We're sorry but you will need to enable Javascript to access all of the features of this site.
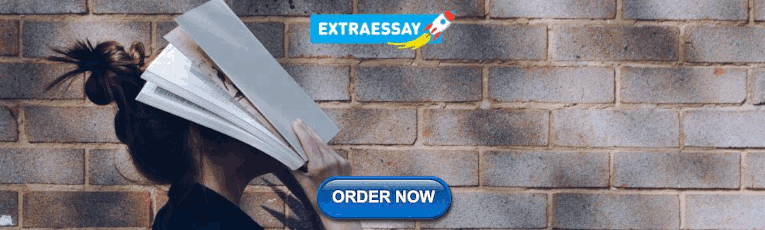
Stanford Online
Clinical trials: design, strategy, and analysis.
SOM-XCHE0030
Stanford School of Medicine , Stanford Center for Health Education
New medical innovations are constantly hitting the market and changing patient care and research - but what goes into creating these technologies? Researchers need to design, conduct, and report on clinical trials to create impactful technologies that can better our understanding of health and medicine. From learning frameworks and reporting methods to determining how to accurately collect, manage, and measure data, you’ll gain the knowledge necessary to understand the components that go into running a successful clinical trial. Whether you’re in a patient-facing role looking to implement research into your professional career or seeking how to better communicate and collaborate with colleagues working in the labs, the clinical trials course gives you the tools you need to find answers for your health and medicine-related inquiries.
Learning Objectives:
- Understand and apply the principles of clinical trial design, such as using frameworks to create research questions and establish study objectives
- Ensure diverse, representative study populations by studying participant selection criteria and recruitment and retention strategies
- Minimize bias and ensure trial reliability and validity through blinding techniques and randomization methods
- Use effective data collection and management techniques to ensure data integrity throughout trials
- Effectively communicate trial findings through an understanding of reporting methods, ethical considerations, and regulatory bodies
Course Outline
Begin your studies of clinical trials with an introduction to the background and rationale, a review of protocol development and trial registration, and an outline of a trial’s phases and stages. | Compare the advantages and disadvantages of different trial types and review three frameworks for clinical trials. |
Learn how to organize a research question using PICOT framework, be able to identify two types of statistical hypotheses, and explore Type I and II errors. | Understand methods for recruitment, retention, and tracking, and dive into the ethical principles and nuances of human subject research. |
Discover the best practices for randomization and blinding for intervention and comparison. | Explore multiple testing methods, outline strategies for controlling Type I errors, and understand the importance of selecting and refining primary outcomes. |
Define Adverse Events (AE) and the roles and responsibilities of the Data and Safety Monitoring Boards (DSMBs). | Dive into Regulatory Framework and the Medical Device Regulatory Strategy. Data Collection, Management, and Sharing Uncover the importance of reproducible research techniques and adequate data checking and cleaning. |
Review CONSORT reporting guidelines and scientific manuscript tips for accurately conveyed information. |
Accreditation In support of improving patient care, Stanford Medicine is jointly accredited by the Accreditation Council for Continuing Medical Education (ACCME), the Accreditation Council for Pharmacy Education (ACPE), and the American Nurses Credentialing Center (ANCC), to provide continuing education for the healthcare team.
Credit Designation American Medical Association (AMA) The Stanford University School of Medicine designates this enduring material for a maximum of 12.00 AMA PRA Category 1 Credits ™. Physicians should claim only the credit commensurate with the extent of their participation in the activity.
View the full accreditation information HERE from Stanford Medicine
Core Competencies
- Trial Phases
- Study Design
- Hypothesis Testing
- Statistical Analysis
Small Groups and Team Programs
Special Pricing
Enroll as a group or team and learn together. We can advise you on the best group options to meet your organization’s training and development goals and provide you with the support needed to streamline the process. Participating together, your group will develop a shared knowledge, language, and mindset to tackle the challenges ahead.
What Our Learners Are Saying
I recently completed the "Clinical Trials: Design, Strategy, and Analysis" course, and it was outstanding. The presenters were not only knowledgeable but also incredibly engaging, making complex concepts easy to understand and directly applicable to real-world scenarios. Highly recommend for anyone looking to deepen their understanding of clinical trials." - Carole-Anne B., Medical Manager
Teaching Team

Regina Nuzzo
Gallaudet University

Kristin Sainani
Epidemiology and Population Health
Kristin Sainani (née Cobb) is an associate professor at Stanford University and also a health and science writer. After receiving an MS in statistics and a PhD in epidemiology from Stanford University, she studied science writing at the University of California, Santa Cruz. She has taught statistics and writing at Stanford for more than a decade and has received several Excellence in Teaching Awards from the graduate program in epidemiology. Dr. Sainani writes about science and health for a range of audiences. She authored the health column Body News for Allure magazine for a decade. She is also the statistical editor for the journal Physical Medicine & Rehabilitation; and she authors a statistics column, Statistically Speaking, for this journal.
You May Also Like

Design and Conduct of Clinical Trials
Stanford School of Medicine

Thinking Critically: Interpreting Randomized Clinical Trials (CME)
SOM-YCME0019

Introduction to Clinical Data
SOM-XCHE0009
Stanford School of Medicine, Stanford Center for Health Education

Clinical Trial Design: From Initial Concept to Regulatory Approval
- Engineering
- Artificial Intelligence
- Computer Science & Security
- Business & Management
- Energy & Sustainability
- Data Science
- Medicine & Health
- Explore All
- Technical Support
- Master’s Application FAQs
- Master’s Student FAQs
- Master's Tuition & Fees
- Grades & Policies
- HCP History
- Graduate Application FAQs
- Graduate Student FAQs
- Graduate Tuition & Fees
- Community Standards Review Process
- Academic Calendar
- Exams & Homework FAQs
- Enrollment FAQs
- Tuition, Fees, & Payments
- Custom & Executive Programs
- Free Online Courses
- Free Content Library
- School of Engineering
- Graduate School of Education
- Stanford Doerr School of Sustainability
- School of Humanities & Sciences
- Stanford Human Centered Artificial Intelligence (HAI)
- Graduate School of Business
- Stanford Law School
- School of Medicine
- Learning Collaborations
- Stanford Credentials
- What is a digital credential?
- Grades and Units Information
- Our Community
- Get Course Updates
Understanding Clinical Data Analysis
Learning Statistical Principles from Published Clinical Research
- © 2017
- Ton J. Cleophas 0 ,
- Aeilko H. Zwinderman 1
Albert Schweitzer Hospital, Department Medicine Albert Schweitzer Hospital, Sliedrecht, The Netherlands
You can also search for this author in PubMed Google Scholar
Dept. Epidemiology and Biostatistics, Academic Medical Center Dept. Epidemiology and Biostatistics, Amsterdam, The Netherlands
- The book uses the best-help-there-is for making the difficult issues understandable by using real data examples rather than hypothetical examples
- Complementarily to real data examples, the book continually gives a philosophical treatise of the basics of the scientific method.
- The book explains all of the novel issues of clinical data analysis from the past few years.
21k Accesses
4 Citations
1 Altmetric
This is a preview of subscription content, log in via an institution to check access.
Access this book
Subscribe and save.
- Get 10 units per month
- Download Article/Chapter or eBook
- 1 Unit = 1 Article or 1 Chapter
- Cancel anytime
- Available as EPUB and PDF
- Read on any device
- Instant download
- Own it forever
- Compact, lightweight edition
- Dispatched in 3 to 5 business days
- Free shipping worldwide - see info
- Durable hardcover edition
Tax calculation will be finalised at checkout
Other ways to access
Licence this eBook for your library
Institutional subscriptions
About this book
This textbook consists of ten chapters, and is a must-read to all medical and health professionals, who already have basic knowledge of how to analyze their clinical data, but still, wonder, after having done so, why procedures were performed the way they were. The book is also a must-read to those who tend to submerge in the flood of novel statistical methodologies, as communicated in current clinical reports, and scientific meetings.
In the past few years, the HOW-SO of current statistical tests has been made much more simple than it was in the past, thanks to the abundance of statistical software programs of an excellent quality. However, the WHY-SO may have been somewhat under-emphasized. For example, why do statistical tests constantly use unfamiliar terms, like probability distributions, hypothesis testing, randomness, normality, scientific rigor, and why are Gaussian curves so hard, and do they make non-mathematicians getting lost all the time? Thebook will cover the WHY-SOs.
Similar content being viewed by others
Biostatistics
Essential Statistical Tests
- Statistical Reasoning
- Hypothesis Testing
- Clinical data analysis
- Statistical methodologies
- Medical Statistics
Table of contents (10 chapters)
Front matter.
- Ton J. Cleophas, Aeilko H. Zwinderman
Randomized and Observational Research
Randomized clinical trials, history, designs, randomized clinical trials, analysis sets, statistical analysis, reporting issues, discrete data analysis, failure time data analysis, quantitative data analysis, subgroup analysis, interim analysis, multiplicity analysis, medical statistics: a discipline at the interface of biology and mathematics, back matter, authors and affiliations.
Ton J. Cleophas
Aeilko H. Zwinderman
About the authors
The authors are well-qualified in their field. Professor Zwinderman is past-president of the International Society of Biostatistics (2012-2015), and Professor Cleophas is past-president of the American College of Angiology (2000-2002). From their expertise they should be able to choose the best-help-there-is for making difficult issues understandable, that is real data examples from the global literature rather than hypothetical examples.
The authors have been working and publishing together for 18 years, and their research can be characterized as a continued effort to demonstrate that clinical data analysis is not mathematics but rather a discipline at the interface of philosophy, biology, and mathematics.
The authors, as professors and teachers in statistics at universities in The Netherlands and France for the most part of their lives, are convinced, that the scientific method of statistical reasoning and hypothesis testing is little used by physicians and otherhealth workers, and they hope, that the current production will help them find the appropriate ways for answering their scientific questions.
Bibliographic Information
Book Title : Understanding Clinical Data Analysis
Book Subtitle : Learning Statistical Principles from Published Clinical Research
Authors : Ton J. Cleophas, Aeilko H. Zwinderman
DOI : https://doi.org/10.1007/978-3-319-39586-9
Publisher : Springer Cham
eBook Packages : Medicine , Medicine (R0)
Copyright Information : Springer International Publishing Switzerland 2017
Hardcover ISBN : 978-3-319-39585-2 Published: 31 August 2016
Softcover ISBN : 978-3-319-81917-4 Published: 14 June 2018
eBook ISBN : 978-3-319-39586-9 Published: 23 August 2016
Edition Number : 1
Number of Pages : X, 234
Number of Illustrations : 119 b/w illustrations, 92 illustrations in colour
Topics : Medicine/Public Health, general
- Publish with us
Policies and ethics
- Find a journal
- Track your research
Thank you for visiting nature.com. You are using a browser version with limited support for CSS. To obtain the best experience, we recommend you use a more up to date browser (or turn off compatibility mode in Internet Explorer). In the meantime, to ensure continued support, we are displaying the site without styles and JavaScript.
- View all journals
- Explore content
- About the journal
- Publish with us
- Sign up for alerts
- Perspective
- Open access
- Published: 26 July 2019
Artificial intelligence and machine learning in clinical development: a translational perspective
- Pratik Shah 1 na1 ,
- Francis Kendall 1 , 2 na1 ,
- Sean Khozin 3 ,
- Ryan Goosen 4 ,
- Jianying Hu 5 ,
- Jason Laramie 6 ,
- Michael Ringel 4 &
- Nicholas Schork 7
npj Digital Medicine volume 2 , Article number: 69 ( 2019 ) Cite this article
73k Accesses
272 Citations
107 Altmetric
Metrics details
- Computer science
- Translational research
Future of clinical development is on the verge of a major transformation due to convergence of large new digital data sources, computing power to identify clinically meaningful patterns in the data using efficient artificial intelligence and machine-learning algorithms, and regulators embracing this change through new collaborations. This perspective summarizes insights, recent developments, and recommendations for infusing actionable computational evidence into clinical development and health care from academy, biotechnology industry, nonprofit foundations, regulators, and technology corporations. Analysis and learning from publically available biomedical and clinical trial data sets, real-world evidence from sensors, and health records by machine-learning architectures are discussed. Strategies for modernizing the clinical development process by integration of AI- and ML-based digital methods and secure computing technologies through recently announced regulatory pathways at the United States Food and Drug Administration are outlined. We conclude by discussing applications and impact of digital algorithmic evidence to improve medical care for patients.
Similar content being viewed by others
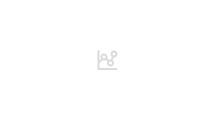
The need for a system view to regulate artificial intelligence/machine learning-based software as medical device
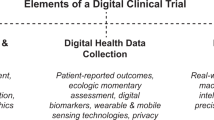
Digitizing clinical trials
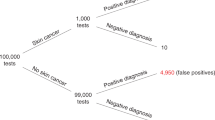
Direct-to-consumer medical machine learning and artificial intelligence applications
Introduction.
Clinical drug development has remained relatively unchanged for the last 30 years. This is due, in part, to uncertainties in regulatory requirements, risk aversion, and skepticism about rapidly emerging, yet largely unproven, technologies (such as machine learning, and wireless health monitoring devices and sensors), and the lack of relevant actionable biomedical data sources and advanced analytics to generate hypotheses that could motivate the development of innovative diagnostics and therapies. Testing new biomedical treatments for safety and efficacy will also require new strategies, since it has been shown that existing therapies often only work for a small number of indicated individuals. The application of emerging digital technologies, such as next-generation sequencing, though, have increased both our understanding of disease mechanisms in larger pool of patients and the potential for developing personalized therapies. For example, the majority of the new molecular entities approved by the U.S. FDA in recent years were designed to target specific aberrations implicated in disease initiation and maintenance—a hallmark of precision medicine—which aims to tailor interventions based on individual characteristics of patients. 1 In this light, an emerging strategy based on co-developing precision diagnostics and therapeutic agents as companion diagnostics for example may produce highly effective drugs with clinical outcomes that greatly exceed standard therapies. 2 , 3
Another key challenge in the clinical development process is linked to reporting the results of most conventional clinical trials of average treatment effects that may not easily translate into making individualized treatment decisions at the routine point-of-care. 4 Promising approaches to overcoming this challenge are more streamlined processes, exploiting new digital clinical endpoints and treatment response biomarkers amenable to close and efficient monitoring (such as circulating tumor DNA), improve safety and efficacy while reducing toxicity and adverse events and greater insights into the patient journey via sensors, and low cost imaging. 5 , 6 , 7 , 8 Securing, standardizing, and enhancing routinely collected EHR data as a source of credible medical evidence based on RWD can facilitate the organization of clinical trials at the point-of-care and should serve to improve the clinical development process. 9
Machine learning and computer vision have enhanced many aspects of human visual perception to identify clinically meaningful patterns in, e.g., imaging data, 10 and neural networks are been used for variety of tasks ranging from medical image segmentation, generation, classification, and prediction of clinical data sets. 11 Broadly academic research labs, biotechnology corporations, and technology companies have been exploring the use of AI and ML in three key areas:
machine-based learning to predict pharmaceutical properties of molecular compounds and targets for drug discovery; 12 , 13
using pattern recognition and segmentation techniques on medical images (from, e.g., retinal scans, pathology slides and body surfaces, bones and internal organs) to enable faster diagnoses and tracking of disease progression; 14 , 15 and generative algorithms for computational augmentation of existing clinical and imaging data sets; 16
developing deep-learning techniques on multimodal data sources such as combining genomic and clinical data to detect new predictive models. 17 , 18
Despite these propositions for the use of ML to accelerate medical research, very few successful use cases have emerged. These limited successes have been attributed to, among other things, insufficient time elapsing since the introduction of relevant technologies and deficiency of current computer science deep learning and related ML models to generalize more complex and realistic medical data sets and tasks. 19 , 20 Other important factors that impede the adoption of AI/ML techniques in therapeutic development include the paucity of large numbers of high-quality labeled data, nascent regulations, and ethical and legal concerns about data sharing. Alternative learning systems that leverage human brain and its neocortex and learn from fewer examples have been proposed as alternatives to deep learning, but have not been widely adopted. 21 Recently, perspectives and commentaries highlighting applications of DNN to imaging data sets, pharmaceutical properties of compounds, clinical diagnoses and genomics, computer vision applications for medical imaging, and applications of Natural Language Processing to EHR have been published. 22 , 23 These predominantly focused on data in primary care or hospital ecosystem and early drug discovery applications, and did not describe use cases and regulatory framework derived from a multi-stakeholder perspective for successful embedding of AI and ML and RWE into the process of clinical development outlined in this perspective.
From March 2017 to December 2018, a series of six broad, cross-institutional workshops were convened at The MIT Media Lab to discuss the current state of AI and ML and RWE usage in clinical development opportunities, challenges, and ways of addressing challenges. Participation was designed to be multidisciplinary and multi-stakeholder, involving leading researchers from academic institutions, leaders from biopharma firms, foundations technology corporations, and regulators to engender broad outlook and cross-functional perspectives. Each two-part workshop was structured as follows: a series of talks outlining current challenges and opportunities and regulatory insights for introducing AI and ML in the clinical development process either as researchers or adopters, followed by a brainstorming session with breakaway groups focusing on specific themes. This manuscript, a consolidated viewpoint on infusion of AI and ML in clinical development, is one of the key outputs of the workshop. We focus on three key themes discussed in the workshops related to development of next-generation medicines by adoption of digital evidence generated by AI and ML: (1) validation and modernizing the clinical trials process, (2) strategies for rational use of AI- and ML-driven learning from real-world data and evidence and, (3) required regulatory oversight for integration, explanation, and de-risking of AI/ML digital analytics in medical care to patients. A glossary is provided as Supplementary Material for explanation of key terms.
MIT Workshops discussed new pathways set up by regulatory agencies for evaluation and adoption of AI and ML in clinical development. For example, in 2016, the 21 st Century Cures Act was signed into law, a significant bipartisan legislative achievement aimed at accelerating the discovery, development, and delivery of new cures and treatments was highlighted. FDA’s current strategic policy places emphasis on leveraging innovation, advancing digital health technologies, and developing next-generation analytical approaches to improve health care, broaden access, and advance public health goals. SaMD and digital health pathways for regulatory approvals for AI, ML, and computer vision algorithms have been set up at FDA. 24 , 25 To date, FDA has cleared or approved several AI/ML-based SaMD. Typically, these have only included algorithms that are locked prior to marketing. For example, FDA has approved diagnostics company IDx’s ML-based software system for autonomous detection of diabetic retinopathy. 26 In addition, Viz.ai’s software, which uses a ML techniques to scan Computed Tomography images for indicators associated with stroke, also obtained the regulatory approval. 27 Other software systems listed included automated detection of atrial fibrillation and coronary calcification scores. 28 , 29 The FDA is also considering the ability of AI/ML-based SaMD for continuously learning and adaptive algorithms that have the potential to adapt and optimize device performance in real time to improve health care for patients in its regulatory framework. More broadly, FDA is using significance of information provided by SaMD to health care decision such as treat or diagnose, drive clinical or inform clinical management as key determinations for regulatory strategies. Pharmaceutical, biotechnology, and startup community participating in the workshops has recognized the potential of AI and ML in the development of personalized medicines and generating evidence motivating new products (summarized in Box 1 ). As examples, Johnson & Johnson Innovation’s life sciences “JLABS” newco incubator currently includes ML startups, such as Analytics 4 Life, A2A Pharmaceuticals, Envisagenics, among others. Precision medicine company GNS Healthcare and Genentech aim to collaboratively discover and validate new oncology drugs and patient response markers. Pfizer and Novartis are each working with IBM Watson Health to facilitate immuno-oncological research and development. Glaxosmithkline recently announced the creation of “Accelerating Therapeutics for Opportunities in Medicine (ATOM)” Consortium to accelerate drug discovery process using ML tools. 30 These early initiatives are either set up as in-house research groups or as public–private partnerships to engender cross-functional teams.
Discussions also focused on RWD-based simulations are now accepted as a reasonable way to inform clinical study design, modeling the impact of different study eligibility criteria, the timing of endpoint assessments, and study timelines at the FDA. 31 Treatment and regulatory decisions are based largely on data obtained from clinical trials and observational real-world data, and evidence from hospitals, EHR, and primary care are considered ancillary. An approach for evaluating RWE for clinical development and regulatory decisions would have the advantage of getting the medicine to the patient more quickly, with a better and fuller understanding of how effective and safe the medicines are in real-world settings. RWD can also inform study site selection, as well as identify patients potentially eligible for trials. Simulation of study control arms, potentially replacing the need to randomize a patient to a control arm in some scenarios, is another successful use of RWD. Authors note emergence and impact of analytics of RWD and RWE in academic biomedical science and health care delivery. For example, large “data lakes” have been created by aggregating information from hospital EHR, creating an opportunity for exploration via AI and ML approaches to identify clinically meaningful patterns. 32 , 33 , 34 These data lakes can be used to and ability to track patients longitudinally. By using RWD and tracking patients longitudinally via EHR for example, necessity for certain traditionally conducted late-phase trials could be reduced or eliminated altogether: one would provide a drug (after it has proven safe and efficacious in phase I and II trials) to patients and keep track of their experience.
Another theme at workshops focused on novel trials designs such as “basket,” “umbrella,” and many adaptive designs have been encouraged by regulatory agencies and can exploit emerging AI and ML techniques. These designs can enroll patients in a trial, profile them (e.g., using DNA sequencing, proteomics, metabolomics, etc.), and then use RWD for matching drugs considered in the trial to the pathologies identified from the profiling. Strategies for matching drugs to patient profiles in these studies can be based on AI and ML analysis of large relevant data sets. AI and ML can further be used to support an electronic version of study data monitoring, thereby ensuring that data are correct and the patients are safe; thus reducing the need for expensive on-site study monitoring. Furthermore, EHR data can be combined with other RWD types, such as genomics and patient-reported concerns, can be mined with AI and ML techniques to create a more comprehensive picture for drug and biomarker discovery. As methods for each of these tasks are determined and refined, computational solutions, including AI and ML, can be implemented to reliably replicate clinical trial activities at scale. These types of clinical trials—which ultimately test intervention “algorithms” such as drug–patient profile matching schemes—are likely to become more pronounced and prevalent in the future, and could be greatly facilitated by leveraging clinical outcomes monitoring and RWD collection. This interaction could also lead to the development of “Clinical Decision Support” tools that provide insight into optimal ways of treating patients. Experience with these tools could lead to further refinements, ultimately providing continuous feedback on their effectiveness.
Recent research at the intersection of computer science and medicine, proactive regulatory landscape, and availability of large data sets offers use cases and promise of testing and delivering faster cures to patients by leveraging sophisticated AI and ML methods (Fig. 1 ). This perspective aims to engage and inform researchers from fields, such as computer science, biology, medicine, engineering, biostatistics, and policy makers, with value of emerging technologies of AI and ML in solving key challenges facing modernization of the current clinical development process. Accordingly, Box 1 lists specific call-to-action, use cases, and considerations for different stakeholders across biotechnology and technology companies, foundations, regulators, and academic institutions discussed in a series of workshops. The authors also note that collaborations between engineering, medical imaging, machine learning, secure computing, and medicine have recently been fostered at various academic institutions across United States showing commitment toward such efforts. For example, MIT Media Lab and United States FDA signed a Memorandum of Understanding “Health 0.0” to engender AI and ML research for computational medicine and clinical development and accompanying regulatory framework to improve health outcomes for patients. Life sciences, biotechnology, foundations, universities, and patient advocacy groups are parts of this ecosystem. Partnerships at MIT with IBM, Abdul Latif Jameel Foundation Clinic, and Stephen A. Schwarzman College of Computing have been launched to support machine-learning research for health care needs. 35 , 36 Partnership in AI-assisted care at Stanford, Center for Artificial Intelligence in Diagnostic Medicine at University of California, Irvine and Center for Clinical Data Sciences at Massachusetts General Hospital and Brigham and Womens Hospital have joined the ecosystem. 37 , 38 , 39 A number of key recommendations and successful use cases and value and challenges facing AI and ML adoption in clinical development outlined in this perspective (summarized in Box I) can thus be implemented for taking advantage of digital algorithmic evidence to improve medical care for patients.
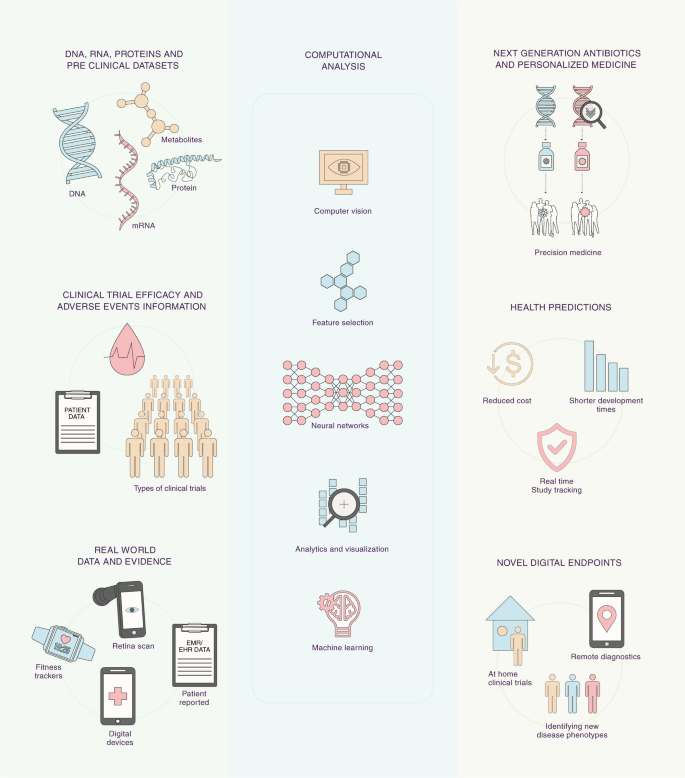
Use cases of artificial intelligence, computer vision, and machine learning in clinical development
Box 1. Key Recommendations, action items, and challenges
Biotechnology industry
Maximize AI and ML opportunities by focusing on data aggregation and easier access to data within and across pharma, biotech, and infotech companies.
Shared data in a noncompetitive manner (such as, e.g., TransCelerate placebo data, DREAM challenge and IBM, Project DataSphere) with multiple parties in the health care ecosystem, including technologists and academics to generate insights faster and collaboratively. 40 , 41 , 42 , 43
Embed computer science experts in relevant initiatives in order to build core capabilities and interdisciplinary teams, as well as leverage advisors from academic research groups.
New research, and academic and education departments focusing on training next generation of AI and ML professionals with experience in learning from clinical data.
Develop ML and AI algorithms capable of continuous learning from multimodal and sparse input data usually found in clinical development.
Host high value data sets in a secure public–private–government sandbox to engender research to infuse AI and ML in clinical development and design new digital medicine tools with an eye toward facilitating collaborations. 44
Develop AI ML toolboxes that are publically available for use by multiple stakeholders. 45
Regulatory agencies
Consider AI and ML techniques as effective tools and aids to drug development. 46
Evaluate the use of real-world evidence, generated by data outside traditional clinical trials from sources such as electronic health records and digital health devices, to support new drugs and health technologies. 47
Expand and promote internal innovations groups to engage actively with academic institutions, other government agencies, and technology companies to improve the clinical development process. 48
Technology corporations
Promote and maintain collaborations with academic groups developing AI and ML tools as such collaborations can also be used to shape the creation of a “future” workforce that can have a bigger impact on health care.
Leverage expertise across the whole spectrum of computing: from hardware designs, including quantum computing, to security, platforms, and services, in order to develop platforms for efficient and agile development.
Challenges and considerations
Limitations of current computer science deep learning models to generalize to complex medical data sets and tasks.
Necessities of high volumes of labeled data sets for training deep learning algorithms
Strategies and regulatory framework for dealing with relevant ethics issues (e.g., patient privacy, retaining anonymity, securing data) 45 and de-risk use of AI- and ML-based clinical prediction and decision support in health care. 49
FDA. Novel Drug Approvals for 2018. https://www.fda.gov/drugs/developmentapprovalprocess/druginnovation/ucm592464.htm (2018).
FDA. Companion Diagnostics. https://www.fda.gov/medicaldevices/productsandmedicalprocedures/invitrodiagnostics/ucm407297.htm (2018).
Jorgensen, J. T. & Hersom, M. Companion diagnostics-a tool to improve pharmacotherapy. Ann. Transl. Med. 4 , 482 (2016).
Article Google Scholar
Rothwell, P. M. Factors that can affect the external validity of randomised controlled trials. PLoS Clin. Trials 1 , e9 (2006).
Bettegowda, C. et al. Detection of circulating tumor DNA in early- and late-stage human malignancies. Sci. Transl. Med. 6 , 224ra224 (2014).
Patel, S., Park, H., Bonato, P., Chan, L. & Rodgers, M. A review of wearable sensors and systems with application in rehabilitation. J. Neuroeng. Rehabil. 9 , 21 (2012).
Shah, P. et al. Technology-enabled examinations of cardiac rhythm, optic nerve, oral health, tympanic membrane, gait and coordination evaluated jointly with routine health screenings: an observational study at the 2015 Kumbh Mela in India. BMJ Open 8 , e018774 (2018).
Yauney, G. & Shah, P. Reinforcement learning with action-derived rewards for chemotherapy and clinical trial dosing regimen selection. Proc. Mach. Learn. Res. 85 , 161–226 (2018).
Sherman, R. E. et al. Real-world evidence—what is it and what can it tell us? N. Engl. J. Med. 375 , 2293–2297 (2016).
Krizhevsky, A., Sutskever, I. & Hinton, G. E. ImageNet classification with deep convolutional neural networks. Commun. ACM 60 , 84–90 (2017).
Topol, E. J. High-performance medicine: the convergence of human and artificial intelligence. Nat. Med . 25 , 44–56 (2019).
Article CAS Google Scholar
Gawehn, E., Hiss, J. A. & Schneider, G. Deep learning in drug discovery. Mol. Inf. 35 , 3–14 (2016).
Vamathevan, J. et al. Applications of machine learning in drug discovery and development. Nat. Rev. Drug Disco . https://doi.org/10.1038/s41573-019-0024-5 (2019).
Esteva, A. et al. Dermatologist-level classification of skin cancer with deep neural networks. Nature 542 , 115–118 (2017).
Gulshan, V. et al. Development and validation of a deep learning algorithm for detection of diabetic retinopathy in retinal fundus photographs. JAMA 316 , 2402–2410 (2016).
Rana, A., Yauney, G., Lowe, A. & Shah, P. Computational histological staining and destaining of prostate core biopsy RGB images with generative adversarial neural networks. 17 th IEEE International Conference on Machine Learning and Applications (ICMLA) . (IEEE, Orlando, FL, USA, 2018). https://doi.org/10.1109/ICMLA.2018.00133 .
Alipanahi, B., Delong, A., Weirauch, M. T. & Frey, B. J. Predicting the sequence specificities of DNA- and RNA-binding proteins by deep learning. Nat. Biotechnol. 33 , 831–838 (2015).
Rajkomar, A. et al. Scalable and accurate deep learning with electronic health records. npj Digit. Med. 1 , 18 (2018).
Marcus, G. Deep learning: a critical appraisal. Preprint at https://ui.adsabs.harvard.edu//#abs/2018arXiv180100631M (2018).
Tu, J. V. Advantages and disadvantages of using artificial neural networks versus logistic regression for predicting medical outcomes. J. Clin. Epidemiol. 49 , 1225–1231 (1996).
George, D. et al. A generative vision model that trains with high data efficiency and breaks text-based CAPTCHAs. Science 358 , https://doi.org/10.1126/science.aag2612 (2017).
Esteva, A. et al. A guide to deep learning in healthcare. Nat. Med. 25 , 24–29 (2019).
Norgeot, B., Glicksberg, B. S. & Butte, A. J. A call for deep-learning healthcare. Nat. Med. 25 , 14–15 (2019).
FDA. Software as a Medical Device. https://www.fda.gov/MedicalDevices/DigitalHealth/SoftwareasaMedicalDevice/ucm20086412.htm (2018).
FDA. Digital Health Innovation Action Plan. https://www.fda.gov/medicaldevices/digitalhealth/ (2018).
FDA. FDA Permits Marketing of Artificial Intelligence-based Device to Detect Certain Diabetes-related Eye Problems. https://www.fda.gov/newsevents/newsroom/pressannouncements/ucm604357.htm (2018).
FDA. FDA Permits Marketing of Clinical Decision Support Software for Alerting Providers of a Potential Stroke in Patients. https://www.fda.gov/newsevents/newsroom/pressannouncements/ucm596575.htm (2018).
FDA. Telephone Electrocardiograph Transmitter and Receiver. https://www.accessdata.fda.gov/scripts/cdrh/cfdocs/cfpcd/classification.cfm?id=815 (2019).
Rogers, S. Zebra Medical Gains FDA Approval for AI-powered Heart Disease Detection. https://venturebeat.com/2018/07/12/zebra-medical-gains-fda-approval-for-ai-powered-heart-disease-detection/ (2018).
Center, U. N. Public-Private Consortium Aims to Cut Preclinical Cancer Drug Discovery from Six Years to Just One. https://www.ucsf.edu/news/2017/10/408841/public-private-consortium-aims-cut-preclinical-cancer-drug-discovery-six-years (2017).
FDA. Framework for FDA’s Real-World Evidence Program. https://www.fda.gov/science-research/science-and-research-special-topics/real-world-evidence (2018).
Bycroft, C. et al. TheUK Biobank resource with deep phenotyping and genomic data. Nature 562 , 203–209 (2018).
Johnson, A. E. et al. MIMIC-III, a freely accessible critical care database. Sci. Data 3 , 160035 (2016).
Herrett, E. et al. Data resource profile: clinical practice research datalink (CPRD). Int J. Epidemiol. 44 , 827–836 (2015).
Office, M. N. Abdul Latif Jameel Clinic for Machine Learning in Health at MIT aims to revolutionize disease prevention, detection, and treatment. http://news.mit.edu/2018/abdul-latif-jameel-clinic-machine-learning-health-0917 (2018).
Office, M. N. FAQ on the Newly Established MIT Stephen A. Schwarzman College of Computing. http://news.mit.edu/2018/faq-mit-stephen-schwarzman-college-of-computing-1015 (2018).
University, S. Partnership in AI-Assisted Care . https://aicare.stanford.edu/index.php (2018).
Office, U. o. C. N. UCI Center to Advance the Use of Artificial Intelligence in Healthcare. http://www.ucihealth.org/news/2018/07/ai-center-for-diagnostic-medicine (2018).
Hospital, M. G. MGH & BWH Center for Clinical Data Science. https://www.massgeneral.org/imaging/research/researchlab.aspx?id=1759 (2018).
Abdallah, K., Hugh-Jones, C., Norman, T., Friend, S. & Stolovitzky, G. The prostate cancer DREAM challenge: a community-wide effort to use open clinical trial data for the quantitative prediction of outcomes in metastatic prostate cancer. Oncologist 20 , 459–460 (2015).
Bertagnolli, M. M. et al. Advantages of a truly open-access data-sharing model. N. Engl. J. Med. 376 , 1178–1181 (2017).
Ferri, M. & Abdallah, K. Preparing for responsible sharing of clinical trial data. N. Engl. J. Med. 370 , 484–485 (2014).
Gill, D. Re-inventing clinical trials through TransCelerate. Nat. Rev. Drug Disco. 13 , 787–788 (2014).
Shah, P. Health 0.0. https://www.media.mit.edu/groups/health-0-0/overview/ (2019).
Office, M. N. MIT Media Lab to Participate in $27 Million Initiative on AI Ethics and Governance. http://news.mit.edu/2017/mit-media-lab-to-participate-in-ai-ethics-and-governance-initiative-0110 (2017).
Gottlieb, S. Transforming FDA’s Approach to Digital Health. https://www.fda.gov/newsevents/speeches/ucm605697.htm (2018).
Gottlieb, S. FDA Budget Matters: A Cross-Cutting Data Enterprise for Real World Evidence. https://blogs.fda.gov/fdavoice/index.php/2018/07/fda-budget-matters-a-cross-cutting-data-enterprise-for-real-world-evidence/ (2018).
Khozin, S., Kim, G. & Pazdur, R. REGULATORY WATCH From big data to smart data: FDA’s INFORMED initiative. Nat. Rev. Drug Disco. 16 , 306–306 (2017).
Office, M. N. IBM and MIT to Pursue Joint Research in Artificial Intelligence, Establish new MIT–IBM Watson AI Lab. http://news.mit.edu/2017/ibm-mit-joint-research-watson-artificial-intelligence-lab-0907 (2017).
Download references
Acknowledgements
Dr. Amy Abernethy for participating in workshops and brainstorming sessions and providing comments while she was at Flatiron Health; Dr. Abernethy’s participation in these activities occurred prior to her appointment as Principal Deputy Commissioner of the Food and Drug Administration. Ryan McCarthy, Joichi Ito at The MIT Media Lab and Joling Mew at Genentech for workshop program management. The MIT Media Lab discretionary funding supported hosting of the workshops by providing space and audiovisual equipment.
Author information
These authors contributed equally: Pratik Shah, Francis Kendall
Authors and Affiliations
Massachusetts Institute of Technology, Media Laboratory, Cambridge, MA, USA
Pratik Shah & Francis Kendall
F. Hoffmann-La Roche AG, Strategic Innovation, San Francisco, CA, USA
Francis Kendall
US Food and Drug Administration, Silver Spring, MD, USA
Sean Khozin
The Boston Consulting Group, Boston, MA, USA
Ryan Goosen & Michael Ringel
IBM Research, Center for Computational Health, New York, NY, USA
Jianying Hu
Novartis Institute of Biomedical Research, Cambridge, MA, USA
Jason Laramie
The Translational Genomics Research Institute, Quantitative Medicine and Systems Biology Phoenix, Phoenix, AZ, USA
Nicholas Schork
You can also search for this author in PubMed Google Scholar
Contributions
Pratik Shah and Francis Kendall contributed equally to conception and workshop leadership, brainstorming sessions and drafting manuscript. Sean Khozin contributed to workshop and brainstorming strategy sessions. Ryan Goosen co-organized brainstorming sessions and created summary documents. Ryan Goosen Amy Abernethy, Jiaying Hu, Sean Khozin, Jason Laramie, Michael Ringel, and Nicholas Schork participated in workshops and brainstorming sessions, wrote individual sections of the paper, and provided general comments.
Corresponding author
Correspondence to Pratik Shah .
Ethics declarations
Competing interests.
The authors declare no competing interests.
Additional information
Publisher’s note: Springer Nature remains neutral with regard to jurisdictional claims in published maps and institutional affiliations.
Supplementary information
Rights and permissions.
Open Access This article is licensed under a Creative Commons Attribution 4.0 International License, which permits use, sharing, adaptation, distribution and reproduction in any medium or format, as long as you give appropriate credit to the original author(s) and the source, provide a link to the Creative Commons license, and indicate if changes were made. The images or other third party material in this article are included in the article’s Creative Commons license, unless indicated otherwise in a credit line to the material. If material is not included in the article’s Creative Commons license and your intended use is not permitted by statutory regulation or exceeds the permitted use, you will need to obtain permission directly from the copyright holder. To view a copy of this license, visit http://creativecommons.org/licenses/by/4.0/ .
Reprints and permissions
About this article
Cite this article.
Shah, P., Kendall, F., Khozin, S. et al. Artificial intelligence and machine learning in clinical development: a translational perspective. npj Digit. Med. 2 , 69 (2019). https://doi.org/10.1038/s41746-019-0148-3
Download citation
Received : 26 February 2019
Accepted : 08 July 2019
Published : 26 July 2019
DOI : https://doi.org/10.1038/s41746-019-0148-3
Share this article
Anyone you share the following link with will be able to read this content:
Sorry, a shareable link is not currently available for this article.
Provided by the Springer Nature SharedIt content-sharing initiative
This article is cited by
Predicting outcomes following lower extremity open revascularization using machine learning.
- Mohammed Al-Omran
Scientific Reports (2024)
P.O.L.A.R. Star: A New Framework Developed and Applied by One Mid-Sized Pharmaceutical Company to Drive Digital Transformation in R&D
- Riccardo Mariani
- Maria Carmela De Vuono
- Diego Ardigò
Pharmaceutical Medicine (2024)
Nanomedicine Advancements: Vanadium Oxide Nanoparticles as a Game-Changer in Antimicrobial and Anticancer Therapies
- Adebayo Efunnuga
- Adeyemi Efunnuga
- Akinola David Olugbemide
BioNanoScience (2024)
Artificial intelligence in medical science: a review
- Simrata Bindra
Irish Journal of Medical Science (1971 -) (2024)
Towards precision medicine based on a continuous deep learning optimization and ensemble approach
- Linyuan Jin
npj Digital Medicine (2023)
Quick links
- Explore articles by subject
- Guide to authors
- Editorial policies
Sign up for the Nature Briefing: Translational Research newsletter — top stories in biotechnology, drug discovery and pharma.


