- Open access
- Published: 05 January 2022
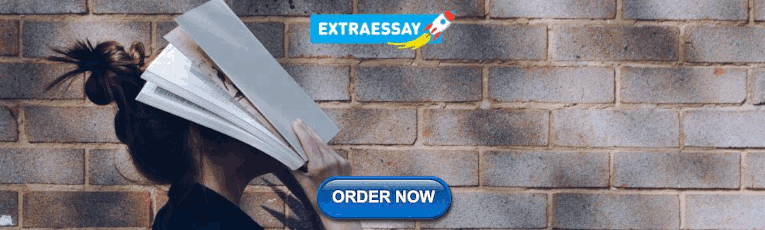
Profiles and predictors of healthcare utilization: using a cluster-analytic approach to identify typical users across conventional, allied and complementary medicine, and self-care
- Daniela Rodrigues Recchia 1 ,
- Holger Cramer 2 , 3 ,
- Jon Wardle 3 ,
- David J. Lee 4 ,
- Thomas Ostermann 1 &
- Romy Lauche 3
BMC Health Services Research volume 22 , Article number: 29 ( 2022 ) Cite this article
3070 Accesses
6 Citations
2 Altmetric
Metrics details
Introduction
The identification of typologies of health care users and their specific characteristics can be performed using cluster analysis. This statistical approach aggregates similar users based on their common health-related behavior. This study aims to examine health care utilization patterns using cluster analysis; and the associations of health care user types with sociodemographic, health-related and health-system related factors.
Cross-sectional data from the 2012 National Health Interview Survey were used. Health care utilization was measured by consultations with a variety of medical, allied and complementary health practitioners or the use of several interventions (exercise, diet, supplementation etc.) within the past 12 months (used vs. not used). A model-based clustering approach based on finite normal mixture modelling, and several indices of cluster fit were determined. Health care utilization within the cluster was analyzed descriptively, and independent predictors of belonging to the respective clusters were analyzed using logistic regression models including sociodemographic, health- and health insurance-related factors.
Nine distinct health care user types were identified, ranging from nearly non-use of health care modalities to over-utilization of medical, allied and complementary health care. Several sociodemographic and health-related characteristics were predictive of belonging to the respective health care user types, including age, gender, health status, education, income, ethnicity, and health care coverage.
Conclusions
Cluster analysis can be used to identify typical health care utilization patterns based on empirical data; and those typologies are related to a variety of sociodemographic and health-related characteristics. These findings on individual differences regarding health care access and utilization can inform future health care research and policy regarding how to improve accessibility of different medical approaches.
Peer Review reports
The use of health services is a very individual process, yet it is shaped by institutional, cultural and social circumstances. Plenty of research has investigated determinants of health care use. Those include contextual characteristics and their influence on health care access [ 1 ], patient segmentation [ 2 ], digital interventions in relation to health behaviors [ 3 ] and the relation of physical activity and healthcare utilization [ 4 ] to predict health care utilization, and improve health care provision while at the same time targeting costs.
Research however often does not include the integrative approach of complementary medicine usage and user’s typology. So far, a comprehensive understanding of the patterns of health care use, and its individual determinants is missing. Examining health care utilization patterns may assist in understanding over- and underutilization, and related factors, and has the potential to shape health care provision, research and policy.
Analysis of health care utilization patterns has been advanced by new statistical methods and increasing computing power to employ more accurate and adequate (big) data analysis methods such as model-based cluster analysis and classification. Such methods have been used before to analyze homeless shelter utilization patterns [ 5 ], dietary [ 6 ] or other health related behavior patterns [ 7 ].
Cluster analysis is a statistical technique used to recognize natural patterns of subjects, i.e. to group them in such way that subjects in one group or cluster are more alike than subjects in different groups or clusters with regards to defined patterns, such as health care utilisation. Similarity of subjects can be defined by various methods and indices including the Euclidean distance between observations or the density estimation of subject distribution. Using this approach, one can identify empirical health care utilization patterns, and define typologies of consumers and their specific characteristics based on the pattern.
To our knowledge no study has determined general health care utilization patterns, this is, determined patterns of conventional, allied medicine, complementary medicine, and self-care in a nationally representative sample. While there is a study on pattern of complementary medicine use in the US-based National Health Interview Survey (NHIS) (2012), it was limited to children with mental health issues [ 8 ].
This study aims to examine NHIS health care utilization patterns in adults using a cluster analytic approach; and the associations of cluster patterns with sociodemographic, health-related and health-system related factors.
The nationally representative NHIS monitors the health status and health care access and utilization of the non-institutionalized US population on a yearly basis including the use of complementary and alternative medicine (CAM) therapies every 5 years. For this analysis data from the Family Core, the Sample Adult Core, and the Adult Complementary and Alternative Medicine questionnaires from 2012 were merged. The more recent NHIS 2017 no longer assessed the totality of CAM modalities, but was limited to a few selected approaches. Thereby, data on the use of common treatment modalities such as acupuncture, osteopathy, supplements or herbal medicine were missing. We therefore chose to investigate the more comprehensive 2012 dataset.
The Family Core and the Sample Adult Core questionnaires collected data on socio-demographic characteristics including age, gender, ethnicity, region, marital status, education, and annual household income; self-perceived general health status, diagnosed conditions and diseases; and health care coverage, access and utilization. The Adult Complementary and Alternative Medicine questionnaire collected data on the use of complementary and alternative medicine.
Health care use in the past 12 months was queried with several question designs, such as whether a practitioner was consulted, or an intervention was used (“During the past 12 months, did you see a practitioner/ use/ practice …?” ). Visits to the dentist and emergency room were queried differently, see Table 1 . All items were coded as or recoded into binary variables (used vs. not used in the past 12 months) for the cluster analysis. A number of complementary and alternative therapies were combined to respond to the lack of observations. For example, all queried herbal medicines were combined into one variable (used herbal medicine vs. did not use herbal medicine); the same was done for non-vitamin supplements, vitamins and minerals, native healers, osteopathy and craniosacral therapy, Tai Chi and qigong, all forms of meditation, exercises and medical diets (see Table 1 ).
A total of 42,366 households were eligible and 34,525 adults provided data (79.7% response rate) [ 9 ]. The final analysis was conducted on 32,017 (75.6%) adults providing complete health care utilization data for all modalities. Population-based estimates were calculated using weights calibrated to the 2010 census-based population estimates for age, gender, and ethnicity of the US civilian non-institutionalized population. By using the population weights the full dataset of 34,525 adults represents a total of 234,9 million US adults.
Statistical analysis
Distribution of frequencies of health care use and sociodemographic data within each cluster are presented as relative percentages (%) in Tables 2 and 3 respectively. To identify possible user typologies and their utilization pattern, the cluster analysis approach was used. This method is able to handle complex data structures and designs in big data scenarios.
The cluster analysis was performed using binary variables only, i.e. all modalities were coded as “ used in the past 12 months” vs. “not used in the past 12 months” , independent of the frequency of use. For the ease of interpretation, cluster analyses based on probabilities are preferred over other models [ 10 , 11 ]; thus, a model-based clustering using finite normal mixture modelling was chosen. This method provides functions for parameter estimation via the Expectation Maximization (EM) algorithm for normal mixture models with the possibility of accounting for different covariance structures and the integration of the Bayesian Information Criterion (BIC) for model selection.
The cluster analysis was performed using the package mclust [ 12 ] for the statistical software R [ 13 ], this package also handles sampling weights if needed. The fitted model was specified as “EII” – spherical, equal volume multivariate mixture , which specifies a spherical distribution with both volume and shape equal enabling the parameters to be better estimated. These parameters consider the within-group covariance matrix.
To determine the statistical fit of the respective cluster solutions the following indices were independently determined: Bayesian Information Criterion (BIC) [ 14 , 15 ], the Dunn Index [ 16 ], Silhouette [ 17 ], the Davies-Bouldin index [ 18 ], and the C-index [ 19 ]. The following order by which the results of the cluster analysis were valuated was used: Silhouette Width, C-index and Dunn-index, BIC and Davies-Bouldin index, these indices are presented at Table 5 .
The cluster solutions also contained information on the probabilities with which the person fit into the cluster. They are based on a classification matrix generated by the cluster analysis. This matrix contains the probability that the observation belongs to each cluster. From this classification matrix the uncertainty values were defined and only those subjects who had a 95% probability of belonging to the respective cluster ( n = 30,251; 87.6%) were considered for further analysis.
Sociodemographic, health related characteristics and health care access were compared between the respective clusters. The variable classes were based on a similar study from NHIS [ 20 ]. The following sociodemographic predictors were considered: age (categories: 18–29; 30–39; 40–49; 50–64, 65 or older), gender (categories: female; male), ethnicity (categories: non-Hispanic White; Hispanic; African American; Asian; Other), US region (categories: West; Northeast; Midwest; South), marital status (categories: not in relationship; in relationship), education (categories: less than college; some college or more), employment (categories: employed, not employed) and annual household income (categories: less than $20,000; $20,000 to $34,999; $35,000–$64,999; $65,000 or more). Additionally, health related factors such as general health status (categories: excellent, very good, good, fair or poor), medical conditions/diseases (no chronic condition, one chronic condition, two chronic conditions, three or more chronic conditions), BMI (categories: < 18.5; 18.5–25; 25.5–30; 30.5 or more), health behaviors such as smoking (categories: non-smoker, smoker), alcohol consumption (categories: alcohol abstainer; light drinker; regular or heavy drinker), and exercise behavior (categories: low level exerciser, moderate level exerciser, high level exerciser); health insurance coverage (categories: no insurance, public health insurance, private health insurance) and the affordability of prescription medication, mental care, dental care, eyeglasses and specialists (categories: could afford, could not afford) were also used as potential predictors.
Backward stepwise regression analyses employing a likelihood-ratio-statistic were conducted for each cluster to determine predictors for belonging in that cluster, and adjusted odds ratios with 95% confidence intervals were calculated. All potential predictors were included in the logistic regression analyses, and a sample size adjusted weight was used considering for design effects. Statistical significance was set at p ≤ 0.05.
The regression models and distributions analyses were performed with Statistical Package for Social Sciences software (IBM SPSS Statistics for Windows, release 22.0. Armonk, NY: IBM Corp.).
A total of 32,017 of 34,525 subjects (92.7%) provided full data on health care use and therefore were selected for the cluster analysis, 30,251 (87.6%) were considered for the estimation of sample representativeness. Based on the population weight the sample of 30,251 sample represents a total of 205.2 million US adults.
Based on the individual health care utilization patterns, the optimal cluster solution as per Silhouette and the C-index was identified as having 9 clusters (Table 5 ), with cluster size ranging from 2067 to 5117 observations. The model fit indices were 0.21 for Silhouette, and 0.24 for C-index, indicating sufficient statistical fit.
The prevalence of health care utilization within each cluster are shown in Table 2 . Sociodemographic, health- and health-insurance related associations for belonging in either cluster can be found in Tables 3 and 4 . A graphic visualization from health care utilization patters within each cluster is presented in Fig. 1 . The following clusters of health care user types have been identified, and analyzed with regards to their characteristics. The respective frequencies are displayed at Table 3 .
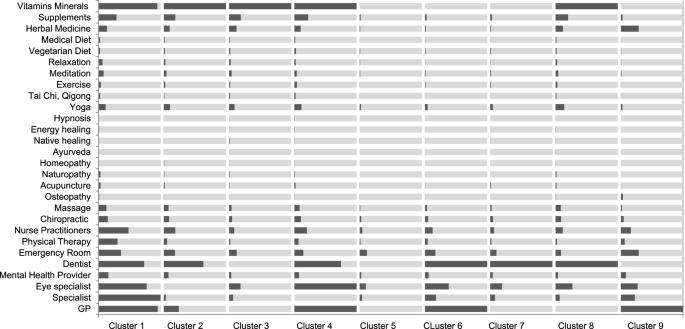
Visualization of health care utilization patterns within each cluster. Within each cluster dark color indicates use in %, bright color non-use. Sizes of clusters are not indicative of actual size
Cluster 1 – “Overutilization of health care ”: White (81.1%), female (57.5%), higher educated (48.9%), and/or older adults (65 years or more; 34%) with higher income > $65,000 (27%), multiple chronic conditions (23.7%), and/or private health insurance (70.3%) more likely over-utilize every health care modality relative to the general population. This includes conventional, allied and complementary health care, and non-provider-based health care (i.e. self-care) (Table 2 ). Region, BMI and frequency of exercise were not relevant variables in the logistic model (Table 4 ).
Cluster 2 – “High users of vitamins and mineral supplementation” : Mid-aged (50–64 years old; 26.3%), female adults (56.7%) living in the Southern region of the country (35.3%) with one chronic condition (57.9%), and/or private health insurance (68.5%) more likely show average health care utilization in general. Relative to the general population they have lower consultation rates with GPs and specialists, and higher utilization of vitamins and mineral supplementation (Table 2 ). Ethnicity, Education, Income, Marital and Health Status, BMI and Smoking habits were not included in the final regression model (Table 4 ).
Cluster 3 – “Underutilization of health care, GP, specialists, ER and dentists” : Single (55%), male (56.1%) adults living in the South (41%) with high school but not college education (52.8%), small income (< $20,000; 36.3%), no chronic conditions (74.1%), and/or average health status (28%), but no health insurance (43.1%) more likely belonged to Cluster 3. Compared to Cluster 1 and 2, adults of Asian ethnicity also more likely belonged to this cluster (6.5%). Adults in this cluster underutilize conventional health care, including visits to GPs, specialists, ER and dentists relative to the general population. In contrast, health care interventions and behavior not associated with visits to a health professional are used on an average level (Table 2 ). This is the only cluster where age was not a relevant variable for the statistical model, together with BMI and Smoking and Drinking habits (Table 4 ).
