Root out friction in every digital experience, super-charge conversion rates, and optimize digital self-service
Uncover insights from any interaction, deliver AI-powered agent coaching, and reduce cost to serve
Increase revenue and loyalty with real-time insights and recommendations delivered to teams on the ground
Know how your people feel and empower managers to improve employee engagement, productivity, and retention
Take action in the moments that matter most along the employee journey and drive bottom line growth
Whatever they’re saying, wherever they’re saying it, know exactly what’s going on with your people
Get faster, richer insights with qual and quant tools that make powerful market research available to everyone
Run concept tests, pricing studies, prototyping + more with fast, powerful studies designed by UX research experts
Track your brand performance 24/7 and act quickly to respond to opportunities and challenges in your market
Explore the platform powering Experience Management
- Free Account
- Product Demos
- For Digital
- For Customer Care
- For Human Resources
- For Researchers
- Financial Services
- All Industries
Popular Use Cases
- Customer Experience
- Employee Experience
- Net Promoter Score
- Voice of Customer
- Customer Success Hub
- Product Documentation
- Training & Certification
- XM Institute
- Popular Resources
- Customer Stories
- Artificial Intelligence
- Market Research
- Partnerships
- Marketplace
The annual gathering of the experience leaders at the world’s iconic brands building breakthrough business results, live in Salt Lake City.
- English/AU & NZ
- Español/Europa
- Español/América Latina
- Português Brasileiro
- REQUEST DEMO
- Experience Management
- What is a survey?
- Close-ended Questions
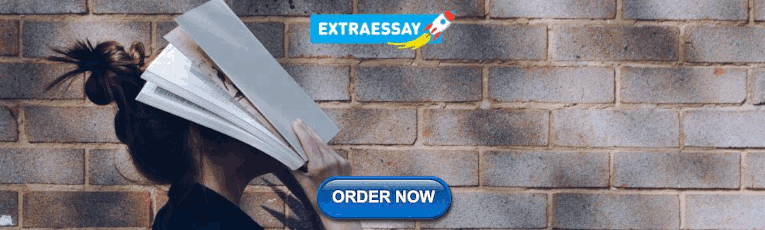
Try Qualtrics for free
Close-ended questions: everything you need to know.
7 min read In this guide, find out how you can use close-ended survey questions to gather quantifiable data and gain easy-to-analyze survey responses.
What is a close-ended question?
If you want to collect survey responses within a limited frame of options, close-ended questions (also closed-ended questions) and their question types are critical.
Close-ended questions ask respondents to choose from a predefined set of responses, typically one-word answers such as “yes/no”, “true/false”, or a set of multiple-choice questions.
For example: “Is the sky blue?” and the respondent then has to choose “Yes/No”.
The purpose of close-ended questions is to gather focused, quantitative data — numbers, dates, or a one-word answer — from respondents as it’s easy to group, compare and analyze.
Also, researchers use close-ended questions because the results are statistically significant and help to show trends and percentages over time. Open-ended questions, on the other hand, provide qualitative data: information that helps you to understand your customers and the context behind their actions.
Ebook: The Qualtrics Handbook of Question Design
A close-ended vs open-ended question
Here are the main differences between open and closed-ended questions:
Open-ended questions | Closed-ended questions |
---|---|
Qualitative | Quantitative |
Contextual | Data-driven |
Personalized | Manufactured |
Exploratory | Focused |
More on open-ended questions
Open-ended questions are survey questions that begin with ‘who / what / where / when / why/ how’. Because there are no fixed responses, respondents have the freedom to answer in their own words — leading to more authentic and meaningful insights for researchers to leverage.
See below the differences between an open and a close-ended question:
Open-ended question requesting qualitative data
What are you feeling right now?
close-ended question requesting quantitative data
On a scale of 1-10 (where 1 is feeling terrible and 10 is feeling amazing), how are you feeling right now?
Both provide data, but the information differs based on the question (what are you feeling versus a numerical scale). With this in mind, researchers can design surveys that are fit-for-purpose depending on requirements.
However, best practice is to use a combination of open and close-ended survey questions. You might start with a close-ended question and follow up with an open-ended one so that respondents can explain their answers.
For example, if you’re curious about your company’s Net Promoter Score (NPS) , you could run a survey that includes these two questions:
- “How likely are you to recommend this product/service on a scale from 0 to 10?” (close-ended question) followed by:
- “Why have you responded this way?” (open-ended question)
This provides both quantitative and qualitative research data — so researchers have the numerical data but also the stories that contextualize how people answer.
Now, let’s look at the advantages and disadvantages of close-ended survey questions for collecting data.
Business advantages to using close-ended questions
- Easier and quicker to answer thanks to pre-populated answer choices.
- Provides measurable and statistically significant stats (quantitative data) that’s easy to analyze
- Gives better understanding of the question through answer options
- Higher response rates (respondents can quickly answer questions)
- Gets rid of irrelevant answers (as they are predetermined)
- Gives answers and data that is easy to compare
- They’re easy to customize
- You can categorize respondents based on answer choices
Business disadvantages to using close-ended questions
- Lacks detailed information (context)
- Can’t get customer opinions or comments (qualitative data)
- Doesn’t cover all possible answers and options
- Choices can often create confusion
- Are sometimes suggestive and therefore leads to bias
- No neutral option for those who don’t have an answer
Having highlighted the advantages and disadvantages of close-ended survey questions for user research, what types of close-ended questions can you use to collect data?
Types of closed questions
Dichotomous questions.
These question types have two options as answers (Di = two or split), which ask the survey participant for a single-word answer. These include:
- True or False
- Agree or Disagree
For example:
- Are you thirsty? Yes or No
- Is the sky blue? True or False
- Is stealing bad? Agree or Disagree.
Multiple-choice questions
Multiple-choice questions form the basis of most research. These questions have several options and are usually displayed as a list of choices, a dropdown menu, or a select box.
Multiple-choice questions also come in different formats:
- Multi-select: Multi-select is used when you want participants to select more than one answer from a list.
- Ranking order: Rank order is used to determine the order of preference for a list of items. This question type is best for measuring your respondents’ attitudes toward something.
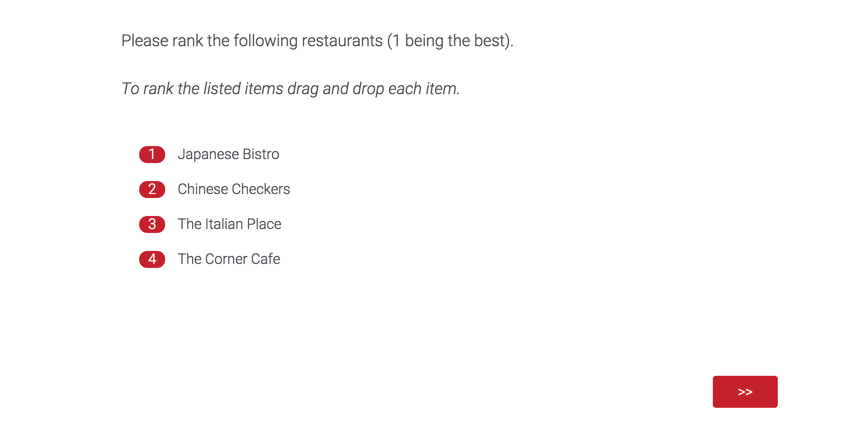
- Rating order or rating scale: Rating questions ask respondents to indicate their levels for aspects such as agreement, satisfaction, or frequency. An example is a Likert scale.
- Matrix table: Matrix questions collect multiple pieces of information in one question. This type provides an effective way to condense your survey or to group similar items into one question.
- Sliders: Sliders let respondents indicate their level of preference with a draggable bar rather than a traditional button or checkbox.
- Simple, direct, comprehensible
- Jargon-free
- Specific and concrete (rather than general and abstract)
- Unambiguous
- Not double-barreled
- Positive, not negative
- Not leading
- Include filter questions
- Easy to read aloud
- Not emotionally charged
- Inclusive of all possible responses
What Qualtrics survey analysis tools can help you?
Whatever your choice of the survey instrument, a good tool will make it easy for your researchers to create and deploy quantitative research studies, as well as empower respondents to answer in the fastest and most authentic ways possible.
There are several things to look out for when considering a survey solution, including:
- Functionality Choose a cloud-based platform that’s optimized for mobile, desktop, tablet, and more.
- Integrations Choose a software tool that plugs straight into your existing systems via APIs.
- Ease of use Choose a software tool that has a user-friendly drag-and-drop interface.
- Statistical analysis tools Choose a software tool that automatically does data analysis, that generates deep insights and future predictions with just a few clicks.
- Dashboards and reporting Choose a software tool that presents your data in dashboards in real-time, giving you clear results of statistical significance and showing strengths and deficiencies.
- The ability to act on results Choose a software tool that helps leaders make strategies and development decisions with greater speed and confidence.
Qualtrics CoreXM has “built-for-purpose” technology that empowers everyone to gather insights and take action. Through a suite of best-in-class analytics tools and an intuitive drag-and-drop interface, you can run end-to-end surveys, market research projects, and more to capture high-quality insights.
Start Creating World Class Surveys
Related resources
Survey vs questionnaire 12 min read, response bias 13 min read, double barreled question 11 min read, likert scales 14 min read, survey research 15 min read, survey bias types 24 min read, post event survey questions 10 min read, request demo.
Ready to learn more about Qualtrics?
Close-Ended Questions: Examples and How to Use Them
- by Alice Ananian
- August 20, 2024
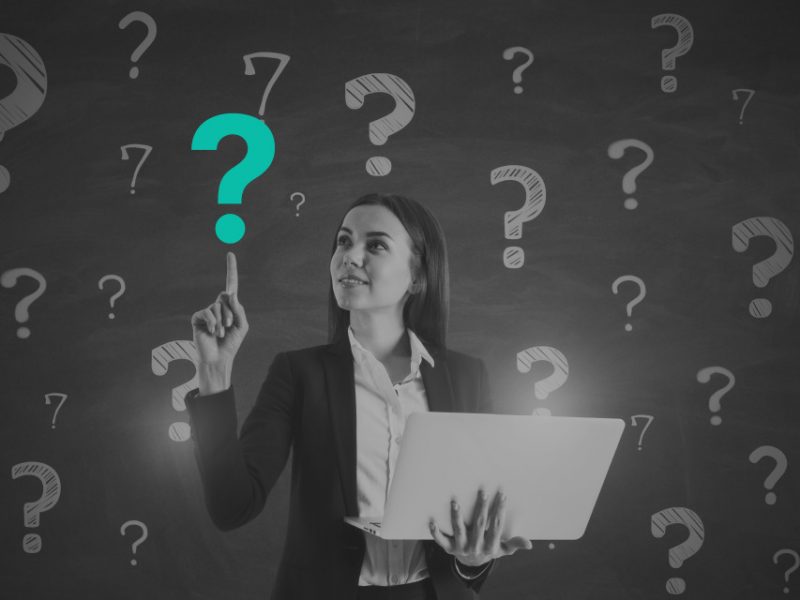
Imagine you’re a detective trying to solve a complex case with a room full of witnesses and time running out. Would you ask each witness to recount their entire day, or fire off a series of quick, specific questions to piece together the puzzle? This scenario illustrates the power of close-ended questions – a vital tool not just for detectives, but for researchers, marketers, educators, and professionals across all fields. In the vast landscape of data collection and analysis , asking the right questions can mean the difference between drowning in a sea of irrelevant information and uncovering precise, actionable insights.
Whether you’re conducting market research , gauging customer satisfaction, or designing an academic study, understanding how to craft and utilize close-ended questions effectively can be a game-changer. In this comprehensive guide, we’ll unlock the potential of close-ended questions, exploring what they are, their benefits, types, and how to use them to supercharge your data collection efforts. Get ready to transform your research from a time-consuming ordeal into a streamlined, efficient process.
What Are Close-Ended Questions?
Close-ended questions, also known as closed-ended questions, are queries that can be answered with a simple “yes” or “no,” or with a specific piece of information. These questions typically have a limited set of possible answers, often presented as multiple choice, rating scales, or dropdown menus. Unlike open-ended questions that allow respondents to provide free-form answers, close-ended questions offer a structured format that’s easy to answer and analyze.
For example:
- “Do you enjoy reading fiction books?” (Yes/No)
- “How often do you exercise?” (Daily/Weekly/Monthly/Never)
- “On a scale of 1-5, how satisfied are you with our service?” (1 = Very Dissatisfied, 5 = Very Satisfied)
These questions provide clear, concise data points that can be quickly collected and analyzed, making them invaluable in many fields.
Benefits of Using Closed-Ended Questions
Close-ended questions offer several advantages that make them a popular choice in various research and data collection scenarios:
Ease of Analysis
The structured nature of close-ended questions makes data analysis straightforward. Responses can be easily quantified, compared, and visualized using statistical tools.
Time-efficient
Both for respondents and researchers, close-ended questions are quicker to answer and process compared to open-ended questions.
Higher Response Rates
The simplicity of close-ended questions often leads to higher response rates, as participants find them less intimidating and time-consuming.
Reduced Ambiguity
With predefined answer options, there’s less room for misinterpretation of responses, leading to more reliable data.
Easier Comparisons
Standardized answers make it simpler to compare responses across different groups or time periods.
Focused Responses
Close-ended questions help keep respondents on topic, ensuring you get the specific information you’re seeking.
Scalability
These questions are ideal for large-scale surveys where processing open-ended responses would be impractical.
Consistency
They provide a uniform frame of reference for all respondents, leading to more consistent data.
By leveraging these benefits, researchers and professionals can gather precise, actionable data to inform decision-making processes across various fields.
Collect Insights From Your True Customers
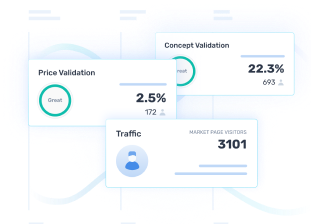
Types of Close-Ended Questions
Close-ended questions come in various forms, each serving a specific purpose in data collection. Understanding these types can help you choose the most appropriate format for your research needs:
Dichotomous Questions: These offer two mutually exclusive options, typically yes/no, true/false, or agree/disagree.
Example: “Have you ever traveled outside your country?” (Yes/No)
Multiple Choice Questions: These provide a list of preset options, from which respondents choose one or more answers.
Example: “Which of the following social media platforms do you use? (Select all that apply)”
- TikTok
Rating Scale Questions: Also known as Likert scale questions, these ask respondents to rate something on a predefined scale.
Example: “How satisfied are you with our customer service?”
1 (Very Dissatisfied) to 5 (Very Satisfied)
Semantic Differential Questions: These use opposite adjectives at each end of a scale, allowing respondents to choose a point between them.
Example: “How would you describe our product?”
Unreliable 1 – 2 – 3 – 4 – 5 Reliable
Ranking Questions: These ask respondents to order a list of items based on preference or importance.
Example: “Rank the following factors in order of importance when choosing a hotel (1 being most important, 4 being least)”
- Price
- Location
- Amenities
- Customer reviews
Dropdown Questions: Similar to multiple choice, but presented in a dropdown menu format, useful for long lists of options.
Example: “In which country were you born?” (Dropdown list of countries)
Understanding these types allows you to choose the most appropriate format for gathering the specific data you need.
Examples of Close-Ended Questions
To illustrate how close-ended questions can be applied across various fields, here are some examples:
Market Research
- “How likely are you to recommend our product to a friend?” (Scale of 0-10)
- “Which of these features is most important to you?” (Multiple choice)
Employee Satisfaction Surveys
- “Do you feel your work is valued by your manager?” (Yes/No)
- “How would you rate your work-life balance?” (Scale of 1-5)
Academic Research
- “What is your highest level of education?” (Dropdown menu)
- “How often do you engage in physical exercise?” (Multiple choice: Daily, Weekly, Monthly, Rarely, Never)
Customer Feedback
- “Was your issue resolved during this interaction?” (Yes/No)
- “How would you rate the quality of our product?” (Scale of 1-5)
Political Polling
- “Do you plan to vote in the upcoming election?” (Yes/No/Undecided)
- “Which of the following issues is most important to you?” (Multiple choice)
Healthcare Surveys
- “Have you been diagnosed with any chronic conditions?” (Yes/No)
- “On a scale of 1-10, how would you rate your current health?”
These examples demonstrate the versatility of close-ended questions across different sectors and research needs.
How to Ask Close-Ended Questions
Crafting effective close-ended questions requires careful consideration. Here are some best practices to follow:
1. Be Clear and Specific: Ensure your question is unambiguous and focuses on a single concept.
Good: “Do you own a car?”
Poor: “Do you own a car or bike?”
2. Provide Exhaustive Options: When using multiple choice, make sure your options cover all possible answers.
Include an “Other” option if necessary.
3. Avoid Leading Questions: Frame your questions neutrally to prevent biasing responses.
Good: “How would you rate our service?”
Poor: “How amazing was our excellent service?”
4. Use Appropriate Scales: Choose scales that match the level of detail you need. A 5-point scale is often sufficient, but you might need a 7 or 10-point scale for more nuanced data.
5. Balance Your Scales: For rating questions, provide an equal number of positive and negative options.
6. Consider Your Audience: Use language and examples that are familiar to your respondents.
7. Pilot Test Your Questions: Before launching a full survey, test your questions with a small group to identify any issues or ambiguities.
By following these guidelines, you can create close-ended questions that yield reliable, actionable data.
When to Use Close-Ended Questions
While close-ended questions are powerful tools, they’re not suitable for every situation. Here are some scenarios where they’re particularly effective:
1. Large-Scale Surveys: When you need to collect data from a large number of respondents quickly and efficiently.
2. Quantitative Research: When you need numerical data for statistical analysis.
3. Benchmarking: When you want to compare results across different groups or time periods.
4. Initial Screening: To quickly identify respondents who meet specific criteria for further research.
5. Customer Satisfaction Measurement: To get a clear picture of customer sentiment using standardized metrics.
6. Performance Evaluations: To gather consistent feedback on employee or process performance.
7. Market Segmentation: To categorize respondents based on demographic or behavioral characteristics.
However, it’s important to note that close-ended questions may not be ideal when:
- You need in-depth, explanatory information
- You’re exploring a new topic and don’t know all possible answer options
- You want to understand the reasoning behind responses
In these cases, open-ended questions or a mix of both types might be more appropriate.
Close-ended questions are a fundamental tool for researchers, marketers, and educators due to their ability to efficiently collect precise data. It’s essential to comprehend the variations of these questions, when to use them, and how to craft them effectively to enhance data collection quality. Remember, while they are effective in many scenarios, they should occasionally be integrated with open-ended questions to achieve a comprehensive view.
Consider your objectives when designing surveys. Are quick, comparable data points necessary, or is there a need for in-depth explanations? The answer will guide your choice between close-ended and open-ended questions or a strategic mix of the two. Mastering the art of asking the right questions is a skill that can significantly improve your research insights, providing you with a powerful tool to make informed decisions and progress your projects.
What is the difference between closed-ended and open-ended questions?
Closed-ended questions provide respondents with a fixed set of options to choose from, while open-ended questions allow respondents to answer in their own words. Here’s a quick comparison:
Closed-ended questions:
- Have predefined answer choices
- Yield quantitative data
- Are easier to analyze statistically
- Take less time to answer
Example: “Do you enjoy reading? (Yes/No)”
Open-ended questions:
- Allow free-form responses
- Yield qualitative data
- Provide more detailed, nuanced information
- Take more time to answer and analyze
Example: “What do you enjoy about reading?”
Each type has its strengths, and many effective surveys use a combination of both to gather comprehensive insights.
Can closed-ended questions be used in qualitative research?
Yes, closed-ended questions can be utilized in qualitative research not only for participant screening, context setting, and data triangulation but also in mixed-methods research and as follow-ups to open-ended inquiries. Despite this, their use should be limited and strategic, as the primary aim of qualitative research is to elicit rich and descriptive responses using open-ended questions.
What tools can help in creating closed-ended questions?
Some of the top tools for creating closed-ended questions include Google Forms, SurveyMonkey , Qualtrics , Typeform , and LimeSurvey . These platforms are user-friendly and offer a range of question types, advanced features, and analysis tools. While these tools simplify the process, high-quality surveys require sound question design principles and subject expertise.
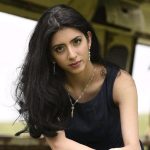
Alice Ananian
Alice has over 8 years experience as a strong communicator and creative thinker. She enjoys helping companies refine their branding, deepen their values, and reach their intended audiences through language.
Related Articles
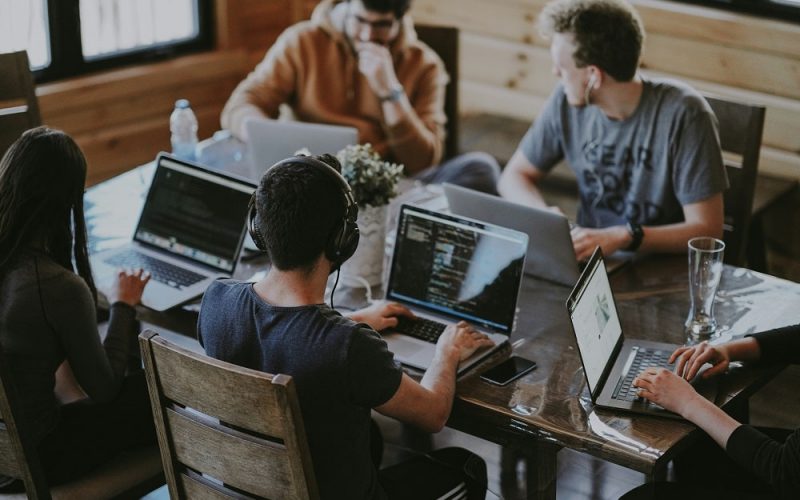
10 Best Startup Incubators Worldwide
- by Arman Khachikyan
- February 8, 2024
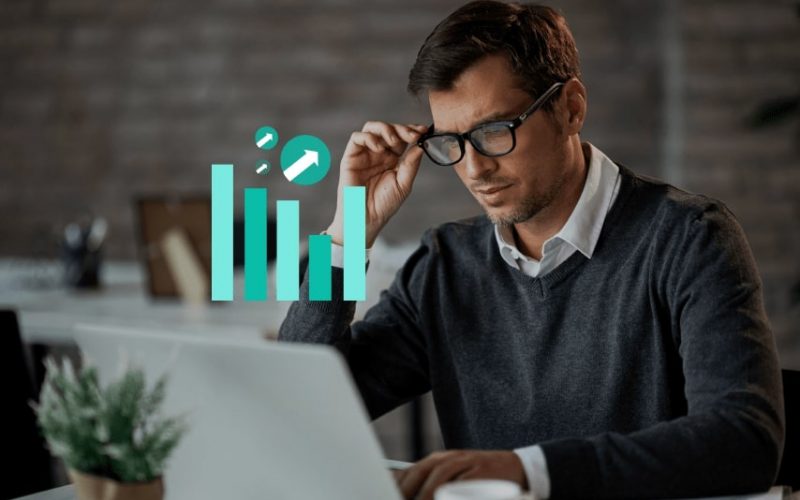
5 Steps to Conduct Market Opportunity Analysis [Example Included]
- June 3, 2024
Close-Ended Questions: Definition, Types, Examples
Appinio Research · 15.12.2023 · 32min read
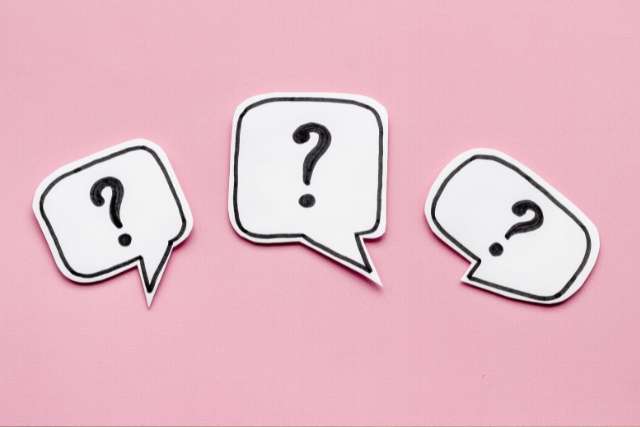
Are you seeking a powerful tool to gather structured data efficiently and gain valuable insights in your research, surveys, or communication? Close-ended questions, characterized by their predefined response options, offer a straightforward and effective way to achieve your goals . In this guide, we'll explore the world of close-ended questions, from their definition and characteristics to best practices, common pitfalls to avoid, and real-world examples. Whether you're a researcher, survey designer, or communicator, mastering close-ended questions will empower you to collect, analyze, and leverage data effectively for informed decision-making.
What are Close-Ended Questions?
Close-ended questions are a fundamental component of surveys, questionnaires , and research instruments. They are designed to gather specific and structured data by offering respondents a limited set of predefined response options.
Characteristics of Close-Ended Questions
Close-ended questions possess several defining characteristics that set them apart from open-ended questions.
- Limited Response Options: Close-ended questions present respondents with a finite set of answer choices, typically in the form of checkboxes, radio buttons, or a predefined list.
- Quantitative Data: The responses to close-ended questions yield quantitative data , making it easier to analyze statistically and draw numerical conclusions.
- Efficiency: They are efficient for data collection, as respondents can select from predetermined options, reducing response time and effort.
- Standardization: Close-ended questions ensure that all respondents receive the same set of questions and response options, promoting consistency in data collection .
- Objective Measurement: The structured nature of close-ended questions helps maintain objectivity in data collection, as personal interpretations are minimized.
Importance of Close-Ended Questions
Close-ended questions play a vital role in various communication contexts, offering several advantages that contribute to their significance.
- Clarity and Precision: Close-ended questions are crafted to elicit specific, focused responses, helping to avoid ambiguity and ensuring that respondents understand the intended query.
- Efficiency in Data Collection: They facilitate efficient data collection in scenarios where time and resources are limited, such as large-scale surveys, market research , or customer feedback.
- Quantitative Analysis: Close-ended responses can be quantified, allowing for statistical analysis, making them indispensable for empirical research and data-driven decision-making.
- Comparative Studies : They enable straightforward comparisons between different groups, individuals, or time periods, contributing to a better understanding of trends and patterns.
- Standardized Research: In academic and scientific research, close-ended questions contribute to the standardization of data collection methods, increasing the reliability of studies.
- Structured Interviews: In structured interviews , close-ended questions help interviewers cover specific topics systematically and consistently, ensuring that all key points are addressed.
- Reduced Respondent Burden: By providing predefined options, close-ended questions simplify the response process, reducing the cognitive load on respondents.
- Quantitative Feedback in Business: In business and customer service, close-ended questions provide numerical feedback that can be used to assess customer satisfaction, product performance, and service quality.
- Public Opinion Polls: Close-ended questions are commonly employed in public opinion polling to gauge public sentiment on various political, social, or economic issues.
Understanding close-ended questions and characteristics, as well as their importance in communication, empowers researchers, survey designers, and communicators to effectively collect and analyze structured data to inform decisions, policies, and strategies.
Types of Close-Ended Questions
Close-ended questions are versatile tools used in surveys and research to gather specific, structured data. Let's explore the various types of close-ended questions to understand their unique characteristics and applications.
Yes/No Questions
Yes/no questions are the simplest form of close-ended questions. Respondents are presented with a binary choice and are required to select either "Yes" or "No." These questions are excellent for gathering clear-cut information and can be used in a variety of research contexts.
For example, in a customer satisfaction survey, you might ask, "Were you satisfied with our service?" with the options "Yes" or "No."
Multiple-Choice Questions
Multiple-choice questions provide respondents with a list of options, and they are asked to select one or more answers from the provided choices. These questions offer flexibility and are ideal when you want to capture a range of possible responses.
For instance, in a product feedback survey, you could ask, "Which of the following features do you find most valuable?" with a list of feature options for respondents to choose from.
Rating Scale Questions
Rating scale questions ask respondents to rate something on a numerical scale. Commonly used scales range from 1 to 5 or 1 to 7, allowing participants to express their opinions or attitudes quantitatively. These questions are widely used in fields such as psychology, marketing, and customer feedback.
For instance, you might use a rating scale question like, "On a scale of 1 to 5, how satisfied are you with our customer service?"
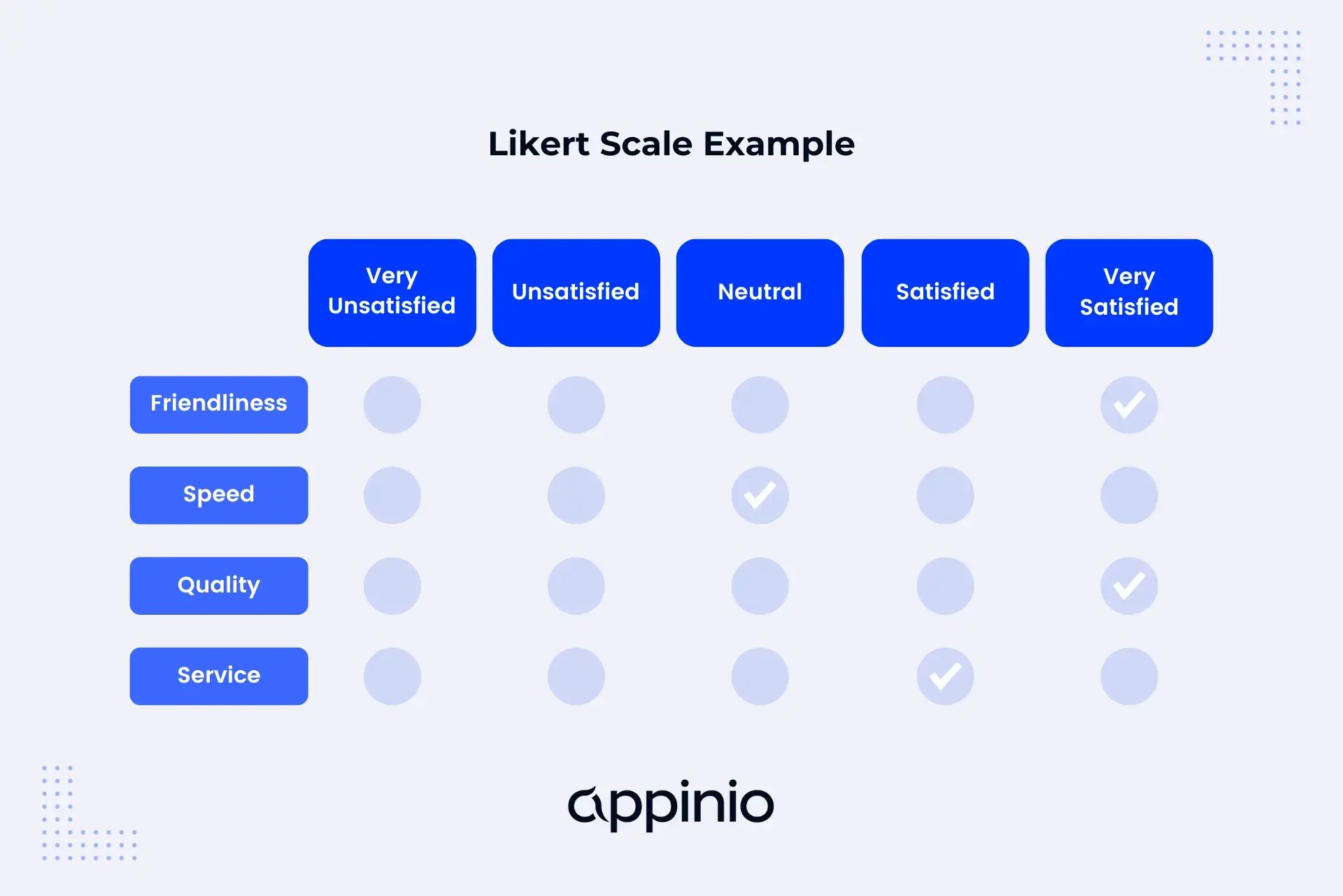
Dichotomous Questions
Dichotomous questions are a subset of yes/no questions but may include more nuanced options beyond a simple "yes" or "no." They provide respondents with two contrasting choices, making them suitable for situations where a binary decision is necessary but requires more detail.
For example, in a political survey, you could ask, "Do you support the proposed policy: strongly support, somewhat support, somewhat oppose, strongly oppose?"
Forced-Choice Questions
Forced-choice questions present respondents with a set of options and require them to select only one choice, eliminating the possibility of choosing multiple answers. These questions are useful when you want to force respondents to make a decision, even if they are indecisive or unsure.
In employee performance evaluations, for instance, you might ask, "Which area should the employee focus on for improvement this quarter?" with a list of specific areas to choose from.
Understanding the characteristics and applications of these close-ended question types will help you design surveys and questionnaires that effectively collect the data you need for your research or decision-making processes.
Why Are Close-Ended Questions Used?
Close-ended questions offer several advantages when incorporated into surveys, questionnaires, and research instruments. These advantages make them a valuable choice for collecting structured data.
- Efficient Data Collection: Close-ended questions streamline the data collection process. Respondents can quickly choose from predefined options, reducing the time and effort required to complete surveys or questionnaires.
- Standardized Responses: With close-ended questions, all respondents receive the same set of questions and response options. This standardization ensures consistency in data collection and simplifies the analysis process.
- Statistical Analysis: Close-ended responses can be easily quantified, making them ideal for statistical analysis. Researchers can use statistical tools to identify patterns , correlations , and trends within the data.
- Ease of Comparison: The structured nature of close-ended questions enables easy comparison of responses across different participants, groups, or time periods. This comparability is particularly valuable in longitudinal studies and market research.
- Reduced Ambiguity: Close-ended questions leave little room for ambiguity in responses, as they provide clear and predefined options. This clarity helps minimize misinterpretation of answers.
- Objective Data: Close-ended questions generate objective data, making it easier to draw conclusions based on quantitative information. This objectivity is especially important in fields like psychology and social sciences.
Disadvantages of Close-Ended Questions
While close-ended questions have their advantages, it's essential to be aware of their limitations and potential drawbacks. Here are some disadvantages associated with using close-ended questions.
- Limited Insight into Participant's Perspective: Close-ended questions may restrict respondents from fully expressing their thoughts, feelings, or experiences. This limitation can lead to a lack of depth in understanding participant perspectives.
- Risk of Bias: The phrasing of close-ended questions can introduce bias. Biased questions may unintentionally influence respondents to select specific responses, leading to skewed results.
- Difficulty Capturing Nuanced Opinions: Some topics require nuanced responses that cannot be adequately captured by predefined answer options. Close-ended questions may oversimplify complex issues.
- Inability to Explore Unforeseen Issues: Close-ended questions limit researchers to the options provided in the questionnaire. They may not account for unforeseen issues or emerging insights that open-ended questions could capture.
- Possible Social Desirability Bias: Respondents may choose answers they believe are socially acceptable or expected, rather than their actual opinions or experiences. This can result in inaccurate data.
- Limited Qualitative Data: Close-ended questions prioritize quantitative data. If qualitative insights are essential for your research, incorporating open-ended questions is necessary to capture detailed narratives.
Understanding both the advantages and disadvantages of close-ended questions is crucial for effective survey and questionnaire design. Depending on your research goals and the nature of the data you seek, you can make informed decisions about when and how to use close-ended questions in your data collection process.
When to Use Close-Ended Questions?
Close-ended questions are a valuable tool in research and surveys, but knowing when to deploy them is essential for effective data collection. Let's explore the various contexts and scenarios in which close-ended questions are particularly advantageous.
Surveys and Questionnaires
Surveys and questionnaires are perhaps the most common and well-suited applications for close-ended questions. They offer several advantages in this context.
- Efficiency: In surveys and questionnaires, respondents often face time constraints. Close-ended questions allow them to provide structured responses quickly, leading to higher completion rates.
- Ease of Data Entry: Close-ended responses are typically easier to process and enter into databases or analysis software. This reduces the chances of data entry errors.
- Comparability: When conducting large-scale surveys, the ability to compare responses across participants becomes crucial. Close-ended questions provide standardized response options for easy comparison.
- Quantitative Data: Surveys often aim to gather quantitative data for statistical analysis. Close-ended questions are well-suited for this purpose, as they generate numerical data that can be analyzed using various statistical techniques.
Quantitative Research
In quantitative research , where the primary goal is to obtain numerical data that can be subjected to statistical analysis , close-ended questions play a significant role. They are favored in quantitative research due to:
- Measurement Precision: Close-ended questions enable precise measurement of specific variables or constructs. Researchers can assign numerical values to responses, facilitating quantitative analysis.
- Hypothesis Testing: Quantitative research often involves hypothesis testing. Close-ended questions provide structured data that can be directly used to test hypotheses and draw statistical inferences.
- Large Sample Sizes: Quantitative research often requires large sample sizes to ensure the reliability of findings. Close-ended questions are efficient in collecting data from a large number of participants.
- Data Consistency: The standardized nature of close-ended questions ensures that all respondents are presented with the same set of options, reducing response variations due to question wording.
Market Research
Market researchers frequently rely on close-ended questions to gather insights into consumer behavior , preferences, and opinions. Close-ended questions are well-suited for market research for the following reasons:
- Comparative Analysis: Close-ended questions make it easy to compare customer responses across different demographics , regions, or time periods. This comparative analysis informs marketing strategies and product development .
- Quantitative Insights: Market research often involves the collection of quantitative data to measure customer satisfaction, brand perception , and market trends. Close-ended questions provide numerical data for analysis.
- Efficiency in Data Processing: Market research often deals with large volumes of data. Close-ended questions simplify data processing and analysis, enabling faster decision-making.
- Benchmarking: Companies use close-ended questions to benchmark their performance against competitors. Standardized response options make it possible to gauge how a company fares in comparison to others in the industry.
As you navigate the intricate world of research and surveys, striking the right balance between close-ended and open-ended questions is key to uncovering valuable insights. To streamline your data collection process and gain a holistic understanding of your research objectives, you should explore the capabilities of Appinio.
Appinio offers versatile tools to help you craft well-rounded surveys, harnessing the power of both structured and open-ended questions. Ready to elevate your research game? Book a demo today and experience how Appinio can enhance your data collection efforts, enabling you to make informed decisions based on comprehensive insights.
Book a Demo
How to Craft Close-Ended Questions?
Designing close-ended questions that yield accurate and meaningful data requires careful consideration of various factors. Let's delve into the fundamental principles and best practices for crafting practical close-ended questions.
Clarity and Simplicity
Clarity and simplicity are fundamental when formulating close-ended questions. Your goal is to make it easy for respondents to understand and respond accurately.
- Use Clear Language: Frame questions using straightforward and clear language. Avoid jargon, technical terms, or complex vocabulary that may confuse respondents.
- Avoid Double-Barreled Questions: Double-barreled questions combine multiple ideas or topics into one question, making it challenging for respondents to provide a precise answer. Split such questions into separate inquiries.
- Keep It Concise: Long and convoluted questions can overwhelm respondents. Keep your questions concise and to the point.
- Use Everyday Language: Ensure that your questions are phrased in a way that resonates with your target audience. Use language they are familiar with to maximize comprehension.
Avoiding Leading Questions
It's crucial to avoid leading questions when crafting close-ended questions. Leading questions can unintentionally influence respondents to provide a specific answer rather than expressing their genuine opinions. To steer clear of them:
- Stay Neutral: Phrase questions in a neutral and unbiased manner. Avoid any language or tone that suggests a preferred answer.
- Balance Positive and Negative Wording: If you have a set of response options that include both positive and negative statements, ensure they are balanced to avoid bias.
- Pretest for Bias: Conduct pretesting with a diverse group of respondents to identify any potential bias in your questions. Adjust questions as needed based on feedback.
Balancing Response Options
Balancing response options is essential to ensure that your close-ended questions provide accurate and comprehensive data.
- Mutually Exclusive Options: Ensure that response options are mutually exclusive, meaning respondents can choose only one option that best aligns with their perspective.
- Exhaustive Choices: Include all relevant response options to cover a full range of possible answers. Leaving out options can lead to incomplete or skewed data.
- Avoiding Overloading: Be cautious not to overload respondents with too many response choices. Striking the right balance between providing choices and avoiding overwhelming respondents is essential.
Pilot Testing
Before deploying your survey or questionnaire, pilot testing is a crucial step to refine your close-ended questions. Pilot testing involves administering the survey to a small group of participants to identify and address any issues.
- Select a Representative Sample : To ensure realistic feedback, choose a sample that closely resembles your target audience .
- Gather Feedback: Collect feedback on your questions' clarity, wording, and comprehensibility. Ask participants if any questions were confusing or if they felt any bias in the questions.
- Iterate and Revise: Based on the feedback received during pilot testing, make necessary revisions to your close-ended questions to improve their quality and effectiveness.
Crafting effective close-ended questions is a skill that improves with practice. By focusing on clarity, neutrality, balance, and thorough testing, you can create questions that elicit reliable and insightful responses from your survey or research participants.
How to Analyze Close-Ended Responses?
Once you've collected close-ended responses in your survey or research, the next crucial step is to analyze and interpret the data effectively. We'll explore the various methods and techniques for making sense of close-ended responses.
Data Cleaning
Data cleaning is an essential preliminary step in the analysis process. It involves identifying and rectifying inconsistencies, errors, or outliers in your close-ended responses.
- Identify Missing Data: Check for missing responses and decide how to handle them—whether by imputing values or excluding incomplete responses from analysis.
- Outlier Detection: Identify outliers in your data that may skew the results. Determine whether outliers are genuine data points or errors that need correction.
- Standardization: Ensure that all data is in a consistent format. This may involve converting responses to a common scale or addressing variations in how respondents answered.
- Data Validation: Validate responses against predefined criteria to ensure accuracy and reliability. Flag any responses that deviate from expected patterns.
- Documentation: Keep a detailed record of the data cleaning process, including the rationale for decisions made. This documentation is crucial for transparency and reproducibility.
Frequency Distribution
Frequency distribution is a fundamental technique for understanding the distribution of responses in your data. It provides an overview of how often participants chose each response option. To create a frequency distribution:
- Tabulate Responses: Count the number of times each response option was selected for each close-ended question.
- Create Frequency Tables: Organize the data into frequency tables, displaying response options and their corresponding frequencies.
- Visualize Data: Visual representations such as bar charts or histograms can help you quickly grasp the distribution of responses.
- Identify Patterns: Examine the frequency distribution for patterns , trends, or anomalies. This step can reveal insights about respondent preferences or tendencies.
Cross-Tabulation
Cross-tabulation is a powerful technique that allows you to explore relationships between two or more variables in your close-ended responses. It's particularly useful for identifying patterns or correlations between variables.
- Select Variables: Choose the variables you want to analyze for relationships. These variables can be from different questions in your survey.
- Create Cross-Tabulation Tables: Create tables that show how responses to one variable relate to responses to another variable. This involves counting how many participants fall into each combination of responses.
- Calculate Percentages: Convert the counts into percentages to understand the proportion of respondents in each category.
- Analyze Patterns: Examine the cross-tabulation tables to identify significant patterns, associations, or trends between variables.
Drawing Conclusions
Drawing conclusions from close-ended responses involves making sense of the data and using it to answer your research questions or hypotheses.
- Statistical Analysis: Depending on your research design, apply statistical tests or techniques to determine the significance of relationships or differences in responses.
- Contextual Understanding: Consider the broader context in which the data was collected. Understand how external factors may have influenced the responses.
- Comparative Analysis: Compare your findings to existing literature or benchmarks to provide context and insights.
- Limitations: Acknowledge any limitations in your analysis, such as sample size , potential bias, or data collection constraints.
- Actionable Insights: Ensure that your conclusions provide actionable insights or recommendations based on the data collected.
- Report Findings: Present your findings in a clear and accessible manner, using visuals, tables, and narrative explanations to convey the results effectively.
Effectively analyzing and interpreting close-ended responses is critical for deriving meaningful insights and making informed decisions in your research or survey projects. By following these steps and techniques, you can unlock the valuable information hidden within your data.
Close-Ended Questions Examples
To better understand how close-ended questions are crafted and used effectively, let's delve into some real-world examples across different fields and scenarios. These examples will illustrate the diversity of close-ended questions and their applications.
Example 1: Customer Satisfaction Survey
Question: On a scale of 1 to 5, how satisfied are you with our product?
- Very Dissatisfied
- Dissatisfied
- Neutral
- Satisfied
- Very Satisfied
This is a classic example of a rating scale question . Respondents are asked to rate their satisfaction level on a numerical scale. The predefined response options allow for quantifiable data collection, making it easy to analyze and track changes in customer satisfaction over time.
Example 2: Employee Feedback
Question: Did you receive adequate training for your new role?
This yes/no question is straightforward and ideal for capturing specific information. It provides a binary response, making it easy to categorize employees who received adequate training from those who did not.
Example 3: Political Opinion Poll
Question: Which political party do you align with the most?
- Democratic Party
- Republican Party
- Independent
- Other (please specify): ________________
This multiple-choice question allows respondents to choose their political affiliation from a set of predefined options. It also provides an "Other" category with an open-ended field for respondents to specify a different affiliation, ensuring inclusivity.
Example 4: Product Feature Prioritization
Question: Please rank the following product features in order of importance, with 1 being the most important and 5 being the least important:
- Price
- Durability
- User-Friendliness
- Performance
- Design
In this example, respondents are asked to rank product features based on their preferences. This type of close-ended question helps gather valuable insights into which features customers prioritize when making purchasing decisions.
Example 5: Healthcare Patient Feedback
Question: How would you rate the quality of care you received during your recent hospital stay?
- Excellent
- Very Good
This rating scale question assesses patient satisfaction with the quality of healthcare services . It allows for the collection of quantitative data that can be analyzed to identify areas for improvement in patient care.
These examples showcase the versatility of close-ended questions in various domains, including customer feedback, employee assessments, political polling, product development, and healthcare. When crafting close-ended questions, consider the specific context, the type of data you need, and the preferences of your target audience to design questions that yield valuable and actionable insights.
Best Practices for Close-Ended Questionnaires
Creating effective close-ended questionnaires and surveys requires attention to detail and adherence to best practices. Here are some tips to ensure your questionnaires yield high-quality data.
- Clear and Concise Language: Use clear, simple, and jargon-free language to ensure respondents understand the questions easily.
- Logical Flow: Organize questions in a logical order, starting with easy-to-answer and non-sensitive questions before moving to more complex or sensitive topics.
- Avoid Double Negatives: Ensure questions are phrased positively, avoiding double negatives that can confuse respondents.
- Provide Clear Instructions: Include clear instructions at the beginning of the questionnaire to guide respondents on how to complete it.
- Use Consistent Scales: If you're using rating scales, keep the scale consistent throughout the questionnaire. For example, if you use a 1-5 scale, maintain that scale for all relevant questions.
- Randomize Response Order: To minimize order bias, consider randomizing the order of response options for multiple-choice questions.
- Pretest Questionnaires: Conduct pilot testing with a small group of participants to identify and rectify any issues with question clarity, wording, or bias.
- Limit Open-Ended Questions: While focusing on close-ended questions, consider including a few strategic open-ended questions to capture qualitative insights where necessary.
Common Close-Ended Questions Mistakes to Avoid
Avoiding common mistakes is essential to ensure the quality and reliability of your close-ended questionnaires. Here are some pitfalls to steer clear of:
- Biased Questions: Avoid framing questions in a way that leads respondents toward a particular answer or opinion.
- Ambiguity: Ensure questions are clear and unambiguous to prevent confusion among respondents.
- Overloading Respondents: Don't overwhelm respondents with too many close-ended questions. Balance with open-ended questions when needed.
- Ignoring Response Options: Ensure that response options cover the full range of possible answers and don't miss out on relevant choices.
- Using Double-Barreled Questions: Avoid combining multiple ideas or topics into a single question, as it can lead to imprecise responses.
- Neglecting Pilot Testing: Skipping pilot testing can result in issues with question wording or formatting that could have been resolved beforehand.
- Lack of Variability: Ensure that response options have sufficient variability to capture nuances in responses. Avoid creating questions with very similar answer choices.
- Failure to Update Questions: Over time, the relevance of certain questions may change. Regularly review and update your questionnaires to reflect current contexts and concerns.
- Neglecting Privacy and Sensitivity: Be mindful of sensitive or personal questions, and provide options for respondents to decline or skip such questions.
By adhering to best practices and avoiding common mistakes, you can design close-ended questionnaires that collect accurate, unbiased, and actionable data while providing a positive experience for respondents.
Close-ended questions are a valuable tool for anyone looking to collect specific, structured data efficiently and accurately. They offer clarity, ease of analysis, and standardization, making them essential in various fields , from research and surveys to business and communication. By understanding the characteristics, best practices, and potential pitfalls of close-ended questions, you can harness their power to gain insights, make informed decisions, and drive positive outcomes in your endeavors. So, whether you're conducting surveys, analyzing customer feedback, or conducting research, remember that well-crafted close-ended questions are your key to unlocking valuable data and understanding your audience or participants better. Start using close-ended questions wisely, and watch your ability to collect, analyze, and act on data soar, ultimately leading you toward more successful and informed outcomes in your professional pursuits.
How to Use Close-Ended Questions for Market Research?
Introducing Appinio , the real-time market research platform that revolutionizes the way you leverage close-ended questions for market research. With Appinio, you can conduct your own market research in minutes, gaining valuable insights for data-driven decision-making.
- Speedy Insights: From questions to insights in minutes. Appinio's lightning-fast platform ensures you get the answers you need when you need them.
- User-Friendly: No need for a PhD in research. Appinio's intuitive platform is designed for everyone, making market research accessible to all.
- Global Reach: Survey your defined target group from over 90 countries, with the ability to narrow down from 1200+ characteristics.
Say goodbye to boring, intimidating, and overpriced market research—Appinio is here to make it exciting, intuitive, and seamlessly integrated into your everyday decision-making.
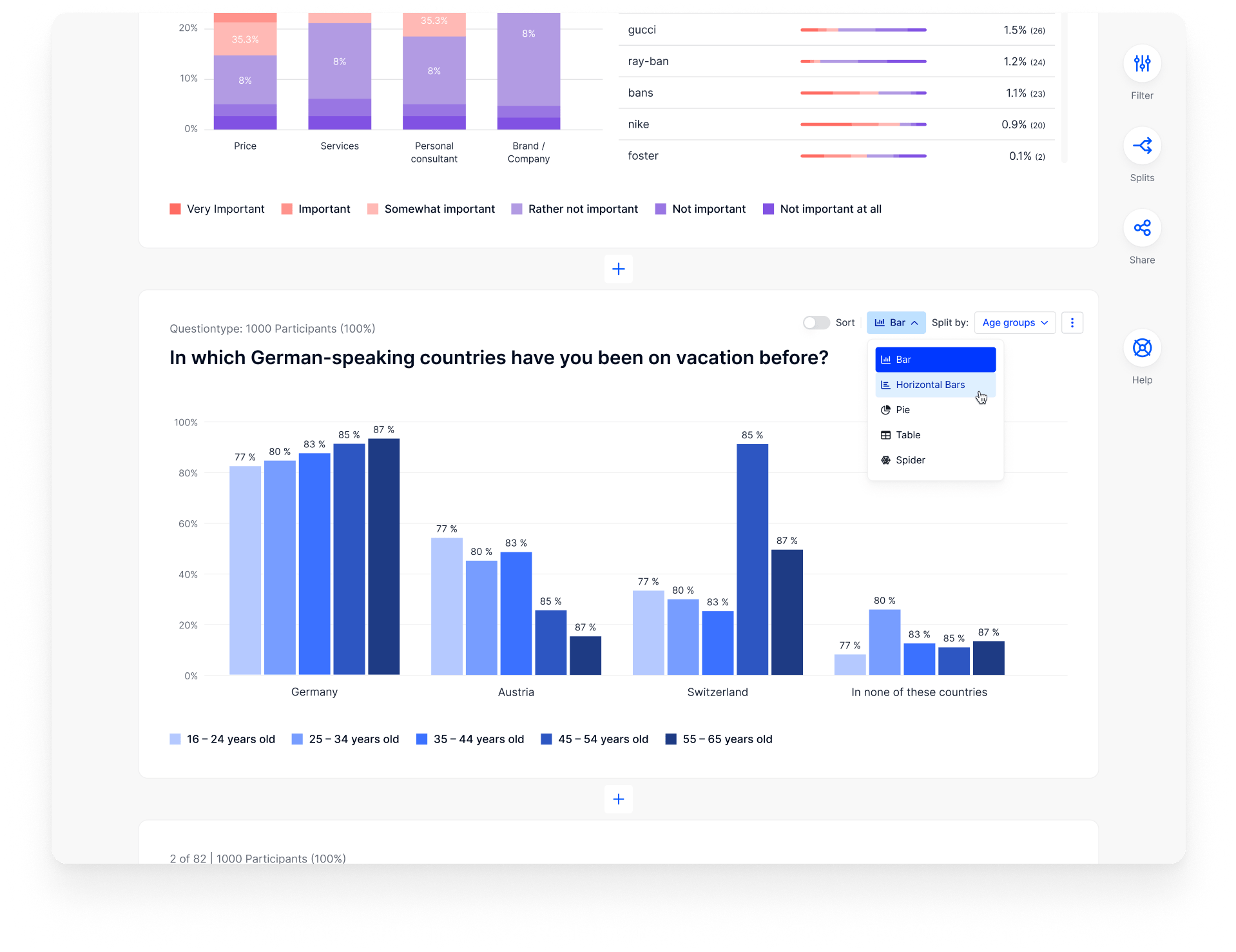
Get free access to the platform!
Join the loop 💌
Be the first to hear about new updates, product news, and data insights. We'll send it all straight to your inbox.
Get the latest market research news straight to your inbox! 💌
Wait, there's more
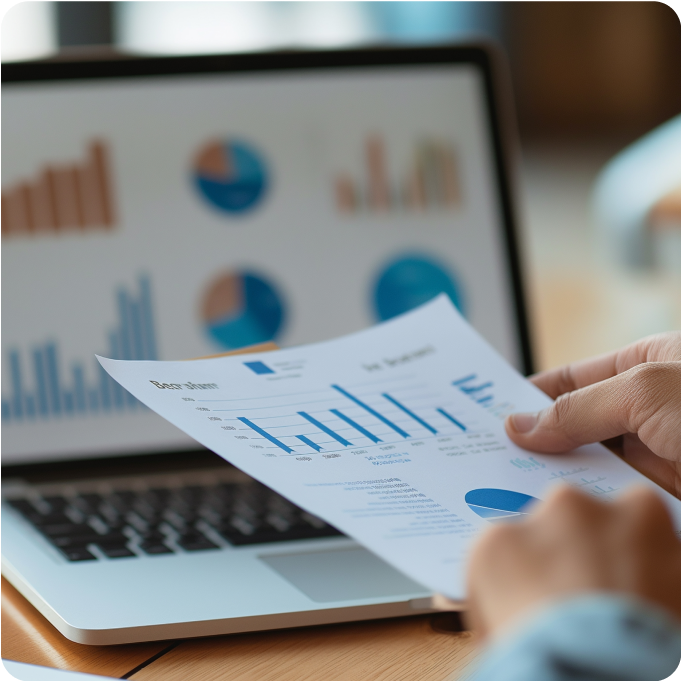
19.09.2024 | 8min read
Track Your Customer Retention & Brand Metrics for Post-Holiday Success
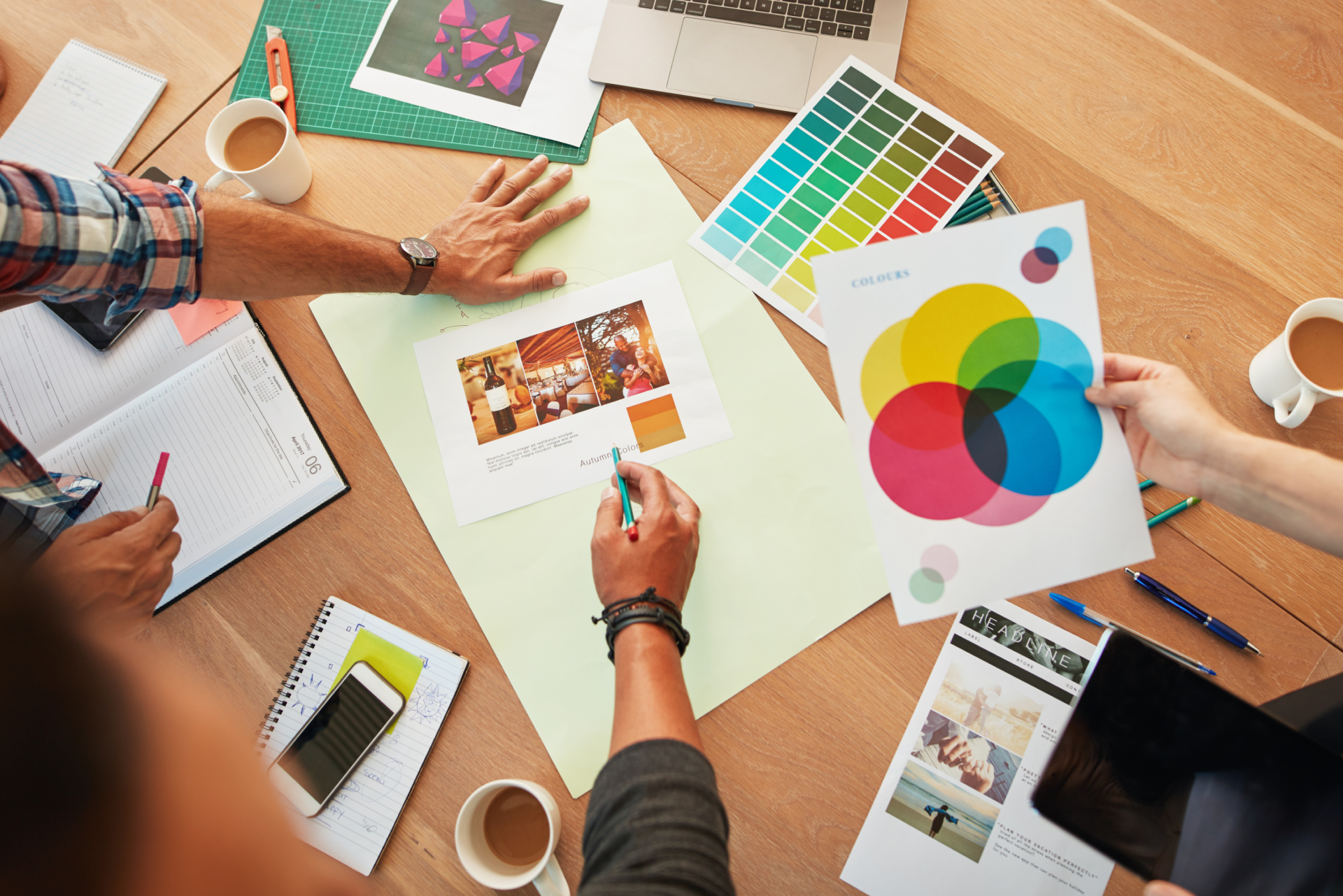
16.09.2024 | 10min read
Creative Checkup – Optimize Advertising Slogans & Creatives for ROI
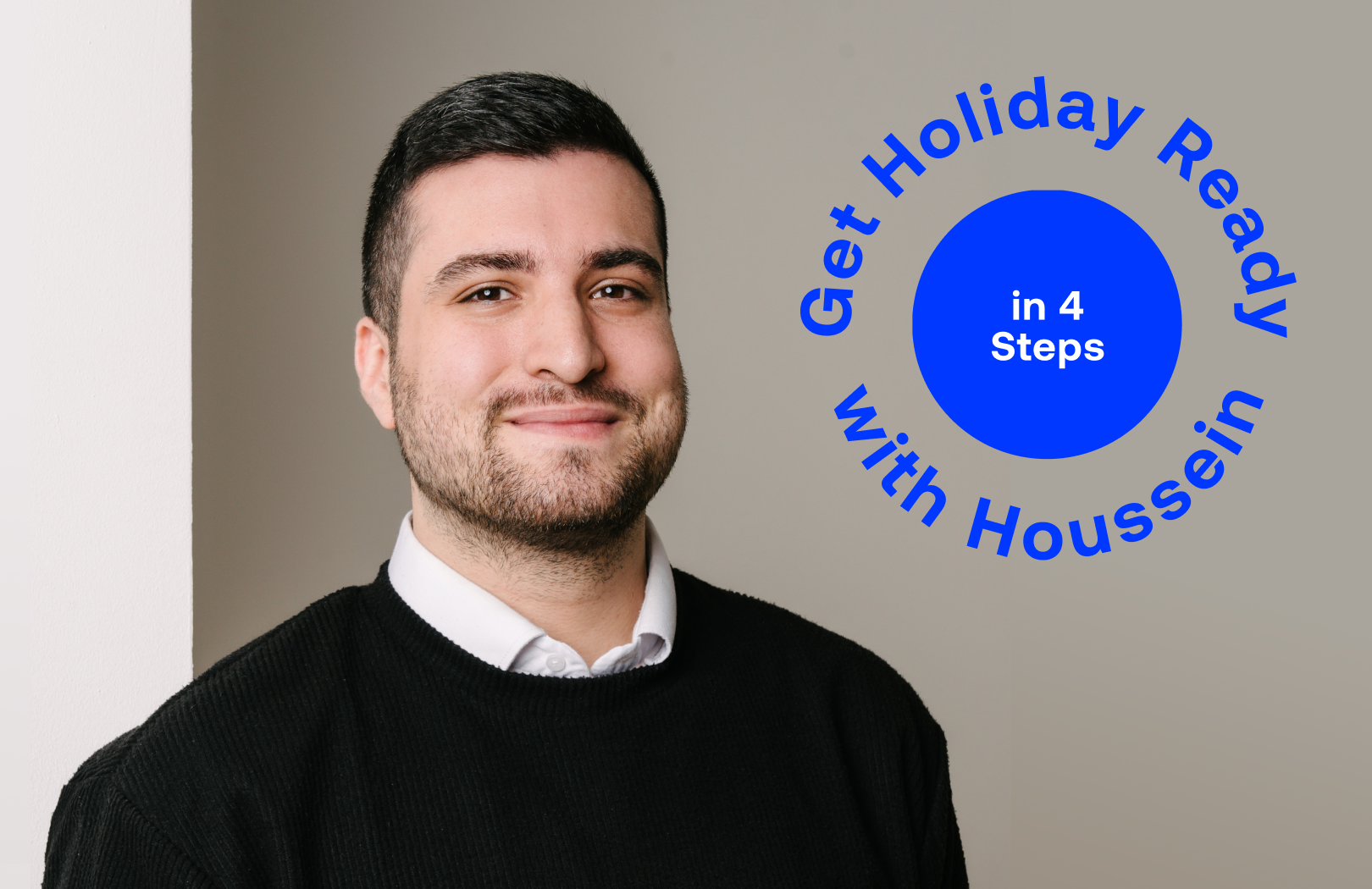
03.09.2024 | 8min read
Get your brand Holiday Ready: 4 Essential Steps to Smash your Q4

