Deepfake generation and detection, a survey
- Published: 08 January 2022
- Volume 81 , pages 6259–6276, ( 2022 )
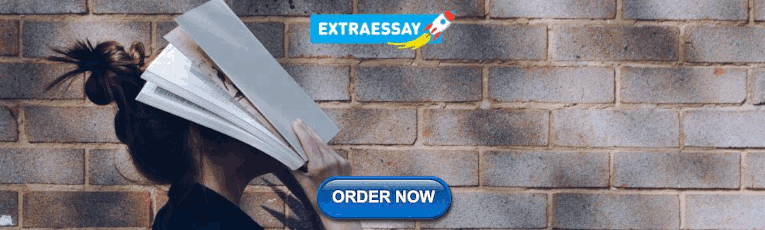
Cite this article
- Tao Zhang ORCID: orcid.org/0000-0001-8242-5691 1 , 2 , 3 , 4
7940 Accesses
42 Citations
2 Altmetric
Explore all metrics
Deepfake refers to realistic, but fake images, sounds, and videos generated by articial intelligence methods. Recent advances in deepfake generation make deepfake more realistic and easier to make. Deepfake has been a signicant threat to national security, democracy, society, and our privacy, which calls for deepfake detection methods to combat potential threats. In the paper, we make a survey on state-ofthe-art deepfake generation methods, detection methods, and existing datasets. Current deepfake generation methods can be classified into face swapping and facial reenactment. Deepfake detection methods are mainly based features and machine learning methods. There are still some challenges for deepfake detection, such as progress on deepfake generation, lack of high quality datasets and benchmark. Future trends on deepfake detection can be efficient, robust and systematical detection methods and high quality datasets.
This is a preview of subscription content, log in via an institution to check access.
Access this article
Price includes VAT (Russian Federation)
Instant access to the full article PDF.
Rent this article via DeepDyve
Institutional subscriptions
Similar content being viewed by others
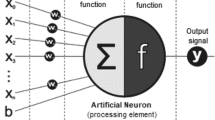
Deep Learning: A Comprehensive Overview on Techniques, Taxonomy, Applications and Research Directions
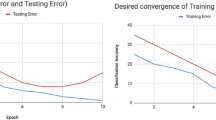
A survey on Image Data Augmentation for Deep Learning
CBAM: Convolutional Block Attention Module
(2018) Malicious deep fake prohibition act of 2018. https://www.congress.gov/bill/115th-congress/senate-bill/3805/text
(2019) Defending each and every person from false appearances by keeping exploitation subject to accountability Act of 2019. https://www.congress.gov/bill/116th-congress/house-bill/3230/text
Afchar D, et al (2018) Mesonet: a compact facial video forgery detection network. In: 2018 IEEE international workshop on information forensics and security (WIFS). IEEE
Agarwal S, et al (2019) Protecting world leaders against deep fakes. In: Proceedings of the IEEE conference on computer vision and pattern recognition workshops
Ajder H, Cavalli F, Patrini G, Cullen L (2019) The state of Deepfakes: Landscape, threats, and impact
Amerini I, Caldelli R (2020) Exploiting prediction error inconsistencies through LSTM-based classifiers to detect deepfake videos. In: Proceedings of the 2020 ACM workshop on information hiding and multimedia security. Association for Computing Machinery: Denver, CO, USA, pp 97–102
Amerini I, et al (2019) Deepfake video detection through optical flow based CNN. In: Proceedings of the IEEE international conference on computer vision workshops
Bayar B, Stamm MC (2016) A deep learning approach to universal image manipulation detection using a new convolutional layer, pp 5–10
Carlini N, Farid H (2020) Evading deepfake-image detectors with white- and black-box attacks. In: 2020 IEEE/CVF conference on computer vision and pattern recognition workshops (CVPRW)
Chan C, et al (2018) Everybody dance now. arXiv:1808.07371
Chang X, et al (2020) Deepfake face image detection based on improved VGG convolutional neural network. In: 2020 39th chinese control conference (CCC)
Chen D et al (2019) Face swapping: realistic image synthesis based on facial landmarks alignment. Mathematical Problems in Engineering 2019:1–11
Google Scholar
Chesney R, Citron DK (2018) Deep fakes: a looming challenge for privacy, democracy, and national security
Chintha A et al (2020) Recurrent convolutional structures for audio spoof and video deepfake detection. IEEE Journal of Selected Topics in Signal Processing 14(5):1024–1037
Article Google Scholar
Chintha A, et al (2020) Leveraging edges and optical flow on faces for Deepfake detection. In: 2020 IEEE international joint conference on biometrics (IJCB)
Choi Y, et al (2018) Stargan: Unified generative adversarial networks for multi-domain image-to-image translation. In: Proceedings of the IEEE conference on computer vision and pattern recognition
Ciftci UA, Demir I, Yin L (2020) How do the hearts of deep fakes beat? Deep fake source detection via interpreting residuals with biological signals. In: 2020 IEEE international joint conference on biometrics (IJCB)
Dang L et al (2018) Deep learning based computer generated face identification using convolutional neural network. Applied Sciences 8(12):2610
DeepFaceLab. Available from: https://github.com/iperov/DeepFaceLab
Deepfake reddit. Available from: https://www.reddit.com/user/Deepfakes/
DeepFakeDetection data. https://github.com/ondyari/FaceForensics/blob/master/dataset/README.md
Deepfakes. Available from: https://github.com/Deepfakes/
Ding X, et al (2019) Swapped face detection using deep learning and subjective assessment. arXiv:1909.04217
Dolhansky B, et al (2019) The deepfake detection challenge (DFDC) preview dataset. arXiv:1910.08854
Dolhansky B, et al (2020) The deepfake detection challenge (DFDC) dataset. arXiv:2006.07397
Du CXT, et al (2020) Efficient-frequency: a hybrid visual forensic framework for facial forgery detection. In: 2020 IEEE symposium series on computational intelligence (SSCI)
Faceapp. Available from: http://www.faceapp.com/
Farid H (2009) Image forgery detection. IEEE Signal Processing Magazine 26(2):16–25
Fernandes S, et al (2019) Predicting heart rate variations of deepfake videos using neural ODE. In: Proceedings of the IEEE international conference on computer vision workshops
Gandhi A, Jain S (2020) Adversarial perturbations fool deepfake detectors. In: 2020 international joint conference on neural networks (IJCNN)
Geng Z, Cao C, Tulyakov S (2019) 3d guided fine-grained face manipulation. In: Proceedings of the IEEE conference on computer vision and pattern recognition
Gera D, Delp EJ (2018) Deepfake video detection using recurrent neural networks. In: 2018 15th IEEE international conference on advanced video and signal based surveillance (AVSS)
Goodfellow I, et al (2014) Generative adversarial nets. In: Advances in neural information processing systems
Gouhara K, Watanabe T, Uchikawa Y (1991) Learning process of recurrent neural networks. In: [Proceedings] 1991 IEEE international joint conference on neural networks
Guan H, et al (2019) MFC datasets: Large-scale benchmark datasets for media forensic challenge evaluation. In: 2019 IEEE winter applications of computer vision workshops (WACVW). IEEE
Guarnera L, Giudice O, Battiato S (2020) Deepfake detection by analyzing convolutional traces. In: 2020 IEEE/CVF conference on computer vision and pattern recognition workshops (CVPRW)
Gupta P, et al (2020) The eyes know it: FakeET-an eye-tracking database to understand Deepfake perception, In: Proceedings of the 2020 international conference on multimodal interaction, Association for Computing Machinery: Virtual Event, Netherlands, pp 519–527
Hearst MA et al (1998) Support vector machines. IEEE Intelligent Systems and their Applications 13(4):18–28
Hochreiter S, Schmidhuber J (1997) Long short-term memory. Neural Computation 9(8):1735–1780
Hsu C-C, Lee C-Y, Zhuang Y-X (2018) Learning to detect fake face images in the wild. In: 2018 international symposium on computer, consumer and control (IS3C). IEEE
Huang Y, et al (2020) FakePolisher: making deepfakes more detection-evasive by shallow reconstruction. In: Proceedings of the 28th ACM international conference on multimedia. Association for Computing Machinery, pp 1217–1226
Huh M, et al (2018) Fighting fake news: Image splice detection via learned self-consistency. In: Proceedings of the European conference on computer vision (ECCV)
Isola P, et al (2017) Image-to-image translation with conditional adversarial networks. In: Proceedings of the IEEE conference on computer vision and pattern recognition
Jung T, Kim S, Kim K (2020) DeepVision: Deepfakes detection using human eye blinking pattern. IEEE Access 8:83144–83154
Karras T, et al (2017) Progressive growing of gans for improved quality, stability, and variation. arXiv:1710.10196
Karras T, Laine S, Aila T (2019) A style-based generator architecture for generative adversarial networks. In: Proceedings of the IEEE conference on computer vision and pattern recognition
Khalid H, Woo SS (2020) OC-FakeDect: classifying deepfakes using one-class variational autoencoder. In: 2020 IEEE/CVF conference on computer vision and pattern recognition workshops (CVPRW)
Kharbat FF, et al (2019) Image feature detectors for deepfake video detection. In: 2019 IEEE/ACS 16th international conference on computer systems and applications (AICCSA)
Khodabakhsh A, Busch C (2020) A generalizable deepfake detector based on neural conditional distribution modelling. In: 2020 international conference of the biometrics special interest group (BIOSIG)
Khodabakhsh A, et al (2018) Fake face detection methods: can they be generalized? In: 2018 international conference of the biometrics special interest group (BIOSIG). IEEE
Kim H et al (2018) Deep video portraits. ACM Transactions on Graphics (TOG) 37(4):163
Korshunova I, et al (2017) Fast face-swap using convolutional neural networks. In: Proceedings of the IEEE international conference on computer vision
Korshunov P, Marcel S (2018) Deepfakes: a new threat to face recognition? assessment and detection. arXiv:1812.08685
Korshunov P, Marcel S (2018) Speaker inconsistency detection in tampered video. In: 2018 26th european signal processing conference (EUSIPCO). IEEE
Korshunov P, Marcel S (2019) Vulnerability assessment and detection of Deepfake videos. In: The 12th IAPR international conference on biometrics (ICB)
LeCun Y, Bengio Y, Hinton G (2015) Deep learning. Nature 521(7553):436–444
Liang T, et al (2020) SDHF: spotting deepfakes with hierarchical features. In: 2020 IEEE 32nd international conference on tools with artificial intelligence (ICTAI)
Li Y, Chang M-C, Lyu S (2018) In ictu oculi: Exposing ai created fake videos by detecting eye blinking. In: 2018 IEEE international workshop on information forensics and security (WIFS). IEEE
Li H, et al (2018) Can forensic detectors identify GAN generated images? In: 2018 Asia-pacific signal and information processing association annual summit and conference (APSIPA ASC)
Li Y, et al (2020) Celeb-DF: A large-scale challenging dataset for deepfake forensics. In: 2020 IEEE/CVF conference on computer vision and pattern recognition (CVPR)
Li Y, Lyu S (2018) Exposing Deepfake videos by detecting face warping artifacts. arXiv:1811.00656
Matern F, Riess C, Stamminger M (2019) Exploiting visual artifacts to expose Deepfakes and face manipulations. In: 2019 IEEE winter applications of computer vision workshops (WACVW)
Mittal T, et al (2020) Emotions Don’t Lie: An audio-visual deepfake detection method using affective cues. In: Proceedings of the 28th ACM international conference on multimedia. Association for Computing Machinery, pp 2823–2832
Mo H, Chen B, Luo W (2018) Fake faces identification via convolutional neural network. In: Proceedings of the 6th ACM workshop on information hiding and multimedia security. ACM
Montserrat DM, et al (2020) Deepfakes detection with automatic face weighting. In: 2020 IEEE/CVF conference on computer vision and pattern recognition workshops (CVPRW)
Nataraj L, et al (2019) Detecting GAN generated fake images using co-occurrence matrices. arXiv:1903.06836
Natsume R, Yatagawa T, Morishima S (2018) RSGAN: face swapping and editing using face and hair representation in latent spaces. arXiv:1804.03447
Neves JC et al (2020) GANprintR: improved fakes and evaluation of the state of the art in face manipulation detection. IEEE Journal of Selected Topics in Signal Processing 14(5):1038–1048
Nguyen HM, Derakhshani R (2020) Eyebrow recognition for identifying Deepfake videos. In: 2020 international conference of the biometrics special interest group (BIOSIG)
Nguyen HH, Yamagishi J, Echizen I (2019) Capsule-forensics: using capsule networks to detect forged images and videos. In: ICASSP 2019-2019 IEEE international conference on acoustics, speech and signal processing (ICASSP). IEEE
Nirkin Y, Keller Y, Hassner T (2019) FSGAN: Subject agnostic face swapping and reenactment. arXiv:1908.05932
Oord Avd, et al (2016) Wavenet: A generative model for raw audio. arXiv:1609.03499
Pan SJ, Yang Q (2009) A survey on transfer learning. IEEE Transactions on Knowledge and Data Engineering 22(10):1345–1359
Patel M, et al (2020) Trans-DF: a transfer learning-based end-to-end deepfake detector. In: 2020 IEEE 5th international conference on computing communication and automation (ICCCA)
Pu J, et al (2020) NoiseScope: detecting deepfake images in a blind setting. In: Annual computer security applications conference. Association for Computing Machinery, Austin, pp 913–927
Pumarola A, et al (2018) Ganimation: Anatomically-aware facial animation from a single image. In: Proceedings of the european conference on computer vision (ECCV)
Rahmouni N, et al (2017) Distinguishing computer graphics from natural images using convolution neural networks. In: 2017 IEEE workshop on information forensics and security (WIFS). IEEE
Rana MS, Sung AH (2020) DeepfakeStack: a deep ensemble-based learning technique for deepfake detection. In: 2020 7th IEEE international conference on cyber security and cloud computing (CSCloud)/2020 6th IEEE international conference on edge computing and scalable cloud (EdgeCom)
Ranjan P, Patil S, Kazi F (2020) Improved generalizability of deep-fakes detection using transfer learning based CNN framework. In: 2020 3rd international conference on information and computer technologies (ICICT)
Rssler A, et al (2018) Faceforensics: A large-scale video dataset for forgery detection in human faces. arXiv:1803.09179
Rssler A, et al (2019) FaceForensics++: learning to detect manipulated facial images. In: 2019 IEEE/CVF international conference on computer vision (ICCV)
Sabir E et al (2019) Recurrent convolutional strategies for face manipulation detection in videos. Interfaces (GUI) 3:1
Thies J et al (2018) Headon: Real-time reenactment of human portrait videos. ACM Transactions on Graphics (TOG) 37(4):164
Thies J, Zollhfer M, Niener M (2019) Deferred neural rendering: image synthesis using neural textures. ACM Trans. Graph 38(4):66
Thies J, et al (2016) Face2face: Real-time face capture and reenactment of rgb videos. In: Proceedings of the IEEE conference on computer vision and pattern recognition
Tursman E, et al (2020) Towards untrusted social video verification to combat deepfakes via face geometry consistency. In: 2020 IEEE/CVF conference on computer vision and pattern recognition workshops (CVPRW)
Wang S-Y, et al (2019) Detecting photoshopped faces by scripting photoshop. arXiv:1906.05856
Xie D, et al (2020) Deepfake detection on publicly available datasets using modified AlexNet. In: 2020 IEEE symposium series on computational intelligence (SSCI)
Yang T, et al (2020) VTD-Net: depth face forgery oriented video tampering detection based on convolutional neural network. In: 2020 39th chinese control conference (CCC)
Yang X, Li Y, Lyu S (2019) Exposing deep fakes using inconsistent head poses. In: 2019 IEEE international conference on acoustics, speech and signal processing (ICASSP)
Yu N, Davis L, Fritz M (2018) Attributing fake images to GANs: Analyzing fingerprints in generated images. arXiv:1811.08180
ZAO. Available from: https://www.zaoapp.net
Zakharov E, et al (2019) Few-shot adversarial learning of realistic neural talking head models. arXiv:1905.08233
Zhao Y et al (2020) Capturing the persistence of facial expression features for deepfake video detection. Springer International Publishing, Cham
Book Google Scholar
Zhao Z, Wang P, Lu W (2020) Detecting deepfake video by learning two-level features with two-stream convolutional neural network. In: Proceedings of the 2020 6th international conference on computing and artificial intelligence. Association for Computing Machinery, pp 291–297
Zhou P, et al (2017) Two-stream neural networks for tampered face detection. In: 2017 IEEE conference on computer vision and pattern recognition workshops (CVPRW). IEEE
Zhuang Y-X, Hsu C-C (2019) Detecting generated image based on a coupled network with two-step pairwise learning. In: 2019 IEEE international conference on image processing (ICIP). IEEE
Zhu K, Wu B, Wang B (2020) Deepfake detection with clustering-based embedding regularization. In: 2020 IEEE fifth international conference on data science in cyberspace (DSC)
Zi B, et al (2020) WildDeepfake: a challenging real-world dataset for deepfake detection. In: Proceedings of the 28th ACM international conference on multimedia. Association for Computing Machinery, pp 2382–2390
Download references
Acknowledgements
This work was supported by Guangxi Key Laboratory of Cryptography and Information Security (GCIS201806), Guangxi Key Laboratory of Trusted Software (No. kx202016), Key Lab of Film and TV Media Technology of Zhejiang Province (No.2020E10015), Guangxi Key Laboratory of Hybrid Computation and IC Design Analysis, Guangxi University for Nationalities(GXIC20-03), Key Laboratory of Oceanographic Big Data Mining & Application of Zhejiang Province(obdma202001). We’d like to thank Zelei Cheng from Purdue University and Yingjie Wang from Virginia Tech for writing assistance, language editing, and proofreading.
Author information
Authors and affiliations.
School of Cyber Science and Technology, Beihang University, Beijing, China
Guangxi Key Laboratory of Cryptography and Information Security, Guilin University of Electronic Technology, Guilin, China
Guangxi Key Laboratory of Trusted Software & Guangxi Key Laboratory of Cryptography and Information Security, Guilin University of Electronic Technology, Guilin, China
Key Lab of Film and TV Media Technology of Zhejiang Province, Hangzhou, China
You can also search for this author in PubMed Google Scholar
Corresponding author
Correspondence to Tao Zhang .
Ethics declarations
Conflicts of interest.
The authors declare that they have no conflict of interest.
Additional information
Publisher's note.
Springer Nature remains neutral with regard to jurisdictional claims in published maps and institutional affiliations.
Rights and permissions
Reprints and permissions
About this article
Zhang, T. Deepfake generation and detection, a survey. Multimed Tools Appl 81 , 6259–6276 (2022). https://doi.org/10.1007/s11042-021-11733-y
Download citation
Received : 27 August 2020
Revised : 02 September 2021
Accepted : 05 November 2021
Published : 08 January 2022
Issue Date : February 2022
DOI : https://doi.org/10.1007/s11042-021-11733-y
Share this article
Anyone you share the following link with will be able to read this content:
Sorry, a shareable link is not currently available for this article.
Provided by the Springer Nature SharedIt content-sharing initiative
- Media forensics
- Find a journal
- Publish with us
- Track your research
Thank you for visiting nature.com. You are using a browser version with limited support for CSS. To obtain the best experience, we recommend you use a more up to date browser (or turn off compatibility mode in Internet Explorer). In the meantime, to ensure continued support, we are displaying the site without styles and JavaScript.
- View all journals
- My Account Login
- Explore content
- About the journal
- Publish with us
- Sign up for alerts
- Open access
- Published: 08 May 2023
Deep fake detection and classification using error-level analysis and deep learning
- Rimsha Rafique 1 ,
- Rahma Gantassi 2 ,
- Rashid Amin 1 , 3 ,
- Jaroslav Frnda 4 , 5 ,
- Aida Mustapha 6 &
- Asma Hassan Alshehri 7
Scientific Reports volume 13 , Article number: 7422 ( 2023 ) Cite this article
19k Accesses
13 Citations
6 Altmetric
Metrics details
- Energy science and technology
- Mathematics and computing
Due to the wide availability of easy-to-access content on social media, along with the advanced tools and inexpensive computing infrastructure, has made it very easy for people to produce deep fakes that can cause to spread disinformation and hoaxes. This rapid advancement can cause panic and chaos as anyone can easily create propaganda using these technologies. Hence, a robust system to differentiate between real and fake content has become crucial in this age of social media. This paper proposes an automated method to classify deep fake images by employing Deep Learning and Machine Learning based methodologies. Traditional Machine Learning (ML) based systems employing handcrafted feature extraction fail to capture more complex patterns that are poorly understood or easily represented using simple features. These systems cannot generalize well to unseen data. Moreover, these systems are sensitive to noise or variations in the data, which can reduce their performance. Hence, these problems can limit their usefulness in real-world applications where the data constantly evolves. The proposed framework initially performs an Error Level Analysis of the image to determine if the image has been modified. This image is then supplied to Convolutional Neural Networks for deep feature extraction. The resultant feature vectors are then classified via Support Vector Machines and K-Nearest Neighbors by performing hyper-parameter optimization. The proposed method achieved the highest accuracy of 89.5% via Residual Network and K-Nearest Neighbor. The results prove the efficiency and robustness of the proposed technique; hence, it can be used to detect deep fake images and reduce the potential threat of slander and propaganda.
Similar content being viewed by others
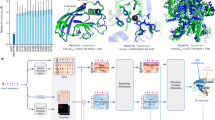
Highly accurate protein structure prediction with AlphaFold
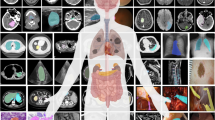
Segment anything in medical images
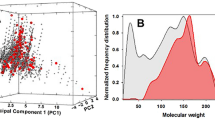
Forecasting vaping health risks through neural network model prediction of flavour pyrolysis reactions
Introduction.
