ENCYCLOPEDIC ENTRY
Population density.
Population density is the concentration of individuals within a species in a specific geographic locale. Population density data can be used to quantify demographic information and to assess relationships with ecosystems, human health, and infrastructure.
Geography, Geographic Information Systems (GIS), Human Geography
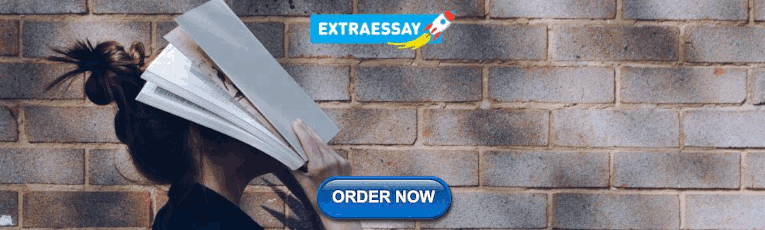
Manhattan Skyline
Studies of human populations often happen at or below the city level in places like Manhattan, which is part of New York City, New York, United States.
Photograph from Lost Horizon Images
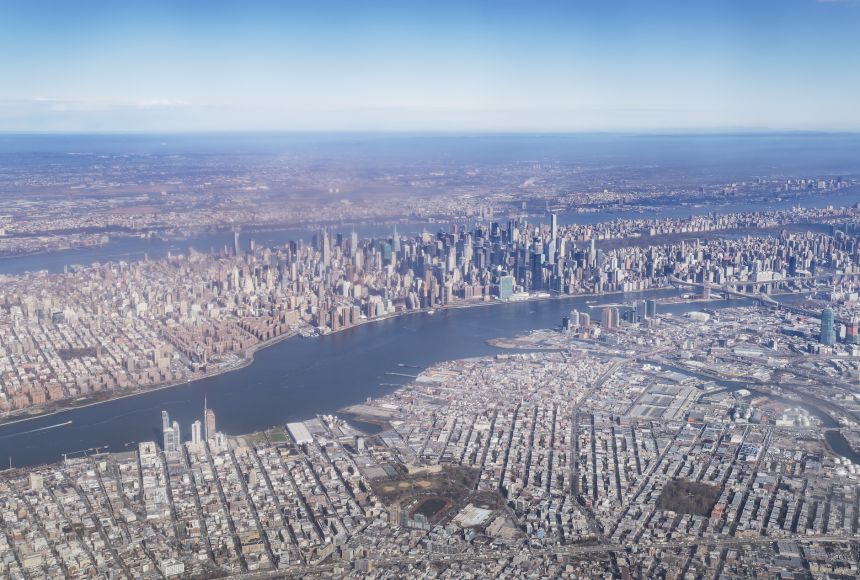
A population is a subgroup of individuals within the same species that are living and breeding within a geographic area. The number of individuals living within that specific location determines the population density, or the number of individuals divided by the size of the area. Population density can be used to describe the location, growth, and migration of many organisms . In the case of humans, population density is often discussed in relation to urbanization , im migration , and population demographics . Globally, statistics related to population density are tracked by the United Nations Statistics Division, and the United States Constitution requires population data to be collected every 10 years, an operation carried out by the U.S. Census Bureau. However, data on human population density at the country level, and even at regional levels, may not be very informative; society tends to form clusters that can be surrounded by sparsely inhabited areas. Therefore, the most useful data describes smaller, more discrete population centers. Dense population clusters generally coincide with geographical locations often referred to as city, or as an urban or metropolitan area; sparsely populated areas are often referred to as rural. These terms do not have globally agreed upon definitions, but they are useful in general discussions about population density and geographic location. Population density data can be important for many related studies, including studies of ecosystems and improvements to human health and infrastructure . For example, the World Health Organization, the U.S. Energy Information Administration, the U.S. Global Change Research Program, and the U.S. Departments of Energy and Agriculture all use population data from the U.S. Census or UN statistics to understand and better predict resource use and health trends. Key areas of study include the following:
- Ecology: how increasing population density in certain areas impacts biodiversity and use of natural resources .
- Epidemiology: how densely populated areas differ with respect to incidence, prevalence, and transmission of infectious disease.
- Infrastructure: how population density drives specific requirements for energy use and the transport of goods.
This list is not inclusive—the way society structures its living spaces affects many other fields of study as well. Scientists have even studied how happiness correlates with population density. A substantial area of study, however, focuses on demographics of populations as they relate to density. Areas of demographic breakdown and study include, but are not limited to:
- age (including tracking of elderly population centers);
- sex (biological classification as male or female); and
- race and ethnic group , or cultural characteristics (ethnic origin and language use).
Media Credits
The audio, illustrations, photos, and videos are credited beneath the media asset, except for promotional images, which generally link to another page that contains the media credit. The Rights Holder for media is the person or group credited.
Production Managers
Program specialists, last updated.
October 19, 2023
User Permissions
For information on user permissions, please read our Terms of Service. If you have questions about how to cite anything on our website in your project or classroom presentation, please contact your teacher. They will best know the preferred format. When you reach out to them, you will need the page title, URL, and the date you accessed the resource.
If a media asset is downloadable, a download button appears in the corner of the media viewer. If no button appears, you cannot download or save the media.
Text on this page is printable and can be used according to our Terms of Service .
Interactives
Any interactives on this page can only be played while you are visiting our website. You cannot download interactives.
Related Resources
- IAS Preparation
- UPSC Preparation Strategy
- World Population Distribution Density and Growth
World Population: Distribution, Density and Growth
The world population, its distribution, density and growth reflect the advancement and development of each geographical zone. The world population has a huge impact on the world economy and climate change.
Being an important topic, world population is discussed widely and is featured in the General Studies I section of the UPSC syllabus.
This article gives a good understanding of the topic, world population from the IAS Exam point of view.
Patterns of Population Distribution in the World
The term population distribution refers to the way people are spaced over the surface of the earth. Broadly, 90% of the world’s population lives in about 10% of the earth’s land area. The 10 most populous countries of the world contribute about 60% of the world’s population (the six countries out of the ten are located in Asia).
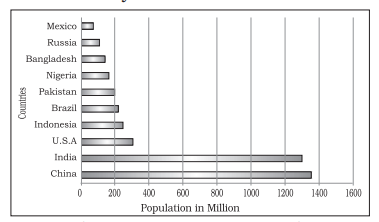
Image source-NCERT
Population Density
Population density is the ratio between the number of people to the area of the land. It is measured in persons per square kilometre or km². The densely populated parts of the world are the north-eastern part of the U.S.A, north-western part of Europe, south, south-east and east Asia. The thinly populated regions of the world are near the north and south poles, the hot and the cold deserts and high rainfall zones near the equator. The population density of India in 2011 was 382 per sq. km.
Density of population = Total Population / Total Area Patterns of population distribution and density give the demographic characteristics of any area.
Factors influencing the distribution of population
The following factors affect the population distribution:
- Geographical factors
- Economic factors
- Social and cultural factors
Geographical Factors-
- Water availability – Water is the most essential factor for the existence of life. It is used for multiple purposes like cooking, drinking, agriculture, etc. People prefer to inhibit areas which have water in abundance, that is why river valleys are among the most densely populated areas of the world.
- Landforms – The Ganga Plains are among the most densely populated areas of the world while mountainous regions of the Himalayas are thinly populated. People prefer flat plains and gentler slopes which are favourable for agricultural purposes, to build better road connectivity and for industries.
- Climate – A climate which is neither too hot nor too cold attracts more people. Areas with very heavy rainfall or extreme and harsh climate have low population density.
- Soil – Areas with fertile, loamy soils are preferred by people as this type of soil supports intensive agriculture.
Economic Factors-
- Minerals -The regions with rich mineral deposits attract industries which in turn generate employment opportunities. People move to such places and make them thickly populated. For example, Katanga Zambia copper belt in Africa.
- Urbanisation – Urban centres offer better employment opportunities, educational and medical facilities, have better means of transport and communication. This results in rural to urban migration and cities become densely populated.
- Industrialisation – Industrial areas provide job opportunities thereby attracting a huge number of people. For example, the Kobe-Osaka region of Japan is densely populated due to the presence of a large number of industries.
Social and Cultural Factors-
Religious or cultural importance also affects the population density of a place. Such places attract more people and if there is social and political unrest in a region, people tend to move away from such places. Sometimes the government offers incentives to people to move to thinly populated areas or move away from overcrowded places.
Population Growth/Population Change
It refers to the change in the number of inhabitants of a territory over a specific period of time. Population growth is expressed either in terms of percentage or absolute numbers. It can be positive as well as negative. Population change indicates the economic development, social upliftment and historical and cultural background of the area.
Some Basic Concepts of Population Geography
- Growth of population – It is the change of population in a particular area between two points of time.
- Growth rate of population – When the change in population is expressed in terms of percentage, it is the growth rate of population.
- Natural growth of population – Natural Growth = Births – Deaths (It is the population increase by the difference between births and deaths in a particular region between two points of time).
- Actual growth of population – Actual Growth = Births – Deaths + In Migration – Out Migration.
- Positive growth of population – This happens when the birth rate is more than the death rate between two points of time or when people from other countries migrate permanently to a region.
- Negative growth of population – This happens when the population decreases between two points of time, either when the birth rate falls below the death rate or people migrate to other regions.
Components of Population Change
There are three components of population change – births, deaths and migration.
- Crude Birth Rate (CBR) – It is expressed as the number of live births in a year per thousand of the population.
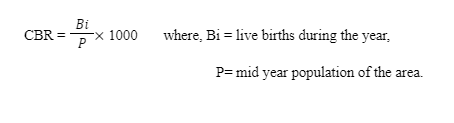
The region’s demographic structure, social advancement and economic development largely affect the mortality rates of that particular region.
3. Migration – The population size also changes by way of migration. Migration refers to the movement of people from one place to the other, the place they move from is called the place of origin and the place they move to is called the place of destination. Migration may be permanent, temporary or even seasonal. The people who move are called migrants. Migrants who move into a new place are called immigrants and migrants who move out of a place are called emigrants. The main purpose of migration is to attain better economic and social life. Factors that influence migration-
- Push factors – The factors like poor living conditions, unemployment, unpleasant climate, political turmoil, epidemics, natural disasters and social-economic backwardness make the place of origin seem less attractive.
- Pull factors – The factors like better living conditions, job opportunities, peace and stability, security of life and property, and pleasant climate make the place of destination more attractive
Trends in Population Growth
During the early periods, the population of the world grew very slowly. The world population exploded in the 18th century after the Industrial Revolution . Technological developments helped in reducing the death rate thereby, paving the way for accelerated population growth. The human population increased more than ten times in the past 500 years.
- In the 20th century itself, population growth has increased four times.
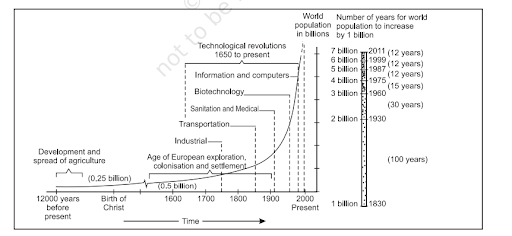
Image Source- NCERT
- The annual population growth rate in India is 1.64%.
Demographic Transition
It is a theory which is used to represent the movement of high birth and death rates as the society progresses from rural agrarian and illiterate to urban industrial and literate society. It studies the relationship between economic development and population growth. This theory can be used to describe and predict the future population of any area. These changes occur in stages which are collectively called a demographic cycle.
- Stage 1 – The first stage is characterised by high birth and death rates, and therefore, population growth is slow. This stage is associated with under-developed, low output and agriculture-dominated conditions. Two hundred years ago, all the countries of the world were in the first stage of demographic transition.
- Stage 2 – It is characterised by a high birth rate and reduced mortality rate leading to an increased population. Improvements in medical care, water supply and sanitation result in decreased death rates.
- Stage 3 – In this stage both birth and death rate decline appreciably. The population is either stable or grows slowly. The population becomes urbanised, literate and has a high technical know-how and controls the family size.
In the present times, different countries are at different stages of demographic transition.
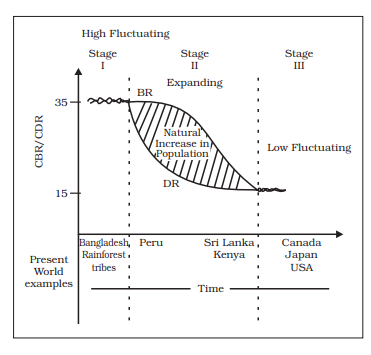
Image Source NCERT
_____________________________________
World Population:- Download PDF Here
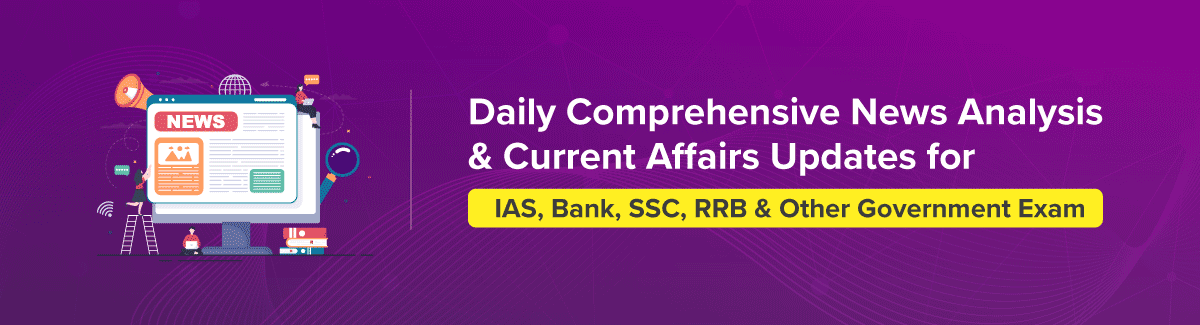
Leave a Comment Cancel reply
Your Mobile number and Email id will not be published. Required fields are marked *
Request OTP on Voice Call
Post My Comment

IAS 2024 - Your dream can come true!
Download the ultimate guide to upsc cse preparation, register with byju's & download free pdfs, register with byju's & watch live videos.
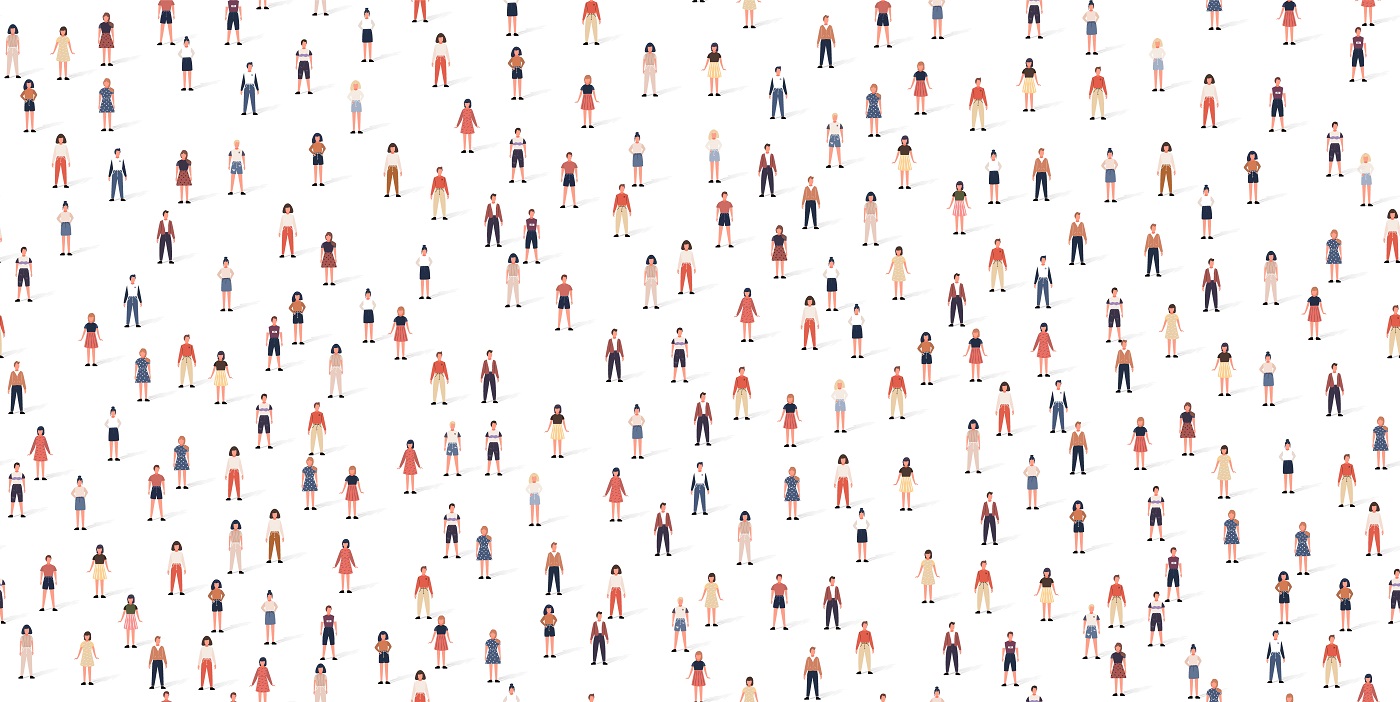
High Population Density: Pros and Cons
Population density refers to the number of people who live in a given area. It is typically measured as residents per square mile or kilometer.
High population density is increasingly common as urban areas become more concentrated. While the world’s population grows and resources are depleted, living in densely populated regions is becoming attractive for many people.
However, high population density has advantages and disadvantages that should be considered when deciding where to live or invest .
In this article, we’ll explore the pros and cons of high population density to help you make an informed decision or simply gain a better understanding of this phenomenon.
Key Takeaways
- High population density refers to the number of people who live in a given area. It has advantages and disadvantages that should be considered when deciding where to live or invest.
- The pros of high population density include access to resources, cultural diversity, economic growth, increased demand for goods and services, and cost efficiency.
- The cons of high population density include limited resources, increased levels of pollution, social problems, and pressure on the natural environment.
- Population density varies greatly depending on the country, region, city, or town.
- It is crucial to weigh all the pros and cons before deciding where to live or invest.
How Does Population Density Differ Around the World?
Population density is not the same everywhere . It varies greatly depending on country, region, city, or town.
The world’s population density is currently estimated to be around 15,3 people per square kilometer .
Some countries, such as Bangladesh (1,301 people per square kilometer) and Singapore (8,274 people per square kilometer), have higher population densities than others, like Canada (4,26 people per square kilometer) and Australia (3,37 people per square kilometer).
The Pros of High Population Density

The main advantages of high population density include:
- Access to Resources – Living in densely populated areas provides access to various resources, such as transportation, healthcare services, and job opportunities not available elsewhere.
This can help improve the quality of life for residents by providing them with greater social and economic security, but only if the resources are properly managed and distributed.
- Cultural Diversity – High population density also increases cultural diversity due to the influx of people from different backgrounds and cultures living together in one region or city.
This can lead to more vibrant communities where culture is celebrated and appreciated, which may contribute positively towards social cohesion and stability.
- Economic Growth – Densely populated regions tend to be economically prosperous as businesses take advantage of larger markets for their products or services.
Increased demand leads companies to develop new technologies or methods that can boost efficiency while reducing costs at the same time. This raises overall productivity levels within the area’s industries, leading toward economic growth.
Additionally, densely packed cities have higher tax revenues due to the large number of citizens paying taxes. It ultimately boosts government income, allowing it to allocate funds to other necessary public works projects like infrastructure upgrades, educational initiatives, etc., further promoting local development endeavors.
- Increased Demand for Goods and Services – High population density leads to higher demand for goods and services, which could benefit local entrepreneurs who can offer what the market requires.
This is likely to increase competition among businesses, each striving to stay ahead of the game, leading them toward greater innovation as they attract consumers by offering differentiated products.
It also means more job opportunities as businesses must hire more staff to meet the increased service demand.
- Cost Efficiency – Living in a densely populated area is often more cost-efficient than living in suburban or rural areas.
This is because it reduces the need for transportation as most things are located within proximity.
As such, people can significantly cut down on their expenses by simply walking or taking public transport instead of using private vehicles, which may incur additional costs over time due to gasoline and maintenance fees, etc., thus allowing them to save money that can be used elsewhere.
The Cons of High Population Density

In addition to its advantages, there are also some disadvantages associated with high population density:
- Limited Resources – High population density may also mean limited resources, such as land, housing, and facilities like schools, hospitals, etc., compared to rural or suburban regions.
If not managed properly, this could lead to overcrowding and an increased risk of disease transmission.
Additionally, it could result in higher prices of goods and services offered in the area since companies must cater to larger markets at once while trying to maintain profit margins.
- Increased Pollution – High population density often leads to increased pollution levels due to the concentration of people living together in one region.
It results from numerous vehicles and factories producing goods necessary for daily life, emitting harmful emissions into the atmosphere, contaminating air quality, which can cause health problems over time.
Waste management can also exacerbate the issue if not addressed properly, leading to an unsanitary environment with a higher risk of disease transmission.
- Social Problems – Large numbers of people living close can also lead to social issues , such as crime, gang activity, and drug use.
When people feel they have no other options or cannot meet their basic needs, they may turn to criminal activities to achieve their goals.
Overcrowding could also make it difficult for authorities to properly monitor what’s happening within the region, making it easier for criminals or gangs to operate without fear of being caught.
- Pressure on Natural Environment – High population density can also put pressure on the natural environment. It’s often necessary to build additional housing, roads, and other infrastructure to accommodate everyone living there.
This could lead to the destruction of forests or other habitats previously used by animals as well as loss of biodiversity due to fragmentation or pollution from human activities.
The Bottom Line
High population density has both advantages and disadvantages that must be taken into consideration when making decisions about where to live or invest. It is crucial to weigh all of the pros and cons before making a decision, as it could significantly impact your quality of life.
Ultimately, each person will need to decide what is best for them regarding their lifestyle preferences, financial resources available, etc., to make the most informed decision possible.
Consider the individual characteristics of each place, as the cons mentioned above are usually the result of poor resource management and other external factors, not the population density per se , so they won’t necessarily be the same everywhere.
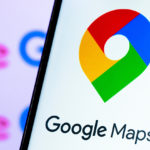
Related articles
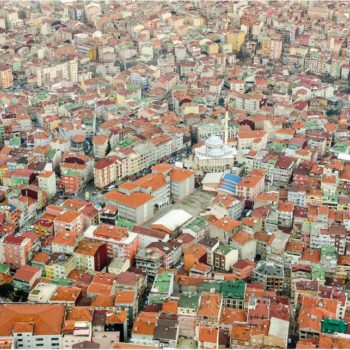
6 Possible Overpopulation Solutions: A Deep Dive
Understand what overpopulation means and explore comprehensive strategies and innovative solutions for overpopulation in the modern era.
Learn the Difference Between Compostable and Biodegradable
Learn how these eco-friendly alternatives differ from traditional plastics. Explore the nuances of compostable and biodegradable materials.
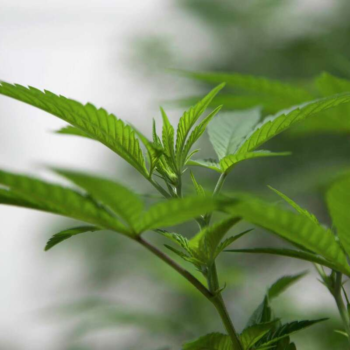
- Energy and Environment
Sustainability in Cannabis Industry
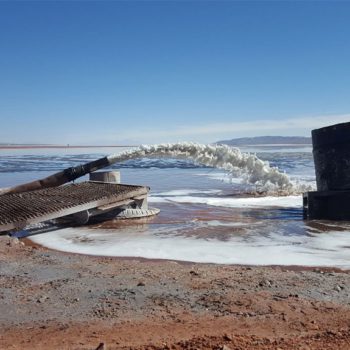
Sustainable Lithium Extraction: How is Lithium Mined and Processed
Discover sustainable lithium extraction methods and how lithium is mined and processed for electric vehicle battery production. Explore responsible extraction techniques from brine and ore sources to support clean energy technologies.
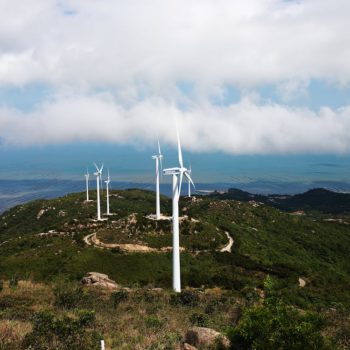
Simple Yet Impactful Tips for Conserving Natural Resources
Discover Simple Yet Impactful Ways to Conserve Natural Resources. Learn how to conserve natural resources effectively and contribute to a sustainable future. Explore eco-friendly tips and practices to minimize your environmental footprint.

How to Purify Water: 5 Methods for Cleaner H2O
Clean, drinkable water is essential for our survival, but it's not always readily available. The quality of water is often compromised, leaving us vulnerable to harmful contaminants. That's where water purification methods come in. In this article, we'll explore five different techniques that can turn questionable water into safe, drinkable water. Whether you prefer the simplicity of boiling or the advanced technology of reverse osmosis, we've got you covered. Get ready to learn how to get cleaner, purer water.
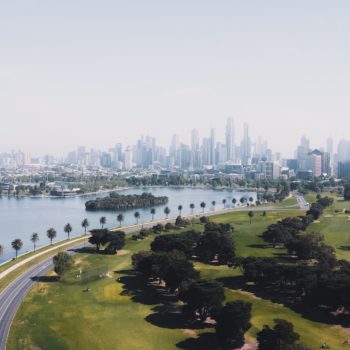
Hitting Green Goals: The 15 Greenest Cities In The World
Discover the 15 greenest cities worldwide and learn from their sustainable practices. From renewable energy to cycling infrastructure, explore how these cities are shaping a greener future for urban living.
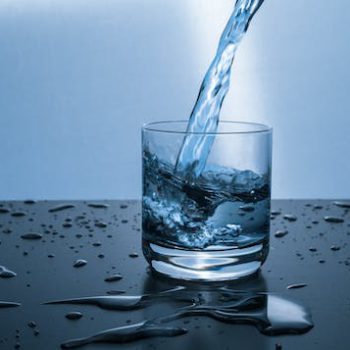
All You Need to Know About Water Filtration Systems and Reducing Plastic Waste
Discover everything about water filtration systems: How they filter water, reduce plastic pollution, and help the environment. Explore also the best way to reduce plastic waste in water filtering.
No Comments
You must be logged in to post a comment.
Weekly Sustainability News!
By subscribing you agree to our Privacy Policy .
Sustainable Review is copyright material. All rights reserved.
Essay on Population for Students and Children
500+ words essay on population.
Population refers to the total number of beings living in a particular area. Population helps us get an estimate of the number of beings and how to act accordingly. For instance, if we know the particular population of a city, we can estimate the number of resources it needs. Similarly, we can do the same for animals. If we look at the human population, we see how it is becoming a cause of concern. In particular, the third world countries suffer the most from population explosion. As it is the resources there are limited and the ever-increasing population just makes it worse. On the other hand, there is a problem of low population in many regions.
India population crisis
India faces a major population crisis due to the growing population. If we were to estimate, we can say that almost 17% of the population of the world lives in India alone. India ranks second in the list of most populated countries.

Furthermore, India is also one of the countries with low literacy rates. This factor contributes largely to the population explosion in India. It is usually seen that the illiterate and poor classes have a greater number of children. This happens mainly because they do not have sufficient knowledge about birth control methods . In addition, more people in a family are equals to more helping hands. This means they have better chances of earning.
Moreover, we also see how these classes practice early marriage. This makes it one of the major reasons for a greater population. People marry off their young daughters to men way older than them for money or to get free from their responsibility. The young girl bears children from an early age and continues to do so for a long time.
As India is facing a shortage of resources, the population crisis just adds on to the problem. It makes it quite hard for every citizen to get an equal share of resources. This makes the poor poorer and the rich richer.
Get the huge list of more than 500 Essay Topics and Ideas
Impact of Population Explosion

Subsequently, pollution levels are on the rise because of the population explosion. As more and more humans are purchasing automobiles, our air is getting polluted. Moreover, the increased need calls for faster rates of industrialization. These industries pollute our water and lands, harming and degrading our quality of life.
In addition, our climate is also facing drastic changes because of human activities. Climate change is real and it is happening. It is impacting our lives very harmfully and must be monitored now. Global warming which occurs mostly due to activities by humans is one of the factors for climate change.
Humans are still able to withstand the climate and adapt accordingly, but animals cannot. This is why wildlife is getting extinct as well.
In other words, man always thinks about his well-being and becomes selfish. He overlooks the impact he is creating on the surroundings. If the population rates continue to rise at this rate, then we won’t be able to survive for long. As with this population growth comes harmful consequences. Therefore, we must take measures to control the population.
{ “@context”: “https://schema.org”, “@type”: “FAQPage”, “mainEntity”: [{ “@type”: “Question”, “name”: “Why is India having a population crisis?”, “acceptedAnswer”: { “@type”: “Answer”, “text”: “India suffers from a population crisis because the literacy rate is very low. This causes other problems which contribute to population growth.” } }, { “@type”: “Question”, “name”: “What is the impact of population explosion?”, “acceptedAnswer”: { “@type”: “Answer”, “text”:”Population explosion has a very harmful impact on wildlife and vegetation. Animals are getting extinct due to it and climate change is also happening.”} }] }
Customize your course in 30 seconds
Which class are you in.

