not manipulated, and an experimental group that does receive the treatment or manipulation. If the experiment includes random assignment, participants have an equal chance of being placed in the control or experimental group. The control group allows the researcher to make a comparison to the experimental group, making a causal statement possible, and stronger.
Within the experimental analysis of behavior (and applied behavior analysis), experimental procedures take on several different forms. In discussing each, understand that we will use the following notations:
A will represent the baseline phase and B will represent the treatment phase.
- A-B design — This is by far the most basic of all designs used in behavior modification and includes just one rotation from baseline to treatment phase and from that we see if the behavior changed in the predicted manner. The issue with this design is that no functional relationship can be established since there is no replication. It is possible that the change occurred not due to the treatment that was used, but due to an extraneous variable , or an unseen and unaccounted for factor on the results and specifically our DV.
- A-B-A-B Reversal Design — In this design, the baseline and treatment phases are implemented twice. After the first treatment phase occurs, the individual(s) is/are taken back to baseline and then the treatment phase is implemented again. Replication is built into this design, allowing for a causal statement, but it may not be possible or ethical to take the person back to baseline after a treatment has been introduced, and one that likely is working well. What if you developed a successful treatment to reduce self-injurious behavior in children or to increase feelings of self-worth? You would want to know if the decrease in this behavior or increase in the positive thoughts was due to your treatment and not extraneous behaviors, but can you take the person back to baseline? Is it ethical to remove a treatment for something potentially harmful to the person? Now let’s say a teacher developed a new way to teach fractions to a fourth-grade class. Was it the educational paradigm or maybe additional help a child received from his/her parents or a tutor that accounts for improvement in performance? Well, we need to take the child back to baseline and see if the strategy works again, but can we? How can the child forget what has been learned already? ABAB Reversal Designs work well at establishing functional relationships if you can take the person back to baseline but are problematic if you cannot. An example of them working well includes establishing a system, such as a token economy (more on this later), to ensure your son does his chores, having success with it, and then taking it away. If the child stops doing chores and only restarts when the token economy is put back into place, then your system works. Note that with time the behavior of doing chores would occur on its own and the token economy would be fazed out.
- Multiple-baseline designs — This design can take on three different forms. In an across-subjects design, there is a baseline and treatment phase for two or more subjects for the same target behavior. For example, an applied behavior analyst is testing a new intervention to reduce disruptions in the classroom. The intervention involves a combination of antecedent manipulations, prompts, social support, differential reinforcement, and time-outs. He uses the intervention on six problematic students in a 6th period math class. Secondly, the across-settings design has a baseline and treatment phase for two or more settings in the same person for which the same behavior is measured. What if this same specialist now tests the intervention with one student but across her other five classes which include social studies, gym, science, English, and shop. Finally, in an across-behaviors design , there is a baseline and treatment phase for two or more different behaviors the same participant makes. The intervention continues to show promise and now the ABA specialist wants to see if it can help the same student but with his problem with procrastination and inability to organize.
- Changing-Criterion Design — In this design, the performance criteria changes as the subject achieves specific goals. The individual may go from having to workout at the gym 2 days a week to 3 days, then 4 days, and then finally 5 days. Once the goal of 2 days a week is met, the criterion changes to 3 days a week. In a learning study, a rat may have to press the lever 5 times to receive a food pellet and then once this is occurring regularly, the schedule changes to 10 times to receive the same food pellet. We are asking the rat to make more behaviors for the same consequence. The changing-criterion design has an A-B design but rules out extraneous variables since the person or animal continues meeting the changing criterion/new goals using the same treatment plan or experimental manipulation. Hence successfully moving from one goal to the next must be due to the strategies that were selected.
2.2.6. Ways We Gather Data
When we record, we need to decide what method we will use. Several strategies are possible to include continuous, product or outcome, and interval. First, in continuous recording, we watch a person or animal continuously throughout an observation period , or time when observations will be made, and all occurrences of the behavior are recorded. This technique allows you to record both frequency and duration. The frequency is reported as a rate, or the number of responses that occur per minute. Duration is the total time the behavior takes from start to finish. You can also record the intensity using a rating scale in which 1 is low intensity and 5 is high intensity. Finally, latency can be recorded by noting how long it took the person to engage in the desirable behavior, or to discontinue a problem behavior, from when the demand was uttered. You can also use real-time recording in which you write down the time when the behavior starts and when it ends, and then do this each time the behavior occurs. You can look at the number of start-stops to get the frequency and then average out the time each start-stop lasted to get the duration. For instance:
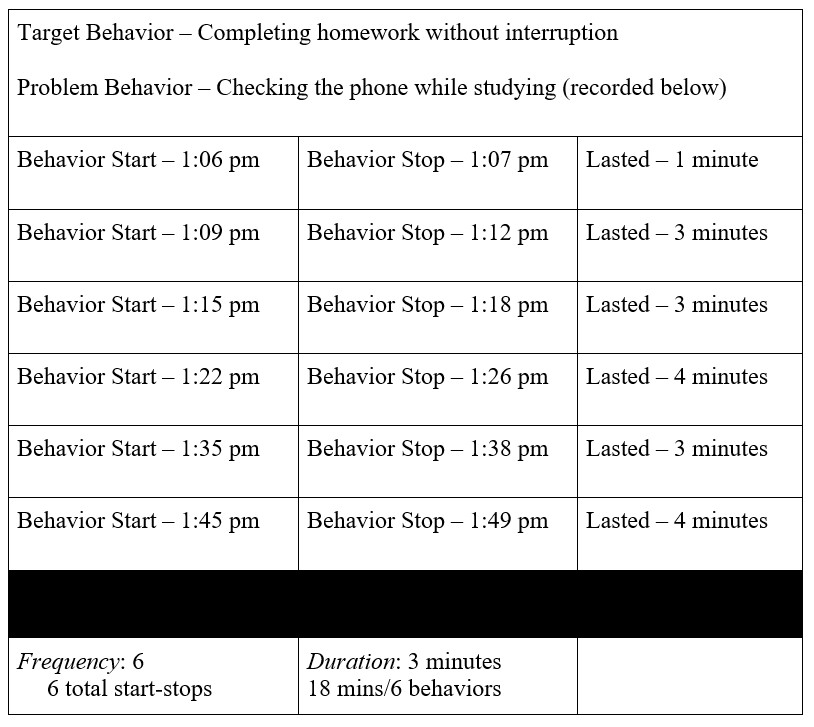
Next is product or outcome recording . This technique can be used when there is a tangible outcome you are interested in, such as looking at how well a student has improved his long division skills by examining his homework assignment or a test. Or you might see if your friend’s plan to keep a cleaner house is working by inspecting his or her house randomly once a week. This will allow you to know if an experimental teaching technique works. It is an indirect assessment method meaning that the observer does not need to be present. You can also examine many types of behaviors. But because the observer is not present, you are not sure if the person did the work himself or herself. It may be that answers were looked up online, cheating occurred as in the case of a test, or someone else did the homework for the student such as a sibling, parent, or friend. Also, you have to make sure you are examining the result/outcome of the behavior and not the behavior itself.
Finally, interval recording occurs when you take the observation period and divide it up into shorter periods of time. The person or animal is observed, and the target behavior recorded based on whether it occurs during the entire interval, called whole interval recording, or some part of the interval, called partial interval recording. With the latter, you are not interested in the dimensions of duration and frequency. We also say the interval recording is continuous if each subsequent interval follows immediately after the current one. Let’s say you are studying students in a classroom. Your observation period is the 50 minutes the student is in his home economics class and you divide it up into ten, 5-minute intervals. If using whole, then the behavior must occur during the entire 5-minute interval. If using partial, it only must occur sometime during the 5-minute interval. You can also use what is called time sample recording in which you divide the observation period into intervals of time but then observe and record during part of each interval (the sample). There are periods of time in between the observation periods in which no observation and recording occur. As such, the recording is discontinuous. This is a useful method since the observer does not have to observe the entire interval and the level of behavior is reported as the percentage of intervals in which the behavior occurred. Also, more than one behavior can be observed.
2.2.7. The Apparatus We Use
What we need to understand next in relation to learning research is what types of apparatus’ are used. As you might expect, the maze is the primary tool and has been so for over 100 years. Through the use of mazes, we can determine general principles about learning that apply to not only animals such as rats, but to human beings too. The standard or classic maze is built on a large platform with vertical walls and a transparent ceiling. The rat begins at a start point or box and moves through the maze until it reaches the end or goal box. There may be a reward at the end such as food or water to encourage the rat to learn the maze. Through the use of such a maze, we can determine how many trials it takes for the rat to reach the goal box without making a mistake. As you will see, in Section 2.3, we can also determine how long it took the rat to run the maze.
An alternative to this design is what is called the T-maze which obtains its name from its characteristic T-structure. The rat begins in a start box and proceeds up the corridor until it reaches a decision point – go left or right. We might discover if rats have a side preference or how fast they can learn if food-deprived the night before. One arm would have a food pellet while the other would not. It is also a great way to distinguish place and response learning (Blodgett & McCutchan, 1947). Some forms of the T-maze have multiple T-junctions in which the rat can make the correct decision and continues in the maze or makes a wrong decision. The rat can use cues in the environment to learn how to correctly navigate the maze and once learned, the rat will make few errors and run through it very quickly (Gentry, Brown, & Lee, 1948; Stone & Nyswander, 1927).
Similar to the T-maze is what is called the Y-maze . Starting in one arm, the rat moves forward and then has to choose one of two arms. The turns are not as sharp as in a T-maze making learning a bit easier. There is also a radial arm maze (Olton, 1987; Olton, Collison, & Werz, 1977) in which a rat starts in the center and can choose to enter any of 8, 12, or 16 spokes radiating out from this central location. It is a great test of short-term memory as the rat has to recall which arms have been visited and which have not. The rat successfully completes the maze when all arms have been visited.
One final maze is worth mentioning. The Morris water maze (Morris, 1984) is an apparatus that includes a large round tub of opaque water. There are two hidden platforms 1-2 cm under the water’s surface. The rat begins on a start platform and swims around until the other platform is located and it stands on it. It utilizes external cues placed outside the maze to find the end platform and run time is the typical dependent measure that is used.
To learn more about rat mazes, please visit: http://ratbehavior.org/RatsAndMazes.htm
Check this Out
Do you want to increase how fast rats learn their way through a multiple T-maze? Research has shown that you can do this by playing Mozart. Rats were exposed in utero plus 60 days to either a complex piece of music in the form of a sonata from Mozart, minimalist music, white noise, or silence. They were then tested over 5 days with 3 trials per day in a multiple T-maze. Results showed that rats exposed to Mozart completed the maze quicker and made fewer errors than the rats in the other conditions. The authors state that exposure to complex music facilitates spatial-temporal learning in rats and this matches results found in humans (Rauscher, Robinson, & Jens, 1998). Another line of research found that when rats were stressed they performed worse in water maze learning tasks than their non-stressed counterparts (Holscher, 1999).
So when you are studying for your quizzes or exams in this class (or other classes), play Mozart and minimize stress. These actions could result in a higher grade.
Outside of mazes, learning researchers may also utilize a Skinner Box . This is a small chamber used to conduct operant conditioning experiments with animals such as rats or pigeons. Inside the chamber, there is a lever for rats to push or a key for pigeons to peck which results in the delivery of food or water. The behavior of pushing or pecking is recorded through electronic equipment which allows for the behavior to be counted or quantified. This device is also called an operant conditioning chamber .
Finally, Edward Thorndike (1898) used a puzzle box to arrive at his law of effect or the idea that an organism will be more likely to repeat a behavior if it produced a satisfying effect in the past than if the effect was negative. This later became the foundation upon which operant conditioning was built. In his experiments, a hungry cat was placed in a box with a plate of fish outside the box. It was close enough that the cat could see and smell it but could not touch it. To get to the food, the cat had to figure out how to escape the box or which mechanism would help it to escape. Once free, the cat would take a bite, be placed back into the box, and then had to work to get out again. Thorndike discovered that the cat was able to get out quicker each time which demonstrated learning.
2.2.8. The Scientific Research Article
In scientific research, it is common practice to communicate the findings of our investigation. By reporting what we found in our study, other researchers can critique our methodology and address our limitations. Publishing allows psychology to grow its knowledge base about human behavior. We can also see where gaps still exist. We move it into the public domain so others can read and comment on it. Scientists can also replicate what we did and possibly extend our work if it is published.
As noted earlier, there are several ways to communicate our findings. We can do so at conferences in the form of posters or oral presentations, through newsletters from APA itself or one of its many divisions or other organizations, or through research journals and specifically scientific research articles. Published journal articles represent a form of communication between scientists and in them, the researchers describe how their work relates to previous research, how it replicates and/or extends this work, and what their work might mean theoretically.
Research articles begin with an abstract or a 150-250-word summary of the entire article. The purpose is to describe the experiment and allows the reader to decide whether he or she wants to read it further. The abstract provides a statement of purpose, overview of the methods, main results, and a brief statement of what these results mean. Keywords are also given that allow for students and other researchers alike to find the article when doing a search.
The abstract is followed by four major sections – Introduction, Method, Results, and Discussion. First, the introduction is designed to provide a summary of the current literature as it relates to the topic. It helps the reader to see how the researcher arrived at their hypothesis and the design of the study. Essentially, it gives the logic behind the decisions that were made.
Next, is the method section. Since replication is a required element of science, we must have a way to share information on our design and sample with readers. This is the essence of the method section and covers three major aspects of a study — the participants, materials or apparatus, and procedure. The reader needs to know who was in the study so that limitations related to the generalizability of the findings can be identified and investigated in the future. The researcher will also state the operational/behavioral definition, describe any groups that were used, identify random sampling or assignment procedures, and provide information about how a scale was scored or if a specific piece of apparatus was used, etc. Think of the method section as a cookbook. The participants are the ingredients, the materials or apparatus are whatever tools are needed, and the procedure is the instructions for how to bake the cake.
Third, is the results section. In this section, the researcher states the outcome of the experiment and whether it was statistically significant or not. The researchers can also present tables and figures. It is here we will find both descriptive and inferential statistics.
Finally, the discussion section starts by restating the main findings and hypothesis of the study. Next, is an interpretation of the findings and what their significance might be. Finally, the strengths and limitations of the study are stated which will allow the researcher to propose future directions or for other researchers to identify potential areas of exploration for their work. Whether you are writing a research paper for a class, preparing an article for publication, or reading a research article, the structure and function of a research article is the same. Understanding this will help you when reading articles in learning and behavior but also note, this same structure is used across disciplines.
- List typical dependent measures used in learning experiments.
- Describe the use of errors as a dependent measure.
- Describe the use of frequency as a dependent measure.
- Describe the use of intensity as a dependent measure.
- Describe the use of duration/run time/speed as a dependent measure.
- Describe the use of latency as a dependent measure.
- Describe the use of topography as a dependent measure.
- Describe the use of rate as a dependent measure.
- Describe the use of fluency as a dependent measure.
As we have learned, experiments include dependent and independent variables. The independent variable is the manipulation we are making while the dependent variable is what is being measured to see the effect of the manipulation. So, what types of DVs might we use in the experimental analysis of behavior or applied behavior analysis? We will cover the following: errors, frequency, intensity, duration, latency, topography, rate, and fluency.
2.3.1. Errors
A very simple measure of learning is to assess the number of errors made. If an animal running a maze has learned the maze, he/she should make fewer errors or mistakes with each trial, compared to say the first trial when many errors were made. The same goes for a child learning how to do multiplication. There will be numerous errors at start and then fewer to none later.
2.3.2. Frequency
Frequency is a measure of how often a behavior occurs. If we want to run more often, we may increase the number of days we run each week from 3 to 5. In terms of behavior modification, I once had a student who wished to decrease the number of times he used expletives throughout the day.
2.3.3. Intensity
Intensity is a measure of how strong the response is. For instance, a person on a treadmill may increase the intensity from 5 mph to 6 mph meaning the belt moves quicker and so the runner will have to move faster to keep up. We might tell children in a classroom to use their inside voices or to speak softer as opposed to their playground voices when they can yell.
2.3.4. Duration/Run Time/Speed
Duration is a measure of how long the behavior lasts. A runner may run more often (frequency), faster (intensity), or may run longer (duration). In the case of the latter, the runner may wish to build endurance and run for increasingly longer periods of time. A parent may wish to decrease the amount of time a child plays video games or is on his/her phone before bed. For rats in a maze, the first few attempts will likely take longer to reach the goal box than later attempts once the path needed to follow is learned. In other words, duration, or run time, will go down which demonstrates learning.
2.3.5. Latency
Latency represents the time it takes for a behavior to follow from the presentation of a stimulus. For instance, if a parent tells a child to take out the trash and he does so 5 minutes later, then the latency for the behavior of walking the trash outside is 5 minutes.
2.3.6. Topography
Topography represents the physical form a behavior takes. For instance, if a child is being disruptive, in what way is this occurring? Could it be the child is talking out of turn, being aggressive with other students, fidgeting in his/her seat, etc? In the case of rats and pushing levers, the mere act of pushing may not be of interest, but which paw is used or how much pressure is applied to the lever?
2.3.7. Rate
Rate is a measure of the change in response over time, or how often a behavior occurs. We may wish the rat to push the lever more times per minute to earn food reinforcement. Initially, the rat was required to push the lever 20 times per minute and now the experimenter requires 35 times per minute to receive a food pellet. In humans, a measure of rate would be words typed per minute. I may start at 20 words per minute but with practice (representing learning) I could type 60 words per minute or more.
2.3.8. Fluency
Though I may type fast, do I type accurately? This is where fluency comes in. Think about a foreign language. If you are fluent you speak it well. So, fluency is a measure of the number of correct responses made per minute. I may make 20 errors per minute of typing but with practice, I not only get quicker (up to 60 words per minute) but more accurate and reduce mistakes measure to 5 errors per minute. A student taking a semester of Spanish may measure learning by how many verbs he can correctly conjugate in a minute. Initially, he could only conjugate 8 verbs per minute but by the end of the semester can conjugate 24.
- Defend the use of animals in research.
- Describe safeguards to protect human research subjects.
2.4.1. Animal Models of Behavior
Learning research frequently uses animal models. According to AnimalResearch.info , animals are used “…when there is a need to find out what happens in the whole, living body, which is far more complex than the sum of its parts. It is difficult, and in most cases simply not yet possible, to replace the use of living animals in research with alternative methods.” They cite four main reasons to use animals. First, to advance scientific understanding such as how living things work to apply that knowledge for the benefit of both humans and animals. They state, “Many basic cell processes are the same in all animals, and the bodies of animals are like humans in the way that they perform many vital functions such as breathing, digestion, movement, sight, hearing, and reproduction.”
Second, animals can serve as models to study disease. For example, “Dogs suffer from cancer, diabetes, cataracts, ulcers and bleeding disorders such as hemophilia, which make them natural candidates for research into these disorders. Cats suffer from some of the same visual impairments as humans.” Therefore, animal models help us to understand how diseases affect the body and how our immune system responds.
Third, animals can be used to develop and test potential treatments for these diseases. As the website says, “Data from animal studies is essential before new therapeutic techniques and surgical procedures can be tested on human patients.”
Finally, animals help protect the safety of people, other animals, and our environment. Before a new medicine can go to market, it must be tested to ensure that the benefits outweigh the harmful effects. Legally and ethically, we have to move away from in vitro testing of tissues and isolated organs to suitable animal models and then testing in humans.
In conducting research with animals, three principles are followed. First, when possible, animals should be replaced with alternative techniques such as cell cultures, tissue engineering, and computer modeling. Second, the number of animals used in research should be reduced to a minimum. We can do this by “re-examining the findings of studies already conducted (e.g. by systematic reviews), by improving animal models, and by use of good experimental design.” Finally, we should refine the way experiments are conducted to reduce any suffering the animals may experience as much as possible. This can include better housing and improving animal welfare. Outside of the obvious benefit to the animals, the quality of research findings can also increase due to reduced stress in the animals. This framework is called the 3Rs.
Please visit: http://www.animalresearch.info/en/
One way to guarantee these principles are followed is through what is called the Institutional Animal Care and Use Committee (IACUC). The IACUC is responsible for the oversight and review of the humane care and use of animals; upholds standards set forth in laws, policies, and guidance; inspects animal housing facilities; approves protocols for use of animals in research, teaching, or education; addresses animal welfare concerns of the public; and reports to the appropriate bodies within a university, accrediting organizations, or government agencies. At times, projects may have to be suspended if found to be noncompliant with the regulations and policies of that institution.
- For more on the IACUC within the National Institutes of Health, please visit: https://olaw.nih.gov/resources/tutorial/iacuc.htm
- For another article on the use of animals in research, please check out the following published in the National Academies Press – https://www.nap.edu/read/10089/chapter/3
- The following is an article published on the ethics of animal research and discusses the 3Rs in more detail – https://www.ncbi.nlm.nih.gov/pmc/articles/PMC2002542/
- And finally, here is a great article published by the Washington State University IACUC on the use of animals in research and teaching at WSU – https://research.wsu.edu/frequently-asked-questions-about-animal-care-and-use-at-washington-state-university/
2.4.2. Human Models of Behavior
Throughout this module, we have seen that it is important for researchers to understand the methods they are using. Equally important, they must understand and appreciate ethical standards in research. As we have seen already in Section 2.3.1, such standards exist for the use of animals in research. The American Psychological Association (APA) identifies high standards of ethics and conduct as one of its four main guiding principles or missions and as it relates to humans. To read about the other three, please visit https://www.apa.org/about/index.aspx . Studies such as Milgram’s obedience study, Zimbardo’s Stanford prison study, and others, have necessitated standards for the use of humans in research. The standards can be broken down in terms of when they should occur during the process of a person participating in the study.
2.4.2.1. Before participating. First, researchers must obtain informed consent or when the person agrees to participate because they are told what will happen to them. They are given information about any risks they face, or potential harm that could come to them, whether physical or psychological. They are also told about confidentiality or the person’s right not to be identified. Since most research is conducted with students taking introductory psychology courses, they have to be given the right to do something other than a research study to likely earn required credits for the class. This is called an alternative activity and could take the form of reading and summarizing a research article. The amount of time taken to do this should not exceed the amount of time the student would be expected to participate in a study.
2.4.2.2. While participating. Participants are afforded the ability to withdraw or the person’s right to exit the study if any discomfort is experienced.
2.4.2.3. After participating . Once their participation is over, participants should be debriefed or when the true purpose of the study is revealed and they are told where to go if they need assistance and how to reach the researcher if they have questions. So, can researchers deceive participants, or intentionally withhold the true purpose of the study from them? According to the APA, a minimal amount of deception is allowed.
Human research must be approved by an Institutional Review Board or IRB. It is the IRB that will determine whether the researcher is providing enough information for the participant to give consent that is truly informed, if debriefing is adequate, and if any deception is allowed or not. According to the Food and Drug Administration (FDA), “The purpose of IRB review is to assure, both in advance and by periodic review, that appropriate steps are taken to protect the rights and welfare of humans participating as subjects in the research. To accomplish this purpose, IRBs use a group process to review research protocols and related materials (e.g., informed consent documents and investigator brochures) to ensure the protection of the rights and welfare of human subjects of research.”
If you would like to learn more about how to use ethics in your research, please read: https://opentext.wsu.edu/carriecuttler/chapter/putting-ethics-into-practice/
To learn more about IRBs, please visit: https://www.fda.gov/RegulatoryInformation/Guidances/ucm126420.htm
Module Recap
That’s it. In Module 2 we discussed the process of research used when studying learning and behavior. We learned about the scientific method and its steps which are universally used in all sciences and social sciences. Our breakdown consisted of six steps but be advised that other authors could combine steps or separate some of the ones in this module. Still, the overall spirit is the same. In the experimental analysis of behavior, we do talk about making a causal statement in the form of an If-Then statement, or respectfully we discuss functional relationships and contingencies. We also define our terms clearly, objectively, and precisely through a behavioral definition. In terms of research designs, psychology uses five main ones and our investigation of learning and behavior focuses on three of those designs, with experiment and observation being the main two. Methods by which we collect data, the apparatus we use, and later, who our participants/subjects are, were discussed. The structure of a research article was outlined which is consistent across disciplines and we covered some typical dependent variables or measures used in the study of learning and behavior. These include errors, frequency, intensity, duration, latency, topography, rate, and fluency.
Armed with this information we begin to explore the experimental analysis of behavior by investigating elicited behaviors and more in Module 3. From this, we will move to a discussion of respondent and then operant conditioning and finally observational learning. Before closing out with complementary cognitive processes we will engage in an exercise to see how the three models complement one another and are not competing with each other.
2nd edition

Share This Book
- Increase Font Size
Learning Strategies That Work
Dr. Mark A. McDaniel shares effective, evidence-based strategies about learning to replace less effective but widely accepted practices.
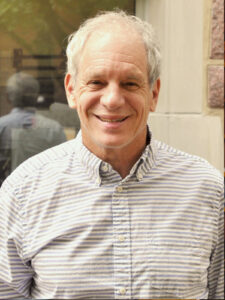
How do we learn and absorb new information? Which learning strategies actually work and which are mere myths?
Such questions are at the center of the work of Mark McDaniel , professor of psychology and the director of the Center for Integrative Research on Cognition, Learning, and Education at Washington University in St. Louis. McDaniel coauthored the book Make it Stick: The Science of Successful Learning .
In this Q&A adapted from a Career & Academic Resource Center podcast episode , McDaniel discusses his research on human learning and memory, including the most effective strategies for learning throughout a lifetime.
Harvard Extension: In your book, you talk about strategies to help students be better learners in and outside of the classroom. You write, “We harbor deep convictions that we learn better through single-minded focus and dogged repetition. And these beliefs are validated time and again by the visible improvement that comes during practice, practice, practice.”
McDaniel: This judgment that repetition is effective is hard to shake. There are cues present that your brain picks up when you’re rereading, when you’re repeating something that give you the metacognitive, that is your judgment about your own cognition, give you the misimpression that you really have learned this stuff well.
Older learners shouldn’t feel that they’re at a definitive disadvantage, because they’re not. Older learners really want to try to leverage their prior knowledge and use that as a basis to structure and frame and understand new information coming in.
And two of the primary cues are familiarity. So as you keep rereading, the material becomes more familiar to you. And we mistakenly judge familiarity as meaning robust learning.
And the second cue is fluency. It’s very clear from much work in reading and cognitive processes during reading that when you reread something at every level, the processes are more fluent. Word identification is more fluent. Parsing the structure of the sentence is more fluent. Extracting the ideas is more fluent. Everything is more fluent. And we misinterpret these fluency cues that the brain is getting. And these are accurate cues. It is more fluent. But we misinterpret that as meaning, I’ve really got this. I’ve really learned this. I’m not going to forget this. And that’s really misleading.
So let me give you another example. It’s not just rereading. It’s situations in, say, the STEM fields or any place where you’ve got to learn how to solve certain kinds of problems. One of the standard ways that instructors present homework is to present the same kind of problem in block fashion. You may have encountered this in your own math courses, your own physics courses.
So for example, in a physics course, you might get a particular type of work problem. And the parameters on it, the numbers might change, but in your homework, you’re trying to solve two or three or four of these work problems in a row. Well, it gets more and more fluid because exactly what formula you have to use. You know exactly what the problem is about. And as you get more fluid, and as we say in the book, it looks like you’re getting better. You are getting better at these problems.
But the issue is that can you remember how to identify which kinds of problems go with which kinds of solutions a week later when you’re asked to do a test where you have all different kinds of problems? And the answer is no, you cannot when you’ve done this block practice. So even though instructors who feel like their students are doing great with block practice and students will feel like they’re doing great, they are doing great on that kind of block practice, but they’re not at all good now at retaining information about what distinguishing features or problems are signaling certain kinds of approaches.
What you want to do is interleave practice in these problems. You want to randomly have a problem of one type and then solve a problem of another type and then a problem of another type. And in doing that, it feels difficult and it doesn’t feel fluent. And the signals to your brain are, I’m not getting this. I’m not doing very well. But in fact, that effort to try to figure out what kinds of approaches do I need for each problem as I encounter a different kind of problem, that’s producing learning. That’s producing robust skills that stick with you.
So this is a seductive thing that we have to, instructors and students alike, have to understand and have to move beyond those initial judgments, I haven’t learned very much, and trust that the more difficult practice schedule really is the better learning.
And I’ve written more on this since Make It Stick . And one of my strong theoretical tenets now is that in order for students to really embrace these techniques, they have to believe that they work for them. Each student has to believe it works for them. So I prepare demonstrations to show students these techniques work for them.
The net result of adopting these strategies is that students aren’t spending more time. Instead they’re spending more effective time. They’re working better. They’re working smarter.
When students take an exam after doing lots of retrieval practice, they see how well they’ve done. The classroom becomes very exciting. There’s lots of buy-in from the students. There’s lots of energy. There’s lots of stimulation to want to do more of this retrieval practice, more of this difficulty. Because trying to retrieve information is a lot more difficult than rereading it. But it produces robust learning for a number of reasons.
I think students have to trust that these techniques, and I think they also have to observe that these techniques work for them. It’s creating better learning. And then as a learner, you are more motivated to replace these ineffective techniques with more effective techniques.
Harvard Extension: You talk about tips for learners , how to make it stick. And there are several methods or tips that you share: elaboration, generation, reflection, calibration, among others. Which of these techniques is best?
McDaniel: It depends on the learning challenges that are faced. So retrieval practice, which is practicing trying to recall information from memory is really super effective if the requirements of your course require you to reproduce factual information.
For other things, it may be that you want to try something like generating understanding, creating mental models. So if your exams require you to draw inferences and work with new kinds of problems that are illustrative of the principles, but they’re new problems you haven’t seen before, a good technique is to try to connect the information into what I would call mental models. This is your representation of how the parts and the aspects fit together, relate together.
It’s not that one technique is better than the other. It’s that different techniques produce certain kinds of outcomes. And depending on the outcome you want, you might select one technique or the other.
I really firmly believe that to the extent that you can make learning fun and to the extent that one technique really seems more fun to you, that may be your go to technique. I teach a learning strategy course and I make it very clear to students. You don’t need to use all of these techniques. Find a couple that really work for you and then put those in your toolbox and replace rereading with these techniques.
Harvard Extension: You reference lifelong learning and lifelong learners. You talk about the brain being plastic, mutability of the brain in some ways, and give examples of how some lifelong learners approach their learning.
McDaniel: In some sense, more mature learners, older learners, have an advantage because they have more knowledge. And part of learning involves relating new information that’s coming into your prior knowledge, relating it to your knowledge structures, relating it to your schemas for how you think about certain kinds of content.
And so older adults have the advantage of having this richer knowledge base with which they can try to integrate new material. So older learners shouldn’t feel that they’re at a definitive disadvantage, because they’re not. Older learners really want to try to leverage their prior knowledge and use that as a basis to structure and frame and understand new information coming in.
Our challenges as older learners is that we do have these habits of learning that are not very effective. We turn to these habits. And if these aren’t such effective habits, we maybe attribute our failures to learn to age or a lack of native ability or so on and so forth. And in fact, that’s not it at all. In fact, if you adopt more effective strategies at any age, you’re going to find that your learning is more robust, it’s more successful, it falls into place.
You can learn these strategies at any age. Successful lifelong learning is getting these effective strategies in place, trusting them, and having them become a habit for how you’re going to approach your learning challenges.
6 Benefits of Connecting with an Enrollment Coach
Thinking about pursuing a degree or certificate at Harvard Extension School? Learn more about how working with an enrollment coach will get you off to a great start.
Harvard Division of Continuing Education
The Division of Continuing Education (DCE) at Harvard University is dedicated to bringing rigorous academics and innovative teaching capabilities to those seeking to improve their lives through education. We make Harvard education accessible to lifelong learners from high school to retirement.
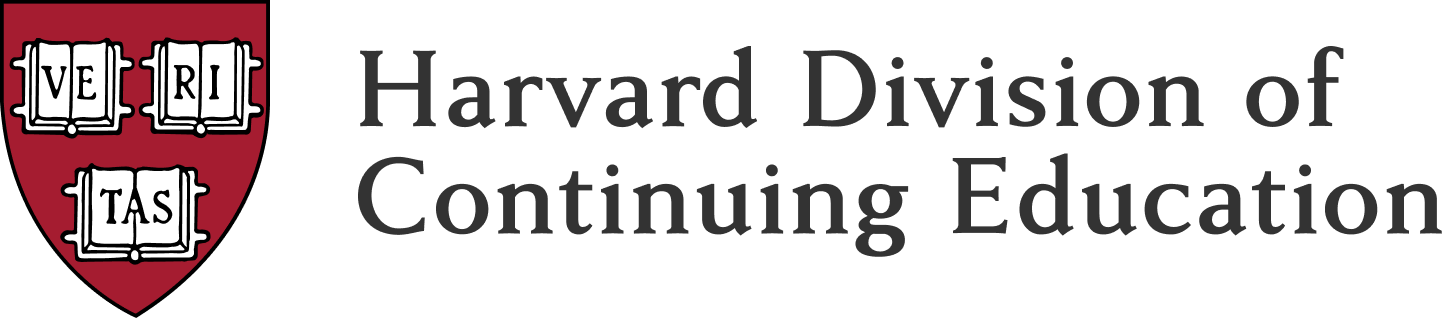
- Tutorial Review
- Open access
- Published: 24 January 2018
Teaching the science of learning
- Yana Weinstein ORCID: orcid.org/0000-0002-5144-968X 1 ,
- Christopher R. Madan 2 , 3 &
- Megan A. Sumeracki 4
Cognitive Research: Principles and Implications volume 3 , Article number: 2 ( 2018 ) Cite this article
245k Accesses
89 Citations
763 Altmetric
Metrics details
The science of learning has made a considerable contribution to our understanding of effective teaching and learning strategies. However, few instructors outside of the field are privy to this research. In this tutorial review, we focus on six specific cognitive strategies that have received robust support from decades of research: spaced practice, interleaving, retrieval practice, elaboration, concrete examples, and dual coding. We describe the basic research behind each strategy and relevant applied research, present examples of existing and suggested implementation, and make recommendations for further research that would broaden the reach of these strategies.
Significance
Education does not currently adhere to the medical model of evidence-based practice (Roediger, 2013 ). However, over the past few decades, our field has made significant advances in applying cognitive processes to education. From this work, specific recommendations can be made for students to maximize their learning efficiency (Dunlosky, Rawson, Marsh, Nathan, & Willingham, 2013 ; Roediger, Finn, & Weinstein, 2012 ). In particular, a review published 10 years ago identified a limited number of study techniques that have received solid evidence from multiple replications testing their effectiveness in and out of the classroom (Pashler et al., 2007 ). A recent textbook analysis (Pomerance, Greenberg, & Walsh, 2016 ) took the six key learning strategies from this report by Pashler and colleagues, and found that very few teacher-training textbooks cover any of these six principles – and none cover them all, suggesting that these strategies are not systematically making their way into the classroom. This is the case in spite of multiple recent academic (e.g., Dunlosky et al., 2013 ) and general audience (e.g., Dunlosky, 2013 ) publications about these strategies. In this tutorial review, we present the basic science behind each of these six key principles, along with more recent research on their effectiveness in live classrooms, and suggest ideas for pedagogical implementation. The target audience of this review is (a) educators who might be interested in integrating the strategies into their teaching practice, (b) science of learning researchers who are looking for open questions to help determine future research priorities, and (c) researchers in other subfields who are interested in the ways that principles from cognitive psychology have been applied to education.
While the typical teacher may not be exposed to this research during teacher training, a small cohort of teachers intensely interested in cognitive psychology has recently emerged. These teachers are mainly based in the UK, and, anecdotally (e.g., Dennis (2016), personal communication), appear to have taken an interest in the science of learning after reading Make it Stick (Brown, Roediger, & McDaniel, 2014 ; see Clark ( 2016 ) for an enthusiastic review of this book on a teacher’s blog, and “Learning Scientists” ( 2016c ) for a collection). In addition, a grassroots teacher movement has led to the creation of “researchED” – a series of conferences on evidence-based education (researchED, 2013 ). The teachers who form part of this network frequently discuss cognitive psychology techniques and their applications to education on social media (mainly Twitter; e.g., Fordham, 2016 ; Penfound, 2016 ) and on their blogs, such as Evidence Into Practice ( https://evidenceintopractice.wordpress.com/ ), My Learning Journey ( http://reflectionsofmyteaching.blogspot.com/ ), and The Effortful Educator ( https://theeffortfuleducator.com/ ). In general, the teachers who write about these issues pay careful attention to the relevant literature, often citing some of the work described in this review.