- Solutions Industry Gaming Automotive Sports and events Education Government Travel & Hospitality Financial Services Healthcare Member Experience Technology Use case AskWhy Communities Audience InsightsHub InstantAnswers Digsite LivePolls Journey Mapping GDPR Positive People Science 360 Feedback Surveys Research Edition
- Resources Blog eBooks Survey Templates Case Studies Training Webinars Help center
Close Ended Questions
Close ended questions and its types are critical for collecting survey responses within a limited range of options. they are the foundation of all statistical analysis techniques applied on questionnaires and surveys..
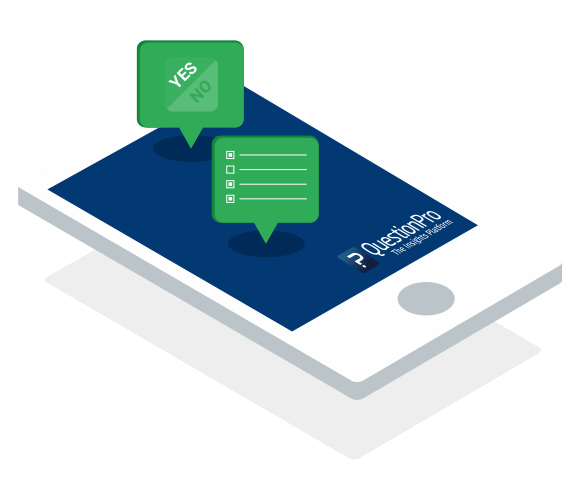
Join over 10 million users

Content Index
- What are Close Ended Questions?
- Types of Close Ended Questions with Examples
When to use Close Ended Questions?
Advantages of close ended questions, what are open ended questions, examples of open ended questions, difference between open ended and closed ended questions.
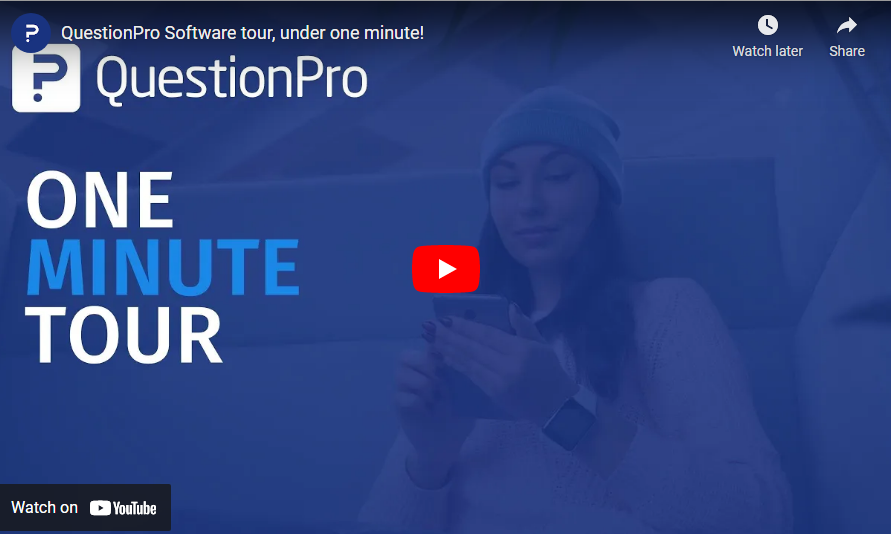
Watch the 1-minute tour
What are Close Ended Questions: Definition
Close ended questions are defined as question types that ask respondents to choose from a distinct set of pre-defined responses, such as “yes/no” or among set multiple choice questions . In a typical scenario, closed-ended questions are used to gather quantitative data from respondents.
Closed-ended questions come in a multitude of forms but are defined by their need to have explicit options for a respondent to select from.
However, one should opt for the most applicable question type on a case-by-case basis, depending on the objective of the survey . To understand more about the close ended questions, let us first know its types.
Types of Close Ended Questions with Survey Examples
Dichotomous question.
These close ended question are indicative questions that can be answered either in one of the two ways, “yes” or “no” or “true” or “false”.
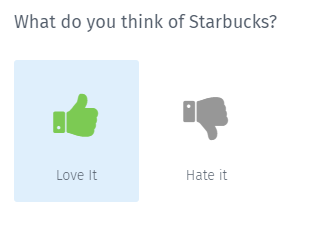
Multiple choice question
Multiple choice closed ended questions are easy and flexible and helps the researcher obtain data that is clean and easy to analyse. It typically consists of stem - the question, correct answer, closest alternative and distractors.
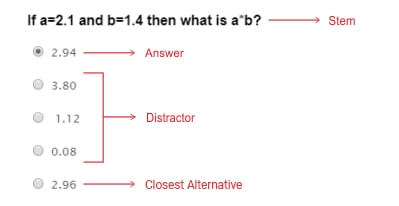
Types of Multiple Choice Questions
1. likert scale multiple choice questions.
These closed ended questions, typically are 5 pointer or above scale questions where the respondent is required to complete the questionnaire that needs them to indicate the extent to which they agree or disagree.
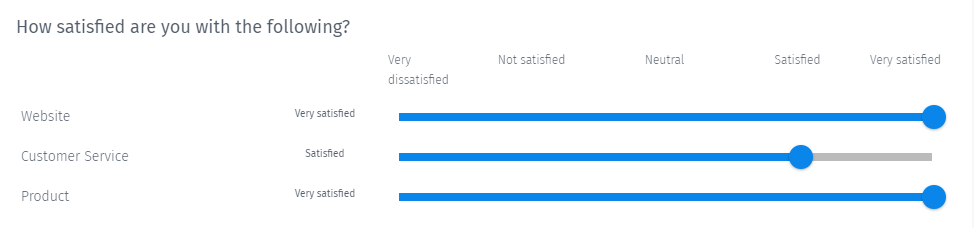
2. Rating Scale Multiple Choice Questions
These close ended survey questions require the respondents to assign a fixed value in response, usually numeric. The number of scale points depends on what sort of questions a researcher is asking.
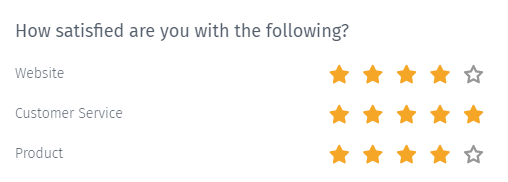
3. Checklist type Multiple Choice Questions
This type of closed ended question expects the respondents to make choices from the many options that have been stated, the respondent can choose one or more options depending on the question being asked.
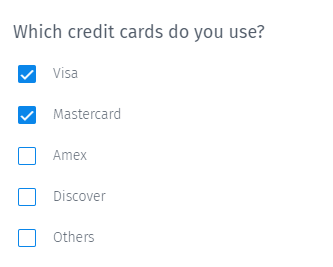
4. Rank Order Multiple Choice Question
These closed ended questions come with multiple options from which the respondent can choose based on their preference from most prefered to least prefered (usually in bullet points).
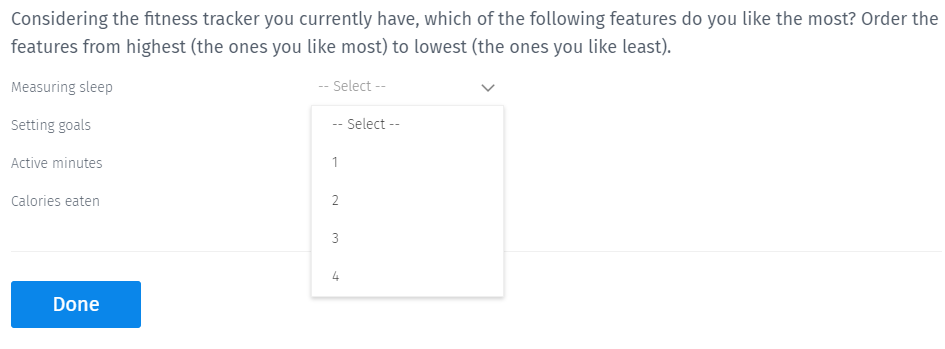
While answering a survey, it is most likely that you may end up answering only close ended questions. There is a specific reason to this, close ended question helps gather actionable, quantitative data. Let’s look at the definitive instances where closed-ended questions are useful.
Closed Ended Questions have very distinct responses, one can use these responses by allocating a value to every answer. This makes it easy to compare responses of different individuals which, in turn, enables statistical analysis of survey findings.
For example: respondents have to rate a product from 1 to 5 (where 1= Horrible, 2=Bad, 3=Average, 4= Good, and 5=Excellent) an average rating of 2.5 would mean the product is below average.
To restrict the responses:
To reduce doubts, to increase consistency and to understand the outlook of a parameter across the respondents close ended questions work the best as they have a specific set of responses, that restricts the respondents and allows the person conducting the survey obtain a more concrete result.
For example, if you ask open ended question “Tell me about your mobile usage”, you will end up receiving a lot of unique responses. Instead one can use close ended question (multiple choice), “How many hours do you use your mobile in a day”, 0-5 hours, 5-10 hours, 10-15 hours. Here you can easily analyse the data form a conclusion saying, “54% of the respondents use their mobile for 0-5 hours a day.”
To conduct surveys on a large scale:
Close ended questions are often asked to collect facts about respondents. They usually take less time to answer. Close ended questions work the best when the sample population of the respondents is large.
For example, if an organization wants to collect information on the gadgets provided to its employees instead of asking a question like, “What gadgets has the organization provided to you?”, it is easier to give the employees specific choice like, laptop, tablet, phone, mouse, others. This way the employees will be able to choose quickly and correctly.
They are easy to understand hence the respondents don’t need to spend much time on reading the questions time and again. Close ended questions are quick to respond to.
When the data is obtained and needs to be compared closed ended question provide better insight.
Since close ended questions are quantifiable, the statistical analysis of the same becomes much easier.
Since the response to the questions are straightforward it is much likely that the respondents will answer on sensitive or even personal questions.
Although, many organizations use open ended questions in their survey, using close ended question is beneficial because closed-ended questions come in a variety of forms. They are usually categorized based on the need to have specific options for the respondents, so that they can select them without any hesitation.
The open-ended questions are questions that cannot be answered by a simple ‘Yes’ or ‘No’, and require respondents to elaborate on their answers. They are textual responses and generally used for qualitative analysis. Responses to these questions are analyzed for their sentiments to understand if the respondent is satisfied or not.
Open-ended questions are typically used to ask comments or suggestions that may not have been covered in the survey questions prior. Survey takers can explain their responses, feedback, experiences, etc. and convey their feelings or concerns.
How can we improve your experience?
Do you have any comments or suggestions?
What would you like to see differently in our product or service?
What are the challenges you have faced while using our product or service?
How can we help you to grow your business?
How can we help you to perform better?
What did you like/dislike most about the event?
The selection between open-ended and closed-ended questions depends mainly on the below factors.
Type of data : Closed-ended questions are used when you need to collect data that will be used for statistical analysis. They collect quantitative data and offer a clear direction of the trends. The statements inferred from the quantitative data are unambiguous and hardly leave any scope for debate. Open-ended questions, on the other hand, collect qualitative data pertaining to emotions and experiences that can be subjective in nature. Qualitative data is used to generate sentiment analysis reports, text analytics, and word cloud report.
Depth of data : Closed-ended questions can be used to questions that collect quantifiable data needed for the primary analysis. To dig into the reasons behind the response, open-ended questions can be used. It will help you understand why respondents gave specific feedback or a rating.
Situation : At times, the options mentioned in the survey do not cover all the possible scenarios. Open-ended questions help to cover this gap and offers freedom to the respondents to convey whatever they want to. Whereas closed-ended questions are simple and easy to answer. It does not take much time to answer them and so are quite respondent-friendly.
Create a free account
- Sample questions
- Sample reports
- Survey logic
- Integrations
- Professional services
- Survey Software
- Customer Experience
- Communities
- Polls Explore the QuestionPro Poll Software - The World's leading Online Poll Maker & Creator. Create online polls, distribute them using email and multiple other options and start analyzing poll results.
- Research Edition
- InsightsHub
- Survey Templates
- Case Studies
- AI in Market Research
- Quiz Templates
- Qualtrics Alternative Explore the list of features that QuestionPro has compared to Qualtrics and learn how you can get more, for less.
- SurveyMonkey Alternative
- VisionCritical Alternative
- Medallia Alternative
- Likert Scale Complete Likert Scale Questions, Examples and Surveys for 5, 7 and 9 point scales. Learn everything about Likert Scale with corresponding example for each question and survey demonstrations.
- Conjoint Analysis
- Net Promoter Score (NPS) Learn everything about Net Promoter Score (NPS) and the Net Promoter Question. Get a clear view on the universal Net Promoter Score Formula, how to undertake Net Promoter Score Calculation followed by a simple Net Promoter Score Example.
- Offline Surveys
- Customer Satisfaction Surveys
- Employee Survey Software Employee survey software & tool to create, send and analyze employee surveys. Get real-time analysis for employee satisfaction, engagement, work culture and map your employee experience from onboarding to exit!
- Market Research Survey Software Real-time, automated and advanced market research survey software & tool to create surveys, collect data and analyze results for actionable market insights.
- GDPR & EU Compliance
- Employee Experience
- Customer Journey
- Executive Team
- In the news
- Testimonials
- Advisory Board
QuestionPro in your language
- Encuestas Online
- Pesquisa Online
- Umfrage Software
- برامج للمسح
Awards & certificates
The experience journal.
Find innovative ideas about Experience Management from the experts
- © 2021 QuestionPro Survey Software | +1 (800) 531 0228
- Privacy Statement
- Terms of Use
- Cookie Settings
Instant insights, infinite possibilities
Closed-ended questions: Overview, uses, and examples
Last updated
20 March 2024
Reviewed by
Do you value the simplicity and elegance of a yes-or-no question?
These simple questions—known as closed-ended questions—are the fundamental tools you can use to collect quantitative data.
In this article, we will cover everything you need to know about closed-ended questions, including the different types, use cases, and best practices to gain easy-to-analyze data you can use to improve your business.
Free template to analyze your survey results
Analyze your survey results in a way that's easy to digest for your clients, colleagues or users.
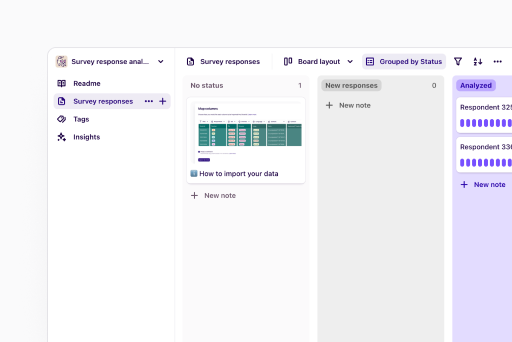
- What are closed-ended questions?
Closed-ended questions encourage the reader to respond with one of the predetermined options you or your team have selected. The most common answer formats for closed-ended questions are yes/no, true/false, or a set list of multiple-choice answers.
Closed-ended questions aim to direct the responder to answer your question in a standardized way that produces controlled quantitative data about your target audience.
For example, the question, “Did you enjoy the latest feature update to our platform?” is a closed-ended question that requires either a “yes” or “no” answer. It will give your team a quick understanding of the overall customer opinion of the update.
Over time, researchers can use closed-ended questions to collect statistical information about your brand or product. These quantitative insights can be incredibly valuable when making future brand decisions, as they give your team a better understanding of your target demographic, their purchasing trends, and more.
- Closed-ended vs. open-ended questions
Depending on the type of information you want to collect from your survey or questionnaire, there are two overarching categories of questions you can use. Each style of question offers unique benefits and needs to be used correctly to produce accurate and helpful insights that your team can use.
Closed-ended questions
Closed-ended questions collect quantitative data and encourage the participant to answer from a list of predetermined options. They help teams collect easily measurable data about a specific metric they’re tracking. Closed-ended questions are rigid, focused, and data-driven.
An example of a closed-ended, data-driven question:
On a scale from 1 to 5 (1 being horrible, and 5 being excellent), how would you rate your recent visit to our store?
Open-ended questions
Open-ended questions collect qualitative data and encourage participants to provide subjective, personal responses based on their experiences and opinions. They help your team collect a more nuanced understanding of a subject, e.g., a particular pain point or positive experience related to a product or service.
These questions are exploratory, encouraging participants to write sentences or paragraphs to share their thoughts.
An example of an open-ended, personalized question:
Tell us about your most recent visit to our store.
Important note: The most effective surveys contain closed and open-ended questions to collect a diverse range of data. Understanding the core benefits of each question style is necessary to pick the right question style for your survey. From there, your team will collect information from your survey to produce helpful insights to improve your work and future projects.
- Types of closed-ended questions
To collect the most accurate data, you need to understand the different types of closed-ended questions. Before you begin your next round of research, consider the following types of survey questions to ensure you collect the correct type of quantitative data.
Dichotomous questions
Dichotomous questions (based on the word “dichotomy” – to be divided into two mutually exclusive categories) refer to questions that have only two possible answers.
Questions that can be answered with “yes/no,” “true/false,” “thumbs up/thumbs down,” or “agree/disagree” are examples of dichotomous questions. They can be incredibly effective for collecting quick, simple participant responses.
While dichotomous questions do not offer the participant options for nuanced responses, these questions are incredibly effective for getting snapshot data about a specific tracking metric.
Examples of dichotomous closed-ended questions include:
Have you heard of [X product] before? (Yes or no)
You have bought a product from [X brand] in the last six months. (True or false)
How was your recent call with our sales team? (Thumbs up or thumbs down)
The price of our premium service tier matches its value. (Agree or disagree)
Rating-scale questions
Rating-scale questions use a pre-set scale of responses to encourage participants to provide feedback on their experiences, opinions, or preferences.
Commonly used in customer experience surveys, the goal of this type of closed-ended question is to collect quantitative data that is standardized and easy to analyze.
Depending on the content of the question you are asking, examples of types of measures you can use for rating questions include a 10-point rating scale, the Likert scale (from strongly disagree to strongly agree), or a rating based on level of satisfaction (very dissatisfied to very satisfied).
As a great way to collect more nuanced information that does not require rigorous analysis, examples of rating-scale closed-ended questions include:
[X customer service worker] was supportive during our call. (Rate from strongly agree to strongly disagree)
On a scale from 1 to 10, (1 being very unlikely and 10 being very likely), how likely are you to recommend our products to a friend?
How satisfied were you with the latest software update? (Rate from very satisfied to very dissatisfied)
Multiple-choice questions
Multiple-choice questions offer the participant a selection of possible answers, but, unlike questions at school, there are no right or wrong answers.
Designed to tread the line between getting more nuanced participant answers but still being easy to analyze and interpret, well-written multiple-choice survey questions must include answers specific to the information you want to collect.
This easy-to-understand survey question style often has a higher engagement rate. Integrating multiple-choice questions into key sections of your survey is a great way to collect information from your target audience.
Examples of multiple-choice closed-ended questions include:
How did you hear about our company? (Answers could include online, from a friend, on social media, etc.)
Which restaurant interests you most for the company Christmas party? (Answers would include the restaurants being considered)
How long have you been using our products or services? (Answers could include month or year ranges)
Important note: In some cases, adding “Other” as a possible answer option for multiple-choice questions may be appropriate if your provided answers do not cover all possible responses. If you choose to do this (which is very common and can be an effective way to collect additional insights), you need to be aware that the question is no longer truly closed-ended. By adding the option to write out their answer, the question becomes more open-ended, which can make the data harder to analyze and less standardized.
Ranking-order questions
Ranking-order questions are a style of closed-ended question that asks the participants to order a list of predetermined answers based on the type of information your team is looking to collect. This is a great tool for collecting information about a list of related options.
Used to gain insights into the preferences or opinions of your participants, these types of questions are still structured and easy to analyze. Additionally, the advantage of using ranking-style closed-ended questions is that you not only discover which option is most in demand but you also gain insights into the overall ordering of all possible options.
Examples of ranking-order closed-ended questions include:
Rank the following product features based on your preferences. (From most likely to use to least likely to use)
Organize these possible new logos. (From your favorite to least favorite)
Rank the following product brands. (From most appealing to least appealing)
- Closed-ended questions pros and cons
Because closed-ended questions produce a certain type of data from your target audience, it is important to understand their advantages and disadvantages before using them in your next survey.
Closed-ended questions can improve your survey because they:
Are quick and easy to fill out
Offer standardized responses for easy analysis
Increase survey response rates
Naturally group participants based on responses
Are customizable to the specific metric you are tracking
Avoid incorrect and irrelevant responses
Reduce participant confusion
Closed-ended questions can be limited because they:
Can lack nuance and personalization
Produce responses with limited context
Can offer choices that alienate participants if not written correctly i.e., there is no response option provided that accurately captures how they think or feel
Are highly susceptible to bias
Can encourage participants to “just pick an answer”
Cannot cover all possible answer options
- When to use closed-ended questions
Like any other data-collection tool, there are specific instances where using closed-ended questions will benefit your team. Consider using closed-ended questions if you need to collect data in the following scenarios:
Surveys with a large sample size
If you’re sending your survey to hundreds or thousands of potential respondents, using closed-ended questions can be a helpful way to increase response rates and simplify your data.
Survey response rates can vary greatly, but even receiving a 10% response rate based on a sample size of multiple thousands of people can result in a ton of analysis work if you ask open-ended questions.
Using well-written, closed-ended questions is a great way to tackle this problem while still collecting meaningful insights that can improve your products and services.
Examples of scenarios where relying on closed-ended questions can be beneficial are:
Sending a customer-experience survey to people who purchased from you in the past month
Collecting demographic information about one of your brand’s customer personas
Surveying your top users about a recent software launch
Quick feedback or check-ins
If you are looking to collect survey results to get a general idea of the current situation, using closed-ended questions can help improve your response rate.
Perfect for employee check-ins or post-purchase customer experience surveys, closed-ended questions can give your team a quick snapshot into the particular metric you’re tracking.
As a great tool for assessing employee satisfaction or doing a quick “vibe check” with your target audience, asking a short closed-ended question like, “Are you happy with the service you receive from our team?” is a great way to collect fast feedback your team can act on.
Time-sensitive inquiries
Do you need to collect some data but you’re on a tight timeline? Improve your response rate and get the insights you need by making your survey quick and easy with closed-ended questions.
Examples of time-sensitive situations perfect for closed-ended questions are:
Asking about a customer’s experience after they connect with your support team
Quickly surveying your team about their current workload before a meeting
Checking for possible tech issues during the first 24 hours of a new feature launch
Measuring customer satisfaction
If you’re looking to gain a better understanding of your customer’s opinions and preferences about your brand, your products or services, or newly launched features, well-written rating-scale questions can be incredibly helpful.
When paired with a few open-ended questions to collect more personalized answers, rating-scale and ranking closed-ended questions can help you collect quantitative data about your customer’s shopping experience, product opinions, and more.
- Analyze closed-ended question data with Dovetail
Closed-ended questions are a helpful tool your team can use when creating a survey or questionnaire to collect specific, easy-to-analyze quantitative data.
Created as a one-stop shop for everything to do with customer insights, Dovetail is a great solution for your research analysis. You will benefit from fast, accurate, and compelling results that will guide your brand’s future decisions.
Should you be using a customer insights hub?
Do you want to discover previous survey findings faster?
Do you share your survey findings with others?
Do you analyze survey data?
Start for free today, add your research, and get to key insights faster
Editor’s picks
Last updated: 4 March 2023
Last updated: 28 June 2024
Last updated: 16 April 2023
Last updated: 20 March 2024
Last updated: 22 February 2024
Last updated: 23 May 2023
Last updated: 21 December 2023
Last updated: 26 July 2023
Last updated: 14 February 2024
Last updated: 11 March 2023
Last updated: 13 May 2024
Last updated: 30 January 2024
Latest articles
Related topics, .css-je19u9{-webkit-align-items:flex-end;-webkit-box-align:flex-end;-ms-flex-align:flex-end;align-items:flex-end;display:-webkit-box;display:-webkit-flex;display:-ms-flexbox;display:flex;-webkit-flex-direction:row;-ms-flex-direction:row;flex-direction:row;-webkit-box-flex-wrap:wrap;-webkit-flex-wrap:wrap;-ms-flex-wrap:wrap;flex-wrap:wrap;-webkit-box-pack:center;-ms-flex-pack:center;-webkit-justify-content:center;justify-content:center;row-gap:0;text-align:center;max-width:671px;}@media (max-width: 1079px){.css-je19u9{max-width:400px;}.css-je19u9>span{white-space:pre;}}@media (max-width: 799px){.css-je19u9{max-width:400px;}.css-je19u9>span{white-space:pre;}} decide what to .css-1kiodld{max-height:56px;display:-webkit-box;display:-webkit-flex;display:-ms-flexbox;display:flex;-webkit-align-items:center;-webkit-box-align:center;-ms-flex-align:center;align-items:center;}@media (max-width: 1079px){.css-1kiodld{display:none;}} build next, decide what to build next, log in or sign up.
Get started for free

Learn and Grow
Close-Ended Questions: +20 Examples, Tips and Comparison

By Caitriona Maria
November 11, 2023
In contrast to open-ended questions, close-ended questions limit the possible responses to a predetermined set of options or a simple “yes” or “no.”
While open-ended questions can provide in-depth and nuanced information, close-ended questions offer a more efficient way to gather specific data and statistics.
See next: Open-Ended Questions: Examples, Tips, and When To Use
Why Use Close-Ended Questions?
Close-ended questions benefit quantitative research, where the goal is to gather numerical data that can be easily analyzed and compared. They also offer a straightforward way to categorize responses and draw conclusions from the data.
Additionally, close-ended questions can be helpful when time or resources are limited, such as in surveys or market research. They allow for quick and uniform data collection, making it easier to draw meaningful insights and make informed decisions, even if the data set is large.
Characteristics of Close-Ended Questions
- Limited possible responses or options
- Easily quantifiable
- Quick and efficient to answer
- Often used in surveys or market research
Examples of Close-Ended Questions
Common close-ended questions include multiple-choice, ranking scale, or binary questions. Here are some examples:
- Which brand of laundry detergent do you prefer from the following options?
- On a scale of 1 to 5, how likely are you to recommend our restaurant?
- Do you prefer online shopping or in-store shopping?
- Are you a vegetarian or a meat-eater?
- Would you be interested in attending our next event?
- Did you find our customer service helpful?
- Would you rather spend your vacation at a beach or in the mountains?
- How much do you typically spend on groceries each week? Select from the range below.
- On a scale of 1 to 10, how satisfied are you with your recent travel experience?
- Do you believe climate change is real?
- Which of the following credit cards do you use?
Tips for Asking Close-Ended Questions
The follow are some tips for crafting and asking close-ended questions.
Keep Them Simple and Direct
When forming close-ended questions, keep them simple and direct. Avoid using complex or ambiguous language that may confuse respondents.
Provide Clear and Specific Response Options
To gather accurate data, provide clear and specific response options for each question.
For example, if asking the question, “How often do you eat out?” provide options such as “Once a week,” “2-3 times a week,” etc.
Avoid Leading or Biased Questions
Leading or loaded questions can unintentionally influence respondents and skew the results. Be mindful of the language used and ensure that all options are neutral.
Use a Mix of Close-Ended and Open-Ended Questions
Combining close-ended and open-ended questions in a survey or research can provide a well-rounded understanding of the topic.
Use close-ended questions and open-ended questions to gather specific data for more detailed insights. Begin with close-ended questions to warm up and engage the respondent.
Test Your Questions Beforehand
Before using close-ended questions in a research setting, it’s helpful to test them with a small group of people and gather feedback. This can help identify any confusing or unclear phrasing that may need to be revised.
Types of Close-Ended Questions
Use a range of close-ended questions to get the responses you are looking for. Here are types of close-ended questions to choose from:
Binary or Dichotomous Questions
These questions offer only two options, such as “ yes ” or “no,” “true” or “false,” making them easy to answer but not very informative.
Examples:
- Do you own a car?
- Have you ever owned health insurance?
Multiple-choice questions
Multiple-choice questions provide a list of options for respondents to choose from. They can offer more variety and depth in responses compared to dichotomous questions.
Examples:
- Which social media platform do you use the most? Select all options that apply.
- How satisfied are you with our customer service? Select from the options below.
Ranking Scale Questions
These questions ask respondents to rank their preferences or opinions on a scale, usually 1-5 or 1-10. They can provide more detailed and nuanced data than dichotomous or multiple-choice questions.
Examples: On a scale of 1-10, how likely are you to recommend our product to others ?
Ranking Order Questions
These questions ask respondents to rank items in order of importance or preference. They can provide valuable insights on what matters most to your target audience .
Examples: Rank the following factors in order of importance when choosing a vacation destination.
Benefits of Close-Ended Questions
Close-ended questions offer several advantages over other forms of questions. Here are some key benefits:
1. Easier to Analyze
Closed-ended questions provide responses that can be easily quantified and analyzed, making identifying patterns and trends in the data simpler.
2. Less Time-Consuming
Since respondents only need to select from predetermined options, closed-ended questions can be quicker to answer, making them ideal for larger surveys.
3. More Objective Responses
Closed-ended questions offer objectivity as the choices are standardized and do not depend on individual interpretation. This can help reduce bias in responses.
Closed-Ended Questions Vs. Open Ended Questions
Open-ended questions allow for more in-depth and varied responses, providing subjective insights. They are useful for understanding thought processes and opinions. However, they can be time-consuming and challenging to analyze.
Common types of open-ended questions include essay, opinion-based, and problem-solving questions.
While open-ended questions are valuable for qualitative research, close-ended questions offer a more efficient and straightforward way to gather specific data and statistics. Researchers can comprehensively understand their target audience by using a mix of both types of questions.
Open-Ended Question Examples
- How do you feel about the current political climate?
- Describe your ideal vacation.
- What challenges have you faced in your career?
- How can we improve our customer service experience?
- Tell us about a time when you had to resolve a conflict.
Close-ended Questions To Ask Customers or Clients
- How often do you use our product/service? Select from the options.
- On a scale of 1-10, how satisfied are you with your experience?
- Which of the following features do you find most useful in our product?
- Are you familiar with our new product line? Yes/No.
- Would you recommend our company to a friend or colleague?
- Which of the following best describes your reason for choosing us as your service provider?
In summary, close-ended questions offer a more direct and efficient way to gather specific information. While they may not provide the depth or nuance of open-ended questions, they can be helpful when the main priorities are time, resources, or quantifiable data.
By crafting clear and well-designed questions, you can gather valuable insights and make informed decisions for your business or research purposes. So, next time you need to gather specific and actionable information, consider using close-ended questions in your research methods.
Caitriona Maria is an education writer and founder of TPR Teaching, crafting inspiring pieces that promote the importance of developing new skills. For 7 years, she has been committed to providing students with the best learning opportunities possible, both domestically and abroad. Dedicated to unlocking students' potential, Caitriona has taught English in several countries and continues to explore new cultures through her travels.
Open-Ended Questions: +20 Examples, Tips and Comparison
Flaws of the leading question: definition, examples and types.
You cannot copy content of this page

Closed Questions Explained
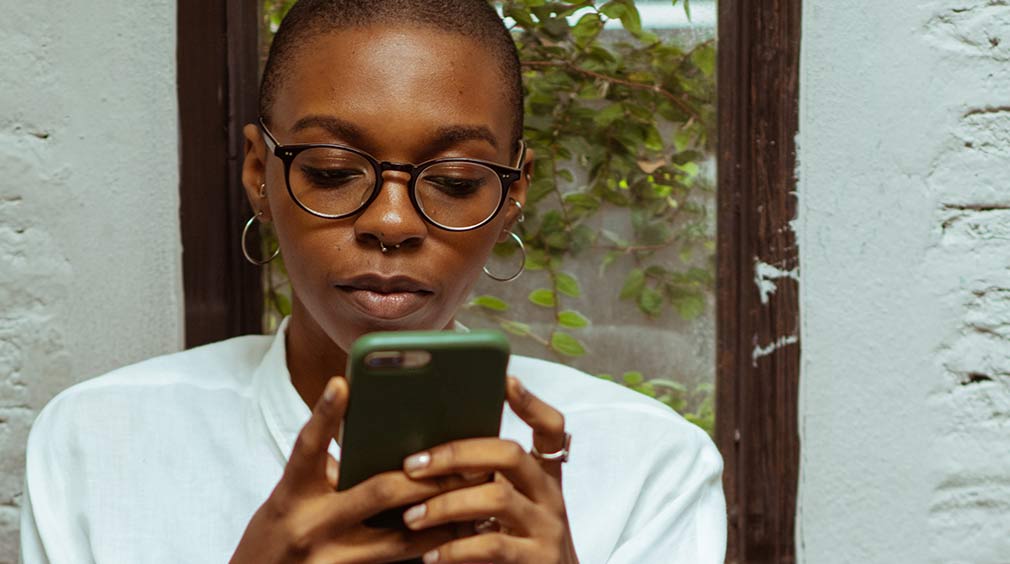
When conducting any form of research, considering the way questions are structured is essential. Carefully designed questionnaires that use the appropriate type of questions are often the key to collecting data successfully. There are broadly two types of questions in research: closed questions and open questions . In this guide, we will explain how closed (aka “close-ended”) questions are used to effectively gather data.
We’ll look into the differences between open questions and their closed siblings, as it’s important to understand how the two approaches differ. We’ll also give some real-world examples of closed questions, and explain when closed ended questions should be used.
What is a closed question?
Closed questions collect quantitative data. They give the respondent a limited amount of options to choose from. They are popular, as quantitative data is easier to analyse than qualitative data.
There are a few versions of close-ended questions, such as those that only allow “yes” or “no” answers, or “correct” or “incorrect” answers to statements. These are classed as dichotomous questions. Alternatively, multiple choice questions are also by definition closed, as response options are limited and respondents must select from a list of choices.
Open vs. closed questions
Open ended questions provide in-depth insights to user behaviour and opinions. This is because the respondent has the opportunity to answer the question freely. They are not restricted to answering from a few limited choices, like with closed questions. Instead, they can write out their answer and explain their reasoning. This is considered to be qualitative data.
While some projects are better suited to closed ended questions, there is always some level of value in open questions. This is because they can provide quality answers, where respondents elaborate on their feelings. Open ended questions provide detailed evidence as to what the consumer wants or expects. However, you may have no way of evaluating these written answers in a proportional way. This means the data is then impractical to analyse.
Closed ended questions, on the other hand, limit the respondent to a predetermined list of response options. Unlike open questions, which open a window for ongoing discussion, closed questions elicit controlled responses.
Examples of closed questions
There are a few ways that close ended questions can be structured. These examples provide a variety of questions that illustrate how different types of closed questions can be formatted. For context, we’ll imagine we are running a hospitality survey with questions regarding a restaurant service.
Multiple choice questions
Multiple choice questions can be designed in a few ways. They often involve the use of scales, as this helps to assign a numerical value to each possible selection. Here are examples of each type of multiple-choice question.
This is where respondents have the option to select the specifics that apply from a list of options. The researcher can then determine how many participants ticked each option and figure out where they should improve on service from there.
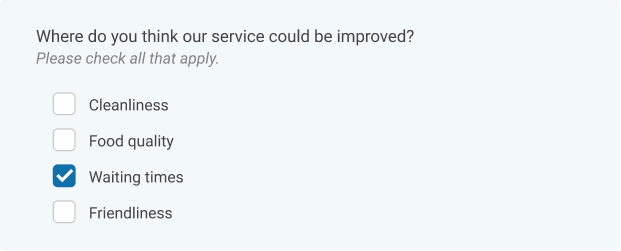
Likert scale:
Likert scales are very easy to use, as the respondent can visually determine just how much they feel about a certain topic. For the researcher, there is usually a number assigned to each option.
For example, the happiest face might be assigned the number 10, while the unhappiest face is 0. This means they can then calculate how happy customers were with each aspect of the restaurant, by adding up the values of each respondent.
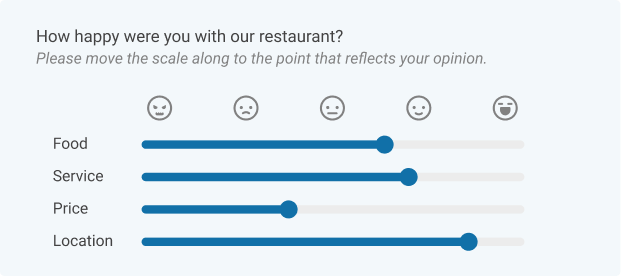
Rating scale:
This is where the respondent rates how they feel about something, usually on a scale of 1-5. Reviews usually use rating scales.

