
An official website of the United States government
The .gov means it’s official. Federal government websites often end in .gov or .mil. Before sharing sensitive information, make sure you’re on a federal government site.
The site is secure. The https:// ensures that you are connecting to the official website and that any information you provide is encrypted and transmitted securely.
- Publications
- Account settings
Preview improvements coming to the PMC website in October 2024. Learn More or Try it out now .
- Advanced Search
- Journal List
- Health Expect
- v.21(6); 2018 Dec
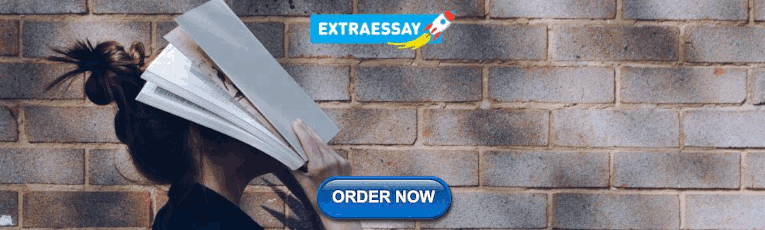
Public and patient involvement in quantitative health research: A statistical perspective
Ailish hannigan.
1 Public and Patient Involvement Research Unit, Graduate Entry Medical School, University of Limerick, Limerick, Ireland
2 Health Research Institute, University of Limerick, Limerick, Ireland
The majority of studies included in recent reviews of impact for public and patient involvement (PPI) in health research had a qualitative design. PPI in solely quantitative designs is underexplored, particularly its impact on statistical analysis. Statisticians in practice have a long history of working in both consultative (indirect) and collaborative (direct) roles in health research, yet their perspective on PPI in quantitative health research has never been explicitly examined.
To explore the potential and challenges of PPI from a statistical perspective at distinct stages of quantitative research, that is sampling, measurement and statistical analysis, distinguishing between indirect and direct PPI.
Conclusions
Statistical analysis is underpinned by having a representative sample, and a collaborative or direct approach to PPI may help achieve that by supporting access to and increasing participation of under‐represented groups in the population. Acknowledging and valuing the role of lay knowledge of the context in statistical analysis and in deciding what variables to measure may support collective learning and advance scientific understanding, as evidenced by the use of participatory modelling in other disciplines. A recurring issue for quantitative researchers, which reflects quantitative sampling methods, is the selection and required number of PPI contributors, and this requires further methodological development. Direct approaches to PPI in quantitative health research may potentially increase its impact, but the facilitation and partnership skills required may require further training for all stakeholders, including statisticians.
1. BACKGROUND
Public and patient involvement (PPI) in health research has been defined as research being carried out “with” or “by” members of the public rather than “to,” “about” or “for” them. 1 PPI covers a diverse range of approaches from “one off” information gathering to sustained partnerships. Tritter's conceptual framework for PPI distinguished between indirect involvement where information is gathered from patients and the public, but they do not have the power to make final decisions and direct involvement where patients and the public take part in the decision‐making. 2
A bibliometric review of the literature reported strong growth in the number of published empirical health research studies with public involvement. 3 In a systematic review of the impact of PPI on health and social care research, Brett et al 4 reported positive impacts at all stages of research from planning and undertaking the study to analysis, dissemination and implementation. The design of the majority of empirical research studies included in both reviews was qualitative (70% of studies in Brett. et al 4 and 73% in Boote et al 3 ). More significant tensions have been reported in community‐academic partnerships that use quantitative methods rather than solely qualitative methods, for example tensions with the community about having and recruiting to a “no intervention” comparison group. 5 Particular challenges for PPI have been reported in the most structured and regulated of quantitative designs, that is a randomized controlled trial (RCT), where there is little opportunity for flexibility once the trial has started 6 and Boote et al 3 concluded that researchers may find it easier to involve the public in qualitative rather than quantitative research.
If the full potential of PPI for health research is to be realized, its potential and challenges in quantitative research require more exploration, particularly the features of quantitative research which are different from qualitative research, for example, sampling, measurement and statistical analysis. Statisticians in practice have a long history of working with a variety of stakeholders in health research and have examined the difference between an indirect or consulting role for the statistician and a more direct, collaborative role, 7 yet their perspective has never been explicitly explored in health research with PPI. The objective of this study therefore was to critically reflect on the potential and challenges for PPI at distinct stages of quantitative research from a statistical perspective, distinguishing between direct and indirect approaches to PPI. 2
2. SAMPLE SIZE AND SELECTION
Quantitative research usually aims to provide precise, unbiased estimates of parameters of interest for the entire population which requires a large, randomly selected sample. Brett et al 4 reported a positive impact of PPI on recruitment in studies, but the representativeness of the sample is as important in quantitative research as sample size. Studies have shown that even when accrual targets have been met, the sample may not be fully representative of the population of interest. In cancer clinical trials, for example, those with health insurance and from higher socio‐economic backgrounds can be over‐represented, while older patients, ethnic minorities and so‐called hard‐to‐reach groups (often with higher cancer mortality rates) are under‐represented. 8 This limits the ability to generalize the results of the trials to all those with cancer. There is evidence that a direct approach to PPI with sustained partnerships between community leaders, primary care providers and clinical trial researchers can be effective in increasing awareness and participation of under‐represented groups in cancer clinical trials 9 , 10 and therefore help to achieve the goal of a population‐representative sample.
Collecting representative health data for some groups in the population may only be possible with their involvement. Marin et al 11 reports on the challenges of identifying an appropriate sampling frame for a health survey of Aboriginal adults in Southern Australia. Access to information identifying Aboriginal dwellings was not publically available, making it difficult to randomly select participants for large population household surveys. Trying to overcome this challenge involved reaching agreement on the process of research for Aboriginal adults with their local communities. An 8‐month consultation process was undertaken with representatives from multiple locations including Aboriginal owned lands in one region; however, it was ultimately agreed that it was culturally inappropriate for the research team to survey this region. The study demonstrated the opportunities for PPI in quantitative research with a representative sample of randomly chosen Aboriginal adults (excluding those resident in one region) ultimately achieved but also the challenges for PPI. The direct approach to involvement in this study, after a lengthy consultation process, resulted in a decision not to carry out the planned sampling and data collection in one region with implications for generalization of results and overall sample size.
Of course, given the importance of representativeness in quantitative research, there may be particular challenges for statisticians and quantitative researchers in accepting the term patient or public representative with some suggesting PPI contributor as a more appropriate term. 6 PPI representative may suggest to a quantitative researcher that an individual patient or member of the public is typical of an often diverse population, yet there is evidence that the opportunities and capacity to be involved as PPI contributors vary by level of education, income, cognitive skills and cultural background. 12 Dudley et al carried out a qualitative study of the impact of PPI in RCTs with patients and researchers from a cohort of RCTs. 6 The types of roles of PPI contributors described by researchers involved in the RCTs were grouped into oversight, managerial and responsive roles. Responsive PPI was described as informal and impromptu with researchers approaching multiple “responsive” PPI contributors as difficulties arose, for example advising on patient information sheets and follow‐up of patients. It was reported that contributions from responsive roles may carry more weight with the researchers in RCTs because it allowed access to a more diverse range of contributors who researchers saw as more “representative” of the target population.
3. MEASUREMENT
Measurement of quantitative data involves decisions about what to measure, how to measure it and how often to measure it with these decisions typically made by the research team. Without the involvement of patients and the public, however, important outcomes for people living with a condition have been missed or overlooked, for example fatigue for people with rheumatoid arthritis 13 or the long‐term effects of therapy for children with asthma. 14
Core outcome sets (COS) are a minimum set of agreed important outcomes to be measured in research on particular illnesses, conditions or treatments to ensure important outcomes are consistently reported and allow the results from multiple studies to be easily combined and compared. Young reported on workshops to explore what principles, methods and strategies that COS developers may need to consider when seeking patient input into the development of a COS. 15 The importance of distinguishing between an indirect role for patients in COS development where patients respond to a consensus survey or a direct role where patients are partners in planning, running and disseminating a COS study was highlighted by delegates in the workshops. While all delegates agreed that participation by patients should be meaningful and on an equal footing with other stakeholders, there was considerable uncertainty on how to achieve this, for example how many patients are needed in the COS development process or what proportion of patients relative to other stakeholders should be included. This raises the issue again of the number and selection of PPI contributors for quantitative researchers, and it was concluded that methodological work was needed to understand the COS development process from the perspective of patients and how the process may be improved for them.
Important considerations in longitudinal research are the number and timing of repeated measurements. From a statistical perspective, measurements on the same subject at different times are almost always correlated, with measurements taken close together in time being more highly correlated than measurements taken far apart in time. Unequal spacing of observation times may be more computationally challenging in statistical analysis of repeated measurements and missing data within subjects over time can be particularly challenging depending on the amount, cause and pattern of missing data. 16 There are therefore important statistical considerations to be taken into account in the design of longitudinal studies but these have to be balanced with input from PPI contributors on appropriate timing and frequency of data collection for potential participants.
Lucas et al reported on how European birth cohorts are engaging and consulting with young birth cohort members. 17 Of the 84 individual cohorts identified, only eight had a mechanism for consulting with parents and three a mechanism for consulting with young people themselves (usually “one off” consultations). Very varied follow‐up rates were reported from 13% to 84% more than 10 years after enrolment for individual data rounds of the birth cohorts. 17 Being motivated to continue to participate may be influenced by whether a participant believes the study is interesting, important, or relevant to them. 18 One of the key strategies for retention in the Australian Aboriginal Birth Cohort study was partnerships with community members with local knowledge who were involved in all phases of the follow‐up. 19 Retention rates of 86% at 11‐year follow‐up and 72% at 18‐year follow‐up were reported which demonstrates the potential of a direct approach to PPI. Ethical approval for the study involved an Aboriginal Ethical Sub‐committee which had the power of veto and a staged consent was used where participants had the right to refuse individual procedures at each wave. As with all missing data, this has implications for the statistical analysis yet only 10% of participants in this study chose to opt out of different assessments at follow‐up.
3.1. Statistical analysis
A report on the impact of PPI found that it had a positive impact at all stages of qualitative research including data analysis but that there was little evidence of its impact on quantitative data analysis. 20 It was concluded this lack of evidence may reflect a lack of involvement rather than an evidence gap. Booth et al 3 also suggested that the public may be more comfortable with interpreting interview and focus group data compared with numeric data. Low levels of numerical and statistical literacy in the general population may contribute to this.
Statistical analysis involves describing the data using appropriate graphical and numerical summaries (descriptive statistics) and using more advanced statistical methods to draw inferences about the population using the data from a sample (statistical inference). Choosing appropriate methods for statistical inference, testing the underlying assumptions and checking the adequacy of the models produced requires advanced statistical training and implementing them typically involves the use of statistical software or programming. Statisticians bring this expertise to quantitative health research and while it is important that the chosen methods are adequately communicated to all stakeholders, replicating this type of expertise in PPI contributors seems like an inefficient use of resources for PPI.
Quantitative data are, however, “not just numbers, they are numbers with a context” 21 and most practising statisticians agree that knowledge of the context is needed to carry out even a purely technical role effectively. 22 While many associate statistical analysis with objectivity, in practice, statisticians routinely use “subjective” external information to guide, for example the decision on what is a meaningful effect size; whether an outlier is an error in data entry or represents an unusual but meaningful observation; and potential issues with measurement of variables and confounding. 23 Gelman and Hennin argue that we should move beyond the discussion of objectivity and subjectivity in statistics and “replace each of them with broader collections of attributes, with objectivity replaced by transparency, consensus, impartiality and correspondence to observable reality, and subjectivity replaced by awareness of multiple perspectives and context dependence.” 23 This debate within statistics is relevant for PPI where the perceived objectivity and standardization of statistical analysis can be used as a reason for lack of involvement.
External information and context are particularly important in statistical modelling where statisticians are often faced with many potential predictors of an outcome. The “best” way of selecting a multivariable model is still unresolved from a statistical perspective, and it is generally agreed that subject matter knowledge, when available, should guide model building. 24 Even when the potential predictors are known, understanding the causal pathways of exposure on an outcome is challenging where the effect of a variable on the outcome can be direct or indirect. Christiaens et al 25 used a causal diagram to visualize the relationship between pain acceptance and personal control of women in labour and the use of pain medication during labour. Their analysis accounted for the maternal care context of the country where the women were giving birth and other characteristics such as age of the woman and duration of labour. The choice of these characteristics was underpinned by a literature review but women who have given birth also have expert knowledge on why they use pain relief and how other variables such as their personal beliefs and social context might influence that decision. 26
Collaborative or participatory modelling is an approach to scientific modelling in areas such as natural resource management which involves all stakeholders in the model building process. Participants can suggest characteristics for inclusion in the model and how they may impact on the outcome. Causal diagrams are then used to create a shared view across stakeholders. 27 Rockman et al 28 concluded, in the context of marine policy, that “participatory modelling has the potential to facilitate and structure discussions between scientists and stakeholders about uncertainties and the quality of the knowledge base. It can also contribute to collective learning, increase legitimacy and advance scientific understanding.”
There is emerging evidence that the importance of PPI in the development and application of modelling in health research is being recognized. Van Voorn 29 discussed the benefits and risks of PPI in health economic modelling of cost‐effectiveness of new drugs and treatment strategies, with public and patients described as the missing stakeholder group in the modelling process. The potential benefits included the expertise that patients could bring to the process, a greater understanding and possible acceptance by patients of the results of the models and improved model validation. The risks included potential patient bias and the increased resources required for training. The number and selection of patients to contribute to the process was also discussed with a suggestion to include patients “who were able to take a neutral view” and “at least five patients that differ significantly in their background,” again highlighting the focus of quantitative researchers on bias and sample size. The role for this type of participatory modelling in informing debate on public health problems is increasingly being recognized, drawing on the experience of its use in other areas where optimal use of limited resources is required to address complex problems in society. 30
4. CONCLUSIONS
Statistical analysis of quantitative data is underpinned by having a representative sample, and there is evidence that a direct approach to PPI can help achieve that by supporting access to and increasing participation of under‐represented groups in the population. The direct approach has also demonstrated its potential in the retention of those recruited over time, thus reducing bias caused by missing data in longitudinal studies. At all stages of statistical analysis, a statistician continuously refers back to the context of the data collected. 22 Lay knowledge of PPI contributors has an important role in providing this context, and there is evidence from other disciplines of the benefits of including this knowledge in analysis to support collective learning and advance scientific understanding.
The direct approach to PPI where patients and the public have the power to make decisions also brings challenges and the statistician needs to be able to clearly communicate the impact of each decision on the scientific rigour and validity of sampling, measurement and analysis to all stakeholders. Decisions made on participation impact on generalizability. Participatory modelling requires facilitation and partnership skills which may require further training for all stakeholders, including statisticians.
The direct and indirect role for PPI contributors mirrors what happens for statisticians in practice. Statisticians can have a consultative role, that is answering a specific statistical question or a collaborative role where a statistician works with others as equal partners to create new knowledge, with professional organizations for statisticians providing guidance and mentorship on moving from consulting to collaboration to leadership roles. 7 , 31 Statisticians therefore bring very relevant experience and understanding for PPI contributors on the ladder of participation in health research. Further exploration is required on the impact of direct compared to indirect involvement in quantitative research, drawing on the evidence base for community‐based participatory research in quantitative designs 9 and the framework for participatory health research and epidemiology. 32 , 33
CONFLICT OF INTERESTS
No conflict of interests.
ACKNOWLEDGEMENTS
Prof. Anne MacFarlane, Public and Patient Involvement Research Unit, University of Limerick, for discussion of ideas and comments on drafts.
Hannigan A. Public and patient involvement in quantitative health research: A statistical perspective . Health Expect . 2018; 21 :939–943. 10.1111/hex.12800 [ PMC free article ] [ PubMed ] [ CrossRef ] [ Google Scholar ]
- Research article
- Open access
- Published: 03 February 2021
A review of the quantitative effectiveness evidence synthesis methods used in public health intervention guidelines
- Ellesha A. Smith ORCID: orcid.org/0000-0002-4241-7205 1 ,
- Nicola J. Cooper 1 ,
- Alex J. Sutton 1 ,
- Keith R. Abrams 1 &
- Stephanie J. Hubbard 1
BMC Public Health volume 21 , Article number: 278 ( 2021 ) Cite this article
4128 Accesses
5 Citations
3 Altmetric
Metrics details
The complexity of public health interventions create challenges in evaluating their effectiveness. There have been huge advancements in quantitative evidence synthesis methods development (including meta-analysis) for dealing with heterogeneity of intervention effects, inappropriate ‘lumping’ of interventions, adjusting for different populations and outcomes and the inclusion of various study types. Growing awareness of the importance of using all available evidence has led to the publication of guidance documents for implementing methods to improve decision making by answering policy relevant questions.
The first part of this paper reviews the methods used to synthesise quantitative effectiveness evidence in public health guidelines by the National Institute for Health and Care Excellence (NICE) that had been published or updated since the previous review in 2012 until the 19th August 2019.The second part of this paper provides an update of the statistical methods and explains how they address issues related to evaluating effectiveness evidence of public health interventions.
The proportion of NICE public health guidelines that used a meta-analysis as part of the synthesis of effectiveness evidence has increased since the previous review in 2012 from 23% (9 out of 39) to 31% (14 out of 45). The proportion of NICE guidelines that synthesised the evidence using only a narrative review decreased from 74% (29 out of 39) to 60% (27 out of 45).An application in the prevention of accidents in children at home illustrated how the choice of synthesis methods can enable more informed decision making by defining and estimating the effectiveness of more distinct interventions, including combinations of intervention components, and identifying subgroups in which interventions are most effective.
Conclusions
Despite methodology development and the publication of guidance documents to address issues in public health intervention evaluation since the original review, NICE public health guidelines are not making full use of meta-analysis and other tools that would provide decision makers with fuller information with which to develop policy. There is an evident need to facilitate the translation of the synthesis methods into a public health context and encourage the use of methods to improve decision making.
Peer Review reports
To make well-informed decisions and provide the best guidance in health care policy, it is essential to have a clear framework for synthesising good quality evidence on the effectiveness and cost-effectiveness of health interventions. There is a broad range of methods available for evidence synthesis. Narrative reviews provide a qualitative summary of the effectiveness of the interventions. Meta-analysis is a statistical method that pools evidence from multiple independent sources [ 1 ]. Meta-analysis and more complex variations of meta-analysis have been extensively applied in the appraisals of clinical interventions and treatments, such as drugs, as the interventions and populations are clearly defined and tested in randomised, controlled conditions. In comparison, public health studies are often more complex in design, making synthesis more challenging [ 2 ].
Many challenges are faced in the synthesis of public health interventions. There is often increased methodological heterogeneity due to the inclusion of different study designs. Interventions are often poorly described in the literature which may result in variation within the intervention groups. There can be a wide range of outcomes, whose definitions are not consistent across studies. Intermediate, or surrogate, outcomes are often used in studies evaluating public health interventions [ 3 ]. In addition to these challenges, public health interventions are often also complex meaning that they are made up of multiple, interacting components [ 4 ]. Recent guidance documents have focused on the synthesis of complex interventions [ 2 , 5 , 6 ]. The National Institute for Health and Care Excellence (NICE) guidance manual provides recommendations across all topics that are covered by NICE and there is currently no guidance that focuses specifically on the public health context.
Research questions
A methodological review of NICE public health intervention guidelines by Achana et al. (2014) found that meta-analysis methods were not being used [ 3 ]. The first part of this paper aims to update and compare, to the original review, the meta-analysis methods being used in evidence synthesis of public health intervention appraisals.
The second part of this paper aims to illustrate what methods are available to address the challenges of public health intervention evidence synthesis. Synthesis methods that go beyond a pairwise meta-analysis are illustrated through the application to a case study in public health and are discussed to understand how evidence synthesis methods can enable more informed decision making.
The third part of this paper presents software, guidance documents and web tools for methods that aim to make appropriate evidence synthesis of public health interventions more accessible. Recommendations for future research and guidance production that can improve the uptake of these methods in a public health context are discussed.
Update of NICE public health intervention guidelines review
Nice guidelines.
The National Institute for Health and Care Excellence (NICE) was established in 1999 as a health authority to provide guidance on new medical technologies to the NHS in England and Wales [ 7 ]. Using an evidence-based approach, it provides recommendations based on effectiveness and cost-effectiveness to ensure an open and transparent process of allocating NHS resources [ 8 ]. The remit for NICE guideline production was extended to public health in April 2005 and the first recommendations were published in March 2006. NICE published ‘Developing NICE guidelines: the manual’ in 2006, which has been updated since, with the most recent in 2018 [ 9 ]. It was intended to be a guidance document to aid in the production of NICE guidelines across all NICE topics. In terms of synthesising quantitative evidence, the NICE recommendations state: ‘meta-analysis may be appropriate if treatment estimates of the same outcome from more than 1 study are available’ and ‘when multiple competing options are being appraised, a network meta-analysis should be considered’. The implementation of network meta-analysis (NMA), which is described later, as a recommendation from NICE was introduced into the guidance document in 2014, with a further update in 2018.
Background to the previous review
The paper by Achana et al. (2014) explored the use of evidence synthesis methodology in NICE public health intervention guidelines published between 2006 and 2012 [ 3 ]. The authors conducted a systematic review of the methods used to synthesise quantitative effectiveness evidence within NICE public health guidelines. They found that only 23% of NICE public health guidelines used pairwise meta-analysis as part of the effectiveness review and the remainder used a narrative summary or no synthesis of evidence at all. The authors argued that despite significant advances in the methodology of evidence synthesis, the uptake of methods in public health intervention evaluation is lower than other fields, including clinical treatment evaluation. The paper concluded that more sophisticated methods in evidence synthesis should be considered to aid in decision making in the public health context [ 3 ].
The search strategy used in this paper was equivalent to that in the previous paper by Achana et al. (2014)[ 3 ]. The search was conducted through the NICE website ( https://www.nice.org.uk/guidance ) by searching the ‘Guidance and Advice List’ and filtering by ‘Public Health Guidelines’ [ 10 ]. The search criteria included all guidance documents that had been published from inception (March 2006) until the 19th August 2019. Since the original review, many of the guidelines had been updated with new documents or merged. Guidelines that remained unchanged since the previous review in 2012 were excluded and used for comparison.
The guidelines contained multiple documents that were assessed for relevance. A systematic review is a separate synthesis within a guideline that systematically collates all evidence on a specific research question of interest in the literature. Systematic reviews of quantitative effectiveness, cost-effectiveness evidence and decision modelling reports were all included as relevant. Qualitative reviews, field reports, expert opinions, surveillance reports, review decisions and other supporting documents were excluded at the search stage.
Within the reports, data was extracted on the types of review (narrative summary, pairwise meta-analysis, network meta-analysis (NMA), cost-effectiveness review or decision model), design of included primary studies (randomised controlled trials or non-randomised studies, intermediate or final outcomes, description of outcomes, outcome measure statistic), details of the synthesis methods used in the effectiveness evaluation (type of synthesis, fixed or random effects model, study quality assessment, publication bias assessment, presentation of results, software). Further details of the interventions were also recorded, including whether multiple interventions were lumped together for a pairwise comparison, whether interventions were complex (made up of multiple components) and details of the components. The reports were also assessed for potential use of complex intervention evidence synthesis methodology, meaning that the interventions that were evaluated in the review were made up of components that could potentially be synthesised using an NMA or a component NMA [ 11 ]. Where meta-analysis was not used to synthesis effectiveness evidence, the reasons for this was also recorded.
Search results and types of reviews
There were 67 NICE public health guidelines available on the NICE website. A summary flow diagram describing the literature identification process and the list of guidelines and their reference codes are provided in Additional files 1 and 2 . Since the previous review, 22 guidelines had not been updated. The results from the previous review were used for comparison to the 45 guidelines that were either newly published or updated.
The guidelines consisted of 508 documents that were assessed for relevance. Table 1 shows which types of relevant documents were available in each of the 45 guidelines. The median number of relevant articles per guideline was 3 (minimum = 0, maximum = 10). Two (4%) of the NICE public health guidelines did not report any type of systematic review, cost-effectiveness review or decision model (NG68, NG64) that met the inclusion criteria. 167 documents from 43 NICE public health guidelines were systematic reviews of quantitative effectiveness, cost-effectiveness or decision model reports and met the inclusion criteria.
Narrative reviews of effectiveness were implemented in 41 (91%) of the NICE PH guidelines. 14 (31%) contained a review that used meta-analysis to synthesise the evidence. Only one (1%) NICE guideline contained a review that implemented NMA to synthesise the effectiveness of multiple interventions; this was the same guideline that used NMA in the original review and had been updated. 33 (73%) guidelines contained cost-effectiveness reviews and 34 (76%) developed a decision model.
Comparison of review types to original review
Table 2 compares the results of the update to the original review and shows that the types of reviews and evidence synthesis methodologies remain largely unchanged since 2012. The proportion of guidelines that only contain narrative reviews to synthesise effectiveness or cost-effectiveness evidence has reduced from 74% to 60% and the proportion that included a meta-analysis has increased from 23% to 31%. The proportion of guidelines with reviews that only included evidence from randomised controlled trials and assessed the quality of individual studies remained similar to the original review.
Characteristics of guidelines using meta-analytic methods
Table 3 details the characteristics of the meta-analytic methods implemented in 24 reviews of the 14 guidelines that included one. All of the reviews reported an assessment of study quality, 12 (50%) reviews included only data from randomised controlled trials, 4 (17%) reviews used intermediate outcomes (e.g. uptake of chlamydia screening rather than prevention of chlamydia (PH3)), compared to the 20 (83%) reviews that used final outcomes (e.g. smoking cessation rather than uptake of a smoking cessation programme (NG92)). 2 (8%) reviews only used a fixed effect meta-analysis, 19 (79%) reviews used a random effects meta-analysis and 3 (13%) did not report which they had used.
An evaluation of the intervention information reported in the reviews concluded that 12 (50%) reviews had lumped multiple (more than two) different interventions into a control versus intervention pairwise meta-analysis. Eleven (46%) of the reviews evaluated interventions that are made up of multiple components (e.g. interventions for preventing obesity in PH47 were made up of diet, physical activity and behavioural change components).
21 (88%) of the reviews presented the results of the meta-analysis in the form of a forest plot and 22 (92%) presented the results in the text of the report. 20 (83%) of the reviews used two or more forms of presentation for the results. Only three (13%) reviews assessed publication bias. The most common software to perform meta-analysis was RevMan in 14 (58%) of the reviews.
Reasons for not using meta-analytic methods
The 143 reviews of effectiveness and cost effectiveness that did not use meta-analysis methods to synthesise the quantitative effectiveness evidence were searched for reasons behind this decision. 70 reports (49%) did not give a reason for not synthesising the data using a meta-analysis and 164 reasons were reported which are displayed in Fig. 1 . Out of the remaining reviews, multiple reasons for not using a meta-analysis were given. 53 (37%) of the reviews reported at least one reason due to heterogeneity. 30 (21%) decision model reports did not give a reason and these are categorised separately. 5 (3%) reviews reported that meta-analysis was not applicable or feasible, 1 (1%) reported that they were following NICE guidelines and 5 (3%) reported that there were a lack of studies.
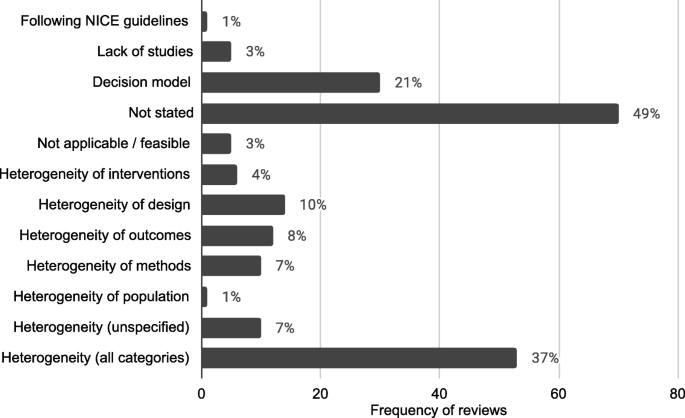
Frequency and proportions of reasons reported for not using statistical methods in quantitative evidence synthesis in NICE PH intervention reviews
The frequency of reviews and guidelines that used meta-analytic methods were plotted against year of publication, which is reported in Fig. 2 . This showed that the number of reviews that used meta-analysis were approximately constant but there is some suggestion that the number of meta-analyses used per guideline increased, particularly in 2018.
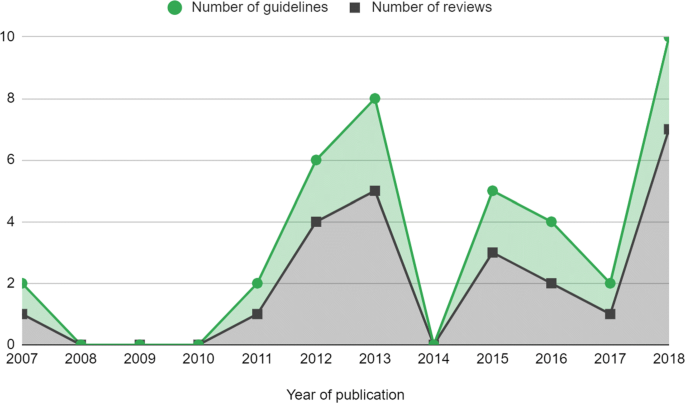
Number of meta-analyses in NICE PH guidelines by year. Guidelines that were published before 2012 had been updated since the previous review by Achana et al. (2014) [ 3 ]
Comparison of meta-analysis characteristics to original review
Table 4 compares the characteristics of the meta-analyses used in the evidence synthesis of NICE public health intervention guidelines to the original review by Achana et al. (2014) [ 3 ]. Overall, the characteristics in the updated review have not much changed from those in the original. These changes demonstrate that the use of meta-analysis in NICE guidelines has increased but remains low. Lumping of interventions still appears to be common in 50% of reviews. The implications of this are discussed in the next section.
Application of evidence synthesis methodology in a public health intervention: motivating example
Since the original review, evidence synthesis methods have been developed and can address some of the challenges of synthesising quantitative effectiveness evidence of public health interventions. Despite this, the previous section shows that the uptake of these methods is still low in NICE public health guidelines - usually limited to a pairwise meta-analysis.
It has been shown in the results above and elsewhere [ 12 ] that heterogeneity is a common reason for not synthesising the quantitative effectiveness evidence available from systematic reviews in public health. Statistical heterogeneity is the variation in the intervention effects between the individual studies. Heterogeneity is problematic in evidence synthesis as it leads to uncertainty in the pooled effect estimates in a meta-analysis which can make it difficult to interpret the pooled results and draw conclusions. Rather than exploring the source of the heterogeneity, often in public health intervention appraisals a random effects model is fitted which assumes that the study intervention effects are not equivalent but come from a common distribution [ 13 , 14 ]. Alternatively, as demonstrated in the review update, heterogeneity is used as a reason to not undertake any quantitative evidence synthesis at all.
Since the size of the intervention effects and the methodological variation in the studies will affect the impact of the heterogeneity on a meta-analysis, it is inappropriate to base the methodological approach of a review on the degree of heterogeneity, especially within public health intervention appraisal where heterogeneity seems inevitable. Ioannidis et al. (2008) argued that there are ‘almost always’ quantitative synthesis options that may offer some useful insights in the presence of heterogeneity, as long as the reviewers interpret the findings with respect to their limitations [ 12 ].
In this section current evidence synthesis methods are applied to a motivating example in public health. This aims to demonstrate that methods beyond pairwise meta-analysis can provide appropriate and pragmatic information to public health decision makers to enable more informed decision making.
Figure 3 summarises the narrative of this part of the paper and illustrates the methods that are discussed. The red boxes represent the challenges in synthesising quantitative effectiveness evidence and refers to the section within the paper for more detail. The blue boxes represent the methods that can be applied to investigate each challenge.
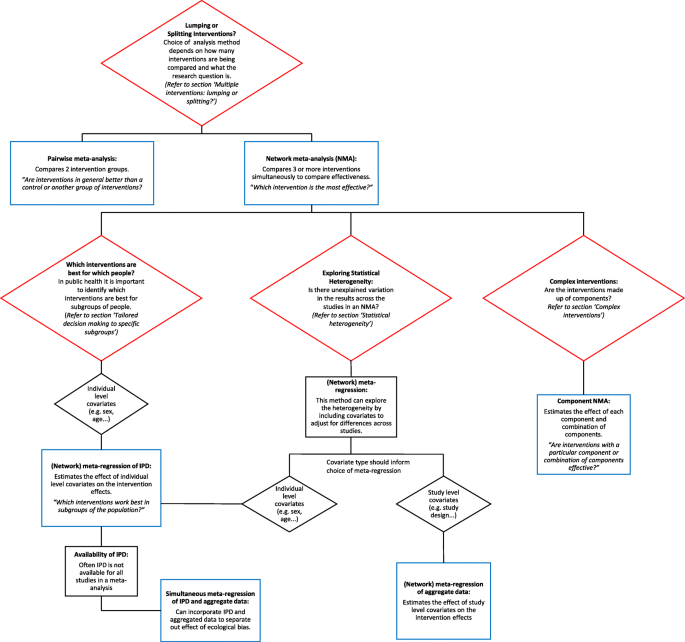
Summary of challenges that are faces in the evidence synthesis of public health interventions and methods that are discussed to overcome these challenges
Evaluating the effect of interventions for promoting the safe storage of cleaning products to prevent childhood poisoning accidents
To illustrate the methodological developments, a motivating example is used from the five year, NIHR funded, Keeping Children Safe Programme [ 15 ]. The project included a Cochrane systematic review that aimed to increase the use of safety equipment to prevent accidents at home in children under five years old. This application is intended to be illustrative of the benefits of new evidence synthesis methods since the previous review. It is not a complete, comprehensive analysis as it only uses a subset of the original dataset and therefore the results are not intended to be used for policy decision making. This example has been chosen as it demonstrates many of the issues in synthesising effectiveness evidence of public health interventions, including different study designs (randomised controlled trials, observational studies and cluster randomised trials), heterogeneity of populations or settings, incomplete individual participant data and complex interventions that contain multiple components.
This analysis will investigate the most effective promotional interventions for the outcome of ‘safe storage of cleaning products’ to prevent childhood poisoning accidents. There are 12 studies included in the dataset, with IPD available from nine of the studies. The covariate, single parent family, is included in the analysis to demonstrate the effect of being a single parent family on the outcome. In this example, all of the interventions are made up of one or more of the following components: education (Ed), free or low cost equipment (Eq), home safety inspection (HSI), and installation of safety equipment (In). A Bayesian approach using WinBUGS was used and therefore credible intervals (CrI) are presented with estimates of the effect sizes [ 16 ].
The original review paper by Achana et al. (2014) demonstrated pairwise meta-analysis and meta-regression using individual and cluster allocated trials, subgroup analyses, meta-regression using individual participant data (IPD) and summary aggregate data and NMA. This paper firstly applies NMA to the motivating example for context, followed by extensions to NMA.
Multiple interventions: lumping or splitting?
Often in public health there are multiple intervention options. However, interventions are often lumped together in a pairwise meta-analysis. Pairwise meta-analysis is a useful tool for two interventions or, alternatively in the presence of lumping interventions, for answering the research question: ‘are interventions in general better than a control or another group of interventions?’. However, when there are multiple interventions, this type of analysis is not appropriate for informing health care providers which intervention should be recommended to the public. ‘Lumping’ is becoming less frequent in other areas of evidence synthesis, such as for clinical interventions, as the use of sophisticated synthesis techniques, such as NMA, increases (Achana et al. 2014) but lumping is still common in public health.
NMA is an extension of the pairwise meta-analysis framework to more than two interventions. Multiple interventions that are lumped into a pairwise meta-analysis are likely to demonstrate high statistical heterogeneity. This does not mean that quantitative synthesis could not be undertaken but that a more appropriate method, NMA, should be implemented. Instead the statistical approach should be based on the research questions of the systematic review. For example, if the research question is ‘are any interventions effective for preventing obesity?’, it would be appropriate to perform a pairwise meta-analysis comparing every intervention in the literature to a control. However, if the research question is ‘which intervention is the most effective for preventing obesity?’, it would be more appropriate and informative to perform a network meta-analysis, which can compare multiple interventions simultaneously and identify the best one.
NMA is a useful statistical method in the context of public health intervention appraisal, where there are often multiple intervention options, as it estimates the relative effectiveness of three or more interventions simultaneously, even if direct study evidence is not available for all intervention comparisons. Using NMA can help to answer the research question ‘what is the effectiveness of each intervention compared to all other interventions in the network?’.
In the motivating example there are six intervention options. The effect of lumping interventions is shown in Fig. 4 , where different interventions in both the intervention and control arms are compared. There is overlap of intervention and control arms across studies and interpretation of the results of a pairwise meta-analysis comparing the effectiveness of the two groups of interventions would not be useful in deciding which intervention to recommend. In comparison, the network plot in Fig. 5 illustrates the evidence base of the prevention of childhood poisonings review comparing six interventions that promote the use of safety equipment in the home. Most of the studies use ‘usual care’ as a baseline and compare this to another intervention. There are also studies in the evidence base that compare pairs of the interventions, such as ‘Education and equipment’ to ‘Equipment’. The plot also demonstrates the absence of direct study evidence between many pairs of interventions, for which the associated treatment effects can be indirectly estimated using NMA.
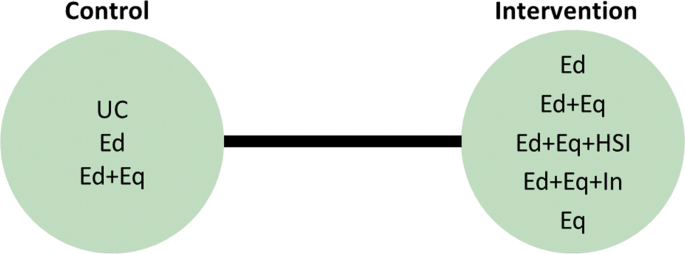
Network plot to illustrate how pairwise meta-analysis groups the interventions in the motivating dataset. Notation UC: Usual care, Ed: Education, Ed+Eq: Education and equipment, Ed+Eq+HSI: Education, equipment, and home safety inspection, Ed+Eq+In: Education, equipment and installation, Eq: Equipment
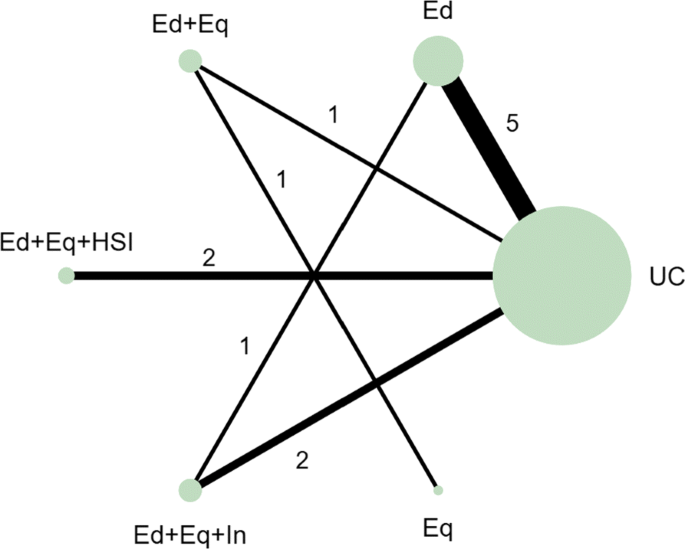
Network plot for the safe storage of cleaning products outcome. Notation UC: Usual care, Ed: Education, Ed+Eq: Education and equipment, Ed+Eq+HSI: Education, equipment, and home safety inspection, Ed+Eq+In: Education, equipment and installation, Eq: Equipment
An NMA was fitted to the motivating example to compare the six interventions in the studies from the review. The results are reported in the ‘triangle table’ in Table 5 [ 17 ]. The top right half of the table shows the direct evidence between pairs of the interventions in the corresponding rows and columns by either pooling the studies as a pairwise meta-analysis or presenting the single study results if evidence is only available from a single study. The bottom left half of the table reports the results of the NMA. The gaps in the top right half of the table arise where no direct study evidence exists to compare the two interventions. For example, there is no direct study evidence comparing ‘Education’ (Ed) to ‘Education, equipment and home safety inspection’ (Ed+Eq+HSI). The NMA, however, can estimate this comparison through the direct study evidence as an odds ratio of 3.80 with a 95% credible interval of (1.16, 12.44). The results suggest that the odds of safely storing cleaning products in the Ed+Eq+HSI intervention group is 3.80 times the odds in the Ed group. The results demonstrate a key benefit of NMA that all intervention effects in a network can be estimated using indirect evidence, even if there is no direct study evidence for some pairwise comparisons. This is based on the consistency assumption (that estimates of intervention effects from direct and indirect evidence are consistent) which should be checked when performing an NMA. This is beyond the scope of this paper and details on this can be found elsewhere [ 18 ].
NMA can also be used to rank the interventions in terms of their effectiveness and estimate the probability that each intervention is likely to be the most effective. This can help to answer the research question ‘which intervention is the best?’ out of all of the interventions that have provided evidence in the network. The rankings and associated probabilities for the motivating example are presented in Table 6 . It can be seen that in this case the ‘education, equipment and home safety inspection’ (Ed+Eq+HSI) intervention is ranked first, with a 0.87 probability of being the best intervention. However, there is overlap of the 95% credible intervals of the median rankings. This overlap reflects the uncertainty in the intervention effect estimates and therefore it is important that the interpretation of these statistics clearly communicates this uncertainty to decision makers.
NMA has the potential to be extremely useful but is underutilised in the evidence synthesis of public health interventions. The ability to compare and rank multiple interventions in an area where there are often multiple intervention options is invaluable in decision making for identifying which intervention to recommend. NMA can also include further literature in the analysis, compared to a pairwise meta-analysis, by expanding the network to improve the uncertainty in the effectiveness estimates.
Statistical heterogeneity
When heterogeneity remains in the results of an NMA, it is useful to explore the reasons for this. Strategies for dealing with heterogeneity involve the inclusion of covariates in a meta-analysis or NMA to adjust for the differences in the covariates across studies [ 19 ]. Meta-regression is a statistical method developed from meta-analysis that includes covariates to potentially explain the between-study heterogeneity ‘with the aim of estimating treatment-covariate interactions’ (Saramago et al. 2012). NMA has been extended to network meta-regression which investigates the effect of trial characteristics on multiple intervention effects. Three ways have been suggested to include covariates in an NMA: single covariate effect, exchangeable covariate effects and independent covariate effects which are discussed in more detail in the NICE Technical Support Document 3 [ 14 ]. This method has the potential to assess the effect of study level covariates on the intervention effects, which is particularly relevant in public health due to the variation across studies.
The most widespread method of meta-regression uses study level data for the inclusion of covariates into meta-regression models. Study level covariate data is when the data from the studies are aggregated, e.g. the proportion of participants in a study that are from single parent families compared to dual parent families. The alternative to study level data is individual participant data (IPD), where the data are available and used as a covariate at the individual level e.g. the parental status of every individual in a study can be used as a covariate. Although IPD is considered to be the gold standard for meta-analysis, aggregated level data is much more commonly used as it is usually available and easily accessible from published research whereas IPD can be hard to obtain from study authors.
There are some limitations to network meta-regression. In our motivating example, using the single parent covariate in a meta-regression would estimate the relative difference in the intervention effects of a population that is made up of 100% single parent families compared to a population that is made up of 100% dual parent families. This interpretation is not as useful as the analysis that uses IPD, which would give the relative difference of the intervention effects in a single parent family compared to a dual parent family. The meta-regression using aggregated data would also be susceptible to ecological bias. Ecological bias is where the effect of the covariate is different at the study level compared to the individual level [ 14 ]. For example, if each study demonstrates a relationship between a covariate and the intervention but the covariate is similar across the studies, a meta-regression of the aggregate data would not demonstrate the effect that is observed within the studies [ 20 ].
Although meta-regression is a useful tool for investigating sources of heterogeneity in the data, caution should be taken when using the results of meta-regression to explain how covariates affect the intervention effects. Meta-regression should only be used to investigate study characteristics, such as the duration of intervention, which will not be susceptible to ecological bias and the interpretation of the results (the effect of intervention duration on intervention effectiveness) would be more meaningful for the development of public health interventions.
Since the covariate of interest in this motivating example is not a study characteristic, meta-regression of aggregated covariate data was not performed. Network meta-regression including IPD and aggregate level data was developed by Samarago et al. (2012) [ 21 ] to overcome the issues with aggregated data network meta-regression, which is discussed in the next section.
Tailored decision making to specific sub-groups
In public health it is important to identify which interventions are best for which people. There has been a recent move towards precision medicine. In the field of public health the ‘concept of precision prevention may [...] be valuable for efficiently targeting preventive strategies to the specific subsets of a population that will derive maximal benefit’ (Khoury and Evans, 2015). Tailoring interventions has the potential to reduce the effect of inequalities in social factors that are influencing the health of the population. Identifying which interventions should be targeted to which subgroups can also lead to better public health outcomes and help to allocate scarce NHS resources. Research interest, therefore, lies in identifying participant level covariate-intervention interactions.
IPD meta-analysis uses data at the individual level to overcome ecological bias. The interpretation of IPD meta-analysis is more relevant in the case of using participant characteristics as covariates since the interpretation of the covariate-intervention interaction is at the individual level rather than the study level. This means that it can answer the research question: ‘which interventions work best in subgroups of the population?’. IPD meta-analyses are considered to be the gold standard for evidence synthesis since it increases the power of the analysis to identify covariate-intervention interactions and it has the ability to reduce the effect of ecological bias compared to aggregated data alone. IPD meta-analysis can also help to overcome scarcity of data issues and has been shown to have higher power and reduce the uncertainty in the estimates compared to analysis including only summary aggregate data [ 22 ].
Despite the advantages of including IPD in a meta-analysis, in reality it is often very time consuming and difficult to collect IPD for all of the studies [ 21 ]. Although data sharing is becoming more common, it remains time consuming and difficult to collect IPD for all studies in a review. This results in IPD being underutilised in meta-analyses. As an intermediate solution, statistical methods have been developed, such as the NMA in Samarago et al. (2012), that incorporates both IPD and aggregate data. Methods that simultaneously include IPD and aggregate level data have been shown to reduce uncertainty in the effect estimates and minimise ecological bias [ 20 , 21 ]. A simulation study by Leahy et al. (2018) found that an increased proportion of IPD resulted in more accurate and precise NMA estimates [ 23 ].
An NMA including IPD, where it is available, was performed, based on the model presented in Samarago et al. (2012) [ 21 ]. The results in Table 7 demonstrates the detail that this type of analysis can provide to base decisions on. More relevant covariate-intervention interaction interpretations can be obtained, for example the regression coefficients for covariate-intervention interactions are the individual level covariate intervention interactions or the ‘within study interactions’ that are interpreted as the effect of being in a single parent family on the effectiveness of each of the interventions. For example, the effect of Ed+Eq compared to UC in a single parent family is 1.66 times the effect of Ed+Eq compared to UC in a dual parent family but this is not an important difference as the credible interval crosses 1. The regression coefficients for the study level covariate-intervention interactions or the ‘between study interactions’ can be interpreted as the relative difference in the intervention effects of a population that is made up of 100% single parent families compared to a population that is made up of 100% dual parent families.
- Complex interventions
In many public health research settings the complex interventions are comprised of a number of components. An NMA can compare all of the interventions in a network as they are implemented in the original trials. However, NMA does not tell us which components of the complex intervention are attributable to this effect. It could be that particular components, or the interacting effect of multiple components, are driving the effectiveness and other components are not as effective. Often, trials have not directly compared every combination of components as there are so many component combination options, it would be inefficient and impractical. Component NMA was developed by Welton et al. (2009) to estimate the effect of each component of the complex interventions and combination of components in a network, in the absence of direct trial evidence and answers the question: ‘are interventions with a particular component or combination of components effective?’ [ 11 ]. For example, for the motivating example, in comparison to Fig. 5 , which demonstrates the interventions that an NMA can estimate effectiveness, Fig. 6 demonstrates all of the possible interventions of which the effectiveness can be estimated in a component NMA, given the components present in the network.
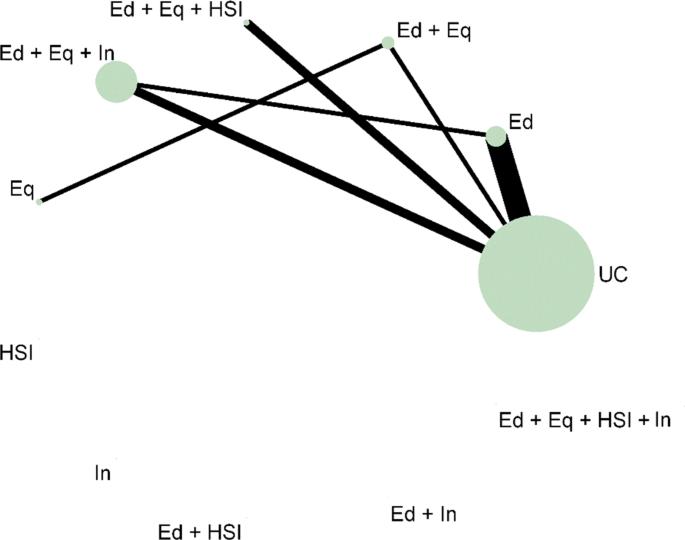
Network plot that illustrates how component network meta-analysis can estimate the effectiveness of intervention components and combinations of components, even when they are not included in the direct evidence. Notation UC: Usual care, Ed: Education, Eq: Equipment, Installation, Ed+Eq: Education and equipment, Ed+HSI: Education and home safety inspection, Ed+In: Education and installation, Eq+HSI: Equipment and home safety inspection, Eq+In: equipment and installation, HSI+In: Home safety inspection and installation, Ed+Eq+HSI: Education, equipment, and home safety inspection, Ed+Eq+In: Education, equipment and installation, Eq+HSI+In: Equipment, home safety inspection and installation, Ed+Eq+HSI+In: Education, equipment, home safety inspection and installation
The results of the analyses of the main effects, two way effects and full effects models are shown in Table 8 . The models, proposed in the original paper by Welton et al. (2009), increase in complexity as the assumptions regarding the component effects relax [ 24 ]. The main effects component NMA assumes that the components in the interventions each have separate, independent effects and intervention effects are the sum of the component effects. The two-way effects models assumes that there are interactions between pairs of the components, so the effects of the interventions are more than the sum of the effects. The full effects model assumes that all of the components and combinations of the components interact. Component NMA did not provide further insight into which components are likely to be the most effective since all of the 95% credible intervals were very wide and overlapped 1. There is a lot of uncertainty in the results, particularly in the 2-way and full effects models. A limitation of component NMA is that there are issues with uncertainty when data is scarce. However, the results demonstrate the potential of component NMA as a useful tool to gain better insights from the available dataset.
In practice, this method has rarely been used since its development [ 24 – 26 ]. It may be challenging to define the components in some areas of public health where many interventions have been studied. However, the use of meta-analysis for planning future studies is rarely discussed and component NMA would provide a useful tool for identifying new component combinations that may be more effective [ 27 ]. This type of analysis has the potential to prioritise future public health research, which is especially useful where there are multiple intervention options, and identify more effective interventions to recommend to the public.
Further methods / other outcomes
The analysis and methods described in this paper only cover a small subset of the methods that have been developed in meta-analysis in recent years. Methods that aim to assess the quality of evidence supporting a NMA and how to quantify how much the evidence could change due to potential biases or sampling variation before the recommendation changes have been developed [ 28 , 29 ]. Models adjusting for baseline risk have been developed to allow for different study populations to have different levels of underlying risk, by using the observed event rate in the control arm [ 30 , 31 ]. Multivariate methods can be used to compare the effect of multiple interventions on two or more outcomes simultaneously [ 32 ]. This area of methodological development is especially appealing within public health where studies assess a broad range of health effects and typically have multiple outcome measures. Multivariate methods offer benefits over univariate models by allowing the borrowing of information across outcomes and modelling the relationships between outcomes which can potentially reduce the uncertainty in the effect estimates [ 33 ]. Methods have also been developed to evaluate interventions with classes or different intervention intensities, known as hierarchical interventions [ 34 ]. These methods were not demonstrated in this paper but can also be useful tools for addressing challenges of appraising public health interventions, such as multiple and surrogate outcomes.
This paper only considered an example with a binary outcome. All of the methods described have also been adapted for other outcome measures. For example, the Technical Support Document 2 proposed a Bayesian generalised linear modelling framework to synthesise other outcome measures. More information and models for continuous and time-to-event data is available elsewhere [ 21 , 35 – 38 ].
Software and guidelines
In the previous section, meta-analytic methods that answer more policy relevant questions were demonstrated. However, as shown by the update to the review, methods such as these are still under-utilised. It is suspected from the NICE public health review that one of the reasons for the lack of uptake of methods in public health could be due to common software choices, such as RevMan, being limited in their flexibility for statistical methods.
Table 9 provides a list of software options and guidance documents that are more flexible than RevMan for implementing the statistical methods illustrated in the previous section to make these methods more accessible to researchers.
In this paper, the network plot in Figs. 5 and 6 were produced using the networkplot command from the mvmeta package [ 39 ] in Stata [ 61 ]. WinBUGS was used to fit the NMA in this paper by adapting the code in the book ‘Evidence Synthesis for Decision Making in Healthcare’ which also provides more detail on Bayesian methods and assessing convergence of Bayesian models [ 45 ]. The model for including IPD and summary aggregate data in an NMA was based on the code in the paper by Saramago et al. (2012). The component NMA in this paper was performed in WinBUGS through R2WinBUGS, [ 47 ] using the code in Welton et al. (2009) [ 11 ].
WinBUGS is a flexible tool for fitting complex models in a Bayesian framework. The NICE Decision Support Unit produced a series of Evidence Synthesis Technical Support Documents [ 46 ] that provide a comprehensive technical guide to methods for evidence synthesis and WinBUGS code is also provided for many of the models. Complex models can also be performed in a frequentist framework. Code and commands for many models are available in R and STATA (see Table 9 ).
The software, R2WinBUGS, was used in the analysis of the motivating example. Increasing numbers of researchers are using R and so packages that can be used to link the two softwares by calling BUGS models in R, packages such as R2WinBUGS, can improve the accessibility of Bayesian methods [ 47 ]. The new R package, BUGSnet, may also help to facilitate the accessibility and improve the reporting of Bayesian NMA [ 48 ]. Webtools have also been developed as a means of enabling researchers to undertake increasingly complex analyses [ 52 , 53 ]. Webtools provide a user-friendly interface to perform statistical analyses and often help in the reporting of the analyses by producing plots, including network plots and forest plots. These tools are very useful for researchers that have a good understanding of the statistical methods they want to implement as part of their review but are inexperienced in statistical software.
This paper has reviewed NICE public health intervention guidelines to identify the methods that are currently being used to synthesise effectiveness evidence to inform public health decision making. A previous review from 2012 was updated to see how method utilisation has changed. Methods have been developed since the previous review and these were applied to an example dataset to show how methods can answer more policy relevant questions. Resources and guidelines for implementing these methods were signposted to encourage uptake.
The review found that the proportion of NICE guidelines containing effectiveness evidence summarised using meta-analysis methods has increased since the original review, but remains low. The majority of the reviews presented only narrative summaries of the evidence - a similar result to the original review. In recent years, there has been an increased awareness of the need to improve decision making by using all of the available evidence. As a result, this has led to the development of new methods, easier application in standard statistical software packages, and guidance documents. Based on this, it would have been expected that their implementation would rise in recent years to reflect this, but the results of the review update showed no such increasing pattern.
A high proportion of NICE guideline reports did not provide a reason for not applying quantitative evidence synthesis methods. Possible explanations for this could be time or resource constraints, lack of statistical expertise, being unaware of the available methods or poor reporting. Reporting guidelines, such as the Preferred Reporting Items for Systematic Reviews and Meta-Analyses (PRISMA), should be updated to emphasise the importance of documenting reasons for not applying methods, as this can direct future research to improve uptake.
Where it was specified, the most common reported reason for not conducting a meta-analysis was heterogeneity. Often in public health, the data is heterogeneous due to the differences between studies in population, design, interventions or outcomes. A common misconception is that the presence of heterogeneity implies that it is not possible to pool the data. Meta-analytic methods can be used to investigate the sources of heterogeneity, as demonstrated in the NMA of the motivating example, and the use of IPD is recommended where possible to improve the precision of the results and reduce the effect of ecological bias. Although caution should be exercised in the interpretation of the results, quantitative synthesis methods provide a stronger basis for making decisions than narrative accounts because they explicitly quantify the heterogeneity and seek to explain it where possible.
The review also found that the most common software to perform the synthesis was RevMan. RevMan is very limited in its ability to perform advanced statistical analyses, beyond that of pairwise meta-analysis, which might explain the above findings. Standard software code is being developed to help make statistical methodology and application more accessible and guidance documents are becoming increasingly available.
The evaluation of public health interventions can be problematic due to the number and complexity of the interventions. NMA methods were applied to a real Cochrane public health review dataset. The methods that were demonstrated showed ways to address some of these issues, including the use of NMA for multiple interventions, the inclusion of covariates as both aggregated data and IPD to explain heterogeneity, and the extension to component network meta-analysis for guiding future research. These analyses illustrated how the choice of synthesis methods can enable more informed decision making by allowing more distinct interventions, and combinations of intervention components, to be defined and their effectiveness estimated. It also demonstrated the potential to target interventions to population subgroups where they are likely to be most effective. However, the application of component NMA to the motivating example has also demonstrated the issues around uncertainty if there are a limited number of studies observing the interventions and intervention components.
The application of methods to the motivating example demonstrated a key benefit of using statistical methods in a public health context compared to only presenting a narrative review – the methods provide a quantitative estimate of the effectiveness of the interventions. The uncertainty from the credible intervals can be used to demonstrate the lack of available evidence. In the context of decision making, having pooled estimates makes it much easier for decision makers to assess the effectiveness of the interventions or identify when more research is required. The posterior distribution of the pooled results from the evidence synthesis can also be incorporated into a comprehensive decision analytic model to determine cost-effectiveness [ 62 ]. Although narrative reviews are useful for describing the evidence base, the results are very difficult to summarise in a decision context.
Although heterogeneity seems to be inevitable within public health interventions due to their complex nature, this review has shown that it is still the main reported reason for not using statistical methods in evidence synthesis. This may be due to guidelines that were originally developed for clinical treatments that are tested in randomised conditions still being applied in public health settings. Guidelines for the choice of methods used in public health intervention appraisals could be updated to take into account the complexities and wide ranging areas in public health. Sophisticated methods may be more appropriate in some cases than simpler models for modelling multiple, complex interventions and their uncertainty, given the limitations are also fully reported [ 19 ]. Synthesis may not be appropriate if statistical heterogeneity remains after adjustment for possible explanatory covariates but details of exploratory analysis and reasons for not synthesising the data should be reported. Future research should focus on the application and dissemination of the advantages of using more advanced methods in public health, identifying circumstances where these methods are likely to be the most beneficial, and ways to make the methods more accessible, for example, the development of packages and web tools.
There is an evident need to facilitate the translation of the synthesis methods into a public health context and encourage the use of methods to improve decision making. This review has shown that the uptake of statistical methods for evaluating the effectiveness of public health interventions is slow, despite advances in methods that address specific issues in public health intervention appraisal and the publication of guidance documents to complement their application.
Availability of data and materials
The dataset supporting the conclusions of this article is included within the article.
Abbreviations
National institute for health and care excellence
- Network meta-analysis
Individual participant data
Home safety inspection
Installation
Credible interval
Preferred reporting items for systematic reviews and meta-analyses
Dias S, Welton NJ, Sutton AJ, Ades A. NICE DSU Technical Support Document 2: A Generalised Linear Modelling Framework for Pairwise and Network Meta-Analysis of Randomised Controlled Trials: National Institute for Health and Clinical Excellence; 2011, p. 98. (Technical Support Document in Evidence Synthesis; TSD2).
Higgins JPT, López-López JA, Becker BJ, et al.Synthesising quantitative evidence in systematic reviews of complex health interventions. BMJ Global Health. 2019; 4(Suppl 1):e000858. https://doi.org/10.1136/bmjgh-2018-000858 .
Article PubMed PubMed Central Google Scholar
Achana F, Hubbard S, Sutton A, Kendrick D, Cooper N. An exploration of synthesis methods in public health evaluations of interventions concludes that the use of modern statistical methods would be beneficial. J Clin Epidemiol. 2014; 67(4):376–90.
Article PubMed Google Scholar
Craig P, Dieppe P, Macintyre S, Michie S, Nazareth I, Petticrew M. Developing and evaluating complex interventions: the new medical research council guidance. Int J Nurs Stud. 2013; 50(5):587–92.
Caldwell DM, Welton NJ. Approaches for synthesising complex mental health interventions in meta-analysis. Evidence-Based Mental Health. 2016; 19(1):16–21.
Melendez-Torres G, Bonell C, Thomas J. Emergent approaches to the meta-analysis of multiple heterogeneous complex interventions. BMC Med Res Methodol. 2015; 15(1):47.
Article CAS PubMed PubMed Central Google Scholar
NICE. NICE: Who We Are. https://www.nice.org.uk/about/who-we-are . Accessed 19 Sept 2019.
Kelly M, Morgan A, Ellis S, Younger T, Huntley J, Swann C. Evidence based public health: a review of the experience of the national institute of health and clinical excellence (NICE) of developing public health guidance in England. Soc Sci Med. 2010; 71(6):1056–62.
NICE. Developing NICE Guidelines: The Manual. https://www.nice.org.uk/process/pmg20/chapter/introduction-and-overview . Accessed 19 Sept 2019.
NICE. Public Health Guidance. https://www.nice.org.uk/guidance/published?type=ph . Accessed 19 Sept 2019.
Welton NJ, Caldwell D, Adamopoulos E, Vedhara K. Mixed treatment comparison meta-analysis of complex interventions: psychological interventions in coronary heart disease. Am J Epidemiol. 2009; 169(9):1158–65.
Ioannidis JP, Patsopoulos NA, Rothstein HR. Reasons or excuses for avoiding meta-analysis in forest plots. BMJ. 2008; 336(7658):1413–5.
Higgins JP, Thompson SG. Quantifying heterogeneity in a meta-analysis. Stat Med. 2002; 21(11):1539–58.
Article Google Scholar
Dias S, Sutton A, Welton N, Ades A. NICE DSU Technical Support Document 3: Heterogeneity: Subgroups, Meta-Regression, Bias and Bias-Adjustment: National Institute for Health and Clinical Excellence; 2011, p. 76.
Kendrick D, Ablewhite J, Achana F, et al.Keeping Children Safe: a multicentre programme of research to increase the evidence base for preventing unintentional injuries in the home in the under-fives. Southampton: NIHR Journals Library; 2017.
Google Scholar
Lunn DJ, Thomas A, Best N, et al.WinBUGS - A Bayesian modelling framework: Concepts, structure, and extensibility. Stat Comput. 2000; 10:325–37. https://doi.org/10.1023/A:1008929526011 .
Dias S, Caldwell DM. Network meta-analysis explained. Arch Dis Child Fetal Neonatal Ed. 2019; 104(1):8–12. https://doi.org/10.1136/archdischild-2018-315224. http://arxiv.org/abs/https://fn.bmj.com/content/104/1/F8.full.pdf.
Dias S, Welton NJ, Sutton AJ, Caldwell DM, Lu G, Ades A. NICE DSU Technical Support Document 4: Inconsistency in Networks of Evidence Based on Randomised Controlled Trials: National Institute for Health and Clinical Excellence; 2011. (NICE DSU Technical Support Document in Evidence Synthesis; TSD4).
Cipriani A, Higgins JP, Geddes JR, Salanti G. Conceptual and technical challenges in network meta-analysis. Ann Intern Med. 2013; 159(2):130–7.
Riley RD, Steyerberg EW. Meta-analysis of a binary outcome using individual participant data and aggregate data. Res Synth Methods. 2010; 1(1):2–19.
Saramago P, Sutton AJ, Cooper NJ, Manca A. Mixed treatment comparisons using aggregate and individual participant level data. Stat Med. 2012; 31(28):3516–36.
Lambert PC, Sutton AJ, Abrams KR, Jones DR. A comparison of summary patient-level covariates in meta-regression with individual patient data meta-analysis. J Clin Epidemiol. 2002; 55(1):86–94.
Article CAS PubMed Google Scholar
Leahy J, O’Leary A, Afdhal N, Gray E, Milligan S, Wehmeyer MH, Walsh C. The impact of individual patient data in a network meta-analysis: an investigation into parameter estimation and model selection. Res Synth Methods. 2018; 9(3):441–69.
Freeman SC, Scott NW, Powell R, Johnston M, Sutton AJ, Cooper NJ. Component network meta-analysis identifies the most effective components of psychological preparation for adults undergoing surgery under general anesthesia. J Clin Epidemiol. 2018; 98:105–16.
Pompoli A, Furukawa TA, Efthimiou O, Imai H, Tajika A, Salanti G. Dismantling cognitive-behaviour therapy for panic disorder: a systematic review and component network meta-analysis. Psychol Med. 2018; 48(12):1945–53.
Rücker G, Schmitz S, Schwarzer G. Component network meta-analysis compared to a matching method in a disconnected network: A case study. Biom J. 2020. https://doi.org/10.1002/bimj.201900339 .
Efthimiou O, Debray TP, van Valkenhoef G, Trelle S, Panayidou K, Moons KG, Reitsma JB, Shang A, Salanti G, Group GMR. GetReal in network meta-analysis: a review of the methodology. Res Synth Methods. 2016; 7(3):236–63.
Salanti G, Del Giovane C, Chaimani A, Caldwell DM, Higgins JP. Evaluating the quality of evidence from a network meta-analysis. PLoS ONE. 2014; 9(7):99682.
Article CAS Google Scholar
Phillippo DM, Dias S, Welton NJ, Caldwell DM, Taske N, Ades A. Threshold analysis as an alternative to grade for assessing confidence in guideline recommendations based on network meta-analyses. Ann Intern Med. 2019; 170(8):538–46.
Dias S, Welton NJ, Sutton AJ, Ades AE. NICE DSU Technical Support Document 5: Evidence Synthesis in the Baseline Natural History Model: National Institute for Health and Clinical Excellence; 2011, p. 29. (NICE DSU Technical Support Document in Evidence Synthesis; TSD5).
Achana FA, Cooper NJ, Dias S, Lu G, Rice SJ, Kendrick D, Sutton AJ. Extending methods for investigating the relationship between treatment effect and baseline risk from pairwise meta-analysis to network meta-analysis. Stat Med. 2013; 32(5):752–71.
Riley RD, Jackson D, Salanti G, Burke DL, Price M, Kirkham J, White IR. Multivariate and network meta-analysis of multiple outcomes and multiple treatments: rationale, concepts, and examples. BMJ (Clinical research ed.) 2017; 358:j3932. https://doi.org/10.1136/bmj.j3932 .
Achana FA, Cooper NJ, Bujkiewicz S, Hubbard SJ, Kendrick D, Jones DR, Sutton AJ. Network meta-analysis of multiple outcome measures accounting for borrowing of information across outcomes. BMC Med Res Methodol. 2014; 14(1):92.
Owen RK, Tincello DG, Keith RA. Network meta-analysis: development of a three-level hierarchical modeling approach incorporating dose-related constraints. Value Health. 2015; 18(1):116–26.
Jansen JP. Network meta-analysis of individual and aggregate level data. Res Synth Methods. 2012; 3(2):177–90.
Donegan S, Williamson P, D’Alessandro U, Garner P, Smith CT. Combining individual patient data and aggregate data in mixed treatment comparison meta-analysis: individual patient data may be beneficial if only for a subset of trials. Stat Med. 2013; 32(6):914–30.
Saramago P, Chuang L-H, Soares MO. Network meta-analysis of (individual patient) time to event data alongside (aggregate) count data. BMC Med Res Methodol. 2014; 14(1):105.
Thom HH, Capkun G, Cerulli A, Nixon RM, Howard LS. Network meta-analysis combining individual patient and aggregate data from a mixture of study designs with an application to pulmonary arterial hypertension. BMC Med Res Methodol. 2015; 15(1):34.
Gasparrini A, Armstrong B, Kenward MG. Multivariate meta-analysis for non-linear and other multi-parameter associations. Stat Med. 2012; 31(29):3821–39.
Chaimani A, Higgins JP, Mavridis D, Spyridonos P, Salanti G. Graphical tools for network meta-analysis in stata. PLoS ONE. 2013; 8(10):76654.
Rücker G, Schwarzer G, Krahn U, König J. netmeta: Network meta-analysis with R. R package version 0.5-0. 2014. R package version 0.5-0. Availiable: http://CRAN.R-project.org/package=netmeta .
van Valkenhoef G, Kuiper J. gemtc: Network Meta-Analysis Using Bayesian Methods. R package version 0.8-2. 2016. Available online at: https://CRAN.R-project.org/package=gemtc .
Lin L, Zhang J, Hodges JS, Chu H. Performing arm-based network meta-analysis in R with the pcnetmeta package. J Stat Softw. 2017; 80(5):1–25. https://doi.org/10.18637/jss.v080.i05 .
Rücker G, Schwarzer G. Automated drawing of network plots in network meta-analysis. Res Synth Methods. 2016; 7(1):94–107.
Welton NJ, Sutton AJ, Cooper N, Abrams KR, Ades A. Evidence Synthesis for Decision Making in Healthcare, vol. 132. UK: Wiley; 2012.
Book Google Scholar
Dias S, Welton NJ, Sutton AJ, Ades AE. Evidence synthesis for decision making 1: introduction. Med Decis Making Int J Soc Med Decis Making. 2013; 33(5):597–606. https://doi.org/10.1177/0272989X13487604 .
Sturtz S, Ligges U, Gelman A. R2WinBUGS: a package for running WinBUGS from R. J Stat Softw. 2005; 12(3):1–16.
Béliveau A, Boyne DJ, Slater J, Brenner D, Arora P. Bugsnet: an r package to facilitate the conduct and reporting of bayesian network meta-analyses. BMC Med Res Methodol. 2019; 19(1):196.
Neupane B, Richer D, Bonner AJ, Kibret T, Beyene J. Network meta-analysis using R: a review of currently available automated packages. PLoS ONE. 2014; 9(12):115065.
White IR. Multivariate random-effects meta-analysis. Stata J. 2009; 9(1):40–56.
Chaimani A, Salanti G. Visualizing assumptions and results in network meta-analysis: the network graphs package. Stata J. 2015; 15(4):905–50.
Owen RK, Bradbury N, Xin Y, Cooper N, Sutton A. MetaInsight: An interactive web-based tool for analyzing, interrogating, and visualizing network meta-analyses using R-shiny and netmeta. Res Synth Methods. 2019; 10(4):569–81. https://doi.org/10.1002/jrsm.1373 .
Freeman SC, Kerby CR, Patel A, Cooper NJ, Quinn T, Sutton AJ. Development of an interactive web-based tool to conduct and interrogate meta-analysis of diagnostic test accuracy studies: MetaDTA. BMC Med Res Methodol. 2019; 19(1):81.
Nikolakopoulou A, Higgins JPT, Papakonstantinou T, Chaimani A, Del Giovane C, Egger M, Salanti G. CINeMA: An approach for assessing confidence in the results of a network meta-analysis. PLoS Med. 2020; 17(4):e1003082. https://doi.org/10.1371/journal.pmed.1003082 .
Viechtbauer W. Conducting meta-analyses in R with the metafor package. J Stat Softw. 2010; 36(3):1–48.
Freeman SC, Carpenter JR. Bayesian one-step ipd network meta-analysis of time-to-event data using royston-parmar models. Res Synth Methods. 2017; 8(4):451–64.
Riley RD, Lambert PC, Staessen JA, Wang J, Gueyffier F, Thijs L, Boutitie F. Meta-analysis of continuous outcomes combining individual patient data and aggregate data. Stat Med. 2008; 27(11):1870–93.
Debray TP, Moons KG, van Valkenhoef G, Efthimiou O, Hummel N, Groenwold RH, Reitsma JB, Group GMR. Get real in individual participant data (ipd) meta-analysis: a review of the methodology. Res Synth Methods. 2015; 6(4):293–309.
Tierney JF, Vale C, Riley R, Smith CT, Stewart L, Clarke M, Rovers M. Individual Participant Data (IPD) Meta-analyses of Randomised Controlled Trials: Guidance on Their Use. PLoS Med. 2015; 12(7):e1001855. https://doi.org/10.1371/journal.pmed.1001855 .
Stewart LA, Clarke M, Rovers M, Riley RD, Simmonds M, Stewart G, Tierney JF. Preferred reporting items for a systematic review and meta-analysis of individual participant data: the prisma-ipd statement. JAMA. 2015; 313(16):1657–65.
StataCorp. Stata Statistical Software: Release 16. College Station: StataCorp LLC; 2019.
Cooper NJ, Sutton AJ, Abrams KR, Turner D, Wailoo A. Comprehensive decision analytical modelling in economic evaluation: a bayesian approach. Health Econ. 2004; 13(3):203–26.
Download references
Acknowledgements
We would like to acknowledge Professor Denise Kendrick as the lead on the NIHR Keeping Children Safe at Home Programme that originally funded the collection of the evidence for the motivating example and some of the analyses illustrated in the paper.
ES is funded by a National Institute for Health Research (NIHR), Doctoral Research Fellow for this research project. This paper presents independent research funded by the National Institute for Health Research (NIHR). The views expressed are those of the authors and not necessarily those of the NHS, the NIHR or the Department of Health and Social Care. The funding bodies played no role in the design of the study and collection, analysis, and interpretation of data and in writing the manuscript.
Author information
Authors and affiliations.
Department of Health Sciences, University of Leicester, Lancaster Road, Leicester, UK
Ellesha A. Smith, Nicola J. Cooper, Alex J. Sutton, Keith R. Abrams & Stephanie J. Hubbard
You can also search for this author in PubMed Google Scholar
Contributions
ES performed the review, analysed the data and wrote the paper. SH supervised the project. SH, KA, NC and AS provided substantial feedback on the manuscript. All authors have read and approved the manuscript.
Corresponding author
Correspondence to Ellesha A. Smith .
Ethics declarations
Ethics approval and consent to participate.
Not applicable.
Consent for publication
Competing interests.
KA is supported by Health Data Research (HDR) UK, the UK National Institute for Health Research (NIHR) Applied Research Collaboration East Midlands (ARC EM), and as a NIHR Senior Investigator Emeritus (NF-SI-0512-10159). The views expressed are those of the author(s) and not necessarily those of the NIHR or the Department of Health and Social Care. KA has served as a paid consultant, providing unrelated methodological advice, to; Abbvie, Amaris, Allergan, Astellas, AstraZeneca, Boehringer Ingelheim, Bristol-Meyers Squibb, Creativ-Ceutical, GSK, ICON/Oxford Outcomes, Ipsen, Janssen, Eli Lilly, Merck, NICE, Novartis, NovoNordisk, Pfizer, PRMA, Roche and Takeda, and has received research funding from Association of the British Pharmaceutical Industry (ABPI), European Federation of Pharmaceutical Industries & Associations (EFPIA), Pfizer, Sanofi and Swiss Precision Diagnostics. He is a Partner and Director of Visible Analytics Limited, a healthcare consultancy company.
Additional information
Publisher’s note.
Springer Nature remains neutral with regard to jurisdictional claims in published maps and institutional affiliations.
Supplementary Information
Additional file 1.
Key for the Nice public health guideline codes. Available in NICEGuidelinesKey.xlsx .
Additional file 2
NICE public health intervention guideline review flowchart for the inclusion and exclusion of documents. Available in Flowchart.JPG .
Rights and permissions
Open Access This article is licensed under a Creative Commons Attribution 4.0 International License, which permits use, sharing, adaptation, distribution and reproduction in any medium or format, as long as you give appropriate credit to the original author(s) and the source, provide a link to the Creative Commons licence, and indicate if changes were made. The images or other third party material in this article are included in the article’s Creative Commons licence, unless indicated otherwise in a credit line to the material. If material is not included in the article’s Creative Commons licence and your intended use is not permitted by statutory regulation or exceeds the permitted use, you will need to obtain permission directly from the copyright holder. To view a copy of this licence, visit http://creativecommons.org/licenses/by/4.0/ . The Creative Commons Public Domain Dedication waiver ( http://creativecommons.org/publicdomain/zero/1.0/ ) applies to the data made available in this article, unless otherwise stated in a credit line to the data.
Reprints and permissions
About this article
Cite this article.
Smith, E.A., Cooper, N.J., Sutton, A.J. et al. A review of the quantitative effectiveness evidence synthesis methods used in public health intervention guidelines. BMC Public Health 21 , 278 (2021). https://doi.org/10.1186/s12889-021-10162-8
Download citation
Received : 22 September 2020
Accepted : 04 January 2021
Published : 03 February 2021
DOI : https://doi.org/10.1186/s12889-021-10162-8
Share this article
Anyone you share the following link with will be able to read this content:
Sorry, a shareable link is not currently available for this article.
Provided by the Springer Nature SharedIt content-sharing initiative
- Meta-analysis
- Systematic review
- Public health
- Decision making
- Evidence synthesis
BMC Public Health
ISSN: 1471-2458
- General enquiries: [email protected]
- - Google Chrome
Intended for healthcare professionals
- My email alerts
- BMA member login
- Username * Password * Forgot your log in details? Need to activate BMA Member Log In Log in via OpenAthens Log in via your institution

