Information
- Author Services
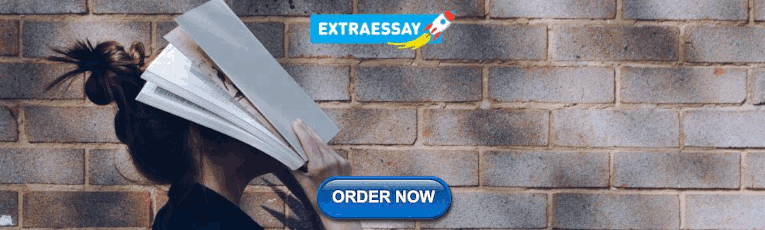
Initiatives
You are accessing a machine-readable page. In order to be human-readable, please install an RSS reader.
All articles published by MDPI are made immediately available worldwide under an open access license. No special permission is required to reuse all or part of the article published by MDPI, including figures and tables. For articles published under an open access Creative Common CC BY license, any part of the article may be reused without permission provided that the original article is clearly cited. For more information, please refer to https://www.mdpi.com/openaccess .
Feature papers represent the most advanced research with significant potential for high impact in the field. A Feature Paper should be a substantial original Article that involves several techniques or approaches, provides an outlook for future research directions and describes possible research applications.
Feature papers are submitted upon individual invitation or recommendation by the scientific editors and must receive positive feedback from the reviewers.
Editor’s Choice articles are based on recommendations by the scientific editors of MDPI journals from around the world. Editors select a small number of articles recently published in the journal that they believe will be particularly interesting to readers, or important in the respective research area. The aim is to provide a snapshot of some of the most exciting work published in the various research areas of the journal.
Original Submission Date Received: .
- Active Journals
- Find a Journal
- Proceedings Series
- For Authors
- For Reviewers
- For Editors
- For Librarians
- For Publishers
- For Societies
- For Conference Organizers
- Open Access Policy
- Institutional Open Access Program
- Special Issues Guidelines
- Editorial Process
- Research and Publication Ethics
- Article Processing Charges
- Testimonials
- Preprints.org
- SciProfiles
- Encyclopedia
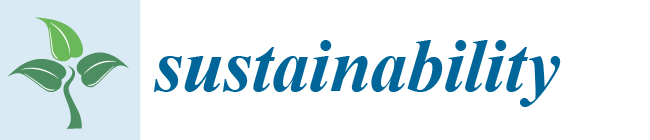
Article Menu
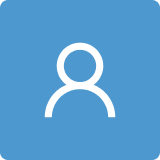
- Subscribe SciFeed
- Recommended Articles
- Google Scholar
- on Google Scholar
- Table of Contents
Find support for a specific problem in the support section of our website.
Please let us know what you think of our products and services.
Visit our dedicated information section to learn more about MDPI.
JSmol Viewer
Assessing the impact of online-learning effectiveness and benefits in knowledge management, the antecedent of online-learning strategies and motivations: an empirical study.
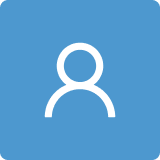
1. Introduction
2. literature review and research hypothesis, 2.1. online-learning self-efficacy terminology, 2.2. online-learning monitoring terminology, 2.3. online-learning confidence in technology terminology, 2.4. online-learning willpower terminology, 2.5. online-learning attitude terminology, 2.6. online-learning motivation terminology, 2.7. online-learning strategies and online-learning effectiveness terminology, 2.8. online-learning effectiveness terminology, 3. research method, 3.1. instruments, 3.2. data analysis and results, 4.1. reliability and validity analysis, 4.2. hypothesis result, 5. discussion, 6. conclusions, 7. limitations and future directions, author contributions, institutional review board statement, informed consent statement, data availability statement, conflicts of interest.
- UNESCO. COVID-19 Educational Disruption and Response ; UNESCO: Paris, France, 2020. [ Google Scholar ]
- Moore, D.R. E-learning and the science of instruction: Proven guidelines for consumers and designers of multimedia learning. Educ. Technol. Res. Dev. 2006 , 54 , 197–200. [ Google Scholar ] [ CrossRef ]
- McDonald, E.W.; Boulton, J.L.; Davis, J.L. E-learning and nursing assessment skills and knowledge–An integrative review. Nurse Educ. Today 2018 , 66 , 166–174. [ Google Scholar ] [ CrossRef ] [ PubMed ]
- Homan, S.R.; Wood, K. Taming the mega-lecture: Wireless quizzing. Syllabus Sunnyvale Chatsworth 2003 , 17 , 23–27. [ Google Scholar ]
- Emran, M.A.; Shaalan, K. E-podium technology: A medium of managing knowledge at al buraimi university college via mlearning. In Proceedings of the 2nd BCS International IT Conference, Abu Dhabi, United Arab Emirates, 9–10 March 2014; pp. 1–4. [ Google Scholar ]
- Tenório, T.; Bittencourt, I.I.; Isotani, S.; Silva, A.P. Does peer assessment in on-line learning environments work? A systematic review of the literature. Comput. Hum. Behav. 2016 , 64 , 94–107. [ Google Scholar ] [ CrossRef ]
- Sheshasaayee, A.; Bee, M.N. Analyzing online learning effectiveness for knowledge society. In Information Systems Design and Intelligent Applications ; Bhateja, V., Nguyen, B., Nguyen, N., Satapathy, S., Le, D.N., Eds.; Springer: Singapore, 2018; pp. 995–1002. [ Google Scholar ]
- Panigrahi, R.; Srivastava, P.R.; Sharma, D. Online learning: Adoption, continuance, and learning outcome—A review of literature. Int. J. Inform. Manag. 2018 , 43 , 1–14. [ Google Scholar ] [ CrossRef ]
- Al-Rahmi, W.M.; Alias, N.; Othman, M.S.; Alzahrani, A.I.; Alfarraj, O.; Saged, A.A. Use of e-learning by university students in Malaysian higher educational institutions: A case in Universiti Teknologi Malaysia. IEEE Access 2018 , 6 , 14268–14276. [ Google Scholar ] [ CrossRef ]
- Al-Rahmi, W.M.; Yahaya, N.; Aldraiweesh, A.A.; Alamri, M.M.; Aljarboa, N.A.; Alturki, U. Integrating technology acceptance model with innovation diffusion theory: An empirical investigation on students’ intention to use E-learning systems. IEEE Access 2019 , 7 , 26797–26809. [ Google Scholar ] [ CrossRef ]
- Gunawan, I.; Hui, L.K.; Ma’sum, M.A. Enhancing learning effectiveness by using online learning management system. In Proceedings of the 6th International Conference on Education and Technology (ICET), Beijing, China, 18–20 June 2021; pp. 48–52. [ Google Scholar ]
- Nguyen, P.H.; Tangworakitthaworn, P.; Gilbert, L. Individual learning effectiveness based on cognitive taxonomies and constructive alignment. In Proceedings of the IEEE Region 10 Conference (Tencon), Osaka, Japan, 16–19 November 2020; pp. 1002–1006. [ Google Scholar ]
- Pee, L.G. Enhancing the learning effectiveness of ill-structured problem solving with online co-creation. Stud. High. Educ. 2020 , 45 , 2341–2355. [ Google Scholar ] [ CrossRef ]
- Kintu, M.J.; Zhu, C.; Kagambe, E. Blended learning effectiveness: The relationship between student characteristics, design features and outcomes. Int. J. Educ. Technol. High. Educ. 2017 , 14 , 1–20. [ Google Scholar ] [ CrossRef ] [ Green Version ]
- Wang, M.H.; Vogel, D.; Ran, W.J. Creating a performance-oriented e-learning environment: A design science approach. Inf. Manag. 2011 , 48 , 260–269. [ Google Scholar ] [ CrossRef ] [ Green Version ]
- Hew, K.F.; Cheung, W.S. Students’ and instructors’ use of massive open online courses (MOOCs): Motivations and challenges. Educ. Res. Rev. 2014 , 12 , 45–58. [ Google Scholar ] [ CrossRef ]
- Bryant, J.; Bates, A.J. Creating a constructivist online instructional environment. TechTrends 2015 , 59 , 17–22. [ Google Scholar ] [ CrossRef ]
- Lee, M.C. Explaining and predicting users’ continuance intention toward e-learning: An extension of the expectation–confirmation model. Comput. Educ. 2010 , 54 , 506–516. [ Google Scholar ] [ CrossRef ]
- Lin, K.M. E-Learning continuance intention: Moderating effects of user e-learning experience. Comput. Educ. 2011 , 56 , 515–526. [ Google Scholar ] [ CrossRef ]
- Huang, E.Y.; Lin, S.W.; Huang, T.K. What type of learning style leads to online participation in the mixed-mode e-learning environment? A study of software usage instruction. Comput. Educ. 2012 , 58 , 338–349. [ Google Scholar ]
- Chu, T.H.; Chen, Y.Y. With good we become good: Understanding e-learning adoption by theory of planned behavior and group influences. Comput. Educ. 2016 , 92 , 37–52. [ Google Scholar ] [ CrossRef ]
- Bandura, A. Self-efficacy: Toward a unifying theory of behavioral change. Psychol. Rev. 1977 , 84 , 191–215. [ Google Scholar ] [ CrossRef ]
- Torkzadeh, G.; Van Dyke, T.P. Development and validation of an Internet self-efficacy scale. Behav. Inform. Technol. 2001 , 20 , 275–280. [ Google Scholar ] [ CrossRef ]
- Saadé, R.G.; Kira, D. Computer anxiety in e-learning: The effect of computer self-efficacy. J. Inform. Technol. Educ. Res. 2009 , 8 , 177–191. [ Google Scholar ] [ CrossRef ] [ Green Version ]
- Tucker, J.; Gentry, G. Developing an E-Learning strategy in higher education. In Proceedings of the SITE 2009–Society for Information Technology & Teacher Education International Conference, Charleston, SC, USA, 2–6 March 2009; pp. 2702–2707. [ Google Scholar ]
- Wang, Y.; Peng, H.M.; Huang, R.H.; Hou, Y.; Wang, J. Characteristics of distance learners: Research on relationships of learning motivation, learning strategy, self-efficacy, attribution and learning results. Open Learn. J. Open Distance Elearn. 2008 , 23 , 17–28. [ Google Scholar ] [ CrossRef ]
- Mahmud, B.H. Study on the impact of motivation, self-efficacy and learning strategies of faculty of education undergraduates studying ICT courses. In Proceedings of the 4th International Postgraduate Research Colloquium (IPRC) Proceedings, Bangkok, Thailand, 29 October 2009; pp. 59–80. [ Google Scholar ]
- Yusuf, M. Investigating relationship between self-efficacy, achievement motivation, and self-regulated learning strategies of undergraduate Students: A study of integrated motivational models. Procedia Soc. Behav. Sci. 2011 , 15 , 2614–2617. [ Google Scholar ] [ CrossRef ] [ Green Version ]
- De la Fuente, J.; Martínez-Vicente, J.M.; Peralta-Sánchez, F.J.; GarzónUmerenkova, A.; Vera, M.M.; Paoloni, P. Applying the SRL vs. ERL theory to the knowledge of achievement emotions in undergraduate university students. Front. Psychol. 2019 , 10 , 2070. [ Google Scholar ] [ CrossRef ] [ PubMed ]
- Ahmadi, S. Academic self-esteem, academic self-efficacy and academic achievement: A path analysis. J. Front. Psychol. 2020 , 5 , 155. [ Google Scholar ]
- Meyen, E.L.; Aust, R.J.; Bui, Y.N. Assessing and monitoring student progress in an E-learning personnel preparation environment. Teach. Educ. Spec. Educ. 2002 , 25 , 187–198. [ Google Scholar ] [ CrossRef ] [ Green Version ]
- Dunlosky, J.; Kubat-Silman, A.K.; Christopher, H. Training monitoring skills improves older adults’ self-paced associative learning. Psychol. Aging 2003 , 18 , 340–345. [ Google Scholar ] [ CrossRef ]
- Zhang, H.J. Research on the relationship between English learning motivation. Self-monitoring and Test Score. Ethnic Educ. Res. 2005 , 6 , 66–71. [ Google Scholar ]
- Rosenberg, M.J. E-Learning: Strategies for Delivering Knowledge in the Digital Age ; McGraw-Hill: New York, NY, USA, 2001. [ Google Scholar ]
- Bhat, S.A.; Bashir, M. Measuring ICT orientation: Scale development & validation. Educ. Inf. Technol. 2018 , 23 , 1123–1143. [ Google Scholar ]
- Achuthan, K.; Francis, S.P.; Diwakar, S. Augmented reflective learning and knowledge retention perceived among students in classrooms involving virtual laboratories. Educ. Inf. Technol. 2017 , 22 , 2825–2855. [ Google Scholar ] [ CrossRef ]
- Hu, X.; Yelland, N. An investigation of preservice early childhood teachers’ adoption of ICT in a teaching practicum context in Hong Kong. J. Early Child. Teach. Educ. 2017 , 38 , 259–274. [ Google Scholar ] [ CrossRef ]
- Fraillon, J.; Ainley, J.; Schulz, W.; Friedman, T.; Duckworth, D. Preparing for Life in a Digital World: The IEA International Computer and Information Literacy Study 2018 International Report ; Springer: New York, NY, USA, 2019. [ Google Scholar ]
- Huber, S.G.; Helm, C. COVID-19 and schooling: Evaluation, assessment and accountability in times of crises—Reacting quickly to explore key issues for policy, practice and research with the school barometer. Educ. Assess. Eval. Account. 2020 , 32 , 237–270. [ Google Scholar ] [ CrossRef ]
- Eickelmann, B.; Gerick, J. Learning with digital media: Objectives in times of Corona and under special consideration of social Inequities. Dtsch. Schule. 2020 , 16 , 153–162. [ Google Scholar ]
- Shehzadi, S.; Nisar, Q.A.; Hussain, M.S.; Basheer, M.F.; Hameed, W.U.; Chaudhry, N.I. The role of e-learning toward students’ satisfaction and university brand image at educational institutes of Pakistan: A post-effect of COVID-19. Asian Educ. Dev. Stud. 2020 , 10 , 275–294. [ Google Scholar ] [ CrossRef ]
- Miller, E.M.; Walton, G.M.; Dweck, C.S.; Job, V.; Trzesniewski, K.; McClure, S. Theories of willpower affect sustained learning. PLoS ONE 2012 , 7 , 38680. [ Google Scholar ] [ CrossRef ] [ PubMed ]
- Moriña, A.; Molina, V.M.; Cortés-Vega, M.D. Voices from Spanish students with disabilities: Willpower and effort to survive university. Eur. J. Spec. Needs Educ. 2018 , 33 , 481–494. [ Google Scholar ] [ CrossRef ]
- Koballa, T.R., Jr.; Crawley, F.E. The influence of attitude on science teaching and learning. Sch. Sci. Math. 1985 , 85 , 222–232. [ Google Scholar ] [ CrossRef ]
- Chao, C.Y.; Chen, Y.T.; Chuang, K.Y. Exploring students’ learning attitude and achievement in flipped learning supported computer aided design curriculum: A study in high school engineering education. Comput. Appl. Eng. Educ. 2015 , 23 , 514–526. [ Google Scholar ] [ CrossRef ]
- Stefan, M.; Ciomos, F. The 8th and 9th grades students’ attitude towards teaching and learning physics. Acta Didact. Napocensia. 2010 , 3 , 7–14. [ Google Scholar ]
- Sedighi, F.; Zarafshan, M.A. Effects of attitude and motivation on the use of language learning strategies by Iranian EFL University students. J. Soc. Sci. Humanit. Shiraz Univ. 2007 , 23 , 71–80. [ Google Scholar ]
- Megan, S.; Jennifer, H.C.; Stephanie, V.; Kyla, H. The relationship among middle school students’ motivation orientations, learning strategies, and academic achievement. Middle Grades Res. J. 2013 , 8 , 1–12. [ Google Scholar ]
- Nasser, O.; Majid, V. Motivation, attitude, and language learning. Procedia Soc. Behav. Sci. 2011 , 29 , 994–1000. [ Google Scholar ]
- Özhan, Ş.Ç.; Kocadere, S.A. The effects of flow, emotional engagement, and motivation on success in a gamified online learning environment. J. Educ. Comput. Res. 2020 , 57 , 2006–2031. [ Google Scholar ] [ CrossRef ]
- Wang, A.P.; Che, H.S. A research on the relationship between learning anxiety, learning attitude, motivation and test performance. Psychol. Dev. Educ. 2005 , 21 , 55–59. [ Google Scholar ]
- Lin, C.H.; Zhang, Y.N.; Zheng, B.B. The roles of learning strategies and motivation in online language learning: A structural equation modeling analysis. Comput. Educ. 2017 , 113 , 75–85. [ Google Scholar ] [ CrossRef ]
- Deschênes, M.F.; Goudreau, J.; Fernandez, N. Learning strategies used by undergraduate nursing students in the context of a digital educational strategy based on script concordance: A descriptive study. Nurse Educ. Today 2020 , 95 , 104607. [ Google Scholar ] [ CrossRef ] [ PubMed ]
- Jerusalem, M.; Schwarzer, R. Self-efficacy as a resource factor in stress appraisal processes. In Self-Efficacy: Thought Control of Action ; Schwarzer, R., Ed.; Hemisphere Publishing Corp: Washington, DC, USA, 1992; pp. 195–213. [ Google Scholar ]
- Zimmerman, B.J. Becoming a self-regulated learner: An overview. Theory Pract. 2002 , 41 , 64–70. [ Google Scholar ] [ CrossRef ]
- Pintrich, P.R.; Smith, D.A.F.; García, T.; McKeachie, W.J. A Manual for the Use of the Motivated Strategies Questionnaire (MSLQ) ; University of Michigan, National Center for Research to Improve Post Secondary Teaching and Learning: Ann Arbor, MI, USA, 1991. [ Google Scholar ]
- Knowles, E.; Kerkman, D. An investigation of students attitude and motivation toward online learning. InSight Collect. Fac. Scholarsh. 2007 , 2 , 70–80. [ Google Scholar ] [ CrossRef ]
- Hair, J.F., Jr.; Black, W.C.; Babin, B.J.; Anderson, R.E. Multivariate Data Analysis: A Global Perspective , 7th ed.; Pearson Education International: Upper Saddle River, NJ, USA, 2010. [ Google Scholar ]
- Fornell, C.; Larcker, D.F. Evaluating structural equation models with unobservable variables and measurement error. J. Mark. Res. 1981 , 18 , 39–50. [ Google Scholar ] [ CrossRef ]
- Hair, J.F., Jr.; Hult, G.T.M.; Ringle, C.; Sarstedt, M. A Primer on Partial Least Squares Structural Equation Modeling (PLS-SEM) ; Sage: Los Angeles, CA, USA, 2016. [ Google Scholar ]
- Kiliç-Çakmak, E. Learning strategies and motivational factors predicting information literacy self-efficacy of e-learners. Aust. J. Educ. Technol. 2010 , 26 , 192–208. [ Google Scholar ] [ CrossRef ] [ Green Version ]
- Zheng, C.; Liang, J.C.; Li, M.; Tsai, C. The relationship between English language learners’ motivation and online self-regulation: A structural equation modelling approach. System 2018 , 76 , 144–157. [ Google Scholar ] [ CrossRef ]
- May, M.; George, S.; Prévôt, P. TrAVis to enhance students’ self-monitoring in online learning supported by computer-mediated communication tools. Int. J. Comput. Inform. Syst. Ind. Manag. Appl. 2011 , 3 , 623–634. [ Google Scholar ]
- Rafart, M.A.; Bikfalvi, A.; Soler, J.; Poch, J. Impact of using automatic E-Learning correctors on teaching business subjects to engineers. Int. J. Eng. Educ. 2019 , 35 , 1630–1641. [ Google Scholar ]
- Lee, P.M.; Tsui, W.H.; Hsiao, T.C. A low-cost scalable solution for monitoring affective state of students in E-learning environment using mouse and keystroke data. In Intelligent Tutoring Systems ; Cerri, S.A., Clancey, W.J., Papadourakis, G., Panourgia, K., Eds.; Springer: Berlin, Germany, 2012; pp. 679–680. [ Google Scholar ]
- Metz, D.; Karadgi, S.S.; Müller, U.J.; Grauer, M. Self-Learning monitoring and control of manufacturing processes based on rule induction and event processing. In Proceedings of the 4th International Conference on Information, Process, and Knowledge Management eKNOW, Valencia, Spain, 21–25 November 2012; pp. 78–85. [ Google Scholar ]
- Fitch, J.L.; Ravlin, E.C. Willpower and perceived behavioral control: Intention-behavior relationship and post behavior attributions. Soc. Behav. Pers. Int. J. 2005 , 33 , 105–124. [ Google Scholar ] [ CrossRef ]
- Sridharan, B.; Deng, H.; Kirk, J.; Brian, C. Structural equation modeling for evaluating the user perceptions of e-learning effectiveness in higher education. In Proceedings of the ECIS 2010: 18th European Conference on Information Systems, Pretoria, South Africa, 7–9 June 2010. [ Google Scholar ]
- Tarhini, A.; Hone, K.; Liu, X. The effects of individual differences on e-learning users’ behaviour in developing countries: A structural equation model. Comput. Hum. Behav. 2014 , 41 , 153–163. [ Google Scholar ] [ CrossRef ] [ Green Version ]
- de Leeuw, R.A.; Logger, D.N.; Westerman, M.; Bretschneider, J.; Plomp, M.; Scheele, F. Influencing factors in the implementation of postgraduate medical e-learning: A thematic analysis. BMC Med. Educ. 2019 , 19 , 300. [ Google Scholar ] [ CrossRef ] [ Green Version ]
- Erenler, H.H.T. A structural equation model to evaluate students’ learning and satisfaction. Comput. Appl. Eng. Educ. 2020 , 28 , 254–267. [ Google Scholar ] [ CrossRef ]
- Fee, K. Delivering E-learning: A complete strategy for design, application and assessment. Dev. Learn. Organ. 2013 , 27 , 40–52. [ Google Scholar ] [ CrossRef ]
- So, W.W.N.; Chen, Y.; Wan, Z.H. Multimedia e-Learning and self-regulated science learning: A study of primary school learners’ experiences and perceptions. J. Sci. Educ. Technol. 2019 , 28 , 508–522. [ Google Scholar ] [ CrossRef ]
Click here to enlarge figure
Variables | Category | Frequency | Percentage |
---|---|---|---|
Gender | Male | 243 | 51.81 |
Female | 226 | 48.19 | |
Education program level | Undergraduate program | 210 | 44.78 |
Master program | 154 | 32.84 | |
Doctoral program | 105 | 22.39 | |
Online learning tools | Smartphone | 255 | 54.37 |
Computer/PC | 125 | 26.65 | |
Tablet | 89 | 18.98 | |
Online learning media | Google Meet | 132 | 28.14 |
Microsoft Teams | 99 | 21.11 | |
Zoom | 196 | 41.79 | |
Others | 42 | 8.96 |
Construct | Measurement Items | Factor Loading/Coefficient (t-Value) | AVE | Composite Reliability | Cronbach’s Alpha |
---|---|---|---|---|---|
Online Learning Benefit (LBE) | LBE1 | 0.88 | 0.68 | 0.86 | 0.75 |
LBE2 | 0.86 | ||||
LBE3 | 0.71 | ||||
Online-learning effectiveness (LEF) | LEF1 | 0.83 | 0.76 | 0.90 | 0.84 |
LEF2 | 0.88 | ||||
LEF3 | 0.90 | ||||
Online-learning motivation (LMT) | LMT1 | 0.86 | 0.77 | 0.91 | 0.85 |
LMT2 | 0.91 | ||||
LMT3 | 0.85 | ||||
Online-learning strategies (LST) | LST1 | 0.90 | 0.75 | 0.90 | 0.84 |
LST2 | 0.87 | ||||
LST3 | 0.83 | ||||
Online-learning attitude (OLA) | OLA1 | 0.89 | 0.75 | 0.90 | 0.84 |
OLA2 | 0.83 | ||||
OLA3 | 0.87 | ||||
Online-learning confidence-in-technology (OLC) | OLC1 | 0.87 | 0.69 | 0.87 | 0.76 |
OLC2 | 0.71 | ||||
OLC3 | 0.89 | ||||
Online-learning monitoring (OLM) | OLM1 | 0.88 | 0.75 | 0.89 | 0.83 |
OLM2 | 0.91 | ||||
OLM3 | 0.79 | ||||
Online-learning self-efficacy (OLS) | OLS1 | 0.79 | 0.64 | 0.84 | 0.73 |
OLS2 | 0.81 | ||||
OLS3 | 0.89 | ||||
Online-learning willpower (OLW) | OLW1 | 0.91 | 0.69 | 0.87 | 0.77 |
OLW2 | 0.84 | ||||
OLW3 | 0.73 |
LBE | LEF | LMT | LST | OLA | OLC | OLM | OLS | OLW | |
---|---|---|---|---|---|---|---|---|---|
LBE | |||||||||
LEF | 0.82 | ||||||||
LMT | 0.81 | 0.80 | |||||||
LST | 0.80 | 0.84 | 0.86 | ||||||
OLA | 0.69 | 0.63 | 0.78 | 0.81 | |||||
OLC | 0.76 | 0.79 | 0.85 | 0.79 | 0.72 | ||||
OLM | 0.81 | 0.85 | 0.81 | 0.76 | 0.63 | 0.83 | |||
OLS | 0.71 | 0.59 | 0.69 | 0.57 | 0.56 | 0.69 | 0.75 | ||
OLW | 0.75 | 0.75 | 0.80 | 0.74 | 0.64 | 0.81 | 0.80 | 0.79 |
LBE | LEF | LMT | LST | OLA | OLC | OLM | OLS | OLW | |
---|---|---|---|---|---|---|---|---|---|
LBE1 | 0.88 | 0.76 | 0.87 | 0.66 | 0.54 | 0.79 | 0.78 | 0.63 | 0.74 |
LBE2 | 0.86 | 0.68 | 0.74 | 0.63 | 0.57 | 0.75 | 0.91 | 0.73 | 0.79 |
LBE3 | 0.71 | 0.54 | 0.59 | 0.71 | 0.63 | 0.55 | 0.50 | 0.36 | 0.53 |
LEF1 | 0.63 | 0.83 | 0.72 | 0.65 | 0.51 | 0.62 | 0.69 | 0.46 | 0.57 |
LEF2 | 0.77 | 0.88 | 0.78 | 0.71 | 0.55 | 0.73 | 0.78 | 0.52 | 0.69 |
LEF3 | 0.72 | 0.90 | 0.80 | 0.83 | 0.57 | 0.72 | 0.76 | 0.58 | 0.69 |
LMT1 | 0.88 | 0.76 | 0.87 | 0.66 | 0.54 | 0.79 | 0.78 | 0.63 | 0.74 |
LMT2 | 0.79 | 0.89 | 0.91 | 0.79 | 0.62 | 0.73 | 0.88 | 0.61 | 0.67 |
LMT3 | 0.72 | 0.65 | 0.85 | 0.77 | 0.89 | 0.72 | 0.67 | 0.59 | 0.69 |
LST1 | 0.61 | 0.63 | 0.68 | 0.90 | 0.78 | 0.64 | 0.57 | 0.39 | 0.57 |
LST2 | 0.74 | 0.59 | 0.72 | 0.87 | 0.78 | 0.68 | 0.61 | 0.48 | 0.63 |
LST3 | 0.72 | 0.90 | 0.80 | 0.83 | 0.57 | 0.72 | 0.76 | 0.58 | 0.69 |
OLA1 | 0.72 | 0.65 | 0.85 | 0.79 | 0.89 | 0.72 | 0.67 | 0.59 | 0.69 |
OLA2 | 0.51 | 0.48 | 0.55 | 0.59 | 0.83 | 0.58 | 0.47 | 0.42 | 0.43 |
OLA3 | 0.52 | 0.44 | 0.55 | 0.70 | 0.87 | 0.55 | 0.43 | 0.39 | 0.47 |
OLC1 | 0.78 | 0.70 | 0.73 | 0.65 | 0.53 | 0.87 | 0.77 | 0.65 | 0.91 |
OLC2 | 0.51 | 0.53 | 0.57 | 0.62 | 0.75 | 0.71 | 0.46 | 0.39 | 0.47 |
OLC3 | 0.81 | 0.73 | 0.78 | 0.69 | 0.55 | 0.89 | 0.80 | 0.66 | 0.75 |
OLM1 | 0.79 | 0.89 | 0.91 | 0.79 | 0.62 | 0.73 | 0.88 | 0.61 | 0.69 |
OLM2 | 0.86 | 0.68 | 0.74 | 0.63 | 0.57 | 0.75 | 0.91 | 0.73 | 0.79 |
OLM3 | 0.69 | 0.55 | 0.57 | 0.47 | 0.39 | 0.67 | 0.79 | 0.61 | 0.73 |
OLS1 | 0.41 | 0.23 | 0.35 | 0.28 | 0.39 | 0.41 | 0.40 | 0.69 | 0.49 |
OLS2 | 0.45 | 0.41 | 0.48 | 0.38 | 0.43 | 0.48 | 0.52 | 0.81 | 0.49 |
OLS3 | 0.75 | 0.66 | 0.72 | 0.60 | 0.49 | 0.69 | 0.77 | 0.89 | 0.82 |
OLW1 | 0.78 | 0.70 | 0.73 | 0.65 | 0.53 | 0.87 | 0.77 | 0.65 | 0.91 |
OLW2 | 0.75 | 0.65 | 0.71 | 0.59 | 0.51 | 0.69 | 0.77 | 0.87 | 0.84 |
OLW3 | 0.57 | 0.49 | 0.54 | 0.59 | 0.57 | 0.57 | 0.53 | 0.39 | 0.73 |
Hypothesis | Path | Standardized Path Coefficient | t-Value | Result |
---|---|---|---|---|
H1 | OLS → LST | 0.29 *** | 2.14 | Accepted |
H2 | OLM → LST | 0.24 *** | 2.29 | Accepted |
H3 | OLC → LST | 0.28 *** | 1.99 | Accepted |
H4 | OLC → LMT | 0.36 *** | 2.96 | Accepted |
H5 | OLW → LMT | 0.26 *** | 2.55 | Accepted |
H6 | OLA → LMT | 0.34 *** | 4.68 | Accepted |
H7 | LMT → LST | 0.71 *** | 4.96 | Accepted |
H8 | LMT → LEF | 0.60 *** | 5.89 | Accepted |
H9 | LST → LEF | 0.32 *** | 3.04 | Accepted |
H10 | LEF → LBE | 0.81 *** | 23.6 | Accepted |
MDPI stays neutral with regard to jurisdictional claims in published maps and institutional affiliations. |
Share and Cite
Hongsuchon, T.; Emary, I.M.M.E.; Hariguna, T.; Qhal, E.M.A. Assessing the Impact of Online-Learning Effectiveness and Benefits in Knowledge Management, the Antecedent of Online-Learning Strategies and Motivations: An Empirical Study. Sustainability 2022 , 14 , 2570. https://doi.org/10.3390/su14052570
Hongsuchon T, Emary IMME, Hariguna T, Qhal EMA. Assessing the Impact of Online-Learning Effectiveness and Benefits in Knowledge Management, the Antecedent of Online-Learning Strategies and Motivations: An Empirical Study. Sustainability . 2022; 14(5):2570. https://doi.org/10.3390/su14052570
Hongsuchon, Tanaporn, Ibrahiem M. M. El Emary, Taqwa Hariguna, and Eissa Mohammed Ali Qhal. 2022. "Assessing the Impact of Online-Learning Effectiveness and Benefits in Knowledge Management, the Antecedent of Online-Learning Strategies and Motivations: An Empirical Study" Sustainability 14, no. 5: 2570. https://doi.org/10.3390/su14052570
Article Metrics
Article access statistics, further information, mdpi initiatives, follow mdpi.
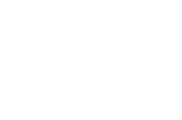
Subscribe to receive issue release notifications and newsletters from MDPI journals
Click through the PLOS taxonomy to find articles in your field.
For more information about PLOS Subject Areas, click here .
Loading metrics
Open Access
Peer-reviewed
Research Article
COVID-19’s impacts on the scope, effectiveness, and interaction characteristics of online learning: A social network analysis
Roles Data curation, Formal analysis, Methodology, Writing – review & editing
¶ ‡ JZ and YD are contributed equally to this work as first authors.
Affiliation School of Educational Information Technology, South China Normal University, Guangzhou, Guangdong, China
Roles Data curation, Formal analysis, Methodology, Writing – original draft
Affiliations School of Educational Information Technology, South China Normal University, Guangzhou, Guangdong, China, Hangzhou Zhongce Vocational School Qiantang, Hangzhou, Zhejiang, China
Roles Data curation, Writing – original draft
Roles Data curation
Roles Writing – original draft
Affiliation Faculty of Education, Shenzhen University, Shenzhen, Guangdong, China
Roles Conceptualization, Supervision, Writing – review & editing
* E-mail: [email protected] (JH); [email protected] (YZ)

- Junyi Zhang,
- Yigang Ding,
- Xinru Yang,
- Jinping Zhong,
- XinXin Qiu,
- Zhishan Zou,
- Yujie Xu,
- Xiunan Jin,
- Xiaomin Wu,
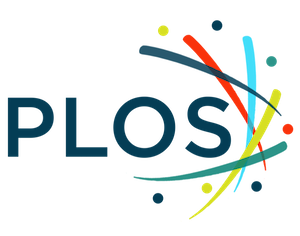
- Published: August 23, 2022
- https://doi.org/10.1371/journal.pone.0273016
- Reader Comments
The COVID-19 outbreak brought online learning to the forefront of education. Scholars have conducted many studies on online learning during the pandemic, but only a few have performed quantitative comparative analyses of students’ online learning behavior before and after the outbreak. We collected review data from China’s massive open online course platform called icourse.163 and performed social network analysis on 15 courses to explore courses’ interaction characteristics before, during, and after the COVID-19 pan-demic. Specifically, we focused on the following aspects: (1) variations in the scale of online learning amid COVID-19; (2a) the characteristics of online learning interaction during the pandemic; (2b) the characteristics of online learning interaction after the pandemic; and (3) differences in the interaction characteristics of social science courses and natural science courses. Results revealed that only a small number of courses witnessed an uptick in online interaction, suggesting that the pandemic’s role in promoting the scale of courses was not significant. During the pandemic, online learning interaction became more frequent among course network members whose interaction scale increased. After the pandemic, although the scale of interaction declined, online learning interaction became more effective. The scale and level of interaction in Electrodynamics (a natural science course) and Economics (a social science course) both rose during the pan-demic. However, long after the pandemic, the Economics course sustained online interaction whereas interaction in the Electrodynamics course steadily declined. This discrepancy could be due to the unique characteristics of natural science courses and social science courses.
Citation: Zhang J, Ding Y, Yang X, Zhong J, Qiu X, Zou Z, et al. (2022) COVID-19’s impacts on the scope, effectiveness, and interaction characteristics of online learning: A social network analysis. PLoS ONE 17(8): e0273016. https://doi.org/10.1371/journal.pone.0273016
Editor: Heng Luo, Central China Normal University, CHINA
Received: April 20, 2022; Accepted: July 29, 2022; Published: August 23, 2022
Copyright: © 2022 Zhang et al. This is an open access article distributed under the terms of the Creative Commons Attribution License , which permits unrestricted use, distribution, and reproduction in any medium, provided the original author and source are credited.
Data Availability: The data underlying the results presented in the study were downloaded from https://www.icourse163.org/ and are now shared fully on Github ( https://github.com/zjyzhangjunyi/dataset-from-icourse163-for-SNA ). These data have no private information and can be used for academic research free of charge.
Funding: The author(s) received no specific funding for this work.
Competing interests: The authors have declared that no competing interests exist.
1. Introduction
The development of the mobile internet has spurred rapid advances in online learning, offering novel prospects for teaching and learning and a learning experience completely different from traditional instruction. Online learning harnesses the advantages of network technology and multimedia technology to transcend the boundaries of conventional education [ 1 ]. Online courses have become a popular learning mode owing to their flexibility and openness. During online learning, teachers and students are in different physical locations but interact in multiple ways (e.g., via online forum discussions and asynchronous group discussions). An analysis of online learning therefore calls for attention to students’ participation. Alqurashi [ 2 ] defined interaction in online learning as the process of constructing meaningful information and thought exchanges between more than two people; such interaction typically occurs between teachers and learners, learners and learners, and the course content and learners.
Massive open online courses (MOOCs), a 21st-century teaching mode, have greatly influenced global education. Data released by China’s Ministry of Education in 2020 show that the country ranks first globally in the number and scale of higher education MOOCs. The COVID-19 outbreak has further propelled this learning mode, with universities being urged to leverage MOOCs and other online resource platforms to respond to government’s “School’s Out, But Class’s On” policy [ 3 ]. Besides MOOCs, to reduce in-person gatherings and curb the spread of COVID-19, various online learning methods have since become ubiquitous [ 4 ]. Though Lederman asserted that the COVID-19 outbreak has positioned online learning technologies as the best way for teachers and students to obtain satisfactory learning experiences [ 5 ], it remains unclear whether the COVID-19 pandemic has encouraged interaction in online learning, as interactions between students and others play key roles in academic performance and largely determine the quality of learning experiences [ 6 ]. Similarly, it is also unclear what impact the COVID-19 pandemic has had on the scale of online learning.
Social constructivism paints learning as a social phenomenon. As such, analyzing the social structures or patterns that emerge during the learning process can shed light on learning-based interaction [ 7 ]. Social network analysis helps to explain how a social network, rooted in interactions between learners and their peers, guides individuals’ behavior, emotions, and outcomes. This analytical approach is especially useful for evaluating interactive relationships between network members [ 8 ]. Mohammed cited social network analysis (SNA) as a method that can provide timely information about students, learning communities and interactive networks. SNA has been applied in numerous fields, including education, to identify the number and characteristics of interelement relationships. For example, Lee et al. also used SNA to explore the effects of blogs on peer relationships [ 7 ]. Therefore, adopting SNA to examine interactions in online learning communities during the COVID-19 pandemic can uncover potential issues with this online learning model.
Taking China’s icourse.163 MOOC platform as an example, we chose 15 courses with a large number of participants for SNA, focusing on learners’ interaction characteristics before, during, and after the COVID-19 outbreak. We visually assessed changes in the scale of network interaction before, during, and after the outbreak along with the characteristics of interaction in Gephi. Examining students’ interactions in different courses revealed distinct interactive network characteristics, the pandemic’s impact on online courses, and relevant suggestions. Findings are expected to promote effective interaction and deep learning among students in addition to serving as a reference for the development of other online learning communities.
2. Literature review and research questions
Interaction is deemed as central to the educational experience and is a major focus of research on online learning. Moore began to study the problem of interaction in distance education as early as 1989. He defined three core types of interaction: student–teacher, student–content, and student–student [ 9 ]. Lear et al. [ 10 ] described an interactivity/ community-process model of distance education: they specifically discussed the relationships between interactivity, community awareness, and engaging learners and found interactivity and community awareness to be correlated with learner engagement. Zulfikar et al. [ 11 ] suggested that discussions initiated by the students encourage more students’ engagement than discussions initiated by the instructors. It is most important to afford learners opportunities to interact purposefully with teachers, and improving the quality of learner interaction is crucial to fostering profound learning [ 12 ]. Interaction is an important way for learners to communicate and share information, and a key factor in the quality of online learning [ 13 ].
Timely feedback is the main component of online learning interaction. Woo and Reeves discovered that students often become frustrated when they fail to receive prompt feedback [ 14 ]. Shelley et al. conducted a three-year study of graduate and undergraduate students’ satisfaction with online learning at universities and found that interaction with educators and students is the main factor affecting satisfaction [ 15 ]. Teachers therefore need to provide students with scoring justification, support, and constructive criticism during online learning. Some researchers examined online learning during the COVID-19 pandemic. They found that most students preferred face-to-face learning rather than online learning due to obstacles faced online, such as a lack of motivation, limited teacher-student interaction, and a sense of isolation when learning in different times and spaces [ 16 , 17 ]. However, it can be reduced by enhancing the online interaction between teachers and students [ 18 ].
