![triac method of essay writing SASC - Student Academic Success Center - UNE [logo]](https://files.uneportfolio.org/wp-content/uploads/sites/5/2022/02/SASCLogoSquare_Smaller-3-e1644936452801.jpeg)
Dr. Eric Drown
Reading and Writing are Superpowers*
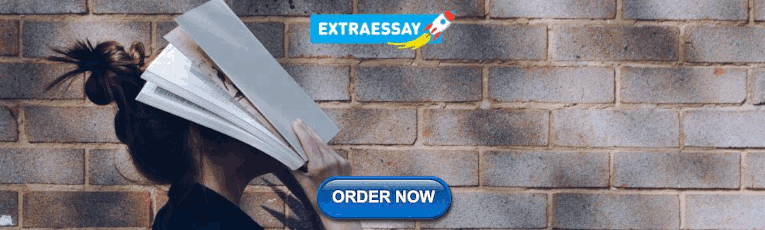
TRIAC Paragraph Structure
Emerging academic writers often wonder how to beef up their paragraphs without adding fluff. TRIAC can help writers explain their ideas in depth. TRIAC paragraphs (or paragraph sequences) feature these elements:
Topic . Start the paragraph by introducing a topic that supports or complicates your thesis – the central problem or idea that the paragraph aims to explore. Better topic sentences function as a mini-thesis and make a claim about the topic.
Restriction . Because there can be many aspects and approaches to a topic, it makes sense to specify the aspect or approach you’re going to take. Follow the topic with sentences that narrow the scope of the topic. In these sentences, you’re rewriting the topic in more specific terms and setting the direction the paragraph will follow.
Illustration/Example/Evidence . Help your reader understand the restricted topic in concrete terms, and give him or her something to think about by offering an example, exhibit, or evidence.
Analysis . Help your reader see your point by looking at the evidence you offered through your eyes. What parts of it are the most significant? How does the evidence support, complicate, or counter the restricted claim about the topic you (or another writer) are making?
Conclusion/Connections . What, having understood your evidence as you see it, should your reader have learned from your paragraph? How can this conclusion be connected to other relevant ideas in your essay?
Here’s an example of a short TRIAC paragraph.
[TOPIC] Many critics worry that the way we use the Internet is reshaping our minds. [RESTRICTION] Their biggest concern is that our shallow-reading habits are fostering inattention and undermining literacy. [ILLUSTRATION/EXAMPLE] For example, in “ Is Google Making Us Stupid? ,” journalist Nicholas Carr worries that the connection-making state of mind promoted by slow, deep reading is giving way to an information-seeking state of mind best adapted to finding separate bits of information. In his view, instead of diving deep into the ocean of ideas, we merely “zip along the surface like a guy on a Jet Ski.” [ ANALYSIS ] Carr rightly points out that our reading habits are certainly changing. It is true that much of our everyday reading feeds our information-seeking appetites. It is also true that it takes work to learn how to read and think slowly and deeply. But his insistence that we are losing our ability to think in a complex way is countered by the slow patient thinking that takes place in activities such as prayer, meditation, and scholarship . [CONCLUSION] While it may indeed take conscious and disciplined effort to learn how to read and think well, today’s students are capable of making that effort, provided that we recognize that, like previous generations, some may need guided practice in the habit.
Visit my Barclay’s Formula or Observation-Implication-Conclusion pages for other useful paragraph structures.
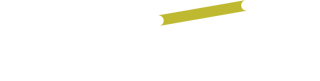
- Services for education institutions
- Academic subject areas
- Peer connection
- Evidence of Studiosity impact
- Case studies from our partners
- Research Hub
- The Tracey Bretag Integrity Prize
- The Studiosity Symposium
- Studiosity for English learners
- Video case studies
- Meet the online team
Academic Advisory Board
Meet the board.
- Social responsibility
- Meet the team
- Join the team
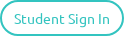
How to structure paragraphs using the PEEL method
Sophia Gardner
Sep 1, 2023
You may have heard of the acronym PEEL for essays, but what exactly does it mean? And how can it help you? We’re here to explain it all, plus give you some tips on how to nail your next essay.
There’s certainly an art to writing essays. If you haven’t written one for a while, or if you would like to hone your academic writing skills, the PEEL paragraph method is an easy way to get your point across in a clear and concise way, that is easily digestible to the reader.
So, what exactly is PEEL ?
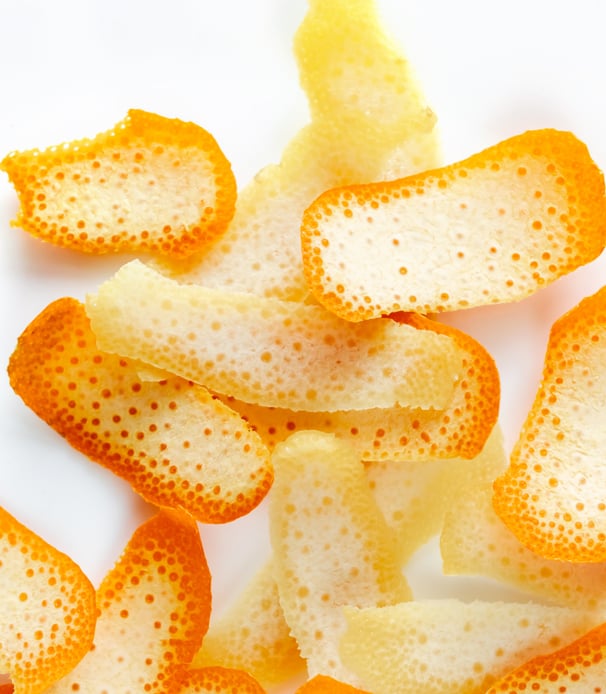
The PEEL paragraph method is a technique used in writing to help structure paragraphs in a way that presents a single clear and focused argument, which links back to the essay topic or thesis statement.
It’s good practice to dedicate each paragraph to one aspect of your argument, and the PEEL structure simplifies this for you.
It allows you to create a paragraph that is easy and accessible for others to understand. Remember, when you’re writing something, it’s not just you who is reading it - you need to consider the reader and how they are going to be digesting this new information.
What does PEEL stand for?
P = Point: start your paragraph with a clear topic sentence that establishes what your paragraph is going to be about. Your point should support your essay argument or thesis statement.
E = Evidence/Example: here you should use a piece of evidence or an example that helps to reaffirm your initial point and develop the argument.
E = Explain: next you need to explain exactly how your evidence/example supports your point, giving further information to ensure that your reader understands its relevance.
L = Link: to finish the paragraph off, you need to link the point you’ve just made back to your essay question, topic, or thesis.
Download a free PEEL paragraph planner below. 👇

Studiosity English specialist Ellen, says says students often underestimate the importance of a well-structured paragraph.
PEEL in practice
Here’s an example of what you might include in a PEEL structured paragraph:
Topic: Should infants be given iPads? Thesis/argument: Infants should not be given iPads.
Point : Infants should not be given iPads, because studies show children under two can face developmental delays if they are exposed to too much screen time.
Evidence/Example: A recent paediatric study showed that infants who are exposed to too much screen time may experience delays in speech development.
Explanation: The reason infants are facing these delays is because screen time is replacing other key developmental activities.
Link: The evidence suggests that infants who have a lot of screen time experience negative consequences in their speech development, and therefore they should not be exposed to iPads at such a young age.
Once you’ve written your PEEL paragraph, do a checklist to ensure you have covered off all four elements of the PEEL structure. Your point should be a clear introduction to the argument you are making in this paragraph; your example or evidence should be strong and relevant (ask yourself, have you chosen the best example?); your explanation should be demonstrate why your evidence is important and how it conveys meaning; and your link should summarise the point you’ve just made and link back to the broader essay argument or topic.
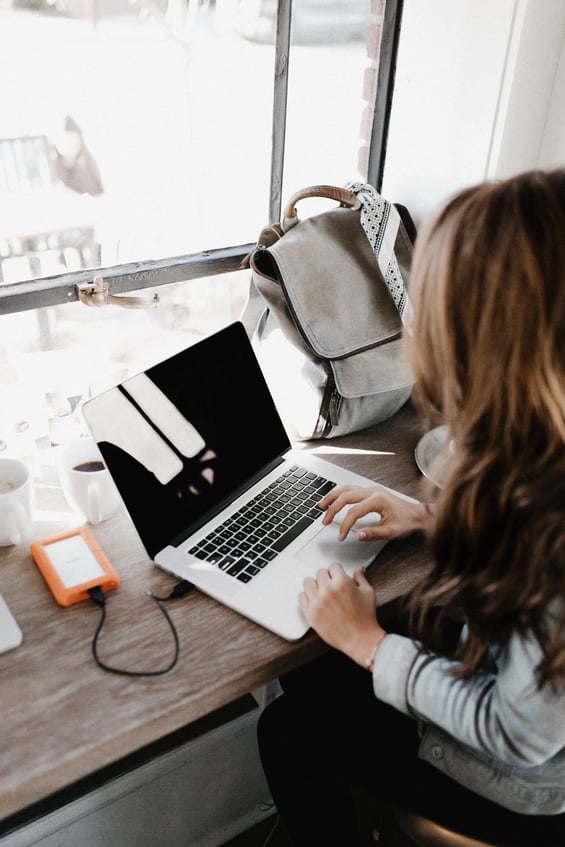
Keep your paragraphs clear, focused, and not too long. If you find your paragraphs are getting lengthy, take a look at how you could split them into multiple paragraphs, and ensure you’re creating a new paragraph for each new idea you introduce to the essay.
Finally, it’s important to always proofread your paragraph. Read it once, twice, and then read it again. Check your paragraph for spelling, grammar, language and sentence flow. A good way to do this is to read it aloud to yourself, and if it sounds clunky or unclear, consider rewriting it.
That’s it! We hope this helps explain the PEEL method and how it can help you with your next essay. 😊
You might also like: Proofreading vs editing: what's the difference? How to get easy marks in an exam 5 study hacks that actually work
Topics: English , Writing , Grammar
About Studiosity
Asking for feedback on your work is an essential part of learning. So when you want to better understand a concept or check you're on the right track, we're here for you.
Find out if you have free access through your institution here .
Recent Posts
Posts by topic.
- Students (85)
- Higher education (67)
- Student Experience (46)
- University (46)
- Education (41)
- online study (34)
- Interview (28)
- Learning (28)
- Tertiary education (28)
- Educators (27)
- Research (24)
- Parents (23)
- English (18)
- High School (18)
- Podcast (17)
- Technology (17)
- students first (17)
- Writing (16)
- Student stories (14)
- Homework (13)
- student wellbeing (13)
- Assignment Help (12)
- Education policy (12)
- Formative feedback (12)
- Literacy (12)
- academic integrity (12)
- Events (11)
- Academic Advisory Board (10)
- Studiosity (10)
- covid19 (10)
- international student (10)
- Australia (9)
- Health and Wellbeing (9)
- Learning trends (9)
- Student satisfaction (9)
- Teaching (9)
- Secondary education (8)
- Equality (7)
- Science (7)
- Student retention (7)
- UK students (7)
- staff wellbeing (7)
- Student support (6)
- UK Higher Education (6)
- academic services (6)
- online learning (6)
- CanHigherEd (5)
- Online Tutoring (5)
- Workload (5)
- belonging (5)
- CVs and cover letters (4)
- Internet (4)
- Mathematics (4)
- Partnerships (4)
- School holidays (4)
- Student performance (4)
- Widening Participation (4)
- student success (4)
- #InthisTogether (3)
- Grammar (3)
- University of Exeter (3)
- teaching & learning (3)
- Charity (2)
- Government (2)
- Mentors (2)
- Primary education (2)
- Subject Specialists (2)
- accessibility (2)
- community (2)
- diversity (2)
- plagiarism prevention (2)
- student stress (2)
- webinar (2)
- Biology (1)
- Careers (1)
- Chemistry (1)
- EU students (1)
- First years (1)
- Indigenous Strategy (1)
- Middle East (1)
- Nutrition (1)
- Teacher (1)
- academic support (1)
- academic writing (1)
- business schools (1)
- choice of language (1)
- dyslexia (1)
- ethical AI (1)
- generativeAI (1)
- job help (1)
- library services (1)
- podcasts (1)
- reflection (1)
- university of west of england (1)
- July 2015 (12)
- March 2020 (11)
- June 2020 (10)
- July 2020 (8)
- September 2020 (8)
- March 2015 (7)
- April 2015 (7)
- October 2019 (7)
- April 2020 (7)
- May 2018 (6)
- April 2019 (6)
- May 2020 (6)
- September 2022 (6)
- June 2015 (5)
- August 2015 (5)
- December 2017 (5)
- March 2018 (5)
- February 2020 (5)
- March 2021 (5)
- June 2021 (5)
- July 2016 (4)
- March 2017 (4)
- October 2017 (4)
- February 2018 (4)
- August 2018 (4)
- May 2019 (4)
- July 2019 (4)
- August 2019 (4)
- March 2024 (4)
- August 2024 (4)
- February 2015 (3)
- May 2015 (3)
- September 2015 (3)
- December 2015 (3)
- January 2016 (3)
- April 2016 (3)
- October 2016 (3)
- December 2016 (3)
- April 2017 (3)
- September 2017 (3)
- April 2018 (3)
- October 2018 (3)
- March 2019 (3)
- January 2020 (3)
- October 2020 (3)
- November 2020 (3)
- June 2022 (3)
- October 2022 (3)
- November 2022 (3)
- August 2023 (3)
- November 2023 (3)
- April 2024 (3)
- July 2024 (3)
- March 2016 (2)
- May 2016 (2)
- August 2016 (2)
- July 2017 (2)
- January 2018 (2)
- November 2018 (2)
- December 2018 (2)
- February 2019 (2)
- June 2019 (2)
- September 2019 (2)
- January 2021 (2)
- February 2021 (2)
- April 2021 (2)
- August 2021 (2)
- September 2021 (2)
- December 2021 (2)
- August 2022 (2)
- February 2023 (2)
- March 2023 (2)
- May 2023 (2)
- December 2023 (2)
- June 2024 (2)
- October 2008 (1)
- August 2013 (1)
- October 2015 (1)
- February 2016 (1)
- June 2016 (1)
- September 2016 (1)
- November 2016 (1)
- January 2017 (1)
- May 2017 (1)
- June 2017 (1)
- August 2017 (1)
- November 2017 (1)
- June 2018 (1)
- September 2018 (1)
- January 2019 (1)
- November 2019 (1)
- December 2019 (1)
- August 2020 (1)
- December 2020 (1)
- May 2021 (1)
- February 2022 (1)
- March 2022 (1)
- July 2022 (1)
- December 2022 (1)
- January 2023 (1)
- June 2023 (1)
- July 2023 (1)
- September 2023 (1)
- October 2023 (1)
- February 2024 (1)
Studiosity is personalised study help, anytime, anywhere. We partner with institutions to extend their core academic skills support online with timely, after-hours help for all their students, at scale - regardless of their background, study mode or location.
Now you can subscribe to our educator newsletter, for insights and free downloads straight to your inbox:

