40–120 ms, during
delay, active/sham
Trigram identification task and visual DMS | Stim during delay impaired identification of trigrams as compared to sham. Stim during delay of DMS decreased memory scanning rates. No impact on accuracy. | | 8 | R/L PPC (P3/P4) | 200 ms of 25 Hz rTMS at 115% rMT, during delay, active/sham | Spatial DMS | Stim to right PC during delay increased RT compared to left stim, but not sham (~561 ms vs. ~522 and ~540 ms). |
| 8 | L DMPFC, DLPFC, VPFC | 10 min of 1 Hz rTMS at 90% rMT, comparison with baseline | Spatial or face DMS (objects and faces) | Stim to DMPFC increased error rate for spatial task compared to baseline (2.88 vs. 1.58). Stim to DLPFC increased error rates for spatial (4.25 vs. 2.21) and face task (3.38 vs. 2.17). Stim to VPFC increased error rates for face task (3.63 vs. 1.96). No impact on RT. |
| 9 | L PFC, PMC, PC (fMRI-guided), homolog regions (control) | 3 s of 15 Hz rTMS at 110% rMT, during delay (second half) | Verbal DMS (1 or 6 letters) | Stim over left PMC (~14.3 vs. 9.5%) but not PC or PFC increased error rate. No impact on RT. |
| 9 | R PPC (P6), premotor cortex (SFG), and DLPFC (F4) | 300 ms of 25 Hz rTMS at 110% rMT, during delay or decision, active/sham | Matching of spatial sequences | Stim over PPC (~29%) and DLPFC (~22%) but not SFG during the delay phase impaired RT. Stim over DLPFC during the decision phase selectively impaired RT (~38%). No impact on accuracy. |
| 17 | R superior Cb | sp TMS at 120% rMT, during delay, active/ non-active trials/ sham | Verbal DMS and motor control task | Stim at the beginning of the delay phase increased RT on correct trials compared to non-active trials, sham, and motor control task. No effect on accuracy. |
| 30 | Left IPL | 3 sp at 120% rMT, during delay (at 1,3,5 s), active/sham control region | Verbal DMS (phonologically similar/ dissimilar pseudo-words and distractors) | Stim during delay improved RT for similar pseudo-words as compared to sham. Accuracy improved marginally. No difference observed between TMS and placebo scores for dissimilar pairs. |
| 44 | Exp. 1: Midline PC (precuneus) or left DLPFC Exp. 2: Midline PC (precuneus) | 100% rMT, active/sham rTMS Exp. 1: 1 or 5 Hz (7 s) or 20 Hz (2 s), during delay Exp. 2: 5 Hz (7 s), during delay or decision | Verbal DMS (1 or 6 letters) | Exp. 1: Only 5 Hz rTMS over PC but not DLPFC during delay phase improved 6-letter RT compared to sham (626 vs. 702 ms, ~11%) and 1-letter RT (491 vs. 542 ms, ~ 9%). Exp. 2: 5 Hz rTMS over PC during delay but not decision phase improved RT by 88 ms. 1-letter accuracy improved during decision phase compared to sham (~97 vs. ~90, ~7%). |
| Exp. 1: 30 Exp. 2: 24 | Exp. 1: R/L DLPFC, SPL, PCG (control) Exp. 2: R/L FEF, IPS, PCG (control) | 3 s of 10 Hz rTMS at 110% rMT, during delay, active/control | Spatial DMS | Exp. 1: Stim over SPL improved RT ~2% as compared to PCG-control (~950 ms vs. ~970 ms). Stim over LH impaired accuracy more as stim over RH (largest effect over DLPFC). Stim was more disruptive if applied contralaterally to the visual field (faster/slower RT for LH/RH stim). Exp. 2: Stim decreased accuracy overall and specifically for contralaterally presented stimuli. |
| 15 (sleep deprived for 48 h s) | BA 19 and midline PC, BA 18 (control), (as localized in fMRI) | 7 s of 5 Hz rTMS at 100%rMT, during delay, active/sham | Visual DMS | Stim to the upper middle occipital region only reduced sleep-deprivation induced RT deficit compared to sham (1026 ms vs. 1169 ms). No impact on accuracy or non-sleep deprived subjects (state-dependency). |
| 24 | R/L DLPFC, SPL, and PCG (control) | 3 s of 10 Hz rTMS at 110% rMT, during decision, active/ control | Spatial DMS (recognition) and recall | Recognition: Stim to right DLPFC resulted in accuracy improvement, stim to left DLPFC led to reduced accuracy. Recall: Stim to right DLPFC resulted in reduced accuracy. No impact of stim over SPL. |
| Exp. 1: 14 Exp. 2: 11 | OC (V1, V2) and vertex | sp TMS at 65% MSO, at beginning or end of delay, compared to baseline | Visual Imagery and visuospatial STM Exp. 1: at end of delay Exp. 2: at beginning of delay | Exp. 1: Stim facilitated both tasks compared to vertex stim and baseline. Exp. 2: Stim impaired STM compared to vertex and baseline but not visual imagery. No impact on accuracy. |
| 32 | R/L DLPFC (F3/F4) | 5 5-s trains of 10 Hz rTMS, ITI 10 s, at 100% rMT, offline, active/sham | Verbal DMS | Stim decreased correct response RT in active (−21%) compared to sham (+0.3%). No impact on accuracy. |
| 52 | R/L PC | 5 Hz rTMS at 100% rMT, during delay (6 s), active/sham | Spatial DMS and attentional control task | Stim over right PC during delay improved RT ~7% compared to sham (~800 ms vs. ~865 ms). Increase of frontal oxygenated hemoglobin during DMS and decrease during control task. |
| 12 | Exp. 1: R/LV5/MT (2 coils) Exp. 2: R/L lateral OC (2 coils) | sp TMS at 120% PT, at 3 s into delay, baseline phosphene | Delayed visual motion discrimination | Exp. 1: Reported phosphene motion was influenced by the motion component of the memory item: enhanced when direction was the same as in baseline phosphene, weakened if opposite direction. Exp. 2: No relation between task and phosphenes after stim of lateral occipital region. |
| Exp. 1: 6 Exp. 3: 6 | MFG area with/without S1 connection | sp TMS at 120% rMT, at 300 or 1200 ms into delay, baseline control | Tactile STM (discrimination) without (Exp. 1) or with (Exp. 3) distraction | Exp. 1: Stim delivered during early but not late delay over MFG regions with connection to S1 decreased RT ~15% compared to baseline (~730 ms vs. ~860 ms). Exp. 3: Distraction prolonged mean RT by 5%. |
| 16 | R DLPFC, combined with fMRI | 3 sp TMS at 110% rMT or 40% rMT (control), during delay | Visual DMS (face or house) with/without distractor interference | Stim (time-locked to distractors) over DLPFC increased activation in posterior areas (that represented stimuli but not distractors) only when distractors were present. |
| 20 | R IFJ (as localized in fMRI), combined with EEG | 10 min of 1 Hz rTMS at 120% rMT offline, active/sham | Visual DMS (motion direction or color of dots) | Stim led to a decline of P1 and accuracy during the first half but not second half of the color condition, no effects during motion condition (P1 modulation predicted accuracy changes). The magnitude of phase locking value in the alpha band (but not beta or gamma) decreased after rTMS. |
Exp. 2 | 9 | L fO (as localized in fMRI in Exp. 1) | 15 min 1 Hz rTMS, offline, adjusted to RMT, active | Visual delayed matching to stimulus class (houses, body parts, faces) | TMS over fO disrupted top-down selective attentional modulation in the occipitotemporal cortex but did not alter bottom-up activation. The fO may play a role in regulating activity levels of representations in posterior brain areas. |
| 12 | MFG area with/without S1 connection | sp TMS at 120% rMT, at 300 ms into delay, baseline control | Tactile STM (discrimination) with tactile or visual distractor | Stim over MFG region with S1 connection followed by tactile (but not visual) distractor decreased RT~4% compared to baseline (~770 ms vs. ~800 ms). |
Exp. 2 | 8 | L SFG and LOC (as localized in fMRI Exp. 1) | 15 min of 1 Hz rTMS at 55% MSO, offline, active/sham | Visual and verbal DMS | Stim to left SFG increased RT for recognition of colored shapes compared to sham. Stim to the LOC increased RT for recognition of written words compared to sham. No impact on accuracy. |
| 20 | R PC, L IFG | 40 s train of cTBS at 80% aMT, offline, active/sham | Object color, angle averaging, and combined task | Stim to right PC or left IFG selectively impaired WM for the combined task, but not single feature tasks as compared to sham. |
| 12 | Lateral OC | sp TMS at 110% PT at 100, 200, or 400 ms into delay, active/ sham | Modified change detection task with low or high memory loads | Stim delivered at 200 ms into the delay phase decreased accuracy for high but not low memory loads in the contralateral visual field compared to sham. |
| | | |
| 14 | R/L DLPFC, Fz (control), combined with PET | 30 s of 4 Hz rTMS at 110% rMT, during task, active/control | Verbal 2-back, 0-back (control) | Stim over either R/L DLPFC reduced accuracy and rCBF in the targeted area as well as afferent networks specific to each hemisphere. Stim to Fz had no effect on WM task. Performance on the control task was not affected by stim. |
| 7 | R/L DLPFC | spTMS at 115% rMT, at 400 ms into delay, active/no TMS | Verbal 3-back | Stim over L DLPFC increased error rate compared to no TMS control (5.4%). No impact of stim over R DLPFC. |
| 35 (5 Exp.: 8, 6, 6, 25, 6) | Exp. series 1: R/L or bilateral temporal (T5/T6) and parietal (P4/P5) Exp. series 2: Bilateral SFG and DLPFC | Uni- or bilateral spTMS at 130% rMT, at 300 or 600 ms, active/ baseline | Spatial 2-back Visual-object 2-back (abstract patterns) | Exp. series 1: Bilateral parietal stim at 300 ms increased RT in visuospatial task compared to temporal (11%) and baseline (20%). Bilateral temporal stim at 300 ms impaired RT in visual-object task. No impact on accuracy. Exp. series 2: Bilateral stim over SFG at 600 ms increased RTs in visuospatial task compared to baseline (11%), whereas bilateral stim over DLPFC at 600 ms interfered in both tasks with accuracy (visuospatial: 10%, visual- object: 13%) and RT (visuospatial: 6%, visual-object: 6%). |
| 12 | R/L DLPFC (F3/F4) | 0.5 s of 20 Hz rTMS at 90% rMT, during encoding or retrieval, active/ sham/baseline | Verbal LTM: Recognition of unrelated/related word pairs after 1 h | Impaired recognition accuracy of unrelated word pairs after stim over R and L DLPFC during encoding and right PFC in retrieval. No impact on RT besides faster RT for related as compared to unrelated words. |
| 6 | R/L MFG, inferior PC | sp TMS at 120% rMT, at 140-500 (at 10 time points, ISI 40 ms) into delay, after every 4th letter, active/control | Exp. 1: Verbal 2-back Exp. 2: Choice reaction (control task) | Impaired accuracy occurred after stim of R PC (180 ms) of L PC (220 ms) and R MFG (220 ms), and L MFG (260 ms). RT was impaired only after L MFG stim (180 ms). No impact on control task. |
| 14 | R/L DLPFC (F3/F4) | 30 s of 4 Hz rTMS at 110% rMT, during task, active/control/ baseline | Verbal 2-back, 0-back (control task) | Stim over L DLPFC led to a shift of BBR towards the SFG and to a positive BBR in anterior parts of the SFG. Stim over R DLPFC led to a shift of the BBR to left posterior and inferior IFG. Baseline measurements indicated a negative BBR in the left MFG and no significant BBR in the right MFG. |
| 16 | HF stim to R/L DLFPC and right Cb, LF stim to L DLPFC | 10-s trains of 1 or 5 Hz rTMS at 90% rMT, 30 s intervals, during encoding and retrieval, active/ baseline | STM (digits forward), WM (digits backward, letter- number sequencing WAIS III), episodic memory (RBMT), verbal fluency | HF stim over L DLFPC impaired verbal episodic memory as compared to HF stim over R DLPFC, LF stim over L DLPFC, and baseline. |
| R: 5 L: 7 | R/L DLPFC, SPL, PCG (control) (as localized with fMRI) | 6 s of 5 Hz rTMS at 100% rMT, during delay, active/control | Verbal STM or WM | Stim over DLPFC impaired accuracy of WM but not STM compared to control. Stim over SPL impaired accuracy of WM and STM. No impact on RT. |
| 8 | L DLPFC, Cz (control) | pp TMS (ISI 100 ms) at .47 T, during delay, active/sham/control | Reading span task (maintain target words) | Stim decreased mean accuracy compared to sham or stim over Cz (10.9% and 7.5%). |
| Exp. 1: 9 Exp. 2: 14 Exp. 3: 9 | R/L DLPFC | 0.5 s of 10 Hz rTMS at 90% rMT, at end of delay, active/sham | Exp. 1: combined verbal/spatial 1-back Exp. 2: combined verbal/spatial 2-back Exp. 3: 2-back with one domain only | R DLPFC stim impaired RT in the verbal condition (~834 ms vs. ~790 and ~803 ms), whereas L DLPFC stim impaired RT in the spatial condition compared to opposite side and sham (792 ms vs. 728 and 737 ms). No impact on accuracy, variation of only one domain, or 1-back task. |
| 12 | R/L DLPFC (F3/F4), inferior PC (P3/P4) | sp TMS at 100% rMT, at 250, 450, 650, or 850 ms into delay, active | Audioverbal 2-back Pitch 2-back | Stim over RH increased RT in the pitch 2-back at 650 and 850 ms (724 and 850 ms vs. 656 ms). Stim over P3 increased RT in the audioverbal 2- back at 450 ms. |
| Exp. 1: 27 Exp. 2: 24 Exp. 3: 18 | R/L DLPFC, interhemispheric sulcus (control) | spTMS at 100% rMT, delivered 300 ms into delay, active/ control | Exp. 1: WM (medium = 3, high = 5) and lexical decision (word/ pseudoword), prospective condition (react to specific words); Exp. 2: prospective condition 1 or 3 words; Exp. 3: with TMS | Stim increased error rates of the PM task more than the WM task and compared to sham. Exp. 1 and 2: Higher PM demand affected WM only at higher loads. Exp. 3: Stim over R/L DLPFC impaired accuracy of PM task regardless of WM load, while effect on WM was marginal. |
| Exp. 1: 8 Exp. 2: 8 | Exp. 1: R/L BA 10 (frontal pole), Cz (control) Exp. 2: L BA 46 (DLPFC), Cz (control) | 20 s of cTBS (3-pulse bursts at 50 Hz every 200 ms) at 80% aMT | Verbal forward/ backward memorization task with simultaneous response to target word (PM task) | Exp. 1: Stim over left BA 10 decreased accuracy in PM compared to Cz stim (58.6% vs. 73.4%). Exp. 2: Stim over left DLPFC had no significant effect on accuracy or RT. |
| | | | |
| 5 | R/L hemisphere (F7/F8, T5/T6, P3/P4, O1/O2) | 5 p of 20 Hz rTMS at 120% rMT, during encoding (at 0, 250, 500, 1000 ms), active/sham | Verbal memory (word recall) | Stim over T5, F7, and F8 at 0 and 250 ms showed highest impairment of recall as compared to sham. Furthermore stim over T5 and F7 at 500 ms impaired recall. Stim over T5 and F7 also impaired the primacy effect. |
| 13 | R/L DLPFC (F3/F4) | 500 ms of 20 Hz rTMS at 90% rMT, during encoding or retrieval, active/ sham/baseline | Visual memory (indoor/outdoor images) | Stim over R DLPFC during retrieval impaired accuracy, while stim over L DLPFC during encoding and over R DLPFC during retrieval impaired discrimination. No impact of R DLPFC stim during encoding and L DLPFC stim during retrieval. |
| 10 | R/L DLPFC, Cz (control) | pp TMS (ISI 60 ms), 120% rMT, during encoding at 180 ms, active/controls/no stim | Visual memory (associate Kanji words and abstract patterns) | Stim during encoding over R DLPFC decreased accuracy compared to stim over L DLPFC. RT was not measured. |
| 15 | R/L IFG | 0.5 s of 20 Hz rTMS at 90% rMT, during encoding, active/ sham/no stim | Verbal (letters) and nonverbal (abstract shapes) memory | Stim over L IFG impaired word recognition, while stim over R IFG impaired image recognition, each as compared to opposite stim (words 20% and images 14%) or sham (words 24% and images 14%). No impact on RT. |
| 12 | L Inferior PFC (guided by fMRI) R inferior PFC and L PC (controls) | 5 p of 7 Hz rTMS at 100% rMT, during encoding, active/ control/no stim | During fMRI: semantic/non- semantic decisions, crosshair fixation During stim: semantic decisions After stim: verbal memory (recognition) | Stim over L PFC increased recognition accuracy compared to non-stim and control (R PFC, L PC). No impact on RT. But, RT for semantic decisions made under L PFC stim was impaired. |
| 10 | L DLPFC | 0.9 Hz rTMS at 110% rMT, during task (192 p per subtest), active/sham | Verbal memory (word recall) Visual memory (facial recognition) | Stim over L DLPFC during task impaired free recall of words but not recognition of faces as compared to sham. |
| 14 | R/L posterior VLPFC, (guided by fMRI) | spTMS at mean 66% MSO, during encoding (btw 250- 600 ms), active/ baseline | Verbal memory (decision if 2/3- syllable word or peusdo-word, then surpise recognition task with confidence judgments) | Stim over L VLPFC impaired word memory (confidence), while stim over R VLPFC facilitated word and pseudo- word memory (confidence, difference strongest at 380 ms). Phonological decision accuracy was facilitated for words and pseudo- words after stim over R VLPFC (strongest at 340 ms). |
| 42 | R/L DLPFC or IPS (P3/P4) | 500 ms rTMS at 20 Hz at 90 or 120% rMT, during encoding, active/sham | Visual memory (indoor/outdoor images), visuospatial attention (Posner, control task) | L DLPFC stim interfered with encoding while R DLPFC stim interfered with retrieval. No impact of stim over IPS on encoding or retrieval even at higher intensity. However, stim over R IPS impaired RT in the attention task. |
| 11 | L OFC (Fp1), L DLPFC (F3) | 20 min of 1 Hz rTMS at 80% rMT, offline, active/sham | Visual memory (neutral, fearful, and happy faces) | Stim over L OFC improved memory for happy faces compared to sham. Stim over L DLPFC improved memory marginally for happy faces compared to sham. |
| 20 | L ATL (between T7/FT7) | 10 min of 1 Hz rTMS at 90% rMT, offline, active/sham | Verbal memory (false memories) | Stim decreased the number of false memories by 36% compared to sham (~3 vs. ~2 errors). |
| Exp. 3: 7 Exp. 4: 13 | Exp. 3: R/L PC (P3/P4), Cz (control) Exp. 4: R/L PC (P3/P4), centroparietal control | 9 pulses of 10 Hz or 14 pulses of 15 Hz rTMS, at 110% rMT, during delay, active/ sham | Visual STM (memorize color of a square presented in one but not other visual hemifield) | 10 Hz rTMS to PC ipsilateral to the stimulus improved visual STM (Exp. 3/4: 40% less false alarms, 37% fewer missed trials), while contralateral stim over PC led to a decrease. No effect of 15 Hz rTMS over PC or 10 Hz rTMS over centroparietal sites. |
| 16 | R/L DLPFC (F3/F4) | ppTMS, ISI 3 ms, 90% rMT, during encoding or retrieval, active/ sham | Verbal (letters) and nonverbal (shapes) memory, under full or divided attention | Stim over L DLPFC impaired recall as compared to stim over R DLPFC under attention encoding (but not as compared to sham). Stim over R DLPFC impaired recall as compared to sham under attention encoding (but not as compared to stim over L DLPFC). |
| 18 | R/L DLPFC | ppTMS, ISI 3 ms, at 90% rMT, during encoding or retrieval, active/ sham | Verbal (letters) and nonverbal (abstract shapes) memory | Stim over L DLPFC during encoding decreased DR as compared to sham and stim over R DLPFC. Stim over the R DLPFC during retrieval decreased DR and hit rate compared to stim over L DLPFC. No significant differences between verbal/nonverbal material. |
| 11 | R/L DLPFC (F3/F4) | ppTMS, ISI 15 ms, at 90% rMT, during encoding or retrieval, active/ sham | Verbal (letters) and nonverbal (abstract shapes) memory | Stim over L DLPFC during encoding improved RT as compared to stim over R DLPFC or sham. Stim over R DLPFC during retrieval improved RT as compared to stim over L DLPFC. More false alarms for shapes than for words occurred after stim over R DLPFC or sham. |
| 13 | R OC (V1) to interfere with lower-L (but not upper-R) quadrant | Priming with 20 trains of 30 pulses at 6 Hz (ITI 25 s) at 45% MSO, 6.7 min of 1 Hz rTMS at 50 MSO, 45 min after session 1 and 2, active/no stim | Visual orientation discrimination (day 1: lower L quadrant, upper R quadrant, day 2: opposite or vice versa) | Stim delivered 45 min after the first and second training session to interfere with lower-L quadrant strongly impaired learning as measured on the next day. This interference occurred only when training of the L visual field was followed by training of the R visual field before TMS and not vice versa. No differences between quadrants at baseline. |