These informal writings, while allowing teachers to explore their approach to teaching practice (Luehmann, 2008 ), give us a unique window into the application of the science of learning to the classroom. By examining these blogs, we can not only observe how basic cognitive research is being applied in the classroom by teachers who are reading it, but also how it is being misapplied, and what questions teachers may be posing that have gone unaddressed in the scientific literature. Throughout this review, we illustrate each strategy with examples of how it can be implemented (see Table 1 and Figs. 1 , 2 , 3 , 4 , 5 , 6 and 7 ), as well as with relevant teacher blog posts that reflect on its application, and draw upon this work to pin-point fruitful avenues for further basic and applied research.
Spaced practice schedule for one week. This schedule is designed to represent a typical timetable of a high-school student. The schedule includes four one-hour study sessions, one longer study session on the weekend, and one rest day. Notice that each subject is studied one day after it is covered in school, to create spacing between classes and study sessions. Copyright note: this image was produced by the authors
a Blocked practice and interleaved practice with fraction problems. In the blocked version, students answer four multiplication problems consecutively. In the interleaved version, students answer a multiplication problem followed by a division problem and then an addition problem, before returning to multiplication. For an experiment with a similar setup, see Patel et al. ( 2016 ). Copyright note: this image was produced by the authors. b Illustration of interleaving and spacing. Each color represents a different homework topic. Interleaving involves alternating between topics, rather than blocking. Spacing involves distributing practice over time, rather than massing. Interleaving inherently involves spacing as other tasks naturally “fill” the spaces between interleaved sessions. Copyright note: this image was produced by the authors, adapted from Rohrer ( 2012 )
Concept map illustrating the process and resulting benefits of retrieval practice. Retrieval practice involves the process of withdrawing learned information from long-term memory into working memory, which requires effort. This produces direct benefits via the consolidation of learned information, making it easier to remember later and causing improvements in memory, transfer, and inferences. Retrieval practice also produces indirect benefits of feedback to students and teachers, which in turn can lead to more effective study and teaching practices, with a focus on information that was not accurately retrieved. Copyright note: this figure originally appeared in a blog post by the first and third authors ( http://www.learningscientists.org/blog/2016/4/1-1 )
Illustration of “how” and “why” questions (i.e., elaborative interrogation questions) students might ask while studying the physics of flight. To help figure out how physics explains flight, students might ask themselves the following questions: “How does a plane take off?”; “Why does a plane need an engine?”; “How does the upward force (lift) work?”; “Why do the wings have a curved upper surface and a flat lower surface?”; and “Why is there a downwash behind the wings?”. Copyright note: the image of the plane was downloaded from Pixabay.com and is free to use, modify, and share
Three examples of physics problems that would be categorized differently by novices and experts. The problems in ( a ) and ( c ) look similar on the surface, so novices would group them together into one category. Experts, however, will recognize that the problems in ( b ) and ( c ) both relate to the principle of energy conservation, and so will group those two problems into one category instead. Copyright note: the figure was produced by the authors, based on figures in Chi et al. ( 1981 )
Example of how to enhance learning through use of a visual example. Students might view this visual representation of neural communications with the words provided, or they could draw a similar visual representation themselves. Copyright note: this figure was produced by the authors
Example of word properties associated with visual, verbal, and motor coding for the word “SPOON”. A word can evoke multiple types of representation (“codes” in dual coding theory). Viewing a word will automatically evoke verbal representations related to its component letters and phonemes. Words representing objects (i.e., concrete nouns) will also evoke visual representations, including information about similar objects, component parts of the object, and information about where the object is typically found. In some cases, additional codes can also be evoked, such as motor-related properties of the represented object, where contextual information related to the object’s functional intention and manipulation action may also be processed automatically when reading the word. Copyright note: this figure was produced by the authors and is based on Aylwin ( 1990 ; Fig. 2 ) and Madan and Singhal ( 2012a , Fig. 3 )
Spaced practice
The benefits of spaced (or distributed) practice to learning are arguably one of the strongest contributions that cognitive psychology has made to education (Kang, 2016 ). The effect is simple: the same amount of repeated studying of the same information spaced out over time will lead to greater retention of that information in the long run, compared with repeated studying of the same information for the same amount of time in one study session. The benefits of distributed practice were first empirically demonstrated in the 19 th century. As part of his extensive investigation into his own memory, Ebbinghaus ( 1885/1913 ) found that when he spaced out repetitions across 3 days, he could almost halve the number of repetitions necessary to relearn a series of 12 syllables in one day (Chapter 8). He thus concluded that “a suitable distribution of [repetitions] over a space of time is decidedly more advantageous than the massing of them at a single time” (Section 34). For those who want to read more about Ebbinghaus’s contribution to memory research, Roediger ( 1985 ) provides an excellent summary.
Since then, hundreds of studies have examined spacing effects both in the laboratory and in the classroom (Kang, 2016 ). Spaced practice appears to be particularly useful at large retention intervals: in the meta-analysis by Cepeda, Pashler, Vul, Wixted, and Rohrer ( 2006 ), all studies with a retention interval longer than a month showed a clear benefit of distributed practice. The “new theory of disuse” (Bjork & Bjork, 1992 ) provides a helpful mechanistic explanation for the benefits of spacing to learning. This theory posits that memories have both retrieval strength and storage strength. Whereas retrieval strength is thought to measure the ease with which a memory can be recalled at a given moment, storage strength (which cannot be measured directly) represents the extent to which a memory is truly embedded in the mind. When studying is taking place, both retrieval strength and storage strength receive a boost. However, the extent to which storage strength is boosted depends upon retrieval strength, and the relationship is negative: the greater the current retrieval strength, the smaller the gains in storage strength. Thus, the information learned through “cramming” will be rapidly forgotten due to high retrieval strength and low storage strength (Bjork & Bjork, 2011 ), whereas spacing out learning increases storage strength by allowing retrieval strength to wane before restudy.
Teachers can introduce spacing to their students in two broad ways. One involves creating opportunities to revisit information throughout the semester, or even in future semesters. This does involve some up-front planning, and can be difficult to achieve, given time constraints and the need to cover a set curriculum. However, spacing can be achieved with no great costs if teachers set aside a few minutes per class to review information from previous lessons. The second method involves putting the onus to space on the students themselves. Of course, this would work best with older students – high school and above. Because spacing requires advance planning, it is crucial that the teacher helps students plan their studying. For example, teachers could suggest that students schedule study sessions on days that alternate with the days on which a particular class meets (e.g., schedule review sessions for Tuesday and Thursday when the class meets Monday and Wednesday; see Fig. 1 for a more complete weekly spaced practice schedule). It important to note that the spacing effect refers to information that is repeated multiple times, rather than the idea of studying different material in one long session versus spaced out in small study sessions over time. However, for teachers and particularly for students planning a study schedule, the subtle difference between the two situations (spacing out restudy opportunities, versus spacing out studying of different information over time) may be lost. Future research should address the effects of spacing out studying of different information over time, whether the same considerations apply in this situation as compared to spacing out restudy opportunities, and how important it is for teachers and students to understand the difference between these two types of spaced practice.
It is important to note that students may feel less confident when they space their learning (Bjork, 1999 ) than when they cram. This is because spaced learning is harder – but it is this “desirable difficulty” that helps learning in the long term (Bjork, 1994 ). Students tend to cram for exams rather than space out their learning. One explanation for this is that cramming does “work”, if the goal is only to pass an exam. In order to change students’ minds about how they schedule their studying, it might be important to emphasize the value of retaining information beyond a final exam in one course.
Ideas for how to apply spaced practice in teaching have appeared in numerous teacher blogs (e.g., Fawcett, 2013 ; Kraft, 2015 ; Picciotto, 2009 ). In England in particular, as of 2013, high-school students need to be able to remember content from up to 3 years back on cumulative exams (General Certificate of Secondary Education (GCSE) and A-level exams; see CIFE, 2012 ). A-levels in particular determine what subject students study in university and which programs they are accepted into, and thus shape the path of their academic career. A common approach for dealing with these exams has been to include a “revision” (i.e., studying or cramming) period of a few weeks leading up to the high-stakes cumulative exams. Now, teachers who follow cognitive psychology are advocating a shift of priorities to spacing learning over time across the 3 years, rather than teaching a topic once and then intensely reviewing it weeks before the exam (Cox, 2016a ; Wood, 2017 ). For example, some teachers have suggested using homework assignments as an opportunity for spaced practice by giving students homework on previous topics (Rose, 2014 ). However, questions remain, such as whether spaced practice can ever be effective enough to completely alleviate the need or utility of a cramming period (Cox, 2016b ), and how one can possibly figure out the optimal lag for spacing (Benney, 2016 ; Firth, 2016 ).
There has been considerable research on the question of optimal lag, and much of it is quite complex; two sessions neither too close together (i.e., cramming) nor too far apart are ideal for retention. In a large-scale study, Cepeda, Vul, Rohrer, Wixted, and Pashler ( 2008 ) examined the effects of the gap between study sessions and the interval between study and test across long periods, and found that the optimal gap between study sessions was contingent on the retention interval. Thus, it is not clear how teachers can apply the complex findings on lag to their own classrooms.
A useful avenue of research would be to simplify the research paradigms that are used to study optimal lag, with the goal of creating a flexible, spaced-practice framework that teachers could apply and tailor to their own teaching needs. For example, an Excel macro spreadsheet was recently produced to help teachers plan for lagged lessons (Weinstein-Jones & Weinstein, 2017 ; see Weinstein & Weinstein-Jones ( 2017 ) for a description of the algorithm used in the spreadsheet), and has been used by teachers to plan their lessons (Penfound, 2017 ). However, one teacher who found this tool helpful also wondered whether the more sophisticated plan was any better than his own method of manually selecting poorly understood material from previous classes for later review (Lovell, 2017 ). This direction is being actively explored within personalized online learning environments (Kornell & Finn, 2016 ; Lindsey, Shroyer, Pashler, & Mozer, 2014 ), but teachers in physical classrooms might need less technologically-driven solutions to teach cohorts of students.
It seems teachers would greatly appreciate a set of guidelines for how to implement spacing in the curriculum in the most effective, but also the most efficient manner. While the cognitive field has made great advances in terms of understanding the mechanisms behind spacing, what teachers need more of are concrete evidence-based tools and guidelines for direct implementation in the classroom. These could include more sophisticated and experimentally tested versions of the software described above (Weinstein-Jones & Weinstein, 2017 ), or adaptable templates of spaced curricula. Moreover, researchers need to evaluate the effectiveness of these tools in a real classroom environment, over a semester or academic year, in order to give pedagogically relevant evidence-based recommendations to teachers.
Interleaving
Another scheduling technique that has been shown to increase learning is interleaving. Interleaving occurs when different ideas or problem types are tackled in a sequence, as opposed to the more common method of attempting multiple versions of the same problem in a given study session (known as blocking). Interleaving as a principle can be applied in many different ways. One such way involves interleaving different types of problems during learning, which is particularly applicable to subjects such as math and physics (see Fig. 2 a for an example with fractions, based on a study by Patel, Liu, & Koedinger, 2016 ). For example, in a study with college students, Rohrer and Taylor ( 2007 ) found that shuffling math problems that involved calculating the volume of different shapes resulted in better test performance 1 week later than when students answered multiple problems about the same type of shape in a row. This pattern of results has also been replicated with younger students, for example 7 th grade students learning to solve graph and slope problems (Rohrer, Dedrick, & Stershic, 2015 ). The proposed explanation for the benefit of interleaving is that switching between different problem types allows students to acquire the ability to choose the right method for solving different types of problems rather than learning only the method itself, and not when to apply it.
Do the benefits of interleaving extend beyond problem solving? The answer appears to be yes. Interleaving can be helpful in other situations that require discrimination, such as inductive learning. Kornell and Bjork ( 2008 ) examined the effects of interleaving in a task that might be pertinent to a student of the history of art: the ability to match paintings to their respective painters. Students who studied different painters’ paintings interleaved at study were more successful on a later identification test than were participants who studied the paintings blocked by painter. Birnbaum, Kornell, Bjork, and Bjork ( 2013 ) proposed the discriminative-contrast hypothesis to explain that interleaving enhances learning by allowing the comparison between exemplars of different categories. They found support for this hypothesis in a set of experiments with bird categorization: participants benefited from interleaving and also from spacing, but not when the spacing interrupted side-by-side comparisons of birds from different categories.
Another type of interleaving involves the interleaving of study and test opportunities. This type of interleaving has been applied, once again, to problem solving, whereby students alternate between attempting a problem and viewing a worked example (Trafton & Reiser, 1993 ); this pattern appears to be superior to answering a string of problems in a row, at least with respect to the amount of time it takes to achieve mastery of a procedure (Corbett, Reed, Hoffmann, MacLaren, & Wagner, 2010 ). The benefits of interleaving study and test opportunities – rather than blocking study followed by attempting to answer problems or questions – might arise due to a process known as “test-potentiated learning”. That is, a study opportunity that immediately follows a retrieval attempt may be more fruitful than when that same studying was not preceded by retrieval (Arnold & McDermott, 2013 ).
For problem-based subjects, the interleaving technique is straightforward: simply mix questions on homework and quizzes with previous materials (which takes care of spacing as well); for languages, mix vocabulary themes rather than blocking by theme (Thomson & Mehring, 2016 ). But interleaving as an educational strategy ought to be presented to teachers with some caveats. Research has focused on interleaving material that is somewhat related (e.g., solving different mathematical equations, Rohrer et al., 2015 ), whereas students sometimes ask whether they should interleave material from different subjects – a practice that has not received empirical support (Hausman & Kornell, 2014 ). When advising students how to study independently, teachers should thus proceed with caution. Since it is easy for younger students to confuse this type of unhelpful interleaving with the more helpful interleaving of related information, it may be best for teachers of younger grades to create opportunities for interleaving in homework and quiz assignments rather than putting the onus on the students themselves to make use of the technique. Technology can be very helpful here, with apps such as Quizlet, Memrise, Anki, Synap, Quiz Champ, and many others (see also “Learning Scientists”, 2017 ) that not only allow instructor-created quizzes to be taken by students, but also provide built-in interleaving algorithms so that the burden does not fall on the teacher or the student to carefully plan which items are interleaved when.
An important point to consider is that in educational practice, the distinction between spacing and interleaving can be difficult to delineate. The gap between the scientific and classroom definitions of interleaving is demonstrated by teachers’ own writings about this technique. When they write about interleaving, teachers often extend the term to connote a curriculum that involves returning to topics multiple times throughout the year (e.g., Kirby, 2014 ; see “Learning Scientists” ( 2016a ) for a collection of similar blog posts by several other teachers). The “interleaving” of topics throughout the curriculum produces an effect that is more akin to what cognitive psychologists call “spacing” (see Fig. 2 b for a visual representation of the difference between interleaving and spacing). However, cognitive psychologists have not examined the effects of structuring the curriculum in this way, and open questions remain: does repeatedly circling back to previous topics throughout the semester interrupt the learning of new information? What are some effective techniques for interleaving old and new information within one class? And how does one determine the balance between old and new information?
Retrieval practice
While tests are most often used in educational settings for assessment, a lesser-known benefit of tests is that they actually improve memory of the tested information. If we think of our memories as libraries of information, then it may seem surprising that retrieval (which happens when we take a test) improves memory; however, we know from a century of research that retrieving knowledge actually strengthens it (see Karpicke, Lehman, & Aue, 2014 ). Testing was shown to strengthen memory as early as 100 years ago (Gates, 1917 ), and there has been a surge of research in the last decade on the mnemonic benefits of testing, or retrieval practice . Most of the research on the effectiveness of retrieval practice has been done with college students (see Roediger & Karpicke, 2006 ; Roediger, Putnam, & Smith, 2011 ), but retrieval-based learning has been shown to be effective at producing learning for a wide range of ages, including preschoolers (Fritz, Morris, Nolan, & Singleton, 2007 ), elementary-aged children (e.g., Karpicke, Blunt, & Smith, 2016 ; Karpicke, Blunt, Smith, & Karpicke, 2014 ; Lipko-Speed, Dunlosky, & Rawson, 2014 ; Marsh, Fazio, & Goswick, 2012 ; Ritchie, Della Sala, & McIntosh, 2013 ), middle-school students (e.g., McDaniel, Thomas, Agarwal, McDermott, & Roediger, 2013 ; McDermott, Agarwal, D’Antonio, Roediger, & McDaniel, 2014 ), and high-school students (e.g., McDermott et al., 2014 ). In addition, the effectiveness of retrieval-based learning has been extended beyond simple testing to other activities in which retrieval practice can be integrated, such as concept mapping (Blunt & Karpicke, 2014 ; Karpicke, Blunt, et al., 2014 ; Ritchie et al., 2013 ).
A debate is currently ongoing as to the effectiveness of retrieval practice for more complex materials (Karpicke & Aue, 2015 ; Roelle & Berthold, 2017 ; Van Gog & Sweller, 2015 ). Practicing retrieval has been shown to improve the application of knowledge to new situations (e.g., Butler, 2010 ; Dirkx, Kester, & Kirschner, 2014 ); McDaniel et al., 2013 ; Smith, Blunt, Whiffen, & Karpicke, 2016 ); but see Tran, Rohrer, and Pashler ( 2015 ) and Wooldridge, Bugg, McDaniel, and Liu ( 2014 ), for retrieval practice studies that showed limited or no increased transfer compared to restudy. Retrieval practice effects on higher-order learning may be more sensitive than fact learning to encoding factors, such as the way material is presented during study (Eglington & Kang, 2016 ). In addition, retrieval practice may be more beneficial for higher-order learning if it includes more scaffolding (Fiechter & Benjamin, 2017 ; but see Smith, Blunt, et al., 2016 ) and targeted practice with application questions (Son & Rivas, 2016 ).
How does retrieval practice help memory? Figure 3 illustrates both the direct and indirect benefits of retrieval practice identified by the literature. The act of retrieval itself is thought to strengthen memory (Karpicke, Blunt, et al., 2014 ; Roediger & Karpicke, 2006 ; Smith, Roediger, & Karpicke, 2013 ). For example, Smith et al. ( 2013 ) showed that if students brought information to mind without actually producing it (covert retrieval), they remembered the information just as well as if they overtly produced the retrieved information (overt retrieval). Importantly, both overt and covert retrieval practice improved memory over control groups without retrieval practice, even when feedback was not provided. The fact that bringing information to mind in the absence of feedback or restudy opportunities improves memory leads researchers to conclude that it is the act of retrieval – thinking back to bring information to mind – that improves memory of that information.
The benefit of retrieval practice depends to a certain extent on successful retrieval (see Karpicke, Lehman, et al., 2014 ). For example, in Experiment 4 of Smith et al. ( 2013 ), students successfully retrieved 72% of the information during retrieval practice. Of course, retrieving 72% of the information was compared to a restudy control group, during which students were re-exposed to 100% of the information, creating a bias in favor of the restudy condition. Yet retrieval led to superior memory later compared to the restudy control. However, if retrieval success is extremely low, then it is unlikely to improve memory (e.g., Karpicke, Blunt, et al., 2014 ), particularly in the absence of feedback. On the other hand, if retrieval-based learning situations are constructed in such a way that ensures high levels of success, the act of bringing the information to mind may be undermined, thus making it less beneficial. For example, if a student reads a sentence and then immediately covers the sentence and recites it out loud, they are likely not retrieving the information but rather just keeping the information in their working memory long enough to recite it again (see Smith, Blunt, et al., 2016 for a discussion of this point). Thus, it is important to balance success of retrieval with overall difficulty in retrieving the information (Smith & Karpicke, 2014 ; Weinstein, Nunes, & Karpicke, 2016 ). If initial retrieval success is low, then feedback can help improve the overall benefit of practicing retrieval (Kang, McDermott, & Roediger, 2007 ; Smith & Karpicke, 2014 ). Kornell, Klein, and Rawson ( 2015 ), however, found that it was the retrieval attempt and not the correct production of information that produced the retrieval practice benefit – as long as the correct answer was provided after an unsuccessful attempt, the benefit was the same as for a successful retrieval attempt in this set of studies. From a practical perspective, it would be helpful for teachers to know when retrieval attempts in the absence of success are helpful, and when they are not. There may also be additional reasons beyond retrieval benefits that would push teachers towards retrieval practice activities that produce some success amongst students; for example, teachers may hesitate to give students retrieval practice exercises that are too difficult, as this may negatively affect self-efficacy and confidence.
In addition to the fact that bringing information to mind directly improves memory for that information, engaging in retrieval practice can produce indirect benefits as well (see Roediger et al., 2011 ). For example, research by Weinstein, Gilmore, Szpunar, and McDermott ( 2014 ) demonstrated that when students expected to be tested, the increased test expectancy led to better-quality encoding of new information. Frequent testing can also serve to decrease mind-wandering – that is, thoughts that are unrelated to the material that students are supposed to be studying (Szpunar, Khan, & Schacter, 2013 ).
Practicing retrieval is a powerful way to improve meaningful learning of information, and it is relatively easy to implement in the classroom. For example, requiring students to practice retrieval can be as simple as asking students to put their class materials away and try to write out everything they know about a topic. Retrieval-based learning strategies are also flexible. Instructors can give students practice tests (e.g., short-answer or multiple-choice, see Smith & Karpicke, 2014 ), provide open-ended prompts for the students to recall information (e.g., Smith, Blunt, et al., 2016 ) or ask their students to create concept maps from memory (e.g., Blunt & Karpicke, 2014 ). In one study, Weinstein et al. ( 2016 ) looked at the effectiveness of inserting simple short-answer questions into online learning modules to see whether they improved student performance. Weinstein and colleagues also manipulated the placement of the questions. For some students, the questions were interspersed throughout the module, and for other students the questions were all presented at the end of the module. Initial success on the short-answer questions was higher when the questions were interspersed throughout the module. However, on a later test of learning from that module, the original placement of the questions in the module did not matter for performance. As with spaced practice, where the optimal gap between study sessions is contingent on the retention interval, the optimum difficulty and level of success during retrieval practice may also depend on the retention interval. Both groups of students who answered questions performed better on the delayed test compared to a control group without question opportunities during the module. Thus, the important thing is for instructors to provide opportunities for retrieval practice during learning. Based on previous research, any activity that promotes the successful retrieval of information should improve learning.
Retrieval practice has received a lot of attention in teacher blogs (see “Learning Scientists” ( 2016b ) for a collection). A common theme seems to be an emphasis on low-stakes (Young, 2016 ) and even no-stakes (Cox, 2015 ) testing, the goal of which is to increase learning rather than assess performance. In fact, one well-known charter school in the UK has an official homework policy grounded in retrieval practice: students are to test themselves on subject knowledge for 30 minutes every day in lieu of standard homework (Michaela Community School, 2014 ). The utility of homework, particularly for younger children, is often a hotly debated topic outside of academia (e.g., Shumaker, 2016 ; but see Jones ( 2016 ) for an opposing viewpoint and Cooper ( 1989 ) for the original research the blog posts were based on). Whereas some research shows clear links between homework and academic achievement (Valle et al., 2016 ), other researchers have questioned the effectiveness of homework (Dettmers, Trautwein, & Lüdtke, 2009 ). Perhaps amending homework to involve retrieval practice might make it more effective; this remains an open empirical question.
One final consideration is that of test anxiety. While retrieval practice can be very powerful at improving memory, some research shows that pressure during retrieval can undermine some of the learning benefit. For example, Hinze and Rapp ( 2014 ) manipulated pressure during quizzing to create high-pressure and low-pressure conditions. On the quizzes themselves, students performed equally well. However, those in the high-pressure condition did not perform as well on a criterion test later compared to the low-pressure group. Thus, test anxiety may reduce the learning benefit of retrieval practice. Eliminating all high-pressure tests is probably not possible, but instructors can provide a number of low-stakes retrieval opportunities for students to help increase learning. The use of low-stakes testing can serve to decrease test anxiety (Khanna, 2015 ), and has recently been shown to negate the detrimental impact of stress on learning (Smith, Floerke, & Thomas, 2016 ). This is a particularly important line of inquiry to pursue for future research, because many teachers who are not familiar with the effectiveness of retrieval practice may be put off by the implied pressure of “testing”, which evokes the much maligned high-stakes standardized tests (e.g., McHugh, 2013 ).
Elaboration
Elaboration involves connecting new information to pre-existing knowledge. Anderson ( 1983 , p.285) made the following claim about elaboration: “One of the most potent manipulations that can be performed in terms of increasing a subject’s memory for material is to have the subject elaborate on the to-be-remembered material.” Postman ( 1976 , p. 28) defined elaboration most parsimoniously as “additions to nominal input”, and Hirshman ( 2001 , p. 4369) provided an elaboration on this definition (pun intended!), defining elaboration as “A conscious, intentional process that associates to-be-remembered information with other information in memory.” However, in practice, elaboration could mean many different things. The common thread in all the definitions is that elaboration involves adding features to an existing memory.
One possible instantiation of elaboration is thinking about information on a deeper level. The levels (or “depth”) of processing framework, proposed by Craik and Lockhart ( 1972 ), predicts that information will be remembered better if it is processed more deeply in terms of meaning, rather than shallowly in terms of form. The leves of processing framework has, however, received a number of criticisms (Craik, 2002 ). One major problem with this framework is that it is difficult to measure “depth”. And if we are not able to actually measure depth, then the argument can become circular: is it that something was remembered better because it was studied more deeply, or do we conclude that it must have been studied more deeply because it is remembered better? (See Lockhart & Craik, 1990 , for further discussion of this issue).
Another mechanism by which elaboration can confer a benefit to learning is via improvement in organization (Bellezza, Cheesman, & Reddy, 1977 ; Mandler, 1979 ). By this view, elaboration involves making information more integrated and organized with existing knowledge structures. By connecting and integrating the to-be-learned information with other concepts in memory, students can increase the extent to which the ideas are organized in their minds, and this increased organization presumably facilitates the reconstruction of the past at the time of retrieval.
Elaboration is such a broad term and can include so many different techniques that it is hard to claim that elaboration will always help learning. There is, however, a specific technique under the umbrella of elaboration for which there is relatively strong evidence in terms of effectiveness (Dunlosky et al., 2013 ; Pashler et al., 2007 ). This technique is called elaborative interrogation, and involves students questioning the materials that they are studying (Pressley, McDaniel, Turnure, Wood, & Ahmad, 1987 ). More specifically, students using this technique would ask “how” and “why” questions about the concepts they are studying (see Fig. 4 for an example on the physics of flight). Then, crucially, students would try to answer these questions – either from their materials or, eventually, from memory (McDaniel & Donnelly, 1996 ). The process of figuring out the answer to the questions – with some amount of uncertainty (Overoye & Storm, 2015 ) – can help learning. When using this technique, however, it is important that students check their answers with their materials or with the teacher; when the content generated through elaborative interrogation is poor, it can actually hurt learning (Clinton, Alibali, & Nathan, 2016 ).
Students can also be encouraged to self-explain concepts to themselves while learning (Chi, De Leeuw, Chiu, & LaVancher, 1994 ). This might involve students simply saying out loud what steps they need to perform to solve an equation. Aleven and Koedinger ( 2002 ) conducted two classroom studies in which students were either prompted by a “cognitive tutor” to provide self-explanations during a problem-solving task or not, and found that the self-explanations led to improved performance. According to the authors, this approach could scale well to real classrooms. If possible and relevant, students could even perform actions alongside their self-explanations (Cohen, 1981 ; see also the enactment effect, Hainselin, Picard, Manolli, Vankerkore-Candas, & Bourdin, 2017 ). Instructors can scaffold students in these types of activities by providing self-explanation prompts throughout to-be-learned material (O’Neil et al., 2014 ). Ultimately, the greatest potential benefit of accurate self-explanation or elaboration is that the student will be able to transfer their knowledge to a new situation (Rittle-Johnson, 2006 ).
The technical term “elaborative interrogation” has not made it into the vernacular of educational bloggers (a search on https://educationechochamberuncut.wordpress.com , which consolidates over 3,000 UK-based teacher blogs, yielded zero results for that term). However, a few teachers have blogged about elaboration more generally (e.g., Hobbiss, 2016 ) and deep questioning specifically (e.g., Class Teaching, 2013 ), just without using the specific terminology. This strategy in particular may benefit from a more open dialog between researchers and teachers to facilitate the use of elaborative interrogation in the classroom and to address possible barriers to implementation. In terms of advancing the scientific understanding of elaborative interrogation in a classroom setting, it would be informative to conduct a larger-scale intervention to see whether having students elaborate during reading actually helps their understanding. It would also be useful to know whether the students really need to generate their own elaborative interrogation (“how” and “why”) questions, versus answering questions provided by others. How long should students persist to find the answers? When is the right time to have students engage in this task, given the levels of expertise required to do it well (Clinton et al., 2016 )? Without knowing the answers to these questions, it may be too early for us to instruct teachers to use this technique in their classes. Finally, elaborative interrogation takes a long time. Is this time efficiently spent? Or, would it be better to have the students try to answer a few questions, pool their information as a class, and then move to practicing retrieval of the information?
Concrete examples
Providing supporting information can improve the learning of key ideas and concepts. Specifically, using concrete examples to supplement content that is more conceptual in nature can make the ideas easier to understand and remember. Concrete examples can provide several advantages to the learning process: (a) they can concisely convey information, (b) they can provide students with more concrete information that is easier to remember, and (c) they can take advantage of the superior memorability of pictures relative to words (see “Dual Coding”).
Words that are more concrete are both recognized and recalled better than abstract words (Gorman, 1961 ; e.g., “button” and “bound,” respectively). Furthermore, it has been demonstrated that information that is more concrete and imageable enhances the learning of associations, even with abstract content (Caplan & Madan, 2016 ; Madan, Glaholt, & Caplan, 2010 ; Paivio, 1971 ). Following from this, providing concrete examples during instruction should improve retention of related abstract concepts, rather than the concrete examples alone being remembered better. Concrete examples can be useful both during instruction and during practice problems. Having students actively explain how two examples are similar and encouraging them to extract the underlying structure on their own can also help with transfer. In a laboratory study, Berry ( 1983 ) demonstrated that students performed well when given concrete practice problems, regardless of the use of verbalization (akin to elaborative interrogation), but that verbalization helped students transfer understanding from concrete to abstract problems. One particularly important area of future research is determining how students can best make the link between concrete examples and abstract ideas.
Since abstract concepts are harder to grasp than concrete information (Paivio, Walsh, & Bons, 1994 ), it follows that teachers ought to illustrate abstract ideas with concrete examples. However, care must be taken when selecting the examples. LeFevre and Dixon ( 1986 ) provided students with both concrete examples and abstract instructions and found that when these were inconsistent, students followed the concrete examples rather than the abstract instructions, potentially constraining the application of the abstract concept being taught. Lew, Fukawa-Connelly, Mejí-Ramos, and Weber ( 2016 ) used an interview approach to examine why students may have difficulty understanding a lecture. Responses indicated that some issues were related to understanding the overarching topic rather than the component parts, and to the use of informal colloquialisms that did not clearly follow from the material being taught. Both of these issues could have potentially been addressed through the inclusion of a greater number of relevant concrete examples.
One concern with using concrete examples is that students might only remember the examples – especially if they are particularly memorable, such as fun or gimmicky examples – and will not be able to transfer their understanding from one example to another, or more broadly to the abstract concept. However, there does not seem to be any evidence that fun relevant examples actually hurt learning by harming memory for important information. Instead, fun examples and jokes tend to be more memorable, but this boost in memory for the joke does not seem to come at a cost to memory for the underlying concept (Baldassari & Kelley, 2012 ). However, two important caveats need to be highlighted. First, to the extent that the more memorable content is not relevant to the concepts of interest, learning of the target information can be compromised (Harp & Mayer, 1998 ). Thus, care must be taken to ensure that all examples and gimmicks are, in fact, related to the core concepts that the students need to acquire, and do not contain irrelevant perceptual features (Kaminski & Sloutsky, 2013 ).
The second issue is that novices often notice and remember the surface details of an example rather than the underlying structure. Experts, on the other hand, can extract the underlying structure from examples that have divergent surface features (Chi, Feltovich, & Glaser, 1981 ; see Fig. 5 for an example from physics). Gick and Holyoak ( 1983 ) tried to get students to apply a rule from one problem to another problem that appeared different on the surface, but was structurally similar. They found that providing multiple examples helped with this transfer process compared to only using one example – especially when the examples provided had different surface details. More work is also needed to determine how many examples are sufficient for generalization to occur (and this, of course, will vary with contextual factors and individual differences). Further research on the continuum between concrete/specific examples and more abstract concepts would also be informative. That is, if an example is not concrete enough, it may be too difficult to understand. On the other hand, if the example is too concrete, that could be detrimental to generalization to the more abstract concept (although a diverse set of very concrete examples may be able to help with this). In fact, in a controversial article, Kaminski, Sloutsky, and Heckler ( 2008 ) claimed that abstract examples were more effective than concrete examples. Later rebuttals of this paper contested whether the abstract versus concrete distinction was clearly defined in the original study (see Reed, 2008 , for a collection of letters on the subject). This ideal point along the concrete-abstract continuum might also interact with development.
Finding teacher blog posts on concrete examples proved to be more difficult than for the other strategies in this review. One optimistic possibility is that teachers frequently use concrete examples in their teaching, and thus do not think of this as a specific contribution from cognitive psychology; the one blog post we were able to find that discussed concrete examples suggests that this might be the case (Boulton, 2016 ). The idea of “linking abstract concepts with concrete examples” is also covered in 25% of teacher-training textbooks used in the US, according to the report by Pomerance et al. ( 2016 ); this is the second most frequently covered of the six strategies, after “posing probing questions” (i.e., elaborative interrogation). A useful direction for future research would be to establish how teachers are using concrete examples in their practice, and whether we can make any suggestions for improvement based on research into the science of learning. For example, if two examples are better than one (Bauernschmidt, 2017 ), are additional examples also needed, or are there diminishing returns from providing more examples? And, how can teachers best ensure that concrete examples are consistent with prior knowledge (Reed, 2008 )?
Dual coding
Both the memory literature and folk psychology support the notion of visual examples being beneficial—the adage of “a picture is worth a thousand words” (traced back to an advertising slogan from the 1920s; Meider, 1990 ). Indeed, it is well-understood that more information can be conveyed through a simple illustration than through several paragraphs of text (e.g., Barker & Manji, 1989 ; Mayer & Gallini, 1990 ). Illustrations can be particularly helpful when the described concept involves several parts or steps and is intended for individuals with low prior knowledge (Eitel & Scheiter, 2015 ; Mayer & Gallini, 1990 ). Figure 6 provides a concrete example of this, illustrating how information can flow through neurons and synapses.
In addition to being able to convey information more succinctly, pictures are also more memorable than words (Paivio & Csapo, 1969 , 1973 ). In the memory literature, this is referred to as the picture superiority effect , and dual coding theory was developed in part to explain this effect. Dual coding follows from the notion of text being accompanied by complementary visual information to enhance learning. Paivio ( 1971 , 1986 ) proposed dual coding theory as a mechanistic account for the integration of multiple information “codes” to process information. In this theory, a code corresponds to a modal or otherwise distinct representation of a concept—e.g., “mental images for ‘book’ have visual, tactual, and other perceptual qualities similar to those evoked by the referent objects on which the images are based” (Clark & Paivio, 1991 , p. 152). Aylwin ( 1990 ) provides a clear example of how the word “dog” can evoke verbal, visual, and enactive representations (see Fig. 7 for a similar example for the word “SPOON”, based on Aylwin, 1990 (Fig. 2 ) and Madan & Singhal, 2012a (Fig. 3 )). Codes can also correspond to emotional properties (Clark & Paivio, 1991 ; Paivio, 2013 ). Clark and Paivio ( 1991 ) provide a thorough review of dual coding theory and its relation to education, while Paivio ( 2007 ) provides a comprehensive treatise on dual coding theory. Broadly, dual coding theory suggests that providing multiple representations of the same information enhances learning and memory, and that information that more readily evokes additional representations (through automatic imagery processes) receives a similar benefit.
