Academia.edu no longer supports Internet Explorer.
To browse Academia.edu and the wider internet faster and more securely, please take a few seconds to upgrade your browser .
Enter the email address you signed up with and we'll email you a reset link.
- We're Hiring!
- Help Center
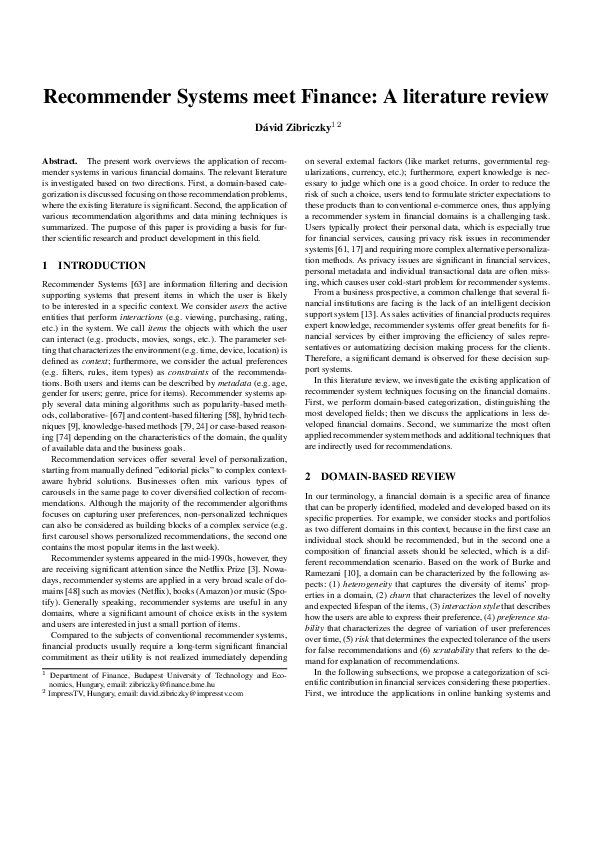
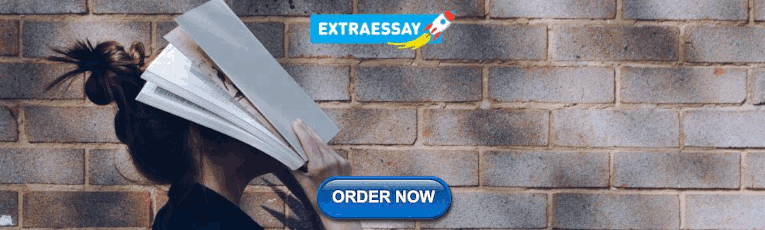
Recommender Systems meet Finance: A literature review

The present work overviews the application of recom-mender systems in various financial domains. The relevant literature is investigated based on two directions. First, a domain-based cate-gorization is discussed focusing on those recommendation problems, where the existing literature is significant. Second, the application of various recommendation algorithms and data mining techniques is summarized. The purpose of this paper is providing a basis for further scientific research and product development in this field.
Related Papers
Paul Blazek
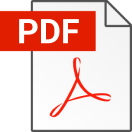
Ricardo Colomo-Palacios
Portfolio selection addresses the problem of how to diversify investments in the most efficient and profitable way possible. Portfolio selection is a field of study that has been broached from several perspectives, including, among others, recommender systems. This paper presents SINVLIO (Semantic INVestment portfoLIO), a tool based on semantic technologies and fuzzy logic techniques that recommends investments grounded in both psychological aspects of the investor and traditional financial parameters of the investments.
Khaled Wassif
Bruno Medeiros Rafael
Private banking is a business area in which the investor requires tailor-made advice. Because of the current market situation, investors are requiring answers to difficult questions and looking for assurance from wealth managers. Private bankers need to have deep knowledge about an innumerable list of products and their characteristics as well as the suitability of each product for the client's characteristics to be able to offer an optimal portfolio according to client expectations.
Recommender Systems Handbook
Robin Burke
Sai Swaroop
Journal of Computer Science IJCSIS
Recommender systems (RS) aim to capture the user behavior by suggesting/recommending users with relevant items or services that they find interesting in. Recommender systems have gained prominence in the field of information technology, e-commerce, etc., by inferring personalized recommendations by effectively pruning from a universal set of choices that directed users to identify content of interest. A number of recommendation methods have been available for generating personalized recommendations, which includes collaborative filtering, content-based filtering, knowledge-based recommendation system and other methods. In addition to these methods, hybrid recommendation systems have been proposed which combines different methods to overcome their corresponding drawbacks. This paper provides the landscape of different recommendation methods and their basic approaches.
Workshop on Recommender …
Gunnar Stevens
IUI-08 Workshop on …
With the rapid development of recommender system technology in recent years, it has become difficult for developers to determine which technology is suitable to a particular context. To alleviate this difficulty, we propose a framework that organizes the space of recommendation ...
RELATED PAPERS
BRAIN: Broad Research in Artificial Intelligence and Neuroscience
Academia EduSoft
Deborah L. McGuinness
International Journal of Pattern Recognition and Artificial Intelligence
Bartosz Gula
Workshop on Recommender Systems
Nicholas Ampazis
Sohaib Ahmad
Workshop on …
Michael Grossniklaus
Rebecca A. Okaka
Becky Okaka
IOSR Journals
Tsvi Kuflik
Neil Hurley
Francesco Ricci
Samar Shilbayeh
Data mining applications for empowering …
Hakikur Rahman
kislay srivastava
Juha Tiihonen
Frans Coenen
Gerhard Leitner
Priyansh Lakkadwala
Sebastián Ventura
OEGAI Journal
Chai Kein Yng
Laszlo Koczy , Dávid Zibriczky
SpringerBriefs in Applied Sciences and Technology (Polimi-SpringerBriefs)
Yashar Deldjoo
Bracha Shapira
Lecture Notes in Business Information Processing
Gilles Hubert
Silvia Milano , Mariarosaria Taddeo
Trends in Applied Intelligent Systems
Leila Etaati
Malika MADENE
Luciana Zaina , Maria Angélica C. A. Cardieri , Jose F Rodrigues-Jr
José Janssen
Ricardo Colomo Palacios
Applied Intelligence
Kostyantyn Shchekotykhin
Nikolaos Polatidis
Wim Waterink
Information Systems Volume 92, September 2020, 101545
Olga Gil HigherEd , Iván Cantador
Mehdi Elahi
Proceedings of the Collaborative European Research Conference 2017
Udo Bleimann
RELATED TOPICS
- We're Hiring!
- Help Center
- Find new research papers in:
- Health Sciences
- Earth Sciences
- Cognitive Science
- Mathematics
- Computer Science
- Academia ©2024
- Survey paper
- Open access
- Published: 03 May 2022
A systematic review and research perspective on recommender systems
- Deepjyoti Roy ORCID: orcid.org/0000-0002-8020-7145 1 &
- Mala Dutta 1
Journal of Big Data volume 9 , Article number: 59 ( 2022 ) Cite this article
67k Accesses
107 Citations
9 Altmetric
Metrics details
Recommender systems are efficient tools for filtering online information, which is widespread owing to the changing habits of computer users, personalization trends, and emerging access to the internet. Even though the recent recommender systems are eminent in giving precise recommendations, they suffer from various limitations and challenges like scalability, cold-start, sparsity, etc. Due to the existence of various techniques, the selection of techniques becomes a complex work while building application-focused recommender systems. In addition, each technique comes with its own set of features, advantages and disadvantages which raises even more questions, which should be addressed. This paper aims to undergo a systematic review on various recent contributions in the domain of recommender systems, focusing on diverse applications like books, movies, products, etc. Initially, the various applications of each recommender system are analysed. Then, the algorithmic analysis on various recommender systems is performed and a taxonomy is framed that accounts for various components required for developing an effective recommender system. In addition, the datasets gathered, simulation platform, and performance metrics focused on each contribution are evaluated and noted. Finally, this review provides a much-needed overview of the current state of research in this field and points out the existing gaps and challenges to help posterity in developing an efficient recommender system.
Introduction
The recent advancements in technology along with the prevalence of online services has offered more abilities for accessing a huge amount of online information in a faster manner. Users can post reviews, comments, and ratings for various types of services and products available online. However, the recent advancements in pervasive computing have resulted in an online data overload problem. This data overload complicates the process of finding relevant and useful content over the internet. The recent establishment of several procedures having lower computational requirements can however guide users to the relevant content in a much easy and fast manner. Because of this, the development of recommender systems has recently gained significant attention. In general, recommender systems act as information filtering tools, offering users suitable and personalized content or information. Recommender systems primarily aim to reduce the user’s effort and time required for searching relevant information over the internet.
Nowadays, recommender systems are being increasingly used for a large number of applications such as web [ 1 , 67 , 70 ], books [ 2 ], e-learning [ 4 , 16 , 61 ], tourism [ 5 , 8 , 78 ], movies [ 66 ], music [ 79 ], e-commerce, news, specialized research resources [ 65 ], television programs [ 72 , 81 ], etc. It is therefore important to build high-quality and exclusive recommender systems for providing personalized recommendations to the users in various applications. Despite the various advances in recommender systems, the present generation of recommender systems requires further improvements to provide more efficient recommendations applicable to a broader range of applications. More investigation of the existing latest works on recommender systems is required which focus on diverse applications.
There is hardly any review paper that has categorically synthesized and reviewed the literature of all the classification fields and application domains of recommender systems. The few existing literature reviews in the field cover just a fraction of the articles or focus only on selected aspects such as system evaluation. Thus, they do not provide an overview of the application field, algorithmic categorization, or identify the most promising approaches. Also, review papers often neglect to analyze the dataset description and the simulation platforms used. This paper aims to fulfil this significant gap by reviewing and comparing existing articles on recommender systems based on a defined classification framework, their algorithmic categorization, simulation platforms used, applications focused, their features and challenges, dataset description and system performance. Finally, we provide researchers and practitioners with insight into the most promising directions for further investigation in the field of recommender systems under various applications.
In essence, recommender systems deal with two entities—users and items, where each user gives a rating (or preference value) to an item (or product). User ratings are generally collected by using implicit or explicit methods. Implicit ratings are collected indirectly from the user through the user’s interaction with the items. Explicit ratings, on the other hand, are given directly by the user by picking a value on some finite scale of points or labelled interval values. For example, a website may obtain implicit ratings for different items based on clickstream data or from the amount of time a user spends on a webpage and so on. Most recommender systems gather user ratings through both explicit and implicit methods. These feedbacks or ratings provided by the user are arranged in a user-item matrix called the utility matrix as presented in Table 1 .
The utility matrix often contains many missing values. The problem of recommender systems is mainly focused on finding the values which are missing in the utility matrix. This task is often difficult as the initial matrix is usually very sparse because users generally tend to rate only a small number of items. It may also be noted that we are interested in only the high user ratings because only such items would be suggested back to the users. The efficiency of a recommender system greatly depends on the type of algorithm used and the nature of the data source—which may be contextual, textual, visual etc.
Types of recommender systems
Recommender systems are broadly categorized into three different types viz. content-based recommender systems, collaborative recommender systems and hybrid recommender systems. A diagrammatic representation of the different types of recommender systems is given in Fig. 1 .
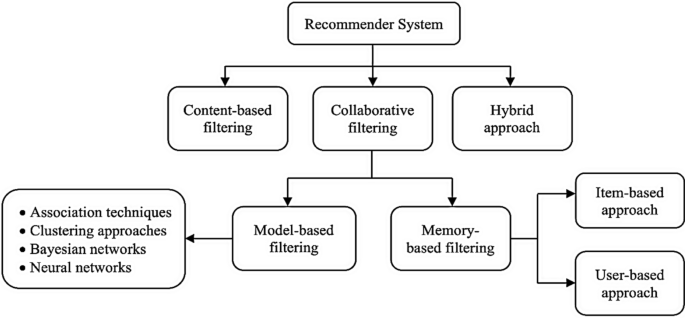
Content-based recommender system
In content-based recommender systems, all the data items are collected into different item profiles based on their description or features. For example, in the case of a book, the features will be author, publisher, etc. In the case of a movie, the features will be the movie director, actor, etc. When a user gives a positive rating to an item, then the other items present in that item profile are aggregated together to build a user profile. This user profile combines all the item profiles, whose items are rated positively by the user. Items present in this user profile are then recommended to the user, as shown in Fig. 2 .
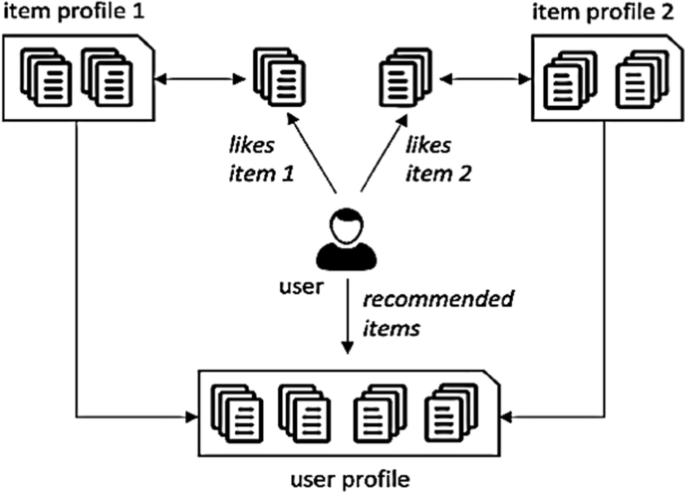
One drawback of this approach is that it demands in-depth knowledge of the item features for an accurate recommendation. This knowledge or information may not be always available for all items. Also, this approach has limited capacity to expand on the users' existing choices or interests. However, this approach has many advantages. As user preferences tend to change with time, this approach has the quick capability of dynamically adapting itself to the changing user preferences. Since one user profile is specific only to that user, this algorithm does not require the profile details of any other users because they provide no influence in the recommendation process. This ensures the security and privacy of user data. If new items have sufficient description, content-based techniques can overcome the cold-start problem i.e., this technique can recommend an item even when that item has not been previously rated by any user. Content-based filtering approaches are more common in systems like personalized news recommender systems, publications, web pages recommender systems, etc.
Collaborative filtering-based recommender system
Collaborative approaches make use of the measure of similarity between users. This technique starts with finding a group or collection of user X whose preferences, likes, and dislikes are similar to that of user A. X is called the neighbourhood of A. The new items which are liked by most of the users in X are then recommended to user A. The efficiency of a collaborative algorithm depends on how accurately the algorithm can find the neighbourhood of the target user. Traditionally collaborative filtering-based systems suffer from the cold-start problem and privacy concerns as there is a need to share user data. However, collaborative filtering approaches do not require any knowledge of item features for generating a recommendation. Also, this approach can help to expand on the user’s existing interests by discovering new items. Collaborative approaches are again divided into two types: memory-based approaches and model-based approaches.
Memory-based collaborative approaches recommend new items by taking into consideration the preferences of its neighbourhood. They make use of the utility matrix directly for prediction. In this approach, the first step is to build a model. The model is equal to a function that takes the utility matrix as input.
Model = f (utility matrix)
Then recommendations are made based on a function that takes the model and user profile as input. Here we can make recommendations only to users whose user profile belongs to the utility matrix. Therefore, to make recommendations for a new user, the user profile must be added to the utility matrix, and the similarity matrix should be recomputed, which makes this technique computation heavy.
Recommendation = f (defined model, user profile) where user profile ∈ utility matrix
Memory-based collaborative approaches are again sub-divided into two types: user-based collaborative filtering and item-based collaborative filtering. In the user-based approach, the user rating of a new item is calculated by finding other users from the user neighbourhood who has previously rated that same item. If a new item receives positive ratings from the user neighbourhood, the new item is recommended to the user. Figure 3 depicts the user-based filtering approach.
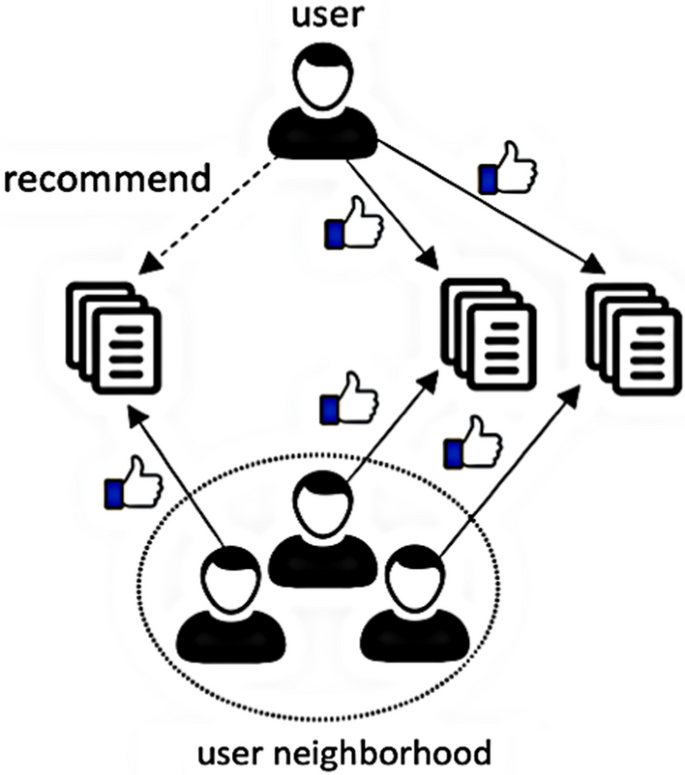
User-based collaborative filtering
In the item-based approach, an item-neighbourhood is built consisting of all similar items which the user has rated previously. Then that user’s rating for a different new item is predicted by calculating the weighted average of all ratings present in a similar item-neighbourhood as shown in Fig. 4 .