- Travelling Essay
- Picnic Essay
- Our Country Essay
- My Parents Essay
- Essay on Favourite Personality
- Essay on Memorable Day of My Life
- Essay on Knowledge is Power
- Essay on Gurpurab
- Essay on My Favourite Season
- Essay on Types of Sports
Leave a Reply Cancel reply
Your email address will not be published. Required fields are marked *
Download the App

- 0 Shopping Cart
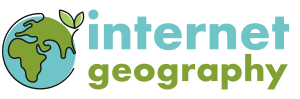
What factors affect population density?
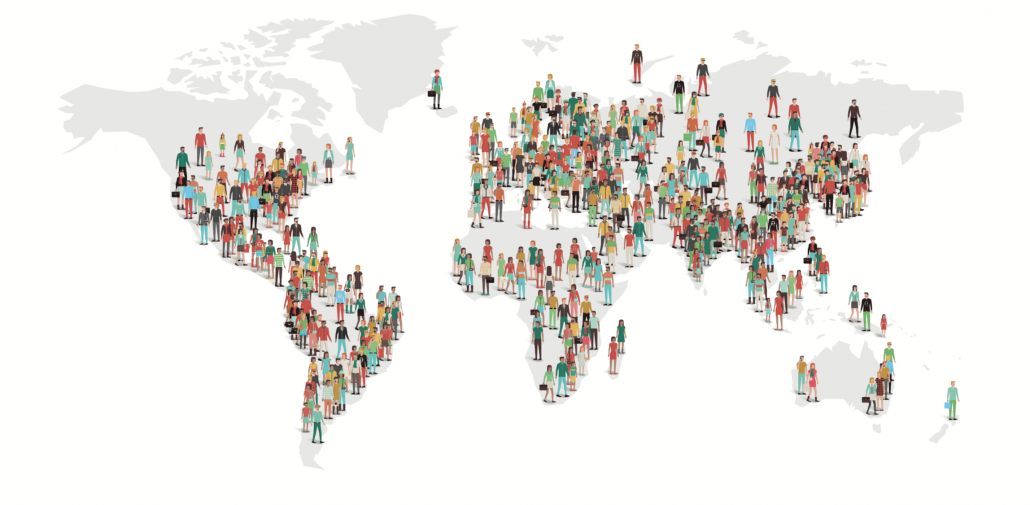
There are a range of human and natural factors that affect population density. The tables below illustrate this.
Further reading: What causes population change?
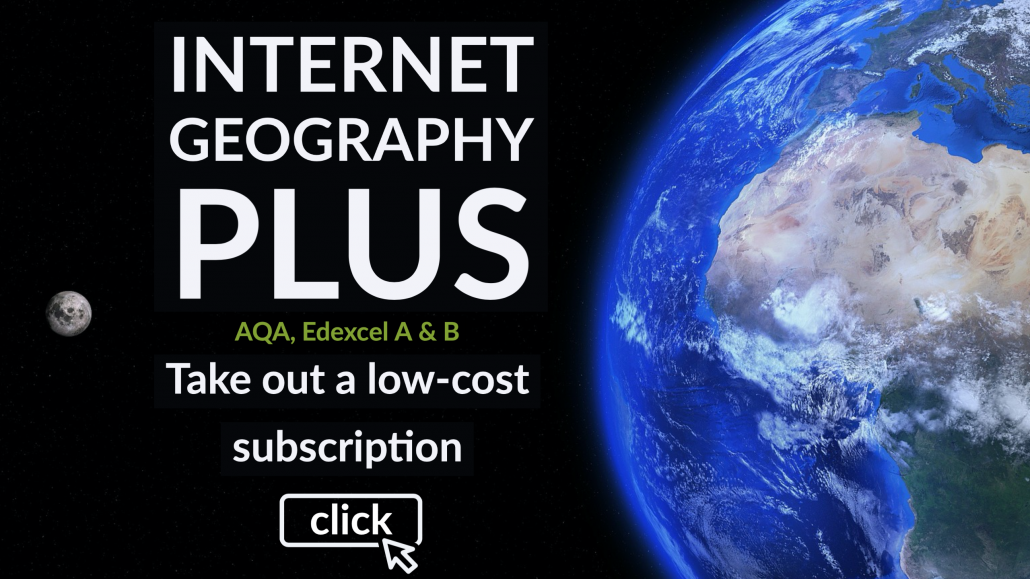
Premium Resources
Please support internet geography.
If you've found the resources on this page useful please consider making a secure donation via PayPal to support the development of the site. The site is self-funded and your support is really appreciated.
Related Topics
Use the images below to explore related GeoTopics.
What is population density?
Topic home, how has world population changed, share this:.
- Click to share on Twitter (Opens in new window)
- Click to share on Facebook (Opens in new window)
- Click to share on Pinterest (Opens in new window)
- Click to email a link to a friend (Opens in new window)
- Click to share on WhatsApp (Opens in new window)
- Click to print (Opens in new window)
If you've found the resources on this site useful please consider making a secure donation via PayPal to support the development of the site. The site is self-funded and your support is really appreciated.
Search Internet Geography
Top posts and pages.

Latest Blog Entries

Pin It on Pinterest
- Click to share
- Print Friendly
One to one maths interventions built for KS4 success
Weekly online one to one GCSE maths revision lessons now available
In order to access this I need to be confident with:
This topic is relevant for:
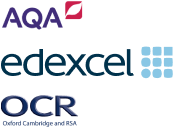
Population Density
Here we will learn about population density, including how to calculate population density and how to solve problems involving population density.
There are also population density worksheets based on Edexcel, AQA and OCR exam questions, along with further guidance on where to go next if you’re still stuck.
What is population density?
Population density is a compound measure that tells us how many people live in an area of a specified size.
If an area has a high population density it is crowded, for example in a city.
If an area has a low population density it is sparsely populated, for example a mountainous region.
To work out population density we need to know the relationship between population density , population (the number of people) and land area .
We can turn this into a formula triangle,
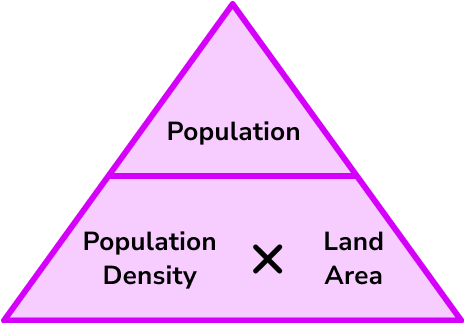
Population density can be used to make population estimates. The units are often people/km^2 (people per square kilometre). Occasionally the units might be people per square mile.
You may study more about world population, population growth, urbanisation and demographics in geography.
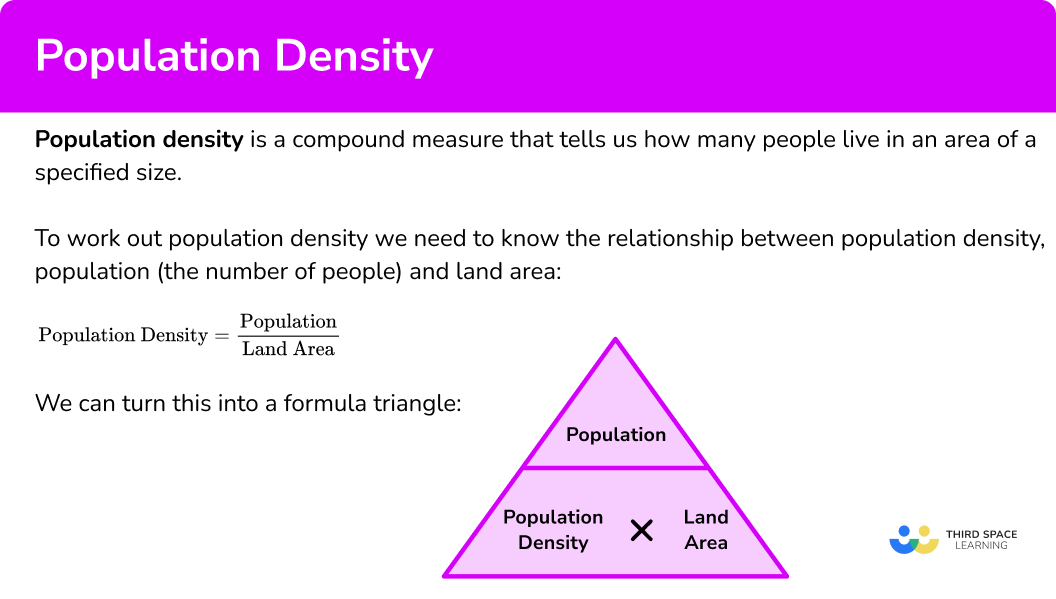
How to use population density
In order to solve problems involving population density:
Check what information we have been given and what we are finding.
Use the formula.
Write down the final answer.
Explain how to use population density
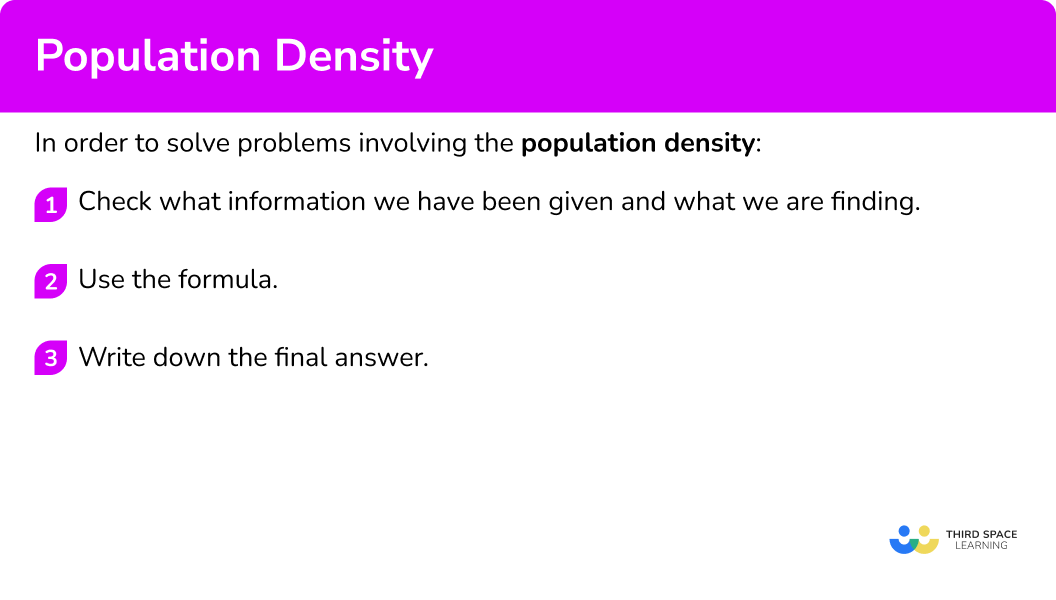
Population density worksheet
Get your free population density worksheet of 20+ questions and answers. Includes reasoning and applied questions.
Related lessons on compound measures
Population density is part of our series of lessons to support revision on compound measures . You may find it helpful to start with the main compound measures lesson for a summary of what to expect, or use the step by step guides below for further detail on individual topics. Other lessons in this series include:
- Compound measures
- Mass density volume
- Pressure force area
- Speed distance time
Population density examples
Example 1: calculating population density.
Malta has a population of 440 \ 000 and an area of 316 \ km^{2}. Calculate the population density of Malta. Give your answer to the nearest integer.
We have been given the population and the land area. We need to find the population density.
2 Use the formula.
The formula is
\text{Population density}=\frac{\text{population}}{\text{land area}} .
We can substitute in the values and work out the population density.
3 Write down the final answer.
The population density of Malta is \bf{1392} people \ per \ km^{2} (to the nearest integer).
Example 2: calculating population density
Australia has a population of 24 \ 900 \ 000 and an area of 7 \ 690 \ 000 \ km^{2}. Calculate the population density of Australia. Give your answer to 3 significant figures.
\text{Population density}=\frac{\text{population}}{\text{land area}}=\frac{24\ 900 \ 000}{7 \ 690 \ 000}=3.2379…
The population density of Australia is \bf{3.24} people \ per \ km^2 (to 3 significant figures).
Example 3: calculating population
Hong Kong has a population density of 6700 \ people/km^2 and an area of 1100 \ km^{2}. Calculate the population of Hong Kong.
We have been given the population density and the land area. We need to find the population.
\begin{aligned} \text{Population density}&=\frac{\text{population}}{\text{land area}}\\\\ 6 \ 700 &=\frac{\text{population}}{1 \ 100}\\\\ \text{Population}&=6 \ 700 \times 1 \ 100\\\\ \text{Population}&=7 \ 370 \ 000 \end{aligned}
The population of Hong Kong is 7 \ 370 \ 000 people.
Example 4: calculating population
Rwanda has a population density of 470 \ people/km^2 and an area of 26 \ 000 \ km^{2}. Calculate the population of Rwanda.
\begin{aligned} \text{Population density}&=\frac{\text{population}}{\text{land area}}\\\\ 470 &=\frac{\text{population}}{26 \ 000}\\\\ \text{Population}&=470 \times 26 \ 000 \\\\ \text{Population}&=12 \ 220 \ 000 \end{aligned}
The population of Rwanda is \bf{12 \ 220 \ 000} people.
Example 5: calculating land area
New York has a population density of 11 \ 300 \ people/km^2 and a population of 8.8 million people. Calculate the land area of New York. Give your answer to 3 significant figures.
We have been given the population density and the population. We need to find the land area
\begin{aligned} \text{Population density}&=\frac{\text{population}}{\text{land area}}\\\\ 11 \ 300 &=\frac{8 \ 800 \ 000}{\text{land area}}\\\\ \text{Land area}&=8 \ 800 \ 000\div 11 \ 300\\\\ \text{Land area}&=778.76… \end{aligned}
The land area of New York is \bf{779} km^2 (to 3 significant figures).
Example 6: calculating land area
Pakistan has a population density of 267 \ people/km^2 and a population of 2.12 \times 10^8 people. Calculate the land area of Pakistan. Give your answer in standard form to 3 significant figures.
We have been given the population density and the population. We need to find the land area.
\begin{aligned} \text{Population density}&=\frac{\text{population}}{\text{land area}}\\\\ 267 &=\frac{2.12\times 10^8}{\text{land area}}\\\\ \text{Land area}&=2.12\times 10^8\div 267\\\\ \text{Land area}&=794 \ 007.49… \end{aligned}
The land area of Pakistan is 7.94 \times 10^5 \ km^2 (to 3 significant figures).
Common misconceptions
- Make sure you use the formula wisely
Make sure you divide or multiply appropriately. When dividing, make sure the population is the dividend (the number being divided).
- Check the units
The units usually involve km^{2}, but another square unit may be used such as miles^2 or m^{2}.
- Check what form the final answer needs to be in
You may be asked to round your answer. You may be asked to write your final answer in standard form, as the numbers involved can be very large.
Practice population density questions
1. Calculate the population density of Nepal given that the population of Nepal is 28 \ 100 \ 000 and the land area is 147 \ 000 \ km^{2}. Give your answer to 3 significant figures.

Population density =\frac{\text{population}}{\text{land area}}=\frac{28 \ 100 \ 00}{147 \ 000}=191.156…
The answer needs rounding to 3 significant figures.
191.156…=191 to 3 significant figures.
So the population density of Nepal is 191 \ people/km^2 .
2. Calculate the population density of Monaco given that the population of Monaco is 3.87 \times 10^4 and the land area is 2 \ km^{2}. Give your answer as an ordinary number to 3 significant figures.
\text{Population density}=\frac{\text{population}}{\text{land area}}=\frac{3.87\times 10^4}{2}=19 \ 350 .
19 \ 350=19 \ 400 to 3 significant figures.
The population density of Monaco is 19 \ 400 \ people/km^{2}.
3. Calculate the population of Nigeria given that the population density of Nigeria is 212 \ people/km^2 and the land area is 924 \ 000 \ km^{2}. Give your answer to the nearest million.
4 million is the number of people who live in Nigeria.
40 million is the number of people who live in Nigeria.
2 million is the number of people who live in Nigeria.
20 million is the number of people who live in Nigeria.
To find the population, multiply the population density by the land area.
\text{Population}=\text{population density}\times \text{land area}= 212\times 924 \ 000=19 \ 588 \ 000 .
The answer needs giving to the nearest million.
19 \ 588 \ 000 is 20 million to the nearest million.
4. Calculate the population of Venezuela given that the population density is 32 \ people/km^2 and the land area of Venezuela is 9.12 \times 10^5 \ km^{2}. Give your answer in standard form correct to 3 significant figures.
2.91 \times 10^6 is the number of people living in Venezuela.
2.91 \times 10^7 is the number of people living in Venezuela.
2.92 \times 10^7 is the number of people living in Venezuela.
2.92 \times 10^6 is the number of people living in Venezuela.
\text{Population}=\text{population density}\times \text{land area}=32\times (9.12\times 10^5)=29 \ 184 \ 000 .
Then we need to write the final answer in standard form and round to 3 significant figures.
29 \ 184 \ 000= 2.9184\times 10^7=2.92\times 10^7 to 3 significant figures. So, the population of Venezuela is 2.92\times 10^7.
5. Calculate the land area of Taiwan when the population density is 652 \ people/km^{2} and the population of Taiwan is 23.6 million. Give your answer to 3 significant figures.
36 \ 200 \ km^2 is the land area of Taiwan.
36 \ 200 \ miles^2 is the land area of Taiwan.
3 \ 620 \ miles^2 is the land area of Taiwan.
3 \ 620 \ km^2 is the land area of Taiwan.
To find the land area, divide the population by the population density.
\text{Land area}=\frac{\text{population}}{\text{population density}}=\frac{23 \ 600 \ 000}{652}=36 \ 196.3… .
Then we need to write the final answer rounded to 3 significant figures.
36 \ 196.3…=36 \ 200 to 3 significant figures. The units for the land area will be km^2 since km^2 was part of the units for the population density.
6. Calculate the land area when the population density of Indonesia is 140 \ people/km^2 and the population of Indonesia 2.68 \times 10^{8}. Give your answer in standard form correct to 3 significant figures.
1.92\times 10^5 \ km^2 is the land area of Indonesia.
1.92\times 10^6 \ km^2 is the land area of Indonesia.
1.91\times 10^6 \ km^2 is the land area of Indonesia.
1.91\times 10^5 \ km^2 is the land area of Indonesia.
1 \ 914 \ 285.7… = 1.9142857…\times 10^6=1.91\times 10^6 to 3 significant figures.
Population density GCSE questions
1. Bangladesh has a population of 161 \ 000 \ 000 people. The population density is 1000 people per km^2. Calculate the area of Bangladesh.
2. Here is some information about the populations and areas of 3 different towns.
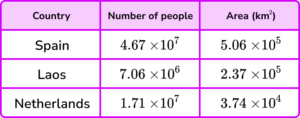
Which country has the highest population density? You must show your workings.
Netherlands has the highest population density.
3. (a) A village has an area of 5 \ km^2 and a population density of 600 \ people \ per \ km^{2}.
Calculate the number of people living in the village.
(b) A new estate is built. It has a population density of 800 \ people \ per \ km^{2}. \ 500 people live in the new estate.
Calculate the overall population density of the village and the new estate.
3000 people
Learning checklist
You have now learned how to:
- Calculate the population density
- Solve problems involving population density
The next lessons are
- Best buy maths
- Scale maths
- Units of measurement
Still stuck?
Prepare your KS4 students for maths GCSEs success with Third Space Learning. Weekly online one to one GCSE maths revision lessons delivered by expert maths tutors.
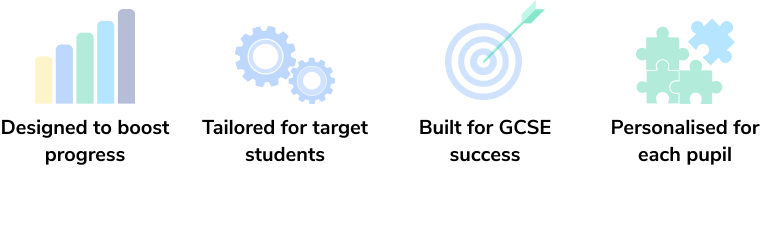
Find out more about our GCSE maths tuition programme.
Privacy Overview
Home — Essay Samples — Nursing & Health — Stress — Population Density and Mental Health

Population Density and Mental Health
- Categories: Anxiety Stress
About this sample

