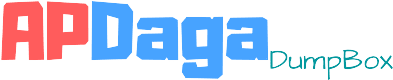
- 🌐 All Sites
- _APDaga DumpBox
- _APDaga Tech
- _APDaga Invest
- _APDaga Videos
- 🗃️ Categories
- _Free Tutorials
- __Python (A to Z)
- __Internet of Things
- __Coursera (ML/DL)
- __HackerRank (SQL)
- __Interview Q&A
- _Artificial Intelligence
- __Machine Learning
- __Deep Learning
- _Internet of Things
- __Raspberry Pi
- __Coursera MCQs
- __Linkedin MCQs
- __Celonis MCQs
- _Handwriting Analysis
- __Graphology
- _Investment Ideas
- _Open Diary
- _Troubleshoots
- _Freescale/NXP
- 📣 Mega Menu
- _Logo Maker
- _Youtube Tumbnail Downloader
- 🕸️ Sitemap
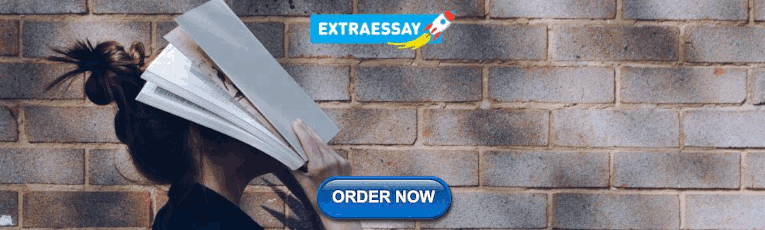
Coursera: Machine Learning (Week 3) [Assignment Solution] - Andrew NG
![Coursera: Machine Learning (Week 3) [Assignment Solution] - Andrew NG Coursera: Machine Learning (Week 3) [Assignment Solution] - Andrew NG](https://blogger.googleusercontent.com/img/b/R29vZ2xl/AVvXsEhTuWdrcTV5DJiQ6-pBw2KhxwwhS3PzeqS7I9l0Fe6om9B4nOJYYXjcNc9xA-Ty7mXjIv97jKR8ivilb3FCkfExpmKRf7L-4wxJO4MJmSrADJbLT8cQsrkp49iX97okifNqUH-y0xAWC_s/w320-h208/Coursera+Machine+Learning+%2528Week+3%2529+%255BAssignment+Solution%255D+-+Andrew+NG.webp)
Recommended Machine Learning Courses: Coursera: Machine Learning Coursera: Deep Learning Specialization Coursera: Machine Learning with Python Coursera: Advanced Machine Learning Specialization Udemy: Machine Learning LinkedIn: Machine Learning Eduonix: Machine Learning edX: Machine Learning Fast.ai: Introduction to Machine Learning for Coders
- ex2.m - Octave/MATLAB script that steps you through the exercise
- ex2 reg.m - Octave/MATLAB script for the later parts of the exercise
- ex2data1.txt - Training set for the first half of the exercise
- ex2data2.txt - Training set for the second half of the exercise
- submit.m - Submission script that sends your solutions to our servers
- mapFeature.m - Function to generate polynomial features
- plotDecisionBoundary.m - Function to plot classifier's decision boundary
- [*] plotData.m - Function to plot 2D classification data
- [*] sigmoid.m - Sigmoid Function
- [*] costFunction.m - Logistic Regression Cost Function
- [*] predict.m - Logistic Regression Prediction Function
- [*] costFunctionReg.m - Regularized Logistic Regression Cost
- Video - YouTube videos featuring Free IOT/ML tutorials
plotData.m :
Sigmoid.m :, costfunction.m :, check-out our free tutorials on iot (internet of things):.
predict.m :
Costfunctionreg.m :, 62 comments.

how could you do this please explain me...

What explanation you want? Please be more specific.
How can i download these files?
You can copy the the code from above code sections.
