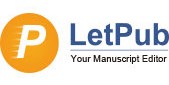
- Language Editing     For Manuscripts    For Response Letter new    For LaTeX    For Annual Review and Tenure    For Books new
- Scientific Editing     For Manuscripts    For Response Letter new
- Grant Editing 
- Translation 
- Publication Support  Journal Recommendation  Manuscript Formatting  Figure Formatting  Data Analysis new  Plagiarism Check  Conference Poster  Plain Language Summary
- Scientific Illustration  Journal Cover Design  Graphical Abstract  Infographic  Custom Illustration
- Scientific Videos  Video Abstract  Explainer Video  Scientific Animation
- Ethics and Confidentiality
- Editorial Certificate
- Testimonials
- Design Gallery
- Institutional Provider
- Publisher Portal
- Brand Localization
- Journal Selector Tool
- Learning Nexus
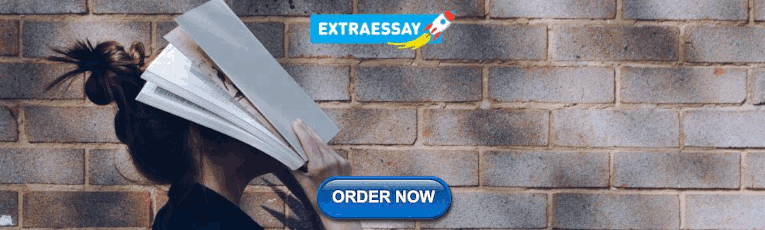
Scientific Journal Selector
COMPUTER SPEECH AND LANGUAGE
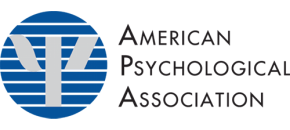
APA has partnered with LetPub to provide a full suite of author services.
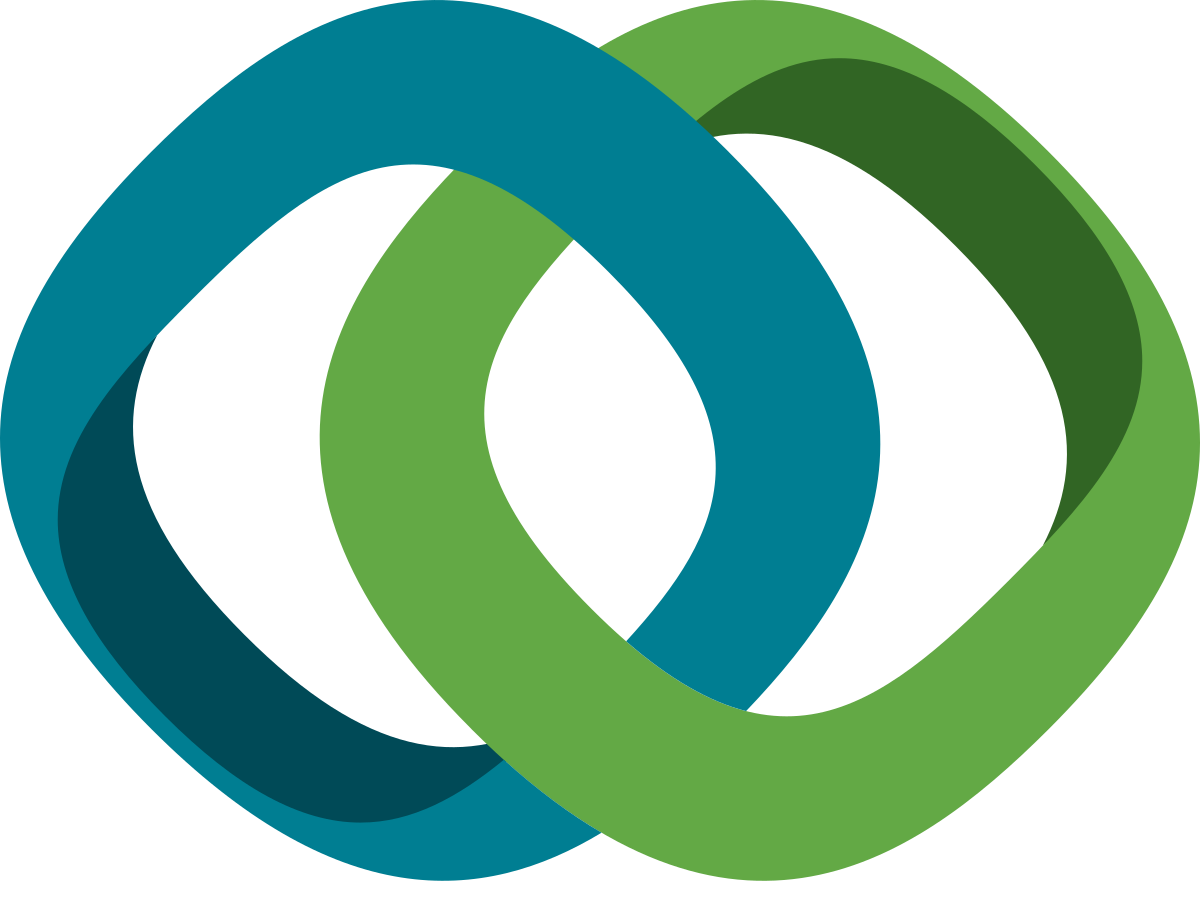
Free Webinar Series Conversations with a Hindawi Editor
Professional Journal Cover Design
Professionally designed and impactful journal cover art. Delivered fast and consistent with journal guidelines.
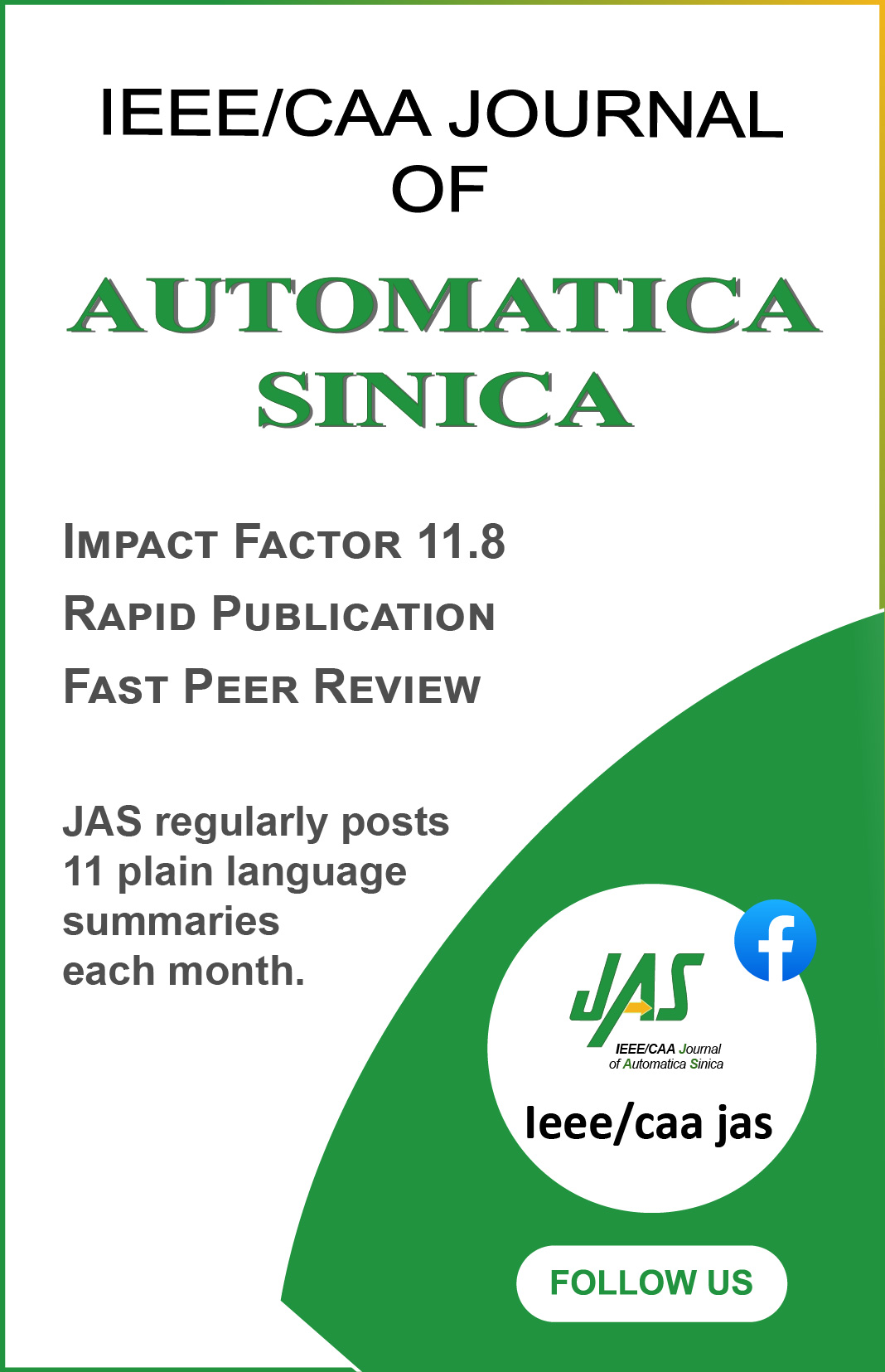
Intentional Space Tag
Contact us
Your name *
Your email *
Your message *
Please fill in all fields and provide a valid email.
© 2010-2024 ACCDON LLC 400 5 th Ave, Suite 530, Waltham, MA 02451, USA Privacy • Terms of Service
© 2010-2024 United States: ACCDON LLC Tel: 1-781-202-9968 Email: [email protected]
Address: 400 5 th Ave, Suite 530, Waltham, Massachusetts 02451, United States
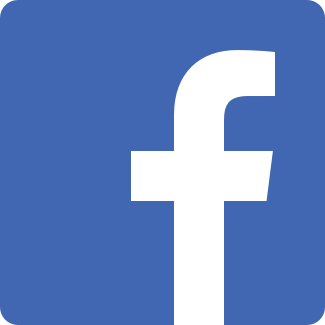

An official website of the United States government
The .gov means it's official. Federal government websites often end in .gov or .mil. Before sharing sensitive information, make sure you're on a federal government site.
The site is secure. The https:// ensures that you are connecting to the official website and that any information you provide is encrypted and transmitted securely.
- Publications
- Account settings
- Browse Titles
NCBI Bookshelf. A service of the National Library of Medicine, National Institutes of Health.

Evidence reviews for computer-based tools for speech and language therapy
Evidence review K
NICE Guideline, No. 236
- Copyright and Permissions
1. Computer-based tools for speech and language therapy
1.1. review question.