ABN 41 114 279 668
Student zone, assignment calculator, calendars and organisers, study survival guides, free practice tests, student faqs, download our mobile app, student sign in, success stories.
Student Reviews & Testimonials
Specialist Sign In
Meet our specialists
Meet the team, media and research, student reviews.
Read more on Google
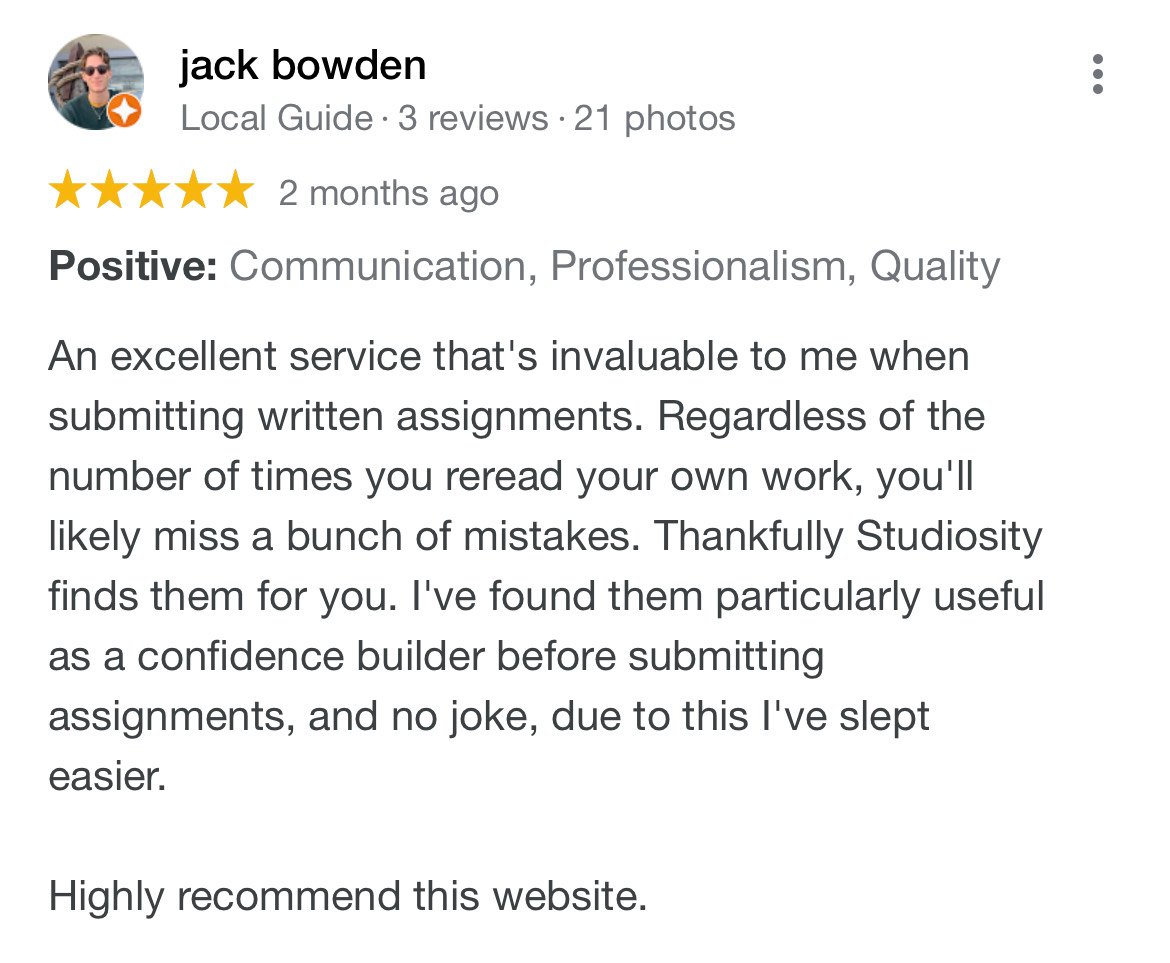
Studiosity acknowledges the Traditional Indigenous Custodians of country throughout Australia, and all lands where we work, and recognises their continuing connection to land, waters, and culture. We pay our respects to Elders past and present.
Contact • FAQ • Privacy • Accessibility • Acceptable Use • Terms of Use AI-for-Learning Polic y • Academic Integrity Policy
Beef Up Critical Thinking and Writing Skills: Comparison Essays
Organizing the Compare-Contrast Essay
- Teaching Resources
- An Introduction to Teaching
- Tips & Strategies
- Policies & Discipline
- Community Involvement
- School Administration
- Technology in the Classroom
- Teaching Adult Learners
- Issues In Education
- Becoming A Teacher
- Assessments & Tests
- Elementary Education
- Secondary Education
- Special Education
- Homeschooling
- M.Ed., Curriculum and Instruction, University of Florida
- B.A., History, University of Florida
The compare/contrast essay is an excellent opportunity to help students develop their critical thinking and writing skills. A compare and contrast essay examines two or more subjects by comparing their similarities and contrasting their differences.
Compare and contrast is high on Bloom's Taxonomy of critical reasoning and is associated with a complexity level where students break down ideas into simpler parts in order to see how the parts relate. For example, in order to break down ideas for comparison or to contrast in an essay, students may need to categorize, classify, dissect, differentiate, distinguish, list, and simplify.
Preparing to Write the Essay
First, students need to select pick comparable objects, people, or ideas and list their individual characteristics. A graphic organizer, like a Venn Diagram or top hat chart, is helpful in preparing to write the essay:
- What is the most interesting topic for comparison? Is the evidence available?
- What is the most interesting topic to contrast? Is the evidence available?
- Which characteristics highlight the most significant similarities?
- Which characteristics highlight the most significant differences?
- Which characteristics will lead to a meaningful analysis and an interesting paper?
A link to 101 compare and contrast essay topics for students provides opportunities for students to practice the similarities and differences such as
- Fiction vs. Nonfiction
- Renting a home vs. Owning a home
- General Robert E. Lee vs General Ulysses S. Grant
Writing the Block Format Essay: A, B, C points vs A, B, C points
The block method for writing a compare and contrast essay can be illustrated using points A, B, and C to signify individual characteristics or critical attributes.
A. history B. personalities C. commercialization
This block format allows the students to compare and contrast subjects, for example, dogs vs. cats, using these same characteristics one at a time.
The student should write the introductory paragraph to signal a compare and contrast essay in order to identify the two subjects and explain that they are very similar, very different or have many important (or interesting) similarities and differences. The thesis statement must include the two topics that will be compared and contrasted.
The body paragraph(s) after the introduction describe characteristic(s) of the first subject. Students should provide the evidence and examples that prove the similarities and/or differences exist, and not mention the second subject. Each point could be a body paragraph. For example,
A. Dog history. B. Dog personalities C. Dog commercialization.
The body paragraphs dedicated to the second subject should be organized in the same method as the first body paragraphs, for example:
A. Cat history. B. Cat personalities. C. Cat commercialization.
The benefit of this format is that it allows the writer to concentrate on one characteristic at a time. The drawback of this format is that there may be some imbalance in treating the subjects to the same rigor of comparing or contrasting.
The conclusion is in the final paragraph, the student should provide a general summary of the most important similarities and differences. The student could end with a personal statement, a prediction, or another snappy clincher.
Point by Point Format: AA, BB, CC
Just as in the block paragraph essay format, students should begin the point by point format by catching the reader's interest. This might be a reason people find the topic interesting or important, or it might be a statement about something the two subjects have in common. The thesis statement for this format must also include the two topics that will be compared and contrasted.
In the point by point format, the students can compare and/or contrast the subjects using the same characteristics within each body paragraph. Here the characteristics labeled A, B, and C are used to compare dogs vs. cats together, paragraph by paragraph.
A. Dog history A Cat history
B. Dog personalities B. Cat personalities
C. Dog commercialization C. Cat commercialization
This format does help students to concentrate on the characteristic(s) which may be may result in a more equitable comparison or contrast of the subjects within each body paragraph(s).
Transitions to Use
Regardless of the format of the essay, block or point-by-point, the student must use transition words or phrases to compare or contrast one subject to another. This will help the essay sound connected and not sound disjointed.
Transitions in the essay for comparison can include:
- in the same way or by the same token
- in like manner or likewise
- in similar fashion
Transitions for contrasts can include:
- nevertheless or nonetheless
- however or though
- otherwise or on the contrary
- in contrast
- notwithstanding
- on the other hand
- at the same time
In the final concluding paragraph, the student should give a general summary of the most important similarities and differences. The student could also end with a personal statement, a prediction, or another snappy clincher.
Part of the ELA Common Core State Standards
The text structure of compare and contrast is so critical to literacy that it is referenced in several of the English Language Arts Common Core State Standards in both reading and writing for K-12 grade levels. For example, the reading standards ask students to participate in comparing and contrasting as a text structure in the anchor standard R.9 :
"Analyze how two or more texts address similar themes or topics in order to build knowledge or to compare the approaches the authors take."
The reading standards are then referenced in the grade level writing standards, for example, as in W7.9
"Apply grade 7 Reading standards to literature (e.g., 'Compare and contrast a fictional portrayal of a time, place, or character and a historical account of the same period as a means of understanding how authors of fiction use or alter history')."
Being able to identify and create compare and contrast text structures is one of the more important critical reasoning skills that students should develop, regardless of grade level.
- How to Teach the Compare and Contrast Essay
- Organizing Compare-Contrast Paragraphs
- Compare-Contrast Prewriting Chart
- 101 Compare and Contrast Essay Topics
- 6 Skills Students Need to Succeed in Social Studies Classes
- How to Teach Topic Sentences Using Models
- Higher Level Thinking: Synthesis in Bloom's Taxonomy
- 25 Essay Topics for American Government Classes
- Bloom's Taxonomy: Analysis Category
- What to Include in a Student Portfolio
- Expository Essay Genre With Suggested Prompts
- 61 General Expository Essay Topic to Practice Academic Writing
- Social Studies Warmups: Exercises to Get Students Thinking
- Cosmos Episode 6 Viewing Worksheet
- 9 Tips for Successful Textbook Adoption
- How Many Electoral Votes Does a Candidate Need to Win?
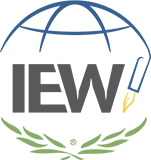
Portable Walls™ for Academic Writing
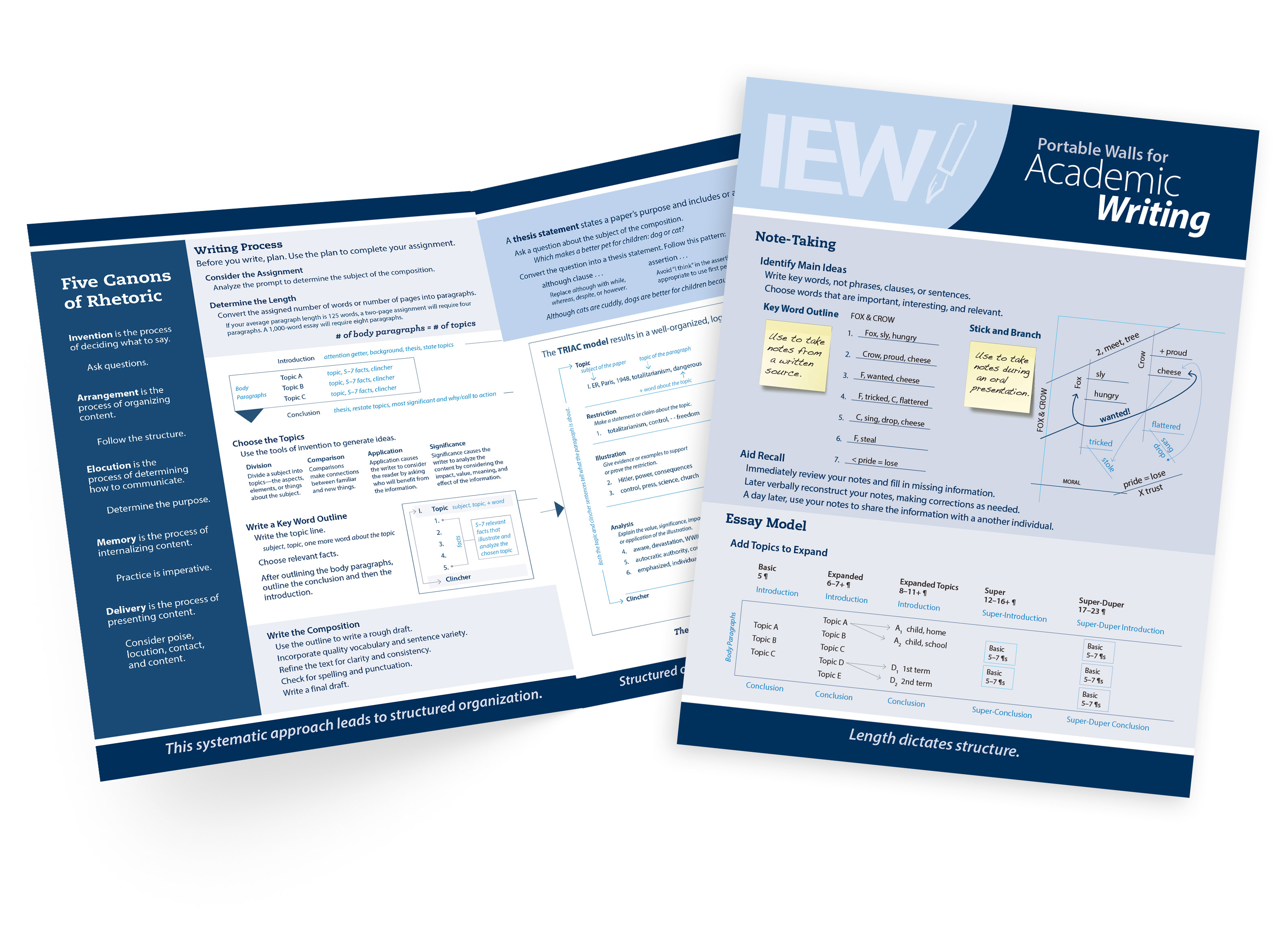
Included in the University-Ready Writing .
Click here to purchase 10 copies of Portable Walls for Academic Writing .
Specifications: 1 three-panel folder
ISBN: 978-1-62341-409-2
Copyright Date: 2024
All rights reserved.
No part of this product may be reproduced, stored in a retrieval system, or transmitted in any form or by any means, electronic, mechanical, photocopying, recording, or otherwise, without the prior written permission of the author, except as provided by U.S.A. copyright law.
Related Products
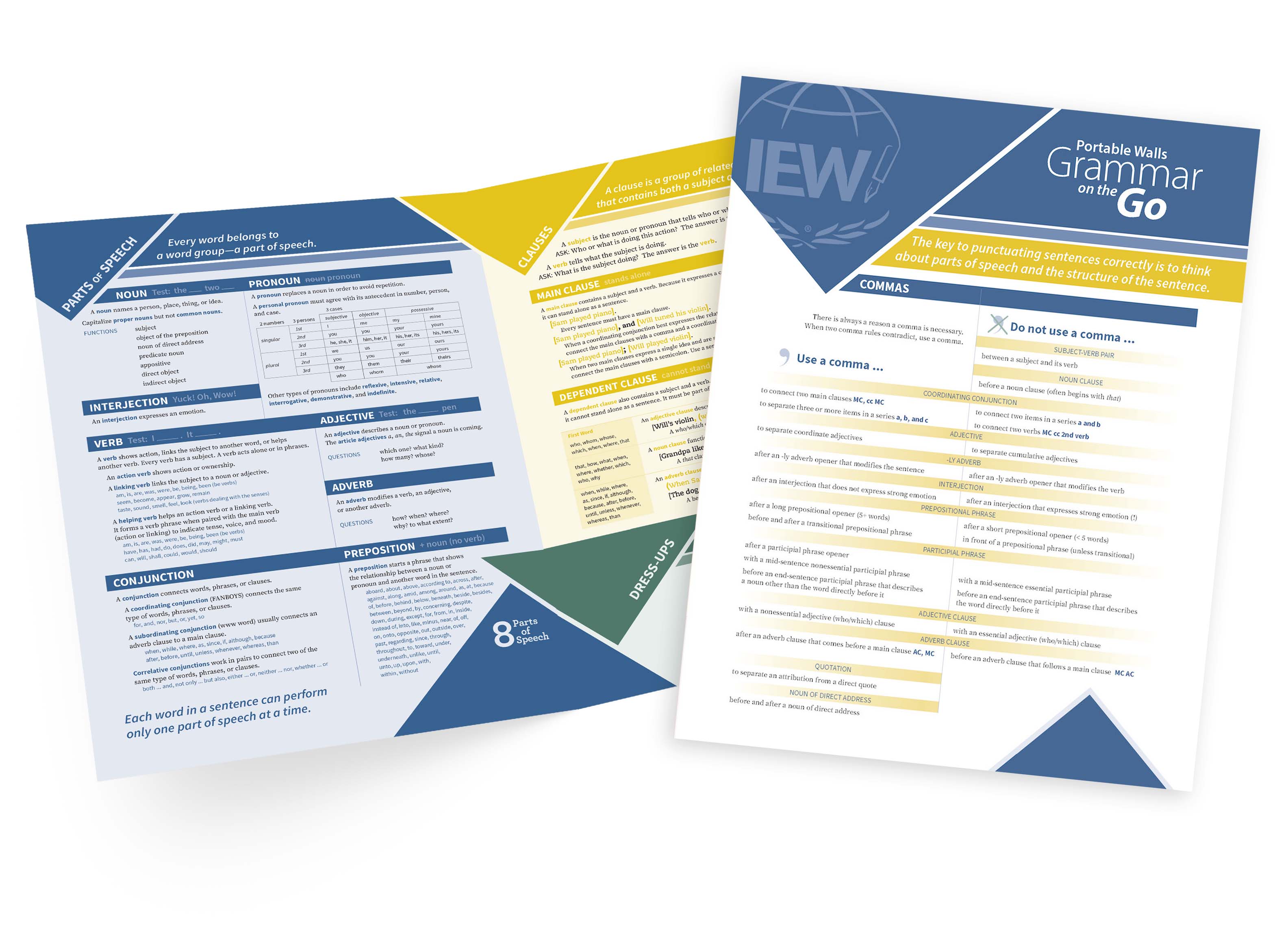
Portable Walls™ Grammar on the Go
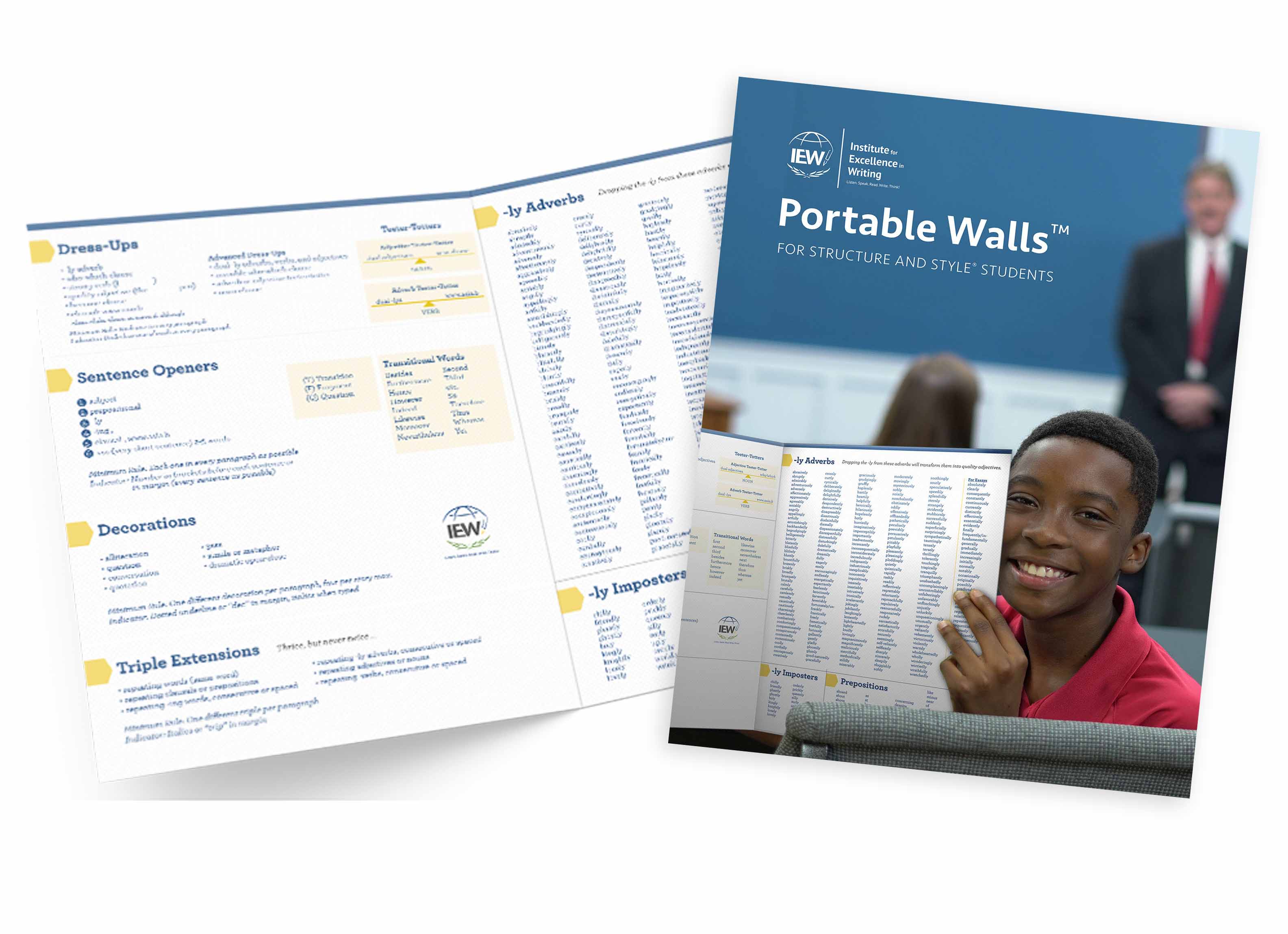
Portable Walls™ for Structure and Style® Students
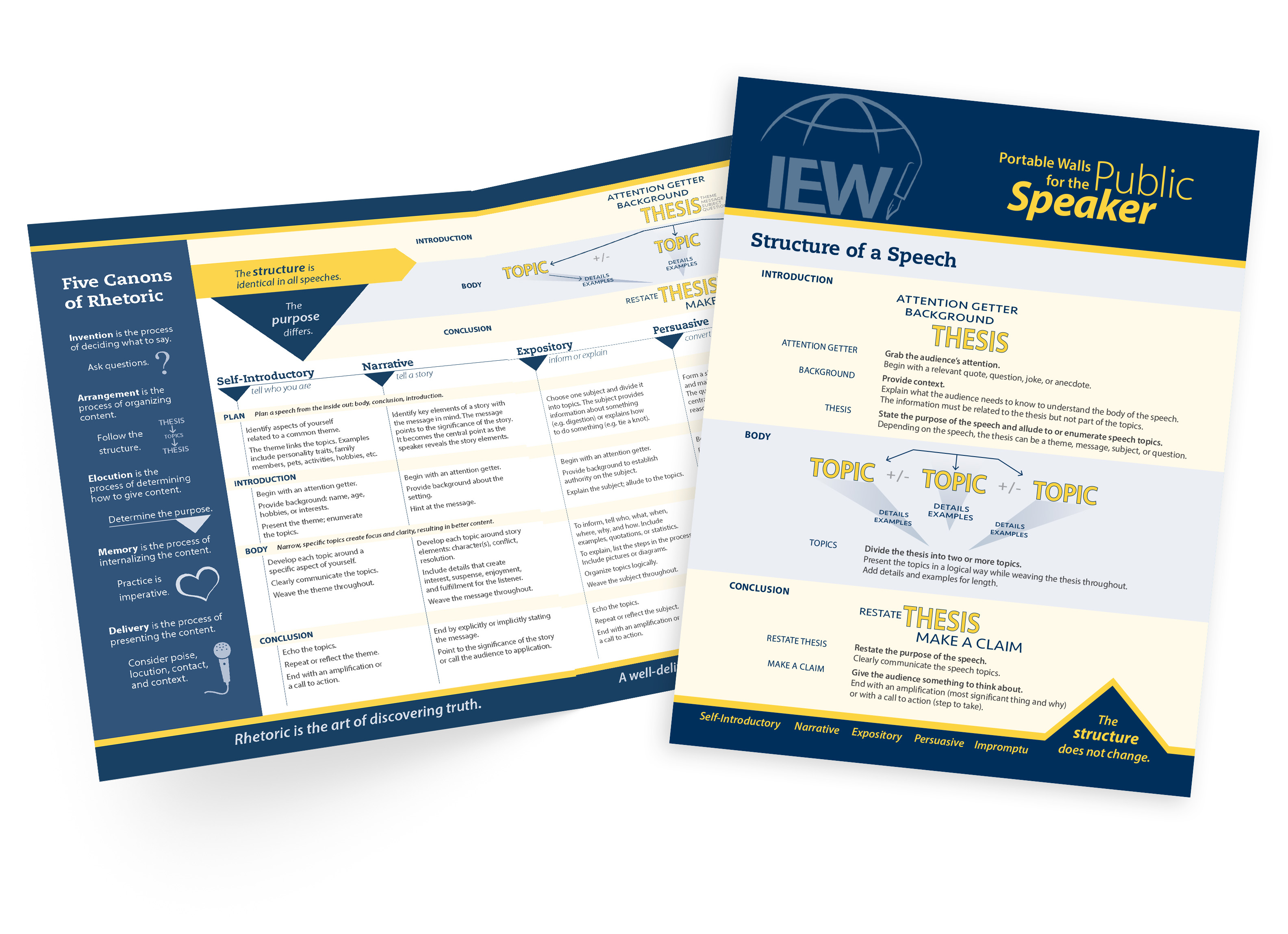
Portable Walls™ for the Public Speaker
![triac method of essay writing University-Ready Writing [Forever Streaming or DVD]](https://storage.googleapis.com/iew-public-production/products/urw-fs_thumb.jpg)
University-Ready Writing [Forever Streaming or DVD]
100% satisfaction guarantee.
We offer a 100% satisfaction, no time limit guarantee on everything we sell.
Essay Papers Writing Online
Tips and tricks for crafting engaging and effective essays.
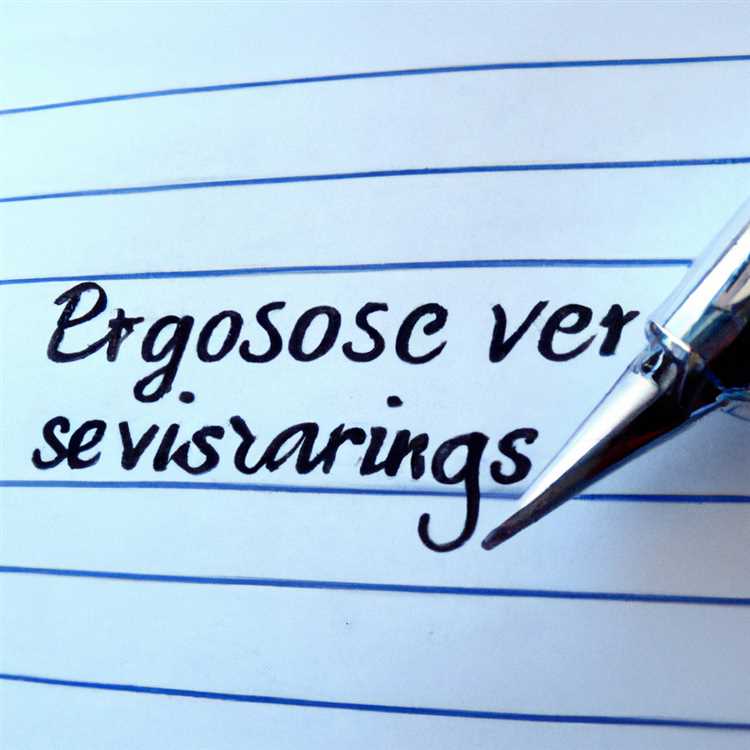
Writing essays can be a challenging task, but with the right approach and strategies, you can create compelling and impactful pieces that captivate your audience. Whether you’re a student working on an academic paper or a professional honing your writing skills, these tips will help you craft essays that stand out.
Effective essays are not just about conveying information; they are about persuading, engaging, and inspiring readers. To achieve this, it’s essential to pay attention to various elements of the essay-writing process, from brainstorming ideas to polishing your final draft. By following these tips, you can elevate your writing and produce essays that leave a lasting impression.
Understanding the Essay Prompt
Before you start writing your essay, it is crucial to thoroughly understand the essay prompt or question provided by your instructor. The essay prompt serves as a roadmap for your essay and outlines the specific requirements or expectations.
Here are a few key things to consider when analyzing the essay prompt:
- Read the prompt carefully and identify the main topic or question being asked.
- Pay attention to any specific instructions or guidelines provided, such as word count, formatting requirements, or sources to be used.
- Identify key terms or phrases in the prompt that can help you determine the focus of your essay.
By understanding the essay prompt thoroughly, you can ensure that your essay addresses the topic effectively and meets the requirements set forth by your instructor.
Researching Your Topic Thoroughly
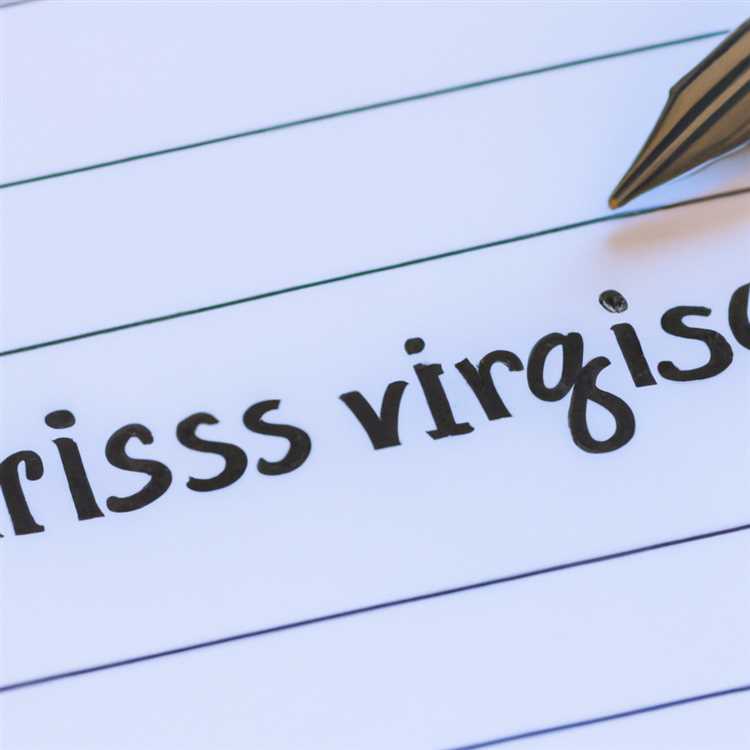
One of the key elements of writing an effective essay is conducting thorough research on your chosen topic. Research helps you gather the necessary information, facts, and examples to support your arguments and make your essay more convincing.
Here are some tips for researching your topic thoroughly:
Don’t rely on a single source for your research. Use a variety of sources such as books, academic journals, reliable websites, and primary sources to gather different perspectives and valuable information. | |
While conducting research, make sure to take detailed notes of important information, quotes, and references. This will help you keep track of your sources and easily refer back to them when writing your essay. | |
Before using any information in your essay, evaluate the credibility of the sources. Make sure they are reliable, up-to-date, and authoritative to strengthen the validity of your arguments. | |
Organize your research materials in a systematic way to make it easier to access and refer to them while writing. Create an outline or a research plan to structure your essay effectively. |
By following these tips and conducting thorough research on your topic, you will be able to write a well-informed and persuasive essay that effectively communicates your ideas and arguments.
Creating a Strong Thesis Statement
A thesis statement is a crucial element of any well-crafted essay. It serves as the main point or idea that you will be discussing and supporting throughout your paper. A strong thesis statement should be clear, specific, and arguable.
To create a strong thesis statement, follow these tips:
- Be specific: Your thesis statement should clearly state the main idea of your essay. Avoid vague or general statements.
- Be concise: Keep your thesis statement concise and to the point. Avoid unnecessary details or lengthy explanations.
- Be argumentative: Your thesis statement should present an argument or perspective that can be debated or discussed in your essay.
- Be relevant: Make sure your thesis statement is relevant to the topic of your essay and reflects the main point you want to make.
- Revise as needed: Don’t be afraid to revise your thesis statement as you work on your essay. It may change as you develop your ideas.
Remember, a strong thesis statement sets the tone for your entire essay and provides a roadmap for your readers to follow. Put time and effort into crafting a clear and compelling thesis statement to ensure your essay is effective and persuasive.
Developing a Clear Essay Structure
One of the key elements of writing an effective essay is developing a clear and logical structure. A well-structured essay helps the reader follow your argument and enhances the overall readability of your work. Here are some tips to help you develop a clear essay structure:
1. Start with a strong introduction: Begin your essay with an engaging introduction that introduces the topic and clearly states your thesis or main argument.
2. Organize your ideas: Before you start writing, outline the main points you want to cover in your essay. This will help you organize your thoughts and ensure a logical flow of ideas.
3. Use topic sentences: Begin each paragraph with a topic sentence that introduces the main idea of the paragraph. This helps the reader understand the purpose of each paragraph.
4. Provide evidence and analysis: Support your arguments with evidence and analysis to back up your main points. Make sure your evidence is relevant and directly supports your thesis.
5. Transition between paragraphs: Use transitional words and phrases to create flow between paragraphs and help the reader move smoothly from one idea to the next.
6. Conclude effectively: End your essay with a strong conclusion that summarizes your main points and reinforces your thesis. Avoid introducing new ideas in the conclusion.
By following these tips, you can develop a clear essay structure that will help you effectively communicate your ideas and engage your reader from start to finish.
Using Relevant Examples and Evidence
When writing an essay, it’s crucial to support your arguments and assertions with relevant examples and evidence. This not only adds credibility to your writing but also helps your readers better understand your points. Here are some tips on how to effectively use examples and evidence in your essays:
- Choose examples that are specific and relevant to the topic you’re discussing. Avoid using generic examples that may not directly support your argument.
- Provide concrete evidence to back up your claims. This could include statistics, research findings, or quotes from reliable sources.
- Interpret the examples and evidence you provide, explaining how they support your thesis or main argument. Don’t assume that the connection is obvious to your readers.
- Use a variety of examples to make your points more persuasive. Mixing personal anecdotes with scholarly evidence can make your essay more engaging and convincing.
- Cite your sources properly to give credit to the original authors and avoid plagiarism. Follow the citation style required by your instructor or the publication you’re submitting to.
By integrating relevant examples and evidence into your essays, you can craft a more convincing and well-rounded piece of writing that resonates with your audience.
Editing and Proofreading Your Essay Carefully
Once you have finished writing your essay, the next crucial step is to edit and proofread it carefully. Editing and proofreading are essential parts of the writing process that help ensure your essay is polished and error-free. Here are some tips to help you effectively edit and proofread your essay:
1. Take a Break: Before you start editing, take a short break from your essay. This will help you approach the editing process with a fresh perspective.
2. Read Aloud: Reading your essay aloud can help you catch any awkward phrasing or grammatical errors that you may have missed while writing. It also helps you check the flow of your essay.
3. Check for Consistency: Make sure that your essay has a consistent style, tone, and voice throughout. Check for inconsistencies in formatting, punctuation, and language usage.
4. Remove Unnecessary Words: Look for any unnecessary words or phrases in your essay and remove them to make your writing more concise and clear.
5. Proofread for Errors: Carefully proofread your essay for spelling, grammar, and punctuation errors. Pay attention to commonly misused words and homophones.
6. Get Feedback: It’s always a good idea to get feedback from someone else. Ask a friend, classmate, or teacher to review your essay and provide constructive feedback.
By following these tips and taking the time to edit and proofread your essay carefully, you can improve the overall quality of your writing and make sure your ideas are effectively communicated to your readers.
Related Post
How to master the art of writing expository essays and captivate your audience, convenient and reliable source to purchase college essays online, step-by-step guide to crafting a powerful literary analysis essay, unlock success with a comprehensive business research paper example guide, unlock your writing potential with writers college – transform your passion into profession, “unlocking the secrets of academic success – navigating the world of research papers in college”, master the art of sociological expression – elevate your writing skills in sociology.
- Network Sites:
- Technical Articles
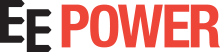
- Join EEPower
- Or sign in with
- iHeartRadio
An Introduction to TRIAC Basics
Join our engineering community sign-in with:, learn about the triode for alternative currents (triac), including its construction, circuit characteristics, applications, and testing procedures..
A thyristor is a common term for a wide variety of semiconductor components used as an electronic switch. Like a mechanical switch, thyristors only have two states: on (conductive) and off (non-conductive). They can also be used, in addition to switching, to adjust the amount of power given to a load.
Thyristors are used mostly with high voltages and currents. The triode for alternative current (TRIAC) and silicon controlled rectifier (SCR) are the most commonly used thyristor devices. This article explores the construction, characteristics, and applications of TRIACs.
What is a TRIAC?
A TRIAC is a bidirectional, three-electrode AC switch that allows electrons to flow in either direction. It is the equivalent of two SCRs connected in a reverse-parallel arrangement with gates connected to each other.
A TRIAC is triggered into conduction in both directions by a gate signal like that of an SCR. TRIACs were designed to provide a means for the development of improved AC power controls.
TRIACs are available in a variety of packaging arrangements. They can handle a wide range of current and voltage. TRIACs generally have relatively low-current capabilities compared to SCRs — they are usually limited to less than 50 A and cannot replace SCRs in high-current applications.
TRIACs are considered versatile because of their ability to operate with positive or negative voltages across their terminals. Since SCRs have a disadvantage of conducting current in only one direction, controlling low power in an AC circuit is better served with the use of a TRIAC.
TRIAC Construction
Although TRIACs and SCRs look alike, their schematic symbols are dissimilar. The terminals of a TRIAC are the gate, terminal 1 (T1), and terminal 2 (T2). See Figure 1.