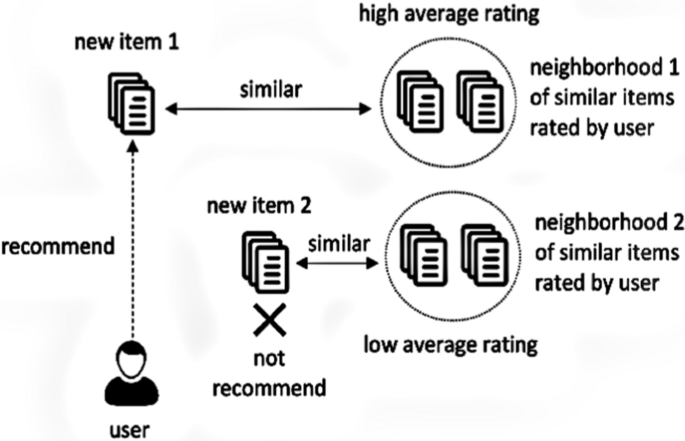
Item-based collaborative filtering
Model-based systems use various data mining and machine learning algorithms to develop a model for predicting the user’s rating for an unrated item. They do not rely on the complete dataset when recommendations are computed but extract features from the dataset to compute a model. Hence the name, model-based technique. These techniques also need two steps for prediction—the first step is to build the model, and the second step is to predict ratings using a function (f) which takes the model defined in the first step and the user profile as input.
Recommendation = f (defined model, user profile) where user profile ∉ utility matrix
Model-based techniques do not require adding the user profile of a new user into the utility matrix before making predictions. We can make recommendations even to users that are not present in the model. Model-based systems are more efficient for group recommendations. They can quickly recommend a group of items by using the pre-trained model. The accuracy of this technique largely relies on the efficiency of the underlying learning algorithm used to create the model. Model-based techniques are capable of solving some traditional problems of recommender systems such as sparsity and scalability by employing dimensionality reduction techniques [ 86 ] and model learning techniques.
Hybrid filtering
A hybrid technique is an aggregation of two or more techniques employed together for addressing the limitations of individual recommender techniques. The incorporation of different techniques can be performed in various ways. A hybrid algorithm may incorporate the results achieved from separate techniques, or it can use content-based filtering in a collaborative method or use a collaborative filtering technique in a content-based method. This hybrid incorporation of different techniques generally results in increased performance and increased accuracy in many recommender applications. Some of the hybridization approaches are meta-level, feature-augmentation, feature-combination, mixed hybridization, cascade hybridization, switching hybridization and weighted hybridization [ 86 ]. Table 2 describes these approaches.
Recommender system challenges
This section briefly describes the various challenges present in current recommender systems and offers different solutions to overcome these challenges.
Cold start problem
The cold start problem appears when the recommender system cannot draw any inference from the existing data, which is insufficient. Cold start refers to a condition when the system cannot produce efficient recommendations for the cold (or new) users who have not rated any item or have rated a very few items. It generally arises when a new user enters the system or new items (or products) are inserted into the database. Some solutions to this problem are as follows: (a) Ask new users to explicitly mention their item preference. (b) Ask a new user to rate some items at the beginning. (c) Collect demographic information (or meta-data) from the user and recommend items accordingly.
Shilling attack problem
This problem arises when a malicious user fakes his identity and enters the system to give false item ratings [ 87 ]. Such a situation occurs when the malicious user wants to either increase or decrease some item’s popularity by causing a bias on selected target items. Shilling attacks greatly reduce the reliability of the system. One solution to this problem is to detect the attackers quickly and remove the fake ratings and fake user profiles from the system.
Synonymy problem
This problem arises when similar or related items have different entries or names, or when the same item is represented by two or more names in the system [ 78 ]. For example, babywear and baby cloth. Many recommender systems fail to distinguish these differences, hence reducing their recommendation accuracy. To alleviate this problem many methods are used such as demographic filtering, automatic term expansion and Singular Value Decomposition [ 76 ].
Latency problem
The latency problem is specific to collaborative filtering approaches and occurs when new items are frequently inserted into the database. This problem is characterized by the system’s failure to recommend new items. This happens because new items must be reviewed before they can be recommended in a collaborative filtering environment. Using content-based filtering may resolve this issue, but it may introduce overspecialization and decrease the computing time and system performance. To increase performance, the calculations can be done in an offline environment and clustering-based techniques can be used [ 76 ].
Sparsity problem
Data sparsity is a common problem in large scale data analysis, which arises when certain expected values are missing in the dataset. In the case of recommender systems, this situation occurs when the active users rate very few items. This reduces the recommendation accuracy. To alleviate this problem several techniques can be used such as demographic filtering, singular value decomposition and using model-based collaborative techniques.
Grey sheep problem
The grey sheep problem is specific to pure collaborative filtering approaches where the feedback given by one user do not match any user neighbourhood. In this situation, the system fails to accurately predict relevant items for that user. This problem can be resolved by using pure content-based approaches where predictions are made based on the user’s profile and item properties.
Scalability problem
Recommender systems, especially those employing collaborative filtering techniques, require large amounts of training data, which cause scalability problems. The scalability problem arises when the amount of data used as input to a recommender system increases quickly. In this era of big data, more and more items and users are rapidly getting added to the system and this problem is becoming common in recommender systems. Two common approaches used to solve the scalability problem is dimensionality reduction and using clustering-based techniques to find users in tiny clusters instead of the complete database.
Methodology
The purpose of this study is to understand the research trends in the field of recommender systems. The nature of research in recommender systems is such that it is difficult to confine each paper to a specific discipline. This can be further understood by the fact that research papers on recommender systems are scattered across various journals such as computer science, management, marketing, information technology and information science. Hence, this literature review is conducted over a wide range of electronic journals and research databases such as ACM Portal, IEEE/IEE Library, Google Scholars and Science Direct [ 88 ].
The search process of online research articles was performed based on 6 descriptors: “Recommender systems”, “Recommendation systems”, “Movie Recommend*”, “Music Recommend*”, “Personalized Recommend*”, “Hybrid Recommend*”. The following research papers described below were excluded from our research:
News articles.
Master’s dissertations.
Non-English papers.
Unpublished papers.
Research papers published before 2011.
We have screened a total of 350 articles based on their abstracts and content. However, only research papers that described how recommender systems can be applied were chosen. Finally, 60 papers were selected from top international journals indexed in Scopus or E-SCI in 2021. We now present the PRISMA flowchart of the inclusion and exclusion process in Fig. 5 .
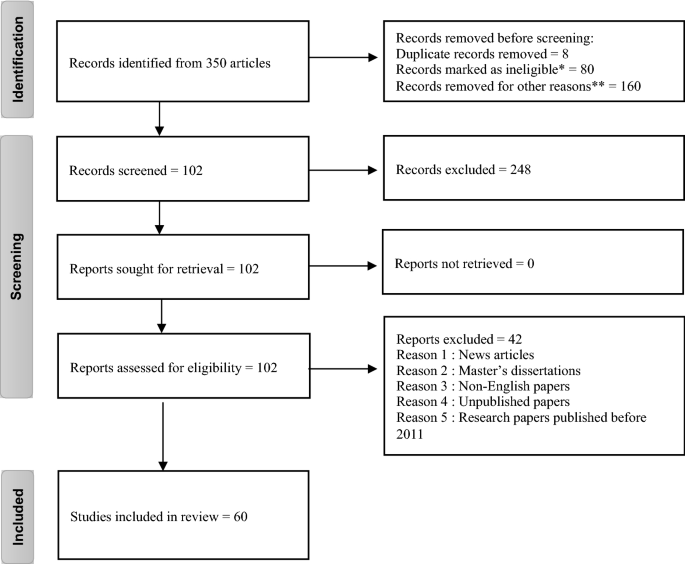
PRISMA flowchart of the inclusion and exclusion process. Abstract and content not suitable to the study: * The use or application of the recommender system is not specified: **
Each paper was carefully reviewed and classified into 6 categories in the application fields and 3 categories in the techniques used to develop the system. The classification framework is presented in Fig. 6 .
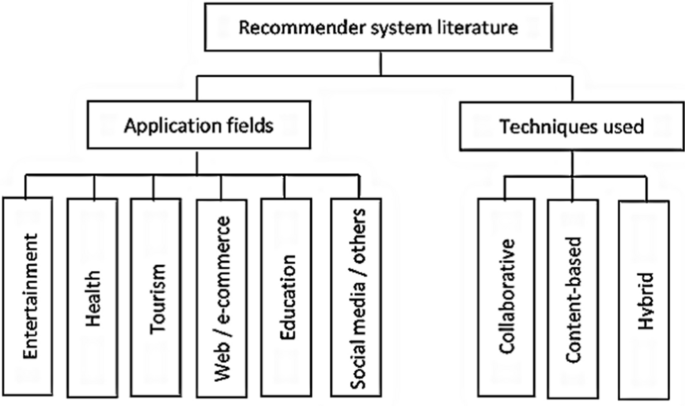
Classification framework
The number of relevant articles come from Expert Systems with Applications (23%), followed by IEEE (17%), Knowledge-Based System (17%) and Others (43%). Table 3 depicts the article distribution by journal title and Table 4 depicts the sector-wise article distribution.
Both forward and backward searching techniques were implemented to establish that the review of 60 chosen articles can represent the domain literature. Hence, this paper can demonstrate its validity and reliability as a literature review.
Review on state-of-the-art recommender systems
This section presents a state-of-art literature review followed by a chronological review of the various existing recommender systems.
Literature review
In 2011, Castellano et al. [ 1 ] developed a “NEuro-fuzzy WEb Recommendation (NEWER)” system for exploiting the possibility of combining computational intelligence and user preference for suggesting interesting web pages to the user in a dynamic environment. It considered a set of fuzzy rules to express the correlations between user relevance and categories of pages. Crespo et al. [ 2 ] presented a recommender system for distance education over internet. It aims to recommend e-books to students using data from user interaction. The system was developed using a collaborative approach and focused on solving the data overload problem in big digital content. Lin et al. [ 3 ] have put forward a recommender system for automatic vending machines using Genetic algorithm (GA), k-means, Decision Tree (DT) and Bayesian Network (BN). It aimed at recommending localized products by developing a hybrid model combining statistical methods, classification methods, clustering methods, and meta-heuristic methods. Wang and Wu [ 4 ] have implemented a ubiquitous learning system for providing personalized learning assistance to the learners by combining the recommendation algorithm with a context-aware technique. It employed the Association Rule Mining (ARM) technique and aimed to increase the effectiveness of the learner’s learning. García-Crespo et al. [ 5 ] presented a “semantic hotel” recommender system by considering the experiences of consumers using a fuzzy logic approach. The system considered both hotel and customer characteristics. Dong et al. [ 6 ] proposed a structure for a service-concept recommender system using a semantic similarity model by integrating the techniques from the view of an ontology structure-oriented metric and a concept content-oriented metric. The system was able to deliver optimal performance when compared with similar recommender systems. Li et al. [ 7 ] developed a Fuzzy linguistic modelling-based recommender system for assisting users to find experts in knowledge management systems. The developed system was applied to the aircraft industry where it demonstrated efficient and feasible performance. Lorenzi et al. [ 8 ] presented an “assumption-based multiagent” system to make travel package recommendations using user preferences in the tourism industry. It performed different tasks like discovering, filtering, and integrating specific information for building a travel package following the user requirement. Huang et al. [ 9 ] proposed a context-aware recommender system through the extraction, evaluation and incorporation of contextual information gathered using the collaborative filtering and rough set model.
In 2012, Chen et al. [ 10 ] presented a diabetes medication recommender model by using “Semantic Web Rule Language (SWRL) and Java Expert System Shell (JESS)” for aggregating suitable prescriptions for the patients. It aimed at selecting the most suitable drugs from the list of specific drugs. Mohanraj et al. [ 11 ] developed the “Ontology-driven bee’s foraging approach (ODBFA)” to accurately predict the online navigations most likely to be visited by a user. The self-adaptive system is intended to capture the various requirements of the online user by using a scoring technique and by performing a similarity comparison. Hsu et al. [ 12 ] proposed a “personalized auxiliary material” recommender system by considering the specific course topics, individual learning styles, complexity of the auxiliary materials using an artificial bee colony algorithm. Gemmell et al. [ 13 ] demonstrated a solution for the problem of resource recommendation in social annotation systems. The model was developed using a linear-weighted hybrid method which was capable of providing recommendations under different constraints. Choi et al. [ 14 ] proposed one “Hybrid Online-Product rEcommendation (HOPE) system” by the integration of collaborative filtering through sequential pattern analysis-based recommendations and implicit ratings. Garibaldi et al. [ 15 ] put forward a technique for incorporating the variability in a fuzzy inference model by using non-stationary fuzzy sets for replicating the variabilities of a human. This model was applied to a decision problem for treatment recommendations of post-operative breast cancer.
In 2013, Salehi and Kmalabadi [ 16 ] proposed an e-learning material recommender system by “modelling of materials in a multidimensional space of material’s attribute”. It employed both content and collaborative filtering. Aher and Lobo [ 17 ] introduced a course recommender system using data mining techniques such as simple K-means clustering and Association Rule Mining (ARM) algorithm. The proposed e-learning system was successfully demonstrated for “MOOC (Massively Open Online Courses)”. Kardan and Ebrahimi [ 18 ] developed a hybrid recommender system for recommending posts in asynchronous discussion groups. The system was built combining both collaborative filtering and content-based filtering. It considered implicit user data to compute the user similarity with various groups, for recommending suitable posts and contents to its users. Chang et al. [ 19 ] adopted a cloud computing technology for building a TV program recommender system. The system designed for digital TV programs was implemented using Hadoop Fair Scheduler (HFC), K-means clustering and k-nearest neighbour (KNN) algorithms. It was successful in processing huge amounts of real-time user data. Lucas et al. [ 20 ] implemented a recommender model for assisting a tourism application by using associative classification and fuzzy logic to predict the context. Niu et al. [ 21 ] introduced “Affivir: An Affect-based Internet Video Recommendation System” which was developed by calculating user preferences and by using spectral clustering. This model recommended videos with similar effects, which was processed to get optimal results with dynamic adjustments of recommendation constraints.
In 2014, Liu et al. [ 22 ] implemented a new route recommendation model for offering personalized and real-time route recommendations for self-driven tourists to minimize the queuing time and traffic jams infamous tourist places. Recommendations were carried out by considering the preferences of users. Bakshi et al. [ 23 ] proposed an unsupervised learning-based recommender model for solving the scalability problem of recommender systems. The algorithm used transitive similarities along with Particle Swarm Optimization (PSO) technique for discovering the global neighbours. Kim and Shim [ 24 ] proposed a recommender system based on “latent Dirichlet allocation using probabilistic modelling for Twitter” that could recommend the top-K tweets for a user to read, and the top-K users to follow. The model parameters were learned from an inference technique by using the differential Expectation–Maximization (EM) algorithm. Wang et al. [ 25 ] developed a hybrid-movie recommender model by aggregating a genetic algorithm (GA) with improved K-means and Principal Component Analysis (PCA) technique. It was able to offer intelligent movie recommendations with personalized suggestions. Kolomvatsos et al. [ 26 ] proposed a recommender system by considering an optimal stopping theory for delivering books or music recommendations to the users. Gottschlich et al. [ 27 ] proposed a decision support system for stock investment recommendations. It computed the output by considering the overall crowd’s recommendations. Torshizi et al. [ 28 ] have introduced a hybrid recommender system to determine the severity level of a medical condition. It could recommend suitable therapies for patients suffering from Benign Prostatic Hyperplasia.
In 2015, Zahálka et al. [ 29 ] proposed a venue recommender: “City Melange”. It was an interactive content-based model which used the convolutional deep-net features of the visual domain and the linear Support Vector Machine (SVM) model to capture the semantic information and extract latent topics. Sankar et al. [ 30 ] have proposed a stock recommender system based on the stock holding portfolio of trusted mutual funds. The system employed the collaborative filtering approach along with social network analysis for offering a decision support system to build a trust-based recommendation model. Chen et al. [ 31 ] have put forward a novel movie recommender system by applying the “artificial immune network to collaborative filtering” technique. It computed the affinity of an antigen and the affinity between an antibody and antigen. Based on this computation a similarity estimation formula was introduced which was used for the movie recommendation process. Wu et al. [ 32 ] have examined the technique of data fusion for increasing the efficiency of item recommender systems. It employed a hybrid linear combination model and used a collaborative tagging system. Yeh and Cheng [ 33 ] have proposed a recommender system for tourist attractions by constructing the “elicitation mechanism using the Delphi panel method and matrix construction mechanism using the repertory grids”, which was developed by considering the user preference and expert knowledge.