In people with aphasia after stroke, what is the clinical and cost effectiveness of computer-based tools to augment speech and language therapy?
1.1.1. Introduction
Speech and language therapy after stroke is provided in hospitals and in the community to help people with resulting communication disorders to improve their speech/language impairment, their ability to communicate and participate in their everyday roles and activities. It is generally accepted that improvement requires practice, and that rehabilitation is more effective in higher doses. Providing therapy and practice opportunities in sufficient dose can be a challenge in clinical practice due to limitations on therapy resources and distance between patients and therapists in some community settings. In addition, people with communication needs often wish to continue to work on their speech/language for longer than therapy is available for and look for alternative ways to support them in doing this. A growing number of computer software programmes, apps and online therapy tools are commercially available (see aphasia therapy software finder https://www.aphasiasoftwarefinder.org ). These tools are used by some therapists and patients to increase therapy practice opportunities either as home practice between therapy sessions or after face-to-face therapy has ended. Computer tools also offer a large range of practice material, practice material can be personalised, and some tools provide useful feedback.
This review has been prompted by publication of new evidence about effectiveness, and by an increasing interest in using computer tools to increase dose and to provide therapy remotely as was required during the COVID-19 pandemic.
1.1.2. Summary of the protocol
PICO characteristics of review question.
For full details see the review protocol in Appendix A .
1.1.3. Methods and process
This evidence review was developed using the methods and process described in Developing NICE guidelines: the manual . Methods specific to this review question are described in the review protocol in Appendix A and the methods document.
Declarations of interest were recorded according to NICE’s conflicts of interest policy .
1.1.4. Effectiveness evidence
1.1.4.1. included studies.
Twenty-two randomised control trial studies (including 2 cross-over trials and 3 quasi-randomised trials) (27 papers) were included in the review; 4 , 6 – 8 , 11 , 13 , 14 , 19 , 20 , 23 – 28 , 33 , 37 , 39 , 42 , 45 , 47 , 48 these are summarised in Table 2 below. Evidence from these studies is summarised in the clinical evidence summary below ( Table 3 ).
3 quasi-randomised trials 6 , 25 , 47 were included. Due to the limited evidence investigating computer-based tools for speech and language therapy, it was agreed to include these studies but ensure that they were downgraded sufficiently for risk of bias due to the randomisation process. Evidence was available for all outcomes apart from carer generic health-related quality of life.
Population factors
The majority of studies included people with aphasia 4 , 6 – 8 , 11 , 13 , 14 , 19 , 20 , 23 – 26 , 33 , 39 , 42 , 47 , 48 . However, studies occasionally included a mixture of people with aphasia or cognitive communication 27 , mixture of people with aphasia or aphasia and apraxia of speech 37 , people with dysarthria 28 or people with apraxia of speech 45 . Severity of communication difficulty was rarely reported, but when it was included people with mild communication difficulties 39 or with a mixture of different severities 37 , 42 . Additionally, the majority of studies included people in the chronic phase after stroke 4 , 6 – 8 , 11 , 13 , 14 , 19 , 25 – 27 , 37 , 39 , 45 , 47 with only occasional studies including people in the subacute phase or a mixture of people in the chronic and subacute phases 20 , 23 , 28 , 33 , 48
Types of computer-based tools
- Word finding therapy 4 , 19 , 37 , 47
- Reading therapy 6 – 8
- Comprehension therapy 14
- Expressive language/communication 26 , 45
- Articulation therapy 28
- Other (cognitive therapy) 23
- Combinations of approaches 11 , 13 , 20 , 24 , 25 , 27 , 33 , 39 , 42 , 48
There was a mixture of therapies being delivered in person 6 , 7 , 11 , 13 , 14 , 20 , 23 , 39 , 42 , remotely 4 , 8 , 24 – 28 , 33 , 37 , 45 , 47 , 48 (implementing telerehabilitation technology) or a combination of both 37 .
Intensity of therapy
- ≤10 hours 27 , 45 , 47
- 11-20 hours 6 , 11 , 20 , 23 , 33
- 21-30 hours 4 , 7 , 25 , 26 , 39 , 48
- ≥30 hours 8 , 13 , 19 , 24 , 42
- Mixed (intensity could be varied) 28
- Not stated/unclear 37
Inconsistency
The majority of outcomes included only one study. However, occasionally meta-analysis was possible. Occasionally this would lead to heterogeneity. This could not be resolved by subgroup or sensitivity analysis, with the majority of outcomes containing an insufficient number of studies to allow valid conclusions on the analyses to be drawn. Therefore, outcomes were downgraded for inconsistency.
See also the study selection flow chart in Appendix C , study evidence tables in Appendix D , forest plots in Appendix E and GRADE tables in Appendix F .
1.1.4.2. Excluded studies
Two Cochrane reviews 3 , 46 were identified and excluded from this review. For Brady 2016 3 this was due to the review including all speech and language therapy studies for people with aphasia, rather than just those that had computer-based tools being implemented. For West 2005 46 this included all speech and language therapy studies for people with apraxia of speech, rather than just those that had computer-based tools being implemented. In both cases, the citation lists of both studies were checked for relevant studies which were included if appropriate.
See the excluded studies list in Appendix J .
1.1.5. Summary of studies included in the effectiveness evidence
Summary of studies included in the evidence review.
See Appendix D for full evidence tables.
1.1.5.1. Summary matrix
Summary matrix of computer-based tools for speech and language therapy compared to each comparison groups.
1.1.6. Summary of the effectiveness evidence
Clinical evidence summary: computer-based tools for speech and language therapy compared to speech and language therapy without computer-based tools (usual care).
Clinical evidence summary: computer-based tools for speech and language therapy compared to social support/stimulation.
Clinical evidence summary: computer-based tools for speech and language therapy compared to no treatment.
Clinical evidence summary: computer-based tools for speech and language therapy compared to placebo.
See Appendix F for full GRADE tables.
1.1.7. Economic evidence
1.1.7.1. included studies.
- the CACTUS trial assessed computer exercises (3 days per week was recommended over 5-month period) that contained a combination of word finding and reading therapies,
- while BIG CACTUS assessed word-finding therapy computer exercises only and recommended that participants practice daily over 6-period.
These studies are summarised in the health economic evidence profile below ( Table 8 ) and the health economic evidence table in Appendix H .
1.1.7.2. Excluded studies
No relevant health economic studies were excluded due to assessment of limited applicability or methodological limitations.
See also the health economic study selection flow chart in Appendix G .
1.1.8. Summary of included economic evidence
Health economic evidence profile: Computer-based tools for speech and language therapy versus usual care.
1.1.9. Economic model
This area was not prioritised for new cost-effectiveness analysis.
1.1.10. Unit costs
Relevant unit costs are provided below to aid consideration of cost effectiveness.
Unit costs of health care professionals who may be involved in delivering interventions involving computer-based tools for speech and language therapy.
- The type of computer tool used varied across studies; Table 10 provides example costs associated with some of the tools that were assessed in the clinical review, with the cost per patient depending on both the type of software and whether multiple licences are purchased at once.
- Variation in method of delivery of therapy sessions: there was a mixture of studies assessing therapies delivered either in person or remotely, with one reporting a combination of both 37 Therapy delivered remotely is considered to be less resource intensive compared to face-to-face therapy.
- The frequency and duration of the intervention being delivered, with sessions ranging from 20-90 minutes, occurring 2-6 days per week, In the included clinical studies, the interventions were delivered for between 4-13 weeks.
- Staff who delivered the intervention varied as studies reported either physiotherapists, occupational therapists, or trained instructors. Palmer 2020 38 reported the use of SLTs and SLT assistants as well as trained volunteers to deliver the intervention.
- Study setting: interventions were conducted in hospitals, community centres, and outpatient rehabilitation centres. Non-clinical settings will incur lower or no costs compared to clinical settings.
- Additional resource use required to deliver the intervention, such as staff-training costs and information or instructional materials. Table 11 shows the summary costs provided in Marshall 2020, 26 which assessed the home-based EVA Park virtual reality program. This study also calculated the total per participant cost for the intervention (assuming 16 participants) was £1,364 when including hardware costs and £114 for an average online attendance (excluding hardware).
Example costs of computer-based tools for the treatment of aphasia.
Summary costs from Marshall 2020.
1.1.11. Evidence statements
Effectiveness/qualitative.
- One cost-utility analysis found that in post-stroke adults with aphasia, computerised word-finding therapy was not cost-effective when compared to usual care alone (ICER of £42,686 per QALY gained) or when compared to attention control plus usual care (ICER of £40,164 per QALY gained). This study was assessed as directly applicable with potentially serious limitations.
- One cost-utility analysis found that in post-stroke adults with aphasia, computerised word-finding and reading therapy was cost-effective when compared to usual care alone (ICER of £3,058 per QALY gained). This study was assessed as partially applicable with potentially serious limitations.
1.1.12. The committee’s discussion and interpretation of the evidence
1.1.12.1. the outcomes that matter most.
The committee included the following outcomes: person/participant generic health-related quality of life, carer generic health-related quality of life, communication outcomes, including: overall language ability, impairment specific measures (such as naming, auditory comprehension, reading, expressive language and speech impairment and activity for people with dysarthria) and functional communication, communication related quality of life, psychological distress (including depression, anxiety and distress) and discontinuation. All outcomes were considered equally important for decision making and therefore have all been rated as critical.
Person/participant health-related quality of life outcomes were considered particularly important as a holistic measure of the impact on the person’s quality of living. However, the committee acknowledged that generic measures may be more responsive to physical changes after stroke and less responsive to communication changes, and this may affect the interpretation of the outcome. In particular, for EQ-5D, the committee noted that there are no subscales specific to communication, which makes it hard to relate to speech and language therapy. In response to this, communication related quality of life scores were also included. Communication outcomes were key to this review as a direct answer to the question. Psychological distress was included as a response to the significant psychological distress that can be experienced by people with communication difficulties that may be resolved by the treatment. Discontinuation was considered as a measure of adherence to the treatment with the acknowledgement that there are unlikely to be significant adverse events as a result of the treatment. Mortality was not considered as it was deemed unlikely to be a result of the treatment. However, if mortality was a reason for discontinuation, then this was highlighted to the committee during their deliberation.
The committee chose to investigate these outcomes at less than 3 months and more than and equal to 3 months, as they considered that there could be a difference in the short term and long term effects of the interventions, in particular for people who have had an acute stroke where effects at less than 3 months could be very different then effects at greater than 3 months. With regards to communication difficulties, this may be seen at 3 months, in contrast to other reviews for this guideline where 6 months was used.