Cluster 4 – “Underutilization of specialists and emergency rooms” : Female (61,2%), higher educated (47.4%), and/or older adults (> 65 years or more; 29.3%) with very high income >$65,000 (26.3%), one or two chronic conditions (42.9%), and/or private health insurance (74.5%) more likely show a high utilization of GPs, eye specialists, manual therapies and self-care. They are however characterized by an under-utilization of specialists visits and visits to the emergency room (Table 2 ). Ethnicity, Region and BMI were not present at the final logistic model (Table 4 ).
Cluster 5 – “Underutilization of every health care modality” : Young (35%), male (64.5%), and/or non-White individuals (52.9%) without high-school education (28.2%), very low income (49.3%), no health insurance (53.4%), and/or no chronic condition (78.9%) more likely show under-utilization of every health care modality (Table 2 ).
Cluster 6 – “ Underutilization of CAM and self-care” : Young or mid-aged (41.5%), male (54.4%), and/or non-White individuals (36.1%), not living in the West (81.5%), having at least high-school education (50.3%), with good to very good health (65.5%) despite one chronic condition (58.4%) more likely show an over-utilization of GPs and dentists, average utilization of other conventional and allied health care, and an under-utilization of CAM and self-care (Table 2 ). Region, Health Status and Smoking habits were not relevant in the model (Table 4 ).
Cluster 7 – “ Underutilization of every health care but dental care”: Very young (35.9%), male (58.8%), and/or non-White individuals (39.8%), not living in the West (78.6%), with no chronic condition (78%) more likely show under-utilization of every health care modality with the exception of dental care (Table 2 ). Education, Income, Marital and Health Status, BMI, Health Insurance and Smoking habits were not relevant (Table 4 ).
Cluster 8 – “Underutilization of every health care modality” : Very young (29.8%), female adults (52.5%), living in the West (27.5%), with higher education (49.1%), very good health (77.5%) and/or no chronic conditions (77.5%) more likely show under-utilization of every health care modality, and over-utilization of dental care and vitamin/mineral supplementation (Table 2 ). Ethnicity, Income, Health Insurance and Alcohol Consumptions were not included in the final model (Table 4 ).
Cluster 9 – “Overutilization of GPs, ER and herbal medicines” : Young to mid-aged (34.3%), male individuals (54.4%) not living in the West (82.4%), with no high-school or college education (71.4%), and/or with very low income (39.6%), poor health status (26.8%) with at least one chronic condition (25.2%), and/or public health insurance (33.9%) more likely show over-utilization of GPs, emergency rooms, and herbal medicines, and under-utilization of all other health care modalities (Table 2 ). For Cluster 9 only Ethnicity was not considered in the logistic model (Table 4 ).
Summary of results
The cluster analysis identified several types of health care user based on their health care utilization patterns. However, no single optimal cluster solution could be consistently favored by all indices. The preferred cluster solution identified 9 types of health care users, who showed significant differences in the utilization patterns with substantial rates of over- and underutilization of certain health care modalities. The regression analysis further found sociodemographic, health and health insurance related factors predictive of being a member of a respective cluster. The presence or non-presence of multiple chronic conditions was the only variable identified in the logistic model to be significant as a predictor for all 9 clusters.
Model fit and indices
The cluster analysis was performed with different numbers of clusters until the optimal final 9-cluster solution was identified. Because the dataset is relatively big, both regarding observations and variables, and the used method (mclust) iterates many parameters and run different estimations, the run time per adjusted model was considerably high (several days considering all models).
The final solution was achieved after evaluating for both statistical model properties – the cluster validation measures in Table 5 , and for the theoretical interpretation of the similarity of characteristics. There was unfortunately not a single solution where all five statistical indices were optimal.
In a review of clustering methods, mclust has shown a better overall cluster performance and ability regarding handling different data types [ 21 ]. This enhances a good level of certainty regarding the chosen method. The method incorporates the complex sampling design of the NHIS enabling a better model adequacy.
Typology of health care users, and their impact
The cluster analysis identified 9 different types of health care users, who showed substantial differences in utilization of certain health care modalities. Members in the first cluster for example showed a substantial overutilization of practically every health care modality, while those in the fifth cluster were using almost no health care at all. Several sociodemographic and health-related characteristics were predictive of belonging into the respective clusters, including age and gender, education, income, ethnic origin, health care coverage, and health status. Several findings deserve attention. Below we summarized the findings into groups of differences and commonalities between the profiles of health care utilization (i.e. the clusters).
Healthy aging
Members of the first cluster showed overutilization of health care, and that might be explained by the fact that they were more likely to be above 50 years of age and to have multiple chronic conditions. Chronic medical conditions are increasingly prevalent among older adults [ 22 ]. However, health status alone cannot fully explain the overutilization of health care modalities. Members in cluster 4, for example, also are more likely to be at the same age range, and to have chronic conditions. However, they are using far less practitioner-based health care modalities as compared to those in cluster 1. Clusters 1 and 4 probably reflect ‘more successful’ and ‘less successful’ pathways to aging. They also represent a substantial proportion of the US population, making this finding even more important given the aging of the US population (and in other industrialized nations). It has been known for some time that a small proportion of Americans account for the majority of healthcare expenditures and there are concerted efforts to better manage this utilization pattern [ 23 ]. Additionally, lifestyle factors that contributed to healthy aging such as non-smoking and social support [ 24 ], physical activity and diet quality [ 25 ] should be in focus of the coordination of public health care.
Clusters 2, 6 and 9 are mid-aged, and are likely to suffer from one or two (early stage) chronic conditions; individuals in cluster 2 strongly use self-care, those in 6 and 9 strongly utilize more conventional practitioner-based health care. We could not surely predict where these individuals would end up in higher age, and only taking action in time might prevent them from ending up in the over-user group.
Cluster 6 shows an underutilization of CAM while an overutilization could not be detected in any cluster. This might indicate that we need to have a closer look at the efficacy and dissemination of CAM in the population. A program that would encourage the use of evidence-based CAM approaches such as the one applied for veterans [ 26 ] could be applied.
Gender influence and self-care
We identified gender differences regarding health- and self-care behavior. Female individuals are more likely to use health-care in general (Cluster 1) or specifically vitamins & minerals supplementation (Cluster 2). On the other hand, males often underutilize health-care (Clusters 3 and 7) and self-care (Cluster 5). Research has shown consistently that men tend to neglect self-care [ 27 ] and engage less in health-related self-care behaviors [ 28 ]. From a public health perspective it is essential to raise awareness for the need of self-care in male populations.
Several factors might limit self-care besides gender: education/health literacy, self-efficacy, access or costs. Barriers need to be identified, and attempts to increase self-care utilization for improving overall health, preventing chronic conditions, and lower the costs associated with health care are needed.
Healthcare coverage
A substantial proportion of participants (43.1% in Cluster 3 and 53.4% in Cluster 5) reported to have no health insurance. Clusters 4 (74.5%), 6 (72.4%) and 8 (72.9%) have the biggest proportion of private health insurance coverage. Other factors associated with significantly higher health-care use or problems when underserving those in need should be identifiable. Health status is reported as very good or excellent for a large proportion of participants in cluster 7 (72.2%) and 8 (77.5%), indicating a probable association with health insurance type.
Preventive, curative or aesthetic intervention
Several health care interventions may not be related to a medical condition, but to preventative or aesthetic needs. Dentist visits for example are often for prevention, or for aesthetic purposes. Men were found to value dental care (Clusters 3 and 7) despite appearing ‘less caring’ about other health-care interventions. Dental care however is expensive, and not surprisingly, the highest frequency of participants who did not utilize dental care as frequently where those having no health insurance (Clusters 3 and 5).
Implications for research
Cluster analyses have been used before in health research, for example in research of homeless shelter utilization patterns [ 5 ]. Several types of homeless people have been identified (e.g. transitionally homeless, episodically homeless, chronically homeless), and those types were associated with different usage patterns.
In this analysis, we used a model-based clustering approach based on finite normal mixture modelling, and the model was evaluated with several indices of cluster fit. This method has shown to be effective in differentiating and clustering individuals in 9 different groups with similar characteristics within and dissimilarities between clusters.
The findings of this cluster analysis may have important implications for health policy. They highlight distinct patterns of health care over- and underutilization associated with age, gender, socio-economical, ethnical and regional differences. By understanding health care utilization, interventional programs and prevention campaigns may be better tailored to specific groups of individuals with specific health care use patterns. Specifically, social inequalities and barriers to health care access can be addressed by tailoring health care to these groups.
The usage of CAM or dental care, where gender differences exist regarding self-care behavior, are areas that require attention. More awareness on the importance of health-care and program development to encourage and enable their utilization are crucial.
Limitations
The findings identified through this analysis must be considered in light of the study limitations. The data were drawn from a cross-sectional survey; as such, the results can only suggest associations for a particular time point. A longitudinal survey would be necessary to document changes in health care utilization patterns over time.
Health care utilization was further queried using binary variables only, i.e. it was only assessed whether participants had used a certain health care intervention or not; and no information on the number of consultations, or the out of pocket expenditure was analyzed. Influence of binary variables on the cluster analyses, and distribution (low prevalence for several interventions) could not be measured regarding its frequency but only regarding its presence. The survey is further based on self-report data and as such there is at risk of recall bias or measurement error.
The decision in favor of the 9-cluster solution was not only based on statistical indices but on theoretical interpretation as well. There were different solutions according to the indices, indicating that there is not one solution, but several possibilities and the selection of cluster specification and its indices was user determined. Unfortunately an optimal single solution, where all 5 indices fit the best, could not be achieved.
Nevertheless, the US National Health Survey is an internationally recognized epidemiological study, and the findings from this study provide useful first insights into the patterns of health care utilization.
In this analysis, we identified 9 types of health-care utilization patterns and their characteristics based on the similarity of their behavior. A model-based clustering approach based on finite normal mixture modelling and cluster fit indices were determined.
The clusters differentiate between health-care user types, ranging from nearly non-use of health care modalities to overutilization pattern of medical, allied and complementary health care. Several sociodemographic and health-related characteristics were predictive of belonging into a respective cluster, including age and gender, health status, education, income, ethnic origin, and health care coverage.
In conclusion, cluster analysis may be useful to identify typical health-care utilization patterns based on empirical data; and those typologies appear to be related to a variety of sociodemographic and health-related characteristics. Those findings can inform future health research and policy.
Availability of data and materials
The datasets analyzed are available at the open source from National Center for Health Statistics: https://www.cdc.gov/nchs/nhis/nhis_2012_data_release.htm .
Andersen RM, Davidson PL, Baumeister SE. Improving access to care. Kominski. 2013;1:33–69.
Low LL, Yan S, Kwan YH, Tan CS, Thumboo J. Assessing the validity of a data driven segmentation approach: a 4 year longitudinal study of healthcare utilization and mortality. PLoS ONE. 2018;13(4):1–15.
Michie S, Yardley L, West R, Patrick K, Greaves F. Developing and evaluating digital interventions to promote behavior change in health and health care: recommendations resulting from an international workshop. J Med Internet Res. 2017;19(6):1–39.
Sari N. Exercise, physical activity and healthcare utilization: a review of literature for older adults. Maturitas. 2011;70(3):285–9.
Article Google Scholar
Lee CT, Gurman D, Ponath C, Tieu L, Riley E, Kushel M. Residential patterns in older homeless adults: results of a cluster analysis. Soc Sci Med. 2016;153:131–40. https://doi.org/10.1016/j.socscimed.2016.02.004 .
Hu FB. Dietary pattern analysis: a new direction in nutritional epidemiology. Curr Opin Lipidol. 2002;13(1):3–9.
Choi SH, Duffy SA. Analysis od health bahvior theories for clustering of health behaviors. J Addict Nurs. 2017;4:203–9.
Wang C, Preisser J, Chung Y, Li K. Complementary and alternative medicine use among children with mental health issues: results from the National Health Interview Survey. BMC Complement Altern Med. 2018;18(1);1–17.
Blackwell DL, Lucas JW, Clarke TC. Summary health statistics for U.S. adults: national health interview survey, 2012. Vital Health Stat. 2014;260:1–161.
Google Scholar
C. Fraley and A. E. Raftery, "MCLUST version 3 for R: Normal mixture modeling and model-based clustering," Technical report, DTIC Document, 2006.
Fraley C, Raftery AE. Model-based methods of classification: using the mclust software in hemometrics. J Stat Sotw. 2007;18(6):1–13.
Scrucca L, Fop M, Murphy TB, Raftery AE. Mclust 5: clustering, classification and density estimation using Gaussian finite mixture models. R J. 2016;8(1):289–317.
R Core Team. R: A language and environment for statistical computing. Vienna: R Core Team; 2020.
Schwarz G. Estimating the dimension of a model. Ann Stat. 1978;6(2):461–4.
Vrieze SI. Model selection and psychological theory: a discussion of the differences between the Akaike information criterion (AIC) and he Bayesian information criterion (BIC). Psychol Methods. 2012;17(2):228–43.
Dunn JC. Well-separated clusters and optimal fuzzy partitions. J Cyberkin. 1974;4(1):95–104.
Rousseeuw PJ. Silhouettes: a graphical aid to the interpretation and validation of cluster analysis. J Comput Appl Math. 1987;20:53–65.
Davies DL, Bouldin DW. A cluster separation measure. IEEE Trans Pattern Anal Mach Intell. 1979;1(2):224–7.