Figure 1. TRIAC terminals include a gate, terminal 1 (T1), and terminal 2 (T2).
There is no designation of anode and cathode. Current may flow in either direction through the main switch terminals, T1 and T2. Terminal 1 is the reference terminal for all voltages. Terminal 2 is the case or metal-mounting tab to which a heat sink can be attached.
TRIAC Triggering Circuit and its Advantages
TRIACs block current in either direction between T1 and T2. A TRIAC can be triggered into conduction in either direction by a momentary positive or negative pulse supplied to the gate.
If the appropriate signal is applied to the TRIAC gate, it conducts electricity. The TRIAC remains off until the gate is triggered at point A. See Figure 2.

Figure 2. A TRIAC remains off until its gate is triggered.
At point A, the trigger circuit pulses the gate, and the TRIAC is turned on, allowing the current to flow.
At point B, the forward current is reduced to zero, and the TRIAC is turned off.
The trigger circuit can be designed to generate a pulse that changes in the positive or negative half-cycle at any point. Consequently, the average current delivered to the load can vary.
One advantage of the TRIAC is that virtually no power is wasted by being converted to heat. Heat is generated when the current is impeded, not when the current is switched off. The TRIAC is either fully ON or fully OFF. It never partially limits current.
Another essential feature of the TRIAC is the absence of a reverse breakdown condition of high voltages and high currents, such as those found in diodes and SCRs.
If the voltage across the TRIAC goes too high, the TRIAC is turned on. Once on, the TRIAC can conduct a reasonably high current.
The Characteristic Curve of TRIACs
The characteristics of a TRIAC are based on T1 as the voltage reference point. The polarities shown for voltage and current are the polarities of T2 with respect to T1.
The polarities shown for the gate are also with respect to T1. See Figure 3.

Figure 3. A TRIAC characteristic curve shows the characteristics of a TRIAC when triggered into conduction.
Again, the TRIAC may be triggered into conduction in either direction by a gate current (IG) of either polarity.
TRIAC Applications
TRIACs are often used instead of mechanical switches because of their versatility. Also, where amperage is low, TRIACs are more economical than back-to-back SCRs.
Single-Phase Motor Starters
Often, a capacitor-start or split-phase motor must operate where arcing of a mechanical cut-out start switch is undesirable or even dangerous. In such cases, the mechanical cut- out start switch can be replaced by a TRIAC. See Figure 4.

Figure 4. A mechanical cut-out start switch may be replaced by a TRIAC.
A TRIAC is able to operate in such dangerous environments because it does not create an arc. The gate and cut-out signal are given to the TRIAC through a current transformer.
As the motor speeds up, the current is reduced in the current transformer, and the transformer no longer triggers the TRIAC. With the TRIAC turned off, the start windings are removed from the circuit.
Testing Procedures for TRIACs
TRIACs should be tested under operating conditions using an oscilloscope. A DMM may be used to make a rough test with the TRIAC out of the circuit. See Figure 5.

Figure 5. A DMM may be used to make a rough test of a TRIAC that is out of the circuit.
To test a TRIAC using a DMM, the following procedure is applied:
- Set the DMM on the Ω scale.
- Connect the negative lead to the main terminal 1.
- Connect the positive lead to the main terminal 2. The DMM should read infinity.
- Short-circuit the gate to main terminal 2 using a jumper wire. The DMM should read almost 0 Ω. The zero reading should remain when the lead is removed.
- Reverse the DMM leads so that the positive lead is on the main terminal 1, and the negative lead is on the main terminal 2. The DMM should read infinity.
- Short-circuit the gate of the TRIAC to main terminal 2 using a jumper wire. The DMM should read almost 0 Ω. The zero reading should remain after the lead is removed.
Related Content
- Introduction to STATCOM Systems
- Introduction to Power Regulation
- Introduction to Battery Emulators
- Back to Capacitor Basics
- Introduction to Transportation Electrification
Learn More About:
- Silicon Controlled Rectifier
- triode for alternative current
- TRIAC circuit
- TRIAC testing

You May Also Like

Online Design Kit Makes EV Battery Design Easier and Faster
by Mike Falter

EV Battery Test System Improves Production Capacity

New Battery Storage Tech Emerges From Iron, Air, Water
by Jake Hertz

EV Charger Test Platform Handles Four Vehicles Simultaneously

Defining Voltage Pulse Test Requirements for Insulation Endurance Assessment
by Benjamin Sahan
Welcome Back
Don't have an EEPower account? Create one now .
Forgot your password? Click here .

Provide details on what you need help with along with a budget and time limit. Questions are posted anonymously and can be made 100% private.

Studypool matches you to the best tutor to help you with your question. Our tutors are highly qualified and vetted.

Your matched tutor provides personalized help according to your question details. Payment is made only after you have completed your 1-on-1 session and are satisfied with your session.
TRIAC method of paragraphing, writing homework help
User Generated
Description
Essay structure:
Introduction (with the article’s title and author’s name) that must contain your thesis
At least three TRIAC body paragraphs (with in-text parenthetical citations of the articles)
A Conclusion
## I uploaded a file for the (TRIAC method of paragraphing).
## every thing is uploaded in files.
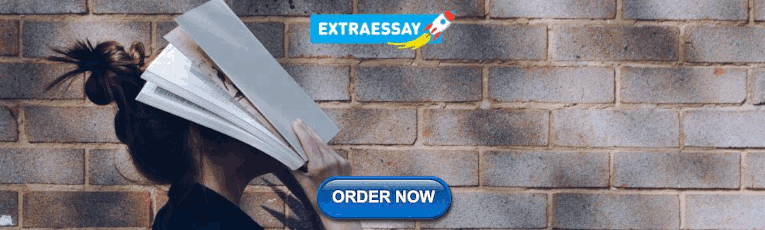
Unformatted Attachment Preview

Explanation & Answer

Attached. Running head: ARGUMENT ANALYSIS 1 Applying the TRIAC Method in Arguments Name Institution ARGUMENT ANALYSIS 2 Applying the TRIAC Method in Arguments There is no shortage of tales of pest control methods that have caused serious problems. Examples of pest control going terribly wrong are abundant in history (Lafrance, 2016). The importation of the importation of the mongoose to the Hawaiian Islands in the 1880s is one such example. Another example is the Australia’s 1930s ill-fated introduction of poisonous toads in an attempt to control the beetle invasions of sugar cane fields is another. These tales are a historical lesson in how complex ecosystems are. In light of the recent Zika virus epidemic, there is a debate on the viability of...