Research showed that interactions contributed to maintaining students’ motivation to continue learning [ 19 ]. Baber argued that interaction played a key role in students’ academic performance and influenced the quality of the online learning experience [ 20 ]. Hodges et al. maintained that well-designed online instruction can lead to unique teaching experiences [ 21 ]. Banna et al. mentioned that using discussion boards, chat sessions, blogs, wikis, and other tools could promote student interaction and improve participation in online courses [ 22 ]. During the COVID-19 pandemic, Mahmood proposed a series of teaching strategies suitable for distance learning to improve its effectiveness [ 23 ]. Lapitan et al. devised an online strategy to ease the transition from traditional face-to-face instruction to online learning [ 24 ]. The preceding discussion suggests that online learning goes beyond simply providing learning resources; teachers should ideally design real-life activities to give learners more opportunities to participate.
As mentioned, COVID-19 has driven many scholars to explore the online learning environment. However, most have ignored the uniqueness of online learning during this time and have rarely compared pre- and post-pandemic online learning interaction. Taking China’s icourse.163 MOOC platform as an example, we chose 15 courses with a large number of participants for SNA, centering on student interaction before and after the pandemic. Gephi was used to visually analyze changes in the scale and characteristics of network interaction. The following questions were of particular interest:
- (1) Can the COVID-19 pandemic promote the expansion of online learning?
- (2a) What are the characteristics of online learning interaction during the pandemic?
- (2b) What are the characteristics of online learning interaction after the pandemic?
- (3) How do interaction characteristics differ between social science courses and natural science courses?
3. Methodology
3.1 research context.
We selected several courses with a large number of participants and extensive online interaction among hundreds of courses on the icourse.163 MOOC platform. These courses had been offered on the platform for at least three semesters, covering three periods (i.e., before, during, and after the COVID-19 outbreak). To eliminate the effects of shifts in irrelevant variables (e.g., course teaching activities), we chose several courses with similar teaching activities and compared them on multiple dimensions. All course content was taught online. The teachers of each course posted discussion threads related to learning topics; students were expected to reply via comments. Learners could exchange ideas freely in their responses in addition to asking questions and sharing their learning experiences. Teachers could answer students’ questions as well. Conversations in the comment area could partly compensate for a relative absence of online classroom interaction. Teacher–student interaction is conducive to the formation of a social network structure and enabled us to examine teachers’ and students’ learning behavior through SNA. The comment areas in these courses were intended for learners to construct knowledge via reciprocal communication. Meanwhile, by answering students’ questions, teachers could encourage them to reflect on their learning progress. These courses’ successive terms also spanned several phases of COVID-19, allowing us to ascertain the pandemic’s impact on online learning.
3.2 Data collection and preprocessing
To avoid interference from invalid or unclear data, the following criteria were applied to select representative courses: (1) generality (i.e., public courses and professional courses were chosen from different schools across China); (2) time validity (i.e., courses were held before during, and after the pandemic); and (3) notability (i.e., each course had at least 2,000 participants). We ultimately chose 15 courses across the social sciences and natural sciences (see Table 1 ). The coding is used to represent the course name.
- PPT PowerPoint slide
- PNG larger image
- TIFF original image
https://doi.org/10.1371/journal.pone.0273016.t001
To discern courses’ evolution during the pandemic, we gathered data on three terms before, during, and after the COVID-19 outbreak in addition to obtaining data from two terms completed well before the pandemic and long after. Our final dataset comprised five sets of interactive data. Finally, we collected about 120,000 comments for SNA. Because each course had a different start time—in line with fluctuations in the number of confirmed COVID-19 cases in China and the opening dates of most colleges and universities—we divided our sample into five phases: well before the pandemic (Phase I); before the pandemic (Phase Ⅱ); during the pandemic (Phase Ⅲ); after the pandemic (Phase Ⅳ); and long after the pandemic (Phase Ⅴ). We sought to preserve consistent time spans to balance the amount of data in each period ( Fig 1 ).
https://doi.org/10.1371/journal.pone.0273016.g001
3.3 Instrumentation
Participants’ comments and “thumbs-up” behavior data were converted into a network structure and compared using social network analysis (SNA). Network analysis, according to M’Chirgui, is an effective tool for clarifying network relationships by employing sophisticated techniques [ 25 ]. Specifically, SNA can help explain the underlying relationships among team members and provide a better understanding of their internal processes. Yang and Tang used SNA to discuss the relationship between team structure and team performance [ 26 ]. Golbeck argued that SNA could improve the understanding of students’ learning processes and reveal learners’ and teachers’ role dynamics [ 27 ].
To analyze Question (1), the number of nodes and diameter in the generated network were deemed as indicators of changes in network size. Social networks are typically represented as graphs with nodes and degrees, and node count indicates the sample size [ 15 ]. Wellman et al. proposed that the larger the network scale, the greater the number of network members providing emotional support, goods, services, and companionship [ 28 ]. Jan’s study measured the network size by counting the nodes which represented students, lecturers, and tutors [ 29 ]. Similarly, network nodes in the present study indicated how many learners and teachers participated in the course, with more nodes indicating more participants. Furthermore, we investigated the network diameter, a structural feature of social networks, which is a common metric for measuring network size in SNA [ 30 ]. The network diameter refers to the longest path between any two nodes in the network. There has been evidence that a larger network diameter leads to greater spread of behavior [ 31 ]. Likewise, Gašević et al. found that larger networks were more likely to spread innovative ideas about educational technology when analyzing MOOC-related research citations [ 32 ]. Therefore, we employed node count and network diameter to measure the network’s spatial size and further explore the expansion characteristic of online courses. Brief introduction of these indicators can be summarized in Table 2 .
https://doi.org/10.1371/journal.pone.0273016.t002
To address Question (2), a list of interactive analysis metrics in SNA were introduced to scrutinize learners’ interaction characteristics in online learning during and after the pandemic, as shown below:
- (1) The average degree reflects the density of the network by calculating the average number of connections for each node. As Rong and Xu suggested, the average degree of a network indicates how active its participants are [ 33 ]. According to Hu, a higher average degree implies that more students are interacting directly with each other in a learning context [ 34 ]. The present study inherited the concept of the average degree from these previous studies: the higher the average degree, the more frequent the interaction between individuals in the network.
- (2) Essentially, a weighted average degree in a network is calculated by multiplying each degree by its respective weight, and then taking the average. Bydžovská took the strength of the relationship into account when determining the weighted average degree [ 35 ]. By calculating friendship’s weighted value, Maroulis assessed peer achievement within a small-school reform [ 36 ]. Accordingly, we considered the number of interactions as the weight of the degree, with a higher average degree indicating more active interaction among learners.
- (3) Network density is the ratio between actual connections and potential connections in a network. The more connections group members have with each other, the higher the network density. In SNA, network density is similar to group cohesion, i.e., a network of more strong relationships is more cohesive [ 37 ]. Network density also reflects how much all members are connected together [ 38 ]. Therefore, we adopted network density to indicate the closeness among network members. Higher network density indicates more frequent interaction and closer communication among students.
- (4) Clustering coefficient describes local network attributes and indicates that two nodes in the network could be connected through adjacent nodes. The clustering coefficient measures users’ tendency to gather (cluster) with others in the network: the higher the clustering coefficient, the more frequently users communicate with other group members. We regarded this indicator as a reflection of the cohesiveness of the group [ 39 ].
- (5) In a network, the average path length is the average number of steps along the shortest paths between any two nodes. Oliveres has observed that when an average path length is small, the route from one node to another is shorter when graphed [ 40 ]. This is especially true in educational settings where students tend to become closer friends. So we consider that the smaller the average path length, the greater the possibility of interaction between individuals in the network.
- (6) A network with a large number of nodes, but whose average path length is surprisingly small, is known as the small-world effect [ 41 ]. A higher clustering coefficient and shorter average path length are important indicators of a small-world network: a shorter average path length enables the network to spread information faster and more accurately; a higher clustering coefficient can promote frequent knowledge exchange within the group while boosting the timeliness and accuracy of knowledge dissemination [ 42 ]. Brief introduction of these indicators can be summarized in Table 3 .
https://doi.org/10.1371/journal.pone.0273016.t003
To analyze Question 3, we used the concept of closeness centrality, which determines how close a vertex is to others in the network. As Opsahl et al. explained, closeness centrality reveals how closely actors are coupled with their entire social network [ 43 ]. In order to analyze social network-based engineering education, Putnik et al. examined closeness centrality and found that it was significantly correlated with grades [ 38 ]. We used closeness centrality to measure the position of an individual in the network. Brief introduction of these indicators can be summarized in Table 4 .
https://doi.org/10.1371/journal.pone.0273016.t004
3.4 Ethics statement
This study was approved by the Academic Committee Office (ACO) of South China Normal University ( http://fzghb.scnu.edu.cn/ ), Guangzhou, China. Research data were collected from the open platform and analyzed anonymously. There are thus no privacy issues involved in this study.
4.1 COVID-19’s role in promoting the scale of online courses was not as important as expected
As shown in Fig 2 , the number of course participants and nodes are closely correlated with the pandemic’s trajectory. Because the number of participants in each course varied widely, we normalized the number of participants and nodes to more conveniently visualize course trends. Fig 2 depicts changes in the chosen courses’ number of participants and nodes before the pandemic (Phase II), during the pandemic (Phase III), and after the pandemic (Phase IV). The number of participants in most courses during the pandemic exceeded those before and after the pandemic. But the number of people who participate in interaction in some courses did not increase.
https://doi.org/10.1371/journal.pone.0273016.g002
In order to better analyze the trend of interaction scale in online courses before, during, and after the pandemic, the selected courses were categorized according to their scale change. When the number of participants increased (decreased) beyond 20% (statistical experience) and the diameter also increased (decreased), the course scale was determined to have increased (decreased); otherwise, no significant change was identified in the course’s interaction scale. Courses were subsequently divided into three categories: increased interaction scale, decreased interaction scale, and no significant change. Results appear in Table 5 .
https://doi.org/10.1371/journal.pone.0273016.t005
From before the pandemic until it broke out, the interaction scale of five courses increased, accounting for 33.3% of the full sample; one course’s interaction scale declined, accounting for 6.7%. The interaction scale of nine courses decreased, accounting for 60%. The pandemic’s role in promoting online courses thus was not as important as anticipated, and most courses’ interaction scale did not change significantly throughout.
No courses displayed growing interaction scale after the pandemic: the interaction scale of nine courses fell, accounting for 60%; and the interaction scale of six courses did not shift significantly, accounting for 40%. Courses with an increased scale of interaction during the pandemic did not maintain an upward trend. On the contrary, the improvement in the pandemic caused learners’ enthusiasm for online learning to wane. We next analyzed several interaction metrics to further explore course interaction during different pandemic periods.
4.2 Characteristics of online learning interaction amid COVID-19
4.2.1 during the covid-19 pandemic, online learning interaction in some courses became more active..
Changes in course indicators with the growing interaction scale during the pandemic are presented in Fig 3 , including SS5, SS6, NS1, NS3, and NS8. The horizontal ordinate indicates the number of courses, with red color representing the rise of the indicator value on the vertical ordinate and blue representing the decline.
https://doi.org/10.1371/journal.pone.0273016.g003
Specifically: (1) The average degree and weighted average degree of the five course networks demonstrated an upward trend. The emergence of the pandemic promoted students’ enthusiasm; learners were more active in the interactive network. (2) Fig 3 shows that 3 courses had increased network density and 2 courses had decreased. The higher the network density, the more communication within the team. Even though the pandemic accelerated the interaction scale and frequency, the tightness between learners in some courses did not improve. (3) The clustering coefficient of social science courses rose whereas the clustering coefficient and small-world property of natural science courses fell. The higher the clustering coefficient and the small-world property, the better the relationship between adjacent nodes and the higher the cohesion [ 39 ]. (4) Most courses’ average path length increased as the interaction scale increased. However, when the average path length grew, adverse effects could manifest: communication between learners might be limited to a small group without multi-directional interaction.
When the pandemic emerged, the only declining network scale belonged to a natural science course (NS2). The change in each course index is pictured in Fig 4 . The abscissa indicates the size of the value, with larger values to the right. The red dot indicates the index value before the pandemic; the blue dot indicates its value during the pandemic. If the blue dot is to the right of the red dot, then the value of the index increased; otherwise, the index value declined. Only the weighted average degree of the course network increased. The average degree, network density decreased, indicating that network members were not active and that learners’ interaction degree and communication frequency lessened. Despite reduced learner interaction, the average path length was small and the connectivity between learners was adequate.
https://doi.org/10.1371/journal.pone.0273016.g004
4.2.2 After the COVID-19 pandemic, the scale decreased rapidly, but most course interaction was more effective.
Fig 5 shows the changes in various courses’ interaction indicators after the pandemic, including SS1, SS2, SS3, SS6, SS7, NS2, NS3, NS7, and NS8.
https://doi.org/10.1371/journal.pone.0273016.g005
Specifically: (1) The average degree and weighted average degree of most course networks decreased. The scope and intensity of interaction among network members declined rapidly, as did learners’ enthusiasm for communication. (2) The network density of seven courses also fell, indicating weaker connections between learners in most courses. (3) In addition, the clustering coefficient and small-world property of most course networks decreased, suggesting little possibility of small groups in the network. The scope of interaction between learners was not limited to a specific space, and the interaction objects had no significant tendencies. (4) Although the scale of course interaction became smaller in this phase, the average path length of members’ social networks shortened in nine courses. Its shorter average path length would expedite the spread of information within the network as well as communication and sharing among network members.
Fig 6 displays the evolution of course interaction indicators without significant changes in interaction scale after the pandemic, including SS4, SS5, NS1, NS4, NS5, and NS6.
https://doi.org/10.1371/journal.pone.0273016.g006
Specifically: (1) Some course members’ social networks exhibited an increase in the average and weighted average. In these cases, even though the course network’s scale did not continue to increase, communication among network members rose and interaction became more frequent and deeper than before. (2) Network density and average path length are indicators of social network density. The greater the network density, the denser the social network; the shorter the average path length, the more concentrated the communication among network members. However, at this phase, the average path length and network density in most courses had increased. Yet the network density remained small despite having risen ( Table 6 ). Even with more frequent learner interaction, connections remained distant and the social network was comparatively sparse.
https://doi.org/10.1371/journal.pone.0273016.t006
In summary, the scale of interaction did not change significantly overall. Nonetheless, some course members’ frequency and extent of interaction increased, and the relationships between network members became closer as well. In the study, we found it interesting that the interaction scale of Economics (a social science course) course and Electrodynamics (a natural science course) course expanded rapidly during the pandemic and retained their interaction scale thereafter. We next assessed these two courses to determine whether their level of interaction persisted after the pandemic.
4.3 Analyses of natural science courses and social science courses
4.3.1 analyses of the interaction characteristics of economics and electrodynamics..
Economics and Electrodynamics are social science courses and natural science courses, respectively. Members’ interaction within these courses was similar: the interaction scale increased significantly when COVID-19 broke out (Phase Ⅲ), and no significant changes emerged after the pandemic (Phase Ⅴ). We hence focused on course interaction long after the outbreak (Phase V) and compared changes across multiple indicators, as listed in Table 7 .
https://doi.org/10.1371/journal.pone.0273016.t007
As the pandemic continued to improve, the number of participants and the diameter long after the outbreak (Phase V) each declined for Economics compared with after the pandemic (Phase IV). The interaction scale decreased, but the interaction between learners was much deeper. Specifically: (1) The weighted average degree, network density, clustering coefficient, and small-world property each reflected upward trends. The pandemic therefore exerted a strong impact on this course. Interaction was well maintained even after the pandemic. The smaller network scale promoted members’ interaction and communication. (2) Compared with after the pandemic (Phase IV), members’ network density increased significantly, showing that relationships between learners were closer and that cohesion was improving. (3) At the same time, as the clustering coefficient and small-world property grew, network members demonstrated strong small-group characteristics: the communication between them was deepening and their enthusiasm for interaction was higher. (4) Long after the COVID-19 outbreak (Phase V), the average path length was reduced compared with previous terms, knowledge flowed more quickly among network members, and the degree of interaction gradually deepened.
The average degree, weighted average degree, network density, clustering coefficient, and small-world property of Electrodynamics all decreased long after the COVID-19 outbreak (Phase V) and were lower than during the outbreak (Phase Ⅲ). The level of learner interaction therefore gradually declined long after the outbreak (Phase V), and connections between learners were no longer active. Although the pandemic increased course members’ extent of interaction, this rise was merely temporary: students’ enthusiasm for learning waned rapidly and their interaction decreased after the pandemic (Phase IV). To further analyze the interaction characteristics of course members in Economics and Electrodynamics, we evaluated the closeness centrality of their social networks, as shown in section 4.3.2.
4.3.2 Analysis of the closeness centrality of Economics and Electrodynamics.
The change in the closeness centrality of social networks in Economics was small, and no sharp upward trend appeared during the pandemic outbreak, as shown in Fig 7 . The emergence of COVID-19 apparently fostered learners’ interaction in Economics albeit without a significant impact. The closeness centrality changed in Electrodynamics varied from that of Economics: upon the COVID-19 outbreak, closeness centrality was significantly different from other semesters. Communication between learners was closer and interaction was more effective. Electrodynamics course members’ social network proximity decreased rapidly after the pandemic. Learners’ communication lessened. In general, Economics course showed better interaction before the outbreak and was less affected by the pandemic; Electrodynamics course was more affected by the pandemic and showed different interaction characteristics at different periods of the pandemic.
(Note: "****" indicates the significant distinction in closeness centrality between the two periods, otherwise no significant distinction).
https://doi.org/10.1371/journal.pone.0273016.g007
5. Discussion
We referred to discussion forums from several courses on the icourse.163 MOOC platform to compare online learning before, during, and after the COVID-19 pandemic via SNA and to delineate the pandemic’s effects on online courses. Only 33.3% of courses in our sample increased in terms of interaction during the pandemic; the scale of interaction did not rise in any courses thereafter. When the courses scale rose, the scope and frequency of interaction showed upward trends during the pandemic; and the clustering coefficient of natural science courses and social science courses differed: the coefficient for social science courses tended to rise whereas that for natural science courses generally declined. When the pandemic broke out, the interaction scale of a single natural science course decreased along with its interaction scope and frequency. The amount of interaction in most courses shrank rapidly during the pandemic and network members were not as active as they had been before. However, after the pandemic, some courses saw declining interaction but greater communication between members; interaction also became more frequent and deeper than before.
5.1 During the COVID-19 pandemic, the scale of interaction increased in only a few courses
The pandemic outbreak led to a rapid increase in the number of participants in most courses; however, the change in network scale was not significant. The scale of online interaction expanded swiftly in only a few courses; in others, the scale either did not change significantly or displayed a downward trend. After the pandemic, the interaction scale in most courses decreased quickly; the same pattern applied to communication between network members. Learners’ enthusiasm for online interaction reduced as the circumstances of the pandemic improved—potentially because, during the pandemic, China’s Ministry of Education declared “School’s Out, But Class’s On” policy. Major colleges and universities were encouraged to use the Internet and informational resources to provide learning support, hence the sudden increase in the number of participants and interaction in online courses [ 46 ]. After the pandemic, students’ enthusiasm for online learning gradually weakened, presumably due to easing of the pandemic [ 47 ]. More activities also transitioned from online to offline, which tempered learners’ online discussion. Research has shown that long-term online learning can even bore students [ 48 ].
Most courses’ interaction scale decreased significantly after the pandemic. First, teachers and students occupied separate spaces during the outbreak, had few opportunities for mutual cooperation and friendship, and lacked a sense of belonging [ 49 ]. Students’ enthusiasm for learning dissipated over time [ 50 ]. Second, some teachers were especially concerned about adapting in-person instructional materials for digital platforms; their pedagogical methods were ineffective, and they did not provide learning activities germane to student interaction [ 51 ]. Third, although teachers and students in remote areas were actively engaged in online learning, some students could not continue to participate in distance learning due to inadequate technology later in the outbreak [ 52 ].
5.2 Characteristics of online learning interaction during and after the COVID-19 pandemic
5.2.1 during the covid-19 pandemic, online interaction in most courses did not change significantly..
The interaction scale of only a few courses increased during the pandemic. The interaction scope and frequency of these courses climbed as well. Yet even as the degree of network interaction rose, course network density did not expand in all cases. The pandemic sparked a surge in the number of online learners and a rapid increase in network scale, but students found it difficult to interact with all learners. Yau pointed out that a greater network scale did not enrich the range of interaction between individuals; rather, the number of individuals who could interact directly was limited [ 53 ]. The internet facilitates interpersonal communication. However, not everyone has the time or ability to establish close ties with others [ 54 ].
In addition, social science courses and natural science courses in our sample revealed disparate trends in this regard: the clustering coefficient of social science courses increased and that of natural science courses decreased. Social science courses usually employ learning approaches distinct from those in natural science courses [ 55 ]. Social science courses emphasize critical and innovative thinking along with personal expression [ 56 ]. Natural science courses focus on practical skills, methods, and principles [ 57 ]. Therefore, the content of social science courses can spur large-scale discussion among learners. Some course evaluations indicated that the course content design was suboptimal as well: teachers paid close attention to knowledge transmission and much less to piquing students’ interest in learning. In addition, the thread topics that teachers posted were scarcely diversified and teachers’ questions lacked openness. These attributes could not spark active discussion among learners.
5.2.2 Online learning interaction declined after the COVID-19 pandemic.
Most courses’ interaction scale and intensity decreased rapidly after the pandemic, but some did not change. Courses with a larger network scale did not continue to expand after the outbreak, and students’ enthusiasm for learning paled. The pandemic’s reduced severity also influenced the number of participants in online courses. Meanwhile, restored school order moved many learning activities from virtual to in-person spaces. Face-to-face learning has gradually replaced online learning, resulting in lower enrollment and less interaction in online courses. Prolonged online courses could have also led students to feel lonely and to lack a sense of belonging [ 58 ].
The scale of interaction in some courses did not change substantially after the pandemic yet learners’ connections became tighter. We hence recommend that teachers seize pandemic-related opportunities to design suitable activities. Additionally, instructors should promote student-teacher and student-student interaction, encourage students to actively participate online, and generally intensify the impact of online learning.
5.3 What are the characteristics of interaction in social science courses and natural science courses?
The level of interaction in Economics (a social science course) was significantly higher than that in Electrodynamics (a natural science course), and the small-world property in Economics increased as well. To boost online courses’ learning-related impacts, teachers can divide groups of learners based on the clustering coefficient and the average path length. Small groups of students may benefit teachers in several ways: to participate actively in activities intended to expand students’ knowledge, and to serve as key actors in these small groups. Cultivating students’ keenness to participate in class activities and self-management can also help teachers guide learner interaction and foster deep knowledge construction.
As evidenced by comments posted in the Electrodynamics course, we observed less interaction between students. Teachers also rarely urged students to contribute to conversations. These trends may have arisen because teachers and students were in different spaces. Teachers might have struggled to discern students’ interaction status. Teachers could also have failed to intervene in time, to design online learning activities that piqued learners’ interest, and to employ sound interactive theme planning and guidance. Teachers are often active in traditional classroom settings. Their roles are comparatively weakened online, such that they possess less control over instruction [ 59 ]. Online instruction also requires a stronger hand in learning: teachers should play a leading role in regulating network members’ interactive communication [ 60 ]. Teachers can guide learners to participate, help learners establish social networks, and heighten students’ interest in learning [ 61 ]. Teachers should attend to core members in online learning while also considering edge members; by doing so, all network members can be driven to share their knowledge and become more engaged. Finally, teachers and assistant teachers should help learners develop knowledge, exchange topic-related ideas, pose relevant questions during course discussions, and craft activities that enable learners to interact online [ 62 ]. These tactics can improve the effectiveness of online learning.
As described, network members displayed distinct interaction behavior in Economics and Electrodynamics courses. First, these courses varied in their difficulty: the social science course seemed easier to understand and focused on divergent thinking. Learners were often willing to express their views in comments and to ponder others’ perspectives [ 63 ]. The natural science course seemed more demanding and was oriented around logical thinking and skills [ 64 ]. Second, courses’ content differed. In general, social science courses favor the acquisition of declarative knowledge and creative knowledge compared with natural science courses. Social science courses also entertain open questions [ 65 ]. Natural science courses revolve around principle knowledge, strategic knowledge, and transfer knowledge [ 66 ]. Problems in these courses are normally more complicated than those in social science courses. Third, the indicators affecting students’ attitudes toward learning were unique. Guo et al. discovered that “teacher feedback” most strongly influenced students’ attitudes towards learning social science courses but had less impact on students in natural science courses [ 67 ]. Therefore, learners in social science courses likely expect more feedback from teachers and greater interaction with others.
6. Conclusion and future work
Our findings show that the network interaction scale of some online courses expanded during the COVID-19 pandemic. The network scale of most courses did not change significantly, demonstrating that the pandemic did not notably alter the scale of course interaction. Online learning interaction among course network members whose interaction scale increased also became more frequent during the pandemic. Once the outbreak was under control, although the scale of interaction declined, the level and scope of some courses’ interactive networks continued to rise; interaction was thus particularly effective in these cases. Overall, the pandemic appeared to have a relatively positive impact on online learning interaction. We considered a pair of courses in detail and found that Economics (a social science course) fared much better than Electrodynamics (a natural science course) in classroom interaction; learners were more willing to partake in-class activities, perhaps due to these courses’ unique characteristics. Brint et al. also came to similar conclusions [ 57 ].
This study was intended to be rigorous. Even so, several constraints can be addressed in future work. The first limitation involves our sample: we focused on a select set of courses hosted on China’s icourse.163 MOOC platform. Future studies should involve an expansive collection of courses to provide a more holistic understanding of how the pandemic has influenced online interaction. Second, we only explored the interactive relationship between learners and did not analyze interactive content. More in-depth content analysis should be carried out in subsequent research. All in all, the emergence of COVID-19 has provided a new path for online learning and has reshaped the distance learning landscape. To cope with associated challenges, educational practitioners will need to continue innovating in online instructional design, strengthen related pedagogy, optimize online learning conditions, and bolster teachers’ and students’ competence in online learning.
- View Article
- Google Scholar
- PubMed/NCBI
- 30. Serrat O. Social network analysis. Knowledge solutions: Springer; 2017. p. 39–43. https://doi.org/10.1007/978-981-10-0983-9_9
- 33. Rong Y, Xu E, editors. Strategies for the Management of the Government Affairs Microblogs in China Based on the SNA of Fifty Government Affairs Microblogs in Beijing. 14th International Conference on Service Systems and Service Management 2017.
- 34. Hu X, Chu S, editors. A comparison on using social media in a professional experience course. International Conference on Social Media and Society; 2013.
- 35. Bydžovská H. A Comparative Analysis of Techniques for Predicting Student Performance. Proceedings of the 9th International Conference on Educational Data Mining; Raleigh, NC, USA: International Educational Data Mining Society2016. p. 306–311.
- 40. Olivares D, Adesope O, Hundhausen C, et al., editors. Using social network analysis to measure the effect of learning analytics in computing education. 19th IEEE International Conference on Advanced Learning Technologies 2019.
- 41. Travers J, Milgram S. An experimental study of the small world problem. Social Networks: Elsevier; 1977. p. 179–197. https://doi.org/10.1016/B978-0-12-442450-0.50018–3
- 43. Okamoto K, Chen W, Li X-Y, editors. Ranking of closeness centrality for large-scale social networks. International workshop on frontiers in algorithmics; 2008; Springer, Berlin, Heidelberg: Springer.
- 47. Ding Y, Yang X, Zheng Y, editors. COVID-19’s Effects on the Scope, Effectiveness, and Roles of Teachers in Online Learning Based on Social Network Analysis: A Case Study. International Conference on Blended Learning; 2021: Springer.
- 64. Boys C, Brennan J., Henkel M., Kirkland J., Kogan M., Youl P. Higher Education and Preparation for Work. Jessica Kingsley Publishers. 1988. https://doi.org/10.1080/03075079612331381467
ORIGINAL RESEARCH article
Insights into students’ experiences and perceptions of remote learning methods: from the covid-19 pandemic to best practice for the future.
- 1 Minerva Schools at Keck Graduate Institute, San Francisco, CA, United States
- 2 Ronin Institute for Independent Scholarship, Montclair, NJ, United States
- 3 Department of Physics, University of Toronto, Toronto, ON, Canada
This spring, students across the globe transitioned from in-person classes to remote learning as a result of the COVID-19 pandemic. This unprecedented change to undergraduate education saw institutions adopting multiple online teaching modalities and instructional platforms. We sought to understand students’ experiences with and perspectives on those methods of remote instruction in order to inform pedagogical decisions during the current pandemic and in future development of online courses and virtual learning experiences. Our survey gathered quantitative and qualitative data regarding students’ experiences with synchronous and asynchronous methods of remote learning and specific pedagogical techniques associated with each. A total of 4,789 undergraduate participants representing institutions across 95 countries were recruited via Instagram. We find that most students prefer synchronous online classes, and students whose primary mode of remote instruction has been synchronous report being more engaged and motivated. Our qualitative data show that students miss the social aspects of learning on campus, and it is possible that synchronous learning helps to mitigate some feelings of isolation. Students whose synchronous classes include active-learning techniques (which are inherently more social) report significantly higher levels of engagement, motivation, enjoyment, and satisfaction with instruction. Respondents’ recommendations for changes emphasize increased engagement, interaction, and student participation. We conclude that active-learning methods, which are known to increase motivation, engagement, and learning in traditional classrooms, also have a positive impact in the remote-learning environment. Integrating these elements into online courses will improve the student experience.
Introduction
The COVID-19 pandemic has dramatically changed the demographics of online students. Previously, almost all students engaged in online learning elected the online format, starting with individual online courses in the mid-1990s through today’s robust online degree and certificate programs. These students prioritize convenience, flexibility and ability to work while studying and are older than traditional college age students ( Harris and Martin, 2012 ; Levitz, 2016 ). These students also find asynchronous elements of a course are more useful than synchronous elements ( Gillingham and Molinari, 2012 ). In contrast, students who chose to take courses in-person prioritize face-to-face instruction and connection with others and skew considerably younger ( Harris and Martin, 2012 ). This leaves open the question of whether students who prefer to learn in-person but are forced to learn remotely will prefer synchronous or asynchronous methods. One study of student preferences following a switch to remote learning during the COVID-19 pandemic indicates that students enjoy synchronous over asynchronous course elements and find them more effective ( Gillis and Krull, 2020 ). Now that millions of traditional in-person courses have transitioned online, our survey expands the data on student preferences and explores if those preferences align with pedagogical best practices.
An extensive body of research has explored what instructional methods improve student learning outcomes (Fink. 2013). Considerable evidence indicates that active-learning or student-centered approaches result in better learning outcomes than passive-learning or instructor-centered approaches, both in-person and online ( Freeman et al., 2014 ; Chen et al., 2018 ; Davis et al., 2018 ). Active-learning approaches include student activities or discussion in class, whereas passive-learning approaches emphasize extensive exposition by the instructor ( Freeman et al., 2014 ). Constructivist learning theories argue that students must be active participants in creating their own learning, and that listening to expert explanations is seldom sufficient to trigger the neurological changes necessary for learning ( Bostock, 1998 ; Zull, 2002 ). Some studies conclude that, while students learn more via active learning, they may report greater perceptions of their learning and greater enjoyment when passive approaches are used ( Deslauriers et al., 2019 ). We examine student perceptions of remote learning experiences in light of these previous findings.
In this study, we administered a survey focused on student perceptions of remote learning in late May 2020 through the social media account of @unjadedjade to a global population of English speaking undergraduate students representing institutions across 95 countries. We aim to explore how students were being taught, the relationship between pedagogical methods and student perceptions of their experience, and the reasons behind those perceptions. Here we present an initial analysis of the results and share our data set for further inquiry. We find that positive student perceptions correlate with synchronous courses that employ a variety of interactive pedagogical techniques, and that students overwhelmingly suggest behavioral and pedagogical changes that increase social engagement and interaction. We argue that these results support the importance of active learning in an online environment.
Materials and Methods
Participant pool.
Students were recruited through the Instagram account @unjadedjade. This social media platform, run by influencer Jade Bowler, focuses on education, effective study tips, ethical lifestyle, and promotes a positive mindset. For this reason, the audience is presumably academically inclined, and interested in self-improvement. The survey was posted to her account and received 10,563 responses within the first 36 h. Here we analyze the 4,789 of those responses that came from undergraduates. While we did not collect demographic or identifying information, we suspect that women are overrepresented in these data as followers of @unjadedjade are 80% women. A large minority of respondents were from the United Kingdom as Jade Bowler is a British influencer. Specifically, 43.3% of participants attend United Kingdom institutions, followed by 6.7% attending university in the Netherlands, 6.1% in Germany, 5.8% in the United States and 4.2% in Australia. Ninety additional countries are represented in these data (see Supplementary Figure 1 ).
Survey Design
The purpose of this survey is to learn about students’ instructional experiences following the transition to remote learning in the spring of 2020.
This survey was initially created for a student assignment for the undergraduate course Empirical Analysis at Minerva Schools at KGI. That version served as a robust pre-test and allowed for identification of the primary online platforms used, and the four primary modes of learning: synchronous (live) classes, recorded lectures and videos, uploaded or emailed materials, and chat-based communication. We did not adapt any open-ended questions based on the pre-test survey to avoid biasing the results and only corrected language in questions for clarity. We used these data along with an analysis of common practices in online learning to revise the survey. Our revised survey asked students to identify the synchronous and asynchronous pedagogical methods and platforms that they were using for remote learning. Pedagogical methods were drawn from literature assessing active and passive teaching strategies in North American institutions ( Fink, 2013 ; Chen et al., 2018 ; Davis et al., 2018 ). Open-ended questions asked students to describe why they preferred certain modes of learning and how they could improve their learning experience. Students also reported on their affective response to learning and participation using a Likert scale.
The revised survey also asked whether students had responded to the earlier survey. No significant differences were found between responses of those answering for the first and second times (data not shown). See Supplementary Appendix 1 for survey questions. Survey data was collected from 5/21/20 to 5/23/20.
Qualitative Coding
We applied a qualitative coding framework adapted from Gale et al. (2013) to analyze student responses to open-ended questions. Four researchers read several hundred responses and noted themes that surfaced. We then developed a list of themes inductively from the survey data and deductively from the literature on pedagogical practice ( Garrison et al., 1999 ; Zull, 2002 ; Fink, 2013 ; Freeman et al., 2014 ). The initial codebook was revised collaboratively based on feedback from researchers after coding 20–80 qualitative comments each. Before coding their assigned questions, alignment was examined through coding of 20 additional responses. Researchers aligned in identifying the same major themes. Discrepancies in terms identified were resolved through discussion. Researchers continued to meet weekly to discuss progress and alignment. The majority of responses were coded by a single researcher using the final codebook ( Supplementary Table 1 ). All responses to questions 3 (4,318 responses) and 8 (4,704 responses), and 2,512 of 4,776 responses to question 12 were analyzed. Valence was also indicated where necessary (i.e., positive or negative discussion of terms). This paper focuses on the most prevalent themes from our initial analysis of the qualitative responses. The corresponding author reviewed codes to ensure consistency and accuracy of reported data.
Statistical Analysis
The survey included two sets of Likert-scale questions, one consisting of a set of six statements about students’ perceptions of their experiences following the transition to remote learning ( Table 1 ). For each statement, students indicated their level of agreement with the statement on a five-point scale ranging from 1 (“Strongly Disagree”) to 5 (“Strongly Agree”). The second set asked the students to respond to the same set of statements, but about their retroactive perceptions of their experiences with in-person instruction before the transition to remote learning. This set was not the subject of our analysis but is present in the published survey results. To explore correlations among student responses, we used CrossCat analysis to calculate the probability of dependence between Likert-scale responses ( Mansinghka et al., 2016 ).
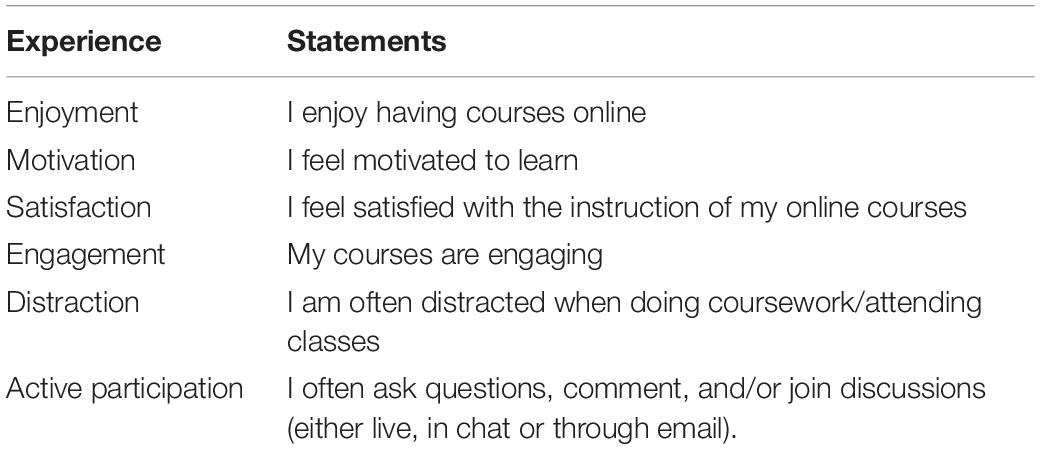
Table 1. Likert-scale questions.
Mean values are calculated based on the numerical scores associated with each response. Measures of statistical significance for comparisons between different subgroups of respondents were calculated using a two-sided Mann-Whitney U -test, and p -values reported here are based on this test statistic. We report effect sizes in pairwise comparisons using the common-language effect size, f , which is the probability that the response from a random sample from subgroup 1 is greater than the response from a random sample from subgroup 2. We also examined the effects of different modes of remote learning and technological platforms using ordinal logistic regression. With the exception of the mean values, all of these analyses treat Likert-scale responses as ordinal-scale, rather than interval-scale data.
Students Prefer Synchronous Class Sessions
Students were asked to identify their primary mode of learning given four categories of remote course design that emerged from the pilot survey and across literature on online teaching: live (synchronous) classes, recorded lectures and videos, emailed or uploaded materials, and chats and discussion forums. While 42.7% ( n = 2,045) students identified live classes as their primary mode of learning, 54.6% ( n = 2613) students preferred this mode ( Figure 1 ). Both recorded lectures and live classes were preferred over uploaded materials (6.22%, n = 298) and chat (3.36%, n = 161).
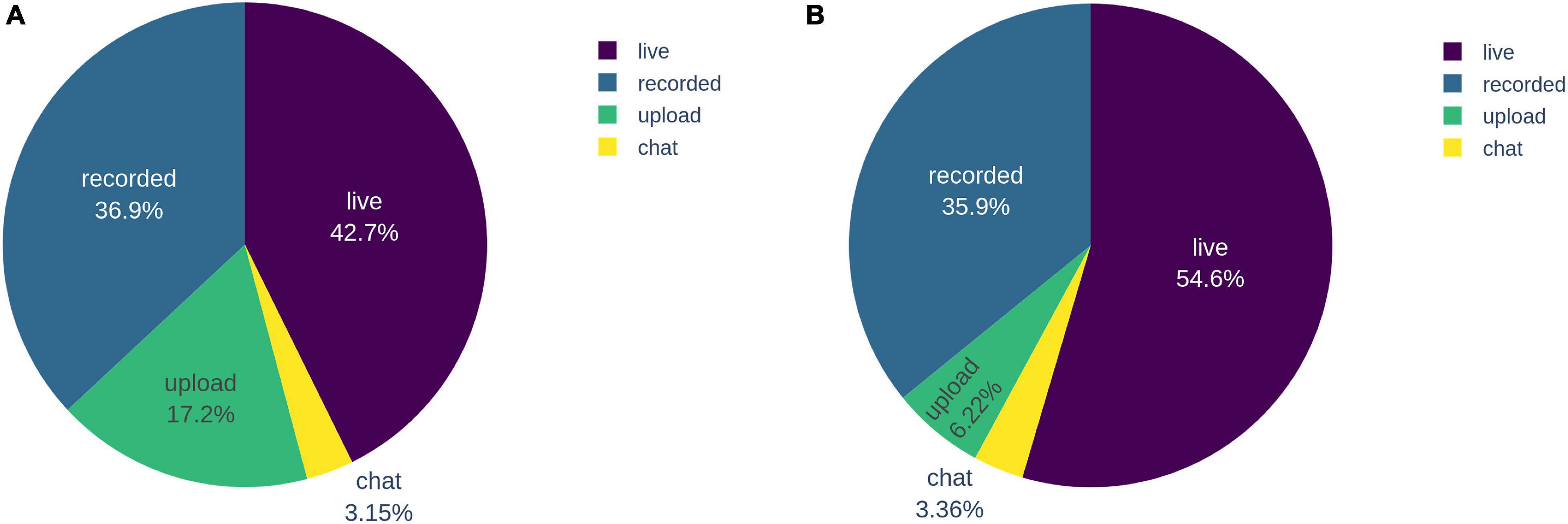
Figure 1. Actual (A) and preferred (B) primary modes of learning.