Article CAS Google Scholar
Hubert LJ, Levin JR. A general statistical framework for assessing categorical clustering in free recall. Psychol Bull. 1976;83(6):1072–80.
Badu E, Agyei-Baffour P, Acheampong IO, Opoku MP, Addai-Donkor K. Households Sociodemographic profile as predictors of health insurance uptake and service utilization: a cross-sectional study in a municipality of Ghana. Adv Public Health. 2018;1–13.
Haughton D, Legrand P, Woolford S. Review of three latent class cluster analysis packages: latent GOLD, poLCA, and MCLUST. Am Stat. 2009;63(1):81–91.
N. C. o. A. NCOA. National Council on Aging NCOA. 2020. [Online]. Available: https://www.ncoa.org/news/resources-for-reporters/get-the-facts/healthy-aging-facts/ .
Stanton MW. The high concentration of U.S. health care expenditures. Res Action. 2006;1–11.
Bosnes I, Nordahl HM, Bosnes O, Myklebust TÅ, Almkvist O. Lifestyle predictors of successul aging: A 20-year prospective HUNT study. PLoS ONE. 2019;14(7);1–12.
Atallah N, Adjibade M, Lelong H, Hercberg S, Galan P, Assmann KE, et al. How healthy lifestyle factors at midlife relate to healthy aging. Nutrients. 2018;10(7):1–10.
Held RF, Santos S, Marki M, Helmer D. Dissemination and implementation of an educational tool for veterans on complementary and alternative medicine: a case study. BMC Complement Altern Med. 2016;1–8.
Mei J, Tian Y, Chai X, Fan X. Gender differences in self-care maintenance and its associations among patients with chronic heart failure. Int J Nurs Sci. 2019;1:58–64.
Abe R, Sakata Y, Nochioka K, Miura M, Oikawa T, Kasahara S, et al. Gender differences in prognostic relevance of self-care behaviors on mortality and hospitalization in patients with heart failure – a report from the CHART-2 study. J Cardiol. 2019;73(5):370–8.
Download references
Acknowledgements
Not applicable.
Open Access funding enabled and organized by Projekt DEAL. This work was produced with no funding.
Author information
Authors and affiliations.
Chair of Research Methods and Statistics in Psychology, Department of Psychology and Psychotherapy, Witten/Herdecke University, Alfred-Herrhausen-Strasse 50, 58448, Witten, Germany
Daniela Rodrigues Recchia & Thomas Ostermann
Department of Internal and Integrative Medicine, Evang. Kliniken Essen-Mitte, Faculty of Medicine, University of Duisburg-Essen, Essen, Germany
Holger Cramer
National Centre for Naturopathic Medicine, Southern Cross University, Lismore, New South Wales, Australia
Holger Cramer, Jon Wardle & Romy Lauche
Chair of Department of Public Health Sciences, University of Miami, Miami, FL, USA
David J. Lee
You can also search for this author in PubMed Google Scholar
Contributions
DRR: conceptualization, methodology, formal analysis, investigation, writing – original draft, visualization. HC, JW, DL & TO: conceptualization, methodology, writing – review & editing, supervision. RL: conceptualization, methodology, formal analysis, investigation, writing – original and review & editing, resources, supervision, project administration. The author(s) read and approved the final manuscript.
Corresponding author
Correspondence to Daniela Rodrigues Recchia .
Ethics declarations
Ethics approval and consent to participate.
All methods were performed in accordance with relevant guidelines and regulations.
Consent for publication
Competing interests.
The authors declare that they have no competing interests.
Additional information
Publisher’s note.
Springer Nature remains neutral with regard to jurisdictional claims in published maps and institutional affiliations.
Rights and permissions
Open Access This article is licensed under a Creative Commons Attribution 4.0 International License, which permits use, sharing, adaptation, distribution and reproduction in any medium or format, as long as you give appropriate credit to the original author(s) and the source, provide a link to the Creative Commons licence, and indicate if changes were made. The images or other third party material in this article are included in the article's Creative Commons licence, unless indicated otherwise in a credit line to the material. If material is not included in the article's Creative Commons licence and your intended use is not permitted by statutory regulation or exceeds the permitted use, you will need to obtain permission directly from the copyright holder. To view a copy of this licence, visit http://creativecommons.org/licenses/by/4.0/ . The Creative Commons Public Domain Dedication waiver ( http://creativecommons.org/publicdomain/zero/1.0/ ) applies to the data made available in this article, unless otherwise stated in a credit line to the data.
Reprints and permissions
About this article
Cite this article.
Recchia, D.R., Cramer, H., Wardle, J. et al. Profiles and predictors of healthcare utilization: using a cluster-analytic approach to identify typical users across conventional, allied and complementary medicine, and self-care. BMC Health Serv Res 22 , 29 (2022). https://doi.org/10.1186/s12913-021-07426-9
Download citation
Received : 04 June 2021
Accepted : 17 December 2021
Published : 05 January 2022
DOI : https://doi.org/10.1186/s12913-021-07426-9
Share this article
Anyone you share the following link with will be able to read this content:
Sorry, a shareable link is not currently available for this article.
Provided by the Springer Nature SharedIt content-sharing initiative
- Complementary medicine
- Cluster analysis
- Healthcare utilization pattern
BMC Health Services Research
ISSN: 1472-6963
- General enquiries: [email protected]
- Research article
- Open access
- Published: 23 July 2020
Utilization of public health care by people with private health insurance: a systematic review and meta-analysis
- Congcong Zhang 1 ,
- Chenwei Fu 1 ,
- Yimin Song 1 ,
- Rong Feng 1 ,
- Xinjuan Wu 2 &
- Yongning Li 1
BMC Public Health volume 20 , Article number: 1153 ( 2020 ) Cite this article
7059 Accesses
7 Citations
4 Altmetric
Metrics details
The objective of this systematic review was to explore the association between private health insurance and health care utilization.
We searched the MEDLINE, EMBASE, and Cochrane Central Register of Controlled Trials (CENTRAL) electronic databases for relevant articles since 2010. Studies were eligible if they described original empirical research on the utilization of public health care by individuals with private health insurance, compared with individuals without private insurance. A pooled measure of association between insurance status with health care utilization was assessed through meta-analysis.
Twenty-six articles were included in the final analysis. We found that patients with private insurance did not use more public health care than people without private insurance ( P < 0.05). According to the subgroup analysis, people with private insurance were more likely to be hospitalized than people with no insurance (OR 1.67; 95% CI, 1.18 to 2.36).
Conclusions
People with private insurance did not increase their use of health care (outpatient services), compared to those without private insurance. Private health insurance coverage may ease the financial burden on patients and on the public health insurance system.
Peer Review reports
As most countries across the world face rapidly escalating health expenditures, exorbitant out-of-pocket payments have resulted in high demand for supplementary private health insurance [ 1 , 2 ]. For instance, in 2015, approximately 80% of households in the United States had to purchase at least one private health insurance plan, and more than 25% of Brazilians had private health insurance in 2019 [ 3 , 4 ].
The role of private health insurance is fiercely debated. Some researchers believe that the use of private health insurance should be encouraged in order to ease the financial burden on patients and on social healthcare systems [ 5 ]. However, others maintain that the use of private health insurance will contribute to the current rapid increase in health expenditures, induce fragmentation of the healthcare system, and aggravate social inequity by increasing the gap in health care utilization between opposite ends of the socioeconomic spectrum [ 6 , 7 ]. One of the critical controversies over private health insurance is its potential impact on health care utilization. If individuals with private health insurance increase their utilization of health care, the result will be inequity in health care utilization between those who purchase private health insurance and those who do not.
Although previous studies have examined the effect of private insurance on the utilization of public health care in specific countries [ 3 , 6 ], no study published to date has systematically investigated the issue on a global scale. However, it is necessary for stakeholders to understand the role that private insurance companies play the use of healthcare services from a macro perspective. The objective of this systematic review was to synthesize available evidence to compare the effect of private health insurance to the effect of having no (private) insurance or public health care insurance on the utilization of health care (inpatient and outpatient resources) among all kinds of patients worldwide.
Search strategy
This systematic review was performed in accordance with the recommendations of the Preferred Reporting Items for Systematic Reviews and Meta-Analyses (PRISMA) guidelines, but the review protocol was not registered [ 8 ]. Two reviewers searched MEDLINE, EMBASE, and the Cochrane Central Register of Controlled Trials (CENTRAL) databases for relevant articles published from January 1, 2010 to June 1, 2019. The search terms used included: “health insurance,” “private or commercial health plan(s),” “private or commercial health insurance,” “private or commercial health company,” “health within six words around the word of utilization or utility,” and “hospital within six words around the word of utilization or utility” (see detailed search strategies in Additional file 1 ). We searched for additional references by cross-checking the reference lists of the studies retrieved and of relevant reviews. We also contacted researchers in the field to identify trials that were eligible for inclusion.
Inclusion and exclusion criteria
We included both prospective and retrospective longitudinal controlled studies in this systematic review. Studies were eligible if they described original empirical research on the utilization of health care by individuals with private health insurance. The eligibility criteria were: 1) original studies (randomized controlled trial, case-control, cohort, cross-sectional, or pre-post); 2) one group of study participants with private health insurance (exposure group); 3) one group of study participants without private insurance (control); 4) utilization of health care [outpatient services: emergency department (ED) visits, clinic visits; inpatient services: length of stay (LOS), hospitalization rate] as an outcome [ 9 ]; 5) publication in the English language in 2010 or later. Reviews, commentaries, protocols, editorials, case reports, qualitative research, and letters were excluded. Studies on diagnostic support (e.g., radiology, clinical pathology) were also excluded. If two articles were found to derive from the same study, only the original study was included. However, if different target outcomes were reported, then both papers were included.
Study selection
Titles and abstracts were first screened for relevance by two independent reviewers, and full-text articles with potential eligibility were downloaded for further assessment. When consensus could not be reached, disagreements were resolved by consulting a third author.
Data collection
Data were collected with an extraction form validated in pilot studies. The data items extracted in this review were as follows: (1) The surname of the first author with the year in which the paper was published; (2) study design; (3) country in which the study was conducted; (4) full report or abstract; (5) target population; (6) target exposure group; (7) target control group; (8) target outcomes [emergency department (ED) visits, clinic visits, length of stay (LOS), and/or hospitalization rate]; (9) the numerical data included the number of visits to the ED, the percentages of visits to the ED, the rates of hospitalization, the rates of outpatient office visits, and the length of inpatient stays (days).
Quality assessment
Risk of bias was assessed independently by two reviewers. We applied the ROBINS I tool to assess the risk of bias among non-randomized intervention studies [ 10 ]. Risk of bias was assessed at the study level, and these results were used to inform a GRADE evidence assessment [ 11 ].
Statistical analysis
We performed meta-analyses of the studies to obtain a pooled estimate for the utilization of health care by individuals with private health insurance, compared with individuals without private health insurance. Odds ratios [with 95% confidence intervals (CIs)] were obtained for the rates of visits to the ED, the percentages of ED visits, and the rates of hospitalization with Review Manager 5.3 software [ 12 ]. Using the same software program, mean differences were obtained for the rates of outpatient office visits and the length of inpatient stay (days). P -values < 0.05 were considered as statistically significant. Between-study heterogeneity was measured using Cochrane’s Q-test and the Higgins I 2 statistic ( P < 0.10 or I 2 > 50%) was considered as statistically significant heterogeneity [ 13 ]. When heterogeneity was present, a random-effect model (Der Simonian and Laird method) was applied. The fixed-effect model was used in the absence of between-study heterogeneity ( P > 0.10 or I 2 < 50%). As sensitivity analysis to confirm the robustness of our results, we performed a subgroup analysis for the control arm of no private health insurance in order to distinguish individuals with no insurance from individuals with public insurance.
A total of 8727 articles were selected by searching the selected electronic databases, and an additional five records were identified by cross-checking the reference lists of retrieved studies or relevant reviews. After excluding duplicates and screening titles and abstracts, we obtained 181 articles for full-text review. We eliminated 155 papers from among the 181 originally identified, based on our inclusion and exclusion criteria. Ultimately, 26 articles were included in the analysis (Fig. 1 ).
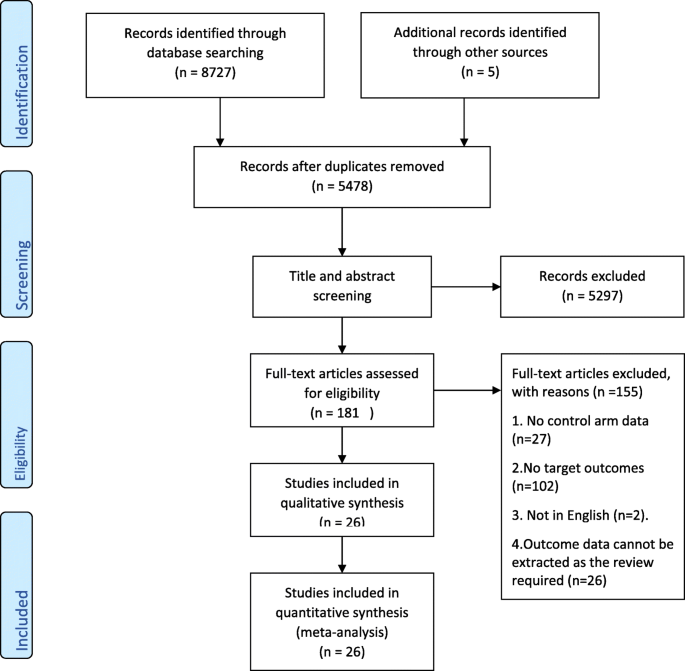
PRISMA flow diagram detailing the search strategy and results
Study characteristics
The basic characteristics and target outcomes of included studies are listed in Table 1 . All included articles ( n = 26) were observational studies, in the form of abstract ( n = 6) or full report ( n = 20). The studies included in the meta-analysis had been conducted in the United States (57.7%, 15/26), Brazil (11.5%, 3/26), South Korea (7.7%, 2/26), Australia (7.7%, 2/26), India (7.7%, 2/26), Japan (3.8%, 1/26), and Germany (3.8%, 1/26). The study populations ranged from healthy controls to patients with specific diseases or medical conditions. Among the 26 studies included, 13 (50%) focusing on the comparison between private insurance and both no insurance and public insurance, 8 (30.8%) on the comparison between private insurance and no public insurance, and 5 studies (19.2%) compared private insurance with a lack of insurance.
