
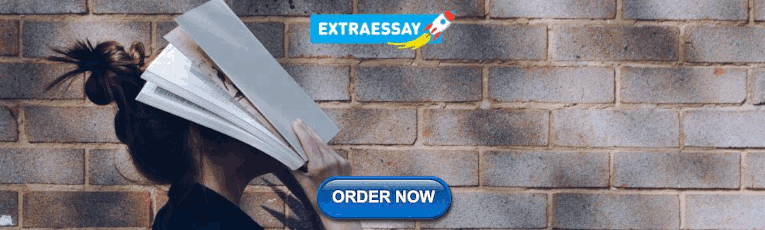
Site Content
Statistics in social work.
An Introduction to Practical Applications
Amy Batchelor
Columbia University Press

Pub Date: November 2019
ISBN: 9780231193276
Format: Paperback
List Price: $30.00 £25.00
Shipping Options
Purchasing options are not available in this country.
ISBN: 9780231193269
Format: Hardcover
List Price: $90.00 £75.00
ISBN: 9780231550222
Format: E-book
List Price: $29.99 £25.00
- EPUB via the Columbia UP App
- PDF via the Columbia UP App
Statistics in Social Work is a practical and effective resource for social work students. Batchelor requires no prior knowledge of statistics from her readers and explains topics in plain language with relatable examples. Most importantly, she offers a social justice perspective that emphasizes and integrates the core value of the social work profession. Ashley Davis, Boston University
This is an excellent introduction to statistics for both students and practitioners in social work—it demystifies terms and procedures and uses real world examples to help the reader to see the everyday applicability of statistical knowledge, whether in practice or in study. John Devaney, coauthor of Quantitative Research Methods for Social Work: Making Social Work Count
About the Author
- Social Work
- Mathematics
- Social Sciences
- Social Work: Research Methods
- Architecture and Design
- Asian and Pacific Studies
- Business and Economics
- Classical and Ancient Near Eastern Studies
- Computer Sciences
- Cultural Studies
- Engineering
- General Interest
- Geosciences
- Industrial Chemistry
- Islamic and Middle Eastern Studies
- Jewish Studies
- Library and Information Science, Book Studies
- Life Sciences
- Linguistics and Semiotics
- Literary Studies
- Materials Sciences
- Mathematics
- Social Sciences
- Sports and Recreation
- Theology and Religion
- Publish your article
- The role of authors
- Promoting your article
- Abstracting & indexing
- Publishing Ethics
- Why publish with De Gruyter
- How to publish with De Gruyter
- Our book series
- Our subject areas
- Your digital product at De Gruyter
- Contribute to our reference works
- Product information
- Tools & resources
- Product Information
- Promotional Materials
- Orders and Inquiries
- FAQ for Library Suppliers and Book Sellers
- Repository Policy
- Free access policy
- Open Access agreements
- Database portals
- For Authors
- Customer service
- People + Culture
- Journal Management
- How to join us
- Working at De Gruyter
- Mission & Vision
- De Gruyter Foundation
- De Gruyter Ebound
- Our Responsibility
- Partner publishers

Your purchase has been completed. Your documents are now available to view.
Statistics in Social Work
An introduction to practical applications.
- Amy Batchelor
- X / Twitter
Please login or register with De Gruyter to order this product.
- Language: English
- Publisher: Columbia University Press
- Copyright year: 2019
- Audience: Professional and scholarly;
- Other: 50 figures
- Published: November 19, 2019
- ISBN: 9780231550222
Social Work Research Methods That Drive the Practice
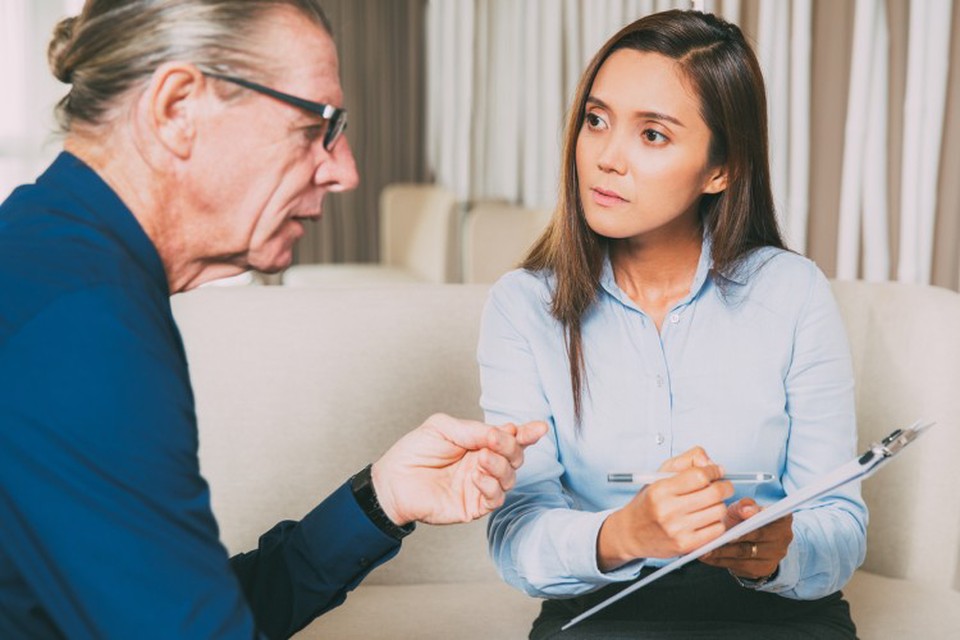
Social workers advocate for the well-being of individuals, families and communities. But how do social workers know what interventions are needed to help an individual? How do they assess whether a treatment plan is working? What do social workers use to write evidence-based policy?
Social work involves research-informed practice and practice-informed research. At every level, social workers need to know objective facts about the populations they serve, the efficacy of their interventions and the likelihood that their policies will improve lives. A variety of social work research methods make that possible.
Data-Driven Work
Data is a collection of facts used for reference and analysis. In a field as broad as social work, data comes in many forms.
Quantitative vs. Qualitative
As with any research, social work research involves both quantitative and qualitative studies.
Quantitative Research
Answers to questions like these can help social workers know about the populations they serve — or hope to serve in the future.
- How many students currently receive reduced-price school lunches in the local school district?
- How many hours per week does a specific individual consume digital media?
- How frequently did community members access a specific medical service last year?
Quantitative data — facts that can be measured and expressed numerically — are crucial for social work.
Quantitative research has advantages for social scientists. Such research can be more generalizable to large populations, as it uses specific sampling methods and lends itself to large datasets. It can provide important descriptive statistics about a specific population. Furthermore, by operationalizing variables, it can help social workers easily compare similar datasets with one another.
Qualitative Research
Qualitative data — facts that cannot be measured or expressed in terms of mere numbers or counts — offer rich insights into individuals, groups and societies. It can be collected via interviews and observations.
- What attitudes do students have toward the reduced-price school lunch program?
- What strategies do individuals use to moderate their weekly digital media consumption?
- What factors made community members more or less likely to access a specific medical service last year?
Qualitative research can thereby provide a textured view of social contexts and systems that may not have been possible with quantitative methods. Plus, it may even suggest new lines of inquiry for social work research.
Mixed Methods Research
Combining quantitative and qualitative methods into a single study is known as mixed methods research. This form of research has gained popularity in the study of social sciences, according to a 2019 report in the academic journal Theory and Society. Since quantitative and qualitative methods answer different questions, merging them into a single study can balance the limitations of each and potentially produce more in-depth findings.
However, mixed methods research is not without its drawbacks. Combining research methods increases the complexity of a study and generally requires a higher level of expertise to collect, analyze and interpret the data. It also requires a greater level of effort, time and often money.
The Importance of Research Design
Data-driven practice plays an essential role in social work. Unlike philanthropists and altruistic volunteers, social workers are obligated to operate from a scientific knowledge base.
To know whether their programs are effective, social workers must conduct research to determine results, aggregate those results into comprehensible data, analyze and interpret their findings, and use evidence to justify next steps.
Employing the proper design ensures that any evidence obtained during research enables social workers to reliably answer their research questions.
Research Methods in Social Work
The various social work research methods have specific benefits and limitations determined by context. Common research methods include surveys, program evaluations, needs assessments, randomized controlled trials, descriptive studies and single-system designs.
Surveys involve a hypothesis and a series of questions in order to test that hypothesis. Social work researchers will send out a survey, receive responses, aggregate the results, analyze the data, and form conclusions based on trends.
Surveys are one of the most common research methods social workers use — and for good reason. They tend to be relatively simple and are usually affordable. However, surveys generally require large participant groups, and self-reports from survey respondents are not always reliable.
Program Evaluations
Social workers ally with all sorts of programs: after-school programs, government initiatives, nonprofit projects and private programs, for example.
Crucially, social workers must evaluate a program’s effectiveness in order to determine whether the program is meeting its goals and what improvements can be made to better serve the program’s target population.
Evidence-based programming helps everyone save money and time, and comparing programs with one another can help social workers make decisions about how to structure new initiatives. Evaluating programs becomes complicated, however, when programs have multiple goal metrics, some of which may be vague or difficult to assess (e.g., “we aim to promote the well-being of our community”).
Needs Assessments
Social workers use needs assessments to identify services and necessities that a population lacks access to.
Common social work populations that researchers may perform needs assessments on include:
- People in a specific income group
- Everyone in a specific geographic region
- A specific ethnic group
- People in a specific age group
In the field, a social worker may use a combination of methods (e.g., surveys and descriptive studies) to learn more about a specific population or program. Social workers look for gaps between the actual context and a population’s or individual’s “wants” or desires.
For example, a social worker could conduct a needs assessment with an individual with cancer trying to navigate the complex medical-industrial system. The social worker may ask the client questions about the number of hours they spend scheduling doctor’s appointments, commuting and managing their many medications. After learning more about the specific client needs, the social worker can identify opportunities for improvements in an updated care plan.
In policy and program development, social workers conduct needs assessments to determine where and how to effect change on a much larger scale. Integral to social work at all levels, needs assessments reveal crucial information about a population’s needs to researchers, policymakers and other stakeholders. Needs assessments may fall short, however, in revealing the root causes of those needs (e.g., structural racism).
Randomized Controlled Trials
Randomized controlled trials are studies in which a randomly selected group is subjected to a variable (e.g., a specific stimulus or treatment) and a control group is not. Social workers then measure and compare the results of the randomized group with the control group in order to glean insights about the effectiveness of a particular intervention or treatment.
Randomized controlled trials are easily reproducible and highly measurable. They’re useful when results are easily quantifiable. However, this method is less helpful when results are not easily quantifiable (i.e., when rich data such as narratives and on-the-ground observations are needed).
Descriptive Studies
Descriptive studies immerse the researcher in another context or culture to study specific participant practices or ways of living. Descriptive studies, including descriptive ethnographic studies, may overlap with and include other research methods:
- Informant interviews
- Census data
- Observation
By using descriptive studies, researchers may glean a richer, deeper understanding of a nuanced culture or group on-site. The main limitations of this research method are that it tends to be time-consuming and expensive.
Single-System Designs
Unlike most medical studies, which involve testing a drug or treatment on two groups — an experimental group that receives the drug/treatment and a control group that does not — single-system designs allow researchers to study just one group (e.g., an individual or family).
Single-system designs typically entail studying a single group over a long period of time and may involve assessing the group’s response to multiple variables.
For example, consider a study on how media consumption affects a person’s mood. One way to test a hypothesis that consuming media correlates with low mood would be to observe two groups: a control group (no media) and an experimental group (two hours of media per day). When employing a single-system design, however, researchers would observe a single participant as they watch two hours of media per day for one week and then four hours per day of media the next week.
These designs allow researchers to test multiple variables over a longer period of time. However, similar to descriptive studies, single-system designs can be fairly time-consuming and costly.
Learn More About Social Work Research Methods
Social workers have the opportunity to improve the social environment by advocating for the vulnerable — including children, older adults and people with disabilities — and facilitating and developing resources and programs.
Learn more about how you can earn your Master of Social Work online at Virginia Commonwealth University . The highest-ranking school of social work in Virginia, VCU has a wide range of courses online. That means students can earn their degrees with the flexibility of learning at home. Learn more about how you can take your career in social work further with VCU.
From M.S.W. to LCSW: Understanding Your Career Path as a Social Worker
How Palliative Care Social Workers Support Patients With Terminal Illnesses
How to Become a Social Worker in Health Care
Gov.uk, Mixed Methods Study
MVS Open Press, Foundations of Social Work Research
Open Social Work Education, Scientific Inquiry in Social Work
Open Social Work, Graduate Research Methods in Social Work: A Project-Based Approach
Routledge, Research for Social Workers: An Introduction to Methods
SAGE Publications, Research Methods for Social Work: A Problem-Based Approach
Theory and Society, Mixed Methods Research: What It Is and What It Could Be
READY TO GET STARTED WITH OUR ONLINE M.S.W. PROGRAM FORMAT?
Bachelor’s degree is required.
VCU Program Helper
This AI chatbot provides automated responses, which may not always be accurate. By continuing with this conversation, you agree that the contents of this chat session may be transcribed and retained. You also consent that this chat session and your interactions, including cookie usage, are subject to our privacy policy .
Want to create or adapt books like this? Learn more about how Pressbooks supports open publishing practices.
Module 4 Chapter 2: Working With Quantitative Descriptive Data
Prior modules introduced quantitative approaches and methods, including study design and data collection methods. This chapter examines what investigators do with collected quantitative data to begin answering their research questions. As you work your way through the contents of this chapter, you will find it helpful to have a basic calculator handy (even one on your smart phone will do). In addition, you are presented with links to the Excel workbook for learning activities that need to be completed using a computer (data analyses do not work on most tablets or phones).
In this chapter you will learn:
- the distinction between univariate and bivariate analyses,
- descriptive statistics with categorical and numeric variables,
- computing and interpreting basic inferential statistics
- understanding graphs, figures, and tables with basic statistics.
Univariate and Bivariate Analyses
The way we think about quantitative variables has to do with how they are operationalized in a particular study. First, let us consider the distinction between univariate and bivariate work with quantitative variables, then explore distinctions between independent and dependent variables. When the goal is to describe a social work problem, diverse population, or social phenomenon along a particular dimension, investigators engage in univariate analyses . The prefix “uni” means one (like a unicycle having one wheel).
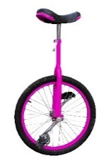
Univariate Analysis . Univariate analysis means that one variable is analyzed at a time; if multiple variables are of interest, univariate analyses are repeated for each. Univariate analysis has the purpose of describing the data variable-by-variable, and are often referred to as descriptive statistics , or simply as “descriptives.” See Table 2-1 for an example of how this might appear in a research report. The example is based on a subset of data about the Safe At Home instrument developed to assess readiness to change among men entering a treatment program to address their intimate partner violence behavior (Begun et al., 2003). The study participants are described in terms of several variables, with the descriptive statistics reported for each variable, one at a time.
Table 2-1. Univariate descriptive data example for N=520* men entering treatment
Back to Basics: Rounding Numbers
Rounding numbers: The note at the bottom of Table 1 mentions “rounding” as a reason why totals might not equal 100%. Rounding is often used when there are more digits than necessary to convey the important message. For example, the fraction 2/3 is the same as 0.666666 with the “6s” repeating indefinitely when 2 is divided by 3. In social work, social science, and behavioral research, it is seldom necessary to go beyond two or three decimal places. We “round up” when the next digit is 5 or higher (5-9) and “round down” when the next digit is less than 5 (0-4). In our example, 0.666666 would be rounded up to 0.67. The decimal version of 1/3, 0.333333 with the “3s” repeating indefinitely, would be rounded down to 0.33. Thus, with slight rounding error being introduced, we might end up with a total slightly lower or higher than 100%.
Bivariate Analysis . When investigators examine the relationship between two variables, they are conducting bivariate analysis (“bi” meaning two, as in a bicycle having two wheels). This is contrasted to the descriptive work using univariate analysis (one variable at a time).
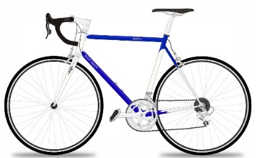
For example, investigators might be interested to know if two variables related to each other (correlated or associated). Turning back to the Safe at Home example (Begun et al., 2003), the variable indicating men’s readiness to change their intimate partner violence behavior was positively correlated with the variable about their assuming responsibility for the behavior: men with higher readiness to change scores were more likely to assume responsibility for their behavior than were men with lower readiness scores. Looking at this pair of variables together, in relation to each other, is an example of bivariate analysis. Other examples of research questions leading to bivariate analysis include: having a history of child maltreatment victimization associated with school absenteeism in a Swedish study (Hagborg, Berglund, & Fahlke, 2018) and mothers’ smoking during pregnancy being associated with children’s behavioral regulation problems (and other variables) at the age of 12 years (Minnes, e Add New t al., 2016).
How Univariate and Bivariate Analyses Are Used: In earlier modules you were introduced to the types of research questions that lend themselves to quantitative approaches (Module 2) and to different kinds of quantitative variables (Module 3). Likewise, the type of data analyses and reports that investigators generate are directly related to the nature of the questions the data answer. Univariate and bivariate analyses are used descriptively. In other words, when the question to be answered calls for a description of a population, social work problem, or social phenomenon, these are useful analyses to conduct and present. Univariate and bivariate analyses help develop a picture of how the numeric values for each quantitative variable are distributed among a sample of study participants. Bivariate analyses are also used to test hypotheses about the relationships between variables—the subject of Chapters 3, 4, and 5 in this module. The next section goes into greater detail as to the nature of descriptive analyses.
