
An official website of the United States government
The .gov means it’s official. Federal government websites often end in .gov or .mil. Before sharing sensitive information, make sure you’re on a federal government site.
The site is secure. The https:// ensures that you are connecting to the official website and that any information you provide is encrypted and transmitted securely.
- Publications
- Account settings
- My Bibliography
- Collections
- Citation manager
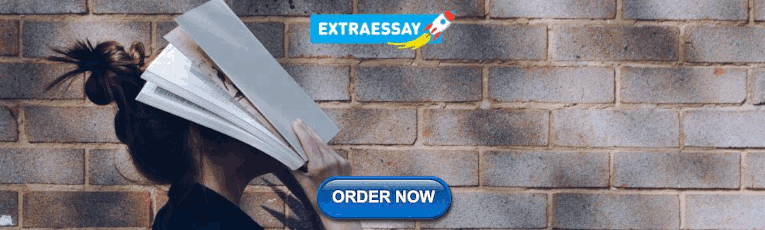
Save citation to file
Email citation, add to collections.
- Create a new collection
- Add to an existing collection
Add to My Bibliography
Your saved search, create a file for external citation management software, your rss feed.
- Search in PubMed
- Search in NLM Catalog
- Add to Search
Qualitative case study data analysis: an example from practice
Affiliation.
- 1 School of Nursing and Midwifery, National University of Ireland, Galway, Republic of Ireland.
- PMID: 25976531
- DOI: 10.7748/nr.22.5.8.e1307
Aim: To illustrate an approach to data analysis in qualitative case study methodology.
Background: There is often little detail in case study research about how data were analysed. However, it is important that comprehensive analysis procedures are used because there are often large sets of data from multiple sources of evidence. Furthermore, the ability to describe in detail how the analysis was conducted ensures rigour in reporting qualitative research.
Data sources: The research example used is a multiple case study that explored the role of the clinical skills laboratory in preparing students for the real world of practice. Data analysis was conducted using a framework guided by the four stages of analysis outlined by Morse ( 1994 ): comprehending, synthesising, theorising and recontextualising. The specific strategies for analysis in these stages centred on the work of Miles and Huberman ( 1994 ), which has been successfully used in case study research. The data were managed using NVivo software.
Review methods: Literature examining qualitative data analysis was reviewed and strategies illustrated by the case study example provided. Discussion Each stage of the analysis framework is described with illustration from the research example for the purpose of highlighting the benefits of a systematic approach to handling large data sets from multiple sources.
Conclusion: By providing an example of how each stage of the analysis was conducted, it is hoped that researchers will be able to consider the benefits of such an approach to their own case study analysis.
Implications for research/practice: This paper illustrates specific strategies that can be employed when conducting data analysis in case study research and other qualitative research designs.
Keywords: Case study data analysis; case study research methodology; clinical skills research; qualitative case study methodology; qualitative data analysis; qualitative research.
PubMed Disclaimer
Similar articles
- Using Framework Analysis in nursing research: a worked example. Ward DJ, Furber C, Tierney S, Swallow V. Ward DJ, et al. J Adv Nurs. 2013 Nov;69(11):2423-31. doi: 10.1111/jan.12127. Epub 2013 Mar 21. J Adv Nurs. 2013. PMID: 23517523
- Rigour in qualitative case-study research. Houghton C, Casey D, Shaw D, Murphy K. Houghton C, et al. Nurse Res. 2013 Mar;20(4):12-7. doi: 10.7748/nr2013.03.20.4.12.e326. Nurse Res. 2013. PMID: 23520707
- Selection, collection and analysis as sources of evidence in case study research. Houghton C, Casey D, Smyth S. Houghton C, et al. Nurse Res. 2017 Mar 22;24(4):36-41. doi: 10.7748/nr.2017.e1482. Nurse Res. 2017. PMID: 28326917
- Qualitative case study methodology in nursing research: an integrative review. Anthony S, Jack S. Anthony S, et al. J Adv Nurs. 2009 Jun;65(6):1171-81. doi: 10.1111/j.1365-2648.2009.04998.x. Epub 2009 Apr 3. J Adv Nurs. 2009. PMID: 19374670 Review.
- Avoiding and identifying errors in health technology assessment models: qualitative study and methodological review. Chilcott J, Tappenden P, Rawdin A, Johnson M, Kaltenthaler E, Paisley S, Papaioannou D, Shippam A. Chilcott J, et al. Health Technol Assess. 2010 May;14(25):iii-iv, ix-xii, 1-107. doi: 10.3310/hta14250. Health Technol Assess. 2010. PMID: 20501062 Review.
- Genital Cosmetic Surgery in Women of Different Generations: A Qualitative Study. Yıldırım Bayraktar BN, Ada G, Hamlacı Başkaya Y, Ilçioğlu K. Yıldırım Bayraktar BN, et al. Aesthetic Plast Surg. 2024 Aug 15. doi: 10.1007/s00266-024-04290-w. Online ahead of print. Aesthetic Plast Surg. 2024. PMID: 39145811
- The lived experiences of fatigue among patients receiving haemodialysis in Oman: a qualitative exploration. Al-Naamani Z, Gormley K, Noble H, Santin O, Al Omari O, Al-Noumani H, Madkhali N. Al-Naamani Z, et al. BMC Nephrol. 2024 Jul 29;25(1):239. doi: 10.1186/s12882-024-03647-2. BMC Nephrol. 2024. PMID: 39075347 Free PMC article.
- How a National Organization Works in Partnership With People Who Have Lived Experience in Mental Health Improvement Programs: Protocol for an Exploratory Case Study. Robertson C, Hibberd C, Shepherd A, Johnston G. Robertson C, et al. JMIR Res Protoc. 2024 Apr 19;13:e51779. doi: 10.2196/51779. JMIR Res Protoc. 2024. PMID: 38640479 Free PMC article.
- Implementation of an office-based addiction treatment model for Medicaid enrollees: A mixed methods study. Treitler P, Enich M, Bowden C, Mahone A, Lloyd J, Crystal S. Treitler P, et al. J Subst Use Addict Treat. 2024 Jan;156:209212. doi: 10.1016/j.josat.2023.209212. Epub 2023 Nov 5. J Subst Use Addict Treat. 2024. PMID: 37935350
- Using the quadruple aim to understand the impact of virtual delivery of care within Ontario community health centres: a qualitative study. Bhatti S, Dahrouge S, Muldoon L, Rayner J. Bhatti S, et al. BJGP Open. 2022 Dec 20;6(4):BJGPO.2022.0031. doi: 10.3399/BJGPO.2022.0031. Print 2022 Dec. BJGP Open. 2022. PMID: 36109022 Free PMC article.
- Search in MeSH
- Citation Manager
NCBI Literature Resources
MeSH PMC Bookshelf Disclaimer
The PubMed wordmark and PubMed logo are registered trademarks of the U.S. Department of Health and Human Services (HHS). Unauthorized use of these marks is strictly prohibited.

Data Analysis Case Study: Learn From Humana’s Automated Data Analysis Project
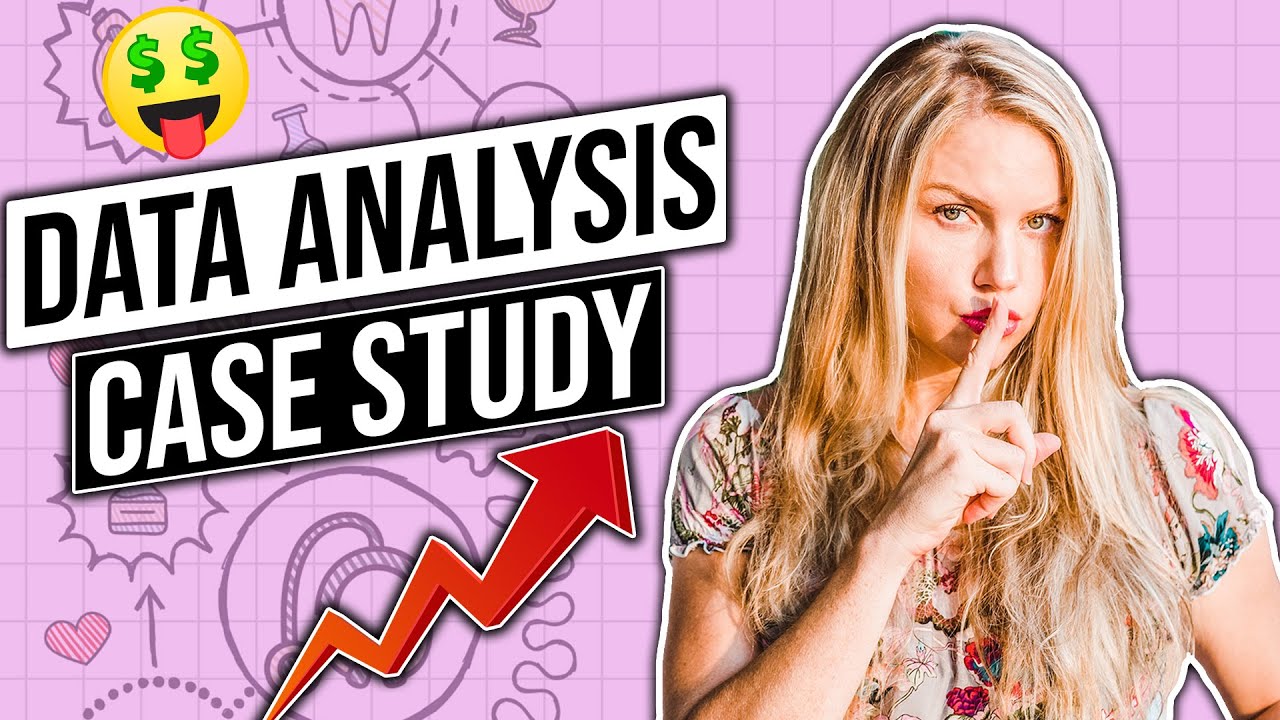
Lillian Pierson, P.E.
Playback speed:
Got data? Great! Looking for that perfect data analysis case study to help you get started using it? You’re in the right place.
If you’ve ever struggled to decide what to do next with your data projects, to actually find meaning in the data, or even to decide what kind of data to collect, then KEEP READING…
Deep down, you know what needs to happen. You need to initiate and execute a data strategy that really moves the needle for your organization. One that produces seriously awesome business results.
But how you’re in the right place to find out..
As a data strategist who has worked with 10 percent of Fortune 100 companies, today I’m sharing with you a case study that demonstrates just how real businesses are making real wins with data analysis.
In the post below, we’ll look at:
- A shining data success story;
- What went on ‘under-the-hood’ to support that successful data project; and
- The exact data technologies used by the vendor, to take this project from pure strategy to pure success
If you prefer to watch this information rather than read it, it’s captured in the video below:
Here’s the url too: https://youtu.be/xMwZObIqvLQ
3 Action Items You Need To Take
To actually use the data analysis case study you’re about to get – you need to take 3 main steps. Those are:
- Reflect upon your organization as it is today (I left you some prompts below – to help you get started)
- Review winning data case collections (starting with the one I’m sharing here) and identify 5 that seem the most promising for your organization given it’s current set-up
- Assess your organization AND those 5 winning case collections. Based on that assessment, select the “QUICK WIN” data use case that offers your organization the most bang for it’s buck
Step 1: Reflect Upon Your Organization
Whenever you evaluate data case collections to decide if they’re a good fit for your organization, the first thing you need to do is organize your thoughts with respect to your organization as it is today.
Before moving into the data analysis case study, STOP and ANSWER THE FOLLOWING QUESTIONS – just to remind yourself:
- What is the business vision for our organization?
- What industries do we primarily support?
- What data technologies do we already have up and running, that we could use to generate even more value?
- What team members do we have to support a new data project? And what are their data skillsets like?
- What type of data are we mostly looking to generate value from? Structured? Semi-Structured? Un-structured? Real-time data? Huge data sets? What are our data resources like?
Jot down some notes while you’re here. Then keep them in mind as you read on to find out how one company, Humana, used its data to achieve a 28 percent increase in customer satisfaction. Also include its 63 percent increase in employee engagement! (That’s such a seriously impressive outcome, right?!)
Step 2: Review Data Case Studies
Here we are, already at step 2. It’s time for you to start reviewing data analysis case studies (starting with the one I’m sharing below). I dentify 5 that seem the most promising for your organization given its current set-up.
Humana’s Automated Data Analysis Case Study
The key thing to note here is that the approach to creating a successful data program varies from industry to industry .
Let’s start with one to demonstrate the kind of value you can glean from these kinds of success stories.
Humana has provided health insurance to Americans for over 50 years. It is a service company focused on fulfilling the needs of its customers. A great deal of Humana’s success as a company rides on customer satisfaction, and the frontline of that battle for customers’ hearts and minds is Humana’s customer service center.
Call centers are hard to get right. A lot of emotions can arise during a customer service call, especially one relating to health and health insurance. Sometimes people are frustrated. At times, they’re upset. Also, there are times the customer service representative becomes aggravated, and the overall tone and progression of the phone call goes downhill. This is of course very bad for customer satisfaction.
Humana wanted to use artificial intelligence to improve customer satisfaction (and thus, customer retention rates & profits per customer).
Humana wanted to find a way to use artificial intelligence to monitor their phone calls and help their agents do a better job connecting with their customers in order to improve customer satisfaction (and thus, customer retention rates & profits per customer ).
In light of their business need, Humana worked with a company called Cogito, which specializes in voice analytics technology.
Cogito offers a piece of AI technology called Cogito Dialogue. It’s been trained to identify certain conversational cues as a way of helping call center representatives and supervisors stay actively engaged in a call with a customer.
The AI listens to cues like the customer’s voice pitch.
If it’s rising, or if the call representative and the customer talk over each other, then the dialogue tool will send out electronic alerts to the agent during the call.
Humana fed the dialogue tool customer service data from 10,000 calls and allowed it to analyze cues such as keywords, interruptions, and pauses, and these cues were then linked with specific outcomes. For example, if the representative is receiving a particular type of cues, they are likely to get a specific customer satisfaction result.
The Outcome
Customers were happier, and customer service representatives were more engaged..
This automated solution for data analysis has now been deployed in 200 Humana call centers and the company plans to roll it out to 100 percent of its centers in the future.
The initiative was so successful, Humana has been able to focus on next steps in its data program. The company now plans to begin predicting the type of calls that are likely to go unresolved, so they can send those calls over to management before they become frustrating to the customer and customer service representative alike.
What does this mean for you and your business?
Well, if you’re looking for new ways to generate value by improving the quantity and quality of the decision support that you’re providing to your customer service personnel, then this may be a perfect example of how you can do so.
Humana’s Business Use Cases
Humana’s data analysis case study includes two key business use cases:
- Analyzing customer sentiment; and
- Suggesting actions to customer service representatives.
Analyzing Customer Sentiment
First things first, before you go ahead and collect data, you need to ask yourself who and what is involved in making things happen within the business.
In the case of Humana, the actors were:
- The health insurance system itself
- The customer, and
- The customer service representative
As you can see in the use case diagram above, the relational aspect is pretty simple. You have a customer service representative and a customer. They are both producing audio data, and that audio data is being fed into the system.
Humana focused on collecting the key data points, shown in the image below, from their customer service operations.
By collecting data about speech style, pitch, silence, stress in customers’ voices, length of call, speed of customers’ speech, intonation, articulation, silence, and representatives’ manner of speaking, Humana was able to analyze customer sentiment and introduce techniques for improved customer satisfaction.
Having strategically defined these data points, the Cogito technology was able to generate reports about customer sentiment during the calls.
Suggesting actions to customer service representatives.
The second use case for the Humana data program follows on from the data gathered in the first case.
In Humana’s case, Cogito generated a host of call analyses and reports about key call issues.
In the second business use case, Cogito was able to suggest actions to customer service representatives, in real-time , to make use of incoming data and help improve customer satisfaction on the spot.
The technology Humana used provided suggestions via text message to the customer service representative, offering the following types of feedback:
- The tone of voice is too tense
- The speed of speaking is high
- The customer representative and customer are speaking at the same time
These alerts allowed the Humana customer service representatives to alter their approach immediately , improving the quality of the interaction and, subsequently, the customer satisfaction.
The preconditions for success in this use case were:
- The call-related data must be collected and stored
- The AI models must be in place to generate analysis on the data points that are recorded during the calls
Evidence of success can subsequently be found in a system that offers real-time suggestions for courses of action that the customer service representative can take to improve customer satisfaction.
Thanks to this data-intensive business use case, Humana was able to increase customer satisfaction, improve customer retention rates, and drive profits per customer.
The Technology That Supports This Data Analysis Case Study
I promised to dip into the tech side of things. This is especially for those of you who are interested in the ins and outs of how projects like this one are actually rolled out.
Here’s a little rundown of the main technologies we discovered when we investigated how Cogito runs in support of its clients like Humana.
- For cloud data management Cogito uses AWS, specifically the Athena product
- For on-premise big data management, the company used Apache HDFS – the distributed file system for storing big data
- They utilize MapReduce, for processing their data
- And Cogito also has traditional systems and relational database management systems such as PostgreSQL
- In terms of analytics and data visualization tools, Cogito makes use of Tableau
- And for its machine learning technology, these use cases required people with knowledge in Python, R, and SQL, as well as deep learning (Cogito uses the PyTorch library and the TensorFlow library)
These data science skill sets support the effective computing, deep learning , and natural language processing applications employed by Humana for this use case.
If you’re looking to hire people to help with your own data initiative, then people with those skills listed above, and with experience in these specific technologies, would be a huge help.
Step 3: S elect The “Quick Win” Data Use Case
Still there? Great!
It’s time to close the loop.
Remember those notes you took before you reviewed the study? I want you to STOP here and assess. Does this Humana case study seem applicable and promising as a solution, given your organization’s current set-up…
YES ▶ Excellent!
Earmark it and continue exploring other winning data use cases until you’ve identified 5 that seem like great fits for your businesses needs. Evaluate those against your organization’s needs, and select the very best fit to be your “quick win” data use case. Develop your data strategy around that.
NO , Lillian – It’s not applicable. ▶ No problem.
Discard the information and continue exploring the winning data use cases we’ve categorized for you according to business function and industry. Save time by dialing down into the business function you know your business really needs help with now. Identify 5 winning data use cases that seem like great fits for your businesses needs. Evaluate those against your organization’s needs, and select the very best fit to be your “quick win” data use case. Develop your data strategy around that data use case.
More resources to get ahead...
Get income-generating ideas for data professionals, are you tired of relying on one employer for your income are you dreaming of a side hustle that won’t put you at risk of getting fired or sued well, my friend, you’re in luck..
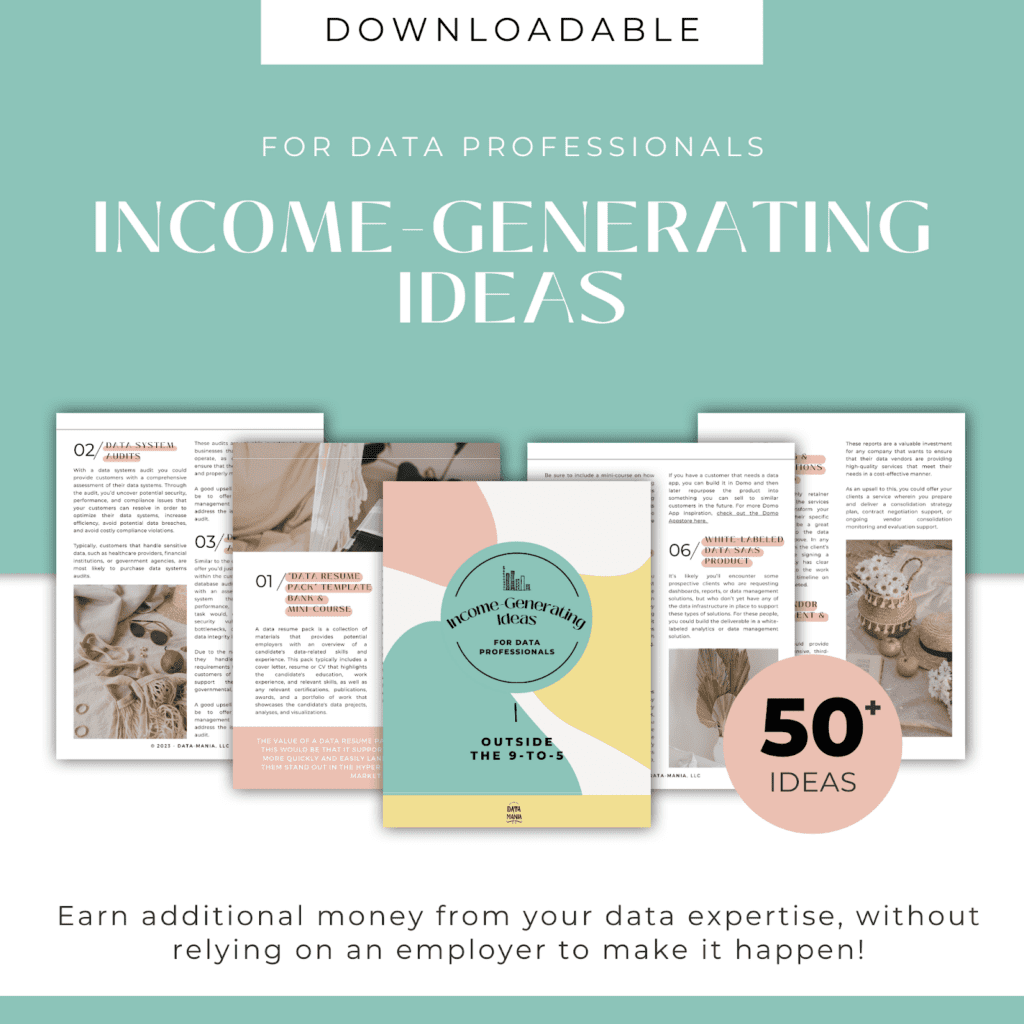
This 48-page listing is here to rescue you from the drudgery of corporate slavery and set you on the path to start earning more money from your existing data expertise. Spend just 1 hour with this pdf and I can guarantee you’ll be bursting at the seams with practical, proven & profitable ideas for new income-streams you can create from your existing expertise. Learn more here!
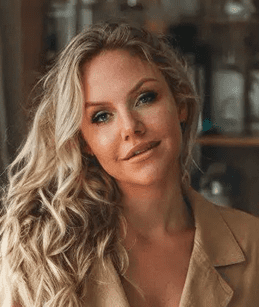
We love helping tech brands gain exposure and brand awareness among our active audience of 530,000 data professionals. If you’d like to explore our alternatives for brand partnerships and content collaborations, you can reach out directly on this page and book a time to speak.
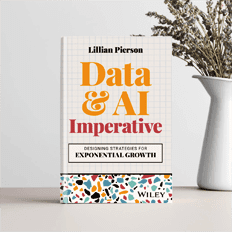
DOES YOUR GROWTH STRATEGY PASS THE AI-READINESS TEST?
I've put these processes to work for Fortune 100 companies, and now I'm handing them to you...