| | | | |
| 12 | Bilateral RA/LA or RC/ LC DLPFC (F3/F4), S | 0.26 mA, intermittent on/off 15 s over 15 min, during task, ref mastoids, active/ sham | Visual STM (modified Sternberg) | Bilateral A and C stim both impaired RT as compared to placebo. No impact on accuracy. |
| 17 | A/C/S, R/L Cb and PFC (btw Fp1/F3 and Fp2/F4) | 2 mA, 15 min, offline, ref deltoid, active/ sham | Numerical STM (modified Sternberg) | C-tDCS over PFC improved RT ~6% compared to sham (~625 ms vs. ~665 ms). No effect after A-tDCS. A-tDCS and C-tDCS blocked RT decrease induced by task repetition. |
| 11 | A./C/S, R inferior PC (P4) | 1.5 mA, 10 min, during learning, ref left cheek, active/sham | Visual STM (recognition and free recall of objects) | C-tDCS selectively impaired WM on recognition tasks versus anodal and sham. No impact on free recall. |
| 14 | A/S, L DLPFC | 1 mA, 10 min, during task, ref SOA, active/sham | Verbal STM (modified Sternberg) | A-tDCS improved RT when distractor was present compared to non- distractor and sham conditions. No impact on accuracy. |
| 12 | A/C/S, R PC (btw P8/ P10), combined with EEG | 1 mA, 30 min, offline, ref btw P7/P9, active/ sham | Spatial DMS | While A-tDCS over RH impaired capacity for contralateral stimuli, C -tDCS improved it. Both A-tDCS and C-tDCS affected capacity for ipsilateral stimuli compared to sham. tDCS altered ERPs (N2, P2, N3) and oscillatory power in the alpha band at posterior electrodes. |
| | | | |
| 15 | A/C/S, L DLPFC (F3),A M1 (control) | 1 mA, 10 min, during task, ref SOA, active/sham/M1 | Verbal 3-back (sequential-letter task) | A-tDCS over L DLPFC improved accuracy by ~10% (21.7 vs. 19.8) and decreased number of errors by ~28% as compared to sham (4.7 vs. 6.9). No impact after C-tDCS over LDLPFC or A-tDCS over M1. No impact on RT. |
| 15 | A/S, L DLPFC (F3) | 1 mA, 30 min, during, ref SOA, active/ sham | Verbal 3-back (assessed 10, 20, and 30 min into stim, and 30 min after) | A-tDCS improved accuracy by 10% (at 20 min), 16% (at 30 min), 14% (at 30 min after) as compared to sham. No impact on error rates or RT. |
| 10 | A, L DLPFC (F3) | 1 mA, 10 min, during task, ref SOA active/ sham/tRNS | Pre and post stimulation: visual STM (one card task, 1-back), WM (2- back) During stimulation: STM (Sternberg) | A-tDCS decreased RT in WM (2-back) for correct responses by ~2% compared to sham. No impact on accuracy. No impact on STM tasks. |
| 12 | A/S, L DLPFC (F3) | 1 or 2 mA, 20 min, during task, ref SOA, active/sham | Verbal 3-back during stim, STM (Sternberg) after stim | During the final 5 min of A-tDCS (2 mA) over L DLPFC RT improved significantly as compared to sham (~581ms vs. ~605.25 and ~629.49 ms). No impact on accuracy. No impact on STM after stim. |
| 16 | A/C/S, L DLPFC (F3), combined with EEG | 1 mA, 15 min, offline, ref mastoid, active/ sham/control | Verbal 2-back (letters) | A-tDCS improved RT as compared to C-tDCS and resulted in amplified oscillatory power in the theta and alpha bands under posterior electrode sites. C-tDCS had opposite effects on EEG measures. No impact on accuracy. |
| 10 | A/S, L DLPFC (F3) | 1 mA, 10 min, during task or offline, ref SOA, active/sham | During stim: verbal 2-back followed by 3-back (letters) Pre/post stim: STM (digit span forward) and WM (digit span backward) | Online A-tDCS improved digit span forward by 5.5% as compared to offline A-tDCS and sham. No information regarding online task outcome. |
| 24 | A/C/S, R/L DLPFC (F3/F4) | 2 mA, 20 min, 15 min before and during task, ref SOA active/ sham | Verbal 2-back Pain percpetion (warm/ cold) | A-tDCS over R DLPFC increased tolerance to heat pain as compared to sham. During C-tDCS over the L DLPFC there were fewer outliers as compared to sham. No significant differences in accuracy (dissociation of analgesic effect from cognitive function). |
| 27 | Bilateral PPC (P3/P4), LAlRC, LC/RA, S | 1.5 mA, 13 min, active/ sham | Verbal STM (1-back) and WM (2-back) | 1-back: LA/RC tDCS abolished practice- dependent improvement in RT as compared to sham (9% vs. 0.65%). 2- back: LC/RA tDCS abolished practice- dependent improvement in RT (9.8% vs. 0.45%) as compared to sham. No impact on error rates. |
| 41 | A /S, R/L DLPFC | 2 mA, 15 min, during task, ref Cz, active/ sham | Verbal n-back (4 levels of WM load), during and after stim | During online stimulation at highest WM loads males benefited from stim over L DLPFC as compared to sham, while females improved after stim over R DLPFC. No impact on RT. Online accuracy scores at the highest WM level was related to post-tDCS recall. |
| | | | |
| 22 | A/C/S, L DLPFC (N= 14) and VI ( = 8) | 1 mA, 10 min, 5 min before and during learning, ref Cz, active/sham, | Probabilistic classification learning | A-tDCS over L DLPFC improved learning compared to sham. No effect after C-stim or stim over V1. |
| 30 (males) | Bilateral RA/LA DLPFC (F3/F4) | 0.26 mA/cm , intermittent on/off 15 s over 30 min, during sleep, active/ sham | Declarative and procedural learning (paired associate word lists and mirror tracing), PANAS/ EWL (mood) | Bilateral anodal tDCS during sleep enhanced word retention compared to sham (35.7 vs. 34.5). No impact when applied during wakefulness and no impact on procedural memory. After active but not sham tDCS positive affect decreased less and feelings of depression decreased. |
| 11 | C/S, L SMG (TP3), R SMG (control) | 1.2 mA, 20 min, offline, ref SOA, active/sham/control | Pitch matching (6/7 tones) | C-tDCS to L SMG affected short-term pitch memory performance (9%) compared to R SMG and sham. |
| 20 | A/C/S, R/L DLPFC (F3/F4) | 1.5 mA, 5 min, during learning, ref mastoid, active/sham | Verbal LTM (VLMT) | C-tDCS to L DLPFC decreased number of words recalled after 25 min compared to sham (12%). No effects on long-term retrieval were found. |
| 30 | Bilateral ATL (T3/T4), (LA/RC), unilateral ATL (LA/RC enlarged electrode), S | 2 mA, 10 min, during encoding and retrieval, active/ sham | False memory (within word categories) | Bilateral and unilateral tDCS reduced false memories (73%) compared to sham. Bilateral tDCS decreased the number of false memories compared to unilateral stim (~1 vs. ~ 2 errors) and compared to sham (~1 vs. ~3.7 errors). |
| 28 | Bilateral RA/LA, DLPFC (F3/F4) | Five 5 min epochs of transcranial slow oscillation stimulation (tSOS, 0.75 Hz), 1 min ISI, 0.517 mA/cm , ref mastoid, active/sham | Verbal and non-verbal paired association, verbal memory (VLMT), number list learning, procedural memory (mirror tracing, finger sequence tapping), control tasks | TSOS during wakefulness induced a local increase in endogenous EEG slow oscillations (0.4-1.2 Hz) and a widespread increase in EEG theta and beta activity. TSOS during learning improved verbal encoding, but not consolidation as assessed 7 h after learning. |
| 96 (divided in diff. stim groups) | Exp. 1–3: A, R inferior PFC (F10) Exp. 4: A, R PC (P4) | 0.6 mA or 2 mA, 30 min, during learning, ref arm, active/control (0.1 mA) | Detection of cues indicative of covert threats | Exp. 1–3: A-tDCS at 2 mA over R inferior PFC improved threat detection sign. more (26.6%) as compared to control (0.1 mA, 14.2%), while forgetting rate over 1 h was similar. Intermediate current strength (0.6 mA) was associated with an intermediate improvement (16.8%). Exp. 4: A-tDCS at 2 mA over R PC improved accuracy sign. more (22.5%) as compared to control (0.1 mA over F10). |
| 36 (12 each condition) | Bilateral ATL, LA/RC, RA/LC, S | 2 mA, 13 min, during task, active/sham | Visual memory (geometric shapes) | LC/RA-tDCS resulted in a improved visual memory (accuracy) by 110% as compared to sham. No change after LA/RC-tDCS. |
| 15 | Bilateral PC, RA/LC, RC/LA, S | 1 mA, 20 min, 6 days, during learning, active/sham | Numerical learning (pseudo-number paired association), changes assessed by numerical tasks (Stroop, number-to- space task) | 6 days of RA/LC-tDCS improved RT in Stroop compared to sham. RC/LA- tDCS impaired performance compared to sham |
| 12 | Bilateral frontotemporal stim between F3/4 and C3/ 4, LA/RC, RA/LC | 1 mA, 20 min, during encodig, active/sham | Visual memory (free recall of images differing in affective arousal and valence) | Bilateral RA/LC-tDCS improved recall of pleasant images compared to unpleasant/neutral images, while bilateral LA/RC-tDCS improved recall of unpleasant images compared to pleasant and neutral images. |
| 13 | A/C, L DLPFC (F3) | 1.5 mA, 1.6 sc, during encoding or delay, ref SOA, active/no stim | Word memorization | A-tDCS during encoding improved accuracy and RT compared to late A-tDCS or no tDCS. C-tDCS during encoding impaired accuracy and RT compared to late C-tDCS or no tDCS. Stim during delay had no effect. |
| 32 | A/C/S, L DLPFC (F3), M1 (C3, control) | 1.5 mA, 20 s A, 30 s C, during encoding or recognition, ref SOA, active/sham | Word memorization | During encoding A-tDCS over DLPFC improved accuracy, while C-tDCS impaired accuracy compared to sham. M1-tDCS had no impact. During recognition C-tDCS impaired recognition compared to sham, while A-tDCS showed a trend towards improvement. |
| 36 (A/S=18, C/S=18) | A/C/S, L DLPFC (F3) | 1 mA, 30 min, 10 min before and during learning, ref SOA, active/sham | Errorless/errorfull learning (word stem completion) | C-tDCS impaired encoding and retrieval after errorful learning compared to errorless learning and sham. No impact of anodal stimulation. |
| 34 (control = 14, early = 11, late = 9) | A, R Inferior PFC (F8) | 2 mA, 30 min, early/ late during learning, ref arm, active/ control (0.1 mA) | Detection of cues indicative of covert threats | A-tDCS (2 mA) improved threat detection compared to control (0.1 mA). A-tDCS was more effective when applied during early learning. |
| 16 | Bilateral RA/LA, DLPFC, (F3, F4) | Theta-tDCS at 5 Hz, 0.517 mA/cm , 5 min, 1 min ISI, during REM or non- REM sleep, ref mastoid, active/sham | Verbal paired association, procedural memory (mirror tracing, finger sequence tapping), mood (PANAS) | Theta-tDCS during non-REM impaired consolidation of verbal memory compared to sham. No effect on consolidation in procedural memory. Stim during REM led to an increase of negative affect. |
| 24 | Bilateral L IPS/SPL (P3), R IPL (P6), LA/ RC, RA/LC (control) | 1 mA, 10 min, active/ control stim/control group | Verbal memory (discrimination of familiar/unfamiliar words) | LA/RC-tDCS improved accuracy, but not RT as compared to control stim. No effect after LC/RA-tDCS. |
| | | |
| 66 (<45 and >50 y) | R/L DLPFC (F3/F4) | 500 ms of 20 Hz at 90% rMT, during encoding and retrieval, active/ sham/baseline | Visuospatial memory (old/new discrimination of images) | Stim over R DLPFC in younger subjects interfered with retrieval more than stim over L DLPFC. This asymmetrical effect dissipated with age as indicated by bilateral interference effects on recognition. Stim of left DLPFC during encoding had a disruptive effect on all subjects which would not comply with the HAROLD model. |
| 39 (>50 y with 1+ y memory complaints) | Bilateral R/L DLPFC combined with baseline and post- TMS fMRI | 10 trains of 10 s rTMS at 5 Hz, ITI 30 s, 80% rMT, offline, active/sham | Face–name association | Stim improved associative memory compared to sham (rate of change: 1.60 vs. -0.63). TMS led to an increase in activation of the right IFG and MFG and occipital areas. |
| 31 (60–81 y), HP and LP | R/L DLPFC | 450 ms of 20 Hz rTMS (ISI 7–8 s), total of 640 pulses, 90% rMT, during encoding or retrieval, active/ sham/baseline | Verbal memory (associated/non- associated word pairs) | The high-performing (HP) group performed better in the experimental task than the low-performing group (LP) (92.0% vs. 78.9%). Stim over L DLPFC affected accuracy more during encoding than during retrieval, but only for unrelated word-pairs in the LP group. No significant differences in RT. Asymmetry as predicted by the HERA model was observed only in LP. |
| 20 (50–80 y, mean 62 y) | A, R TPC | 1 mA, 20 min, during learning, active/ sham | Object location learning, immediate and delayed (1 week later) free-recall | Anodal stim improved delayed correct free-recall responses compared to sham (24% vs. 8.5%), but not immediate recall (34% vs. 28.8%). No significant differences in RT. |
| | | |
| 15 | R/L DLPFC (btw F3/F4 and F/7/F8), 1 session | 600 ms of 20 Hz TMS at 90% rMT, during encoding, active/ sham | Visuoverbal object and action naming | Stimulation over L and R DLPFC improved accuracy in action naming as compared to sham stimulation. Object naming did not improve significantly. |
| 24 | R/L DLPFC, 1 session | 500 ms of 20 Hz TMS at 90% rMT, during encoding, active/ sham | Visuoverbal object and action naming | Stimulation over L and R DLPFC improved accuracy in action naming but not object naming as compared to sham stimulation in the mild AD group. Improved naming accuracy for both classes of stimuli was only found in moderate-to-severely impaired patients. |
| 10 | A/C/S, bilateral TPC (LA/RA, LC/RC, S), 1 session per condition | 1.5 mA, 15 min, offline, active/sham/baseline | Verbal memory and visual attention | A-tDCS improved accuracy, while C-tDCS decreased performance as compared to baseline. No impact on visual attention. |
| 10 | A/S, L DLPFC (F3), L temporal cortex (T7) | 2 mA, 30 min, A/S, during task, ref SOA, active/sham | Visual STM, WM (digit span backward), Stroop | Accuracy in visual memory improved during A-tDCS over L DLPFC (18%) and temporal cortex (14%) as compared to sham. No effect on WM and Stroop. |
| 10 | L DLPFC, 20 sessions without training vs. 10 sessions placebo | 25 min, 2 s of 20 Hz (ITI 28 s) at 100% rMT, offline, active/sham/baseline | Various tests for memory, executive functions, and language | Improvement of auditory sentence comprehension as compared to baseline and placebo training; no effect on other cognitive or langauge functions. |
| 8 | 6 regions, 3 per day (Broca, Wernicke, R DLPFC and R-pSAC, L-pSAC, l-DLPFC), (30 sessions with training) | 20 2-s trains of 10 Hz per area (=1200 pulses per day), 90% MT (frontal areas), 110% MT (other areas), active/ baseline | Training tasks: attention, memory, language | ADAS-cog improved by approx. 4 points after training and was maintained at 4.5 months follow-up. CGIC improved by 1.0 and 1.6 points, respectively. MMSE, ADAS-ADL, Hamilton improved, but not significantly. No change in NPI. |
| 15 | A/S, bilateral (RA/LA) temporal cortex (T3/ T4), 5 sessions | 30 min, 2 mA, ref deltoid, offline, active/sham | Visual STM, visual attention, MMSE, ADAS-Cog | A-tDCS improved memory performance by 8.99% from baseline compared to sham (−2.62%). No impact on visual attention or other cognitive measures. |
Haffen et al. (2011) | 1 | L DLPFC, 10 sessions | 20 min of 5-s trains of 10 Hz (ITI 25 s), 100% rMT, offline, active | Verbal memory (Memory Impairment Screen, free and cued recall), Isaacs Set Test, TMT, picture naming, copying, MMSE | Stimulation improved episodic memory task performance and speed performance. Improvements were still seen 1 month later, however scores returned to baseline by 5 months. ADL improvements reported by wife. |
| 45 | R/L DLPFC, 5 sessions without training | Group 1: ~10 min of 5-s trains of 20 Hz (ITI 25 s), 90% rMT per DLPFC Group 2: ~ 16 min of 1 Hz rTMS, 100% rMT, ~16 min per DLPFC (2000 p) | MMSE, IADL, GDS | Mild to moderate AD patients (20 Hz) showed improved scores on all rating scales as compared to the 1-Hz and sham groups. Although improvements were present at 1 month, scores returned to near baseline level by 3 months. |
| | | |
| 25 (PD & depression) | L DLPFC, 10 sessions without training | 40 trains of 5 s of 15 Hz, 110% rMT and fluoxetine (20 mg/day), offline, active/sham/baseline | NP (TMT, WCST, Stroop, HVOT, CPM, WM): before treatment, after 2 and 8 weeks | Both the fluoxetine and rTMS groups showed significant improvement in Stroop (colored words), Hooper, and WCST (perseverative errors), and in depression rates. No significant effects on other cognitive functions. |
| 18 | A/S, L DLPFC, M1 (control), 1 session per protocol | 20 min, 1 or 2 mA, 20 min, during task, ref SOA, active/ sham/control | Verbal 3-back | Accuracy in 3-back task after stimulation with 2 mA (20.1%) as well as error frequency (35.3%) improved significantly more as compared to stim with 1 mA, stim over M1, or baseline. |
| | | |
| 10 (RH stroke), 1–4 months poststroke | A/S, L DLPFC (F3), 1 to session per protocol | 30 min of 2 mA, online (25 min after starting stimulation), ref SOA, active/sham/ baseline | Verbal 2-back before and at 25 min tDCS onset | A-tDCS improved recognition accuracy as compared to sham. No impact on RT. |
A, C, S, anodal, cathodal, sham; ADAS-Cog, Alzheimer’s Disease Assessment Scale – Cognitive subscale; aMT, active motor threshold; ATL, anterior temporal lobe; BA, Brodmann’s area; BBR, brain-behavior relationship; Cb, cerebellum; CGIC, Clinical Global Impression of Change; CPM, colored progressive matrices; cTBS, continuous theta-burst stimulation; Cz, vertex; DLPFC, dorsolateral prefrontal cortex; DMS, delayed match-to-sample; DMPFC, dorsomedial prefrontal cortex; DR, discrimination rate; EF, executive functions; ERP, event-related potential; Exp., experiment; FEF, frontal eye fields; FL, frontal lobe; fO, frontal operculum; Fz, frontal midline; GDS, Geriatric Depression Scale; HF, high frequency; HVOT, Hooper Visual Organization Test; IADL, Instrumental Activities of Daily Living; IFG, inferior frontal gyrus; IFJ, inferior frontal junction; IPL, inferior parietal lobule; IPS, intraparietal sulcus; ITI, intertrain interval; L, left; LA/RA, left anodal/right anodal; LC/RC, left cathodal/ right cathodal; LF, low frequency; LH, left hemisphere; LOC, lateral occipital cortex; M1, primary motor cortex; MFG, middle frontal gyrus; MMSE, Mini Mental State Examination; MSO, maximum stimulator output; NP, neuropsychological; NPI, neuropsychiatric inventory; OC, occipital cortex; OFC, orbitofrontal cortex; p, pulse; PANAS, positive and negative symptoms scale; PC, parietal cortex; PCG, postcentral gyrus; PD, Parkinson’s disease; PFC, prefrontal cortex; PL, parietal lobule; PM, prospective memory; PMC, premotor cortex; PPC, posterior parietal cortex; ppTMS, paired-pulse transcranial magnetic stimulation; R, right; RBMT, Rivermead Behavioural Memory Test; rCBF, regional cerebral blood flow; ref, reference; RH, right hemisphere; rMT, resting motor threshold; R-pSAC and L-pSAC, right and left parietal somatosensory association cortex; RT, reaction time; rTMS, repetitive transcranial magnetic stimulation; S1, primary somatosensory cortex; SFG, superior frontal gyrus; sign., significant; SMG, supramarginal gyrus; SOA, supraorbital area; sp, single pulse; SPL, superior parietal lobule; stim, stimulation; STM, short-term memory; T, tesla; TL, temporal lobe; TMT, trail making test; TPC, temporoparietal cortex; tRNS, transcranial random noise stimulation; TSOS, transcranial slow oscillation stimulation; VAT, visual attention task; VLPFC, ventrolateral prefrontal cortex; VPFC, ventral prefrontal cortex; VFT, verbal fluency test; VRT, visual recognition task; WAIS, Wechsler Adult Intelligence Scale; WCST, Wisconsin Card Sorting Test; WM, working memory; y, years.