Univariate Descriptive Analysis
Here we explore ways that investigators might analyze specific types of variables in order to help answer their research questions. First, our focus is on descriptive statistics: statistics that help describe populations and groups, or the dimensions of social work problems and social phenomena. As we work through these Module 4 materials, you may find that the question “Statistics or sadistics?” asked in the book Statistics for people who (think they) hate statistics (Salkind, 2017) changes to the statement “Statistics, not sadistics!”
Descriptive Statistics
Descriptive statistics help develop the picture of a situation. Since the point of variables is that their values vary across individual cases, investigators need to understand the way those values are distributed across a population or in a study sample. The three main features about distribution are of great interest to investigators:
- central tendency, and
These three features are reported for one variable at a time (univariate analysis). The descriptive statistics reported about a variable depend on the type of variable: categorical, ordinal, and interval variables that you learned about in Module 3. In Module 3 you also learned about descriptive research: quantitative descriptive research reports the results of descriptive statistical analyses. Descriptive analyses are reported for variables used in quantitative exploratory and explanatory studies as background for understanding the additional (bivariate and other) statistical analyses reported.
Frequency Analysis: Categorical Variables
The first descriptive analyses are concerned with frequency. Frequency is a count of how much, how often, or how many, depending on what the variable was measuring.
Frequency Count. Counting “how many” is among the simplest descriptive statistics. In the Safe At Home example above (Table 2-1), investigators wanted to know how many men participating in the study entered batterer treatment voluntarily and how many were mandated to enter treatment by court order (nonvoluntary clients). As you can see in Table 2-2, the total number of participating clients was 520 (this would be written as N=520, where the capital N reflects total sample size). In addition, you can see that the study included 96 “voluntary” clients and 424 “nonvoluntary” clients (this is sometimes written as n=96 and n=424, where the lower case “n” is about a group within the larger, full set where we used “N”). Not only do we see that more clients entered nonvoluntarily, we can calculate how many more nonvoluntary clients there were: 424 – 96 = 328 more nonvoluntary than voluntary clients participated in the study.
Table 2-2. Frequency report for voluntary and nonvoluntary participants.
Interactive Excel Workbook Activities
Complete the following Workbook Activity:
- SWK 3401.4-2.1 Frequency Counts
Pie-Chart. The pie-chart is a useful tool for graphically presenting frequency data. It is a quick, easily-interpreted visual device. Here is a sample pie chart depicting the nonvoluntary and voluntary client frequency (and proportion) data described in Table 2-2.
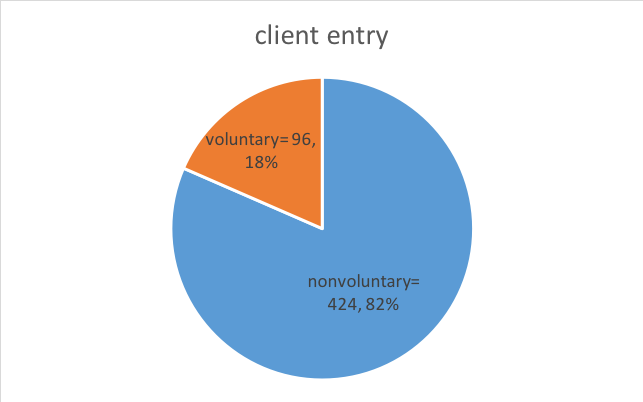
Frequency, Proportion, or Percentage. The investigators in our example not only wished to know how many voluntary and nonvoluntary clients were involved in their study, they also wanted to consider their relative proportions . You might wonder why it matters since they already know how many men were in each group, and that there were 328 more involuntary than voluntary clients in the study. It matters because the investigators wish to evaluate how meaningful that difference might be. A difference of 328 clients is proportionately large in a sample of 520, but the same 328 would be proportionately small among a very large sample such as 5,200.
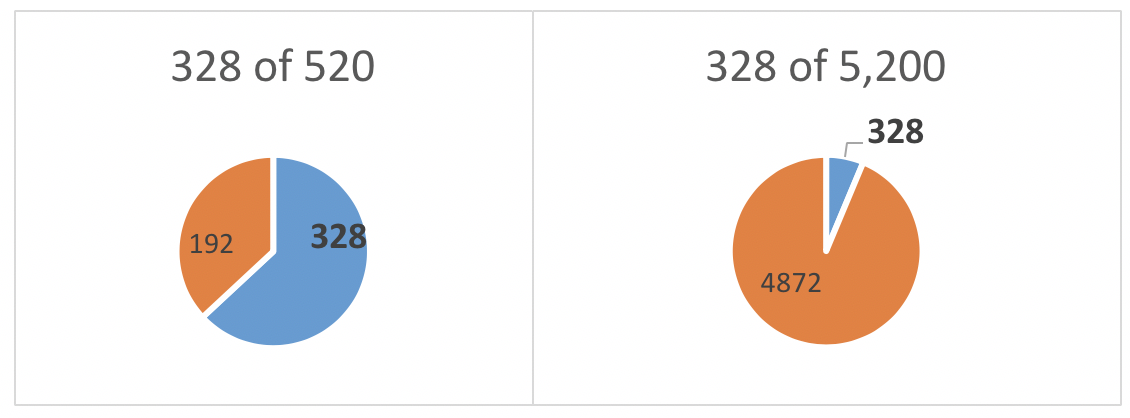
Back to Basics: Converting Fractions to Decimal Units
Mathematical operations are simpler when fractions are expressed in decimals. To make the conversion, think about what the fraction stands for: a top number (numerator) over a bottom number (denominator) means to divide the numerator by the denominator. Example: 3/4 means divide 3 by 4, so 3/4 = .75
A proportion is simply a fraction, or ratio, calculated by dividing the number in each group (fraction numerator) by the total number in the whole sample (fraction denominator). To simplify interpretation, the fraction is often converted to percent—the proportion of 100% represented in the fraction.
- For nonvoluntary clients, this would be computed as: (424/520) x 100%, which comes out to be (.815) x 100%, or 81.5%.
- For voluntary clients, this would be computed as: (96/520) x 100%, which comes out to be (.185) x 100%, or 18.5%.
The investigators provided these percentages in Table 2-2; the percentages total to 100% (81.5% + 18.5% = 100%). You can see that the two groups were not equivalent in actual size, relative proportion, or percentages of the total sample. In Chapters 4 and 5 you will learn about statistical analysis approaches that allow investigators to test if the size of the observed difference is statistically significant.
- SWK 3401.4-2.2 Proportion and Percentage
Univariate Central Tendency Analysis: Numeric Variables
Knowing the frequency with which certain categories or levels of a categorical variable are reported in the data is useful information, as witnessed above. Frequency counts are less useful in understanding data for a numeric variable, however. For example, in the Safe At Home study, it would be difficult to interpret information about the participants’ ages: 3 were age 18, 7 were age 19, 17 were age 20, 8 were age 21, 14 were age 22, and so forth for all 520 individuals between 18 to 72 years. A more useful way of looking at these numeric (interval type) data is to consider how the study participants’ values for age were distributed and how any individual’s values for age might differ from the rest of the group. Ideally, we would like to see a meaningful summary of the data.
The concept of central tendency refers to a single value that summarizes numeric data by characterizing the “center” values of a data set—a single value around which individuals’ values tend to cluster. Central tendency helps make sense of the variability observed among participants on a numeric variable. The most commonly used central tendency ways to summarize data are:
- median, and
For example, within a classroom of public school students we might see many different values for the variable “number of days absent” during a school year. Most states in the U.S. require 180 student instruction days in the school year. Let’s consider a hypothetical example (see Table 2-3) where hypothetically:
- we have a class of 28 students
- the number of days absent for each student ranges from 0 to 49 out of the year’s 180 days
- the total number of absent days for the class members combined was 194.
Table 2-3: Number of days absent for each student in (hypothetical) class.
Back to Basics: Computing and Average or Mean Value
Step 1. Add up the values for each member of the group on the variable of interest Step 2. Divide that total by the number of values there were in the group (how many individual members contributed to that total). Step 3. The result is the “average” value for the group, also called the “mean” value (written as a capital, italicized M ).
Our goal is to find a single central tendency value that helps represent the group on this days absent variable; summarizing the variable without having to rely on all 28 values at once. Let’s look at each of the common central tendency options.
Mean. The first central tendency indicator we consider is the mean. You might recognize this as numeric average; average and mean for a set of values are the same thing. The mean is computed by adding up all the values, then dividing by the actual number of values. In our example, we would first find the sum of the number of days absent across all the students together (194), then divide that total by the number of students contributing to that total (N=28). This would be the mean value for our students on the variable for number of days absent: 194 divided by 28 (194/28) = 6.93 with rounding. We would report this as M =6.93.
- SWK 3401.4-2.3 Computing a Mean
Median . The median is the next common central tendency feature, and it can be thought of as the half-way point in a distribution of values. In other words, it is the point where half of the values are higher and half of the values are lower. Another name for the median is the 50th percentile—since 50% is halfway to 100%. In other words, the 50th percentile is the value where 50% (half) of the scores are lower and the other 50% (other half) are higher. In our classroom absenteeism example, the median value is 3 days: three is the point where half of the students had more and half had fewer days absent. We can easily compute that half of the 28 students is 14, so we identify the median point as where the lowest 14 values are included and all of the higher values are excluded—14 students had scores of 3 or less. We would report this information as: Mdn=3 .
Mode. The last common measure of central tendency to consider is the mode. Sometimes it is useful to know the most common value for a variable. This is simply a frequency count—how many times did each value appear in our data. For our student absenteeism data, the most common value was 0—six of our students did not miss any days of school (see Table 2-4). This would be written as Mode=0.
Table 2-4. Frequency of each value for days absent example.
- SWK 3401.4-2.4 Computing Descriptive Statistics (Mean, Median, Standard Deviation)
Comparing Mean, Median, and Mode. Why wouldn’t we always use the mean to describe the variable of interest? The answer is demonstrated in our student absenteeism example: even a few extreme values (sometimes called outliers ) can seriously distort or skew the picture. The most common value (mode) was 0 but we had a couple of students with some really high values compared to the others: 26 and 49. When we add those two extreme values into the total number of absent days to compute the group mean, they have a big impact on the total (194): the mean for the 28 students was 6.93 days absent. Without those two extreme values, the total would have been 119, making the mean for the 26 remaining students (28 – 2 = 26) only 4.6 days (119/26=4.6). This mean of 4.6 days is considerably less than the mean of 6.93 days we observed with these two extreme students included. Thus, you can see that the two extreme values had a powerful skewing impact on the mean.
As a real-world example, consider data about the 2017 incomes (salary plus bonuses) for 1,999 NFL professional football players. The range was $27,353 to $23,943,600—a difference between 5-figure and 8-figure incomes! In 2017:
- mean player income was $1,489,042 (we would report this as M =$1,489,042),
- median income was $687,500 ( Mdn =$687,500), and
- mode income was $465,000.
The mean and median incomes are very different, and the mean differs markedly from the mode, as well. This NFL player income example demonstrates what data look like when great disparities exist between individuals across a group or population—because the mean is highly sensitive to extreme values, there exist large differences between the mean, the median, and the mode. This is an important social work and social justice issue in poverty, health, incarceration, and other rates where large disparities between population groups exist.
In a relatively small sample (like our 28-student classroom), one or two extreme values made a big difference between the mean and median. Think about the mean income of people in a room where everyone is a social work student learning about social justice issues. Then, think about what would happen to that mean if Bill Gates arrived as a guest speaker invited to talk about social justice on a global scale. Just that one person would drastically change the summary picture based on mean income. However, the median would not really shift much; Bill Gates would be only one person out of the total number of people in the room; the halfway point is not affected much by one person being extreme, since the median is a frequency count of people on either side of the central midpoint value regardless of how far out the values are spread. The mode also is not affected since Bill Gates’ one extreme value is certainly not going to be the most common value (otherwise it would not be extreme). This all leads to a discussion of the importance in understanding the nature of variance as it relates to distribution of values for a variable.
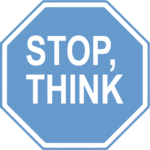
Take a moment to complete the following activity.
Univariate Data Distribution: Numeric Variables
Central tendency is about how the set of values for a specific variable are similar, how they cluster around a mean, median, or mode value. However, investigators are also interested in how individual values differ. This is a curiosity about how those values are distributed or spread across a population or sample: the range and variance of the values. These elements are explained below, along with the more commonly reported standard deviation .
Range. Turning once again to our school absenteeism example, we can see that there exist very great differences in the numbers of days absent among the students in this class. The minimum value observed was 0 and the maximum value observed was 49. The range of values in a set of data is computed as the highest value minus the lowest value. In our example, the range would be 49 – 0 = 49 days absent. It is just a coincidence that the range in our example equals the highest value: this is only because our lowest value was 0 (if the lowest value was different from zero, the range would be different from the highest value).
The range has its greatest meaning when we understand the context in which it exists: a 49-point range is large for the possible 180 days but would not be if the context for possible values was 1,800 instead. Therefore, it becomes helpful to consider the range in ratio or percent absent days, giving the range greater meaning. This is calculated as the observed value divided by the possible value for the ratio, then multiplying by 100% to determine percent.
- Missing 0 of 180 days possible is a division (ratio) problem: (0/180) x 100% = 0 x 100% = 0% days absent;
- Missing 49 of 180 days possible is computed as (49/180) x 100% = 27.2 x 100% = 27.2% days absent.
- Thus, the range is 0% to 27.2% days absent.
Back to Basics: Subtracting Negative Numbers
Computing the range can be a little tricky if the lowest value is a negative number and the highest value is a positive number–unless you remember that subtracting a negative number is the same as adding it as a positive number (the two negative signs cancel each other out). For example: if the lowest value is -4 and the highest value is 26, then the range would be computed as: (26) – (-4) = (26) + (4) = 30
Variance . One way of thinking about variability in the data is to compare each individual’s score to the mean for the group—how far from that central, summary value each individual’s value falls. When individuals’ scores are clustered very close to the mean, the variance is small; when individuals’ scores are spread far from the mean, the variance is large.
This sequence of steps describes how variance is computed in a sample.
- Step 1. Compute the mean of all the values together (overall mean).
- Step 2. Compute the distance of each individual value from that mean—this becomes a subtraction problem where the mean is subtracted from each individual score, showing the “difference” between the individual score and the group mean.
- Step 3. Multiply each of those distance values by itself (square the difference value). Why bother with this step, you might wonder? This is done to resolve potential problems with negative numbers from our earlier subtraction step. Some values were smaller than the mean and some were larger than the mean—when we subtract the mean from those smaller values, we end up with a negative number. In our next step (step 4) we are going to add the computed distances together. If we add in negative numbers, we are essentially subtracting values from the total which does not give an accurate picture of the total distances from the mean. To solve this negative number problem, we capitalize on the fact that multiplying two negative numbers together gives a positive value. Therefore, if we multiply a negative value by itself (square a negative difference score) we get a positive distance value (the distance squared).
- Step 4. This step is where we add up the squared distance values to get a single total (sum) of the squared distances.
Back to Basics: Multiplying With Negative Numbers
Remember that multiplying two numbers with the same sign results in a positive sign number, whether those two signs are both positive or both negative. So, when multiplying two negative numbers, the negative signs cancel each other out.
positive x positive = positive positive x negative = negative negative x negative = positive
Here is what it looks like with our school absenteeism example.
- Step 1. We know that the mean is 6.93 from our earlier discussion about means.
- (2 – 6.93) = -4.93 (a negative number).
- (-4.93)2= (-4.93) x (-4.93) = 24.3 (rounding)
- Step 4. After computing each student’s squared difference, we add them up (sum or total).
- Step 5. We take that total value and divide it by one less than the number of students contributing data: (28-1) = 27. The value we end up with for variance in the class is 109.25 (see Table 2-5).
Table 2-5. Computing variance in student absenteeism example: (2949.86)/(27)=109.25
Standard Deviation
Working with variances can be unwieldy, for two reasons. A practical reason is that the numbers can become very large, making them difficult to work with. Another practical reason is that variance is a bit difficult to interpret. Remember when we calculated the square of the differences in days absent minus the mean? We started with the unit of analysis being days absent. By squaring the values, our units are now the square of the difference in days absent. That just is not very intuitive. Instead, we find it easier to convert the figure back to the unit of days of absent. To do this is straightforward math: we take the square root of the variance of the squared distances. This gets both the value and the units back to a more interpretable, practical place.
- Square root of 109.254 is 10.452 (using the square root calculator on www.math.com )
- Cross-check this yourself: multiply 10.452 x 10.452 and see what result you get—it is slightly different in the third decimal place because of rounding.
- Thus, the standard deviation in our example is reported as: sd =10.45 days absent.
The way to think about standard deviation is that the greater a standard of deviation is, the more variable the values are—the more they spread rather than cluster around the mean. This is an important aspect of understanding the distribution of values on the variable of interest. A graph is also very helpful.
Graphing Numeric Variables
The way the numeric values of a variable are distributed can be visualized using what is called a histogram . The histogram is a graph where the x -axis represents the set of observed values for a specific variable (a univariate analysis), and the y -axis represents how many times each of those values was observed in the data (see Figure 2-1). You can see from the height of the bars that the frequencies begin to taper off after about 4 days absent and how far from the rest of the class those few extreme students are situated.
Figure 2-1. Histogram depicting distribution of days absent data, mean, and median.