In addition to a preference for live classes, students whose primary mode was synchronous were more likely to enjoy the class, feel motivated and engaged, be satisfied with instruction and report higher levels of participation ( Table 2 and Supplementary Figure 2 ). Regardless of primary mode, over two-thirds of students reported they are often distracted during remote courses.

Table 2. The effect of synchronous vs. asynchronous primary modes of learning on student perceptions.
Variation in Pedagogical Techniques for Synchronous Classes Results in More Positive Perceptions of the Student Learning Experience
To survey the use of passive vs. active instructional methods, students reported the pedagogical techniques used in their live classes. Among the synchronous methods, we identify three different categories ( National Research Council, 2000 ; Freeman et al., 2014 ). Passive methods (P) include lectures, presentations, and explanation using diagrams, white boards and/or other media. These methods all rely on instructor delivery rather than student participation. Our next category represents active learning through primarily one-on-one interactions (A). The methods in this group are in-class assessment, question-and-answer (Q&A), and classroom chat. Group interactions (F) included classroom discussions and small-group activities. Given these categories, Mann-Whitney U pairwise comparisons between the 7 possible combinations and Likert scale responses about student experience showed that the use of a variety of methods resulted in higher ratings of experience vs. the use of a single method whether or not that single method was active or passive ( Table 3 ). Indeed, students whose classes used methods from each category (PAF) had higher ratings of enjoyment, motivation, and satisfaction with instruction than those who only chose any single method ( p < 0.0001) and also rated higher rates of participation and engagement compared to students whose only method was passive (P) or active through one-on-one interactions (A) ( p < 0.00001). Student ratings of distraction were not significantly different for any comparison. Given that sets of Likert responses often appeared significant together in these comparisons, we ran a CrossCat analysis to look at the probability of dependence across Likert responses. Responses have a high probability of dependence on each other, limiting what we can claim about any discrete response ( Supplementary Figure 3 ).
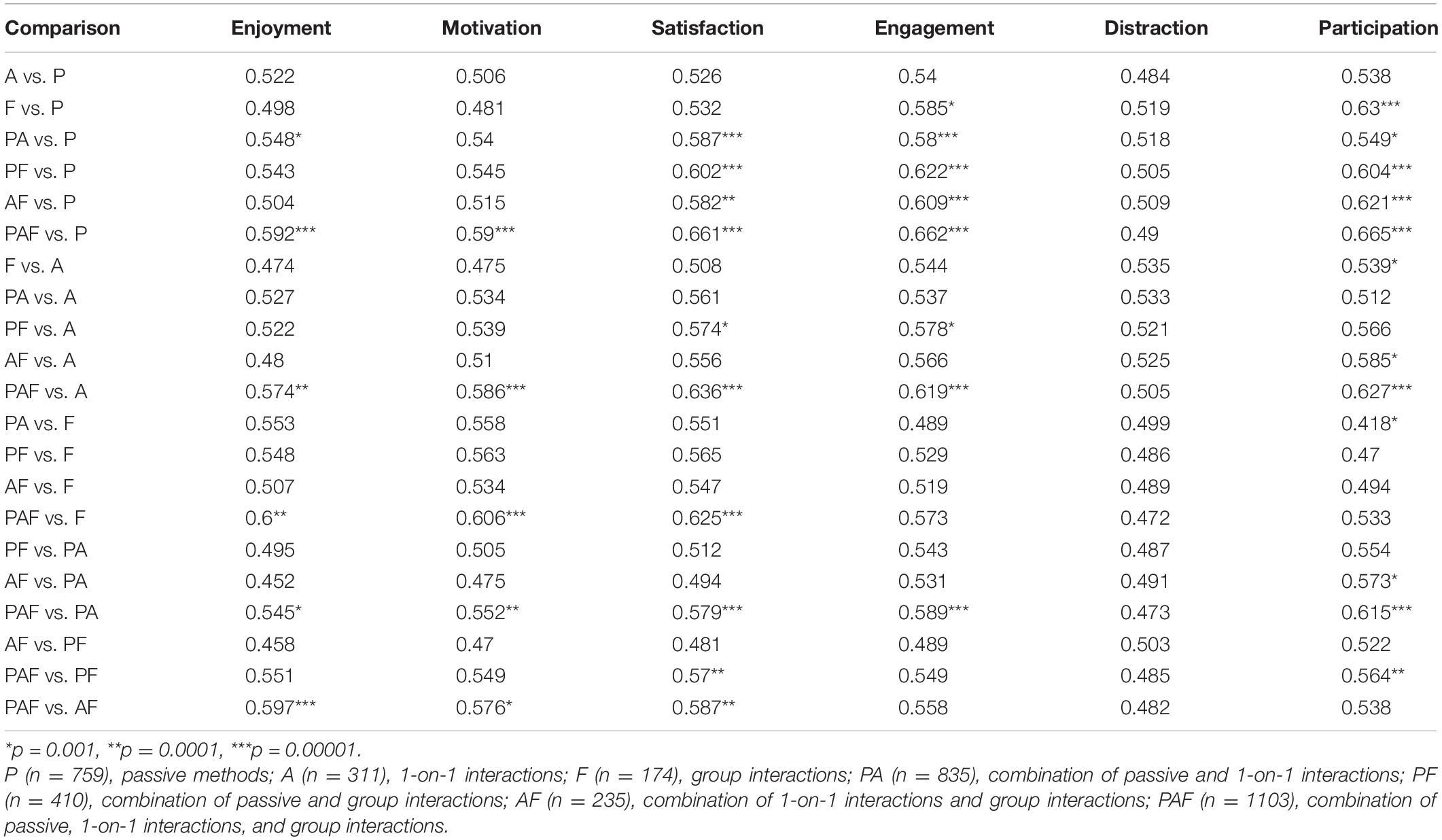
Table 3. Comparison of combinations of synchronous methods on student perceptions. Effect size (f).
Mann-Whitney U pairwise comparisons were also used to check if improvement in student experience was associated with the number of methods used vs. the variety of types of methods. For every comparison, we found that more methods resulted in higher scores on all Likert measures except distraction ( Table 4 ). Even comparison between four or fewer methods and greater than four methods resulted in a 59% chance that the latter enjoyed the courses more ( p < 0.00001) and 60% chance that they felt more motivated to learn ( p < 0.00001). Students who selected more than four methods ( n = 417) were also 65.1% ( p < 0.00001), 62.9% ( p < 0.00001) and 64.3% ( p < 0.00001) more satisfied with instruction, engaged, and actively participating, respectfully. Therefore, there was an overlap between how the number and variety of methods influenced students’ experiences. Since the number of techniques per category is 2–3, we cannot fully disentangle the effect of number vs. variety. Pairwise comparisons to look at subsets of data with 2–3 methods from a single group vs. 2–3 methods across groups controlled for this but had low sample numbers in most groups and resulted in no significant findings (data not shown). Therefore, from the data we have in our survey, there seems to be an interdependence between number and variety of methods on students’ learning experiences.

Table 4. Comparison of the number of synchronous methods on student perceptions. Effect size (f).
Variation in Asynchronous Pedagogical Techniques Results in More Positive Perceptions of the Student Learning Experience
Along with synchronous pedagogical methods, students reported the asynchronous methods that were used for their classes. We divided these methods into three main categories and conducted pairwise comparisons. Learning methods include video lectures, video content, and posted study materials. Interacting methods include discussion/chat forums, live office hours, and email Q&A with professors. Testing methods include assignments and exams. Our results again show the importance of variety in students’ perceptions ( Table 5 ). For example, compared to providing learning materials only, providing learning materials, interaction, and testing improved enjoyment ( f = 0.546, p < 0.001), motivation ( f = 0.553, p < 0.0001), satisfaction with instruction ( f = 0.596, p < 0.00001), engagement ( f = 0.572, p < 0.00001) and active participation ( f = 0.563, p < 0.00001) (row 6). Similarly, compared to just being interactive with conversations, the combination of all three methods improved five out of six indicators, except for distraction in class (row 11).
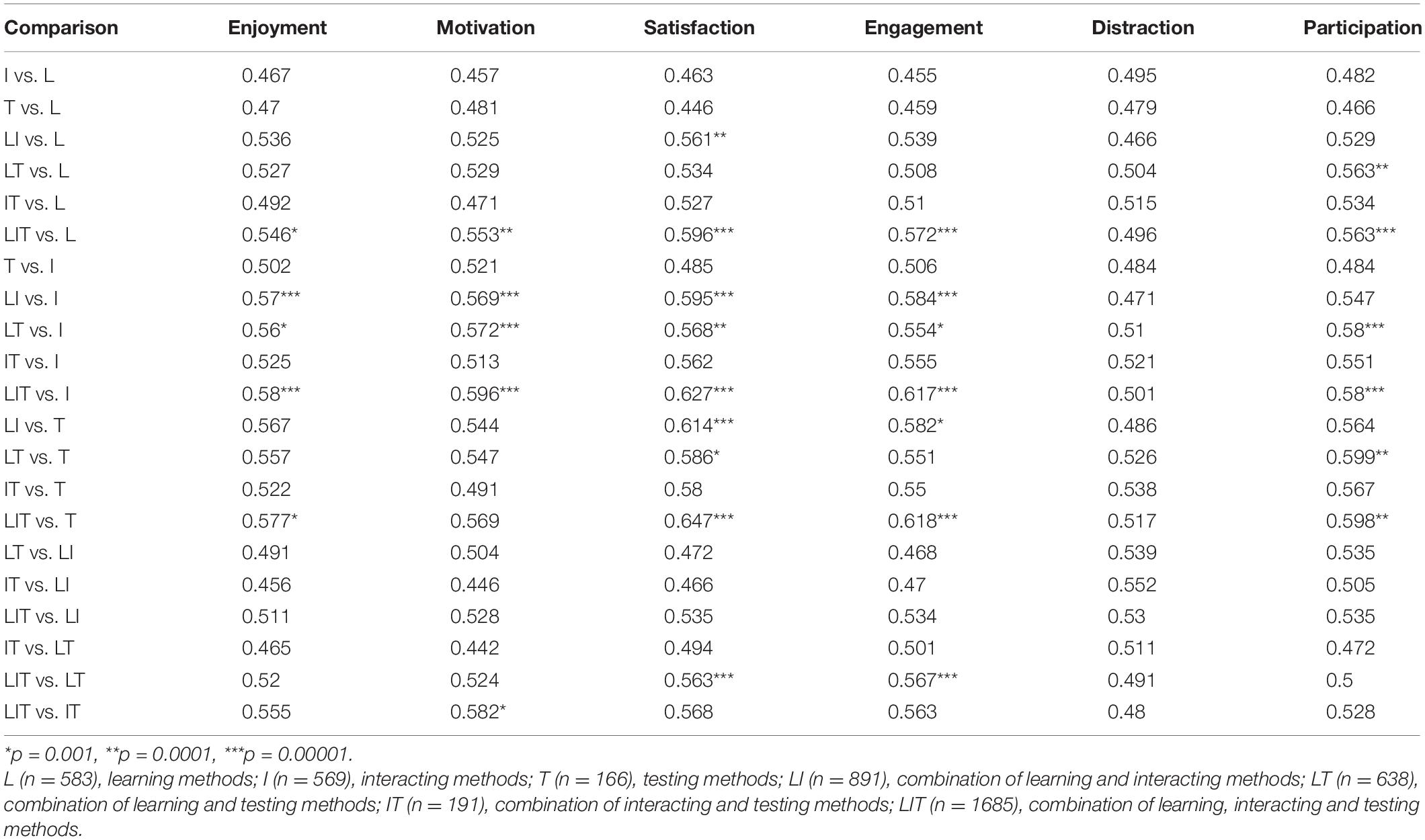
Table 5. Comparison of combinations of asynchronous methods on student perceptions. Effect size (f).
Ordinal logistic regression was used to assess the likelihood that the platforms students used predicted student perceptions ( Supplementary Table 2 ). Platform choices were based on the answers to open-ended questions in the pre-test survey. The synchronous and asynchronous methods used were consistently more predictive of Likert responses than the specific platforms. Likewise, distraction continued to be our outlier with no differences across methods or platforms.
Students Prefer In-Person and Synchronous Online Learning Largely Due to Social-Emotional Reasoning
As expected, 86.1% (4,123) of survey participants report a preference for in-person courses, while 13.9% (666) prefer online courses. When asked to explain the reasons for their preference, students who prefer in-person courses most often mention the importance of social interaction (693 mentions), engagement (639 mentions), and motivation (440 mentions). These students are also more likely to mention a preference for a fixed schedule (185 mentions) vs. a flexible schedule (2 mentions).
In addition to identifying social reasons for their preference for in-person learning, students’ suggestions for improvements in online learning focus primarily on increasing interaction and engagement, with 845 mentions of live classes, 685 mentions of interaction, 126 calls for increased participation and calls for changes related to these topics such as, “Smaller teaching groups for live sessions so that everyone is encouraged to talk as some people don’t say anything and don’t participate in group work,” and “Make it less of the professor reading the pdf that was given to us and more interaction.”
Students who prefer online learning primarily identify independence and flexibility (214 mentions) and reasons related to anxiety and discomfort in in-person settings (41 mentions). Anxiety was only mentioned 12 times in the much larger group that prefers in-person learning.
The preference for synchronous vs. asynchronous modes of learning follows similar trends ( Table 6 ). Students who prefer live classes mention engagement and interaction most often while those who prefer recorded lectures mention flexibility.

Table 6. Most prevalent themes for students based on their preferred mode of remote learning.
Student Perceptions Align With Research on Active Learning
The first, and most robust, conclusion is that incorporation of active-learning methods correlates with more positive student perceptions of affect and engagement. We can see this clearly in the substantial differences on a number of measures, where students whose classes used only passive-learning techniques reported lower levels of engagement, satisfaction, participation, and motivation when compared with students whose classes incorporated at least some active-learning elements. This result is consistent with prior research on the value of active learning ( Freeman et al., 2014 ).
Though research shows that student learning improves in active learning classes, on campus, student perceptions of their learning, enjoyment, and satisfaction with instruction are often lower in active-learning courses ( Deslauriers et al., 2019 ). Our finding that students rate enjoyment and satisfaction with instruction higher for active learning online suggests that the preference for passive lectures on campus relies on elements outside of the lecture itself. That might include the lecture hall environment, the social physical presence of peers, or normalization of passive lectures as the expected mode for on-campus classes. This implies that there may be more buy-in for active learning online vs. in-person.
A second result from our survey is that student perceptions of affect and engagement are associated with students experiencing a greater diversity of learning modalities. We see this in two different results. First, in addition to the fact that classes that include active learning outperform classes that rely solely on passive methods, we find that on all measures besides distraction, the highest student ratings are associated with a combination of active and passive methods. Second, we find that these higher scores are associated with classes that make use of a larger number of different methods.
This second result suggests that students benefit from classes that make use of multiple different techniques, possibly invoking a combination of passive and active methods. However, it is unclear from our data whether this effect is associated specifically with combining active and passive methods, or if it is associated simply with the use of multiple different methods, irrespective of whether those methods are active, passive, or some combination. The problem is that the number of methods used is confounded with the diversity of methods (e.g., it is impossible for a classroom using only one method to use both active and passive methods). In an attempt to address this question, we looked separately at the effect of number and diversity of methods while holding the other constant. Across a large number of such comparisons, we found few statistically significant differences, which may be a consequence of the fact that each comparison focused on a small subset of the data.
Thus, our data suggests that using a greater diversity of learning methods in the classroom may lead to better student outcomes. This is supported by research on student attention span which suggests varying delivery after 10–15 min to retain student’s attention ( Bradbury, 2016 ). It is likely that this is more relevant for online learning where students report high levels of distraction across methods, modalities, and platforms. Given that number and variety are key, and there are few passive learning methods, we can assume that some combination of methods that includes active learning improves student experience. However, it is not clear whether we should predict that this benefit would come simply from increasing the number of different methods used, or if there are benefits specific to combining particular methods. Disentangling these effects would be an interesting avenue for future research.
Students Value Social Presence in Remote Learning
Student responses across our open-ended survey questions show a striking difference in reasons for their preferences compared with traditional online learners who prefer flexibility ( Harris and Martin, 2012 ; Levitz, 2016 ). Students reasons for preferring in-person classes and synchronous remote classes emphasize the desire for social interaction and echo the research on the importance of social presence for learning in online courses.
Short et al. (1976) outlined Social Presence Theory in depicting students’ perceptions of each other as real in different means of telecommunications. These ideas translate directly to questions surrounding online education and pedagogy in regards to educational design in networked learning where connection across learners and instructors improves learning outcomes especially with “Human-Human interaction” ( Goodyear, 2002 , 2005 ; Tu, 2002 ). These ideas play heavily into asynchronous vs. synchronous learning, where Tu reports students having positive responses to both synchronous “real-time discussion in pleasantness, responsiveness and comfort with familiar topics” and real-time discussions edging out asynchronous computer-mediated communications in immediate replies and responsiveness. Tu’s research indicates that students perceive more interaction with synchronous mediums such as discussions because of immediacy which enhances social presence and support the use of active learning techniques ( Gunawardena, 1995 ; Tu, 2002 ). Thus, verbal immediacy and communities with face-to-face interactions, such as those in synchronous learning classrooms, lessen the psychological distance of communicators online and can simultaneously improve instructional satisfaction and reported learning ( Gunawardena and Zittle, 1997 ; Richardson and Swan, 2019 ; Shea et al., 2019 ). While synchronous learning may not be ideal for traditional online students and a subset of our participants, this research suggests that non-traditional online learners are more likely to appreciate the value of social presence.
Social presence also connects to the importance of social connections in learning. Too often, current systems of education emphasize course content in narrow ways that fail to embrace the full humanity of students and instructors ( Gay, 2000 ). With the COVID-19 pandemic leading to further social isolation for many students, the importance of social presence in courses, including live interactions that build social connections with classmates and with instructors, may be increased.
Limitations of These Data
Our undergraduate data consisted of 4,789 responses from 95 different countries, an unprecedented global scale for research on online learning. However, since respondents were followers of @unjadedjade who focuses on learning and wellness, these respondents may not represent the average student. Biases in survey responses are often limited by their recruitment techniques and our bias likely resulted in more robust and thoughtful responses to free-response questions and may have influenced the preference for synchronous classes. It is unlikely that it changed students reporting on remote learning pedagogical methods since those are out of student control.
Though we surveyed a global population, our design was rooted in literature assessing pedagogy in North American institutions. Therefore, our survey may not represent a global array of teaching practices.
This survey was sent out during the initial phase of emergency remote learning for most countries. This has two important implications. First, perceptions of remote learning may be clouded by complications of the pandemic which has increased social, mental, and financial stresses globally. Future research could disaggregate the impact of the pandemic from students’ learning experiences with a more detailed and holistic analysis of the impact of the pandemic on students.
Second, instructors, students and institutions were not able to fully prepare for effective remote education in terms of infrastructure, mentality, curriculum building, and pedagogy. Therefore, student experiences reflect this emergency transition. Single-modality courses may correlate with instructors who lacked the resources or time to learn or integrate more than one modality. Regardless, the main insights of this research align well with the science of teaching and learning and can be used to inform both education during future emergencies and course development for online programs that wish to attract traditional college students.
Global Student Voices Improve Our Understanding of the Experience of Emergency Remote Learning
Our survey shows that global student perspectives on remote learning agree with pedagogical best practices, breaking with the often-found negative reactions of students to these practices in traditional classrooms ( Shekhar et al., 2020 ). Our analysis of open-ended questions and preferences show that a majority of students prefer pedagogical approaches that promote both active learning and social interaction. These results can serve as a guide to instructors as they design online classes, especially for students whose first choice may be in-person learning. Indeed, with the near ubiquitous adoption of remote learning during the COVID-19 pandemic, remote learning may be the default for colleges during temporary emergencies. This has already been used at the K-12 level as snow days become virtual learning days ( Aspergren, 2020 ).
In addition to informing pedagogical decisions, the results of this survey can be used to inform future research. Although we survey a global population, our recruitment method selected for students who are English speakers, likely majority female, and have an interest in self-improvement. Repeating this study with a more diverse and representative sample of university students could improve the generalizability of our findings. While the use of a variety of pedagogical methods is better than a single method, more research is needed to determine what the optimal combinations and implementations are for courses in different disciplines. Though we identified social presence as the major trend in student responses, the over 12,000 open-ended responses from students could be analyzed in greater detail to gain a more nuanced understanding of student preferences and suggestions for improvement. Likewise, outliers could shed light on the diversity of student perspectives that we may encounter in our own classrooms. Beyond this, our findings can inform research that collects demographic data and/or measures learning outcomes to understand the impact of remote learning on different populations.
Importantly, this paper focuses on a subset of responses from the full data set which includes 10,563 students from secondary school, undergraduate, graduate, or professional school and additional questions about in-person learning. Our full data set is available here for anyone to download for continued exploration: https://dataverse.harvard.edu/dataset.xhtml?persistentId= doi: 10.7910/DVN/2TGOPH .
Data Availability Statement
The raw data supporting the conclusions of this article will be made available by the authors, without undue reservation.
Ethics Statement
Ethical review and approval was not required for the study on human participants in accordance with the local legislation and institutional requirements. The patients/participants provided their written informed consent to participate in this study.
Author Contributions
GS: project lead, survey design, qualitative coding, writing, review, and editing. TN: data analysis, writing, review, and editing. CN and PB: qualitative coding. JW: data analysis, writing, and editing. CS: writing, review, and editing. EV and KL: original survey design and qualitative coding. PP: data analysis. JB: original survey design and survey distribution. HH: data analysis. MP: writing. All authors contributed to the article and approved the submitted version.
Conflict of Interest
The authors declare that the research was conducted in the absence of any commercial or financial relationships that could be construed as a potential conflict of interest.
Acknowledgments
We want to thank Minerva Schools at KGI for providing funding for summer undergraduate research internships. We also want to thank Josh Fost and Christopher V. H.-H. Chen for discussion that helped shape this project.
Supplementary Material
The Supplementary Material for this article can be found online at: https://www.frontiersin.org/articles/10.3389/feduc.2021.647986/full#supplementary-material
Aspergren, E. (2020). Snow Days Canceled Because of COVID-19 Online School? Not in These School Districts.sec. Education. USA Today. Available online at: https://www.usatoday.com/story/news/education/2020/12/15/covid-school-canceled-snow-day-online-learning/3905780001/ (accessed December 15, 2020).
Google Scholar
Bostock, S. J. (1998). Constructivism in mass higher education: a case study. Br. J. Educ. Technol. 29, 225–240. doi: 10.1111/1467-8535.00066
CrossRef Full Text | Google Scholar
Bradbury, N. A. (2016). Attention span during lectures: 8 seconds, 10 minutes, or more? Adv. Physiol. Educ. 40, 509–513. doi: 10.1152/advan.00109.2016
PubMed Abstract | CrossRef Full Text | Google Scholar
Chen, B., Bastedo, K., and Howard, W. (2018). Exploring best practices for online STEM courses: active learning, interaction & assessment design. Online Learn. 22, 59–75. doi: 10.24059/olj.v22i2.1369
Davis, D., Chen, G., Hauff, C., and Houben, G.-J. (2018). Activating learning at scale: a review of innovations in online learning strategies. Comput. Educ. 125, 327–344. doi: 10.1016/j.compedu.2018.05.019
Deslauriers, L., McCarty, L. S., Miller, K., Callaghan, K., and Kestin, G. (2019). Measuring actual learning versus feeling of learning in response to being actively engaged in the classroom. Proc. Natl. Acad. Sci. 116, 19251–19257. doi: 10.1073/pnas.1821936116
Fink, L. D. (2013). Creating Significant Learning Experiences: An Integrated Approach to Designing College Courses. Somerset, NJ: John Wiley & Sons, Incorporated.
Freeman, S., Eddy, S. L., McDonough, M., Smith, M. K., Okoroafor, N., Jordt, H., et al. (2014). Active learning increases student performance in science, engineering, and mathematics. Proc. Natl. Acad. Sci. 111, 8410–8415. doi: 10.1073/pnas.1319030111
Gale, N. K., Heath, G., Cameron, E., Rashid, S., and Redwood, S. (2013). Using the framework method for the analysis of qualitative data in multi-disciplinary health research. BMC Med. Res. Methodol. 13:117. doi: 10.1186/1471-2288-13-117
Garrison, D. R., Anderson, T., and Archer, W. (1999). Critical inquiry in a text-based environment: computer conferencing in higher education. Internet High. Educ. 2, 87–105. doi: 10.1016/S1096-7516(00)00016-6
Gay, G. (2000). Culturally Responsive Teaching: Theory, Research, and Practice. Multicultural Education Series. New York, NY: Teachers College Press.
Gillingham, and Molinari, C. (2012). Online courses: student preferences survey. Internet Learn. 1, 36–45. doi: 10.18278/il.1.1.4
Gillis, A., and Krull, L. M. (2020). COVID-19 remote learning transition in spring 2020: class structures, student perceptions, and inequality in college courses. Teach. Sociol. 48, 283–299. doi: 10.1177/0092055X20954263
Goodyear, P. (2002). “Psychological foundations for networked learning,” in Networked Learning: Perspectives and Issues. Computer Supported Cooperative Work , eds C. Steeples and C. Jones (London: Springer), 49–75. doi: 10.1007/978-1-4471-0181-9_4
Goodyear, P. (2005). Educational design and networked learning: patterns, pattern languages and design practice. Australas. J. Educ. Technol. 21, 82–101. doi: 10.14742/ajet.1344
Gunawardena, C. N. (1995). Social presence theory and implications for interaction and collaborative learning in computer conferences. Int. J. Educ. Telecommun. 1, 147–166.
Gunawardena, C. N., and Zittle, F. J. (1997). Social presence as a predictor of satisfaction within a computer mediated conferencing environment. Am. J. Distance Educ. 11, 8–26. doi: 10.1080/08923649709526970
Harris, H. S., and Martin, E. (2012). Student motivations for choosing online classes. Int. J. Scholarsh. Teach. Learn. 6, 1–8. doi: 10.20429/ijsotl.2012.060211
Levitz, R. N. (2016). 2015-16 National Online Learners Satisfaction and Priorities Report. Cedar Rapids: Ruffalo Noel Levitz, 12.
Mansinghka, V., Shafto, P., Jonas, E., Petschulat, C., Gasner, M., and Tenenbaum, J. B. (2016). CrossCat: a fully Bayesian nonparametric method for analyzing heterogeneous, high dimensional data. J. Mach. Learn. Res. 17, 1–49. doi: 10.1007/978-0-387-69765-9_7
National Research Council (2000). How People Learn: Brain, Mind, Experience, and School: Expanded Edition. Washington, DC: National Academies Press, doi: 10.17226/9853
Richardson, J. C., and Swan, K. (2019). Examining social presence in online courses in relation to students’ perceived learning and satisfaction. Online Learn. 7, 68–88. doi: 10.24059/olj.v7i1.1864
Shea, P., Pickett, A. M., and Pelz, W. E. (2019). A Follow-up investigation of ‘teaching presence’ in the suny learning network. Online Learn. 7, 73–75. doi: 10.24059/olj.v7i2.1856
Shekhar, P., Borrego, M., DeMonbrun, M., Finelli, C., Crockett, C., and Nguyen, K. (2020). Negative student response to active learning in STEM classrooms: a systematic review of underlying reasons. J. Coll. Sci. Teach. 49, 45–54.
Short, J., Williams, E., and Christie, B. (1976). The Social Psychology of Telecommunications. London: John Wiley & Sons.
Tu, C.-H. (2002). The measurement of social presence in an online learning environment. Int. J. E Learn. 1, 34–45. doi: 10.17471/2499-4324/421
Zull, J. E. (2002). The Art of Changing the Brain: Enriching Teaching by Exploring the Biology of Learning , 1st Edn. Sterling, VA: Stylus Publishing.
Keywords : online learning, COVID-19, active learning, higher education, pedagogy, survey, international
Citation: Nguyen T, Netto CLM, Wilkins JF, Bröker P, Vargas EE, Sealfon CD, Puthipiroj P, Li KS, Bowler JE, Hinson HR, Pujar M and Stein GM (2021) Insights Into Students’ Experiences and Perceptions of Remote Learning Methods: From the COVID-19 Pandemic to Best Practice for the Future. Front. Educ. 6:647986. doi: 10.3389/feduc.2021.647986
Received: 30 December 2020; Accepted: 09 March 2021; Published: 09 April 2021.
Reviewed by:
Copyright © 2021 Nguyen, Netto, Wilkins, Bröker, Vargas, Sealfon, Puthipiroj, Li, Bowler, Hinson, Pujar and Stein. This is an open-access article distributed under the terms of the Creative Commons Attribution License (CC BY) . The use, distribution or reproduction in other forums is permitted, provided the original author(s) and the copyright owner(s) are credited and that the original publication in this journal is cited, in accordance with accepted academic practice. No use, distribution or reproduction is permitted which does not comply with these terms.
*Correspondence: Geneva M. Stein, [email protected]
Disclaimer: All claims expressed in this article are solely those of the authors and do not necessarily represent those of their affiliated organizations, or those of the publisher, the editors and the reviewers. Any product that may be evaluated in this article or claim that may be made by its manufacturer is not guaranteed or endorsed by the publisher.
- Open access
- Published: 16 September 2021
Online learning during COVID-19 produced equivalent or better student course performance as compared with pre-pandemic: empirical evidence from a school-wide comparative study
- Meixun Zheng 1 ,
- Daniel Bender 1 &
- Cindy Lyon 1
BMC Medical Education volume 21 , Article number: 495 ( 2021 ) Cite this article
215k Accesses
95 Citations
119 Altmetric
Metrics details
The COVID-19 pandemic forced dental schools to close their campuses and move didactic instruction online. The abrupt transition to online learning, however, has raised several issues that have not been resolved. While several studies have investigated dental students’ attitude towards online learning during the pandemic, mixed results have been reported. Additionally, little research has been conducted to identify and understand factors, especially pedagogical factors, that impacted students’ acceptance of online learning during campus closure. Furthermore, how online learning during the pandemic impacted students’ learning performance has not been empirically investigated. In March 2020, the dental school studied here moved didactic instruction online in response to government issued stay-at-home orders. This first-of-its-kind comparative study examined students’ perceived effectiveness of online courses during summer quarter 2020, explored pedagogical factors impacting their acceptance of online courses, and empirically evaluated the impact of online learning on students’ course performance, during the pandemic.
The study employed a quasi-experimental design. Participants were 482 pre-doctoral students in a U.S dental school. Students’ perceived effectiveness of online courses during the pandemic was assessed with a survey. Students’ course grades for online courses during summer quarter 2020 were compared with that of a control group who received face-to-face instruction for the same courses before the pandemic in summer quarter 2019.
Survey results revealed that most online courses were well accepted by the students, and 80 % of them wanted to continue with some online instruction post pandemic. Regression analyses revealed that students’ perceived engagement with faculty and classmates predicted their perceived effectiveness of the online course. More notably, Chi Square tests demonstrated that in 16 out of the 17 courses compared, the online cohort during summer quarter 2020 was equally or more likely to get an A course grade than the analogous face-to-face cohort during summer quarter 2019.
Conclusions
This is the first empirical study in dental education to demonstrate that online courses during the pandemic could achieve equivalent or better student course performance than the same pre-pandemic in-person courses. The findings fill in gaps in literature and may inform online learning design moving forward.
Peer Review reports
Introduction
Research across disciplines has demonstrated that well-designed online learning can lead to students’ enhanced motivation, satisfaction, and learning [ 1 , 2 , 3 , 4 , 5 , 6 , 7 ]. A report by the U.S. Department of Education [ 8 ], based on examinations of comparative studies of online and face-to-face versions of the same course from 1996 to 2008, concluded that online learning could produce learning outcomes equivalent to or better than face-to-face learning. The more recent systematic review by Pei and Wu [ 9 ] provided additional evidence that online learning is at least as effective as face-to-face learning for undergraduate medical students.
To take advantage of the opportunities presented by online learning, thought leaders in dental education in the U.S. have advocated for the adoption of online learning in the nation’s dental schools [ 10 , 11 , 12 ]. However, digital innovation has been a slow process in academic dentistry [ 13 , 14 , 15 ]. In March 2020, the COVID-19 pandemic brought unprecedented disruption to dental education by necessitating the need for online learning. In accordance with stay-at-home orders to prevent the spread of the virus, dental schools around the world closed their campuses and moved didactic instruction online.
The abrupt transition to online learning, however, has raised several concerns and question. First, while several studies have examined dental students’ online learning satisfaction during the pandemic, mixed results have been reported. Some studies have reported students’ positive attitude towards online learning [ 15 , 16 , 17 , 18 , 19 , 20 ]. Sadid-Zadeh et al. [ 18 ] found that 99 % of the surveyed dental students at University of Buffalo, in the U.S., were satisfied with live web-based lectures during the pandemic. Schlenz et al. [ 15 ] reported that students in a German dental school had a favorable attitude towards online learning and wanted to continue with online instruction in their future curriculum. Other studies, however, have reported students’ negative online learning experience during the pandemic [ 21 , 22 , 23 , 24 , 25 , 26 ]. For instance, dental students at Harvard University felt that learning during the pandemic had worsened and engagement had decreased [ 23 , 24 ]. In a study with medical and dental students in Pakistan, Abbasi et al. [ 21 ] found that 77 % of the students had negative perceptions about online learning and 84 % reported reduced student-instructor interactions.
In addition to these mixed results, little attention has been given to factors affecting students’ acceptance of online learning during the pandemic. With the likelihood that online learning will persist post pandemic [ 27 ], research in this area is warranted to inform online course design moving forward. In particular, prior research has demonstrated that one of the most important factors influencing students’ performance in any learning environment is a sense of belonging, the feeling of being connected with and supported by the instructor and classmates [ 28 , 29 , 30 , 31 ]. Unfortunately, this aspect of the classroom experience has suffered during school closure. While educational events can be held using a video conferencing system, virtual peer interaction on such platforms has been perceived by medical trainees to be not as easy and personal as physical interaction [ 32 ]. The pandemic highlights the need to examine instructional strategies most suited to the current situation to support students’ engagement with faculty and classmates.
Furthermore, there is considerable concern from the academic community about the quality of online learning. Pre-pandemic, some faculty and students were already skeptical about the value of online learning [ 33 ]. The longer the pandemic lasts, the more they may question the value of online education, asking: Can online learning during the pandemic produce learning outcomes that are similar to face-to-face learning before the pandemic? Despite the documented benefits of online learning prior to the pandemic, the actual impact of online learning during the pandemic on students’ academic performance is still unknown due to reasons outlined below.
On one hand, several factors beyond the technology used could influence the effectiveness of online learning, one of which is the teaching context [ 34 ]. The sudden transition to online learning has posed many challenges to faculty and students. Faculty may not have had adequate time to carefully design online courses to take full advantage of the possibilities of the online format. Some faculty may not have had prior online teaching experience and experienced a deeper learning curve when it came to adopting online teaching methods [ 35 ]. Students may have been at the risk of increased anxiety due to concerns about contracting the virus, on time graduation, finances, and employment [ 36 , 37 ], which may have negatively impacted learning performance [ 38 ]. Therefore, whether online learning during the pandemic could produce learning outcomes similar to those of online learning implemented during more normal times remains to be determined.
Most existing studies on online learning in dental education during the pandemic have only reported students’ satisfaction. The actual impact of the online format on academic performance has not been empirically investigated. The few studies that have examined students’ learning outcomes have only used students’ self-reported data from surveys and focus groups. According to Kaczmarek et al. [ 24 ], 50 % of the participating dental faculty at Harvard University perceived student learning to have worsened during the pandemic and 70 % of the students felt the same. Abbasi et al. [ 21 ] reported that 86 % of medical and dental students in a Pakistan college felt that they learned less online. While student opinions are important, research has demonstrated a poor correlation between students’ perceived learning and actual learning gains [ 39 ]. As we continue to navigate the “new normal” in teaching, students’ learning performance needs to be empirically evaluated to help institutions gauge the impact of this grand online learning experiment.
Research purposes
In March 2020, the University of the Pacific Arthur A. Dugoni School of Dentistry, in the U.S., moved didactic instruction online to ensure the continuity of education during building closure. This study examined students’ acceptance of online learning during the pandemic and its impacting factors, focusing on instructional practices pertaining to students’ engagement/interaction with faculty and classmates. Another purpose of this study was to empirically evaluate the impact of online learning during the pandemic on students’ actual course performance by comparing it with that of a pre-pandemic cohort. To understand the broader impact of the institutional-wide online learning effort, we examined all online courses offered in summer quarter 2020 (July to September) that had a didactic component.
This is the first empirical study in dental education to evaluate students’ learning performance during the pandemic. The study aimed to answer the following three questions.
How well was online learning accepted by students, during the summer quarter 2020 pandemic interruption?
How did instructional strategies, centered around students’ engagement with faculty and classmates, impact their acceptance of online learning?
How did online learning during summer quarter 2020 impact students’ course performance as compared with a previous analogous cohort who received face-to-face instruction in summer quarter 2019?
This study employed a quasi-experimental design. The study was approved by the university’s institutional review board (#2020-68).
Study context and participants
The study was conducted at the Arthur A. Dugoni School of Dentistry, University of the Pacific. The program runs on a quarter system. It offers a 3-year accelerated Doctor of Dental Surgery (DDS) program and a 2-year International Dental Studies (IDS) program for international dentists who have obtained a doctoral degree in dentistry from a country outside the U.S. and want to practice in the U.S. Students advance throughout the program in cohorts. IDS students take some courses together with their DDS peers. All three DDS classes (D1/DDS 2023, D2/DDS 2022, and D3/DDS 2021) and both IDS classes (I1/IDS 2022 and I2/IDS 2021) were invited to participate in the study. The number of students in each class was: D1 = 145, D2 = 143, D3 = 143, I1 = 26, and I2 = 25. This resulted in a total of 482 student participants.
During campus closure, faculty delivered remote instruction in various ways, including live online classes via Zoom @ [ 40 ], self-paced online modules on the school’s learning management system Canvas @ [ 41 ], or a combination of live and self-paced delivery. For self-paced modules, students studied assigned readings and/or viewings such as videos and pre-recorded slide presentations. Some faculty also developed self-paced online lessons with SoftChalk @ [ 42 ], a cloud-based platform that supports the inclusion of gamified learning by insertion of various mini learning activities. The SoftChalk lessons were integrated with Canvas @ [ 41 ] and faculty could monitor students’ progress. After students completed the pre-assigned online materials, some faculty held virtual office hours or live online discussion sessions for students to ask questions and discuss key concepts.
Data collection and analysis
Student survey.
Students’ perceived effectiveness of summer quarter 2020 online courses was evaluated by the school’s Office of Academic Affairs in lieu of the regular course evaluation process. A total of 19 courses for DDS students and 10 courses for IDS students were evaluated. An 8-question survey developed by the researchers (Additional file 1 ) was administered online in the last week of summer quarter 2020. Course directors invited student to take the survey during live online classes. The survey introduction stated that taking the survey was voluntary and that their anonymous responses would be reported in aggregated form for research purposes. Students were invited to continue with the survey if they chose to participate; otherwise, they could exit the survey. The number of students in each class who took the survey was as follows: D1 ( n = 142; 98 %), D2 ( n = 133; 93 %), D3 ( n = 61; 43 %), I1 ( n = 23; 88 %), and I2 ( n = 20; 80 %). This resulted in a total of 379 (79 %) respondents across all classes.