Rank order:
This multiple-choice question allows respondents to assign a value to a specific list of options. When translating rank order data into a graph or chart, researchers can use these numerical values to do so.
In this example, the researcher would be able to determine which option is most important to customers by adding all the values associated with each option. They could then see which option had the lowest total score – this would be the most important aspect of dining out for the selection of participants that responded.
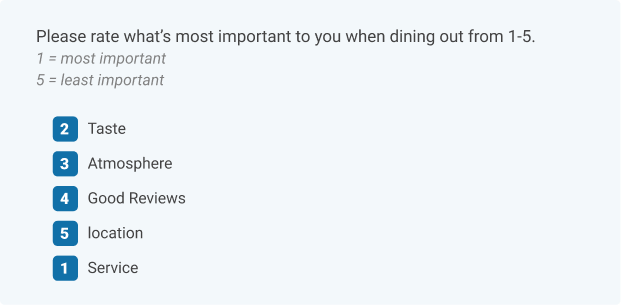
Dichotomous questions
Dichotomous questions will give two options. They can include yes or no answers, to true or false statements. Dichotomous questions are the easiest for customers to answer, however they may feel limited with their options and wish to explain their choice further.
This is where open questions are perhaps a good choice. In this example, the researcher could open up further discussion by adding a section that says: “Please explain why?”

Advantages of closed questions
Many researchers find using closed-ended questions to be advantageous over open-ended questions. The major advantage of close-ended questions comes down to one simple detail – closed questions collect quantitative data. Quantitative data is data that is numerical, and can therefore easily be turned into percentages, charts, and graphs.
Here are the main benefits of close ended questions:
- Data from closed questions can be interpreted into graphs, charts and percentages: This gives researchers a visual insight into consumer behaviours and thoughts.
- Closed questions save time and money: Analysing qualitative data into numerical (quantitative) data takes up a lot of time and resources. Primary research can be costly, so closed questions are often the preferred choice.
- Closed ended questions are easier for respondents, too: Having limited options to answer with means participants aren’t overthinking their responses. Closed questions are also easier to understand, as they’re usually worded in simpler terms.
- Data from close-ended questions can be easily compared and sorted into categories: This helps with the analysis of data. It can therefore help researchers make well-informed conclusions that are backed up by the research.
When to use closed questions
Determining which type of questions to use can get complicated. First, you need to consider the reasons behind designing your questionnaire. You should use close-ended questions if:
You want to convert opinions or behaviours into numerical data
Open questions leave a lot of room for useless information. Instead, you can ask them to rate a product (for example) on a scale of 1 – 10 (a closed ended question). By using the close-ended question option, you are allocating each opinion with a number. This enables you to analyse data clearly.
You want to collect reliable, consistent data
Closed questions allow you to choose the responses that can be made by participants beforehand. This means you are prompting participants to be specific in their answers, giving clear results.
You have a large number of participants
If everyone responded with written, descriptive opinions, insights would become so jumbled they’re basically worthless. In large studies, involving a sizeable sample size , using close ended questions can help you analyse and stratify data with ease.
In conclusion
Conducting any form of research involves coming up with suitable methodologies. Selected approaches should support the ease of collecting data. Where questionnaires are concerned, the way questions are designed is a crucial consideration. Closed questions are a great, reliable way of collecting large amounts of quantitative data that can then be organised and analysed with ease.
Despite this, open questions can provide very valuable information. Perhaps the respondent has a concern that cannot be explained within a closed question. In these cases, open questions can be beneficial, as they provide deeper insights. However, close-ended questions are much easier to quantify and analyse. If you have a limited time frame or budget, closed ended questions may be the better option. Closed questions can also provide more reliable, conclusive data.
Whether you use closed, open, or a mixture of both styles of questions will depend on the type of data you’re trying to collect. Always consider the expectations you have for the outcomes of your research, before deciding which type of questions to use.
Solutions to meet any survey need
Ready to get started, discover our enterprise survey software, survey uses, survey distribution, survey templates, comparisons, useful links.
- Close Ended Questions: Definition, Types + Examples

Close-ended questions are question formats that provoke a simple response from a respondent. They are designed such there isn’t much thought into the single word answer. An example of a close ended question is, “Are you hungry?”.
Individuals generally enjoy talking about themselves. If you give then an opportunity, you’ll be surprised how much information they’ll disclose to you. However, close-ended questions seek the exact opposite. Rather than seek to hear all they have to say, these questions target specifics.
Close-ended questions are better suited to quantitative research , where the respondents answer your questions in a manner such that they’re less likely to disengage
What is a close ended question
A closed-ended question, by definition, is a question that could be answered with a one-word answer or a simple “yes” or “no.” In research, a closed-ended question refers to any question in which participants are provided with options to choose a response from.
In a search for statistically significant stats? closed-ended questions are your best bet.
Close-ended questions allow a limited number of responses and are ideal for surveys because you get higher response rates when users don’t have to type so much.
Types & Examples of Close Ended Questions
Dichotomous or true/false questions .
The true and false questions basically consist of a question and TWO answer options. Many a time, the answer options used are ‘True and False’. You can, nevertheless, use other options, such as ‘Yes’ and ‘No’, ‘I Agree’ and ‘I Disagree’.
- Examples of a true/false close ended question includes;
For each of the following statements, indicate True or False
- The sun rises in the east and sets in the west.
- Regression coefficients have a sum of 0.
- Printers can be connected directly to a computer network
Multiple Choice Questions
A multiple-choice question is one in which provides respondents with multiple answer options. In examinations, a multiple-choice question contains a set of alternatives or possible answers that contain one that is the best answer to the question and a number of distractors that are plausible but incorrect answers to the question.
Multichoice can be divided into two; one preferred answer per question (Radio Choice) and the ability to choose more than one option (CheckBoxes).
- Close Ended Question Example on Radio Choice
What is the name of the incumbent president of the United States?
Which of these cities is situated in the United States?
Rating Scale Choice Questions
A rating scale is a subset of the multiple-choice question which is widely used to gather opinions that provide relative information about a specific topic. Most researchers use a rating scale when they mean to associate a qualitative measure with the various aspects of a product or feature.
Examples of Rating Scale Close Ended Questions
- How difficult (1) or easy (5) was it to log in to the app? (1=Very difficult, 5=Very easy)
- How disinterested (1) or interested (5) are you in purchasing Nike boots? (1=Not at all interested, 5=Extremely interested)
- Please rate your agreement with the following statement: “I understand who this product is for.” (1=Strongly disagree, 5=Strongly agree)
Rank Order Choice Questions
Rank order questions are basically multiple-choice questions represented in a single column format. They are close ended questions that allow respondents to evaluate multiple row items in relation to one column item or a question in a ranking survey and then rank the row items.
- Examples of Rank Order Closed Questions
- Please rank these toppings on a scale of 1 to 5. With 1 being your favorite.
- Please rank the following in order of importance from 1 to 4, where 1 is most important to you and 4 is least important to you.
- Cleanliness
- Ease of packing
- Friendliness of staff
- Speed of service
- Please rank (1 to 4) the following in order of interest.
- Snowboarding
Use of Close Ended Questions
- Surveys/Questionnaires
Close-ended questions are used on Surveys and questionnaires to collect quantitative information from respondents on a particular phenomenon. In surveys, a closed-ended question is made up of pre-populated answer choices for the respondent to choose from.
Close-ended questions are used for administering examinations to students to test their understanding of a given course or subject. In examinations, close ended questions could come in a multitude of forms, including multiple-choice, drop down, checkboxes, and ranking questions.
Close-ended questions are often asked to collect fast facts about your interviewee. They usually take less time to answer. Close-ended questions work best when the number of interviewees is large. Closed-ended questions, in this case, are those which can be answered by a simple “yes” or “No”. Even though ideally for interviews, open ended questions are better.
Close-ended questions are ideal for research. For a researcher looking for an easier and quicker way for respondents to answer, it’s ideal to employ close ended questions. The answers from different respondents are easier to compare, code and statistically analyze. The response choices can also clarify question meaning for respondents.
When to Choose Close Ended Questions Over Open-Ended Questions
Close-ended questions generally look for specific facts and only require a one-word answer which may be a yes or a no. If you’re looking to find out specific information, a close ended question is your best show. If you patronize open ended questions, you might have a problem with information overload.
Close-ended questions also help you make a decision quickly saving you a lot of time. The reason is that the information you’ve collected is quantitative in nature and as such, can be quickly analyzed. When there is a large amount of information to collect, close ended questions work better. They save time and ultimately cost.
Why Formplus is the best data collection tool for asking close ended Questions
Formplus is a powerful platform to create forms that collect data online and offline(beta) . With an easy to use online form builder and a variety of intuitive features that make data collection seamless. Here are a few features that assist you in collecting data for close ended questions.
- Dropbox/Microsoft Onedrive Integration
Store files received from your form with Formplus unlimited storage or in your preferred cloud storage option (Google Drive, Microsoft OneDrive and Dropbox are currently available). With unlimited file uploads, you can submit files, photos, or videos via your online forms without any restriction to the size or number of files that can be uploaded.
- Workflows & Approvals
With Formplus, you can automate monotonous and repetitive tasks by creating digital workflows and adding approvals or review process to your forms so you and other members of your team can automatically review submissions. After reviewing the workflows, the team members can easily approve submissions. This helps you to save time and be more productive.
- Radio Choice
Preparing a survey/questionnaire, use the Radio choice to ask your respondents to choose a single option from a shortlist. Radio Choice should always be used when asking close ended questions.
- Multiple Select & Checkboxes
The Checkbox field allows you to add options to your form for your respondents to select from. This field is best used for surveys with questions requiring more than one answer, unlike the Radio field which is useful when you want your respondents to select only one answer.
- Rating Scale Feature
With ratings on Scale, Stars, Hearts, Smileys, and Matrix, you can assign weights to each answer choice. The Matrix rating is the most ideal and is used for a closed-ended question that asks respondents to evaluate one or more row items using the same set of column choices.
- Logic & Calculation to Measure Quantitativeness
Logic & calculating form feature field allows you to perform simple mathematical operations on your forms such as addition, subtraction, multiplication, and division. This feature is especially useful in order forms to display the details of surveys. All you need to do is assign values to the options you have used in your choice-fields before they can be calculated.
- Export Data as CSV or PDF
With this feature, you can export all submitted responses to your form as a CSV file. You can also download the submitted responses as a Docx file and/or PDF.
- Formplus Analysis
With Form analytics, you can gather useful insights from forms. The Analytics dashboard reveals information like the total form views, unique views, abandonment rate, conversion rate, the average time it takes to complete a questionnaire/survey, top devices, and the countries your form views are from. Using Reports, you can get an overview of the data submitted to your form.
- Intro & Post Submission Message
With this Formplus feature, you can customize the intro and final message that will be displayed to your form users before and after they have filled and successfully submitted your form.
Advantages of Closed-Ended Questions over Open-Ended Questions
- Easy and quick to answer
While Close-ended questions are easy and quick to answer because of the introduction of options for the respondent, open ended questions require more thought, introspection and are generally more time-consuming.
- Response choice can clarify the question text for the respondent
The response choice provides clarity on the expected answer for the question asked as is the case of close ended questions. Open-ended questions may be ambiguous and difficult to understand to the recipient and as such discourage a response or lead to abandonment.
- Improves consistency of responses
With open ended questions, you can somewhat marshall the respondents to maintain consistency in their responses by asking follow-up questions for confirmation. The same cannot be achieved with open ended questions which are mostly qualitative in nature and allows the respondents freedom of expression.
- Easy to compare with other respondents or questionnaires
Open-ended questions are a researchers dream. You can easily make comparisons between sets of respondents. The same cannot be said of open ended questions, because no two respondents can have the exact same opinion on a particular question asked.
- Less costly to analyze
Open-ended questions save you a fortune when it comes to analyzing the information collected from your respondents. Even though analyzing open ended questions helps you to empathize with your audience and gather essential insights, It takes a lot of time and expenses to execute.
- Motivates respondents to answer
Closed ended questions are easier to complete than open ended questions. This is because, closed-ended questions layout all of the possible answers, removing respondents’ task of coming up with their own responses.
- Lets you categorize respondents
In other words, they allow you to conduct demographic studies. Closed ended questions on gender, age, employment status, and any other demographic information they’d like to know could be added to your survey.
Disadvantages of close ended Questions
- In some cases, a close ended question may not have the exact answer the respondent wants to give.
- The respondents might be influenced by the options available.
- The respondents may select answers most similar to true response, even though it is different.
- The number of available options may confuse the respondent.
- Respondents who don’t have an opinion may answer anyway.
- A close ended question doesn’t give information about whether or not the respondent actually understood the question asked.
On the whole, it is important to note that Close-ended questions are best used when you want a short, direct answer to a very specific question. In reality, most closed-ended questions can easily be turned into open ended questions with a few minor tweaks here and there.
Closed-ended questions aren’t just simple questions that anyone can quickly answer merely because they require a yes or no answer. Close-ended questions may also be complicated sometimes. If you’ve ever filled out a multiple-choice form, you can relate. But they are indeed a lot easier to analyze than open ended questions.
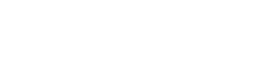
Connect to Formplus, Get Started Now - It's Free!
- close ended
- close ended question examples
- close ended questions
- close ended surveys
- close open ended questions
- closed question examples
- closed questions
- busayo.longe
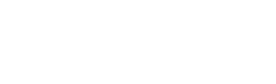
You may also like:
Open vs Close-Ended Question: 13 Key Differences
Simple guide on the difference between close and open ended questions. Where and how to use them.