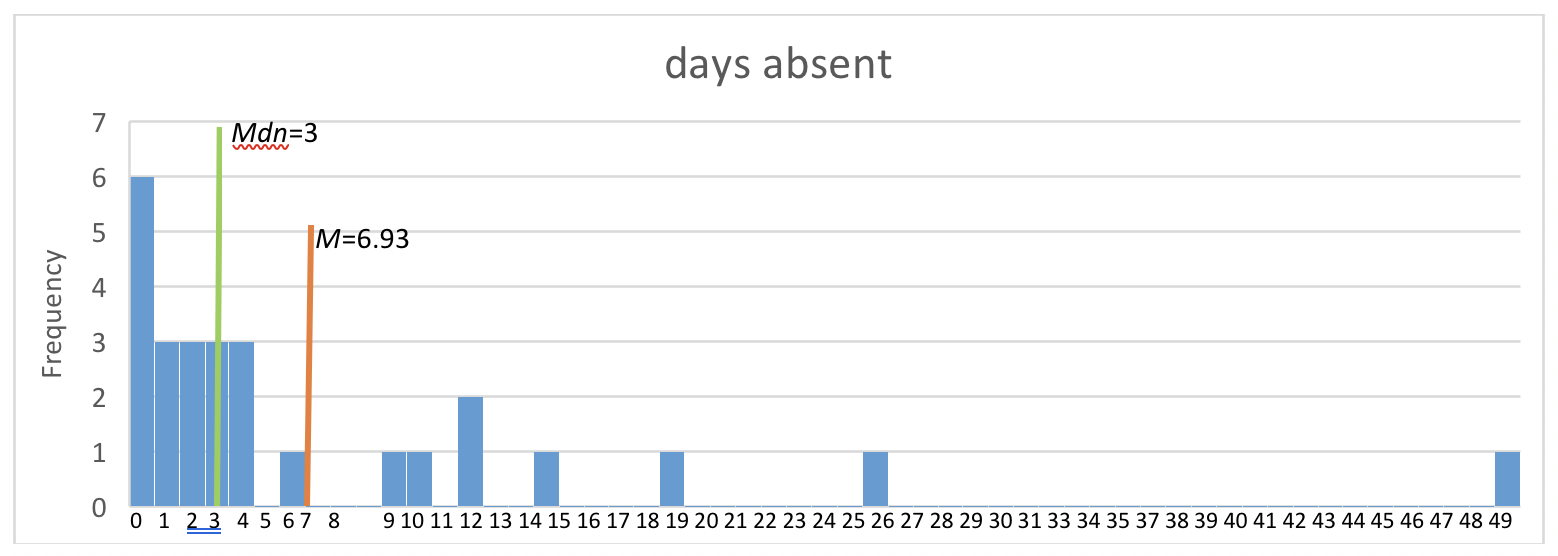
Another clue about the degree of spread or variability is the relative size of the sample’s standard deviation compared to the sample mean. In our example, the sd =10.45 is greater than the M =6.93. This indicates that a great deal of spread exists in the data. This observation relates to understanding the characteristics of normal distribution.
NORMAL DISTRIBUTION
You have probably been exposed to at least the general concept of the bell curve or normal curve at some point in your education. A normal distribution curve is sometimes called a “bell” curve because of its shape: high in the middle, low in the “tails,” and even on both sides of center. Bell-shaped curves do not necessarily meet all criteria of a normal distribution curve—you will see examples in this chapter. We focus on the true normal curve at this point, a fundamental concept related to many of the statistics used in social work, social science, and behavioral research.
Our in-depth exploration of its importance in understanding data begins with the picture of a normal distribution curve (Figure 2-2). The type of variable mapped onto a normal distribution curve is an interval or continuous variable—the units are equal intervals across a continuum.
Figure 2-2. Generic normal distribution curve (histogram).
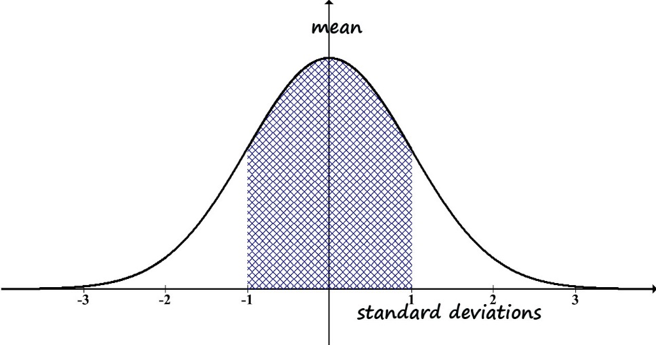
- The mean is the center of the curve.
- The observed data values are distributed equally on either side of the mean—the right and left sides are mirror images. This goes along with the mean being in the center; the right and left ends are called “tails” of the distribution curve.
- The median is equal to the mean. This also goes along with the mean being in the center of the distribution.
- 68% are within one standard deviation of the mean (34% on each side of the mean)—see the grid-marked area under the curve in Figure 2-3.
- Combining all values observed for 2 standard deviations either side of the mean will include a total of 95% of the values (all of those within 1 standard deviation plus those from 1 to 2 standard deviations from the mean).
- Combining all values within 3 standard deviations of the mean accounts for 99% of the values.
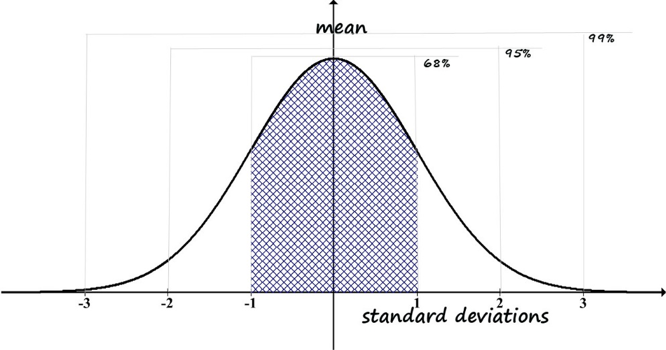
Percentile Scores. You may wonder how this relates to information such as someone having a 95th percentile score on some measure. Look at the right-side tail, out at 2 standard deviations from the mean. You can see that very few scores are way out in the tail beyond that point—in other words, it is rare to be more than 2 standard deviations away from the mean, and exceedingly rare to be more than 3 standard deviations away.
We saw that 95% of the scores fall between -2 and +2 standard deviations. This, however, is NOT the 95th percentile score. The reason it is not has to do with the definition of the 95th percentile: it is the point with 95% of the observed scores falling below and only 5% of the observed scores falling above. In this case, at 2 standard deviation above the mean (+2) we have 95% of scores in the -2 to +2 standard deviation range, which means 5% of scores are outside the range (100% – 95% = 5%). But, these are not all on one tail; there are those scores in the -2 to -3 standard deviation range to contend with. Those scores are also below the point we identified for being 2 standard deviations above the mean. So, using the +2-standard deviation value as our decision criterion, we would actually have half of the left-over 5% (5%/2 = 2.5%), plus the original 95%. This means 2 standard deviations above the mean is the 97.5 percentile (95% + 2.5% = 97.5%). The trick is to keep in mind which standard deviation range is relevant to the question being asked—is it about a range around the mean or is it about a range up to a standard deviation criterion value (see Figure 2-4)?
Figure 2-4. Computation of percentile for +2 SD.
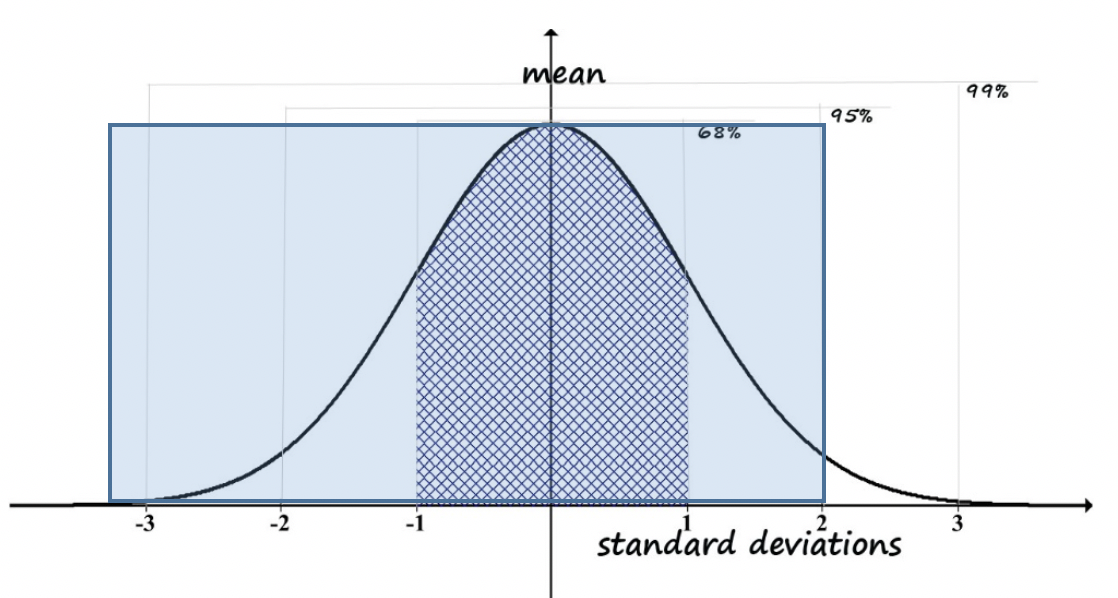
Here is another example to consider. Imagine that policy makers want to provide services to individuals whose IQ scores are equal to or less than 1 standard deviation below the mean (this would be written as: ≤ -1 SD). This decision concerns one tail of the distribution—those at the far left (bottom) of the range, not the mirror tail on the right (top) of the range. If you wanted to determine what percentile would be covered, you would start with figuring out the total percent that fall outside of the -1 to +1 standard deviation range. That would be 100% minus the 68% that ARE in the range: 100% – 68% = 32% outside of the range. We also do not want to include those individuals in the range greater than 1 standard deviation above the mean (> +1 SD). They account for half of the number who are outside the -1 to +1 standard deviation range, which was 32%. So, we are going to subtract half of 32% (32%/2 is 16%) and we are left with only 16% of individuals falling below 1 standard deviation being eligible for the services (see Figure 2-5).
Figure 2-5. Computation of percentile for -1 SD.
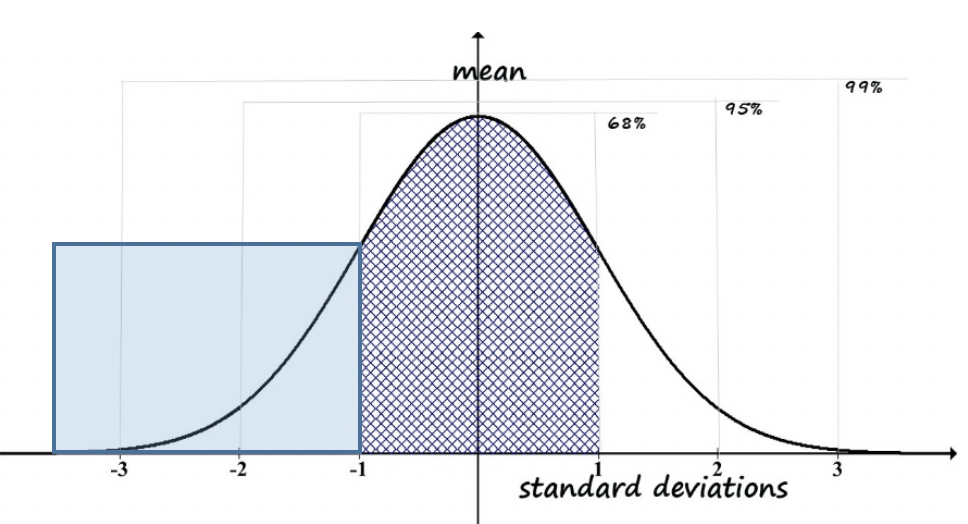
In fact, this scenario became an issue in one state during the 1970s. IQ scores are presumed to distribute on a normal curve with a mean of 100 across the population, and each standard deviation defined by 26 points on the test. As a cost-saving plan, the state determined that services to persons with intellectual developmental disabilities would be offered to individuals whose IQ scores were 68 or below. Previously, the criterion value had been 70. Rather than having “cured” individuals whose scores were between 68 and 70, the state simply ceased providing services to them—several thousand individuals no longer met criteria.
Next, let’s consider the real-world example of NFL player salaries mentioned earlier. Looking at the histogram, it does not seem to be very normally distributed. First, the mean does not seem to be in the middle, the bars to the right and left of the mean are not mirror images, and the median is very much lower than the mean—they are not even close to equal (see Figure 2-6). These are ways in which distribution on a variable fails to be normal.
Figure 2-6. Histogram of NFL player salaries.
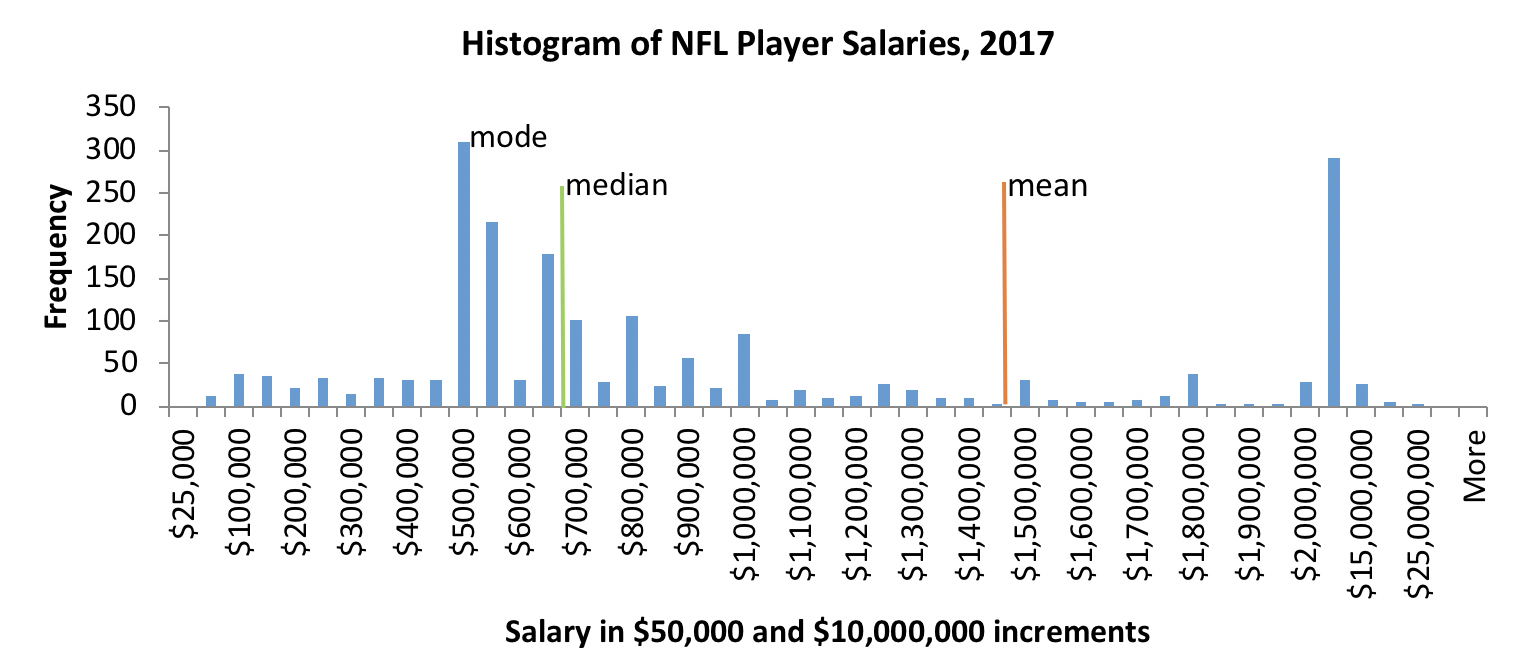
Bimodal Curve. Another way in which distributions are non-normal is when we see a bimodal curve. The normal, or “bell” curve, has only one peak or hump in the data. Sometimes, we get a curve that has two humps. If there had been more players in the 400,000 to 600,000 range we might have seen a second “hump” after the first one at around 500,000 to 600,000.
This scenario is “scary” in statistical terms, because it means that our assumption of normal distribution, the basis of many statistical analyses, has been violated. If you ever read The Little Prince (de Saint-Exupéry, 1943), you might understand the scary picture better. The Little Prince shows his scary picture (Drawing Number one) to the adults, who say a hat is not scary at all.
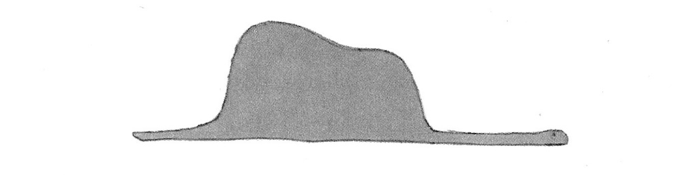
But, the picture is scary because it is not a picture of a hat, it is a picture of a snake who ate an elephant.
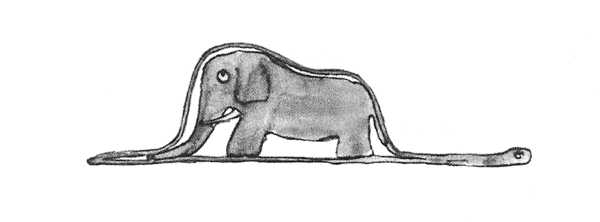
To statisticians, a bimodal curve is a scary picture—it has two “humps.” In other words, there exists a second peak in the data and this needs to be explored. As an example for explaining the bimodal curve in The Little Prince’s Drawing Number One, perhaps the curve is showing salaries where the left peak (lower salaries) is for women and the right peak (higher salaries) is for men; or, it could be about salary differences for two different raceial groups; or, it could depict an inequity or disparity in the frequency for some other variable.