An official website of the United States government
The .gov means it’s official. Federal government websites often end in .gov or .mil. Before sharing sensitive information, make sure you’re on a federal government site.
The site is secure. The https:// ensures that you are connecting to the official website and that any information you provide is encrypted and transmitted securely.
- Publications
- Account settings
- My Bibliography
- Collections
- Citation manager
Save citation to file
Email citation, add to collections.
- Create a new collection
- Add to an existing collection
Add to My Bibliography
Your saved search, create a file for external citation management software, your rss feed.
- Search in PubMed
- Search in NLM Catalog
- Add to Search
An overview of commonly used statistical methods in clinical research
Affiliations.
- 1 Center for Surgical Outcomes Research, The Research Institute at Nationwide Children's Hospital, Columbus, OH, USA.
- 2 Department of Surgery, Children's Mercy Hospital, 2401 Gillham Road, Kansas City, MO 64108, USA. Electronic address: [email protected].
- PMID: 30473041
- DOI: 10.1053/j.sempedsurg.2018.10.008
Statistics plays an essential role in clinical research by providing a framework for making inferences about a population of interest. In order to interpret research datasets, clinicians involved in clinical research should have an understanding of statistical methodology. This article provides a brief overview of statistical methods that are frequently used in clinical research studies. Descriptive and inferential methods, including regression modeling and propensity scores, are discussed, with focus on the rationale, assumptions, strengths, and limitations to their application.
Keywords: Descriptive statistics; Inferential statistics; Propensity scores; Regression analysis; Survival analysis.
Copyright © 2018 Elsevier Inc. All rights reserved.
PubMed Disclaimer
Similar articles
- Study design: the basics. Lim HJ, Hoffmann RG. Lim HJ, et al. Methods Mol Biol. 2007;404:1-17. doi: 10.1007/978-1-59745-530-5_1. Methods Mol Biol. 2007. PMID: 18450042 Review.
- Evaluating statistics in clinical trials: making the unintelligible intelligible. Gilmore SJ. Gilmore SJ. Australas J Dermatol. 2008 Nov;49(4):177-84; quiz 185-6. doi: 10.1111/j.1440-0960.2008.00465_1.x. Australas J Dermatol. 2008. PMID: 18855776 Review.
- Biostatistics primer: part 2. Overholser BR, Sowinski KM. Overholser BR, et al. Nutr Clin Pract. 2008 Feb;23(1):76-84. doi: 10.1177/011542650802300176. Nutr Clin Pract. 2008. PMID: 18203967
- Statistical problems in medical research. Okeh UM. Okeh UM. East Afr J Public Health. 2009 Apr;6 Suppl(1):1-7. doi: 10.4314/eajph.v6i3.45762. East Afr J Public Health. 2009. PMID: 20088069
- Biostatistics primer: part I. Overholser BR, Sowinski KM. Overholser BR, et al. Nutr Clin Pract. 2007 Dec;22(6):629-35. doi: 10.1177/0115426507022006629. Nutr Clin Pract. 2007. PMID: 18042950 Review.
- Type and approach of hysterectomy and oncological survival of women with stage II cancer of endometrium: a large retrospective cohort study. Shuai X, Xiao D, Han B, Du Y. Shuai X, et al. Front Oncol. 2024 May 13;14:1404831. doi: 10.3389/fonc.2024.1404831. eCollection 2024. Front Oncol. 2024. PMID: 38803540 Free PMC article.
- Say farewell to bland regression reporting: Three forest plot variations for visualizing linear models. Fries J, Oberleiter S, Pietschnig J. Fries J, et al. PLoS One. 2024 Feb 2;19(2):e0297033. doi: 10.1371/journal.pone.0297033. eCollection 2024. PLoS One. 2024. PMID: 38306346 Free PMC article.
- Correlation between hypertensive retinopathy and fetal outcomes in patients with preeclampsia in a tertiary care hospital: A prospective cohort study. Jacob N, Kasturi N, Deb AK, Maurya DK. Jacob N, et al. Indian J Ophthalmol. 2023 Mar;71(3):910-915. doi: 10.4103/IJO.IJO_2995_22. Indian J Ophthalmol. 2023. PMID: 36872708 Free PMC article.
- Genotyping Helicobacter pylori and fgf7 gene expression in gastric cancer. Hedayati MA, Khani D, Bashiri H. Hedayati MA, et al. Mol Biol Rep. 2022 Sep;49(9):8827-8834. doi: 10.1007/s11033-022-07732-3. Epub 2022 Jul 7. Mol Biol Rep. 2022. PMID: 35799083
- A systematic review and meta-analysis of the effect of transitional care interventions on the prognosis of patients with heart failure. Lin X, Ji R, Wang X, Xin R, Chen Q. Lin X, et al. J Thorac Dis. 2022 Apr;14(4):1164-1171. doi: 10.21037/jtd-22-102. J Thorac Dis. 2022. PMID: 35572902 Free PMC article.
Publication types
- Search in MeSH
LinkOut - more resources
Full text sources.
- Elsevier Science
- MedlinePlus Health Information
- Citation Manager
NCBI Literature Resources
MeSH PMC Bookshelf Disclaimer
The PubMed wordmark and PubMed logo are registered trademarks of the U.S. Department of Health and Human Services (HHS). Unauthorized use of these marks is strictly prohibited.
Handbook home
- Search the Handbook
- Undergraduate courses
- Graduate courses
- Research courses
- Undergraduate subjects
- Graduate subjects
- Research subjects
- Breadth Tracks
- CAPS Login - Staff only
- Data Analysis in Clinical Research
Data Analysis in Clinical Research (CLRS90010)
Graduate coursework Points: 12.5 On Campus (Parkville)
View full page
About this subject
Contact information.
Email: [email protected]
Phone: + 61 3 8344 0149
Contact hours : https://unimelb.edu.au/professional-development/contact-us
Please refer to the LMS for up-to-date subject information, including assessment and participation requirements, for subjects being offered in 2020.
Availability | |
---|---|
Fees |
Data analysis methods are an integral part of modern clinical research. They are powerful techniques that enable researchers to draw meaningful conclusions from data collected through observation, survey, or experimentation.
However, data analysis is a huge discipline with different paradigms, schools of thought and alternative methodologies. Therefore consideration of the appropriate methods used must be undertaken when designing a study and selecting variables and groups.
This subject introduces students to the basic principles of qualitative and quantitative data analysis techniques. It will provide a functional grounding in the theoretical concepts behind each type of analysis, as well as exploration of the interpretation of data and the difference, where applicable, between clinical vs statistical significance.
Intended learning outcomes
On completion of this subject students should be able to:
- describe the theoretical concepts behind a range of qualitative and quantitative data analysis techniques
- compare and contrast the strengths and weaknesses of different qualitative and quantitative data analysis techniques
- describe a strategy for selecting an appropriate data analysis technique based on the study design selected and/or research data collected
- competently perform a range of basic data analysis techniques using appropriate analysis software and interpret analysis output/s
- provide a rationale for the importance of statistical power and perform power calculations
- identify and discuss the key elements associated with ensuring data integrity including storage, management, collation and coding
- critically compare and contrast statistical vs clinical significance and its relevance to clinical practice
- demonstrate confidence in discussing the validity of data analysis outcomes reported in the scientific literature.
Generic skills
- to engage with unfamiliar problems and identify relevant data analysis strategies
- to construct and express logical arguments and to work in abstract or general terms to increase the clarity and efficiency of data analysis
- communicate advanced data analysis concepts in written and oral form;
- the ability to comprehend complex data analysis information
- exercise responsibility for their own learning;
- manage their time effectively.
Last updated: 3 November 2022
The Role of Clinical Data and Clinical Data Science
November 24, 2020
Clinical data and its analysis are critical to clinical research. Ensuring the overall quality of clinical data is then paramount to ensuring quality care and appropriate decision-making in the medical and healthcare fields.
What constitutes clinical data, what should you look for in that data, and what resources are there to manage data and data analysis and ensure their quality?
Let’s take a look.
What is Clinical Data?
Clinical data is information gathered for the broad purpose of clinical research on the micro-level (patient care) to the macro-level (broad applications within a health system). Clinical data can be collected in a number of different ways:
- Electronic Health Records : These records, typically only available within a hospital system, are essentially a patient’s digital history. They include everything from the patient’s last round of diagnostics to any medications they are taking and everything in between.
- Patient/Disease Registries : These registries follow specific patient populations based on certain diseases and conditions. Information related to these populations is gathered to then inform further research, and ideally, improve patient outcomes. The National Program of Cancer Registries , for example, gathers data from local entities to allow for a more unified response to cancer research.
- Clinical Trial Data : This is data gathered as part of a clinical trial, which is research around new drug applications, treatment methods, device testing, and other applications where data gathering is necessary to determine patient outcomes.
Clinical data has broad applications in the healthcare industry. It's important to know what to look for in that data, particularly when working with data gathering as part of clinical research.
What to Look for in Clinical Data
Before collecting and reviewing clinical data it is critically important to understand the goal or objective of the data. It must be understood what the data is trying to show and what question the data is trying to answer.
Once the objective of the data is understood, clinical data must be reviewed for potential biases. Objective evidence is an absolute must in making major decisions pertaining to public health and safety. Biases can come in many forms, and a successful clinical trial is designed in such a way to minimize all potential biases.
A common technique to obtain unbiased data is to blind the study participants as well as the researchers conducting the study. This ensures all data points recorded are objective and no participants or researchers are trying to push any of their own objectives into the data).
Another common technique to obtain unbiased data is to randomize the study participants to receive either the investigational product or to receive the comparator product. This ensures the researchers do not purposely give the investigational product to the healthier subjects with a higher probability of seeing treatment success.
Data structures are critically important for FDA submissions. Most submissions to the FDA (including NDAs and BLAs) require specific structures of data known as CDISC datasets. CDISC datasets are required for submission to the agency as they allow for a common structure of data across all clinical trials run and across all sponsors conducting clinical trials.
Data Trends
Data trends should be reviewed to ensure the data being collected in the clinical trial are meaningful and represent real-life expectations. As an example of data trends, a single site within a clinical trial may be responsible for over 75% of all adverse events recorded in the study, despite only enrolling 10% of all study subjects. Noticing a data trend such as this can lead the clinical study teams to review this specific site’s procedures and ensure the study is being conducted correctly.
How Clinical Data Sciences Can Help
Clinical data sciences supports the collection, management, and analysis of clinical data.
What is Clinical Data Science?
Clinical data science links the methods and insights of data science with clinical data. Clinical data scientists then work within clinical trials to ensure sound data management and analysis.
Clinical Data Science is made up of the three following functions:
Biostatistics
The Biostatistician is responsible for the analysis of the clinical trial data. At the end of the day, the FDA speaks the language of statistics and clinical trial results must be conveyed in the form of confidence intervals and p-values. It is the responsibility of the biostatistician to determine which analysis should be conducted, the number of subjects needed to be enrolled to conduct statistically meaningful analyses and how these analysis results should be conveyed to the FDA to meet all regulatory requirements.
Clinical Programming
A clinical programmer on a data science team is responsible for building the programs that will create datasets for further analysis and finding pathways for transferring that data based on the client’s needs. As discussed above, for many applications submitted to the FDA, a special structure of dataset known as CDISC is required. The clinical programming team is responsible for creating these specific data structures to the agency.
Clinical Data Management
The clinical data manager is responsible for the capture and cleaning of the clinical data. The data manager communicates with both the statisticians and the programming team to ensure the data needed for CDISC datasets and for final analysis is collected in a meaningful and unbiased way.
How Clinical Data Science Can Benefit Your Project
ProPharma Group has extensive clinical data experience , including a recent clinical data management project around creating an Electronic Data Capture System ( EDC ) to capture data, creating CDISC datasets and conducting the final statistical analysis for FDA submission for a midsized pharmaceutical client.
Our data science teams work to support clients with everything from where to begin in their clinical data analysis, to identifying pathways to solutions-based outcomes, to analyzing data with efficacy and efficiency.
Contact ProPharma to see how we can support you with your next clinical management project.
TAGS: Clinical Research Solutions
Related Articles
January 5, 2021
Data Integrity in Clinical Research: Audit Trail Review as a Key Tool
Data Integrity in Clinical Research: Audit Trail Review as a Key Tool A recent position paper from the eClinical Forum and the Society for Clinical Data Management highlighted the value of audit...
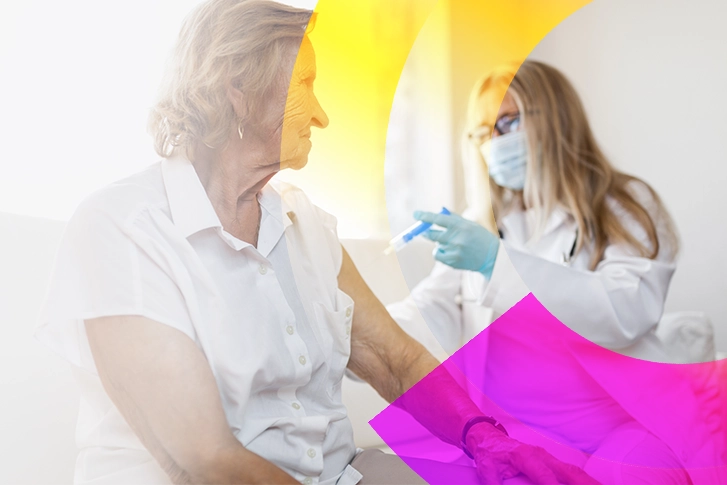
July 17, 2023
Why Decentralized Clinical Trials are the Future of Clinical Research
Decentralized clinical trials (DCTs) have emerged as a transformative approach in clinical research, offering unique benefits that challenge the traditional models. However, deciding whether to...
March 26, 2018
First Clinical Study Report Data Published for FDA Pilot Project
On Monday, March 19th, the FDA officially began a new Clinical Data Summary Pilot Program. This program is intended to “assess the feasibility of using a different format to provide required...
- Open access
- Published: 24 August 2024
Mixed effects models but not t-tests or linear regression detect progression of apathy in Parkinson’s disease over seven years in a cohort: a comparative analysis
- Anne-Marie Hanff 1 , 2 , 3 , 4 ,
- Rejko Krüger 1 , 2 , 5 ,
- Christopher McCrum 4 ,
- Christophe Ley 6 on behalf of
BMC Medical Research Methodology volume 24 , Article number: 183 ( 2024 ) Cite this article
200 Accesses
2 Altmetric
Metrics details
Introduction
While there is an interest in defining longitudinal change in people with chronic illness like Parkinson’s disease (PD), statistical analysis of longitudinal data is not straightforward for clinical researchers. Here, we aim to demonstrate how the choice of statistical method may influence research outcomes, (e.g., progression in apathy), specifically the size of longitudinal effect estimates, in a cohort.
In this retrospective longitudinal analysis of 802 people with typical Parkinson’s disease in the Luxembourg Parkinson's study, we compared the mean apathy scores at visit 1 and visit 8 by means of the paired two-sided t-test. Additionally, we analysed the relationship between the visit numbers and the apathy score using linear regression and longitudinal two-level mixed effects models.
Mixed effects models were the only method able to detect progression of apathy over time. While the effects estimated for the group comparison and the linear regression were smaller with high p -values (+ 1.016/ 7 years, p = 0.107, -0.056/ 7 years, p = 0.897, respectively), effect estimates for the mixed effects models were positive with a very small p -value, indicating a significant increase in apathy symptoms by + 2.345/ 7 years ( p < 0.001).
The inappropriate use of paired t-tests and linear regression to analyse longitudinal data can lead to underpowered analyses and an underestimation of longitudinal change. While mixed effects models are not without limitations and need to be altered to model the time sequence between the exposure and the outcome, they are worth considering for longitudinal data analyses. In case this is not possible, limitations of the analytical approach need to be discussed and taken into account in the interpretation.
Peer Review reports
In longitudinal studies: “an outcome is repeatedly measured, i.e., the outcome variable is measured in the same subject on several occasions.” [ 1 ]. When assessing the same individuals over time, the different data points are likely to be more similar to each other than measurements taken from other individuals. Consequently, the application of special statistical techniques is required, which take into account the fact that the repeated observations of each subject are correlated [ 1 ]. Parkinson’s disease (PD) is a heterogeneous neurodegenerative disorder resulting in a wide variety of motor and non-motor symptoms including apathy, defined as a disorder of motivation, characterised by reduced goal-directed behaviour and cognitive activity and blunted affect [ 2 ]. Apathy increases over time in people with PD [ 3 ]. Specifically, apathy has been associated with the progressive denervation of ascending dopaminergic pathways in PD [ 4 , 5 ] leading to dysfunctions of circuits implicated in reward-related learning [ 5 ].
T-tests are often misused to analyse changes over time [ 6 ]. Consequently, we aim to demonstrate how the choice of statistical method may influence research outcomes, specifically the size and interpretation of longitudinal effect estimates in a cohort. Thus, the findings are intended for illustrative and educational purposes related to the statistical methodology. In a retrospective analysis of data from the Luxembourg Parkinson's study, a nation-wide, monocentric, observational, longitudinal-prospective dynamic cohort [ 7 , 8 ], we assess change in apathy using three different statistical approaches (paired t-test, linear regression, mixed effects model). We defined the following target estimand: In people diagnosed with PD, what is the change in the apathy score from visit 1 to visit 8? To estimate this change, we formulated the statistical hypothesis as follows:
While apathy was the dependent variable, we included the visit number as an independent variable (linear regression, mixed effects model) and as a grouping variable (paired t-test). The outcome apathy was measured by the discrete score from the Starkstein apathy scale (0 – 42, higher = worse) [ 9 ], a scale recommended by the Movement Disorders Society [ 10 ]. This data was obtained from the National Centre of Excellence in Research on Parkinson's disease (NCER-PD). The establishment of data collection standards, completion of the questionnaires at home at the participants’ convenience, mobile recruitment team for follow-up visits or standardized telephone questionnaire with a reduced assessment were part of the efforts in the primary study to address potential sources of bias [ 7 , 8 ]. Ethical approval was provided by the National Ethics Board (CNER Ref: 201,407/13). We used data from up to eight visits, which were performed annually between 2015 and 2023. Among the participants are people with typical PD and PD dementia (PDD), living mostly at home in Luxembourg and the Greater Region (geographically close areas of the surrounding countries Belgium, France, and Germany). People with atypical PD were excluded. The sample at the date of data export (2023.06.22) consisted of 802 individuals of which 269 (33.5%) were female. The average number of observations was 3.0. Fig. S1 reports the numbers of individuals at each visit while the characteristics of the participants are described in Table 1 .
As illustrated in the flow diagram (Fig. 1 ), the sample analysed from the paired t-test is highly selective: from the 802 participants at visit 1, the t-test only included 63 participants with data from visit 8. This arises from the fact that, first, we analyse the dataset from a dynamic cohort, i.e., the data at visit 1 were not collected at the same time point. Thus, 568 of the 802 participants joined the study less than eight years before, leading to only 234 participants eligible for the eighth yearly visit. Second, after excluding non-participants at visit 8 due to death ( n = 41) and other reasons ( n = 130), only 63 participants at visit 8 were left. To discuss the selective study population of a paired t-test, we compared the characteristics (age, education, age at diagnosis, apathy at visit 1) of the remaining 63 participants at visit 8 (included in the paired t-test) and the 127 non-participants at visit 8 (excluded from the paired t-test) [ 12 ].
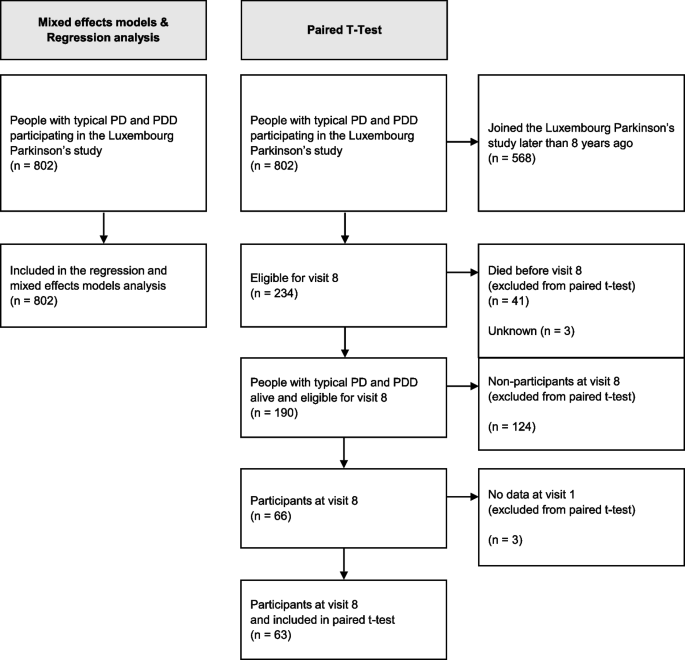
Flow diagram of patient recruitment
The paired two-sided t-test compared the mean apathy score at visit 1 with the mean apathy score at the visit 8. We attract the reader’s attention to the fact that this implies a rather small sample size as it includes only those people with data from the first and 8th visit. The linear regression analysed the relationship between the visit number and the apathy score (using the “stats” package [ 13 ]), while we performed longitudinal two-level mixed effects models analysis with a random intercept on subject level, a random slope for visit number and the visit number as fixed effect (using the “lmer”-function of the “lme4”-package [ 14 ]). The latter two approaches use all available data from all visits while the paired t-test does not. We illustrated the analyses in plots with the function “plot_model” of the R package sjPlot [ 15 ]. We conducted data analysis using R version 3.6.3 [ 13 ] and the R syntax for all analyses is provided on the OSF project page ( https://doi.org/ https://doi.org/10.17605/OSF.IO/NF4YB ).
Panel A in Fig. 2 illustrates the means and standard deviations of apathy for all participants at each visit, while the flow-chart (Fig. S1 ) illustrates the number of participants at each stage. On average, we see lower apathy scores at visit 8 compared to visit 1 (higher score = worse). By definition, the paired t-test analyses pairs, and in this case, only participants with complete apathy scores at visit 1 and visit 8 are included, reducing the total analysed sample to 63 pairs of observations. Consequently, the t-test compares mean apathy scores in a subgroup of participants with data at both visits leading to different observations from Panel A, as illustrated and described in Panel B: the apathy score has increased at visit 8, hence symptoms of apathy have worsened. The outcome of the t-test along with the code is given in Table 2 . Interestingly, the effect estimates for the increase in apathy were not statistically significant (+ 1.016 points, 95%CI: -0.225, 2.257, p = 0.107). A possible reason for this non-significance is a loss of statistical power due to a small sample size included in the paired t-test. To visualise the loss of information between visit 1 and visit 8, we illustrated the complex individual trajectories of the participants in Fig. 3 . Moreover, as described in Table S1 in the supplement, the participants at visit 8 (63/190) analysed in the t-test were inherently significantly different compared to the non-participants at visit 8 (127/190): they were younger, had better education, and most importantly their apathy scores at visit 1 were lower. Consequently, those with the better overall situation kept coming back while this was not the case for those with a worse outcome at visit 1, which explains the observed (non-significant) increase. This may result in a biased estimation of change in apathy when analysed by the compared statistical methods.
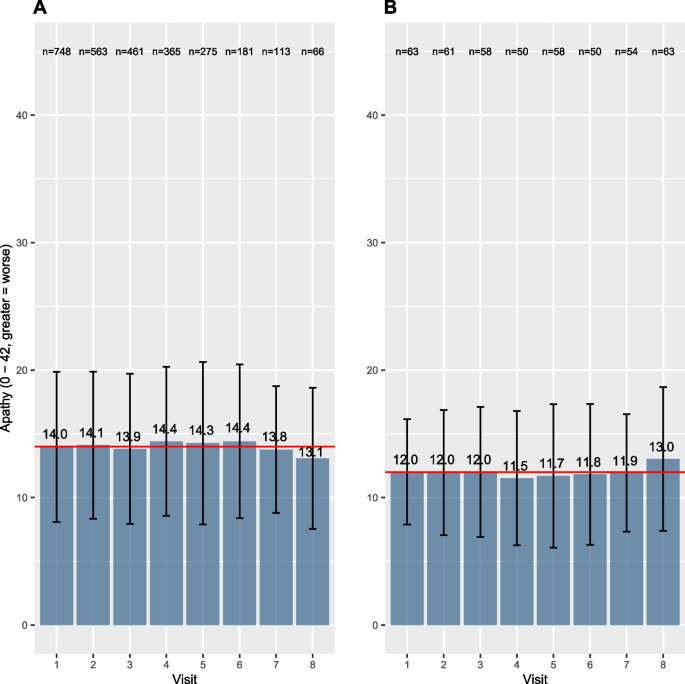
Bar charts illustrating apathy scores (means and standard deviations) per visit (Panel A: all participants, Panel B: subgroup analysed in the t-test). The red line indicates the mean apathy at visit 1
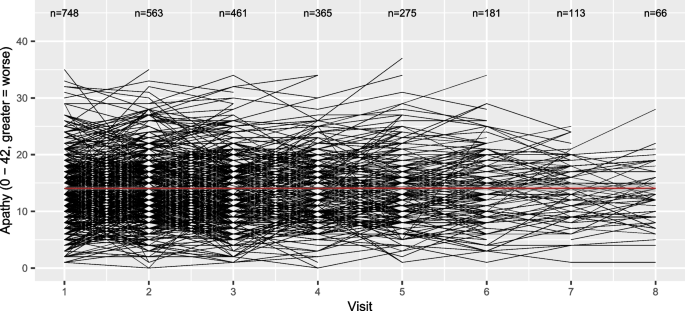
Scatterplot illustrating the individual trajectories. The red line indicates the regression line
From the results in Table 2 , we see that the linear regression coefficient, representing change in apathy symptoms per year, is not significantly different from zero, indicating no change over time. One possible explanation is the violation of the assumption of independent observations for linear regressions. On the contrary, the effect estimates for the linear mixed effects models indicated a significant increase in apathy symptoms from visit 1 to visit 8 by + 2.680 points (95%CI: 1.880, 3.472, p < 0.001). Consequently, mixed effects models were the only method able to detect an increase in apathy symptoms over time and choosing mixed effect models for the analysis of longitudinal data reduces the risk of false negative results. The differences in the effect sizes are also reflected in the regression lines in Panel A and B of Fig. 4 .
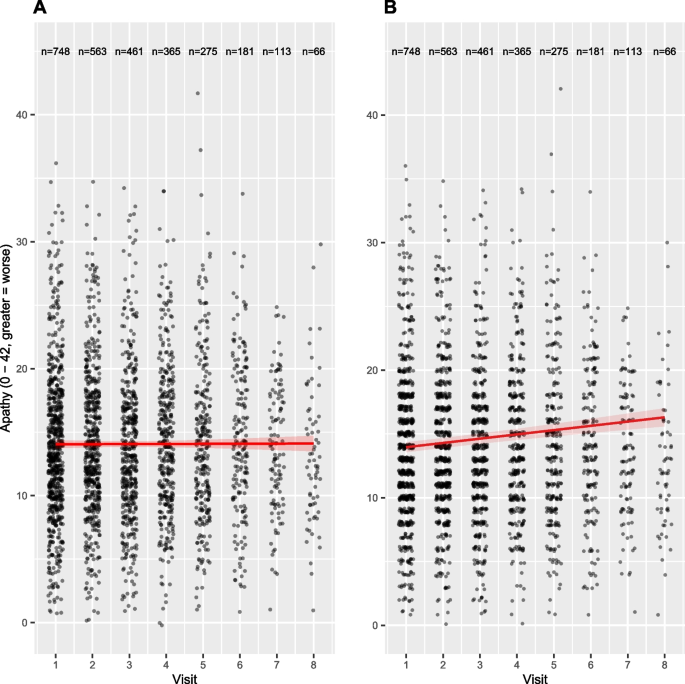
Scatterplot illustrating the relationship between visit number and apathy. Apathy measured by a whole number interval scale, jitter applied on x- and y-axis to illustrate the data points (Panel A: Linear regression, Panel B: Linear mixed effects model). The red line indicates the regression line
The effect sizes differed depending on the choice of the statistical method. Thus, the paired t-test and the linear regression resulted in an output that would lead to different interpretations than the mixed effects models. More specifically, compared to the t-test and linear regression (which indicated non-significant changes in apathy of only + 1.016, -0.064 points from visit 1 to visit 8, respectively), the linear mixed effects models found an increase of + 2.680 points from visit 1 to visit 8 on the apathy scale. This increase is more than twice as high as indicated by the t-test and suggests linear mixed models is a more sensitive approach to detect meaningful changes perceived by people with PD over time.
Mixed effects models are a valuable tool in longitudinal data analysis as these models expand upon linear regression models by considering the correlation among repeated measurements within the same individuals through the estimation of a random intercept [ 1 , 16 , 17 ]. Specifically, to account for correlation between observations, linear mixed effects models use random effects to explicitly model the correlation structure, thus removing correlation from the error term. A random slope in addition to a random intercept allows both the rate of change and the mean value to vary by participant, capturing individual differences. This distinguishes them from group comparisons or standard linear regressions, in which such explicit modelling of correlation is not possible. Thus, the linear regression not considering correlation among the repeated observations leads to an underestimation of longitudinal change, explaining the smaller effect sizes and insignificant results of the regression. By including random effects, linear mixed effects models can better capture the variability within the data.
Another common challenge in longitudinal studies is missing data. Compared to the paired t-test and regression, the mixed effects models can also include participants with missing data at single visits and account for the individual trajectories of each participant as illustrated in Fig. 2 [ 18 ]. Although multiple imputation could increase the sample size, those results need to be interpreted with caution in case the data is not missing at random [ 18 , 19 ]. Note that we do not further elaborate here on this topic since this is a separate issue to statistical method comparison. Finally, assumptions of the different statistical methods need to be respected. The paired t-test assumes a normal distribution, homogeneity of variance and pairs of the same individuals in both groups [ 20 , 21 ]. While mixed effects models don’t rely on independent observations as it is the case for linear regression, all other assumptions for standard linear regression analysis (e.g., linearity, homoscedasticity, no multicollinearity) also hold for mixed effects model analyses. Thus, additional steps, e.g., check for linearity of the relationships or data transformations are required before the analysis of clinical research questions [ 17 ].
While mixed effects models are not without limitations and need to be altered to model the time sequence between the exposure and the outcome [ 1 ], they are worth considering for longitudinal data analyses. Thus, assuming an increase of apathy over time [ 3 ], mixed effects models were the only method able to detect statistically significant changes in the defined estimand, i.e., the change in apathy from visit 1 to visit 8. Possible reasons are a loss of statistical power due to a small sample size included in the paired t-test and the violence of the assumption of independent observations for linear regressions. Specifically, the effects estimated for the group comparison and the linear regression were smaller with high p -values, indicating a statistically insignificant change in apathy over time. The effect estimates for the mixed effects models were positive with a very small p -value, indicating a statistically significant increase in apathy symptoms from visit 1 to visit 8 in line with clinical expectations. Mixed effects models can be used to estimate different types of longitudinal effects while an inappropriate use of paired t-tests and linear regression to analyse longitudinal data can lead to underpowered analyses and an underestimation of longitudinal change and thus clinical significance. Therefore, researchers should more often consider mixed effects models for longitudinal analyses. In case this is not possible, limitations of the analytical approach need to be discussed and taken into account in the interpretation.
Availability of data and materials
The LUXPARK database used in this study was obtained from the National Centre of Excellence in Research on Parkinson’s disease (NCER-PD). NCER-PD database are not publicly available as they are linked to the Luxembourg Parkinson’s study and its internal regulations. The NCER-PD Consortium is willing to share its available data. Its access policy was devised based on the study ethics documents, including the informed consent form approved by the national ethics committee. Requests for access to datasets should be directed to the Data and Sample Access Committee by email at [email protected].
The code is available on OSF ( https://doi.org/10.17605/OSF.IO/NF4YB )
Abbreviations
Parkinson's disease
Null hypothesis
Alternative hypothesis
Parkinson's disease dementia
National Centre of Excellence in Research on Parkinson's disease
Open Science Framework
Confidence Interval
Twisk JWR. Applied Longitudinal Data Analysis for Epidemiology. A Practical Guide: Cambridge University Press; 2013.
Book Google Scholar
Levy R, Dubois B. Apathy and the functional anatomy of the prefrontal cortex-basal ganglia circuits. Cereb Cortex. 2006;16(7):916–28.
Article PubMed Google Scholar
Poewe W, Seppi K, Tanner CM, Halliday GM, Brundin P, Volkmann J, et al. Parkinson disease. Nat Rev Dis Primers. 2017;3:17013.
Pagonabarraga J, Kulisevsky J, Strafella AP, Krack P. Apathy in Parkinson’s disease: clinical features, neural substrates, diagnosis, and treatment. Lancet Neurol. 2015;14(5):518–31.
Drui G, Carnicella S, Carcenac C, Favier M, Bertrand A, Boulet S, Savasta M. Loss of dopaminergic nigrostriatal neurons accounts for the motivational and affective deficits in Parkinson’s disease. Mol Psychiatry. 2014;19(3):358–67.
Article CAS PubMed Google Scholar
Liang G, Fu W, Wang K. Analysis of t-test misuses and SPSS operations in medical research papers. Burns Trauma. 2019;7:31.
Article PubMed PubMed Central Google Scholar
Hipp G, Vaillant M, Diederich NJ, Roomp K, Satagopam VP, Banda P, et al. The Luxembourg Parkinson’s Study: a comprehensive approach for stratification and early diagnosis. Front Aging Neurosci. 2018;10:326.
Pavelka L, Rawal R, Ghosh S, Pauly C, Pauly L, Hanff A-M, et al. Luxembourg Parkinson’s study -comprehensive baseline analysis of Parkinson’s disease and atypical parkinsonism. Front Neurol. 2023;14:1330321.
Starkstein SE, Mayberg HS, Preziosi TJ, Andrezejewski P, Leiguarda R, Robinson RG. Reliability, validity, and clinical correlates of apathy in Parkinson’s disease. J Neuropsychiatry Clin Neurosci. 1992;4(2):134–9.
Leentjens AF, Dujardin K, Marsh L, Martinez-Martin P, Richard IH, Starkstein SE, et al. Apathy and anhedonia rating scales in Parkinson’s disease: critique and recommendations. Mov Disord. 2008;23(14):2004–14.
Goetz CG, Tilley BC, Shaftman SR, Stebbins GT, Fahn S, Martinez-Martin P, et al. Movement Disorder Society-sponsored revision of the Unified Parkinson’s Disease Rating Scale (MDS-UPDRS): scale presentation and clinimetric testing results. Mov Disord. 2008;23(15):2129–70.
Little RJA. A test of missing completely at random for multivariate data with missing values. J Am Stat Assoc. 1988;83(404):1198–202.
Article Google Scholar
R Core Team. R: A language and environment for statistical computing Vienna: R Foundation for Statistical Computing; 2023. Available from: https://www.R-project.org/ .
Bates D, Maechler M, Bolker B, Walker S. Fitting linear mixed-effects models using lme4. J Stat Softw. 2015;67:1–48.
Lüdecke D. sjPlot: Data Visualization for Statistics in Social Science. 2022 [R package version 2.8.11]. Available from: https://CRAN.R-project.org/package=sjPlot .
Twisk JWR. Applied Multilevel Analysis: A Practical Guide for Medical Researchers. Cambridge: Cambridge University Press; 2006.
Twisk JWR. Applied Mixed Model Analysis. New York: A Practical Guide; 2019.
Long DJ. Longitudinal data analysis for the behavioral sciences using R. United States of America: SAGE; 2012.
Google Scholar
Twisk JWR, de Boer M, de Vente W, Heymans M. Multiple imputation of missing values was not necessary before performing a longitudinal mixed-model analysis. J Clin Epidemiol. 2013;66(9):1022–8.
Student. The probable error of a mean. Biometrika. 1908;6(1):1–25.
Polit DF. Statistics and Data Analysis for Nursing Research. England: Pearson; 2014.
Download references
Acknowledgements
We would like to thank all participants of the Luxembourg Parkinson’s Study for their important support of our research. Furthermore, we acknowledge the joint effort of the National Centre of Excellence in Research on Parkinson’s Disease (NCER-PD) Consortium members from the partner institutions Luxembourg Centre for Systems Biomedicine, Luxembourg Institute of Health, Centre Hospitalier de Luxembourg, and Laboratoire National de Santé generally contributing to the Luxembourg Parkinson’s Study as listed below:
Geeta ACHARYA 2, Gloria AGUAYO 2, Myriam ALEXANDRE 2, Muhammad ALI 1, Wim AMMERLANN 2, Giuseppe ARENA 1, Michele BASSIS 1, Roxane BATUTU 3, Katy BEAUMONT 2, Sibylle BÉCHET 3, Guy BERCHEM 3, Alexandre BISDORFF 5, Ibrahim BOUSSAAD 1, David BOUVIER 4, Lorieza CASTILLO 2, Gessica CONTESOTTO 2, Nancy DE BREMAEKER 3, Brian DEWITT 2, Nico DIEDERICH 3, Rene DONDELINGER 5, Nancy E. RAMIA 1, Angelo Ferrari 2, Katrin FRAUENKNECHT 4, Joëlle FRITZ 2, Carlos GAMIO 2, Manon GANTENBEIN 2, Piotr GAWRON 1, Laura Georges 2, Soumyabrata GHOSH 1, Marijus GIRAITIS 2,3, Enrico GLAAB 1, Martine GOERGEN 3, Elisa GÓMEZ DE LOPE 1, Jérôme GRAAS 2, Mariella GRAZIANO 7, Valentin GROUES 1, Anne GRÜNEWALD 1, Gaël HAMMOT 2, Anne-Marie HANFF 2, 10, 11, Linda HANSEN 3, Michael HENEKA 1, Estelle HENRY 2, Margaux Henry 2, Sylvia HERBRINK 3, Sascha HERZINGER 1, Alexander HUNDT 2, Nadine JACOBY 8, Sonja JÓNSDÓTTIR 2,3, Jochen KLUCKEN 1,2,3, Olga KOFANOVA 2, Rejko KRÜGER 1,2,3, Pauline LAMBERT 2, Zied LANDOULSI 1, Roseline LENTZ 6, Laura LONGHINO 3, Ana Festas Lopes 2, Victoria LORENTZ 2, Tainá M. MARQUES 2, Guilherme MARQUES 2, Patricia MARTINS CONDE 1, Patrick MAY 1, Deborah MCINTYRE 2, Chouaib MEDIOUNI 2, Francoise MEISCH 1, Alexia MENDIBIDE 2, Myriam MENSTER 2, Maura MINELLI 2, Michel MITTELBRONN 1, 2, 4, 10, 12, 13, Saïda MTIMET 2, Maeva Munsch 2, Romain NATI 3, Ulf NEHRBASS 2, Sarah NICKELS 1, Beatrice NICOLAI 3, Jean-Paul NICOLAY 9, Fozia NOOR 2, Clarissa P. C. GOMES 1, Sinthuja PACHCHEK 1, Claire PAULY 2,3, Laure PAULY 2, 10, Lukas PAVELKA 2,3, Magali PERQUIN 2, Achilleas PEXARAS 2, Armin RAUSCHENBERGER 1, Rajesh RAWAL 1, Dheeraj REDDY BOBBILI 1, Lucie REMARK 2, Ilsé Richard 2, Olivia ROLAND 2, Kirsten ROOMP 1, Eduardo ROSALES 2, Stefano SAPIENZA 1, Venkata SATAGOPAM 1, Sabine SCHMITZ 1, Reinhard SCHNEIDER 1, Jens SCHWAMBORN 1, Raquel SEVERINO 2, Amir SHARIFY 2, Ruxandra SOARE 1, Ekaterina SOBOLEVA 1,3, Kate SOKOLOWSKA 2, Maud Theresine 2, Hermann THIEN 2, Elodie THIRY 3, Rebecca TING JIIN LOO 1, Johanna TROUET 2, Olena TSURKALENKO 2, Michel VAILLANT 2, Carlos VEGA 2, Liliana VILAS BOAS 3, Paul WILMES 1, Evi WOLLSCHEID-LENGELING 1, Gelani ZELIMKHANOV 2,3
1 Luxembourg Centre for Systems Biomedicine, University of Luxembourg, Esch-sur-Alzette, Luxembourg
2 Luxembourg Institute of Health, Strassen, Luxembourg
3 Centre Hospitalier de Luxembourg, Strassen, Luxembourg
4 Laboratoire National de Santé, Dudelange, Luxembourg
5 Centre Hospitalier Emile Mayrisch, Esch-sur-Alzette, Luxembourg
6 Parkinson Luxembourg Association, Leudelange, Luxembourg
7 Association of Physiotherapists in Parkinson's Disease Europe, Esch-sur-Alzette, Luxembourg
8 Private practice, Ettelbruck, Luxembourg
9 Private practice, Luxembourg, Luxembourg
10 Faculty of Science, Technology and Medicine, University of Luxembourg, Esch-sur-Alzette, Luxembourg
11 Department of Epidemiology, CAPHRI School for Public Health and Primary Care, Maastricht University Medical Centre+, Maastricht, the Netherlands
12 Luxembourg Center of Neuropathology, Dudelange, Luxembourg
13 Department of Life Sciences and Medicine, University of Luxembourg, Esch-sur-Alzette, Luxembourg
This work was supported by grants from the Luxembourg National Research Fund (FNR) within the National Centre of Excellence in Research on Parkinson's disease [NCERPD(FNR/NCER13/BM/11264123)]. The funding body played no role in the design of the study and collection, analysis, interpretation of data, and in writing the manuscript.
Author information
Authors and affiliations.
Transversal Translational Medicine, Luxembourg Institute of Health, Strassen, Luxembourg
Anne-Marie Hanff & Rejko Krüger
Translational Neurosciences, Luxembourg Centre for Systems Biomedicine, University of Luxembourg, Esch-Sur-Alzette, Luxembourg
Department of Epidemiology, CAPHRI Care and Public Health Research Institute, Maastricht University Medical Centre+, Maastricht, The Netherlands
Anne-Marie Hanff
Department of Nutrition and Movement Sciences, NUTRIM School of Nutrition and Translational Research in Metabolism, Maastricht University Medical Centre+, Maastricht, The Netherlands
Anne-Marie Hanff & Christopher McCrum
Parkinson Research Clinic, Centre Hospitalier du Luxembourg, Luxembourg, Luxembourg
Rejko Krüger
Department of Mathematics, University of Luxembourg, Esch-Sur-Alzette, Luxembourg
Christophe Ley
You can also search for this author in PubMed Google Scholar
- Geeta Acharya
- , Gloria Aguayo
- , Myriam Alexandre
- , Muhammad Ali
- , Wim Ammerlann
- , Giuseppe Arena
- , Michele Bassis
- , Roxane Batutu
- , Katy Beaumont
- , Sibylle Béchet
- , Guy Berchem
- , Alexandre Bisdorff
- , Ibrahim Boussaad
- , David Bouvier
- , Lorieza Castillo
- , Gessica Contesotto
- , Nancy de Bremaeker
- , Brian Dewitt
- , Nico Diederich
- , Rene Dondelinger
- , Nancy E. Ramia
- , Angelo Ferrari
- , Katrin Frauenknecht
- , Joëlle Fritz
- , Carlos Gamio
- , Manon Gantenbein
- , Piotr Gawron
- , Laura georges
- , Soumyabrata Ghosh
- , Marijus Giraitis
- , Enrico Glaab
- , Martine Goergen
- , Elisa Gómez de Lope
- , Jérôme Graas
- , Mariella Graziano
- , Valentin Groues
- , Anne Grünewald
- , Gaël Hammot
- , Anne-Marie Hanff
- , Linda Hansen
- , Michael Heneka
- , Estelle Henry
- , Margaux Henry
- , Sylvia Herbrink
- , Sascha Herzinger
- , Alexander Hundt
- , Nadine Jacoby
- , Sonja Jónsdóttir
- , Jochen Klucken
- , Olga Kofanova
- , Rejko Krüger
- , Pauline Lambert
- , Zied Landoulsi
- , Roseline Lentz
- , Laura Longhino
- , Ana Festas Lopes
- , Victoria Lorentz
- , Tainá M. Marques
- , Guilherme Marques
- , Patricia Martins Conde
- , Patrick May
- , Deborah Mcintyre
- , Chouaib Mediouni
- , Francoise Meisch
- , Alexia Mendibide
- , Myriam Menster
- , Maura Minelli
- , Michel Mittelbronn
- , Saïda Mtimet
- , Maeva Munsch
- , Romain Nati
- , Ulf Nehrbass
- , Sarah Nickels
- , Beatrice Nicolai
- , Jean-Paul Nicolay
- , Fozia Noor
- , Clarissa P. C. Gomes
- , Sinthuja Pachchek
- , Claire Pauly
- , Laure Pauly
- , Lukas Pavelka
- , Magali Perquin
- , Achilleas Pexaras
- , Armin Rauschenberger
- , Rajesh Rawal
- , Dheeraj Reddy Bobbili
- , Lucie Remark
- , Ilsé Richard
- , Olivia Roland
- , Kirsten Roomp
- , Eduardo Rosales
- , Stefano Sapienza
- , Venkata Satagopam
- , Sabine Schmitz
- , Reinhard Schneider
- , Jens Schwamborn
- , Raquel Severino
- , Amir Sharify
- , Ruxandra Soare
- , Ekaterina Soboleva
- , Kate Sokolowska
- , Maud Theresine
- , Hermann Thien
- , Elodie Thiry
- , Rebecca Ting Jiin Loo
- , Johanna Trouet
- , Olena Tsurkalenko
- , Michel Vaillant
- , Carlos Vega
- , Liliana Vilas Boas
- , Paul Wilmes
- , Evi Wollscheid-Lengeling
- & Gelani Zelimkhanov
Contributions
A-MH: Conceptualization, Methodology, Formal analysis, Investigation, Visualization, Project administration, Writing – original draft, Writing – review & editing. RK: Conceptualization, Methodology, Funding, Resources, Supervision, Project administration, Writing – review & editing. CMC: Conceptualization, Methodology, Supervision, Writing – original draft, Writing – review & editing. CL: Conceptualization, Methodology, Writing – original draft, Writing – review & editing.
Corresponding author
Correspondence to Anne-Marie Hanff .
Ethics declarations
Ethics approval and consent to participate.
The study involved human participants, was reviewed and obtained approval from the National Ethics Board Comité National d’Ethique de Recherche (CNER Ref: 201407/13). The study was performed in accordance with the Declaration of Helsinki and patients/participants provided their written informed consent to participate in this study. We confirm that we have read the Journal’s position on issues involved in ethical publication and affirm that this work is consistent with those guidelines.
Consent for publication
Competing interests.
The authors declare no competing interests.
Additional information
Publisher’s note.
Springer Nature remains neutral with regard to jurisdictional claims in published maps and institutional affiliations.
Supplementary Information
Supplementary material 1., rights and permissions.
Open Access This article is licensed under a Creative Commons Attribution 4.0 International License, which permits use, sharing, adaptation, distribution and reproduction in any medium or format, as long as you give appropriate credit to the original author(s) and the source, provide a link to the Creative Commons licence, and indicate if changes were made. The images or other third party material in this article are included in the article's Creative Commons licence, unless indicated otherwise in a credit line to the material. If material is not included in the article's Creative Commons licence and your intended use is not permitted by statutory regulation or exceeds the permitted use, you will need to obtain permission directly from the copyright holder. To view a copy of this licence, visit http://creativecommons.org/licenses/by/4.0/ . The Creative Commons Public Domain Dedication waiver ( http://creativecommons.org/publicdomain/zero/1.0/ ) applies to the data made available in this article, unless otherwise stated in a credit line to the data.
Reprints and permissions
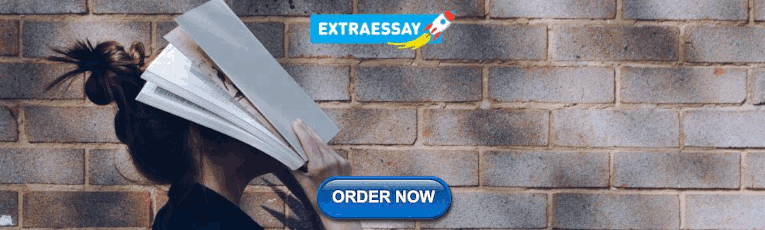
About this article
Cite this article.
Hanff, AM., Krüger, R., McCrum, C. et al. Mixed effects models but not t-tests or linear regression detect progression of apathy in Parkinson’s disease over seven years in a cohort: a comparative analysis. BMC Med Res Methodol 24 , 183 (2024). https://doi.org/10.1186/s12874-024-02301-7
Download citation
Received : 21 March 2024
Accepted : 01 August 2024
Published : 24 August 2024
DOI : https://doi.org/10.1186/s12874-024-02301-7
Share this article
Anyone you share the following link with will be able to read this content:
Sorry, a shareable link is not currently available for this article.
Provided by the Springer Nature SharedIt content-sharing initiative
- Cohort studies
- Epidemiology
- Disease progression
- Lost to follow-up
- Statistical model
BMC Medical Research Methodology
ISSN: 1471-2288
- General enquiries: [email protected]
- Article Information
Data Sharing Statement
- As Ozempic’s Popularity Soars, Here’s What to Know About Semaglutide and Weight Loss JAMA Medical News & Perspectives May 16, 2023 This Medical News article discusses chronic weight management with semaglutide, sold under the brand names Ozempic and Wegovy. Melissa Suran, PhD, MSJ
- Patents and Regulatory Exclusivities on GLP-1 Receptor Agonists JAMA Special Communication August 15, 2023 This Special Communication used data from the US Food and Drug Administration to analyze how manufacturers of brand-name glucagon-like peptide 1 (GLP-1) receptor agonists have used patent and regulatory systems to extend periods of market exclusivity. Rasha Alhiary, PharmD; Aaron S. Kesselheim, MD, JD, MPH; Sarah Gabriele, LLM, MBE; Reed F. Beall, PhD; S. Sean Tu, JD, PhD; William B. Feldman, MD, DPhil, MPH
- What to Know About Wegovy’s Rare but Serious Adverse Effects JAMA Medical News & Perspectives December 12, 2023 This Medical News article discusses Wegovy, Ozempic, and other GLP-1 receptor agonists used for weight management and type 2 diabetes. Kate Ruder, MSJ
- GLP-1 Receptor Agonists and Gastrointestinal Adverse Events—Reply JAMA Comment & Response March 12, 2024 Ramin Rezaeianzadeh, BSc; Mohit Sodhi, MSc; Mahyar Etminan, PharmD, MSc
- GLP-1 Receptor Agonists and Gastrointestinal Adverse Events JAMA Comment & Response March 12, 2024 Karine Suissa, PhD; Sara J. Cromer, MD; Elisabetta Patorno, MD, DrPH
- GLP-1 Receptor Agonist Use and Risk of Postoperative Complications JAMA Research Letter May 21, 2024 This cohort study evaluates the risk of postoperative respiratory complications among patients with diabetes undergoing surgery who had vs those who had not a prescription fill for glucagon-like peptide 1 receptor agonists. Anjali A. Dixit, MD, MPH; Brian T. Bateman, MD, MS; Mary T. Hawn, MD, MPH; Michelle C. Odden, PhD; Eric C. Sun, MD, PhD
- Glucagon-Like Peptide-1 Receptor Agonist Use and Risk of Gallbladder and Biliary Diseases JAMA Internal Medicine Original Investigation May 1, 2022 This systematic review and meta-analysis of 76 randomized clinical trials examines the effects of glucagon-like peptide-1 receptor agonist use on the risk of gallbladder and biliary diseases. Liyun He, MM; Jialu Wang, MM; Fan Ping, MD; Na Yang, MM; Jingyue Huang, MM; Yuxiu Li, MD; Lingling Xu, MD; Wei Li, MD; Huabing Zhang, MD
- Cholecystitis Associated With the Use of Glucagon-Like Peptide-1 Receptor Agonists JAMA Internal Medicine Research Letter October 1, 2022 This case series identifies cases reported in the US Food and Drug Administration Adverse Event Reporting System of acute cholecystitis associated with use of glucagon-like peptide-1 receptor agonists that did not have gallbladder disease warnings in their labeling. Daniel Woronow, MD; Christine Chamberlain, PharmD; Ali Niak, MD; Mark Avigan, MDCM; Monika Houstoun, PharmD, MPH; Cindy Kortepeter, PharmD
See More About
Select your interests.
Customize your JAMA Network experience by selecting one or more topics from the list below.
- Academic Medicine
- Acid Base, Electrolytes, Fluids
- Allergy and Clinical Immunology
- American Indian or Alaska Natives
- Anesthesiology
- Anticoagulation
- Art and Images in Psychiatry
- Artificial Intelligence
- Assisted Reproduction
- Bleeding and Transfusion
- Caring for the Critically Ill Patient
- Challenges in Clinical Electrocardiography
- Climate and Health
- Climate Change
- Clinical Challenge
- Clinical Decision Support
- Clinical Implications of Basic Neuroscience
- Clinical Pharmacy and Pharmacology
- Complementary and Alternative Medicine
- Consensus Statements
- Coronavirus (COVID-19)
- Critical Care Medicine
- Cultural Competency
- Dental Medicine
- Dermatology
- Diabetes and Endocrinology
- Diagnostic Test Interpretation
- Drug Development
- Electronic Health Records
- Emergency Medicine
- End of Life, Hospice, Palliative Care
- Environmental Health
- Equity, Diversity, and Inclusion
- Facial Plastic Surgery
- Gastroenterology and Hepatology
- Genetics and Genomics
- Genomics and Precision Health
- Global Health
- Guide to Statistics and Methods
- Hair Disorders
- Health Care Delivery Models
- Health Care Economics, Insurance, Payment
- Health Care Quality
- Health Care Reform
- Health Care Safety
- Health Care Workforce
- Health Disparities
- Health Inequities
- Health Policy
- Health Systems Science
- History of Medicine
- Hypertension
- Images in Neurology
- Implementation Science
- Infectious Diseases
- Innovations in Health Care Delivery
- JAMA Infographic
- Law and Medicine
- Leading Change
- Less is More
- LGBTQIA Medicine
- Lifestyle Behaviors
- Medical Coding
- Medical Devices and Equipment
- Medical Education
- Medical Education and Training
- Medical Journals and Publishing
- Mobile Health and Telemedicine
- Narrative Medicine
- Neuroscience and Psychiatry
- Notable Notes
- Nutrition, Obesity, Exercise
- Obstetrics and Gynecology
- Occupational Health
- Ophthalmology
- Orthopedics
- Otolaryngology
- Pain Medicine
- Palliative Care
- Pathology and Laboratory Medicine
- Patient Care
- Patient Information
- Performance Improvement
- Performance Measures
- Perioperative Care and Consultation
- Pharmacoeconomics
- Pharmacoepidemiology
- Pharmacogenetics
- Pharmacy and Clinical Pharmacology
- Physical Medicine and Rehabilitation
- Physical Therapy
- Physician Leadership
- Population Health
- Primary Care
- Professional Well-being
- Professionalism
- Psychiatry and Behavioral Health
- Public Health
- Pulmonary Medicine
- Regulatory Agencies
- Reproductive Health
- Research, Methods, Statistics
- Resuscitation
- Rheumatology
- Risk Management
- Scientific Discovery and the Future of Medicine
- Shared Decision Making and Communication
- Sleep Medicine
- Sports Medicine
- Stem Cell Transplantation
- Substance Use and Addiction Medicine
- Surgical Innovation
- Surgical Pearls
- Teachable Moment
- Technology and Finance
- The Art of JAMA
- The Arts and Medicine
- The Rational Clinical Examination
- Tobacco and e-Cigarettes
- Translational Medicine
- Trauma and Injury
- Treatment Adherence
- Ultrasonography
- Users' Guide to the Medical Literature
- Vaccination
- Venous Thromboembolism
- Veterans Health
- Women's Health
- Workflow and Process
- Wound Care, Infection, Healing
Others Also Liked
- Download PDF
- X Facebook More LinkedIn
Sodhi M , Rezaeianzadeh R , Kezouh A , Etminan M. Risk of Gastrointestinal Adverse Events Associated With Glucagon-Like Peptide-1 Receptor Agonists for Weight Loss. JAMA. 2023;330(18):1795–1797. doi:10.1001/jama.2023.19574
Manage citations:
© 2024
- Permissions
Risk of Gastrointestinal Adverse Events Associated With Glucagon-Like Peptide-1 Receptor Agonists for Weight Loss
- 1 Faculty of Medicine, University of British Columbia, Vancouver, British Columbia, Canada
- 2 StatExpert Ltd, Laval, Quebec, Canada
- 3 Department of Ophthalmology and Visual Sciences and Medicine, University of British Columbia, Vancouver, Canada
- Medical News & Perspectives As Ozempic’s Popularity Soars, Here’s What to Know About Semaglutide and Weight Loss Melissa Suran, PhD, MSJ JAMA
- Special Communication Patents and Regulatory Exclusivities on GLP-1 Receptor Agonists Rasha Alhiary, PharmD; Aaron S. Kesselheim, MD, JD, MPH; Sarah Gabriele, LLM, MBE; Reed F. Beall, PhD; S. Sean Tu, JD, PhD; William B. Feldman, MD, DPhil, MPH JAMA
- Medical News & Perspectives What to Know About Wegovy’s Rare but Serious Adverse Effects Kate Ruder, MSJ JAMA
- Comment & Response GLP-1 Receptor Agonists and Gastrointestinal Adverse Events—Reply Ramin Rezaeianzadeh, BSc; Mohit Sodhi, MSc; Mahyar Etminan, PharmD, MSc JAMA
- Comment & Response GLP-1 Receptor Agonists and Gastrointestinal Adverse Events Karine Suissa, PhD; Sara J. Cromer, MD; Elisabetta Patorno, MD, DrPH JAMA
- Research Letter GLP-1 Receptor Agonist Use and Risk of Postoperative Complications Anjali A. Dixit, MD, MPH; Brian T. Bateman, MD, MS; Mary T. Hawn, MD, MPH; Michelle C. Odden, PhD; Eric C. Sun, MD, PhD JAMA
- Original Investigation Glucagon-Like Peptide-1 Receptor Agonist Use and Risk of Gallbladder and Biliary Diseases Liyun He, MM; Jialu Wang, MM; Fan Ping, MD; Na Yang, MM; Jingyue Huang, MM; Yuxiu Li, MD; Lingling Xu, MD; Wei Li, MD; Huabing Zhang, MD JAMA Internal Medicine
- Research Letter Cholecystitis Associated With the Use of Glucagon-Like Peptide-1 Receptor Agonists Daniel Woronow, MD; Christine Chamberlain, PharmD; Ali Niak, MD; Mark Avigan, MDCM; Monika Houstoun, PharmD, MPH; Cindy Kortepeter, PharmD JAMA Internal Medicine
Glucagon-like peptide 1 (GLP-1) agonists are medications approved for treatment of diabetes that recently have also been used off label for weight loss. 1 Studies have found increased risks of gastrointestinal adverse events (biliary disease, 2 pancreatitis, 3 bowel obstruction, 4 and gastroparesis 5 ) in patients with diabetes. 2 - 5 Because such patients have higher baseline risk for gastrointestinal adverse events, risk in patients taking these drugs for other indications may differ. Randomized trials examining efficacy of GLP-1 agonists for weight loss were not designed to capture these events 2 due to small sample sizes and short follow-up. We examined gastrointestinal adverse events associated with GLP-1 agonists used for weight loss in a clinical setting.
We used a random sample of 16 million patients (2006-2020) from the PharMetrics Plus for Academics database (IQVIA), a large health claims database that captures 93% of all outpatient prescriptions and physician diagnoses in the US through the International Classification of Diseases, Ninth Revision (ICD-9) or ICD-10. In our cohort study, we included new users of semaglutide or liraglutide, 2 main GLP-1 agonists, and the active comparator bupropion-naltrexone, a weight loss agent unrelated to GLP-1 agonists. Because semaglutide was marketed for weight loss after the study period (2021), we ensured all GLP-1 agonist and bupropion-naltrexone users had an obesity code in the 90 days prior or up to 30 days after cohort entry, excluding those with a diabetes or antidiabetic drug code.
Patients were observed from first prescription of a study drug to first mutually exclusive incidence (defined as first ICD-9 or ICD-10 code) of biliary disease (including cholecystitis, cholelithiasis, and choledocholithiasis), pancreatitis (including gallstone pancreatitis), bowel obstruction, or gastroparesis (defined as use of a code or a promotility agent). They were followed up to the end of the study period (June 2020) or censored during a switch. Hazard ratios (HRs) from a Cox model were adjusted for age, sex, alcohol use, smoking, hyperlipidemia, abdominal surgery in the previous 30 days, and geographic location, which were identified as common cause variables or risk factors. 6 Two sensitivity analyses were undertaken, one excluding hyperlipidemia (because more semaglutide users had hyperlipidemia) and another including patients without diabetes regardless of having an obesity code. Due to absence of data on body mass index (BMI), the E-value was used to examine how strong unmeasured confounding would need to be to negate observed results, with E-value HRs of at least 2 indicating BMI is unlikely to change study results. Statistical significance was defined as 2-sided 95% CI that did not cross 1. Analyses were performed using SAS version 9.4. Ethics approval was obtained by the University of British Columbia’s clinical research ethics board with a waiver of informed consent.
Our cohort included 4144 liraglutide, 613 semaglutide, and 654 bupropion-naltrexone users. Incidence rates for the 4 outcomes were elevated among GLP-1 agonists compared with bupropion-naltrexone users ( Table 1 ). For example, incidence of biliary disease (per 1000 person-years) was 11.7 for semaglutide, 18.6 for liraglutide, and 12.6 for bupropion-naltrexone and 4.6, 7.9, and 1.0, respectively, for pancreatitis.
Use of GLP-1 agonists compared with bupropion-naltrexone was associated with increased risk of pancreatitis (adjusted HR, 9.09 [95% CI, 1.25-66.00]), bowel obstruction (HR, 4.22 [95% CI, 1.02-17.40]), and gastroparesis (HR, 3.67 [95% CI, 1.15-11.90) but not biliary disease (HR, 1.50 [95% CI, 0.89-2.53]). Exclusion of hyperlipidemia from the analysis did not change the results ( Table 2 ). Inclusion of GLP-1 agonists regardless of history of obesity reduced HRs and narrowed CIs but did not change the significance of the results ( Table 2 ). E-value HRs did not suggest potential confounding by BMI.
This study found that use of GLP-1 agonists for weight loss compared with use of bupropion-naltrexone was associated with increased risk of pancreatitis, gastroparesis, and bowel obstruction but not biliary disease.
Given the wide use of these drugs, these adverse events, although rare, must be considered by patients who are contemplating using the drugs for weight loss because the risk-benefit calculus for this group might differ from that of those who use them for diabetes. Limitations include that although all GLP-1 agonist users had a record for obesity without diabetes, whether GLP-1 agonists were all used for weight loss is uncertain.
Accepted for Publication: September 11, 2023.
Published Online: October 5, 2023. doi:10.1001/jama.2023.19574
Correction: This article was corrected on December 21, 2023, to update the full name of the database used.
Corresponding Author: Mahyar Etminan, PharmD, MSc, Faculty of Medicine, Departments of Ophthalmology and Visual Sciences and Medicine, The Eye Care Center, University of British Columbia, 2550 Willow St, Room 323, Vancouver, BC V5Z 3N9, Canada ( [email protected] ).
Author Contributions: Dr Etminan had full access to all of the data in the study and takes responsibility for the integrity of the data and the accuracy of the data analysis.
Concept and design: Sodhi, Rezaeianzadeh, Etminan.
Acquisition, analysis, or interpretation of data: All authors.
Drafting of the manuscript: Sodhi, Rezaeianzadeh, Etminan.
Critical review of the manuscript for important intellectual content: All authors.
Statistical analysis: Kezouh.
Obtained funding: Etminan.
Administrative, technical, or material support: Sodhi.
Supervision: Etminan.
Conflict of Interest Disclosures: None reported.
Funding/Support: This study was funded by internal research funds from the Department of Ophthalmology and Visual Sciences, University of British Columbia.
Role of the Funder/Sponsor: The funder had no role in the design and conduct of the study; collection, management, analysis, and interpretation of the data; preparation, review, or approval of the manuscript; and decision to submit the manuscript for publication.
Data Sharing Statement: See Supplement .
- Register for email alerts with links to free full-text articles
- Access PDFs of free articles
- Manage your interests
- Save searches and receive search alerts
- Open access
- Published: 22 August 2024
Unveiling the unique role of TSPAN7 across tumors: a pan-cancer study incorporating retrospective clinical research and bioinformatic analysis
- Bingnan Lu 1 na1 ,
- Yifan Liu 1 na1 ,
- Yuntao Yao 1 na1 ,
- Dawei Zhu 2 na1 ,
- Xiangmin Zhang 3 ,
- Keqin Dong 1 ,
- Xiao Xu 2 ,
- Donghao Lv 1 ,
- Zihui Zhao 1 ,
- Haoyu Zhang 1 ,
- Xinyue Yang 1 ,
- Wenjia Fu 1 ,
- Runzhi Huang 4 ,
- Jianwei Cao 1 , 2 ,
- Jian Chu 3 ,
- Xiuwu Pan 1 &
- Xingang Cui 1
Biology Direct volume 19 , Article number: 72 ( 2024 ) Cite this article
84 Accesses
Metrics details
TSPAN7 is an important factor in tumor progression. However, the precise function of TSPAN7 and its role in pan-cancer are not clear.
Based on Xinhua cohort incorporating 370 patients with kidney neoplasm, we conducted differential expression analysis by immunohistochemistry between tumor and normal tissues, and explored correlations of TSPAN7 with patients’ survival. Subsequently, we conducted a pan-cancer study, and successively employed differential expression analysis, competing endogenous RNA (ceRNA) analysis, protein-protein interaction (PPI) analysis, correlation analysis of TSPAN7 with clinical characteristics, tumor purity, tumor genomics, tumor immunity, and drug sensitivity. Last but not least, gene set enrichment analysis was applied to identify enriched pathways of TSPAN7.
In Xinhua cohort, TSPAN7 expression was significantly up-regulated (P-value = 0.0019) in tumor tissues of kidney neoplasm patients. High TSPAN7 expression was associated with decreases in overall survival (OS) (P-value = 0.009) and progression-free survival (P-value = 0.009), and it was further revealed as an independent risk factor for OS (P-value = 0.0326, HR = 5.66, 95%CI = 1.155–27.8). In pan-cancer analysis, TSPAN7 expression was down-regulated in most tumors, and it was associated with patients’ survival, tumor purity, tumor genomics, tumor immunity, and drug sensitivity. The ceRNA network and PPI network of TSPAN7 were also constructed. Last but not least, the top five enriched pathways of TSPAN7 in various tumors were identified.
TSPAN7 served as a promising biomarker of various tumors, especially kidney neoplasms, and it was closely associated with tumor purity, tumor genomics, tumor immunology, and drug sensitivity in pan-cancer level.
Introduction
Tetraspanins (TSPANs) are a protein family with 33 mammalian members that consists of four transmembrane segments, a large extracellular loop, a small extracellular loop, and a small intracellular loop [ 1 , 2 , 3 ]. Large amounts of evidence have demonstrated TSPANs as a facilitator of tumor development [ 4 ]. Runzhi Huang et al. discovered the important role of TSPANs in pan-cancer [ 5 ]. Notably, it has been found that TSPAN8 could increase tumor cell stemness through the activation of sonic Hedgehog pathway in breast cancer [ 6 ]. Apart from this, high expression of TSPAN13 could induce the CXCR4/CXCL12 signaling transduction, and increase the homing or migration of leukemia stem cells towards bone marrow niche [ 7 ]. In addition, TSPAN1 was related to the chemotherapy resistance of tumor cells [ 8 ]. Above all, TSPANs function in multiple steps of tumor development and tumor therapy.
TSPAN7 is a member of TSPANs located in chromosome Xp11.4 in humans, and exhibits a significant expression in the cerebral cortex and hippocampal region [ 9 , 10 ]. Although other proteins of TSPAN family have been widely reported to be related to tumor development, there are relatively limited studies about TSPAN7’s roles in tumors. Marco Gerlinger et al. identified TSPAN7 as a predictive factor of poor cancer-specific survival in clear cell renal cell carcinoma [ 11 ]. Besides, in osteosarcoma, it has been discovered that TSPAN7 could stimulate the invasion of malignant cells by triggering FAK-Src-Ras-ERK1/2 signaling pathway and generating epithelial-mesenchymal transition (EMT) [ 12 ]. In glioma, the expressions of PD-1, PD-L1, and CTLA-4 were negatively correlated with the expression level of TSPAN7, suggesting it as a potential target of immunotherapy [ 13 ]. Although these studies preliminarily highlighted the pivotal role of TSPAN7 in a single kind of tumor, few studies have focused on the general function of TSPAN7 across various tumors.
Some previous studies have already identified certain genes as biomarkers in cancer progression at a pan-cancer level [ 14 , 15 ]. Thus, we intended to carry out a pan-cancer study to comprehensively illustrate the complicated functions of TSPAN7 in tumors. First of all, based on a large retrospective cohort of kidney neoplasm, we discovered the differential expression level of TSPAN7 between tumor and normal tissues, and identified TSPAN7 as a promising prognostic biomarker for kidney neoplasm patients. Subsequently, we questioned if TSPAN7 also played a vital role in other tumors, and conducted a pan-cancer study. Based on The Cancer Genome Atlas (TCGA), we revealed the differential expression of TSPAN7 at a transcription level across 33 cancer types, and further constructed the competing endogenouse RNA (ceRNA) network and protein-protein interaction (PPI) network of TSPAN7. Then, we studied the clinical correlations of TSPAN7, as well as its relationships with tumor purity, tumor genomics, tumor immunity, and drug sensitivity. Last but not least, we conducted functional enrichment analysis of TSPAN7, to preliminarily reveal the molecular mechanism of TSPAN7’s role in tumor development.
Materials and methods
Data source.
Our study was approved by the Ethics Committee of Xinhua Hospital Affiliated to Shanghai Jiao Tong University School of Medicine. 370 patients with kidney neoplasm, who received surgery from 2016 to 2018 were selected to constitute a large retrospective cohort. We conducted follow-up on March, 2021. Tumor and normal tissues of these patients were extracted for immunohistochemical (IHC) staining and scoring to reveal the expression of TSPAN7 in kidney neoplasm at a proteomic level. The TSPAN7 expression data, the patients’ clinical data, the single nucleotide variation (SNV) data, the copy number variation (CNV) data, and the methylation data across 33 cancer types were obtained from TCGA database ( https://tcga-data.nci.nih.gov/tcga/ ). The data of microRNAs (miRNAs) derived from DIANA-microT ( https://dianalab.e-ce.uth.gr/microt_webserver/#/ ) [ 16 ], miRWalk ( http://mirwalk.umm.uni-heidelberg.de/ ) [ 17 ], miRDB ( https://mirdb.org/ ) [ 18 ], miRcode ( http://www.mircode.org/ ) [ 19 ], and Encyclopedia of RNA Interactomes (ENCORI) ( https://rnasysu.com/encori/ ) databases [ 20 ]. The data of long noncoding RNAs (lncRNAs) was from ENCORI database as well. The protein-protein interaction data came from STRING database ( https://cn.string-db.org/ ) [ 21 ]. The microsatellite instability (MSI) scores derived from Sameek Roychowdhury et al.’s study [ 22 ]. The genetic alteration profiles of various pan-cancer studies were obtained from cBioPortal ( https://www.cbioportal.org/ ) [ 23 ]. Besides, we also retrieved the immune cell infiltration data from Tumor Immune Estimation Resource 2.0 (TIMER2.0) ( http://timer.cistrome.org/ ) [ 24 ], and searched for the data concerned with cytotoxic T-lymphocyte (CTL) infiltration and function from the Tumor Immune Dysfunction and Exclusion (TIDE) database ( http://tide.dfci.harvard.edu/ ) [ 25 ]. The data concerned drug sensitivity was obtained from Genomics of Drug Sensitivity in Cancer (GDSC) ( https://www.cancerrxgene.org/ ) [ 26 ], The Cancer Therapeutics Response Portal (CTRP) ( https://pubmed.ncbi.nlm.nih.gov/23993102/ ) [ 27 , 28 , 29 ], and CellMiner database ( https://discover.nci.nih.gov/cellminer/home.do ) [ 30 , 31 ]. Last but not least, the data concerning signaling pathways came from the Kyoto Encyclopedia of Genes and Genomes (KEGG) ( https://www.genome.jp/kegg/ ) [ 32 ].
Immunohistochemical staining and scoring
Tumor and normal tissues of 370 patients with kidney neoplasm were preliminarily fixed, embedded in paraffin and then sliced into tissue sections. Then, the sections were dewaxed and rehydrated, and Ethylene Diamine Tetraacetic Acid (EDTA) (pH = 9.0) buffer was employed for the antigen-retrieval process. After that, the samples were treated with 3% hydrogen peroxide solution to block endogenous hydrogen peroxide. 5% goat serum was then added to prevent the non-specific antibody binding. Afterwards, we successively added primary antibodies (TSPAN7, proteintech, 18695-1-AP, 1: 50) and secondary antibodies marked with enzyme Horseradish Peroxidase (HRP). Then, Diaminobenzidine (DAB), the specific substrate for HRP, was then added for color development. Besides, haematoxylin was utilized to enhance nuclear contrast. Ultimately, a series of alcohol solution with graded concentrations were used to dehydrate the samples, and all tissue sections were observed under a light microscope. Two pathologists were invited to independently evaluate the slides. The conflicted results were carefully checked by a third pathologist. As TSPAN7 is mainly expressed in cytoplasm and membranes [ 4 ], the staining of these locations under the light microscope was regarded as positive staining. The staining intensity score was defined as: 0 for negative, 1 for weak, 2 for moderate, and 3 for intense. The score for the percentage of positive cells was defined as: 0 for 0%, 1 for 1–10%, 2 for 11–40%, 3 for 41–70%, and 4 for 71–100%. The IHC score was the product of the staining intensity score and score for the percentage of positive cells, ranging from 0 to 12.
Clinical correlation analysis of TSPAN7 in kidney neoplasm
We collected the clinical data of 370 patients with kidney neoplasm in the retrospective cohort, and data concerning age, gender, histopathological classification, Fuhrman nuclear stage, TNM stage, clinical stage, progression after treatment, progression free survival (PFS), and overall survival (OS) were put into further analysis. The differential IHC scores of TSPAN7 in tumor and normal tissues were visualized in violin plot based on “ggviolin” function from “ggpubr” package (version 0.6.0, https://rpkgs.datanovia.com/ggpubr/ ). The “surv_cutpoint” function from “survminer” R package (version 0.4.9, https://rpkgs.datanovia.com/survminer/index.html ) was applied to identify the cut-off value of the IHC score of TSPAN7, and divided the patients into two subgroups. Kaplan-Meier (K-M) survival analysis was then applied to explore the associations of TSPAN7 with the OS and PFS of patients with kidney neoplasm. Besides, we also used the method of Chi-square test to explore the relationships between TSPAN7 and other clinicopathological variables based on “chisq.test” function from “rstatix” package (version 0.7.2, https://rpkgs.datanovia.com/rstatix/ ) as well as “ggstatsplot” package (version 0.12.3, https://indrajeetpatil.github.io/ggstatsplot/ , https://github.com/IndrajeetPatil/ggstatsplot ). Subsequently, we incorporated demographic variables including “age”, “gender”, some important clinicopathological variables in the clinical practice of kidney neoplasms including “KIRC (yes or no)”, “Fuhrman nuclear stage”, “T stage”, “progression after treatment”, as well as “IHC score of TSPAN7 in tumor tissues” to conduct multivariable Cox regression analysis based on “coxph” function from “survival” package (version 3.7-0, https://github.com/therneau/survival ). 76 patients lacking any of the above data were excluded, and 294 patients were ultimately included in the analysis. The forest plots and nomograms concerning the OS of patients with kidney neoplasm were then constructed, and the risk scores were calculated as follows:
Risk Score = β 1 × Variable 1 + β 2 × Variable 2 + …… + β n × Variable n .
β referred to the regression coefficient determined by the multivariable Cox regression model. n referred to the number of variables.
Based on the median value of risk scores, we divided the patients into the high- and low-risk subgroup, and visualized the OS of patients within the two subgroups, respectively, in the form of scatter plots and K-M survival curves. Finally, we employed residual analysis, calibration curve analysis, and Receiver Operating Characteristic (ROC) analysis to diagnose the multivariable Cox regression model (“survminer” package, “survival” package, and “timeROC” package (version 0.4, https://CRAN.R-project.org/package=timeROC )).
Differential expression analysis of TSPAN7 in pan-cancer
“ggpubr” R package was applied to reveal the differential expression of TSPAN7 between tumor and normal tissues across 33 cancer types in TCGA (Wilcox test). The expression of TSPAN7 was illustrated in the boxplot.
Competing endogenouse RNA (ceRNA) network analysis of TSPAN7 in pan-cancer
First of all, we identified miRNAs targeting TSPAN7 based on DIANA-microT, miRWalk, miRDB, and miRcode databases, and took the intersections of them as key miRNAs. Then, we explored the correlations between the expressions of key miRNAs and TSPAN7 in pan-cancer based on ENCORI database. Afterwards, we identified the target lncRNAs of key miRNAs as well, and constructed the ceRNA network of TSPAN7, visualized in the form of Cytoscape network and Sankey diagram.
Protein-protein interaction (PPI) analysis of TSPAN7 in pan-cancer
We investigated possible interactions of TSPAN7 with other proteins based on the STRING database, and exhibited the results in a protein-protein interaction (PPI) network.
Clinical correlation analysis of TSPAN7 in pan-cancer
Based on the data of TSPAN7 expression and patients’ survival data including OS, PFS, disease free survival (DFS), and disease specific survival (DSS), we successively conducted K-M survival analysis and univariable Cox regression analysis to explore the associations of TSPAN7 with patients’ survival in pan-cancer based on “survival” R package. Besides, we also displayed TSPAN7 expression in diverse stages of cancer, and used Wilcox test to reveal the correlations between TSPAN7 and clinical stages. Moreover, for four types of tumors that commonly metastasized to bones, including BLCA, BRCA, MESO, and PRAD, we conducted differential expression analysis between the primary and metastatic tumor to discover the potential role of TSPAN7 in tumor metastasis. Last but not least, we also exhibited the differential expression level of TSPAN7 across four different molecular subtypes of BRCA and the corresponding normal tissues, suggesting TSPAN7’s value in tumor heterogeneity.
Estimation of STromal and Immune cells in MAlignant tumors using expression data (ESTIMATE) analysis
Tumors are usually consisted of tumor cells and the adjacent tumor microenvironment (TME), and TME is composed of stromal cells, immune cells, etc. [ 33 , 34 ]. In our study, the ESTIMATE algorithm was employed to calculate the immune scores and stromal scores of 33 types of cancers in TCGA [ 35 ]. Subsequently, Spearman correlation analysis was conducted between TSPAN7 expression and immune scores or stromal scores. Generally speaking, more abundant immune and stromal components there were, lower the tumor purity was. Tumor purity was negatively correlated with immune scores and stromal scores. Similarly, the immune cell infiltration was also evaluated by the ESTIMATE algorithm, and correlations of TSPAN7 expression with immune infiltration were tested by Spearman correlation analysis as well.
Tumor mutation burden (TMB) analysis, microsatellite instability (MSI) analysis, cBioPortal analysis, and gene set cancer analysis (GSCA)
The TMB in 33 types of cancers was tested by “maftools” based on the TCGA Whole Exome Sequencing (WES) data [ 36 ], and the MSI scores of them came from Sameek Roychowdhury et al.’s study [ 22 ]. Subsequently, we used the method of Spearman correlation analysis to explore the relationships between TSPAN7 expression and TMB or MSI, visualizing the results in two radar maps. cBioPortal was applied to further reveal the genomic relevance of TSPAN7 ( https://www.cbioportal.org/ ) [ 23 ]. We selected 10 pan-cancer studies as our database [ 37 , 38 , 39 , 40 , 41 , 42 , 43 , 44 , 45 , 46 ], and analyzed CNVs as well as mutation sites of TSPAN7. Afterwards, based on GSCA platform, we further identified the mutation profiles of TSPAN7 concerning SNV, CNV, and methylation, and explored the survival differences among patients with various TSPAN7 mutation types.
Co-expression analysis of TSPAN7 and immune gene in pan-cancer
Across 33 cancer types in TCGA, we used Spearman correlation analysis to analyze the correlations between TSPAN7 expression and 47 immune-related genes, including TNFRSF9, CD44, CD86, etc. The above results were illustrated in a heatmap, with the yellow triangle referring to the P-value, and the red or blue triangle referring to the correlation coefficient.
Immune infiltration analysis
TIMER2.0 used methods of CIBERSORT, TIMER, quanTIseq, xCell, MCP-counter, and EPIC algorithm to estimate the immune cell infiltration [ 24 ]. Subsequently, we used the method of Spearman correlation analysis to discover the associations between TSPAN7 and immune cell infiltration.
Cytotoxic T-lymphocyte infiltration and survival analysis
We used TIDE to explore correlations of TSPAN7 with CTLs and patients’ survival [ 25 ]. Based on the E-MTAB-179 ( https://www.ebi.ac.uk/biostudies/arrayexpress/studies/E-MTAB-179 ), GSE12417_GPL570 [ 47 ], METABRIC [ 48 ], TCGA [ 49 ] database, we first exhibited the relevance of TSPAN7 with CTLs in the form of scatter plots and fitting curves. Then, we divided the samples into high- or low-TSPAN7 subgroups based on the median value of TSPAN7 expression, as well as separating the samples into high- or low-CTL subgroups based on the expressions of CTL biomarkers, including PRF1, CD8A, CD8B, GZMA, and GZMB. Finally, we obtained the K-M survival curves of these subgroups, to further reveal the impact of TSPAN7 on CTLs’ function.
Drug sensitivity prediction
We collected the half maximal inhibitory concentration (IC50) data and the corresponding mRNA expression of TSPAN7 in certain cell lines from GDSC, CTRP, and CellMiner database. For GDSC and CTRP database, Pearson correlation analysis was conducted between TSPAN7 expression and IC50 of certain small molecule drugs based on GSCA online platform. For CellMiner database, Pearson correlation analysis was conducted between TSPAN7 expression and Z scores of certain drugs based on “cor.test” function. Higher Z scores indicated higher drug sensitivity.
Gene set enrichment analysis (GSEA)
We applied GSEA to reveal the enriched KEGG pathways related to TSPAN7 expression across 33 cancer types in TCGA. Specifically, we first divided the pan-cancer samples from TCGA database into high- and low-TSPAN7 subgroups based on the median TSPAN7 expression, and re-ordered these samples according to the fold change of differential expressions. Then, we conducted functional enrichment analysis by comparing the gene expression profiles of certain KEGG pathways [ 32 ] with the gene expression profiles of our pan-cancer samples through the clusterProfiler R package (version 4.12.0, https://yulab-smu.top/biomedical-knowledge-mining-book/(docs) https://doi.org/10.1016/j.xinn.2021.100141(paper) ) [ 50 ]. The cancer types with P-value < 0.05 were selected and the top five KEGG pathways with the highest enrichment scores of them were exhibited, respectively.
Statistical analysis
The statistical analysis was conducted with R version 4.3.2 software. Continuous variables having a normal distribution were described by mean ± standard deviation, while continuous variables with an abnormal distribution were described by median (range). Two-tailed P-values < 0.05 and false discovery rate (FDR) < 0.05 were regarded as statistically significant.
Demographic information and clinical features of the cohort including 370 patients with kidney neoplasm
The comprehensive analysis steps of our study were illustrated in Fig. 1 A and Figure S1 . We selected 370 patients with kidney neoplasm, who received surgery from 2018 to 2022 to constitute a large retrospective cohort. The inclusion and exclusion criteria of the clinical cohort were shown in Fig. 1 B. Table S1 illustrated the demographic information and clinical features of the cohort. As for the histopathological classification of neoplasm, 88.92% of the patients were diagnosed with KIRC, while 4.86%, 2.16%, and 4.05% of them were diagnosed with KIRP, KICH, and other kidney neoplasms, respectively. Considering the Fuhrman nuclear stage, patients with stage 1, 2, 3, 4 accounted for 18.65%, 63.24%, 13.78%, and 4.32% of all patients, separately. Besides, the majority of the patients were diagnosed with kidney neoplasm of an early clinical stage, as “Stage 1” (76.22%) accounted for the majority of the pathological sections. Regarding the patients’ survival, 74.59% of them were alive, and most of the patients were alive without the progression of the disease (69.73%).