24/7 Study Help
Stuck on a study question? Our verified tutors can answer all questions, from basic math to advanced rocket science !

Similar Content
Related tags.
writing assignment Industrial Revolution spiritual classis Maquiladora philosophy Common Core Standards formatting christian bible personality types Europe’s Cold War essay science
Their Eyes Were Watching God
by Zora Neale Hurston
by Chaim Potok
Unf*ck Yourself
by Gary John Bishop
Sharp Objects
by Gillian Flynn
Death on the Nile
by Agatha Christie
Sense And Sensibility
by Jane Austen
12 Rules for Life
by Jordan Peterson
Slaughterhouse Five
by Kurt Vonnegut
by Ayn Rand

working on a study question?

Studypool is powered by Microtutoring TM
Copyright © 2024. Studypool Inc.
Studypool is not sponsored or endorsed by any college or university.
Ongoing Conversations

Access over 35 million study documents through the notebank

Get on-demand Q&A study help from verified tutors

Read 1000s of rich book guides covering popular titles

Sign up with Google

Sign up with Facebook
Already have an account? Login
Login with Google
Login with Facebook
Don't have an account? Sign Up
Linking essay-writing tests using many-facet models and neural automated essay scoring
- Original Manuscript
- Open access
- Published: 20 August 2024
Cite this article
You have full access to this open access article
- Masaki Uto ORCID: orcid.org/0000-0002-9330-5158 1 &
- Kota Aramaki 1
76 Accesses
Explore all metrics
For essay-writing tests, challenges arise when scores assigned to essays are influenced by the characteristics of raters, such as rater severity and consistency. Item response theory (IRT) models incorporating rater parameters have been developed to tackle this issue, exemplified by the many-facet Rasch models. These IRT models enable the estimation of examinees’ abilities while accounting for the impact of rater characteristics, thereby enhancing the accuracy of ability measurement. However, difficulties can arise when different groups of examinees are evaluated by different sets of raters. In such cases, test linking is essential for unifying the scale of model parameters estimated for individual examinee–rater groups. Traditional test-linking methods typically require administrators to design groups in which either examinees or raters are partially shared. However, this is often impractical in real-world testing scenarios. To address this, we introduce a novel method for linking the parameters of IRT models with rater parameters that uses neural automated essay scoring technology. Our experimental results indicate that our method successfully accomplishes test linking with accuracy comparable to that of linear linking using few common examinees.
Similar content being viewed by others
Rater-Effect IRT Model Integrating Supervised LDA for Accurate Measurement of Essay Writing Ability
Integration of Automated Essay Scoring Models Using Item Response Theory
Robust Neural Automated Essay Scoring Using Item Response Theory
Avoid common mistakes on your manuscript.
Introduction
The growing demand for assessing higher-order skills, such as logical reasoning and expressive capabilities, has led to increased interest in essay-writing assessments (Abosalem, 2016 ; Bernardin et al., 2016 ; Liu et al., 2014 ; Rosen & Tager, 2014 ; Schendel & Tolmie, 2017 ). In these assessments, human raters assess the written responses of examinees to specific writing tasks. However, a major limitation of these assessments is the strong influence that rater characteristics, including severity and consistency, have on the accuracy of ability measurement (Bernardin et al., 2016 ; Eckes, 2005 , 2023 ; Kassim, 2011 ; Myford & Wolfe, 2003 ). Several item response theory (IRT) models that incorporate parameters representing rater characteristics have been proposed to mitigate this issue (Eckes, 2023 ; Myford & Wolfe, 2003 ; Uto & Ueno, 2018 ).
The most prominent among them are many-facet Rasch models (MFRMs) (Linacre, 1989 ), and various extensions of MFRMs have been proposed to date (Patz & Junker, 1999 ; Patz et al., 2002 ; Uto & Ueno, 2018 , 2020 ). These IRT models have the advantage of being able to estimate examinee ability while accounting for rater effects, making them more accurate than simple scoring methods based on point totals or averages.
However, difficulties can arise when essays from different groups of examinees are evaluated by different sets of raters, a scenario often encountered in real-world testing. For instance, in academic settings such as university admissions, individual departments may use different pools of raters to assess essays from specific applicant pools. Similarly, in the context of large-scale standardized tests, different sets of raters may be allocated to various test dates or locations. Thus, when applying IRT models with rater parameters to account for such real-world testing cases while also ensuring that ability estimates are comparable across groups of examinees and raters, test linking becomes essential for unifying the scale of model parameters estimated for each group.
Conventional test-linking methods generally require some overlap of examinees or raters across the groups being linked (Eckes, 2023 ; Engelhard, 1997 ; Ilhan, 2016 ; Linacre, 2014 ; Uto, 2021a ). For example, linear linking based on common examinees, a popular linking method, estimates the IRT parameters for shared examinees using data from each group. These estimates are then used to build a linear regression model, which adjusts the parameter scales across groups. However, the design of such overlapping groups can often be impractical in real-world testing environments.
To facilitate test linking in these challenging environments, we introduce a novel method that leverages neural automated essay scoring (AES) technology. Specifically, we employ a cutting-edge deep neural AES method (Uto & Okano, 2021 ) that can predict IRT-based abilities from examinees’ essays. The central concept of our linking method is to construct an AES model using the ability estimates of examinees in a reference group, along with their essays, and then to apply this model to predict the abilities of examinees in other groups. An important point is that the AES model is trained to predict examinee abilities on the scale established by the reference group. This implies that the trained AES model can predict the abilities of examinees in other groups on the ability scale established by the reference group. Therefore, we use the predicted abilities to calculate the linking coefficients required for linear linking and to perform a test linking. In this study, we conducted experiments based on real-world data to demonstrate that our method successfully accomplishes test linking with accuracy comparable to that of linear linking using few common examinees.
It should be noted that previous studies have attempted to employ AES technologies for test linking (Almond, 2014 ; Olgar, 2015 ), but their focus has primarily been on linking tests with varied writing tasks or a mixture of essay tasks and objective items, while overlooking the influence of rater characteristics. This differs from the specific scenarios and goals that our study aims to address. To the best of our knowledge, this is the first study that employs AES technologies to link IRT models incorporating rater parameters for writing assessments without the need for common examinees and raters.
Setting and data
In this study, we assume scenarios in which two groups of examinees respond to the same writing task and their written essays are assessed by two distinct sets of raters following the same scoring rubric. We refer to one group as the reference group , which serves as the basis for the scale, and the other as the focal group , whose scale we aim to align with that of the reference group.
Let \(u^{\text {ref}}_{jr}\) be the score assigned by rater \(r \in \mathcal {R}^{\text {ref}}\) to the essay of examinee \(j \in \mathcal {J}^{\text {ref}}\) , where \(\mathcal {R}^{\text {ref}}\) and \(\mathcal {J}^{\text {ref}}\) denote the sets of raters and examinees in the reference group, respectively. Then, a collection of scores for the reference group can be defined as
where \(\mathcal{K} = \{1,\ldots ,K\}\) represents the rating categories, and \(-1\) indicates missing data.
Similarly, a collection of scores for the focal group can be defined as
where \(u^{\text {foc}}_{jr}\) indicates the score assigned by rater \(r \in \mathcal {R}^{\text {foc}}\) to the essay of examinee \(j \in \mathcal {J}^{\text {foc}}\) , and \(\mathcal {R}^{\text {foc}}\) and \(\mathcal {J}^{\text {foc}}\) represent the sets of raters and examinees in the focal group, respectively.
The primary objective of this study is to apply IRT models with rater parameters to the two sets of data, \(\textbf{U}^{\text {ref}}\) and \(\textbf{U}^{\text {foc}}\) , and to establish IRT parameter linking without shared examinees and raters: \(\mathcal {J}^{\text {ref}} \cap \mathcal {J}^{\text {foc}} = \emptyset \) and \(\mathcal {R}^{\text {ref}} \cap \mathcal {R}^{\text {foc}} = \emptyset \) . More specifically, we seek to align the scale derived from \(\textbf{U}^{\text {foc}}\) with that of \(\textbf{U}^{\text {ref}}\) .
- Item response theory
IRT (Lord, 1980 ), a test theory grounded in mathematical models, has recently gained widespread use in various testing situations due to the growing prevalence of computer-based testing. In objective testing contexts, IRT makes use of latent variable models, commonly referred to as IRT models. Traditional IRT models, such as the Rasch model and the two-parameter logistic model, give the probability of an examinee’s response to a test item as a probabilistic function influenced by both the examinee’s latent ability and the item’s characteristic parameters, such as difficulty and discrimination. These IRT parameters can be estimated from a dataset consisting of examinees’ responses to test items.
However, traditional IRT models are not directly applicable to essay-writing test data, where the examinees’ responses to test items are assessed by multiple human raters. Extended IRT models with rater parameters have been proposed to address this issue (Eckes, 2023 ; Jin and Wang, 2018 ; Linacre, 1989 ; Shin et al., 2019 ; Uto, 2023 ; Wilson & Hoskens, 2001 ).
Many-facet Rasch models and their extensions
The MFRM (Linacre, 1989 ) is the most commonly used IRT model that incorporates rater parameters. Although several variants of the MFRM exist (Eckes, 2023 ; Myford & Wolfe, 2004 ), the most representative model defines the probability that the essay of examinee j for a given test item (either a writing task or prompt) i receives a score of k from rater r as
where \(\theta _j\) is the latent ability of examinee j , \(\beta _{i}\) represents the difficulty of item i , \(\beta _{r}\) represents the severity of rater r , and \(d_{m}\) is a step parameter denoting the difficulty of transitioning between scores \(m-1\) and m . \(D = 1.7\) is a scaling constant used to minimize the difference between the normal and logistic distribution functions. For model identification, \(\sum _{i} \beta _{i} = 0\) , \(d_1 = 0\) , \(\sum _{m = 2}^{K} d_{m} = 0\) , and a normal distribution for the ability \(\theta _j\) are assumed.
Another popular MFRM is one in which \(d_{m}\) is replaced with \(d_{rm}\) , a rater-specific step parameter denoting the severity of rater r when transitioning from score \(m-1\) to m . This model is often used to investigate variations in rating scale criteria among raters caused by differences in the central tendency, extreme response tendency, and range restriction among raters (Eckes, 2023 ; Myford & Wolfe, 2004 ; Qiu et al., 2022 ; Uto, 2021a ).
A recent extension of the MFRM is the generalized many-facet model (GMFM) (Uto & Ueno, 2020 ) Footnote 1 , which incorporates parameters denoting rater consistency and item discrimination. GMFM defines the probability \(P_{ijrk}\) as
where \(\alpha _i\) indicates the discrimination power of item i , and \(\alpha _r\) indicates the consistency of rater r . For model identification, \(\prod _{r} \alpha _i = 1\) , \(\sum _{i} \beta _{i} = 0\) , \(d_{r1} = 0\) , \(\sum _{m = 2}^{K} d_{rm} = 0\) , and a normal distribution for the ability \(\theta _j\) are assumed.
In this study, we seek to apply the aforementioned IRT models to data involving a single test item, as detailed in the Setting and data section. When there is only one test item, the item parameters in the above equations become superfluous and can be omitted. Consequently, the equations for these models can be simplified as follows.
MFRM with rater-specific step parameters (referred to as MFRM with RSS in the subsequent sections):
Note that the GMFM can simultaneously capture the following typical characteristics of raters, whereas the MFRM and MFRM with RSS can only consider a subset of these characteristics.
Severity : This refers to the tendency of some raters to systematically assign higher or lower scores compared with other raters regardless of the actual performance of the examinee. This tendency is quantified by the parameter \(\beta _r\) .
Consistency : This is the extent to which raters maintain their scoring criteria consistently over time and across different examinees. Consistent raters exhibit stable scoring patterns, which make their evaluations more reliable and predictable. In contrast, inconsistent raters show varying scoring tendencies. This characteristic is represented by the parameter \(\alpha _r\) .
Range Restriction : This describes the limited variability in scores assigned by a rater. Central tendency and extreme response tendency are special cases of range restriction. This characteristic is represented by the parameter \(d_{rm}\) .
For details on how these characteristics are represented in the GMFM, see the article (Uto & Ueno, 2020 ).
Based on the above, it is evident that both the MFRM and MFRM with RSS are special cases of the GMFM. Specifically, the GMFM with constant rater consistency corresponds to the MFRM with RSS. Moreover, the MFRM with RSS that assumes no differences in the range restriction characteristic among raters aligns with the MFRM.
When the aforementioned IRT models are applied to datasets from multiple groups composed of different examinees and raters, such as \(\textbf{U}^{\text {red}}\) and \(\textbf{U}^{\text {foc}}\) , the scales of the estimated parameters generally differ among them. This discrepancy arises because IRT permits arbitrary scaling of parameters for each independent dataset. An exception occurs when it is feasible to assume equality in between-test distributions of examinee abilities and rater parameters (Linacre, 2014 ). However, real-world testing conditions may not always satisfy this assumption. Therefore, if the aim is to compare parameter estimates between different groups, test linking is generally required to unify the scale of model parameters estimated from each individual group’s dataset.
One widely used approach for test linking is linear linking . In the context of the essay-writing test considered in this study, implementing linear linking necessitates designing two groups so that there is some overlap in examinees between them. With this design, IRT parameters for the shared examinees are estimated individually for each group. These estimates are then used to construct a linear regression model for aligning the parameter scales across groups, thereby rendering them comparable. We now introduce the mean and sigma method (Kolen & Brennan, 2014 ; Marco, 1977 ), a popular method for linear linking, and illustrate the procedures for parameter linking specifically for the GMFM, as defined in Eq. 7 , because both the MFRM and the MFRM with RSS can be regarded as special cases of the GMFM, as explained earlier.
To elucidate this, let us assume that the datasets corresponding to the reference and focal groups, denoted as \(\textbf{U}^{\text {ref}}\) and \(\textbf{U}^{\text {foc}}\) , contain overlapping sets of examinees. Furthermore, let us assume that \(\hat{\varvec{\theta }}^{\text {foc}}\) , \(\hat{\varvec{\alpha }}^{\text {foc}}\) , \(\hat{\varvec{\beta }}^{\text {foc}}\) , and \(\hat{\varvec{d}}^{\text {foc}}\) are the GMFM parameters estimated from \(\textbf{U}^{\text {foc}}\) . The mean and sigma method aims to transform these parameters linearly so that their scale aligns with those estimated from \(\textbf{U}^{\text {ref}}\) . This transformation is guided by the equations
where \(\tilde{\varvec{\theta }}^{\text {foc}}\) , \(\tilde{\varvec{\alpha }}^{\text {foc}}\) , \(\tilde{\varvec{\beta }}^{\text {foc}}\) , and \(\tilde{\varvec{d}}^{\text {foc}}\) represent the scale-transformed parameters for the focal group. The linking coefficients are defined as
where \({\mu }^{\text {ref}}\) and \({\sigma }^{\text {ref}}\) represent the mean and standard deviation (SD) of the common examinees’ ability values estimated from \(\textbf{U}^{\text {ref}}\) , and \({\mu }^{\text {foc}}\) and \({\sigma }^{\text {foc}}\) represent those values obtained from \(\textbf{U}^{\text {foc}}\) .
This linear linking method is applicable when there are common examinees across different groups. However, as discussed in the introduction, arranging for multiple groups with partially overlapping examinees (and/or raters) can often be impractical in real-world testing environments. To address this limitation, we aim to facilitate test linking without the need for common examinees and raters by leveraging AES technology.
Automated essay scoring models
Many AES methods have been developed over recent decades and can be broadly categorized into either feature-engineering or automatic feature extraction approaches (Hussein et al., 2019 ; Ke & Ng, 2019 ). The feature-engineering approach predicts essay scores using either a regression or classification model that employs manually designed features, such as essay length and the number of spelling errors (Amorim et al., 2018 ; Dascalu et al., 2017 ; Nguyen & Litman, 2018 ; Shermis & Burstein, 2002 ). The advantages of this approach include greater interpretability and explainability. However, it generally requires considerable effort in developing effective features to achieve high scoring accuracy for various datasets. Automatic feature extraction approaches based on deep neural networks (DNNs) have recently attracted attention as a means of eliminating the need for feature engineering. Many DNN-based AES models have been proposed in the last decade and have achieved state-of-the-art accuracy (Alikaniotis et al., 2016 ; Dasgupta et al., 2018 ; Farag et al., 2018 ; Jin et al., 2018 ; Mesgar & Strube, 2018 ; Mim et al., 2019 ; Nadeem et al., 2019 ; Ridley et al., 2021 ; Taghipour & Ng, 2016 ; Uto, 2021b ; Wang et al., 2018 ). In the next section, we introduce the most widely used DNN-based AES model, which utilizes Bidirectional Encoder Representations from Transformers (BERT) (Devlin et al., 2019 ).
BERT-based AES model
BERT, a pre-trained language model developed by Google’s AI language team, achieved state-of-the-art performance in various natural language processing (NLP) tasks in 2019 (Devlin et al., 2019 ). Since then, it has frequently been applied to AES (Rodriguez et al., 2019 ) and automated short-answer grading (Liu et al., 2019 ; Lun et al., 2020 ; Sung et al., 2019 ) and has demonstrated high accuracy.
BERT is structured as a multilayer bidirectional transformer network, where the transformer is a neural network architecture designed to handle ordered sequences of data using an attention mechanism. See Ref. (Vaswani et al., 2017 ) for details of transformers.
BERT undergoes training in two distinct phases, pretraining and fine-tuning . The pretraining phase utilizes massive volumes of unlabeled text data and is conducted through two unsupervised learning tasks, specifically, masked language modeling and next-sentence prediction . Masked language modeling predicts the identities of words that have been masked out of the input text, while next-sequence prediction predicts whether two given sentences are adjacent.
Fine-tuning is required to adapt a pre-trained BERT model for a specific NLP task, including AES. This entails retraining the BERT model using a task-specific supervised dataset after initializing the model parameters with pre-trained values and augmenting with task-specific output layers. For AES applications, the addition of a special token, [CLS] , at the beginning of each input is required. Then, BERT condenses the entire input text into a fixed-length real-valued hidden vector referred to as the distributed text representation , which corresponds to the output of the special token [CLS] (Devlin et al., 2019 ). AES scores can thus be derived by feeding the distributed text representation into a linear layer with sigmoid activation , as depicted in Fig. 1 . More formally, let \( \varvec{h} \) be the distributed text representation. The linear layer with sigmoid activation is defined as \(\sigma (\varvec{W}\varvec{h}+\text{ b})\) , where \(\varvec{W}\) is a weight matrix and \(\text{ b }\) is a bias, both learned during the fine-tuning process. The sigmoid function \(\sigma ()\) maps its input to a value between 0 and 1. Therefore, the model is trained to minimize an error loss function between the predicted scores and the gold-standard scores, which are normalized to the [0, 1] range. Moreover, score prediction using the trained model is performed by linearly rescaling the predicted scores back to the original score range.
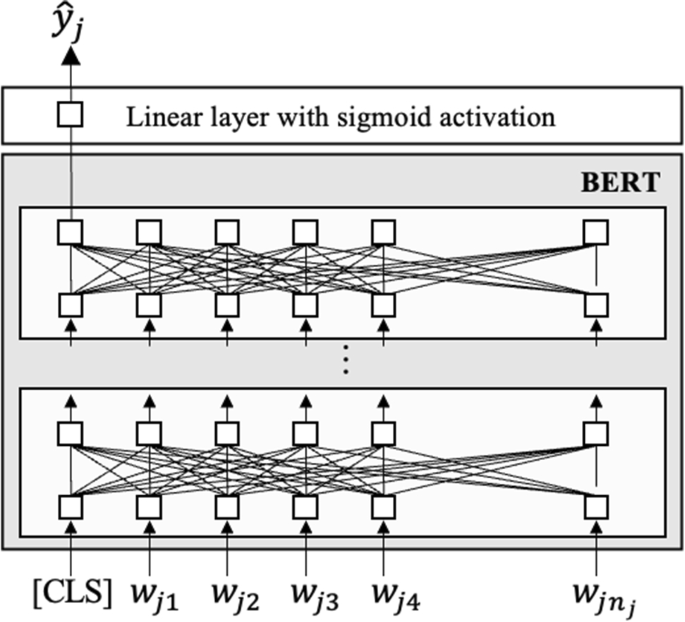
BERT-based AES model architecture. \(w_{jt}\) is the t -th word in the essay of examinee j , \(n_j\) is the number of words in the essay, and \(\hat{y}_{j}\) represents the predicted score from the model
Problems with AES model training
As mentioned above, to employ BERT-based and other DNN-based AES models, they must be trained or fine-tuned using a large dataset of essays that have been graded by human raters. Typically, the mean-squared error (MSE) between the predicted and the gold-standard scores serves as the loss function for model training. Specifically, let \(y_{j}\) be the normalized gold-standard score for the j -th examinee’s essay, and let \(\hat{y}_{j}\) be the predicted score from the model. The MSE loss function is then defined as
where J denotes the number of examinees, which is equivalent to the number of essays, in the training dataset.