Despite the many differences between studies, the growing literature summarized in Table 55.1 is providing important novel insights in the neurobiology of human learning and memory, and illustrates the power of NBS in this area of cognitive neuroscience.
S hort-term memory
Prefrontal areas undoubtedly play an important role in STM processes. However, one of the questions that NBS studies are helping address relates to the organization of information processing streams. Is processing of STM supported through a domain-specific segregation (spatial, object, verbal processing) or rather through a processing segregation (encoding, maintenance, storage)?
Processing segregation
Most studies examining this question have used a delayed match-to-sample task and applied stimulation during either the delay period or the decision period ( Fig. 55.2 ). High-frequency TMS applied over the parietal cortex during the delay period can improve STM function ( Kessels et al., 2000 ; Kirschen et al., 2006 ; Luber et al., 2007 ; Yamanaka et al., 2010 ), but some studies found it to impair STM ( Koch et al., 2005 ; Postle et al., 2006 ). In either case, the effects seem specific to the delay period, since parietal TMS during the decision phase has not been found to impact STM ( Luber et al., 2007 ; Hamidi et al., 2009 ). The question whether DLPFC also plays a role during the delay phase has not been answered yet. Although some TMS studies support DLPFC participation ( Pascual-Leone and Hallett, 1994 ; Koch et al., 2005 ), others have found no impact when stimulating DLPFC during the delay phase ( Herwig et al., 2003 ; Postle et al., 2006 ; Hamidi et al., 2008 ; Sandrini et al., 2008 ). On the other hand, high-frequency TMS over the DLPFC during the decision period impairs STM functions ( Koch et al., 2005 ; Hamidi et al., 2009 ). Therefore, although further studies are needed, findings suggest a dissociation between parietal and prefrontal areas, playing primary roles in delay and decision phases, respectively. These findings are supportive of the notions of posteroanterior temporal gradient in memory processing: parietal regions coming online first and prefrontal regions contributing to later subprocesses. Chronometric TMS experimental designs enable such notions to be directly tested further.

Schematic summary of findings from studies investigating the impact on short-term memory after stimulation over the left or right prefrontal cortex, parietal areas, or the cerebellum during the delay (green) or the decision period (orange).
Mottaghy et al. (2003a) conducted the first such experiment ( Fig. 55.3 ), albeit focusing on verbal WM. They used single-pulse TMS to explore the temporal dynamics of left and right inferior parietal and DLPFC involvement in verbal WM in six healthy volunteers. TMS was applied at 10 different time points 140–500 ms into the delay period of a 2-back verbal WM task. Precise and consistent targeting of a given cortical brain region was assured by using frameless stereotactic neuronavigation. A choice reaction task was used as a control task. Interference with task accuracy was induced by TMS earlier in the parietal cortex than in the PFC, and earlier over the right than the left hemisphere. This suggests a propagation of information flow from posterior to anterior cortical sites, converging in the left PFC. Significant interference with reaction time was observed after 180 ms with left PFC stimulation. These effects were not observed in the control task, underlining the task specificity of our results. Hamidi and colleagues (2009) also examined the roles of right and left DLPFC in recall and recognition. They found that right DLPFC stimulation impaired accuracy in delayed recall, while enhancing accuracy in delayed recognition. On the other hand, left DLPFC stimulation impaired delayed recognition. Therefore, it seems clear that TMS, in repetitive and chronometric single-pulse experimental designs, can provide valuable insights into the functional segregation of core subprocesses of STM.

Accuracy in the 2-back task as a function of the time of transcranial magnetic stimulation (TMS). TMS interference peaked at 180 ms at the right inferior parietal cortex, at 220 ms at the left inferior parietal cortex and right middle frontal gyrus (MFG), and at 260 ms at the left MFG (all p < 0.05). This study illustrates the chronometry of causal contributions of different brain regions to memory processing. (Modified from Mottaghy et al., 2003a , by permission of the authors.)
Domain-specific segregation
Mottaghy et al. (2002b) , in another pioneering study ( Fig. 55.4 ) used TMS to show that functional and modality-specific segregation need not be mutually exclusive. They applied low-frequency rTMS to explore the functional organization of STM by selectively disrupting the left dorsomedial PFC (DMPFC), DLPFC, or ventral PFC (VPFC). They applied a 10-min 1-Hz rTMS train before assessing spatial or nonspatial (face recognition) delayed-response performance. Spatial task performance was impaired after rTMS to DMPFC, whereas nonspatial task performance was impaired after rTMS to VPFC. Disruption of the DLPFC affected the performance in both tasks. This finding reveals a task-related segregation of processing streams along prefrontal structures. More recent studies have confirmed the utility of TMS to offer empirical support for modality-specific segregation. For example, Soto et al. (2012) combined evidence from fMRI and rTMS to demonstrate that verbal and nonverbal memories interact with attention functions independently: whereas rTMS to the superior frontal gyrus disrupted STM effects from colored shapes, rTMS to the lateral occipital cortex disrupted effects from written words. Finally, Morgan and colleagues (2013) used TMS to reveal the neural substrates for integration of segregated features of STM processes. They investigated STM for colors, orientations, and combinations of these, and found that continuous TBS (cTBS) over the right parietal cortex or left inferior frontal gyrus selectively impaired STM for combinations but not for single features. Therefore, functional coupling between frontal and parietal areas appears to be critical to bind modality-specific segregated processes.

Study exploring the segregation of memory processes in prefrontal cortex. Two alternative models were proposed based on the data. ( A ) There might be two different, nonoverlapping, functionally segregated regions within the prefrontal cortex (PFC) that are domain-specific (S, spatial domain; F, face domain). Repetitive transcranial magnetic stimulation (TMS) over the dorsomedial PFC (DMPFC) interferes only with the processing of the spatial information. Dorsolateral PFC (DLPFC) stimulation might have induced overlapping interference of two adjacent domain-specific areas, whereas the ventral PFC (VPFC) led only to interference with the processing of the face stimuli. ( B ) The DMPFC and the VPFC interference effects can be explained in the same manner as in proposal ( A ); however, the performance deterioration over the DLPFC in this model might be explained by the interference with information processing of a common module (C) that is employed during both types of stimulus. (Modified from Mottaghy et al., 2002b , by permission of the authors.)
Frontoparietal binding
Frontoparietal interactions in memory formation and maintenance appear to be dynamic and NBS studies – particularly studies combining TMS with MRI or EEG – help gaining critical insights in this regard.
In the motor domain, frontoparietal interactions seem to be particularly important in the early phase of learning, as has been shown in a recent study combining TMS and EEG ( Karabanov et al., 2012 ). In the nonmotor domain, a recent TMS–fMRI study ( Feredoes et al., 2011 ) found that DLPFC contribution to maintenance of stimuli in STM is highly dynamic depending on the presence or absence of distractors. In the presence of distractors, DLPFC changes its communication with posterior regions to support maintenance. These results are supported by tDCS studies that assign the DLPFC an important role in STM in the presence of distractors ( Gladwin et al., 2012 ; Meiron and Lavidor, 2013 ). Zanto and colleagues (2011) combined EEG with 1-Hz rTMS to the right inferior frontal junction to investigate the contribution of the prefrontal cortex in top-down modulation of visual processing and STM in a delayed-match-to-sample task. They found that EEG patterns from posterior electrodes, which are associated with the distinction of task-relevant and -irrelevant stimuli during early encoding, were diminished after TMS, which again predicted a subsequent decrease in STM accuracy. Subjects with stronger frontoposterior functional connectivity furthermore showed greater disruption. Higo and colleagues (2011) combined offline TMS over the frontal junction with subsequent fMRI to explore the same question. They also observed a TMS-induced decrease of effects in posterior regions depending on task relevance/irrelevance. The inferior frontal junction may therefore control the causal connection between early attentional processes and subsequent STM performance, and may regulate the level of activity of representations in posterior brain areas depending on their relevance/irrelevance for response selection.
It could be hypothesized that the interaction between frontal and posterior areas during the delay period secures the maintenance of information, especially if this information needs to be protected from distracting information. These processes may be related to the regulation and reactivation of patterns that were active during encoding. Accordingly, frontal areas might recruit neuronal assemblies and regulate their activity in posterior areas in order to protect and actively maintain information. Such activations may be most prominent at the beginning of the delay period and decrease gradually.
Other brain regions involved in STM
Frontal and parietal areas are undoubtedly the most explored areas in STM. Although it has been debated in the literature, there is some evidence that the cerebellum may also be involved in STM. When Desmond and colleagues (2005) applied single-pulse TMS (at 120% resting motor threshold) over the right superior cerebellum at the beginning of the delay phase, they found an increased reaction time but no change in accuracy for correct trials in the Sternberg task. This is in agreement with a tDCS study that probed the cerebellum and found an abolishment of practice-dependent improvements in reaction time after anodal as well as cathodal tDCS in a Sternberg task ( Ferrucci et al., 2008 ).
Last, but not least, cortical areas implied in sensory processing are also believed to be involved in STM of sensory information, which may be guided through attentional processes. A number of TMS studies have shown a role of visual cortex with visual STM and WM (see review by Postle et al., 2006 ). A few studies have furthermore investigated the tactile domain. Application of TMS to the visual cortex during the delay phase of STM tasks results in a decrease of accuracy in the targeted visual field for high memory loads ( Van de Ven et al., 2012 ) or a decrease in memory scanning rates ( Beckers and Hömberg, 1991 ). The effect of TMS was furthermore shown to be different if applied at the beginning (inhibitory) or at the end (facilitatory) of the delay period in both a visual STM task and imagery ( Cattaneo et al., 2009 ). This is an elegant application of state-dependency TMS experimental designs ( Silvanto and Pascual-Leone, 2008 ). Although neurons implicated in encoding are highly active at the onset of the retention period, TMS might preferentially activate neurons not involved in encoding, thereby reducing the signal-to-noise ratio of the memory trace, and impair behavior.
In the tactile domain, a TMS study using single-pulse stimulation over the middle frontal gyrus (MFG) during the early maintenance period led to a decrease in reaction time in a tactile STM task, even in the presence of a distracting stimulus ( Hannula et al., 2010 ). In a follow-up study, the same group investigated whether this improvement only occurs when the interference is tactile, or whether MFG creates a more general top-down suppression ( Savolainen et al., 2011 ). Their results showed that TMS did not lead to facilitation when a visual interference was presented, but only when the interfering stimulus was also tactile.
These and other findings (e.g., Silvanto and Cattaneo, 2010 ) suggest that sensory brain areas involved in early, modality-specific, processing of perceptual stimuli contribute to the formation and maintenance of STM representations through an interaction with the attentional system. In this context, TMS can help elaborate the chronology of memory processes and contributions of state-dependent processes.
W orking memory
WM has been investigated with NBS in a growing number of studies. As for STM, most of these studies have explored the roles of DLPFC and parietal areas, trying to find an answer to the question of whether information is separately processed with regard to domain or functional subprocess ( Fig. 55.5 ). In addition, some studies have examined the question of whether the same areas that participate in STM are also active in WM tasks.

Schematic summary of findings from studies investigating the impact on verbal (red) or nonverbal (blue) working memory (WM) after stimulation over the left or right prefrontal cortex (PFC), parietal areas, or cerebellum.
Verbal and nonverbal WM in DLPFC
Again building on pioneering work from Mottaghy et al. (2000) , most researchers have found an impairment of verbal WM after stimulation of the left DLPFC ( Mull and Seyal, 2001 ; Mottaghy et al., 2000 , 2003a ; Postle et al., 2006 ; Osaka et al., 2007 ) and after stimulation of the right DLPFC ( Mottaghy et al., 2003a ; Postle et al., 2006 ; Sandrini et al., 2008 ). However, some studies failed to find such effects ( Mull and Seyal, 2001 ; Rami et al., 2003 ; Imm et al., 2008 ; Sandrini et al., 2008 ).
The role of DLPFC in nonverbal WM has been studied much less ( Oliveri et al., 2001 ; Imm et al., 2008 ; Sandrini et al., 2008 ). Sandrini and colleagues (2008) tried to clarify domain- and process-specific contributions of the DLPFC. They presented physically identical stimuli (letters in different spatial locations) in a 1-back task (STM) and a 2-back task (WM). Furthermore, they presented the 2-back task with stimuli of both or just one domain. A short train of 10-Hz rTMS was applied at the end of the delay period between stimuli. They found interference only during the 2-back task, and only when stimuli from both domains were presented. Interestingly, performance in the letter task was impaired after rTMS over the right DLPFC, whereas performance in the location task was impaired after rTMS over the left DLPFC. These results were interpreted as an interference effect on control mechanisms (central executive) in the sense of the suppression of task-irrelevant information. The same hypothesis has been put forward with regard to the protection of memory contents in STM ( Feredoes et al., 2011 ; Higo et al., 2011 ; Zanto et al., 2011 ), according to which an interaction between frontal and posterior areas during the delay period secures the maintenance of information, especially in the presence of distractors.
Further experiments have aimed at dissecting the role of DLPFC in WM in order to find out whether domain- or process-specific models should be favored, and others have examined the role of interactions between DLPFC and other brain areas. Combination of TMS with brain imaging has proven quite valuable in this context. Mottaghy and colleagues (2000) found that performance in a verbal WM (2-back) task was significantly diminished after rTMS (30-second train of 4-Hz rTMS) to the left but also the right DLPFC (F3/F4). Importantly, by combining TMS with PET, they showed that TMS-altered performance in the WM task was associated with a reduction in regional cerebral blood flow (rCBF) at the stimulation site and in distant areas as assessed with PET. In an elegant follow-up TMS–PET study, the same authors ( Mottaghy et al., 2003b ) showed that at baseline (in the absence of TMS) there was a negative correlation between rCBF in the left (but not the right) DLPFC and WM task performance. Application of rTMS to the left or the right DLPFC could disrupt WM performance, but appeared to do so on the basis of different distributed impact on a bihemispheric network of frontal and parietal regions: whereas rTMS over the left DLPFC led to changes in rCBF in the directly targeted left DLPFC and the contralateral right PFC, rTMS over the right DLPFC led to more distributed changes involving not only bihemispheric prefrontal, but also parietal areas ( Fig. 55.6B ). Regardless of the differential network impact of the right or left stimulation, the behavioral consequences of rTMS were always related to the impact onto left DLPFC rCBF. This study highlights a number of important findings of relevance for future studies on NBS in memory and learning. First, it shows that rTMS to different nodes of a given brain network can exert differential impact onto said brain network. More recently, Eldaief et al. (2011) have expanded on this line of inquiry combining resting-state fMRI with TMS to examine brain network dynamics. Second, the study shows that network dynamics are modified by behavioral engagement. In other words, it might be possible to learn about mechanisms of memory and learning by examining how the impact of TMS onto a given brain network is modulated by the behavioral state. Finally, the study illustrates that brain stimulation can affect behavior by disrupting a computation in the targeted brain region (as in the case of left DLPFC rTMS) or by disrupting function of a brain regions reached via trans-synaptic network impact (as in the case of rTMS to the right DLPFC altering left DLPFC via interhemispheric connections). This later finding is important in the interpretation of brain stimulation results in general, and illustrates the power of studies integrating brain stimulation with neuroimaging in exploring causal relations between brain activity and behavior ( Fig. 55.6A ). In a later study, Mottaghy and colleagues (2003a) applied single-pulse TMS at different time points after stimulus presentation to probe the temporal dynamics of parietal and prefrontal contributions in verbal WM. With this approach they were able to add chronometric information to their prior findings. They showed that single-pulse TMS could interfere with task accuracy earlier in the parietal than in the PFC, and earlier over the right than left hemisphere. This indicates an information flow from posterior to anterior converging in the left PFC. These series of studies reveal that both hemispheres contribute to WM, but that the computation performed by the left PFC is critical in verbal WM.

Transcranial magnetic stimulation–positron emission tomography (TMS–PET) study of the neurobiological substrates of working memory. ( A ) The impact of TMS on behavior relies on activity changes in local and distributed brain networks. The combination of TMS with brain imaging techniques, such as EEG, fMRI, PET, and EMG, allows us to detect correlations between these activity changes and behavior. Moreover, it allows study of the impact of state dependency on stimulation outcome. ( B ) Positive (green) and negative (red) correlation between regional cerebral blood flow (rCBF) and performance in the 2-back working memory (WM) task (1) without application of repetitive TMS (rTMS), (2) with rTMS delivered over the left middle frontal gyrus (MFG), and (3) with rTMS applied over the right MFG. While rTMS over the left MFG has a local impact, which is correlated with behavior, rTMS over the right MFG has an impact on a distributed network, including homologous areas. Importantly, also in the case of stimulation over the right MFG, activity changes in the left MFG but not right MFG are correlated with behavioral output. This key finding shows that the effect of TMS can be achieved by a direct effect on underlying areas, but also through trans-synaptic effects (e.g., in homologous areas). The combination of TMS with imaging techniques is crucial in order to identify neural substrates associated with behavioral output. (Modified from Mottaghy et al., 2003b , by permission of the authors.)
