- Search Menu
- Sign in through your institution
- Advance articles
- Editor's Choice
- Virtual Issue archive
- The Pitelka Award
- Author Guidelines
- Submission Site
- Open Access
- About Behavioral Ecology
- About the International Society for Behavioral Ecology
- Editorial Board
- Advertising and Corporate Services
- Journals Career Network
- Self-Archiving Policy
- Journals on Oxford Academic
- Books on Oxford Academic
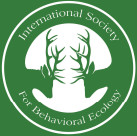
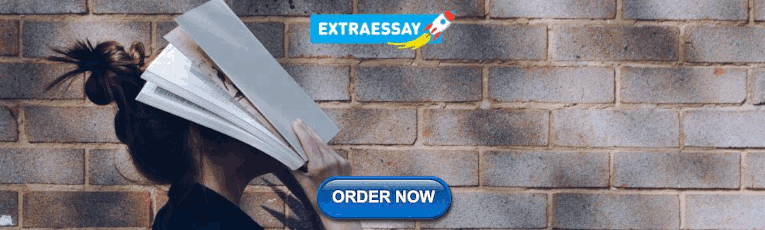
Article Contents
Ssh: critical and compatible predictions, ssh in polygynous mating systems with biparental care, review of empirical studies, conclusions.
- < Previous
Testing the sexy son hypothesis—a research framework for empirical approaches
- Article contents
- Figures & tables
- Supplementary Data
Thomas Huk, Wolfgang Winkel, Testing the sexy son hypothesis—a research framework for empirical approaches, Behavioral Ecology , Volume 19, Issue 2, March 2008, Pages 456–461, https://doi.org/10.1093/beheco/arm150
- Permissions Icon Permissions
Sexual selection by direct and/or indirect benefits as well as sexual conflict determines the evolution of animal mating systems (e.g., Andersson and Simmons 2006 ). In polygynous mating systems, the female preference of apparently useless or deleterious male characteristics can be understood if a correlation between preferred male characteristics and direct benefits for the female exists (see polygyny threshold model, e.g., Orians 1969 ), such as improved paternal care or territorial quality (e.g., Price et al. 1993 ; Iwasa and Pomiankowski 1999 ). This issue is particularly interesting in facultative polygynous mating systems with biparental care because the female's choice of a polygynous male as a mate is most often accompanied by a reduction in paternal care by this mate (for a review, see Ligon 1999 ). Several studies (e.g., Johnson et al. 1994 ; Pribil and Searcy 2001 ; Moreno et al. 2002 ; but see Both 2002 ) have observed a reduction in offspring number and/or offspring performance in females mated with a polygynous male, thus raising doubt in the relevancy of the polygyny threshold model (see, e.g., Orians 1969 ) in these instances. More generally, females of most species do not seem to take any appreciable direct benefits of their mate choice ( Kirkpatrick and Ryan 1991 ; Andersson 1994 ).
Since the 1970s, indirect benefits are discussed as a possibility to explain female choice ( Kirkpatrick and Ryan 1991 ; Andersson and Simmons 2006 ). Weatherhead and Robertson (1979) proposed the sexy son hypothesis (SSH) as an additional explanation for the establishment of polygyny. According to this hypothesis, females are assumed to compensate for their direct inferior breeding success by the increased number of descendents produced by their sexy—and therefore polygynous—sons (see Table 1 ). The idea of focusing on fitness advantages as a result of male attractiveness is similar to the “Fisherian runaway selection process” (see, e.g., Andersson and Simmons 2006 ). The “good genes” model (see Table 1 ), that is, the idea of an indirect benefit due to the male's genetic quality, is a second, complementary hypothesis that is discussed as a possibility to explain female choice by indirect benefits ( Andersson and Simmons 2006 ; Charmantier and Sheldon 2006 ). Both hypothesized mechanisms, SSH and good genes, belong to the same continuum of indirect selection ( Kokko et al. 2002 ; Radwan 2002 ). SSH as well as good genes postulate that indirect benefits due to male characteristics in a specific species will be similar for all females of this species (see also Table 1 ). Compatible genes, on the other hand, try to explain intraspecific differences in female mate choice (see Table 1 ). According to this theory, each individual female tries to enhance its fitness by choosing a male with a genetic pattern that is compatible with its own genetic configuration (e.g., Neff and Pitcher 2005 ).
Definitions of major concepts
Definitions |
Sexual conflict: Sexual conflict refers to intersexual conflict. It describes the diverging interests in males and females to optimize their fitness. From the point of few of one sex, this is done best if the mate cares for the young thus freeing resources (e.g., time and energy) that can be invested in additional offspring. Regarding to polygynous mating systems, sexual conflict means the optimization of male reproductive success by having mated with multiple females although reproductive success of a polygynously mated female is reduced. |
Good genes: Good genes refer to the assumption of an additive indirect genetic benefit that increases offspring quality. Offspring quality, that is, viability, leads to a compensation of any inferior direct reproductive success, that is, fewer offspring. |
Fisherian runaway selection: Fisherian runaway selection assumes indirect genetic benefits in mating decisions. This process assumes that sexual selection for a specific trait was at first correlated with a small increase in viability. During evolution, the female preference for this trait becomes exaggerated while the good genes effect loses its importance. At the end, sexual selection is driven only by the attractiveness of this trait and not by any additive genetic effect that is relevant for natural selection. |
Sexy son hypothesis: The sexy son hypothesis (SSH) is closely related to the good genes assumption and the fisherian runaway selection process. SSH, too, assumes indirect genetic benefits that are able to compensate any inferior direct reproductive success, that is, fewer offspring. The main difference between good genes and SSH is that SSH assumes an indirect effect due to the attractiveness of the sons whereas good genes focus on viability in sons and daughters. However, attractiveness is not specified and can be every trait that increases a male's probability to become polygynous. |
Compatible genes: Compatible genes also belong to indirect genetic benefits that increase the genetic quality of offspring. Whereas good genes generally lead to positive effects, compatible genes are beneficial only for specific individuals. That is, a positive effect depends on the interplay of the individual genetic architecture of both mates. |
Definitions |
Sexual conflict: Sexual conflict refers to intersexual conflict. It describes the diverging interests in males and females to optimize their fitness. From the point of few of one sex, this is done best if the mate cares for the young thus freeing resources (e.g., time and energy) that can be invested in additional offspring. Regarding to polygynous mating systems, sexual conflict means the optimization of male reproductive success by having mated with multiple females although reproductive success of a polygynously mated female is reduced. |
Good genes: Good genes refer to the assumption of an additive indirect genetic benefit that increases offspring quality. Offspring quality, that is, viability, leads to a compensation of any inferior direct reproductive success, that is, fewer offspring. |
Fisherian runaway selection: Fisherian runaway selection assumes indirect genetic benefits in mating decisions. This process assumes that sexual selection for a specific trait was at first correlated with a small increase in viability. During evolution, the female preference for this trait becomes exaggerated while the good genes effect loses its importance. At the end, sexual selection is driven only by the attractiveness of this trait and not by any additive genetic effect that is relevant for natural selection. |
Sexy son hypothesis: The sexy son hypothesis (SSH) is closely related to the good genes assumption and the fisherian runaway selection process. SSH, too, assumes indirect genetic benefits that are able to compensate any inferior direct reproductive success, that is, fewer offspring. The main difference between good genes and SSH is that SSH assumes an indirect effect due to the attractiveness of the sons whereas good genes focus on viability in sons and daughters. However, attractiveness is not specified and can be every trait that increases a male's probability to become polygynous. |
Compatible genes: Compatible genes also belong to indirect genetic benefits that increase the genetic quality of offspring. Whereas good genes generally lead to positive effects, compatible genes are beneficial only for specific individuals. That is, a positive effect depends on the interplay of the individual genetic architecture of both mates. |
Another approach to understand mating behavior that results in deleterious female reproductive success, such as offspring number or performance, is the idea of sexual conflict (see Table 1 ; Gavrilets et al. 2001 ; Cameron et al. 2003 ; Arnqvist and Rowe 2005 ; Andersson and Simmons 2006 ; Lessells 2006 ; Parker 2006 ; Rice et al. 2006 ). Sexual conflict is driven by the opposed reproductive interests in females and males that result in sexually antagonistic behavior and coevolving adaptations. Whereas the traditional hypotheses of sexual selection, such as the polygyny threshold model and SSH, discuss the importance of direct versus indirect benefits, sexual conflict considers the possibility that polygynous males are not beneficial to females, neither directly nor indirectly. For example, Arnqvist and Kirkpatrick (2005) demonstrated that extrapair copulations, which do not lead to any direct advantages such as additional food resources or shelter, in socially monogamous birds are in line with sexual conflict assumptions as indirect benefits are much smaller than the direct negative selection caused by depressed paternal investment by social males.
The present study will focus on SSH because SSH as well as the good genes assumption are based on the relative magnitude of direct and indirect effects of mate choice and thus have become relevant to the recent debate of whether sexual conflict can result in sexually antagonistic selection. SSH developed by Weatherhead and Robertson (1979) , further called “narrow-sense SSH,” refers to mating systems with biparental care. In these mating systems, females mated with a polygynous male normally receive less assistance than females mated with a monogamous male (see, e.g., Ligon 1999 ) and thus suffer from direct fitness consequences that have to be (over)compensated by their sexy sons. On the other hand, “broad-sense SSH” encompasses polygyny and promiscuous mating systems with and without biparental care. Alatalo (1998) argues that the costs of any additional choice may be so minor that female choice for honestly signaling males, that is good genes, may evolve even if the indirect benefits on offspring quality are small. A similar argument can be given for SSH if mates of attractive males do not suffer any direct fitness consequences.
Since it was first proposed by Weatherhead and Robertson (1979) , SSH has been discussed vividly. Several theoretical studies either support ( Weatherhead 1994 ; Kokko et al. 2002 ; McNamara et al. 2003 ) or attempt to argue against SSH (e.g., Kirkpatrick 1985 ; Wagner 1994 ; Alatalo and Ratti 1995 ). Recent sophisticated empirical studies dealing with SSH have reached contrasting results (compare Gwinner and Schwabl 2005 ; Head et al. 2005 ; Orteiza et al. 2005 ; Gustafsson and Qvarnström 2006 ; Huk and Winkel 2006 ). However, these studies differ in terms of the explicit research questions they ask and thus highlight the importance of questioning precisely what factors should be measured. The aim of the present paper is to shed light on the contrasting empirical results by analyzing the appropriateness of various research questions in empirical tests of SSH and in so doing to differentiate between “critical predictions” and “compatible predictions” (see Table 2 ). Critical predictions should be studied in future work as they address topics that are at the core of the predictions of SSH. Critical predictions are therefore able to validate or invalidate SSH as a hypothesis explaining female choice. Compatible predictions are aspects that can be discussed in the light of this hypothesis without directly addressing the critical predictions. Thus, compatible predictions only test for “by-product” predictions that can neither validate nor invalidate SSH.