The analysis flow and the results of immunohistochemical (IHC) staining and scoring. ( A ) The workflow highlighted the important steps of our analysis. ( B ) The inclusion and exclusion criteria of the retrospective cohort were illustrated. ( C ) The IHC scores of TSPAN7 in kidney neoplasm tissues were higher than those in normal tissues (P-value = 0.0019). ( D ) Several IHC staining results of kidney neoplasm tissues and normal tissues were exhibited. Abbreviations: ceRNA, competing endogenouse RNA; PPI, protein-protein interaction
Immunohistochemical staining and scoring of TSPAN7 in kidney neoplasm tissues and normal tissues
IHC staining was employed on the tissue sections obtained from the 370 patients in the cohort (Fig. 1 B). The mean values of the IHC scores of TSPAN7 in tumor and normal tissues were 10.45 and 9.77, respectively, suggesting an up-regulation of TSPAN7 protein expression in kidney neoplasm (P-value = 0.0019) (Table S1 and Fig. 1 C). The IHC scores were graded as 0–9 (low), 10–12 (high) by the cut-off value (Figure S2 A). Specifically, the distribution of tumor tissue sections within low- and high-IHC score subgroups was 31.62% and 67.84% while the distribution of normal tissues sections was 33.51% and 48.65%, separately (Table S1 ). In Fig. 1 D, we exhibited several IHC staining pictures of the tumor and normal tissue sections from the cohort.
Clinical correlations of TSPAN7 in kidney neoplasm
The specific clinicopathological data of each patient was shown in the heatmap in Fig. 2 A. Then, through K-M survival analysis, we discovered that TSPAN7 protein expression in tumor tissues harbored significant correlation with the OS (P-value = 0.009) and PFS (P-value = 0.009) of patients with kidney neoplasm, and high TSPAN7 protein expression usually led to poor clinical outcomes (Fig. 2 B-C). Through Chi-square tests, we further revealed that the IHC scores of TSPAN7 in tumor tissues were significantly associated with three variables, including the IHC scores of TSPAN7 in normal tissues (P-value < 0.001), PFS classification (P-value < 0.05), and OS classification (P-value < 0.01) (Figure S2 B and Table S1 ).
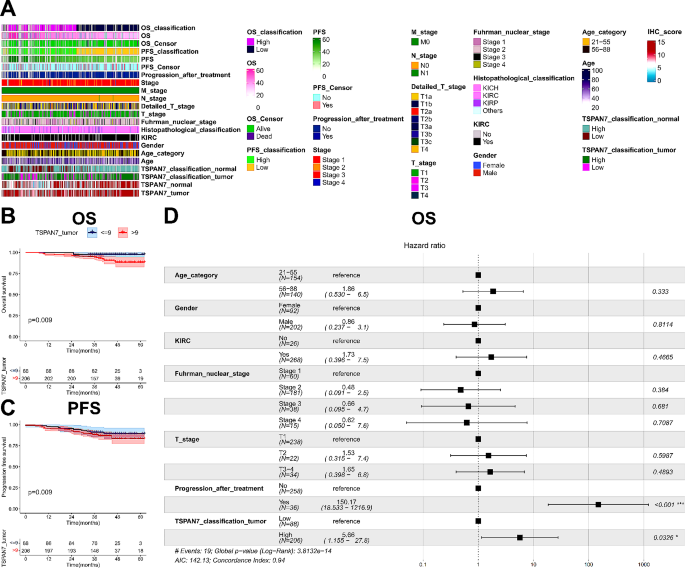
Exploring the clinical correlation of TSPAN7 in kidney neoplasm
( A ) The demographic information and clinical features of the cohort including 370 patients with kidney neoplasm were illustrated in the heatmap. ( B - C ) The Kaplan-Meier survival curves showed that high expression of TSPAN7 was usually associated with lower overall survival (OS) (P-value = 0.009) and progression free survival (PFS) (P-value = 0.009) in kidney neoplasm. ( D ) High IHC scores of TSPAN7 in tumor tissues served as a risk factor of OS (hazard ratio (HR) = 5.66, confidential interval (CI) = 1.155–27.8, P-value = 0.0326) in kidney neoplasm. *, P-value < 0.05; **, P-value < 0.01; ***, P-value < 0.001. Abbreviations: OS, overall survival; PFS, progression free survival; KIRC, Kidney clear cell carcinoma; KICH, kidney chromophobe; KIRP, kidney renal papillary cell carcinoma.
Subsequently, we incorporated seven variables to conduct multivariable Cox regression analysis. From the forest plot, we could identify high TSPAN7 protein expression as an independent risk factor of the OS of patients with kidney neoplasm. The hazard ratio (HR) value of high IHC scores of TSPAN7 in tumor tissues within the multivariable Cox regression model was 5.66, with confidential interval (CI) = 1.155–27.8, P-value = 0.0326 (Fig. 2 D). The nomogram was also constructed (Figure S2 C). Based on the median value of the risk scores, we separated the patients into high- or low-risk subgroups (Figure S2 D). The survival time, OS censor, and risk score of each patient were shown in the scatter plot (Figure S2 E). In the K-M survival analysis, patients in the low-risk subgroup usually harbored better prognosis (P-value < 0.001) (Figure S2 F). Last but not least, residual analysis, calibration curves, and ROC were applied for model diagnosis. Through residual analysis, the proportional hazards assumption of the multivariable Cox regression model was tested (Figure S2 G-H). In the calibration curves, the observed OS was approximately consistent with the predicted OS at 1 year, 2 years, 3 years, 4 years, and 5 years (Figure S2 I). Notably, in the ROC analysis, the area under the curve (AUC) for OS at 1 year, 2 years, 3 years, 4 years, and 5 years were 0.971, 0,975, 0,939, 0.982, and 0.980, respectively, suggesting the outstanding prognostic prediction value of the multivariable Cox regression models (Figure S2 J).
Differential expression, ceRNA network, and PPI network of TSPAN7 in pan-cancer
Considering the differential expression and strong clinical correlations of TSPAN7 in kidney neoplasm, we further questioned if TSPAN7 also played a vital role in pan-cancer. The abbreviations of 33 cancer types in TCGA were shown in Table S2 . At the transcriptomic level, TSPAN7 expression in tumor tissues was lower than that in normal tissues in BLCA, BRCA, CESC, COAD, GBM, HNSC, KIRC, KIRP, LIHC, LUAD, LUSC, PRAD, READ, SARC, STAD, THCA, and UCEC. The results were opposite when considering KICH and PCPG (Fig. 3 A). Subsequently, we searched for TSPAN7-targeted miRNAs from DIANA-microT, miRWalk, miRDB, and miRcode databases, and identified hsa-miR-140, hsa-miR-302 as key TSPAN7-targeted miRNAs by taking the intersections of all miRNAs (Figure S3 A). The expression level of hsa-miR-140-3p, hsa-miR-140-5p, hsa-miR-302a-3p, hsa-miR-302a-5p, hsa-miR-302b-3p, hsa-miR-302b-5p, hsa-miR-302c-3p harbored close correlations with TSPAN7 in various cancers (Figure S3B). Finally, integrated with hsa-miR-140-targeting- and hsa-miR-302-targeting lncRNAs, we revealed the TSPAN7-miRNAs-lncRNAs interactions and constructed the ceRNA network of TSPAN7 (Figure S3 C and Fig. 3 B). Moreover, we also investigated the possible interactions of TSPAN7 with other proteins. At the proteomic level, TSPAN7 was closely associated with OPHN1, IL1RAPL1, GRIA3, PICK1, GRIA2, HSPA5, TSPAN32, CD81, ITGB1, IGSF3, etc. (Fig. 3 C). The ceRNA and PPI network might indicate the possible mechanisms of TSPAN7’s role in cancer progression.
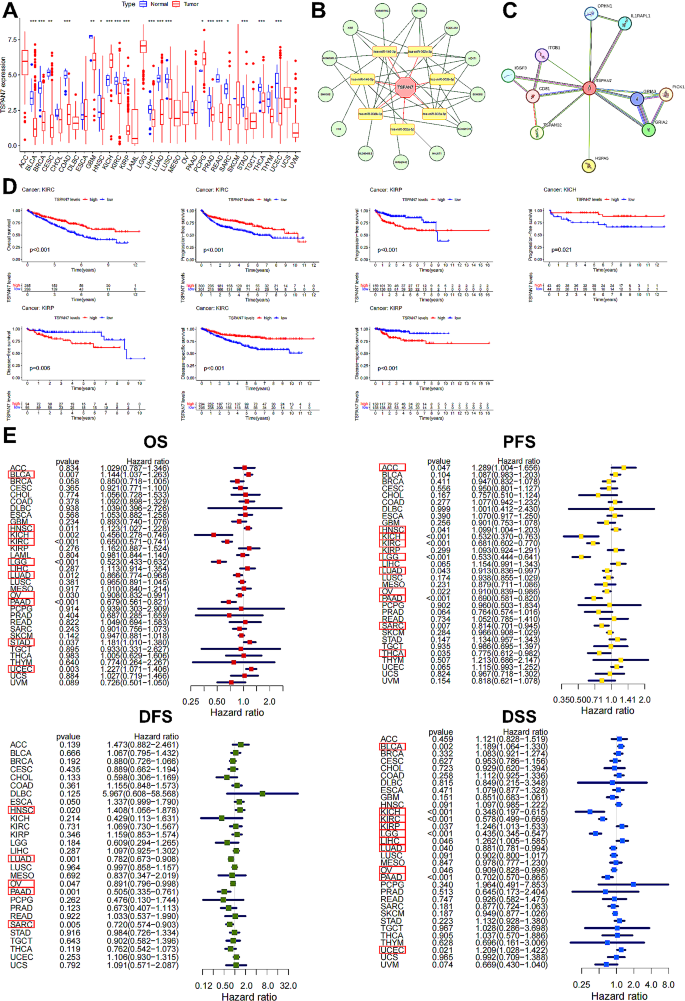
Differential expression, protein–protein interaction (PPI) network, and survival analysis of TSPAN7 in pan-cancer. ( A ) The expression of TSPAN7 in tumor was down-regulated in BLCA (P-value < 0.001), BRCA (P-value < 0.001), CESC (P-value < 0.01), COAD (P-value < 0.001), GBM (P-value < 0.01), HNSC (P-value < 0.05), KIRC (P-value < 0.001), KIRP (P-value < 0.001), LIHC (P-value < 0.001), LUAD (P-value < 0.001), LUSC (P-value < 0.001), PRAD (P-value < 0.001), READ (P-value < 0.001), SARC (P-value < 0.05), STAD (P-value < 0.001), THCA (P-value < 0.001), and UCEC (P-value < 0.001), however, up-regulated in KICH (P-value < 0.001) and PCPG (P-value < 0.05). ( B ) The ceRNA network was constructed by Cytoscape, illustrating the possible TSPAN7-miRNAs-lncRNAs interactions. ( C ) The PPI network illustrated the close association of TSPAN7 with OPHN1, IL1RAPL1, GRIA3, PICK1, GRIA2, HSPA5, TSPAN32, CD81, ITGB1, and IGSF3.. ( D ) The Kaplan-Meier survival curves showed that low expression of TSPAN7 was associated with low probability of OS in KIRC (P-value < 0.001). Besides, low expression of TSPAN7 was also correlated with lower PFS in KICH (P-value = 0.021), KIRC (P-value < 0.001), but higher PFS in KIRP (P-value < 0.001). Moreover, TSPAN7 was negatively related to the disease free survival (DFS) of patients in KIRP (P-value = 0.006). As for disease specific survival (DSS), patients with high expression of TSPAN7 usually harbored lower DSS in KIRP (P-value < 0.001), but higher DSS in KIRC (P-value < 0.001). ( E ) TSPAN7 served as a risk factor of OS in BLCA (HR = 1.144, CI = 1.037–1.263, P-value = 0.007), HNSC (HR = 1.123, CI = 1.027–1.228, P-value = 0.011), STAD (HR = 1.181, CI = 1.010–1.380, P-value = 0.037), UCEC (HR = 1.227, CI = 1.071–1.406, P-value = 0.003), however, served as a protective factor in KICH (HR = 0.456, CI = 0.278–0.746, P-value = 0.002), KIRC (HR = 0.650, CI = 0.571–0.741, P-value < 0.001), LGG (HR = 0.523, CI = 0.433–0.632, P-value < 0.001), LUAD (HR = 0.866, CI = 0.774–0.968, P-value = 0.012), OV (HR = 0.908, CI = 0.832–0.991, P-value = 0.030), PAAD (HR = 0.679, CI = 0.561–0.821, P-value < 0.001). As for PFS, TSPAN7 acted as a risk factor of ACC (HR = 1.289, CI = 1.004–1.656, P-value = 0.047), HNSC (HR = 1.099 CI = 1.004–1.203, P-value = 0.041), while played an opposite role in KICH (HR = 0.532, CI = 0.370–0.763, P-value < 0.001), KIRC (HR = 0.681, CI = 0.602–0.770, P-value < 0.001), LGG (HR = 0.533, CI = 0.444–0.641, P-value < 0.001), LUAD (HR = 0.913, CI = 0.836–0.997, P-value = 0.043), OV (HR = 0.910, CI = 0.839–0.986, P-value = 0.022), PAAD (HR = 0.690, CI = 0.581–0.820, P-value < 0.001), SARC (HR = 0.814, CI = 0.701–0.945, P-value = 0.007), and THCA (HR = 0.775, CI = 0.612–0.982, P-value = 0.035). Moreover, TSPAN7 was protective of DFS in LUAD (HR = 0.782, CI = 0.673–0.908, P-value = 0.001), OV (HR = 0.891, CI = 0.796–0.998, P-value = 0.047), PAAD (HR = 0.505, CI = 0.335–0.761, P-value = 0.001), and SARC (HR = 0.720, CI = 0.574–0.903, P-value = 0.005), but served as a risk factor of HNSC (HR = 1.408, CI = 1.056–1.878, P-value = 0.020). As for DSS, while TSPAN7 was a risk factor in BLCA (HR = 1.189, CI = 1.064–1.330, P-value = 0.002), KIRP (HR = 1.246, CI = 1.013–1.533, P-value = 0.037), LIHC (HR = 1.262, CI = 1.005–1.585, P-value = 0.046), and UCEC (HR = 1.209, CI = 1.028–1.422, P-value = 0.021), it played a protective role in KICH (HR = 0.348, CI = 0.197–0.615, P-value < 0.001), KIRC (HR = 0.578, CI = 0.499–0.669, P-value < 0.001), LGG (HR = 0.435, CI = 0.345–0.547, P-value < 0.001), LUAD (HR = 0.881, CI = 0.781–0.994, P-value = 0.040), OV (HR = 0.909, CI = 0.828–0.998, P-value = 0.046), and PAAD (HR = 0.702, CI = 0.570–0.865, P-value < 0.001). *, P-value < 0.05; **, P-value < 0.01; ***, P-value < 0.001. Abbreviations: DFS, disease free survival; DSS, disease specific survival
Clinical correlations of TSPAN7 in pan-cancer
We further studied the associations between TSPAN7 and patients’ survival in pan-cancer. Through K-M survival analysis, we discovered that low TSPAN7 expression was typically associated with decreased OS in KIRC, LGG, LUAD, and PAAD. Besides, patients with lower expression level of TSPAN7 usually harbored lower PFS in KICH, KIRC, LGG, PAAD, SARC, and THCA, except for ACC and KIRP. As for DFS, TSPAN7 expression was negatively associated with the DFS of patients in DLBC, KIRP, but was positively correlated with LUAD, PAAD, and SARC. Moreover, TSPAN7 was a prognostic prediction index of low DSS in KIRP and LIHC. The results were opposite in KIRC, LGG, and PAAD (Fig. 3 D and Figure S4 ). Subsequently, the univariable Cox regression analysis was applied, and the results were shown in the forest plots. TSPAN7 served as a risk factor in BLCA, HNSC, STAD, and UCEC, but was a protective factor in KICH, KIRC, LGG, LUAD, OV, and PAAD. As for PFS, TSPAN7 was a risk factor of ACC, HNSC, while served as a protective factor of KICH, KIRC, LGG, LUAD, OV, PAAD, SARC, and THCA. In addition, TSPAN7 was a protective factor of DFS in LUAD, OV, PAAD, and SARC, but a risk factor of HNSC. As for DSS, the role of TSPAN7 was two-sided. While it was a risk factor in BLCA, KIRP, LIHC, and UCEC, it functioned as a protective role in KICH, KIRC, LGG, LUAD, OV, and PAAD (Fig. 3 E).
Besides, TSPAN7 was positively correlated with the clinical stage of BLCA, KIRP, and MESO, but was negatively associated with that of BRCA, KIRC, and THCA (Figure S5 A). For four types of tumors which usually metastasized to bones, including BLCA, BRCA, MESO, and PRAD, the expression level of TSPAN7 did not show a statistical difference between the primary and metastatic tumor (Figure S5 B). Last but not least, the expression of TSPAN7 varied significantly among different molecular subtypes of BRCA (Figure S5 C).
The correlations of TSPAN7 with tumor purity in pan-cancer
To further reveal the molecular mechanism of TSPAN7’s role in the development of pan-cancer, we conducted the following research. First of all, we used the ESTIMATE algorithm to calculate the immune score and stromal score of tumors. Generally speaking, TSPAN7 was negatively correlated with immune scores in most types of cancers, including ACC, GBM, KIRC, LGG, LUSC, OV, and THCA, except for BRCA, KIRP, PAAD, and PRAD. However, TSPAN7 harbored a positive correlation with stromal scores in most cancer types, including BLCA, BRCA, CESC, HNSC, KIRP, LAML, PAAD, PRAD, STAD, TGCT, and THYM, except for ACC, GBM, LGG (Fig. 4 A).