Risk of bias
We evaluated risk of bias for all full reports included in the meta-analysis ( n = 20) with the ROBINS I tool. We did not assess the risk of bias in abstracts because there was insufficient information for the evaluation of methodological quality. Figure 2 a shows the risk of bias for each cohort. Evaluations for each domain are shown in Fig. 2 b. These figures did not include studies reported as abstracts only.
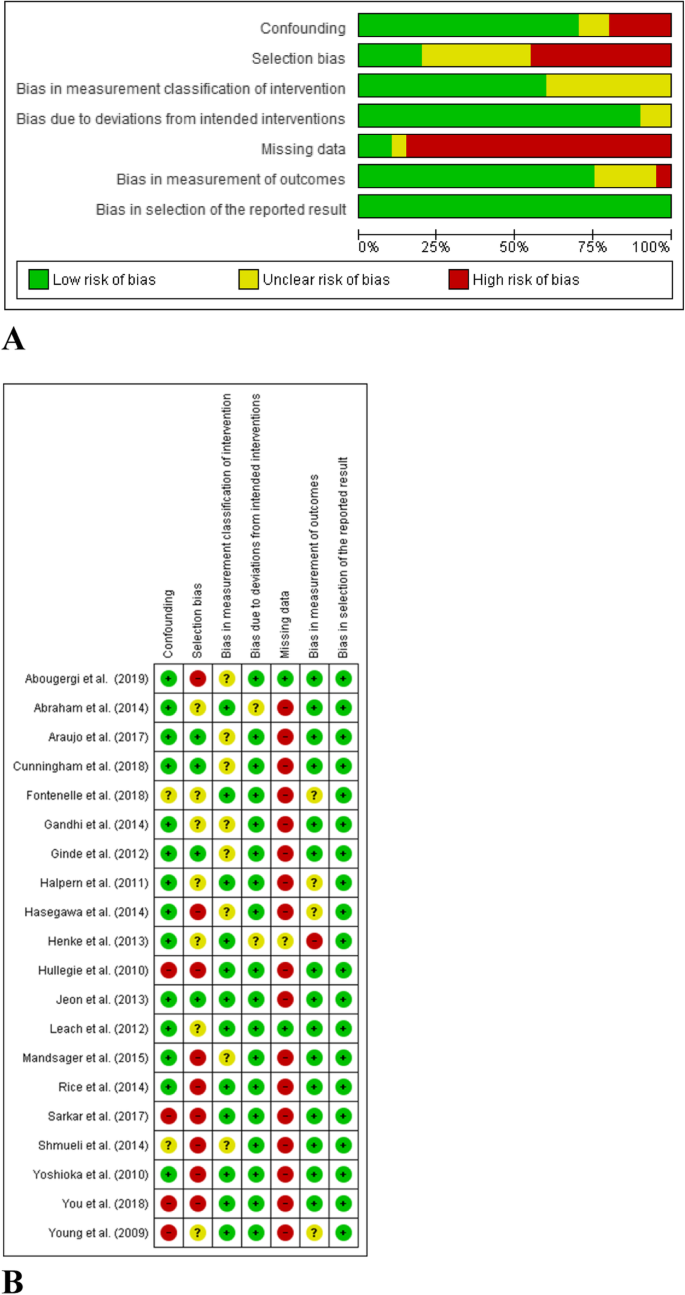
Risk of bias assessment. a Risk of bias graph: review authors’ judgements about each risk of bias item presented as percentages across all included full reported studies ( n = 20). b Risk of bias summary: review authors’ judgements about each risk of bias item for each included full reported study
Utilization of outpatient services
All detailed data extraction results can be found in Additional file 1 .
We used the data from 5 studies, which collectively included > 500,000,000 participants, to determine the odds ratio (OR) for a comparison of the rates of ED visits among people with private insurance, compared to people without private insurance [ 15 , 19 , 20 , 25 , 34 ]. The pooled results yielded an OR of 1.01 (95% CI 0.58–1.76) (Fig. 3 a). There was no significant difference between people with private insurance and people without private insurance in the rate of ED visits. The results of subgroup analysis showed that this OR was similar for people with public insurance and people with no insurance (Fig. 4 a).
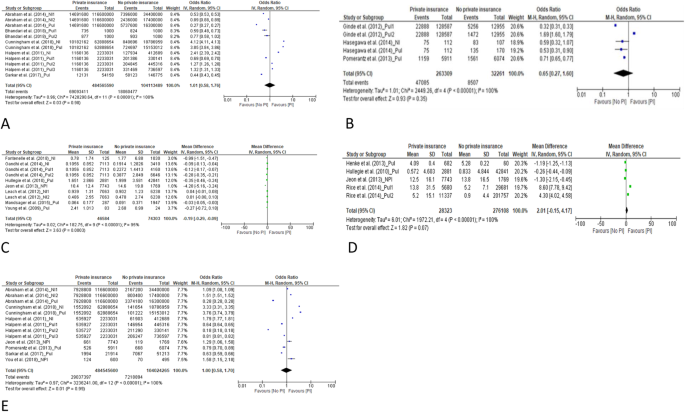
Forest plots of the total pooling results. a for comparison of the rates of emergency department visits between private insurance and no private insurance. b for comparison of the percentages of emergency department visits between those with private insurance and those without private insurance. c for comparison of the rates of outpatient office visits between private insurance and no private insurance. d for comparison of the rates of outpatient office visits between private insurance and no private insurance. e for comparison of the length of inpatient stay (days) between those with private insurance and those without private insurance
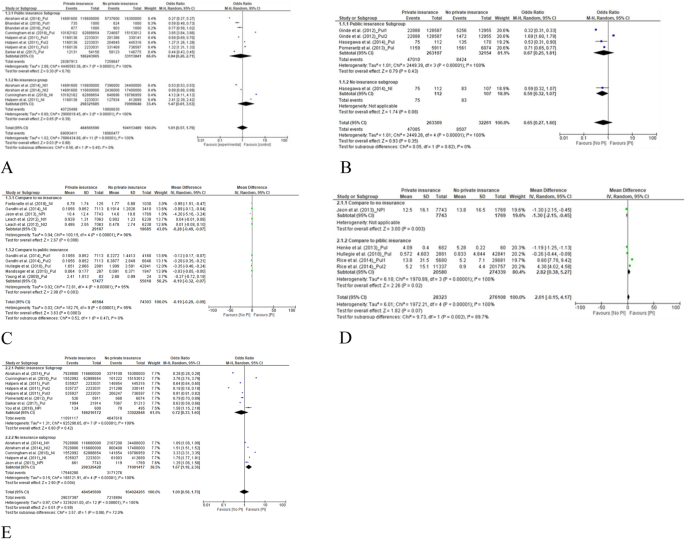
Forest plots of subgroup analysis according to the control of public insurance and no health insurance. a for comparison of the rates of emergency department visits between private insurance and no private insurance. b for comparison of the percentages of emergency department visits between those with private insurance and those without private insurance. c for comparison of the rates of outpatient office visits between private insurance and no private insurance. d Comparison of the length of inpatient stay (days) between those with private insurance and those without private insurance. e Comparison of the rates of hospitalization between individuals with private insurance and those with no private insurance
Three included studies, which included 285,570 participants, reported the percentage of study participants who had visited the ED [ 24 , 26 , 32 ]. The proportion of those with private insurance who visited the ED was similar to the proportion of people without private insurance who visited the ED. The OR for pooled results was 0.65 (95% CI 0.27–1.60). See Fig. 3 b.
The results of subgroup analysis (Fig. 4 b) showed that there was no significant difference between the percentage of people with private insurance who visited the ED and either those with public insurance or those with no insurance at all.
Rate of outpatient office visits
We used the data from 7 studies, which included 120,887 participants, to determine the mean difference in the rate of outpatient office visits between people with private insurance and people without private insurance. After pooling the results, the mean difference was − 0.19 (95% CI − 0.29 to − 0.09) (see forest plot in Fig. 3 c). People with private insurance were significantly less likely to visit the hospital as outpatients than people without private insurance. According to the subgroup analysis, people with private insurance were less likely to visit the outpatient office, compared to people with public insurance, and also compared to people without insurance ( P < 0.05) (see forest plot in Fig. 4 c). In Additional file 1 , we present the data pertaining to outpatient visits that could not be included in the meta-analysis (9 articles) [ 15 , 16 , 17 , 18 , 21 , 25 , 32 , 37 , 38 ]. The favorable results (more outpatient visits) for both people with private insurance and people without private insurance were reported.
Utilization of inpatient services
Inpatient los.
We used the data from 4 studies, which included 304,431 participants, to determine the mean difference in LOS (days) between people with private insurance and people without private insurance [ 27 , 28 , 29 , 33 ]. The mean difference in pooled results was 2.01 (95% CI − 0.15 to 4.17, Fig. 3 d). There was no significant difference between people with private insurance and people without private insurance in terms of inpatient LOS.
According to the subgroup analysis (Fig. 4 d), compared to people with public insurance, people with private insurance were more likely to stay longer in the hospital (mean difference (days) = 2.82, 95% CI 0.38–5.27). While there was only one study left for compared to people without private insurance with the results of mean difference of LOS (− 1.30, 95% CI − 2.15 to − 0.45), which means the favorite result (longer of LOS) for people without private insurance.
In Additional file 1 , we list the data for the mean difference in LOS from 6 articles that could not be included in the meta-analysis [ 14 , 16 , 19 , 25 , 35 , 36 ]. Favorable results (longer LOS) for both people with private insurance and people without private insurance were reported.
Rate of hospitalization
We used the data from 7 studies, which included > 500,000,000 participants in determining the OR for the rate of hospitalization among those with private insurance, compared with those without private insurance [ 15 , 20 , 25 , 29 , 32 , 34 , 38 ]. The OR for the pooled results was 1.00 (95% CI 0.58–1.70) (see forest plot in Fig. 3 e). There was no significant difference in the rate of hospitalization between people with private insurance and people without private insurance.
According to the subgroup analysis (Fig. 4 e), those with public insurance and those with private insurance had similar rates of hospitalization (OR = 0.72, 95% CI 0.33–1.60). Compared to people with no insurance, people with private insurance were more likely to be hospitalized (OR 1.67; 95% CI, 1.18–2.36).
The certainty of the evidence (GRADE)
The certainty of the evidence ranged from low to moderate. The observational study design meant the GRADE rating started as moderate certainty (Table 2 ), and almost all studies (except Abougergi et al. 2019) were missing data. Furthermore, we considered it likely that possible biases and confounding factors would have had a significant impact on the results presented in abstract form only.
In this systematic review, we investigated whether people with private insurance were more likely to utilize health care than those without private insurance. According to the results of the meta-analysis, the utilization was similar between those with and those without private health insurance. For the target outcome of outpatient office visits, people with private insurance were less likely to visit the outpatient office than people without private insurance (mean difference = − 0.19 (95% CI − 0.29 to − 0.09)). In theory, people with private insurance should have more access to health care. However, our results indicate that there was no significant increase in the consumption of healthcare services among individuals with private health insurance. In one of the dimensions examined, those with private health insurance coverage actually used fewer of the health care available to them. One possible explanation is that the utilization of medical services was more directly correlated with the need for the service than with insurance coverage, as suggested by previous studies [ 40 , 41 , 42 ]. Private health insurance coverage does not appear to increase the utilization of health care and may ease the financial burdens on patients and social health insurance plans.
The results of subgroup analysis to identify differences between those without insurance and those with public insurance showed that most results were consistent with the total pooled results. For LOS, people with private insurance were more likely to stay longer in the hospital, compared to people with public insurance (mean difference (days) = 2.82, 95% CI 0.38–5.27). With regard to the rate of hospitalization, compared to people without any insurance, people with private insurance were more likely to be hospitalized (OR 1.67; 95% CI, 1.18–2.36). As inpatient services are more tightly linked to medical necessity than outpatient services, these results reflect the potential for private insurance to relieve patients’ financial burden.
To our best knowledge, this systematic review is the first review to assess the impact of private insurance coverage on the utilization of health care across the globe. This study strictly followed the standards for systematic reviews, including explicit eligibility criteria, duplicated independent assessments of eligibility, and a comprehensive literature search. One limitation of this study was that more than half of the included studies were conducted in the United States, which restricted the external validity of the results. Another limitation of this review is that the results may have been confounded by selection bias due to divergences in methodology among health care systems. Next, the evidence of this present study has temporal limitations. Studies on this topic were conducted prior to 2010. However, we restricted the search period to years from 2010 onward in order to focus our investigation on current insurance policy. Finally, as there is no standardized tool for the assessment of abstract quality, all abstracts included in the review were not graded in terms of quality. This fact may limit the ability of other researchers to extrapolate from the results reported here. Additional studies will be necessary to explore these issues.