In 2016, Liao et al. [ 34 ] proposed a recommender model for online customers using a rough set association rule. The model computed the probable behavioural variations of online consumers and provided product category recommendations for e-commerce platforms. Li et al. [ 35 ] have suggested a movie recommender system based on user feedback collected from microblogs and social networks. It employed the sentiment-aware association rule mining algorithm for recommendations using the prior information of frequent program patterns, program metadata similarity and program view logs. Wu et al. [ 36 ] have developed a recommender system for social media platforms by aggregating the technique of Social Matrix Factorization (SMF) and Collaborative Topic Regression (CTR). The model was able to compute the ratings of users to items for making recommendations. For improving the recommendation quality, it gathered information from multiple sources such as item properties, social networks, feedback, etc. Adeniyi et al. [ 37 ] put forward a study of automated web-usage data mining and developed a recommender system that was tested in both real-time and online for identifying the visitor’s or client’s clickstream data.
In 2017, Rawat and Kankanhalli [ 38 ] have proposed a viewpoint recommender system called “ClickSmart” for assisting mobile users to capture high-quality photographs at famous tourist places. Yang et al. [ 39 ] proposed a gradient boosting-based job recommendation system for satisfying the cost-sensitive requirements of the users. The hybrid algorithm aimed to reduce the rate of unnecessary job recommendations. Lee et al. [ 40 ] proposed a music streaming recommender system based on smartphone activity usage. The proposed system benefitted by using feature selection approaches with machine learning techniques such as Naive Bayes (NB), Support Vector Machine (SVM), Multi-layer Perception (MLP), Instance-based k -Nearest Neighbour (IBK), and Random Forest (RF) for performing the activity detection from the mobile signals. Wei et al. [ 41 ] have proposed a new stacked denoising autoencoder (SDAE) based recommender system for cold items. The algorithm employed deep learning and collaborative filtering method to predict the unknown ratings.
In 2018, Li et al. [ 42 ] have developed a recommendation algorithm using Weighted Linear Regression Models (WLRRS). The proposed system was put to experiment using the MovieLens dataset and it presented better classification and predictive accuracy. Mezei and Nikou [ 43 ] presented a mobile health and wellness recommender system based on fuzzy optimization. It could recommend a collection of actions to be taken by the user to improve the user’s health condition. Recommendations were made considering the user’s physical activities and preferences. Ayata et al. [ 44 ] proposed a music recommendation model based on the user emotions captured through wearable physiological sensors. The emotion detection algorithm employed different machine learning algorithms like SVM, RF, KNN and decision tree (DT) algorithms to predict the emotions from the changing electrical signals gathered from the wearable sensors. Zhao et al. [ 45 ] developed a multimodal learning-based, social-aware movie recommender system. The model was able to successfully resolve the sparsity problem of recommender systems. The algorithm developed a heterogeneous network by exploiting the movie-poster image and textual description of each movie based on the social relationships and user ratings.
In 2019, Hammou et al. [ 46 ] proposed a Big Data recommendation algorithm capable of handling large scale data. The system employed random forest and matrix factorization through a data partitioning scheme. It was then used for generating recommendations based on user rating and preference for each item. The proposed system outperformed existing systems in terms of accuracy and speed. Zhao et al. [ 47 ] have put forward a hybrid initialization method for social network recommender systems. The algorithm employed denoising autoencoder (DAE) neural network-based initialization method (ANNInit) and attribute mapping. Bhaskaran and Santhi [ 48 ] have developed a hybrid, trust-based e-learning recommender system using cloud computing. The proposed algorithm was capable of learning online user activities by using the Firefly Algorithm (FA) and K-means clustering. Afolabi and Toivanen [ 59 ] have suggested an integrated recommender model based on collaborative filtering. The proposed model “Connected Health for Effective Management of Chronic Diseases”, aimed for integrating recommender systems for better decision-making in the process of disease management. He et al. [ 60 ] proposed a movie recommender system called “HI2Rec” which explored the usage of collaborative filtering and heterogeneous information for making movie recommendations. The model used the knowledge representation learning approach to embed movie-related information gathered from different sources.
In 2020, Han et al. [ 49 ] have proposed one Internet of Things (IoT)-based cancer rehabilitation recommendation system using the Beetle Antennae Search (BAS) algorithm. It presented the patients with a solution for the problem of optimal nutrition program by considering the objective function as the recurrence time. Kang et al. [ 50 ] have presented a recommender system for personalized advertisements in Online Broadcasting based on a tree model. Recommendations were generated in real-time by considering the user preferences to minimize the overhead of preference prediction and using a HashMap along with the tree characteristics. Ullah et al. [ 51 ] have implemented an image-based service recommendation model for online shopping based random forest and Convolutional Neural Networks (CNN). The model used JPEG coefficients to achieve an accurate prediction rate. Cai et al. [ 52 ] proposed a new hybrid recommender model using a many-objective evolutionary algorithm (MaOEA). The proposed algorithm was successful in optimizing the novelty, diversity, and accuracy of recommendations. Esteban et al. [ 53 ] have implemented a hybrid multi-criteria recommendation system concerned with students’ academic performance, personal interests, and course selection. The system was developed using a Genetic Algorithm (GA) and aimed at helping university students. It combined both course information and student information for increasing system performance and the reliability of the recommendations. Mondal et al. [ 54 ] have built a multilayer, graph data model-based doctor recommendation system by exploiting the trust concept between a patient-doctor relationship. The proposed system showed good results in practical applications.
In 2021, Dhelim et al. [ 55 ] have developed a personality-based product recommending model using the techniques of meta path discovery and user interest mining. This model showed better results when compared to session-based and deep learning models. Bhalse et al. [ 56 ] proposed a web-based movie recommendation system based on collaborative filtering using Singular Value Decomposition (SVD), collaborative filtering and cosine similarity (CS) for addressing the sparsity problem of recommender systems. It suggested a recommendation list by considering the content information of movies. Similarly, to solve both sparsity and cold-start problems Ke et al. [ 57 ] proposed a dynamic goods recommendation system based on reinforcement learning. The proposed system was capable of learning from the reduced entropy loss error on real-time applications. Chen et al. [ 58 ] have presented a movie recommender model combining various techniques like user interest with category-level representation, neighbour-assisted representation, user interest with latent representation and item-level representation using Feed-forward Neural Network (FNN).
Comparative chronological review
A comparative chronological review to compare the total contributions on various recommender systems in the past 10 years is given in Fig. 7 .
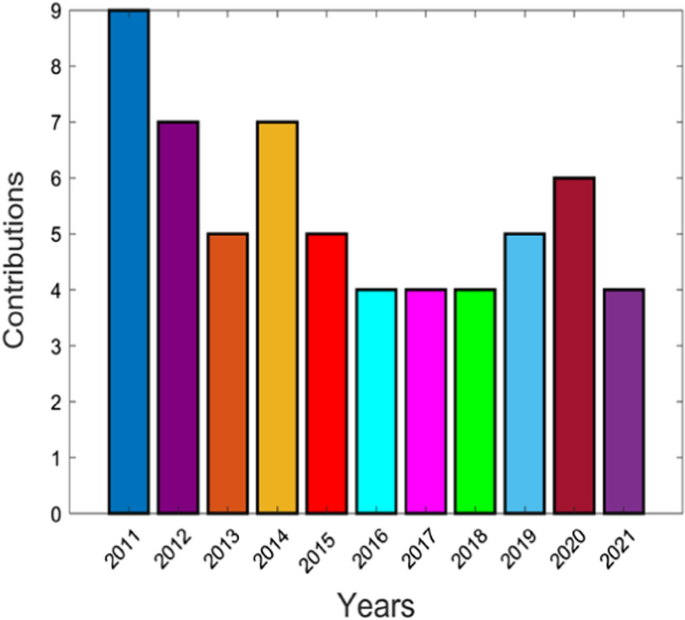
Comparative chronological review of recommender systems under diverse applications
This review puts forward a comparison of the number of research works proposed in the domain of recommender systems from the year 2011 to 2021 using various deep learning and machine learning-based approaches. Research articles are categorized based on the recommender system classification framework as shown in Table 5 . The articles are ordered according to their year of publication. There are two key concepts: Application fields and techniques used. The application fields of recommender systems are divided into six different fields, viz. entertainment, health, tourism, web/e-commerce, education and social media/others.
Algorithmic categorization, simulation platforms and applications considered for various recommender systems
This section analyses different methods like deep learning, machine learning, clustering and meta-heuristic-based-approaches used in the development of recommender systems. The algorithmic categorization of different recommender systems is given in Fig. 8 .
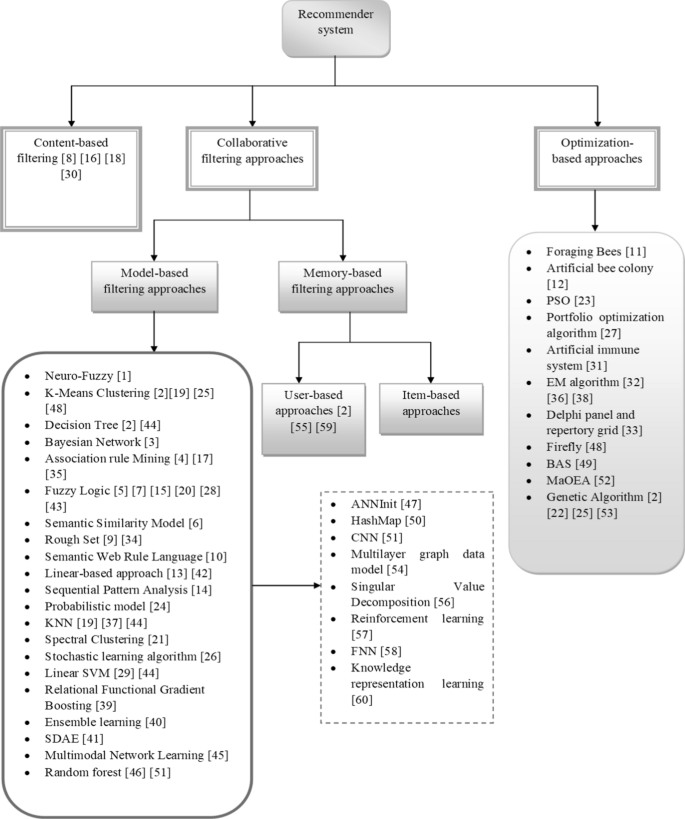
Algorithmic categorization of different recommender systems
Categorization is done based on content-based, collaborative filtering-based, and optimization-based approaches. In [ 8 ], a content-based filtering technique was employed for increasing the ability to trust other agents and for improving the exchange of information by trust degree. In [ 16 ], it was applied to enhance the quality of recommendations using the account attributes of the material. It achieved better performance concerning with F1-score, recall and precision. In [ 18 ], this technique was able to capture the implicit user feedback, increasing the overall accuracy of the proposed model. The content-based filtering in [ 30 ] was able to increase the accuracy and performance of a stock recommender system by using the “trust factor” for making decisions.
Different collaborative filtering approaches are utilized in recent studies, which are categorized as follows:
Model-based techniques
Neuro-Fuzzy [ 1 ] based technique helps in discovering the association between user categories and item relevance. It is also simple to understand. K-Means Clustering [ 2 , 19 , 25 , 48 ] is efficient for large scale datasets. It is simple to implement and gives a fast convergence rate. It also offers automatic recovery from failures. The decision tree [ 2 , 44 ] technique is easy to interpret. It can be used for solving the classic regression and classification problems in recommender systems. Bayesian Network [ 3 ] is a probabilistic technique used to solve classification challenges. It is based on the theory of Bayes theorem and conditional probability. Association Rule Mining (ARM) techniques [ 4 , 17 , 35 ] extract rules for projecting the occurrence of an item by considering the existence of other items in a transaction. This method uses the association rules to create a more suitable representation of data and helps in increasing the model performance and storage efficiency. Fuzzy Logic [ 5 , 7 , 15 , 20 , 28 , 43 ] techniques use a set of flexible rules. It focuses on solving complex real-time problems having an inaccurate spectrum of data. This technique provides scalability and helps in increasing the overall model performance for recommender systems. The semantic similarity [ 6 ] technique is used for describing a topological similarity to define the distance among the concepts and terms through ontologies. It measures the similarity information for increasing the efficiency of recommender systems. Rough set [ 9 , 34 ] techniques use probability distributions for solving the challenges of existing recommender models. Semantic web rule language [ 10 ] can efficiently extract the dataset features and increase the model efficiency. Linear programming-based approaches [ 13 , 42 ] are employed for achieving quality decision making in recommender models. Sequential pattern analysis [ 14 ] is applied to find suitable patterns among data items. This helps in increasing model efficiency. The probabilistic model [ 24 ] is a famous tool to handle uncertainty in risk computations and performance assessment. It offers better decision-making capabilities. K-nearest neighbours (KNN) [ 19 , 37 , 44 ] technique provides faster computation time, simplicity and ease of interpretation. They are good for classification and regression-based problems and offers more accuracy. Spectral clustering [ 21 ] is also called graph clustering or similarity-based clustering, which mainly focuses on reducing the space dimensionality in identifying the dataset items. Stochastic learning algorithm [ 26 ] solves the real-time challenges of recommender systems. Linear SVM [ 29 , 44 ] efficiently solves the high dimensional problems related to recommender systems. It is a memory-efficient method and works well with a large number of samples having relative separation among the classes. This method has been shown to perform well even when new or unfamiliar data is added. Relational Functional Gradient Boosting [ 39 ] technique efficiently works on the relational dependency of data, which is useful for statical relational learning for collaborative-based recommender systems. Ensemble learning [ 40 ] combines the forecast of two or more models and aims to achieve better performance than any of the single contributing models. It also helps in reducing overfitting problems, which are common in recommender systems.
SDAE [ 41 ] is used for learning the non-linear transformations with different filters for finding suitable data. This aids in increasing the performance of recommender models. Multimodal network learning [ 45 ] is efficient for multi-modal data, representing a combined representation of diverse modalities. Random forest [ 46 , 51 ] is a commonly used approach in comparison with other classifiers. It has been shown to increase accuracy when handling big data. This technique is a collection of decision trees to minimize variance through training on diverse data samples. ANNInit [ 47 ] is a type of artificial neural network-based technique that has the capability of self-learning and generating efficient results. It is independent of the data type and can learn data patterns automatically. HashMap [ 50 ] gives faster access to elements owing to the hashing methodology, which decreases the data processing time and increases the performance of the system. CNN [ 51 ] technique can automatically fetch the significant features of a dataset without any supervision. It is a computationally efficient method and provides accurate recommendations. This technique is also simple and fast for implementation. Multilayer graph data model [ 54 ] is efficient for real-time applications and minimizes the access time through mapping the correlation as edges among nodes and provides superior performance. Singular Value Decomposition [ 56 ] can simplify the input data and increase the efficiency of recommendations by eliminating the noise present in data. Reinforcement learning [ 57 ] is efficient for practical scenarios of recommender systems having large data sizes. It is capable of boosting the model performance by increasing the model accuracy even for large scale datasets. FNN [ 58 ] is one of the artificial neural network techniques which can learn non-linear and complex relationships between items. It has demonstrated a good performance increase when employed in different recommender systems. Knowledge representation learning [ 60 ] systems aim to simplify the model development process by increasing the acquisition efficiency, inferential efficiency, inferential adequacy and representation adequacy. User-based approaches [ 2 , 55 , 59 ] specialize in detecting user-related meta-data which is employed to increase the overall model performance. This technique is more suitable for real-time applications where it can capture user feedback and use it to increase the user experience.
Optimization-based techniques
The Foraging Bees [ 11 ] technique enables both functional and combinational optimization for random searching in recommender models. Artificial bee colony [ 12 ] is a swarm-based meta-heuristic technique that provides features like faster convergence rate, the ability to handle the objective with stochastic nature, ease for incorporating with other algorithms, usage of fewer control parameters, strong robustness, high flexibility and simplicity. Particle Swarm Optimization [ 23 ] is a computation optimization technique that offers better computational efficiency, robustness in control parameters, and is easy and simple to implement in recommender systems. Portfolio optimization algorithm [ 27 ] is a subclass of optimization algorithms that find its application in stock investment recommender systems. It works well in real-time and helps in the diversification of the portfolio for maximum profit. The artificial immune system [ 31 ]a is computationally intelligent machine learning technique. This technique can learn new patterns in the data and optimize the overall system parameters. Expectation maximization (EM) [ 32 , 36 , 38 ] is an iterative algorithm that guarantees the likelihood of finding the maximum parameters when the input variables are unknown. Delphi panel and repertory grid [ 33 ] offers efficient decision making by solving the dimensionality problem and data sparsity issues of recommender systems. The Firefly algorithm (FA) [ 48 ] provides fast results and increases recommendation efficiency. It is capable of reducing the number of iterations required to solve specific recommender problems. It also provides both local and global sets of solutions. Beetle Antennae Search (BAS) [ 49 ] offers superior search accuracy and maintains less time complexity that promotes the performance of recommendations. Many-objective evolutionary algorithm (MaOEA) [ 52 ] is applicable for real-time, multi-objective, search-related recommender systems. The introduction of a local search operator increases the convergence rate and gets suitable results. Genetic Algorithm (GA) [ 2 , 22 , 25 , 53 ] based techniques are used to solve the multi-objective optimization problems of recommender systems. They employ probabilistic transition rules and have a simpler operation that provides better recommender performance.