Words: 620 |
Published: Mar 25, 2024
Words: 620 | Page: 1 | 4 min read
Table of contents
Introduction, stress and population density, anxiety and population density, social alienation and population density, mechanisms and moderating factors.
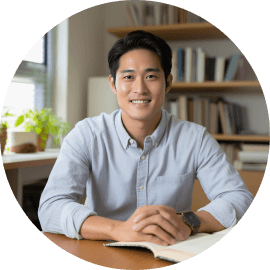
Cite this Essay
Let us write you an essay from scratch
- 450+ experts on 30 subjects ready to help
- Custom essay delivered in as few as 3 hours
Get high-quality help
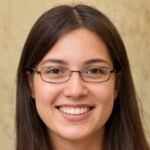
Verified writer
- Expert in: Nursing & Health
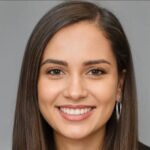
+ 120 experts online
By clicking “Check Writers’ Offers”, you agree to our terms of service and privacy policy . We’ll occasionally send you promo and account related email
No need to pay just yet!
Related Essays
6 pages / 2555 words
4 pages / 1906 words
1 pages / 430 words
3 pages / 1705 words
Remember! This is just a sample.
You can get your custom paper by one of our expert writers.
121 writers online
Still can’t find what you need?
Browse our vast selection of original essay samples, each expertly formatted and styled
Related Essays on Stress
Well, today I’d like to share with you what social anxiety is, the symptoms and who are most affected by it, and how to relax when your anxiety starts to kick in. To begin, let me first explain what social anxiety is. Social [...]
American Heart Association. (2018, April 4). Stress and Heart Health. American Heart Association. https://www.sleepeducation.org/sleep-topics/stress-and-sleep
Entering college is a significant milestone in one's life, marked by excitement, anticipation, and the promise of new beginnings. However, for many students, including myself, it can also be a time of heightened anxiety. In this [...]
Adolescence is a pivotal period in an individual's life, marked by significant physical, emotional, and psychological changes. It is a time of transition from childhood to adulthood and is often accompanied by a myriad of [...]
In today’s world, stress is unavoidable especially for people who work or study in universities. Studying in a university is very stressful for most of students especially for those who come from another country with different [...]
Stress is a universal experience that impacts individuals from all walks of life, regardless of age, gender, or social background. From the pressures of work and school to personal relationships and financial obligations, the [...]
Related Topics
By clicking “Send”, you agree to our Terms of service and Privacy statement . We will occasionally send you account related emails.
Where do you want us to send this sample?
By clicking “Continue”, you agree to our terms of service and privacy policy.
Be careful. This essay is not unique
This essay was donated by a student and is likely to have been used and submitted before
Download this Sample
Free samples may contain mistakes and not unique parts
Sorry, we could not paraphrase this essay. Our professional writers can rewrite it and get you a unique paper.
Please check your inbox.
We can write you a custom essay that will follow your exact instructions and meet the deadlines. Let's fix your grades together!
Get Your Personalized Essay in 3 Hours or Less!
We use cookies to personalyze your web-site experience. By continuing we’ll assume you board with our cookie policy .
- Instructions Followed To The Letter
- Deadlines Met At Every Stage
- Unique And Plagiarism Free
- Free Samples
- Premium Essays
- Editing Services Editing Proofreading Rewriting
- Extra Tools Essay Topic Generator Thesis Generator Citation Generator GPA Calculator Study Guides Donate Paper
- Essay Writing Help
- About Us About Us Testimonials FAQ
Essays on population density
- Studentshare
- Population Density
- TERMS & CONDITIONS
- PRIVACY POLICY
- COOKIES POLICY
Relationship Between Population and Economic Growth Analytical Essay
- To find inspiration for your paper and overcome writer’s block
- As a source of information (ensure proper referencing)
- As a template for you assignment
Introduction
Relationship between population and economic growth.
Economic growth is dependent on a wide array of factors that range from technology to capital. Of particular interest is the rate of population growth vis-à-vis economic growth and development. Various economic models have attempted to establish the relationship between different demographic dynamics and economic growth (Barro 1991, p. 87).
While some economists postulate that economic development slows down in periods of high population growth rates, others argue that high population growth boosts economic growth. This paper discusses such factors as age structure, level of economic development, population density and rates of poverty in addition to their impacts on economy. Besides, the paper utilizes various theoretical frameworks that establish the correlation of population dynamics and economic growth.
The relationship between population and economic growth has many perspectives. Various economists have developed models to explain the correlation between population and economic growth (Gonzalo 1994, p. 67). Malthus was among classical economists who attempted to explain the relationship. He claimed that increase in population could surpass the level of production in an economy leading to poverty (Jones 2002, p. 45).
The rationale is that population grows in geometrical way while productivity improves in an arithmetic rate. This does not only lead to poverty but also inefficiencies and diminished productivity. To that end, it is important to highlight that the relationship between population and economic growth has elicited heated debate (Weil 2009, p. 71).
Some schools of thoughts believe that there is a positive correlation between population and economic growth. Opponents argue that population growth complements the economy in the sense that there is a high number of skilled and talented laborers in an economy (Jayati 2013, p. 81). This in turn leads to increased productivity and production of goods and services across entire economic spectrum.
Other theorists have attempted to distinguish economic growth and population dynamics. Neo-classical theorists such as Solow differentiate various aspects of societies in order to establish accurate correlations and relationships (Thirlwall 1994, p. 132). In particular, Solow claims that high population growth in a steady state is likely to reduce disposable income and income per capital. Nonetheless, population growth does not affect the rate of economic growth in steady states (Jayati 2013, p. 76).
Consequently, Solow argues that the rate of population growth will be equal to the rate of economic growth in steady states. While neo-classical theorists classify states and nations in order to understand interplay between demographic dynamics and economy, other models use age structure of a nation to predict the effects of population growth/decrease in an economy.
According to Jones (2002, p. 67), industrialized nations have distinct age structures when compared to developing economies and nations. While majority of the population has reached adulthood in industrialized countries, children and young people constitute highest majority of developing countries’ population.
On the one hand, age structure in industrialized nations is major cause for skewed relationship between population growth and economy (Aliber 2001, p. 36). For instance, United Nations Report on global population growth indicates that aging will be a major driver of economic slowdown in many industrialized nations in the world by 2050 (Eastwood & Lipton 2001, p. 33).
The reason is that high number of working population will age out leading to a deceleration in productivity. It is important to point out that young population will not be in a position to meet the needs of the aging population.
This will not only lead to high rate of dependency but also high cost of caring for the aged populace (Weil 2009, p. 87). The government will also increase its funding towards social welfare programs to take care of its aging population.
In a country where government is attempting to cut down its expenditure, aging population will lead to slower economic growth because of high rate of dependency. To that end, population growth in such countries as Britain and United States is likely to accelerate economic growth and prosperity.
On the other hand, age structures in developing countries impede economic progress in third world. The rationale is that population growth will exert pressure on already scarce economic resources. As elucidated by Kohler & Kohler (2002, p. 56), high rates of population growth as witnessed in many African countries will lead to unsustainable economic development and growth. This is because many African economies are dependent on cultivation and agriculture (Jones 2002, p. 62).
An increase in population will imply that expansive agricultural activities and extraction of resources will increase at the expense of environment. It may also lead to deforestation and increased degradation of natural resources.
To that end, environmental degradation leads to agricultural stagnation that in turn affects the economy in a negative way (McCulloch & Baulch 2000, p. 102). Malthus asserts that social expenditure by government on young age structure at the expense of capital investment will also stall the economy (Weil 2009, p. 102).
While many economists argue that high population growth rates in developing countries have a counterproductive effect on the economy, it is apparent that increase in population is important for developing countries. According to Murthi & Drèze (2001, p. 45), a rise in population growth in developing countries implies that the economy will benefit from diverse workforce and vibrant labor market. In countries that have adopted labor-intensive model of production, population growth boosts productivity.
In such countries as China and India, the high rate of population growth has complemented economic growth rate (Drèze & Sen 1995, p. 38). In addition, it is essential to mention that a high rate of population growth offsets the risk of increased dependency emanating from the aging population (Kremer & Chen 2002, p. 34-89). As such, population growth plays a significant in enhancing steady development and economic growth in third world.
Kremer & Chen (2002, p. 56) articulate that population growth has a direct relationship with poverty rates in a country. Undoubtedly, level of poverty is a major indicator of economic growth and development in a country. Bourguignon (2001, p. 112) says that countries with high population growth rates have high rates of fertility.
However, fertility rates are dependent on incomes, literacy and inequalities that typify an economy. For instance, countries that experience high rates of income inequalities exhibit fertility discrepancies between poor uneducated people and rich and literate people (O’Sullivan 2003, p. 57).
The rationale is that affluent members of society tend to bear few children, which increases their likelihood of becoming literate and wealthy. Conversely, poor and probably uneducated members of the society have high fertility rates implying that they have many children who join vicious cycle of poverty (Lee 2003, p. 75).
To this end, high population growth rate in countries that have alarming levels of income inequalities tends to increase levels of poverty in the population. Governments therefore increase their social expenditure on poverty eradication and health at the expense of capital investments. This implies that population growth in income unequal countries is not only detrimental for the economy but it also threatens social stability.
Population density is another parameter that theorists use to establish correlation between population growth and economic growth. It refers to the number of people living within one square kilometer. Malthus asserts that countries with low population density experience many challenges (Kelley & Schmidt 1995, p. 67). At the outset, innovation and technology tend to spread slowly owing low contact between social institutions, individuals and economic institutions (Meier 1995, p. 34).
Due to little contact that is apparent in sparsely populated societies, negative ethnicity increases and persists for a long period. Besides, governments of such societies face constraints in provision of public goods such as infrastructure and electricity due to minimal and expensive interaction with the rest of the world (Solow 1956, p. 67).
On the other hand, high population density allows fast and efficient flow of innovation, creativity and technology leading to increase in uptake of modern ways of production (Kelley & Schmidt 2005, p. 56). This does not only boost economic growth but also enhances capital investments. It is important to highlight that high population density may also lead to environmental degradation and other effects of overutilization of resources.
In summary, population growth has a direct relationship with economic growth. Various economists classify different nations according to levels of economic development (developed or developing), population density, age -structure and income inequalities in order to establish the apparent correlation.
Population growth leads to economic growth in industrialized nations that have high population density. However, economic growth stalls in poor and unequal countries due to high rates of poverty and sparse population.
Aliber, M 2001, ‘An Overview of the Incidence and Nature of Chronic Poverty in South Africa’, Chronic Poverty Research Centre, vol. 4 no. 3, pp. 2-56.
Barro, R 1991, ‘Economic Growth in a Cross Section of Countries’, Quarterly Journal of Economics, vol. 67 no. 3, pp. 73-123.
Bourguignon, F 2001, The Distributional Effects of Growth: Micro vs. Macro Approaches, Delta Pres: Paris.
Drèze, J & Sen, A 1995, India: Economic Development and Social Opportunity, Clarendon Press: New York.
Eastwood, R & Lipton, M 2001, ‘Pro-poor Growth and Pro-growth Poverty Reduction: Meaning, Evidence, and Policy Implications’, Asian Development Review, vol. 6 no. 4, pp. 19: 1-37.
Gonzalo, J 1994, ‘Five Alternative Methods of Estimating Long-Run Equilibrium Relationships’, Journal of Econometrics, vol. 60 no 1, pp. 19-94.
Jayati, G 2013, Too Much of the Same: Development and Cooperation , McGraw Hill Publishers: New York.
Jones, C 2002, Introduction to Economic Growth, McGraw Hill: New York.
Kelley, C & Schmidt, M 1995, Aggregate Population and Economic Growth Correlations: The Role of the Components of Demographic Change’, Demography, vol. 32 no. 4, pp. 35-78.
Kelley, C & Schmidt, M 2005, ‘Evolution of Recent Economic-Demographic Modeling: A Synthesis’, Journal of Population Economics, vol. 18 no. 2, pp, 20- 34.
Kohler, P & Kohler, I 2002, ‘Fertility Decline in Russia in the Early and Mid-1990s: The Role of Economic Uncertainty and Labor Market Crises’, European Journal of Population, vol. 18 no. 6, pp. 56-189.
Kremer, M & Chen, D 2002, ‘Income Distribution Dynamics with Endogenous Fertility’, Journal of Economic Growth , vol. 7 no. 3, pp. 227-258.
Lee, D 2003, ‘The Demographic Transition: Three Centuries of Fundamental Change’, Journal of Economic Perspectives, vol. 17 no. 4, pp. 45-92.
McCulloch, N & Baulch, B 2000, ‘Simulating the Impact of Policy on Chronic and Transitory Poverty in Rural Pakistan’, Journal of Development Studies, vol. 36 no. 6, pp. 100-130.
Meier, G 1995, Leading Issues in Economic Development, Oxford University Press: New York.
Murthi, M & Drèze, J 2001, ‘Fertility, Education, and Development: Evidence from India’, Population and Development Review, vol. 27 no. 3, pp. 33-63.
O’Sullivan, A 2003, Economics: Principles in Action, Pearson Prentice Hall: New Jersey.
Solow, M 1956, ‘A Contribution to the Theory of Economic Growth’, Quarterly Journal of Economics, vol. 70 no. 2, pp. 45-134.
Thirlwall, P 1994, Growth and Development, Basingstoke: Macmillan Press: Basingstoke.
Weil, D 2009, Economic Growth, Pearson-Addison Wesley: New Jersey.
- Life Expectancy and Fertility Rate Connection
- "Bank of England Holds Rates Steady" by Anderson
- The Evolution of Industrialized Workers in Chicago
- Sustaining Australia's Rate of Economic Growth
- Comparative studies of real estate industry: Hong Kong and Singapore
- The Effect of Market-Type Procurement on the Public Sector
- The Worst of the Global Financial Crisis Is Still To Come
- The Business Environment of India
- Chicago (A-D)
- Chicago (N-B)
IvyPanda. (2019, June 12). Relationship Between Population and Economic Growth. https://ivypanda.com/essays/relationship-between-population-and-economic-growth/
"Relationship Between Population and Economic Growth." IvyPanda , 12 June 2019, ivypanda.com/essays/relationship-between-population-and-economic-growth/.
IvyPanda . (2019) 'Relationship Between Population and Economic Growth'. 12 June.
IvyPanda . 2019. "Relationship Between Population and Economic Growth." June 12, 2019. https://ivypanda.com/essays/relationship-between-population-and-economic-growth/.
1. IvyPanda . "Relationship Between Population and Economic Growth." June 12, 2019. https://ivypanda.com/essays/relationship-between-population-and-economic-growth/.
Bibliography
IvyPanda . "Relationship Between Population and Economic Growth." June 12, 2019. https://ivypanda.com/essays/relationship-between-population-and-economic-growth/.
Thank you for visiting nature.com. You are using a browser version with limited support for CSS. To obtain the best experience, we recommend you use a more up to date browser (or turn off compatibility mode in Internet Explorer). In the meantime, to ensure continued support, we are displaying the site without styles and JavaScript.
- View all journals
- My Account Login
- Explore content
- About the journal
- Publish with us
- Sign up for alerts
- Open access
- Published: 27 September 2023
Research on the relationship between population distribution pattern and urban industrial facility agglomeration in China
- Peng Zeng 1 &
- Cheng Zong 2
Scientific Reports volume 13 , Article number: 16225 ( 2023 ) Cite this article
2237 Accesses
3 Citations
Metrics details
- Population dynamics
- Scientific data
- Socioeconomic scenarios
- Sustainability
Investigating the impact of industrial facility agglomeration on population distribution provides valuable insights for advancing urban and regional development, as well as aiding in planning, forecasting, and achieving regional equilibrium. However, there remains a notable gap in understanding the influence and mechanisms of industrial facility agglomeration on population distribution, particularly when considering different industry types and diverse regions comprehensively. Additionally, conventional panel data used to assess industrial facility agglomeration are constrained by limitations in coverage and timeliness. In contrast, Point of Interest (POI) data offers a superior solution with its real-time, fine-grained, and innovative advantages. This study utilizes real-time and fine-grained POI data in conjunction with the LandScan population raster dataset to precisely assess industrial facility agglomeration in 352 administrative units at the prefecture level and above in China. The key findings of this research can be summarized as follows: (1) factors influencing urban population growth rates have evolved, with increased significance attributed to Government Agencies and Social Groups, alongside a consistent impact from Science, Education, and Cultural Services. (2) The correlation between industrial facility agglomerations and population growth rates displayed linear relationships in 2015 and 2021, with varying strengths and directional shifts. (3) Regional disparities in industrial facility agglomeration patterns underscore the necessity for customized strategies to optimize industrial structures, foster innovation-driven sectors, and promote sustainable population growth.
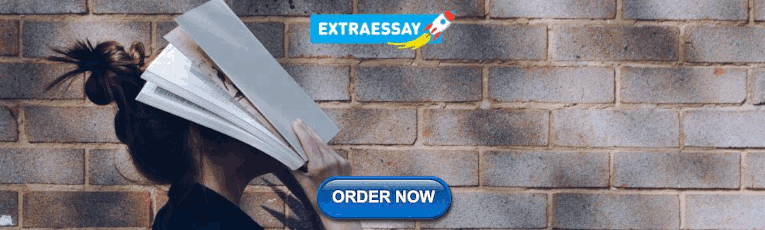
Similar content being viewed by others
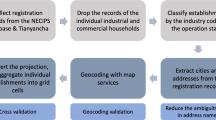
A gridded establishment dataset as a proxy for economic activity in China
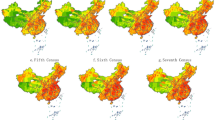
Spatial and temporal changes in population distribution and population projection at county level in China
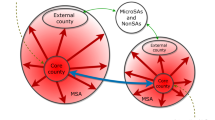
Spatial structure of city population growth
Introduction.
In recent decades, rapid economic development and urbanization have emerged as global phenomena with far-reaching implications for population distribution and industrial dynamics 1 . These transformative processes extend beyond the borders of any single nation and have profound consequences for societies and economies worldwide 2 . This study delves into the intricate interplay between these forces, focusing on China, a nation that has experienced remarkable demographic and economic shifts between 2015 and 2021. China's rapid economic development and urbanization over the past few decades have profoundly affected population distribution and industrial development. Between 2015 and 2021, China experienced significant demographic and economic shifts characterized by a consistent yet declining population growth rate 3 . Concurrently, the nation experienced dynamic shifts in its economic landscape, including a transition towards a more service-oriented economy, intensified technological innovation, and evolving trade dynamics. These economic developments posed significant challenges and opportunities, influencing labor markets, income distribution, and industrial structure 4 . Recognizing the multifaceted nature of these changes, the Chinese government embarked on policy revisions to address demographic and economic concerns. This comprehensive approach centralizes population dynamics and urban industrial development within the broader framework of social and economic development 5 .
Furthermore, China's population distribution displayed notable regional disparities, rapid urbanization, an aging demographic, and decelerated growth during this period. Eastern coastal regions witnessed high population densities due to an influx of rural laborers and migrants, while western regions experienced lower population densities 6 . Urbanization resulted in declining rural populations and an expanding urban–rural divide 7 , 8 . The aging population posed challenges to social security and the labor market, while internal population mobility transformed due to new policies, reforms, and rural economic development 9 . Notably, despite introducing the two-child policy in 2015, persistently low fertility rates persisted, influenced by cultural beliefs and economic conditions 10 .
Given the dynamic interplay between demographic shifts and industrial development, this study focuses on the intricate relationship between urban industrial facility agglomeration and population distribution in China from 2015 to 2021. The primary objectives are to (1) unveil distinctive population distribution patterns and industrial facility agglomeration features across various regions, (2) investigate the diverse impacts of different industrial facility agglomerations on urban population size, and (3) scrutinize the intricate relationship between industrial facility agglomeration and population distribution to develop a more comprehensive theoretical framework.
This study employs various quantitative analysis techniques to rigorously investigate the relationship between population distribution patterns and urban industrial facility agglomeration in China from 2015 to 2021. Kernel density analysis is initially employed to describe the spatial distribution characteristics of the population and industrial facility agglomeration 11 . Subsequently, spatial autocorrelation analysis is utilized to assess the degree of spatial association between population distribution and industrial facility agglomeration. Finally, multiple regression analysis is applied to unveil the relationship between industrial facility agglomeration and urban population size 12 and to evaluate the heterogeneous impact of different industrial facility agglomerations on urban population size 13 .
Literature review
Industrial facility agglomeration refers to the spatial concentration of industrial activities 14 , with its intellectual roots tracing back to the seminal works of Marshall 15 and Weber 16 . However, it gained significant prominence and theoretical grounding within the framework of New Economic Geography, mainly due to the influential contributions of Krugman 17 , particularly his introduction of the concept of increasing returns to scale, which has fundamentally shaped our understanding of urban industrial facility agglomeration.
The significance of urban industrial facility agglomeration extends across multiple dimensions of urban development. Empirical evidence from Nielsen et al. 18 underscores its role in enhancing production efficiency. Furthermore, studies by Liao and Li 19 emphasize its contribution to fostering innovation, bolstering urban competitiveness, and catalyzing regional development. Indeed, urban industrial facility agglomeration plays a pivotal role in comprehending the dynamics of urban economic growth, optimizing spatial structures, fortifying competitive advantages, and advancing the cause of sustainable development, as highlighted in research conducted by Huang et al. 20 and Chen et al. 21 .
The scholarly exploration of industrial facility agglomeration and its relationship with urban population size has encompassed diverse perspectives and research dimensions. Previous investigations have unraveled the intricate interplay between population mobility and urban industrial development, examined the influence of population distribution on urban industrial structure, and assessed the role of urban industrial facility agglomeration in shaping population distribution patterns 22 .
Primarily, research in this domain has delved into the interaction between industrial facility agglomeration and urban population size, frequently employing classical models such as the push–pull population migration theory 23 . A notable finding from this body of research highlights the pivotal role of human capital as a driver of industrial advancement. Population agglomeration, initially driven by economic development, encounters constraints owing to increasing demands for human capital 24 . Secondly, investigations have scrutinized the influence of urban population size on industrial facility agglomeration. Within this context, labor dynamics have exhibited a "cumulative causation" effect, with the net impact of urban scale on industrial facility agglomeration being significantly positive in the short term but potentially diminishing over the medium to long term 25 . Finally, research efforts have explored the mechanisms underpinning urban population agglomeration. While industrial facility agglomeration drives population agglomeration, uncertainties linger regarding its impact on urban population size 13 , 26 . For instance, the agglomeration of secondary industry facilities has boosted population size growth, while tertiary industries and industrial structure sophistication may suppress population growth 27 . Additionally, industrial facility agglomeration can sometimes lead to agglomeration diseconomies, potentially hindering productivity growth 28 , 29 .
However, it is essential to acknowledge that existing research in this field exhibits certain limitations, primarily related to geographical scope and research periods. Most studies have focused on national-level or representative city-level analyses, often overlooking regional disparities 30 , 31 . Furthermore, limited attention has been paid to the crucial period between 2015 and 2021, during which China witnessed significant shifts in population distribution patterns and industrial facility agglomeration characteristics 32 .
This study contributes significantly to the field by introducing innovative perspectives and addressing noteworthy research gaps. Specifically, it departs from conventional panel data techniques and employs groundbreaking methods utilizing POI data to measure industrial facility agglomeration. This approach provides real-time and granular data, enabling precise evaluations of industrial concentration within diverse urban settings. By effectively overcoming the limitations of traditional data, such as inadequate coverage, delayed updates, and the absence of fine-grained information, this pioneering application of POI data offers fresh insights and invaluable analytical tools for comprehending urban development and facilitating effective urban planning. Furthermore, this study delves into the intricate relationship between various forms of urban industrial facility agglomeration and urban population growth rates across 352 administrative units at or above the prefecture level throughout the country, focusing on the eastern, central, and western regions. This unique and meticulous regional approach delivers a comprehensive understanding and comparative analysis of how urban industrial facility agglomeration influences population growth across distinct regions, uncovering the nuances and distinctive attributes of the eastern, central, and western regions 33 . Consequently, this study equips local governments and urban planners with targeted development strategies and policy recommendations tailored to their contexts 34 .
Methods and data
This study employs statistical and spatial analysis techniques to explore the evolution of population distribution patterns and their relationship with industrial facility agglomeration. The overall method consists of four main steps: data collection, data processing, analysis, and interpretation of results. The methodological innovation of this research lies in integrating multiple techniques, such as the Gini coefficient, Moran's I, the geographical detectors, and multiple regression analysis, which together provide a comprehensive understanding of the spatial distribution and correlation of data. This approach is valuable in guiding decision-making in geography, urban planning, and environmental protection. The overall technical flow chart of this paper is shown in Fig. 1 .
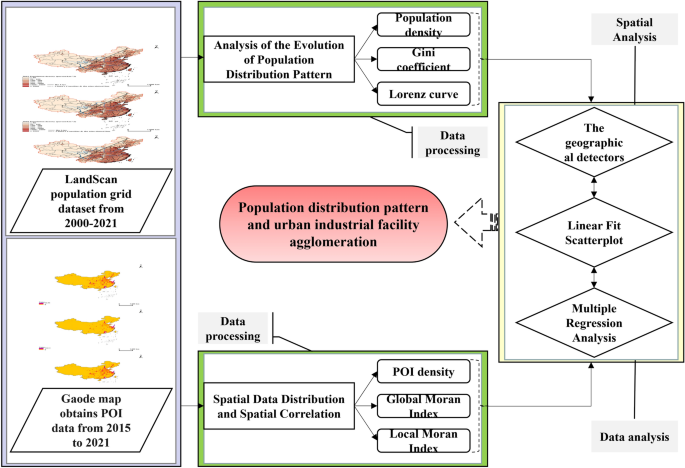
Research method and data processing flowchart. A flowchart illustrating the research methodology and data processing steps. Created using Microsoft® PowerPoint® 2019MSO (Version 2308 Build 16.0.16731.20182) 64-bit.
Analysis of the evolution of population distribution pattern
The Gini coefficient is a statistical measure employed to evaluate inequality levels and can be applied to quantify the disparities in population distribution patterns. In this study, we rank the population density of each province and the autonomous region at the prefecture-level and higher administrative units and establish a Lorenz curve for population distribution using cumulative percentages of population and area. Specifically, we organize all spatial units within region \(Z\) in descending order based on their population density, with the sequence of spatial units represented as \(\left( {i = 1,2,3 \ldots n} \right)\) . Subsequently, we calculate the proportion of the population in unit \(i\) relative to the total population ( \(P_{i}\) ) and the proportion of the area within the unit relative to the total area ( \(W_{i}\) ). The Gini coefficient ( \(G_{Z}\) ) is determined using the following formula:
In this equation, \(Q_{i} = \sum\nolimits_{k = 1}^{i} {W_{k} }\) denotes the cumulative percentage of the area.
Spatial data distribution and spatial correlation
Moran's I and the geographical detectors are tools utilized to study the spatial distribution and correlation of spatial data. Moran's I is a spatial autocorrelation statistical method that measures spatial data's degree of spatial clustering 35 . It reflects whether the observed values in a region have spatial correlation, i.e., whether they are close or far apart in space. The larger the value of Moran's I, the stronger the spatial correlation, and vice versa. The geographical detectors can further explore the spatial relationships behind Moran's I and determine these relationships' causes and influencing factors 36 . It helps researchers identify hotspot areas in space, i.e., areas with significant spatial clustering properties, while also revealing spatial heterogeneity, i.e., the differences and diversity between different regions in space. The core idea behind the geographical detectors is based on the assumption that if an independent variable significantly impacts a dependent variable, then the spatial distribution of the independent and dependent variables should be similar. Therefore, Moran's I and the geographical detectors can complement each other and be used together to gain a deeper understanding of the distribution and correlation of spatial data, providing support and guidance for decision-making in fields such as geography, urban planning, and environmental protection.
Spatial autocorrelation refers to the degree of similarity between adjacent regions in geographic space in terms of a particular attribute. The primary purpose of spatial autocorrelation is to reveal the mutual relationships between spatial units and the patterns of spatial structure 37 . The calculation methods for spatial autocorrelation include global and local spatial autocorrelation. Based on the results of the analysis of the impact of industrial facility agglomeration on population distribution patterns, this article used global Moran's I to reveal the overall spatial correlation trend in China 38 and local Moran's I to clarify the spatial agglomeration degree and heterogeneity of each prefecture-level administrative unit 39 .
The z-score standardization method is commonly used in spatial autocorrelation analysis because it can better handle customarily distributed data. Standardizing data using the z-score method converts data into dimensionless data, and the formula for z-score standardization is as follows:
In this formula, \(z\) represents the standardized data, \(x\) represents the original data, \(\mu\) represents the mean of the original data, and \(\sigma\) represents the standard deviation of the original data 40 .
The geographical detectors are based on the theory of spatial heterogeneity and diagnose a research object's spatial or attribute heterogeneity or coupling to reveal the mechanisms and major driving factors 41 . Using factor detectors, this study analyzed the explanatory power of various industrial facility agglomerations for the population growth rate of prefecture-level and higher administrative units, and the analytical model is as follows:
In this formula, \(N_{h}\) and \(\mu_{h}^{2}\) represent the sample size and variance of layer \(h\left( {h = 1, 2, \ldots , R} \right)\) , \(K\) represents the total sample size, and \(q\) represents the explanatory power of each detector factor for the population growth rate of prefecture-level and higher administrative units, ranging from 0 to 1. A larger \(q\) value indicates a stronger explanatory power of the detector factor for its spatial distribution, while a smaller \(q\) value indicates weaker explanatory power 42 . In this study, 12 detector factors representing industrial facility agglomeration were selected: Catering Services (A), Famous tourist sites (B), Company (C), Shopping service (D), Financial insurance service (E), Science, education and cultural services (F), Business residence (G), Domestic services (H), Sports and Leisure Services (I), Healthcare services (J), Government agencies and social groups (K), and Accommodation service (L). The k-means classification algorithm was used to classify these factors 22 , 43 .
Explanatory models and variables
This study employed multiple regression analysis to investigate the relationship between urban population growth rate and agglomeration of industrial facilities. Multiple regression analysis is a standard statistical technique used to examine the effects of multiple independent variables on a dependent variable while controlling for other variables. The dependent variable in this study was the urban population growth rate, and the independent variables were the agglomeration levels of 12 types of urban industrial facilities 44 . A residual normality test, variance inflation factor test, and stepwise regression analysis were conducted to ensure the validity and accuracy of the model. The hypothesis that residuals follow a normal distribution was initially confirmed by observing p values and figures. Subsequently, multicollinearity issues were examined by computing each independent variable's variance inflation factor. Lastly, stepwise regression analysis was conducted to obtain a final regression model that contains significant independent variables. This approach helped to identify the most influential independent variables, eliminate insignificant ones, and improve the model's predictability and interpretability. The benchmark econometric model used in this study is as follows:
Here, \(growth_{it}\) represents the population growth rate in region \(i\) during period \(t\) , and \(A_{{IA_{it} }}\) represents the agglomeration level of industry type \(A\) in region \(i\) and period \(t\) . A total of 12 industry types \(\left( {A, B, \ldots , L} \right)\) were examined as independent variables 45 .
Data source and calculation method
The population data utilized in this study were obtained from the LandScan population grid dataset with a resolution of 1 km from 2015 to 2021. The LandScan population dataset was developed by the Oak Ridge National Laboratory and provided by East View Cartographic. LandScan is a social standard for publishing global population data using innovative GIS and remote sensing methods. It is the most accurate and reliable global population dynamic statistical analysis database based on geographical location, with distribution models and optimal resolution.
POI, an abbreviation for "Point of Interest," refers to any meaningful point on a map that is not geographically significant, such as shops, bars, gas stations, hospitals, and stations 46 . The POI dataset used in this study from 2015 to 2021 was obtained by crawling the Amap API interface. In the specific operation, it was necessary to first apply for an Amap API KEY and obtain the "POI classification code table." The final results of this study were generated by separate tables for different POI types, with table fields including longitude, latitude, number of retrievals, POI category name, POI sub-category name, POI name, POI code, and administrative unit name, totaling eight items. The specific POI data information is shown in Table 1 .
Our study necessitated the seamless integration of two diverse datasets: POI data and population raster data. This integration was executed with precision using ArcGIS software, encompassing a structured, step-by-step approach within a geospatial context.
Step 1 Our journey commenced with population data initially in raster format. Employing ArcGIS, we conducted georeferencing for spatial alignment, data extraction for relevant information, and, when required, conversion to vector format. This transformation yielded vector polygons representing precise geographic regions. Step 2 Concurrently, the POI dataset underwent rigorous preprocessing using ArcGIS. Data cleansing rectified inconsistencies, spatial clustering optimized data representation, and attribute refinement enhanced overall data accuracy. Step 3 ArcGIS facilitated spatial joins, linking the population data (in vector polygons) with the POI dataset. This step precisely associated each POI with its corresponding geographic area. Step 4 The final phase utilized ArcGIS's toolkit to merge and attribute relevant data fields from both datasets systematically. This meticulous process ensured the accurate linkage of each POI to the geographic area defined by the population data.
Research result
Evolution trend of population distribution pattern in china.
The changing trend of China's population distribution pattern is closely tied to the facility agglomeration of urban industrial facilities, which significantly impacts population dynamics 47 . The accelerated pace of economic development and urbanization has made the influence of urban industrial facility agglomeration increasingly pronounced. This phenomenon leads to the concentration of industries in specific regions, such as the Yangtze River Delta, the Pearl River Delta, and the Beijing-Tianjin-Hebei region, resulting in distinct industrial facility agglomeration zones 25 . Moreover, the size of urban areas attracts more industrial investment and facility construction, creating a positive feedback loop between population growth and industrial development. Therefore, understanding the impact of urban industrial facility agglomeration is vital for comprehending the evolving trend of China's population distribution pattern. Building upon this understanding, this paper offers a comprehensive analysis of China's population distribution pattern changing in urban industrial facility agglomeration. The findings of this study provide a scientific basis for formulating policies that can promote regional economic development 7 , 8 .
Population spatial distribution characteristics
The Hu Line has played a crucial role in identifying China's population distribution pattern, dividing the country into the southeast and northwest regions. This line serves as a boundary, revealing significant disparities in population distribution. The densely populated areas are primarily located in the east, while the west remains sparsely populated (Fig. 2 ). Over the years, China has witnessed substantial population migration, predominantly occurring in the southeast. Meanwhile, the northwest has experienced higher population growth due to higher birth rates and lower emigration rates. The proportion of the population in the northwest has gradually increased from 5.77% in 1982 to 6.5% in 2020. The seventh national population census in 2020 confirms the influence of natural geographical environments on population distribution stability. From 2015 to 2021, areas with a population density of over 1000 person/km 2 were predominantly found in the southeast, while only Lanzhou, Yinchuan, and Shihezi in the northwest had a density of over 300 person/km 2 .
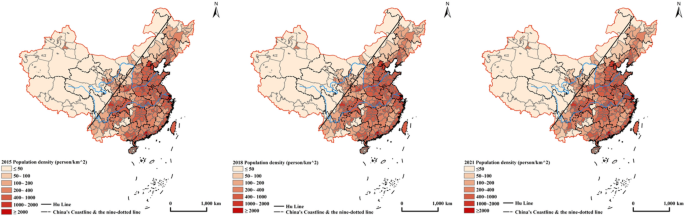
Distribution pattern of population density in China from 2015 to 2021. This map displays the distribution pattern of population density in China from 2015 to 2021. Generated using ArcGIS Desktop 10.8 (ArcMap 10.8, Release Version 10.8, Product Version 10.7.0.10450).
The southeast side of the Hu Line, encompassing coastal areas such as Guangdong, Zhejiang, and Jiangsu, exhibits higher population density. In contrast, the northwest side, including Tibet, Xinjiang, and Qinghai, has a lower population density due to climatic and natural conditions. The eastern coastal areas have experienced higher urbanization rates, reflecting the process of population migration from rural to urban areas. The eastern region's higher urbanization rate has contributed to its relatively higher economic and social development. However, from 2015 to 2021, the aging population issue worsened, particularly in the eastern region, due to declining fertility rates and increasing life expectancy 48 . Population mobility remains high in the western region, driven by disparities in economic development between the western and eastern coastal areas 49 . Many young individuals from the western region seek better opportunities in the eastern coastal cities, resulting in a continuous decrease in the western region's population.
From 2015 to 2021, China experienced continued large-scale population migration, with some notable shifts compared to previous years. Urbanization, rising production costs, stricter management policies for migrant workers, and evolving economic and demographic structures have influenced population migration patterns. These changes have affected the prevalence of rural migrant workers, prompting some to return to their hometowns or seek opportunities elsewhere. In certain areas, stricter management policies have constrained population mobility. Furthermore, rapid economic growth and urbanization have led to aging populations and declining population figures in some cities. Conversely, economic development in the western regions has attracted population inflows. These factors collectively contribute to the transformation of population migration patterns.
Characteristics of population distribution equilibrium changes
The Gini coefficient is a practical indicator widely used to assess population distribution inequality in China. This study utilizes rasterized population data from 2000 to 2021 to calculate the Gini coefficient, revealing minor fluctuations but an overall upward trend (Fig. 3 ). This indicates a growing imbalance in population distribution. Efforts to foster balanced distribution include developing the Western regions' economy, improving infrastructure connectivity, and encouraging regional coordinated development. Addressing this imbalance requires persistent implementation of effective policies.
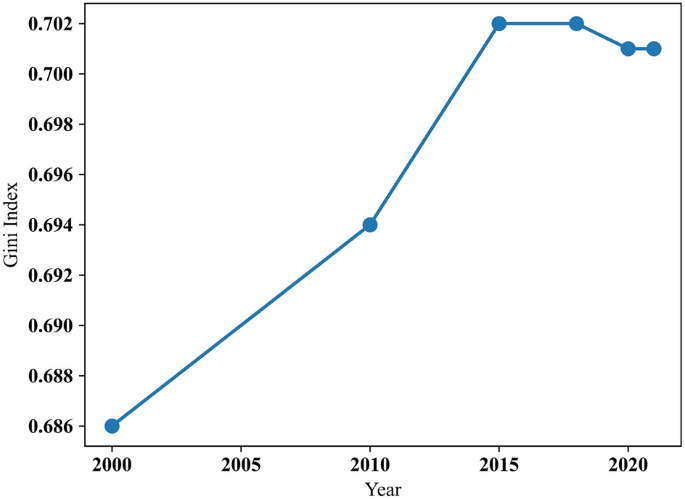
Gini coefficient of population distribution in China from 2000 to 2021.
Regional disparities in economic development have intensified population distribution imbalances. Rapid economic growth in the eastern regions attracted migrants while lagging development in the west led to population outflows. Variations in natural geographical conditions also contribute to these imbalances, with the east offering favorable conditions and the west experiencing lower population densities due to limiting factors.
Analyzing specific periods, the Gini coefficient increased between 2000 and 2010 due to rapid economic development and urbanization. Between 2010 and 2015, the growth slowed as the government supported western and central-western regions, curbing inequality. From 2015 to 2018, stability was observed due to regional development efforts. Between 2018 and 2021, a slight decrease occurred as the government focused on coordinated development, and inland cities and western regions experienced growth.