Empirical studies dealing with compatible or critical predictions
Critical predictions (exhaustive) supportive of SSH | No support of SSH |
: Correlational study in pied flycatcher ( ); polygynous pairing in primary females resulted in sons with a higher probability of becoming polygynous. Moreover, a small but significant inferior direct reproductive success was compensated in terms of the number of grandchildren | : Correlational study in pied flycatcher ( ); polygynous pairing did not increase attractiveness in sons of secondary females, that is, the number of sons becoming polygynous. Moreover, females without male assistance were not able to compensate inferior direct reproductive success in terms of the number of grandchildren |
: Correlational study in the collared flycatcher ( ); polygynous pairing, that is, primary and secondary females with male assistance, resulted in less attractive sons and fewer grandchildren as compared with females mated with a monogamous male | |
: Experimental study in ; male descendents of attractive males were 30% more successful on average at securing mates than male descendents of unattractive males | : Experimental study in ; male descendents of attractive and unattractive fathers did not differ in productivity |
: Experimental study in the house cricket ( ); females mated to attractive males incur a substantial survival cost that is predominantly outweighed by their attractive sons | : Experimental study in ; a multigeneration fitness assay found that indirect effects through sexy sons could not recoup the direct costs |
: Experimental study in red flour beetles ( ); females mating with multiple males (2–16 males) enhanced the relative fitness of F1 males in comparison with singly mated females. F1 males from mothers with 16 different males inseminated more females than F1 males from mothers with a single partner. Thus, polyandry enhances the chance to get a mate with superior genes, that is, genes that increase the attractiveness of their sons | : Experimental study in the Mediterranean fruit fly ( ); father/son correlation and sibling analysis showed no heritable component to male copulatory success |
: Quantitative genetics in the field cricket ( ); no positive genetic correlation between paternity in sons and polyandry in daughters as predicted by the “sexy sperm” hypothesis | |
Compatible predictions (not exhaustive) supportive of SSH | No support of SSH |
: Experimental study in the pied flycatcher ( ); females preferred males with increased UV plumage coloration when the effect of territory quality is controlled for | : Correlational study in the American kestrel ( ); no male trait was clearly related to the time males had to wait before obtaining females |
: Experimental study in the pied flycatcher ( ); females trade between male mating status and male attractiveness | |
: Experimental study in the blue tit ( ); positive relationship between the proportion of sons in a male's brood and measures of ultraviolet plumage coloration | : Correlational study in the blue tit ( ); no relationship between male color ornamentation and brood sex ratio |
: Correlational study in the blue tit ( ); males with relatively brighter UV coloration produced a greater proportion of sons in their broods | : Experimental study in the sand lizard ( ); negative relationship between proportion of sons in a male's brood and male coloration |
: Correlational study in the blue tit ( ); positive relationship between the length of the strophe bout and the proportion of sons in their broods | : Experimental study in the red junglefowl ( ); no evidence for a female bias in the sex ratio of their brood in response to either their own condition or the attractiveness of the males with whom they copulate |
: Experimental study in the peafowl ( ); females control the primary sex ratio of their offspring in response to paternal attractiveness | and : Correlational study in the great reed warbler ( ); primary females had a higher proportion of sons in their broods than secondary females. According to SSH, primary and secondary females should adjust their sex ratio in favor of sons |
: Experimental study with captive canaries ( ); females exposed to playback of more attractive songs produced larger eggs that are normally more likely to hatch male offspring | |
showed heritability of male attractiveness in stickleback | : Experimental study in the blue tit ( ); variation in plumage coloration is only weakly heritable, and a positive genetic covariation between parental color and offspring fitness was not observed |
: Correlational study in zebra finches ( ); sons of attractive males have higher song rates | |
: Correlational/experimental study in the European starling ( ); sons of polygynous fathers defended more nest-boxes and produced more courtship songs | |
: Experimental study in the great tit ( ): plumage trait associated with attractiveness in males was heritable |
Critical predictions (exhaustive) supportive of SSH | No support of SSH |
: Correlational study in pied flycatcher ( ); polygynous pairing in primary females resulted in sons with a higher probability of becoming polygynous. Moreover, a small but significant inferior direct reproductive success was compensated in terms of the number of grandchildren | : Correlational study in pied flycatcher ( ); polygynous pairing did not increase attractiveness in sons of secondary females, that is, the number of sons becoming polygynous. Moreover, females without male assistance were not able to compensate inferior direct reproductive success in terms of the number of grandchildren |
: Correlational study in the collared flycatcher ( ); polygynous pairing, that is, primary and secondary females with male assistance, resulted in less attractive sons and fewer grandchildren as compared with females mated with a monogamous male | |
: Experimental study in ; male descendents of attractive males were 30% more successful on average at securing mates than male descendents of unattractive males | : Experimental study in ; male descendents of attractive and unattractive fathers did not differ in productivity |
: Experimental study in the house cricket ( ); females mated to attractive males incur a substantial survival cost that is predominantly outweighed by their attractive sons | : Experimental study in ; a multigeneration fitness assay found that indirect effects through sexy sons could not recoup the direct costs |
: Experimental study in red flour beetles ( ); females mating with multiple males (2–16 males) enhanced the relative fitness of F1 males in comparison with singly mated females. F1 males from mothers with 16 different males inseminated more females than F1 males from mothers with a single partner. Thus, polyandry enhances the chance to get a mate with superior genes, that is, genes that increase the attractiveness of their sons | : Experimental study in the Mediterranean fruit fly ( ); father/son correlation and sibling analysis showed no heritable component to male copulatory success |
: Quantitative genetics in the field cricket ( ); no positive genetic correlation between paternity in sons and polyandry in daughters as predicted by the “sexy sperm” hypothesis | |
Compatible predictions (not exhaustive) supportive of SSH | No support of SSH |
: Experimental study in the pied flycatcher ( ); females preferred males with increased UV plumage coloration when the effect of territory quality is controlled for | : Correlational study in the American kestrel ( ); no male trait was clearly related to the time males had to wait before obtaining females |
: Experimental study in the pied flycatcher ( ); females trade between male mating status and male attractiveness | |
: Experimental study in the blue tit ( ); positive relationship between the proportion of sons in a male's brood and measures of ultraviolet plumage coloration | : Correlational study in the blue tit ( ); no relationship between male color ornamentation and brood sex ratio |
: Correlational study in the blue tit ( ); males with relatively brighter UV coloration produced a greater proportion of sons in their broods | : Experimental study in the sand lizard ( ); negative relationship between proportion of sons in a male's brood and male coloration |
: Correlational study in the blue tit ( ); positive relationship between the length of the strophe bout and the proportion of sons in their broods | : Experimental study in the red junglefowl ( ); no evidence for a female bias in the sex ratio of their brood in response to either their own condition or the attractiveness of the males with whom they copulate |
: Experimental study in the peafowl ( ); females control the primary sex ratio of their offspring in response to paternal attractiveness | and : Correlational study in the great reed warbler ( ); primary females had a higher proportion of sons in their broods than secondary females. According to SSH, primary and secondary females should adjust their sex ratio in favor of sons |
: Experimental study with captive canaries ( ); females exposed to playback of more attractive songs produced larger eggs that are normally more likely to hatch male offspring | |
showed heritability of male attractiveness in stickleback | : Experimental study in the blue tit ( ); variation in plumage coloration is only weakly heritable, and a positive genetic covariation between parental color and offspring fitness was not observed |
: Correlational study in zebra finches ( ); sons of attractive males have higher song rates | |
: Correlational/experimental study in the European starling ( ); sons of polygynous fathers defended more nest-boxes and produced more courtship songs | |
: Experimental study in the great tit ( ): plumage trait associated with attractiveness in males was heritable |
A major reason for the different conclusions reached by empirical studies dealing with SSH is that they differ both in terms of the variables investigated and of the different treatment factors compared. How best to test SSH empirically? The major emerging critical prediction is that indirect effects, that is, genes delivered by the male, do increase lifetime reproductive success in sons. Therefore, females of attractive males, which in narrow-sense SSH suffer from inferior direct fitness estimates, in the long run achieve the same fitness as females mated with a monogamous male ( Weatherhead and Robertson 1979 ). This prediction, however, occurs also in the good genes hypothesis (see Andersson and Simmons 2006 ; Charmantier and Sheldon 2006 ). According to SSH, it is their sexy sons who are assumed to be responsible for this compensation as they inherit their father's genes which in turn enable them to become polygynous themselves, thus increasing the number of grandchildren produced. SSH therefore predicts that females mated with a polygynous male rear sons that become polygynous themselves. More specifically, the number of polygynous breeding attempts should be higher in sons descending from females mated with a polygamous male than in sons descending from females mated with a monogamous male. Investigation of this prediction would clarify whether the underlying assumption of SSH, that is heritability of attractiveness, is valid. This would be, however, no direct evidence for the compensation of any direct inferior fitness consequences. To test SSH, the aforementioned second critical prediction must also be investigated, that is, a fitness (over)compensation with respect to the number of F 1+ x descendents.
SSH as well as the good genes hypothesis predict that the number of grand-offspring is similar or higher in females mated with a polygynous male than in females mated with a monogamous male. Whereas a greater number of grand-offspring would be in line with SSH, a lower number would be an argument against this hypothesis. In case of a similar reproductive success, it is rather difficult to distinguish between 1) instances that observed no significant differences in fitness due to the fact that there really were no differences—as proposed by SSH—and 2) empirical studies that failed to detect a difference in the number of grandchildren, although females mated with a polygynous male in fact could not compensate direct inferior reproductive success. In long-lived species with several subsequent breeding events, another important parameter is the consequence of mating behavior for any further breeding success in the future, that is, the risk to suffer from sexually transmitted diseases especially in females mating with polygynous males (see Thrall et al. 2000 ). SSH studies on long-lived species, therefore, have to investigate not only the number of grandchildren of the actual breeding event but also possible subsequent differences in female longevity or offspring performance in any further breeding attempts (for the possibility of an increase in longevity in polygynously mated females, see also Garamszegi et al. 2004 ).
However, only few empirical studies have to date focused on these critical predictions. In particular, lifetime reproductive success, measured in terms of the number of grand-offspring produced, has rarely been investigated (see Hunt et al. 2004 ).
In addition to these critical predictions, further research predictions that encompass the theoretical concept of SSH can be made. These compatible predictions deal with topics that rely on the validity of the critical predictions mentioned above. Females have to be able to detect males that will pass on genes that increase the probability of rearing sons that will become polygynous during their own reproductive phase. Various studies demonstrate that females are able to detect “attractive” males by secondary sexual characteristics such as ornamentation (e.g., Gustafsson et al. 1995 ; Sheldon et al. 1999 ; Siitari et al. 2002 ). As a second compatible prediction, one should expect that females trade mate choice between male attractiveness and pairing status in species with biparental care as pairing status is an indicator of male assistance in these species. Third, if a female mated with a polygynous male rears sexy sons that are able to compensate any direct inferior reproductive success, one might argue that such females could be expected to shift their brood sex ratio toward producing more males than females ( Burley 1981 ). Similarly, one should expect that sons of polygynous (thus attractive) males should exhibit behavior that increases their probability to become polygynous themselves.
Especially interesting are female decision opportunities in species with short-term pair-bond, that is, species in which females and males choose their partner at the beginning of the actual reproduction period, in which fitness is mainly attributed to habitat quality, male assistance during the rearing of offspring, and heritable male attractiveness. For convenience, a facultative bigynous mating system is assumed to make female mate choice decisions more explicit. At the beginning of the reproduction period, all males are unmated. Females should therefore select males on the basis of habitat quality and attractiveness; they should primarily select attractive males occupying a superior habitat in order to increase their reproductive success. In the subsequent breeding season, unpaired females have ideally to decide whether 1) to mate with these superior (but already mated) males, thus becoming a secondary female sharing their male with the already existing primary female, or to mate with a still unmated male that is either 2) less attractive or 3) one which occupies a territory of lower quality (but see, e.g., Searcy and Yasukawa 1989 for alternative no-compensation models). In the latter case, these females do not have to share their monogamous mate. In order to test SSH, only females of the categories 1) and 2) should be compared with test SSH. A comparison between 1) and 3), on the other hand, is not appropriate for testing SSH as fitness differences are due to differences in territorial differences. A good system may be, therefore, a facultative polygynous species that does not defend feeding territories, thus making territorial differences less important.
When testing SSH, it is important to analyze the reason for a mate choice decision in favor of a polygamous male. Is the choice based on the attractiveness of the male or is it based on the quality of the male's territory? It should be stressed that in the case of a positive correlation between territorial quality and male attractiveness, it is quite difficult to investigate SSH as it is not possible to distinguish fitness consequences between these 2 confounding factors in the field, that is territorial quality and male attractiveness. In this case, only experimental approaches can solve this problem by controlling territorial quality in the laboratory or by cross fostering of whole or partial broods (see, e.g., Hadfield et al. 2006 ). If habitat quality and male attractiveness are independent, that is, female choice in the sense of runaway selection, females should select an already mated male for 2 reasons: the already mated male occupies a superior habitat or is more attractive than the unpaired male. As one cannot rule out in most cases that territorial quality and male attractiveness are at least partially correlated, studies focusing on SSH experimental approaches, for example, cross fostering of whole or partial broods between females with different mating status and in territories of different quality, have to be preferred over correlational studies as they allow to break down correlations between territorial quality and male attractiveness.
As regards female perspective in mate choice, 3 categories can, therefore, be distinguished for females breeding in territories of similar quality: females mated with a monogamous male, primary females, and secondary females. As primary females, as a rule, receive more male assistance ( Ligon 1999 ), their direct reproductive success is less affected than that of secondary females. Primary females therefore combine a pattern of relatively high direct male assistance with the supposed indirect benefits due to the genetic constitution of their attractive males (however, it is important not to consider primary females mated with a less attractive male occupying a superior habitat, see above). According to this combination, a comparison of primary females and females mated with a monogamous male should exhibit not only more polygynous sons in primary females but also a significantly higher number of grand-offspring as differences in direct reproductive success should be of minor importance.
What have we learned from existing empirical studies? Different empirical studies demonstrated that female mating decisions may be explained by the good genes model of sexual selection. It was demonstrated that attractive males confer disease resistance to their offspring ( Barber et al. 2001 ) or increase offspring viability ( Hasselquist 1998 ; Byers and Waits 2006 ; Forsman and Hagman 2006 ). Whereas good genes may support fitness of sons and/or daughters, SSH predicts fitness advantages in sons only. According to Alatalo and Lundberg (1986) , SSH should not apply in the model species Ficedula hypoleuca due to poor phenotypic quality of nestlings of secondary females. In their study, they supported their assumption by estimation of lifetime reproductive success. Contrary, Johnson et al. (1994) concluded that secondary females in the house wren ( Troglodytes aedon ) may recoup fitness losses in the next generation if male mating success is at least moderately heritable. However, both studies did not measure critical predictions of SSH directly.
A review of empirical studies revealed that compatible predictions of SSH were investigated by a rather large variety of studies. The majority of these studies have not been intended to look especially at SSH because compatible predictions of SSH do play a role in other theoretical concepts as well. Although each compatible prediction was supported by empirical results, the pattern is not unequivocal because several studies observed pattern that contradict compatible SSH predictions (see Table 2 ).
Empirical studies dealing with critical predictions of SSH are rare. These empirical approaches used either birds or insects as model species, whereas studies on other taxa are missing (see, e.g., Barbosa and Magurran 2006 ). Two correlational studies in the closely related collared flycatcher ( Ficedula albicollis ) and pied flycatcher ( F. hypoleuca ) revealed no support for narrow-sense SSH, that is, no compensation of inferior reproductive success via sexy sons (see Table 2 ; Gustafsson and Qvarnström 2006 ; Huk and Winkel 2006 ). However, in the pied flycatcher, this result held true for secondary females, whereas in primary females, that is, females with a relatively weak inferior direct reproductive success, the pattern was compatible with SSH (see Table 2 ). Recent studies, however, that did not look at the number of grand-offspring came to contrasting results ( Both 2002 ; Garamszegi et al. 2004 ).
To sum up, it can be concluded that empirical studies dealing with critical predictions to date only partially support SSH; that is, only studies with rather small direct fitness consequences are compatible with critical SSH predictions. Contrary, the demonstration of compensation of considerable lower direct reproductive success via a heritable genetic effect of male attractiveness, and hence male mating status in sons, is not demonstrated until now. Thus, facultative polygyny in biparental species seems to be best explained by sexual conflict. Approaches derived from quantitative genetic models of mate choice came to similar results ( Kirkpatrick and Barton 1997 ; Charmantier and Sheldon 2006 ; Hadfield et al. 2006 ; Qvarnström et al. 2006 ). Recent studies therefore support the position that inferior direct reproductive success cannot be overcompensated by a “sexy son” effect (e.g., Kirkpatrick 1985 ). Thus, attractiveness of sexy sons and its resulting fitness advantages seem to be of minor biological effect.
The deception hypothesis, that is, the assumption that males hide their mating status and deceive females into polygyny in polyterritorial species ( Alatalo et al. 1982 ; Temrin and Arak 1989 ; Ligon 1999 ), is an alternative no-compensation explanation that fits into sexual conflict assumptions. Slagsvold and Dale (1994) found little support for deception hypothesis while observing pied flycatcher behavior. They instead suggest that female pied flycatchers are able to detect male mating status but sometimes settle with mated males because of cost of searching thus doing “the best of a bad job.”
The impact of extrapair copulations for female choice decisions in the light of SSH has already been discussed by Wagner (1994) and Weatherhead (1984) . As extrapair copulations allow females to benefit from “sexy genes” of attractive males even if they are not the primary or secondary female, differences between females mated with a monogamous male and primary or secondary females can be assumed to be mitigated. Thus, differences in territorial quality will become more important in these cases, highlighting the importance of comparing only females breeding in habitats of similar quality. A more precise approach would be to genetically detect male paternity (see Andersson and Simmons 2006 ).