- Marketing Optimization Toolkit
- CMO Portfolio
- Fractional CMO Services
- Marketing Consulting
- The Power Hour
- Integrated Leader
- Advisory Support
- VIP Strategy Intensive
- MBA Strategy
Get In Touch
- 1-407-852-8511
- [email protected]
Privacy Overview
DISCOVER UNTAPPED PROFITS IN YOUR MARKETING EFFORTS TODAY!
If you’re ready to reach your next level of growth.
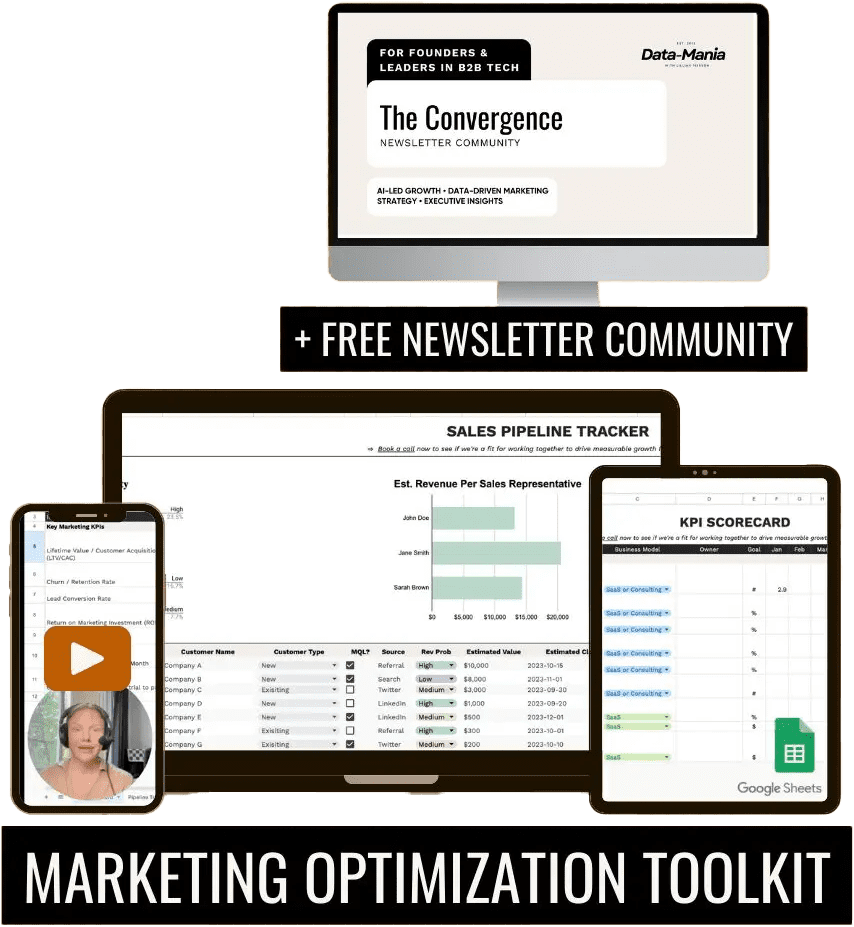
- Privacy Policy

Home » Case Study – Methods, Examples and Guide
Case Study – Methods, Examples and Guide
Table of Contents

A case study is a research method that involves an in-depth examination and analysis of a particular phenomenon or case, such as an individual, organization, community, event, or situation.
It is a qualitative research approach that aims to provide a detailed and comprehensive understanding of the case being studied. Case studies typically involve multiple sources of data, including interviews, observations, documents, and artifacts, which are analyzed using various techniques, such as content analysis, thematic analysis, and grounded theory. The findings of a case study are often used to develop theories, inform policy or practice, or generate new research questions.
Types of Case Study
Types and Methods of Case Study are as follows:
Single-Case Study
A single-case study is an in-depth analysis of a single case. This type of case study is useful when the researcher wants to understand a specific phenomenon in detail.
For Example , A researcher might conduct a single-case study on a particular individual to understand their experiences with a particular health condition or a specific organization to explore their management practices. The researcher collects data from multiple sources, such as interviews, observations, and documents, and uses various techniques to analyze the data, such as content analysis or thematic analysis. The findings of a single-case study are often used to generate new research questions, develop theories, or inform policy or practice.
Multiple-Case Study
A multiple-case study involves the analysis of several cases that are similar in nature. This type of case study is useful when the researcher wants to identify similarities and differences between the cases.
For Example, a researcher might conduct a multiple-case study on several companies to explore the factors that contribute to their success or failure. The researcher collects data from each case, compares and contrasts the findings, and uses various techniques to analyze the data, such as comparative analysis or pattern-matching. The findings of a multiple-case study can be used to develop theories, inform policy or practice, or generate new research questions.
Exploratory Case Study
An exploratory case study is used to explore a new or understudied phenomenon. This type of case study is useful when the researcher wants to generate hypotheses or theories about the phenomenon.
For Example, a researcher might conduct an exploratory case study on a new technology to understand its potential impact on society. The researcher collects data from multiple sources, such as interviews, observations, and documents, and uses various techniques to analyze the data, such as grounded theory or content analysis. The findings of an exploratory case study can be used to generate new research questions, develop theories, or inform policy or practice.
Descriptive Case Study
A descriptive case study is used to describe a particular phenomenon in detail. This type of case study is useful when the researcher wants to provide a comprehensive account of the phenomenon.
For Example, a researcher might conduct a descriptive case study on a particular community to understand its social and economic characteristics. The researcher collects data from multiple sources, such as interviews, observations, and documents, and uses various techniques to analyze the data, such as content analysis or thematic analysis. The findings of a descriptive case study can be used to inform policy or practice or generate new research questions.
Instrumental Case Study
An instrumental case study is used to understand a particular phenomenon that is instrumental in achieving a particular goal. This type of case study is useful when the researcher wants to understand the role of the phenomenon in achieving the goal.
For Example, a researcher might conduct an instrumental case study on a particular policy to understand its impact on achieving a particular goal, such as reducing poverty. The researcher collects data from multiple sources, such as interviews, observations, and documents, and uses various techniques to analyze the data, such as content analysis or thematic analysis. The findings of an instrumental case study can be used to inform policy or practice or generate new research questions.
Case Study Data Collection Methods
Here are some common data collection methods for case studies:
Interviews involve asking questions to individuals who have knowledge or experience relevant to the case study. Interviews can be structured (where the same questions are asked to all participants) or unstructured (where the interviewer follows up on the responses with further questions). Interviews can be conducted in person, over the phone, or through video conferencing.
Observations
Observations involve watching and recording the behavior and activities of individuals or groups relevant to the case study. Observations can be participant (where the researcher actively participates in the activities) or non-participant (where the researcher observes from a distance). Observations can be recorded using notes, audio or video recordings, or photographs.
Documents can be used as a source of information for case studies. Documents can include reports, memos, emails, letters, and other written materials related to the case study. Documents can be collected from the case study participants or from public sources.
Surveys involve asking a set of questions to a sample of individuals relevant to the case study. Surveys can be administered in person, over the phone, through mail or email, or online. Surveys can be used to gather information on attitudes, opinions, or behaviors related to the case study.
Artifacts are physical objects relevant to the case study. Artifacts can include tools, equipment, products, or other objects that provide insights into the case study phenomenon.
How to conduct Case Study Research
Conducting a case study research involves several steps that need to be followed to ensure the quality and rigor of the study. Here are the steps to conduct case study research:
- Define the research questions: The first step in conducting a case study research is to define the research questions. The research questions should be specific, measurable, and relevant to the case study phenomenon under investigation.
- Select the case: The next step is to select the case or cases to be studied. The case should be relevant to the research questions and should provide rich and diverse data that can be used to answer the research questions.
- Collect data: Data can be collected using various methods, such as interviews, observations, documents, surveys, and artifacts. The data collection method should be selected based on the research questions and the nature of the case study phenomenon.
- Analyze the data: The data collected from the case study should be analyzed using various techniques, such as content analysis, thematic analysis, or grounded theory. The analysis should be guided by the research questions and should aim to provide insights and conclusions relevant to the research questions.
- Draw conclusions: The conclusions drawn from the case study should be based on the data analysis and should be relevant to the research questions. The conclusions should be supported by evidence and should be clearly stated.
- Validate the findings: The findings of the case study should be validated by reviewing the data and the analysis with participants or other experts in the field. This helps to ensure the validity and reliability of the findings.
- Write the report: The final step is to write the report of the case study research. The report should provide a clear description of the case study phenomenon, the research questions, the data collection methods, the data analysis, the findings, and the conclusions. The report should be written in a clear and concise manner and should follow the guidelines for academic writing.
Examples of Case Study
Here are some examples of case study research:
- The Hawthorne Studies : Conducted between 1924 and 1932, the Hawthorne Studies were a series of case studies conducted by Elton Mayo and his colleagues to examine the impact of work environment on employee productivity. The studies were conducted at the Hawthorne Works plant of the Western Electric Company in Chicago and included interviews, observations, and experiments.
- The Stanford Prison Experiment: Conducted in 1971, the Stanford Prison Experiment was a case study conducted by Philip Zimbardo to examine the psychological effects of power and authority. The study involved simulating a prison environment and assigning participants to the role of guards or prisoners. The study was controversial due to the ethical issues it raised.
- The Challenger Disaster: The Challenger Disaster was a case study conducted to examine the causes of the Space Shuttle Challenger explosion in 1986. The study included interviews, observations, and analysis of data to identify the technical, organizational, and cultural factors that contributed to the disaster.
- The Enron Scandal: The Enron Scandal was a case study conducted to examine the causes of the Enron Corporation’s bankruptcy in 2001. The study included interviews, analysis of financial data, and review of documents to identify the accounting practices, corporate culture, and ethical issues that led to the company’s downfall.
- The Fukushima Nuclear Disaster : The Fukushima Nuclear Disaster was a case study conducted to examine the causes of the nuclear accident that occurred at the Fukushima Daiichi Nuclear Power Plant in Japan in 2011. The study included interviews, analysis of data, and review of documents to identify the technical, organizational, and cultural factors that contributed to the disaster.
Application of Case Study
Case studies have a wide range of applications across various fields and industries. Here are some examples:
Business and Management
Case studies are widely used in business and management to examine real-life situations and develop problem-solving skills. Case studies can help students and professionals to develop a deep understanding of business concepts, theories, and best practices.
Case studies are used in healthcare to examine patient care, treatment options, and outcomes. Case studies can help healthcare professionals to develop critical thinking skills, diagnose complex medical conditions, and develop effective treatment plans.
Case studies are used in education to examine teaching and learning practices. Case studies can help educators to develop effective teaching strategies, evaluate student progress, and identify areas for improvement.
Social Sciences
Case studies are widely used in social sciences to examine human behavior, social phenomena, and cultural practices. Case studies can help researchers to develop theories, test hypotheses, and gain insights into complex social issues.
Law and Ethics
Case studies are used in law and ethics to examine legal and ethical dilemmas. Case studies can help lawyers, policymakers, and ethical professionals to develop critical thinking skills, analyze complex cases, and make informed decisions.
Purpose of Case Study
The purpose of a case study is to provide a detailed analysis of a specific phenomenon, issue, or problem in its real-life context. A case study is a qualitative research method that involves the in-depth exploration and analysis of a particular case, which can be an individual, group, organization, event, or community.
The primary purpose of a case study is to generate a comprehensive and nuanced understanding of the case, including its history, context, and dynamics. Case studies can help researchers to identify and examine the underlying factors, processes, and mechanisms that contribute to the case and its outcomes. This can help to develop a more accurate and detailed understanding of the case, which can inform future research, practice, or policy.
Case studies can also serve other purposes, including:
- Illustrating a theory or concept: Case studies can be used to illustrate and explain theoretical concepts and frameworks, providing concrete examples of how they can be applied in real-life situations.
- Developing hypotheses: Case studies can help to generate hypotheses about the causal relationships between different factors and outcomes, which can be tested through further research.
- Providing insight into complex issues: Case studies can provide insights into complex and multifaceted issues, which may be difficult to understand through other research methods.
- Informing practice or policy: Case studies can be used to inform practice or policy by identifying best practices, lessons learned, or areas for improvement.
Advantages of Case Study Research
There are several advantages of case study research, including:
- In-depth exploration: Case study research allows for a detailed exploration and analysis of a specific phenomenon, issue, or problem in its real-life context. This can provide a comprehensive understanding of the case and its dynamics, which may not be possible through other research methods.
- Rich data: Case study research can generate rich and detailed data, including qualitative data such as interviews, observations, and documents. This can provide a nuanced understanding of the case and its complexity.
- Holistic perspective: Case study research allows for a holistic perspective of the case, taking into account the various factors, processes, and mechanisms that contribute to the case and its outcomes. This can help to develop a more accurate and comprehensive understanding of the case.
- Theory development: Case study research can help to develop and refine theories and concepts by providing empirical evidence and concrete examples of how they can be applied in real-life situations.
- Practical application: Case study research can inform practice or policy by identifying best practices, lessons learned, or areas for improvement.
- Contextualization: Case study research takes into account the specific context in which the case is situated, which can help to understand how the case is influenced by the social, cultural, and historical factors of its environment.
Limitations of Case Study Research
There are several limitations of case study research, including:
- Limited generalizability : Case studies are typically focused on a single case or a small number of cases, which limits the generalizability of the findings. The unique characteristics of the case may not be applicable to other contexts or populations, which may limit the external validity of the research.
- Biased sampling: Case studies may rely on purposive or convenience sampling, which can introduce bias into the sample selection process. This may limit the representativeness of the sample and the generalizability of the findings.
- Subjectivity: Case studies rely on the interpretation of the researcher, which can introduce subjectivity into the analysis. The researcher’s own biases, assumptions, and perspectives may influence the findings, which may limit the objectivity of the research.
- Limited control: Case studies are typically conducted in naturalistic settings, which limits the control that the researcher has over the environment and the variables being studied. This may limit the ability to establish causal relationships between variables.
- Time-consuming: Case studies can be time-consuming to conduct, as they typically involve a detailed exploration and analysis of a specific case. This may limit the feasibility of conducting multiple case studies or conducting case studies in a timely manner.
- Resource-intensive: Case studies may require significant resources, including time, funding, and expertise. This may limit the ability of researchers to conduct case studies in resource-constrained settings.
About the author
Muhammad Hassan
Researcher, Academic Writer, Web developer
You may also like