Hi Akshay, Please may I have theses files as well: ex2.m ex2 reg.m ex2data1.txt ex2data2.txt submit.m mapFeature.m plotDecisionBoundary.m
You can get those files from Coursera assignments. I don't have those with me now.
can you please tell me what you did by this grad = (1/m)* (X'*(h_x-y));

its the simplified version of derivative term d/d0*j0 which we call gradient. check the formula once and you will understand it
this means:- take the transpose of feature matrix X(i.e X') and multiply it with the difference of matrices h_x and y i.e the matrix with sigmoid outputs and the result matrix(y). Finally multiply the end product with 1/m , where m is the number of training examples. This is the vectorized implementation of the code that's actually way more lengthier to implement using loops.
Hi, can you please explain the predict function?
In this gradient decent the number of iteration are not specified so how is the gradient decent working? can someone please explain?
I used the exact code at the end but I'm still getting 65/100 not able to figure out the reason
Did you figure out the reason yet?
Hi !! why didn't you use sum() function for grad even why formula contains that ?
sum() is used for the summation in the formula. But here while coding for grad computation: grad = (1/m)* (X'*(h_x-y)); Here We are doing matrix multiplication which itself consist of "sum of product". So, no need of external sum function. Please try to do it on paper by yourself, you will get clear idea. Thanks
we have learned that Z= theta transpose X then why are using Z=X multiplied by theta in the above codes ?
When we are calculating z(small z) for a single sample, then it is z=theta' * x. (here small x) But When you do the same computation for all the samples at the same time then we call it as Z (Capital Z). Z = X * theta. (Here Capital X) Try to do it using pen-paper, you will get clear understanding.
Hii, thanks for your help mr. Akshay. I had this one doubt about predict.m function: I tried coding for predict function in the following way: h_x = sigmoid(X*theta); if (0<=h_x<0.5) p=0; elseif (0.5<=h_x<=1) p=1; endif I know I did it in a long way but the accuracy that I am getting 60.00. Your code gave me the accuracy 89.00. Can you please help me understand what's wrong with this and what's the exact difference between your code and mines'?
P is a matrix with dimensions m x 1. Solution: You can put your code in a "for" loop and check the value of each element in h_x and accordingly set the value of each element in p. It will work.

hey bro it says z not defined why???
Hi, I think you are doing this assignment in Octave and that's why you are facing this issue. Chethan Bhandarkar has provided solution for it. Please check it out: https://www.apdaga.com/2018/06/coursera-machine-learning-week-2.html?showComment=1563986935868#c4682866656714070064 Thanks

I have copy the exact code for plotData.m , and all the others program worked very well but I am still getting 70/100. Can you tel what's the problem ?
Can you tell me , how can I run "ex2" script in console ?
hi I want to clarify few things from you, I have read in regression, these are few important points which have not been covered in andrew ng regression topic, how to find how significant your variable is, significance of p value and R^2 (R-square) values. I would like to know more about them. kindly share some sources.
HI, The line code reg_term = (lambda/(2*m)) * sum(theta(2:end).^2); in costFunctionReg function, can you explain more about this part theta(2:end) , what does it mean and how did you deduce it,
sir,please explain me predict.m function I used for i=1:size(X,1) if sigmoid(X*theta)>=0.5 p=sigmoid(X*theta); end as well as, h_x = sigmoid(X*theta); for i=1:size(X,1) if (0<=h_x<0.5) p=0; elseif (0.5<=h_x<=1) p=1; end but i am getting 40 accuracy it is working only with your code.why sir?
Hi there, I am trying the the same code as yours of sigmoid() function but each time it is getting an error saying that 'z' undefined near line 6 column 18 error: called from sigmoid at line 6 column 5 what to do please help me out..
Hello Akshay, It'd be great if you kindly share the code for "fminunc" in this week's files(wherever needed), coz i don't understand that particular function well, neither did i get its solution anywhere else on internet.
Hi Ankit, Sorry but I don't have the code for "fminunc".
grad(2:end) = (1/m)* (X(:,2:end)'*(h_x-y))+(lambda/m)*theta(2:end); can u please explain this..
Hey it says my plot is empty can someone help?