Here, note that a large-scale training dataset is often created by assigning a few raters from a pool of potential raters to each essay to reduce the scoring burden and to increase scoring reliability. In such cases, the gold-standard score for each essay is commonly determined by averaging the scores given by multiple raters assigned to that essay. However, as discussed in earlier sections, these straightforward average scores are highly sensitive to rater characteristics. When training data includes rater bias effects, an AES model trained on that data can show decreased performance as a result of inheriting these biases (Amorim et al., 2018 ; Huang et al., 2019 ; Li et al., 2020 ; Wind et al., 2018 ). An AES method that uses IRT has been proposed to address this issue (Uto & Okano, 2021 ).
AES method using IRT
The main idea behind the AES method using IRT (Uto & Okano, 2021 ) is to train an AES model using the ability value \(\theta _j\) estimated by IRT models with rater parameters, such as MFRM and its extensions, from the data given by multiple raters for each essay, instead of a simple average score. Specifically, AES model training in this method occurs in two steps, as outlined in Fig. 2 .
Estimate the IRT-based abilities \(\varvec{\theta }\) from a score dataset, which includes scores given to essays by multiple raters.
Train an AES model given the ability estimates as the gold-standard scores. Specifically, the MSE loss function for training is defined as
where \(\hat{\theta }_j\) represents the AES’s predicted ability of the j -th examinee, and \(\theta _{j}\) is the gold-standard ability for the examinee obtained from Step 1. Note that the gold-standard scores are rescaled into the range [0, 1] by applying a linear transformation from the logit range \([-3, 3]\) to [0, 1]. See the original paper (Uto & Okano, 2021 ) for details.
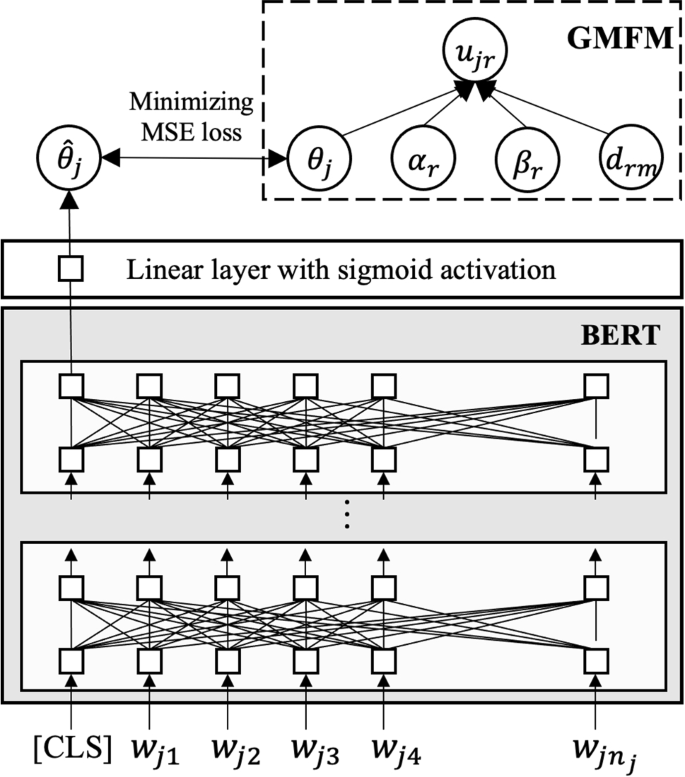
Architecture of a BERT-based AES model that uses IRT
A trained AES model based on this method will not reflect bias effects because IRT-based abilities \(\varvec{\theta }\) are estimated while removing rater bias effects.
In the prediction phase, the score for an essay from examinee \(j^{\prime }\) is calculated in two steps.
Predict the IRT-based ability \(\theta _{j^{\prime }}\) for the examinee using the trained AES model, and then linearly rescale it to the logit range \([-3, 3]\) .
Calculate the expected score \(\mathbb {E}_{r,k}\left[ P_{j^{\prime }rk}\right] \) , which corresponds to an unbiased original-scaled score, given \(\theta _{j'}\) and the rater parameters. This is used as a predicted essay score in this method.
This method originally aimed to train an AES model while mitigating the impact of varying rater characteristics present in the training data. A key feature, however, is its ability to predict an examinee’s IRT-based ability from their essay texts. Our linking approach leverages this feature to enable test linking without requiring common examinees and raters.
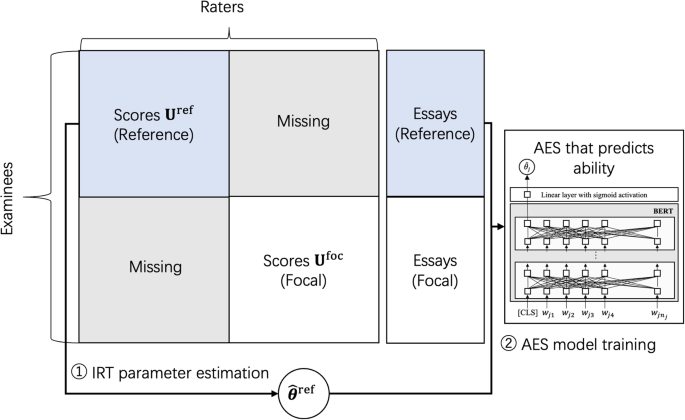
Outline of our proposed method, steps 1 and 2
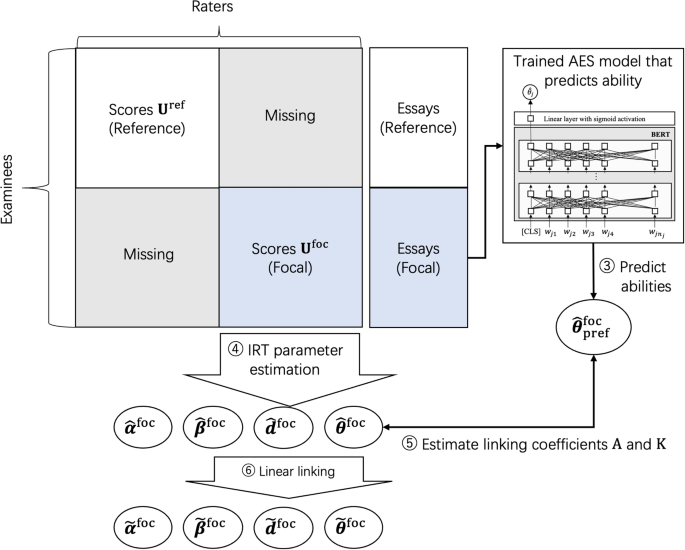
Outline of our proposed method, steps 3–6
Proposed method
The core idea behind our method is to develop an AES model that predicts examinee ability using score and essay data from the reference group, and then to use this model to predict the abilities of examinees in the focal group. These predictions are then used to estimate the linking coefficients for a linear linking. An outline of our method is illustrated in Figs. 3 and 4 . The detailed steps involved in the procedure are as follows.
Estimate the IRT model parameters from the reference group’s data \(\textbf{U}^{\text {ref}}\) to obtain \(\hat{\varvec{\theta }}^{\text {ref}}\) indicating the ability estimates of the examinees in the reference group.
Use the ability estimates \(\hat{\varvec{\theta }}^{\text {ref}}\) and the essays written by the examinees in the reference group to train the AES model that predicts examinee ability.
Use the trained AES model to predict the abilities of examinees in the focal group by inputting their essays. We designate these AES-predicted abilities as \(\hat{\varvec{\theta }}^{\text {foc}}_{\text {pred}}\) from here on. An important point to note is that the AES model is trained to predict ability values on the parameter scale aligned with the reference group’s data, meaning that the predicted abilities for examinees in the focal group follow the same scale.
Estimate the IRT model parameters from the focal group’s data \(\textbf{U}^{\text {foc}}\) .
Calculate the linking coefficients A and K using the AES-predicted abilities \(\hat{\varvec{\theta }}^{\text {foc}}_{\text {pred}}\) and the IRT-based ability estimates \(\hat{\varvec{\theta }}^{\text {foc}}\) for examinees in the focal group as follows.
where \({\mu }^{\text {foc}}_{\text {pred}}\) and \({\sigma }^{\text {foc}}_{\text {pred}}\) represent the mean and the SD of the AES-predicted abilities \(\hat{\varvec{\theta }}^{\text {foc}}_{\text {pred}}\) , respectively. Furthermore, \({\mu }^{\text {foc}}\) and \({\sigma }^{\text {foc}}\) represent the corresponding values for the IRT-based ability estimates \(\hat{\varvec{\theta }}^{\text {foc}}\) .
Apply linear linking based on the mean and sigma method given in Eq. 8 using the above linking coefficients and the parameter estimates for the focal group obtained in Step 4. This procedure yields parameter estimates for the focal group that are aligned with the scale of the parameters of the reference group.
As described in Step 3, the AES model used in our method is trained to predict examinee abilities on the scale derived from the reference data \(\textbf{U}^{\text {ref}}\) . Therefore, the abilities predicted by the trained AES model for the examinees in the focal group, denoted as \(\hat{\varvec{\theta }}^{\text {foc}}_{\text {pred}}\) , also follow the ability scale derived from the reference data. Consequently, by using the AES-predicted abilities, we can infer the differences in the ability distribution between the reference and focal groups. This enables us to estimate the linking coefficients, which then allows us to perform linear linking based on the mean and sigma method. Thus, our method allows for test linking without the need for common examinees and raters.
It is important to note that the current AES model for predicting examinees’ abilities does not necessarily offer sufficient prediction accuracy for individual ability estimates. This implies that their direct use in mid- to high-stakes assessments could be problematic. Therefore, we focus solely on the mean and SD values of the ability distribution based on predicted abilities, rather than using individual predicted ability values. Our underlying assumption is that these AES models can provide valuable insights into differences in the ability distribution across various groups, even though the individual predictions might be somewhat inaccurate, thereby substantiating their utility for test linking.
Experiments
In this section, we provide an overview of the experiments we conducted using actual data to evaluate the effectiveness of our method.
Actual data
We used the dataset previously collected in Uto and Okano ( 2021 ). It consists of essays written in English by 1805 students from grades 7 to 10 along with scores from 38 raters for these essays. The essays originally came from the ASAP (Automated Student Assessment Prize) dataset, which is a well-known benchmark dataset for AES studies. The raters were native English speakers recruited from Amazon Mechanical Turk (AMT), a popular crowdsourcing platform. To alleviate the scoring burden, only a few raters were assigned to each essay, rather than having all raters evaluate every essay. Rater assignment was conducted based on a systematic links design (Shin et al., 2019 ; Uto, 2021a ; Wind & Jones, 2019 ) to achieve IRT-scale linking. Consequently, each rater evaluated approximately 195 essays, and each essay was graded by four raters on average. The raters were asked to grade the essays using a holistic rubric with five rating categories, which is identical to the one used in the original ASAP dataset. The raters were provided no training before the scoring process began. The average Pearson correlation between the scores from AMT raters and the ground-truth scores included in the original ASAP dataset was 0.70 with an SD of 0.09. The minimum and maximum correlations were 0.37 and 0.81, respectively. Furthermore, we also calculated the intraclass correlation coefficient (ICC) between the scores from each AMT rater and the ground-truth scores. The average ICC was 0.60 with an SD of 0.15, and the minimum and maximum ICCs were 0.29 and 0.79, respectively. The calculation of the correlation coefficients and ICC for each AMT rater excluded essays that the AMT rater did not assess. Furthermore, because the ground-truth scores were given as the total scores from two raters, we divided them by two in order to align the score scale with the AMT raters’ scores.
For further analysis, we also evaluated the ICC among the AMT raters as their interrater reliability. In this analysis, missing value imputation was required because all essays were evaluated by a subset of AMT raters. Thus, we first applied multiple imputation with predictive mean matching to the AMT raters’ score dataset. In this process, we generated five imputed datasets. For each imputed dataset, we calculated the ICC among all AMT raters. Finally, we aggregated the ICC values from each imputed dataset to calculate the mean ICC and its SD. The results revealed a mean ICC of 0.43 with an SD of 0.01.
These results suggest that the reliability of raters is not necessarily high. This variability in scoring behavior among raters underscores the importance of applying IRT models with rater parameters. For further details of the dataset see Uto and Okano ( 2021 ).
Experimental procedures
Using this dataset, we conducted the following experiment for three IRT models with rater parameters, MFRM, MFRM with RSS, and GMFM, defined by Eqs. 5 , 6 , and 7 , respectively.
We estimated the IRT parameters from the dataset using the No-U-Turn sampler-based Markov chain Monte Carlo (MCMC) algorithm, given the prior distributions \(\theta _j, \beta _r, d_m, d_{rm} \sim N(0, 1)\) , and \(\alpha _r \sim LN(0, 0.5)\) following the previous work (Uto & Ueno, 2020 ). Here, \( N(\cdot , \cdot )\) and \(LN(\cdot , \cdot )\) indicate normal and log-normal distributions with mean and SD values, respectively. The expected a posteriori (EAP) estimator was used as the point estimates.
We then separated the dataset randomly into two groups, the reference group and the focal group, ensuring no overlap of examinees and raters between them. In this separation, we selected examinees and raters in each group to ensure distinct distributions of examinee abilities and rater severities. Various separation patterns were tested and are listed in Table 1 . For example, condition 1 in Table 1 means that the reference group comprised randomly selected high-ability examinees and low-severity raters, while the focal group comprised low-ability examinees and high-severity raters. Condition 2 provided a similar separation but controlled for narrower variance in rater severity in the focal group. Details of the group creation procedures can be found in Appendix A .
Using the obtained data for the reference and focal groups, we conducted test linking using our method, the details of which are given in the Proposed method section. In it, the IRT parameter estimations were carried out using the same MCMC algorithm as in Step 1.
We calculated the Root Mean Squared Error (RMSE) between the IRT parameters for the focal group, which were linked using our proposed method, and their gold-standard parameters. In this context, the gold-standard parameters were obtained by transforming the scale of the parameters estimated from the entire dataset in Step 1 so that it aligned with that of the reference group. Specifically, we estimated the IRT parameters using data from the reference group and collected those estimated from the entire dataset in Step 1. Then, using the examinees in the reference group as common examinees, we applied linear linking based on the mean and sigma method to adjust the scale of the parameters estimated from the entire dataset to match that of the reference group.
For comparison, we also calculated the RMSE between the focal group’s IRT parameters, obtained without applying the proposed linking, and their gold-standard parameters. This functions as the worst baseline against which the results of the proposed method are compared. Additionally, we examined other baselines that use linear linking based on common examinees. For these baselines, we randomly selected five or ten examinees from the reference group, who were assigned scores by at least two focal group’s raters in the entire dataset. The scores given to these selected examinees by the focal group’s raters were then merged with the focal group’s data, where the added examinees worked as common examinees between the reference and focal groups. Using this data, we examined linear linking using common examinees. Specifically, we estimated the IRT parameters from the data of the focal group with common examinees and applied linear linking based on the mean and sigma method using the ability estimates of the common examinees to align its scale with that of the reference group. Finally, we calculated the RMSE between the linked parameter estimates for the examinees and raters belonging only to the original focal group and their gold-standard parameters. Note that this common examinee approach operates under more advantageous conditions compared with the proposed linking method because it can utilize larger samples for estimating the parameters of raters in the focal group.
We repeated Steps 2–5 ten times for each data separation condition and calculated the average RMSE for four cases: one in which our proposed linking method was applied, one without linking, and two others where linear linkings using five and ten common examinees were applied.
The parameter estimation program utilized in Steps 1, 4, and 5 was implemented using RStan (Stan Development Team, 2018 ). The EAP estimates were calculated as the mean of the parameter samples obtained from 2,000 to 5,000 periods using three independent chains. The AES model was developed in Python, leveraging the PyTorch library Footnote 2 . For the AES model training in Step 3, we randomly selected \(90\%\) of the data from the reference group to serve as the training set, with the remaining \(10\%\) designated as the development set. We limited the maximum number of steps for training the AES model to 800 and set the maximum number of epochs to 800 divided by the number of mini-batches. Additionally, we employed early stopping based on the performance on the development set. The AdamW optimization algorithm was used, and the mini-batch size was set to 8.
MCMC statistics and model fitting
Before delving into the results of the aforementioned experiments, we provide some statistics related to the MCMC-based parameter estimation. Specifically, we computed the Gelman–Rubin statistic \(\hat{R}\) (Gelman et al., 2013 ; Gelman & Rubin, 1992 ), a well-established diagnostic index for convergence, as well as the effective sample size (ESS) and the number of divergent transitions for each IRT model during the parameter estimation phase in Step 1. Across all models, the \(\hat{R}\) statistics were below 1.1 for all parameters, indicating convergence of the MCMC runs. Furthermore, as shown in the first row of Table 2 , our ESS values for all parameters in all models exceeded the criterion of 400, which is considered sufficiently large according to Zitzmann and Hecht ( 2019 ). We also observed no divergent transitions in any of the cases. These results support the validity of the MCMC-based parameter estimation.
Furthermore, we evaluated the model – data fit for each IRT model during the parameter estimation step in Step 1. To assess this fit, we employed the posterior predictive p value ( PPP -value) (Gelman et al., 2013 ), a commonly used metric for evaluating the model–data fit in Bayesian frameworks (Nering & Ostini, 2010 ; van der Linden, 2016 ). Specifically, we calculated the PPP -value using an averaged standardized residual, a conventional metric for IRT model fit in non-Bayesian settings, as a discrepancy function, similar to the approach in Nering and Ostini ( 2010 ); Tran ( 2020 ); Uto and Okano ( 2021 ). A well-fitted model yields a PPP -value close to 0.5, while poorly fitted models exhibit extreme low or high values, such as those below 0.05 or above 0.95. Additionally, we calculated two information criteria, the widely applicable information criterion (WAIC) (Watanabe, 2010 ) and the widely applicable Bayesian information criterion (WBIC) (Watanabe, 2013 ). The model that minimizes these criteria is considered optimal.
The last three rows in Table 2 shows the results. We can see that the PPP -value for GMFM is close to 0.5, indicating a good fit to the data. In contrast, the other models exhibit high values, suggesting a poor fit to the data. Furthermore, among the three IRT models evaluated, GMFM exhibits the lowest WAIC and WBIC values. These findings suggest that GMFM offers the best fit to the data, corroborating previous work that investigated the same dataset using IRT models (Uto & Okano, 2021 ). We provide further discussion about the model fit in the Analysis of rater characteristics section given later.
According to these results, the following section focuses on the results for GMFM. Note that we also include the results for MFRM and MFRM with RSS in Appendix B , along with the open practices statement.
Effectiveness of our proposed linking method
The results of the aforementioned experiments for GMFM are shown in Table 3 . In the table, the Unlinked row represents the average RMSE between the focal group’s IRT parameters without applying our linking method and their gold-standard parameters. Similarly, the Linked by proposed method row represents the average RMSE between the focal group’s IRT parameters after applying our linking method and their gold-standard parameters. The rows labeled Linked by five/ten common examinees represent the results for linear linking using common examinees.
A comparison of the results from the unlinked condition and the proposed method reveals that the proposed method improved the RMSEs for the ability and rater severity parameters, namely, \(\theta _j\) and \(\beta _r\) , which we intentionally varied between the reference and focal groups. The degree of improvement is notably substantial when the distributional differences between the reference and focal groups are large, as is the case in Conditions 1–5. On the other hand, for Conditions 6–8, where the distributional differences are relatively minor, the improvements are also smaller in comparison. This is because the RMSEs for the unlinked parameters are already lower in these conditions than in Conditions 1–5. Nonetheless, it is worth emphasizing that the RMSEs after employing our linking method are exceptionally low in Conditions 6–8.
Furthermore, the table indicates that the RMSEs for the step parameters and rater consistency parameters, namely, \(d_{rm}\) and \(\alpha _r\) , also improved in many cases, while the impact of applying our linking method is relatively small for these parameters compared with the ability and rater severity parameters. This is because we did not intentionally vary their distribution between the reference and focal groups, and thus their distribution differences were smaller than those for the ability and rater severity parameters, as shown in the next section.
Comparing the results from the proposed method and linear linking using five common examinees, we observe that the proposed method generally exhibits lower RMSE values for the ability \(\theta _j\) and the rater severity parameters \(\beta _r\) , except for conditions 2–3. Furthermore, when comparing the proposed method with linear linking using ten common examinees, it achieves superior performance in conditions 4–8 and slightly lower performance in conditions 1–3 for \(\theta _j\) and \(\beta _r\) , while the differences are more minor overall than those observed when comparing the proposed method with the condition of five common examinees. Note that the reasons why the proposed method tends to show lower performance for conditions 1–3 are as follows.
The proposed method utilizes fewer samples to estimate the rater parameters compared with the linear linking method using common examinees.
In situations where distributional differences between the reference and focal groups are relatively large, as in conditions 1–3, constructing an accurate AES model for the focal group becomes challenging due to the limited overlap in the ability value range. We elaborate on this point in the next section.
Furthermore, in terms of the rater consistency parameter \(\alpha _r\) and the step parameter \(d_{rm}\) , the proposed method typically shows lower RMSE values compared with linear linking using common examinees. We attribute this to the fact that the performance of the linking method using common examinees is highly dependent on the choice of common examinees, which can sometimes result in significant errors in these parameters. This issue is also further discussed in the next section.
These results suggest that our method can perform linking with comparable accuracy to linear linking using few common examinees, even in the absence of common examinees and raters. Additionally, as reported in Tables 15 and 16 in Appendix B , both MFRM and MFRM with RSS also exhibit a similar tendency, further validating the effectiveness of our approach regardless of the IRT models employed.
Detailed analysis
Analysis of parameter scale transformation using the proposed method.