Phenomenology – Methods, Examples and Guide

Focus Groups – Steps, Examples and Guide

Descriptive Research Design – Types, Methods and...

Mixed Methods Research – Types & Analysis

Survey Research – Types, Methods, Examples

Explanatory Research – Types, Methods, Guide

FOR EMPLOYERS
Top 10 real-world data science case studies.
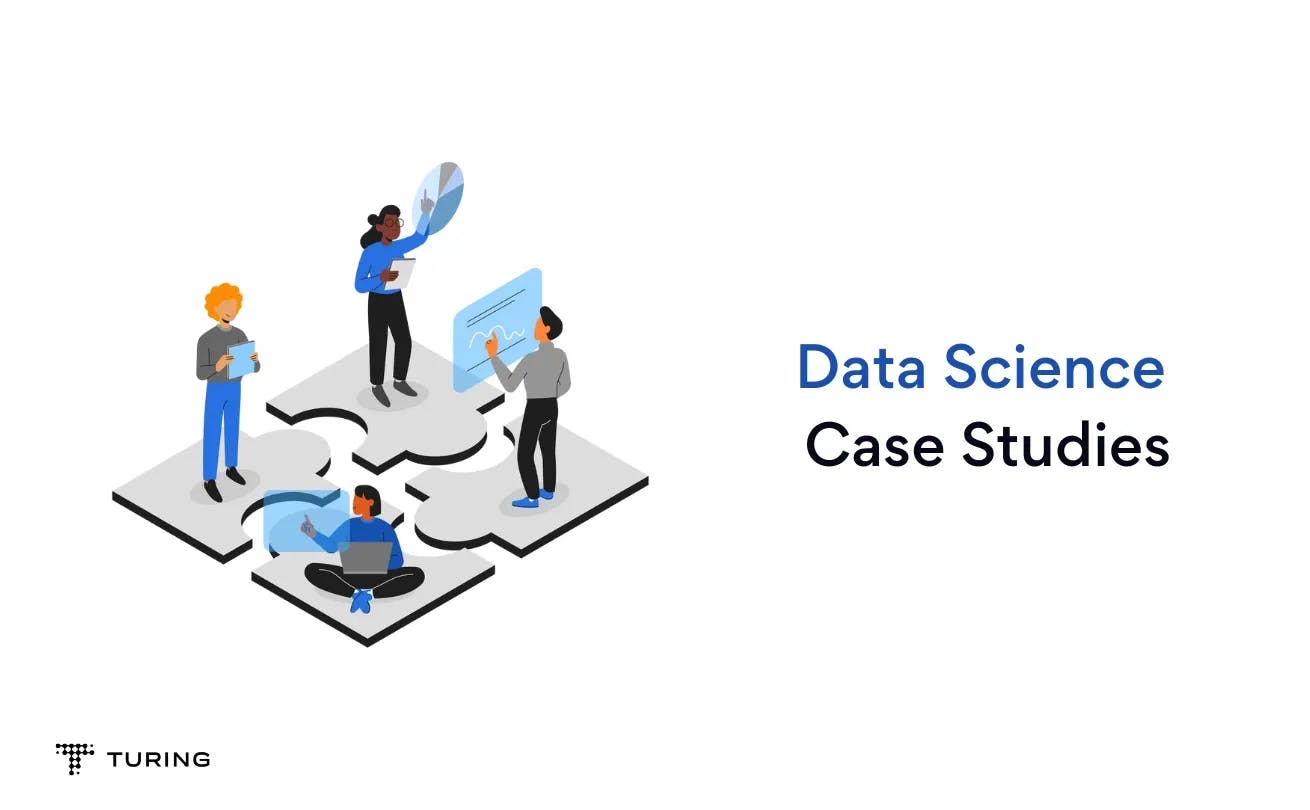
Aditya Sharma
Aditya is a content writer with 5+ years of experience writing for various industries including Marketing, SaaS, B2B, IT, and Edtech among others. You can find him watching anime or playing games when he’s not writing.
Frequently Asked Questions
Real-world data science case studies differ significantly from academic examples. While academic exercises often feature clean, well-structured data and simplified scenarios, real-world projects tackle messy, diverse data sources with practical constraints and genuine business objectives. These case studies reflect the complexities data scientists face when translating data into actionable insights in the corporate world.
Real-world data science projects come with common challenges. Data quality issues, including missing or inaccurate data, can hinder analysis. Domain expertise gaps may result in misinterpretation of results. Resource constraints might limit project scope or access to necessary tools and talent. Ethical considerations, like privacy and bias, demand careful handling.
Lastly, as data and business needs evolve, data science projects must adapt and stay relevant, posing an ongoing challenge.
Real-world data science case studies play a crucial role in helping companies make informed decisions. By analyzing their own data, businesses gain valuable insights into customer behavior, market trends, and operational efficiencies.
These insights empower data-driven strategies, aiding in more effective resource allocation, product development, and marketing efforts. Ultimately, case studies bridge the gap between data science and business decision-making, enhancing a company's ability to thrive in a competitive landscape.
Key takeaways from these case studies for organizations include the importance of cultivating a data-driven culture that values evidence-based decision-making. Investing in robust data infrastructure is essential to support data initiatives. Collaborating closely between data scientists and domain experts ensures that insights align with business goals.
Finally, continuous monitoring and refinement of data solutions are critical for maintaining relevance and effectiveness in a dynamic business environment. Embracing these principles can lead to tangible benefits and sustainable success in real-world data science endeavors.
Data science is a powerful driver of innovation and problem-solving across diverse industries. By harnessing data, organizations can uncover hidden patterns, automate repetitive tasks, optimize operations, and make informed decisions.
In healthcare, for example, data-driven diagnostics and treatment plans improve patient outcomes. In finance, predictive analytics enhances risk management. In transportation, route optimization reduces costs and emissions. Data science empowers industries to innovate and solve complex challenges in ways that were previously unimaginable.
Hire remote developers
Tell us the skills you need and we'll find the best developer for you in days, not weeks.