Job Evaluation: Definition, Methods + [Form Template]
Everything you need to know about job evaluation. Importance, types, methods and question examples
Open Ended Questions: Definition + [30 Questionnaire Examples]
Ultimate guide to understanding open ended questions, examples, advantages and questionnaire examples in surveys & research
25 Great NPS Survey Question Examples
This article outlines 25 great NPS survey questions to help you gather feedback from your customers
Formplus - For Seamless Data Collection
Collect data the right way with a versatile data collection tool. try formplus and transform your work productivity today..
We use cookies
This website uses cookies to provide better user experience and user's session management. By continuing visiting this website you consent the use of these cookies.
ChartExpo Survey
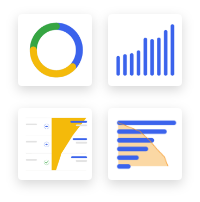
Closed-Ended Questions: Definition, Types & Examples
Embark on a journey of structured inquiry with the illuminating guide to “Closed-Ended Questions Examples”.
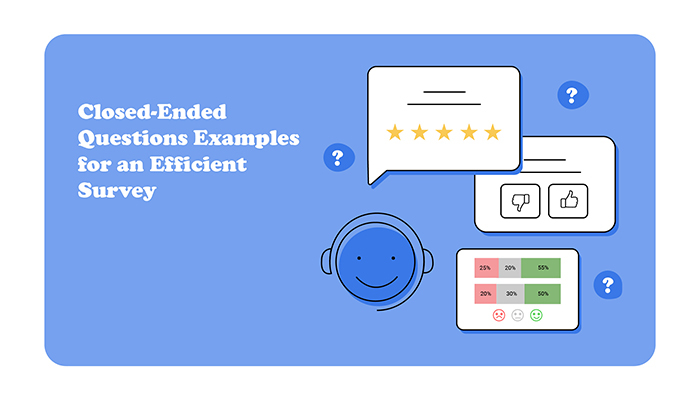
In the landscape of survey creation and data collection, closed-ended questionnaires serve as confident architects, providing a clear and efficient path to gathering specific responses.
Think of closed-ended questions as the sprinters of the survey world – quick, snappy, and laser-focused. Like a well-orchestrated symphony, they cut through the noise, sparing respondents the agony of lengthy musings.
You’ve come to the right place if you’re tired of long-winded responses and yearn for efficiency.
Here, we’ll explore various closed-ended question examples suitable for your surveys. These examples encompass a variety of domains, including customer satisfaction, market research, employee feedback, and more.
Ultimately, you will create well-structured and efficient surveys that yield valuable insights.
This article is your gateway to understanding the power of closed-ended questions through diverse examples, where each query is designed with purpose and precision.
Table of Contents:
What are closed-ended questions, when to use closed-ended questions, types of closed-ended questions with examples, purpose of using closed-ended survey questions, 16 best closed-ended questions examples, how to craft a closed-ended questionnaire, how to examine closed-ended questions, tips for using closed-ended questions, advantages of closed-ended questions, disadvantages of closed-ended questions.
First…
Definition: Closed-ended questions are a type of survey question or interview questions that offer predefined answer options. They limit respondents to choose from specific responses, such as “yes” or “no,” multiple-choice options, or rating scales. These questions are designed to elicit quick and concise answers, making data analysis more straightforward and efficient.
Closed-ended questions are well-suited for various situations and research scenarios due to their structured and objective nature. Here are some of the scenarios on when to use closed-ended questions:
- Surveys with large sample sizes: Closed-ended questions are ideal for surveys involving many respondents. They simplify survey data collection and analysis processes, making managing and interpreting responses from a large population easier.
- Quantitative research: If your study aims to gather numerical data and statistical insights, closed-ended questions are the way to go. The predefined response options allow for easy quantification and analysis of the data. Consequently, it enables you to identify trends and patterns efficiently.
- Objective data collection: Closed-ended questions are advantageous when seeking factual and objective information. Providing set response choices minimizes the risk of subjective interpretations, ensuring consistency in data collection.
- Comparison and ranking: Closed-ended questions are valuable for comparative analyses . They facilitate straightforward data organization and comparison. For instance, when asking participants to rank their preferences or compare various options.
- Limited response options: When you limit respondents’ answers to specific choices, closed-ended questions are the obvious choice. They ensure respondents choose from the available options, avoiding ambiguous or open-ended responses.
In survey research, closed-ended questionnaires, presenting predefined response choices, are commonly utilized. They are structured and efficient for gathering specific information. Below are different classifications of closed-ended questionnaire types:
Multiple Choice Questions (MCQs)
Survey takers opt from a prearranged list of choices. Example: “Which of the following social media platforms do you use?
- LinkedIn”
Likert Scale Questions
Rate your level of alignment or dissent with the presented statement. Example: Kindly indicate your degree of agreement or disagreement regarding the quality of service received.
- Strongly Agree
- Strongly Disagree”
Semantic Differential Scale Questions
Respondents rate something on a scale between two polar adjectives. Example: “Please rate the quality of the product: Excellent [ ] [ ] [ ] [ ] [ ] Poor”
Dichotomous Questions
Queries typically offer binary choices, often between ‘affirmative’ and ‘negative’. Example: “Have you purchased our product in the last six months?
Ranking Questions
Respondents rank options in order of preference. Example: “Please rank the following factors in order of importance when choosing a smartphone:
- Battery Life
- Camera Quality
- Brand Reputation”
Checklist Questions
Respondents select multiple options from a list. Example: “Please select all the items you purchased in the last month:
- Cheese”
Numeric Response Questions
Respondents provide a numerical response. Example: “Please indicate your daily social media usage by selecting the appropriate time frame:”
Why closed-ended questions?
- Standardization: Closed-ended questions provide a structured format with predetermined answer options. This uniformity ensures that all respondents are presented with the same choices. As a result, it eliminates ambiguity and potential bias in the data collection process. Consequently, it makes it easier to compare and interpret responses.
- Quantitative data: Closed-ended questions typically involve numerical scales or multiple-choice options . Thus, the data collected is quantitative. As a result, the responses can be easily quantified, measured, and analyzed statistically.
- Ease of response: Respondents find closed-ended questions easy to answer as they don’t require lengthy explanations or open-ended responses. This simplicity encourages higher response rates and reduces the risk of participants skipping questions.
- Comparability: Closed-ended questions enable straightforward comparisons between different respondents or groups. With standardized response options, you can directly compare how different population segments feel or think. As a result, this comparability aids in drawing meaningful conclusions and identifying significant differences.
- Efficient analysis: The structured nature of closed-ended questions streamlines the data analysis process, including self-service analytics . You can quickly categorize and quantify the responses, making spotting patterns and drawing conclusions easier. Moreover, this efficiency saves time and resources for researchers.
Unlock the power of precision as you capture laser-focused responses with these closed-ended question examples.
- Very satisfied
- Dissatisfied
- Very dissatisfied
- Not likely at all
- Very likely
- 5 or more times
- Yes, very satisfied
- Yes, somewhat satisfied
- No, somewhat dissatisfied
- No, very dissatisfied
- Less than 6 months
- 6 months-12 months
- More than 1 year
- More than 2 years
- TV advertisement
- Social media
- Word of mouth
- Online search
- Print media
- Occasionally
- In-person visit
- Self-help documentation
Let’s say you want to conduct a customer satisfaction survey for a footwear brand. You want your survey to include the closed-ended survey questions below.
- How satisfied are you with the style and design of the footwear?
- How satisfied are you with the fit and size options available for the footwear?
- How satisfied are you with the value for money offered by the footwear?
Below are the response options associated with each question:
- Extremely Dissatisfied
- Extremely Satisfied
Follow the steps below to create a survey using Google Forms .
- Open Google Forms and sign in with your Google account.
- Click the “ Blank ” button to create a new form.
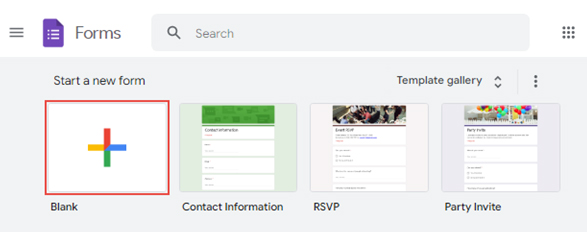
- Give your form a title and description that reflects your brand or purpose.
- Choose the type of question you want to use, such as multiple-choice, checkbox, or short answer.
- Type your question in the “Untitled Question” section for multiple-choice questions . Then write “Option 1” and choose “Add option” for more options.
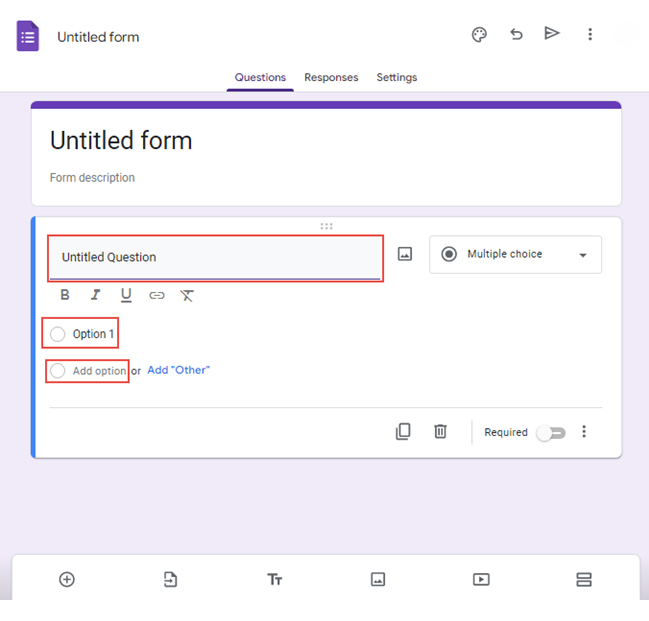
- You can customize the look and feel of your survey to match your brand or theme.
- Preview the form before sharing it with your target audience.
- Click the share button to distribute it to your target audience.
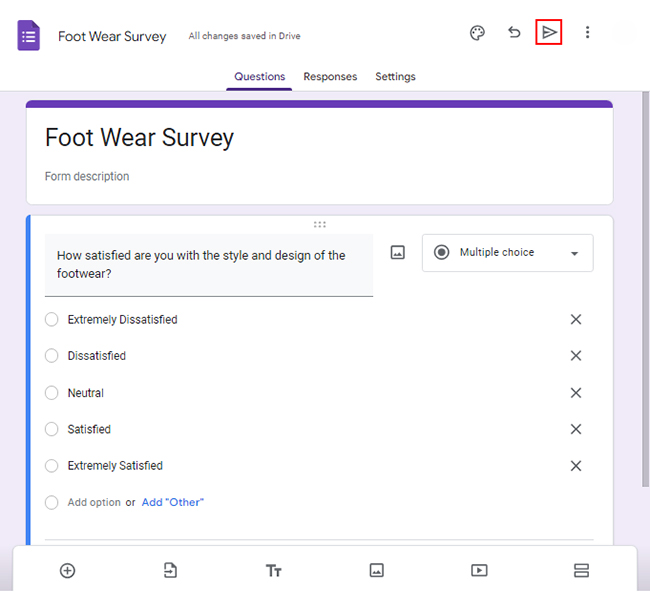
- Once you collect enough responses, click the “ Link to Sheets ” button.
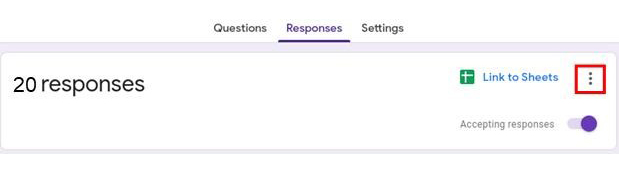
- Download the responses as a .csv file from the drop-down menu.
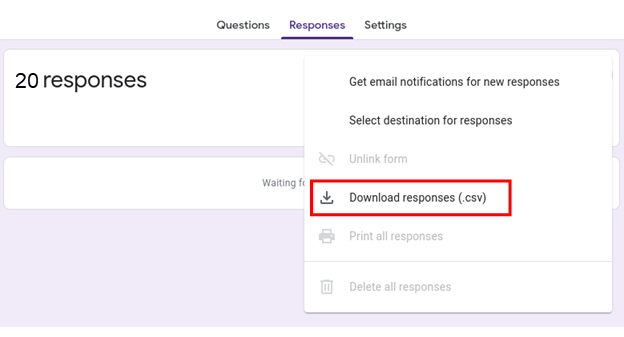
Excel is undoubtedly a popular choice for data analysis , offering many features and functionalities. However, when it comes to visualizing large datasets, Excel has its limitations.
With overwhelming data, creating data visualizations conveying the key insights becomes increasingly challenging.
That’s where ChartExpo comes in.
ChartExpo seamlessly integrates with Excel, providing a user-friendly interface and a wide array of visualization options. With ChartExpo, you can transform raw data into stunning visual representations, making data presentation and analysis easier.
Let’s learn how to Install ChartExpo in Excel.
- Open your Excel application.
- Open the worksheet and click the “ Insert ” menu.
- You’ll see the “ My Apps ” option.
- In the office Add-ins window, click “ Store ” and search for ChartExpo on my Apps Store.
- Click the “ Add ” button to install ChartExpo in your Excel.
ChartExpo charts are available both in Google Sheets and Microsoft Excel. Please use the following CTA’s to install the tool of your choice and create beautiful visualizations in a few clicks in your favorite tool.
Assume your closed-ended survey yields the data table below.
10-13-2023 17:47:33 | Extremely Dissatisfied | Neutral | Dissatisfied |
10-13-2023 17:47:33 | Extremely Dissatisfied | Satisfied | Neutral |
10-13-2023 17:47:33 | Extremely Dissatisfied | Dissatisfied | Extremely Satisfied |
10-13-2023 17:47:33 | Neutral | Extremely Satisfied | Dissatisfied |
10-13-2023 17:47:33 | Satisfied | Extremely Dissatisfied | Dissatisfied |
10-13-2023 17:47:33 | Dissatisfied | Dissatisfied | Extremely Satisfied |
10-13-2023 17:47:33 | Extremely Satisfied | Extremely Dissatisfied | Neutral |
10-13-2023 17:47:33 | Dissatisfied | Satisfied | Extremely Dissatisfied |
10-13-2023 17:47:33 | Satisfied | Dissatisfied | Satisfied |
10-13-2023 17:47:33 | Dissatisfied | Neutral | Extremely Satisfied |
10-13-2023 17:47:33 | Extremely Dissatisfied | Dissatisfied | Extremely Dissatisfied |
10-13-2023 17:47:34 | Extremely Dissatisfied | Extremely Dissatisfied | Satisfied |
10-13-2023 17:47:35 | Extremely Satisfied | Extremely Satisfied | Extremely Satisfied |
10-13-2023 17:47:36 | Satisfied | Extremely Satisfied | Extremely Satisfied |
10-13-2023 17:47:37 | Neutral | Extremely Dissatisfied | Extremely Dissatisfied |
10-13-2023 17:47:38 | Neutral | Extremely Dissatisfied | Extremely Satisfied |
10-13-2023 17:47:39 | Satisfied | Satisfied | Dissatisfied |
10-13-2023 17:47:40 | Satisfied | Satisfied | Neutral |
10-13-2023 17:47:41 | Extremely Dissatisfied | Extremely Satisfied | Dissatisfied |
10-13-2023 17:47:42 | Satisfied | Extremely Dissatisfied | Neutral |
This table contains example data. Expect many responses and questions in real life.
- To get started with ChartExpo, install ChartExpo in Excel .
- Now Click on My Apps from the INSERT menu.
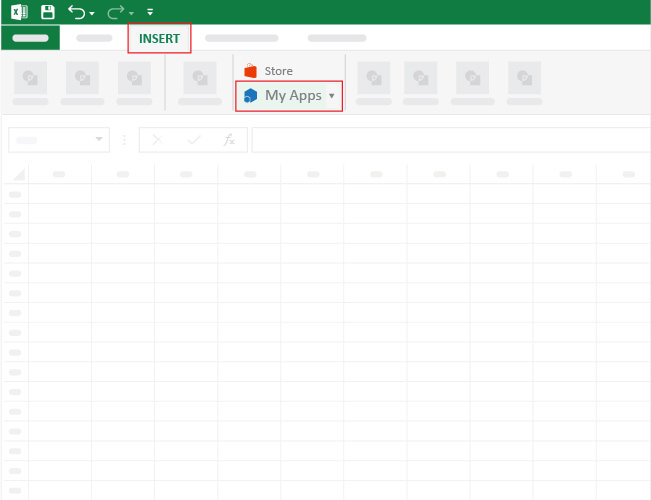
- Choose ChartExpo from My Apps , then click Insert.
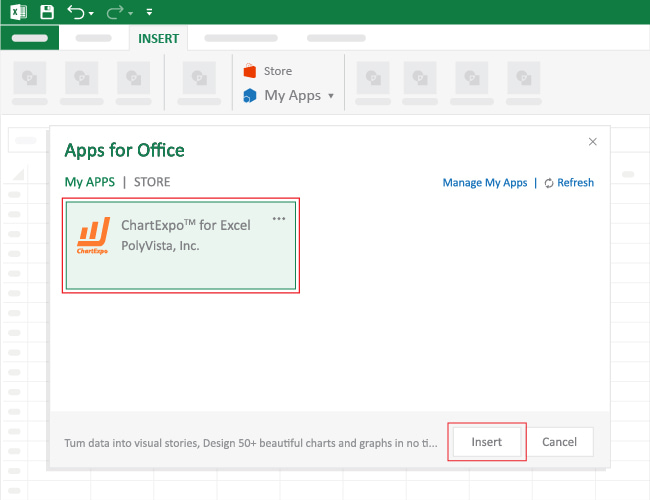
- Once it loads, choose the “ Likert Scale Chart ” from the charts list.
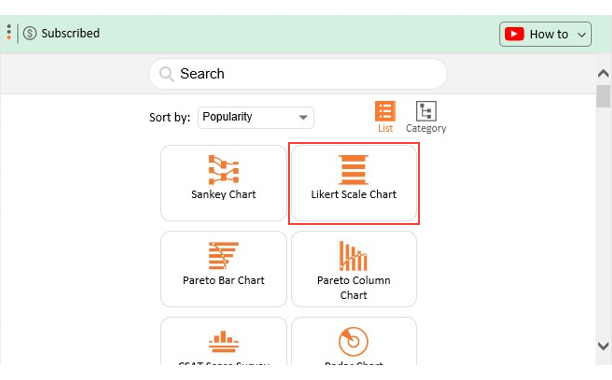
- Click the “ Create Chart From Selection ” button after selecting the data from the sheet, as shown.
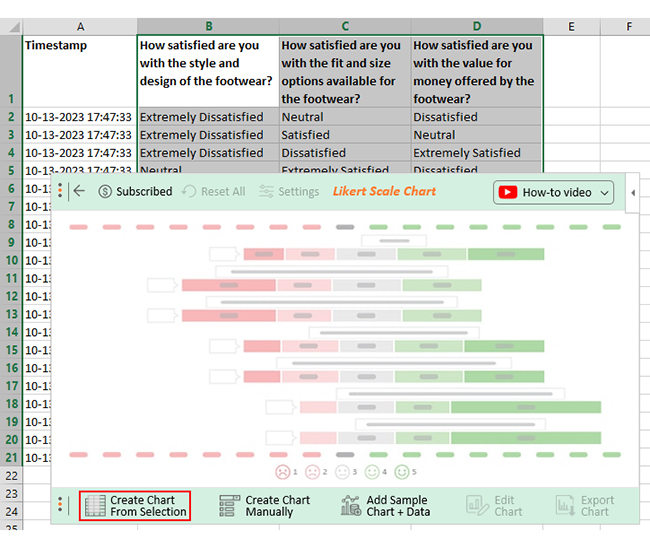
- When you click the “ Create Chart From Selection ” button, you have to map responses with numbers manually. The Likert scale has this arrangement:
- Extremely Dissatisfied = 1
- Dissatisfied = 2
- Neutral = 3
- Satisfied = 4
- Extremely Satisfied = 5
- Once all is set, click the “ Create Chart ” button.
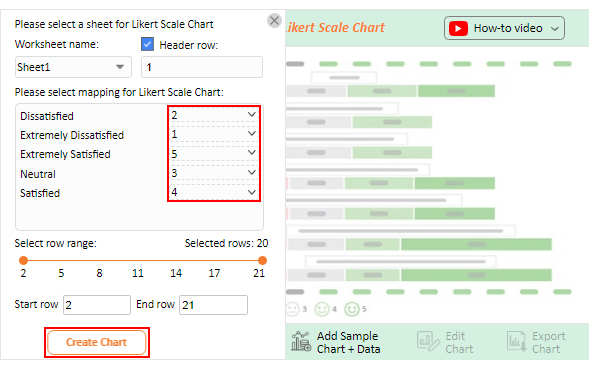
- ChartExpo will generate the visualization below for you.
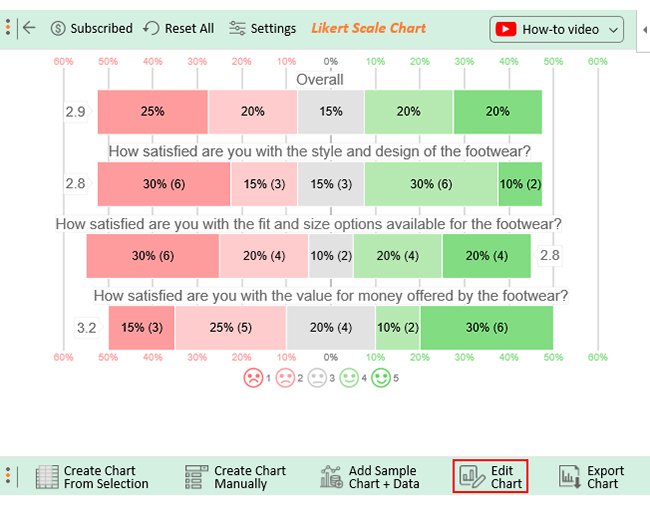
- If you want to have the chart’s title, click Edit Chart , as shown in the above image.
- Click the pencil icon next to the Chart Header to change the title.
- It will open the properties dialog. Under the Text section, you can add a heading in Line 1 and enable Show .
- Give the appropriate title of your chart and click the Apply button.
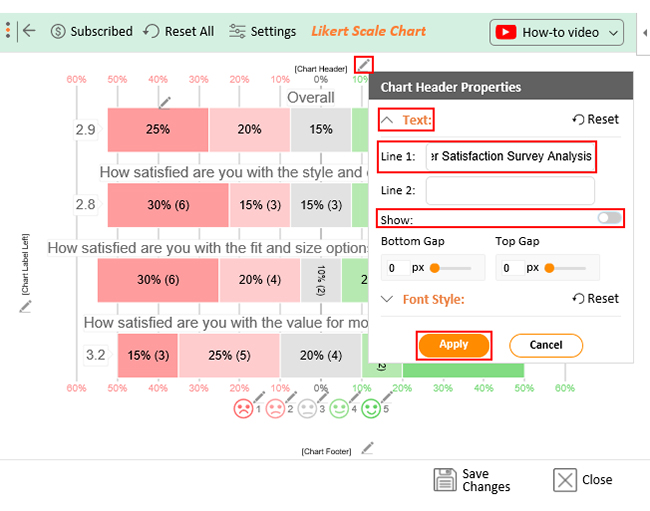
- Let’s say you want to add text responses instead of numbers against every emoji.
- Click the pencil icon next to the respective emoji. Expand the “ Label ” properties and write the required text. Then click the “ Apply All ” button.
- Click the “ Save Changes ” button to persist the changes.
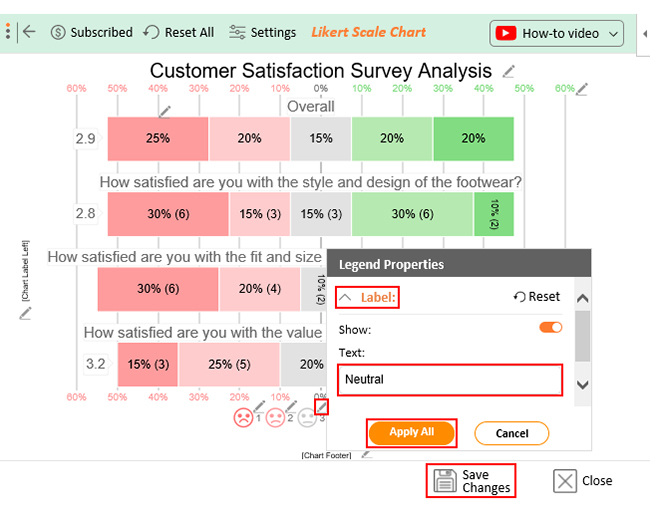
- Your final chart will appear below.
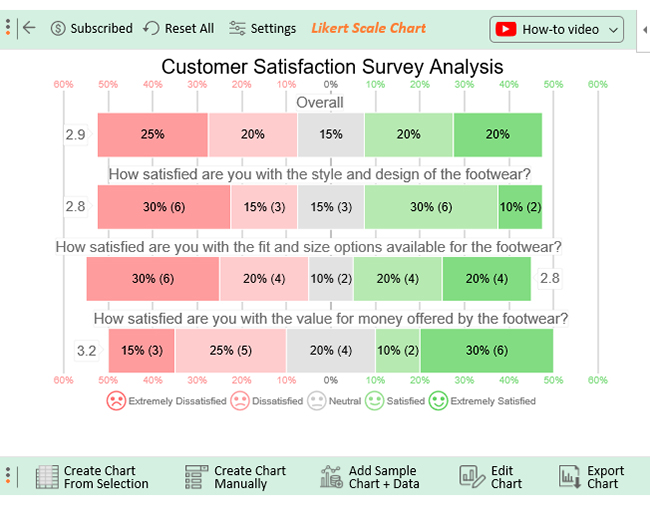
- 40% of customers express satisfaction with the style and design of the footwear. 45% express dissatisfaction and 15% remain neutral.
- 40% are satisfied with the fit and size options available for the footwear, while 50% are dissatisfied.
- 40% are satisfied with the value for money the footwear offers, with 40% expressing dissatisfaction.
- 40% of customers report being satisfied with the footwear, with 20% extremely satisfied.
- 45% of customers report dissatisfaction, with 25% extremely dissatisfied
- 15% remain neutral.
Let’s say you manage a school. You want to gather feedback from students on various issues like the quality of teaching. You have created a survey with the closed-ended questionnaire below;
- How satisfied are you with the quality of teaching?
- How satisfied are you with the academic resources?
- How satisfied are you with the administrative services?
- How satisfied are you with the availability of extracurricular activities?
Students respond to each question using the response scale below.
Assume your survey yields the data table below.
10-13-2023 17:47:33 | Neutral | Dissatisfied | Dissatisfied | Delighted |
10-13-2023 17:47:33 | Delighted | Delighted | Frustrated | Neutral |
10-13-2023 17:47:33 | Satisfied | Dissatisfied | Delighted | Dissatisfied |
10-13-2023 17:47:33 | Delighted | Delighted | Delighted | Satisfied |
10-13-2023 17:47:33 | Neutral | Satisfied | Neutral | Satisfied |
10-13-2023 17:47:33 | Satisfied | Neutral | Delighted | Delighted |
10-13-2023 17:47:33 | Delighted | Frustrated | Delighted | Frustrated |
10-13-2023 17:47:33 | Dissatisfied | Delighted | Delighted | Satisfied |
10-13-2023 17:47:33 | Frustrated | Neutral | Satisfied | Delighted |
10-13-2023 17:47:33 | Satisfied | Satisfied | Satisfied | Delighted |
10-13-2023 17:47:33 | Delighted | Satisfied | Frustrated | Satisfied |
10-13-2023 17:47:34 | Satisfied | Frustrated | Dissatisfied | Frustrated |
10-13-2023 17:47:35 | Satisfied | Satisfied | Neutral | Delighted |
10-13-2023 17:47:36 | Delighted | Dissatisfied | Dissatisfied | Delighted |
10-13-2023 17:47:37 | Dissatisfied | Frustrated | Delighted | Delighted |
10-13-2023 17:47:38 | Delighted | Dissatisfied | Satisfied | Frustrated |
10-13-2023 17:47:39 | Delighted | Neutral | Frustrated | Satisfied |
10-13-2023 17:47:40 | Neutral | Dissatisfied | Frustrated | Delighted |
10-13-2023 17:47:41 | Dissatisfied | Delighted | Dissatisfied | Satisfied |
10-13-2023 17:47:42 | Satisfied | Neutral | Dissatisfied | Dissatisfied |
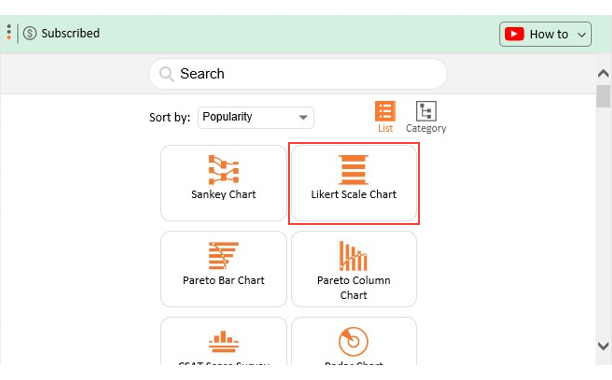
- Frustrated = 1
- Delighted = 5
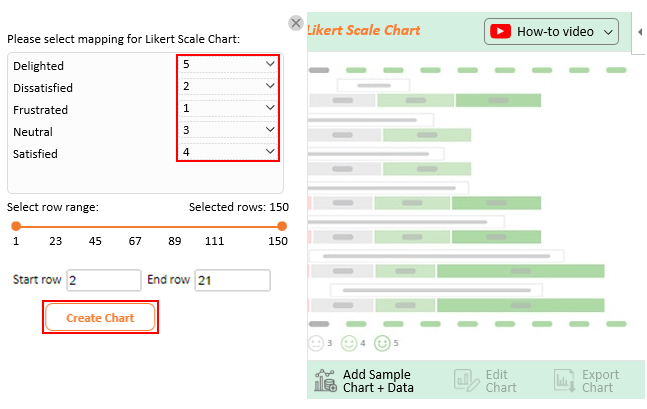
- 35% of students expressed their delight with the quality of teaching, while 30% indicated satisfaction. On the other hand, 15% were dissatisfied, 5% frustrated, and 15% neutral.
- Regarding academic resources, 40% were content, while 25% expressed dissatisfaction and 15% were frustrated.
- Regarding administrative services, 30% were delighted, while 20% were frustrated.
- Regarding extracurricular activities, 70% expressed gratification, while 25% felt unhappy.
- 31% of students reported being delighted with school.
- 24% expressed satisfaction.
- 14% felt frustrated.
- 19% were dissatisfied.
- 13% remained neutral.
Here are some tips for effectively using closed-ended questions:
Be Clear and Concise
Ensure that your closed-ended questions are straightforward to understand, avoiding ambiguity or confusion.
Use Simple Language
Keep the language of your questions simple and accessible to all respondents, regardless of their background or education level.
Limit the Number of Options
Provide a reasonable number of response options to prevent overwhelming responses and encourage accurate responses.
Offer Balanced Response Options
Ensure that the response options provided cover the full range of possible answers without bias or leading the respondent to a specific answer.
Randomize Response Order
If relevant, consider shuffling the order of response options to mitigate the possibility of response bias influenced by the presentation order.
Avoid Double-Barreled Questions
Each question should address only one specific aspect to avoid confusion and ensure clarity in responses.
Pilot Test Questions
Before administering your survey, pilot test your closed-ended questions with a small sample group to identify any potential issues or areas for improvement.
Consider Using Likert Scales
Move beyond simple agree/disagree. Likert scales empower respondents. They can choose from a range of options, reflecting their exact level of agreement or disagreement with a statement.
Keep Response Formats Consistent
Ensure uniformity in the response format, whether it be checkboxes or radio buttons, to enhance respondent navigation ease.
Mix Closed-Ended with Open-Ended Questions
Craft your survey with a mix of question formats! Include closed-ended options for selection and open-ended prompts for elaboration. This approach facilitates a comprehensive analysis by gathering both statistical data and in-depth insights. This approach gathers both numerical data and in-depth responses, providing a clearer picture.
Here are some advantages of using closed-ended questions:
Closed-ended questions facilitate swift responses, rendering them effective tools for collecting data from a broad spectrum of respondents.
Standardization
Closed-ended questions generate responses that maintain organization and consistency, thereby facilitating analysis and comparison.
Ease of Analysis
Closed-ended responses can be easily quantified and analyzed using statistical methods, allowing for straightforward interpretation.
Reduced Bias
Closed-ended questions reduce the likelihood of interviewer bias or influence since respondents choose from pre-established options.
Increased Respondent Comfort
Respondents may feel more comfortable answering closed-ended questions since they are not required to generate their responses.
Suitable for Sensitive Topics
Closed-ended questions prove advantageous in collecting data on sensitive subjects, offering respondents the choice to offer discreet responses.
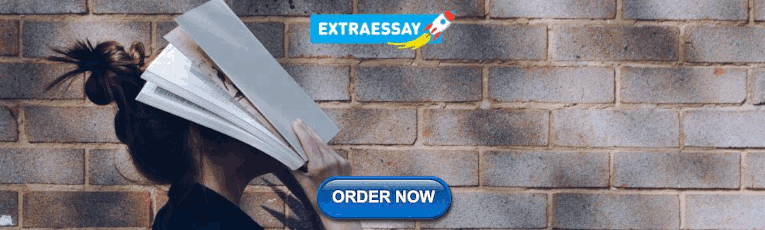
Facilitation of Survey Design
By structuring surveys and questionnaires, closed-ended questions ensure that the data collection process remains clear and coherent.
Enhanced Data Accuracy
The provision of specific response options in closed-ended questions helps enhance data accuracy by minimizing the possibility of ambiguous or misunderstood responses.
Minimized Missing Data
Closed-ended questions generally necessitate respondents to choose a response, which decreases occurrences of missing data in contrast to open-ended queries.
Increased Response Rate
The straightforwardness and conciseness of closed-ended questions can boost response rates as they impose lesser cognitive load on participants.
Here are some disadvantages of using closed-ended questions:
Limited response options
The finite options presented in closed-ended questions can limit respondents’ ability to convey nuanced or complex perspectives.
Lack of depth
Closed-ended questions may yield responses that lack the depth and detail required, thus restricting the richness of the collected data.
Potential for bias
The predefined response options in closed-ended questions may reflect the biases or assumptions of the questionnaire designer, leading to biased results.
Difficulty in capturing unexpected responses
Closed-ended questions may not capture unexpected or unanticipated responses, potentially overlooking valuable insights.
What are closed-ended questions?
Closed-ended questions are a type of survey or interview questions that offer predefined answer options. Respondents choose from specific responses, such as “yes” or “no,” multiple-choice options, or rating scales.
What are closed-ended questionnaires?
Closed-ended questionnaires are surveys or data collection instruments primarily composed of closed-ended questions. These questionnaires use specific answer choices, restricting respondents to select from the provided options. As a result, this allows for straightforward data analysis and quantification.
What are 2 examples of closed-ended questions?
- Very Likely
- Somewhat Likely
- Somewhat Unlikely
- Very Unlikely
What is a good example of a closed-ended question?
“How likely are you to recommend our product on a scale of 1 to 10?” This closed-ended question quantitatively measures customer satisfaction and the likelihood of a recommendation.
Why use closed-ended questions?
Closed-ended questions offer standardized data collection, quick analysis, and ease of response. They provide specific answer options, facilitating quantitative data gathering. As a result, they make comparisons between respondents or groups more straightforward.
Closed-ended questions serve as powerful allies in the realm of efficient surveys. They streamline data collection by offering predetermined response options, ensuring standardized and concise answers.
The closed-ended questionnaire examples above showcase their versatility across various domains. From measuring customer satisfaction and conducting market research to gathering valuable employee feedback. Moreover, the structured nature of closed-ended questions allows for easy categorization and quantification of responses. This makes it easier to analyze the data and draw meaningful conclusions.
With a closed-ended questionnaire, you can obtain quantifiable data that simplifies analysis and enables easy comparison between different groups. This approach boosts response rates due to simplicity and expedites the overall research process.
When complemented with ChartExpo, the power of closed-ended questionnaires reaches new heights. ChartExpo’s user-friendly interface empowers you to transform survey data into visually stunning and insightful charts effortlessly. This breathes life into closed-ended survey responses, making data interpretation a breeze.
Go forth, armed with these examples and ChartExpo, and unlock your surveys’ full potential of closed-ended questions.
Happy surveying.
How much did you enjoy this article?
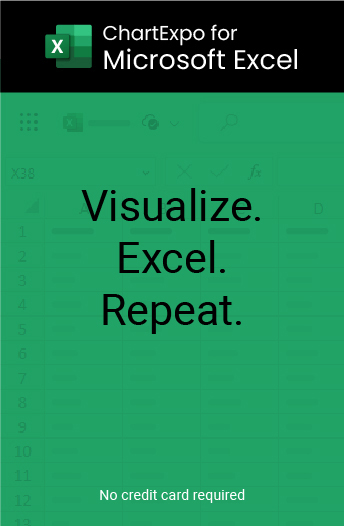
Related articles
Chart Elements in Excel for Effective Formatting
Understand chart elements in Excel to transform raw data into insightful visuals. Learn how customizing titles, axes, & legends leads to clear & impactful charts.
What Does DEI Stands for in Business & Corporate Culture?
Discover what DEI stands for and why it matters in business. Learn how Diversity, Equity, and Inclusion initiatives create fair, inclusive, & innovative workplaces.
Impact vs. Effort Matrix for Effective Planning and Strategy
Discover the Impact vs. Effort Matrix, a tool for prioritizing tasks. Maximize productivity by identifying high-impact projects that require less effort and quick wins.
Thurstone Scales: Key Concepts and Practical Insights
Unlock the potential of Thurstone scales for accurate attitude measurement. Learn their applications in research, from public opinion surveys to market analysis.
Electric Vehicle Sales Statistics: Analyzing Market Growth
Discover how Electric Vehicle Sales Statistics highlight global market expansion, government incentives, and future mobility trends in this analysis of EV growth.
8 Close Ended Questions Examples for Better Market Research
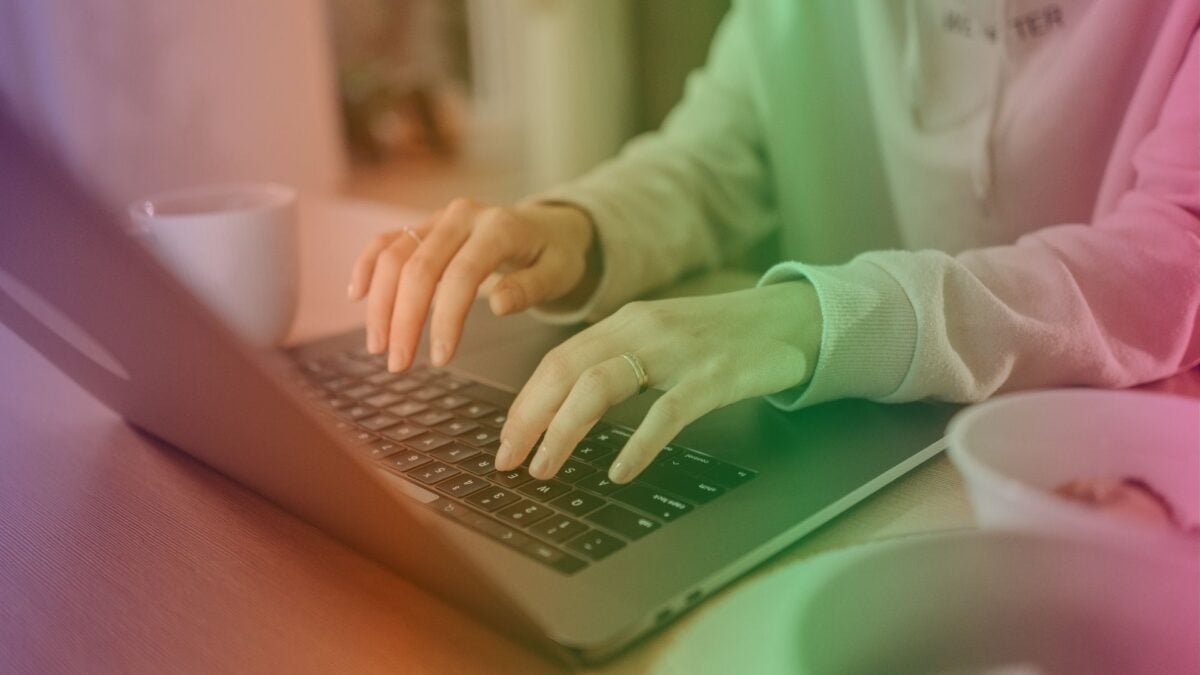
With surveys, the thing is: it’s all about asking the right questions. In our guide, we’re diving into the art of formulating perfect, close ended questions.
Multiple choice magic, the clarity of single word answers, and the power of quantitative data – it’s all here.
Ready to give your survey a serious accuracy boost? Let’s get into it!
What are close ended questions?
Close ended questions in surveys provide a limited number of choices, like multiple choice or rating scales.
Unlike open ended ones, they limit responses to predetermined choices, which makes data collection and statistical analysis much easier. Ideal for quantifiable data, they’re user-friendly and save time. You’ve got direct insight without needing respondents to elaborate or asking many additional questions.
When to use close ended questions?
If you have some doubts, we’ve come up with a few examples.
#1 Quantitative data collection
Close ended questions are great when you need to collect quantifiable data for statistical analysis. Multiple choice or rating scale questions in surveys allow for easy aggregation of responses.
🟩 Great for: companies looking to analyze customer feedback or opinions in a structured, numerical way. It gives you more precise data, which helps a lot in making smart business choices.
#2 Time-efficient surveys
When survey time is limited, close ended questions are the way to go. They require less time to complete compared to open ended questions, as respondents choose from a predetermined list of answers.
🟩 Great for: quick feedback collection, for instance, in customer satisfaction surveys where every second counts.
#3 Large-scale response analysis
For surveys with many respondents, closed ended questions simplify the analysis process. Use limited response options like ‘agree or disagree’ or a Likert scale to make it simpler to compare and see the differences in answers across a broad audience.
🟩 Great for: market research where understanding general trends is more relevant than gathering detailed individual insights.
#4 Clarity and consistency in responses
Closed ended questions provide clarity and consistency in survey responses. By limiting answers to a specific set of choices, they reduce the ambiguity that can arise from open ended responses.
🟩 Great for: times when you need simple answers, like figuring out what products people prefer or how satisfied customers are.
8 Examples of close ended questions
Now that you know when to use close ended questions, let’s see what their types are and how you can use them not only in a close ended survey, but also to collect data from survey respondents… anywhere you want.
01 Multiple choice questions
Multiple choice questions are standard in close ended surveys. They give respondents a bunch of predetermined answers, which makes it more convenient to collect and analyze data.
Closed-ended questions are your go-to when you need to lead to statistical analysis, especially to see common opinions or choices. They’re helpful for crunching numbers, but they might not get as deep into details as open-ended questions do.
Example: What is your favorite type of cuisine? a) Italian b) Chinese c) Mexican d) Indian
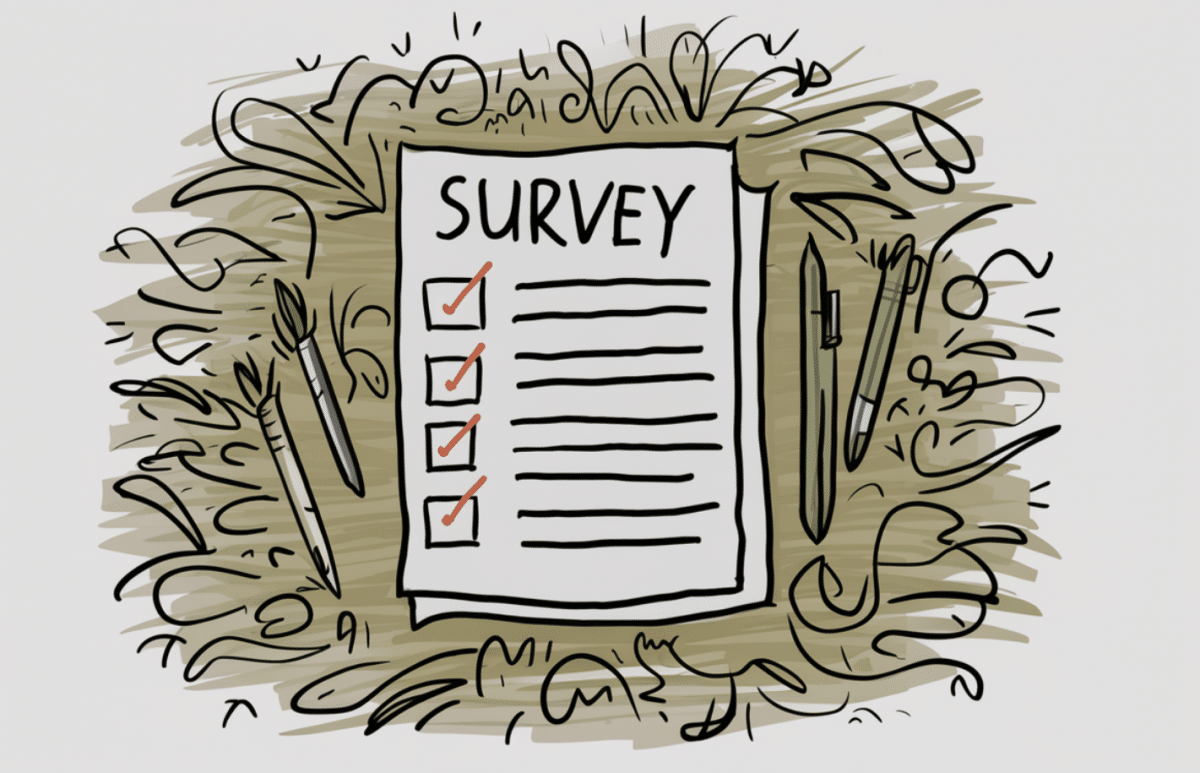
02 Likert scale questions
Likert scale questions are used to measure attitudes or opinions. They typically range from ‘strongly agree’ to ‘strongly disagree’ and provide a nuanced view of respondents’ feelings. You can use it in customer feedback surveys, as they quantify subjective responses, making them easier to analyze .
Example: How satisfied are you with our customer service? 1 – Not at all satisfied, 2 – Slightly satisfied, 3 – Neutral, 4 – Satisfied, 5 – Very satisfied
03 Dichotomous Questions
They’re usually framed as ‘Yes/No’ or ‘True/False’ and provide you with straightforward, decisive answers. Dichotomous questions are suitable for collecting clear-cut data or when detailed answers are not practical or necessary.
However, they might not capture the nuances of respondents’ opinions as effectively as multiple choice questions .
Example: Do you currently use our product? Yes/No
04 Rating scale questions
They let respondents rate something on a scale, for instance from 1 to 5 or 1 to 10. Using them can be a great way to find out how satisfied people are with a product or service or to compare different parts of it. You get numbers that are easy to work with, but they might not tell you why someone gave a certain rating.
Example: On a scale of 1 to 10, how would you rate the quality of our product?
05 Demographic questions
Gathering specific details like age, gender, or job? These are called demographic questions and are later used to divide up survey responses and get to know what different groups of people prefer or how they behave.
Conduct them in a way that respects people’s privacy and makes sure they’re relevant to what the survey is trying to find out.
Example: What is your age group? a) Under 20 b) 21-30 c) 31-40 d) 41-50 e) Over 50
When designing demographic questions, especially those related to age, precision is must-have for categorizing your respondents correctly. A solid age calculator can help ensure that respondents are grouped accurately.
06 Single answer questions
If you want respondents to choose one thing, like their favorite part of a product, opt for choosing single answer questions. Analyzing answers takes less time, but it might not show all the details of respondents’ likes and dislikes as well as multiple choice questions can.
Example: Which feature of our app do you find most useful? a) Navigation b) Notifications c) Customization options d) Social sharing
07 Closed ended personal questions
Closed ended personal questions ask about personal preferences or experiences but limit responses to predefined options. They’re less intrusive than open ended personal questions and are easier for respondents to answer.
Example: How often do you exercise per week? a) Not at all b) 1-2 times c) 3-4 times d) More than 4 times
08 Binary outcome questions
Similarly to dichotomous ones, they provide two opposite choices, like ‘satisfied/unsatisfied.’
Perfect for quick insights into specific issues. But here’s the thing – they lack the depth and range of responses that other closed ended question types, like multiple choice or rating scales, might provide.
Example: Was our website easy to navigate? Yes/No
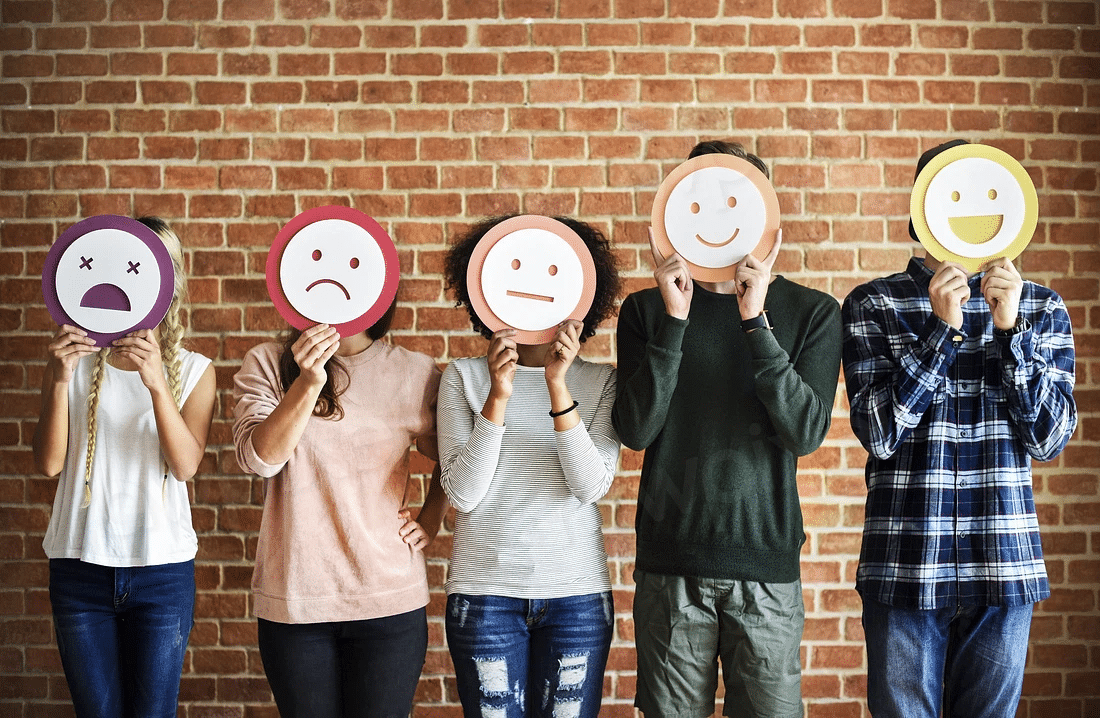
Good Practices for Close Ended Questions
How to ask your survey questions to get a set of responses answered that is satisfying for you? Read our best practices.
1. Take advantage of software for creating surveys
Try using Surveylab . It’s got handy tools to assist you in creating questions easily, ready-made answer options, and simple templates . The tool also sorts and analyzes answers for you, so you can understand your results faster.
Without any hassle, your survey will be exactly what you’ve been looking for.
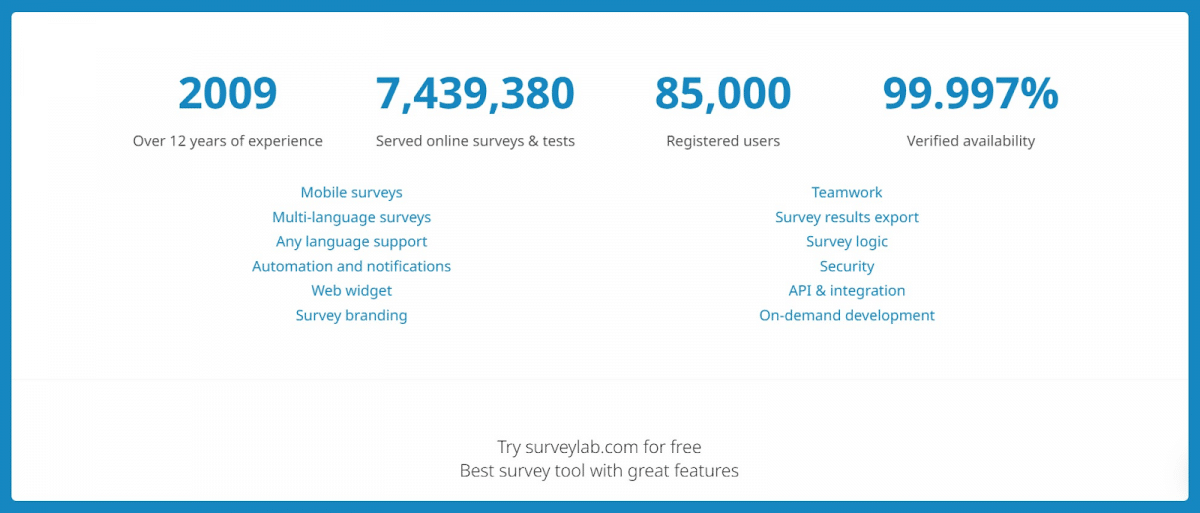
2. Collect quantitative data with precision
Design closed-ended questions carefully to effectively collect quantitative data. You should use a few clear and specific answer choices, so respondents can easily pick the one that fits best for them. Such a method is key for statistical analysis because it gives clean, number-based data that’s simple to understand and compare.
3. Create effective multiple choice questions
A well-formulated, easy-to-follow multiple choice question may decide if the respondents are going to continue taking surveys or not. That’s why you should take special care in designing those. Double-check if the multiple options cover all potential answers so respondents aren’t pushed into choosing alternatives that don’t truly reflect their views.
4. Use rating scales for detailed feedback
Rating scale questions allow respondents to express their opinions on a scale and give more context than a single word answer. That’s why they’re invaluable for collecting accurate feedback in a quantifiable format. It’s quite often used to measure customer satisfaction or attitudes towards a product or service.
5. Limit responses for clearer data
Want to obtain clearer and more consistent data? Maybe limiting responses to close ended questions can help.
Respondents may find the survey less difficult to take, and the data is quicker to interpret for companies. Once you just need simple, direct answers, limited responses really come in handy.
6. Balance qualitative insights with quantitative data
While close ended questions are intended to collect quantitative data , they can also provide qualitative insights. Carefully designing questions and answer choices, you can glean a richer understanding from the responses. Consider this balance, especially in case you’re trying to figure out why people behave the way they do.
📰 See 10 tricks to help you build better surveys .
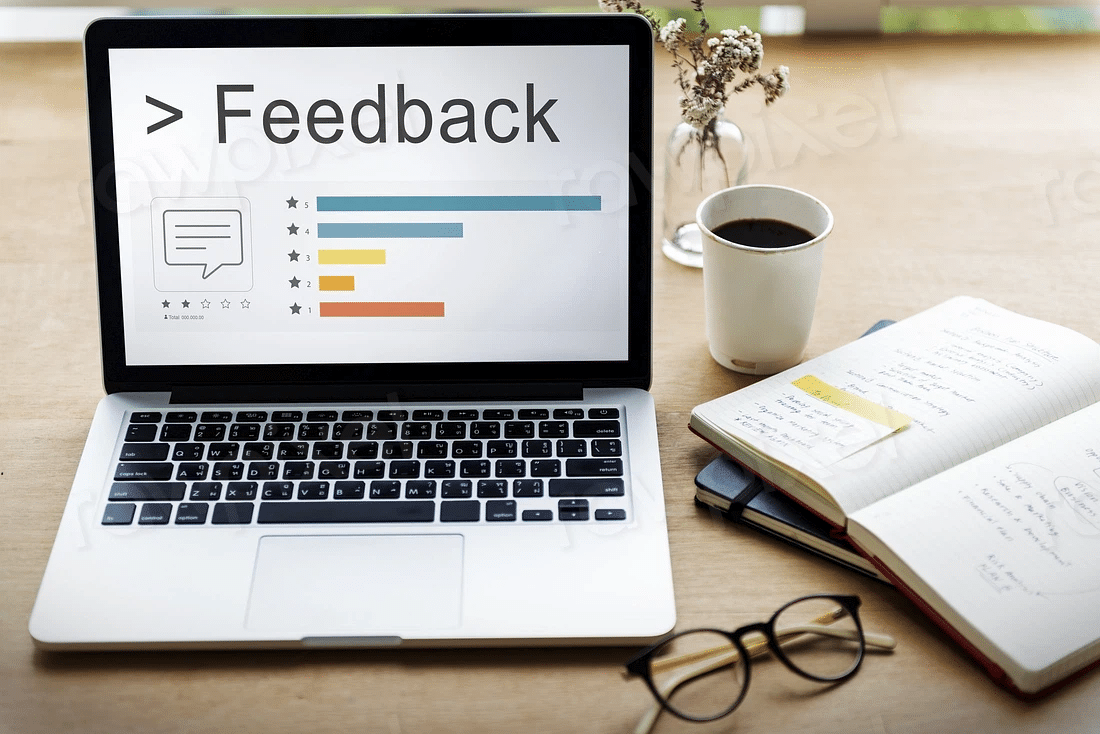
When is a close-ended question not the right idea?
Looking for in-depth, qualitative data ? Maybe you should look for something else than close ended questions.
If the goal is to understand complex opinions, feelings, or experiences, let respondents answer freely in their own words. Open ended questions are more suitable in these scenarios since they allow a richer qualitative analysis.
Questions, where respondents answer using their own words might be an option for a company to uncover the subtle details in customer responses. Closed-ended questions have their perks, like making analysis simpler and responses clearer. But, they might not catch all the insights that you can get from open-ended questions. It’s true when you apply statistical analysis techniques.
The choice between open ended and closed ended questions in surveys makes a huge difference.
Knowing when to use different types of questions is a must for any company that wants to collect relevant information. The decision on which type to use depends on what the survey aims to achieve and the kind of information that’s needed to help make good decisions.
Closed ended questions, with their structured answer options, lead to straightforward analysis and clear data, which is beneficial for companies looking to quantify customer feedback. They shine in scenarios where responses can be easily categorized, like in multiple choice questions or rating scale questions.
However, when the goal is to get more information into the thoughts and feelings of customers, open ended questions take the lead. Respondents can answer what they want, providing richer, more nuanced insights.
Sign up for Surveylab , and create powerful surveys with both closed and open ended questions with ease.
FAQ on close-ended questions
Any doubts on the topic? Let’s go through the frequently asked questions.
Close ended questions are the type where you give specific answers to choose from. These answers can be yes or no, multiple choice, or a rating scale. They make it easy to collect and analyze data because all responses are standard.
It is not good to use close ended questions when you want detailed feedback or opinions. They limit how much respondents can share. If you want to understand feelings or complex views, open ended questions are better.
Keep them simple and clear. Ensure that the choices cover all possible answers so respondents don’t feel stuck. Also, using tools like SurveyLab can help you design, administer, and analyze surveys more efficiently.
Try SurveyLab for free Best survey tool with great features
14 days trial | view complete list of features
Open-Ended vs. Closed Questions in User Research
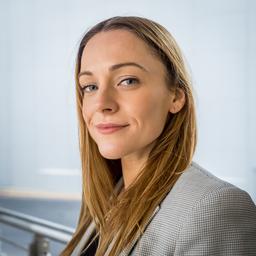
January 26, 2024 2024-01-26
- Email article
- Share on LinkedIn
- Share on Twitter
When conducting user research, asking questions helps you uncover insights. However, how you ask questions impacts what and how much you can discover .
In This Article:
Open-ended vs. closed questions, why asking open-ended questions is important, how to ask open-ended questions.
There are two types of questions we can use in research studies: open-ended and closed.
Open-ended questions allow participants to give a free-form text answer. Closed questions (or closed-ended questions) restrict participants to one of a limited set of possible answers.
Open-ended questions encourage exploration of a topic; a participant can choose what to share and in how much detail. Participants are encouraged to give a reasoned response rather than a one-word answer or a short phrase.
Examples of open-ended questions include:
- Walk me through a typical day.
- Tell me about the last time you used the website.
- What are you thinking?
- How did you feel about using the website to do this task?
Note that the first two open-ended questions are commands but act as questions. These are common questions asked in user interviews to get participants to share stories. Questions 3 and 4 are common questions that a usability-test facilitator may ask during and after a user attempts a task, respectively.
Closed questions have a short and limited response. Examples of closed questions include:
- What’s your job title?
- Have you used the website before?
- Approximately, how many times have you used the website?
- When was the last time you used the website?
Strictly speaking, questions 3 and 4 would only be considered “closed” if they were accompanied by answer options, such as (a) never, (b) once, (c) two times or more. This is because the number of times and days could be infinite. That being said, in UX, we treat questions like these as closed questions.
In the dialog between a facilitator and a user below, closed questions provide a short, clarifying response, while open-ended questions result in the user describing an experience.
T | |
Using Closed Questions in Surveys
Closed questions are heavily utilized in surveys because the responses can be analyzed statistically (and surveys are usually a quantitative exercise). When used in surveys, they often take the form of multiple-choice questions or rating-scale items , rather than open-text questions. This way, the respondent has the answer options provided, and researchers can easily quantify how popular certain responses are. That being said, some closed questions could be answered through an open-text field to provide a better experience for the respondent. Consider the following closed questions:
- In which industry do you work?
- What is your gender?
Both questions could be presented as multiple-choice questions in a survey. However, the respondent might find it more comfortable to share their industry and gender in a free-text field if they feel the survey does not provide an option that directly aligns with their situation or if there are too many options to review.
Another reason closed questions are used in surveys is that they are much easier to answer than open-ended ones. A survey with many open-ended questions will usually have a lower completion rate than one with more closed questions.
Using Closed Questions in Interviews and Usability Tests
Closed questions are used occasionally in interviews and usability tests to get clarification and extra details. They are often used when asking followup questions. For example, a facilitator might ask:
- Has this happened to you before?
- When was the last time this happened?
- Was this a different time than the time you mentioned previously?
Closed questions help facilitators gather important details. However, they should be used sparingly in qualitative research as they can limit what you can learn.
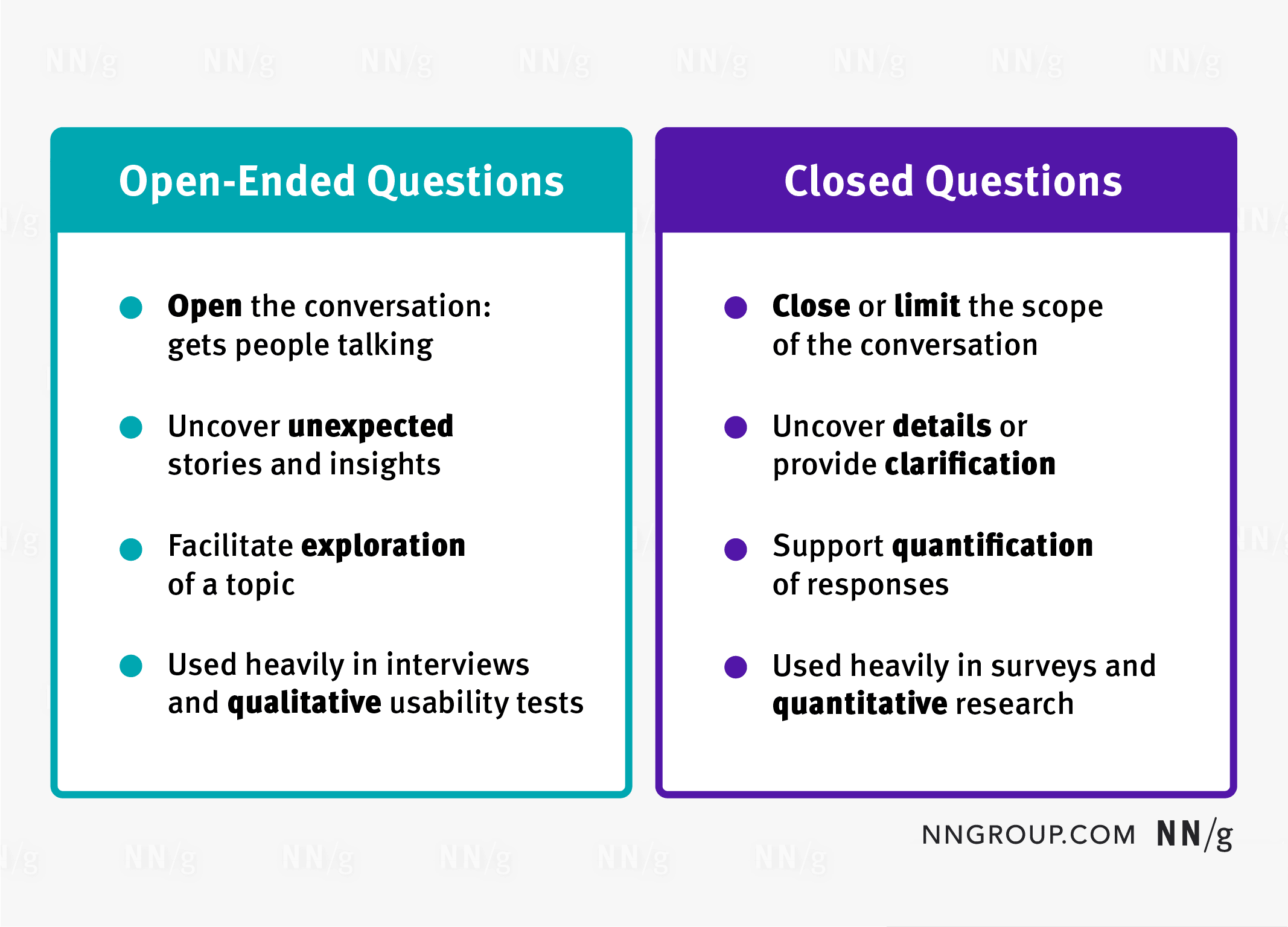
The greatest benefit of open-ended questions is that they allow you to find more than you anticipate. You don’t know what you don’t know. People may share motivations you didn’t expect and mention behaviors and concerns you knew nothing about. When you ask people to explain things, they often reveal surprising mental models , problem-solving strategies, hopes, and fears.
On the other hand, closed questions stop the conversation. If an interviewer or usability-test facilitator were to ask only closed questions, the conversation would be stilted and surface-level. The facilitator might not learn important things they didn’t think to ask because closed questions eliminate surprises: what you expect is what you get.
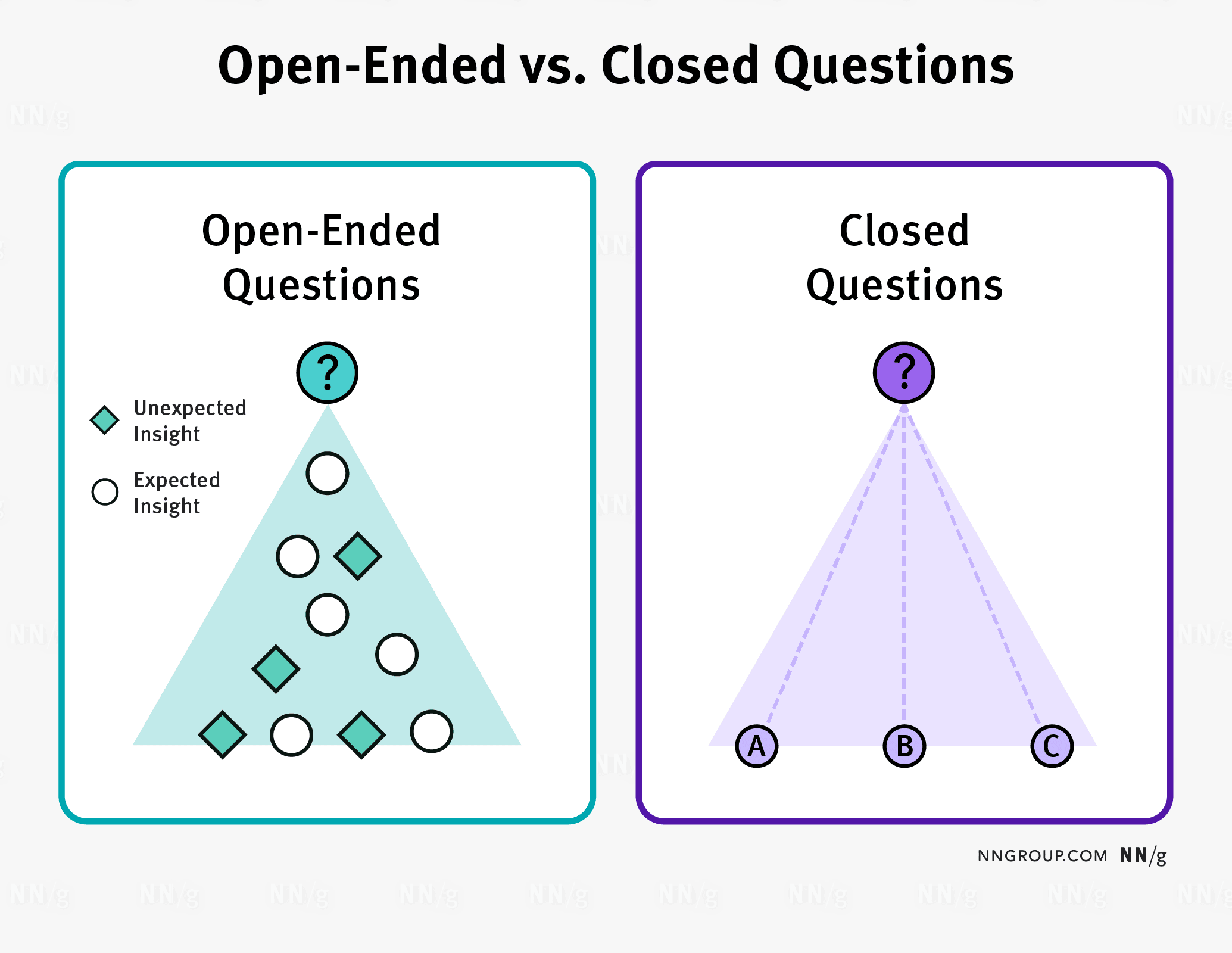
Closed Questions Can Sometimes Be Leading
When you ask closed questions, you may accidentally reveal what you’re interested in and prime participants to volunteer only specific information. This is why researchers use the funnel technique , where the session or followup questions begin with broad, open-ended questions before introducing specific, closed questions.
Not all closed questions are leading. That being said, it’s easy for a closed question to become leading if it suggests an answer.
The table below shows examples of leading closed questions . Reworking a question so it’s not leading often involves making it open-ended, as shown in column 2 of the table below.
One way to spot a leading, closed question is to look at how the question begins. Leading closed questions often start with the words “did,” “was,” or “is.” Open-ended questions often begin with “how” or “what.”
New interviewers and usability-test facilitators often struggle to ask enough open-ended questions. A new interviewer might be tempted to ask many factual, closed questions in quick succession, such as the following:
- Do you have children?
- Do you work?
- How old are you?
- Do you ever [insert behavior]?
However, these questions could be answered in response to a broad, open-ended question like Tell me a bit about yourself .
When constructing an interview guide for a user interview, try to think of a broad, open-ended version of a closed question that might get the participant talking about the question you want answered, like in the example above.
When asking questions in a usability test, try to favor questions that begin with “how,” or “what,” over “do,” or “did” like in the table below.
|
|
Another tip to help you ask open-ended questions is to use one of the following question stems :
- Walk me through [how/what]...
- Tell me a bit about…
- Tell me about a time where…
Finally, you can ask open-ended questions when probing. Probing questions are open-ended and are used in response to what a participant shares. They are designed to solicit more information. You can use the following probing questions in interviews and usability tests.
- Tell me more about that.
- What do you mean by that?
- Can you expand on that?
- What do you think about that?
- Why do you think that?
Ask open-ended questions in conversations with users to discover unanticipated answers and important insights. Use closed questions to gather additional small details, gain clarification, or when you want to analyze responses quantitatively.
Related Topics
- Research Methods Research Methods
Learn More:
Please accept marketing cookies to view the embedded video. https://www.youtube.com/watch?v=LpV3tMy_WZ0
Open vs. Closed Questions in User Research
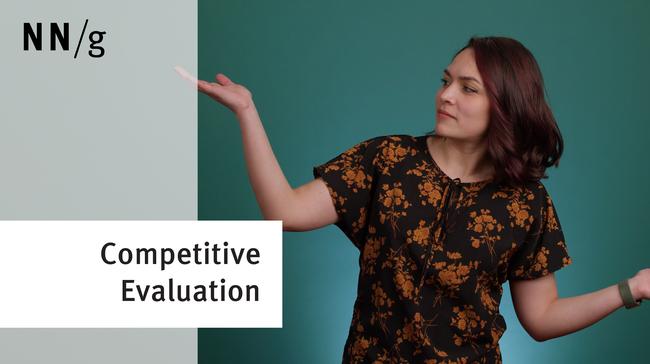
Competitive Reviews vs. Competitive Research
Therese Fessenden · 4 min
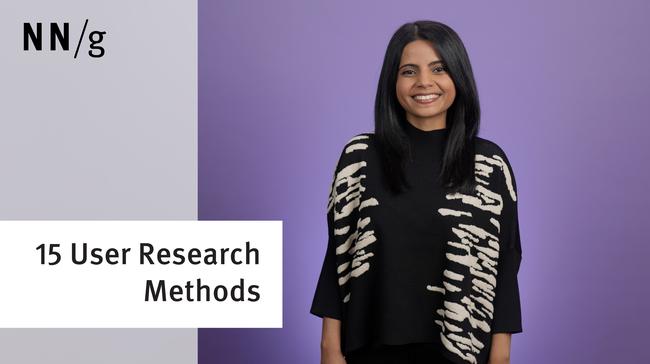
15 User Research Methods to Know Beyond Usability Testing
Samhita Tankala · 3 min
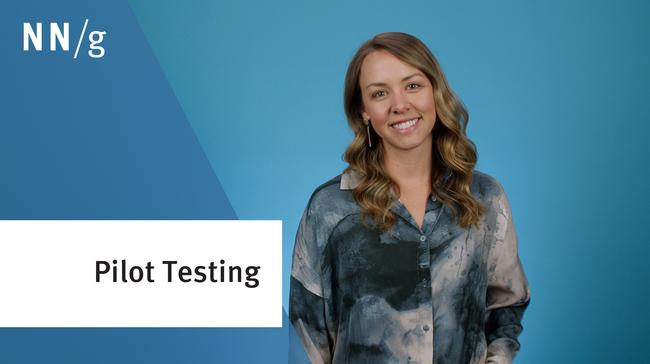
Always Pilot Test User Research Studies
Kim Flaherty · 3 min
Related Articles:
Field Studies Done Right: Fast and Observational
Jakob Nielsen · 3 min
Should You Run a Survey?
Maddie Brown · 6 min
The Funnel Technique in Qualitative User Research
Maria Rosala and Kate Moran · 7 min
Card Sorting: Pushing Users Beyond Terminology Matches
Samhita Tankala · 5 min
Card Sorting: Uncover Users' Mental Models for Better Information Architecture
Samhita Tankala and Katie Sherwin · 11 min
The Diverge-and-Converge Technique for UX Workshops
Therese Fessenden · 6 min
Frequently asked questions
What’s the difference between closed-ended and open-ended questions.
Closed-ended, or restricted-choice, questions offer respondents a fixed set of choices to select from. These questions are easier to answer quickly.
Open-ended or long-form questions allow respondents to answer in their own words. Because there are no restrictions on their choices, respondents can answer in ways that researchers may not have otherwise considered.
Frequently asked questions: Methodology
Attrition refers to participants leaving a study. It always happens to some extent—for example, in randomized controlled trials for medical research.
Differential attrition occurs when attrition or dropout rates differ systematically between the intervention and the control group . As a result, the characteristics of the participants who drop out differ from the characteristics of those who stay in the study. Because of this, study results may be biased .
Action research is conducted in order to solve a particular issue immediately, while case studies are often conducted over a longer period of time and focus more on observing and analyzing a particular ongoing phenomenon.
Action research is focused on solving a problem or informing individual and community-based knowledge in a way that impacts teaching, learning, and other related processes. It is less focused on contributing theoretical input, instead producing actionable input.
Action research is particularly popular with educators as a form of systematic inquiry because it prioritizes reflection and bridges the gap between theory and practice. Educators are able to simultaneously investigate an issue as they solve it, and the method is very iterative and flexible.
A cycle of inquiry is another name for action research . It is usually visualized in a spiral shape following a series of steps, such as “planning → acting → observing → reflecting.”
To make quantitative observations , you need to use instruments that are capable of measuring the quantity you want to observe. For example, you might use a ruler to measure the length of an object or a thermometer to measure its temperature.
Criterion validity and construct validity are both types of measurement validity . In other words, they both show you how accurately a method measures something.
While construct validity is the degree to which a test or other measurement method measures what it claims to measure, criterion validity is the degree to which a test can predictively (in the future) or concurrently (in the present) measure something.
Construct validity is often considered the overarching type of measurement validity . You need to have face validity , content validity , and criterion validity in order to achieve construct validity.
Convergent validity and discriminant validity are both subtypes of construct validity . Together, they help you evaluate whether a test measures the concept it was designed to measure.
- Convergent validity indicates whether a test that is designed to measure a particular construct correlates with other tests that assess the same or similar construct.
- Discriminant validity indicates whether two tests that should not be highly related to each other are indeed not related. This type of validity is also called divergent validity .
You need to assess both in order to demonstrate construct validity. Neither one alone is sufficient for establishing construct validity.
- Discriminant validity indicates whether two tests that should not be highly related to each other are indeed not related
Content validity shows you how accurately a test or other measurement method taps into the various aspects of the specific construct you are researching.
In other words, it helps you answer the question: “does the test measure all aspects of the construct I want to measure?” If it does, then the test has high content validity.
The higher the content validity, the more accurate the measurement of the construct.
If the test fails to include parts of the construct, or irrelevant parts are included, the validity of the instrument is threatened, which brings your results into question.
Face validity and content validity are similar in that they both evaluate how suitable the content of a test is. The difference is that face validity is subjective, and assesses content at surface level.
When a test has strong face validity, anyone would agree that the test’s questions appear to measure what they are intended to measure.
For example, looking at a 4th grade math test consisting of problems in which students have to add and multiply, most people would agree that it has strong face validity (i.e., it looks like a math test).
On the other hand, content validity evaluates how well a test represents all the aspects of a topic. Assessing content validity is more systematic and relies on expert evaluation. of each question, analyzing whether each one covers the aspects that the test was designed to cover.
A 4th grade math test would have high content validity if it covered all the skills taught in that grade. Experts(in this case, math teachers), would have to evaluate the content validity by comparing the test to the learning objectives.
Snowball sampling is a non-probability sampling method . Unlike probability sampling (which involves some form of random selection ), the initial individuals selected to be studied are the ones who recruit new participants.
Because not every member of the target population has an equal chance of being recruited into the sample, selection in snowball sampling is non-random.
Snowball sampling is a non-probability sampling method , where there is not an equal chance for every member of the population to be included in the sample .
This means that you cannot use inferential statistics and make generalizations —often the goal of quantitative research . As such, a snowball sample is not representative of the target population and is usually a better fit for qualitative research .
Snowball sampling relies on the use of referrals. Here, the researcher recruits one or more initial participants, who then recruit the next ones.
Participants share similar characteristics and/or know each other. Because of this, not every member of the population has an equal chance of being included in the sample, giving rise to sampling bias .
Snowball sampling is best used in the following cases:
- If there is no sampling frame available (e.g., people with a rare disease)
- If the population of interest is hard to access or locate (e.g., people experiencing homelessness)
- If the research focuses on a sensitive topic (e.g., extramarital affairs)
The reproducibility and replicability of a study can be ensured by writing a transparent, detailed method section and using clear, unambiguous language.
Reproducibility and replicability are related terms.
- Reproducing research entails reanalyzing the existing data in the same manner.
- Replicating (or repeating ) the research entails reconducting the entire analysis, including the collection of new data .
- A successful reproduction shows that the data analyses were conducted in a fair and honest manner.
- A successful replication shows that the reliability of the results is high.
Stratified sampling and quota sampling both involve dividing the population into subgroups and selecting units from each subgroup. The purpose in both cases is to select a representative sample and/or to allow comparisons between subgroups.
The main difference is that in stratified sampling, you draw a random sample from each subgroup ( probability sampling ). In quota sampling you select a predetermined number or proportion of units, in a non-random manner ( non-probability sampling ).
Purposive and convenience sampling are both sampling methods that are typically used in qualitative data collection.
A convenience sample is drawn from a source that is conveniently accessible to the researcher. Convenience sampling does not distinguish characteristics among the participants. On the other hand, purposive sampling focuses on selecting participants possessing characteristics associated with the research study.
The findings of studies based on either convenience or purposive sampling can only be generalized to the (sub)population from which the sample is drawn, and not to the entire population.
Random sampling or probability sampling is based on random selection. This means that each unit has an equal chance (i.e., equal probability) of being included in the sample.
On the other hand, convenience sampling involves stopping people at random, which means that not everyone has an equal chance of being selected depending on the place, time, or day you are collecting your data.
Convenience sampling and quota sampling are both non-probability sampling methods. They both use non-random criteria like availability, geographical proximity, or expert knowledge to recruit study participants.
However, in convenience sampling, you continue to sample units or cases until you reach the required sample size.
In quota sampling, you first need to divide your population of interest into subgroups (strata) and estimate their proportions (quota) in the population. Then you can start your data collection, using convenience sampling to recruit participants, until the proportions in each subgroup coincide with the estimated proportions in the population.
A sampling frame is a list of every member in the entire population . It is important that the sampling frame is as complete as possible, so that your sample accurately reflects your population.
Stratified and cluster sampling may look similar, but bear in mind that groups created in cluster sampling are heterogeneous , so the individual characteristics in the cluster vary. In contrast, groups created in stratified sampling are homogeneous , as units share characteristics.
Relatedly, in cluster sampling you randomly select entire groups and include all units of each group in your sample. However, in stratified sampling, you select some units of all groups and include them in your sample. In this way, both methods can ensure that your sample is representative of the target population .
A systematic review is secondary research because it uses existing research. You don’t collect new data yourself.
The key difference between observational studies and experimental designs is that a well-done observational study does not influence the responses of participants, while experiments do have some sort of treatment condition applied to at least some participants by random assignment .
An observational study is a great choice for you if your research question is based purely on observations. If there are ethical, logistical, or practical concerns that prevent you from conducting a traditional experiment , an observational study may be a good choice. In an observational study, there is no interference or manipulation of the research subjects, as well as no control or treatment groups .
It’s often best to ask a variety of people to review your measurements. You can ask experts, such as other researchers, or laypeople, such as potential participants, to judge the face validity of tests.
While experts have a deep understanding of research methods , the people you’re studying can provide you with valuable insights you may have missed otherwise.
Face validity is important because it’s a simple first step to measuring the overall validity of a test or technique. It’s a relatively intuitive, quick, and easy way to start checking whether a new measure seems useful at first glance.
Good face validity means that anyone who reviews your measure says that it seems to be measuring what it’s supposed to. With poor face validity, someone reviewing your measure may be left confused about what you’re measuring and why you’re using this method.
Face validity is about whether a test appears to measure what it’s supposed to measure. This type of validity is concerned with whether a measure seems relevant and appropriate for what it’s assessing only on the surface.
Statistical analyses are often applied to test validity with data from your measures. You test convergent validity and discriminant validity with correlations to see if results from your test are positively or negatively related to those of other established tests.
You can also use regression analyses to assess whether your measure is actually predictive of outcomes that you expect it to predict theoretically. A regression analysis that supports your expectations strengthens your claim of construct validity .
When designing or evaluating a measure, construct validity helps you ensure you’re actually measuring the construct you’re interested in. If you don’t have construct validity, you may inadvertently measure unrelated or distinct constructs and lose precision in your research.
Construct validity is often considered the overarching type of measurement validity , because it covers all of the other types. You need to have face validity , content validity , and criterion validity to achieve construct validity.
Construct validity is about how well a test measures the concept it was designed to evaluate. It’s one of four types of measurement validity , which includes construct validity, face validity , and criterion validity.
There are two subtypes of construct validity.
- Convergent validity : The extent to which your measure corresponds to measures of related constructs
- Discriminant validity : The extent to which your measure is unrelated or negatively related to measures of distinct constructs
Naturalistic observation is a valuable tool because of its flexibility, external validity , and suitability for topics that can’t be studied in a lab setting.
The downsides of naturalistic observation include its lack of scientific control , ethical considerations , and potential for bias from observers and subjects.
Naturalistic observation is a qualitative research method where you record the behaviors of your research subjects in real world settings. You avoid interfering or influencing anything in a naturalistic observation.
You can think of naturalistic observation as “people watching” with a purpose.
A dependent variable is what changes as a result of the independent variable manipulation in experiments . It’s what you’re interested in measuring, and it “depends” on your independent variable.
In statistics, dependent variables are also called:
- Response variables (they respond to a change in another variable)
- Outcome variables (they represent the outcome you want to measure)
- Left-hand-side variables (they appear on the left-hand side of a regression equation)
An independent variable is the variable you manipulate, control, or vary in an experimental study to explore its effects. It’s called “independent” because it’s not influenced by any other variables in the study.
Independent variables are also called:
- Explanatory variables (they explain an event or outcome)
- Predictor variables (they can be used to predict the value of a dependent variable)
- Right-hand-side variables (they appear on the right-hand side of a regression equation).
As a rule of thumb, questions related to thoughts, beliefs, and feelings work well in focus groups. Take your time formulating strong questions, paying special attention to phrasing. Be careful to avoid leading questions , which can bias your responses.
Overall, your focus group questions should be:
- Open-ended and flexible
- Impossible to answer with “yes” or “no” (questions that start with “why” or “how” are often best)
- Unambiguous, getting straight to the point while still stimulating discussion
- Unbiased and neutral
A structured interview is a data collection method that relies on asking questions in a set order to collect data on a topic. They are often quantitative in nature. Structured interviews are best used when:
- You already have a very clear understanding of your topic. Perhaps significant research has already been conducted, or you have done some prior research yourself, but you already possess a baseline for designing strong structured questions.
- You are constrained in terms of time or resources and need to analyze your data quickly and efficiently.
- Your research question depends on strong parity between participants, with environmental conditions held constant.
More flexible interview options include semi-structured interviews , unstructured interviews , and focus groups .
Social desirability bias is the tendency for interview participants to give responses that will be viewed favorably by the interviewer or other participants. It occurs in all types of interviews and surveys , but is most common in semi-structured interviews , unstructured interviews , and focus groups .
Social desirability bias can be mitigated by ensuring participants feel at ease and comfortable sharing their views. Make sure to pay attention to your own body language and any physical or verbal cues, such as nodding or widening your eyes.
This type of bias can also occur in observations if the participants know they’re being observed. They might alter their behavior accordingly.
The interviewer effect is a type of bias that emerges when a characteristic of an interviewer (race, age, gender identity, etc.) influences the responses given by the interviewee.
There is a risk of an interviewer effect in all types of interviews , but it can be mitigated by writing really high-quality interview questions.
A semi-structured interview is a blend of structured and unstructured types of interviews. Semi-structured interviews are best used when:
- You have prior interview experience. Spontaneous questions are deceptively challenging, and it’s easy to accidentally ask a leading question or make a participant uncomfortable.
- Your research question is exploratory in nature. Participant answers can guide future research questions and help you develop a more robust knowledge base for future research.
An unstructured interview is the most flexible type of interview, but it is not always the best fit for your research topic.
Unstructured interviews are best used when:
- You are an experienced interviewer and have a very strong background in your research topic, since it is challenging to ask spontaneous, colloquial questions.
- Your research question is exploratory in nature. While you may have developed hypotheses, you are open to discovering new or shifting viewpoints through the interview process.
- You are seeking descriptive data, and are ready to ask questions that will deepen and contextualize your initial thoughts and hypotheses.
- Your research depends on forming connections with your participants and making them feel comfortable revealing deeper emotions, lived experiences, or thoughts.
The four most common types of interviews are:
- Structured interviews : The questions are predetermined in both topic and order.
- Semi-structured interviews : A few questions are predetermined, but other questions aren’t planned.
- Unstructured interviews : None of the questions are predetermined.
- Focus group interviews : The questions are presented to a group instead of one individual.
Deductive reasoning is commonly used in scientific research, and it’s especially associated with quantitative research .
In research, you might have come across something called the hypothetico-deductive method . It’s the scientific method of testing hypotheses to check whether your predictions are substantiated by real-world data.
Deductive reasoning is a logical approach where you progress from general ideas to specific conclusions. It’s often contrasted with inductive reasoning , where you start with specific observations and form general conclusions.
Deductive reasoning is also called deductive logic.
There are many different types of inductive reasoning that people use formally or informally.
Here are a few common types:
- Inductive generalization : You use observations about a sample to come to a conclusion about the population it came from.
- Statistical generalization: You use specific numbers about samples to make statements about populations.
- Causal reasoning: You make cause-and-effect links between different things.
- Sign reasoning: You make a conclusion about a correlational relationship between different things.
- Analogical reasoning: You make a conclusion about something based on its similarities to something else.
Inductive reasoning is a bottom-up approach, while deductive reasoning is top-down.
Inductive reasoning takes you from the specific to the general, while in deductive reasoning, you make inferences by going from general premises to specific conclusions.
In inductive research , you start by making observations or gathering data. Then, you take a broad scan of your data and search for patterns. Finally, you make general conclusions that you might incorporate into theories.
Inductive reasoning is a method of drawing conclusions by going from the specific to the general. It’s usually contrasted with deductive reasoning, where you proceed from general information to specific conclusions.
Inductive reasoning is also called inductive logic or bottom-up reasoning.
A hypothesis states your predictions about what your research will find. It is a tentative answer to your research question that has not yet been tested. For some research projects, you might have to write several hypotheses that address different aspects of your research question.
A hypothesis is not just a guess — it should be based on existing theories and knowledge. It also has to be testable, which means you can support or refute it through scientific research methods (such as experiments, observations and statistical analysis of data).
Triangulation can help:
- Reduce research bias that comes from using a single method, theory, or investigator
- Enhance validity by approaching the same topic with different tools
- Establish credibility by giving you a complete picture of the research problem
But triangulation can also pose problems:
- It’s time-consuming and labor-intensive, often involving an interdisciplinary team.
- Your results may be inconsistent or even contradictory.
There are four main types of triangulation :
- Data triangulation : Using data from different times, spaces, and people
- Investigator triangulation : Involving multiple researchers in collecting or analyzing data
- Theory triangulation : Using varying theoretical perspectives in your research
- Methodological triangulation : Using different methodologies to approach the same topic
Many academic fields use peer review , largely to determine whether a manuscript is suitable for publication. Peer review enhances the credibility of the published manuscript.
However, peer review is also common in non-academic settings. The United Nations, the European Union, and many individual nations use peer review to evaluate grant applications. It is also widely used in medical and health-related fields as a teaching or quality-of-care measure.
Peer assessment is often used in the classroom as a pedagogical tool. Both receiving feedback and providing it are thought to enhance the learning process, helping students think critically and collaboratively.
Peer review can stop obviously problematic, falsified, or otherwise untrustworthy research from being published. It also represents an excellent opportunity to get feedback from renowned experts in your field. It acts as a first defense, helping you ensure your argument is clear and that there are no gaps, vague terms, or unanswered questions for readers who weren’t involved in the research process.
Peer-reviewed articles are considered a highly credible source due to this stringent process they go through before publication.
In general, the peer review process follows the following steps:
- First, the author submits the manuscript to the editor.
- Reject the manuscript and send it back to author, or
- Send it onward to the selected peer reviewer(s)
- Next, the peer review process occurs. The reviewer provides feedback, addressing any major or minor issues with the manuscript, and gives their advice regarding what edits should be made.
- Lastly, the edited manuscript is sent back to the author. They input the edits, and resubmit it to the editor for publication.
Exploratory research is often used when the issue you’re studying is new or when the data collection process is challenging for some reason.
You can use exploratory research if you have a general idea or a specific question that you want to study but there is no preexisting knowledge or paradigm with which to study it.
Exploratory research is a methodology approach that explores research questions that have not previously been studied in depth. It is often used when the issue you’re studying is new, or the data collection process is challenging in some way.
Explanatory research is used to investigate how or why a phenomenon occurs. Therefore, this type of research is often one of the first stages in the research process , serving as a jumping-off point for future research.
Exploratory research aims to explore the main aspects of an under-researched problem, while explanatory research aims to explain the causes and consequences of a well-defined problem.
Explanatory research is a research method used to investigate how or why something occurs when only a small amount of information is available pertaining to that topic. It can help you increase your understanding of a given topic.
Clean data are valid, accurate, complete, consistent, unique, and uniform. Dirty data include inconsistencies and errors.
Dirty data can come from any part of the research process, including poor research design , inappropriate measurement materials, or flawed data entry.
Data cleaning takes place between data collection and data analyses. But you can use some methods even before collecting data.
For clean data, you should start by designing measures that collect valid data. Data validation at the time of data entry or collection helps you minimize the amount of data cleaning you’ll need to do.
After data collection, you can use data standardization and data transformation to clean your data. You’ll also deal with any missing values, outliers, and duplicate values.
Every dataset requires different techniques to clean dirty data , but you need to address these issues in a systematic way. You focus on finding and resolving data points that don’t agree or fit with the rest of your dataset.
These data might be missing values, outliers, duplicate values, incorrectly formatted, or irrelevant. You’ll start with screening and diagnosing your data. Then, you’ll often standardize and accept or remove data to make your dataset consistent and valid.
Data cleaning is necessary for valid and appropriate analyses. Dirty data contain inconsistencies or errors , but cleaning your data helps you minimize or resolve these.
Without data cleaning, you could end up with a Type I or II error in your conclusion. These types of erroneous conclusions can be practically significant with important consequences, because they lead to misplaced investments or missed opportunities.
Data cleaning involves spotting and resolving potential data inconsistencies or errors to improve your data quality. An error is any value (e.g., recorded weight) that doesn’t reflect the true value (e.g., actual weight) of something that’s being measured.
In this process, you review, analyze, detect, modify, or remove “dirty” data to make your dataset “clean.” Data cleaning is also called data cleansing or data scrubbing.
Research misconduct means making up or falsifying data, manipulating data analyses, or misrepresenting results in research reports. It’s a form of academic fraud.
These actions are committed intentionally and can have serious consequences; research misconduct is not a simple mistake or a point of disagreement but a serious ethical failure.
Anonymity means you don’t know who the participants are, while confidentiality means you know who they are but remove identifying information from your research report. Both are important ethical considerations .
You can only guarantee anonymity by not collecting any personally identifying information—for example, names, phone numbers, email addresses, IP addresses, physical characteristics, photos, or videos.
You can keep data confidential by using aggregate information in your research report, so that you only refer to groups of participants rather than individuals.
Research ethics matter for scientific integrity, human rights and dignity, and collaboration between science and society. These principles make sure that participation in studies is voluntary, informed, and safe.
Ethical considerations in research are a set of principles that guide your research designs and practices. These principles include voluntary participation, informed consent, anonymity, confidentiality, potential for harm, and results communication.
Scientists and researchers must always adhere to a certain code of conduct when collecting data from others .
These considerations protect the rights of research participants, enhance research validity , and maintain scientific integrity.
In multistage sampling , you can use probability or non-probability sampling methods .
For a probability sample, you have to conduct probability sampling at every stage.
You can mix it up by using simple random sampling , systematic sampling , or stratified sampling to select units at different stages, depending on what is applicable and relevant to your study.
Multistage sampling can simplify data collection when you have large, geographically spread samples, and you can obtain a probability sample without a complete sampling frame.
But multistage sampling may not lead to a representative sample, and larger samples are needed for multistage samples to achieve the statistical properties of simple random samples .
These are four of the most common mixed methods designs :
- Convergent parallel: Quantitative and qualitative data are collected at the same time and analyzed separately. After both analyses are complete, compare your results to draw overall conclusions.
- Embedded: Quantitative and qualitative data are collected at the same time, but within a larger quantitative or qualitative design. One type of data is secondary to the other.
- Explanatory sequential: Quantitative data is collected and analyzed first, followed by qualitative data. You can use this design if you think your qualitative data will explain and contextualize your quantitative findings.
- Exploratory sequential: Qualitative data is collected and analyzed first, followed by quantitative data. You can use this design if you think the quantitative data will confirm or validate your qualitative findings.
Triangulation in research means using multiple datasets, methods, theories and/or investigators to address a research question. It’s a research strategy that can help you enhance the validity and credibility of your findings.
Triangulation is mainly used in qualitative research , but it’s also commonly applied in quantitative research . Mixed methods research always uses triangulation.
In multistage sampling , or multistage cluster sampling, you draw a sample from a population using smaller and smaller groups at each stage.
This method is often used to collect data from a large, geographically spread group of people in national surveys, for example. You take advantage of hierarchical groupings (e.g., from state to city to neighborhood) to create a sample that’s less expensive and time-consuming to collect data from.
No, the steepness or slope of the line isn’t related to the correlation coefficient value. The correlation coefficient only tells you how closely your data fit on a line, so two datasets with the same correlation coefficient can have very different slopes.
To find the slope of the line, you’ll need to perform a regression analysis .
Correlation coefficients always range between -1 and 1.
The sign of the coefficient tells you the direction of the relationship: a positive value means the variables change together in the same direction, while a negative value means they change together in opposite directions.
The absolute value of a number is equal to the number without its sign. The absolute value of a correlation coefficient tells you the magnitude of the correlation: the greater the absolute value, the stronger the correlation.
These are the assumptions your data must meet if you want to use Pearson’s r :
- Both variables are on an interval or ratio level of measurement
- Data from both variables follow normal distributions
- Your data have no outliers
- Your data is from a random or representative sample
- You expect a linear relationship between the two variables
Quantitative research designs can be divided into two main categories:
- Correlational and descriptive designs are used to investigate characteristics, averages, trends, and associations between variables.
- Experimental and quasi-experimental designs are used to test causal relationships .
Qualitative research designs tend to be more flexible. Common types of qualitative design include case study , ethnography , and grounded theory designs.
A well-planned research design helps ensure that your methods match your research aims, that you collect high-quality data, and that you use the right kind of analysis to answer your questions, utilizing credible sources . This allows you to draw valid , trustworthy conclusions.
The priorities of a research design can vary depending on the field, but you usually have to specify:
- Your research questions and/or hypotheses
- Your overall approach (e.g., qualitative or quantitative )
- The type of design you’re using (e.g., a survey , experiment , or case study )
- Your sampling methods or criteria for selecting subjects
- Your data collection methods (e.g., questionnaires , observations)
- Your data collection procedures (e.g., operationalization , timing and data management)
- Your data analysis methods (e.g., statistical tests or thematic analysis )
A research design is a strategy for answering your research question . It defines your overall approach and determines how you will collect and analyze data.
Questionnaires can be self-administered or researcher-administered.
Self-administered questionnaires can be delivered online or in paper-and-pen formats, in person or through mail. All questions are standardized so that all respondents receive the same questions with identical wording.
Researcher-administered questionnaires are interviews that take place by phone, in-person, or online between researchers and respondents. You can gain deeper insights by clarifying questions for respondents or asking follow-up questions.
You can organize the questions logically, with a clear progression from simple to complex, or randomly between respondents. A logical flow helps respondents process the questionnaire easier and quicker, but it may lead to bias. Randomization can minimize the bias from order effects.
A questionnaire is a data collection tool or instrument, while a survey is an overarching research method that involves collecting and analyzing data from people using questionnaires.
The third variable and directionality problems are two main reasons why correlation isn’t causation .
The third variable problem means that a confounding variable affects both variables to make them seem causally related when they are not.
The directionality problem is when two variables correlate and might actually have a causal relationship, but it’s impossible to conclude which variable causes changes in the other.
Correlation describes an association between variables : when one variable changes, so does the other. A correlation is a statistical indicator of the relationship between variables.
Causation means that changes in one variable brings about changes in the other (i.e., there is a cause-and-effect relationship between variables). The two variables are correlated with each other, and there’s also a causal link between them.
While causation and correlation can exist simultaneously, correlation does not imply causation. In other words, correlation is simply a relationship where A relates to B—but A doesn’t necessarily cause B to happen (or vice versa). Mistaking correlation for causation is a common error and can lead to false cause fallacy .
Controlled experiments establish causality, whereas correlational studies only show associations between variables.
- In an experimental design , you manipulate an independent variable and measure its effect on a dependent variable. Other variables are controlled so they can’t impact the results.
- In a correlational design , you measure variables without manipulating any of them. You can test whether your variables change together, but you can’t be sure that one variable caused a change in another.
In general, correlational research is high in external validity while experimental research is high in internal validity .
A correlation is usually tested for two variables at a time, but you can test correlations between three or more variables.
A correlation coefficient is a single number that describes the strength and direction of the relationship between your variables.
Different types of correlation coefficients might be appropriate for your data based on their levels of measurement and distributions . The Pearson product-moment correlation coefficient (Pearson’s r ) is commonly used to assess a linear relationship between two quantitative variables.
A correlational research design investigates relationships between two variables (or more) without the researcher controlling or manipulating any of them. It’s a non-experimental type of quantitative research .
A correlation reflects the strength and/or direction of the association between two or more variables.
- A positive correlation means that both variables change in the same direction.
- A negative correlation means that the variables change in opposite directions.
- A zero correlation means there’s no relationship between the variables.
Random error is almost always present in scientific studies, even in highly controlled settings. While you can’t eradicate it completely, you can reduce random error by taking repeated measurements, using a large sample, and controlling extraneous variables .
You can avoid systematic error through careful design of your sampling , data collection , and analysis procedures. For example, use triangulation to measure your variables using multiple methods; regularly calibrate instruments or procedures; use random sampling and random assignment ; and apply masking (blinding) where possible.
Systematic error is generally a bigger problem in research.
With random error, multiple measurements will tend to cluster around the true value. When you’re collecting data from a large sample , the errors in different directions will cancel each other out.
Systematic errors are much more problematic because they can skew your data away from the true value. This can lead you to false conclusions ( Type I and II errors ) about the relationship between the variables you’re studying.
Random and systematic error are two types of measurement error.
Random error is a chance difference between the observed and true values of something (e.g., a researcher misreading a weighing scale records an incorrect measurement).
Systematic error is a consistent or proportional difference between the observed and true values of something (e.g., a miscalibrated scale consistently records weights as higher than they actually are).
On graphs, the explanatory variable is conventionally placed on the x-axis, while the response variable is placed on the y-axis.
- If you have quantitative variables , use a scatterplot or a line graph.
- If your response variable is categorical, use a scatterplot or a line graph.
- If your explanatory variable is categorical, use a bar graph.
The term “ explanatory variable ” is sometimes preferred over “ independent variable ” because, in real world contexts, independent variables are often influenced by other variables. This means they aren’t totally independent.
Multiple independent variables may also be correlated with each other, so “explanatory variables” is a more appropriate term.
The difference between explanatory and response variables is simple:
- An explanatory variable is the expected cause, and it explains the results.
- A response variable is the expected effect, and it responds to other variables.
In a controlled experiment , all extraneous variables are held constant so that they can’t influence the results. Controlled experiments require:
- A control group that receives a standard treatment, a fake treatment, or no treatment.
- Random assignment of participants to ensure the groups are equivalent.
Depending on your study topic, there are various other methods of controlling variables .
There are 4 main types of extraneous variables :
- Demand characteristics : environmental cues that encourage participants to conform to researchers’ expectations.
- Experimenter effects : unintentional actions by researchers that influence study outcomes.
- Situational variables : environmental variables that alter participants’ behaviors.
- Participant variables : any characteristic or aspect of a participant’s background that could affect study results.
An extraneous variable is any variable that you’re not investigating that can potentially affect the dependent variable of your research study.
A confounding variable is a type of extraneous variable that not only affects the dependent variable, but is also related to the independent variable.
In a factorial design, multiple independent variables are tested.
If you test two variables, each level of one independent variable is combined with each level of the other independent variable to create different conditions.
Within-subjects designs have many potential threats to internal validity , but they are also very statistically powerful .
Advantages:
- Only requires small samples
- Statistically powerful
- Removes the effects of individual differences on the outcomes
Disadvantages:
- Internal validity threats reduce the likelihood of establishing a direct relationship between variables
- Time-related effects, such as growth, can influence the outcomes
- Carryover effects mean that the specific order of different treatments affect the outcomes
While a between-subjects design has fewer threats to internal validity , it also requires more participants for high statistical power than a within-subjects design .
- Prevents carryover effects of learning and fatigue.
- Shorter study duration.
- Needs larger samples for high power.
- Uses more resources to recruit participants, administer sessions, cover costs, etc.
- Individual differences may be an alternative explanation for results.
Yes. Between-subjects and within-subjects designs can be combined in a single study when you have two or more independent variables (a factorial design). In a mixed factorial design, one variable is altered between subjects and another is altered within subjects.
In a between-subjects design , every participant experiences only one condition, and researchers assess group differences between participants in various conditions.
In a within-subjects design , each participant experiences all conditions, and researchers test the same participants repeatedly for differences between conditions.
The word “between” means that you’re comparing different conditions between groups, while the word “within” means you’re comparing different conditions within the same group.
Random assignment is used in experiments with a between-groups or independent measures design. In this research design, there’s usually a control group and one or more experimental groups. Random assignment helps ensure that the groups are comparable.
In general, you should always use random assignment in this type of experimental design when it is ethically possible and makes sense for your study topic.
To implement random assignment , assign a unique number to every member of your study’s sample .
Then, you can use a random number generator or a lottery method to randomly assign each number to a control or experimental group. You can also do so manually, by flipping a coin or rolling a dice to randomly assign participants to groups.
Random selection, or random sampling , is a way of selecting members of a population for your study’s sample.
In contrast, random assignment is a way of sorting the sample into control and experimental groups.
Random sampling enhances the external validity or generalizability of your results, while random assignment improves the internal validity of your study.
In experimental research, random assignment is a way of placing participants from your sample into different groups using randomization. With this method, every member of the sample has a known or equal chance of being placed in a control group or an experimental group.
“Controlling for a variable” means measuring extraneous variables and accounting for them statistically to remove their effects on other variables.
Researchers often model control variable data along with independent and dependent variable data in regression analyses and ANCOVAs . That way, you can isolate the control variable’s effects from the relationship between the variables of interest.
Control variables help you establish a correlational or causal relationship between variables by enhancing internal validity .
If you don’t control relevant extraneous variables , they may influence the outcomes of your study, and you may not be able to demonstrate that your results are really an effect of your independent variable .
A control variable is any variable that’s held constant in a research study. It’s not a variable of interest in the study, but it’s controlled because it could influence the outcomes.
Including mediators and moderators in your research helps you go beyond studying a simple relationship between two variables for a fuller picture of the real world. They are important to consider when studying complex correlational or causal relationships.
Mediators are part of the causal pathway of an effect, and they tell you how or why an effect takes place. Moderators usually help you judge the external validity of your study by identifying the limitations of when the relationship between variables holds.
If something is a mediating variable :
- It’s caused by the independent variable .
- It influences the dependent variable
- When it’s taken into account, the statistical correlation between the independent and dependent variables is higher than when it isn’t considered.
A confounder is a third variable that affects variables of interest and makes them seem related when they are not. In contrast, a mediator is the mechanism of a relationship between two variables: it explains the process by which they are related.
A mediator variable explains the process through which two variables are related, while a moderator variable affects the strength and direction of that relationship.
There are three key steps in systematic sampling :
- Define and list your population , ensuring that it is not ordered in a cyclical or periodic order.
- Decide on your sample size and calculate your interval, k , by dividing your population by your target sample size.
- Choose every k th member of the population as your sample.
Systematic sampling is a probability sampling method where researchers select members of the population at a regular interval – for example, by selecting every 15th person on a list of the population. If the population is in a random order, this can imitate the benefits of simple random sampling .
Yes, you can create a stratified sample using multiple characteristics, but you must ensure that every participant in your study belongs to one and only one subgroup. In this case, you multiply the numbers of subgroups for each characteristic to get the total number of groups.
For example, if you were stratifying by location with three subgroups (urban, rural, or suburban) and marital status with five subgroups (single, divorced, widowed, married, or partnered), you would have 3 x 5 = 15 subgroups.
You should use stratified sampling when your sample can be divided into mutually exclusive and exhaustive subgroups that you believe will take on different mean values for the variable that you’re studying.
Using stratified sampling will allow you to obtain more precise (with lower variance ) statistical estimates of whatever you are trying to measure.
For example, say you want to investigate how income differs based on educational attainment, but you know that this relationship can vary based on race. Using stratified sampling, you can ensure you obtain a large enough sample from each racial group, allowing you to draw more precise conclusions.
In stratified sampling , researchers divide subjects into subgroups called strata based on characteristics that they share (e.g., race, gender, educational attainment).
Once divided, each subgroup is randomly sampled using another probability sampling method.
Cluster sampling is more time- and cost-efficient than other probability sampling methods , particularly when it comes to large samples spread across a wide geographical area.
However, it provides less statistical certainty than other methods, such as simple random sampling , because it is difficult to ensure that your clusters properly represent the population as a whole.
There are three types of cluster sampling : single-stage, double-stage and multi-stage clustering. In all three types, you first divide the population into clusters, then randomly select clusters for use in your sample.
- In single-stage sampling , you collect data from every unit within the selected clusters.
- In double-stage sampling , you select a random sample of units from within the clusters.
- In multi-stage sampling , you repeat the procedure of randomly sampling elements from within the clusters until you have reached a manageable sample.
Cluster sampling is a probability sampling method in which you divide a population into clusters, such as districts or schools, and then randomly select some of these clusters as your sample.
The clusters should ideally each be mini-representations of the population as a whole.
If properly implemented, simple random sampling is usually the best sampling method for ensuring both internal and external validity . However, it can sometimes be impractical and expensive to implement, depending on the size of the population to be studied,
If you have a list of every member of the population and the ability to reach whichever members are selected, you can use simple random sampling.
The American Community Survey is an example of simple random sampling . In order to collect detailed data on the population of the US, the Census Bureau officials randomly select 3.5 million households per year and use a variety of methods to convince them to fill out the survey.
Simple random sampling is a type of probability sampling in which the researcher randomly selects a subset of participants from a population . Each member of the population has an equal chance of being selected. Data is then collected from as large a percentage as possible of this random subset.
Quasi-experimental design is most useful in situations where it would be unethical or impractical to run a true experiment .
Quasi-experiments have lower internal validity than true experiments, but they often have higher external validity as they can use real-world interventions instead of artificial laboratory settings.
A quasi-experiment is a type of research design that attempts to establish a cause-and-effect relationship. The main difference with a true experiment is that the groups are not randomly assigned.
Blinding is important to reduce research bias (e.g., observer bias , demand characteristics ) and ensure a study’s internal validity .
If participants know whether they are in a control or treatment group , they may adjust their behavior in ways that affect the outcome that researchers are trying to measure. If the people administering the treatment are aware of group assignment, they may treat participants differently and thus directly or indirectly influence the final results.
- In a single-blind study , only the participants are blinded.
- In a double-blind study , both participants and experimenters are blinded.
- In a triple-blind study , the assignment is hidden not only from participants and experimenters, but also from the researchers analyzing the data.
Blinding means hiding who is assigned to the treatment group and who is assigned to the control group in an experiment .
A true experiment (a.k.a. a controlled experiment) always includes at least one control group that doesn’t receive the experimental treatment.
However, some experiments use a within-subjects design to test treatments without a control group. In these designs, you usually compare one group’s outcomes before and after a treatment (instead of comparing outcomes between different groups).
For strong internal validity , it’s usually best to include a control group if possible. Without a control group, it’s harder to be certain that the outcome was caused by the experimental treatment and not by other variables.
An experimental group, also known as a treatment group, receives the treatment whose effect researchers wish to study, whereas a control group does not. They should be identical in all other ways.
Individual Likert-type questions are generally considered ordinal data , because the items have clear rank order, but don’t have an even distribution.
Overall Likert scale scores are sometimes treated as interval data. These scores are considered to have directionality and even spacing between them.
The type of data determines what statistical tests you should use to analyze your data.
A Likert scale is a rating scale that quantitatively assesses opinions, attitudes, or behaviors. It is made up of 4 or more questions that measure a single attitude or trait when response scores are combined.
To use a Likert scale in a survey , you present participants with Likert-type questions or statements, and a continuum of items, usually with 5 or 7 possible responses, to capture their degree of agreement.
In scientific research, concepts are the abstract ideas or phenomena that are being studied (e.g., educational achievement). Variables are properties or characteristics of the concept (e.g., performance at school), while indicators are ways of measuring or quantifying variables (e.g., yearly grade reports).
The process of turning abstract concepts into measurable variables and indicators is called operationalization .
There are various approaches to qualitative data analysis , but they all share five steps in common:
- Prepare and organize your data.
- Review and explore your data.
- Develop a data coding system.
- Assign codes to the data.
- Identify recurring themes.
The specifics of each step depend on the focus of the analysis. Some common approaches include textual analysis , thematic analysis , and discourse analysis .
There are five common approaches to qualitative research :
- Grounded theory involves collecting data in order to develop new theories.
- Ethnography involves immersing yourself in a group or organization to understand its culture.
- Narrative research involves interpreting stories to understand how people make sense of their experiences and perceptions.
- Phenomenological research involves investigating phenomena through people’s lived experiences.
- Action research links theory and practice in several cycles to drive innovative changes.
Hypothesis testing is a formal procedure for investigating our ideas about the world using statistics. It is used by scientists to test specific predictions, called hypotheses , by calculating how likely it is that a pattern or relationship between variables could have arisen by chance.
Operationalization means turning abstract conceptual ideas into measurable observations.
For example, the concept of social anxiety isn’t directly observable, but it can be operationally defined in terms of self-rating scores, behavioral avoidance of crowded places, or physical anxiety symptoms in social situations.
Before collecting data , it’s important to consider how you will operationalize the variables that you want to measure.
When conducting research, collecting original data has significant advantages:
- You can tailor data collection to your specific research aims (e.g. understanding the needs of your consumers or user testing your website)
- You can control and standardize the process for high reliability and validity (e.g. choosing appropriate measurements and sampling methods )
However, there are also some drawbacks: data collection can be time-consuming, labor-intensive and expensive. In some cases, it’s more efficient to use secondary data that has already been collected by someone else, but the data might be less reliable.
Data collection is the systematic process by which observations or measurements are gathered in research. It is used in many different contexts by academics, governments, businesses, and other organizations.
There are several methods you can use to decrease the impact of confounding variables on your research: restriction, matching, statistical control and randomization.
In restriction , you restrict your sample by only including certain subjects that have the same values of potential confounding variables.
In matching , you match each of the subjects in your treatment group with a counterpart in the comparison group. The matched subjects have the same values on any potential confounding variables, and only differ in the independent variable .
In statistical control , you include potential confounders as variables in your regression .
In randomization , you randomly assign the treatment (or independent variable) in your study to a sufficiently large number of subjects, which allows you to control for all potential confounding variables.
A confounding variable is closely related to both the independent and dependent variables in a study. An independent variable represents the supposed cause , while the dependent variable is the supposed effect . A confounding variable is a third variable that influences both the independent and dependent variables.
Failing to account for confounding variables can cause you to wrongly estimate the relationship between your independent and dependent variables.
To ensure the internal validity of your research, you must consider the impact of confounding variables. If you fail to account for them, you might over- or underestimate the causal relationship between your independent and dependent variables , or even find a causal relationship where none exists.
Yes, but including more than one of either type requires multiple research questions .
For example, if you are interested in the effect of a diet on health, you can use multiple measures of health: blood sugar, blood pressure, weight, pulse, and many more. Each of these is its own dependent variable with its own research question.
You could also choose to look at the effect of exercise levels as well as diet, or even the additional effect of the two combined. Each of these is a separate independent variable .
To ensure the internal validity of an experiment , you should only change one independent variable at a time.
No. The value of a dependent variable depends on an independent variable, so a variable cannot be both independent and dependent at the same time. It must be either the cause or the effect, not both!
You want to find out how blood sugar levels are affected by drinking diet soda and regular soda, so you conduct an experiment .
- The type of soda – diet or regular – is the independent variable .
- The level of blood sugar that you measure is the dependent variable – it changes depending on the type of soda.
Determining cause and effect is one of the most important parts of scientific research. It’s essential to know which is the cause – the independent variable – and which is the effect – the dependent variable.
In non-probability sampling , the sample is selected based on non-random criteria, and not every member of the population has a chance of being included.
Common non-probability sampling methods include convenience sampling , voluntary response sampling, purposive sampling , snowball sampling, and quota sampling .
Probability sampling means that every member of the target population has a known chance of being included in the sample.
Probability sampling methods include simple random sampling , systematic sampling , stratified sampling , and cluster sampling .
Using careful research design and sampling procedures can help you avoid sampling bias . Oversampling can be used to correct undercoverage bias .
Some common types of sampling bias include self-selection bias , nonresponse bias , undercoverage bias , survivorship bias , pre-screening or advertising bias, and healthy user bias.
Sampling bias is a threat to external validity – it limits the generalizability of your findings to a broader group of people.
A sampling error is the difference between a population parameter and a sample statistic .
A statistic refers to measures about the sample , while a parameter refers to measures about the population .
Populations are used when a research question requires data from every member of the population. This is usually only feasible when the population is small and easily accessible.
Samples are used to make inferences about populations . Samples are easier to collect data from because they are practical, cost-effective, convenient, and manageable.
There are seven threats to external validity : selection bias , history, experimenter effect, Hawthorne effect , testing effect, aptitude-treatment and situation effect.
The two types of external validity are population validity (whether you can generalize to other groups of people) and ecological validity (whether you can generalize to other situations and settings).
The external validity of a study is the extent to which you can generalize your findings to different groups of people, situations, and measures.
Cross-sectional studies cannot establish a cause-and-effect relationship or analyze behavior over a period of time. To investigate cause and effect, you need to do a longitudinal study or an experimental study .
Cross-sectional studies are less expensive and time-consuming than many other types of study. They can provide useful insights into a population’s characteristics and identify correlations for further research.
Sometimes only cross-sectional data is available for analysis; other times your research question may only require a cross-sectional study to answer it.
Longitudinal studies can last anywhere from weeks to decades, although they tend to be at least a year long.
The 1970 British Cohort Study , which has collected data on the lives of 17,000 Brits since their births in 1970, is one well-known example of a longitudinal study .
Longitudinal studies are better to establish the correct sequence of events, identify changes over time, and provide insight into cause-and-effect relationships, but they also tend to be more expensive and time-consuming than other types of studies.
Longitudinal studies and cross-sectional studies are two different types of research design . In a cross-sectional study you collect data from a population at a specific point in time; in a longitudinal study you repeatedly collect data from the same sample over an extended period of time.
Longitudinal study | Cross-sectional study |
---|---|
observations | Observations at a in time |
Observes the multiple times | Observes (a “cross-section”) in the population |
Follows in participants over time | Provides of society at a given point |
There are eight threats to internal validity : history, maturation, instrumentation, testing, selection bias , regression to the mean, social interaction and attrition .
Internal validity is the extent to which you can be confident that a cause-and-effect relationship established in a study cannot be explained by other factors.
In mixed methods research , you use both qualitative and quantitative data collection and analysis methods to answer your research question .
The research methods you use depend on the type of data you need to answer your research question .
- If you want to measure something or test a hypothesis , use quantitative methods . If you want to explore ideas, thoughts and meanings, use qualitative methods .
- If you want to analyze a large amount of readily-available data, use secondary data. If you want data specific to your purposes with control over how it is generated, collect primary data.
- If you want to establish cause-and-effect relationships between variables , use experimental methods. If you want to understand the characteristics of a research subject, use descriptive methods.
A confounding variable , also called a confounder or confounding factor, is a third variable in a study examining a potential cause-and-effect relationship.
A confounding variable is related to both the supposed cause and the supposed effect of the study. It can be difficult to separate the true effect of the independent variable from the effect of the confounding variable.
In your research design , it’s important to identify potential confounding variables and plan how you will reduce their impact.
Discrete and continuous variables are two types of quantitative variables :
- Discrete variables represent counts (e.g. the number of objects in a collection).
- Continuous variables represent measurable amounts (e.g. water volume or weight).
Quantitative variables are any variables where the data represent amounts (e.g. height, weight, or age).
Categorical variables are any variables where the data represent groups. This includes rankings (e.g. finishing places in a race), classifications (e.g. brands of cereal), and binary outcomes (e.g. coin flips).
You need to know what type of variables you are working with to choose the right statistical test for your data and interpret your results .
You can think of independent and dependent variables in terms of cause and effect: an independent variable is the variable you think is the cause , while a dependent variable is the effect .
In an experiment, you manipulate the independent variable and measure the outcome in the dependent variable. For example, in an experiment about the effect of nutrients on crop growth:
- The independent variable is the amount of nutrients added to the crop field.
- The dependent variable is the biomass of the crops at harvest time.
Defining your variables, and deciding how you will manipulate and measure them, is an important part of experimental design .
Experimental design means planning a set of procedures to investigate a relationship between variables . To design a controlled experiment, you need:
- A testable hypothesis
- At least one independent variable that can be precisely manipulated
- At least one dependent variable that can be precisely measured
When designing the experiment, you decide:
- How you will manipulate the variable(s)
- How you will control for any potential confounding variables
- How many subjects or samples will be included in the study
- How subjects will be assigned to treatment levels
Experimental design is essential to the internal and external validity of your experiment.
I nternal validity is the degree of confidence that the causal relationship you are testing is not influenced by other factors or variables .
External validity is the extent to which your results can be generalized to other contexts.
The validity of your experiment depends on your experimental design .
Reliability and validity are both about how well a method measures something:
- Reliability refers to the consistency of a measure (whether the results can be reproduced under the same conditions).
- Validity refers to the accuracy of a measure (whether the results really do represent what they are supposed to measure).
If you are doing experimental research, you also have to consider the internal and external validity of your experiment.
A sample is a subset of individuals from a larger population . Sampling means selecting the group that you will actually collect data from in your research. For example, if you are researching the opinions of students in your university, you could survey a sample of 100 students.
In statistics, sampling allows you to test a hypothesis about the characteristics of a population.
Quantitative research deals with numbers and statistics, while qualitative research deals with words and meanings.
Quantitative methods allow you to systematically measure variables and test hypotheses . Qualitative methods allow you to explore concepts and experiences in more detail.
Methodology refers to the overarching strategy and rationale of your research project . It involves studying the methods used in your field and the theories or principles behind them, in order to develop an approach that matches your objectives.
Methods are the specific tools and procedures you use to collect and analyze data (for example, experiments, surveys , and statistical tests ).
In shorter scientific papers, where the aim is to report the findings of a specific study, you might simply describe what you did in a methods section .
In a longer or more complex research project, such as a thesis or dissertation , you will probably include a methodology section , where you explain your approach to answering the research questions and cite relevant sources to support your choice of methods.
Ask our team
Want to contact us directly? No problem. We are always here for you.
- Email [email protected]
- Start live chat
- Call +1 (510) 822-8066
- WhatsApp +31 20 261 6040
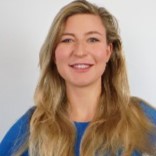
Our team helps students graduate by offering:
- A world-class citation generator
- Plagiarism Checker software powered by Turnitin
- Innovative Citation Checker software
- Professional proofreading services
- Over 300 helpful articles about academic writing, citing sources, plagiarism, and more
Scribbr specializes in editing study-related documents . We proofread:
- PhD dissertations
- Research proposals
- Personal statements
- Admission essays
- Motivation letters
- Reflection papers
- Journal articles
- Capstone projects
Scribbr’s Plagiarism Checker is powered by elements of Turnitin’s Similarity Checker , namely the plagiarism detection software and the Internet Archive and Premium Scholarly Publications content databases .
The add-on AI detector is powered by Scribbr’s proprietary software.
The Scribbr Citation Generator is developed using the open-source Citation Style Language (CSL) project and Frank Bennett’s citeproc-js . It’s the same technology used by dozens of other popular citation tools, including Mendeley and Zotero.
You can find all the citation styles and locales used in the Scribbr Citation Generator in our publicly accessible repository on Github .
All You Need to Know About Closed-Ended and Open-Ended Questions