The correlations of TSPAN7 with tumor purity and tumor genomics in pan-cancer. ( A ) The scatter plots and the corresponding fitting curves illustrated the correlations of TSPAN7 with the immune score and stromal score in pan-cancer. TSPAN7 harbored a negative correlation with the immune scores in most types of tumors, including ACC ( R = -0.59, P-value < 0.001), GBM ( R = -0.36, P-value < 0.001), KIRC ( R = -0.34, P-value < 0.001), LGG ( R = -0.53, P-value < 0.001), LUSC ( R = -0.18, P-value < 0.001), OV ( R = -0.3, P-value < 0.001), and THCA ( R = -0.27, P-value < 0.001), except for BRCA ( R = 0.31, P-value < 0.001), KIRP ( R = 0.21, P-value < 0.001), PAAD ( R = 0.32, P-value < 0.001), and PRAD ( R = 0.53, P-value < 0.001). As for stromal scores, TSPAN7 was positively correlated with the stromal scores in most types of cancers, including BLCA ( R = 0.23, P-value < 0.001), BRCA ( R = 0.44, P-value < 0.001), CESC ( R = 0.21, P-value < 0.001), HNSC ( R = 0.2, P-value < 0.001), KIRP ( R = 0.41, P-value < 0.001), LAML ( R = 0.27, P-value < 0.001), PAAD ( R = 0.27, P-value < 0.001), PRAD ( R = 0.69, P-value < 0.001), STAD ( R = 0.37, P-value < 0.001), TGCT ( R = 0.6, P-value < 0.001), and THYM ( R = 0.45, P-value < 0.001), except for ACC ( R = -0.44, P-value < 0.001), GBM ( R = -0.4, P-value < 0.001), LGG ( R = -0.44, P-value < 0.001). ( B ) The radar map highlighted the negative correlation of TSPAN7 with TMB in BLCA (P-value < 0.001), BRCA (P-value < 0.001), COAD (P-value < 0.001), DLBC (P-value < 0.05), ESCA (P-value < 0.001), KIRC (P-value < 0.001), KIRP (P-value < 0.001), LGG (P-value < 0.001), LIHC (P-value < 0.05), LUAD (P-value < 0.001), PAAD (P-value < 0.001), PRAD (P-value < 0.001), READ (P-value < 0.05), SARC (P-value < 0.001), STAD (P-value < 0.001), THCA (P-value < 0.001), UCEC (P-value < 0.001), and UCS (P-value < 0.05). ( C ) The radar map highlighted the negative correlation of TSPAN7 with MSI in BLCA (P-value < 0.05), COAD (P-value < 0.001), SARC (P-value < 0.05), SKCM (P-value < 0.05), STAD (P-value < 0.001), and UCEC (P-value < 0.001). ( D ) The major copy number alterations of TSPAN7 were shallow deletion. ( E ) The mutation site of TSPAN7 was exhibited in the plot. *, P-value < 0.05; **, P-value < 0.01; ***, P-value < 0.001
The correlations of TSPAN7 with tumor genomics in pan-cancer
As for genomics, in Fig. 4 B, we could find that TSPAN7 was negatively associated with TMB in most types of cancers, including BLCA, BRCA, COAD, DLBC, ESCA, KIRC, KIRP, LGG, LIHC, LUAD, PAAD, PRAD, READ, SARC, STAD, THCA, UCEC, and UCS. In the MSI radar map, we also identified the negative association between TSPAN7 and MSI in BLCA, COAD, SARC, SKCM, STAD, and UCEC (Fig. 4 C). Besides, through cBioPortal analysis, we also discovered the main type of CNVs of TSPAN7 as shallow deletion (Fig. 4 D). The mutation site of TSPAN7 was also exhibited in Fig. 4 E. Employing GSCA, we further explored the SNV, CNV, and methylation profiles of TSPAN7. The main SNV type of TSPAN7 across various cancer types were “C > T” (Figure S6 A), and there were no statistically significant differences between the survival of SNV and wildtype TSPAN7 (Figure S6 B). The CNV of TSPAN7 was negatively correlated with its mRNA expression in KIRP and SARC, but was positively associated with its mRNA expression in PAAD, PRAD, and OV (Figure S6 C). Regarding the CNV subtypes, we observed more hetezygous amplification of TSPAN7 in ACC, KIRP, SARC, UCS, and more hetezygous deletion in CHOL, ESCA, KICH, OV (Figure S6 D). Besides, there were also more homozygous amplification of TSPAN7 in DLBC, SARC, UCS, but more homozygous deletion in ESCA, HNSC, OV (Figure S6 E). As for patients’ survival, the CNV of TSPAN7 was significantly correlated with OS in KIRP, PAAD, PRAD, and UCEC, with PFS in KIRP, PAAD, THYM, and UCEC, with DSS in KIRP, LUAD, PAAD, PRAD, THCA, and UCEC, with DFS in COAD, KIRP, and UCEC (Figure S6 F). In consideration of methylation, it had negative correlations with the mRNA expression of TSPAN7 in CESC, COAD, ESCA, HNSC, KICH, KIRC, LAML, LUAD, LUSC, PCPG, SKCM, STAD, OV, UCEC, UCS, UVM, except for BRCA (Figure S6 G). The methylation of TSPAN7 served as a risk factor of OS in PAAD, of PFS in KIRP, PRAD, of DSS in PAAD, however, served as a protective factor of OS in COAD, MESO, of PFS in COAD, of DSS in COAD, ESCA, STAD (Figure S6 H).
The correlations of TSPAN7 with tumor immunity in pan-cancer
In the following studies, we explored the associations of TSPAN7 with tumor immunology and immunotherapy. Among 33 types of cancer in TCGA database, TSPAN7 showed a positive co-expression with both the immune inhibitory genes and stimulatory genes in BRCA, KIRP, LAML, LIHC, PAAD, PRAD, STAD, etc., but was negatively correlated with them in ACC, GBM, KICH, KIRC, LGG, LUSC, OV, THCA, UCEC, etc. The immune correlations of TSPAN7 in pan-cancer were two-sided and complicated (Fig. 5 A).
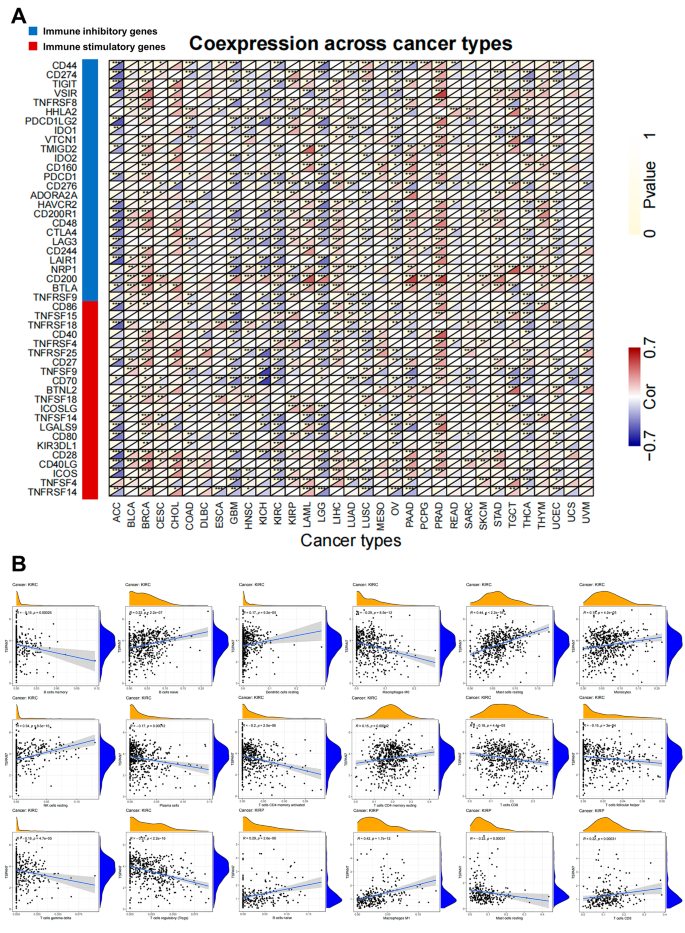
The correlations of TSPAN7 with immune gene sets and immune cell infiltration in pan-cancer. ( A ) TSPAN7 was positively associated with both the immune inhibitory genes and stimulatory genes in BRCA, KIRP, LAML, LIHC, PAAD, PRAD, STAD, etc., but showed a negative correlation with them in ACC, GBM, KICH, KIRC, LGG, LUSC, OV, THCA, UCEC, etc. ( B ) TSPAN7 was negatively associated with the infiltration of B cells memory ( R = -0.16, P-value < 0.001), macrophages M0 ( R = -0.29, P-value < 0.001), plasma cells ( R = -0.17, P-value < 0.001), T cells CD4 memory activated ( R = -0.2, P-value < 0.001), T cells CD8 ( R = -0.18, P-value < 0.001), T cells follicular helper ( R = -0.16, P-value < 0.001), T cells gamma delta ( R = -0.18, P-value < 0.001), and T cells regulatory ( R = -0.37, P-value < 0.001), but positively associated with the infiltration of B cells naïve ( R = 0.22, P-value < 0.001), dendritic cells resting ( R = 0.17, P-value < 0.001), mast cells resting ( R = 0.44, P-value < 0.001), monocytes ( R = 0.18, P-value < 0.001), NK cells resting ( R = 0.34, P-value < 0.001), and T cells CD4 memory resting ( R = 0.15, P-value < 0.001) in KIRC. TSPAN7 was positively associated with the infiltration of B cells naïve ( R = 0.29, P-value < 0.001), macrophages M1 ( R = 0.42, P-value < 0.001), and T cells CD8 ( R = 0.22, P-value < 0.001), except for mast cells resting ( R = -0.22, P-value < 0.001) in KIRP. *, P-value < 0.05; **, P-value < 0.01; ***, P-value < 0.001
Then, we used the ESTIMATE algorithm as well as the TIMER2.0 database to further discover the associations of TSPAN7 with immune cell infiltration. Notably, TSPAN7 played an immune inhibiting role in most types of cancers, especially for KIRC, GBM, THCA, and UCEC. In KIRC, TSPAN7 exhibited a significantly negative correlation with the infiltration of macrophages (macrophages M0), myeloid cells, B cells (B cells memory, plasma cells), and T cells (T cells CD4 memory activated, T cells CD8, T cells follicular helper, T cells gamma delta, T cells regulatory), but a weak positive correlation with NK cell infiltration (NK cell resting). In GBM, TSPAN7 was negatively associated with the infiltration of macrophages, myeloid cells, CD8 + T cells, but was positively associated with B cells. In THCA, TSPAN7 was negatively associated with the infiltration of macrophages, myeloid cells (dendritic cells activated, monocytes), and T cells (T cells CD4 memory activated, T cells regulatory). In UCEC, TSPAN7 also harbored a negative correlation with the infiltration of macrophages, myeloid cells, and T cells (T cells CD4 memory activated). However, in KIRP, TSPAN7 seemed to played an opposite role, as tumors with high TSPAN7 expression were enriched with macrophages (macrophages M1), B cells (B cells naïve), and CD8 + T cells (T cells CD8). Interestingly, TSPAN7 was strongly positively correlated with endothelial cells and cancer-associated fibroblasts in almost all types of tumors (Fig. 5 B, Figure S7 , and Figure S8 ).
CTLs were one of the most important components in tumor immunity. Thus, we retrieved the TIDE database to further explore the association of TSPAN7 with CTLs (Figure S9 A). From Figure S9 B, we could find that TSPAN7 was positively correlated with CTLs in BRCA, but was negatively correlated in UCEC. Regarding the survival status, patients with higher TSPAN7 expression usually harbored better prognosis in LAML, but had worse prognosis in UCEC (Figure S9 C). However, there was no statistical difference between the survival of patients with high- or low-CTL infiltration in the high- and low-TSPAN7 subgroups, indicating that TSPAN7 was not statistically associated with the CTL dysfunction phenotype in our study (Figure S9 D).
The correlations of TSPAN7 with drug sensitivity in pan-cancer
Ulteriorly, we discovered that TSPAN7 expression had significant correlation with drug sensitivity in pan-cancer based on GDSC (Fig. 6 A), CTRP (Fig. 6 B), and CellMiner database (Fig. 6 C). Notably, high expression of TSPAN7 was related with high drug sensitivity of most drugs, specifically for Navitoclax and Vorinostat, which served as the top associated drugs in all three databases. Exceptionally, the drug sensitivity of Dasatinib was found to be negatively correlated with TSPAN7 expression. The above results suggested that TSPAN7 might function in drug therapies of cancers and TSPAN7-target treatment was promising in improve the therapeutic effect of anti-cancer drugs.
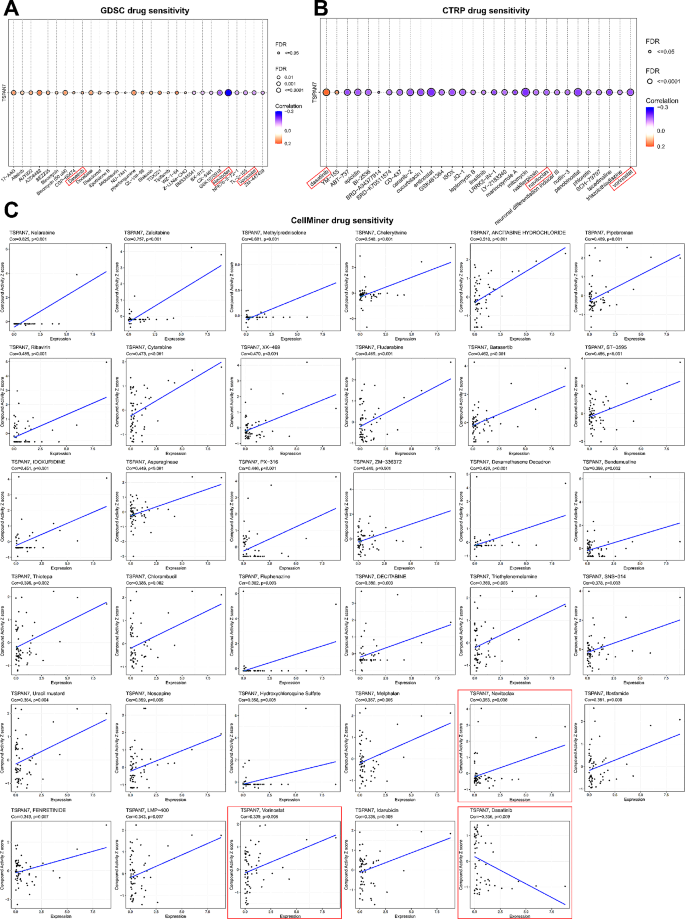
The correlations of TSPAN7 with drug sensitivity in pan-cancer. ( A ) In GDSC database, TSPAN7 expression was positively correlated with IC50 of 17-AAG, Afatinib, AUY922, AZD6482, BEZ235, Bleomycin, Bleomycin (50 μm), CGP-60,474, Dasatinib, Docetaxel, Elesclomol, Epothilone B, Midostaurin, NU-7441, piperlongumine, QL-VIII-58, Shikonin, TGX221, Tipifarnib, WZ-1-84, Z-LLNle-CHO, but was negatively correlated with IC50 of BMS345541, BX-912, CX-5461, GSK1070916, NPK76-II-72-1, TL-2-105, Vorinostat, ZM-447,439. ( B ) In CTRP database, TSPAN7 expression was positively correlated with IC50 of Dasatinib, YM-155, but was negatively correlated with IC50 of ABT-737, Apicidin, BI-2536, BRD-A94377914, BRD-K70511574, CD-437, Ceranib-2, Cucurbitacin I, Entinostat, GSK461364, ISOX, JQ-1, Leptomycin B, Linsitinib, LRRK2-IN-1, LY-2,183,240, Marinopyrrole A, Mitomycin, Nakiterpiosin, Navitoclax, Neuronal differentiation inducer III, Nutlin-3, Panobinostat, Phloretin, SCH-79,797, Tacedinaline, Triazolothiadiazine, Vorinostat. ( C ) In CellMiner database, TSPAN7 expression was positively correlated with compound activity of Nelarabine ( R = 0.825, P-value < 0.001), Zalcitabine ( R = 0.757, P-value < 0.001), Methylprednisolone ( R = 0.681, P-value < 0.001), Chelerythrine ( R = 0.548, P-value < 0.001), ANCITABINE HYDROCHLORIDE ( R = 0.510, P-value < 0.001), Pipobroman ( R = 0.489, P-value < 0.001), Ribavirin ( R = 0.485, P-value < 0.001), Cytarabine ( R = 0.473, P-value < 0.001), XK-469 ( R = 0.470, P-value < 0.001), Fludarabine ( R = 0.469, P-value < 0.001), Barasertib ( R = 0.462, P-value < 0.001), ST-3595 ( R = 0.456, P-value < 0.001), IDOXURIDINE ( R = 0.451, P-value < 0.001), Asparaginase ( R = 0.449, P-value < 0.001), PX-316 ( R = 0.446, P-value < 0.001), ZM-336,372 ( R = 0.440, P-value < 0.001), Dexamethasone Decadron ( R = 0.429, P-value < 0.001), Bendamustine ( R = 0.399, P-value < 0.01), Thiotepa ( R = 0.396, P-value < 0.01), Chlorambucil ( R = 0.388, P-value < 0.01), Fluphenazine ( R = 0.382, P-value < 0.01), DECITABINE ( R = 0.380, P-value < 0.01), Triethylenemelamine( R = 0.380, P-value < 0.01), SNS-314 ( R = 0.378, P-value < 0.01), Uracil mustard ( R = 0.364, P-value < 0.01), Noscapine ( R = 0.359, P-value < 0.01), Hydroxychloroquine Sulfate ( R = 0.358, P-value < 0.01), Melphalan ( R = 0.357, P-value < 0.01), Navitoclax ( R = 0.353, P-value < 0.01), Ifosfamide ( R = 0.351, P-value < 0.01), FENRETINIDE ( R = 0.343, P-value < 0.01), LMP-400 ( R = 0.343, P-value < 0.01), Vorinostat ( R = 0.339, P-value < 0.01), Idarubicin ( R = 0.338, P-value < 0.01), Dasatinib ( R = -0.336, P-value < 0.01). Abbreviations: GDSC, Genomics of Drug Sensitivity in Cancer; CTRP, The Cancer Therapeutics Response Portal; Cor, correlation coefficient
Functional enrichment analysis of TSPAN7 in pan-cancer through GSEA
To further explore the role of TSPAN7 in pan-cancer, we applied GSEA to identify the enriched KEGG pathways in high-TSPAN7 subgroups. Generally speaking, “Calcium signaling pathway”, “Cytosolic DNA sensing pathway”, “Drug metabolism cytochrome P450”, “Metabolism of xenobiotics by cytochrome P450”, “Neuroactive ligand receptor interaction”, “Olfactory transduction”, “Regulation of autophagy”, and “RIG I like receptor signaling pathway” were the most common KEGG pathways in our analysis. Specifically, “Calcium signaling pathway” was up-regulated in KIRP, PRAD, PAAD, and UVM. “Drug metabolism cytochrome P450” and “Metabolism of xenobiotics by cytochrome P450” was up-regulated in TGCT, KIRP, LUAD, and HNSC. “Neuroactive ligand receptor interaction” was up-regulated in KIRP, PAAD, UVM, and SARC. “Olfactory transduction” was down-regulated in CESC, STAD, SARC, but up-regulated in UVM. “Cytosolic DNA sensing pathway”, “Regulation of autophagy” and “RIG I like receptor signaling pathway” was down-regulated in BLCA, CESC, PAAD, and UCS. These enriched KEGG pathways might suggest the molecular biological functions of TSPAN7 in pan-cancer (Fig. 7 and Figure S10 ).
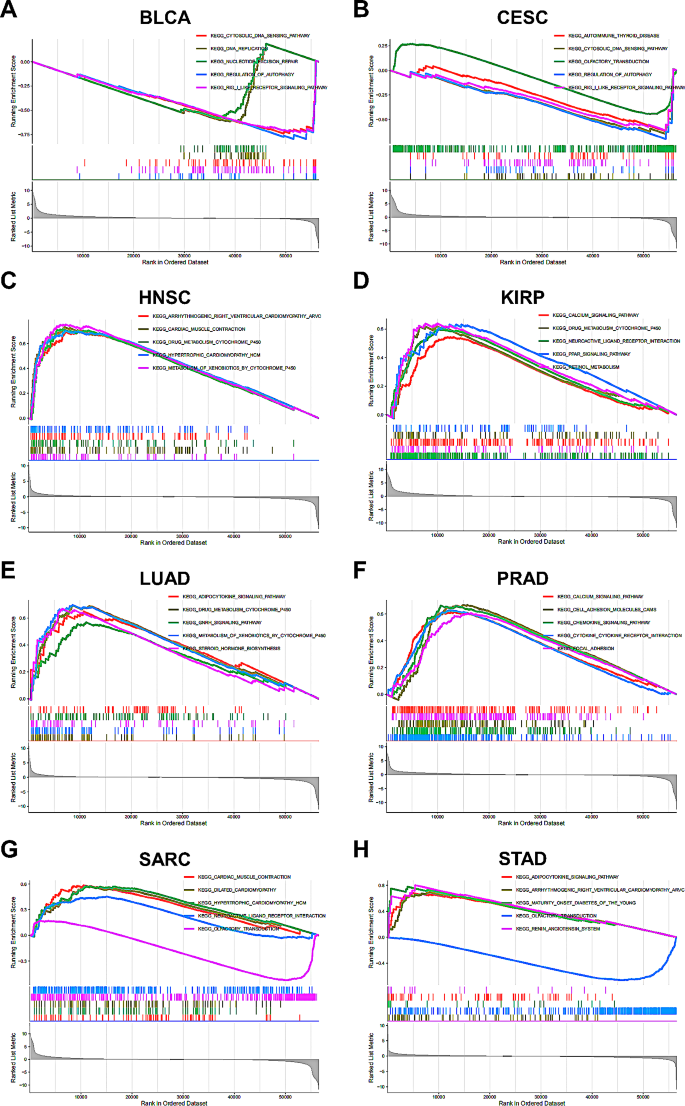
The enriched signaling pathways of TSPAN7 in pan-cancer. ( A ) In BLCA, the top five enriched down-regulated KEGG pathways were “Cytosolic DNA sensing pathway”, “DNA replication”, “Nucleotide excision repair”, “Regulation of autophagy”, and “RIG I like receptor signaling pathway”. ( B ) In CESC, the top five enriched down-regulated KEGG pathways were “Autoimmune thyroid disease”, “Cytosolic DNA sensing pathway”, “Olfactory transduction”, “Regulation of autophagy”, and “RIG I like receptor signaling pathway”. ( C ) In HNSC, the top five enriched up-regulated KEGG pathways were “Arrhythmogenic right ventricular cardiomyopathy_ARVC”, “Cardiac muscle contraction”, “Hypertrophic cardiomyopathy_HCM”, “Drug metabolism cytochrome P450”, and “Metabolism of xenobiotics by cytochrome P450”. ( D ) In KIRP, the top five enriched up-regulated KEGG pathways were “Calcium signaling pathway”, “Drug metabolism cytochrome P450”, “Neuroactive ligand receptor interaction”, “PPAR signaling pathway”, and “Retinol metabolism”. ( E ) In LUAD, the top five enriched up-regulated KEGG pathways were “Adipocytokine signaling pathway”, “Drug metabolism cytochrome P450”, “GnRH signaling pathway”, “Metabolism of xenobiotics by cytochrome P450”, and “Steroid hormone biosynthesis”. ( F ) In PRAD, the top five enriched up-regulated KEGG pathways were “Calcium signaling pathway”, “Cell adhesion molecules_CAMs”, “Chemokine signaling pathway”, “Cytokine-cytokine receptor interaction”, and “focal adhesion”. ( G ) In SARC, the four enriched up-regulated KEGG pathways were “Cardiac muscle contraction”, “Dilated cardiomyopathy”, “Hypertrophic cardiomyopathy_HCM”, and “Neuroactive ligand receptor interaction”. However, “Olfactory transduction” was down-regulated in SARC. ( H ) In STAD, the four enriched up-regulated KEGG pathways were “Adipocytokine signaling pathway”, “Arrhythmogenic right ventricular cardiomyopathy_ARVC”, “Maturity onset diabetes of the young”, and “Renin angiotensin system”. However, “Olfactory transduction” was down-regulated in STAD
TSPAN7, a member of TSPANs, is found to be closely associated with tumor progression. Several studies have reported TSPAN7’s role in tumor cell invasion, metastasis, and immunotherapy [ 11 , 12 , 13 ]. However, the previous studies have contradictory results, and the exact function of TSPAN7 in tumor development is not clear enough. Thus, we conducted a pan-cancer study of TSPAN7 in order to comprehensively reveal the unique role of TSPAN7 across various types of tumors. First of all, we employed IHC staining on a large retrospective cohort consisted of 370 patients with kidney neoplasm, and discovered the differential expression of TSPAN7 between tumor and normal tissues. Clinical correlation analyses were then applied, suggesting the potential function of TSPAN7 in the development of kidney neoplasm. Subsequently, we questioned if TSPAN7 also harbored an important role in pan-cancer level, and successively conducted differential expression analysis, ceRNA analysis, PPI analysis, correlation analysis of TSPAN7 with patients’ survival, clinical stages, tumor purity, tumor genomics, tumor immunology, and drug sensitivity. Last but not least, we also conducted GSEA to discover the enriched signaling pathways of TSPAN7, indicating the possible molecular mechanisms of TSPAN7 in pan-cancer.
TSPAN7 might serve as a potential biomarker across various types of tumors
Previous studies have reported the prognostic prediction value of TSPAN7 in several tumor types. Low TSPAN7 expression was found to be related to poor prognosis in BRCA, GBM, LUAD, KIRC, LIHC, PDAC, SARC, BLCA, and multiple myeloma [ 13 , 51 , 52 , 53 , 54 , 55 , 56 , 57 , 58 ]. However, in STAD and osteosarcoma, oppositely, TSPAN7 could promote the progression of tumor, and was usually correlated with worse clinical outcomes [ 12 , 59 ]. In our study, through IHC staining, we discovered an up-regulation of TSPAN7 in kidney neoplasm tissues compared to normal ones. High TSPAN7 expression was predictive of shorter OS and PFS of patients with kidney neoplasm. In pan-cancer, TSPAN7 expression in tumor tissues was lower than normal tissues in most types of tumors, including BLCA, BRCA, CESC, COAD, GBM, HNSC, KIRC, KIRP, LIHC, LUAD, LUSC, PRAD, READ, SARC, STAD, THCA, and UCEC, except for KICH and PCPG at the transcriptomic level. From the K-M survival analysis and univariable Cox regression analysis, we further identified TSPAN7 as a protective factor of OS in KIRC, LGG, LUAD, PAAD, of PFS in KICH, KIRC, LGG, PAAD, SARC, THCA, of DFS in LUAD, PAAD, SARC, of DSS in KIRC, LGG, PAAD, but as a risk factor of PFS in ACC, of DSS in KIRP, LIHC. Besides, TSPAN7 was also positively correlated with the clinical stage of BLCA, KIRP, and MESO, but was negatively associated with that of BRCA, KIRC, and THCA. Although the function of TSPAN7 in various types of tumors was two-sided, our study still indicated TSPAN7 as a promising biomarker in pan-cancer.
In addition, TSPAN7 might also serve as a potential therapeutic biomarker in various cancers. In our study, we found that high TSPAN7 expression was of significant correlation with high drug sensitivity of Navitoclax and Vorinostat, but oppositely associated to low drug sensitivity of Dasatinib. Navitoclax was a double antagonist of BCL-2 and BCL-XL, and had been approved for patients with 17p-deficient chronic lymphocytic leukemia who had received at least one therapy type [ 60 ]. The application of Navitoclax in other solid tumors, especially small cell lung cancer, was also under clinical research [ 61 ]. Besides, Vorinostat was a FDA-approved drug for cutaneous T-cell lymphoma [ 62 ], and “Vorinostat plus other drugs” therapy was gradually catching more researchers’ attention in treating Hodgkin lymphoma [ 63 , 64 ]. Last but not least, Dasatinib, a BCR-ABL inhibitor [ 65 ], had been widely studied in treatment of metastatic breast cancer [ 66 ], small cell lung cancer [ 67 ], non-clear cell renal cell carcinoma [ 68 ], etc. However, there were no previous studies reporting the correlations between TSPAN7 and the sensitivity of these drugs. Pharmaceutical research concerned TSPAN7 in pan-cancer seemed to be a promising research direction in the future.
Further, we constructed the ceRNA and PPI network to suggest the possible mechanism of TSPAN7 in cancer progression. In ceRNA network, hsa-miR-140 and hsa-miR-302 were identified as two key miRNA family closely associated with TSPAN7. Although there were not previous studies directly reporting the correlations between the two miRNA families and TSPAN7, both of them played an important role in the development of cancers. Especially for hsa-miR-140, it was found that hsa-miR-140 could not only inhibit the proliferation, migration, and invasion of gastric cancer, but also function in the chemoresistance of pancreatic ductal adenocarcinoma [ 69 , 70 ]. Among the target lncRNAs of key miRNAs, KCNQ1OT1 stands out as the most widely associated one. It was found that KCNQ1OT1 took part in the progression of cholangiocarcinoma through the regulation of miR-140-5p/SOX4 axis [ 71 ]. In the PPI network, we found TSPAN7 was possibly interacted with IGSF3, IL1RAPL1, PICK1, GRIA2, and GRIA3 (known interactions from curated databases or experiments), especially for PICK1 with the highest predicted score. However, there were not previous studies of TSPAN7 with the above potential protein partners in the field of cancers. Maria Passafaro et al. reported that TSPAN7 could regulate excitatory synapse development and AMPAR trafficking through PICK1 in X-linked intellectual disability [ 72 ]. Deeper investigations of the ceRNA and PPI network are conducive to reveal the possible mechanisms of TSPAN7 in cancer progression.
TSPAN7 was possibly associated with immune infiltration and immunotherapy across various types of tumors
Moreover, we explored the correlations of TSPAN7 with tumor purity, tumor genomics, and tumor immunology. Notably, TSPAN7 was found to have a close correlation with immune infiltration and immunotherapy across various types of tumors. Previous studies have validated our findings. In GBM, TSPAN7 was negatively associated with the infiltration of cancer-associated M2 macrophages, and the expression of CTLA-4, PD-1, and PD-L1 [ 13 ]. In BRCA, TSPAN7 was reported to be associated with the T and B cell infiltration as well as the occurrence of tertiary lymphoid structures, which was predictive of the therapeutic effect of immune checkpoint inhibitors [ 51 ]. In our study, we discovered that TSPAN7 was positively co-expressed with both the immune inhibitory genes and stimulatory genes in BRCA, KIRP, LAML, LIHC, PAAD, PRAD, STAD, and negatively correlated with them in ACC, GBM, KICH, KIRC, LGG, LUSC, OV, THCA, UCEC. Considering immune cell infiltration, TSPAN7 usually functioned as an immune inhibitory factor in pan-cancer, especially for KIRC, GBM, THCA, and UCEC. Among these four types of tumors, TSPAN7 was mostly negatively associated with the infiltration of macrophages, myeloid cells, and T cells. Macrophages were reported to stimulate angiogenesis, malignant cell invasion, and inhibit the anti-tumor immunity, thus promoting the initiation and progression of tumor [ 73 ]. However, T cells usually served as a tumor-killing actor, and were associated with favorable clinical outcomes. Among T cells, CD4 + T cells could communicate with B cells, antigen presenting cells (APCs), and other immune cells, in order to facilitate their anti-tumor functions [ 74 , 75 ]. Most importantly, CD4 + T cells could contribute to the proliferation and differentiation of CD8 + T cells, and enhance the tumor-killing role of CD8 + T cells through various ways [ 76 , 77 ]. CTLs, the main subtypes of CD8 + T cells, were of the greatest importance in tumor immunity. CTLs could secrete perforin and apoptosis-related cytokines to kill tumor cells [ 78 ]. The above results suggested the close relationships between TSPAN7 and macrophages, myeloid cells, T cells, and identified TSPAN7 as a promising immunotherapeutic target for tumors. Apart from this, it was noteworthy that TSPAN7 was positively associated with the infiltration of endothelial cells and cancer-associated fibroblasts in almost all cancer types in TCGA. Although there was no study reporting the relationship between TSPAN7 and cancer-associated fibroblasts, TSPAN7 was found to harbor a far higher expression within endothelial cells than tumor cells in KIRC [ 53 ]. In BRCA, TSPAN7 was reported to be associated with the formation of high endothelial venules, which could recruit naïve lymphocytes to lymph nodes and enhance the anti-tumor immunity [ 51 ].
Nonetheless, CTL dysfunction and exclusion were the main reason for the failure of anti-tumor immunity [ 79 ]. Thus, we conducted our subsequent analysis among BRCA, SKCM, UCEC, LAML, and neuroblastoma based on the TIDE database. We discovered that patients with high TSPAN7 expression were usually accompanied by enriched CTLs in BRCA, but low infiltration of CTLs in UCEC. However, there were no statistical correlations between TSPAN7 and CTL dysfunction.
Apart from this, in our study, we also found that TSPAN7 was closely associated with tumor genomics. TSPAN7 exhibited a negative correlation with TMB in most cancer types including BLCA, BRCA, COAD, DLBC, ESCA, KIRC, KIRP, LGG, LIHC, LUAD, PAAD, PRAD, READ, SARC, STAD, THCA, UCEC, UCS, and was negatively correlated with MSI in BLCA, COAD, SARC, SKCM, STAD, UCS. Besides, we also revealed the close relationships between TSPAN7 and genomics instability in pan-cancer through cBioPortal analysis and GSCA. Notably, the genomic instability of tumors was of close associations with immunotherapy. It has been reported that tumors with large amounts of somatic mutations driven by mismatch-repair defects were more sensitive to PD-1 targeted therapy [ 80 , 81 ], and MSI might serve as a reliable biomarker for the therapeutic effect of PD-1 blockade [ 82 ]. TSPAN7 possibly played a role in tumor immunotherapy by regulating tumor genomics.
Then, we used GESA and identified several enriched signaling pathways of TSPAN7, which were possibly associated with its role in tumor immunity across various types of tumors. “Cytosolic DNA sensing pathway” is involved in the recognition of double-stranded cytosolic DNA, and functioned in the innate immune system [ 83 ]. Cyclic GMP-AMP synthase (cGAS) is one of the DNA sensors in the pathway, and could activate STING to initiate type I interferon (IFN) response, which could promote the maturity of dendritic cells and the presentation of tumor antigens towards T cells [ 84 , 85 ]. Besides, the activation of cGAS/STING is also associated with PD-L1 expression [ 86 ]. However, the activation of “Neuroactive ligand receptor interaction” is associated with the inhibition of “Cytosolic DNA sensing pathway”, and could lead to the reduction of CD8 + T cell infiltration in COAD [ 87 ]. The recognition of DNA could also be indirectly accomplished by RNA sensor RIG-I through “RIG I like receptor signaling pathway” [ 88 ]. The “RIG I like receptor signaling pathway” could enhance the function of Vγ9Vδ2 T cells [ 89 ], and induce apoptosis, pyroptosis, and autophagy of tumor cells [ 90 , 91 , 92 ]. The above signaling pathways had close correlation with immunity, and might be interacted with TSPAN7’s role of anti-tumor immunity across various types of tumors. In spite of this, there have not been previous studies directly illustrating the correlations of TSPAN7 with these signaling pathways. Further studies should be focused on revealing the precise molecular mechanisms of TSPAN7’s role in pan-cancer.
Limitations of the study
In spite of this, there were still some limitations in our study. Firstly, all 370 patients with kidney neoplasm were from Xinhua Hospital Affiliated to Shanghai Jiao Tong University School of Medicine. The findings of our study were not validated by patients from other medical centers. Besides, the pan-cancer data of our study was from public databases like TCGA, cBioPortal, TIMER2.0, TIDE, etc. The majority of these samples derived from patients in Western countries, which might lead to the racial deviance of our study. Last but not least, our study only preliminarily identified TSPAN7 as a promising biomarker in pan-cancer, and discovered its relationships with tumor purity, tumor genomics, tumor immunology, and drug sensitivity. As studies concerned TSPAN7 in tumors were still in the initial stages, we did not deeply investigate the molecular mechanisms of it, and more studies need to be conducted in the future to unveil the precise regulatory role of TSPAN7 in tumor progression.
In summary, we comprehensively studied the unique role of TSPAN7 in tumor progression across various types of tumors based on a large retrospective cohort of kidney neoplasm and subsequently online databases in pan-cancer level. Our study identified TSPAN7 as a promising biomarker of various tumors, and illustrated its close relationships with tumor purity, tumor genomics, tumor immunology, and drug sensitivity. Last but not least, we preliminarily explored the enriched signaling pathways of TSPAN7 in different types of tumors. In the future, more efforts need to be made to unveil the precise molecular mechanism of TSPAN7’s role in tumor progression.
Data availability
The datasets generated and/or analyzed during the study are available in TCGA database ( https://tcga-data.nci.nih.gov/tcga/ ), DIANA-microT database ( https://dianalab.e-ce.uth.gr/microt_webserver/#/ ), miRWalk database ( http://mirwalk.umm.uni-heidelberg.de/ ), miRDB database ( https://mirdb.org/ ), miRcode database ( http://www.mircode.org/ ), ENCORI database ( https://rnasysu.com/encori/ ), STRING database ( https://cn.string-db.org/ ), cBioPortal database ( https://www.cbioportal.org/ ), TIMER2.0 ( http://timer.cistrome.org/ ) database, TIDE database ( http://tide.dfci.harvard.edu/ ), GDSC ( https://www.cancerrxgene.org/ ), CTRP ( https://pubmed.ncbi.nlm.nih.gov/23993102/ ), CellMiner database ( https://discover.nci.nih.gov/cellminer/home.do ) and KEGG database ( https://www.genome.jp/kegg/ ).
Abbreviations
tetraspanin
epithelial-mesenchymal transition
The Cancer Genome Atlas
competing endogenouse RNA
protein-protein interaction
immunohistochemical
single nucleotide variation
copy number variation
Encyclopedia of RNA Interactomes
long noncoding RNA
microsatellite instability
Tumor Immune Estimation Resource
cytotoxic T-lymphocyte
Tumor Immune Dysfunction and Exclusion
Genomics of Drug Sensitivity in Cancer
The Cancer Therapeutics Response Portal
Kyoto Encyclopedia of Genes and Genomes
Ethylene Diamine Tetraacetic Acid
Horseradish Peroxidase
Diaminobenzidine
overall survival
progression free survival
Kaplan-Meier
Receiver Operating Characteristic
disease free survival
disease specific survival
Estimation of STromal and Immune cells in MAlignant Tumors using Expression data
tumor microenvironment
tumor mutation burden
gene set cancer analysis
Whole Exome Sequencing
half maximal inhibitory concentration
gene set enrichment analysis
false discovery rate
hazard ratio
area under the curve
antigen presenting cell
cyclic GMP-AMP synthase
Luan M, et al. The peptide mimicking small extracellular loop domain of CD82 inhibits tumor cell migration, adhesion and induces apoptosis by inhibiting integrin mediated signaling. Biochem Biophys Res Commun. 2018;503(4):2206–11.
Article CAS PubMed Google Scholar
Charrin S, et al. Lateral organization of membrane proteins: tetraspanins spin their web. Biochem J. 2009;420(2):133–54.
Hemler ME. Tetraspanin functions and associated microdomains. Nat Rev Mol Cell Biol. 2005;6(10):801–11.
Hemler ME. Tetraspanin proteins promote multiple cancer stages. Nat Rev Cancer. 2014;14(1):49–60.
Huang R, et al. The role of tetraspanins pan-cancer. iScience. 2022;25(8):104777.
Article CAS PubMed PubMed Central Google Scholar
Zhu R, et al. TSPAN8 promotes cancer cell stemness via activation of sonic hedgehog signaling. Nat Commun. 2019;10(1):2863.
Article PubMed PubMed Central Google Scholar
Li Y, et al. TET2-mediated mRNA demethylation regulates leukemia stem cell homing and self-renewal. Cell Stem Cell. 2023;30(8):1072–e109010.
Garcia-Mayea Y, et al. TSPAN1, a novel tetraspanin member highly involved in carcinogenesis and chemoresistance. Biochim Biophys Acta Rev Cancer. 2022;1877(1):188674.
Hosokawa Y, et al. Molecular cloning of a cDNA encoding mouse A15, a member of the transmembrane 4 superfamily, and its preferential expression in brain neurons. Neurosci Res. 1999;35(4):281–90.
Piluso G, et al. Assessment of de novo copy-number variations in Italian patients with schizophrenia: detection of putative mutations involving regulatory enhancer elements. World J Biol Psychiatry. 2019;20(2):126–36.
Article PubMed Google Scholar
Gulati S, et al. Systematic evaluation of the prognostic impact and intratumour heterogeneity of clear cell renal cell carcinoma biomarkers. Eur Urol. 2014;66(5):936–48.
Shao S, et al. Tetraspanin 7 promotes osteosarcoma cell invasion and metastasis by inducing EMT and activating the FAK-Src-Ras-ERK1/2 signaling pathway. Cancer Cell Int. 2022;22(1):183.
Chen L, et al. Functional characterization of TSPAN7 as a novel indicator for immunotherapy in glioma. Front Immunol. 2023;14:1105489.
Wang M, et al. N6AMT1 is a novel potential diagnostic, prognostic and immunotherapy response biomarker in pan-cancer. Aging. 2023;15(13):6526–44.
Ye Y, et al. Role of ARRB1 in prognosis and immunotherapy: a Pan-cancer analysis. Front Mol Biosci. 2022;9:1001225.
Tastsoglou S, et al. DIANA-microT 2023: including predicted targets of virally encoded miRNAs. Nucleic Acids Res. 2023;51(W1):W148–53.
Sticht C, et al. miRWalk: an online resource for prediction of microRNA binding sites. PLoS ONE. 2018;13(10):e0206239.
Chen Y, Wang X. miRDB: an online database for prediction of functional microRNA targets. Nucleic Acids Res. 2020;48(D1):D127–31.
Jeggari A, Marks DS, Larsson E. miRcode: a map of putative microRNA target sites in the long non-coding transcriptome. Bioinformatics. 2012;28(15):2062–3.
Li JH, et al. starBase v2.0: decoding miRNA-ceRNA, miRNA-ncRNA and protein-RNA interaction networks from large-scale CLIP-Seq data. Nucleic Acids Res. 2014;42(Database issue):D92–7.
Szklarczyk D, et al. The STRING database in 2023: protein-protein association networks and functional enrichment analyses for any sequenced genome of interest. Nucleic Acids Res. 2023;51(D1):D638–46.
Bonneville R et al. Landscape of Microsatellite Instability Across 39 Cancer Types JCO Precis Oncol, 2017. 2017.
Gao JJ et al. Integrative Analysis of Complex Cancer Genomics and Clinical profiles using the cBioPortal. Sci Signal, 2013. 6(269).
Li T, et al. TIMER2.0 for analysis of tumor-infiltrating immune cells. Nucleic Acids Res. 2020;48(W1):W509–14.
Jiang P, et al. Signatures of T cell dysfunction and exclusion predict cancer immunotherapy response. Nat Med. 2018;24(10):1550–8.
Yang W, et al. Genomics of Drug Sensitivity in Cancer (GDSC): a resource for therapeutic biomarker discovery in cancer cells. Nucleic Acids Res. 2012;41(D1):D955–61.
Rees MG, et al. Correlating chemical sensitivity and basal gene expression reveals mechanism of action. Nat Chem Biol. 2016;12(2):109–16.
Seashore-Ludlow B, et al. Harnessing connectivity in a large-scale small-molecule sensitivity dataset. Cancer Discov. 2015;5(11):1210–23.
Basu A, et al. An interactive resource to identify cancer genetic and lineage dependencies targeted by small molecules. Cell. 2013;154(5):1151–61.
Shankavaram UT, et al. CellMiner: a relational database and query tool for the NCI-60 cancer cell lines. BMC Genomics. 2009;10(1):277.
Reinhold WC, et al. CellMiner: a web-based suite of genomic and pharmacologic tools to explore transcript and drug patterns in the NCI-60 Cell Line Set. Cancer Res. 2012;72(14):3499–511.
Kanehisa M, Goto S. KEGG: kyoto encyclopedia of genes and genomes. Nucleic Acids Res. 2000;28(1):27–30.
Xiao Y, Yu D. Tumor microenvironment as a therapeutic target in cancer. Pharmacol Ther. 2021;221:107753.
Elhanani O, Ben-Uri R, Keren L. Spatial profiling technologies illuminate the tumor microenvironment. Cancer Cell. 2023;41(3):404–20.
Yoshihara K, et al. Inferring tumour purity and stromal and immune cell admixture from expression data. Nat Commun. 2013;4:2612.
Mayakonda A, et al. Maftools: efficient and comprehensive analysis of somatic variants in cancer. Genome Res. 2018;28(11):1747–56.
Campbell PJ, et al. Pan-cancer analysis of whole genomes. Nature. 2020;578(7793):82–.
Article Google Scholar
Zehir A, et al. Mutational landscape of metastatic cancer revealed from prospective clinical sequencing of 10,000 patients. Nat Med. 2017;23(6):703–13.
Robinson DR, et al. Integrative clinical genomics of metastatic cancer. Nature. 2017;548(7667):297–303.
Miao D, et al. Genomic correlates of response to immune checkpoint blockade in microsatellite-stable solid tumors. Nat Genet. 2018;50(9):1271–81.
Hyman DM, et al. HER kinase inhibition in patients with HER2- and HER3-mutant cancers. Nature. 2018;554(7691):189–94.
Samstein RM, et al. Tumor mutational load predicts survival after immunotherapy across multiple cancer types. Nat Genet. 2019;51(2):202–6.
Rosen EY, et al. TRK fusions are enriched in cancers with uncommon histologies and the absence of canonical driver mutations. Clin Cancer Res. 2020;26(7):1624–32.
Bolton KL, et al. Cancer therapy shapes the fitness landscape of clonal hematopoiesis. Nat Genet. 2020;52(11):1219–26.
Wu L, et al. Landscape of somatic alterations in large-scale solid tumors from an Asian population. Nat Commun. 2022;13(1):4264.
Nguyen B, et al. Genomic characterization of metastatic patterns from prospective clinical sequencing of 25,000 patients. Cell. 2022;185(3):563–e57511.
Barrett T, et al. NCBI GEO: archive for functional genomics data sets–update. Nucleic Acids Res. 2013;41(Database issue):D991–5.
CAS PubMed Google Scholar
Curtis C, et al. The genomic and transcriptomic architecture of 2,000 breast tumours reveals novel subgroups. Nature. 2012;486(7403):346–52.
Weinstein JN, et al. The Cancer Genome Atlas Pan-cancer analysis project. Nat Genet. 2013;45(10):1113–20.
Yu G, et al. clusterProfiler: an R package for comparing biological themes among gene clusters. Omics. 2012;16(5):284–7.
Sawada J, et al. Molecular signature of Tumor-Associated High Endothelial venules that can predict breast Cancer survival. Cancer Immunol Res. 2022;10(4):468–81.
Yang J, et al. Identification and validation of a novel cuproptosis-related stemness signature to predict prognosis and immune landscape in lung adenocarcinoma by integrating single-cell and bulk RNA-sequencing. Front Immunol. 2023;14:1174762.
Wuttig D, et al. CD31, EDNRB and TSPAN7 are promising prognostic markers in clear-cell renal cell carcinoma revealed by genome-wide expression analyses of primary tumors and metastases. Int J Cancer. 2012;131(5):E693–704.
Qi Y, et al. Expression and function of transmembrane 4 superfamily proteins in digestive system cancers. Cancer Cell Int. 2020;20:314.
Cheong CM, et al. Tetraspanin 7 (TSPAN7) expression is upregulated in multiple myeloma patients and inhibits myeloma tumour development in vivo. Exp Cell Res. 2015;332(1):24–38.
Luo L, et al. A new 7-gene survival score assay for pancreatic cancer patient prognosis prediction. Am J Cancer Res. 2021;11(2):495–512.
CAS PubMed PubMed Central Google Scholar
Gu HY, et al. Risk score based on expression of five novel genes predicts survival in soft tissue sarcoma. Aging. 2020;12(4):3807–27.
Yu X, et al. TSPAN7 exerts Anti-tumor effects in bladder Cancer through the PTEN/PI3K/AKT pathway. Front Oncol. 2020;10:613869.
Oh S et al. Genomic and transcriptomic characterization of gastric Cancer with bone metastasis. Cancer Res Treat, 2023.
Ashkenazi A, et al. From basic apoptosis discoveries to advanced selective BCL-2 family inhibitors. Nat Rev Drug Discov. 2017;16(4):273–84.
Gandhi L, et al. Phase I study of Navitoclax (ABT-263), a novel Bcl-2 family inhibitor, in patients with small-cell lung cancer and other solid tumors. J Clin Oncol. 2011;29(7):909–16.
Siegel D, et al. Vorinostat in solid and hematologic malignancies. J Hematol Oncol. 2009;2:31.
Mei M, et al. Pembrolizumab plus Vorinostat induces responses in patients with Hodgkin lymphoma refractory to prior PD-1 blockade. Blood. 2023;142(16):1359–70.
Janku F, et al. Safety and Efficacy of Vorinostat Plus Sirolimus or Everolimus in patients with relapsed refractory Hodgkin Lymphoma. Clin Cancer Res. 2020;26(21):5579–87.
Olivieri A, Manzione L. Dasatinib: a new step in molecular target therapy. Ann Oncol. 2007;18(Suppl 6):vi42–6.
Fornier MN, et al. A phase I study of dasatinib and weekly paclitaxel for metastatic breast cancer. Ann Oncol. 2011;22(12):2575–81.
Miller AA, et al. A phase II study of dasatinib in patients with chemosensitive relapsed small cell lung cancer (Cancer and Leukemia Group B 30602). J Thorac Oncol. 2010;5(3):380–4.
Lue HW, et al. Cabozantinib and Dasatinib synergize to induce tumor regression in non-clear cell renal cell carcinoma. Cell Rep Med. 2021;2(5):100267.
Fang Z, et al. Mir-140-5p suppresses the proliferation, migration and invasion of gastric cancer by regulating YES1. Mol Cancer. 2017;16(1):139.
Lu J, et al. FTO-mediated LINC01134 stabilization to promote chemoresistance through miR-140-3p/WNT5A/WNT pathway in PDAC. Cell Death Dis. 2023;14(11):713.
Sun H, et al. Dysregulation of KCNQ1OT1 promotes cholangiocarcinoma progression via miR-140-5p/SOX4 axis. Arch Biochem Biophys. 2018;658:7–15.
Bassani S, et al. The X-linked intellectual disability protein TSPAN7 regulates excitatory synapse development and AMPAR trafficking. Neuron. 2012;73(6):1143–58.
Cassetta L, Pollard JW. Targeting macrophages: therapeutic approaches in cancer. Nat Rev Drug Discov. 2018;17(12):887–904.
Bevan MJ. Helping the CD8(+) T-cell response. Nat Rev Immunol. 2004;4(8):595–602.
Castellino F, Germain RN. Cooperation between CD4 + and CD8 + T cells: when, where, and how. Annu Rev Immunol. 2006;24:519–40.
Laidlaw BJ, Craft JE, Kaech SM. The multifaceted role of CD4(+) T cells in CD8(+) T cell memory. Nat Rev Immunol. 2016;16(2):102–11.
Ahrends T, et al. CD4(+) T cell help confers a cytotoxic T cell Effector Program including coinhibitory receptor downregulation and increased tissue invasiveness. Immunity. 2017;47(5):848–e8615.
St Paul M, Ohashi PS. The roles of CD8(+) T cell subsets in Antitumor Immunity. Trends Cell Biol. 2020;30(9):695–704.
van der Leun AM, Thommen DS, Schumacher TN. CD8(+) T cell states in human cancer: insights from single-cell analysis. Nat Rev Cancer. 2020;20(4):218–32.
Le DT, et al. PD-1 blockade in tumors with Mismatch-Repair Deficiency. N Engl J Med. 2015;372(26):2509–20.
Le DT, et al. Mismatch repair deficiency predicts response of solid tumors to PD-1 blockade. Science. 2017;357(6349):409–13.
Dudley JC, et al. Microsatellite instability as a biomarker for PD-1 blockade. Clin Cancer Res. 2016;22(4):813–20.
Yu L, Liu P. Cytosolic DNA sensing by cGAS: regulation, function, and human diseases. Signal Transduct Target Therapy. 2021;6(1):170.
Article CAS Google Scholar
Hopfner KP, Hornung V. Molecular mechanisms and cellular functions of cGAS-STING signalling. Nat Rev Mol Cell Biol. 2020;21(9):501–21.
Sprooten J, Agostinis P, Garg AD. Type I interferons and dendritic cells in cancer immunotherapy. Int Rev Cell Mol Biol. 2019;348:217–62.
Grabosch S, et al. Cisplatin-induced immune modulation in ovarian cancer mouse models with distinct inflammation profiles. Oncogene. 2019;38(13):2380–93.
Yang Y, et al. Inhibition of neuroactive ligand-receptor interaction pathway can enhance immunotherapy response in colon cancer: an in silico study. Expert Rev Anticancer Ther. 2023;23(11):1205–15.
Onomoto K, Onoguchi K, Yoneyama M. Regulation of RIG-I-like receptor-mediated signaling: interaction between host and viral factors. Cell Mol Immunol. 2021;18(3):539–55.
Li J, et al. Gastric cancer derived exosomal THBS1 enhanced Vγ9Vδ2 T-cell function through activating RIG-I-like receptor signaling pathway in a N6-methyladenosine methylation dependent manner. Cancer Lett. 2023;576:216410.
Wu Y, et al. The anticancer functions of RIG-I-like receptors, RIG-I and MDA5, and their applications in cancer therapy. Transl Res. 2017;190:51–60.
Elion DL, et al. Therapeutically active RIG-I agonist induces immunogenic Tumor Cell killing in breast cancers. Cancer Res. 2018;78(21):6183–95.
Hou P, et al. A novel selective autophagy receptor, CCDC50, delivers K63 polyubiquitination-activated RIG-I/MDA5 for degradation during viral infection. Cell Res. 2021;31(1):62–79.
Download references
Acknowledgements
We thank TCGA database, DIANA-microT database, miRWalk database, miRDB database, miRcode database, ENCORI database, STRING database, cBioportal database, TIMER 2.0 database, TIDE database, GDSC database, CTRP database, and CellMiner database for allowing us to use their data.
This work was sponsored by the National Natural Science Foundation of China (No. 82330094, 82173265, 82072806); Leading health talents of Shanghai Municipal Health Commission (2022LJ002); Shanghai Rising-Star Program (23QC1401400); Shanghai Rising-Star Program (Sailing Special Program) (23YF1458400); the Natural Science Foundation of Shanghai (23ZR1441300); 2022 medical health key project of Science and Technology Commission of Baoshan District (2023-E-37); Baoshan District Health Commission Key Subject Construction Project (BSZK-2023-A06); Hospital Funded Clinical Research, Xinhua Hospital Affiliated to Shanghai Jiao Tong University School of Medicine (21XHDB06); Jiaxing Science and Technology Plan Project (No. 2022-2). The funders had no role in study design, data collection and analysis, decision to publish, or preparation of the manuscript.
Author information
Bingnan Lu, Yifan Liu, Yuntao Yao and Dawei Zhu contributed equally to this work and shared first authorship.
Authors and Affiliations
Department of Urology, Xinhua Hospital, Shanghai Jiao Tong University School of Medicine, No.1665 Kongjiang Road, Shanghai, 200092, China
Bingnan Lu, Yifan Liu, Yuntao Yao, Keqin Dong, Donghao Lv, Zihui Zhao, Haoyu Zhang, Xinyue Yang, Wenjia Fu, Jianwei Cao, Xiuwu Pan & Xingang Cui
Department of Urology, the Second People’s Hospital of Pinghu, Zhejiang, 314200, China
Dawei Zhu, Xiao Xu & Jianwei Cao
Department of Urology, Shanghai Baoshan Luodian Hospital, Shanghai, 201908, China
Xiangmin Zhang & Jian Chu
Department of Burn Surgery, the First Affiliated Hospital of Naval Medical University, Shanghai, 200433, China
Runzhi Huang
You can also search for this author in PubMed Google Scholar
Contributions
X.P. and X.C. conceptualized the project. K.D. and X.P. collected the data. B.L., Y.L., Y.Y., and R.H. conducted data analysis. B.L., X.Z., D.L., Z.Z., H.Z., X.Y., and W.F. wrote the original draft of the manuscript. Y.L., Y.Y., J.C., X.P., and X.C. reviewed the manuscript. B.L., Y.L., Y.Y., D.Z., X.X., R.H., J.C., J.C., X.P., and X.C. revised the manuscript. R.H., J.C., J.C., X.P., and X.C. acquired the funding.
Corresponding authors
Correspondence to Runzhi Huang , Jianwei Cao , Jian Chu , Xiuwu Pan or Xingang Cui .
Ethics declarations
Ethics approval and consent to participate.
Our study was approved by the Ethics Committee of Xinhua Hospital Affiliated to Shanghai Jiao Tong University School of Medicine (XHEC-C-2021-145-1). All authors confirmed that the whole study was carried out in accordance with relevant guidelines.
Competing interests
The authors declare no competing interests.
Additional information
Publisher’s note.
Springer Nature remains neutral with regard to jurisdictional claims in published maps and institutional affiliations.
Electronic supplementary material
Below is the link to the electronic supplementary material.
Supplementary Material 1
Supplementary material 2, rights and permissions.
Open Access This article is licensed under a Creative Commons Attribution-NonCommercial-NoDerivatives 4.0 International License, which permits any non-commercial use, sharing, distribution and reproduction in any medium or format, as long as you give appropriate credit to the original author(s) and the source, provide a link to the Creative Commons licence, and indicate if you modified the licensed material. You do not have permission under this licence to share adapted material derived from this article or parts of it. The images or other third party material in this article are included in the article’s Creative Commons licence, unless indicated otherwise in a credit line to the material. If material is not included in the article’s Creative Commons licence and your intended use is not permitted by statutory regulation or exceeds the permitted use, you will need to obtain permission directly from the copyright holder. To view a copy of this licence, visit http://creativecommons.org/licenses/by-nc-nd/4.0/ .
Reprints and permissions
About this article
Cite this article.
Lu, B., Liu, Y., Yao, Y. et al. Unveiling the unique role of TSPAN7 across tumors: a pan-cancer study incorporating retrospective clinical research and bioinformatic analysis. Biol Direct 19 , 72 (2024). https://doi.org/10.1186/s13062-024-00516-8
Download citation
Received : 28 April 2024
Accepted : 08 August 2024
Published : 22 August 2024
DOI : https://doi.org/10.1186/s13062-024-00516-8
Share this article
Anyone you share the following link with will be able to read this content:
Sorry, a shareable link is not currently available for this article.
Provided by the Springer Nature SharedIt content-sharing initiative
- Kidney neoplasm
- Tumor immunology
Biology Direct
ISSN: 1745-6150
- General enquiries: [email protected]
This paper is in the following e-collection/theme issue:
Published on 26.8.2024 in Vol 26 (2024)
Evaluation of a Natural Language Processing Approach to Identify Diagnostic Errors and Analysis of Safety Learning System Case Review Data: Retrospective Cohort Study
Authors of this article:

Original Paper
- Azade Tabaie 1, 2 , PhD ;
- Alberta Tran 3 , RN, CCRN, PhD ;
- Tony Calabria 3 , MA, CPHQ, CSSBB ;
- Sonita S Bennett 1 , MSc ;
- Arianna Milicia 4 , BSc ;
- William Weintraub 5, 6 , MACC, MD ;
- William James Gallagher 6, 7 , MD ;
- John Yosaitis 6, 8 , MD ;
- Laura C Schubel 4 , MPH ;
- Mary A Hill 9, 10 , MS ;
- Kelly Michelle Smith 9, 10 , PhD ;
- Kristen Miller 4, 6 , MSPH, MSL, CPPS, DrPH
1 Center for Biostatistics, Informatics, and Data Science, MedStar Health Research Institute, Washington, DC, United States
2 Department of Emergency Medicine, Georgetown University School of Medicine, Washington, DC, United States
3 Department of Quality and Safety, MedStar Health Research Institute, Washington, DC, United States
4 National Center for Human Factors in Healthcare, MedStar Health Research Institute, Washington, DC, United States
5 Population Health, MedStar Health Research Institute, Washington, DC, United States
6 Georgetown University School of Medicine, Washington, DC, United States
7 Family Medicine Residency Program, MedStar Health Georgetown-Washington Hospital Center, Washington, DC, United States
8 MedStar Simulation Training & Education Lab (SiTEL), MedStar Institute for Innovation, Washington, DC, United States
9 Institute of Health Policy, Management & Evaluation, University of Toronto, Toronto, ON, Canada
10 Michael Garron Hospital, Toronto, ON, Canada
Corresponding Author:
Azade Tabaie, PhD
Center for Biostatistics, Informatics, and Data Science
MedStar Health Research Institute
3007 Tilden Street NW
Washington, DC, 20008
United States
Phone: 1 202 244 9810
Email: [email protected]
Background: Diagnostic errors are an underappreciated cause of preventable mortality in hospitals and pose a risk for severe patient harm and increase hospital length of stay.
Objective: This study aims to explore the potential of machine learning and natural language processing techniques in improving diagnostic safety surveillance. We conducted a rigorous evaluation of the feasibility and potential to use electronic health records clinical notes and existing case review data.
Methods: Safety Learning System case review data from 1 large health system composed of 10 hospitals in the mid-Atlantic region of the United States from February 2016 to September 2021 were analyzed. The case review outcome included opportunities for improvement including diagnostic opportunities for improvement. To supplement case review data, electronic health record clinical notes were extracted and analyzed. A simple logistic regression model along with 3 forms of logistic regression models (ie, Least Absolute Shrinkage and Selection Operator, Ridge, and Elastic Net) with regularization functions was trained on this data to compare classification performances in classifying patients who experienced diagnostic errors during hospitalization. Further, statistical tests were conducted to find significant differences between female and male patients who experienced diagnostic errors.
Results: In total, 126 (7.4%) patients (of 1704) had been identified by case reviewers as having experienced at least 1 diagnostic error. Patients who had experienced diagnostic error were grouped by sex: 59 (7.1%) of the 830 women and 67 (7.7%) of the 874 men. Among the patients who experienced a diagnostic error, female patients were older (median 72, IQR 66-80 vs median 67, IQR 57-76; P =.02), had higher rates of being admitted through general or internal medicine (69.5% vs 47.8%; P =.01), lower rates of cardiovascular-related admitted diagnosis (11.9% vs 28.4%; P =.02), and lower rates of being admitted through neurology department (2.3% vs 13.4%; P =.04). The Ridge model achieved the highest area under the receiver operating characteristic curve (0.885), specificity (0.797), positive predictive value (PPV; 0.24), and F 1 -score (0.369) in classifying patients who were at higher risk of diagnostic errors among hospitalized patients.
Conclusions: Our findings demonstrate that natural language processing can be a potential solution to more effectively identifying and selecting potential diagnostic error cases for review and therefore reducing the case review burden.
Introduction
Diagnostic errors are an underappreciated cause of preventable mortality in hospitals, estimated to affect a quarter million hospital inpatients, and account for an estimated 40,000-80,000 deaths annually in the United States [ 1 ]. These errors pose a risk for severe patient harm [ 2 , 3 ], increase hospital length of stay [ 4 ], and made up 22% and accounted for US $5.7 billion of paid malpractice claims in hospitalized patients throughout a nearly 13-year period [ 5 ]. In their analysis of malpractice claims occurring in the US National Practitioner Database from 1999 to 2011, Gupta et al [ 5 ] found that diagnosis-related paid claims were most likely to be associated with death and cost (following surgery); among diagnosis-related paid claims, failure to diagnose was the most common subtype and was more likely than other types to be associated with mortality. Several factors have been proposed as contributors to inpatient diagnostic errors including time constraints related to the concurrent care of multiple patients, unpredictable workflows, distractions, and competing priorities for trainees. From their systematic review and meta-analysis, Gunderson et al [ 2 ] estimate that 250,000 diagnostic adverse events occur annually among hospitalized patients in the United States, and this is likely an underestimation of the problem due to several challenges in diagnostic error measurement [ 6 ].
Challenges in identifying and measuring diagnostic errors occur due to the evolving and iterative nature of the diagnostic process, making it difficult to determine when, if at all, a correct or more specific diagnosis could have been established by clinicians to start the appropriate treatment [ 6 ]. Since its landmark report, Improving Diagnosis in Health Care , the National Academies of Science, Engineering, and Medicine (NASEM) has produced a common understanding of diagnostic error that includes accuracy, timeliness, and communication of the explanation to the patient or patient’s family member [ 3 ]. Diagnostic errors often involve missed opportunities related to various aspects of the diagnostic process [ 7 - 9 ] and diagnostic adverse events resulting in harm [ 10 ]. However, many hospitals currently do not capture or include surveillance for diagnostic errors, despite having robust systems in place to report and analyze patient safety issues [ 6 , 11 , 12 ].
A crucial first step to improving diagnosis in hospitals is the creation of programs to identify, analyze, and learn from diagnostic errors. Ongoing efforts by the Agency for Health Care Research and Quality have supported pragmatic measurement approaches for health organizations to build a diagnostic safety program and identify and learn from diagnostic errors such as those described in the Measure Dx resource [ 9 ]. One proposed and promising solution for hospitals to improve diagnostic surveillance is to build on existing efforts to collect patient safety data, root cause analyses, or other forms of case reviews for quality improvement purposes. Cases that have already been reviewed or investigated in the organization for general patient safety and quality purposes may be able to inform or be rereviewed for information and learning opportunities specific to diagnostic safety. Widely used case-based learning methodologies in particular, such as the “Learning From Every Death” initiative developed at Mayo Clinic [ 13 ] used both nationally and worldwide, offer an excellent opportunity for hospitals to augment their existing quality and safety efforts and support diagnostic safety.
Clinical notes in electronic health records (EHRs) written by health providers in free-text format are rich sources of a patient’s diagnoses and care trajectory through hospitalization time. Approaches to processing free text, such as through natural language processing (NLP) and machine learning (ML), have demonstrated significant opportunities to improve quality and safety within health care organizations in diverse applications [ 14 - 16 ] such as cancer research [ 17 , 18 ] and infection prediction [ 19 ] to sleep issues [ 20 ] and neurological outcome prediction [ 21 ]. Besides its use in the diagnostic process, ML models proved to have added benefits when used in diagnostic error identification [ 22 , 23 ]. However, despite significant progress and evidence about the use of these ML and NLP approaches to improve patient safety, the use of ML and NLP approaches to diagnostic safety and surveillance has largely remained untapped. A 2022 study demonstrates how an academic medical center’s implementation of an NLP-based algorithm to flag safety event reports for manual review enabled early detection of emerging diagnostic risks from large volumes of safety reports, and was among the first to apply an NLP approach to safety event reports to facilitate identification of COVID-19 related diagnostic errors [ 24 ]. Meanwhile, progress in the use of data mining approaches to develop electronic trigger tools offers promising methods to detect potential diagnostic events, promote organizational learning, and support the monitoring of data prospectively to identify patients at high risk for future adverse events [ 25 ]. To our knowledge, however, NLP has not yet been applied to case review data to facilitate the identification of diagnostic errors and understand its features and sources.
While free-text formatted clinical notes provide unique opportunities to incorporate ML models, the lack of reliable labels to represent diagnostic errors often limits the use of clinical notes for diagnostic safety surveillance efforts. The opportunity to train ML and NLP algorithms to identify diagnostic errors and opportunities depends on the collation of EHR data with existing efforts to identify diagnostic errors such as through case review findings from the Safety Learning System (SLS). To further explore the potential for this approach to be used to improve diagnostic safety surveillance, a rigorous evaluation of the feasibility and potential of using EHR and existing case review data is needed.
We hypothesized that ML and NLP methods can be applied to train models based on available case review data to examine content potentially related to diagnostic errors within EHR clinical notes. These approaches automatically identify features or information from free text using controlled vocabularies, rule sets, reference dictionaries, or lexicons.
Data Sets and Case Review Approach
We analyzed SLS data from 1 large health system comprised of 10 hospitals in the mid-Atlantic region of the United States. The SLS is one example of a holistic case review methodology delivered by health care organizations in the United States and globally. Established in 2015, the SLS builds upon the Mayo Clinic Mortality Review System of Huddleston et al [ 13 ] to review and analyze EHR data from patient mortality cases to find safety issues that could be found and mitigated. This approach was designed to enhance current quality improvement projects done within health organizations, providing a perspective and strategy based on the Safety II lens and rooted in the belief that every death provides an opportunity to improve care. With a Safety II lens, participating organizations use a holistic case review methodology designed to identify vulnerabilities in systems and processes of care delivery. Reviewers identify and translate these into different categories and labels to (1) define and quantify types of process of care and system failures contributing to adverse outcomes (errors) and (2) identify the components of the process of care and system failures that when fixed will improve performance (opportunities for improvement [OFIs]).
To ensure a sufficient cross-sampling of patients across different specialties and areas, patients are selected for case reviews at this health system based on their primary provider service line category (eg, medicine, surgery, etc) and hospital length of stay; patients in primary and ambulatory care settings are not included for case review selection. The case review process occurs according to the standardized SLS methodology and recommendations [ 13 , 26 ], and between at least 1 physician and 1 nurse within the health system who have both received training in the SLS approach. The case review outcome and identification of OFIs, including diagnostic OFIs, relies on the reviewer’s consensus of any findings and through multiple multidisciplinary and multispecialty meetings that may involve a committee Chair member, clinical department leader, or escalation to other leadership.
We obtained SLS data from February 2016 to September 2021; data in later years were available but not included because of key changes to the case selection process made during and in response to the COVID-19 pandemic. All hospitalized adult patients older than 18 years were included for analysis, regardless of their hospitalization outcome (eg, mortality or discharge location). Pediatric and neonatal patients were excluded.
Ethical Considerations
The original data collection and study protocol was approved by the institutional review board (00001245) at MedStar Health Research Institute on August 26, 2019.
Data Extraction
Medical record number, encounter number, length of stay, age, date of birth, sex, diagnosis at the time of admission (ie, ICD-10 [ International Statistical Classification of Diseases, Tenth Revision ] diagnosis codes), mortality, OFI categories (eg, delayed or missed diagnosis and diagnostic opportunities), number of identified OFIs and diagnosis issues (eg, the accuracy of diagnosis and confirmation or fixation bias) were the features and patient identifiers which were extracted from SLS data [ 13 , 26 ].
Because chart reviews generally occur at a single point in time within the patient’s care trajectory, they often do not contain information or details of the patient’s full hospital course. However, clinical notes written by health care providers are rich sources of patient’s health status throughout their hospitalization period [ 27 - 29 ]. Therefore, to supplement these chart review data, we additionally extracted and included all clinical notes from the EHR for patients who could be matched by patient identifiers (eg, encounter number and date of birth).
Coding Diagnostic Errors
Case reviewers can select any number of labels to describe a diagnosis issue or an OFI identified and agreed upon by consensus. For this study, diagnostic errors were defined by the available features from chart review pertaining to diagnosis and impacting the timeliness, accuracy, or communication of a diagnosis. Our definition of diagnostic errors was limited to the categories identified during chart reviews and recorded within the SLS data set; therefore, our diagnostic error definition does not include all aspects of the definition developed by the NASEM report [ 3 ]. Table 1 describes the SLS categories and values that were labeled as diagnostic errors and used to train our classification models. Patients were coded as having experienced a diagnostic error if one or more of the conditions listed in Table 1 were identified in their SLS case review.
Feature from chart reviews | Value to indicate diagnostic error |
OFI category | Delayed or missed diagnosis |
OFI category | Diagnostic opportunities |
Diagnosis issues | accuracy of diagnosis |
Diagnosis issues | Accuracy of interpretation of laboratory or test results |
Diagnosis issues | Squirrel (red herring lab or test results) |
Diagnosis issues | Confirmation or fixation bias |
Diagnosis issues | Appropriateness of chosen tests or equipment given the patient’s differential diagnosis |
a OFI: opportunity for improvement.
NLP Approach
We used an NLP approach on critical incident reporting system data to explore the features and risk of diagnostic error among hospitalized patients.
Features From Free-Text Data
Descriptive statistical analyses were performed to identify any differences among age, length of stay, and mortality between the female and male patients who had experienced diagnostic errors.
All EHR clinical notes were transformed to lowercase. Extra white spaces, numbers, punctuations, and stop words were removed and words were stemmed. The term frequency-inverse document frequency (TF-IDF) matrix was calculated for each clinical note using the bag-of-words from the preprocessed EHR clinical notes [ 30 ]. TF-IDF is a statistical measure that evaluates how relevant a word is to a document in a collection of documents and is a popular method to translate free text to numerical features in training ML models. The TF-IDF of a word in a document is calculated by multiplying 2 metrics: the number of times a word appeared in a document and the inverse document frequency of the word across a set of documents. TF-IDF is computationally efficient and easy to interpret. We excluded the most frequent words that had appeared in more than 95% of the EHR clinical notes, as these frequent words do not provide information to help with the classification. Moreover, we excluded the rare words that appeared in less than 5% of the EHR clinical notes [ 31 ].
In a TF-IDF matrix, the number of rows corresponds to the unique patients, and the number of columns represents the unique words found in EHR clinical notes. There are numerous unique words used in EHR clinical notes; therefore, the TF-IDF approach provides a high-dimensional input matrix for the classification task. The high-dimensional input matrix can lead to training inaccurate classifiers. To overcome that issue, we used the chi-square statistical test to select the most relevant words to identify diagnostic errors; therefore, if P values associated with a word (also called a feature) are less than .05, that word is selected and included in the feature matrix to train ML classification models.
Classification Models
In lieu of an existing model with the same objective in the literature, a simple logistic regression model was trained as the baseline classifier to identify patients within SLS data who were at higher risk of diagnostic error. Moreover, 3 forms of logistic regression models with regularization functions were trained on this data to compare classification performances and identify the best-performing model [ 32 ]: Least Absolute Shrinkage and Selection Operator (LASSO), Ridge, and Elastic Net.
- LASSO: for a more accurate prediction, LASSO regularization is used with a logistic regression model. The LASSO procedure encourages simple, sparse models which has fewer parameters in a way that the estimated coefficient of features with less effect will be set to zero. This characteristic makes LASSO well-suited for models showing high levels of multicollinearity or variable selection and parameter elimination is needed. LASSO is also called L1 regularization.
- Ridge: also called L2 regularization, Ridge is a regularization method used for models suffering from multicollinearity or high-dimensional feature space. Ridge regularization keeps all the features regardless of their effect on the model. However, it pushes the estimated coefficient of features with less effect toward zero to minimize their effect on the classification outcome. This characteristic of Ridge makes it well-suited when most features impact the outcome variable.
- Elastic Net: a logistic regression model with Elastic Net regularization is a weighted combination of LASSO (L1) and Ridge (L2) regularizations [ 33 ]. Elastic Net can remove the effect of the insignificant features by setting their estimated coefficient to zero and lower the effect of the less significant features by pushing their estimated coefficient toward zero while adding more weights to the more important features. From implementation and interpretation aspects, the Elastic Net model is simple to use. Such characteristics make this model an accepted baseline in ML-based studies [ 34 ].
The hyperparameters of the 3 classification models were optimized through cross-validation. All the analyses were conducted using Python 3 (Python Software Foundation).
Classification Performance Metrics
We calculated 7 common performance metrics reported for binary classifiers to compare the performance of the 4 classification models: area under receiver operating characteristics curve (AUROC), sensitivity or recall or true positive rate, specificity or true negative rate, positive predictive value (PPV) or precision, negative predictive value (NPV), F 1 -score, and area under precision-recall curve (AUPRC). The 7 metrics take values between 0 and 1. Values closer to 1 indicate a well-performing classifier. Multimedia Appendix 1 presents the definition of the performance metrics used in this study. Figure 1 presents the summary of the methods used in this analysis.