The Ultimate Guide to Qualitative Research - Part 1: The Basics
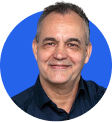
- Introduction and overview
- What is qualitative research?
- What is qualitative data?
- Examples of qualitative data
- Qualitative vs. quantitative research
- Mixed methods
- Qualitative research preparation
- Theoretical perspective
- Theoretical framework
- Literature reviews
Research question
- Conceptual framework
- Conceptual vs. theoretical framework
Data collection
- Qualitative research methods
- Focus groups
- Observational research
What is a case study?
Applications for case study research, what is a good case study, process of case study design, benefits and limitations of case studies.
- Ethnographical research
- Ethical considerations
- Confidentiality and privacy
- Power dynamics
- Reflexivity
Case studies
Case studies are essential to qualitative research , offering a lens through which researchers can investigate complex phenomena within their real-life contexts. This chapter explores the concept, purpose, applications, examples, and types of case studies and provides guidance on how to conduct case study research effectively.
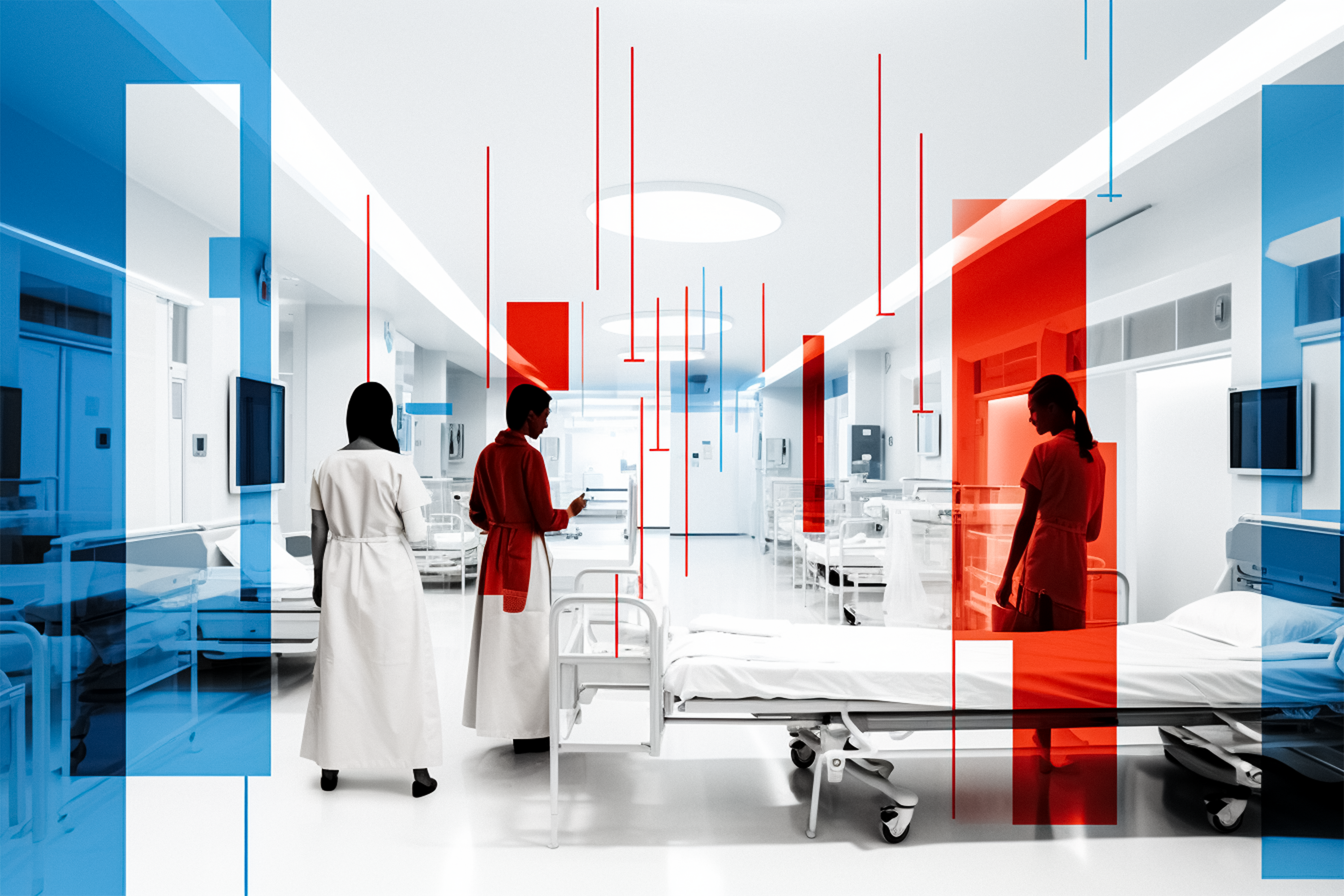
Whereas quantitative methods look at phenomena at scale, case study research looks at a concept or phenomenon in considerable detail. While analyzing a single case can help understand one perspective regarding the object of research inquiry, analyzing multiple cases can help obtain a more holistic sense of the topic or issue. Let's provide a basic definition of a case study, then explore its characteristics and role in the qualitative research process.
Definition of a case study
A case study in qualitative research is a strategy of inquiry that involves an in-depth investigation of a phenomenon within its real-world context. It provides researchers with the opportunity to acquire an in-depth understanding of intricate details that might not be as apparent or accessible through other methods of research. The specific case or cases being studied can be a single person, group, or organization – demarcating what constitutes a relevant case worth studying depends on the researcher and their research question .
Among qualitative research methods , a case study relies on multiple sources of evidence, such as documents, artifacts, interviews , or observations , to present a complete and nuanced understanding of the phenomenon under investigation. The objective is to illuminate the readers' understanding of the phenomenon beyond its abstract statistical or theoretical explanations.
Characteristics of case studies
Case studies typically possess a number of distinct characteristics that set them apart from other research methods. These characteristics include a focus on holistic description and explanation, flexibility in the design and data collection methods, reliance on multiple sources of evidence, and emphasis on the context in which the phenomenon occurs.
Furthermore, case studies can often involve a longitudinal examination of the case, meaning they study the case over a period of time. These characteristics allow case studies to yield comprehensive, in-depth, and richly contextualized insights about the phenomenon of interest.
The role of case studies in research
Case studies hold a unique position in the broader landscape of research methods aimed at theory development. They are instrumental when the primary research interest is to gain an intensive, detailed understanding of a phenomenon in its real-life context.
In addition, case studies can serve different purposes within research - they can be used for exploratory, descriptive, or explanatory purposes, depending on the research question and objectives. This flexibility and depth make case studies a valuable tool in the toolkit of qualitative researchers.
Remember, a well-conducted case study can offer a rich, insightful contribution to both academic and practical knowledge through theory development or theory verification, thus enhancing our understanding of complex phenomena in their real-world contexts.
What is the purpose of a case study?
Case study research aims for a more comprehensive understanding of phenomena, requiring various research methods to gather information for qualitative analysis . Ultimately, a case study can allow the researcher to gain insight into a particular object of inquiry and develop a theoretical framework relevant to the research inquiry.
Why use case studies in qualitative research?
Using case studies as a research strategy depends mainly on the nature of the research question and the researcher's access to the data.
Conducting case study research provides a level of detail and contextual richness that other research methods might not offer. They are beneficial when there's a need to understand complex social phenomena within their natural contexts.
The explanatory, exploratory, and descriptive roles of case studies
Case studies can take on various roles depending on the research objectives. They can be exploratory when the research aims to discover new phenomena or define new research questions; they are descriptive when the objective is to depict a phenomenon within its context in a detailed manner; and they can be explanatory if the goal is to understand specific relationships within the studied context. Thus, the versatility of case studies allows researchers to approach their topic from different angles, offering multiple ways to uncover and interpret the data .
The impact of case studies on knowledge development
Case studies play a significant role in knowledge development across various disciplines. Analysis of cases provides an avenue for researchers to explore phenomena within their context based on the collected data.
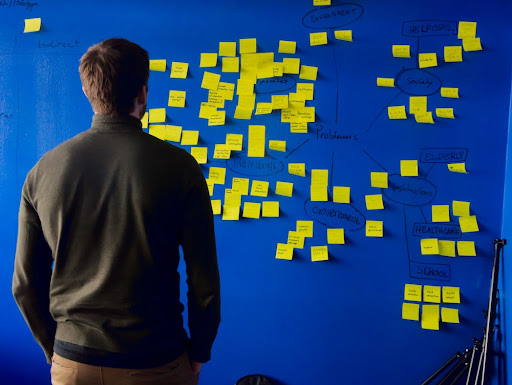
This can result in the production of rich, practical insights that can be instrumental in both theory-building and practice. Case studies allow researchers to delve into the intricacies and complexities of real-life situations, uncovering insights that might otherwise remain hidden.
Types of case studies
In qualitative research , a case study is not a one-size-fits-all approach. Depending on the nature of the research question and the specific objectives of the study, researchers might choose to use different types of case studies. These types differ in their focus, methodology, and the level of detail they provide about the phenomenon under investigation.
Understanding these types is crucial for selecting the most appropriate approach for your research project and effectively achieving your research goals. Let's briefly look at the main types of case studies.
Exploratory case studies
Exploratory case studies are typically conducted to develop a theory or framework around an understudied phenomenon. They can also serve as a precursor to a larger-scale research project. Exploratory case studies are useful when a researcher wants to identify the key issues or questions which can spur more extensive study or be used to develop propositions for further research. These case studies are characterized by flexibility, allowing researchers to explore various aspects of a phenomenon as they emerge, which can also form the foundation for subsequent studies.
Descriptive case studies
Descriptive case studies aim to provide a complete and accurate representation of a phenomenon or event within its context. These case studies are often based on an established theoretical framework, which guides how data is collected and analyzed. The researcher is concerned with describing the phenomenon in detail, as it occurs naturally, without trying to influence or manipulate it.
Explanatory case studies
Explanatory case studies are focused on explanation - they seek to clarify how or why certain phenomena occur. Often used in complex, real-life situations, they can be particularly valuable in clarifying causal relationships among concepts and understanding the interplay between different factors within a specific context.
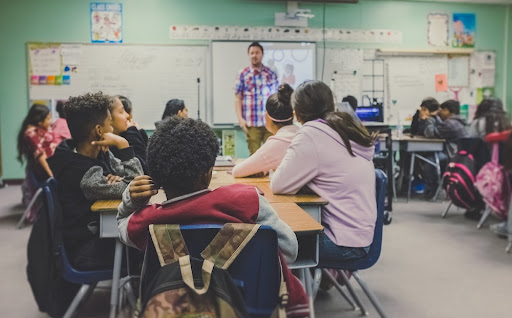
Intrinsic, instrumental, and collective case studies
These three categories of case studies focus on the nature and purpose of the study. An intrinsic case study is conducted when a researcher has an inherent interest in the case itself. Instrumental case studies are employed when the case is used to provide insight into a particular issue or phenomenon. A collective case study, on the other hand, involves studying multiple cases simultaneously to investigate some general phenomena.
Each type of case study serves a different purpose and has its own strengths and challenges. The selection of the type should be guided by the research question and objectives, as well as the context and constraints of the research.
The flexibility, depth, and contextual richness offered by case studies make this approach an excellent research method for various fields of study. They enable researchers to investigate real-world phenomena within their specific contexts, capturing nuances that other research methods might miss. Across numerous fields, case studies provide valuable insights into complex issues.
Critical information systems research
Case studies provide a detailed understanding of the role and impact of information systems in different contexts. They offer a platform to explore how information systems are designed, implemented, and used and how they interact with various social, economic, and political factors. Case studies in this field often focus on examining the intricate relationship between technology, organizational processes, and user behavior, helping to uncover insights that can inform better system design and implementation.
Health research
Health research is another field where case studies are highly valuable. They offer a way to explore patient experiences, healthcare delivery processes, and the impact of various interventions in a real-world context.
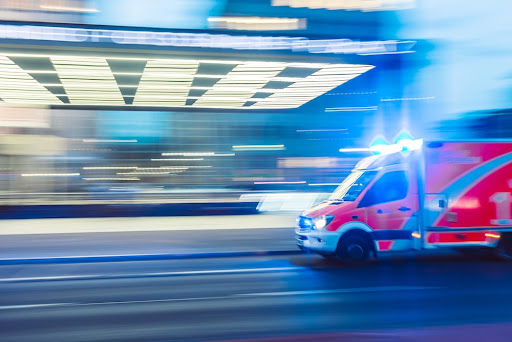
Case studies can provide a deep understanding of a patient's journey, giving insights into the intricacies of disease progression, treatment effects, and the psychosocial aspects of health and illness.
Asthma research studies
Specifically within medical research, studies on asthma often employ case studies to explore the individual and environmental factors that influence asthma development, management, and outcomes. A case study can provide rich, detailed data about individual patients' experiences, from the triggers and symptoms they experience to the effectiveness of various management strategies. This can be crucial for developing patient-centered asthma care approaches.
Other fields
Apart from the fields mentioned, case studies are also extensively used in business and management research, education research, and political sciences, among many others. They provide an opportunity to delve into the intricacies of real-world situations, allowing for a comprehensive understanding of various phenomena.
Case studies, with their depth and contextual focus, offer unique insights across these varied fields. They allow researchers to illuminate the complexities of real-life situations, contributing to both theory and practice.
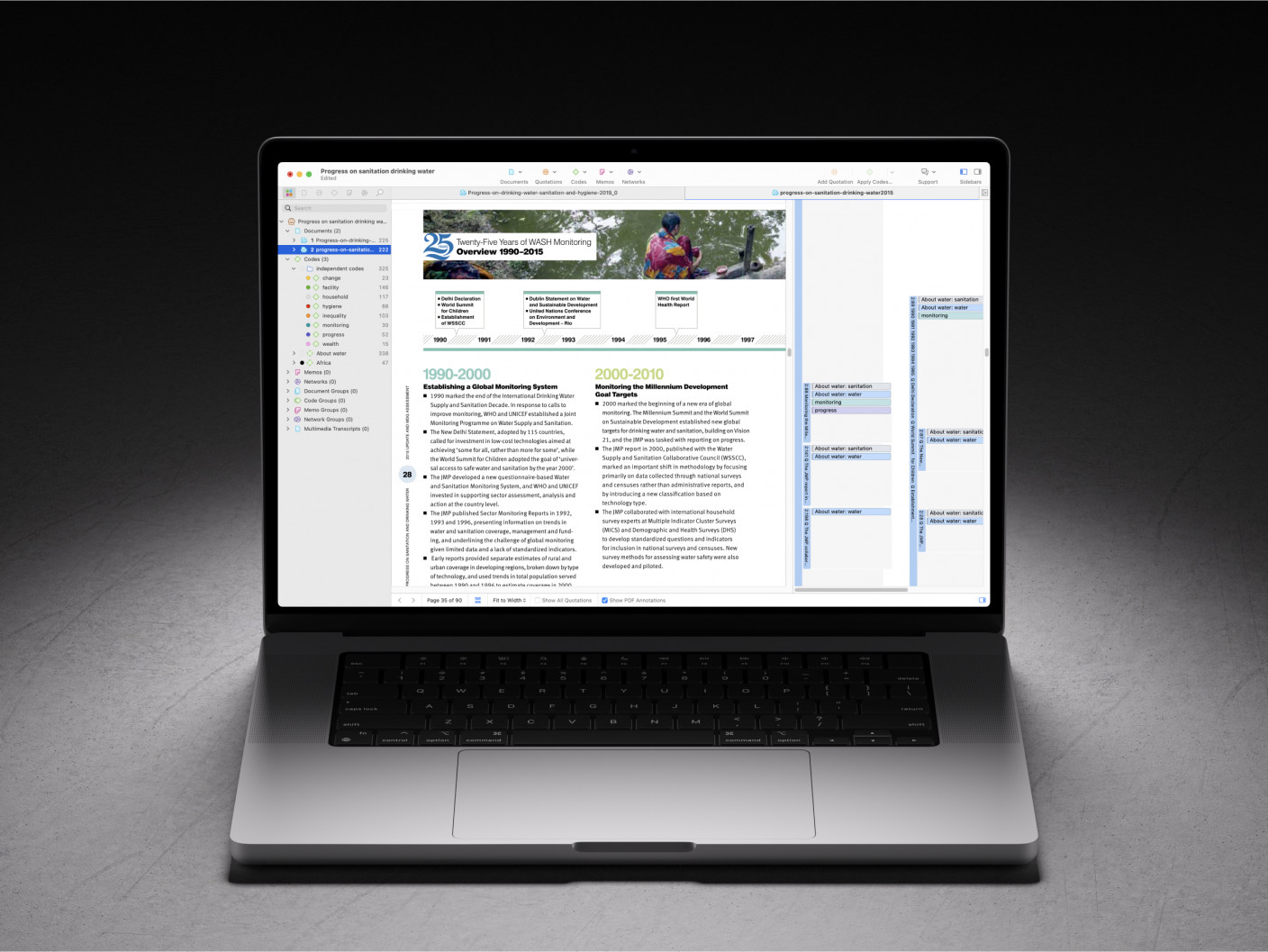
Whatever field you're in, ATLAS.ti puts your data to work for you
Download a free trial of ATLAS.ti to turn your data into insights.
Understanding the key elements of case study design is crucial for conducting rigorous and impactful case study research. A well-structured design guides the researcher through the process, ensuring that the study is methodologically sound and its findings are reliable and valid. The main elements of case study design include the research question , propositions, units of analysis, and the logic linking the data to the propositions.
The research question is the foundation of any research study. A good research question guides the direction of the study and informs the selection of the case, the methods of collecting data, and the analysis techniques. A well-formulated research question in case study research is typically clear, focused, and complex enough to merit further detailed examination of the relevant case(s).
Propositions
Propositions, though not necessary in every case study, provide a direction by stating what we might expect to find in the data collected. They guide how data is collected and analyzed by helping researchers focus on specific aspects of the case. They are particularly important in explanatory case studies, which seek to understand the relationships among concepts within the studied phenomenon.
Units of analysis
The unit of analysis refers to the case, or the main entity or entities that are being analyzed in the study. In case study research, the unit of analysis can be an individual, a group, an organization, a decision, an event, or even a time period. It's crucial to clearly define the unit of analysis, as it shapes the qualitative data analysis process by allowing the researcher to analyze a particular case and synthesize analysis across multiple case studies to draw conclusions.
Argumentation
This refers to the inferential model that allows researchers to draw conclusions from the data. The researcher needs to ensure that there is a clear link between the data, the propositions (if any), and the conclusions drawn. This argumentation is what enables the researcher to make valid and credible inferences about the phenomenon under study.
Understanding and carefully considering these elements in the design phase of a case study can significantly enhance the quality of the research. It can help ensure that the study is methodologically sound and its findings contribute meaningful insights about the case.
Ready to jumpstart your research with ATLAS.ti?
Conceptualize your research project with our intuitive data analysis interface. Download a free trial today.
Conducting a case study involves several steps, from defining the research question and selecting the case to collecting and analyzing data . This section outlines these key stages, providing a practical guide on how to conduct case study research.
Defining the research question
The first step in case study research is defining a clear, focused research question. This question should guide the entire research process, from case selection to analysis. It's crucial to ensure that the research question is suitable for a case study approach. Typically, such questions are exploratory or descriptive in nature and focus on understanding a phenomenon within its real-life context.
Selecting and defining the case
The selection of the case should be based on the research question and the objectives of the study. It involves choosing a unique example or a set of examples that provide rich, in-depth data about the phenomenon under investigation. After selecting the case, it's crucial to define it clearly, setting the boundaries of the case, including the time period and the specific context.
Previous research can help guide the case study design. When considering a case study, an example of a case could be taken from previous case study research and used to define cases in a new research inquiry. Considering recently published examples can help understand how to select and define cases effectively.
Developing a detailed case study protocol
A case study protocol outlines the procedures and general rules to be followed during the case study. This includes the data collection methods to be used, the sources of data, and the procedures for analysis. Having a detailed case study protocol ensures consistency and reliability in the study.
The protocol should also consider how to work with the people involved in the research context to grant the research team access to collecting data. As mentioned in previous sections of this guide, establishing rapport is an essential component of qualitative research as it shapes the overall potential for collecting and analyzing data.
Collecting data
Gathering data in case study research often involves multiple sources of evidence, including documents, archival records, interviews, observations, and physical artifacts. This allows for a comprehensive understanding of the case. The process for gathering data should be systematic and carefully documented to ensure the reliability and validity of the study.
Analyzing and interpreting data
The next step is analyzing the data. This involves organizing the data , categorizing it into themes or patterns , and interpreting these patterns to answer the research question. The analysis might also involve comparing the findings with prior research or theoretical propositions.
Writing the case study report
The final step is writing the case study report . This should provide a detailed description of the case, the data, the analysis process, and the findings. The report should be clear, organized, and carefully written to ensure that the reader can understand the case and the conclusions drawn from it.