The most challenging part about designing any survey is to identify and create the right set of questions. Typically, there are two types of survey questions – open-ended questions and closed-ended questions.
Let’s take a closer look at the following questions:
- What are closed-ended and open-ended questions?
- When do we use closed-ended and open-ended questions?
- What are some examples of the two question types?
What Are Closed-Ended and Open-Ended Questions?
Closed-ended questions ask the respondent to choose from a discrete set of responses, such as between “yes/no” or among a multiple-choice list. Open-ended questions , on the other hand, capture the respondents’ opinions and comments without suggesting a preset list of answers.
Typically, closed-ended questions are used to gather facts about the respondent, while open-ended questions help gain their opinions or feedback. However, you should pick the most applicable question type on a case-by-case basis, depending on the objective of your survey.
Why Use Closed-Ended Questions?
Most questions in a survey are closed ended because they help gather actionable, quantitative data. Let’s look at specific instances where closed-ended questions are useful.
Gain Quantitative Insights
Since closed-ended questions have discrete responses, you can analyze these responses by assigning a number or a value to every answer. This makes it easy to compare responses of different individuals which, in turn, enables statistical analysis of survey findings.
For example, if respondents were to rate a product from 1 to 5 (where 1=Horrible, 2=Bad, 3=Average, 4=Good, and 5=Excellent), an average rating of 2.5 would suggest that the product is perceived as below average. Further, a high standard deviation would imply that people’s perceptions are extreme.
Here’s why quantitative research is indispensable to your study.
Limit the Set of Responses
Closed-ended questions have a specific set of responses. Limiting the scope of possible responses helps remove ambiguity, ensures consistency, and allows the study of distribution of a certain parameter across the population.
For example, if you ask the open-ended question “Tell me about your internet usage”, you will end up with lots of unique responses (such as “2 hours per day”, “all the time”, “when I feel like it”) that cannot be analyzed easily. Instead, you can use the multiple choice question “How many hours per week do you use the internet?” with response options like “0-5 hours”, “5-10 hours”, and so on. Then you can easily analyze the data and report a clear result like “63% of respondents use the internet less than 5 hours per week”.
Conduct Large-Scale Surveys
Since closed-ended questions typically gather facts about a respondent, they take less time to respond to and are preferable when the size of the sample population is quite large.
For example, the Census of India gathers information on household assets using a multiple-choice list with the names of items (such as fan, AC, mobile phone, landline connection, 2-wheeler, 4-wheeler, and so on). The respondents can quickly tick all the items that they own in this list, rather than recalling all items they own and listing them in an open-ended question. Saving even one minute per survey can save weeks of time for the Census, which surveys 97-98% of India’s population.
Examples of Closed-Ended Questions
Dichotomous.
Do you think this product would be useful?
Multiple Choice
What are the top reasons for you to purchase a product?
- Ease of use
- Please specify: ________________
Please rate your use of this product from 1 to 6:
- [2] Twice a week
- [3] Once a week
- [4] Twice a month
- [5] Once every six months
Likert Scale
Indicate your agreement with the following statement by circling the number on the scale which most closely represents your opinion.
Do you believe adding this additional feature will make this product useful?
- [1] Strongly disagree
- [2] Disagree
- [3] No opinion
- [5] Strongly agree
A tabular Likert scale is useful when multiple questions have the same set of options.