People with private insurance did not increase their utilization of health care (outpatient services), compared to those without private insurance. Private health insurance coverage may ease the financial burdens on patients and on the public health insurance system.
Availability of data and materials
The datasets used during the current study are available from the corresponding author on reasonable request.
Abbreviations
Preferred Reporting Items for Systematic Reviews and Meta-Analyses
Cochrane Central Register of Controlled Trials
Emergency department
Length of stay
Confidence intervals
Tamblyn R, Laprise R, Hanley JA, Abrahamowicz M, Scott S, Mayo N, et al. Adverse events associated with prescription drug cost-sharing among poor and elderly persons. Jama. 2001;285:421–9.
Article CAS PubMed Google Scholar
Manning WG, Newhouse JP, Duan N, Keeler EB, Leibowitz A, Marquis MS. Health insurance and the demand for medical care: evidence from a randomized experiment. Am Econ Rev. 1987;77:251–77.
CAS PubMed Google Scholar
Fontenelle LF, Sarti TD, Camargo MBJ, Maciel ELN, Barros AJD. Utilization of the Brazilian public health system by privately insured individuals: a literature review. Cad Saude Publica. 2019;35:e00004118.
Article PubMed Google Scholar
Barnett JC, Vornovitsky MS. Health insurance coverage in the United States: 2015. Washington, DC: US Government Printing Office; 2016.
Google Scholar
Iglehart JK. Canada's health care system. In: Mass Medical Soc; 1986.
Sohn M, Jung M. Effects of public and private health insurance on medical service utilization in the National Health Insurance System: national panel study in the Republic of Korea. BMC Health Serv Res. 2016;16:503.
Article PubMed PubMed Central Google Scholar
Bodenheimer T. Should we abolish the private health insurance industry? Int J Health Serv. 1990;20:199–220.
Moher D, Liberati A, Tetzlaff J, Altman DG. Preferred reporting items for systematic reviews and meta-analyses: the PRISMA statement. Int J Surg. 2010;8:336–41.
Carrasquillo O. Health Care Utilization. In: Gellman MD, Turner JR, editors. Encyclopedia of Behavioral Medicine. New York: Springer New York; 2013. p. 909–10.
Sterne JA, Hernan MA, Reeves BC, Savovic J, Berkman ND, Viswanathan M, et al. ROBINS-I: a tool for assessing risk of bias in non-randomised studies of interventions. Bmj. 2016;355:i4919.
Guyatt GH, Oxman AD, Vist GE, Kunz R, Falck-Ytter Y, Alonso-Coello P, et al. GRADE: an emerging consensus on rating quality of evidence and strength of recommendations. Bmj. 2008;336:924–6.
Manager R: Version 5.0. In . : The Nordic Cochrane Centre, The Cochrane Collaboration Copenhagen, Denmark; 2008.
DerSimonian R, Laird N. Meta-analysis in clinical trials. Control Clin Trials. 1986;7:177–88.
Abougergi MS, Avila P, Saltzman JR. Impact of insurance status and race on outcomes in Nonvariceal upper gastrointestinal hemorrhage: a nationwide analysis. J Clin Gastroenterol. 2019;53:E12–E8.
Abraham JM. How might the affordable care Act's coverage expansion provisions influence demand for medical care? Milbank Q. 2014;92:63–87.
Abraham PS, Greene M. The impact of different types of payer on healthcare resource utilization and costs among cancer patients in India. Value Health. 2017;20(5):A131.
Abraham PS, Greene M, Eguale T, Rodriguez-Monguio R, Seoane-Vazquez E. Impact of payer on healthcare resource utilization and costs among breast cancer patients in India. Value Health. 2017;20(5):A128.
Araujo MEA, Silva MT, Galvao TF, Pereira MG. Prevalence of health services usage and associated factors in the Amazon region of Brazil: A population-based cross-sectional study. BMJ Open. 2017;7(11) (no pagination).
Bhandari NR, Chopra DA, Kathe N, Moore G, Lewis K, Li C, et al. Contrasting utilization of inpatient hospitalizations and emergency department visits between medicaid and commercially insured arkansans. Value Health. 2018;21(Supplement 1):S131.
Cunningham P, Sheng Y. Trends in preventable inpatient and emergency department utilization in California between 2012 and 2015. Med Care. 2018;56:544–50.
Dabbous FM, Dorey J, Thokagevistk K, Toumi M. Impact of health insurance status on health care resource utilization among diabetic patients in the United States. Value Health. 2014;17(3):A252.
Article Google Scholar
Fontenelle LF, Camargo MBJ, Bertoldi AD, Goncalves H, Maciel ELN, Barros AJD. Utilization of basic health units of FHS according to private health insurance. Revista de Saude Publica. 2018;52:55.
Gandhi SO, Grant LP, Sabik LM. Trends in nonemergent use of emergency departments by health insurance status. Med Care Res Rev. 2014;71:496–521.
Ginde AA, Lowe RA, Wiler JL. Health insurance status change and emergency department use among US adults. Arch Intern Med. 2012;172:642–7.
Halpern MT, Renaud JM, Vickrey BG. Impact of insurance status on access to care and out-of-pocket costs for U.S. individuals with epilepsy. Epilepsy Behav. 2011;22:483–9.
Hasegawa K, Sullivan AF, Tovar Hirashima E, Gaeta TJ, Fee C, Turner SJ, et al. A Multicenter Observational Study of US Adults with Acute Asthma: Who Are the Frequent Users of the Emergency Department? J Allergy Clin Immunol: In Pract. 2014;2:733–40.e3.
Henke RM, Maeda JL, Marder WD, Friedman BS, Wong HS. Medicare and commercial inpatient resource use: impact of hospital competition. Am J Manag Care. 2013;19:e238–e48.
PubMed Google Scholar
Hullegie P, Klein TJ. The effect of private health insurance on medical care utilization and self-assessed health in Germany. Health Econ. 2010;19:1048–62.
Jeon B, Kwon S. Effect of private health insurance on health care utilization in a universal public insurance system: a case of South Korea. Health Policy. 2013;113:69–76.
Leach LS, Butterworth P, Whiteford H. Private health insurance, mental health and service use in Australia. Aust N Z J Psychiatry. 2012;46:468–75.
Mandsager P, Lebrun-Harris LA, Sripipatana A. Health center patients’ insurance status and healthcare use prior to implementation of the affordable care act. Am J Prev Med. 2015;49:545–52.
Pomerantz D, Machado M, DiBonaventura M. The impact of private versus public insurance on health status, work productivity and health care utilization for individuals residing in Brazil. Value Health. 2013;16(3):A76.
Rice JB, Desai U, Cummings AK, Birnbaum HG, Skornicki M, Parsons NB. Burden of diabetic foot ulcers for medicare and private insurers. Diabetes Care. 2014;37:651–8.
Sarkar M, Earley ER, Asti L, Chisolm DJ. Differences in health care needs, health care utilization, and health care outcomes among children with special health care needs in Ohio: a comparative analysis between Medicaid and private insurance. J Public Health Manag Pract. 2017;23:e1–9.
Shmueli A, Savage E. Private and public patients in public hospitals in Australia. Health Policy. 2014;115:189–95.
Terveen DC, Hsu BS, Tufty GT. The impact of insurance status on hospital resource utilization in pediatric ophthalmic inpatients. J AAPOS. 2015;19(4):e65.
Yoshioka Y, Tamiya N, Kashiwagi M, Sato M, Okubo I. Comparison of public and private care management agencies under public long-term care insurance in Japan: a cross-sectional study. Geriatr Gerontol Int. 2010;10:48–55.
You CH, Choi JH, Kang S, Oh EH, Kwon YD. Association between supplementary private health insurance and visits to physician offices versus hospital outpatient departments among adults with diabetes in the universal public insurance system. PLoS ONE. 2018;13(4) (no pagination).
Young A, Ruble L, McGrew J. Public vs. private insurance: cost, use, accessibility, and outcomes of services for children with autism spectrum disorders. Res Autism Spectr Disord. 2009;3:1023–33.
Geil P, Million A, Rotte R, Zimmermann KF. Economic incentives and hospitalization in Germany. J Appl Econ. 1997;12:295–311.
Riphahn RT, Wambach A, Million A. Incentive effects in the demand for health care: a bivariate panel count data estimation. J Appl Econ. 2003;18:387–405.
Jürges H. Health insurance status and physician behavior in Germany. Schmollers Jahr. 2009;129:297.
Download references
Acknowledgments
I am grateful to my department leaders and my family for their great encouragement, support and help to this project.
This research did not receive any specific grant from funding agencies in the public, commercial, or not-for-profit sectors.
Author information
Authors and affiliations.
Department of International Medical Servicers, Peking Union Medical College Hospital, No.1 Shuaifuyuan, Wangfujing Dongcheng District, Beijing, 100730, China
Congcong Zhang, Chenwei Fu, Yimin Song, Rong Feng & Yongning Li
Department of Nursing, Peking Union Medical College Hospital, No.1 Shuaifuyuan, Wangfujing Dongcheng District, Beijing, 100730, China
You can also search for this author in PubMed Google Scholar
Contributions
CZ was responsible for the search strategy, designing, testing of the data extraction form, and writing the initial draft. YL and XW provided input for the design, analyses and reporting. XW was responsible for the conception, designing of the survey and critical review the final draft. CZ and CF were involved in designing and testing of the data extraction form. CZ, CF, YS and RF contributed to quality checking of the data and the manuscript writing. All authors contributed to the protocol and approved the final manuscript.
Corresponding authors
Correspondence to Xinjuan Wu or Yongning Li .
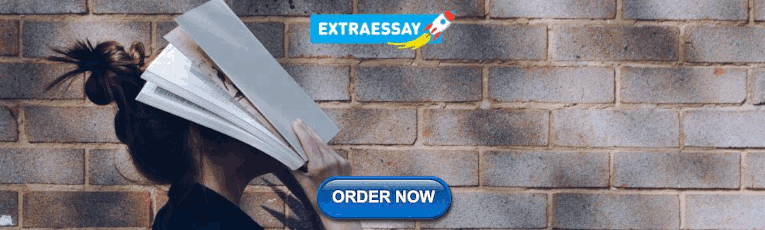
Ethics declarations
Ethics approval and consent to participate.
The present project is a systematic review based on review of publicly reported literature, which does not require ethics review.
Consent for publication
Not applicable.
Competing interests
The authors declare that they have no competing interests.
Additional information
Publisher’s note.
Springer Nature remains neutral with regard to jurisdictional claims in published maps and institutional affiliations.
Supplementary information
Additional file 1., rights and permissions.
Open Access This article is licensed under a Creative Commons Attribution 4.0 International License, which permits use, sharing, adaptation, distribution and reproduction in any medium or format, as long as you give appropriate credit to the original author(s) and the source, provide a link to the Creative Commons licence, and indicate if changes were made. The images or other third party material in this article are included in the article's Creative Commons licence, unless indicated otherwise in a credit line to the material. If material is not included in the article's Creative Commons licence and your intended use is not permitted by statutory regulation or exceeds the permitted use, you will need to obtain permission directly from the copyright holder. To view a copy of this licence, visit http://creativecommons.org/licenses/by/4.0/ . The Creative Commons Public Domain Dedication waiver ( http://creativecommons.org/publicdomain/zero/1.0/ ) applies to the data made available in this article, unless otherwise stated in a credit line to the data.
Reprints and permissions
About this article
Cite this article.
Zhang, C., Fu, C., Song, Y. et al. Utilization of public health care by people with private health insurance: a systematic review and meta-analysis. BMC Public Health 20 , 1153 (2020). https://doi.org/10.1186/s12889-020-08861-9
Download citation
Received : 20 January 2020
Accepted : 06 May 2020
Published : 23 July 2020
DOI : https://doi.org/10.1186/s12889-020-08861-9
Share this article
Anyone you share the following link with will be able to read this content:
Sorry, a shareable link is not currently available for this article.
Provided by the Springer Nature SharedIt content-sharing initiative
- Private health insurance
- Health care utilization
- Systematic review
- Meta-analysis
BMC Public Health
ISSN: 1471-2458
- Submission enquiries: [email protected]
- General enquiries: [email protected]
Childhood Obesity and Health Care Utilization: Empirical Evidence from Austrian Administrative Data
Affiliations.
- 1 Institute of Health Sciences, University of Leeds School of Medicine, Leeds, United Kingdom.
- 2 Department of Economics, Johannes Kepler University Linz, Linz, Austria.
- 3 Christian Doppler Laboratory for Aging, Health, and the Labor Market, Johannes Kepler University Linz, Linz, Austria.
- 4 New Zealand Work Research Institute, Auckland University of Technology, Auckland, New Zealand.
- PMID: 36103290
- DOI: 10.1089/chi.2022.0011
Background: Increases in pediatric obesity have been associated with higher levels of health care utilization. There is currently a lack of knowledge on the therapeutic drivers of increased health care use. Objective: To examine the association between different measures of health care utilization and BMI among children. Methods: We linked cross-sectional administrative data from a regional health insurance fund in Austria with objectively measured BMI from routine school health examinations in 6-15-year-old children ( n = 13,493). Differences in probabilities of annual health care utilization (drug prescriptions by therapeutic classification, physician visits by medical specialty, and hospitalizations) were compared between children with normal weight, overweight, or obesity using Probit regressions. Results: Children with obesity had a 1.6 and 8.6 percentage points (pp) higher probability of outpatient doctor visits and prescribed medication, respectively (all p < 0.05). Children with overweight were intermediate. There was a higher probability of consulting a general practitioner, pediatrician, or orthopedist, and higher levels of prescribing for children with obesity across most common drug groups. Children with obesity were ∼40% more likely to receive medication for musculoskeletal and for mental health problems. This was reflected in orthopedic clinic appointments but not in psychology clinics. There were no major differences by gender or age, or parental socioeconomic status. Conclusions: Our data show clear and objective evidence of higher health care utilization by children with obesity. This highlights the importance of policy interventions to curb obesity in children and young people.