Skew and Kurtosis. Two other ways that a variable might be distributed in a non-normal way is that it is skewed to one side or the other in relation to the mean, and/or the peak of the bell is either too tall or too flat to represent those probabilities we mentioned earlier (e.g., 68% being within one standard deviation of the mean). The first of these is called skew and the second is called kurtosis. A curve with a lot of skew fails to look normal because the curve is no longer symmetrical on both sides of the mean. This is how skewed curves might look:
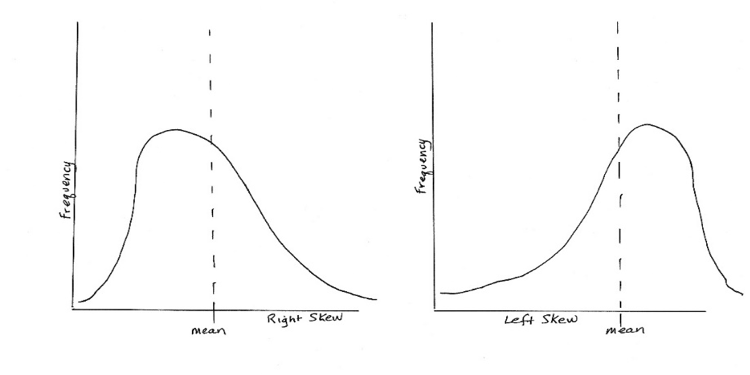
- If the value for skewness is less than -1.0 or greater than +1.0, far from zero, the distribution is highly skewed (asymmetrical or non-normally distributed).
- If the value for skewness is between -1.0 and -0.5, or it is between +.5 and +1.0, then the distribution is considered to be moderately skewed (asymmetrical).
- If the value for skewness is between -0.5 and +0.5 (including zero), the distribution is considered relatively symmetrical.

Kurtosis, on the other hand, has to do with how much of the distribution is sitting out in the tail or tails instead of being in the center “peak” area compared to a normal distribution. On one hand, there may be too few cases in the tails (clustered too much in the “peak”); on the other hand, there may be too many cases in the tails (spread away from the “peak”). This is how curves with kurtosis might look:
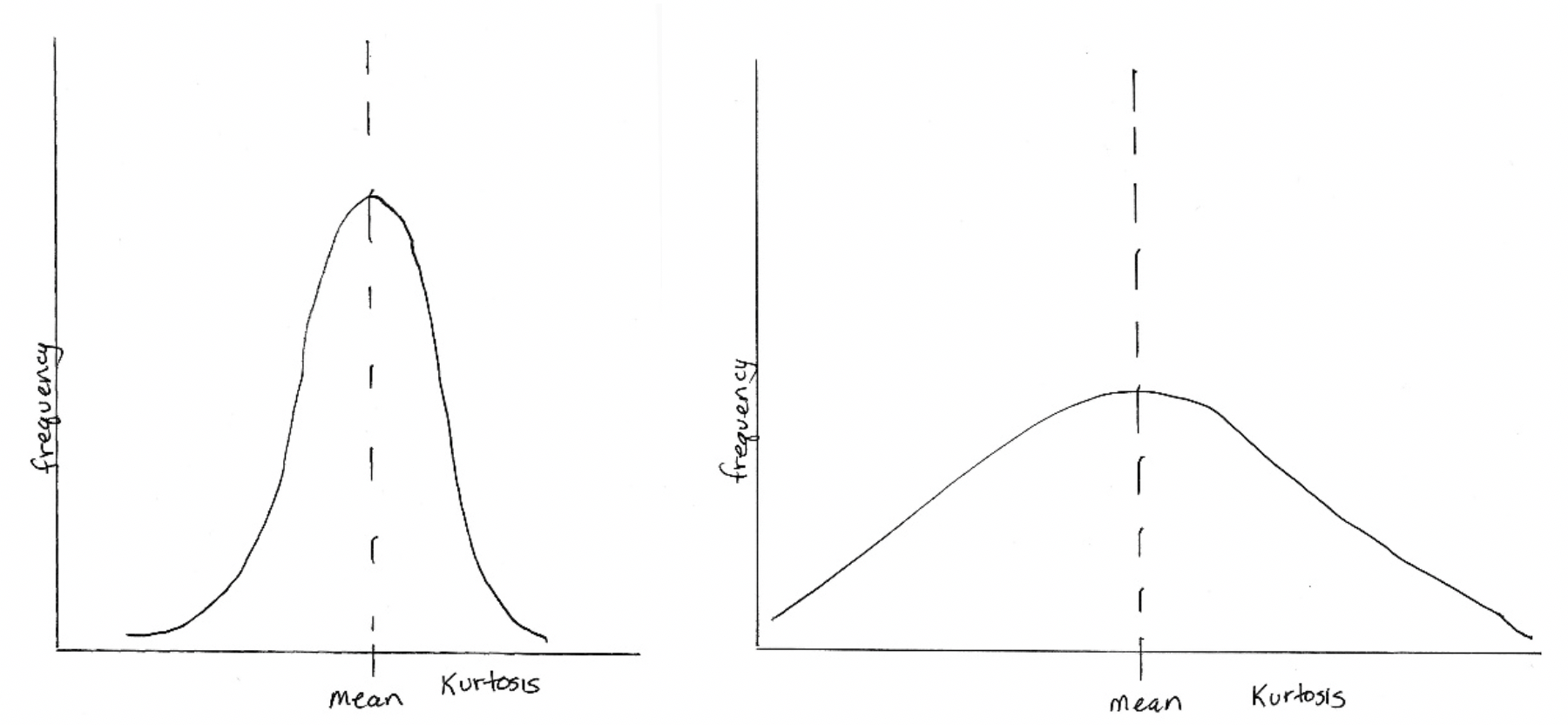
Kurtosis values are a bit more complicated to interpret than skewness values. When we learn about statistical tests of significance we can revisit the issue. In general, kurtosis is considered to be problematic when the value falls outside of the range from -7.0 to +7.0 (for social work, social science, and behavioral research). Some scholars argue for applying criteria with a tighter range, from -2.0 to +2.0.
The website called Seeking Alpha ( α, https://seekingalpha.com/article/2100123-invest-like-taleb-why-skewness-matters ) explains the importance of skew and kurtosis by using an example from Nassim Taleb (2012):
Suppose you placed your grandmother in a room with an average temperature of 70 degrees. For the first hour, the temperature will be -10. For the second hour, it will be 140 degrees. In this case, it looks as though you will end up with no grandmother, a funeral, and possibly an inheritance.
(Note: for our purposes, degrees Celsius were translated into degrees Fahrenheit to make the point from the original example clear.) The website goes on to state:
While this example is rather extreme, it does show one important principle that is highly relevant…It is dispersion around the mean that matters, not necessarily the mean itself.
- SWK 3401.4-2.5 Understanding Skew and Kurtosis
Understanding Graphical Representations: Categorical Variables
There exist at least 3 different ways of presenting the very same information about categorical variables: as text (words) only, as a table of information, or in graphs/figures. We can compare these approaches using a real-world data example: suicide rates by age group, as reported by the Centers for Disease Control and Prevention (CDC, 2017). A “rate” is similar to a frequency: rather than being an actual count (frequency), the frequencies are converted into population proportions. So, we often see a rate described as some number per 1,000 (or per 10,000, or per 100,000) of the population, instead of seeing the actual number of individuals.
Text: During 2016, middle-aged adults between the ages of 45 and 54 represented the group with the highest suicide rate (19.7 per 100,000 persons in the population). This was closely followed by individuals aged 85 or older (19.0 per 100,000), adults aged 55 to 64 (18.7), and older adults in the 75 to 84 age range (18.2 per 100,000). Next were adults in the 35 to 44 age group (17.4 per 100,000), adults in the 65 to 74 age range (16.9 per 100,000), and adults aged 25 to 34 (16.5 per 100,000). The suicide rate among adolescents and young/emerging adults aged 15 to 24 was somewhat lower (13.2 per 100,000), and the very lowest rate occurred among persons under the age of 15 (1.1 per 100,000). The rate for all ages combined was 13.42 per 100,000 persons in the population (age-adjusted).
Table: Table 2-6 presents the same information described in the text above, but much more succinctly.
Table 2-6. Suicide rate per 100,000 by age group during 2016.
Bar Charts: The same information is presented once again in Figure 2-6, but graphically this time, where you can visually compare the height of the bars representing each age group.
Figure 2-6. Suicide rate bar chart.
Chapter Summary
The emphasis in this chapter surrounded univariate descriptive statistics. You learned about describing frequency and percentage results. Then, you learned about the “Central Tendency” statistics most commonly used in social work, social, and behavioral research: mean, median, and mode. You also learned about the statistics concerned with how data are distributed: variance and standard deviation. Related to the issue of data distribution, you learned about normal distribution (and histograms), as well as the nature of skew and kurtosis in relation to non-normal distribution. Finally, you witnessed the power of graphs and table to present univariate statistics information compared to written text. In the next chapter, we begin to explore bivariate analyses.
Social Work 3401 Coursebook Copyright © by Dr. Audrey Begun is licensed under a Creative Commons Attribution-NonCommercial-NoDerivatives 4.0 International License , except where otherwise noted.
Share This Book
- Skip to header navigation
- Skip to primary navigation
- Skip to main content
- Skip to primary sidebar
- Skip to footer
- Member login
International Federation of Social Workers
Global Online conference
Social Work
- Urban Studies
- Victorian Literature
- Browse All Subjects
How to Subscribe
- Free Trials
In This Article Expand or collapse the "in this article" section Social Work Research Methods
Introduction.
- History of Social Work Research Methods
- Feasibility Issues Influencing the Research Process
- Measurement Methods
- Existing Scales
- Group Experimental and Quasi-Experimental Designs for Evaluating Outcome
- Single-System Designs for Evaluating Outcome
- Program Evaluation
- Surveys and Sampling
- Introductory Statistics Texts
- Advanced Aspects of Inferential Statistics
- Qualitative Research Methods
- Qualitative Data Analysis
- Historical Research Methods
- Meta-Analysis and Systematic Reviews
- Research Ethics
- Culturally Competent Research Methods
- Teaching Social Work Research Methods
Related Articles Expand or collapse the "related articles" section about
About related articles close popup.
Lorem Ipsum Sit Dolor Amet
Vestibulum ante ipsum primis in faucibus orci luctus et ultrices posuere cubilia Curae; Aliquam ligula odio, euismod ut aliquam et, vestibulum nec risus. Nulla viverra, arcu et iaculis consequat, justo diam ornare tellus, semper ultrices tellus nunc eu tellus.
- Community-Based Participatory Research
- Economic Evaluation
- Evidence-based Social Work Practice
- Evidence-based Social Work Practice: Finding Evidence
- Evidence-based Social Work Practice: Issues, Controversies, and Debates
- Experimental and Quasi-Experimental Designs
- Impact of Emerging Technology in Social Work Practice
- Implementation Science and Practice
- Interviewing
- Measurement, Scales, and Indices
- Meta-analysis
- Occupational Social Work
- Postmodernism and Social Work
- Qualitative Research
- Research, Best Practices, and Evidence-based Group Work
- Social Intervention Research
- Social Work Profession
- Systematic Review Methods
- Technology for Social Work Interventions
Other Subject Areas
Forthcoming articles expand or collapse the "forthcoming articles" section.
- Child Welfare Effectiveness
- Rare and Orphan Diseases and Social Work Practice
- Unaccompanied Immigrant and Refugee Children
- Find more forthcoming articles...
- Export Citations
- Share This Facebook LinkedIn Twitter
Social Work Research Methods by Allen Rubin LAST REVIEWED: 14 December 2009 LAST MODIFIED: 14 December 2009 DOI: 10.1093/obo/9780195389678-0008
Social work research means conducting an investigation in accordance with the scientific method. The aim of social work research is to build the social work knowledge base in order to solve practical problems in social work practice or social policy. Investigating phenomena in accordance with the scientific method requires maximal adherence to empirical principles, such as basing conclusions on observations that have been gathered in a systematic, comprehensive, and objective fashion. The resources in this entry discuss how to do that as well as how to utilize and teach research methods in social work. Other professions and disciplines commonly produce applied research that can guide social policy or social work practice. Yet no commonly accepted distinction exists at this time between social work research methods and research methods in allied fields relevant to social work. Consequently useful references pertaining to research methods in allied fields that can be applied to social work research are included in this entry.
This section includes basic textbooks that are used in courses on social work research methods. Considerable variation exists between textbooks on the broad topic of social work research methods. Some are comprehensive and delve into topics deeply and at a more advanced level than others. That variation is due in part to the different needs of instructors at the undergraduate and graduate levels of social work education. Most instructors at the undergraduate level prefer shorter and relatively simplified texts; however, some instructors teaching introductory master’s courses on research prefer such texts too. The texts in this section that might best fit their preferences are by Yegidis and Weinbach 2009 and Rubin and Babbie 2007 . The remaining books might fit the needs of instructors at both levels who prefer a more comprehensive and deeper coverage of research methods. Among them Rubin and Babbie 2008 is perhaps the most extensive and is often used at the doctoral level as well as the master’s and undergraduate levels. Also extensive are Drake and Jonson-Reid 2007 , Grinnell and Unrau 2007 , Kreuger and Neuman 2006 , and Thyer 2001 . What distinguishes Drake and Jonson-Reid 2007 is its heavy inclusion of statistical and Statistical Package for the Social Sciences (SPSS) content integrated with each chapter. Grinnell and Unrau 2007 and Thyer 2001 are unique in that they are edited volumes with different authors for each chapter. Kreuger and Neuman 2006 takes Neuman’s social sciences research text and adapts it to social work. The Practitioner’s Guide to Using Research for Evidence-based Practice ( Rubin 2007 ) emphasizes the critical appraisal of research, covering basic research methods content in a relatively simplified format for instructors who want to teach research methods as part of the evidence-based practice process instead of with the aim of teaching students how to produce research.
Drake, Brett, and Melissa Jonson-Reid. 2007. Social work research methods: From conceptualization to dissemination . Boston: Allyn and Bacon.
This introductory text is distinguished by its use of many evidence-based practice examples and its heavy coverage of statistical and computer analysis of data.
Grinnell, Richard M., and Yvonne A. Unrau, eds. 2007. Social work research and evaluation: Quantitative and qualitative approaches . 8th ed. New York: Oxford Univ. Press.
Contains chapters written by different authors, each focusing on a comprehensive range of social work research topics.
Kreuger, Larry W., and W. Lawrence Neuman. 2006. Social work research methods: Qualitative and quantitative applications . Boston: Pearson, Allyn, and Bacon.
An adaptation to social work of Neuman's social sciences research methods text. Its framework emphasizes comparing quantitative and qualitative approaches. Despite its title, quantitative methods receive more attention than qualitative methods, although it does contain considerable qualitative content.
Rubin, Allen. 2007. Practitioner’s guide to using research for evidence-based practice . Hoboken, NJ: Wiley.
This text focuses on understanding quantitative and qualitative research methods and designs for the purpose of appraising research as part of the evidence-based practice process. It also includes chapters on instruments for assessment and monitoring practice outcomes. It can be used at the graduate or undergraduate level.
Rubin, Allen, and Earl R. Babbie. 2007. Essential research methods for social work . Belmont, CA: Thomson Brooks Cole.
This is a shorter and less advanced version of Rubin and Babbie 2008 . It can be used for research methods courses at the undergraduate or master's levels of social work education.
Rubin, Allen, and Earl R. Babbie. Research Methods for Social Work . 6th ed. Belmont, CA: Thomson Brooks Cole, 2008.
This comprehensive text focuses on producing quantitative and qualitative research as well as utilizing such research as part of the evidence-based practice process. It is widely used for teaching research methods courses at the undergraduate, master’s, and doctoral levels of social work education.
Thyer, Bruce A., ed. 2001 The handbook of social work research methods . Thousand Oaks, CA: Sage.
This comprehensive compendium includes twenty-nine chapters written by esteemed leaders in social work research. It covers quantitative and qualitative methods as well as general issues.
Yegidis, Bonnie L., and Robert W. Weinbach. 2009. Research methods for social workers . 6th ed. Boston: Allyn and Bacon.
This introductory paperback text covers a broad range of social work research methods and does so in a briefer fashion than most lengthier, hardcover introductory research methods texts.
back to top
Users without a subscription are not able to see the full content on this page. Please subscribe or login .
Oxford Bibliographies Online is available by subscription and perpetual access to institutions. For more information or to contact an Oxford Sales Representative click here .
- About Social Work »
- Meet the Editorial Board »
- Adolescent Depression
- Adolescent Pregnancy
- Adolescents
- Adoption Home Study Assessments
- Adult Protective Services in the United States
- African Americans
- Aging out of foster care
- Aging, Physical Health and
- Alcohol and Drug Abuse Problems
- Alcohol and Drug Problems, Prevention of Adolescent and Yo...
- Alcohol Problems: Practice Interventions
- Alcohol Use Disorder
- Alzheimer's Disease and Other Dementias
- Anti-Oppressive Practice
- Asian Americans
- Asian-American Youth
- Autism Spectrum Disorders
- Baccalaureate Social Workers
- Behavioral Health
- Behavioral Social Work Practice
- Bereavement Practice
- Bisexuality
- Brief Therapies in Social Work: Task-Centered Model and So...
- Bullying and Social Work Intervention
- Canadian Social Welfare, History of
- Case Management in Mental Health in the United States
- Central American Migration to the United States
- Child Maltreatment Prevention
- Child Neglect and Emotional Maltreatment
- Child Poverty
- Child Sexual Abuse
- Child Welfare
- Child Welfare and Child Protection in Europe, History of
- Child Welfare and Parents with Intellectual and/or Develop...
