
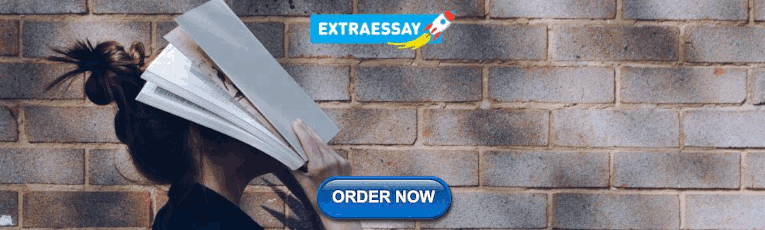
QUT ePrints
- Advanced search
- Thesis search
Quick links
- Deposit research now
- Academic profiles
- Research Data Finder
- Research Support Services
- Repository statistics
QUT ePrints hosts 140,000 research works with 140,000 downloads in the past week.

Indigenous Australian Research Excellence
- Browse research
- TEQSA Provider ID: PRV12079 (Australian University)
- CRICOS No. 00213J
- ABN 83 791 724 622
- Accessibility
- Right to Information
- skip navigation
- Privacy Policy
- search search
- Campusplan Campus Map
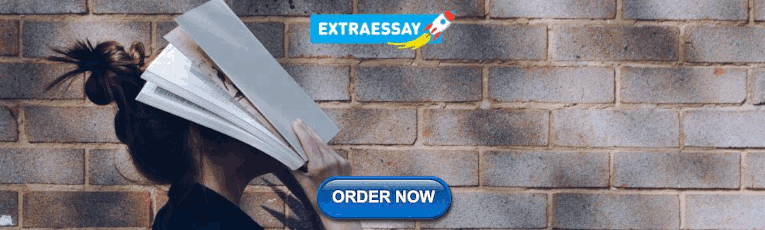
IISM | Digital Service Innovation Prof. Dr. Gerhard Satzger
Write your thesis with us, digital innovations: aais.
Take a look at our research department: Applied AI in Services
Digital Innovations: DSDI
Take a look at our research department: Digital Service Design & Innovation
Startups coached by DSI
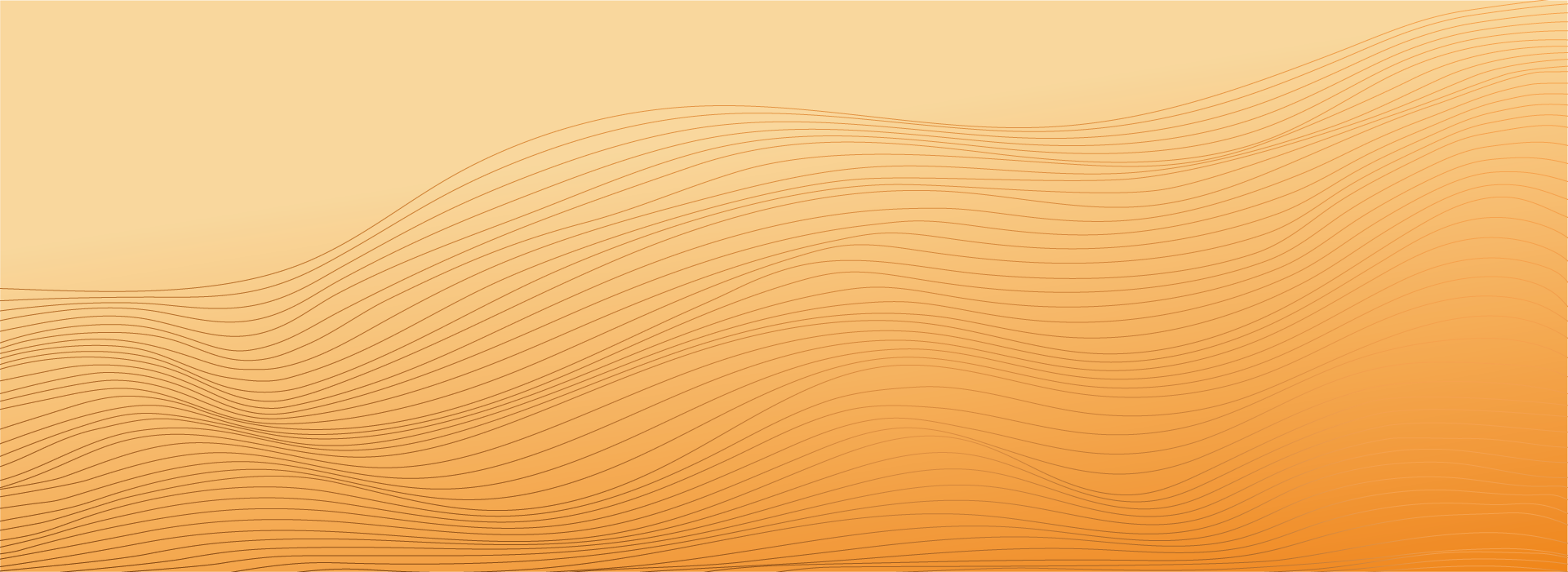
Our research group "Digital Service Innovation" (DSI) is focusing on designing, developing, and evaluating novel digital services and service business models based on the use of data and AI. Established in 2008 in cooperation with IBM, we build on a proven "industry-on-campus" concept bringing industry experience to KIT - and put a particular emphasis on connecting theory and practical application. We are part of both the Institute of Information Systems and Marketing (IISM) as well as the interdisciplinary Karlsruhe Digital Service Research & Innovation Hub (KSRI) .