The evidence for this question was limited, with some outcomes not being reported. No study investigated the effects of interventions on carer generic health-related quality of life and the anxiety and distress sections of psychological distress. Outcomes were reported at both less than 3 months and more than and equal to 3 months.
1.1.12.2. The quality of the evidence
Twenty randomised controlled trial studies (including 1 cross-over trial and three quasi-randomised trials) were included in the review. The 3 quasi-randomised trials were included due to the limited evidence investigating computer-based tools for speech and language therapy. However, the limitations produced by the study design was reflected in the risk of bias assessment. Non-randomised studies were considered for this review. However, none were identified that fulfilled the protocol criteria.
The quality of the evidence ranged from high to very low quality, most of the evidence being of low quality. Outcomes were commonly downgraded due to risk of bias (mainly due to bias arising from the randomisation process, bias due to deviations from the intended intervention and bias due to missing outcome data) and imprecision. No outcomes were affected by indirectness.
Some outcomes were downgraded for inconsistency. However, this was less common as meta-analysis was not possible for the majority of outcomes, with only 1 study being included in most outcomes. Where heterogeneity was identified, subgroup and sensitivity analyses did not resolve this mainly due to the limited number of studies making it not possible to form valid subgroups. In general, the majority of studies included people with aphasia, with a minority including people with dysarthria, people with apraxia of speech and a combination of people with other communication difficulties and aphasia. The majority of studies included people in the chronic phase after stroke, with only occasional studies including people in the subacute phase. The types of computer-based tools used varied across the studies, with the majority including a combination of approaches. There was a mixture of therapies being delivered in person and being delivered remotely. The amount of therapy varied between studies ranging from less than and equal to 10 hours to more than and equal to 30 hours.
The majority of the studies included a small number of participants (the majority including 10 to 20 participants in each study arm), while few studies included a larger number of participants (at most around 100 participants in each study arm).
These factors introduced additional uncertainty in the results. The effects on risk of bias did not appear to influence the direction of the effect in the trials. The committee took all these factors into account when interpreting the evidence.
The committee concluded that the evidence was of sufficient quality to make recommendations. They acknowledged the varied quality of the evidence and the heterogeneity in the interventions being compared in this analysis. They committee noted the study size and variations that may occur from studies conducted outside of an NHS-based healthcare setting. However, a large multi-site NIHR funded study 37 recently took place in the United Kingdom which included a health economic analysis. The study reported the use of a word finding computer-based therapy compared to social support/stimulation and speech and language therapy without computer-based tools. The study reported many of the outcomes included in this review and was of low risk of bias. Therefore, the committee gave this study greater consideration in their decision making.
1.1.12.2.1. Computer-based tools compared to speech and language therapy without computer-based tools
The majority of identified evidence was considered to be categorised in this comparison. When compared to speech and language therapy without computer-based tools, 39 outcomes were reported that ranged between high and very low quality. Where downgraded, outcomes were commonly downgraded due to risk of bias (due to a mixture of bias arising from the randomisation process, bias due to deviations from the intended intervention, bias due to missing outcome data and bias in measurement of the outcome) and imprecision. Two outcomes were downgraded for inconsistency due to the outcomes including a mixture of studies reporting zero events in at least 1 study arm and studies reporting events in both study arms.
1.1.12.2.2. Computer-based tools compared to social support/stimulation
When compared to social support/stimulation, 7 outcomes were reported that ranged from high to very low quality. When downgraded, outcomes were commonly downgraded due to risk of bias (due to bias arising from the randomisation process) and imprecision. Two outcomes were downgraded for inconsistency either as heterogeneity was observed and not resolved by sensitivity analysis or subgroup analysis or that the outcome included a mixture of studies reporting zero events in at least 1 study arm and studies reporting events in both study arms.
1.1.12.2.3. Computer-based tools compared to no treatment
When compared to no treatment, 11 outcomes were reported that ranged from low to very low quality, with the majority being of very low quality. Outcomes were commonly downgraded due to risk of bias (due to a mixture of bias arising from the randomisation process, bias due to deviations from the intended interventions, bias due to missing outcome data and bias in measurement of the outcome) and imprecision. Two outcomes were downgraded for inconsistency as heterogeneity was observed and not resolved by sensitivity analysis or subgroup analysis.
1.1.12.2.4. Computer-based tools compared to placebo
When compared to placebo, 5 outcomes were reported that ranged from low to very low quality, with the majority being of very low quality. Outcomes were commonly downgraded due to risk of bias (due to a mixture of bias arising from the randomisation process, bias due to deviations from the intended interventions, bias due to missing outcome data and bias in measurement of the outcome) and imprecision. One outcome was downgraded for inconsistency either as heterogeneity was observed and not resolved by sensitivity analysis or subgroup analysis.
1.1.12.3. Benefits and harms
1.1.12.3.1. key uncertainties.
The committee agreed that there was significant heterogeneity in the interventions included in the analysis, reflecting the complexity and range of speech and language therapy needs that can be targeted by computerised therapy. The interventions varied from computer programs aiming to deliver speech and language therapy to telerehabilitation approaches aiming to support speech and language therapist to deliver therapy over long distances. A subgroup analysis for remote delivery compared to in person delivery of therapy did not resolve any heterogeneity in the analysis. Furthermore, the types of computer programs used to deliver therapy varied significantly. While some focussed on specific methods of therapy (for example: word finding therapy) others included a mixture of approaches aiming for more holistic delivery of therapy. A subgroup analysis for the method of therapy did not resolve any heterogeneity in the analysis.
- Speech and language therapy with computer-based tools compared to equal amounts of therapy without computer-based tools (intensity and duration matched)
- Speech and language therapy with computer-based tools in addition to speech and language therapy delivered in person compared to in person delivery only (usual care with additional computer-based tools)
The committee noted that computer-based tools for speech and language therapy would most likely not be used as the only speech and language therapy for a person. Speech and language therapy with computer-based tools can often allow for training in activities where repetition is required, but it is often harder to adapt to the person’s needs. The approach can make it harder for the person after stroke to feel they are receiving adequate attention if it is not adequately supported by a health care professional or is not person centred, and this may reduce their motivation to continue with the computer therapy. The committee noted that personalisation was possible with some computer software, but this will incur additional costs for staff to be involved with this process (including additional time with people to discuss how the therapy is going). The approaches used in the studies varied.
The committee noted that the evidence included mostly small studies with very few participants and so it was difficult to make firm conclusions about the efficacy of the intervention. The majority of interventions appeared to include components of word finding, but there were very few interventions looking at other methods of therapy. In addition, the majority of evidence was for people with aphasia with very few studies involving people with other types of speech and language difficulties (such as dysarthria and apraxia of speech). The committee agreed that additional research with larger sample sizes, computerised therapy focussed on other aspects of speech and language impairment, and ways to support use of new speech and language skills in everyday communication situations would be important for future work.
1.1.12.3.2. Computer-based tools compared to speech and language therapy without computer-based tools, social support/stimulation, no treatment and placebo
When compared to speech and language therapy without computer-based tools, clinically important benefits were seen for psychological distress – depression and discontinuation at less than 3 months and more than and equal to 3 months. Unclear effects where some outcomes indicated a clinically important benefit of computer-based tools, while others indicated no clinically important difference was seen for naming at less than 3 months and more than and equal to 3 months and expressive language at more than and equal to 3 months. An unclear effect where some outcomes indicated a clinically important benefit of computer-based tools (including 30 participants), while others indicated a clinically important benefit of speech and language therapy without computer-based tools (including 198 participants) was seen for person/participant generic health-related quality of life at more than and equal to 3 months. No clinically important difference was seen for overall language ability, reading functional communication and communication related quality of life at less than 3 months and more than and equal to 3 months and auditory comprehension, expressive language, speech impairment – dysarthria and activity – dysarthria at less than 3 months. An unclear effect where some outcomes indicated no clinically important difference, while others indicated a clinically important benefit of speech and language therapy without computer-based tools was seen for auditory comprehension at more than and equal to 3 months.
When compared to social support/stimulation, clinically important benefits were seen for naming at less than 3 months and more than and equal to 3 months. No clinically important difference was seen in person/participant generic health-related quality of life, functional communication and communication related quality of life at more than and equal to 3 months and discontinuation at less than 3 months and more than and equal to 3 months. When compared to no treatment, clinically important benefits were seen for naming and communicated related quality of life at less than 3 months. No clinically important difference was seen in overall language ability, auditory comprehension, expressive language, functional communication, depression and discontinuation at less than 3 months. When compared to placebo, no clinically important difference was seen for overall language ability at less than 3 months and more than and equal to 3 months and naming at less than 3 months. Clinically important harms of computer-based tools were seen in discontinuation at less than 3 months and more than and equal to 3 months.
The committee noted that the evidence was complicated to examine due to the variety of computer-based tools being meta-analysed that were examining different techniques. The intervention of note had a high degree of interventional complexity that made it complicated to fully understand using this analysis. However, the committee weighed up the benefits and the harms from the evidence available. Benefits were seen in naming therapies that were either focussed on word finding or included word finding as a component. The committee noted that this was realistic but highlighted that this did not necessarily make a difference on a person’s ability to communicate. They noted that word finding may be useful for finding specific words, but not necessarily to use those words in communication and required extra support to put those words into context. No clinically important differences were seen in functional communication, which may indicate that the ability to use words in context may not have been achieved with these therapies.
The committee noted that the outcome reported for person/participant generic health-related quality of life was EQ-5D, that did not specifically include a subscale for communication. Due to this, it is difficult to conclude that the interventions are or are not effective based on this outcome. Therefore, the committee did not give the outcome a large weighting in their decision when making recommendations.
The committee considered the clinically important harm in discontinuation when computer-based tools were compared to placebo. People dropped out for unclear reasons during the first 2 weeks of therapy in 1 study in the group using computer-based tools, which may reflect dissatisfaction with the computer-based therapy though this is uncertain. Weighing up this evidence against the potential evidence of benefits, the committee decided that the evidence of benefit outweighed the potential for harm from this. If people found that computer-based tools were not suitable for them then they could work with their therapist to explore other methods of therapy, including methods that do not use computer-based tools.
The committee agreed that computer-based tools for speech and language therapy should be used as an adjunct to speech and language therapy, not alone. There was insufficient evidence of clinically important changes in anything except in improving word finding. Most of the evidence was from small studies and it was not possible to make recommendations, either positive or negative, for other uses of computer-based speech and language tools. Based on this they agreed that computer-based tools could be considered where word finding is an important aim for the person after stroke and they should be used as an adjunct to therapy delivered by a speech and language therapist. However, there should also be additional research with larger sample sizes investigating the other potential uses of computer-based tools for speech and language therapy to gain a complete understanding of the effect of the interventions.