- Child Welfare, Immigration and
- Child Welfare Practice with LGBTQ Youth and Families
- Children of Incarcerated Parents
- Christianity and Social Work
- Chronic Illness
- Clinical Social Work Practice with Adult Lesbians
- Clinical Social Work Practice with Males
- Cognitive Behavior Therapies with Diverse and Stressed Pop...
- Cognitive Processing Therapy
- Cognitive-Behavioral Therapy
- Community Development
- Community Policing
- Community-Needs Assessment
- Comparative Social Work
- Computational Social Welfare: Applying Data Science in Soc...
- Conflict Resolution
- Council on Social Work Education
- Counseling Female Offenders
- Criminal Justice
- Crisis Interventions
- Cultural Competence and Ethnic Sensitive Practice
- Culture, Ethnicity, Substance Use, and Substance Use Disor...
- Dementia Care
- Dementia Care, Ethical Aspects of
- Depression and Cancer
- Development and Infancy (Birth to Age Three)
- Differential Response in Child Welfare
- Digital Storytelling for Social Work Interventions
- Direct Practice in Social Work
- Disabilities
- Disability and Disability Culture
- Domestic Violence Among Immigrants
- Early Pregnancy and Parenthood Among Child Welfare–Involve...
- Eating Disorders
- Ecological Framework
- Elder Mistreatment
- End-of-Life Decisions
- Epigenetics for Social Workers
- Ethical Issues in Social Work and Technology
- Ethics and Values in Social Work
- European Institutions and Social Work
- European Union, Justice and Home Affairs in the
- Evidence-based Social Work Practice: Issues, Controversies...
- Families with Gay, Lesbian, or Bisexual Parents
- Family Caregiving
- Family Group Conferencing
- Family Policy
- Family Services
- Family Therapy
- Family Violence
- Fathering Among Families Served By Child Welfare
- Fetal Alcohol Spectrum Disorders
- Field Education
- Financial Literacy and Social Work
- Financing Health-Care Delivery in the United States
- Forensic Social Work
- Foster Care
- Foster care and siblings
- Gender, Violence, and Trauma in Immigration Detention in t...
- Generalist Practice and Advanced Generalist Practice
- Grounded Theory
- Group Work across Populations, Challenges, and Settings
- Group Work, Research, Best Practices, and Evidence-based
- Harm Reduction
- Health Care Reform
- Health Disparities
- Health Social Work
- History of Social Work and Social Welfare, 1900–1950
- History of Social Work and Social Welfare, 1950-1980
- History of Social Work and Social Welfare, pre-1900
- History of Social Work from 1980-2014
- History of Social Work in China
- History of Social Work in Northern Ireland
- History of Social Work in the Republic of Ireland
- History of Social Work in the United Kingdom
- HIV/AIDS and Children
- HIV/AIDS Prevention with Adolescents
- Homelessness
- Homelessness: Ending Homelessness as a Grand Challenge
- Homelessness Outside the United States
- Human Needs
- Human Trafficking, Victims of
- Immigrant Integration in the United States
- Immigrant Policy in the United States
- Immigrants and Refugees
- Immigrants and Refugees: Evidence-based Social Work Practi...
- Immigration and Health Disparities
- Immigration and Intimate Partner Violence
- Immigration and Poverty
- Immigration and Spirituality
- Immigration and Substance Use
- Immigration and Trauma
- Impaired Professionals
- Indigenous Peoples
- Individual Placement and Support (IPS) Supported Employmen...
- In-home Child Welfare Services
- Intergenerational Transmission of Maltreatment
- International Human Trafficking
- International Social Welfare
- International Social Work
- International Social Work and Education
- International Social Work and Social Welfare in Southern A...
- Internet and Video Game Addiction
- Interpersonal Psychotherapy
- Intervention with Traumatized Populations
- Intimate-Partner Violence
- Juvenile Justice
- Kinship Care
- Korean Americans
- Latinos and Latinas
- Law, Social Work and the
- LGBTQ Populations and Social Work
- Mainland European Social Work, History of
- Major Depressive Disorder
- Management and Administration in Social Work
- Maternal Mental Health
- Medical Illness
- Men: Health and Mental Health Care
- Mental Health
- Mental Health Diagnosis and the Addictive Substance Disord...
- Mental Health Needs of Older People, Assessing the
- Mental Health Services from 1990 to 2023
- Mental Illness: Children
- Mental Illness: Elders
- Microskills
- Middle East and North Africa, International Social Work an...
- Military Social Work
- Mixed Methods Research
- Moral distress and injury in social work
- Motivational Interviewing
- Multiculturalism
- Native Americans
- Native Hawaiians and Pacific Islanders
- Neighborhood Social Cohesion
- Neuroscience and Social Work
- Nicotine Dependence
- Organizational Development and Change
- Pain Management
- Palliative Care
- Palliative Care: Evolution and Scope of Practice
- Pandemics and Social Work
- Parent Training
- Personalization
- Person-in-Environment
- Philosophy of Science and Social Work
- Physical Disabilities
- Podcasts and Social Work
- Police Social Work
- Political Social Work in the United States
- Positive Youth Development
- Postsecondary Education Experiences and Attainment Among Y...
- Post-Traumatic Stress Disorder (PTSD)
- Practice Interventions and Aging
- Practice Interventions with Adolescents
- Practice Research
- Primary Prevention in the 21st Century
- Productive Engagement of Older Adults
- Profession, Social Work
- Program Development and Grant Writing
- Promoting Smart Decarceration as a Grand Challenge
- Psychiatric Rehabilitation
- Psychoanalysis and Psychodynamic Theory
- Psychoeducation
- Psychometrics
- Psychopathology and Social Work Practice
- Psychopharmacology and Social Work Practice
- Psychosocial Framework
- Psychosocial Intervention with Women
- Psychotherapy and Social Work
- Race and Racism
- Readmission Policies in Europe
- Redefining Police Interactions with People Experiencing Me...
- Rehabilitation
- Religiously Affiliated Agencies
- Reproductive Health
- Restorative Justice
- Risk Assessment in Child Protection Services
- Risk Management in Social Work
- Rural Social Work in China
- Rural Social Work Practice
- School Social Work
- School Violence
- School-Based Delinquency Prevention
- Services and Programs for Pregnant and Parenting Youth
- Severe and Persistent Mental Illness: Adults
- Sexual and Gender Minority Immigrants, Refugees, and Asylu...
- Sexual Assault
- Single-System Research Designs
- Social and Economic Impact of US Immigration Policies on U...
- Social Development
- Social Insurance and Social Justice
- Social Justice and Social Work
- Social Movements
- Social Planning
- Social Policy
- Social Policy in Denmark
- Social Security in the United States (OASDHI)
- Social Work and Islam
- Social Work and Social Welfare in East, West, and Central ...
- Social Work and Social Welfare in Europe
- Social Work Education and Research
- Social Work Leadership
- Social Work Luminaries: Luminaries Contributing to the Cla...
- Social Work Luminaries: Luminaries contributing to the fou...
- Social Work Luminaries: Luminaries Who Contributed to Soci...
- Social Work Regulation
- Social Work Research Methods
- Social Work with Interpreters
- Solution-Focused Therapy
- Strategic Planning
- Strengths Perspective
- Strengths-Based Models in Social Work
- Supplemental Security Income
- Survey Research
- Sustainability: Creating Social Responses to a Changing En...
- Syrian Refugees in Turkey
- Task-Centered Practice
- Technology Adoption in Social Work Education
- Technology, Human Relationships, and Human Interaction
- Technology in Social Work
- Terminal Illness
- The Impact of Systemic Racism on Latinxs’ Experiences with...
- Transdisciplinary Science
- Translational Science and Social Work
- Transnational Perspectives in Social Work
- Transtheoretical Model of Change
- Trauma-Informed Care
- Triangulation
- Tribal child welfare practice in the United States
- United States, History of Social Welfare in the
- Universal Basic Income
- Veteran Services
- Vicarious Trauma and Resilience in Social Work Practice wi...
- Vicarious Trauma Redefining PTSD
- Victim Services
- Virtual Reality and Social Work
- Welfare State Reform in France
- Welfare State Theory
- Women and Macro Social Work Practice
- Women's Health Care
- Work and Family in the German Welfare State
- Workforce Development of Social Workers Pre- and Post-Empl...
- Working with Non-Voluntary and Mandated Clients
- Young and Adolescent Lesbians
- Youth at Risk
- Youth Services
- Privacy Policy
- Cookie Policy
- Legal Notice
- Accessibility
Powered by:
- [66.249.64.20|91.193.111.216]
- 91.193.111.216
- University of Michigan Library
- Research Guides
Social Work
- Statistics for Social Work
- Community Change
- Global Social Work Practice
- Interpersonal Practice in Integrated Health, Mental Health, and Substance Abuse
- Management & Leadership
- Policy & Political Social Work
- Program Evaluation and Applied Research
- Social Work Practice with Older Adults and Families from a Lifespan Perspective
- Welfare of Children & Families
- Article Databases
- Finding Electronic Materials
- Tips for Searching Library Resources
- Data for Social Work
- Anti-racist & Decolonized Social Work
- APA Style, 7th Edition
- Reference Works
- How To's for Students
Demographics and General Statistics
General Statistics & Demographics
- ProQuest Statistical Insight Provides indexes, abstracts and some full-text to federal, state and international statistics. Many of the items indexed are available on the web once you find a title within this database.
- Sage Data Provides access to statistical information produced by U.S. Federal agencies, private companies, and intergovernmental organizations. Create maps and reports from data you select from over 640 datasets. Subjects include health, crime, population, finance, industry, and marketing.
- ProQuest Statistical Abstract of the United States ProQuest has taken over the publication of this annually published statistical resource after the United States discontinued publication in 2012.
- Social Explorer Social Explorer provides easy access to demographic information about the United States. It includes U.S. Census data from 1790 to present as well as data on religions and churches in the U.S. Users can easily create maps or reports within this library database.
- Simply Map Simply Map is another library database with data from the U.S. Census, Center for Disease Control & Prevention and other sources. Create maps or reports for specific geographic areas using this tool.
- Mapping the Measure of America The American Human Development Project, affiliated with the Social Science Research Council developed this tool which shows data on health, education and income in easy to understand graphics.
- Poverty in the United States - National Poverty Center Helpful information on finding information on poverty from the National Poverty Center-University of Michigan.
- Statista Statista includes ready-made statistical charts, graphs and tables on marketing, demographics, communication, technology, politics, health, leisure and public opinion polls. About 20 percent of the data in Statista comes from government sources like the World Bank and the U.S. Census. The rest comes from industry, marketing, and trade groups. The tables, charts and graphs can be downloaded as images or into Microsoft Powerpoint and Excel.
Child & Family Statistics
Child & Family
- Child Welfare Information Gateway - Statistics Reports, databases and demographics on children, fostercare and families in the U.S.
- Kids Count Data Center An important source of data on child well-being on the state and city level. This site, sponsored by the Annie E. Casey Foundation provides data on teen births, children in poverty, prenatal risk factors, as well as demographic, health, education and family well-being data.
- How Many Children are Poor? -- Institute for Research on Poverty A clear explaination of child poverty with links to a number of useful resources for statistics and additional information.
- Children's Defense Fund The Children Defense Fund Research Library provides U.S. Census data related to children, state data related to children and a database for finding publications and information on children at risk.
Sexual Orientation Statistics
Sexual Orientation
- The Williams Institute, UCLA School of Law
- U.S. Census site - Same Sex Couples
- Movement Advancement Project, LGBT Populations
Statistics on Racial, Ethnic & Special Populations
Racial, Ethnic and Special Population Groups
- Arab American Institute
- Pew Hispanic Center
- The Schotts Foundation 50 State Report on Black Males & Education, 2010
- National Center for Veterans Analysis & Statistics
- Disability Statistics
- Immigration Policy Center - Economic & Political Impact of Immigrants, by state
Federal Government Sources
- Committee on Ways & Means U.S. House of Representative's Green Book Annual data and reports on federal programs such as Medicare, Social Security, Supplementary Security Income, Social Services Block Grants, Child Care & Child Welfare
- FedStats A portal to statistical information produced by the Federal Government agencies.
State & Local Statistics
State and Local Statistics
- South East Michigan Council of Governments (SEMCOG) Data, maps and aerial images of southeastern Michigan communities. This site offers community profile information listed by city, county or the entire area of southeast Michigan.
- Michigan.gov Statistics & Demographic Data Links to U.S. Census and Demographic data for Michigan
- Michigan School Data The state of Michigan's Center for Educational Performance & Information provides data on dropout rates, MEAP scores and other measures for early childhood, K-12, post-secondary students and the connections between education and education work and wages
- Kids Count - Michigan Data on the state of Michigan and many Michigan cities related to the well-being of Michigan children.
- Kids Count in Michigan - Michigan League for Human Services Additional sources of information published by the Michigan League for Human Services, Michigan's Children and the Annie E. Casey Foundation
- Michigan Labor Market Information Michigan state, county and city unemployment rates, industrial information, wage information and more.
- City of Ann Arbor Data Catalog Links to data collected by the city of Ann Arbor which can be mapped using Google Earth or ArcGIS.
- Data Driven Detroit Data Driven Detroit (D3) provides accessible, high-quality information and analysis to drive informed decision-making to strenthen communities in Southeast Michigan. The site provides U.S. Census data mapped to neighborhood levels.
- Michigan County Health Rankings A project sponsored by the Robert Wood Johnson Foundation in collaboration with the University of Wisconsin Population Health Institute, these rankings include statistics on morbidity, mortality, health behavior, clinical care and economic factors related to health at the county level.
Health Statistics
- University of Maryland Health Science & Health Services Library Guide Links to a number of health and health care statistics by subject.
- University of Michigan Taubman Library Health Statistics Guide A useful guide to finding health statistics of specific topics, such as by population, environmental health, health indicators, specific disease statistics and more.
- Center for Disease Control & Prevention - Data & Statistics by Topic Lists over 30 diseases and health issues with links on data and statistics for each one.
- Simply Analytics Simply Map is another library database with data from the U.S. Census, Center for Disease Control & Prevention and other sources. Create maps or reports for specific geographic areas using this tool.
- Michigan County Health Rankings A projecty sponsored by the Robert Wood Johnson Foundation in collaboration with the University of Wisconsin Population Health Institute, these rankings include statistics on morbidity, mortality, health behavior, clinical care and economic factors related to health at the county level.
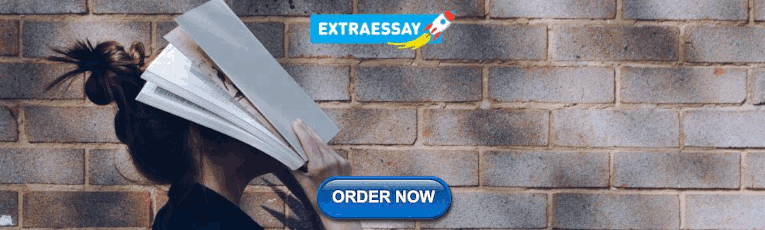
Poverty Statistics
- Current Population Survey A monthly survey conducted by the U.S. Census Bureau and the Bureau of Labor Statistics
- How Many Children Are Poor? - Institute for Research on Poverty
- Poverty in the United States - National Poverty Center
- National Center for Children in Poverty (NCCP) This organization provides many online data tools along with state profiles for demographics, early childhood, adolescent and family economic security statistics.
- Annual Homeless Assessment Report (AHAR) HUD's annual report to Congress estimating the extent of homelessness in the U.S. and assistance programs.
- Homelessness Data Exchange Reports Annual county and city data on homlessness from the years 2007 through 2009 prepared by the Department of Housing and Urban Development (HUD)
- Washtenaw Housing Alliance - Blueprint Progress Report 2004-2011
Crime Statistics
- CrimeMapping.com Select a city and date range to map the number and types of crimes committed over that time period.
- FBI Uniform Crime Reports A compilation of annual crime statistics for the United States
- Bureau of Justice Statistics (BJS) Provides statistics on corrections, federal law enforcement, courts, victims of crime and types of crime. This site also offeres several special data analysis tools.
Data or Statistics?
What's the difference between data and statistics .

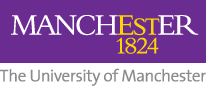
Social Statistics
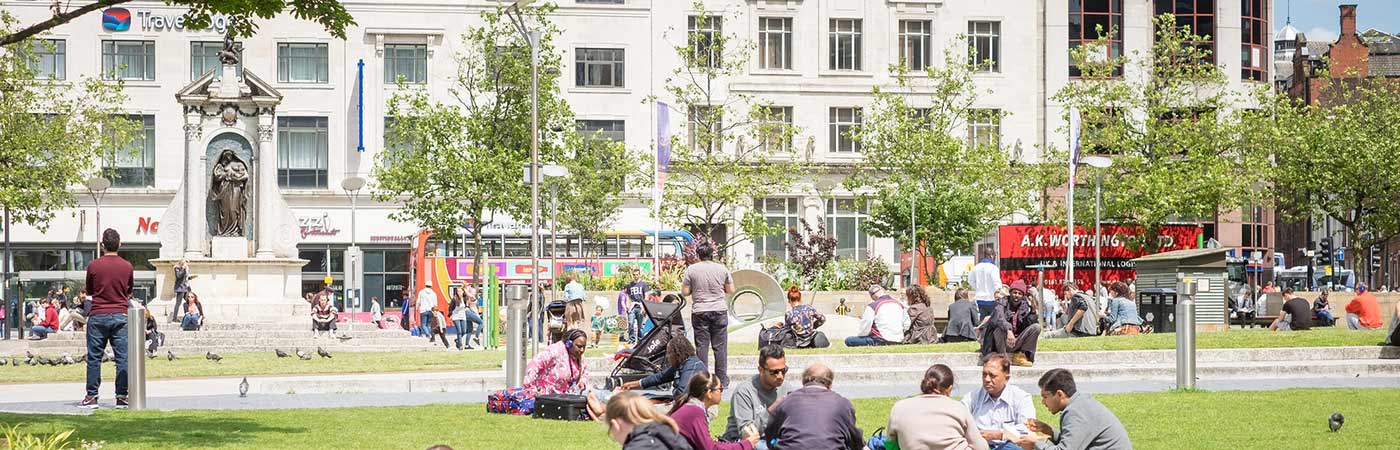
What is Social Statistics?