In this section, we detail how our method transforms the parameter scale. To demonstrate this, we first summarize the mean and SD values of the gold-standard parameters for both the reference and focal groups in Table 4 . The values in the table are averages calculated from ten repetitions of the experimental procedures. The table shows that the mean and SD values of both examinee ability and rater severity vary significantly between the reference and focal groups following our intended settings, as outlined in Table 1 . Additionally, the mean and SD values for the rater consistency parameter \(\alpha _r\) and the rater-specific step parameters \(d_{rm}\) also differ slightly between the groups, although we did not intentionally alter them.
Second, the averaged values of the means and SDs of the parameters, estimated solely from either the reference or the focal group’s data over ten repetitions, are presented in Table 5 . The table reveals that the estimated parameters for both groups align with a normal distribution centered at nearly zero, despite the actual ability distributions differing between the groups. This phenomenon arises because IRT permits arbitrary scaling of parameters for each independent dataset, as mentioned in the Linking section. This leads to differences in the parameter scale for the focal group compared with their gold-standard values, thereby highlighting the need for parameter linking.
Next, the first two rows of Table 6 display the mean and SD values of the ability estimates for the focal group’s examinees, as predicted by the BERT-based AES model. In the table, the RMSE row indicates the RMSE between the AES-predicted ability values and the gold-standard ability values for the focal groups. The Linking Coefficients row presents the linking coefficients calculated based on the AES-predicted abilities. As with the abovementioned tables, these values are also averages over ten experimental repetitions. According to the table, for Conditions 6–8, where the distributional differences between the groups are relatively minor, both the mean and SD estimates align closely with those of the gold-standard parameters. In contrast, for Conditions 1–5, where the distributional differences are more pronounced, the mean and SD estimates tend to deviate from the gold-standard values, highlighting the challenges of parameter linking under such conditions.
In addition, as indicated in the RMSE row, the AES-predicted abilities may lack accuracy under specific conditions, such as Conditions 1, 2, and 3. This inaccuracy could arise because the AES model, trained on the reference group’s data, could not cover the ability range of the focal group due to significant differences in the ability distribution between the groups. Note that even in cases where the mean and SD estimates are relatively inaccurate, these values are closer to the gold-standard ones than those estimated solely from the focal group’s data. This leads to meaningful linking coefficients, which transform the focal group’s parameters toward the scale of their gold-standard values.
Finally, Table 7 displays the averaged values of the means and SDs of the focal group’s parameters obtained through our linking method over ten repetitions. Note that the mean and SD values of the ability estimates are the same as those reported in Table 6 because the proposed method is designed to align them. The table indicates that the differences in the mean and SD values between the proposed method and the gold-standard condition, shown in Table 4 , tend to be smaller compared with those between the unlinked condition, shown in Table 5 , and the gold-standard. To verify this point more precisely, Table 8 shows the average absolute differences in the mean and SD values of the parameters for the focal groups between the proposed method and the gold-standard condition, as well as those between the unlinked condition and the gold-standard. These values were calculated by averaging the absolute differences in the mean and SD values obtained from each of the ten repetitions, unlike the simple absolute differences in the values reported in Tables 4 and 7 . The table shows that the proposed linking method tends to derive lower values, especially for \(\theta _j\) and \(\beta _r\) , than the unlinked condition. Furthermore, this tendency is prominent for conditions 6–8 in which the distributional differences between the focal and reference groups are relatively small. These trends are consistent with the cases for which our method revealed high linking performance, detailed in the previous section.
In summary, the above analyses suggest that although the AES model’s predictions may not always be perfectly accurate, they can offer valuable insights into scale differences between the reference and focal groups, thereby facilitating successful IRT parameter linking without common examinees and raters.
We now present the distributions of examinee ability and rater severity for the focal group, comparing their gold-standard values with those before and after the application of the linking method. Figures 5 , 6 , 7 , 8 , 9 , 10 , 11 , and 12 are illustrative examples for the eight data-splitting conditions. The gray bars depict the distributions of the gold-standard parameters, the blue bars represent those of the parameters estimated from the focal group’s data, the red bars signify those of the parameters obtained using our linking method, and the green bars indicate the ability distribution as predicted by the BERT-based AES. The upper part of the figure presents results for examinee ability \(\theta _j\) and the lower part presents those for rater severity \(\beta _r\) .
The blue bars in these figures reveal that the parameters estimated from the focal group’s data exhibit distributions with different locations and/or scales compared with their gold-standard values. Meanwhile, the red bars reveal that the distributions of the parameters obtained through our linking method tend to align closely with those of the gold-standard parameters. This is attributed to the fact that the ability distributions for the focal group given by the BERT-based AES model, as depicted by the green bars, were informative for performing linear linking.
Analysis of the linking method based on common examinees
For a detailed analysis of the linking method based on common examinees, Table 9 reports the averaged values of means and SDs of the focal groups’ parameter estimates obtained by the linking method based on five and ten common examinees for each condition. Furthermore, Table 10 shows the average absolute differences between these values and those from the gold standard condition. Table 10 shows that an increase in the number of common examinees tends to lower the average absolute differences, which is a reasonable trend. Furthermore, comparing the results with those of the proposed method reported in Table 8 , the proposed method tends to achieve smaller absolute differences in conditions 4–8 for \(\theta _j\) and \(\beta _r\) , which is consistent with the tendency of the linking performance discussed in the “Effectiveness of our proposed linking method” section.
Note that although the mean and SD values in Table 9 are close to those of the gold-standard parameters shown in Table 4 , this does not imply that linear linking based on five or ten common examinees achieves high linking accuracy for each repetition. To explain this, Table 11 shows the means of the gold-standard ability values for the focal group and their estimates obtained from the proposed method and the linking method based on ten common examinees, for each of ten repetitions under condition 8. This table also shows the absolute differences between the estimated ability means and the corresponding gold-standard means.
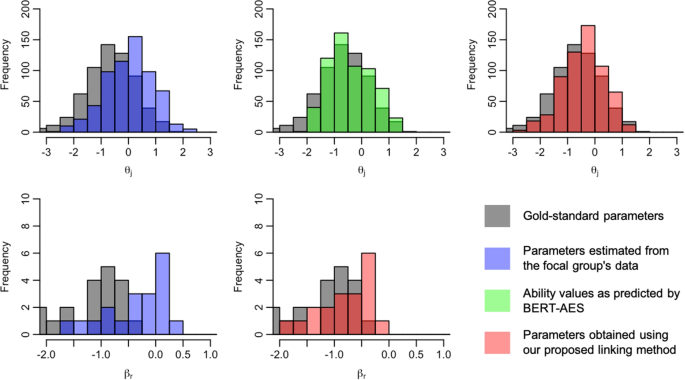
Example of ability and rater severity distributions for the focal group under data-splitting condition 1
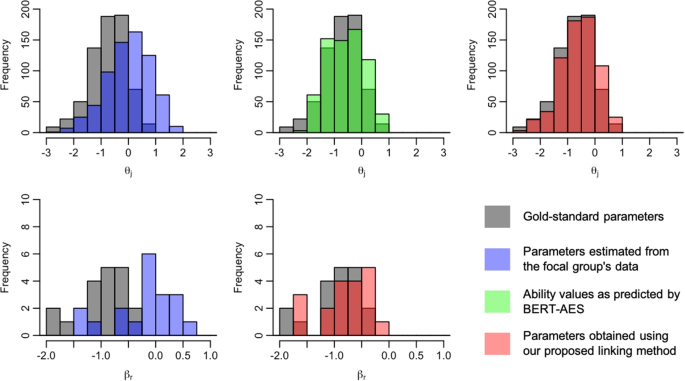
Example of ability and rater severity distributions for the focal group under data-splitting condition 2
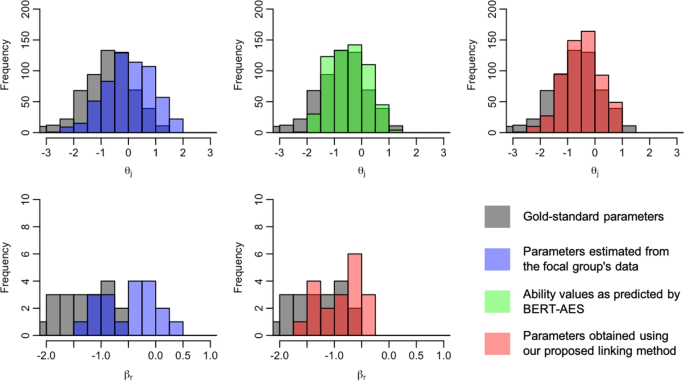
Example of ability and rater severity distributions for the focal group under data-splitting condition 3
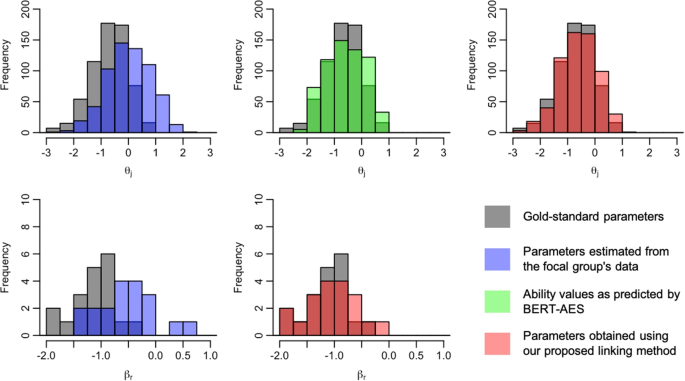
Example of ability and rater severity distributions for the focal group under data-splitting condition 4
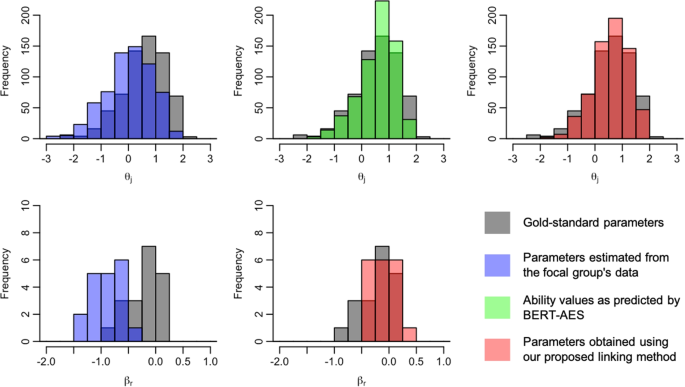
Example of ability and rater severity distributions for the focal group under data-splitting condition 5
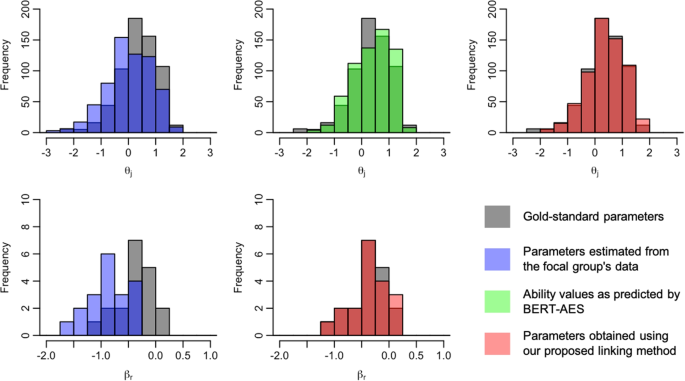
Example of ability and rater severity distributions for the focal group under data-splitting condition 6
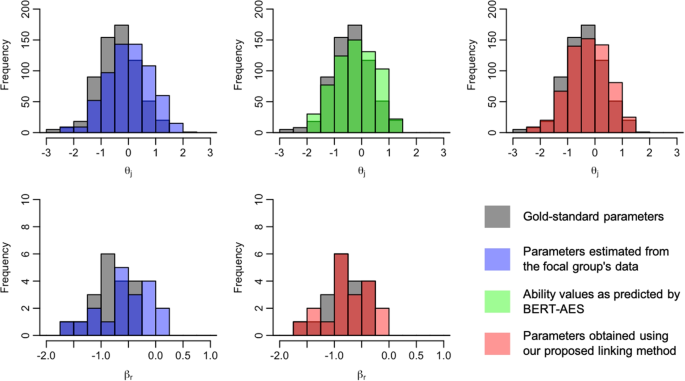
Example of ability and rater severity distributions for the focal group under data-splitting condition 7
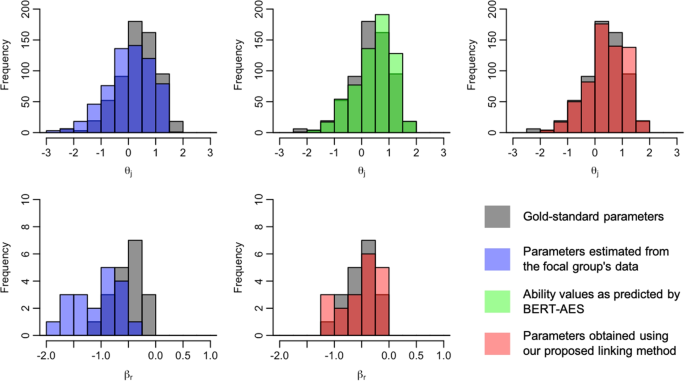
Example of ability and rater severity distributions for the focal group under data-splitting condition 8
The table shows that the results of the proposed method are relatively stable, consistently revealing low absolute differences for every repetition. In contrast, the results of linear linking based on ten common examinees vary significantly across repetitions, resulting in large absolute differences for some repetitions. These results yield a smaller average absolute difference for the proposed method compared with linear linking based on ten common examinees. However, in terms of the absolute difference in the averaged ability means, linear linking based on ten common examinees shows a smaller difference ( \(|0.38-0.33| = 0.05\) ) compared with the proposed method ( \(|0.38-0.46| = 0.08\) ). This occurs because the results of linear linking based on ten common examinees for ten repetitions fluctuate around the ten-repetition average of the gold standard, thereby canceling out the positive and negative differences. However, this does not imply that linear linking based on ten common examinees achieves high linking accuracy for each repetition. Thus, it is reasonable to interpret the average of the absolute differences calculated for each of the ten repetitions, as reported in Tables 8 and 10 .
This greater variability in performance of the linking method based on common examinees also relates to the tendency of the proposed method to show lower RMSE values for the rater consistency parameter \(\alpha _r\) and the step parameters \(d_{rm}\) compared with linking based on common examinees, as mentioned in the Effectiveness of our proposed linking method section. In that section, we mentioned that this is due to the fact that linear linking based on common examinees is highly dependent on the selection of common examinees, which can sometimes lead to significant errors in these parameters.
To confirm this point, Table 12 displays the SD of RMSEs calculated from ten repetitions of the experimental procedures for both the proposed method and linear linking using ten common examinees. The table indicates that the linking method using common examinees tends to exhibit larger SD values overall, suggesting that this linking method sometimes becomes inaccurate, as we also exemplified in Table 11 . This variability also implies that the estimation of the linking coefficient can be unstable.
Furthermore, the tendency of having larger SD values in the common examinee approach is particularly pronounced for the step parameters at the extreme categories, namely, \(d_{r2}\) and \(d_{r5}\) . We consider this comes from the instability of linking coefficients and the fact that the step parameters for the extreme categories tend to have large absolute values (see Table 13 for detailed estimates). Linear linking multiplies the step parameters by a linking coefficient A , although applying an inappropriate linking coefficient to larger absolute values can have a more substantial impact than when applied to smaller values. We concluded that this is why the RMSEs of the step difficulty parameters in the common examinee approach were deteriorated compared with those in the proposed method. The same reasoning would be applicable to the rater consistency parameter, given that it is distributed among positive values with a mean over one. See Table 13 for details.
Prerequisites of the proposed method
As demonstrated thus far, the proposed method can perform IRT parameter linking without the need for common examinees and raters. As outlined in the Introduction section, certain testing scenarios may encounter challenges or incur significant costs in assembling common examinees or raters. Our method provides a viable solution in these situations. However, it does come with specific prerequisites and inherent costs.
The prerequisites of our proposed method are as follows.
The same essay writing task is offered to both the reference and focal groups, and the written essays for it are scored by different groups of raters using the same rubric.
Raters will function identically across both the reference and focal groups, and the established scales can be adjusted through linear transformations. This implies that there are no systematic differences in scoring that are correlated with the groups but are unrelated to the measured construct, such as differential rater functioning (Leckie & Baird, 2011 ; Myford & Wolfe, 2009 ; Uto, 2023 ; Wind & Guo, 2019 ).
The ability ranges of the reference and focal groups require some overlap because the ability prediction accuracy of the AES decreases as the differences in the ability distributions between the groups increases, as discussed in the Detailed analysis section. This is a limitation of this approach, which requires future studies to overcome.
The reference group consists of a sufficient number of examinees for training AES models using their essays as training data.
Related to the fourth point, we conducted an additional experiment to investigate the number of samples required to train AES models. In this experiment, we assessed the ability prediction accuracy of the BERT-based AES model used in this study by varying the number of training samples. The detailed experimental procedures are outlined below.
Estimate the ability of all 1805 examinees from the entire dataset based on the GMFM.
Randomly split the examinees into 80% (1444) and 20% (361) groups. The 20% subset, consisting of examinees’ essays and their ability estimates, was used as test data to evaluate the ability prediction accuracy of the AES model trained through the following steps.
The 80% subset was further divided into 80% (1155) and 20% (289) groups. Here, the essays and ability estimates of the 80% subset were used as the training data, while those of the 20% served as development data for selecting the optimal epoch.
Train the BERT-based AES model using the training data and select the optimal epoch that minimizes the RMSE between the predicted and gold-standard ability values for the development set.
Use the trained AES model at the optimal epoch to evaluate the RMSE between the predicted and gold-standard ability values for the test data.
Randomly sample 50, 100, 200, 300, 500, 750, and 1000 examinees from the training data created in Step 3.
Train the AES model using each sampled set as training data, and select the optimal epoch using the same development data as before.
Use the trained AES model to evaluate the RMSE for the same test data as before.
Repeat Steps 2–8 five times and calculate the average RMSE for the test data.
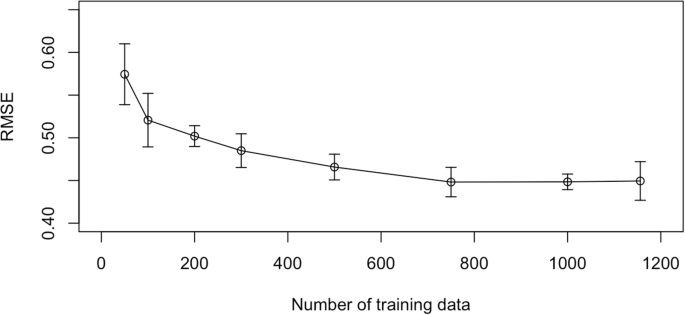
Relationship between the number of training samples and the ability prediction accuracy of AES
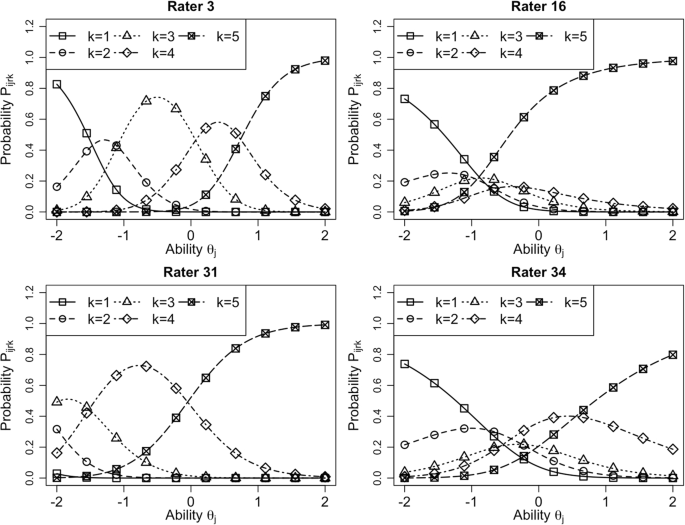
Item response curves of four representative raters found in experiments using actual data
Figure 13 displays the results. The horizontal axis represents the number of training samples, and the vertical axis shows the RMSE values. Each plot illustrates the average RMSE, with error bars indicating the SD ranges. The results demonstrate that larger sample sizes enhance the accuracy of the AES model. Furthermore, while the RMSE decreases significantly when the sample size is small, the improvements tend to plateau beyond 500 samples. This suggests that, for this dataset, approximately 500 samples would be sufficient to train the AES model with reasonable accuracy. However, note that the required number of samples may vary depending on the essay tasks. A detailed analysis of the relationship between the required number of samples and the characteristics of essay writing tasks is planned for future work.
An inherent cost associated with the proposed method is the computational expense required to construct the BERT-based AES model. Specifically, a computer with a reasonably powerful GPU is necessary to efficiently train the AES model. In this study, for example, we utilized an NVIDIA Tesla T4 GPU on Google Colaboratory. To elaborate on the computational expense, we calculated the computation times and costs for the above experiment under a condition where 1155 training samples were used. Consequently, training the AES model with 1155 samples, including evaluating the RMSE for the development set of 289 essays in each epoch, took approximately 10 min in total. Moreover, it required about 10 s to predict the abilities of 361 examinees from their essays using the trained model. The computational units consumed on Google Colaboratory for both training and inference amounted to 0.44, which corresponds to approximately $0.044. These costs and the time required are significantly smaller than what is required for human scoring.
Analysis of rater characteristics
The MCMC statistics and model fitting section demonstrated that the GMFM provides a better fit to the actual data compared with the MFRM and MFRM with RSS. To explain this, Table 13 shows the rater parameters estimated by the GMFM using the entire dataset. Additionally, Fig. 14 illustrates the item response curves (IRCs) for raters 3, 16, 31, and 34, where the horizontal axis represents the ability \(\theta _j\) , and the vertical axis depicts the response probability for each category.
The table and figure reveal that the raters exhibit diverse and unique characteristics in terms of severity, consistency, and range restriction. For instance, Rater 3 demonstrates nearly average values for all parameters, indicating standard rating characteristics. In contrast, Rater 16 exhibits a pronounced extreme response tendency, as evidenced by higher \(d_{r2}\) and lower \(d_{r5}\) values. Additionally, Rater 31 is characterized by a low severity score, generally preferring higher scores (four and five). Rater 34 exhibits a low consistency value \(\alpha _r\) , which results in minimal variation in response probabilities among categories. This indicates that the rater is likely to assign different ratings to essays of similar quality.
As detailed in the Item Response Theory section, the GMFM can capture these variations in rater severity, consistency, and range restriction simultaneously, while the MFRM and MFRM with RSS can consider only its subsets. We can infer that this capability, along with the large variety of rater characteristics, contributed to the superior model fit of the GMFM compared with the other models.
It is important to note that, the proposed method is also useful for facilitating linking for MFRM and MFRM with RSS, even though the model fits for them were relatively worse, as well as for the GMFM, which we mentioned earlier and is shown in Appendix B .
Effect of using cloud workers as raters
As we detailed in the Actual data section, we used scores given by untrained non-expert cloud workers instead of expert raters. A concern with using raters from cloud workers without adequate training is the potential for greater variability in rating characteristics compared with expert raters. This variability is evidenced by the diverse correlations between the raters’ scores and their ground truth, reported in the Actual data section, and the large variety of rater parameters discussed above. These observations suggest the importance of the following two strategies for ensuring reliable essay scoring when employing crowd workers as raters.
Assigning a larger number of raters to each essay than would typically be used with expert raters.
Estimating the standardized essay scores while accounting for differences in rater characteristics, potentially through the use of IRT models that incorporate rater parameters, which we used in this study.
In this study, we propose a novel IRT-based linking method for essay-writing tests that uses AES technology to enable parameter linking based on IRT models with rater parameters across multiple groups in which neither examinees nor raters are shared. Specifically, we use a deep neural AES method capable of predicting IRT-based examinee abilities based on their essays. The core concept of our approach involves developing an AES model to predict examinee abilities using data from a reference group. This AES model is then applied to predict the abilities of examinees in the focal group. These predictions are used to estimate the linking coefficients required for linear linking. Experimental results with real data demonstrate that our method successfully accomplishes test linking with accuracy comparable to that of linear linking using few common examinees.
In our experiments, we compared the linking performance of the proposed method with linear linking based on the mean and sigma method using only five or ten common examinees. However, such a small number of common examinees is generally insufficient for accurate linear linking and thus leads to unstable estimation of linking coefficients, as discussed in the “Analysis of the linking method based on common examinees” section. Although this study concluded that our method could perform linking with accuracy comparable to that of linear linking using few common examinees, further detailed evaluations of our method involving comparisons with various conventional linking methods using different numbers of common examinees and raters will be the target of future work.
Additionally, our experimental results suggest that although the AES model may not provide sufficient predictive accuracy for individual examinee abilities, it does tend to yield reasonable mean and SD values for the ability distribution of focal groups. This lends credence to our assumption stated in the Proposed method section that AES models incorporating IRT can offer valuable insights into differences in ability distribution across various groups, thereby validating their utility for test linking. This result also supports the use of the mean and sigma method for linking. While concurrent calibration, another common linking method, requires highly accurate individual AES-predicted abilities to serve as anchor values, linear linking through the mean and sigma method necessitates only the mean and SD of the ability distribution. Given that the AES model can provide accurate estimates for these statistics, successful linking can be achieved, as shown in our experiments.