I am facing this type of problem in matlab , what can i do ? how to fix that n where ?? 'fminunc' requires Optimization Toolbox. Error in ex2 (line 99) fminunc(@(t)(costFunction(t, X, y)), initial_theta, options);
In sigmoid error in line 6 (the preallocated value assigned to variable 'g' might be unused) what should i do
How's value of 'g' is unused. 'g' is nothing but output of sigmoid function. If you are getting some msg, it must be warning not error. So, don't worry about it, keep it as it is. (But I don't think you should get any kind of warning like this). line 6, is called initialization of variable.
Hi Akshay can you please explain why we use this X(:,2:end) and theta(2:end) instead of plain X and theta??
It's because as per the theory in videos, We don't apply regularization on theta_0. Regularization is applied from theta_1 onwards. and that's why 2 gradients. 1st corresponding to theta_0 and other for theta_1 onwards.
And also why use two gradents?
Good day sir, im new in this course...i could not fully understand the assignment in week 3...as i enter my code...i think still in error..
please explain the predict function
Predict function is fairly simple. You have implemented your gradient and now you just have to predict whether the answer will be 1 or 0... So, what will you do is check for the result > 0.5. If it is above the 0.5, then prediction will be true (1), otherwise false (0)
@Hassan Ashas Thank you very much for your explanation.
costfuntion is not returning the scalar value, it is returning the 1*100 matrix.
Hello Akshay, I keep getting this error for the costFunctionReg.m file: syntax error >>> reg_term = (lambda/2*m)) * sum(theta(2:end).^2); ^ What is the problem here I do not understand. Thank you
Opening and closing brackets are not matching you code. NOTE: check the brackets are "2*m" YOUR CODE: reg_term = (lambda/2*m)) * sum(theta(2:end).^2); WORKING CODE: reg_term = (lambda/(2*m)) * sum(theta(2:end).^2);
Hello Akshay, While computing cost function I am getting so many outputs
You should only get [J, grad] as a output of costFunction & costFunctionReg.
Error - theta may not be defined , predict function
hi i have a doubt i took theta as [zeros(n+1),1] it is giving me 0 and i cant submit the assignment can you specify initial value of theta and theta and values of X. i am totally confused
nothing is working here every time it is showing >> plotData error: 'y' undefined near line 14 column 12 error: called from plotData at line 14 column 5 >>
J = (1 / m) * sum ((- y. * Log (h_x)) - ((1-y). * Log (1-h_x))) the log representation in this equation means ln isn't it? So, shouldn't we write it as log (1-h_x) / log (10).
I made it this way: function [J, grad] = costFunctionReg(theta, X, y, lambda) %COSTFUNCTIONREG Compute cost and gradient for logistic regression with regularization % J = COSTFUNCTIONREG(theta, X, y, lambda) computes the cost of using % theta as the parameter for regularized logistic regression and the % gradient of the cost w.r.t. to the parameters. % Initialize some useful values m = length(y); % number of training examples % You need to return the following variables correctly J = 0; grad = zeros(size(theta)); % ====================== YOUR CODE HERE ====================== % Instructions: Compute the cost of a particular choice of theta. % You should set J to the cost. % Compute the partial derivatives and set grad to the partial % derivatives of the cost w.r.t. each parameter in theta [J, grad] = costFunction(theta, X, y); feats = theta(2:end); J = J + lambda / (2 * m) * (feats' * feats); grad(2:end) = grad(2:end) + lambda / m * feats; % ============================================================= end
My question is about the solved subroutine 'plotDecisionBoundary.m' Line 20 : plot_y I didn't understand the definition of this Infact how this particular code helped to plot the decision boundary! Please explain..
so in cost function grad is basically you doing gradient descent right? but what is the use of 1/m? i'm really confused sorry
While calculating cost function, we are doing sum (summation) operation over 'm' samples. And then dividing it by 'm' in order to scale the output (as a scaling factor).