Social statistics is the use of statistics to study human behaviour and social environments. Social statistics data is information or knowledge on an individual, object or event.
Understanding society
Social statistics are a means of investigating and testing research questions and policy impacts across different areas of people’s lives. These observations can help our understanding of society. Social Statisticians are concerned with such questions as:
- How are populations growing?
- Are wealthy people happier?
- Is society becoming more tolerant of diversity?
- How do people cope with financial hardship?
- Do people with higher qualifications earn more?
- Does volunteering increase your sense of wellbeing?
What are statistics?
Statistics are numbers, summaries of patterns and can also be probabilities.
Statistical analysis can include the design and collection of data, its interpretation and presentation.
Social statistics and quantitative data analysis are key tools for understanding society and social change. We can try to capture people’s attitudes and map patterns in behaviour and circumstances using numbers and also describe how people and populations change.
What is data?
Data can be numerical values or text, sounds or images, memories or perceptions.
Often the concept of data suggests information that has been through some kind of processing and having a structure. However, many examples of new types of data have very different and often unstructured formats; for example, millions of tweets or thousands of PDFs of public documents.
Huge quantities of data on people, organisations and social groups are collected each day, across the world. As social statisticians, it is our role to analyse and make sense of the huge volumes and sources of data using hypothesis-driven social research.
Social statistics in practice

Social statistics are used by The United Nations Social Statistics Division to analyse differences among social groups and countries, covering such issues as housing, health, education, conditions of work and employment.
The Division pay special attention to the study of conditions of special population groups, including children, the elderly, the unemployed, and people with disabilities.
Compare the facts
Social statistics are also used to compare data from before and after a policy intervention.
For example, we need statistics to measure poverty in the first place and we then may want to assess the impact and costs of a policy providing financial support to families living in poverty.
Patterns and relations
Statistical analysis techniques can be used to explore patterns and underlying relationships in data sets, such as:
- in relation to people’s responses to multiple questions in a survey;
- to take account of aspects of people's circumstances such as the unemployment rates of where they live;
- the educational standards of the class and/or school they are studying in;
- to measure change through longitudinal surveys where people are interviewed at different points during their lives.
My master's degree provides a good foundation of statistical and research methods and I have really enjoyed the course units on more advanced statistical methods. Emily Buehler / MSc Social Research Methods and Statistics student
Statistical testing and modelling techniques can be used to generalise from small samples to larger populations, for example:
- predicting the outcome of an election;
- tracking attitudes towards the economy in a country.
Probability tests can be used to identify the key factor(s) associated with a particular outcome or behaviour. For example, are older people more likely to be worried about being a victim of crime than younger people once you have taken account of their family status, education, job and the type of area they live in?
If you want to learn more about studying Social Statistics at The University of Manchester please visit our Courses page to explore our undergraduate courses, master's degrees and PhD programme, or find out what's been happening in the Social Statistics department on our News page .
Further reading
- The Guardian: Data blog
- Radical Statistics Group - Using statistics to support progressive social change
- Ben Goldacre’s Bad Science
Useful organisations
- Royal Statistical Society
- Office for National Statistics
- UK Statistics Authority
- United Nations Statistics Commission
- World Health Organisation
Discover more
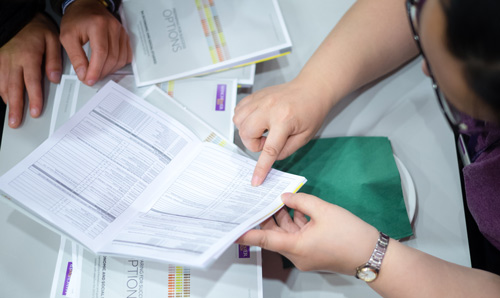
Social Statistics courses
Explore our undergraduate and master's degree options, and PhD opportunities.
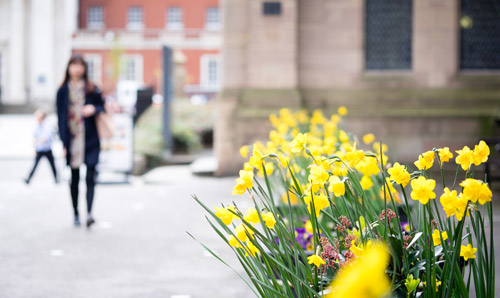
Why study here?
Both our research and our teaching rank consistently highly.

Student spotlights
Find out why students chose Manchester to study social statistics.
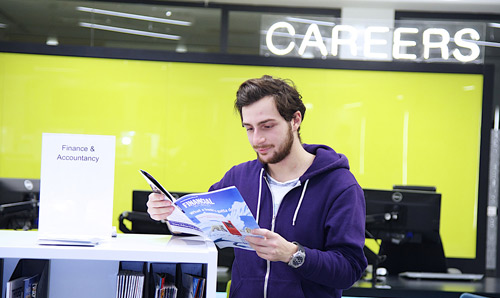
Careers and employability
Find out where social statistics can take you in the world of work and academia.
Q-Step at Manchester
Q-Step at Manchester offers new courses and training to support the career development of future social science researchers.
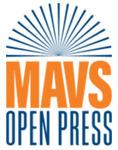
Want to create or adapt books like this? Learn more about how Pressbooks supports open publishing practices.
5.3 Levels of measurement
Learning objectives.
- Define and provide examples for the four levels of measurement
Now that we have figured out how to define, or conceptualize, our terms we’ll need to think about operationalizing them. Operationalization is the process by which researchers conducting quantitative research spell out precisely how a concept will be measured. It involves identifying the specific research procedures we will use to gather data about our concepts. This of course requires that we know what research method(s) we will employ to learn about our concepts, and we’ll examine specific research methods later on in the text. For now, let’s take a broad look at how operationalization works. We can then revisit how this process works when we examine specific methods of data collection in later chapters. Remember, operationalization is only a process in quantitative research. Measurement in qualitative research will be discussed at the end of this section.
Levels of measurement
When social scientists measure concepts, they sometimes use the language of variables and attributes (also called values ). A variable refers to a phenomenon that can vary. It can be thought of as a grouping of several characteristics. For example, hair color could be a variable because it has varying characteristics. Attributes are the characteristics that make up a variable. For example, the variable hair color would contain attributes like blonde, brown, black, red, gray, etc.
A variable’s attributes determine its level of measurement . There are four possible levels of measurement: nominal , ordinal , interval , and ratio . The first two levels of measurement are categorical , meaning their attributes are categories rather than numbers. The latter two levels of measurement are continuous , meaning their attributes are numbers, not categories.
Nominal level of measurement
Hair color is an example of a nominal level of measurement. Nominal measures are categorical, and those categories cannot be mathematically ranked. There is no ranking order between hair colors. They are simply different. That is what constitutes a nominal level of measurement. Gender and race are also measured at the nominal level.
When using nominal level of measurement in research, it is very important to assign the attributes of potential answers very precisely. The attributes need to be exhaustive and mutually exclusive . Let’s think about the attributes contained in the variable hair color . Black, brown, blonde, and red are common colors. But, if we listed only these attributes, people with gray hair wouldn’t fit anywhere. That means our attributes were not exhaustive. Exhaustiveness means that all possible attributes are listed. We may have to list a lot of colors before we can meet the criteria of exhaustiveness. Clearly, there is a point at which trying to achieve exhaustiveness can get to be too much. If a person insists that their hair color is light burnt sienna , it is not your responsibility to list that as an option. Rather, that person could reasonably be described as brown-haired. Perhaps listing a category for other color would suffice to make our list of colors exhaustive.
What about a person who has multiple hair colors at the same time, such as red and black? They would fall into multiple attributes. This violates the rule of mutual exclusivity , in which a person cannot fall into two different attributes. Instead of listing all of the possible combinations of colors, perhaps you might include a list of attributes like all black , all brown , all blonde , all red , multi-color, other to include people with more than one hair color, but keep everyone in only one category.
The discussion of hair color elides an important point with measurement—reification. You should remember reification from our previous discussion in this chapter. For many years, the attributes for gender were male and female. Now, our understanding of gender has evolved to encompass more attributes including transgender, non-binary, or genderqueer. We shouldn’t confuse our labeling of attributes or measuring of a variable with the objective truth “out there.” Another example could be children of parents from different races were often classified as one race or another in the past, even if they identified with both cultures equally. The option for bi-racial or multi-racial on a survey not only more accurately reflects the racial diversity in the real world but validates and acknowledges people who identify in that manner.
Ordinal level of measurement
Unlike nominal-level measures, attributes at the ordinal level can be rank ordered. For example, someone’s degree of satisfaction in their romantic relationship can be ordered by rank. That is, you could say you are not at all satisfied, a little satisfied, moderately satisfied, or highly satisfied. Note that even though these have a rank order to them (not at all satisfied is certainly worse than highly satisfied), we cannot calculate a mathematical distance between those attributes. We can simply say that one attribute of an ordinal-level variable is more or less than another attribute.
This can get a little confusing when using Likert scales . If you have ever taken a customer satisfaction survey or completed a course evaluation for school, you are familiar with Likert scales. “On a scale of 1-5, with one being the lowest and 5 being the highest, how likely are you to recommend our company to other people?” Sound familiar? Likert scales use numbers but only as a shorthand to indicate what attribute (highly likely, somewhat likely, etc.) the person feels describes them best. You wouldn’t say you are “2” more likely to recommend the company. But you could say you are not very likely to recommend the company.
Ordinal-level attributes must also be exhaustive and mutually exclusive, as with nominal-level variables.
Interval level of measurement
At the interval level, the distance between attributes is known to be equal. Interval measures are also continuous, meaning their attributes are numbers, rather than categories. IQ scores are interval level, as are temperatures. Interval-level variables are not particularly common in social science research, but their defining characteristic is that we can say how much more or less one attribute differs from another. We cannot, however, say with certainty what the ratio of one attribute is in comparison to another. For example, it would not make sense to say that 50 degrees is half as hot as 100 degrees. But we can say it is 50 degrees cooler than 100. At the interval level, attributes must also be exhaustive and mutually exclusive.
Ratio level of measurement
Finally, at the ratio level, attributes can be rank ordered, the distance between attributes is equal, and attributes have a true zero point. Thus, with these variables, we can say what the ratio of one attribute is in comparison to another. Examples of ratio-level variables include age and years of education. We know, for example, that a person who is 12 years old is twice as old as someone who is 6 years old. Just like all other levels of measurement, at the ratio level, attributes must be mutually exclusive and exhaustive.
The differences between each level of measurement are visualized in Table 5.1.
Key Takeaways
- In social science, our variables can be one of four different levels of measurement: nominal, ordinal, interval, or ratio.
- Categorical measures- a measure with attributes that are categories
- Continuous measures- a measures with attributes that are numbers
- Exhaustiveness- all possible attributes are listed
- Interval level- a level of measurement that is continuous, can be rank ordered, is exhaustive and mutually exclusive, and for which the distance between attributes is known to be equal
- Likert scales- ordinal measures that use numbers as a shorthand (e.g., 1=highly likely, 2=somewhat likely, etc.) to indicate what attribute the person feels describes them best
- Mutual exclusivity- a person cannot identify with two different attributes simultaneously
- Nominal- level of measurement that is categorical and those categories cannot be mathematically ranked, though they are exhaustive and mutually exclusive
- Ordinal- level of measurement that is categorical, those categories can be rank ordered, and they are exhaustive and mutually exclusive
- Ratio level- level of measurement in which attributes are mutually exclusive and exhaustive, attributes can be rank ordered, the distance between attributes is equal, and attributes have a true zero point
- Variable- refers to a grouping of several characteristics
Foundations of Social Work Research Copyright © 2020 by Rebecca L. Mauldin is licensed under a Creative Commons Attribution-NonCommercial-ShareAlike 4.0 International License , except where otherwise noted.
Share This Book
- USC Libraries
- Research Guides
- Social Work *
- Data & Statistics
Social Work *: Data & Statistics
- Search Operators
- Developing a Search Strategy
- Scholarly and Popular Sources
- Reading a scholarly research article effectively
- Evaluating Your Sources - How do I know this is reliable?
- Organizing your research with Citation Managers
- Library Services
- Full Text Articles
- Find Dissertations
- Find Books and E-books
- Using Google Scholar
- Find Newspaper Articles
- Finding Grey Literature in Social Work
- What are journal impact factors?
- How do you find a journal impact factor?
- Workshops and Webinars
- Antiracist/Anti-oppression Resources
- Evidence-based Practice Resources
- Tests & Measurements
- Treatment Planners
- Where do I find background information on a topic?
- What is a Policy Brief?
- Topic Specific Statistics and Data
- Documentaries, Therapy Demonstrations, Training Videos
- Distance Learning
- Writing Resources This link opens in a new window
- Course Guides
- DSW (Doctor of Social Work) Resources
- Faculty Resources
- Alumni Resources
- APA 7th Edition
Finding, Using and Managing Data
- Data Reference Toolkit by Andy Rutkowski Last Updated Jan 11, 2024 310 views this year
- Statistics & Data by Eimmy Solis Last Updated May 14, 2024 1885 views this year
Finding Statistics and Data
Data is the raw information that is analyzed to create statistics, or interpreted data. Data can be numerical, or qualitative (interviews, opinion data, etc).

- Roper Center The Roper Center maintains an archive of public opinion survey data--including the General Social Survey (1972-2008). Allows users to search survey questions or download data directly. Users will need to enter their USC email address to access data.
- National Council on Disabilility NCD is an independent federal agency charged with advising the President, Congress, and other federal agencies regarding policies, programs, practices, and procedures that affect people with disabilities.
International/Global Data and Statistics
- International Education Statistics National Center for Education Statistics (NCES), Institute of Education Sciences (IES)
- Pan American Health Organization PAHO is the specialized international health agency for the Americas. It works with countries throughout the region to improve and protect people's health
- UNICEF Statistics United Nations International Children's Emergency Fund (UNICEF)
- United Nations Statistics Provides data on international trade, national accounts, energy, industry, environment and demographic and social statistics gathered from national and international sources.
- World Health Organization WHO's annual World Health Statistics reports present the most recent health statistics for the WHO Member States.
- Inter-university Consortium for Political and Social Research This link opens in a new window The Inter-university Consortium for Political and Social Research (ICPSR), established in 1962, is an integral part of the infrastructure of social science research. more... less... ICPSR maintains and provides access to a vast archive of social science data for research and instruction.
Finding Community Demographics
- U.S. Census Bureau Data The Census Bureau is the leading source of quality data about the nation's people and economy. You have two options to start your search on the landing page of data.census.gov: a free-form single search bar or an advanced search filtering experience.
- Census Data Tutorials These tutorials are from the U.S. Census Bureau and will help with navigating the new database.
- Homelessness Data These raw data sets contain Point-in-Time (PIT) estimates and national PIT estimates of homelessness as well as national estimates of homelessness by state and estimates of chronic homelessness from 2007 - 2019
- Pew Research Center Pew Research Center is a nonpartisan fact tank that informs the public about the issues, attitudes and trends shaping the world.
- Social Explorer This link opens in a new window Visually display demographic data in the U.S. from 1790-present at a variety of geographic levels. Create reports, maps and animations that can be downloaded.
View the videos below for more info on how to use social explorer.
Open and Free Data
- CHHS Open Data California Health and Human Services (CHHS) Open Data Portal
- Data.gov Data.gov is the homepage for the U.S. Government's open data. You will find Federal, state, and local data.
- HealthData.gov This information includes clinical care provider quality information, nationwide health service provider directories, databases of the latest medical and scientific knowledge, consumer product data, community health performance information, government spending data.
- Journal of Open Psychology Data The Journal of Open Psychology Data (JOPD) features peer reviewed data papers describing psychology datasets with high reuse potential. Data papers may describe data from unpublished work, including replication research, or from papers published previously in a traditional journal.
- Kids Count Data Center Annie E. Casey Foundation database including over 100 indicators of child well-being, including economic status, health, safety, and risk factors on the local community, city, state, and national level.
- Los Angeles Open Data A portal to find information, insights and analysis from the city of Los Angeles.
- openICPSR openICPSR provides a place for researchers to archive, store and share their data. All data included in OpenICPSR is free for public use and primarily focuses on behavioral health and social sciences.
Los Angeles and California

- California Department of Education, Data and Statistics Data and statistics collected from California schools and learning support resources to identify trends and educational needs and to measure performance
- California Department of Rehabilitation The California Department of Rehabilitation (DOR) is an employment and independent living resource for people with disabilities.
- California Department of Social Services (CDSS) Data Portal California Department of Social Services (CDSS)
- Healthy City Healthycity.org is an online platform for accessing data, creating maps, and working collaboratively. It allows users to theme and filter data, conduct research, and connect with their communities. From the Advancement Project California.
- Los Angeles Homeless Services Authority
- Know Your Community: LA City The City of Los Angeles hub for information, data, and resources.
- << Previous: What is a Policy Brief?