Search form
- Advanced search
- Search responses
- Search blogs
- Using data for...
Using data for improvement
Read the full collection.
- Related content
- Peer review
- Amar Shah , chief quality officer and consultant forensic psychiatrist, national improvement lead for the Mental Health Safety Improvement Programme
- East London NHS Foundation Trust, London, E1 8DE, UK
- amarshah{at}nhs.net @DrAmarShah
What you need to know
Both qualitative and quantitative data are critical for evaluating and guiding improvement
A family of measures, incorporating outcome, process, and balancing measures, should be used to track improvement work
Time series analysis, using small amounts of data collected and displayed frequently, is the gold standard for using data for improvement
We all need a way to understand the quality of care we are providing, or receiving, and how our service is performing. We use a range of data in order to fulfil this need, both quantitative and qualitative. Data are defined as “information, especially facts and numbers, collected to be examined and considered and used to help decision-making.” 1 Data are used to make judgements, to answer questions, and to monitor and support improvement in healthcare ( box 1 ). The same data can be used in different ways, depending on what we want to know or learn.
Defining quality improvement 2
Quality improvement aims to make a difference to patients by improving safety, effectiveness, and experience of care by:
Using understanding of our complex healthcare environment
Applying a systematic approach
Designing, testing, and implementing changes using real-time measurement for improvement
Within healthcare, we use a range of data at different levels of the system:
Patient level—such as blood sugar, temperature, blood test results, or expressed wishes for care)
Service level—such as waiting times, outcomes, complaint themes, or collated feedback of patient experience
Organisation level—such as staff experience or financial performance
Population level—such as mortality, quality of life, employment, and air quality.
This article outlines the data we need to understand the quality of care we are providing, what we need to capture to see if care is improving, how to interpret the data, and some tips for doing this more effectively.
Sources and selection criteria
This article is based on my experience of using data for improvement at East London NHS Foundation Trust, which is seen as one of the world leaders in healthcare quality improvement. Our use of data, from trust board to clinical team, has transformed over the past six years in line with the learning shared in this article. This article is also based on my experience of teaching with the Institute for Healthcare Improvement, which guides and supports quality improvement efforts across the globe.
What data do we need?
Healthcare is a complex system, with multiple interdependencies and an array of factors influencing outcomes. Complex systems are open, unpredictable, and continually adapting to their environment. 3 No single source of data can help us understand how a complex system behaves, so we need several data sources to see how a complex system in healthcare is performing.
Avedis Donabedian, a doctor born in Lebanon in 1919, studied quality in healthcare and contributed to our understanding of using outcomes. 4 He described the importance of focusing on structures and processes in order to improve outcomes. 5 When trying to understand quality within a complex system, we need to look at a mix of outcomes (what matters to patients), processes (the way we do our work), and structures (resources, equipment, governance, etc).
Therefore, when we are trying to improve something, we need a small number of measures (ideally 5-8) to help us monitor whether we are moving towards our goal. Any improvement effort should include one or two outcome measures linked explicitly to the aim of the work, a small number of process measures that show how we are doing with the things we are actually working on to help us achieve our aim, and one or two balancing measures ( box 2 ). Balancing measures help us spot unintended consequences of the changes we are making. As complex systems are unpredictable, our new changes may result in an unexpected adverse effect. Balancing measures help us stay alert to these, and ought to be things that are already collected, so that we do not waste extra resource on collecting these.
Different types of measures of quality of care
Outcome measures (linked explicitly to the aim of the project).
Aim— To reduce waiting times from referral to appointment in a clinic
Outcome measure— Length of time from referral being made to being seen in clinic
Data collection— Date when each referral was made, and date when each referral was seen in clinic, in order to calculate the time in days from referral to being seen
Process measures (linked to the things you are going to work on to achieve the aim)
Change idea— Use of a new referral form (to reduce numbers of inappropriate referrals and re-work in obtaining necessary information)
Process measure— Percentage of referrals received that are inappropriate or require further information
Data collection— Number of referrals received that are inappropriate or require further information each week divided by total number of referrals received each week
Change idea— Text messaging patients two days before the appointment (to reduce non-attendance and wasted appointment slots)
Process measure— Percentage of patients receiving a text message two days before appointment
Data collection— Number of patients each week receiving a text message two days before their appointment divided by the total number of patients seen each week
Process measure— Percentage of patients attending their appointment
Data collection— Number of patients attending their appointment each week divided by the total number of patients booked in each week
Balancing measures (to spot unintended consequences)
Measure— Percentage of referrers who are satisfied or very satisfied with the referral process (to spot whether all these changes are having a detrimental effect on the experience of those referring to us)
Data collection— A monthly survey to referrers to assess their satisfaction with the referral process
Measure— Percentage of staff who are satisfied or very satisfied at work (to spot whether the changes are increasing burden on staff and reducing their satisfaction at work)
Data collection— A monthly survey for staff to assess their satisfaction at work
How should we look at the data?
This depends on the question we are trying to answer. If we ask whether an intervention was efficacious, as we might in a research study, we would need to be able to compare data before and after the intervention and remove all potential confounders and bias. For example, to understand whether a new treatment is better than the status quo, we might design a research study to compare the effect of the two interventions and ensure that all other characteristics are kept constant across both groups. This study might take several months, or possibly years, to complete, and would compare the average of both groups to identify whether there is a statistically significant difference.
This approach is unlikely to be possible in most contexts where we are trying to improve quality. Most of the time when we are improving a service, we are making multiple changes and assessing impact in real-time, without being able to remove all confounding factors and potential bias. When we ask whether an outcome has improved, as we do when trying to improve something, we need to be able to look at data over time to see how the system changes as we intervene, with multiple tests of change over a period. For example, if we were trying to improve the time from a patient presenting in the emergency department to being admitted to a ward, we would likely be testing several different changes at different places in the pathway. We would want to be able to look at the outcome measure of total time from presentation to admission on the ward, over time, on a daily basis, to be able to see whether the changes made lead to a reduction in the overall outcome. So, when looking at a quality issue from an improvement perspective, we view smaller amounts of data but more frequently to see if we are improving over time. 2
What is best practice in using data to support improvement?
Best practice would be for each team to have a small number of measures that are collectively agreed with patients and service users as being the most important ways of understanding the quality of the service being provided. These measures would be displayed transparently so that all staff, service users, and patients and families or carers can access them and understand how the service is performing. The data would be shown as time series analysis, to provide a visual display of whether the service is improving over time. The data should be available as close to real-time as possible, ideally on a daily or weekly basis. The data should prompt discussion and action, with the team reviewing the data regularly, identifying any signals that suggest something unusual in the data, and taking action as necessary.
The main tools used for this purpose are the run chart and the Shewhart (or control) chart. The run chart ( fig 1 ) is a graphical display of data in time order, with a median value, and uses probability-based rules to help identify whether the variation seen is random or non-random. 2 The Shewhart (control) chart ( fig 2 ) also displays data in time order, but with a mean as the centre line instead of a median, and upper and lower control limits (UCL and LCL) defining the boundaries within which you would predict the data to be. 6 Shewhart charts use the terms “common cause variation” and “special cause variation,” with a different set of rules to identify special causes.