Descriptive Summary
In total, there were 2184 unique patient records within SLS data from February 2016 to September 2021. EHR clinical notes were cross-matched, extracted, and included in analyses for 1704 (78%) of these SLS patient records. Of those patients with cross-matched EHR data, 126 (7.4%) patients had been identified by case reviewers as having experienced at least 1 diagnostic error. A total number of 20,848 EHR clinical notes associated with the 1704 unique patients were used in this study.
Patients who had experienced diagnostic errors were grouped by sex: 59 (7.1%) of the 830 women and 67 (7.7%) of the 874 men in the larger cross-matched sample had been found to have a diagnostic error. Table 2 presents the descriptive statistics between female and male patient groups. We applied the Wilcoxon rank sum test for numerical features (ie, age and length of stay), and the chi-square test for mortality rate, admission diagnosis, and admission department or specialty. Patients in the female group were older than the male group by a median of 72 (IQR 66-80) versus a median of 67 (IQR 57-76; P =.02). Compared to the male group, female patients who experienced diagnostic error had higher rates of being admitted through general or internal medicine (69.5% vs 47.8%; P =.01), lower rates of cardiovascular-related admitted diagnosis (11.9% vs 28.4%; P =.02), and lower rates of being admitted through neurology department (2.3% vs 13.4%; P =.04). We observed no differences between groups in mortality rates and length of stay.
Patients who experienced diagnostic error | All patients | |||||||||
Female group (n=59) | Male group (n=67) | Female group (n=830) | Male group (n=874) | |||||||
Age (in years), median (IQR) | 72 (66-80) | 67 (57-76) | 72 (62-83) | 69 (59-79) | ||||||
African American | 38 (64) | 42 (62) | 429 (51.7) | 429 (51.7) | ||||||
Asian | 0 (0) | 0 (0) | 12 (1.4) | 12 (1.4) | ||||||
Multiple | 0 (0) | 0 (0) | 2 (0.2) | 2 (0.2) | ||||||
Not recorded | 4 (6) | 2 (2.9) | 30 (3.6) | 30 (3.6) | ||||||
White | 11 (18) | 21 (31.3) | 310 (37.3) | 310 (37.3) | ||||||
Other | 6 (10) | 2 (2.9) | 47 (5.7) | 47 (5.7) | ||||||
Length of stay in days, median (IQR) | 4 (6-10) | 4 (8-14) | 7 (4-12) | 8 (4-12) | ||||||
Count | 25 (42) | 29 (43) | 456 (54.9) | 459 (52.5) | ||||||
General or internal medicine or hospitalist | 41 (69) | 32 (47) | 427 (51.4) | 389 (44.5) | ||||||
Cardiology | 5 (8) | 12 (17) | 99 (11.9) | 131 (14.9) | ||||||
Critical care | 6 (10) | 6 (8) | 117 (14.1) | 142 (16.2) | ||||||
Neurology | 2 (3) | 9 (13) | 75 (9) | 90 (10.3) | ||||||
Pulmonary | 1 (1) | 1 (1) | 22 (2.6) | 31 (3.5) | ||||||
Other | 4 (6) | 7 (10) | 90 (10.8) | 91 (10.4) | ||||||
Cardiovascular | 7 (11) | 19 (28) | 154 (18.6) | 167 (19.1) | ||||||
Respiratory | 7 (11) | 5 (7) | 88 (10.6) | 69 (7.9) | ||||||
Sepsis | 7 (11) | 4 (5) | 65 (7.8) | 63 (7.2) | ||||||
Altered mental status | 1 (1) | 2 (2) | 36 (4.3) | 28 (3.2) | ||||||
Diabetes | 1 (1) | 1 (1) | 6 (0.7) | 3 (0.3) | ||||||
Other | 23 (38) | 21 (31) | 244 (29.4) | 270 (30.9) | ||||||
General care | 54 (91) | 60 (89) | 144 (17.3) | 179 (20.5) | ||||||
Critical care | 5 (8.5) | 7 (10) | 686 (82.7) | 695 (79.5) | ||||||
categories, n (%) | ||||||||||
Delayed or missed diagnosis | 43 (72) | 46 (68) | 43 (5.2) | 46 (5.3) | ||||||
Diagnostic opportunities | 15 (25) | 16 (23) | 15 (1.8) | 16 (1.8) | ||||||
Accuracy of diagnosis | 1 (1) | 4 (6) | 1 (0.1) | 4 (0.5) | ||||||
Accuracy of interpretation of laboratory or test results | 0 (0) | 0 (0) | 0 (0) | 0 (0) | ||||||
Squirrel (red herring lab or test results) | 0 (0) | 1 (1) | 0 (0) | 1 (0.1) | ||||||
Confirmation or fixation bias | 0 (0) | 0 (0) | 0 (0) | 0 (0) | ||||||
Appropriateness of chosen tests or equipment given patient’s differential diagnosis | 1 (1) | 0 (0) | 1 (0.1) | 0 (0) | ||||||
Critical care | 15 (25) | 22 (32) | 273 (32.9) | 318 (36.4) | ||||||
Emergency department | 17 (28) | 18 (26) | 81 (9.8) | 76 (8.7) | ||||||
General care | 27 (45) | 27 (40) | 290 (34.9) | 285 (32.6) |
Classification Models’ Performance
Clinical notes were preprocessed for TF-IDF feature calculation. The bag-of-words included 2227 words, and each word was considered a feature (see Table S1 in Multimedia Appendix 2 for the top 100 words). We found that abscess, ascend, abnormality, scant, pair, and prefer were the top 5 features with the highest positive estimated coefficient (0.42 to 0.28); post, select, gave, muscl, hours, and unrespons were the top 5 features with the highest negative coefficients (–0.35 to –0.26). After applying the chi-square test, 250 features with a P value less than .05 were selected for the modeling process. All 4 ML classifiers were trained using the 250 selected features.
Table 3 presents the performances of the simple logistic regression and 3 regularized logistic regression models (LASSO, Ridge, and Elastic Net). The Ridge model achieved the highest AUROC (0.885), specificity (0.797), PPV (0.24), NPV (0.981), and F 1 -score (0.369) in classifying patients who were at higher risk of diagnostic errors among hospitalized patients in SLS system. The simple logistic regression model obtained the highest AUPRC (0.537). The simple logistic regression model classified all patients as the ones with diagnostic errors; therefore, it achieved a sensitivity of 1, and specificity and NPV of 0.
Figures 2 and 3 present the receiver operating characteristics curves and precision-recall curves for the 4 classifiers in this study. Models that give ROC curves closer to the top-left corner indicate a better performance. The AUROC values represent the probability that a patient who experienced a diagnostic error, chosen at random, is ranked higher by the Ridge model than a randomly chosen patient who did not experience a diagnostic error. The higher value of AUPRC indicates that the Ridge model can identify patients who experienced diagnostic errors more precisely with fewer false positives compared to LASSO and Elastic Net models.
Simple logistic regression | LASSO | Ridge | Elastic Net | |
AUROC | 0.5 | 0.846 | 0.885 | 0.859 |
Sensitivity | 1.0 | 0.802 | 0.802 | 0.802 |
Specificity | 0 | 0.733 | 0.797 | 0.742 |
Positive predictive value | 0.074 | 0.193 | 0.24 | 0.199 |
Negative predictive value | 0 | 0.979 | 0.981 | 0.979 |
-score | 0.138 | 0.312 | 0.369 | 0.319 |
AUPRC | 0.537 | 0.361 | 0.491 | 0.411 |
a LASSO: Least Absolute Shrinkage and Selection Operator.
b AUROC: area under receiver operating characteristics curve.
c AUPRC: area under precision-recall curve.