In the last decade, social media content such as photographs and movies has grown exponentially online due to inexpensive devices such as smartphones, cameras, and computers. The rise in social media applications has enabled people to quickly share this content across the platforms, drastically increasing online content, and providing easy access. At the same time, we have seen enormous progress in complex yet efficient machine learning (ML) and Deep Learning (DL) algorithms that can be deployed for manipulating audiovisual content to disseminate misinformation and damage the reputation of people online. We now live in such times where spreading disinformation can be easily used to sway peoples’ opinions and can be used in election manipulation or defamation of any individual. Deep fake creation has evolved dramatically in recent years, and it might be used to spread disinformation worldwide, posing a serious threat soon. Deep fakes are synthesized audio and video content generated via AI algorithms. Using videos as evidence in legal disputes and criminal court cases is standard practice. The authenticity and integrity of any video submitted as evidence must be established. Especially when deep fake generation becomes more complex, this is anticipated to become a difficult task.
The following categories of deep fake videos exist: face-swap, synthesis, and manipulation of facial features. In face-swap deep fakes, a person's face is swapped with that of the source person to create a fake video to target a person for the activities they have not committed 1 , which can tarnish the reputation of the person 2 . In another type of deep fake called lip-synching, the target person’s lips are manipulated to alter the movements according to a certain audio track. The purpose of lip-syncing is to simulate the victim's attacker's voice by having someone talk in that voice. With puppet-master, deep fakes are produced by imitating the target's facial expressions, eye movements, and head movements. Using fictitious profiles, this is done to propagate false information on social media. Last but not least, deep audio fakes or voice cloning is used to manipulate an individual's voice that associates something with the speaker they haven’t said in actual 1 , 3 .
The importance of discovering the truth in the digital realm has therefore increased. Dealing with deep fakes is significantly more difficult because they are mostly utilized for harmful objectives and virtually anyone can now produce deep fakes utilizing the tools already available. Many different strategies have been put out so far to find deep fakes. Since most are also based on deep learning, a conflict between bad and good deep learning applications has developed 4 . Hence, to solve this problem, the United States Defense Advanced Research Projects Agency (DARPA) launched a media forensics research plan to develop fake digital media detection methods 5 . Moreover, in collaboration with Microsoft, Facebook also announced an AI-based deep fake detection challenge to prevent deep fakes from being used to deceive viewers 6 .
Over the past few years, several researchers have explored Machine Learning and Deep Learning (DL) areas to detect deep fakes from audiovisual media. The ML-based algorithms use labor-intensive and erroneous manual feature extraction before the classification phase. As a result, the performance of these systems is unstable when dealing with bigger databases. However, DL algorithms automatically carry out these tasks, which have proven tremendously helpful in various applications, including deep fake detection. Convolutional neural network (CNN), one of the most prominent DL models, is frequently used due to its state-of-the-art performance that automatically extracts low-level and high-level features from the database. Hence, these methods have drawn the researcher’s interest in scientists across the globe 7 .
Despite substantial research on the subject of deep fakes detection, there is always potential for improvement in terms of efficiency and efficacy. It may be noted that the deep fake generation techniques are improving quickly, thus resulting in increasingly challenging datasets on which previous techniques may not perform effectively. The motivation behind developing automated DL based deep fake detection systems is to mitigate the potential harm caused by deep fake technology. Deep fake content can deceive and manipulate people, leading to serious consequences, such as political unrest, financial fraud, and reputational damage. The development such systems can have significant positive impacts on various industries and fields. These systems also improve the trust and reliability of media and online content. As deep fake technology becomes more sophisticated and accessible, it is important to have reliable tools to distinguish between real and fake content. Hence, developing a robust system to detect deep fakes from media has become very necessary in this age of social media. This paper is a continuation of to study provided by Rimsha et al. 8 . The paper compares the performance of CNN architectures such as AlexNet and VGG16 to detect if the image is real of has been digitally altered. The main contributions of this study are as follows:
In this study, we propose a novel deep fake detection and classification method employing DL and ML-based methods.
The proposed framework preprocesses the image by resizing it according to CNN’s input layer and then performing Error Level Analysis to find any digital manipulation on a pixel level.
The resultant ELA image is supplied to Convolutional Neural Networks, i.e., GoogLeNet, ResNet18 and SqueezeNet, for deep feature extraction.
Extensive experiments are conducted to find the optimal hyper-parameter setting by hyper-parameter tuning.
The performance of the proposed technique is evaluated on the publically available dataset for deep fake detection
Related work
The first ever deep fake was developed in 1860, when a portrait of southern leader John Calhoun was expertly altered for propaganda by swapping his head out for the US President. These manipulations are typically done by splicing, painting, and copy-moving the items inside or between two photos. The appropriate post-processing processes are then used to enhance the visual appeal, scale, and perspective coherence. These steps include scaling, rotating, and color modification 9 , 10 . A range of automated procedures for digital manipulation with improved semantic consistency are now available in addition to these conventional methods of manipulation due to developments in computer graphics and ML/DL techniques. Modifications in digital media have become relatively affordable due to widely available software for developing such content. The manipulation is in digital media is increasing at a very fast pace which requires development of such algorithms to robustly detect and analyze such content to find the difference between right and wrong 11 , 12 , 13 .
Despite being a relatively new technology, deep fake has been the topic of investigation. In recent years, there had been a considerable increase in deep fake articles towards the end of 2020. Due to the advent of ML and DL-based techniques, many researchers have developed automated algorithms to detect deep fakes from audiovisual content. These techniques have helped in finding out the real and fake content easily. Deep learning is well renowned for its ability to represent complicated and high-dimensional data 11 , 14 . Matern et al. 15 employed detected deep fakes from Face Forensics dataset using Multilayered perceptron (MLP) with an AUC of 0.85. However, the study considers facial images with open eyes only. Agarwal et al. 16 extracted features using Open Face 2 toolkit and performed classification via SVM. The system obtained 93% AUC; however, the system provides incorrect results when a person is not facing camera. The authors in Ciftci et al. 17 extracted medical signal features and performed classification via CNN with 97% accuracy. However, the system is computationally complex due to a very large feature vector. In their study, Yang et al. 18 extracted 68-D facial landmarks using DLib and classified these features via SVM. The system obtained 89% ROC. However, the system is not robust to blurred and requires a preprocessing stage. Rossle et al. 19 employed SVM + CNN for feature classification and a Co-Occurrence matrix for feature extraction. The system attained 90.29% accuracy on Face Forensics dataset. However, the system provides poor results on compressed videos. McCloskey et al. 20 developed a deep fake detector by using the dissimilarity of colors between real camera and synthesized and real image samples. The SVM classifier was trained on color based features from the input samples. However, the system may struggle on non-preprocessed and blurry images.
A Hybrid Multitask Learning Framework with a Fire Hawk Optimizer for Arabic Fake News Detection aims to address the issue of identifying fake news in the Arabic language. The study proposes a hybrid approach that leverages the power of multiple tasks to detect fake news more accurately and efficiently. The framework uses a combination of three tasks, namely sentence classification, stance detection, and relevance prediction, to determine the authenticity of the news article. The study also suggests the use of the Fire Hawk Optimizer algorithm, a nature-inspired optimization algorithm, to fine-tune the parameters of the framework. This helps to improve the accuracy of the model and achieve better performance. The Fire Hawk Optimizer is an efficient and robust algorithm that is inspired by the hunting behavior of hawks. It uses a global and local search strategy to search for the optimal solution 21 . The authors in 22 propose a Convolution Vision Transformer (CVT) architecture that differs from CNN in that it relies on a combination of attention mechanisms and convolution operations, making it more effective in recognizing patterns within images.The CVT architecture consists of multi-head self-attention and multi-layer perceptron (MLP) layers. The self-attention layer learns to focus on critical regions of the input image without the need for convolution operations, while the MLP layer helps to extract features from these regions. The extracted features are then forwarded to the output layer to make the final classification decision. However, the system is computationally expensive due to deep architecture. Guarnera et al. 23 identified deep fake images using Expectation Maximization for extracting features and SVM, KNN, LDA as classification methods. However, the system fails in recognizing compressed images. Nguyen et al. 24 proposed a CNN based architecture to detect deep fake content and obtained 83.7% accuracy on Face Forensics dataset. However, the system is unable to generalize well on unseen cases. Khalil et al. 25 employed Local Binary Patterns (LBP) for feature extraction and CNN and Capsule Network for deep fake detection. The models were trained on Deep Fake Detection Challenge-Preview dataset and tested on DFDC-Preview and Celeb- DF datasets. A deep fake approach developed by Afchar et al. 26 employed MesoInception-4 and achieved 81.3% True Positive Rate via Face Forensics dataset.
However, the system requires preprocessing before feature extraction and classification. Hence, results in a low overall performance on low-quality videos. Wang et al. 27 evaluated the performance of Residual Networks on deep fake classification. The authors employed ResNet and ResNeXt, on videos from Face forensics dataset. In another study by Stehouwer et al. 28 , the authors presented a CNN based approach for deep fake content detection that achieved 99% overall accuracy on Diverse Fake Face Dataset. However, the system is computationally expensive due to a very large size feature vector. Despite significant progress, existing DL algorithms are computationally expensive to train and require high-end GPUs or specialized hardware. This can make it difficult for researchers and organizations with limited resources to develop and deploy deep learning models. Moreover, some of the existing DL algorithms are prone to overfitting, which occurs when the model becomes too complex and learns to memorize the training data rather than learning generalizable patterns. This can result in poor performance on new, unseen data. The limitations in the current methodologies prove there is still a need to develop a robust and efficient deep fake detection and classification method using ML and DL based approaches.
Proposed methodology
This section discusses the proposed workflow employed for deep fakes detection. The workflow diagram of our proposed framework is illustrated in Fig. 1 . The proposed system comprises of three core steps (i) image preprocessing by resizing the image according to CNN’s input layer and then generating Error Level Analysis of the image to determine pixel level alterations (ii) deep feature extraction via CNN architectures (iii) classification via SVM and KNN by performing hyper-parameter optimization.
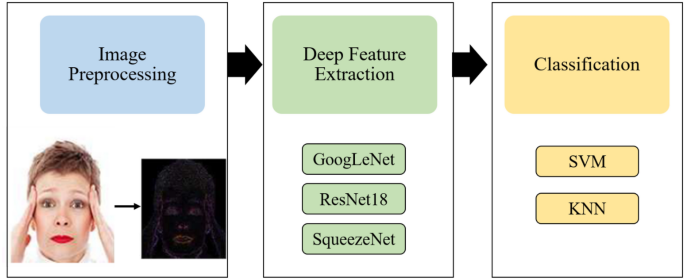
Workflow diagram of the proposed method.
(i) Error level analysis
Error level analysis, also known as ELA, is a forensic technique used to identify image segments with varying compression levels. By measuring these compression levels, the method determines if an image has undergone digital editing. This technique works best on .JPG images as in that case, the entire image pixels should have roughly the same compression levels and may vary in case of tampering 29 , 30 .
JPEG (Joint Photographic Experts Group) is a technique for the lossy compression of digital images. A data compression algorithm discards (loses) some of the data to compress it. The compression level could be used as an acceptable compromise between image size and image quality. Typically, the JPEG compression ratio is 10:1. The JPEG technique uses 8 × 8 pixel image grids independently compressed. Any matrices larger than 8 × 8 are more difficult to manipulate theoretically or are not supported by the hardware, whereas any matrices smaller than 8 × 8 lack sufficient information.
Consequently, the compressed images are of poor quality. All 8 × 8 grids for unaltered images should have a same error level, allowing for the resave of the image. Given that uniformly distributed faults are throughout the image, each square should deteriorate roughly at the same pace. The altered grid in a modified image should have a higher error potential than the rest 31 .
ELA. The image is resaved with 95% error rate, and the difference between the two images is computed. This technique determines if there is any change in cells by checking whether the pixels are at their local minima 8 , 32 . This helps determine whether there is any digital tampering in the database. The ELA is computed on our database, as shown in Fig. 2 .
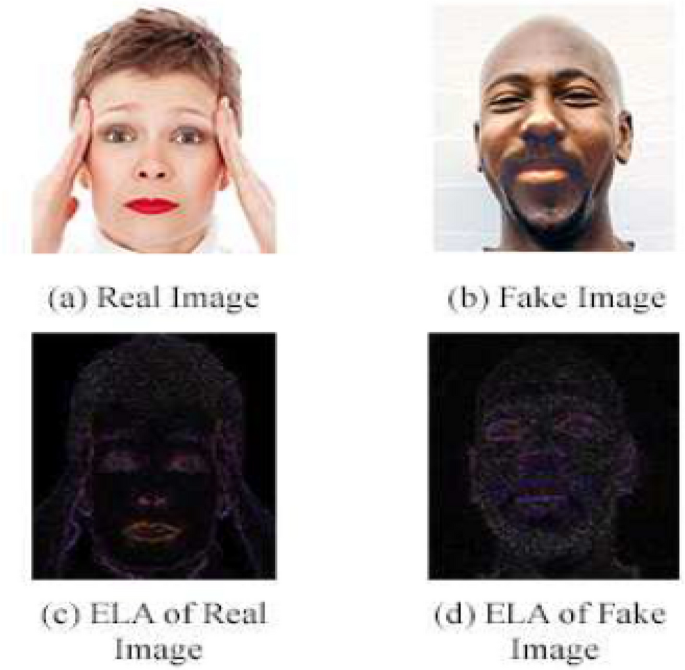
Result of ELA on dataset images.
(ii) Feature extraction using convolutional neural networks
The discovery of CNN has raised its popularity among academics and motivated them to work through difficult problems that they had previously given up on. Researchers have designed several CNN designs in recent years to deal with multiple challenges in various research fields, including deep fake detection. The general architecture of CNN as shown in Fig. 3 , is usually made up of many layers stacked on top of one another. The architecture of CNN consists of a feature extraction module composed of convolutional layers to learn the features and pooling layers reduce image dimensionality. Secondly, it consists of a module comprising a fully connected (FC) layer to classify an image 33 , 34 .
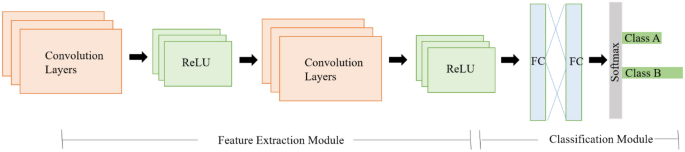
General CNN architecture.
The image is input using the input layer passed down to convolution for deep feature extraction. This layer learns the visual features from the image by preserving the relationship between its pixels. This mathematical calculation is performed on an image matrix using filter/kernel of the specified size 35 . The max-pooling layer reduces the image dimensions. This process helps increase the training speed and reduce the computational load for the next stages 36 . Some networks might include normalization layers, i.e., batch normalization or dropout layer. Batch normalization layer stabilizes the network training performance by performing standardization operations on the input to mini-batches. Whereas, the dropout layer randomly drops some nodes to reduce the network complexity, increasing the network performance 37 , 38 . The last layers of the CNN include an FC layer with a softmax probability function. FC layer stores all the features extracted from the previous phases. These features are then supplied to classifiers for image classification 38 . Since CNN architectures can extract significant features without any human involvement, hence, we used pre-trained CNNs such as GoogLeNet 39 , ResNet18 31 , and SqueezeNet 40 in this study. It may be noted that developing and training a deep learning architecture from scratch is not only a time-consuming task but requires resources for computation; hence we use pre-trained CNN architectures as deep feature extractors in our proposed framework.
Microsoft introduced Residual Network (ResNet) architecture in 2015 that consists of several Convolution Layers of kernel size 3 × 3, an FC layer followed by an additional softmax layer for classification. Because they use shortcut connections that skip one or more levels, residual networks are efficient and low in computational cost 41 . Instead of anticipating that every layer stack will instantly match a specified underlying mapping, the layers fit a residual mapping. As a result of the resulting outputs being added to those of the stacked layers, these fast connections reduce loss of value during training. This functionality also aids in training the algorithm considerably faster than conventional CNNs.
Furthermore, this mapping has no parameters because it transfers the output to the next layer. The ResNet architecture outperformed other CNNs by achieving the lowest top 5% error rate in a classification job, which is 3.57% 31 , 42 . The architecture of ResNet50 is shown in Fig. 4 43 .
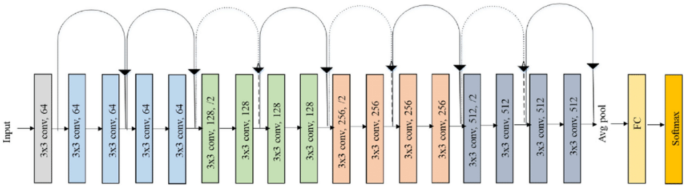
ResNet18 architecture 44 .
SqueezNet was developed by researchers at UC Berkeley and Stanford University that is a very lightweight and small architecture. The smaller CNN architectures are useful as they require less communication across servers in distributed training. Moreover, these CNNs also train faster and require less memory, hence are not computationally expensive compared to conventional deep CNNs. By modifying the architecture, the researchers claim that SqueezeNet can achieve AlexNet level accuracy via a smaller CNN 45 . Because an 1 × 1 filter contains 9× fewer parameters than a 3 × 3 filter, the 3 × 3 filters in these modifications have been replaced with 1 × 1 filters. Furthermore, the number of input channels is reduced to 3 × 3 filters via squeeze layers, which lowers the overall number of parameters.
Last but not least, the downsampling is carried out very late in the network so the convolution layers’ large activation maps which is said to increase classification accuracy 40 . Developed by Google researchers, GoogLeNet is a 22-layer deep convolutional neural network that uses a 1 × 1 convolution filter size, global average pooling and an input size of 224 × 224 × 3. The architecture of GoogLeNet is shown in Fig. 5 . To increase the depth of the network architecture, the convolution filter size is reduced to 1 × 1. Additionally, the network uses global average pooling towards the end of the architecture, which inputs a 7 × 7 feature map and averages it to an 1 × 1 feature map. This helps reduce trainable parameters and enhances the system's performance. A dropout regularization of 0.7 is also used in the architecture, and the features are stored in an FC layer 39 .
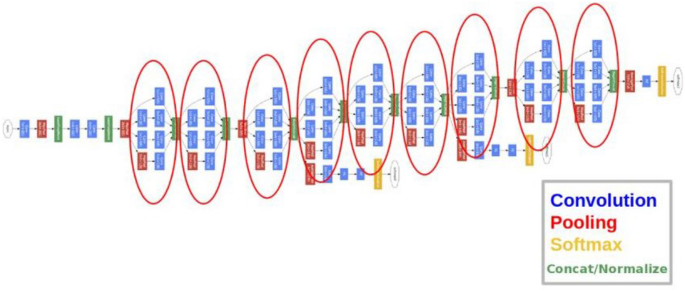
GoogLeNet architecture 46 .
CNNs extract features from images hierarchically using convolutional, pooling, and fully connected layers. The features extracted by CNNs can be broadly classified into two categories: low-level features and high-level features. Low-level features include edges, corners, and intensity variations. CNNs can detect edges by convolving the input image with a filter that highlights the edges in the image. They can also detect corners by convolving the input image with a filter that highlights the corners. Morever, CNNs can extract color features by convolving the input image with filters that highlight specific colors. On the other hand, high-level features include texture, objects, and contextual and hierarchical features. Textures from images are detected by convolving the input image with filters that highlight different textures. The CNNs detect objects by convolving the input image with filters highlighting different shapes. Whereas, contextual features are extracted by considering the relationships between different objects in the image. Finally, the CNNs can learn to extract hierarchical features by stacking multiple convolutional layers on top of each other. The lower layers extract low-level features, while the higher layers extract high-level features.
(iii) Classification via support vector machines and k-nearest neighbors
We classified the deep CNN features via SVM and KNN classifiers in this phase. KNN has gained much popularity in the research community in classification and regression tasks since it outperforms many other existing classifiers due to its simplicity and robustness. KNN calculates the distance between a test sample (k) with its neighbours and then groups the k test sample to its nearest neighbour. The KNN classifier is shown in Fig. 6
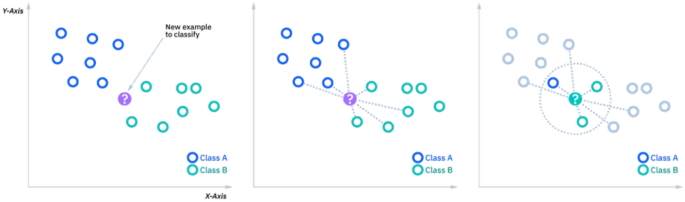
The second classifier used in this study is SVM, a widely popular classifier used frequently in many research fields because of its faster speeds and superior prediction outcomes even on a minimal dataset. The classifier finds the plane with the largest margin that separates the two classes. The wider the margin better is the classification performance of the classifier 30 , 47 . Figure 7 A depicts potential hyperplanes for a particular classification problem, whereas Fig. 7 B depicts the best hyperplane determined by SVM for that problem.