As empirical studies focusing on critical SSH predictions are rare, future research is needed: ideally, the comparison of future fitness consequences between females that choose to be secondary females with the success they would have had if they had been paired with a monogamous male. As this is principally not possible, future research should therefore focus on a comparison of total fitness, that is, number of grand-offspring, between females mated with a monogamous male and primary females as well as between females mated with a monogamous male and secondary females while controlling for possible confounding variables such as timing of reproduction, habitat quality, and frequency of interactions with males. This total fitness measure should also incorporate female and offspring survival. Furthermore, these studies should use genetic proof of paternity as social matings are generally only rather poor measures of the numbers of offspring produced (e.g., Griffith et al. 2002 ). A fruitful avenue would be experimental approaches such as cross fostering of whole or partial broods (see, e.g., Hadfield et al. 2006 ) between females with different mating status.
We thank Ben Sheldon as well as anonymous referees for valuable comments.
Google Scholar
Month: | Total Views: |
---|---|
November 2016 | 4 |
December 2016 | 8 |
January 2017 | 8 |
February 2017 | 25 |
March 2017 | 23 |
April 2017 | 20 |
May 2017 | 32 |
June 2017 | 12 |
July 2017 | 5 |
August 2017 | 12 |
September 2017 | 16 |
October 2017 | 26 |
November 2017 | 49 |
December 2017 | 205 |
January 2018 | 166 |
February 2018 | 147 |
March 2018 | 260 |
April 2018 | 280 |
May 2018 | 184 |
June 2018 | 197 |
July 2018 | 142 |
August 2018 | 134 |
September 2018 | 97 |
October 2018 | 72 |
November 2018 | 161 |
December 2018 | 190 |
January 2019 | 217 |
February 2019 | 156 |
March 2019 | 223 |
April 2019 | 255 |
May 2019 | 210 |
June 2019 | 149 |
July 2019 | 184 |
August 2019 | 124 |
September 2019 | 160 |
October 2019 | 155 |
November 2019 | 225 |
December 2019 | 226 |
January 2020 | 168 |
February 2020 | 209 |
March 2020 | 198 |
April 2020 | 243 |
May 2020 | 119 |
June 2020 | 145 |
July 2020 | 95 |
August 2020 | 127 |
September 2020 | 167 |
October 2020 | 176 |
November 2020 | 219 |
December 2020 | 161 |
January 2021 | 146 |
February 2021 | 160 |
March 2021 | 182 |
April 2021 | 191 |
May 2021 | 220 |
June 2021 | 145 |
July 2021 | 126 |
August 2021 | 136 |
September 2021 | 103 |
October 2021 | 186 |
November 2021 | 187 |
December 2021 | 160 |
January 2022 | 207 |
February 2022 | 170 |
March 2022 | 282 |
April 2022 | 229 |
May 2022 | 229 |
June 2022 | 159 |
July 2022 | 172 |
August 2022 | 163 |
September 2022 | 210 |
October 2022 | 288 |
November 2022 | 241 |
December 2022 | 260 |
January 2023 | 203 |
February 2023 | 179 |
March 2023 | 224 |
April 2023 | 271 |
May 2023 | 232 |
June 2023 | 213 |
July 2023 | 192 |
August 2023 | 238 |
September 2023 | 162 |
October 2023 | 180 |
November 2023 | 285 |
December 2023 | 235 |
January 2024 | 203 |
February 2024 | 150 |
March 2024 | 285 |
April 2024 | 225 |
May 2024 | 235 |
June 2024 | 188 |
July 2024 | 96 |
August 2024 | 71 |
Email alerts
Citing articles via.
- Recommend to your Library
Affiliations
- Online ISSN 1465-7279
- Copyright © 2024 International Society of Behavioral Ecology
- About Oxford Academic
- Publish journals with us
- University press partners
- What we publish
- New features
- Open access
- Institutional account management
- Rights and permissions
- Get help with access
- Accessibility
- Advertising
- Media enquiries
- Oxford University Press
- Oxford Languages
- University of Oxford
Oxford University Press is a department of the University of Oxford. It furthers the University's objective of excellence in research, scholarship, and education by publishing worldwide
- Copyright © 2024 Oxford University Press
- Cookie settings
- Cookie policy
- Privacy policy
- Legal notice
This Feature Is Available To Subscribers Only
Sign In or Create an Account
This PDF is available to Subscribers Only
For full access to this pdf, sign in to an existing account, or purchase an annual subscription.
- Bipolar Disorder
- Therapy Center
- When To See a Therapist
- Types of Therapy
- Best Online Therapy
- Best Couples Therapy
- Managing Stress
- Sleep and Dreaming
- Understanding Emotions
- Self-Improvement
- Healthy Relationships
- Student Resources
- Personality Types
- Sweepstakes
- Guided Meditations
- Verywell Mind Insights
- 2024 Verywell Mind 25
- Mental Health in the Classroom
- Editorial Process
- Meet Our Review Board
- Crisis Support
How to Write a Great Hypothesis
Hypothesis Definition, Format, Examples, and Tips
Verywell / Alex Dos Diaz
- The Scientific Method
Hypothesis Format
Falsifiability of a hypothesis.
- Operationalization
Hypothesis Types
Hypotheses examples.
- Collecting Data
A hypothesis is a tentative statement about the relationship between two or more variables. It is a specific, testable prediction about what you expect to happen in a study. It is a preliminary answer to your question that helps guide the research process.
Consider a study designed to examine the relationship between sleep deprivation and test performance. The hypothesis might be: "This study is designed to assess the hypothesis that sleep-deprived people will perform worse on a test than individuals who are not sleep-deprived."
At a Glance
A hypothesis is crucial to scientific research because it offers a clear direction for what the researchers are looking to find. This allows them to design experiments to test their predictions and add to our scientific knowledge about the world. This article explores how a hypothesis is used in psychology research, how to write a good hypothesis, and the different types of hypotheses you might use.
The Hypothesis in the Scientific Method
In the scientific method , whether it involves research in psychology, biology, or some other area, a hypothesis represents what the researchers think will happen in an experiment. The scientific method involves the following steps:
- Forming a question
- Performing background research
- Creating a hypothesis
- Designing an experiment
- Collecting data
- Analyzing the results
- Drawing conclusions
- Communicating the results
The hypothesis is a prediction, but it involves more than a guess. Most of the time, the hypothesis begins with a question which is then explored through background research. At this point, researchers then begin to develop a testable hypothesis.
Unless you are creating an exploratory study, your hypothesis should always explain what you expect to happen.
In a study exploring the effects of a particular drug, the hypothesis might be that researchers expect the drug to have some type of effect on the symptoms of a specific illness. In psychology, the hypothesis might focus on how a certain aspect of the environment might influence a particular behavior.
Remember, a hypothesis does not have to be correct. While the hypothesis predicts what the researchers expect to see, the goal of the research is to determine whether this guess is right or wrong. When conducting an experiment, researchers might explore numerous factors to determine which ones might contribute to the ultimate outcome.
In many cases, researchers may find that the results of an experiment do not support the original hypothesis. When writing up these results, the researchers might suggest other options that should be explored in future studies.
In many cases, researchers might draw a hypothesis from a specific theory or build on previous research. For example, prior research has shown that stress can impact the immune system. So a researcher might hypothesize: "People with high-stress levels will be more likely to contract a common cold after being exposed to the virus than people who have low-stress levels."
In other instances, researchers might look at commonly held beliefs or folk wisdom. "Birds of a feather flock together" is one example of folk adage that a psychologist might try to investigate. The researcher might pose a specific hypothesis that "People tend to select romantic partners who are similar to them in interests and educational level."
Elements of a Good Hypothesis
So how do you write a good hypothesis? When trying to come up with a hypothesis for your research or experiments, ask yourself the following questions:
- Is your hypothesis based on your research on a topic?
- Can your hypothesis be tested?
- Does your hypothesis include independent and dependent variables?
Before you come up with a specific hypothesis, spend some time doing background research. Once you have completed a literature review, start thinking about potential questions you still have. Pay attention to the discussion section in the journal articles you read . Many authors will suggest questions that still need to be explored.
How to Formulate a Good Hypothesis
To form a hypothesis, you should take these steps:
- Collect as many observations about a topic or problem as you can.
- Evaluate these observations and look for possible causes of the problem.
- Create a list of possible explanations that you might want to explore.
- After you have developed some possible hypotheses, think of ways that you could confirm or disprove each hypothesis through experimentation. This is known as falsifiability.
In the scientific method , falsifiability is an important part of any valid hypothesis. In order to test a claim scientifically, it must be possible that the claim could be proven false.
Students sometimes confuse the idea of falsifiability with the idea that it means that something is false, which is not the case. What falsifiability means is that if something was false, then it is possible to demonstrate that it is false.
One of the hallmarks of pseudoscience is that it makes claims that cannot be refuted or proven false.
The Importance of Operational Definitions
A variable is a factor or element that can be changed and manipulated in ways that are observable and measurable. However, the researcher must also define how the variable will be manipulated and measured in the study.
Operational definitions are specific definitions for all relevant factors in a study. This process helps make vague or ambiguous concepts detailed and measurable.
For example, a researcher might operationally define the variable " test anxiety " as the results of a self-report measure of anxiety experienced during an exam. A "study habits" variable might be defined by the amount of studying that actually occurs as measured by time.
These precise descriptions are important because many things can be measured in various ways. Clearly defining these variables and how they are measured helps ensure that other researchers can replicate your results.
Replicability
One of the basic principles of any type of scientific research is that the results must be replicable.
Replication means repeating an experiment in the same way to produce the same results. By clearly detailing the specifics of how the variables were measured and manipulated, other researchers can better understand the results and repeat the study if needed.
Some variables are more difficult than others to define. For example, how would you operationally define a variable such as aggression ? For obvious ethical reasons, researchers cannot create a situation in which a person behaves aggressively toward others.
To measure this variable, the researcher must devise a measurement that assesses aggressive behavior without harming others. The researcher might utilize a simulated task to measure aggressiveness in this situation.
Hypothesis Checklist
- Does your hypothesis focus on something that you can actually test?
- Does your hypothesis include both an independent and dependent variable?
- Can you manipulate the variables?
- Can your hypothesis be tested without violating ethical standards?
The hypothesis you use will depend on what you are investigating and hoping to find. Some of the main types of hypotheses that you might use include:
- Simple hypothesis : This type of hypothesis suggests there is a relationship between one independent variable and one dependent variable.
- Complex hypothesis : This type suggests a relationship between three or more variables, such as two independent and dependent variables.
- Null hypothesis : This hypothesis suggests no relationship exists between two or more variables.
- Alternative hypothesis : This hypothesis states the opposite of the null hypothesis.
- Statistical hypothesis : This hypothesis uses statistical analysis to evaluate a representative population sample and then generalizes the findings to the larger group.
- Logical hypothesis : This hypothesis assumes a relationship between variables without collecting data or evidence.
A hypothesis often follows a basic format of "If {this happens} then {this will happen}." One way to structure your hypothesis is to describe what will happen to the dependent variable if you change the independent variable .
The basic format might be: "If {these changes are made to a certain independent variable}, then we will observe {a change in a specific dependent variable}."
A few examples of simple hypotheses:
- "Students who eat breakfast will perform better on a math exam than students who do not eat breakfast."
- "Students who experience test anxiety before an English exam will get lower scores than students who do not experience test anxiety."
- "Motorists who talk on the phone while driving will be more likely to make errors on a driving course than those who do not talk on the phone."
- "Children who receive a new reading intervention will have higher reading scores than students who do not receive the intervention."
Examples of a complex hypothesis include:
- "People with high-sugar diets and sedentary activity levels are more likely to develop depression."
- "Younger people who are regularly exposed to green, outdoor areas have better subjective well-being than older adults who have limited exposure to green spaces."
Examples of a null hypothesis include:
- "There is no difference in anxiety levels between people who take St. John's wort supplements and those who do not."
- "There is no difference in scores on a memory recall task between children and adults."
- "There is no difference in aggression levels between children who play first-person shooter games and those who do not."
Examples of an alternative hypothesis:
- "People who take St. John's wort supplements will have less anxiety than those who do not."
- "Adults will perform better on a memory task than children."
- "Children who play first-person shooter games will show higher levels of aggression than children who do not."
Collecting Data on Your Hypothesis
Once a researcher has formed a testable hypothesis, the next step is to select a research design and start collecting data. The research method depends largely on exactly what they are studying. There are two basic types of research methods: descriptive research and experimental research.
Descriptive Research Methods
Descriptive research such as case studies , naturalistic observations , and surveys are often used when conducting an experiment is difficult or impossible. These methods are best used to describe different aspects of a behavior or psychological phenomenon.
Once a researcher has collected data using descriptive methods, a correlational study can examine how the variables are related. This research method might be used to investigate a hypothesis that is difficult to test experimentally.
Experimental Research Methods
Experimental methods are used to demonstrate causal relationships between variables. In an experiment, the researcher systematically manipulates a variable of interest (known as the independent variable) and measures the effect on another variable (known as the dependent variable).
Unlike correlational studies, which can only be used to determine if there is a relationship between two variables, experimental methods can be used to determine the actual nature of the relationship—whether changes in one variable actually cause another to change.
The hypothesis is a critical part of any scientific exploration. It represents what researchers expect to find in a study or experiment. In situations where the hypothesis is unsupported by the research, the research still has value. Such research helps us better understand how different aspects of the natural world relate to one another. It also helps us develop new hypotheses that can then be tested in the future.
Thompson WH, Skau S. On the scope of scientific hypotheses . R Soc Open Sci . 2023;10(8):230607. doi:10.1098/rsos.230607
Taran S, Adhikari NKJ, Fan E. Falsifiability in medicine: what clinicians can learn from Karl Popper [published correction appears in Intensive Care Med. 2021 Jun 17;:]. Intensive Care Med . 2021;47(9):1054-1056. doi:10.1007/s00134-021-06432-z
Eyler AA. Research Methods for Public Health . 1st ed. Springer Publishing Company; 2020. doi:10.1891/9780826182067.0004
Nosek BA, Errington TM. What is replication ? PLoS Biol . 2020;18(3):e3000691. doi:10.1371/journal.pbio.3000691
Aggarwal R, Ranganathan P. Study designs: Part 2 - Descriptive studies . Perspect Clin Res . 2019;10(1):34-36. doi:10.4103/picr.PICR_154_18
Nevid J. Psychology: Concepts and Applications. Wadworth, 2013.
By Kendra Cherry, MSEd Kendra Cherry, MS, is a psychosocial rehabilitation specialist, psychology educator, and author of the "Everything Psychology Book."
- More from M-W
- To save this word, you'll need to log in. Log In
Definition of hypothesis
Did you know.
The Difference Between Hypothesis and Theory
A hypothesis is an assumption, an idea that is proposed for the sake of argument so that it can be tested to see if it might be true.