Interestingly, involvement of DLPFC, regardless of stimulus modality, has been shown in an often-cited study using bilateral single-pulse stimulation during a 2-back task ( Oliveri et al., 2001 ). Early temporal stimulation (300 ms) increased reaction time for object-related WM, whereas early parietal stimulation and late stimulation (600 ms) over the superior frontal gyrus increased reaction time for spatial WM. However, late DLPFC stimulation interfered with both tasks and not only with RT, but also with accuracy. These results relate to the discrimination of a dorsal (“where”) and ventral (“what”) pathway and again information flow from parietotemporal to frontal areas. They indicate that there might not only exist a bilateral involvement of the DLPFC in verbal WM, but that DLPFC might be active irrespective of stimulus material, unlike other prefrontal regions that may be segregated (see e.g., Mottaghy et al., 2002b ). Segregation in posterior areas seems to be easier to pinpoint, and is concordant with the view that both hemispheres are implicated in spatial and object WM tasks ( Smith and Jonides, 1997 ). The research that has been done up to date generally points into the direction of favoring a process-specific model for DLPFC, whereas other areas of the prefrontal or parietal cortex may be modality-based. Possibly, WM operations relying on DLPFC, such as selective attention and other executive processes (e.g., the inhibition of task-irrelevant stimuli), are independent of modality ( Smith and Jonides, 1999 ) and play a role in both STM and WM. The combination of fMRI and EEG with TMS may help us to disentangle further the interactions of DLPFC and other prefrontal and parietal areas to WM functions.
P rospective memory
Prospective memory is tightly connected with other memory subcomponents (see Fig. 55.1 ), which makes it difficult to single out its processes. Perhaps this challenge accounts for the fact that few studies to date have explored prospective memory using NBS. One study ( Basso et al., 2010 ) investigated whether verbal WM and prospective memory are based on common or separate processes. In a first experiment participants had to accomplish tasks with low, medium, or high WM load. In the prospective condition, subjects had to react whenever a specific word appeared. In a second experiment the prospective conditions included 1 or 3 prospective words. A higher prospective memory demand interfered with the WM task only at higher loads, whereas WM activity did not affect prospective memory performance. If both processes were part of the same system one might expect a trade-off. In a third experiment single-pulse TMS was applied to the left and right DLPFC in order to test the notion that WM and prospective memory rely on distinct systems. TMS to the DLPFC increased error rates in the prospective memory task, whereas the effect on the WM task was only marginal. No difference between hemispheres was detected. The authors concluded that WM and prospective memory may not be based on the same memory system. However, it is hard to rule out that prospective memory may require resources (including in part WM resources) and may thus be easier to disrupt with TMS. More complex TMS designs, such as input–output designs with TMS applied at various intensity and timings, seem necessary to explore this issue further.
Costa and coworkers (2011) investigated the effects of cTBS (80% active motor threshold) on prospective memory. Stimulation over left Brodmann area (BA) 10 (frontal pole) resulted in impaired accuracy as compared with stimulation over Cz. In a second experiment they did not find a significant difference after cTBS over left BA46 (DLPFC) and Cz. They concluded that the left BA10 is important for prospective memory processes. This is in accordance with a neuroimaging study ( Koechlin et al., 1999 ) that tried to dissociate the roles of frontopolar and DLPF cortices in prospective memory. Costa and colleagues employed a fairly novel TMS paradigm (cTBS) and tackled a complicated memory construct (prospective memory). However, this important, innovative study also illustrates one important challenge for all studies using NBS in memory: it is ultimately critical to have separate empirical demonstration of the impact of brain stimulation on brain function, and on behavior. In fact, ideally, one would want to apply TMS, measure the behavioral impact and the impact on brain physiology, and then correlate one with the other (see Fig. 55.6A ). Costa and coworkers (like most investigators using TMS or tDCS in studies of memory) placed the TMS coil over the scalp overlaying the brain regions they wanted to target (frontal pole or DLPFC). They then assumed that the TMS impact on brain activity would be maximal in the underlying cortex. They assessed the impact of TMS onto prospective memory and assumed that said impact must reflect the consequence of TMS-induced change in activity in the targeted brain region. There is a risk of circular logic in this approach: “If TMS over a given region has a predicted impact onto a given memory process, then I have shown that said brain region was affected by TMS and that it plays said role in memory.” Obviously, independent empirical demonstration of these two steps would be important and the use of NBS in studies of memory, or studies of cognitive functions in general, should aim to achieve such experimental discrimination.
E ncoding, consolidation, retrieval
Some studies have applied rTMS during the encoding phase and support the critical role of the PFC in such memory processes. Stimulation of the left DLPFC during the encoding phase has been found to affect both verbal ( Grafman et al., 1994 ; Rami et al., 2003 ; Sandrini et al., 2003 ; Flöel et al., 2004 ; Skrdlantová et al., 2005 ; Blanchet et al., 2010 ; Gagnon et al., 2010 , 2011 ) and nonverbal ( Rossi et al., 2001 , 2004 ; Blanchet et al., 2010 ; Gagnon et al., 2010 , 2011 ) memory. However, a few studies have reported an impact on memory functions after stimulating right frontal areas during the encoding phase of verbal ( Grafman et al., 1994 ; Sandrini et al., 2003 ; Kahn et al., 2005 ; Blanchet et al., 2010 ; Machizawa et al., 2010 ) or nonverbal ( Epstein et al., 2002 ; Flöel et al., 2004 ; Blanchet et al., 2010 ) memory functions. Some investigators did not find any effects after stimulating right frontal cortex ( Rami et al., 2003 ; Köhler et al., 2004 ). No effects have been found after stimulating parietal ( Köhler et al., 2004 ; Rossi et al., 2006 ) or occipital cortex ( Grafman et al., 1994 ). Only one study reported impairment after stimulating the temporal cortex ( Grafman et al., 1994 ).
Fewer studies have applied TMS during the retrieval phase of memories. Stimulation of the right DLPFC during the retrieval phase appears to be associated with an impact on both verbal ( Sandrini et al., 2003 ; Gagnon et al., 2010 , 2011 ) and nonverbal ( Rossi et al., 2001 , 2004 ; Gagnon et al., 2010 , 2011 ) memory. No studies have reported an effect after stimulation of the left hemisphere during the retrieval phase.
Several studies have used NBS to reveal the important role of the ventrolateral PFC (VLPFC) in the formation of long-term memory ( Grafman et al., 1994 ; Flöel et al., 2004 ; Köhler et al., 2004 ; Machizawa et al., 2010 ), and it has been suggested that VLPFC may be material-specific whereas DLPFC is not. Further studies are needed to shed light on these mechanisms.
Recent studies by Gagnon and colleagues explicitly addressed the assumptions of the HERA model ( Blanchet et al., 2010 ; Gagnon et al., 2010 , 2011 ) and tried to shed light on the contribution of left and right DLPFC in encoding and retrieval of verbal as well as nonverbal information. These are particularly important studies as they illustrate the value of TMS in the systematic testing of key aspects of a well formulated cognitive conceptual model. It is this type of experimental approach that can fully leverage the advantages of TMS in studies of memory and learning. In a first study, Gagnon et al. (2010) applied paired-pulse TMS (interstimulus interval (ISI) 3 ms) over the left or right DLPFC during encoding or retrieval of verbal (words) and nonverbal stimuli (random shapes). They found that left and right DLPFC play different roles in encoding and retrieval irrespective of stimulus type: stimulation of the left DLPFC during encoding resulted in discrimination deficits, whereas stimulation of the right DLFPC during retrieval resulted in a reduced hit and disrimination rate. In a follow-up study they applied paired-pulse TMS with a longer ISI (15 ms) to promote facilitation (rather than cortical suppression) to the left and right DLPFC during encoding or retrieval of verbal (words) and nonverbal stimuli (random shapes) ( Gagnon et al., 2011 ). They found a facilitation of reaction times during encoding (left DLPFC) and retrieval (right DLPFC) regardless of the type of material presented. These results are consistent with other TMS studies ( Rossi et al., 2001 , 2006 ; Rami et al., 2003 ) and provide experimental support for the HERA model, which proposes that the left PFC is more involved in semantic retrieval and episodic encoding than the right PFC, whereas the right PFC is involved in episodic retrieval ( Tulving et al., 1994 ). This hemispheric asymmetry seems to uphold for both verbal and nonverbal material ( Haxby et al., 2000 ; Blanchet et al., 2010 ).
USING NONINVASIVE BRAIN STIMULATION AS A DIAGNOSTIC TOOL
In addition to uses in cognitive neuroscience, it is worth considering the potential utility of NBS in clinical neuroscience as a diagnostic tool. Diagnostic applications of NBS are appealing as they are noninvasive and can be applied safely to various patient populations across the lifespan, if appropriate precautions are taken and guidelines are followed ( Rossi et al., 2009 ). TMS has an excellent temporal resolution and its spatial resolution is superior to tDCS, which are important advantages in diagnostic applications and make TMS a superior tool to probe brain reactivity and brain connectivity.
To date, TMS has not been established as a diagnostic tool. However, if we define carefully the areas of need in specific patient populations, we may be able to complement currently used test measures, which rely mainly on behavioral assessments ( Rost et al., 2008 ; Sigurdardottir et al., 2009 ; Gialanella, 2011 ; Wagle et al., 2011 ).
As for motor dysfunctions, nonmotor memory functions could be characterized by changes in the excitation/inhibition (E/I) balance and cortical plasticity in specific brain areas, which could be assessed with TMS–EEG measures ( Thut and Pascual-Leone, 2010 ). Changes of such neurophysiological measures over the time-course of cognitive rehabilitation, during normal and pathological aging, or in response to treatment of disease could help us establishing neurophysiological biomarkers indicative of functional improvements. Such measures could not only be helpful to differentiate across pathological entities, but may also disentangle underlying causes of memory dysfunctions on an individual level. Finally, this information could help develop novel and improve existing interventions in order to improve memory functions.
In the memory domain there are several questions worth exploring with TMS as a diagnostic tool: (1) What is the pathogenesis of present memory problems? (2) Who is at risk of developing memory problems and what kind of memory problems? (3) Who is likely to benefit from a given behavioral/physiologic/pharmacological intervention?
Identify the pathogenesis of memory problems
Depending on the etiology, the pathogenesis of an individual patient’s memory problem can be vastly different and be affected by many factors including age, environmental, and genetic predispositions. Regardless of etiology, though, one can also aim to identify the proximal, neural dysfunction that accounts for a given memory deficit. TMS can be applied to gain insights at both these levels of inquiry.
Single- and paired-pulse TMS measures may reveal changes in connectivity or altered network dynamics and link those to specific memory functions. Advanced combined technologies such as TMS–EEG or TMS–MRI allow us to utilize TMS-induced cortical evoked potentials or TMS-induced blood oxygen level-dependent (BOLD) fMRI changes as neural measures of brain activity in specific brain regions or networks to relate to behavioral memory measures.
rTMS paradigms, for example intermittent and continuous TBS stimulation (iTBS and cTBS), can be used to obtain indices of cortical plasticity that appear related to long-term potentiation and depression (LTP and LTD)- like induction of synaptic plasticity. Such paradigms can be used to evaluate cortical plasticity in neural structures thought to support memory processes and may allow us to draw conclusions regarding the pathogenesis of a memory problem. For example, a cortical lesion within a widespread memory network could not only have a direct impact on memory functions caused by this particular lesion but could also lead to indirect deficits due to disconnection of the lesioned area with another memory hub. TMS measures could inform us about acute processes as well as adaptive or maladaptive changes characteristic of chronic processes that lead to memory dysfunctions ( Pascual-Leone et al., 2011 ).
Identify risk for developing memory problems
Another major area of interest lies in the possible use of TMS as a physiological biomarker, which could indicate the individual risk of developing memory dysfunctions with age and predict what kind of memory problems could be expected in certain populations. Cognitive decline including memory functions presents a critical hallmark of aging ( Morrison and Baxter, 2012 ). Early changes in neuroplasticity and neurophysiological circuits indicated by TMS measures, such as short-latency afferent inhibition (SAI), could constitute biomarkers for the development of neurodegenerative disorders ( Freitas et al., 2011b ). Risk identification with this approach requires the integration of numerous factors associated with causal and formal pathomechanisms, including age-related changes, but also, for example, changes related to systemic diseases, such as diabetes mellitus, that may indirectly have an impact on brain physiology and plasticity. TMS could be a valuable tool to identify these factors and consequently help guide and implement early interventions in populations at risk.
Another approach is using TMS measures to identify risks related to interventions that could result in brain lesions or dysfunctions. For example, consider neurosurgical interventions: presurgical detailed knowledge about functional contributions of brain areas to be resected can critically inform surgical approaches and minimize the risk. In this context, the Wada test can be used to determine hemispheric language dominance prior to brain surgery ( Wada and Rasmussen, 1960 ). However, this test has a non-negligible risk of complications and discomfort for the patient and does not allow precise functional localization. Neuronavigated TMS can provide detailed information regarding functional anatomy of the targeted brain area and is potentially valuable for presurgical planning not only in regard to language dominance ( Pascual-Leone et al., 1991 ; Devlin and Watkins, 2007 ), but also in regard to memory ( Grafman et al., 1994 ). Such noninvasive neuronavigated TMS cortical mapping appears to correlate well with direct cortical stimulation (DCS) results and seems to be more precise than fMRI, which is the most widely used technique today ( Krieg et al., 2012 ). As DCS is limited to intraoperative use, presurgical TMS might also save operation time by guiding intraoperative DCS.
Predicting benefit from a given intervention/medication
Cognitive rehabilitation consists in assessment-based therapeutic interventions aiming to reduce disability and promote functional recovery. Functional changes are achieved through various intervention methods targeting restitution, compensation, and adaptation ( Cicerone et al., 2000 ). But how can we determine whether a given therapeutic intervention will have a beneficial effect for an individual patient?
TMS measures may be used not only to track but also to predict intervention-related neuroplastic changes within memory networks. Moreover, TMS measures can inform us about the functionality of specific neurophysiological circuits implicated in memory functions and may be indicative of how well an individual will profit from a given pharmacological intervention. For instance, acetylcholine (ACh) is a neurotransmitter that plays a crucial role in synaptic plasticity and memory functions, and ACh imbalances have been associated with memory deficits in patients with Alzheimer’s disease (AD) ( Davies and Maloney, 1976 ; Coyle et al., 1983 ). Deficits in cholinergic circuits can be counteracted with pharmacological interventions involving acetylcholine esterase (AChE) inhibitors. SAI is a TMS measure that is indicative of cholinergic circuits in the motor cortex ( Di Lazzaro et al., 2000 ) and is altered in patients with AD (for a review see Freitas et al., 2011a ). SAI may even be useful to differentiate dementia subtypes ( Di Lazzaro et al., 2006 , 2008 ) and may be used as an indicator of who will profit from AChE inhibitors. Short-latency intracortical inhibition (SICI) and the cortical silent period (cSP) are thought to reflect the excitability of inhibitory γ-aminobutyric acid (GABA)ergic circuits ( Hallett, 2000 ) and were also found to be abnormal in patients with AD. However, the relationship of these TMS measures with specific memory dysfunctions is less clear ( Freitas et al., 2011a ). Notably, studies up to date have relied on TMS measures from the motor cortex. However, the combination of TMS with EEG may enable us to find more precise TMS biomarkers by exploring neurophysiological changes outside the motor cortex.
MODULATING LEARNING AND MEMORY
The interest in the augmentation of cognitive functions reaches far back into the history of modern humanity. The use of memory techniques, for instance in order to improve rhetorical skills, was already promoted by Marcus Tullius Cicero (“De Oratore”, Book II, 55 bc ). One of these methods, the “Cicero Memory Method” (Method of loci), a simple memory enhancement method that uses visualization to structure information, is still in use today. The pursuit of cognitive augmentation has since led researchers to take advantage of technical developments in order to achieve a better outcome. In the past decade, scientists have therefore started investigating the impact of various NBS techniques on memory functions.
Learning is a prerequisite for the formation of memory traces and is thought to be dependent on synaptic plasticity mediated by LTP and LTD, which also represent key mechanisms in the effects of NBS on brain functions. This has not only rendered NBS valuable for the investigation of neuroplastic processes associated with learning and memory but also promotes it as a valuable tool to enhance memory functions.
Although TMS is used mostly for diagnostic purposes and the investigation of brain structures contributing to specific functions, tDCS is more often applied to enhance brain functions.
Healthy subjects
In the past decade, researchers have begun examining the effects of WM training on neural correlates and concomitant performance ( Jaeggi et al., 2008 ). These studies have shown that not only can WM capacity be increased via constructive training but also that said training increases the density of cortical D1 dopamine receptors in prefrontal regions ( McNab et al., 2009 ). The neurobiological substrate of WM is an ongoing topic of research; however, prefrontal regions are believed to be critically involved. Consistent with such notions, studies exploring the potential for NBS to enhance WM have focused on the prefrontal cortex, generally the DLPFC, and the majority have used verbal WM tasks. In most studies subjects were asked to practice STM or WM tasks concurrently to tDCS, and their WM abilities were assessed either during or afterwards.
Compared with sham stimulation, tDCS with the anode over the left DLPFC (and the cathode right supraorbitally) has been repeatedly reported to enhance WM in healthy subjects ( Fregni et al., 2005 ; Ohn et al., 2008 ; Mulquiney et al., 2011 ; Teo et al., 2011 ; Zaehle et al., 2011 ). Some researchers have suggested that increasing stimulation intensity ( Teo et al., 2011 ) or duration ( Ohn et al., 2008 ) might lead to more robust effects. Only one study has reported no memory improvement following tDCS with the anode over the left DLPFC ( Mylius et al., 2012 ), and one study reported improvement in STM but not in WM ( Andrews et al., 2011 ). The only study applying tDCS with the anode over the right DLPFC showed no WM effect ( Mylius et al., 2012 ). On the other hand, tDCS with the cathode over the left DLPFC (and the anode right supraorbitally) yielded diverse results in different studies, ranging from memory benefits ( Mylius et al., 2012 ), to no effects ( Fregni et al., 2005 ), and even negative effects ( Zaehle et al., 2011 ). The study by Zaehle et al. (2011) is of particular interest as the authors reported that the negative effects of tDCS with the cathode over the left DLPFC were associated with decreased electroencephalographic power in theta and alpha bands over posterior (parietal) regions. On the other hand, the authors found that improved WM following tDCS with the anode over the left DLPFC was associated with increased power in alpha and theta EEG bands over parietal regions. This study illustrates the potential of studies combining behavioral and neurophysiological outcome measures, and suggests the critical role of corticocortical interactions in memory enhancement. It has been proposed that a more distributed network may subserve WM functions with the posterior parietal cortex (PPC) playing an important role ( Mottaghy et al., 2002a ; Collette et al., 2006 ). Stimulation might disrupt activity in a given cortical region and thus release activity in a distant connected node, resulting in paradoxical facilitation ( Najib and Pascual-Leone, 2011 ). The specific nature of the stimulation seems important, although, for example, random noise stimulation over the left DLPFC showed no effects ( Mulquiney et al., 2011 ).
In order to explore further the role of parietal structures in WM, Sandrini and colleagues (2012) applied bilateral stimulation over the PPC during a 1-back (STM) or a 2-back (WM) task. They found a double dissociation, with STM being impaired after left-anodal/right-cathodal and WM being impaired after left-cathodal/right-anodal stimulation. They concluded that this dissociation might be due to differential processing strategies in STM and WM. However, the effects might have been mediated by impact on attentional (rather than memory) processes given the fact that only response time, and not accuracy, was affected. Future studies will need to investigate further the contribution of parietal areas and their interaction with prefrontal areas to WM enhancement.
Further studies could examine the duration of effects, the likely synergistic effect of cognitive training with tDCS, or the applicability of tDCS or other NBS methods to enhance WM across the age span, from children to elderly. However, all such studies need carefully to weigh risk–benefit considerations, and should be informed by a thoughtful discussion of the ethical connotations of such enhancement approaches ( Rossi et al., 2009 ; Hamilton et al, 2011 ; Horvath et al., 2011 ).