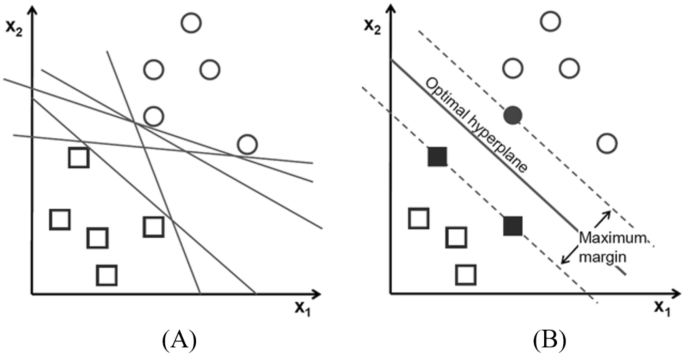
Possible SVM hyperplanes 30 .
Results and discussion
This study uses a publicly accessible dataset compiled by Yonsei University's Computational Intelligence and Photography Lab. The real and fake face database from Yonsei University's Computational Intelligence and Photography Lab is a dataset that contains images of both real and fake human faces. The dataset was designed for use in the research and development of facial recognition and verification systems, particularly those designed to detect fake or manipulated images. Each image in the dataset is labelled as either real or fake, and the dataset also includes additional information about the image, such as the age, gender, and ethnicity of the subject, as well as the manipulation technique used for fake images. Moreover, the images contain different faces, split by the eyes, nose, mouth, or entire face. The manipulated images further subdivided into three categories: easy, mid, and hard images as shown in Fig. 8 48 .
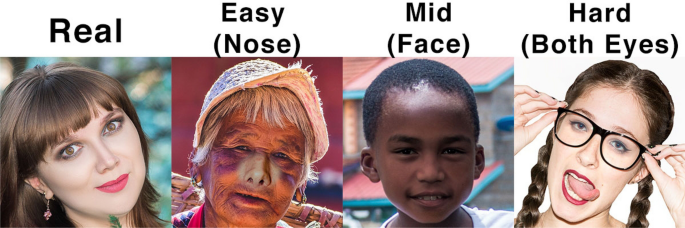
Image samples from the dataset showing real and edited images.
Evaluation metrics
Evaluation metrics are used in machine learning to measure the performance of a model. Machine learning models are designed to learn from data and make predictions or decisions based on that data. It is important to evaluate the performance of a model to understand how well it is performing and to make necessary improvements. One of the most commonly used techniques is a confusion matrix, a table to evaluate the performance of a classification model by comparing the actual and predicted classes for a set of test data. It is a matrix of four values: true positives (TP), false positives (FP), true negatives (TN), and false negatives (FN). The proposed framework is evaluated using accuracy, precision, recall, and f1-score. Even though accuracy is a widely used metric, but is suitable in the case of a balanced dataset; hence, we also evaluated our proposed methods using F1-Score that combines both recall and precision into a single metric. All the evaluation metrics that we used to assess our models are calculated from Eq. ( 1 ) to Eq. ( 4 ).
Proposed method results
The escalating problems with deep fakes have made researchers more interested in media forensics in recent years. Deep fake technology has various applications in the media sector, including lip sync, face swapping, and de-aging humans. Although advances in DL and deep fake technology have various beneficial applications in business, entertainment, and the film industry, they can serve harmful goals and contribute to people's inability to believe what's true 49 , 50 . Hence, finding the difference between real and fake has become vital in this age of social media. Finding deep fake content via the human eye has become more difficult due to progress in deep fake creation technologies. Hence, a robust system must be developed to classify these fake media without human intervention accurately.
In this study, we propose a novel and robust architecture to detect and classify deep fake images using ML and DL-based techniques. The proposed framework employs a preprocessing approach to find ELA. ELA helps find if any portion of the image has been altered by analyzing the image on a pixel level. These images are then supplied to deep CNN architectures (SqueezeNet, ResNet18 & GoogLeNet) to extract deep features. The deep features are then classified via SVM and KNN. The results obtained from ResNet’s confusion matrix and ML classifiers is shown in Fig. 9 . The feature vector achieved highest accuracy of 89.5% via KNN. We tested our various hyper-parameters for both classifiers before reaching the conclusion. The proposed method achieved 89.5% accuracy via KNN on Correlation as a distance metric and total 881 neighbors. SVM achieved 88.6% accuracy on Gaussian Kernel with a 2.3 scale.
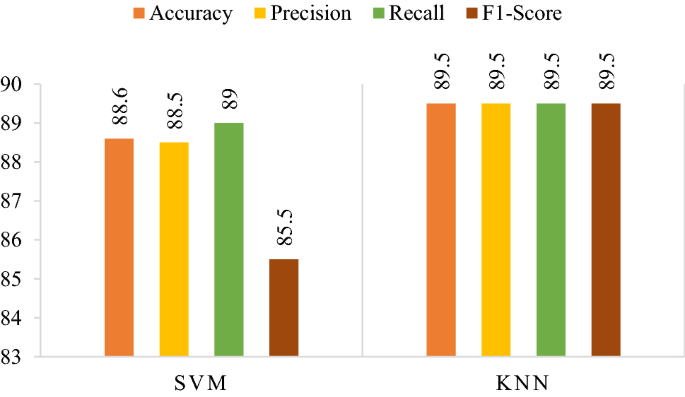
Results obtained from ResNet18's confusion matrix.
Hyperparameter optimization is the process of selecting the best set of hyperparameters for automated algorithms. Optimization is crucial for models because the model's performance depends on the choice of hyperparameters. We optimized parameters such as kernel functions, scale, no. of neighbors, distance metrics, etc., for KNN and SVM. The results obtained from the best parametric settings for different feature vectors are highlighted in bold text and shown in Table 1 . Confusion matrices of both (a) SVM and (b) KNN are illustrated in Fig. 10 .
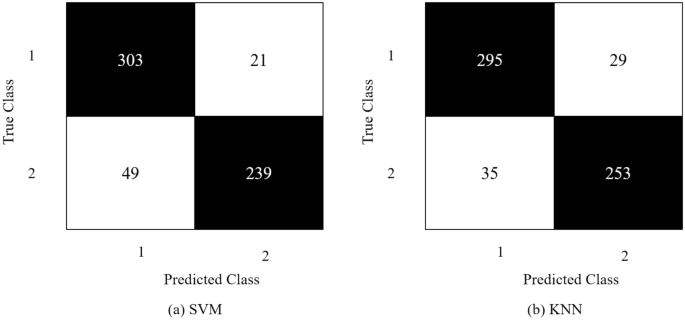
ResNet18's confusion matrix via ( a ) SVM, ( b ) KNN.
Moreover, the feature vector obtained from GoogLeNet’s obtained the highest accuracy of 81% via KNN on Chebyshev as a distance metric with a total number of 154 neighbours. The SVM classified the feature vector with 80.9% accuracy on Gaussian kernel with a 0.41 kernel scale. The tested and optimal metrics (highlighted in bold) are mentioned in Table 2 . Detailed results in other evaluation metrics are mentioned in Fig. 11 , whereas Fig. 12 shows its confusion matrices.
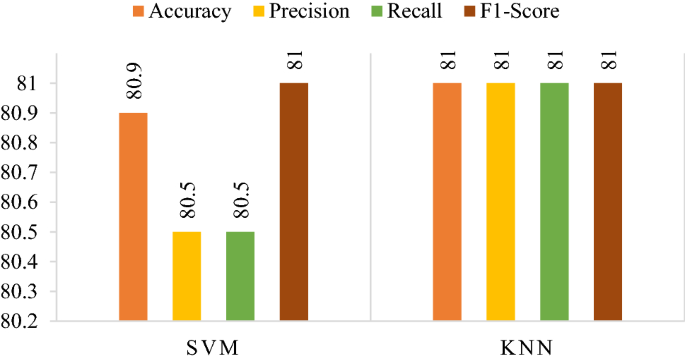
GoogLeNet’s results in terms of ACC, PRE, REC and F1-Score.
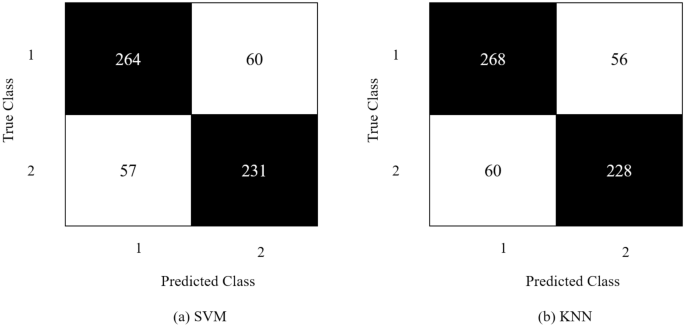
Confusion matrix obtained from GoogLeNet.
SVM and KNN classified the feature vector from SqueezeNet via 69.4% and 68.8%, respectively. The classifiers were evaluated on different parameters, as mentioned in Table 3 and achieved maximum performance on the parameters highlighted in bold text. The results in accuracy, precision, recall and f1-score are mentioned in Fig. 13 . The confusion matrix is shown in Fig. 14 .
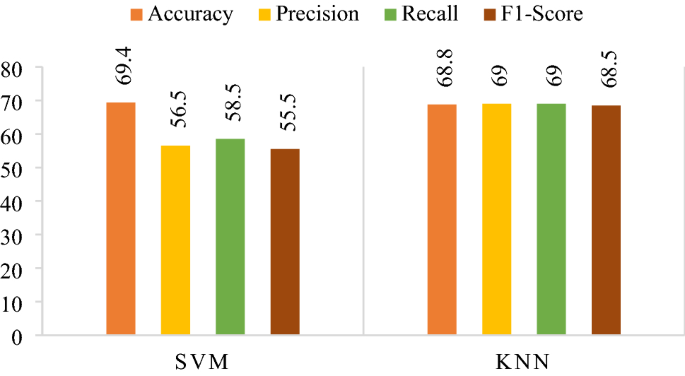
Results obtained from SqueezeNet's confusion matrices.
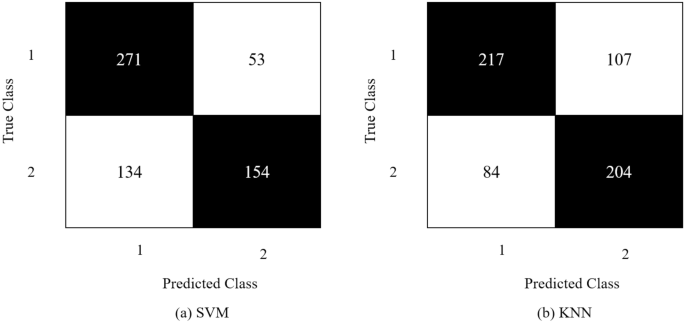
Confusion matrix obtained from SqueezeNet.
Comparison with state-of-the-art methods
This paper proposes a novel architecture to detect and classify deep fake images via DL and ML-based techniques. The proposed framework initially preprocesses the image to generate ELA, which helps determine if the image has been digitally manipulated. The resultant ELA image is then fed to CNN architectures such as GoogLeNet, ResNet18 and ShuffleNet for deep feature extraction. The classification is then performed via SVM and KNN. The proposed method achieved highest accuracy of 89.5% via ResNet18 and KNN. Residual Networks are very efficient and lightweight and perform much better than many other traditional classifiers due to their robust feature extraction and classification techniques. The detailed comparison is shown in Table 4 . Mittal et al. 51 employed Alex Net for deepfake detection. However, the study resulted in a very poor performance. Chandani et al. 50 used a residual network framework to detect deep fake images. Similary, MLP and Meso Inception 4 by Matern et al. 15 and Afchar et al. 26 obtained more than 80% accuracy respectively. Despite being a deep CNN, Residual Networks perform much faster due to their shortcut connections which also aids in boosting the system’s performance. Hence, the proposed method performed much better on the features extracted from ResNet18.
Deep faking is a new technique widely deployed to spread disinformation and hoaxes amongst the people. Even while not all deep fake contents are malevolent, they need to be found because some threaten the world. The main goal of this research was to discover a trustworthy method for identifying deep fake images. Many researchers have been working tirelessly to detect deep fake content using a variety of approaches. However, the importance of this study lies in its use of DL and ML based methods to obtain good results. This study presents a novel framework to detect and classify deep fake images more accurately than many existing systems. The proposed method employs ELA to preprocess images and detect manipulation on a pixel level. The ELA generated images are then supplied to CNNs for feature extraction. These deep features are finally classified using SVM and KNN. The proposed technique achieved highest accuracy of 89.5% via ResNet18’s feature vector & SVM classifier. The results prove the robustness of the proposed method; hence, the system can detect deep fake images in real time. However, the proposed method is developed using image based data. In the future, we will investigate several other CNN architectures on video-based deep fake datasets. We also aim to acquire real life deep fake dataset from the people in our community and use ML and DL techniques to distinguish between deep fake images and regular images to make it more useful and robust. It is worth mentioning that the ground-breaking work will have a significant influence on our society. Using this technology, fake victims can rapidly assess whether the images are real or fake. People will continue to be cautious since our work will enable them to recognize the deep fake image.
Data availability
The datasets used and/or analysed during the current study are available from the corresponding author on reasonable request.
Boylan, J. F. Will Deep-Fake Technology Destroy Democracy (The New York Times, 2018).
Google Scholar
Harwell, D. Scarlett Johansson on fake AI-generated sex videos:‘Nothing can stop someone from cutting and pasting my image’. J. Washigton Post 31 , 12 (2018).
Masood, M. et al. Deepfakes generation and detection: State-of-the-art, open challenges, countermeasures, and way forward. Appl. Intell. 53 , 1–53 (2022).
Amin, R., Al Ghamdi, M. A., Almotiri, S. H. & Alruily, M. Healthcare techniques through deep learning: Issues, challenges and opportunities. IEEE Access 9 , 98523–98541 (2021).
Article Google Scholar
Turek, M.J. Defense Advanced Research Projects Agency . https://www.darpa.mil/program/media-forensics . Media Forensics (MediFor). Vol. 10 (2019).
Schroepfer, M. J. F. Creating a data set and a challenge for deepfakes. Artif. Intell. 5 , 263 (2019).
Kibriya, H. et al. A Novel and Effective Brain Tumor Classification Model Using Deep Feature Fusion and Famous Machine Learning Classifiers . Vol. 2022 (2022).
Rafique, R., Nawaz, M., Kibriya, H. & Masood, M. DeepFake detection using error level analysis and deep learning. in 2021 4th International Conference on Computing & Information Sciences (ICCIS) . 1–4 (IEEE, 2021).
Güera, D. & Delp, E.J. Deepfake video detection using recurrent neural networks. in 2018 15th IEEE International Conference on Advanced Video and Signal Based Surveillance (AVSS) . 1–6 (IEEE, 2018).
Aleem, S. et al. Machine learning algorithms for depression: Diagnosis, insights, and research directions. Electronics 11 (7), 1111 (2022).
Pavan Kumar, M. & Jayagopal, P. Generative adversarial networks: A survey on applications and challenges. Int. J. Multimed. Inf. 10 (1), 1–24 (2021).
Mansoor, M. et al. A machine learning approach for non-invasive fall detection using Kinect. Multimed. Tools Appl. 81 (11), 15491–15519 (2022).
Thies, J., Zollhofer, M., Stamminger, M., Theobalt, C. & Nießner, M. Face2face: Real-time face capture and reenactment of rgb videos. in Proceedings of the IEEE Conference on Computer Vision and Pattern Recognition . 2387–2395 (2016).
Shad, H.S. et al. Comparative Analysis of Deepfake Image Detection Method Using Convolutional Neural Network. Vol. 2021 (2021).
Matern, F., Riess, C. & Stamminger, M. Exploiting visual artifacts to expose deepfakes and face manipulations. in 2019 IEEE Winter Applications of Computer Vision Workshops (WACVW) . 83–92 (IEEE, 2019).
Agarwal, S., Farid, H., Gu, Y., He, M., Nagano, K. & Li, H. Protecting world leaders against deep fakes. in CVPR Workshops . Vol. 1. 38 (2019).
Ciftci, U. A., Demir, I. & Yin, L. Fakecatcher: Detection of Synthetic Portrait Videos Using Biological Signals (Google Patents, 2021).
Yang, X., Li, Y. & Lyu, S. Exposing deep fakes using inconsistent head poses. in ICASSP 2019–2019 IEEE International Conference on Acoustics, Speech and Signal Processing (ICASSP) 8261–8265. (IEEE, 2019).
Rossler, A., Cozzolino, D., Verdoliva, L., Riess, C., Thies, J. & Nießner, M. Faceforensics++: Learning to detect manipulated facial images. in Proceedings of the IEEE/CVF International Conference on Computer Vision . 1–11 (2019).
McCloskey, S. & Albright, M. Detecting GAN-generated imagery using saturation cues. in 2019 IEEE International Conference on Image Processing (ICIP) . 4584–4588. (IEEE, 2019).
Abd Elaziz, M., Dahou, A., Orabi, D.A., Alshathri, S., Soliman, E.M. & Ewees, A.A.J.M. A Hybrid Multitask Learning Framework with a Fire Hawk Optimizer for Arabic Fake News Detection. Vol. 11(2). 258 (2023).
Wodajo, D. & Atnafu, S.J.A.P.A. Deepfake Video Detection Using Convolutional Vision Transformer (2021).
Guarnera, L., Giudice, O. & Battiato, S. Deepfake detection by analyzing convolutional traces. in Proceedings of the IEEE/CVF Conference on Computer Vision and Pattern Recognition Workshops . 666–667 (2020).
Nguyen, H.H., Fang, F., Yamagishi, J. & Echizen, I. Multi-task learning for detecting and segmenting manipulated facial images and videos. in 2019 IEEE 10th International Conference on Biometrics Theory, Applications and Systems (BTAS) . 1–8. (IEEE, 2019).
Khalil, S.S., Youssef, S.M. & Saleh, S.N.J.F.I. iCaps-Dfake: An Integrated Capsule-Based Model for Deepfake Image and Video Detection . Vol. 13(4). 93 (2021).
Afchar, D., Nozick, V., Yamagishi, J. & Echizen, I. Mesonet: A compact facial video forgery detection network. in 2018 IEEE International Workshop on Information Forensics and Security (WIFS) . 1–7 (IEEE, 2018).
Wang, Y. & Dantcheva, A. A video is worth more than 1000 lies. Comparing 3DCNN approaches for detecting deepfakes. in 2020 15th IEEE International Conference on Automatic Face and Gesture Recognition (FG 2020) . 515–519. (IEEE, 2020).
Cozzolino, D., Thies, J., Rössler, A., Riess, C., Nießner, M. & Verdoliva, L.J.A.P.A. Forensictransfer: Weakly-Supervised Domain Adaptation for Forgery Detection (2018).
Huang, G., Liu, Z., Van Der Maaten, L., & Weinberger, K.Q. Densely connected convolutional networks. in Proceedings of the IEEE Conference on Computer Vision and Pattern Recognition . 4700–4708 (2017).
LeCun, Y., Bengio, Y. & Hinton, G. Deep learning. Nature 521 (7553), 436–444 (2015).
Article ADS CAS PubMed Google Scholar
He, K., Zhang, X., Ren, S. & Sun, J. Deep residual learning for image recognition. in Proceedings of the IEEE Conference on Computer Vision and Pattern Recognition . 770–778 (2016).
Nida, N., Irtaza, A. & Ilyas, N. Forged face detection using ELA and deep learning techniques. in 2021 International Bhurban Conference on Applied Sciences and Technologies (IBCAST) . 271–275 (IEEE, 2021).
Kibriya, H., Masood, M., Nawaz, M., Rafique, R. & Rehman, S. Multiclass brain tumor classification using convolutional neural network and support vector machine. in 2021 Mohammad Ali Jinnah University International Conference on Computing (MAJICC) . 1–4 (IEEE, 2021).
Kibriya, H., Masood, M., Nawaz, M. & Nazir, T. J. M. T. Multiclass classification of brain tumors using a novel CNN architecture. Multimed. Tool Appl. 81 , 1–17 (2022).
Salman, F. M. & Abu-Naser, S. S. Classification of real and fake human faces using deep learning. IJAER 6 (3), 1–14 (2022).
Anaraki, A. K., Ayati, M. & Kazemi, F. J. Magnetic resonance imaging-based brain tumor grades classification and grading via convolutional neural networks and genetic algorithms. Information 39 (1), 63–74 (2019).
Albawi, S., Mohammed, T.A. & Al-Zawi, S. Understanding of a convolutional neural network. in 2017 International Conference on Engineering and Technology (ICET) . 1–6 (IEEE, 2017).
O'Shea, K. & Nash, R. J. An Introduction to Convolutional Neural Networks (2015).
Szegedy, C. et al. Going deeper with convolutions. in Proceedings of the IEEE Conference on Computer Vision and Pattern Recognition . 1–9 (2015).
Iandola, F.N., Han, S., Moskewicz, M.W., Ashraf, K., Dally, W.J. & Keutzer, K.J. SqueezeNet : AlexNet-Level Accuracy with 50× Fewer Parameters and< 0.5 MB Model Size (2016).
He, K., Zhang, X., Ren, S. & Sun, J. Deep residual learning for image recognition. in Proceedings of the IEEE Conference on Computer Vision and Pattern Recognition , Las Vegas, USA. 770–778 (2016).
Introduction to Residual Networks . https://www.geeksforgeeks.org/introduction-to-residual-networks/ (2020).
Ali, L. et al. Performance evaluation of deep CNN-based crack detection and localization techniques for concrete structures. Sensors 21 (5), 1688 (2021).
Article ADS PubMed PubMed Central Google Scholar
Ramzan, F. et al. A deep learning approach for automated diagnosis and multi-class classification of Alzheimer’s disease stages using resting-state fMRI and residual neural networks. J. Med. Syst. 44 (2), 1–16 (2020).
Article MathSciNet Google Scholar
Mancini, M., Costante, G., Valigi, P. & Ciarfuglia, T.A. Fast robust monocular depth estimation for obstacle detection with fully convolutional networks. in 2016 IEEE/RSJ International Conference on Intelligent Robots and Systems (IROS) . 4296–4303 (IEEE, 2016).
Kasim, N., Rahman, N., Ibrahim, Z. & Mangshor, N. A. Celebrity face recognition using deep learning. Indonesian J. Electr. Eng. Comput. Sci. 12 (2), 476–481 (2018).
Rezgui, D. & Lachiri, Z. ECG biometric recognition using SVM-based approach. IEEJ Trans. Electr. Electron. Eng. 11 , S94–S100 (2016).
Y. U. Computational Intelligence and Photography Lab. Real-and-Fake-Face-Detection (2019).
Tolosana, R., Romero-Tapiador, S., Fierrez, J. & Vera-Rodriguez, R. Deepfakes evolution: Analysis of facial regions and fake detection performance. in International Conference on Pattern Recognition . 442–456 (Springer, 2016).
Mehra, A. Deepfake Detection Using Capsule Networks with Long Short-Term Memory Networks (University of Twente, 2020).
Mittal, H., Saraswat, M., Bansal, J.C. & Nagar, A. Fake-face image classification using improved quantum-inspired evolutionary-based feature selection method. in 2020 IEEE Symposium Series on Computational Intelligence (SSCI) . 989–995 (IEEE, 2020).
Chandani, K. & Arora, M. Automatic facial forgery detection using deep neural networks. in Advances in Interdisciplinary Engineering . 205–214 (Springer, 2021).
Lee, S., Tariq, S., Shin, Y. & Woo, S. S. Detecting handcrafted facial image manipulations and GAN-generated facial images using Shallow-FakeFaceNet. Appl. Soft Comput. 105 , 107256 (2021).
Download references
This research was supported by the Ministry of Education, Youth and Sports of the Czech Republic under the grant SP2023/007 conducted by VSB—Technical University of Ostrava.
Author information
Authors and affiliations.
Department of Computer Science, University of Engineering and Technology, Taxila, Pakistan, 47050
Rimsha Rafique & Rashid Amin
Department of Electrical Engineering, Chonnam National University, Gwangju, 61186, South Korea
Rahma Gantassi
Department of Computer Science, University of Chakwal, Chakwal, 48800, Pakistan
Rashid Amin
Department of Quantitative Methods and Economic Informatics, Faculty of Operation and Economics of Transport and Communications, University of Zilina, 01026, Zilina, Slovakia
Jaroslav Frnda
Department of Telecommunications, Faculty of Electrical Engineering and Computer Science, VSB Technical University of Ostrava, 70800, Ostrava, Czech Republic
Faculty of Applied Sciences and Technology, Universiti Tun Hussein Onn Malaysia, KM1 Jalan Pagoh, 84600, Pagoh, Johor, Malaysia
Aida Mustapha
Durma College of Science and Humanities, Shaqra University, Shaqra, 11961, Saudi Arabia
Asma Hassan Alshehri
You can also search for this author in PubMed Google Scholar
Contributions
All the authors contributed equally.
Corresponding author
Correspondence to Rashid Amin .
Ethics declarations
Competing interests.
The authors declare no competing interests.
Additional information
Publisher's note.
Springer Nature remains neutral with regard to jurisdictional claims in published maps and institutional affiliations.
Rights and permissions
Open Access This article is licensed under a Creative Commons Attribution 4.0 International License, which permits use, sharing, adaptation, distribution and reproduction in any medium or format, as long as you give appropriate credit to the original author(s) and the source, provide a link to the Creative Commons licence, and indicate if changes were made. The images or other third party material in this article are included in the article's Creative Commons licence, unless indicated otherwise in a credit line to the material. If material is not included in the article's Creative Commons licence and your intended use is not permitted by statutory regulation or exceeds the permitted use, you will need to obtain permission directly from the copyright holder. To view a copy of this licence, visit http://creativecommons.org/licenses/by/4.0/ .
Reprints and permissions
About this article
Cite this article.
Rafique, R., Gantassi, R., Amin, R. et al. Deep fake detection and classification using error-level analysis and deep learning. Sci Rep 13 , 7422 (2023). https://doi.org/10.1038/s41598-023-34629-3
Download citation
Received : 26 December 2022
Accepted : 04 May 2023
Published : 08 May 2023
DOI : https://doi.org/10.1038/s41598-023-34629-3
Share this article
Anyone you share the following link with will be able to read this content:
Sorry, a shareable link is not currently available for this article.
Provided by the Springer Nature SharedIt content-sharing initiative
This article is cited by
Image forgery detection: comprehensive review of digital forensics approaches.
- Satyendra Singh
- Rajesh Kumar
Journal of Computational Social Science (2024)
By submitting a comment you agree to abide by our Terms and Community Guidelines . If you find something abusive or that does not comply with our terms or guidelines please flag it as inappropriate.
Quick links
- Explore articles by subject
- Guide to authors
- Editorial policies
Sign up for the Nature Briefing: AI and Robotics newsletter — what matters in AI and robotics research, free to your inbox weekly.