A typical run chart
- Download figure
- Open in new tab
- Download powerpoint

A typical Shewhart (or control) chart
Is it just about numbers?
We need to incorporate both qualitative and quantitative data to help us learn about how the system is performing and to see if we improve over time. Quantitative data express quantity, amount, or range and can be measured numerically—such as waiting times, mortality, haemoglobin level, cash flow. Quantitative data are often visualised over time as time series analyses (run charts or control charts) to see whether we are improving.
However, we should also be capturing, analysing, and learning from qualitative data throughout our improvement work. Qualitative data are virtually any type of information that can be observed and recorded that is not numerical in nature. Qualitative data are particularly useful in helping us to gain deeper insight into an issue, and to understand meaning, opinion, and feelings. This is vital in supporting us to develop theories about what to focus on and what might make a difference. 7 Examples of qualitative data include waiting room observation, feedback about experience of care, free-text responses to a survey.
Using qualitative data for improvement
One key point in an improvement journey when qualitative data are critical is at the start, when trying to identify “What matters most?” and what the team’s biggest opportunity for improvement is. The other key time to use qualitative data is during “Plan, Do, Study, Act” (PDSA) cycles. Most PDSA cycles, when done well, rely on qualitative data as well as quantitative data to help learn about how the test fared compared with our original theory and prediction.
Table 1 shows four different ways to collect qualitative data, with advantages and disadvantages of each, and how we might use them within our improvement work.
Different ways to collect qualitative data for improvement
- View inline
Tips to overcome common challenges in using data for improvement?
One of the key challenges faced by healthcare teams across the globe is being able to access data that is routinely collected, in order to use it for improvement. Large volumes of data are collected in healthcare, but often little is available to staff or service users in a timescale or in a form that allows it to be useful for improvement. One way to work around this is to have a simple form of measurement on the unit, clinic, or ward that the team own and update. This could be in the form of a safety cross 8 or tally chart. A safety cross ( fig 3 ) is a simple visual monthly calendar on the wall which allows teams to identify when a safety event (such as a fall) occurred on the ward. The team simply colours in each day green when no fall occurred, or colours in red the days when a fall occurred. It allows the team to own the data related to a safety event that they care about and easily see how many events are occurring over a month. Being able to see such data transparently on a ward allows teams to update data in real time and be able to respond to it effectively.

Example of a safety cross in use
A common challenge in using qualitative data is being able to analyse large quantities of written word. There are formal approaches to qualitative data analyses, but most healthcare staff are not trained in these methods. Key tips in avoiding this difficulty are ( a ) to be intentional with your search and sampling strategy so that you collect only the minimum amount of data that is likely to be useful for learning and ( b ) to use simple ways to read and theme the data in order to extract useful information to guide your improvement work. 9 If you want to try this, see if you can find someone in your organisation with qualitative data analysis skills, such as clinical psychologists or the patient experience or informatics teams.
Education into practice
What are the key measures for the service that you work in?
Are these measures available, transparently displayed, and viewed over time?
What qualitative data do you use in helping guide your improvement efforts?
How patients were involved in the creation of this article
Service users are deeply involved in all quality improvement work at East London NHS Foundation Trust, including within the training programmes we deliver. Shared learning over many years has contributed to our understanding of how best to use all types of data to support improvement. No patients have had input specifically into this article.
This article is part of a series commissioned by The BMJ based on ideas generated by a joint editorial group with members from the Health Foundation and The BMJ , including a patient/carer. The BMJ retained full editorial control over external peer review, editing, and publication. Open access fees and The BMJ ’s quality improvement editor post are funded by the Health Foundation.
Competing interests: I have read and understood the BMJ Group policy on declaration of interests and have no relevant interests to declare.
Provenance and peer review: Commissioned; externally peer reviewed.
This is an Open Access article distributed in accordance with the Creative Commons Attribution Non Commercial (CC BY-NC 4.0) license, which permits others to distribute, remix, adapt, build upon this work non-commercially, and license their derivative works on different terms, provided the original work is properly cited and the use is non-commercial. See: http://creativecommons.org/licenses/by-nc/4.0/ .
- ↵ Cambridge University Press. Cambridge online dictionary , 2008. https://dictionary.cambridge.org/ .
- Provost LP ,
- Braithwaite J
- Neuhauser D
- Donabedian A
- Mohammed MA
- Davidoff F ,
- Dixon-Woods M ,
- Leviton L ,
- ↵ Flynn M. Quality & Safety—The safety cross system: simple and effective. https://www.inmo.ie/MagazineArticle/PrintArticle/11155 .
Loading metrics
Open Access
Peer-reviewed
Research Article
Assessing the impact of healthcare research: A systematic review of methodological frameworks
Roles Conceptualization, Data curation, Formal analysis, Funding acquisition, Investigation, Methodology, Validation, Writing – original draft, Writing – review & editing
Affiliation Centre for Patient Reported Outcomes Research, Institute of Applied Health Research, College of Medical and Dental Sciences, University of Birmingham, Birmingham, United Kingdom

Roles Conceptualization, Formal analysis, Funding acquisition, Methodology, Project administration, Supervision, Validation, Writing – review & editing
* E-mail: [email protected]
Roles Data curation, Formal analysis, Methodology, Validation, Writing – review & editing
Roles Formal analysis, Methodology, Supervision, Validation, Writing – review & editing
- Samantha Cruz Rivera,
- Derek G. Kyte,
- Olalekan Lee Aiyegbusi,
- Thomas J. Keeley,
- Melanie J. Calvert
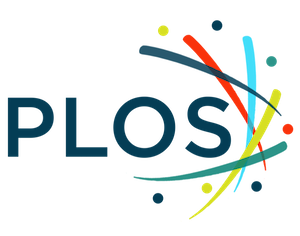
- Published: August 9, 2017
- https://doi.org/10.1371/journal.pmed.1002370
- Reader Comments
Increasingly, researchers need to demonstrate the impact of their research to their sponsors, funders, and fellow academics. However, the most appropriate way of measuring the impact of healthcare research is subject to debate. We aimed to identify the existing methodological frameworks used to measure healthcare research impact and to summarise the common themes and metrics in an impact matrix.
Methods and findings
Two independent investigators systematically searched the Medical Literature Analysis and Retrieval System Online (MEDLINE), the Excerpta Medica Database (EMBASE), the Cumulative Index to Nursing and Allied Health Literature (CINAHL+), the Health Management Information Consortium, and the Journal of Research Evaluation from inception until May 2017 for publications that presented a methodological framework for research impact. We then summarised the common concepts and themes across methodological frameworks and identified the metrics used to evaluate differing forms of impact. Twenty-four unique methodological frameworks were identified, addressing 5 broad categories of impact: (1) ‘primary research-related impact’, (2) ‘influence on policy making’, (3) ‘health and health systems impact’, (4) ‘health-related and societal impact’, and (5) ‘broader economic impact’. These categories were subdivided into 16 common impact subgroups. Authors of the included publications proposed 80 different metrics aimed at measuring impact in these areas. The main limitation of the study was the potential exclusion of relevant articles, as a consequence of the poor indexing of the databases searched.
Conclusions
The measurement of research impact is an essential exercise to help direct the allocation of limited research resources, to maximise research benefit, and to help minimise research waste. This review provides a collective summary of existing methodological frameworks for research impact, which funders may use to inform the measurement of research impact and researchers may use to inform study design decisions aimed at maximising the short-, medium-, and long-term impact of their research.
Author summary
Why was this study done.
- There is a growing interest in demonstrating the impact of research in order to minimise research waste, allocate resources efficiently, and maximise the benefit of research. However, there is no consensus on which is the most appropriate tool to measure the impact of research.
- To our knowledge, this review is the first to synthesise existing methodological frameworks for healthcare research impact, and the associated impact metrics by which various authors have proposed impact should be measured, into a unified matrix.
What did the researchers do and find?
- We conducted a systematic review identifying 24 existing methodological research impact frameworks.
- We scrutinised the sample, identifying and summarising 5 proposed impact categories, 16 impact subcategories, and over 80 metrics into an impact matrix and methodological framework.
What do these findings mean?
- This simplified consolidated methodological framework will help researchers to understand how a research study may give rise to differing forms of impact, as well as in what ways and at which time points these potential impacts might be measured.
- Incorporating these insights into the design of a study could enhance impact, optimizing the use of research resources.
Citation: Cruz Rivera S, Kyte DG, Aiyegbusi OL, Keeley TJ, Calvert MJ (2017) Assessing the impact of healthcare research: A systematic review of methodological frameworks. PLoS Med 14(8): e1002370. https://doi.org/10.1371/journal.pmed.1002370
Academic Editor: Mike Clarke, Queens University Belfast, UNITED KINGDOM
Received: February 28, 2017; Accepted: July 7, 2017; Published: August 9, 2017
Copyright: © 2017 Cruz Rivera et al. This is an open access article distributed under the terms of the Creative Commons Attribution License , which permits unrestricted use, distribution, and reproduction in any medium, provided the original author and source are credited.
Data Availability: All relevant data are within the paper and supporting files.
Funding: Funding was received from Consejo Nacional de Ciencia y Tecnología (CONACYT). The funders had no role in study design, data collection and analysis, decision to publish, or preparation of the manuscript ( http://www.conacyt.mx/ ).
Competing interests: I have read the journal's policy and the authors of this manuscript have the following competing interests: MJC has received consultancy fees from Astellas and Ferring pharma and travel fees from the European Society of Cardiology outside the submitted work. TJK is in full-time paid employment for PAREXEL International.
Abbreviations: AIHS, Alberta Innovates—Health Solutions; CAHS, Canadian Academy of Health Sciences; CIHR, Canadian Institutes of Health Research; CINAHL+, Cumulative Index to Nursing and Allied Health Literature; EMBASE, Excerpta Medica Database; ERA, Excellence in Research for Australia; HEFCE, Higher Education Funding Council for England; HMIC, Health Management Information Consortium; HTA, Health Technology Assessment; IOM, Impact Oriented Monitoring; MDG, Millennium Development Goal; NHS, National Health Service; MEDLINE, Medical Literature Analysis and Retrieval System Online; PHC RIS, Primary Health Care Research & Information Service; PRISMA, Preferred Reporting Items for Systematic Reviews and Meta-Analyses; PROM, patient-reported outcome measures; QALY, quality-adjusted life year; R&D, research and development; RAE, Research Assessment Exercise; REF, Research Excellence Framework; RIF, Research Impact Framework; RQF, Research Quality Framework; SDG, Sustainable Development Goal; SIAMPI, Social Impact Assessment Methods for research and funding instruments through the study of Productive Interactions between science and society
Introduction
In 2010, approximately US$240 billion was invested in healthcare research worldwide [ 1 ]. Such research is utilised by policy makers, healthcare providers, and clinicians to make important evidence-based decisions aimed at maximising patient benefit, whilst ensuring that limited healthcare resources are used as efficiently as possible to facilitate effective and sustainable service delivery. It is therefore essential that this research is of high quality and that it is impactful—i.e., it delivers demonstrable benefits to society and the wider economy whilst minimising research waste [ 1 , 2 ]. Research impact can be defined as ‘any identifiable ‘benefit to, or positive influence on the economy, society, public policy or services, health, the environment, quality of life or academia’ (p. 26) [ 3 ].
There are many purported benefits associated with the measurement of research impact, including the ability to (1) assess the quality of the research and its subsequent benefits to society; (2) inform and influence optimal policy and funding allocation; (3) demonstrate accountability, the value of research in terms of efficiency and effectiveness to the government, stakeholders, and society; and (4) maximise impact through better understanding the concept and pathways to impact [ 4 – 7 ].
Measuring and monitoring the impact of healthcare research has become increasingly common in the United Kingdom [ 5 ], Australia [ 5 ], and Canada [ 8 ], as governments, organisations, and higher education institutions seek a framework to allocate funds to projects that are more likely to bring the most benefit to society and the economy [ 5 ]. For example, in the UK, the 2014 Research Excellence Framework (REF) has recently been used to assess the quality and impact of research in higher education institutions, through the assessment of impact cases studies and selected qualitative impact metrics [ 9 ]. This is the first initiative to allocate research funding based on the economic, societal, and cultural impact of research, although it should be noted that research impact only drives a proportion of this allocation (approximately 20%) [ 9 ].
In the UK REF, the measurement of research impact is seen as increasingly important. However, the impact element of the REF has been criticised in some quarters [ 10 , 11 ]. Critics deride the fact that REF impact is determined in a relatively simplistic way, utilising researcher-generated case studies, which commonly attempt to link a particular research outcome to an associated policy or health improvement despite the fact that the wider literature highlights great diversity in the way research impact may be demonstrated [ 12 , 13 ]. This led to the current debate about the optimal method of measuring impact in the future REF [ 10 , 14 ]. The Stern review suggested that research impact should not only focus on socioeconomic impact but should also include impact on government policy, public engagement, academic impacts outside the field, and teaching to showcase interdisciplinary collaborative impact [ 10 , 11 ]. The Higher Education Funding Council for England (HEFCE) has recently set out the proposals for the REF 2021 exercise, confirming that the measurement of such impact will continue to form an important part of the process [ 15 ].
With increasing pressure for healthcare research to lead to demonstrable health, economic, and societal impact, there is a need for researchers to understand existing methodological impact frameworks and the means by which impact may be quantified (i.e., impact metrics; see Box 1 , 'Definitions’) to better inform research activities and funding decisions. From a researcher’s perspective, understanding the optimal pathways to impact can help inform study design aimed at maximising the impact of the project. At the same time, funders need to understand which aspects of impact they should focus on when allocating awards so they can make the most of their investment and bring the greatest benefit to patients and society [ 2 , 4 , 5 , 16 , 17 ].
Box 1. Definitions
- Research impact: ‘any identifiable benefit to, or positive influence on, the economy, society, public policy or services, health, the environment, quality of life, or academia’ (p. 26) [ 3 ].
- Methodological framework: ‘a body of methods, rules and postulates employed by a particular procedure or set of procedures (i.e., framework characteristics and development)’ [ 18 ].
- Pathway: ‘a way of achieving a specified result; a course of action’ [ 19 ].
- Quantitative metrics: ‘a system or standard of [quantitative] measurement’ [ 20 ].
- Narrative metrics: ‘a spoken or written account of connected events; a story’ [ 21 ].
Whilst previous researchers have summarised existing methodological frameworks and impact case studies [ 4 , 22 – 27 ], they have not summarised the metrics for use by researchers, funders, and policy makers. The aim of this review was therefore to (1) identify the methodological frameworks used to measure healthcare research impact using systematic methods, (2) summarise common impact themes and metrics in an impact matrix, and (3) provide a simplified consolidated resource for use by funders, researchers, and policy makers.
Search strategy and selection criteria
Initially, a search strategy was developed to identify the available literature regarding the different methods to measure research impact. The following keywords: ‘Impact’, ‘Framework’, and ‘Research’, and their synonyms, were used during the search of the Medical Literature Analysis and Retrieval System Online (MEDLINE; Ovid) database, the Excerpta Medica Database (EMBASE), the Health Management Information Consortium (HMIC) database, and the Cumulative Index to Nursing and Allied Health Literature (CINAHL+) database (inception to May 2017; see S1 Appendix for the full search strategy). Additionally, the nonindexed Journal of Research Evaluation was hand searched during the same timeframe using the keyword ‘Impact’. Other relevant articles were identified through 3 Internet search engines (Google, Google Scholar, and Google Images) using the keywords ‘Impact’, ‘Framework’, and ‘Research’, with the first 50 results screened. Google Images was searched because different methodological frameworks are summarised in a single image and can easily be identified through this search engine. Finally, additional publications were sought through communication with experts.
Following Preferred Reporting Items for Systematic Reviews and Meta-Analyses (PRISMA) guidelines (see S1 PRISMA Checklist ), 2 independent investigators systematically screened for publications describing, evaluating, or utilising a methodological research impact framework within the context of healthcare research [ 28 ]. Papers were eligible if they included full or partial methodological frameworks or pathways to research impact; both primary research and systematic reviews fitting these criteria were included. We included any methodological framework identified (original or modified versions) at the point of first occurrence. In addition, methodological frameworks were included if they were applicable to the healthcare discipline with no need of modification within their structure. We defined ‘methodological framework’ as ‘a body of methods, rules and postulates employed by a particular procedure or set of procedures (i.e., framework characteristics and development)’ [ 18 ], whereas we defined ‘pathway’ as ‘a way of achieving a specified result; a course of action’ [ 19 ]. Studies were excluded if they presented an existing (unmodified) methodological framework previously available elsewhere, did not explicitly describe a methodological framework but rather focused on a single metric (e.g., bibliometric analysis), focused on the impact or effectiveness of interventions rather than that of the research, or presented case study data only. There were no language restrictions.
Data screening
Records were downloaded into Endnote (version X7.3.1), and duplicates were removed. Two independent investigators (SCR and OLA) conducted all screening following a pilot aimed at refining the process. The records were screened by title and abstract before full-text articles of potentially eligible publications were retrieved for evaluation. A full-text screening identified the publications included for data extraction. Discrepancies were resolved through discussion, with the involvement of a third reviewer (MJC, DGK, and TJK) when necessary.
Data extraction and analysis
Data extraction occurred after the final selection of included articles. SCR and OLA independently extracted details of impact methodological frameworks, the country of origin, and the year of publication, as well as the source, the framework description, and the methodology used to develop the framework. Information regarding the methodology used to develop each methodological framework was also extracted from framework webpages where available. Investigators also extracted details regarding each framework’s impact categories and subgroups, along with their proposed time to impact (‘short-term’, ‘mid-term’, or ‘long-term’) and the details of any metrics that had been proposed to measure impact, which are depicted in an impact matrix. The structure of the matrix was informed by the work of M. Buxton and S. Hanney [ 2 ], P. Buykx et al. [ 5 ], S. Kuruvila et al. [ 29 ], and A. Weiss [ 30 ], with the intention of mapping metrics presented in previous methodological frameworks in a concise way. A consensus meeting with MJC, DGK, and TJK was held to solve disagreements and finalise the data extraction process.
Included studies
Our original search strategy identified 359 citations from MEDLINE (Ovid), EMBASE, CINAHL+, HMIC, and the Journal of Research Evaluation, and 101 citations were returned using other sources (Google, Google Images, Google Scholar, and expert communication) (see Fig 1 ) [ 28 ]. In total, we retrieved 54 full-text articles for review. At this stage, 39 articles were excluded, as they did not propose new or modified methodological frameworks. An additional 15 articles were included following the backward and forward citation method. A total of 31 relevant articles were included in the final analysis, of which 24 were articles presenting unique frameworks and the remaining 7 were systematic reviews [ 4 , 22 – 27 ]. The search strategy was rerun on 15 May 2017. A further 19 publications were screened, and 2 were taken forward to full-text screening but were ineligible for inclusion.
- PPT PowerPoint slide
- PNG larger image
- TIFF original image
https://doi.org/10.1371/journal.pmed.1002370.g001
Methodological framework characteristics
The characteristics of the 24 included methodological frameworks are summarised in Table 1 , 'Methodological framework characteristics’. Fourteen publications proposed academic-orientated frameworks, which focused on measuring academic, societal, economic, and cultural impact using narrative and quantitative metrics [ 2 , 3 , 5 , 8 , 29 , 31 – 39 ]. Five publications focused on assessing the impact of research by focusing on the interaction process between stakeholders and researchers (‘productive interactions’), which is a requirement to achieve research impact. This approach tries to address the issue of attributing research impact to metrics [ 7 , 40 – 43 ]. Two frameworks focused on the importance of partnerships between researchers and policy makers, as a core element to accomplish research impact [ 44 , 45 ]. An additional 2 frameworks focused on evaluating the pathways to impact, i.e., linking processes between research and impact [ 30 , 46 ]. One framework assessed the ability of health technology to influence efficiency of healthcare systems [ 47 ]. Eight frameworks were developed in the UK [ 2 , 3 , 29 , 37 , 39 , 42 , 43 , 45 ], 6 in Canada [ 8 , 33 , 34 , 44 , 46 , 47 ], 4 in Australia [ 5 , 31 , 35 , 38 ], 3 in the Netherlands [ 7 , 40 , 41 ], and 2 in the United States [ 30 , 36 ], with 1 model developed with input from various countries [ 32 ].
https://doi.org/10.1371/journal.pmed.1002370.t001
Methodological framework development
The included methodological frameworks varied in their development process, but there were some common approaches employed. Most included a literature review [ 2 , 5 , 7 , 8 , 31 , 33 , 36 , 37 , 40 – 46 ], although none of them used a recognised systematic method. Most also consulted with various stakeholders [ 3 , 8 , 29 , 31 , 33 , 35 – 38 , 43 , 44 , 46 , 47 ] but used differing methods to incorporate their views, including quantitative surveys [ 32 , 35 , 43 , 46 ], face-to-face interviews [ 7 , 29 , 33 , 35 , 37 , 42 , 43 ], telephone interviews [ 31 , 46 ], consultation [ 3 , 7 , 36 ], and focus groups [ 39 , 43 ]. A range of stakeholder groups were approached across the sample, including principal investigators [ 7 , 29 , 43 ], research end users [ 7 , 42 , 43 ], academics [ 3 , 8 , 39 , 40 , 43 , 46 ], award holders [ 43 ], experts [ 33 , 38 , 39 ], sponsors [ 33 , 39 ], project coordinators [ 32 , 42 ], and chief investigators [ 31 , 35 ]. However, some authors failed to identify the stakeholders involved in the development of their frameworks [ 2 , 5 , 34 , 41 , 45 ], making it difficult to assess their appropriateness. In addition, only 4 of the included papers reported using formal analytic methods to interpret stakeholder responses. These included the Canadian Academy of Health Sciences framework, which used conceptual cluster analysis [ 33 ]. The Research Contribution [ 42 ], Research Impact [ 29 ], and Primary Health Care & Information Service [ 31 ] used a thematic analysis approach. Finally, some authors went on to pilot their framework, which shaped refinements on the methodological frameworks until approval. Methods used to pilot the frameworks included a case study approach [ 2 , 3 , 30 , 32 , 33 , 36 , 40 , 42 , 44 , 45 ], contrasting results against available literature [ 29 ], the use of stakeholders’ feedback [ 7 ], and assessment tools [ 35 , 46 ].
Major impact categories
1. primary research-related impact..
A number of methodological frameworks advocated the evaluation of ‘research-related impact’. This encompassed content related to the generation of new knowledge, knowledge dissemination, capacity building, training, leadership, and the development of research networks. These outcomes were considered the direct or primary impacts of a research project, as these are often the first evidenced returns [ 30 , 62 ].
A number of subgroups were identified within this category, with frameworks supporting the collection of impact data across the following constructs: ‘research and innovation outcomes’; ‘dissemination and knowledge transfer’; ‘capacity building, training, and leadership’; and ‘academic collaborations, research networks, and data sharing’.
1 . 1 . Research and innovation outcomes . Twenty of the 24 frameworks advocated the evaluation of ‘research and innovation outcomes’ [ 2 , 3 , 5 , 7 , 8 , 29 – 39 , 41 , 43 , 44 , 46 ]. This subgroup included the following metrics: number of publications; number of peer-reviewed articles (including journal impact factor); citation rates; requests for reprints, number of reviews, and meta-analysis; and new or changes in existing products (interventions or technology), patents, and research. Additionally, some frameworks also sought to gather information regarding ‘methods/methodological contributions’. These advocated the collection of systematic reviews and appraisals in order to identify gaps in knowledge and determine whether the knowledge generated had been assessed before being put into practice [ 29 ].
1 . 2 . Dissemination and knowledge transfer . Nineteen of the 24 frameworks advocated the assessment of ‘dissemination and knowledge transfer’ [ 2 , 3 , 5 , 7 , 29 – 32 , 34 – 43 , 46 ]. This comprised collection of the following information: number of conferences, seminars, workshops, and presentations; teaching output (i.e., number of lectures given to disseminate the research findings); number of reads for published articles; article download rate and number of journal webpage visits; and citations rates in nonjournal media such as newspapers and mass and social media (i.e., Twitter and blogs). Furthermore, this impact subgroup considered the measurement of research uptake and translatability and the adoption of research findings in technological and clinical applications and by different fields. These can be measured through patents, clinical trials, and partnerships between industry and business, government and nongovernmental organisations, and university research units and researchers [ 29 ].
1 . 3 . Capacity building , training , and leadership . Fourteen of 24 frameworks suggested the evaluation of ‘capacity building, training, and leadership’ [ 2 , 3 , 5 , 8 , 29 , 31 – 35 , 39 – 41 , 43 ]. This involved collecting information regarding the number of doctoral and postdoctoral studentships (including those generated as a result of the research findings and those appointed to conduct the research), as well as the number of researchers and research-related staff involved in the research projects. In addition, authors advocated the collection of ‘leadership’ metrics, including the number of research projects managed and coordinated and the membership of boards and funding bodies, journal editorial boards, and advisory committees [ 29 ]. Additional metrics in this category included public recognition (number of fellowships and awards for significant research achievements), academic career advancement, and subsequent grants received. Lastly, the impact metric ‘research system management’ comprised the collection of information that can lead to preserving the health of the population, such as modifying research priorities, resource allocation strategies, and linking health research to other disciplines to maximise benefits [ 29 ].
1 . 4 . Academic collaborations , research networks , and data sharing . Lastly, 10 of the 24 frameworks advocated the collection of impact data regarding ‘academic collaborations (internal and external collaborations to complete a research project), research networks, and data sharing’ [ 2 , 3 , 5 , 7 , 29 , 34 , 37 , 39 , 41 , 43 ].
2. Influence on policy making.
Methodological frameworks addressing this major impact category focused on measurable improvements within a given knowledge base and on interactions between academics and policy makers, which may influence policy-making development and implementation. The returns generated in this impact category are generally considered as intermediate or midterm (1 to 3 years). These represent an important interim stage in the process towards the final expected impacts, such as quantifiable health improvements and economic benefits, without which policy change may not occur [ 30 , 62 ]. The following impact subgroups were identified within this category: ‘type and nature of policy impact’, ‘level of policy making’, and ‘policy networks’.
2 . 1 . Type and nature of policy impact . The most common impact subgroup, mentioned in 18 of the 24 frameworks, was ‘type and nature of policy impact’ [ 2 , 7 , 29 – 38 , 41 – 43 , 45 – 47 ]. Methodological frameworks addressing this subgroup stressed the importance of collecting information regarding the influence of research on policy (i.e., changes in practice or terminology). For instance, a project looking at trafficked adolescents and women (2003) influenced the WHO guidelines (2003) on ethics regarding this particular group [ 17 , 21 , 63 ].
2 . 2 . Level of policy impact . Thirteen of 24 frameworks addressed aspects surrounding the need to record the ‘level of policy impact’ (international, national, or local) and the organisations within a level that were influenced (local policy makers, clinical commissioning groups, and health and wellbeing trusts) [ 2 , 5 , 8 , 29 , 31 , 34 , 38 , 41 , 43 – 47 ]. Authors considered it important to measure the ‘level of policy impact’ to provide evidence of collaboration, coordination, and efficiency within health organisations and between researchers and health organisations [ 29 , 31 ].
2 . 3 . Policy networks . Five methodological frameworks highlighted the need to collect information regarding collaborative research with industry and staff movement between academia and industry [ 5 , 7 , 29 , 41 , 43 ]. A policy network emphasises the relationship between policy communities, researchers, and policy makers. This relationship can influence and lead to incremental changes in policy processes [ 62 ].
3. Health and health systems impact.
A number of methodological frameworks advocated the measurement of impacts on health and healthcare systems across the following impact subgroups: ‘quality of care and service delivering’, ‘evidence-based practice’, ‘improved information and health information management’, ‘cost containment and effectiveness’, ‘resource allocation’, and ‘health workforce’.
3 . 1 . Quality of care and service delivery . Twelve of the 24 frameworks highlighted the importance of evaluating ‘quality of care and service delivery’ [ 2 , 5 , 8 , 29 – 31 , 33 – 36 , 41 , 47 ]. There were a number of suggested metrics that could be potentially used for this purpose, including health outcomes such as quality-adjusted life years (QALYs), patient-reported outcome measures (PROMs), patient satisfaction and experience surveys, and qualitative data on waiting times and service accessibility.
3 . 2 . Evidence-based practice . ‘Evidence-based practice’, mentioned in 5 of the 24 frameworks, refers to making changes in clinical diagnosis, clinical practice, treatment decisions, or decision making based on research evidence [ 5 , 8 , 29 , 31 , 33 ]. The suggested metrics to demonstrate evidence-based practice were adoption of health technologies and research outcomes to improve the healthcare systems and inform policies and guidelines [ 29 ].
3 . 3 . Improved information and health information management . This impact subcategory, mentioned in 5 of the 24 frameworks, refers to the influence of research on the provision of health services and management of the health system to prevent additional costs [ 5 , 29 , 33 , 34 , 38 ]. Methodological frameworks advocated the collection of health system financial, nonfinancial (i.e., transport and sociopolitical implications), and insurance information in order to determine constraints within a health system.
3 . 4 . Cost containment and cost-effectiveness . Six of the 24 frameworks advocated the subcategory ‘cost containment and cost-effectiveness’ [ 2 , 5 , 8 , 17 , 33 , 36 ]. ‘Cost containment’ comprised the collection of information regarding how research has influenced the provision and management of health services and its implication in healthcare resource allocation and use [ 29 ]. ‘Cost-effectiveness’ refers to information concerning economic evaluations to assess improvements in effectiveness and health outcomes—for instance, the cost-effectiveness (cost and health outcome benefits) assessment of introducing a new health technology to replace an older one [ 29 , 31 , 64 ].
3 . 5 . Resource allocation . ‘Resource allocation’, mentioned in 6frameworks, can be measured through 2 impact metrics: new funding attributed to the intervention in question and equity while allocating resources, such as improved allocation of resources at an area level; better targeting, accessibility, and utilisation; and coverage of health services [ 2 , 5 , 29 , 31 , 45 , 47 ]. The allocation of resources and targeting can be measured through health services research reports, with the utilisation of health services measured by the probability of providing an intervention when needed, the probability of requiring it again in the future, and the probability of receiving an intervention based on previous experience [ 29 , 31 ].
3 . 6 . Health workforce . Lastly, ‘health workforce’, present in 3 methodological frameworks, refers to the reduction in the days of work lost because of a particular illness [ 2 , 5 , 31 ].
4. Health-related and societal impact.
Three subgroups were included in this category: ‘health literacy’; ‘health knowledge, attitudes, and behaviours’; and ‘improved social equity, inclusion, or cohesion’.
4 . 1 . Health knowledge , attitudes , and behaviours . Eight of the 24 frameworks suggested the assessment of ‘health knowledge, attitudes, behaviours, and outcomes’, which could be measured through the evaluation of levels of public engagement with science and research (e.g., National Health Service (NHS) Choices end-user visit rate) or by using focus groups to analyse changes in knowledge, attitudes, and behaviour among society [ 2 , 5 , 29 , 33 – 35 , 38 , 43 ].
4 . 2 . Improved equity , inclusion , or cohesion and human rights . Other methodological frameworks, 4 of the 24, suggested capturing improvements in equity, inclusion, or cohesion and human rights. Authors suggested these could be using a resource like the United Nations Millennium Development Goals (MDGs) (superseded by Sustainable Development Goals [SDGs] in 2015) and human rights [ 29 , 33 , 34 , 38 ]. For instance, a cluster-randomised controlled trial in Nepal, which had female participants, has demonstrated the reduction of neonatal mortality through the introduction of maternity health care, distribution of delivery kits, and home visits. This illustrates how research can target vulnerable and disadvantaged groups. Additionally, this research has been introduced by the World Health Organisation to achieve the MDG ‘improve maternal health’ [ 16 , 29 , 65 ].
4 . 3 . Health literacy . Some methodological frameworks, 3 of the 24, focused on tracking changes in the ability of patients to make informed healthcare decisions, reduce health risks, and improve quality of life, which were demonstrably linked to a particular programme of research [ 5 , 29 , 43 ]. For example, a systematic review showed that when HIV health literacy/knowledge is spread among people living with the condition, antiretroviral adherence and quality of life improve [ 66 ].
5. Broader economic impacts.
Some methodological frameworks, 9 of 24, included aspects related to the broader economic impacts of health research—for example, the economic benefits emerging from the commercialisation of research outputs [ 2 , 5 , 29 , 31 , 33 , 35 , 36 , 38 , 67 ]. Suggested metrics included the amount of funding for research and development (R&D) that was competitively awarded by the NHS, medical charities, and overseas companies. Additional metrics were income from intellectual property, spillover effects (any secondary benefit gained as a repercussion of investing directly in a primary activity, i.e., the social and economic returns of investing on R&D) [ 33 ], patents granted, licences awarded and brought to the market, the development and sales of spinout companies, research contracts, and income from industry.
The benefits contained within the categories ‘health and health systems impact’, ‘health-related and societal impact’, and ‘broader economic impacts’ are considered the expected and final returns of the resources allocated in healthcare research [ 30 , 62 ]. These benefits commonly arise in the long term, beyond 5 years according to some authors, but there was a recognition that this could differ depending on the project and its associated research area [ 4 ].
Data synthesis
Five major impact categories were identified across the 24 included methodological frameworks: (1) ‘primary research-related impact’, (2) ‘influence on policy making’, (3) ‘health and health systems impact’, (4) ‘health-related and societal impact’, and (5) ‘broader economic impact’. These major impact categories were further subdivided into 16 impact subgroups. The included publications proposed 80 different metrics to measure research impact. This impact typology synthesis is depicted in ‘the impact matrix’ ( Fig 2 and Fig 3 ).
CIHR, Canadian Institutes of Health Research; HTA, Health Technology Assessment; PHC RIS, Primary Health Care Research & Information Service; RAE, Research Assessment Exercise; RQF, Research Quality Framework.
https://doi.org/10.1371/journal.pmed.1002370.g002
AIHS, Alberta Innovates—Health Solutions; CAHS, Canadian Institutes of Health Research; IOM, Impact Oriented Monitoring; REF, Research Excellence Framework; SIAMPI, Social Impact Assessment Methods for research and funding instruments through the study of Productive Interactions between science and society.
https://doi.org/10.1371/journal.pmed.1002370.g003
Commonality and differences across frameworks
The ‘Research Impact Framework’ and the ‘Health Services Research Impact Framework’ were the models that encompassed the largest number of the metrics extracted. The most dominant methodological framework was the Payback Framework; 7 other methodological framework models used the Payback Framework as a starting point for development [ 8 , 29 , 31 – 35 ]. Additional methodological frameworks that were commonly incorporated into other tools included the CIHR framework, the CAHS model, the AIHS framework, and the Exchange model [ 8 , 33 , 34 , 44 ]. The capture of ‘research-related impact’ was the most widely advocated concept across methodological frameworks, illustrating the importance with which primary short-term impact outcomes were viewed by the included papers. Thus, measurement of impact via number of publications, citations, and peer-reviewed articles was the most common. ‘Influence on policy making’ was the predominant midterm impact category, specifically the subgroup ‘type and nature of policy impact’, in which frameworks advocated the measurement of (i) changes to legislation, regulations, and government policy; (ii) influence and involvement in decision-making processes; and (iii) changes to clinical or healthcare training, practice, or guidelines. Within more long-term impact measurement, the evaluations of changes in the ‘quality of care and service delivery’ were commonly advocated.
In light of the commonalities and differences among the methodological frameworks, the ‘pathways to research impact’ diagram ( Fig 4 ) was developed to provide researchers, funders, and policy makers a more comprehensive and exhaustive way to measure healthcare research impact. The diagram has the advantage of assorting all the impact metrics proposed by previous frameworks and grouping them into different impact subgroups and categories. Prospectively, this global picture will help researchers, funders, and policy makers plan strategies to achieve multiple pathways to impact before carrying the research out. The analysis of the data extraction and construction of the impact matrix led to the development of the ‘pathways to research impact’ diagram ( Fig 4 ). The diagram aims to provide an exhaustive and comprehensive way of tracing research impact by combining all the impact metrics presented by the different 24 frameworks, grouping those metrics into different impact subgroups, and grouping these into broader impact categories.
NHS, National Health Service; PROM, patient-reported outcome measure; QALY, quality-adjusted life year; R&D, research and development.
https://doi.org/10.1371/journal.pmed.1002370.g004
This review has summarised existing methodological impact frameworks together for the first time using systematic methods ( Fig 4 ). It allows researchers and funders to consider pathways to impact at the design stage of a study and to understand the elements and metrics that need to be considered to facilitate prospective assessment of impact. Users do not necessarily need to cover all the aspects of the methodological framework, as every research project can impact on different categories and subgroups. This review provides information that can assist researchers to better demonstrate impact, potentially increasing the likelihood of conducting impactful research and reducing research waste. Existing reviews have not presented a methodological framework that includes different pathways to impact, health impact categories, subgroups, and metrics in a single methodological framework.
Academic-orientated frameworks included in this review advocated the measurement of impact predominantly using so-called ‘quantitative’ metrics—for example, the number of peer-reviewed articles, journal impact factor, and citation rates. This may be because they are well-established measures, relatively easy to capture and objective, and are supported by research funding systems. However, these metrics primarily measure the dissemination of research finding rather than its impact [ 30 , 68 ]. Whilst it is true that wider dissemination, especially when delivered via world-leading international journals, may well lead eventually to changes in healthcare, this is by no means certain. For instance, case studies evaluated by Flinders University of Australia demonstrated that some research projects with non-peer-reviewed publications led to significant changes in health policy, whilst the studies with peer-reviewed publications did not result in any type of impact [ 68 ]. As a result, contemporary literature has tended to advocate the collection of information regarding a variety of different potential forms of impact alongside publication/citations metrics [ 2 , 3 , 5 , 7 , 8 , 29 – 47 ], as outlined in this review.
The 2014 REF exercise adjusted UK university research funding allocation based on evidence of the wider impact of research (through case narrative studies and quantitative metrics), rather than simply according to the quality of research [ 12 ]. The intention was to ensure funds were directed to high-quality research that could demonstrate actual realised benefit. The inclusion of a mixed-method approach to the measurement of impact in the REF (narrative and quantitative metrics) reflects a widespread belief—expressed by the majority of authors of the included methodological frameworks in the review—that individual quantitative impact metrics (e.g., number of citations and publications) do not necessary capture the complexity of the relationships involved in a research project and may exclude measurement of specific aspects of the research pathway [ 10 , 12 ].
Many of the frameworks included in this review advocated the collection of a range of academic, societal, economic, and cultural impact metrics; this is consistent with recent recommendations from the Stern review [ 10 ]. However, a number of these metrics encounter research ‘lag’: i.e., the time between the point at which the research is conducted and when the actual benefits arise [ 69 ]. For instance, some cardiovascular research has taken up to 25 years to generate impact [ 70 ]. Likewise, the impact may not arise exclusively from a single piece of research. Different processes (such as networking interactions and knowledge and research translation) and multiple individuals and organisations are often involved [ 4 , 71 ]. Therefore, attributing the contribution made by each of the different actors involved in the process can be a challenge [ 4 ]. An additional problem associated to attribution is the lack of evidence to link research and impact. The outcomes of research may emerge slowly and be absorbed gradually. Consequently, it is difficult to determine the influence of research in the development of a new policy, practice, or guidelines [ 4 , 23 ].
A further problem is that impact evaluation is conducted ‘ex post’, after the research has concluded. Collecting information retrospectively can be an issue, as the data required might not be available. ‘ex ante’ assessment is vital for funding allocation, as it is necessary to determine the potential forthcoming impact before research is carried out [ 69 ]. Additionally, ex ante evaluation of potential benefit can overcome the issues regarding identifying and capturing evidence, which can be used in the future [ 4 ]. In order to conduct ex ante evaluation of potential benefit, some authors suggest the early involvement of policy makers in a research project coupled with a well-designed strategy of dissemination [ 40 , 69 ].
Providing an alternate view, the authors of methodological frameworks such as the SIAMPI, Contribution Mapping, Research Contribution, and the Exchange model suggest that the problems of attribution are a consequence of assigning the impact of research to a particular impact metric [ 7 , 40 , 42 , 44 ]. To address these issues, these authors propose focusing on the contribution of research through assessing the processes and interactions between stakeholders and researchers, which arguably take into consideration all the processes and actors involved in a research project [ 7 , 40 , 42 , 43 ]. Additionally, contributions highlight the importance of the interactions between stakeholders and researchers from an early stage in the research process, leading to a successful ex ante and ex post evaluation by setting expected impacts and determining how the research outcomes have been utilised, respectively [ 7 , 40 , 42 , 43 ]. However, contribution metrics are generally harder to measure in comparison to academic-orientated indicators [ 72 ].
Currently, there is a debate surrounding the optimal methodological impact framework, and no tool has proven superior to another. The most appropriate methodological framework for a given study will likely depend on stakeholder needs, as each employs different methodologies to assess research impact [ 4 , 37 , 41 ]. This review allows researchers to select individual existing methodological framework components to create a bespoke tool with which to facilitate optimal study design and maximise the potential for impact depending on the characteristic of their study ( Fig 2 and Fig 3 ). For instance, if researchers are interested in assessing how influential their research is on policy making, perhaps considering a suite of the appropriate metrics drawn from multiple methodological frameworks may provide a more comprehensive method than adopting a single methodological framework. In addition, research teams may wish to use a multidimensional approach to methodological framework development, adopting existing narratives and quantitative metrics, as well as elements from contribution frameworks. This approach would arguably present a more comprehensive method of impact assessment; however, further research is warranted to determine its effectiveness [ 4 , 69 , 72 , 73 ].
Finally, it became clear during this review that the included methodological frameworks had been constructed using varied methodological processes. At present, there are no guidelines or consensus around the optimal pathway that should be followed to develop a robust methodological framework. The authors believe this is an area that should be addressed by the research community, to ensure future frameworks are developed using best-practice methodology.
For instance, the Payback Framework drew upon a literature review and was refined through a case study approach. Arguably, this approach could be considered inferior to other methods that involved extensive stakeholder involvement, such as the CIHR framework [ 8 ]. Nonetheless, 7 methodological frameworks were developed based upon the Payback Framework [ 8 , 29 , 31 – 35 ].
Limitations
The present review is the first to summarise systematically existing impact methodological frameworks and metrics. The main limitation is that 50% of the included publications were found through methods other than bibliographic databases searching, indicating poor indexing. Therefore, some relevant articles may not have been included in this review if they failed to indicate the inclusion of a methodological impact framework in their title/abstract. We did, however, make every effort to try to find these potentially hard-to-reach publications, e.g., through forwards/backwards citation searching, hand searching reference lists, and expert communication. Additionally, this review only extracted information regarding the methodology followed to develop each framework from the main publication source or framework webpage. Therefore, further evaluations may not have been included, as they are beyond the scope of the current paper. A further limitation was that although our search strategy did not include language restrictions, we did not specifically search non-English language databases. Thus, we may have failed to identify potentially relevant methodological frameworks that were developed in a non-English language setting.
In conclusion, the measurement of research impact is an essential exercise to help direct the allocation of limited research resources, to maximise benefit, and to help minimise research waste. This review provides a collective summary of existing methodological impact frameworks and metrics, which funders may use to inform the measurement of research impact and researchers may use to inform study design decisions aimed at maximising the short-, medium-, and long-term impact of their research.
Supporting information
S1 appendix. search strategy..
https://doi.org/10.1371/journal.pmed.1002370.s001
S1 PRISMA Checklist. Preferred Reporting Items for Systematic Reviews and Meta-Analyses (PRISMA) checklist.
https://doi.org/10.1371/journal.pmed.1002370.s002
Acknowledgments
We would also like to thank Mrs Susan Bayliss, Information Specialist, University of Birmingham, and Mrs Karen Biddle, Research Secretary, University of Birmingham.
- View Article
- PubMed/NCBI
- Google Scholar
- 3. HEFCE. REF 2014: Assessment framework and guidance on submissions 2011 [cited 2016 15 Feb]. Available from: http://www.ref.ac.uk/media/ref/content/pub/assessmentframeworkandguidanceonsubmissions/GOS%20including%20addendum.pdf .
- 8. Canadian Institutes of Health Research. Developing a CIHR framework to measure the impact of health research 2005 [cited 2016 26 Feb]. Available from: http://publications.gc.ca/collections/Collection/MR21-65-2005E.pdf .
- 9. HEFCE. HEFCE allocates £3.97 billion to universities and colleges in England for 2015–1 2015. Available from: http://www.hefce.ac.uk/news/newsarchive/2015/Name,103785,en.html .
- 10. Stern N. Building on Success and Learning from Experience—An Independent Review of the Research Excellence Framework 2016 [cited 2016 05 Aug]. Available from: https://www.gov.uk/government/uploads/system/uploads/attachment_data/file/541338/ind-16-9-ref-stern-review.pdf .
- 11. Matthews D. REF sceptic to lead review into research assessment: Times Higher Education; 2015 [cited 2016 21 Apr]. Available from: https://www.timeshighereducation.com/news/ref-sceptic-lead-review-research-assessment .
- 12. HEFCE. The Metric Tide—Report of the Independent Review of the Role of Metrics in Research Assessment and Management 2015 [cited 2016 11 Aug]. Available from: http://www.hefce.ac.uk/media/HEFCE,2014/Content/Pubs/Independentresearch/2015/The,Metric,Tide/2015_metric_tide.pdf .
- 14. LSE Public Policy Group. Maximizing the impacts of your research: A handbook for social scientists. http://www.lse.ac.uk/government/research/resgroups/LSEPublicPolicy/Docs/LSE_Impact_Handbook_April_2011.pdf . London: LSE; 2011.
- 15. HEFCE. Consultation on the second Research Excellence Framework. 2016.
- 18. Merriam-Webster Dictionary 2017. Available from: https://www.merriam-webster.com/dictionary/methodology .
- 19. Oxford Dictionaries—pathway 2016 [cited 2016 19 June]. Available from: http://www.oxforddictionaries.com/definition/english/pathway .
- 20. Oxford Dictionaries—metric 2016 [cited 2016 15 Sep]. Available from: https://en.oxforddictionaries.com/definition/metric .
- 21. WHO. WHO Ethical and Safety Guidelines for Interviewing Trafficked Women 2003 [cited 2016 29 July]. Available from: http://www.who.int/mip/2003/other_documents/en/Ethical_Safety-GWH.pdf .
- 31. Kalucy L, et al. Primary Health Care Research Impact Project: Final Report Stage 1 Adelaide: Primary Health Care Research & Information Service; 2007 [cited 2016 26 Feb]. Available from: http://www.phcris.org.au/phplib/filedownload.php?file=/elib/lib/downloaded_files/publications/pdfs/phcris_pub_3338.pdf .
- 33. Canadian Academy of Health Sciences. Making an impact—A preferred framework and indicators to measure returns on investment in health research 2009 [cited 2016 26 Feb]. Available from: http://www.cahs-acss.ca/wp-content/uploads/2011/09/ROI_FullReport.pdf .
- 39. HEFCE. RAE 2008—Guidance in submissions 2005 [cited 2016 15 Feb]. Available from: http://www.rae.ac.uk/pubs/2005/03/rae0305.pdf .
- 41. Royal Netherlands Academy of Arts and Sciences. The societal impact of applied health research—Towards a quality assessment system 2002 [cited 2016 29 Feb]. Available from: https://www.knaw.nl/en/news/publications/the-societal-impact-of-applied-health-research/@@download/pdf_file/20021098.pdf .
- 48. Weiss CH. Using social research in public policy making: Lexington Books; 1977.
- 50. Kogan M, Henkel M. Government and research: the Rothschild experiment in a government department: Heinemann Educational Books; 1983.
- 51. Thomas P. The Aims and Outcomes of Social Policy Research. Croom Helm; 1985.
- 52. Bulmer M. Social Science Research and Government: Comparative Essays on Britain and the United States: Cambridge University Press; 2010.
- 53. Booth T. Developing Policy Research. Aldershot, Gower1988.
- 55. Kalucy L, et al Exploring the impact of primary health care research Stage 2 Primary Health Care Research Impact Project Adelaide: Primary Health Care Research & Information Service (PHCRIS); 2009 [cited 2016 26 Feb]. Available from: http://www.phcris.org.au/phplib/filedownload.php?file=/elib/lib/downloaded_files/publications/pdfs/phcris_pub_8108.pdf .
- 56. CHSRF. Canadian Health Services Research Foundation 2000. Health Services Research and Evidence-based Decision Making [cited 2016 February]. Available from: http://www.cfhi-fcass.ca/migrated/pdf/mythbusters/EBDM_e.pdf .
- 58. W.K. Kellogg Foundation. Logic Model Development Guide 2004 [cited 2016 19 July]. Available from: http://www.smartgivers.org/uploads/logicmodelguidepdf.pdf .
- 59. United Way of America. Measuring Program Outcomes: A Practical Approach 1996 [cited 2016 19 July]. Available from: https://www.bttop.org/sites/default/files/public/W.K.%20Kellogg%20LogicModel.pdf .
- 60. Nutley S, Percy-Smith J and Solesbury W. Models of research impact: a cross sector review of literature and practice. London: Learning and Skills Research Centre 2003.
- 61. Spaapen J, van Drooge L. SIAMPI final report [cited 2017 Jan]. Available from: http://www.siampi.eu/Content/SIAMPI_Final%20report.pdf .
- 63. LSHTM. The Health Risks and Consequences of Trafficking in Women and Adolescents—Findings from a European Study 2003 [cited 2016 29 July]. Available from: http://www.oas.org/atip/global%20reports/zimmerman%20tip%20health.pdf .
- 70. Russell G. Response to second HEFCE consultation on the Research Excellence Framework 2009 [cited 2016 04 Apr]. Available from: http://russellgroup.ac.uk/media/5262/ref-consultation-response-final-dec09.pdf .
- Get the Job
- Resumes and CVs
- Applications
- Cover Letters
- Professional References
Professional Licenses and Exams
- Get a Promotion
- Negotiation
- Professional Ethics
- Professionalism
- Dealing with Coworkers
- Dealing with Bosses
Communication Skills
Managing the office, disabilities, harassment and discrimination, unemployment.
- Career Paths
- Compare Careers
- Switching Careers
- Training and Certifications
- Start a Company
- Internships and Apprenticeships
- Entry Level Jobs
- College Degrees
Growth Trends for Related Jobs
What are the benefits of quantitative research in health care.