In the scientific method, the hypothesis is constructed before any applicable research has been done, apart from a basic background review. You ask a question, read up on what has been studied before, and then form a hypothesis.
A hypothesis is usually tentative; it's an assumption or suggestion made strictly for the objective of being tested.
A theory , in contrast, is a principle that has been formed as an attempt to explain things that have already been substantiated by data. It is used in the names of a number of principles accepted in the scientific community, such as the Big Bang Theory . Because of the rigors of experimentation and control, it is understood to be more likely to be true than a hypothesis is.
In non-scientific use, however, hypothesis and theory are often used interchangeably to mean simply an idea, speculation, or hunch, with theory being the more common choice.
Since this casual use does away with the distinctions upheld by the scientific community, hypothesis and theory are prone to being wrongly interpreted even when they are encountered in scientific contexts—or at least, contexts that allude to scientific study without making the critical distinction that scientists employ when weighing hypotheses and theories.
The most common occurrence is when theory is interpreted—and sometimes even gleefully seized upon—to mean something having less truth value than other scientific principles. (The word law applies to principles so firmly established that they are almost never questioned, such as the law of gravity.)
This mistake is one of projection: since we use theory in general to mean something lightly speculated, then it's implied that scientists must be talking about the same level of uncertainty when they use theory to refer to their well-tested and reasoned principles.
The distinction has come to the forefront particularly on occasions when the content of science curricula in schools has been challenged—notably, when a school board in Georgia put stickers on textbooks stating that evolution was "a theory, not a fact, regarding the origin of living things." As Kenneth R. Miller, a cell biologist at Brown University, has said , a theory "doesn’t mean a hunch or a guess. A theory is a system of explanations that ties together a whole bunch of facts. It not only explains those facts, but predicts what you ought to find from other observations and experiments.”
While theories are never completely infallible, they form the basis of scientific reasoning because, as Miller said "to the best of our ability, we’ve tested them, and they’ve held up."
- proposition
- supposition
hypothesis , theory , law mean a formula derived by inference from scientific data that explains a principle operating in nature.
hypothesis implies insufficient evidence to provide more than a tentative explanation.
theory implies a greater range of evidence and greater likelihood of truth.
law implies a statement of order and relation in nature that has been found to be invariable under the same conditions.
Examples of hypothesis in a Sentence
These examples are programmatically compiled from various online sources to illustrate current usage of the word 'hypothesis.' Any opinions expressed in the examples do not represent those of Merriam-Webster or its editors. Send us feedback about these examples.
Word History
Greek, from hypotithenai to put under, suppose, from hypo- + tithenai to put — more at do
1641, in the meaning defined at sense 1a
Phrases Containing hypothesis
- counter - hypothesis
- nebular hypothesis
- null hypothesis
- planetesimal hypothesis
- Whorfian hypothesis
Articles Related to hypothesis
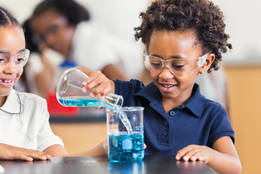
This is the Difference Between a...
This is the Difference Between a Hypothesis and a Theory
In scientific reasoning, they're two completely different things
Dictionary Entries Near hypothesis
hypothermia
hypothesize
Cite this Entry
“Hypothesis.” Merriam-Webster.com Dictionary , Merriam-Webster, https://www.merriam-webster.com/dictionary/hypothesis. Accessed 26 Aug. 2024.
Kids Definition
Kids definition of hypothesis, medical definition, medical definition of hypothesis, more from merriam-webster on hypothesis.
Nglish: Translation of hypothesis for Spanish Speakers
Britannica English: Translation of hypothesis for Arabic Speakers
Britannica.com: Encyclopedia article about hypothesis
Subscribe to America's largest dictionary and get thousands more definitions and advanced search—ad free!
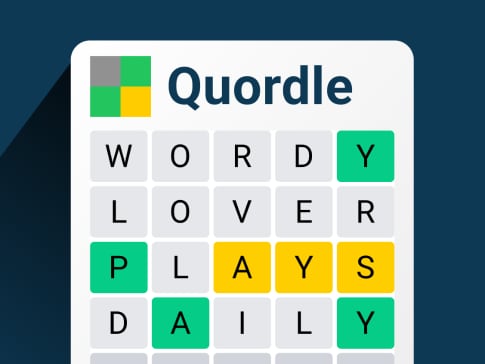
Can you solve 4 words at once?
Word of the day.
See Definitions and Examples »
Get Word of the Day daily email!
Popular in Grammar & Usage
Plural and possessive names: a guide, 31 useful rhetorical devices, more commonly misspelled words, why does english have so many silent letters, your vs. you're: how to use them correctly, popular in wordplay, 8 words for lesser-known musical instruments, it's a scorcher words for the summer heat, 7 shakespearean insults to make life more interesting, birds say the darndest things, 10 words from taylor swift songs (merriam's version), games & quizzes.
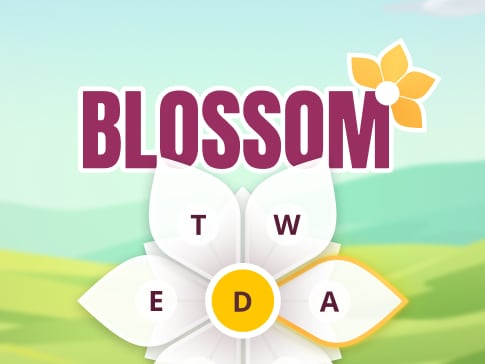
BREAKING: Special counsel Jack Smith appeals decision by Florida judge to dismiss Trump's classified documents case
What is a nonverbal learning disorder? Tim Walz’s son Gus’ condition, explained
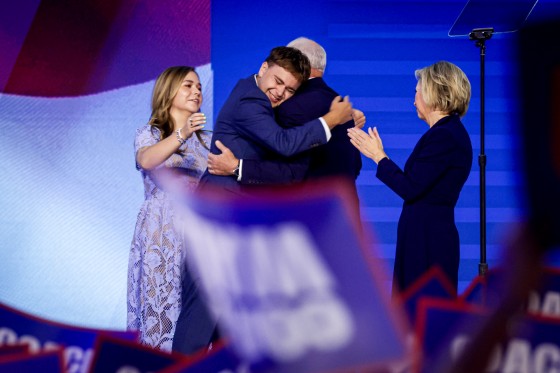
Gus Walz stole the show Wednesday when his father, Minnesota Gov. Tim Walz, officially accepted the vice presidential nomination on the third night of the Democratic National Convention.
The 17-year-old stood up during his father’s speech and said, “That’s my dad,” later adding, “I love you, Dad.”
The governor and his wife, Gwen Walz, revealed in a People interview that their son was diagnosed with nonverbal learning disability as a teenager.
A 2020 study estimated that as many as 2.9 million children and adolescents in North America have nonverbal learning disability, or NVLD, which affects a person’s spatial-visual skills.
The number of people who receive a diagnosis is likely much smaller than those living with the disability, said Santhosh Girirajan, the T. Ming Chu professor of biochemistry and molecular biology and professor of genomics at Penn State.
“These individuals are very intelligent and articulate well verbally, but they are typically clumsy with motor and spatial coordination,” he told NBC News. “It’s called a learning disorder because there are a lot of cues other than verbal cues that are necessary for us to keep information in our memory.”
People with NVLD often struggle with visual-spatial skills, such as reading a map, following directions, identifying mathematical patterns, remembering how to navigate spaces or fitting blocks together. Social situations can also be difficult.
“Body language and some of the things we think about with day-to-day social norms, they may not be able to catch those,” Girirajan said.
Unlike other learning disabilities such as dyslexia, signs of the disability typically don't become apparent until adolescence.
Early in elementary school, learning is focused largely on memorization — learning words or performing straightforward mathematical equations, at which people with NVLD typically excel. Social skills are also more concrete, such as playing a game of tag at recess.
“But as you get older, there’s a lot more subtlety, like sarcasm, that you have to understand in social interactions, that these kids might not understand,” said Laura Phillips, senior director and senior neuropsychologist of the Learning and Development Center at the Child Mind Institute, a nonprofit organization in New York.
In her own practice, she typically sees adolescents with NVLD, who usually have an average or above average IQ, when school demands more integrated knowledge and executive functioning, such as reading comprehension or integrating learning between subjects. They also usually seek help for something else, usually anxiety or depression, which are common among people with NVLD.
Sometimes misdiagnosed as autism
Amy Margolis, director of the Environment, Brain, and Behavior Lab at Columbia University, is part of a group of researchers that is beginning to call the disability “developmental visual-spatial disorder” in an effort to better describe how it affects people who have it.
People with NVLD are “very much verbal,” Margolis said, contrary to what the name suggests.
The learning disability is sometimes misdiagnosed as autism spectrum disorder. Margolis led a 2019 study that found that although kids with autism spectrum disorder and NVLD often have overlapping traits, the underlying neurobiology — that is, what’s happening in their brains to cause these traits — is unique between the two conditions.
Margolis is trying to get NVLD recognized by the DSM-5, the handbook health care providers use to diagnose mental health conditions. Without such official recognition, people with NVLD can struggle to get the resources they need, such as special class placements or extra support in school.
“Without an officially recognized diagnosis, it’s hard for parents to understand how to seek information, and then communicate to other people what kinds of things might be challenging for their kid,” Phillips said, adding that widespread awareness is key to helping these families navigate NVLD.
Kaitlin Sullivan is a contributor for NBCNews.com who has worked with NBC News Investigations. She reports on health, science and the environment and is a graduate of the Craig Newmark Graduate School of Journalism at City University of New York.

- Cambridge Dictionary +Plus
Meaning of hypothesis in English
Your browser doesn't support HTML5 audio
- abstraction
- accepted wisdom
- afterthought
- anthropocentrism
- determinist
- non-dogmatic
- non-empirical
- social Darwinism
- supersensible
- the domino theory
hypothesis | American Dictionary
Hypothesis | business english, examples of hypothesis, translations of hypothesis.
Get a quick, free translation!
Word of the Day
bank holiday
an official holiday when banks and most businesses are closed for a day

Trial, judge, and jury: talking about what happens when a criminal is caught

Learn more with +Plus
- Recent and Recommended {{#preferredDictionaries}} {{name}} {{/preferredDictionaries}}
- Definitions Clear explanations of natural written and spoken English English Learner’s Dictionary Essential British English Essential American English
- Grammar and thesaurus Usage explanations of natural written and spoken English Grammar Thesaurus
- Pronunciation British and American pronunciations with audio English Pronunciation
- English–Chinese (Simplified) Chinese (Simplified)–English
- English–Chinese (Traditional) Chinese (Traditional)–English
- English–Dutch Dutch–English
- English–French French–English
- English–German German–English
- English–Indonesian Indonesian–English
- English–Italian Italian–English
- English–Japanese Japanese–English
- English–Norwegian Norwegian–English
- English–Polish Polish–English
- English–Portuguese Portuguese–English
- English–Spanish Spanish–English
- English–Swedish Swedish–English
- Dictionary +Plus Word Lists
- English Noun
- American Noun
- Business Noun
- Translations
- All translations
To add hypothesis to a word list please sign up or log in.
Add hypothesis to one of your lists below, or create a new one.
{{message}}
Something went wrong.
There was a problem sending your report.
Research Hypothesis In Psychology: Types, & Examples
Saul McLeod, PhD
Editor-in-Chief for Simply Psychology
BSc (Hons) Psychology, MRes, PhD, University of Manchester
Saul McLeod, PhD., is a qualified psychology teacher with over 18 years of experience in further and higher education. He has been published in peer-reviewed journals, including the Journal of Clinical Psychology.
Learn about our Editorial Process
Olivia Guy-Evans, MSc
Associate Editor for Simply Psychology
BSc (Hons) Psychology, MSc Psychology of Education
Olivia Guy-Evans is a writer and associate editor for Simply Psychology. She has previously worked in healthcare and educational sectors.
On This Page:
A research hypothesis, in its plural form “hypotheses,” is a specific, testable prediction about the anticipated results of a study, established at its outset. It is a key component of the scientific method .
Hypotheses connect theory to data and guide the research process towards expanding scientific understanding
Some key points about hypotheses:
- A hypothesis expresses an expected pattern or relationship. It connects the variables under investigation.
- It is stated in clear, precise terms before any data collection or analysis occurs. This makes the hypothesis testable.
- A hypothesis must be falsifiable. It should be possible, even if unlikely in practice, to collect data that disconfirms rather than supports the hypothesis.
- Hypotheses guide research. Scientists design studies to explicitly evaluate hypotheses about how nature works.
- For a hypothesis to be valid, it must be testable against empirical evidence. The evidence can then confirm or disprove the testable predictions.
- Hypotheses are informed by background knowledge and observation, but go beyond what is already known to propose an explanation of how or why something occurs.
Predictions typically arise from a thorough knowledge of the research literature, curiosity about real-world problems or implications, and integrating this to advance theory. They build on existing literature while providing new insight.
Types of Research Hypotheses
Alternative hypothesis.
The research hypothesis is often called the alternative or experimental hypothesis in experimental research.
It typically suggests a potential relationship between two key variables: the independent variable, which the researcher manipulates, and the dependent variable, which is measured based on those changes.
The alternative hypothesis states a relationship exists between the two variables being studied (one variable affects the other).
A hypothesis is a testable statement or prediction about the relationship between two or more variables. It is a key component of the scientific method. Some key points about hypotheses:
- Important hypotheses lead to predictions that can be tested empirically. The evidence can then confirm or disprove the testable predictions.
In summary, a hypothesis is a precise, testable statement of what researchers expect to happen in a study and why. Hypotheses connect theory to data and guide the research process towards expanding scientific understanding.
An experimental hypothesis predicts what change(s) will occur in the dependent variable when the independent variable is manipulated.
It states that the results are not due to chance and are significant in supporting the theory being investigated.
The alternative hypothesis can be directional, indicating a specific direction of the effect, or non-directional, suggesting a difference without specifying its nature. It’s what researchers aim to support or demonstrate through their study.
Null Hypothesis
The null hypothesis states no relationship exists between the two variables being studied (one variable does not affect the other). There will be no changes in the dependent variable due to manipulating the independent variable.
It states results are due to chance and are not significant in supporting the idea being investigated.
The null hypothesis, positing no effect or relationship, is a foundational contrast to the research hypothesis in scientific inquiry. It establishes a baseline for statistical testing, promoting objectivity by initiating research from a neutral stance.