Subscribe to the PwC Newsletter
Join the community, add a new evaluation result row, deepfake detection.
141 papers with code • 5 benchmarks • 18 datasets
DeepFake Detection is the task of detecting fake videos or images that have been generated using deep learning techniques. Deepfakes are created by using machine learning algorithms to manipulate or replace parts of an original video or image, such as the face of a person. The goal of deepfake detection is to identify such manipulations and distinguish them from real videos or images.
Description source: DeepFakes: a New Threat to Face Recognition? Assessment and Detection
Image source: DeepFakes: a New Threat to Face Recognition? Assessment and Detection

Benchmarks Add a Result

Most implemented papers
Faceforensics++: learning to detect manipulated facial images.
ondyari/FaceForensics • 25 Jan 2019
In particular, the benchmark is based on DeepFakes, Face2Face, FaceSwap and NeuralTextures as prominent representatives for facial manipulations at random compression level and size.
Taming Transformers for High-Resolution Image Synthesis

We demonstrate how combining the effectiveness of the inductive bias of CNNs with the expressivity of transformers enables them to model and thereby synthesize high-resolution images.
MesoNet: a Compact Facial Video Forgery Detection Network
This paper presents a method to automatically and efficiently detect face tampering in videos, and particularly focuses on two recent techniques used to generate hyper-realistic forged videos: Deepfake and Face2Face.
Celeb-DF: A Large-scale Challenging Dataset for DeepFake Forensics
danmohaha/celeb-deepfakeforensics • CVPR 2020
AI-synthesized face-swapping videos, commonly known as DeepFakes, is an emerging problem threatening the trustworthiness of online information.
The DeepFake Detection Challenge (DFDC) Dataset
In addition to Deepfakes, a variety of GAN-based face swapping methods have also been published with accompanying code.
Unmasking DeepFakes with simple Features
cc-hpc-itwm/DeepFakeDetection • 2 Nov 2019
In this work, we present a simple way to detect such fake face images - so-called DeepFakes.
Face X-ray for More General Face Forgery Detection
neverUseThisName/Face-X-Ray • CVPR 2020
For this reason, face X-ray provides an effective way for detecting forgery generated by most existing face manipulation algorithms.
Combining EfficientNet and Vision Transformers for Video Deepfake Detection
Traditionally, Convolutional Neural Networks (CNNs) have been used to perform video deepfake detection, with the best results obtained using methods based on EfficientNet B7.
Analyzing Fairness in Deepfake Detection With Massively Annotated Databases
pterhoer/DeepFakeAnnotations • 11 Aug 2022
In this work, we investigate factors causing biased detection in public Deepfake datasets by (a) creating large-scale demographic and non-demographic attribute annotations with 47 different attributes for five popular Deepfake datasets and (b) comprehensively analysing attributes resulting in AI-bias of three state-of-the-art Deepfake detection backbone models on these datasets.
DeepFakes and Beyond: A Survey of Face Manipulation and Fake Detection
The free access to large-scale public databases, together with the fast progress of deep learning techniques, in particular Generative Adversarial Networks, have led to the generation of very realistic fake content with its corresponding implications towards society in this era of fake news.
Deepfake Detection through Deep Learning
Ieee account.
- Change Username/Password
- Update Address
Purchase Details
- Payment Options
- Order History
- View Purchased Documents
Profile Information
- Communications Preferences
- Profession and Education
- Technical Interests
- US & Canada: +1 800 678 4333
- Worldwide: +1 732 981 0060
- Contact & Support
- About IEEE Xplore
- Accessibility
- Terms of Use
- Nondiscrimination Policy
- Privacy & Opting Out of Cookies
A not-for-profit organization, IEEE is the world's largest technical professional organization dedicated to advancing technology for the benefit of humanity. © Copyright 2024 IEEE - All rights reserved. Use of this web site signifies your agreement to the terms and conditions.