Each of these steps is crucial in ensuring that the case study research is rigorous, reliable, and provides valuable insights about the case.
The type, depth, and quality of data in your study can significantly influence the validity and utility of the study. In case study research, data is usually collected from multiple sources to provide a comprehensive and nuanced understanding of the case. This section will outline the various methods of collecting data used in case study research and discuss considerations for ensuring the quality of the data.
Interviews are a common method of gathering data in case study research. They can provide rich, in-depth data about the perspectives, experiences, and interpretations of the individuals involved in the case. Interviews can be structured , semi-structured , or unstructured , depending on the research question and the degree of flexibility needed.
Observations
Observations involve the researcher observing the case in its natural setting, providing first-hand information about the case and its context. Observations can provide data that might not be revealed in interviews or documents, such as non-verbal cues or contextual information.
Documents and artifacts
Documents and archival records provide a valuable source of data in case study research. They can include reports, letters, memos, meeting minutes, email correspondence, and various public and private documents related to the case.
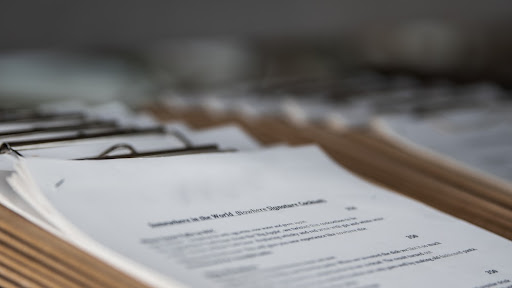
These records can provide historical context, corroborate evidence from other sources, and offer insights into the case that might not be apparent from interviews or observations.
Physical artifacts refer to any physical evidence related to the case, such as tools, products, or physical environments. These artifacts can provide tangible insights into the case, complementing the data gathered from other sources.
Ensuring the quality of data collection
Determining the quality of data in case study research requires careful planning and execution. It's crucial to ensure that the data is reliable, accurate, and relevant to the research question. This involves selecting appropriate methods of collecting data, properly training interviewers or observers, and systematically recording and storing the data. It also includes considering ethical issues related to collecting and handling data, such as obtaining informed consent and ensuring the privacy and confidentiality of the participants.
Data analysis
Analyzing case study research involves making sense of the rich, detailed data to answer the research question. This process can be challenging due to the volume and complexity of case study data. However, a systematic and rigorous approach to analysis can ensure that the findings are credible and meaningful. This section outlines the main steps and considerations in analyzing data in case study research.
Organizing the data
The first step in the analysis is organizing the data. This involves sorting the data into manageable sections, often according to the data source or the theme. This step can also involve transcribing interviews, digitizing physical artifacts, or organizing observational data.
Categorizing and coding the data
Once the data is organized, the next step is to categorize or code the data. This involves identifying common themes, patterns, or concepts in the data and assigning codes to relevant data segments. Coding can be done manually or with the help of software tools, and in either case, qualitative analysis software can greatly facilitate the entire coding process. Coding helps to reduce the data to a set of themes or categories that can be more easily analyzed.
Identifying patterns and themes
After coding the data, the researcher looks for patterns or themes in the coded data. This involves comparing and contrasting the codes and looking for relationships or patterns among them. The identified patterns and themes should help answer the research question.
Interpreting the data
Once patterns and themes have been identified, the next step is to interpret these findings. This involves explaining what the patterns or themes mean in the context of the research question and the case. This interpretation should be grounded in the data, but it can also involve drawing on theoretical concepts or prior research.
Verification of the data
The last step in the analysis is verification. This involves checking the accuracy and consistency of the analysis process and confirming that the findings are supported by the data. This can involve re-checking the original data, checking the consistency of codes, or seeking feedback from research participants or peers.
Like any research method , case study research has its strengths and limitations. Researchers must be aware of these, as they can influence the design, conduct, and interpretation of the study.
Understanding the strengths and limitations of case study research can also guide researchers in deciding whether this approach is suitable for their research question . This section outlines some of the key strengths and limitations of case study research.
Benefits include the following:
- Rich, detailed data: One of the main strengths of case study research is that it can generate rich, detailed data about the case. This can provide a deep understanding of the case and its context, which can be valuable in exploring complex phenomena.
- Flexibility: Case study research is flexible in terms of design , data collection , and analysis . A sufficient degree of flexibility allows the researcher to adapt the study according to the case and the emerging findings.
- Real-world context: Case study research involves studying the case in its real-world context, which can provide valuable insights into the interplay between the case and its context.
- Multiple sources of evidence: Case study research often involves collecting data from multiple sources , which can enhance the robustness and validity of the findings.
On the other hand, researchers should consider the following limitations:
- Generalizability: A common criticism of case study research is that its findings might not be generalizable to other cases due to the specificity and uniqueness of each case.
- Time and resource intensive: Case study research can be time and resource intensive due to the depth of the investigation and the amount of collected data.
- Complexity of analysis: The rich, detailed data generated in case study research can make analyzing the data challenging.
- Subjectivity: Given the nature of case study research, there may be a higher degree of subjectivity in interpreting the data , so researchers need to reflect on this and transparently convey to audiences how the research was conducted.
Being aware of these strengths and limitations can help researchers design and conduct case study research effectively and interpret and report the findings appropriately.
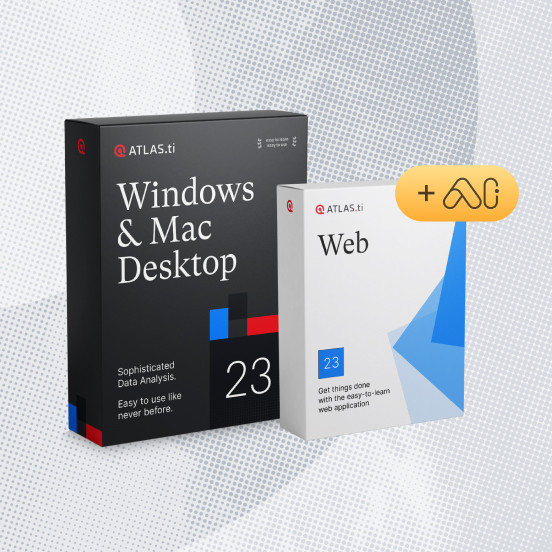
Ready to analyze your data with ATLAS.ti?
See how our intuitive software can draw key insights from your data with a free trial today.
The 7 Most Useful Data Analysis Methods and Techniques
Data analytics is the process of analyzing raw data to draw out meaningful insights. These insights are then used to determine the best course of action.
When is the best time to roll out that marketing campaign? Is the current team structure as effective as it could be? Which customer segments are most likely to purchase your new product?
Ultimately, data analytics is a crucial driver of any successful business strategy. But how do data analysts actually turn raw data into something useful? There are a range of methods and techniques that data analysts use depending on the type of data in question and the kinds of insights they want to uncover.
You can get a hands-on introduction to data analytics in this free short course .
In this post, we’ll explore some of the most useful data analysis techniques. By the end, you’ll have a much clearer idea of how you can transform meaningless data into business intelligence. We’ll cover:
- What is data analysis and why is it important?
- What is the difference between qualitative and quantitative data?
- Regression analysis
- Monte Carlo simulation
- Factor analysis
- Cohort analysis
- Cluster analysis
- Time series analysis
- Sentiment analysis
- The data analysis process
- The best tools for data analysis
- Key takeaways
The first six methods listed are used for quantitative data , while the last technique applies to qualitative data. We briefly explain the difference between quantitative and qualitative data in section two, but if you want to skip straight to a particular analysis technique, just use the clickable menu.
1. What is data analysis and why is it important?
Data analysis is, put simply, the process of discovering useful information by evaluating data. This is done through a process of inspecting, cleaning, transforming, and modeling data using analytical and statistical tools, which we will explore in detail further along in this article.
Why is data analysis important? Analyzing data effectively helps organizations make business decisions. Nowadays, data is collected by businesses constantly: through surveys, online tracking, online marketing analytics, collected subscription and registration data (think newsletters), social media monitoring, among other methods.
These data will appear as different structures, including—but not limited to—the following:
The concept of big data —data that is so large, fast, or complex, that it is difficult or impossible to process using traditional methods—gained momentum in the early 2000s. Then, Doug Laney, an industry analyst, articulated what is now known as the mainstream definition of big data as the three Vs: volume, velocity, and variety.
- Volume: As mentioned earlier, organizations are collecting data constantly. In the not-too-distant past it would have been a real issue to store, but nowadays storage is cheap and takes up little space.
- Velocity: Received data needs to be handled in a timely manner. With the growth of the Internet of Things, this can mean these data are coming in constantly, and at an unprecedented speed.
- Variety: The data being collected and stored by organizations comes in many forms, ranging from structured data—that is, more traditional, numerical data—to unstructured data—think emails, videos, audio, and so on. We’ll cover structured and unstructured data a little further on.
This is a form of data that provides information about other data, such as an image. In everyday life you’ll find this by, for example, right-clicking on a file in a folder and selecting “Get Info”, which will show you information such as file size and kind, date of creation, and so on.
Real-time data
This is data that is presented as soon as it is acquired. A good example of this is a stock market ticket, which provides information on the most-active stocks in real time.
Machine data
This is data that is produced wholly by machines, without human instruction. An example of this could be call logs automatically generated by your smartphone.
Quantitative and qualitative data
Quantitative data—otherwise known as structured data— may appear as a “traditional” database—that is, with rows and columns. Qualitative data—otherwise known as unstructured data—are the other types of data that don’t fit into rows and columns, which can include text, images, videos and more. We’ll discuss this further in the next section.
2. What is the difference between quantitative and qualitative data?
How you analyze your data depends on the type of data you’re dealing with— quantitative or qualitative . So what’s the difference?
Quantitative data is anything measurable , comprising specific quantities and numbers. Some examples of quantitative data include sales figures, email click-through rates, number of website visitors, and percentage revenue increase. Quantitative data analysis techniques focus on the statistical, mathematical, or numerical analysis of (usually large) datasets. This includes the manipulation of statistical data using computational techniques and algorithms. Quantitative analysis techniques are often used to explain certain phenomena or to make predictions.
Qualitative data cannot be measured objectively , and is therefore open to more subjective interpretation. Some examples of qualitative data include comments left in response to a survey question, things people have said during interviews, tweets and other social media posts, and the text included in product reviews. With qualitative data analysis, the focus is on making sense of unstructured data (such as written text, or transcripts of spoken conversations). Often, qualitative analysis will organize the data into themes—a process which, fortunately, can be automated.
Data analysts work with both quantitative and qualitative data , so it’s important to be familiar with a variety of analysis methods. Let’s take a look at some of the most useful techniques now.
3. Data analysis techniques
Now we’re familiar with some of the different types of data, let’s focus on the topic at hand: different methods for analyzing data.
a. Regression analysis
Regression analysis is used to estimate the relationship between a set of variables. When conducting any type of regression analysis , you’re looking to see if there’s a correlation between a dependent variable (that’s the variable or outcome you want to measure or predict) and any number of independent variables (factors which may have an impact on the dependent variable). The aim of regression analysis is to estimate how one or more variables might impact the dependent variable, in order to identify trends and patterns. This is especially useful for making predictions and forecasting future trends.
Let’s imagine you work for an ecommerce company and you want to examine the relationship between: (a) how much money is spent on social media marketing, and (b) sales revenue. In this case, sales revenue is your dependent variable—it’s the factor you’re most interested in predicting and boosting. Social media spend is your independent variable; you want to determine whether or not it has an impact on sales and, ultimately, whether it’s worth increasing, decreasing, or keeping the same. Using regression analysis, you’d be able to see if there’s a relationship between the two variables. A positive correlation would imply that the more you spend on social media marketing, the more sales revenue you make. No correlation at all might suggest that social media marketing has no bearing on your sales. Understanding the relationship between these two variables would help you to make informed decisions about the social media budget going forward. However: It’s important to note that, on their own, regressions can only be used to determine whether or not there is a relationship between a set of variables—they don’t tell you anything about cause and effect. So, while a positive correlation between social media spend and sales revenue may suggest that one impacts the other, it’s impossible to draw definitive conclusions based on this analysis alone.
There are many different types of regression analysis, and the model you use depends on the type of data you have for the dependent variable. For example, your dependent variable might be continuous (i.e. something that can be measured on a continuous scale, such as sales revenue in USD), in which case you’d use a different type of regression analysis than if your dependent variable was categorical in nature (i.e. comprising values that can be categorised into a number of distinct groups based on a certain characteristic, such as customer location by continent). You can learn more about different types of dependent variables and how to choose the right regression analysis in this guide .
Regression analysis in action: Investigating the relationship between clothing brand Benetton’s advertising expenditure and sales
b. Monte Carlo simulation
When making decisions or taking certain actions, there are a range of different possible outcomes. If you take the bus, you might get stuck in traffic. If you walk, you might get caught in the rain or bump into your chatty neighbor, potentially delaying your journey. In everyday life, we tend to briefly weigh up the pros and cons before deciding which action to take; however, when the stakes are high, it’s essential to calculate, as thoroughly and accurately as possible, all the potential risks and rewards.
Monte Carlo simulation, otherwise known as the Monte Carlo method, is a computerized technique used to generate models of possible outcomes and their probability distributions. It essentially considers a range of possible outcomes and then calculates how likely it is that each particular outcome will be realized. The Monte Carlo method is used by data analysts to conduct advanced risk analysis, allowing them to better forecast what might happen in the future and make decisions accordingly.