Most scientific research will follow one of two approaches - it can be either qualitative or quantitative. Health care research is often based on quantitative methods in which, by definition, information is quantifiable. That is, the variables used in research are measured and recorded as numerical data that can be analyzed by means of statistical tools. The use of quantitative research in health care has several benefits.
The main strength of quantitative methods is in their usefulness in producing factual and reliable outcome data. After the effects of a given drug or treatment have been tested on a sample population, the statistic record of the observed outcomes will provide objective results generalizable to larger populations. The statistical methods associated with quantitative research are well suited for figuring out ways to maximize dependent variables on the basis of independents, which translates into a capability for identifying and applying the interventions that can maximize the quality and quantity of life for a patient.
Reductionism
Quantitative researchers are often accused of reductionism; they take a complex phenomena and reduce them to a few essential numbers, loosing every nuance in the process. However, this reductionism is a two-edged sword with a very significant benefit. By reducing health cases to their essentials, a very large number of them can be taken into consideration for any given study. Large, statistically representative samples that would be unfeasible in qualitative studies can be easily analyzed using quantitative methods.
Evidence-Based Health Research
Given the benefits of quantitative methods in health care, evidence-based medicine seeks to use scientific methods to determine which drugs and procedures are best for treating diseases. At the core of evidence-based practice is the systematic and predominantly quantitative review of randomized controlled trials. Because quantitative researchers tend to use similar statistical methods, experiments and trials performed in different institutions and at different times and places can be aggregated together in large meta-analysis. Thus, quantitative research on health care can build on previous studies, accumulating a body of evidence regarding the effectiveness of different treatments.
Mixed Methods
Evidence-based medicine, and quantitative methods overall, are sometimes accused of leading to "cookbook" medicine. Some of the phenomena of interest to health researchers are of a qualitative nature and, almost by definition, inaccessible to quantitative tools -- for example, the lived experiences of the patient, his social interactions or his perspective of the doctor-patient interaction. However, judicious researchers can find a combination of qualitative and quantitative approaches so the strengths of each method reinforce those of the other. For instance, qualitative methods can be used for the creative generation of hypotheses or research questions, adding a human touch to the rigorous quantitative approach.
Related Articles
Pros & cons of descriptive research →.

Types of Medical Scientists →
What is a hypothesis →.

The Duties of a Biochemist →

Pros & Cons of Evidence-Based Practice →
Career Objectives of Biochemists →

- "Research Design: Qualitative, Quantitative, and Mixed Methods Approaches" ; John W. Creswell; 2009
- Health Promotion Practice; "Appraising Quantitative Research in Health Education: Guidelines for Public Health Educators"; Leonard Jack Jr et al; 2010
- British Medical Journal; "Evidence Based Medicine: What it is and What it isn't"; David L Sackett et al; 1996
Alan Valdez started his career reviewing video games for an obscure California retailer in 2003 and has been writing weekly articles on science and technology for Grupo Reforma since 2006. He got his Bachelor of Science in engineering from Monterrey Tech in 2003 and moved to the U.K., where he is currently doing research on competitive intelligence applied to the diffusion of innovations.
bymuratdeniz/E+/GettyImages
- Job Descriptions
- Law Enforcement Job Descriptions
- Administrative Job Descriptions
- Healthcare Job Descriptions
- Sales Job Descriptions
- Fashion Job Descriptions
- Education Job Descriptions
- Salary Insights
- Journalism Salaries
- Healthcare Salaries
- Military Salaries
- Engineering Salaries
- Teaching Salaries
- Accessibility
- Privacy Notice
- Cookie Notice
- Copyright Policy
- Contact Us
- Find a Job
- Manage Preferences
- California Notice of Collection
- Terms of Use
Quantitative Research
- Reference work entry
- First Online: 13 January 2019
- Cite this reference work entry
- Leigh A. Wilson 2 , 3
4589 Accesses
4 Citations
Quantitative research methods are concerned with the planning, design, and implementation of strategies to collect and analyze data. Descartes, the seventeenth-century philosopher, suggested that how the results are achieved is often more important than the results themselves, as the journey taken along the research path is a journey of discovery. High-quality quantitative research is characterized by the attention given to the methods and the reliability of the tools used to collect the data. The ability to critique research in a systematic way is an essential component of a health professional’s role in order to deliver high quality, evidence-based healthcare. This chapter is intended to provide a simple overview of the way new researchers and health practitioners can understand and employ quantitative methods. The chapter offers practical, realistic guidance in a learner-friendly way and uses a logical sequence to understand the process of hypothesis development, study design, data collection and handling, and finally data analysis and interpretation.
This is a preview of subscription content, log in via an institution to check access.
Access this chapter
- Available as PDF
- Read on any device
- Instant download
- Own it forever
- Available as EPUB and PDF
- Durable hardcover edition
- Dispatched in 3 to 5 business days
- Free shipping worldwide - see info
Tax calculation will be finalised at checkout
Purchases are for personal use only
Institutional subscriptions
Babbie ER. The practice of social research. 14th ed. Belmont: Wadsworth Cengage; 2016.
Google Scholar
Descartes. Cited in Halverston, W. (1976). In: A concise introduction to philosophy, 3rd ed. New York: Random House; 1637.
Doll R, Hill AB. The mortality of doctors in relation to their smoking habits. BMJ. 1954;328(7455):1529–33. https://doi.org/10.1136/bmj.328.7455.1529 .
Article Google Scholar
Liamputtong P. Research methods in health: foundations for evidence-based practice. 3rd ed. Melbourne: Oxford University Press; 2017.
McNabb DE. Research methods in public administration and nonprofit management: quantitative and qualitative approaches. 2nd ed. New York: Armonk; 2007.
Merriam-Webster. Dictionary. http://www.merriam-webster.com . Accessed 20th December 2017.
Olesen Larsen P, von Ins M. The rate of growth in scientific publication and the decline in coverage provided by Science Citation Index. Scientometrics. 2010;84(3):575–603.
Pannucci CJ, Wilkins EG. Identifying and avoiding bias in research. Plast Reconstr Surg. 2010;126(2):619–25. https://doi.org/10.1097/PRS.0b013e3181de24bc .
Petrie A, Sabin C. Medical statistics at a glance. 2nd ed. London: Blackwell Publishing; 2005.
Portney LG, Watkins MP. Foundations of clinical research: applications to practice. 3rd ed. New Jersey: Pearson Publishing; 2009.
Sheehan J. Aspects of research methodology. Nurse Educ Today. 1986;6:193–203.
Wilson LA, Black DA. Health, science research and research methods. Sydney: McGraw Hill; 2013.
Download references
Author information
Authors and affiliations.
School of Science and Health, Western Sydney University, Penrith, NSW, Australia
Leigh A. Wilson
Faculty of Health Science, Discipline of Behavioural and Social Sciences in Health, University of Sydney, Lidcombe, NSW, Australia
You can also search for this author in PubMed Google Scholar
Corresponding author
Correspondence to Leigh A. Wilson .
Editor information
Editors and affiliations.
Pranee Liamputtong
Rights and permissions
Reprints and permissions
Copyright information
© 2019 Springer Nature Singapore Pte Ltd.
About this entry
Cite this entry.
Wilson, L.A. (2019). Quantitative Research. In: Liamputtong, P. (eds) Handbook of Research Methods in Health Social Sciences. Springer, Singapore. https://doi.org/10.1007/978-981-10-5251-4_54
Download citation
DOI : https://doi.org/10.1007/978-981-10-5251-4_54
Published : 13 January 2019
Publisher Name : Springer, Singapore
Print ISBN : 978-981-10-5250-7
Online ISBN : 978-981-10-5251-4
eBook Packages : Social Sciences Reference Module Humanities and Social Sciences Reference Module Business, Economics and Social Sciences
Share this entry
Anyone you share the following link with will be able to read this content:
Sorry, a shareable link is not currently available for this article.
Provided by the Springer Nature SharedIt content-sharing initiative
- Publish with us
Policies and ethics
- Find a journal
- Track your research
How Has Quantitative Analysis Changed Health Care?
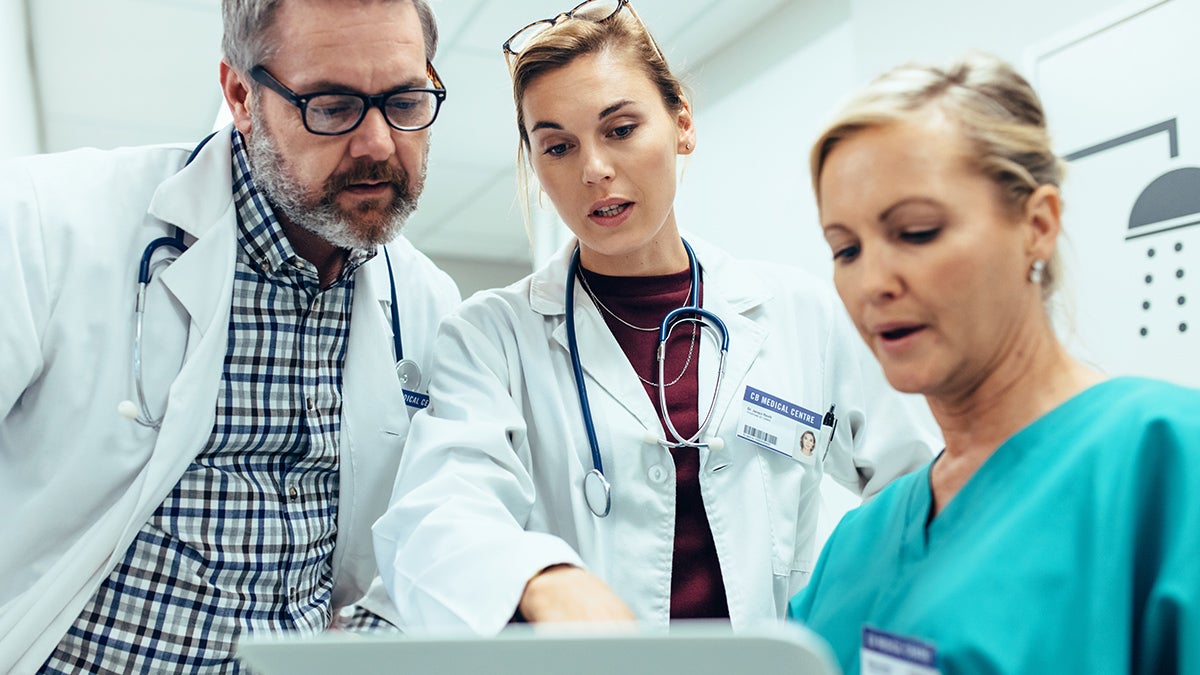
Fill out the form below and we'll email you more information about UCF's online healthcare programs.
- Name * First Last
- Degree * Autism Spectrum Disorders Executive Master of Health Administration, EMHA Forensic Science, MS Fundraising Gender Studies Health Informatics and Information Management, BS Health Services Administration, BS Healthcare Simulation Healthcare Systems Engineering Certificate Healthcare Systems Engineering, MS Integrative General Studies, BGS Interdisciplinary Studies – Diversity Studies Leadership Track, BA Interdisciplinary Studies, BA/BS Master of Public Administration, MPA Master of Science in Healthcare Informatics Master of Social Work Online Nonprofit Management Nonprofit Management, MNM Nursing Education Nursing Practice, DNP, Advanced Track Nursing Practice, DNP, Executive Track Nursing, BS Nursing, BSN to PhD Nursing, MSN Nursing, PhD Project Engineering Psychology, BS Public Administration Research Administration Certificate Research Administration, MRA Systems Engineering
Privacy Notice
In health care, groundbreaking solutions often follow a new capacity for measurement and pattern finding. For example, developing the ability to measure blood glucose levels led to better treatments of diabetes. Florence Nightingale changed nursing forever with her careful measurements of hospital care outcomes. Today, we’re in the midst of an even more significant change in the health care industry: Troves of data are mixing with technologies newly powerful enough to adequately analyze them. As a result, the unprecedented pattern-finding power of quantitative analysis is remaking the health care industry.
Quantitative analysis refers to the process of using complex mathematical or statistical modeling to make sense of data and potentially to predict behavior. Though quantitative analysis is well-established in the fields of economics and finance, cutting-edge quantitative analysis has only recently become possible in health care. Some experts insist that the unfurling of QA in health care will radically change the industry—and how all of us maintain our health and are treated when we’re sick.
It will be up to professionals in the transforming field of health care information technology to make the most of the opportunities borne from these expanding data sets. But what are a few of the specific ways in which quantitative analysis could improve health care?
Stronger Research
Dr. Richard Biehl, former education coordinator of the online Master of Science in Health Care Systems Engineering program at the University of Central Florida, explains that QA stands to change the face of research in the health care field, because, suddenly, it may become very easy to test the strength of correlations between thousands of variables with the touch of a button. In other words, no researcher will need to make the concerted decision to build a study around a question such as “Is this particular allele driving lipid metabolism?” Powerful analytical tools driven by QA will be able to point researchers in the direction of promising correlations between variables they might not have realized were linked.
“We used to get the data to support our research; now we’re getting the data to suggest our research,” Dr. Biehl says. “That’s very, very different.”
The upshot? The field of health care research will become a much more targeted and efficient space—and more likely to regularly uncover lifesaving treatments.
Saving Time, Money, and Lives Through Efficiency and Safety
New QA tools will decrease wait times and call patients into doctors’ offices only when a visit is necessary. As more and more data is crunched to determine, for example, what bodily indicators tend to precede a heart attack, the provider (who will be monitoring the patient’s vital signs via wearable devices) will be able to alert the patient when his or her indicators are trending in a worrisome direction. That means paying for fewer checkup appointments when one is healthy.
Even more importantly, QA tools will allow health care professionals to decrease the impact of human error in prescribing medication and invasive health care procedures. More data can save lives by uncovering complicated patterns (in physiology, DNA, diet, or lifestyle) that help explain why certain medications can prove dangerous for some.
Making Sure Supply Meets Demand
Certain geographic locations and clinical specialties are already facing doctor shortages as mergers and acquisitions reform the health care landscape and financial difficulties force providers to close their doors. But by filling in the picture of oversupply and undersupply around the country, QA can help providers plug holes where they need to.
“Making sure there’s an adequate supply of health care in the right places, in the right specialties, and at the right times, is a health care systems engineering challenge,” Dr. Biehl says.
Amid all the exciting possibilities, QA’s application to health care is still newer than other industries and faces challenges. This type of analysis requires that variables be recorded as numerical data so that they can be analyzed with statistical tools—a format that health care has struggled to conform with, as much of its outcome data is recorded as “positive” or “negative.”
Additionally, QA statistical tools work best when fed with huge amounts of data, as more data makes for clearer patterns and stronger conclusions. Big data analysts at corporations such as Amazon and Google—where every click is tracked and measured—have been collecting unprecedented amounts of data to feed into complex statistical tools for years. Health care has yet to catch up, but it likely will once more wearable technology options, such as expanded versions of Fitbit devices and trackers embedded in up-and-coming “internet of things” appliances, track more and more users’ every move, bite, and night of sleep.
“Once we start collecting all this personal, wearable data from people, health care will start to look more like the Googles and Amazons of the world,” Dr. Biehl says. “We’ll have hundreds of millions of people collecting tens of thousands of data points a day. We’ll finally have big data; we’re heading in that direction.”
Additional Resources
https://www.worldcat.org/wcpa/servlet/DCARead?standardNo=0787971642&standardNoType=1&excerpt=true https://bizfluent.com/info-8168865-benefits-quantitative-research-health-care.html https://www.ruralhealthinfo.org/community-health/rural-toolkit/4/quantitative-qualitative https://www.dotmed.com/news/story/37262
UCF’s Online Healthcare Degrees
- Autism Spectrum Disorders
- Executive Master of Health Administration, EMHA
- Forensic Science, MS
- Fundraising
- Gender Studies
- Health Informatics and Information Management, BS
- Health Services Administration, BS
- Healthcare Simulation
- Healthcare Systems Engineering Certificate
- Healthcare Systems Engineering, MS
- Integrative General Studies, BGS
- Interdisciplinary Studies – Diversity Studies Leadership Track, BA
- Interdisciplinary Studies, BA/BS
- Master of Public Administration, MPA
- Master of Science in Healthcare Informatics
- Master of Social Work Online
- Nonprofit Management
- Nonprofit Management, MNM
- Nursing Education
- Nursing Practice, DNP, Advanced Track
- Nursing Practice, DNP, Executive Track
- Nursing, BS
- Nursing, BSN to PhD
- Nursing, MSN
- Nursing, PhD
- Project Engineering
- Psychology, BS
- Public Administration
- Research Administration Certificate
- Research Administration, MRA
- Systems Engineering
You May Also Enjoy
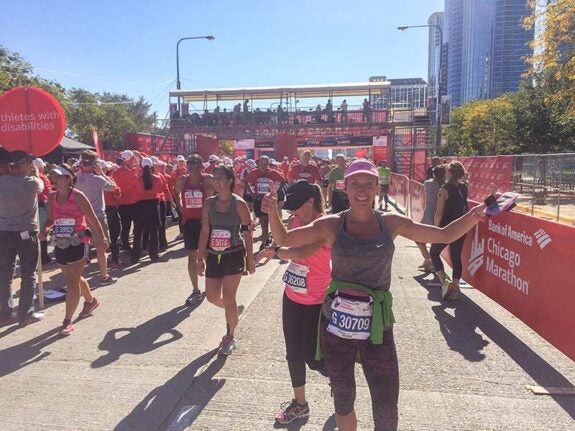
- Open access
- Published: 03 March 2015
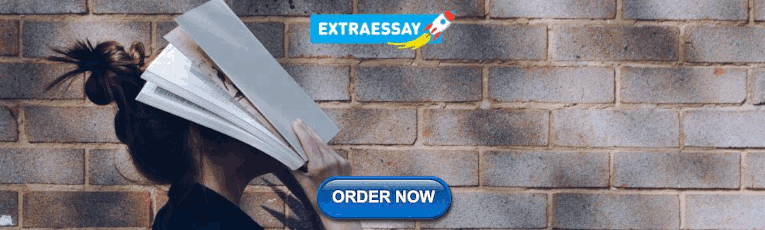
Health research improves healthcare: now we have the evidence and the chance to help the WHO spread such benefits globally
- Stephen R Hanney 1 &
- Miguel A González-Block 2
Health Research Policy and Systems volume 13 , Article number: 12 ( 2015 ) Cite this article
12k Accesses
21 Citations
16 Altmetric
Metrics details
There has been a dramatic increase in the body of evidence demonstrating the benefits that come from health research. In 2014, the funding bodies for higher education in the UK conducted an assessment of research using an approach termed the Research Excellence Framework (REF). As one element of the REF, universities and medical schools in the UK submitted 1,621 case studies claiming to show the impact of their health and other life sciences research conducted over the last 20 years. The recently published results show many case studies were judged positively as providing examples of the wide range and extensive nature of the benefits from such research, including the development of new treatments and screening programmes that resulted in considerable reductions in mortality and morbidity.
Analysis of specific case studies yet again illustrates the international dimension of progress in health research; however, as has also long been argued, not all populations fully share the benefits. In recognition of this, in May 2013 the World Health Assembly requested the World Health Organization (WHO) to establish a Global Observatory on Health Research and Development (R&D) as part of a strategic work-plan to promote innovation, build capacity, improve access, and mobilise resources to address diseases that disproportionately affect the world’s poorest countries.
As editors of Health Research Policy and Systems ( HARPS ), we are delighted that our journal has been invited to help inform the establishment of the WHO Global Observatory through a Call for Papers covering a range of topics relevant to the Observatory, including topics on which HARPS has published articles over the last few months, such as approaches to assessing research results, measuring expenditure data with a focus on R&D, and landscape analyses of platforms for implementing R&D. Topics related to research capacity building may also be considered. The task of establishing a Global Observatory on Health R&D to achieve the specified objectives will not be easy; nevertheless, this Call for Papers is well timed – it comes just at the point where the evidence of the benefits from health research has been considerably strengthened.
The start of 2015 sees a dramatic increase in the body of evidence demonstrating the benefits arising from health research. Throughout 2014, the higher education funding bodies in the UK conducted an assessment of research, termed the Research Excellence Framework (REF), in which, for the first time, account was taken of the impact on society of the research undertaken. As part of this, UK universities and medical schools produced 1,621 case studies that aimed to show the benefits, such as improved healthcare, arising from examples of their health and other life sciences research conducted over the last 20 years. Panels of experts, including leading academics from many countries, published their assessments of these case studies in December 2014 [ 1 ], with the full case studies and an analysis of the results being made public in January 2015 [ 2 , 3 ].
As we recently anticipated [ 4 ], the expert panels concluded that the case studies did indeed overwhelmingly illustrate the wide range and extensive nature of the benefits from health research. Main Panel A covered the range of life sciences and its overview report states: “ MPA [Main Panel A] believes that the collection of impact case studies provide a unique and powerful illustration of the outstanding contribution that research in the fields covered by this panel is making to health, wellbeing, wealth creation and society within and beyond the UK ” [ 3 ], p. 1. The section of the report covering public health and health services research also notes that: “ Outstanding examples included cases focused on national screening programmes for the selection and early diagnosis of conditions ” [ 3 ], p. 30. In their section of the report, the international experts say of the REF2014: “ It is the boldest, largest, and most comprehensive exercise of its kind of any country’s assessment of its science ” [ 3 ], p. 20.
The REF2014 is therefore attracting wide international attention. Indeed, some of the methods used are already informing studies in other countries, including, for example, an innovative assessment recently published in Health Research Policy and Systems ( HARPS ) identifying the beneficial effects made on healthcare policies and practice in Australia by intervention studies funded by the National Health and Medical Research Council [ 5 ].
The REF also illustrates that, even when focusing on the research from one country, there are examples of studies in which there has been international collaboration and which have built on research conducted elsewhere. For example, one REF case study on screening describes how a major UK randomised controlled trial of screening for abdominal aortic aneurysms (AAA) involving 67,800 men [ 6 , 7 ] was the most significant trial globally. The trial provided the main evidence for the policy to introduce national screening programmes for AAA for men reaching 65 throughout the UK [ 2 ]. The importance of this trial lay partly in its size, given that it accounted for over 50% of the men included in the meta-analyses performed in the 2007 Cochrane review [ 8 ] and the 2009 practice guideline from the US Society for Vascular Surgery [ 9 ]. Nevertheless, two of the three smaller studies that were also included in these two meta-analyses came from outside the UK, specifically from Denmark [ 10 ] and Australia [ 11 ].
Moreover, a recent paper published in HARPS also included descriptions of how the research contributing to new interventions often comes from more than one country. These accounts are included in a separate set of seven extensive case studies constructed to illustrate innovative ways to measure the time that can elapse between research being conducted and its translation into improved health [ 12 ]. While being a separate set of case studies, one of them does, nevertheless, explore the international timelines involved in research on screening for AAA, and, in addition to highlighting the key role of the UK research, it also highlights that the pioneering first screening study using ultrasound had been conducted in 1983 on 73 patients in a US Army medical base [ 13 ].
These case studies therefore further reinforce the well-established argument that health research progress often involves contributions from various countries. However, as has long been argued, not all populations fully share the benefits. In recognition of this, in May 2013, the World Health Assembly requested the World Health Organization (WHO), in its resolution 66.22, to establish a Global Observatory on Health Research and Development as part of a strategic work-plan to promote innovation, build capacity, improve access, and mobilise resources to address diseases that disproportionately affect the world’s poorest countries [ 14 ].
As editors of HARPS , we are delighted that our journal has been invited to help inform the establishment of the WHO Global Observatory by publishing a series of papers whose publication costs will be funded by the WHO. In support of this WHO initiative, Taghreed Adam, John-Arne Røttingen, and Marie-Paule Kieny recently published a Call for Papers for this series [ 15 ], which can be accessed through the HARPS webpage.
The aim of the series is “ to contribute state-of-the-art knowledge and innovative approaches to analyse, interpret, and report on health R&D information… [and] to serve as a key resource to inform the future WHO-convened coordination mechanism, which will be utilized to generate evidence-informed priorities for new R&D investments to be financed through a proposed new global financing and coordination mechanism for health R&D ” [ 15 ], p. 1. The Call for Papers covers a range of topics relevant to the aims of the Global Observatory. These include ones on which HARPS has published articles in the last few months, such as approaches to assessing research results, as seen in the Australian article described above [ 5 ]; papers measuring expenditure data with a focus on R&D, as described in a recent Commentary by Young et al. [ 16 ]; and landscape analyses of platforms for implementing R&D, as described in the article by Ongolo-Zogo et al. [ 17 ], analysing knowledge translation platforms in Cameroon and Uganda, and partially in the article by Yazdizadeh et al. [ 18 ], relaying lessons learnt from knowledge networks in Iran.
Adam et al. also make clear that the topics listed in the Call for Papers are examples and that the series editors are also willing to consider other areas [ 15 ]. Indeed, in the Introduction to the Call for Papers, the importance of capacity building is highlighted. This, too, is a topic described in recent papers in HARPS , such as those by Ager and Zarowsky [ 19 ], analysing the experiences of the Health Research Capacity Strengthening initiative’s Global Learning program of work across sub-Saharan Africa, and by Hunter et al. [ 20 ], describing needs assessment to strengthen capacity in water and sanitation research in Africa.
Finally, as we noted in our earlier editorial [ 4 ], the World Health Report 2013: Health Research for Universal Coverage showed how the demonstration of the benefits from health research could be a strong motivation for further funding of such research. As the Report states, “ adding impetus to do more research is a growing body of evidence on the returns on investments … there is mounting quantitative proof of the benefits of research to health, society and the economy ” [ 21 ]. We noted, too, that since the Report’s publication in 2013, there had been further examples from many countries of the benefits from medical research. The REF2014 in the UK signifies an additional major boost to the evidence that a wide range of health research does contribute to improved health and other social benefits. The results of such evaluations highlight the appropriateness of the WHO’s actions in attempting to ensure all populations share the benefits of health research endeavours by creating the Global Observatory on Health Research and Development. This will not be an easy task, but we welcome the opportunity afforded by the current Call for Papers for researchers and other stakeholders to engage with this process and influence it [ 15 ].
Abbreviations
Abdominal aortic aneurysms
Health Research Policy and Systems
Main Panel A
Research and development
Research Excellence Framework
- World Health Organization
Higher Education Funding Council for England. Research Excellence Framework 2014: The results. 2014. http://www.ref.ac.uk/pubs/201401/ . Accessed 20 Feb 2015.
Higher Education Funding Council for England. Research Excellence Framework 2014: Results and submissions. 2015. http://results.ref.ac.uk/ . Accessed 20 Feb 2015.
Higher Education Funding Council for England. REF 2014 Panel overview reports: Main Panel A and sub-panels 1–6. 2015. http://www.ref.ac.uk/media/ref/content/expanel/member/Main%20Panel%20A%20overview%20report.pdf . Accessed 20 Feb 2015.
Hanney SR, González-Block MA. Four centuries on from Bacon: progress in building health research systems to improve health systems? Health Res Policy Syst. 2014;12:56.
Article PubMed PubMed Central Google Scholar
Cohen G, Schroeder J, Newson R, King L, Rychetnik L, Milat AJ, et al. Does health intervention research have real world policy and practice impacts: testing a new impact assessment tool. Health Res Policy Syst. 2015;13:3.
Scott RAP, Ashton HA, Buxton M, Day NE, Kim LG, Marteau TM, et al. The Multicentre Aneurysm Screening Study (MASS) into the effect of abdominal aortic aneurysm screening on mortality in men: a randomised controlled trial. Lancet. 2002;360:1531–9.
Article PubMed Google Scholar
Buxton M, Ashton H, Campbell H, Day NE, Kim LG, Marteau TM, et al. Multicentre aneurysm screening study (MASS): cost effectiveness analysis of screening for abdominal aortic aneurysms based on four year results from randomised controlled trial. BMJ. 2002;325:1135–8.
Article Google Scholar
Cosford PA, Leng GC, Thomas J: Screening for abdominal aortic aneurysm. Cochrane Database Syst Rev 2007; (2)CD002945.
Chaikof EL, Brewster DC, Dalman RL, Makaroun MS, Illig KA, Sicard GA, et al. The care of patients with an abdominal aortic aneurysm: the Society for Vascular Surgery practice guidelines. J Vasc Surg. 2009;50(4 Suppl):S2–49.
Lindholt JS, Juul S, Fasting H, Henneberg EW. Hospital costs and benefits of screening for abdominal aortic aneurysms. Results from a randomised population screening trial. Eur J Vasc Endovasc Surg. 2002;23:55–60.
Article CAS PubMed Google Scholar
Norman PE, Jamrozik K, Lawrence-Brown MM, Le MT, Spencer CA, Tuohy RJ, et al. Population based randomised controlled trial on impact of screening on mortality from abdominal aortic aneurysm. BMJ. 2004;329(7477):1259–64.
Hanney SR, Castle-Clarke S, Grant J, Guthrie S, Henshall C, Mestre-Ferrandiz J, et al. How long does biomedical research take? Studying the time taken between biomedical and health research and its translation into products, policy, and practice. Health Res Policy Syst. 2015;13:1.
Cabellon S, Moncrief CL, Pierre DR, Cavenaugh DG. Incidence of abdominal aortic aneurysms in patients with artheromatous arterial disease. Am J Surg. 1983;146:575–6.
World Health Organization. WHA resolution 66.22: Follow up of the report of the Consultative Expert Working Group on Research and Development: financing and coordination. Geneva: WHO; 2013. http://www.who.int/phi/resolution_WHA-66.22.pdf . Accessed 20 Feb 2015.
Google Scholar
Adam T, Røttingen JA, Kieny MP. Informing the establishment of the WHO Global Observatory on Health Research and Development: a call for papers. Health Res Policy Syst. 2015;13:9.
Young AJ, Terry RF, Røttingen JA, Viergever RF. Global trends in health research and development expenditures – the challenge of making reliable estimates for international comparison. Health Res Policy Syst. 2015;13:7.
Ongolo-Zogo P, Lavis JN, Tomson G, Sewankambo NK. Climate for evidence informed health system policymaking in Cameroon and Uganda before and after the introduction of knowledge translation platforms: a structured review of governmental policy documents. Health Res Policy Syst. 2015;13:2.
Yazdizadeh B, Majdzadeh R, Alami A, Amrolalaei S. How can we establish more successful knowledge networks in developing countries? Lessons learnt from knowledge networks in Iran. Health Res Policy Syst. 2014;12:63.
Ager A, Zarowsky C. Balancing the personal, local, institutional, and global: multiple case study and multidimensional scaling analysis of African experiences in addressing complexity and political economy in health research capacity strengthening. Health Res Policy Syst. 2015;13:5.
Hunter PR, Abdelrahman SH, Antwi-Agyei P, Awuah E, Cairncross S, Chappell E, et al. Needs assessment to strengthen capacity in water and sanitation research in Africa: experiences of the African SNOWS consortium. Health Res Policy Syst. 2014;12:68.
The World Health Organization. The World Health Report 2013: Research for Universal Health Coverage. Geneva: WHO; 2013.
Download references
Acknowledgements
The authors thank Bryony Soper for most helpful comments on an earlier draft.
Author information
Authors and affiliations.
Health Economics Research Group, Brunel University London, Kingston Lane, Uxbridge, UB8 3PH, UK
Stephen R Hanney
Universidad Anáhuac, Av. Universidad Anáhuac 46, Lomas Anáhuac, 52786 Huixquilucan, Mexico City, Mexico
Miguel A González-Block
You can also search for this author in PubMed Google Scholar
Corresponding author
Correspondence to Stephen R Hanney .
Additional information
Competing interests.
The authors are co-Chief Editors of Health Research Policy and Systems.
Rights and permissions
This article is published under license to BioMed Central Ltd. This is an Open Access article distributed under the terms of the Creative Commons Attribution License ( http://creativecommons.org/licenses/by/4.0 ), which permits unrestricted use, distribution, and reproduction in any medium, provided the original work is properly credited. The Creative Commons Public Domain Dedication waiver ( http://creativecommons.org/publicdomain/zero/1.0/ ) applies to the data made available in this article, unless otherwise stated.
Reprints and permissions
About this article
Cite this article.
Hanney, S.R., González-Block, M.A. Health research improves healthcare: now we have the evidence and the chance to help the WHO spread such benefits globally. Health Res Policy Sys 13 , 12 (2015). https://doi.org/10.1186/s12961-015-0006-y
Download citation
Received : 20 February 2015
Accepted : 20 February 2015
Published : 03 March 2015
DOI : https://doi.org/10.1186/s12961-015-0006-y
Share this article
Anyone you share the following link with will be able to read this content:
Sorry, a shareable link is not currently available for this article.
Provided by the Springer Nature SharedIt content-sharing initiative
- Assessing research benefits
- Capacity building
- Diseases of poorest countries
- Global health
- Global Observatory
- Platforms for research implementation
- Research expenditure
- Screening for abdominal aortic aneurysms
- World Health Report 2013
ISSN: 1478-4505
- Submission enquiries: Access here and click Contact Us
- General enquiries: [email protected]
- Fact sheets
- Facts in pictures
Publications
- Questions and answers
- Tools and toolkits
- HIV and AIDS
- Hypertension
- Mental disorders
- Top 10 causes of death
- All countries
- Eastern Mediterranean
- South-East Asia
- Western Pacific
- Data by country
- Country presence
- Country strengthening
- Country cooperation strategies
- News releases
- Feature stories
- Press conferences
- Commentaries
- Photo library
- Afghanistan
- Cholera
- Coronavirus disease (COVID-19)
- Greater Horn of Africa
- Israel and occupied Palestinian territory
- Disease Outbreak News
- Situation reports
- Weekly Epidemiological Record
- Surveillance
- Health emergency appeal
- International Health Regulations
- Independent Oversight and Advisory Committee
- Classifications
- Data collections
- Global Health Estimates
- Mortality Database
- Sustainable Development Goals
- Health Inequality Monitor
- Triple Billion
- Data collection tools
- Global Health Observatory
- Insights and visualizations
- COVID excess deaths
- World Health Statistics
- Partnerships
- Committees and advisory groups
- Collaborating centres
- Technical teams
- Organizational structure
- Initiatives
- General Programme of Work
- WHO Academy
- Investment case
- WHO Foundation
- External audit
- Financial statements
- Internal audit and investigations
- Programme Budget
- Results reports
- Governing bodies
- World Health Assembly
- Executive Board
- Member States Portal
- Health topics /
Research is indispensable for resolving public health challenges – whether it be tackling diseases of poverty, responding to rise of chronic diseases, or ensuring that mothers have access to safe delivery practices.
Likewise, shared vulnerability to global threats, such as severe acute respiratory syndrome, Ebola virus disease, Zika virus and avian influenza has mobilized global research efforts in support of enhancing capacity for preparedness and response. Research is strengthening surveillance, rapid diagnostics and development of vaccines and medicines.
Public-private partnerships and other innovative mechanisms for research are concentrating on neglected diseases in order to stimulate the development of vaccines, drugs and diagnostics where market forces alone are insufficient.
Research for health spans 5 generic areas of activity:
- measuring the magnitude and distribution of the health problem;
- understanding the diverse causes or the determinants of the problem, whether they are due to biological, behavioural, social or environmental factors;
- developing solutions or interventions that will help to prevent or mitigate the problem;
- implementing or delivering solutions through policies and programmes; and
- evaluating the impact of these solutions on the level and distribution of the problem.
High-quality research is essential to fulfilling WHO’s mandate for the attainment by all peoples of the highest possible level of health. One of the Organization’s core functions is to set international norms, standards and guidelines, including setting international standards for research.
Under the “WHO strategy on research for health”, the Organization works to identify research priorities, and promote and conduct research with the following 4 goals:
- Capacity - build capacity to strengthen health research systems within Member States.
- Priorities - support the setting of research priorities that meet health needs particularly in low- and middle-income countries.
- Standards - develop an enabling environment for research through the creation of norms and standards for good research practice.
- Translation - ensure quality evidence is turned into affordable health technologies and evidence-informed policy.
- Prequalification of medicines by WHO
- Global Observatory on Health R&D
- Global Observatory on Health Research and Development
- Implementation research toolkit
- Ethics in implementation research: participant's guide
- Ethics in implementation research: facilitator's guide
- Ethics in epidemics, emergencies and disasters: Research, surveillance and patient care: WHO training manual
- WHA58.34 Ministerial Summit on Health Research
- WHA60.15 WHO's role and responsibilities in health research
- WHA63.21 WHO's role and responsibilities in health research
- EB115/30 Ministerial Summit on Health Research: report by the Secretariat
- Science division
WHO advisory group convenes its first meeting on responsible use of the life sciences in Geneva
Challenging harmful masculinities and engaging men and boys in sexual and reproductive health
Stakeholders convene in Uganda on responsible use of the life sciences
The Technical Advisory Group on the Responsible Use of the Life Sciences and Dual-Use Research meets for the first time

WHO Science Council meeting, Geneva, Switzerland, 30-31 January 2024: report
This is a visual summary of the meeting of the WHO Science Council which took place on 30 and 31 January 2024.