Muje 55 marks hi aa rahe he mane code bhi sahi likha he phir bhi...logistic regression cost and regularised logistic regression gradient dono me 0 marks he..
i really confused in assignment, i enjoyed all the stuff that prof.Ng doing buat why it turns out to become nightmare when im face the programming assignment? In the cosfunctionreg.m why you put grad(1) = (1/m)* (X(:,1)'*(h_x-y)); whats this mean? grad(2:end) = (1/m)* (X(:,2:end)'*(h_x-y))+(lambda/m)*theta(2:end); what grad(2:end) mean?
These 2 lines are for calcuating gradient with regularization. since we don't add regularization term to 1st entry. (we have to write 2 seperate lines of code for it)
Hi dear Akshay. I'm trying to submit week 3 assignment but I keep seeing the error: !! Submission failed: unexpected error: Error: File: costFunctionReg.m Line: 22 Column: 3 Invalid expression. Check for missing or extra characters. Can you help me out?
I am getting a syntax error in exercise "CostfunctionReg.m" at grad(1) = (1/m)* (X(:,1)'*(h_x-y)); please tell me why am i getting this error. yes i am running it in octave but please don't tell me to go through the another link . please just tell me the issue.
!! Submission failed: Index exceeds array bounds. Function: getResponse LineNumber: 132of submitWithConfiguration
Here in the cost function though y and log(h_x) both have the same dimensions (mx1), how the dot product is possible between them?
We are not doing the dot product of y and log(h_x) while calculating cost function. Multiplication represented by dot astrix (.*) means element wise multiplication in matlab. Eg. -y.*log(h_x) Please check the code once again.
Our website uses cookies to improve your experience. Learn more
Contact form
Instantly share code, notes, and snippets.
mGalarnyk / machineLearningWeek3Quiz1.md
- Download ZIP
- Star ( 2 ) 2 You must be signed in to star a gist
- Fork ( 2 ) 2 You must be signed in to fork a gist
- Embed Embed this gist in your website.
- Share Copy sharable link for this gist.
- Clone via HTTPS Clone using the web URL.
- Learn more about clone URLs
- Save mGalarnyk/a890c3d6bb42923cb0543c7d7809cb77 to your computer and use it in GitHub Desktop.
Machine Learning Week 3 Quiz 1 (Logistic Regression) Stanford Coursera
Github repo for the Course: Stanford Machine Learning (Coursera) Quiz Needs to be viewed here at the repo (because the image solutions cant be viewed as part of a gist)
Answer | Explanation |
---|---|
Our estimate for P(y=0|x;θ) is 0.8. | P(y=0|x;θ) = 1 - P(y = 1| x; θ); the former is 1 - 0.2 = 0.8 |
Our estimate for P(y=1|x;θ) is 0.2. | h (x) = 0.2 |

Answer | Explanation |
---|---|
J(θ) will be a convex function, so gradient descent should converge to the global minimum. | none |
Adding polynomial features (e.g., instead using h (x) = g(θ + θ x + θ x2 + θ x + θ x x + θ x )) could increase how well we can fit the training data | Adding new features can only improve the fit on the training set: since setting θ = θ = θ = 0 makes the hypothesis the same as the original one, gradient descent will use those features (by making the corresponding non-zero) only if doing so improves the training set fit |
Answer | Explanation |
---|---|
The cost function J(θ) for logistic regression trained with examples is always greater than or equal to zero. | The cost for any example x is always ≥ 0 since it is the negative log of a quantity less than one. The cost function J(θ) is a summation over the cost for each eample, so the cost function itself must be greater than or equal to zero. |
The sigmoid function is never greater than one | none |

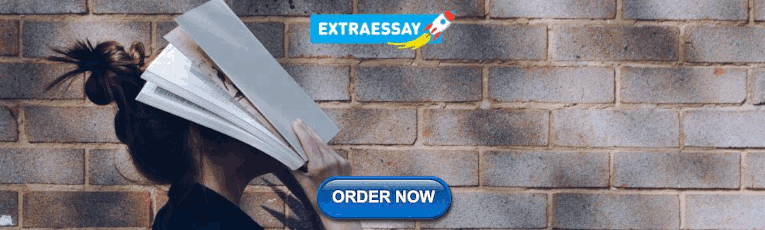
chukwurah498 commented Sep 3, 2020
There ought to be an explanation why you felt these are answers. While I agree on some , there must be explanation as the instructor does.