Our conviction
We strongly believe that digitalization will radically transform the way value is generated for businesses and individuals and drive the "servitization" of businesses. Information and communication technology will be the "glue" for service systems in which several partners cooperate to jointly create value. In a business context, this means that innovation concepts have to elevate above the scope of the single enterprise and comprise customers, partners, or whole ecosystems.
We are fascinated by the opportunitities that digitalization holds for service innovation. Our research focuses on concepts, methods and tools to design novel services and entire business models. At the same time, we run an integrated teaching and training concept within the KSRI to educate future leaders for a global, services-led world. Based on a solid understanding of service systems, students are developing economic and technical competences essential to successfully engineer and manage IT-based services. Courses are usually taught in English.
Our expertise
We specialize in analyzing and designing user-centric and IT-based services and evaluating them in practice – while tightly connecting business and technology. Our current research is organized in two labs – in which we add interdisciplinary and domain expertise from other KSRI groups and partners.
Digital Service Design & Innovation : We conceive and apply methods, techniques and tools for the design and innovation of digital services. We provide knowledge and guidance to leverage emerging technologies such as AI, Analytics, Blockchain, IoT for impactful services, business models and service systems - emphasizing a human perspective. Current topics include Design Thinking-based Innovation Processes, Digital User Engagement, and Data- and AI-based Services and Business Models.
Applied Artificial Intelligence (AI) in Services : We utilize artificial intelligence and, in particular machine learning, to conceive, build and evaluate innovative digital services – with a particular focus on developing "system" solutions across organizations and on complementing human and machine capabilities. Current topics include AI in Service Systems, IoT-based (Smart) Services, and Human-AI Collaboration, in particular topics of complementary team performance, fairness, and appropriate reliance on AI.
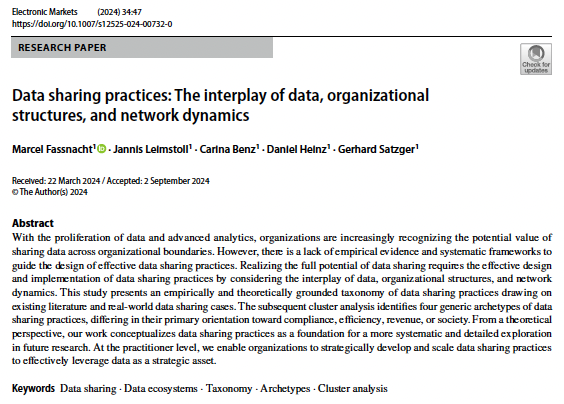
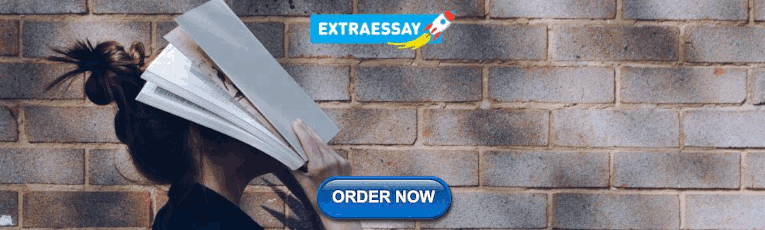
COMMENTS
This paper reports on the findings of a process mining case study conducted at an Australian University that had espoused a digital transformation initiative to improve PhD student journeys.
Process mining is a technique that uses the event logs of a system to discover, monitor, and improve process models. Analyzing these models can provide insights into a system’s behavior.
This article introduces process mining as a new research field and summarizes the guiding principles and challenges described in the manifesto.
This PhD research project focuses on conceptualising the notion of impact in the process mining domain. Process mining is a field of techniques that automatically discover process models and monitor performance indicators, …
We are looking for prospective PhD candidates to work on various topic of Process Science (Process Data Analysis – Process Mining, Process Querying, Machine Learning-based …
Providing domain knowledge for process mining with ReWOO-based agents. Max Vogt, Peter Van der Putten and Hajo A. Reijers Towards an Ethogram of Exploratory Process Mining Behavior.
The IEEE Task Force for Process Mining is happy to announce the 2024 edition of the Best Process Mining PhD Dissertation Award. The award will be delivered during the 6th …
Process Mining (PM), a specialized form of data-driven process analytics, enables the extraction of detailed insights regarding process behavior, process performance, confor-mance of …
Subject: Process Mining in Manufacturing: A Causal AI Approach. Type: Master's thesis. Date: Vergeben. Supervisor: Joshua Holstein. Background: The landscape of modern manufacturing …