Paivio and Csapo ( 1973 ) suggest that verbal and imaginal codes have independent and additive effects on memory recall. Using visuals to improve learning and memory has been particularly applied to vocabulary learning (Danan, 1992 ; Sadoski, 2005 ), but has also shown success in other domains such as in health care (Hartland, Biddle, & Fallacaro, 2008 ). To take advantage of dual coding, verbal information should be accompanied by a visual representation when possible. However, while the studies discussed all indicate that the use of multiple representations of information is favorable, it is important to acknowledge that each representation also increases cognitive load and can lead to over-saturation (Mayer & Moreno, 2003 ).
Given that pictures are generally remembered better than words, it is important to ensure that the pictures students are provided with are helpful and relevant to the content they are expected to learn. McNeill, Uttal, Jarvin, and Sternberg ( 2009 ) found that providing visual examples decreased conceptual errors. However, McNeill et al. also found that when students were given visually rich examples, they performed more poorly than students who were not given any visual example, suggesting that the visual details can at times become a distraction and hinder performance. Thus, it is important to consider that images used in teaching are clear and not ambiguous in their meaning (Schwartz, 2007 ).
Further broadening the scope of dual coding theory, Engelkamp and Zimmer ( 1984 ) suggest that motor movements, such as “turning the handle,” can provide an additional motor code that can improve memory, linking studies of motor actions (enactment) with dual coding theory (Clark & Paivio, 1991 ; Engelkamp & Cohen, 1991 ; Madan & Singhal, 2012c ). Indeed, enactment effects appear to primarily occur during learning, rather than during retrieval (Peterson & Mulligan, 2010 ). Along similar lines, Wammes, Meade, and Fernandes ( 2016 ) demonstrated that generating drawings can provide memory benefits beyond what could otherwise be explained by visual imagery, picture superiority, and other memory enhancing effects. Providing convergent evidence, even when overt motor actions are not critical in themselves, words representing functional objects have been shown to enhance later memory (Madan & Singhal, 2012b ; Montefinese, Ambrosini, Fairfield, & Mammarella, 2013 ). This indicates that motoric processes can improve memory similarly to visual imagery, similar to memory differences for concrete vs. abstract words. Further research suggests that automatic motor simulation for functional objects is likely responsible for this memory benefit (Madan, Chen, & Singhal, 2016 ).
When teachers combine visuals and words in their educational practice, however, they may not always be taking advantage of dual coding – at least, not in the optimal manner. For example, a recent discussion on Twitter centered around one teacher’s decision to have 7 th Grade students replace certain words in their science laboratory report with a picture of that word (e.g., the instructions read “using a syringe …” and a picture of a syringe replaced the word; Turner, 2016a ). Other teachers argued that this was not dual coding (Beaven, 2016 ; Williams, 2016 ), because there were no longer two different representations of the information. The first teacher maintained that dual coding was preserved, because this laboratory report with pictures was to be used alongside the original, fully verbal report (Turner, 2016b ). This particular implementation – having students replace individual words with pictures – has not been examined in the cognitive literature, presumably because no benefit would be expected. In any case, we need to be clearer about implementations for dual coding, and more research is needed to clarify how teachers can make use of the benefits conferred by multiple representations and picture superiority.
Critically, dual coding theory is distinct from the notion of “learning styles,” which describe the idea that individuals benefit from instruction that matches their modality preference. While this idea is pervasive and individuals often subjectively feel that they have a preference, evidence indicates that the learning styles theory is not supported by empirical findings (e.g., Kavale, Hirshoren, & Forness, 1998 ; Pashler, McDaniel, Rohrer, & Bjork, 2008 ; Rohrer & Pashler, 2012 ). That is, there is no evidence that instructing students in their preferred learning style leads to an overall improvement in learning (the “meshing” hypothesis). Moreover, learning styles have come to be described as a myth or urban legend within psychology (Coffield, Moseley, Hall, & Ecclestone, 2004 ; Hattie & Yates, 2014 ; Kirschner & van Merriënboer, 2013 ; Kirschner, 2017 ); skepticism about learning styles is a common stance amongst evidence-informed teachers (e.g., Saunders, 2016 ). Providing evidence against the notion of learning styles, Kraemer, Rosenberg, and Thompson-Schill ( 2009 ) found that individuals who scored as “verbalizers” and “visualizers” did not perform any better on experimental trials matching their preference. Instead, it has recently been shown that learning through one’s preferred learning style is associated with elevated subjective judgements of learning, but not objective performance (Knoll, Otani, Skeel, & Van Horn, 2017 ). In contrast to learning styles, dual coding is based on providing additional, complementary forms of information to enhance learning, rather than tailoring instruction to individuals’ preferences.
Genuine educational environments present many opportunities for combining the strategies outlined above. Spacing can be particularly potent for learning if it is combined with retrieval practice. The additive benefits of retrieval practice and spacing can be gained by engaging in retrieval practice multiple times (also known as distributed practice; see Cepeda et al., 2006 ). Interleaving naturally entails spacing if students interleave old and new material. Concrete examples can be both verbal and visual, making use of dual coding. In addition, the strategies of elaboration, concrete examples, and dual coding all work best when used as part of retrieval practice. For example, in the concept-mapping studies mentioned above (Blunt & Karpicke, 2014 ; Karpicke, Blunt, et al., 2014 ), creating concept maps while looking at course materials (e.g., a textbook) was not as effective for later memory as creating concept maps from memory. When practicing elaborative interrogation, students can start off answering the “how” and “why” questions they pose for themselves using class materials, and work their way up to answering them from memory. And when interleaving different problem types, students should be practicing answering them rather than just looking over worked examples.
But while these ideas for strategy combinations have empirical bases, it has not yet been established whether the benefits of the strategies to learning are additive, super-additive, or, in some cases, incompatible. Thus, future research needs to (a) better formalize the definition of each strategy (particularly critical for elaboration and dual coding), (b) identify best practices for implementation in the classroom, (c) delineate the boundary conditions of each strategy, and (d) strategically investigate interactions between the six strategies we outlined in this manuscript.
Aleven, V. A., & Koedinger, K. R. (2002). An effective metacognitive strategy: learning by doing and explaining with a computer-based cognitive tutor. Cognitive Science, 26 , 147–179.
Article Google Scholar
Anderson, J. R. (1983). A spreading activation theory of memory. Journal of Verbal Learning and Verbal Behavior, 22 , 261–295.
Arnold, K. M., & McDermott, K. B. (2013). Test-potentiated learning: distinguishing between direct and indirect effects of tests. Journal of Experimental Psychology: Learning, Memory, and Cognition, 39 , 940–945.
PubMed Google Scholar
Aylwin, S. (1990). Imagery and affect: big questions, little answers. In P. J. Thompson, D. E. Marks, & J. T. E. Richardson (Eds.), Imagery: Current developments . New York: International Library of Psychology.
Google Scholar
Baldassari, M. J., & Kelley, M. (2012). Make’em laugh? The mnemonic effect of humor in a speech. Psi Chi Journal of Psychological Research, 17 , 2–9.
Barker, P. G., & Manji, K. A. (1989). Pictorial dialogue methods. International Journal of Man-Machine Studies, 31 , 323–347.
Bauernschmidt, A. (2017). GUEST POST: two examples are better than one. [Blog post]. The Learning Scientists Blog . Retrieved from http://www.learningscientists.org/blog/2017/5/30-1 . Accessed 25 Dec 2017.
Beaven, T. (2016). @doctorwhy @FurtherEdagogy @doc_kristy Right, I thought the whole point of dual coding was to use TWO codes: pics + words of the SAME info? [Tweet]. Retrieved from https://twitter.com/TitaBeaven/status/807504041341308929 . Accessed 25 Dec 2017.
Bellezza, F. S., Cheesman, F. L., & Reddy, B. G. (1977). Organization and semantic elaboration in free recall. Journal of Experimental Psychology: Human Learning and Memory, 3 , 539–550.
Benney, D. (2016). (Trying to apply) spacing in a content heavy subject [Blog post]. Retrieved from https://mrbenney.wordpress.com/2016/10/16/trying-to-apply-spacing-in-science/ . Accessed 25 Dec 2017.
Berry, D. C. (1983). Metacognitive experience and transfer of logical reasoning. Quarterly Journal of Experimental Psychology, 35A , 39–49.
Birnbaum, M. S., Kornell, N., Bjork, E. L., & Bjork, R. A. (2013). Why interleaving enhances inductive learning: the roles of discrimination and retrieval. Memory & Cognition, 41 , 392–402.
Bjork, R. A. (1999). Assessing our own competence: heuristics and illusions. In D. Gopher & A. Koriat (Eds.), Attention and peformance XVII. Cognitive regulation of performance: Interaction of theory and application (pp. 435–459). Cambridge, MA: MIT Press.
Bjork, R. A. (1994). Memory and metamemory considerations in the training of human beings. In J. Metcalfe & A. Shimamura (Eds.), Metacognition: Knowing about knowing (pp. 185–205). Cambridge, MA: MIT Press.
Bjork, R. A., & Bjork, E. L. (1992). A new theory of disuse and an old theory of stimulus fluctuation. From learning processes to cognitive processes: Essays in honor of William K. Estes, 2 , 35–67.
Bjork, E. L., & Bjork, R. A. (2011). Making things hard on yourself, but in a good way: creating desirable difficulties to enhance learning. Psychology and the real world: Essays illustrating fundamental contributions to society , 56–64.
Blunt, J. R., & Karpicke, J. D. (2014). Learning with retrieval-based concept mapping. Journal of Educational Psychology, 106 , 849–858.
Boulton, K. (2016). What does cognitive overload look like in the humanities? [Blog post]. Retrieved from https://educationechochamberuncut.wordpress.com/2016/03/05/what-does-cognitive-overload-look-like-in-the-humanities-kris-boulton-2/ . Accessed 25 Dec 2017.
Brown, P. C., Roediger, H. L., & McDaniel, M. A. (2014). Make it stick . Cambridge, MA: Harvard University Press.
Book Google Scholar
Butler, A. C. (2010). Repeated testing produces superior transfer of learning relative to repeated studying. Journal of Experimental Psychology: Learning, Memory, and Cognition, 36 , 1118–1133.
Caplan, J. B., & Madan, C. R. (2016). Word-imageability enhances association-memory by recruiting hippocampal activity. Journal of Cognitive Neuroscience, 28 , 1522–1538.
Article PubMed Google Scholar
Cepeda, N. J., Pashler, H., Vul, E., Wixted, J. T., & Rohrer, D. (2006). Distributed practice in verbal recall tasks: a review and quantitative synthesis. Psychological Bulletin, 132 , 354–380.
Cepeda, N. J., Vul, E., Rohrer, D., Wixted, J. T., & Pashler, H. (2008). Spacing effects in learning a temporal ridgeline of optimal retention. Psychological Science, 19 , 1095–1102.
Chi, M. T., De Leeuw, N., Chiu, M. H., & LaVancher, C. (1994). Eliciting self-explanations improves understanding. Cognitive Science, 18 , 439–477.
Chi, M. T., Feltovich, P. J., & Glaser, R. (1981). Categorization and representation of physics problems by experts and novices. Cognitive Science, 5 , 121–152.
CIFE. (2012). No January A level and other changes. Retrieved from http://www.cife.org.uk/cife-general-news/no-january-a-level-and-other-changes/ . Accessed 25 Dec 2017.
Clark, D. (2016). One book on learning that every teacher, lecturer & trainer should read (7 reasons) [Blog post]. Retrieved from http://donaldclarkplanb.blogspot.com/2016/03/one-book-on-learning-that-every-teacher.html . Accessed 25 Dec 2017.
Clark, J. M., & Paivio, A. (1991). Dual coding theory and education. Educational Psychology Review, 3 , 149–210.
Class Teaching. (2013). Deep questioning [Blog post]. Retrieved from https://classteaching.wordpress.com/2013/07/12/deep-questioning/ . Accessed 25 Dec 2017.
Clinton, V., Alibali, M. W., & Nathan, M. J. (2016). Learning about posterior probability: do diagrams and elaborative interrogation help? The Journal of Experimental Education, 84 , 579–599.
Coffield, F., Moseley, D., Hall, E., & Ecclestone, K. (2004). Learning styles and pedagogy in post-16 learning: a systematic and critical review . London: Learning & Skills Research Centre.
Cohen, R. L. (1981). On the generality of some memory laws. Scandinavian Journal of Psychology, 22 , 267–281.
Cooper, H. (1989). Synthesis of research on homework. Educational Leadership, 47 , 85–91.
Corbett, A. T., Reed, S. K., Hoffmann, R., MacLaren, B., & Wagner, A. (2010). Interleaving worked examples and cognitive tutor support for algebraic modeling of problem situations. In Proceedings of the Thirty-Second Annual Meeting of the Cognitive Science Society (pp. 2882–2887).
Cox, D. (2015). No stakes testing – not telling students their results [Blog post]. Retrieved from https://missdcoxblog.wordpress.com/2015/06/06/no-stakes-testing-not-telling-students-their-results/ . Accessed 25 Dec 2017.
Cox, D. (2016a). Ditch revision. Teach it well [Blog post]. Retrieved from https://missdcoxblog.wordpress.com/2016/01/09/ditch-revision-teach-it-well/ . Accessed 25 Dec 2017.
Cox, D. (2016b). ‘They need to remember this in three years time’: spacing & interleaving for the new GCSEs [Blog post]. Retrieved from https://missdcoxblog.wordpress.com/2016/03/25/they-need-to-remember-this-in-three-years-time-spacing-interleaving-for-the-new-gcses/ . Accessed 25 Dec 2017.
Craik, F. I. (2002). Levels of processing: past, present… future? Memory, 10 , 305–318.
Craik, F. I., & Lockhart, R. S. (1972). Levels of processing: a framework for memory research. Journal of Verbal Learning and Verbal Behavior, 11 , 671–684.
Danan, M. (1992). Reversed subtitling and dual coding theory: new directions for foreign language instruction. Language Learning, 42 , 497–527.
Dettmers, S., Trautwein, U., & Lüdtke, O. (2009). The relationship between homework time and achievement is not universal: evidence from multilevel analyses in 40 countries. School Effectiveness and School Improvement, 20 , 375–405.
Dirkx, K. J., Kester, L., & Kirschner, P. A. (2014). The testing effect for learning principles and procedures from texts. The Journal of Educational Research, 107 , 357–364.
Dunlosky, J. (2013). Strengthening the student toolbox: study strategies to boost learning. American Educator, 37 (3), 12–21.
Dunlosky, J., Rawson, K. A., Marsh, E. J., Nathan, M. J., & Willingham, D. T. (2013). Improving students’ learning with effective learning techniques: promising directions from cognitive and educational psychology. Psychological Science in the Public Interest, 14 , 4–58.
Ebbinghaus, H. (1913). Memory (HA Ruger & CE Bussenius, Trans.). New York: Columbia University, Teachers College. (Original work published 1885) . Retrieved from http://psychclassics.yorku.ca/Ebbinghaus/memory8.htm . Accessed 25 Dec 2017.
Eglington, L. G., & Kang, S. H. (2016). Retrieval practice benefits deductive inference. Educational Psychology Review , 1–14.
Eitel, A., & Scheiter, K. (2015). Picture or text first? Explaining sequential effects when learning with pictures and text. Educational Psychology Review, 27 , 153–180.
Engelkamp, J., & Cohen, R. L. (1991). Current issues in memory of action events. Psychological Research, 53 , 175–182.
Engelkamp, J., & Zimmer, H. D. (1984). Motor programme information as a separable memory unit. Psychological Research, 46 , 283–299.
Fawcett, D. (2013). Can I be that little better at……using cognitive science/psychology/neurology to plan learning? [Blog post]. Retrieved from http://reflectionsofmyteaching.blogspot.com/2013/09/can-i-be-that-little-better-atusing.html . Accessed 25 Dec 2017.
Fiechter, J. L., & Benjamin, A. S. (2017). Diminishing-cues retrieval practice: a memory-enhancing technique that works when regular testing doesn’t. Psychonomic Bulletin & Review , 1–9.
Firth, J. (2016). Spacing in teaching practice [Blog post]. Retrieved from http://www.learningscientists.org/blog/2016/4/12-1 . Accessed 25 Dec 2017.
Fordham, M. [mfordhamhistory]. (2016). Is there a meaningful distinction in psychology between ‘thinking’ & ‘critical thinking’? [Tweet]. Retrieved from https://twitter.com/mfordhamhistory/status/809525713623781377 . Accessed 25 Dec 2017.
Fritz, C. O., Morris, P. E., Nolan, D., & Singleton, J. (2007). Expanding retrieval practice: an effective aid to preschool children’s learning. The Quarterly Journal of Experimental Psychology, 60 , 991–1004.
Gates, A. I. (1917). Recitation as a factory in memorizing. Archives of Psychology, 6.
Gick, M. L., & Holyoak, K. J. (1983). Schema induction and analogical transfer. Cognitive Psychology, 15 , 1–38.
Gorman, A. M. (1961). Recognition memory for nouns as a function of abstractedness and frequency. Journal of Experimental Psychology, 61 , 23–39.
Hainselin, M., Picard, L., Manolli, P., Vankerkore-Candas, S., & Bourdin, B. (2017). Hey teacher, don’t leave them kids alone: action is better for memory than reading. Frontiers in Psychology , 8 .
Harp, S. F., & Mayer, R. E. (1998). How seductive details do their damage. Journal of Educational Psychology, 90 , 414–434.
Hartland, W., Biddle, C., & Fallacaro, M. (2008). Audiovisual facilitation of clinical knowledge: A paradigm for dispersed student education based on Paivio’s dual coding theory. AANA Journal, 76 , 194–198.
Hattie, J., & Yates, G. (2014). Visible learning and the science of how we learn . New York: Routledge.
Hausman, H., & Kornell, N. (2014). Mixing topics while studying does not enhance learning. Journal of Applied Research in Memory and Cognition, 3 , 153–160.
Hinze, S. R., & Rapp, D. N. (2014). Retrieval (sometimes) enhances learning: performance pressure reduces the benefits of retrieval practice. Applied Cognitive Psychology, 28 , 597–606.
Hirshman, E. (2001). Elaboration in memory. In N. J. Smelser & P. B. Baltes (Eds.), International encyclopedia of the social & behavioral sciences (pp. 4369–4374). Oxford: Pergamon.
Chapter Google Scholar
Hobbiss, M. (2016). Make it meaningful! Elaboration [Blog post]. Retrieved from https://hobbolog.wordpress.com/2016/06/09/make-it-meaningful-elaboration/ . Accessed 25 Dec 2017.
Jones, F. (2016). Homework – is it really that useless? [Blog post]. Retrieved from http://www.learningscientists.org/blog/2016/4/5-1 . Accessed 25 Dec 2017.
Kaminski, J. A., & Sloutsky, V. M. (2013). Extraneous perceptual information interferes with children’s acquisition of mathematical knowledge. Journal of Educational Psychology, 105 (2), 351–363.
Kaminski, J. A., Sloutsky, V. M., & Heckler, A. F. (2008). The advantage of abstract examples in learning math. Science, 320 , 454–455.
Kang, S. H. (2016). Spaced repetition promotes efficient and effective learning policy implications for instruction. Policy Insights from the Behavioral and Brain Sciences, 3 , 12–19.
Kang, S. H. K., McDermott, K. B., & Roediger, H. L. (2007). Test format and corrective feedback modify the effects of testing on long-term retention. European Journal of Cognitive Psychology, 19 , 528–558.
Karpicke, J. D., & Aue, W. R. (2015). The testing effect is alive and well with complex materials. Educational Psychology Review, 27 , 317–326.
Karpicke, J. D., Blunt, J. R., Smith, M. A., & Karpicke, S. S. (2014). Retrieval-based learning: The need for guided retrieval in elementary school children. Journal of Applied Research in Memory and Cognition, 3 , 198–206.
Karpicke, J. D., Lehman, M., & Aue, W. R. (2014). Retrieval-based learning: an episodic context account. In B. H. Ross (Ed.), Psychology of Learning and Motivation (Vol. 61, pp. 237–284). San Diego, CA: Elsevier Academic Press.
Karpicke, J. D., Blunt, J. R., & Smith, M. A. (2016). Retrieval-based learning: positive effects of retrieval practice in elementary school children. Frontiers in Psychology, 7 .
Kavale, K. A., Hirshoren, A., & Forness, S. R. (1998). Meta-analytic validation of the Dunn and Dunn model of learning-style preferences: a critique of what was Dunn. Learning Disabilities Research & Practice, 13 , 75–80.
Khanna, M. M. (2015). Ungraded pop quizzes: test-enhanced learning without all the anxiety. Teaching of Psychology, 42 , 174–178.
Kirby, J. (2014). One scientific insight for curriculum design [Blog post]. Retrieved from https://pragmaticreform.wordpress.com/2014/05/05/scientificcurriculumdesign/ . Accessed 25 Dec 2017.
Kirschner, P. A. (2017). Stop propagating the learning styles myth. Computers & Education, 106 , 166–171.
Kirschner, P. A., & van Merriënboer, J. J. G. (2013). Do learners really know best? Urban legends in education. Educational Psychologist, 48 , 169–183.
Knoll, A. R., Otani, H., Skeel, R. L., & Van Horn, K. R. (2017). Learning style, judgments of learning, and learning of verbal and visual information. British Journal of Psychology, 108 , 544-563.
Kornell, N., & Bjork, R. A. (2008). Learning concepts and categories is spacing the “enemy of induction”? Psychological Science, 19 , 585–592.
Kornell, N., & Finn, B. (2016). Self-regulated learning: an overview of theory and data. In J. Dunlosky & S. Tauber (Eds.), The Oxford Handbook of Metamemory (pp. 325–340). New York: Oxford University Press.
Kornell, N., Klein, P. J., & Rawson, K. A. (2015). Retrieval attempts enhance learning, but retrieval success (versus failure) does not matter. Journal of Experimental Psychology: Learning, Memory, and Cognition, 41 , 283–294.
Kraemer, D. J. M., Rosenberg, L. M., & Thompson-Schill, S. L. (2009). The neural correlates of visual and verbal cognitive styles. Journal of Neuroscience, 29 , 3792–3798.
Article PubMed PubMed Central Google Scholar
Kraft, N. (2015). Spaced practice and repercussions for teaching. Retrieved from http://nathankraft.blogspot.com/2015/08/spaced-practice-and-repercussions-for.html . Accessed 25 Dec 2017.
Learning Scientists. (2016a). Weekly Digest #3: How teachers implement interleaving in their curriculum [Blog post]. Retrieved from http://www.learningscientists.org/blog/2016/3/28/weekly-digest-3 . Accessed 25 Dec 2017.
Learning Scientists. (2016b). Weekly Digest #13: how teachers implement retrieval in their classrooms [Blog post]. Retrieved from http://www.learningscientists.org/blog/2016/6/5/weekly-digest-13 . Accessed 25 Dec 2017.
Learning Scientists. (2016c). Weekly Digest #40: teachers’ implementation of principles from “Make It Stick” [Blog post]. Retrieved from http://www.learningscientists.org/blog/2016/12/18-1 . Accessed 25 Dec 2017.
Learning Scientists. (2017). Weekly Digest #54: is there an app for that? Studying 2.0 [Blog post]. Retrieved from http://www.learningscientists.org/blog/2017/4/9/weekly-digest-54 . Accessed 25 Dec 2017.
LeFevre, J.-A., & Dixon, P. (1986). Do written instructions need examples? Cognition and Instruction, 3 , 1–30.
Lew, K., Fukawa-Connelly, T., Mejí-Ramos, J. P., & Weber, K. (2016). Lectures in advanced mathematics: Why students might not understand what the mathematics professor is trying to convey. Journal of Research in Mathematics Education, 47 , 162–198.
Lindsey, R. V., Shroyer, J. D., Pashler, H., & Mozer, M. C. (2014). Improving students’ long-term knowledge retention through personalized review. Psychological Science, 25 , 639–647.
Lipko-Speed, A., Dunlosky, J., & Rawson, K. A. (2014). Does testing with feedback help grade-school children learn key concepts in science? Journal of Applied Research in Memory and Cognition, 3 , 171–176.
Lockhart, R. S., & Craik, F. I. (1990). Levels of processing: a retrospective commentary on a framework for memory research. Canadian Journal of Psychology, 44 , 87–112.
Lovell, O. (2017). How do we know what to put on the quiz? [Blog Post]. Retrieved from http://www.ollielovell.com/olliesclassroom/know-put-quiz/ . Accessed 25 Dec 2017.
Luehmann, A. L. (2008). Using blogging in support of teacher professional identity development: a case study. The Journal of the Learning Sciences, 17 , 287–337.
Madan, C. R., Glaholt, M. G., & Caplan, J. B. (2010). The influence of item properties on association-memory. Journal of Memory and Language, 63 , 46–63.
Madan, C. R., & Singhal, A. (2012a). Motor imagery and higher-level cognition: four hurdles before research can sprint forward. Cognitive Processing, 13 , 211–229.
Madan, C. R., & Singhal, A. (2012b). Encoding the world around us: motor-related processing influences verbal memory. Consciousness and Cognition, 21 , 1563–1570.
Madan, C. R., & Singhal, A. (2012c). Using actions to enhance memory: effects of enactment, gestures, and exercise on human memory. Frontiers in Psychology, 3 .
Madan, C. R., Chen, Y. Y., & Singhal, A. (2016). ERPs differentially reflect automatic and deliberate processing of the functional manipulability of objects. Frontiers in Human Neuroscience, 10 .
Mandler, G. (1979). Organization and repetition: organizational principles with special reference to rote learning. In L. G. Nilsson (Ed.), Perspectives on Memory Research (pp. 293–327). New York: Academic Press.
Marsh, E. J., Fazio, L. K., & Goswick, A. E. (2012). Memorial consequences of testing school-aged children. Memory, 20 , 899–906.
Mayer, R. E., & Gallini, J. K. (1990). When is an illustration worth ten thousand words? Journal of Educational Psychology, 82 , 715–726.
Mayer, R. E., & Moreno, R. (2003). Nine ways to reduce cognitive load in multimedia learning. Educational Psychologist, 38 , 43–52.
McDaniel, M. A., & Donnelly, C. M. (1996). Learning with analogy and elaborative interrogation. Journal of Educational Psychology, 88 , 508–519.
McDaniel, M. A., Thomas, R. C., Agarwal, P. K., McDermott, K. B., & Roediger, H. L. (2013). Quizzing in middle-school science: successful transfer performance on classroom exams. Applied Cognitive Psychology, 27 , 360–372.
McDermott, K. B., Agarwal, P. K., D’Antonio, L., Roediger, H. L., & McDaniel, M. A. (2014). Both multiple-choice and short-answer quizzes enhance later exam performance in middle and high school classes. Journal of Experimental Psychology: Applied, 20 , 3–21.
McHugh, A. (2013). High-stakes tests: bad for students, teachers, and education in general [Blog post]. Retrieved from https://teacherbiz.wordpress.com/2013/07/01/high-stakes-tests-bad-for-students-teachers-and-education-in-general/ . Accessed 25 Dec 2017.
McNeill, N. M., Uttal, D. H., Jarvin, L., & Sternberg, R. J. (2009). Should you show me the money? Concrete objects both hurt and help performance on mathematics problems. Learning and Instruction, 19 , 171–184.
Meider, W. (1990). “A picture is worth a thousand words”: from advertising slogan to American proverb. Southern Folklore, 47 , 207–225.
Michaela Community School. (2014). Homework. Retrieved from http://mcsbrent.co.uk/homework-2/ . Accessed 25 Dec 2017.
Montefinese, M., Ambrosini, E., Fairfield, B., & Mammarella, N. (2013). The “subjective” pupil old/new effect: is the truth plain to see? International Journal of Psychophysiology, 89 , 48–56.
O’Neil, H. F., Chung, G. K., Kerr, D., Vendlinski, T. P., Buschang, R. E., & Mayer, R. E. (2014). Adding self-explanation prompts to an educational computer game. Computers In Human Behavior, 30 , 23–28.
Overoye, A. L., & Storm, B. C. (2015). Harnessing the power of uncertainty to enhance learning. Translational Issues in Psychological Science, 1 , 140–148.
Paivio, A. (1971). Imagery and verbal processes . New York: Holt, Rinehart and Winston.
Paivio, A. (1986). Mental representations: a dual coding approach . New York: Oxford University Press.
Paivio, A. (2007). Mind and its evolution: a dual coding theoretical approach . Mahwah: Erlbaum.
Paivio, A. (2013). Dual coding theory, word abstractness, and emotion: a critical review of Kousta et al. (2011). Journal of Experimental Psychology: General, 142 , 282–287.
Paivio, A., & Csapo, K. (1969). Concrete image and verbal memory codes. Journal of Experimental Psychology, 80 , 279–285.
Paivio, A., & Csapo, K. (1973). Picture superiority in free recall: imagery or dual coding? Cognitive Psychology, 5 , 176–206.
Paivio, A., Walsh, M., & Bons, T. (1994). Concreteness effects on memory: when and why? Journal of Experimental Psychology: Learning, Memory, and Cognition, 20 , 1196–1204.
Pashler, H., McDaniel, M., Rohrer, D., & Bjork, R. (2008). Learning styles: concepts and evidence. Psychological Science in the Public Interest, 9 , 105–119.
Pashler, H., Bain, P. M., Bottge, B. A., Graesser, A., Koedinger, K., McDaniel, M., & Metcalfe, J. (2007). Organizing instruction and study to improve student learning. IES practice guide. NCER 2007–2004. National Center for Education Research .
Patel, R., Liu, R., & Koedinger, K. (2016). When to block versus interleave practice? Evidence against teaching fraction addition before fraction multiplication. In Proceedings of the 38th Annual Meeting of the Cognitive Science Society, Philadelphia, PA .
Penfound, B. (2017). Journey to interleaved practice #2 [Blog Post]. Retrieved from https://fullstackcalculus.com/2017/02/03/journey-to-interleaved-practice-2/ . Accessed 25 Dec 2017.
Penfound, B. [BryanPenfound]. (2016). Does blocked practice/learning lessen cognitive load? Does interleaved practice/learning provide productive struggle? [Tweet]. Retrieved from https://twitter.com/BryanPenfound/status/808759362244087808 . Accessed 25 Dec 2017.
Peterson, D. J., & Mulligan, N. W. (2010). Enactment and retrieval. Memory & Cognition, 38 , 233–243.
Picciotto, H. (2009). Lagging homework [Blog post]. Retrieved from http://blog.mathedpage.org/2013/06/lagging-homework.html . Accessed 25 Dec 2017.
Pomerance, L., Greenberg, J., & Walsh, K. (2016). Learning about learning: what every teacher needs to know. Retrieved from http://www.nctq.org/dmsView/Learning_About_Learning_Report . Accessed 25 Dec 2017.
Postman, L. (1976). Methodology of human learning. In W. K. Estes (Ed.), Handbook of learning and cognitive processes (Vol. 3). Hillsdale: Erlbaum.
Pressley, M., McDaniel, M. A., Turnure, J. E., Wood, E., & Ahmad, M. (1987). Generation and precision of elaboration: effects on intentional and incidental learning. Journal of Experimental Psychology: Learning, Memory, and Cognition, 13 , 291–300.
Reed, S. K. (2008). Concrete examples must jibe with experience. Science, 322 , 1632–1633.
researchED. (2013). How it all began. Retrieved from http://www.researched.org.uk/about/our-story/ . Accessed 25 Dec 2017.
Ritchie, S. J., Della Sala, S., & McIntosh, R. D. (2013). Retrieval practice, with or without mind mapping, boosts fact learning in primary school children. PLoS One, 8 (11), e78976.
Rittle-Johnson, B. (2006). Promoting transfer: effects of self-explanation and direct instruction. Child Development, 77 , 1–15.
Roediger, H. L. (1985). Remembering Ebbinghaus. [Retrospective review of the book On Memory , by H. Ebbinghaus]. Contemporary Psychology, 30 , 519–523.
Roediger, H. L. (2013). Applying cognitive psychology to education translational educational science. Psychological Science in the Public Interest, 14 , 1–3.
Roediger, H. L., & Karpicke, J. D. (2006). The power of testing memory: basic research and implications for educational practice. Perspectives on Psychological Science, 1 , 181–210.
Roediger, H. L., Putnam, A. L., & Smith, M. A. (2011). Ten benefits of testing and their applications to educational practice. In J. Mester & B. Ross (Eds.), The psychology of learning and motivation: cognition in education (pp. 1–36). Oxford: Elsevier.
Roediger, H. L., Finn, B., & Weinstein, Y. (2012). Applications of cognitive science to education. In Della Sala, S., & Anderson, M. (Eds.), Neuroscience in education: the good, the bad, and the ugly . Oxford, UK: Oxford University Press.
Roelle, J., & Berthold, K. (2017). Effects of incorporating retrieval into learning tasks: the complexity of the tasks matters. Learning and Instruction, 49 , 142–156.
Rohrer, D. (2012). Interleaving helps students distinguish among similar concepts. Educational Psychology Review, 24(3), 355–367.
Rohrer, D., Dedrick, R. F., & Stershic, S. (2015). Interleaved practice improves mathematics learning. Journal of Educational Psychology, 107 , 900–908.
Rohrer, D., & Pashler, H. (2012). Learning styles: Where’s the evidence? Medical Education, 46 , 34–35.
Rohrer, D., & Taylor, K. (2007). The shuffling of mathematics problems improves learning. Instructional Science, 35 , 481–498.
Rose, N. (2014). Improving the effectiveness of homework [Blog post]. Retrieved from https://evidenceintopractice.wordpress.com/2014/03/20/improving-the-effectiveness-of-homework/ . Accessed 25 Dec 2017.
Sadoski, M. (2005). A dual coding view of vocabulary learning. Reading & Writing Quarterly, 21 , 221–238.
Saunders, K. (2016). It really is time we stopped talking about learning styles [Blog post]. Retrieved from http://martingsaunders.com/2016/10/it-really-is-time-we-stopped-talking-about-learning-styles/ . Accessed 25 Dec 2017.
Schwartz, D. (2007). If a picture is worth a thousand words, why are you reading this essay? Social Psychology Quarterly, 70 , 319–321.
Shumaker, H. (2016). Homework is wrecking our kids: the research is clear, let’s ban elementary homework. Salon. Retrieved from http://www.salon.com/2016/03/05/homework_is_wrecking_our_kids_the_research_is_clear_lets_ban_elementary_homework . Accessed 25 Dec 2017.
Smith, A. M., Floerke, V. A., & Thomas, A. K. (2016). Retrieval practice protects memory against acute stress. Science, 354 , 1046–1048.
Smith, M. A., Blunt, J. R., Whiffen, J. W., & Karpicke, J. D. (2016). Does providing prompts during retrieval practice improve learning? Applied Cognitive Psychology, 30 , 784–802.
Smith, M. A., & Karpicke, J. D. (2014). Retrieval practice with short-answer, multiple-choice, and hybrid formats. Memory, 22 , 784–802.
Smith, M. A., Roediger, H. L., & Karpicke, J. D. (2013). Covert retrieval practice benefits retention as much as overt retrieval practice. Journal of Experimental Psychology: Learning, Memory, and Cognition, 39 , 1712–1725.
Son, J. Y., & Rivas, M. J. (2016). Designing clicker questions to stimulate transfer. Scholarship of Teaching and Learning in Psychology, 2 , 193–207.
Szpunar, K. K., Khan, N. Y., & Schacter, D. L. (2013). Interpolated memory tests reduce mind wandering and improve learning of online lectures. Proceedings of the National Academy of Sciences, 110 , 6313–6317.
Thomson, R., & Mehring, J. (2016). Better vocabulary study strategies for long-term learning. Kwansei Gakuin University Humanities Review, 20 , 133–141.
Trafton, J. G., & Reiser, B. J. (1993). Studying examples and solving problems: contributions to skill acquisition . Technical report, Naval HCI Research Lab, Washington, DC, USA.
Tran, R., Rohrer, D., & Pashler, H. (2015). Retrieval practice: the lack of transfer to deductive inferences. Psychonomic Bulletin & Review, 22 , 135–140.
Turner, K. [doc_kristy]. (2016a). My dual coding (in red) and some y8 work @AceThatTest they really enjoyed practising the technique [Tweet]. Retrieved from https://twitter.com/doc_kristy/status/807220355395977216 . Accessed 25 Dec 2017.
Turner, K. [doc_kristy]. (2016b). @FurtherEdagogy @doctorwhy their work is revision work, they already have the words on a different page, to compliment not replace [Tweet]. Retrieved from https://twitter.com/doc_kristy/status/807360265100599301 . Accessed 25 Dec 2017.