The Gini coefficient ranges from 0 to 1, with 0 indicating equitable distribution and 1 representing uneven distribution. The Lorenz curve visually represents distributional inequality, with the Gini coefficient derived from it. China's Lorenz curve for population distribution demonstrates significant inequality driven by economic development, urbanization, and migration. The curve shows concentration in economically developed eastern coastal regions and large cities.
The Gini coefficient decreased slightly by 2018 and 2021, reflecting a modest reduction in population distribution imbalance 50 . Efforts to bolster regional coordination and develop central and western regions contributed to this change. However, the imbalance persists, albeit with limited improvement. Economic transition and ongoing regional development strategies contribute to the decreasing trend 51 . The specific Lorenz curve for China's population distribution is depicted in Fig. 4 .
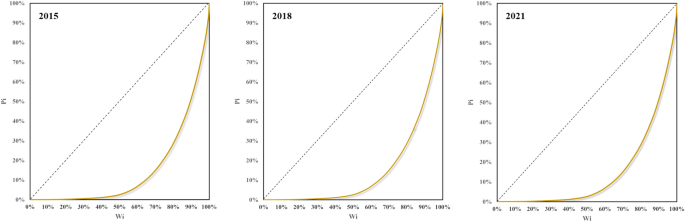
Lorenz curve of population distribution in China from 2015 to 2021.
Spatial analysis of urban industrial facility agglomeration
Amidst globalization and urbanization, urban industrial facility agglomeration has emerged as a critical driver of economic development and urban competitiveness. Industrial facility agglomeration fosters communication and collaboration among industries, enhancing production efficiency and stimulating employment, innovation, and regional development. To uncover the intrinsic relationship between urban industrial facility agglomeration and urban population growth rates, this study utilizes spatial analysis methods to examine the influence of spatial distribution characteristics and agglomeration degrees of 12 industry types on urban population growth rates.
In conjunction with POI data, this paper employs a kernel density algorithm to characterize these industry types and analyze the spatial distribution patterns of various urban industries across China, as depicted in Fig. 5 . Observations from the 3D kernel density maps of the 12 industries in 2015 and 2021 reveal the following trends.
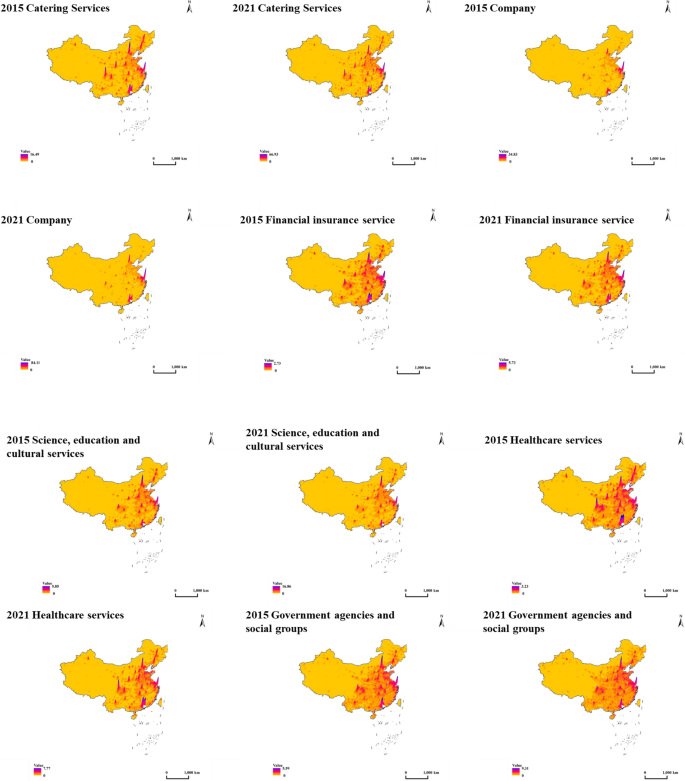
3D kernel density map of industrial facilities in each city in 2015 and 2021. A 3D kernel density map representing the spatial distribution of industrial facilities in various cities for the years 2015 and 2021. Created using ArcGIS Desktop 10.8 (ArcScene 10.8, Release Version 10.8, Product Version 10.7.0.10450).
To sum up, between 2015 and 2021, the high-density regions across various industries were primarily concentrated in the Beijing-Tianjin-Hebei, Yangtze River Delta, and Pearl River Delta areas. These regions play a crucial role in China's economic development and are characterized by high output value and population density. Beijing and Shanghai consistently emerged as the top industry categories' top performers, reflecting their positions as China's political and economic hubs 52 . Furthermore, cities like Chengdu and Chongqing highly competed in specific industry sectors.
Global autocorrelation analysis
In this section, we utilized the Global Moran's I index to calculate the spatial autocorrelation of 12 types of industrial facilities across China in 2015, 2018, and 2021. Each industry type exhibited significant spatial autocorrelation at all three time points, indicating that industrial facilities in each region were highly correlated with adjacent geographic units. The changes in the Global Moran's I value from 2015 to 2021 are presented in Table 2 .
The spatial autocorrelation of the 12 types of industrial facilities displayed significant spatial clustering at all three time points. Notably, the Company type had the strongest spatial correlation with Global Moran's I values of 0.223 (in 2015), 0.237 (in 2018), and 0.231 (in 2021). This suggests that the spatial distribution of Company type industrial facilities exhibited the strongest correlation across China during the study period. Additionally, Famous tourist sites, Shopping service, and Financial insurance service types also exhibited relatively high Global Moran's I value of 0.167 (in 2021), 0.199 (in 2021), and 0.167 (in 2021), respectively. The z-values of all industries at the three-time points were significantly greater than zero, and the p-values were zero, indicating that the Global Moran's I was highly significant, and spatial autocorrelation was significant across these industries 53 . These results demonstrate that the spatial distribution of the 12 types of industrial facilities in China exhibited a certain degree of clustering during the study period, which may be attributed to the economic development level, industrial structure, and policy orientation of different regions in China 54 .
By comparing the Global Moran's I value of 12 industries across China in 2015, 2018, and 2021, this article can analyze the changing trends in spatial autocorrelation. Catering Services showed a consistent year-on-year increase at all three time points, indicating a continuous rise in the spatial agglomeration of catering facilities. From 2015 to 2018, Famous tourist sites displayed a decreasing trend but slightly rebounded from 2018 to 2021, suggesting a fluctuation in the spatial agglomeration of tourist attractions yet remaining relatively stable overall. The Global Moran's I value of Company facilities increased from 2015 to 2021, indicating an overall increase in the spatial agglomeration of company facilities 55 . Similarly, Shopping service, Financial insurance service, Science, education and cultural services, Domestic services, Sports and Leisure Services, Healthcare services, and Government agencies and social groups all showed an increasing trend in their Global Moran's I value from 2015 to 2021, indicating an overall increase in the spatial agglomeration of facilities in these industries 56 . However, Business residence showed an overall decreasing trend from 2015 to 2021, indicating a reduction in the spatial agglomeration of commercial and residential facilities. Accommodation services also decreased from 2015 to 2021, indicating decreased spatial agglomeration of accommodation facilities.
Local autocorrelation analysis
This section uses the Local Moran's I index to compute the spatial autocorrelation of 12 industrial facilities across China and examine the interrelationships among various industry types among administrative units at or above the prefectural level. The LISA map is an indicator that assesses the degree of similarity and dissimilarity and its significance between spatial unit attributes and neighboring units. LISA clustering maps depict four distinct types of spatial autocorrelation relationships: High-High (H–H), Low–Low (L–L), Low–High (L–H), and High–Low (H–L). H–H type represents a high level of industrial facility agglomeration in the study area and its adjacent regions. In contrast, the L–L type indicates a low level of industrial facility agglomeration in the study area and its surrounding areas. The L–H type suggests that the industrial facility agglomeration level is low in the study area but high in its surrounding areas. In contrast, the H–L type indicates a high industrial facility agglomeration level in the study area but low in its surrounding areas. By calculating the Moran's I value of industrial facility agglomeration distribution among administrative units at or above the prefectural level in 2015 and 2021 and drawing LISA clustering maps based on Z-test values ( P = 0.05), readers can observe the changes and trends of industrial facility agglomeration as shown in Fig. 6 . An in-depth analysis of data for the 12 types of industries reveals that the number of prefectural and higher-level units for each type of industry generally exhibits an increasing trend. Moreover, the Beijing-Tianjin-Hebei, the Yangtze River Delta, and the Pearl River Delta regions show high agglomeration levels in most industries. However, regions such as Tibet, Qinghai, Xinjiang, Gansu, southwest Inner Mongolia, southern Guangxi, and Hainan exhibit relatively low agglomeration levels in most industries. Further research into the factors that affect the spatial agglomeration of facilities in various industries, such as policy changes, regional development strategies, industrial structure adjustments, and population mobility, can provide targeted recommendations for urban planning, industrial policy development, and regional economic development.
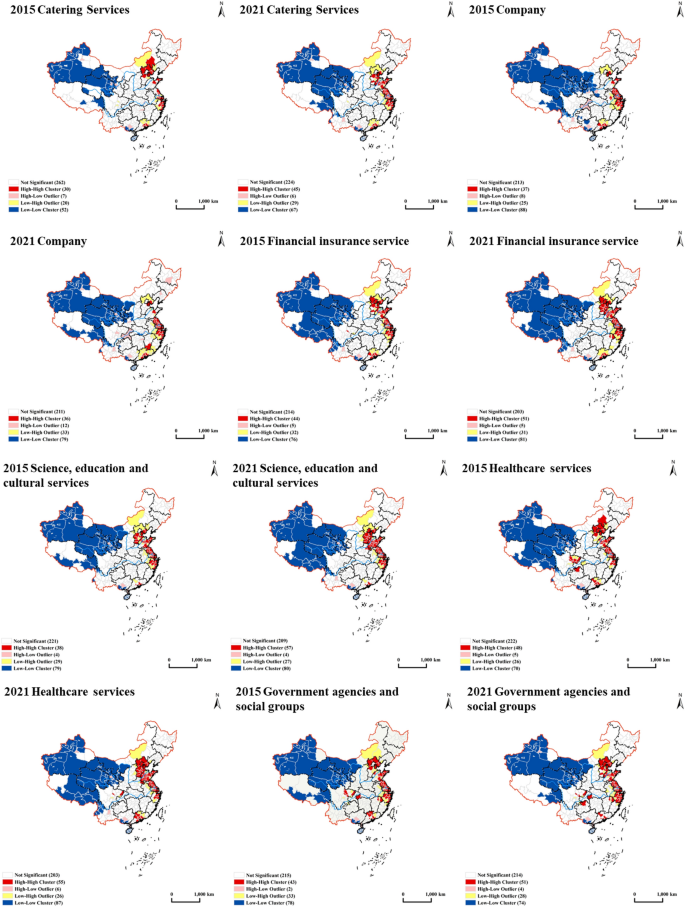
LISA map of industrial facility agglomeration in China in 2015 and 2021. This map visualizes the Local Indicators of Spatial Association for the agglomeration of industrial facilities in China during 2015 and 2021. Produced with ArcGIS Desktop 10.8 (ArcMap 10.8, Release Version 10.8, Product Version 10.7.0.10450).
In conclusion, the spatial autocorrelation relationships of various industries underwent significant changes between 2015 and 2021. In general, the number of H–H level prefectural and higher-level administrative units increased in the Beijing-Tianjin-Hebei, Yangtze River Delta, and Pearl River Delta regions, indicating an increase in agglomeration. Conversely, the number of H–L level units is relatively small and mainly concentrated in central and northeastern China's provincial capitals or central cities. The distribution of L–H level units is uneven among industries, primarily concentrated in the northern part of Hebei and southeastern Anhui. In contrast, the number of L–L level units is relatively high and mainly distributed in areas with lower economic development levels, such as Tibet, Qinghai, and Xinjiang. A comparative analysis of the 2015 and 2021 data shows that industry facility agglomeration tends to concentrate, particularly in economically developed regions. This trend is closely linked to policy support, infrastructure construction, and market demand. However, some relatively underdeveloped regions still face challenges in industrial development. Therefore, more support is necessary for these areas in the future to promote industry development and spatial optimization.
Spatial heterogeneity analysis of population distribution and industrial facility agglomeration
This study used the geographical detectors method to analyze the agglomeration of various industries and its impact on urban population growth rates. Table 3 presents the results of the factor detector for 2015 and 2021, including q-statistic values and p values. The findings reveal significant disparities in the influence of different factors on population growth rates between the two periods. Note that “A B C …” represents different types of POIs (A, B, C, …, L).
In 2015, Science, Education, and Cultural Services (F), Business Residence (G), and Financial Insurance Services (E) had a substantial impact on urban population growth rates (q = 0.217, q = 0.183, and q = 0.171, respectively, all with p < 0.001). This suggests that these factors played a significant role in shaping population growth in 2015. However, in 2021, Science, Education, and Cultural Services (F) and Government Agencies and Social Groups (K) emerged as the key influencers (q = 0.092 and q = 0.096, respectively, both with p < 0.001). These findings indicate that the factors influencing population growth rates have evolved over time, with Science, Education, and Cultural Services and Government Agencies and Social Groups gaining increasing importance.
It is worth noting that certain factors had a relatively low impact on population growth rates in both periods. For example, Domestic Services (H) had a q-statistic of 0.162 in 2015, which decreased to 0.055 in 2021. Similarly, Accommodation Services (L) had a q-statistic of 0.123 in 2015, further decreasing to 0.038 in 2021. These results suggest that these factors have a minor influence on urban population growth, with varying degrees of impact across different periods.
The analysis reveals a shift in the key factors influencing urban population growth rates over time. Government Agencies and Social Groups gained significance, while factors like Business Residence and Financial Insurance Services exhibited a weaker impact. Notably, Science, Education, and Cultural Services consistently strongly influenced both periods. This emphasizes the crucial role of education, scientific research, and government policies in shaping population distribution and industry facility agglomeration dynamics.
The evolution and influence of China's industrial facility agglomeration on the shape of population distribution pattern
This study examines the impact of industrial facility agglomeration on population distribution patterns in China from 2015 to 2021. Using a linear regression model and data visualization techniques, the relationships between urban industrial facilities and population growth rates are analyzed 57 . The findings reveal linear relationships between industrial facility agglomerations and population growth rates, with variations in strength and direction between the two time periods (Fig. 7 ). Please note that the Population Growth Rate is calculated as follows: (End-of-Year Population − Beginning-of-Year Population)/Beginning-of-Year Population × 100%. In 2015, Financial Insurance Services had a significant impact on population growth rates. However, this influence weakened in 2021 due to the deceleration of overall population growth and the emergence of other influential factors. The correlation coefficients between industrial facility agglomerations and population growth rates shifted from positive to negative during this period, reflecting changes in China's population policies and economic structure 58 . Notably, the relationships between Famous Tourist Sites, Science, Education and Cultural Services, Government Agencies and Social Groups, and population growth rates weakened in 2021, attributed to government initiatives promoting industrial transformation and upgrading 59 . Emerging industries, high-tech, and service sectors have gained more attractiveness, while traditional industrial facilities have comparatively diminished appeal.
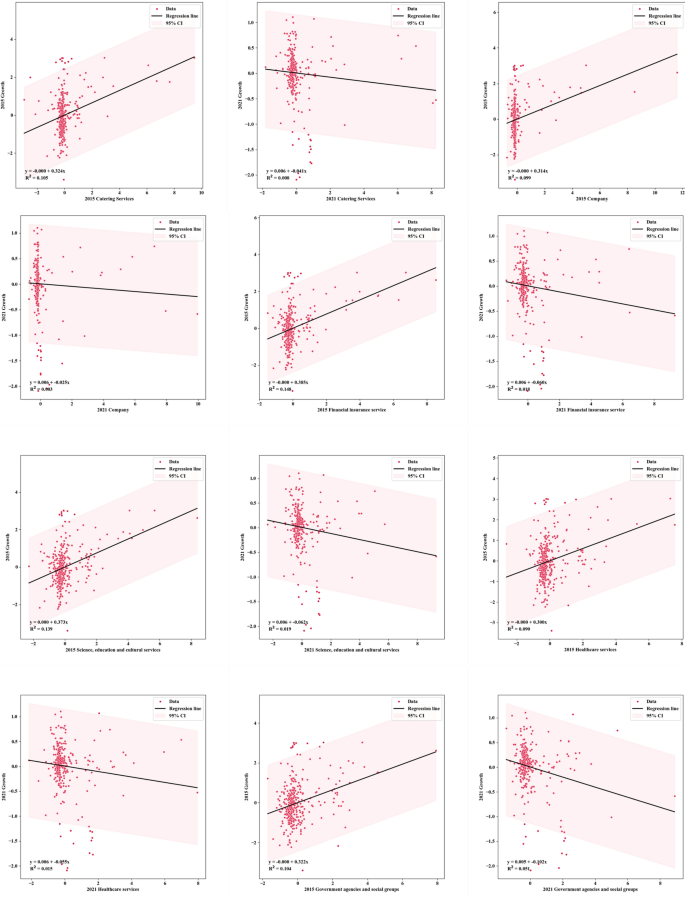
Scatter plot of China’s urban population growth rate and urban industrial facility agglomeration in 2015 and 2021.
This analysis examines the relationship between urban industrial facility agglomeration and urban population growth in different regions of China. The focus is on the Catering Services industry as a case study (Fig. 8 ), with supplementary materials providing information on other industries.
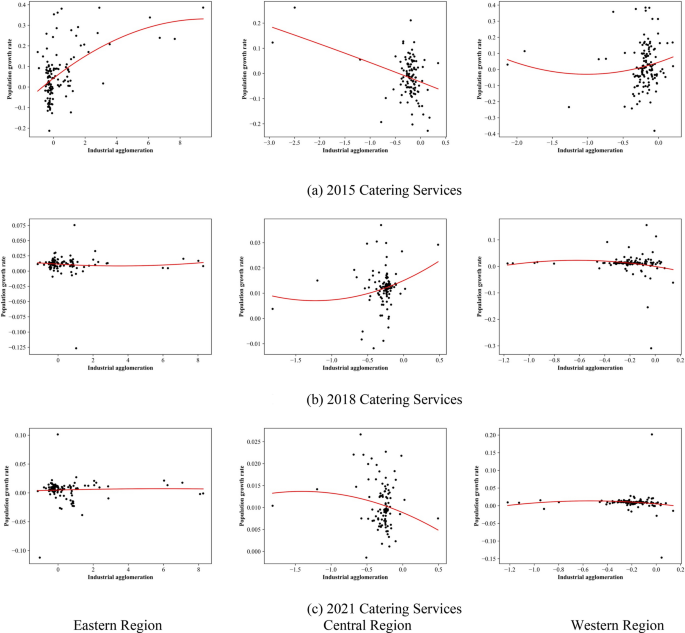
The relationship between industrial facility agglomeration and population growth by dividing China into eastern region, central region, and western region.
In 2015, the eastern region showed high industrial facility agglomeration in Catering Services, positively correlated with population growth. The central region had lower industrial facility agglomeration, negatively correlated with population growth. The western region had low industrial facility agglomeration, with an insignificant correlation to population growth. By 2018, the eastern region maintained high industrial facility agglomeration in Catering Services, but the correlation with population growth was unclear. The central region showed low industrial facility agglomeration, positively correlated with population growth. The western region maintained low industrial facility agglomeration, with an insignificant correlation to population growth. In 2021, the eastern region still had high industrial facility agglomeration in Catering Services, but the relationship with population growth was unclear. The central region had low industrial facility agglomeration, negatively correlated with population growth. The western region had low industrial facility agglomeration, with an insignificant correlation to population growth.
Overall, industrial facility agglomeration for other industries had specific characteristics. In the eastern region, agglomeration concentrated between (0, 2), while in the central and western regions, it concentrated between (− 0.5, 0). Various factors influenced these patterns:
Eastern Region Rapid economic growth and urbanization led to industry saturation, resulting in small changes in agglomeration. Urbanization and population aging slowed population growth and weakened the correlation with agglomeration. Economic restructuring towards the service and innovation sectors is crucial for sustainable growth.
Central Region Lower economic development and social resources limited population growth compared to the eastern region. Attracting advantageous industries from the east can improve employment and economic development. Industries like Company, Science, Education, Business Residence, and Accommodation Services performed significantly.
Western Region Initially reliant on natural resource development, the region faced limitations and weakened agglomeration. Strengthening industrial diversification and fostering innovation-driven development is necessary. Industries like Famous Tourist Sites, Company, Financial Insurance Services, and Science, Education and Cultural Services performed remarkably. Focus on tourism, business support, financial services, and science, education, and culture investments can promote growth.
This study conducted a multiple regression analysis on the relationship between urban population growth rates and agglomerations of industrial facilities in China. Data from 2015, 2018, and 2021 were analyzed, and comprehensive results can be found in Table 4 .
In 2015, after controlling for other factors, Financial Insurance Services agglomeration showed a significantly positive correlation with urban population growth rates. On the other hand, Business Residence and Accommodation Services agglomerations had significantly negative correlations. These findings suggest that Financial Insurance Services were attractive to urban populations in 2015, while Business Residence and Accommodation Services were less appealing. The regression model explained about 16.6% of the variation in population growth rates (R 2 = 0.166).
In 2018, the regression results showed a significantly negative correlation between Company agglomeration and urban population growth rates. Financial Insurance Services and Science, Education, and Cultural Services agglomerations had significant positive and negative correlations. This indicates that Financial Insurance Services remained attractive to urban populations in 2018, while Companies and Science, Education, and Cultural Services were less appealing. The regression model explained about 4.7% of the variation in population growth rates (R 2 = 0.047).
In 2021, Catering Services, Companies, Government Agencies, and Social Groups agglomerations were significantly correlated with urban population growth rates. Business Residence and Domestic Services agglomerations had significantly negative correlations. This suggests that Catering Services, Companies, Government Agencies, and Social Groups were highly appealing to urban populations in 2021, while Business Residence and Domestic Services were less enticing. The regression model explained about 5.3% of the variation in population growth rates (R 2 = 0.053).
Over the study period, the relationships between population growth rates and agglomerations of industrial facilities in China's administrative units experienced notable changes. Financial Insurance Services consistently attracted urban populations, while the appeal of other industries varied. This highlights the impact of industrial restructuring and transformation on population distribution patterns during China's urban development. As China's economy advances and its industrial structure evolves, high-value-added industries and service sectors will be crucial in shaping future urban population distribution. Therefore, the government must prioritize optimizing industrial facility layouts, promoting industrial restructuring and transformation, and fostering sustainable urban development to address these evolving population patterns.
The findings of this study offer unique insights into the intricate relationship between urban industrial facility agglomeration and population growth in China. By leveraging the LandScan population dataset and the POI spatial dataset, along with mature spatial analysis techniques and multiple regression models, this study breaks new ground in understanding the dynamics of population distribution and industrial facility agglomeration.
One of the distinctive contributions of this study lies in its examination of the evolving factors influencing urban population growth rates. While previous studies have focused on traditional determinants, this research highlights the increasing significance of Government Agencies and Social Groups alongside the consistent impact of Science, Education, and Cultural Services.
Furthermore, this study unveils the shifting correlations between industrial facility agglomerations and population growth rates. The linear relationships observed in 2015 and 2021, albeit with variations in strength and direction, highlight the nuanced nature of these connections. This demonstrates that many factors influence population growth in China, and industrial facility agglomeration is just one piece of the puzzle. These findings challenge conventional assumptions and underscore the need for a comprehensive understanding of the complex interplay between industrial facility agglomeration, population dynamics, and other contextual factors.
The regional disparities in industrial facility agglomeration patterns identified in this study further underscore its significance. The unique characteristics and development trajectories of China's eastern, central, and western regions call for tailored strategies to optimize industrial structures, foster innovation-driven industries, and promote sustainable population growth. This regional approach to urban development is crucial for addressing the diverse challenges and harnessing the opportunities presented by China's evolving economic landscape.
Moreover, the innovative use of the LandScan population and POI spatial datasets sets this study apart. These datasets provide comprehensive, real-time, and fine-grained information, enabling a more nuanced understanding of population distribution and industrial facility agglomeration dynamics. The integration of mature spatial analysis techniques and multiple regression models enhances the findings' accuracy and reliability, contributing to this research's methodological innovation.
While the study offers valuable insights, it acknowledges certain limitations that future research could address. For a more comprehensive prediction and explanation of population growth rates, future studies should consider additional factors like infrastructure, the policy environment, social welfare, and educational resources 60 . It would also be beneficial to examine the interplay among different industrial facility agglomerations and assess their collective impact on urban development and population growth. Furthermore, analyzing the effects of various city types and regional disparities on the relationship between industrial facility agglomeration and population growth rates could shed light on spatial heterogeneity in population distribution and industrial facility agglomeration 61 . Additionally, future research could explore potential nonlinear relationships using nonlinear or multivariate regression models 62 .
This study employs the LandScan population dataset and the POI spatial dataset, in conjunction with a range of population spatial analysis techniques and multiple regression models, to examine the interplay between population distribution patterns and industrial facility agglomeration in 352 Chinese administrative units at the prefecture level and above. The primary findings are as follows:
Factors influencing urban population growth rates varied between 2015 and 2021, with Government Agencies and Social Groups gaining significance. Science, Education, and Cultural Services consistently influenced population growth, highlighting the importance of education, research, and government policies in the relationship between population distribution and industrial facility agglomeration.
The correlation between industrial facility agglomerations and urban population growth rates exhibited linear relationships in 2015 and 2021, but the strength and direction of these relationships differed. Correlation coefficients shifted from positive to negative, indicating changes in China's population growth rate and the influence of other factors.
Industrial facility agglomeration patterns varied across regions, with the eastern region experiencing rapid economic growth and urbanization, resulting in saturated industries. The central region faced economic development and resource limitations, while the western region relied on natural resources but required diversification. The focus should be optimizing industrial structure, fostering innovation-driven industries, and developing service sectors to achieve sustainable population growth and economic development 63 .
Based on the study's findings, the following specific development strategies and policy recommendations are proposed for local governments and planners:
Promote the transition to high-value-added sectors Encourage shifting from traditional manufacturing industries to sectors with higher value and innovation potential, such as technology, research and development, and knowledge-intensive services. This can be achieved by providing targeted investments, incentives, and supportive policies that facilitate the growth and development of innovation-driven enterprises. Emphasizing a diversified and technologically advanced industrial landscape will create employment opportunities, attract skilled workers, and drive sustainable population growth.
Address regional disparities Tailor strategies to each region's unique characteristics and challenges. In the eastern region, focus on optimizing the existing industrial structure and transitioning towards service-oriented and innovation-driven sectors. The central region should attract advantageous industries from the eastern region, expand employment opportunities, and enhance economic development. In the western region, diversify the industrial base, reduce dependence on natural resources, and promote emerging industries. These approaches will foster balanced regional development and sustainable population growth.
Establish a harmonious relationship between industrial facility agglomeration and population growth Prioritize measures that optimize the layout of industrial facilities to enhance efficiency and minimize environmental impacts. Support green and sustainable urban planning practices, including providing quality public services, infrastructure development, and creating livable urban environments. Increase investments in education, research, and cultural sectors to promote human capital development and attract talent. Strengthen social welfare systems to address income disparities and improve urban residents' overall quality of life. These efforts will ensure that population growth is accompanied by enhanced livability and improved well-being.
Previous research on population distribution and industrial facility agglomeration in China has emphasized economic factors as the primary drivers of urban population growth. These studies often established a stable positive correlation between industrial facility agglomerations and population growth rates, attributing urbanization and economic development as the key factors. While this study aligns with this fundamental understanding, it provides significant contributions and novel insights that differentiate it from existing research:
Evolving Nature of the Relationship Existing studies have generally treated the relationship between industrial facility agglomeration and population growth as relatively static. In contrast, this research reveals a dynamic and evolving relationship. We demonstrate that the influence of industrial agglomerations on population growth rates has shifted over the study period, moving from a predominantly positive correlation to a more complex and nuanced interaction.
Changing Role of Non-Economic Factors Previous research often neglected or downplayed the role of non-economic factors, such as government policies and social dynamics, in shaping population distribution patterns. This study, however, highlights the increasing significance of these factors. Government Agencies and Social Groups have emerged as influential determinants of urban population growth, indicating a shift in the landscape of influence away from purely economic considerations.
Necessity for Tailored Regional Strategies Prior studies have recognized regional disparities in population distribution and industrial agglomeration, but this research underscores the need for region-specific strategies. This article provides concrete evidence of how different regions within China exhibit varying patterns of industrial facility agglomeration, necessitating tailored approaches. This regional differentiation emphasizes that a one-size-fits-all policy approach may not effectively address each region's unique challenges and opportunities.
Emphasis on Diversified, Innovation-Driven Industries A notable distinction in this study is the emphasis on the need for diversified, innovation-driven industries to foster sustainable population growth. Previous research tended to focus primarily on traditional manufacturing industries. We advocate for transitioning to high-value-added sectors, such as technology, research and development, and knowledge-intensive services, aligning with global trends toward knowledge-based economies.
The differences between this study and previous research can be attributed to several factors. Firstly, from 2015 to 2021, the study period captures a period of rapid socio-economic transformation in China, leading to shifts in population dynamics and industrial structures. Additionally, this research's comprehensive dataset and advanced spatial analysis techniques enable a more nuanced understanding of the interplay between factors. Lastly, this study emphasizes interdisciplinary perspectives, integrating economic, social, and policy dimensions to offer a holistic view of the subject.
In conclusion, this study underscores the dynamic interplay between urban industrial agglomeration and population growth in China, revealing the evolving nature of the underlying factors. The influence of government agencies, social groups, and sectors such as science, education, and cultural services has grown over time. The correlations between industrial agglomeration and population growth rates have exhibited notable shifts in intensity and direction, mirroring changes in China's overall population growth rate and the escalating impact of additional determinants. Furthermore, regional disparities in industrial agglomeration patterns underscore the necessity for tailored strategies, optimizing industrial structures, cultivating innovation-driven sectors, and fostering sustainable population growth in different regions. As China advances its economy and continues industrial restructuring, policymakers must accord priority to reconfiguring industrial facility layouts, facilitating industrial transformation, and propelling sustainable urban development to accommodate the ever-evolving patterns of population distribution. By implementing these measures, China can effectively navigate the complexities of urbanization and establish a harmonious relationship between industrial agglomeration and population growth in the future.
Data availability
The datasets used and/or analysed during the current study available from the corresponding author on reasonable request.
Jarzebski, M. P. et al. Ageing and population shrinking: Implications for sustainability in the urban century. NPJ Urban Sustain. 1 (1), 17 (2021).
Article Google Scholar
Folke, C. et al. Our future in the Anthropocene biosphere. Ambio 50 , 834–869 (2021).
Article PubMed PubMed Central Google Scholar
Ye, J., Chen, Z. & Peng, B. Is the demographic dividend diminishing in China? Evidence from population aging and economic growth during 1990–2015. Rev. Dev. Econ. 25 (4), 2255–2274 (2021).
Zhang, X., Guo, F. & Zhai, Z. China’s demographic future under the new two-child policy. Popul. Res. Policy Rev. 38 , 537–563 (2019).
Liang, L., Wang, Z. & Li, J. The effect of urbanization on environmental pollution in rapidly developing urban agglomerations. J. Clean. Prod. 237 , 117649 (2019).
Qiao, L., Li, Y., Liu, Y. & Yang, R. The spatio-temporal change of China’s net floating population at county scale from 2000 to 2010. Asia Pac. Viewpoint 57 (3), 365–378 (2016).
Chen, M., Gong, Y., Li, Y., Lu, D. & Zhang, H. Population distribution and urbanization on both sides of the Hu Huanyong Line: Answering the Premier’s question. J. Geogr. Sci. 26 , 1593–1610 (2016).
Chen, M., Liu, W. & Lu, D. Challenges and the way forward in China’s new-type urbanization. Land Use Policy 55 , 334–339 (2016).
Xi, Y., Qiang, L., Zhengdong, H. & Renzhong, G. Characterising population spatial structure change in Chinese cities. Cities 123 , 103555 (2022).
Deng, X. & Yu, M. Does the marginal child increase household debt?—Evidence from the new fertility policy in China. Int. Rev. Financ. Anal. 77 , 101870 (2021).
Yang, Q. & He, L. Spatiotemporal changes in population distribution and socioeconomic development in China from 1950 to 2010. Arab. J. Geosci. 10 , 1–16 (2017).
Zaborovskaia, O., Nadezhina, O. & Avduevskaya, E. The impact of digitalization on the formation of human capital at the regional level. J. Open Innov. Technol. Market Complex. 6 (4), 184 (2020).
Li, W. et al. Does producer services agglomeration improve urban green development performance of the Yangtze River Economic Belt in China?. Ecol. Ind. 145 , 109581 (2022).
Liu, K., Liu, X., Long, H., Wang, D. & Zhang, G. Spatial agglomeration and energy efficiency: Evidence from China’s manufacturing enterprises. J. Clean. Prod. 380 , 135109 (2022).
Marshall, A. Principles of Economics 8th edn. (Macmillan, London, 1890) ( Published in 1920 ).
MATH Google Scholar
Weber, A. Theory of the Location of Industries (University of Chicago Press, Chicago, 1962).
Google Scholar
Krugman, P. Increasing returns and economic geography. J. Political Econ. 99 (3), 483–499 (1991).
Nielsen, B. B., Asmussen, C. G., Weatherall, C. D. & Lyngemark, D. H. Marshall vs Jacobs agglomeration and the micro-location of foreign and domestic firms. Cities 117 , 103322 (2021).
Liao, B. & Li, L. Spatial division of labor, specialization of green technology innovation process and urban coordinated green development: Evidence from China. Sustain. Cities Soc. 80 , 103778 (2022).
Huang, D., Liu, Z., Zhao, X. & Zhao, P. Emerging polycentric megacity in China: An examination of employment subcenters and their influence on population distribution in Beijing. Cities 69 , 36–45 (2017).
Chen, Q., Guan, X. & Huan, T. C. The spatial agglomeration productivity premium of hotel and catering enterprises. Cities 112 , 103113 (2021).
Lan, T., Shao, G., Xu, Z., Tang, L. & Sun, L. Measuring urban compactness based on functional characterization and human activity intensity by integrating multiple geospatial data sources. Ecol. Ind. 121 , 107177 (2021).
Gao, J., Song, G. & Sun, X. Does labor migration affect rural land transfer? Evidence from China. Land Use Policy 99 , 105096 (2020).
Wang, X. & Wang, Q. Research on the impact of green finance on the upgrading of China’s regional industrial structure from the perspective of sustainable development. Resour. Policy 74 , 102436 (2021).
Wang, W., Gong, J., Wang, Y. & Shen, Y. Exploring the effects of rural site conditions and household livelihood capitals on agricultural land transfers in China. Land Use Policy 108 , 105523 (2021).
Ning, L., Wang, F. & Li, J. Urban innovation, regional externalities of foreign direct investment and industrial agglomeration: Evidence from Chinese cities. Res. Policy 45 (4), 830–843 (2016).
Yang, Z., Song, T. & Chahine, T. Spatial representations and policy implications of industrial co-agglomerations, a case study of Beijing. Habitat Int. 55 , 32–45 (2016).
Henderson, J. V. Marshall’s scale economies. J. Urban Econ. 53 (1), 1–28 (2003).
Lin, H. L., Li, H. Y. & Yang, C. H. Agglomeration and productivity: Firm-level evidence from China’s textile industry. China Econ. Rev. 22 (3), 313–329 (2011).
Lu, C. et al. Mapping urban spatial structure based on poi (point of interest) data: A case study of the central city of Lanzhou, China. ISPRS Int. J. Geo-Inf. 9 (2), 92 (2020).
Yu, Z. & Liu, X. Urban agglomeration economies and their relationships to built environment and socio-demographic characteristics in Hong Kong. Habitat Int. 117 , 102417 (2021).
Tonne, C. et al. Defining pathways to healthy sustainable urban development. Environ. Int. 146 , 106236 (2021).
Article PubMed Google Scholar
Lang, W., Chen, T. & Li, X. A new style of urbanization in China: Transformation of urban rural communities. Habitat Int. 55 , 1–9 (2016).
Wang, L. & Xue, C. Spatio-temporal characteristics and influencing factors of urban floating population in China from 2011 to 2015. Chin. J. Popul. Resour. Environ. 17 (4), 359–373 (2019).
Article CAS Google Scholar
Chen, X. & Chang, C. P. Fiscal decentralization, environmental regulation, and pollution: a spatial investigation. Environ. Sci. Pollut. Res. 27 (25), 31946–31968 (2020).
Hou, M., Deng, Y. & Yao, S. Coordinated relationship between urbanization and grain production in China: Degree measurement, spatial differentiation and its factors detection. J. Clean. Prod. 331 , 129957 (2022).
Zhang, J., Zhang, K. & Zhao, F. Research on the regional spatial effects of green development and environmental governance in China based on a spatial autocorrelation model. Struct. Change Econ. Dyn. 55 , 1–11 (2020).
Article ADS Google Scholar
Bisello, A., Antoniucci, V. & Marella, G. Measuring the price premium of energy efficiency: A two-step analysis in the Italian housing market. Energy Build. 208 , 109670 (2020).
Liu, M. Y. et al. Spatial and temporal clustering analysis of tuberculosis in the mainland of China at the prefecture level, 2005–2015. Infect. Dis. Poverty 7 (1), 1–10 (2018).
Gao, W. et al. Impact of 3D modeling behavior patterns on the creativity of sustainable building design through process mining. Autom. Constr. 150 , 104804 (2023).
Li, Z., Hu, M. & Wang, Z. The space-time evolution and driving forces of county economic growth in China from 1998 to 2015. Growth Change 51 (3), 1203–1223 (2020).
Zhao, R., Zhan, L., Yao, M. & Yang, L. A geographically weighted regression model augmented by Geodetector analysis and principal component analysis for the spatial distribution of PM2.5. Sustain. Cities Soc. 56 , 102106 (2020).
Bi, H. & Ye, Z. Exploring ridesourcing trip patterns by fusing multi-source data: A big data approach. Sustain. Cities Soc. 64 , 102499 (2021).
Endri, E., Sari, A. K., Budiasih, Y., Yuliantini, T. & Kasmir, K. Determinants of profit growth in food and beverage companies in Indonesia. J. Asian Finance Econ. Bus. 7 (12), 739–748 (2020).
Manning, R. L. Is the insurance aspect of producer liability valued by consumers? Liability changes and childhood vaccine consumption. J. Risk Uncertain. 13 , 37–51 (1996).
Article MATH Google Scholar
Naji, H. A., Xue, Q., Zhu, H. & Li, T. Forecasting taxi demands using generative adversarial networks with multi-source data. Appl. Sci. 11 (20), 9675 (2021).
Ouyang, X., Xu, J., Li, J., Wei, X. & Li, Y. Land space optimization of urban-agriculture-ecological functions in the Changsha-Zhuzhou-Xiangtan Urban Agglomeration, China. Land Use Policy 117 , 106112 (2022).
Ren, Y., Tian, Y. & Zhang, C. Investigating the mechanisms among industrial agglomeration, environmental pollution and sustainable industrial efficiency: A case study in China. Environ. Dev. Sustain. 24 (11), 12467–12493 (2022).
Ma, F., Li, J., Ma, H. & Sun, Y. Evaluation of the regional financial efciency based on SBM-Shannon entropy model. Procedia Comput. Sci. 199 , 954–961 (2022).
Merabet, G. H. et al. Intelligent building control systems for thermal comfort and energy-efficiency: A systematic review of artificial intelligence-assisted techniques. Renew. Sustain. Energy Rev. 144 , 110969 (2021).
Yu, H. The Guangdong-Hong Kong-Macau greater bay area in the making: Development plan and challenges. Camb. Rev. Int. Aff. 34 (4), 481–509 (2021).
Wu, Y., Wei, Y. D., Li, H. & Liu, M. Amenity, firm agglomeration, and local creativity of producer services in Shanghai. Cities 120 , 103421 (2022).
Su, J., Yin, H. & Kong, F. Ecological networks in response to climate change and the human footprint in the Yangtze River Delta urban agglomeration, China. Landsc. Ecol. 36 , 2095–2112 (2021).
Escudero, V. & Mourelo, E. L. Understanding the Drivers of the Youth Labour Market in Kenya 203–228 (Springer, Berlin, 2014).
Xue, L. et al. Dynamic projection of ecological risk in the Manas River basin based on terrain gradients. Sci. Total Environ. 653 , 283–293 (2019).
Article ADS CAS PubMed Google Scholar
Ives, B., Cossick, K. & Adams, D. Amazon Go: Disrupting retail?. J. Inf. Technol. Teach. Cases 9 (1), 2–12 (2019).
Leavey, A., Zwaigenbaum, L., Heavner, K. & Burstyn, I. Gestational age at birth and risk of autism spectrum disorders in Alberta, Canada. J. Pediatr. 162 (2), 361–368 (2013).
Yang, J. et al. Understanding land surface temperature impact factors based on local climate zones. Sustain. Cities Soc. 69 , 102818 (2021).
Maryam, J., Banday, U. J. & Mittal, A. Trade intensity and revealed comparative advantage: An analysis of Intra-BRICS trade. Int. J. Emerg. Mark. 13 (5), 1182–1195 (2018).
Yu, H., Xie, W., Sun, L. & Wang, Y. Identifying the regional disparities of ecosystem services from a supply-demand perspective. Resour. Conserv. Recycl. 169 , 105557 (2021).
Du, Y., Deng, F. & Liao, F. A model framework for discovering the spatio-temporal usage patterns of public free-floating bike-sharing system. Transp. Res. Part C Emerg. Technol. 103 , 39–55 (2019).
Guo, S. & Ma, H. Does industrial agglomeration promote high-quality development of the Yellow River Basin in China? Empirical test from the moderating effect of environmental regulation. Growth Change 52 (4), 2040–2070 (2021).
Yang, R., Liu, Y., Long, H. & Qiao, L. Spatio-temporal characteristics of rural settlements and land use in the Bohai Rim of China. J. Geogr. Sci. 25 , 559–572 (2015).
Download references
This research was supported by Guangxi Natural Science Foundation Program (Grant No. 2020GXNSFAA297228). This study was funded by National Social Science Fund of China (No. 20&ZD157).
Author information
Authors and affiliations.
School of Ethnology and Sociology, Guangxi Minzu University, Nanning, 530006, Guangxi, China
School of Economics, Guangxi Minzu University, Nanning, 530006, Guangxi, China
You can also search for this author in PubMed Google Scholar
Contributions
Z.P.validation, resources, supervision, funding acquisition, conceptualization. Z.C.: Conceptualization, methodology, software, formal analysis, investigation, data collection, writing-original draft, preparation, writing-review and editing, visualization; all authors have read and agreed to the published version of the manuscript.
Corresponding author
Correspondence to Cheng Zong .
Ethics declarations
Competing interests.
The authors declare no competing interests.
Additional information
Publisher's note.
Springer Nature remains neutral with regard to jurisdictional claims in published maps and institutional affiliations.
Supplementary Information
Supplementary information 1., supplementary information 2., supplementary information 3., supplementary information 4., supplementary information 5., supplementary information 6., supplementary information 7., supplementary information 8., rights and permissions.
Open Access This article is licensed under a Creative Commons Attribution 4.0 International License, which permits use, sharing, adaptation, distribution and reproduction in any medium or format, as long as you give appropriate credit to the original author(s) and the source, provide a link to the Creative Commons licence, and indicate if changes were made. The images or other third party material in this article are included in the article's Creative Commons licence, unless indicated otherwise in a credit line to the material. If material is not included in the article's Creative Commons licence and your intended use is not permitted by statutory regulation or exceeds the permitted use, you will need to obtain permission directly from the copyright holder. To view a copy of this licence, visit http://creativecommons.org/licenses/by/4.0/ .
Reprints and permissions
About this article
Cite this article.
Zeng, P., Zong, C. Research on the relationship between population distribution pattern and urban industrial facility agglomeration in China. Sci Rep 13 , 16225 (2023). https://doi.org/10.1038/s41598-023-43376-4
Download citation
Received : 27 May 2023
Accepted : 22 September 2023
Published : 27 September 2023
DOI : https://doi.org/10.1038/s41598-023-43376-4
Share this article
Anyone you share the following link with will be able to read this content:
Sorry, a shareable link is not currently available for this article.
Provided by the Springer Nature SharedIt content-sharing initiative
This article is cited by
Exploring the impact of geographic factors on urban financial innovation in china: insights from the banking, insurance, and securities industries.
- Ziling Huang
- Xiaofeng Wang
Journal of the Knowledge Economy (2024)
By submitting a comment you agree to abide by our Terms and Community Guidelines . If you find something abusive or that does not comply with our terms or guidelines please flag it as inappropriate.
Quick links
- Explore articles by subject
- Guide to authors
- Editorial policies
Sign up for the Nature Briefing: AI and Robotics newsletter — what matters in AI and robotics research, free to your inbox weekly.