- Next: Topic Specific Statistics and Data >>
- Last Updated: Apr 11, 2024 10:03 AM
- URL: https://libguides.usc.edu/socialwork
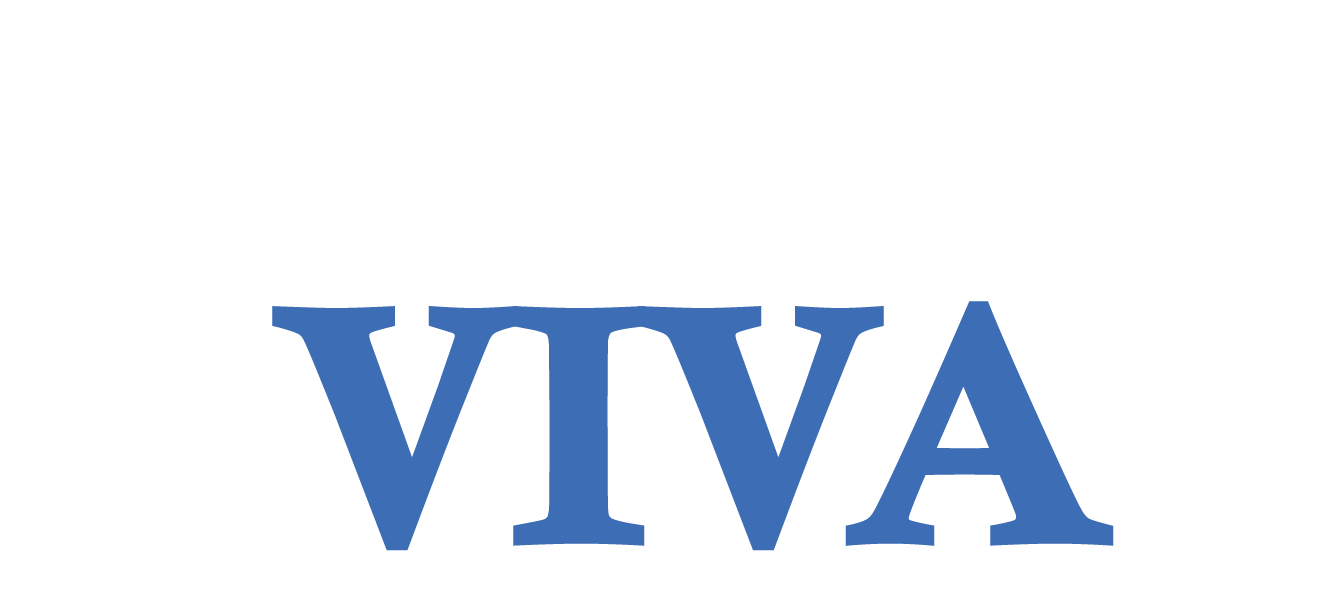
Want to create or adapt books like this? Learn more about how Pressbooks supports open publishing practices.
23 14. Univariate analysis
Chapter outline.
- Where do I start with quantitative data analysis? (12 minute read time)
- Measures of central tendency (17 minute read time, including 5-minute video)
- Frequencies and variability (13 minute read time)
People often dread quantitative data analysis because – oh no – it’s math. And true, you’re going to have to work with numbers. For years, I thought I was terrible at math, and then I started working with data and statistics, and it turned out I had a real knack for it. (I have a statistician friend who claims statistics is not math, which is a math joke that’s way over my head, but there you go.) This chapter, and the subsequent quantitative analysis chapters, are going to focus on helping you understand descriptive statistics and a few statistical tests, NOT calculate them (with a couple of exceptions). Future research classes will focus on teaching you to calculate these tests for yourself. So take a deep breath and clear your mind of any doubts about your ability to understand and work with numerical data.

In this chapter, we’re going to discuss the first step in analyzing your quantitative data: univariate data analysis. Univariate data analysis is a quantitative method in which a variable is examined individually to determine its distribution , or “the way the scores are distributed across the levels of that variable” (Price et. al, Chapter 12.1, para. 2). When we talk about levels , what we are talking about are the possible values of the variable – like a participant’s age, income or gender. (Note that this is different than our earlier discussion in Chaper 10 of levels of measurement , but the level of measurement of your variables absolutely affects what kinds of analyses you can do with it.) Univariate analysis is n on-relational , which just means that we’re not looking into how our variables relate to each other. Instead, we’re looking at variables in isolation to try to understand them better. For this reason, univariate analysis is best for descriptive research questions.
So when do you use univariate data analysis? Always! It should be the first thing you do with your quantitative data, whether you are planning to move on to more sophisticated statistical analyses or are conducting a study to describe a new phenomenon. You need to understand what the values of each variable look like – what if one of your variables has a lot of missing data because participants didn’t answer that question on your survey? What if there isn’t much variation in the gender of your sample? These are things you’ll learn through univariate analysis.
14.1 Where do I start with quantitative data analysis?
Learning objectives.
Learners will be able to…
- Define and construct a data analysis plan
- Define key data management terms – variable name, data dictionary, primary and secondary data, observations/cases
No matter how large or small your data set is, quantitative data can be intimidating. There are a few ways to make things manageable for yourself, including creating a data analysis plan and organizing your data in a useful way. We’ll discuss some of the keys to these tactics below.
The data analysis plan
As part of planning for your research, and to help keep you on track and make things more manageable, you should come up with a data analysis plan. You’ve basically been working on doing this in writing your research proposal so far. A data analysis plan is an ordered outline that includes your research question, a description of the data you are going to use to answer it, and the exact step-by-step analyses, that you plan to run to answer your research question. This last part – which includes choosing your quantitative analyses – is the focus of this and the next two chapters of this book.
A basic data analysis plan might look something like what you see in Table 14.1. Don’t panic if you don’t yet understand some of the statistical terms in the plan; we’re going to delve into them throughout the next few chapters. Note here also that this is what operationalizing your variables and moving through your research with them looks like on a basic level.
An important point to remember is that you should never get stuck on using a particular statistical method because you or one of your co-researchers thinks it’s cool or it’s the hot thing in your field right now. You should certainly go into your data analysis plan with ideas, but in the end, you need to let your research question and the actual content of your data guide what statistical tests you use. Be prepared to be flexible if your plan doesn’t pan out because the data is behaving in unexpected ways.
Managing your data
Whether you’ve collected your own data or are using someone else’s data, you need to make sure it is well-organized in a database in a way that’s actually usable. “Database” can be kind of a scary word, but really, I just mean an Excel spreadsheet or a data file in whatever program you’re using to analyze your data (like SPSS, SAS, or r). (I would avoid Excel if you’ve got a very large data set – one with millions of records or hundreds of variables – because it gets very slow and can only handle a certain number of cases and variables, depending on your version. But if your data set is smaller and you plan to keep your analyses simple, you can definitely get away with Excel.) Your database or data set should be organized with variables as your columns and observations/cases as your rows. For example, let’s say we did a survey on ice cream preferences and collected the following information in Table 14.2:
There are a few key data management terms to understand:
- Variable name : Just what it sounds like – the name of your variable. Make sure this is something useful, short and, if you’re using something other than Excel, all one word. Most statistical programs will automatically rename variables for you if they aren’t one word, but the names are usually a little ridiculous and long.
- Observations/cases : The rows in your data set. In social work, these are often your study participants (people), but can be anything from census tracts to black bears to trains. When we talk about sample size, we’re talking about the number of observations/cases. In our mini data set, each person is an observation/case.
- Primary data : Data you have collected yourself.
- Secondary data : Data someone else has collected that you have permission to use in your research. For example, for my student research project in my MSW program, I used data from a local probation program to determine if a shoplifting prevention group was reducing the rate at which people were re-offending. I had data on who participated in the program and then received their criminal history six months after the end of their probation period. This was secondary data I used to determine whether the shoplifting prevention group had any effect on an individual’s likelihood of re-offending.
- Data dictionary (sometimes called a code book) : This is the document where you list your variable names, what the variables actually measure or represent, what each of the values of the variable mean if the meaning isn’t obvious (i.e., if there are numbers assigned to gender), the level of measurement and anything special to know about the variables (for instance, the source if you mashed two data sets together). If you’re using secondary data, the data dictionary should be available to you.
When considering what data you might want to collect as part of your project, there are two important considerations that can create dilemmas for researchers. You might only get one chance to interact with your participants, so you must think comprehensively in your planning phase about what information you need and collect as much relevant data as possible. At the same time, though, especially when collecting sensitive information, you need to consider how onerous the data collection is for participants and whether you really need them to share that information. Just because something is interesting to us doesn’t mean it’s related enough to our research question to chase it down. Work with your research team and/or faculty early in your project to talk through these issues before you get to this point. And if you’re using secondary data , make sure you have access to all the information you need in that data before you use it.
Let’s take that mini data set we’ve got up above and I’ll show you what your data dictionary might look like in Table 14.3.
Key Takeaways
- Getting organized at the beginning of your project with a data analysis plan will help keep you on track. Data analysis plans should include your research question, a description of your data, and a step-by-step outline of what you’re going to do with it.
- Be flexible with your data analysis plan – sometimes data surprises us and we have to adjust the statistical tests we are using.
- Always make a data dictionary or, if using secondary data, get a copy of the data dictionary so you (or someone else) can understand the basics of your data.
- Make a data analysis plan for your project. Remember this should include your research question, a description of the data you will use, and a step-by-step outline of what you’re going to do with your data once you have it, including statistical tests (non-relational and relational) that you plan to use. You can do this exercise whether you’re using quantitative or qualitative data! The same principles apply.
- Make a data dictionary for the data you are proposing to collect as part of your study. You can use the example above as a template.
14.2 Measures of central tendency
- Explain measures of central tendency – mean, median and mode – and when to use them to describe your data
- Explain the importance of examining the range of your data
- Apply the appropriate measure of central tendency to a research problem or question
A measure of central tendency is one number that can give you an idea about the distribution of your data. The video below gives a more detailed introduction to central tendency. Then we’ll talk more specifically about our three measures of central tendency – mean, median and mode.
One quick note: the narrator in the video mentions skewness and kurtosis . Basically, these refer to a particular shape for a distribution when you graph it out.e.That gets into some more advanced multivariate analysis that we aren’t tackling in this book, so just file them away for a more advanced class, if you ever take on.
There are three key measures of central tendency, which we’ll go into now.
The mean , also called the average, is calculated by adding all your cases and dividing the sum by the number of cases. You’ve undoubtedly calculated a mean at some point in your life. The mean is the most widely used measure of central tendency because it’s easy to understand and calculate. It can only be used with interval/ratio variables, like age, test scores or years of post-high school education. (If you think about it, using it with a nominal or ordinal variable doesn’t make much sense – why do we care about the average of our numerical values we assigned to certain races?)
The biggest drawback of using the mean is that it’s extremely sensitive to outliers , or extreme values in your data. And the smaller your data set is, the more sensitive your mean is to these outliers. One thing to remember about outliers – they are not inherently bad, and can sometimes contain really important information. Don’t automatically discard them because they skew your data.
Let’s take a minute to talk about how to locate outliers in your data. If your data set is very small, you can just take a look at it and see outliers. But in general, you’re probably going to be working with data sets that have at least a couple dozen cases, which makes just looking at your values to find outliers difficult. The best way to quickly look for outliers is probably to make a scatter plot with excel or whatever database management program you’re using.
Let’s take a very small data set as an example. Oh hey, we had one before! I’ve re-created it in Table 14.5. We’re going to add some more cases to it so it’s a little easier to illustrate what we’re doing.
Let’s say we’re interested in knowing more about the distribution of participant age. Let’s see a scatterplot of age (Figure 14.1). On our y-axis (the vertical one) is the value of age, and on our x-axis (the horizontal one) is the frequency of each age, or the number of times it appears in our data set.
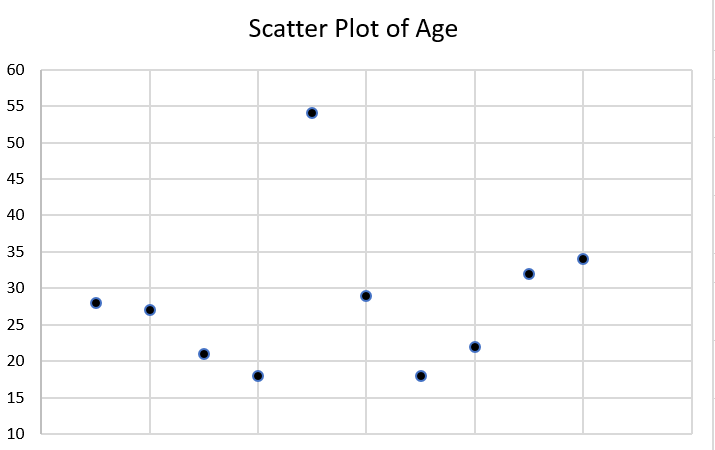
Do you see any outliers in the scatter plot? There is one participant who is significantly older than the rest at age 54. Let’s think about what happens when we calculate our mean with and without that outlier. Complete the two exercises below by using the ages listed in our mini-data set in this section.
Next, let’s try it without the outlier.
With our outlier, the average age of our participants is 28, and without it, the average age is 25. That might not seem enormous, but it illustrates the effects of outliers on the mean.
Just because Tom is an outlier at age 54 doesn’t mean you should exclude him. The most important thing about outliers is to think critically about them and how they could affect your analysis. Finding outliers should prompt a couple of questions. First, could the data have been entered incorrectly? Is Tom actually 24, and someone just hit the “5” instead of the “2” on the number pad? What might be special about Tom that he ended up in our group, given how that he is different? Are there other relevant ways in which Tom differs from our group (is he an outlier in other ways)? Does it really matter than Tom is much older than our other participants? If we don’t think age is a relevant factor in ice cream preferences, then it probably doesn’t. If we do, then we probably should have made an effort to get a wider range of ages in our participants.
The median (also called the 50th percentile) is the middle value when all our values are placed in numerical order. If you have five values and you put them in numerical order, the third value will be the median. When you have an even number of values, you’ll have to take the average of the middle two values to get the median. So, if you have 6 values, the average of values 3 and 4 will be the median. Keep in mind that for large data sets, you’re going to want to use either Excel or a statistical program to calculate the median – otherwise, it’s nearly impossible logistically.
Like the mean, you can only calculate the median with interval/ratio variables, like age, test scores or years of post-high school education. The median is also a lot less sensitive to outliers than the mean. While it can be more time intensive to calculate, the median is preferable in most cases to the mean for this reason. It gives us a more accurate picture of where the middle of our distribution sits in most cases. In my work as a policy analyst and researcher, I rarely, if ever, use the mean as a measure of central tendency. Its main value for me is to compare it to the median for statistical purposes. So get used to the median, unless you’re specifically asked for the mean. (When we talk about t- tests in the next chapter, we’ll talk about when the mean can be useful.)
Let’s go back to our little data set and calculate the median age of our participants (Table 14.6).
Remember, to calculate the median, you put all the values in numerical order and take the number in the middle. When there’s an even number of values, take the average of the two middle values.
What happens if we remove Tom, the outlier?
With Tom in our group, the median age is 27.5, and without him, it’s 27. You can see that the median was far less sensitive to him being included in our data than the mean was.
The mode of a variable is the most commonly occurring value. While you can calculate the mode for interval/ratio variables, it’s mostly useful when examining and describing nominal or ordinal variables. Think of it this way – do we really care that there are two people with an income of $38,000 per year, or do we care that these people fall into a certain category related to that value, like above or below the federal poverty level?
Let’s go back to our ice cream survey (Table 14.7).
We can use the mode for a few different variables here: gender, hometown and fav_ice_cream. The cool thing about the mode is that you can use it for numeric/quantitative and text/quantitative variables.
So let’s find some modes. For hometown – or whether the participant’s hometown is the one in which the survey was administered or not – the mode is 0, or “no” because that’s the most common answer. For gender, the mode is 0, or “female.” And for fav_ice_cream, the mode is Chocolate, although there’s a lot of variation there. Sometimes, you may have more than one mode, which is still useful information.
One final thing I want to note about these three measures of central tendency: if you’re using something like a ranking question or a Likert scale, depending on what you’re measuring, you might use a mean or median, even though these look like they will only spit out ordinal variables. For example, say you’re a car designer and want to understand what people are looking for in new cars. You conduct a survey asking participants to rank the characteristics of a new car in order of importance (an ordinal question). The most commonly occurring answer – the mode – really tells you the information you need to design a car that people will want to buy. On the flip side, if you have a scale of 1 through 5 measuring a person’s satisfaction with their most recent oil change, you may want to know the mean score because it will tell you, relative to most or least satisfied, where most people fall in your survey. To know what’s most helpful, think critically about the question you want to answer and about what the actual values of your variable can tell you.
- The mean is the average value for a variable, calculated by adding all values and dividing the total by the number of cases. While the mean contains useful information about a variable’s distribution, it’s also susceptible to outliers, especially with small data sets.
- In general, the mean is most useful with interval/ratio variables.
- The median , or 50th percentile, is the exact middle of our distribution when the values of our variable are placed in numerical order. The median is usually a more accurate measurement of the middle of our distribution because outliers have a much smaller effect on it.
- In general, the median is only useful with interval/ratio variables.
- The mode is the most commonly occurring value of our variable. In general, it is only useful with nominal or ordinal variables.
- Say you want to know the income of the typical participant in your study. Which measure of central tendency would you use? Why?
- Find an interval/ratio variable and calculate the mean and median. Make a scatter plot and look for outliers.
- Find a nominal variable and calculate the mode.
14.3 Frequencies and variability
- Define descriptive statistics and understand when to use these methods.
- Produce and describe visualizations to report quantitative data.