A limitation of this study is that our method is designed for test situations where a single essay writing item is administered to multiple groups, each comprising different examinees and raters. Consequently, the method is not directly applicable for linking multiple tests that offer different items. Developing an extension of our approach to accommodate such test situations is one direction for future research. Another involves evaluating the effectiveness of our method using other datasets. To the best of our knowledge, there are no open datasets that include examinee essays along with scores from multiple assigned raters. Therefore, we plan to develop additional datasets and to conduct further evaluations. Further investigation of the impact of the AES model’s accuracy on linking performance is also warranted.
Availability of data and materials
The data and materials from our experiments are available at https://github.com/AI-Behaviormetrics/LinkingIRTbyAES.git . This includes all experimental results and a sample dataset.
Code availability
The source code for our linking method, developed in R and Python, is available in the same GitHub repository.
The original paper referred to this model as the generalized MFRM. However, in this paper, we refer to it as GMFM because it does not strictly belong to the family of Rasch models.
https://pytorch.org/
Abosalem, Y. (2016). Assessment techniques and students’ higher-order thinking skills. International Journal of Secondary Education, 4 (1), 1–11. https://doi.org/10.11648/j.ijsedu.20160401.11
Article Google Scholar
Alikaniotis, D., Yannakoudakis, H., & Rei, M. (2016). Automatic text scoring using neural networks. Proceedings of the annual meeting of the association for computational linguistics (pp. 715–725).
Almond, R. G. (2014). Using automated essay scores as an anchor when equating constructed response writing tests. International Journal of Testing, 14 (1), 73–91. https://doi.org/10.1080/15305058.2013.816309
Amorim, E., Cançado, M., & Veloso, A. (2018). Automated essay scoring in the presence of biased ratings. Proceedings of the annual conference of the north american chapter of the association for computational linguistics (pp. 229–237).
Bernardin, H. J., Thomason, S., Buckley, M. R., & Kane, J. S. (2016). Rater rating-level bias and accuracy in performance appraisals: The impact of rater personality, performance management competence, and rater accountability. Human Resource Management, 55 (2), 321–340. https://doi.org/10.1002/hrm.21678
Dascalu, M., Westera, W., Ruseti, S., Trausan-Matu, S., & Kurvers, H. (2017). ReaderBench learns Dutch: Building a comprehensive automated essay scoring system for Dutch language. Proceedings of the international conference on artificial intelligence in education (pp. 52–63).
Dasgupta, T., Naskar, A., Dey, L., & Saha, R. (2018). Augmenting textual qualitative features in deep convolution recurrent neural network for automatic essay scoring. Proceedings of the workshop on natural language processing techniques for educational applications (pp. 93–102).
Devlin, J., Chang, M. -W., Lee, K., & Toutanova, K. (2019). BERT: Pre-training of deep bidirectional transformers for language understanding. Proceedings of the annual conference of the north American chapter of the association for computational linguistics: Human language technologies (pp. 4171–4186).
Eckes, T. (2005). Examining rater effects in TestDaF writing and speaking performance assessments: A many-facet Rasch analysis. Language Assessment Quarterly, 2 (3), 197–221. https://doi.org/10.1207/s15434311laq0203_2
Eckes, T. (2023). Introduction to many-facet Rasch measurement: Analyzing and evaluating rater-mediated assessments . Peter Lang Pub. Inc.
Engelhard, G. (1997). Constructing rater and task banks for performance assessments. Journal of Outcome Measurement, 1 (1), 19–33.
PubMed Google Scholar
Farag, Y., Yannakoudakis, H., & Briscoe, T. (2018). Neural automated essay scoring and coherence modeling for adversarially crafted input. Proceedings of the annual conference of the north American chapter of the association for computational linguistics (pp. 263–271).
Gelman, A., Carlin, J., Stern, H., Dunson, D., Vehtari, A., & Rubin, D. (2013). Bayesian data analysis (3rd ed.). Taylor & Francis.
Book Google Scholar
Gelman, A., & Rubin, D. B. (1992). Inference from iterative simulation using multiple sequences. Statistical Science, 7 (4), 457–472. https://doi.org/10.1214/ss/1177011136
Huang, J., Qu, L., Jia, R., & Zhao, B. (2019). O2U-Net: A simple noisy label detection approach for deep neural networks. Proceedings of the IEEE international conference on computer vision .
Hussein, M. A., Hassan, H. A., & Nassef, M. (2019). Automated language essay scoring systems: A literature review. PeerJ Computer Science, 5 , e208. https://doi.org/10.7717/peerj-cs.208
Article PubMed PubMed Central Google Scholar
Ilhan, M. (2016). A comparison of the results of many-facet Rasch analyses based on crossed and judge pair designs. Educational Sciences: Theory and Practice, 16 (2), 579–601. https://doi.org/10.12738/estp.2016.2.0390
Jin, C., He, B., Hui, K., & Sun, L. (2018). TDNN: A two-stage deep neural network for prompt-independent automated essay scoring. Proceedings of the annual meeting of the association for computational linguistics (pp. 1088–1097).
Jin, K. Y., & Wang, W. C. (2018). A new facets model for rater’s centrality/extremity response style. Journal of Educational Measurement, 55 (4), 543–563. https://doi.org/10.1111/jedm.12191
Kassim, N. L. A. (2011). Judging behaviour and rater errors: An application of the many-facet Rasch model. GEMA Online Journal of Language Studies, 11 (3), 179–197.
Google Scholar
Ke, Z., & Ng, V. (2019). Automated essay scoring: A survey of the state of the art. Proceedings of the international joint conference on artificial intelligence (pp. 6300–6308).
Kolen, M. J., & Brennan, R. L. (2014). Test equating, scaling, and linking . New York: Springer.
Leckie, G., & Baird, J. A. (2011). Rater effects on essay scoring: A multilevel analysis of severity drift, central tendency, and rater experience. Journal of Educational Measurement, 48 (4), 399–418. https://doi.org/10.1111/j.1745-3984.2011.00152.x
Li, S., Ge, S., Hua, Y., Zhang, C., Wen, H., Liu, T., & Wang, W. (2020). Coupled-view deep classifier learning from multiple noisy annotators. Proceedings of the association for the advancement of artificial intelligence (vol. 34, pp. 4667–4674).
Linacre, J. M. (1989). Many-faceted Rasch measurement . MESA Press.
Linacre, J. M. (2014). A user’s guide to FACETS Rasch-model computer programs .
Liu, O. L., Frankel, L., & Roohr, K. C. (2014). Assessing critical thinking in higher education: Current state and directions for next-generation assessment. ETS Research Report Series, 2014 (1), 1–23. https://doi.org/10.1002/ets2.12009
Liu, T., Ding, W., Wang, Z., Tang, J., Huang, G. Y., & Liu, Z. (2019). Automatic short answer grading via multiway attention networks. Proceedings of the international conference on artificial intelligence in education (pp. 169–173).
Lord, F. (1980). Applications of item response theory to practical testing problems . Routledge.
Lun, J., Zhu, J., Tang, Y., & Yang, M. (2020). Multiple data augmentation strategies for improving performance on automatic short answer scoring. Proceedings of the association for the advancement of artificial intelligence (vol. 34, pp. 13389–13396).
Marco, G. L. (1977). Item characteristic curve solutions to three intractable testing problems. Journal of Educational Measurement, 14 (2), 139–160.
Mesgar, M., & Strube, M. (2018). A neural local coherence model for text quality assessment. Proceedings of the conference on empirical methods in natural language processing (pp. 4328–4339).
Mim, F. S., Inoue, N., Reisert, P., Ouchi, H., & Inui, K. (2019). Unsupervised learning of discourse-aware text representation for essay scoring. Proceedings of the annual meeting of the association for computational linguistics: Student research workshop (pp. 378–385).
Myford, C. M., & Wolfe, E. W. (2003). Detecting and measuring rater effects using many-facet Rasch measurement: Part I. Journal of Applied Measurement, 4 (4), 386–422.
Myford, C. M., & Wolfe, E. W. (2004). Detecting and measuring rater effects using many-facet Rasch measurement: Part II. Journal of Applied Measurement, 5 (2), 189–227.
Myford, C. M., & Wolfe, E. W. (2009). Monitoring rater performance over time: A framework for detecting differential accuracy and differential scale category use. Journal of Educational Measurement, 46 (4), 371–389. https://doi.org/10.1111/j.1745-3984.2009.00088.x
Nadeem, F., Nguyen, H., Liu, Y., & Ostendorf, M. (2019). Automated essay scoring with discourse-aware neural models. Proceedings of the workshop on innovative use of NLP for building educational applications (pp. 484–493).
Nering, M. L., & Ostini, R. (2010). Handbook of polytomous item response theory models . Evanston, IL, USA: Routledge.
Nguyen, H. V., & Litman, D. J. (2018). Argument mining for improving the automated scoring of persuasive essays. Proceedings of the association for the advancement of artificial intelligence (Vol. 32).
Olgar, S. (2015). The integration of automated essay scoring systems into the equating process for mixed-format tests [Doctoral dissertation, The Florida State University].
Patz, R. J., & Junker, B. (1999). Applications and extensions of MCMC in IRT: Multiple item types, missing data, and rated responses. Journal of Educational and Behavioral Statistics, 24 (4), 342–366. https://doi.org/10.3102/10769986024004342
Patz, R. J., Junker, B. W., Johnson, M. S., & Mariano, L. T. (2002). The hierarchical rater model for rated test items and its application to large-scale educational assessment data. Journal of Educational and Behavioral Statistics, 27 (4), 341–384. https://doi.org/10.3102/10769986027004341
Qiu, X. L., Chiu, M. M., Wang, W. C., & Chen, P. H. (2022). A new item response theory model for rater centrality using a hierarchical rater model approach. Behavior Research Methods, 54 , 1854–1868. https://doi.org/10.3758/s13428-021-01699-y
Article PubMed Google Scholar
Ridley, R., He, L., Dai, X. Y., Huang, S., & Chen, J. (2021). Automated cross-prompt scoring of essay traits. Proceedings of the association for the advancement of artificial intelligence (vol. 35, pp. 13745–13753).
Rodriguez, P. U., Jafari, A., & Ormerod, C. M. (2019). Language models and automated essay scoring. https://doi.org/10.48550/arXiv.1909.09482 . arXiv:1909.09482
Rosen, Y., & Tager, M. (2014). Making student thinking visible through a concept map in computer-based assessment of critical thinking. Journal of Educational Computing Research, 50 (2), 249–270. https://doi.org/10.2190/EC.50.2.f
Schendel, R., & Tolmie, A. (2017). Beyond translation: adapting a performance-task-based assessment of critical thinking ability for use in Rwanda. Assessment & Evaluation in Higher Education, 42 (5), 673–689. https://doi.org/10.1080/02602938.2016.1177484
Shermis, M. D., & Burstein, J. C. (2002). Automated essay scoring: A cross-disciplinary perspective . Routledge.
Shin, H. J., Rabe-Hesketh, S., & Wilson, M. (2019). Trifactor models for Multiple-Ratings data. Multivariate Behavioral Research, 54 (3), 360–381. https://doi.org/10.1080/00273171.2018.1530091
Stan Development Team. (2018). RStan: the R interface to stan . R package version 2.17.3.
Sung, C., Dhamecha, T. I., & Mukhi, N. (2019). Improving short answer grading using transformer-based pre-training. Proceedings of the international conference on artificial intelligence in education (pp. 469–481).
Taghipour, K., & Ng, H. T. (2016). A neural approach to automated essay scoring. Proceedings of the conference on empirical methods in natural language processing (pp. 1882–1891).
Tran, T. D. (2020). Bayesian analysis of multivariate longitudinal data using latent structures with applications to medical data. (Doctoral dissertation, KU Leuven).
Uto, M. (2021a). Accuracy of performance-test linking based on a many-facet Rasch model. Behavior Research Methods, 53 , 1440–1454. https://doi.org/10.3758/s13428-020-01498-x
Uto, M. (2021b). A review of deep-neural automated essay scoring models. Behaviormetrika, 48 , 459–484. https://doi.org/10.1007/s41237-021-00142-y
Uto, M. (2023). A Bayesian many-facet Rasch model with Markov modeling for rater severity drift. Behavior Research Methods, 55 , 3910–3928. https://doi.org/10.3758/s13428-022-01997-z
Uto, M., & Okano, M. (2021). Learning automated essay scoring models using item-response-theory-based scores to decrease effects of rater biases. IEEE Transactions on Learning Technologies, 14 (6), 763–776. https://doi.org/10.1109/TLT.2022.3145352
Uto, M., & Ueno, M. (2018). Empirical comparison of item response theory models with rater’s parameters. Heliyon, Elsevier , 4 (5), , https://doi.org/10.1016/j.heliyon.2018.e00622
Uto, M., & Ueno, M. (2020). A generalized many-facet Rasch model and its Bayesian estimation using Hamiltonian Monte Carlo. Behaviormetrika, Springer, 47 , 469–496. https://doi.org/10.1007/s41237-020-00115-7
van der Linden, W. J. (2016). Handbook of item response theory, volume two: Statistical tools . Boca Raton, FL, USA: CRC Press.
Vaswani, A., Shazeer, N., Parmar, N., Uszkoreit, J., Jones, L., Gomez, A. N., & Polosukhin, I. (2017). Attention is all you need. Advances in neural information processing systems (pp. 5998–6008).
Wang, Y., Wei, Z., Zhou, Y., & Huang, X. (2018). Automatic essay scoring incorporating rating schema via reinforcement learning. Proceedings of the conference on empirical methods in natural language processing (pp. 791–797).
Watanabe, S. (2010). Asymptotic equivalence of Bayes cross validation and widely applicable information criterion in singular learning theory. Journal of Machine Learning Research, 11 , 3571–3594. https://doi.org/10.48550/arXiv.1004.2316
Watanabe, S. (2013). A widely applicable Bayesian information criterion. Journal of Machine Learning Research, 14 (1), 867–897. https://doi.org/10.48550/arXiv.1208.6338
Wilson, M., & Hoskens, M. (2001). The rater bundle model. Journal of Educational and Behavioral Statistics, 26 (3), 283–306. https://doi.org/10.3102/10769986026003283
Wind, S. A., & Guo, W. (2019). Exploring the combined effects of rater misfit and differential rater functioning in performance assessments. Educational and Psychological Measurement, 79 (5), 962–987. https://doi.org/10.1177/0013164419834613
Wind, S. A., & Jones, E. (2019). The effects of incomplete rating designs in combination with rater effects. Journal of Educational Measurement, 56 (1), 76–100. https://doi.org/10.1111/jedm.12201
Wind, S. A., Wolfe, E. W., Jr., G.E., Foltz, P., & Rosenstein, M. (2018). The influence of rater effects in training sets on the psychometric quality of automated scoring for writing assessments. International Journal of Testing, 18 (1), 27–49. https://doi.org/10.1080/15305058.2017.1361426
Zitzmann, S., & Hecht, M. (2019). Going beyond convergence in Bayesian estimation: Why precision matters too and how to assess it. Structural Equation Modeling: A Multidisciplinary Journal, 26 (4), 646–661. https://doi.org/10.1080/10705511.2018.1545232
Download references
This work was supported by Japan Society for the Promotion of Science (JSPS) KAKENHI Grant Numbers 19H05663, 21H00898, and 23K17585.
Author information
Authors and affiliations.
The University of Electro-Communications, Tokyo, Japan
Masaki Uto & Kota Aramaki
You can also search for this author in PubMed Google Scholar
Corresponding author
Correspondence to Masaki Uto .
Ethics declarations
Conflict of interest.
The authors declare that they have no conflicts of interest.
Ethics approval
Not applicable
Consent to participate
Consent for publication.
All authors agreed to publish the article.
Open Practices Statement
All results presented from our experiments for all models, including MFRM, MFRM with RSS, and GMFM, as well as the results for each repetition, are available for download at https://github.com/AI-Behaviormetrics/LinkingIRTbyAES.git . This repository also includes programs for performing our linking method, along with a sample dataset. These programs were developed using R and Python, along with RStan and PyTorch. Please refer to the README file for information on program usage and data format details.
Additional information
Publisher's note.
Springer Nature remains neutral with regard to jurisdictional claims in published maps and institutional affiliations.
Appendix A: Data splitting procedures
In this appendix, we explain the detailed procedures used to construct the reference group and the focal group while aiming to ensure distinct distributions of examinee abilities and rater severities, as outlined in experimental Procedure 2 in the Experimental procedures section.
Let \(\mu ^{\text {all}}_\theta \) and \(\sigma ^{\text {all}}_\theta \) be the mean and SD of the examinees’ abilities estimated from the entire dataset in Procedure 1 of the Experimental procedures section. Similarly, let \(\mu ^{\text {all}}_\beta \) and \(\sigma ^{\text {all}}_\beta \) be the mean and SD of the rater severity parameter estimated from the entire dataset. Using these values, we set target mean and SD values of abilities and severities for both the reference and focal groups. Specifically, let \(\acute{\mu }^{\text {ref}}_{\theta }\) and \(\acute{\sigma }^{\text {ref}}_{\theta }\) denote the target mean and SD for the abilities of examinees in the reference group, and \(\acute{\mu }^{\text {ref}}_{\beta }\) and \(\acute{\sigma }^{\text {ref}}_{\beta }\) be those for the rater severities in the reference group. Similarly, let \(\acute{\mu }^{\text {foc}}_{\theta }\) , \(\acute{\sigma }^{\text {foc}}_{\theta }\) , \(\acute{\mu }^{\text {foc}}_{\beta }\) , and \(\acute{\sigma }^{\text {foc}}_{\beta }\) represent the target mean and SD for the examinee abilities and rater severities in the focal group. Each of the eight conditions in Table 1 uses these target values, as summarized in Table 14 .
Given these target means and SDs, we constructed the reference and focal groups for each condition through the following procedure.
Prepare the entire set of examinees and raters along with their ability and severity estimates. Specifically, let \(\hat{\varvec{\theta }}\) and \(\hat{\varvec{\beta }}\) be the collections of ability and severity estimates, respectively.
Randomly sample a value from the normal distribution \(N(\acute{\mu }^{\text {ref}}_\theta , \acute{\sigma }^{\text {ref}}_\theta )\) , and choose an examinee with \(\hat{\theta }_j \in \hat{\varvec{\theta }}\) nearest to the sampled value. Add the examinee to the reference group, and remove it from the remaining pool of examinee candidates \(\hat{\varvec{\theta }}\) .
Similarly, randomly sample a value from \(N(\acute{\mu }^{\text {ref}}_\beta ,\acute{\sigma }^{\text {ref}}_\beta )\) , and choose a rater with \(\hat{\beta }_j \in \hat{\varvec{\beta }}\) nearest to the sampled value. Then, add the rater to the reference group, and remove it from the remaining pool of rater candidates \(\hat{\varvec{\beta }}\) .
Repeat Steps 2 and 3 for the focal group, using \(N(\acute{\mu }^{\text {foc}}_\theta , \) \(\acute{\sigma }^{\text {foc}}_\theta )\) and \(N(\acute{\mu }^{\text {foc}}_\beta ,\acute{\sigma }^{\text {foc}}_\beta )\) as the sampling distributions.
Continue to repeat Steps 2, 3, and 4 until the pools \(\hat{\varvec{\theta }}\) and \(\hat{\varvec{\beta }}\) are empty.
Given the examinees and raters in each group, create the data for the reference group \(\textbf{U}^{\text {ref}}\) and the focal group \(\textbf{U}^{\text {foc}}\) .
Remove examinees from each group, as well as their data, if they have received scores from only one rater, thereby ensuring that each examinee is graded by at least two raters.
Appendix B: Experimental results for MFRM and MFRM with RSS
The experiments discussed in the main text focus on the results obtained from GMFM, as this model demonstrated the best fit to the dataset. However, it is important to note that our linking method is not restricted to GMFM and can also be applied to other models, including MFRM and MFRM with RSS. Experiments involving these models were carried out in the manner described in the Experimental procedures section, and the results are shown in Tables 15 and 16 . These tables reveal trends similar to those observed for GMFM, validating the effectiveness of our linking method under the MFRM and MFRM with RSS as well.
Rights and permissions
Open Access This article is licensed under a Creative Commons Attribution 4.0 International License, which permits use, sharing, adaptation, distribution and reproduction in any medium or format, as long as you give appropriate credit to the original author(s) and the source, provide a link to the Creative Commons licence, and indicate if changes were made. The images or other third party material in this article are included in the article’s Creative Commons licence, unless indicated otherwise in a credit line to the material. If material is not included in the article’s Creative Commons licence and your intended use is not permitted by statutory regulation or exceeds the permitted use, you will need to obtain permission directly from the copyright holder. To view a copy of this licence, visit http://creativecommons.org/licenses/by/4.0/ .
Reprints and permissions
About this article
Uto, M., Aramaki, K. Linking essay-writing tests using many-facet models and neural automated essay scoring. Behav Res (2024). https://doi.org/10.3758/s13428-024-02485-2
Download citation
Accepted : 26 July 2024
Published : 20 August 2024
DOI : https://doi.org/10.3758/s13428-024-02485-2
Share this article
Anyone you share the following link with will be able to read this content:
Sorry, a shareable link is not currently available for this article.
Provided by the Springer Nature SharedIt content-sharing initiative
- Writing assessment
- Many-facet Rasch models
- IRT linking
- Automated essay scoring
- Educational measurement
- Find a journal
- Publish with us
- Track your research