Keywords: children; health care need; health care utilization; obesity; outpatient visits; prescribed medication.
Publication types
- Research Support, Non-U.S. Gov't
- Austria / epidemiology
- Cross-Sectional Studies
- Patient Acceptance of Health Care
- Pediatric Obesity* / epidemiology
- Pediatric Obesity* / therapy
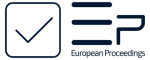
- Publishing Policies
- For Organizers/Editors
- For Authors
- For Peer Reviewers

The Effect Of Health Insurance On Health Care Utilization: Evidence From Malaysia

The link between health insurance ownership and over-utilization of health care services are highly debated. This paper examines the effect of insurance ownership on hospital utilization within the context of Malaysia. The study employs the data from Malaysia National Health and Morbidity Survey III and 14,223 respondents are selected for this purpose. Malaysia provides new evidence from an emerging market where voluntary purchase of private health insurance co-exists with almost free public health care. Logit model is used to identify the effect of insurance ownership on the probability of utilizing health care while the zero truncated Poisson model are used to identify the role of insurance ownership on the intensity of utilization. From the analysis, it is found that insurance ownership determines access to health care but it does not influence frequency of use. The findings suggest moral hazard problem may not be prevalent in the Malaysian health insurance market. Thus, institutionalizing health insurance through initiative such as national health insurance may be a sustainable program and give better access to care for the public. Keywords: Health Insurance Utilization Moral Hazard Poisson Model Malaysia
Introduction
The link between health insurance ownership and over-utilization of health care services is highly debated. Some scholars argue that health insurance can induce moral hazard problem ( Pauly, 1986 ). The moral hazard problem arises when an individual is indifferent on spending additional health care since the price paid during consumption is zero due to the reimbursement by the insurance company. Previous work examining the decision by an individual to purchase health insurance has provided conflicting evidence of the effect of ownership on health care utilization ( Cheng & Chiang, 1998 ; Lopez-Nicolas, 1998 ; Shen, 2013 ). In the case of Taiwan, when the National Health Insurance (NHI) Program was introduced in year 1995, the use of medical care has increased ( Cheng & Chiang, 1998 ). Empirical studies on the effect of insurance ownership within Malaysia context are quite limited except Kefeli and Jones ( 2012 ), Samsudin, Jamil and Zulhaid ( 2012 ) and Wan Abdullah and Ng ( 2009 ).
This study differs from Kefeli and Jones ( 2012 ) as it uses different empirical estimation and with some differences in selection of variables. While Samsudin, Jamil and Zulhaid ( 2012 ) focuses on the elderly this study covers individuals above 18 years. Unlike Wan Abdullah and Ng ( 2009 ) who analyzed individuals subscribed to employer sponsored health insurance this study uses individuals who purchased health insurance directly from the insurance company. Moreover, the result from other countries may be less applicable to Malaysia as the health care financing systems are different. It is expected that the existence of health insurance may induce demand thus those who own health insurance are more likely to use and utilize more health care.
The findings from this study are valuable to policymakers as ensuring access to health care is a policy issue. Can ownership of health insurance improve access to care? In addition, this study is of interest to health insurance providers as existence of moral hazard either by the policyholder or the medical providers may jeopardize the sustainability of a health insurance program.
The remainder of this article is organized as follows. The next section reviews the theoretical foundation and the literatures in the field. Then the methods employed in this study are discussed followed by the results and discussion. The final section concludes.
Theoretical background and literature review
A large and growing body of literature has investigated the effect of health insurance on health care use. Scholars have realized that spending on health care, and the utility that it generates, plays a central role in determining the value of health insurance. For an expected utility maximizing consumer, the demand for health insurance and health care is interdependence. Individual who expects to consume more health care will be more likely to buy health insurance or purchase more comprehensive health insurance coverage.
The utilization of health services can be explained using the Anderson Model which divides the factors that influence utilization into three main categories ( Anderson, 1995 ). First, the predisposing factors which consist of inherent factors that exist within individuals. Examples are age, gender and education level and economic activity. Second, the enabling factors which are family means to obtain health care services. Examples are income, health insurance and distance to hospital. Lastly, the presence of need either perceived by individuals or required by medical providers. Example is individual health status.
As an enabling factor, insurance variable is often included in studies that are based on mixed health care financing system, as in the United States ( Manning, Newhouse, Duan, Keeler, Leibowitz & Marquis, 1987 ; Munkin & Trivedi, 2003 ). Manning et. al (1987) found that there is no significant difference in utilization of health care services between the healthy and the sickly. However, Sarma and Simpson ( 2006 ) found that insurance has significantly determined doctor utilisation for healthy users and insignificantly for the less healthy in the Canadian health care.
Some studies found that utilization of health care is not different between the insured and uninsured or between types of insurance ownership ( Lopez-Nicolas, 1998 ; Nandakumar, Chawla & Khan, 2000]. In other studies, in which the datasets are based on tax-financed system, such as Italy, UK, Sweden, Portugal and Spain, the role of insurance status in health care demand is not prominent. Empirical research in Jordan found that health insurance ownership does not affect the probability of health care use but it significantly influence the intensity of use (Ekman, 2007). While in Indonesia, Hidayat and Pokhrel ( 2010 ) found no evidence that insurance ownership influences future outpatient visits. In Australia, the use of medical care has increased sharply among the elderly when Medicare was introduced ( Cameron, Trivedi, Milne & Piggott, 1988 ).
Data were extracted from the Malaysian National Health and Morbidity Survey (NHMS) III. NHMS III reported that 18.8% of the respondents owned some type of medical and health insurance (MHI). This study uses 14,223 cases of individuals above age of 18. The definition and summary statistics of variables used are explained in Table 1 .
Empirical specification
The objective of the analysis is divided into twofold where the first is to identify the role of insurance ownership on access to inpatient care and second to identify its role on the frequency of visits.

The probability density function is,
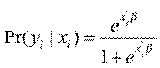
The second analysis involves the use of count data in modelling the frequency of hospitalization. We use both standard Poisson (one-part) and two-part Poisson models (TPM) or sometimes known as hurdle model for this purpose. We start with standard Poisson model where hospitalization, Y, is specified as below:

where y i is the realised hospitalization for individual i and x i is a vector of characteristics of individual i , assumed to be exogenous, that determine y i . At this stage, no assumption has been made to distinguish different decision processes between the contact decision and the frequency of hospitalization. Suppose the number of occurrences for y i given x i , is Poisson distributed with density:
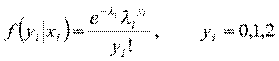
The next approach involves two-part process, where the first part represents access and the second part signifies the frequency of subsequent use after the first access. Two-part model is used in several studies and is considered this study due to the large number of zero-count (non-users) in the sample [4, 6, 15]. Strong assumption, however, has to be made that the hospitalization episodes are related to the same spell of illness. The first part involves logit model as its modelling access to care and the second part utilizes zero truncated Poisson model, where it only counts observation more than 0. The density function of these two distinct processes of health care demand, y for individual i can be described as:
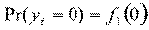
Results and discussion
Table 2 presents the estimates for inpatient care from logit, Poisson zero-truncated Poisson model (TPM). The TPM model consists of logit and zero-truncated Poisson. Prior to further discussion, we use two selection criteria, namely Akaike Information Criteria (AIC) and Bayesian Information Criteria (BIC) to select the best count model which could explain the frequency of hospitalization. Both AIC and BIC consistently prefer TPM than single stage Poisson model. Since TPM estimates determinants of access and frequency at once, therefore the following discussion on access and frequency of hospitalization would be based on this model.
The results show that health insurance ownership is significant in determining access but has no influence on subsequent use. The fact that health insurance ownership influence access to care provides evidence for the needs to promote health insurance ownership either private or public to ensure better access to care for all individuals. More importantly, the finding shows no evidence of moral hazard as health insurance ownership does not influence the intensity of health care use. In addition, as the model assumes that the subsequent health care visits are related to the same illnesses, then the finding also suggests that there is no evidence of moral hazard by the medical providers.
Other significant variables that determine access are age, marital status, household size, health status and income while only health status influences intensity of care. The findings indicate that individual who is married and from a household with a larger number of members are more likely to access health care. Perhaps the spouse and the family members become enabling factors pushing the individual to seek for care. The likelihood of access also increases with the increase in age. As health status is controlled, this may indicates that older individual are more risk averse or are more anxious about their health and thus seek more care.
As expected, the health status influences both the health access and frequency of use. Those with bad health status are more likely to seek care and have higher intensity of use. Interestingly, those with higher income are less likely to access health care. It may reflect that individuals with higher income have greater resources at their disposal to avoid inpatient treatment perhaps through better healthy lifestyle.
In the Malaysian context, extending health insurance coverage may increase health care access, in particular to inpatient services. This study finds no evidence of increase in intensity of inpatient care use by health insurance owners thus eliminating the presence of moral hazard behavior either from the insured or the medical providers. Thus, institutionalizing health insurance through initiative such as national health insurance may be a sustainable program and give better access to care for the public.
- Andersen, R. M. (1995). Revisiting the behavioral model and access to care: does it matter? Journal of Health and Social Behavior, 36(1), 1-10
- Cameron, A. C., Trivedi, P. K., Milne, F. & Piggott, J. (1988). A microeconometric model of the demand for health care and health insurance in Australia. Review of Economic Studies, 85-106.
- Chan, S. (2013). Determinants of health care decisions: insurance, utilization, and expenditures. The Review of Economics and Statistics, 95(1), 142-153.
- Cheng, S. H. & Chiang, T. L. (1998). Disparity of medical care utilization among different health insurance schemes in Taiwan. Social Science Medicine, 47(5), 613-620.
- Deb, P. & Trivedi, P. K. (2002). The structure of demand for health care: latent class versus two-part models. Journal of Health Economics, 21(4), 601-625.
- Ekman, B. (2007). The impact of health insurance on outpatient utilization and expenditure: evidence from one middle-income country using national household survey data. Health Research Policy and Systems, 5, 6.
- Friedman, B. & Savage, L. J. (1948). The utility analysis of choices involving risk. The Journal of Political Economy, 56(4), 279-304.
- Gerdtham, U. G. (1997). Equity in health care utilization: further tests based on hurdle models and Swedish micro data. Health Economics, 6(3), 303-319.
- Hidayat, B. & Pokhrel, S. (2010). The selection of an appropriate count data model for modelling health insurance and health care demand: case of Indonesia. International Journal of Environment Research and Public Health, 7, 9-27.
- Kefeli, Z. & Jones, G. (2012). Moral hazard and the impact of private health insurance on the utilization of health care in Malaysia. Jurnal Ekonomi Malaysia, 46(2), 159-175.
- Lopez-Nicolas, A. (1998). Unobserved heterogeneity and censoring in the demand for health care. Health Economics, 7(5), 429-437.
- Manning, W. G., Newhouse, J. P., Duan, N., Keeler, E. B., Leibowitz, A. & Marquis, M. S. (1987). Health insurance and the demand for medical care: evidence from a randomized experiment. American Economic Review, 77(3), 251-277.
- Munkin, M. K. & Trivedi, P. K. (2003). Bayesian analysis of a self-selection model with multiple outcomes using simulation-based estimation: an application to the demand for healthcare. Journal of Econometrics, 114(2), 197-220.
- Nandakumar, A. K., Chawla, M. & Khan, M. (2000). Utilization of outpatient care in Egypt and its implications for the role of government in health care provision. World Development, 28(1), 187-196.
- Nyman, J. A. (2006). Evaluating health insurance: a review of the theoretical foundations. The Geneva Papers, 31, 720-738.
- Pauly, M. V. (1986). Taxation, health insurance and market failure in the medical economy. Journal of Economic Literature, 24(2), 629-675.
- Samsuddin, S., Jamil, N. & Zulhaid, N. H. (2012). Health care utilisation in Kedah: a microeconometric analysis. OIDA International Journal of Sustainable Development, 4(5), 45-52.
- Sarma, S. & Simpson, W. (2006). A microeconometric analysis of Canadian health care utilization. Health Economics, 15(3), 219-239.
- Wan Abdullah, W. R. & Ng, K. E. (2009). Health insurance and health services utilisations: evidence from employer-based health insurance in Malaysia. Working Paper Series 2009-25. Universiti Malaya.
Copyright information

About this article
Publication date.
22 August 2016
Article Doi
https://doi.org/10.15405/epsbs.2016.08.51
978-1-80296-013-6
Future Academy
Print ISBN (optional)
Edition number.
1st Edition
Sociology, work, labour, organizational theory, organizational behaviour, social impact, environmental issues
Cite this article as:
Abu-Bakar, A., Samsudin, S., Regupathi, A., & Aljunid, S. M. (2016). The Effect Of Health Insurance On Health Care Utilization: Evidence From Malaysia. In B. Mohamad (Ed.), Challenge of Ensuring Research Rigor in Soft Sciences, vol 14. European Proceedings of Social and Behavioural Sciences (pp. 362-368). Future Academy. https://doi.org/10.15405/epsbs.2016.08.51
We care about your privacy
We use cookies or similar technologies to access personal data, including page visits and your IP address. We use this information about you, your devices and your online interactions with us to provide, analyse and improve our services. This may include personalising content or advertising for you. You can find out more in our privacy policy and cookie policy and manage the choices available to you at any time by going to ‘Privacy settings’ at the bottom of any page.