Valle, A., Regueiro, B., Núñez, J. C., Rodríguez, S., Piñeiro, I., & Rosário, P. (2016). Academic goals, student homework engagement, and academic achievement in elementary school. Frontiers in Psychology, 7 .
Van Gog, T., & Sweller, J. (2015). Not new, but nearly forgotten: the testing effect decreases or even disappears as the complexity of learning materials increases. Educational Psychology Review, 27 , 247–264.
Wammes, J. D., Meade, M. E., & Fernandes, M. A. (2016). The drawing effect: evidence for reliable and robust memory benefits in free recall. Quarterly Journal of Experimental Psychology, 69 , 1752–1776.
Weinstein, Y., Gilmore, A. W., Szpunar, K. K., & McDermott, K. B. (2014). The role of test expectancy in the build-up of proactive interference in long-term memory. Journal of Experimental Psychology: Learning, Memory, and Cognition, 40 , 1039–1048.
Weinstein, Y., Nunes, L. D., & Karpicke, J. D. (2016). On the placement of practice questions during study. Journal of Experimental Psychology: Applied, 22 , 72–84.
Weinstein, Y., & Weinstein-Jones, F. (2017). Topic and quiz spacing spreadsheet: a planning tool for teachers [Blog Post]. Retrieved from http://www.learningscientists.org/blog/2017/5/11-1 . Accessed 25 Dec 2017.
Weinstein-Jones, F., & Weinstein, Y. (2017). Topic spacing spreadsheet for teachers [Excel macro]. Zenodo. http://doi.org/10.5281/zenodo.573764 . Accessed 25 Dec 2017.
Williams, D. [FurtherEdagogy]. (2016). @doctorwhy @doc_kristy word accompanying the visual? I’m unclear how removing words benefit? Would a flow chart better suit a scientific exp? [Tweet]. Retrieved from https://twitter.com/FurtherEdagogy/status/807356800509104128 . Accessed 25 Dec 2017.
Wood, B. (2017). And now for something a little bit different….[Blog post]. Retrieved from https://justateacherstandinginfrontofaclass.wordpress.com/2017/04/20/and-now-for-something-a-little-bit-different/ . Accessed 25 Dec 2017.
Wooldridge, C. L., Bugg, J. M., McDaniel, M. A., & Liu, Y. (2014). The testing effect with authentic educational materials: a cautionary note. Journal of Applied Research in Memory and Cognition, 3 , 214–221.
Young, C. (2016). Mini-tests. Retrieved from https://colleenyoung.wordpress.com/revision-activities/mini-tests/ . Accessed 25 Dec 2017.
Download references
Acknowledgements
Not applicable.
YW and MAS were partially supported by a grant from The IDEA Center.
Availability of data and materials
Author information, authors and affiliations.
Department of Psychology, University of Massachusetts Lowell, Lowell, MA, USA
Yana Weinstein
Department of Psychology, Boston College, Chestnut Hill, MA, USA
Christopher R. Madan
School of Psychology, University of Nottingham, Nottingham, UK
Department of Psychology, Rhode Island College, Providence, RI, USA
Megan A. Sumeracki
You can also search for this author in PubMed Google Scholar
Contributions
YW took the lead on writing the “Spaced practice”, “Interleaving”, and “Elaboration” sections. CRM took the lead on writing the “Concrete examples” and “Dual coding” sections. MAS took the lead on writing the “Retrieval practice” section. All authors edited each others’ sections. All authors were involved in the conception and writing of the manuscript. All authors gave approval of the final version.
Corresponding author
Correspondence to Yana Weinstein .
Ethics declarations
Ethics approval and consent to participate, consent for publication, competing interests.
YW and MAS run a blog, “The Learning Scientists Blog”, which is cited in the tutorial review. The blog does not make money. Free resources on the strategies described in this tutorial review are provided on the blog. Occasionally, YW and MAS are invited by schools/school districts to present research findings from cognitive psychology applied to education.
Publisher’s Note
Springer Nature remains neutral with regard to jurisdictional claims in published maps and institutional affiliations.
Rights and permissions
Open Access This article is distributed under the terms of the Creative Commons Attribution 4.0 International License ( http://creativecommons.org/licenses/by/4.0/ ), which permits unrestricted use, distribution, and reproduction in any medium, provided you give appropriate credit to the original author(s) and the source, provide a link to the Creative Commons license, and indicate if changes were made.
Reprints and permissions
About this article
Cite this article.
Weinstein, Y., Madan, C.R. & Sumeracki, M.A. Teaching the science of learning. Cogn. Research 3 , 2 (2018). https://doi.org/10.1186/s41235-017-0087-y
Download citation
Received : 20 December 2016
Accepted : 02 December 2017
Published : 24 January 2018
DOI : https://doi.org/10.1186/s41235-017-0087-y
Share this article
Anyone you share the following link with will be able to read this content:
Sorry, a shareable link is not currently available for this article.
Provided by the Springer Nature SharedIt content-sharing initiative
Methodologies of Research on Learning (Overview Article)
- Reference work entry
- pp 2255–2260
- Cite this reference work entry
- Norbert M. Seel 2
337 Accesses
Paradigms of learning research ; Scientific method
“Good methodology is essential to good science” (Simon and Kaplan 1989 , p. 20).
The term “methodology” refers to the theoretical analysis of research methods in a discipline that are generally considered appropriate for the inquiry of relevant or important issues. It may refer to a set of methods or procedures or to the rationale which underlies a particular study relative to the applied scientific method , which basically consists of the collection of data through observation and/or experimentation, and the formulation and testing of theoretically sound hypotheses. The choice of a particular methodology is often determined by a paradigm , i.e., the goals and interests which scientists strive for.
Theoretical Background
Every discipline that maintains a theoretically sound interpretation of its fundamental statements depends on both the applied terminology and methodology. However, this is to a large extent dependent...
This is a preview of subscription content, log in via an institution to check access.
Access this chapter
- Available as PDF
- Read on any device
- Instant download
- Own it forever
- Available as EPUB and PDF
- Durable hardcover edition
- Dispatched in 3 to 5 business days
- Free shipping worldwide - see info
Tax calculation will be finalised at checkout
Purchases are for personal use only
Institutional subscriptions
Bronfenbrenner, U. (1978). Ansätze zu einer experimentellen Ökologie menschlicher Entwicklung. In R. Oerter (Ed.), Entwicklung als lebenslanger Prozeß (S (pp. 33–65). Hamburg: Hoffmann und Campe.
Google Scholar
Campbell, D. T., & Stanley, J. C. (1965). Experimental and quasi-experimental designs for research on teaching. In N. L. Gage (Ed.), Handbook of research on teaching (2nd ed., pp. 171–246). Chicago: Rand MnNally.
Cook, T. D., & Campbell, D. T. (1979). Quasi-experimentation: Design & analysis issues for field settings . Chicago: Rand McNally.
Creswell, J. W. (1998). Qualitative inquiry and research design: Choosing among five traditions . Thousand Oaks: Sage.
Creswell, J. W. (2005). Educational research. Planning, conducting, and evaluating quantitative and qualitative research (2nd ed.). Upper Saddle River: Pearson.
Goddard, W., & Melville, S. (2001). Research methodology. An introduction (2nd ed.). Lansdowne: Juta & Comp.
Halle, T., Forry, N., Hair, E., Perper, K., Wandner, L., Wessel, J., & Vick, J. (2009). Disparities in early learning and development: Lessons from the early childhood longitudinal study – birth cohort (ECLS-B) . Washington, DC: Child Trends.
Kuhn, T. A. (1970). The structure of scientific revolutions (2nd ed.). Chicago: University of Chicago Press.
Kumar, R. (2005). Research methodology. A step-by-step guide for beginners . Thousand Oaks: Sage.
Norman, D. A. (1981). Twelve issues for cognitive science. In D. A. Norman (Ed.), Perspectives on cognitive science (pp. 265–295). Norwood: Ablex.
Pedhazur, E. J., & Pedhazur Schmelkin, L. (1991). Measurement, design, and analysis: An integrated approach . Hillsdale: Lawrence Erlbaum.
Saldana, J. (2003). Longitudinal qualitative research: Analyzing change through time . Walnut Creek: AltaMira Press.
Shadish, W., Cook, T., & Campbell, D. (2002). Experimental and quasi-experimental designs for generalized causal inference . Boston: Houghton-Mifflin.
Simon, H. A., & Kaplan, C. A. (1989). Foundations of cognitive science. In M. I. Posner (Ed.), Foundations of cognitive science (pp. 1–47). Cambridge, MA: MIT Press.
Download references
Author information
Authors and affiliations.
Department of Education, University of Freiburg, Rempartstr. 11, 3. OG, 79098, Freiburg, Germany
Norbert M. Seel ( Faculty of Economics and Behavioral Sciences )
You can also search for this author in PubMed Google Scholar
Corresponding author
Correspondence to Norbert M. Seel .
Editor information
Editors and affiliations.
Faculty of Economics and Behavioral Sciences, Department of Education, University of Freiburg, 79085, Freiburg, Germany
Norbert M. Seel
Rights and permissions
Reprints and permissions
Copyright information
© 2012 Springer Science+Business Media, LLC
About this entry
Cite this entry.
Seel, N.M. (2012). Methodologies of Research on Learning (Overview Article). In: Seel, N.M. (eds) Encyclopedia of the Sciences of Learning. Springer, Boston, MA. https://doi.org/10.1007/978-1-4419-1428-6_924
Download citation
DOI : https://doi.org/10.1007/978-1-4419-1428-6_924
Publisher Name : Springer, Boston, MA
Print ISBN : 978-1-4419-1427-9
Online ISBN : 978-1-4419-1428-6
eBook Packages : Humanities, Social Sciences and Law
Share this entry
Anyone you share the following link with will be able to read this content:
Sorry, a shareable link is not currently available for this article.
Provided by the Springer Nature SharedIt content-sharing initiative
- Publish with us
Policies and ethics
- Find a journal
- Track your research
Thank you for visiting nature.com. You are using a browser version with limited support for CSS. To obtain the best experience, we recommend you use a more up to date browser (or turn off compatibility mode in Internet Explorer). In the meantime, to ensure continued support, we are displaying the site without styles and JavaScript.
- View all journals
- Explore content
- About the journal
- Publish with us
- Sign up for alerts
- Review Article
- Published: 02 August 2022
The science of effective learning with spacing and retrieval practice
- Shana K. Carpenter ORCID: orcid.org/0000-0003-0784-9026 1 ,
- Steven C. Pan ORCID: orcid.org/0000-0001-9080-5651 2 &
- Andrew C. Butler ORCID: orcid.org/0000-0002-6367-0795 3 , 4
Nature Reviews Psychology volume 1 , pages 496–511 ( 2022 ) Cite this article
7472 Accesses
25 Citations
562 Altmetric
Metrics details
- Learning and memory
Research on the psychology of learning has highlighted straightforward ways of enhancing learning. However, effective learning strategies are underused by learners. In this Review, we discuss key research findings on two specific learning strategies: spacing and retrieval practice. We focus on how these strategies enhance learning in various domains across the lifespan, with an emphasis on research in applied educational settings. We also discuss key findings from research on metacognition — learners’ awareness and regulation of their own learning. The underuse of effective learning strategies by learners could stem from false beliefs about learning, lack of awareness of effective learning strategies or the counter-intuitive nature of these strategies. Findings in learner metacognition highlight the need to improve learners’ subjective mental models of how to learn effectively. Overall, the research discussed in this Review has important implications for the increasingly common situations in which learners must effectively monitor and regulate their own learning.
This is a preview of subscription content, access via your institution
Access options
Subscribe to this journal
Receive 12 digital issues and online access to articles
55,14 € per year
only 4,60 € per issue
Buy this article
- Purchase on Springer Link
- Instant access to full article PDF
Prices may be subject to local taxes which are calculated during checkout
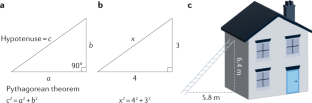
Similar content being viewed by others
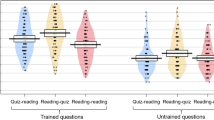
Does pre-testing promote better retention than post-testing?
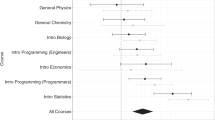
Real-world effectiveness of a social-psychological intervention translated from controlled trials to classrooms
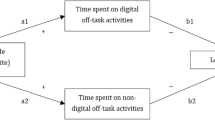
Learning in hybrid classes: the role of off-task activities
Witherby, A. E. & Tauber, S. K. The current status of students’ note-taking: why and how do students take notes? J. Appl. Res. Mem. Cogn. 8 , 139–153 (2019).
Article Google Scholar
Feitosa de Moura, V., Alexandre de Souza, C. & Noronha Viana, A. B. The use of massive open online courses (MOOCs) in blended learning courses and the functional value perceived by students. Comput. Educ. 161 , 104077 (2021).
Hew, K. F. & Cheung, W. S. Students’ and instructors’ use of massive open online courses (MOOCs): motivations and challenges. Educ. Res. Rev. 12 , 45–58 (2014).
Adesope, O. O., Trevisan, D. A. & Sundararajan, N. Rethinking the use of tests: a meta-analysis of practice testing. Rev. Educ. Res. 87 , 659–701 (2017).
Carpenter, S. K. in Learning and Memory: A Comprehensive Reference 2nd edn (ed. Byrne, J. H.) 465–485 (Academic, 2017).
Carpenter, S. K. Distributed practice or spacing effect. Oxford Research Encyclopedia of Education https://oxfordre.com/education/view/10.1093/acrefore/9780190264093.001.0001/acrefore-9780190264093-e-859 (2020).
Yang, C., Luo, L., Vadillo, M. A., Yu, R. & Shanks, D. R. Testing (quizzing) boosts classroom learning: a systematic and meta-analytic review. Psychol. Bull. 147 , 399–435 (2021).
Article PubMed Google Scholar
Agarwal, P. K., Nunes, L. D. & Blunt, J. R. Retrieval practice consistently benefits student learning: a systematic review of applied research in schools and classrooms. Educ. Psychol. Rev. 33 , 1409–1453 (2021).
Cepeda, N. J., Pashler, H., Vul, E., Wixted, J. T. & Rohrer, D. Distributed practice in verbal recall tasks: a review and quantitative synthesis. Psychol. Bull. 132 , 354–380 (2006).
Chi, M. T. H. & Ohlsson, S. in The Cambridge Handbook of Thinking and Reasoning 371–399 (Cambridge Univ. Press, 2005).
Bransford, J. D. & Schwartz, D. L. Chapter 3: Rethinking transfer: a simple proposal with multiple implications. Rev. Res. Educ. 24 , 61–100 (1999).
Google Scholar
Barnett, S. M. & Ceci, S. J. When and where do we apply what we learn?: a taxonomy for far transfer. Psychol. Bull. 128 , 612–637 (2002).
Ebbinghaus, H. Über das Gedächtnis: Untersuchungen zur experimentellen Psychologie [German] (Duncker & Humblot, 1885).
Vlach, H. A., Sandhofer, C. M. & Kornell, N. The spacing effect in children’s memory and category induction. Cognition 109 , 163–167 (2008).
Jackson, C. E., Maruff, P. T. & Snyder, P. J. Massed versus spaced visuospatial memory in cognitively healthy young and older adults. Alzheimer’s Dement. 9 , S32–S38 (2013).
Emeny, W. G., Hartwig, M. K. & Rohrer, D. Spaced mathematics practice improves test scores and reduces overconfidence. Appl. Cognit. Psychol. 35 , 1082–1089 (2021). This study demonstrates significant benefits of spacing over massed learning on 11–12-year-old students’ mathematics knowledge .
Vlach, H. A. & Sandhofer, C. M. Distributing learning over time: the spacing effect in children’s acquisition and generalization of science concepts: spacing and generalization. Child. Dev. 83 , 1137–1144 (2012).
Article PubMed PubMed Central Google Scholar
Foot-Seymour, V., Foot, J. & Wiseheart, M. Judging credibility: can spaced lessons help students think more critically online? Appl. Cognit. Psychol. 33 , 1032–1043 (2019). This study demonstrates significant long-term benefits of spacing on 9–12-year-old children’s ability to evaluate the credibility of information on websites .
Rohrer, D., Dedrick, R. F., Hartwig, M. K. & Cheung, C.-N. A randomized controlled trial of interleaved mathematics practice. J. Educ. Psychol. 112 , 40–52 (2020).
Yazdani, M. A. & Zebrowski, E. Spaced reinforcement: an effective approach to enhance the achievement in plane geometry. J. Math. Sci . 7 , 37–43 (2006).
Samani, J. & Pan, S. C. Interleaved practice enhances memory and problem-solving ability in undergraduate physics. npj Sci. Learn. 6 , 32 (2021). This study demonstrates significant benefits of distributing homework problems on retention and transfer of university students’ physics knowledge over an academic term .
Raman, M. et al. Teaching in small portions dispersed over time enhances long-term knowledge retention. Med. Teach. 32 , 250–255 (2010).
Moulton, C.-A. E. et al. Teaching surgical skills: what kind of practice makes perfect?: a randomized, controlled trial. Ann. Surg. 244 , 400–409 (2006).
Van Dongen, K. W., Mitra, P. J., Schijven, M. P. & Broeders, I. A. M. J. Distributed versus massed training: efficiency of training psychomotor skills. Surg. Tech. Dev. 1 , e17 (2011).
Spruit, E. N., Band, G. P. H. & Hamming, J. F. Increasing efficiency of surgical training: effects of spacing practice on skill acquisition and retention in laparoscopy training. Surg. Endosc. 29 , 2235–2243 (2015).
Lyle, K. B., Bego, C. R., Hopkins, R. F., Hieb, J. L. & Ralston, P. A. S. How the amount and spacing of retrieval practice affect the short- and long-term retention of mathematics knowledge. Educ. Psychol. Rev. 32 , 277–295 (2020).
Kapler, I. V., Weston, T. & Wiseheart, M. Spacing in a simulated undergraduate classroom: long-term benefits for factual and higher-level learning. Learn. Instr. 36 , 38–45 (2015).
Sobel, H. S., Cepeda, N. J. & Kapler, I. V. Spacing effects in real-world classroom vocabulary learning. Appl. Cognit. Psychol. 25 , 763–767 (2011).
Carpenter, S. K., Pashler, H. & Cepeda, N. J. Using tests to enhance 8th grade students’ retention of US history facts. Appl. Cognit. Psychol. 23 , 760–771 (2009). This study finds that spacing and retrieval practice can improve eighth- grade students’ knowledge of history facts across a 9-month period .
Cepeda, N. J., Vul, E., Rohrer, D., Wixted, J. T. & Pashler, H. Spacing effects in learning: a temporal ridgeline of optimal retention. Psychol. Sci. 19 , 1095–1102 (2008).
Delaney, P. F., Spirgel, A. S. & Toppino, T. C. A deeper analysis of the spacing effect after “deep” encoding. Mem. Cogn. 40 , 1003–1015 (2012).
Hintzman, D. L., Block, R. A. & Summers, J. J. Modality tags and memory for repetitions: locus of the spacing effect. J. Verbal Learn. Verbal Behav. 12 , 229–238 (1973).
Glenberg, A. M. Component-levels theory of the effects of spacing of repetitions on recall and recognition. Mem. Cogn. 7 , 95–112 (1979).
Verkoeijen, P. P. J. L., Rikers, R. M. J. P. & Schmidt, H. G. Detrimental influence of contextual change on spacing effects in free recall. J. Exp. Psychol. Learn. Mem. Cogn. 30 , 796–800 (2004).
Benjamin, A. S. & Tullis, J. What makes distributed practice effective? Cognit. Psychol. 61 , 228–247 (2010).
Thios, S. J. & D’Agostino, P. R. Effects of repetition as a function of study-phase retrieval. J. Verbal Learn. Verbal Behav. 15 , 529–536 (1976).
Smolen, P., Zhang, Y. & Byrne, J. H. The right time to learn: mechanisms and optimization of spaced learning. Nat. Rev. Neurosci. 17 , 77–88 (2016).
Goossens, N. A. M. C., Camp, G., Verkoeijen, P. P. J. L., Tabbers, H. K. & Zwaan, R. A. Spreading the words: a spacing effect in vocabulary learning. J. Cognit. Psychol. 24 , 965–971 (2012).
Zulkiply, N., McLean, J., Burt, J. S. & Bath, D. Spacing and induction: application to exemplars presented as auditory and visual text. Learn. Instr. 22 , 215–221 (2012).
Küpper-Tetzel, C. E. & Erdfelder, E. Encoding, maintenance, and retrieval processes in the lag effect: a multinomial processing tree analysis. Memory 20 , 37–47 (2012).
Verkoeijen, P. P. J. L., Rikers, R. M. J. P. & Schmidt, H. G. Limitations to the spacing effect: demonstration of an inverted U-shaped relationship between interrepetition spacing and free recall. Exp. Psychol. 52 , 257–263 (2005).
Randler, C., Kranich, K. & Eisele, M. Block scheduled versus traditional biology teaching—an educational experiment using the water lily. Instr. Sci. 36 , 17–25 (2008).
Abbott, E. E. On the analysis of the factor of recall in the learning process. Psychol. Rev. Monogr. Suppl. 11 , 159–177 (1909).
Roediger, H. L. & Butler, A. C. The critical role of retrieval practice in long-term retention. Trends Cognit. Sci. 15 , 20–27 (2011).
Rowland, C. A. The effect of testing versus restudy on retention: a meta-analytic review of the testing effect. Psychol. Bull. 140 , 1432–1463 (2014).
Pan, S. C. & Rickard, T. C. Transfer of test-enhanced learning: meta-analytic review and synthesis. Psychol. Bull. 144 , 710–756 (2018).
Sheffield, E. & Hudson, J. You must remember this: effects of video and photograph reminders on 18-month-olds’ event memory. J. Cogn. Dev. 7 , 73–93 (2006).
Fazio, L. K. & Marsh, E. J. Retrieval-based learning in children. Curr. Dir. Psychol. Sci. 28 , 111–116 (2019). This brief review highlights evidence that retrieval practice can benefit learning as early as infancy .
Coane, J. H. Retrieval practice and elaborative encoding benefit memory in younger and older adults. J. Appl. Res. Mem. Cogn. 2 , 95–100 (2013).
Bahrick, H. P., Bahrick, L. E., Bahrick, A. S. & Bahrick, P. E. Maintenance of foreign language vocabulary and the spacing effect. Psychol. Sci. 4 , 316–321 (1993). This classic study demonstrates benefits of spaced retrieval practice (successive relearning) on the learning of foreign language vocabulary in adults over a period of 5 years .
Bahrick, H. P. & Phelps, E. Retention of Spanish vocabulary over 8 years. J. Exp. Psychol. Learn. Mem. Cogn. 13 , 344–349 (1987).
Kulhavy, R. W. & Stock, W. A. Feedback in written instruction: the place of response certitude. Educ. Psychol. Rev. 1 , 279–308 (1989).
Pan, S. C., Hutter, S. A., D’Andrea, D., Unwalla, D. & Rickard, T. C. In search of transfer following cued recall practice: the case of process-based biology concepts. Appl. Cogn. Psychol. 33 , 629–645 (2019).
Pashler, H., Cepeda, N. J., Wixted, J. T. & Rohrer, D. When does feedback facilitate learning of words? J. Exp. Psychol. Learn. Mem. Cogn. 31 , 3–8 (2005).
Kang, S. H. K., McDermott, K. B. & Roediger, H. L. Test format and corrective feedback modify the effect of testing on long-term retention. Eur. J. Cognit. Psychol. 19 , 528–558 (2007).
Jaeger, A., Eisenkraemer, R. E. & Stein, L. M. Test-enhanced learning in third-grade children. Educ. Psychol. 35 , 513–521 (2015).
Pan, S. C., Rickard, T. C. & Bjork, R. A. Does spelling still matter — and if so, how should it be taught? Perspectives from contemporary and historical research. Educ. Psychol. Rev. 33 , 1523–1552 (2021).
Jones, A. C. et al. Beyond the rainbow: retrieval practice leads to better spelling than does rainbow writing. Educ. Psychol. Rev. 28 , 385–400 (2016).
McDermott, K. B., Agarwal, P. K., D’Antonio, L., Roediger, H. L. & McDaniel, M. A. Both multiple-choice and short-answer quizzes enhance later exam performance in middle and high school classes. J. Exp. Psychol. Appl. 20 , 3–21 (2014).
Roediger, H., Agarwal, P., McDaniel, M. & McDermott, K. Test-enhanced learning in the classroom: long-term improvements from quizzing. J. Exp. Psychol. Appl. 17 , 382–395 (2011).
Bobby, Z. & Meiyappan, K. “Test-enhanced” focused self-directed learning after the teaching modules in biochemistry. Biochem. Mol. Biol. Educ. 46 , 472–477 (2018).
Pan, S. C. et al. Online and clicker quizzing on jargon terms enhances definition-focused but not conceptually focused biology exam performance. CBE Life Sci. Educ. 18 , ar54 (2019).
Thomas, A. K., Smith, A. M., Kamal, K. & Gordon, L. T. Should you use frequent quizzing in your college course? Giving up 20 minutes of lecture time may pay off. J. Appl. Res. Mem. Cogn. 9 , 83–95 (2020).
Lyle, K. B. & Crawford, N. A. Retrieving essential material at the end of lectures improves performance on statistics exams. Teach. Psychol. 38 , 94–97 (2011).
Larsen, D. P., Butler, A. C. & Roediger, H. L. III Comparative effects of test-enhanced learning and self-explanation on long-term retention. Med. Educ. 47 , 674–682 (2013).
Eglington, L. G. & Kang, S. H. K. Retrieval practice benefits deductive inference. Educ. Psychol. Rev. 30 , 215–228 (2018).
Butler, A. C. Repeated testing produces superior transfer of learning relative to repeated studying. J. Exp. Psychol. Learn. Mem. Cogn. 36 , 1118–1133 (2010). This study demonstrates that retrieval practice can promote the ability to answer inferential questions involving a new knowledge domain (far transfer) .
Brabec, J. A., Pan, S. C., Bjork, E. L. & Bjork, R. A. True–false testing on trial: guilty as charged or falsely accused? Educ. Psychol. Rev. 33 , 667–692 (2021).
McDaniel, M. A., Wildman, K. M. & Anderson, J. L. Using quizzes to enhance summative-assessment performance in a web-based class: an experimental study. J. Appl. Res. Mem. Cogn. 1 , 18–26 (2012).
Rawson, K. A., Dunlosky, J. & Sciartelli, S. M. The power of successive relearning: improving performance on course exams and long-term retention. Educ. Psychol. Rev. 25 , 523–548 (2013).
Morris, P. E. & Fritz, C. O. The name game: using retrieval practice to improve the learning of names. J. Exp. Psychol. Appl. 6 , 124–129 (2000).
Smith, M. A., Roediger, H. L. & Karpicke, J. D. Covert retrieval practice benefits retention as much as overt retrieval practice. J. Exp. Psychol. Learn. Mem. Cogn. 39 , 1712–1725 (2013).
Rummer, R., Schweppe, J., Gerst, K. & Wagner, S. Is testing a more effective learning strategy than note-taking? J. Exp. Psychol. Appl. 23 , 293–300 (2017).
Karpicke, J. D. & Blunt, J. R. Retrieval practice produces more learning than elaborative studying with concept mapping. Science 331 , 772–775 (2011).
Ebersbach, M., Feierabend, M. & Nazari, K. B. B. Comparing the effects of generating questions, testing, and restudying on students’ long-term recall in university learning. Appl. Cognit. Psychol. 34 , 724–736 (2020).
Roelle, J. & Nückles, M. Generative learning versus retrieval practice in learning from text: the cohesion and elaboration of the text matters. J. Educ. Psychol. 111 , 1341–1361 (2019).
Endres, T., Carpenter, S., Martin, A. & Renkl, A. Enhancing learning by retrieval: enriching free recall with elaborative prompting. Learn. Instr. 49 , 13–20 (2017).
Glover, J. A. The ‘testing’ phenomenon: not gone but nearly forgotten. J. Educ. Psychol. 81 , 392–399 (1989).
Karpicke, J. D., Lehman, M. & Aue, W. R. in Psychology of Learning and Motivation Vol. 61 Ch. 7 (ed. Ross, B. H.) 237–284 (Academic, 2014).
Carpenter, S. K. Cue strength as a moderator of the testing effect: the benefits of elaborative retrieval. J. Exp. Psychol. Learn. Mem. Cogn. 35 , 1563–1569 (2009).
Carpenter, S. K. Semantic information activated during retrieval contributes to later retention: support for the mediator effectiveness hypothesis of the testing effect. J. Exp. Psychol. Learn. Mem. Cogn. 37 , 1547–1552 (2011).
Rickard, T. C. & Pan, S. C. A dual memory theory of the testing effect. Psychon. Bull. Rev. 25 , 847–869 (2018).
Bjork, R. A. Retrieval as a Memory Modifier: An Interpretation of Negative Recency and Related Phenomena (CiteSeer X , 1975).
Arnold, K. M. & McDermott, K. B. Test-potentiated learning: distinguishing between direct and indirect effects of tests. J. Exp. Psychol. Learn. Mem. Cogn. 39 , 940–945 (2013).
Roediger, H. L. & Karpicke, J. D. The power of testing memory: basic research and implications for educational practice. Perspect. Psychol. Sci. 1 , 181–210 (2006). This review details the history of psychology research on the retrieval practice effect and is contributing heavily to the resurgence of researcher interest in the topic .
Carpenter, S. K. Testing enhances the transfer of learning. Curr. Dir. Psychol. Sci. 21 , 279–283 (2012).
Pan, S. C. & Agarwal, P. K. Retrieval Practice and Transfer of Learning: Fostering Students’ Application of Knowledge (Univ. of California, 2018).
Tran, R., Rohrer, D. & Pashler, H. Retrieval practice: the lack of transfer to deductive inferences. Psychon. Bull. Rev. 22 , 135–140 (2015).
Wissman, K. T., Zamary, A. & Rawson, K. A. When does practice testing promote transfer on deductive reasoning tasks? J. Appl. Res. Mem. Cogn. 7 , 398–411 (2018).
van Gog, T. & Sweller, J. Not new, but nearly forgotten: the testing effect decreases or even disappears as the complexity of learning materials increases. Educ. Psychol. Rev. 27 , 247–264 (2015).
Carpenter, S. K., Endres, T. & Hui, L. Students’ use of retrieval in self-regulated learning: implications for monitoring and regulating effortful learning experiences. Educ. Psychol. Rev. 32 , 1029–1054 (2020).
Yeo, D. J. & Fazio, L. K. The optimal learning strategy depends on learning goals and processes: retrieval practice versus worked examples. J. Educ. Psychol. 111 , 73–90 (2019).
Peterson, D. J. & Wissman, K. T. The testing effect and analogical problem-solving. Memory 26 , 1460–1466 (2018).
Hostetter, A. B., Penix, E. A., Norman, M. Z., Batsell, W. R. & Carr, T. H. The role of retrieval practice in memory and analogical problem-solving. Q. J. Exp. Psychol. 72 , 858–871 (2019).
Karpicke, J. D., Blunt, J. R., Smith, M. A. & Karpicke, S. S. Retrieval-based learning: the need for guided retrieval in elementary school children. J. Appl. Res. Mem. Cogn. 3 , 198–206 (2014).
Smith, M. A. & Karpicke, J. D. Retrieval practice with short-answer, multiple-choice, and hybrid tests. Memory 22 , 784–802 (2014).
Latimier, A., Peyre, H. & Ramus, F. A meta-analytic review of the benefit of spacing out retrieval practice episodes on retention. Educ. Psychol. Rev. 33 , 959–987 (2021).
Higham, P. A., Zengel, B., Bartlett, L. K. & Hadwin, J. A. The benefits of successive relearning on multiple learning outcomes. J. Educ. Psychol. https://doi.org/10.1037/edu0000693 (2021).
Hopkins, R. F., Lyle, K. B., Hieb, J. L. & Ralston, P. A. S. Spaced retrieval practice increases college students’ short- and long-term retention of mathematics knowledge. Educ. Psychol. Rev. 28 , 853–873 (2016).
Bahrick, H. P. Maintenance of knowledge: questions about memory we forgot to ask. J. Exp. Psychol. Gen. 108 , 296–308 (1979).
Rawson, K. A. & Dunlosky, J. Successive relearning: an underexplored but potent technique for obtaining and maintaining knowledge. Curr. Dir. Psychol. Sci. https://doi.org/10.1177/09637214221100484 (2022). This brief review discusses the method of successive relearning — an effective learning technique that combines spacing and retrieval — and its benefits .
Rawson, K. A. & Dunlosky, J. When is practice testing most effective for improving the durability and efficiency of student learning? Educ. Psychol. Rev. 24 , 419–435 (2012).
Janes, J. L., Dunlosky, J., Rawson, K. A. & Jasnow, A. Successive relearning improves performance on a high-stakes exam in a difficult biopsychology course. Appl. Cognit. Psychol. 34 , 1118–1132 (2020).
Rawson, K. A., Dunlosky, J. & Janes, J. L. All good things must come to an end: a potential boundary condition on the potency of successive relearning. Educ. Psychol. Rev. 32 , 851–871 (2020).
Rawson, K. A. & Dunlosky, J. Optimizing schedules of retrieval practice for durable and efficient learning: how much is enough? J. Exp. Psychol. Gen. 140 , 283–302 (2011).
Flavell, J. H. Metacognition and cognitive monitoring: a new area of cognitive–developmental inquiry. Am. Psychol. 34 , 906–911 (1979). This classic paper introduces ideas that are now foundational to research on metacognition .
Kuhn, D. Metacognition matters in many ways. Educ. Psychol. 57 , 73–86 (2021).
Norman, E. et al. Metacognition in psychology. Rev. Gen. Psychol. 23 , 403–424 (2019).
Was, C. A. & Al-Harthy, I. S. Persistence of overconfidence in young children: factors that lead to more accurate predictions of memory performance. Eur. J. Dev. Psychol. 15 , 156–171 (2018).
Forsberg, A., Blume, C. L. & Cowan, N. The development of metacognitive accuracy in working memory across childhood. Dev. Psychol. 57 , 1297–1317 (2021).
Kuhn, D. Metacognitive development. Curr. Dir. Psychol. Sci . 9 , 178-181 (2000).
Bell, P. & Volckmann, D. Knowledge surveys in general chemistry: confidence, overconfidence, and performance. J. Chem. Educ. 88 , 1469–1476 (2011).
Saenz, G. D., Geraci, L. & Tirso, R. Improving metacognition: a comparison of interventions. Appl. Cognit. Psychol. 33 , 918–929 (2019).
Morphew, J. W. Changes in metacognitive monitoring accuracy in an introductory physics course. Metacogn. Learn. 16 , 89–111 (2021).
Geller, J. et al. Study strategies and beliefs about learning as a function of academic achievement and achievement goals. Memory 26 , 683–690 (2018).
Kornell, N. & Bjork, R. A. The promise and perils of self-regulated study. Psychon. Bull. Rev. 14 , 219–224 (2007).
Yan, V. X., Thai, K.-P. & Bjork, R. A. Habits and beliefs that guide self-regulated learning: do they vary with mindset? J. Appl. Res. Mem. Cogn. 3 , 140–152 (2014).
Rivers, M. L. Metacognition about practice testing: a review of learners’ beliefs, monitoring, and control of test-enhanced learning. Educ. Psychol. Rev. 33 , 823–862 (2021).
Carpenter, S. K. et al. Students’ use of optional online reviews and its relationship to summative assessment outcomes in introductory biology. LSE 16 , ar23 (2017).
Corral, D., Carpenter, S. K., Perkins, K. & Gentile, D. A. Assessing students’ use of optional online lecture reviews. Appl. Cognit. Psychol. 34 , 318–329 (2020).
Blasiman, R. N., Dunlosky, J. & Rawson, K. A. The what, how much, and when of study strategies: comparing intended versus actual study behaviour. Memory 25 , 784–792 (2017).
Karpicke, J. D., Butler, A. C. & Roediger, H. L. III Metacognitive strategies in student learning: do students practise retrieval when they study on their own? Memory 17 , 471–479 (2009).