Sorry, something went wrong.
Coursera - Predictive Modeling Analytics - Week 3
Matt girard.
This document will walk through the Week 3 assignment for the Coursera Predictive Modeling Analytics course. This course focuses on classification using Logistic Regression.
The following libraries are used:
Reading and Preparing Data
Per the assignment, we will continue working with the customer rewards program dataset. The code below reads in the data and creates the two binary dummy columns as called for by the assignment.
Training initial model
Next, we train a Logistic Regression model using Reward as the target variable and our dummy grocery and discount variables as our predictors. Note that family is set to "binomial" meaning that our output/target is between 0 and 1.
Confusion Matrix
To display our confusion matrix, we use the predict function to get probability predictions for our data, then use ifelse to create discrete predictions that align with our desired binary output.
For this, we use a standard 0.5 as our cutoff.
Data Partitioning
The second part of the assignment calls for data partition using a 60/40 split for training and testing sets respectively.
Note: Due to variations in how caret or R’s random number generator work compared to XLMiner, you will need to use XLMiner to get the exact answers required to pass the assignment’s automated grader.
Partitioning can be done using a variety of methods, in a commented section I use R’s sample.int function to generate a vector of random indecies that I can use to subset my data, using the size parameter to limit the numbers selected to match the desired split size. We negate this set using the - operator to get our testing set.
An easier method is to use the createDataPartition method offered by caret which allows you to specify the percent using the p argument, as well as a vector to partition by. In this case I used Reward , which will ensure that the distribution of Reward in our training and testing sets is the same as the original.
To check distributions among the different datasets I create a function, get_dist , to aid in prepapring the summary.
I then plot the results using ggplot. Alternatively, we can print to console using dcast.
Both methods show that our target variable distirbution is the same across all datasets.
We then train our model using the training dataset and display the summary.
With the model trained, we get predictions for our test set and create a confusion matrix.
As the assignment suggests, we can modify our cutoff to be 0.3 instead of the standard 0.5. However, this ends up giving us all positive predictions.
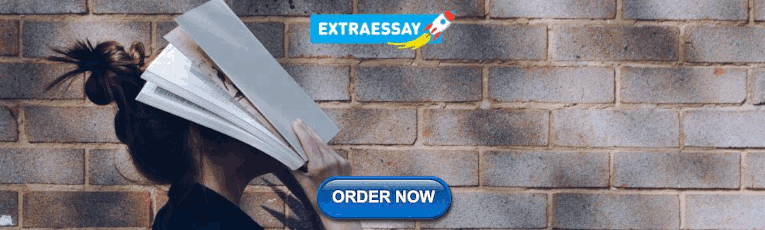
COMMENTS
Week 2. Practice quiz: Gradient descent in practice; Practice quiz: Multiple linear regression; Optional Labs. Numpy Vectorization; Multi Variate Regression; Feature Scaling; Feature Engineering; Sklearn Gradient Descent; Sklearn Normal Method; Programming Assignment. Linear Regression; Week 3. Practice quiz: Cost function for logistic regression
62. Logistic regression and apply it to two different datasets. I have recently completed the Machine Learning course from Coursera by Andrew NG. While doing the course we have to go through various quiz and assignments. Here, I am sharing my solutions for the weekly assignments throughout the course. These solutions are for reference only.
If you are unable to complete the Coursera machine learning week 3 Assignment Logistic regression Ex 2 then this video is for you, compact and perfect method...
%COSTFUNCTIONREG Compute cost and gradient for logistic regression with regularization % J = COSTFUNCTIONREG(theta, X, y, lambda) computes the cost of using % theta as the parameter for regularized logistic regression and the
Machine-Learning---Week 3: Logistic Regression and Regularized Logistic Regression Exercises. This repository is to keep track of my solutions to the Machine Learning course by Andrew Ng on Coursera. About.