The survey questions were on a 4-point scale, ranging from Strongly Disagree (1 point), Disagree (2 points), Agree (3 points), and Strongly Agree (4 points). Students were asked to rate each online course by responding to four statements: “ I could fully engage with the instructor and classmates in this course”; “The online format of this course supported my learning”; “Overall this online course is effective.”, and “ I would have preferred face-to-face instruction for this course ”. For the first three survey questions, a higher mean score indicated a more positive attitude toward the online course. For the fourth question “ I would have preferred face-to-face instruction for this course ”, a higher mean score indicated that more students would have preferred face-to-face instruction for the course. Two additional survey questions asked students to select their preferred online delivery method for fully online courses during the pandemic from three given choices (synchronous online/live, asynchronous online/self-paced, and a combination of both), and to report whether they wanted to continue with some online instruction post pandemic. Finally, two open-ended questions at the end of the survey allowed students to comment on the aspects of online format that they found to be helpful and to provide suggestion for improvement. For the purpose of this study, we focused on the quantitative data from the Likert-scale questions.
Descriptive data such as the mean scores were reported for each course. Regression analyses were conducted to examine the relationship between instructional strategies focusing on students’ engagement with faculty and classmates, and their overall perceived effectiveness of the online course. The independent variable was student responses to the question “ I could fully engage with the instructor and classmates in this course ”, and the dependent variable was their answer to the question “ Overall, this online course is effective .”
Student course grades
Using Chi-square tests, student course grade distributions (A, B, C, D, and F) for summer quarter 2020 online courses were compared with that of a previous cohort who received face-to-face instruction for the same course in summer quarter 2019. Note that as a result of the school’s pre-doctoral curriculum redesign implemented in July 2019, not all courses offered in summer quarter 2020 were offered in the previous year in summer quarter 2019. In other words, some of the courses offered in summer quarter 2020 were new courses offered for the first time. Because these new courses did not have a previous face-to-face version to compare to, they were excluded from data analysis. For some other courses, while course content remained the same between 2019 and 2020, the sequence of course topics within the course had changed. These courses were also excluded from data analysis.
After excluding the aforementioned courses, it resulted in a total of 17 “comparable” courses that were included in data analysis (see the subsequent section). For these courses, the instructor, course content, and course goals were the same in both 2019 and 2020. The assessment methods and grading policies also remained the same through both years. For exams and quizzes, multiple choice questions were the dominating format for both years. While some exam questions in 2020 were different from 2019, faculty reported that the overall exam difficulty level was similar. The main difference in assessment was testing conditions. The 2019 cohort took computer-based exams in the physical classroom with faculty proctoring, and the 2020 cohort took exams at home with remote proctoring to ensure exam integrity. The remote proctoring software monitored the student during the exam through a web camera on their computer/laptop. The recorded video file flags suspicious activities for faculty review after exam completion.
Students’ perceived effectiveness of online learning
Table 1 summarized data on DDS students’ perceived effectiveness of each online course during summer quarter 2020. For the survey question “ Overall, this online course is effective ”, the majority of courses received a mean score that was approaching or over 3 points on the 4-point scale, suggesting that online learning was generally well accepted by students. Despite overall positive online course experiences, for many of the courses examined, there was an equal split in student responses to the question “ I would have preferred face-to-face instruction for this course .” Additionally, for students’ preferred online delivery method for fully online courses, about half of the students in each class preferred a combination of synchronous and asynchronous online learning (see Fig. 1 ). Finally, the majority of students wanted faculty to continue with some online instruction post pandemic: D1class (110; 78.60 %), D2 class (104; 80 %), and D3 class (49; 83.10 %).
While most online courses received favorable ratings, some variations did exist among courses. For D1 courses, “ Anatomy & Histology ” received lower ratings than others. This could be explained by its lab component, which didn’t lend itself as well to the online format. For D2 courses, several of them received lower ratings than others, especially for the survey question on students’ perceived engagement with faculty and classmates.
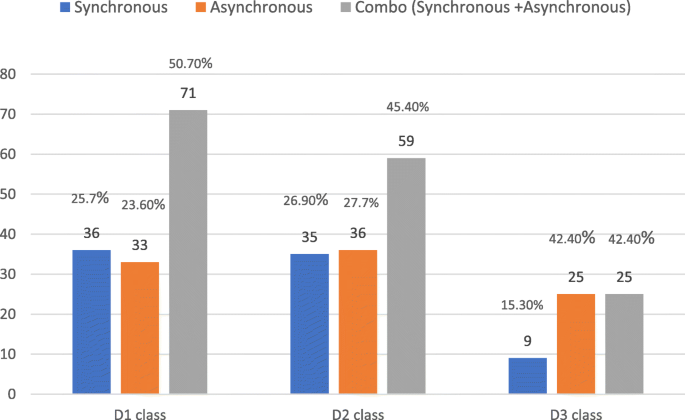
DDS students’ preferred online delivery method for fully online courses
Table 2 summarized IDS students’ perceived effectiveness of each online course during summer quarter 2020. For the survey question “ Overall, this online course is effective ”, all courses received a mean score that was approaching or over 3 points on a 4-point scale, suggesting that online learning was well accepted by students. For the survey question “ I would have preferred face-to-face instruction for this course ”, for most online courses examined, the percentage of students who would have preferred face-to-face instruction was similar to that of students who preferred online instruction for the course. Like their DDS peers, about half of the IDS students in each class also preferred a combination of synchronous and asynchronous online delivery for fully online courses (See Fig. 2 ). Finally, the majority of IDS students (I1, n = 18, 81.80 %; I2, n = 16, 84.20 %) wanted to continue with some online learning after the pandemic is over.
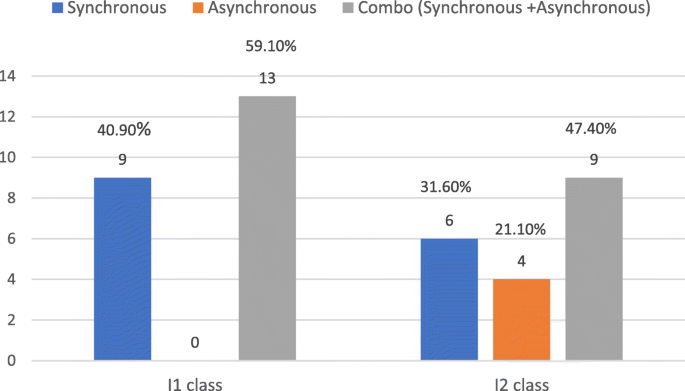
IDS students’ preferred online delivery method for fully online courses
Factors impacting students’ acceptance of online learning
For all 19 online courses taken by DDS students, regression analyses indicated that there was a significantly positive relationship between students’ perceived engagement with faculty and classmates and their perceived effectiveness of the course. P value was 0.00 across all courses. The ranges of effect size (r 2 ) were: D1 courses (0.26 to 0.50), D2 courses (0.39 to 0.650), and D3 courses (0.22 to 0.44), indicating moderate to high correlations across courses.
For 9 out of the 10 online courses taken by IDS students, there was a positive relationship between students’ perceived engagement with faculty and classmates and their perceived effectiveness of the course. P value was 0.00 across courses. The ranges of effect size were: I1 courses (0.35 to 0.77) and I2 courses (0.47 to 0.63), indicating consistently high correlations across courses. The only course in which students’ perceived engagement with faculty and classmates didn’t predict perceived effective of the course was “ Integrated Clinical Science III (ICS III) ”, which the I2 class took together with their D3 peers.
Impact of online learning on students’ course performance
Chi square test results (Table 3 ) indicated that in 4 out of the 17 courses compared, the online cohort during summer quarter 2020 was more likely to receive an A grade than the face-to-face cohort during summer quarter 2019. In 12 of the courses, the online cohort were equally likely to receive an A grade as the face-to-face cohort. In the remaining one course, the online cohort was less likely to receive an A grade than the face-to-face cohort.
Students’ acceptance of online learning during the pandemic
Survey results revealed that students had generally positive perceptions about online learning during the pandemic and the majority of them wanted to continue with some online learning post pandemic. Overall, our findings supported several other studies in dental [ 18 , 20 ], medical [ 43 , 44 ], and nursing [ 45 ] education that have also reported students’ positive attitudes towards online learning during the pandemic. In their written comments in the survey, students cited enhanced flexibility as one of the greatest benefits of online learning. Some students also commented that typing questions in the chat box during live online classes was less intimidating than speaking in class. Others explicitly stated that not having to commute to/from school provided more time for sleep, which helped with self-care and mental health. Our findings are in line with previous studies which have also demonstrated that online learning offered higher flexibility [ 46 , 47 ]. Meanwhile, consistent with findings of other researchers [ 19 , 21 , 46 ], our students felt difficulty engaging with faculty and classmates in several online courses.
There were some variations among individual courses in students’ acceptance of the online format. One factor that could partially account for the observed differences was instructional strategies. In particular, our regression analysis results demonstrated a positive correlation between students’ perceived engagement with faculty and classmates and their perceived overall effectiveness of the online course. Other aspects of course design might also have influenced students’ overall rating of the online course. For instance, some D2 students commented that the requirements of the course “ Integrated Case-based Seminars (ICS II) ” were not clear and that assessment did not align with lecture materials. It is important to remember that communicating course requirements clearly and aligning course content and assessment are principles that should be applied in any course, whether face-to-face or online. Our results highlighted the importance of providing faculty training on basic educational design principles and online learning design strategies. Furthermore, the nature of the course might also have impacted student ratings. For example, D1 course “ Anatomy and Histology ” had a lab component, which did not lend itself as well to the online format. Many students reported that it was difficult to see faculty’s live demonstration during Zoom lectures, which may have resulted in a lower student satisfaction rating.
As for students’ preferred online delivery method for fully online courses during the pandemic, about half of them preferred a combination of synchronous and asynchronous online learning. In light of this finding, as we continue with remote learning until public health directives allow a return to campus, we will encourage faculty to integrate these two online delivery modalities. Finally, in view of the result that over 80 % of the students wanted to continue with some online instruction after the pandemic, the school will advocate for blended learning in the post-pandemic world [ 48 ]. For future face-to-face courses on campus after the pandemic, faculty are encouraged to deliver some content online to reduce classroom seat time and make learning more flexible. Taken together, our findings not only add to the overall picture of the current situation but may inform learning design moving forward.
Role of online engagement and interaction
To reiterate, we found that students’ perceived engagement with faculty and classmates predicted their perceived overall effectiveness of the online course. This aligns with the larger literature on best practices in online learning design. Extensive research prior to the pandemic has confirmed that the effectiveness of online learning is determined by a number of factors beyond the tools used, including students’ interactions with the instructor and classmates [ 49 , 50 , 51 , 52 ]. Online students may feel isolated due to reduced or lack of interaction [ 53 , 54 ]. Therefore, in designing online learning experiences, it is important to remember that learning is a social process [ 55 ]. Faculty’s role is not only to transmit content but also to promote the different types of interactions that are an integral part of the online learning process [ 33 ]. The online teaching model in which faculty uploads materials online but teach it in the same way as in the physical classroom, without special effort to engage students, doesn’t make the best use of the online format. Putting the “sage on the screen” during a live class meeting on a video conferencing system is not different from “sage on the stage” in the physical classroom - both provide limited space for engagement. Such one-way monologue devalues the potentials that online learning presents.
In light of the critical role that social interaction plays in online learning, faculty are encouraged to use the interactive features of online learning platforms to provide clear channels for student-instructor and student-student interactions. In the open-ended comments, students highlighted several instructional strategies that they perceived to be helpful for learning. For live online classes, these included conducting breakout room activities, using the chat box to facilitate discussions, polling, and integrating gameplay with apps such as Kahoot! @ [ 56 ]. For self-paced classes, students appreciated that faculty held virtual office hours or subsequent live online discussion sessions to reinforce understanding of the pre-assigned materials.
Quality of online education during the pandemic
This study provided empirical evidence in dental education that it was possible to ensure the continuity of education without sacrificing the quality of education provided to students during forced migration to distance learning upon building closure. To reiterate, in all but one online course offered in summer quarter 2020, students were equally or more likely to get an A grade than the face-to-face cohort from summer quarter 2019. Even for courses that had less student support for the online format (e.g., the D1 course “ Anatomy and Histology ”), there was a significant increase in the number of students who earned an A grade in 2020 as compared with the previous year. The reduced capacity for technical training during the pandemic may have resulted in more study time for didactic content. Overall, our results resonate with several studies in health sciences education before the pandemic that the quality of learning is comparable in face-to-face and online formats [ 9 , 57 , 58 ]. For the only course ( Integrated Case-based Seminars ICS II) in which the online cohort had inferior performance than the face-to-face cohort, as mentioned earlier, students reported that assessment was not aligned with course materials and that course expectations were not clear. This might explain why students’ course performance was not as strong as expected.
Limitations
This study used a pre-existing control group from the previous year. There may have been individual differences between students in the online and the face-to-face cohorts, such as motivation, learning style, and prior knowledge, that could have impacted the observed outcomes. Additionally, even though course content and assessment methods were largely the same in 2019 and 2020, changes in other aspects of the course could have impacted students’ course performance. Some faculty may have been more compassionate with grading (e.g., more flexible with assignment deadlines) in summer quarter 2020 given the hardship students experienced during the pandemic. On the other hand, remote proctoring in summer quarter 2020 may have heightened some students’ exam anxiety knowing that they were being monitored through a webcam. The existence and magnitude of effect of these factors needs to be further investigated.
This present study only examined the correlation between students’ perceived online engagement and their perceived overall effectiveness of the online course. Other factors that might impact their acceptance of the online format need to be further researched in future studies. Another future direction is to examine how students’ perceived online engagement correlates with their actual course performance. Because the survey data collected for our present study are anonymous, we cannot match students’ perceived online engagement data with their course grades to run this additional analysis. It should also be noted that this study was focused on didactic online instruction. Future studies might examine how technical training was impacted during the COVID building closure. It was also out of the scope of this study to examine how student characteristics, especially high and low academic performance as reflected by individual grades, affects their online learning experience and performance. We plan to conduct a follow-up study to examine which group of students are most impacted by the online format. Finally, this study was conducted in a single dental school, and so the findings may not be generalizable to other schools and disciplines. Future studies could be conducted in another school or disciplines to compare results.
This study revealed that dental students had generally favorable attitudes towards online learning during the COVID-19 pandemic and that their perceived engagement with faculty and classmates predicted their acceptance of the online course. Most notably, this is the first study in dental education to demonstrate that online learning during the pandemic could achieve similar or better learning outcomes than face-to-face learning before the pandemic. Findings of our study could contribute significantly to the literature on online learning during the COVID-19 pandemic in health sciences education. The results could also inform future online learning design as we re-envision the future of online learning.
Availability of data and materials
The datasets used and/or analyzed during the current study are available from the corresponding author on reasonable request.
Bello G, Pennisi MA, Maviglia R, Maggiore SM, Bocci MG, Montini L, et al. Online vs live methods for teaching difficult airway management to anesthesiology residents. Intensive Care Med. 2005; 31 (4): 547–552.
Article Google Scholar
Ruiz JG, Mintzer MJ, Leipzig RM. The impact of e-learning in medical education. Acad Med. 2006; 81(3): 207–12.
Kavadella A, Tsiklakis K, Vougiouklakis G, Lionarakis A. Evaluation of a blended learning course for teaching oral radiology to undergraduate dental students. Eur J Dent Educ. 2012; 16(1): 88–95.
de Jong N, Verstegen DL, Tan FS, O’Connor SJ. A comparison of classroom and online asynchronous problem-based learning for students undertaking statistics training as part of a public health master’s degree. Adv Health Sci Educ. 2013; 18(2):245–64.
Hegeman JS. Using instructor-generated video lectures in online mathematics coursesimproves student learning. Online Learn. 2015;19(3):70–87.
Gaupp R, Körner M, Fabry G. Effects of a case-based interactive e-learning course on knowledge and attitudes about patient safety: a quasi-experimental study with third-year medical students. BMC Med Educ. 2016; 16(1):172.
Zheng M, Bender D, Reid L, Milani J. An interactive online approach to teaching evidence-based dentistry with Web 2.0 technology. J Dent Educ. 2017; 81(8): 995–1003.
Means B, Toyama Y, Murphy R, Bakia M, Jones K. Evaluation of evidence-based practices in online learning: A meta-analysis and review of online learning studies. U.S. Department of Education, Office of Planning, Evaluation and Policy Development. Washington D.C. 2009.
Google Scholar
Pei L, Wu H. Does online learning work better than offline learning in undergraduate medical education? A systematic review and meta-analysis. Med Educ Online. 2019; 24(1):1666538.
Andrews KG, Demps EL. Distance education in the U.S. and Canadian undergraduate dental curriculum. J Dent Educ. 2003; 67(4):427–38.
Kassebaum DK, Hendricson WD, Taft T, Haden NK. The dental curriculum at North American dental institutions in 2002–03: a survey of current structure, recent innovations, and planned changes. J Dent Educ. 2004; 68(9):914–931.
Haden NK, Hendricson WD, Kassebaum DK, Ranney RR, Weinstein G, Anderson EL, et al. Curriculum changes in dental education, 2003–09. J Dent Educ. 2010; 74(5):539–57.
DeBate RD, Cragun D, Severson HH, Shaw T, Christiansen S, Koerber A, et al. Factors for increasing adoption of e-courses among dental and dental hygiene faculty members. J Dent Educ. 2011; 75 (5): 589–597.
Saeed SG, Bain J, Khoo E, Siqueira WL. COVID-19: Finding silver linings for dental education. J Dent Educ. 2020; 84(10):1060–1063.
Schlenz MA, Schmidt A, Wöstmann B, Krämer N, Schulz-Weidner N. Students’ and lecturers’ perspective on the implementation of online learning in dental education due to SARS-CoV-2 (COVID-19): a cross-sectional study. BMC Med Educ. 2020;20(1):1–7.
Donn J, Scott JA, Binnie V, Bell A. A pilot of a virtual Objective Structured Clinical Examination in dental education. A response to COVID-19. Eur J Dent Educ. 2020; https://doi.org/10.1111/eje.12624
Hung M, Licari FW, Hon ES, Lauren E, Su S, Birmingham WC, Wadsworth LL, Lassetter JH, Graff TC, Harman W, et al. In an era of uncertainty: impact of COVID-19 on dental education. J Dent Educ. 2020; 85 (2): 148–156.
Sadid-Zadeh R, Wee A, Li R, Somogyi‐Ganss E. Audience and presenter comparison of live web‐based lectures and traditional classroom lectures during the COVID‐19 pandemic. J Prosthodont. 2020. doi: https://doi.org/10.1111/jopr.13301
Wang K, Zhang L, Ye L. A nationwide survey of online teaching strategies in dental education in China. J Dent Educ. 2020; 85 (2): 128–134.
Rad FA, Otaki F, Baqain Z, Zary N, Al-Halabi M. Rapid transition to distance learning due to COVID-19: Perceptions of postgraduate dental learners and instructors. PLoS One. 2021; 16(2): e0246584.
Abbasi S, Ayoob T, Malik A, Memon SI. Perceptions of students regarding E-learning during Covid-19 at a private medical college. Pak J Med Sci. 2020; 3 6 : 57–61.
Al-Azzam N, Elsalem L, Gombedza F. A cross-sectional study to determine factors affecting dental and medical students’ preference for virtual learning during the COVID-19 outbreak. Heliyon. 6(12). 2020. doi: https://doi.org/10.1016/j.heliyon.2020.e05704
Chen E, Kaczmarek K, Ohyama H. Student perceptions of distance learning strategies during COVID-19. J Dent Educ. 2020. doi: https://doi.org/10.1002/jdd.12339
Kaczmarek K, Chen E, Ohyama H. Distance learning in the COVID-19 era: Comparison of student and faculty perceptions. J Dent Educ. 2020. https://doi.org/10.1002/jdd.12469
Sarwar H, Akhtar H, Naeem MM, Khan JA, Waraich K, Shabbir S, et al. Self-reported effectiveness of e-learning classes during COVID-19 pandemic: A nation-wide survey of Pakistani undergraduate dentistry students. Eur J Dent. 2020; 14 (S01): S34-S43.
Al-Taweel FB, Abdulkareem AA, Gul SS, Alshami ML. Evaluation of technology‐based learning by dental students during the pandemic outbreak of coronavirus disease 2019. Eur J Dent Educ. 2021; 25(1): 183–190.
Elangovan S, Mahrous A, Marchini L. Disruptions during a pandemic: Gaps identified and lessons learned. J Dent Educ. 2020; 84 (11): 1270–1274.
Goodenow C. Classroom belonging among early adolescent students: Relationships to motivation and achievement. J Early Adolesc.1993; 13(1): 21–43.
Goodenow C. The psychological sense of school membership among adolescents: Scale development and educational correlates. Psychol Sch. 1993; 30(1): 79–90.
St-Amand J, Girard S, Smith J. Sense of belonging at school: Defining attributes, determinants, and sustaining strategies. IAFOR Journal of Education. 2017; 5(2):105–19.
Peacock S, Cowan J. Promoting sense of belonging in online learning communities of inquiry at accredited courses. Online Learn. 2019; 23(2): 67–81.
Chan GM, Kanneganti A, Yasin N, Ismail-Pratt I, Logan SJ. Well‐being, obstetrics and gynecology and COVID‐19: Leaving no trainee behind. Aust N Z J Obstet Gynaecol. 2020; 60(6): 983–986.
Hodges C, Moore S, Lockee B, Trust T, Bond A. The difference between emergency remote teaching and online learning. Educause Review. 2020; 2 7 , 1–12.
Means B, Bakia M, Murphy R. Learning online: What research tells us about whether, when and how. Routledge. 2014.
Iyer P, Aziz K, Ojcius DM. Impact of COVID-19 on dental education in the United States. J Dent Educ. 2020; 84(6): 718–722.
Machado RA, Bonan PRF, Perez DEDC, Martelli JÚnior H. 2020. COVID-19 pandemic and the impact on dental education: Discussing current and future perspectives. Braz Oral Res. 2020; 34: e083.
Wu DT, Wu KY, Nguyen TT, Tran SD. The impact of COVID-19 on dental education in North America-Where do we go next? Eur J Dent Educ. 2020; 24(4): 825–827.
de Oliveira Araújo FJ, de Lima LSA, Cidade PIM, Nobre CB, Neto MLR. Impact of Sars-Cov-2 and its reverberation in global higher education and mental health. Psychiatry Res. 2020; 288:112977. doi: https://doi.org/10.1016/j.psychres.2020.112977
Persky AM, Lee E, Schlesselman LS. Perception of learning versus performance as outcome measures of educational research. Am J Pharm Educ. 2020; 8 4 (7): ajpe7782.
Zoom @ . Zoom Video Communications , San Jose, CA, USA. https://zoom.us/
Canvas @ . Instructure, INC. Salt Lake City, UT, USA. https://www.instructure.com/canvas
SoftChalk @ . SoftChalk LLC . San Antonio, TX, USA. https://www.softchalkcloud.com/
Agarwal S, Kaushik JS. Student’s perception of online learning during COVID pandemic. Indian J Pediatr. 2020; 87: 554–554.
Khalil R, Mansour AE, Fadda WA, Almisnid K, Aldamegh M, Al-Nafeesah A, et al. The sudden transition to synchronized online learning during the COVID-19 pandemic in Saudi Arabia: a qualitative study exploring medical students’ perspectives. BMC Med Educ. 2020; 20(1): 1–10.
Riley E, Capps N, Ward N, McCormack L, Staley J. Maintaining academic performance and student satisfaction during the remote transition of a nursing obstetrics course to online instruction. Online Learn. 2021; 25(1), 220–229.
Amir LR, Tanti I, Maharani DA, Wimardhani YS, Julia V, Sulijaya B, et al. Student perspective of classroom and distance learning during COVID-19 pandemic in the undergraduate dental study program Universitas Indonesia. BMC Med Educ. 2020; 20(1):1–8.
Dost S, Hossain A, Shehab M, Abdelwahed A, Al-Nusair L. Perceptions of medical students towards online teaching during the COVID-19 pandemic: a national cross-sectional survey of 2721 UK medical students. BMJ Open. 2020; 10(11).
Graham CR, Woodfield W, Harrison JB. A framework for institutional adoption and implementation of blended learning in higher education. Internet High Educ. 2013; 18 : 4–14.
Sing C, Khine M. An analysis of interaction and participation patterns in online community. J Educ Techno Soc. 2006; 9(1): 250–261.
Bernard RM, Abrami PC, Borokhovski E, Wade CA, Tamim RM, Surkes MA, et al. A meta-analysis of three types of interaction treatments in distance education. Rev Educ Res. 2009; 79(3): 1243–1289.
Fedynich L, Bradley KS, Bradley J. Graduate students’ perceptions of online learning. Res High Educ. 2015; 27.
Tanis CJ. The seven principles of online learning: Feedback from faculty and alumni on its importance for teaching and learning. Res Learn Technol. 2020; 28 . https://doi.org/10.25304/rlt.v28.2319
Dixson MD. Measuring student engagement in the online course: The Online Student Engagement scale (OSE). Online Learn. 2015; 19 (4).
Kwary DA, Fauzie S. Students’ achievement and opinions on the implementation of e-learning for phonetics and phonology lectures at Airlangga University. Educ Pesqui. 2018; 44 .
Vygotsky LS. Mind in society: The development of higher psychological processes. Cambridge (MA): Harvard University Press. 1978.
Kahoot! @ . Oslo, Norway. https://kahoot.com/
Davis J, Chryssafidou E, Zamora J, Davies D, Khan K, Coomarasamy A. Computer-based teaching is as good as face to face lecture-based teaching of evidence-based medicine: a randomised controlled trial. BMC Med Educ. 2007; 7(1): 1–6.
Davis J, Crabb S, Rogers E, Zamora J, Khan K. Computer-based teaching is as good as face to face lecture-based teaching of evidence-based medicine: a randomized controlled trial. Med Teach. 2008; 30(3): 302–307.
Download references
Acknowledgements
Not applicable.
Authors’ information
MZ is an Associate Professor of Learning Sciences and Senior Instructional Designer at School of Dentistry, University of the Pacific. She has a PhD in Education, with a specialty on learning sciences and technology. She has dedicated her entire career to conducting research on online learning, learning technology, and faculty development. Her research has resulted in several peer-reviewed publications in medical, dental, and educational technology journals. MZ has also presented regularly at national conferences.
DB is an Assistant Dean for Academic Affairs at School of Dentistry, University of the Pacific. He has an EdD degree in education, with a concentration on learning and instruction. Over the past decades, DB has been overseeing and delivering faculty pedagogical development programs to dental faculty. His research interest lies in educational leadership and instructional innovation. DB has co-authored several peer-reviewed publications in health sciences education and presented regularly at national conferences.
CL is Associate Dean of Oral Healthcare Education, School of Dentistry, University of the Pacific. She has a Doctor of Dental Surgery (DDS) degree and an EdD degree with a focus on educational leadership. Her professional interest lies in educational leadership, oral healthcare education innovation, and faculty development. CL has co-authored several publications in peer-reviewed journals in health sciences education and presented regularly at national conferences.
Author information
Authors and affiliations.
Office of Academic Affairs, Arthur A. Dugoni School of Dentistry, University of the Pacific, CA, San Francisco, USA
Meixun Zheng, Daniel Bender & Cindy Lyon
You can also search for this author in PubMed Google Scholar
Contributions
MZ analyzed the data and wrote the initial draft of the manuscript. DB and CL both provided assistance with research design, data collection, and reviewed and edited the manuscript. The author(s) read and approved the final manuscript.
Corresponding author
Correspondence to Meixun Zheng .
Ethics declarations
Ethics approval and consent to participate.
The study was approved by the institutional review board at University of the Pacific in the U.S. (#2020-68). Informed consent was obtained from all participants. All methods were carried out in accordance with relevant guidelines and regulations.
Consent for publication
Competing interests.
The authors declare that they have no competing interests.
Additional information
Publisher’s note.
Springer Nature remains neutral with regard to jurisdictional claims in published maps and institutional affiliations.
Supplementary Information
Additional file 1:.
Survey of online courses during COVID-19 pandemic.
Rights and permissions
Open Access This article is licensed under a Creative Commons Attribution 4.0 International License, which permits use, sharing, adaptation, distribution and reproduction in any medium or format, as long as you give appropriate credit to the original author(s) and the source, provide a link to the Creative Commons licence, and indicate if changes were made. The images or other third party material in this article are included in the article's Creative Commons licence, unless indicated otherwise in a credit line to the material. If material is not included in the article's Creative Commons licence and your intended use is not permitted by statutory regulation or exceeds the permitted use, you will need to obtain permission directly from the copyright holder. To view a copy of this licence, visit http://creativecommons.org/licenses/by/4.0/ . The Creative Commons Public Domain Dedication waiver ( http://creativecommons.org/publicdomain/zero/1.0/ ) applies to the data made available in this article, unless otherwise stated in a credit line to the data.
Reprints and permissions
About this article
Cite this article.
Zheng, M., Bender, D. & Lyon, C. Online learning during COVID-19 produced equivalent or better student course performance as compared with pre-pandemic: empirical evidence from a school-wide comparative study. BMC Med Educ 21 , 495 (2021). https://doi.org/10.1186/s12909-021-02909-z
Download citation
Received : 31 March 2021
Accepted : 26 August 2021
Published : 16 September 2021
DOI : https://doi.org/10.1186/s12909-021-02909-z
Share this article
Anyone you share the following link with will be able to read this content:
Sorry, a shareable link is not currently available for this article.
Provided by the Springer Nature SharedIt content-sharing initiative
- Dental education
- Online learning
- COVID-19 pandemic
- Instructional strategies
- Interaction
- Learning performance
BMC Medical Education
ISSN: 1472-6920
- General enquiries: [email protected]
Impact of online classes on the satisfaction and performance of students during the pandemic period of COVID 19
- Published: 21 April 2021
- Volume 26 , pages 6923–6947, ( 2021 )
Cite this article
- Ram Gopal 1 ,
- Varsha Singh 1 &
- Arun Aggarwal ORCID: orcid.org/0000-0003-3986-188X 2
631k Accesses
251 Citations
25 Altmetric
Explore all metrics
The aim of the study is to identify the factors affecting students’ satisfaction and performance regarding online classes during the pandemic period of COVID–19 and to establish the relationship between these variables. The study is quantitative in nature, and the data were collected from 544 respondents through online survey who were studying the business management (B.B.A or M.B.A) or hotel management courses in Indian universities. Structural equation modeling was used to analyze the proposed hypotheses. The results show that four independent factors used in the study viz. quality of instructor, course design, prompt feedback, and expectation of students positively impact students’ satisfaction and further student’s satisfaction positively impact students’ performance. For educational management, these four factors are essential to have a high level of satisfaction and performance for online courses. This study is being conducted during the epidemic period of COVID- 19 to check the effect of online teaching on students’ performance.
Explore related subjects
- Artificial Intelligence
- Digital Education and Educational Technology
Avoid common mistakes on your manuscript.
1 Introduction
Coronavirus is a group of viruses that is the main root of diseases like cough, cold, sneezing, fever, and some respiratory symptoms (WHO, 2019 ). Coronavirus is a contagious disease, which is spreading very fast amongst the human beings. COVID-19 is a new sprain which was originated in Wuhan, China, in December 2019. Coronavirus circulates in animals, but some of these viruses can transmit between animals and humans (Perlman & Mclntosh, 2020 ). As of March 282,020, according to the MoHFW, a total of 909 confirmed COVID-19 cases (862 Indians and 47 foreign nationals) had been reported in India (Centers for Disease Control and Prevention, 2020 ). Officially, no vaccine or medicine is evaluated to cure the spread of COVID-19 (Yu et al., 2020 ). The influence of the COVID-19 pandemic on the education system leads to schools and colleges’ widespread closures worldwide. On March 24, India declared a country-wide lockdown of schools and colleges (NDTV, 2020 ) for preventing the transmission of the coronavirus amongst the students (Bayham & Fenichel, 2020 ). School closures in response to the COVID-19 pandemic have shed light on several issues affecting access to education. COVID-19 is soaring due to which the huge number of children, adults, and youths cannot attend schools and colleges (UNESCO, 2020 ). Lah and Botelho ( 2012 ) contended that the effect of school closing on students’ performance is hazy.
Similarly, school closing may also affect students because of disruption of teacher and students’ networks, leading to poor performance. Bridge ( 2020 ) reported that schools and colleges are moving towards educational technologies for student learning to avoid a strain during the pandemic season. Hence, the present study’s objective is to develop and test a conceptual model of student’s satisfaction pertaining to online teaching during COVID-19, where both students and teachers have no other option than to use the online platform uninterrupted learning and teaching.
UNESCO recommends distance learning programs and open educational applications during school closure caused by COVID-19 so that schools and teachers use to teach their pupils and bound the interruption of education. Therefore, many institutes go for the online classes (Shehzadi et al., 2020 ).
As a versatile platform for learning and teaching processes, the E-learning framework has been increasingly used (Salloum & Shaalan, 2018 ). E-learning is defined as a new paradigm of online learning based on information technology (Moore et al., 2011 ). In contrast to traditional learning academics, educators, and other practitioners are eager to know how e-learning can produce better outcomes and academic achievements. Only by analyzing student satisfaction and their performance can the answer be sought.
Many comparative studies have been carried out to prove the point to explore whether face-to-face or traditional teaching methods are more productive or whether online or hybrid learning is better (Lockman & Schirmer, 2020 ; Pei & Wu, 2019 ; González-Gómez et al., 2016 ; González-Gómez et al., 2016 ). Results of the studies show that the students perform much better in online learning than in traditional learning. Henriksen et al. ( 2020 ) highlighted the problems faced by educators while shifting from offline to online mode of teaching. In the past, several research studies had been carried out on online learning to explore student satisfaction, acceptance of e-learning, distance learning success factors, and learning efficiency (Sher, 2009 ; Lee, 2014 ; Yen et al., 2018 ). However, scant amount of literature is available on the factors that affect the students’ satisfaction and performance in online classes during the pandemic of Covid-19 (Rajabalee & Santally, 2020 ). In the present study, the authors proposed that course design, quality of the instructor, prompt feedback, and students’ expectations are the four prominent determinants of learning outcome and satisfaction of the students during online classes (Lee, 2014 ).
The Course Design refers to curriculum knowledge, program organization, instructional goals, and course structure (Wright, 2003 ). If well planned, course design increasing the satisfaction of pupils with the system (Almaiah & Alyoussef, 2019 ). Mtebe and Raisamo ( 2014 ) proposed that effective course design will help in improving the performance through learners knowledge and skills (Khan & Yildiz, 2020 ; Mohammed et al., 2020 ). However, if the course is not designed effectively then it might lead to low usage of e-learning platforms by the teachers and students (Almaiah & Almulhem, 2018 ). On the other hand, if the course is designed effectively then it will lead to higher acceptance of e-learning system by the students and their performance also increases (Mtebe & Raisamo, 2014 ). Hence, to prepare these courses for online learning, many instructors who are teaching blended courses for the first time are likely to require a complete overhaul of their courses (Bersin, 2004 ; Ho et al., 2006 ).
The second-factor, Instructor Quality, plays an essential role in affecting the students’ satisfaction in online classes. Instructor quality refers to a professional who understands the students’ educational needs, has unique teaching skills, and understands how to meet the students’ learning needs (Luekens et al., 2004 ). Marsh ( 1987 ) developed five instruments for measuring the instructor’s quality, in which the main method was Students’ Evaluation of Educational Quality (SEEQ), which delineated the instructor’s quality. SEEQ is considered one of the methods most commonly used and embraced unanimously (Grammatikopoulos et al., 2014 ). SEEQ was a very useful method of feedback by students to measure the instructor’s quality (Marsh, 1987 ).
The third factor that improves the student’s satisfaction level is prompt feedback (Kinicki et al., 2004 ). Feedback is defined as information given by lecturers and tutors about the performance of students. Within this context, feedback is a “consequence of performance” (Hattie & Timperley, 2007 , p. 81). In education, “prompt feedback can be described as knowing what you know and what you do not related to learning” (Simsek et al., 2017 , p.334). Christensen ( 2014 ) studied linking feedback to performance and introduced the positivity ratio concept, which is a mechanism that plays an important role in finding out the performance through feedback. It has been found that prompt feedback helps in developing a strong linkage between faculty and students which ultimately leads to better learning outcomes (Simsek et al., 2017 ; Chang, 2011 ).
The fourth factor is students’ expectation . Appleton-Knapp and Krentler ( 2006 ) measured the impact of student’s expectations on their performance. They pin pointed that the student expectation is important. When the expectations of the students are achieved then it lead to the higher satisfaction level of the student (Bates & Kaye, 2014 ). These findings were backed by previous research model “Student Satisfaction Index Model” (Zhang et al., 2008 ). However, when the expectations are students is not fulfilled then it might lead to lower leaning and satisfaction with the course. Student satisfaction is defined as students’ ability to compare the desired benefit with the observed effect of a particular product or service (Budur et al., 2019 ). Students’ whose grade expectation is high will show high satisfaction instead of those facing lower grade expectations.
The scrutiny of the literature show that although different researchers have examined the factors affecting student satisfaction but none of the study has examined the effect of course design, quality of the instructor, prompt feedback, and students’ expectations on students’ satisfaction with online classes during the pandemic period of Covid-19. Therefore, this study tries to explore the factors that affect students’ satisfaction and performance regarding online classes during the pandemic period of COVID–19. As the pandemic compelled educational institutions to move online with which they were not acquainted, including teachers and learners. The students were not mentally prepared for such a shift. Therefore, this research will be examined to understand what factors affect students and how students perceived these changes which are reflected through their satisfaction level.
This paper is structured as follows: The second section provides a description of theoretical framework and the linkage among different research variables and accordingly different research hypotheses were framed. The third section deals with the research methodology of the paper as per APA guideline. The outcomes and corresponding results of the empirical analysis are then discussed. Lastly, the paper concludes with a discussion and proposes implications for future studies.
2 Theoretical framework
Achievement goal theory (AGT) is commonly used to understand the student’s performance, and it is proposed by four scholars Carole Ames, Carol Dweck, Martin Maehr, and John Nicholls in the late 1970s (Elliot, 2005 ). Elliott & Dweck ( 1988 , p11) define that “an achievement goal involves a program of cognitive processes that have cognitive, affective and behavioral consequence”. This theory suggests that students’ motivation and achievement-related behaviors can be easily understood by the purpose and the reasons they adopted while they are engaged in the learning activities (Dweck & Leggett, 1988 ; Ames, 1992 ; Urdan, 1997 ). Some of the studies believe that there are four approaches to achieve a goal, i.e., mastery-approach, mastery avoidance, performance approach, and performance-avoidance (Pintrich, 1999 ; Elliot & McGregor, 2001 ; Schwinger & Stiensmeier-Pelster, 2011 , Hansen & Ringdal, 2018 ; Mouratidis et al., 2018 ). The environment also affects the performance of students (Ames & Archer, 1988 ). Traditionally, classroom teaching is an effective method to achieve the goal (Ames & Archer, 1988 ; Ames, 1992 ; Clayton et al., 2010 ) however in the modern era, the internet-based teaching is also one of the effective tools to deliver lectures, and web-based applications are becoming modern classrooms (Azlan et al., 2020 ). Hence, following section discuss about the relationship between different independent variables and dependent variables (Fig. 1 ).
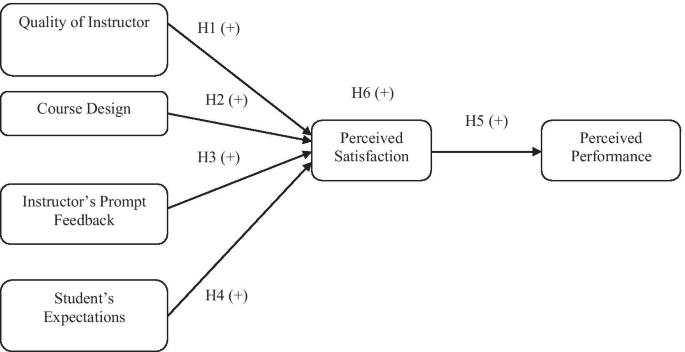
Proposed Model
3 Hypotheses development
3.1 quality of the instructor and satisfaction of the students.