Semantic Differential Scale
Indicate your opinion about this additional feature:

Rank these cities in the order of where you’d like to live. (“1” indicates the highest preference, “5” indicates the lowest preference.)
Which of the following would you like to see in a cafeteria? (Check all that apply)
- Cold sandwiches
- Hot sandwiches
- Soups and salads
Why Use Open-Ended Questions?
Open-ended questions help identify additional concerns or opinions from the respondent that have not been captured by closed-ended questions in the survey. Let’s look at some particular instances when open-ended questions are useful.
Small Surveys
Open-ended questions are useful for surveys where you want to gather detailed opinions from a small set of people. For example, an HR personnel looking to improve the quality of communication between employees can include open-ended questions to gain critical insights on communication barriers in a team.
Pilot Studies
A pilot or preliminary research study helps you test your survey, identify and fix data quality issues, and better understand your survey audience before conducting a large-scale study. Open-ended questions are useful in pilots, since they can help you learn which questions to ask and how to structure them in your final survey.
For example, before conducting a large-scale survey on a particular product with a sample size of 5,000 respondents, you can conduct a pilot survey with a sample size of 50 respondents. The insights from this sample study can be used to design an appropriate questionnaire for the larger sample.
Here are 11 tips you should know before you pilot your survey.
Comments Section
It is usually a good idea in any survey to leave an open-ended comments section at the end of a survey. A comments section is important to gain other opinions that may be useful for your research. For example, if your survey consists mainly of closed-ended questions on the features of a product, you can add an open-ended question at the end asking for the respondent’s opinion on any additional features that they might like or prefer in the product.
Examples of Open-Ended Questions
- What are some of the most important decisions you’ve made related to your child’s education?
- What is your opinion about the current political situation?
- How would you react to these outcomes?
- What is your definition of success?
Although open-ended questions allow survey participants to elaborate on a particular issue, closed-ended questions are essential for uncovering quantitative insights from large samples of the population. It is probably best to use a combination of the two, with more closed-ended survey questions for large-scale surveys.
Photo by Gradikaa on Unsplash
Note : This article was originally published on 23 May 2016, then refreshed and updated on 09 October 2017.

Amrutha is a technology evangelist by profession and prolific blogger by choice. She is deeply passionate about writing on social issues. She believes in finding and executing structured solutions to the modern day environment and societal problems. In her free time she enjoys playing badminton and practicing Ashtanga yoga.
Related Posts

3 Myths About Paper-Based Data Collection

A Complete Guide to Quantitative Research Methods

18 Data Validations That Will Help You Collect Accurate Data
Dear Sir/ Madam, Greetings from Seva Mandir, Really a very good information about close and open ended questions for any survey. For the first time I read about this in detail and it clears my concepts. Thanks Dr. Kusum Mathur, Incharge Health Programmes, Seva Mandir Udaipur
Thank you for this very important ebook that ghive us all the tools to succeed our open-ended questions, etc. Big up to you. Mamadou Faye, from Senegal
It’s a good compiled information for every social student
very very good and very useful guide knowledge
Write A Comment Cancel Reply
Save my name, email, and website in this browser for the next time I comment.
This site uses Akismet to reduce spam. Learn how your comment data is processed .
- The Future of the Modern Data Stack in 2023
- The Third-Generation Data Catalog Primer
- The Secrets of a Modern Data Leader
- The Ultimate Guide to Evaluating Data Lineage
- How Active Metadata Helps Modern Organizations Embrace the DataOps Way
- Inside Atlan
Type above and press Enter to search. Press Esc to cancel.
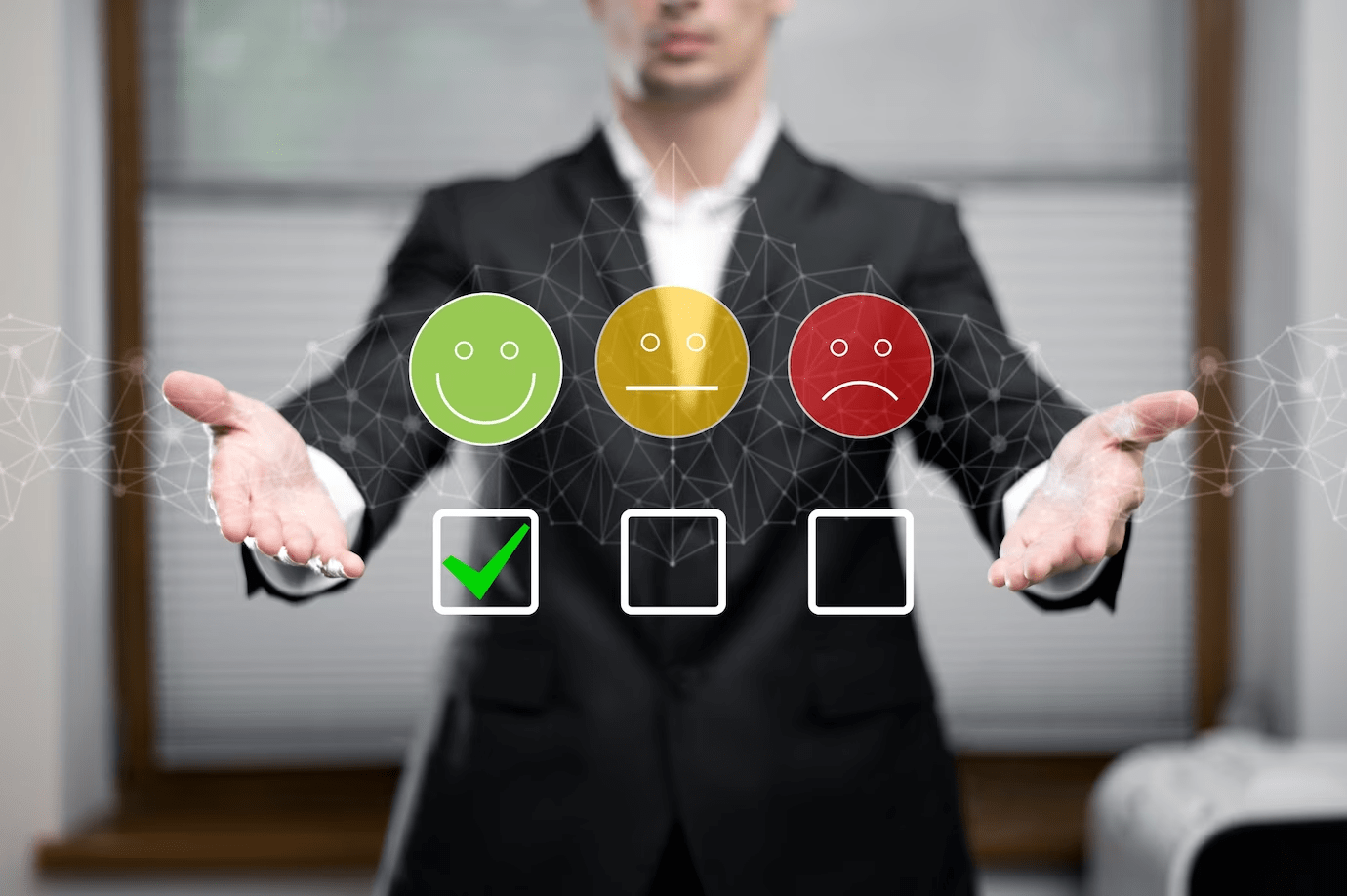
Close-Ended Questions: Benefits, Use Cases, And Examples
What are close-ended questions, close-ended question types, pros and cons of close-ended questions, when to use close-ended questions, how to create effective close-ended questions, 5 closed-ended questions examples, close-ended vs. open-ended questions - which ones are better for me, how can fullsession's tools help you gather customer feedback, install your first website feedback form right now, fullsession pricing plans, faqs in relation to close-ended questions.
Close-ended questions are the "essence" of each survey and poll, as they can bring the numbers and help you gain more data-based insights and eliminate all the guesswork.
In this article, we'll see what close-ended questions are, go through the main types and use cases, and finish up with some valuable examples that can help you make your customers loyal advocates of the brand.
Close-ended questions are a type of inquiry that limits respondents to a set of predefined answers, allowing for straightforward, concise responses. These questions are often formatted as yes/no, multiple-choice, or rating scale queries.
They are particularly useful in surveys, polls, and research contexts where statistical analysis is required, as they allow researchers to gather quantitative data that is easy to measure and compare. Their structure shines when clarity is key because they leave little room for misinterpretation, as a straightforward question gets a straightforward answer.
Digging deeper into their design reveals why these questions work well in surveys and polls. Like multiple-choice tests back in school, they offer limited responses—A, B, C, or D—and who doesn't remember filling out those Scantron sheets?
In practice, this means asking something like "Do you use social media daily?" The possible answers could be 'Yes' or 'No'. This binary setup makes tallying results faster. And can lift the survey response rate, too!
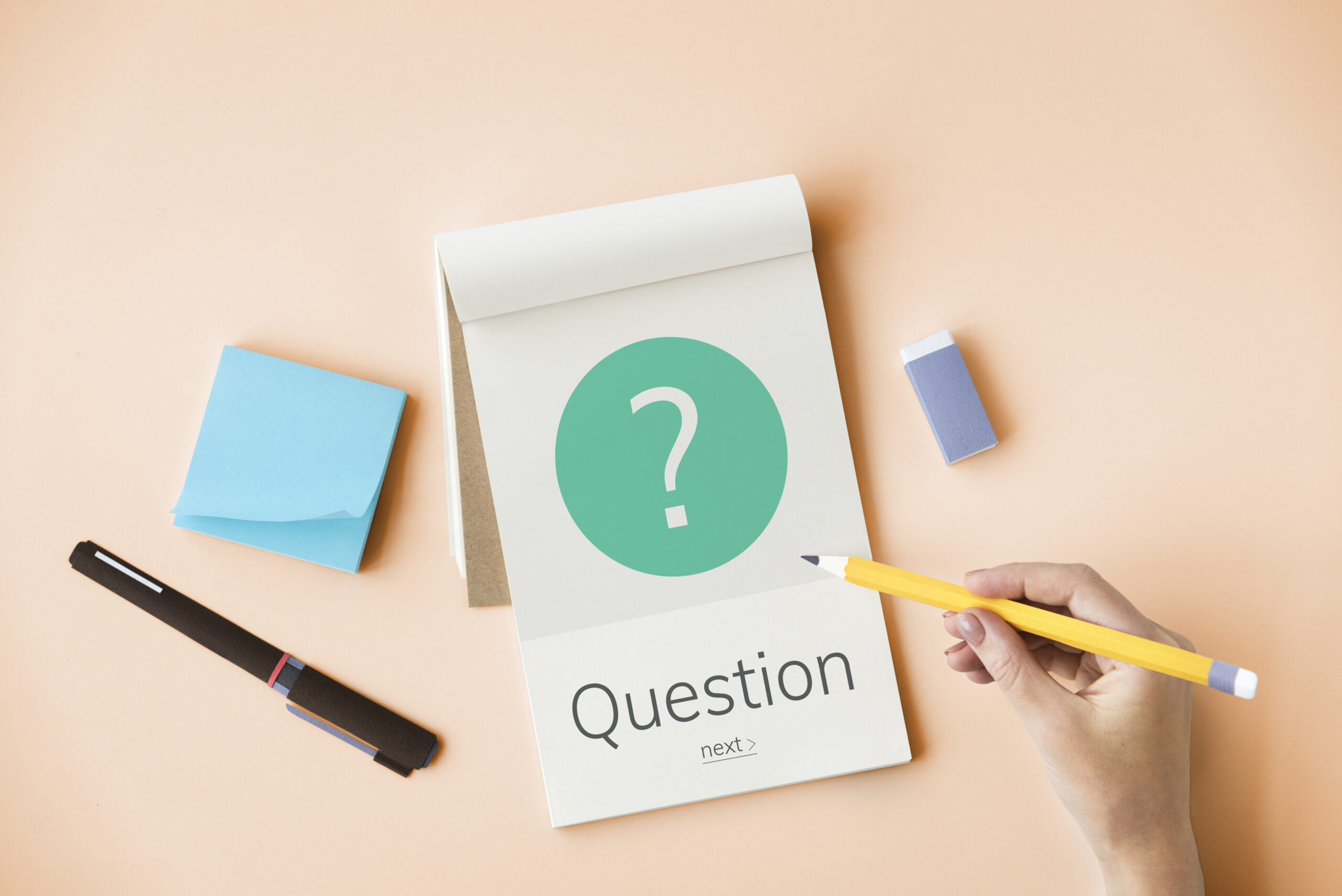
Closed-ended questions are an essential tool in research, surveys , and various forms of assessments. They provide a limited set of options for respondents, ensuring ease of answering and simplicity in data analysis. There are several types of closed-ended questions, each serving different purposes:
- Yes/No Questions: The simplest form of closed-ended questions, they require respondents to choose between 'Yes' or 'No'. These questions are useful for gathering straightforward, binary responses.
- Multiple Choice Questions (MCQs): These questions offer respondents a list of predefined choices and ask them to select one or more that apply. MCQs are versatile and can be used to gather information on preferences, opinions, and behaviors.
- Rating Scale Questions: Often used to measure attitudes or the intensity of feelings, these questions ask respondents to rate their responses on a scale. The scale could be numerical (e.g., 1 to 5) or descriptive (e.g., from 'Strongly Agree' to 'Strongly Disagree').
- Likert Scale Questions: A specific type of rating scale, Likert scales measure how strongly respondents agree or disagree with a statement. This format is widely used in surveys to gauge public opinion or attitudes.
- Rank Order Questions: These questions ask respondents to rank a set of items in order of preference or importance. This type is useful when the goal is to understand preferences or priorities among multiple options.
- Dichotomous Close-Ended Questions: Similar to yes/no questions, dichotomous questions offer two opposing options like 'True/False' or 'Agree/Disagree'. They are straightforward and easy to analyze.
- Semantic Differential Scale: This type uses a scale with two opposite adjectives at each end (e.g., 'Happy-Sad'). Respondents choose where their opinion falls on this continuum.
Closed-ended questions are widely used in various data-gathering activities for their specific benefits and drawbacks.
- Higher Response Rate: Closed-ended questions tend to yield higher response rates as they are quick and easy for respondents to answer, especially in large-scale surveys.
- Ease of Data Analysis: The uniformity of answers makes it easy to collect quantitive data, analyze it properly, and generate statistics and graphs.
- Simplification of Data Collection: These questions streamline the data collection process, making it possible to automate responses, which is particularly beneficial in quantitative research.
- Efficient Answer Options: They provide predefined options that can reduce the ambiguity in responses and minimize the effort required to interpret answers.
- Limited Insight: Respondents are restricted to the provided options, which can limit the depth of understanding and insight into their true opinions or experiences.
- Potential for Misinterpretation: The fixed nature of answer choices may not fully capture the nuance of respondents' feelings or thoughts, leading to possible misinterpretation of the data.
- Bias in Question Formation: The way questions and answer options are framed can introduce bias, influencing the responses and skewing the results.
Polls and surveys are like the bread and butter of close-ended questions; they get straight to the point. But that's not all these little gems are good for.
1. In Product Reviews
Imagine you've just launched a new sneaker line, and you want quick customer feedback . Closed-ended questions work wonders here because they give customers an easy way to express their satisfaction without needing a novel on "The Comforts of Modern Footwear." A simple 'Yes' or 'No' does the trick, which lets you collect quantitative data, and review it (and take measures, of course!)
But why stop at yes or no? Ratings on a scale from 1 to 5 can turn vague feelings into actionable data faster than you can say "market research." And when it comes time for analysis, quantitative responses have your back more reliably than caffeine during finals week.
2. During Live Polling Events
You're in the middle of a webinar. Your audience is scattered across different time zones but united by one thing: curiosity about what others think. Here come close-ended survey questions swooping in. They let participants cast their votes swiftly without interrupting their snack break, which would be a win-win situation if there ever was one.
This real-time engagement isn't just cool, it's also insightful. With immediate results popping up on screens everywhere, trends emerge before attendees even sign off. That means instant gratification with insights included—like getting your pie (chart) and eating it too.
3. To Improve Customer Service Interactions
In customer service interactions, using multiple choice close-ended questions can significantly streamline the process. By replacing blank fields with structured options, customers can swiftly pinpoint their issues, allowing service representatives to identify and resolve queries more efficiently.
You can reduce ambiguity and direct the conversations to a quicker path to satisfactory resolutions. It's particularly effective in automated customer service systems where users can select from a menu of common problems, expediting the help process.
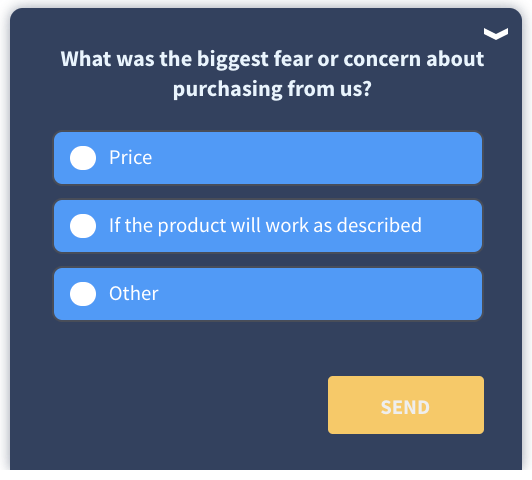
Creating closed-ended questions is just as important as your customers' input. You want questions to come out consistent and satisfying every time. Let's see how you can make your questions hit the sweet spot.
- Keep it simple - use clear, concise language
- Avoid leading or biased wording that might sway answers
- Offer balanced choices if using multiple-choice format
- Make sure options are mutually exclusive to avoid confusion
- Pilot test your survey questions to iron out any wrinkles before going live
Pose a simple question, and you might just unlock the treasure trove of data you've been after. That's the magic of close-ended questions, they're like keys that open up chests full of insightful nuggets (minus the pirate ship). So here are five shiny examples to stash in your survey toolkit.
1. Are You Satisfied with Our Service?
This straightforward yes-or-no question is a quick way for businesses to gauge customer satisfaction. A majority of positive responses indicate customer contentment, while negative feedback signals the need for immediate improvement.
Suppose it's mostly thumbs-up, great. If not, it’s time to fix what's broken, and quickly.
2. How Likely Are You to Recommend Us to a Friend or a Colleague?
This one’s got clout because it goes beyond mere satisfaction. It taps into loyalty and brand advocacy. It often pops up as part of Net Promoter Score surveys, where each response slots customers into promoters, passives, or detractors categories. It's super handy for measuring growth potential.
Spoiler alert: High scores mean you’re probably doing something right.
3. Did You Find What You Were Looking For On Our Website?
As a digital version of "Can I help find something?", this question is crucial for finding out usability issues lurking on websites.
When users hit 'No', it's clear there's homework to do, like refining navigation menus or improving search functions, to stop visitors from bouncing away from your website (which may crush your business.)
4. Which Feature Do You Value Most In Our Product?
Talk about getting straight to the point, this query puts product features under the microscope and asks users directly which one steals their heart (metaphorically speaking). The answers line up neatly like ducks in a row for easy analysis and can be pivotal when deciding where to funnel those development dollars next quarter.
Bonus points if all fingers point towards your latest release. That means kudos all around.
5. In The Last 12 Months, How Often Have You Used Our Product/Service?
This frequency check isn’t about patting on the back. It gets down to how integral your offering has become in people’s lives. Whether they're super fans or casual acquaintances with your product/service, it becomes crystal clear once responses roll in.
So remember these questions as nifty tools at your disposal, each crafted carefully so you can dive deep into discussions, spark meaningful conversations, and unlock new insights. They're here to help guide you through any professional scenario with ease.
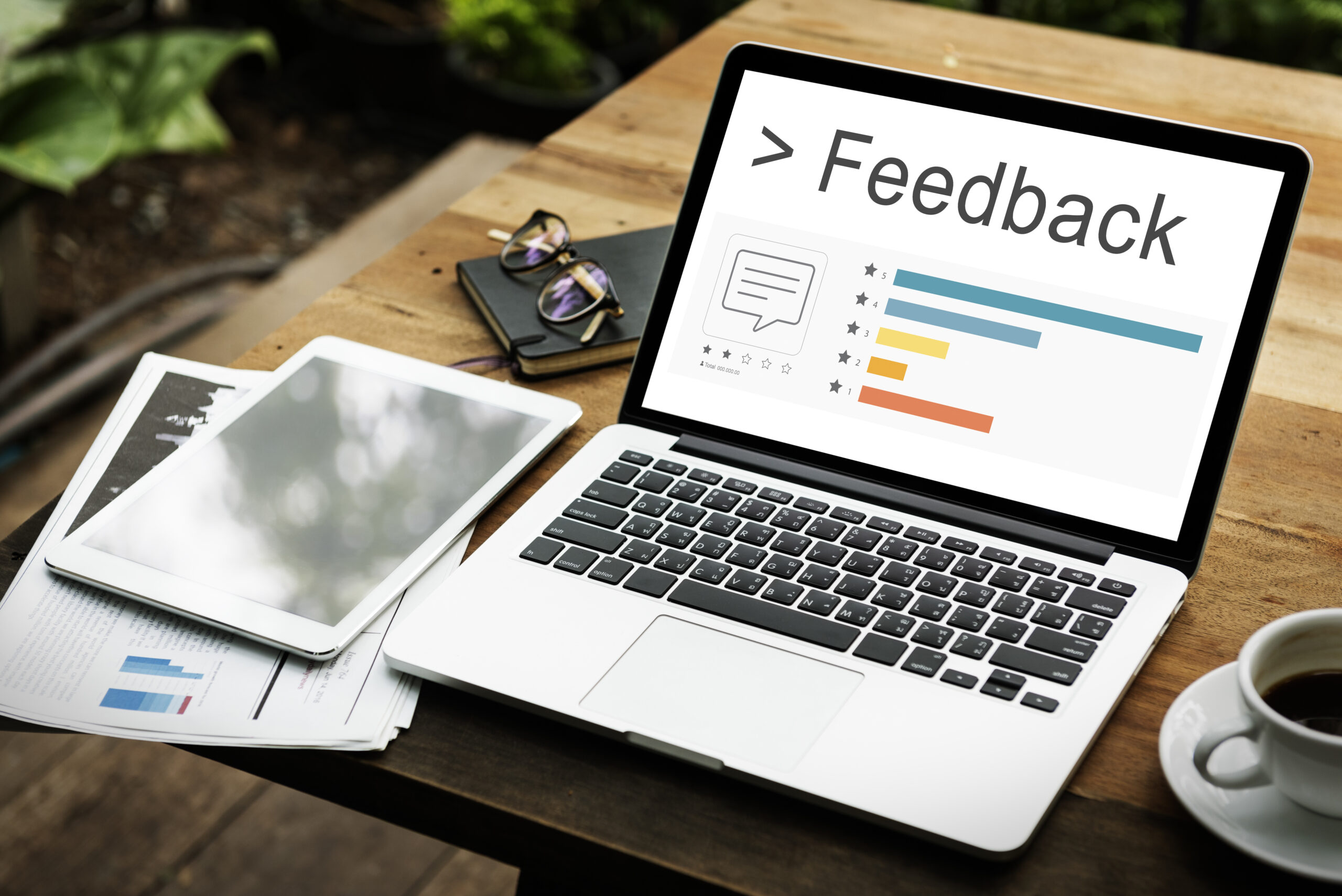
Understanding the differences between close-ended and open-ended questions is fundamental in designing effective surveys, interviews, and research studies. Each type serves distinct purposes and provides varied depths of insight.
1. Nature of Responses
Close-ended questions provide quantifiable data during your online survey, with responses limited to predefined options such as 'yes' or 'no', multiple-choice, or scales. Such structure makes it straightforward to answer and analyze statistically.
In contrast, open-ended questions invite respondents to answer freely (in their own words) offering richer, qualitative feedback that can uncover motivations, feelings, and detailed opinions.
2. Analysis and Interpretations
The responses from close-ended questions are easier to tabulate and translate into charts or graphs, facilitating a more objective analysis.
Open-ended responses, however, require more nuanced interpretation and coding to identify themes and patterns, providing subjective but detailed insights.
3. Context and Depth
Closed-ended questions are efficient for obtaining specific information and are ideal when the research question is clear-cut. However, they can miss the context and depth that open-ended questions can elicit.
Open-ended questions are invaluable when exploring new areas where the range of possible responses is not known beforehand, allowing for a more exploratory approach.
FullSession offers a range of feedback tools that can help you gather feedback and make your customers happy. You can use feedback forms and feedback buttons, all of which you can find on the FullSession's dashboard, which you can also customize.
Why is this so important? Feedback tools let you get direct thoughts from your customers. Then, you can understand what they like and dislike, what they would change, and what works for them perfectly.
Steps to Collecting User Feedback with FullSession
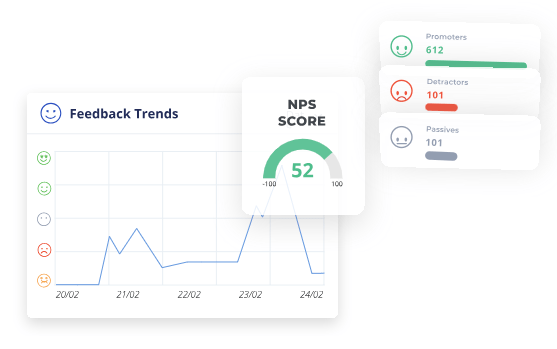
Here's a step-by-step guide on how to gather customer feedback with FullSession's tools :
- Locate the ‘New Feedback’ widget at the top-right corner of the page.
- Add a name and description to the form for easy identification later.
- Adjust language, reactions, colors, and position. Preview the form before publishing, and use the reset button if needed.
- Set up the feedback form's flow, choosing questions about the user's feelings or requesting contact details.
- Target the form's audience based on the devices they use using the FullSession tracking code. Choose whether to collect feedback from all pages or a specific one.
- Choose the feedback delivery method, such as your email inbox, but keep in mind the daily response limit.
- Review all configured steps before activating the feedback form. Once you're satisfied, activate the form to start receiving customer feedback.
It takes less than 5 minutes to set up your first website or app feedback form, with FullSession , and it's completely free!
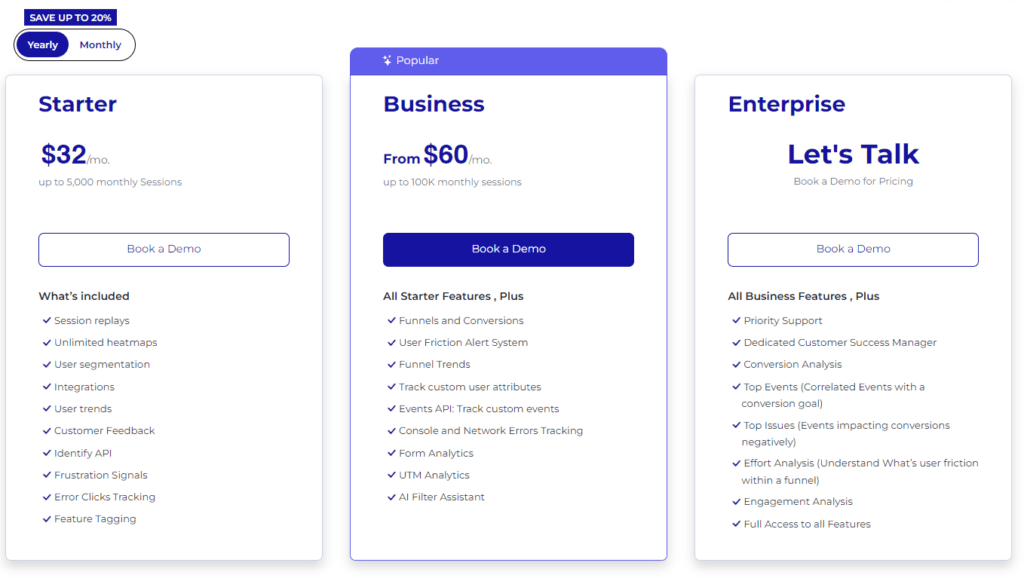
Here are more details on each plan.
- The Starter plan costs $39/month or $32/year and allows you to monitor up to 5,000 monthly sessions with up to 6 months of data storage.
- The Business plan costs $75/month or $60/year and helps you to track and analyze up to 100,000 monthly sessions with up to 12 months of data storage.
- The Enterprise plan has custom pricing and offers customizable sessions plus full access to all features.
Book a demo today .
Close-ended questions can add a lot of value to your business. They are unbiased and can give you a clear picture of what you're about to expect from customers.
Nail them down right, clear, unbiased, and they'll serve up the goods without fail. Just know when to pair them with their open-ended questions, so you get the whole story, rather than a part of it.
FullSession can give you all the feedback tools to improve customer satisfaction, bringing more clients to your company. Book a demo now.
What is an example of a close-ended question?
"Did you enjoy the meal?" qualifies as a classic close-ended question, which aims for a simple Yes/No answer.
What is the meaning of close-ended?
Close-ended refers to questions designed for quick, often one-word answers – like just yes or no, true or false.
Why use close-ended questions?
Close-ended questions require quick answers, so they are a good option in case you need to get as many survey participants as possible. You can also use them if you need valuable data for a presentation where you can show numbers.
What are open-ended and closed-ended questions?
Closed-ended and open-ended questions are both used in many satisfaction surveys. Their goal is to provide a detailed look at quantitative and qualitative data, draw conclusions, and act accordingly.
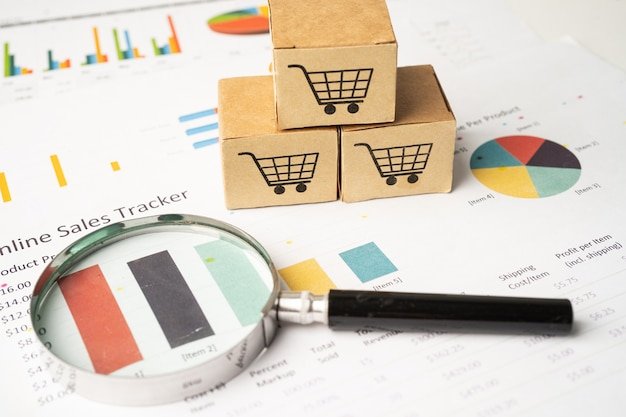

An official website of the United States government
The .gov means it’s official. Federal government websites often end in .gov or .mil. Before sharing sensitive information, make sure you’re on a federal government site.
The site is secure. The https:// ensures that you are connecting to the official website and that any information you provide is encrypted and transmitted securely.
- Publications
- Account settings
The PMC website is updating on October 15, 2024. Learn More or Try it out now .
- Advanced Search
- Journal List

Comparing the use of open and closed questions for Web-based measures of the continued-influence effect
Saoirse connor desai.
Department of Psychology, City, University of London, Northampton Square, London, EC1V 0HB UK
Stian Reimers
Associated data.
Open-ended questions, in which participants write or type their responses, are used in many areas of the behavioral sciences. Although effective in the lab, they are relatively untested in online experiments, and the quality of responses is largely unexplored. Closed-ended questions are easier to use online because they generally require only single key- or mouse-press responses and are less cognitively demanding, but they can bias the responses. We compared the data quality obtained using open and closed response formats using the continued-influence effect (CIE), in which participants read a series of statements about an unfolding event, one of which is unambiguously corrected later. Participants typically continue to refer to the corrected misinformation when making inferential statements about the event. We implemented this basic procedure online (Exp. 1 A, n = 78), comparing standard open-ended responses to an alternative procedure using closed-ended responses (Exp. 1 B, n = 75). Finally, we replicated these findings in a larger preregistered study (Exps. 2A and 2B, n = 323). We observed the CIE in all conditions: Participants continued to refer to the misinformation following a correction, and their references to the target misinformation were broadly similar in number across open- and closed-ended questions. We found that participants’ open-ended responses were relatively detailed (including an average of 75 characters for inference questions), and almost all responses attempted to address the question. The responses were faster, however, for closed-ended questions. Overall, we suggest that with caution it may be possible to use either method for gathering CIE data.
Electronic supplementary material
The online version of this article (10.3758/s13428-018-1066-z) contains supplementary material, which is available to authorized users.
Over the past decade, many areas of research that have traditionally been conducted in the lab have moved to using Web-based data collection (e.g., Peer, Brandimarte, Samat, & Acquisti, 2017 ; Simcox & Fiez, 2014 ; Stewart, Chandler, & Paolacci, 2017 ; Wolfe, 2017 ). Collecting data online has many advantages for researchers, including ease and speed of participant recruitment and a broader demographic of participants, relative to lab-based students.
Part of the justification for this shift has been the finding that the data quality from Web-based studies is comparable to that obtained in the lab: The vast majority of Web-based studies have replicated existing findings (e.g., Crump, McDonnell, & Gureckis, 2013 ; Germine et al., 2012 ; Zwaan et al., 2017 ). However, the majority of these studies have been in areas in which participants make single key- or mouse-press responses to stimuli. Less well explored are studies using more open-ended responses, in which participants write their answers to questions. These types of question are useful for assessing recall rather than recognition and for examining spontaneous responses that are unbiased by experimenter expectations, and as such may be unavoidable for certain types of research.
There are reasons to predict that typed responses might be of lower quality for open-ended than for closed-ended questions. Among the few studies that have failed to replicate online have been those that have required high levels of attention and engagement (Crump et al., 2013 ), and typing is both time-consuming and more physically effortful than pointing and clicking. Relatedly, participants who respond on mobile devices might struggle to make meaningful typed responses without undue effort.
Thus, researchers who typically run their studies with open-ended questions in the lab, and who wish to move to running them online, have two options. Either they can retain the open-ended question format and hope that the online participants are at least as diligent as those in the lab, or they can use closed-ended questions in place of open-ended questions, but with the risk that participants will respond differently or draw on different memory or reasoning processes to answer the questions. We examined the relative feasibility of these two options by using the continued-influence effect , a paradigm that (a) is a relatively well-used memory and reasoning task, (b) has traditionally used open-ended questions, and (c) is one that we have experience with running in the lab.
The continued-influence effect
The continued-influence effect of misinformation refers to the consistent finding that misinformation continues to influence people’s beliefs and reasoning even after it has been corrected (Chan, Jones, Hall Jamieson, & Albarracín, 2017 ; Ecker, Lewandowsky, & Apai, 2011b ; Ecker, Lewandowsky, Swire, & Chang, 2011a ; Ecker, Lewandowsky, & Tang, 2010 ; Gordon, Brooks, Quadflieg, Ecker, & Lewandowsky, 2017 ; Guillory & Geraci, 2016 ; Johnson & Seifert, 1994 ; Rich & Zaragoza, 2016 ; Wilkes & Leatherbarrow, 1988 ; for a review, see Lewandowsky, Ecker, Seifert, Schwarz, & Cook, 2012 ). Misinformation can have a lasting effect on people’s reasoning, even when they demonstrably remember that the information has been corrected (Johnson & Seifert, 1994 ) and are given prior warnings about the persistence of misinformation (Ecker et al., 2010 ).
In the experimental task used to study the continued-influence effect (CIE), participants are presented with a series of 10–15 sequentially presented statements describing an unfolding event. Target (mis)information that allows inferences to be drawn about the cause of the event is presented early in the sequence and is later corrected. Participants’ inferential reasoning and factual memory based on the event report are then assessed through a series of open-ended questions.
For example, in Johnson and Seifert ( 1994 ), participants read a story about a warehouse fire in which the target (mis)information implies that carelessly stored flammable materials (oil paint and gas cylinders) are a likely cause of the fire. Later in the story, some participants learned that no such materials had actually been stored in the warehouse, and therefore that they could not have caused the fire. The ensuing questionnaire included indirect inference questions (e.g., “what could have caused the explosions?”), as well as direct questions probing recall of the literal content of the story (e.g., “what was the cost of the damage done?”). The responses to inference questions were coded in order to measure whether the misinformation had been appropriately updated (no oil paint and gas cylinders were present in the warehouse). The responses were categorized according to whether they were consistent with the explanation implied by the target (mis)information 1 (e.g., “exploding gas cylinders”) or were not (e.g., “electrical short circuit”).
In a typical CIE experiment, performance on a misinformation-followed-by-correction condition is usually compared to one or more baselines: a condition in which the misinformation is presented but is not then retracted (no-correction condition) or a condition in which the misinformation is never presented (no-misinformation condition). The former control condition allows for assessment of the retraction’s effectiveness; the latter arguably shows whether the correction reduces reference to misinformation to a level comparable to never having been exposed to the misinformation (but see below).
The key finding from CIE studies is that people continue to use the misinformation to answer the inference questions, even though it has been corrected. The most consistent pattern of findings is that references to previously corrected misinformation are elevated relative to a no-misinformation condition, and are either below, or in some cases indistinguishable from, references in the no-correction condition.
Using open- and closed-ended questions online
With only a few exceptions (Guillory & Geraci, 2013 , 2016 ; Rich & Zaragoza, 2016 ), research concerning reliance on misinformation has used open-ended questions administered in the lab (see Capella, Ophir, & Sutton, 2018 , for an overview of approaches to measuring misinformation beliefs). There are several good reasons for using such questions, particularly on memory-based tasks that involve the comprehension or recall of previously studied text. First, the responses to open-ended questions are constructed rather than suggested by response options, and so avoid bias introduced by suggesting responses to participants. Second, open-ended questions also allow participants to give detailed responses about complex stimuli and permit a wide range of possible responses. Open-ended questions also resemble cued-recall tasks, which mostly depend on controlled retrieval processes (Jacoby, 1996 ) and provide limited retrieval cues (Graesser, Ozuru, & Sullins, 2010 ). These factors are particularly important for memory-based tasks wherein answering the questions requires the active generation of previously studied text (Ozuru, Briner, Kurby, & McNamara, 2013 ).
For Web-based testing, these advantages are balanced against the potential reduction in data quality when participants have to type extensive responses. The evidence concerning written responses is mixed. Grysman ( 2015 ) found that participants on the Amazon Mechanical Turk (AMT) wrote shorter self-report event narratives than did college participants completing online surveys, typing in the presence of a researcher, or giving verbal reports. Conversely, Behrend, Sharek, Meade, and Wiebe ( 2011 ) found no difference in the amounts written in free-text responses between university-based and AMT respondents.
A second potential effect concerns missing data: Participants have anecdotally reported to us that they did not enjoy typing open-ended responses. Open-ended questions could particularly discourage participants with lower levels of literacy or certain disabilities from expressing themselves in the written form, which could in turn increase selective dropout from some demographic groups (Berinsky, Margolis, & Sances, 2014 ). As well as losing whole participant datasets, open-ended questions in Web surveys could also result in more individual missing data points than closed-ended questions do (Reja, Manfreda, Hlebec, & Vehovar, 2003 ).
The alternative to using open-ended questions online is using closed-ended questions. These have many advantages, particularly in a context where there is less social pressure to perform diligently. However, response options can also inform participants about the researcher’s knowledge and expectations about the world and suggest a range of reasonable responses (Schwarz, Hippler, Deutsch, & Strack, 1985 ; Schwarz, Knauper, Hippler, Neumann, & Clark, 1991 ; Schwarz, Strack, Müller, & Chassein, 1988 ). There is also empirical evidence to suggest that open- and closed-end responses are supported by different cognitive (Frew, Whynes, & Wolstenholme, 2003 ; Frew, Wolstenholme, & Whynes, 2004 ) or memory (Khoe, Kroll, Yonelinas, Dobbins, & Knight, 2000 ; see Yonelinas, 2002 , for a review) processes. A straightforward conversion of open- to closed-ended questions might therefore be impractical for testing novel scientific questions in a given domain.
The latter caveat may be particularly relevant for the CIE. Repeated statements are easier to process and are subsequently perceived as more truthful than new statements (Ecker, Lewandowsky, Swire, & Chang, 2011a ; Fazio, Brashier, Payne, & Marsh, 2015 ; Moons, Mackie, & Garcia-Marques, 2009 ). Therefore, repeating misinformation in the response options could activate automatic (familiarity-based) rather than strategic (recollection-based) retrieval of studied text, which may not reflect how people reason about misinformation in the real world. Conversely, presenting corrections that explicitly repeat misinformation is more effective at reducing misinformation effects than is presenting corrections that avoid repetition (Ecker, Hogan, & Lewandowsky, 2017 ). As such, substituting closed-ended for open-ended questions might have unpredictable consequences.
Overview of experiments
The overarching aim of the experiments reported here was to examine open- and closed-ended questions in Web-based memory and inference research. The more specific goals were (1) to establish whether a well-known experimental task that elicits responses with open-ended questions would replicate online, and (2) to explore the feasibility of converting open-ended questions to the type of closed-ended questions more typically seen online. To achieve these goals, two experiments were designed to replicate the CIE. Experiments 1 A and 1 B used the same experimental stimuli and subset of questions as in Johnson and Seifert ( 1994 , Exp. 3A), wherein participants read a report about a warehouse fire and answered questions that assessed inferential reasoning about the story, factual accuracy, and the ability to recall the correction or control information (critical information). Experiments 1 A and 2 A employed standard open-ended measures, whereas a closed-ended analogue was used in Experiments 1 B and 2 B. Although they are reported as separate experiments, both Experiments 1 A and 1 B were run concurrently as one study, as were Experiments 2 A and 2 B, with participants being randomly allocated to each experiment, as well as to the experimental conditions within each experiment.
Experiment 1A
Participants.
A power analysis using the effect size observed in previous research using the same stimuli and experimental design (Johnson & Seifert, 1994 ; effect size obtained from the means in Exp. 3A) indicated that a minimum of 69 participants were required ( f = 0.39, 1– β = .80, α = .05). In total, 78 US-based participants (50 males, 28 females; between 19 and 62 years of age, M = 31.78, SD = 10.10) were recruited via AMT. Only participants with a Human Intelligence Task (HIT) approval rating greater than or equal to 99% were recruited for the experiment, to ensure high-quality data without having to include attentional check questions (Peer, Vosgerau, & Acquisti, 2014 ). The participants were paid $2, and the median completion time was 11 min.
Stimuli and design
The experiment was programmed in Adobe Flash (Reimers & Stewart, 2007 , 2015 ). Participants read one of three versions of a fictional news report about a warehouse fire, which consisted of 15 discrete messages. The stimuli were identical to those used in Johnson and Seifert ( 1994 , Exp. 3A). Figure Figure1 1 illustrates how the message content was varied across the experimental conditions, as well as the message presentation format. The effect of the correction information on reference to the target (mis)information was assessed between groups; participants were randomly assigned to one of three experimental groups: no correction ( n = 32), correction ( n = 21), and alternative explanation ( n = 25).