Hamman, D., Berthelot, J., Saia, J. & Crowley, E. Teachers’ coaching of learning and its relation to students’ strategic learning. J. Educ. Psychol. 92 , 342–348 (2000).
Kistner, S. et al. Promotion of self-regulated learning in classrooms: investigating frequency, quality, and consequences for student performance. Metacogn. Learn. 5 , 157–171 (2010).
Morehead, K., Rhodes, M. G. & DeLozier, S. Instructor and student knowledge of study strategies. Memory 24 , 257–271 (2016).
Pomerance, L., Greenberg, J. & Walsh, K. Learning about Learning: What Every New Teacher Needs to Know (National Council on Teacher Quality, 2016).
Dinsmore, D. L., Alexander, P. A. & Loughlin, S. M. Focusing the conceptual lens on metacognition, self-regulation, and self-regulated learning. Educ. Psychol. Rev. 20 , 391–409 (2008). This conceptual review paper explores the relationship between metacognition, self-regulation and self-regulated learning .
Winne, P. H. in Handbook of Self-regulation of Learning and Performance 2nd edn 36–48 (Routledge/Taylor & Francis, 2018).
Pintrich, P. R. A conceptual framework for assessing motivation and self-regulated learning in college students. Educ. Psychol. Rev. 16 , 385–407 (2004).
Zimmerman, B. J. Self-efficacy: an essential motive to learn. Contemp. Educ. Psychol. 25 , 82–91 (2000).
McDaniel, M. A. & Butler, A. C. in Successful Remembering and Successful Forgetting: A Festschrift in Honor of Robert A. Bjork 175–198 (Psychology Press, 2011).
Bjork, R. A., Dunlosky, J. & Kornell, N. Self-regulated learning: beliefs, techniques, and illusions. Annu. Rev. Psychol. 64 , 417–444 (2013). This review provides an overview of the cognitive psychology perspective on the metacognition of strategy planning and use .
Nelson, T. O. & Narens, L. in Psychology of Learning and Motivation Vol. 26 (ed. Bower, G. H.) 125–173 (Academic, 1990).
Fiechter, J. L., Benjamin, A. S. & Unsworth, N. in The Oxford Handbook of Metamemory (eds Dunlosky, J. & Tauber, S. K.) 307–324 (Oxford Univ. Press, 2016).
Efklides, A. Interactions of metacognition with motivation and affect in self-regulated learning: the MASRL model. Educ. Psychol. 46 , 6–25 (2011).
Zimmerman, B. J. in Handbook of Self-regulation (eds Boekaerts, M. & Pintrich, P. R.) 13–39 (Academic, 2000). This paper lays out a prominent theory of self-regulated learning and exemplifies the educational psychology perspective on the metacognition of strategy planning and use .
Wolters, C. A. Regulation of motivation: evaluating an underemphasized aspect of self-regulated learning. Educ. Psychol. 38 , 189–205 (2003).
Wolters, C. A. & Benzon, M. Assessing and predicting college students’ use of strategies for the self-regulation of motivation. J. Exp. Educ. 18 , 199–221 (2013).
Abel, M. & Bäuml, K.-H. T. Would you like to learn more? Retrieval practice plus feedback can increase motivation to keep on studying. Cognition 201 , 104316 (2020).
Kang, S. H. K. & Pashler, H. Is the benefit of retrieval practice modulated by motivation? J. Appl. Res. Mem. Cogn. 3 , 183–188 (2014).
Vermunt, J. D. & Verloop, N. Congruence and friction between learning and teaching. Learn. Instr. 9 , 257–280 (1999).
Coertjens, L., Donche, V., De Maeyer, S., Van Daal, T. & Van Petegem, P. The growth trend in learning strategies during the transition from secondary to higher education in Flanders. High. Educ.: Int. J. High. Education Educ. Plan. 3 , 499–518 (2017).
Severiens, S., Ten Dam, G. & Van Hout Wolters, B. Stability of processing and regulation strategies: two longitudinal studies on student learning. High. Educ. 42 , 437–453 (2001).
Watkins, D. & Hattie, J. A longitudinal study of the approaches to learning of Austalian tertiary students. Hum. Learn. J. Practical Res. Appl. 4 , 127–141 (1985).
Russell, J. M., Baik, C., Ryan, A. T. & Molloy, E. Fostering self-regulated learning in higher education: making self-regulation visible. Act. Learn. Higher Educ . 23 , 97–113 (2020).
Schraw, G. Promoting general metacognitive awareness. Instr. Sci. 26 , 113–125 (1998).
Lundeberg, M. A. & Fox, P. W. Do laboratory findings on test expectancy generalize to classroom outcomes? Rev. Educ. Res. 61 , 94–106 (1991).
Rivers, M. L. & Dunlosky, J. Are test-expectancy effects better explained by changes in encoding strategies or differential test experience? J. Exp. Psychol. Learn. Mem. Cognn. 47 , 195–207 (2021).
Chi, M. in Handbook of Research on Conceptual Change (ed. Vosniadou, S.) 61–82 (Lawrence Erlbaum, 2009).
Susser, J. A. & McCabe, J. From the lab to the dorm room: metacognitive awareness and use of spaced study. Instr. Sci. 41 , 345–363 (2013).
Yan, V. X., Bjork, E. L. & Bjork, R. A. On the difficulty of mending metacognitive illusions: a priori theories, fluency effects, and misattributions of the interleaving benefit. J. Exp. Psychol. Gen. 145 , 918–933 (2016).
Ariel, R. & Karpicke, J. D. Improving self-regulated learning with a retrieval practice intervention. J. Exp. Psychol.Appl. 24 , 43–56 (2018).
Biwer, F., oude Egbrink, M. G. A., Aalten, P. & de Bruin, A. B. H. Fostering effective learning strategies in higher education — a mixed-methods study. J. Appl. Res. Mem. Cogn. 9 , 186–203 (2020).
McDaniel, M. A. & Einstein, G. O. Training learning strategies to promote self-regulation and transfer: the knowledge, belief, commitment, and planning framework. Perspect. Psychol. Sci. 15 , 1363–1381 (2020). This paper provides a framework for training students on how to use learning strategies .
Cleary, A. M. et al. Wearable technology for automatizing science-based study strategies: reinforcing learning through intermittent smartwatch prompting. J. Appl. Res. Mem. Cogn. 10 , 444–457 (2021).
Fazio, L. K. Repetition increases perceived truth even for known falsehoods. Collabra: Psychology 6 , 38 (2020).
Kozyreva, A., Lewandowsky, S. & Hertwig, R. Citizens versus the Internet: confronting digital challenges with cognitive tools. Psychol. Sci. Public. Interest. 21 , 103–156 (2020).
Pennycook, G. & Rand, D. G. The psychology of fake news. Trends Cognit. Sci. 25 , 388–402 (2021).
Ecker, U. K. H. et al. The psychological drivers of misinformation belief and its resistance to correction. Nat. Rev. Psychol. 1 , 13–29 (2022).
Toppino, T. C., Kasserman, J. E. & Mracek, W. A. The effect of spacing repetitions on the recognition memory of young children and adults. J. Exp. Child. Psychol. 51 , 123–138 (1991).
Childers, J. B. & Tomasello, M. Two-year-olds learn novel nouns, verbs, and conventional actions from massed or distributed exposures. Dev. Psychol. 38 , 967–978 (2002).
Lotfolahi, A. R. & Salehi, H. Spacing effects in vocabulary learning: young EFL learners in focus. Cogent Education 4 , 1287391 (2017).
Ambridge, B., Theakston, A. L., Lieven, E. V. M. & Tomasello, M. The distributed learning effect for children’s acquisition of an abstract syntactic construction. Cognit. Dev. 21 , 174–193 (2006).
Schutte, G. M. et al. A comparative analysis of massed vs. distributed practice on basic math fact fluency growth rates. J. Sch. Psychol. 53 , 149–159 (2015).
Küpper-Tetzel, C. E., Erdfelder, E. & Dickhäuser, O. The lag effect in secondary school classrooms: enhancing students’ memory for vocabulary. Instr. Sci. 42 , 373–388 (2014).
Bloom, K. C. & Shuell, T. J. Effects of massed and distributed practice on the learning and retention of second-language vocabulary. J. Educ. Res. 74 , 245–248 (1981).
Grote, M. G. Distributed versus massed practice in high school physics. Sch. Sci. Math. 95 , 97 (1995).
Minnick, B. Can spaced review help students learn brief forms? J. Educ. Bus. 44 , 146–148 (1969).
Dobson, J. L., Perez, J. & Linderholm, T. Distributed retrieval practice promotes superior recall of anatomy information. Anat. Sci. Educ. 10 , 339–347 (2017).
Kornell, N. & Bjork, R. A. Learning concepts and categories: is spacing the “enemy of induction”? Psychol. Sci. 19 , 585–592 (2008).
Rawson, K. A. & Kintsch, W. Rereading effects depend on time of test. J. Educ. Psychol. 97 , 70–80 (2005).
Butler, A. C., Marsh, E. J., Slavinsky, J. P. & Baraniuk, R. G. Integrating cognitive science and technology improves learning in a STEM classroom. Educ. Psychol. Rev. 26 , 331–340 (2014).
Carpenter, S. K. & DeLosh, E. L. Application of the testing and spacing effects to name learning. Appl. Cognit. Psychol. 19 , 619–636 (2005).
Pan, S. C., Tajran, J., Lovelett, J., Osuna, J. & Rickard, T. C. Does interleaved practice enhance foreign language learning? The effects of training schedule on Spanish verb conjugation skills. J. Educ. Psychol. 111 , 1172–1188 (2019).
Miles, S. W. Spaced vs. massed distribution instruction for L2 grammar learning. System 42 , 412–428 (2014).
Rohrer, D. & Taylor, K. The effects of overlearning and distributed practise on the retention of mathematics knowledge. Appl. Cognit. Psychol. 20 , 1209–1224 (2006).
Wahlheim, C. N., Dunlosky, J. & Jacoby, L. L. Spacing enhances the learning of natural concepts: an investigation of mechanisms, metacognition, and aging. Mem. Cogn. 39 , 750–763 (2011).
Simmons, A. L. Distributed practice and procedural memory consolidation in musicians’ skill learning. J. Res. Music. Educ. 59 , 357–368 (2012).
Ebersbach, M. & Barzagar Nazari, K. Implementing distributed practice in statistics courses: benefits for retention and transfer. J. Appl. Res. Mem. Cogn. 9 , 532–541 (2020).
Kornell, N. Optimising learning using flashcards: spacing is more effective than cramming. Appl. Cognit. Psychol. 23 , 1297–1317 (2009).
Bouzid, N. & Crawshaw, C. M. Massed versus distributed wordprocessor training. Appl. Ergon. 18 , 220–222 (1987).
Lin, Y., Cheng, A., Grant, V. J., Currie, G. R. & Hecker, K. G. Improving CPR quality with distributed practice and real-time feedback in pediatric healthcare providers—a randomized controlled trial. Resuscitation 130 , 6–12 (2018).
Terenyi, J., Anksorus, H. & Persky, A. M. Impact of spacing of practice on learning brand name and generic drugs. Am. J. Pharm. Educ. 82 , 6179 (2018).
Kerfoot, B. P., DeWolf, W. C., Masser, B. A., Church, P. A. & Federman, D. D. Spaced education improves the retention of clinical knowledge by medical students: a randomised controlled trial. Med. Educ. 41 , 23–31 (2007).
Kornell, N., Castel, A. D., Eich, T. S. & Bjork, R. A. Spacing as the friend of both memory and induction in young and older adults. Psychol. Aging 25 , 498–503 (2010).
Leite, C. M. F., Ugrinowitsch, H., Carvalho, M. F. S. P. & Benda, R. N. Distribution of practice effects on older and younger adults’ motor-skill learning ability. Hum. Mov. 14 , 20–26 (2013).
Balota, D. A., Duchek, J. M. & Paullin, R. Age-related differences in the impact of spacing, lag, and retention interval. Psychol. Aging 4 , 3–9 (1989).
Kliegl, O., Abel, M. & Bäuml, K.-H. T. A (preliminary) recipe for obtaining a testing effect in preschool children: two critical ingredients. Front. Psychol. 9 , 1446 (2018).
Fritz, C. O., Morris, P. E., Nolan, D. & Singleton, J. Expanding retrieval practice: an effective aid to preschool children’s learning. Q. J. Exp. Psychol. 60 , 991–1004 (2007).
Rohrer, D., Taylor, K. & Sholar, B. Tests enhance the transfer of learning. J. Exp. Psychol. Learn. Mem. Cogn. 36 , 233–239 (2010).
Lipowski, S. L., Pyc, M. A., Dunlosky, J. & Rawson, K. A. Establishing and explaining the testing effect in free recall for young children. Dev. Psychol. 50 , 994–1000 (2014).
Wartenweiler, D. Testing effect for visual-symbolic material: enhancing the learning of Filipino children of low socio-economic status in the public school system. Int. J. Res. Rev . 20 , 74–93 (2011).
Karpicke, J. D., Blunt, J. R. & Smith, M. A. Retrieval-based learning: positive effects of retrieval practice in elementary school children. Front. Psychol. 7 , 350 (2016).
Metcalfe, J., Kornell, N. & Son, L. K. A cognitive-science based programme to enhance study efficacy in a high and low risk setting. Eur. J. Cognit. Psychol. 19 , 743–768 (2007).
Rowley, T. & McCrudden, M. T. Retrieval practice and retention of course content in a middle school science classroom. Appl. Cognit. Psychol. 34 , 1510–1515 (2020).
McDaniel, M. A., Agarwal, P. K., Huelser, B. J., McDermott, K. B. & Roediger, H. L. Test-enhanced learning in a middle school science classroom: the effects of quiz frequency and placement. J. Educ. Psychol. 103 , 399–414 (2011).
Nungester, R. J. & Duchastel, P. C. Testing versus review: effects on retention. J. Educ. Psychol. 74 , 18–22 (1982).
Dirkx, K. J. H., Kester, L. & Kirschner, P. A. The testing effect for learning principles and procedures from texts. J. Educ. Res. 107 , 357–364 (2014).
Marsh, E. J., Agarwal, P. K. & Roediger, H. L. Memorial consequences of answering SAT II questions. J. Exp. Psychol. Appl. 15 , 1–11 (2009).
Chang, C., Yeh, T. & Barufaldi, J. P. The positive and negative effects of science concept tests on student conceptual understanding. Int. J. Sci. Educ. 32 , 265–282 (2010).
Grimaldi, P. J. & Karpicke, J. D. Guided retrieval practice of educational materials using automated scoring. J. Educ. Psychol. 106 , 58–68 (2014).
Pan, S. C., Gopal, A. & Rickard, T. C. Testing with feedback yields potent, but piecewise, learning of history and biology facts. J. Educ. Psychol. 108 , 563–575 (2016).
Darabi, A., Nelson, D. W. & Palanki, S. Acquisition of troubleshooting skills in a computer simulation: worked example vs. conventional problem solving instructional strategies. Comput. Hum. Behav. 23 , 1809–1819 (2007).
Kang, S. H. K., Gollan, T. H. & Pashler, H. Don’t just repeat after me: retrieval practice is better than imitation for foreign vocabulary learning. Psychon. Bull. Rev. 20 , 1259–1265 (2013).
Carpenter, S. K. & Pashler, H. Testing beyond words: using tests to enhance visuospatial map learning. Psychon. Bull. Rev. 14 , 474–478 (2007).
Carpenter, S. K. & Kelly, J. W. Tests enhance retention and transfer of spatial learning. Psychon. Bull. Rev. 19 , 443–448 (2012).
Kang, S. H. K., McDaniel, M. A. & Pashler, H. Effects of testing on learning of functions. Psychon. Bull. Rev. 18 , 998–1005 (2011).
Jacoby, L. L., Wahlheim, C. N. & Coane, J. H. Test-enhanced learning of natural concepts: effects on recognition memory, classification, and metacognition. J. Exp. Psychol. Learn. Mem. Cogn. 36 , 1441–1451 (2010).
McDaniel, M. A., Anderson, J. L., Derbish, M. H. & Morrisette, N. Testing the testing effect in the classroom. Eur. J. Cognit. Psychol. 19 , 494–513 (2007).
Foss, D. J. & Pirozzolo, J. W. Four semesters investigating frequency of testing, the testing effect, and transfer of training. J. Educ. Psychol. 109 , 1067–1083 (2017).
Wong, S. S. H., Ng, G. J. P., Tempel, T. & Lim, S. W. H. Retrieval practice enhances analogical problem solving. J. Exp. Educ. 87 , 128–138 (2019).
Pan, S. C., Rubin, B. R. & Rickard, T. C. Does testing with feedback improve adult spelling skills relative to copying and reading? J. Exp. Psychol. Appl. 21 , 356–369 (2015).
Coppens, L., Verkoeijen, P. & Rikers, R. Learning Adinkra symbols: the effect of testing. J. Cognit. Psychol. 23 , 351–357 (2011).
Zaromb, F. M. & Roediger, H. L. The testing effect in free recall is associated with enhanced organizational processes. Mem. Cogn. 38 , 995–1008 (2010).
Carpenter, S. K., Pashler, H. & Vul, E. What types of learning are enhanced by a cued recall test? Psychon. Bull. Rev. 13 , 826–830 (2006).
Pan, S. C., Wong, C. M., Potter, Z. E., Mejia, J. & Rickard, T. C. Does test-enhanced learning transfer for triple associates? Mem. Cogn. 44 , 24–36 (2016).
Butler, A. C. & Roediger, H. L. Testing improves long-term retention in a simulated classroom setting. Eur. J. Cognit. Psychol. 19 , 514–527 (2007).
Dobson, J. L. & Linderholm, T. Self-testing promotes superior retention of anatomy and physiology information. Adv. Health Sci. Educ. 20 , 149–161 (2015).
Kromann, C. B., Jensen, M. L. & Ringsted, C. The effect of testing on skills learning. Med. Educ. 43 , 21–27 (2009).
Baghdady, M., Carnahan, H., Lam, E. W. N. & Woods, N. N. Test-enhanced learning and its effect on comprehension and diagnostic accuracy. Med. Educ. 48 , 181–188 (2014).
Freda, N. M. & Lipp, M. J. Test-enhanced learning in competence-based predoctoral orthodontics: a four-year study. J. Dental Educ. 80 , 348–354 (2016).
Tse, C.-S., Balota, D. A. & Roediger, H. L. The benefits and costs of repeated testing on the learning of face–name pairs in healthy older adults. Psychol. Aging 25 , 833–845 (2010).
Meyer, A. N. D. & Logan, J. M. Taking the testing effect beyond the college freshman: benefits for lifelong learning. Psychol. Aging 28 , 142–147 (2013).
Guran, C.-N. A., Lehmann-Grube, J. & Bunzeck, N. Retrieval practice improves recollection-based memory over a seven-day period in younger and older adults. Front. Psychol. 10 , 2997 (2020).
McCabe, J. Metacognitive awareness of learning strategies in undergraduates. Mem. Cogn. 39 , 462–476 (2011).
Carpenter, S. K., Witherby, A. E. & Tauber, S. K. On students’ (mis)judgments of learning and teaching effectiveness. J. Appl. Res. Mem. Cogn. 9 , 137–151 (2020). This review discusses the factors underlying faulty metacognition, and how they can mislead students’ judgements of their own learning as well as the quality of effective teaching .
Chi, M. T. H., Bassok, M., Lewis, M. W., Reimann, P. & Glaser, R. Self-explanations: how students study and use examples in learning to solve problems. Cognit. Sci. 13 , 145–182 (1989).
Gurung, R. A. R. How do students really study (and does it matter)? Teach. Psychol. 32 , 238–241 (2005).
Deslauriers, L., McCarty, L. S., Miller, K., Callaghan, K. & Kestin, G. Measuring actual learning versus feeling of learning in response to being actively engaged in the classroom. Proc. Natl Acad. Sci. USA 116 , 19251–19257 (2019).
Hartwig, M. K., Rohrer, D. & Dedrick, R. F. Scheduling math practice: students’ underappreciation of spacing and interleaving. J. Exp. Psychol. Appl. 28 , 100–113 (2022).
Carpenter, S. K., King-Shepard, Q., & Nokes-Malach, T. J. in In Their Own Words: What Scholars Want You to Know About Why and How to Apply the Science of Learning in Your Academic Setting (eds Overson, C., Hakala, C., Kordonowy, L. & Benassi, V.) (American Psychological Association, in the press).
Kirk-Johnson, A., Galla, B. M. & Fraundorf, S. H. Perceiving effort as poor learning: the misinterpreted-effort hypothesis of how experienced effort and perceived learning relate to study strategy choice. Cognit. Psychol. 115 , 101237 (2019).
Fisher, O. & Oyserman, D. Assessing interpretations of experienced ease and difficulty as motivational constructs. Motiv. Sci. 3 , 133–163 (2017).
Schiefele, U. Interest, learning, and motivation. Educ. Psychol. 26 , 299–323 (1991).
Simons, J., Dewitte, S. & Lens, W. The role of different types of instrumentality in motivation, study strategies, and performance: know why you learn, so you’ll know what you learn! Br. J. Educ. Psychol. 74 , 343–360 (2004).
Pan, S. C., Sana, F., Samani, J., Cooke, J. & Kim, J. A. Learning from errors: students’ and instructors’ practices, attitudes, and beliefs. Memory 28 , 1105–1122 (2020).
Download references
Acknowledgements
This material is based upon work supported by the James S. McDonnell Foundation 21st Century Science Initiative in Understanding Human Cognition, Collaborative Grant 220020483. The authors thank C. Phua for assistance with verifying references.
Author information
Authors and affiliations.
Department of Psychology, Iowa State University, Ames, IA, USA
Shana K. Carpenter
Department of Psychology, National University of Singapore, Singapore City, Singapore
- Steven C. Pan
Department of Education, Washington University in St. Louis, St. Louis, MO, USA
Andrew C. Butler
Department of Psychological and Brain Sciences, Washington University in St. Louis, St. Louis, MO, USA
You can also search for this author in PubMed Google Scholar
Contributions
All authors contributed to the design of the article. S.K.C. drafted the sections on measuring learning, spacing, successive relearning and future directions; S.C.P. drafted the section on retrieval practice, developed the figures and drafted the tables; A.C.B. drafted the section on metacognition. All authors edited and approved the final draft of the complete manuscript.
Corresponding author
Correspondence to Shana K. Carpenter .
Ethics declarations
Competing interests.
The authors declare no competing interests.
Peer review
Peer review information.
Nature Reviews Psychology thanks Veronica Yan, who co-reviewed with Brendan Schuetze; Mirjam Ebersbach; and Nate Kornell for their contribution to the peer review of this work.
Additional information
Publisher’s note.
Springer Nature remains neutral with regard to jurisdictional claims in published maps and institutional affiliations.
Rights and permissions
Springer Nature or its licensor holds exclusive rights to this article under a publishing agreement with the author(s) or other rightsholder(s); author self-archiving of the accepted manuscript version of this article is solely governed by the terms of such publishing agreement and applicable law.
Reprints and permissions
About this article
Cite this article.
Carpenter, S.K., Pan, S.C. & Butler, A.C. The science of effective learning with spacing and retrieval practice. Nat Rev Psychol 1 , 496–511 (2022). https://doi.org/10.1038/s44159-022-00089-1
Download citation
Accepted : 23 June 2022
Published : 02 August 2022
Issue Date : September 2022
DOI : https://doi.org/10.1038/s44159-022-00089-1
Share this article
Anyone you share the following link with will be able to read this content:
Sorry, a shareable link is not currently available for this article.
Provided by the Springer Nature SharedIt content-sharing initiative
This article is cited by
Optimizing self-organized study orders: combining refutations and metacognitive prompts improves the use of interleaved practice.
- Felicitas Biwer
- Anique de Bruin
npj Science of Learning (2024)
Improved Soft-Skill Competencies of ABA Professionals Following Training and Coaching: A Feasibility Study
- Zahava L. Friedman
- Daphna El Roy
- Angela Broff
Behavior and Social Issues (2024)
A Computational Model of School Achievement
- Brendan A. Schuetze
Educational Psychology Review (2024)
Emerging and Future Directions in Test-Enhanced Learning Research
- John Dunlosky
- Kim Ouwehand
Happy Together? On the Relationship Between Research on Retrieval Practice and Generative Learning Using the Case of Follow-Up Learning Tasks
- Julian Roelle
- Tino Endres
- Alexander Renkl
Educational Psychology Review (2023)
Quick links
- Explore articles by subject
- Guide to authors
- Editorial policies
Sign up for the Nature Briefing newsletter — what matters in science, free to your inbox daily.

- Open supplemental data
- Reference Manager
- Simple TEXT file
People also looked at
Systematic review article, a meta-analysis of ten learning techniques.
- Science of Learning Research Centre, Graduate School of Education, University of Melbourne, Melbourne, VIC, Australia
This article outlines a meta-analysis of the 10 learning techniques identified in Dunlosky et al. (2013a), and is based on 242 studies, 1,619 effects, 169,179 unique participants, with an overall mean of 0.56. The most effective techniques are Distributed Practice and Practice Testing and the least effective (but still with relatively high effects) are Underlining and Summarization. A major limitation was that the majority of studies in the meta-analysis were based on surface or factual outcomes, and there is caution needed when applying these findings to deeper and more relational outcomes. Other important moderators included the presence of feedback or not, near or far transfer, and the effects were much greater for lower than higher ability students. It is recommended that more attention be paid to when, under what conditions, each technique can be used, and how they can best be taught.
Introduction
While the purpose of schooling may change over time and differ across jurisdictions, the mechanisms by which human learning occurs arguably are somewhat more universal. Learning techniques actions that learners themselves can take to enhance their learning–have attracted considerable research interest in recent years ( Edwards et al., 2014 ). This is unsurprising given the direct, practical applicability of such research, and its relevance to students, educators and school leaders alike.
A major, thorough, and important review of various learning techniques has created much interest. Dunlosky et al. (2013a) reviewed 10 learning techniques and a feature of their review is their careful analyses of possible moderators to the conclusions about the effectiveness of these learning techniques, such as learning conditions (e.g., study alone or in groups), student characteristics (e.g., age, ability), materials (e.g., simple concepts or problem-based analyses), and criterion tasks (different outcome measures). This article uses this review as a basis for conducting a meta-analysis on these authors’ references to add another perspective of the magnitude of the various learning techniques and how they are affected by various moderators.
Dunlosky et al. (2013a) , claim to have conducted an exhaustive search of the literature, relied on previous empirical reviews of learning techniques, and applied a robust set of selection criteria before selecting final 10 techniques. These criteria included that the technique could be implemented by students without assistance, there was sufficient empirical evidence to support at least a preliminary assessment of efficacy, and there was robust evidence to identify the generalizability of its benefits across four categories of variables materials, learning conditions, student characteristics and criterion tasks. Indeed the authors’ mastery of the literature is most evident throughout the article.
The authors then categorised the 10 techniques into three groups based on whether they considered them having high, medium or low support for their effectiveness in enhancing learning. Categorised as “high” support were Practice Testing (self-testing or taking practice tests on to-be-learned material) and Distributed Practice (implementing a schedule of practice that spreads out study activities over time in contrast to massed or ‘crammed’ practice). Categorised as “moderate” support were Elaborative Interrogation (generating an explanation of a fact or concept), Self-Explanation (where the student explains how new information is related to already-known information) and Interleaved Practice (implementing a schedule of practice mixing different kinds of problems within a single study session). Finally, categorised as “low” support were Summarization (writing summaries of to-be-learned texts), Highlighting/Underlining (marking potentially important portions of to-be-learned materials whilst reading), Keyword Mnemonic (generating keywords and mental imagery to associate verbal materials), Imagery use (attempting to form mental images of text materials while reading or listening) and Re-Reading (restudying text material again after an initial reading). In an accompanying article, Dunlosky et al. (2013b) claimed that some of these low support techniques (that students use a lot) have “failed to help students of all sorts” ( p . 20), the benefits can be short lived, they may not be widely applicable, the benefits are relatively limited, and they do not provide “bang for the buck” ( p . 21).
Practice Testing is one of the two techniques with the highest utility. This must be distinguished from high stakes testing: Practice Testing instead involves any activity where the student practices retrieval of to-be-learned information, reproduces that information in some form, and evaluates the correctness of that reproduction against an accepted ‘correct’ answer. Any discrepancy between the produced and “correct” information then forms a type of feedback that the learner uses to modify their understanding. Practice tests can include a range of activities that students can conduct on their own, such as completing questions from textbooks or previous exams, or even self-generated flashcards. According to Dunlosky et al. (2013a) , such testing helps increase the likelihood that target information can be retrieved from long-term memory and it helps students mentally organize information that supports better retention and test performance. This effect is strong regardless of test form (multiple choice or essay), even when the format of the practice test does not match the format of the criterion test, and it is effective for all ages of student. Practice Testing works well even when it is massed, but is even more effective when it is spaced over time. It does not place high demand on time, is easy to learn to do (but some basic instruction on how to most effectively use practice tests helps), is so much better than unguided restudy, and so much more effective when there is feedback about the practice test outputs (which also enhances confidence in performance).
Many studies have shown that practice spread out over time (spaced) is much more effective than practice over a short time period (massed)–this is what is meant by Distributed Practice. Most students need three to four opportunities to learn something ( Nuthall, 2007 ) but these learning opportunities are more effective if they are distributed over time, rather than delivered in one massed session: that is, spaced practice, not skill and drill, spread out not crammed, and longer inter-study intervals are more effective than shorter. There have been four meta-analyses of Spaced vs. Massed practices involving about 300 studies, with an average effect of 0.60 ( Donovan and Radosevich, 1999 ; Cepeda et al., 2006 ; Janiszewski et al., 2003 ; Lee and Genovese 1988 ). Cepeda et al. (2008) showed that for almost all retention intervals, memory performance increases sharply with the length of the spacing interval. But at a certain spacing interval, optimal test performance is reached, and from that interval onwards, performance declines but only to a limited degree. But they also note that this does not take into account the absolute level of performance, which decreases as the retention interval increases. Further, Spaced Practice is more effective for deeper than surface processing, and for all ages. Rowland (2014) completed a meta-analysis on 61 studies investigating the effect of testing vs. restudy on retention. He found a high effect size ( d = 0.50) supporting the testing over restudy, and the effects were greater for recall than for recognition tasks. The educational message is to review previously covered material in subsequent units of work, time tests regularly and not all at the end (which encourages cramming and massed practice), and given that students tend to rate learning higher after massed, educate them as to the benefits of spaced practice and show them those benefits.
Elaborative Interrogation, Self-Explanation, and Interleaved Practice received moderate support. Elaborative Interrogation involves asking “Why” questions (“Why does it make sense that” “Why is this true”) and a major purpose is to integrate new information with existing prior knowledge. The effects are higher when elaborations are precise rather than imprecise, when prior knowledge is higher than lower, and when elaborations are self-generated rather than provided. A constraint of the method is that is more applicable to surface than to deep understanding. Self-explanation involves students explaining some aspect of their processing during learning. It works across task domains, across ages, but may require training, and can take some time to implement. Interleaved Practice involves alternating study practice of different kinds of items, problems, and even subject domains rather than blocking study. The claim is that Interleaving leads to better discrimination of different kinds of problems, more attention to the actual question or problem posed, and as above there is better learning from Spaced than Mass Practice. The research evidence base is currently small, and it is not clear how to break tasks in an optimal manner so as to interleave them.
There is mixed and often low support, claimed Dunlosky et al. (2013a) , for Summarization, Highlighting, Keyword Mnemonic, Imagery Use for text learning, and Re-Reading. Summarization involves students writing summaries of to-be-learned texts with the aim of capturing the main points and excluding unimportant or repetitive material. The generality and accuracy of the summary are important moderators, and it is not clear whether it is better to summarize smaller pieces of a text (more frequent Summarization) or to capture more of the text in a larger summary (less frequent Summarization). Younger and less able students are not as good at Summarization, it is better when the assessments are performance or generative and not closed or multiple choice tests, and it can require extensive training to use optimally. Highlighting and Underlining are simple to use, do not require training, and demand hardly any additional time beyond the reading of the text. It is more effective when professionals do the highlighting, then for the student doing the highlighting, and least for reading other student’s highlights. It may be detrimental to later ability to make inferences; overall it does little to boost performance. The Keyword Mnemonic involves associating some imagery with the word or concept to be learned. The method requires generating images that can be difficult for younger and less able students, and there is evidence is may not produce durable retention. Similarly Imagery Use is of low utility. This method involves students mentally imaging or drawing pictures of the content using simple and clear mental images. It too is more constrained to imagery-friendly materials, and memory capacity. Re-Reading is very common. It is more effective when the Re-Reading is spaced and not massed, the effects seem to decrease beyond the second reading, is better for factual recall than for developing comprehension, and it is not clear it is effective with students below college age.
A follow-up and more teacher accessible article by Dunlosky et al. (2013b) asks why students do not learn about the best techniques for learning. Perhaps, the authors suggest, it is because curricula are developed to highlight content rather than how to effectively acquire it; and it may be because many recent textbooks used in teacher education courses fail to adequately cover the most effective techniques or how to teach students to use them. They noted that employing the best techniques will only be effective if students are motivated to use them correctly but teaching students to guide their learning of content using effective techniques will allow them to successfully learn throughout their lifetime. Some of the authors’ tips include: give a low-stakes quiz at the beginning of each class and focus on the most important material; give a cumulative exam that encourages students to re-study the most important material in a distributed fashion; encourage students to develop a “study planner” so they can distribute their study throughout a class and rely less on cramming; encourage students to use practice retrieval when studying instead of passively re-reading their books and notes; encourage students to elaborate on what they are reading, such as by asking “why” questions; mix up problems from earlier classes so students can practice identifying problems and their solutions; and tell students that highlighting is fine but only in the beginning of their learning journey.
The Dunlosky et al. (2013a) , review shows a high level of care of selection of articles, an expansiveness of the review, an attention to generalizability and moderators, and is sophisticated in its conclusions. There are two aspects of the this research that the current paper aims to address. First, Dunlosky et al. (2013a) relied on a traditional literature review method and did not include any estimates of the effect-sizes of their various techniques, nor did they indicate the magnitude of their terms high, medium, and low. One of the purposes of this article is to provide these empirical estimates. Second, the authors did not empirically evaluate the moderators of the 10 learning techniques, such as Deep vs. Surface learning, Far vs. Near Transfer, or age/grade level of learner. An aim of this paper is to analyze the effects of each of the 10 techniques with respect to these and other potential moderators.
Research syntheses aim to summarise past research by estimating effect-sizes from multiple, separate studies that address, in this case, 10 major learning techniques. The data is based on the 399 studies referenced in Dunlosky et al. (2013a) . We removed all non-empirical studies, and any studies that did not report sufficient data for the calculation of a Cohen’s d . This resulted in 242 studies being included in the meta-analysis, many of which contained data for multiple effect sizes, resulting in 1,620 cases for which a Cohen’s d was calculated (see Figure 1 ).
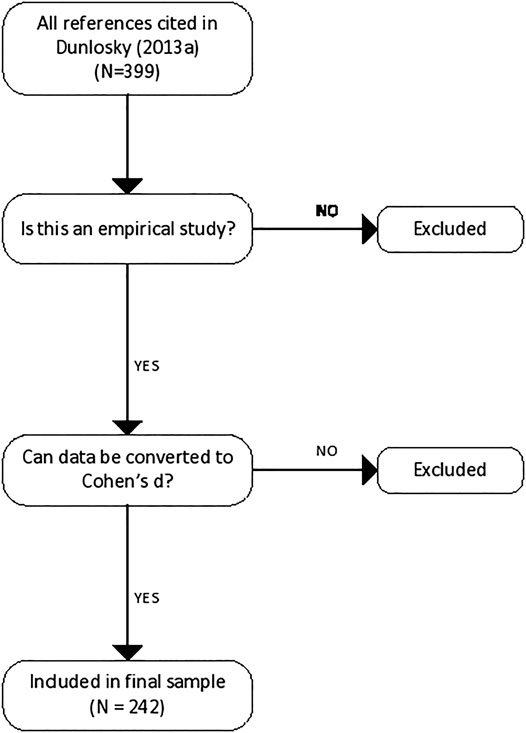
FIGURE 1 . Flow diagram of articles used in the meta-analysis.