Quality of instructor with high fanaticism on student’s learning has a positive impact on their satisfaction. Quality of instructor is one of the most critical measures for student satisfaction, leading to the education process’s outcome (Munteanu et al., 2010 ; Arambewela & Hall, 2009 ; Ramsden, 1991 ). Suppose the teacher delivers the course effectively and influence the students to do better in their studies. In that case, this process leads to student satisfaction and enhances the learning process (Ladyshewsky, 2013 ). Furthermore, understanding the need of learner by the instructor also ensures student satisfaction (Kauffman, 2015 ). Hence the hypothesis that the quality of instructor significantly affects the satisfaction of the students was included in this study.
H1: The quality of the instructor positively affects the satisfaction of the students.
3.2 Course design and satisfaction of students
The course’s technological design is highly persuading the students’ learning and satisfaction through their course expectations (Liaw, 2008 ; Lin et al., 2008 ). Active course design indicates the students’ effective outcomes compared to the traditional design (Black & Kassaye, 2014 ). Learning style is essential for effective course design (Wooldridge, 1995 ). While creating an online course design, it is essential to keep in mind that we generate an experience for students with different learning styles. Similarly, (Jenkins, 2015 ) highlighted that the course design attributes could be developed and employed to enhance student success. Hence the hypothesis that the course design significantly affects students’ satisfaction was included in this study.
H2: Course design positively affects the satisfaction of students.
3.3 Prompt feedback and satisfaction of students
The emphasis in this study is to understand the influence of prompt feedback on satisfaction. Feedback gives the information about the students’ effective performance (Chang, 2011 ; Grebennikov & Shah, 2013 ; Simsek et al., 2017 ). Prompt feedback enhances student learning experience (Brownlee et al., 2009 ) and boosts satisfaction (O'donovan, 2017 ). Prompt feedback is the self-evaluation tool for the students (Rogers, 1992 ) by which they can improve their performance. Eraut ( 2006 ) highlighted the impact of feedback on future practice and student learning development. Good feedback practice is beneficial for student learning and teachers to improve students’ learning experience (Yorke, 2003 ). Hence the hypothesis that prompt feedback significantly affects satisfaction was included in this study.
H3: Prompt feedback of the students positively affects the satisfaction.
3.4 Expectations and satisfaction of students
Expectation is a crucial factor that directly influences the satisfaction of the student. Expectation Disconfirmation Theory (EDT) (Oliver, 1980 ) was utilized to determine the level of satisfaction based on their expectations (Schwarz & Zhu, 2015 ). Student’s expectation is the best way to improve their satisfaction (Brown et al., 2014 ). It is possible to recognize student expectations to progress satisfaction level (ICSB, 2015 ). Finally, the positive approach used in many online learning classes has been shown to place a high expectation on learners (Gold, 2011 ) and has led to successful outcomes. Hence the hypothesis that expectations of the student significantly affect the satisfaction was included in this study.
H4: Expectations of the students positively affects the satisfaction.
3.5 Satisfaction and performance of the students
Zeithaml ( 1988 ) describes that satisfaction is the outcome result of the performance of any educational institute. According to Kotler and Clarke ( 1986 ), satisfaction is the desired outcome of any aim that amuses any individual’s admiration. Quality interactions between instructor and students lead to student satisfaction (Malik et al., 2010 ; Martínez-Argüelles et al., 2016 ). Teaching quality and course material enhances the student satisfaction by successful outcomes (Sanderson, 1995 ). Satisfaction relates to the student performance in terms of motivation, learning, assurance, and retention (Biner et al., 1996 ). Mensink and King ( 2020 ) described that performance is the conclusion of student-teacher efforts, and it shows the interest of students in the studies. The critical element in education is students’ academic performance (Rono, 2013 ). Therefore, it is considered as center pole, and the entire education system rotates around the student’s performance. Narad and Abdullah ( 2016 ) concluded that the students’ academic performance determines academic institutions’ success and failure.
Singh et al. ( 2016 ) asserted that the student academic performance directly influences the country’s socio-economic development. Farooq et al. ( 2011 ) highlights the students’ academic performance is the primary concern of all faculties. Additionally, the main foundation of knowledge gaining and improvement of skills is student’s academic performance. According to Narad and Abdullah ( 2016 ), regular evaluation or examinations is essential over a specific period of time in assessing students’ academic performance for better outcomes. Hence the hypothesis that satisfaction significantly affects the performance of the students was included in this study.
H5: Students’ satisfaction positively affects the performance of the students.
3.6 Satisfaction as mediator
Sibanda et al. ( 2015 ) applied the goal theory to examine the factors persuading students’ academic performance that enlightens students’ significance connected to their satisfaction and academic achievement. According to this theory, students perform well if they know about factors that impact on their performance. Regarding the above variables, institutional factors that influence student satisfaction through performance include course design and quality of the instructor (DeBourgh, 2003 ; Lado et al., 2003 ), prompt feedback, and expectation (Fredericksen et al., 2000 ). Hence the hypothesis that quality of the instructor, course design, prompts feedback, and student expectations significantly affect the students’ performance through satisfaction was included in this study.
H6: Quality of the instructor, course design, prompt feedback, and student’ expectations affect the students’ performance through satisfaction.
H6a: Students’ satisfaction mediates the relationship between quality of the instructor and student’s performance.
H6b: Students’ satisfaction mediates the relationship between course design and student’s performance.
H6c: Students’ satisfaction mediates the relationship between prompt feedback and student’s performance.
H6d: Students’ satisfaction mediates the relationship between student’ expectations and student’s performance.
4.1 Participants
In this cross-sectional study, the data were collected from 544 respondents who were studying the management (B.B.A or M.B.A) and hotel management courses. The purposive sampling technique was used to collect the data. Descriptive statistics shows that 48.35% of the respondents were either MBA or BBA and rests of the respondents were hotel management students. The percentages of male students were (71%) and female students were (29%). The percentage of male students is almost double in comparison to females. The ages of the students varied from 18 to 35. The dominant group was those aged from 18 to 22, and which was the under graduation student group and their ratio was (94%), and another set of students were from the post-graduation course, which was (6%) only.
4.2 Materials
The research instrument consists of two sections. The first section is related to demographical variables such as discipline, gender, age group, and education level (under-graduate or post-graduate). The second section measures the six factors viz. instructor’s quality, course design, prompt feedback, student expectations, satisfaction, and performance. These attributes were taken from previous studies (Yin & Wang, 2015 ; Bangert, 2004 ; Chickering & Gamson, 1987 ; Wilson et al., 1997 ). The “instructor quality” was measured through the scale developed by Bangert ( 2004 ). The scale consists of seven items. The “course design” and “prompt feedback” items were adapted from the research work of Bangert ( 2004 ). The “course design” scale consists of six items. The “prompt feedback” scale consists of five items. The “students’ expectation” scale consists of five items. Four items were adapted from Bangert, 2004 and one item was taken from Wilson et al. ( 1997 ). Students’ satisfaction was measure with six items taken from Bangert ( 2004 ); Wilson et al. ( 1997 ); Yin and Wang ( 2015 ). The “students’ performance” was measured through the scale developed by Wilson et al. ( 1997 ). The scale consists of six items. These variables were accessed on a five-point likert scale, ranging from 1(strongly disagree) to 5(strongly agree). Only the students from India have taken part in the survey. A total of thirty-four questions were asked in the study to check the effect of the first four variables on students’ satisfaction and performance. For full details of the questionnaire, kindly refer Appendix Tables 6 .
The study used a descriptive research design. The factors “instructor quality, course design, prompt feedback and students’ expectation” were independent variables. The students’ satisfaction was mediator and students’ performance was the dependent variable in the current study.
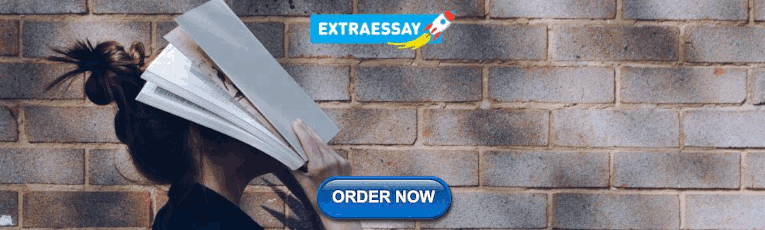
4.4 Procedure
In this cross-sectional research the respondents were selected through judgment sampling. They were informed about the objective of the study and information gathering process. They were assured about the confidentiality of the data and no incentive was given to then for participating in this study. The information utilizes for this study was gathered through an online survey. The questionnaire was built through Google forms, and then it was circulated through the mails. Students’ were also asked to write the name of their college, and fifteen colleges across India have taken part to fill the data. The data were collected in the pandemic period of COVID-19 during the total lockdown in India. This was the best time to collect the data related to the current research topic because all the colleges across India were involved in online classes. Therefore, students have enough time to understand the instrument and respondent to the questionnaire in an effective manner. A total of 615 questionnaires were circulated, out of which the students returned 574. Thirty responses were not included due to the unengaged responses. Finally, 544 questionnaires were utilized in the present investigation. Male and female students both have taken part to fill the survey, different age groups, and various courses, i.e., under graduation and post-graduation students of management and hotel management students were the part of the sample.
5.1 Exploratory factor analysis (EFA)
To analyze the data, SPSS and AMOS software were used. First, to extract the distinct factors, an exploratory factor analysis (EFA) was performed using VARIMAX rotation on a sample of 544. Results of the exploratory analysis rendered six distinct factors. Factor one was named as the quality of instructor, and some of the items were “The instructor communicated effectively”, “The instructor was enthusiastic about online teaching” and “The instructor was concerned about student learning” etc. Factor two was labeled as course design, and the items were “The course was well organized”, “The course was designed to allow assignments to be completed across different learning environments.” and “The instructor facilitated the course effectively” etc. Factor three was labeled as prompt feedback of students, and some of the items were “The instructor responded promptly to my questions about the use of Webinar”, “The instructor responded promptly to my questions about general course requirements” etc. The fourth factor was Student’s Expectations, and the items were “The instructor provided models that clearly communicated expectations for weekly group assignments”, “The instructor used good examples to explain statistical concepts” etc. The fifth factor was students’ satisfaction, and the items were “The online classes were valuable”, “Overall, I am satisfied with the quality of this course” etc. The sixth factor was performance of the student, and the items were “The online classes has sharpened my analytic skills”, “Online classes really tries to get the best out of all its students” etc. These six factors explained 67.784% of the total variance. To validate the factors extracted through EFA, the researcher performed confirmatory factor analysis (CFA) through AMOS. Finally, structural equation modeling (SEM) was used to test the hypothesized relationships.
5.2 Measurement model
The results of Table 1 summarize the findings of EFA and CFA. Results of the table showed that EFA renders six distinct factors, and CFA validated these factors. Table 2 shows that the proposed measurement model achieved good convergent validity (Aggarwal et al., 2018a , b ). Results of the confirmatory factor analysis showed that the values of standardized factor loadings were statistically significant at the 0.05 level. Further, the results of the measurement model also showed acceptable model fit indices such that CMIN = 710.709; df = 480; CMIN/df = 1.481 p < .000; Incremental Fit Index (IFI) = 0.979; Tucker-Lewis Index (TLI) = 0.976; Goodness of Fit index (GFI) = 0.928; Adjusted Goodness of Fit Index (AGFI) = 0.916; Comparative Fit Index (CFI) = 0.978; Root Mean Square Residual (RMR) = 0.042; Root Mean Squared Error of Approximation (RMSEA) = 0.030 is satisfactory.
The Average Variance Explained (AVE) according to the acceptable index should be higher than the value of squared correlations between the latent variables and all other variables. The discriminant validity is confirmed (Table 2 ) as the value of AVE’s square root is greater than the inter-construct correlations coefficient (Hair et al., 2006 ). Additionally, the discriminant validity existed when there was a low correlation between each variable measurement indicator with all other variables except with the one with which it must be theoretically associated (Aggarwal et al., 2018a , b ; Aggarwal et al., 2020 ). The results of Table 2 show that the measurement model achieved good discriminate validity.
5.3 Structural model
To test the proposed hypothesis, the researcher used the structural equation modeling technique. This is a multivariate statistical analysis technique, and it includes the amalgamation of factor analysis and multiple regression analysis. It is used to analyze the structural relationship between measured variables and latent constructs.
Table 3 represents the structural model’s model fitness indices where all variables put together when CMIN/DF is 2.479, and all the model fit values are within the particular range. That means the model has attained a good model fit. Furthermore, other fit indices as GFI = .982 and AGFI = 0.956 be all so supportive (Schumacker & Lomax, 1996 ; Marsh & Grayson, 1995 ; Kline, 2005 ).
Hence, the model fitted the data successfully. All co-variances among the variables and regression weights were statistically significant ( p < 0.001).
Table 4 represents the relationship between exogenous, mediator and endogenous variables viz—quality of instructor, prompt feedback, course design, students’ expectation, students’ satisfaction and students’ performance. The first four factors have a positive relationship with satisfaction, which further leads to students’ performance positively. Results show that the instructor’s quality has a positive relationship with the satisfaction of students for online classes (SE = 0.706, t-value = 24.196; p < 0.05). Hence, H1 was supported. The second factor is course design, which has a positive relationship with students’ satisfaction of students (SE = 0.064, t-value = 2.395; p < 0.05). Hence, H2 was supported. The third factor is Prompt feedback, and results show that feedback has a positive relationship with the satisfaction of the students (SE = 0.067, t-value = 2.520; p < 0.05). Hence, H3 was supported. The fourth factor is students’ expectations. The results show a positive relationship between students’ expectation and students’ satisfaction with online classes (SE = 0.149, t-value = 5.127; p < 0.05). Hence, H4 was supported. The results of SEM show that out of quality of instructor, prompt feedback, course design, and students’ expectation, the most influencing factor that affect the students’ satisfaction was instructor’s quality (SE = 0.706) followed by students’ expectation (SE =5.127), prompt feedback (SE = 2.520). The factor that least affects the students’ satisfaction was course design (2.395). The results of Table 4 finally depicts that students’ satisfaction has positive effect on students’ performance ((SE = 0.186, t-value = 2.800; p < 0.05). Hence H5 was supported.
Table 5 shows that students’ satisfaction partially mediates the positive relationship between the instructor’s quality and student performance. Hence, H6(a) was supported. Further, the mediation analysis results showed that satisfaction again partially mediates the positive relationship between course design and student’s performance. Hence, H6(b) was supported However, the mediation analysis results showed that satisfaction fully mediates the positive relationship between prompt feedback and student performance. Hence, H6(c) was supported. Finally, the results of the Table 5 showed that satisfaction partially mediates the positive relationship between expectations of the students and student’s performance. Hence, H6(d) was supported.
6 Discussion
In the present study, the authors evaluated the different factors directly linked with students’ satisfaction and performance with online classes during Covid-19. Due to the pandemic situation globally, all the colleges and universities were shifted to online mode by their respective governments. No one has the information that how long this pandemic will remain, and hence the teaching method was shifted to online mode. Even though some of the educators were not tech-savvy, they updated themselves to battle the unexpected circumstance (Pillai et al., 2021 ). The present study results will help the educators increase the student’s satisfaction and performance in online classes. The current research assists educators in understanding the different factors that are required for online teaching.
Comparing the current research with past studies, the past studies have examined the factors affecting the student’s satisfaction in the conventional schooling framework. However, the present study was conducted during India’s lockdown period to identify the prominent factors that derive the student’s satisfaction with online classes. The study also explored the direct linkage between student’s satisfaction and their performance. The present study’s findings indicated that instructor’s quality is the most prominent factor that affects the student’s satisfaction during online classes. This means that the instructor needs to be very efficient during the lectures. He needs to understand students’ psychology to deliver the course content prominently. If the teacher can deliver the course content properly, it affects the student’s satisfaction and performance. The teachers’ perspective is critical because their enthusiasm leads to a better online learning process quality.
The present study highlighted that the second most prominent factor affecting students’ satisfaction during online classes is the student’s expectations. Students might have some expectations during the classes. If the instructor understands that expectation and customizes his/her course design following the student’s expectations, then it is expected that the students will perform better in the examinations. The third factor that affects the student’s satisfaction is feedback. After delivering the course, appropriate feedback should be taken by the instructors to plan future courses. It also helps to make the future strategies (Tawafak et al., 2019 ). There must be a proper feedback system for improvement because feedback is the course content’s real image. The last factor that affects the student’s satisfaction is design. The course content needs to be designed in an effective manner so that students should easily understand it. If the instructor plans the course, so the students understand the content without any problems it effectively leads to satisfaction, and the student can perform better in the exams. In some situations, the course content is difficult to deliver in online teaching like the practical part i.e. recipes of dishes or practical demonstration in the lab. In such a situation, the instructor needs to be more creative in designing and delivering the course content so that it positively impacts the students’ overall satisfaction with online classes.
Overall, the students agreed that online teaching was valuable for them even though the online mode of classes was the first experience during the pandemic period of Covid-19 (Agarwal & Kaushik, 2020 ; Rajabalee & Santally, 2020 ). Some of the previous studies suggest that the technology-supported courses have a positive relationship with students’ performance (Cho & Schelzer, 2000 ; Harasim, 2000 ; Sigala, 2002 ). On the other hand, the demographic characteristic also plays a vital role in understanding the online course performance. According to APA Work Group of the Board of Educational Affairs ( 1997 ), the learner-centered principles suggest that students must be willing to invest the time required to complete individual course assignments. Online instructors must be enthusiastic about developing genuine instructional resources that actively connect learners and encourage them toward proficient performances. For better performance in studies, both teachers and students have equal responsibility. When the learner faces any problem to understand the concepts, he needs to make inquiries for the instructor’s solutions (Bangert, 2004 ). Thus, we can conclude that “instructor quality, student’s expectation, prompt feedback, and effective course design” significantly impact students’ online learning process.
7 Implications of the study
The results of this study have numerous significant practical implications for educators, students and researchers. It also contributes to the literature by demonstrating that multiple factors are responsible for student satisfaction and performance in the context of online classes during the period of the COVID-19 pandemic. This study was different from the previous studies (Baber, 2020 ; Ikhsan et al., 2019 ; Eom & Ashill, 2016 ). None of the studies had examined the effect of students’ satisfaction on their perceived academic performance. The previous empirical findings have highlighted the importance of examining the factors affecting student satisfaction (Maqableh & Jaradat, 2021 ; Yunusa & Umar, 2021 ). Still, none of the studies has examined the effect of course design, quality of instructor, prompt feedback, and students’ expectations on students’ satisfaction all together with online classes during the pandemic period. The present study tries to fill this research gap.
The first essential contribution of this study was the instructor’s facilitating role, and the competence he/she possesses affects the level of satisfaction of the students (Gray & DiLoreto, 2016 ). There was an extra obligation for instructors who taught online courses during the pandemic. They would have to adapt to a changing climate, polish their technical skills throughout the process, and foster new students’ technical knowledge in this environment. The present study’s findings indicate that instructor quality is a significant determinant of student satisfaction during online classes amid a pandemic. In higher education, the teacher’s standard referred to the instructor’s specific individual characteristics before entering the class (Darling-Hammond, 2010 ). These attributes include factors such as instructor content knowledge, pedagogical knowledge, inclination, and experience. More significantly, at that level, the amount of understanding could be given by those who have a significant amount of technical expertise in the areas they are teaching (Martin, 2021 ). Secondly, the present study results contribute to the profession of education by illustrating a realistic approach that can be used to recognize students’ expectations in their class effectively. The primary expectation of most students before joining a university is employment. Instructors have agreed that they should do more to fulfill students’ employment expectations (Gorgodze et al., 2020 ). The instructor can then use that to balance expectations to improve student satisfaction. Study results can be used to continually improve and build courses, as well as to make policy decisions to improve education programs. Thirdly, from result outcomes, online course design and instructors will delve deeper into how to structure online courses more efficiently, including design features that minimize adversely and maximize optimistic emotion, contributing to greater student satisfaction (Martin et al., 2018 ). The findings suggest that the course design has a substantial positive influence on the online class’s student performance. The findings indicate that the course design of online classes need to provide essential details like course content, educational goals, course structure, and course output in a consistent manner so that students would find the e-learning system beneficial for them; this situation will enable students to use the system and that leads to student performance (Almaiah & Alyoussef, 2019 ). Lastly, the results indicate that instructors respond to questions promptly and provide timely feedback on assignments to facilitate techniques that help students in online courses improve instructor participation, instructor interaction, understanding, and participation (Martin et al., 2018 ). Feedback can be beneficial for students to focus on the performance that enhances their learning.
Author information
Authors and affiliations.
Chitkara College of Hospitality Management, Chitkara University, Chandigarh, Punjab, India
Ram Gopal & Varsha Singh
Chitkara Business School, Chitkara University, Chandigarh, Punjab, India
Arun Aggarwal
You can also search for this author in PubMed Google Scholar
Corresponding author
Correspondence to Arun Aggarwal .
Ethics declarations
Ethics approval.
Not applicable.
Conflict of interest
The authors declare no conflict of interest, financial or otherwise.
Additional information
Publisher’s note.
Springer Nature remains neutral with regard to jurisdictional claims in published maps and institutional affiliations.
Rights and permissions
Reprints and permissions
About this article
Gopal, R., Singh, V. & Aggarwal, A. Impact of online classes on the satisfaction and performance of students during the pandemic period of COVID 19. Educ Inf Technol 26 , 6923–6947 (2021). https://doi.org/10.1007/s10639-021-10523-1
Download citation
Received : 07 December 2020
Accepted : 22 March 2021
Published : 21 April 2021
Issue Date : November 2021
DOI : https://doi.org/10.1007/s10639-021-10523-1
Share this article
Anyone you share the following link with will be able to read this content:
Sorry, a shareable link is not currently available for this article.
Provided by the Springer Nature SharedIt content-sharing initiative
- Quality of instructor
- Course design
- Instructor’s prompt feedback
- Expectations
- Student’s satisfaction
- Perceived performance
Advertisement
- Find a journal
- Publish with us
- Track your research

An official website of the United States government
The .gov means it’s official. Federal government websites often end in .gov or .mil. Before sharing sensitive information, make sure you’re on a federal government site.
The site is secure. The https:// ensures that you are connecting to the official website and that any information you provide is encrypted and transmitted securely.
- Publications
- Account settings
Preview improvements coming to the PMC website in October 2024. Learn More or Try it out now .
- Advanced Search
- Journal List
- Springer Nature - PMC COVID-19 Collection

Academic and emotional effects of online learning during the COVID-19 pandemic on engineering students
Rosó baltà-salvador.
1 Department of Graphic and Design Engineering, Universitat Politècnica de Catalunya C, Colom 11, 08222 Terrassa, Spain
Noelia Olmedo-Torre
2 Department of Graphic and Design Engineering, Universitat Politècnica de Catalunya Av, Eduard Maristany 16, 08019 Barcelona, Spain
Marta Peña
3 Department of Mathematics, Universitat Politècnica de Catalunya Av. Diagonal 647, 08028 Barcelona, Spain
Ana-Inés Renta-Davids
4 Department of Pedagogy, Universitat Rovira i Virgili Ctra. de Valls, 43007 Tarragona, Spain
Associated Data
The data and materials that support the findings of this study are available from the corresponding author upon reasonable request.
The unprecedented situation of the COVID-19 pandemic has caused the closure of universities worldwide and has forced the transition to online learning. This exceptional context compels us to understand students' experience with online learning. Previous literature identifies relevant factors that intervene in the online education experience and can affect students' academic development. One of the main concerns is the students' mental health, given the lockdown restrictions under which classes have been conducted. Furthermore, the impact of the prolonged lockdown and the pandemic fatigue on university students and their academic experience is still unclear. This study delves into engineering undergraduate students’ online education experience during the COVID-19 pandemic and its emotional impact across time. With this aim, a questionnaire was distributed to second, third, and fourth-year engineering undergraduate students at two time points, approximately six months apart. The results show significant differences in students' connection with other students and teachers, workspace conditions, and boredom between time points. Besides, the findings indicate significant correlations between academic development and quality of online classes, adaptation of the course, workspace conditions, and connection with other students and teachers, and also between students' emotions and connection with other students and teachers. Finally, the study identifies best practices carried out during online teaching that will be of value for future courses and engineering education beyond the pandemic situation, amongst which those related to effective communication with teachers stand out.
Introduction
The COVID-19 pandemic has posed an unprecedented challenge in education, leading to the suspension of face-to-face teaching (UNESCO, 2020 ). This change has been particularly challenging in university undergraduate engineering degrees since much of the learning process is based on practical applications, laboratory classes, and direct contact with teachers and other students. Being an exceptional and novel situation, the potential impact of the health crisis and the prolonged lockdown on students' academic development and emotional state is still unknown.
Recent work has identified some variables that intervene in the online education process, such as the correct adaptation of teaching to the online format, including classes, assessment methods, and teacher support (Alnusairat et al., 2020 ; Linh & Trang, 2020 ); the quality of the classes received (Al-Balas et al., 2020 ; Amir et al., 2020 ); the conditions of students' workspace (Gelles et al., 2020 ; Son et al., 2020 ); and the connection with other students and teachers (Elmer et al., 2020 ; Radu et al., 2020 ). However, there is a knowledge gap in how these variables are related to students' academic development and whether having been in a prolonged lockdown might have affected them.
Furthermore, educational and psychological research has raised concerns about students' mental health as they have had to suddenly switch to online learning systems and follow classes under lockdown restrictions. Investigations pointed out that students have experienced an increase in stress, anxiety, and depression (Aslan et al., 2020 ; Odriozola-González et al., 2020 ; Saravanan et al., 2020 ; Son et al., 2020 ), and have felt some negative feelings intensified, such as fear, worry, or boredom (Aristovnik et al., 2020 ; Son et al., 2020 ). Several studies have highlighted the protective effect that the connection with the rest of the academic community can have on anxiety, depression, and stress (Elmer et al., 2020 ; Magson et al., 2021 ; Procentese et al., 2020 ). Although mental health during the COVID-19 pandemic has received much attention in the academic field, studies have focused on analyzing adverse mental states such as depression, stress, or anxiety, but a broader perspective on the emotional state of the students, including a wider range of emotions and considering positive emotions such as calm or trust, is still missing.
Within this context, the present study carried out at the Polytechnic University of Catalonia (UPC), Spain, investigates how the COVID-19 pandemic and the lockdown has affected the academic experience of students and their emotional state across time. Two measurements taken six months apart were compared to detect changes potentially caused by a prolonged public health crisis and lockdown, providing new knowledge about pandemic fatigue in university students. Moreover, we assessed the correlations between the variables related to online education and the academic development of students to understand how the change to online teaching may have affected the performance of engineering students. Also, based on Plutchik’s theoretical framework on emotions (Plutchik, 1994 ), a study has been carried out to understand the impact of the pandemic on students' emotional state and how the connection with other students and teachers can help not only to reduce adverse emotions, but also to enhance positive emotions such as calm or confidence. To the best of our knowledge, no prior studies on the impact of the COVID-19 pandemic on engineering university students have considered such a wide spectrum of emotions and have observed the impact of being in contact with the rest of the academic community on these emotions. Furthermore, our research is novel as it encompasses the different variables involved in online education identified in previous studies, and conducts a longitudinal study to understand the impact of time and pandemic fatigue on these variables and students' academic development. Finally, this investigation identifies best practices carried out during distance teaching that can help improve the online learning experience in engineering studies beyond the pandemic situation.
Understanding the academic and emotional effects of the pandemic on engineering students is essential for several reasons. There is a growing trend for universities to offer online courses. However, in engineering, this transition is still a challenge since traditional engineering studies are based fundamentally on the practical application of scientific and technological principles. The unexpected situation generated by COVID-19 has forced engineering universities to offer their studies online, even for students who would not have proactively chosen to learn online. Therefore, it is an opportunity to analyze students' academic experience in distance engineering studies since they are rarely offered online. This information will contribute to the design of remote engineering courses to make them more accessible. On the other hand, the pandemic's scope during the next few years is unclear, so it is essential to understand its impact on learning to develop support actions for students. Also, all these lessons will be relevant if we face a similar situation that requires the confinement of the population in the coming years.
Impact of COVID-19 on students’ learning experience
The COVID-19 pandemic caused by the SARS-CoV-2 virus began in late 2019 and spread around the world rapidly within months (Du Toit, 2020 ; Zhou et al., 2020 ). On March 11th, 2020, The World Health Organization (WHO) declared the public health emergency caused by the new coronavirus an international pandemic. Due to the high transmission rate of SARS-CoV-2, most countries took measures to stop the spread, including the blockade of cities, strict implementation of contact isolation, and strict medical system precaution (World Health Organization, 2021 ). This reduction in population mobility caused higher education institutions to cancel in-person classes and move towards remote learning (UNESCO, 2020 ). In the case of traditional engineering studies, this posed a significant challenge since it is a field in which a large part of the curriculum is based on the practical application of knowledge and relies heavily on face-to-face practical and laboratory classes (Jacques et al., 2020 ). This unexpected change in the teaching format has forced engineering students to adapt to new ways of learning under the conditions of the health crisis, potentially affecting their academic development. Furthermore, while previous research has revealed the impact of online education on those students who proactively chose distance learning, the current pandemic situation allows studying the impact on all students and in degrees that are generally not available online, as it is the case for engineering studies. Due to the exceptional nature of the situation, the effect of online classes derived from the emergence of COVID-19 on students is yet unknown, so work providing empirical data is crucial to understand the scope of its impact and to be able to propose support actions for students.
Although literature is still limited in this regard, several studies have tried to explain the impact of the rapid transition to online models during COVID-19 on students’ academic development. However, the results of these investigations are not homogeneous and show remarkable differences in the results. These variations may be due to the start and end dates of the academic years and school holidays, the timing and impact of the pandemic in each country, and the corresponding measures implemented to manage the health crisis. For example, in some countries face-to-face classes were suspended from the beginning of the pandemic while others were less restrictive and just reduced face-to-face teaching or postponed the beginning of the semester (Gonzalez et al., 2020 ). These contrasts can also be related to other factors like the differences between academic fields, the resources available for students, and the methodologies implemented by teachers during online teaching, among others. This lack of consistency opens the door to new studies that provide complementary evidence which might allow for a better understanding of the impact of online teaching during the COVID-19 pandemic on university students from different countries and academic fields.
To study the way in which the lockdown and distance teaching have affected engineering students during the COVID-19 pandemic, it is necessary to identify the variables that intervene in the online educational experience. Previous research in psychology and education has identified four relevant constructs in distance education, which have also received attention in studies on remote education in the context of the COVID-19 pandemic.
First, previous research on online teaching highlighted the courses’ quality as a significant factor in students’ satisfaction and learning (Piccoli et al., 2001 ; Sun et al., 2008 ) and pointed out that the effective switch toward online teaching models is influenced by the perceived quality of the classes (Ibrahim et al., 2013 ). When designing online courses, classes cannot simply be transferred from a face-to-face to an online environment. Content, pedagogy, methodology, and technology need to be adapted for successful online teaching (Aristovnik et al., 2020 ). The quality of online teaching has received considerable attention in studies on the effects of COVID-19 on higher education. In some of the studies conducted during the lockdown, university students reported low satisfaction with the quality of online teaching (Al-Balas et al., 2020 ; Alnusairat et al., 2020 ) and higher learning satisfaction in face-to-face learning than in distance learning (Amir et al., 2020 ; Linh & Trang, 2020 ). According to the UNESCO ( 2020 ), this disaffection with the online classes stems from the fact that the content offered was never designed within the framework of a distance course but instead tried to make up for the absence of face-to-face classes with virtual classes without sufficient preparation. However, the results of other studies showed that students were satisfied with the overall e-learning provided thus far in their university studies (Aristovnik et al., 2020 ; Jacques et al., 2020 ; Puljak et al., 2020 ). More specifically, in a study with a sample that included engineering students, 85% of the respondents indicated that online teaching quality during the COVID-19 pandemic was good or very good (Radu et al., 2020 ). Since the quality of teaching is one of the main constructs in the evaluation of distance teaching, it has been included in this research as a study variable. Also, as there is a lack of consistency in the results of previous studies regarding the satisfaction with the online course’s quality during COVID-19, it is necessary to provide more data from samples of engineering students.
Second, there are considerable differences in results among research regarding specific aspects, such as classes, exams, or teachers’ support, in adaptation to distance learning during the COVID-19 pandemic. Nevertheless, the results differ across the investigations. On the one hand, previous research reported students’ low satisfaction with the support received from their teachers (Alnusairat et al., 2020 ) and less satisfaction with the classes and assessment methods in distance education compared to classroom learning (Linh & Trang, 2020 ). This low satisfaction with how teaching was adapted to the online format is associated with an increase in the perceived workload (Alnusairat et al., 2020 ; Gelles et al., 2020 ; Son et al., 2020 ). Furthermore, Khalil et al. ( 2020 ) pointed out that the issues related to the implementation and quality of online courses can become barriers to the engagement and acquisition of knowledge. On the other hand, there are studies in university settings in which students were satisfied with the teacher support received and the content of their online classes during the COVID-19 pandemic (Jacques et al., 2020 ; Rodríguez-Rodríguez et al., 2020 ). Also, in Puljak et al.’s ( 2020 ) investigation, students reported that the assessment methods and materials used in their classes during the lockdown were tailored to e-learning. Similar to the courses’ quality, current studies show contradictory results regarding student satisfaction on how their courses were adapted to the remote format. Moreover, adaptation to online teaching was a highly discussed construct during the COVID-19 health crisis due to the short time in which classes, evaluation methods, and teachers' support and guidance had to be readjusted to the online format (UNESCO, 2020 ).
Third, another major challenge on distance education are the feelings of isolation and disconnection in online courses due to lack of face-to-face contact with other students and teachers (Mcinnerney & Roberts, 2004 ). Numerous studies prior to the COVID-19 pandemic indicated that interactions with other students and teachers were essential for student satisfaction and played a decisive role in academic development and students’ achievements (Arbaugh, 2000 ; Hong, 2002 ; Mcinnerney & Roberts, 2004 ; Piccoli et al., 2001 ; Sun et al., 2008 ). Hence, when designing online courses, the interaction mechanisms must be considered to offer enriching and thriving learning environments. Concerning the perceived connection with other students and teachers during the lockdown, previous research indicated that students felt less connected with fellow students and teachers than in face-to-face education (Al-Balas et al., 2020 ; Puljak et al., 2020 ; Son et al., 2020 ). Overall, university students indicated that they have missed in-person contact with other students and professors during the lockdown (Puljak et al., 2020 ) and that communication has been more complicated than in face-to-face education (Alnusairat et al., 2020 ; Amir et al., 2020 ; Radu et al., 2020 ). In Elmer et al. ( 2020 ) investigation on students enrolled in engineering and natural science programs, students reported fewer study partners and felt significantly more socially isolated. Also, in the study by Tang et al. ( 2020 ) on undergraduate students from engineering majors, almost 70% thought that they had not communicated often with their teachers from the online courses during the pandemic. This lack of contact is worrisome since social contact and socialization routines are part of the daily experience of higher education students and can affect their academic development (UNESCO, 2020 ). In the context of online classes during the pandemic, a greater connection with the community has been related to greater self-efficacy and engagement and lower academic stress (Luan et al., 2020 ; Procentese et al., 2020 ). Connection with fellow students and teachers is one of the variables that has received the most attention in the academic community concerning the experience in online education. Social isolation of students during the pandemic has further increased the importance of this factor and hence, we have included connection with other students and teachers as a variable in this study.
Finally, although it had not received much attention in distance education literature before the pandemic, learning environment conditions, its ergonomics, and access to a quality internet connection are additional and indeed important variables to consider in distance learning. A workspace that does not offer the appropriate conditions represents a risk factor for comfort, well-being, and students’ academic performance (Braat-Eggen et al., 2017 ; Hviid et al., 2020 ; Parvez et al., 2019 ; Zhong et al., 2019 ). The unexpected change to online education due to the COVID-19 pandemic has made researchers and academic staff wonder whether students were prepared to take classes from their home and whether they had an adequate workspace, equipment, and facilities for effective learning. In addition, the lockdown situation has prevented students from going to libraries or study halls and, in many cases, has forced family members to share the spaces of their houses, which might have worsened student workplace conditions by increasing noise and distractions (Driessen et al., 2020 ). In several studies, university students reported that their home has been a distractive environment and mentioned that they were more prone to be interrupted by roommates or family members (Gelles et al., 2020 ; Kyne & Thompson, 2020 ; Son et al., 2020 ). Moreover, Realyvásquez-Vargas et al. ( 2020 ) pointed out that environmental factors such as noise, temperature, and lighting had a significant effect on university students’ academic performance during online classes in the pandemic context. These issues were associated with more difficulties in focusing and concentrating while learning (Amir et al., 2020 ; Son et al., 2020 ) and can become a barrier to the acquisition of knowledge through online courses (Khalil et al., 2020 ). Another drawback identified in online classes during the pandemic has been inadequate internet access (Al-Balas et al., 2020 ; Alnusairat et al., 2020 ; Amir et al., 2020 ; Aristovnik et al., 2020 ; Kyne & Thompson, 2020 ). Several studies have reported that a significant percentage of university students, especially those from disadvantaged families, have had problems accessing internet services (Aristovnik et al., 2020 ). Due to the unexpected lockdown situation and the rapid transition to online learning, students did not have time to adapt their workspace, which may have had an impact on their academic development. Thus, we included workspace conditions as a variable for this study.
All these factors should be considered when designing online courses to avoid detrimental effects on students’ academic development (Braat-Eggen et al., 2017 ; Hviid et al., 2020 ; Mcinnerney & Roberts, 2004 ; Piccoli et al., 2001 ; Sun et al., 2008 ; Zhong et al., 2019 ). Numerous studies predicted that the change in teaching methods during the pandemic affected students’ academic development and their outcomes, although the results are inconsistent. Some of the studies have shown that confinement had positively affected students’ academic performance and their learning efficiency (Gonzalez et al., 2020 ; Khalil et al., 2020 ). The study by Jacques et al. ( 2020 ) carried out with engineering students showed that distance learning did not reduce students’ performance and that the grades obtained were similar to those expected in face-to-face teaching. Contrarily, previous research revealed that students perceived worse performance upon face-to-face classes being canceled (Aristovnik et al., 2020 ) and showed concerns about the negative impact that the pandemic situation will have on their academic outcomes (Nassr et al., 2020 ; Son et al., 2020 ). The study by Hamann et al. ( 2020 ) suggested that students who followed the course entirely online were significantly less likely to be successful than students who also, or exclusively, attended face-to-face courses during the pandemic. Furthermore, Tang et al. ( 2020 ) indicated in their study during the COVID-19 lockdown that students felt generally dissatisfied with the effects of online engineering courses on their learning.
Based on these assumptions, we expect to find a positive correlation between students' academic development with the variables of quality of teaching, adaptation of the classes to the online format, connection with other students and professors, and workspace conditions (Hypothesis 1). Since many of the studies done so far in the context of the pandemic have identified these variables separately, few correlational studies have analyzed their associations and identified the factors that may have had the greatest impact on students' academic development during COVID-19. Knowing how the variables related to the online experience have affected the performance of the students will allow us to identify relevant points that should be considered in the design of online courses. To complement this information, we also identified the best practices carried out by instructors in online teaching during the pandemic that have helped students transition to this new learning environment. This information can help instructors and institutions improve online teaching beyond the pandemic situation. This approach is aligned with the conclusions of Anderson et al. ( 2011 ), which showed that receiving feedback from students about the online lessons is vital to improve the courses offered.
Emotional effects of COVID-19
Studies before the COVID-19 pandemic already reported the negative psychological effects that lockdown can cause on people (Blendon et al., 2004 ). Quarantine is often described as an unpleasant experience for those who suffer it, and can involve uncertainty about the situation and boredom (Brooks et al., 2020 ; Cava et al., 2005 ). It is also associated with significant psychological distress, depressive symptoms, post-traumatic stress, and aversive emotional states such as anger, confusion, anguish, disgust, fear, or nervousness, among others (Brooks et al., 2020 ; Hawryluck et al., 2004 ). In the studies carried out during the COVID-19 lockdown, university students reported negative effects on their mental health and emotions. Generally, students have experienced an increase in their stress, anxiety, and depression during the COVID-19 pandemic (Aslan et al., 2020 ; Odriozola-González et al., 2020 ; Saravanan et al., 2020 ; Son et al., 2020 ). Besides, they reported feeling some negative emotions intensified, such as fear, worry, or boredom (Aristovnik et al., 2020 ; Son et al., 2020 ).