Features and challenges
The features and challenges of the existing recommender models are given in Table 6 .
Simulation platforms
The various simulation platforms used for developing different recommender systems with different applications are given in Fig. 9 .
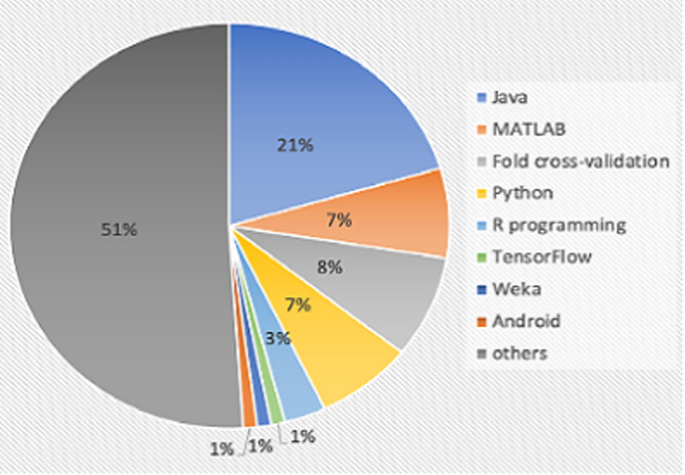
Simulation platforms used for developing different recommender systems
Here, the Java platform is used in 20% of the contributions, MATLAB is implemented in 7% of the contributions, different fold cross-validation are used in 8% of the contributions, 7% of the contributions are utilized by the python platform, 3% of the contributions employ R-programming and 1% of the contributions are developed by Tensorflow, Weka and Android environments respectively. Other simulation platforms like Facebook, web UI (User Interface), real-time environments, etc. are used in 50% of the contributions. Table 7 describes some simulation platforms commonly used for developing recommender systems.
Application focused and dataset description
This section provides an analysis of the different applications focused on a set of recent recommender systems and their dataset details.
Recent recommender systems were analysed and found that 11% of the contributions are focused on the domain of healthcare, 10% of the contributions are on movie recommender systems, 5% of the contributions come from music recommender systems, 6% of the contributions are focused on e-learning recommender systems, 8% of the contributions are used for online product recommender systems, 3% of the contributions are focused on book recommendations and 1% of the contributions are focused on Job and knowledge management recommender systems. 5% of the contributions concentrated on social network recommender systems, 10% of the contributions are focused on tourist and hotels recommender systems, 6% of the contributions are employed for stock recommender systems, and 3% of the contributions contributed for video recommender systems. The remaining 12% of contributions are miscellaneous recommender systems like Twitter, venue-based recommender systems, etc. Similarly, different datasets are gathered for recommender systems based on their application types. A detailed description is provided in Table 8 .
Performance analysis of state-of-art recommender systems
The performance evaluation metrics used for the analysis of different recommender systems is depicted in Table 9 . From the set of research works, 35% of the works use recall measure, 16% of the works employ Mean Absolute Error (MAE), 11% of the works take Root Mean Square Error (RMSE), 41% of the papers consider precision, 30% of the contributions analyse F1-measure, 31% of the works apply accuracy and 6% of the works employ coverage measure to validate the performance of the recommender systems. Moreover, some additional measures are also considered for validating the performance in a few applications.
Research gaps and challenges
In the recent decade, recommender systems have performed well in solving the problem of information overload and has become the more appropriate tool for multiple areas such as psychology, mathematics, computer science, etc. [ 80 ]. However, current recommender systems face a variety of challenges which are stated as follows, and discussed below:
Deployment challenges such as cold start, scalability, sparsity, etc. are already discussed in Sect. 3.
Challenges faced when employing different recommender algorithms for different applications.
Challenges in collecting implicit user data
Challenges in handling real-time user feedback.
Challenges faced in choosing the correct implementation techniques.
Challenges faced in measuring system performance.
Challenges in implementing recommender system for diverse applications.
Numerous recommender algorithms have been proposed on novel emerging dimensions which focus on addressing the existing limitations of recommender systems. A good recommender system must increase the recommendation quality based on user preferences. However, a specific recommender algorithm is not always guaranteed to perform equally for different applications. This encourages the possibility of employing different recommender algorithms for different applications, which brings along a lot of challenges. There is a need for more research to alleviate these challenges. Also, there is a large scope of research in recommender applications that incorporate information from different interactive online sites like Facebook, Twitter, shopping sites, etc. Some other areas for emerging research may be in the fields of knowledge-based recommender systems, methods for seamlessly processing implicit user data and handling real-time user feedback to recommend items in a dynamic environment.
Some of the other research areas like deep learning-based recommender systems, demographic filtering, group recommenders, cross-domain techniques for recommender systems, and dimensionality reduction techniques are also further required to be studied [ 83 ]. Deep learning-based recommender systems have recently gained much popularity. Future research areas in this field can integrate the well-performing deep learning models with new variants of hybrid meta-heuristic approaches.
During this review, it was observed that even though recent recommender systems have demonstrated good performance, there is no single standardized criteria or method which could be used to evaluate the performance of all recommender systems. System performance is generally measured by different evaluation matrices which makes it difficult to compare. The application of recommender systems in real-time applications is growing. User satisfaction and personalization play a very important role in the success of such recommender systems. There is a need for some new evaluation criteria which can evaluate the level of user satisfaction in real-time. New research should focus on capturing real-time user feedback and use the information to change the recommendation process accordingly. This will aid in increasing the quality of recommendations.
Conclusion and future scope
Recommender systems have attracted the attention of researchers and academicians. In this paper, we have identified and prudently reviewed research papers on recommender systems focusing on diverse applications, which were published between 2011 and 2021. This review has gathered diverse details like different application fields, techniques used, simulation tools used, diverse applications focused, performance metrics, datasets used, system features, and challenges of different recommender systems. Further, the research gaps and challenges were put forward to explore the future research perspective on recommender systems. Overall, this paper provides a comprehensive understanding of the trend of recommender systems-related research and to provides researchers with insight and future direction on recommender systems. The results of this study have several practical and significant implications:
Based on the recent-past publication rates, we feel that the research of recommender systems will significantly grow in the future.
A large number of research papers were identified in movie recommendations, whereas health, tourism and education-related recommender systems were identified in very few numbers. This is due to the availability of movie datasets in the public domain. Therefore, it is necessary to develop datasets in other fields also.
There is no standard measure to compute the performance of recommender systems. Among 60 papers, 21 used recall, 10 used MAE, 25 used precision, 18 used F1-measure, 19 used accuracy and only 7 used RMSE to calculate system performance. Very few systems were found to excel in two or more matrices.
Java and Python (with a combined contribution of 27%) are the most common programming languages used to develop recommender systems. This is due to the availability of a large number of standard java and python libraries which aid in the development process.
Recently a large number of hybrid and optimizations techniques are being proposed for recommender systems. The performance of a recommender system can be greatly improved by applying optimization techniques.
There is a large scope of research in using neural networks and deep learning-based methods for developing recommender systems. Systems developed using these methods are found to achieve high-performance accuracy.
This research will provide a guideline for future research in the domain of recommender systems. However, this research has some limitations. Firstly, due to the limited amount of manpower and time, we have only reviewed papers published in journals focusing on computer science, management and medicine. Secondly, we have reviewed only English papers. New research may extend this study to cover other journals and non-English papers. Finally, this review was conducted based on a search on only six descriptors: “Recommender systems”, “Recommendation systems”, “Movie Recommend*”, “Music Recommend*”, “Personalized Recommend*” and “Hybrid Recommend*”. Research papers that did not include these keywords were not considered. Future research can include adding some additional descriptors and keywords for searching. This will allow extending the research to cover more diverse articles on recommender systems.
Availability of data and materials
Not applicable.
Castellano G, Fanelli AM, Torsello MA. NEWER: A system for neuro-fuzzy web recommendation. Appl Soft Comput. 2011;11:793–806.
Article Google Scholar
Crespo RG, Martínez OS, Lovelle JMC, García-Bustelo BCP, Gayo JEL, Pablos PO. Recommendation system based on user interaction data applied to intelligent electronic books. Computers Hum Behavior. 2011;27:1445–9.
Lin FC, Yu HW, Hsu CH, Weng TC. Recommendation system for localized products in vending machines. Expert Syst Appl. 2011;38:9129–38.
Wang SL, Wu CY. Application of context-aware and personalized recommendation to implement an adaptive ubiquitous learning system. Expert Syst Appl. 2011;38:10831–8.
García-Crespo Á, López-Cuadrado JL, Colomo-Palacios R, González-Carrasco I, Ruiz-Mezcua B. Sem-Fit: A semantic based expert system to provide recommendations in the tourism domain. Expert Syst Appl. 2011;38:13310–9.
Dong H, Hussain FK, Chang E. A service concept recommendation system for enhancing the dependability of semantic service matchmakers in the service ecosystem environment. J Netw Comput Appl. 2011;34:619–31.
Li M, Liu L, Li CB. An approach to expert recommendation based on fuzzy linguistic method and fuzzy text classification in knowledge management systems. Expert Syst Appl. 2011;38:8586–96.
Lorenzi F, Bazzan ALC, Abel M, Ricci F. Improving recommendations through an assumption-based multiagent approach: An application in the tourism domain. Expert Syst Appl. 2011;38:14703–14.
Huang Z, Lu X, Duan H. Context-aware recommendation using rough set model and collaborative filtering. Artif Intell Rev. 2011;35:85–99.
Chen RC, Huang YH, Bau CT, Chen SM. A recommendation system based on domain ontology and SWRL for anti-diabetic drugs selection. Expert Syst Appl. 2012;39:3995–4006.
Mohanraj V, Chandrasekaran M, Senthilkumar J, Arumugam S, Suresh Y. Ontology driven bee’s foraging approach based self-adaptive online recommendation system. J Syst Softw. 2012;85:2439–50.
Hsu CC, Chen HC, Huang KK, Huang YM. A personalized auxiliary material recommendation system based on learning style on facebook applying an artificial bee colony algorithm. Comput Math Appl. 2012;64:1506–13.
Gemmell J, Schimoler T, Mobasher B, Burke R. Resource recommendation in social annotation systems: A linear-weighted hybrid approach. J Comput Syst Sci. 2012;78:1160–74.
Article MathSciNet Google Scholar
Choi K, Yoo D, Kim G, Suh Y. A hybrid online-product recommendation system: Combining implicit rating-based collaborative filtering and sequential pattern analysis. Electron Commer Res Appl. 2012;11:309–17.
Garibaldi JM, Zhou SM, Wang XY, John RI, Ellis IO. Incorporation of expert variability into breast cancer treatment recommendation in designing clinical protocol guided fuzzy rule system models. J Biomed Inform. 2012;45:447–59.
Salehi M, Kmalabadi IN. A hybrid attribute–based recommender system for e–learning material recommendation. IERI Procedia. 2012;2:565–70.
Aher SB, Lobo LMRJ. Combination of machine learning algorithms for recommendation of courses in e-learning System based on historical data. Knowl-Based Syst. 2013;51:1–14.
Kardan AA, Ebrahimi M. A novel approach to hybrid recommendation systems based on association rules mining for content recommendation in asynchronous discussion groups. Inf Sci. 2013;219:93–110.
Chang JH, Lai CF, Wang MS, Wu TY. A cloud-based intelligent TV program recommendation system. Comput Electr Eng. 2013;39:2379–99.
Lucas JP, Luz N, Moreno MN, Anacleto R, Figueiredo AA, Martins C. A hybrid recommendation approach for a tourism system. Expert Syst Appl. 2013;40:3532–50.
Niu J, Zhu L, Zhao X, Li H. Affivir: An affect-based Internet video recommendation system. Neurocomputing. 2013;120:422–33.
Liu L, Xu J, Liao SS, Chen H. A real-time personalized route recommendation system for self-drive tourists based on vehicle to vehicle communication. Expert Syst Appl. 2014;41:3409–17.
Bakshi S, Jagadev AK, Dehuri S, Wang GN. Enhancing scalability and accuracy of recommendation systems using unsupervised learning and particle swarm optimization. Appl Soft Comput. 2014;15:21–9.
Kim Y, Shim K. TWILITE: A recommendation system for twitter using a probabilistic model based on latent Dirichlet allocation. Inf Syst. 2014;42:59–77.
Wang Z, Yu X, Feng N, Wang Z. An improved collaborative movie recommendation system using computational intelligence. J Vis Lang Comput. 2014;25:667–75.
Kolomvatsos K, Anagnostopoulos C, Hadjiefthymiades S. An efficient recommendation system based on the optimal stopping theory. Expert Syst Appl. 2014;41:6796–806.
Gottschlich J, Hinz O. A decision support system for stock investment recommendations using collective wisdom. Decis Support Syst. 2014;59:52–62.
Torshizi AD, Zarandi MHF, Torshizi GD, Eghbali K. A hybrid fuzzy-ontology based intelligent system to determine level of severity and treatment recommendation for benign prostatic hyperplasia. Comput Methods Programs Biomed. 2014;113:301–13.
Zahálka J, Rudinac S, Worring M. Interactive multimodal learning for venue recommendation. IEEE Trans Multimedia. 2015;17:2235–44.
Sankar CP, Vidyaraj R, Kumar KS. Trust based stock recommendation system – a social network analysis approach. Procedia Computer Sci. 2015;46:299–305.
Chen MH, Teng CH, Chang PC. Applying artificial immune systems to collaborative filtering for movie recommendation. Adv Eng Inform. 2015;29:830–9.
Wu H, Pei Y, Li B, Kang Z, Liu X, Li H. Item recommendation in collaborative tagging systems via heuristic data fusion. Knowl-Based Syst. 2015;75:124–40.
Yeh DY, Cheng CH. Recommendation system for popular tourist attractions in Taiwan using delphi panel and repertory grid techniques. Tour Manage. 2015;46:164–76.
Liao SH, Chang HK. A rough set-based association rule approach for a recommendation system for online consumers. Inf Process Manage. 2016;52:1142–60.
Li H, Cui J, Shen B, Ma J. An intelligent movie recommendation system through group-level sentiment analysis in microblogs. Neurocomputing. 2016;210:164–73.
Wu H, Yue K, Pei Y, Li B, Zhao Y, Dong F. Collaborative topic regression with social trust ensemble for recommendation in social media systems. Knowl-Based Syst. 2016;97:111–22.
Adeniyi DA, Wei Z, Yongquan Y. Automated web usage data mining and recommendation system using K-Nearest Neighbor (KNN) classification method. Appl Computing Inform. 2016;12:90–108.
Rawat YS, Kankanhalli MS. ClickSmart: A context-aware viewpoint recommendation system for mobile photography. IEEE Trans Circuits Syst Video Technol. 2017;27:149–58.
Yang S, Korayem M, Aljadda K, Grainger T, Natarajan S. Combining content-based and collaborative filtering for job recommendation system: A cost-sensitive Statistical Relational Learning approach. Knowl-Based Syst. 2017;136:37–45.
Lee WP, Chen CT, Huang JY, Liang JY. A smartphone-based activity-aware system for music streaming recommendation. Knowl-Based Syst. 2017;131:70–82.
Wei J, He J, Chen K, Zhou Y, Tang Z. Collaborative filtering and deep learning based recommendation system for cold start items. Expert Syst Appl. 2017;69:29–39.
Li C, Wang Z, Cao S, He L. WLRRS: A new recommendation system based on weighted linear regression models. Comput Electr Eng. 2018;66:40–7.
Mezei J, Nikou S. Fuzzy optimization to improve mobile health and wellness recommendation systems. Knowl-Based Syst. 2018;142:108–16.
Ayata D, Yaslan Y, Kamasak ME. Emotion based music recommendation system using wearable physiological sensors. IEEE Trans Consum Electron. 2018;64:196–203.
Zhao Z, Yang Q, Lu H, Weninger T. Social-aware movie recommendation via multimodal network learning. IEEE Trans Multimedia. 2018;20:430–40.
Hammou BA, Lahcen AA, Mouline S. An effective distributed predictive model with matrix factorization and random forest for big data recommendation systems. Expert Syst Appl. 2019;137:253–65.