The publication dates of the articles ranged from 1929 to 2014, with half being published since 1996. Most participants were undergraduates (65%), but also included secondary (11%), primary (13%), adults (2%), and early childhood (9%). Most were chosen from the average range of abilities (86%), while 7% were categorised low ability and 7% high ability. The participants were mainly North Americans (86%), and also included Europeans (11%), and Australians (3%).
All articles were coded by the two authors, and independent colleagues were asked to re-code a sample of 30 (about 10%) to estimate inter-rater reliability. This resulted in a kappa value of 0 89, which gives much confidence in the dependability of the coding.
For each study, three sets of moderators were coded. The first set of moderators included attributes of the article: quality of the journal (h-index), year of publication (to assess any changes in effectiveness as more research has been added into the literature), and sample size. The second set of moderators included attributes of the students: ability level of the students (low, average, and high), country of the study, grade levels of the student (pre and primary, high, Univ, adults). The third set of moderators included attributes of the design: whether the outcome was near or far transfer (e.g., was the learner tested on criterion tasks that differed from the training tasks or did the effect of the technique improve the student learning in a different subject domain), the depth of the outcome (Surface or content-specific vs. Deep or more generalizable learning), how delayed was the testing from the actual study (under 1 day, or 2 + days), the learning domain of the content of the study or measure (e.g., cognitive, non-cognitive).
The design of most studies include experimental compared to control group (91%), longitudinal (pre-post, time series) 6.5%, and within subject designs (2.4%). Most learning outcomes were classified as Surface (93%) and the other 7% Deep. The post-tests were predominantly completed very soon after the intervention - 74% completed in 1 day or less, 17% from 2 to 7 days, 3.3% from 8 days to month, 0.4% from 1 to 3 months, and 0.2% from 4 months to 7 years.
We used two major methods for calculating Cohen’s d from the various statistics published in the studies. First, standardized mean differences ( N = 1,203 effects) involved subtracting the mean of the control group from the mean of the experimental group, then dividing by an estimate of the pooled standard deviation, as follows.-
The standard errors of the effect size (ES) were calculated as follows,
We adjusted the effect sizes (ES) according to Hedges and Olkin, (1985) to account for bias in sample sizes, according to this
Second, F-statistics (for two groups only) were converted using:
The Standard Error was calculated using:
In all cases, therefore, a positive effect meant that the learning technique had a positive impact on learning.
The distribution of effect sizes and sample sizes was examined to determine if any were statistical outliers. Grubbs (1950) test was applied (see also Barnett and Lewis, 1994 ). If outliers were identified, these values were set at the value of their next nearest neighbour. We used inverse variance weighted procedures to calculate average effect sizes across all comparisons ( Borenstein et al., 2005 ). Also, 95% confidence intervals were calculated for average effects. Possible moderators (e.g., grade level, duration of the treatment) of the DBP to student outcome relationship were tested using homogeneity analyses ( Hedges and Olkin, 1985 ; Cooper et al., 2019 ). The analyses were carried out to determine whether a) the variance in a group of individual effect sizes varies more than predicted by sampling error and/or b) multiple groups of average effect sizes vary more than predicted by sampling error.
Rather than opt for a single model of error, we conducted the overall analyses twice, once employing fixed-error assumptions and once employing random-error assumptions (see Hedges and Vevea, 1998 , for a discussion of fixed and random effects). This sensitivity analysis allowed us to examine the effects of the different assumptions (fixed or random) on the findings. If, for example, a moderator is found to be significant under a random-effects assumption but not significant under a fixed effects assumption, then this suggests a limit on the generalizability of the inferences of the moderator. All statistical processes were conducted using the Comprehensive Meta-Analysis software package ( Borenstein et al., 2005 ).
The examination of heterogeneity of the effect size distributions within each outcome category was conducted using the Q statistic and the I 2 statistic ( Borenstein et al., 2009 ). To calculate Q and I 2 , we entered the corrected effect-sizes for every case, along with the SE (calculated as above) and generated homogeneity data. Due to the substantive variability within the studies, even in the case of a non-significant Q test, when I 2 was different from zero, moderation analyses were carried out through subgroup analysis ( Lipsey and Wilson, 2001 ). As all hypothesized moderators were operationalized as categorical variables, these analyses were performed primarily through subgroup analyses using a mixed-effects model.
Table 1 shows a comprehensive analysis of the collected data. For the 242 studies, we calculated a total of 1,619 effects which related to 169,179 unique participants. The overall mean assuming a fixed model was 0.56 (SD = 0.81, SEM = 0.072, skewness 1.3, kurtosis = 5.64); the overall mean assuming a random model was 0.56 (SE = 0.016). The overall mean at the study level was 0.77 (SE = 0.049). The fixed effects model assumes that all studies in the meta-analysis share a common true effect size, whereas the random effects model assumes that the studies were drawn from populations that differ from each other in ways that could impact on the treatment effect. Given that the means estimated under the two models are similar we proceed to use only one (the random model) in subsequent analyses.
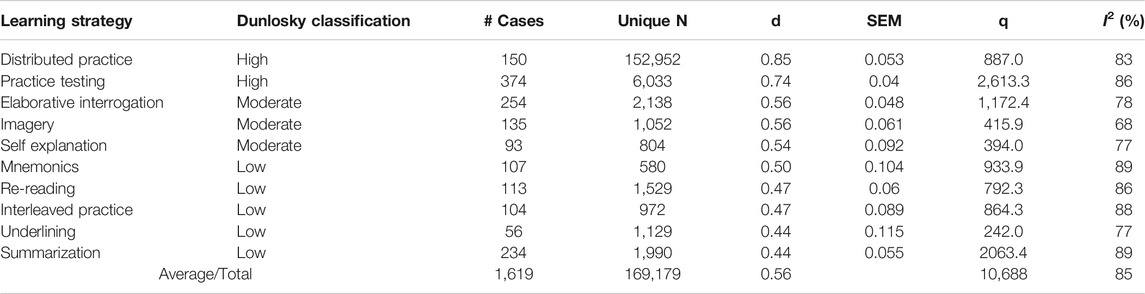
TABLE 1 . Summary of effects for each learning strategy.
The distribution of all effects is presented in Figure 1 and the studies, their attributes, and study effect-size are presented in Table 1 . It is clear that there is much variance among these effects ( Q = 10,688.2, I 2 = 84.87). The I 2 a measure of the degree of inconsistency in the studies’ results; and this I 2 of 85% shows that most of the variability across studies is due to heterogeneity rather than chance. Thus, the search for moderators is critical to understanding which learning techniques work best in which situations.
Table 2 shows an overall summary of effects moderated by the learning domain. The effects correspond with the classification of High, Moderate, and Low by Dunlosky et al. (2013a) , but it is noted that Low is still relatively above the average of most meta-analysis in education – Hattie, (2009) , Hattie, (2012) , Hattie, (2015) reported an average effect-size of 0.40 from over 1,200 meta-analyses relating to achievement outcomes. All techniques analyzed in the current study had an ES of over 0.40.
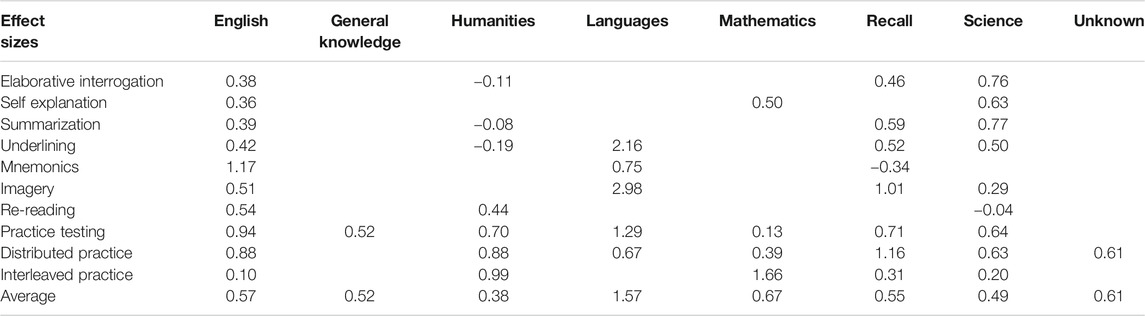
TABLE 2 . Effect Sizes moderated by the Learning Domain.
Moderator Analyses
Year of publication.
There was no relation between the magnitude of the effects and the year of the study ( r = 0.08, df = 236, p = 0.25) indicating that the effects of the learning technique have not changed over time (from 1929 to 2015).
Learning Domain
The vast majority of the evidence is based on measurements of academic achievement: 222 of the 242 studies (91.7%) and 1,527 of the 1,619 effects (94.3%). English or Reading was the basis for 85 of the studies (35.1%) and 546 of the effects (33.7%), and Science 41 of the studies (16.9%) and 336 of the effects (20.8%). There was considerable variation in the effect sizes of these domains, as shown in Table 3 .
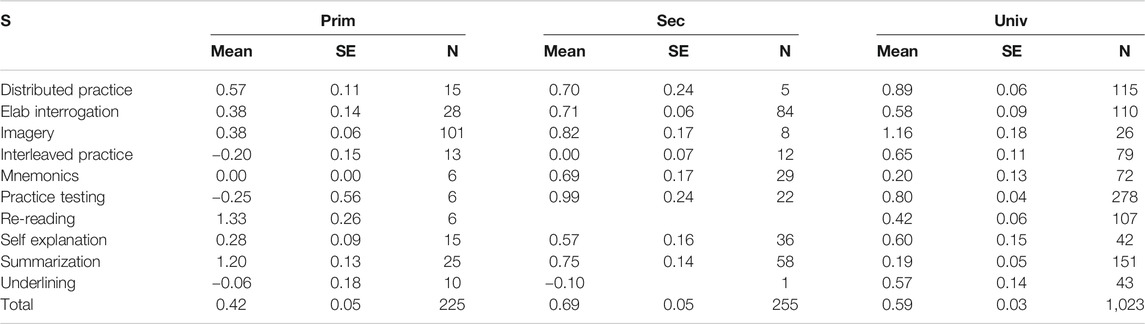
TABLE 3 . Effect sizes moderated by grade level.
Near vs. Far Transfer
If the study measured an effect on performance on a task similar to the task used in the experiment, it was classified as measuring Near transfer, alternatively if the transfer was to another dissimilar context it was classified as Far transfer. There were so few Far transfer effects that the information is not broken into the 10 learning techniques. Overall, the effects on Near ( d = 0.61, SE = 0.052, N = 197) are much greater than the effects on Far ( d = 0.39, SE = -0.002, N = 1,385).
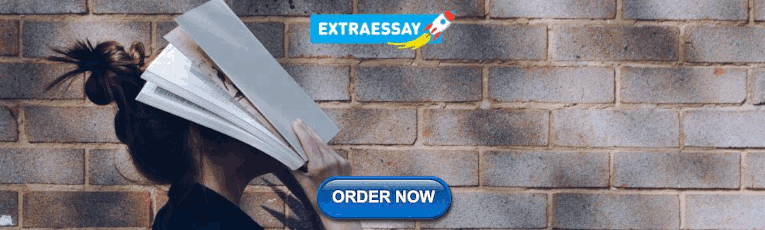
Depth of Learning
The effects were higher for Surface ( d = 0.60, SE = 0.021, N = 1,473) than for Deep processing ( d = 0.26, SE = 0.064, N = 109).
Grade Level
The effects moderated by grade level of the participants are presented in Table 4 . All students had higher effects on summarization, distributed practice, imagery use, and re-reading, primary students had lower effects on interleaved practice, mnemonics, self-explanation, and practice testing. Both primary and secondary students had lower effects on Underlining.
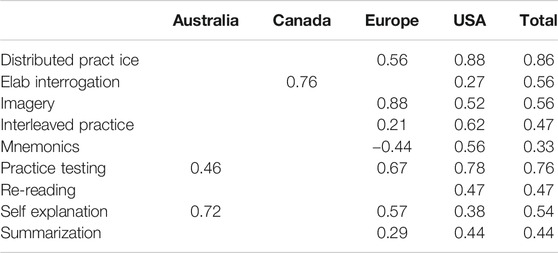
TABLE 4 . Effect size moderated by Country of first author.
Each study was coded for the country where the study was conducted. Where that information was not made clear in the article, the first author’s country of employment was used. Of the 242 studies, 187 (77.3%) were from USA, 20 (8.3%) were from Canada, 27 (11.1%) from Europe: United Kingdom, Denmark, France, Germany, Italy, Netherlands), 7 (2.9%) from Australia and 1 (0.4%) from Iran making a total North American proportion of 207 (85.6%). Other than the drop for Europe in Mnemonics, Interleaved Practice and Summarisation there is not a great difference by country.
Ability Level
Almost all studies referred to participants as being either “Low” “Normal” or “High” ability. This language has been continued in the collection and analysis of the data, however in the body of the paper the terms “Low”, “Average” and “High” ability have been used instead. In all cases, these categories aligned with percentiles of the normal distribution for academic scores. Of the 242 studies, only six investigated High ability students, and only 13 Low ability. Across all techniques, the mean effect on High ability students was -0.11 (SE = 0.10, N = 28) for Low ability students was 0.47, SE = 0.15, N = 58. The High ability students had negative effects for Interleaved Practice and Summarization.
Studies predominantly measured only very short-term effects, the exception being the three learning techniques focused on practice effects (Practice Testing, Distributed Practice and Interleaved Practice). Most (68%) where evaluated within a day (usually immediately). There were no overall differences relating to the effects less than a day (d = 0.58, SE = 0.025, N = 1,073), > 1 day and < 1 week ( d = 0.59, SE = 0.057, N = 204), > 1 week and < 1 month ( d = 0.56, SE = 0.058, N = 228), < 1 month and less than 6 months ( d = 0.51, SE = 0.082, N = 64).
Journal Impact Factor
The published Impact factor for each journal was sourced from that Journal’s website. Where a multiple-year (usually 5 years) average h-index was provided, that was used in preference to a single (the most recent) year (PhD theses were left blank). The average impact factor is 2.80 (SE = 3.29), which relative to Journals in educational psychology indicates that the overall quality of Journals is quite high. Across all 10 learning techniques, there was a moderate positive correlation between effect size and Journal Impact Factor, r (235) = 0.24, < 0.001. Thus the effect-sizes were slightly higher in the more highly cited Journals.
Discussion and Conclusion
The purpose of the current study was twofold: to provide empirical estimates of the effectiveness of the 10 learning techniques, and second, to empirically evaluate a range of their potential moderators. The major conclusion from the meta-analysis is a confirmation of the major findings in Dunlosky et al. (2013a) . They rated the effects by High, Moderate, and Low and there was much correspondence between their ratings and the actual effect-sizes: High in the meta-analysis was > 0.70, Moderate between 0.54 and 0.69, and Low < 0.53. This meta-analysis, however, shows the arbitrariness of these ratings, as some of the low effects were very close estimates to the moderate. mnemonics, re-reading and interleaved practice were all within 0.06 of the moderate category and these techniques may have similar importance to those Dunlosky et al. (2013a) classified as Moderate. Certainly they should not be dismissed as ineffective. Even the lowest learning techniques (Underlining and Summarization (both d = 0.44) are sufficiently effective to be included in a student’s toolbox of techniques.
The rating method into High, Medium, and Low was matched by the findings of the meta-analysis, but Table 2 shows the usual difficulties of such arbitrary (but not capricious) cut scores. Mnemonics ( d = 0.50) is close to Self-Explanation ( d = 0.54), although there is a clear separation between Moderate (Elaborative Interrogation d = 0.56) and Practice Testing ( d = 0.74). All have a sufficient positive effect to consider by students choosing learning techniques, and it may be that there is a more optimal stage during the learning process to choose the high techniques related to consolidating learning, and the low techniques related to first encountering new material and ideas. It may also be that techniques are affected by whether the tasks are more relevant to memory vs. those that are relevant to comprehension. Many of the techniques in the authors’ list of 10 are more related to the former than the latter.
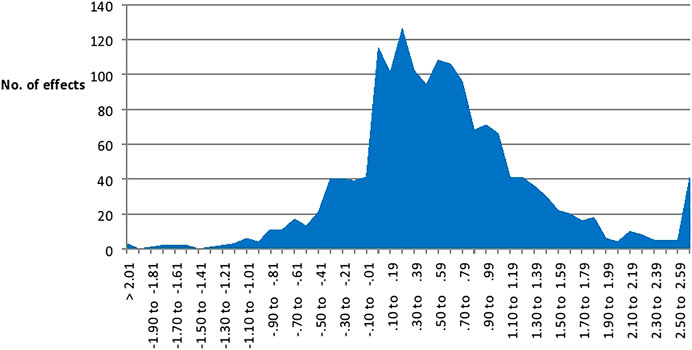
FIGURE 2 . Distribution of effects.
The technique with the lowest overall effect was Summarization. Dunlosky et al. (2013a) note that it is difficult to draw general conclusions about its efficacy, it is likely a family of techniques, and should not be confused with mere copying. They noted that it is easy to learn and use, training typically helps improve the effect (but such training may need to be extensive), but suggest other techniques might better serve the students. In their other article ( Dunlosky et al., 2013b ), the authors classified Summarization as among the “less useful techniques” that “have not fared so well when considered with an eye toward effectiveness” ( p . 19). They also noted that a critical moderator for the effectiveness of all techniques is the student’s motivation to use them correctly. This meta-analysis shows that Summarization, while among the less effective of the 10 under review, still has a sufficiently high impact to be considered worthwhile in the student’s arsenal of learning techniques, and with training could be among the more easier to use techniques.
One of the sobering aspects of this meta-analysis is the finding that the majority of studies are based on Surface learning of factual, academic content, measure learning almost immediately after the technique has been used, and only measure Near transfer. This limits the generalisability of the Dunlosky et al. (2013a) review and this meta-analysis and there may well be different learning techniques that optimise deeper learning, non-academic learning, or more intensive learning that requires longer retention periods and Far transfer. The verdict is still out on the effectiveness and identification of the optimal techniques in these latter conditions. It should be noted, however, that this may be not only a criticism of the current research on learning techniques but could well be the same criticism of student experiences in most classrooms. Too many modern classrooms are still dominated by a preponderance of surface learning, teachers asking low level questions demanding content answers, and assessments privileging surface knowledge ( Tyack & Cuban, 1995 ). Thus the 10 techniques may remain optimal for many current classrooms.
The implication for teachers is not that these learning techniques should be implemented as stand-alone “learning interventions” or fostered through study skills courses. They can be used, however, within a teaching process to maximise the surface and deeper outcomes of a series of lessons. For example, Practice Testing is among the top two techniques but it would be a mistake to then make claims that there should be more testing, especially high-stakes testing! Dunlosky et al. (2013a) concluded that more Practice Testing is better, should be spaced not massed, works with all ages, levels of ability, and across all levels of cognitive complexity. A major moderator is whether the practice tests are accompanied by feedback or not. “The advantage of Practice Testing with feedback over restudy is extremely robust. Practice Testing with feedback also consistently outperforms Practice Testing alone” ( p . 35). If students continue to practice wrong answers, errors or misconceptions, then these will be successfully learnt and become high-confidence errors; hence the power of feedback. It is not the frequency of testing that matters, but the skill in using practice testing to learn and consolidate knowledge and ideas.
There are still many unanswered questions that need further attention. First, there is a need to develop a more overarching model of learning techniques to situate these 10 and the many other learning techniques. For example, we have developed a model that argues that various learning techniques can be optimised at certain phases of learning from Surface to Deep to Transfer, from acquiring and consolidating knowledge and understanding, and involves three inputs and outputs -knowing, dispositions, and motivations; which we call the skill, the will, and the thrill ( Hattie and Donoghue, 2016 ). Memorisation and Practice Testing, for example, can be shown to be effective in the consolidating of surface knowing but not effective without first acquiring surface knowing. Problem based learning is relatively ineffective for promoting surface but more effective at the deeper understanding, and thus should be optimal after it has been shown students have sufficient surface knowledge to then work through problem based methods.
Second, it was noted above that the preponderance of current studies (and perhaps classrooms) favour Surface and Near learning and care should be taken to not generalise the results of either the original review or our meta-analysis to when Deep and Far learning is desired. Third, it is likely, as the original authors hint, having a toolbox of optimal learning techniques may be most effective, but we suggest that there may need to be a higher sense of self-regulation to know when to use them. Fourth, as the authors noted, it is likely that motivation and emotions are involved in the selection, persistence with, and effectiveness of using the learning techniques, so attention to these matters is imperative for many students. Fifth, given the extensive and robust evidence for the efficacy of these learning techniques, an important avenue of future research may centre on the value in teaching them to both teachers and students. Can these techniques be taught, and if so, how? Need they be taught in the context of specific content? In what ways can the emerging field of educational neuroscience inform these questions?
Third, Dunlosky and Rawson (2015) noted that more recent research may influence some of these findings. For example, he noted that while Interleaving was a “Low” technique, there have since been many studies demonstrating the benefits of Interleaving. For example, Carvalho and Goldstone (2015) found that the way information is ordered impacts learning and that this influence is modulated by the demands of the study task; in particular whether learning is active or passive. Learners in the active study condition tend to look for features that discriminate between categories, and these features are easier to detect when categories frequently alternate (i.e., using Interleaving). Learners in the passive study condition are more likely to look for features that consistently appear within one category’s examples, and these features are easier to detect when categories rarely alternate.
A significant limitation of the current study is that no publications beyond 2014 have been meta-analysed. Notwithstanding, the authors are unaware of any more recent study that contradicts any of our findings. Accordingly, the study represents a comprehensive and valid quantitative review of research published between 1929 and 2014, one that complements and underpins Dunlosky et al. (2013a) qualitative review.
Concluding Remarks
The major contribution from Dunlosky et al. (2013a) , and supported by the findings from this study is to highlight the relative importance of learning techniques, to identify and allow for the optimal moderators, and clearly more defensible models are needed that take into account the demands of the task, the timing of the intervention, and the role of learning techniques within content domains. Future research that examines the impact of these (and other) moderators, and incorporates findings into theoretical and conceptual models, is much needed.
Author Contributions
JH conceived study, wrote article with GD. GD found and coded all article, worked on analyses, contributed to writing.
Conflict of Interest
The authors declare that the research was conducted in the absence of any commercial or financial relationships that could be construed as a potential conflict of interest.
Barnett, V., and Lewis, T. (1994). Outliers in statistical data . New York, NY: Wiley .
Borenstein, M., Cooper, H., Hedges, L., and Valentine, J. (2009). Effect sizes for continuous data. Handbook Res. Synth. Meta-Anal. 2, 221–235. doi:10.7758/9781610448864.4
Google Scholar
Borenstein, M., Hedges, L., Higgins, J., and Rothstein, H. (2005). Comprehensive meta-analysis version 2 . Englewood, NJ: Biostat .
Carvalho, P. F., and Goldstone, R. L. (2015). The benefits of interleaved and blocked study: different tasks benefit from different schedules of study. Psychon. Bull. Rev. 22 (1), 281–288. doi:10.3758/s13423-014-0676-4
PubMed Abstract | CrossRef Full Text | Google Scholar
Cepeda, N. J., Pashler, H., Vul, E., Wixted, J. T., and Rohrer, D. (2006). Distributed practice in verbal recall tasks: a review and quantitative synthesis. Psychol. Bull. 132 (3), 354. doi:10.1037/0033-2909.132.3.354
Cepeda, N. J., Vul, E., Rohrer, D., Wixted, J. T., and Pashler, H. (2008). Spacing effects in learning: a temporal ridgeline of optimal retention. Psychol. Sci. 19 (11), 1095–1102. doi:10.1111/j.1467-9280.2008.02209.x
H. Cooper, L. V. Hedges, and J. C. Valentine (Editors) (2019). The handbook of research synthesis and meta-analysis (Newyork, NY: Russell Sage Foundation ).
Donovan, J. J., and Radosevich, D. J. (1999). A meta-analytic review of the distribution of practice effect: now you see it, now you don’t. J. Appl. Psychol. 84 (5), 795. doi:10.1037/0021-9010.84.5.795
CrossRef Full Text | Google Scholar
Dunlosky, J., Rawson, K. A., Marsh, E. J., Nathan, M. J., and Willingham, D. T. (2013a). Improving students’ learning with effective learning techniques: promising directions from cognitive and educational psychology. Psychol. Sci. Public Interest 14 (1), 4–58. doi:10.1177/1529100612453266
Dunlosky, J., Rawson, K. A., Marsh, E. J., Nathan, M. J., and Willingham, D. T. (2013b). What works, what doesn’t. Sci. Am. Mind 24 (4), 46–53. doi:10.1038/scientificamericanmind0913-46
Dunlosky, J., and Rawson, K. A. (2015). Practice tests, spaced practice, and successive relearning: tips for classroom use and for guiding students' learning. Scholarship Teach. Learn. Psychol. 1 (1), 72. doi:10.1037/stl0000024
Edwards, A. J., Weinstein, C. E., Goetz, E. T., and Alexander, P. A. (2014). Learning and study techniques: issues in assessment, instruction, and evaluation . Amsterdam, The Netherland: Elsevier .
Grubbs, F. E. (1950). Sample criteria for testing outlying observations. Ann. Math. Statist. 21 (1), 27–58. doi:10.1214/aoms/1177729885
Hattie, J. A., and Donoghue, G. M. (2016). Learning techniques: a synthesis and conceptual model. Npj Sci. Learn. 1, 16013. doi:10.1038/npjscilearn.2016.13
Hattie, J. (2015). The applicability of Visible Learning to higher education. Scholarship Teach. Learn. Psychol. 1 (1), 79. doi:10.1037/stl0000021
Hattie, J. (2012). Visible learning for teachers: maximizing impact on learning . England, United Kingdom: Routledge .
Hattie, J. (2009). Visible learning: a synthesis of over 800 meta-analyses relating to achievement . England, United Kingdom: Routledge .
Hedges, L. V., and Olkin, I. (1985). Statistical methods for meta-analysis . Cambridge, MA: Academic Press .
Hedges, L. V. and Vevea, J. L. (1998). Fixed-and randomeffects models in meta-analysis. Psychol. Meth. 3, 486.
Janiszewski, C., Noel, H., and Sawyer, A. G. (2003). A meta-analysis of the spacing effect in verbal learning: implications for research on advertising repetition and consumer memory. J. Consum. Res. 30 (1), 138–149. doi:10.1086/374692
Lee, T. D., and Genovese, E. D. (1988). Distribution of practice in motor skill acquisition: learning and performance effects reconsidered. Res. Q. Exerc. Sport 59 (4), 277–287. doi:10.1080/02701367.1988.10609373
Lipsey, M. W. and Wilson, D. B. (2001). Practical meta-analysis. Newbury Park, CA, United States: SAGE publications, Inc.
Nuthall, G. (2007). The hidden lives of learners . Wellington, New Zealand: NZCER Press.
Rowland, C. A. (2014). The effect of testing versus restudy on retention: a meta-analytic review of the testing effect. Psychol. Bull. 140 (6), 1432. doi:10.1037/a0037559
Tyack, D. B., and Cuban, L. (1995). Tinkering toward utopia . Cambridge, MA: Harvard University Press .
Keywords: meta-analysis, learning strategies, transfer of learning, learning technique, surface and deep learning
Citation: Donoghue GM and Hattie JAC (2021) A Meta-Analysis of Ten Learning Techniques. Front. Educ. 6:581216. doi: 10.3389/feduc.2021.581216
Received: 08 July 2020; Accepted: 08 February 2021; Published: 31 March 2021.
Reviewed by:
Copyright © 2021 Donoghue and Hattie. This is an open-access article distributed under the terms of the Creative Commons Attribution License (CC BY). The use, distribution or reproduction in other forums is permitted, provided the original author(s) and the copyright owner(s) are credited and that the original publication in this journal is cited, in accordance with accepted academic practice. No use, distribution or reproduction is permitted which does not comply with these terms.
*Correspondence: Gregory M. Donoghue, [email protected]
- Utility Menu

- ARC Scheduler
- Student Employment
- Effective Learning Practices
Learning at college requires processing and retaining a high volume of information across various disciplines and subjects at the same time, which can be a daunting task, especially if the information is brand new. In response, college students try out varied approaches to their learning – often drawing from their high school experiences and modeling what they see their peers doing. While it’s great to try different styles and approaches to learning and studying for your courses, it's smart to incorporate into your daily habits some learning practices that are backed up by current research.
Below are some effective learning practices suggested by research in the cognitive and learning sciences:
Take ownership of your educational experience.
As an engaged learner, it is important to take an active, self-directed role in your academic experience. Taking agency might feel new to you. In high school, you might have felt like you had little control over your learning experience, so transitioning to an environment where you are implicitly expected to be in the driver’s seat can be disorienting.
A shift in your mindset regarding your agency, however, can make a big difference in your ability to learn effectively and get the results you want out of your courses.
Here are four concrete actions you can take to assert ownership over your education :
- Attend office hours . Come prepared with questions for your instructor about lectures, readings, or other aspects of the course.
- Schedule meetings with administrators and faculty to discuss your academic trajectory and educational goals. You might meet with your academic adviser, course heads, or the Director of Undergraduate Studies (DUS) in your concentration.
- Identify areas for growth and development based on your academic goals. Then, explore opportunities to shape and further refine your skills in those areas.
- Advocate for support, tools, equipment, or considerations that address your learning needs.
Seek out opportunities for active learning.
Many courses include opportunities for active and engaged learning within their structure. Take advantage of those opportunities in order to enhance your understanding of the material. If such opportunities are not built into the course structure, you can develop your own active learning strategies, including joining study groups and using other active studying techniques. Anytime you grapple actively with your course material, rather than taking it in passively, you’re engaging in active learning. By doing so, you are increasing your retention of key course concepts.
One particularly effective way to help yourself stay focused and engaged in the learning process is to cultivate learning communities, such as accountability groups and study groups. Working in the company of other engaged learners can help remind you why you love learning or why you chose a particular course, concentration, research project, or field of study. Those reminders can re-energize and refocus your efforts.
Practice study strategies that promote deep learning.
In an attempt to keep up with the demands of college, many students learn concepts just in time for assessment benchmarks (tests, exams, and quizzes). The problem with this methodology is that, for many disciplines (and especially in STEM), the concepts build on one another. Students survive the course only to be met at the final with concepts from the first quiz that they have forgotten long ago. This is why deep learning is important. Deep learning occurs when students use study strategies that ensure course ideas and concepts are embedded into long-term, rather than just short-term, memory. Building your study plans and review sessions in a way that helps create a conceptual framing of the material will serve you now and in the long run.
Here are some study strategies that promote deep learning:
Concept Mapping : A concept map is a visualization of knowledge that is organized by the relationships between the topics. At its core, it is made of concepts that are connected together by lines (or arrows) that are labeled with the relationship between the concepts.
Collaboration : You don’t have to go it alone. In fact, research on learning suggests that it’s best not to. Using study groups, ARC accountability hours, office hours, question centers, and other opportunities to engage with your peers helps you not only test your understanding but also learn different approaches to tackling the material.
Self-test : Quiz yourself about the material you need to know with your notes put away. Refamiliarize yourself with the answers to questions you get wrong, wait a few hours, and then try asking yourself again. Use practice tests provided by your courses or use free apps to create quizzes for yourself.
Create a connection : As you try to understand how all the concepts and ideas from your course fit together, try to associate new information with something you already know. Making connections can help you create a more holistic picture of the material you’re learning.
Teach someone (even yourself!) : Try teaching someone the concept you’re trying to remember. You can even try to talk to yourself about it! Vocalizing helps activate different sensory processes, which can enhance memory and help you embed concepts more deeply.
Interleave : We often think we’ll do best if we study one subject for long periods of time, but research contradicts this. Try to work with smaller units of time (a half-hour to an hour) and switch up your subjects. Return to concepts you studied earlier at intervals to ensure you learned them sufficiently.
Be intentional about getting started and avoiding procrastination.
When students struggle to complete tasks and projects, their procrastination is not because of laziness, but rather because of the anxiety and negative emotions that accompany starting the task. Understanding what conditions promote or derail your intention to begin a task can help you avoid procrastinating.
Consider the following tips for getting started:
Eat the Frog : The frog is that one thing you have on your to-do list that you have absolutely no motivation to do and that you’re most likely to procrastinate on. Eating the frog means to just do it, as the first thing you do, and get it over with. If you don’t, odds are that you’ll procrastinate all day. With that one task done, you will experience a sense of accomplishment at the beginning of your day and gain some momentum that will help you move through the rest of your tasks.
Pomodoro Technique : Sometimes, we can procrastinate because we’re overwhelmed by the sheer amount of time we expect it will take to complete a task. But, while it might feel hard to sit down for several hours to work on something, most of us feel we can easily work for a half hour on almost any task. Enter the Pomodoro Technique! When faced with any large task or series of tasks, break the work down into short, timed intervals (25 minutes or so) that are spaced out by short breaks (5 minutes). Working in short intervals trains your brain to focus for manageable periods of time and helps you stay on top of deadlines. With time, the Pomodoro Technique can even help improve your attention span and concentration. Pomodoro is a cyclical system. You work in short sprints, which makes sure you’re consistently productive. You also get to take regular breaks that bolster your motivation and get you ready for your next pomodoro.
Distraction Pads : Sometimes we stop a task that took us a lot of time to get started on because we get distracted by something else. To avoid this, have a notepad beside you while working, and every time you get distracted with a thought, write it down, then push it aside for later. Distracting thoughts can be anything from remembering that you still have another assignment to complete to daydreaming about your next meal. Later on in the day, when you have some free time, you can review your distraction pad to see if any of those thoughts are important and need to be addressed.
Online Apps : It can be hard to rely on our own force of will to get ourselves to start a task, so consider using an external support. There are many self-control apps available for free online (search for "self-control apps"). Check out a few and decide on one that seems most likely to help you eliminate the distractions that can get in the way of starting and completing your work.
Engage in metacognition.
An effective skill for learning is metacognition. Metacognition is the process of “thinking about thinking” or reflecting on personal habits, knowledge, and approaches to learning. Engaging in metacognition enables students to become aware of what they need to do to initiate and persist in tasks, to evaluate their own learning strategies, and to invest the adequate mental effort to succeed. When students work at being aware of their own thinking and learning, they are more likely to recognize patterns and to intentionally transfer knowledge and skills to solve increasingly complex problems. They also develop a greater sense of self-efficacy.
Mentally checking in with yourself while you study is a great metacognitive technique for assessing your level of understanding. Asking lots of “why,” “how,” and “what” questions about the material you’re reviewing helps you to be reflective about your learning and to strategize about how to tackle tricky material. If you know something, you should be able to explain to yourself how you know it. If you don’t know something, you should start by identifying exactly what you don’t know and determining how you can find the answer.
Metacognition is important in helping us overcome illusions of competence (our brain’s natural inclination to think that we know more than we actually know). All too often students don’t discover what they really know until they take a test. Metacognition helps you be a better judge of how well you understand your course material, which then enables you to refine your approach to studying and better prepare for tests.
Accordion style
- Assessing Your Understanding
- Building Your Academic Support System
- Common Class Norms
- First-Year Students
- How to Prepare for Class
- Interacting with Instructors
- Know and Honor Your Priorities
- Memory and Attention
- Minimizing Zoom Fatigue
- Note-taking
- Office Hours
- Perfectionism
- Scheduling Time
- Senior Theses
- Study Groups
- Tackling STEM Courses
- Test Anxiety

An official website of the United States government
The .gov means it’s official. Federal government websites often end in .gov or .mil. Before sharing sensitive information, make sure you’re on a federal government site.
The site is secure. The https:// ensures that you are connecting to the official website and that any information you provide is encrypted and transmitted securely.
- Publications
- Account settings
Preview improvements coming to the PMC website in October 2024. Learn More or Try it out now .
- Advanced Search
- Journal List
- Am J Pharm Educ
- v.73(1); 2009 Feb 19
Learning Styles: A Review of Theory, Application, and Best Practices
Much pedagogical research has focused on the concept of “learning styles.” Several authors have proposed that the ability to typify student learning styles can augment the educational experience. As such, instructors might tailor their teaching style so that it is more congruent with a given student's or class of students' learning style. Others have argued that a learning/teaching style mismatch encourages and challenges students to expand their academic capabilities. Best practice might involve offering courses that employ a variety of teaching styles. Several scales are available for the standardization of learning styles. These scales employ a variety of learning style descriptors and are sometimes criticized as being measures of personality rather than learning style. Learning styles may become an increasingly relevant pedagogic concept as classes increase in size and diversity. This review will describe various learning style instruments as well as their potential use and limitations. Also discussed is the use of learning style theory in various concentrations including pharmacy.