Despite the proven adverse effects that lockdown can have on people, in cases like a public health emergency due to an infectious disease, imposing measures on the population to stop the spread of such disease is needed. Studying which elements can minimize the negative impact and aversive feelings during isolation are of great importance in this context. Some studies have indicated that contact with the academic community can act as a protector and decrease the negative impact of lockdown on students’ mental health (Elmer et al., 2020 ; Magson et al., 2021 ; Procentese et al., 2020 ). Others have shown that the lack of relationships and connection with other students and teachers is associated with an increase in academic stress (Zurlo et al., 2020 ).
Most studies during the COVID-19 pandemic on the emotional state of university students have only analyzed negative emotional states such as anxiety, stress, or depression. However, there is a lack of research with a more global perspective on the emotional state of university students that also includes positive emotions to study whether these have decreased during the pandemic. In the study of emotions, psychological theories have proposed some dimensions to measure people’s emotional state, although they have hardly been contemplated in studies on the emotional impact of the COVID-19 pandemic. One of the best known and widely used is Plutchik’s ( 1994 ) theory, which presents eight emotional dimensions in opposite pairs. Compared with other theories such as Ekman ( 1992 ) or Parrot ( 2001 ), Plutchik’s framework is well-founded in psychological studies, presents a good balance between positive and negative emotions, and offers a broader subset of emotional dimensions (Wang et al., 2019 ), the aforementioned reasons being why it has been used as a reference to measure the emotions of the students in this study.
Based on the results of the previous literature, we hypothesize that there is an association between the perceived connection with other students and teachers and the emotional state of the students, so a greater connection with other students and teachers will be associated with a decrease in negative emotions and an increase in positive emotions (Hypothesis 2).
Effects of pandemic fatigue
One of the great unknowns related to the impact of the COVID-19 pandemic are the effects of a prolonged lockdown situation. The entire world population has been exposed to a state of exceptionality generated by the COVID-19 pandemic that has required the implementation of invasive measures with unprecedented impact on daily lives. When these measures are prolonged for an extended period of time, it can cause what is known as pandemic fatigue, which is the mental exhaustion caused by a public health crisis and the restrictions derived from it. This state can affect the mental health of those who suffer it, causing boredom, demotivation, alienation, and hopelessness (World Health Organization & Regional Office for Europe, 2020 ). Given the unusual nature of the situation, the literature on the impact of the pandemic and the lockdown implemented is still limited, and there is little evidence to indicate whether the academic and psychological effects of lockdown are greater at the beginning of the pandemic because of the uncertainty of the situation or may become more significant as the pandemic continues due to the feeling of burnout during the prolonged lockdown (Canet-Juric et al., 2020 ). Additionally, most longitudinal studies in the context of the COVID-19 pandemic in university students assessed only the psychological impact, so more research is required to analyze the academic impact. As Odriozola-González et al. ( 2020 ) pointed out, more longitudinal studies are needed to analyze the long-term impact of the COVID-19 pandemic and to draw conclusions about the cause and effect relationships between the variables involved.
In the current literature there is substantial debate about the detrimental effects of pandemic fatigue. In particular, there are two major views: some longitudinal studies supported the theory that adverse effects have intensified as time in lockdown increased and showed significant increments in negative symptoms such as depression, anxiety, or stress (Ausín et al., 2021 ; Cecchini et al., 2021 ; Gopal et al., 2020 ). In contrast, other studies argued that the effects did not increase over time and, in any case, were are greater at the beginning of the pandemic due to uncertainty and fear of the unknown situation (Canet-Juric et al., 2020 ). Regarding this theory, the study by Ramos-Morcillo et al. ( 2020 ) identified two phases as a pandemic progresses. The first is the so-called shock phase, which occurs during the first weeks, and disorientation and mental performance decreases, along with the ability to concentrate. The second is the normalization phase, in which conditions of confinement start to be assimilated, and the new everyday life is normalized. Thus, based on this second theory, we hypothesize that there is an association between time in lockdown and academic experience, and students will have a worst online learning experience at the beginning of lockdown (Hypothesis 3). We also expect an association between the time in lockdown and students’ emotional state, and we hypothesize that negative feelings will be greater at the beginning of lockdown, and positive feelings will be greater as time progresses (Hypothesis 4). Knowing when the impact of a confinement situation or health crisis is greater and which feelings increase over time will help to develop support plans for students and plan corrective measures in similar situations like climate, political, or security crises that can restrict people’s movement and prevent regular university access.
Context of the current study
In the current study carried out at the UPC, we made two measurements at different time points and compared data collected in spring and fall semesters, 2020, to evaluate the effects of prolonged lockdown and pandemic fatigue on the educational experience and emotional state of engineering students. Regarding the restrictions derived from the pandemic in Spain, the first state of alarm was decreed on March 14th, and the free movement of citizens was limited to essential activities resulting in the confinement of the population in their places of residence and the suspension of face-to-face education. Consequently, educational institutions had to switch the teaching to the online format, and many held the classes and academic activities remotely until the end of the course in June 2020. Due to the increase in cases, on October 16th, all face-to-face activity in universities was suspended again, although many higher education institutions had already started the academic year online after the summer. On October 25th, after exceeding half a million infected countywide, the second state of alarm was established to face the pandemic’s second wave. In this case, a curfew was imposed between 10 p.m. and 6 a.m., and later the population was confined again in their municipalities and social gatherings were restricted.
Participants
The participants of this study were students enrolled in the second, third, and fourth-year of the Bachelor’s Degree in Industrial Design and Product Development Engineering. First-year students were excluded from the sample due to the short time they had been in engineering studies and the inability to compare the impact of the lockdown on their academic activity with previous courses. All students enrolled in the other three courses were invited to participate, that is, a total of 339 students, 168 at Time 1 (T1) and 171 at Time 2 (T2). The required sample size calculated based on a confidence level of 95% and a margin of error of 5% was 181 students for the total sample, 119 for each measurement. Finally, a total of 254 students participated in the study, 122 at T1 and 132 at T2, so the study sample meets the intended size. Participants were self-selected and no incentive was given to students to participate in the study. The protection of personal data was duly taken into account, ensuring that all recipients agreed to receive communications. Both anonymity of the participants and confidentiality of the data to be collected were guaranteed. Data did not include personal characteristics of the students. Table Table1 1 shows the academic year and the conditions in which the participants took the online classes during the pandemic, type of residence and workspace conditions.
Academic course and lockdown conditions of the participants
Baseline characteristic | Time 1 | Time 2 | ||
---|---|---|---|---|
% | % | |||
Academic course | ||||
Second-year | 22 | 18.0 | 41 | 31.1 |
Third-year | 62 | 50.8 | 52 | 39.4 |
Fourth-year | 38 | 31.1 | 39 | 29.5 |
Residence | ||||
Family home | 119 | 97.5 | 112 | 85 |
Student flat | 3 | 2.5 | 12 | 9 |
University residence | 0 | 0 | 8 | 6 |
Workspace | ||||
Personal bedroom | 79 | 64.8 | 92 | 69.7 |
Home office | 25 | 20.5 | 18 | 13.6 |
Common area | 18 | 14.8 | 22 | 16.7 |
Due to the exceptional nature of the situation a questionnaire was designed for this study with measures derived from previous literature and adapted to fit the research context. In previous investigations on the impact of online teaching on students' academic experience during the COVID-19 pandemic, these constructs were measured using questionnaires addressed to students. In these questionnaires, students were asked about their perception of the quality of teaching (Al-Balas et al., 2020 ; Alnusairat et al., 2020 ; Amir et al., 2020 ; Aristovnik et al., 2020 ; Linh & Trang, 2020 ; Puljak et al., 2020 ; Radu et al., 2020 ), the adaptation of classes to the online format (Al-Balas et al., 2020 ; Alnusairat et al., 2020 ; Aristovnik et al., 2020 ; Linh & Trang, 2020 ; Puljak et al., 2020 ; Rodríguez-Rodríguez et al., 2020 ), the perceived connection with other students and teachers (Al-Balas et al., 2020 ; Alnusairat et al., 2020 ; Amir et al., 2020 ; Linh & Trang, 2020 ; Puljak et al., 2020 ; Radu et al., 2020 ; Tang et al., 2020 ), their workspace conditions (Al-Balas et al., 2020 ; Amir et al., 2020 ; Driessen et al., 2020 ; Kyne & Thompson, 2020 ; Linh & Trang, 2020 ; Realyvásquez-Vargas et al., 2020 ), and the impact on students' academic development (Al-Balas et al., 2020 ; Aristovnik et al., 2020 ; Linh & Trang, 2020 ; Realyvásquez-Vargas et al., 2020 ; Tang et al., 2020 ). These investigations have been used as a foundation to build the measuring instrument for this study.
The quality of the online classes that students received during the lockdown was assessed with a 4-point scale (1 = Very bad to 4 = Very good). To measure students’ perceptions about the adaptation of the course to the online format, a set of 4 items was designed (α = .76) rated on a 4-point scale (1 = Strongly disagree to 4 = Strongly agree). Students were asked to indicate if the classes and the assessment methods had been adapted correctly to the online format, if they had been able to follow the course correctly, and if they had received the necessary support from teachers. To assess the level of connection that students felt with other students and teachers a set of 4 items was designed (α = .65) rated on a 4-point scale (1 = Strongly disagree to 4 = Strongly agree). Students were asked to indicate if they felt connected with other students and teachers and if they had missed having contact with fellow students and teachers. To measure the workspace conditions, students were asked about the place and the type of room from where they followed the online classes with two multiple-choice questions of three options each (e.g., from a student flat) and an open field. Students also had to indicate if their workspace conditions had been suitable on a 4-point scale (1 = Strongly disagree to 4 = Strongly agree). To determine how students perceived the impact of the switch to online classes on their academic development, they were asked to rate on a 3-point scale (1 = It has worsened to 3 = It has improved) if they believed that their academic development had been affected by online teaching.
To measure the emotions students felt during online classes while they were in lockdown, a measure based on Plutchik’s ( 1994 ) wheel of emotions was designed using a multiple-choice question. Students were asked to select their feelings during the lockdown from 8 options adapted from Plutchik’s classification.
Finally, an optional open question was added in which students could share if there was something that teachers did during the online teaching that helped them especially.
For the present study, students completed the exact same questionnaire at two points, approximately six months apart. The first time the questionnaire was distributed was on June 3rd, 2020 (T1), during the spring semester and almost three months after the first lockdown was established and all classes went online. Consequently, students had a reasonable exposure to the online learning experience and the lockdown to answer the questionnaire. The second measurement was made in the fall semester on November 18th, 2020 (T2), approximately one month after the second state of alarm was decreed and the new lockdown was applied to face the second COVID-19 outbreak. Therefore, both measurements were made while students were taking classes online.
The questionnaire, which was anonymous and drawn up using Google Forms, was sent by email together with a motivational letter explaining the purpose of the study. Both times, students had up to 1 week to complete it, and took approximately fifteen minutes to finish. Participants were recruited via email messages sent by the authors of this research and faculty members, and were encouraged to answer all the questions accordingly to their opinions. Before being sent, the questionnaire was submitted to a validation process to identify whether it omitted some question areas, determine whether the questions were clear and well-formulated, and detect possible errors in its preparation.
No outliers were identified, and no missing values were found either since all the questions in the questionnaire were mandatory. Descriptive statistics and frequency analyses were applied to characterize the sample. Spearman's rank correlation coefficient was used to test the first hypothesis on how the variables related to the online academic experience affected students' academic development. Although the intercorrelations were calculated between all the variables of the online academic experience, the analysis was done using academic development as the independent variable and the variables of connection with other students and teachers, workspace conditions, quality of online classes, and adaptation of the course as dependent variables. To evaluate our second hypothesis about whether the connection with other students and teachers can affect students' emotional state, a Chi-Square Test for Independence was performed using connection with other students and teachers as the independent variable and emotions as the dependent variables. To fulfill the assumption concerning the minimum expected cell frequency, the measure of connection with other students and teachers was transformed into a dichotomous variable combining the negative and positive values, respectively. To assess our third hypothesis on how the time in lockdown affected the academic experience of the students, we performed a Mann-Whitney U Test using the time in which the measurements were made as the independent variable and the variables of academic development, connection with other students and teachers, workspace conditions, quality of online classes, and adaptation of the course as dependent variables. Since the emotion variables were dichotomous, for the fourth hypothesis about how time in lockdown affected the emotional state of the students, a Chi-Square Test for Independence was carried out using the time in which the measurements were taken as the independent variable and the different emotions as dependent variables. In the analyzes, p < 0.05 was considered statistically significant, and the effect size was assessed using Cohen’s ( 1988 ) criteria. For the qualitative data, an abductive methodology was used to identify the codes. First, half of the dataset was analyzed, and a preliminary code list was obtained. Next, the entire dataset was processed with the identified codes, and the rest of the codes emerged from the data iteratively, adding new codes if practices not identified in the preliminary list were found.
Descriptive analyses indicated that about half of the students reported that the quality of the online classes received during the pandemic was bad or very bad (T1 = 54.1%, T2 = 46.2%), and more than a half thought that their academic development worsened during online classes compared to face-to-face classes (T1 = 66.4%, T2 = 68.9%). Also, more than half of the students indicated that their workspace conditions had been adequate, especially at T2 (T1 = 58.2%, T2 = 73.5%). Regarding the single items that compose the variable adaptation of the course variable, over half of the students reported that classes were correctly adapted to the online format (T1 = 57.4%, T2 = 68.2%) and that the evaluation methods were also properly adapted (T1 = 56.6%, T2 = 54.5%). Furthermore, the majority indicated that they had been able to follow the course correctly (T1 = 63.9%, T2 = 78.8%) and had the necessary support from teachers (T1 = 57.4%, T2 = 67.4%). Remarkable differences can be observed between the two time points regarding the 4-items that compose the variable connection with other students and teachers. Students felt less connected to other students and teachers in T2 than in T1 (T1 = 67.2%, T2 = 27.3%; and T1 = 51.6%, T2 = 40.1%; respectively) and the vast majority missed having contact with other students and teachers (T1 = 82.0%, T2 = 95.5%; and T1 = 81.1%, T2 = 91.7%; respectively).
As shown in Table Table2, 2 , the workspace conditions in which students took the online classes improved significantly from T1 to T2 with a small effect size. On the other hand, the perceived connection with fellow students and teachers worsened significantly from T1 to T2 with a medium effect size, so that in T2 they felt less connected with other students and teachers. Although the quality of online classes and the adaptation of the course improved at T2 compared with T1, no significant differences were found.
Descriptive Statistics and Mann-Whitney Test at Time 1 and Time 2
Variables | No. of items | Range | Time 1 | Time 2 | |||||
---|---|---|---|---|---|---|---|---|---|
Quality of online classes | 1 | 1-4 | 2.5 | 0.7 | 2.5 | 0.7 | 7540 | 0.97 | .334 |
Academic development | 1 | 1-3 | 1.4 | 0.6 | 1.4 | 0.6 | 7909 | -0.30 | .765 |
Workspace conditions | 1 | 1-4 | 2.7 | 0.8 | 2.9 | 0.7 | 6864 | 2.23 | .026 |
Adaptation of the course | 4 | 1-4 | 2.6 | 0.6 | 2.7 | 0.5 | 7146 | 1.57 | .116 |
Connection with other students and teachers | 4 | 1-4 | 2.2 | 0.5 | 1.8 | 0.5 | 4361 | -6.38 | .000 |
At both times, correlations between academic development and quality of online classes and adaptation to the course were relatively high and positive with a medium effect size (see Table Table3). 3 ). Also, the workspace conditions had a positive significant correlation with academic development with a small effect at T1 and T2. Therefore, those students who perceived a better quality and adaptation of the online classes and had better workspace conditions were those who reported a better academic development. On the other hand, academic development had a significant positive correlation with connection with other students and teachers with a small effect size at T2, the time point when they felt more disconnected with fellow students and teachers.
Intercorrelations for Study Variables at Time 1 and Time 2
Variable | 1 | 2 | 3 | 4 | 5 |
---|---|---|---|---|---|
1. Academic development | - | .176 | .403*** | .358*** | .237** |
2. Connection with other students and teachers | .282** | - | .274** | .340*** | .118 |
3. Quality of online classes | .496*** | .265** | - | .409*** | .258** |
4. Adaptation of the course | .435*** | .298** | .571*** | - | .313*** |
5. Workspace conditions | .243** | .188* | .423*** | .393*** |
The results for the Time 1 sample ( n = 122) are shown above the diagonal. The results for the Time 2 sample ( n = 132) are shown below the diagonal
* p < .05; ** p < .01; *** p < .001
As illustrated in Fig. 1 , the emotions that students felt the most during the lockdown were discouragement (22.6% T1, 23.0% T2), boredom (T1 = 17.5%, T2 = 21.8%), confusion (T1 = 18.3%, T2 = 15.7%), worry (T1 = 15.4%, T2 = 14.3%), and annoyance (T1 = 10.8%, T2 = 10.3%). Contrary, the least common were vigilance (T1 = 8.2%, T2 = 7.3%), calm (T1 = 5.7%, T2 = 6.1%), and trust (T1 = 1.5%, T2 = 1.6%). Regarding the differences between T1 and T2, students felt slightly less worried and confused at T2, although no statistically significant difference was found (X2 (1, N = 254) = 0.12, p = .728, phi = -.030; and X2 (1, N = 254) = 1.13, p = .288, phi = -.075; respectively). Besides, they felt more bored at T2 than T1, with a statistically significant difference (X2 (1, N = 254) = 5.30, p = .021, phi = .153) with a small effect.

Changes in Students’ Emotions between Time 1 and Time 2
As shown in Table Table4, 4 , a significant association with small effect was found between the connection with other students and teachers and the emotions felt by the students, with the exception of boredom, which did not present significant differences. Those students who felt more connected with other students and teachers were more likely to feel calm and trust. Otherwise, those students who felt more disconnected were the ones who felt more worry, confusion, discouragement, annoyance, and vigilance.
Chi-Square Results for Connection with Other Students and Teachers in Students’ Emotions
Variable | Disconnected | Connected | |||||
---|---|---|---|---|---|---|---|
% | % | ||||||
Calm | 30 | 15.3% | 18 | 31.0% | 6.23 | .013 | .169 |
Worry | 101 | 51.5% | 20 | 34.5% | 4.55 | .033 | -.143 |
Confusion | 115 | 58.7% | 23 | 39.7% | 5.78 | .016 | -.160 |
Discouragement | 153 | 78.1% | 33 | 56.9% | 9.18 | .002 | -.201 |
Boredom | 127 | 64.8% | 34 | 58.6% | 0.49 | .482 | -.054 |
Annoyance | 74 | 37.8% | 12 | 20.7% | 5.08 | .024 | -.151 |
Trust | 5 | 2.6% | 8 | 13.8% | 9.45 | .002 | .214 |
Vigilance | 55 | 28.1% | 8 | 13.8% | 4.15 | .042 | -.139 |
The open question regarding best practices carried out during the online teaching received 117 responses, 63 from T1 and 54 from T2. From these responses, 21 best practices were identified, plus the “other practices” code (Table (Table5). 5 ). As some answers referred to more than one code, the total number of best practices identified ( n = 182) is greater than the total number of responses. The codes were classified into four categories: communication, classes, course adaptation to the online format, and teachers.
Best Practices in Online Teaching During COVID-19 Lockdown
Category | Code | % | |
---|---|---|---|
Communication | Instant messaging | 10 | 5.3 |
9 | 4.8 | ||
Forum | 2 | 1.1 | |
Social networks | 2 | 1.1 | |
Classes | Record and share classes | 37 | 19.6 |
Individual or small group tutoring | 18 | 9.5 | |
Sessions to resolve doubts | 9 | 4.8 | |
Live problem solving | 3 | 1.6 | |
Synchronous learning | 3 | 1.6 | |
Asynchronous learning | 3 | 1.6 | |
Course adaptation to the online format | Provide solved problems | 10 | 5.3 |
Provide support videos | 10 | 5.3 | |
Adapt the statement of the projects | 5 | 2.6 | |
Provide extra support documentation | 5 | 2.6 | |
Make assignment deadlines more flexible | 4 | 2.1 | |
Small workgroups | 4 | 2.1 | |
Adapt evaluation methods | 4 | 2.1 | |
Follow-up activities (as questionnaires or discussions) | 3 | 1.6 | |
Teachers | Fast answers | 20 | 10.6 |
Supportive attitude | 19 | 10.1 | |
Frequent contact | 2 | 1.1 | |
Other practices | 7 | 3.7 | |
Total | 189 | 100 |
The COVID-19 pandemic has brought a global change in educational systems, forcing the transition from face-to-face to online learning due to the restrictions and lockdowns imposed in most countries. This study has examined university engineering students’ academic experience and the emotional impact of online education during the COVID-19 pandemic using a longitudinal approach. The results provide novel information and extend prior research on the impact of distance education during the COVID-19 lockdown on engineering students.
Effects of the prolonged lockdown on students’ learning experience
The majority of students in our sample reported that their academic development worsened during online learning, and a high percentage considered that the online teaching they received was of a bad quality. Therefore, students have perceived a negative impact regarding the change to online teaching during COVID-19 in their academic experience, especially in their academic development and the quality of the teaching they have received. These findings are consistent with some studies on university students during the COVID-19 pandemic regarding the quality of teaching received (Al-Balas et al., 2020 ; Alnusairat et al., 2020 ; Amir et al., 2020 ; Linh & Trang, 2020 ) and the impact on students’ learning outcomes (Aristovnik et al., 2020 ; Nassr et al., 2020 ; Son et al., 2020 ; Tang et al., 2020 ). As UNESCO ( 2020 ) has pointed out, this may be because the change towards online learning has been sudden and consequently the content offered in the classes was not designed to be taught in an online course and online classes were given with limited preparation.
Contrary to our hypothesis (Hypothesis 3), there are no significant differences between T1 and T2 regarding the quality of online classes, the adaptation of teaching to the online format, and the academic development of students. However, we observed a slight increase in the perception of the online classes’ quality and the adaptation of the course, though this difference is not statistically significant. These results suggest that although there may have been an improvement in some aspects of teaching, probably due to enhancements introduced by teachers in the subjects after the first months of the pandemic, these have not been enough to make a significant change in students’ perception. On the other hand, our data show significant differences between T1 and T2 on the workspace conditions and the perceived contact with fellow students and professors. The conditions of the workspace have significantly improved as time progressed in lockdown. This result suggests that students, foreseeing that the new course would also be online, prepared their workspace conditions to suit their needs. As UNESCO ( 2020 ) argues, student's expectations differ if they expect to enroll from the beginning in a distance course or a regular course. So, it is relevant that academic institutions inform students in advance on possible changes in teaching, so that they can adapt their expectations and prepare properly. Contrary to what was expected, the perception of connection with other students and teachers is significantly lower at T2, so the feeling of isolation worsens significantly as the time in lockdown passes and online classes become regular. Students who reported having missed the contact with other students at T2 reach 95.5% and with teachers 91.7%. Similarly, previous studies have highlighted the lack of social contact and the feelings of isolation and disconnection during the COVID-19 lockdown (Al-Balas et al., 2020 ; Elmer et al., 2020 ; Puljak et al., 2020 ; Radu et al., 2020 ; Son et al., 2020 ). These studies indicate that students have felt less connected to the academic community than in face-to-face teaching (Al-Balas et al., 2020 ; Puljak et al., 2020 ; Son et al., 2020 ). Our findings reveal that not only do students feel less connected to their peers and teachers in online learning compared to face-to-face classes, but also that this feeling increases as time in lockdown lengthens. This finding reaffirms the importance of social contact and communication mechanisms in online education and suggests that if these mechanisms are not properly implemented in the online education systems, the lack of social contact and its negative effects on students’ educational experience may get worse (Luan et al., 2020 ; Procentese et al., 2020 ).
The results of this study indicate that the effects of the prolonged lockdown may impact differently on the variables involved in the online educational experience, and raise new hypotheses regarding the impact of a prolonged lockdown. On the one hand, the impact on variables related to pedagogical aspects of online teaching, such as quality and adaptation of the course, may be more negative at the beginning of the pandemic due to the uncertainty of the situation (Canet-Juric et al., 2020 ; Ramos-Morcillo et al., 2020 ). However, these aspects may improve as time passes due to teaching improvements on the online practices and students’ preparation of their workspace environment foreseeing that they will continue the classes remotely (Scull et al., 2020 ). This hypothesis is supported by studies such as Van Nuland et al. ( 2020 ), which indicates that many teachers have been asked, almost overnight, to implement classes remotely in response to the consequences of the COVID-19 pandemic. Many of these teachers had no or little prior experience in online teaching and that they lacked the pedagogical content knowledge needed for online teaching. As the pandemic has progressed, teachers and universities have been adapting and have implemented several innovations to improve the online teaching experience of students (Scull et al., 2020 ). On the other hand, the variables related to the social aspects of the academic experience, such as the contact with other students and teachers, may be negatively affected by the feeling of burnout as time in lockdown passes (Ausín et al., 2021 ; Cecchini et al., 2021 ; Gopal et al., 2020 ). As other studies have reported, the lockdown situation has triggered the feeling of isolation and disconnection of students (Al-Balas et al., 2020 ; Puljak et al., 2020 ; Son et al., 2020 ), feelings already identified in pedagogical research on the challenges of e-learning (Mcinnerney & Roberts, 2004 ). Understandably, this feeling strengthens while students remain locked in their homes, and as the time without seeing their classmates and teachers increases. Therefore, it will be crucial for academic institutions and faculty members to put mechanisms in place that help students feel connected to each other and to their teachers, such as discussion forums or instant messaging channels (Moorhouse, 2020 ; Rosenberg & Asterhan, 2018 ). The study by Scull et al. ( 2020 ) shares some learnings from universities and teachers that might help strengthen the connection and communication with students during the pandemic, such as providing channels through which they can ask for help or by opening the debate to more personal day-to-day issues to relax the atmosphere and enhance engagement.
In line with what was predicted in our first hypothesis (Hypothesis 1), we find significant relationships between academic development and the rest of the variables related to the online academic experience of students. Specifically, students’ academic development is associated with the quality of the classes received, the adaptation of the courses to the distance format, and the workspace conditions. Also, a positive correlation was found in T2 between students’ academic development and contact with other students and teachers. Previous literature already pointed out the relationship between the variables studied and the academic development of students (Braat-Eggen et al., 2017 ; Hviid et al., 2020 ; Mcinnerney & Roberts, 2004 ; Piccoli et al., 2001 ; Sun et al., 2008 ; Zhong et al., 2019 ). In this line, our results confirm these relationships between the variables under the conditions of a pandemic and lockdown in engineering students. Considering the direction of the correlations, offering students classes of high quality, adapting the class contents, assessing teaching methods properly, and giving support from teachers to the online format relate to a better academic development. Therefore, it is important to offer teachers training and pedagogical tools needed to provide adequate distance teaching (Van Nuland et al., 2020 ). Moreover, having better workspace conditions can enhance students’ academic development. In this regard, academic institutions can ask students about their conditions and offer support, for example, temporarily borrowing computers from the university or granting access to online learning platforms through mobile phones (UNESCO et al., 2020 ).
Furthermore, previous literature has focused on the positive effects that contact with teachers and other students can have on academic development, such as greater self-efficacy and learning engagement, and less academic stress (Luan et al., 2020 ; Procentese et al., 2020 ). Our study suggests that having good connections with other students and teachers is not necessarily associated with academic development (as observed at T1), but if this lack of contact is extended in time (as observed at T2), it may negatively impact students’ academic development. This new finding supports the previous observation that it is necessary to work on the relationships between students themselves and with their teachers in online learning; otherwise, if these connections are lacking, it may have a negative effect on the educational experience and students’ outcomes.
Emotional impact of prolonged lockdown
Students’ most-reported emotions during the lockdown are discouragement, boredom, confusion, and worry, all of them negative emotions. On the contrary, those less prevalent are the positive emotions of calm and trust. Despite previous articles mainly focusing on negative emotions (Aslan et al., 2020 ; Odriozola-González et al., 2020 ; Saravanan et al., 2020 ; Son et al., 2020 ), future studies on students’ mental health should also include positive feelings to understand to what extent these decrease due to the pandemic situation. Assessing positive feelings during a public health crisis is essential as it has been shown that positive emotions can help maintain and improve human mental health (Yamaguchi et al., 2020 ). Thus, using a theoretical framework as Plutchik's ( 1994 ), which includes a broad subset of emotional dimensions, can give a more detailed picture of the emotional state of students and can help detect which feelings teachers and academic institutions need to reinforce, such as students' confidence and calm. Based on this information, institutions can work on messages and communications towards their students to counteract the emotional impact and enhance these positive emotions (Heffner et al., 2021 ).
Contrary to what was expected regarding the differences in the emotional state of the students as the lockdown elapses (Hypothesis 2), most emotions do not show significant changes from T1 to T2. However, worry and confusion are less reported in T2. The fact that students feel more concerned and confused at the beginning of confinement is aligned with the phases of the pandemic identified by Ramos-Morcillo et al. ( 2020 ), in which there is a first phase when disorientation prevails due to the novelty of the situation and a second phase as time progresses when the situation is assimilated and normalized. The only emotion that shows a significant increase from T1 to T2 is boredom, a feeling also present in other studies in the context of the COVID-19 pandemic (Aristovnik et al., 2020 ; Son et al., 2020 ). Although this result is not consistent with our hypothesis, it is aligned with the effect of mental exhaustion and demotivation the WHO ( 2020 ) claims that pandemic fatigue can cause. These results suggest that burnout does not affect all emotional states in the same way, and while some may not increase as time progresses or even decrease, such as worry or confusion, others such as boredom may increase (Canet-Juric et al., 2020 ). This information is relevant for teachers and academic institutions to understand how their students feel in each phase of the pandemic and to adapt the type of support provided at each moment.
In line with hypothesis 4 and the results of prior research (Elmer et al., 2020 ; Magson et al., 2021 ; Procentese et al., 2020 ), the connection with other students and teachers is correlated with students’ emotions. As expected, connection with others acts as a protector and, as the perception of contact with the academic community increases, the negative feelings of worry, confusion, discouragement, annoyance, and vigilance decrease. Besides, it has also been found that contact with fellow students and teachers is positively associated with the feelings of calm and trust, which suggests that social contact is not only a protector against negative emotions, but that it can also enhance positive emotions. Since positive emotions are the least reported by students during the lockdown, it is essential to identify which actions can enhance these emotions to improve students' mood. This finding reinforces the need to create alternative ways to stay in contact, so students can feel more connected with their classmates and teachers. Fostering social contact and communication will improve students’ mental health during online learning in the public health crisis and, as indicated by other studies, even beyond the pandemic situation (Holen et al., 2018 ).
Best practices in online teaching
In addition to validating the hypotheses raised, this study explores best practices carried out by the teaching staff during distance classes that have helped engineering students during online teaching. Although these practices have been identified in the context of the COVID-19 pandemic during the lockdown, they can also be initiatives of interest for regular teaching in university studies or in the design of online courses beyond the pandemic. Concerning the communication between students and teachers, the two most mentioned communication channels are instant messages and emails. Students have highly appreciated those teachers who have opened instant messaging channels such as WhatsApp to communicate with them in a faster and more accessible way. During the classes, the practice that helped students the most was the recording of the online classes allowing students to review them later. Besides, individual or small group video calls with the professor to clarify doubts more closely and in a more comfortable environment were also highly appreciated. Regarding the course adaptation, the two most relevant initiatives have been sharing problems already solved so students have guidelines to self-correct their exercises and sharing support videos with complementary explanations to the subjects’ content. Finally, it was highly appreciated that teachers gave quick answers to students’ questions and that they had a supportive attitude and were attentive to students’ needs. Some students reported on teachers having asked them for feedback to know how they were doing and improve their classes accordingly. Previous research has already highlighted the importance for teachers of receiving feedback from students in order to improve teaching (Anderson et al., 2011 ). The results of our study suggest that the benefits of the feedback are bi-directional, and it is not only helpful for teachers, but also makes students feel heard and valued. Previous research indicates that communication between students and teachers can be more complicated online than in face-to-face teaching (Alnusairat et al., 2020 ; Amir et al., 2020 ; Radu et al., 2020 ). Our study supports this claim, showing that most of the outstanding initiatives have been those practices that allowed students to solve their doubts more efficiently, such as faster communication methods, individual or small group tutoring sessions or having problems already solved in order to self-solve possible doubts autonomously. Although other studies already identified some of the initiatives undertaken by teachers to improve the experience during online teaching (Scull et al., 2020 ), our work collects those practices most valued from the students’ perspective and the ones that have been most helpful for them.
Limitations and future directions
The results of this study should be interpreted in the context of some limitations, which can be addressed in future research.
The study was conducted during an exceptional public health crisis, so it is not easily replicable. Also, the results of this study are influenced by the actions to face the COVID-19 pandemic taken by both the state and local governments and the academic institution in which the study was carried out. The measures adopted by the different countries and universities have differed, adapting them to the possibilities and characteristics of each case (Gonzalez et al., 2020 ). These differences may make the results of this study difficult to extrapolate to other countries or university degrees in which different solutions to the COVID-19 crisis have been implemented. For this reason, while it is expected that some of the results may be of value beyond the pandemic situation, it will be necessary to validate their applicability in other contexts.
Participants of this research were recruited from second, third and fourth year from a specific engineering degree, so the lack of random sampling and the representation of a student population limited to one engineering degree seem to be a limitation in generalizing the results to all engineering studies or all academic courses. As the field of engineering is vast and there are many different specializations, it would be interesting to expand the study and validate the results in other engineering degrees and in other courses. For example, the academic experience of first-year students who have started their studies in a pandemic situation may be different from those students from second, third and fourth year. It would also be interesting to extend the sample to other universities and countries since the impact of the COVID-19 pandemic has not been the same everywhere. Furthermore, we have only included students in the study sample and not teachers. Perspectives of teachers regarding the switch to online learning would be valuable and should be explored in future studies. The samples of this study have been treated as independent. However, since it is a longitudinal study, it would be interesting to validate the observations with paired samples to compare the changes between time points on an individual basis.
Regarding the instruments used in the research, one limitation is that we created a new questionnaire for this study. Although the questions are based on previous studies, we could not find a similar questionnaire in the literature that incorporated all the measurements. Also, the reliability value of the scale used to measure the contact with other students and teachers is a bit low (α = 0.65) although Cronbach’s alpha is quite sensitive to the number of items in the scale, and short scales often have low Cronbach alpha values. Future research should validate these results and the instrument used and expand the number of items of the proposed scales to improve the scale’s reliability. Moreover, we did not collect participants’ personal data, such as gender or demographic information to preserve participants’ anonymity and favor the predisposition of students to answer the questionnaire.
As the study was conducted using a questionnaire, the results studied are based on students’ perceptions. However, perceptions do not always match reality. For example, while in our study students indicated that their academic development worsened during online teaching, the study of Jacques et al. ( 2020 ) found no differences between the grades of engineering students in online education and those expected in a face-to-face teaching. Thus, it would be interesting for future research to compare students’ perceptions regarding their academic development with their academic qualifications to validate the impact of the lockdown and distance classes on students’ outcomes. Also, we phrased the questions to collect the opinion of students regarding the majority of teachers and courses. We acknowledge that they may be differences between different teachers and courses that may impact individual experiences. However, we were interested in analyzing overall collective students’ opinion towards the online learning experience.
Despite these limitations, the results of this study offer valuable information on the academic and emotional effects that online learning during the COVID-19 pandemic had on engineering students and raises new hypotheses which can be examined in more detail in subsequent work. Moreover, a more specific analysis can be carried out to know how much of the variance in academic development can be explained by the quality of the classes, the adaptation of the teaching to the online format, the conditions of students’ workspace and the connection with other students and teachers.
Conclusions
The findings of this study highlight that the majority of students were not satisfied with the quality of their online education enforced during the lockdown due to the COVID-19 pandemic, and that they believe that it has negatively affected their academic performance. Moreover, students' academic development is correlated with the quality of the teaching, the adaptation of the assignments, workspace conditions, and the contact with other students and professors. Regarding their emotional state, students reported feeling discouragement, boredom, confusion, and worry to a greater extent, and calm and trust to a lesser extent. Except for boredom, all emotions are associated with the connection with classmates and teachers perceived by students, hence the students who have perceived a higher level of connection are those who reported more positive emotions and less negative ones. Additionally, we find significant improvements as time in lockdown elapsed regarding the students’ workspace conditions, while perceived contact with other students and teachers and boredom worsened significantly as the pandemic progressed. These results indicate that it is necessary to consider how the courses should be adapted to the online format since their quality and their correct adaptation will have an impact on the students' academic development. Furthermore, it is essential to work on connection and communication mechanisms among students and between students and teachers since these can improve students’ emotional state. These conclusions, along with the good practices that teachers have carried out during online classes in the pandemic and that we have identified in this study, will hopefully help in the design of future online courses and in the implementation of support plans to improve the student learning experience and their emotional state.
Acknowledgements
The authors wish to thank all the students from the Bachelor's Degree in Industrial Design and Product Development Engineering of the UPC who took part in answering the questionnaire and the teachers who facilitated the distribution of the questionnaire.
Authors’ contributions
Conceptualization, R.B.-S.; Methodology, R.B.-S., N.O.-T. and M.P.; Software, R.B.-S and A.-I.R.-D.; Validation, N.O.-T. and M.P.; Formal analysis, R.B.-S and A.-I.R.-D.; Investigation, R.B.-S.; Resources, N.O.-T. and M.P.; Writing—original draft preparation, R.B.-S., N.O.-T., M.P. and A.-I.R.-D.; Writing—review and editing, R.B.-S, N.O.-T., M.P. and A.-I.R.-D.; Supervision, R.B.-S., N.O.-T. and M.P. All authors have read and agreed to the published version of the manuscript.
This research received no external funding.
Availability of data and material
Declarations.
Informed consent was obtained from all individual participants included in the study.
The participants have consented to the submission of the case report to the journal.
The authors declare no conflict of interest.
Publisher's Note
Springer Nature remains neutral with regard to jurisdictional claims in published maps and institutional affiliations.
Contributor Information
Rosó Baltà-Salvador, Email: [email protected] .
Noelia Olmedo-Torre, Email: [email protected] .
Marta Peña, Email: [email protected] .
Ana-Inés Renta-Davids, Email: [email protected] .