Zhao J, Geng X, Zhou J, Sun Q, Xiao Y, Zhang Z, Fu Z. Attribute mapping and autoencoder neural network based matrix factorization initialization for recommendation systems. Knowl-Based Syst. 2019;166:132–9.
Bhaskaran S, Santhi B. An efficient personalized trust based hybrid recommendation (TBHR) strategy for e-learning system in cloud computing. Clust Comput. 2019;22:1137–49.
Han Y, Han Z, Wu J, Yu Y, Gao S, Hua D, Yang A. Artificial intelligence recommendation system of cancer rehabilitation scheme based on IoT technology. IEEE Access. 2020;8:44924–35.
Kang S, Jeong C, Chung K. Tree-based real-time advertisement recommendation system in online broadcasting. IEEE Access. 2020;8:192693–702.
Ullah F, Zhang B, Khan RU. Image-based service recommendation system: A JPEG-coefficient RFs approach. IEEE Access. 2020;8:3308–18.
Cai X, Hu Z, Zhao P, Zhang W, Chen J. A hybrid recommendation system with many-objective evolutionary algorithm. Expert Syst Appl. 2020. https://doi.org/10.1016/j.eswa.2020.113648 .
Esteban A, Zafra A, Romero C. Helping university students to choose elective courses by using a hybrid multi-criteria recommendation system with genetic optimization. Knowledge-Based Syst. 2020;194:105385.
Mondal S, Basu A, Mukherjee N. Building a trust-based doctor recommendation system on top of multilayer graph database. J Biomed Inform. 2020;110:103549.
Dhelim S, Ning H, Aung N, Huang R, Ma J. Personality-aware product recommendation system based on user interests mining and metapath discovery. IEEE Trans Comput Soc Syst. 2021;8:86–98.
Bhalse N, Thakur R. Algorithm for movie recommendation system using collaborative filtering. Materials Today: Proceedings. 2021. https://doi.org/10.1016/j.matpr.2021.01.235 .
Ke G, Du HL, Chen YC. Cross-platform dynamic goods recommendation system based on reinforcement learning and social networks. Appl Soft Computing. 2021;104:107213.
Chen X, Liu D, Xiong Z, Zha ZJ. Learning and fusing multiple user interest representations for micro-video and movie recommendations. IEEE Trans Multimedia. 2021;23:484–96.
Afolabi AO, Toivanen P. Integration of recommendation systems into connected health for effective management of chronic diseases. IEEE Access. 2019;7:49201–11.
He M, Wang B, Du X. HI2Rec: Exploring knowledge in heterogeneous information for movie recommendation. IEEE Access. 2019;7:30276–84.
Bobadilla J, Serradilla F, Hernando A. Collaborative filtering adapted to recommender systems of e-learning. Knowl-Based Syst. 2009;22:261–5.
Russell S, Yoon V. Applications of wavelet data reduction in a recommender system. Expert Syst Appl. 2008;34:2316–25.
Campos LM, Fernández-Luna JM, Huete JF. A collaborative recommender system based on probabilistic inference from fuzzy observations. Fuzzy Sets Syst. 2008;159:1554–76.
Funk M, Rozinat A, Karapanos E, Medeiros AKA, Koca A. In situ evaluation of recommender systems: Framework and instrumentation. Int J Hum Comput Stud. 2010;68:525–47.
Porcel C, Moreno JM, Herrera-Viedma E. A multi-disciplinar recommender system to advice research resources in University Digital Libraries. Expert Syst Appl. 2009;36:12520–8.
Bobadilla J, Serradilla F, Bernal J. A new collaborative filtering metric that improves the behavior of recommender systems. Knowl-Based Syst. 2010;23:520–8.
Ochi P, Rao S, Takayama L, Nass C. Predictors of user perceptions of web recommender systems: How the basis for generating experience and search product recommendations affects user responses. Int J Hum Comput Stud. 2010;68:472–82.
Olmo FH, Gaudioso E. Evaluation of recommender systems: A new approach. Expert Syst Appl. 2008;35:790–804.
Zhen L, Huang GQ, Jiang Z. An inner-enterprise knowledge recommender system. Expert Syst Appl. 2010;37:1703–12.
Göksedef M, Gündüz-Öğüdücü S. Combination of web page recommender systems. Expert Syst Appl. 2010;37(4):2911–22.
Shao B, Wang D, Li T, Ogihara M. Music recommendation based on acoustic features and user access patterns. IEEE Trans Audio Speech Lang Process. 2009;17:1602–11.
Shin C, Woo W. Socially aware tv program recommender for multiple viewers. IEEE Trans Consum Electron. 2009;55:927–32.
Lopez-Carmona MA, Marsa-Maestre I, Perez JRV, Alcazar BA. Anegsys: An automated negotiation based recommender system for local e-marketplaces. IEEE Lat Am Trans. 2007;5:409–16.
Yap G, Tan A, Pang H. Discovering and exploiting causal dependencies for robust mobile context-aware recommenders. IEEE Trans Knowl Data Eng. 2007;19:977–92.
Meo PD, Quattrone G, Terracina G, Ursino D. An XML-based multiagent system for supporting online recruitment services. IEEE Trans Syst Man Cybern. 2007;37:464–80.
Khusro S, Ali Z, Ullah I. Recommender systems: Issues, challenges, and research opportunities. Inform Sci Appl. 2016. https://doi.org/10.1007/978-981-10-0557-2_112 .
Blanco-Fernandez Y, Pazos-Arias JJ, Gil-Solla A, Ramos-Cabrer M, Lopez-Nores M. Providing entertainment by content-based filtering and semantic reasoning in intelligent recommender systems. IEEE Trans Consum Electron. 2008;54:727–35.
Isinkaye FO, Folajimi YO, Ojokoh BA. Recommendation systems: Principles, methods and evaluation. Egyptian Inform J. 2015;16:261–73.
Yoshii K, Goto M, Komatani K, Ogata T, Okuno HG. An efficient hybrid music recommender system using an incrementally trainable probabilistic generative model. IEEE Trans Audio Speech Lang Process. 2008;16:435–47.
Wei YZ, Moreau L, Jennings NR. Learning users’ interests by quality classification in market-based recommender systems. IEEE Trans Knowl Data Eng. 2005;17:1678–88.
Bjelica M. Towards TV recommender system: experiments with user modeling. IEEE Trans Consum Electron. 2010;56:1763–9.
Setten MV, Veenstra M, Nijholt A, Dijk BV. Goal-based structuring in recommender systems. Interact Comput. 2006;18:432–56.
Adomavicius G, Tuzhilin A. Toward the next generation of recommender systems: a survey of the state-of-the-art and possible extensions. IEEE Trans Knowl Data Eng. 2005;17:734–49.
Symeonidis P, Nanopoulos A, Manolopoulos Y. Providing justifications in recommender systems. IEEE Transactions on Systems, Man, and Cybernetics - Part A: Systems and Humans. 2009;38:1262–72.
Zhan J, Hsieh C, Wang I, Hsu T, Liau C, Wang D. Privacy preserving collaborative recommender systems. IEEE Trans Syst Man Cybernet. 2010;40:472–6.
Burke R. Hybrid recommender systems: survey and experiments. User Model User-Adap Inter. 2002;12:331–70.
Article MATH Google Scholar
Gunes I, Kaleli C, Bilge A, Polat H. Shilling attacks against recommender systems: a comprehensive survey. Artif Intell Rev. 2012;42:767–99.
Park DH, Kim HK, Choi IY, Kim JK. A literature review and classification of recommender systems research. Expert Syst Appl. 2012;39:10059–72.
Download references
Acknowledgements
We thank our colleagues from Assam Down Town University who provided insight and expertise that greatly assisted this research, although they may not agree with all the interpretations and conclusions of this paper.
No funding was received to assist with the preparation of this manuscript.
Author information
Authors and affiliations.
Department of Computer Science & Engineering, Assam Down Town University, Panikhaiti, Guwahati, 781026, Assam, India
Deepjyoti Roy & Mala Dutta
You can also search for this author in PubMed Google Scholar
Contributions
DR carried out the review study and analysis of the existing algorithms in the literature. MD has been involved in drafting the manuscript or revising it critically for important intellectual content. Both authors read and approved the final manuscript.
Corresponding author
Correspondence to Deepjyoti Roy .
Ethics declarations
Ethics approval and consent to participate, consent for publication, competing interests.
On behalf of all authors, the corresponding author states that there is no conflict of interest.
Additional information
Publisher's note.
Springer Nature remains neutral with regard to jurisdictional claims in published maps and institutional affiliations.
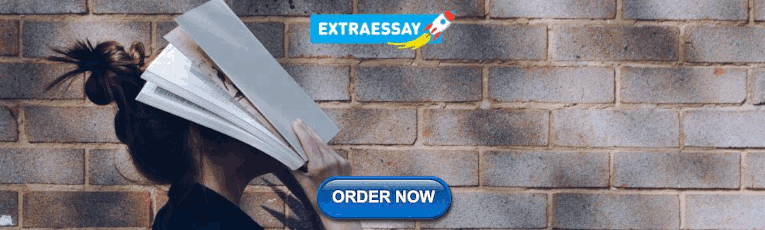
Rights and permissions
Open Access This article is licensed under a Creative Commons Attribution 4.0 International License, which permits use, sharing, adaptation, distribution and reproduction in any medium or format, as long as you give appropriate credit to the original author(s) and the source, provide a link to the Creative Commons licence, and indicate if changes were made. The images or other third party material in this article are included in the article's Creative Commons licence, unless indicated otherwise in a credit line to the material. If material is not included in the article's Creative Commons licence and your intended use is not permitted by statutory regulation or exceeds the permitted use, you will need to obtain permission directly from the copyright holder. To view a copy of this licence, visit http://creativecommons.org/licenses/by/4.0/ .
Reprints and permissions
About this article
Cite this article.
Roy, D., Dutta, M. A systematic review and research perspective on recommender systems. J Big Data 9 , 59 (2022). https://doi.org/10.1186/s40537-022-00592-5
Download citation
Received : 04 October 2021
Accepted : 28 March 2022
Published : 03 May 2022
DOI : https://doi.org/10.1186/s40537-022-00592-5
Share this article
Anyone you share the following link with will be able to read this content:
Sorry, a shareable link is not currently available for this article.
Provided by the Springer Nature SharedIt content-sharing initiative
- Recommender system
- Machine learning
- Content-based filtering
- Collaborative filtering
- Deep learning
Recommender Systems in Financial Trading: Using machine-based conviction analysis in an explainable AI investment framework
Traditionally, assets are selected for inclusion in a portfolio (long or short) by human analysts. Teams of human portfolio managers (PMs) seek to weigh and balance these securities using optimisation methods and other portfolio construction processes. Often, human PMs consider human analyst recommendations against the backdrop of the analyst’s recommendation track record and the applicability of the analyst to the recommendation they provide. Many firms regularly ask analysts to provide a ”conviction” level on their recommendations. In the eyes of PMs, understanding a human analyst’s track record has typically come down to basic spread sheet tabulation or, at best, a ”virtual portfolio” paper trading book to keep track of results of recommendations.
Analysts’ conviction around their recommendations and their ”paper trading” track record are two crucial workflow components between analysts and portfolio construction. Many human PMs may not even appreciate that they factor these data points into their decision-making logic. This chapter explores how Artificial Intelligence (AI) can be used to replicate these two steps and bridge the gap between AI data analytics and AI-based portfolio construction methods. This field of AI is referred to as Recommender Systems (RS). This chapter will further explore what metadata that RS systems functionally supply to downstream systems and their features.
Keywords: Recommender systems, algorithmic trading, AI investment tools, explainable AI
1 Introduction
Definition: Recommender systems (RS) ‘are information filtering and decision supporting systems that present items in which the user is likely to be interested in a specific context. 1 1 1 N K Liu and KK Lee, ‘An intelligent business advisor system for stock investment’, Expert Systems, 14(3), 129–139, (1997).
A Recommender system is a form of AI and is typically a standalone piece of software or computer code. Recommender systems (RS) are designed to ”recommend” a course of action to the end decision maker, or more commonly, another piece of software that makes further analysis. RS’s are typically helpful when dealing with a data problem of selecting from a universe of items that is many orders of magnitude larger than the number of items a user wishes to select. For example, if PM’s theoretical investible universe covers some 1000 – 5000 listed securities, a typical portfolio they manage may contain 100 or few stocks, a small percentage of the universe of all possible choices.
Recommnder systems were initially a niche application of a broader, earlier form of AI, Expert Systems, which came to prominence in the 1980s. The academic field proliferated following the publication of the ”Handbook of Applied Expert Systems” in 1997. One of the first academic works by Lui and Lee of Hong Kong University in 1997 considered a system that was an ”intelligent business advisor system for stock investment and was widely implemented and offered a list of features for analysing and picking stocks, based on user preferences which the authors noted needed to be supplied by the end investor. RS shot to prominence more broadly with the Netflix Prize, a competition with USD $1m in prize money for a solution to produce the best collaborative filtering algorithm for Netflix, then an online DVD rental platform. The prize was finally won in 2009. Since then, many online consumer tools in areas such as music (Spotify), shopping (Amazon) and news media have continued to advance the field. Extensive academic work exists in the field of applied RS as early as the 2000s, with meta review studies being published as early as 2016 covering over 100 papers [ 16 ] .
2 Common Types of RS AI and their mainstream adoption
Four common subtypes of RS AI exist: collaborative filtering, content-based filtering, knowledge-based systems and case-based systems. Knowledge-based systems are the most applicable and widely used subtypes in asset selection and institutional investing.
AI which, rather than using specific data’s inherent or apparent features, a meta-model and user responses classify data. A basic example might be that the AI utilises public tweets or blog posts that mention the stock rather than using the listing location as a data field.
uses the inherent features of an item and incorporates past items selected by a user that are filtered based on the same inherent features. These typically have lower predictive powers in financial settings and rely upon multiple criteria decision analysis (MCDA) techniques. As the name suggests, users must extensively specify these systems and explain why they made previous stock choices.
uses predefined ”cases” stipulated by users. These are then applied to other time periods of evaluation.
The most widely implemented form of RS in finance. These are complex systems that have collected and systematised expert humans’ knowledge and human experts’ decision-making processes. They often involve highly technical analysis components that seek insight from large data sets, e.g., trading price data of single stocks. Additionally, these systems use ranking and sorting methods to produce personalised recommendations based on user specifications, training data, historical versions of the same software and currently available market data.
In the past five years, there has been a significant increase in the number of available AI-based software packages and platforms capable of taking proprietary client data and forming bespoke recommendations. Many tools have long tried to incorporate other forms of AI into their recommendation engines including fuzzy logic methods, artificial neural networks (at the post recommendation analysis phase) and even methods such as support vector machines when trying to filter recommendations with the added usage of data such as news information. Machine Learning techniques can also be applied to the calibration methods within recommendation systems. The key message to take away here is that many AI methods can be applied sequentially or within a broader AI method. In the following section I present a fictional case study of a senior PM at a hedge fund (or investment firm) to illustrate the applicability of AI methods applied to individual asset classes.
3 Features, details, and design
To help illustrate a complex implementation, I will make use of a fictional case study. I will focus on how a firm of trading and investment professionals, utilise teams of internal analysts to develop strategies on individual assets and implement a RS. For ease of discussion, I will assume we are talking about an equity trader, and the asset class that they look at are Asian and European equities. This case study could apply to any listed security market.
3.1 An example of a Senior PM at a hedge fund or investment firm and their journey with RSs
A typical day would involve a PM either directly or in conjunction with a small team of 1 -5 analysts running analysis and internal algorithms on the securities in the universe that make up her trading mandate. For this example, where we use Asian and European equities, liquidity and market capitalisation constraints are crucial considerations, dictating our PM’s starting universe of possible securities. As is most common, PMs will tend to focus on either specific sectors or regions. For many reasons related to capital constraints and regulation, they will most likely look at firms with market capitalisations above some minimum threshold. This clearly defined universe will have other consequences; it will dictate the data the PM can use in standard software and the risk limits they can use. Suppose our PM has the mandate to trade long and short. In that case, the availability of stock borrow for cover of short positions will also form the initial stock universe.
A team of analysts will typically run in-house scripts, code, spreadsheets, or even internal programs looking for patterns and previously identified trading strategies against the universe of securities. Some PMs will have this process automated internally; others who trade with a lower frequency will look to have human analysts build ”high conviction” lists for proposed long and short trades. Except for the sophistication of the automation of these steps defined here, little in this workflow has changed in over two decades in a PM’s day to day role.