INTRODUCTION
The diversity of students engaged in higher education continues to expand. Students come to colleges with varied ethnic and cultural backgrounds, from a multitude of training programs and institutions, and with differing learning styles. 1 Coupled with this increase in diversification has been a growth in distance education programs and expansions in the types of instructional media used to deliver information. 2 , 3 These changes and advances in technology have led many educators to reconsider traditional, uniform instruction methods and stress the importance of considering student learning styles in the design and delivery of course content. 4 - 5 Mismatches between an instructor's style of teaching and a student's method of learning have been cited as potential learning obstacles within the classroom and as a reason for using a variety of teaching modalities to deliver instruction. 6 - 8 The concept of using a menu of teaching modalities is based on the premise that at least some content will be presented in a manner suited to every type of learner within a given classroom or course. Some research has focused on profiling learning types so that instructors have a better understanding of the cohort of students they are educating. 7 - 8 This information can be used to guide the selection of instruction modalities employed in the classroom. Limited research has also focused on describing and characterizing composite learning styles and patterns for students in various concentrations of study (eg, medicine, engineering). 5 , 6 , 9 This review will describe the potential utility and limitations in assessing learning styles.
LEARNING STYLES
A benchmark definition of “learning styles” is “characteristic cognitive, effective, and psychosocial behaviors that serve as relatively stable indicators of how learners perceive, interact with, and respond to the learning environment. 10 Learning styles are considered by many to be one factor of success in higher education. Confounding research and, in many instances, application of learning style theory has begat the myriad of methods used to categorize learning styles. No single commonly accepted method currently exists, but alternatively several potential scales and classifications are in use. Most of these scales and classifications are more similar than dissimilar and focus on environmental preferences, sensory modalities, personality types, and/or cognitive styles. 11 Lack of a conceptual framework for both learning style theory and measurement is a common and central criticism in this area. In 2004 the United Kingdom Learning and Skills Research Center commissioned a report intended to systematically examine existing learning style models and instruments. In the commission report, Coffield et al identified several inconsistencies in learning style models and instruments and cautioned educators with regards to their use. 12 The authors also outlined a suggested research agenda for this area.
Alternatively, many researchers have argued that knowledge of learning styles can be of use to both educators and students. Faculty members with knowledge of learning styles can tailor pedagogy so that it best coincides with learning styles exhibited by the majority of students. 4 Alternatively, students with knowledge of their own preferences are empowered to use various techniques to enhance learning, which in turn may impact overall educational satisfaction. This ability is particularly critical and useful when an instructor's teaching style does not match a student's learning style. Compounding the issue of learning styles in the classroom has been the movement in many collegiate environments to distance and/or asynchronous education. 2 , 3 This shift in educational modality is inconsistent with the learning models with which most older students and adult learners are accustomed from their primary and high school education. 3 , 13 , 14 Alternatively, environmental influences and more widespread availability of technological advances (eg, personal digital assistants, digital video, the World Wide Web, wireless Internet) may make younger generations of students more comfortable with distance learning. 15 - 17
LEARNING STYLES INSTRUMENTS
As previously stated, several models and measures of learning styles have been described in the literature. Kolb proposed a model involving a 4-stage cyclic structure that begins with a concrete experience, which lends to a reflective observation and subsequently an abstract conceptualization that allows for active experimentation. 18 Kolb's model is associated with the Learning Style Inventory instrument (LSI). The LSI focuses on learner's preferences in terms of concrete versus abstract, and action versus reflection. Learners are subsequently described as divergers, convergers, assimilators, or accommodators.
Honey and Mumford developed an alternative instrument known as the Learning Style Questionnaire (LSQ). 6 Presumably, the LSQ has improved validity and predictive accuracy compared to the LSI. The LSQ describes 4 distinct types of learners: activists (learn primarily by experience), reflectors (learn from reflective observation), theorists (learn from exploring associations and interrelationships), and pragmatics (learn from doing or trying things with practical outcomes). The LSQ has been more widely used and studied in management and business settings and its applicability to academia has been questioned. 6 An alternative to the LSQ, the Canfield Learning Style Inventory (CLSI) describes learning styles along 4 dimensions. 19 These dimensions include conditions for learning, area of interest, mode of learning, and conditions for performance. Analogous to the LSQ, applicability of the CLSI to academic settings has been questioned. Additionally, some confusion surrounding scoring and interpretation of certain result values also exists.
Felder and Silverman introduced a learning style assessment instrument that was specifically designed for classroom use and was first applied in the context of engineering education. 20 The instrument consists of 44 short items with a choice between 2 responses to each sentence. Learners are categorized in 4 dichotomous areas: preference in terms of type and mode of information perception (sensory or intuitive; visual or verbal), approaches to organizing and processing information (active or reflective), and the rate at which students progress towards understanding (sequential or global). The instrument associated with the model is known as the Index of Learning Survey (ILS). 21 The ILS is based on a 44-item questionnaire and outputs a preference profile for a student or an entire class. The preference profile is based on the 4 previously defined learning dimensions. The ILS has several advantages over other instruments including conciseness and ease of administration (in both a written and computerized format). 20 , 21 No published data exist with regards to the use of the ILS in populations of pharmacy students or pharmacists. Cook described a study designed to examine the reliability of the ILS for determining learning styles among a population of internal medicine residents. 20 The researchers administered the ILS twice and the Learning Style Type Indicator (LSTI) once to 138 residents (86 men, 52 women). The LSTI has been previously compared to the ILS by several investigators. 8 , 19 Cook found that the Cronbach's alpha scores for the ILS and LSTI ranged from 0.19 to 0.69. They preliminarily concluded that the ILS scores were reliable and valid among this cohort of residents, particularly within the active-reflective and sensing-intuitive domains. In a separate study, Cook et al attempted to evaluate convergence and discrimination among the ILS, LSI, and another computer-based instrument known as the Cognitive Styles Analysis (CSA). 11 The cohort studied consisted of family medicine and internal medicine residents as well as first- and third-year medical students. Eighty-nine participants completed all 3 instruments, and responses were analyzed using calculated Pearson's r and Cronbach's α. The authors found that the ILS active-reflective and sensing-intuitive scores as well as the LSI active-reflective scores were valid in determining learning styles. However, the ILS sequential-global domain failed to correlate well with other instruments and may be flawed, at least in this given population. The authors advised the use of caution when interpreting scores without a strong knowledge of construct definitions and empirical evidence.
Several other instruments designed to measure personality indexes or psychological types may overlap and describe learning styles in nonspecific fashions. One example of such an indicator is the Myers-Briggs Index. 6 While some relation between personality indexes and learning styles may exist, the use of instruments intended to describe personality to characterize learning style has been criticized by several authors. Therefore, the use of these markers to measure learning styles is not recommended. 6 The concept of emotional intelligence is another popular way to characterize intellect and learning capacity but similarly should not be misconstrued as an effective means of describing learning styles. 23
Several authors have proposed correlations between culture and learning styles. 6 , 24 This is predicated on the concept that culture influences environmental perceptions which, in turn, to some degree determine the way in which information is processed and organized. The storage, processing, and assimilation methods for information contribute to how new knowledge is learned. Culture also plays a role in conditioning and reinforcing learning styles and partially explains why teaching methods used in certain parts of the world may be ineffective or less effective when blindly transplanted to another locale. 6 , 24 Teachers should be aware of this phenomenon and the influence it has on the variety of learning styles that are present in classrooms. This is especially true in classrooms that have a large contingency of international students. Such classrooms are becoming increasingly common as more and more schools expand their internationalization efforts. 25
The technological age may also be influencing the learning styles of younger students and emerging generations of learners. The Millennial Generation has been described as more technologically advanced than their Generation X counterparts, with higher expectations for the use of computer-aided media in the classroom. 15 , 16 , 26 Younger students are accustomed to enhanced visual images associated with various computer- and television-based games and game systems. 16 , 26 Additionally, video technology is increasingly becoming “transportable” in the way of mobile computing, MP3 devices, personal digital video players, and other technologies. 26 All of these advances have made visual images more pervasive and common within industrialized nations.
APPLYING LEARNING STYLES TO THE CLASSROOM
As class sizes increase, so do the types and numbers of student learning styles. Also, as previously mentioned, internationalization and changes in the media culture may affect the spectrum of classroom learning styles as well. 24 , 25 Given the variability in learning styles that may exist in a classroom, some authors suggested that students should adapt their learning styles to coincide with a given instruction style. 6 , 27 This allows instructors to dictate the methods used to instruct in the classroom. This approach also allows instructors to “teach from their strengths,” with little consideration to other external factors such as learning style of students. While convenient, this unilateral approach has been criticized for placing all of the responsibility for aligning teaching and learning on the student. When the majority of information is presented in formats that are misaligned with learning styles, students may spend more time manipulating material than they do in comprehending and applying the information. Additionally, a unilaterally designed classroom may reinforce a “do nothing” approach among faculty members. 6 Alternatively, a teaching style-learning style mismatch might challenge students to adjust, grow intellectually, and learn in more integrated ways. However, it may be difficult to predict which students have the baseline capacity to adjust, particularly when significant gaps in knowledge of a given subject already exist or when the learner is a novice to the topic being instructed. 6 , 27 This might be especially challenging within professional curricula where course load expectations are significant.
Best practice most likely involves a teaching paradigm which addresses and accommodates multiple dimensions of learning styles that build self-efficacy. 27 Instructing in a way that encompasses multiple learning styles gives the teacher an opportunity to reach a greater extent of a given class, while also challenging students to expand their range of learning styles and aptitudes at a slower pace. This may avoid lost learning opportunities and circumvent unnecessary frustration from both the teacher and student. For many instructors, multi-style teaching is their inherent approach to learning, while other instructors more commonly employ unilateral styles. Learning might be better facilitated if instructors were cognizant of both their teaching styles and the learning styles of their students. An understanding and appreciation of a given individual's teaching style requires self-reflection and introspection and should be a component of a well-maintained teaching portfolio. Major changes or modifications to teaching styles might not be necessary in order to effectively create a classroom atmosphere that addresses multiple learning styles or targets individual ones. However, faculty members should be cautious to not over ambitiously, arbitrarily, or frivolously design courses and activities with an array of teaching modalities that are not carefully connected, orchestrated, and delivered.
Novice learners will likely be more successful when classrooms, either by design or by chance, are tailored to their learning style. However, the ultimate goal is to instill within students the skills to recognize and react to various styles so that learning is maximized no matter what the environment. 28 This is an essential skill for an independent learner and for students in any career path.
Particular consideration of learning styles might be given to asynchronous courses and other courses where a significant portion of time is spent online. 29 As technology advances and classroom sizes in many institutions become increasingly large, asynchronous instruction is becoming more pervasive. In many instances, students who have grown accustomed to technological advances may prefer asynchronous courses. Online platforms may inherently affect learning on a single dimension (visual or auditory). Most researchers who have compared the learning styles of students enrolled in online versus traditional courses have found no correlations between the learning styles and learning outcomes of cohorts enrolled in either course type. Johnson et al compared learning style profiles to student satisfaction with either online or face-to-face study groups. 30 Forty-eight college students participated in the analysis. Learning styles were measured using the ILS. Students were surveyed with regard to their satisfaction with various study group formats. These results were then correlated to actual performance on course examinations. Active and visual learners demonstrated a significant preference for face-to-face study groups. Alternatively, students who were reflective learners demonstrated a preference for online groups. Likely due to the small sample size, none of these differences achieved statistical significance. The authors suggest that these results are evidence for courses employing hybrid teaching styles that reach as many different students as possible. Cook et al studied 121 internal medicine residents and also found no association (p > 0.05) between ILS-measured learning styles and preferences for learning formats (eg, Web-based versus paper-based learning modules). 31 Scores on assessment questions related to learning modules administered to the residents were also not statistically correlated with learning styles.
Cook et al examined the effectiveness of adapting Web-based learning modules to a given learner's style. 32 The investigators created 2 versions of a Web-based instructional module on complementary and alternative medications. One version of the modules directed the learner to “active” questions that provided learners immediate and comprehensive feedback, while the other version involved “reflective” questions that directed learners back to the case content for answers. Eighty-nine residents were randomly matched or mismatched based on their active-reflective learning styles (as determined by ILS) to either the “active” or “reflective” test version. Posttest scores for either question type among mismatched subjects did not differ significantly ( p = 0.97), suggesting no interaction between learning styles and question types. The authors concluded from this small study that learning styles had no influence on learning outcomes. The study was limited in its lack of assessment of baseline knowledge, motivation, or other characteristics. Also, the difficulty of the assessment may not have been sufficient enough to distinguish a difference and/or “mismatched” learners may have automatically adapted to the information they received regardless of type.
STUDIES OF PHARMACY STUDENTS
There are no published studies that have systematically examined the learning styles of pharmacy students. Pungente et al collected some learning styles data as part of a study designed to evaluate how first-year pharmacy students' learning styles influenced preferences toward different activities associated with problem-based learning (PBL). 33 One hundred sixteen first-year students completed Kolb's LSI. Learning styles were then matched to responses from a survey designed to assess student preferences towards various aspects of PBL. The majority of students were classified by the LSI as being accommodators (36.2%), with a fairly even distribution of styles among remaining students (19.8% assimilators, 22.4% convergers, 21.6% divergers). There was a proportional distribution of learning styles among a convenience sample of pharmacy students. Divergers were the least satisfied with the PBL method of instruction, while convergers demonstrated the strongest preference for this method of learning. The investigators proposed that the next step might be to correlate learning styles and PBL preferences with actual academic success.
Limited research correlating learning styles to learning outcomes has hampered the application of learning style theory to actual classroom settings. Complicating research is the plethora of different learning style measurement instruments available. Despite these obstacles, efforts to better define and utilize learning style theory is an area of growing research. A better knowledge and understanding of learning styles may become increasingly critical as classroom sizes increase and as technological advances continue to mold the types of students entering higher education. While research in this area continues to grow, faculty members should make concentrated efforts to teach in a multi-style fashion that both reaches the greatest extent of students in a given class and challenges all students to grow as learners.
New Research Shows Learning Is More Effective When Active
By Aaron Aupperlee aaupperlee(through)cmu.edu
- School of Computer Science
- aaupperlee(through)cmu.edu
— Related Content —
New intelligent science stations change maker spaces, new ai enables teachers to rapidly develop intelligent tutoring systems, revolutionizing education.

NCRM delivers training and resources at core and advanced levels, covering quantitative, qualitative, digital, creative, visual, mixed and multimodal methods
20th Anniversary Impact Prize
Tell us how NCRM has helped you to make an impact
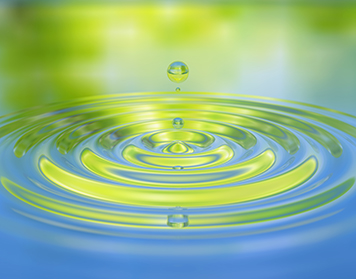
Short courses
Browse our calendar of training courses and events
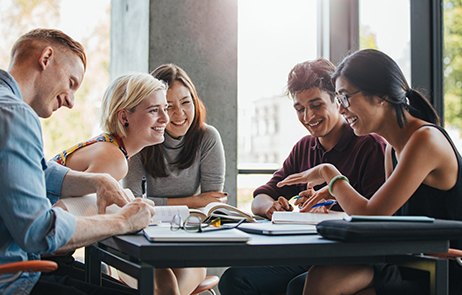
Featured training course
3-4 June 2024
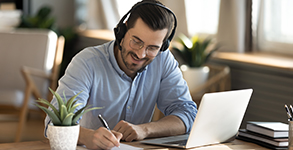
Our resources
NCRM hosts a huge range of online resources, including video tutorials and podcasts, plus an extensive publications catalogue.
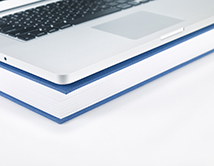
Online tutorials
Access more than 80 free research methods tutorials
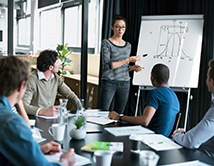
Resources for trainers
Browse our materials for teachers of research methods
Methods News
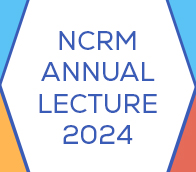
NCRM annual lecture to explore AI in social research
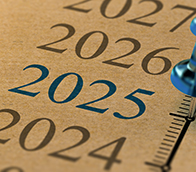
NCRM funding extended until end of 2025
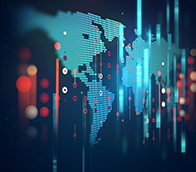
Briefings outline societal changes impacting methods
Help | Advanced Search
Computer Science > Machine Learning
Title: rlhf workflow: from reward modeling to online rlhf.
Abstract: We present the workflow of Online Iterative Reinforcement Learning from Human Feedback (RLHF) in this technical report, which is widely reported to outperform its offline counterpart by a large margin in the recent large language model (LLM) literature. However, existing open-source RLHF projects are still largely confined to the offline learning setting. In this technical report, we aim to fill in this gap and provide a detailed recipe that is easy to reproduce for online iterative RLHF. In particular, since online human feedback is usually infeasible for open-source communities with limited resources, we start by constructing preference models using a diverse set of open-source datasets and use the constructed proxy preference model to approximate human feedback. Then, we discuss the theoretical insights and algorithmic principles behind online iterative RLHF, followed by a detailed practical implementation. Our trained LLM, SFR-Iterative-DPO-LLaMA-3-8B-R, achieves impressive performance on LLM chatbot benchmarks, including AlpacaEval-2, Arena-Hard, and MT-Bench, as well as other academic benchmarks such as HumanEval and TruthfulQA. We have shown that supervised fine-tuning (SFT) and iterative RLHF can obtain state-of-the-art performance with fully open-source datasets. Further, we have made our models, curated datasets, and comprehensive step-by-step code guidebooks publicly available. Please refer to this https URL and this https URL for more detailed information.
Submission history
Access paper:.
- HTML (experimental)
- Other Formats
References & Citations
- Google Scholar
- Semantic Scholar
BibTeX formatted citation

Bibliographic and Citation Tools
Code, data and media associated with this article, recommenders and search tools.
- Institution
arXivLabs: experimental projects with community collaborators
arXivLabs is a framework that allows collaborators to develop and share new arXiv features directly on our website.
Both individuals and organizations that work with arXivLabs have embraced and accepted our values of openness, community, excellence, and user data privacy. arXiv is committed to these values and only works with partners that adhere to them.
Have an idea for a project that will add value for arXiv's community? Learn more about arXivLabs .
- Biology & Environment
- Clean Energy
- Fusion & Fission
- Physical Sciences
- National Security
- Neutron Science
- Supercomputing
- User Facilities
- Educational Programs
- Procurement
- Small Business Programs
- Leadership Team
- Visiting ORNL
- Fact Sheets
- Virtual Tour
Exploiting Machine Learning in Multiscale Modelling of Materials...
Recent developments in efficient machine learning algorithms have spurred significant interest in the materials community. The inherently complex and multiscale problems in Materials Science and Engineering pose a formidable challenge. The present scenario of machine learning research in Materials Science has a clear lacunae, where efficient algorithms are being developed as a separate endeavour, while such methods are being applied as ‘black-box’ models by others. The present article aims to discuss pertinent issues related to the development and application of machine learning algorithms for various aspects of multiscale materials modelling. The authors present an overview of machine learning of equivariant properties, machine learning-aided statistical mechanics, the incorporation of ab initio approaches in multiscale models of materials processing and application of machine learning in uncertainty quantification. In addition to the above, the applicability of Bayesian approach for multiscale modelling will be discussed. Critical issues related to the multiscale materials modelling are also discussed.
Researchers
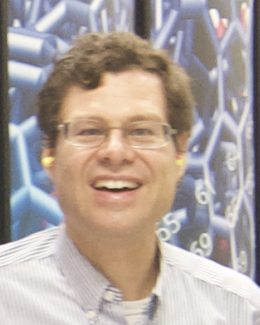
Organizations
- Open access
- Published: 10 May 2024
Cross-site validation of lung cancer diagnosis by electronic nose with deep learning: a multicenter prospective study
- Meng-Rui Lee 1 , 2 ,
- Mu-Hsiang Kao 3 ,
- Ya-Chu Hsieh 3 ,
- Min Sun 3 ,
- Kea-Tiong Tang 3 ,
- Jann-Yuan Wang 1 ,
- Chao-Chi Ho 1 ,
- Jin-Yuan Shih 1 &
- Chong-Jen Yu 1 , 2
Respiratory Research volume 25 , Article number: 203 ( 2024 ) Cite this article
262 Accesses
1 Altmetric
Metrics details
Although electronic nose (eNose) has been intensively investigated for diagnosing lung cancer, cross-site validation remains a major obstacle to be overcome and no studies have yet been performed.
Patients with lung cancer, as well as healthy control and diseased control groups, were prospectively recruited from two referral centers between 2019 and 2022. Deep learning models for detecting lung cancer with eNose breathprint were developed using training cohort from one site and then tested on cohort from the other site. Semi-Supervised Domain-Generalized (Semi-DG) Augmentation (SDA) and Noise-Shift Augmentation (NSA) methods with or without fine-tuning was applied to improve performance.
In this study, 231 participants were enrolled, comprising a training/validation cohort of 168 individuals (90 with lung cancer, 16 healthy controls, and 62 diseased controls) and a test cohort of 63 individuals (28 with lung cancer, 10 healthy controls, and 25 diseased controls). The model has satisfactory results in the validation cohort from the same hospital while directly applying the trained model to the test cohort yielded suboptimal results (AUC, 0.61, 95% CI: 0.47─0.76). The performance improved after applying data augmentation methods in the training cohort (SDA, AUC: 0.89 [0.81─0.97]; NSA, AUC:0.90 [0.89─1.00]). Additionally, after applying fine-tuning methods, the performance further improved (SDA plus fine-tuning, AUC:0.95 [0.89─1.00]; NSA plus fine-tuning, AUC:0.95 [0.90─1.00]).
Our study revealed that deep learning models developed for eNose breathprint can achieve cross-site validation with data augmentation and fine-tuning. Accordingly, eNose breathprints emerge as a convenient, non-invasive, and potentially generalizable solution for lung cancer detection.
Clinical trial registration
This study is not a clinical trial and was therefore not registered.
Introduction
Lung cancer remains a predominant cause of cancer-related mortality worldwide, accounting for an estimated 2.2 million new cases and 1.8 million deaths in 2020 [ 1 ]. In its early stages, lung cancer often presents no symptoms, making it challenging to detect during routine health examinations. Although low-dose computed tomography (CT) of chest has been employed for lung cancer screening to facilitate earlier diagnosis and reduce mortality, a significant number of lung cancer patients remain undiagnosed until the disease has advance [ 2 ]. Furthermore, low-dose CT of chest has its limitations, including high cost, radiation exposure, and limited availability in many clinics. Consequently, there is a pressing need for a non-invasive, cost-effective, and readily accessible screening tool for early detection of lung cancer.
Electronic nose (eNose) is a novel device using sensors to generate breathprints that reflect patterns of volatile organic compounds [ 3 ]. eNose has the advantage of being non-invasive, easy to operate, short turnaround time and point-of-care. eNose has been applied in diagnosis of various diseases, encompassing communicable diseases such as COVID-19, tuberculosis and non-communicable diseases including diabetes and cancer. eNose has also been investigated in lung cancer diagnosis and treatment monitoring in previous studies.
Earlier studies evaluating eNose in lung cancer detection were mainly single center and compare between lung cancer and healthy control [ 4 ]. Previous studies also have shortcomings of lack of validation, especially cross-site validation [ 5 ]. While breathomics are prone to change in environment, external validation remains a major obstacle to clinical application. While more recent studies usually include a multicenter design of recruiting participants, cross site and independent validation were still not readily available [ 6 , 7 ].
On the other hand, algorithms for eNose breathprint analysis is also in evolution [ 8 ]. Deep learning involving convoluted neural network (CNN) is novel and emerging technique for breathprint analysis [ 8 , 9 ]. Some analytic approaches such as transfer learning and data augmentation have been applied in other aspects of biomedical imaging researches [ 10 ]. These methods could potentially propagate sample size, enhance performance and ameliorate the drop of performance in domain shift [ 11 , 12 ]. Most eNose studies have not yet incorporated this into analytic methods of eNose breathprint for lung cancer identification.
This study, therefore, aimed to validate eNose breathprint for lung cancer diagnosis in a cross-site setting with deep learning techniques including data augmentation and fine-tuning incorporated into the analytic methods. We aimed to expand generalizability of eNose breathprint in lung cancer diagnosis and advance eNose further in clinical practice.
Patient selection and study setting
This study was conducted prospectively at two facilities: the National Taiwan University Hospital (NTUH; test cohort, S2, site 2) and its Hsin-Chu branch (NTUH-HC; training/validation cohort, S1, site 1), both of which are referral centers for individuals with lung cancer and lung cancer suspects in Taiwan. The NTUH, a 2300-bed medical center in northern Taiwan, and the NTUH-HC, a regional hospital located 60 km away with a 700-bed capacity, have actively participated in eNose breathprint studies. The personnel at these institutions are well-acquainted with the eNose collection process and equipment operation. The institutional review boards (IRB) of participating hospitals approved this study (IRB no. 202112057RINB, 108-011-E). Inform consent was obtained from all participants who agreed to participate in this study.
For this study, we enlisted participants from three groups: individuals diagnosed with lung cancer, healthy controls, and diseased controls with either structural lung diseases confirmed on chest CTs or spirometry-confirmed chronic obstructive pulmonary disease. We confirmed the absence of lung cancer in the diseased control group through chest CT imaging and follow-up evaluations. During a two-year follow-up period, all control participants, encompassing both healthy and diseased controls, remained free from lung cancer.
Definition of diseases and data collection
For lung cancer patients, pathological confirmation was required for establishing the diagnosis. The stage was classified according to the 8th edition of the American Joint Committee on Cancer staging system for lung cancer [ 13 ]. We collected the data from a prospectively maintained database and medical records. Comorbidities included chronic obstructive pulmonary disease (COPD), asthma, diabetes mellitus (DM), and end-stage renal disease (ESRD). For healthy participants, a screening interview was performed to exclude underlying lung diseases and smoking habits. Chest x-rays of healthy participants, if available, were also reviewed to exclude structural lung disease. For diseased controls, participants must have either structural lung diseases confirmed on chest computed tomography or spirometry-confirmed chronic obstructive pulmonary disease.
Breath sample collection
The breath sample collection process has been described in our previous study [ 9 ]. Briefly, the breath sampling system included a one-way VBMax™ filter and two one-litre multi-layer foil gas sampling bags. Participants fasted for 4 h and avoided smoking and alcohol before testing. Each individual took a deep breath, then used the blow-to-breath sampling system connected to two Robert Clamps: the first collecting dead space air (not analyzed) and the second collecting end-tidal breath for analysis.
Breath analysis using eNose
The eNose system, developed by SEXTANT (Enosim Bio-Tech Co., Ltd., Hsinchu City, Taiwan), builds upon previous work and incorporates a total of 14 metal-oxide gas sensors. This system, which also includes flow meters and temperature and humidity sensors, is designed to work seamlessly with the necessary interface circuits. Leveraging Metal-Oxide-Semiconductor (MOS) gas sensors sourced from Figaro USA, Inc. and Nissha FIS, Inc., the SEXTANT system operates based on oxidation-reduction sensing mechanisms. These sensors have been enhanced with different materials to optimize both selectivity and sensitivity in detecting various gases [ 9 ]. A video describing the process of breath analysis using eNose is also available as Additional File 1 : Supplementary Video.
CNN model construction
For eNose breathprint, we first pre-processed the raw data of eNose into 14-channel \(16\times 16\) images and use a parallelizable calculation model, the convolution neural network, as the training model. We chose the rectified linear units (ReLUs) as the activation function to improve the training speed, and applied three layers of CNN to extract binary output from input images. Positive and negative outputs refer to whether this patient has lung cancer or not, respectively. The structure of CNN is shown in Additional File 2 : Figure S1
Data augmentation and fine-tuning
In this study, we applied two methods of data augmentation including Semi-supervised Domain Generalized (Semi-DG) Augmentation (SDA) and Noise-Shift Augmentation (NSA) methods. In SDA, Fourier transformation was applied and while in the NSA, we added Gaussian noise to the breathprint and performed a backward shift operation [ 14 , 15 , 16 , 17 ]. The detailed techniques of data augmentation were described in Additional File 3 : Supplementary File, Additional File 4 : Figure S2 and Additional File 5 : Figure S3 . We augmented eNose breathprint at an 1:1 ratio.
For fine-tuning, we first trained the model on the training cohort to obtain the initial weight of the model. Then, we used 10 test cohort to fine-tune the model to obtain new model weights. We chose to fine-tune our dataset using 10 samples based on our previous study, where we aimed to use a small proportion of our dataset, approximately 10–20% of the samples, for tuning [ 18 ]. We also conducted another analysis using 20 samples but observed only marginal improvement in the results. Additionally, data used for fine-tuning were separated from the test data and not used for testing.
Dataset definition and analytic flow
The training cohort was divided at 7:3 ratio, with 70% used for model training and the remaining 30% for model validation, according to time frame of recruitment. For the analysis, data augmentation (at a 1:1 ratio) was applied to the training portion. After training and validation, the model was tested with or without fine-tuning on the test dataset. The rest of the test dataset served to evaluate the model’s performance. The detailed process was described in Fig. 1 .
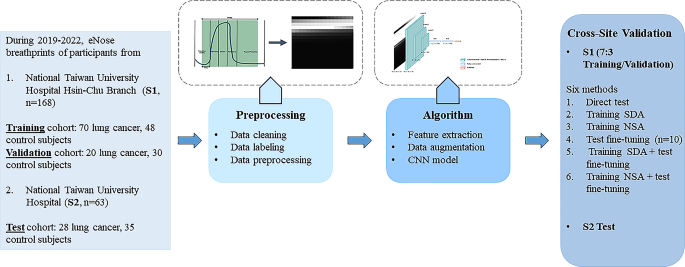
Flowchart and analytic flow. CNN, convoluted neural network; NSA, noise-shift augmentation; SDA, semi-supervised domain generalized augmentation
Statistical analysis
All variables were presented as either numbers (percentages) or as the mean ± standard deviation, depending on their nature. For categorical variables, the chi-square test was employed. For continuous variables, either the student’s t-test or the one-way analysis of variance (ANOVA) was used for comparison. To evaluate the model’s performance, we assessed accuracy, sensitivity, and specificity. Additionally, the area under the receiver operating characteristic (AU-ROC) curves were constructed to showcase the model’s performance. Confidence intervals (CI) were provided for analysis using the bootstrapping procedure. For the machine learning method, we used the scikit-learn package (version 0.23.2) in Python (version 3.8.5). All p-values were two-sided, with statistical significance set at p < 0.05.
Demographics of participants
A total of 231 participants were enrolled (168 in the training/validation cohort (Site 1, National Taiwan University Hospital Hsin-Chu branch cohort) and 63 in the test cohort (Site 2, National Taiwan University Hospital cohort)). Table 1 . describes the demographic data of all participants in the training, validation and test cohort. In the training cohort (S1), there were 70 (59.3%) lung cancer patients and 48 (33.9%) non-lung cancer control subjects (including 10 healthy control and 38 diseased control). In the validation cohort (S1), there were 20 (40%) lung cancer and 30 (60%) control subjects (including 6 healthy control and 24 diseased control). On the other hand, there were 28 (44.4%) lung cancer and 35 (55.6%) non lung cancer patients (including 10 healthy control and 25 diseased control) in the test cohort (S2).
In the training cohort, the smoking status were different between the lung cancer and control subjects. In the validation test, the demographic data were similar between the lung cancer and control subjects. In the test cohort, there is a slight female preponderance not reaching statistical significance in the lung cancer subjects compared with the control subjects (Table 1 ).
For the lung cancer patients in training/validation cohort, 70 (77.8%) were adenocarcinoma, 12 (13.3%) were squamous cell carcinoma, 4 (4.4%) were small cell lung cancer while 4 (4.4%) were other histology type. In the test cohort, 15 (53.6%) were adenocarcinoma, 4 (14.3%) were squamous cell carcinoma, 4 (14.3%) were small cell lung cancer while 5 (17.9%) were other histology type. The distribution of histology type was different in the training/validation cohort and test cohort ( p = 0.0165). For cancer stage, the two cohorts were not different ( p = 0.5444) while the majority was stage IV cancer patients (Additional File 3 : Table S1 ).
eNose breathprints PCA
Figure 2 illustrates the PCA plots of breathprints in this study. Breathprints from the two individual sites were distinct. Within each site, the breathprints of both the lung cancer and non-lung cancer groups were interspersed and scattered.
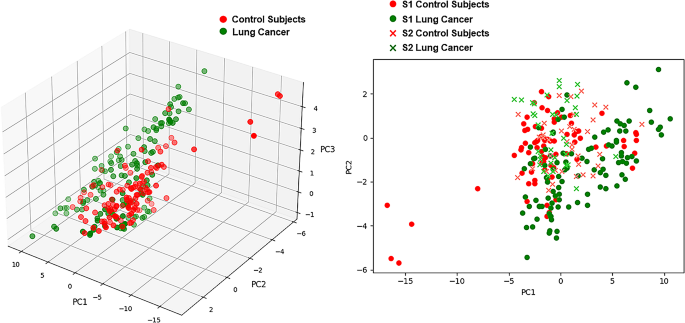
Principal component analysis plots of eNose breathprints
Performance of eNose
In the validation cohort (S1), the performance of eNose achieved an AUC of 0.89 (95% CI:0.84─0.93) with sensitivity of 0.90 (95% CI:0.85─0.95) and specificity of 0.83 (95% CI:0.73─0.87). While applying to the test cohort (S2), the performance was suboptimal with an AUC of 0.61 (95% CI:0.47─0.76), sensitivity of 0.43 (95% CI:0.36─0.50), specificity 0.43 (95% CI:0.37─0.54). With SDA, the AUC improved to 0.89 (95% CI: 0.81─0.97) with sensitivity of 0.82 (95% CI: 0.75─0.86) and specificity of 0.69 (95% CI: 0.60─0.80). With NSA, the AUC improved to 0.90 (95% CI: 0.83─0.98) with sensitivity of 0.82 (95% CI:0.75─0.86) and specificity of 0.69 (95% CI: 0.60─0.80). Applying fine-tuning, the AUC improved to 0.83 (95% CI: 0.72─0.94) and sensitivity of 0.78 (95% CI:0.70─0.83) and specificity of 0.6 (95% CI: 0.53─0.73). With SDA and fine-tuning, the performance further improved to AUC of 0.95 (95% CI: 0.89─1.00), sensitivity of 0.91 (95% CI: 0.83─0.96) and specificity of 0.77 (95% CI: 0.67─0.90). With NSA and fine-tuning, the performance also improved to AUC of 0.95 (95% CI: 0.90─1.00), sensitivity of 0.91 (95% CI: 0.83─0.96) and specificity of 0.77 (95% CI: 0.67─0.90) (Table 2 ). The AU-ROC of the test cohort (S2) is illustrated in Fig. 3 .
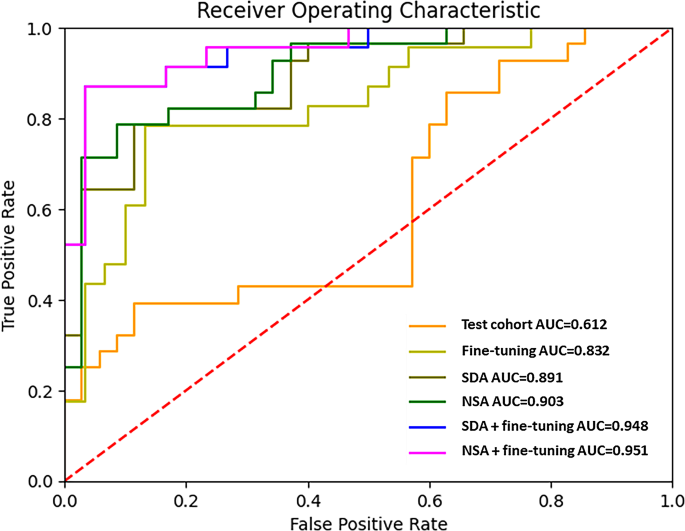
Area under the receiver operating characteristic curve of the test cohort (S2). AUC, area under the receiver operating characteristic curve; NSA, noise-shift augmentation; SDA, semi-supervised domain generalized augmentation
Reversing the training/validation and test cohort (the training/validation cohort (S1) then became the test cohort, while the test cohort (S2) became the training validation cohort), we found that the performance of eNose achieved an AUC of 0.91 (95% CI: 0.81─1.00) with sensitivity of 0.89 (95% CI: 0.80─1.00) and specificity of 0.80 (95% CI: 0.60─1.00) in the new validation cohort. Again, the performance was unsatisfactory in the test cohort with an AUC of 0.56 (95% CI: 0.44─0.73), sensitivity of 0.63 (95% CI: 0.52─0.76), specificity 0.54 (95% CI: 0.48─0.60). SDA or NSA plus fine-tuning both achieved an AUC of 0.84 (95% CI: 0.78─0.90), sensitivity of 0.82 (95% CI: 0.73─0.90) and specificity of 0.79 (95% CI: 0.70─0.89) (Additional File 3 : Table S2 ). The AU-ROC of the test cohort (S1) is illustrated in Additional File 6 : Supplementary Fig. 4 .
