
An official website of the United States government
The .gov means it’s official. Federal government websites often end in .gov or .mil. Before sharing sensitive information, make sure you’re on a federal government site.
The site is secure. The https:// ensures that you are connecting to the official website and that any information you provide is encrypted and transmitted securely.
- Publications
- Account settings
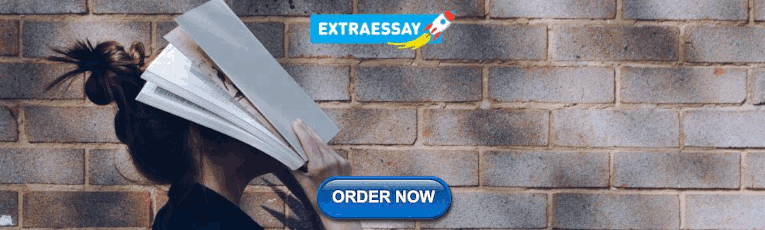
Trending Articles
- Streptococcus anginosus promotes gastric inflammation, atrophy, and tumorigenesis in mice. Fu K, et al. Cell. 2024. PMID: 39461336 No abstract available.
- Tobacco Smoke Plays an Important Role in Initiation and Development of Lung Cancer by Promoting the Characteristics of Cancer Stem Cells. Lu L, et al. Cancer Manag Res. 2020. PMID: 33116833 Free PMC article. Review.
- Amino acid is a major carbon source for hepatic lipogenesis. Liao Y, et al. Cell Metab. 2024. PMID: 39461344
- Global, regional, and national burden of injuries, and burden attributable to injuries risk factors, 1990 to 2019: results from the Global Burden of Disease study 2019. GBD 2019 Injuries Collaborators. Public Health. 2024. PMID: 39454232
- CAR-Macrophage Therapy Alleviates Myocardial Ischemia-Reperfusion Injury. Wang J, et al. Circ Res. 2024. PMID: 39465245
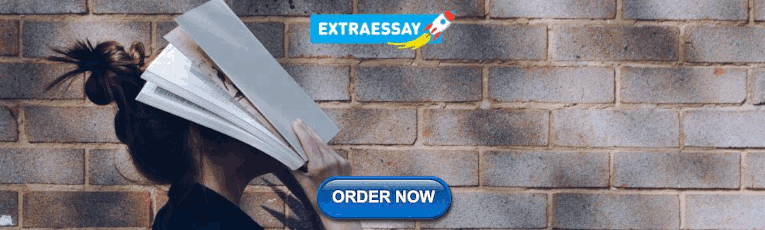
Latest Literature
- Ann Oncol (1)
- Gastroenterology (2)
- J Am Acad Dermatol (5)
- J Am Coll Cardiol (3)
- J Biol Chem (9)
- J Neurosci (2)
- Spine (Phila Pa 1976) (5)
NCBI Literature Resources
MeSH PMC Bookshelf Disclaimer
The PubMed wordmark and PubMed logo are registered trademarks of the U.S. Department of Health and Human Services (HHS). Unauthorized use of these marks is strictly prohibited.
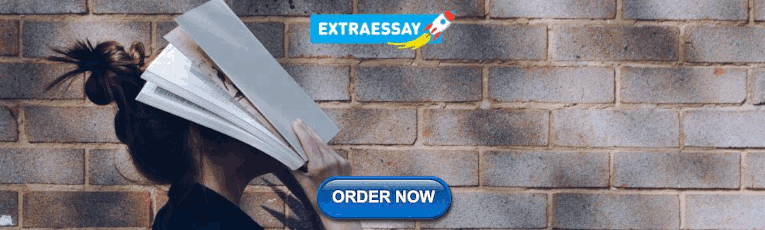
IMAGES
VIDEO
COMMENTS
repeating the essence of a research study to see whether the results can be reliably reproduced. Study with Quizlet and memorize flashcards containing terms like longitudinal study, random …
Research methods tell the researcher how to collect and analyse data, e.g. through interviews, questionnaires, or statistical methods. Thus, a research strategy offers high-level guidance, …
This article offers a methodological jus-tification for most similar case designs, explains the benefits of using match-ing for qualitative case selection, shows how existing matching …
Matching is a technique used to avoid confounding in a study design. In a cohort study this is done by ensuring an equal distribution among exposed and unexposed of the variables …
Matching Methods. Matching is a process that aims to close back doors - potential sources of bias - by constructing comparison groups that are similar according to a set of matching …
False positive matches are common but can be recognized because they typically (i) involve unimportant residues in the target (39), (ii) are not reciprocated back to the query (40), and (iii)...
Handout 2-3 Matching (Research Strategies) Log in. Sign up. Get a hint. Studying the same group of individuals over a long period of time. Click the card to flip.
a research technique used to obtain an in-depth analysis of a person, group, or phenomenon. Correlational Study. a research project used to discover a suggest relationship that exists …