An official website of the United States government
The .gov means it’s official. Federal government websites often end in .gov or .mil. Before sharing sensitive information, make sure you’re on a federal government site.
The site is secure. The https:// ensures that you are connecting to the official website and that any information you provide is encrypted and transmitted securely.
- Publications
- Account settings
Preview improvements coming to the PMC website in October 2024. Learn More or Try it out now .
- Advanced Search
- Journal List

Deepfakes Generation and Detection: A Short Survey
Associated data.
Data sharing not applicable.
Advancements in deep learning techniques and the availability of free, large databases have made it possible, even for non-technical people, to either manipulate or generate realistic facial samples for both benign and malicious purposes. DeepFakes refer to face multimedia content, which has been digitally altered or synthetically created using deep neural networks. The paper first outlines the readily available face editing apps and the vulnerability (or performance degradation) of face recognition systems under various face manipulations. Next, this survey presents an overview of the techniques and works that have been carried out in recent years for deepfake and face manipulations. Especially, four kinds of deepfake or face manipulations are reviewed, i.e., identity swap, face reenactment, attribute manipulation, and entire face synthesis. For each category, deepfake or face manipulation generation methods as well as those manipulation detection methods are detailed. Despite significant progress based on traditional and advanced computer vision, artificial intelligence, and physics, there is still a huge arms race surging up between attackers/offenders/adversaries (i.e., DeepFake generation methods) and defenders (i.e., DeepFake detection methods). Thus, open challenges and potential research directions are also discussed. This paper is expected to aid the readers in comprehending deepfake generation and detection mechanisms, together with open issues and future directions.
1. Introduction
It is estimated that 1.8 billion images and videos per day are uploaded to online services, including social and professional networking sites [ 1 ]. However, approximately 40% to 50% of these images and videos appear to be manipulated [ 2 ] for benign reasons (e.g., images retouched for magazine covers) or adversarial purposes (e.g., propaganda or misinformation campaigns). In particular, human face image/video manipulation is a serious issue menacing the integrity of information on the Internet and face recognition systems since faces play a central role in human interactions and biometrics-based person identification. Therefore, plausible manipulations in face samples can critically subvert trust in digital communications and security applications (e.g., law enforcement).
DeepFakes refer to multimedia content that has been digitally altered or synthetically created using deep learning models [ 3 ]. Deepfakes are the results of face swapping, enactment/animation of facial expressions, and/or digitally generated audio or non-existing human faces. In contrast, face manipulation involves modifying facial attributes such as age, gender, ethnicity, morphing, attractiveness, skin color or texture, hair color, style or length, eyeglass, makeup, mustache, emotion, beard, pose, gaze, mouth open or closed, eye color, injury and effects of drug use [ 4 , 5 ], and adding imperceptible perturbations (i.e., adversarial examples), as shown in Figure 1 . The readily-available face editing apps (e.g., FaceApp [ 6 ], ZAO [ 7 ], Face Swap Live [ 8 ], Deepfake web [ 9 ], AgingBooth [ 10 ], PotraitPro Studio [ 11 ], Reface [ 12 ], Audacity [ 13 ], Soundforge [ 14 ], Adobe Photoshop [ 15 ]), and Deep Neural network (DNN) source codes [ 16 , 17 ] have enabled even non-experts and non-technical people to create sophisticated deepfakes and altered face samples, which are difficult to be detected by human examiners and current image/video analysis forensics tools.

Examples of different face manipulations: original samples (first row) and manipulated samples (second row).
Deepfakes are expected to advance present disinformation and misinformation sources to the next level, which could be exploited by trolls, bots, conspiracy theorists, hyperpartisan media, and foreign governments; thus, deepfakes could be fake news 2.0. Deepfakes can be used for productive applications such as realistic dubbing of foreign video films [ 18 ] or historical figure reanimation for education [ 19 ]. Deepfakes can also be used for destructive applications such as the use of fake pornographic videos to damage a person’s reputation or to blackmail them [ 20 ], manipulating elections [ 21 ], creating warmongering situations [ 22 ], generating political or religious unrest via fake speeches [ 23 ], causing chaos in financial markets [ 24 ], or identity theft [ 25 ]. It is easy to notice that the number of malevolent exploitations of deepfakes chiefly dominates the benevolent ones. In fact, not only have recent advances made creating a deepfake with just a still image [ 26 ], but also deepfakes are successfully being misused by cybercriminals in the real world. For instance, an audio deepfake was utilized to scam a CEO out of $243,000 [ 27 ]. The issue of deepfakes and face manipulations is getting compounded as they can negatively affect the automated face recognition system (AFRS). For instance, studies have shown that AFRS’s error rates can reach up to 95% under deepfakes [ 28 ], 50–99% under morphing [ 29 ], 17.08% under makeup manipulation [ 30 ], 17.05–99.77% under partial face tampering [ 31 ], 40–74% under digital beautification [ 32 ], 93.82% under adversarial examples [ 33 ], and 67% under GANs generated synthetic samples [ 34 ]. Similarly, automated speaker verification’s accuracy drops to 40% from 98% under adversarial examples [ 35 ].
There exist many deepfake and face manipulation detection methods. However, a systematic analysis shows that the majority of them have low generalization capability, i.e., their performances drop drastically when they encounter a novel deepfake/manipulation type that was not used during the training stage, as also demonstrated in [ 36 , 37 , 38 , 39 , 40 ]. Also, prior studies considered deepfake detection a reactive defense mechanism and not as a battle between the attackers (i.e., deepfake generation methods) and the defenders (i.e., deepfake detection methods) [ 41 , 42 , 43 ]. Therefore, there is a crucial gap between academic deepfake solutions and real-world scenarios or requirements. For instance, the foregoing works are usually lagging in the robustness of the systems against adversarial attacks [ 44 ], decision explainability [ 45 ], and real-time mobile deepfake detection [ 46 ].
The study of deepfake generation and detection, in recent years, is gathering much more momentum in the computer vision and machine learning community. There exist some review papers on this topic (e.g., [ 5 , 24 , 47 , 48 ]), but they are focused mainly on deepfake or synthetic samples using generative adversarial networks. Moreover, most survey articles (e.g., [ 4 , 49 , 50 ]) were mainly written from an academic point of view and not from a practical development point of view. Also, they did not cover the advent of very recent face manipulation methods and new deepfake generation and detection techniques. Thus, this paper provides a concise but comprehensive overview from both theoretical and practical points of view to furnish the reader with an intellectual grasp as well as to facilitate the progression of novel and more resilient techniques. For example, publicly available apps, codes, or software information can be easily accessed or downloaded for further development and use. All in all, this paper presents an overview of current deepfake and face manipulation techniques by covering four kinds of deepfake or face manipulation. The four main types of manipulation are identity swap, face reenactment, attribute manipulation, and entire face synthesis, where every category manipulation generation and such manipulation detection methods are summarized. Furthermore, open challenges and potential future directions (e.g., robust deepfake detection systems against adversarial attacks using multistream and filtering schemes) that need to be addressed in this evolving field of deepfakes are highlighted. The main objectives of this article are to complement earlier survey papers with recent advancements, to impart to the reader a deeper understanding of the deepfake creation and detection domain, and to use this article as ground truth to develop novel algorithms for deepfake and face manipulation generation and detection systems.
The rest of the article is organized as follows. Section 2 presents deepfake and face manipulation generation as well as detection techniques. In Section 3 , the open issues and potential future directions of deepfake generation and detection are discussed. The conclusions are described in Section 4 .
2. Deepfake Generation and Detection
We can broadly define deepfake as “believable audio-, visual- or multimedia generated by deep neural networks”. Deepfake/face manipulation can be categorized into four main groups: identity swap, face reenactment, attribute manipulation, and entire face synthesis [ 47 ], as shown in Figure 2 . Several works have been conducted on different types of deepfake/face manipulation generation and detection. However, in the following subsections, we have included representative studies based on their novelty, foundational idea, and/or performance. Also, studies have been incorporated to represent the most up-to-date research works depicting the state-of-the-art in deepfake generation and detection.

Real and fake examples of each deepfake/face manipulation group. The fake sample in “Entire face synthesis” group is obtained from the method in [ 81 ].
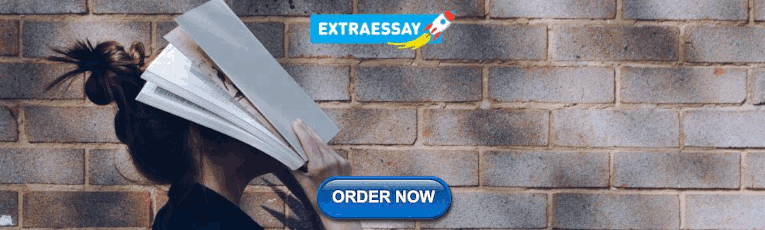
2.1. Identity Swap
Here, an overview of existing identity swap or face swap (i.e., replacing a person’s face with another person’s face) generation and detection methods is presented.
2.1.1. Identity Swap Generation
This consists of replacing the face of a person in the target image/video with the face of another person in the source image/video [ 51 ]. For example, Korshunova et al. [ 52 ] developed a face-swapping method using Convolutional Neural Networks (CNNs). While Nirkin et al. [ 53 ] proposed a technique using a standard fully convolutional network in unconstrained settings. Mahajan et al. [ 54 ] presented a face swap procedure for privacy protection. Wang et al. [ 55 ] presented a real-time face-swapping method. Natsume et al. [ 56 ] proposed a region-separative generative adversarial network (RSGAN) for face swapping and editing. Other interesting face swamping methods can be seen in [ 28 , 57 , 58 , 59 , 60 , 61 ].
2.1.2. Identity Swap Detection
Ample studies have been conducted on identity swap deepfake detection. For instance, Koopman et al. [ 62 ] analyzed photo response non-uniformity (PRNU) for detection. Also, warping artifacts [ 63 ], eye blinking [ 64 ], optical flow with CNNs [ 65 ], heart rate [ 66 ], image quality [ 28 ], local image textures [ 37 ], long short-term memory (LSTM) and recurrent neural network (RNN) [ 67 ], multi-LSTM and blockchain [ 68 ], clustering [ 69 ], context [ 70 ], compression artifacts [ 71 ], metric learning [ 72 ], CNN ensemble [ 73 ], Identity-aware [ 74 ], transformers [ 75 ], audio-visual dissonance [ 76 ], and multi-attentional [ 77 ] features were used. Very few works have been focused on deepfake detection method’s explainability (e.g., [ 78 ]) and generalization capability (e.g., work of Bekci et al. in [ 38 ] and Aneja et al. [ 79 ] work using zero-shot learning). Recently, S. Liu et al. [ 80 ] proposed a block shuffling learning method to detect deepfakes, where the image is divided into blocks, and using random shuffling where intra-block and inter-block-based features are extracted.
2.2. Face Reenactment
Here, an overview of prior face reenactment (i.e., changing the facial expression of the individual) generation and detection techniques is provided.
2.2.1. Face Reenactment Generation
This consists of replacing the facial expression of a person in the target image/video with the facial expression of another person in the source image/video [ 47 ]. It is also known as expression swap or puppet master. For instance, Thies et al. [ 82 ] developed real-time face reenactment RGB video streams. Whereas encoder-decoder, RNN, unified landmark converter with geometry-aware generator, GANs, and task-agnostic GANs-based schemes were designed by Kim et al. [ 83 ], Nirkin et al. [ 84 ], Zhang et al. [ 85 ], Doukas et al. [ 86 ], and Cao et al. [ 87 ], respectively.
2.2.2. Face Reenactment Detection
Face reenactment detection methods were designed by Cozzolino et al. [ 88 ] using CNNs; Matern et al. [ 89 ] using visual features with logistic regression and MLP; Rossler et al. [ 90 ] using mesoscopic, steganalysis, and CNN features; Sabir et al. [ 91 ] using RNN; Amerini et al. [ 65 ] using Optical Flow + CNNs; Kumar et al. [ 92 ] using multistream CNNs; and Wang et al. [ 93 ] using 3DCNN. In contrast, Zhao et al. [ 94 ] designed a spatiotemporal network, which can utilize complementary global and local information. In particular, the framework uses a spatial module for the global information, and the local information module extracts features from patches selected by attention layers.
2.3. Attribute Manipulation
Here, an overview of existing attribute manipulation or face retouching, or face editing (i.e., altering certain face attributes such as skin tone, age, and gender) generation and detection techniques is presented.
2.3.1. Attribute Manipulation Generation
This consists of modifying some facial attributes, e.g., color of hair/skin, gender, age, adding glasses [ 95 , 96 , 97 ]. It is also known as face editing or face retouching. Xiao et al. [ 98 ] presented a multi-attribute manipulation GANs-based system. Moreover, spatial attention in GANs [ 99 ], variational autoencoder (VAE) + GANs [ 100 ], multi-domain GANs [ 101 ], geometry-aware GANs [ 102 ], mask-guided GANs [ 103 ], 3D face morphable model [ 104 ], and GIMP animation [ 105 ] based methods have been designed.
2.3.2. Attribute Manipulation Detection
In [ 36 ], authors studied the efficacy of different deep learning models’ efficacy for attribute manipulation detection. The Deep Boltzmann machine by Bharati et al. [ 106 ], CNN by Dang et al. [ 107 ], LBP + landmarks + CNNs by Rathgeb et al. [ 108 ], adaptive manipulation traces by Guo et al. [ 109 ], encoder-decoder by Mazaheri et al. [ 110 ], facial boundary features by Kim et al. [ 111 ], and PRNU by Scherhag et al. [ 112 ] were exploited.
2.4. Entire Face Synthesis
Here, an overview of prior entire face synthesis (i.e., creating non-existent face samples) generation and detection techniques is provided.
2.4.1. Entire Face Synthesis Generation
This consists of generating entire non-existent face images [ 113 , 114 , 115 ]. Berthelot et al. [ 116 ] developed boundary equilibrium GANs to create synthetic faces. Similarly, various approaches have been devised, e.g., coupled GANs [ 117 ], invertible convolution [ 118 ], U-Net [ 119 ], from speech to face GANs [ 120 ], high-resolution deep convolutional GANs [ 121 ], interactive anycost GANs [ 122 ], and structured disentanglement framework for face generation and editing [ 123 ].
2.4.2. Entire Face Synthesis Detection
Many studies have also focused on entire face synthesis detection. For example, McCloskey et al. [ 124 ] presented a color cues-based system. While GAN fingerprint + CNNs [ 125 ], PRNU [ 126 ], co-occurrence matrices [ 127 ], neuron behaviors [ 128 ], incremental learning + CNNs [ 129 ], and self-attention mechanism [ 130 ] were also utilized. Table 1 presents a summary of deepfake and face manipulation generation and detection techniques. Guo et al. [ 131 ] showed that GANs-generated faces could be detected by analyzing the irregular pupil shapes, which may be caused by the lack of physiological constraints in the GANs models.
Representative works on deepfake and face manipulation generation and detection techniques. SWR = successful swap rate; MS-SSIM = multi-scale structural similarity; Acc = accuracy; LL = Logloss; AUC = area under the curve; CL = contextual loss; RMSE = root mean square error; AU = Facial action unit; CSIM = Cosine Similarity between IMage embeddings; EER = Equal error rate; FID = Frechet inception distance; AP = Average Precision; KID = kernel inception distance; PSNR = Peak Signal-to-Noise Ratio.
3. Open Issues and Research Directions
Although great efforts have been made in devising deepfake generation and detection, there are several issues yet to be addressed successfully. In the following, some of them are discussed.
3.1. Generalization Capability
It is easy to notice in the literature that most of the existing deepfake detection frameworks’ performances decrease remarkably when tested under deepfakes, manipulations, or databases that were not used for the training. Thus, detecting unknown novel deepfakes or deepfake generation tools is yet a big challenge. The generalization capability of deepfake detectors is vital for dependable precision and public trust in the information being shared online. Some preliminary generalization solutions have been proposed, but their ability to tackle novel emerging deepfakes is still an open issue.
3.2. Explainability of Deepfake Detectors
There is a lack of work on the deepfake detection framework’s interpretability and dependability. Most deep-learning-based deepfake or face manipulation detection methods in the literature usually do not explain the reason behind the final detection outcome. It is mainly due to deep learning techniques being the black box in nature. Current deepfake or face manipulation detectors only give a label, confidence percentage, or fakeness probability score but not the insight description of results. Such a description would be useful to know why the detector made a certain decision. Also, deepfake or face manipulation (e.g., applying digital makeup) can be performed either for benign or malicious intentions. Nonetheless, present deepfake or face manipulation detection techniques cannot distinguish the intent. For deepfake detection framework’s interpretability and dependability, various advanced combinations of techniques such as fuzzy inference systems [ 187 ], layer-wise relevance propagation [ 188 ], and the Neural Additive Model [ 189 ] could be helpful.
3.3. Next-Generation Deepfake and Face Manipulation Generators
Improved deepfake and face manipulation generation techniques will help develop more advanced and generalized deepfake detection methods. Some of the shortcomings of current datasets and generation methods are the lack of ultra-high-resolution samples (e.g., existing methods are usually generating 1014 × 1024 resolution samples, which is not sufficient for the next generation of deepfakes), limited face attribution manipulations (i.e., face attribute manipulation types are dependent on the training set, thereby manipulation characteristics and attributes are limited, and novel attributes cannot be generated), video continuity problem (i.e., the deepfake/face manipulation, especially identity swap, techniques neglects the continuation of video frames as well as physiological signals), and no obvious deepfake/face manipulations (i.e., present databases are not composed of obvious fake samples such as a human face with three eyes).
3.4. Vulnerability to Adversarial Attacks
Recent studies have shown that deep learning-based deepfake and face manipulation detection methods are vulnerable to adversarial examples [ 44 ]. Though current detectors are capable of handling several degradations (e.g., compression and noise), their accuracy goes to extremely low levels under adversarial attacks. Thus, next-generation techniques should be not only able to tackle deepfakes but also adversarial examples. To this aim, developing various multistream and filtering schemes could be effective.
3.5. Mobile Deepfake Detector
The neural networks-based deepfake detection methods, which are capable of attaining remarkable accuracy, are mostly unsuited for mobile platforms/applications owing to the huge number of parameters and computational cost. Compressed, yet effective, deep learning-based detection systems, which could be used on mobile and wearable devices, will greatly help counteract deepfakes and fake news.
3.6. Lack of Large-Scale ML-Generated Databases
Most studies on AI-synthesized face sample detection compiled their own database with various GANs. Thereby, different published studies have different performances on GANs samples, because the quality of GANs-generated samples varies and are mostly unknown. Several public GANs-generated fake face sample databases should be produced to help the advancement of this demanding research field.
3.7. Reproducible Research
In machine learning and the deepfake research community, the reproducible results trend should be urged by furnishing the public with large datasets with larger human scores/reasons, experimental setups, and open-source tools/codes. It will surely aid in outlining the true progress in the field and avoid overestimation of the performances by the developed methods.
4. Conclusions
AI-synthesized or digitally manipulated face samples, commonly known as DeepFakes, are a significant challenge threatening the dependability of face recognition systems and the integrity of information on the Internet. This paper provides a survey on recent advances in deepfake and facial manipulation generation and detection. Despite noticeable progress, there are several issues remaining to be resolved to attain highly effective and generalized generation and defense techniques. Thus, this article discussed some of the open challenges and research opportunities. The field of deepfakes still has to go a long way for dependable deepfake and face manipulation detection frameworks, which will need interdisciplinary research efforts in various domains, such as machine learning, computer vision, human vision, psychophysiology, etc. All in all, this survey may be utilized as a ground truth for developing novel AI-based algorithms for deepfake generation and detection. Also, it is hoped that this survey paper will motivate budding scientists, practitioners, researchers, and engineers to consider deepfakes as their domain of study.
Funding Statement
This research received no external funding.
Institutional Review Board Statement
Not applicable.
Informed Consent Statement
Data availability statement, conflicts of interest.
The authors declare no conflict of interest.
Disclaimer/Publisher’s Note: The statements, opinions and data contained in all publications are solely those of the individual author(s) and contributor(s) and not of MDPI and/or the editor(s). MDPI and/or the editor(s) disclaim responsibility for any injury to people or property resulting from any ideas, methods, instructions or products referred to in the content.
Advertisement
Supported by
OpenAI Releases ‘Deepfake’ Detector to Disinformation Researchers
The prominent A.I. start-up is also joining an industrywide effort to spot content made with artificial intelligence.
- Share full article