Principal Findings
Our contribution is 2-fold; first, we integrated 2 data sources that are currently used by and available to many organizations across the United States, SLS and EHR data, to explore the use of ML and NLP algorithms to help identify diagnostic errors among hospitalized patients. Although case review methodologies offer rich insights into systems errors and OFIs, the predefined pull-down menus and structured data labels typically do not capture all the necessary clinical and contextual details that are considered by reviewers. Therefore, a large portion of these case review data are stored in free-text narratives that typically record key information and judgments decided upon by the multidisciplinary reviewers. However, given persistent issues of staff shortage and lack of time in health care settings, it is becoming increasingly important to lower the burden of systematic EHR data reviews for health care providers while maintaining the review systems in place. Second, any developed ML and NLP approaches can potentially be incorporated to generate a diagnostic error risk score for each patient. The predicted risk score can be used in identifying and prioritizing patients for focused chart reviews, thus lowering the burden of systematic EHR data reviews for health care providers while maintaining the review systems in place.
To our knowledge, this study is the first attempt to apply and test several different ML classification models to identify diagnostic errors within routinely collected organizational case review data. Despite a substantial body of literature about the prevalence of diagnostic errors in hospital settings, current efforts to identify diagnostic errors generally rely on reviews of patient cases and data by clinical or quality teams that often are resource-intensive. ML classification models and NLP techniques offer an opportunity to generate diagnostic error risk scores to sort through large data sets and identify signals of potential diagnostic errors that can be flagged for further review. However, these classification models require a high number of observations (and identified diagnostic errors) to perform well, which might not be feasible for health organizations that are just beginning to identify diagnostic errors or may have limited personnel and efforts to perform high numbers of case reviews. In this study, we accessed nearly 2000 patient records (and of those, only 126 cases of diagnostic errors), which is considered to be a limited data sample size in the field of ML. However, techniques, such as feature selection and n-fold cross-validations, can potentially be approaches to address small sample size challenges [ 35 ].
Using the results of the simple logistic regression model as the baseline performance, we found that 3 regularization functions, namely LASSO, Ridge, and Elastic Net, boosted the performance of the baseline model. The Ridge model outperformed the rest of the models in terms of multiple performance metrics: AUROC of 0.885, specificity of 0.797, PPV of 0.24, NPV of 0.981, and F 1 -score of 0.369. The Ridge algorithm tries to keep all features in the model even the features with a slight effect on the classification outcome. Since the patterns pointing at a diagnostic error were subtle in the clinical notes, even a small effect of a feature on the model’s classification outcome could be important for the classification model to learn. On the other hand, the LASSO algorithm rigorously removes features that have a small effect on the classification outcome. The Elastic Net model is a weighted combination of LASSO and Ridge. The performance results presented in Table 3 show that the values achieved by the Elastic Net model lie between those of the LASSO and Ridge models.
Insights From Diagnostic Errors Within Free-Text Clinical Notes
We did not find the free text formatted clinical notes in the EHR to reflect any sort of direct language around diagnostic errors. Our analysis identified no use of the terms misdiagnosis, missed diagnosis, or diagnostic error within clinical notes, finding instead more subtle signals pointing at diagnostic errors such as “there may be a chance of misreading the test,” or “insufficient data to make a diagnosis.” Our findings demonstrate that NLP algorithms can be used to identify such patterns and find the associations between diagnostic errors and the subtle signals in the clinical notes. A natural extension of this work can focus on using other feature extraction methods, such as Bidirectional Encoder Representations from Transformers contextualized word embeddings, and explore the use of the pretrained language models for this objective.
We found that the presence of terms, such as abscess, abnormality, “cp” (chest pain) , and dialysis in a patient’s EHR clinical note were associated with reviewer-identified diagnostic errors ( Multimedia Appendix 2 ). Misinterpretation of chest pain, specifically among female patients, has the potential to cause a cardiovascular-related diagnosis error [ 36 ]. Patients with chronic kidney disease are at higher risk of cardiovascular complications [ 37 ]. Missing such risk for a patient who is on dialysis, adds to the risk of diagnostic error.
Clinical and System Implications Around Diagnostic Inequity
Diagnostic inequity is defined as “the presence of preventable unwarranted variations in diagnostic process among population groups that are socially, economically, demographically, or geographically disadvantaged” [ 38 ]. Despite persistent and well-documented disparities in health care access and outcomes across different population groups, few studies have examined the association between diagnostic errors and health care disparities [ 39 ]. Recent evidence supports the notion that variation in diagnostic error rates across demographic groups may exist, particularly across sex. A systematic review of diagnostic errors in the emergency department, for example, found that female sex and non-White race were often associated with increased risk for diagnostic errors across several clinical conditions in emergency settings [ 40 ]. In cardiovascular medicine, a national cohort study of acute myocardial infarctions found that women were nearly twice as likely as men to receive the wrong initial diagnosis following signs of a heart attack [ 41 ]. Despite efforts to understand and reduce disparities in diagnosis and treatment, women not only continue to be understudied, underdiagnosed, and undertreated in cardiovascular medicine [ 42 ] but also may experience longer lengths of time to diagnosis than men in most patterns of disease diagnosis [ 43 ].
The analysis of case review data and other system-based data (eg, patient safety events or incident reporting) by subsets offer an opportunity to identify events in vulnerable patient populations and help sensitize clinicians to potential biases within the diagnostic process. To explore sex differences in diagnostic errors within our case review data, we statistically compared demographic and clinical differences between female and male patients who had been identified in case reviews as having experienced diagnostic error or errors. We found that of those patients who had experienced diagnostic error or errors, the female group of patients were older, had higher rates of being admitted through general or internal medicine or hospitalist (vs specialty) departments, and had lower rates of having a cardiovascular diagnosis on admission. These preliminary results of this study revealed unexpected differences between male and female diagnostic error groups, offering novel insights that warrant further investigation to fully understand the mechanisms underlying these relationships and their implications for clinical decision-making and practice. Future uses of NLP can potentially support clinical and system-based approaches to capture and increase the evidence around structural biases or disparities in diagnoses. Individual cases from these types of data sources could be used as example narratives to engage clinicians and improve clinician learning, contributing to the development of tailored clinician and systemic interventions that can improve quality and equity throughout the diagnostic process.
Limitations
This study has several limitations. Our definition of diagnostic errors was limited to the categories and labels used within the SLS data set, reviewer interpretations of cases (subject to reviewer bias), and does not include all aspects of the definition developed by the NASEM report [ 3 ]. Despite several continued differences in definitions of diagnostic error in the peer-reviewed literature [ 8 ], we recommend that quality and safety teams within health systems use the NASEM definition for diagnostic error—including errors in communicating the diagnosis to the patient—to develop any definitions, categories, or labels used in their case review and surveillance initiatives. Although a time-consuming task, future studies could consider EHR data chart reviews to have the ground truth for the diagnostic error cases and add to the accuracy of the data set used for training the ML classifiers. Additionally, due to staffing challenges and shifting organizational priorities, case review selection varies by hospital and has changed over time, resulting in a relatively small sample size and also introducing the potential for bias. Our data came from a single health system and may reflect the specific language, culture, and practices occurring within the system and therefore may not be similar to that of other health systems. To enhance the external validity and generalizability of results, future efforts and research studies should consider the random selection of cases to evaluate both diagnostic and general quality issues within the organization; studies with larger sample sizes can build on our preliminary findings and test differences between clinical subgroups. Finally, our classification models were developed and evaluated based on a retrospective cohort from EHR; therefore, the performance may deteriorate when the method is applied to real-time data. Further work or future studies should be conducted to prospectively validate the models.
Conclusions
We performed an NLP approach and compared 4 techniques to classify patients who were at a higher risk of experiencing diagnostic error during hospitalization. Our findings demonstrate that NLP can be a potential solution to more effectively identifying and selecting potential diagnostic error cases for review, and therefore, reducing the case review burden.
Acknowledgments
This work was supported by the Agency for Health Care Research and Quality (grant 5R18HS027280-02).
Conflicts of Interest
None declared.
Binary classification performance metrics.
The Estimated Coefficient from the Ridge Model.
- Newman-Toker DE, Wang Z, Zhu Y, Nassery N, Saber Tehrani AS, Schaffer AC, et al. Rate of diagnostic errors and serious misdiagnosis-related harms for major vascular events, infections, and cancers: toward a national incidence estimate using the "Big Three". Diagnosis (Berl). Feb 23, 2021;8(1):67-84. [ FREE Full text ] [ CrossRef ] [ Medline ]
- Gunderson CG, Bilan VP, Holleck JL, Nickerson P, Cherry BM, Chui P, et al. Prevalence of harmful diagnostic errors in hospitalised adults: a systematic review and meta-analysis. BMJ Qual Saf. Dec 2020;29(12):1008-1018. [ CrossRef ] [ Medline ]
- Ball JR, Miller BT, Balogh EP. Improving Diagnosis in Health Care. Washington, DC. National Academies Press; 2015:472.
- Hautz WE, Kämmer JE, Hautz SC, Sauter TC, Zwaan L, Exadaktylos AK, et al. Diagnostic error increases mortality and length of hospital stay in patients presenting through the emergency room. Scand J Trauma Resusc Emerg Med. May 08, 2019;27(1):54. [ FREE Full text ] [ CrossRef ] [ Medline ]
- Gupta A, Snyder A, Kachalia A, Flanders S, Saint S, Chopra V. Malpractice claims related to diagnostic errors in the hospital. BMJ Qual Saf. Aug 09, 2017;27(1):53-60. [ CrossRef ] [ Medline ]
- Zwaan L, Singh H. Diagnostic error in hospitals: finding forests not just the big trees. BMJ Qual Saf. 2020;29(12):961-964. [ CrossRef ] [ Medline ]
- Singh H. Editorial: helping health care organizations to define diagnostic errors as missed opportunities in diagnosis. Jt Comm J Qual Patient Saf. 2014;40(3):99-101. [ CrossRef ] [ Medline ]
- Giardina TD, Hunte H, Hill MA, Heimlich SL, Singh H, Smith KM. Defining diagnostic error: a scoping review to assess the impact of the national academies' report improving diagnosis in health care. J Patient Saf. 2022;18(8):770-778. [ FREE Full text ] [ CrossRef ] [ Medline ]
- Measure Dx: a resource to identify, analyze, and learn from diagnostic safety events. AHRQ: Agency for Healthcare Research and Quality. URL: https://www.ahrq.gov/patient-safety/settings/multiple/measure-dx.html [accessed 2023-06-22]
- Zwaan L, de Bruijne M, Wagner C, Thijs A, Smits M, van der Wal G, et al. Patient record review of the incidence, consequences, and causes of diagnostic adverse events. Arch Intern Med. 2010;170(12):1015-1021. [ CrossRef ] [ Medline ]
- Singh H. Diagnostic errors: moving beyond 'no respect' and getting ready for prime time. BMJ Qual Saf. 2013;22(10):789-792. [ FREE Full text ] [ CrossRef ] [ Medline ]
- Zwaan L, El-Kareh R, Meyer AND, Hooftman J, Singh H. Advancing diagnostic safety research: results of a systematic research priority setting exercise. J Gen Intern Med. 2021;36(10):2943-2951. [ FREE Full text ] [ CrossRef ] [ Medline ]
- Huddleston JM, Diedrich DA, Kinsey GC, Enzler MJ, Manning DM. Learning from every death. J Patient Saf. 2014;10(1):6-12. [ CrossRef ] [ Medline ]
- Arnaud E, Elbattah M, Gignon M, Dequen G. NLP-based prediction of medical specialties at hospital admission using triage notes. 2021. Presented at: IEEE 9th International Conference on Healthcare Informatics (ICHI); August 09-12, 2021; Victoria, British Columbia, Canada. [ CrossRef ]
- Bansal A, Saraswat B, Sharma B, Nayan S, Kathuria K. Data extraction and integration from unstructured electronic health records. 2023. Presented at: 2023 3rd International Conference on Technological Advancements in Computational Sciences (ICTACS); November 01-03, 2023; Tashkent, Uzbekistan. [ CrossRef ]
- Sezgin E, Hussain S, Rust S, Huang Y. Extracting medical information from free-text and unstructured patient-generated health data using natural language processing methods: feasibility study with real-world data. JMIR Form Res. 2023;7:e43014. [ FREE Full text ] [ CrossRef ] [ Medline ]
- Asri H, Mousannif H, Moatassime HA, Noel T. Using machine learning algorithms for breast cancer risk prediction and diagnosis. Procedia Comput Sci. 2016;83:1064-1069. [ CrossRef ]
- Goldenberg SL, Nir G, Salcudean SE. A new era: artificial intelligence and machine learning in prostate cancer. Nat Rev Urol. 2019;16(7):391-403. [ CrossRef ] [ Medline ]
- Henry KE, Adams R, Parent C, Soleimani H, Sridharan A, Johnson L, et al. Factors driving provider adoption of the TREWS machine learning-based early warning system and its effects on sepsis treatment timing. Nat Med. 2022;28(7):1447-1454. [ CrossRef ] [ Medline ]
- Nasiri S, Gari D. Attentive adversarial network for large-scale sleep staging. 2020. Presented at: Proceedings of the 5th Machine Learning for Healthcare Conference, PMLR; September, 2020:457-478; Atlanta, GA, USA. URL: https://proceedings.mlr.press/v126/nasiri20a.html
- Tabaie A, Nemati S, Allen JW, Chung C, Queiroga F, Kuk W, et al. Assessing contribution of higher order clinical risk factors to prediction of outcome in aneurysmal subarachnoid hemorrhage patients. AMIA Annu Symp Proc. 2019;2019:848-856. [ FREE Full text ] [ Medline ]
- Cheheltani R, King N, Lee S, North B, Kovarik D, Evans-Molina C, et al. Predicting misdiagnosed adult-onset type 1 diabetes using machine learning. Diabetes Res Clin Pract. 2022;191:110029. [ FREE Full text ] [ CrossRef ] [ Medline ]
- Hercus C, Hudaib A. Delirium misdiagnosis risk in psychiatry: a machine learning-logistic regression predictive algorithm. BMC Health Serv Res. 2020;20(1):151. [ FREE Full text ] [ CrossRef ] [ Medline ]
- Shen L, Levie A, Singh H, Murray K, Desai S. Harnessing event report data to identify diagnostic error during the COVID-19 pandemic. Jt Comm J Qual Patient Saf. 2022;48(2):71-80. [ FREE Full text ] [ CrossRef ] [ Medline ]
- Murphy DR, Meyer AN, Sittig DF, Meeks DW, Thomas EJ, Singh H. Application of electronic trigger tools to identify targets for improving diagnostic safety. BMJ Qual Saf. 2019;28(2):151-159. [ FREE Full text ] [ CrossRef ] [ Medline ]
- The safety learning system®. Discover the Hidden Causes of Patient Harm. URL: http://hbhealthcaresafety.org/safety-learning-system/ [accessed 2023-06-22]
- Alsentzer E, Murphy J, Boag W, Weng W, Jindi D, Naumann T, et al. Publicly available clinical BERT embeddings. 2019. Presented at: Proceedings of the 2nd Clinical Natural Language Processing Workshop; June, 2019; Minneapolis, Minnesota, USA. [ CrossRef ]
- Huang K, Altosaar A, Ranganath R. ClinicalBERT: modeling clinical notes and predicting hospital readmission. ArXiv. Preprint posted online on April 10, 2019. 2019:190405342. [ CrossRef ]
- Zhang D, Thadajarassiri J, Sen C, Rundensteiner E. Time-aware transformer-based network for clinical notes series prediction. 2020. Presented at: Proceedings of the 5th Machine Learning for Healthcare Conference; September, 2020; Worcester, MA, USA. URL: https://proceedings.mlr.press/v126/zhang20c/zhang20c.pdf
- Jones KS. A statistical interpretation of term specificity and its application in retrieval. J Doc. 1972;28(1):11-21. [ CrossRef ]
- Tabaie A, Sengupta S, Pruitt ZM, Fong A. A natural language processing approach to categorise contributing factors from patient safety event reports. BMJ Health Care Inform. 2023;30(1):e100731. [ FREE Full text ] [ CrossRef ] [ Medline ]
- Aheto JMK, Duah HO, Agbadi P, Nakua EK. A predictive model, and predictors of under-five child malaria prevalence in Ghana: how do LASSO, Ridge and Elastic Net regression approaches compare? Prev Med Rep. 2021;23:101475. [ FREE Full text ] [ CrossRef ] [ Medline ]
- Zou H, Trevor H. Regularization and variable selection via the Elastic Net. J R Stat Soc Series B Stat Methodol. 2005;67(2):301-320. [ CrossRef ]
- Lee SJ, Weinberg BD, Gore A, Banerjee I. A scalable natural language processing for inferring BT-RADS categorization from unstructured brain magnetic resonance reports. J Digit Imaging. 2020;33(6):1393-1400. [ FREE Full text ] [ CrossRef ] [ Medline ]
- Masino AJ, Harris MC, Forsyth D, Ostapenko S, Srinivasan L, Bonafide CP, et al. Machine learning models for early sepsis recognition in the neonatal intensive care unit using readily available electronic health record data. PLoS One. 2019;14(2):e0212665. [ FREE Full text ] [ CrossRef ] [ Medline ]
- Mehta PK, Bess C, Elias-Smale S, Vaccarino V, Quyyumi A, Pepine CJ, et al. Gender in cardiovascular medicine: chest pain and coronary artery disease. Eur Heart J. 2019;40(47):3819-3826. [ FREE Full text ] [ CrossRef ] [ Medline ]
- Jankowski J, Floege J, Fliser D, Böhm M, Marx N. Cardiovascular disease in chronic kidney disease: pathophysiological insights and therapeutic options. Circulation. 2021;143(11):1157-1172. [ FREE Full text ] [ CrossRef ] [ Medline ]
- Giardina TD, Woodard LD, Singh H. Advancing diagnostic equity through clinician engagement, community partnerships, and connected care. J Gen Intern Med. 2023;38(5):1293-1295. [ FREE Full text ] [ CrossRef ] [ Medline ]
- Ibrahim SA, Pronovost PJ. Diagnostic errors, health disparities, and artificial intelligence: a combination for health or harm? JAMA Health Forum. 2021;2(9):e212430. [ FREE Full text ] [ CrossRef ] [ Medline ]
- Newman-Toker DE, Peterson SM, Badihian S, Hassoon A, Nassery N, Parizadeh D, et al. Diagnostic Errors in the Emergency Department: A Systematic Review [internet]. Rockville (MD). Agency for Healthcare Research and Quality; 2022.
- Wu J, Gale CP, Hall M, Dondo TB, Metcalfe E, Oliver G, et al. Editor's choice—impact of initial hospital diagnosis on mortality for acute myocardial infarction: a national cohort study. Eur Heart J Acute Cardiovasc Care. 2018;7(2):139-148. [ FREE Full text ] [ CrossRef ] [ Medline ]
- Burgess S. Understudied, under-recognized, underdiagnosed, and undertreated: sex-based disparities in cardiovascular medicine. Circ Cardiovasc Interv. 2022;15(2):e011714. [ CrossRef ] [ Medline ]
- Sun TY, Hardin J, Nieva HR, Natarajan K, Cheng R, Ryan P, et al. Large-scale characterization of gender differences in diagnosis prevalence and time to diagnosis. MedRxiv. Preprint posted online on October 16, 2023. 2023. [ FREE Full text ] [ CrossRef ] [ Medline ]
Abbreviations
area under precision-recall curve |
area under receiver operating characteristic curve |
electronic health record |
International Statistical Classification of Diseases, Tenth Revision |
Least Absolute Shrinkage and Selection Operator |
machine learning |
National Academies of Science, Engineering, and Medicine |
natural language processing |
negative predictive value |
opportunity for improvement |
positive predictive value |
Safety Learning System |
term frequency-inverse document frequency |
Edited by S Ma, T Leung; submitted 17.07.23; peer-reviewed by D Chrimes, M Elbattah; comments to author 18.01.24; revised version received 21.03.24; accepted 20.06.24; published 26.08.24.
©Azade Tabaie, Alberta Tran, Tony Calabria, Sonita S Bennett, Arianna Milicia, William Weintraub, William James Gallagher, John Yosaitis, Laura C Schubel, Mary A Hill, Kelly Michelle Smith, Kristen Miller. Originally published in the Journal of Medical Internet Research (https://www.jmir.org), 26.08.2024.
This is an open-access article distributed under the terms of the Creative Commons Attribution License (https://creativecommons.org/licenses/by/4.0/), which permits unrestricted use, distribution, and reproduction in any medium, provided the original work, first published in the Journal of Medical Internet Research (ISSN 1438-8871), is properly cited. The complete bibliographic information, a link to the original publication on https://www.jmir.org/, as well as this copyright and license information must be included.