- Al-Balas M, Al-Balas HI, Jaber HM, Obeidat K, Al-Balas H, Aborajooh EA, Al-Taher R, Al-Balas B. Distance learning in clinical medical education amid COVID-19 pandemic in Jordan: Current situation, challenges, and perspectives. BMC Medical Education. 2020; 20 :341. doi: 10.1186/s12909-020-02257-4. [ PMC free article ] [ PubMed ] [ CrossRef ] [ Google Scholar ]
- Alnusairat, S., Al Maani, D., & Al-Jokhadar, A. (2020). Architecture students’ satisfaction with and perceptions of online design studios during COVID-19 lockdown: the case of Jordan universities. International Journal of Architectural Research , ahead - of - p . 10.1108/ARCH-09-2020-0195
- Amir LR, Tanti I, Maharani DA, Wimardhani YS, Julia V, Sulijaya B, Puspitawati R. Student perspective of classroom and distance learning during COVID-19 pandemic in the undergraduate dental study program Universitas Indonesia. BMC Medical Education. 2020; 20 :392. doi: 10.1186/s12909-020-02312-0. [ PMC free article ] [ PubMed ] [ CrossRef ] [ Google Scholar ]
- Anderson D, Imdieke S, Standerford NS. Feedback Please: Studying Self in the Online Classroom. International Journal. 2011; 4 (1):3–15. [ Google Scholar ]
- Arbaugh JB. Virtual Classroom Characteristics and Student Satisfaction with Internet-Based MBA Courses. Journal of Management Education. 2000; 24 (1):32–54. doi: 10.1177/105256290002400104. [ CrossRef ] [ Google Scholar ]
- Aristovnik A, Keržič D, Ravšelj D, Tomaževič N, Umek L. Impacts of the COVID-19 pandemic on life of higher education students: A global perspective. Sustainability. 2020; 12 (20):8438. doi: 10.3390/su12208438. [ PMC free article ] [ PubMed ] [ CrossRef ] [ Google Scholar ]
- Aslan I, Ochnik D, Çınar O. Exploring perceived stress among students in Turkey during the covid-19 pandemic. International Journal of Environmental Research and Public Health. 2020; 17 (23):8961. doi: 10.3390/ijerph17238961. [ PMC free article ] [ PubMed ] [ CrossRef ] [ Google Scholar ]
- Ausín B, González-Sanguino C, Castellanos MÁ, Muñoz M. Gender-related differences in the psychological impact of confinement as a consequence of COVID-19 in Spain. Journal of Gender Studies. 2021; 30 (1):29–38. doi: 10.1080/09589236.2020.1799768. [ CrossRef ] [ Google Scholar ]
- Blendon RJ, Benson JM, DesRoches CM, Raleigh E, Taylor-Clark K. The public’s response to severe acute respiratory syndrome in Toronto and the United States. Clinical Infectious Diseases. 2004; 38 (7):925–931. doi: 10.1086/382355. [ PubMed ] [ CrossRef ] [ Google Scholar ]
- Braat-Eggen PE, van Heijst A, Hornikx M, Kohlrausch A. Noise disturbance in open-plan study environments: a field study on noise sources, student tasks and room acoustic parameters. Ergonomics. 2017; 60 (9):1297–1314. doi: 10.1080/00140139.2017.1306631. [ PubMed ] [ CrossRef ] [ Google Scholar ]
- Brooks SK, Webster RK, Smith LE, Woodland L, Wessely S, Greenberg N, Rubin GJ. The psychological impact of quarantine and how to reduce it: rapid review of the evidence. The Lancet. 2020; 395 (10227):912–920. doi: 10.1016/S0140-6736(20)30460-8. [ PMC free article ] [ PubMed ] [ CrossRef ] [ Google Scholar ]
- Canet-Juric L, Andrés ML, del Valle M, López-Morales H, Poó F, Galli JI, Yerro M, Urquijo S. A Longitudinal Study on the Emotional Impact Cause by the COVID-19 Pandemic Quarantine on General Population. Frontiers in Psychology. 2020; 11 :565688. doi: 10.3389/fpsyg.2020.565688. [ PMC free article ] [ PubMed ] [ CrossRef ] [ Google Scholar ]
- Cava MA, Fay KE, Beanlands HJ, McCay EA, Wignall R. The experience of quarantine for individuals affected by SARS in Toronto. Public Health Nursing. 2005; 22 (5):398–406. doi: 10.1111/j.0737-1209.2005.220504.x. [ PubMed ] [ CrossRef ] [ Google Scholar ]
- Cecchini, J. A., Carriedo, A., Fernández-Río, J., Méndez-Giménez, A., González, C., Sánchez-Martínez, B., & Rodríguez-González, P. (2021). A longitudinal study on depressive symptoms and physical activity during the Spanish lockdown. International Journal of Clinical and Health Psychology , 21 (1). 10.1016/j.ijchp.2020.09.001 [ PMC free article ] [ PubMed ]
- Cohen, J. (1988). Statistical Power Analysis for the Behavioral Sciences (2nd edn) . Lawrence Erlbaum Associates. 10.4324/9780203771587
- Driessen E, Beatty A, Stokes A, Wood S, Ballen C. Learning principles of evolution during a crisis: An exploratory analysis of student barriers one week and one month into the COVID-19 pandemic. Ecology and Evolution. 2020; 10 (22):12431–12436. doi: 10.1002/ece3.6741. [ PMC free article ] [ PubMed ] [ CrossRef ] [ Google Scholar ]
- Du Toit, A. (2020). Outbreak of a novel coronavirus. Nature Reviews Microbiology , 18 (3), 123. 10.1038/s41579-020-0332-0 [ PMC free article ] [ PubMed ]
- Ekman P. An Argument for Basic Emotions. Cognition and Emotion. 1992; 6 (3):169–200. doi: 10.1080/02699939208411068. [ CrossRef ] [ Google Scholar ]
- Elmer T, Mepham K, Stadtfeld C. Students under lockdown: Comparisons of students’ social networks and mental health before and during the COVID-19 crisis in Switzerland. PLoS ONE. 2020; 15 (7):e0236337. doi: 10.1371/journal.pone.0236337. [ PMC free article ] [ PubMed ] [ CrossRef ] [ Google Scholar ]
- Gelles LA, Lord SM, Hoople GD, Chen DA, Mejia JA. Compassionate flexibility and self-discipline: Student adaptation to emergency remote teaching in an integrated engineering energy course during COVID-19. Education Sciences. 2020; 10 :304. doi: 10.3390/educsci10110304. [ CrossRef ] [ Google Scholar ]
- Gonzalez T, De la Rubia MA, Hincz KP, Comas-Lopez M, Subirats L, Fort S, Sacha GM. Influence of COVID-19 confinement on students’ performance in higher education. PLoS ONE. 2020; 15 (10):e0239490. doi: 10.1371/journal.pone.0239490. [ PMC free article ] [ PubMed ] [ CrossRef ] [ Google Scholar ]
- Gopal A, Sharma AJ, Subramanyam MA. Dynamics of psychological responses to COVID-19 in India: A longitudinal study. PLoS ONE. 2020; 15 (10):e0240650. doi: 10.1371/journal.pone.0240650. [ PMC free article ] [ PubMed ] [ CrossRef ] [ Google Scholar ]
- Hamann K, Glazier RA, Wilson BM, Pollock PH. Online teaching, student success, and retention in political science courses. European Political Science. 2020 doi: 10.1057/s41304-020-00282-x. [ CrossRef ] [ Google Scholar ]
- Hawryluck L, Gold WL, Robinson S, Pogorski S, Galea S, Styra R. SARS control and psychological effects of quarantine, Toronto. Canada. Emerging Infectious Diseases. 2004; 10 (7):1206–1212. doi: 10.3201/eid1007.030703. [ PMC free article ] [ PubMed ] [ CrossRef ] [ Google Scholar ]
- Heffner J, Vives M-L, FeldmanHall O. Emotional responses to prosocial messages increase willingness to self-isolate during the COVID-19 pandemic. Personality and Individual Differences. 2021; 170 :110420. doi: 10.1016/j.paid.2020.110420. [ PMC free article ] [ PubMed ] [ CrossRef ] [ Google Scholar ]
- Holen S, Waaktaar T, Sagatun Å. A Chance Lost in the Prevention of School Dropout? Teacher-Student Relationships Mediate the Effect of Mental Health Problems on Noncompletion of Upper-Secondary School. Scandinavian Journal of Educational Research. 2018; 62 (5):737–753. doi: 10.1080/00313831.2017.1306801. [ CrossRef ] [ Google Scholar ]
- Hong KS. Relationships between students’ and instructional variables with satisfaction and learning from a Web-based course. Internet and Higher Education. 2002; 5 (3):267–281. doi: 10.1016/S1096-7516(02)00105-7. [ CrossRef ] [ Google Scholar ]
- Hviid CA, Pedersen C, Dabelsteen KH. A field study of the individual and combined effect of ventilation rate and lighting conditions on pupils’ performance. Building and Environment. 2020; 171 :106608. doi: 10.1016/j.buildenv.2019.106608. [ CrossRef ] [ Google Scholar ]
- Ibrahim A, Al Kaabi A, El Zaatari W. Teacher resistance to educational change in the United Arab Emirates. International Journal of Research Studies in Education. 2013; 2 (3):25–36. doi: 10.5861/ijrse.2013.254. [ CrossRef ] [ Google Scholar ]
- Jacques S, Ouahabi A, Lequeu T. Remote Knowledge Acquisition and Assessment During the COVID-19 Pandemic. International Journal of Engineering Pedagogy. 2020; 10 (6):120. doi: 10.3991/ijep.v10i6.16205. [ CrossRef ] [ Google Scholar ]
- Khalil R, Mansour AE, Fadda WA, Almisnid K, Aldamegh M, Al-Nafeesah A, Alkhalifah A, Al-Wutayd O. The sudden transition to synchronized online learning during the COVID-19 pandemic in Saudi Arabia: A qualitative study exploring medical students’ perspectives. BMC Medical Education. 2020; 20 :285. doi: 10.1186/s12909-020-02208-z. [ PMC free article ] [ PubMed ] [ CrossRef ] [ Google Scholar ]
- Kyne SH, Thompson CD. The COVID cohort: Student transition to university in the face of a global pandemic. Journal of Chemical Education. 2020; 97 (9):3381–3385. doi: 10.1021/acs.jchemed.0c00769. [ CrossRef ] [ Google Scholar ]
- Linh PD, Trang TN. Pandemic, social distancing, and social work education: students’ satisfaction with online education in Vietnam. Social Work Education. 2020; 39 (8):1074–1083. doi: 10.1080/02615479.2020.1823365. [ CrossRef ] [ Google Scholar ]
- Luan L, Hong J-C, Cao M, Dong Y, Hou X. Exploring the role of online EFL learners’ perceived social support in their learning engagement: a structural equation model. Interactive Learning Environments. 2020 doi: 10.1080/10494820.2020.1855211. [ CrossRef ] [ Google Scholar ]
- Magson NR, Freeman JYA, Rapee RM, Richardson CE, Oar EL, Fardouly J. Risk and Protective Factors for Prospective Changes in Adolescent Mental Health during the COVID-19 Pandemic. Journal of Youth and Adolescence. 2021; 50 :44–57. doi: 10.1007/s10964-020-01332-9. [ PMC free article ] [ PubMed ] [ CrossRef ] [ Google Scholar ]
- Mcinnerney JM, Roberts TS. Online Learning: Social Interaction and the Creation of a Sense of Community. Journal of Educational Technology & Society. 2004; 7 (3):73–81. [ Google Scholar ]
- Moorhouse BL. Adaptations to a face-to-face initial teacher education course ‘forced’ online due to the COVID-19 pandemic. Journal of Education for Teaching. 2020; 46 (4):1–3. doi: 10.1080/02607476.2020.1755205. [ CrossRef ] [ Google Scholar ]
- Nassr RM, Aborujilah A, Aldossary DA, Aldossary AAA. Understanding Education Difficulty During COVID-19 Lockdown: Reports on Malaysian University Students’ Experience. IEEE Access. 2020; 8 :186939–186950. doi: 10.1109/access.2020.3029967. [ PMC free article ] [ PubMed ] [ CrossRef ] [ Google Scholar ]
- Odriozola-González P, Planchuelo-Gómez Á, Irurtia MJ, de Luis-García R. Psychological effects of the COVID-19 outbreak and lockdown among students and workers of a Spanish university. Psychiatry Research. 2020; 290 :113108. doi: 10.1016/j.psychres.2020.113108. [ PMC free article ] [ PubMed ] [ CrossRef ] [ Google Scholar ]
- Parrott WG. Emotions in social psychology. Psychology Press; 2001. [ Google Scholar ]
- Parvez MS, Rahman A, Tasnim N. Ergonomic mismatch between students anthropometry and university classroom furniture. Theoretical Issues in Ergonomics Science. 2019; 20 (5):603–631. doi: 10.1080/1463922X.2019.1617909. [ CrossRef ] [ Google Scholar ]
- Piccoli G, Ahmad R, Ives B. Web-based virtual learning environments: A research framework and a preliminary assessment of effectiveness in basic it skills training. MIS Quarterly: Management Information Systems. 2001; 25 (4):401–426. doi: 10.2307/3250989. [ CrossRef ] [ Google Scholar ]
- Plutchik, R. (1994). The Psychology and Biology of Emotion . Harper Collins College Publishers.
- Procentese F, Capone V, Caso D, Donizzetti AR, Gatti F. Academic community in the face of emergency situations: Sense of responsible togetherness and sense of belonging as protective factors against academic stress during covid-19 outbreak. Sustainability. 2020; 12 (22):9718. doi: 10.3390/su12229718. [ CrossRef ] [ Google Scholar ]
- Puljak L, Čivljak M, Haramina A, Mališa S, Čavić D, Klinec D, Aranza D, Mesarić J, Skitarelić N, Zoranić S, Majstorović D, Neuberg M, Mikšić Š, Ivanišević K. Attitudes and concerns of undergraduate university health sciences students in Croatia regarding complete switch to e-learning during COVID-19 pandemic: a survey. BMC Medical Education. 2020; 20 :416. doi: 10.1186/s12909-020-02343-7. [ PMC free article ] [ PubMed ] [ CrossRef ] [ Google Scholar ]
- Radu MC, Schnakovszky C, Herghelegiu E, Ciubotariu VA, Cristea I. The impact of the COVID-19 pandemic on the quality of educational process: A student survey. International Journal of Environmental Research and Public Health. 2020; 17 (21):7770. doi: 10.3390/ijerph17217770. [ PMC free article ] [ PubMed ] [ CrossRef ] [ Google Scholar ]
- Ramos-Morcillo AJ, Leal-Costa C, Moral-García JE, Ruzafa-Martínez M. Experiences of nursing students during the abrupt change from face-to-face to e-learning education during the first month of confinement due to COVID-19 in Spain. International Journal of Environmental Research and Public Health. 2020; 17 (15):5519. doi: 10.3390/ijerph17155519. [ PMC free article ] [ PubMed ] [ CrossRef ] [ Google Scholar ]
- Realyvásquez-Vargas, A., Maldonado-Macías, A. A., Arredondo-Soto, K. C., Baez-Lopez, Y., Carrillo-Gutiérrez, T., & Hernández-Escobedo, G. (2020). The Impact of Environmental Factors on Academic Performance of University Students Taking Online Classes during the COVID-19 Pandemic in Mexico. Sustainability , 12 (21), 9194. https://www.mdpi.com/2071-1050/12/21/9194
- Rodríguez-Rodríguez E, Sánchez-Paniagua M, Sanz-Landaluze J, Moreno-Guzmán M. Analytical Chemistry Teaching Adaptation in the COVID-19 Period: Experiences and Students’ Opinion. Journal of Chemical Education. 2020; 97 (9):2556–2564. doi: 10.1021/acs.jchemed.0c00923. [ CrossRef ] [ Google Scholar ]
- Rosenberg, H., & Asterhan, C. S. C. (2018). “WhatsApp, Teacher?” Student Perspectives on Teacher-Student WhatsApp Interactions in Secondary Schools. Journal of Information Technology Education Research , 4081 , 205–226. 10.28945/4081
- Saravanan C, Mahmoud I, Elshami W, Taha MH. Knowledge, Anxiety, Fear, and Psychological Distress About COVID-19 Among University Students in the United Arab Emirates. Frontiers in Psychiatry. 2020; 11 :582189. doi: 10.3389/fpsyt.2020.582189. [ PMC free article ] [ PubMed ] [ CrossRef ] [ Google Scholar ]
- Scull J, Phillips M, Sharma U, Garnier K. Innovations in teacher education at the time of COVID19: an Australian perspective. Journal of Education for Teaching. 2020; 46 (4):497–506. doi: 10.1080/02607476.2020.1802701. [ CrossRef ] [ Google Scholar ]
- Son C, Hegde S, Smith A, Wang X, Sasangohar F. Effects of COVID-19 on college students’ mental health in the United States: Interview survey study. Journal of Medical Internet Research. 2020; 22 (9):e21279. doi: 10.2196/21279. [ PMC free article ] [ PubMed ] [ CrossRef ] [ Google Scholar ]
- Sun PC, Tsai RJ, Finger G, Chen YY, Yeh D. What drives a successful e-Learning? An empirical investigation of the critical factors influencing learner satisfaction. Computers and Education. 2008; 50 (4):1183–1202. doi: 10.1016/j.compedu.2006.11.007. [ CrossRef ] [ Google Scholar ]
- Tang T, Abuhmaid AM, Olaimat M, Oudat DM, Aldhaeebi M, Bamanger E. Efficiency of flipped classroom with online-based teaching under COVID-19. Interactive Learning Environments. 2020 doi: 10.1080/10494820.2020.1817761. [ CrossRef ] [ Google Scholar ]
- UNESCO. (2020). COVID-19 and higher education: Today and tomorrow. Impact analysis, policy responses and recommendations . http://www.iesalc.unesco.org/en/wp-content/uploads/2020/04/COVID-19-EN-090420-2.pdf
- UNESCO, UNICEF, & The World Bank. (2020). What Have We Learnt? : Overview of Findings from a Survey of Ministries of Education on National Responses to COVID-19 . https://openknowledge.worldbank.org/handle/10986/34700
- Van Nuland S, Mandzuk D, Tucker Petrick K, Cooper T. COVID-19 and its effects on teacher education in Ontario: a complex adaptive systems perspective. Journal of Education for Teaching. 2020; 46 (4):442–451. doi: 10.1080/02607476.2020.1803050. [ CrossRef ] [ Google Scholar ]
- Wang X, Tang L, (Rebecca), & Kim, E. More than words: Do emotional content and linguistic style matching matter on restaurant review helpfulness? International Journal of Hospitality Management. 2019; 77 :438–447. doi: 10.1016/j.ijhm.2018.08.007. [ CrossRef ] [ Google Scholar ]
- World Health Organization. (2021). Looking back at a year that changed the world. Who’s Response To COVID-19 . https://www.who.int/publications/m/item/looking-back-at-a-year-that-changed-the-world-who-s-response-to-covid-19
- World Health Organization & Regional Office for Europe. (2020). Pandemic fatigue: reinvigorating the public to prevent COVID-19: policy framework for supporting pandemic prevention and management . https://apps.who.int/iris/handle/10665/337574
- Yamaguchi K, Takebayashi Y, Miyamae M, Komazawa A, Yokoyama C, Ito M. Role of Focusing on the Positive Side During COVID-19 Outbreak: Mental Health Perspective From Positive Psychology. Psychological Trauma: Theory, Research, Practice, and Policy. 2020; 12 (S1):S49–S50. doi: 10.1037/tra0000807. [ PubMed ] [ CrossRef ] [ Google Scholar ]
- Zhong, L., Yuan, J., & Fleck, B. (2019). Indoor environmental quality evaluation of lecture classrooms in an institutional building in a cold climate. Sustainability , 11 (23). 10.3390/su11236591
- Zhou P, Yang X. Lou, Wang XG, Hu B, Zhang L, Zhang W, Si HR, Zhu Y, Li B, Huang CL, Chen HD, Chen J, Luo Y, Guo H, Jiang R. Di, Liu MQ, Chen Y, Shen XR, Wang X, Shi ZL. A pneumonia outbreak associated with a new coronavirus of probable bat origin. Nature. 2020; 579 (7798):270–273. doi: 10.1038/s41586-020-2012-7. [ PMC free article ] [ PubMed ] [ CrossRef ] [ Google Scholar ]
- Zurlo MC, Volta Cattaneo Della, M. F., & Vallone, F. COVID-19 Student Stress Questionnaire: Development and Validation of a Questionnaire to Evaluate Students’ Stressors Related to the Coronavirus Pandemic Lockdown. Frontiers in Psychology. 2020; 11 :576758. doi: 10.3389/fpsyg.2020.576758. [ PMC free article ] [ PubMed ] [ CrossRef ] [ Google Scholar ]
Application of the UTAUT model to understand learning behavior using Online Video Conference media for RPL students
New citation alert added.
This alert has been successfully added and will be sent to:
You will be notified whenever a record that you have chosen has been cited.
To manage your alert preferences, click on the button below.
New Citation Alert!
Please log in to your account
Information & Contributors
Bibliometrics & citations, index terms.
Social and professional topics
Professional topics
Computing education
Adult education
Recommendations
Examining students' intention to continue using blogs for learning.
This study is designed to investigate factors influencing undergraduate students' continuance intention to use blogs for learning in a management information systems course. Constructs from three theoretical frameworks, i.e., social-cognitive theory, ...
Using the UTAUT model to understand students’ usage of e-learning systems in developing countries
Research on information systems has identified a variety of factors across a range of adoption models that determine their acceptance. In this research, the unified theory of acceptance and use of technology (UTAUT), which integrates determinants ...
Towards a Japanese Language Learning Process Based on Japanese Dubbing -- A Case Study on University Students
The purpose of this paper was to examine the factors influencing adoption of Japanese language learning process based on Japanese dubbing in university students. According to the process of Japanese dubbing, this paper extended activity playfulness to ...
Information
Published in.

Association for Computing Machinery
New York, NY, United States
Publication History
Permissions, check for updates, author tags.
- Online Learning
- Online Video Conference
- Recognition of Prior Learning
- Technology Acceptance Model
- Research-article
- Refereed limited
Funding Sources
- EQUITY Program, Lembaga Pengelola Dana Pendidikan (LPDP), Ministry of Finance, Indonesia
Contributors
Other metrics, bibliometrics, article metrics.
- 0 Total Citations
- 0 Total Downloads
- Downloads (Last 12 months) 0
- Downloads (Last 6 weeks) 0
View Options
Login options.
Check if you have access through your login credentials or your institution to get full access on this article.
Full Access
View options.
View or Download as a PDF file.
View online with eReader .
HTML Format
View this article in HTML Format.
Share this Publication link
Copying failed.
Share on social media
Affiliations, export citations.
- Please download or close your previous search result export first before starting a new bulk export. Preview is not available. By clicking download, a status dialog will open to start the export process. The process may take a few minutes but once it finishes a file will be downloadable from your browser. You may continue to browse the DL while the export process is in progress. Download
- Download citation
- Copy citation
We are preparing your search results for download ...
We will inform you here when the file is ready.
Your file of search results citations is now ready.
Your search export query has expired. Please try again.
Thank you for visiting nature.com. You are using a browser version with limited support for CSS. To obtain the best experience, we recommend you use a more up to date browser (or turn off compatibility mode in Internet Explorer). In the meantime, to ensure continued support, we are displaying the site without styles and JavaScript.
- View all journals
- Explore content
- About the journal
- Publish with us
- Sign up for alerts
- Open access
- Published: 13 September 2024
Does participating in online communities enhance the effectiveness and experience of micro-learning? Evidence from a randomized control trial
- Jiawen Zhu ORCID: orcid.org/0000-0002-9260-6151 1 ,
- Yiran Zhao ORCID: orcid.org/0000-0002-3559-3032 3 &
- Miaoting Cheng 4
Humanities and Social Sciences Communications volume 11 , Article number: 1198 ( 2024 ) Cite this article
Metrics details
- Science, technology and society
In the age of information explosion, people are increasingly accustomed to acquiring knowledge during fragmented periods of time, which has contributed to the growing popularity of micro-learning. However, when micro-learning takes place in non-formal or informal settings, it can be easily disrupted and may lack interaction, negatively impacting the learning experience and knowledge acquisition. This study examined the effect of learning communities on knowledge acquisition and learning experience in non-formal micro-learning settings. An 8-module micro-learning course was designed, and 80 participants were divided into an experimental group with a learning community and a control group without one. All participants completed a pre-test and post-test. The results showed significant improvements in post-test scores for both groups, with no notable difference in knowledge acquisition between them. Learners who took notes and repeatedly reviewed the learning content tended to have higher post-test scores. In addition, differences between the groups in terms of mental effort and satisfaction were insignificant. By the end of the course, the control group expressed a stronger desire to join a learning community. Community learners who made significant progress reported enjoying learning within the community, while those who did not regularly check community messages and experienced a decrease in test scores reported that excessive messaging caused distress. These findings have implications for course designers and researchers aiming to enhance micro-learning through online learning communities.
Similar content being viewed by others
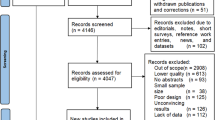
Exploring learning outcomes, communication, anxiety, and motivation in learning communities: a systematic review
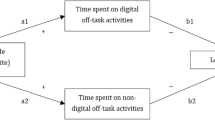
Learning in hybrid classes: the role of off-task activities
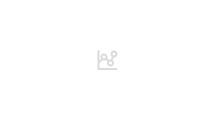
Exploring students’ beliefs about web-based collaborative learning and their practices: a qualitative case study of university English-as-a-foreign-language readers
Introduction.
In the age of information explosion, people are increasingly getting used to searching for information and acquiring knowledge in their spare and fragmented time. Individuals are now more inclined to engage with concise, bite-sized content (Emerson and Berge, 2018 ; Sankaranarayanan et al., 2023 ). Microlearning has emerged as a pivotal instructional strategy to address this shift and reduce the cognitive load, offering access to relatively small learning units. Microlearning is an instructional unit that enables a short engagement in an activity intentionally designed to elicit a specific outcome from the participant (Kapp and Defelice, 2019 , p. 21).
Microlearning’s application spans formal, non-formal, and informal learning settings, where its design plays a crucial role (Jahnke et al., 2020 ). Beyond content creation, establishing an effective learning mechanism is essential (Buchem and Hamelmann, 2010 ). Zhang and West ( 2020 ) emphasized the importance of integrating microlearning with learner interactions, including peer-to-peer, learner-to-expert, and learner-to-content engagements.
Despite recognizing interactions in learning communities as beneficial to microlearning, which is aligned with the Community of Practice theory (Wenger et al., 2002 ), integrating these learning communities with microlearning strategies remains insufficiently explored. Although Emerson and Berge ( 2018 ) advocated for leveraging online communities to enhance informal learning through microlearning modules, and Göschlberger ( 2017 ) identified social media as a potent tool for fostering learner communication, comprehensive strategies for their effective integration are scarce.
This gap is particularly pronounced in settings where microlearning occurs in informal or non-formal contexts, often characterized by learners engaging in self-directed learning in their own time, occasionally isolated from peer support. Challenges such as information overload and difficulties discerning online content further intensify the need for structured guidance (Lu et al., 2019 ). Additionally, there is a noted scarcity of research evaluating the effectiveness of microlearning within learning communities and perceptions by learners (Lee et al., 2021 ; McNeill and Fitch, 2023 ; Taylor and Hung, 2022 ), leaving unanswered questions about the role of online communities in facilitating microlearning and enhancing the learning experience. Specifically, how does using online learning communities in microlearning affect learners’ knowledge acquisition and learning experience?
In order to address these gaps, this study examines the impact of online learning communities on microlearning within a social media-based, non-formal learning context. It aims to elucidate how these communities can be integrated with microlearning strategies and to develop improved materials and activities for community-based microlearning. Through this exploration, the study contributes novel insights into the design and implementation of microlearning environments, establishing a foundation for future academic research and practical applications in digital learning spaces.
Related work
Many individuals feel pressed for time to learn in the fast-paced modern world. At the same time, there is a growing emphasis on professional development and lifelong learning. Against this backdrop, microlearning has emerged as a growing trend in lifelong learning (Giurgiu, 2017 ). Interest in microlearning has surged recently, evidenced by the substantial increase in publications on this topic, as it has garnered significant attention from scholars in the instructional design and technology disciplines (Kohnke et al., 2024 ; Sankaranarayanan et al., 2023 ). Concise learning content enables individuals to efficiently use their limited and fragmented time to access needed information.
Microlearning can be implemented in formal, informal, and non-formal settings. Most research on microlearning focuses on K-12, higher education, or corporate training contexts (Sankaranarayanan et al., 2023 ). Microlearning often serves as supplementary material to traditional classroom content. Teachers often chunk learning materials for students through infographics (Ozdamlı et al., 2016 ) or flashcards (Edge et al., 2012 ) and provide timely feedback. Students who adapt to the microlearning approach often find it a better learning experience than traditional face-to-face learning (Mohammed et al., 2018 ).
Reflecting its versatile nature, microlearning extends beyond traditional school settings. Corporations can make training content accessible to their employees through microlearning lessons. This form of work-based short-term training is known as microtraining (Buchem and Hamelmann, 2010 ). Such microlearning reduces training pressure and increases companies’ competitive advantage (Dolasinski and Reynolds, 2020 ). In addition, this learning approach does not require employees to be in a specific location or at a specific time during training, eliminating the time and physical space constraints for employees and reducing training costs.
Microlearning is often used in non-formal and informal forms, implying that learners are in a more spontaneous state to acquire knowledge. They may complete microlearning online by independently searching for information or taking some online microlearning courses. Scholars generally agree that microlearning can help learners acquire information, reduce cognitive load, achieve high satisfaction, and have a good learning experience (Buchem and Hamelmann, 2010 ; Giurgiu, 2017 ). However, studies have yet to explore the impact of peer interaction in a community on microlearning.
Unlike microlearning, which occurs in formal and corporate training settings, microlearning in non-formal and informal settings expects learners to learn spontaneously. However, research indicates that effective learning involves consuming content to replicate expert knowledge and creating content through social interaction and exploration (Buchem and Hamelmann, 2010 ). By transforming their role from consumer to producer, learners are more motivated and take greater responsibility for achieving their learning goals. This role transformation, in turn, requires learners to be more proactive in interacting with others and applying the information they learn, thus making it easier to acquire knowledge. Abed et al. ( 2024 ) and Wang et al. ( 2017 ) support that there were significant differences in scores between learners who actively interacted with the instructor and those who did not respond to instructor messages.
Online learning communities provide an environment for learners to communicate with others. They are commonly used in formal learning settings, with positive outcomes (Wu et al., 2017 ). Online learning communities promote collaboration among learners and enhance their competencies in a particular area. Based on the Community of Practice theory (Wenger et al., 2002 ), our study explored the impact of microlearning in a community on learners’ knowledge acquisition and learning experience. Researchers have found that learners who learn in communities have improved learning performance and achieve higher satisfaction (Jiménez-Zarco et al., 2015 ). Additionally, these communities facilitate microlearning beyond the classroom setting. Such learning communities are often linked to professional development (Chen et al., 2014 ). Learners are self-driven to communicate and share in learning communities.
However, the impact of joining learning communities on learners’ knowledge acquisition and learning experience in microlearning has yet to be conclusively determined. A case study showed that most learners preferred to learn independently rather than in a group, and many felt that they enjoyed receiving information more than producing it (Buchem and Hamelmann, 2010 ). Speily and Kardan ( 2018 ) also pointed out that most learners in online learning communities remained latent, and learners from different backgrounds caused a decrease in communication and information sharing. Lu et al. ( 2019 ) and Kumar et al. ( 2023 ) also noted that too much online information might affect learners’ information recognition. Given the diverse backgrounds of microlearning learners in online learning communities and the incredible amount of information generated by many learning communities on today’s social media platforms, it still needs to be determined how microlearning with learning communities impacts knowledge acquisition and learning experience. Therefore, this study investigates the impact of applying online learning communities in microlearning on learners’ knowledge acquisition and learning experience.
Research design
This study employed a mixed-methods sequential explanatory design. This approach was driven by the objective of comprehensively understanding the impact of microlearning in online communities. It allows for an initial quantitative analysis of learning outcomes, followed by qualitative investigations to explore the dynamics behind these outcomes. First, a randomized control trial (RCT) involving 100 learners openly recruited from Chinese-language online communities was conducted to evaluate the effectiveness of community-based microlearning on knowledge acquisition and learning experiences. Semi-structured interviews were then conducted with 10 participants randomly selected from the experimental and control groups (five from each group) to elucidate their learning experiences at the end of the experiment.
Participants
We recruited a diverse group of 100 participants online for the RCT. The eligibility criteria included learners older than 18 interested in the course content. At the end of the RCT, 20 participants dropped out due to time constraints or finding that the course content needed to meet their expectations. Therefore, the data analysis was conducted on 80 participants. This group, which included 13 males and 67 females, had an average age of 24.8 years. Undergraduates accounted for 16.25%, while graduate students comprised 46.25%.
Ten participants were recruited from the 80 learners for interviews. Considering their groups, age, pre-test and post-test scores, and community preferences, five learners from the experimental group and five from the control group were selected for the interviews. Their demographic information is shown in Table 1 .
Learning materials
The course design was under Gagné's ( 1985 ) learning theory. Gagné's framework identifies nine instructional events that, when effectively applied, significantly enhance the learning process. This framework was chosen for its comprehensive approach to structuring educational content, particularly its emphasis on sequencing information and providing conditions conducive to learning. By aligning the course with Gagné's principles, such as gaining attention, informing learners of objectives, stimulating recall of prior knowledge, and providing guidance for learning, we aimed to optimize the effectiveness of the microlearning modules.
The learning materials consisted of an 8-module microlearning course on conducting interviews in research studies, with 20 microlearning course videos. Each video was 3–10 min in length. The instructor appeared in the top-right corner of each video (see Fig. 1 ). The course content, specifically designed to explain qualitative research methods through the lens of interviews, included a comprehensive overview of the course, preparation strategies for conducting interviews, essential tools, various types of interviews, interview formats, procedural steps, and critical considerations. This curriculum aims to equip learners with a thorough understanding of conducting qualitative interviews as a research method.
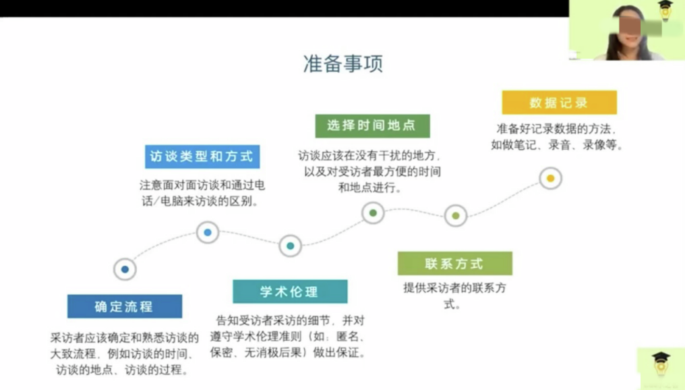
A screenshot of the course video.
Each microlearning video typically concluded with 1–3 reflection questions. Sample questions were like, “Would your interview design be better suited to using focus group interviews or in-depth interviews?” or “What types of questions are appropriate for your research project?” The reflection questions help learners review and deepen their understanding of the course content. They could also answer the questions and send their answers to the instructor (for both groups) or the community (for the experimental group only) to discuss with the instructor or community members. Learning materials were sent to each learner on WeChat as a link through a private message (for the control group) or a group message (for the experimental group). The course lasted 20 days, with one microlearning video sent to students daily. However, the instructor did not force learners to study one lesson per day on time. In other words, learners could study at any time or anywhere. If a learner did not post anything in the group or contact the instructor by private message for more than five days, i.e., no interactive behaviors, the instructor would remind them of learning via private messages.
Data collection
The experiment was conducted online in the summer of 2022. Before data collection, all participants had to sign an informed consent form detailing the study’s purpose and agreeing to the use of their data. A pre-test on knowledge and a pre-course survey were conducted, with 80 participants completing both. Participants were then randomly assigned to two equal-sized groups: the experimental and the control. A balance test was conducted, and there were no significant differences between the groups in any of the variables measured in the pre-test and pre-survey.
Both groups received the same instructions from the same instructor using identical learning materials delivered over WeChat, a mobile chat app widely used in China. For the experimental group, a WeChat group chat was created to form a learning community where regular peer interaction activities were organized. Participants voluntarily engaged in discussions within the community. In the control group, participants could interact with the instructor individually. In this RCT, participants took an 8-module course on interview research methods. After the course, 80 participants completed a knowledge test and a learning experience survey. The attrition rate was 20%, with twenty participants (10 from each group) dropping out due to time constraints.
Given the data attrition, the researcher used t-tests and non-parametric tests to explore differences between dropouts and remaining participants. Although there was data attrition, no significant differences were found between the remaining participants in the experimental and control groups in terms of gender, age, pre-test scores, and prior knowledge. Moreover, the attrition did not significantly affect the data analysis for the follow-up study. There were no significant differences between the experimental and control groups regarding gender and age, suggesting that the study did not suffer from significant attrition bias.
Tests and surveys were designed and distributed using Tencent Survey, a widely used online survey platform in China. Before the course, learners completed a pre-course survey and a pre-knowledge test. All answers to the 20 questions were covered in the course materials. The pre-course survey collected learners’ demographic information, such as age, gender, and year of study. After the course, learners were given a link to complete the post-survey and post-test. The post-survey inquired about learners’ learning habits, satisfaction, mental effort, and preference for learning within an online community. The post-knowledge test was identical to the pre-knowledge test. Experimental group learners who joined the community were additionally asked questions about their sense of community. Interview data were collected and recorded after the post-survey. Ten semi-structured interviews were conducted through Tencent Meeting.
Measurement methods
Knowledge acquisition variable: knowledge test.
The knowledge test consisted of 20 multiple-choice questions about interview research methods, and the content of the knowledge test in the pre-and post-test was the same. The knowledge test was designed by the researchers and examined by two experts in educational technology for content validity. A pilot test was conducted among learners with and without previous learning experience using interview research methods. The learners with previous learning experience in interview research methods scored higher than those with no previous learning experience in interview research methods. Their feedback was used to refine the test. Scores for the pre-test and post-test were calculated on the number of correct answers in the pre-and post-tests. The total score was 20 points, respectively. The pre-test had acceptable internal consistency ( N = 100, KR-20 = 0.69), and the post-test reached good internal consistency ( N = 80, KR-20 = 0.73).
Learning experience variables
Satisfaction.
Learners’ learning experience satisfaction was measured on the post-test using a satisfaction scale adapted from Ritzhaupt et al., ( 2008 ) study. We translated it into Chinese. It is a five-point scale with 9 questions and two bipolar adjectives on both sides. For example, on the left side is the description “obscure” and on the right side is the description “clear”. The satisfaction data has good internal consistency, with a Cronbach’s alpha ( α ) equals to 0.85. The mean score of the 9 items was calculated.
Mental effort
Learners completed a 9-point scale for self-reported mental effort during multimedia learning (Paas, 1992 ) on the post-test. The mental effort scale ranges from “very, very low mental effort” to “very, very high mental effort”. The self-reported mental effort scale was coded from 1 to 9, with higher scores indicating more mental effort required by the learners.
Sense of community
A sense of community scale, adapted from the one produced by Rovai ( 2002 ), was used to collect learners’ perceptions of learning in communities on the post-test. This 5-point Likert scale contains 20 items, which were divided into two factors by Rovai ( 2002 ), i.e., connectedness and learning. The sense of community scale was coded as: for statements 1, 2, 3, 6, 7, 11, 12, 13, 15, 16, and 19, strongly agree = 4, agree = 3, neutral = 2, disagree = 1, and strongly disagree = 0. The remaining items were inverted: strongly agree = 0, agree = 1, neutral = 2, disagree = 3, and strongly disagree = 4 (Rovai, 2002 ). The scores of each subscale 10 items were added together, and mean scores were calculated. Learners with higher scores had more positive attitudes towards the community. Both the connectedness and learning factor in the sense of community scale had good internal consistency with Cronbach’s alpha of 0.76 and 0.80, respectively. The overall course community scale had good internal consistency ( α = 0.84).
Preference for learning in a community
We also asked participants’ preferences for learning in a community. In the post-survey, we asked learners if they preferred to learn in a community after the 8-module course. Learners could express their opinions by choosing “yes” or “no”. Their answers with “yes” were coded as 1, and “no” was coded as 0.
Data analysis methods
A paired sample t -test was conducted to examine whether the micro-learning course was effective in enhancing learner knowledge of interview methods for all the participants. Then, to explore the impact of applying an online learning community in microlearning on learners’ knowledge acquisition, a one-way ANCOVA was conducted to examine whether there were significant differences in the post-test scores between the experimental and control groups while controlling for pre-test scores. Next, to investigate the effect of the online learning community on the learning experience, one-way ANOVA tests were conducted to analyze whether the two groups differed significantly in mental effort, preference for learning in a community, and satisfaction. Since only the experimental group had a community, there was only data from the experimental group on the sense of community. A descriptive analysis was conducted to analyze their experience and sense of community.
Interview data was transcribed in Chinese and then translated into English. We browsed the interview transcripts and extracted key information that indicated learners’ knowledge acquisition and learning experience. Then, we reviewed and reported what we found in the results.
In this section, we present our findings. We used the letter + number for interview results to refer to interviewees. For example, “E1” refers to an interviewee from the experimental group, while “C2” refers to an interviewee from the control group.
Descriptive data
Table 2 shows the means and standard deviations for experimental and control group learners by gender, age, prior knowledge, pre-test, post-test, mental effort, and satisfaction. These measures were comparable between the groups. Learners who did not study in a community reported higher post-test scores but required slightly higher mental effort to complete the course.