WHO Technical Advisory Group on the Responsible Use of the Life Sciences and Dual-Use Research (TAG-RULS...
The Technical Advisory Group on the Responsible Use of the Life Sciences and Dual-Use Research (TAG-RULS DUR) was established in November 2023 to provide...

Target product profile to detect "Dracunculus medinensis" presence in environmental samples
Dracunculiasis, also known as Guinea-worm disease, is caused by infection with the parasitic nematode (the Guinea worm). In May 1986, the Thirty-ninth...

Target product profile to detect prepatent "Dracunculus medinensis" infections in animals
Dracunculiasis, also known as Guinea-worm disease, is caused by infection with the parasitic nematode Dracunculus medinensis (the Guinea worm). In May...
Coordinating R&D on antimicrobial resistance
Ensuring responsible use of life sciences research
Optimizing research and development processes for accelerated access to health products
Prioritizing diseases for research and development in emergency contexts
Promoting research on Buruli ulcer
Research in maternal, perinatal, and adolescent health
Undertaking health law research
Feature story
One year on, Global Observatory on Health R&D identifies striking gaps and inequalities

Video: Open access to health: WHO joins cOAlition S

Video: Multisectional research on sleeping sickness in Tanzania in the context of climate change
Related health topics
Clinical trials
Global health ethics
Health Laws
Intellectual property and trade
Related links
Research and Development Blueprint
WHO Collaborating Centres
R&D Blueprint for Action to Prevent Epidemics
International Clinical Trials Registry Platform
Log in using your username and password
- Search More Search for this keyword Advanced search
- Latest content
- Current issue
- Write for Us
- BMJ Journals
You are here
- Volume 21, Issue 4
- How to appraise quantitative research
- Article Text
- Article info
- Citation Tools
- Rapid Responses
- Article metrics
This article has a correction. Please see:
- Correction: How to appraise quantitative research - April 01, 2019

- Xabi Cathala 1 ,
- Calvin Moorley 2
- 1 Institute of Vocational Learning , School of Health and Social Care, London South Bank University , London , UK
- 2 Nursing Research and Diversity in Care , School of Health and Social Care, London South Bank University , London , UK
- Correspondence to Mr Xabi Cathala, Institute of Vocational Learning, School of Health and Social Care, London South Bank University London UK ; cathalax{at}lsbu.ac.uk and Dr Calvin Moorley, Nursing Research and Diversity in Care, School of Health and Social Care, London South Bank University, London SE1 0AA, UK; Moorleyc{at}lsbu.ac.uk
https://doi.org/10.1136/eb-2018-102996
Statistics from Altmetric.com
Request permissions.
If you wish to reuse any or all of this article please use the link below which will take you to the Copyright Clearance Center’s RightsLink service. You will be able to get a quick price and instant permission to reuse the content in many different ways.
Introduction
Some nurses feel that they lack the necessary skills to read a research paper and to then decide if they should implement the findings into their practice. This is particularly the case when considering the results of quantitative research, which often contains the results of statistical testing. However, nurses have a professional responsibility to critique research to improve their practice, care and patient safety. 1 This article provides a step by step guide on how to critically appraise a quantitative paper.
Title, keywords and the authors
The authors’ names may not mean much, but knowing the following will be helpful:
Their position, for example, academic, researcher or healthcare practitioner.
Their qualification, both professional, for example, a nurse or physiotherapist and academic (eg, degree, masters, doctorate).
This can indicate how the research has been conducted and the authors’ competence on the subject. Basically, do you want to read a paper on quantum physics written by a plumber?
The abstract is a resume of the article and should contain:
Introduction.
Research question/hypothesis.
Methods including sample design, tests used and the statistical analysis (of course! Remember we love numbers).
Main findings.
Conclusion.
The subheadings in the abstract will vary depending on the journal. An abstract should not usually be more than 300 words but this varies depending on specific journal requirements. If the above information is contained in the abstract, it can give you an idea about whether the study is relevant to your area of practice. However, before deciding if the results of a research paper are relevant to your practice, it is important to review the overall quality of the article. This can only be done by reading and critically appraising the entire article.
The introduction
Example: the effect of paracetamol on levels of pain.
My hypothesis is that A has an effect on B, for example, paracetamol has an effect on levels of pain.
My null hypothesis is that A has no effect on B, for example, paracetamol has no effect on pain.
My study will test the null hypothesis and if the null hypothesis is validated then the hypothesis is false (A has no effect on B). This means paracetamol has no effect on the level of pain. If the null hypothesis is rejected then the hypothesis is true (A has an effect on B). This means that paracetamol has an effect on the level of pain.
Background/literature review
The literature review should include reference to recent and relevant research in the area. It should summarise what is already known about the topic and why the research study is needed and state what the study will contribute to new knowledge. 5 The literature review should be up to date, usually 5–8 years, but it will depend on the topic and sometimes it is acceptable to include older (seminal) studies.
Methodology
In quantitative studies, the data analysis varies between studies depending on the type of design used. For example, descriptive, correlative or experimental studies all vary. A descriptive study will describe the pattern of a topic related to one or more variable. 6 A correlational study examines the link (correlation) between two variables 7 and focuses on how a variable will react to a change of another variable. In experimental studies, the researchers manipulate variables looking at outcomes 8 and the sample is commonly assigned into different groups (known as randomisation) to determine the effect (causal) of a condition (independent variable) on a certain outcome. This is a common method used in clinical trials.
There should be sufficient detail provided in the methods section for you to replicate the study (should you want to). To enable you to do this, the following sections are normally included:
Overview and rationale for the methodology.
Participants or sample.
Data collection tools.
Methods of data analysis.
Ethical issues.
Data collection should be clearly explained and the article should discuss how this process was undertaken. Data collection should be systematic, objective, precise, repeatable, valid and reliable. Any tool (eg, a questionnaire) used for data collection should have been piloted (or pretested and/or adjusted) to ensure the quality, validity and reliability of the tool. 9 The participants (the sample) and any randomisation technique used should be identified. The sample size is central in quantitative research, as the findings should be able to be generalised for the wider population. 10 The data analysis can be done manually or more complex analyses performed using computer software sometimes with advice of a statistician. From this analysis, results like mode, mean, median, p value, CI and so on are always presented in a numerical format.
The author(s) should present the results clearly. These may be presented in graphs, charts or tables alongside some text. You should perform your own critique of the data analysis process; just because a paper has been published, it does not mean it is perfect. Your findings may be different from the author’s. Through critical analysis the reader may find an error in the study process that authors have not seen or highlighted. These errors can change the study result or change a study you thought was strong to weak. To help you critique a quantitative research paper, some guidance on understanding statistical terminology is provided in table 1 .
- View inline
Some basic guidance for understanding statistics
Quantitative studies examine the relationship between variables, and the p value illustrates this objectively. 11 If the p value is less than 0.05, the null hypothesis is rejected and the hypothesis is accepted and the study will say there is a significant difference. If the p value is more than 0.05, the null hypothesis is accepted then the hypothesis is rejected. The study will say there is no significant difference. As a general rule, a p value of less than 0.05 means, the hypothesis is accepted and if it is more than 0.05 the hypothesis is rejected.
The CI is a number between 0 and 1 or is written as a per cent, demonstrating the level of confidence the reader can have in the result. 12 The CI is calculated by subtracting the p value to 1 (1–p). If there is a p value of 0.05, the CI will be 1–0.05=0.95=95%. A CI over 95% means, we can be confident the result is statistically significant. A CI below 95% means, the result is not statistically significant. The p values and CI highlight the confidence and robustness of a result.
Discussion, recommendations and conclusion
The final section of the paper is where the authors discuss their results and link them to other literature in the area (some of which may have been included in the literature review at the start of the paper). This reminds the reader of what is already known, what the study has found and what new information it adds. The discussion should demonstrate how the authors interpreted their results and how they contribute to new knowledge in the area. Implications for practice and future research should also be highlighted in this section of the paper.
A few other areas you may find helpful are:
Limitations of the study.
Conflicts of interest.
Table 2 provides a useful tool to help you apply the learning in this paper to the critiquing of quantitative research papers.
Quantitative paper appraisal checklist
- 1. ↵ Nursing and Midwifery Council , 2015 . The code: standard of conduct, performance and ethics for nurses and midwives https://www.nmc.org.uk/globalassets/sitedocuments/nmc-publications/nmc-code.pdf ( accessed 21.8.18 ).
- Gerrish K ,
- Moorley C ,
- Tunariu A , et al
- Shorten A ,
Competing interests None declared.
Patient consent Not required.
Provenance and peer review Commissioned; internally peer reviewed.
Correction notice This article has been updated since its original publication to update p values from 0.5 to 0.05 throughout.
Linked Articles
- Miscellaneous Correction: How to appraise quantitative research BMJ Publishing Group Ltd and RCN Publishing Company Ltd Evidence-Based Nursing 2019; 22 62-62 Published Online First: 31 Jan 2019. doi: 10.1136/eb-2018-102996corr1
Read the full text or download the PDF:
- Research article
- Open access
- Published: 01 December 2006
Using quantitative and qualitative data in health services research – what happens when mixed method findings conflict? [ISRCTN61522618]
- Suzanne Moffatt 1 ,
- Martin White 1 ,
- Joan Mackintosh 1 &
- Denise Howel 1
BMC Health Services Research volume 6 , Article number: 28 ( 2006 ) Cite this article
84k Accesses
127 Citations
16 Altmetric
Metrics details
In this methodological paper we document the interpretation of a mixed methods study and outline an approach to dealing with apparent discrepancies between qualitative and quantitative research data in a pilot study evaluating whether welfare rights advice has an impact on health and social outcomes among a population aged 60 and over.
Quantitative and qualitative data were collected contemporaneously. Quantitative data were collected from 126 men and women aged over 60 within a randomised controlled trial. Participants received a full welfare benefits assessment which successfully identified additional financial and non-financial resources for 60% of them. A range of demographic, health and social outcome measures were assessed at baseline, 6, 12 and 24 month follow up. Qualitative data were collected from a sub-sample of 25 participants purposively selected to take part in individual interviews to examine the perceived impact of welfare rights advice.
Separate analysis of the quantitative and qualitative data revealed discrepant findings. The quantitative data showed little evidence of significant differences of a size that would be of practical or clinical interest, suggesting that the intervention had no impact on these outcome measures. The qualitative data suggested wide-ranging impacts, indicating that the intervention had a positive effect. Six ways of further exploring these data were considered: (i) treating the methods as fundamentally different; (ii) exploring the methodological rigour of each component; (iii) exploring dataset comparability; (iv) collecting further data and making further comparisons; (v) exploring the process of the intervention; and (vi) exploring whether the outcomes of the two components match.
The study demonstrates how using mixed methods can lead to different and sometimes conflicting accounts and, using this six step approach, how such discrepancies can be harnessed to interrogate each dataset more fully. Not only does this enhance the robustness of the study, it may lead to different conclusions from those that would have been drawn through relying on one method alone and demonstrates the value of collecting both types of data within a single study. More widespread use of mixed methods in trials of complex interventions is likely to enhance the overall quality of the evidence base.
Combining quantitative and qualitative methods in a single study is not uncommon in social research, although, 'traditionally a gulf is seen to exist between qualitative and quantitative research with each belonging to distinctively different paradigms'. [ 1 ] Within health research there has, more recently, been an upsurge of interest in the combined use of qualitative and quantitative methods, sometimes termed mixed methods research [ 2 ] although the terminology can vary. [ 3 ] Greater interest in qualitative research has come about for a number of reasons: the numerous contributions made by qualitative research to the study of health and illness [ 4 – 6 ]; increased methodological rigor [ 7 ] within the qualitative paradigm, which has made it more acceptable to researchers or practitioners trained within a predominantly quantitative paradigm [ 8 ]; and, because combining quantitative and qualitative methods may generate deeper insights than either method alone. [ 9 ] It is now widely recognised that public health problems are embedded within a range of social, political and economic contexts. [ 10 ] Consequently, a range of epidemiological and social science methods are employed to research these complex issues. [ 11 ] Further legitimacy for the use of qualitative methods alongside quantitative has resulted from the recognition that qualitative methods can make an important contribution to randomised controlled trials (RCTs) evaluating complex health service interventions. There is published work on the various ways that qualitative methods are being used in RCTs (e.g. [ 12 , 13 ] but little on how they can optimally enhance the usefulness and policy relevance of trial findings. [ 14 , 15 ]
A number of mixed methods publications outline the various ways in which qualitative and quantitative methods can be combined. [ 1 , 2 , 9 , 16 ] For the purposes of this paper with its focus on mixed methods in the context of a pilot RCT, the significant aspects of mixed methods appear to be: purpose, process and, analysis and interpretation. In terms of purpose, qualitative research may be used to help identify the relevant variables for study [ 17 ], develop an instrument for quantitative research [ 18 ], to examine different questions (such as acceptability of the intervention, rather than its outcome) [ 19 ]; and to examine the same question with different methods (using, for example participant observation or in depth interviews [ 1 ]). Process includes the priority accorded to each method and ordering of both methods which may be concurrent, sequential or iterative. [ 20 ] Bryman [ 9 ] points out that, 'most researchers rely primarily on a method associated with either quantitative or qualitative methods and then buttress their findings with a method associated with the other tradition' (p128). Both datasets may be brought together at the 'analysis/interpretation' phase, often known as 'triangulation' [ 21 ]. Brannen [ 1 ] suggests that most researchers have taken this to mean more than one type of data, but she stresses that Denzin's original conceptualisation involved methods, data, investigators or theories. Bringing different methods together almost inevitably raises discrepancies in findings and their interpretation. However, the investigation of such differences may be as illuminating as their points of similarity. [ 1 , 9 ]
Although mixed methods are now widespread in health research, quantitative and qualitative methods and results are often published separately. [ 22 , 23 ] It is relatively rare to see an account of the methodological implications of the strategy and the way in which both methods are combined when interpreting the data within a particular study. [ 1 ] A notable exception is a study showing divergence between qualitative and quantitative findings of cancer patients' quality of life using a detailed case study approach to the data. [ 13 ]
By presenting quantitative and qualitative data collected within a pilot RCT together, this paper has three main aims: firstly, to demonstrate how divergent quantitative and qualitative data led us to interrogate each dataset more fully and assisted in the interpretation process, producing a greater research yield from each dataset; secondly, to demonstrate how combining both types of data at the analysis stage produces 'more than the sum of its parts'; and thirdly, to emphasise the complementary nature of qualitative and quantitative methods in RCTs of complex interventions. In doing so, we demonstrate how the combination of quantitative and qualitative data led us to conclusions different from those that would have been drawn through relying on one or other method alone.
The study that forms the basis of this paper, a pilot RCT to examine the impact of welfare rights advice in primary care, was funded under the UK Department of Health's Policy Research Programme on tackling health inequalities, and focused on older people. To date, little research has been able to demonstrate how health inequalities can be tackled by interventions within and outside the health sector. Although living standards have risen among older people, a common experience of growing old is worsening material circumstances. [ 24 ] In 2000–01 there were 2.3 million UK pensioners living in households with below 60 per cent of median household income, after housing costs. [ 25 ] Older people in the UK may be eligible for a number of income- or disability-related benefits (the latter could be non-financial such as parking permits or adaptations to the home), but it has been estimated that approximately one in four (about one million) UK pensioner households do not claim the support to which they are entitled. [ 26 ] Action to facilitate access to and uptake of welfare benefits has taken place outside the UK health sector for many years and, more recently, has been introduced within parts of the health service, but its potential to benefit health has not been rigorously evaluated. [ 27 – 29 ]
There are a number of models of mixed methods research. [ 2 , 16 , 30 ] We adopted a model which relies of the principle of complementarity, using the strengths of one method to enhance the other. [ 30 ] We explicitly recognised that each method was appropriate for different research questions. We undertook a pragmatic RCT which aimed to evaluate the health effects of welfare rights advice in primary care among people aged over 60. Quantitative data included standardised outcome measures of health and well-being, health related behaviour, psycho-social interaction and socio-economic status ; qualitative data used semi-structured interviews to explore participants' views about the intervention, its outcome, and the acceptability of the research process.
Following an earlier qualitative pilot study to inform the selection of appropriate outcome measures [ 31 ], contemporaneous quantitative and qualitative data were collected. Both datasets were analysed separately and neither compared until both analyses were complete. The sampling strategy mirrored the embedded design; probability sampling for the quantitative study and theoretical sampling for the qualitative study, done on the basis of factors identified in the quantitative study.
Approval for the study was obtained from Newcastle and North Tyneside Joint Local Research Ethics Committee and from Newcastle Primary Care Trust.
The intervention
The intervention was delivered by a welfare rights officer from Newcastle City Council Welfare Rights Service in participants' own homes and comprised a structured assessment of current welfare status and benefits entitlement, together with active assistance in making claims where appropriate over the following six months, together with necessary follow-up for unresolved claims.
Quantitative study
The design presented ethical dilemmas as it was felt problematic to deprive the control group of welfare rights advice, since there is adequate evidence to show that it leads to significant financial gains. [ 32 ] To circumvent this dilemma, we delivered welfare rights advice to the control group six months after the intervention group. A single-blinded RCT with allocation of individuals to intervention (receipt of welfare rights consultation immediately) and control condition (welfare rights consultation six months after entry into the trial) was undertaken.
Four general practices located at five surgeries across Newcastle upon Tyne took part. Three of the practices were located in the top ten per cent of most deprived wards in England using the Index of Multiple Deprivation (two in the top one percent – ranked 30 th and 36 th most deprived); the other practice was ranked 3,774 out of a total of 8,414 in England. [ 33 ]
Using practice databases, a random sample of 100 patients aged 60 years or over from each of four participating practices was invited to take part in the study. Only one individual per household was allowed to participate in the trial, but if a partner or other adult household member was also eligible for benefits, they also received welfare rights advice. Patients were excluded if they were permanently hospitalised or living in residential or nursing care homes.
Written informed consent was obtained at the baseline interview. Structured face to face interviews were carried out at baseline, six, 12 and 24 months using standard scales covering the areas of demographics, mental and physical health (SF36) [ 34 ], Hospital Anxiety and Depression Scale (HADS) [ 35 ], psychosocial descriptors (e.g. Social Support Questionnaire [ 36 ] and the Self-Esteem Inventory, [ 37 ], and socioeconomic indicators (e.g. affordability and financial vulnerability). [ 38 ] Additionally, a short semi-structured interview was undertaken at 24 months to ascertain the perceived impact of additional resources for those who received them.
All health and welfare assessment data were entered onto customised MS Access databases and checked for quality and completeness. Data were transferred to the Statistical Package for the Social Sciences (SPSS) v11.0 [ 39 ] and STATA v8.0 for analysis. [ 40 ]
Qualitative study
The qualitative findings presented in this paper focus on the impact of the intervention. The sampling frame was formed by those (n = 96) who gave their consent to be contacted during their baseline interview for the RCT. The study sample comprised respondents from intervention and control groups purposively selected to include those eligible for the following resources: financial only; non-financial only; both financial and non financial; and, none. Sampling continued until no new themes emerged from the interviews; until data 'saturation' was reached. [ 21 ]
Initial interviews took place between April and December 2003 in participants' homes after their welfare rights assessment; follow-up interviews were undertaken in January and February 2005. The semi-structured interview schedule covered perceptions of: impact of material and/or financial benefits; impact on mental and/or physical health; impact on health related behaviours; social benefits; and views about the link between material resources and health. All participants agreed to the interview being audio-recorded. Immediately afterwards, observational field notes were made. Interviews were transcribed in full.
Data analysis largely followed the framework approach. [ 41 ] Data were coded, indexed and charted systematically; and resulting typologies discussed with other members of the research team, 'a pragmatic version of double coding'. [ 42 ] Constant comparison [ 43 ] and deviant case analysis [ 44 ] were used since both methods are important for internal validation. [ 7 , 42 ] Finally, sets of categories at a higher level of abstraction were developed.
A brief semi-structured interview was undertaken (by JM) with all participants who received additional resources. These interview data explored the impact data of additional resources on all of those who received them, not just the qualitative sub-sample. The data were independently coded by JM and SM using the same coding frame. Discrepant codes were examined by both researchers and a final code agreed.
One hundred and twenty six people were recruited into the study; there were 117 at 12 month follow-up and 109 at 24 months (five deaths, one moved, the remainder declined).
Table 1 shows the distribution of financial and non-financial benefits awarded as a result of the welfare assessments. Sixty percent of participants were awarded some form of welfare benefit, and just over 40% received a financial benefit. Some households received more than one type of benefit.
Table 2 compares the quantitative and qualitative sub-samples on a number of personal, economic, health and lifestyle factors at baseline. Intervention and control groups were comparable.
Table 3 compares outcome measures by award group, i.e. no award, non-financial and financial and shows only small differences between the mean changes across each group, none of which were statistically significant. Other analyses of the quantitative data compared the changes seen between baseline and six months (by which time the intervention group had received the welfare rights advice but the control group had not) and found little evidence of differences between the intervention and control groups of any practical importance. The only statistically significant difference between the groups was a small decrease in financial vulnerability in the intervention group after six months. [ 45 ]
There was little evidence for differences in health and social outcomes measures as a result of the receipt of welfare advice of a size that would be of major practical or clinical interest. However, this was a pilot study, with only the power to detect large differences if they were present. One reason for a lack of difference may be that the scales were less appropriate for older people and did not capture all relevant outcomes. Another reason for the lack of differences may be that insufficient numbers of people had received their benefits for long enough to allow any health outcomes to have changed when comparisons were made. Fourteen per cent of participants found to be eligible for financial benefits had not started receiving their benefits by the time of the first follow-up interview after their benefit assessment (six months for intervention, 12 months for control); and those who had, had only received them for an average of 2 months. This is likely to have diluted any impact of the intervention effect, and might account, to some extent, for the lack of observed effect.
Twenty five interviews were completed, fourteen of whom were from the intervention group. Ten participants were interviewed with partners who made active contributions. Twenty two follow-up interviews were undertaken between twelve and eighteen months later (three individuals were too ill to take part).
Table 1 (fifth column) shows that 14 of the participants in the qualitative study received some financial award. The median income gain was (€84, $101) (range £10 (€15, $18) -£100 (€148, $178)) representing a 4%-55% increase in weekly income. 18 participants were in receipt of benefit, either as a result of the current intervention or because of claims made prior to this study.
By the follow-up (FU) interviews all but one participant had been receiving their benefits for between 17 and 31 months. The intervention was viewed positively by all interviewees irrespective of outcome. However, for the fourteen participants who received additional financial resources the impact was considerable and accounts revealed a wide range of uses for the extra money. Participants' accounts revealed four linked categories, summarised on Table 4 . Firstly, increased affordability of necessities , without which maintaining independence and participating in daily life was difficult. This included accessing transport, maintaining social networks and social activities, buying better quality food, stocking up on food, paying bills, preventing debt and affording paid help for household activities. Secondly, occasional expenses such as clothes, household equipment, furniture and holidays were more affordable. Thirdly, extra income was used to act as a cushion against potential emergencies and to increase savings . Fourthly, all participants described the easing of financial worries as bringing ' peace of mind' .
Without exception, participants were of the view that extra money or resources would not improve existing health problems. The reasons behind these strongly held views about individual health conditions was generally that their poor health was attributed to specific health conditions and a combination of family history or fate, which were immune to the effects of money. Most participants had more than one chronic condition and felt that because of these conditions, plus their age, additional money would have no effect.
However, a number of participants linked the impact of the intervention with improved ways of coping with their conditions because of what the extra resources enabled them to do:
Mrs T: Having money is not going to improve his health, we could win the lottery and he would still have his health problems.
Mr T: No, but we don't need to worry if I wanted .... Well I mean I eat a lot of honey and I think it's very good, very healthful for you ... at one time we couldn't have afforded to buy these things. Now we can go and buy them if I fancy something, just go and get it where we couldn't before .
Mrs T: Although the Attendance Allowance is actually his [partners], it's made me relax a bit more ... I definitely worry less now (N15, female, 62 and partner)
Despite the fact that no-one expected their own health conditions to improve, most people believed that there was a link between resources and health in a more abstract sense, either because they experienced problems affording necessities such as healthy food or maintaining adequate heat in their homes, or because they empathised with those who lacked money. Participants linked adequate resources to maintaining health and contributing to a sense of well-being.
Money does have a lot to do with health if you are poor. It would have a lot to do with your health ... I don't buy loads and loads of luxuries, but I know I can go out and get the food we need and that sort of thing. I think that money is a big part of how a house, or how people in that house are . (N13, female, 72)
Comparing the results from the two datasets
When the separate analyses of the quantitative and qualitative datasets after the 12 month follow-up structured interviews were completed, the discrepancy in the findings became apparent. The quantitative study showed little evidence of a size that would be of practical or clinical interest, suggesting that the intervention had no impact on these outcome measures. The qualitative study found a wide-ranging impact, indicating that the intervention had a positive effect. The presence of such inter-method discrepancy led to a great deal of discussion and debate, as a result of which we devised six ways of further exploring these data.
(i) Treating the methods as fundamentally different
This process of simultaneous qualitative and quantitative dataset interrogation enables a deeper level of analysis and interpretation than would be possible with one or other alone and demonstrates how mixed methods research produces more than the sum of its parts. It is worth emphasising however, that it is not wholly surprising that each method comes up with divergent findings since each asked different, but related questions, and both are based on fundamentally different theoretical paradigms. Brannen [ 1 ] and Bryman [ 9 ] argue that it is essential to take account of these theoretical differences and caution against taking a purely technical approach to the use of mixed methods, a simple 'bolting together' of techniques. [ 17 ] Combining the two methods for crossvalidation (triangulation) purposes is not a viable option because it rests on the premise that both methods are examining the same research problem. [ 1 ] We have approached the divergent findings as indicative of different aspects of the phenomena in question and searched for reasons which might explain these inconsistencies. In the approach that follows, we have treated the datasets as complementary, rather than attempt to integrate them, since each approach reflects a different view on how social reality ought to be studied.
(ii) Exploring the methodological rigour of each component
It is standard practice at the data analysis and interpretation phases of any study to scrutinise methodological rigour. However, in this case, we had another dataset to use as a yardstick for comparison and it became clear that our interrogation of each dataset was informed to some extent by the findings of the other. It was not the case that we expected to obtain the same results, but clearly the divergence of our findings was of great interest and made us more circumspect about each dataset. We began by examining possible reasons why there might be problems with each dataset individually, but found ourselves continually referring to the results of the other study as a benchmark for comparison.
With regard to the quantitative study, it was a pilot, of modest sample size, and thus not powered to detect small differences in the key outcome measures. In addition there were three important sources of dilution effects: firstly, only 63% of intervention group participants received some type of financial award; secondly, we found that 14% of those in the trial eligible for financial benefits did not receive their money until after the follow up assessments had been carried out; and thirdly, many had received their benefits for only a short period, reducing the possibility of detecting any measurable effects at the time of follow-up. All of these factors provide some explanation for the lack of a measurable effect between intervention and control group and between those who did and did not receive additional financial resources.
The number of participants in the qualitative study who received additional financial resources as a result of this intervention was small (n = 14). We would argue that the fieldwork, analysis and interpretation [ 46 ] were sufficiently transparent to warrant the degree of methodological rigour advocated by Barbour [ 7 , 17 ] and that the findings were therefore an accurate reflection of what was being studied. However, there still remained the possibility that a reason for the discrepant findings was due to differences between the qualitative sub-sample and the parent sample, which led us to step three.
(iii) Exploring dataset comparability
We compared the qualitative and quantitative samples on a number of social and economic factors (Table 2 ). In comparison to the parent sample, the qualitative sub-sample was slightly older, had fewer men, a higher proportion with long-term limiting illness, but fewer current smokers. However, there was nothing to indicate that such small differences would account for the discrepancies. There were negligible differences in SF-36 (Physical and Mental) and HAD (Anxiety and Depression) scores between the groups at baseline, which led us to discount the possibility that those in the quantitative sub sample were markedly different to the quantitative sample on these outcome measures.
(iv) Collection of additional data and making further comparisons
The divergent findings led us to seek further funding to undertake collection of additional quantitative and qualitative data at 24 months. The quantitative and qualitative follow-up data verified the initial findings of each study. [ 45 ] We also collected a limited amount of qualitative data on the perceived impact of resources, from all participants who had received additional resources. These data are presented in figure 1 which shows the uses of additional resources at 24 month follow-up for 35 participants (N = 35, 21 previously in quantitative study only, 14 in both). This dataset demonstrates that similar issues emerged for both qualitative and quantitative datasets: transport, savings and 'peace of mind' emerged as key issues, but the data also showed that the additional money was used on a wide range of items. This follow-up confirmed the initial findings of each study and further, indicated that the perceived impact of the additional resources was the same for a larger sample than the original qualitative sub-sample, further confirming our view that the positive findings extended beyond the fourteen participants in the qualitative sub-sample, to all those receiving additional resources.
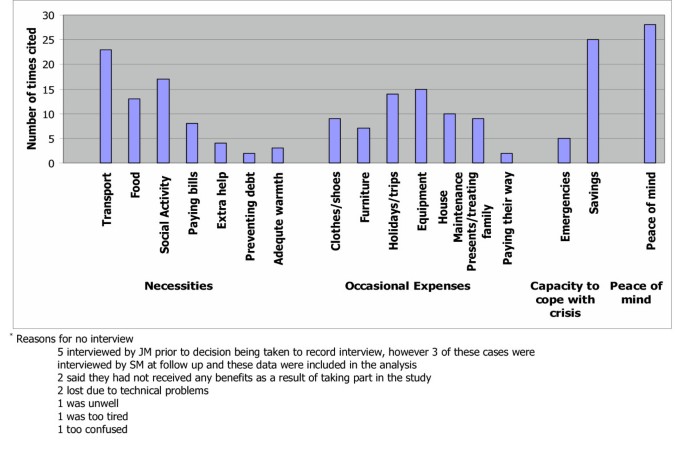
Use of additional resources at 2 year follow up (N = 35)*.
(v) Exploring whether the intervention under study worked as expected
The qualitative study revealed that many participants had received welfare benefits via other services prior to this study, revealing the lack of a 'clean slate' with regard to the receipt of benefits, which we had not anticipated. We investigated this further in the quantitative dataset and found that 75 people (59.5%) had received benefits prior to the study; if the first benefit was on health grounds, a later one may have been because their health had deteriorated further.
(vi) Exploring whether the outcomes of the quantitative and qualitative components match
'Probing certain issues in greater depth' as advocated by Bryman (p134) [ 1 ] focussed our attention on the outcome measures used in the quantitative part of the study and revealed several challenges. Firstly, the qualitative study revealed a number of dimensions not measured by the quantitative study, such as, 'maintaining independence' which included affording paid help, increasing and improving access to facilities and managing better within the home. Secondly, some of the measures used with the intention of capturing dimensions of mental health did not adequately encapsulate participants' accounts of feeling 'less stressed' and 'less depressed' by financial worries. Probing both datasets also revealed congruence along the dimension of physical health. No differences were found on the SF36 physical scale and participants themselves did not expect an improvement in physical health (for reasons of age and chronic health problems). The real issue would appear to be measuring ways in which older people are better able to cope with existing health problems and maintain their independence and quality of life, despite these conditions.
Qualitative study results also led us to look more carefully at the quantitative measures we used. Some of the standardised measures were not wholly applicable to a population of older people. Mallinson [ 47 ] also found this with the SF36 when she demonstrated some of its limitations with this age group, as well as how easy it is to, 'fall into the trap of using questionnaires like a form of laboratory equipment and forget that ... they are open to interpretation'. The data presented here demonstrate the difficulties of trying to capture complex phenomena quantitatively. However, they also demonstrate the usefulness of having alternative data forms on which to draw whether complementary (where they differ but together generate insights) or contradictory (where the findings conflict). [ 30 ] In this study, the complementary and contradictory findings of the two datasets proved useful in making recommendations for the design of a definitive study.
Many researchers understand the importance, indeed the necessity, of combining methods to investigate complex health and social issues. Although quantitative research remains the dominant paradigm in health services research, qualitative research has greater prominence than before and is no longer, as Barbour [ 42 ] points out regarded as the 'poor relation to quantitative research that it has been in the past' (p1019). Brannen [ 48 ] argues that, despite epistemological differences there are 'more overlaps than differences'. Despite this, there is continued debate about the authority of each individual mode of research which is not surprising since these different styles, 'take institutional forms, in relation to cultures of and markets for knowledge' (p168). [ 49 ] Devers [ 50 ] points out that the dominance of positivism, especially within the RCT method, has had an overriding influence on the criteria used to assess research which has had the inevitable result of viewing qualitative studies unfavourably. We advocate treating qualitative and quantitative datasets as complementary rather than in competition for identifying the true version of events. This, we argue, leads to a position which exploits the strengths of each method and at the same time counters the limitations of each. The process of interpreting the meaning of these divergent findings has led us to conclude that much can be learned from scientific realism [ 51 ]which has 'sought to position itself as a model of scientific explanation which avoids the traditional epistemological poles of positivism and relativism' (p64). This stance enables investigators to take account of the complexity inherent in social interventions and reinforces, at a theoretical level, the problems of attempting to measure the impact of a social intervention via experimental means. However, the current focus on evidence based health care [ 52 ] now includes public health [ 53 , 54 ] and there is increased attention paid to the results of trials of public health interventions, attempting as they do, to capture complex social phenomena using standardised measurement tools. We would argue that at the very least, the inclusion of both qualitative and quantitative elements in such studies, is essential and ultimately more cost-effective, increasing the likelihood of arriving at a more thoroughly researched and better understood set of results.
The findings of this study demonstrate how the use of mixed methods can lead to different and sometimes conflicting accounts. This, we argue, is largely due to the outcome measures in the RCT not matching the outcomes emerging from the qualitative arm of the study. Instead of making assumptions about the correct version, we have reported the results of both datasets together rather than separately, and advocate six steps to interrogate each dataset more fully. The methodological strategy advocated by this approach involves contemporaneous qualitative and quantitative data collection, analysis and reciprocal interrogation to inform interpretation in trials of complex interventions. This approach also indicates the need for a realistic appraisal of quantitative tools. More widespread use of mixed methods in trials of complex interventions is likely to enhance the overall quality of the evidence base.
Brannen J: Mixing Methods: qualitative and quantitative research. 1992, Aldershot, Ashgate
Google Scholar
Tashakkori A, Teddlie C: Handbook of Mixed Methods in Social and Behavioural Research. 2003, London, Sage
Morgan DL: Triangulation and it's discontents: Developing pragmatism as an alternative justification for combining qualitative and quantitative methods. Cambridge, 11-12 July.. 2005.
Pill R, Stott NCH: Concepts of illness causation and responsibility: some preliminary data from a sample of working class mothers. Social Science and Medicine. 1982, 16: 43-52. 10.1016/0277-9536(82)90422-1.
Article CAS PubMed Google Scholar
Scambler G, Hopkins A: Generating a model of epileptic stigma: the role of qualitative analysis. Social Science and Medicine. 1990, 30: 1187-1194. 10.1016/0277-9536(90)90258-T.
Townsend A, Hunt K, Wyke S: Managing multiple morbidity in mid-life: a qualitative study of attitudes to drug use. BMJ. 2003, 327: 837-841. 10.1136/bmj.327.7419.837.
Article PubMed PubMed Central Google Scholar
Barbour RS: Checklists for improving rigour in qualitative research: the case of the tail wagging the dog?. British Medical Journal. 2001, 322: 1115-1117.
Article CAS PubMed PubMed Central Google Scholar
Pope C, Mays N: Qualitative Research in Health Care. 2000, London, BMJ Books
Bryman A: Quantity and Quality in Social Research. 1995, London, Routledge
Ashton J: Healthy cities. 1991, Milton Keynes, Open University Press
Baum F: Researching Public Health: Behind the Qualitative-Quantitative Methodological Debate. Social Science and Medicine. 1995, 40: 459-468. 10.1016/0277-9536(94)E0103-Y.
Donovan J, Mills N, Smith M, Brindle L, Jacoby A, Peters T, Frankel S, Neal D, Hamdy F: Improving design and conduct of randomised trials by embedding them in qualitative research: (ProtecT) study. British Medical Journal. 2002, 325: 766-769.
Cox K: Assessing the quality of life of patients in phase I and II anti-cancer drug trials: interviews versus questionnaires. Social Science and Medicine. 2003, 56: 921-934. 10.1016/S0277-9536(02)00100-4.
Article PubMed Google Scholar
Lewin S: Mixing methods in complex health service randomised controlled trials: research 'best practice'. Mixed Methods in Health Services Research Conference 23rd November 2004, Sheffield University.
Cresswell JW: Mixed methods research and applications in intervention studies.: ; Cambridge, July 11-12. 2005.
Cresswell JW: Research Design. Qualitative, Quantitative and Mixed Methods Approaches. 2003, London, Sage
Barbour RS: The case for combining qualitative and quanitative approaches in health services research. Journal of Health Services Research and Policy. 1999, 4: 39-43.
Gabriel Z, Bowling A: Quality of life from the perspectives of older people. Ageing & Society. 2004, 24: 675-691. 10.1017/S0144686X03001582.
Article Google Scholar
Koops L, Lindley RL: Thrombolysis for acute ischaemic stroke: consumer involvement in design of new randomised controlled trial. BMJ. 2002, 325: 415-418. 10.1136/bmj.325.7361.415.
O'Cathain A, Nicholl J, Murphy E: Making the most of mixed methods. Mixed Methods in Health Services Research Conference 23rd November 2004, Sheffield University.
Denzin NK: The Research Act. 1978, New York, McGraw-Hill Book Company
Roberts H, Curtis K, Liabo K, Rowland D, DiGuiseppi C, Roberts I: Putting public health evidence into practice: increasing the prevalance of working smoke alarms in disadvantaged inner city housing. Journal of Epidemiology & Community Health. 2004, 58: 280-285. 10.1136/jech.2003.007948.
Article CAS Google Scholar
Rowland D, DiGuiseppi C, Roberts I, Curtis K, Roberts H, Ginnelly L, Sculpher M, Wade A: Prevalence of working smoke alarms in local authority inner city housing: randomised controlled trial. British Medical Journal. 2002, 325: 998-1001.
Vincent J: Old Age. 2003, London, Routledge
Chapter Google Scholar
Department for Work and Pensions: Households below average income statistics 2000/01. 2002, London, Department for Work and Pensions
National Audit Office: Tackling Pensioner Pverty: Encouraging take-up of entitlements. 2002, London, National Audit Office
Paris JAG, Player D: Citizens advice in general practice. British Medical Journal. 1993, 306: 1518-1520.
Abbott S: Prescribing welfare benefits advice in primary care: is it a health intervention, and if so, what sort?. Journal of Public Health Medicine. 2002, 24: 307-312. 10.1093/pubmed/24.4.307.
Harding R, Sherr L, Sherr A, Moorhead R, Singh S: Welfare rights advice in primary care: prevalence, processes and specialist provision. Family Practice. 2003, 20: 48-53. 10.1093/fampra/20.1.48.
Morgan DL: Practical strategies for combining qualitative and quantitative methods: applications to health research. Qualitative Health Research. 1998, 8: 362-376.
Moffatt S, White M, Stacy R, Downey D, Hudson E: The impact of welfare advice in primary care: a qualitative study. Critical Public Health. 2004, 14: 295-309. 10.1080/09581590400007959.
Thomson H, Hoskins R, Petticrew M, Ogilvie D, Craig N, Quinn T, Lindsey G: Evaluating the health effects of social interventions. British Medical Journal. 2004, 328: 282-285.
Department for the Environment TR: Measuring Multiple Deprivation at the Small Area Level: The Indices of Deprivation. 2000, London, Department for the Envrionment, Trransport and the Regions
Ware JE, Sherbourne CD: The MOS 36 item short form health survey (SF-36). Conceptual framework and item selection. Medical Care. 1992, 30: 473-481.
Snaith RP, Zigmond AS: The hospital anxiety and depression scale. Acta Psychiatrica Scandinivica. 1983, 67: 361-370.
Sarason I, Carroll C, Maton K: Assessing social support: the social support questionnaire. Journal of Personality and Social Psychology. 1983, 44: 127-139. 10.1037//0022-3514.44.1.127.
Ward R: The impact of subjective age and stigma on older persons. Journal of Gerontology. 1977, 32: 227-232.
Ford G, Ecob R, Hunt K, Macintyre S, West P: Patterns of class inequality in health through the lifespan: class gradients at 15, 35 and 55 years in the west of Scotland. Social Science and Medicine. 1994, 39: 1037-1050. 10.1016/0277-9536(94)90375-1.
SPSS.: v. 11.0 for Windows [program]. 2003, Chicago, Illinois.
STATA.: Statistical Software [program]. 8.0 version. 2003, Texas, College Station
Ritchie J, Lewis J: Qualitative Research Practice. A Guide for Social Scientists. 2003, London, Sage
Barbour RS: The Newfound Credibility of Qualitative Research? Tales of Technical Essentialism and Co-Option. Qualitative Health Research. 2003, 13: 1019-1027. 10.1177/1049732303253331.
Silverman D: Doing qualitative research. 2000, London, Sage
Clayman SE, Maynard DW: Ethnomethodology and conversation analysis. Situated Order: Studies in the Social Organisation of Talk and Embodied Activities. Edited by: Have PT and Psathas G. 1994, Washington, D.C., University Press of America
White M, Moffatt S, Mackintosh J, Howel D, Sandell A, Chadwick T, Deverill M: Randomised controlled trial to evaluate the health effects of welfare rights advice in primary health care: a pilot study. Report to the Department of Health, Policy Research Programme. 2005, Newcastle upon Tyne, University of Newcastle upon Tyne
Moffatt S: "All the difference in the world". A qualitative study of the perceived impact of a welfare rights service provided in primary care. 2004, , University College London
Mallinson S: Listening to reposndents: a qualitative assessment of the Short-Form 36 Health Status Questionnaire. Social Science and Medicine. 2002, 54: 11-21. 10.1016/S0277-9536(01)00003-X.
Brannen J: Mixing Methods: The Entry of Qualitative and Quantitative Approaches into the Research Process. International Journal of Social Research Methodology. 2005, 8: 173-184. 10.1080/13645570500154642.
Green A, Preston J: Editorial: Speaking in Tongues- Diversity in Mixed Methods Research. International Journal of Social Research Methodology. 2005, 8: 167-171. 10.1080/13645570500154626.
Devers KJ: How will we know "good" qualitative research when we see it? Beginning the dialogue in Health Services Research. Health Services Research. 1999, 34: 1153-1188.
CAS PubMed PubMed Central Google Scholar
Pawson R, Tilley N: Realistic Evaluation. 2004, London, Sage
Miles A, Grey JE, Polychronis A, Price N, Melchiorri C: Current thinking in the evidence-based health care debate. Journal of Evaluation in Clinical Practice. 2003, 9: 95-109. 10.1046/j.1365-2753.2003.00438.x.
Pencheon D, Guest C, Melzer D, Gray JAM: Oxford Handbook of Public Health Practice. 2001, Oxford, Oxford University Press
Wanless D: Securing Good Health for the Whole Population. 2004, London, HMSO
Pre-publication history
The pre-publication history for this paper can be accessed here: http://www.biomedcentral.com/1472-6963/6/28/prepub
Download references
Acknowledgements
We wish to thank: Rosemary Bell, Jenny Dover and Nick Whitton from Newcastle upon Tyne City Council Welfare Rights Service; all the participants and general practice staff who took part; and for their extremely helpful comments on earlier drafts of this paper, Adam Sandell, Graham Scambler, Rachel Baker, Carl May and John Bond. We are grateful to referees Alicia O'Cathain and Sally Wyke for their insightful comments. The views expressed in this paper are those of the authors and not necessarily those of the Department of Health.
Author information
Authors and affiliations.
Public Health Research Group, School of Population & Health Sciences, Faculty of Medical Sciences, William Leech Building, Framlington Place, Newcastle upon Tyne, NE2 4HH, UK
Suzanne Moffatt, Martin White, Joan Mackintosh & Denise Howel
You can also search for this author in PubMed Google Scholar
Corresponding author
Correspondence to Suzanne Moffatt .
Additional information
Competing interests.
The author(s) declare that they have no competing interests.
Authors' contributions
SM and MW had the original idea for the study, and with the help of DH, Adam Sandell and Nick Whitton developed the proposal and gained funding. JM collected the data for the quantitative study, SM designed and collected data for the qualitative study. JM, DH and MW analysed the quantitative data, SM analysed the qualitative data. All authors contributed to interpretation of both datasets. SM wrote the first draft of the paper, JM, MW and DH commented on subsequent drafts. All authors have read and approved the final manuscript.
Authors’ original submitted files for images
Below are the links to the authors’ original submitted files for images.
Authors’ original file for figure 1
Rights and permissions.
Open Access This article is published under license to BioMed Central Ltd. This is an Open Access article is distributed under the terms of the Creative Commons Attribution License ( https://creativecommons.org/licenses/by/2.0 ), which permits unrestricted use, distribution, and reproduction in any medium, provided the original work is properly cited.
Reprints and permissions
About this article
Cite this article.
Moffatt, S., White, M., Mackintosh, J. et al. Using quantitative and qualitative data in health services research – what happens when mixed method findings conflict? [ISRCTN61522618]. BMC Health Serv Res 6 , 28 (2006). https://doi.org/10.1186/1472-6963-6-28
Download citation
Received : 29 September 2005
Accepted : 08 March 2006
Published : 01 December 2006
DOI : https://doi.org/10.1186/1472-6963-6-28
Share this article
Anyone you share the following link with will be able to read this content:
Sorry, a shareable link is not currently available for this article.
Provided by the Springer Nature SharedIt content-sharing initiative
- Mixed Method
- Welfare Benefit
- Mixed Method Research
- Divergent Finding
- Financial Vulnerability
BMC Health Services Research
ISSN: 1472-6963
- General enquiries: [email protected]