Manage My Preferences
You have control over your personal data. For more detailed information about your personal data, please see our Privacy Policy and Cookie Policy .
These cookies are essential in order to enable you to move around the site and use its features, such as accessing secure areas of the site. Without these cookies, services you have asked for cannot be provided.
Third-party advertising and social media cookies are used to (1) deliver advertisements more relevant to you and your interests; (2) limit the number of times you see an advertisement; (3) help measure the effectiveness of the advertising campaign; and (4) understand people’s behavior after they view an advertisement. They remember that you have visited a site and quite often they will be linked to site functionality provided by the other organization. This may impact the content and messages you see on other websites you visit.
Advertisement
Predictors of utilization of public healthcare facilities in India: an empirical assessment
- Original Article
- Published: 16 June 2023
Cite this article
- Shahid Hamid Raina ORCID: orcid.org/0000-0001-7534-3866 nAff1 ,
- Khursheed Hussain Dar ORCID: orcid.org/0000-0002-3925-0758 2 ,
- Samir-ul Hassan 3 &
- Masroor Ahmad 2
157 Accesses
Explore all metrics
As the government of India has been undertaking various initiatives since 2005 (launch year of National Rural Health Mission) to increase the utilization of public healthcare facilities, it is very important to investigate the factors governing their utilization. Therefore, the purpose of this study was to explore the determinants of utilization of public healthcare facilities in India.
Subject and methods
The data for the study were collected using a comprehensive structured interview schedule. Data were collected from 399 outpatients of district hospitals of the erstwhile state of Jammu and Kashmir, India. A logit model was used to study the variables determining the use of public healthcare facilities.
The results showed that distance and waiting time are negatively related to utilization, whereas satisfaction with hospital services and provision of free medicines are positively related and significantly increase the odds in favour of utilization of public hospitals. It was found that more than 60% of outpatients belonged to groups below the poverty line (BPL) and Antodaya Anna Yojna (AAY) families, but most of them had to spend out of pocket for medicines and diagnostic tests.
The findings suggest that service delivery and provision of free medicines significantly increase the odds in favour of utilization whereas delayed services and distance decrease the odds in favour of utilization.
Implications
The outcomes of this study are significant to achieve universal health coverage. Our study indicates that user fees should be abolished at least for economically disadvantaged patients, and the availability of medicines should be improved significantly to increase the utilization of public helathcare facilities as envisaged in National Health Policy, 2017.
This is a preview of subscription content, log in via an institution to check access.
Access this article
Price includes VAT (Russian Federation)
Instant access to the full article PDF.
Rent this article via DeepDyve
Institutional subscriptions
Similar content being viewed by others
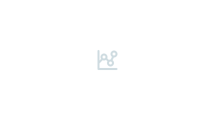
Demand for health care service and associated factors among patients in the community of Tsegedie District, Northern Ethiopia
The effect of the national health insurance scheme (nhis) on health service delivery in mission facilities in ghana: a retrospective study.
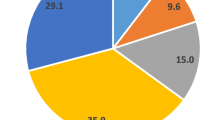
Utilisation of rural primary health centers for outpatient services - a study based on Rajasthan, India
Data availability.
Data is available
Code availability
Not applicable
Awoke MA, Negin J, Moller J, Farell P, Yawson AE, Biritwum RB, Kowal P (2017) Predictors of public and private healthcare utilization and associated health system responsiveness among older adults in Ghana. Glob Health Action 10(1):1301723
Article PubMed PubMed Central Google Scholar
Bagchi T, Das A, Dawad S, Dalal K (2020) Non-utilization of public healthcare facilities during sickness: a national study in India. J Public Health:1-9
Bajpai N, Sachs JD, Dholakia R (2009) Improving access, service delivery and efficiency of the public health system in rural India: mid-term evaluation of the national rural health mission. Working Paper 37, Centre for Globalization and Sustainable Development. The Earth Institute at Columbia University, New York
Google Scholar
Bakeera SK, Wamala SP, Galea S, Peterson S, Pariyo GW (2009) Community perceptions and factors influencing utilization of health services in Uganda. Int J Equity Health 8:1–12
Article Google Scholar
Balla S, Sk MI, Ambade M, Hossain B (2022) Distress financing in coping with out-of-pocket expenditure for maternity care in India. BMC Health Serv Res 22(1):1–4
Banerjee S (2021) Determinants of rural-urban differential in healthcare utilization among the elderly population in India. BMC Public Health 21(1):1–8
Berry LL, Bendapudi N (2007) Health care: a fertile field for service research. J Ser Res 10(2):111–122
Chandra S, Patwardhan K (2018) Allopathic, Ayush and informal medical practitioners in rural India — a prescription for change. J Ayurveda Integ Med 9:143–150
Chaudhury N, Hammer J, Kremer M, Muralidharan K, Rogers FH (2006) Missing in action: teacher and health worker absence in developing countries. J Econ Perspect 20:91–116
Article PubMed Google Scholar
Dalal K, Dawad S (2009) Non-utilization of public health care facilities: examining the reasons through a national study of women in India. Rural Remote Health 9:1–10
Das J, Holla A, Das V, Mohanan M, Chan B, Tabak D (2011) The quality of medical care in India: evidence from a standardized patient study in two states. Working Paper, Washington, DC : World Bank.
Dey DK, Mishra V (2014) Determinants of choice of healthcare services utilization: empirical evidence from India. Indian J Commun Health 26:356–363
Dey S, Nambiar D, Lakshmi JK, Sheikh K, Reddy KS (2012) Health of the elderly in India: challenges of access and affordability. In Aging in Asia: Findings from new and emerging data initiatives 2012. National Academies Press (US)
Filippi V, Ronsmans C, Campbell OM, Graham WJ, Mills A, Borghi J, Osrin D (2006) Maternal health in poor countries: the broader context and a call for action. The Lancet 368:1535–1541
Gage AJ, Guirlène CM (2006) Effects of the physical accessibility of maternal health services on their use in rural Haiti. Population Stud 60:271–288
Gujarati DN (2009) Basic econometrics. Tata McGraw-Hill Education, New Delhi
Indian Council of Medical Research (ICMR), Public Health Foundation of India, Institute for Health Metrics and Evaluation (2017) India — the India State-Level Disease Burden Initiative
International Institute for Population Sciences (IIPS) (2020) National Programme for Health Care of Elderly (NPHCE), MoHFW, Harvard T. H. Chan School of Public Health (HSPH) and the University of Southern California (USC). 2020. Longitudinal Ageing Study in India (LASI) Wave 1, 2017–18, India Report, International Institute for Population Sciences, Mumbai. https://www.iipsindia.ac.in/sites/default/files/LASI_India_Report_2020_compressed.pdf
Katyal A, Singh PV, Bergkvist S, Samarth A, Rao M (2015) Private sector participation in delivering tertiary health care: a dichotomy of access and affordability across two Indian states. Health Policy Plann 30:23–31
Malhi R, Goel D, Gambhir RS, Brar P, Behal D, Bhardwaj A (2020) RashtriyaSwasthyaBimaYojana (RSBY) and outpatient coverage. J Family Med Primary Care 9(2):459
Ministry of Statistics and Programme Implementation (2015) National sample survey, 71st round (2014). Government of India, New Delhi
Mishra R, Chatterjee R, Rao S (2003) India health report. Oxford University Press
Mohanty SK, Pathak PK (2009) Rich–poor gap in utilization of reproductive and child health services in India, 1992–2005. J Biosocial Sci 41:381–398
Article CAS Google Scholar
Pati S, Agrawal S, Swain S, Lee JT, Vellakkal S, Hussain MA, Millett C (2014) Non communicable disease multimorbidity and associated health care utilization and expenditures in India: cross-sectional study. BMC Health Serv Res 14(1):1–9
Rao PH (2012) The private health sector in India: A framework for improving the quality of care. ASCI J Manag 41(2):14–39
Reddy K (2011) High level expert group (HLEG) report on universal health coverage (UHC) for India. Public Health Foundation of India at New Delhi, India
Rout SK (2015) Utilization of outpatient care services in Odisha: factors determining the choice of public or private health care facility. J Health Manag 17:381–393
Rout SK, Sahu KS, Mahapatra S (2019) Utilization of health care services in public and private healthcare in India: Causes and determinants. International J Healthcare Manag 14: 509-516.
Rout SK, Sahu KS, Mahapatra S (2021) Utilization of health care services in public and private healthcare in India: causes and determinants. Int J Healthcare Manag 14(2):509–516
Selvaraj S,Farooqui HH, Karan A (2018) Quantifying the financial burden of households' out-of-pocket payments on medicines in India: a repeated cross-sectional analysis of National Sample Survey data, 1994–2014
Srivastava S, Karan AK, Bhan N, Mukhopadhya I (2022) World Health Organization. India: health system review. Health Syst Trans 11(1)
Sunil TS, Rajaram S, Zottarelli LK (2006) Do individual and program factors matter in the utilization of maternal care services in rural India? A theoretical approach. Social Sci Med 62:1943–1957
Zeng Y, Wan Y, Yuan Z, Fang Y (2021) Healthcare-seeking behavior among Chinese older adults: patterns and predictive factors. Int J Environ Res Public Health 18(6):2969
Download references
Author information
Shahid Hamid Raina
Present address: Department of Social Sciences, Vellore Institute of Technology, Vellore, Tamil Nadu, India
Authors and Affiliations
Department of Economics, Central University of Kashmir, Ganderbal, J& K, India
Khursheed Hussain Dar & Masroor Ahmad
Department of Commerce, NMIMS, Mumbai, India
Samir-ul Hassan
You can also search for this author in PubMed Google Scholar
Contributions
Shahid Hamid Raina conceived the study. He collected the data that was necessary for the execution of the study. He also wrote the introduction part of the paper.
Khursheed Hussain Dar analyzed the data and also did the first revision of the manuscript.
Samir-ul –Hassan wrote the discussion part of the paper and also did the second revision of the paper.
Masroor Ahmad wrote the literature review for the paper and also proofread the manuscript before submission.
Corresponding author
Correspondence to Shahid Hamid Raina .
Ethics declarations
Ethical statement.
The study did not require any ethical approval. However, prior consent was taken from the administrators of the surveyed health facilities for interviewing the patients. Further, verbal consent of respondents was taken for participating in the survey. The personal information of the respondents was kept completely confidential.
Ethics approval
Not Applicable. However, the respondents were informed about the purpose of the study. Prior consent was taken for them for participating in the study.
Consent for publication
Conflict of interest.
The authors declare that there is no conflict of interest involved.
Additional information
Publisher’s note.
Springer Nature remains neutral with regard to jurisdictional claims in published maps and institutional affiliations.
Rights and permissions
Springer Nature or its licensor (e.g. a society or other partner) holds exclusive rights to this article under a publishing agreement with the author(s) or other rightsholder(s); author self-archiving of the accepted manuscript version of this article is solely governed by the terms of such publishing agreement and applicable law.
Reprints and permissions
About this article
Raina, S.H., Dar, K.H., Hassan, Su. et al. Predictors of utilization of public healthcare facilities in India: an empirical assessment. J Public Health (Berl.) (2023). https://doi.org/10.1007/s10389-023-01970-w
Download citation
Received : 23 December 2022
Accepted : 29 May 2023
Published : 16 June 2023
DOI : https://doi.org/10.1007/s10389-023-01970-w
Share this article
Anyone you share the following link with will be able to read this content:
Sorry, a shareable link is not currently available for this article.
Provided by the Springer Nature SharedIt content-sharing initiative
- Utilization
- Find a journal
- Publish with us
- Track your research
Academia.edu no longer supports Internet Explorer.
To browse Academia.edu and the wider internet faster and more securely, please take a few seconds to upgrade your browser .
Enter the email address you signed up with and we'll email you a reset link.
- We're Hiring!