Differences in the acquisition of knowledge
Without considering grouping, we used a paired sample t -test to analyze learners’ knowledge acquisition through 8-module microlearning. The result showed that the learners’ post-test scores ( M = 15.48) were significantly higher than the pre-test ( M = 12.83), t (79) = 9.657, p < 0.001. This suggests that the course successfully enhanced participants’ knowledge about interview methods.
A one-way ANCOVA, with the pre-test scores controlled, was conducted to examine whether having an online learning community would further enhance knowledge acquisition. Results indicated no significant difference in the post-test scores between the experimental and control groups ( F (1, 76) = 0.257, p = 0.614). Joining a community had no significant effect on learners’ knowledge acquisition.
Findings from the interview data were in tandem with those from quantitative analysis. Learners who progressed through the course and those whose test scores slid existed in both groups. Interviews were used to explore further their mastery of the course content, which revealed that the inclusion of learners in a community did not have minimal effects on their mastery but that the way they learned played a more critical role. Learners who repeatedly studied the microlearning content and took notes during the learning process usually had higher post-test scores than pre-test scores. For example, E3, who scored 13 points on the pre-test, took notes using her iPad and scored 20 points on the post-test. Moreover, C4 mentioned that she took notes in her notebook as she watched the video. If she forgot some learning points, she would go back and watch the learning content again. C4 got 14 points on the pre-test but scored 20 points on the post-test. During the interviews, they reported that they could clearly recall the content of each module. For instance, E3 said, “Sometimes the instructor would ask us in the video if we remembered the content mentioned in the previous course. If I did not remember the content, I would immediately find the previous course video to make sure I remembered it.” C4 said, “I would find a quiet time to watch the microlearning videos without interruptions and record the content of the lessons. In this way, I could open my notes for review during the weeks.”
Other learners reported that in the learning process, they studied repeatedly to practice what they learned and searched information online for what they did not understand. For example, C1 shared an experience:
During the microlearning course, I happened to need to use the interview research method. So, I used interview methods over and over again to collect the data I needed. The course really helped me a lot…… When I encountered something I could not understand, I would go to literature and collect more information online, so I could successfully collect the interview data .
Learners whose post-test scores were lower than their pre-test scores indicated in the interviews that they might not listen well enough during online microlearning. When asked what they remembered about the course content, they only gave the general course framework or remembered only the content of a particular module that the instructor repeatedly emphasized. For instance, E1 admitted, “ Sorry, I do not really remember exactly what I learned; I just remember that the instructor focused on the steps of the interview research method and that the teacher said it many times .”
Differences in the learning experience
Learning experiences in this study included the levels of mental effort that learners believed they needed to invest in the 8-module course, their preferences for learning in a community, and their satisfaction with the learning process. One-way ANOVA was conducted to determine whether the groups had significant differences regarding their learning experience. Experimental group learners who studied in the community also reported their sense of community in the post-survey.
Though the descriptive data showed that learners from the control group ( M = 4.55) required slightly more mental effort than learners from the experimental group ( M = 4.38), there was no significant difference between the two groups according to one-way ANOVA results on their self-reported mental effort score, F (1,78) = 0.226, p = 0.636 (see Table 3 ).
Both groups of learners indicated in the interviews that the microlearning content was relatively easy. Learner E5 said, “The microlearning lessons were all relatively short in length, no more than 10 min, as I remember. The instructor sent us one lesson per day, so I did not need to spend much effort to complete the course.” Learner C4 also said, “The microlearning videos were shorter, and I could keep track of learning on my own; therefore, I sometimes accumulated a few days of learning content together.” She said that she liked this self-directed learning process. Learned E2 noted, “I checked the community information to see what my peers were discussing to deepen my understanding of the course content.”
Community preferences
Interestingly, there was a significant difference between the experimental and control group learners on their community preferences, F (1,78) = 6.27, p = 0.01 (see Table 3 ). Based on the descriptive data, learners who did not join a community ( M = 0.82) were more likely to prefer having a learning community than learners in a community ( M = 0.57).
Through the interviews, we found that learners had too many chat groups (learning communities) on WeChat, where they received hundreds of community messages every day, resulting in their ignoring the information. Learners in the experimental group mentioned that they did not check the community messages daily. They only skimmed through the information and may not have made much of an impression. Learner E4 said, “I took time out of my busy day to study the microlearning course. Since there are so many group messages, I often choose to mute them so that I am not disturbed by too many messages. But this may also lead to me missing much information.” Besides, some learners in the experimental group reported that community learning enabled them to enhance learning. Learner E1 said, “Sometimes there were learners in the group who asked questions that happened to be confusing to me as well, and I think it is very rewarding to learn in a community.”
Moreover, learners in the control group were often excited about community learning, even though they did not join the community during the experiment. Learner C3 told us, “I think I would have learned more if there had been a community.” However, when asked if they would choose to interact in a community if they had joined a community at the beginning of the course, many of the control group learners indicated that they probably would not have. Learner C2 explained, “I prefer to watch others speak, but I would probably not choose to speak in a community.” Learner C4 also noted, “I would be more apprehensive about speaking in public when there are many learners in a community. I am afraid of saying the wrong things.” Of course, not every learner from the control group would like to learn in a community. Learner C5 refused to learn in a community and thought that she would most likely not check the group messages often. She believed that the important thing about microlearning for her was understanding the course content and that the discussion part was not essential to her.
We found no significant difference between the experimental and control groups on their satisfaction scores, F (1,78) = 0.297, p = 0.587 (see Table 3 ). Based on the descriptive data, learners who did not join a community ( M = 4.08) and those who were in a community ( M = 4.01) were similar in terms of satisfaction.
Both groups expressed high satisfaction with the microlearning course. They perceived that the microlearning content was easy to understand, the course content was useful, and the instructor would help them during the learning process. Learners in the control group mentioned that the daily private messages from the instructor made them feel valued (see Fig. 2 ). Learner C1 said, “I always felt like the instructor I received messages from every day was a robot until one day I asked a question, and the instructor answered it patiently. I was so excited that I communicated more with my instructor since that day.” Some learners in the experimental group reported that learning in a community positively impacted their learning experience. Learner E1 mentioned that she learned a lot from examples shared by other more experienced learners in the community; “They were like course assistants. Reading the messages about their experiences related to interviews helped me understand the interview research method.”
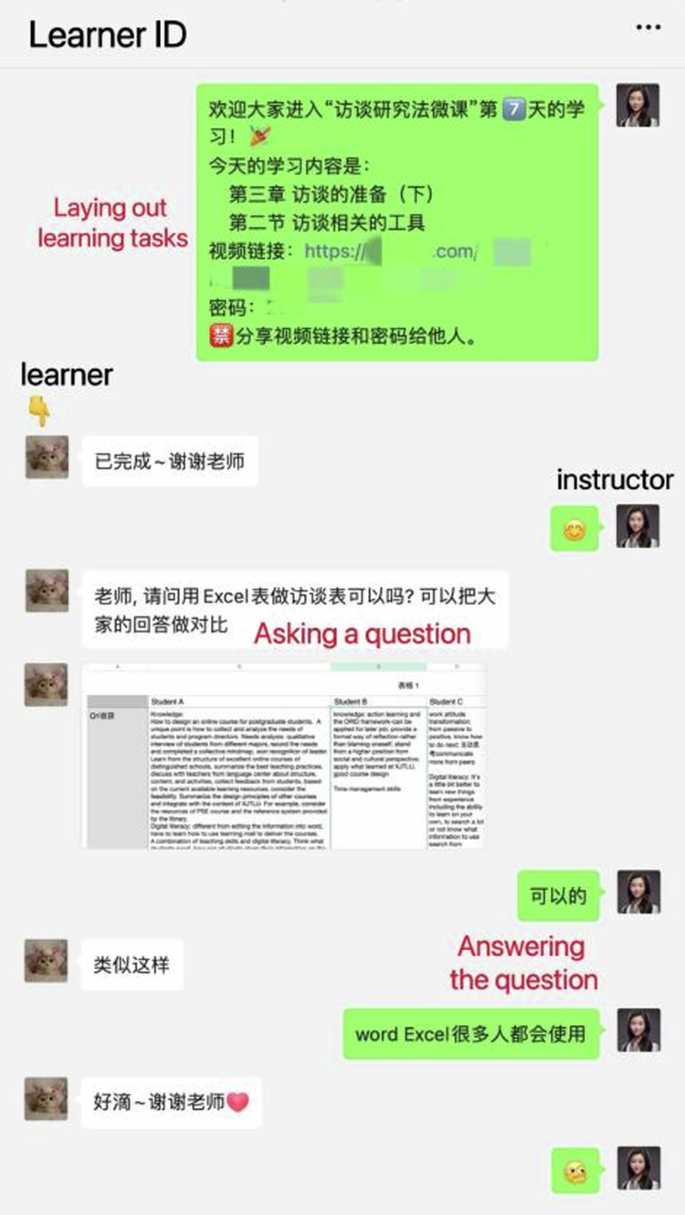
A screenshot of private messages between a learner and the instructor.
Forty learners in the experimental group reported their sense of community in the post-survey. Table 4 reports the mean and standard deviation of their sense of community scores.
Community learners who made significant progress in their performance mentioned that they enjoyed learning in the community. Communication in the community helped them better understand the learning materials, and the community provided good opportunities for them to learn comfortably (see Fig. 3 ). “I think sometimes other learners’ questions were also my questions. It made me happy to see the questions being answered in the community,” said E2. E3 explained, “I was able to see the perspective of my peers thinking through their responses. When they answered the same question from different angles, I was able to think about the same question from various perspectives. It is interesting.” E2 and E5 mentioned that by checking the community messages, they were able to discover some points they had overlooked in the study process, which helped them fill in the knowledge gaps. At the same time, E2 also raised the problem of missing information due to the large number of WeChat community messages and hoped that the managers or instructors could provide collated key information.
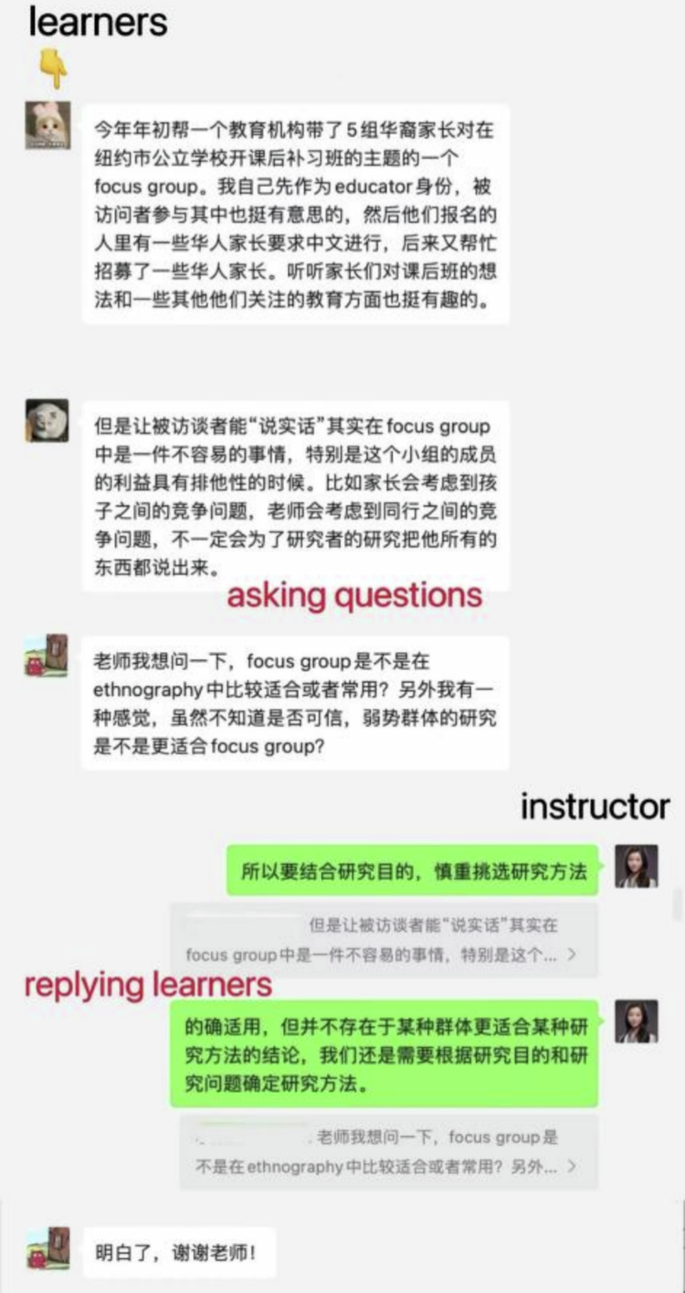
A screenshot of discussions in the community.
However, some learners did not check the community messages very often, and their test scores dropped. For instance, E4 said, “I do not think the presence of a community has much impact on me, and I do not really read the group messages anyway. Sometimes, too many group messages are a nuisance to me.” E1, when asked if she checked community messages, said, “I have so many communities with too much information to read that I usually muted them. But this also caused me to sometimes forget to check the group messages and miss the key information.” At the same time, she also mentioned that even if she checked the group messages, she did not remember any key information. She had not posted anything nor connected with anyone in the community.
In this study, we examined the effectiveness of community-based microlearning on knowledge acquisition and learning experiences. In particular, we engaged 80 learners, 40 of whom were in the community and another 40 who were not. This section revisits the research question with two aspects, knowledge acquisition, and learning experience, and discusses the implications.
Knowledge acquisition
The majority of participants in this study demonstrated significant improvements in learning performance through microlearning. This finding is consistent with the existing microlearning research that has reported increased knowledge (Lee et al., 2021 ). The concise format of microlearning effectively breaks down complex content into digestible chunks, facilitating clearer and easier comprehension. This also makes it easier for learners to grasp the main points. Microlearning has been recognized as a potent instructional approach or intervention across various educational contexts, including higher education (online, hybrid, and blended courses), corporate training, and professional development for K-12 teachers (Sankaranarayanan et al., 2023 ). Using microlearning as an intervention is crucial because it provides a focused, efficient, and adaptable learning experience tailored to the unique needs of learners in these diverse settings. Yet, the presence of a learning community did not significantly influence knowledge acquisition among learners. This contrasts the findings of Jiménez-Zarco et al. ( 2015 ), who found that learning in a community improved learners’ learning performance. This outcome may stem from the inherent characteristics of microlearning itself. Jiménez-Zarco et al. ( 2015 ) did not provide specific learning materials; learners joined a virtual community of practice to obtain the information they needed selectively. In contrast, our study provided learning materials and aimed to help learners master the basic knowledge points of interview research methods through micro-lessons. While learners might prefer a more relaxed and informal learning setting, it could result in a less serious engagement with microlearning content. Disengaged learning combined with too much online information may disrupt learners’ receptivity to the information. This is also similar to Lu et al. ( 2019 ) findings, which reported that overwhelming information in the online environment sometimes hinders learners’ recognition in response to online information.
Interview data revealed the seriousness with which learners approached the course content, and their learning choices significantly impacted knowledge acquisition. When learners listened carefully, took notes, and selectively played back course content, they were able actually to remember more information and receive higher test scores. Kauffman et al. ( 2011 ) found that learners with high self-monitoring prompts used matrix note-taking devices and improved learning achievement in online learning environments. In addition, playing back the course video means reviewing the learning content, which could help learners deepen their impression of the knowledge points and thus achieve higher test scores. Instructors can encourage note-taking during microlearning sessions and assist learners in reviewing content to enhance retention.
Learning experience
Despite the lack of a statistically significant difference in mental effort between groups, descriptive data indicated that community participants expended marginally less effort to complete the course compared to their non-community counterparts, perhaps because the community dissolved the cognitive load that would otherwise have to be undertaken by each learner in the experimental group. Jung et al. ( 2015 ) supported this finding that under low cognitive load conditions, an individual can adequately process activities, and learning collaboratively may generate the costs of recombination and coordination. Microlearning requires low mental effort because it is concise. Therefore, the mental effort required in microlearning is low, so whether or not learners were in a community did not significantly affect mental effort.
There was no significant difference in learning satisfaction between the two groups of learners, with most expressing satisfaction with the microlearning process. However, learners who completed the post-test demonstrated higher course satisfaction, while those who were less satisfied may have discontinued their participation earlier. Angelino et al. ( 2007 ) noted that online learning had higher dropout rates, ranging from 10 to 20 percent. Unlike traditional learning classes, learners engage in spontaneous learning in informal and non-formal settings. The microlearning activities they participated in gave them greater autonomy (Kohnke et al., 2024 ), making them more likely to discontinue learning when they encountered problems with time limits or when the content did not meet their expectations. This finding provides insight to community managers and micro-course educators that creating a community does not mean that learners will be more satisfied and that it is important to explore how to use course design to retain learners.
We found that learners who were not in a community were eager to learn in a community, but learners who were in a community might ignore or choose to mute community messages. As mentioned by Lu et al. ( 2019 ) and Kumar et al. ( 2023 ), for learners, too much information in the online environment may have a negative impact on learning. Chane et al. ( 2022 ) also noted that students preferred to receive personal attention from the teachers. In our study, the instructor sent private messages to the control group learners, and those who received the instructor’s private messages might be able to get noticed; in contrast, learners in the experimental group might miss the instructor’s public message sent to the entire community if they chose to mute the community message reminder and thus were unable to feel the instructor’s attention to them. Although learners in the control groups expressed the urge to join a community, they also received additional personal attention from the instructor. Although learners in the control group without learning communities wished to have an opportunity to communicate with others, they also expressed uncertainty when asked if they would post in an online community. Whether or not they would post in a community is influenced by many factors. A large number of messages already in the communities may cause learners to be reluctant to post in the community (Nguyen, 2021 ; Nonnecke et al., 2006 ). Beaudoin ( 2002 ) also noted that learners were often apprehensive about speaking in public. When they were unsure that their responses were helpful, they usually chose not to post.
Community learners’ sense of community may be polarized. Learners who were actively engaged in community learning and had improved their test scores enjoyed their learning in the community. They were able to learn about the perspectives of their peers from the community information, which helped them fill in the knowledge gaps (Schreurs, 2014 ). For learners who did not check the community information frequently or even mentioned in the interview that they did not need the community, they often chose to mute the community and received lower test scores. The community information may cause information overload for them. Kuo et al. ( 2017 ) also supported this finding. They found that the sense of community and perceived collaborative learning contributed significantly to learning, and most students in the groups had a positive sense of community. Moreover, Speily and Kardan ( 2018 ) mentioned that learners in online learning communities had different backgrounds, which might result in information not being applicable to all learners and learners being reluctant to share in the community. This is one of the reasons why some of the interviewees mentioned that they were reluctant to post in a community when the community is large and lacks connections. If group members are familiar with each other, they may find it easier to communicate within the group. High familiarity could contribute to online collaboration and give group members a more positive sense of community (Janssen et al., 2009 ). This suggests that community managers and educators should consider forming multiple small groups based on learners’ backgrounds to foster a more cohesive and supportive online learning environment. Kohnke et al. ( 2024 ) emphasized the importance of enhancing interactions in microlearning activities, suggesting that learners can feel more connected and engaged by improving the quality and frequency of these interactions. This helps to reduce the number of learners in each community, create communities with closer relationships, and promote community members to post in the community and gain a more positive sense of community (Speily and Kardan, 2018 ).
During the interviews, some learners expressed a desire to summarize and organize the information in the community into a document so that everyone could review the messages that had been discussed. In their study, Yang et al. ( 2004 ) indicated that creating a document through collaboration could motivate learners to share knowledge in an online learning community, help them sort out the relationships between knowledge points, and contribute to knowledge acquisition. This is also a good suggestion for microlearning designers and educators. Especially in social media-based microlearning, learners are often distracted by redundant information. Providing collaborative documents and encouraging learners to edit and contribute may motivate them to engage better in microlearning, facilitate tracking their learning progress, and keep them from missing out on important community discussions.
In light of these findings, it becomes imperative for course designers to meticulously consider the composition and management of online learning communities to optimize learner knowledge acquisition and learning experience. Specifically, designing the format of microlearning that is responsive to the learners’ feedback and preferences, as uncovered in our qualitative insights, can significantly enrich the learning experience. For researchers, these results highlight the critical need for further exploration into the effective integration of community features within microlearning environments. This study, therefore, not only contributes to the existing body of knowledge by providing empirical evidence on the efficacy of online learning communities in microlearning settings but also paves the way for future research to explore educational designs that cater to the evolving needs of learners.
Limitations and future studies
Some limitations of this study are listed as follows: First, the number of learners who participated was relatively limited. The attrition rate of the RCT was 20%. Future research could use the same learning materials designed for this study to recruit more learners and validate the experimental findings. Researchers could also take measures to prevent attrition and conduct sensitivity analysis after attrition. Second, we did not include questions about how learners chose to learn, such as whether they took notes during learning and re-played the study videos in our post-survey design. Future studies could include these questions in the experimental design to find evidence of what we found in the interview data. Third, learners were required to complete the post-test immediately after the 8-module microlearning course. Future studies can implement several tests during and long after the course to understand the changes in learners’ knowledge acquisition.
Microlearning, the latest lifelong learning trend, has attracted the public’s attention. Although many studies have been done on microlearning, researchers lack empirical findings on the impact of online learning communities on microlearning learners regarding knowledge acquisition and learning experience. Through its empirical exploration, this study illuminates the nuanced role of online learning communities in enhancing microlearning, focusing on knowledge acquisition and the learner’s experience. The conclusions of this study underline critical implications for educators, researchers, and microlearning designers, underscoring the paramount importance of delivering personalized learning experiences in the design of microlearning courses. It highlights the necessity of preemptively gathering data on learners’ preferences and their informational absorption capacity to tailor the micro-courses effectively. Furthermore, the study advises against overcrowding online learning communities, suggesting instead that community managers should foster interactive opportunities and prevent excessive lurking by learners. Significantly, the findings caution that providing online learning communities does not automatically enhance the learning experience. There is a vital need for a focused approach to providing personalized microlearning features, ensuring they align with individual learner profiles. This expanded understanding not only enriches the learning experience but also marks a significant stride in optimizing the efficacy of online learning environments through targeted, learner-centered strategies.
Data availability
Data will be made available from the corresponding author upon reasonable request.
Abed LG, Abed MG, Shackelford TK (2024) Does student-instructor interaction in universities influence academic attainment? The context of Saudi Arabia. Innov Educ Teach Int 61(2):303–314. https://doi.org/10.1080/14703297.2023.2173270
Article Google Scholar
Angelino LM, Williams FK, Natvig D (2007) Strategies to engage online students and reduce attrition rates. J Educ Online 4(2)
Beaudoin MF (2002) Learning or lurking?: tracking the “invisible” online student. Internet High Educ 5(2):147–155. https://doi.org/10.1016/S1096-7516(02)00086-6
Buchem I, Hamelmann H (2010) Microlearning: a strategy for ongoing professional development. ELearn Pap 21(7):1–15
Google Scholar
Chane R, Gupta G, Kaur J, Kumar M (2022) Transition to online teaching during covid times: a comparative study of online education and traditional offline education from A 1st-year medical student’s perspective. Int J 5(3):789
Chen HL, Fan HL, Tsai CC (2014) The role of community trust and altruism in knowledge sharing: an investigation of a virtual community of teacher professionals. Educ Technol Soc 17(3):168–179. https://www.jstor.org/stable/jeductechsoci.17.3.168
Dolasinski MJ, Reynolds J (2020) Microlearning: a new learning model. J Hosp Tour Res 44(3):551–561. https://doi.org/10.1177/1096348020901579
Edge D, Fitchett S, Whitney M, Landay J (2012) MemReflex: adaptive flashcards for mobile microlearning. Paper presented at the proceedings of the 14th international conference on human–computer interaction with mobile devices and services, pp. 431–440
Emerson LC, Berge ZL (2018) Microlearning: knowledge management applications and competency-based training in the workplace. Knowl Manag E-Learn 10(2):125–132. https://doi.org/10.34105/j.kmel.2018.10.008
Gagné R (1985) The conditions of learning and theory of instruction, 4th edn. Holt, Rinehart and Winston
Giurgiu L (2017) Microlearning an evolving elearning trend. Sci Bull-Nicolae Balcescu Land Forces Acad 22(1):18–23
Göschlberger B (2017) Social microlearning motivates learners to pursue higher-level cognitive objectives. In: Vincenti G, Bucciero A, Helfert M, Glowatz M (eds) E-learning, E-education, and online training, vol 180. Springer International Publishing, pp. 201–208
Jahnke I, Lee YM, Pham M, He H, Austin L (2020) Unpacking the Inherent design principles of mobile microlearning. Technol Knowl Learn 25(3):585–619. https://doi.org/10.1007/s10758-019-09413-w
Janssen J, Erkens G, Kirschner PA, Kanselaar G (2009) Influence of group member familiarity on online collaborative learning. Comput Hum Behav 25(1):161–170. https://doi.org/10.1016/j.chb.2008.08.010
Jiménez-Zarco AI, González-González I, Saigí-Rubió F, Torrent-Sellens J (2015) The co-learning process in healthcare professionals: assessing user satisfaction in virtual communities of practice. Comput Hum Behav 51:1303–1313. https://doi.org/10.1016/j.chb.2014.11.057
Jung I, Kudo M, Choi SK (2015) Towards effective and less stressful online collaborative learning: Strategies to promote engagement while minimizing unnecessary cognitive load and stress. In: Badrul HK, Mohamed A (eds) International Handbook of E-learning, vol 1. Routledge, New York, pp. 115–126
Kapp KM, Defelice RA (2019) Microlearning: short and Sweet. American Society for Training and Development
Kauffman DF, Zhao R, Yang YS (2011) Effects of online note taking formats and self-monitoring prompts on learning from online text: using technology to enhance self-regulated learning. Contemp Educ Psychol 36(4):313–322. https://doi.org/10.1016/j.cedpsych.2011.04.001
Kohnke L, Foung D, Zou D (2024) Microlearning: a new normal for flexible teacher professional development in online and blended learning. Educ Inf Technol 29(4):4457–4480. https://doi.org/10.1007/s10639-023-11964-6
Kumar A, Shankar A, Tiwari AK, Hong HJ (2023) Understanding dark side of online community engagement: an innovation resistance theory perspective. Inf Syst e-Bus Manag 1–27. https://doi.org/10.1007/s10257-023-00633-3
Kuo YC, Belland BR, Kuo YT (2017) Learning through blogging: students’ perspectives in collaborative blog-enhanced learning communities. Educ Technol Soc 20(2):37–50. https://www.jstor.org/stable/90002162
Lee YM, Jahnke I, Austin L (2021) Mobile microlearning design and effects on learning efficacy and learner experience. Educ Technol Res Dev 69(2):885–915. https://doi.org/10.1007/s11423-020-09931-w
Lu Y, Mao X, Wang T, Yin G, Li Z, Wang W (2019) Studying in the ‘bazaar’: an exploratory study of crowdsourced learning in GitHub. IEEE Access 7:58930–58944. https://doi.org/10.1109/ACCESS.2019.2915247
McNeill L, Fitch D (2023) Microlearning through the Lens of Gagne’s nine events of instruction: a qualitative study. TechTrends 67(3):521–533. https://doi.org/10.1007/S11528-022-00805-X
Article PubMed Google Scholar
Mohammed GS, Wakil K, Nawroly SS (2018) The effectiveness of microlearning to improve students’ learning ability. Int J Educ Res Rev 3(3):32–38. https://doi.org/10.24331/ijere.415824
Nguyen TM (2021) Four-dimensional model: a literature review in online organisational knowledge sharing. VINE J Inf Knowl Manag Syst 51(1):109–138. https://doi.org/10.1108/VJIKMS-05-2019-0077
Nonnecke B, Andrews D, Preece J (2006) Non-public and public online community participation: needs, attitudes and behavior. Electron Commer Res 6(1):7–20. https://doi.org/10.1007/s10660-006-5985-x
Ozdamlı F, Kocakoyun S, Sahin T, Akdag S (2016) Statistical reasoning of impact of infographics on education. Procedia Comput Sci 102:370–377. https://doi.org/10.1016/j.procs.2016.09.414
Paas F. G (1992) Training strategies for attaining transfer of problem-solving skill in statistics: A cognitive-load approach. J Educ Psychol 84(4):429
Ritzhaupt AD, Gomes ND, Barron AE (2008) The effects of time-compressed audio and verbal redundancy on learner performance and satisfaction. Comput Hum Behav 24(5):2434–2445. https://doi.org/10.1016/j.chb.2008.02.017
Rovai AP (2002) Sense of community, perceived cognitive learning, and persistence in asynchronous learning networks. Internet High Educ 5(4):319–332. https://doi.org/10.1016/S1096-7516(02)00130-6
Sankaranarayanan R, Leung J, Abramenka-Lachheb V, Seo G, Lachheb A (2023) Microlearning in diverse contexts: a bibliometric analysis. TechTrends 67(2):260–276. https://doi.org/10.1007/s11528-022-00794-x
Schreurs B (2014) Analysing learning ties to stimulate continuous professional development in the workplace. In: Hodgson V, de Laat M, McConnell D, Ryberg Th (eds) The design, experience and practice of networked learning. Springer, Cham, Switzerland, pp. 207–224
Speily ORB, Kardan AA (2018) Increasing information reposting behavior in online learning community. Educ Technol Soc 21(4):100–110. https://www.jstor.org/stable/26511541
Taylor AD, Hung W (2022) The effects of microlearning: a scoping review. Educ Technol Res Dev 70(2):363–395. https://doi.org/10.1007/S11423-022-10084-1
Wang J, Gao F, Li J, Zhang J, Li S, Xu GT, Xu L, Chen J, Lu L (2017) The usability of WeChat as a mobile and interactive medium in student‐centered medical teaching. Biochem Mol Biol Educ 45(5):421–425. https://doi.org/10.1002/bmb.21065
Article CAS PubMed Google Scholar
Wenger E, McDermott R, Snyder WM (2002) Cultivating communities of practice: a guide to managing knowledge. Harvard Business School Press, Boston
Wu WCV, Chen JS, Yang JC (2017) Creating an online learning community in a flipped classroom to enhance EFL learners’ oral proficiency. Educ Technol Soc 20(2):142–157. https://www.jstor.org/stable/90002170
Yang SJH, Chen IYL, Shao NWY (2004) Ontology enabled annotation and knowledge management for collaborative learning in virtual learning community. Educ Technol Soc 7(4):70–81. https://www.jstor.org/stable/jeductechsoci.7.4.70
ADS Google Scholar
Zhang J, West RE (2020) Designing microlearning instruction for professional development through a competency based approach. TechTrends 64(2):310–318. https://doi.org/10.1007/s11528-019-00449-4
Download references
Acknowledgements
We would like to thank the research participants who generously agreed to participate in this study and share their time and experience. This work is supported by the Chenguang Program of Shanghai Education Development Foundation and Shanghai Municipal Education Commission and the Peak Discipline Construction Project of Education at East China Normal University.
Author information
Authors and affiliations.
Faculty of Education, East China Normal University, Shanghai, China
School of Library and Information Management, Emporia State University, Emporia, KS, USA
Department of Educational Studies, Academy of Future Education, Xi’an Jiaotong-Liverpool University, Suzhou, China
Department of Educational Technology, Faculty of Education, Shenzhen University, Shenzhen, China
Miaoting Cheng
You can also search for this author in PubMed Google Scholar
Contributions
J.Z.: corresponding author; conceptualization, data collection and analysis, writing, and editing. H.H.: data collection, methodology, review, and editing. Y.Z.: methodology, review, and editing. M.C.: microlearning course design.
Corresponding author
Correspondence to Jiawen Zhu .
Ethics declarations
Competing interests.
The authors declare no competing interests.
Ethical approval
Approval was obtained from the ethics committee of East China Normal University (HR074-2020). The procedures used in this study adhere to the tenets of the Declaration of Helsinki.
Informed Consent
Informed consent was obtained from individual participants before the summer of 2022 ended. All the participants are older than 18.
Additional information
Publisher’s note Springer Nature remains neutral with regard to jurisdictional claims in published maps and institutional affiliations.
Rights and permissions
Open Access This article is licensed under a Creative Commons Attribution-NonCommercial-NoDerivatives 4.0 International License, which permits any non-commercial use, sharing, distribution and reproduction in any medium or format, as long as you give appropriate credit to the original author(s) and the source, provide a link to the Creative Commons licence, and indicate if you modified the licensed material. You do not have permission under this licence to share adapted material derived from this article or parts of it. The images or other third party material in this article are included in the article’s Creative Commons licence, unless indicated otherwise in a credit line to the material. If material is not included in the article’s Creative Commons licence and your intended use is not permitted by statutory regulation or exceeds the permitted use, you will need to obtain permission directly from the copyright holder. To view a copy of this licence, visit http://creativecommons.org/licenses/by-nc-nd/4.0/ .
Reprints and permissions
About this article
Cite this article.
Zhu, J., He, H., Zhao, Y. et al. Does participating in online communities enhance the effectiveness and experience of micro-learning? Evidence from a randomized control trial. Humanit Soc Sci Commun 11 , 1198 (2024). https://doi.org/10.1057/s41599-024-03719-6
Download citation
Received : 04 August 2023
Accepted : 05 September 2024
Published : 13 September 2024
DOI : https://doi.org/10.1057/s41599-024-03719-6
Share this article
Anyone you share the following link with will be able to read this content:
Sorry, a shareable link is not currently available for this article.
Provided by the Springer Nature SharedIt content-sharing initiative
Quick links
- Explore articles by subject
- Guide to authors
- Editorial policies

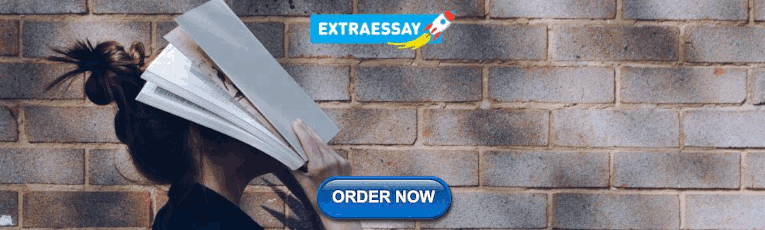
IMAGES
VIDEO
COMMENTS
The purpose of this study is to determine the effect size of online education on academic achievement. Before determining the effect sizes in the study, the probability of publication bias of this meta-analysis study was analyzed by using the funnel plot, Orwin's Safe N Analysis, Duval and Tweedie's Trip and Fill Analysis, and Egger's Regression Test.
This study aims at determining the effect size of online education, which has been highly used since the beginning of the pandemic, on students' academic achievement in different courses by using a meta-analysis method. Meta-analysis is a synthesis method that enables gathering of several study results accurately and efficiently, and getting ...
The COVID-19 pandemic highlighted how distance learning and the special case of online learning would continue to be used in all formal educational settings. Research and best practices on distance and online learning have been implemented in several distance courses (Seaman et al., 2018).
This systematic analysis examines effectiveness research on online and blended learning from schools, particularly relevant during the Covid-19 pandemic, and also educational games, computer-supported cooperative learning (CSCL) and computer-assisted instruction (CAI), largely used in schools but with potential for outside school.
ARTICLE Online vs in-person learning in higher education: effects on student achievement and recommendations for leadership Bandar N. Alarifi1 & Steve Song2 This study is a comparative analysis ...
Online learning is one of the educational solutions for students during the COVID-19 pandemic. Worldwide, most universities have shifted much of their learning frameworks to an online learning model to limit physical interaction between people and slow the spread of COVID-19. The effectiveness of online learning depends on many factors, including student and instructor self-efficacy, attitudes ...
Given the dearth of research on the academic impact of online learning, especially in light of Covid-19 in the educational arena, the present study aims to address that gap by assessing the ...
Metrics. The coronavirus pandemic has forced students and educators across all levels of education to rapidly adapt to online learning. The impact of this — and the developments required to make ...
Zhang et al. (2022) implemented a bibliometric review to provide a holistic view of research on online learning in higher education during the COVID-19 pandemic period. They concluded that the majority of research focused on identifying the use of strategies and technologies, psychological impacts brought by the pandemic, and student perceptions.
1. Introduction. Online learning has been on the increase in the last two decades. In the United States, though higher education enrollment has declined, online learning enrollment in public institutions has continued to increase (Allen & Seaman, 2017), and so has the research on online learning.There have been review studies conducted on specific areas on online learning such as innovations ...
The COVID-19 outbreak brought online learning to the forefront of education. Scholars have conducted many studies on online learning during the pandemic, but only a few have performed quantitative comparative analyses of students' online learning behavior before and after the outbreak. We collected review data from China's massive open online course platform called icourse.163 and ...
Nashville, TN 3720 3 USA. t [email protected]. Abstract. The physical "brick and mortar" classroom is starting to lose its monopoly as the place of. learning. The Internet has made ...
This result is consistent with prior research on the value of active learning (Freeman et al., 2014). Though research shows that student learning improves in active learning classes, on campus, student perceptions of their learning, enjoyment, and satisfaction with instruction are often lower in active-learning courses (Deslauriers et al., 2019 ...
Research across disciplines has demonstrated that well-designed online learning can lead to students' enhanced motivation, satisfaction, and learning [1,2,3,4,5,6,7].]. A report by the U.S. Department of Education [], based on examinations of comparative studies of online and face-to-face versions of the same course from 1996 to 2008, concluded that online learning could produce learning ...
We use a sample of 395 s‐year students taking a macroeconomics module in the Economics department to compare the effects of F2F and online learning on students' performance using a range of assessments. ... Review of Educational Research, 74 (3), 379-439 ... As reported in 355 research reports, summaries and papers. North Carolina State ...
International Journal of Learning, Teach ing and Educational Research. Vol. 22, No. 2, pp. 148-164, February 2023 ... 3.3.1 Positive effects of online learning stra tegy on students' performance.
online classes could affect the academic performance of students. This paper seeks to study the. impact of online learning on the academic performance of university students and to determine. whether education systems should increase the amount of online learning for traditional in-class. subjects.
The COVID-19 pandemic led to an abrupt shift from in-person to virtual instruction in the spring of 2020. We use two complementary difference-in-differences frameworks: one that leverages within-instructor-by-course variation on whether students started their spring 2020 courses in person or online and another that incorporates student fixed effects.
Online learning is defined as an educational strategy in which the learner is geographically distant from the teacher, and the entire educational process is conducted across the Internet and communication networks (Ali Ta'amneh, 2021). Despite the advantages of online learning, there are still numerous challenges for students, administration ...
The aim of the study is to identify the factors affecting students' satisfaction and performance regarding online classes during the pandemic period of COVID-19 and to establish the relationship between these variables. The study is quantitative in nature, and the data were collected from 544 respondents through online survey who were studying the business management (B.B.A or M.B.A) or ...
Introduction. The COVID-19 pandemic has posed an unprecedented challenge in education, leading to the suspension of face-to-face teaching (UNESCO, 2020).This change has been particularly challenging in university undergraduate engineering degrees since much of the learning process is based on practical applications, laboratory classes, and direct contact with teachers and other students.
1. Introduction. Online learning has been on the increase in the last two decades. In the United States, though higher education enrollment has declined, online learning enrollment in public institutions has continued to increase (Allen & Seaman, 2017), and so has the research on online learning.There have been review studies conducted on specific areas on online learning such as innovations ...
The Covid-19 pandemic has spread across the globe, causing educational institutions to shut down. As a result, classes have been held online. This study investigated the impact of online learning ...
Online learning intentions then influence RPL students' actual online learning in online lectures using online video conferencing. On the other hand, perceived usefulness, perceived enjoyment, and social influence have no effect on RPL students' online learning intentions. This research uses the Unified Theory of Acceptance and Use of ...
Research design. This study employed a mixed-methods sequential explanatory design. This approach was driven by the objective of comprehensively understanding the impact of microlearning in online ...
Teaching presence. Teaching presence refers to the design, facilitation, and direction of cognitive and social processes in order to realize meaningful learning outcomes (Anderson et al., Citation 2001).There is growing evidence that teaching presence positively correlates with students' online learning experiences (e.g. Caskurlu et al., Citation 2021; Martin et al., Citation 2022).
PDF | Background Inquiry-based learning (IBL) is a student-centred pedagogical approach that promotes critical thinking, creativity, and active... | Find, read and cite all the research you need ...