Many statistical methods are tailored to test the null hypothesis, determining the likelihood of observed results if no true effect exists.
This dual-hypothesis approach provides clarity, ensuring that research intentions are explicit, and fosters consistency across scientific studies, enhancing the standardization and interpretability of research outcomes.
Nondirectional Hypothesis
A non-directional hypothesis, also known as a two-tailed hypothesis, predicts that there is a difference or relationship between two variables but does not specify the direction of this relationship.
It merely indicates that a change or effect will occur without predicting which group will have higher or lower values.
For example, “There is a difference in performance between Group A and Group B” is a non-directional hypothesis.
Directional Hypothesis
A directional (one-tailed) hypothesis predicts the nature of the effect of the independent variable on the dependent variable. It predicts in which direction the change will take place. (i.e., greater, smaller, less, more)
It specifies whether one variable is greater, lesser, or different from another, rather than just indicating that there’s a difference without specifying its nature.
For example, “Exercise increases weight loss” is a directional hypothesis.
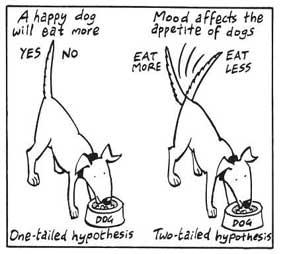
Falsifiability
The Falsification Principle, proposed by Karl Popper , is a way of demarcating science from non-science. It suggests that for a theory or hypothesis to be considered scientific, it must be testable and irrefutable.
Falsifiability emphasizes that scientific claims shouldn’t just be confirmable but should also have the potential to be proven wrong.
It means that there should exist some potential evidence or experiment that could prove the proposition false.
However many confirming instances exist for a theory, it only takes one counter observation to falsify it. For example, the hypothesis that “all swans are white,” can be falsified by observing a black swan.
For Popper, science should attempt to disprove a theory rather than attempt to continually provide evidence to support a research hypothesis.
Can a Hypothesis be Proven?
Hypotheses make probabilistic predictions. They state the expected outcome if a particular relationship exists. However, a study result supporting a hypothesis does not definitively prove it is true.
All studies have limitations. There may be unknown confounding factors or issues that limit the certainty of conclusions. Additional studies may yield different results.
In science, hypotheses can realistically only be supported with some degree of confidence, not proven. The process of science is to incrementally accumulate evidence for and against hypothesized relationships in an ongoing pursuit of better models and explanations that best fit the empirical data. But hypotheses remain open to revision and rejection if that is where the evidence leads.
- Disproving a hypothesis is definitive. Solid disconfirmatory evidence will falsify a hypothesis and require altering or discarding it based on the evidence.
- However, confirming evidence is always open to revision. Other explanations may account for the same results, and additional or contradictory evidence may emerge over time.
We can never 100% prove the alternative hypothesis. Instead, we see if we can disprove, or reject the null hypothesis.
If we reject the null hypothesis, this doesn’t mean that our alternative hypothesis is correct but does support the alternative/experimental hypothesis.
Upon analysis of the results, an alternative hypothesis can be rejected or supported, but it can never be proven to be correct. We must avoid any reference to results proving a theory as this implies 100% certainty, and there is always a chance that evidence may exist which could refute a theory.
How to Write a Hypothesis
- Identify variables . The researcher manipulates the independent variable and the dependent variable is the measured outcome.
- Operationalized the variables being investigated . Operationalization of a hypothesis refers to the process of making the variables physically measurable or testable, e.g. if you are about to study aggression, you might count the number of punches given by participants.
- Decide on a direction for your prediction . If there is evidence in the literature to support a specific effect of the independent variable on the dependent variable, write a directional (one-tailed) hypothesis. If there are limited or ambiguous findings in the literature regarding the effect of the independent variable on the dependent variable, write a non-directional (two-tailed) hypothesis.
- Make it Testable : Ensure your hypothesis can be tested through experimentation or observation. It should be possible to prove it false (principle of falsifiability).
- Clear & concise language . A strong hypothesis is concise (typically one to two sentences long), and formulated using clear and straightforward language, ensuring it’s easily understood and testable.
Consider a hypothesis many teachers might subscribe to: students work better on Monday morning than on Friday afternoon (IV=Day, DV= Standard of work).
Now, if we decide to study this by giving the same group of students a lesson on a Monday morning and a Friday afternoon and then measuring their immediate recall of the material covered in each session, we would end up with the following:
- The alternative hypothesis states that students will recall significantly more information on a Monday morning than on a Friday afternoon.
- The null hypothesis states that there will be no significant difference in the amount recalled on a Monday morning compared to a Friday afternoon. Any difference will be due to chance or confounding factors.
More Examples
- Memory : Participants exposed to classical music during study sessions will recall more items from a list than those who studied in silence.
- Social Psychology : Individuals who frequently engage in social media use will report higher levels of perceived social isolation compared to those who use it infrequently.
- Developmental Psychology : Children who engage in regular imaginative play have better problem-solving skills than those who don’t.
- Clinical Psychology : Cognitive-behavioral therapy will be more effective in reducing symptoms of anxiety over a 6-month period compared to traditional talk therapy.
- Cognitive Psychology : Individuals who multitask between various electronic devices will have shorter attention spans on focused tasks than those who single-task.
- Health Psychology : Patients who practice mindfulness meditation will experience lower levels of chronic pain compared to those who don’t meditate.
- Organizational Psychology : Employees in open-plan offices will report higher levels of stress than those in private offices.
- Behavioral Psychology : Rats rewarded with food after pressing a lever will press it more frequently than rats who receive no reward.

The Good Genes Hypothesis
- Living reference work entry
- First Online: 13 January 2023
- Cite this living reference work entry
- Urszula M. Marcinkowska 2
401 Accesses
23 Altmetric
Honest signalling
The good genes hypothesis proposes that the characteristics preferred by females are a signal of males’ ability to pass on genes which will increase the survival and reproductive success of the offspring sired with a male possessing them.
Introduction
The good genes hypothesis (GGH) was formulated by evolutionary biologist W.D. Hamilton and behavioral ecologist M. Zuk ( 1982 ). It proposes that the characteristics preferred by females are a signal of males’ ability to pass on genes (coding that certain characteristic) which will increase the survival and reproductive success of the offspring sired with a male possessing them. In such case, sexual selection would lead to the increase of the proportion of genes (coding the attractive trait) that are beneficial under prevailing conditions. In other words, good genes increase fitness of the offspring. In an evolutionary and ecological understanding individual’s fitness depends on how many copies of own...
This is a preview of subscription content, log in via an institution to check access.
Access this chapter
Institutional subscriptions
Achorn, A. M., & Rosenthal, G. G. (2019). It’s not about him: Mismeasuring ‘good genes’ in sexual selection. Trends in Ecology & Evolution, 35 (3), 206–219. https://doi.org/10.1016/j.tree.2019.11.007
Boothroyd, L. G., Scott, I., Gray, A. W., Coombes, C. I., & Pound, N. (2013). Male facial masculinity as a Cue to health outcomes. Evolutionary Psychology, 11 (5), 1044–1058. https://doi.org/10.1177/147470491301100508
Article Google Scholar
Foo, Y. Z., Simmons, L. W., & Rhodes, G. (2017). Predictors of facial attractiveness and health in humans. Scientific Reports, 7 . https://doi.org/10.1038/srep39731
Hakkarainen, T. J., Krams, I., Coetzee, V., Skrinda, I., Kecko, S., Krama, T., … & Rantala, M. J. (2021). MHC class II heterozygosity associated with attractiveness of men and women. Evolutionary Psychology , 19 (1). https://doi.org/10.1177/1474704921991994 .
Hamilton, W. D., & Zuk, M. (1982). Heritable true fitness and bright birds: A role for parasites? Science, 218 (4570), 384–387. https://doi.org/10.1126/science.7123238
Marcinkowska, U. M., Ziomkiewicz, A., Kleisner, K., Galbarczyk, A., Klimek, M., Sancilio, A., … & Bribiescas, R. G. (2020). Oxidative stress as a hidden cost of attractiveness in postmenopausal women. Scientific Reports, 10 (1). https://doi.org/10.1038/s41598-020-76627-9 .
Prum, R. O. (2010). The Lande-Kirkpatrick mechanism is the null model of evolution by intersexual selection: Implications for meaning, honesty, and design in intersexual signals. Evolution, 64 (11), 3085–3100.
Rantala, M. J., Coetzee, V., Moore, F. R., Skrinda, I., Kecko, S., Krama, T., … & Krams, I. (2013). Adiposity, compared with masculinity, serves as a more valid Cue to immunocompetence in human mate choice. Proceedings of the Royal Society B-Biological Sciences, 280 (1751). https://doi.org/10.1098/rspb.2012.2495 .
Scott, I. M. L., Clark, A. P., Boothroyd, L. G., & Penton-Voak, I. S. (2013). Do Men’s faces really signal heritable immunocompetence? Behavioral Ecology, 24 (3), 579–589. https://doi.org/10.1093/beheco/ars092
Stephen, I. D., Hiew, V., Coetzee, V., Tiddeman, B. P., & Perrett, D. I. (2017). Facial shape analysis identifies valid Cues to aspects of physiological health in Caucasian, Asian, and African populations. Frontiers in Psychology, 8 . https://doi.org/10.3389/fpsyg.2017.01883
Weatherhead, P., & Robertson, R. (1979). Offspring quality and the polygyny threshold: “The sexy son hypothesis”. The American Naturalist, 113 (2), 201–208. https://doi.org/10.1086/283379
Zahavi, A. (1975). Mate selection—A selection for a handicap. Journal of Theoretical Biology, 53 (1), 205–214. https://doi.org/10.1016/0022-5193(75)90111-3
Zaidi, A. A., White, J. D., Mattern, B. C., Liebowitz, C. R., Puts, D. A., Claes, P., & Shriver, M. D. (2019). Facial masculinity does not appear to be a condition-dependent male ornament and does not reflect MHC heterozygosity in humans. Proceedings of the National Academy of Sciences of the United States of America, 116 (5), 1633–1638. https://doi.org/10.1073/pnas.1808659116
Download references
Author information
Authors and affiliations.
Institute of Public Health, Jagiellonian University Medical College, Cracow, Poland
Urszula M. Marcinkowska
You can also search for this author in PubMed Google Scholar
Editor information
Editors and affiliations.
Department of Psychology, Oakland University, ROCHESTER, MN, USA
Todd K. Shackelford
Section Editor information
Department of Psychology, Oakland University, Rochester, MI, USA
Oakland University, Rochester, MI, USA
Gavin Vance
Department of Psychology, Oakland University, Rochester Hills, MI, USA
Madeleine K. Meehan
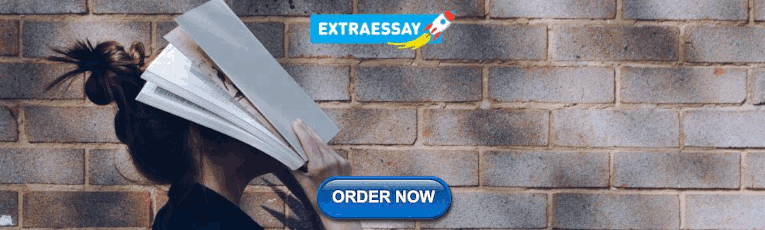
Rights and permissions
Reprints and permissions
Copyright information
© 2023 Springer Nature Switzerland AG
About this entry
Cite this entry.
Marcinkowska, U.M. (2023). The Good Genes Hypothesis. In: Shackelford, T.K. (eds) Encyclopedia of Sexual Psychology and Behavior. Springer, Cham. https://doi.org/10.1007/978-3-031-08956-5_1081-1
Download citation
DOI : https://doi.org/10.1007/978-3-031-08956-5_1081-1
Received : 29 August 2022
Accepted : 29 August 2022
Published : 13 January 2023
Publisher Name : Springer, Cham
Print ISBN : 978-3-031-08956-5
Online ISBN : 978-3-031-08956-5
eBook Packages : Springer Reference Behavioral Science and Psychology Reference Module Humanities and Social Sciences Reference Module Business, Economics and Social Sciences
- Publish with us
Policies and ethics
- Find a journal
- Track your research
Definition of a Hypothesis
What it is and how it's used in sociology
- Key Concepts
- Major Sociologists
- News & Issues
- Research, Samples, and Statistics
- Recommended Reading
- Archaeology
A hypothesis is a prediction of what will be found at the outcome of a research project and is typically focused on the relationship between two different variables studied in the research. It is usually based on both theoretical expectations about how things work and already existing scientific evidence.
Within social science, a hypothesis can take two forms. It can predict that there is no relationship between two variables, in which case it is a null hypothesis . Or, it can predict the existence of a relationship between variables, which is known as an alternative hypothesis.
In either case, the variable that is thought to either affect or not affect the outcome is known as the independent variable, and the variable that is thought to either be affected or not is the dependent variable.
Researchers seek to determine whether or not their hypothesis, or hypotheses if they have more than one, will prove true. Sometimes they do, and sometimes they do not. Either way, the research is considered successful if one can conclude whether or not a hypothesis is true.
Null Hypothesis
A researcher has a null hypothesis when she or he believes, based on theory and existing scientific evidence, that there will not be a relationship between two variables. For example, when examining what factors influence a person's highest level of education within the U.S., a researcher might expect that place of birth, number of siblings, and religion would not have an impact on the level of education. This would mean the researcher has stated three null hypotheses.
Alternative Hypothesis
Taking the same example, a researcher might expect that the economic class and educational attainment of one's parents, and the race of the person in question are likely to have an effect on one's educational attainment. Existing evidence and social theories that recognize the connections between wealth and cultural resources , and how race affects access to rights and resources in the U.S. , would suggest that both economic class and educational attainment of the one's parents would have a positive effect on educational attainment. In this case, economic class and educational attainment of one's parents are independent variables, and one's educational attainment is the dependent variable—it is hypothesized to be dependent on the other two.
Conversely, an informed researcher would expect that being a race other than white in the U.S. is likely to have a negative impact on a person's educational attainment. This would be characterized as a negative relationship, wherein being a person of color has a negative effect on one's educational attainment. In reality, this hypothesis proves true, with the exception of Asian Americans , who go to college at a higher rate than whites do. However, Blacks and Hispanics and Latinos are far less likely than whites and Asian Americans to go to college.
Formulating a Hypothesis
Formulating a hypothesis can take place at the very beginning of a research project , or after a bit of research has already been done. Sometimes a researcher knows right from the start which variables she is interested in studying, and she may already have a hunch about their relationships. Other times, a researcher may have an interest in a particular topic, trend, or phenomenon, but he may not know enough about it to identify variables or formulate a hypothesis.