- Help Center
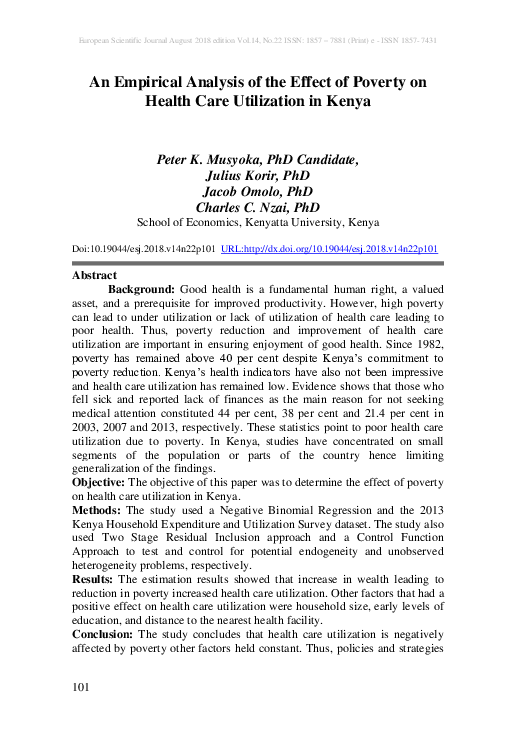
An Empirical Analysis of the Effect of Poverty on Health Care Utilization in Kenya

Background: Good health is a fundamental human right, a valued asset, and a prerequisite for improved productivity. However, high poverty can lead to under utilization or lack of utilization of health care leading to poor health. Thus, poverty reduction and improvement of health care utilization are important in ensuring enjoyment of good health. Since 1982, poverty has remained above 40 per cent despite Kenya's commitment to poverty reduction. Kenya's health indicators have also not been impressive and health care utilization has remained low. Evidence shows that those who fell sick and reported lack of finances as the main reason for not seeking medical attention constituted 44 per cent, 38 per cent and 21.4 per cent in 2003, 2007 and 2013, respectively. These statistics point to poor health care utilization due to poverty. In Kenya, studies have concentrated on small segments of the population or parts of the country hence limiting generalization of the findings. Objective: The objective of this paper was to determine the effect of poverty on health care utilization in Kenya. Methods: The study used a Negative Binomial Regression and the 2013 Kenya Household Expenditure and Utilization Survey dataset. The study also used Two Stage Residual Inclusion approach and a Control Function Approach to test and control for potential endogeneity and unobserved heterogeneity problems, respectively. Results: The estimation results showed that increase in wealth leading to reduction in poverty increased health care utilization. Other factors that had a positive effect on health care utilization were household size, early levels of education, and distance to the nearest health facility. Conclusion: The study concludes that health care utilization is negatively affected by poverty other factors held constant. Thus, policies and strategies
Related Papers
Public Health Research
Peter Musyoka
One of the most important social-goal world-over is the achievement of good health. This is because, apart from been a fundamental right, it is also an invaluable asset and a prerequisite for improved productivity. However, the benefits associated with good health status may not be enjoyed in the presence of high poverty rates. Thus, poverty reduction is important in ensuring enjoyment of good health. In Kenya, despite the government’s effort to reduce poverty and improve health status of her citizens, poverty has remained high and health indicators have not been impressive either. This paper, therefore, sought to establish the effect of poverty on health status in Kenya. The study used Ordered Probit and the 2013 Kenya Household Expenditure and Utilization Survey dataset to achieve its objective. Estimation results indicate poverty reduction increased the probability of reporting own health as being very good and reduced that of reporting poor health, ceteris paribus. Increase in h...
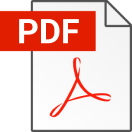
Unpublished research paper
chris james
European Scientific Journal
International Journal of Academic Research in Business and Social Sciences
DR. MARTINE ODHIAMBO OLECHE
BMC Health Services Research
Purity Njagi
Background The assessment of unmet need is one way to gauge inequities in access to healthcare services. While there are multiple reasons for unmet need, financial barriers are a major reason particularly in low- and middle-income countries where healthcare systems do not offer financial protection. Moreover, accessibility and affordability are paramount in achieving universal health coverage. This study examines the extent of unmet need in Kenya due to financial barriers, the associated determinants, and the influence of regional variations. Methods We use data from the 2013 Kenya household health expenditure and utilization (KHHEUS) cross sectional survey. Self-reported unmet need due to lack of money and high costs of care is used to compute the outcome of interest. A multilevel regression model is employed to assess the determinants of cost-related unmet need, confounding for the effect of variations at the regional level. Results Cost-related barriers are the main cause of unme...
Pauline Kamau
ObjectiveTo determine the prevalence of unmet need for primary healthcare and associated individual and household-level factors in Kenya.DesignThe data for this study are drawn from the 2016 Kenya Integrated Household Budget Survey (KIHBS). A multistage sampling technique involving a systematic selection of clusters at the national level and final selection of households was used.SettingThis study was conducted in Kenya. The KIHBS is a nationally representative survey on a wide range of indicators to assess the progress made in improving the living standards of the population at the national level.ParticipantsA total of 9447 households comprising 15 539 household members who reported a sickness or injury over the 4 weeks preceding this survey were included in this study. The study respondents comprised of the household heads.Primary outcome measureThe primary outcome of this study is unmet need for primary healthcare defined as an unexpressed demand for primary healthcare following ...
Journal of Economics and Sustainable Development
Wanja Douglas
European Scientific Journal ESJ
Health inputs are critical in attaining a healthy nation and improving health outcomes. Kenya, like other developing countries, grapples with limited health expenditures and poor population health indicators. Specifically, Kenya is yet to achieve the allocation of least 15% of the government’s annual budget to improve the health sector as enshrined in the Abuja Declaration. Though there is an improvement with regards to infant mortality rate decreasing from 96.6 per 1, 000 live birth in 1970 to 30.6 per 1, 000 live birth in 2018. This indicator of population health outcome is currently far below the Sustainable Development Goals (SDGs) target of reducing the under five mortality rate to as low as 12 deaths per 1,000 live births by 2030. The literature suggests that increase in government’s budgetary allocation to the health sector can improve country’s health outcomes. Evidence on the impact of health expenditures on health outcomes is mixed and limited in developing countries. This study aims to analyze the impact of public health expenditures on health outcomes, among other control variables in Kenya. The study uses time series data from 1970 to 2018. The variables are found to be integrated of different orders suggesting the choice of Autoregressive Distributed Lag (ARDL) model. ARDL provides a useful link between long run equilibrium relationships and short run disequilibrium dynamics is estimated. The ARDL bounds test suggests presence of cointegration thus leading to the estimation of Error Correction Model (ECM). The findings suggest that improvements in public health expenditures enhance health outcomes in Kenya. The control variablesthat are found to be important determinants of infant mortality rate in Kenya include the national income and number of hospital beds per 100, 000. The study recommends that Kenyan government should increase annual budgetary allocation to health sector. Such increase is likely to lead to investments in physical and human capital in the health sector thus translating to improved health outcomes in Kenya.
Annals of Global Health
Esther Nderitu
Journal of Healthcare
Brian Odhiambo
RELATED PAPERS
Richin Surana
EFISIENSI - KAJIAN ILMU ADMINISTRASI
Sutirman Sutirman
Kathryn Reyes
American journal of human genetics
Juliann Paolicchi
Estudios De Traduccion
Salud María Jarilla Bravo
International journal of Sudan research
Elsiddig Elsheikh
Transplantation
Deanna Toews
Eventledelse
Harry Arne Solberg
Scientific reports
Andrew Mason
Organic Geochemistry
Dawit Solomon
Jurnal Agrotek Tropika
Suskandini Ratih DIRMAWATI
Patient Preference and Adherence
Susan Brandis
École nationale d'administration publique (ENAP) / Université de Sherbrooke
Louis Demers
Human Pathology
Sherry Chiu
David Faitelson
Néstor Camino
keon hughes
Powder Technology
Javier Bilbao
Saeed Hassanzade
Estudios Internacionales
Edward Gonzalez
International Journal of Infection
Reza kalantari
Ocean Engineering
Han Ligteringen
International journal of sport, exercise and health research
Jakub Dziura
Ananda Gubbi
RELATED TOPICS
- We're Hiring!
- Help Center
- Find new research papers in:
- Health Sciences
- Earth Sciences
- Cognitive Science
- Mathematics
- Computer Science
- Academia ©2024
- Country / Region Change

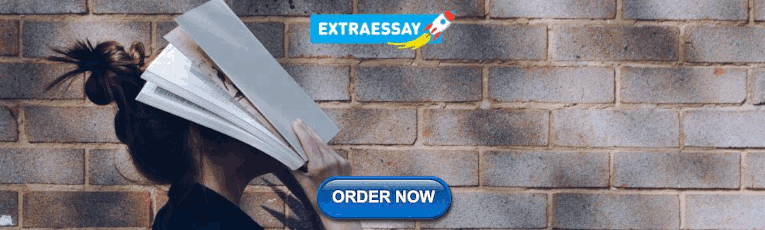
IMAGES
VIDEO
COMMENTS
The delivery of healthcare contributes toward individual, community, and population level health outcomes. 3 Therefore, research efforts to understand the health service utilization process are important to equitably deliver high-quality health services to all individuals, communities, and populations. To guide health services research, theoretical models to explain health service utilization ...
Estabrooks (1999b) identified no empirical methods in the health literature that attempted to map the field. As a result, she mapped factors hindering research utilization in nursing using bibliometric methods. ... Patterns of research utilization on patient care units. Implementation Science, 3, 31. 10.1186/1748-5908-3-31 [PMC free article ...
The identification of typologies of health care users and their specific characteristics can be performed using cluster analysis. This statistical approach aggregates similar users based on their common health-related behavior. This study aims to examine health care utilization patterns using cluster analysis; and the associations of health care user types with sociodemographic, health-related ...
The objective of this systematic review was to explore the association between private health insurance and health care utilization. We searched the MEDLINE, EMBASE, and Cochrane Central Register of Controlled Trials (CENTRAL) electronic databases for relevant articles since 2010. Studies were eligible if they described original empirical research on the utilization of public health care by ...
A decrease in health care utilization or a shift of inpatient activity to primary care as a result of more appropriate care is conceivable (Kringos et al., 2016), but so too is an increase in utilization due to the identification of previously unmet patient needs (Damery et al., 2016; Rummery, 2009). 4 DATA 4.1 Data sources and key variables
An estimated 40-70 percent of patients who receive care through Veterans Health Administration (VHA) facilities also receive care from non‐VA community providers (Hynes et al. 2007; Liu et al. 2010, 2011a; U.S. Department of Veterans Affairs 2011; Humensky et al. 2012).This proportion is likely to grow in light of the 2014 Veterans Choice Act, which stipulates specific conditions under ...
The broad objective of the study is to synthesize the empirical research studies on health issues of elderly, status of health care utilization and expenditure on health care among elderly in India. ... Health care utilization of elderly. The healthcare utilization has been extensively studied around the world [7, 22, 32, 35, 54]. Globally, ...
Empirical-Based Typology of Health Care Utilization by Medicare Eligible Veterans. Empirical-Based Typology of Health Care Utilization by Medicare Eligible Veterans. Health Serv Res. 2018 Dec;53 Suppl 3 (Suppl Suppl 3):5181-5200. doi: 10.1111/1475-6773.12995. Epub 2018 Jun 12.
The demographic change towards a larger proportion of older individuals challenges universal health care systems in sustaining high-quality care and universal coverage without budget expansions. To build valuable predictions of the economic burden from population ageing, it is crucial to understand the determinants of individual-level health care expenditures. Often, the focus has been on the ...
Background: Increases in pediatric obesity have been associated with higher levels of health care utilization. There is currently a lack of knowledge on the therapeutic drivers of increased health care use. Objective: To examine the association between different measures of health care utilization and BMI among children.Methods: We linked cross-sectional administrative data from a regional ...
Introduction. Health service utilization has been described as the product of interactions between healthcare professionals, the generation of health services, and patients. 1 At the most basic level, health service utilization is determined by the need and supply of health services. 2 However, health service utilization is significantly more complex and multidimensional with many underlying ...
The link between health insurance ownership and over-utilization of health care services are highly debated. ... Sweden, Portugal and Spain, the role of insurance status in health care demand is not prominent. Empirical research in Jordan found that health insurance ownership does not affect the probability of health care use but it ...
The committee was tasked with identifying factors that influence a person's use of health-care services, including poverty and level of urbanization. This chapter will address those factors. The committee has organized the beginning of the chapter around individual and societal determinants of health-care utilization, including factors that affect the need for care, the propensity to use ...
Measuring Health Care Utilization in Medicare Advantage Encounter Data: Methods, Estimates, and Considerations for Research Prepared for the Centers for Medicare & Medicaid Services ... We also address the following research questions through the empirical analysis: 1. What was the average utilization of health care services by MA enrollees for ...
Banerjee S (2021) Determinants of rural-urban differential in healthcare utilization among the elderly population in India. BMC Public Health 21(1):1-8. Article Google Scholar Berry LL, Bendapudi N (2007) Health care: a fertile field for service research. J Ser Res 10(2):111-122
However, high poverty. can lead to under utilization or lack of utiliz ation of health car e leading to. poor health. Thus, poverty reduction and improvement of health care. utilization are ...
Other factors that had a positive effect on health care utilization were household size, early levels of education, and distance to the nearest health facility. Conclusion: The study concludes that health care utilization is negatively affected by poverty other factors held constant. Thus, policies and strategies
Boris B Gorbunov currently works at the Institute of Biomedical Engineering of National Research University of Electronic Technology (Russia, Moscow, Zelenograd). Boris does research in ...
Urban-rural disparities in health care utilization among Chinese adults from 1993 to 2011: BMC Health Services Research ... these attempts have already greatly pushed forward the evaluation of the effectiveness of China's healthcare policy. More empirical research on and evidence related to these fields are clearly needed to illuminate the ...
Propagating knowledge related to folic acid (OR = 0.252, 95% CI 0.197 - 0.323) and directing pregnant women to use folic acid (OR = 0.168, 95% CI 0.096 - 0.296) by health care providers were also ...
NMT Institute of Nano and Microsystem Technology. AMT Institute of Advanced Materials and Technologies. BMS Institute of Biomedical Systems. ILPE Institute of Linguistic and Pedagogical Education. IМО Institute of International Education. SSEIT Institute of Systems and Software Engineering and Information Technology.
Quantitative measures used in empirical evaluations of mental health policy implementation: A systematic review ... Harnessing implementation science to increase the impact of health equity research. Medical Care, 55 (Suppl 9 2), S16-S23. 10.1097/MLR.0000000000000769 ... Health Research Policy And Systems, 16 (1), 81-81. 10.1186/s12961-018 ...
Readout No. E44 - Contribution of Diagnostics to Total Medical Care/Healthcare Readout No. E43 - Watching the Environmental and Society with Measurements Readout No. E42 - More Efficient Testing on Automotive Development, Improving the Accuracy of Fuel Consumption Measurement Readout No. E41 - Application Readout No. E40 - Application