An official website of the United States government
The .gov means it’s official. Federal government websites often end in .gov or .mil. Before sharing sensitive information, make sure you’re on a federal government site.
The site is secure. The https:// ensures that you are connecting to the official website and that any information you provide is encrypted and transmitted securely.
- Publications
- Account settings
Preview improvements coming to the PMC website in October 2024. Learn More or Try it out now .
- Advanced Search
- Journal List
- J Thorac Dis
- v.6(12); 2014 Dec
Big data and clinical research: perspective from a clinician
Introduction.
The 21 st century is an era of information technology and people faces information explosion with large amount of data. The term big data has been defined in Wikepedia as: big data is the term for a collection of data sets so large and complex that it becomes difficult to process using on-hand database management tools or traditional data processing applications. In all areas of disciplines, such a big data may help to explore underlying mechanisms of varieties of phenomenon, and further facilitate decision-making. For example, in the 2012 USA presidential election, data-driven decision-making played a huge role in helping Obama win ( 1 ). In biomedical field, big data also begin to show its important role. Medical decision-making is becoming more and more dependent on data analysis, rather than conventional experience and intuition ( 2 ).
The present paper will focus on how to do clinical research by using big data. Firstly, I will review some basic concepts of clinical research and some characteristics of big data. Next, I will take some examples to illustrate how to address clinical uncertainty via data mining. The aim of the article is to provide more insights into clinical research based on big data in the hope that more people will take initiatives to conduct further investigations into clinical big data. I cannot list all detailed technical issues in this article, for which I will provide more references. Interested investigators may take these references as tutorials to guide them through the difficult journal of data exploration.
Bewilderedness in the current clinical research
It is well known that clinical research can be generally categorized into interventional and observational studies. The former is an experimental study that certain interventions will be given to participants. The most commonly performed randomized controlled trial (RCT) belongs to this kind of study. RCT usually has strict inclusion and exclusion criteria for participants. The procedure of randomization is employed to balance potential confounding factors between treatment and control arms. RCTs and/or systematic review involving high quality RCTs are the gold standard for clinical guidelines. However, with more and more RCTs being conducted, its limitations have arisen. In the area of sepsis and critical care medicine, we have compared the results obtained from RCTs and observational studies, and found that interventional effects obtained from these two types of study were quite different ( 3 , 4 ). Biological efficacy, a measurement of effect size under strict experimental condition, can be obtained by RCT. However, this biological efficacy may be attenuated or even not take place at real world setting. In this circumstance the biological efficacy cannot be translated into clinical efficacy ( 5 ). Clinical effectiveness is the most relevant to clinicians. The next question goes to why biological efficacy cannot be translated to clinical effectiveness. The plausible explanation is that RCTs are usually conducted with strict experimental design. There is a long list of inclusion and exclusion criteria. The participants are highly selected to exclude potential confounders. The protocol of intervention is also strictly defined with respect to timing, dosing and sequence of procedure. However, in reality, the condition as defined in RCTs cannot be fulfilled. Patients are usually complicated by comorbidities such as renal dysfunction, hypertension, and congestive heart failure. The timing of drug administration may be delayed due to admission at busy hours. As a result, it is probably that we treat our patients based on knowledge derived from a minority of highly selected patients. That is to say, the conclusion from RCTs may not be generalizable to “real world” patients.
Although there is no solution to above-mentioned limitations of current clinical research, big data may provide some insights into future direction of clinical trials ( 6 , 7 ). Wang and colleagues have proposed that big-data clinical trial (BCT) may become a mainstay type of clinical research and complement RCT in an important way ( 8 , 9 ). Big data in clinical study refers to the information collected using electronic database. These data come from daily routine clinical practice without modification or screening with strict inclusion and exclusion criteria, therefore retaining its real-world features ( 10 ). The advantage of BCT is that the result directly reflects clinical effectiveness. Big data in medical or epidemiological research generally consists electronic medical record system, administrative registry for chronic and infectious diseases and medical insurance system ( 11 ). As clinicians, our research may focused on EMR system which consists information on demographics, laboratory findings, microbiology data, medical order, procedures, surgery and clinical outcomes ( 12 ). However, big data is not a panacea without limitations. BCT is a kind of observational study in nature and has inherent limitations of its kind. For example, the observed and un-observed baseline characteristics cannot be well balanced. The conclusion may not be generalizable to other institutions if data were collected from single center. Such limitation can be addressed with advanced statistical method such as random effects model and bootstrap estimation of coefficient.
How to do clinical research with big data: an example from multiparameter intelligent monitoring in intensive care II (MIMIC-II)
This section will take MIMIC-II as an example to illustrate how to incorporate big data into clinical research ( 13 ). The flow chart of analysis is shown in Figure 1 .

Flow chart of exploring MIMIC-II database. SQL, structural query language; MIMIC-II, multiparameter intelligent monitoring in intensive care II.
MIMIC-IIis an open access database comprising clinical data of ICU patients from Beth Israel Deaconess Medical Center ( http://physionet.org/mimic2/ ). The database is consistently updating with current version of 2.6 that contains >30,000 ICU patients from 2001 to 2008 ( 14 ). MIMIC-II consists clinical data and high resolution waveform.
Gaining access to MIMIC-II
The investigator should register a username via on the website, and then apply for the access to database. An online training course named “protecting human research participants” should be completed and a certification number will be assigned to individual investigator ( Figure 2 ). With this certification number, one is qualified to apply for the access to database. After a couple of days, the whole database is accessible to you and data analysis can be performed according to one’s interests.

Certificate of completion of the training cause “protecting human research participants”. NIH, provided by National Institute of Health.
Conceiving clinical research ideas
With such a huge amount of clinical data, the next question is how to conceive clinical research ideas. Firstly, I would like to enumerate several types of clinical research by using big data ( Table 1 ). The first one involves exploration of risk factors, for which high resolution data are usually required for confounding controls. Multivariable models, stratification and propensity score analysis are useful tool for such kind of analysis. The second involves assessment of effectiveness of interventions. Similarly, this type of study requires high-resolution data to control for confounding factors. Bias associated with selective treatment may play an important role and should be considered in study design. The third involves prediction model building, which aims to fit a model for future prediction. The forth type is assessment of cost-effectiveness of health policy.
Types of studies | Examples (research question) | Requirement for clinical data | Note |
---|---|---|---|
Risk factor evaluation (independency) | Is urine output on ICU entry associated with mortality outcome? | High resolution (other risk factors should be provided) | Multivariable model, stratified analysis and propensity score analysis can be employed |
Effectiveness of intervention | Will PiCCO monitoring improve outcome of patients with septic shock? | High resolution (including a large number of confounding factors) | Intervention may be given for patients with different conditions. These conditions should be controlled to avoid “selective treatment” |
Prediction model | Prediction model for ICU delirium | Moderate resolution (general description of risk factors) | The predictive value of whole model is stressed, rather than a single risk factor |
Epidemiological study | The incidence and prevalence of catheter-related blood stream infection in ICU | Low resolution | A simple description is enough and no risk factor adjustment is required |
Implementation and efficacy of healthcare policy | Is the policy of screening and controlling hypertension effective in lowering cardiovascular event rate? | Low resolution | No complex clinical data are required |
† , resolution of clinical data refers to the intensity of data recording. For example, the study on urine output requires it be recorded on hourly basis. The resolution is higher for hourly urine output than daily urine output. For risk factor analysis, every covariate should be completely recorded. Otherwise, the resolution is not enough if some covariates are missing in the dataset.
Another key issue is how to conceive research ideas that can be addressed with database. There are two approaches: one is to perform data mining according to your research question, and the other is to adapt your research question to the database. Sometimes these two approaches may be used simultaneously. In a study investigating the association of lactate and clinical outcome ( 15 ), we planed to explore lactate measured on ICU entry at the outset. However, after data mining we found that lactate was usually measured repeatedly and decision was made to explore the trend of lactate (lactate clearance). The study protocol was adapted accordingly.
To conceive research idea based on your data is another way. One can perform statistical description for a dataset, by using traditional central tendency (mean, median) and discrete tendency (full range, 95% confidence interval). Graphical demonstration may be particularly helpful. For example, contour plot may help to explore associations between three variables; histogram can be used to explore distribution of a variable. However, someone may contend that a peek at dataset before drafting a study protocol may introduce bias (e.g., the problem of multiple testing and selective reporting are of this kind). That is to say, twenty times statistical testing for association will result in one with P<0.05 among independently generated random variables. I acknowledge this limitation, but such study can still be used as hypothesis generating and provide rationale for further explorations.
Another type of study is to investigate simple and easily obtainable parameters. By using such parameters, your study and ideas can be addressed by using varieties of database. Our study group has previously performed association study involving urine output and mortality ( 16 ). Because urine output is an essential but easily obtainable variable, it should be recorded in all kinds of ICUs. There is no reason to omit this recording, just like all other vital signs. We were confident at the outset that urine output must be carefully recorded in MIMIC-II, and the study was expected to conduct smoothly. Such simple variables included temperature, electrolytes and heart rate. In another study we investigated the association of ionized calcium and mortality ( 17 ). However, studies involving simple variables are usually criticized as being lack of novelty, and this may be the most important reason to reject our paper.
Data extraction
There is a small version of the MIMIC-II that can be accessed via query builder ( https://mimic2app.csail.mit.edu/querybuilder/ ). A limited number of rows can be exported from this version, and thus it is primarily used for testing structural query language (SQL). I found that it is useful for preliminary exploration of the data. I was doing research on brain natriuretic peptide (BNP) when I first encounter the MIMIC-II ( 18 , 19 ), the first idea come to my mind is to use this big data to establish a linkage between BNP and clinical outcome. However, when I started to explore the database I found that the information on BNP was scarce. In the end I realized that the payment for medical insurance in USA is closely monitored and not every one was indicated to have BNP measured (e.g., BNP is only covered by insurance when it is used to determine the cause of respiratory distress in emergency department). Query builder can be accessed via windows operating system that is convenient for most Chinese users, whereas the whole database can only be extracted via virtual machine on Ubuntu operating system.
Access to the whole MIMIC-II database requires the users to have some basic knowledge on virtual machine and Ubuntu operating system. The downloaded package compressed file with suffix “.tar”, taking disc space of 30 G. The file can be directly imported into the virtual box (Oracle). After entering username and password, one gains access to the Ubuntu operating system. The username and password are mimic2 and 2CIMIM_2v6, respectively. Pgadmin is the most popular and feature rich open Source administration and development platform for PostgreSQL, it will be opened to extract data under Ubuntu operating system.
Some investigators may want to export data for further analysis under Windows system. Data transferring between different operating systems can be performed via email. The file extracted has suffix of .cvs, which can be imported into Stata statistical package. Other statistical software such as SAS and SPSS also support this format.
Data processing using Stata
The author is more familiar with Stata statistical package in data processing, and the following section will introduce some steps in data processing with Stata.
Data processing using Stata is consisted of data management and statistical analysis. In my experience around 80% time and energy are spent on data management. This step included several aspects: (I) to generate new variables, for example, one wants to transform continuous variable age into binary variable (e.g., old vs. young); (II) variable checking, the sum module can check for some senseless values (e.g., age =200); (III) to transform string variable into numerical variable, or vise versa; (IV) combination of dataset. Different types of variable are stored in different relational tables. They should be combined into one dataset for the purpose of statistical analysis. Stata provided very useful modules such as merge and append for this purpose. Statistical analysis in based on correctly performed data management.
One advantage of Stata is its ability to record complete process of data analysis. Data analysis can be performed in three ways: windows pull-down menu, command input in command window and do-file. I suggest that data analysis be performed by using do-file, because it is able to record the whole process of how data is managed and analyzed. This will facilitate replication of analysis and made revisions. Furthermore, cooperation among researchers also requires do-file. The other two methods (e.g., windows pull-down menu and command input in command window) are mainly used to test a stata syntax or to facilitate draw graphs with complex options.
A complete dual flow chart of data analysis using stata is shown in Figure 3 . I suggest split data analysis into two parts: data management and statistical analysis. The left column shows data management that will make changes to the dataset in memory. Dataset generated by using do-file will be stored in memory with suffix of “.dta”. The right column simply performs statistical analysis and will not significantly change the dataset.

Dual flow chart of data management and statistical analysis by using STATA.
Using structural query language (SQL) to extract data
An important step in preparing data for analysis is data extraction by using SQL. Efficient use of SQL will save a lot of time and disc space. Some tasks previously mentioned during data management can be performed at the step of data extraction. For example, one intends to restrict data analysis to adult population. This can be performed by using stata command “if”, or using SQL “where” clause. I prefer to use stata for data management because it tracks the process of data management. However, it is at the discretion of investigator.
A simple SQL syntax can be written as: Select variable name from table name where conditions , where variable name refers to variables contained in relational tables. The asterisk “*” can replace the variable name if all variables are expected to be extracted. The table name refers to the name of the relational table. The conditions are a set of expressions used to select observations fulfilling certain criteria. For example, the expression “age >65” can be used if your target population is old people. For complex SQL syntax, readers can see other textbooks on SQL such as the language of SQL: How to Access Data in Relational Databases edited by Larry Rockoff and SQL Cookbook by Anthony Molinaro.
Conclusions
The exploration of big data is a process of trial and error. Someone may feel that it is a task of distress, but I feel it is a hard way filled with success and surprise. The secret of human disease may lurk under the vast ocean of big data, waiting us to decode and understand them. The article is not intended to serve as a step-by-step guidance on using big data but to inspire people who are interested in doing exploration on it. In China, it is possible to establish our own high quality big data because we have the largest population in the world.
Acknowledgements
Disclosure: The author declares no conflict of interest.
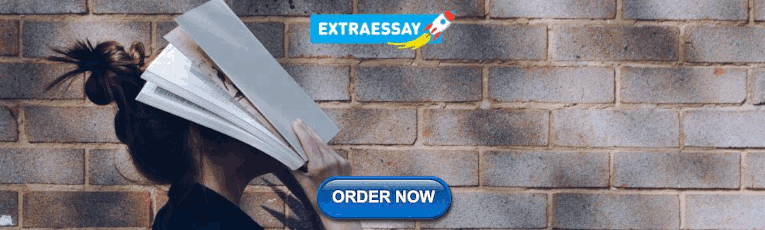
COMMENTS
Statistical analysis is an essential technique that enables a medical research practitioner to draw meaningful inference from their data analysis. Improper application of study design and data analysis may render insufficient and improper results and conclusion. Converting a medical problem into a statistical hypothesis with appropriate ...
As a repercussion, the scientific process cycle is broken, leaving researchers who want to reuse prior results with three options: 1. Re-run the analysis if the code and original source data are ...
This article is a practical guide to conducting data analysis in general literature reviews. The general literature review is a synthesis and analysis of published research on a relevant clinical issue, and is a common format for academic theses at the bachelor's and master's levels in nursing, physiotherapy, occupational therapy, public health and other related fields.
Clinical Data Management (CDM) is a critical phase in clinical research, which leads to generation of high-quality, reliable, and statistically sound data from clinical trials. This helps to produce a drastic reduction in time from drug development to marketing. Team members of CDM are actively involved in all stages of clinical trial right ...
Clinical research can be completed in two major steps: study designing and study reporting. Three study designs should be planned in sequence and iterated until properly refined: theoretical design, data collection design, and statistical analysis design.
1 Introduction. Statistics is a branch of mathematics that focuses on collection, presentation, analysis, and interpretation of numerical and categorical data. It is widely used in any discipline where data are collected and conclusions are drawn from them. In particular, medicine makes enormous use of statistics.
Begin your studies of clinical trials with an introduction to the background and rationale, a review of protocol development and trial registration, and an outline of a trial's phases and stages. Trial Design. Compare the advantages and disadvantages of different trial types and review three frameworks for clinical trials.
Four textbooks complementary to the current production and written by the same authors are Statistics applied to clinical studies 5th edition, 2012, Machine learning in medicine a complete overview, 2015, SPSS for starters and 2nd levelers 2nd edition, 2015, Clinical Data Analysis on a Pocket Calculator 2nd edition, 2016, all of them edited by ...
While data management has broad applications (and meaning) across many fields and industries, in clinical research the term data management is frequently used in the context of clinical trials. 1 ...
Here we'll provide an intuitive understanding of clinical research results. So this isn't a comprehensive statistics course - rather it offers a practical orientation to the field of medical research and commonly used statistical analysis. ... If a research question is evaluated through the collection of data points and statistical analysis ...
Analysis and learning from publically available biomedical and clinical trial data sets, real-world evidence from sensors, and health records by machine-learning architectures are discussed.
Keywords: Design; sampling; study. Statistical analysis is an essential technique that enables a medical research practitioner to draw meaningful inference from their data analysis. Improper application of study design and data analysis may render insufficient and improper results and conclusion. Converting a medical problem into a s ….
In order to interpret research datasets, clinicians involved in clinical research should have an understanding of statistical methodology. This article provides a brief overview of statistical methods that are frequently used in clinical research studies. Descriptive and inferential methods, including regression modeling and propensity scores ...
There are 6 modules in this course. This course presents critical concepts and practical methods to support planning, collection, storage, and dissemination of data in clinical research. Understanding and implementing solid data management principles is critical for any scientific domain. Regardless of your current (or anticipated) role in the ...
This course aims to teach the concepts of clinical data models and common data models. Upon completion of this course, learners will be able to interpret and evaluate data model designs using Entity-Relationship Diagrams (ERDs), differentiate between data models and articulate how each are used to support clinical care and data science, and create SQL statements in Google BigQuery to query the ...
This open access book comprehensively covers the fundamentals of clinical data science, focusing on data collection, modelling and clinical applications. Topics covered in the first section on data collection include: data sources, data at scale (big data), data stewardship (FAIR data) and related privacy concerns. Aspects of predictive modelling using techniques such as classification ...
Data analysis methods are an integral part of modern clinical research. They are powerful techniques that enable researchers to draw meaningful conclusions from data collected through observation, survey, or experimentation. However, data analysis is a huge discipline with different paradigms, schools of thought and alternative methodologies.
8. Minitab. 9. Statistica. 10. Excel. Kolabtree freelance statistician Kingsley Ukwuoma writes about the top statistical tools used in medical research and clinical data analysis . There was a time when validating experiments through data was done fully using manual computation.
The Role of Clinical Data and Clinical Data Science. November 24, 2020. Clinical data and its analysis are critical to clinical research. Ensuring the overall quality of clinical data is then paramount to ensuring quality care and appropriate decision-making in the medical and healthcare fields. What constitutes clinical data, what should you ...
Abstract. Data are a key resource for modern societies and expected to improve quality, accessibility, affordability, safety, and equity of health care. Dental care and research are currently transforming into what we term data dentistry, with 3 main applications: 1) medical data analysis uses deep learning, allowing one to master unprecedented ...
INTRODUCTION. Efficient evidence generation would integrate data capture for clinical practice and research through the effective use of electronic health records (EHRs). 1-3 Data elements within EHRs are not always structured or interoperable, requiring manual curation, which is costly and time-consuming. 4-7 Case report forms (CRFs) and EHRs remain disconnected by incompatible design and a ...
Ths intensive course is designed to meet the requirements for research methods training in most ACGME-accredited fellowship training programs by covering issues related to developing a research question, synthesizing the literature, study design, data collection, questionnaire design, data management, sample size planning, data analysis ...
Introduction While there is an interest in defining longitudinal change in people with chronic illness like Parkinson's disease (PD), statistical analysis of longitudinal data is not straightforward for clinical researchers. Here, we aim to demonstrate how the choice of statistical method may influence research outcomes, (e.g., progression in apathy), specifically the size of longitudinal ...
Ethics approval was obtained by the University of British Columbia's clinical research ethics board with a waiver of informed consent. Results ... collection, management, analysis, and interpretation of the data; preparation, review, or approval of the manuscript; and decision to submit the manuscript for publication. Data Sharing Statement ...
Other major steps of clinical research include the approval of IRBs, conduction and supervision of the research, data review, and analysis. Successful clinical research includes various essential elements like a letter of intent which is the evidence that supports the interest of the researcher to conduct drug research, timeline, funding source ...
Background TSPAN7 is an important factor in tumor progression. However, the precise function of TSPAN7 and its role in pan-cancer are not clear. Methods Based on Xinhua cohort incorporating 370 patients with kidney neoplasm, we conducted differential expression analysis by immunohistochemistry between tumor and normal tissues, and explored correlations of TSPAN7 with patients' survival ...
Journal of Obstetrics and Gynaecology Research is a leading OBGYN journal publishing original research, case reports and reviews in obstetrics and gynecology. Abstract Aim The objective of this meta-analysis was to evaluate obstetric outcomes in gestational diabetes mellitus (GDM) patients treated with flexible management based on intrauterine ...
The Journal of Clinical Apheresis publishes articles dealing with the basic research and clinical applications of hemapheresis and transfusion medicine. ... After analysis, the p values of all data in this study were greater than .05, ... (Since it is a meta-analysis, it necessitates that the research objects in the literature be consistent ...
We conducted a rigorous evaluation of the feasibility and potential to use electronic health records clinical notes and existing case review data. Methods: Safety Learning System case review data from 1 large health system composed of 10 hospitals in the mid-Atlantic region of the United States from February 2016 to September 2021 were analyzed.
As clinicians, our research may focused on EMR system which consists information on demographics, laboratory findings, microbiology data, medical order, procedures, surgery and clinical outcomes ( 12 ). However, big data is not a panacea without limitations. BCT is a kind of observational study in nature and has inherent limitations of its kind.