Band 4+: the diagram illustrates the process of lithography, a method of printing newspapers
The flow-chart demonstrates the stage called “lithoprint”a process used for printing newspapers.
There are four main stages in the process of this, beginning with the white plate is lifted onto the rollers and the completed paper is transferred onto the paper
Firstly, the plate is lifted through rollers which provides by the mix of water and chemical. Second, the plate passes through a set of ink rollers in order to the ink will stick full of the image area. Thirdly, the plate through the transfer and blanket cylinders combine with impression cylinder to paper pressed here. After that, the paper is dried with hot and cold air and putting on the delivery pile
Check Your Answer On This Topic?
Generate a band-9 sample answer, overall band score, task response, coherence & cohesion, lexical resource, grammatical range & accuracy, answers on the same topic:, the diagram illustrates the process of lithography, a method of printing newspapers.
The given diagram below depicts an intricate process called “lithography” which is used for imprinting newspapers. Generally, this is a linear process involving six main stages, commencing with the implementing water and ink and culminating with dehydrating printed images via hot and cool air of the transfer cylinders. In the first stage, blank paper is […]
The diagram illustrates the process of how to print newspapers. Overall, the process involves six main stages, starting with putting the paper in the machine and ending with drying it by hot and cold air. First, the paper is rolled through the plate cylinder to absorb the water from the roller. Secondly, it is gone […]
The diagram illustrates the process of how to print newspapers. Overall the process involves 6 main stage, starting with putting the paper in the machine and ending with drying it by hot and cold air. First, the paper is rolled through the plate cylinder to absorb the water from the roller. Secondly, it is gone […]
The diagram illustrates how a method of printing newspapers called lithography is processed. The process includes several steps, in which the paper is processed through a number of cylinders. Firstly, the paper is pressed through a plate cylinder, where it is damped by water from the rollers. Secondly, it is passed through the ink rollers […]
Other Topics:
The diagram shows the design of a modern landfill for household waste. summarize the information by selecting and reporting the main features and make comparisons where relevant..
The picture illustrates how a current landfill is constructed for the disposal of domestic waster. From an overall perspective, this artificial process involves a number of distinct stages, beginning from excavation a large hole, following by further constructed and installed drainage system, then it come to using stage and ending with the formation gas come […]
The diagram below shows the various stages involved in publishing a book. Summarise the information by selecting and reporting the main features, and make comparisons where relevant.
The flowchart illustrates the process by which books are published. Overall, it is evident that there are nine stages in the diagram, starting from writing books and ending with distributing them to different bookshops. Notably, the process through which a book is published takes approximately 18-24 months and can be divided into three main phases. […]
The flow chart illustrates the process by which a book is published. Overall, the process entails nine steps, starting with writing a book and ending with distributing it to bookshops. It is also clear that the process is divided into two main stages: writing a book in an appropriate way and publishing it. Notably, a […]
The flowchart illustrates the process by which books are published. Overall, it is evident that there are 9 stages in the diagram, starting from writing books and ending with distributing them to different bookshops. Notably, the process through which a book is published takes approximately 18-24 months and can be divided into three main phases. […]
The diagram shows the design of a modern landfill for household waste. Summarize the information by selecting and reporting the main features, and make comparisons where relevant.
The given diagram illustrated a blueprint of a modern landfill for household wastes Overall, there are 3 main designs of a landfill: the construction, the working method, and the closure. It can be seen that a two-layer base in which household refuse is kept and toxic liquids are removed from the landfill by the use […]
Plans & Pricing
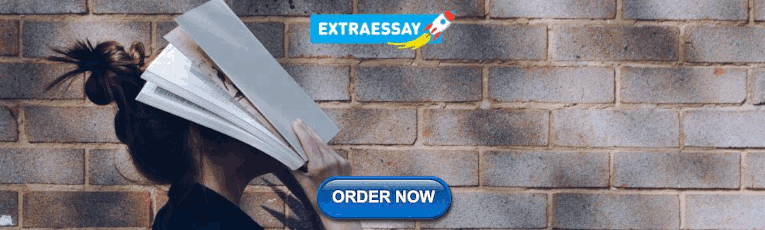
IMAGES
COMMENTS
The TRIAC Model. The TRIAC model refers to a writing method for organizing and developing paragraphs requiring evidence and analysis. The TRIAC is beneficial for: Approaching prompt-based writing assignments in which students are required to draw on their prior knowledge to write a compelling, cohesive composition (Unit 7).
TRIAC can help writers explain their ideas in depth. TRIAC paragraphs (or paragraph sequences) feature these elements: Topic. Start the paragraph by introducing a topic that supports or complicates your thesis - the central problem or idea that the paragraph aims to explore. Better topic sentences function as a mini-thesis and make a claim ...
In writing, it is important to begin by making your point clear and concise. We do this through a thesis statement when considering an essay, and through a topic sentence when considering a paragraph. In the upcoming weeks, we will be working on building solid, evidence-supported paragraphs using the TRIAC method.
TRIAC: Paragraph and Paper Organization TRIAC is a writing pattem you can use at the paragraph level for strong organization and effective argument. The same components in TRIAC paragraphs can give entire papers stronger organization. TRIAC has five parts: T Topic Sentence - The first sentence introduces the subject of a paragraph, essentially serving as a miniature thesis statement. R ...
Then state what aspects of the subject you are going to write about and prove. Strategy for Success: Begin with your second-‐strongest point as Key Term 1. Use your weakest point in the middle of your essay as Key Term 2. Finish with your strongest point as Key Term 3.
In an informal essay, it may be fine to stop there. In formal academic writing, however, the reader will still be waiting for your analysis of the evidence you've given. A paragraph like this is often followed by another paragraph in which that evidence is discussed... and that second paragraph often follows a basic or TRIAC structure.
The TRIAC Model A simple method for organizing and developing paragraphs that require evidence and analysis.
WHAT ARE PARAGRAPHS? Paragraphs group related sentences around one main point, so the paragraphs can work together to prove the larger argument (the thesis) in an essay. Paragraphs provide visual breaks between ideas and signal a progression of ideas in the essay.
B Paragraphs 4-6 in body How the Russian Revolution encouraged or thwarted innovation If you use the block method, do not append two disconnected essays to an introductory thesis. The B block, or second half of your essay, should refer to the A block, or first half, and make clear points of comparison whenever comparisons are relevant.
TRIAC paragraph structure Learn with flashcards, games, and more — for free.
Might also evaluate the connections made. Keep in mind. you are possibly setting up to move smoothly onto the next paragraph. Final sentence or two. Remember. 1. TRIAC can be extended into an essay if the analysis runs long. 2. The illustration and analysis sections can be repeated several times.
The PEEL paragraph method is a technique used in writing to help structure paragraphs in a way that presents a single clear and focused argument, which links back to the essay topic or thesis statement. It's good practice to dedicate each paragraph to one aspect of your argument, and the PEEL structure simplifies this for you.
Teach two different formats of compare and contrast essays, point-by-point or block, in order to sharpen critical thinking and writing skills.
Portable Walls for Academic Writing is a quick-reference guide that aids students as they plan to write précis (summaries) and essays of varying lengths. It includes a clear explanation of the TRIAC model-a template for organized, logical paragraphs. It also provides examples of note-taking methods for written sources and oral presentations.
Learn how to master the art of writing essays with our comprehensive guide, covering tips, techniques, and strategies for crafting compelling and impactful essays on any topic.
I hope you will be, too. As you learn about this method of formatting a paragraph, remember that just as the Classical Oration format is just one method of developing an essay, the TRIAC is just one method out of many of developing a paragraph. The letters in the made-up term "TRIAC" stand for parts in a paragraph.
Learn about the triode for alternative currents (TRIAC), including its construction, circuit characteristics, applications, and testing procedures.
The essay contains several grammatical errors, including subject-verb agreement and pronoun usage, which affect the clarity and fluency of the writing. Essays On The Same Topic: Some think newspapers are the best method for reading the news while others think other media is better.
Writing assistance materials essay writing triac 11:56 am the letters in the term stand for parts in paragraph. these parts might be sentences or they could be
Essay structure: Introduction (with the article's title and author's name) that must contain your thesis At least three TRIAC body paragraphs (with in-text parenthetical citations of the articles)A ConclusionNote:-## I uploaded a file for the (TRIAC method of paragraphing). ## every thing is uploaded in files.
For essay-writing tests, challenges arise when scores assigned to essays are influenced by the characteristics of raters, such as rater severity and consistency. Item response theory (IRT) models incorporating rater parameters have been developed to tackle this issue, exemplified by the many-facet Rasch models. These IRT models enable the estimation of examinees' abilities while accounting ...
The image details the lithography process for printing newspapers: beginning with dampening rollers applying water, followed by ink rollers applying ink onto a plate cylinder, which transfers the inked image to a blanket cylinder; the impression cylinder presses paper against the blanket cylinder to transfer the image; finally, printed images pass through a series of transfer cylinders to be ...