By Cade Metz and Tiffany Hsu
Reporting from San Francisco
As experts warn that images, audio and video generated by artificial intelligence could influence the fall elections , OpenAI is releasing a tool designed to detect content created by its own popular image generator, DALL-E . But the prominent A.I. start-up acknowledges that this tool is only a small part of what will be needed to fight so-called deepfakes in the months and years to come.
On Tuesday, OpenAI said it would share its new deepfake detector with a small group of disinformation researchers so they could test the tool in real-world situations and help pinpoint ways it could be improved.
“This is to kick-start new research,” said Sandhini Agarwal, an OpenAI researcher who focuses on safety and policy. “That is really needed.”
OpenAI said its new detector could correctly identify 98.8 percent of images created by DALL-E 3, the latest version of its image generator. But the company said the tool was not designed to detect images produced by other popular generators like Midjourney and Stability.
Because this kind of deepfake detector is driven by probabilities, it can never be perfect. So, like many other companies, nonprofits and academic labs, OpenAI is working to fight the problem in other ways.
Like the tech giants Google and Meta, the company is joining the steering committee for the Coalition for Content Provenance and Authenticity, or C2PA, an effort to develop credentials for digital content. The C2PA standard is a kind of “nutrition label” for images, videos, audio clips and other files that shows when and how they were produced or altered — including with A.I.
OpenAI also said it was developing ways of “watermarking” A.I.-generated sounds so they could easily be identified in the moment. The company hopes to make these watermarks difficult to remove.
Anchored by companies like OpenAI, Google and Meta, the A.I. industry is facing increasing pressure to account for the content its products make. Experts are calling on the industry to prevent users from generating misleading and malicious material — and to offer ways of tracing its origin and distribution.
In a year stacked with major elections around the world, calls for ways to monitor the lineage of A.I. content are growing more desperate. In recent months, audio and imagery have already affected political campaigning and voting in places including Slovakia, Taiwan and India.
OpenAI’s new deepfake detector may help stem the problem, but it won’t solve it. As Ms. Agarwal put it: In the fight against deepfakes, “there is no silver bullet.”
Cade Metz writes about artificial intelligence, driverless cars, robotics, virtual reality and other emerging areas of technology. More about Cade Metz
Tiffany Hsu reports on misinformation and disinformation and its origins, movement and consequences. She has been a journalist for more than two decades. More about Tiffany Hsu
Explore Our Coverage of Artificial Intelligence
News and Analysis
News Corp, the Murdoch-owned empire of publications like The Wall Street Journal and The New York Post, announced that it had agreed to a deal with OpenAI to share its content to train and service A.I. chatbots.
The Silicon Valley company Nvidia was again lifted by sales of its A.I. chips , but it faces growing competition and heightened expectations.
Researchers at the A.I. company Anthropic claim to have found clues about the inner workings of large language models, possibly helping to prevent their misuse and to curb their potential threats.
The Age of A.I.
D’Youville University in Buffalo had an A.I. robot speak at its commencement . Not everyone was happy about it.
A new program, backed by Cornell Tech, M.I.T. and U.C.L.A., helps prepare lower-income, Latina and Black female computing majors for A.I. careers.
Publishers have long worried that A.I.-generated answers on Google would drive readers away from their sites. They’re about to find out if those fears are warranted, our tech columnist writes .
A new category of apps promises to relieve parents of drudgery, with an assist from A.I. But a family’s grunt work is more human, and valuable, than it seems.
The Global Struggle Against Deepfake Technology: A Critical Overview

[EDRM Editor’s Note: This article was first published here on May 14, 2024 , and EDRM is grateful to Rob Robinson, Editor and Managing Director of ComplexDiscovery , for permission to republish.]
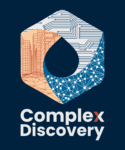
Complex Discovery Editor’s Note: The rapid evolution of deepfake technology, a sophisticated byproduct of artificial intelligence, has emerged as a significant challenge within digital media landscapes, affecting sectors from entertainment to politics. This article delves into the multifaceted efforts to mitigate the misuse of deepfakes—highlighting the crucial roles played by tech giants like Microsoft and OpenAI, and governmental bodies worldwide. As these entities pioneer technologies and regulations to combat the proliferation of AI-generated misinformation, the discourse also encompasses ethical concerns, legal ramifications, and the broader societal impacts. For professionals in cybersecurity, information governance, and eDiscovery, understanding these developments is paramount, not only to navigate the complexities of digital content authenticity but also to bolster defenses against the potential threats posed by AI innovations.
Deepfake technology, a byproduct of advanced artificial intelligence (AI), has rapidly transformed the landscape of digital media, presenting new challenges in the fight against misinformation. This technology, capable of generating highly realistic fake or altered digital content, is making waves across various sectors, from entertainment to politics, raising ethical concerns and sparking debates about the authenticity of digital content.
The fight against deepfake technology is multifaceted, involving technological innovation, legal and ethical considerations, and international cooperation. Rob Robinson, ComplexDiscovery.
As the technology grows more sophisticated, entities like Microsoft, OpenAI, and various government agencies are at the forefront of combating its misuse. For instance, Microsoft has launched initiatives to detect and mitigate the effects of deepfakes, particularly in the context of political misinformation. Similarly, OpenAI, known for its cutting-edge AI research, has introduced a tool that can distinguish between AI-generated images and real ones with an impressive accuracy rate of 99%.
Notable personalities such as Warren Buffett and Narendra Modi have voiced their concerns about the potential dangers of deepfake technology. Buffett likened AI’s impact to that of nuclear technology, suggesting it has the power to cause significant harm if not properly managed. On the other hand, Modi has identified misinformation spread through deepfakes as a significant threat in the context of India’s elections, labeling the 2024 general elections as India’s first AI election.
Efforts to regulate and control the misuse of deepfake technology extend beyond individual companies to geopolitical considerations. The U.S. government, through agencies like the Department of Defense and the Federal Trade Commission (FTC), is investing in research and setting regulations to prevent deepfakes from being used for fraud or to undermine democracy.
In Europe, the EU Digital Services Act proposes strict measures to hold online platforms accountable for the content they host, including deepfakes. This legislation is part of a broader effort to create an enforcement ecosystem that addresses the complexities introduced by AI-generated content.
The battle against deepfake technology is a global one, with countries from the United States to China and South Korea implementing measures to protect the integrity of digital content. While the potential benefits of AI in enhancing digital media and user experiences are vast, the imperative to safeguard against its misuse is clear. The collective effort from tech giants, regulatory bodies, and international bodies is crucial to ensuring that the future of digital media remains secure and trustworthy.
On the legal front, lawmakers in various countries are considering legislation specifically targeting the malicious use of deepfakes. These laws aim to criminalize the creation and distribution of deepfakes intended to harm individuals, spread false information, or interfere with democratic processes. Legal experts emphasize the importance of balancing these measures with the protection of free speech and innovation in AI technology. Rob Robinson, ComplexDiscovery.
The proliferation of deepfake technology has also led to the development of new academic fields and research initiatives aimed at understanding and countering its effects. Universities and research institutions worldwide are dedicating resources to study the psychological impact of deepfakes, the technical aspects of their creation, and the societal implications of their spread. These studies are critical in developing more effective detection tools and educating the public about the risks associated with deepfake content.
Furthermore, the entertainment industry is grappling with the ethical implications of using deepfake technology. While it offers filmmakers and content creators new avenues for creativity, such as de-aging actors or resurrecting deceased performers, it also raises questions about consent and the authenticity of artistic expression. Industry guidelines and ethical standards are being discussed to navigate these challenges responsibly.
On the legal front, lawmakers in various countries are considering legislation specifically targeting the malicious use of deepfakes. These laws aim to criminalize the creation and distribution of deepfakes intended to harm individuals, spread false information, or interfere with democratic processes. Legal experts emphasize the importance of balancing these measures with the protection of free speech and innovation in AI technology.
In the realm of cybersecurity, deepfakes pose a unique threat to personal and national security. Cybercriminals can use deepfake technology to impersonate individuals in video calls, create convincing phishing campaigns, or spread disinformation to destabilize economies and societies. As a result, cybersecurity firms are developing advanced detection algorithms and training their systems to recognize and flag deepfake content, enhancing the security of digital communications and information.
The fight against deepfake technology is multifaceted, involving technological innovation, legal and ethical considerations, and international cooperation. As AI continues to evolve, so too will the strategies to mitigate its potential harms. The ongoing dialogue among tech companies, governments, civil society, and the public is essential in shaping a digital future that harnesses the benefits of AI while protecting against its risks.
News Sources
- The Deepfake Menance – AI’s Double-Edged Sword in Digital Era
- Meeting the moment: combating AI deepfakes in elections through today’s new tech accord
- OpenAI Introduces Advanced ‘Deepfake’ Detector to Combat Misinformation
- Fighting Election Deepfakes: Microsoft, OpenAI Pump $2 Million Fund Aims to Counter AI Misinformation
- Deepfakes and Democracy: Combatting Disinformation in the 2024 Election
Additional Reading
- U.S. Proposes New AI Export Controls Amid Security Concerns
- From Silicon Valley to Sacramento: California’s Bold AI Regulatory Framework
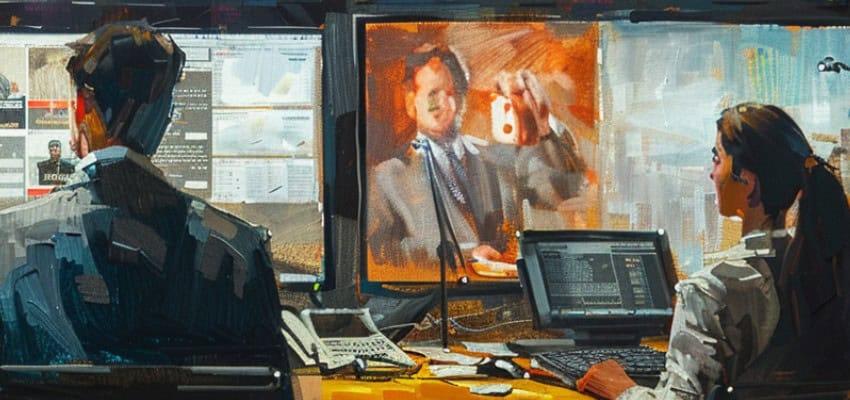
Latest Posts
- [Webinar] "My AI Did It!" Is No Excuse for Unethical or Unprofessional Conduct: Consideration of recent case law and ethics opinions. - June 28th, 1:00 pm - 2:30 pm ET
- [Webinar] Get your SaaS in Gear with Cloud-Based Remote Data Collection - June 25th, 1:00 pm - 1:45 pm ET
- Illumination Zone: Episode 168 | John Burchfield sits down with Kaylee & Mary
- StubHub: Modification of ESI Protocol and Denial of Sanctions – Performance Was Impossible
- [Webinar] Public Access or Not: What Can and Cannot Be Protected from Disclosure in Litigation - June 18th, 1:00 pm - 2:00 pm ET
See more »
Refine your interests »
Written by:
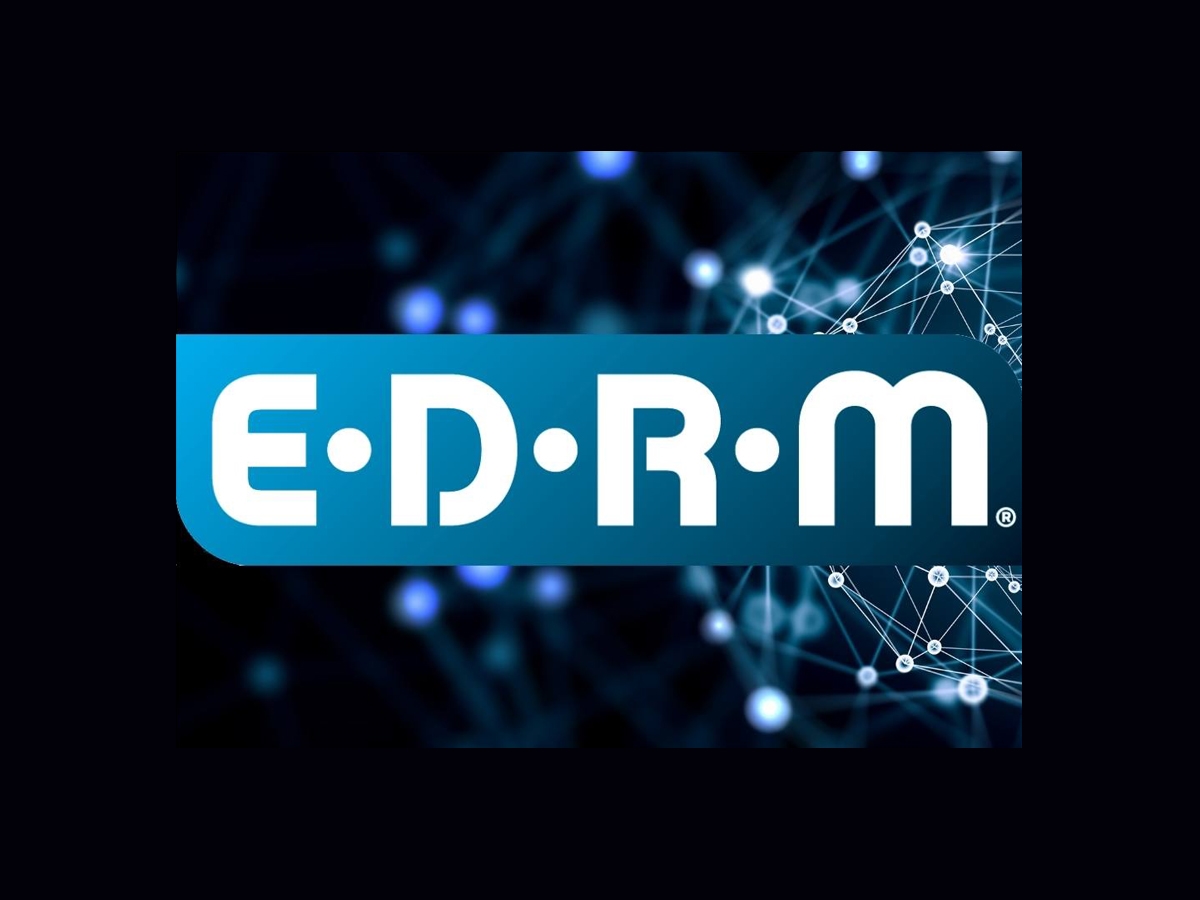
PUBLISH YOUR CONTENT ON JD SUPRA NOW
- Increased visibility
- Actionable analytics
- Ongoing guidance
Published In:
Edrm - electronic discovery reference model on:.
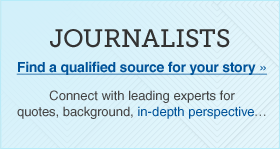
"My best business intelligence, in one easy email…"
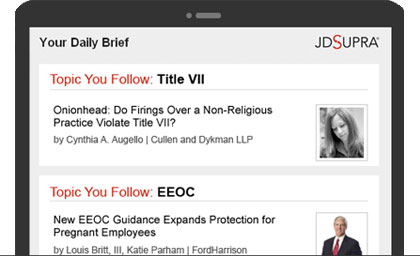
Fooled by AI? These firms sell deepfake detection that’s ‘REAL 100%.’
A surge of companies now claim to offer hyper-accurate detection services, but their capabilities are largely untested.
In a world desperate not to be fooled by artificial intelligence, Deep Media is a rising star.
The Bay Area start-up — which claims it can identify eerily lifelike, AI-created images, audio and video with 99 percent accuracy — has won at least five military contracts worth nearly $2 million since late 2022, including a $1.25 million deal to develop a custom detector to help the Air Force counter Russian and Chinese information warfare. Last month, its CEO, Rijul Gupta, testified before a Senate subcommittee about the threat AI “deepfakes” pose to U.S. elections .
But despite Deep Media’s growing prominence, the company’s sole machine learning engineer graduated from college two years ago — with an undergraduate degree in astrophysics, according to his LinkedIn profile. Meanwhile, a review of other employee LinkedIn profiles suggests the company has no PhDs, AI specialists or forensic scientists on staff — a lack of subject matter expertise that, experts say, suggests a disconnect from the fast-moving deepfake research community.
Gupta is “essentially saying, ‘Trust me.’ And that doesn’t work in science,” said Wael Abd-Almageed, a Clemson University professor who studies deepfakes.
Whatever its bona fides, Deep Media is part of a growing segment of start-ups pitching themselves as a bulwark against the flood of fake content that has risen alongside new, easy-to-use AI tools. Fears that synthetic media could disrupt elections and threaten national security have left institutions such as Congress, the military and the media desperately searching for a trustworthy technical fix to identify fake content. Last week, ChatGPT maker OpenAI announced that it is developing its own detection tool.
But while detection is an increasingly crucial and sought-after service, its capabilities are largely untested. The methods used to create deepfakes are constantly evolving, meaning detection tools built in a lab don’t always work in the wild, academics and researchers say. A test of several publicly accessible, free or low-cost deepfake detectors by the Reuters Institute for the Study of Journalism in April found that most could be fooled by the same techniques they were supposed to spot.
GET CAUGHT UP Summarized stories to quickly stay informed

How Florida’s abortion law is affecting East Coast abortion clinics

In France, two young politicians are in a bitter fight over the future of Europe
Schools that never needed AC are now overheating. Fixes will cost billions.