The continued-influence effect task: Messages 1–5 provide general information about the event, beginning with the fire being reported. The target (mis)information is presented at Message 6 and is then corrected, for correction and correction + alternative explanation groups, at Message 13. The correction + alternative explanation group then receive information providing a substitute account of the fire to “fill the gap” left by invalidating the misinformation. This condition usually leads to a robust reduction in reference to the misinformation
The target (mis)information, implying that carelessly stored oil paint and gas cylinders played a role in the fire, was presented at Message 6. This information was then corrected at Message 13 for the two conditions featuring a correction. Information implying that the fire was actually the result of arson (alternative explanation group) was presented at Message 14; the other two experimental groups merely learned that the storage hall contained stationery materials. The other messages provided further details about the incident and were identical in all three experimental conditions.
The questionnaire following the statements consisted of three question blocks: inference, factual, and critical information recall. The question order was randomized within the inference and factual blocks, but not in the correction recall block, in which the questions were presented in a predefined order: Inference questions (e.g., “What was a possible cause of the fumes”) were presented first, followed by factual questions (e.g., “What business was the firm in?”), and then critical information recall questions (e.g., “What was the point of the second message from Police Investigator Lucas?”).
There were three dependent measures: (1) reference to the target (mis)information in the inference questions, (2) factual recall, and (3) critical information recall. The first dependent measure assessed the extent to which the misinformation influenced interpretation of the news report, whereas the second assessed memory for the literal content of the report. The final measure specifically assessed understanding and accurate recall of the critical information that appeared at Message 13 (see Fig. Fig.1). 1 ). Although not all groups received a correction, the participants in all experimental groups were asked these questions so that the questions would not differ between the conditions. The stimuli were piloted on a small group of participants to check their average completion time and obtain feedback about the questionnaire. Following the pilot, the number of questions included in the inference and factual blocks was reduced from ten to six, because participants felt some questions were repetitive.
Participants clicked on a link in AMT to enter the experimental site. After seeing details about the experiment, giving consent, and receiving detailed instructions, they were told that they would not be able to backtrack and that each message would appear for a minimum of 10 s before they could move on to the next message.
Immediately after reading the final statement, participants were informed that they would see a series of inference-based questions. They were told to type their responses in the text box provided, giving as much detail as necessary and writing in full sentences; that they should write at least 25 characters to be able to continue to the next question; and that they should answer questions on the basis of their understanding of the report and of industrial fires in general. After this they were informed that they would answer six factual questions, which then followed. Next, participants were instructed to answer the two critical information recall questions on the basis of what they remembered from the report. After completing the questionnaire, participants were asked to provide their sex, age, and highest level of education.
Coding of responses
The main dependent variable extracted from responses to the inference questions was “reference to target (mis)information.” References that explicitly stated, or strongly implied, that oil paint and gas cylinders caused or contributed to the fire were scored a 1; otherwise, responses were scored as 0. Table Table1 1 shows an example of a response that was coded as a reference to target (mis)information and an example of a response that was not coded as such. There were several examples of references to flammable items that did not count as references to the corrected information. For example, stating that the fire spread quickly “Because there were a lot of flammable things in the shop” would not be counted as a reference to the corrected information, since there was no specific reference to gas, paint, liquids, substances, or the fact that they were (allegedly) in the closet. The maximum individual score across the inference questions was 6. The responses to factual questions were scored for accuracy; correct or partially correct responses were scored 1, and incorrect responses were scored 0. Again, the maximum factual score was 6. We also examined critical information recall, to check participants’ awareness of either the correction to the misinformation or the control message, computed using two questions that assessed awareness and accuracy for the critical information that appeared at Message 13. This meant that the correct response depended on correction information condition. For the participants in the no-correction group, the correct response was that the injured firefighters had been released from hospital, and for the two conditions featuring a correction, this was a correction of the target (mis)information.
Example of response codings in Experiment 1
Question | Example of a Response Scored 1 | Example of a Response Scored 0 |
---|---|---|
Why did the fire spread so quickly? | Fire spread quickly due to gas cylinder explosion. Gas cylinders were stored inside the closet | The fire occurred in a stationery warehouse that housed envelopes and bales of paper that could easily ignite |
Intercoder reliability
All participants’ responses to the inference, factual, and correction recall questions were independently coded by two trained coders. Interrater agreement was .88, and Cohen’s Κ = .76 ± .02, indicating a high level of agreement between coders; both measures are higher than the benchmark values of .7 and .6 (Krippendorff, 2012 ; Landis & Koch, 1977 ), respectively, and there was no systematic bias between raters, χ 2 = 0.29, p = . 59.
Inference responses
The overall effect of the correction information on references to the target (mis)information was significant, F (2, 75) = 10.73, p < .001, η p 2 = .22 [.07, .36]. Dunnett multiple comparison tests (shown in panel A of Fig. Fig.2) 2 ) revealed that a correction or a correction with an alternative explanation significantly reduced reference to the target (mis)information in response to the inference questions.

Effects of correction information on the numbers of (A) references to the target (mis)information in Experiment 1 A, (B) references to the target misinformation in Experiment 1 B, (C) accurately recalled facts in Experiment 1 A, and (D) accurately recalled facts in Experiment 1 B. Error bars represent 95% confidence intervals of the means. The brackets represent Dunnett’s multiple comparison tests (which account for unequal group sizes) for significant omnibus tests. The dashed lines represent the means after excluding participants who did not recall the critical information (i.e., scored 0 on the first critical information recall question asking what the point of the second message from Police Investigator Lucas was)
A Bayesian analysis using the BayesFactor package in R and default priors (Morey & Rouder, 2015 ) was performed to examine the relative predictive success of the comparisons between conditions. The BF 10 for the first comparison 28.93, indicating strong evidence (Lee & Wagenmakers, 2014 ) in favor of the alternative that there was a difference between the no correction and correction groups. The BF 10 for the comparison between the no-correction and alternative-explanation groups was 209.03, again indicating very strong evidence in favor of the alternative. The BF 10 was 0.36 for the final comparison between the correction and alternative-explanation groups, indicating anecdotal evidence in favor of the null.
The Bayes factor analysis was mostly consistent with the p values and effect sizes. Both conditions featuring a correction led to a decrease in references to the target (mis)information, but the data for the two conditions featuring a correction cannot distinguish between the null hypothesis and previous findings (i.e., that an alternative explanation substantially reduces reference to misinformation, as compared to a correction alone).
Factual responses
Factual responses were examined to establish whether the differences in references to the (mis)information could be explained by memory for the literal content of the report. Overall, participants accurately recalled similar numbers of correct details across the correction information conditions (Fig. (Fig.2C), 2 C), and the omnibus test was not significant, F (2, 75) = 0.78, p = .46, η p 2 = .02.
Response quality
Participants were required to write a minimum of 25 characters in response to the questions. The number of characters written was examined as a measure of response quality. Participants wrote between 36% and 64% more, on average, than the minimum required 25 characters in response to the inference ( M = 69.45, SD = 40.49), factual ( M = 39.09, SD = 15.85), and critical information recall ( M = 66.72, SD = 42.76) questions. There was—unsurprisingly—a positive correlation between time taken to complete the study and number of characters written, r (76) = .31, p = .007.
Experiment 1B
In Experiment 1B we examined the feasibility of converting open-ended questions to a comparable closed-ended form.
Seventy-five US-based (46 male, 29 female; between 18 and 61 years of age, M = 34.31, SD = 10.54) participants were recruited from AMT. The participants were paid $2; the median completion time was 9 min.
Design, stimuli, and procedure
Experiment 1 B used the same story/newsfeed stimuli and high-level design as Experiment 1 A; participants were randomly assigned to one of three experimental conditions: no correction ( n = 33), correction ( n = 22), or alternative explanation ( n = 20). The only difference between the experiments was that closed-ended questions were used in the subsequent questionnaire. Figure Figure3 3 shows how participants had to respond to inference and factual questions. For the inferential questions, points were allocated to response alternatives that corresponded to four possible explanations. For example, when answering the question “What could have caused the explosions?,” participants could allocate points to a misinformation-consistent option (e.g., “Fire came in contact with compressed gas cylinders”), an alternative-explanation-consistent option (e.g., “Steel drums filled with liquid accelerants”), an option that was plausible given the story details but that was not explicitly stated (e.g., “Volatile compounds in photocopiers caught on fire”), or an option that was inconsistent with the story details (e.g., “Cooking equipment caught on fire”).

Screenshots of how the inference (left) and factual (right) questions and response options were presented to participants. Participants used the red arrow features to allocate points to the response alternatives in response to the inference questions. The factual questions were answered by selecting the “correct” option based on the information in the report
The response options were chosen in this way to give participants the opportunity to provide more nuanced responses than would be possible using multiple-choice or true/false alternatives. This approach allowed the participants who were presented with misinformation and then a correction to choose an explanation that was consistent with the story but did not make use of the target (mis)information. If the CIE were observed in response to closed-ended questions, then the number of points allocated to misinformation-consistent options in the conditions featuring a correction should be non-zero. The accuracy on factual questions was measured using four-alternative forced choice multiple-choice questions, in which participants responded by choosing the correct answer from a set of four possible options. The order of presentation for the response alternatives for inference and factual questions was randomized across participants. The critical informatin recall questions were open-ended, and participants gave free-text responses in the same manner as Experiment 1 A.
Individual inference, factual, and critical information recall scores (an analysis of the critical information recall responses is shown in the additional analyses in the supplemental materials ) were calculated for each participant. Since the maximum number of points that could be allocated to a given option explanation theme for each question was 10, the maximum inference score for an individual participant was 60. The maximum factual score was 6, and the maximum critical information recall score was 2. Critical information recall questions were open-ended, and responses were coded using the same criteria as in Experiment 1 A.
A one-way analysis of variance (ANOVA) on reference to the target (mis)information revealed a significant effect of correction information, F (2, 72) = 9.39, p < .001, η p 2 = .21 [.05, .35]. Overall, the pattern of results for reference to the target (mis)information in response to closed-ended questions was very similar to that in Experiment 1 A (Fig. (Fig.2B). 2B ). Although a correction with an alternative explanation significantly reduced reference to the target (mis)information, a correction on its own did not. The difference between the two conditions featuring a correction was also not significant.
The BF 10 was 1.02 for the first comparison, between the no-correction and correction groups, indicating anecdotal evidence in favor of the alternative, or arbitrary evidence for either hypothesis. The BF 10 was 250.81 for the second comparison, between the no-correction and alternative-explanation groups, indicating strong evidence for the alternative. The BF 10 was 4.22 for the final comparison, indicating substantial evidence in favor of the alternative.
The Bayes factor analysis was mostly consistent with the p values and effect sizes, except that the Bayes factor for the comparison between the correction and alternative-explanation conditions suggested an effect, whereas the p value did not.
Analysis of the factual scores indicated a significant difference between the correction information groups, F (2, 72) = 5.30, p = .007, η p 2 = .13 [.01, .26]. Figure Figure2D 2 D shows that the average number of factually correct details recalled from the report was significantly lower in the correction condition than in the no-correction group but not than in the alternative-explanation group. The poorer overall performance on factual questions for the correction group was mainly attributable to incorrect responses to two questions. The first of these questions asked about the contents of the closet that had reportedly contained flammable materials, before the fire; the second asked about the time the fire was put out. Only a third (23% in the correction and 25% in the alternative-explanation group) answered the question about the contents of the closet correctly (i.e., that the storeroom was empty before the fire), whereas 86% of the no-correction group correctly responded that oil paint and gas cylinders were in the storeroom before the fire. This is perhaps unsurprising: The correct answer for the no-correction condition (“paint and gas cylinders”) was more salient and unambiguous than the correct answer for the other two conditions (“The storage closet was empty before the fire”).
The results for Experiments 1 A and 1 B suggest that both open- and closed-ended questions can successfully be used in online experiments with AMT to measure differences in references to misinformation in a standard continued-influence experiment. There was a clear CIE of misinformation in all conditions of both experiments—a correction reduced, but did not come near eliminating, reference to misinformation in inference questions. In both experiments, references to target (mis)information were significantly lower in the correction + alternative than in the no-correction condition, with the correction condition lying between those two extremes (see Fig. Fig.2A 2 A and B). Although the patterns of significant results were slightly different (correction condition was significantly below no correction in Exp. 1 A but not in Exp. 1 B), this is consistent the variability seen across experiments using the CIE, in that some researchers have found a reduction in references to (mis)information following a correction (Connor Desai & Reimers, 2017 ; Ecker, Lewandowsky, & Apai, 2011b ; Ecker et al., 2010 ), but others have found no significant reduction (Johnson & Seifert, 1994 ).
With regard to motivation, we found that the vast majority of participants wrote reasonable responses to the open-ended questions. The answers were of a considerable length for the question, with participants usually typing substantially more than the minimum number of characters required. We found that the absolute numbers of references to the misinformation were comparable to those found in existing studies. That said, the open-ended questions had to be coded by hand, and for participants the median completion time was 18% longer in Experiment 1 A (11 min) than in Experiment 1 B (9 min). This disparity in completion times only serves to emphasize that using closed-ended questions streamlines the data collection process relative to open-ended questions.
Taken as a whole, these findings show that reasonably complex experimental tasks that traditionally require participants to construct written responses can be implemented online using either the same type of open-ended questions or comparable closed-ended questions.
Rationale for Experiments 2 A and 2 B
The results of Experiments 1 A and 1 B are promising with regard to using open-ended questions in online research in general, and to examining phenomena such as the CIE specifically. However, they have some limitations. The most salient limitation was the sample size. Although the numbers of participants in the different conditions were comparable to those in many lab-based studies of the CIE, the sample size was nonetheless small. One of the advantages of using Web-based procedures is that it is relatively straightforward to recruit large numbers of participants, so in Experiments 2 A and 2 B we replicated the key conditions of the previous studies with twice as many participants. We also preregistered the method, directional hypotheses, and analysis plan (including planned analyses, data stopping rule, and exclusion criteria) prior to data collection; this information can be found at https://osf.io/cte3g/ .
We also used this opportunity to include a second baseline condition. Several CIE experiments have included control conditions in some form that makes it possible to see whether references to the cause suggested by the misinformation following its correction are not only greater than zero, but greater than the references to the same cause if the misinformation is never presented. In this study we did not believe that such a condition would be very informative, because the strictness of the coding criteria meant that it would be unlikely that participants would spontaneously suggest paint or gas cylinders as contributing to the fire. 2
Instead, Experiments 2 A and 2 B included a more directly comparable control group for whom a correction was presented without the initial target (mis)information. According to the mental-model-updating account of the CIE, event information is integrated into a mental model that is updated when new information becomes available. Corrections may be poorly encoded or retrieved because they threaten the model’s internal coherence (Ecker et al., 2010 ; Johnson & Seifert, 1994 ; Johnson-Laird, 1980 ). If the CIE arises because of a mental-model-updating failure, then presenting the misinformation only as part of a correction should not result in a CIE, because there would not be an opportunity to develop a mental model involving the misinformation. On the other hand, participants might continue to refer to the misinformation for more superficial reasons: If the cause presented in the misinformation were available in memory and recalled without the context of its being corrected, then presenting the misinformation as part of the correction should lead to a CIE comparable to those in other conditions.
In these experiments, we repeated the no-correction and correction conditions from Experiments 1 A and 1 B. In place of the correction + alternative condition, however, we had the no-mention condition, which was the same as the correction condition except that we replaced the target (mis)information with a filler statement (“Message 6—4:30 a.m. Message received from Police Investigator Lucas saying that they have urged local residents to keep their windows and doors shut”). The wording of the correction message for this condition stated that “a closet reportedly containing cans of oil paint and gas cylinders had actually been empty before the fire” rather than referring simply to “the closet,” so that the participants would not think they had missed some earlier information.
Beyond this, the general setup for Experiments 2 A and 2 B was the same as that for Experiments 1 A and 1 B, except in the following respects: We included an instruction check (which appeared immediately after the initial instructions and immediately before the warehouse fire report was presented) that tested participants’ comprehension of the instructions via three multiple-choice questions. Participants were not excluded because of this check, but they were not allowed to proceed to the main experiment until they had answered all three questions correctly, consistent with Crump et al.’s ( 2013 ) recommendations. Because Adobe Flash, which we had used for Experiments 1 A and 1 B, is being deprecated and is increasingly hard to use for Web-based research, we implemented Experiments 2 A and 2 B using Qualtrics, which led to some superficial changes in the implementation. Most notable was that the point-allocation method for closed-ended inference questions required participants to type numbers of points to allocate, rather than adjusting the values using buttons.
The sample size was also doubled in this second set of experiments.
Experiment 2A
In all, 157 US- and UK-based participants (91 male, 66 female; between 18 and 64 years of age, M = 33.98, SD = 10.57) were recruited using AMT. 3 The median completion time was 16 min and participants, and were paid $1.25. 4
Design and procedure
Participants were randomly assigned to one of three experimental conditions: misinformation + no correction ( n = 52), misinformation + correction ( n = 52), or no misinformation + correction ( n = 53).
Participants’ responses to the inference, factual, and critical information recall 5 questions were coded by one trained coder, and 10% ( n = 16) of the responses were independently coded by a second trained coder. The interrater agreement was 1 and Cohen’s K = 1 ± 0, indicating, surprisingly, perfect agreement between the coders.
Participants produced similar numbers of references to the target (mis)information across correction information conditions (Fig. (Fig.4A), 4 A), and the omnibus test was not significant, F (2, 154) = 0.62, p = . 54, η p 2 = .01 [.00, .05]. Unlike in Experiment 1 A, a correction did not significantly reduce the number of references to the target (mis)information relative to a control group who did not receive a correction. Moreover, participants who were not presented with the initial misinformation but did receive a correction message, made a number of misinformation references similar to those for participants who were first exposed to the misinformation.

Effects of correction information on the numbers of (A) references to the target (mis)information in Experiment 2 A, (B) references to the target (mis)information in Experiment 2 B, (C) accurately recalled facts in Experiment 2 A, and (D) accurately recalled facts in Experiment 2 B. Error bars represent 95% confidence intervals of the means. The brackets represent Tukey multiple comparison tests when the omnibus test was significant. The dashed lines represent the means for the restricted sample of participants who did not answer the first critical information recall question correctly
Participants’ ability to accurately recall details from the report differed across correction information conditions (Fig. (Fig.4C), 4 C), F (2, 154) = 8.12, p < .001, η p 2 = .10 [.02, .18]. Tukey’s test for multiple comparisons revealed that the group who received a correction without the initial misinformation recalled significantly fewer details from the report than did the group who saw the uncorrected misinformation, but the other differences were nonsignificant, p s > .05.
Participants wrote between 48% and 69% more, on average, than the minimum of 25 required characters in response to the inference ( M = 80.76, SD = 56.38), factual ( M = 48.15, SD = 24.86), and critical information recall ( M = 75.56, SD = 47.05) questions. We found a positive correlation between the time taken to complete the study and the number of characters written, r (155) = .34, p < .0001, showing that the participants who took longer wrote more.
Experiment 2B
A total of 166 US- and UK-based participants (100 male, 66 female; between 18 and 62 years of age, M = 35.04, SD = 10.36) were recruited using AMT. 6 Participants were paid $1.25; their median completion time was 13 min.
Experiment 2 B used the same high-level design and procedure as Experiment 2 A. The responses were closed-ended and made in the same way as in Experiment 1 B. Participants were randomly assigned to one of three experimental conditions: misinformation + no correction ( n = 54), misinformation + correction ( n = 56), or no misinformation + correction ( n = 56).
We found a significant effect of correction information on references to the target (mis)information for closed-ended measures (Fig. (Fig.4B), 4 B), F (2, 163) = 26.90, p < .001, η p 2 = .25 [.14, .35]. Tukey-adjusted multiple comparisons further revealed that the group exposed to misinformation and its correction, and the group who saw only the correction without the initial misinformation, made significantly fewer references to the target (mis)information than did the uncorrected misinformation condition. The two groups who received correction information did not differ significantly.
Participants’ responses to the factual questions also showed a significant effect of correction information condition (Fig. (Fig.4D), 4 D), F (2, 163) = 4.70, p = .01, η p 2 = .05 [.00, .13]. Tukey’s tests revealed that the factual responses from participants in the condition featuring a correction without the initial misinformation were significantly lower than those from the group who saw uncorrected misinformation. The other differences were not significant ( p s > .1). A closer inspection of the individual answers revealed that incorrect responses for the no misinformation + correction group were mainly attributable to the question asking about the contents of the closet before the fire.
Dropout analysis
Of the 375 people who started the study, only 323 fully completed it (dropout rate 13%). Of those who completed the study, four (1.23%) were excluded prior to the analysis because they gave nonsense open-ended responses (e.g., “21st century fox, the biggest movie in theatre”). The majority of participants who dropped out did so immediately after entering their worker ID and before being assigned to a condition (41%). Of the remaining dropout participants who were assigned to a condition, 27% were assigned to one of the open-ended conditions and dropped out during the first question block. A further 16% were assigned to one of the closed-ended conditions and dropped out when asked to answer the open-ended critical information recall questions. The remaining 14% were assigned to a closed-ended condition and dropped out as soon as they reached the first question block. The dropout breakdown suggests that many people dropped out because they were unhappy about having to give open-ended responses. Some participants who were assigned to the closed-ended conditions dropped out when faced with open-ended questions, despite the fact that the progress bar showed that they had almost completed the study.
Experiments 2 A and 2 B again showed clear evidence of a CIE. As in Experiments 1 A and 1 B, participants continued to refer to the misinformation after it had been corrected. Also consistent with the previous two experiments, the effects of a correction differed slightly across conditions. This time the reduction in references to the (mis)information was significant for the closed-ended questions, but not for the open-ended questions. As we noted earlier, this is consistent with findings that a correction sometimes reduces references to misinformation relative to no correction, and sometimes it does not (Connor Desai & Reimers, 2017 ; Ecker et al., 2010 ).
Experiments 2 A and 2 B also included a novel control condition in which participants were not exposed to the initial misinformation but were exposed to its correction. Contrary to expectations, the new condition resulted in a number of references to the target (mis)information that was statistically equivalent to that in the group who were exposed to both the misinformation and its correction. This finding suggests that the CIE might not reflect a model-updating failure, but rather a decontextualized recall process.
General discussion
In four experiments we examined the feasibility of collecting data on the CIE online, comparing the efficacy of using traditional open-ended questions versus adapting the task to use closed-ended questions. For both types of elicitation procedures, we observed clear CIEs: Following an unambiguous correction of earlier misinformation, participants continued to refer to the misinformation when answering inferential questions. As such, these studies provide clear evidence that both open-ended and closed-ended questions can be used in online experiments.
Across all four studies we found that participants continued to use misinformation that had been subsequently corrected. This occurred even though a majority of participants recalled the correction. We found mixed results when examining whether a correction had any effect at all in reducing references to misinformation. Experiments using similar designs have both found (Ecker, Lewandowsky, & Apai, 2011b ; Ecker et al., 2010 ) and failed to find (Johnson & Seifert, 1994 ) an effect of a correction. Overall, we found limited evidence for an effect of a correction for the open-ended questions, but substantial evidence for an effect of a correction using closed-ended questions. For open-ended questions, it appears that any effect of a correction on reference to misinformation—at least using this scenario—is relatively small, and would be hard to detect consistently using the small sample sizes that have traditionally been used in this area. This may explain the variability in findings in the literature.
A correction with an alternative explanation appeared (at least numerically) to be more effective in reducing reliance on misinformation than a correction alone. Furthermore, given that Experiment 1 B’s results were actually more consistent with the original finding (Johnson & Seifert, 1994 ), the differences between past and present work are most likely unsystematic and therefore unrelated to the online testing environment or question type.
Finally, with regard to the main results, in Experiments 2 A and 2 B we found using a novel condition, that misinformation that was only presented as part of a correction had as much of a continuing influence effect as misinformation presented early in a series of statements and only later corrected. This has both theoretical and practical implications. Theoretically, it suggests that—under some circumstances—the CIE may not be the result of participants’ unwillingness to give up an existing mental model without an alternative explanation (Ecker, Lewandowsky, & Apai, 2011b ; Ecker, Lewandowsky, Swire, & Chang, 2011a ; Johnson & Seifert, 1994 ). Instead, it might be that participants search their memory for possible causes when asked inferential questions, but fail to retrieve the information correcting the misinformation.
Open- and closed-ended questions and the CIE
The pattern of results in response to inference questions was qualitatively very similar across both open and closed ended questions. This finding is particularly interesting in light of the fact that responses to open and closed questions might be supported by different underlying retrieval processes (Fisher, Brewer, & Mitchell, 2009 ; Ozuru et al., 2013 ; Shapiro, 2006 ). Crucially, the response options used in Experiments 1 B and 2 B required participants to make a more considered judgment than multiple-choice or yes/no questions, which may have encouraged recall rather than a familiarity-based heuristic. It is also interesting that participants still referred to the incorrect misinformation despite the fact that another response option was consistent with the report, although this was not explicitly stated.
Another important observation was that we found an effect of correction information on responses to closed-ended factual questions, but not to open questions. The difference between conditions is significant, because it was partly attributable to a question that probed participants’ verbatim memory of the correction. Many participants in both conditions featuring a correction answered this question incorrectly, despite the fact that the options clearly distinguished between the correct and incorrect answers, given what participants had read. This question asked what the contents of the closet were before the fire, so it not hard to see why participants who continued to rely on the misinformation might have answer this question incorrectly. The fact that there were differences between the conditions highlights the importance of carefully wording questions and response options in order to avoid bias.
It is also worth noting that floor effects were not observed (i.e., the misinformation was still influential for both groups that received a correction), despite the fact that the present study did not include a distractor task and that participants answered the inference questions directly after reading the news report (and so, theoretically, should have had better memory for the report details).
A brief note on the use of closed-ended questions and response alternatives: There is the possibility that presenting a closed list of options reminded participants of the arson materials explanation and inhibited responses consistent with the oil paint and gas cylinders explanation. Also, the closed list of options that repeated the misinformation could have increased its familiarity, making it more likely to be accepted as true (e.g., Ecker, Lewandowsky, Swire, & Chang, 2011a ). For the group that received a simple correction, the other options had not been explicitly stated in the story. These participants may not have fully read or understood the question block instructions, and therefore perceived the task as choosing the option that had appeared in the story, irrespective of the correction. In contrast, the participants in the alternative-explanation group were able to better detect the discrepancy between the misinformation and its correction, because of the option alluding to arson materials. Although the response alternatives provided a plausible response that was consistent with the details of the fire story, none of the options made it possible to rule out that participants just did not consider the correction when responding. The response alternatives provided forced the participants to choose one from among four explanations, which may not have reflected their understanding of the event, but nonetheless was the option that was most consistent with what they had read. This explanation is also consistent with previous studies showing that the response options chosen by the researcher can be used by the participants to infer which information the participant considers relevant (Schwarz et al., 1985 ; Schwarz et al., 1991 ).
Open- and closed-ended questions in Web-based research
As well as looking directly at the CIE, we also examined the extent to which participants recruited via Amazon Mechanical Turk could provide high-quality data from open-ended questions. We found high levels of diligence—participants typed much more than was required in order to give full answers to the questions, they spent more time reading statements than was required, and—with a small number of exceptions—they engaged well with the task and attempted to answer the questions set.
We found that dropout did increase, however, when participants had to give open-ended responses. This may suggest that some participants dislike typing open-ended responses, to the extent that they choose not to participate. (It could be that participants find it too much effort, or that they do not feel confident giving written answers, or that it feels more personal having to type an answer oneself.) Alternatively, it may be that some participants, because of the device they were using, would struggle to provide open-ended responses, and so dropped out when faced with open-ended questions. Either way, it is striking that we had over 4% of the participants in Experiment 2 B who read all the statements and gave answers to all the closed-ended questions, but then dropped out when asked to type their responses to the final two critical information recall questions. There are ethical implications of having participants spend 10 min on a task before dropping out, so the requirement for typed answers should be presented prominently before participants begin the experiment.
We found that participants’ recall of the correction for the misinformation was worse than in previous lab-based studies. We found that only a little over half of participants across the conditions in our study correctly reported the correction when prompted. This figure is poor when compared to the figures of 95% (correction) and 75% (alternative explanation) found in Johnson and Seifert’s ( 1994 , Exp. 3A) laboratory-based experiment. It is possible that this was the result of poor attention and recall of the correction, but we believe it was more likely a response issue, in which participants retained the information but did not realize that they were being asked to report it when asked whether they were aware of any inconsistencies or corrections. (In other unpublished research, we have found that simply labeling the relevant statement “Correction:” greatly increased participants’ reference to it when asked about any corrections.) Although this did not affect the CIE, in future research we would recommend making the instructions for the critical information recall questions particularly clear and explicit. This advice would, we imagine, generalize to any questions that might be ambiguous and would require a precise answer.
In choosing whether to use open-ended questions or to adapt them to closed-ended questions for use online, there are several pros and cons to weigh up. Open-ended questions allow for a consistency of methodology with traditional lab-based approaches—meaning there is no risk of participants switching to using different strategies or processes, as they might with closed-ended questions. We have shown that participants generally engage well and give good responses to open-ended questions. It is also much easier to spot and exclude participants who respond with minimal effort, since their written answers tend to be nonsense or copied and pasted from elsewhere. For closed-ended responses, attention or consistency checks or other measures of participant engagement are more likely to be necessary. That said, closed-ended questions are, we have found, substantially faster to complete, meaning that researchers on a budget could test more participants or ask more questions; such questions require no time to manually code; participants are less likely to drop out with them; and—at least in the area of research used here—they provide results comparable to those from open-ended questions.
In conclusion, the continued-influence effect can be added to the existing list of psychological findings that have been successfully replicated online. Data obtained online are of sufficiently high quality to allow examining original research questions and are comparable to data collected in the laboratory. Furthermore, the influence of misinformation can be examined using closed-ended questions with direct choices between options. Nevertheless, as with any methodological tool, researchers should proceed with caution and ensure that sufficient piloting is conducted prior to extensive testing. More generally, the research reported here suggests that open-ended written responses can be collected via the Web and Amazon Mechanical Turk.
Author note
We thank Cassandra Springate for help with coding the data.
This analysis differed from the preregistered confirmatory analysis. We planned to compare the conditions using t-tests but instead used chi-squared tests for the following reason. The second question (“Were you aware of any corrections or contradictions in the story you read”) was only relevant to the conditions featuring initial misinformation and its correction. We wanted to be able to compare all three conditions so only used the first question which was applicable to all three conditions. Accordingly, we used chi-square tests to test for dependence between correction information condition and recall of critical information. (DOCX 64 kb)
1 We use the term (mis)information throughout to refer to the original statement presented early in a CIE study that is later corrected. We parenthesize the (mis) because in some control conditions the information is not corrected, meaning that it cannot be considered misinformation from those participants’ perspective.
2 There was also a conceptual issue concerning whether references to the cause presented in the misinformation should be compared across correction and no-mention conditions. In the former case, the correction ruled out the cause; in the latter, the cause would still be possible.
3 Three of the participants were recruited from Prolific Academic. Data was collected from 159 participants but two of the participants were excluded because they gave nonsense answers to the questions (e.g., “because the wind is blow, love is fall, I think it is very interesting”).
4 The modal completion time in Experiments 1 and 2 was below 10 min, so the fee was reduced so that participants were paid the equivalent of the federal minimum wage in the US ($7.25).
5 Critical information recall is referred to as correction recall in the preregistration document submitted for the second set of studies reported. We changed the name of this variable to reflect the fact that a correction was not presented in the no-correction condition.
6 The recruited number of participants differed from stopping rule specified in the preregistration. In total, 168 participants were recruited for the closed-ended condition, due to an error. Ultimately we decided to include the extra participants in the analysis rather than exclude their data. However, the responses from two participants were excluded: one because the participant took the HIT twice, and another because the participant provided nonsense answers to the open-ended questions at the end of the study.
- Behrend TS, Sharek DJ, Meade AW, Wiebe EN. The viability of crowdsourcing for survey research. Behavior Research Methods. 2011; 43 :800–813. doi: 10.3758/s13428-011-0081-0. [ PubMed ] [ CrossRef ] [ Google Scholar ]
- Berinsky AJ, Margolis MF, Sances MW. Separating the shirkers from the workers? Making sure respondents pay attention on self-administered surveys. American Journal of Political Science. 2014; 58 :739–753. doi: 10.1111/ajps.12081. [ CrossRef ] [ Google Scholar ]
- Cappella JN, Ophir Y, Sutton J. The importance of measuring knowledge in the age of misinformation and challenges in the tobacco domain. In: Southwell BG, Thorson EA, Sheble L, editors. Misinformation and mass audiences. Austin, TX: University of Texas Press; 2018. pp. 51–70. [ Google Scholar ]
- Chan MS, Jones CR, Hall Jamieson K, Albarracín D. Debunking: A meta-analysis of the psychological efficacy of messages countering misinformation. Psychological Science. 2017; 28 :1531–1546. doi: 10.1177/0956797617714579. [ PMC free article ] [ PubMed ] [ CrossRef ] [ Google Scholar ]
- Connor Desai S, Reimers S. But where’s the evidence? The effect of explanatory corrections on inferences about false information. In: Gunzelmann G, Howes A, Tenbrink T, Davelaar E, editors. Proceedings of the 39th Annual Conference of the Cognitive Science Society. Austin, TX: Cognitive Science Society; 2017. pp. 1824–1829. [ Google Scholar ]
- Crump MJC, McDonnell JV, Gureckis TM. Evaluating Amazon’s Mechanical Turk as a tool for experimental behavioral research. PLoS ONE. 2013; 8 :e57410. doi: 10.1371/journal.pone.0057410. [ PMC free article ] [ PubMed ] [ CrossRef ] [ Google Scholar ]
- Ecker UKH, Hogan JL, Lewandowsky S. Reminders and repetition of misinformation: Helping or hindering its retraction? Journal of Applied Research in Memory and Cognition. 2017; 6 :185–192. doi: 10.1016/j.jarmac.2017.01.014. [ CrossRef ] [ Google Scholar ]
- Ecker UKH, Lewandowsky S, Apai J. Terrorists brought down the plane!—No, actually it was a technical fault: processing corrections of emotive information. Quarterly Journal of Experimental Psychology. 2011; 64 :283–310. doi: 10.1080/17470218.2010.497927. [ PubMed ] [ CrossRef ] [ Google Scholar ]
- Ecker UKH, Lewandowsky S, Swire B, Chang D. Correcting false information in memory: Manipulating the strength of misinformation encoding and its retraction. Psychonomic Bulletin & Review. 2011; 18 :570–578. doi: 10.3758/s13423-011-0065-1. [ PubMed ] [ CrossRef ] [ Google Scholar ]
- Ecker UKH, Lewandowsky S, Tang DTW. Explicit warnings reduce but do not eliminate the continued influence of misinformation. Memory & Cognition. 2010; 38 :1087–1100. doi: 10.3758/MC.38.8.1087. [ PubMed ] [ CrossRef ] [ Google Scholar ]
- Fazio LK, Brashier NM, Payne BK, Marsh EJ. Knowledge does not protect against illusory truth. Journal of Experimental Psychology: General. 2015; 144 :993–1002. doi: 10.1037/xge0000098. [ PubMed ] [ CrossRef ] [ Google Scholar ]
- Fisher RP, Brewer N, Mitchell G. The relation between consistency and accuracy of eyewitness testimony: Legal versus cognitive explanations. In: Bull R, Valentine T, Williamson T, editors. Handbook of psychology of investigative interviewing: Current developments and future directions. Hoboken, NJ: Wiley; 2009. pp. 121–136. [ Google Scholar ]
- Frew EJ, Whynes DK, Wolstenholme JL. Eliciting willingness to pay: Comparing closed-ended with open-ended and payment scale formats. Medical Decision Making. 2003; 23 :150–159. doi: 10.1177/0272989X03251245. [ PubMed ] [ CrossRef ] [ Google Scholar ]
- Frew EJ, Wolstenholme JL, Whynes DK. Comparing willingness-to-pay: Bidding game format versus open-ended and payment scale formats. Health Policy. 2004; 68 :289–298. doi: 10.1016/j.healthpol.2003.10.003. [ PubMed ] [ CrossRef ] [ Google Scholar ]
- Germine L, Nakayama K, Duchaine BC, Chabris CF, Chatterjee G, Wilmer JB. Is the Web as good as the lab? Comparable performance from Web and lab in cognitive/perceptual experiments. Psychonomic Bulletin & Review. 2012; 19 :847–857. doi: 10.3758/s13423-012-0296-9. [ PubMed ] [ CrossRef ] [ Google Scholar ]
- Gordon A, Brooks JCW, Quadflieg S, Ecker UKH, Lewandowsky S. Exploring the neural substrates of misinformation processing. Neuropsychologia. 2017; 106 :216–224. doi: 10.1016/j.neuropsychologia.2017.10.003. [ PubMed ] [ CrossRef ] [ Google Scholar ]
- Graesser A, Ozuru Y, Sullins J. What is a good question? In: McKeown M, Kucan G, editors. Bringing reading research to life. New York, NY: Guilford; 2010. pp. 112–141. [ Google Scholar ]
- Grysman A. Collecting narrative data on Amazon’s Mechanical Turk. Applied Cognitive Psychology. 2015; 29 :573–583. doi: 10.1002/acp.3140. [ CrossRef ] [ Google Scholar ]
- Guillory JJ, Geraci L. Correcting erroneous inferences in memory: The role of source credibility. Journal of Applied Research in Memory and Cognition. 2013; 2 :201–209. doi: 10.1016/j.jarmac.2013.10.001. [ CrossRef ] [ Google Scholar ]
- Guillory JJ, Geraci L. The persistence of erroneous information in memory: The effect of valence on the acceptance of corrected information. Applied Cognitive Psychology. 2016; 30 :282–288. doi: 10.1002/acp.3183. [ CrossRef ] [ Google Scholar ]
- Jacoby LL. Dissociating automatic and consciously controlled effects of study/test compatibility. Journal of Memory and Language. 1996; 35 :32–52. doi: 10.1006/jmla.1996.0002. [ CrossRef ] [ Google Scholar ]
- Johnson HM, Seifert CM. Sources of the continued influence effect: When misinformation in memory affects later inferences. Journal of Experimental Psychology: Learning, Memory, and Cognition. 1994; 20 :1420–1436. [ Google Scholar ]
- Johnson-Laird PN. Mental models in cognitive science. Cognitive Science. 1980; 4 :71–115. doi: 10.1207/s15516709cog0401_4. [ CrossRef ] [ Google Scholar ]
- Khoe W, Kroll NE, Yonelinas AP, Dobbins IG, Knight RT. The contribution of recollection and familiarity to yes–no and forced-choice recognition tests in healthy subjects and amnesics. Neuropsychologia. 2000; 38 :1333–1341. doi: 10.1016/S0028-3932(00)00055-5. [ PubMed ] [ CrossRef ] [ Google Scholar ]
- Krippendorff K. Content analysis: An introduction to its methodology. New York, NY: Sage; 2012. [ Google Scholar ]
- Landis JR, Koch GG. The measurement of observer agreement for categorical data. Biometrics. 1977; 33 :159–174. doi: 10.2307/2529310. [ PubMed ] [ CrossRef ] [ Google Scholar ]
- Lee MD, Wagenmakers E-J. Bayesian cognitive modeling: A practical course. Cambridge, UK: Cambridge University Press; 2014. [ Google Scholar ]
- Lewandowsky S, Ecker UKH, Seifert CM, Schwarz N, Cook J. Misinformation and its correction: Continued influence and successful debiasing. Psychological Science in the Public Interest. 2012; 13 :106–131. doi: 10.1177/1529100612451018. [ PubMed ] [ CrossRef ] [ Google Scholar ]
- Moons WG, Mackie DM, Garcia-Marques T. The impact of repetition-induced familiarity on agreement with weak and strong arguments. Journal of Personality and Social Psychology. 2009; 96 :32–44. doi: 10.1037/a0013461. [ PubMed ] [ CrossRef ] [ Google Scholar ]
- Morey, R. D., & Rouder, J. N. (2015). BayesFactor: Computation of Bayes factors for common designs. Retrieved from https://cran.r-project.org/package=BayesFactor
- Ozuru Y, Briner S, Kurby CA, McNamara DS. Comparing comprehension measured by multiple-choice and open-ended questions. Canadian Journal of Experimental Psychology. 2013; 67 :215–227. doi: 10.1037/a0032918. [ PubMed ] [ CrossRef ] [ Google Scholar ]
- Peer E, Brandimarte L, Samat S, Acquisti A. Beyond the Turk: Alternative platforms for crowdsourcing behavioral research. Journal of Experimental Social Psychology. 2017; 70 :153–163. doi: 10.1016/j.jesp.2017.01.006. [ CrossRef ] [ Google Scholar ]
- Peer E, Vosgerau J, Acquisti A. Reputation as a sufficient condition for data quality on Amazon Mechanical Turk. Behavior Research Methods. 2014; 46 :1023–1031. doi: 10.3758/s13428-013-0434-y. [ PubMed ] [ CrossRef ] [ Google Scholar ]
- Reimers S, Stewart N. Adobe Flash as a medium for online experimentation: A test of reaction time measurement capabilities. Behavior Research Methods. 2007; 39 :365–370. doi: 10.3758/BF03193004. [ PubMed ] [ CrossRef ] [ Google Scholar ]
- Reimers S, Stewart N. Presentation and response timing accuracy in Adobe Flash and HTML5/JavaScript Web experiments. Behavior Research Methods. 2015; 47 :309–327. doi: 10.3758/s13428-014-0471-1. [ PMC free article ] [ PubMed ] [ CrossRef ] [ Google Scholar ]
- Reja U, Manfreda KL, Hlebec V, Vehovar V. Open-ended vs. close-ended questions in Web questionnaires. Developments in Applied Statistics. 2003; 19 :159–177. [ Google Scholar ]
- Rich PR, Zaragoza MS. The continued influence of implied and explicitly stated misinformation in news reports. Journal of Experimental Psychology: Learning, Memory, and Cognition. 2016; 42 :62–74. [ PubMed ] [ Google Scholar ]
- Schwarz N, Hippler HJ, Deutsch B, Strack F. Response scales—Effects of category range on reported behavior and comparative judgments. Public Opinion Quarterly. 1985; 49 :388–395. doi: 10.1086/268936. [ CrossRef ] [ Google Scholar ]
- Schwarz N, Knauper B, Hippler HJ, Neumann B, Clark L. Rating scales: Numeric values may change the meaning of scale labels. Public Opinion Quarterly. 1991; 55 :570–582. doi: 10.1086/269282. [ CrossRef ] [ Google Scholar ]
- Schwarz N, Strack F, Müller G, Chassein B. The range of response alternatives may determine the meaning of the question: Further evidence on informative functions of response alternatives. Social Cognition. 1988; 6 :107–117. doi: 10.1521/soco.1988.6.2.107. [ CrossRef ] [ Google Scholar ]
- Shapiro, L. R. (2006). The effects of question type and eyewitness temperament on accuracy and quantity of recall for a simulated misdemeanor crime. Emporia State Research Studies, 43 , 1–7.
- Simcox T, Fiez JA. Collecting response times using amazon mechanical turk and adobe flash. Behavior Research Methods. 2014; 46 :95–111. doi: 10.3758/s13428-013-0345-y. [ PMC free article ] [ PubMed ] [ CrossRef ] [ Google Scholar ]
- Stewart N, Chandler J, Paolacci G. Crowdsourcing samples in cognitive science. Trends in Cognitive Sciences. 2017; 21 :736–748. doi: 10.1016/j.tics.2017.06.007. [ PubMed ] [ CrossRef ] [ Google Scholar ]
- Wilkes AL, Leatherbarrow M. Editing episodic memory following the identification of error. Quarterly Journal of Experimental Psychology. 1988; 40A :361–387. doi: 10.1080/02724988843000168. [ CrossRef ] [ Google Scholar ]
- Wolfe CR. Twenty years of Internet-based research at SCiP: A discussion of surviving concepts and new methodologies. Behavior Research Methods. 2017; 49 :1615–1620. doi: 10.3758/s13428-017-0858-x. [ PubMed ] [ CrossRef ] [ Google Scholar ]
- Yonelinas AP. The nature of recollection and familiarity: A review of 30 years of research. Journal of Memory and Language. 2002; 46 :441–517. doi: 10.1006/jmla.2002.2864. [ CrossRef ] [ Google Scholar ]
- Zwaan, R. A., Pecher, D., Paolacci, G., Bouwmeester, S., Verkoeijen, P., Dijkstra, K., & Zeelenberg, R. (2017). Participant nonnaiveté and the reproducibility of cognitive psychology. Psychonomic Bulletin & Review . 10.3758/s13423-017-1348-y [ PubMed ]
Open-ended vs. closed-ended questions in survey
Published on, open-ended vs. closed-ended questions.
In today’s rapidly evolving workforce development and education sectors, organizations must be equipped to gather and respond to real-time feedback. As AI-driven changes reshape the market, it’s critical to clearly communicate the value of your programs and their impact on stakeholders. However, many organizations face challenges in capturing and conveying the full scope of their success, often leading to a disconnect and missed opportunities for growth.
A key to overcoming this challenge lies in designing the right questions—questions that capture both the qualitative and quantitative dimensions of your work. Well-crafted surveys, featuring a blend of open-ended and closed-ended questions, are powerful tools in this process. Open-ended questions allow you to gather personal insights and experiences, while closed-ended questions enable you to measure program outcomes and effectiveness.
By designing surveys that generate both narrative and numerical data, organizations can:
- Capture genuine stakeholder voices: Understand the deeper needs and expectations of those engaged with your programs.
- Demonstrate clear program outcomes: Showcase measurable results that provide a comprehensive view of your program’s effectiveness.
- Strengthen stakeholder engagement: Build trust by presenting a compelling, data-driven story that motivates continued participation and support.
This article will guide you through the art of crafting effective questions, enabling you to design surveys that provide the insights needed to adapt and thrive in today’s fast-paced environment. With quicker feedback and meaningful data, you can demonstrate the success of your initiatives and secure the backing you need for continued progress.
Diverse Closed-ended vs. Open-ended Questions for Actionable Insights
Closed-ended Questions | Open-ended Questions |
---|---|
On a scale of 0-10, how likely are you to recommend our program to a friend or colleague? | What specific aspects of our program would you highlight when recommending it to others? If you wouldn't recommend it, what improvements would make you more likely to do so? |
Rate your agreement with the statement: "The training provided by our organization has significantly improved my job skills." (1: Strongly Disagree, 2: Disagree, 3: Neutral, 4: Agree, 5: Strongly Agree) | Which specific skills from our training have you applied in your work? Can you provide an example of how these skills have impacted your job performance? |
How satisfied are you with the support provided by our organization? (Very Unsatisfied, Unsatisfied, Neutral, Satisfied, Very Satisfied) | What aspects of our support have been most valuable to you, and what areas could be improved to better meet your needs? |
Has your household income increased as a result of participating in our microfinance program? | How has our microfinance program impacted your financial situation? Please describe any changes in your income, savings, or overall financial stability. |
Which of the following best describes the primary benefit you've experienced from our health education program? (a) Improved personal hygiene practices (b) Better understanding of nutrition (c) Increased access to healthcare services (d) Enhanced mental health awareness | Can you elaborate on how the health education program has influenced your daily life and overall well-being? Please provide specific examples of changes you've made. |
On a scale of 1-7, how empowered do you feel to make positive changes in your community after participating in our leadership workshop? (1: Not at all empowered, 7: Extremely empowered) | In what ways has our leadership workshop empowered you to make changes in your community? Please describe any initiatives or actions you've taken as a result. |
How would you characterize the environmental impact of our sustainable agriculture project? Harmful 1 - 2 - 3 - 4 - 5 Beneficial | What specific changes have you observed in local agricultural practices and the environment since the implementation of our sustainable agriculture project? |
Defining Open-Ended and Closed-Ended Questions
Open-ended questions allow respondents to provide unrestricted, qualitative responses in their own words. These questions typically begin with phrases like "how," "what," or "why," encouraging respondents to express their thoughts, feelings, and opinions freely. Examples of open-ended questions include "What factors influenced your decision?" or "How do you feel about this product?"
On the other hand, closed-ended questions offer respondents a set of predetermined response options to choose from. These questions often require a simple "yes" or "no" answer or ask respondents to select from a list of predefined options. Closed-ended questions are designed to elicit specific, quantitative responses and are often used to gather structured data efficiently. Examples of closed-ended questions include "Did you purchase this product?" or "Which of the following options best describes your experience?"
Open Ended vs Closed Ended Survey
Step 1: survey design, quantitative question (nps):.
"On a scale of 0-10, how likely are you to recommend our scholarship program to a friend or colleague?"
Qualitative Question:
"Please explain how the scholarship has impacted your academic journey and future prospects."
Step 2: Data Collection
Collect responses from scholarship recipients through online surveys or interviews.
Step 3: Data Analysis and Pattern Recognition
Qualitative responses:, inductive analysis (automated pattern recognition):, deductive analysis (key insights):.
- Financial relief is the most prevalent theme, mentioned by all respondents and strongly correlated with high NPS scores (9.2 average).
- Supporting family financial struggles, though less frequent (33%), shows the highest correlation with NPS (9.8 average), indicating a significant impact on those facing family-related financial challenges.
- Avoidance of debt is a key factor, mentioned by 66% of respondents and associated with high NPS scores (9.5 average).
- Campus engagement, while mentioned less frequently, is associated with high NPS scores (9.3 average), suggesting that the scholarship enables a more fulfilling college experience beyond academics.
- Stress reduction and addressing commuting difficulties, though mentioned less often, still show a positive impact on NPS scores, indicating the scholarship's holistic effect on students' lives.
Step 4: Sopact Sense Generated Impact Story
"Our scholarship program is transforming lives with remarkable efficiency. With an NPS of 25, we're not just providing financial aid; we're catalyzing academic success and personal growth. The 100% mention rate of financial relief, coupled with an average NPS of 9.2 for this theme, underscores the program's core impact.
Notably, while only 33% of recipients mentioned support for family financial struggles, this aspect correlates with the highest average NPS of 9.8. This reveals the profound impact on students from challenging financial backgrounds. Additionally, the 66% who highlighted debt avoidance gave an average NPS of 9.5, demonstrating the long-term financial benefits of our program.
Beyond finances, we're fostering a more engaged student body. The 33% who mentioned increased campus engagement gave an average NPS of 9.3, indicating that our support extends to enriching the overall college experience. By addressing diverse needs from stress reduction to commuting difficulties, we're creating a comprehensive support system that resonates deeply with our recipients.
This data-driven analysis shows that our scholarship program isn't just meeting needs; it's exceeding expectations and creating loyal advocates. We're not only changing individual lives but potentially reshaping the landscape of higher education accessibility and student success."
Step 5: Actionable Insights
Based on the analysis, here are key actionable insights to improve the scholarship program:
1. Expand Family Financial Support Services
Insight: Supporting family financial struggles showed the highest NPS correlation (9.8).
Action: Develop a complementary program offering financial literacy workshops and resources for recipients' families. This could include budgeting tools, financial planning sessions, and information on additional support services.
2. Enhance Debt Avoidance Strategies
Insight: 66% of recipients mentioned debt avoidance, with a high NPS correlation (9.5).
Action: Partner with financial institutions to provide low-interest loan options or loan forgiveness programs for any remaining educational expenses. Offer targeted financial counseling to help students minimize overall debt burden.
3. Boost Campus Engagement Opportunities
Insight: Campus engagement, while mentioned by 33%, had a high NPS correlation (9.3).
Action: Allocate a portion of the scholarship funds specifically for campus activity fees or club memberships. Create a mentorship program pairing scholarship recipients with alumni or upperclassmen to encourage greater campus involvement.
4. Address Commuting Challenges
Insight: Commuting difficulties were mentioned by 33% of recipients, with a lower NPS correlation (8.5).
Action: Explore partnerships with local transportation services to offer discounted passes for scholarship recipients. Consider allocating additional funds for recipients with significant commuting challenges to support housing closer to campus.
5. Implement Stress Reduction Programs
Insight: Stress reduction was noted by 33% of recipients, with an NPS correlation of 8.7.
Advantages of Open-Ended Questions
One of the primary advantages of open-ended questions is their ability to capture rich, detailed responses. By allowing respondents to elaborate on their thoughts and experiences, open-ended questions provide researchers with a deeper understanding of complex issues. These responses can reveal unexpected insights, uncovering nuances that closed-ended questions may overlook.
Moreover, open-ended questions empower respondents to express themselves in their own words, fostering a sense of ownership and authenticity in their responses. This approach can lead to more honest and insightful feedback, as respondents feel valued and heard throughout the research process.
Additionally, open-ended questions are versatile and adaptable to various research contexts. They can be used to explore a wide range of topics and are particularly well-suited for exploratory research, hypothesis generation, and qualitative data analysis. Researchers can gain valuable insights into respondents' perspectives, attitudes, and behaviors, providing a holistic view of the subject under study.
Advantages of Closed-Ended Questions
Closed-ended questions offer several advantages, particularly in terms of data collection efficiency and analysis. By providing predefined response options, these questions enable researchers to gather standardized data quickly and easily. This structured approach streamlines the data collection process, allowing researchers to collect large volumes of data efficiently.
Moreover, closed-ended questions facilitate quantitative analysis, as the responses can be easily categorized and quantified. Researchers can use statistical techniques to analyze and interpret the data, identifying patterns, trends, and correlations with precision. This quantitative approach is particularly valuable for making data-driven decisions, evaluating hypotheses, and measuring the effectiveness of interventions or treatments.
Additionally, closed-ended questions minimize respondent burden by providing clear and concise response options. Respondents can answer these questions quickly, reducing survey fatigue and improving overall response rates. This efficiency is especially beneficial in large-scale research studies or surveys conducted in time-sensitive environments.
Open and Closed Ended Questions For Deep Insight
While both open-ended and closed-ended questions offer distinct advantages, choosing the right approach depends on the research objectives, context, and target audience. In many cases, a combination of both types of questions may be most effective, allowing researchers to gather comprehensive data while balancing the need for depth and efficiency.
For exploratory research or when seeking in-depth insights into complex phenomena, open-ended questions are invaluable. They encourage respondents to share their perspectives openly, uncovering nuanced details and diverse viewpoints. Researchers can use qualitative analysis techniques, such as thematic coding or content analysis, to identify patterns and themes within the data, enriching their understanding of the subject matter.
In contrast, closed-ended questions are well-suited for research scenarios that require standardized data collection and quantitative analysis. They enable researchers to measure attitudes, behaviors, and preferences systematically, facilitating comparisons across groups or time periods. Statistical analysis techniques, such as descriptive statistics or inferential tests, can be applied to closed-ended data to draw meaningful conclusions and make evidence-based recommendations.
Insightful Question Process for Actionable Insights
Background | Closed-ended Question | Open-ended Follow-up | Impact Dimension | Potential Analysis & Actionable Insight |
---|---|---|---|---|
How often do you feel comfortable discussing your mental health with your assigned mentor? a) Always b) Sometimes c) Rarely d) Never e) I don't have an assigned mentor | What factors contribute to your comfort level in discussing mental health with your mentor? | Age, Gender, Duration in program | ||
Since joining our microfinance program, my ability to meet my household's basic needs has: 1: Significantly decreased 2: Slightly decreased 3: Remained the same 4: Slightly increased 5: Significantly increased | Can you describe specific ways in which the microfinance program has affected your household's financial situation? | Loan amount, Business type, Rural/Urban location | ||
After participating in our environmental workshop, my daily actions are: Harmful to the environment 1 - 2 - 3 - 4 - 5 Beneficial to the environment | What specific environmentally friendly actions have you incorporated into your daily routine since the workshop? | Education level, Age group, Workshop attendance frequency | ||
Are you currently employed in the field you were trained for? If No: Which best describes your situation? a) Employed in a different field b) Unemployed but searching c) Not seeking employment d) Pursuing further education | How has the training program influenced your career path, regardless of your current employment status? | Training field, Time since program completion, Previous work experience |
Difference between open and closed ended questions
Open-ended and closed-ended questions are two fundamental types of questions used in surveys, interviews, and research studies. Each type has its own strengths and weaknesses, and the choice between them depends on the goals of the data collection process.
Open-ended vs Closed-ended Questions
Comparison table.
Feature | Open-Ended Questions | Closed-Ended Questions |
---|---|---|
Response Type | Descriptive, detailed | Predefined options |
Data Type | Qualitative | Quantitative |
Analysis | Complex, time-consuming | Simple, quick |
Depth of Insight | High, rich detail | Low, limited detail |
Ease of Response | Requires more effort | Easy, quick |
Use Case | Exploring new topics, understanding experiences | Measuring specific variables, comparing responses |
Risk of Bias | Lower, as responses are freeform | Higher, depends on how options are framed |
Open-ended Questions
Open-ended questions allow respondents to answer in their own words, providing rich, detailed responses. They are excellent for exploring new topics or gathering in-depth information about experiences and opinions.
Closed-ended Questions
Closed-ended questions provide respondents with a set of predefined answer options to choose from. They are ideal for collecting quantitative data and making comparisons across respondents or over time.
Pros and Cons
Pros of open-ended questions.
- Provide rich, detailed insights
- Allow for unexpected responses
- Useful for exploring new topics
- Lower risk of bias in responses
Cons of Open-ended Questions
- Time-consuming to analyze
- May be difficult to quantify
- Require more effort from respondents
- Can lead to irrelevant responses
When to Use Each Type
Choose open-ended questions when you need detailed insights, are exploring new topics, or want to understand complex experiences. Opt for closed-ended questions when you need quantifiable data, want to make direct comparisons, or are dealing with a large sample size.
In practice, many surveys and research studies use a combination of both types to balance the need for detailed insights with the ability to quantify and compare responses efficiently.
Open-Ended Questions in Surveys
Open-ended questions are a cornerstone of qualitative research, providing a window into the deeper thoughts, feelings, and motivations of respondents. They are particularly effective when you need nuanced insights or varied perspectives that structured data cannot capture. To craft effective open-ended questions:
Focus on the Specific : Tailor questions to gather specific information that aligns with the research objectives. For instance, instead of asking "What do you think about our product?" refine it to "What specific features do you like most about our product, and why?"