Advertisement
Qualitative vs. Quantitative: Key Differences in Research Types
- Share Content on Facebook
- Share Content on LinkedIn
- Share Content on Flipboard
- Share Content on Reddit
- Share Content via Email
Let's say you want to learn how a group will vote in an election. You face a classic decision of gathering qualitative vs. quantitative data.
With one method, you can ask voters open-ended questions that encourage them to share how they feel, what issues matter to them and the reasons they will vote in a specific way. With the other, you can ask closed-ended questions, giving respondents a list of options. You will then turn that information into statistics.
Neither method is more right than the other, but they serve different purposes. Learn more about the key differences between qualitative and quantitative research and how you can use them.
What Is Qualitative Research?
What is quantitative research, qualitative vs. quantitative research: 3 key differences, benefits of combining qualitative and quantitative research.
Qualitative research aims to explore and understand the depth, context and nuances of human experiences, behaviors and phenomena. This methodological approach emphasizes gathering rich, nonnumerical information through methods such as interviews, focus groups , observations and content analysis.
In qualitative research, the emphasis is on uncovering patterns and meanings within a specific social or cultural context. Researchers delve into the subjective aspects of human behavior , opinions and emotions.
This approach is particularly valuable for exploring complex and multifaceted issues, providing a deeper understanding of the intricacies involved.
Common qualitative research methods include open-ended interviews, where participants can express their thoughts freely, and thematic analysis, which involves identifying recurring themes in the data.
Examples of How to Use Qualitative Research
The flexibility of qualitative research allows researchers to adapt their methods based on emerging insights, fostering a more organic and holistic exploration of the research topic. This is a widely used method in social sciences, psychology and market research.
Here are just a few ways you can use qualitative research.
- To understand the people who make up a community : If you want to learn more about a community, you can talk to them or observe them to learn more about their customs, norms and values.
- To examine people's experiences within the healthcare system : While you can certainly look at statistics to gauge if someone feels positively or negatively about their healthcare experiences, you may not gain a deep understanding of why they feel that way. For example, if a nurse went above and beyond for a patient, they might say they are content with the care they received. But if medical professional after medical professional dismissed a person over several years, they will have more negative comments.
- To explore the effectiveness of your marketing campaign : Marketing is a field that typically collects statistical data, but it can also benefit from qualitative research. For example, if you have a successful campaign, you can interview people to learn what resonated with them and why. If you learn they liked the humor because it shows you don't take yourself too seriously, you can try to replicate that feeling in future campaigns.
Types of Qualitative Data Collection
Qualitative data captures the qualities, characteristics or attributes of a subject. It can take various forms, including:
- Audio data : Recordings of interviews, discussions or any other auditory information. This can be useful when dealing with events from the past. Setting up a recording device also allows a researcher to stay in the moment without having to jot down notes.
- Observational data : With this type of qualitative data analysis, you can record behavior, events or interactions.
- Textual data : Use verbal or written information gathered through interviews, open-ended surveys or focus groups to learn more about a topic.
- Visual data : You can learn new information through images, photographs, videos or other visual materials.
Quantitative research is a systematic empirical investigation that involves the collection and analysis of numerical data. This approach seeks to understand, explain or predict phenomena by gathering quantifiable information and applying statistical methods for analysis.
Unlike qualitative research, which focuses on nonnumerical, descriptive data, quantitative research data involves measurements, counts and statistical techniques to draw objective conclusions.
Examples of How to Use Quantitative Research
Quantitative research focuses on statistical analysis. Here are a few ways you can employ quantitative research methods.
- Studying the employment rates of a city : Through this research you can gauge whether any patterns exist over a given time period.
- Seeing how air pollution has affected a neighborhood : If the creation of a highway led to more air pollution in a neighborhood, you can collect data to learn about the health impacts on the area's residents. For example, you can see what percentage of people developed respiratory issues after moving to the neighborhood.
Types of Quantitative Data
Quantitative data refers to numerical information you can measure and count. Here are a few statistics you can use.
- Heights, yards, volume and more : You can use different measurements to gain insight on different types of research, such as learning the average distance workers are willing to travel for work or figuring out the average height of a ballerina.
- Temperature : Measure in either degrees Celsius or Fahrenheit. Or, if you're looking for the coldest place in the universe , you may measure in Kelvins.
- Sales figures : With this information, you can look at a store's performance over time, compare one company to another or learn what the average amount of sales is in a specific industry.
Quantitative and qualitative research methods are both valid and useful ways to collect data. Here are a few ways that they differ.
- Data collection method : Quantitative research uses standardized instruments, such as surveys, experiments or structured observations, to gather numerical data. Qualitative research uses open-ended methods like interviews, focus groups or content analysis.
- Nature of data : Quantitative research involves numerical data that you can measure and analyze statistically, whereas qualitative research involves exploring the depth and richness of experiences through nonnumerical, descriptive data.
- Sampling : Quantitative research involves larger sample sizes to ensure statistical validity and generalizability of findings to a population. With qualitative research, it's better to work with a smaller sample size to gain in-depth insights into specific contexts or experiences.
You can simultaneously study qualitative and quantitative data. This method , known as mixed methods research, offers several benefits, including:
- A comprehensive understanding : Integration of qualitative and quantitative data provides a more comprehensive understanding of the research problem. Qualitative data helps explain the context and nuances, while quantitative data offers statistical generalizability.
- Contextualization : Qualitative data helps contextualize quantitative findings by providing explanations into the why and how behind statistical patterns. This deeper understanding contributes to more informed interpretations of quantitative results.
- Triangulation : Triangulation involves using multiple methods to validate or corroborate findings. Combining qualitative and quantitative data allows researchers to cross-verify results, enhancing the overall validity and reliability of the study.
This article was created in conjunction with AI technology, then fact-checked and edited by a HowStuffWorks editor.
Please copy/paste the following text to properly cite this HowStuffWorks.com article:
An Assessment on the Level of Effectiveness of Using Office Technology as to the Health Care Services in the Barangay Novaliches Proper District V, Quezon City
Vol.4, no.1.
- Rechel Lorete Bestlink College of the Philippines
- John Mark Baldoza Bestlink College of the Philippines
- Clara Jane Javier Bestlink College of the Philippines
- Ma Bienne Ramiro Bestlink College of the Philippines
This quantitative research study was conducted to demonstrate the Assessment of the Level of Effectiveness of using Office Technology in the barangay healthcare staff, medical officer, and medical encoder as to the healthcare services in the barangay Novaliches Proper District V, Quezon City. The researchers aimed to provide knowledge to the readers about the information and important ideas about the effectiveness of healthcare employees when it comes to using office technology.
The first question aimed to answer how well a male and female group of respondents assess the level of effectiveness of using Office Technology for Health Care Services in Barangay Novaliches Proper. Based on the data gathered, the majority answered in the indicator of records management, which is that employees can create backups of files and important documents.
For the second question, based on the data gathered, the highest weighted mean is in the indicator of Emergency response. It implies that by using office technology, some healthcare employees can improve their efficiency in response to the emergency of their constituents.
How to Cite
- Endnote/Zotero/Mendeley (RIS)
Similar Articles
- Gerson M. Seda, Kim Mark A. Rebutaso, Carl Francis SD. Furigay, Jhon Paul V. Domingo, The Effects of Social Media in the Study Habit of 4th Year Criminology Students of Bestlink College, Bulacan Campus , Ascendens Asia Singapore – Bestlink College of the Philippines Journal of Multidisciplinary Research: Vol. 3 No. 1C (2022): AASg-BCP-JMRA_Vol3_No1C_June2022
- John Acobera, Kenneth T. Dionisio, Arvie T. Galit, Lloyd S. Malaque, Alfred Olmar, Denise Anne G. Osorio, Mscrim (Op), Development of Physical Agility Test in Criminology Department at Bestlink College of the Philippines , Ascendens Asia Singapore – Bestlink College of the Philippines Journal of Multidisciplinary Research: Vol. 1 No. 1 (2019): Ascendens Asia Singapore – Bestlink College of the Philippines Journal of Multidisciplinary Research Abstracts, Vol.1, No.1, March 2019
- Christian James Sicat, Erole Elma, Michael Tejero, Jessa Mae Jacobe, The Effectiveness of Instilling Students’ Discipline for Criminology Students: An Assessment , Ascendens Asia Singapore – Bestlink College of the Philippines Journal of Multidisciplinary Research: Vol. 4 No. 1 (2023): AASg-BCP-JMRA_Vol4_No1_August2023
- Delycelle Cae Cano, Carol Fenollar, Paula Mamaril, Shainna Milano, Rhose Anne Tugano, Riando D. Mosqueda, Ph.D. CRIM, The Causes and Effects of Abused Use of Alcoholic Beverages Among Criminology Students: Towards A Guide , Ascendens Asia Singapore – Bestlink College of the Philippines Journal of Multidisciplinary Research: Vol. 1 No. 1 (2019): Ascendens Asia Singapore – Bestlink College of the Philippines Journal of Multidisciplinary Research Abstracts, Vol.1, No.1, March 2019
- Mikko Z. Andres, Rocky Victor M. Arguelles, Jan Bryan R. Barrios, John Patrick J. Boral, Jhon Mark E. Poblete, Impact of Pandemic Towards on On-The-Job Training of Criminology Students of Bestlink College of the Philippines Bulacan Campus , Ascendens Asia Singapore – Bestlink College of the Philippines Journal of Multidisciplinary Research: Vol. 3 No. 1C (2022): AASg-BCP-JMRA_Vol3_No1C_June2022
- Mikaella Origenes, Lovely Lanario, Jinkie Pegarido, Heizel Santos, Influence of Motivation Among First-Year Students of Bestlink College of the Philippines Taking BS Criminology , Ascendens Asia Singapore – Bestlink College of the Philippines Journal of Multidisciplinary Research: Vol. 4 No. 1 (2023): AASg-BCP-JMRA_Vol4_No1_August2023
- Greolo Arnaldo, Jimboy Geslani, Gabriel Arcega, Leilanie Cusack, Leilanie Cusack, Thesis the Impact of Media’s Publication of Suspects’ Identity on Privacy, Security, and Threat Perception: An Assestment , Ascendens Asia Singapore – Bestlink College of the Philippines Journal of Multidisciplinary Research: Vol. 4 No. 1 (2023): AASg-BCP-JMRA_Vol4_No1_August2023
- Clarisse B. May-as, Christine C. Arnesto, Chrissa T. Fevidal, Ma, Elizabeth P. Lagto, Ma.Elena O. Mendoza, The Efficacy of Different Learning Style in Online Class of 4th Year Criminology Students at Bestlink College of the Philippines - Bulacan Campus , Ascendens Asia Singapore – Bestlink College of the Philippines Journal of Multidisciplinary Research: Vol. 3 No. 1C (2022): AASg-BCP-JMRA_Vol3_No1C_June2022
- Christian R. Aguirre, Richard Andrade, Niño Arvie N. Galsim, Mark L. Antoque, The Effects of COVID-19 Pandemic on the Academic Performance of 4th Year Criminology Students of Bestlink College of the Philippines (Bulacan Campus) , Ascendens Asia Singapore – Bestlink College of the Philippines Journal of Multidisciplinary Research: Vol. 3 No. 1A (2022): AASg-BCP-JMRA_Vol3_No1A_June2022
- Avegail Galanto, Christy Colas, Gloribelle Millevo, Honey Glazelle Sobrenilla, Perspective of Criminology Students in Anti-illegal Drug Operations Conducted by Philippine National Police Towards Awareness Campaign Plan , Ascendens Asia Singapore – Bestlink College of the Philippines Journal of Multidisciplinary Research: Vol. 4 No. 1 (2023): AASg-BCP-JMRA_Vol4_No1_August2023
1 2 3 4 5 6 7 8 9 10 > >>
You may also start an advanced similarity search for this article.
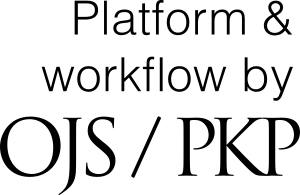
Numbers, Facts and Trends Shaping Your World
Read our research on:
Full Topic List
Regions & Countries
Publications
- Our Methods
- Short Reads
- Tools & Resources
Read Our Research On:
Internet & Technology
6 facts about americans and tiktok.
62% of U.S. adults under 30 say they use TikTok, compared with 39% of those ages 30 to 49, 24% of those 50 to 64, and 10% of those 65 and older.
Many Americans think generative AI programs should credit the sources they rely on
Americans’ use of chatgpt is ticking up, but few trust its election information, whatsapp and facebook dominate the social media landscape in middle-income nations, sign up for our internet, science, and tech newsletter.
New findings, delivered monthly
Electric Vehicle Charging Infrastructure in the U.S.
64% of Americans live within 2 miles of a public electric vehicle charging station, and those who live closest to chargers view EVs more positively.
When Online Content Disappears
A quarter of all webpages that existed at one point between 2013 and 2023 are no longer accessible.
A quarter of U.S. teachers say AI tools do more harm than good in K-12 education
High school teachers are more likely than elementary and middle school teachers to hold negative views about AI tools in education.
Teens and Video Games Today
85% of U.S. teens say they play video games. They see both positive and negative sides, from making friends to harassment and sleep loss.
Americans’ Views of Technology Companies
Most Americans are wary of social media’s role in politics and its overall impact on the country, and these concerns are ticking up among Democrats. Still, Republicans stand out on several measures, with a majority believing major technology companies are biased toward liberals.
22% of Americans say they interact with artificial intelligence almost constantly or several times a day. 27% say they do this about once a day or several times a week.
About one-in-five U.S. adults have used ChatGPT to learn something new (17%) or for entertainment (17%).
Across eight countries surveyed in Latin America, Africa and South Asia, a median of 73% of adults say they use WhatsApp and 62% say they use Facebook.
5 facts about Americans and sports
About half of Americans (48%) say they took part in organized, competitive sports in high school or college.
REFINE YOUR SELECTION
Research teams, signature reports.
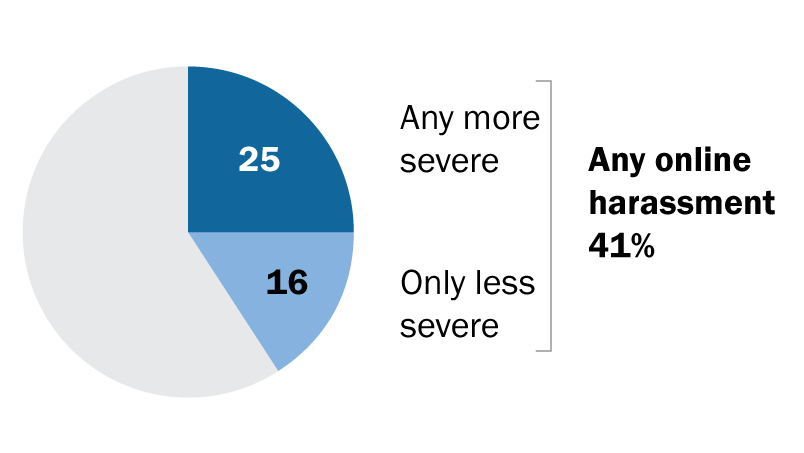
The State of Online Harassment
Roughly four-in-ten Americans have experienced online harassment, with half of this group citing politics as the reason they think they were targeted. Growing shares face more severe online abuse such as sexual harassment or stalking
Parenting Children in the Age of Screens
Two-thirds of parents in the U.S. say parenting is harder today than it was 20 years ago, with many citing technologies – like social media or smartphones – as a reason.
Dating and Relationships in the Digital Age
From distractions to jealousy, how Americans navigate cellphones and social media in their romantic relationships.
Americans and Privacy: Concerned, Confused and Feeling Lack of Control Over Their Personal Information
Majorities of U.S. adults believe their personal data is less secure now, that data collection poses more risks than benefits, and that it is not possible to go through daily life without being tracked.
Americans and ‘Cancel Culture’: Where Some See Calls for Accountability, Others See Censorship, Punishment
Social media fact sheet, digital knowledge quiz, video: how do americans define online harassment.
1615 L St. NW, Suite 800 Washington, DC 20036 USA (+1) 202-419-4300 | Main (+1) 202-857-8562 | Fax (+1) 202-419-4372 | Media Inquiries
Research Topics
- Email Newsletters
ABOUT PEW RESEARCH CENTER Pew Research Center is a nonpartisan fact tank that informs the public about the issues, attitudes and trends shaping the world. It conducts public opinion polling, demographic research, media content analysis and other empirical social science research. Pew Research Center does not take policy positions. It is a subsidiary of The Pew Charitable Trusts .
© 2024 Pew Research Center
17 Social Media Metrics You Need to Track in 2024 [BENCHMARKS]
Pin down the social media metrics that really matter and learn how to track them to build a winning social media presence.
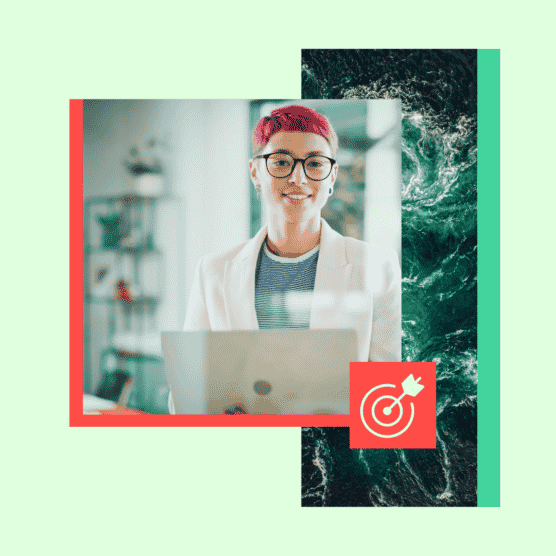
Table of Contents
Social media metrics allow you to track every little detail of your social media performance. This is great for honing your strategy, but it can also lead to information overload.
Here, we’ve selected the top 17 metrics you need to track to really understand your social success and understand where you can improve. Where available, we’ve included benchmarks that will help you set realistic performance goals.
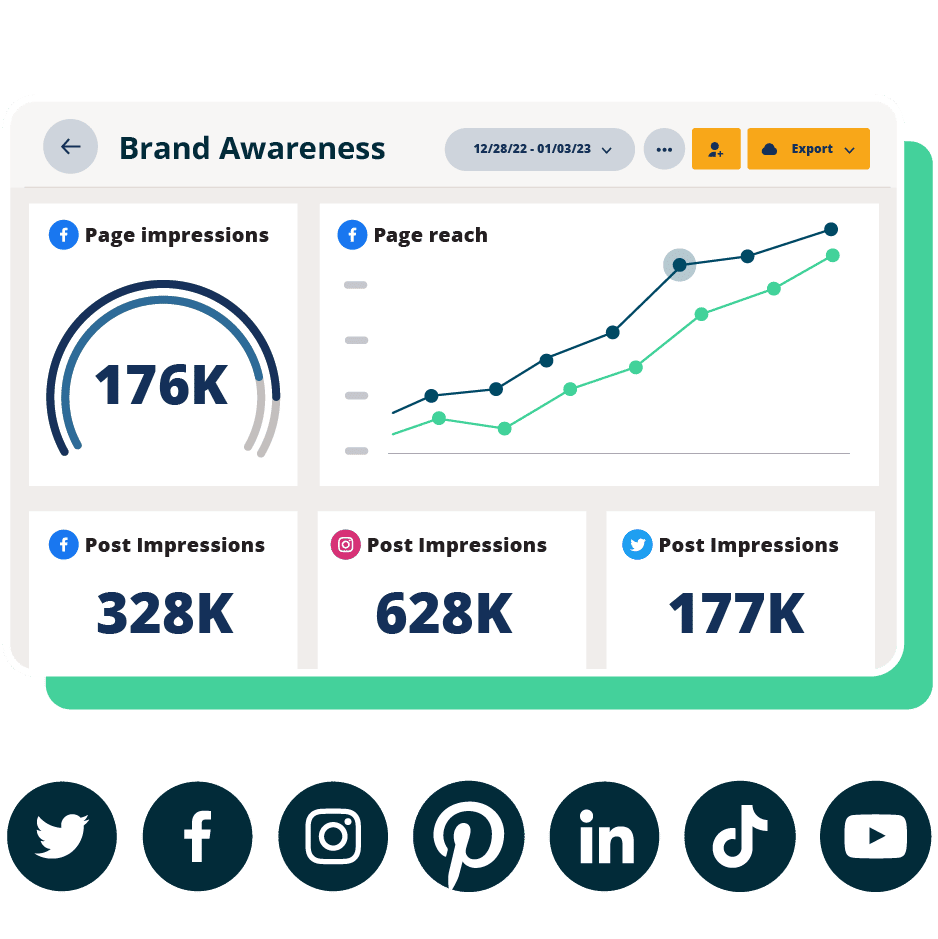
Beautiful reports. Clear data. Actionable insights to help you grow faster.
What are social media metrics, and why are they important?
Social media metrics are data points that measure how well your social media strategy is performing — and help you understand how you can improve. They are like scorecards for your online posts and interactions, showing how many people saw, liked, shared, or commented on your content. Social media metrics also reveal how much effort and money you’re spending, and how much you’re getting in return.
This is not about vanity (or vanity metrics). Social media strategic planning and analysis require you to track metrics to understand what’s happening with your business in the social sphere.
Without metrics, you can’t create an informed strategy. You can’t tie your social media efforts to real business goals or prove your success. And you can’t spot downward trends that might require a change in strategy.
Keep reading for a complete list of social media metrics to track in 2023.
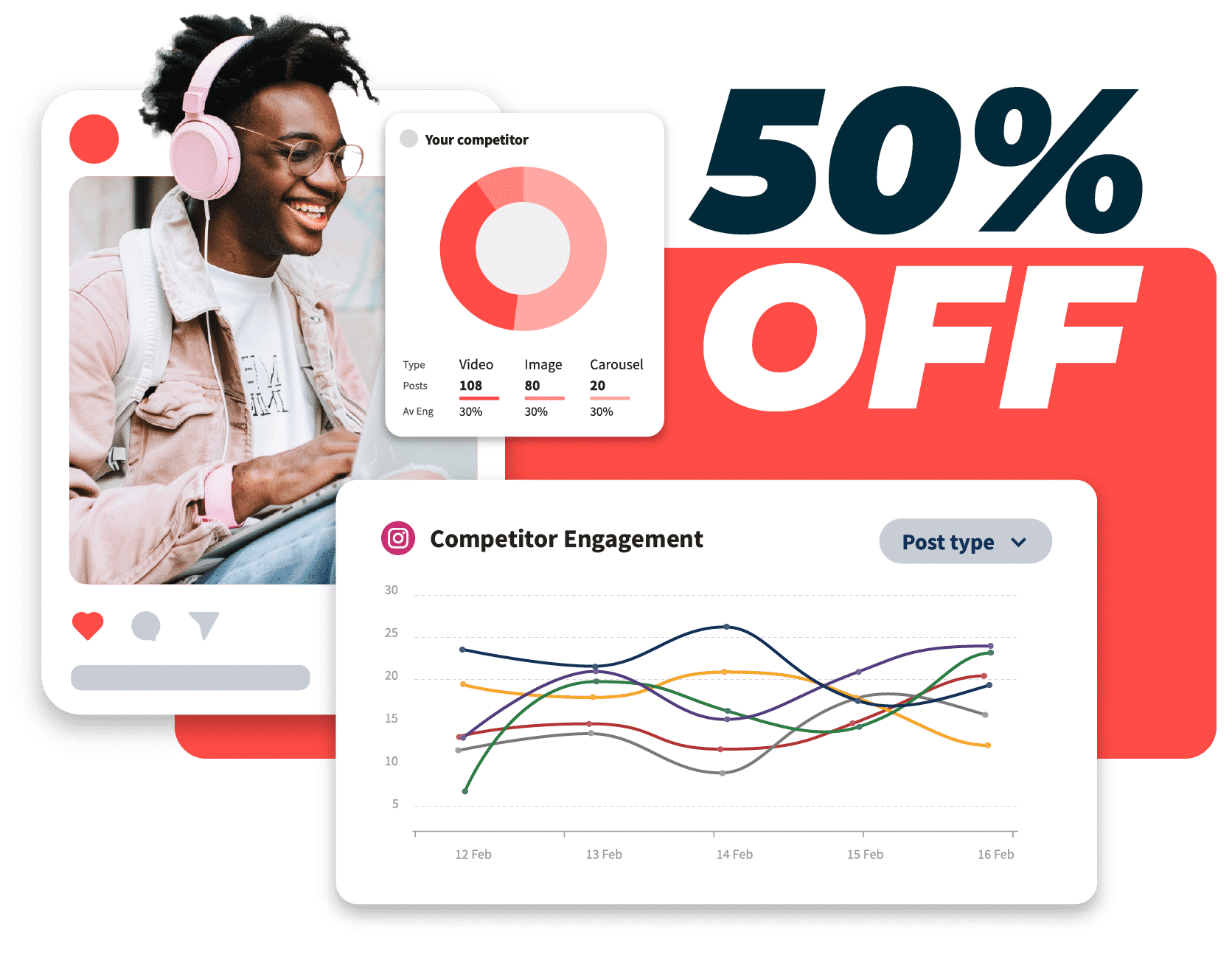
Get the all-in-one social media performance engine for HALF OFF.
(This price won’t be around for long!)
Social media engagement metrics
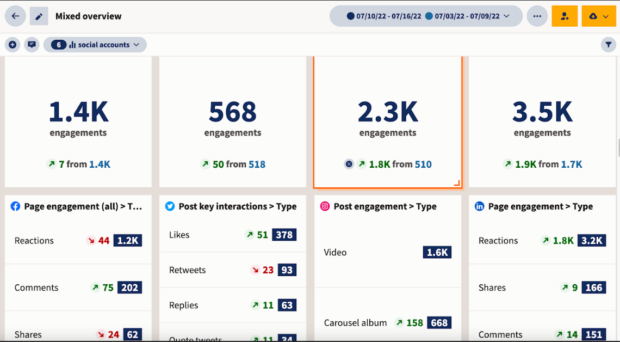
Social media engagement metrics show how often people interact with your content. These are valuable metrics to track for a couple of reasons. First, engagement shows that your audience is interested enough in the content you post to take some kind of social action.
Second, engagement sends powerful signals to the social media algorithms , which can help expand your reach.
1. Engagement rate
Engagement rate measures the number of engagements (reactions, comments and shares) your content gets as a percentage of your audience.
How you define “audience” may vary. You might want to calculate engagement relative to your number of followers. But remember that not all your followers will see each post. Plus, you might get engagement from people who don’t (yet) follow you.
So, there are multiple ways to calculate engagement. So many, in fact, that we dedicated a whole blog post to the many ways to measure engagement rate .
One of the most common ways is to add up your total likes, comments, shares, and saves, and divide the total by your number of followers. Then multiply by 100 to get a percentage.
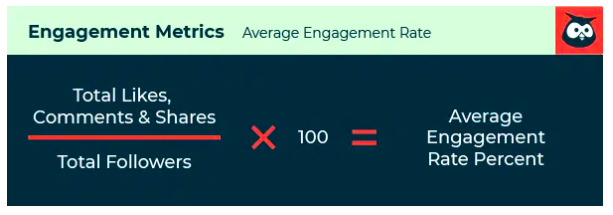
You can also use our free engagement rate calculator to measure your engagement rate by post, account, or campaign.
Note: If you’re calculating your account’s total engagement, include information about all your posts (e.g total number of posts published, total number of likes, and so on). If you’re calculating the engagement rate of a specific campaign, only include the details of the posts that were part of the campaign.
Instagram post engagement rate benchmarks:
- Education: 2.03%
- Financial services: 1.69%
- Government: 1.96%
- Healthcare/Wellness: 2.24%
- Travel/hospitality/leisure: 1.73%
2. Amplification rate
Amplification Rate is the ratio of shares per post to the number of overall followers.
Coined by Avinash Kaushik , author and digital marketing evangelist at Google, amplification is “the rate at which your followers take your content and share it through their networks.”
Basically, the higher your amplification rate, the more your followers are expanding your reach for you.
To calculate amplification rate, divide a post’s total number of shares by your total number of followers. Multiply by 100 to get your amplification rate as a percentage.
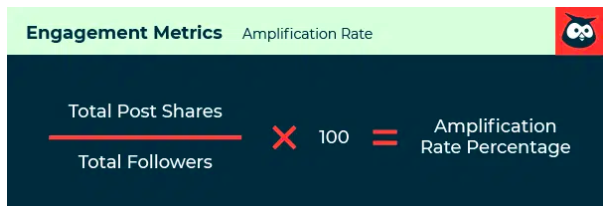
Facebook amplification rate benchmarks:
- Education: 0.05%
- Financial services: 0.06%
- Government: 0.06%
- Healthcare/Wellness: 0.08%
- Travel/hospitality/leisure: 0.03%
3. Virality rate
Virality rate is similar to amplification rate in that it measures how much your content is shared. However, virality rate calculates shares as a percentage of impressions rather than as a percentage of followers.
Remember that every time someone shares your content, it achieves a fresh set of impressions via their audience. So virality rate measures how your content is spreading exponentially.
To calculate virality rate, divide a post’s number of shares by its impressions. Multiply by 100 to get your virality rate as a percentage.
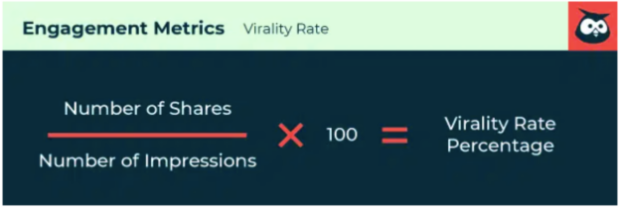
Social media awareness metrics
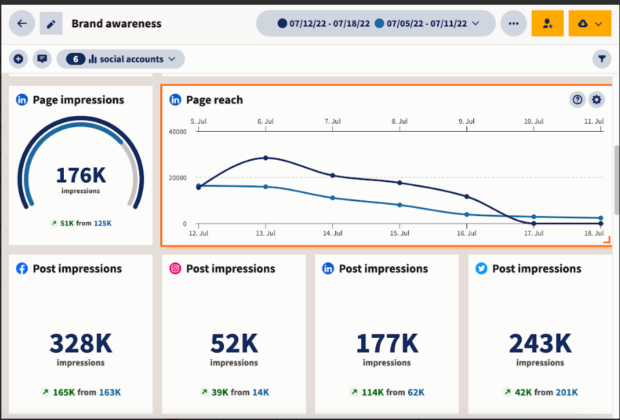
Social media brand awareness metrics show how many people see your content and how much attention your brand gets on social media .
Reach is simply the number of people who see your content. It’s a good idea to monitor your average reach, as well as the reach of each individual post, story, or video. You can also measure the reach for your page/profile overall.
A valuable subset of this metric is to look at what percentage of your reach is made up of followers vs. non-followers. If a lot of non-followers are seeing your content, that means it’s being shared or doing well in the algorithms, or both.
Facebook page reach benchmarks (30 days):
- Education: 273K
- Financial services: 164K
- Government: 497K
- Healthcare/Wellness: 170K
- Travel/hospitality/leisure: 366K
5. Impressions
Impressions indicate the number of times people saw your content. You can measure impressions by post, as well as the overall number of impressions on your social media profile.
Impressions can be higher than reach because the same person might look at your content more than once.
An especially high level of impressions compared to reach means people are looking at a post multiple times. Do some digging to see if you can understand why it’s so sticky.
Facebook page impressions benchmarks (30 days):
- Education: 374K
- Financial services: 223K
- Government: 646K
- Healthcare/Wellness: 223K
- Travel/hospitality/leisure: 485K
6. Video views
Each social network determines what counts as a “view” a little differently, but usually, even a few seconds of watch time counts as a “view.”
So, video views is basically a good at-a-glance indicator of how many people have seen at least the start of your video.
Instagram three-second video view benchmarks:
- Education: 192.77
- Financial services: 48.42
- Government: 1.1K
- Healthcare/Wellness: 393.85
- Travel/hospitality/leisure: 259.28
7. Video completion rate
Video views are great, but they only let you know that someone started to watch your video. So how often do people actually watch your videos all the way through to the end? Video completion rate is a good indicator that you’re creating quality content that connects with your audience.
Video completion rate is also a key signal to many social media algorithms , so this is a good one to focus on improving.
8. Audience growth rate
Audience growth rate measures how many new followers your brand gets on social media within a certain amount of time.
It’s not a simple count of your new followers. Instead, it measures your new followers as a percentage of your total audience. So when you’re just starting out, getting 10 or 100 new followers in a month can give you a high growth rate.
But once you have a larger existing audience, you need more new followers to maintain that momentum.
To calculate your audience growth rate, track your net new followers (on each social media platform) over a reporting period. Then divide that number by your total audience (on each platform) and multiply by 100 to get your audience growth rate percentage.
Social media metrics 1.png
Facebook audience growth rate benchmarks:
- Education: -0.81%
- Financial services: -0.72%
- Government: -0.32%
- Healthcare/Wellness: -1.64%
- Travel/hospitality/leisure: -2.65%
Social media marketing metrics
9. click-through rate (ctr).
Click-through rate, or CTR, indicates how often people click a link in one of your posts to access additional content. That could be anything from a blog post to your online store.
CTR gives you a sense of how many people saw your social content and wanted to know more. It’s a good indicator of how well different types of content promote your brand on social.
To calculate CTR, divide the total number of clicks for a post by the total number of impressions. Multiply by 100 to get your CTR as a percentage.
10. Conversion rate
Conversion rate measures how often your social content starts the process to a conversion event like a subscription, download, or sale. This is one of the most important social media marketing metrics because it shows the value of your social media campaigns (organic and paid) as a means of feeding your funnel.
UTM parameters are the key to making your social conversions trackable. Learn all about how they work in our blog post on using UTM parameters to track social success .
Once you’ve added your UTMs, calculate conversion rate by dividing the number of conversions by the number of clicks.
11. Cost-per-click (CPC)
Cost-per-click, or CPC, is the amount you pay per individual click on a social ad.
Knowing the lifetime value of a customer for your business, or even the average order value, will help you put this number in important context.
A higher lifetime value of a customer combined with a high conversion rate means you can afford to spend more per click to get visitors to your website in the first place.
You don’t need to calculate CPC: You can find it in the analytics for the social network where you’re running your ad.
12. Cost per thousand impressions (CPM)
Cost per thousand impressions, or CPM, is exactly what it sounds like. It’s the cost you pay for every thousand impressions of your social media ad.
CPM is all about views, not actions.
Again, there’s nothing to calculate here—just import the data from your social network’s analytics.
Bonus: Get a free social media report template to easily and effectively present your social media performance to key stakeholders.
Social customer service metrics
13. average response time.
Response time is a metric that measures how long it takes for your customer service team to respond to queries that come in through social channels. It’s the social media equivalent of time spent on hold.
Using AI customer service bots can significantly reduce response time for many simple requests.
If you’re using a social customer service tool like Hootsuite Inbox l, you can add response time directly to your analytics report.
Otherwise, you can calculate it manually by adding up the total amount of time taken for an initial response to customer queries and dividing it by the number of queries.
14. Customer satisfaction (CSAT) score
Of course, customer service metrics are not just about response times and response rates. CSAT (customer satisfaction score), is a metric that measures how happy people are with your product or service.
Usually, the CSAT score is based on one, straightforward question: How would you rate your overall level of satisfaction? In this case, it’s used to measure the level of satisfaction with your social customer service.
It’s the reason why so many brands ask you to rate your experience with a customer service agent after it’s over. And that’s exactly how you can measure it too.
Create a one-question survey asking your customers to rate their satisfaction with your customer service and send it via the same social channel used for the service interaction. This is a great use for bots .
Add up all the scores and divide the sum by the number of responses. Then multiply by 100 to get your CSAT score as a percentage.
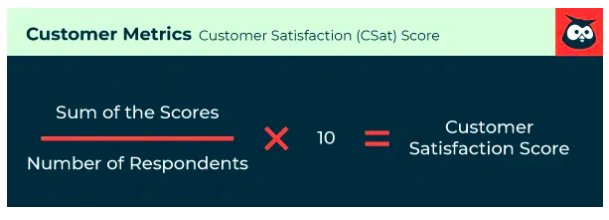
15. Net promoter score (NPS)
Net promoter score , or NPS, is a metric that measures customer loyalty.
Unlike CSAT, NPS is good at predicting future customer relationships. It is based on one—and only one—specifically phrased question: How likely is it that you would recommend our [company/product/service] to a friend?
Customers are asked to answer on a scale of zero to 10. Based on their response, each customer is grouped into one of three categories:
- Detractors: 0–6 score range
- Passives: 7–8 score range
- Promoters: 9–10 score range
NPS is unique in that it measures customer satisfaction as well as the potential for future sales, which has made it a valuable, go-to metric for organizations of all sizes.
To calculate NPS, subtract the number of promoters from the number of detractors.
Divide the result by the total number of respondents and multiply by 100 to get your NPS.
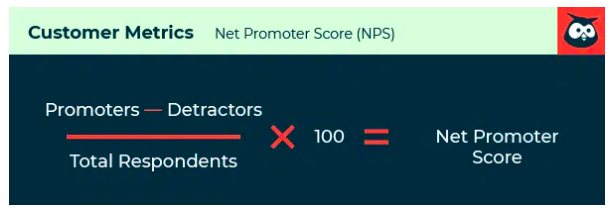
For more details, check out our post that dives deep into customer service metrics .
Other important social media metrics
16. social share of voice (ssov).
Social share of voice measures how many people are talking about your brand on social media compared to your competitors. How much of the social conversation in your industry is all about you?
Mentions can be either:
- Direct (tagged—e.g., “@Hootsuite”)
- Indirect (untagged—e.g., “hootsuite”)
SSoV is, essentially, competitive analysis: how visible—and, therefore, relevant—is your brand in the market?
To calculate it, add up every mention of your brand on social across all networks. Do the same for your competitors. Add both sets of mentions together to get a total number of mentions for your industry. Divide your brand mentions by the industry total, then multiply by 100 to get your SSoV as a percentage.
17. Social sentiment
Whereas SSoV tracks your share of the social conversation, social sentiment tracks the feelings and attitudes behind the conversation. When people talk about you online, are they saying positive or negative things?
Calculating social sentiment requires some help from a social media metrics tool that can process and categorize language and context. We’ve got a whole post on how to measure sentiment effectively .
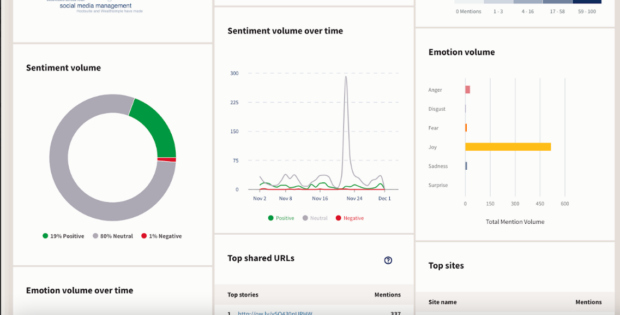
Source: Hootsuite Analytics
How to set up a social media metrics dashboard
Each social network has a built-in social media metrics tracker through which you can find much of the raw data you need to calculate and track your social media success.
However, this is a somewhat cumbersome way to track your social metrics. Jumping between accounts takes time, and learning different networks’ native analytics tools can be confusing. That said, these tools are free to use, so they can be a good entry point to tracking social metrics.
We’ve got lots of guides to help you understand the individual native analytics tools:
- Twitter Analytics
- Meta Business Suite (Facebook and Instagram)
- TikTok Analytics
- LinkedIn Analytics
- Pinterest Analytics
If you need to present your results to your boss or other stakeholders, you can manually input the data from all platforms into a report. We’ve created a free social media report template you can use to track your data over time and present your findings.
Or, you could track all your social media metrics from Twitter, Instagram, Facebook, TikTok, Pinterest, and LInkedIn all in one place and easily create custom reports with a social media metrics tool like Hootsuite .
Here’s how to use Hootsuite Analytics to set up a social media metrics dashboard that calculates and measures your metrics for you.
- Log into your Hootsuite dashboard and head to the Analytics tab.
- Click New Report . Scroll through the various reporting options and templates to create a custom report template based on the metrics you can most about. Note that once you add these metrics to your social media metrics dashboard, you don’t need to remember the formulas anymore because Hootsuite will calculate them for you.
- Head to the Benchmarking section on Analytics and click Competitive Analysis . Choose your social profiles and add competitors to compare your performance to the competition.
- Also under the Benchmarking section, click on Industry , then choose your industry to benchmark your performance against your industry as a whole. This is the tool we used to gather the benchmarks listed throughout this post.
- Track your social media customer service metrics using the Team Activity tab.
Here’s a video that runs through some of the most important ways you can use the metrics in this post – and in your Hootsuite Analytics dashboard – to answer real business-oriented questions related to your social media performance.
Track your social media performance and squeeze more out of your marketing budget with Hootsuite. Publish your posts and analyze the results in the same, easy-to-use dashboard. Try it free today.
All your social media analytics in one place . Use Hootsuite to see what’s working and where to improve performance.
Become a better social marketer.
Get expert social media advice delivered straight to your inbox.
Christina Newberry is an award-winning writer and editor whose greatest passions include food, travel, urban gardening, and the Oxford comma—not necessarily in that order.
Related Articles
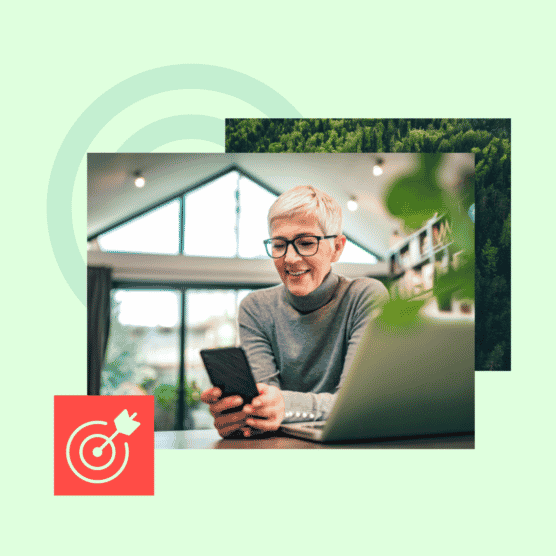
How to Create a Social Media Report [Free Template Included]
A comprehensive social media report proves the value of your social marketing plan. It shows what you’ve accomplished, backed up by data.
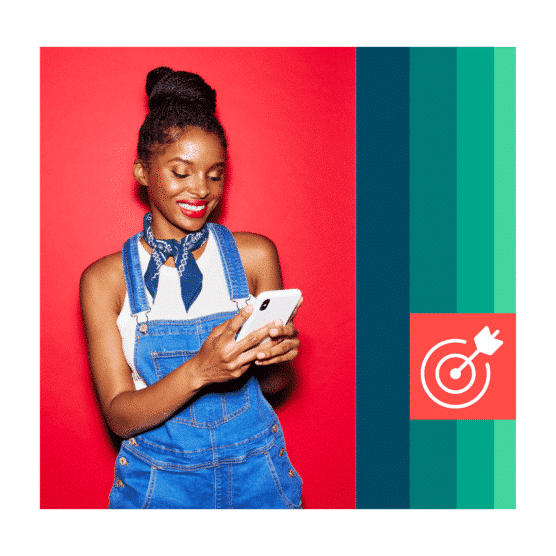
What Are Social Media KPIs? 25 Examples + How to Track Them
Setting smart social media KPIs will help your team focus on the metrics that really matter and track performance over time.
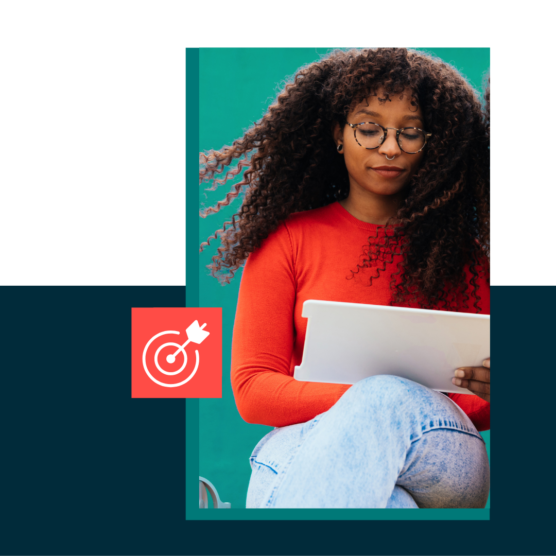
21 of the Best Social Media Analytics Tools for 2024
Are you a social media marketer who wants to better focus your time, effort, and budget? It’s time for some new social media analytics tools!
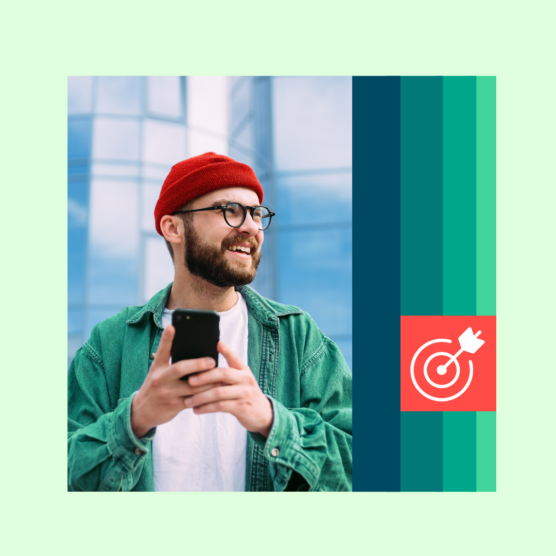
How to Prove (and Improve!) Your Social Media ROI
Learn how to calculate your social media ROI — aka the return on investment from your social media activities and expenses.
Information
- Author Services
Initiatives
You are accessing a machine-readable page. In order to be human-readable, please install an RSS reader.
All articles published by MDPI are made immediately available worldwide under an open access license. No special permission is required to reuse all or part of the article published by MDPI, including figures and tables. For articles published under an open access Creative Common CC BY license, any part of the article may be reused without permission provided that the original article is clearly cited. For more information, please refer to https://www.mdpi.com/openaccess .
Feature papers represent the most advanced research with significant potential for high impact in the field. A Feature Paper should be a substantial original Article that involves several techniques or approaches, provides an outlook for future research directions and describes possible research applications.
Feature papers are submitted upon individual invitation or recommendation by the scientific editors and must receive positive feedback from the reviewers.