1.1.12.4. Cost effectiveness and resource use
The economic evidence review included 2 published studies with relevant comparisons. These studies were economic evaluations of a pilot feasibility trial (CACTUS) and a randomised controlled trial (Big CACTUS) of the StepByStep computer program, respectively - both of which were included in the clinical review. The StepByStep software allowed for participants to receive supported self-managed intensive speech practice at home. Both studies were UK model-based cost-utility analyses with lifetime horizons, although the interventions differed slightly as described in the following paragraphs.
The CACTUS trial compared the StepByStep approach (computer exercises, support from an SLT and a volunteer who practiced carryover activities face to face) to usual stimulation, which included activities that provided general language stimulation, such as communication support groups and conversation, as well as reading and writing activities. The analysis included a three-state Markov model with month-long cycles, whereby participants could transition from their initial aphasia health state to a response state (defined as a ≥17% increase in proportion of words named correctly at 5 months), or to death. Patients in the response state could relapse to the aphasia state or die. Utility weights were assigned to response and no response states to estimate QALYs, which were measured using a pictorial version of EQ-5D-3L (adapted for this study to be accessible to patients with aphasia) collected at baseline and at 5-and 8-months. 5-month utility data was then extrapolated to a lifetime horizon with 0.08% monthly relapse rate applied. Intervention costs included computers and microphones provided to participants, as well StepByStep software and training for speech and language therapists (SLTs). Healthcare resource use between both groups was also compared using patient and carer diaries collected at 5 months post-randomisation. After 5 months, resource use costs were assumed to be the same for both groups by applying 5-month resource use estimates collected from the control group. The results of the CACTUS trial suggested that StepByStep was cost-effective, with an incremental cost of only £437 for an incremental QALY gain of 0.14, producing an incremental cost-effectiveness ratio (ICER) of £3,058 per QALY gained. Probabilistic sensitivity analyses also suggested that the probability of the intervention being cost-effective was 75.8% at a £20,000 threshold. However, deterministic sensitivity analyses found that the base case results were sensitive to utility gain (for example, utility gain of ≤0.01 resulted in ICER of >£20,000) and relapse rate parameters (for example, relapse rate of >30% resulted in ICER of >£20,000).
This study was assessed as partially applicable for this review, as 2010-unit costs may not reflect the current UK NHS context and the year in which resource use estimates were collected was not reported. Potentially serious limitations were also identified, as the lifetime model was based on an RCT with a short follow up (8 months) and focused on one piece of software which limits interpretation for the wider evidence base identified in the clinical review. Additional limitations included: resource use estimates were taken from a self-reported questionnaire not from a systematic review; utility of non-responders assumed to be equal for both trial groups, overlooking the possibility that non-responder utility scores could be lower in the intervention group; the definition of a “good response” was arbitrarily defined, and how the accessible version of the EQ-5D-3L questionnaire is yet to be validated, although this did allow for utility scores to be elicited directly from people with aphasia. Finally, it should be noted that the sample size of the CACTUS trial was small (n=34) and aimed to assess the feasibility of a rigorous RCT of a self-managed computer therapy. Therefore, it cannot be expected to provide conclusive cost-effectiveness results.
For this reason, an economic evaluation of Big CACTUS trial was conducted. The trial compared the StepByStep program to both usual care and an attention control arm, who received puzzle books and monthly supportive telephone calls plus usual care. The StepByStep intervention was delivered both remote and in-person, supported by volunteers and SLT assistants. The Markov model included with 3-month cycles where all participants begin in the ‘aphasia’ health state but differed from the model used in the CACTUS trial, as it included two tunnel heath states for ‘good response’ (defined as a ≥10% increase in words correctly found on a naming test and/or a 0.5 increase on the Therapy Outcomes Measures activity scale) at 6 and 9 months from baseline. No new responses were assumed to occur after 12 months – participants either remained in the ‘good response (12 months and beyond), relapsed to the ‘Aphasia’ health state or die. People in the ‘Aphasia’ health state at 12 months either remain in that health state or die. Utility weights were assigned to response and no response states to estimate QALYs, which were measured using an adapted pictorial version of EQ-5D-5L collected at baseline, 6, 9 and 12 months. EQ-5D-5L scores were also mapped to EQ-5D-5L using an algorithm by Van Hout 2012 44 . The relapse rate observed between 9 and 12 months was assumed to remain constant for the remainder of the modelled period, hence it was assumed that good responses were lost over time. Only intervention costs were incorporated into the model, which included hardware and software costs (computers, including StepByStep software licences, headphones, puzzle books), SLT training costs and volunteer time/travel costs for SLTs and SLT assistants.
The results found that StepByStep was not cost-effective when compared to usual care, as the QALY gain associated with the intervention was small (0.017) relative to the incremental cost (£733), resulting in an ICER of £42,686 per QALY gained. The same result was found when the intervention was compared to the active control group (£40,165 per QALY gained). The active control group was also dominated by usual care, having higher costs (£695) and lower QALYs (−0.0001). The probability that usual care was cost-effective was 56% at a £20,000 threshold, compared to 22% for both the active control and StepByStep groups. The only cost-effective result identified for the StepByStep intervention was when only patient subgroups with moderate word finding difficulties were assessed, which reported an ICER of £13,673 per QALY gained when compared to the active control group, and £21,262 per QALY gained for StepByStep compared to usual care alone. Alternative costing assumptions (including the inclusion of volunteer costs) did not change conclusions on cost-effectiveness. The study was deemed as directly applicable with potentially serious limitations for the following reasons: This lifetime model was based on an RCT with a short follow up (12 months) and assessed a single piece of software; the health-related quality of life benefit of a “good response” for the StepByStep intervention was small and uncertain; only direct intervention costs were included as Big CACTUS did not collect data on wider resource use (due to the CACTUS pilot study reporting no important differences in indirect resource use) and the how the accessible version of the EQ-5D-5L questionnaire is yet to be validated.
In addition to the economic evidence, unit costs of computer-based tools and health care professionals that were reported in the clinical studies were presented to aid committee discussion. Additional resource use would be required for computer-based therapy, and variation in resource use across studies reported in the clinical review highlighted the uncertainty towards the potential resource impact of these interventions on the NHS. For example, the cost per patient for these tools depends on both the type of software and whether multiple licences are purchased at once. The intervention setting would also affect the resource impact, as the clinical studies reported interventions that were conducted in hospitals, community centres, and outpatient rehabilitation centres, as well as those that were delivered remotely. Non-clinical settings will incur lower or no costs compared to clinical settings, while remote-based therapies are considered to be less resource intensive compared to face-to-face therapy. Differences in the frequency and duration of therapy delivery were also reported, with sessions ranging from 20-90 minutes, occurring 2-6 days per week, for a total of 4-13 weeks. Staff who delivered the intervention varied as studies reported using physiotherapists, occupational therapists, or trained instructors. The Big CACTUS RCT also reported the use of SLTs and SLT assistants as well as trained volunteers to deliver the intervention. Studies also reported other various resource use requirements, such as staff-training costs and information or instructional materials.
The committee discussed economic evidence, noting that the results of the two included studies could not be used to reflect the cost-effectiveness of the wider evidence base as they assessed a single computer program that required substantial resource use in terms of hardware and software costs compared to other interventions identified in the clinical review. Neither version of the StepByStep program is widely available as part of current practice which would increase the resource impact if recommended. Further uncertainty of the cost-effectiveness was raised when considering the variation in the delivery and resource use requirements of the interventions reported in the clinical studies. The committee agreed that there would be a resource impact for providing computer-based therapy as this is not routinely used in current practice.
Although the clinical studies varied in quality, with significant uncertainty due to the complexity of the interventions, clinically important benefits were seen for naming when interventions focused on or included word finding as a component. This led the committee to agree that computer-based interventions aimed at improving naming skills may be useful as additional therapy, as the majority of studies provided computer-based therapy in addition to face-to-face speech and language therapy. The committee also specified that such interventions should be adapted to the needs of the person (for example, word finding activities that include terms which are important to the user). Considering the uncertainty of the clinical evidence and limited economic evidence, the committee proposed a ‘consider’ recommendation for computer-based therapy programmes tailored to individual goals in relation to naming in addition to face-to-face speech and language therapy.
1.1.12.5. Other factors the committee took into account
The committee noted the potential inequity of using programs that are only available in English and notes that there will be some people who cannot access this due to speaking other languages. They noted the complexities for multilingual people who may have therapy focussed on their use of English instead of including all languages that a person may speak. Computer-based tools may exacerbate this inequity in care and so the committee highlighted that it is important to consider all languages that a person speaks and providing holistic support for the person.
The committee noted that computer-based tools may not be accessible for all people, dependent on multiple factors including their access to technology due to cost and computer literacy. Hospitals may be able to lend out technology and provide additional support to people to use it, but it was noted that there may be a geographic variation in the effect of this with a greater requirement for technology to be leant out in areas where there is greater socioeconomic deprivation.
The committee agreed that computer-based tools should not be used as the only speech and language therapy someone should be offered, and that all people who require speech and language therapy should receive support from a speech and language therapist. However, there is currently insufficient available speech therapist time in many Stroke Units, and computer-based tools could be an important means of increasing the intensity of therapy someone could receive (see Evidence review E ).
The committee noted that there could be wider effect on psychological outcomes. Some outcomes for evidence were not available for this review, such as outcomes on psychological distress for group-based computer-based tools. The committee discussed how this may help with psychological wellbeing by integrating with other people after stroke.
1.1.13. Recommendations supported by this evidence review
This evidence review supports recommendation 1.12.8 and the research recommendation on computer-based speech and language therapy.
1.1.14. References
Appendix a. review protocols.