Whether NBS can enhance STM in normal subjects is less clear. Studies show less consistent results. This could in part be due to the fact that basic STM tasks are easy for healthy subjects, which leads to ceiling effects. More recent studies have applied adapted tasks, which, however, makes it difficult to compare across studies. Most studies, similar to the literature on WM, have targeted the DLPFC. Two recent studies reported beneficial effects of tDCS with the anode over the DLPFC for an STM task with additional distractors ( Gladwin et al., 2012 ; Meiron and Lavidor, 2013 ). One study found a gender-dependent improvement in accuracy, with male subjects profiting more from left DLPFC stimulation and female subjects profiting more from right DLPFC stimulation, but only if distractor loads were high ( Meiron and Lavidor, 2013 ). The other study used a modified Sternberg task, which introduced additional distractor stimuli during the delay period ( Gladwin et al., 2012 ). These workers found significant reaction time improvements after stimulation of the left DLPFC. Compared with these studies, Marshall et al. (2005) applied tDCS with either two anodes or two cathodes over DLPFC, with the reference electrodes positioned over the mastoids, and found deleterious effects of STM. This may indicate that the introduction of distractors to an STM task changes underlying neurobiological processes and enables enhancement effects. Improvements after tDCS may be due to either improved selective attention or more successful inhibition of distracting information. Indeed, a recent TMS study has shown that the role of the DLPFC in STM tasks seems to be dependent on the presence of distractors. The stronger the distraction, the more prominent the frontoparietal interactions become, in order to protect relevant memory representations ( Feredoes et al., 2011 ).
Studies in which investigators stimulated parietal areas have yielded partly opposing results. This is true of studies using tDCS and those employing TMS. Regarding TMS experiments, some show worsened STM ( Koch et al., 2005 ; Postle et al, 2006 ), while the other report improved STM ( Hamidi et al., 2008 ; Yamanaka et al., 2010 ) after high-frequency parietal stimulation during the delay period. As for tDCS experiments, Berryhill et al. (2010) found impairment in recognition, but not free recall, after tDCS with the cathode over the right parietal cortex (and the anode over the left cheek), whereas Heimrath and coworkers (2012) , positioning the cathode over the right parietal cortex (and the anode over the contralateral homologous area), found an improved capacity in a delayed match-to-sample task after tDCS when stimuli were presented in the left visual hemifield (STM for stimuli presented in the left hemifield decreased). Interestingly, Heimrath et al. used concurrent tDCS and EEG, and found a decrease in oscillatory power in the alpha band after cathodal stimulation. As alpha activity is assumed to reflect inhibition of distractors ( Klimesch, 1999 ), the authors suggested that this measure might indicate memory performance. This study again illustrates the potential of experiments combining behavioral and neurophysiological outcome measures with NBS.
Finally, one study probed the cerebellum and found an abolishment of practice-dependent improvements in response time in a Sternberg task, regardless of whether the anode or the cathode was placed over the cerebellum (and the other electrode over the vertex) ( Ferrucci et al., 2008 ). The contribution of the cerebellum to STM was also probed with single-pulse TMS by Desmond and colleagues (2005) , who also found a negative effect on response time in the Sternberg task. Whether other cerebellar stimulation paradigms can induce an enhancement of STM remains unexplored.
G eneral memory and learning
Researchers attempting to enhance learning processes have targeted various neural regions. Such diverse approaches again render it difficult to single out a pattern regarding stimulatory condition, mechanisms, and outcome. Most studies have applied tDCS during the learning phase, and most have targeted the left DLPFC or other left prefrontal areas. Generally, studies report memory improvement following tDCS with the anode over DLPFC ( Kincses et al., 2004 ; Javadi and Walsh, 2012 ; Javadi et al., 2012 ) or other prefrontal areas ( De Vries et al., 2010 ), and worsening memory after tDCS with the cathode over DLPFC ( Elmer et al., 2009 ; Hammer et al., 2011 ; Javadi and Walsh, 2012 ; Javadi et al., 2012 ) or other prefrontal areas ( Vines et al., 2006 ). However, in interpreting their results, investigators have often made the overly simplistic assumption that the effects of tDCS can be accounted for by the neurobiological effect of one of the electrodes, the anode enhancing and the cathode suppressing activity in the brain area under them. Yet, it is important to remember that tDCS is not monopolar and that all electrodes are active. Thus the brain is exposed to a flow of current with opposite faradizing effects of the anode and the cathode. Therefore, to speak of anodal tDCS or cathodal tDCS is inaccurate.
Few studies have targeted right prefrontal areas. One study reported no effects in an episodic verbal memory task after tDCS with either anode or cathode over the right prefrontal region ( Elmer et al., 2009 ). Two studies showed that the learning process of threat detection in a virtual reality environment and the time required to learn this skill can be improved following tDCS with the anode over the right prefrontal ( Bullard et al., 2011 ; Clark et al., 2012 ) or right parietal region ( Clark et al., 2012 ). Furthermore, Bullard and colleagues (2011) found that applying tDCS at the beginning of the learning phase significantly enhanced learning in comparison with findings in experienced learners (after 1 hour of training).
Bilateral stimulation (anode and cathode over homologous areas of either hemisphere) has been applied in a few studies ( Marshall et al., 2004 , 2011 ; Boggio et al., 2009 ; Chi et al., 2010 ; Cohen Kadosh et al., 2010 ; Penolazzi et al., 2010 ; Jacobson et al., 2012 ). Jacobson and coworkers (2012) applied bilateral tDCS (anodal left, cathodal right, or vice versa) over the parietal lobe during encoding. They found improved verbal memory only when the anode was placed over the left hemisphere and the cathode over the right hemisphere. Another study investigating the contribution of the parietal cortex to numerical learning applied bilateral tDCS during a training phase of 6 days ( Cohen Kadosh et al., 2010 ). While right-anodal/left-cathodal stimulation improved learning significantly, right-cathodal/left-anodal stimulation decreased learning compared with sham tDCS.
Penolazzi and colleagues (2010) applied bilateral tDCS (anode left and cathode right, or vice versa) over the frontotemporal cortex during encoding and found facilitated recall of pleasant images after right-anodal/left-cathodal tDCS, whereas left-anodal/right-cathodal tDCS facilitated recall of unpleasant images. These results support a theoretical model (specific valence hypothesis) according to which the right and left hemispheres are specialized in the processing of unpleasant and pleasant stimuli respectively. Another group applying bilateral stimulation (anodal left, cathodal right, or vice versa) over the anterior temporal lobe assessed visual memory ( Chi et al., 2010 ) and also reported an improvement in memorizing different types of shape after right-anodal/left-cathodal stimulation, but no effects when applying an inverse stimulation pattern.
One set of studies has investigated effects of bilateral anodal stimulation over DLPFC during sleep and wakefulness. In their first study, Marshall and colleagues (2004) reported an improvement of memory consolidation when applying intermittent (on/off 15 seconds) anodal tDCS simultaneously over both DLPFCs during slow-wave (nonrapid eye movement, non-REM) sleep but not during wakefulness. In a second study they investigated state-dependent effects, and found enhanced theta activity when transcranial slow oscillation stimulation (tSOS) was applied during wakefulness ( Kirov et al., 2009 ). Memory enhancement occurred only when tSOS was applied during learning, but not after learning. In their third study, Marshall and colleagues (2011) applied anodal theta-tDCS (tDCS oscillating at 5 Hz) during REM sleep and non-REM sleep, which led to increased gamma-band activity and decreased memory consolidation respectively. The data from these studies illustrate the potential of transcranial current stimulation at specific stimulation frequencies selectively to modulate specific brain oscillations. This NBS method provides an interesting approach for investigating the relation between cortical brain rhythms, sleep-related processes, and memory functions.
Some studies have reported apparently contradictory results, highlighting the need for further investigation of the mechanisms of action underlying tDCS and TMS. Boggio et al. (2009) found decreased “false memories” utilizing anodal tDCS over the left anterior temporal lobe, or bilateral (left-anodal/right-cathodal) tDCS. However, the same researchers reported a nearly identical effect after applying 1-Hz rTMS over the same region, a protocol that is believed to suppress activity of the targeted brain area ( Gallate et al., 2009 ). Of course, it is possible that the behavioral effect might be related to trans-synaptic network effects, rather than being mediated by the targeted brain region. Indeed, a study using single-pulse TMS reported a facilitatory effect on verbal memory after stimulating the right inferior PFC ( Kahn et al., 2005 ), presumably due to interhemispheric paradoxical facilitation effects. This would be consistent with another study that found an improvement in verbal memory after stimulating the left inferior PFC with 7-Hz rTMS bursts ( Köhler et al., 2004 ). Furthermore, a paired-pulse protocol known to induce facilitatory effects led to memory improvements after stimulation of the left and right DLPFC in verbal as well as nonverbal episodic memory. The combination of stimulation techniques and other methods, such as EEG and fMRI, allows their inherent advantages to be combined to help answer these open questions.
Elderly healthy subjects
Basic memory research includes mostly young and healthy subjects. However, one of the key topics in the domain of NBS research concerns the changes of interhemispheric balance and the increased compensatory recruitment of brain areas with aging. As memory represents an overarching topic for the elderly, it is crucial to promote research that investigates these changes and provides information as to how to enhance memory functions. Furthermore, research with healthy elderly subjects is vital if we want to translate it into the clinical setting, as patients with memory deficits are mostly older. A newly emerging field has started to investigate memory enhancement in elderly subjects and underlying models ( Rossi et al., 2004 ; Solé-Padullés et al., 2006 ; Manenti et al., 2011 ; Flöel et al., 2012 ).
The “Hemispheric Asymmetry Reduction in Older Adults” (HAROLD) model states that prefrontal activity during cognitive performance becomes less lateralized with advancing age ( Cabeza, 2002 ). Manenti and colleagues investigated the differential assumptions of the HERA model (young subjects) and the HAROLD model (elderly subjects), suggesting that hemispheric asymmetry is reduced with age. Interestingly, they could show that low-performing elderly subjects continue showing prefrontal asymmetry, whereas high-performing elderly individuals show reduced asymmetry indicative of compensatory mechanisms ( Manenti et al., 2011 ).
Although lateralized activations within the PFC can be observed in younger subjects during episodic memory tasks ( Rossi et al., 2001 ), this asymmetry vanishes progressively with advancing age, as indicated by bilateral interference effects ( Rossi et al., 2004 ).
Conversely, the predominance of left DLPFC effect during encoding was not abolished in older subjects, indicating its causal role for encoding along the lifespan. However, this study did not differentiate between high- and low-performing subjects. Another study supported the assumption that higher performance is associated with more bilateral recruitment of brain areas and that stimulation may be able to promote the recruitment of additional brain areas to compensate for age-related decline. Solé-Padullés and colleagues (2006) found improved performance in associative learning after 5-Hz offline rTMS, which was accompanied by additional recruitment of right prefrontal and bilateral posterior brain regions.
A tDCS study showed improvements in spatial learning and memory in elderly subjects (mean 62 years) when stimulating during encoding ( Flöel et al., 2012 ). Anodal stimulation over the right temporoparietal cortex improved free recall 1 week later compared with sham stimulation. No immediate learning differences were observed, which indicates that retention (less decay) rather than encoding was affected by the stimulation.
To summarize, several studies have found different results following the stimulation of the DLPFC in young and elderly healthy subjects in accordance with the HAROLD model ( Cabeza, 2002 ). These differences could be due to changes in interhemispheric balance and recruitment of different brain areas for the same tasks, which could arise due to compensatory mechanisms. It remains to be further elucidated whether these changes reflect local or distributed mechanisms, whether compensatory recruitment of additional brain areas is associated with higher performance levels and could be enhanced by NBS.
Compared with the wealth of studies that have been done with healthy and mostly young subjects, studies on patients are rather sparse (see Table 55.1 ). The evidence is encouraging and calls for further investigation of the combined application of NBS and neuropsychological therapy. Besides behavioral measures, these studies should ideally include other measurements, such as assessment of brain plasticity or memory-specific neurophysiological outcomes. The work on patients with stroke is very preliminary, and more studies with larger patient numbers and better control of lesion location are needed. In one crossover, sham-controlled study, Jo et al. (2009) applied tDCS with the anode over the left DLPFC (and the cathode over the contralateral supraorbital area) in a 2-back task to 10 patients with unilateral, right-hemispheric, ischemic, or hemorrhagic strokes (1–4 months poststroke). After a single stimulation session, performance accuracy but not reaction time improved significantly. Enhancement of memory functions has been more extensively investigated in patients with AD and Parkinson’s disease (PD). These findings provide evidence that NBS could be a safe and useful tool in restoring/compensating brain functions through activation of primary and compensatory networks that underlie memory functions.
A lzheimer’s disease
A few studies have demonstrated effects of NBS on cognitive functions in AD (6 TMS, 3 tDCS). The first studies that used NBS in AD looked primarily at language and not memory functions. Cotelli and colleagues used rTMS (20 Hz) over the left and right DLPFC and reported positive effects for both hemispheres. They applied a single online session of rTMS in two crossover, sham-controlled studies ( Cotelli et al., 2006 , 2008 ). In the first study they reported improved accuracy in action naming, but not object naming, for all patients ( Cotelli et al., 2006 ). In the second study they could replicate the positive results for action naming; however, object naming also improved significantly, although only in moderately to severely impaired patients ( Cotelli et al., 2008 ). The authors hypothesized that the lack of improvement in object naming may be due to a ceiling effect. Furthermore, the bilateral effect could have been due to compensatory activation of right hemispheric resources.
In a third placebo-controlled study the same authors tested various functions, including memory, executive functions, and language in patients with moderate AD ( Cotelli et al., 2011 ). This study entailed 4 weeks of daily sessions of 20-Hz rTMS to the left DLPFC. Although they found significant improvements in sentence comprehension after 10 sessions (with no further improvement after 20 sessions), they did not find any improvements in memory and executive functions ( Cotelli et al., 2011 ). This lack of improvement could be due to the fact that the patients were not doing any specific concomitant cognitive training. Alternatively, the lack of memory effects could be related to the targeted brain region.
Bentwich and colleagues (2011) interleaved cognitive training and rTMS (10 Hz) during 30 sessions while stimulating six different brain regions (Broca, Wernicke, right and left DLPFC and parietal cortices). During each session three of these regions were stimulated while patients did cognitive tasks that were developed to fit each of these regions. Improvements in cognitive functions were significant, as measured using the cognitive subscale of the Alzheimer’s Disease Assessment Scale (ADAS-Cog), and were maintained for 4.5 months after the training. A case report ( Haffen et al., 2012 ) showed an improvement in episodic memory (free recall) and processing speed following 10 sessions of rTMS (10 Hz) over the left DLPFC. These are open trials and, obviously, sham-controlled interventions are needed. However, the results are promising and warrant follow-up. In a sham-controlled trial, Ahmed and colleagues (2012) assigned 45 patients with AD to three different treatment groups to study the effects of high- or low-frequency rTMS (20 Hz, 1 Hz), or sham stimulation. Patients received treatment on 5 consecutive days without combined cognitive training. Mildly to moderately impaired patients receiving high-frequency rTMS improved significantly on all scales (Mini Mental State Examination (MMSE), Instrumental Daily Living Activity Scale, Geriatric Depression Scale), and maintained these improvements for 3 months. However, severely impaired patients did not respond to the treatment.
Two crossover studies applied tDCS for one session and reported improvements in visual recognition memory following stimulation of the left DLPFC and temporoparietal cortex (TPC) ( Boggio et al., 2009 ), and in word recognition following stimulation of the bilateral TPC ( Ferrucci et al., 2008 ). In the first study, the authors applied 15 minutes of anodal, cathodal, and sham stimulation over bilateral TPC on three different sessions in patients with mild AD. While anodal tDCS led to an improvement, cathodal stimulation led to impairments in word recognition. No effects were observed in a visual attention task ( Ferrucci et al., 2008 ). In the second study, mildly to moderately impaired AD patients received anodal tDCS over the left DLPFC, the left TPC, or sham stimulation. Stimulation over both DLFPC and TPC resulted in a significant improvement in visual recognition. No effects were observed on selective attention or a visual delayed match-to-sample task.
Possibly, tDCS-induced changes in cholinergic activity contributed to these improvements. A recent study reported a significant change of SAI (ISI 2 ms) in the motor cortex of healthy subjects after anodal stimulation, while the resting motor threshold and amplitudes of motor evoked potentials did not change ( Scelzo et al., 2011 ). This could explain the positive impact of tDCS on memory functions in the above-mentioned studies. Future studies measuring behavioral along with neurophysiological effects and exploring correlations between them would be desirable.
P arkinson’s disease
Two studies have applied TMS or tDCS with the aim of improving cognitive functioning in PD. The first study compared the effects of active or sham rTMS and fluoxetine or placebo in patients with PD with concurrent depression ( Boggio et al., 2005 ). The authors applied 15-Hz rTMS over the left DLPFC for 10 daily sessions, and assessed cognitive functions at baseline, and 2 and 8 weeks after the treatment. Treatments were not combined with cognitive training or psychotherapy. After 2 weeks both interventions led to similar improvements in the Stroop Test and the Wisconsin Card Sorting Test (executive functions), and the Hooper (visuospatial functions). Furthermore, depression rates improved significantly in both groups. However, no improvements were reported in STM or WM (digits forward and backward). Eight weeks after treatment, these improvements declined slightly but remained significant.
The second study found improved accuracy in a 3-back task during a single session of anodal tDCS over the left DLPFC. Improvement was significant at a stimulation intensity of 2 mA but not at 1 mA ( Boggio et al., 2006 ).
Cognitive impairments in PD are often associated with depression symptoms, which occur in about 35% of patients. Furthermore, dementia is common in these patients with a point prevalence of 30% ( Aarsland and Kurz, 2010 ). Further studies are needed to investigate underlying processes leading to cognitive impairments. Moreover, studies should evaluate the efficacy of repetitive NBS in combination with cognitive training for this patient population.
A quickly growing number of studies is using NBS applications to study the underlying neurobiological substrates of memory functions, to investigate the use of TMS as a diagnostic tool, and the application of NBS to enhance memory functions. To date, most studies have used TMS to probe underlying memory processes and their causal and temporal relationships, whereas TMS, tDCS, and other forms of transcranial current stimulation are being used to enhance memory functions in healthy as well as patient populations. The combination of NBS with other methods, such as EEG and fMRI, enables the measurement of behavioral along with neurophysiological effects; the exploration of correlations between them is desirable to advance our neurobiological understanding and optimize future interventions.
ACKNOWLEDGMENTS
A.P.-L. serves on the scientific advisory boards for Nexstim, Neuronix, Starlab Neuroscience, Neosync, and Novavision, and is listed as an inventor on several issued and pending patents on the real-time integration of transcranial magnetic stimulation (TMS) with electroencephalography (EEG) and magnetic resonance imaging (MRI). Work on this study was supported by grants from the National Center for Research Resources: Harvard Clinical and Translational Science Center/Harvard Catalyst (UL1 RR025758), and investigator-initiated grants from Nexstim Inc. and Neuronix. A.-K.B. was supported by the Young Academics Support of the University of Zurich, Switzerland. K.R. was supported by the Dean’s Summer Research Award Grant, Harvard University.