Whenever a hypothesis is formulated, the most important thing is to be precise about what one's variables are, what the nature of the relationship between them might be, and how one can go about conducting a study of them.
Updated by Nicki Lisa Cole, Ph.D
- What It Means When a Variable Is Spurious
- Understanding Path Analysis
- Pilot Study in Research
- Simple Random Sampling
- Exploitation
- What Is Multiculturalism? Definition, Theories, and Examples
- Convenience Samples for Research
- What Is Cultural Capital? Do I Have It?
- What Does Consumerism Mean?
- Visualizing Social Stratification in the U.S.
- What Is Symbolic Interactionism?
- What Is Cultural Hegemony?
- Understanding Stratified Samples and How to Make Them
- What Is Groupthink? Definition and Examples
- What Is Ethnography?
- What Is a Reference Group?
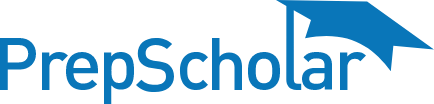
Choose Your Test
- Search Blogs By Category
- College Admissions
- AP and IB Exams
- GPA and Coursework
What Is a Hypothesis and How Do I Write One?
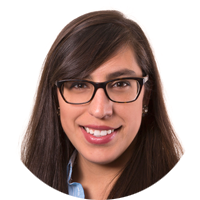
General Education
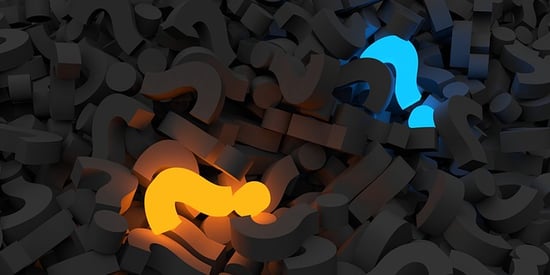
Think about something strange and unexplainable in your life. Maybe you get a headache right before it rains, or maybe you think your favorite sports team wins when you wear a certain color. If you wanted to see whether these are just coincidences or scientific fact, you would form a hypothesis, then create an experiment to see whether that hypothesis is true or not.
But what is a hypothesis, anyway? If you’re not sure about what a hypothesis is--or how to test for one!--you’re in the right place. This article will teach you everything you need to know about hypotheses, including:
- Defining the term “hypothesis”
- Providing hypothesis examples
- Giving you tips for how to write your own hypothesis
So let’s get started!
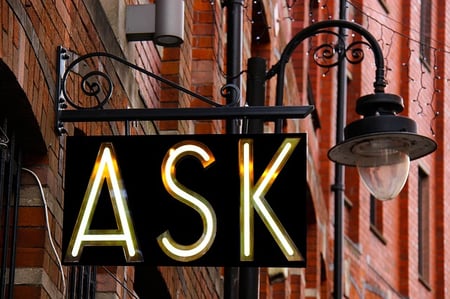
What Is a Hypothesis?
Merriam Webster defines a hypothesis as “an assumption or concession made for the sake of argument.” In other words, a hypothesis is an educated guess . Scientists make a reasonable assumption--or a hypothesis--then design an experiment to test whether it’s true or not. Keep in mind that in science, a hypothesis should be testable. You have to be able to design an experiment that tests your hypothesis in order for it to be valid.
As you could assume from that statement, it’s easy to make a bad hypothesis. But when you’re holding an experiment, it’s even more important that your guesses be good...after all, you’re spending time (and maybe money!) to figure out more about your observation. That’s why we refer to a hypothesis as an educated guess--good hypotheses are based on existing data and research to make them as sound as possible.
Hypotheses are one part of what’s called the scientific method . Every (good) experiment or study is based in the scientific method. The scientific method gives order and structure to experiments and ensures that interference from scientists or outside influences does not skew the results. It’s important that you understand the concepts of the scientific method before holding your own experiment. Though it may vary among scientists, the scientific method is generally made up of six steps (in order):
- Observation
- Asking questions
- Forming a hypothesis
- Analyze the data
- Communicate your results
You’ll notice that the hypothesis comes pretty early on when conducting an experiment. That’s because experiments work best when they’re trying to answer one specific question. And you can’t conduct an experiment until you know what you’re trying to prove!
Independent and Dependent Variables
After doing your research, you’re ready for another important step in forming your hypothesis: identifying variables. Variables are basically any factor that could influence the outcome of your experiment . Variables have to be measurable and related to the topic being studied.
There are two types of variables: independent variables and dependent variables. I ndependent variables remain constant . For example, age is an independent variable; it will stay the same, and researchers can look at different ages to see if it has an effect on the dependent variable.
Speaking of dependent variables... dependent variables are subject to the influence of the independent variable , meaning that they are not constant. Let’s say you want to test whether a person’s age affects how much sleep they need. In that case, the independent variable is age (like we mentioned above), and the dependent variable is how much sleep a person gets.
Variables will be crucial in writing your hypothesis. You need to be able to identify which variable is which, as both the independent and dependent variables will be written into your hypothesis. For instance, in a study about exercise, the independent variable might be the speed at which the respondents walk for thirty minutes, and the dependent variable would be their heart rate. In your study and in your hypothesis, you’re trying to understand the relationship between the two variables.
Elements of a Good Hypothesis
The best hypotheses start by asking the right questions . For instance, if you’ve observed that the grass is greener when it rains twice a week, you could ask what kind of grass it is, what elevation it’s at, and if the grass across the street responds to rain in the same way. Any of these questions could become the backbone of experiments to test why the grass gets greener when it rains fairly frequently.
As you’re asking more questions about your first observation, make sure you’re also making more observations . If it doesn’t rain for two weeks and the grass still looks green, that’s an important observation that could influence your hypothesis. You'll continue observing all throughout your experiment, but until the hypothesis is finalized, every observation should be noted.
Finally, you should consult secondary research before writing your hypothesis . Secondary research is comprised of results found and published by other people. You can usually find this information online or at your library. Additionally, m ake sure the research you find is credible and related to your topic. If you’re studying the correlation between rain and grass growth, it would help you to research rain patterns over the past twenty years for your county, published by a local agricultural association. You should also research the types of grass common in your area, the type of grass in your lawn, and whether anyone else has conducted experiments about your hypothesis. Also be sure you’re checking the quality of your research . Research done by a middle school student about what minerals can be found in rainwater would be less useful than an article published by a local university.
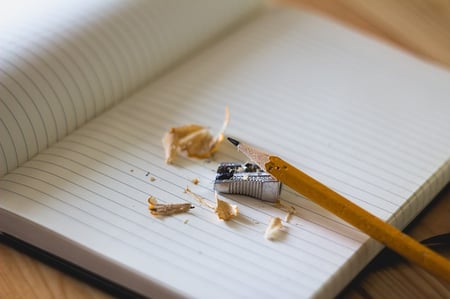
Writing Your Hypothesis
Once you’ve considered all of the factors above, you’re ready to start writing your hypothesis. Hypotheses usually take a certain form when they’re written out in a research report.
When you boil down your hypothesis statement, you are writing down your best guess and not the question at hand . This means that your statement should be written as if it is fact already, even though you are simply testing it.
The reason for this is that, after you have completed your study, you'll either accept or reject your if-then or your null hypothesis. All hypothesis testing examples should be measurable and able to be confirmed or denied. You cannot confirm a question, only a statement!
In fact, you come up with hypothesis examples all the time! For instance, when you guess on the outcome of a basketball game, you don’t say, “Will the Miami Heat beat the Boston Celtics?” but instead, “I think the Miami Heat will beat the Boston Celtics.” You state it as if it is already true, even if it turns out you’re wrong. You do the same thing when writing your hypothesis.
Additionally, keep in mind that hypotheses can range from very specific to very broad. These hypotheses can be specific, but if your hypothesis testing examples involve a broad range of causes and effects, your hypothesis can also be broad.
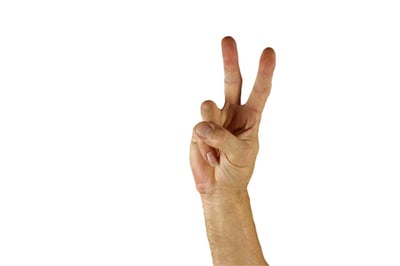
The Two Types of Hypotheses
Now that you understand what goes into a hypothesis, it’s time to look more closely at the two most common types of hypothesis: the if-then hypothesis and the null hypothesis.
#1: If-Then Hypotheses
First of all, if-then hypotheses typically follow this formula:
If ____ happens, then ____ will happen.
The goal of this type of hypothesis is to test the causal relationship between the independent and dependent variable. It’s fairly simple, and each hypothesis can vary in how detailed it can be. We create if-then hypotheses all the time with our daily predictions. Here are some examples of hypotheses that use an if-then structure from daily life:
- If I get enough sleep, I’ll be able to get more work done tomorrow.
- If the bus is on time, I can make it to my friend’s birthday party.
- If I study every night this week, I’ll get a better grade on my exam.
In each of these situations, you’re making a guess on how an independent variable (sleep, time, or studying) will affect a dependent variable (the amount of work you can do, making it to a party on time, or getting better grades).
You may still be asking, “What is an example of a hypothesis used in scientific research?” Take one of the hypothesis examples from a real-world study on whether using technology before bed affects children’s sleep patterns. The hypothesis read s:
“We hypothesized that increased hours of tablet- and phone-based screen time at bedtime would be inversely correlated with sleep quality and child attention.”
It might not look like it, but this is an if-then statement. The researchers basically said, “If children have more screen usage at bedtime, then their quality of sleep and attention will be worse.” The sleep quality and attention are the dependent variables and the screen usage is the independent variable. (Usually, the independent variable comes after the “if” and the dependent variable comes after the “then,” as it is the independent variable that affects the dependent variable.) This is an excellent example of how flexible hypothesis statements can be, as long as the general idea of “if-then” and the independent and dependent variables are present.
#2: Null Hypotheses
Your if-then hypothesis is not the only one needed to complete a successful experiment, however. You also need a null hypothesis to test it against. In its most basic form, the null hypothesis is the opposite of your if-then hypothesis . When you write your null hypothesis, you are writing a hypothesis that suggests that your guess is not true, and that the independent and dependent variables have no relationship .
One null hypothesis for the cell phone and sleep study from the last section might say:
“If children have more screen usage at bedtime, their quality of sleep and attention will not be worse.”
In this case, this is a null hypothesis because it’s asking the opposite of the original thesis!
Conversely, if your if-then hypothesis suggests that your two variables have no relationship, then your null hypothesis would suggest that there is one. So, pretend that there is a study that is asking the question, “Does the amount of followers on Instagram influence how long people spend on the app?” The independent variable is the amount of followers, and the dependent variable is the time spent. But if you, as the researcher, don’t think there is a relationship between the number of followers and time spent, you might write an if-then hypothesis that reads:
“If people have many followers on Instagram, they will not spend more time on the app than people who have less.”
In this case, the if-then suggests there isn’t a relationship between the variables. In that case, one of the null hypothesis examples might say:
“If people have many followers on Instagram, they will spend more time on the app than people who have less.”
You then test both the if-then and the null hypothesis to gauge if there is a relationship between the variables, and if so, how much of a relationship.
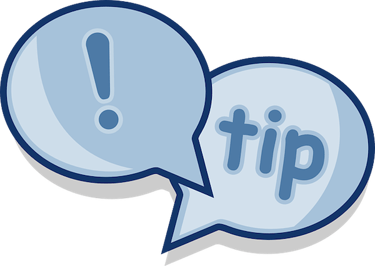
4 Tips to Write the Best Hypothesis
If you’re going to take the time to hold an experiment, whether in school or by yourself, you’re also going to want to take the time to make sure your hypothesis is a good one. The best hypotheses have four major elements in common: plausibility, defined concepts, observability, and general explanation.
#1: Plausibility
At first glance, this quality of a hypothesis might seem obvious. When your hypothesis is plausible, that means it’s possible given what we know about science and general common sense. However, improbable hypotheses are more common than you might think.
Imagine you’re studying weight gain and television watching habits. If you hypothesize that people who watch more than twenty hours of television a week will gain two hundred pounds or more over the course of a year, this might be improbable (though it’s potentially possible). Consequently, c ommon sense can tell us the results of the study before the study even begins.
Improbable hypotheses generally go against science, as well. Take this hypothesis example:
“If a person smokes one cigarette a day, then they will have lungs just as healthy as the average person’s.”
This hypothesis is obviously untrue, as studies have shown again and again that cigarettes negatively affect lung health. You must be careful that your hypotheses do not reflect your own personal opinion more than they do scientifically-supported findings. This plausibility points to the necessity of research before the hypothesis is written to make sure that your hypothesis has not already been disproven.
#2: Defined Concepts
The more advanced you are in your studies, the more likely that the terms you’re using in your hypothesis are specific to a limited set of knowledge. One of the hypothesis testing examples might include the readability of printed text in newspapers, where you might use words like “kerning” and “x-height.” Unless your readers have a background in graphic design, it’s likely that they won’t know what you mean by these terms. Thus, it’s important to either write what they mean in the hypothesis itself or in the report before the hypothesis.
Here’s what we mean. Which of the following sentences makes more sense to the common person?
If the kerning is greater than average, more words will be read per minute.
If the space between letters is greater than average, more words will be read per minute.
For people reading your report that are not experts in typography, simply adding a few more words will be helpful in clarifying exactly what the experiment is all about. It’s always a good idea to make your research and findings as accessible as possible.
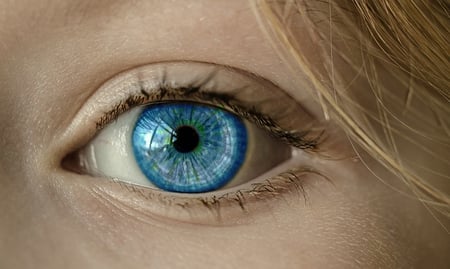
Good hypotheses ensure that you can observe the results.
#3: Observability
In order to measure the truth or falsity of your hypothesis, you must be able to see your variables and the way they interact. For instance, if your hypothesis is that the flight patterns of satellites affect the strength of certain television signals, yet you don’t have a telescope to view the satellites or a television to monitor the signal strength, you cannot properly observe your hypothesis and thus cannot continue your study.
Some variables may seem easy to observe, but if you do not have a system of measurement in place, you cannot observe your hypothesis properly. Here’s an example: if you’re experimenting on the effect of healthy food on overall happiness, but you don’t have a way to monitor and measure what “overall happiness” means, your results will not reflect the truth. Monitoring how often someone smiles for a whole day is not reasonably observable, but having the participants state how happy they feel on a scale of one to ten is more observable.