Review protocol for the clinical and cost-effectiveness of computer-based tools to augment speech and language therapy in people with aphasia after stroke (PDF, 244K)
Health economic review protocol (PDF, 145K)
Appendix B. Literature search strategies
B.1. Clinical search literature search strategy (PDF, 180K)
B.2. Health Economics literature search strategy (PDF, 181K)
Appendix C. Effectiveness evidence study selection
Figure 1. Flow chart of clinical study selection for the review of computer-based tools for speech and language therapy (PDF, 246K)
Appendix D. Effectiveness evidence
Download PDF (1.0M)
Appendix E. Forest plots
E.1. Computer-based tools for speech and language therapy compared to speech and language therapy without computer-based tools (usual care) (PDF, 242K)
E.2. Computer-based tools for speech and language therapy compared to social support/stimulation (PDF, 179K)
E.3. Computer-based tools for speech and language therapy compared to no treatment (PDF, 194K)
E.4. Computer-based tools for speech and language therapy compared to placebo (PDF, 170K)
Appendix F. GRADE tables
Table 14. Clinical evidence profile: computer-based tools for speech and language therapy compared to speech and language therapy without computer-based tools (usual care) (PDF, 280K)
Table 15. Clinical evidence profile: computer-based tools for speech and language therapy compared to social support/stimulation (PDF, 188K)
Table 16. Clinical evidence profile: computer-based tools for speech and language therapy compared to no treatment (PDF, 220K)
Table 17. Clinical evidence profile: computer-based tools for speech and language therapy compared to placebo (PDF, 198K)
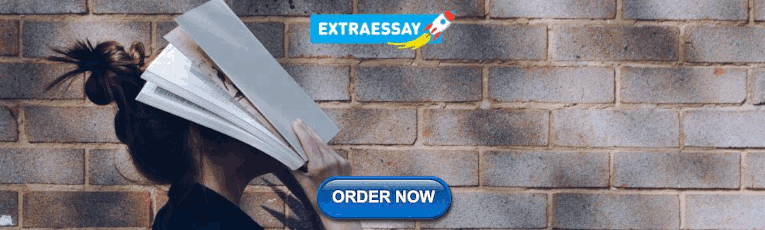
Appendix G. Economic evidence study selection
Figure 1. Flow chart of health economic study selection for the guideline (PDF, 193K)
Appendix H. Economic evidence tables
Download PDF (187K)
Table 18. Cost-effectiveness results from base-case and secondary analyses from Latimer 2021 – computerised therapy plus usual care compared to usual care alone, and compared to attention control plus usual care (PDF, 134K)
Download PDF (208K)
Appendix I. Health economic model
Modelling was not prioritised for this question.
Appendix J. Excluded studies
Clinical studies, table 19 studies excluded from the clinical review.
View in own window
Health Economic studies
Download PDF (159K)
Appendix K. Research recommendations – full details
K.1. Research recommendation (PDF, 202K)
Evidence reviews underpinning recommendation 1.12.8 and research recommendations in the NICE guideline
These evidence reviews were developed by NICE
Disclaimer : The recommendations in this guideline represent the view of NICE, arrived at after careful consideration of the evidence available. When exercising their judgement, professionals are expected to take this guideline fully into account, alongside the individual needs, preferences and values of their patients or service users. The recommendations in this guideline are not mandatory and the guideline does not override the responsibility of healthcare professionals to make decisions appropriate to the circumstances of the individual patient, in consultation with the patient and/or their carer or guardian.
Local commissioners and/or providers have a responsibility to enable the guideline to be applied when individual health professionals and their patients or service users wish to use it. They should do so in the context of local and national priorities for funding and developing services, and in light of their duties to have due regard to the need to eliminate unlawful discrimination, to advance equality of opportunity and to reduce health inequalities. Nothing in this guideline should be interpreted in a way that would be inconsistent with compliance with those duties.
NICE guidelines cover health and care in England. Decisions on how they apply in other UK countries are made by ministers in the Welsh Government , Scottish Government , and Northern Ireland Executive . All NICE guidance is subject to regular review and may be updated or withdrawn.
- Cite this Page Evidence reviews for computer-based tools for speech and language therapy: Stroke rehabilitation in adults (update): Evidence review K. London: National Institute for Health and Care Excellence (NICE); 2023 Oct. (NICE Guideline, No. 236.)
- PDF version of this title (2.9M)
In this Page
- Computer-based tools for speech and language therapy
Other titles in this collection
- NICE Evidence Reviews Collection
Related NICE publication
- Stroke rehabilitation in adults
Supplemental NICE documents
- Economic model: Cost-utility analysis: intensity of physiotherapy rehabilitation (PDF)
- Economic model: Cost-utility analysis of botulinum toxin A to reduce spasticity (PDF)
- Methods (PDF)
Related information
- PMC PubMed Central citations
- PubMed Links to PubMed
Similar articles in PubMed
- Computerised speech and language therapy or attention control added to usual care for people with long-term post-stroke aphasia: the Big CACTUS three-arm RCT. [Health Technol Assess. 2020] Computerised speech and language therapy or attention control added to usual care for people with long-term post-stroke aphasia: the Big CACTUS three-arm RCT. Palmer R, Dimairo M, Latimer N, Cross E, Brady M, Enderby P, Bowen A, Julious S, Harrison M, Alshreef A, et al. Health Technol Assess. 2020 Apr; 24(19):1-176.
- Suicidal Ideation. [StatPearls. 2024] Suicidal Ideation. Harmer B, Lee S, Rizvi A, Saadabadi A. StatPearls. 2024 Jan
- Identifying the Active Ingredients of a Computerized Speech and Language Therapy Intervention for Poststroke Aphasia: Multiple Methods Investigation Alongside a Randomized Controlled Trial. [JMIR Rehabil Assist Technol. 2...] Identifying the Active Ingredients of a Computerized Speech and Language Therapy Intervention for Poststroke Aphasia: Multiple Methods Investigation Alongside a Randomized Controlled Trial. Harrison M, Palmer R, Cooper C. JMIR Rehabil Assist Technol. 2023 Dec 5; 10:e47542. Epub 2023 Dec 5.
- Review A systematic review of nursing rehabilitation of stroke patients with aphasia. [J Clin Nurs. 2010] Review A systematic review of nursing rehabilitation of stroke patients with aphasia. Poslawsky IE, Schuurmans MJ, Lindeman E, Hafsteinsdóttir TB. J Clin Nurs. 2010 Jan; 19(1-2):17-32.
- Review Speech and language therapy for aphasia following stroke. [Cochrane Database Syst Rev. 2000] Review Speech and language therapy for aphasia following stroke. Greener J, Enderby P, Whurr R. Cochrane Database Syst Rev. 2000; (2):CD000425.
Recent Activity
- Evidence reviews for computer-based tools for speech and language therapy Evidence reviews for computer-based tools for speech and language therapy
Your browsing activity is empty.
Activity recording is turned off.
Turn recording back on
Connect with NLM
National Library of Medicine 8600 Rockville Pike Bethesda, MD 20894
Web Policies FOIA HHS Vulnerability Disclosure
Help Accessibility Careers
Search form
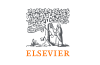
Computer Speech And Language
You may order single or multiple copies of back and recent journal issues. If you are an Author wishing to obtain a printed copy of the journal issue featuring your article, or you require a printed copy for research, review or add to your library, the process is easy –
- Select your journal volume and issue.
- Select the required quantity in the Review cart page
- Provide the shipping details and process the payment.
- Average production time is approx. 2 weeks.
- Your shipping options and general shipping times are: DHL for international - 2-5 postal days and UPS for domestic – 1-6 business days depending on delivery address. . We can track your shipment status at any time.
Suggestions or feedback?
MIT News | Massachusetts Institute of Technology
- Machine learning
- Social justice
- Black holes
- Classes and programs
Departments
- Aeronautics and Astronautics
- Brain and Cognitive Sciences
- Architecture
- Political Science
- Mechanical Engineering
Centers, Labs, & Programs
- Abdul Latif Jameel Poverty Action Lab (J-PAL)
- Picower Institute for Learning and Memory
- Lincoln Laboratory
- School of Architecture + Planning
- School of Engineering
- School of Humanities, Arts, and Social Sciences
- Sloan School of Management
- School of Science
- MIT Schwarzman College of Computing
Natural language boosts LLM performance in coding, planning, and robotics
Press contact :.
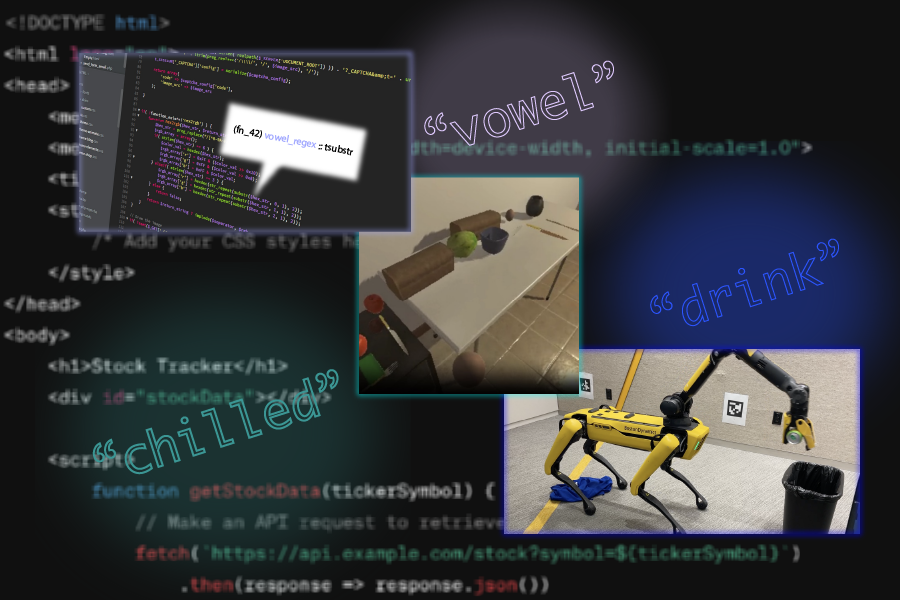
Previous image Next image
Large language models (LLMs) are becoming increasingly useful for programming and robotics tasks, but for more complicated reasoning problems, the gap between these systems and humans looms large. Without the ability to learn new concepts like humans do, these systems fail to form good abstractions — essentially, high-level representations of complex concepts that skip less-important details — and thus sputter when asked to do more sophisticated tasks. Luckily, MIT Computer Science and Artificial Intelligence Laboratory (CSAIL) researchers have found a treasure trove of abstractions within natural language. In three papers to be presented at the International Conference on Learning Representations this month, the group shows how our everyday words are a rich source of context for language models, helping them build better overarching representations for code synthesis, AI planning, and robotic navigation and manipulation. The three separate frameworks build libraries of abstractions for their given task: LILO (library induction from language observations) can synthesize, compress, and document code; Ada (action domain acquisition) explores sequential decision-making for artificial intelligence agents; and LGA (language-guided abstraction) helps robots better understand their environments to develop more feasible plans. Each system is a neurosymbolic method, a type of AI that blends human-like neural networks and program-like logical components. LILO: A neurosymbolic framework that codes Large language models can be used to quickly write solutions to small-scale coding tasks, but cannot yet architect entire software libraries like the ones written by human software engineers. To take their software development capabilities further, AI models need to refactor (cut down and combine) code into libraries of succinct, readable, and reusable programs. Refactoring tools like the previously developed MIT-led Stitch algorithm can automatically identify abstractions, so, in a nod to the Disney movie “Lilo & Stitch,” CSAIL researchers combined these algorithmic refactoring approaches with LLMs. Their neurosymbolic method LILO uses a standard LLM to write code, then pairs it with Stitch to find abstractions that are comprehensively documented in a library. LILO’s unique emphasis on natural language allows the system to do tasks that require human-like commonsense knowledge, such as identifying and removing all vowels from a string of code and drawing a snowflake. In both cases, the CSAIL system outperformed standalone LLMs, as well as a previous library learning algorithm from MIT called DreamCoder, indicating its ability to build a deeper understanding of the words within prompts. These encouraging results point to how LILO could assist with things like writing programs to manipulate documents like Excel spreadsheets, helping AI answer questions about visuals, and drawing 2D graphics.