In the early 2000s, many stock exchanges started to increase the availability of their market trading data and allow computers to link to their electronic order books directly and explicitly. Those equity exchanges that were completely voice-driven moved to electronic trading screens and dealer interfaces. Those largely screen-based exchanges invested heavily in the necessary hardware to support faster data access and trading capabilities. What has resulted is a sea of market data. By the late 2000s and into the 2010s, many firms had grown internal specialised teams of programmers and analysts. These teams are highly skilled at fast data analysis and produced robust in-house technology infrastructure to repeatably and reliably automate the daily data analysis process. However, the output of many of these firms that still maintained traditional PMs skills was to produce information for a human PM to digest, synthesise and ultimately use to make discretionary trading decisions. A small but highly successful group of quantitative funds flourished from the mid-2000s onward and specialised in technology. Firms traditionally focused on discretionary trading now have PMs who deal with an ever-increasing deluge of semi-processed market information each day. Gradually, as firms acknowledged the increasing size and challenge of garnering insight from partially processed data, a sort of “virtual” analyst evolved, which technically is a form of RS. The financial market journey parallels many of the similar advances made in non-financial market products, such as consumer software used in online shopping or digital media around the same time.
As a human team of analysts would present their high conviction buy and sell recommendations to our hypothetical PM, people build RS to produce virtual analyst recommendations. Systems began to develop organically from outsourced software providers as early as 2010 and are increasingly popular and well adopted today. One key reason for the increasing adoption of RS is the advent of a field of AI called Explainable AI, or Causal AI. This academic field focuses on what a RS and AI system need to show an end-user to elicit trust in their virtual recommendation. Many currently available software packages rely heavily on graphs and visualisation techniques to illustrate intermediate steps in the overall recommendation process. Such methods appear to be users’ preferred format for gaining trust in otherwise black box AI systems in this field.
Several firms are dedicated to cloud-based AI algorithms looking at stock price information. Hedge funds and asset managers who use these cloud-based software packages can log in and run proprietary or generic AI trading strategies on single stocks or baskets. Many firms will do a similar thing across data from other industries regarding medical clinical trials and online sales data from consumer goods platforms. These cloud-based AI firms looking at stock information require the PM to design their algorithm within their framework, import their data, or use existing options. The output of these systems is what differentiates this group of RS from other forms of software: they provide relative recommendations, come with some supporting information about the decision and usually some heuristic interpretation of the recommendation.
A key feature is RS by design rarely, if ever, recommended a course of action such as “buy” or “sell”. So, what does a RS output look like? A list of buys ranked by some pre-agreed analysis method from highest conviction to lowest conviction. Some systems will enrich the idea of “conviction” to help explain the system’s idea of a human notion of confidence. For example, a sample track record may be applied to each conviction on each stock that made it into a ranked suggestion list of “buys” – where the conviction of the algorithm producing the recommendation may show a “hit ratio” of that algorithm on that stock over the past 100 days. You can begin to see that many practical forms of conviction analysis can be done here. For example, perhaps the PM might want to know how the algorithm has performed on a given stock over the past 10 days, 50 days, and 100 days because that aligns with the PM’s macro view on the current markets. Similarly, a PM with a long-term trading strategy may only be interested in a conviction analysis of 100 or more days. Some may look for time period analysis that follows a geometric progression or even a much-beloved Fibonacci number sequence. Just like single stock technical trading styles favour different technical indicators for different market environments, commonly available RS have many varieties of preloaded and embedded AI-based algorithms – though they tend to be knowledge-based AI systems.
Returning to our PM, they may seek to use an in-house implementation of a RS, uploaded and calibrated to her specific macro or market views, and within the risk management framework constraints of her trading mandate. The PM might request a ranked list of high conviction stock trading suggestions and high conviction short trading suggestions to meet the market-neutral nature of her overall portfolio constraint (noting that long and short portfolios may not guarantee any in and of themselves market neutrality). they might even have sector or country limits that they incorporated into her RS to receive a ranked and sorted recommendation list that has factored these into their recommendations. They may also use one of several commercially available third-party cloud-based software providers rather than an in-house software solution. Once received, our PM will typically follow one of two courses of action; the first is to review these recommendations manually (using human intuition) or, the second, to import the recommendations (along with associated metadata) into another technology system. Whilst external software platforms for recommendations are a new invention, older styles of in-house software that seamlessly passed data from a RS into other downstream internal systems are still far more common.
3.2 Dealing with a RSs set of recommendations: what next?
Turning again to our PM, assuming they are one of the more sophisticated PMs, looking to make use of every piece of data at their disposal, now a different analysis process occurs. The research comes into play in various fields of old and new AI. Older fields of RS or expert systems can form a basis of rigid “if this, then that” rules that run over these recommendations to refine a list of trades long and short. Systems like these have been covered in academic literature from the 1970s. Several large, prestigious, and long-established hedge funds imply they use these methods in addition to high-frequency trading firms (though with slightly different applications).
More recently, however, as AI research has increased and explainable AI, causal AI and even “ethical AI” have grown in importance and relevance, newer methods of dealing with the output from algorithmic trading recommendation systems have emerged. These fields have all been spurred along by increasing human adoption of AI in people’s personal lives (think AI-enabled home devices, online grocery shopping apps and ride-sharing apps that seem to know where you are travelling to before you even type in an address). In addition, increasingly, regulatory, and legal responsibilities are being placed on AI. Namely, human end-users of AI interim recommendations and decisions need to be comfortable with those decisions as they, in many jurisdictions, carry the legal responsibility for those trading decisions. Hence, humans need to understand the recommendations made and essentially own the risk that taking decisions based on them brings.
So, what might an example be? Returning to our busy PM, her ranked, sorted list of long and short high conviction stock recommendations would be loaded up into different pieces of software. This downstream software will typically fall into three broad groups –
Risk management – pre-trade analysis
Portfolio management – pre-trade analysis
Pure alpha analysis – PM specific further analysis
As experience in modern portfolio management will attest, today’s money management firms have a plethora of internal software – everything from books and records systems to order management systems and at least one compliance and one risk management software package. Many firms may use up to 15 different software packages in a given day, all with specific niche applications that remain crucial to any investment process’s efficient and compliant running. A straightforward example of this is the need in many jurisdictions to source stock borrow for a short position before placing a trade that will take a specific trading account into a short position. Firstly, finding the required stock borrow can bring market challenges (such as telegraphing to other firms the desire to short a holding), not to mention the administrative challenge of making sure it is internally assigned to the correct PM’s trading account. These are all burdensome processes, without which our PM may find herself non-compliant in certain jurisdictions. What is more crucial to appreciate here is that the PM will need more information than simply a list of ranked buy and sell recommendations to put into “downstream” systems. These additional details are commonly referred to as “metadata” and are crucial for the correct technical functioning of independent technology software such as below;
3.2.1 Risk management system analysis
The PM might analyse the impact of recommendations on the risk characteristics of her current portfolio. Any sound risk system will incorporate “what if” scenario analysis. Perhaps the PM may have a more manual process for incorporating a dummy portfolio to look at its risk features and how it would have performed historically. More than the possible returns of the portfolio, the use of risk systems would allow the PM to check that by incorporating the recommendations, no strict risk limits and mandate restrictions would be broken. More technically advanced trading firms, or those readers with a strong high frequency trading (HFT) background, might perform this analysis in a systematic automated process through database manipulation and other scripting/programmatic methods. Essentially the same outcome is sort: how the recommended ranked and sorted list of buy and sell ideas would affect the current holdings of the PM.
In terms of “metadata” needed to perform these stages, the ranked and sorted list of recommendations really would need to pass appropriate identification tickers for securities and some way to turn that into either a proposed number of shares to buy or sell, or more as a percentage of the ideal portfolio the PM is trying to target. Special care needs to be taken here in the choice of stock price used to calculate either, as prices need to be synchronous with the risk system data and the analysis periods used in the initial recommendation systems. This detail is often missed and can produce a sense of “forsight” of a model that can bias more automated backtesting processes.
3.2.2 Portfolio management – pre-trade analysis
Here, the PM would use similar data fed to a risk system to evaluate the performance attributes of the proposed buys and sells. Depending on the sophistication of the PM and the software used, this may include shadow profit and loss (PNL) forecasting and even parsimonious methods for shadow PNL forecast attribution. Whilst outside the scope of this chapter, both outcomes will ideally need to know more about how a buy or sell recommendation was ranked in the conviction recommendations.
This brings us to a crucial aspect of Explainable AI in particular – how does one piece of AI explain to a human (or even a second piece of software that a human uses to visualise it) why it ranked its recommendations the way it did? For those unfamiliar with this type of analysis, it’s important to understand how basic AI programs operate when performing linear program analysis (i.e., simple ”if this, then that” logic). The AI software can quickly explain how it arrived at its decisions because it can essentially retrace the path it took through all the conditional statements (”ifs” and ”else’s”) to reach a particular conclusion or ranking.
It can even output all the various maths calculations it used to come up with some mark or score, and then the way it thought about ranking them (e.g., biggest to smallest or smallest to biggest, or even removing outliers then taking some ordered list). However, the methodologies here are almost endless; the point is that in older AI forms, the methods are linear and easy to describe in a tree diagram or “list of steps”.
We run into more difficulty with more advances and modern forms of nonlinear AI; that is to say, they do not follow a clear linear path from input to output, with the same steps repeated for the same inputs every time. For those of you not familiar with nonlinear AI methods, you may have heard of things such as Machine Learning, neural networks, random forest, deep learning algorithms and fuzzy logic – to name a few. In their most straightforward format, in an attempt to be smarter than a simple list of “if this then that” commands, AI has developed to think more like humans naturally do (which is associative) and to think in entirely unstructured unintuitive ways (e.g., neural networks, random connections tested over large amounts of history). Newer methods can be compelling as they introduce an element of uncertainty and artificially “lateral” thinking. This “lateral” thinking component is a strength and weakness, making such AI hard to explain and potentially untrustworthy to a human user. The following section will deal with practical examples of these issues.
3.2.3 Decision Support Systems - alpha analysis and PM specific further analysis
The third use case for recommendation systems output is for our PM to upload the ranked and sorted lists into an alpha analysis program that provides decision support services. Alpha analysis programs can be as simple as a spreadsheet or as complex as several commercially available AI supercomputer frameworks (e.g., IBM Watson or TensorFlow).
Her team of analysts could even produce the program in Python or C++. The location or type of program matters little; what is critical here is the reason for analysis and the methods utilised to look at the recommendations from “many angles”. The straightforward idea is to try and take the recommendations and correlate them independently to other macro factors such as a currency rate or interest rate curve, looking to identify a relationship with independent variables. This process itself could be done as a form of machine learning. However, most experienced PMs would be concerned about taking a series of recommendations and arbitrarily testing them for correlations to other variables where little or no use of the actual variable was used in coming up with a recommendation – this would be fertile ground for discovering spurious correlations. More likely, as we saw in our discussion on the Portfolio management software, the PM would want the recommendation software to pass along metadata that allowed more extensive analysis in a testing environment controlled by the PM. Extensive back testing is some of the tests they might run beyond those already completed in a risk and portfolio setting. So how would a nonlinear AI explain its own decisions? Welcome to the world of explainable AI. One critical method is a counterfactual explanation, another more practical process is paper trading records.
3.3 How to explain RS outputs, how to explain AI-led recommendations
The field of explainable AI is a huge and growing one. In practice, there remains little consensus on the ultimate “perfect” explanation. Instead, practically speaking, people look to things that have tended to replicate existing frameworks of explanation in other domains. For example, when a senior PM looks at her team of PMs and analysts, they might naturally recall their profit and loss records, their trading decisions around specific events, and when her analysts and PMs got decisions very right and very wrong. They might also recall the over-exuberance of analysts who presented investment decision cases with high degrees of certainty, only to be wrong-footed by the market a few days later as some unexpected implicit assumption no longer held. Depending on her level of experience and that of her team, they may recall live examples of how people traded, and decisions made over particularly turbulent market events such as the 2008 Global Financial Crisis or Greek bond default and the COVID pandemic. These examples can be easily replicated with AI by asking the RS to produce a paper track record of its various recommendations over time. These track records created within the recommended system (or outside of it by comparing many days’ worth of recommendations) provide Machine Learning algorithms to data-mine and learn and adapt decision-making layers.
Many forms of AI are primarily done without the interference of human users, others require training data and others still like to verify aspects of their processes at various points in time with factual human-verified inputs. These latter classes can be thought of as reinforcement and supervised learning methods. One simple outcome of all this analysis might be that our PM’s internal alpha system can gauge how best to interpret the recommendations from the initial RS program. Such interpretations might include, but would not be limited to, thinking about how good the RS sorting and ranking methods are, and if, for example, the top recommendations might end up putting the PM into already crowded trades or positions with existing very high short interest. These ‘insights’ would require much data and Machine Learning calibration and may or may not be stable through time. Understanding these insights and their weaknesses will become our PM and her team’s core skill and chief value-add.
4 Big Data tools and RS utilisation
The process described features increasing amounts of data. The very concept of time series analysis is such that by virtue of the process described above existing for six months, there is an additional day of history at each additional day. Added to this is the issue that RS that impart or directly rely on some training data or data needed for lookback periods, are notorious for using sizeable historical data sets. Furthermore, depending on the investment horizon and risk management framework of the investment manager in question, modelling intraday data for investments may be necessary. If the RS focuses on investment idea generation, trading implementation, and best execution analysis, significantly more market data will be needed. Any analysis of order execution or intraday risk management intervention would typically require very granular trading information, possibly down to individual tick by tick analysis of the securities in question.
All this adds up to a considerable amount of data. Different components of the analysis process utilise different aspects of data and, potentially, different time granularity of data. Returning to a PM and her team, setting aside the technical challenges of such systems, humans have a strong preference of being about to verify data inputs visually. Many advanced tools now exist for data visualisation. What is perhaps novel in an RS is the need to visualise raw data inputs (e.g., price trends) and intermediate variable states and intermediate decisions. As discussed above, not all forms of AI used by RSs can provide transparency (visual or otherwise) that will satisfy a human or replicate current processes. At this point, visualisation tools will aim to interpret the various mathematical methods of explanation that nonlinear AI models will need.
As any good programmer will know, one should never re-invent the wheel when commoditised code exisits that can be quickly and efficiently sourced external to a firm (e.g. repos on Github). The past decade has seen an explosion in outsourced data analysis firms, many focusing on finance and robustly providing the exact commoditised analysis processes that I mention here. Much like the early 1990s saw the advent of sophisticated statistical analysis programs, and even the extensive expansion of capability within Microsoft Excel, from around 2012, there has been a similar trend in analysis software from portfolio return attribution analysis through to outsourced Machine Learning tools and database AI tools. Many of these early outsourced analysis platforms were high-quality but initially struggled with client adoption. Next, I will detail some of the reasons for this and how overcoming such obstacles has directly affected how RS work.
5 Considerations and complications of outsourced analysis platforms.
We have already seen the evolving need for more robust and industrial-strength analysis platforms to understand, interpret, and evaluate AI decisions, particularly those from RS in portfolio management settings.
Outsourced software, which has grown in popularity and capability, has always provided significant value to those firms willing to use them. However, their adoption has only recently gained pace, with many of these software firms announcing prominent venture capital and investor support alone in the past 12 months.
There are four key categories in terms of practical considerations a PM or their firm might make before allowing the use of such outsourced software.
Intellectual Property (IP): Protection and Creation . Any use of a technical system tends to remain the user’s IP; however, it is the fundamental nature of AI that some degree of learning from one users’ needs is used to enhance the overall product (for other users). The only way to avoid this would be to have a standalone in-house system - but then again, your firm might not benefit from general product development long term. Practically speaking, this can be addressed by segmenting the work done in one disparate system to another.
Robustness and support . A key feature of external software is the size of the team and the technical advantage of specialised IT support. Even small analytic firms often have teams of engineers and programmers who outnumber even a large group at a single financial institution. The increasingly specialised nature of analytic technology means that external software can be a key leverage point for smaller PMs and investment managers.
Integration and quality control . There is always a point at which the end-user no longer has visibility around the software when using external software. Also, many internal software packages at large investment firms remain obscured to the end-user. In the case of Machine Learning, there is a component of proprietary implementation of ubiquitous processes that complicates the picture. External software providers need to keep some aspects of their code proprietary. Hence, integration and quality control for the PM or end-user becomes essential. Internal IT staff to a PM’s firm may change their role to monitor outsourced software for changes rather than maintaining in-house software packages. Again, such monitoring tools exist and are pretty standard in cyber security software.
6 Trends and future development
I have outlined here many directions that AI in RS are taking, such as explainability and methods of garnering the “trust” of human users. In researching this chapter two such trends were most notable; the method systems are using to garner trust, and the people who are most frequently using such systems.
Human users of RS software today appear to be demanding more and more visualisation of key data components and intermediate analysis. This is leading many software providers to develop highly specialised and visually appealing user interfaces. The actual data that appears to be displayed, at the request of the user, is not necessarily the most important data used by the RS, but rather reflects the interest of users into specific data.
Furthermore, external RS software today seems targeted to the large number of discretionary managers and “traditional” asset investors. This contrasts with many forms of specialised AI software which rely on users having advanced understanding of AI. RS software targeted at discretionary managers is potentially going to “bridge the gap” between the vast majority of non-technically trained investment staff and the increasing power of AI applied to investing. It remains to be seen if software firms are able to provide user experiences that can be acceptable to their clients without any loss of power of the AI behind their investment recommendations.