Win up to 100% Scholarship
- UPSC Online
- UPSC offline and Hybrid
- UPSC Optional Coaching
- UPPCS Online
- BPSC Online
- MPSC Online
- MPPSC Online
- WBPSC Online
- OPSC Online
- UPPCS Offline Coaching
- BPSC Offline Coaching
- UPSC Test Series
- State PSC Test Series
- DAILY CURRENT AFFAIRS
- SUBJECT WISE CURRENT AFFAIRS
- DAILY EDITORIAL ANALYSIS
- DAILY CURRENT AFFAIRS QUIZ
- Daily Prelims(MCQs) Practice
- Daily Mains Answer Writing
- Free Resources
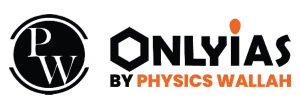
- Offline Centers
- NCERT Notes
- UDAAN Notes
- UPSC Syllabus
- UPSC Prelims PYQs
- UPSC Mains PYQs
- Prelims Preparation
NCERT NOTES
Elevate your upsc preparation with ncert notes – because every word matters on your journey to success..
- Indian Economy
- Physical Geography
- Indian Society
- Science & Tech
- Human Geography
- Art & Culture
.png)
Population Dynamics: Distribution, Density, & Growth In India

Population Dynamics – Distribution, Density, and Growth Population dynamics weave a tale far beyond mere numbers . They encapsulate a country’s socio-economic progression , significantly swayed by geographic intricacies. With India accommodating 17.5% of global inhabitants on a mere 2.4% of the world’s terrain, the dramatic interplay between geography and demography becomes evident.
This intricate study, spanning distribution, density, and growth, deciphers not just the current socio-economic state, but also potential trajectories. , human capital, resources, and characteristics in societal development.
- People make and utilize resources; people are resources themselves with varying qualities.
- Example, coal becomes a resource when technology is invented to obtain it.
Enroll now for UPSC Online Course
- It provides a point of reference for understanding other elements.
- ‘ Resources’, ‘calamities’, and ‘disasters’ derive significance in relation to human beings.
- Human beings are both producers and consumers of earth’s resources.
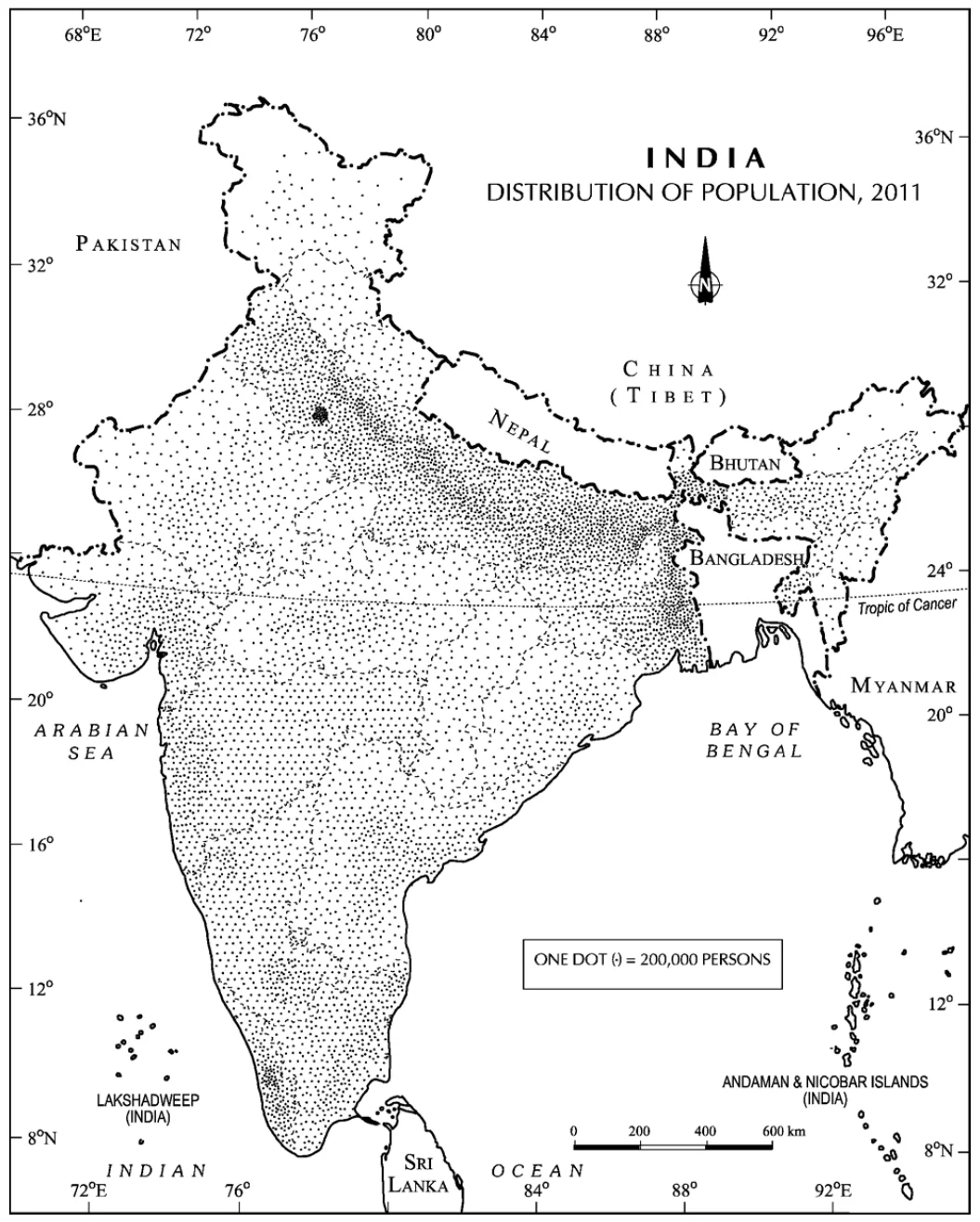
India-Distribution of Population
- The Census of India offers insights regarding the population of the country.
Population Dynamics in India: Demographic Landscapes, Global Shifts, and the Strain of Overpopulation
- India’s Demographic Landscape: India is the second most populous country after China with a population of 1,210 million (2011).
- According to the United Nations data, India has surpassed China to become world’s most populous nation with 142.86 crore people in 2023 )
- India’s population surpasses the combined population of North America, South America, and Australia.
- The Strain of Overpopulation: A large population puts pressure on limited resources and leads to socio-economic challenges.
- Initiatives in India, such as the ‘ Beti Bachao–Beti Padhao’ campaign , add unique threads to this demographic fabric .
- However, it’s pivotal to place these narratives against the global backdrop , highlighting universal shifts in population, density, development, and human capital strategy.
- From Metropolises to Countrysides: Exploring Population Dynamics Across bustling global metropolises to serene rural landscapes, the saga of human advancement and potential is ceaselessly unfolding.
India’s Population Dynamics: Size, Distribution, Density, Growth, and Composition
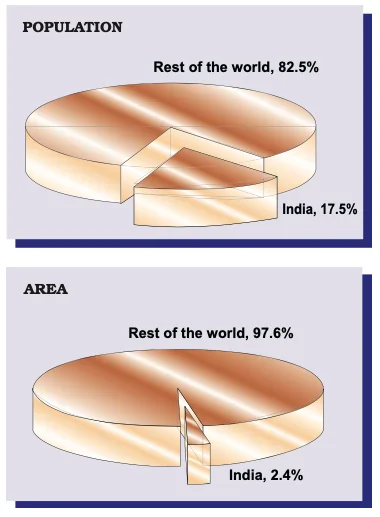
India’s Share of World’s Area and Population
India’s Population Dynamics by Numbers (as of March 2011, Refer to Figure):
- Total population of India is 1,210.6 million.
- India represents 17.5% of the world’s population.
- Population is distributed unevenly across 3.28 million square km (2.4% of the world’s area).
Global Population Dynamics: Patterns, Concentrations, and Disparities Across the Earth’s Landscape
- Population distribution refers to the pattern of how people are spread across the earth.
- South and South-east Asia, Europe, and north-eastern North America are categorized as crowded regions.
- Sparsely Populated Areas: Exploring Population Dynamics in high latitude regions, tropical deserts, high mountains, and equatorial forests.
- Roughly three-quarters of the global population resides in Asia and Africa.
- 60% of the world’s population is found in just 10 countries, all having over 100 million inhabitants. (Refer to Figure)
Enroll now for UPSC Online Classes
Geographical, Social, Cultural, and Economic Factors in Population Distribution
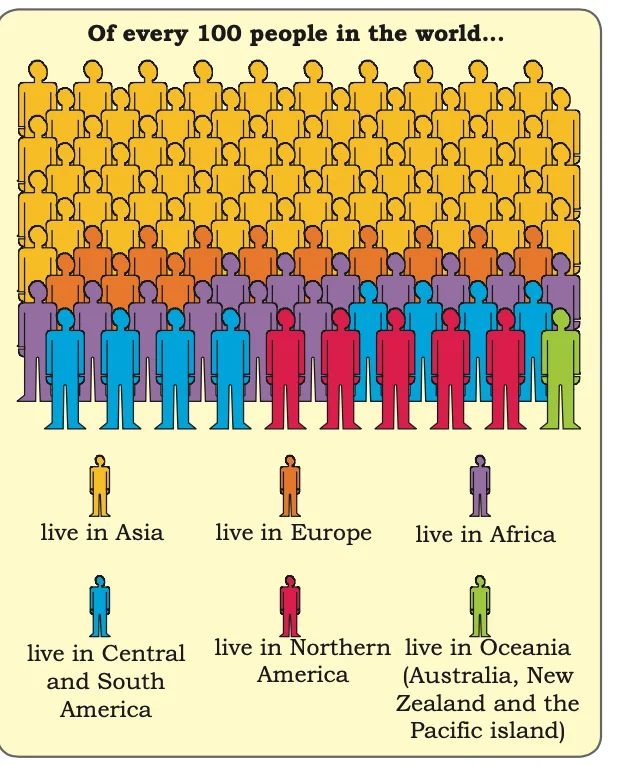
World Population by Continents
- Topography: Plains are preferred over mountains and plateaus due to suitability for various human activities.
- Climate: Extreme climatic regions like Sahara and polar regions are less populated.
- Soil: Fertile lands, such as the Ganga and Brahmaputra plains, attract higher populations.
- Water: Areas with abundant freshwater sources, like river valleys, are densely populated.
- Minerals: Mineral-rich regions like diamond mines in South Africa and oil reserves in the Middle East have higher population densities.
- Social: Areas with better amenities like housing, education, and health facilities have more people.
- Cultural: Cities with religious or cultural importance, like Varanasi and Vatican City, draw more population.
- Economic: Industrial regions, providing job opportunities, like Osaka and Mumbai, are densely populated.
State-Wise Population Dynamics in India
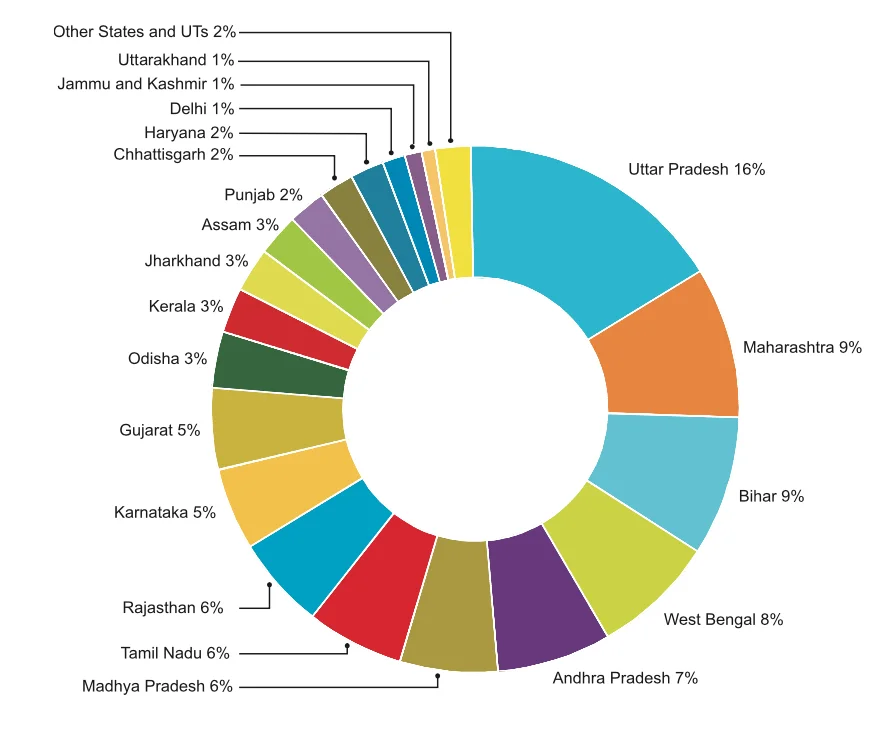
State-wise distribution of Population in india
India showcases a diverse and uneven distribution of its population , largely driven by a blend of physical, socio-economic, and historical factors.
- Uttar Pradesh, being the most populous state, accommodates about 16% of India’s total population, amounting to 199 million individuals.
- Conversely, sparsely populated areas like Sikkim and Lakshadweep have a population of 0.6 million and 64,429 , respectively.
- Surprisingly, vast states like Jammu & Kashmir, Arunachal Pradesh, and Uttarakhand house a minimal percentage of the population despite their expansive territories.
- Following Uttar Pradesh, Maharashtra, Bihar, and West Bengal have significant population counts.
- Ten states , which include U.P., Maharashtra, Bihar, West Bengal, Andhra Pradesh, Tamil Nadu, Madhya Pradesh, Rajasthan, Karnataka, and Gujarat, encompass approximately 76% of the nation’s inhabitants.
- Half of the nation’s populace resides within five pivotal states : Uttar Pradesh, Maharashtra, Bihar, West Bengal, and Andhra Pradesh.
- Rajasthan, India’s largest state in terms of land area , comprises 5.5% of the total national population.
- Physical Factors: Predominantly regions with favorable climates , accessible terrain, and adequate water sources, such as the North Indian Plains, coastal plains, and deltas, are densely populated.
- Metropolises like Delhi, Mumbai, and Kolkata have burgeoned due to urbanization and industrial growth.
- Major cities like Delhi, Mumbai, and Kolkata have burgeoned due to reasons of industrialization and urbanization, acting as magnets drawing individuals in search of opportunities.
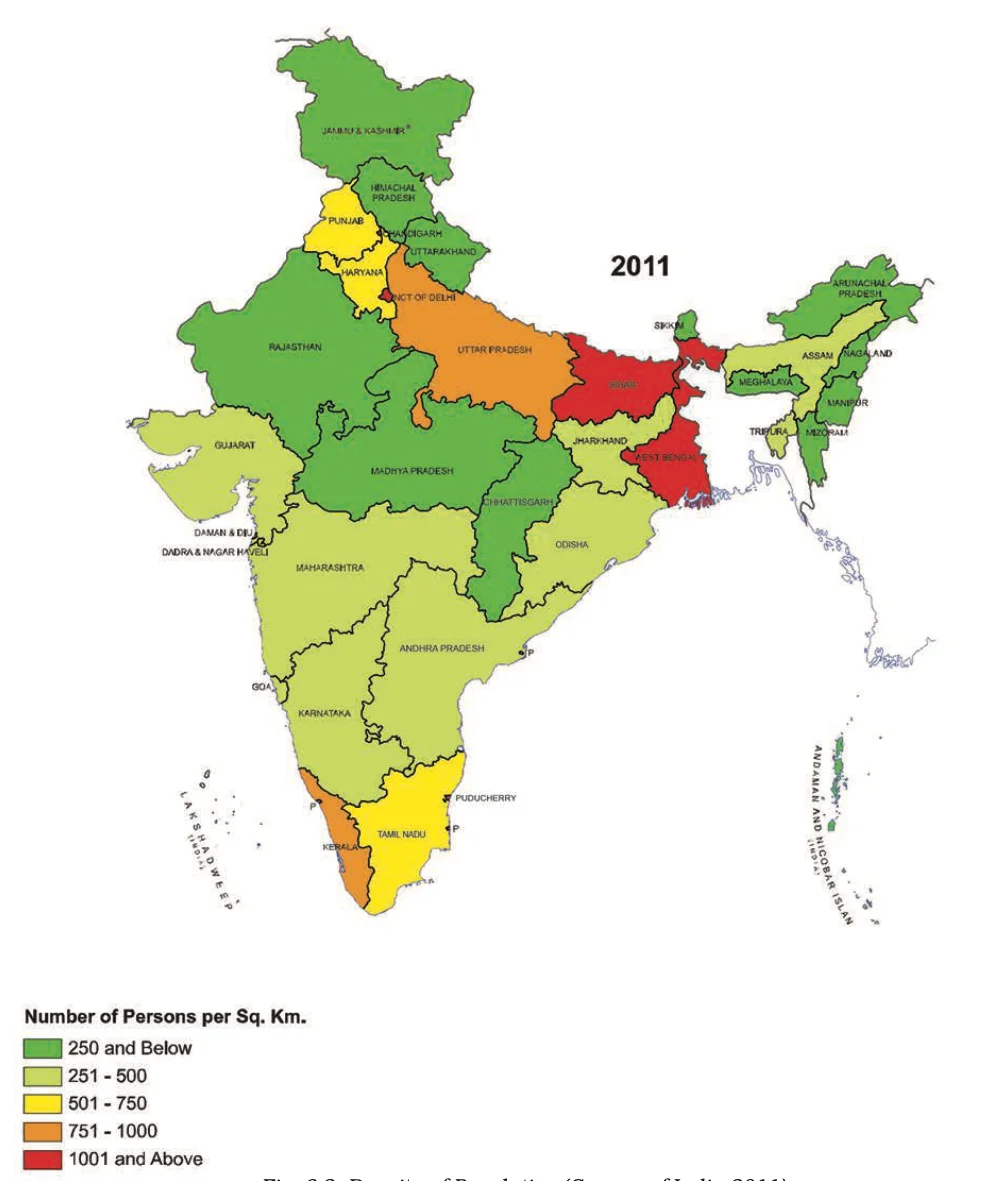
Density of Population (Census of India 2011)
Significance of Density Metrics in Understanding Population Pressure on Cultivable Land
India’s Population Dynamics: Standing as one of the world’s most densely populated countries, with only Bangladesh and Japan having a higher average population density.
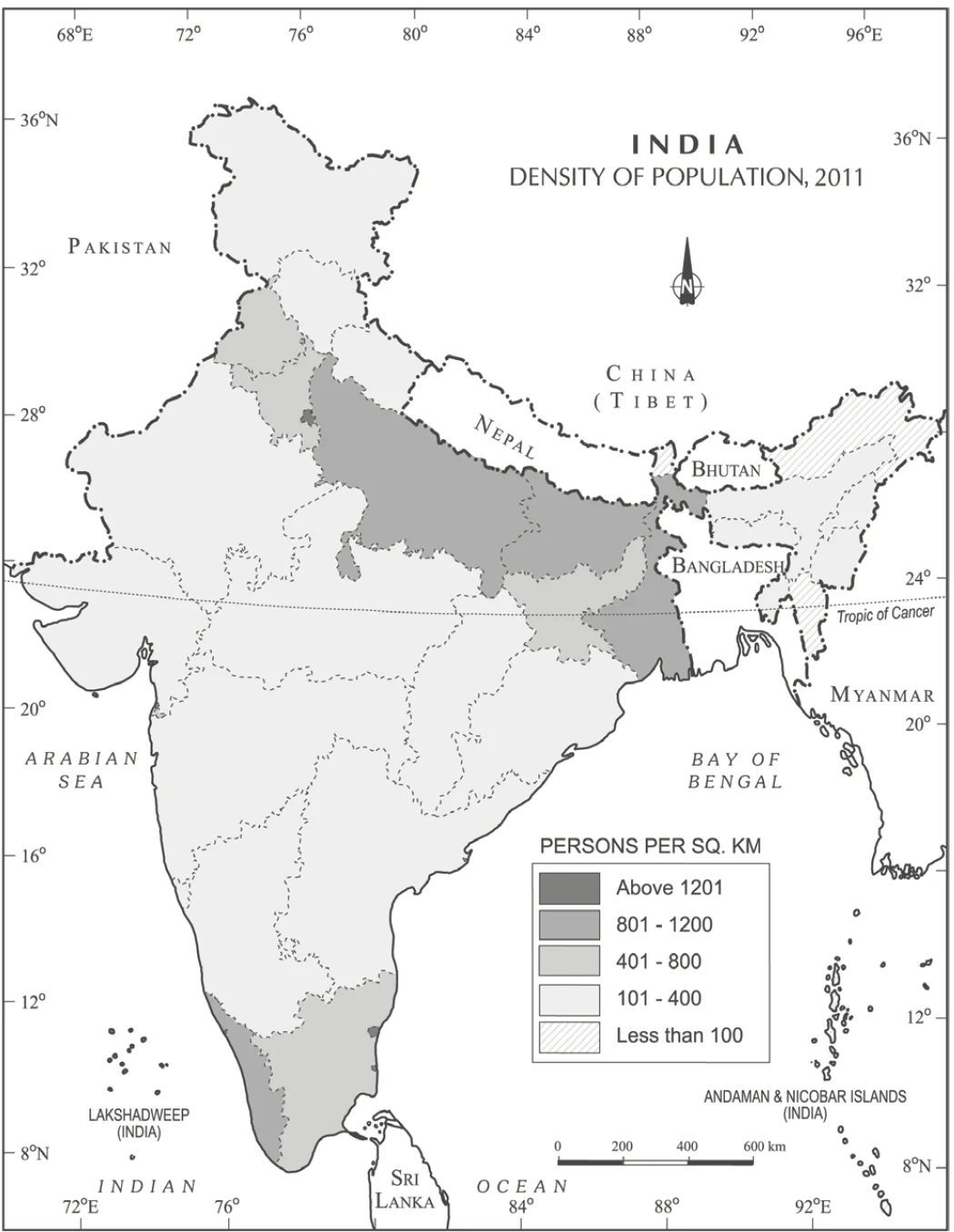
India- Density of Population
- Statistics & Trends:
- As of 2011, the overall population density of India reached 382 persons per sq km, showing a significant rise from 117 persons per sq km in 1951.
- Variations Across States:
- Bihar boasts the highest density , with 1,102 persons/sq km.
- In contrast, Arunachal Pradesh registers the lowest with just 17 persons/sq km.
- Other noteworthy states in terms of density include West Bengal with 1,029 persons/sq km, Uttar Pradesh with 828 , Kerala with 859, and Tamil Nadu with 555.
- The National Capital Territory of Delhi stands out with a staggering density of 11,297 persons/sq km.
- Moderation in density is seen in states like Assam and Gujarat , while Himalayan and North-eastern states (barring Assam) record low figures .
- Additionally, most Union Territories exhibit very high population densities.
- Understanding Density Metrics:
- The physiological Density: It is computed by dividing the total population by the net cultivated area.
- The Agricultural Density: It is determined by dividing the agricultural population by the net cultivable area, where the agricultural population encompasses cultivators, agricultural laborers, and their families.
- Importance of Density Metrics: These densities, especially the physiological and agricultural ones , are pivotal as they provide insights into the population pressure exerted on the available cultivable land.
Factors Shaping Low, Moderate, and High Densities in Different Regions and States
Table: Factors Influencing Population Density
Conclusion The population dynamics significantly influence the patterns of density across regions. Factors such as terrain, climate, and soil fertility play a crucial role. Recognizing these dynamics is essential for informed policy-making and sustainable development in diverse geographical contexts.
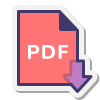
UPDATED :
Recommended For You
Latest comments, the most learning platform.
Learn From India's Best Faculty
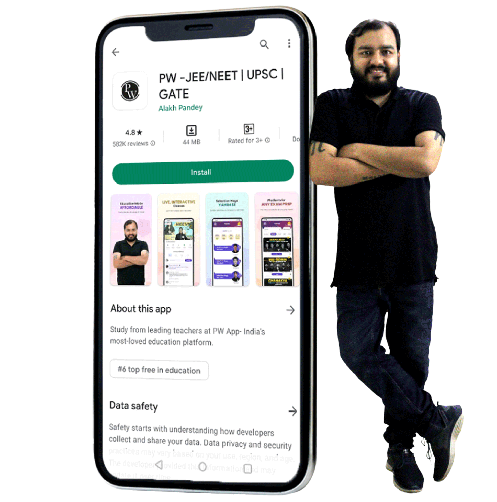
Our Courses
Our initiatives, beginner’s roadmap, quick links.
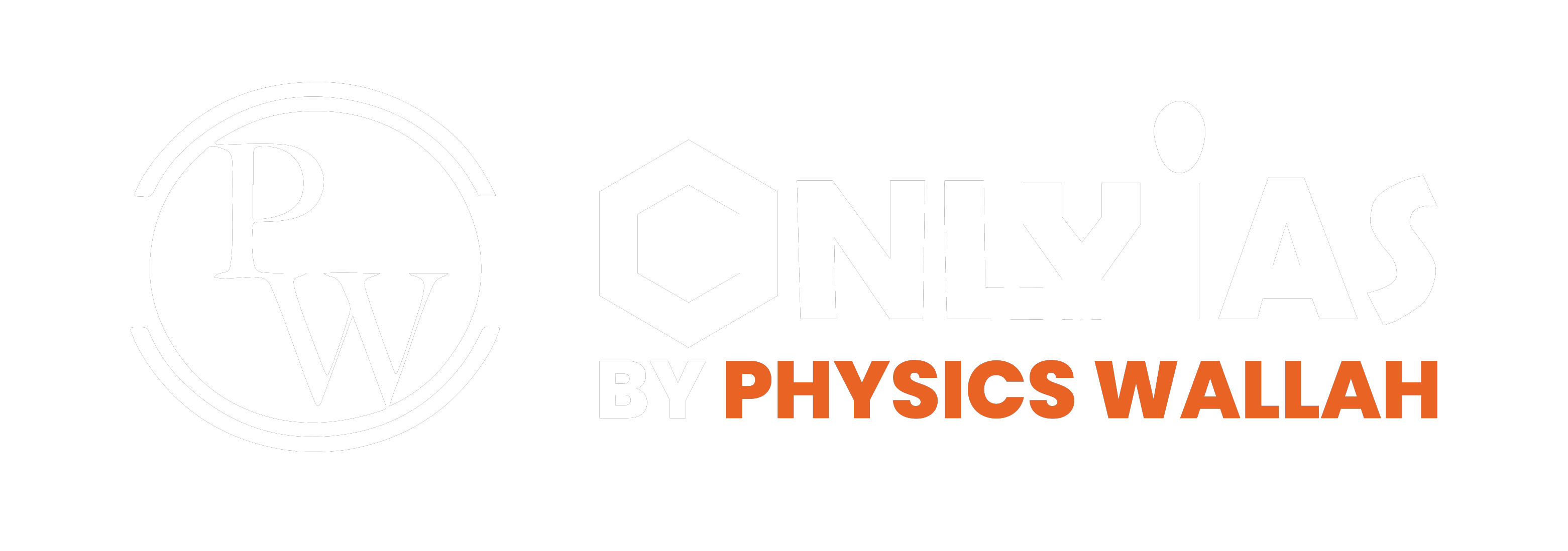
PW-Only IAS came together specifically to carry their individual visions in a mission mode. Infusing affordability with quality and building a team where maximum members represent their experiences of Mains and Interview Stage and hence, their reliability to better understand and solve student issues.
Subscribe our Newsletter
Sign up now for our exclusive newsletter and be the first to know about our latest Initiatives, Quality Content, and much more.
Contact Details
G-Floor,4-B Pusha Road, New Delhi, 110060
- +91 9920613613
- [email protected]
Download Our App
Biginner's roadmap, suscribe now form, fill the required details to get early access of quality content..
Join Us Now
(Promise! We Will Not Spam You.)
CURRENT AF.
<div class="new-fform">
Select centre Online Mode Hybrid Mode PWonlyIAS Delhi (ORN) PWonlyIAS Delhi (MN) PWonlyIAS Lucknow PWonlyIAS Patna Other
Select course UPSC Online PSC ONline UPSC + PSC ONLINE UPSC Offline PSC Offline UPSC+PSC Offline UPSC Hybrid PSC Hybrid UPSC+PSC Hybrid Other
</div>
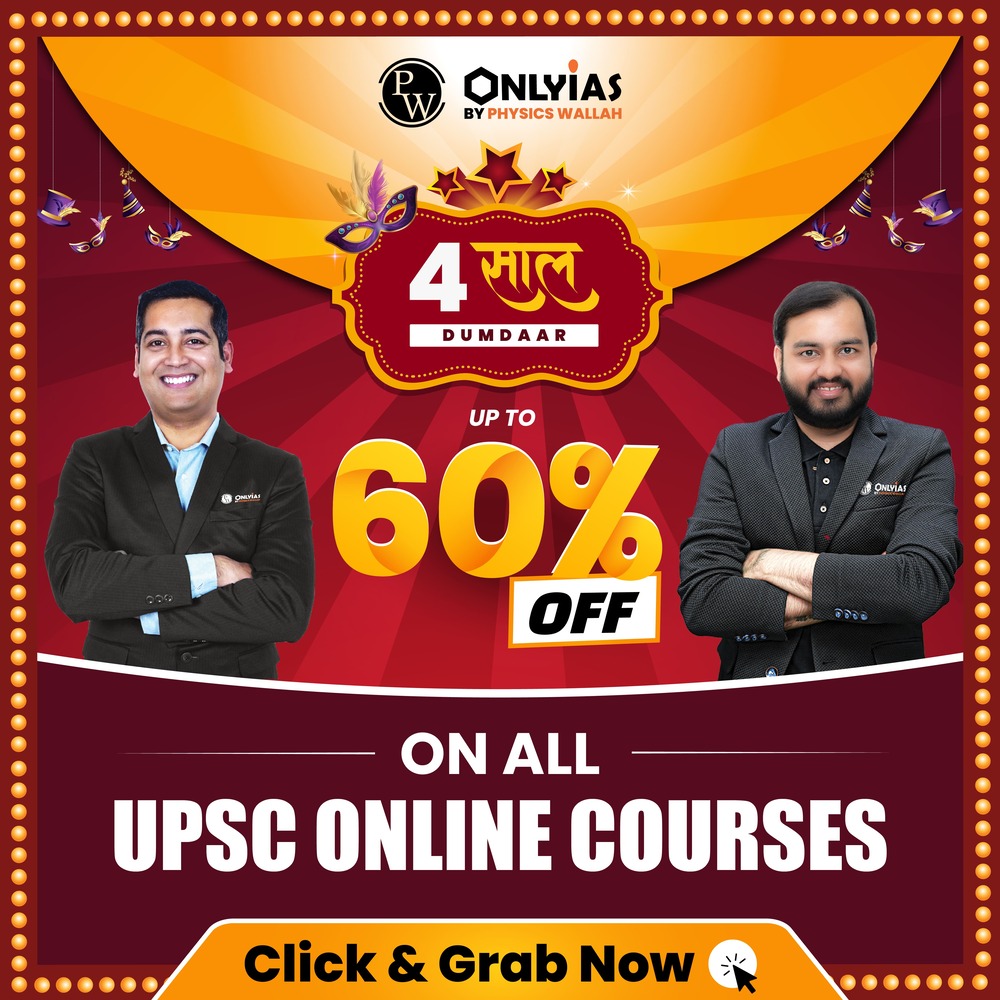