“Language models prefer to work with functions that are named in natural language,” says Gabe Grand SM '23, an MIT PhD student in electrical engineering and computer science, CSAIL affiliate, and lead author on the research. “Our work creates more straightforward abstractions for language models and assigns natural language names and documentation to each one, leading to more interpretable code for programmers and improved system performance.”
When prompted on a programming task, LILO first uses an LLM to quickly propose solutions based on data it was trained on, and then the system slowly searches more exhaustively for outside solutions. Next, Stitch efficiently identifies common structures within the code and pulls out useful abstractions. These are then automatically named and documented by LILO, resulting in simplified programs that can be used by the system to solve more complex tasks.
The MIT framework writes programs in domain-specific programming languages, like Logo, a language developed at MIT in the 1970s to teach children about programming. Scaling up automated refactoring algorithms to handle more general programming languages like Python will be a focus for future research. Still, their work represents a step forward for how language models can facilitate increasingly elaborate coding activities. Ada: Natural language guides AI task planning Just like in programming, AI models that automate multi-step tasks in households and command-based video games lack abstractions. Imagine you’re cooking breakfast and ask your roommate to bring a hot egg to the table — they’ll intuitively abstract their background knowledge about cooking in your kitchen into a sequence of actions. In contrast, an LLM trained on similar information will still struggle to reason about what they need to build a flexible plan. Named after the famed mathematician Ada Lovelace, who many consider the world’s first programmer, the CSAIL-led “Ada” framework makes headway on this issue by developing libraries of useful plans for virtual kitchen chores and gaming. The method trains on potential tasks and their natural language descriptions, then a language model proposes action abstractions from this dataset. A human operator scores and filters the best plans into a library, so that the best possible actions can be implemented into hierarchical plans for different tasks. “Traditionally, large language models have struggled with more complex tasks because of problems like reasoning about abstractions,” says Ada lead researcher Lio Wong, an MIT graduate student in brain and cognitive sciences, CSAIL affiliate, and LILO coauthor. “But we can combine the tools that software engineers and roboticists use with LLMs to solve hard problems, such as decision-making in virtual environments.”
When the researchers incorporated the widely-used large language model GPT-4 into Ada, the system completed more tasks in a kitchen simulator and Mini Minecraft than the AI decision-making baseline “Code as Policies.” Ada used the background information hidden within natural language to understand how to place chilled wine in a cabinet and craft a bed. The results indicated a staggering 59 and 89 percent task accuracy improvement, respectively. With this success, the researchers hope to generalize their work to real-world homes, with the hopes that Ada could assist with other household tasks and aid multiple robots in a kitchen. For now, its key limitation is that it uses a generic LLM, so the CSAIL team wants to apply a more powerful, fine-tuned language model that could assist with more extensive planning. Wong and her colleagues are also considering combining Ada with a robotic manipulation framework fresh out of CSAIL: LGA (language-guided abstraction). Language-guided abstraction: Representations for robotic tasks Andi Peng SM ’23, an MIT graduate student in electrical engineering and computer science and CSAIL affiliate, and her coauthors designed a method to help machines interpret their surroundings more like humans, cutting out unnecessary details in a complex environment like a factory or kitchen. Just like LILO and Ada, LGA has a novel focus on how natural language leads us to those better abstractions. In these more unstructured environments, a robot will need some common sense about what it’s tasked with, even with basic training beforehand. Ask a robot to hand you a bowl, for instance, and the machine will need a general understanding of which features are important within its surroundings. From there, it can reason about how to give you the item you want.
In LGA’s case, humans first provide a pre-trained language model with a general task description using natural language, like “bring me my hat.” Then, the model translates this information into abstractions about the essential elements needed to perform this task. Finally, an imitation policy trained on a few demonstrations can implement these abstractions to guide a robot to grab the desired item. Previous work required a person to take extensive notes on different manipulation tasks to pre-train a robot, which can be expensive. Remarkably, LGA guides language models to produce abstractions similar to those of a human annotator, but in less time. To illustrate this, LGA developed robotic policies to help Boston Dynamics’ Spot quadruped pick up fruits and throw drinks in a recycling bin. These experiments show how the MIT-developed method can scan the world and develop effective plans in unstructured environments, potentially guiding autonomous vehicles on the road and robots working in factories and kitchens.
“In robotics, a truth we often disregard is how much we need to refine our data to make a robot useful in the real world,” says Peng. “Beyond simply memorizing what’s in an image for training robots to perform tasks, we wanted to leverage computer vision and captioning models in conjunction with language. By producing text captions from what a robot sees, we show that language models can essentially build important world knowledge for a robot.” The challenge for LGA is that some behaviors can’t be explained in language, making certain tasks underspecified. To expand how they represent features in an environment, Peng and her colleagues are considering incorporating multimodal visualization interfaces into their work. In the meantime, LGA provides a way for robots to gain a better feel for their surroundings when giving humans a helping hand.
An “exciting frontier” in AI
“Library learning represents one of the most exciting frontiers in artificial intelligence, offering a path towards discovering and reasoning over compositional abstractions,” says assistant professor at the University of Wisconsin-Madison Robert Hawkins, who was not involved with the papers. Hawkins notes that previous techniques exploring this subject have been “too computationally expensive to use at scale” and have an issue with the lambdas, or keywords used to describe new functions in many languages, that they generate. “They tend to produce opaque 'lambda salads,' big piles of hard-to-interpret functions. These recent papers demonstrate a compelling way forward by placing large language models in an interactive loop with symbolic search, compression, and planning algorithms. This work enables the rapid acquisition of more interpretable and adaptive libraries for the task at hand.” By building libraries of high-quality code abstractions using natural language, the three neurosymbolic methods make it easier for language models to tackle more elaborate problems and environments in the future. This deeper understanding of the precise keywords within a prompt presents a path forward in developing more human-like AI models. MIT CSAIL members are senior authors for each paper: Joshua Tenenbaum, a professor of brain and cognitive sciences, for both LILO and Ada; Julie Shah, head of the Department of Aeronautics and Astronautics, for LGA; and Jacob Andreas, associate professor of electrical engineering and computer science, for all three. The additional MIT authors are all PhD students: Maddy Bowers and Theo X. Olausson for LILO, Jiayuan Mao and Pratyusha Sharma for Ada, and Belinda Z. Li for LGA. Muxin Liu of Harvey Mudd College was a coauthor on LILO; Zachary Siegel of Princeton University, Jaihai Feng of the University of California at Berkeley, and Noa Korneev of Microsoft were coauthors on Ada; and Ilia Sucholutsky, Theodore R. Sumers, and Thomas L. Griffiths of Princeton were coauthors on LGA. LILO and Ada were supported, in part, by MIT Quest for Intelligence, the MIT-IBM Watson AI Lab, Intel, U.S. Air Force Office of Scientific Research, the U.S. Defense Advanced Research Projects Agency, and the U.S. Office of Naval Research, with the latter project also receiving funding from the Center for Brains, Minds and Machines. LGA received funding from the U.S. National Science Foundation, Open Philanthropy, the Natural Sciences and Engineering Research Council of Canada, and the U.S. Department of Defense.
Share this news article on:
Related links.
- Jacob Andreas
- Computer Science and Artificial Intelligence Laboratory (CSAIL)
- MIT Language and Intelligence Group
- Center for Brains, Minds, and Machines
- Department of Electrical Engineering and Computer Science
- Department of Brain and Cognitive Sciences
- Department of Aeronautics and Astronautics
Related Topics
- Computer science and technology
- Artificial intelligence
- Natural language processing
- Electrical Engineering & Computer Science (eecs)
- Programming
- Human-computer interaction
- Computer vision
- Brain and cognitive sciences
- Programming languages
- Center for Brains Minds and Machines
- Quest for Intelligence
- MIT-IBM Watson AI Lab
- National Science Foundation (NSF)
- Department of Defense (DoD)
- Defense Advanced Research Projects Agency (DARPA)
Related Articles
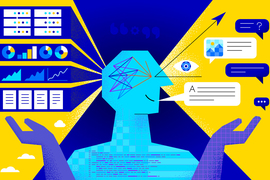
Large language models use a surprisingly simple mechanism to retrieve some stored knowledge
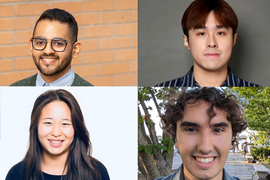
Reasoning and reliability in AI
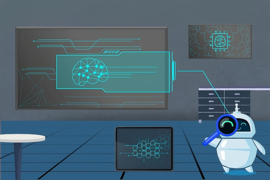
AI agents help explain other AI systems
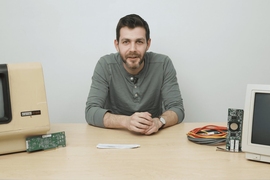
3 Questions: Jacob Andreas on large language models
Previous item Next item
More MIT News
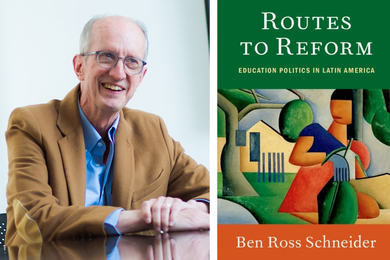
Trying to make the grade
Read full story →
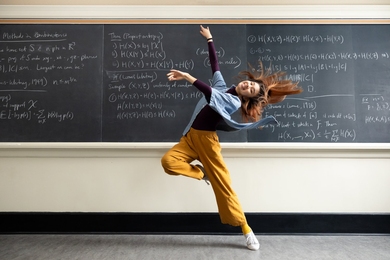
Janabel Xia: Algorithms, dance rhythms, and the drive to succeed
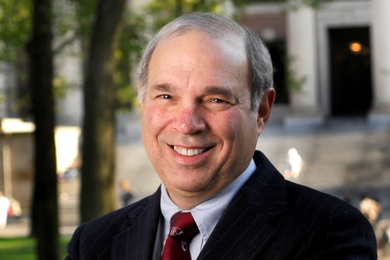
Jonathan Byrnes, MIT Center for Transportation and Logistics senior lecturer and visionary in supply chain management, dies at 75
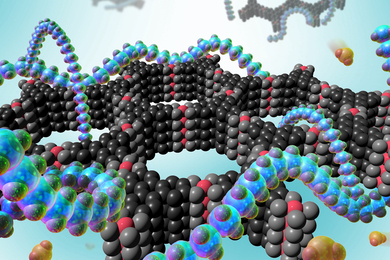
Researchers develop a detector for continuously monitoring toxic gases
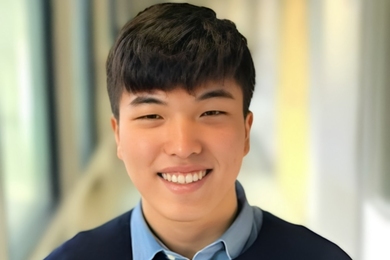
The beauty of biology
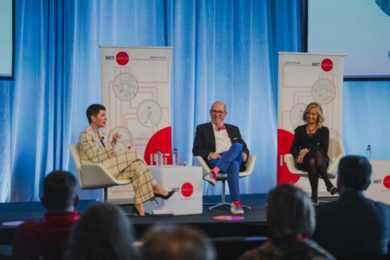
Navigating longevity with industry leaders at MIT AgeLab PLAN Forum
- More news on MIT News homepage →
Massachusetts Institute of Technology 77 Massachusetts Avenue, Cambridge, MA, USA
- Map (opens in new window)
- Events (opens in new window)
- People (opens in new window)
- Careers (opens in new window)
- Accessibility
- Social Media Hub
- MIT on Facebook
- MIT on YouTube
- MIT on Instagram
- HPCWire - ORNL Researchers Develop an AI-driven Tool for Nea...