R eferences
- Aarsland D, Kurz MW. The epidemiology of dementia associated with Parkinson disease. J Neurol Sci. 2010; 289 :18–22. [ PubMed ] [ Google Scholar ]
- Ahmed MA, Darwish ES, Khedr EM, et al. Effects of low versus high frequencies of repetitive transcranial magnetic stimulation on cognitive function and cortical excitability in Alzheimer’s dementia. J Neurol. 2012; 259 :83–92. [ PubMed ] [ Google Scholar ]
- Albouy G, Sterpenich V, Balteau E, et al. Both the hippocampus and striatum are involved in consolidation of motor sequence memory. Neuron. 2008; 58 :261–272. [ PubMed ] [ Google Scholar ]
- Andrews SC, Hoy KE, Enticott PG, et al. Improving working memory: the effect of combining cognitive activity and anodal transcranial direct current stimulation to the left dorsolateral prefrontal cortex. Brain Stimul. 2011; 4 :84–89. [ PubMed ] [ Google Scholar ]
- Atance CM, O’Neill DK. Episodic future thinking. Trends Cogn Sci. 2001; 5 :533–539. [ PubMed ] [ Google Scholar ]
- Baddeley AD. Working Memory. Clarendon; Oxford: 1986. [ Google Scholar ]
- Baddeley AD. The episodic buffer: a new component of working memory? Trends Cogn Sci. 2000; 4 :417–423. [ PubMed ] [ Google Scholar ]
- Baddeley AD, Hitch G. Working memory. In: Bower GA, editor. Recent Advances in Learning and Motivation. Vol. 8. Academic Press; New York: 1974. pp. 47–90. [ Google Scholar ]
- Bailey N, Areni CS. Background music as a quasi clock in retrospective duration judgments. Percept Mot Skills. 2006; 102 :435–444. [ PubMed ] [ Google Scholar ]
- Basso D, Ferrari M, Palladino P. Prospective memory and working memory: asymmetrical effects during frontal lobe TMS stimulation. Neuropsychologia. 2010; 48 :3282–3290. [ PubMed ] [ Google Scholar ]
- Beckers G, Hömberg V. Impairment of visual perception and visual short term memory scanning by transcranial magnetic stimulation of occipital cortex. Exp Brain Res. 1991; 87 :421–432. [ PubMed ] [ Google Scholar ]
- Bentwich J, Dobronevsky E, Aichenbaum S, et al. Beneficial effect of repetitive transcranial magnetic stimulation combined with cognitive training for the treatment of Alzheimer’s disease: a proof of concept study. J Neural Transm. 2011; 118 :463–471. [ PubMed ] [ Google Scholar ]
- Berryhill ME, Wencil EB, Branch Coslett H, et al. A selective working memory impairment after transcranial direct current stimulation to the right parietal lobe. Neurosci Lett. 2010; 479 :312–316. [ PMC free article ] [ PubMed ] [ Google Scholar ]
- Blanchet S, Gagnon G, Schneider C. The contribution of the dorsolateral prefrontal cortex in full and divided encoding: a paired-pulse transcranial magnetic stimulation study. Behav Neurol. 2010; 23 :107–115. [ PMC free article ] [ PubMed ] [ Google Scholar ]
- Boggio PS, Fregni F, Bermpohl F, et al. Effect of repetitive TMS and fluoxetine on cognitive function in patients with Parkinson’s disease and concurrent depression. Mov Disord. 2005; 20 :1178–1184. [ PubMed ] [ Google Scholar ]
- Boggio PS, Ferrucci R, Rigonatti SP, et al. Effects of transcranial direct current stimulation on working memory in patients with Parkinson’s disease. J Neurol Sci. 2006; 249 :31–38. [ PubMed ] [ Google Scholar ]
- Boggio PS, Khoury LP, Martins DC, et al. Temporal cortex direct current stimulation enhances performance on a visual recognition memory task in Alzheimer disease. J Neurol Neurosurg Psychiatry. 2009; 80 :444–447. [ PubMed ] [ Google Scholar ]
- Boggio PS, Ferrucci R, Mameli F, et al. Prolonged visual memory enhancement after direct current stimulation in Alzheimer’s disease. Brain Stimul. 2012; 5 :223–230. [ PubMed ] [ Google Scholar ]
- Boyer P. Evolutionary economics of mental time travel? Trends Cogn Sci. 2008; 12 :219–224. [ PubMed ] [ Google Scholar ]
- Brashers-Krug T, Shadmehr R, Bizzi E. Consolidation in human motor memory. Nature. 1996; 382 :252–255. [ PubMed ] [ Google Scholar ]
- Buckner RL, Koutstaal W, Schacter DL, et al. Functional-anatomic study of episodic retrieval using fMRI. I. Retrieval effort versus retrieval success. Neuroimage. 1998; 7 :151–162. [ PubMed ] [ Google Scholar ]
- Bullard LM, Browning ES, Clark VP, et al. Transcranial direct current stimulation’s effect on novice versus experienced learning. Exp Brain Res. 2011; 213 :9–14. [ PubMed ] [ Google Scholar ]
- Buonomano DV. Decoding temporal information: a model based on short-term synaptic plasticity. J Neurosci. 2000; 20 :1129–1141. [ PMC free article ] [ PubMed ] [ Google Scholar ]
- Burgess PW, Gonen-Yaacovi G, Volle E. Functional neuroimaging studies of prospective memory: what have we learnt so far? Neuropsychologia. 2011; 49 :2246–2257. [ PubMed ] [ Google Scholar ]
- Bütefisch CM, Khurana V, Kopylev L, et al. Enhancing encoding of a motor memory in the primary motor cortex by cortical stimulation. J Neurophysiol. 2004; 91 :2110–2116. [ PubMed ] [ Google Scholar ]
- Cabeza R. Hemispheric asymmetry reduction in older adults: the HAROLD model. Psychol Aging. 2002; 17 :85–100. [ PubMed ] [ Google Scholar ]
- Cabeza R, Nyberg L. Neural bases of learning and memory: functional neuroimaging evidence. Curr Opin Neurol. 2000; 13 :415–421. [ PubMed ] [ Google Scholar ]
- Cabeza R, Ciaramelli E, Olson IR, et al. The parietal cortex and episodic memory: an attentional account. Nat Rev Neurosci. 2008; 9 :613–625. [ PMC free article ] [ PubMed ] [ Google Scholar ]
- Cabeza R, Mazuz YS, Stokes J, et al. Overlapping parietal activity in memory and perception: evidence for the attention to memory model. J Cogn Neurosci. 2011; 23 :3209–3217. [ PMC free article ] [ PubMed ] [ Google Scholar ]
- Cattaneo Z, Vecchi T, Pascual-Leone A, et al. Contrasting early visual cortical activation states causally involved in visual imagery and short-term memory. Eur J Neurosci. 2009; 30 :1393–1400. [ PubMed ] [ Google Scholar ]
- Cave CB, Squire LR. Intact verbal and nonverbal short-term memory following damage to the human hippocampus. Hippocampus. 1992; 2 :151–163. [ PubMed ] [ Google Scholar ]
- Censor N, Cohen LG. Using repetitive transcranial magnetic stimulation to study the underlying neural mechanisms of human motor learning and memory. J Physiol. 2011; 589 :21–28. [ PMC free article ] [ PubMed ] [ Google Scholar ]
- Chi RP, Fregni F, Snyder AW. Visual memory improved by non-invasive brain stimulation. Brain Res. 2010; 1353 :168–175. [ PubMed ] [ Google Scholar ]
- Ciaramelli E, Grady CL, Moscovitch M. Top-down and bottom-up attention to memory: a hypothesis (AtoM) on the role of the posterior parietal cortex in memory retrieval. Neuropsychologia. 2008; 46 :1828–1851. [ PubMed ] [ Google Scholar ]
- Cicerone KD, Dahlberg C, Kalmar K, et al. Evidence-based cognitive rehabilitation: recommendations for clinical practice. Arch Phys Med Rehabil. 2000; 81 :1596–1615. [ PubMed ] [ Google Scholar ]
- Clark VP, Coffman BA, Mayer AR, et al. TDCS guided using fMRI significantly accelerates learning to identify concealed objects. Neuroimage. 2012; 59 :117–128. [ PMC free article ] [ PubMed ] [ Google Scholar ]
- Classen J, Liepert J, Wise SP, et al. Rapid plasticity of human cortical movement representation induced by practice. J Neurophysiol. 1998; 79 :1117–1123. [ PubMed ] [ Google Scholar ]
- Cohen NJ, Eichenbaum H. The theory that wouldn’t die: a critical look at the spatial mapping theory of hippocampal function. Hippocampus. 1991; 1 :265–268. [ PubMed ] [ Google Scholar ]
- Cohen NJ, Squire LR. Preserved learning and retention of pattern-analyzing skill in amnesia: dissociation of knowing how and knowing that. Science. 1980; 210 :207–210. [ PubMed ] [ Google Scholar ]
- Cohen Kadosh R, Soskic S, Iuculano T, et al. Modulating neuronal activity produces specific and long-lasting changes in numerical competence. Curr Biol. 2010; 20 :2016–2020. [ PMC free article ] [ PubMed ] [ Google Scholar ]
- Collette F, Hogge M, Salmon E, et al. Exploration of the neural substrates of executive functioning by functional neuroimaging. Neuroscience. 2006; 139 :209–221. [ PubMed ] [ Google Scholar ]
- Costa A, Oliveri M, Barban F, et al. Keeping memory for intentions: a cTBS investigation of the frontopolar cortex. Cereb Cortex. 2011; 21 :2696–2703. [ PubMed ] [ Google Scholar ]
- Cotelli M, Manenti R, Cappa SF, et al. Effect of transcranial magnetic stimulation on action naming in patients with Alzheimer disease. Arch Neurol. 2006; 63 :1602–1604. [ PubMed ] [ Google Scholar ]
- Cotelli M, Manenti R, Cappa SF, et al. Transcranial magnetic stimulation improves naming in Alzheimer disease patients at different stages of cognitive decline. Eur J Neurol. 2008; 15 :1286–1292. [ PubMed ] [ Google Scholar ]
- Cotelli M, Calabria M, Manenti R, et al. Improved language performance in Alzheimer disease following brain stimulation. J Neurol Neurosurg Psychiatry. 2011; 82 :794–797. [ PubMed ] [ Google Scholar ]
- Coyle JT, Price DL, DeLong MR. Alzheimer’s disease: a disorder of cortical cholinergic innervation. Science. 1983; 219 :1184–1190. [ PubMed ] [ Google Scholar ]
- Davies P, Maloney AJ. Selective loss of central cholinergic neurons in Alzheimer’s disease. Lancet. 1976; 2 :1403. [ PubMed ] [ Google Scholar ]
- De Gennaro L, Fratello F, Marzano C, et al. Cortical plasticity induced by transcranial magnetic stimulation during wakefulness affects electroencephalogram activity during sleep. PLoS One. 2008; 3 :e2483. [ PMC free article ] [ PubMed ] [ Google Scholar ]
- Desmond JE, Chen SHA, Shieh PB. Cerebellar transcranial magnetic stimulation impairs verbal working memory. Ann Neurol. 2005; 58 :553–560. [ PubMed ] [ Google Scholar ]
- de Vries MH, Barth ACR, Maiworm S, et al. Electrical stimulation of Broca’s area enhances implicit learning of an artificial grammar. J Cogn Neurosci. 2010; 22 :2427–2436. [ PubMed ] [ Google Scholar ]
- De Weerd P, Reithler J, van de Ven V, et al. Posttraining transcranial magnetic stimulation of striate cortex disrupts consolidation early in visual skill learning. J Neurosci. 2012; 32 :1981–1988. [ PMC free article ] [ PubMed ] [ Google Scholar ]
- D’Esposito M. From cognitive to neural models of working memory. Philos Trans R Soc Lond B Biol Sci. 2007; 362 :761–772. [ PMC free article ] [ PubMed ] [ Google Scholar ]
- Devlin JT, Watkins KE. Stimulating language: insights from TMS. Brain. 2007; 130 :610–622. [ PMC free article ] [ PubMed ] [ Google Scholar ]
- Di Lazzaro V, Oliviero A, Profice P, et al. Muscarinic receptor blockade has differential effects on the excitability of intracortical circuits in the human motor cortex. Exp Brain Res. 2000; 135 :455–461. [ PubMed ] [ Google Scholar ]
- Di Lazzaro V, Pilato F, Dileone M, et al. In vivo cholinergic circuit evaluation in frontotemporal and Alzheimer dementias. Neurology. 2006; 66 :1111–1113. [ PubMed ] [ Google Scholar ]
- Di Lazzaro V, Pilato F, Dileone M, et al. In vivo functional evaluation of central cholinergic circuits in vascular dementia. Clin Neurophysiol. 2008; 119 :2494–2500. [ PubMed ] [ Google Scholar ]
- Dobbins IG, Foley H, Schacter DL, et al. Executive control during episodic retrieval: multiple prefrontal processes subserve source memory. Neuron. 2002; 35 :989–996. [ PubMed ] [ Google Scholar ]
- Dudai Y. The neurobiology of consolidations, or, how stable is the engram? Annu Rev Psychol. 2004; 55 :51–86. [ PubMed ] [ Google Scholar ]
- Eldaief MC, Halko MA, Buckner RL, et al. Transcranial magnetic stimulation modulates the brain’s intrinsic activity in a frequency-dependent manner. Proc Natl Acad Sci U S A. 2011; 108 :21229–21234. [ PMC free article ] [ PubMed ] [ Google Scholar ]
- Eldridge LL, Engel SA, Zeineh MM, et al. A dissociation of encoding and retrieval processes in the human hippocampus. J Neurosci. 2005; 25 :3280–3286. [ PMC free article ] [ PubMed ] [ Google Scholar ]
- Ellis J, Kvavilashvili L, Milne A. Experimental tests of prospective remembering: the influence of cue-event frequency on performance. Br J Psychol. 1999; 90 :9–23. [ PubMed ] [ Google Scholar ]
- Elmer S, Burkard M, Renz B, et al. Direct current induced short-term modulation of the left dorsolateral prefrontal cortex while learning auditory presented nouns. Behav Brain Funct. 2009; 5 :29. [ PMC free article ] [ PubMed ] [ Google Scholar ]
- Epstein CM, Sekino M, Yamaguchi K, et al. Asymmetries of prefrontal cortex in human episodic memory: effects of transcranial magnetic stimulation on learning abstract patterns. Neurosci Lett. 2002; 320 :5–8. [ PubMed ] [ Google Scholar ]
- Feredoes E, Heinen K, Weiskopf N, et al. Causal evidence for frontal involvement in memory target maintenance by posterior brain areas during distracter interference of visual working memory. Proc Natl Acad Sci U S A. 2011; 108 :17510–17515. [ PMC free article ] [ PubMed ] [ Google Scholar ]
- Ferrucci R, Mameli F, Guidi I, et al. Transcranial direct current stimulation improves recognition memory in Alzheimer disease. Neurology. 2008; 71 :493–498. [ PubMed ] [ Google Scholar ]
- Fletcher PC, Henson RN. Frontal lobes and human memory: insights from functional neuroimaging. Brain. 2001; 124 :849–881. [ PubMed ] [ Google Scholar ]
- Flöel A, Poeppel D, Buffalo EA, et al. Prefrontal cortex asymmetry for memory encoding of words and abstract shapes. Cereb Cortex. 2004; 14 :404–409. [ PubMed ] [ Google Scholar ]
- Flöel A, Suttorp W, Kohl O, et al. Non-invasive brain stimulation improves object-location learning in the elderly. Neurobiol Aging. 2012; 33 :1682–1689. [ PubMed ] [ Google Scholar ]
- Fregni F, Boggio PS, Nitsche M, et al. Anodal transcranial direct current stimulation of prefrontal cortex enhances working memory. Exp Brain Res. 2005; 166 :23–30. [ PubMed ] [ Google Scholar ]
- Freitas C, Mondragón-Llorca H, Pascual-Leone A. Noninvasive brain stimulation in Alzheimer’s disease: systematic review and perspectives for the future. Exp Gerontol. 2011a; 46 :611–627. [ PMC free article ] [ PubMed ] [ Google Scholar ]
- Freitas C, Perez J, Knobel M, et al. Changes in cortical plasticity across the lifespan. Front Aging Neurosci. 2011b; 3 :5. [ PMC free article ] [ PubMed ] [ Google Scholar ]
- Gagnon G, Blanchet S, Grondin S, et al. Paired-pulse transcranial magnetic stimulation over the dorsolateral prefrontal cortex interferes with episodic encoding and retrieval for both verbal and non-verbal materials. Brain Res. 2010; 1344 :148–158. [ PubMed ] [ Google Scholar ]
- Gagnon G, Schneider C, Grondin S, et al. Enhancement of episodic memory in young and healthy adults: a paired-pulse TMS study on encoding and retrieval performance. Neurosci Lett. 2011; 488 :138–142. [ PubMed ] [ Google Scholar ]
- Gallate J, Chi R, Ellwood S, et al. Reducing false memories by magnetic pulse stimulation. Neurosci Lett. 2009; 449 :151–154. [ PubMed ] [ Google Scholar ]
- Gazzaley A, Nobre AC. Top-down modulation: bridging selective attention and working memory. Trends Cogn Sci. 2012; 16 :129–135. [ PMC free article ] [ PubMed ] [ Google Scholar ]
- Gialanella B. Aphasia assessment and functional outcome prediction in patients with aphasia after stroke. J Neurol. 2011; 258 :343–349. [ PubMed ] [ Google Scholar ]
- Gladwin TE, den Uyl TE, Fregni FF, et al. Enhancement of selective attention by tDCS: interaction with interference in a Sternberg task. Neurosci Lett. 2012; 512 :33–37. [ PubMed ] [ Google Scholar ]
- Grafman J, Pascual-Leone A, Alway D, et al. Induction of a recall deficit by rapid-rate transcranial magnetic stimulation. Neuroreport. 1994; 5 :1157–1160. [ PubMed ] [ Google Scholar ]
- Haffen E, Chopard G, Pretalli J-B, et al. A case report of daily left prefrontal repetitive transcranial magnetic stimulation (rTMS) as an adjunctive treatment for Alzheimer disease. Brain Stimul. 2012; 5 :264–266. [ PubMed ] [ Google Scholar ]
- Hallett M. Transcranial magnetic stimulation and the human brain. Nature. 2000; 406 :147–150. [ PubMed ] [ Google Scholar ]
- Halsband U, Lange RK. Motor learning in man: a review of functional and clinical studies. J Physiol Paris. 2006; 99 :414–424. [ PubMed ] [ Google Scholar ]
- Hamidi M, Tononi G, Postle BR. Evaluating frontal and parietal contributions to spatial working memory with repetitive transcranial magnetic stimulation. Brain Res. 2008; 1230 :202–210. [ PMC free article ] [ PubMed ] [ Google Scholar ]
- Hamidi M, Tononi G, Postle BR. Evaluating the role of prefrontal and parietal cortices in memory-guided response with repetitive transcranial magnetic stimulation. Neuropsychologia. 2009; 47 :295–302. [ PMC free article ] [ PubMed ] [ Google Scholar ]
- Hamilton R, Messing S, Chatterjee A. Rethinking the thinking cap: ethics of neural enhancement using noninvasive brain stimulation. Neurology. 2011; 76 :187–193. [ PMC free article ] [ PubMed ] [ Google Scholar ]
- Hammer A, Mohammadi B, Schmicker M, et al. Errorless and errorful learning modulated by transcranial direct current stimulation. BMC Neurosci. 2011; 12 :72. [ PMC free article ] [ PubMed ] [ Google Scholar ]
- Hannula DE, Tranel D, Cohen NJ. The long and the short of it: relational memory impairments in amnesia, even at short lags. J Neurosci. 2006; 26 :8352–8359. [ PMC free article ] [ PubMed ] [ Google Scholar ]
- Hannula H, Neuvonen T, Savolainen P, et al. Increasing top-down suppression from prefrontal cortex facilitates tactile working memory. Neuroimage. 2010; 49 :1091–1098. [ PubMed ] [ Google Scholar ]
- Hartje W, Poeck K. Klinische Neuropsychologie. 5th Thieme; Stuttgart: 2002. [ Google Scholar ]
- Haxby JV, Petit L, Ungerleider LG, et al. Distinguishing the functional roles of multiple regions in distributed neural systems for visual working memory. Neuroimage. 2000; 11 :145–156. [ PubMed ] [ Google Scholar ]
- Heimrath K, Sandmann P, Becke A, et al. Behavioral and electrophysiological effects of transcranial direct current stimulation of the parietal cortex in a visuo-spatial working memory task. Front Psychiatry. 2012; 3 :56. [ PMC free article ] [ PubMed ] [ Google Scholar ]
- Herwig U, Abler B, Schönfeldt-Lecuona C, et al. Verbal storage in a premotor-parietal network: evidence from fMRI-guided magnetic stimulation. Neuroimage. 2003; 20 :1032–1041. [ PubMed ] [ Google Scholar ]
- Higo T, Mars RB, Boorman ED, et al. Distributed and causal influence of frontal operculum in task control. Proc Natl Acad Sci U S A. 2011; 108 :4230–4235. [ PMC free article ] [ PubMed ] [ Google Scholar ]