So how does Monte Carlo simulation work, and what can it tell us? To run a Monte Carlo simulation, you’ll start with a mathematical model of your data—such as a spreadsheet. Within your spreadsheet, you’ll have one or several outputs that you’re interested in; profit, for example, or number of sales. You’ll also have a number of inputs; these are variables that may impact your output variable. If you’re looking at profit, relevant inputs might include the number of sales, total marketing spend, and employee salaries. If you knew the exact, definitive values of all your input variables, you’d quite easily be able to calculate what profit you’d be left with at the end. However, when these values are uncertain, a Monte Carlo simulation enables you to calculate all the possible options and their probabilities. What will your profit be if you make 100,000 sales and hire five new employees on a salary of $50,000 each? What is the likelihood of this outcome? What will your profit be if you only make 12,000 sales and hire five new employees? And so on. It does this by replacing all uncertain values with functions which generate random samples from distributions determined by you, and then running a series of calculations and recalculations to produce models of all the possible outcomes and their probability distributions. The Monte Carlo method is one of the most popular techniques for calculating the effect of unpredictable variables on a specific output variable, making it ideal for risk analysis.
Monte Carlo simulation in action: A case study using Monte Carlo simulation for risk analysis
c. Factor analysis
Factor analysis is a technique used to reduce a large number of variables to a smaller number of factors. It works on the basis that multiple separate, observable variables correlate with each other because they are all associated with an underlying construct. This is useful not only because it condenses large datasets into smaller, more manageable samples, but also because it helps to uncover hidden patterns. This allows you to explore concepts that cannot be easily measured or observed—such as wealth, happiness, fitness, or, for a more business-relevant example, customer loyalty and satisfaction.
Let’s imagine you want to get to know your customers better, so you send out a rather long survey comprising one hundred questions. Some of the questions relate to how they feel about your company and product; for example, “Would you recommend us to a friend?” and “How would you rate the overall customer experience?” Other questions ask things like “What is your yearly household income?” and “How much are you willing to spend on skincare each month?”
Once your survey has been sent out and completed by lots of customers, you end up with a large dataset that essentially tells you one hundred different things about each customer (assuming each customer gives one hundred responses). Instead of looking at each of these responses (or variables) individually, you can use factor analysis to group them into factors that belong together—in other words, to relate them to a single underlying construct. In this example, factor analysis works by finding survey items that are strongly correlated. This is known as covariance . So, if there’s a strong positive correlation between household income and how much they’re willing to spend on skincare each month (i.e. as one increases, so does the other), these items may be grouped together. Together with other variables (survey responses), you may find that they can be reduced to a single factor such as “consumer purchasing power”. Likewise, if a customer experience rating of 10/10 correlates strongly with “yes” responses regarding how likely they are to recommend your product to a friend, these items may be reduced to a single factor such as “customer satisfaction”.
In the end, you have a smaller number of factors rather than hundreds of individual variables. These factors are then taken forward for further analysis, allowing you to learn more about your customers (or any other area you’re interested in exploring).
Factor analysis in action: Using factor analysis to explore customer behavior patterns in Tehran
d. Cohort analysis
Cohort analysis is a data analytics technique that groups users based on a shared characteristic , such as the date they signed up for a service or the product they purchased. Once users are grouped into cohorts, analysts can track their behavior over time to identify trends and patterns.
So what does this mean and why is it useful? Let’s break down the above definition further. A cohort is a group of people who share a common characteristic (or action) during a given time period. Students who enrolled at university in 2020 may be referred to as the 2020 cohort. Customers who purchased something from your online store via the app in the month of December may also be considered a cohort.
With cohort analysis, you’re dividing your customers or users into groups and looking at how these groups behave over time. So, rather than looking at a single, isolated snapshot of all your customers at a given moment in time (with each customer at a different point in their journey), you’re examining your customers’ behavior in the context of the customer lifecycle. As a result, you can start to identify patterns of behavior at various points in the customer journey—say, from their first ever visit to your website, through to email newsletter sign-up, to their first purchase, and so on. As such, cohort analysis is dynamic, allowing you to uncover valuable insights about the customer lifecycle.
This is useful because it allows companies to tailor their service to specific customer segments (or cohorts). Let’s imagine you run a 50% discount campaign in order to attract potential new customers to your website. Once you’ve attracted a group of new customers (a cohort), you’ll want to track whether they actually buy anything and, if they do, whether or not (and how frequently) they make a repeat purchase. With these insights, you’ll start to gain a much better understanding of when this particular cohort might benefit from another discount offer or retargeting ads on social media, for example. Ultimately, cohort analysis allows companies to optimize their service offerings (and marketing) to provide a more targeted, personalized experience. You can learn more about how to run cohort analysis using Google Analytics .
Cohort analysis in action: How Ticketmaster used cohort analysis to boost revenue
e. Cluster analysis
Cluster analysis is an exploratory technique that seeks to identify structures within a dataset. The goal of cluster analysis is to sort different data points into groups (or clusters) that are internally homogeneous and externally heterogeneous. This means that data points within a cluster are similar to each other, and dissimilar to data points in another cluster. Clustering is used to gain insight into how data is distributed in a given dataset, or as a preprocessing step for other algorithms.
There are many real-world applications of cluster analysis. In marketing, cluster analysis is commonly used to group a large customer base into distinct segments, allowing for a more targeted approach to advertising and communication. Insurance firms might use cluster analysis to investigate why certain locations are associated with a high number of insurance claims. Another common application is in geology, where experts will use cluster analysis to evaluate which cities are at greatest risk of earthquakes (and thus try to mitigate the risk with protective measures).
It’s important to note that, while cluster analysis may reveal structures within your data, it won’t explain why those structures exist. With that in mind, cluster analysis is a useful starting point for understanding your data and informing further analysis. Clustering algorithms are also used in machine learning—you can learn more about clustering in machine learning in our guide .
Cluster analysis in action: Using cluster analysis for customer segmentation—a telecoms case study example
f. Time series analysis
Time series analysis is a statistical technique used to identify trends and cycles over time. Time series data is a sequence of data points which measure the same variable at different points in time (for example, weekly sales figures or monthly email sign-ups). By looking at time-related trends, analysts are able to forecast how the variable of interest may fluctuate in the future.
When conducting time series analysis, the main patterns you’ll be looking out for in your data are:
- Trends: Stable, linear increases or decreases over an extended time period.
- Seasonality: Predictable fluctuations in the data due to seasonal factors over a short period of time. For example, you might see a peak in swimwear sales in summer around the same time every year.
- Cyclic patterns: Unpredictable cycles where the data fluctuates. Cyclical trends are not due to seasonality, but rather, may occur as a result of economic or industry-related conditions.
As you can imagine, the ability to make informed predictions about the future has immense value for business. Time series analysis and forecasting is used across a variety of industries, most commonly for stock market analysis, economic forecasting, and sales forecasting. There are different types of time series models depending on the data you’re using and the outcomes you want to predict. These models are typically classified into three broad types: the autoregressive (AR) models, the integrated (I) models, and the moving average (MA) models. For an in-depth look at time series analysis, refer to our guide .
Time series analysis in action: Developing a time series model to predict jute yarn demand in Bangladesh
g. Sentiment analysis
When you think of data, your mind probably automatically goes to numbers and spreadsheets.
Many companies overlook the value of qualitative data, but in reality, there are untold insights to be gained from what people (especially customers) write and say about you. So how do you go about analyzing textual data?
One highly useful qualitative technique is sentiment analysis , a technique which belongs to the broader category of text analysis —the (usually automated) process of sorting and understanding textual data.
With sentiment analysis, the goal is to interpret and classify the emotions conveyed within textual data. From a business perspective, this allows you to ascertain how your customers feel about various aspects of your brand, product, or service.
There are several different types of sentiment analysis models, each with a slightly different focus. The three main types include:
Fine-grained sentiment analysis
If you want to focus on opinion polarity (i.e. positive, neutral, or negative) in depth, fine-grained sentiment analysis will allow you to do so.
For example, if you wanted to interpret star ratings given by customers, you might use fine-grained sentiment analysis to categorize the various ratings along a scale ranging from very positive to very negative.
Emotion detection
This model often uses complex machine learning algorithms to pick out various emotions from your textual data.
You might use an emotion detection model to identify words associated with happiness, anger, frustration, and excitement, giving you insight into how your customers feel when writing about you or your product on, say, a product review site.
Aspect-based sentiment analysis
This type of analysis allows you to identify what specific aspects the emotions or opinions relate to, such as a certain product feature or a new ad campaign.
If a customer writes that they “find the new Instagram advert so annoying”, your model should detect not only a negative sentiment, but also the object towards which it’s directed.
In a nutshell, sentiment analysis uses various Natural Language Processing (NLP) algorithms and systems which are trained to associate certain inputs (for example, certain words) with certain outputs.
For example, the input “annoying” would be recognized and tagged as “negative”. Sentiment analysis is crucial to understanding how your customers feel about you and your products, for identifying areas for improvement, and even for averting PR disasters in real-time!
Sentiment analysis in action: 5 Real-world sentiment analysis case studies
4. The data analysis process
In order to gain meaningful insights from data, data analysts will perform a rigorous step-by-step process. We go over this in detail in our step by step guide to the data analysis process —but, to briefly summarize, the data analysis process generally consists of the following phases:
Defining the question
The first step for any data analyst will be to define the objective of the analysis, sometimes called a ‘problem statement’. Essentially, you’re asking a question with regards to a business problem you’re trying to solve. Once you’ve defined this, you’ll then need to determine which data sources will help you answer this question.
Collecting the data
Now that you’ve defined your objective, the next step will be to set up a strategy for collecting and aggregating the appropriate data. Will you be using quantitative (numeric) or qualitative (descriptive) data? Do these data fit into first-party, second-party, or third-party data?
Learn more: Quantitative vs. Qualitative Data: What’s the Difference?
Cleaning the data
Unfortunately, your collected data isn’t automatically ready for analysis—you’ll have to clean it first. As a data analyst, this phase of the process will take up the most time. During the data cleaning process, you will likely be:
- Removing major errors, duplicates, and outliers
- Removing unwanted data points
- Structuring the data—that is, fixing typos, layout issues, etc.
- Filling in major gaps in data
Analyzing the data
Now that we’ve finished cleaning the data, it’s time to analyze it! Many analysis methods have already been described in this article, and it’s up to you to decide which one will best suit the assigned objective. It may fall under one of the following categories:
- Descriptive analysis , which identifies what has already happened
- Diagnostic analysis , which focuses on understanding why something has happened
- Predictive analysis , which identifies future trends based on historical data
- Prescriptive analysis , which allows you to make recommendations for the future
Visualizing and sharing your findings
We’re almost at the end of the road! Analyses have been made, insights have been gleaned—all that remains to be done is to share this information with others. This is usually done with a data visualization tool, such as Google Charts, or Tableau.
Learn more: 13 of the Most Common Types of Data Visualization
To sum up the process, Will’s explained it all excellently in the following video:
5. The best tools for data analysis
As you can imagine, every phase of the data analysis process requires the data analyst to have a variety of tools under their belt that assist in gaining valuable insights from data. We cover these tools in greater detail in this article , but, in summary, here’s our best-of-the-best list, with links to each product:
The top 9 tools for data analysts
- Microsoft Excel
- Jupyter Notebook
- Apache Spark
- Microsoft Power BI
6. Key takeaways and further reading
As you can see, there are many different data analysis techniques at your disposal. In order to turn your raw data into actionable insights, it’s important to consider what kind of data you have (is it qualitative or quantitative?) as well as the kinds of insights that will be useful within the given context. In this post, we’ve introduced seven of the most useful data analysis techniques—but there are many more out there to be discovered!
So what now? If you haven’t already, we recommend reading the case studies for each analysis technique discussed in this post (you’ll find a link at the end of each section). For a more hands-on introduction to the kinds of methods and techniques that data analysts use, try out this free introductory data analytics short course. In the meantime, you might also want to read the following:
- The Best Online Data Analytics Courses for 2024
- What Is Time Series Data and How Is It Analyzed?
- What is Spatial Analysis?
10 Real World Data Science Case Studies Projects with Example
Top 10 Data Science Case Studies Projects with Examples and Solutions in Python to inspire your data science learning in 2023.
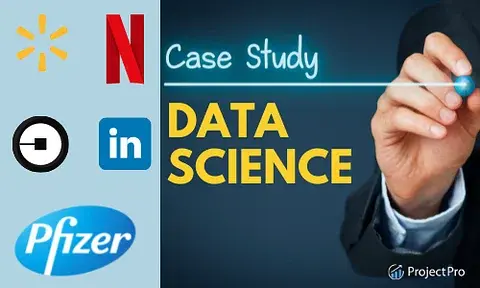
BelData science has been a trending buzzword in recent times. With wide applications in various sectors like healthcare , education, retail, transportation, media, and banking -data science applications are at the core of pretty much every industry out there. The possibilities are endless: analysis of frauds in the finance sector or the personalization of recommendations on eCommerce businesses. We have developed ten exciting data science case studies to explain how data science is leveraged across various industries to make smarter decisions and develop innovative personalized products tailored to specific customers.