- The Mirage - AI Streamlines Cancer Pathology Reporting at Oak R...
- HPC Wire - OLCF’s Summit Supercomputer Lives for Another Year...
- WATE - NASA uses Oak Ridge National Lab’s supercomputers ...
- InsideHPC - Exascale’s New Software Frontier: E3SM-MMF
- Staff Directory
Search the Site

Going Big: World’s Fastest Computer Takes On Large Language Modeling
Frontier could fuel next generation of AI for open science
A team led by researchers at the Department of Energy’s Oak Ridge National Laboratory explored training strategies for one of the largest artificial intelligence models to date with help from the world’s fastest supercomputer.
The findings could help guide training for a new generation of AI models for scientific research.
The study led by ORNL’s Sajal Dash, Feiyi Wang and Prasanna Balaprakash employed Frontier , the world’s first exascale supercomputer, to run the initial stages of training on a large language model similar to OpenAI’s ChatGPT . The research team used a set of test data to project how models with 22 billion, 175 billion, and 1 trillion parameters, or variables, could run across 128 and later 384 of Frontier’s more than 9,400 nodes. The team didn’t attempt to train a full model to completion.
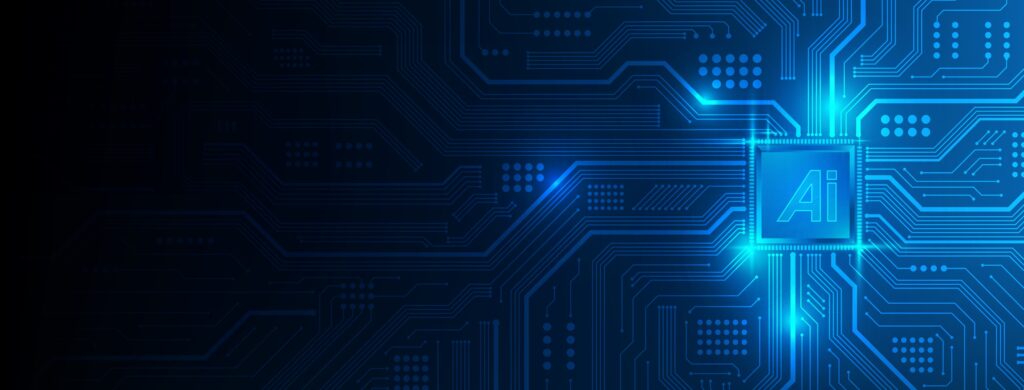
A team led by OLCF researchers used Frontier to explore training strategies for one of the largest artificial intelligence models to date. Credit: Getty Images
“This study and our findings aren’t so much a manual as a potential set of guidelines for users training a large model,” Dash said. “They can draw from our experience to decide how to use Frontier’s resources to train their particular model and make the most effective use of their allotted computing time.”
The team presented the study at the International Supercomputing Conference High Performance 2024 in May in Hamburg, Germany. Fellow scientists Isaac Lyngaas, Junqi Yin, Xiao Wang and Guojing Cong of ORNL and Romaine Egele of Paris-Saclay University also collaborated on the study.
The study focused less on model development than on pinpointing the most efficient ways to exploit the graphics processing units, or GPUs, that power Frontier and similar supercomputers and putting them to work training an AI. Each of Frontier’s nodes relies on four AMD MI250X GPUs for a total of more than 38,000 GPUs.
The training ran for a few hours on about 100 million tokens — basic units of text such as words and characters — of test data. That’s about a ten-thousandth of the necessary data to train a trillion-parameter model to completion and an even smaller fraction of the necessary time.
The research team used the data from those runs to calculate how a trillion-parameter model might perform if trained to completion on Frontier.
“This study was largely an exercise to show we can train this particular size of model on Frontier at this particular scale with this particular level of efficiency,” Wang said. “We didn’t get anywhere near the finish line of a complete large language model.”
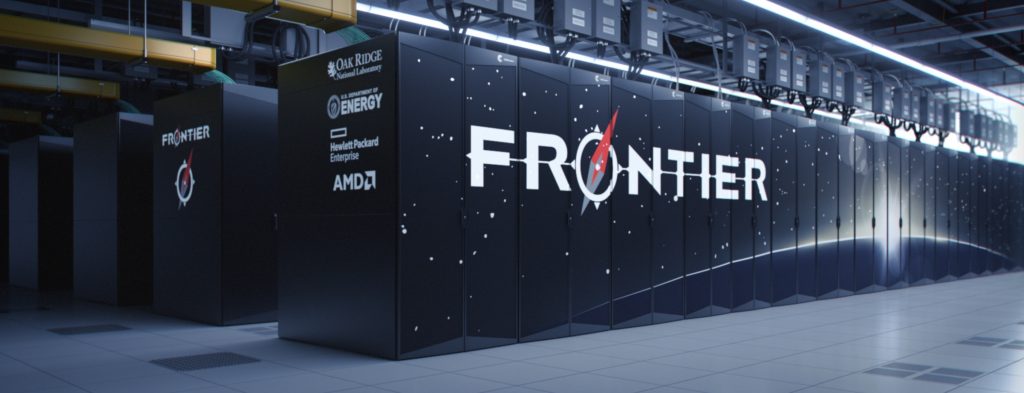
An artist’s rendering of the Frontier supercomputer. Credit: Sibling Rivalry/HPE
Large language models loosely mimic the human brain in their ability to learn and recognize patterns in words and numbers and to improve on that learning over time with additional training. The goal: design a model that can absorb and adjust the lessons learned on training data and apply that knowledge consistently and accurately to new, unfamiliar data and tasks.
The vast datasets and powerful processors needed for such training have remained mostly out of reach of scholars and in the possession of private companies, which tend to guard those resources as proprietary and set strict conditions for use. Those conditions typically limit research opportunities and don’t allow results to be easily verified.
But leadership-class supercomputers like Frontier, which awards computing time to scientific researchers through the DOE’s Innovative and Novel Computational Impact on Theory and Experiment program , could enable a new generation of AI models to be trained more quickly if scientists find the right approach.
“Traditionally, this process has relied on expert knowledge or on trial and error,” said Prasanna Balaprakash, ORNL’s director of AI programs. “One of the highlights of our work in this study is the automation of identifying high-performing strategies among a vast array of options. We leveraged DeepHyper, an open-source scalable tuning software, to automatically determine the optimal settings. We plan to extend this automated approach to fine-tune system-level performance and enhance efficiency at an extreme scale. Furthermore, we have democratized our methodologies and software for the benefit of the scientific community. This strategy ensures that our insights are widely accessible for future research on training large AI foundation models in science.”
The larger the model and its training datasets, the better its performance — but also the higher its demand for computational power. Training a trillion-parameter large language model from the initial stages to completion without optimizations would take months even at Frontier’s world-leading speeds.
The ORNL study examined approaches to data parallelism — a process used by supercomputers like Frontier to break a large problem into smaller problems to reach a solution more quickly — to train AI and how to port that training across proprietary frameworks of GPUs made by competing vendors.
“It’s about finding the best combination of training strategies while getting the best throughput,” Dash said. “Most deep-learning frameworks target the GPUs made by NVIDIA rather than the GPUs made by AMD that power Frontier. We wanted to see if existing models could run on Frontier, how to make the best use of Frontier’s computing power and how to make that level of performance possible across GPU platforms.
“We can’t train a model this size on a single GPU or a single node, for example, and every time we cross the barrier between nodes that requires more communication that consumes more time. How do we slice up the model across GPUs so that we can fit and train the model without losing too much time and energy communicating between nodes?”
The researchers found a blend of parallelism strategies worked best when tailored to the computing platform but said their work’s far from finished.
“The efficiency we achieved on Frontier with this model was decent but not decent enough,” Wang said. “At extreme scale, we achieved 30% efficiency — which means we left about 70% of Frontier’s computing power on the floor. We need much more optimization to make the machine more efficient at this scale.”
The team’s next steps include training a model further with peer-reviewed scientific data across more nodes.
Support for this research came from the DOE Office of Science’s Advanced Scientific Computing Research program and ORNL’s AI Initiative. The OLCF is a DOE Office of Science user facility.
UT-Battelle manages ORNL for DOE’s Office of Science, the single largest supporter of basic research in the physical sciences in the United States. DOE’s Office of Science is working to address some of the most pressing challenges of our time. For more information, visit https://energy.gov/science .