- OUR CENTERS Bangalore Delhi Lucknow Mysuru Srinagar Dharwad Hyderabad
Call us @ 08069405205

Search Here

- An Introduction to the CSE Exam
- Personality Test
- Annual Calendar by UPSC-2024
- Common Myths about the Exam
- About Insights IAS
- Our Mission, Vision & Values
- Director's Desk
- Meet Our Team
- Our Branches
- Careers at Insights IAS
- Daily Current Affairs+PIB Summary
- Insights into Editorials
- Insta Revision Modules for Prelims
- Current Affairs Quiz
- Static Quiz
- Current Affairs RTM
- Insta-DART(CSAT)
- Insta 75 Days Revision Tests for Prelims 2024
- Secure (Mains Answer writing)
- Secure Synopsis
- Ethics Case Studies
- Insta Ethics
- Weekly Essay Challenge
- Insta Revision Modules-Mains
- Insta 75 Days Revision Tests for Mains
- Secure (Archive)
- Anthropology
- Law Optional
- Kannada Literature
- Public Administration
- English Literature
- Medical Science
- Mathematics
- Commerce & Accountancy
- Monthly Magazine: CURRENT AFFAIRS 30
- Content for Mains Enrichment (CME)
- InstaMaps: Important Places in News
- Weekly CA Magazine
- The PRIME Magazine
- Insta Revision Modules-Prelims
- Insta-DART(CSAT) Quiz
- Insta 75 days Revision Tests for Prelims 2022
- Insights SECURE(Mains Answer Writing)
- Interview Transcripts
- Previous Years' Question Papers-Prelims
- Answer Keys for Prelims PYQs
- Solve Prelims PYQs
- Previous Years' Question Papers-Mains
- UPSC CSE Syllabus
- Toppers from Insights IAS
- Testimonials
- Felicitation
- UPSC Results
- Indian Heritage & Culture
- Ancient Indian History
- Medieval Indian History
- Modern Indian History
- World History
- World Geography
- Indian Geography
- Indian Society
- Social Justice
- International Relations
- Agriculture
- Environment & Ecology
- Disaster Management
- Science & Technology
- Security Issues
- Ethics, Integrity and Aptitude

- Indian Heritage & Culture
- Enivornment & Ecology

- How to Study Art & Culture?
- What is Art and Culture? What is the difference between the two?
- Indus Civilization
- Evolution of rock-cut architecture in India
- Important rock-cut caves
- The contribution of Pallavas to Rock-cut architecture
- Comparision of art form found at Ellora and Mahabalipuram
- Buddhist Architecture
- Early Temples in India
- Basic form of Hindu temple
- Dravida style of temple architecture
- Nagara Style or North India Temple style
- Vesara style of temple architecture
- Characteristic features of Indo-Islamic form of architecture
- Styles of Islamic architecture in the Indian subcontinent
- Types of buildings in Islamic architecture in the Indian subcontinent
- Evolution of this form of architecture during the medieval period
- Modern Architecture
- Post-Independence architecture
- Indus Civilization Sculpture
- Bharhut Sculptures
- Sanchi Sculptures
- Gandhara School of Sculpture
- Mathura School of Sculpture
- Amaravati School of Sculpture
- Gupta Sculpture
- Medieval School of Sculpture
- Modern Indian Sculpture
- Pre Historic Painting
- Mural Paintings & Cave Paintings
- Pala School
- Mughal Paintings
- Bundi School of Painting
- Malwa School
- Mewar School
- Basohli School
- Kangra School
- Decanni School of Painting
- Madhubani Paintings or Mithila paintings
- Pattachitra
- Kalighat Painting
- Modern Indian Paintings
- Personalities Associated to Paintings
- Christianity
- Zoroastrianism
- Six Schools of Philosophy
- Lokayata / Charvaka
- Hindustani Music
- Carnatic Music
- Folk Music Tradition
- Modern Music
- Personalities associated with Music
- Bharatanatyam
- Mohiniattam
- Folk Dances
- Modern Dance in India
- Sanskrit Theatre
- Folk Theatre
- Modern Theatre
- Personalities associated with Theatre
- History of Puppetry
- String Puppetry
- Shadow Puppetry
- Rod Puppetry
- Glove Puppetry
- Indian Cinema and Circus
- Shankaracharya
- Ramanujacharya (1017-1137AD)
- Madhvacharya
- Vallabhacharya
- Kabir (1440-1510 AD)
- Guru Nanak (1469-1538 AD)
- Chaitanya Mahaprabhu
- Shankar Dev
- Purandaradasa
- Samard Ramdas
- Classical Languages
- Scheduled Languages
- Literature in Ancient India
- Buddhist and Jain Literature
- Tamil (Sangam) Literature
- Malayalam Literature
- Telugu Literature
- Medieval Literature
- Modern Literature
- Important characteristics of Fairs and Festivals of India
- Some of the major festivals that are celebrated in India
- Art & Crafts
- Ancient Science & Technology
- Medieval Science & Technology
- Famous Personalities in Science & Technology
- Tangible Cultural Heritage
- Intangible Cultural Heritage
- Cultural Heritage Sites
- Natural Heritage Sites
- Important Institutions
- Important programmes related to promotion and preservation of Indian heritage
- Ochre Colored Pottery (OCP)
- Black and Red Ware (BRW)
- Painted Grey-Ware (PGW)
- Northern Black Polished Ware (NBPW)
- Origin of Martial arts in India
- Various forms of Martial arts in India
- Northern Mountains
- Peninsular Plateau
- Islands of India
- Himalayan Drainage System
- Peninsular Drainage System
- Other Small River System
- Factors Determining Climate of India
- Spatial and temporal distribution of temperature & pressure
- Variability
- Rainfall distribution
- Climatic Regions of India
- Classification of Soils
- Problems of Soil
- Soil Conservation
- Forests and their distribution
- Forest Conservation
- Agriculture typology
- Major crops grown in India
- Agro-Climatic Regions
- Land Reforms and Land use pattern
- Animal Husbandry, Fisheries and Aquaculture
- Availability and potential
- Lakes, rivers, dams
- Power Projects and irrigation projects
- Interlinking of rivers
- Classification
- Distribution
- Conventional
- WIND ENERGY
- Population and Growth trends – density, sex ratio, literacy, tribes and racial groups in India
- Rural and Urban Settlements
- INDUSTRIES – TYPES
- Locational factors
- Water transport
- Shipping and sea-ports
- Air transport
- Ramsar sites
- National Parks
Home » Indian Geography » Indian Economic and Human Geography » Population and Growth trends – density, sex ratio, literacy, tribes and racial groups in India
Population and Growth trends – density, sex ratio, literacy, tribes and racial groups in India
Introduction
- A population is a distinct group of individuals, whether that group comprises a nation or a group of people with a common characteristic
- India covers only 2.4% of the land area of the world, but it is home to about 17.5% of the world population
- After 2030 , India is expected to be the most populous country in the world
- The below diagrammatic representation gives an idea of India’s dominant position, among the ten most populous countries of the world:

- A census count offers a spectrum of population at a particular point in time, covering a wide range of demographic, social and economic attributes of population
- The first complete and synchronous census covering the entire country and providing vital demographic data was conducted in 1881
- Since then, census has been conducted regularly after every 10 years
- The 2011 census represents the fifteenth census of India, as reckoned from 1872 and seventh after Independence
Growth of Population: Basic concepts
- This is the net change in population between two points of time, and is expressed in percentage
- The difference between the natural birth-rate and death-rate is called the natural growth of population
- This growth of population is caused by migration of people
- When birth rates are higher than death rates, or when people migrate in, it results in positive growth
- This happens, when birth rates are lower than death rates; and when people migrate out
Population growth in India
- These significant turning points are the census years of 1921, 1951 and 1981
- During most of the 19th century, India witnessed sporadic, irregular and slow growth of population which drifted to 20th century until 1921
- Thus, the population growth during this period can be termed as stagnant , when compared to the growth rates during consequent periods
- The high mortality during this period was the result of large scale deaths due to epidemics of influenza, plague, small pox, cholera, etc.
- In addition, food shortages, loss of Indian lives during first World War, emigration of people to Africa contributed to lesser population growth rate as well
- In fact, the census of 1921 recorded a negative growth rate of -0.31%, which happened only once throughout the demographic history of India
- It is because of this decline in population, that the year 1921 is called the ‘Demographic Divide’ in the demographic history of India
- Thus, this period is called the period of steady growth rate
- The decline of death rates during this time, can be attributed to the distribution system as well, where the improved transportation delivered timely supply of food, to drought and famine stricken areas
- Hence, population growth during this period is called the mortality induced growth
- After 1951. there was a steep fall in the mortality rate, but the fertility remained stubbornly high
- Therefore, this period experienced very high rate of population growth and is often referred to as the Period of Population explosion
- The living conditions of people improved enormously, and death rates declined
- Thus, this was fertility induced growth during this period
- Although the rate of growth was still very high, it started declining after 1981
- This declining trend marks the beginning of the new era in the country’s demographic history
- The declining trend of birth rates during this period, were due to the official efforts of the state in birth control and people’s own inclination for smaller families
- Although India’s population growth rate continues to decline since 1971( the year recorded highest ever growth rate of 2.48%/annum), yet India’s population growth rate is much higher as compared to that of China, USA, Brazil etc.