Subgroup analysis
In subgroup analysis (Fig. 4 ), we found that patients aged above 65-year-old had worse eNose performance compared with age less than 65-year-old (Accuracy: 0.76, 0.64─0.92 vs. 0.89, 0.79─1.00). While female and male patients had similar performance, the eNose had performed less satisfactory among those who ever or actively smoked than never smokers (Accuracy: 0.77, 95% CI: 0.59─0.91 vs. 0.87, 95% CI: 0.74─0.97). The performance was also best in the healthy control (accuracy: 1.00, 95% CI:0.89─1.00), followed by lung cancer patients (accuracy: 0.91, 95% CI:0.64─1.00) and diseased control patients (accuracy: 0.67, 95% CI:0.57─0.80). Among different histology types of lung cancer, the eNOSE correctly identifies all adenocarcinoma, SCLC, SqCC but incorrectly identifies two of the four lung cancer patients with other histologic classification.
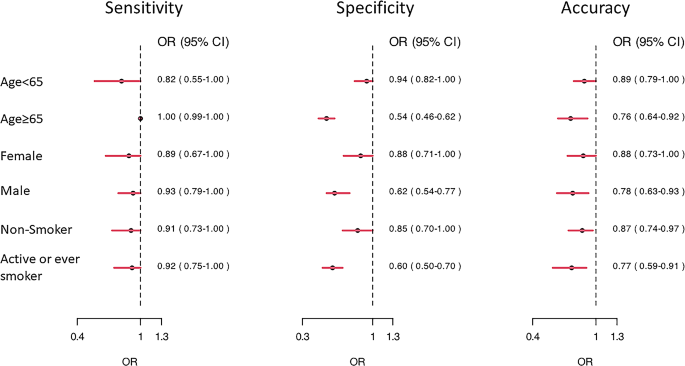
Forest plot of subgroup analysis. OR, odds ratio
Two patients were in their early stage (stage I and II) in the test cohort and they were all correctly classified as lung cancer (100%, 2/2). Also, the accuracy rate was 83.3% (5/6) for stage III lung cancer and 93.3% (14/15) for stage IV lung cancer in the test cohort.
Also, our model correctly identified 16 out of 17 (94.1%) lung cancer patients under treatment and 5 out of 6 (83.3%) fresh lung cancer patients not yet receiving anti-cancer treatment.
Detailed subgroup analysis of age, smoking status and comorbidities were further described in Additional File 3 : Table S3 .
In our study, we found that combining deep learning with transfer learning and data augmentation enables eNose to effectively tackle cross-site validation challenges. Using an eNose model trained at one site directly on another led to suboptimal results. Yet, by utilizing data augmentation and transfer learning, the eNose’s performance notably improved, achieving an AUC exceeding 0.9. As a result, electronic noses can accurately differentiate between lung cancer patients and those without the condition.
Breathomics has undergone extensive research for the purpose of detecting lung cancer. This approach is grounded in the theory that lung cancer patients may exhibit distinct metabolites and exhaled volatile organic compounds (VOCs) compared to persons without lung cancer [ 19 ]. In one prior investigation also conducted in the same participating hospital, the authors employed selected ion flow tube mass spectrometry (SIFT-MS) to identify and quantify 116 VOCs. Subsequently, the authors developed a predictive model for determining the likelihood of lung cancer based on quantitative VOC measurements. This approach yielded a commendable AUC and accuracy, with further enhancements achieved through the adjustment of confounding VOC effects [ 20 ]. It is worth noting, however, that this earlier study remained limited to a single-center setting and lacked external validation.
Cross-site validation of electronic nose has always been an important issue to be overcome. In earlier studies, the differentiation between lung cancer and non-lung cancer patients was performed without validation [ 4 ]. Some studies split one single cohort into training and validation part [ 5 , 21 , 22 ]. In one study, for instance, 199 participants were randomly split into an 80% training cohort and 20% validation cohort. A classification accuracy of 79% was subsequently attained by using XGBoost method [ 22 ]. In another study, by including 60 patients with lung cancer and 107 controls and assigning participants either to training or blinded validation cohort, the blinded validation cohort yielded diagnostic accuracy of 86%, sensitivity of 88% and specificity of 86%. For this approach, one may refer to the results of 86% accuracy obtained in our validation cohort.
Other studies used pooled data from multi-cohorts and then randomly split into training and validation cohort. In one study including multi-center cohorts with total of 575 patients, 376 patients were assigned to the training cohort and 199 patients assigned to the validation cohort. The training model then achieved an AUC-ROC of 0.79 (0.72–0.85) with a sensitivity of 88.2% and specificity of 48.3% in the validation. The study further achieved a better performance after integrating clinical data [ 6 ]. These approaches, however, do not really tackle with the issue of cross-site validation.
Cross-site validation is crucial due to several challenges associated with the generation of eNose breathprints. One significant challenge is the pervasive influence of environmental VOCs, which are constantly inhaled and participate in metabolic processes. This can modify the VOCs exhaled in human breath, subsequently affecting the generation of breathprints [ 20 , 23 ]. Another challenge stems from the device itself, encompassing issues such as sensor drift and the complexities of achieving absolute calibration [ 24 ]. Although the PCA plot revealed a distinct breathprint distribution, it also highlighted the challenges of achieving cross-site validation. Our study indicated that using data-augmentation techniques could significantly reduce the load of data collection and improved model performance. With combination of fine-tuning using data from individual sites, the performance of eNose further improved. Importantly, in our research, we only utilized a small portion of the test dataset for fine-tuning, making a clinical approach feasible.
The appropriate selection of a control group is paramount in ensuring the validity of research findings. Differentiating between healthy individuals and those diagnosed with lung cancer may seem straightforward. However, such differentiation may not encapsulate the complexities of real-world scenarios. To enhance the representativeness of our study, we incorporated individuals with other pulmonary conditions into our control cohort. While smoking is predominantly identified as a primary risk factor for lung cancer among Caucasians, another distinct demographic—non-smoking Asian females with lung adenocarcinoma—emerges as notably susceptible [ 25 ]. In an effort to account for this, our control group integrated patients with structural lung disease primary consisting of bronchiectasis. Additionally, patients with COPD were incorporated into our cohort. By combining different groups with healthy people, we believe our control group more closely matches the variety of individuals with lung screenings in real life.
Subgroup analysis revealed that the eNose exhibited less satisfactory performance in elderly participants and smokers. This finding holds particular significance, as elderly participants often present with a higher prevalence of comorbidities compared to their younger counterparts. These comorbidities may have introduced complexity into the eNose breathprint profiles [ 26 ]. It is noteworthy that elderly patients constitute an emerging demographic among lung cancer patients, and early lung cancer detection could enhance the feasibility of surgical interventions and further improvement of performance of eNose may be warranted [ 27 ]. Additionally, it’s worth highlighting that eNose demonstrated less satisfactory performance in the smoker subgroup. This finding was consistent with our previous which also found inferior performance in the smoker group [ 9 ]. Considering smoking remains a major risk factor for lung cancer [ 28 ], Detecting lung cancer in individuals who smoke or have chronic obstructive pulmonary disease is crucial for early intervention and treatment of lung cancer [ 29 ]. Therefore, our findings highlight areas of weakness that need to be strengthened in our eNose device. eNose technology simulates the human olfactory system.
In real environments, gas mixtures can be influenced by numerous factors, such as environmental volatile organic compounds and humidity. Therefore, data enhancement methods are valuable as they can simulate these variations, making the model more adaptable and reducing the need for extensive data collection. Common data enhancement techniques for eNose encompass noise addition, data rotation and translation, and synthetic data generation. For instance, a study with focus on eNose’s classification of alternative herbal medicines employed several data enhancement strategies to minimize the heavy dependency on training materials [ 17 ]. One method involved augmenting the training dataset by adding Gaussian noise and data shifting [ 17 ]. In another study exploring the use of eNose to identify ripe tomatoes, the collected gas’s concentration value was converted into a grayscale value, synthesized into a grayscale image, and then augmented using methods such as cropping and zooming [ 30 ]. These data augmentation techniques successfully improved the performance of eNose.
There were studies utilizing data augmentation methods in human disease research to enhance domain generalization, bolster model robustness, and minimize overfitting risks. For instance, one study employed a continuous frequency domain spatial interpolation approach for data augmentation, achieving state-of-the-art results in retinal fundus and prostate magnetic resonance imaging segmentations [ 31 ]. More recently, another study explored six data augmentation techniques for electromyography signals: trial averaging, time slice recombination, frequency slice recombination, noise addition, cropping, and the use of a variational autoencoder. This research aimed to enrich data diversity, enabling the model to better adapt to real-world variations, thereby boosting its robustness and domain generalization. Subsequently, the model’s accuracy improved by 3% and 12% on two motor imagery datasets [ 32 ].
Fine-tuning was used in our study to improve the versatility of our model. Fine-tuning is one of the domain adaptation techniques which can help the model better adapt to the features and distribution of new data and improve the performance of the model in new environments [ 33 ]. In one landscape study, a deep learning model was pre-trained on the ImageNet dataset, being fine-tuned and applied to different medical imaging data. The pre-trained model was successfully applied to retinal optical coherence tomography and pneumonia diagnosis [ 34 ]. In our previous studies, we also successfully demonstrated the capability of fine-tuning in improving model performance on external cohort [ 10 , 18 ].
We did not have information on potential confounding various such as BMI, alcohol intake, and dietary habits for our study participants. Although BMI is less frequently reported to affect the results of eNose breathprints, it can be associated with other diseases, such as diabetes, that may lead to distinct breathprints [ 35 ]. Dietary habits have previously been reported to influence VOC metabolites [ 36 ]. Lifestyle has also been noted to affect fecal VOCs [ 37 ]. On the other hand, one study investigating the impact of food intake on eNose breathprints suggested that the impact would be significant if the food intake occurred very recently, and two hours might be sufficient to avoid food-induced alterations in eNose breathprints [ 38 ]. In our study, we requested that participants fast for four hours prior to testing. However, the impact of the aforementioned factors may still warrant special attention and could be evaluated in future studies.
Our study has limitations. Firstly, the majority of lung cancer patients we studied in the study were in advanced stages, limiting the validation of eNose performance in early-stage lung cancer. Though the case number is limited, we have corrected identified two early stage lung cancer in our test cohort. Another limitation concerns transfer learning, which still necessitates some samples from the test cohort, potentially leading to inconvenience. While using data augmentation without fine-tuning yielded satisfactory results, fine-tuning can be viewed as a means to further optimize these results. Also, the study was confined to a Taiwanese population and the generalizability of the findings to other ethnicities remains uncertain. Finally, the reduced performance of eNose among elderly individuals and smokers also necessitates further investigation and strategies for improvement.
In conclusion, our study has shown that cross-site validation of the electronic nose for diagnosing lung cancer is attainable. Data augmentation and fine-tuning have demonstrated to be crucial methods for improving the performance when applying the eNose across different sites. Consequently, the electronic nose holds promise as a valuable tool for accurately identifying lung cancer patients in clinical practice. Future researches were warranted to further assess the generalization of eNose, minimize influence of confounding factors and validate eNose in early-stage lung cancer, diverse populations as well as high-risk groups.
Data availability
All data will be available upon reasonable request. Part of this study has been presented in IEEE BioSensors 2023 conference.
Abbreviations
Electronic Nose
Semi-supervised Domain-generalized Augmentation
Noise-Shift Augmentation
Area Under the Curve
Area Under the Receiver Operating Characteristic
Computed Tomography
Convoluted Neural Network
National Taiwan University Hospital
National Taiwan University Hospital Hsin-Chu branch
Chronic Obstructive Pulmonary Disease
Diabetes Mellitus
End-Stage Renal Disease
Metal-Oxide-Semiconductor
Rectified Linear Units
Confidence Intervals
Principal Component Analysis
Small Cell Lung Cancer
Squamous Cell Carcinoma
Volatile Organic Compounds
Selected Ion Flow Tube Mass Spectrometry
Sharma R. Mapping of global, regional and national incidence, mortality and mortality-to-incidence ratio of lung cancer in 2020 and 2050. Int J Clin Oncol. 2022;27:665–75.
Article PubMed PubMed Central Google Scholar
Jonas DE, Reuland DS, Reddy SM, Nagle M, Clark SD, Weber RP, et al. Screening for Lung Cancer with Low-Dose Computed Tomography: updated evidence report and systematic review for the US Preventive Services Task Force. JAMA. 2021;325:971–87.
Article PubMed Google Scholar
van der Sar IG, Wijbenga N, Nakshbandi G, Aerts J, Manintveld OC, Wijsenbeek MS, et al. The smell of lung disease: a review of the current status of electronic nose technology. Respir Res. 2021;22:246.
Di Natale C, Macagnano A, Martinelli E, Paolesse R, D’Arcangelo G, Roscioni C, et al. Lung cancer identification by the analysis of breath by means of an array of non-selective gas sensors. Biosens Bioelectron. 2003;18:1209–18.
Machado RF, Laskowski D, Deffenderfer O, Burch T, Zheng S, Mazzone PJ, et al. Detection of lung cancer by sensor array analyses of exhaled breath. Am J Respir Crit Care Med. 2005;171:1286–91.
Kort S, Brusse-Keizer M, Schouwink H, Citgez E, de Jongh FH, van Putten JWG, et al. Diagnosing Non-small Cell Lung Cancer by Exhaled Breath profiling using an electronic nose: a Multicenter Validation Study. Chest. 2023;163:697–706.
de Vries R, Farzan N, Fabius T, De Jongh FHC, Jak PMC, Haarman EG, et al. Prospective detection of early lung Cancer in patients with COPD in regular care by electronic nose analysis of exhaled breath. Chest. 2023;164:1315–24.
Chen H, Huo D, Zhang J. Gas recognition in E-Nose system: a review. IEEE Trans Biomed Circuits Syst. 2022;16:169–84.
Lee MR, Huang HL, Huang WC, Wu SY, Liu PC, Wu JC, et al. Electronic nose in differentiating and ascertaining clinical status among patients with pulmonary nontuberculous mycobacteria: a prospective multicenter study. J Infect. 2023;87:255–8.
Liu CJ, Tsai CC, Kuo LC, Kuo PC, Lee MR, Wang JY, et al. A deep learning model using chest X-ray for identifying TB and NTM-LD patients: a cross-sectional study. Insights Imaging. 2023;14:67.
Garcea F, Serra A, Lamberti F, Morra L. Data augmentation for medical imaging: a systematic literature review. Comput Biol Med. 2023;152:106391.
Kim HE, Cosa-Linan A, Santhanam N, Jannesari M, Maros ME, Ganslandt T. Transfer learning for medical image classification: a literature review. BMC Med Imaging. 2022;22:69.
Detterbeck FC, Boffa DJ, Kim AW, Tanoue LT. The eighth edition lung cancer stage classification. Chest. 2017;151:193–203.
Yao HHX, Li X. Enhancing pseudo label quality for semi-supervised domain-generalized medical image segmentation. In Proceedings of the AAAI Conference on Artificial Intelligence. 2022;36:3099–3107.
Stéphane M. A Wavelet Tour of Signal Processing the sparse way. 3rd ed. Elsevier; 2009.
N BR. The Fourier transform and its applications. New York: McGraw-Hill; 1978.
Google Scholar
Li Liu XZ, Wu R, Guan X, Wang Z, Zhang W, Pilanci M, et al. a Case in Alternative Herbal Medicine Discrimination With Electronic Nose. IEEE Sens J. 2021;21:22995–3005. Boost AI Power: Data Augmentation Strategies With Unlabeled Data and Conformal Prediction,.
Yu KL, Tseng YS, Yang HC, Liu CJ, Kuo PC, Lee MR, et al. Deep learning with test-time augmentation for radial endobronchial ultrasound image differentiation: a multicentre verification study. BMJ Open Respir Res. 2023;10:e001602.
Jia Z, Zhang H, Ong CN, Patra A, Lu Y, Lim CT, et al. Detection of Lung Cancer: concomitant volatile Organic compounds and Metabolomic Profiling of Six Cancer Cell lines of different histological origins. ACS Omega. 2018;3:5131–40.
Article CAS PubMed PubMed Central Google Scholar
Tsou PH, Lin ZL, Pan YC, Yang HC, Chang CJ, Liang SK, et al. Exploring volatile Organic compounds in Breath for High-Accuracy Prediction of Lung Cancer. Cancers (Basel). 2021;13:1431.
van de Goor R, van Hooren M, Dingemans AM, Kremer B, Kross K. Training and validating a Portable Electronic nose for Lung Cancer Screening. J Thorac Oncol. 2018;13:676–81.
V AB, Subramoniam M, Mathew L. Detection of COPD and Lung Cancer with electronic nose using ensemble learning methods. Clin Chim Acta. 2021;523:231–8.
Article Google Scholar
Beauchamp J. Inhaled today, not gone tomorrow: pharmacokinetics and environmental exposure of volatiles in exhaled breath. J Breath Res. 2011;5:037103.
Article CAS PubMed Google Scholar
Harper WJ. The strengths and weaknesses of the electronic nose. Adv Exp Med Biol. 2001;488:59–71.
Saito S, Espinoza-Mercado F, Liu H, Sata N, Cui X, Soukiasian HJ. Current status of research and treatment for non-small cell lung cancer in never-smoking females. Cancer Biol Ther. 2017;18:359–68.
Temerdashev AZ, Gashimova EM, Porkhanov VA, Polyakov IS, Perunov DV, Dmitrieva EV. Non-invasive lung Cancer Diagnostics through metabolites in Exhaled Breath: influence of the Disease variability and comorbidities. Metabolites. 2023;13:203.
Blanco R, Maestu I, de la Torre MG, Cassinello A, Nunez I. A review of the management of elderly patients with non-small-cell lung cancer. Ann Oncol. 2015;26:451–63.
Walser T, Cui X, Yanagawa J, Lee JM, Heinrich E, Lee G, et al. Smoking and lung cancer: the role of inflammation. Proc Am Thorac Soc. 2008;5:811–5.
Choi E, Ding VY, Luo SJ, Ten Haaf K, Wu JT, Aredo JV, et al. Risk model-based lung Cancer screening and racial and ethnic disparities in the US. JAMA Oncol. 2023;9:1640–8.
Anticuando MK, D DCKR, Padilla D. Electronic Nose and Deep Learning Approach in Identifying Ripe Lycopersicum esculentum L. TomatoFruit. In 13th International Conference on Computing Communication and Networking Technologies (ICCCNT). pp. 1–6; 2022:1–6.
Liu QCC, Qin J, Dou Q, Heng PA, Feddg. Federated domain generalization on medical image segmentation via episodic learning in continuous frequency space. n Proceedings of the IEEE/CVF Conference on Computer Vision and Pattern Recognition 2021:1013–1023.
George O, Smith R, Madiraju P, Yahyasoltani N, Ahamed SI. Data augmentation strategies for EEG-based motor imagery decoding. Heliyon. 2022;8:e10240.
Sundaresan V, Zamboni G, Dinsdale NK, Rothwell PM, Griffanti L, Jenkinson M. Comparison of domain adaptation techniques for white matter hyperintensity segmentation in brain MR images. Med Image Anal. 2021;74:102215.
Kermany DS, Goldbaum M, Cai W, Valentim CCS, Liang H, Baxter SL et al. Identifying Medical Diagnoses and Treatable Diseases by Image-Based Deep Learning. Cell. 2018;172:1122–1131.
Gudino-Ochoa A, Garcia-Rodriguez JA, Ochoa-Ornelas R, Cuevas-Chavez JI, Sanchez-Arias DA. Noninvasive diabetes detection through human breath using TinyML-Powered E-Nose. Sens (Basel). 2024;24:1294.
Article CAS Google Scholar
Ajibola OA, Smith D, Spanel P, Ferns GA. Effects of dietary nutrients on volatile breath metabolites. J Nutr Sci. 2013;2:e34.
Bosch S, Lemmen JP, Menezes R, van der Hulst R, Kuijvenhoven J, Stokkers PC, et al. The influence of lifestyle factors on fecal volatile organic compound composition as measured by an electronic nose. J Breath Res. 2019;13:046001.
Dragonieri S, Quaranta VN, Portacci A, Ahroud M, Di Marco M, Ranieri T, et al. Effect of Food Intake on Exhaled Volatile Organic compounds Profile analyzed by an electronic nose. Molecules. 2023;28:5755.
Download references
Acknowledgements
We would like to thank all the participants who agreed to take part in this study. The authors would like to thank the Data Science Statistical Cooperation Center of Academia Sinica (AS-CFII-111-215) for statistical support.
This study was funded by National Taiwan University Hospital Hsin-Chu Branch (109-HCH034) and National Tsing-Hua University (110F7MAHE1).
Author information
Authors and affiliations.
Department of Internal Medicine, National Taiwan University Hospital, Taipei, Taiwan
Meng-Rui Lee, Jann-Yuan Wang, Chao-Chi Ho, Jin-Yuan Shih & Chong-Jen Yu
Department of Internal Medicine, National Taiwan University Hospital Hsin-Chu Branch, Hsin-Chu, Taiwan
Meng-Rui Lee & Chong-Jen Yu
Department. of Electrical Engineering, National Tsing Hua University, No. 101, Sec. 2, Kuang-Fu Road, Hsinchu, 30013, Taiwan
Mu-Hsiang Kao, Ya-Chu Hsieh, Min Sun & Kea-Tiong Tang
You can also search for this author in PubMed Google Scholar
Contributions
M.R.L., K.T.T. and M.S. designed all the experiments. M.R.L., M.H.K. and Y.C.H. conducted the experiments and analyzed and interpreted the results. K.T.T, M.S., J.Y.W., C.C.H., J.Y.S. and C.J.Y. supervised the project. M.R.L., M.H.K. and Y.C.H. prepared the manuscript. M.R.L., K.T.T, M.S., J.Y.W., C.C.H.,J.Y.S and C.J.Y. reviewed and edited the manuscript. All the authors have read and agreed to the published version of the manuscript.
Corresponding authors
Correspondence to Min Sun or Kea-Tiong Tang .
Ethics declarations
Ethics approval and consent to participate.
The institutional review boards (IRB) of participating hospitals approved this study (IRB no. 202112057RINB, 108-011-E). Inform consent was obtained from all participants who agreed to participate in this study.
Competing for publication
Not applicable.
Competing interests
The original eNose technology of Enosim Bio-tech Co., Ltd. was licensed by National Tsing Hua University and this technology was owned by K.T.T. who serves as a faculty member at National Tsing Hua University’s department of electrical engineering. K.T.T. also received advisory fee from Enosim biotech.
Additional information
Publisher’s note.
Springer Nature remains neutral with regard to jurisdictional claims in published maps and institutional affiliations.
Electronic supplementary material
Below is the link to the electronic supplementary material.
Supplementary Material 1
Supplementary material 2, supplementary material 3, supplementary material 4, supplementary material 5, supplementary material 6, rights and permissions.
Open Access This article is licensed under a Creative Commons Attribution 4.0 International License, which permits use, sharing, adaptation, distribution and reproduction in any medium or format, as long as you give appropriate credit to the original author(s) and the source, provide a link to the Creative Commons licence, and indicate if changes were made. The images or other third party material in this article are included in the article’s Creative Commons licence, unless indicated otherwise in a credit line to the material. If material is not included in the article’s Creative Commons licence and your intended use is not permitted by statutory regulation or exceeds the permitted use, you will need to obtain permission directly from the copyright holder. To view a copy of this licence, visit http://creativecommons.org/licenses/by/4.0/ . The Creative Commons Public Domain Dedication waiver ( http://creativecommons.org/publicdomain/zero/1.0/ ) applies to the data made available in this article, unless otherwise stated in a credit line to the data.
Reprints and permissions
About this article
Cite this article.
Lee, MR., Kao, MH., Hsieh, YC. et al. Cross-site validation of lung cancer diagnosis by electronic nose with deep learning: a multicenter prospective study. Respir Res 25 , 203 (2024). https://doi.org/10.1186/s12931-024-02840-z
Download citation
Received : 29 January 2024
Accepted : 06 May 2024
Published : 10 May 2024
DOI : https://doi.org/10.1186/s12931-024-02840-z
Share this article
Anyone you share the following link with will be able to read this content:
Sorry, a shareable link is not currently available for this article.
Provided by the Springer Nature SharedIt content-sharing initiative
- Electronic nose
- Cross-site validation
- Lung cancer
- Breathprint
- Deep learning
- Data augmentation
Respiratory Research
ISSN: 1465-993X
- General enquiries: [email protected]
Researchers in Portugal develop an image analysis AI platform to boost worldwide research
A team of researchers from the Instituto Gulbenkian de Ciência (IGC) in Portugal, together with Åbo Akademi University in Finland, the AI4Life consortium, and other collaborators, have developed an innovative open-source platform called DL4MicEverywhere. The paper, "DL4MicEverywhere: Deep learning for microscopy made flexible, shareable, and reproducible," was published in the journal Nature Methods .
This platform provides life scientists with easy access to advanced artificial intelligence (AI) for the analysis of microscopy images. It enables other researchers, regardless of their computational expertise, to easily train and use deep learning models on their own data.
Deep learning, a subfield of AI, has revolutionized the analysis of large and complex microscopy datasets, allowing scientists to automatically identify, track and analyze cells and subcellular structures. However, the lack of computing resources and AI expertise prevents some researchers in life sciences from taking advantage of these powerful techniques in their own work.
DL4MicEverywhere addresses these challenges by providing an intuitive interface for researchers to use deep learning models on any experiment that requires image analysis and in diverse computing infrastructures, from simple laptops to high-performance clusters.
"Our platform establishes a bridge between AI technological advances and biomedical research," said Ivan Hidalgo-Cenamor, first author of the study and researcher at IGC.
"With it, regardless of their expertise in AI, researchers gain access to cutting-edge microscopy methods, enabling them to automatically analyze their results and potentially discover new biological insights."
The DL4MicEverywhere platform builds upon the team's previous work, ZeroCostDL4Mic, to allow the training and use of models across various computational environments. The platform also includes a user-friendly interface and expands the collection of available methodologies that users can apply to common microscopy image analysis tasks.
"DL4MicEverywhere aims to democratize AI for microscopy by promoting community contributions and adhering to FAIR principles for scientific research software—making resources findable, accessible, interoperable and reusable," explained Dr. Estibaliz Gómez-de-Mariscal, co-lead of the study and researcher at IGC.
"We hope this platform will empower researchers worldwide to harness these powerful techniques in their work, regardless of their resources or expertise."
The development of DL4MicEverywhere is a great example of the collaborative environment in science. First, it was developed with the purpose of allowing any researcher worldwide to take advantage of the most advanced technologies in microscopy, contributing to accelerate scientific discoveries. Second, it was made possible only through an international collaboration of experts in computer science, image analysis, and microscopy, with key contributions from the AI4Life consortium.
The project was co-led by Ricardo Henriques at IGC and Guillaume Jacquemet at Åbo Akademi University.
"This work represents an important milestone in making AI more accessible and reusable for the microscopy community," said Professor Jacquemet. "By enabling researchers to share their models and analysis pipelines easily, we can accelerate discoveries and enhance reproducibility in biomedical research."
"DL4MicEverywhere has the potential to be transformative for the life sciences," added Professor Henriques. "It aligns with our vision in AI4Life to develop sustainable AI solutions that empower researchers and drive innovation in health care and beyond."
The DL4MicEverywhere platform is freely available as an open-source resource, reflecting the teams' commitment to open science and reproducibility. The researchers believe that by lowering the barriers to advanced microscopy image analysis, DL4MicEverywhere will enable breakthrough discoveries in fields ranging from basic cell biology to drug discovery and personalized medicine.
More information: DL4MicEverywhere: deep learning for microscopy made flexible, shareable and reproducible, Nature Methods (2024). DOI: 10.1038/s41592-024-02295-6
Provided by Instituto Gulbenkian de Ciencia
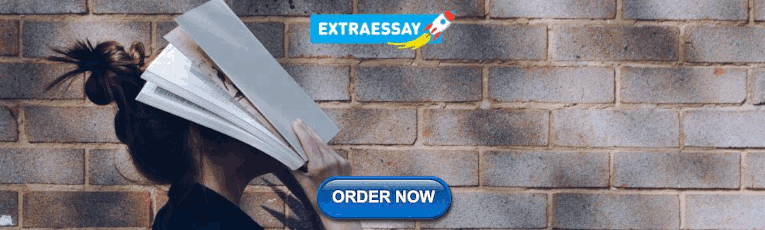
IMAGES
VIDEO
COMMENTS
In many ways, learning research methods is learning how to see and make these decisions." The choice of methods varies by discipline, by the kind of phenomenon being studied and the data being used to study it, by the technology available, and more. This guide is an introduction, but if you don't see what you need here, always contact your ...
Module 2 will cover the critical issue of how research is conducted in the experimental analysis of behavior. To do this, we will discuss the scientific method, research designs, the apparatus we use, how we collect data, and dependent measures used to show that learning has occurred. We also will break down the structure of a research article ...
It's creating better learning. And then as a learner, you are more motivated to replace these ineffective techniques with more effective techniques. Harvard Extension: You talk about tips for learners, how to make it stick. And there are several methods or tips that you share: elaboration, generation, reflection, calibration, among others.
This MOOC is about demystifying research and research methods. It will outline the fundamentals of doing research, aimed primarily, but not exclusively, at the postgraduate level. It places the student experience at the centre of our endeavours by engaging learners in a range of robust and challenging discussions and exercises befitting SOAS ...
The effectiveness of research-based learning. Conducting one's own research project involves various cognitive, behavioural, and affective experiences (Lopatto, Citation 2009, 29), which in turn lead to a wide range of benefits associated with RBL. RBL is associated with long-term societal benefits because it can foster scientific careers: Students participating in RBL reported a greater ...
The science of learning has made a considerable contribution to our understanding of effective teaching and learning strategies. However, few instructors outside of the field are privy to this research. In this tutorial review, we focus on six specific cognitive strategies that have received robust support from decades of research: spaced practice, interleaving, retrieval practice, elaboration ...
Definition. "Good methodology is essential to good science". (Simon and Kaplan 1989, p. 20). The term "methodology" refers to the theoretical analysis of research methods in a discipline that are generally considered appropriate for the inquiry of relevant or important issues. It may refer to a set of methods or procedures or to the ...
Alexander Renkl. Educational Psychology Review (2023) Research on the psychology of learning has highlighted straightforward ways of enhancing learning. However, effective learning strategies are ...
This article outlines a meta-analysis of the 10 learning techniques identified in Dunlosky et al. (2013a), and is based on 242 studies, 1,619 effects, 169,179 unique participants, with an overall mean of 0.56. The most effective techniques are Distributed Practice and Practice Testing and the least effective (but still with relatively high effects) are Underlining and Summarization. A major ...
Effective Learning Practices. Learning at college requires processing and retaining a high volume of information across various disciplines and subjects at the same time, which can be a daunting task, especially if the information is brand new. In response, college students try out varied approaches to their learning - often drawing from ...
Teaching Research Methods: Learning by Doing. June 2009. Journal of Public Affairs Education 15 (2) DOI: 10.1080/15236803.2009.12001557. Authors: Noe Alexander Aguado. University of North Alabama ...
Re-Theorising Learning and Research Methods in Learning Research explores the latest developments in the field of learning theory, offering an overview of emerging methods and demonstrating how recent research contributes to furthering understanding of learning. This book illustrates how theory and methods inform one another, facilitating advancements in the field, while addressing the ways in ...
To this end, teaching-learning methods that involve research can be useful tools to increase interest and encourage the search for knowledge. Research is a vital component of undergraduate education and can play a key role in students' learning, their higher education experience, and the development of general skills. ...
Introduction. In the UK and elsewhere a perceived capacity problem for social science research (Biesta, Allan, and Edwards Citation 2011) is being addressed by provision of postgraduate, course-based research methods training.There is an implicit assumption that academics will deliver courses on research methodology and methods and students completing them will become competent (or at least ...
Research methods are specific procedures for collecting and analyzing data. Developing your research methods is an integral part of your research design. When planning your methods, there are two key decisions you will make. First, decide how you will collect data. Your methods depend on what type of data you need to answer your research question:
The authors of the present review were charged with determining whether these practices are supported by scientific evidence. We concluded that any credible validation of learning-styles-based instruction requires robust documentation of a very particular type of experimental finding with several necessary criteria. First, students must be divided into groups on the basis of their learning ...
LEARNING STYLES. A benchmark definition of "learning styles" is "characteristic cognitive, effective, and psychosocial behaviors that serve as relatively stable indicators of how learners perceive, interact with, and respond to the learning environment. 10 Learning styles are considered by many to be one factor of success in higher education. . Confounding research and, in many instances ...
The research also found that effective active learning methods use not only hands-on and minds-on approaches, but also hearts-on, providing increased emotional and social support. Interest in active learning grew as the COVID-19 pandemic challenged educators to find new ways to engage students.
NCRM delivers research methods training, produces learning resources, conducts research and supports methodological innovation
Although studies found this method to be useful in certain situations, it didn't have a lot of practical use. Surprisingly, re-reading is another learning method that's deemed useless by researchers. Although repetition is the key to learning, [6] research suggests that rereading isn't much of a useful repetition method.
The seven types of learning. New Zealand educator Neil Fleming developed the VARK model in 1987. It's one of the most common methods to identify learning styles. Fleming proposed four primary learning preferences—visual, auditory, reading/writing, and kinesthetic. The first letter of each spells out the acronym (VARK).
158 10 Teaching Learning Methods. 6. The class of students is divided into subgroups, according to ideas, for debate. A variation at this stage is a debate in a large group, critically analysing ...
We present the workflow of Online Iterative Reinforcement Learning from Human Feedback (RLHF) in this technical report, which is widely reported to outperform its offline counterpart by a large margin in the recent large language model (LLM) literature. However, existing open-source RLHF projects are still largely confined to the offline learning setting. In this technical report, we aim to ...
Online learning represents a family of machine learning methods, where a learner attempts to tackle some predictive (or any type of decision-making) task by learning from a sequence of data instances one by one at each ...
Conclusion. Retrieval practice with feedback improved young students' (6 and 7 years old) ability to learn pictures. Mirroring the typical results that we often see with older children and adult learners, feedback makes retrieval practice particularly effective, and retrieval practice is more beneficial compared to other strategies if the goal is longer-term learning.
In conclusion, we tackle the deployment challenges associated with object detection tasks in power system equipment maintenance by presenting YOLO-DFT, an approach method in data fusion and transfer learning. The method delves into the construction of a cloud-based data fusion strategy to produce large-scale human and bird detection datasets ...
The inherently complex and multiscale problems in Materials Science and Engineering pose a formidable challenge. The present scenario of machine learning research in Materials Science has a clear lacunae, where efficient algorithms are being developed as a separate endeavour, while such methods are being applied as 'black-box' models by others.
Background Although electronic nose (eNose) has been intensively investigated for diagnosing lung cancer, cross-site validation remains a major obstacle to be overcome and no studies have yet been performed. Methods Patients with lung cancer, as well as healthy control and diseased control groups, were prospectively recruited from two referral centers between 2019 and 2022. Deep learning ...
The paper, "DL4MicEverywhere: Deep learning for microscopy made flexible, shareable, and reproducible," was published in the journal Nature Methods. Technology News