Editor’s Choice articles are based on recommendations by the scientific editors of MDPI journals from around the world. Editors select a small number of articles recently published in the journal that they believe will be particularly interesting to readers, or important in the respective research area. The aim is to provide a snapshot of some of the most exciting work published in the various research areas of the journal.
Original Submission Date Received: .
- Active Journals
- Find a Journal
- Proceedings Series
- For Authors
- For Reviewers
- For Editors
- For Librarians
- For Publishers
- For Societies
- For Conference Organizers
- Open Access Policy
- Institutional Open Access Program
- Special Issues Guidelines
- Editorial Process
- Research and Publication Ethics
- Article Processing Charges
- Testimonials
- Preprints.org
- SciProfiles
- Encyclopedia
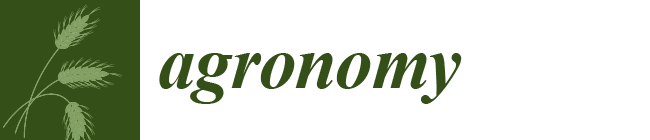
Article Menu
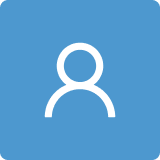
- Subscribe SciFeed
- Recommended Articles
- Google Scholar
- on Google Scholar
- Table of Contents
Find support for a specific problem in the support section of our website.
Please let us know what you think of our products and services.
Visit our dedicated information section to learn more about MDPI.
JSmol Viewer
Quantitative approaches in assessing soil organic matter dynamics for sustainable management.
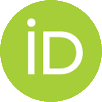
1. Introduction
Importance and overview of the advances in evaluating soil organic matter, 2. conceptualization and terminology, 3. overview of measurement techniques for soil organic matter assessment, 3.1. som fractionation, 3.2. quantitative techniques for som measurement, 3.3. qualitative techniques for som measurement, 3.4. lability and stability of organic matter in soils, 4. modeling as a tool for sustainable soil organic matter assessment and management, 4.1. analytical models, 4.1.1. hénin and dupuis’s model, 4.1.2. hénin et al.’s model, 4.1.3. kortleven’s model, 4.1.4. kolenbrander’s model, 4.1.5. godshalk’s model (1977), 4.1.6. jansen’s model, 4.1.7. yin’s model, 4.1.8. andrén and kätterer’s model (icbm), 4.1.9. andriulo et al.’s model, 4.1.10. somm model, 4.2. simulation models, 4.2.1. pernas’s model (1975), 4.2.2. the century model, 4.2.3. rothc model, 4.2.4. van veen and paul’s model (1981), 4.2.5. dnd model, 4.2.6. daycent model, 4.2.7. yasso model, 4.2.8. animo model, 4.2.9. candy model, 4.2.10. root zone water quality model, 4.2.11. papran model, 4.2.12. ncsoil model, 4.2.13. daisy model, 4.2.14. sundial model, 4.2.15. ecosys model, 4.2.16. apsim model, 5. a synopsis of the strengths, limitations, and applications of some analytical and simulation-based soil organic matter modeling approaches in understanding and predicting som dynamics.
Models | Strengths | Limitations | Applications |
---|---|---|---|
Analytical Models | Easily understandable and interpretable. Requires limited data and information. Suitable for small-scale studies. Can be used to predict long-term SOM dynamics. | Limited scope of applicability. Assumptions are often oversimplified and sometimes unrealistic. Inability to capture the complexity of real-world | Predicting decomposition rate, transformation, lability, and stability of SOM. Quantifying the impact of management practices on SOM. Evaluating the effects of climate change and land-use changes on SOM |
Hénin and Dupuis model (1945) | Provides a simple and intuitive representation of soil organic matter dynamics. Applicable for different soil types. Can be used to estimate SOM turnover time. | Ignoring environmental factors during SOM decomposition. | Useful as a historical reference for the development of soil carbon models. Can be used as a baseline model for more complex soil carbon models. Predicting carbon and nitrogen mineralization rates |
Kortleven Model (1963) | Can be used for predicting nitrogen mineralization. Simple and easy to use. | Assumptions are oversimplified. Ignores the impact of environmental factors | Predicting nitrogen mineralization and SOM decomposition rates in different soils |
Kolenbrander Model (1969) | Suitable for estimating nitrogen immobilization rate. Incorporates environmental factors such as pH and temperature. | Assumes a constant microbial biomass. Limited scope of applicability | Predicting nitrogen immobilization rate and SOM decomposition rate under different management practices and soil conditions |
Godshalk Model (1977) | Simple and easy to use. Applicable for predicting carbon and nitrogen mineralization. | Assumes constant environmental conditions. Limited scope of applicability | Predicting SOM decomposition rates in different soils under varying environmental conditions |
Jansen Model (1984) | Incorporates the effects of temperature and moisture. Suitable for estimating long-term SOM dynamics | Limited scope of applicability, Assumes a constant microbial biomass | Predicting SOM decomposition and mineralization rates, and carbon balance under varying environmental conditions. Assessing management practices in mitigating climate change. |
ICBM (Introductory Carbon Balance Model), Andrén and Kätterer model (1997) | Accounts for the effects of temperature and moisture. Suitable for predicting long-term SOM dynamics. Account for carbon balance at various spatial scales, from individual plants to entire ecosystems. It incorporates a detailed understanding of plant physiology and ecosystem processes. | Requires a lot of detailed input data and parameters (vegetation characteristics, climate, and soil properties). Limited scope of applicability and its calibration can be complex and time-consuming. | Predicting SOM decomposition and mineralization rates under different management practices and environmental conditions |
Andriulo Model (1999) | Incorporates temperature and moisture effects. Suitable for predicting long-term SOM dynamics. | Limited scope of applicability. Assumes a constant microbial biomass | Predicting SOM decomposition and mineralization rates under different management practices and environmental conditions |
SOMM (Soil Organic Matter Mineralization) model | Incorporates a detailed understanding of microbial ecology and soil organic matter dynamics. It can simulate the decomposition and mineralization of different fractions of organic matter, including labile and recalcitrant pools. Suitable for predicting long-term SOM dynamics. | Model calibration can be complex, as it requires detailed information on soil characteristics and microbial processes. Input data requirements can be high, including detailed information on soil texture, structure, and water content. | Useful for understanding and predicting the effects of management practices, such as tillage, fertilization, and crop rotation, on soil organic matter dynamics. Can be used to assess the impacts of climate change on soil organic matter mineralization rates. Predicting SOM dynamics under different land-use scenarios |
Sauerbeck and Gonzalez model (1977) | Simple and easy to use, with few input data requirements. Can be used to estimate soil carbon turnover rates and the decomposition of different soil organic matter fractions. | Does not account for the effects of environmental factors, such as temperature and moisture, on soil organic matter decomposition. Assumes a fixed rate of carbon loss from the soil organic matter pool, which may not reflect actual soil carbon dynamics. | Useful for comparing the turnover rates of different soil organic matter fractions and estimating the potential impact of changes in management practices on soil carbon storage. Can be used as a baseline model for more complex soil carbon models. |
Yang Model (1996) | Can be used for predicting long-term SOM dynamics. Accounts for the effects of temperature and moisture. | Limited scope of applicability. Assumes a constant microbial biomass | Predicting SOM dynamics under different land use and management practices |
Simulation Models | Can capture the complexity of real-world systems. Can incorporate various environmental factors. Can be used to simulate various management practices | Require large amounts of input data and parameters. Difficult to interpret and explain. Limited to specific soil types | Predicting SOM dynamics at large spatial and temporal scales. Evaluating the effects of climate change and land-use changes on SOM. Predicting the impact of different management practices on SOM |
Pernas model (1975) | Provides a simple and intuitive representation of soil organic matter dynamics and mineralization. Can be used to estimate the decomposition rates of different soil organic matter fractions. | Assumes that soil organic matter decomposes at a constant rate, which does not reflect actual soil carbon dynamics. Does not account for the effects of environmental factors, such as temperature and moisture, on soil organic matter decomposition. Limited scope of applicability. | Useful as a historical reference for the development of soil carbon models. Can be used as a baseline model for more complex soil carbon models. Predicting SOM decomposition and mineralization rates under different environmental conditions |
CENTURY model | Accounts for the effects of environmental factors, such as temperature, moisture, and land use, on soil organic matter decomposition. Can simulate the impacts of different management practices on soil carbon storage. Can simulate soil carbon dynamics over long time scales (e.g., centuries). | Requires a large amount of input data, including soil properties, climate data, and management practices. Can be computationally intensive, particularly when simulating large spatial and temporal scales. May not accurately represent soil carbon dynamics in certain soil types or regions. | Widely used in global climate models and to evaluate the impacts of land use and management on soil carbon storage. Can be used to develop management strategies to enhance soil carbon storage and mitigate climate change. |
RothC model | Accounts for the effects of temperature, moisture, and soil properties on soil organic matter decomposition. Can be used to simulate soil carbon dynamics under different management practices. Can simulate soil carbon dynamics over long time scales (e.g., centuries). Includes an option to incorporate soil respiration measurements to calibrate the model. | Requires input data on soil properties, climate data, and management practices. Can be computationally intensive, particularly when simulating large spatial and temporal scales. May not accurately represent soil carbon dynamics in certain soil types or regions. | Used to evaluate the impacts of land use and management on soil carbon storage. Can be used to develop management strategies to enhance soil carbon storage and mitigate climate change. |
Van Veen and Paul model (1981) | Can predict SOM dynamics under different management practices | Limited scope of applicability. Assumes a constant microbial biomass | Predicting SOM dynamics under different management practices and environmental conditions |
DNDC Model | Can simulate the effects of climate change and land-use changes | Requires large amounts of input data and parameters. Model structure is complex and difficult to modify. | Predicting SOM dynamics under different land-use and climate scenarios |
DayCent Model | Suitable for predicting SOM dynamics under different management practices and environmental conditions | Requires large amounts of input data and parameters. Model structure is complex and difficult to modify | Predicting SOM dynamics under different management practices and environmental conditions |
Yasso model | Accounts for the effects of temperature, moisture, and litter quality on soil organic matter decomposition. Can simulate the impacts of different management practices on soil carbon storage. Can simulate soil carbon dynamics over long time scales (e.g., centuries). Includes an option to incorporate field measurements to calibrate the model. | Requires input data on litter quality, climate data, and management practices. May not accurately represent soil carbon dynamics in certain soil types or regions. Does not explicitly account for the effects of soil properties on soil carbon dynamics. | Widely used in global carbon cycle models and to evaluate the impacts of land use and management on soil carbon storage. Can be used to develop management strategies to enhance soil carbon storage and mitigate climate change. |
ANIMO model | Can simulate the effects of different land use and management practices on soil carbon dynamics. Includes options to account for the effects of climate change and elevated atmospheric CO on soil carbon storage. Can simulate soil carbon dynamics over long time scales (e.g., centuries). Includes an option to incorporate field measurements to calibrate the model. | Requires input data on soil properties, climate data, and management practices. May not accurately represent soil carbon dynamics in certain soil types or regions. Does not account for the effects of soil biota on soil carbon dynamics. | Widely used in global carbon cycle models and to evaluate the impacts of land use and management on soil carbon storage. Can be used to develop management strategies to enhance soil carbon storage and mitigate climate change. |
CANDY model | Can simulate the effects of different land use and management practices on soil carbon dynamics. Accounts for the effects of temperature, moisture, and litter quality on soil organic matter decomposition. Can simulate soil carbon dynamics over long time scales (e.g., centuries). Includes an option to incorporate field measurements to calibrate the model. | Requires input data on soil properties, climate data, and management practices. May not accurately represent soil carbon dynamics in certain soil types or regions. Does not explicitly account for the effects of soil biota on soil carbon dynamics. | Widely used in global carbon cycle models and to evaluate the impacts of land use and management on soil carbon storage. Can be used to develop management strategies to enhance soil carbon storage and mitigate climate change. |
Root Zone Water Quality Model | Can simulate the transport and fate of nutrients, pesticides, and other contaminants in soil and groundwater. Accounts for the effects of soil properties, land use, and management practices on soil water and solute transport. Allows for the evaluation of management strategies to reduce non-point-source pollution. Includes user-friendly interface and graphical output. | Requires input data on soil properties, crop management practices, and hydrologic conditions. Does not explicitly account for the effects of soil biota on nutrient cycling and pollutant degradation. May not accurately represent soil water and solute transport in certain soil types or regions. | Widely used by researchers, consultants, and policymakers to assess the impacts of agricultural management practices on water quality. Can be used to evaluate the effectiveness of best management practices (BMPs) to reduce non-point source pollution. |
PAPRAN Model | Simulates the growth and production of annual pastures under different climatic and management conditions. Accounts for the effects of rainfall, temperature, and nitrogen availability on pasture growth and quality. Can be used to optimize fertilization and grazing management practices to maximize pasture productivity and quality. Allows for the assessment of the potential impact of climate change on pasture production. | Does not account for the effects of other environmental factors, such as soil fertility and pests, on pasture growth and quality. May require calibration to local conditions to accurately represent pasture growth and quality. | Can be used by farmers and land managers to optimize pasture management practices and improve productivity. Can be used to assess the impact of climate change on pasture production and inform adaptation strategies. |
NCSOIL Model | Accounts for the interactions between carbon and nitrogen cycles in soil. Simulates the mineralization, immobilization, and nitrification of soil organic matter and nitrogen. Allows for the evaluation of the impact of management practices and environmental factors on soil carbon and nitrogen dynamics. | Requires detailed information on soil properties and management practices to accurately simulate soil carbon and nitrogen dynamics. May not accurately represent the effects of other environmental factors, such as temperature and moisture, on soil carbon and nitrogen dynamics. | Can be used to optimize management practices to increase soil carbon sequestration and reduce nitrogen losses. Can be used to assess the potential impact of climate change on soil carbon and nitrogen dynamics and inform adaptation strategies. |
DAISY Model | Accounts for the aerobic and anaerobic microbial activity in soil. Simulates the decomposition and mineralization of soil organic matter, nitrogen transformations, and soil water dynamics. Can be used to simulate the effects of management practices, such as irrigation and fertilization, on soil carbon and nitrogen dynamics. | Requires detailed information on soil properties and management practices to accurately simulate soil carbon and nitrogen dynamics. May not accurately represent the effects of other environmental factors, such as temperature and moisture, on soil carbon and nitrogen dynamics. | Can be used to optimize management practices to increase soil carbon sequestration and reduce nitrogen losses. Can be used to assess the potential impact of climate change on soil carbon and nitrogen dynamics and inform adaptation strategies. |
SUNDIAL Model | Simulates the dynamics of carbon, nitrogen, phosphorus, and water in agricultural landscapes. Accounts for multiple environmental factors, such as temperature, precipitation, and soil properties, that affect nutrient cycling. Can be used to simulate the effects of management practices, such as crop rotation and fertilizer application, on nutrient cycling and water quality. | Requires detailed information on soil properties, climate, and management practices to accurately simulate nutrient cycling and water quality. May not accurately represent the effects of other environmental factors, such as land-use changes, on nutrient cycling and water quality. | Can be used to optimize management practices to improve nutrient cycling and water quality in agricultural landscapes. Can be used to assess the potential impact of climate change and land-use changes on nutrient cycling and water quality and inform adaptation and mitigation strategies. |
ECOSYS Model | Simulates the exchange of carbon, water, and energy between the land surface and the atmosphere. Accounts for multiple environmental factors, such as temperature, precipitation, and soil properties, that affect ecosystem processes. Can be used to simulate the effects of management practices, such as land-use change and vegetation management, on ecosystem processes and carbon sequestration. | Requires detailed information on soil properties, climate, and vegetation characteristics to accurately simulate ecosystem processes. May not accurately represent the effects of other environmental factors, such as nutrient availability and disturbance regimes, on ecosystem processes. | Can be used to assess the potential for carbon sequestration in different ecosystems and under different management practices. Can be used to inform land-use planning and policy development aimed at mitigating climate change. |
APSIM Model | Can simulate a wide range of agricultural production systems, including crops, pastures, and livestock. Accounts for multiple environmental factors, such as soil properties, climate, and management practices, that affect crop growth and yield. Includes modules for simulating soil water and nutrient dynamics, crop growth and development, and pest and disease interactions. | Requires detailed information on soil properties, climate, and management practices to accurately simulate crop growth and yield. May not accurately represent the effects of extreme weather events or other unpredictable environmental factors on crop production. | Can be used to assess the effects of different management practices, such as crop rotation and irrigation, on crop growth and yield. Can be used to evaluate the potential impacts of climate change on agricultural production and inform adaptation strategies. |
NICCCE (Nitrogen isotopes and carbon cycling in coniferous ecosystems) | The model integrates carbon and nitrogen cycles and explicitly considers the effects of isotopic fractionation, allowing for the analysis of isotopic patterns in the soil and vegetation. The model can be used to simulate the impacts of changes in environmental conditions (e.g., temperature, precipitation, nitrogen deposition) on carbon and nitrogen dynamics in coniferous ecosystems. The model has been extensively tested and validated against field measurements, demonstrating its ability to accurately predict carbon and nitrogen dynamics in coniferous ecosystems. | The model has only been tested in coniferous ecosystems, so its applicability to other ecosystem types is unclear. The model requires a large amount of input data, including site-specific parameters such as soil texture and vegetation characteristics, which can be time-consuming and costly to collect. The model assumes that all carbon and nitrogen inputs and outputs are isotopically distinct, which may not always be the case in the real world. | The model can be used to investigate the impacts of environmental changes on carbon and nitrogen cycling in coniferous ecosystems, including the effects of climate change, nitrogen deposition, and forest management practices. The model can be used to explore the isotopic patterns in soil and vegetation to gain insights into the sources and cycling of carbon and nitrogen in coniferous ecosystems. The model can be used to develop management strategies for coniferous ecosystems that aim to optimize carbon and nitrogen sequestration and reduce greenhouse gas emissions. |
EPIC (Erosion Productivity Impact Calculator) Model | Integrates various processes, including erosion, climate, soil, and crop management, to simulate soil and crop productivity. Incorporates spatial variability of soil properties and weather data to improve accuracy of simulations. Allows for simulating long-term effects of land-use changes and management practices on soil and crop productivity. Has been widely used and tested in various regions across the world. | Data-intensive and requires input data for various variables, which can be difficult to obtain. Calibration of model parameters can be time-consuming and may require extensive field measurements. Requires expertise in modeling and agricultural sciences to use and interpret results. Does not account for all soil and crop processes, such as nutrient cycling and root growth, and may require additional models for more comprehensive analyses. | Used for a wide range of applications, including crop management, land-use planning, and environmental impact assessments. Used in various regions across the world for predicting crop yields and environmental impacts. Used for evaluating the impacts of climate change and extreme weather events on crop productivity. Used for assessing the economic and environmental impacts of agricultural practices and policies |
Osnabruck Model | Considers soil organic matter decomposition and nutrient cycling processes in detail. Accounts for the impact of management practices on soil organic matter dynamics. Applicable to various soil types and climatic conditions. Allows for the simulation of different plant species and cropping systems. | Requires input data on soil properties and management practices that can be time-consuming and costly to collect. Limited validation and testing under different environmental conditions. Does not account for the influence of soil microorganisms on soil organic matter dynamics. | Can be used to evaluate the effects of different management practices on soil organic matter and nutrient cycling. Useful in predicting the long-term impacts of land-use and management changes on soil quality. Can aid in developing sustainable agricultural practices. |
Verberne model | Includes management practices such as tillage, crop rotation, and fertilization. Accounts for different types of organic matter and their decomposition rates. Incorporates environmental factors such as temperature and moisture | Limited validation in certain regions and soil types. Requires input data that may not always be readily available | Assessing the impacts of management practices on soil organic matter dynamics. Predicting the effects of environmental changes on soil carbon storage |
6. Conclusions and Future Perspectives
Author contributions, institutional review board statement, informed consent statement, data availability statement, conflicts of interest.
- Fageria, N.K. Role of Soil Organic Matter in Maintaining Sustainability of Cropping Systems. Commun. Soil Sci. Plant Anal. 2012 , 43 , 2063–2113. [ Google Scholar ] [ CrossRef ]
- Manlay, R.J.; Feller, C.; Swift, M.J. Historical Evolution of Soil Organic Matter Concepts and Their Relationships with the Fertility and Sustainability of Cropping Systems. Agric. Ecosyst. Environ. 2007 , 119 , 217–233. [ Google Scholar ] [ CrossRef ]
- Voltr, V.; Menšík, L.; Hlisnikovský, L.; Hruška, M.; Pokorný, E.; Pospíšilová, L. The Soil Organic Matter in Connection with Soil Properties and Soil Inputs. Agronomy 2021 , 11 , 779. [ Google Scholar ] [ CrossRef ]
- Janker, J.; Mann, S. Understanding the Social Dimension of Sustainability in Agriculture: A Critical Review of Sustainability Assessment Tools. Environ. Dev. Sustain. 2020 , 22 , 1671–1691. [ Google Scholar ] [ CrossRef ]
- Lal, R. Reducing Carbon Footprints of Agriculture and Food Systems. Carbon Footpr. 2022 , 1 , 3. [ Google Scholar ] [ CrossRef ]
- Oberč, B.P.; Arroyo, S.A. Approaches to Sustainable Agriculture: Exploring the Pathways towards the Future of Farming ; IUCN EURO: Brussels, Belgium, 2020; ISBN 978-2-8317-2054-8. [ Google Scholar ]
- Lal, R. Soil Conservation and Ecosystem Services. Int. Soil Water Conserv. Res. 2014 , 2 , 36–47. [ Google Scholar ] [ CrossRef ] [ Green Version ]
- Darwish, O.H.; Persaud, N.; Martens, D.C. Effect of Long-Term Application of Animal Manure on Physical Properties of Three Soils. Plant Soil 1995 , 176 , 289–295. [ Google Scholar ] [ CrossRef ]
- Leroy, A.K.; Walter, F.; Brinks, E.; Bigiel, F.; de Blok, W.J.G.; Madore, B.; Thornley, M.D. The Star Formation Efficiency in Nearby Galaxies: Measuring Where Gas Forms Stars Effectively. Astron. J. 2008 , 136 , 2782–2845. [ Google Scholar ] [ CrossRef ] [ Green Version ]
- Shepherd, M.A.; Harrison, R.; Webb, J. Managing Soil Organic Matter—Implications for Soil Structure on Organic Farms. Soil Use Manag. 2006 , 18 , 284–292. [ Google Scholar ] [ CrossRef ]
- Stockmann, U.; Adams, M.A.; Crawford, J.W.; Field, D.J.; Henakaarchchi, N.; Jenkins, M.; Minasny, B.; McBratney, A.B.; Courcelles, V.d.R.d.; Singh, K.; et al. The Knowns, Known Unknowns and Unknowns of Sequestration of Soil Organic Carbon. Agric. Ecosyst. Environ. 2013 , 164 , 80–99. [ Google Scholar ] [ CrossRef ]
- von Lützow, M.; Kögel-Knabner, I.; Ludwig, B.; Matzner, E.; Flessa, H.; Ekschmitt, K.; Guggenberger, G.; Marschner, B.; Kalbitz, K. Stabilization Mechanisms of Organic Matter in Four Temperate Soils: Development and Application of a Conceptual Model. Z. Pflanzenernähr. Bodenk. 2008 , 171 , 111–124. [ Google Scholar ] [ CrossRef ]
- von Lützow, M.; Kögel-Knabner, I.; Ekschmitt, K.; Flessa, H.; Guggenberger, G.; Matzner, E.; Marschner, B. SOM Fractionation Methods: Relevance to Functional Pools and to Stabilization Mechanisms. Soil Biol. Biochem. 2007 , 39 , 2183–2207. [ Google Scholar ] [ CrossRef ]
- Bot, A.; Benites, J. The Importance of Soil Organic Matter: Key to Drought-Resistant Soil and Sustained Food Production ; FAO Soils Bulletin; Food and Agriculture Organization of the United Nations: Rome, Italy, 2005; ISBN 978-92-5-105366-9. [ Google Scholar ]
- Auzins, A.; Brokking, P.; Jürgenson, E.; Lakovskis, P.; Paulsson, J.; Romanovs, A.; Valčiukienė, J.; Viesturs, J.; Weninger, K. Land Resource Management Policy in Selected European Countries. Land 2022 , 11 , 2280. [ Google Scholar ] [ CrossRef ]
- Kopecký, M.; Kolář, L.; Perná, K.; Váchalová, R.; Mráz, P.; Konvalina, P.; Murindangabo, Y.T.; Ghorbani, M.; Menšík, L.; Dumbrovský, M. Fractionation of Soil Organic Matter into Labile and Stable Fractions. Agronomy 2021 , 12 , 73. [ Google Scholar ] [ CrossRef ]
- Moudrý, J.; Bernas, J.; Moudrý, J.; Konvalina, P.; Ujj, A.; Manolov, I.; Stoeva, A.; Rembialkowska, E.; Stalenga, J.; Toncea, I.; et al. Agroecology Development in Eastern Europe—Cases in Czech Republic, Bulgaria, Hungary, Poland, Romania, and Slovakia. Sustainability 2018 , 10 , 1311. [ Google Scholar ] [ CrossRef ] [ Green Version ]
- Navarro-Pedreño, J.; Almendro-Candel, M.B.; Zorpas, A.A. The Increase of Soil Organic Matter Reduces Global Warming, Myth or Reality? Sci 2021 , 3 , 18. [ Google Scholar ] [ CrossRef ]
- Sainepo, B.M.; Gachene, C.K.; Karuma, A. Assessment of Soil Organic Carbon Fractions and Carbon Management Index under Different Land Use Types in Olesharo Catchment, Narok County, Kenya. Carbon Balance Manag. 2018 , 13 , 4. [ Google Scholar ] [ CrossRef ] [ Green Version ]
- Vonk, W.J.; van Ittersum, M.K.; Reidsma, P.; Zavattaro, L.; Bechini, L.; Guzmán, G.; Pronk, A.; Spiegel, H.; Steinmann, H.H.; Ruysschaert, G.; et al. European Survey Shows Poor Association between Soil Organic Matter and Crop Yields. Nutr. Cycl. Agroecosyst. 2020 , 118 , 325–334. [ Google Scholar ] [ CrossRef ]
- Achard, F.K. Chemische Utersunchungen des Tort. Crell’s Chem. Ann. 1786 , 2 , 391–403. [ Google Scholar ]
- Hochheimer, C.F. Chemische Mineralogie Oder Vollständige Geschichte der Analytischen Untersuchung Der Fossilien: Erster Band, Welcher Die Untersuchung Der Unmetallischen Fossilien Enthält. In Barth ; Nabu Press: Berlin, Germany, 1792; Volume 1. [ Google Scholar ]
- Zaccone, C.; Plaza, C.; Ciavatta, C.; Miano, T.M.; Shotyk, W. Advances in the Determination of Humification Degree in Peat since: Applications in Geochemical and Paleoenvironmental Studies. Earth-Sci. Rev. 2018 , 185 , 163–178. [ Google Scholar ] [ CrossRef ]
- Lundegårdh, H. Carbon Dioxide Evolution of Soil and Crop Growth. Soil Sci. 1927 , 23 , 417–453. [ Google Scholar ] [ CrossRef ]
- McDowell, W.H.; Likens, G.E. Origin, Composition, and Flux of Dissolved Organic Carbon in the Hubbard Brook Valley. Ecol. Monogr. 1988 , 58 , 177–195. [ Google Scholar ] [ CrossRef ]
- De Nobili, M. Comment on “Humic Substances Extracted by Alkali Are Invalid Proxies for the Dynamics and Functions of Organic Matter in Terrestrial and Aquatic Ecosystems,” by Kleber and Lehmann (2019). J. Environ. Qual. 2019 , 48 , 787–789. [ Google Scholar ] [ CrossRef ]
- Hayes, M.H.B.; Swift, R.S.; Wardle, R.E.; Brown, J.K. Humic Materials from an Organic Soil: A Comparison of Extractants and of Properties of Extracts. Geoderma 1975 , 13 , 231–245. [ Google Scholar ] [ CrossRef ]
- Kolář, L.; Moudry, J.; Kopecky, M. The Book of Humus ; ZERA: Croatia, Czech Republic, 2014; ISBN 978-80-87226-34-6. [ Google Scholar ]
- Lawes, J. On the Application of Different Manures to Different Crops and on their Proper Distribution on the Farm. Priv. Publ. Cited Dyke 1997 , 1 , 1847–1863. [ Google Scholar ]
- Lehmann, J.; Kleber, M. The Contentious Nature of Soil Organic Matter. Nature 2015 , 528 , 60–68. [ Google Scholar ] [ CrossRef ]
- Liebig, J. Die Organische Chemie in Ihrer Anwendung Auf Agricultur Und Physiologie/von Justus Liebig … ; Vieweg: Braunschweig, Germany, 1841. [ Google Scholar ]
- Maroušek, J.; Bartoš, P.; Filip, M.; Kolář, L.; Konvalina, P.; Maroušková, A.; Moudrý, J.; Peterka, J.; Šál, J.; Šoch, M.; et al. Advances in the Agrochemical Utilization of Fermentation Residues Reduce the Cost of Purpose-Grown Phytomass for Biogas Production. Energy Sources Part A Recovery Util. Environ. Eff. 2020 , 42 , 1–11. [ Google Scholar ] [ CrossRef ]
- Müller, P.E. Studien Über Die Natürlichen Humusformen und Deren Einwirkung auf Vegetation und Boden ; Salzwasser Verlag: Paderborn, Germany, 1887; ISBN 978-3-8460-4117-8. [ Google Scholar ]
- Schmidt, M.W.I.; Torn, M.S.; Abiven, S.; Dittmar, T.; Guggenberger, G.; Janssens, I.A.; Kleber, M.; Kögel-Knabner, I.; Lehmann, J.; Manning, D.A.C.; et al. Persistence of Soil Organic Matter as an Ecosystem Property. Nature 2011 , 478 , 49–56. [ Google Scholar ] [ CrossRef ] [ PubMed ] [ Green Version ]
- Schnitzer, M. Selected Methods for the Characterization of Soil Humic Substances. In Humic Substances in Soil and Crop Sciences: Selected Readings ; Soil Science Society of America: Madison, WI, USA, 2015; pp. 65–89. ISBN 978-0-89118-874-2. [ Google Scholar ]
- Weber, J.; Chen, Y.; Jamroz, E.; Miano, T. Preface: Humic Substances in the Environment. J. Soils Sediments 2018 , 18 , 2665–2667. [ Google Scholar ] [ CrossRef ] [ Green Version ]
- Francaviglia, R.; Baffi, C.; Nassisi, A.; Cassinari, C.; Farina, R. Use of the “Rothc” Model to Simulate Soil Organic Carbon Dynamics on a Silty-Loam Inceptisol in Northern Italy under Different Fertilization Practices. EQA Int. Environ. Qual. 2013 , 11 , 17–28. [ Google Scholar ] [ CrossRef ]
- Jenny, H. Factors of Soil Formation: A System of Quantitative Pedology ; Dover: New York, NY, USA, 1994; ISBN 978-0-486-68128-3. [ Google Scholar ]
- Waksman, S.A. Humus Origin, Chemical Composition, and Importance in Nature. Soil Sci. 1936 , 41 , 395. [ Google Scholar ] [ CrossRef ]
- Hénin, S.; Gwendal, M.; Turc, L. Un Aspect de La Dynamique des Matieres Organiques Du Sol. Comptes Rendus Hebd. Séances Acad. Sci. 1959 , 248 , 138–141. [ Google Scholar ]
- Henri, L. Bilan de La Matière Organique Du Sol. Le Modèle de Hénin. In Mélanges offerts à Stéphane Hénin ; The Research Institute for Development: Marseille, France, 1993. [ Google Scholar ]
- Parnas, H. Model for Decomposition of Organic Material by Microorganisms. Soil Biol. Biochem. 1975 , 7 , 161–169. [ Google Scholar ] [ CrossRef ]
- Falloon, P.; Smith, P. Modelling Soil Carbon Dynamics. In Soil Carbon Dynamics ; Kutsch, W.L., Bahn, M., Heinemeyer, A., Eds.; Cambridge University Press: Cambridge, UK, 2010; Volume 1, pp. 221–244. ISBN 978-0-521-86561-6. [ Google Scholar ]
- IRD France. Mélanges Stéphane Hénin: Sol, Agronomie, Environnement Jubilé Scientifique, Paris, 25 Septembre 1990 ; Éd. de l’ORSTOM: Paris, France, 1993; ISBN 978-2-7099-1141-2. [ Google Scholar ]
- Kortleven, H. Quantitative Aspects of Humus Accumulation and Decomposition. In Versl Landbk Onderz ; Pudoc: Wageningen, The Netherlands, 1963; Volume 69.1. [ Google Scholar ]
- MacLeod, M.; Nersessian, N.J. Building Simulations from the Ground Up: Modeling and Theory in Systems Biology. Philos. Sci. 2013 , 80 , 533–556. [ Google Scholar ] [ CrossRef ] [ Green Version ]
- Zeigler, B.P.; Nicolau de França, B.B.; Graciano Neto, V.V.; Hill, R.R.; Champagne, L.E.; Ören, T. History of Simulation. In Body of Knowledge for Modeling and Simulation ; Ören, T., Zeigler, B.P., Tolk, A., Eds.; Simulation Foundations, Methods and Applications; Springer International Publishing: Cham, Switzerland, 2023; pp. 413–434. ISBN 978-3-031-11084-9. [ Google Scholar ]
- Basu, M.; Pande, M.; Bhadoria, P.B.S.; Mahapatra, S.C. Potential Fly-Ash Utilization in Agriculture: A Global Review. Progress Nat. Sci. 2009 , 19 , 1173–1186. [ Google Scholar ] [ CrossRef ]
- Campbell, E.E.; Paustian, K. Current Developments in Soil Organic Matter Modeling and the Expansion of Model Applications: A Review. Environ. Res. Lett. 2015 , 10 , 123004. [ Google Scholar ] [ CrossRef ]
- Powlson, D.S.; Smith, P.; Smith, J.U. (Eds.) Evaluation of Soil Organic Matter Models: Using Existing Long-Term Datasets ; Springer: Berlin/Heidelberg, Germany, 1996; ISBN 978-3-642-64692-8. [ Google Scholar ]
- Kolář, L.; Kužel, S.; Horáček, J.; Čechová, V.; Borová-Batt, J.; Peterka, J. Labile Fractions of Soil Organic Matter, Their Quantity and Quality. Plant Soil Environ. 2009 , 55 , 245–251. [ Google Scholar ] [ CrossRef ] [ Green Version ]
- Shirato, Y.; Yokozawa, M. Acid Hydrolysis to Partition Plant Material into Decomposable and Resistant Fractions for Use in the Rothamsted Carbon Model. Soil Biol. Biochem. 2006 , 38 , 812–816. [ Google Scholar ] [ CrossRef ]
- Tirol-Padre, A.; Ladha, J.K. Assessing the Reliability of Permanganate-Oxidizable Carbon as an Index of Soil Labile Carbon. Soil Sci. Soc. Am. J. 2004 , 68 , 969–978. [ Google Scholar ] [ CrossRef ]
- Váchalová, R.; Borová-Batt, J.; Kolář, L.; Váchal, J. Selectivity of Ion Exchange as a Sign of Soil Quality. Commun. Soil Sci. Plant Anal. 2014 , 45 , 2673–2679. [ Google Scholar ] [ CrossRef ]
- Walkley, A. A Critical Examination of a Rapid Method for Determining Organic Carbon in Soils—Effect of Variations in Digestion Conditions and of Inorganic Soil Constituents. Soil Sci. 1947 , 63 , 251–264. [ Google Scholar ] [ CrossRef ]
- Aranda, V.; Ayora-Cañada, M.J.; Domínguez-Vidal, A.; Martín-García, J.M.; Calero, J.; Delgado, R.; Verdejo, T.; González-Vila, F.J. Effect of Soil Type and Management (Organic vs. Conventional) on Soil Organic Matter Quality in Olive Groves in a Semi-Arid Environment in Sierra Mágina Natural Park (S Spain). Geoderma 2011 , 164 , 54–63. [ Google Scholar ] [ CrossRef ]