Coursera : Machine Learning Week 3 Programming Assignment: Logistics Regression Solutions | Stanford University.Logistics Regression Assignment Machine Learn...
assignment. You should now submit your solutions. 1.2.2 Cost function and gradient Now you will implement the cost function and gradient for logistic regression. Complete the code in costFunction.m to return the cost and gradient. Recall that the cost function in logistic regression is J( ) = 1 m Xm i=1 (y(i) log(h (x i))) (1 y(i))log(1 h (x (i)));
Week three programming assignment: logistic regression. The first problem in this week's programming assignment was about student admittance to university. Given two exam scores for students, we were tasked with predicting whether a given student got into a particular university or not.
Answer Explanation; The cost function J(θ) for logistic regression trained with examples is always greater than or equal to zero. The cost for any example x (i) is always ≥ 0 since it is the negative log of a quantity less than one. The cost function J(θ) is a summation over the cost for each eample, so the cost function itself must be greater than or equal to zero.
This document will walk through the Week 3 assignment for the Coursera Predictive Modeling Analytics course. This course focuses on classification using Logistic Regression. Libraries. ... Next, we train a Logistic Regression model using Reward as the target variable and our dummy grocery and discount variables as our predictors.
This video contains logistic regression quiz 1 of week 3.....Follow me on Instagram :-https://www.instagram.com/kumarabhi512/Please like and subscribe to my ...
Study with Quizlet and memorize flashcards containing terms like Logit Transformation 1. Scatterplot, Logit Transformation 2. Average of y per x grouped, Logit Transformation 3. Scatterplot and more.
Logistic Regression is also called a sigmoid function, which maps real numbers into probabilities, range in [0, 1]. Hence, the value of sigmoid function means how certain the data belongs to a ...
Use logistic regression, naïve Bayes, and word vectors to implement sentiment analysis, complete analogies, and translate words, and use locality sensitive hashing for approximate nearest neighbors. Use dynamic programming, hidden Markov models, and word embeddings to autocorrect misspelled words, autocomplete partial sentences, and identify ...
Study with Quizlet and memorize flashcards containing terms like D, A, A and more.
Logistic regression is one of the algorithms used for Classification types of problems and not for regression. For classification problems, the linear function won't fit so naturally.
Week 3 Lecture Notes ML:Logistic Regression. Now we are switching from regression problems to classication problems. Don't be confused by the name "Logistic Regression"; it is named that way for historical reasons and is actually an approach to classication problems, not regression problems. Binary Classication
This assignment is a part of Machine Learning course by Prof. Andrew Ng on Coursera. Programming Exercise 2: Logistic Regression. (machine-learning-ex2 week 3) Description of the contents of the repo: ex2.m - Octave/MATLAB script that steps you through the exercise ex2.
Regression models, a subset of linear models, are the most important statistical analysis tool in a data scientist's toolkit. This course covers regression analysis, least squares and inference using regression models. Special cases of the regression model, ANOVA and ANCOVA will be covered as well. Analysis of residuals and variability will ...
In summary, here are 10 of our most popular logistic regression courses. Logistic Regression in R for Public Health: Imperial College London. Predictive Modeling with Logistic Regression using SAS : SAS. Logistic Regression and Prediction for Health Data: University of Michigan. Logistic Regression with Python and Numpy: Coursera Project Network.
It provides a broad introduction to modern machine learning, including supervised learning (multiple linear regression, logistic regression, neural networks, and decision trees), unsupervised learning (clustering, dimensionality reduction, recommender systems), and some of the best practices used in Silicon Valley for artificial intelligence ...
Python Lesson 1: Categorical Explanatory Variables with More Than Two Categories • 6 minutes. Lesson 2: A Few Things to Keep in Mind • 2 minutes. SAS Lesson 3: Logistic Regression for a Binary Response Variable, pt 1 • 7 minutes. SAS Lesson 4: Logistic Regression for a Binary Response Variable, pt. 2 • 4 minutes.
Solutions of Deep Learning Specialization by Andrew Ng on Coursera - coursera-deep-learning-solutions/A - Neural Networks and Deep Learning/week 2/Logistic_Regression_with_a_Neural_Network_mindset_v6a.html at master · muhac/coursera-deep-learning-solutions