Indian Population growth in tune with classical theory of Demographic transition
- During most of the 19th century, India witnessed a fluctuating but stagnant growth of population, which drifted into the 20th century until 1921
- Thereafter, the country passed through all phases of demographic transition and is now widely believed to have entered the final phase, which is characterized by declining fertility
- However, the UN Department of Economics and Social Affairs has estimated that India’s population will continue to increase till 2050, after which it will start decreasing by the end of 21st century
Spatio-Temporal Variations in Population growth
- Hence the need to assess the spatio-temporal variations in population growth
- The phenomenon of low growth has spread beyond the boundaries of the southern states during 2001-11, where in addition to Andhra Pradesh, Tamilnadu and Karnataka in the south, Himachal Pradesh and Punjab in the north, West Bengal and Odisha in the east and Maharashtra in the west have registered growth rate between 11-16% in 2001-11
- Among smaller states and Union territories , Dadar and Nagar Haveli, Daman & Diu registered the highest growth rate of 55.5% and 53.54% respectively, between 2001-11
- A glaring down trend in the growth rate has been observed in Nagaland , where there has been a steep fall in growth rate from 64.53% in 1991-2001, to negative growth rate of -0.47% in 2011 census
- This state has reached high level of demographic transition and can be compare to the advanced countries of Europe and America
- Other small states with higher growth rate are Meghalaya (27%) and Arunachal Pradesh(25%)

Growth of Child Population in Inida
- Child population in the age group of 0-6 years has special significance in our demographic scene, because this segment of population determines the future course of trends in population growth when it reaches the reproductive age
- According to the 2011 census figures, the total number of children in the age-group 0-6 years is 158.8 million
- Five states namely Uttar Pradesh, Bihar, Maharashtra, Madhya Pradesh and Rajasthan have the largest number of children constituting 52% of India’s child population
CENSUS 2021
- The Census of 2021 in India, will be a digital one
- It will be used to collect data by school teachers who will double up as enumerators during the Census exercise
- The ministry also said that a Census portal has been developed
- This is going to be 16th Census as reckoned from 1872 and eighth after Independence
- The individual will fill in the required details with the help of relevant codes for each field. After self-enumeration is done, an identification number will be sent on the registered number provided by the individual
- The same ID number can be shared with the enumerator, which will help the official to sync the data automatically
- However, The field activities related to thecensus 2021 exercise in the country have been postponed due to the COVID-19 pandemic
Density of Population
- It is expressed as the number of people per unit area
- According to the census of 2011 ,the population density of India is 382 people/square km
- The main cause of worry is that the Indian population density has been consistently increasing since 1921
State level Patterns in India
For the sake of convenience, the spatial distribution of population density is classified into following categories
- Areas having 100 person/sq.km and less, are included in this category
- The states and UT under this category, along with the factors are as below:
- Areas having population density of 101-250 persons per sq.km are included in this class
- This class includes those areas having 251-500 people/sq.km
- These are areas having population density of 501-1000 people/sq.km
- Areas having more than 1000 persons per sq.km fall under this category
- Other UT in this category are Lakshadweep(2013), Daman & Diu(2169), Puducherry(2548), Chandigarh(9592)
The Map indicating the varying population density in India

- One of the most important aspects of India’s population is its uneven distribution
- While on the other hand, there are virtually demographic deserts in high mountains, arid lands, thickly forested areas and in some remote corners of the country
Major factors influencing the distribution and density of population
- This is a potent factor which influences the concentration and growth of population
- It is because of this reason, that Himalayas which occupy 13% of India’s land area, support only 1-2% of country’s population
- Also, the great plain of North India which cover less than one-fourth of country’s land area, is home to more than half of India’s population
- The twin elements of rainfall and temperature , play an important role in determining the population of an area
- Extremes of climate aren’t favorable, while a moderate climate favors population concentration
- As we move from Ganga-Brahmaputra delta in the east, to the Thar desert in the west, the amount of rainfall and density of population decreases
- An exception to this, is the north-western region of India comprising of Punjab, Haryana and Western Uttar Pradesh, where high population concentration is evident because of the developed irrigation facilities
- Since India is a tropical country , temperature is fairly high and does not play as important role as is done by rainfall except in extreme areas
- This factor plays an important role in an overwhelmingly agricultural country like India
- Similarly, the coastal plains with fertile soils have high population density
- On other hand, deserts, mountains with infertile soil have lesser densities
- Water is a basic necessity for irrigation, industries, transport and domestic use
- And rivers are a greatest source of fresh water
- Hence, most population is concentrated in river valleys
- The higher population densities in the Chhota Nagpur plateau region, and adjoining regions of Odisha are largely due to availability of minerals
- Industrial growth offers massive employment opportunities and acts as a great magnet to attract people, resulting in higher densities
- Major causes of high density in West Bengal, Bihar, Jharkhand, Odisha, Maharashtra and Gujarat is the phenomenal growth of Industries in these states
- The northern plains of India has a dense network of transport routes and hence is a densely populated region
- The peninsular plateau has moderate network of transport routes, and is moderately populated area
- The Himalayan region badly lacks transport facilities, and is sparsely populated
- All urban areas are marked by high density of population, as evident in cities of Bangalore, Chennai, Mumbai and Delhi
Distribution of Population in India
- These five states account for about half of the country’s population
- According to the 2011 Census
- Sikkim has the smallest population among all the Indian states
- Delhi with 16.75 million has the largest population among all the Union territories
- The complete scenario of population distribution in Indian states is as indicated below:

- The Population Commission of United Nations considers the ability, to both read and write a simple message with understanding in any language, a sufficient basis for classifying a person as Literate
- It was decided at the 1991 Census that all Children in the age group 0-6, would be treated as illiterate by definition and the population aged seven years and above only would be classified as literate or illiterate
- It should be noted clearly that, it is not necessary that to be treated as literate, a person should have received any formal education or acquired any minimum educational standard
- Crude Literacy rate = ((No of literate persons)/Total population)*100
- Effective literacy rate = ((Number of Literate persons aged 7 and above)/Population aged 7 and above)*100
- Here, Effective literacy rate and literacy rate will be used interchangeably
Literacy rate-Trends
- The corresponding figures for male and female are 82.14% and 65.46% respectively
- Overall improvement – 9.21%
- Improvement of literacy rate in male – 6.88%
- Improvement of literacy rate in female – 11.79%
- Literacy rate in urban areas was higher 87.7% than rural areas with 73.5%, according to 2011 Census

Literates and Illiterates by Gender
- One of the interesting feature of Census 2011 is that out of total literates added during the decade, females out number males
- The decadal(from 2001-2011) increase in number of literates among males is 31.98%; while the corresponding increase in case of females is of 49.1%
- The above two changes are a clear indication of the fact that gender gap in literacy is shrinking in the country
- Bihar(73.39%) state has recorded the lowest male literacy rate
- Rajasthan(52.66%) state has recorded the lowest female literacy rate
Regional Variations in Literacy Rates
- Kerala ranks first in the country with a literacy rate of 93.91%, closely followed by Lakshadweep (92.28%) and Mizoram(91.58%)
- Bihar with a literacy rate of 63.82% ranks last in the country, preceded by Arunachal Pradesh (66.95%) and Rajasthan(67.06%)
- The gap in literacy rates of males and females is lowest in Meghalaya (3.1 percentage points) and less than 5 percentage points in the States of Kerala and Mizoram and between 5 to 10 percentage points in A&N Island, Chandigarh, Goa, Lakshadweep Nagaland, Punjab and Tripura
- The gap in literacy rates of males and females is highest in the State of Rajasthan (27.1 percentage points) and much more in the States of Chhattisgarh, Dadra & Nagar Haveli, Jammu & Kashmir, Jharkhand and Uttar Pradesh

Measures taken in India towards increasing Literacy rate
- Education of Minorities: Article 30 of the Indian Constitution gives all minorities the right to establish and administer institutions of their own choice
- Free and Compulsory Education: The Constitution of India (u/a 41, 45 and 46 of the Directive Principles of State Policy) instructs the state to ensure that all citizens receive free education
- The program was launched in 2001, and it aims to universalise education and improves its quality by time-bound implementation strategy and context-specific planning. It includes children from all social classes
- This plan was launched in 1995 to provide mid-day meals to children studying in primary class. The main objective of creating this scheme was to eliminate classroom hunger of children and to increase attendance and enrolment of children at schools
- The Right to Education (RTE) Act was enacted in 2009, and this Act made education for every child between 6 and 14 years a fundamental right
- The National Education Policy 2020, aims to achieve 100% youth and adult literacy.
Efforts needed to take India’s Literacy rate to greater heights
- We should focus on revamping curriculum and pedagogy to bring modern and innovative elements within it and making it a lot more rigorous.
- Create a national discourse and imperative around the importance of good quality school leadership. This will help in improving and maintaining school quality , nurturing a learning culture within schools, maintaining teacher motivation, ensuring respect for and involvement of all stakeholders
- Work on expanding the idea of good education. There is need to extend it beyond rote learning of concepts. It should largely focus on cognitive development to a belief that values the uniqueness of a child and the celebration of different definitions of ‘intelligence’.
- Extend the scope. With the Right to Education (RTE) Act now making primary education compulsory, there is need look at extending its scope to include pre-primary education (which is not there in all states).
- Our present day population is a conglomeration of people belonging to different racial groups with different backgrounds
- These people entered India from different parts of the world, at different points in time, adopting various land and water routes
- Almost all the major races of the world are visible in India, as a result of which the country is said to have a varied and diverse ethnic composition
Racial groups, from which the present day population of the country has been derived
- Also, they have expressed their view that Negroid people migrated to India from Africa and established their language on the soil of India
- The tribes like the Angami Nagas in the North-East, and Badgis in Rajmahal Hills in Jharkhand possess their traits
- The race is characterized by short stature, dark chocolate brown skin, woolly hair, bulbous forehead, broad flat nose and slightly protruding jaws
- These are believed to have come to India from the East Mediterranean area(Palestine), soon after the Negritos
- Presently, they constitute the bulk of the population in many isolated parts of central and southern India
- The Bhils, Kols, Badagas, Korwas, Mundas, Bhumjis of the highlands of the Central India and the Chenchus, Kurumbas, Malayans and Yeruvas of South India may all be treated as their representatives as well
- According to some Anthropologists, these people on their arrival pushed, displaced and supplanted the Negritos to shift to more inaccessible, remote and less hospitable areas, where they are found even today
- Their other physical characteristics are bulbous forehead, broad flat nose and slightly protruding jaws
- It is believed that China is the homeland of the Mongoloid race, from where they were pushed southward into the Malaya Peninsula and Indonesia
- They entered India through the passes in the northern and eastern mountains
- Presently, they occupy large areas in Ladakh, Sikkim, Arunachal Pradesh and other parts of East India
- Their physical characteristics include a round and broad head, face with very high cheek bones and a long flat nose, with little or no hair on the face and the body
- The tribes of Garo, Khasi, Jaintia, Lipchas, Chakmas, Naga belong to this race
- Paleo-Mongoloids – They were the first of the Mongoloids who came to India. These people are settled mainly in the border areas of the Himalayas. They are found mostly in Assam and the adjacent states
- Tibeto-Mongoloids – These people came from Tibet and are settled mainly in Bhutan, Sikkim, areas of north-western Himalayas and beyond the Himalayas in which Ladakh and Baltistan are included.
- This racial stock came to India from eastern Mediterranean region or South West Asia
- They are believed to have migrated during the third and second millennium BC
- Their physical characteristics include medium stature, dark skin and long head
- In all probability, they first settled in North-western India and started practicing agriculture there; post which they were pushed into central and southern India by subsequent immigrants
- Presently, they form the bulk of population of south India and a considerable proportion in northern India
- The Mediterranean were the chief architect of the Indus Valley civilization as is evident from the excavations of Mohenjo Daro and Harappa
- These are characterized by Broad heads
- Coorgis and Parsis are their representatives in India
- Alpinoids , who came to India along the route passing through Baluchistan, Sind, Kathiawar, Gujarat, Maharashtra,, Karnataka and Tamilnadu
- Dinarics , who followed the Ganga Valley and its delta as their route to enter India
- Armenoids , who came through Chitral, Gilgit, Kashmir and Nepal to enter India
- They spoke the Aryan language and migrated to India sometime during the second millennium BC
- They are a predominant type in Punjab, Haryana and Rajasthan
- The main characteristics of this race are long head, fair complexion, well developed nose and a well-built strong body

INTRODUCTION
- It is an important social indicator to measure the extent of prevailing equity between males and females at a given point in time
- It also becomes important for various types of planning and for the analysis of other demographic characteristics such as mortality, migration, marital status, economic characteristics, etc.
- SEX RATIO is defined as ”number of females per 1000 males in the population”
- Thus, a sex ratio of 1000 implies complete parity between the two sexes
- Ratios above 1000 indicate excess of females over males; those below 1000 indicate a deficit of females
- Differences in mortality rates and life expectancy for women and men. Women, on average, live longer than men. This means that all else being equal, we would expect females to account for slightly more than half of the total population.
- In the absence of selective abortion practices, births in a given population are typically male-biased – the chances of having a boy are very slightly higher than having a girl.
- Migration can also affect the sex ratio of the population. If in some countries there is a significant amount of imported male-dominant labour, all else being equal, we would expect males to account for more than half of the total population
SEX-RATIO IN INDIA
- According to figures of 2011 Census, out of 1210.1 million population, 623.7 million are male and 586.4 million are females
- This suggests that the number of females is quite less as compared to males
- In other words, the sex ratio in the country had always remained unfavorable to females
- The sex-ratio was 933 in the previous census 2001.
- This is a worldwide phenomenon and India is no exception to this
- According to the findings of the Census of India, the imbalance in the number of males and females starts in the beginning
- It is now a well-established law of nature, that the males exceed females at the time of birth
- Many demographers believe that left to its own, this is an unalterable constant
- The preference for male child, leads to sex determination tests and the resultant termination of pregnancy in case fetus happens to be a female
- The pre-conception and pre-natal diagnostic Technique(Prohibition of Sex Selection) Act, has failed to yield the desired result, because of its inherent loopholes and faulty implementation
- With small family norms , many young couples do not go for a second child, if the first child happens to be a male

State level patterns of Sex Ratio
- During the post-Independence period from 1951 to 2011, sex ratio in rural India has decreased from 965 to 946 and increased from 860 to 929 in urban India
- At all India level, the sex ratio has decreased from 946 in 1951 to 943 in 2011
- Notable increase has been recorded in Andaman & Nicobar Islands, (40.16%), Delhi (13.02%), Assam (10.34%) and West Bengal (9.82%). Contrary to this, 16 States/UTs have recorded significant decrease in Sex ratio.
- Notable decrease in sex ratio have been recorded in Daman & Diu (45.03%), Dadra & Nagar Haveli (18.19%), Goa (13.71%), Lakshadweep (9.25%) and Bihar (8.21%)

- the lowest sex ratio in India is in Haryana , where sex ratio is only 879 whereas the highest sex ratio is in Kerala (1084)
- If we talk about the sex ratio in the union territories; Puducherry has highest sex ratio of 1037 while Daman and Diu has lowest sex ratio (618) among all the union territories of India
- Position of the bottom five is occupied by the five Union Territories of India i.e. Andaman and Nicobar Islands, Delhi, Chandigarh, Dadra and Nagar Haveli and Daman and Diu.
- Puducherry and Lakshadweep are the only two union territories which have sex ratio of more than 900 females per thousand males
- In 2011, in age groups 0-6, 0-19, 15-45 and 60+, sex ratio has been observed as 918, 908, 944 and 1033 respectively

Child Sex Ratio
- The Child Sex Ratio is defined as the number of females per 1000 males in the age group 0–6 years.
- In the census 2001 the child sex ratio of India was 927 which declined to 918 in the census 2011

- Among the Union Territories of India; Andaman and Nicobar Islands has the highest child sex ratio i.e.968 per thousand males
- The current child sex ratio is very critical for any demographic set up because, it is this sex ratio that will determine the overall sex ratio in the coming years
- Son preference and the belief that it is only the son who can perform the last rites, that lineage and inheritance runs through the male line, sons will look after parents in old age, men are the bread winners etc.
- Exorbitant dowry demand is another reason for female foeticide/infanticide
- Small family norm coupled with easy availability of sex determination tests may be a catalyst in the declining child sex ratio, further facilitated by easy availability of Pre-conception sex selection facilities.

Consequences of Low Child Sex Ratio
- This has led to a situation where, apart from the ingrained son preference, people don’t want girls all the more as they feel that it is difficult to keep them safe
- In a study done by the Centre for Social Research in Haryana, fear of violence is a cause for female foeticide
- Having lesser women of marriageable age will mean that a significant proportion of men will have to delay their marriage
- The reduced demographic share of women, in democratic regimes would translate into a weaker political voice in public decision-making, a trend that could be reinforced by women’s lessened involvement in non-domestic activities, such as outside employment and civil life
The steps taken to improve the sex ratio in India
- Complete ban under law on sex determination during pregnancy under the PCPDNT Act
- Declaring 24th January as the National Girl Child Day in 2012
- Sabla scheme launched on the International Women’s day in 2011, aims at enabling self-development and empowerment of adolescent girls, improving their health and nutrition status; and spreading awareness about health, hygiene, nutrition, reproductive health, family and child care
- Prevention of gender biased sex selective elimination
- Ensuring survival & protection of the girl child
- Ensuring education and participation of the girl child
- Rolling out campaigns on sensitisation towards women and children
- Effective implementation of the existing women- and children-related policies
- Improving women’s status in the society, could help change the bias for son preference
- Investing on education and economic prosperity could help empower women and reduce gender gap
- They are generally called Adivasi , implying original inhabitants
- Before the introduction of caste system during the Brahminic age, people were divided into various tribes
- A tribe was a homogenous and self-contained unit without any hierarchical discrimination
- For example, the Gonds are a Scheduled tribe in Madhya Pradesh, and are Scheduled caste in Uttar Pradesh
- Article 366 (25) defines scheduled tribes as “such tribes or tribal communities or parts of or groups within such tribes or tribal communities as are deemed under Article 342 to be Scheduled Tribes for the purposes of this constitution”.
- Article 342 of the Indian Constitution provides for specification of tribes or tribal communities, which are deemed to be for the purposes of the Constitution of Scheduled tribes, in relation to that state or Union Territory
Growth of Tribal Population
- The demographic study of tribal population has suffered seriously, due to the adoption of arbitrary criteria for ‘scheduling’ the tribes
- The modification order of 1956, enumerated more tribal people, and in total they accounted for 23% of total population of the country at that time
- 97% of them live in rural areas and 10.03% in urban areas
- The decadal population growth of the tribals from Census 2001 to 2011 has been 23.66% against the 17.69% of the entire population
- The sex ratio for the overall population is 940 females per 1000 males and that of Scheduled Tribes 990 females per thousand males
- There has been a rapid natural growth of tribal population
- Additions have been made to the list of Scheduled tribes time and again
Distribution of Scheduled Tribes
- Thus, most of tribal communities live in hilly and forested tracts and other remote areas of the country
- However, their interaction with non-tribal people after Independence has changed the scenario to some extent
State level patterns
- While as much as 94.43% of total population in Mizoram and 94.79% in Lakshadweep belong to Scheduled Tribes
- Nagaland (86.48%)
- Meghalaya (86.15%)
- Arunachal Pradesh (68.79%)
- Gujarat, Assam, Rajasthan, Jammu-Kashmir region and Goa are the four major states in which more than 10% of the population belong to Scheduled tribes
- Roughly one-third of the Scheduled tribes population of India lives in the states of Madhya Pradesh, Maharashtra and Odisha
- Going by absolute numbers, the Scheduled Tribes population was the highest in Madhya Pradesh, followed by Maharashtra, Odisha, Rajasthan, Gujarat, Jharkhand and so on

The complete list of name of tribes, along with their location in India, can be found on the below link: tribal.nic.in
Tribal Economy
- The dominant economies of the tribes are:
- The main tribes which practice these professions are the Raji in Uttar Pradesh; Kharia, Birhor, Korwa, Pariha and Birgias in Jharkhand; Kuki in West Bengal; Bhil in Rajasthan; Konyak and Naga in Assam, Meghalaya, Nagaland and Arunachal Pradesh; etc.
- After 2-3 years, the fertility of the soil is reduced and the farmer shifts to another piece of land
- It is called Jhum in North-East India, Kumari in Western Ghats, Watra in South-East Rajasthan; penda, bewar, dahia and deppa in different parts of Chhattisgarh and Madhya Pradesh
- Lumbering involved obtaining wood from forests
- Main tribes involved in shifting cultivation and lumbering are Bhutias in Uttar Pradesh; Korwa in Jharkhand; Bhil in Maharashtra and Gujarat; Naga, Chakmas, Garo, Notia in North eastern states; etc.
- This is generally practiced along with Animal Husbandry
- Main tribes adopting these are Tharu, Bhotias in Uttar Pradesh; Santhal, Polia and Bhumji in West Bengal; Irula in Tamilnadu; Bhil in Maharashtra; etc.
Challenges faced by Indian tribes
- With the advent of industrialisation in India and the discovery of mineral and other resources in tribal inhabited areas, the tribal pockets were thrown open to outsiders and state control replaced tribal control.
- With the impetus to the development process after independence, pressure on land and forests increased
- This resulted in loss of ownership rights over land, owing to chronic indebtedness, unscrupulous landlords, moneylenders, contractors and officials
- With the concepts of protected forests and national forests gaining currency, the tribals felt themselves uprooted from their cultural moorings and with no secure means of livelihood.
- As per Census 2011, literacy rate of Scheduled Tribes (STs) was 59%
- The factors which inhibit the tribals from taking to education are superstitions and prejudices, extreme poverty, nomadic lifestyle of certain tribes, lack of interest in alien subjects taught through an alien language and a lack of suitable teachers and other facilities in the tribal areas.
- Because of economic backwardness and insecure livelihood, the tribals face health problems, such as prevalence of disease, like malaria, cholera, tuberculosis, diarrhoea and jaundice, problems associated with malnutrition like iron deficiency and anaemia, high infant mortality rates, low levels of life expectancy, etc.
- The degradation of the natural environment, particularly through the destruction of forests and a rapidly shrinking resource base, has had its impact on the status of women
- The opening of the tribal belts to mining, industries and commercialisation has exposed tribal men and women to the ruthless operations of the market economy, giving rise to consumerism and to commoditisation of women.
Measures taken towards Tribal Development
- Article 342 lays down that the President may by public notification, specify the tribes or tribal communities or part of or groups within tribes or tribal communities or parts which shall for the purpose of this Constitution deemed to be Scheduled Tribes
- Article 164 provides for a Ministry of Tribal Welfare in each of the State of Bihar, Madhya Pradesh and Orissa which have large concentration of Scheduled Tribes population
- Article 244 provides for the inclusion of a Fifth Schedule in the Constitution for incorporating provisions for the administration of Scheduled Areas and Tribes of the States which have sizeable tribal population (other than those of Assam)
- Article 275 provides for the grant of special funds by the Union Government to State Government for promoting the welfare of Scheduled Tribes and providing them with a better administration.
- Under Article 330 and 332 of the Indian Constitution, seats have been reserved for Scheduled Tribes in Lok Sabha and state Vidhan Sabhas.
- Following the introduction of Panchayati Raj, Suitable safeguards have been provided for proper representation of the members of the Scheduled Tribes by reserving seats for them in the Gram Panchayats, Block Panchayats, District Panchayats etc.
- Exemption in age limits
- Relaxation in the standard of suitability
- Under Article 338 of Indian Constitution a Commissioner has been appointed by the President of India. The main duty of the Commissioner is
- to investigate all matters relating to the safeguards for Scheduled Castes and Scheduled Tribes under the Constitution and
- to report the President on working of these safeguards.
- The scheme of Mechanism for Marketing of Minor Forest Produce (MFP) through Minimum Support Price (MSP) and Development of Value Chain for MFP covers various activities including procurement of MFPs when their market price falls below their notified MSP, setting up/expansion of storage facilities, expanding the knowledge base on MFP, training for sustainable collection, value addition, etc.
- Pre Matric Scholarship Scheme for ST students
- Post Matric Scholarship Scheme for ST students
- National Overseas Scholarship for ST students for studying abroad.
- National Fellowship and Scholarship for Higher Education of ST students
- Grants-in-aid to Voluntary Organisations Working for welfare of STs
- Strengthening Education among ST Girls in Low Literacy Districts
- Development of Particularly Vulnerable Tribal Groups (PVTGs)
- Special Central Assistance(SCA) to Tribal Sub-Scheme(TSS)
- Grants-in-aid to Tribal Research Institutes
- Research Information & Mass Education, Tribal Festival and Others