You Might Also Like
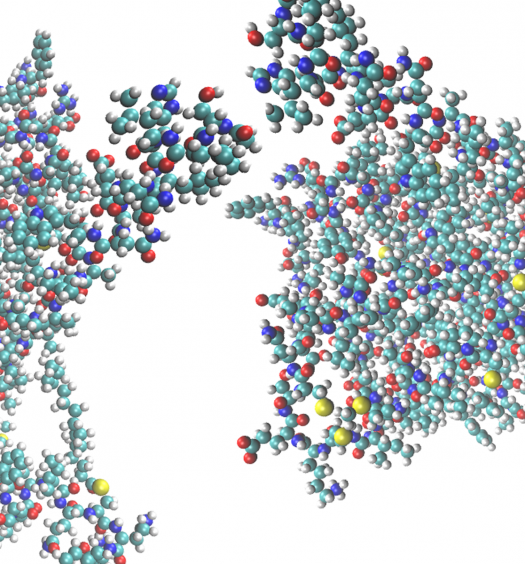
Machine Learning for Better Drug Design
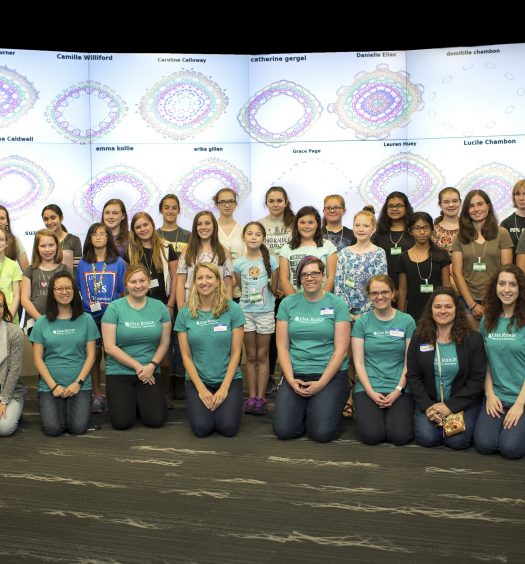
OLCF Staff Reach Students through Coding Events

Meet the NCCS and OLCF Director: Arjun Shankar

Frontier Search for Lightweight, Flexible Alloys Wins Gordon Bell Prize

Breaking Benchmarks: Frontier Supercomputer Sets New Standard in Molecular Simulation

How Hot Is Too Hot in Fusion?

Something in the Water Does Not Compute
May 14, 2024
May 13, 2024
May 6, 2024

ORNL and SLAC Team Up for Breakthrough Biology Projects

Summit Helps Forge Stronger Flights
April 30, 2024
Oak Ridge Leadership Computing Facility
- Application Software Development
- Data Management
- File and Storage Systems
- Non-Volatile Memory
- Operational Efficiency
- Outreach and Education
- Scientific Research
- System Architecture
Oak Ridge National Laboratory is managed by UT-Battelle for the US Department of Energy.
- DOE Office of Science
- Battelle.org
- Accessibility
Oak Ridge Leadership Computing Facility One Bethel Valley Rd P.O. Box 2008 Oak Ridge, TN 37831
Support Email: [email protected]
Quick Links
- Publications
Connect with OLCF

- Our History
- Director’s Letter
- OLCF Groups
- Industry Partnership Program
- OLCF User Group
- Visiting & Tours
- Compute Systems
- Data & Visualization Resources
- Computer Science
- Earth Science
- Engineering
- Materials Science
- Nuclear Energy
- Getting Started
- System User Guides
- OLCF Policy Guide
- Documents & Forms
- User Assistance
- OLCF in the News
- Center Reports
- Media Assets
Helpful Links
- Acknowledgement Statement
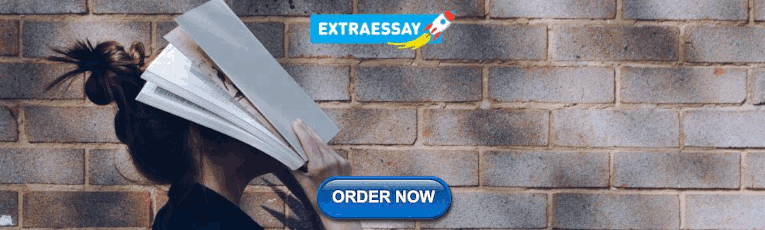
IMAGES
VIDEO
COMMENTS
About the journal. Computer Speech & Language publishes reports of original research related to the recognition, understanding, production, coding and mining of speech and language. The speech and language sciences have a long history, but it is only relatively recently that large-scale implementation of and experimentation with complex models ...
Trends and developments in automatic speech recognition research. Douglas O'Shaughnessy. Article 101538. View PDF. Article preview. Read the latest articles of Computer Speech & Language at ScienceDirect.com, Elsevier's leading platform of peer-reviewed scholarly literature.
Computer Speech and Language Review Speed, Peer-Review Duration, Revision Process, Time from Submission to 1st Editorial/Reviewer Decision & Time from Submission to Acceptance/Publication. Forum. Toolbox . ... Based on the Computer Speech and Language Review Speed Feedback System, it takes authors - days to get the first editorial decision.
Corrigendum to 'Unsupervised sign language validation process based on hand-motion parameter clustering' <Computer Speech & Language Volume 71, January 2022, 101256>. Mehrez Boulares, Ahmed Barnawi. Article 101319.
Computer Speech and Language. Search within CSPL. Search Search. Home; Browse by Title; Periodicals; Computer Speech and Language; Computer Speech and Language. Volume 85, Issue C. Apr 2024. Read More. Academic Press Ltd. 24-28 Oval Rd. London NW1 7DX; United Kingdom; Get Alerts for this Periodical Alerts. Share on.
Frequency and time resolution can be adjusted ... Abstract . Automatic speaker verification systems are found to be vulnerable to spoof attacks such as voice conversion, text-to-speech, and replayed speech. As the security of biometric systems is vital, many countermeasures have been developed for spoofed ...
COMPUTER SPEECH AND LANGUAGE ... Return to Search. APA has partnered with LetPub to provide a full suite of author services. Learn more *Review Duration >12 Week(s), or Invited contributions *Competitiveness Easy. CiteScore 8.8 . CiteScore Rank. Subject Area Rank Percentile; Category: Mathematics
Computer Speech and Language Acceptance Rate Acceptance Rate. The acceptance rate for an academic journal is dependent upon the relative demand for publishing in a particular journal, the peer review processes in place, the mix of invited and unsolicited submissions, and time to publication, among others.
Review of diarization techniques belonging to the proposed taxonomy. ... Conv-tasnet: Surpassing ideal time-frequency magnitude masking for speech separation, 27 (8) (2019) 1256 ... Computer Speech and Language Volume 72, Issue C. Mar 2022. 479 pages. ISSN: 0885-2308. Issue's Table of Contents.
Native language ability, exposure time, ... and optimize computer speech recognition models (Bhatt et al., 2021) in order to identify speech variations in a more intelligent, accurate, and exhaustive manner. ... Connected speech in neurodegenerative language disorders: a review. Front. Psychol. 8:269. doi: 10.3389/fpsyg.2017.00269, ...
Language and Speech is a peer-reviewed journal which provides an international forum for communication among researchers in the disciplines that contribute to our understanding of human production, perception, processing, learning, use, and disorders of speech and language. The journal accepts reports of original research in all these areas. Interdisciplinary submissions are e
The link between screen time and speech and language development is not straightforward, and several factors need to be considered. ... 85.4% have a laptop/computer, 43.2% have a tablet, 16.7% have a streaming television or device, and 18.8% have a smart watch. These statistics are important as it assists in understanding the percentage of ...
An analysis of observation length requirements for machine understanding of human behaviors from spoken language. Sandeep Nallan Chakravarthula, Brian R.W. Baucom, Shrikanth Narayanan, Panayiotis Georgiou. Article 101162.
Speaker diarization is a task to label audio or video recordings with classes that correspond to speaker identity, or in short, a task to identify "who spoke when". In the early years, speaker diarization algorithms were developed for speech recognition on multispeaker audio recordings to enable speaker adaptive processing.
1.1.4.2. Excluded studies. Two Cochrane reviews 3, 46 were identified and excluded from this review. For Brady 2016 3 this was due to the review including all speech and language therapy studies for people with aphasia, rather than just those that had computer-based tools being implemented. For West 2005 46 this included all speech and language therapy studies for people with apraxia of speech ...
Computer Speech And Language. You may order single or multiple copies of back and recent journal issues. If you are an Author wishing to obtain a printed copy of the journal issue featuring your article, or you require a printed copy for research, review or add to your library, the process is easy -. Select your journal volume and issue.
Presents an in-depth analysis of the state of the art in analysis of real-time MRI data of speech production. Describes with examples a unified, graphical taxonomy in which these methods can be understood and extended. Highlights a representative list of linguistic, speech and clinical applications which leverage these analysis techniques.
Exploring intrinsic information content models for addressing the issues of traditional semantic measures to evaluate verb similarity. M. Krishna Siva Prasad, Poonam Sharma. Article 101280. View PDF. Article preview. select article Local and non-local dependency learning and emergence of rule-like representations in speech data by deep ...
NIST SRE 2000 (Disk-8), often referred to as the CALL-HOME dataset, is the most widely used dataset for speaker diarization in recent papers. This dataset contains 500 ses-sions of multilingual telephonic speech. Each session has two to seven speakers with two dominant speakers in each (33) conversation.
Scaling up automated refactoring algorithms to handle more general programming languages like Python will be a focus for future research. Still, their work represents a step forward for how language models can facilitate increasingly elaborate coding activities. Ada: Natural language guides AI task planning
Speech and voice play a crucial role in self-expression and communication. They are used for interacting with virtual assistants, conveying emotions, and establishing identity. Addressing security and privacy concerns in speech communication is increasingly important for various sectors, including commercial, forensic, and government applications.
We have categorized speech GANs based on application areas: speech synthesis, speech enhancement & conversion, and data augmentation in automatic speech recognition and emotion speech recognition systems. This review also includes a summary of the data sets and evaluation metrics commonly used in speech GANs.
The study led by ORNL's Sajal Dash, Feiyi Wang and Prasanna Balaprakash employed Frontier, the world's first exascale supercomputer, to run the initial stages of training on a large language model similar to OpenAI's ChatGPT. The research team used a set of test data to project how models with 22 billion, 175 billion, and 1 trillion ...
Some speech recognition vendors (like Google at the time of writing this review) do not even report filled pauses (which are typically very strong turn-holding cues) in the user's speech. Meena et al. (2014) explored the effect of ASR errors on their IPU-based model. Whereas prosody had very little contribution to the performance when the ASR ...
Dubey and Kumar, 2017 Dubey R.K., Kumar A., Multiple time-instances features of degraded speech for single ended quality measurement, ... Computer Speech and Language Volume 79, Issue C. Apr 2023. 126 pages. ISSN: 0885-2308. Issue's Table of Contents. Elsevier Ltd. Sponsors. In-Cooperation.