- Horvath JC, Perez JM, Forrow L, et al. Transcranial magnetic stimulation: a historical evaluation and future prognosis of therapeutically relevant ethical concerns. J Med Ethics. 2011; 37 :137–143. [ PubMed ] [ Google Scholar ]
- Huber R, Ghilardi MF, Massimini M, et al. Local sleep and learning. Nature. 2004; 430 :78–81. [ PubMed ] [ Google Scholar ]
- Huber R, Ghilardi MF, Massimini M, et al. Arm immobilization causes cortical plastic changes and locally decreases sleep slow wave activity. Nat Neurosci. 2006; 9 :1169–1176. [ PubMed ] [ Google Scholar ]
- Imm J-H, Kang E, Youn T, et al. Different hemispheric specializations for pitch and audioverbal working memory. Neuroreport. 2008; 19 :99–103. [ PubMed ] [ Google Scholar ]
- Jacobson L, Goren N, Lavidor M, et al. Oppositional transcranial direct current stimulation (tDCS) of parietal substrates of attention during encoding modulates episodic memory. Brain Res. 2012; 1439 :66–72. [ PubMed ] [ Google Scholar ]
- Jaeggi SM, Buschkuehl M, Etienne A, et al. On how high performers keep cool brains in situations of cognitive overload. Cogn Affect Behav Neurosci. 2007; 7 :75–89. [ PubMed ] [ Google Scholar ]
- Jaeggi SM, Buschkuehl M, Jonides J, et al. Improving fluid intelligence with training on working memory. Proc Natl Acad Sci U S A. 2008; 105 :6829–6833. [ PMC free article ] [ PubMed ] [ Google Scholar ]
- James W. Principles of Psychology. Henry Holt; New York: 1890. [ Google Scholar ]
- Javadi AH, Walsh V. Transcranial direct current stimulation (tDCS) of the left dorsolateral prefrontal cortex modulates declarative memory. Brain Stimul. 2012; 5 :231–241. [ PubMed ] [ Google Scholar ]
- Javadi AH, Cheng P, Walsh V. Short duration transcranial direct current stimulation (tDCS) modulates verbal memory. Brain Stimul. 2012; 5 :468–474. [ PubMed ] [ Google Scholar ]
- Jo JM, Kim YH, Ko MH, et al. Enhancing the working memory of stroke patients using tDCS. Am J Phys Med Rehabil. 2009; 88 :404–409. [ PubMed ] [ Google Scholar ]
- Jonides J, Lewis RL, Nee DE, et al. The mind and brain of short-term memory. Annu Rev Psychol. 2008; 59 :193–224. [ PMC free article ] [ PubMed ] [ Google Scholar ]
- Kahn I, Pascual-Leone A, Theoret H, et al. Transient disruption of ventrolateral prefrontal cortex during verbal encoding affects subsequent memory performance. J Neurophysiol. 2005; 94 :688–698. [ PubMed ] [ Google Scholar ]
- Karabanov A, Jin S-H, Joutsen A, et al. Timing-dependent modulation of the posterior parietal cortex–primary motor cortex pathway by sensorimotor training. J Neurophysiol. 2012; 107 :3190–3199. [ PMC free article ] [ PubMed ] [ Google Scholar ]
- Karmarkar UR, Buonomano DV. Timing in the absence of clocks: encoding time in neural network states. Neuron. 2007; 53 :427–438. [ PMC free article ] [ PubMed ] [ Google Scholar ]
- Karni A, Meyer G, Rey-Hipolito C, et al. The acquisition of skilled motor performance: fast and slow experience-driven changes in primary motor cortex. Proc Natl Acad Sci U S A. 1998; 95 :861–868. [ PMC free article ] [ PubMed ] [ Google Scholar ]
- Kessels RP, d’Alfonso AA, Postma A, et al. Spatial working memory performance after high-frequency repetitive transcranial magnetic stimulation of the left and right posterior parietal cortex in humans. Neurosci Lett. 2000; 287 :68–70. [ PubMed ] [ Google Scholar ]
- Kincses TZ, Antal A, Nitsche MA, et al. Facilitation of probabilistic classification learning by transcranial direct current stimulation of the prefrontal cortex in the human. Neuropsychologia. 2004; 42 :113–117. [ PubMed ] [ Google Scholar ]
- Kirov R, Weiss C, Siebner HR, et al. Slow oscillation electrical brain stimulation during waking promotes EEG theta activity and memory encoding. Proc Natl Acad Sci U S A. 2009; 106 :15460–15465. [ PMC free article ] [ PubMed ] [ Google Scholar ]
- Kirschen MP, Davis-Ratner MS, Jerde TE, et al. Enhancement of phonological memory following transcranial magnetic stimulation (TMS) Behav Neurol. 2006; 17 :187–194. [ PMC free article ] [ PubMed ] [ Google Scholar ]
- Klimesch W. EEG alpha and theta oscillations reflect cognitive and memory performance: a review and analysis. Brain Res Brain Res Rev. 1999; 29 :169–195. [ PubMed ] [ Google Scholar ]
- Koch G, Oliveri M, Torriero S, et al. rTMS evidence of different delay and decision processes in a fronto-parietal neuronal network activated during spatial working memory. Neuroimage. 2005; 24 :34–39. [ PubMed ] [ Google Scholar ]
- Koechlin E, Basso G, Pietrini P, et al. The role of the anterior prefrontal cortex in human cognition. Nature. 1999; 399 :148–151. [ PubMed ] [ Google Scholar ]
- Köhler S, Paus T, Buckner RL, et al. Effects of left inferior prefrontal stimulation on episodic memory formation: a two-stage fMRI-rTMS study. J Cogn Neurosci. 2004; 16 :178–188. [ PubMed ] [ Google Scholar ]
- Korman M, Doyon J, Doljansky J, et al. Daytime sleep condenses the time course of motor memory consolidation. Nat Neurosci. 2007; 10 :1206–1213. [ PubMed ] [ Google Scholar ]
- Krieg SM, Shiban E, Buchmann N, et al. Utility of presurgical navigated transcranial magnetic brain stimulation for the resection of tumors in eloquent motor areas. J Neurosurg. 2012; 116 :994–1001. [ PubMed ] [ Google Scholar ]
- Lepore FE. Harvey Cushing, Gordon Holmes, and the neurological lessons of World War I. Arch Neurol. 1994; 51 :711–722. [ PubMed ] [ Google Scholar ]
- Luber B, Kinnunen LH, Rakitin BC, et al. Facilitation of performance in a working memory task with rTMS stimulation of the precuneus: frequency- and time-dependent effects. Brain Res. 2007; 1128 :120–129. [ PubMed ] [ Google Scholar ]
- Luber B, Stanford AD, Bulow P, et al. Remediation of sleep-deprivation-induced working memory impairment with fMRI-guided transcranial magnetic stimulation. Cereb Cortex. 2008; 18 :2077–2085. [ PMC free article ] [ PubMed ] [ Google Scholar ]
- Luria AR. The Man With a Shattered World: The History of a Brain Wound. Harvard University Press; Cambridge, MA: 1972. [ Google Scholar ]
- Machizawa MG, Kalla R, Walsh V, et al. The time course of ventrolateral prefrontal cortex involvement in memory formation. J Neurophysiol. 2010; 103 :1569–1579. [ PMC free article ] [ PubMed ] [ Google Scholar ]
- Manenti R, Cotelli M, Miniussi C. Successful physiological aging and episodic memory: a brain stimulation study. Behav Brain Res. 2011; 216 :153–158. [ PubMed ] [ Google Scholar ]
- Markowitsch HJ, Staniloiu A. The impairment of recollection in functional amnesic states. Cortex. 2013; 49 :1494–1510. [ PubMed ] [ Google Scholar ]
- Marshall L, Mölle M, Hallschmid M, et al. Transcranial direct current stimulation during sleep improves declarative memory. J Neurosci. 2004; 24 :9985–9992. [ PMC free article ] [ PubMed ] [ Google Scholar ]
- Marshall L, Mölle M, Siebner HR, et al. Bifrontal transcranial direct current stimulation slows reaction time in a working memory task. BMC Neurosci. 2005; 6 :23. [ PMC free article ] [ PubMed ] [ Google Scholar ]
- Marshall L, Kirov R, Brade J, et al. Transcranial electrical currents to probe EEG brain rhythms and memory consolidation during sleep in humans. PloS One. 2011; 6 :e16905. [ PMC free article ] [ PubMed ] [ Google Scholar ]
- Martin SJ, Grimwood PD, Morris RG. Synaptic plasticity and memory: an evaluation of the hypothesis. Annu Rev Neurosci. 2000; 23 :649–711. [ PubMed ] [ Google Scholar ]
- McGaugh JL. Memory – a century of consolidation. Science. 2000; 287 :248–251. [ PubMed ] [ Google Scholar ]
- McNab F, Varrone A, Farde L, et al. Changes in cortical dopamine D1 receptor binding associated with cognitive training. Science. 2009; 323 :800–802. [ PubMed ] [ Google Scholar ]
- Meiron O, Lavidor M. Unilateral prefrontal direct current stimulation effects are modulated by working memory load and gender. Brain Stimul. 2013; 6 :440–447. [ PubMed ] [ Google Scholar ]
- Miller EK, Cohen JD. An integrative theory of prefrontal cortex function. Annu Rev Neurosci. 2001; 24 :167–202. [ PubMed ] [ Google Scholar ]
- Miniussi C, Thut G. Combining TMS and EEG offers new prospects in cognitive neuroscience. Brain Topogr. 2010; 22 :249–256. [ PubMed ] [ Google Scholar ]
- Missonnier P, Leonards U, Gold G, et al. A new electrophysiological index for working memory load in humans. Neuroreport. 2003; 14 :1451–1455. [ PubMed ] [ Google Scholar ]
- Missonnier P, Gold G, Leonards U, et al. Aging and working memory: early deficits in EEG activation of posterior cortical areas. J Neural Transm. 2004; 111 :1141–1154. [ PubMed ] [ Google Scholar ]
- Mitchell KJ, Johnson MK. Source monitoring 15 years later: what have we learned from fMRI about the neural mechanisms of source memory? Psychol Bull. 2009; 135 :638–677. [ PMC free article ] [ PubMed ] [ Google Scholar ]
- Morgan HM, Jackson MC, van Koningsbruggen MG, et al. Frontal and parietal theta burst TMS impairs working memory for visual-spatial conjunctions. Brain Stimul. 2013; 6 :122–129. [ PMC free article ] [ PubMed ] [ Google Scholar ]
- Morrison JH, Baxter MG. The ageing cortical synapse: hallmarks and implications for cognitive decline. Nat Rev Neurosci. 2012; 13 :240–250. [ PMC free article ] [ PubMed ] [ Google Scholar ]
- Moscovitch M, Nadel L, Winocur G, et al. The cognitive neuroscience of remote episodic, semantic and spatial memory. Curr Opin Neurobiol. 2006; 16 :179–190. [ PubMed ] [ Google Scholar ]
- Mottaghy FM, Krause BJ, Kemna LJ, et al. Modulation of the neuronal circuitry subserving working memory in healthy human subjects by repetitive transcranial magnetic stimulation. Neurosci Lett. 2000; 280 :167–170. [ PubMed ] [ Google Scholar ]
- Mottaghy FM, Döring T, Müller-Gärtner H-W, et al. Bilateral parieto-frontal network for verbal working memory: an interference approach using repetitive transcranial magnetic stimulation (rTMS) Eur J Neurosci. 2002a; 16 :1627–1632. [ PubMed ] [ Google Scholar ]
- Mottaghy FM, Gangitano M, Sparing R, et al. Segregation of areas related to visual working memory in the prefrontal cortex revealed by rTMS. Cereb Cortex. 2002b; 12 :369–375. [ PubMed ] [ Google Scholar ]
- Mottaghy FM, Gangitano M, Krause BJ, et al. Chronometry of parietal and prefrontal activations in verbal working memory revealed by transcranial magnetic stimulation. Neuroimage. 2003a; 18 :565–575. [ PubMed ] [ Google Scholar ]
- Mottaghy FM, Pascual-Leone A, Kemna LJ, et al. Modulation of a brain–behavior relationship in verbal working memory by rTMS. Brain Res Cogn Brain Res. 2003b; 15 :241–249. [ PubMed ] [ Google Scholar ]
- Muellbacher W, Ziemann U, Wissel J, et al. Early consolidation in human primary motor cortex. Nature. 2002; 415 :640–644. [ PubMed ] [ Google Scholar ]
- Mull BR, Seyal M. Transcranial magnetic stimulation of left prefrontal cortex impairs working memory. Clin Neurophysiol. 2001; 112 :1672–1675. [ PubMed ] [ Google Scholar ]
- Mulquiney PG, Hoy KE, Daskalakis ZJ, et al. Improving working memory: exploring the effect of transcranial random noise stimulation and transcranial direct current stimulation on the dorsolateral prefrontal cortex. Clin Neurophysiol. 2011; 122 :2384–2389. [ PubMed ] [ Google Scholar ]
- Mylius V, Jung M, Menzler K, et al. Effects of transcranial direct current stimulation on pain perception and working memory. Eur J Pain. 2012; 16 :974–982. [ PubMed ] [ Google Scholar ]
- Nadel L, Samsonovich A, Ryan L, et al. Multiple trace theory of human memory: computational, neuroimaging, and neuropsychological results. Hippocampus. 2000; 10 :352–368. [ PubMed ] [ Google Scholar ]
- Najib U, Pascual-Leone A. Paradoxical functional facilitation with noninvasive brain stimulation. In: Kapur N, Pascual-Leone A, Ramachandran V, et al., editors. The Paradoxical Brain. Cambridge University Press; Cambridge: 2011. pp. 234–260. [ Google Scholar ]
- Nitsche MA, Cohen LG, Wassermann EM, et al. Transcranial direct current stimulation: state of the art 2008. Brain Stimul. 2008; 1 :206–223. [ PubMed ] [ Google Scholar ]
- Noulhiane M, Pouthas V, Hasboun D, et al. Role of the medial temporal lobe in time estimation in the range of minutes. Neuroreport. 2007; 18 :1035–1038. [ PubMed ] [ Google Scholar ]
- Ohn SH, Park C-I, Yoo W-K, et al. Time-dependent effect of transcranial direct current stimulation on the enhancement of working memory. Neuroreport. 2008; 19 :43–47. [ PubMed ] [ Google Scholar ]
- Okuda J, Fujii T, Yamadori A, et al. Participation of the prefrontal cortices in prospective memory: evidence from a PET study in humans. Neurosci Lett. 1998; 253 :127–130. [ PubMed ] [ Google Scholar ]
- Okuda J, Fujii T, Ohtake H, et al. Differential involvement of regions of rostral prefrontal cortex (Brodmann area 10) in time- and event-based prospective memory. Int J Psychophysiol. 2007; 64 :233–246. [ PubMed ] [ Google Scholar ]
- Oliveri M, Turriziani P, Carlesimo GA, et al. Parietofrontal interactions in visual-object and visual-spatial working memory: evidence from transcranial magnetic stimulation. Cereb Cortex. 2001; 11 :606–618. [ PubMed ] [ Google Scholar ]
- Olson IR, Moore KS, Stark M, et al. Visual working memory is impaired when the medial temporal lobe is damaged. J Cogn Neurosci. 2006a; 18 :1087–1097. [ PubMed ] [ Google Scholar ]
- Olson IR, Page K, Moore KS, et al. Working memory for conjunctions relies on the medial temporal lobe. J Neurosci. 2006b; 26 :4596–4601. [ PMC free article ] [ PubMed ] [ Google Scholar ]
- Osaka N, Otsuka Y, Hirose N, et al. Transcranial magnetic stimulation (TMS) applied to left dorsolateral prefrontal cortex disrupts verbal working memory performance in humans. Neurosci Lett. 2007; 418 :232–235. [ PubMed ] [ Google Scholar ]
- Paller KA. Cross-cortical consolidation as the core defect in amnesia: prospects for hypothesis-testing with neuropsychology and neuroimaging. In: Squire LR, Schacter DL, editors. Neuropsychology of Memory. 3rd Guilford Press; New York: 2002. pp. 73–87. [ Google Scholar ]
- Paller KA, Wagner AD. Observing the transformation of experience into memory. Trends Cogn Sci. 2002; 6 :93–102. [ PubMed ] [ Google Scholar ]
- Pascual-Leone A, Hallett M. Induction of errors in a delayed response task by repetitive transcranial magnetic stimulation of the dorsolateral prefrontal cortex. Neuroreport. 1994; 5 :2517–2520. [ PubMed ] [ Google Scholar ]
- Pascual-Leone A, Gates JR, Dhuna A. Induction of speech arrest and counting errors with rapid-rate transcranial magnetic stimulation. Neurology. 1991; 41 :697–702. [ PubMed ] [ Google Scholar ]
- Pascual-Leone A, Bartres-Faz D, Keenan JP. Transcranial magnetic stimulation: studying the brain–behaviour relationship by induction of “virtual lesions” Philos Trans R Soc Lond B Biol Sci. 1999; 354 :1229–1238. [ PMC free article ] [ PubMed ] [ Google Scholar ]
- Pascual-Leone A, Walsh V, Rothwell J. Transcranial magnetic stimulation in cognitive neuroscience – virtual lesion, chronometry, and functional connectivity. Curr Opin Neurobiol. 2000; 10 :232–237. [ PubMed ] [ Google Scholar ]
- Pascual-Leone A, Freitas C, Oberman L, et al. Characterizing brain cortical plasticity and network dynamics across the age-span in health and disease with TMS-EEG and TMS-fMRI. Brain Topogr. 2011; 24 :302–315. [ PMC free article ] [ PubMed ] [ Google Scholar ]
- Penolazzi B, Di Domenico A, Marzoli D, et al. Effects of transcranial direct current stimulation on episodic memory related to emotional visual stimuli. PloS One. 2010; 5 :e10623. [ PMC free article ] [ PubMed ] [ Google Scholar ]
- Postle BR, Ferrarelli F, Hamidi M, et al. Repetitive transcranial magnetic stimulation dissociates working memory manipulation from retention functions in the prefrontal, but not posterior parietal, cortex. J Cogn Neurosci. 2006; 18 :1712–1722. [ PubMed ] [ Google Scholar ]
- Preston G, Anderson E, Silva C, et al. Effects of 10 Hz rTMS on the neural efficiency of working memory. J Cogn Neurosci. 2009; 22 :447–456. [ PMC free article ] [ PubMed ] [ Google Scholar ]
- Rami L, Gironell A, Kulisevsky J, et al. Effects of repetitive transcranial magnetic stimulation on memory subtypes: a controlled study. Neuropsychologia. 2003; 41 :1877–1883. [ PubMed ] [ Google Scholar ]
- Rammsayer TH, Hennig J, Haag A, et al. Effects of noradrenergic activity on temporal information processing in humans. Q J Exp Psychol B. 2001; 54 :247–258. [ PubMed ] [ Google Scholar ]
- Ranganath C, D’Esposito M. Medial temporal lobe activity associated with active maintenance of novel information. Neuron. 2001; 31 :865–873. [ PubMed ] [ Google Scholar ]
- Rasch B, Büchel C, Gais S, et al. Odor cues during slow-wave sleep prompt declarative memory consolidation. Science. 2007; 315 :1426–1429. [ PubMed ] [ Google Scholar ]
- Reynolds JR, West R, Braver T. Distinct neural circuits support transient and sustained processes in prospective memory and working memory. Cereb Cortex. 2009; 19 :1208–1221. [ PMC free article ] [ PubMed ] [ Google Scholar ]
- Robertson EM. The serial reaction time task: implicit motor skill learning? J Neurosci. 2007; 27 :10073–10075. [ PMC free article ] [ PubMed ] [ Google Scholar ]
- Robertson EM. From creation to consolidation: a novel framework for memory processing. PLoS Biol. 2009; 7 :e19. [ PMC free article ] [ PubMed ] [ Google Scholar ]
- Robertson EM, Cohen DA. Understanding consolidation through the architecture of memories. Neuroscientist. 2006; 12 :261–271. [ PubMed ] [ Google Scholar ]
- Robertson EM, Pascual-Leone A, Miall RC. Current concepts in procedural consolidation. Nat Rev Neurosci. 2004; 5 :576–582. [ PubMed ] [ Google Scholar ]
- Robertson EM, Press DZ, Pascual-Leone A. Off-line learning and the primary motor cortex. J Neurosci. 2005; 25 :6372–6378. [ PMC free article ] [ PubMed ] [ Google Scholar ]
- Rossi S, Cappa SF, Babiloni C, et al. Prefrontal cortex in long-term memory: an “interference” approach using magnetic stimulation. Nat Neurosci. 2001; 4 :948–952. [ PubMed ] [ Google Scholar ]
- Rossi S, Miniussi C, Pasqualetti P, et al. Age-related functional changes of prefrontal cortex in long-term memory: a repetitive transcranial magnetic stimulation study. J Neurosci. 2004; 24 :7939–7944. [ PMC free article ] [ PubMed ] [ Google Scholar ]