Walmart Sales Forecasting Data Science Project
Downloadable solution code | Explanatory videos | Tech Support
Table of Contents
Data science case studies in retail , data science case study examples in entertainment industry , data analytics case study examples in travel industry , case studies for data analytics in social media , real world data science projects in healthcare, data analytics case studies in oil and gas, what is a case study in data science, how do you prepare a data science case study, 10 most interesting data science case studies with examples.
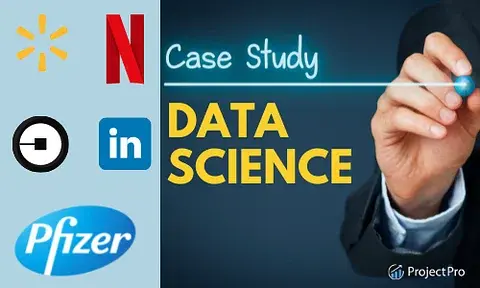
So, without much ado, let's get started with data science business case studies !
With humble beginnings as a simple discount retailer, today, Walmart operates in 10,500 stores and clubs in 24 countries and eCommerce websites, employing around 2.2 million people around the globe. For the fiscal year ended January 31, 2021, Walmart's total revenue was $559 billion showing a growth of $35 billion with the expansion of the eCommerce sector. Walmart is a data-driven company that works on the principle of 'Everyday low cost' for its consumers. To achieve this goal, they heavily depend on the advances of their data science and analytics department for research and development, also known as Walmart Labs. Walmart is home to the world's largest private cloud, which can manage 2.5 petabytes of data every hour! To analyze this humongous amount of data, Walmart has created 'Data Café,' a state-of-the-art analytics hub located within its Bentonville, Arkansas headquarters. The Walmart Labs team heavily invests in building and managing technologies like cloud, data, DevOps , infrastructure, and security.
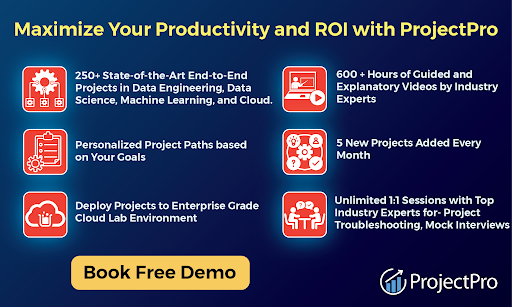
Walmart is experiencing massive digital growth as the world's largest retailer . Walmart has been leveraging Big data and advances in data science to build solutions to enhance, optimize and customize the shopping experience and serve their customers in a better way. At Walmart Labs, data scientists are focused on creating data-driven solutions that power the efficiency and effectiveness of complex supply chain management processes. Here are some of the applications of data science at Walmart:
i) Personalized Customer Shopping Experience
Walmart analyses customer preferences and shopping patterns to optimize the stocking and displaying of merchandise in their stores. Analysis of Big data also helps them understand new item sales, make decisions on discontinuing products, and the performance of brands.
ii) Order Sourcing and On-Time Delivery Promise
Millions of customers view items on Walmart.com, and Walmart provides each customer a real-time estimated delivery date for the items purchased. Walmart runs a backend algorithm that estimates this based on the distance between the customer and the fulfillment center, inventory levels, and shipping methods available. The supply chain management system determines the optimum fulfillment center based on distance and inventory levels for every order. It also has to decide on the shipping method to minimize transportation costs while meeting the promised delivery date.
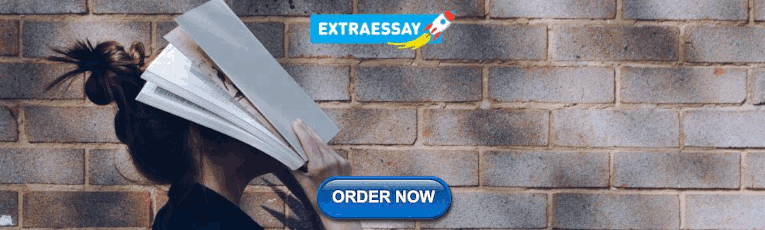
Here's what valued users are saying about ProjectPro
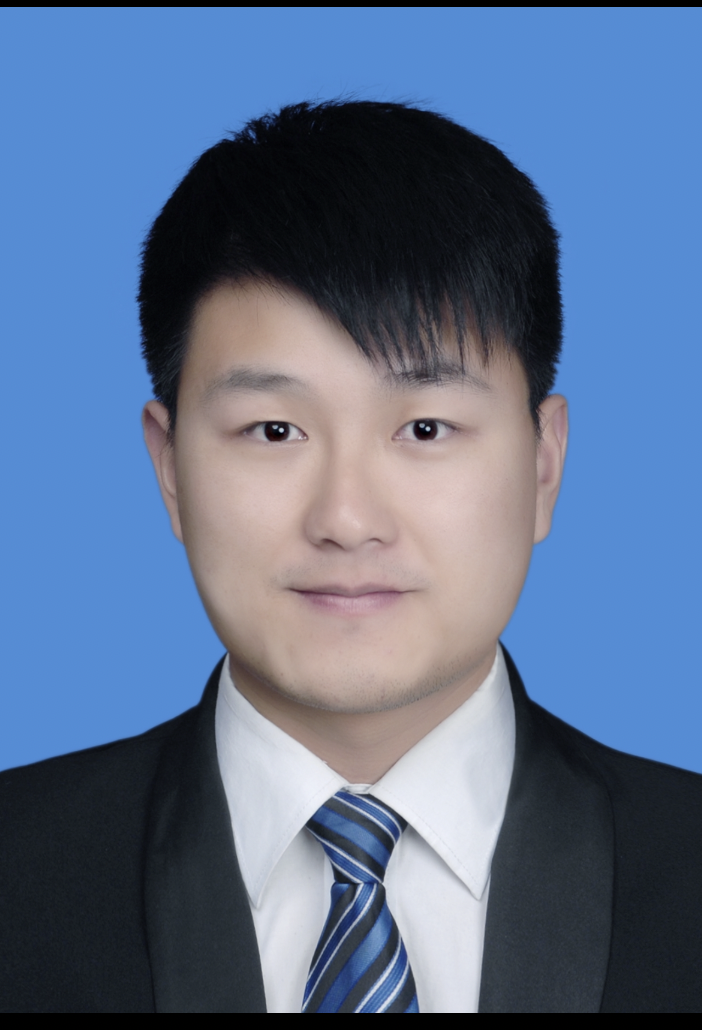
Graduate Research assistance at Stony Brook University
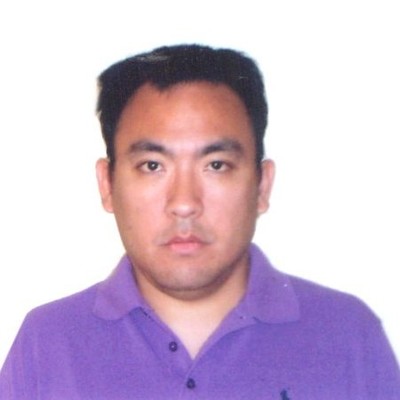
Tech Leader | Stanford / Yale University
Not sure what you are looking for?
iii) Packing Optimization
Also known as Box recommendation is a daily occurrence in the shipping of items in retail and eCommerce business. When items of an order or multiple orders for the same customer are ready for packing, Walmart has developed a recommender system that picks the best-sized box which holds all the ordered items with the least in-box space wastage within a fixed amount of time. This Bin Packing problem is a classic NP-Hard problem familiar to data scientists .
Whenever items of an order or multiple orders placed by the same customer are picked from the shelf and are ready for packing, the box recommendation system determines the best-sized box to hold all the ordered items with a minimum of in-box space wasted. This problem is known as the Bin Packing Problem, another classic NP-Hard problem familiar to data scientists.
Here is a link to a sales prediction data science case study to help you understand the applications of Data Science in the real world. Walmart Sales Forecasting Project uses historical sales data for 45 Walmart stores located in different regions. Each store contains many departments, and you must build a model to project the sales for each department in each store. This data science case study aims to create a predictive model to predict the sales of each product. You can also try your hands-on Inventory Demand Forecasting Data Science Project to develop a machine learning model to forecast inventory demand accurately based on historical sales data.
Get Closer To Your Dream of Becoming a Data Scientist with 70+ Solved End-to-End ML Projects
Amazon is an American multinational technology-based company based in Seattle, USA. It started as an online bookseller, but today it focuses on eCommerce, cloud computing , digital streaming, and artificial intelligence . It hosts an estimate of 1,000,000,000 gigabytes of data across more than 1,400,000 servers. Through its constant innovation in data science and big data Amazon is always ahead in understanding its customers. Here are a few data analytics case study examples at Amazon:
i) Recommendation Systems
Data science models help amazon understand the customers' needs and recommend them to them before the customer searches for a product; this model uses collaborative filtering. Amazon uses 152 million customer purchases data to help users to decide on products to be purchased. The company generates 35% of its annual sales using the Recommendation based systems (RBS) method.
Here is a Recommender System Project to help you build a recommendation system using collaborative filtering.
ii) Retail Price Optimization
Amazon product prices are optimized based on a predictive model that determines the best price so that the users do not refuse to buy it based on price. The model carefully determines the optimal prices considering the customers' likelihood of purchasing the product and thinks the price will affect the customers' future buying patterns. Price for a product is determined according to your activity on the website, competitors' pricing, product availability, item preferences, order history, expected profit margin, and other factors.
Check Out this Retail Price Optimization Project to build a Dynamic Pricing Model.
iii) Fraud Detection
Being a significant eCommerce business, Amazon remains at high risk of retail fraud. As a preemptive measure, the company collects historical and real-time data for every order. It uses Machine learning algorithms to find transactions with a higher probability of being fraudulent. This proactive measure has helped the company restrict clients with an excessive number of returns of products.
You can look at this Credit Card Fraud Detection Project to implement a fraud detection model to classify fraudulent credit card transactions.
New Projects
Let us explore data analytics case study examples in the entertainment indusry.
Ace Your Next Job Interview with Mock Interviews from Experts to Improve Your Skills and Boost Confidence!
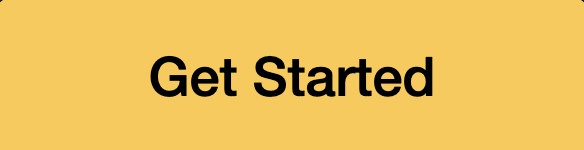
Netflix started as a DVD rental service in 1997 and then has expanded into the streaming business. Headquartered in Los Gatos, California, Netflix is the largest content streaming company in the world. Currently, Netflix has over 208 million paid subscribers worldwide, and with thousands of smart devices which are presently streaming supported, Netflix has around 3 billion hours watched every month. The secret to this massive growth and popularity of Netflix is its advanced use of data analytics and recommendation systems to provide personalized and relevant content recommendations to its users. The data is collected over 100 billion events every day. Here are a few examples of data analysis case studies applied at Netflix :
i) Personalized Recommendation System
Netflix uses over 1300 recommendation clusters based on consumer viewing preferences to provide a personalized experience. Some of the data that Netflix collects from its users include Viewing time, platform searches for keywords, Metadata related to content abandonment, such as content pause time, rewind, rewatched. Using this data, Netflix can predict what a viewer is likely to watch and give a personalized watchlist to a user. Some of the algorithms used by the Netflix recommendation system are Personalized video Ranking, Trending now ranker, and the Continue watching now ranker.
ii) Content Development using Data Analytics
Netflix uses data science to analyze the behavior and patterns of its user to recognize themes and categories that the masses prefer to watch. This data is used to produce shows like The umbrella academy, and Orange Is the New Black, and the Queen's Gambit. These shows seem like a huge risk but are significantly based on data analytics using parameters, which assured Netflix that they would succeed with its audience. Data analytics is helping Netflix come up with content that their viewers want to watch even before they know they want to watch it.
iii) Marketing Analytics for Campaigns
Netflix uses data analytics to find the right time to launch shows and ad campaigns to have maximum impact on the target audience. Marketing analytics helps come up with different trailers and thumbnails for other groups of viewers. For example, the House of Cards Season 5 trailer with a giant American flag was launched during the American presidential elections, as it would resonate well with the audience.
Here is a Customer Segmentation Project using association rule mining to understand the primary grouping of customers based on various parameters.
Get FREE Access to Machine Learning Example Codes for Data Cleaning , Data Munging, and Data Visualization
In a world where Purchasing music is a thing of the past and streaming music is a current trend, Spotify has emerged as one of the most popular streaming platforms. With 320 million monthly users, around 4 billion playlists, and approximately 2 million podcasts, Spotify leads the pack among well-known streaming platforms like Apple Music, Wynk, Songza, amazon music, etc. The success of Spotify has mainly depended on data analytics. By analyzing massive volumes of listener data, Spotify provides real-time and personalized services to its listeners. Most of Spotify's revenue comes from paid premium subscriptions. Here are some of the examples of case study on data analytics used by Spotify to provide enhanced services to its listeners:
i) Personalization of Content using Recommendation Systems
Spotify uses Bart or Bayesian Additive Regression Trees to generate music recommendations to its listeners in real-time. Bart ignores any song a user listens to for less than 30 seconds. The model is retrained every day to provide updated recommendations. A new Patent granted to Spotify for an AI application is used to identify a user's musical tastes based on audio signals, gender, age, accent to make better music recommendations.
Spotify creates daily playlists for its listeners, based on the taste profiles called 'Daily Mixes,' which have songs the user has added to their playlists or created by the artists that the user has included in their playlists. It also includes new artists and songs that the user might be unfamiliar with but might improve the playlist. Similar to it is the weekly 'Release Radar' playlists that have newly released artists' songs that the listener follows or has liked before.
ii) Targetted marketing through Customer Segmentation
With user data for enhancing personalized song recommendations, Spotify uses this massive dataset for targeted ad campaigns and personalized service recommendations for its users. Spotify uses ML models to analyze the listener's behavior and group them based on music preferences, age, gender, ethnicity, etc. These insights help them create ad campaigns for a specific target audience. One of their well-known ad campaigns was the meme-inspired ads for potential target customers, which was a huge success globally.
iii) CNN's for Classification of Songs and Audio Tracks
Spotify builds audio models to evaluate the songs and tracks, which helps develop better playlists and recommendations for its users. These allow Spotify to filter new tracks based on their lyrics and rhythms and recommend them to users like similar tracks ( collaborative filtering). Spotify also uses NLP ( Natural language processing) to scan articles and blogs to analyze the words used to describe songs and artists. These analytical insights can help group and identify similar artists and songs and leverage them to build playlists.
Here is a Music Recommender System Project for you to start learning. We have listed another music recommendations dataset for you to use for your projects: Dataset1 . You can use this dataset of Spotify metadata to classify songs based on artists, mood, liveliness. Plot histograms, heatmaps to get a better understanding of the dataset. Use classification algorithms like logistic regression, SVM, and Principal component analysis to generate valuable insights from the dataset.
Explore Categories
Below you will find case studies for data analytics in the travel and tourism industry.
Airbnb was born in 2007 in San Francisco and has since grown to 4 million Hosts and 5.6 million listings worldwide who have welcomed more than 1 billion guest arrivals in almost every country across the globe. Airbnb is active in every country on the planet except for Iran, Sudan, Syria, and North Korea. That is around 97.95% of the world. Using data as a voice of their customers, Airbnb uses the large volume of customer reviews, host inputs to understand trends across communities, rate user experiences, and uses these analytics to make informed decisions to build a better business model. The data scientists at Airbnb are developing exciting new solutions to boost the business and find the best mapping for its customers and hosts. Airbnb data servers serve approximately 10 million requests a day and process around one million search queries. Data is the voice of customers at AirBnB and offers personalized services by creating a perfect match between the guests and hosts for a supreme customer experience.
i) Recommendation Systems and Search Ranking Algorithms
Airbnb helps people find 'local experiences' in a place with the help of search algorithms that make searches and listings precise. Airbnb uses a 'listing quality score' to find homes based on the proximity to the searched location and uses previous guest reviews. Airbnb uses deep neural networks to build models that take the guest's earlier stays into account and area information to find a perfect match. The search algorithms are optimized based on guest and host preferences, rankings, pricing, and availability to understand users’ needs and provide the best match possible.
ii) Natural Language Processing for Review Analysis
Airbnb characterizes data as the voice of its customers. The customer and host reviews give a direct insight into the experience. The star ratings alone cannot be an excellent way to understand it quantitatively. Hence Airbnb uses natural language processing to understand reviews and the sentiments behind them. The NLP models are developed using Convolutional neural networks .
Practice this Sentiment Analysis Project for analyzing product reviews to understand the basic concepts of natural language processing.
iii) Smart Pricing using Predictive Analytics
The Airbnb hosts community uses the service as a supplementary income. The vacation homes and guest houses rented to customers provide for rising local community earnings as Airbnb guests stay 2.4 times longer and spend approximately 2.3 times the money compared to a hotel guest. The profits are a significant positive impact on the local neighborhood community. Airbnb uses predictive analytics to predict the prices of the listings and help the hosts set a competitive and optimal price. The overall profitability of the Airbnb host depends on factors like the time invested by the host and responsiveness to changing demands for different seasons. The factors that impact the real-time smart pricing are the location of the listing, proximity to transport options, season, and amenities available in the neighborhood of the listing.
Here is a Price Prediction Project to help you understand the concept of predictive analysis which is widely common in case studies for data analytics.
Uber is the biggest global taxi service provider. As of December 2018, Uber has 91 million monthly active consumers and 3.8 million drivers. Uber completes 14 million trips each day. Uber uses data analytics and big data-driven technologies to optimize their business processes and provide enhanced customer service. The Data Science team at uber has been exploring futuristic technologies to provide better service constantly. Machine learning and data analytics help Uber make data-driven decisions that enable benefits like ride-sharing, dynamic price surges, better customer support, and demand forecasting. Here are some of the real world data science projects used by uber:
i) Dynamic Pricing for Price Surges and Demand Forecasting
Uber prices change at peak hours based on demand. Uber uses surge pricing to encourage more cab drivers to sign up with the company, to meet the demand from the passengers. When the prices increase, the driver and the passenger are both informed about the surge in price. Uber uses a predictive model for price surging called the 'Geosurge' ( patented). It is based on the demand for the ride and the location.
ii) One-Click Chat
Uber has developed a Machine learning and natural language processing solution called one-click chat or OCC for coordination between drivers and users. This feature anticipates responses for commonly asked questions, making it easy for the drivers to respond to customer messages. Drivers can reply with the clock of just one button. One-Click chat is developed on Uber's machine learning platform Michelangelo to perform NLP on rider chat messages and generate appropriate responses to them.
iii) Customer Retention
Failure to meet the customer demand for cabs could lead to users opting for other services. Uber uses machine learning models to bridge this demand-supply gap. By using prediction models to predict the demand in any location, uber retains its customers. Uber also uses a tier-based reward system, which segments customers into different levels based on usage. The higher level the user achieves, the better are the perks. Uber also provides personalized destination suggestions based on the history of the user and their frequently traveled destinations.
You can take a look at this Python Chatbot Project and build a simple chatbot application to understand better the techniques used for natural language processing. You can also practice the working of a demand forecasting model with this project using time series analysis. You can look at this project which uses time series forecasting and clustering on a dataset containing geospatial data for forecasting customer demand for ola rides.
Explore More Data Science and Machine Learning Projects for Practice. Fast-Track Your Career Transition with ProjectPro
7) LinkedIn
LinkedIn is the largest professional social networking site with nearly 800 million members in more than 200 countries worldwide. Almost 40% of the users access LinkedIn daily, clocking around 1 billion interactions per month. The data science team at LinkedIn works with this massive pool of data to generate insights to build strategies, apply algorithms and statistical inferences to optimize engineering solutions, and help the company achieve its goals. Here are some of the real world data science projects at LinkedIn:
i) LinkedIn Recruiter Implement Search Algorithms and Recommendation Systems
LinkedIn Recruiter helps recruiters build and manage a talent pool to optimize the chances of hiring candidates successfully. This sophisticated product works on search and recommendation engines. The LinkedIn recruiter handles complex queries and filters on a constantly growing large dataset. The results delivered have to be relevant and specific. The initial search model was based on linear regression but was eventually upgraded to Gradient Boosted decision trees to include non-linear correlations in the dataset. In addition to these models, the LinkedIn recruiter also uses the Generalized Linear Mix model to improve the results of prediction problems to give personalized results.
ii) Recommendation Systems Personalized for News Feed
The LinkedIn news feed is the heart and soul of the professional community. A member's newsfeed is a place to discover conversations among connections, career news, posts, suggestions, photos, and videos. Every time a member visits LinkedIn, machine learning algorithms identify the best exchanges to be displayed on the feed by sorting through posts and ranking the most relevant results on top. The algorithms help LinkedIn understand member preferences and help provide personalized news feeds. The algorithms used include logistic regression, gradient boosted decision trees and neural networks for recommendation systems.
iii) CNN's to Detect Inappropriate Content
To provide a professional space where people can trust and express themselves professionally in a safe community has been a critical goal at LinkedIn. LinkedIn has heavily invested in building solutions to detect fake accounts and abusive behavior on their platform. Any form of spam, harassment, inappropriate content is immediately flagged and taken down. These can range from profanity to advertisements for illegal services. LinkedIn uses a Convolutional neural networks based machine learning model. This classifier trains on a training dataset containing accounts labeled as either "inappropriate" or "appropriate." The inappropriate list consists of accounts having content from "blocklisted" phrases or words and a small portion of manually reviewed accounts reported by the user community.
Here is a Text Classification Project to help you understand NLP basics for text classification. You can find a news recommendation system dataset to help you build a personalized news recommender system. You can also use this dataset to build a classifier using logistic regression, Naive Bayes, or Neural networks to classify toxic comments.
Get confident to build end-to-end projects
Access to a curated library of 250+ end-to-end industry projects with solution code, videos and tech support.
Pfizer is a multinational pharmaceutical company headquartered in New York, USA. One of the largest pharmaceutical companies globally known for developing a wide range of medicines and vaccines in disciplines like immunology, oncology, cardiology, and neurology. Pfizer became a household name in 2010 when it was the first to have a COVID-19 vaccine with FDA. In early November 2021, The CDC has approved the Pfizer vaccine for kids aged 5 to 11. Pfizer has been using machine learning and artificial intelligence to develop drugs and streamline trials, which played a massive role in developing and deploying the COVID-19 vaccine. Here are a few data analytics case studies by Pfizer :
i) Identifying Patients for Clinical Trials
Artificial intelligence and machine learning are used to streamline and optimize clinical trials to increase their efficiency. Natural language processing and exploratory data analysis of patient records can help identify suitable patients for clinical trials. These can help identify patients with distinct symptoms. These can help examine interactions of potential trial members' specific biomarkers, predict drug interactions and side effects which can help avoid complications. Pfizer's AI implementation helped rapidly identify signals within the noise of millions of data points across their 44,000-candidate COVID-19 clinical trial.
ii) Supply Chain and Manufacturing
Data science and machine learning techniques help pharmaceutical companies better forecast demand for vaccines and drugs and distribute them efficiently. Machine learning models can help identify efficient supply systems by automating and optimizing the production steps. These will help supply drugs customized to small pools of patients in specific gene pools. Pfizer uses Machine learning to predict the maintenance cost of equipment used. Predictive maintenance using AI is the next big step for Pharmaceutical companies to reduce costs.
iii) Drug Development
Computer simulations of proteins, and tests of their interactions, and yield analysis help researchers develop and test drugs more efficiently. In 2016 Watson Health and Pfizer announced a collaboration to utilize IBM Watson for Drug Discovery to help accelerate Pfizer's research in immuno-oncology, an approach to cancer treatment that uses the body's immune system to help fight cancer. Deep learning models have been used recently for bioactivity and synthesis prediction for drugs and vaccines in addition to molecular design. Deep learning has been a revolutionary technique for drug discovery as it factors everything from new applications of medications to possible toxic reactions which can save millions in drug trials.
You can create a Machine learning model to predict molecular activity to help design medicine using this dataset . You may build a CNN or a Deep neural network for this data analyst case study project.
Access Data Science and Machine Learning Project Code Examples
9) Shell Data Analyst Case Study Project
Shell is a global group of energy and petrochemical companies with over 80,000 employees in around 70 countries. Shell uses advanced technologies and innovations to help build a sustainable energy future. Shell is going through a significant transition as the world needs more and cleaner energy solutions to be a clean energy company by 2050. It requires substantial changes in the way in which energy is used. Digital technologies, including AI and Machine Learning, play an essential role in this transformation. These include efficient exploration and energy production, more reliable manufacturing, more nimble trading, and a personalized customer experience. Using AI in various phases of the organization will help achieve this goal and stay competitive in the market. Here are a few data analytics case studies in the petrochemical industry:
i) Precision Drilling
Shell is involved in the processing mining oil and gas supply, ranging from mining hydrocarbons to refining the fuel to retailing them to customers. Recently Shell has included reinforcement learning to control the drilling equipment used in mining. Reinforcement learning works on a reward-based system based on the outcome of the AI model. The algorithm is designed to guide the drills as they move through the surface, based on the historical data from drilling records. It includes information such as the size of drill bits, temperatures, pressures, and knowledge of the seismic activity. This model helps the human operator understand the environment better, leading to better and faster results will minor damage to machinery used.
ii) Efficient Charging Terminals
Due to climate changes, governments have encouraged people to switch to electric vehicles to reduce carbon dioxide emissions. However, the lack of public charging terminals has deterred people from switching to electric cars. Shell uses AI to monitor and predict the demand for terminals to provide efficient supply. Multiple vehicles charging from a single terminal may create a considerable grid load, and predictions on demand can help make this process more efficient.
iii) Monitoring Service and Charging Stations
Another Shell initiative trialed in Thailand and Singapore is the use of computer vision cameras, which can think and understand to watch out for potentially hazardous activities like lighting cigarettes in the vicinity of the pumps while refueling. The model is built to process the content of the captured images and label and classify it. The algorithm can then alert the staff and hence reduce the risk of fires. You can further train the model to detect rash driving or thefts in the future.
Here is a project to help you understand multiclass image classification. You can use the Hourly Energy Consumption Dataset to build an energy consumption prediction model. You can use time series with XGBoost to develop your model.
10) Zomato Case Study on Data Analytics
Zomato was founded in 2010 and is currently one of the most well-known food tech companies. Zomato offers services like restaurant discovery, home delivery, online table reservation, online payments for dining, etc. Zomato partners with restaurants to provide tools to acquire more customers while also providing delivery services and easy procurement of ingredients and kitchen supplies. Currently, Zomato has over 2 lakh restaurant partners and around 1 lakh delivery partners. Zomato has closed over ten crore delivery orders as of date. Zomato uses ML and AI to boost their business growth, with the massive amount of data collected over the years from food orders and user consumption patterns. Here are a few examples of data analyst case study project developed by the data scientists at Zomato:
i) Personalized Recommendation System for Homepage
Zomato uses data analytics to create personalized homepages for its users. Zomato uses data science to provide order personalization, like giving recommendations to the customers for specific cuisines, locations, prices, brands, etc. Restaurant recommendations are made based on a customer's past purchases, browsing history, and what other similar customers in the vicinity are ordering. This personalized recommendation system has led to a 15% improvement in order conversions and click-through rates for Zomato.
You can use the Restaurant Recommendation Dataset to build a restaurant recommendation system to predict what restaurants customers are most likely to order from, given the customer location, restaurant information, and customer order history.
ii) Analyzing Customer Sentiment
Zomato uses Natural language processing and Machine learning to understand customer sentiments using social media posts and customer reviews. These help the company gauge the inclination of its customer base towards the brand. Deep learning models analyze the sentiments of various brand mentions on social networking sites like Twitter, Instagram, Linked In, and Facebook. These analytics give insights to the company, which helps build the brand and understand the target audience.
iii) Predicting Food Preparation Time (FPT)
Food delivery time is an essential variable in the estimated delivery time of the order placed by the customer using Zomato. The food preparation time depends on numerous factors like the number of dishes ordered, time of the day, footfall in the restaurant, day of the week, etc. Accurate prediction of the food preparation time can help make a better prediction of the Estimated delivery time, which will help delivery partners less likely to breach it. Zomato uses a Bidirectional LSTM-based deep learning model that considers all these features and provides food preparation time for each order in real-time.
Data scientists are companies' secret weapons when analyzing customer sentiments and behavior and leveraging it to drive conversion, loyalty, and profits. These 10 data science case studies projects with examples and solutions show you how various organizations use data science technologies to succeed and be at the top of their field! To summarize, Data Science has not only accelerated the performance of companies but has also made it possible to manage & sustain their performance with ease.
FAQs on Data Analysis Case Studies
A case study in data science is an in-depth analysis of a real-world problem using data-driven approaches. It involves collecting, cleaning, and analyzing data to extract insights and solve challenges, offering practical insights into how data science techniques can address complex issues across various industries.
To create a data science case study, identify a relevant problem, define objectives, and gather suitable data. Clean and preprocess data, perform exploratory data analysis, and apply appropriate algorithms for analysis. Summarize findings, visualize results, and provide actionable recommendations, showcasing the problem-solving potential of data science techniques.
|
|
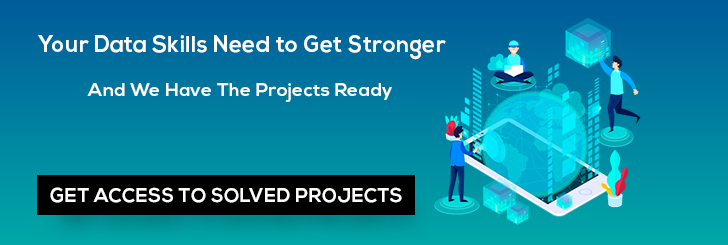
About the Author