As the adoption of AI, systems, analytics, and broadly based recommendation engines increases, many people look to quantify the uplift in returns that such tools can provide. Whilst looking to see what an AI trading system would have traded, and its resulting profit and loss record over a period is helpful; the bigger picture is fast becoming which firms can afford not to use any AI systems at all. Such has been the adoption by investors and consumers alike to the power and potential of AI that many firms now appear to feel they need to say they are using some forms of it to remain relevant and current to the landscape. This chapter has shown the difference between merely purchasing a license to some AI-enabled data platform and the actual integration of recommendation-based AI systems in a portfolio management context.
8 Acknowledgments
I would like to express my appreciation to all those who provided me the possibility to complete this report. A special note of thanks to Dr Middleweek, (University of Technology Sydney), for her careful review and Professor Walsh (Scientia Professor of AI, University of New South Wales) for his thoughtful suggestions.
- [1] Robert Andrews, Joachim Diederich, and Alan Tickle. Survey and critique of techniques for extracting rules from trained artificial neural networks. Knowledge Based Systems , 1995.
- [2] José D. Bermúdez, José Vicente Segura, and Enriqueta Vercher. A fuzzy ranking strategy for portfolio selection applied to the spanish stock market. 2007 IEEE International Fuzzy Systems Conference , 2007.
- [3] Haim Bodek. A case study in regulatory arbitrage and information asymmetry: High frequency trading and the post only intermarket sweep order. Global Algorithmic Capital Markets , 2018.
- [4] WILLIAM BROCK, JOSEF LAKONISHOK, and BLAKE LeBARON. Simple technical trading rules and the stochastic properties of stock returns. The Journal of Finance , 47(5):1731–1764, 1992.
- [5] Wojtek Buczynski, Fabio Cuzzolin, and Barbara J. Sahakian. A review of machine learning experiments in equity investment decision-making: why most published research findings do not live up to their promise in real life. Journal of data science , 2021.
- [6] Robin Burke. Knowledge-based recommender systems. Encyclopedia of library and information systems , 69, 05 2000.
- [7] Thira Chavarnakul and David Enke. Intelligent technical analysis based equivolume charting for stock trading using neural networks. Expert Systems with Applications , 34(2):1004–1017, 2008.
- [8] Roberto Confalonieri, Ludovik Coba, Benedikt Wagner, and Tarek R. Besold. A historical perspective of explainable artificial intelligence. Wiley Interdisciplinary Reviews-Data Mining and Knowledge Discovery , 2020.
- [9] Thomas G. Dietterich. Ensemble methods in machine learning. Multiple Classifier Systems , 2000.
- [10] Manuel Fernández-Delgado, E. Cernadas, Senén Barro, Dinani Gomes Amorim, and Dinani Amorim. Do we need hundreds of classifiers to solve real world classification problems. Journal of Machine Learning Research , 2014.
- [11] Daniel Gallego and Gabriel Huecas. An empirical case of a context-aware mobile recommender system in a banking environment. 2012 Third FTRA International Conference on Mobile, Ubiquitous, and Intelligent Computing , 2012.
- [12] Tomer Geva and Jacob Zahavi. Empirical evaluation of an automated intraday stock recommendation system incorporating both market data and textual news. Decis. Support Syst. , 2014.
- [13] Israel González-Carrasco, Ricardo Colomo-Palacios, Jose Luis Lopez-Cuadrado, Ángel García-Crespo, and Belén Ruiz-Mezcua. Pb-advisor: A private banking multi-investment portfolio advisor. Information Sciences , 2012.
- [14] M. Hassani Goodarzi. A web-based implementation of a portfolio advisor system based on fuzzy expert systems. In IEEE 6th Int. Conf. Information and Communication Technology and System ICTS , pages 15–22, 2010.
- [15] Deqiang Han, X. Rong Li, and Shaoyi Liang. Design of dynamic multiple classifier systems based on belief functions. Proceedings of the 16th International Conference on Information Fusion , 2013.
- [16] Chen He, Denis Parra, and Katrien Verbert. Interactive recommender systems: A survey of the state of the art and future research challenges and opportunities. Expert Systems with Applications , 56:9–27, 2016.
- [17] Tin Kam Ho, Jonathan J. Hull, and Sargur N. Srihari. Decision combination in multiple classifier systems. IEEE Transactions on Pattern Analysis and Machine Intelligence , 1994.
- [18] Yong Hu, Kang Liu, Xiangzhou Zhang, Lijun Su, EWT Ngai, and Mei Liu. Application of evolutionary computation for rule discovery in stock algorithmic trading: A literature review. Applied Soft Computing , 36:534–551, 2015.
- [19] Yea S Huang and Ching Y Suen. The behavior-knowledge space method for combination of multiple classifiers. In IEEE computer society conference on computer vision and pattern recognition , pages 347–347. Institute of Electrical Engineers Inc (IEEE), 1993.
- [20] Y.S. Huang and C.Y. Suen. A method of combining multiple experts for the recognition of unconstrained handwritten numerals. IEEE Transactions on Pattern Analysis and Machine Intelligence , 17(1):90–94, 1995.
- [21] Jay Liebowitz. The handbook of applied expert systems . cRc Press, 2019.
- [22] Jay Liebowitz and Jay Liebowitz. The handbook of applied expert systems. null , 1997.
- [23] N. K. Liu and K. K. Lee. An intelligent business advisor system for stock investment. Expert Systems , 1997.
- [24] Chao Ma, Chao Ma, Xun Liang, and Xun Liang. Online mining in unstructured financial information: An empirical study in bulletin news. 2015 12th International Conference on Service Systems and Service Management (ICSSSM) , 2015.
- [25] Robi Polikar. Ensemble based systems in decision making. IEEE Circuits and systems magazine , 6(3):21–45, 2006.
- [26] Lior Rokach, Guy Shani, Bracha Shapira, Eyal Chapnik, and Gali Siboni. Recommending insurance riders. SAC ’13 , 2013.
- [27] Omer Sagi and Lior Rokach. Ensemble learning: A survey. Wiley Interdisciplinary Reviews-Data Mining and Knowledge Discovery , 8(4), 2018.
- [28] Badrul M. Sarwar, George Karypis, Joseph A. Konstan, and John Riedl. Item-based collaborative filtering recommendation algorithms. WWW ’01 , 2001.
- [29] Robert E Schapire. The strength of weak learnability. Machine learning , 5(2):197–227, 1990.
- [30] AA Spanjersberg. Combination of different systems for the recognition of handwritten digits. In Proc. 2nd Int. Joint Conf. on Pattern Recognition, Copenhagen , pages 208–209, 1974.
- [31] Ching Y. Suen, Louisa Lam, and Louisa Lam. Multiple classifier combination methodologies for different output levels. Multiple Classifier Systems , 2000.
- [32] James Surowiecki. The wisdom of crowds. Nature , 01 2005.
- [33] Mona Taghavi, Kaveh Bakhtiyari, and Edgar Scavino. Agent-based computational investing recommender system. RecSys , 2013.
- [34] David H. Wolpert. Stacked generalization. Neural Networks , 5(2):241–259, 1992.
- [35] David H Wolpert and William G Macready. No free lunch theorems for optimization. IEEE transactions on evolutionary computation , 1(1):67–82, 1997.
- [36] Chukiat Worasucheep. Ensemble classifier for stock trading recommendation. Applied Artificial Intelligence , 36(1):2001178, 2022.
- [37] Zhiyong Zhang, Chuan Shi, Sulan Zhang, and Zhongzhi Shi. Stock time series forecasting using support vector machines employing analyst recommendations. ISNN , 2006.
- [38] Zili Zhang and Chengqi Zhang. An agent-based hybrid intelligent system for financial investment planning. PRICAI , 2002.
- [39] Dávid Zibriczky and Dávid Zibriczky. Recommender systems meet finance: a literature review. FINREC , 2016.
- [40] Ángel García-Crespo, Jose Luis Lopez-Cuadrado, Israel González-Carrasco, Ricardo Colomo-Palacios, and Belén Ruiz-Mezcua. Sinvlio: Using semantics and fuzzy logic to provide individual investment portfolio recommendations. Knowledge Based Systems , 2012.
To read this content please select one of the options below:
Please note you do not have access to teaching notes, recommender systems: a systematic review of the state of the art literature and suggestions for future research.
ISSN : 0368-492X
Article publication date: 15 March 2018
Issue publication date: 2 May 2018
This paper aims to identify, evaluate and integrate the findings of all relevant and high-quality individual studies addressing one or more research questions about recommender systems and performing a comprehensive study of empirical research on recommender systems that have been divided into five main categories. To achieve this aim, the authors use systematic literature review (SLR) as a powerful method to collect and critically analyze the research papers. Also, the authors discuss the selected recommender systems and its main techniques, as well as their benefits and drawbacks in general.
Design/methodology/approach
In this paper, the SLR method is utilized with the aim of identifying, evaluating and integrating the findings of all relevant and high-quality individual studies addressing one or more research questions about recommender systems and performing a comprehensive study of empirical research on recommender systems that have been divided into five main categories. Also, the authors discussed recommender system and its techniques in general without a specific domain.
The major developments in categories of recommender systems are reviewed, and new challenges are outlined. Furthermore, insights on the identification of open issues and guidelines for future research are provided. Also, this paper presents the systematical analysis of the recommender system literature from 2005. The authors identified 536 papers, which were reduced to 51 primary studies through the paper selection process.
Originality/value
This survey will directly support academics and practical professionals in their understanding of developments in recommender systems and its techniques.
- Recommender system
- Collaborative filtering
- Demographic filtering
Alyari, F. and Jafari Navimipour, N. (2018), "Recommender systems: A systematic review of the state of the art literature and suggestions for future research", Kybernetes , Vol. 47 No. 5, pp. 985-1017. https://doi.org/10.1108/K-06-2017-0196
Emerald Publishing Limited
Copyright © 2018, Emerald Publishing Limited
Related articles
We’re listening — tell us what you think, something didn’t work….
Report bugs here
All feedback is valuable
Please share your general feedback
Join us on our journey
Platform update page.
Visit emeraldpublishing.com/platformupdate to discover the latest news and updates
Questions & More Information
Answers to the most commonly asked questions here
A survey on recommendation systems for financial services
- Published: 03 March 2022
- Volume 81 , pages 16761–16781, ( 2022 )
Cite this article
- Marwa Sharaf 1 ,
- Ezz El-Din Hemdan 1 ,
- Ayman El-Sayed 1 &
- Nirmeen A. El-Bahnasawy 1
1184 Accesses
15 Citations
Explore all metrics
Recently, there is difficulty in extracting useful information from huge online information due to the rapid growth of the internet. Therefore, the Recommendation system (RS) is needed for the improvement of many services such as entertainment, e-commerce, healthcare, and financial services. It is an effective tool in the service industry, as it is used for guiding users to an interesting thing from the large space of random things. A recommendation system can discover patterns in input movements and generating system recommendations based on the patterns, thus it can significantly supplement the decision-making process of a stock trader. So, there are many methods for the recommendation process such as collaborative filtering, content-based and hybrid recommendations. Recommendation algorithm can be selected based on the existing research problem. This paper presents a review of the recommendation system, its types, and its applications. Then, this paper concentrated on the finance recommendation system, its operation, and its different finance sectors.
This is a preview of subscription content, log in via an institution to check access.
Access this article
Price includes VAT (Russian Federation)
Instant access to the full article PDF.
Rent this article via DeepDyve
Institutional subscriptions
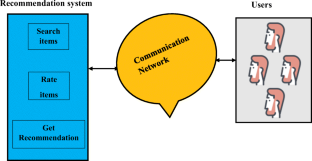
Similar content being viewed by others
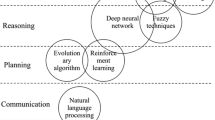
Artificial intelligence in recommender systems
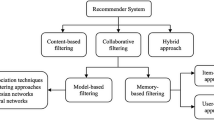
A systematic review and research perspective on recommender systems
Recommender Systems: Techniques, Applications, and Challenges
Agarwal V, Vijayalakshmi A (2019) Recommender system for surplus stock clearance. Int J Electrical Comput Eng 9(5):3813
Google Scholar
Al-Bashiri H, Abdulgabber MA, Romli A, Kahtan H (2018) An improved memory-based collaborative filtering method based on the TOPSIS technique. PLoS One 13(10):e0204434
Article Google Scholar
Alhijawi B, Kilani Y (2020) A collaborative filtering recommender system using genetic algorithm. Inf Process Manag 57(6):102310
Ali F, Islam SR, Kwak D, Khan P, Ullah N, Yoo SJ, Kwak KS (2018) Type-2 fuzzy ontology–aided recommendation systems for IoT–based healthcare. Comput Commun 119:138–155
Alshammari G, Jorro-Aragoneses JL, Kapetanakis S, Polatidis N, Petridis M (2019) A switching approach that improves prediction accuracy for long tail recommendations. In proceedings of SAI intelligent systems conference (pp. 18-28). Springer, Cham.
Atauchi PD, Nedel L, Galante R (2019) Broker-insights: An interactive and visual recommendation system for insurance brokerage. In computer graphics international conference (pp. 155-166). Springer, Cham.
Babu MSP, Kumar BRS (2011) An implementation of the user-based collaborative filtering algorithm. IJCSIT) Int J Comp Sci Inform Technol 2(3):1283–1286
Barathy R, Chitra P (2020) Applying matrix factorization in collaborative filtering recommender systems. In 2020 6th international conference on advanced computing and communication systems (ICACCS) (pp. 635-639). IEEE.
Barros M, Moitinho A, Couto FM (2020) Hybrid semantic recommender system for chemical compounds. In European conference on information retrieval (pp. 94-101). Springer, Cham.
Barua U, Hossain M, Arefin MS (2019) Real estate recommendation using historical data and surrounding environments. Int J Inform Eng Electron Bus 11(5):33–39
Benzarti I, Mili H, Paillard A (2019) A content based e-commerce recommendation approach under the Veristic framework. In international conference on e-business engineering (pp. 495-514). Springer, Cham.
Civan Z, Simsek GG, Akay EC (2020) Identifying the systemically important banks of Turkey with the CoVaR method. Heliyon 6(9):e04790
Dara S, Chowdary CR, Kumar C (2020) A survey on group recommender systems. J Intell Inf Syst 54(2):271–295
Da'u A, Salim N, Rabiu I, Osman A (2020) Recommendation system exploiting aspect-based opinion mining with deep learning method. Inf Sci 512:1279–1292
De Campos LM, Fernández-Luna JM, Huete JF, Rueda-Morales MA (2010) Combining content-based and collaborative recommendations: a hybrid approach based on Bayesian networks. Int J Approx Reason 51(7):785–799
Desirena G, Diaz A, Desirena J, Moreno I, Garcia D (2019) Maximizing customer lifetime value using stacked neural networks: An insurance industry application. In 2019 18th IEEE international conference on machine learning and applications (ICMLA) (pp. 541-544). IEEE.
Ding X, Yu W, Xie Y, Liu S (2020) Efficient model-based collaborative filtering with fast adaptive PCA. arXiv preprint arXiv:2009.02251 .
Dooms S, De Pessemier T, Martens L (2015) Offline optimization for user-specific hybrid recommender systems. Multimed Tools Appl 74(9):3053–3076
Erkek M, Çayırlı K, Taş H, Hepsen A, Aytekin T (2020) Recommendation systems applied in Turkish real estate market. J Comput Modell 10(1):1–10
Fu Z, Xian Y, Zhang Y, Zhang Y (2020) Tutorial on conversational recommendation systems. In fourteenth ACM conference on recommender systems (pp. 751-753).
Ghazanfar M, Prugel-Bennett A (2010) An improved switching hybrid recommender system using naive Bayes classifier and collaborative filtering
Ghazanfar M, Prugel-Bennett A (2010) Building switching hybrid recommender system using machine learning classifiers and collaborative filtering. IAENG Int J Comput Sci 37(3)
Guo Y, Zhou Y, Hu X, Cheng W (2019) Research on recommendation of insurance products based on random Forest. In 2019 international conference on machine learning, big data and business intelligence (MLBDBI) (pp. 308-311). IEEE.
Henrique BM, Sobreiro VA, Kimura H (2019) Literature review: machine learning techniques applied to financial market prediction. Expert Syst Appl 124:226–251
Isinkaye FO, Folajimi YO, Ojokoh BA (2015) Recommendation systems: principles, methods and evaluation. Egyptian Inform J 16(3):261–273
Kaššák O, Kompan M, Bieliková M (2016) Personalized hybrid recommendation for group of users: top-N multimedia recommender. Inf Process Manag 52(3):459–477
Katarya R, Verma OP (2017) An effective collaborative movie recommender system with cuckoo search. Egyptian Inform J 18(2):105–112
Khan Z, Iltaf N, Afzal H, Abbas H (2020) DST-HRS: a topic driven hybrid recommender system based on deep semantics. Comput Commun 156:183–191
Kiran R, Kumar P, Bhasker B (2020) DNNRec: a novel deep learning based hybrid recommender system. Expert Syst Appl 144:113054
Kowsher M, Prottasha NJ, Tahabilder A, Islam MB (2020) Machine learning based recommendation Systems for the Mode of childbirth. In international conference on cyber security and computer science (pp. 295-306). Springer, Cham.