- Rossi S, Pasqualetti P, Zito G, et al. Prefrontal and parietal cortex in human episodic memory: an interference study by repetitive transcranial magnetic stimulation. Eur J Neurosci. 2006; 23 :793–800. [ PubMed ] [ Google Scholar ]
- Rossi S, Hallett M, Rossini PM, et al. Safety, ethical considerations, and application guidelines for the use of transcranial magnetic stimulation in clinical practice and research. Clin Neurophysiol. 2009; 120 :2008–2039. [ PMC free article ] [ PubMed ] [ Google Scholar ]
- Rost NS, Smith EE, Chang Y, et al. Prediction of functional outcome in patients with primary intracerebral hemorrhage: the FUNC score. Stroke. 2008; 39 :2304–2309. [ PubMed ] [ Google Scholar ]
- Sandrini M, Cappa SF, Rossi S, et al. The role of prefrontal cortex in verbal episodic memory: rTMS evidence. J Cogn Neurosci. 2003; 15 :855–861. [ PubMed ] [ Google Scholar ]
- Sandrini M, Rossini PM, Miniussi C. Lateralized contribution of prefrontal cortex in controlling task-irrelevant information during verbal and spatial working memory tasks: rTMS evidence. Neuropsychologia. 2008; 46 :2056–2063. [ PubMed ] [ Google Scholar ]
- Sandrini M, Fertonani A, Cohen LG, et al. Double dissociation of working memory load effects induced by bilateral parietal modulation. Neuropsychologia. 2012; 50 :396–402. [ PMC free article ] [ PubMed ] [ Google Scholar ]
- Sauseng P, Klimesch W, Heise KF, et al. Brain oscillatory substrates of visual short-term memory capacity. Curr Biol. 2009; 19 :1846–1852. [ PubMed ] [ Google Scholar ]
- Savolainen P, Carlson S, Boldt R, et al. Facilitation of tactile working memory by top-down suppression from prefrontal to primary somatosensory cortex during sensory interference. Behav Brain Res. 2011; 219 :387–390. [ PubMed ] [ Google Scholar ]
- Scelzo E, Giannicola G, Rosa M, et al. Increased short latency afferent inhibition after anodal transcranial direct current stimulation. Neurosci Lett. 2011; 498 :167–170. [ PubMed ] [ Google Scholar ]
- Schendan HE, Searl MM, Melrose RJ, et al. An FMRI study of the role of the medial temporal lobe in implicit and explicit sequence learning. Neuron. 2003; 37 :1013–1025. [ PubMed ] [ Google Scholar ]
- Schutter DJLG, van Honk J. Increased positive emotional memory after repetitive transcranial magnetic stimulation over the orbitofrontal cortex. J Psychiatry Neurosci. 2006; 31 :101–104. [ PMC free article ] [ PubMed ] [ Google Scholar ]
- Scoville WB, Milner B. Loss of recent memory after bilateral hippocampal lesions. J Neurol Neurosurg Psychiatry. 1957; 20 :11–21. [ PMC free article ] [ PubMed ] [ Google Scholar ]
- Seidler RD, Purushotham A, Kim S-G, et al. Cerebellum activation associated with performance change but not motor learning. Science. 2002; 296 :2043–2046. [ PubMed ] [ Google Scholar ]
- Shallice T, Warrington EK. Independent functioning of verbal memory stores: a neuropsychological study. Q J Exp Psychol. 1970; 22 :261–273. [ PubMed ] [ Google Scholar ]
- Shimamura AP. Episodic retrieval and the cortical binding of relational activity. Cogn Affect Behav Neurosci. 2011; 11 :277–291. [ PubMed ] [ Google Scholar ]
- Shimamura AP, Wickens TD. Superadditive memory strength for item and source recognition: the role of hierarchical relational binding in the medial temporal lobe. Psychol Rev. 2009; 116 :1–19. [ PubMed ] [ Google Scholar ]
- Sigurdardottir S, Andelic N, Roe C, et al. Cognitive recovery and predictors of functional outcome 1 year after traumatic brain injury. J Int Neuropsychol Soc. 2009; 15 :740–750. [ PubMed ] [ Google Scholar ]
- Silvanto J, Cattaneo Z. Transcranial magnetic stimulation reveals the content of visual short-term memory in the visual cortex. Neuroimage. 2010; 50 :1683–1689. [ PMC free article ] [ PubMed ] [ Google Scholar ]
- Silvanto J, Pascual-Leone A. State-dependency of transcranial magnetic stimulation. Brain Topogr. 2008; 21 :1–10. [ PMC free article ] [ PubMed ] [ Google Scholar ]
- Silvanto J, Pascual-Leone A. Why the assessment of causality in brain–behavior relations requires brain stimulation. J Cogn Neurosci. 2012; 24 :775–777. [ PubMed ] [ Google Scholar ]
- Simons JS, Spiers HJ. Prefrontal and medial temporal lobe interactions in long-term memory. Nat Rev Neurosci. 2003; 4 :637–648. [ PubMed ] [ Google Scholar ]
- Skrdlantová L, Horácek J, Dockery C, et al. The influence of low-frequency left prefrontal repetitive transcranial magnetic stimulation on memory for words but not for faces. Physiol Res. 2005; 54 :123–128. [ PubMed ] [ Google Scholar ]
- Smith EE, Jonides J. Working memory: a view from neuroimaging. Cogn Psychol. 1997; 33 :5–42. [ PubMed ] [ Google Scholar ]
- Smith EE, Jonides J. Storage and executive processes in the frontal lobes. Science. 1999; 283 :1657–1661. [ PubMed ] [ Google Scholar ]
- Solé-Padullés C, Bartrés-Faz D, Junqué C, et al. Repetitive transcranial magnetic stimulation effects on brain function and cognition among elders with memory dysfunction. A randomized sham-controlled study. Cereb Cortex. 2006; 16 :1487–1493. [ PubMed ] [ Google Scholar ]
- Soto D, Rotshtein P, Hodsoll J, et al. Common and distinct neural regions for the guidance of selection by visuo-verbal information held in memory: converging evidence from fMRI and rTMS. Hum Brain Mapp. 2012; 33 :105–120. [ PMC free article ] [ PubMed ] [ Google Scholar ]
- Squire LR. The medial temporal region and memory consolidation: a new hypothesis. In: Weingartner H, Parder E, editors. Memory Consolidation. Erlbaum; Hillsdale, NJ: 1984. pp. 185–210. [ Google Scholar ]
- Squire LR. Memory and Brain. Oxford University Press; New York: 1987. [ Google Scholar ]
- Squire LR. Memory systems of the brain: a brief history and current perspective. Neurobiol Learn Mem. 2004; 82 :171–177. [ PubMed ] [ Google Scholar ]
- Squire LR, Zola SM. Episodic memory, semantic memory, and amnesia. Hippocampus. 1998; 8 :205–211. [ PubMed ] [ Google Scholar ]
- Sternberg S. High-speed scanning in human memory. Science. 1966; 153 :652–654. [ PubMed ] [ Google Scholar ]
- Teo F, Hoy KE, Daskalakis ZJ, et al. Investigating the role of current strength in tDCS modulation of working memory performance in healthy controls. Front Psychiatry. 2011; 2 :45. [ PMC free article ] [ PubMed ] [ Google Scholar ]
- Thut G, Pascual-Leone A. A review of combined TMS-EEG studies to characterize lasting effects of repetitive TMS and assess their usefulness in cognitive and clinical neuroscience. Brain Topogr. 2010; 22 :219–232. [ PMC free article ] [ PubMed ] [ Google Scholar ]
- Tononi G, Cirelli C. Sleep and synaptic homeostasis: a hypothesis. Brain Res Bull. 2003; 62 :143–150. [ PubMed ] [ Google Scholar ]
- Tononi G, Cirelli C. Sleep function and synaptic homeostasis. Sleep Med Rev. 2006; 10 :49–62. [ PubMed ] [ Google Scholar ]
- Tulving E. Episodic memory: from mind to brain. Annu Rev Psychol. 2002; 53 :1–25. [ PubMed ] [ Google Scholar ]
- Tulving E, Kapur S, Craik FI, et al. Hemispheric encoding/ retrieval asymmetry in episodic memory: positron emission tomography findings. Proc Natl Acad Sci U S A. 1994; 91 :2016–2020. [ PMC free article ] [ PubMed ] [ Google Scholar ]
- Ungerleider LG, Doyon J, Karni A. Imaging brain plasticity during motor skill learning. Neurobiol Learn Mem. 2002; 78 :553–564. [ PubMed ] [ Google Scholar ]
- van de Ven V, Jacobs C, Sack AT. Topographic contribution of early visual cortex to short-term memory consolidation: a transcranial magnetic stimulation study. J Neurosci. 2012; 32 :4–11. [ PMC free article ] [ PubMed ] [ Google Scholar ]
- Vines BW, Schnider NM, Schlaug G. Testing for causality with transcranial direct current stimulation: pitch memory and the left supramarginal gyrus. Neuroreport. 2006; 17 :1047–1050. [ PMC free article ] [ PubMed ] [ Google Scholar ]
- Wada J, Rasmussen T. Intracarotid injection of sodium amytal for the lateralization of cerebral speech dominance. J Neurosurg. 1960; 106 :1117–1133. 1960. [ PubMed ] [ Google Scholar ]
- Wagle J, Farner L, Flekkoy K, et al. Early post-stroke cognition in stroke rehabilitation patients predicts functional outcome at 13 months. Dement Geriatr Cogn Disord. 2011; 31 :379–387. [ PubMed ] [ Google Scholar ]
- Wagner AD, Shannon BJ, Kahn I, et al. Parietal lobe contributions to episodic memory retrieval. Trends Cogn Sci. 2005; 9 :445–453. [ PubMed ] [ Google Scholar ]
- Wagner T, Valero-Cabre A, Pascual-Leone A. Noninvasive human brain stimulation. Annu Rev Biomed Eng. 2007; 9 :527–565. [ PubMed ] [ Google Scholar ]
- Wagner U, Gais S, Haider H, et al. Sleep inspires insight. Nature. 2004; 427 :352–355. [ PubMed ] [ Google Scholar ]
- Walker MP. A refined model of sleep and the time course of memory formation. Behav Brain Sci. 2005; 28 :51–64. [ PubMed ] [ Google Scholar ]
- Walker MP, Brakefield T, Morgan A, et al. Practice with sleep makes perfect: sleep-dependent motor skill learning. Neuron. 2002; 35 :205–211. [ PubMed ] [ Google Scholar ]
- Walsh V, Pascual-Leone A. Transcranial Magnetic Stimulation: A Neurochronometrics of Mind. MIT Press; Cambridge, MA: 2003. [ Google Scholar ]
- Watter S, Geffen GM, Geffen LB. The n-back as a dual-task: P300 morphology under divided attention. Psychophysiology. 2001; 38 :998–1003. [ PubMed ] [ Google Scholar ]
- Wheaton LA, Hallett M. Ideomotor apraxia: a review. J Neurol Sci. 2007; 260 :1–10. [ PubMed ] [ Google Scholar ]
- Wheeler ME, Petersen SE, Buckner RL. Memory’s echo: vivid remembering reactivates sensory-specific cortex. Proc Natl Acad Sci U S A. 2000; 97 :11125–11129. [ PMC free article ] [ PubMed ] [ Google Scholar ]
- Wittmann M. The inner experience of time. Philos Trans R Soc Lond B Biol Sci. 2009; 364 :1955–1967. [ PMC free article ] [ PubMed ] [ Google Scholar ]
- Yamanaka K, Yamagata B, Tomioka H, et al. Transcranial magnetic stimulation of the parietal cortex facilitates spatial working memory: near-infrared spectroscopy study. Cereb Cortex. 2010; 20 :1037–1045. [ PubMed ] [ Google Scholar ]
- Zaehle T, Sandmann P, Thorne JD, et al. Transcranial direct current stimulation of the prefrontal cortex modulates working memory performance: combined behavioural and electrophysiological evidence. BMC Neurosci. 2011; 12 :2. [ PMC free article ] [ PubMed ] [ Google Scholar ]
- Zanto TP, Rubens MT, Thangavel A, et al. Causal role of the prefrontal cortex in top-down modulation of visual processing and working memory. Nat Neurosci. 2011; 14 :656–661. [ PMC free article ] [ PubMed ] [ Google Scholar ]
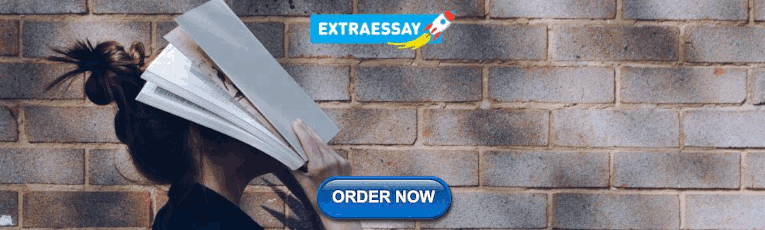
IMAGES
COMMENTS
Abstract. Memory retrieval involves the interaction between external sensory or internally generated cues and stored memory traces (or engrams) in a process termed 'ecphory'. While ecphory has been examined in human cognitive neuroscience research, its neurobiological foundation is less understood. To the extent that ecphory involves ...
In 1966, Tulving and Pearlstone 1 reported a highly influential finding that profoundly altered the direction of subsequent research on memory in ways that few papers do. Up until this point ...
This paper explores memory from a cognitive neuroscience perspective and examines associated neural mechanisms. It examines the different types of memory: working, declarative, and non-declarative, and the brain regions involved in each type. The paper highlights the role of different brain regions, such as the prefrontal cortex in working ...
Yet there is little research on how individual differences in personality traits and working memory capacity moderate the size of the retrieval-practice benefits. The current study is a conceptual replication of a previous study, further investigating whether the testing effect is sensitive to individual differences in the personality traits ...
Our portrayal of memory retrieval as a double-edged sword and characterization of the effects as 'positive' and 'negative' might ultimately be too simplistic. The human cognitive system ...
The reviewed studies indicate that stress does impair retrieval, particularly when induced with the TSST, in the afternoon, up to 45 minutes before the onset of the final memory test, in healthy young men. These results may inform future research on the impact of stress-induced cortisol surges on memory retrieval.
Memory and learning are interdependent processes that involve encoding, storage, and retrieval. Especially memory retrieval is a fundamental cognitive ability to recall memory traces and update stored memory with new information. For effective memory retrieval and learning, the memory must be stabilized from short-term memory to long-term memory. Hence, it is necessary to understand the ...
Engram neurons are typically defined as neurons that were active during both encoding and retrieval of memory. Emerging evidence has illustrated, however, that some neurons play a critical role in ...
Moreover, memory retrieval is closely related to other cognitive processes, such as working memory and memory consolidation. The papers included in this Research Topic examine the role of memory retrieval in memory strengthening and reconsolidation, factors affecting memory retrieval, and the cellular mechanisms underlying retrieval.
Retrieval practice (RP) refers to the learning technique in which participants study stimuli and take three subsequent recall tests. Study practice (SP) refers to the learning technique in which participants study stimuli four Tufts University, 490 Boston Avenue, Medford, MA 02155, USA. times.Tests occurred on the day after learning.
More than a decade of research has supported a robust consensus: Acute stress impairs memory retrieval. We aimed to determine whether a highly effective learning technique could strengthen memory against the negative effects of stress. To bolster memory, we used retrieval practice, or the act of taking practice tests.
Introduction: The experience of stressful events can alter brain structures involved in memory encoding, storage and retrieval. Here we review experimental research assessing the impact of the stress-related hormone cortisol on long-term memory retrieval. Method: A comprehensive literature search was conducted on PubMed, Web of Science and PsycNet databases with the following terms: "stress ...
Human memory processes can b e classi ed as the abil ity of the mind to. understand, retain, a nd successfully recall i nformation. The role of retention. is to store encoded events and ...
Episodic retrieval allows people to access memories from the past to guide current thoughts and decisions. In many real-world situations, retrieval occurs under conditions of acute stress, either elicited by the retrieval task or driven by other, unrelated concerns. Memory under such conditions may be hindered, as acute stress initiates a ...
Memory is the process of retaining of knowledge over a period for the function of affecting future actions.[] From a historical standpoint, the area of memory research from 1870 to 1920 was focused mainly on human memory.[] The book: The Principles of Psychology written by famous psychologist William James suggested that there is a difference between memory and habit.[]
The negative effect of stress on retrieval could be mimicked by administering a GR agonist and blocked by the cortisol synthesis inhibitor metyrapone in rodents, which suggests a GR-dependent ...
Memory of episodes (events) is at the core of psychological research on memory. This type of memory encompasses events that an individual has experienced and thus is a collection of experiences that occurred at a specific time and place (Tulving, Citation 1972, Citation 1983, Citation 2002).The retrieval of information is typically different for verbally encoded events versus those encoded in ...
Lab research has tested predictions from the working memory theory. First, consistent with the theory, other dual tasks that compete with memory retrieval also work, including vertical eye movements, counting backward, attentional breathing, and playing the computer game Tetris.
In line with traditional memory frameworks, several compensatory and instructional techniques are applied to improve memory acquisition and retrieval (i.e., visual imagery, external aids, spaced ...
This result clearly calls for a reconsideration and broadening of how the temporal aspect of memory retrieval should be measured in future studies on cues related to memory accuracy. As noted in the introduction, research suggests that people generally find it difficult to judge the accuracy of others' memories (Lindholm, 2005, 2008a,b). An ...
Scientific papers of a large scale on the Internet encompass a wealth of data and knowledge, attracting the attention of numerous researchers. To fully utilize these knowledge, Retrieval-Augmented Large Language Models (LLMs) usually leverage large-scale scientific corpus to train and then retrieve relevant passages from external memory to ...
PHYSIOLOGY OF MEMORY CONSOLIDATION DURING SLEEP. Behavioral studies of memory consolidation during sleep have produced ample evidence of superior retrieval of various types of information after a period of sleep compared to a period of wake (e.g., Karni et al. 1994, Plihal & Born 1997, Walker et al. 2002, Tucker et al. 2006).In rodents, consistent evidence has suggested a causal role for rapid ...
Rather, retrieval changes memory, as illustrated by the wealth of research establishing that retrieval can improve memory for the retrieved information 1,2,3,4. However, when retrieving encoded ...
5.3 Future research work. In order to improve the model's adaptability and performance, one of our future research directions is to work on enhancing the adaptability to camera viewpoint changes and diverse scenes. This can be done by introducing more multi-view data for training to improve the generalisation ability of the model.
MTL plays its part in memory retrieval by reinstating these features (Eldridge et al., 2005; Moscovitch et al, 2006). ... Basic memory research includes mostly young and healthy subjects. However, one of the key topics in the domain of NBS research concerns the changes of interhemispheric balance and the increased compensatory recruitment of ...