In writing your hypothesis, always keep in mind how you'll execute the experiment.
#4: Generalizability
Perhaps you’d like to study what color your best friend wears the most often by observing and documenting the colors she wears each day of the week. This might be fun information for her and you to know, but beyond you two, there aren’t many people who could benefit from this experiment. When you start an experiment, you should note how generalizable your findings may be if they are confirmed. Generalizability is basically how common a particular phenomenon is to other people’s everyday life.
Let’s say you’re asking a question about the health benefits of eating an apple for one day only, you need to realize that the experiment may be too specific to be helpful. It does not help to explain a phenomenon that many people experience. If you find yourself with too specific of a hypothesis, go back to asking the big question: what is it that you want to know, and what do you think will happen between your two variables?
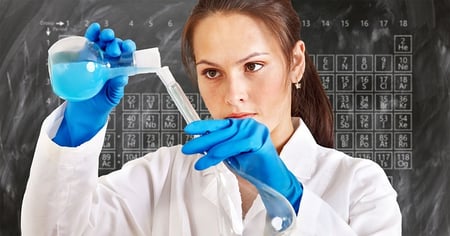
Hypothesis Testing Examples
We know it can be hard to write a good hypothesis unless you’ve seen some good hypothesis examples. We’ve included four hypothesis examples based on some made-up experiments. Use these as templates or launch pads for coming up with your own hypotheses.
Experiment #1: Students Studying Outside (Writing a Hypothesis)
You are a student at PrepScholar University. When you walk around campus, you notice that, when the temperature is above 60 degrees, more students study in the quad. You want to know when your fellow students are more likely to study outside. With this information, how do you make the best hypothesis possible?
You must remember to make additional observations and do secondary research before writing your hypothesis. In doing so, you notice that no one studies outside when it’s 75 degrees and raining, so this should be included in your experiment. Also, studies done on the topic beforehand suggested that students are more likely to study in temperatures less than 85 degrees. With this in mind, you feel confident that you can identify your variables and write your hypotheses:
If-then: “If the temperature in Fahrenheit is less than 60 degrees, significantly fewer students will study outside.”
Null: “If the temperature in Fahrenheit is less than 60 degrees, the same number of students will study outside as when it is more than 60 degrees.”
These hypotheses are plausible, as the temperatures are reasonably within the bounds of what is possible. The number of people in the quad is also easily observable. It is also not a phenomenon specific to only one person or at one time, but instead can explain a phenomenon for a broader group of people.
To complete this experiment, you pick the month of October to observe the quad. Every day (except on the days where it’s raining)from 3 to 4 PM, when most classes have released for the day, you observe how many people are on the quad. You measure how many people come and how many leave. You also write down the temperature on the hour.
After writing down all of your observations and putting them on a graph, you find that the most students study on the quad when it is 70 degrees outside, and that the number of students drops a lot once the temperature reaches 60 degrees or below. In this case, your research report would state that you accept or “failed to reject” your first hypothesis with your findings.
Experiment #2: The Cupcake Store (Forming a Simple Experiment)
Let’s say that you work at a bakery. You specialize in cupcakes, and you make only two colors of frosting: yellow and purple. You want to know what kind of customers are more likely to buy what kind of cupcake, so you set up an experiment. Your independent variable is the customer’s gender, and the dependent variable is the color of the frosting. What is an example of a hypothesis that might answer the question of this study?
Here’s what your hypotheses might look like:
If-then: “If customers’ gender is female, then they will buy more yellow cupcakes than purple cupcakes.”
Null: “If customers’ gender is female, then they will be just as likely to buy purple cupcakes as yellow cupcakes.”
This is a pretty simple experiment! It passes the test of plausibility (there could easily be a difference), defined concepts (there’s nothing complicated about cupcakes!), observability (both color and gender can be easily observed), and general explanation ( this would potentially help you make better business decisions ).
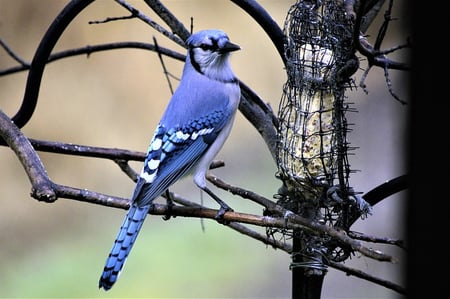
Experiment #3: Backyard Bird Feeders (Integrating Multiple Variables and Rejecting the If-Then Hypothesis)
While watching your backyard bird feeder, you realized that different birds come on the days when you change the types of seeds. You decide that you want to see more cardinals in your backyard, so you decide to see what type of food they like the best and set up an experiment.
However, one morning, you notice that, while some cardinals are present, blue jays are eating out of your backyard feeder filled with millet. You decide that, of all of the other birds, you would like to see the blue jays the least. This means you'll have more than one variable in your hypothesis. Your new hypotheses might look like this:
If-then: “If sunflower seeds are placed in the bird feeders, then more cardinals will come than blue jays. If millet is placed in the bird feeders, then more blue jays will come than cardinals.”
Null: “If either sunflower seeds or millet are placed in the bird, equal numbers of cardinals and blue jays will come.”
Through simple observation, you actually find that cardinals come as often as blue jays when sunflower seeds or millet is in the bird feeder. In this case, you would reject your “if-then” hypothesis and “fail to reject” your null hypothesis . You cannot accept your first hypothesis, because it’s clearly not true. Instead you found that there was actually no relation between your different variables. Consequently, you would need to run more experiments with different variables to see if the new variables impact the results.
Experiment #4: In-Class Survey (Including an Alternative Hypothesis)
You’re about to give a speech in one of your classes about the importance of paying attention. You want to take this opportunity to test a hypothesis you’ve had for a while:
If-then: If students sit in the first two rows of the classroom, then they will listen better than students who do not.
Null: If students sit in the first two rows of the classroom, then they will not listen better or worse than students who do not.
You give your speech and then ask your teacher if you can hand out a short survey to the class. On the survey, you’ve included questions about some of the topics you talked about. When you get back the results, you’re surprised to see that not only do the students in the first two rows not pay better attention, but they also scored worse than students in other parts of the classroom! Here, both your if-then and your null hypotheses are not representative of your findings. What do you do?
This is when you reject both your if-then and null hypotheses and instead create an alternative hypothesis . This type of hypothesis is used in the rare circumstance that neither of your hypotheses is able to capture your findings . Now you can use what you’ve learned to draft new hypotheses and test again!
Key Takeaways: Hypothesis Writing
The more comfortable you become with writing hypotheses, the better they will become. The structure of hypotheses is flexible and may need to be changed depending on what topic you are studying. The most important thing to remember is the purpose of your hypothesis and the difference between the if-then and the null . From there, in forming your hypothesis, you should constantly be asking questions, making observations, doing secondary research, and considering your variables. After you have written your hypothesis, be sure to edit it so that it is plausible, clearly defined, observable, and helpful in explaining a general phenomenon.
Writing a hypothesis is something that everyone, from elementary school children competing in a science fair to professional scientists in a lab, needs to know how to do. Hypotheses are vital in experiments and in properly executing the scientific method . When done correctly, hypotheses will set up your studies for success and help you to understand the world a little better, one experiment at a time.
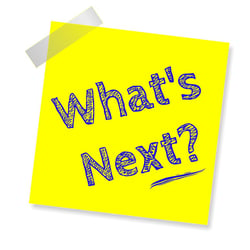
What’s Next?
If you’re studying for the science portion of the ACT, there’s definitely a lot you need to know. We’ve got the tools to help, though! Start by checking out our ultimate study guide for the ACT Science subject test. Once you read through that, be sure to download our recommended ACT Science practice tests , since they’re one of the most foolproof ways to improve your score. (And don’t forget to check out our expert guide book , too.)
If you love science and want to major in a scientific field, you should start preparing in high school . Here are the science classes you should take to set yourself up for success.
If you’re trying to think of science experiments you can do for class (or for a science fair!), here’s a list of 37 awesome science experiments you can do at home
Trending Now
How to Get Into Harvard and the Ivy League
How to Get a Perfect 4.0 GPA
How to Write an Amazing College Essay
What Exactly Are Colleges Looking For?
ACT vs. SAT: Which Test Should You Take?
When should you take the SAT or ACT?
Get Your Free
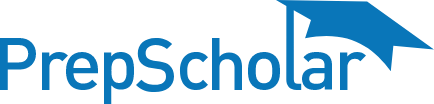
Find Your Target SAT Score
Free Complete Official SAT Practice Tests
How to Get a Perfect SAT Score, by an Expert Full Scorer
Score 800 on SAT Math
Score 800 on SAT Reading and Writing
How to Improve Your Low SAT Score
Score 600 on SAT Math
Score 600 on SAT Reading and Writing
Find Your Target ACT Score
Complete Official Free ACT Practice Tests
How to Get a Perfect ACT Score, by a 36 Full Scorer
Get a 36 on ACT English
Get a 36 on ACT Math
Get a 36 on ACT Reading
Get a 36 on ACT Science
How to Improve Your Low ACT Score
Get a 24 on ACT English
Get a 24 on ACT Math
Get a 24 on ACT Reading
Get a 24 on ACT Science
Stay Informed
Get the latest articles and test prep tips!
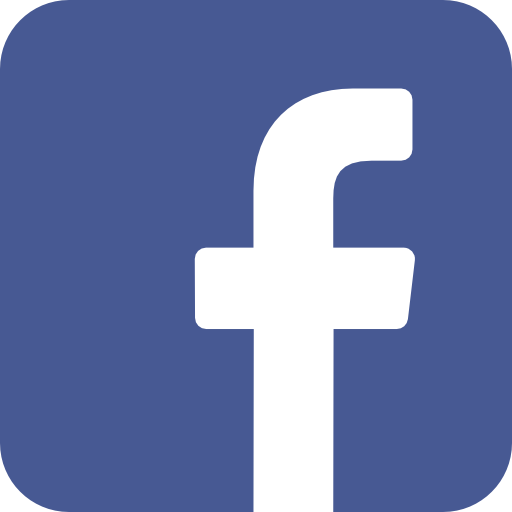
Ashley Sufflé Robinson has a Ph.D. in 19th Century English Literature. As a content writer for PrepScholar, Ashley is passionate about giving college-bound students the in-depth information they need to get into the school of their dreams.
Ask a Question Below
Have any questions about this article or other topics? Ask below and we'll reply!
Teach yourself statistics
Hypothesis Test: Difference Between Means
This lesson explains how to conduct a hypothesis test for the difference between two means. The test procedure, called the two-sample t-test , is appropriate when the following conditions are met:
- The sampling method for each sample is simple random sampling .
- The samples are independent .
- Each population is at least 20 times larger than its respective sample .
- The population distribution is normal.
- The population data are symmetric , unimodal , without outliers , and the sample size is 15 or less.
- The population data are slightly skewed , unimodal, without outliers, and the sample size is 16 to 40.
- The sample size is greater than 40, without outliers.
This approach consists of four steps: (1) state the hypotheses, (2) formulate an analysis plan, (3) analyze sample data, and (4) interpret results.
State the Hypotheses
Every hypothesis test requires the analyst to state a null hypothesis and an alternative hypothesis . The hypotheses are stated in such a way that they are mutually exclusive. That is, if one is true, the other must be false; and vice versa.
The table below shows three sets of null and alternative hypotheses. Each makes a statement about the difference d between the mean of one population μ 1 and the mean of another population μ 2 . (In the table, the symbol ≠ means " not equal to ".)
Set | Null hypothesis | Alternative hypothesis | Number of tails |
---|---|---|---|
1 | μ - μ = d | μ - μ ≠ d | 2 |
2 | μ - μ d | μ - μ < d | 1 |
3 | μ - μ d | μ - μ > d | 1 |
The first set of hypotheses (Set 1) is an example of a two-tailed test , since an extreme value on either side of the sampling distribution would cause a researcher to reject the null hypothesis. The other two sets of hypotheses (Sets 2 and 3) are one-tailed tests , since an extreme value on only one side of the sampling distribution would cause a researcher to reject the null hypothesis.
When the null hypothesis states that there is no difference between the two population means (i.e., d = 0), the null and alternative hypothesis are often stated in the following form.
H o : μ 1 = μ 2
H a : μ 1 ≠ μ 2
Formulate an Analysis Plan
The analysis plan describes how to use sample data to accept or reject the null hypothesis. It should specify the following elements.
- Significance level. Often, researchers choose significance levels equal to 0.01, 0.05, or 0.10; but any value between 0 and 1 can be used.
- Test method. Use the two-sample t-test to determine whether the difference between means found in the sample is significantly different from the hypothesized difference between means.
Analyze Sample Data
Using sample data, find the standard error, degrees of freedom, test statistic, and the P-value associated with the test statistic.
SE = sqrt[ (s 1 2 /n 1 ) + (s 2 2 /n 2 ) ]
DF = (s 1 2 /n 1 + s 2 2 /n 2 ) 2 / { [ (s 1 2 / n 1 ) 2 / (n 1 - 1) ] + [ (s 2 2 / n 2 ) 2 / (n 2 - 1) ] }
t = [ ( x 1 - x 2 ) - d ] / SE
- P-value. The P-value is the probability of observing a sample statistic as extreme as the test statistic. Since the test statistic is a t statistic, use the t Distribution Calculator to assess the probability associated with the t statistic, having the degrees of freedom computed above. (See sample problems at the end of this lesson for examples of how this is done.)
Interpret Results
If the sample findings are unlikely, given the null hypothesis, the researcher rejects the null hypothesis. Typically, this involves comparing the P-value to the significance level , and rejecting the null hypothesis when the P-value is less than the significance level.
Test Your Understanding
In this section, two sample problems illustrate how to conduct a hypothesis test of a difference between mean scores. The first problem involves a two-tailed test; the second problem, a one-tailed test.
Problem 1: Two-Tailed Test
Within a school district, students were randomly assigned to one of two Math teachers - Mrs. Smith and Mrs. Jones. After the assignment, Mrs. Smith had 30 students, and Mrs. Jones had 25 students.
At the end of the year, each class took the same standardized test. Mrs. Smith's students had an average test score of 78, with a standard deviation of 10; and Mrs. Jones' students had an average test score of 85, with a standard deviation of 15.
Test the hypothesis that Mrs. Smith and Mrs. Jones are equally effective teachers. Use a 0.10 level of significance. (Assume that student performance is approximately normal.)