Descriptive statistics refer to a set of techniques for summarizing and displaying data. We’ve already been through the measures of central tendency, (which are considered descriptive statistics) which got their own chapter because they’re such a big topic. Now, we’re going to talk about other descriptive statistics and ways to visually represent data.
Frequency tables
One way to display the distribution of a variable is in a frequency table . Table 14.2, for example, is a frequency table showing a hypothetical distribution of scores on the Rosenberg Self-Esteem Scale for a sample of 40 college students. The first column lists the values of the variable—the possible scores on the Rosenberg scale—and the second column lists the frequency of each score. This table shows that there were three students who had self-esteem scores of 24, five who had self-esteem scores of 23, and so on. From a frequency table like this, one can quickly see several important aspects of a distribution, including the range of scores (from 15 to 24), the most and least common scores (22 and 17, respectively), and any extreme scores that stand out from the rest.
There are a few other points worth noting about frequency tables. First, the levels listed in the first column usually go from the highest at the top to the lowest at the bottom, and they usually do not extend beyond the highest and lowest scores in the data. For example, although scores on the Rosenberg scale can vary from a high of 30 to a low of 0, Table 14.8 only includes levels from 24 to 15 because that range includes all the scores in this particular data set. Second, when there are many different scores across a wide range of values, it is often better to create a grouped frequency table, in which the first column lists ranges of values and the second column lists the frequency of scores in each range. Table 14.9, for example, is a grouped frequency table showing a hypothetical distribution of simple reaction times for a sample of 20 participants. In a grouped frequency table, the ranges must all be of equal width, and there are usually between five and 15 of them. Finally, frequency tables can also be used for nominal or ordinal variables, in which case the levels are category labels. The order of the category labels is somewhat arbitrary, but they are often listed from the most frequent at the top to the least frequent at the bottom.
A histogram is a graphical display of a distribution. It presents the same information as a frequency table but in a way that is grasped more quickly and easily. The histogram in Figure 14.2 presents the distribution of self-esteem scores in Table 14.8. The x- axis (the horizontal one) of the histogram represents the variable and the y- axis (the vertical one) represents frequency. Above each level of the variable on the x- axis is a vertical bar that represents the number of individuals with that score. When the variable is quantitative, as it is in this example, there is usually no gap between the bars. When the variable is nominal or ordinal, however, there is usually a small gap between them. (The gap at 17 in this histogram reflects the fact that there were no scores of 17 in this data set.)
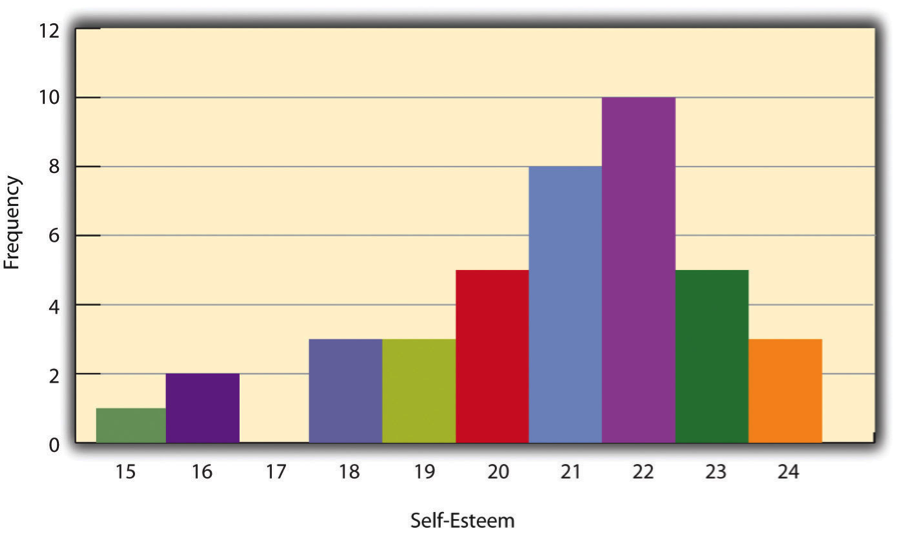
Distribution shapes
When the distribution of a quantitative variable is displayed in a histogram, it has a shape. The shape of the distribution of self-esteem scores in Figure 14.2 is typical. There is a peak somewhere near the middle of the distribution and “tails” that taper in either direction from the peak. The distribution of Figure 14.2 is unimodal , meaning it has one distinct peak, but distributions can also be bimodal , as in Figure 14.3, meaning they have two distinct peaks. Figure 14.3, for example, shows a hypothetical bimodal distribution of scores on the Beck Depression Inventory. I know we talked about the mode mostly for nominal or ordinal variables, but you can actually use histograms to look at the distribution of interval/ratio variables, too, and still have a unimodal or bimodal distribution even if you aren’t calculating a mode. Distributions can also have more than two distinct peaks, but these are relatively rare in social work research.
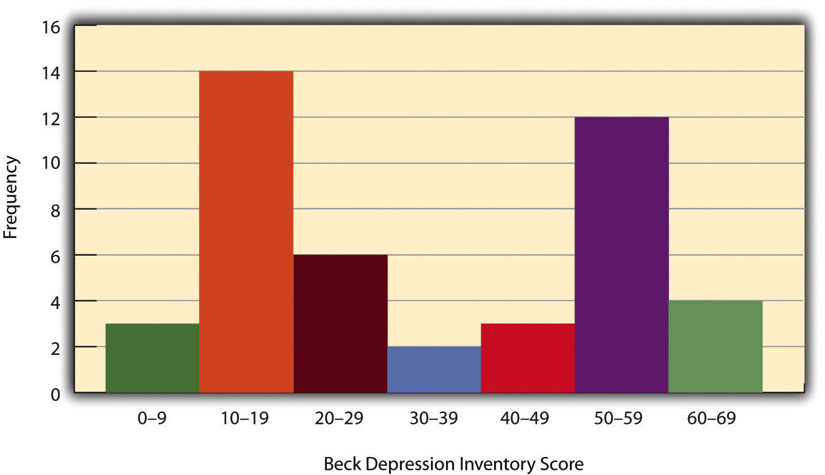
Another characteristic of the shape of a distribution is whether it is symmetrical or skewed. The distribution in the center of Figure 14.4 is symmetrical . Its left and right halves are mirror images of each other. The distribution on the left is negatively skewed , with its peak shifted toward the upper end of its range and a relatively long negative tail. The distribution on the right is positively skewed, with its peak toward the lower end of its range and a relatively long positive tail.

Range: A simple measure of variability
The variability of a distribution is the extent to which the scores vary around their central tendency. Consider the two distributions in Figure 14.5, both of which have the same central tendency. The mean, median, and mode of each distribution are 10. Notice, however, that the two distributions differ in terms of their variability. The top one has relatively low variability, with all the scores relatively close to the center. The bottom one has relatively high variability, with the scores are spread across a much greater range.
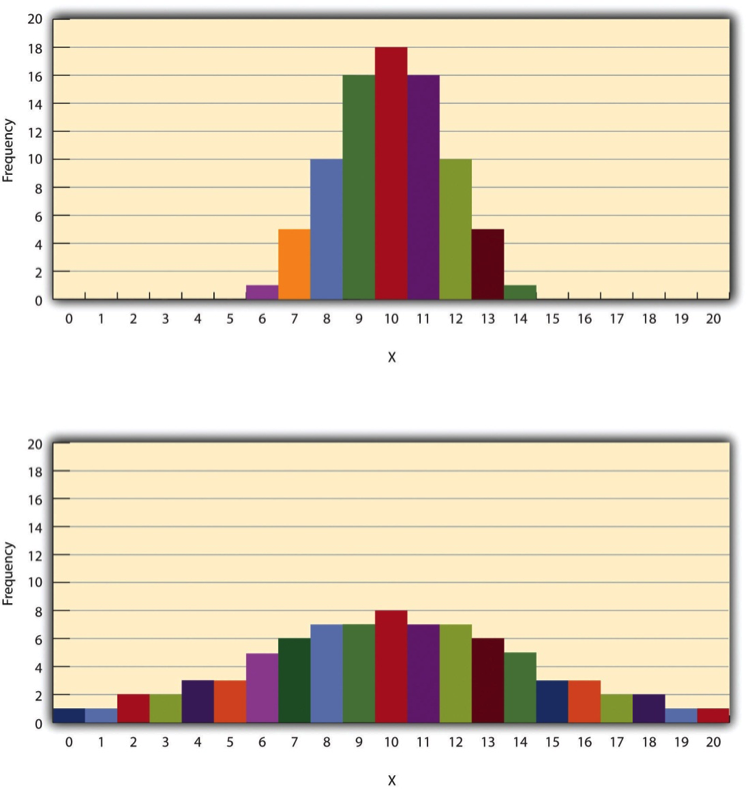
One simple measure of variability is the range , which is simply the difference between the highest and lowest scores in the distribution. The range of the self-esteem scores in Table 12.1, for example, is the difference between the highest score (24) and the lowest score (15). That is, the range is 24 − 15 = 9. Although the range is easy to compute and understand, it can be misleading when there are outliers. Imagine, for example, an exam on which all the students scored between 90 and 100. It has a range of 10. But if there was a single student who scored 20, the range would increase to 80—giving the impression that the scores were quite variable when in fact only one student differed substantially from the rest.
- Descriptive statistics are a way to summarize and display data, and are essential to understand and report your data.
- A frequency table is useful for nominal and ordinal variables and is needed to produce a histogram
- A histogram is a graphic representation of your data that shows how many cases fall into each level of your variable.
- Variability is important to understand in analyzing your data because studying a phenomenon that does not vary for your population does not provide a lot of information.
- Think about the dependent variable in your project. What would you do if you analyzed its variability for people of different genders, and there was very little variability?
- What do you think it would mean if the distribution of the variable were bimodal?
Univariate data analysis is a quantitative method in which a variable is examined individually to determine its distribution.
the way the scores are distributed across the levels of that variable.
The possible values of the variable - like a participant's age, income or gender.
Referring to data analysis that doesn't examine how variables relate to each other.
An ordered outline that includes your research question, a description of the data you are going to use to answer it, and the exact analyses, step-by-step, that you plan to run to answer your research question.
The process of determining how to measure a construct that cannot be directly observed
A group of statistical techniques that examines the relationship between at least three variables
The name of your variable.
The rows in your data set. In social work, these are often your study participants (people), but can be anything from census tracts to black bears to trains.
Data someone else has collected that you have permission to use in your research.
This is the document where you list your variable names, what the variables actually measure or represent, what each of the values of the variable mean if the meaning isn't obvious.
One number that can give you an idea about the distribution of your data.
Also called the average, the mean is calculated by adding all your cases and dividing the total by the number of cases.
Extreme values in your data.
A graphical representation of data where the y-axis (the vertical one along the side) is your variable's value and the x-axis (the horizontal one along the bottom) represents the individual instance in your data.
The value in the middle when all our values are placed in numerical order. Also called the 50th percentile.
The most commonly occurring value of a variable.
A technique for summarizing and presenting data.
A table that lays out how many cases fall into each level of a varible.
a graphical display of a distribution.
A distribution with one distinct peak when represented on a histogram.
A distribution with two distinct peaks when represented on a histogram.
A distribution with a roughly equal number of cases on either side of the median.
A distribution where cases are clustered on one or the other side of the median.
The extent to which the levels of a variable vary around their central tendency (the mean, median, or mode).
The difference between the highest and lowest scores in the distribution.
Graduate research methods in social work Copyright © 2020 by Matthew DeCarlo, Cory Cummings, Kate Agnelli is licensed under a Creative Commons Attribution-NonCommercial-ShareAlike 4.0 International License , except where otherwise noted.
Share This Book
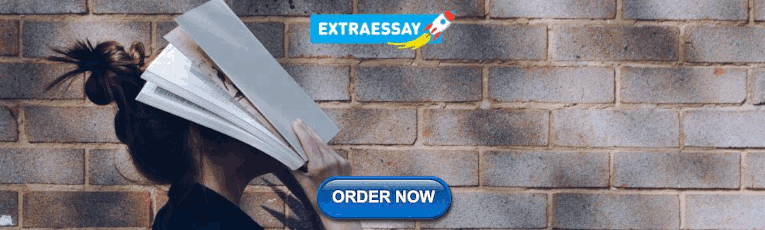
IMAGES
VIDEO
COMMENTS
Statistics in Social Work guides students through concepts and procedures from descriptive statistics and correlation to hypothesis testing and inferential statistics. Besides presenting key concepts, it focuses on real-world examples that students will encounter in a social work practice. Using concrete examples from a variety of potential ...
Statistics in Social Work guides students through concepts and procedures from descriptive statistics and correlation to hypothesis testing and inferential statistics. Besides presenting key concepts, it focuses on real-world examples that students will encounter in a social work practice. Using concrete illustrations from a variety of ...
John Devaney, coauthor of Quantitative Research Methods for Social Work: Making Social Work Count: This is an excellent introduction to statistics for both students and practitioners in social work—it demystifies terms and procedures and uses real world examples to help the reader to see the everyday applicability of statistical knowledge, whether in practice or in study.
Social work researchers will send out a survey, receive responses, aggregate the results, analyze the data, and form conclusions based on trends. Surveys are one of the most common research methods social workers use — and for good reason. They tend to be relatively simple and are usually affordable.
Step 1. Compute the mean of all the values together (overall mean). Step 2. Compute the distance of each individual value from that mean—this becomes a subtraction problem where the mean is subtracted from each individual score, showing the "difference" between the individual score and the group mean.
Social workers, central bureaucracy and evidence-based practice, European Journal of Social Work, 14:3, 323-337. Review author: Ian Harris is a lecturer in social work and teaches at the University of Essex. He specialises in curriculum design and professional education particularly in the practice of assessing risks, vulnerabilities and ...
the social work professional literature several years ago. It took the form of a heated debate between educators. Gould and Kim (1976, p. 50) reported their research findings on "the effects of sex on salary differentials" between social work faculty. In a critique of the Gould and Kim research as well as other research
Understanding statistical concepts is essential for social work professionals. It is key to understanding research and reaching evidence-based decisions in your own practice—but that is only the beginning. If you understand statistics, you can determine the best interventions for your clients. You can use new tools to monitor and evaluate the progress of your client or team.
Written by a social worker for social work students, this is a nuts and bolts guide to statistics that presents complex calculations and concepts in clear, easy-to-understand language. It includes numerous examples, data sets, and issues that students will encounter in social work practice. The first section introduces basic concepts and terms ...
Statistics in Social Work. : Amy Batchelor. Columbia University Press, Nov 19, 2019 - Education - 191 pages. Understanding statistical concepts is essential for social work professionals. It is key to understanding research and reaching evidence-based decisions in your own practice--but that is only the beginning.
Social work research means conducting an investigation in accordance with the scientific method. The aim of social work research is to build the social work knowledge base in order to solve practical problems in social work practice or social policy. Investigating phenomena in accordance with the scientific method requires maximal adherence to ...
Statista. Statista includes ready-made statistical charts, graphs and tables on marketing, demographics, communication, technology, politics, health, leisure and public opinion polls. About 20 percent of the data in Statista comes from government sources like the World Bank and the U.S. Census.
Social statistics are used by The United Nations Social Statistics Division to analyse differences among social groups and countries, covering such issues as housing, health, education, conditions of work and employment. The Division pay special attention to the study of conditions of special population groups, including children, the elderly ...
Statistics in Social Research Sociology 350 MW 10:05-11:25 Room: MCMED 521 Professor K.MacKenzie Department of Sociology Leacock 435, (514) 398-4400 extension 00017 [email protected] Office Hours: TTh 2:00 to 3:45 Teaching Assistants: Angela Kalyta, Deepa Jahagirdar Office Hours TBA Course Description
A variable's attributes determine its level of measurement. There are four possible levels of measurement: nominal, ordinal, interval, and ratio. The first two levels of measurement are categorical, meaning their attributes are categories rather than numbers. The latter two levels of measurement are continuous, meaning their attributes are ...
Social work is acknowledged to be a high-stress profession that involves working with people in distressing circumstances and complex life situations such as those experiencing abuse, domestic violence, substance misuse, and crime (Stanley & Mettilda, 2016).It has been observed that important sources of occupational stress for social workers include excessive workload, working overtime ...
Finding Statistics and Data. Data is the raw information that is analyzed to create statistics, or interpreted data. Data can be numerical, or qualitative (interviews, opinion data, etc). Sage Data (formerly Data-Planet) is an interactive database that allows users to create tables, maps, and figures from a variety data sources covering banking ...
Introduction. Measuring social work is complicated and it is difficult to know whether the desired change being measured is a consequence of social work or whether the change has been influenced by factors outside the social services' control (Cree, Jain, and Hillen Citation 2019).Introducing management and control methods that focus on performance measurement, like New Public Management ...
The history of social work education may have also contributed to making it difficult for those teaching on university social work courses to engage routinely in research (Orme and Powell, 2007).
Univariate data analysis is a quantitative method in which a variable is examined individually to determine its distribution, or "the way the scores are distributed across the levels of that variable" (Price et. al, Chapter 12.1, para. 2).
Abstract: The main objective of the article is to orient the possibilities of using statistical methods both in. education and in research in the areas of Social Work. Social Work and related ...
The report, The Social Work Profession: Findings from Three Years of Surveys of New Social Workers, presents a comprehensive view of where and how social workers are serving clients and communities across the country. The report provides insights into new social workers' demographic and educational backgrounds, the types of jobs they are ...
Social work research is regarded as the systematic use of research concepts, methods, techniques and strategies to provide information related to the objectives of social work programmes and practices. Thus the unit of analysis of social work research could be individuals, groups, families or programme of the agency.