- Our Mission, Vision & Values
- Director’s Desk
- Commerce & Accountancy
- Previous Years’ Question Papers-Prelims
- Previous Years’ Question Papers-Mains
- Environment & Ecology
- Science & Technology
We use cookies to enhance our website for you. Proceed if you agree to this policy or learn more about it.
- Essay Database >
- Essays Examples >
- Essay Topics
Essays on Population Density
49 samples on this topic
To some college goers, crafting Population Density papers comes easy; others require the help of various types. The WowEssays.com directory includes professionally crafted sample essays on Population Density and relevant issues. Most definitely, among all those Population Density essay examples, you will find a piece that resonates with what you perceive as a worthy paper. You can be sure that literally every Population Density work presented here can be used as a bright example to follow in terms of general structure and writing different parts of a paper – introduction, main body, or conclusion.
If, however, you have a hard time coming up with a good Population Density essay or don't have even a minute of extra time to browse our sample collection, our free essay writer service can still be of great assistance to you. The thing is, our writers can tailor a sample Population Density paper to your personal needs and particular requirements within the pre-set period. Buy college essays today!
Land Use Impacts Essay Sample
Introduction
Population Density In Canada: Exemplar Report To Follow
Epidemic mathematical model research papers example.
Mathematical model.
In 1985, Kallen, Arcuri and Murray (377-393) presented a simple mathematical model for the spread of the rabies epidemics to approximate the minimum width of a break and enable the control of the spatial progression of rabies.
Features and assumptions of the model.
Free Human Population Growth And The Environment Essay: Top-Quality Sample To Follow
Example of essay on environmental studies.
Critical Assessment of an Intervention for Sustainability
Learn To Craft Research Papers On How Global Warming Impacts Food Security, Malnutrition And/Or Hunger In The World With This Example
Marketing research: area code 02108 essay template for faster writing.
Area code 02108 is located close to the center of Massachusetts, Boston. The area can be described as the Boston, Cambridge, and Quincy area (Nielsen). It falls within Suffolk County and has a total area of 0.14 square miles with no water area (Nielsen). The area has several factors regarding demographic and economic factors to support diverse marketing potentials since these factors can support a variety of businesses.
Demographics of area 02108
Course Work On Tammy Clothing
Urban growth and development course work.
1. Define a MSA A metropolitan statistical area refers to an urbanized area which may be a county or a group of counties containing diverse population of not less than 50000 people. This area is associated with commuting effects, high social and economic integration.
Example Of Essay On Profile Of Two Nova Scotia Communities
Halifax Regional Municipality
It’s the capital of the Nova Scotia Province in Canada. This paper will be the community profile which will be followed by a succinct comparison of social economic characteristics that have been linked to health status or accessibility or utilization of health services with that of Cape Breton. Halifax is a major economic hub in Canada. The city has been made popular by the concentration of companies and government services which run the city. The high population as will be depicted in this profile will explain the reasons behind its rapid growth.
Free The Effects Of Population Density And Noise On Individuals Essay Sample
Good example of canadian health care system research paper.
A Cost-Benefit Analysis of Provincial Health Services
Free Essay On The City Of Clermont, Florida
Currency essay sample.
In this article “What makes a great city great? An American Perspective” H.V. Savitch (43) asserts that to know what makes a city great a simple approach of using 4Cs can be used. This 4Cs include currency, cosmopolitanism, concentration and charisma. Additionally, he notes that these 4Cs are critical in differentiating a great city from ordinary cities.
Good Example Of Robbins Called Upon Readers Not To Stand Aside Of The Problem Essay
Final Integration Paper
Good Example Of Business Plan On Target Market And Segmentation
Free research paper on overcrowding problems in todays prisons, good essay about urban sprawl and relations to obesity, free essay on geography questions, defining the problem and research methods literature review sample, free research paper about chinas one child policy.
This research paper examines China’s national policy of restricting families to producing only one child, using research based on credible internet sources. The paper includes discussion of why and when the policy was implemented, its effect on the people of China and China as a nation, and whether it is a policy that should be or will be continued.
The Research
Good Research Paper About Other
Haiti vs. Dominican Republic
Sample Essay On Why The Earth Axis Is 23.5
Why The Earth Axis Is 23.5°?
Film Analysis: Do The Right Thing Movie Review Examples
Geographic questions and hypotheses report sample.
Part I: figure I
Good Domestic And International Factors Essay Example
Free research paper on the effects of population density and noise paper, difference and similarity between north america and southeast asia creative writing examples, term paper on new urbanism, term paper on environmental health promotion, research paper on opening a business in ireland.
Executive Summary
Essay On Solar Cells Are Not Effective Replacements For Fossil Fuels
Free research paper on effect of population increase on economic growth across particular groups of nations, controlling overpopulation: a one child policy for australia essay example, example of the mexican state of oaxaca essay.
Mesoamerica is that geographical region which covers parts of both North America, the Caribbean and a portion of South America, bisected by the tropic of cancer. As instructed, a state of the Republic of Mexico i.e. Oaxaca is selected for analysis.
OAXACA (PHYSICAL FEATURES)
Research Paper On Operational Sex Ratio And Density Do Not Affect Directional Selection On Male Sexual
Small business - exam case study examples.
Question 1a
Key Words Research Paper Sample
Poverty and unemployment increases crime rates. When poverty and unemployment occur together with other factors like high population density, low income rates and minority group, the levels of crimes are even higher. Poverty and unemployment results to increase stress levels which may cause mental disturbance, a common cause of crimes. Poverty and unemployment also results to dishonesty which prompts people to indulge in offenses. Crime is also an alternative source of living for the poor and the unemployed.
Unemployment Mental illness Crime Dishonesty
Paolo Volpi Research Paper Examples
Example of the effects of population density and noise essay.
The Effects of Population Density and Noise
Example Of Changing Patterns Of Consumption Research Paper
Free case study on review of gis in housing and the effect of educational level, term paper on sexual selection of red deer the complexity of choosing and winning a mate, hunan province research paper, term paper on territoriality privacy and personal space.
The world's populace increases by approximately 80 million people each year, according to the population reserve website, Population Connection. And with most of this inhabitants occupying comparatively small urban areas, it is not astonishing that population density is a mounting cause of concern. And while there are many unconstructive impacts of high population densities, there are also a few constructive ones.
State Of Crime In Hawaii Research Paper
Crime remains to be the most important issue on the national and international agenda according to many polls. This has been witnessed by the United States commitment to end crime and terrorism that has resulted to diplomatic problems between the United States, Iraq and Afghanistan. People of all races are concerned about the safety of their lives and property. It’s the wish of all mankind that governments, legislators and law enforcers develop an effective and efficient strategy to ensure safety for all by reducing crime rates.
Admission Essay on Demographics of Japan
The demographic characteristics of the Japan’s population include ethnicity, population density, population health, education level, religious affiliations, economic status, and other aspects of the population (Lie 22).
As of March 2009, the population of Japan was 127,076,184, making it the world's tenth most highly populated country. Japans population density was 336 individuals per square kilometer (Hughes 45).
275 words = 1 page double-spaced
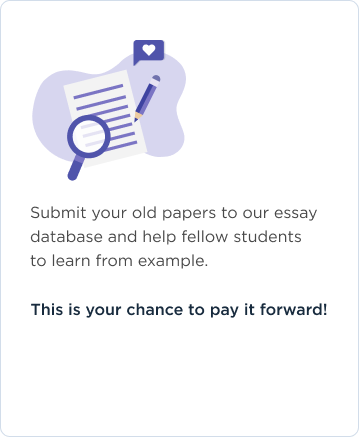
Password recovery email has been sent to [email protected]
Use your new password to log in
You are not register!
By clicking Register, you agree to our Terms of Service and that you have read our Privacy Policy .
Now you can download documents directly to your device!
Check your email! An email with your password has already been sent to you! Now you can download documents directly to your device.
or Use the QR code to Save this Paper to Your Phone
The sample is NOT original!
Short on a deadline?
Don't waste time. Get help with 11% off using code - GETWOWED
No, thanks! I'm fine with missing my deadline
- Search Menu
- Sign in through your institution
- Advance Articles
- Editor's Choice
- Braunwald's Corner
- ESC Guidelines
- EHJ Dialogues
- Issue @ a Glance Podcasts
- CardioPulse
- Weekly Journal Scan
- European Heart Journal Supplements
- Year in Cardiovascular Medicine
- Asia in EHJ
- Most Cited Articles
- ESC Content Collections
- Author Guidelines
- Submission Site
- Why publish with EHJ?
- Open Access Options
- Submit from medRxiv or bioRxiv
- Author Resources
- Self-Archiving Policy
- Read & Publish
- Advertising and Corporate Services
- Advertising
- Reprints and ePrints
- Sponsored Supplements
- Journals Career Network
- About European Heart Journal
- Editorial Board
- About the European Society of Cardiology
- ESC Publications
- War in Ukraine
- ESC Membership
- ESC Journals App
- Developing Countries Initiative
- Dispatch Dates
- Terms and Conditions
- Journals on Oxford Academic
- Books on Oxford Academic
Article Contents
Declarations, apob triumphs once more over ldl-c and non-hdl-c in risk prediction: ready for guidelines.

The opinions expressed in this article are not necessarily those of the Editors of the European Heart Journal or of the European Society of Cardiology.
- Article contents
- Figures & tables
- Supplementary Data
Martin Bødtker Mortensen, ApoB triumphs once more over LDL-C and non-HDL-C in risk prediction: ready for guidelines?, European Heart Journal , 2024;, ehae257, https://doi.org/10.1093/eurheartj/ehae257
- Permissions Icon Permissions

Apolipoprotein B is the most accurate marker of cholesterol-driven risk. Three distinct yet highly correlated cholesterol measures are recommended by guidelines to assess cholesterol-driven risk: (1) apolipoprotein B (apoB), (2) non-high densty lipoprotein cholesterol (non-HDL-C) and (3) low-density lipoprotein cholesterol (LDL-C). Sniderman et al . demonstrate in the UK Biobank that for a given non-HDL-C or LDL-C, variances in apoB levels are present. These differences can lead to clinically significant disparities in risk for affected patients.
This editorial refers to ‘Discordance among apoB, non-high-density lipoprotein cholesterol, and triglycerides: implications for cardiovascular prevention’, by A. D. Sniderman et al ., https://doi.org/10.1093/eurheartj/ehae258 .
Accurately predicting atherosclerotic cardiovascular disease (ASCVD) risk is a crucial aspect of daily medical practice, as it underpins decisions regarding lifelong preventive medication, including lipid-lowering therapies, for millions of patients worldwide. Given that atherosclerosis primarily stems from the accumulation of lipoproteins, and consequently cholesterol, in the arterial intima, it is unsurprising that cholesterol-related information plays a pivotal role in cardiovascular risk prediction and clinical decision-making. 1 This is evident in all guideline-recommended risk prediction models for assessing 10-year ASCVD risk, such as the Systematic Coronary Risk Evaluation (SCORE) endorsed by the European Society of Cardiology (ESC), the Pooled Cohort Equations (PCE) recommended by the American College of Cardiology (ACC) and the American Heart Association (AHA), and the QRISK model endorsed by the National Institute for Health and Care Excellence (NICE) guidelines. 2–6 However, despite decades of research and the undeniable role of cholesterol in atherogenesis, the optimal metric for capturing cholesterol-driven risk remains unsettled. Notably, the cholesterol information utilized in the aforementioned risk calculators varies. Although the SCORE2 model recommended by ESC employs non–high-density lipoprotein cholesterol (non-HDL-C) to assess cholesterol-driven risk, the PCE recommended by ACC/AHA guidelines adopts a different approach by utilizing data on both total cholesterol (where higher values increase risk) and high-density lipoprotein cholesterol (HDL-C, where higher values lower risk) in risk estimation, whereas QRISK includes a ratio of total cholesterol to HDL-C (where a higher ratio increases risk).
Why do the risk prediction models not agree on which cholesterol metric to include? This is because the choice of cholesterol metric in the models does not significantly alter risk prediction or risk stratification on a population level. This is explained by the fact that analyses like c-statistics, which measure a model’s ability to correctly rank patients by risk, along with other traditional statistical analyses of model performance, are minimally influenced by the inclusion of specific cholesterol metrics, as most information on absolute risk in a population is primarily determined by age, sex and smoking status. 7 Does this mean that cholesterol-mediated risk is not important? Not at all. The importance of assessing cholesterol-related risk extends far beyond merely differentiating 10-year ASCVD risk on a population level. Indeed, it provides highly valuable insights regarding the individual patient in terms of 10-year risk and relative risk compared to other patients of similar age and sex. It also offers information on lifetime risk, on what drives the risk in the patient (cholesterol- versus non-cholesterol–driven risk), on how to optimally treat risk in the patient, and serves as an important tool to monitor treatment efficacy and identify patients who continue to have high residual cholesterol risk despite lipid-lowering therapies.
To date, low-density lipoprotein cholesterol (LDL-C) remains the most widely used standard for assessing cholesterol-related risk in individual patients. 8 This preference stems partly from the focus on reducing LDL-C levels in most randomized controlled trials of lipid-lowering therapies, including statins, ezetimibe, proprotein convertase subtilisin/kexin type 9 (PCSK-9) inhibitors and bempedoic acid, given that these treatments most markedly lower this lipoprotein. However, despite being the major cholesterol-fraction in most patients, LDL-C is an imperfect measure of cholesterol-related risk. Indeed, cholesterol carried by triglyceride-rich lipoproteins such as very-low-density lipoprotein (VLDL) and its remnants, referred to as remnant cholesterol, is overlooked when LDL-C alone is assessed ( Graphical Abstract , left panel). This is a major problem as various studies, ranging from Mendelian randomization studies to observational research, have demonstrated that remnant cholesterol is an important causal risk factor for ASCVD, with an association with future ASCVD events at least as strong as that of LDL-C. 9 , 10 Consequently, patients with average or even low LDL-C but elevated remnant cholesterol may be inaccurately classified as having low cholesterol-related risk based solely on LDL-C, when in reality they face high cholesterol-driven risk. In response to this issue, the assessment of non-HDL-C has garnered some attention in both ESC and ACC/AHA guidelines in recent years as it accounts for both LDL-C and remnant cholesterol, offering a more comprehensive view of cholesterol-related risk in patients. 2 , 4 However, it has also become apparent that focusing solely on cholesterol concentrations may not capture the entirety of cholesterol-related risk, because it overlooks factors such as the number and size of cholesterol-containing lipoproteins, which play important roles for entry and entrapment of lipoproteins within the arterial intima ( Graphical Abstract , left panel). Indeed, the number of atherogenic lipoprotein particles circulating in the bloodstream can vary significantly, even with similar overall cholesterol levels. A high number of small, cholesterol-depleted particles is more atherogenic than a low number of cholesterol-enriched lipoproteins at comparable cholesterol levels. 11 Importantly, because all atherogenic lipoprotein particles, including VLDL and its remnants, LDL, and lipoprotein(a), each carry a single apolipoprotein B (apoB) molecule, quantification of apoB directly estimates the number of circulating atherogenic lipoproteins. Like non-HDL-C, numerous studies have demonstrated that apoB serves as a superior risk marker for ASCVD compared to LDL-C.
Thus, we are now at a decisive moment. How can we facilitate a transition in clinical practice towards a broader focus on cholesterol-driven risk beyond simply LDL-C for the benefit of the patients? The answer lies within guideline recommendations. Although recent European and American guidelines on treatment of dyslipidaemia, including the 2019 ESC/EAS guidelines for the management of dyslipidaemia, include suggestions for measuring non-HDL-C and apoB, they still remain secondary to assessment of LDL-C (i.e. serving as risk-enhancers in ACC/AHA guidelines) and are primarily reserved for patients with diabetes, metabolic syndrome or moderate hypertriglyceridaemia where deviations from LDL-C levels are more likely. This ‘equipoise’ likely explains why most physicians continue to prioritize LDL-C despite ample evidence showing that both non-HDL-C and apoB are superior risk markers. However, what remains uncertain, potentially impeding clearer guideline recommendations, is the preference between apoB and non-HDL-C. Are they equally good, or does one excell over the other? Several studies have found no significant difference between apoB and non-HDL-C when compared directly. 12–14 Hence, the 2021 ESC guidelines on Cardiovascular Disease Prevention noted that ‘Non-HDL-C levels contain, in essence, the same information as a measurement of apoB plasma concentration’, leading them to refrain from recommending apoB assessment. 2 The strong correlation between apoB and non-HDL-C often results in concordance, diluting any potential superiority when they disagree (i.e. one is higher than expected based on the other). The majority of prior studies have failed to address this, and have therefore been insufficient in determining the superior lipid metric when discrepancies arise among these correlated lipid parameters.
In this issue of the European Heart Journal , Sniderman et al . present important new data that position apoB ahead of non-HDL-C and LDL-C as the best marker of cholesterol-driven risk. 15 Leveraging the UK Biobank data set encompassing 502 413 participants, the authors excluded individuals with prevalent cardiovascular disease, those on lipid-lowering therapy and those lacking lipid or risk factor information, leaving 293 876 participants for analysis. Median apoB was 1.05 g/l (0.90–1.20), while it was 3.66 mmol/l for LDL-C and 4.33 (3.69–5.03) for non-HDL cholesterol. First, Sniderman et al . confirmed in the UK Biobank what we already knew before. There is a high degree of correlation between apoB, LDL-C and non-HDL-C with Pearson correlation coefficient of r = 0.96 for both apoB versus LDL-C and apoB versus non-HDL-C. Thus, when one is high (i.e. apoB), there is a high likelihood that the other measure is also high (i.e. LDL-C). This finding is not surprising, and is the main reason that apoB fails to demonstrate meaningful superiority over LDL-C or non-HDL-c when traditional statistical methods are applied. However, the authors proceeded to demonstrate that despite this high correlation, considerable variance in apoB persists for a given value of LDL-C or non-HDL-C. Although this variance may have limited impact on population-level risk prediction, it holds significant importance for individual patient risk assessment if such variance translates into clinically relevant risk differences. Indeed, employing clever residual analyses, Sniderman et al . convincingly illustrate apoB’s superiority not only to LDL-C but also to non-HDL-C, showing clinically meaningful differences in absolute risk when apoB deviates from LDL-C or non-HDL-C values. Crucially, the opposite scenario was not observed; risk remained unchanged when LDL-C or non-HDL-C disagreed with apoB. What does this finding signify? It underscores apoB as the premier marker for cholesterol-driven risk, suggesting that once apoB is accounted for, knowing LDL-C or non-HDL-C values does not provide additional predictive information. This latter point is particularly significant as it emphasizes that, contrary to common belief, non-HDL-C does not fully encompass all the information conveyed by apoB.
Where do we stand now? There is now overwhelming evidence from numerous studies conducted across multiple independent cohorts that apoB surpasses LDL-C as a superior marker for ASCVD risk. The present study elegantly reaffirms this assertion and extends the findings to suggest that apoB also outperforms non-HDL-C. It is now time for future guideline update to acknowledge this; non-HDL-C is superior to LDL-C and apoB is the best choice among them all. The integration of apoB into clinical practice will ultimately rely on treating physicians, but clearer guideline recommendations would represent a pivotal first step towards its adoption for improved assessment of cholesterol-driven risk.
In conclusion, the era of focusing solely on LDL-C for assessing cholesterol-driven risk is coming to an end. The analyses conducted by Sniderman et al . offer important insights into the superiority of apoB over LDL-C and non-HDL-C. They demonstrate that significant variance in apoB exists despite a high correlation with LDL-C or non-HDL-C levels, and that these discrepancies translate into clinically meaningful differences in risk for those patients who are affected ( Graphical Abstract , right panel). These findings advocate for the integration of apoB as a central component in ASCVD risk prediction within future guidelines for dyslipidaemia management and cardiovascular disease prevention. Although this transition necessitates an adjustment period for physicians to learn how to assess and interpret apoB values, it will eventually streamline and simplify risk prediction and management. Indeed, other lipid markers appear to offer limited additional information once apoB has been assessed, underscoring apoB’s comprehensive capture of cholesterol-driven risk in the individual patient.
Disclosure of Interest
M.B.M. has received lecture fees from Amgen, AstraZeneca, Sanofi and NovoNordisk.
Bentzon JF , Otsuka F , Virmani R , Falk E . Mechanisms of plaque formation and rupture . Circ Res 2014 ; 114 : 1852 – 66 . https://doi.org/10.1161/CIRCRESAHA.114.302721
Google Scholar
Visseren FLJ , Mach F , Smulders YM , Carballo D , Koskinas KC , Bäck M , et al. 2021 ESC guidelines on cardiovascular disease prevention in clinical practice . Eur Heart J 2021 ; 42 : 3227 – 337 . https://doi.org/10.1093/eurheartj/ehab484
Mach F , Baigent C , Catapano AL , Koskinas KC , Casula M , Badimon L , et al. 2019 ESC/EAS guidelines for the management of dyslipidaemias: lipid modification to reduce cardiovascular risk . Eur Heart J 2020 ; 41 : 111 – 88 . https://doi.org/10.1093/eurheartj/ehz455
Grundy SM , Stone NJ , Bailey AL , Beam C , Birtcher KK , Blumenthal RS , et al. 2018 AHA/ACC/AACVPR/AAPA/ABC/ACPM/ADA/AGS/APhA/ASPC/NLA/PCNA guideline on the management of blood cholesterol: a report of the American College of Cardiology/American Heart Association task force on clinical practice guidelines . Circulation 2019 ; 139 : e1082 – 143 . https://doi.org/10.1161/CIR.0000000000000625
National Institute for Health and Care Excellence (NICE) clinical guideline CG181 . Lipid Modification—Cardiovascular Risk Assessment and the Modification of Blood Lipids for the Primary and Secondary Prevention of Cardiovascular Disease . National Clinical Guideline Centre , 2014 .
Google Preview
Hippisley-Cox J , Coupland C , Brindle P . Development and validation of QRISK3 risk prediction algorithms to estimate future risk of cardiovascular disease: prospective cohort study . BMJ 2017 ; 357 : j2099 . https://doi.org/10.1136/bmj.j2099
Pencina MJ , Navar AM , Wojdyla D , Sanchez RJ , Khan I , Elassal J , et al. Quantifying importance of major risk factors for coronary heart disease . Circulation 2019 ; 139 : 1603 – 11 . https://doi.org/10.1161/CIRCULATIONAHA.117.031855
Mortensen MB , Nordestgaard B . Comparison of five major guidelines for statin use in primary prevention . Ann Intern Med 2018 ; 169 : 67 – 8 . https://doi.org/10.7326/L18-0182
Varbo A , Benn M , Tybjærg-Hansen A , Jørgensen AB , Frikke-Schmidt R , Nordestgaard BG . Remnant cholesterol as a causal risk factor for ischemic heart disease . J Am Coll Cardiol 2013 ; 61 : 427 – 36 . https://doi.org/10.1016/j.jacc.2012.08.1026
Ginsberg HN , Packard CJ , Chapman MJ , Borén J , Aguilar-Salinas CA , Averna M , et al. Triglyceride-rich lipoproteins and their remnants: metabolic insights, role in atherosclerotic cardiovascular disease, and emerging therapeutic strategies—a consensus statement from the European Atherosclerosis Society . Eur Heart J 2021 ; 42 : 4791 – 806 . https://doi.org/10.1093/eurheartj/ehab551
Sniderman AD , Thanassoulis G , Glavinovic T , Navar AM , Pencina M , Catapano A , et al. Apolipoprotein B particles and cardiovascular disease: a narrative review . JAMA Cardiol 2019 ; 4 : 1287 – 95 . https://doi.org/10.1001/jamacardio.2019.3780
Emerging Risk Factors Collaboration ; Di Angelantonio E , Sarwar N , Perry P , Kaptoge S , Ray KK , et al. Major lipids, apolipoproteins, and risk of vascular disease . JAMA 2009 ; 302 : 1993 – 2000 . https://doi.org/10.1001/jama.2009.1619
Emerging Risk Factors Collaboration ; Di Angelantonio E , Gao P , Pennells L , Kaptoge S , Caslake M , et al. Lipid-related markers and cardiovascular disease prediction . JAMA 2012 ; 307 : 2499 – 506 . https://doi.org/10.1001/jama.2012.6571
Welsh C , Celis-Morales CA , Brown R , Mackay DF , Lewsey J , Mark PB , et al. Comparison of conventional lipoprotein tests and apolipoproteins in the prediction of cardiovascular disease . Circulation 2019 ; 140 : 542 – 52 . https://doi.org/10.1161/CIRCULATIONAHA.119.041149
Sniderman AD , Dufresne L , Pencina KM , Bilgic S , Thanassoulis G , Pencina MJ . Discordance among apoB, non–high-density lipoprotein cholesterol, and triglycerides: implications for cardiovascular prevention . Eur Heart J 2024 :ehae258. https://doi.org/10.1093/eurheartj/ehae258
Author notes
Email alerts, companion article.
- Discordance among apoB, non–high-density lipoprotein cholesterol, and triglycerides: implications for cardiovascular prevention
Citing articles via
Looking for your next opportunity, affiliations.
- Online ISSN 1522-9645
- Print ISSN 0195-668X
- Copyright © 2024 European Society of Cardiology
- About Oxford Academic
- Publish journals with us
- University press partners
- What we publish
- New features
- Open access
- Institutional account management
- Rights and permissions
- Get help with access
- Accessibility
- Media enquiries
- Oxford University Press
- Oxford Languages
- University of Oxford
Oxford University Press is a department of the University of Oxford. It furthers the University's objective of excellence in research, scholarship, and education by publishing worldwide
- Copyright © 2024 Oxford University Press
- Cookie settings
- Cookie policy
- Privacy policy
- Legal notice
This Feature Is Available To Subscribers Only
Sign In or Create an Account
This PDF is available to Subscribers Only
For full access to this pdf, sign in to an existing account, or purchase an annual subscription.
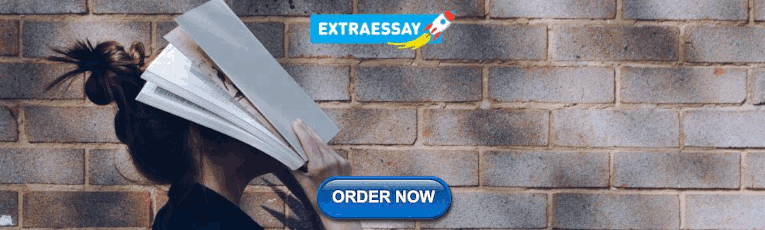
IMAGES
VIDEO
COMMENTS
We will write a custom essay on your topic. Population density is an important aspect because it guides experts in their endeavour to enhance planning and other undertakings that manifest in social contexts. It helps in monitoring and evaluation of population and social trends within society. Through studies on population, experts deduce ...
A population is a subgroup of individuals within the same species that are living and breeding within a geographic area. The number of individuals living within that specific location determines the population density, or the number of individuals divided by the size of the area. Population density can be used to describe the location, growth, and migration of many organisms.
Physical factors that affect population density include water supply, climate, relief (shape of the land), vegetation, soils and availability of natural resources and energy. Human factors that affect population density include social, political and economic factors. Mountainous areas make it difficult to construct buildings and roads.
The term population distribution refers to the way people are spaced over the surface of the earth. Broadly, 90% of the world's population lives in about 10% of the earth's land area. The 10 most populous countries of the world contribute about 60% of the world's population (the six countries out of the ten are located in Asia).
Population density is not the same everywhere.It varies greatly depending on country, region, city, or town. The world's population density is currently estimated to be around 15,3 people per square kilometer.. Some countries, such as Bangladesh (1,301 people per square kilometer) and Singapore (8,274 people per square kilometer), have higher population densities than others, like Canada (4 ...
Effects of Population Density Research Paper. Population density is defined as the number of people living in a specific geographical area (Rylander, 2006). The higher the population density there is, the higher human activity that geographical activity will have. This explains why densely populated areas exert more pressure on the immediate ...
500+ Words Essay on Population. Population refers to the total number of beings living in a particular area. Population helps us get an estimate of the number of beings and how to act accordingly. For instance, if we know the particular population of a city, we can estimate the number of resources it needs.
Population density is the number of people per unit of area, usually transcribed as "per square kilometer" or square mile, and which may include or exclude, for example, areas of water or glaciers. Commonly this is calculated for a county, city, country, another territory or the entire world . The world's population is around 8,000,000,000 [3 ...
Population density Population density describes the number of people living in a given area, usually a km². It can be used on a variety of scales from continents, to countries to regions within a country. It is calculated by dividing the total population of one of these by the total area. On any population density map of the world it is ...
There are a range of human and natural factors that affect population density. The tables below illustrate this. Physical Factors. High Density. Low Density. Relief. (shape and height of land) Low land which is flat e.g. Ganges Valley in India. High land that is mountainous e.g. Himalayas.
Essay # 2.Distribution of World's Population: In terms of continents and countries the world's population is very ill-balanced. More than half of the world's people live in Asia (excluding the U.S.S.R.) which accounts for only one-fifth of the world's land area, while North, Central and South America together, occupying more than a quarter of the land surface, have only one-seventh ...
Population density is becoming a more and more prevalent issue in the contemporary world, as we continue to push the Earth's carrying capacity to dangerous levels. The fallout of this strain our growing worldwide population is putting on the Earth is potentially enormous and many believe that we are already permanently harming our planet.
Example 1: calculating population density. Malta has a population of 440 \ 000 440 000 and an area of 316 \ km^ {2}. 316 km2. Calculate the population density of Malta. Give your answer to the nearest integer. Check what information we have been given and what we are finding.
This essay has explored the relationship between population density and mental health issues, focusing on stress, anxiety, and social alienation. The evidence presented highlights the detrimental effects of crowding on mental well-being. As urbanization continues to shape our world, addressing the challenges posed by high population densities ...
The U.S. Census Bureau (2017) estimates that crude birth and mortality rates in the EU are about equal at 10 per thousand people suggesting that the natural rate of population growth is zero. With net migration at two per thousand people, the EU did realize a positive population growth rate of 0.2%.
5 pages (1682 words) , Download 2. Free. This paper ''Effects of Population Density and Noise on Individuals'' tells that Population Density is a socio-economic term that refers to 'the number of people in a defined jurisdiction, about the size of the area that they occupy' (Last 2002, 941).
Population density is another parameter that theorists use to establish correlation between population growth and economic growth. It refers to the number of people living within one square kilometer. Malthus asserts that countries with low population density experience many challenges (Kelley & Schmidt 1995, p. 67).
From 2015 to 2021, areas with a population density of over 1000 person/km 2 were predominantly found in the southeast, while only Lanzhou, Yinchuan, and Shihezi in the northwest had a density of ...
India's Share of World's Area and Population. India's Population Dynamics by Numbers (as of March 2011, Refer to Figure): Total population of India is 1,210.6 million. India represents 17.5% of the world's population. Population is distributed unevenly across 3.28 million square km (2.4% of the world's area).
THOMAS MALTHUS, AN ESSAY ON THE PRINCIPLE OF POPULATION (1798)1 Thomas Robert Malthus (1766-1834) was an English priest, economist, and demographer best known for his theory of unsustainable population growth, which contradicted more optimistic but widely held views. In his anonymously published An
Introduction. A population is a distinct group of individuals, whether that group comprises a nation or a group of people with a common characteristic; India with a total population of 1,210.2 million according to 2011 census figures, and is the second most populous country of the world, next only to China. India covers only 2.4% of the land area of the world, but it is home to about 17.5% of ...
The effects of population density and noise 3 Essay. The Effects of Population Density and Noise Mary J House Psy 460 September 29, 2014 Ricky Fenwick The Effects of Population Density and Noise Humans are aware and in tune with their environments in many different ways, and interact with them in most everything they do.
Free Research Paper On The Effects Of Population Density And Noise Paper. 1. Describe the concepts of territoriality, privacy, and personal space. The traditional explanation of territoriality was emphasized on the marking off and protection of a physical border line from invasion by those of similar kind.
Importantly, socioeconomic variables accounted for 25 % of the influence on global urban green space, with a positive effect of human development index (HDI) and a negative effect of population density. HDI in relation to urban green space has not previously been tested globally, and our study shows that significantly affects urban greenspace.
To date, low-density lipoprotein cholesterol (LDL-C) remains the most widely used standard for assessing cholesterol-related risk in individual patients. 8 This preference stems partly from the focus on reducing LDL-C levels in most randomized controlled trials of lipid-lowering therapies, including statins, ezetimibe, proprotein convertase ...