Solution: The solution to this problem takes four steps: (1) state the hypotheses, (2) formulate an analysis plan, (3) analyze sample data, and (4) interpret results. We work through those steps below:
State the hypotheses. The first step is to state the null hypothesis and an alternative hypothesis.
Null hypothesis: μ 1 - μ 2 = 0
Alternative hypothesis: μ 1 - μ 2 ≠ 0
- Formulate an analysis plan . For this analysis, the significance level is 0.10. Using sample data, we will conduct a two-sample t-test of the null hypothesis.
SE = sqrt[(s 1 2 /n 1 ) + (s 2 2 /n 2 )]
SE = sqrt[(10 2 /30) + (15 2 /25] = sqrt(3.33 + 9)
SE = sqrt(12.33) = 3.51
DF = (10 2 /30 + 15 2 /25) 2 / { [ (10 2 / 30) 2 / (29) ] + [ (15 2 / 25) 2 / (24) ] }
DF = (3.33 + 9) 2 / { [ (3.33) 2 / (29) ] + [ (9) 2 / (24) ] } = 152.03 / (0.382 + 3.375) = 152.03/3.757 = 40.47
t = [ ( x 1 - x 2 ) - d ] / SE = [ (78 - 85) - 0 ] / 3.51 = -7/3.51 = -1.99
where s 1 is the standard deviation of sample 1, s 2 is the standard deviation of sample 2, n 1 is the size of sample 1, n 2 is the size of sample 2, x 1 is the mean of sample 1, x 2 is the mean of sample 2, d is the hypothesized difference between the population means, and SE is the standard error.
Since we have a two-tailed test , the P-value is the probability that a t statistic having 40 degrees of freedom is more extreme than -1.99; that is, less than -1.99 or greater than 1.99.
We use the t Distribution Calculator to find P(t < -1.99) is about 0.027.
- If you enter 1.99 as the sample mean in the t Distribution Calculator, you will find the that the P(t ≤ 1.99) is about 0.973. Therefore, P(t > 1.99) is 1 minus 0.973 or 0.027. Thus, the P-value = 0.027 + 0.027 = 0.054.
- Interpret results . Since the P-value (0.054) is less than the significance level (0.10), we cannot accept the null hypothesis.
Note: If you use this approach on an exam, you may also want to mention why this approach is appropriate. Specifically, the approach is appropriate because the sampling method was simple random sampling, the samples were independent, the sample size was much smaller than the population size, and the samples were drawn from a normal population.
Problem 2: One-Tailed Test
The Acme Company has developed a new battery. The engineer in charge claims that the new battery will operate continuously for at least 7 minutes longer than the old battery.
To test the claim, the company selects a simple random sample of 100 new batteries and 100 old batteries. The old batteries run continuously for 190 minutes with a standard deviation of 20 minutes; the new batteries, 200 minutes with a standard deviation of 40 minutes.
Test the engineer's claim that the new batteries run at least 7 minutes longer than the old. Use a 0.05 level of significance. (Assume that there are no outliers in either sample.)
Null hypothesis: μ 1 - μ 2 <= 7
Alternative hypothesis: μ 1 - μ 2 > 7
where μ 1 is battery life for the new battery, and μ 2 is battery life for the old battery.
- Formulate an analysis plan . For this analysis, the significance level is 0.05. Using sample data, we will conduct a two-sample t-test of the null hypothesis.
SE = sqrt[(40 2 /100) + (20 2 /100]
SE = sqrt(16 + 4) = 4.472
DF = (40 2 /100 + 20 2 /100) 2 / { [ (40 2 / 100) 2 / (99) ] + [ (20 2 / 100) 2 / (99) ] }
DF = (20) 2 / { [ (16) 2 / (99) ] + [ (2) 2 / (99) ] } = 400 / (2.586 + 0.162) = 145.56
t = [ ( x 1 - x 2 ) - d ] / SE = [(200 - 190) - 7] / 4.472 = 3/4.472 = 0.67
where s 1 is the standard deviation of sample 1, s 2 is the standard deviation of sample 2, n 1 is the size of sample 1, n 2 is the size of sample 2, x 1 is the mean of sample 1, x 2 is the mean of sample 2, d is the hypothesized difference between population means, and SE is the standard error.
Here is the logic of the analysis: Given the alternative hypothesis (μ 1 - μ 2 > 7), we want to know whether the observed difference in sample means is big enough (i.e., sufficiently greater than 7) to cause us to reject the null hypothesis.
Interpret results . Suppose we replicated this study many times with different samples. If the true difference in population means were actually 7, we would expect the observed difference in sample means to be 10 or less in 75% of our samples. And we would expect to find an observed difference to be more than 10 in 25% of our samples Therefore, the P-value in this analysis is 0.25.
Words and phrases
Personal account.
- Access or purchase personal subscriptions
- Get our newsletter
- Save searches
- Set display preferences
Institutional access
Sign in with library card
Sign in with username / password
Recommend to your librarian
Institutional account management
Sign in as administrator on Oxford Academic
hypothesis noun
- Hide all quotations
What does the noun hypothesis mean?
There are seven meanings listed in OED's entry for the noun hypothesis , two of which are labelled obsolete. See ‘Meaning & use’ for definitions, usage, and quotation evidence.
Entry status
OED is undergoing a continuous programme of revision to modernize and improve definitions. This entry has not yet been fully revised.
How common is the noun hypothesis ?
1750 | 18 |
1760 | 13 |
1770 | 19 |
1780 | 18 |
1790 | 18 |
1800 | 18 |
1810 | 18 |
1820 | 20 |
1830 | 19 |
1840 | 19 |
1850 | 20 |
1860 | 19 |
1870 | 24 |
1880 | 22 |
1890 | 21 |
1900 | 19 |
1910 | 21 |
1920 | 21 |
1930 | 22 |
1940 | 22 |
1950 | 35 |
1960 | 45 |
1970 | 55 |
1980 | 52 |
1990 | 44 |
2000 | 37 |
2010 | 37 |
How is the noun hypothesis pronounced?
British english, u.s. english, where does the noun hypothesis come from.
Earliest known use
The earliest known use of the noun hypothesis is in the late 1500s.
OED's earliest evidence for hypothesis is from 1596, in the writing of Earl of Essex.
hypothesis is a borrowing from Greek.
Etymons: Greek ὑπόθεσις .
Nearby entries
- hypothecarious, adj. 1726–
- hypothecary, adj. 1656–
- hypothecate, v. 1693–
- hypothecation, n. 1681–
- hypothecative, adj. 1856–
- hypothecator, n. 1828–
- hypothecium, n. 1866–
- hypothenar, adj. 1706–
- hypothermia, n. 1886–
- hypothermic, adj. 1898–
- hypothesis, n. 1596–
- hypothesist, n. 1788–
- hypothesize, v. 1738–
- hypothesizer, n. 1833–
- hypothetic, adj. & n. a1680–
- hypothetical, adj. & n. 1588–
- hypothetically, adv. 1628–
- hypothetico-deductive, adj. 1912–
- hypothetico-deductively, adv. 1953–
- hypothetico-disjunctive, adj. & n. a1856–
- hypothetist, n. 1852–
Thank you for visiting Oxford English Dictionary
To continue reading, please sign in below or purchase a subscription. After purchasing, please sign in below to access the content.
Meaning & use
Pronunciation, compounds & derived words, entry history for hypothesis, n..
hypothesis, n. was first published in 1899; not yet revised.
hypothesis, n. was last modified in July 2023.
Revision of the OED is a long-term project. Entries in oed.com which have not been revised may include:
- corrections and revisions to definitions, pronunciation, etymology, headwords, variant spellings, quotations, and dates;
- new senses, phrases, and quotations which have been added in subsequent print and online updates.
Revisions and additions of this kind were last incorporated into hypothesis, n. in July 2023.
Earlier versions of this entry were published in:
OED First Edition (1899)
- Find out more
OED Second Edition (1989)
- View hypothesis in OED Second Edition
Please submit your feedback for hypothesis, n.
Please include your email address if you are happy to be contacted about your feedback. OUP will not use this email address for any other purpose.
Citation details
Factsheet for hypothesis, n., browse entry.
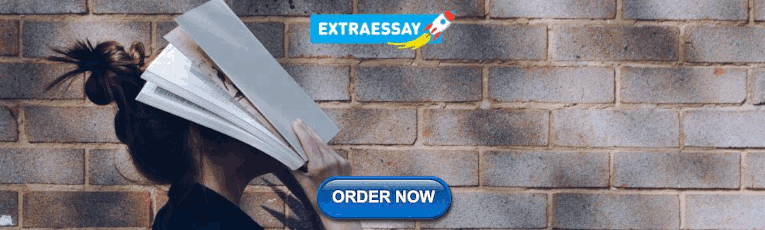
IMAGES
COMMENTS
The sexy son hypothesis in evolutionary biology and sexual selection, proposed by Patrick J. Weatherhead and Raleigh J. Robertson of Queen's University in Kingston, Ontario in 1979, [ 1] states that a female's ideal mate choice among potential mates is one whose genes will produce males with the best chance of reproductive success.
Definition. The sexy son hypothesis provides a theoretical framework to explain the evolution of social polygyny by female choice (Weatherhead and Robertson 1979 ). In some circumstances, a female would benefit in long-term fitness (number of grand-offspring) by forming a pair with an already-mated male instead that with an unmated competitor.
The sexy son hypothesis also implies that a potential mate's capability as a parental care-giver are irrelevant to his value as the potential father or the female's descendant. Introduction Darwin's theory with regard to the process of sexual selection is the foundation of current theories on the evolution of mating systems.
Weatherhead and Robertson (1979) proposed the sexy son hypothesis (SSH) as an additional explanation for the establishment of polygyny. According to this hypothesis, females are assumed to compensate for their direct inferior breeding success by the increased number of descendents produced by their sexy—and therefore polygynous—sons (see ...
Evolutionary aesthetics refers to evolutionary psychology theories in which the basic aesthetic preferences of Homo sapiens are argued to have evolved in order to enhance survival and reproductive success. [1]Based on this theory, things like color preference, preferred mate body ratios, shapes, emotional ties with objects, and many other aspects of the aesthetic experience can be explained ...
Sexy Son Hypothesis definition: The hypothesis, in evolutionary biology , that a female animal 's optimal choice among potential mates is a male whose genes will produce male offspring with the best chance of reproductive success , irrespective of the mate's capacity as a caregiver or any other direct benefits he can offer.
Sexual selection creates colourful differences between sexes in Goldie's bird-of-paradise.Male above; female below. Painting by John Gerrard Keulemans.. Sexual selection is a mode of natural selection in which members of one biological sex choose mates of the other sex to mate with (intersexual selection), and compete with members of the same sex for access to members of the opposite sex ...
Definition The sexy son hypothesis provides a theoretical framework to explain the evolution of social polygyny by female choice (Weatherhead and Robertson 1979). In some circumstances, a ... The Sexy Son Hypothesis in Humans Albeit the SSH was ideated for socially polyga-mous mating systems, where a female forms a breeding association with a ...
The alternative hypothesis is a claim implied by the research question and is an inequality. The alternative hypothesis states that population mean is greater than (>), less than (<), or not equal (≠) to the assumed value in the null hypothesis. When a test involves a single population mean, alternative hypothesis will be one of the following:
A hypothesis is a tentative statement about the relationship between two or more variables. It is a specific, testable prediction about what you expect to happen in a study. It is a preliminary answer to your question that helps guide the research process. Consider a study designed to examine the relationship between sleep deprivation and test ...
hypothesis: [noun] an assumption or concession made for the sake of argument. an interpretation of a practical situation or condition taken as the ground for action.
The governor and his wife, Gwen Walz, revealed in a People interview that their son was diagnosed with nonverbal learning disability as a teenager.. A 2020 study estimated that as many as 2.9 ...
Mate choice is highly visible in lek mating.Here, black grouse males gather in a quagmire and the females then arrive and observe the male before choosing one. Mate choice is one of the primary mechanisms under which evolution can occur. It is characterized by a "selective response by animals to particular stimuli" which can be observed as behavior. [1] In other words, before an animal engages ...
HYPOTHESIS definition: 1. an idea or explanation for something that is based on known facts but has not yet been proved…. Learn more.
Examples. A research hypothesis, in its plural form "hypotheses," is a specific, testable prediction about the anticipated results of a study, established at its outset. It is a key component of the scientific method. Hypotheses connect theory to data and guide the research process towards expanding scientific understanding.
The good genes hypothesis (GGH) was formulated by evolutionary biologist W.D. Hamilton and behavioral ecologist M. Zuk ().It proposes that the characteristics preferred by females are a signal of males' ability to pass on genes (coding that certain characteristic) which will increase the survival and reproductive success of the offspring sired with a male possessing them.
A hypothesis is a prediction of what will be found at the outcome of a research project and is typically focused on the relationship between two different variables studied in the research. It is usually based on both theoretical expectations about how things work and already existing scientific evidence. Within social science, a hypothesis can ...
Definition: Hypothesis is an educated guess or proposed explanation for a phenomenon, based on some initial observations or data. It is a tentative statement that can be tested and potentially proven or disproven through further investigation and experimentation. Hypothesis is often used in scientific research to guide the design of experiments ...
Merriam Webster defines a hypothesis as "an assumption or concession made for the sake of argument.". In other words, a hypothesis is an educated guess. Scientists make a reasonable assumption--or a hypothesis--then design an experiment to test whether it's true or not.
Fisherian runaway or runaway selection is a sexual selection mechanism proposed by the mathematical biologist Ronald Fisher in the early 20th century, to account for the evolution of ostentatious male ornamentation by persistent, directional female choice. [ 1][ 2][ 3] An example is the colourful and elaborate peacock plumage compared to the ...
Step 1: Determine the hypotheses. The hypotheses for a difference in two population means are similar to those for a difference in two population proportions. The null hypothesis, H 0, is again a statement of "no effect" or "no difference.". H 0: μ 1 - μ 2 = 0, which is the same as H 0: μ 1 = μ 2. The alternative hypothesis, H a ...
The first step is to state the null hypothesis and an alternative hypothesis. Null hypothesis: μ 1 - μ 2 = 0. Alternative hypothesis: μ 1 - μ 2 ≠ 0. Note that these hypotheses constitute a two-tailed test. The null hypothesis will be rejected if the difference between sample means is too big or if it is too small.
late 1500s. The earliest known use of the noun hypothesis is in the late 1500s. OED's earliest evidence for hypothesis is from 1596, in the writing of Earl of Essex. hypothesis is a borrowing from Greek. Etymons: Greek ὑπόθεσις.