invite detailed and descriptive answers. Consider using phrases such as "describe in detail," "explain how," or "what led you to," which guide respondents to delve deeper into their experiences and reasoning. This approach not only enriches the data but also brings forth the nuances of personal narratives and insights.
Equally crucial is the formulation of questions that remain neutral, avoiding any language that might steer respondents toward a specific answer. This practice is fundamental to preserving the objectivity of the data collected, ensuring that the insights gained are a genuine reflection of the respondent's thoughts and not influenced by the wording of the question.
Moreover, a well-designed survey question should accommodate a spectrum of perspectives. By framing questions broadly enough, researchers can capture a diverse array of responses, thereby reflecting the varied experiences and opinions of all participants. This inclusivity enriches the data set and provides a more comprehensive understanding of the surveyed group.
In gathering open-ended responses, the goal is to create a platform where each voice and experience can articulate itself freely and distinctly. This approach not only respects the diversity of the respondent pool but also enhances the depth and breadth of the insights gained from the research.

Before launching a survey broadly, it’s crucial to test the open-ended questions with a smaller, representative group. This preliminary phase aims to ensure that the questions effectively elicit the type of responses anticipated. Observing how these respondents interact with the questions provides invaluable insights, allowing for adjustments and refinements to the survey based on actual feedback. This iterative process helps fine-tune the questions to better capture the depth and variety of data needed.
To streamline and enhance this process, employing a modern qualitative data analytics platform like Sopact Sense can be transformative. Sopact Sense dramatically reduces the time and effort traditionally required for data analysis, condensing months of work into mere minutes. With its advanced capabilities, it offers 30 times better accuracy, facilitating both inductive and deductive analysis approaches. Researchers can utilize bottom-up pattern analysis to identify emerging themes without prior assumptions, or apply top-down strategies to test specific hypotheses or code responses post-collection.
Furthermore, Sopact Sense enables detailed demographic filtering, empowering researchers to dissect data layers and uncover genuine causality and correlations. This capability is particularly valuable in understanding how different groups perceive and respond to various issues, enhancing the overall quality and applicability of the research outcomes. By integrating such advanced tools into the survey design and testing phase, researchers can achieve a more dynamic, responsive, and precise exploration of the data they collect.

To ensure that analytics efforts align closely with key organizational goals and foster actionable outcomes at an individual stakeholder level, it’s essential to craft a narrative that integrates both qualitative and quantitative data. This approach allows for a comprehensive understanding of how initiatives are impacting stakeholders, highlighting necessary adjustments and future strategies.
Creating a Comprehensive Narrative:
- Identify and Prioritize Goals: Start by clearly identifying the most crucial goals for the analytics project. Determine what success looks like for each goal and how it aligns with broader organizational objectives.
- Collect Qualitative and Quantitative Data: Use a mixed-methods approach to data collection. Qualitative data can be gathered through open-ended survey questions, interviews, and focus groups that explore stakeholders' feelings, experiences, and suggestions for improvement. Quantitative data should be collected through structured surveys, performance metrics, and other measurable indicators.
- Pre and Post Analysis: If applicable, conduct a pre-intervention analysis to establish a baseline, followed by a post-intervention analysis to measure changes. This comparative analysis can highlight the direct impacts of specific changes and help in communicating these changes effectively.
- Narrate the Story of Each Goal: For each key goal, create a narrative that weaves together the qualitative insights with the quantitative results. This story should outline what was initially found, what changes were implemented, and how these changes influenced the outcomes. Emphasize both the successes and the areas needing improvement.
- Detail Actionable Insights: Based on the narrative, extract actionable insights specific to each stakeholder group. Detail what steps will be taken to address the issues uncovered in the analysis. This might include strategic adjustments, resource reallocations, or new initiatives.
- Communicate Changes and Impact: Use the narratives to communicate with stakeholders about the changes made and their impacts. This communication should be clear and tailored to the audience, ensuring that each stakeholder understands how the findings relate to them and what future actions are planned.
- Plan for Continuous Improvement: Establish a plan for ongoing monitoring and evaluation based on the narrative outcomes. This plan should include regular check-ins and updates to the data collection and analysis processes to ensure they remain aligned with the organization's evolving needs and goals.
By meticulously linking each story to the most important analytics goals and utilizing a narrative that blends qualitative depth with quantitative rigor, organizations can not only achieve a more thorough understanding of their impact but also engage stakeholders in a meaningful way that promotes sustained improvement and strategic decision-making.
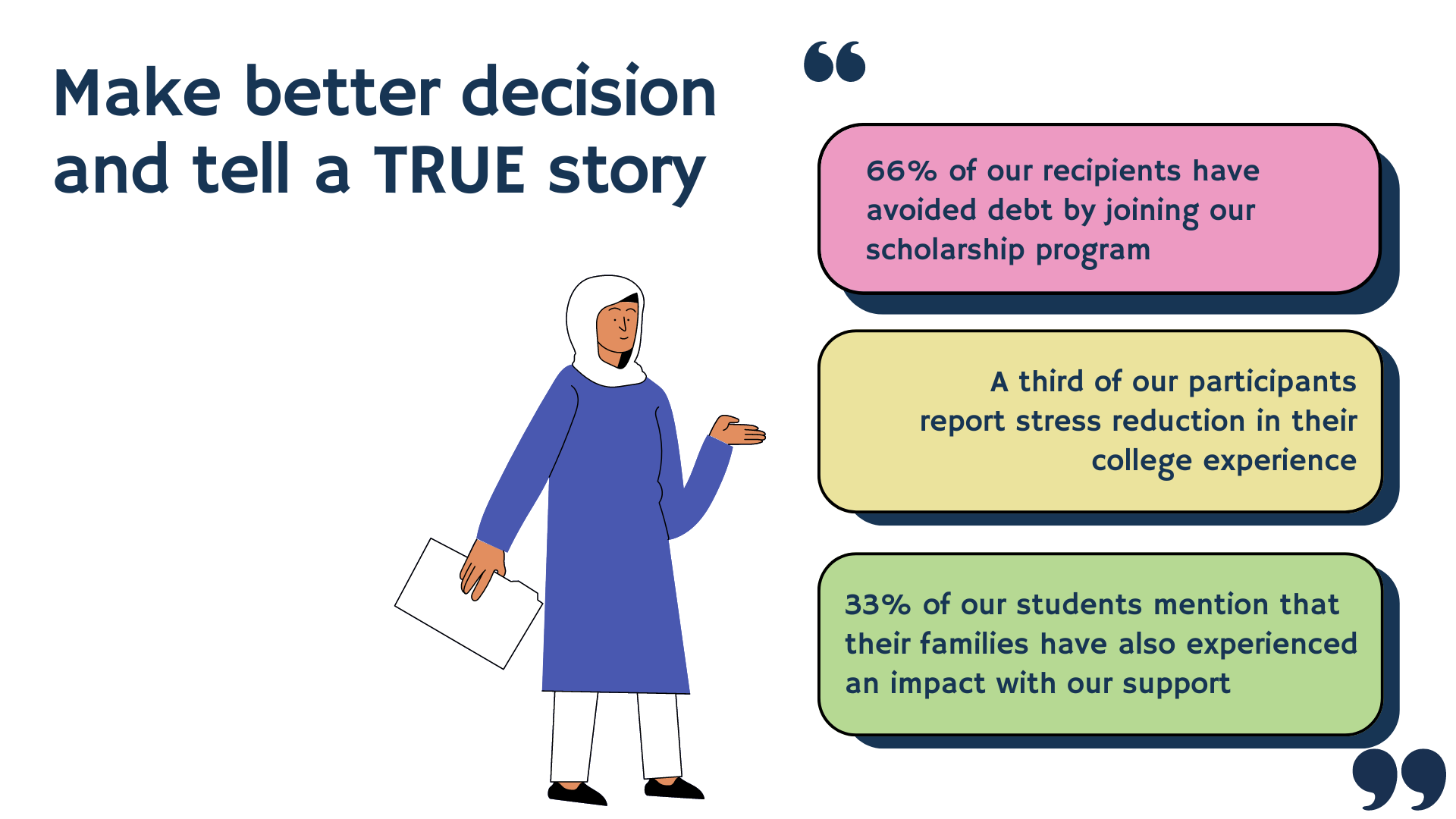
Closed-Ended Questions in Surveys
Creating closed-ended questions for surveys involves a meticulous design process to ensure that the quantitative data collected is accurate, clear, and meaningful. These types of questions are pivotal for confirming hypotheses, measuring trends, and obtaining data that are straightforward to analyze statistically. Here’s how to enhance the effectiveness of closed-ended questions in your surveys:
1. Define Clear Options
To begin, it’s crucial that each closed-ended question provides specific, mutually exclusive categories. This step is essential to cover all possible responses, thus eliminating any potential ambiguity or overlap. For example, if you're asking about frequency of service usage, your options should range clearly from 'Never' to 'Daily' without any vague terms in between. This clarity ensures that the data you collect can be analyzed straightforwardly, leading to more reliable insights.
2. Balance the Scales
Using balanced rating scales, such as Likert scales, can significantly enhance the quality of the data gathered. These scales should offer an equal number of options on either side of a neutral option (if one is included) to ensure an unbiased distribution of responses. For instance, a satisfaction survey might use a scale from 'Very Dissatisfied' to 'Very Satisfied' with 'Neither Satisfied nor Dissatisfied' as a midpoint. This balance helps in minimizing response biases, providing a more accurate picture of respondent sentiments.
3. Keep It Simple
Each question should be formulated to be as clear and straightforward as possible. The language used needs to be simple enough that respondents do not require additional information or context to give an answer. This approach reduces the risk of misinterpretation and ensures that responses are based on the respondents’ true opinions and experiences. An example might be using "Do you agree that the customer service was helpful?" instead of a more complex phrasing that could confuse the respondent.
4. Include an 'Other' Option
Sometimes, even well-designed questions might not capture all possible respondent experiences. In such cases, including an 'Other' option with a space for respondents to specify their answer can be invaluable. This option acts as a safety net, capturing data that might otherwise be missed and offering insights that could lead to new discoveries or considerations in your analysis.
5. Pre-test Your Questions
Before deploying the survey to a larger audience, conduct a pre-test with a small, representative group. This testing helps ensure that the questions are understood as intended and that all potential responses are adequately covered. Gather feedback on the clarity of the questions and the adequacy of the response options. Use this feedback to make necessary adjustments, refining your survey to better meet its objectives.
Putting It All Together
When these elements are carefully integrated into the design of closed-ended questions, the resulting data becomes a powerful tool for statistical analysis and decision-making. These questions not only streamline the data collection process but also enhance the precision and applicability of the insights gained. By rigorously crafting and testing your closed-ended questions, you ensure that the survey effectively measures the intended variables and yields high-quality data that can support robust conclusions and strategic actions.
Open and Closed-Ended Questions in Survey Design
Incorporating both open and closed-ended questions in surveys can significantly enhance the data collection process by melding the depth of qualitative feedback with the quantitative data's scope. This dual approach proves invaluable in multifaceted research areas, where understanding the underlying reasons behind behaviors, decisions, or preferences is key.
Sequential Integration : Begin with closed-ended questions to collect broad data, then follow up with open-ended questions to delve into specific areas of interest more deeply. This technique helps provide a contextual backdrop for the quantitative findings through rich qualitative insights.
Parallel Integration : Simultaneously employ open and closed-ended questions regarding the same subject within a survey. This strategy captures a wide array of data, offering both statistical comprehensiveness and insightful qualitative depth.
Iterative Design : Utilize initial survey responses to refine or introduce new questions that probe deeper into significant themes that arise. This responsive design allows the survey to evolve based on real-time insights, making it highly adaptive to the research needs.
By strategically combining these approaches, researchers can leverage the strengths of both qualitative and quantitative methodologies, resulting in more rounded and actionable data.
For more detailed strategies on mixed-method surveys, refer to the guide provided by Sopact. Learn more about mixed-method surveys at Sopact .

In conclusion, the choice between open-ended and closed-ended questions is a critical consideration in research design and data collection. Each type of question offers unique advantages and limitations, influencing the depth, efficiency, and quality of insights obtained. By understanding the strengths and weaknesses of both approaches and selecting the most appropriate method for each research context, researchers can maximize the value of their findings and generate meaningful contributions to their field.
Looking for something else?
Still need help.

Open-Ended vs Closed-Ended Questions: Choosing the Right Type for Your Survey

When designing a survey, one of the key decisions to make is whether to use open-ended or closed-ended questions. Open-ended questions allow respondents to provide free-form answers in their own words. Closed-ended questions, on the other hand, provide a set of predefined responses for respondents to choose from. In this article, we'll explore the differences between these questions, and provide examples of when to use each type of question.
Open-Ended Questions
Open-ended questions provide respondents with a space to write their own answers, rather than selecting from a predetermined list of responses. These questions allow respondents to elaborate on their thoughts and feelings about a topic, and can provide valuable insights into attitudes and opinions.
One of the key benefits of open-ended questions is that they allow researchers to gather more in-depth and nuanced responses from respondents. For example, if you were conducting a survey about customer satisfaction with a new product, you might ask an open-ended question like, "What do you like best about the product?" This question gives respondents the opportunity to share specific details about why they enjoyed using the product, providing valuable feedback for product improvement.

However, there are also some downsides to using open-ended questions. Because respondents must write their own answers, open-ended questions tend to take longer to complete. This may lead to lower response rates. Additionally, analyzing open-ended responses can be time-consuming and complex, requiring researchers to code and categorize answers to identify common themes.
Here are some examples of open-ended questions:
● What areas of our customer service could be improved in your opinion?
● What features or improvements would you like to see in our product offerings?
● What makes our marketing stand out compared to other companies?
● What values do you think are most important to our company?
● What areas do you feel you need more support or training in to improve your performance?
Closed-Ended Questions
Closed-ended questions, on the other hand, provide respondents with a set of predefined responses to choose from. These questions are typically used for gathering quantitative data. They allow researchers to collect data that can be easily analyzed and compared across respondents.
One of the benefits of closed-ended questions is that they allow researchers to easily compare responses across a large sample size. For example, if you were conducting a survey about course evaluation, you might ask a closed-ended question like, "How would you rate the overall quality of the course?" This question would provide a clear set of options (such as "Excellent," "Very Good," "Good," "Fair,"or "Poor"), allowing for easy analysis of the results.

However, closed-ended questions can also have limitations. Because respondents are limited to a set of predefined responses, closed-ended questions may not allow for the same level of nuance and detail as open-ended questions. Additionally, closed-ended questions may not capture all of the potential responses a respondent might provide, leading to incomplete data.
Here are some examples of closed-ended questions:
● How often do you exercise each week?
- 2-3 times per week
- Once a week
- Once a month
● How likely are you to purchase our product or service?
- Extremely likely
- Extremely unlikely
● How long have you been with the company?
- Less than 1 year
- 1-2 years
- 3-5 years
- 6-10 years
- More than 10 years
When to Use Each Type
When deciding whether to use open-ended or closed-ended questions in a survey, it's important to consider the research question and the goals of the survey. Open-ended questions are generally best suited for qualitative research, where in-depth insights and detailed feedback are important. Closed-ended questions are better suited for quantitative research, where standardized data is needed for comparison and analysis.
In general, mixing open-ended and closed-ended questions tend to be the most effective, as they provide a balance of qualitative and quantitative data. For example, a customer satisfaction survey might include both open-ended questions about what customers liked or disliked about a product, as well as closed-ended questions about overall satisfaction or likelihood to recommend the product.
Conclusion
In summary, open-ended questions and closed-ended questions each have their own strengths and limitations. A well-designed survey will likely include a mix of both types of questions, providing a more complete picture of the attitudes and opinions of the respondents.

—— You might also like ——

To better assist you, please provide your contact information and we will get back to you as soon as possible.
For proactive communication, you can use the email address below to get in touch with us.

What are Close Ended Questions? Examples and Tips
- September 5, 2020
- Survey Design
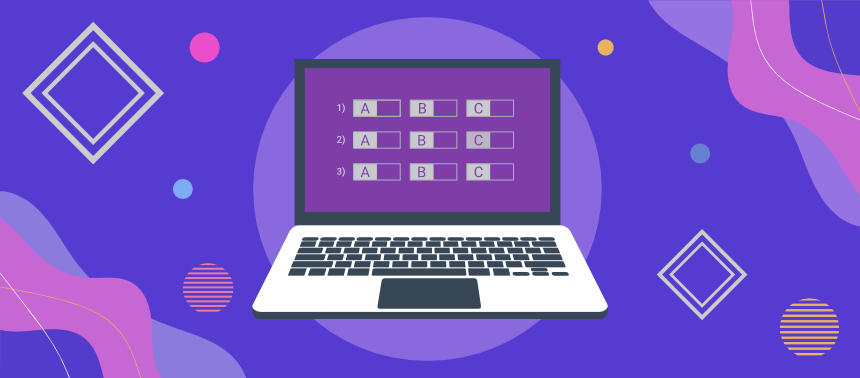
What is a close-ended question?
Close-ended questions are those that start with ‘Can’, ‘Did’, ‘Will’ or ‘Have’. Most commonly, they take the form of multiple-choice questions, where respondents choose from a set list of answers.
You would use closed-ended questions to collect quantitative data. From which you’d determine some ‘statistical significance’. They’re usually simpler than their open-ended counterparts, allowing respondents to quickly answer.
Examples of close-ended questions
Typically, closed questions will have a one-word answer, such as ‘yes’ or ‘no. In these cases you would ask questions like:
- Do you like our service?
- Is London the capital of England?
- Can you run 5 kilometres?
- Have you enjoyed the event?
However, there are examples of close-ended questions that require answers other than yes or no.
- What year were you born?
- On a scale of 1-10, how satisfied are you?
- Which university did you attend?
- How often do use public transport?
Open-ended questions
Open questions ask participants to write unique responses, which are free form. They’re more suited to exploratory research that looks to describe a subject based on trends and patterns. However, they require more effort and time to answer.
Examples of open-ended questions
- What did/ didn’t you like about our service?
- Which aspects of the event were you most satisfied with?
- How would you change our product?
- What did you expect to happen?
Learn more about open-ended questions .
Differences between open-ended and close-ended questions
The difference between open-ended and closed-ended questions lies in the data they collect. Closed questions collect data that can be used to draw generalized conclusions based on statistical analysis.
Open-ended questions ask respondents to describe a subject. You’d then look for trends and patterns in the responses you’ve collected. Think of these question styles as ‘tasks’ with different outputs.
However, the quality of data you collect depends on the way you write questions. E.g. If you write leading questions, your data will not be accurate. Learn how to write survey questions .
Advantages of closed-ended questions
- People can answer quickly
- Easier to understand and interpret
- Results are easy to analyze
- Answer options provide context to questions
- People are more likely to answer sensitive questions
- Respondents can’t give irrelevant answers
- You can include an Other text box with close-ended questions if a respondent wants to provide a unique answer
People will feel less friction when answering closed questions.
Disadvantages of closed-ended questions
Conversely, here are a few problems with using closed questions:
- Your answer lists can provoke choices that participants otherwise wouldn’t make
- Some respondents may feel that none of the set answers reflects their own opinion or experience. In these cases, they may choose to skip the question or even select an answer at random
- Too many answer choices may deter or confuse respondents. So, you should only provide the most important and relevant options. See our article on writing survey answers
- It’s difficult to identify those who misunderstand a question and choose the wrong answer as a result
- The format of close-ended questions may be too simple for complex issues. Especially if a respondent wants to provide more detail on a subject
- To identify ‘statistical significance’ in results, you’ll need to collect a larger data set
As close-ended questions have specific answers, they must be consistent and clear. Any ambiguity can affect the way people answer and can lead to different types of response bias .
Tips for using close-ended questions
1. become an expert but write questions for those who aren’t.
It’s important to fully understand your research topic to ask the right questions and provide the right answers. It’s also your job to translate what you know into terms that are understandable for respondents.
If they can’t understand your questions or answers, then the data you collect will be at risk.
2. Keep questions simple and clear
Your questions should be specific and concise. The longer and more complex questions are, the more likely participants are to misinterpret or disengage.
3. Ensure answer choices are exclusive and exhaustive
Ensure that the answer choices for closed questions are both exhaustive and exclusive.
Exhaustive answer lists are those that provide the entire range of choices (e.g. Very Unsatisfied – Very Satisfied).
Exclusive answer lists ensure no choices share intent or meaning. The most common form of this is where numbered groups overlap, e.g. 18-25, 25-35, 35-45. If a participant is 25 they won’t know whether to select 18-25 or 25-35.
However, words with similar or indistinguishable meanings can also cause issues, e.g. ‘Fine’ and ‘Satisfactory’.
4. Only provide relevant answers
You should be able to anticipate what kind of answers respondents will give for each question.
If you can’t, your question may be too broad or complex.
Closed-ended question examples for surveys
Below are a few examples of closed questions in surveys. The examples are from the most common survey types. Including market research , employee satisfaction and event feedback .
Market research surveys
- Would you recommend our product/ service?
- How helpful was our customer service?
- Please rate our service:
- How likely are you to purchase from us again?
Employee satisfaction surveys
- How satisfied are you with the level of communication in your department?
- Do you feel you use your skills and abilities to their fullest in your role?
- Are your goals clearly defined?
- Do you know what your KPIs are?
- Do you have a good work/ life balance?
Event feedback surveys
- Will you be attending the event?
- Was the event good value for money?
- How could we improve the check-in process?
- Was there enough time to network/ meet other guests?
Close-ended questions limit respondents to answer choices provided by the researcher. They’re an effective means of collecting quantitative data , but do not explore the meaning or intent of participant responses.
However, open-ended and closed questions can be used in tandem. By doing so, you’re able to collect qualitative data alongside the statistical responses.
This will give you a more well-rounded understanding of your respondents. As you not only learn what they think but also give context to their choices with the open feedback.
We’d suggest you begin a line of questioning with a few close-ended questions. Then follow up with an open-ended question to provide context.
Related Posts
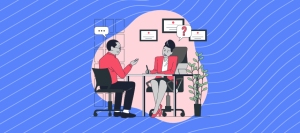
Create the Perfect Digital Marketing Client Questionnaire
- March 6, 2024
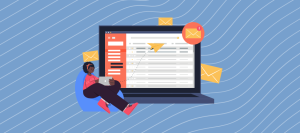
Email Surveys 101: The Guide to Success
- December 21, 2023
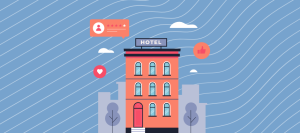
25 Hotel Survey Questions to Improve Guest Satisfaction
- November 7, 2023
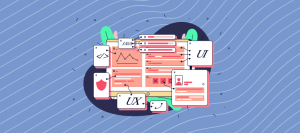
User Experience Survey Questions, Methods & More
- July 14, 2023
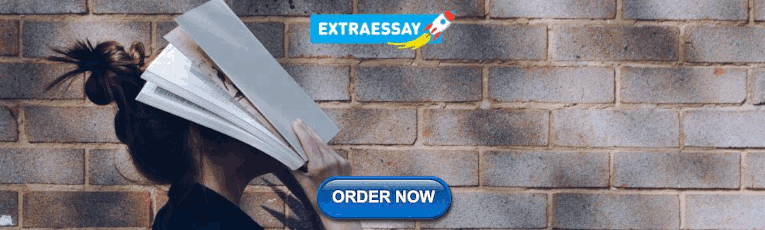
COMMENTS
The purpose of close-ended questions is to gather focused, quantitative data — numbers, dates, or a one-word answer — from respondents as it's easy to group, compare and analyze. Also, researchers use close-ended questions because the results are statistically significant and help to show trends and percentages over time.
When to Use Close-Ended Questions. While close-ended questions are powerful tools, they're not suitable for every situation. Here are some scenarios where they're particularly effective: 1. Large-Scale Surveys: When you need to collect data from a large number of respondents quickly and efficiently. 2.
Hypothesis Testing: Quantitative research often involves hypothesis testing. Close-ended questions provide structured data that can be directly used to test hypotheses and draw statistical inferences. Large Sample Sizes: Quantitative research often requires large sample sizes to ensure the reliability of findings.
Close ended questions are defined as question types that ask respondents to choose from a distinct set of pre-defined responses, such as "yes/no" or among set multiple choice questions. In a typical scenario, closed-ended questions are used to gather quantitative data from respondents. Closed-ended questions come in a multitude of forms but ...
Closed-ended questions are a helpful tool your team can use when creating a survey or questionnaire to collect specific, easy-to-analyze quantitative data. As a great tool for quick check-in surveys or increasing your engagement rates on larger-scale projects, well-written closed-ended questions can give you incredibly helpful data that can be ...
Close-ended questions allow you to create surveys that are easy to answer for respondents. In turn, it will be easier for you to collect quantitative data and conduct statistical analysis on any particular aspect you want to study.. Unlike open-ended questions, these questions only require a one-word answer or a selection from a pre-determined list of answer choices.
Close-ended questions benefit quantitative research, where the goal is to gather numerical data that can be easily analyzed and compared. They also offer a straightforward way to categorize responses and draw conclusions from the data. ... Use close-ended questions and open-ended questions to gather specific data for more detailed insights ...
When conducting any form of research, considering the way questions are structured is essential. Carefully designed questionnaires that use the appropriate type of questions are often the key to collecting data successfully. There are broadly two types of questions in research: closed questions and open questions.In this guide, we will explain how closed (aka "close-ended") questions are ...
Close-ended questions are better suited to quantitative research, where the respondents answer your questions in a manner such that they're less likely to disengage What is a close ended question. A closed-ended question, by definition, is a question that could be answered with a one-word answer or a simple "yes" or "no."
Here are some of the scenarios on when to use closed-ended questions: ... Quantitative research: If your study aims to gather numerical data and statistical insights, closed-ended questions are the way to go. The predefined response options allow for easy quantification and analysis of the data. Consequently, it enables you to identify trends ...
Here are a few close-ended questions to know: 1. Dichotomous questions. Dichotomous questions, by nature, can only be answered in one of two ways (i.e., a dichotomy is the division between two things that are opposites or entirely different). Common answers to dichotomous questions include "yes" or "no"; "true" or "false ...
01 Multiple choice questions. Multiple choice questions are standard in close ended surveys. They give respondents a bunch of predetermined answers, which makes it more convenient to collect and analyze data. Closed-ended questions are your go-to when you need to lead to statistical analysis, especially to see common opinions or choices.
An open-ended question allows respondents total freedom in their responses, while a close-ended question produces quantifiable data. Learn how to use both. Get started. When constructing a survey, researchers have two main question forms to choose from. They can select close-ended questions to generate structured, quantifiable data.
Open-Ended vs. Closed Questions. There are two types of questions we can use in research studies: open-ended and closed. Open-ended questions allow participants to give a free-form text answer. Closed questions (or closed-ended questions) restrict participants to one of a limited set of possible answers.. Open-ended questions encourage exploration of a topic; a participant can choose what to ...
Closed-ended, or restricted-choice, questions offer respondents a fixed set of choices to select from. These questions are easier to answer quickly. Open-ended or long-form questions allow respondents to answer in their own words. Because there are no restrictions on their choices, respondents can answer in ways that researchers may not have ...
Most questions in a survey are closed ended because they help gather actionable, quantitative data. Let's look at specific instances where closed-ended questions are useful. Gain Quantitative Insights. Since closed-ended questions have discrete responses, you can analyze these responses by assigning a number or a value to every answer.
Close-ended questions are a type of inquiry that limits respondents to a set of predefined answers, allowing for straightforward, concise responses. These questions are often formatted as yes/no, multiple-choice, or rating scale queries. They are particularly useful in surveys, polls, and research contexts where statistical analysis is required ...
In choosing whether to use open-ended questions or to adapt them to closed-ended questions for use online, there are several pros and cons to weigh up. Open-ended questions allow for a consistency of methodology with traditional lab-based approaches—meaning there is no risk of participants switching to using different strategies or processes ...
Open-ended questions allow you to gather personal insights and experiences, while closed-ended questions enable you to measure program outcomes and effectiveness. By designing surveys that generate both narrative and numerical data, organizations can: Capture genuine stakeholder voices: Understand the deeper needs and expectations of those ...
Open-ended questions are generally best suited for qualitative research, where in-depth insights and detailed feedback are important. Closed-ended questions are better suited for quantitative research, where standardized data is needed for comparison and analysis. In general, mixing open-ended and closed-ended questions tend to be the most ...
Close-ended questions are those that start with 'Can', 'Did', 'Will' or 'Have'. Most commonly, they take the form of multiple-choice questions, where respondents choose from a set list of answers. You would use closed-ended questions to collect quantitative data. From which you'd determine some 'statistical significance'.