World Central Kitchen draws criticism for its neutrality in Gaza
A Black rising star lost his elite orchestra job. He won’t go quietly.
Nonetheless, about 40 young companies now offer deepfake detection services, with some claiming startling levels of accuracy: Originality.ai promises “99% AI CONTENT DETECTION ACCURACY.” AI Voice Detector says its tool can “ensure authenticity in important situations,” including court cases. GPTZero says it “convincingly surpasses all notable competitor AI detection services.” And to demonstrate its prowess, Kroop AI shows two seemingly identical cartoon faces on its website, one labeled “REAL 100%” and the other “FAKE 100%.”
Kroop AI CEO Jyoti Joshi said the cartoons are meant to illustrate how high-quality deepfakes can look strikingly realistic, adding that her company’s tool excels at picking up “subtle signatures” left by generative AI. Other companies said their detection results should not be read as the final word, with AI Voice Detector CEO Abdellah Azzouzi saying his company encourages users “to investigate more … after getting the results.”
And though Originality.ai claims 99 percent accuracy, CEO Jon Gillham wrote in a statement to The Washington Post that such claims “should not be believed” unless they’re supported by test results. Pointing to a company blog post , Gillham said his company discloses its detector’s results on five third-party data sets, as well as testing results for other detectors.
According to the analytics firm PitchBook, venture capitalists invested $200 million in deepfake start-ups globally in 2023 and the first quarter of 2024, up from $6.6 million in 2017. Those figures cover deepfake creation companies as well as detectors, but some of the biggest deals were for tools to identify AI-manipulated media. Still, spending on deepfake detection is paltry compared with the $29 billion invested in generative AI deals last year, per PitchBook, including funding for popular tools that can be abused to make deceptive images, clone voices or edit video.
With wars raging in Ukraine and Gaza and more than 4 billion people eligible to vote in elections this year, forensic scholars say the surge of detection start-ups — particularly those boasting near-perfect accuracy — risks throwing gasoline on the growing AI fire by providing a false sense of certainty about the legitimacy of some visual and audio content, while eroding public confidence in authentic pieces of media.
The potential security risks from labeling a piece of content “fake” are mounting. In October, the tech news site 404 Media reported that the free tool AI or Not marked an image the Israeli government claimed showed atrocities committed by Hamas as fake, contrary to expert opinion.
AI or Not CEO Anatoly Kvitnitsky said that, of a few dozen images related to the Hamas attack, “We got all of them right except this one.” AI or Not has since improved its tool, Kvitnitsky said, but he acknowledged that the mistake was concerning.
“I’m actually born in Ukraine and Jewish,” he said, “so it did hit home when we got some of this wrong.”
Deep Media also has found evidence of AI manipulation where others have not. Last month, Gupta told The Post that his company’s tool detected a “high likelihood” that a closely scrutinized video of Catherine, Princess of Wales, announcing her cancer diagnosis had been manipulated with AI.
Experts including Clemson’s Abd-Almageed, Brown University misinformation researcher Claire Wardle and Hao Li, the CEO of AI video effects company Pinscreen, said they found no evidence that the video, which was filmed by the BBC in March, was manipulated with AI.
In a more recent interview, Gupta told The Post that the company stands by its analysis. Gupta also defended the company’s level of AI expertise. Gupta, who graduated from Yale with a degree in biochemical engineering, said he personally has 15 years of experience in machine learning, beginning when he was 15 years old. He added that Deep Media employs experienced contractors, including people working toward their PhDs, but declined to name them.
“AI is a rapidly evolving technology that requires deepfake detection AI models to keep up with bad actors,” Gupta said in a statement, noting that Deep Media has trained and tested numerous detection models.
“Deepfake detection is a very difficult problem,” Gupta said in the interview, “and Deep Media is working tirelessly to continue to advance our technology.”
‘Can I trust it or not?’
Forensic scholar Hany Farid, a professor at the University of California at Berkeley, told The Post he’s spoken with four different developers this month who boast near-perfect detection rates. But he said start-ups can boost their numbers by grading themselves on a curve: They often train detection models on a particular set of deepfakes and then evaluate their ability to identify the same type of fakes.
In the real world, deceptive media may not exhibit the patterns AI has learned to detect, Farid said, adding that a more reliable approach requires a nimble combination of computer programming, physics, open-source intelligence and forensics.
Farid’s own detection start-up, Get Real Labs, uses machine learning, but also analyzes elements including shadows, geometry, compression settings and results of reverse-image searches, he said. Eventually, the company might achieve the accuracy to summarize its results in a “final answer” for clients, he said, but the science isn’t there yet.
“If you start to say, ‘We can, with high precision, tell whether something is real or fake,’ and you get it wrong, you are part of the problem,” Farid said. “In fact, you are arguably worse than the disease.”
This gap between lab and real-world results makes it difficult to evaluate competing detectors, Abd-Almageed said. And it makes the slew of entrepreneurs pivoting into deepfake detection as a hot start-up sector, akin to crypto or AI itself, particularly concerning, Farid said.
While boastful claims from eager start-ups are nothing new in Silicon Valley, Raquel Vázquez Llorente, head of technology threats and opportunities for the nonprofit Witness, said conclusions about content such as “80 percent AI-generated” can mislead the public.
“What the hell does that mean?” she said at a recent conference on generative AI and the law . “Can I trust it or not?”
Because deepfake detection is particularly urgent in an election year, Oren Etzioni, co-founder of the Allen Institute for AI, recently founded the nonprofit TrueMedia.org , which is dedicated to developing a trustworthy detection tool that it offers free to fact-checkers.
The Pentagon also is moving faster to keep pace with technological advancements. It has made itself more welcoming to tech start-ups by modernizing its acquisition process and loosening eligibility restrictions for its Small Business Innovation Research development fund. Known as SBIR, the three-stage program finances promising ideas, develops the technology and, finally, puts the technology into production. Most start-ups never make it to the third phase.
All five of Deep Media’s publicly listed military contracts were SBIR grants. Three have ended and a fourth ends in July, while the $1.25 million AI detection deal with the Air Force — now in phase two of SBIR — ends in November.
In a statement, Pentagon spokesperson Jeff Jurgensen said the Defense Department evaluates every SBIR proposal by “considering factors such as technical merit, defense requirements, and commercial potential,” looking for small businesses that might appeal to private investors, who have access to more capital.
99 percent accurate
Before focusing on deepfake detection, Deep Media was in the business of creating synthetic media. The company released what it called a universal translator to dub videos and launched a series of content creation apps, including Babble, CopyCat.ai , DubSync and PolyTalk. Two of the company’s smaller military contracts were for AI translation services.
“The reason that our deepfake detectors work — the reason we have Pentagon contracts — is because we’ve been pioneering the generative side for such a long time,” Gupta told The Post.
In previous press interviews, Gupta has claimed a 99 percent accuracy rate in identifying deepfakes. He amended that somewhat in his interview with The Post, saying he is committed to offering clients at least 95 percent accuracy, even on “the latest and greatest, highest-quality, hardest-to-detect deepfakes.”
Gupta said he tries to avoid misleading numbers, acknowledging that “people can fool you when they talk about accuracy.” If 10 images in a universe of 1,000 are fake, the model can declare everything real and be 99 percent accurate, he said. But in reality, he said, “That number is meaningless, right?”
In talking with The Post, Gupta repeatedly cited a $25 million, three-year cooperative research agreement for deepfake data generation and detection with the Air Force Research Laboratory as evidence of the company’s credibility. A copy of the contract reviewed by The Post shows that 60 percent of the $25 million value comes from resources provided by Deep Media.
The agreement previously has been cast as a $25 million contract, but Gupta acknowledged “that might have been misrepresented” in the press. The agreement does not provide “revenue coming into the company,” he said, “but it is supporting our AI research.”
Meanwhile, the advisory firm that helped Deep Media obtain its military contracts has since sued the company for failing to pay its bills. The lawsuit, filed in federal court in March by Stonegardens Advisory, also alleges that Deep Media falsely claimed Stonegardens’s managing member, a Marine veteran, as a Deep Media executive on its website and in marketing materials, as well as onstage at SXSW and Air Force events. Stonegardens declined to comment on the case.
Gupta declined to address the lawsuit’s claims. He told The Post that Deep Media began applying for military contracts because he fears that manipulative synthetic media could destabilize American society.
“If our government doesn’t have a deepfake detector,” Gupta said, “our government doesn’t know what’s real and what’s fake.”
A previous version of this article incorrectly referred to Kroop AI CEO Jyoti Joshi as a him. The article has been corrected.

Help | Advanced Search
Computer Science > Computer Vision and Pattern Recognition
Title: deepfake detection: a comprehensive study from the reliability perspective.
Abstract: The mushroomed Deepfake synthetic materials circulated on the internet have raised serious social impact to politicians, celebrities, and every human being on earth. In this survey, we provide a thorough review of the existing Deepfake detection studies from the reliability perspective. Reliability-oriented research challenges of the current Deepfake detection research domain are defined in three aspects, namely, transferability, interpretability, and robustness. While solutions have been frequently addressed regarding the three challenges, the general reliability of a detection model has been barely considered, leading to the lack of reliable evidence in real-life usages and even for prosecutions on Deepfake-related cases in court. We, therefore, introduce a model reliability study metric using statistical random sampling knowledge and the publicly available benchmark datasets to review the reliability of the existing detection models on arbitrary Deepfake candidate suspects. Case studies are further executed to justify the real-life Deepfake cases including different groups of victims with the help of the reliably qualified detection models as reviewed in this survey. Reviews and experiments upon the existing approaches provide informative discussions and future research directions of Deepfake detection.
Submission history
Access paper:.
- Other Formats
References & Citations
- Google Scholar
- Semantic Scholar
BibTeX formatted citation