Kumar NP, Fan Z (2015) Hybrid user-item based collaborative filtering. Procedia Comput Sci 60:1453–1461
Kumar PV, Reddy VR (2014) A survey on recommender systems (RSS) and its applications. Int J Innov Res Comput Commun Eng 2(8):5254–5260
Lampropoulos AS, Lampropoulou PS, Tsihrintzis GA (2012) A cascade-hybrid music recommender system for mobile services based on musical genre classification and personality diagnosis. Multimed Tools Appl 59(1):241–258
Lesage L, Deaconu M, Lejay A, Meira JA, Nichil G (2020) A recommendation system for car insurance. Eur Actuar J 10:1–22
Article MathSciNet MATH Google Scholar
Levinas CA (2014) An analysis of memory based collaborative filtering recommender systems with improvement proposals. Master's thesis, Universitat Politècnica de Catalunya
Li C, Wang Z, Cao S, He L (2018) WLRRS: a new recommendation system based on weighted linear regression models. Comput Electrical Eng 66:40–47
Lian M, Li J (2020) Financial product recommendation system based on transformer. In 2020 IEEE 4th information technology, networking, electronic and automation control conference (ITNEC) (Vol. 1, pp. 2547-2551). IEEE.
Liu C, Wang H (2019) Stock recommendation based on depth BRNN and bi-LSTM. In 2019 4th international conference on mechanical, control and computer engineering (ICMCCE) (pp. 751-7514). IEEE.
Logesh R, Subramaniyaswamy V, Malathi D, Sivaramakrishnan N, Vijayakumar V (2020) Enhancing recommendation stability of collaborative filtering recommender system through bio-inspired clustering ensemble method. Neural Comput amp; Applic 32(7):2141–2164
Lops P, Jannach D, Musto C, Bogers T, Koolen M (2019) Trends in content-based recommendation. User Model User-Adap Inter 29(2):239–249
Mathew PS, Pillai AS (2015) Big data solutions in healthcare: problems and perspectives. In 2015 international conference on innovations in information, embedded and communication systems (ICIIECS) (pp. 1-6). IEEE.
Mican D, Sitar-Tăut DA, Moisescu OI (2020) Perceived usefulness: a silver bullet to assure user data availability for online recommendation systems. Decision Support Syst 139:113420
Mokarrama MJ, Khatun S, Arefin MS (2020) A content-based recommender system for choosing universities. Turkish J Electrical Eng Comput Sci 28(4):2128–2142
Montesi G, Papiro G, Fazzini M, Ronga A (2020) Stochastic optimization system for Bank reverse stress testing. JRisk Financial Manag 13(8):174
Narayanan M, Cherukuri AK (2016) A study and analysis of recommendation systems for location-based social network (LBSN) with big data. IIMB Manag Rev 28(1):25–30
Nawi RM, Noah SM, Zakaria LQ (2020) Evaluation of group modelling strategy in model-based collaborative filtering recommendation. Int J Mach Learn Comput 10(2):330–338
Nguyen NC, Duong HC, Nguyen HT, Chen SS, Le HQ, Ngo HH, … Bui XT (2020) Forward osmosis–membrane distillation hybrid system for desalination using mixed trivalent draw solution. J Membrane Sci 603:118029
Nieves EH (2020) New approach to recommend banking products through a hybrid recommender system. In international symposium on ambient intelligence (pp. 262-266). Springer, Cham.
Ortiz Viso B (2020) Evolutionary approach in recommendation Systems for Complex Structured Objects. In fourteenth ACM conference on recommender systems (pp. 776-781).
Oyebode O, Orji R (2020) A hybrid recommender system for product sales in a banking environment. J Banking Financial Technol 4:1–11
Pan Y, He F, Yu H (2019) A novel enhanced collaborative autoencoder with knowledge distillation for top-N recommender systems. Neurocomputing 332:137–148
Pan Y, He F, Yu H (2020) Learning social representations with deep autoencoder for recommender system. World Wide Web 23(4):2259–2279
Pan Y, He F, Yu H (2020) A correlative denoising autoencoder to model social influence for top-N recommender system. Front Comput Sci 14(3):1–13
Rana SP, Dey M, Prieto J, Dudley S (2020) Content-based health recommender systems. Recommender system with machine learning and artificial intelligence: practical tools and applications in medical, agricultural and other industries, 215.
Rehman F, Masood H, Ul-Hasan A, Nawaz R, Shafait F (2019) An intelligent context aware recommender system for real-estate. In Mediterranean conference on pattern recognition and artificial intelligence (pp. 177-191). Springer, Cham.
Ricci F, Rokach L, Shapira B (2011) Introduction to recommender systems handbook, In recommender systems handbook (pp. 1–35). Springer, Boston, MA
Riyahi M, Sohrabi MK (2020) Providing effective recommendations in discussion groups using a new hybrid recommender system based on implicit ratings and semantic similarity. Electron Commer Res Appl 40:100938
Sabbah T, Selamat A, Selamat MH, Ibrahim R, Fujita H (2016) Hybridized term-weighting method for dark web classification. Neurocomputing 173:1908–1926
Sezgin E, Özkan S (2013) A systematic literature review on health recommender systems. In 2013 E-health and bioengineering conference (EHB) (pp. 1-4). IEEE.
Shah J, Sahu L (2014) A survey of various hybrid based recommendation method. Int J Advanc Res Comput Sci Software Eng 4:369–371
Shao B, Li X, Bian G (2020) A survey of research hotspots and frontier trends of recommendation systems from the perspective of knowledge graph. Expert Syst Appl 113764
Sharifihosseini A (2019) A case study for presenting Bank recommender systems based on bon card transaction data. In 2019 9th international conference on computer and knowledge engineering (ICCKE) (pp. 72-77). IEEE.
Soares M, Viana P (2015) Tuning metadata for better movie content-based recommendation systems. Multimed Tools Appl 74(17):7015–7036
Sun Y, Fang M, Wang X (2018) A novel stock recommendation system using Guba sentiment analysis. Pers Ubiquit Comput 22(3):575–587
Tas H, Sumnu HE, Gokoz B, Aytekin T Dev Hybrid Real Estate Recomm Syst
Vismayaa V, Pooja KR, Alekhya A, Malavika CN, Nair BB, Kumar PN (2020) Classifier based stock trading recommender systems for Indian stocks: An empirical evaluation. Comput Econ 55(3):901–923
Wang H, Ma S, Dai HN, Imran M, Wang T (2020) Blockchain-based data privacy management with nudge theory in open banking. Futur Gener Comput Syst 110:812–823
Wang W, Mishra KK (2018) A novel stock trading prediction and recommendation system. Multimed Tools Appl 77(4):4203–4215
Wei S, Ye N, Zhang S, Huang X, Zhu J (2012) Item-based collaborative filtering recommendation algorithm combining item category with interestingness measure. In 2012 international conference on computer science and service system (pp. 2038-2041). IEEE.
Wen H, Zhang J, Lin Q, Yang K, Huang P (2019) Multi-level deep cascade trees for conversion rate prediction in recommendation system. In proceedings of the AAAI conference on artificial intelligence (Vol. 33, pp. 338-345).
Xia H, Wei X, An W, Zhang ZJ, Sun Z (2020) Design of electronic-commerce recommendation systems based on outlier mining. Electron Markets. 1-17.
Xue J, Zhu E, Liu Q, Yin J (2018) Group recommendation based on financial social network for robo-advisor. IEEE Access 6:54527–54535
Yadav S, Nagpal S (2018) An improved collaborative filtering based recommender system using bat algorithm. Procedia Comput Sci 132:1795–1803
Yin C, Shi L, Sun R, Wang J (2020) Improved collaborative filtering recommendation algorithm based on differential privacy protection. J Supercomput 76(7):5161–5174
Ying Y, Chen L, Chen G (2017) A temporal-aware POI recommendation system using context-aware tensor decomposition and weighted HITS. Neurocomputing 242:195–205
Yu K, Xu X, Schwaighofer A, Tresp V, Kriegel HP (2002) Removing redundancy and inconsistency in memory-based collaborative filtering. In conference on information and knowledge management: proceedings of the eleventh international conference on information and knowledge management (Vol. 4, no. 09, pp. 52-59).
Yu K, Schwaighofer A, Tresp V, Xu X, Kriegel HP (2004) Probabilistic memory-based collaborative filtering. IEEE Trans Knowl Data Eng 16(1):56–69
Zhang Q, Zhang D, Lu J, Zhang G, Qu W, Cohen M (2019) A recommender system for cold-start items: a case study in the real estate industry. In 2019 IEEE 14th international conference on intelligent systems and knowledge engineering (ISKE) (pp. 1185-1192). IEEE.
Zhang X, Zhou Y, Ma Y, Chen BC, Zhang L, Agarwal D (2016) Glmix: generalized linear mixed models for large-scale response prediction. In proceedings of the 22nd ACM SIGKDD international conference on knowledge discovery and data mining (pp. 363-372).
Zhang Y, Qiu M, Tsai CW, Hassan MM, Alamri A (2015) Health-CPS: healthcare cyber-physical system assisted by cloud and big data. IEEE Syst J 11(1):88–95
Zheng Z, Gao Y, Yin L, Rabarison MK (2019) Modeling and analysis of a stock-based collaborative filtering algorithm for the Chinese stock market. Expert Syst Appl 162:113006
Zibriczky D (2016). Recommender systems meet finance: a literature review
Zuo T, Zhu S, Lu J (2020) A hybrid recommender system combing singular value decomposition and linear mixed model. In science and information conference (pp. 347-362). Springer, Cham.
Download references
Author information
Authors and affiliations.
Computer Science and Engineering Department, Faculty of Electronic Engineering, Menoufia University, Menoufia, Egypt
Marwa Sharaf, Ezz El-Din Hemdan, Ayman El-Sayed & Nirmeen A. El-Bahnasawy
You can also search for this author in PubMed Google Scholar
Corresponding author
Correspondence to Ezz El-Din Hemdan .
Additional information
Publisher’s note.
Springer Nature remains neutral with regard to jurisdictional claims in published maps and institutional affiliations.
Rights and permissions
Reprints and permissions
About this article
Sharaf, M., Hemdan, E.ED., El-Sayed, A. et al. A survey on recommendation systems for financial services. Multimed Tools Appl 81 , 16761–16781 (2022). https://doi.org/10.1007/s11042-022-12564-1
Download citation
Received : 29 December 2020
Revised : 12 May 2021
Accepted : 31 January 2022
Published : 03 March 2022
Issue Date : May 2022
DOI : https://doi.org/10.1007/s11042-022-12564-1
Share this article
Anyone you share the following link with will be able to read this content:
Sorry, a shareable link is not currently available for this article.
Provided by the Springer Nature SharedIt content-sharing initiative
- Recommendation systems
- Collaborative-filtering
- Hybrid recommender
- Content-based
- Financial services
- And stock market
- Find a journal
- Publish with us
- Track your research
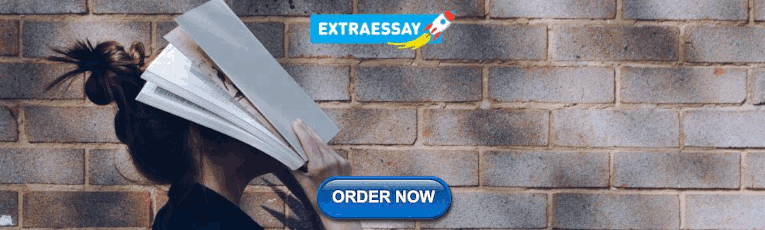
IMAGES
VIDEO
COMMENTS
The present work overviews the application of recommender systems in various financial domains and proposes a domain-based categorization focusing on those recommendation problems, where the existing literature is significant. The present work overviews the application of recommender systems in various financial domains. The relevant literature is investigated based on two directions. First, a ...
Abstract. The present work overviews the application of recommender systems in various financial domains. The relevant literature is investigated based on two directions. First, a domain-based ...
Recommender Systems meet Finance: A literature review Dávid Zibriczky1 2 Abstract. The present work overviews the application of recommender systems in various financial domains. ... Compared to the subjects of conventional recommender systems, financial products usually require a long-term significant financial commitment as their utility is ...
Recommender Systems meet Finance: A literature review ... recommender system techniques focusing on the financial domains. First, we perform domain-based categorization, distinguishing the most developed fields; then we discuss the applications in less de-veloped financial domains. Second, we summarize the most often
1. Introduction. Recommender Systems (RSs) (Jannach et al., 2010) have become an integral part of many modern online services, for example, on Amazon, Netflix, YouTube, or Spotify.Typically, the recommendations provided by the system are designed to serve certain user needs. On the mentioned e-commerce and media streaming sites, for example, these systems support users in navigating large ...
The present work overviews the application of recom-mender systems in various financial domains. The relevant literature is investigated based on two directions. First, a domain-based cate-gorization is discussed focusing on those recommendation
Recommender systems are efficient tools for filtering online information, which is widespread owing to the changing habits of computer users, personalization trends, and emerging access to the internet. Even though the recent recommender systems are eminent in giving precise recommendations, they suffer from various limitations and challenges like scalability, cold-start, sparsity, etc. Due to ...
DB Schenker - Cited by 571 - recommender systems - machine learning - data science - asset pricing - risk estimation ... Recommender systems meet finance: a literature review. D Zibriczky12. Proc. 2nd Int. Workshop Personalization Recommender Syst, 1-10, 2016. 54:
Long-tailed financial recommender systems, are attracting attention for their many applications in online services. ... David Zibriczky. 2016, Recommender Systems meet Finance: A literature review. Conference: 2nd International Workshop on Personalization and Recommender Systems in Financial Services. In: Bari, Italy. Vol. Jun 17, 2016.
Therefore, a Recommender System can be seen as an Information Retrieval system that can respond to an implicit user's query. The RS draw this implicit user's query based on a user's profile that can be created using some semantic or statistic knowledge. In this paper, we provide an in-depth literature review on main RS approaches.
Literature Review of Recommendation Systems. January 2021. DOI: 10.4018/978-1-7998-4339-9.ch009. In book: Transdisciplinary Perspectives on Risk Management and Cyber Intelligence (pp.119-129 ...
Recommender systems (RS) are designed to "recommend" a course of action to the end decision maker, or more commonly, another piece of software that makes further analysis. ... Recommender systems meet finance: a literature review. FINREC, 2016. [40] Ángel García-Crespo, Jose Luis Lopez-Cuadrado, Israel González-Carrasco, ...
A survey of existing publications seems to be a good way to meet that need. In this paper, we perform a literature review of existing research on open data, recommender systems, and social trust, with the intention to illustrate both the relationships and gaps between these three domains and discuss directions for future work. ... Li, C. Scopus ...
To achieve this aim, the authors use systematic literature review (SLR) as a powerful method to collect and critically analyze the research papers. Also, the authors discuss the selected recommender systems and its main techniques, as well as their benefits and drawbacks in general.,In this paper, the SLR method is utilized with the aim of ...
1 Introduction. A recommender system is a tool to supervise the user to a useful item based on his preference. It is a subclass from data filtering systems [ 33 ]. It is software that enables the user to achieve the best items for use [ 57 ]. It plays a key role in information filtering and achieving a useful one.
Multi-objective optimization with recommender systems is an emerging field that aims to help businesses and researchers make better decisions by simultaneously optimizing multiple objectives. The field combines the power of optimization algorithms and RSs to find solutions that satisfy conflicting objectives.
Recommender systems are the engine of online advertising. Not only do they suggest movies, music, or romantic partners, but they also are used to select which advertisements to show to users. This paper reviews the basics of recommender system methodology and then looks at the emerging arena of active recommender systems.
6. 7 Domain-based review / Real estate Real estate: a property consisting of the land, its natural resources and the buildings on it • Goal: Personalized recommendation based on multi-criteria for one purchase • Applications: › Online real estate search application › Multi-criteria journey-aware recommender › Fuzzy Expert System for real estate recommendation Heterogeneity Churn Rate ...
This paper discusses the valuable role recommender systems may play in cybersecurity. First, a comprehensive presentation of recommender system types is presented, as well as their advantages and disadvantages, possible applications and security concerns. Then, the paper collects and presents the state of the art concerning the use of recommender systems in cybersecurity; both the existing ...
To do so, a SLR research methodology was followed in which 25 competence-based recommender systems related to learning or instruction environments were classified according to multiple criteria. We evaluate the role of competences in these proposals and enumerate the emerging challenges.
Course recommender systems play an increasingly pivotal role in the educational landscape, driving personalization and informed decision-making for students. However, these systems face significant challenges, including managing a large and dynamic decision space and addressing the cold start problem for new students. This article endeavors to provide a comprehensive review and background to ...