- Don, A.; Schumacher, J.; Freibauer, A. Impact of Tropical Land-Use Change on Soil Organic Carbon Stocks—A Meta-Analysis: Soil Organic Carbon and Land-Use Change. Glob. Chang. Biol. 2011 , 17 , 1658–1670. [ Google Scholar ] [ CrossRef ] [ Green Version ]
- Laganière, J.; Augusto, L.; Hatten, J.A.; Spielvogel, S. Editorial: Vegetation Effects on Soil Organic Matter in Forested Ecosystems. Front. For. Glob. Chang. 2022 , 4 , 828701. [ Google Scholar ] [ CrossRef ]
- Plante, A.; Conant, R.T. Soil Organic Matter Dynamics, Climate Change Effects. In Global Environmental Change ; Springer: Dordrecht, The Netherlands, 2014; pp. 317–323. ISBN 978-94-007-5783-7. [ Google Scholar ]
- Reijonen, I.; Metzler, M.; Hartikainen, H. Impact of Soil PH and Organic Matter on the Chemical Bioavailability of Vanadium Species: The Underlying Basis for Risk Assessment. Environ. Pollut. 2016 , 210 , 371–379. [ Google Scholar ] [ CrossRef ]
- Vittori Antisari, L.; Trenti, W.; De Feudis, M.; Bianchini, G.; Falsone, G. Soil Quality and Organic Matter Pools in a Temperate Climate (Northern Italy) under Different Land Uses. Agronomy 2021 , 11 , 1815. [ Google Scholar ] [ CrossRef ]
- Wang, S.; Wang, X.; Ouyang, Z. Effects of Land Use, Climate, Topography and Soil Properties on Regional Soil Organic Carbon and Total Nitrogen in the Upstream Watershed of Miyun Reservoir, North China. J. Environ. Sci. 2012 , 24 , 387–395. [ Google Scholar ] [ CrossRef ]
- Bot, A.; Benites, J. Conservation Agriculture: Case Studies in Latin America and Africa ; FAO Soils Bulletin; Food and Agriculture Organization of the United Nations: Rome, Italy, 2001; ISBN 978-92-5-104625-8. [ Google Scholar ]
- Curry, J.P.; Good, J.A. Soil Faunal Degradation and Restoration. In Soil Restoration ; Advances in Soil Science; Springer: New York, NY, USA, 1992; Volume 17, pp. 171–215. ISBN 978-1-4612-7684-5. [ Google Scholar ]
- Murindangabo, Y.T.; Kopecký, M.; Konvalina, P. Adoption of Conservation Agriculture in Rwanda: A Case Study of Gicumbi District Region. Agronomy 2021 , 11 , 1732. [ Google Scholar ] [ CrossRef ]
- Ayanlaja, S.A.; Sanwo, J.O. Management of Soil Organic Matter in the Farming Systems of the Low Land Humid Tropics of West Africa: A Review. Soil Technol. 1991 , 4 , 265–279. [ Google Scholar ] [ CrossRef ]
- Hijbeek, R.; Pronk, A.A.; van Ittersum, M.K.; ten Berge, H.F.M.; Bijttebier, J.; Verhagen, A. What Drives Farmers to Increase Soil Organic Matter? Insights from the Netherlands. Soil Use Manag. 2018 , 34 , 85–100. [ Google Scholar ] [ CrossRef ] [ Green Version ]
- Ramesh, T.; Bolan, N.S.; Kirkham, M.B.; Wijesekara, H.; Kanchikerimath, M.; Srinivasa Rao, C.; Sandeep, S.; Rinklebe, J.; Ok, Y.S.; Choudhury, B.U.; et al. Soil Organic Carbon Dynamics: Impact of Land Use Changes and Management Practices: A Review. In Advances in Agronomy ; Elsevier: Amsterdam, The Netherlands, 2019; Volume 156, pp. 1–107. ISBN 978-0-12-817598-9. [ Google Scholar ]
- Blair, G.; Lefroy, R.; Lisle, L. Soil Carbon Fractions Based on Their Degree of Oxidation, and the Development of a Carbon Management Index for Agricultural Systems. Aust. J. Agric. Res. 1995 , 46 , 1459. [ Google Scholar ] [ CrossRef ]
- Bernoux, M.; da Conceição Santana Carvalho, M.; Volkoff, B.; Cerri, C.C. Brazil’s Soil Carbon Stocks. Soil Sci. Soc. Am. J. 2002 , 66 , 888–896. [ Google Scholar ] [ CrossRef ]
- Sisti, C.P.J.; dos Santos, H.P.; Kohhann, R.; Alves, B.J.R.; Urquiaga, S.; Boddey, R.M. Change in Carbon and Nitrogen Stocks in Soil under 13 Years of Conventional or Zero Tillage in Southern Brazil. Soil Tillage Res. 2004 , 76 , 39–58. [ Google Scholar ] [ CrossRef ]
- Cavani, L. Identification of Organic Matter from Peat, Leonardite and Lignite Fertilisers Using Humification Parameters and Electrofocusing. Bioresour. Technol. 2003 , 86 , 45–52. [ Google Scholar ] [ CrossRef ]
- Ciavatta, C.; Manunza, B.; Montecchio, D.; Govi, M.; Gessa, C. Chemical Parameters to Evaluate the Stabilization Level of the Organic Matter During Composting. In The Science of Composting ; de Bertoldi, M., Sequi, P., Lemmes, B., Papi, T., Eds.; Springer: Dordrecht, The Netherlands, 1996; pp. 1109–1112. ISBN 978-94-010-7201-4. [ Google Scholar ]
- De Nobili, M.; Petrussi, F. Humification Index (HI) as Evaluation of the Stabilization Degree during Composting. J. Ferment. Technol. 1988 , 66 , 577–583. [ Google Scholar ] [ CrossRef ]
- Roberts, C.A.; Workman, J.; Reeves, J.B. (Eds.) Near-Infrared Spectroscopy in Agriculture ; Agronomy Monographs; American Society of Agronomy; Crop Science Society of America; Soil Science Society of America: Madison, WI, USA, 2004; ISBN 978-0-89118-236-8. [ Google Scholar ]
- Sequi, P.; Nobili, M.D.; Leita, L.; Cercignani, G. A New Index of Humification. Agrochimica 1986 , 30 , 175–179. [ Google Scholar ]
- Simpson, A.J.; Simpson, M.J. Nuclear Magnetic Resonance Analysis of Natural Organic Matter. In Biophysico-Chemical Processes Involving Natural Nonliving Organic Matter in Environmental Systems ; Senesi, N., Xing, B., Huang, P.M., Eds.; John Wiley & Sons, Inc.: Hoboken, NJ, USA, 2009; pp. 589–650. ISBN 978-0-470-49495-0. [ Google Scholar ]
- Magdoff, F.; Van Es, H. Building Soils for Better Crops: Ecological Management for Healthy Soils , Handbook Series; 4th ed.; Sustainable Agriculture Research & Education: College Park, MD, USA, 2021; ISBN 978-1-888626-19-3. [ Google Scholar ]
- Gregorich, E.G.; Beare, M.H.; McKim, U.F.; Skjemstad, J.O. Chemical and Biological Characteristics of Physically Uncomplexed Organic Matter. Soil Sci. Soc. Am. J. 2006 , 70 , 975–985. [ Google Scholar ] [ CrossRef ]
- Parsons, J.W. Soil Organic Matter. Nature 1962 , 194 , 324–325. [ Google Scholar ] [ CrossRef ]
- Fiedler, S.R.; Buczko, U.; Jurasinski, G.; Glatzel, S. Soil Respiration after Tillage under Different Fertiliser Treatments—Implications for Modelling and Balancing. Soil Tillage Res. 2015 , 150 , 30–42. [ Google Scholar ] [ CrossRef ]
- Shibu, M.E.; Leffelaar, P.A.; Van Keulen, H.; Aggarwal, P.K. Quantitative Description of Soil Organic Matter Dynamics—A Review of Approaches with Reference to Rice-Based Cropping Systems. Geoderma 2006 , 137 , 1–18. [ Google Scholar ] [ CrossRef ]
- Jastrow, J.; Six, J. Organic Matter Turnover. In Encyclopedia of Soil Science ; Marcel Dekker: New York, NY, USA, 2002; Volume 2, pp. 936–942. ISBN 978-0-8493-3830-4. [ Google Scholar ]
- Jastrow, J.D.; Amonette, J.E.; Bailey, V.L. Mechanisms Controlling Soil Carbon Turnover and Their Potential Application for Enhancing Carbon Sequestration. Clim. Chang. 2007 , 80 , 5–23. [ Google Scholar ] [ CrossRef ]
- Jenkinson, D.S.; Rayner, J.H. The Turnover of Soil Organic Matter in Some of the Rothamsted Classical Experiment. Soil Sci. 1977 , 123 , 298–305. [ Google Scholar ] [ CrossRef ]
- Golchin, A.; Oades, J.; Skjemstad, J.; Clarke, P. Study of Free and Occluded Particulate Organic Matter in Soils by Solid State 13C Cp/MAS NMR Spectroscopy and Scanning Electron Microscopy. Soil Res. 1994 , 32 , 285. [ Google Scholar ] [ CrossRef ]
- John, B.; Yamashita, T.; Ludwig, B.; Flessa, H. Storage of Organic Carbon in Aggregate and Density Fractions of Silty Soils under Different Types of Land Use. Geoderma 2005 , 128 , 63–79. [ Google Scholar ] [ CrossRef ]
- Scrimgeour, C. Soil Sampling and Methods of Analysis , 2nd ed.; Carter, M.R., Gregorich, E.G., Eds.; CRC Press: Boca Raton, FL, USA, 2008; p. 1224. ISBN 978-0-8593-3586-0. [ Google Scholar ]
- Bossuyt, H.; Six, J.; Hendrix, P.F. Protection of Soil Carbon by Microaggregates within Earthworm Casts. Soil Biol. Biochem. 2005 , 37 , 251–258. [ Google Scholar ] [ CrossRef ]
- Apesteguia, M.; Plante, A.F.; Virto, I. Methods Assessment for Organic and Inorganic Carbon Quantification in Calcareous Soils of the Mediterranean Region. Geoderma Reg. 2018 , 12 , 39–48. [ Google Scholar ] [ CrossRef ]
- Nelson, D.W.; Sommers, L.E. Total Carbon, Organic Carbon, and Organic Matter. In SSSA Book Series ; Soil Science Society of America; American Society of Agronomy: Madison, WI, USA, 2018; pp. 961–1010. ISBN 978-0-89118-866-7. [ Google Scholar ]
- Shamrikova, E.V.; Kondratenok, B.M.; Tumanova, E.A.; Vanchikova, E.V.; Lapteva, E.M.; Zonova, T.V.; Lu-Lyan-Min, E.I.; Davydova, A.P.; Libohova, Z.; Suvannang, N. Transferability between Soil Organic Matter Measurement Methods for Database Harmonization. Geoderma 2022 , 412 , 115547. [ Google Scholar ] [ CrossRef ]
- Chang, C.-W.; Laird, D.A.; Mausbach, M.J.; Hurburgh, C.R. Near-Infrared Reflectance Spectroscopy-Principal Components Regression Analyses of Soil Properties. Soil Sci. Soc. Am. J. 2001 , 65 , 480–490. [ Google Scholar ] [ CrossRef ] [ Green Version ]
- Zornoza, R.; Guerrero, C.; Mataix-Solera, J.; Scow, K.M.; Arcenegui, V.; Mataix-Beneyto, J. Near Infrared Spectroscopy for Determination of Various Physical, Chemical and Biochemical Properties in Mediterranean Soils. Soil Biol. Biochem. 2008 , 40 , 1923–1930. [ Google Scholar ] [ CrossRef ] [ Green Version ]
- Brevik, E.C.; Burgess, L.C. (Eds.) Soils and Human Health ; CRC Press: Boca Raton, FL, USA, 2012; ISBN 978-1-4398-4455-7. [ Google Scholar ]
- Gregorich, E.G.; Carter, M.R.; Angers, D.A.; Monreal, C.M.; Ellert, B.H. Towards a Minimum Data Set to Assess Soil Organic Matter Quality in Agricultural Soils. Can. J. Soil. Sci. 1994 , 74 , 367–385. [ Google Scholar ] [ CrossRef ] [ Green Version ]
- Lal, R. Soil Carbon Sequestration to Mitigate Climate Change. Geoderma 2004 , 123 , 1–22. [ Google Scholar ] [ CrossRef ]
- Bongiorno, G. Novel Soil Quality Indicators for the Evaluation of Agricultural Management Practices: A Biological Perspective. Front. Agr. Sci. Eng. 2020 , 7 , 257. [ Google Scholar ] [ CrossRef ] [ Green Version ]
- Cavalieri-Polizeli, K.M.V.; Marcolino, F.C.; Tormena, C.A.; Keller, T.; de Moraes, A. Soil Structural Quality and Relationships with Root Properties in Single and Integrated Farming Systems. Front. Environ. Sci. 2022 , 10 , 901302. [ Google Scholar ] [ CrossRef ]
- Andriuzzi, W.S. Ecological Functions of Earthworms in Soil ; Wageningen University: Wageningen, The Netherlands, 2015; ISBN 978-94-6257-417-5. [ Google Scholar ]
- Zhang, G.; Xie, Z. Soil Surface Roughness Decay under Different Topographic Conditions. Soil Tillage Res. 2019 , 187 , 92–101. [ Google Scholar ] [ CrossRef ]
- Steffens, M.; Zeh, L.; Rogge, D.M.; Buddenbaum, H. Quantitative Mapping and Spectroscopic Characterization of Particulate Organic Matter Fractions in Soil Profiles with Imaging VisNIR Spectroscopy. Sci. Rep. 2021 , 11 , 16725. [ Google Scholar ] [ CrossRef ]
- Freitas, V.D.S.; de Babos, D.V.; Guedes, W.N.; Silva, F.P.; de Lima Tozo, M.L.; Martin-Neto, L.; Milori, D.M.B.P.; Villas-Boas, P.R. Assessing Soil Organic Matter Quality with Laser-Induced Fluorescence (LIFS) and Its Correlation to Soil Carbon Stock. In Proceedings of the Latin America Optics and Photonics (LAOP) Conference 2022, Pernambuco, Brazil, 7–11 August 2022; Optica Publishing Group: Recife, Brazil, 2022; p. W3B.5. [ Google Scholar ]
- Gao, J.; Liu, L.; Shi, Z.; Lv, J. Characteristics of Fluorescent Dissolved Organic Matter in Paddy Soil Amended with Crop Residues After Column (0–40 Cm) Leaching. Front. Environ. Sci. 2022 , 10 , 766795. [ Google Scholar ] [ CrossRef ]
- Leinweber, P.; Schulten, H.-R. Dynamics of Soil Organic Matter Studied by Pyrolysis—Field Ionization Mass Spectrometry. J. Anal. Appl. Pyrolysis 1993 , 25 , 123–136. [ Google Scholar ] [ CrossRef ]
- Brezinski, K.; Gorczyca, B. An Overview of the Uses of High Performance Size Exclusion Chromatography (HPSEC) in the Characterization of Natural Organic Matter (NOM) in Potable Water, and Ion-Exchange Applications. Chemosphere 2019 , 217 , 122–139. [ Google Scholar ] [ CrossRef ] [ PubMed ]
- Bahureksa, W.; Tfaily, M.M.; Boiteau, R.M.; Young, R.B.; Logan, M.N.; McKenna, A.M.; Borch, T. Soil Organic Matter Characterization by Fourier Transform Ion Cyclotron Resonance Mass Spectrometry (FTICR MS): A Critical Review of Sample Preparation, Analysis, and Data Interpretation. Environ. Sci. Technol. 2021 , 55 , 9637–9656. [ Google Scholar ] [ CrossRef ]
- Kujawinski, E. Electrospray Ionization Fourier Transform Ion Cyclotron Resonance Mass Spectrometry (ESI FT-ICR MS): Characterization of Complex Environmental Mixtures. Environ. Forensics 2002 , 3 , 207–216. [ Google Scholar ] [ CrossRef ]
- Kwiatkowska-Malina, J. Qualitative and Quantitative Soil Organic Matter Estimation for Sustainable Soil Management. J. Soils Sediments 2018 , 18 , 2801–2812. [ Google Scholar ] [ CrossRef ] [ Green Version ]
- Parton, W.J.; Del Grosso, S.J.; Plante, A.F.; Adair, E.C.; Lutz, S.M. Modeling the Dynamics of Soil Organic Matter and Nutrient Cycling. In Soil Microbiology, Ecology and Biochemistry ; Elsevier: Amsterdam, The Netherlands, 2015; pp. 505–537. ISBN 978-0-12-415955-6. [ Google Scholar ]
- Hénin, S.; Dupuis, M. Essai de Bilan de La Matière Organique Du Sol ; Chaix: Dudod, India, 1945. [ Google Scholar ]
- Roussel, O.; Bourmeau, E.; Walter, C. Evaluation Du Déficit En Matière Organique des Sols Français et Des Besoins Potentiels En Amendements Organiques. Etude Et Gest. Des Sols 2001 , 8 , 65–81. [ Google Scholar ]
- Remy, J.C.; Marin-Laflèche, A. L’analyse de terre: Réalisation d’un Programme d’interprétation Automatique. Ann. Agron. 1974 , 25 , 607–632. [ Google Scholar ]
- Kolenbrander, G.J. De Bepaling van de Waarde van Verschillende Soorten Organische Stof Ten Aanzien van Hun Effect Op Het Humusgehalte Bij Bouwland ; Inst. Bodemvruchtb: Haren, The Netherlands, 1969; Volume 17, pp. 69–88. [ Google Scholar ]
- Godshalk, G.L. Decomposition of Aquatic Plants in Lakes. Aquat. Bot. 1977 , 5 , 329–354. [ Google Scholar ] [ CrossRef ]
- Saunders, G.W. Transformation of Artificial Detritus in Lake Water. Mem. Ist. Ital. Idrobiol. Dott. Marco Marchi 1972 , 29 , 261–288. [ Google Scholar ]
- Bunnell, F.L.; Tait, D.E.N.; Flanagan, P.W.; Van Clever, K. Microbial Respiration and Substrate Weight Loss—I. Soil Biol. Biochem. 1977 , 9 , 33–40. [ Google Scholar ] [ CrossRef ]
- Minderman, G. Addition, Decomposition and Accumulation of Organic Matter in Forests. J. Ecol. 1968 , 56 , 355. [ Google Scholar ] [ CrossRef ]
- Janssen, B.H. Nitrogen Mineralization in Relation to C:N Ratio and Decomposability of Organic Materials. In Progress in Nitrogen Cycling Studies ; Springer: Dordrecht, The Netherlands, 1996; pp. 69–75. ISBN 978-94-010-6292-3. [ Google Scholar ]
- Janssen, B.H. A Simple Method for Calculating Decomposition and Accumulation of ‘Young’ Soil Organic Matter. Plant Soil 1984 , 76 , 297–304. [ Google Scholar ] [ CrossRef ]
- Yin, C.; Huang, D. A Model of Litter Decomposition and Accumulation in Grassland Ecosystems. Ecol. Model. 1996 , 84 , 75–80. [ Google Scholar ] [ CrossRef ]
- Andrén, O.; Kätterer, T. ICBM: The Introductory Carbon Balance Model for Exploration of Soil Carbon Balances. Ecol. Appl. 1997 , 7 , 1226–1236. [ Google Scholar ] [ CrossRef ]
- Andriulo, A.; Mary, B.; Guerif, J. Modelling Soil Carbon Dynamics with Various Cropping Sequences on the Rolling Pampas. Agronomie 1999 , 19 , 365–377. [ Google Scholar ] [ CrossRef ]
- Mary, B.; Guérif, J. Intérêts et Limites des Modèles de Prévision de l’évolution Des Matières Organiques et de l’azote Dans Le Sol. Cah. Agric. 1994 , 3 , 247–257. [ Google Scholar ]
- Chertov, O.G.; Komarov, A.S. SOMM: A Model of Soil Organic Matter Dynamics. Ecol. Model. 1997 , 94 , 177–189. [ Google Scholar ] [ CrossRef ]
- Lawrence, C.R.; Neff, J.C.; Schimel, J.P. Does Adding Microbial Mechanisms of Decomposition Improve Soil Organic Matter Models? A Comparison of Four Models Using Data from a Pulsed Rewetting Experiment. Soil Biol. Biochem. 2009 , 41 , 1923–1934. [ Google Scholar ] [ CrossRef ]
- Parton, W.J.; Anderson, D.W.; Cole, C.V.; Stewart, J.W.B. Simulation of Soil Organic Matter Formations and Mineralization in Semiarid Agroecosystems. In Nutrient Cycling in Agricultural Ecosystems ; Lowrance, R.R., Todd, R.L., Asmussen, L.E., Eds.; Springer: Berlin/Heidelberg, Germany, 1983; Volume 23, pp. 533–550. [ Google Scholar ]
- Parton, W.J.; Ojima, D.S.; Cole, C.V.; Schimel, D.S. A General Model for Soil Organic Matter Dynamics: Sensitivity to Litter Chemistry, Texture and Management. In SSSA Special Publications ; Bryant, R.B., Arnold, R.W., Eds.; Soil Science Society of America: Madison, WI, USA, 2015; pp. 147–167. ISBN 978-0-89118-934-3. [ Google Scholar ]
- Parton, W.J.; Stewart, J.W.B.; Cole, C.V. Dynamics of C, N, P and S in Grassland Soils: A Model. Biogeochemistry 1988 , 5 , 109–131. [ Google Scholar ] [ CrossRef ]
- Parton, W.J.; Ojima, D.S.; Schimel, D.S. Model ArchiveCENTURY: Modeling Ecosystem Responses to Climate Change, Version 4 (VEMAP 1995) ; ORNL DAAC: Oak Ridge, TN, USA, 2005. [ CrossRef ]
- Jenkinson, D.S.; Coleman, K. RothC-26.3—A Model for the Turnover of Carbon in Soil. In Evaluation of Soil Organic Matter Models ; Springer: Berlin/Heidelberg, Germany, 1996; pp. 237–246. ISBN 978-3-642-64692-8. [ Google Scholar ]
- Hart, P.B.S. Effects of Soil Type and Past Cropping on the Nitrogen Supplying Ability of Arable Soils. Ph.D. Thesis, University of Reading, Reading, UK, 1984. [ Google Scholar ]
- Veen, J.A.V.; Paul, E.A. Organic Carbon Dynamics in Grassland Soils. 1. Background Information and Computer Simulation. Can. J. Soil. Sci. 1981 , 61 , 185–201. [ Google Scholar ] [ CrossRef ] [ Green Version ]
- Li, C. The DNDC Model. In Evaluation of Soil Organic Matter Models ; Springer: Berlin/Heidelberg, Germany, 1996; pp. 263–267. ISBN 978-3-642-64692-8. [ Google Scholar ]
- Giltrap, D.L.; Li, C.; Saggar, S. DNDC: A Process-Based Model of Greenhouse Gas Fluxes from Agricultural Soils. Agric. Ecosyst. Environ. 2010 , 136 , 292–300. [ Google Scholar ] [ CrossRef ]
- Gilmanov, T.G.; Parton, W.J.; Ojima, D.S. Testing the ‘CENTURY’ Ecosystem Level Model on Data Sets from Eight Grassland Sites in the Former USSR Representing a Wide Climatic/Soil Gradient. Ecol. Model. 1997 , 96 , 191–210. [ Google Scholar ] [ CrossRef ]
- Necpálová, M.; Anex, R.P.; Fienen, M.N.; Del Grosso, S.J.; Castellano, M.J.; Sawyer, J.E.; Iqbal, J.; Pantoja, J.L.; Barker, D.W. Understanding the DayCent Model: Calibration, Sensitivity, and Identifiability through Inverse Modeling. Environ. Model. Softw. 2015 , 66 , 110–130. [ Google Scholar ] [ CrossRef ] [ Green Version ]
- Parton, W.J.; Hartman, M.; Ojima, D.; Schimel, D. DAYCENT and Its Land Surface Submodel: Description and Testing. Glob. Planet. Chang. 1998 , 19 , 35–48. [ Google Scholar ] [ CrossRef ]
- Mäkelä, J.; Arppe, L.; Fritze, H.; Heinonsalo, J.; Karhu, K.; Liski, J.; Oinonen, M.; Straková, P.; Viskari, T. Implementation and Initial Calibration of Carbon-13 Soil Organic Matter Decomposition in the Yasso Model. Biogeosciences 2022 , 19 , 4305–4313. [ Google Scholar ] [ CrossRef ]
- Liski, J.; Palosuo, T.; Peltoniemi, M.; Sievänen, R. Carbon and Decomposition Model Yasso for Forest Soils. Ecol. Model. 2005 , 189 , 168–182. [ Google Scholar ] [ CrossRef ]
- Jenkinson, D.S.; Adams, D.E.; Wild, A. Model Estimates of CO2 Emissions from Soil in Response to Global Warming. Nature 1991 , 351 , 304–306. [ Google Scholar ] [ CrossRef ]
- McGechan, M.B.; Hooda, P.S. Modelling Water Pollution by Leached Soluble Phosphorus, Part 1: Calibration of the ANIMO Model. Biosyst. Eng. 2010 , 106 , 138–146. [ Google Scholar ] [ CrossRef ]
- Rijtema, P.E.; Kroes, J.G. Some Results of Nitrogen Simulations with the Model ANIMO. Fertil. Res. 1991 , 27 , 189–198. [ Google Scholar ] [ CrossRef ]
- Franko, U. Modelling Approaches of Soil Organic Matter Turnover within the CANDY System. In Evaluation of Soil Organic Matter Models ; Powlson, D.S., Smith, P., Smith, J.U., Eds.; Springer: Berlin/Heidelberg, Germany, 1996; pp. 247–254. ISBN 978-3-642-64692-8. [ Google Scholar ]
- Huggins, D.R.; Singer, J.W.; Horwath, W.R. CANDY Model: A Spatially Explicit Model of Carbon, Nitrogen, and Energy Dynamics in Agroecosystems. Soil Sci. Soc. Am. J. 1991 , 55 , 175–183. [ Google Scholar ]
- Hanson, J.D.; Ahuja, L.R.; Shaffer, M.D.; Rojas, K.W.; DeCoursey, D.G.; Farahani, H.; Johnson, K. RZWQM: Simulating the Effects of Management on Water Quality and Crop Production. Agric. Syst. 1998 , 57 , 161–195. [ Google Scholar ] [ CrossRef ]
- Simulation of Nitrogen Behaviour of Soil-Plant Systems: Papers of a Workshop Models for the Behaviour of Nitrogen in Soil and Uptake by Plant …, Wageningen, the Netherlands, January 28–February 1, 1980 ; Frissel, M.J.; Veen, J.A. (Eds.) Centrum voor Landbouwpublikaties en Landbouwdocumentatie, Pudoc: Wageningen, The Netherlands, 1981; ISBN 978-90-220-0735-8. [ Google Scholar ]
- Seligman, N.; Keulen, H. A Simulation Model of Annual Pasture Production Limited by Rainfall and Nitrogen. In Simulation of Nitrogen Behaviour of Soil-Plant Systems ; Pudoc: Wageningen, The Netherlands, 1980; pp. 192–221. [ Google Scholar ]
- Molina, J.A.E.; Clapp, C.E.; Shaffer, M.J.; Chichester, F.W.; Larson, W.E. NCSOIL, A Model of Nitrogen and Carbon Transformations in Soil: Description, Calibration, and Behavior. Soil Sci. Soc. Am. J. 1983 , 47 , 85–91. [ Google Scholar ] [ CrossRef ]
- Hansen, S.; Abrahamsen, P.; Petersen, C.T.; Styczen, M. Daisy: Model Use, Calibration, and Validation. Trans. ASABE 2012 , 55 , 1317–1335. [ Google Scholar ] [ CrossRef ]
- Glendining, M.J.; Bailey, N.J.; Smith, J.U.; Addiscott, T.M.; Smith, P. SUNDIAL-FRS User Guide ; Version 1.0.; MAFF London/IACR-Rothamsted, Harpenden: Harpenden, UK, 1998. [ Google Scholar ]
- Grant, R.F. Changes in Soil Organic Matter under Different Tillage and Rotation: Mathematical Modeling in Ecosys. Soil Sci. Soc. Am. J. 1997 , 61 , 1159–1175. [ Google Scholar ] [ CrossRef ]
- Grant, R.F.; Mekonnen, Z.A.; Riley, W.J.; Wainwright, H.M.; Graham, D.; Torn, M.S. Mathematical Modelling of Arctic Polygonal Tundra with Ecosys : 1. Microtopography Determines How Active Layer Depths Respond to Changes in Temperature and Precipitation: Active Layer Depth in Polygonal Tundra. J. Geophys. Res. Biogeosci. 2017 , 122 , 3161–3173. [ Google Scholar ] [ CrossRef ]
- McCown, R.L.; Hammer, G.L.; Hargreaves, J.N.G.; Holzworth, D.P.; Freebairn, D.M. APSIM: A Novel Software System for Model Development, Model Testing and Simulation in Agricultural Systems Research. Agric. Syst. 1996 , 50 , 255–271. [ Google Scholar ] [ CrossRef ]
- Keating, B.A.; Carberry, P.S.; Hammer, G.L.; Probert, M.E.; Robertson, M.J.; Holzworth, D.; Huth, N.I.; Hargreaves, J.N.G.; Meinke, H.; Hochman, Z.; et al. An Overview of APSIM, a Model Designed for Farming Systems Simulation. Eur. J. Agron. 2003 , 18 , 267–288. [ Google Scholar ] [ CrossRef ] [ Green Version ]
- Parton, W.J. Ecosystem Model Comparisons: Science or Fantasy World? In Evaluation of Soil Organic Matter Models: Using Existing Long-Term Datasets 1996 ; Springer: Berlin/Heidelberg, Germany, 1996. [ Google Scholar ]
- Meki, M.N.; Kiniry, J.R.; Behrman, K.D.; Pawlowski, M.N.; Crow, S.E. The Role of Simulation Models in Monitoring Soil Organic Carbon Storage and Greenhouse Gas Mitigation Potential in Bioenergy Cropping Systems. In CO 2 Sequestration and Valorization ; Esteves, V., Ed.; InTech: Rijeka, Croatia, 2014; Chapter 9; ISBN 978-953-51-1225-9. [ Google Scholar ]
- Le Noë, J.; Manzoni, S.; Abramoff, R.; Bölscher, T.; Bruni, E.; Cardinael, R.; Ciais, P.; Chenu, C.; Clivot, H.; Derrien, D.; et al. Soil Organic Carbon Models Need Independent Time-Series Validation for Reliable Prediction. Commun. Earth Environ. 2023 , 4 , 158. [ Google Scholar ] [ CrossRef ]
- Krull, E.S.; Baldock, J.A.; Skjemstad, J.O. Importance of Mechanisms and Processes of the Stabilisation of Soil Organic Matter for Modelling Carbon Turnover. Funct. Plant Biol. 2003 , 30 , 207. [ Google Scholar ] [ CrossRef ]
Terminology | Description |
---|---|
Litter | Organic material onto the soil surface excluding mineral residues. |
Microbial biomass | The organic matter that is from the dead or cells of living microbial organisms. |
Primary soil organic matter | Soil organic elements that are (or are not) partly decomposed and have not humified yet, comprising dead roots, other plant parts, and soil organisms. They consist of less stable fractions to biodegradability, they are highly oxidizable, and their cation exchange capacity is negligible. |
Labile SOM | Actively decomposing free fractions of SOM. |
Free SOM | Labile fractions of SOM with a high rate of decomposition. |
Free light fraction SOM | Free SOM density fractionation gives free light fraction SOM and then occluded SOM fractions. The free light fraction is that from the organic matter of the outer surface of soil aggregates or pseudo-aggregates. They are more labile than occluded fractions. |
Occluded SOM | The organic matter trapped inside aggregates is fractionated, resulting from ultrasonic disintegration of soil stable aggregates, leaving out heavy fractions or organominerals. They are more stable and their conversion time may range from decades to centuries. They are degradable once out of aggregates. |
Organomineral SOM fractions | The SOM found in minerals form organomineral complexes. They are more stable and their conversion time may range from decades to centuries. |
Particulate organic matter | Organic material corresponding to particle sizes of 53–2000 μm (Detritus, Litter of plants …) |
Stable SOM | Resistant, passive, inert fractions of SOM to decomposition |
Humus | Humified soil organic elements with stable fractions to biodegradation and oxidation or hydrolysis and with high cation exchange capacity. They remain in the soil after macro-organic matter and dissolved organic matter are removed. They are amorphous colloidal particles less than 53 μm. |
Non-humic biomolecules | They are biopolymers including polysaccharides and sugars, proteins and amino acids, fats, waxes, other lipids, and lignin. |
Humin | Insoluble part of organic matter after extraction of aqueous base soluble part. Alkaline-solution-insoluble organic material. |
Humic Acid | Alkaline-solution-soluble organic materials, which precipitate on acidification of the alkaline extracts. |
Fulvic Acid | Organic materials that are both soluble in alkaline solution and acidic solution of the alkaline extracts |
Dissolved organic matter | Organic compounds that are soluble in water. They are less than 0.45 μm and are mostly found in soil solution |
Resistant or Inert organic matter | They are organic materials with very long chains of carbon (heavily carbonized). They are materials of high carbon content like charcoal, charred plant materials, graphite, and coal and have a very long turnover time |
Organic matter | Biomaterial under different levels of decomposition or decaying process. |
Organic matter fractions | Measurable organic matter components. |
Organic matter pool (stock) | Theoretically separated, kinetically delineated components of soil organic matter. |
Carbon turnover | The average time taken for carbon mineralization and transformation in terrestrial ecosystem from one pool to another |
Decomposition and transformation | Physical breakdown and chemical transformation of complex organic substrate into simpler components molecules. |
Humification | Process of humic substances’ formation from organic materials. |
SOM modeling | Process of using mathematical models to analyze and simulate the changes of organic matter in the soil. |
The statements, opinions and data contained in all publications are solely those of the individual author(s) and contributor(s) and not of MDPI and/or the editor(s). MDPI and/or the editor(s) disclaim responsibility for any injury to people or property resulting from any ideas, methods, instructions or products referred to in the content. |
Share and Cite
Murindangabo, Y.T.; Kopecký, M.; Konvalina, P.; Ghorbani, M.; Perná, K.; Nguyen, T.G.; Bernas, J.; Baloch, S.B.; Hoang, T.N.; Eze, F.O.; et al. Quantitative Approaches in Assessing Soil Organic Matter Dynamics for Sustainable Management. Agronomy 2023 , 13 , 1776. https://doi.org/10.3390/agronomy13071776
Murindangabo YT, Kopecký M, Konvalina P, Ghorbani M, Perná K, Nguyen TG, Bernas J, Baloch SB, Hoang TN, Eze FO, et al. Quantitative Approaches in Assessing Soil Organic Matter Dynamics for Sustainable Management. Agronomy . 2023; 13(7):1776. https://doi.org/10.3390/agronomy13071776
Murindangabo, Yves Theoneste, Marek Kopecký, Petr Konvalina, Mohammad Ghorbani, Kristýna Perná, Thi Giang Nguyen, Jaroslav Bernas, Sadia Babar Baloch, Trong Nghia Hoang, Festus Onyebuchi Eze, and et al. 2023. "Quantitative Approaches in Assessing Soil Organic Matter Dynamics for Sustainable Management" Agronomy 13, no. 7: 1776. https://doi.org/10.3390/agronomy13071776
Article Metrics
Article access statistics, further information, mdpi initiatives, follow mdpi.
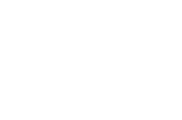
Subscribe to receive issue release notifications and newsletters from MDPI journals
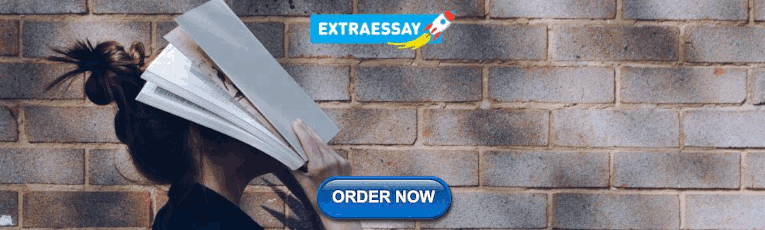
IMAGES
VIDEO
COMMENTS
2. SAMPLE SIZE AND SELECTION. Quantitative research usually aims to provide precise, unbiased estimates of parameters of interest for the entire population which requires a large, randomly selected sample. Brett et al 4 reported a positive impact of PPI on recruitment in studies, but the representativeness of the sample is as important in quantitative research as sample size.
Background Identifying determinants of health and understanding their role in health production constitutes an important research theme. We aimed to document the state of recent multi-country research on this theme in the literature. Methods We followed the PRISMA-ScR guidelines to systematically identify, triage and review literature (January 2013—July 2019).
The complexity of public health interventions create challenges in evaluating their effectiveness. There have been huge advancements in quantitative evidence synthesis methods development (including meta-analysis) for dealing with heterogeneity of intervention effects, inappropriate 'lumping' of interventions, adjusting for different populations and outcomes and the inclusion of various ...
We use a range of data in order to fulfil this need, both quantitative and qualitative. Data are defined as "information, especially facts and numbers, collected to be examined and considered and used to help decision-making." 1 Data are used to make judgements, to answer questions, and to monitor and support improvement in healthcare ( box ...
Quantitative metrics: 'a system or standard of [quantitative] measurement' ... These represent an important interim stage in the process towards the final expected impacts, such as quantifiable health improvements and economic benefits, ... et al. Primary Health Care Research Impact Project: Final Report Stage 1 Adelaide: Primary Health ...
This paper gives an overview of the main quantitative research designs relevant to nursing and healthcare. It outlines some strengths and weaknesses of the designs, provides examples to illustrate the different designs and examines some of the relevant statistical concepts.
Abstract. Quantitative research is the foundation for evidence-based global health practice and interventions. Preparing health research starts with a clear research question to initiate the study, careful planning using sound methodology as well as the development and management of the capacity and resources to complete the whole research cycle.
Evidence-Based Health Research. Given the benefits of quantitative methods in health care, evidence-based medicine seeks to use scientific methods to determine which drugs and procedures are best for treating diseases. At the core of evidence-based practice is the systematic and predominantly quantitative review of randomized controlled trials.
Quantitative research methods are concerned with the planning, design, and implementation of strategies to collect and analyze data. Descartes, the seventeenth-century philosopher, suggested that how the results are achieved is often more important than the results themselves, as the journey taken along the research path is a journey of discovery. . High-quality quantitative research is ...
Quantitative analysis refers to the process of using complex mathematical or statistical modeling to make sense of data and potentially to predict behavior. Though quantitative analysis is well-established in the fields of economics and finance, cutting-edge quantitative analysis has only recently become possible in health care.
There has been a dramatic increase in the body of evidence demonstrating the benefits that come from health research. In 2014, the funding bodies for higher education in the UK conducted an assessment of research using an approach termed the Research Excellence Framework (REF). As one element of the REF, universities and medical schools in the UK submitted 1,621 case studies claiming to show ...
Evidence-based practice includes, in part, implementation of the findings of well-conducted quality research studies. So being able to critique quantitative research is an important skill for nurses. Consideration must be given not only to the results of the study but also the rigour of the research. Rigour refers to the extent to which the researchers worked to enhance the quality of the studies.
The use of research questionnaires or surveys in nursing is a long standing tradition, dating back to the 1960s (Logan, 1966) and 1970s (Oberst, 1978), when the scientific discipline emerged.This type of tool enables nursing researchers to gather primary data from a specific population, whether it is patients, carers, nurses, or other stakeholders to address gaps in the existing evidence base ...
Health research entails systematic collection or analysis of data with the intent to develop generalizable knowledge to understand health challenges and mount an improved response to them. The full spectrum of health research spans five generic areas of activity: measuring the health problem; understanding its cause(s); elaborating solutions; translating the solutions or evidence into policy ...
Title, keywords and the authors. The title of a paper should be clear and give a good idea of the subject area. The title should not normally exceed 15 words 2 and should attract the attention of the reader. 3 The next step is to review the key words. These should provide information on both the ideas or concepts discussed in the paper and the ...
Combining quantitative and qualitative methods in a single study is not uncommon in social research, although, 'traditionally a gulf is seen to exist between qualitative and quantitative research with each belonging to distinctively different paradigms'. [] Within health research there has, more recently, been an upsurge of interest in the combined use of qualitative and quantitative methods ...
Quantitative research design is defined as a research method used in various disciplines, including social sciences, psychology, economics, and market research. It aims to collect and analyze numerical data to answer research questions and test hypotheses. Quantitative research design offers several advantages, including the ability to ...
This method, known as mixed methods research, offers several benefits, including: A comprehensive understanding: Integration of qualitative and quantitative data provides a more comprehensive understanding of the research problem. Qualitative data helps explain the context and nuances, while quantitative data offers statistical generalizability.
Written by Coursera Staff • Updated on Apr 19, 2024. Data analysis is the practice of working with data to glean useful information, which can then be used to make informed decisions. "It is a capital mistake to theorize before one has data. Insensibly one begins to twist facts to suit theories, instead of theories to suit facts," Sherlock ...
The Nuclear Research Foundation School Certificate Integrated, Volume 1. Book ... A Quantitative Outlook. Book • 2014. Academic Voices. ... Accelerator Health Physics. Book • 1973. Acceptance and Commitment Therapy. The Clinician's Guide for Supporting Parents. Book
This quantitative research study was conducted to demonstrate the Assessment of the Levelof Effectiveness of using Office Technology in the barangay healthcare staff, medical officer,and medical encoder as to the healthcare services in the barangay Novaliches ProperDistrict V, Quezon City. The researchers aimed to provide knowledge to the readers aboutthe information and important ideas about ...
Americans' Views of Technology Companies. Most Americans are wary of social media's role in politics and its overall impact on the country, and these concerns are ticking up among Democrats. Still, Republicans stand out on several measures, with a majority believing major technology companies are biased toward liberals. short readsApr 3, 2024.
Research and insights that will help guide you to success on social. Connect. ... Healthcare/Wellness: 0.08%; Travel/hospitality/leisure: 0.03%; 3. Virality rate ... download, or sale. This is one of the most important social media marketing metrics because it shows the value of your social media campaigns (organic and paid) as a means of ...
The aim of this study was to provide an overview of the approaches and methods used to assess the dynamics of soil organic matter (SOM). This included identifying relevant processes that describe and estimate SOM decomposition, lability, and humification for the purpose of sustainable management. Various existing techniques and models for the qualitative and quantitative assessment of SOM were ...