Bibliographic and Citation Tools
Code, data and media associated with this article, recommenders and search tools.
- Institution
arXivLabs: experimental projects with community collaborators
arXivLabs is a framework that allows collaborators to develop and share new arXiv features directly on our website.
Both individuals and organizations that work with arXivLabs have embraced and accepted our values of openness, community, excellence, and user data privacy. arXiv is committed to these values and only works with partners that adhere to them.
Have an idea for a project that will add value for arXiv's community? Learn more about arXivLabs .
- Sustainability
- Latest News
- News Reports
- Documentaries & Shows
- TV Schedule
- CNA938 Live
- Radio Schedule
- Singapore Parliament
- Mental Health
- Interactives
- Entertainment
- Style & Beauty
- Experiences
- Remarkable Living
- Send us a news tip
- Events & Partnerships
- Business Blueprint
- Health Matters
- The Asian Traveller
Trending Topics
Follow our news, recent searches, in focus: 'at the click of a button' – the scourge of deepfake ai porn, advertisement.
Some Singapore MPs were among dozens of victims to receive letters that had images of their faces superimposed on obscene pictures. The number of people potentially at risk of image-based sexual abuse increases exponentially with the advent of generative AI, says a women's rights group.
There has been an "explosion of deepfakes" in recent years, says Ms Sugidha Nithiananthan, a director at women's rights group AWARE. (Illustration: CNA/Clara Ho)
This audio is AI-generated.
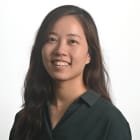
SINGAPORE: The letters were sent to their workplaces – in them, their face superimposed onto an obscene photo of a man and a woman in an “intimate and compromising” position.
Contact this email address, or else, the letter threatened.
Those who contacted the email address were ordered to transfer money to a bank account, or compromising photographs and videos would be leaked and exposed on social media.
One 50-year-old victim transferred S$20,000 (US$14,700).
Victims of deepfake porn can feel helpless and powerless, said Dr Hanita Assudani, a clinical psychologist at Alliance Counselling.
“What has happened is somebody has used something that is part of me – my face, my pictures, my features – without my consent,” she added.
“The intrusiveness is present. It depends on each individual, but the level of intrusiveness, the shock, the humiliation, the shame, and the social consequences of it can be very similar to somebody who experienced a sexual assault.”
Some victims feel unsafe as their trust was broken, she said.
“I’m definitely concerned about anything that will take away consent and power from individuals, violate people’s boundaries,” said Dr Hanita.
There has been an “explosion of deepfakes” in recent years, said Ms Sugidha Nithiananthan, director of advocacy and research communications at the Association of Women for Action and Research (AWARE).
She pointed to a report by identity verification platform Sumsub that said the Asia Pacific region has seen a 1,530 per cent increase in deepfakes from 2022 to 2023.
“Mirroring trends in sexual violence, the non-consensual creation of sexually explicit material with deepfake technology is largely targeted at women,” she added.
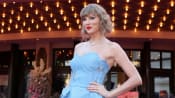
Commentary: Taylor Swift deepfake explicit images are a warning anyone can be targeted
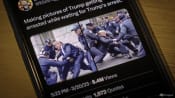
Deepfake porn could be a growing problem amid AI race
But it is not just low-quality, black-and-white photos printed on paper that are a concern. Once online, these deepfake images travel quickly and wide, being replicated on websites and platforms.
A quick search on Google by CNA showed multiple results, including one site that offered to fulfil requests to generate deepfake porn images of local celebrities and influencers.
Superstar Taylor Swift, social media influencer Bobby Althoff and actress Natalie Portman are among celebrities who have reportedly been victims of deepfake AI porn.
In September last year, a man in South Korea was sentenced to more than two years in prison for using AI to create 360 virtual child abuse images .
Some people who view such pornographic material eventually think about creating them. A report by Home Security Heroes, a US company focused on preventing identity theft, found that deepfake pornography makes up 98 per cent of all deepfake videos online.
About 48 per cent of the 1,522 men surveyed had viewed deepfake pornography at least once, with “technological curiosity” being claimed as the top reason for doing so.
Twenty per cent of respondents considered learning how to create deepfakes.
“One in 10 respondents admitted to attempting the creation of deepfake pornography featuring either celebrities or individuals known to them,” the report said.
CREATING A DEEPFAKE "WITHIN MINUTES"
Between March and mid-April, the police received more than 70 reports of victims receiving extortion letters with manipulated obscene photos.
Among the victims were Foreign Affairs Minister Vivian Balakrishnan and Members of Parliament Tan Wu Meng, Edward Chia and Yip Hon Weng.
“With advancements to photo and video editing technology, including the use of Artificial Intelligence (AI) powered tools, manipulated photographs and videos may increasingly be used for extortion,” the police said in April.
Most of the victims’ photographs appeared to have been obtained from public sources, said the police.
Mr Chia warned that with readily available tools, “anyone can create deepfake content within minutes”.
"This can pose a significant threat to our social fabric. Unchecked, this can affect our public standing and those we love.”
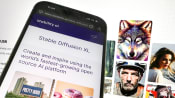
AI-generated child sexual abuse images could flood the internet. A watchdog is calling for action
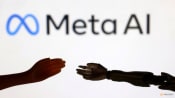
Meta oversight board reviews handling of AI-created celebrity porn
The number of people potentially at risk of image-based sexual abuse increases exponentially with the advent of generative AI, said AWARE’s Ms Sugidha.
“Anyone who has had a photo of them posted online could be a possible victim.
“This makes detecting deepfakes particularly challenging, especially for individuals who have never shared intimate images and may not be actively looking out for such misuse, thus allowing perpetrators to evade detection,” she added.
Associate Professor Terence Sim of the National University of Singapore’s Centre for Trusted Internet and Community said AI-generated porn is “certainly a very pressing issue”.
“The bad guys are really coming up with ways to exploit this and then scam people,” he said.
“Various kinds (of people) - celebrities, politicians, even your normal man on the street. Ordinary folks like you and me.”
There is a range of tools that can be used to generate obscene photos and videos of varying quality.
It is possible to create a realistic deepfake video using just one high-resolution picture of the victim and a video that you want to superimpose the victim’s face onto, sometimes known as a “driving video”.
In a Talking Point episode, Prof Sim showed how he used AI-powered face swapping tool FaceFusion to create a deepfake of host Steven Chia within minutes.
“All I need is an actor that sort of looks like the victim,” he said.
If the “driving video” shows an actor in a compromising position, it can be digitally manipulated to show the victim’s face instead of the actor’s face.
Assoc Prof Sim said that as of now, most deepfakes are of “talking heads”, which refers to a close-up that focuses on the face of a target.
“You don’t even need the full body. It’s very easy for an actor to create this.
“Imagine, I want to fake Donald Trump, I can just wear clothes like Donald Trump, put the Oval Office or US flag behind me. That’s fairly easy to do.”
Installing FaceFusion manually requires knowledge of programming language Python, and is “not for the faint hearted”, he said.
But an automatic installer can be purchased for US$20. Once installed, the user interface is quite intuitive.
Upload a picture and a video, and the AI does most of the work. There are sliders and buttons to make adjustments to the deepfake video to make it as realistic as possible.
Those that are easier to use will likely produce less realistic results, he said.
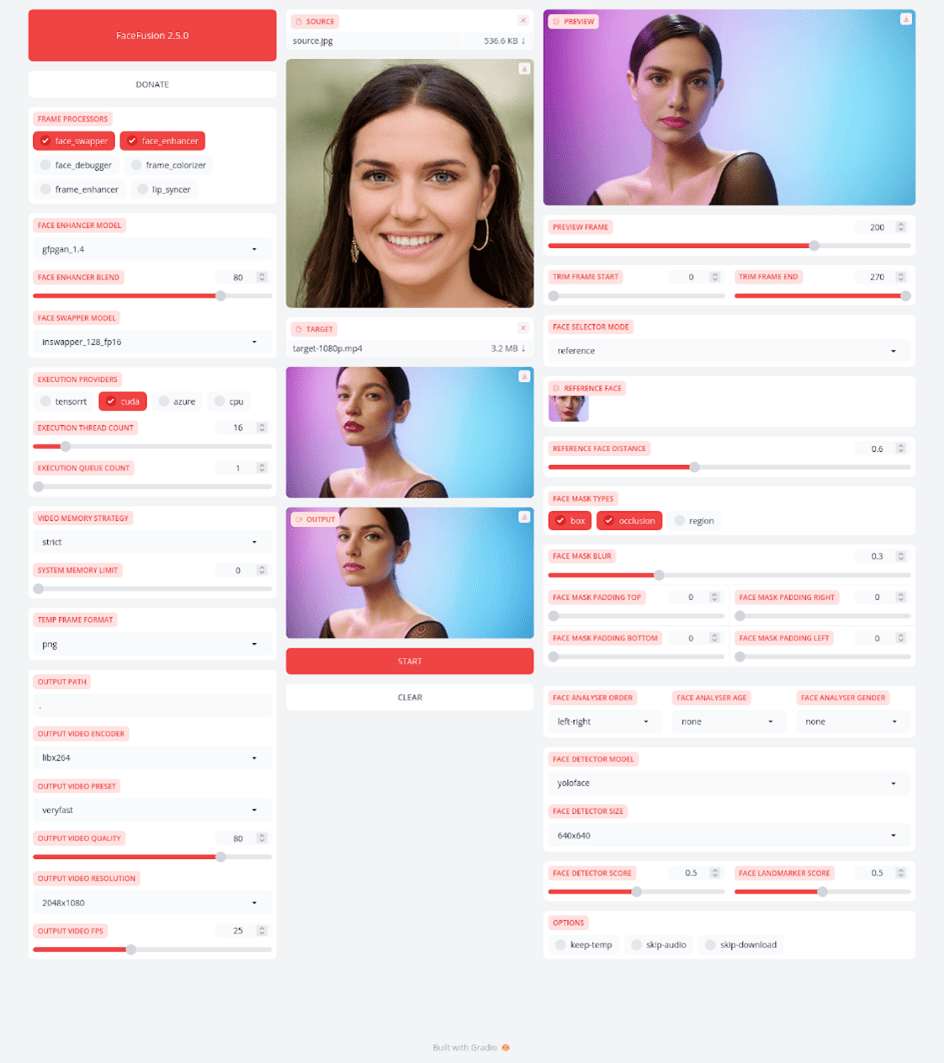
Mr Yip told CNA last month that the images in the letter were not sophisticated and low quality. It was in black and white and not well-rendered.
But it would not take long for someone to pick up the skills required to create a better deepfake, including online.
Associate Professor Cheung Ngai-Man of the Singapore University of Technology and Design (SUTD) estimated that it would take about a week to learn what is needed to create a fairly realistic deepfake.
Face-swapping does not require specialised equipment either. A computer with “more high-end” specifications would allow a user to render these images to be more realistic, he said, adding that such computers are quite accessible.
The technology is continually advancing, with real-time generation of deepfakes getting closer to reality.
In April, Microsoft unveiled its VASA-1 program, which produces “lifelike audio-driven talking faces generated in real time”.
It only needs one photo and a one-minute audio clip to generate a realistic talking head. Some other audio AI tools require much longer audio input of 10 to 15 minutes.
The accessibility and ease with which deepfakes can be created is a concern for AI experts.
“The truth is that these kind of tricks or blackmailing threats have been there ever since Photoshop came about, in fact maybe even earlier,” said SUTD’s Assistant Professor Roy Lee.
“What is getting us worried is how easy (it is). Last time you might need to be a Photoshop expert to basically do it right and make it look realistic.
“Right now, it’s just at the click of a button … it’s making things easier and it’s making things more realistic because generative AI is improving its performance,” he said.
Add the fact that photos and videos are disseminated far and wide on the internet, and the concern becomes greater.
“With all the positive things that come with the internet and generative AI, there is always a flipside,” he said. “If you’re abusing it then it’s more widespread, it’s more realistic.”
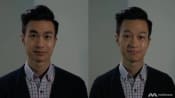
Deepfakes, nudes and the threat to national security
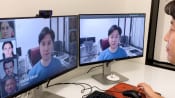
Deep dive into deepfakes: Frighteningly real and sometimes used for the wrong things
Remove all photos from social media.
How can people protect against their faces being used in deepfake porn?
“Remove all your stuff from social media,” said NUS’ Assoc Prof Sim. “That’s certainly one way.”
But he acknowledged that it was not something everyone could do. For some people – such as celebrities, influencers or small business owners – social media is their livelihood.
Asst Prof Lee said that as people become more aware of deepfakes, they may decide to stay off social media and not post pictures or information about themselves.
Some of his friends who are more conscious and tech savvy have already done so. They also steer clear of using their faces as their WhatsApp or Telegram profile pictures.
Others are willing to accept the risk of posting their pictures online.
“It’s an individual choice,” said Asst Prof Lee.
The group he is concerned about are those who post their pictures online without being aware of the risks that come with generative AI.
But as society becomes more educated on AI, people may realise how common deepfakes are.
“I believe that people will get smarter and … if there’s a video circulating of a friend in a compromised position, no one will believe that,” he said.
“If you reach that stage as a society, you become more aware of information verification, be more savvy about how information is created and all - then there is less worry.”
Experts are also working on improving the detection of deepfakes. One way to do so is for people to use “invisible watermarks” on photos and videos.
If the video or photo has been tampered with, analysis will reveal that the watermark has been broken and the contents are not to be trusted.
While this could work to help protect trusted media entities, it is less useful in the context of AI-generated porn since people are less likely to watermark their personal photos.
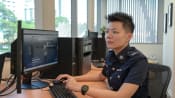
‘The audio gets to you’: What it takes for Singapore police to investigate child porn cases
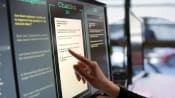
Singapore proposes generative AI framework
Can the law keep up.
Mr Cory Wong, director at Invictus Law Corporation, said there is no law at the moment that is specific to AI.
“It’s still always criminalising the human aspect of it, like what you did with that AI-generated image.”
Clicking on the “generate” button would not be an offence, he said. But if the image generated is offensive, then possessing it would be an offence, said Mr Wong.
“You don’t just generate it in a vacuum,” he said. “You generate it and then you store it somewhere.”
People who possess obscene materials can be jailed for up to three months, fined, or both. Those caught transmitting obscene material face the same penalties.
Mr Chooi Jing Yen, a criminal lawyer at Eugene Thuraisingam LLP, said possession of an obscene deepfake video could also be an offence under the Films Act.
Offenders can be fined up to S$20,000 (US$15,000), jailed for up to six months, or both.
He also said that obscene materials do not necessarily need to be human. "I don’t think that the mere fact (that) it’s AI-created means that it can’t be (obscene)," he added.
Depending on the specifics of the case, there could be other charges.
“A single act can attract a charge under extortion, it can also attract a charge under Section 377BA - it’s not specific to AI but it’s an offence or insulting somebody’s modesty by showing them a picture,” said Mr Chooi.
“The offence could also be under the Protection of Harassment Act, so there are a few routes to it. But extortion is probably the harshest one because it does carry a mandatory minimum prison sentence.”
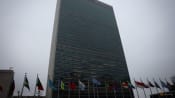
UN adopts first global artificial intelligence resolution
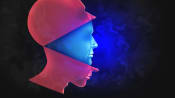
AI in Southeast Asia: As new frontier opens in scams and cyberbullying, authorities wage high-tech battle
Laws specific to deepfake AI pornography will soon be introduced in other countries.
Australia’s federal government announced in May that it will introduce legislation to ban the creation and non-consensual distribution of deepfake pornography.
The UK’s Ministry of Justice also announced in April a new law that criminalises the creation of sexually explicit deepfakes without consent.
The new law also means that if the creator has no intent to share it but wants to “cause alarm, humiliation or distress to the victim”, they will also be prosecuted.
Asked if there is a need to develop legislation for AI-related crimes in Singapore, Mr Chooi said that the technology is still evolving.
“You wouldn’t want to be passing laws every month or every few years because the space moves very quickly. You end up with a very piecemeal kind of situation and people are also all confused,” he said.
Mr Wong said that having specific laws might be good, but there may be too many things to cover.
“It’s really the use of AI or the misuse of AI that is the problem, and I think it’s very difficult to try to legislate every single thing,” he said.
There is also the potential problem of under-legislation, where the law is too wide.
“And then you realise that actually (the) law is a bit defective,” he said.
“Right now, with the existing (laws) - whether it’s Penal Code, whether it’s Miscellaneous Offences Act - they are sufficiently wide to capture all this, then maybe give it a bit more time to see how we will actually criminalise AI-related offences.”
If there is some AI misuse not captured within Singapore's current legislation, that would be the tipping point to push for more legislation, Mr Wong added.
Another scenario would be where the maximum sentences under existing provisions do not seem proportionate to the crime, according to Mr Chooi. In such a case, lawmakers may need to look into introducing new laws.
“I think generally that requires a certain number of such cases to surface first,” he said.
“When we punish people, and people are prosecuted and sentenced, one of the aims is general deterrence, which is to send a message to the public,” Mr Chooi added.
“If it’s not that prevalent then there’s no strong reason to do that.”
In response to CNA's queries, the Attorney-General’s Chambers said it has not prosecuted cases related to AI-generated pornography in Singapore.
“Such offences are relatively new, made possible by the recent evolution of AI technology,” a spokesperson said.
Although people are certainly generating obscene images, the lawyers said they have not personally encountered cases where someone is charged with doing so.
When asked why this is the case, Mr Wong said it first needs to be reported to the authorities. He gave the example of someone superimposing a face onto a naked body.
“Let’s say I don’t even know about it, or if anyone who sees it knows this is probably fake, then they may not take that first step of reporting it,” he said.
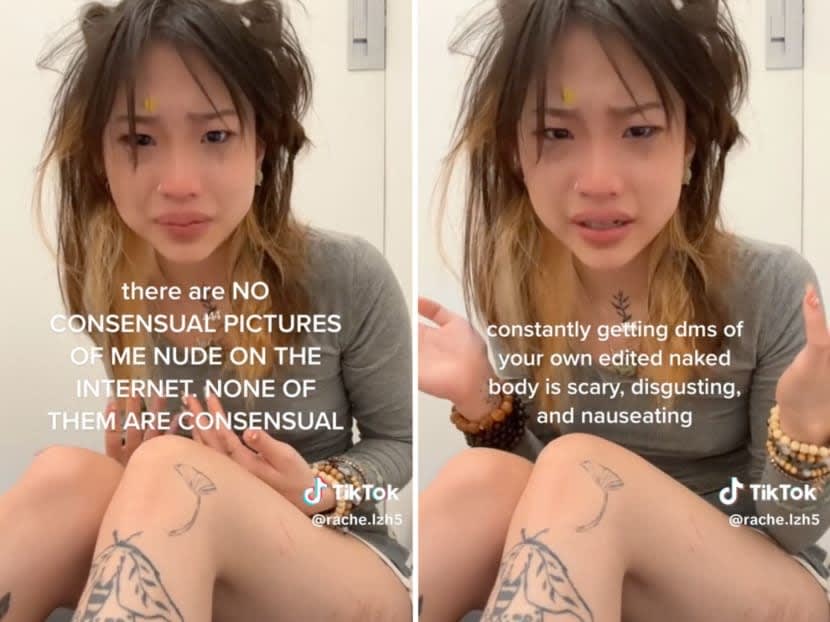
A HEAVY EMOTIONAL TOLL
Last year, a TikTok user named Rachel opened up about her harrowing experience of people using AI tools to generate fake nude images of her, before sending these pictures to her and other online users.
She had received a direct message on Instagram containing fake nude images of her. The anonymous user had used photos on her public profile and ran them through a “deepfake AI program” to create the obscene pictures.
In a TikTok video, she talked about receiving the message and said she had ignored it.
She then received dozens of messages containing AI-generated nudes of herself, this time from other users.
In a later video, she can be seen crying and asking her abusers to stop creating such images, calling them “rapists”.
When CNA searched for her TikTok username on Google, she appeared to have taken down her account. Several porn websites also appear on the first page of the results.
Dr Hanita of Alliance Counselling said that when someone’s photo has been misused, they may not know whether a crime has been committed and whether the police can help them.
People around them may not be able to empathise or relate.
“Then you start thinking, is what I’m going through real? Is it a big deal? Because the crime feels very distant, they take my photo and use it another way. Is it real?
“But I will say it’s very real .... I think the person may not know how to go about reporting the crime, what else to do.”
Some victims develop symptoms of post-traumatic stress disorder, anxiety or depression.
The police told CNA that victim care officers, who have received training from police psychologists, can provide emotional and practical support to those who have been assessed to need it.
Dr Hanita said some victims may feel ashamed and want to keep things to themselves, or end up blaming themselves.
“The first thing is to remember that this is not your fault,” she said. “It’s your image and however you choose to use it, it has been used in another way.
“Even if you take a sexy photo of yourself and put it (online), you choose that this photo be used in this way – the social media way – not to be used in another way.”
Friends and family members can support victims emotionally and practically, such as by going with them to report the crime and helping to collect evidence to hand to the police.
“Don’t chide the person. Respect them, provide empathy, compassion for what they are going through,” she said. “That can be very helpful and powerful.”
She called on people to work together as well, by reporting images or accounts that seem suspicious, even if they do not know the person involved.
“As a community, that’s what we can do to step up vigilance. To know that this is not okay, it’s not funny … for somebody’s images to be used in a sexual manner, or to put them down or humiliate them.”
Related Topics
Also worth reading, this browser is no longer supported.
We know it's a hassle to switch browsers but we want your experience with CNA to be fast, secure and the best it can possibly be.
To continue, upgrade to a supported browser or, for the finest experience, download the mobile app.
Upgraded but still having issues? Contact us
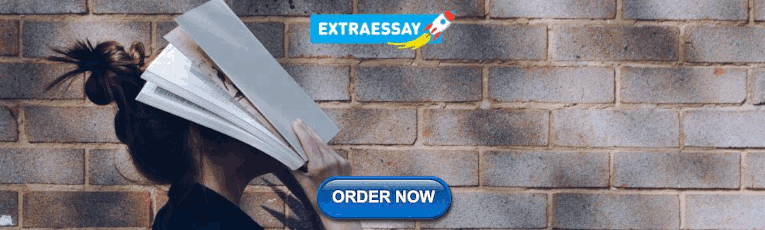
IMAGES
VIDEO
COMMENTS
To provide an updated overview of the research works in Deepfake detection, we conduct a systematic literature review (SLR) in this paper, summarizing 112 relevant articles from 2018 to 2020 that presented a variety of methodologies. We analyze them by grouping them into four different categories: deep learning-based techniques, classical ...
While asking such questions is good practice in experimental research (Thorson et al., 2012), we did not employ them for two reasons. First, Rössler et al. (2018) show that most users have limited ability to discern between a deepfake and an authentic video, so manipulation checks would have been biased by a high degree of guessing. Second, we ...
The purpose of Mirsky and Lee is to provide a deeper understanding of deepfake creation and detection, identify the shortcomings of current defense solutions, and highlight areas requiring further research. The paper discusses prevention and mitigation as countermeasures for deepfake generation.
During this process, theoretical essays, legal and literature reviews, and studies dealing exclusively with technical issues were excluded, as the goal was to synthesize empirical research on deepfakes. ... For example, Meta and Google have created large public deepfake datasets to advance research on deepfake detection, which were used in ...
Deepfakes are the product. of artificial intelligence (AI) applications that merge, combine, replace, and superimpose images and video. clips to create fake videos that appear authentic (Maras ...
with the problems raised by Deepfake. T o provide an updated overview of the research works in Deepfake. detection, we conduct a systematic literature review (SLR) in this paper, summarizing 112 ...
The field research for Deepfake social impact research is emerging and this paper brings more insights drawn from a methodical, subject focused and distribution point of view.
The existing surveys have mainly focused on the detection of deepfake images and videos. This paper provides a comprehensive review and detailed analysis of existing tools and machine learning (ML) based approaches for deepfake generation and the methodologies used to detect such manipulations for both audio and visual deepfakes. For each ...
Hence, this review paper aims to i. deliver a more precise overview of the different types of deepfake as well as their available generation tools and technology, ii. present the recent progress of deepfake detection methods with the open-source deepfake dataset, and iii. outline potential opportunities, research trends of deepfake in terms of ...
In this article, we explore the creation and detection of deepfakes and provide an in-depth view as to how these architectures work. The purpose of this survey is to provide the reader with a deeper understanding of (1) how deepfakes are created and detected, (2) the current trends and advancements in this domain, (3) the shortcomings of the ...
Deepfake is a technology dedicated to creating highly realistic facial images and videos under specific conditions, which has significant application potential in fields such as entertainment, movie production, digital human creation, to name a few. With the advancements in deep learning, techniques primarily represented by Variational Autoencoders and Generative Adversarial Networks have ...
Catching the deepfake alarming problem, research community has focused on developing deepfake detection algorithms and numerous results have been reported. This paper has reviewed the state-of-the-art methods and a summary of typical approaches is provided in Table 2. It is noticeable that a battle between those who use advanced machine ...
the number of deepfake papers has increased signifi-cantly in recent years (Fig. 1). Although the obtained numbers of deepfake papers may be lower than actual numbers but the research trend of this topic is obviously increasing. There have been existing survey papers about creat-ing and detecting deepfakes, presented in [19, 20, 32].
Various research papers have extensively explored the deepfakes, categorizing methods at multiple levels. Tolsana et al. [ 9 ] have divided the deepfake techniques into four categories, limited to image and video media without explaining the detection mechanism based on the feature representation.
Deepfake refers to realistic, but fake images, sounds, and videos generated by articial intelligence methods. Recent advances in deepfake generation make deepfake more realistic and easier to make. Deepfake has been a signicant threat to national security, democracy, society, and our privacy, which calls for deepfake detection methods to combat potential threats. In the paper, we make a survey ...
This paper proposes an automated method to classify deep fake images by employing Deep Learning and Machine Learning based methodologies. ... Mittal et al. 51 employed Alex Net for deepfake ...
DeepFake Detection. 137 papers with code • 5 benchmarks • 18 datasets. DeepFake Detection is the task of detecting fake videos or images that have been generated using deep learning techniques. Deepfakes are created by using machine learning algorithms to manipulate or replace parts of an original video or image, such as the face of a person.
Much research has been devoted to developing detection methods to reduce the potential negative impact of deepfakes. Application of neural networks and deep learning is one approach. In this paper, we consider the deepfake detection technologies Xception and MobileNet as two approaches for classification tasks to automatically detect deepfake ...
This paper presents a survey of algorithms used to create deepfakes and, more importantly, methods proposed to detect deepfakes in the literature to date. We present extensive discussions on challenges, research trends and directions related to deepfake technologies. By reviewing the background of deepfakes and state-of-the-art deepfake ...
Thus, open challenges and potential research directions are also discussed. This paper is expected to aid the readers in comprehending deepfake generation and detection mechanisms, together with open issues and future directions. Keywords: DeepFakes, digital face manipulations, ... In machine learning and the deepfake research community, the ...
A Systematic Review on Deepfake Technology. March 2023. DOI: 10.1007/978-981-19-7615-5_55. In book: Proceedings of Data Analytics and Management (pp.669-685) Authors: Ehtiram Raza Khan. Jamia ...
OpenAI said its new detector could correctly identify 98.8 percent of images created by DALL-E 3, the latest version of its image generator. But the company said the tool was not designed to ...
The proliferation of deepfake technology has also led to the development of new academic fields and research initiatives aimed at understanding and countering its effects.
11 min. In a world desperate not to be fooled by artificial intelligence, Deep Media is a rising star. The Bay Area start-up — which claims it can identify eerily lifelike, AI-created images ...
The mushroomed Deepfake synthetic materials circulated on the internet have raised serious social impact to politicians, celebrities, and every human being on earth. In this survey, we provide a thorough review of the existing Deepfake detection studies from the reliability perspective. Reliability-oriented research challenges of the current Deepfake detection research domain are defined in ...
A strong fraud detection system that makes use of the synergies between Random Forest, NLTK, NLTK (Natural Language Toolkit), ML (Machine Learning), ML (Machine Learning) and recurrent neural networks approaches is presented. Recognizing fraudulent activity on social media has grown in importance in the digital realm. Facebook has 11% of monthly active users who are duplicates (about 150 ...
A Systematic Review on Deepfake Technology. Abstract. The 21st century has seen technology become an integral part of human survival. Living. standards have been affected as the technology ...
The number of deepfake attacks (successful ones at that) is phenomenal. According to a Sumsub Research report conducted last year, global deepfake incidents have surged tenfold from 2022 to 2023, with North America having the highest deepfake attack growth of 1740 percent.
One 50-year-old victim transferred S$20,000 (US$14,700). Victims of deepfake porn can feel helpless and powerless, said Dr Hanita Assudani, a clinical psychologist at Alliance Counselling. "What ...
Review Paper on Deepfake Video Detection using Neural Networks. April 2024. International Journal of Advanced Research in Science Communication and Technology. April 2024. DOI: 10.48175/IJARSCT ...