ProjectPro is the only online platform designed to help professionals gain practical, hands-on experience in big data, data engineering, data science, and machine learning related technologies. Having over 270+ reusable project templates in data science and big data with step-by-step walkthroughs,

© 2024
© 2024 Iconiq Inc.
Privacy policy
User policy
Write for ProjectPro
Top 20 Analytics Case Studies in 2024
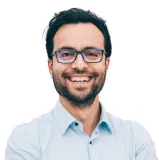
Although the potential of Big Data and business intelligence are recognized by organizations, Gartner analyst Nick Heudecker says that the failure rate of analytics projects is close to 85%. Uncovering the power of analytics improves business operations, reduces costs, enhances decision-making , and enables the launching of more personalized products.
In this article, our research covers:
How to measure analytics success?
What are some analytics case studies.
According to Gartner CDO Survey, the top 3 critical success factors of analytics projects are:
- Creation of a data-driven culture within the organization,
- Data integration and data skills training across the organization,
- And implementation of a data management and analytics strategy.
The success of the process of analytics depends on asking the right question. It requires an understanding of the appropriate data required for each goal to be achieved. We’ve listed 20 successful analytics applications/case studies from different industries.
During our research, we examined that partnering with an analytics consultant helps organizations boost their success if organizations’ tech team lacks certain data skills.
Enterprise | Industry of End User | Business Function | Type of Analytics | Description | Results | Analytics Vendor or Consultant |
---|---|---|---|---|---|---|
Fitbit | Health/ Fitness | Consumer Products | IoT Analytics | Better lifestyle choices for users. | Bernard Marr&Co. | |
Dominos | Food | Marketing | Marketing Analytics | Increased monthly revenue by 6%. | Google Analytics 360 and DBI | |
Brian Gravin Diamond | Luxury/ Jewelry | Sales | Sales Analytics | Improving their online sales by understanding user pre-purchase behaviour. | New line of designs in the website contributed to 6% boost in sales. | Google Analytics Enhanced Ecommerce |
* | Marketing Automation | Marketing | Marketing Analytics | Conversions improved by the rate of 10x | Google Analytics and Marketo | |
Build.com | Home Improvement Retail | Sales | Retail Analytics | Providing dynamic online pricing analysis and intelligence | Increased sales & profitability Better, faster pricing decisions | Numerator Pricing Intel and Numerator |
Ace Hardware | Hardware Retail | Sales | Pricing Analytics | Increased exact and ‘like’ matches by 200% across regional markets. | Numerator Pricing Intel and Numerator | |
SHOP.COM | Online Comparison in Retail | Supply Chain | Retail Analytics | increased supply chain and onboarding process efficiencies. | 57% growth in drop ship orders | SPS Commerce Analytics and SPS Commerce |
Bayer Crop Science | Agriculture | Operations | Edge Analytics/IoT Analytics | Faster decision making to help farmers optimize growing conditions | AWS IoT Analytics AWS Greengrass | |
Farmers Edge | Agriculture | Operations | Edge Analytics | Collecting data from edge in real-time | Better farm management decisions that maximize productivity and profitability. | Microsoft Azure IoT Edge |
Lufthansa | Transportation | Operations | Augmented Analytics/Self-service reporting | Increase in the company’s efficiency by 30% as data preparation and report generation time has reduced. | Tableau | |
Walmart | Retail | Operations | Graph Analytics | Increased revenue by improving customer experience | Neo4j | |
Cerved | Risk Analysis | Operations | Graph Analytics | Neo4j | ||
Nextplus | Communication | Sales/ Marketing | Application Analytics | With Flurry, they analyzed every action users perform in-app. | Boosted conversion rate 5% in one month | Flurry |
Telenor | Telco | Maintenance | Application Analytics | Improved customer experience | AppDynamics | |
Cepheid | Molecular diagnostics | Maintenance | Application Analytics | Eliminating the need for manual SAP monitoring. | AppDynamics | |
* | Telco | HR | Workforce Analytics | Finding out what technical talent finds most and least important. | Improved employee value proposition | Crunchr |
Hostelworld | Vacation | Customer experience | Marketing Analytics | 500% higher engagement across websites and social | Adobe Analytics | |
Phillips | Retail | Marketing | Marketing Analytics | Testing ‘Buy’ buttons increased clicks by 20%. | Adobe | |
* | Insurance | Security | Behavioral Analytics/Security Analytics | Identifying anomalous events such as privileged account logins from | Securonix | |
Under Armour | Retail | Operations | Retail Analytics | IBM Watson |
*Vendors have not shared the client name
For more on analytics
If your organization is willing to implement an analytics solution but doesn’t know where to start, here are some of the articles we’ve written before that can help you learn more:
- AI in analytics: How AI is shaping analytics
- Edge Analytics in 2022: What it is, Why it matters & Use Cases
- Application Analytics: Tracking KPIs that lead to success
Finally, if you believe that your business would benefit from adopting an analytics solution, we have data-driven lists of vendors on our analytics hub and analytics platforms
We will help you choose the best solution tailored to your needs:
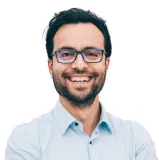
Next to Read
14 case studies of manufacturing analytics in 2024, iot analytics: benefits, challenges, use cases & vendors [2024].
Your email address will not be published. All fields are required.
Related research
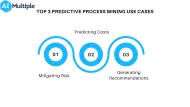
Predictive Process Mining in '24: Top 3 use cases & case studies
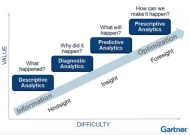
What is Analytics? How is it Evolving in 2024?
Data Topics
- Data Architecture
- Data Literacy
- Data Science
- Data Strategy
- Data Modeling
- Governance & Quality
- Education Resources For Use & Management of Data
Case Study: Executing an Effective Data Strategy
Few tasks are more logistically and technologically daunting than providing air, land, and sea transportation for the U.S. military across the entire world. Yet that is precisely the mission of the United States Transportation Command, or USTRANSCOM. According to a Congressional Research Service report, on any given day USTRANSCOM conducts over 240 air missions, sends […]
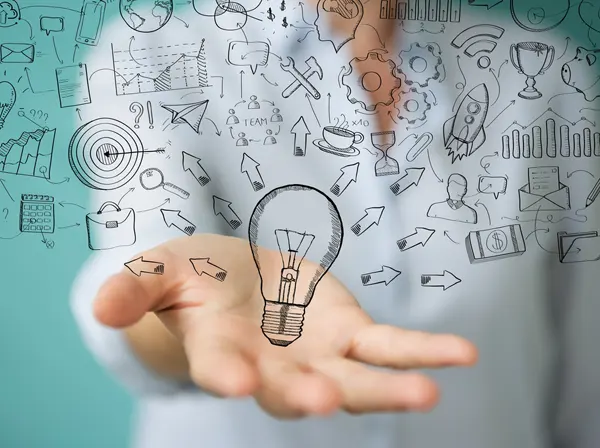
Few tasks are more logistically and technologically daunting than providing air, land, and sea transportation for the U.S. military across the entire world. Yet that is precisely the mission of the United States Transportation Command, or USTRANSCOM. According to a Congressional Research Service report , on any given day USTRANSCOM conducts over 240 air missions, sends 1,500 ground shipments, and has 20 ships underway, as well as aiding in humanitarian relief efforts and transporting patients who need aeromedical evacuation.

Asking the Right Data Strategy Questions
First and foremost, the Data Strategy nests under the company’s broader organizational vision. The organization’s vision is the basis of the strategy, the strategy guides the creation of goals, and goals are achieved through objectives.
The organization must ask itself: Is the current status quo good enough to survive? Do the data and digital strategies align with the organization’s goals ? And do you hope to gain or maintain a strategic advantage over your peers? Having an effective Data Strategy allows the government to make better strategic and tactical decisions. “Especially in our military and government world, we need to make decisions quickly, and they need to be accurate,” said McLean.
Having an effective Data Strategy also helps organizations reap the benefits of data in the first place: better-informed decision-making, understanding customers and trends, providing better products, improving internal operations, and creating additional revenue.
“In the government, we don’t make money. But we sure as heck really want to look at how we can become more efficient, to find ways to do things faster, better, cheaper,” said McLean.
In the particular case of USTRANSCOM, there were a variety of specific reasons that a Data Strategy was necessary, including the need to:
- Advance decision-making
- Mature as a data-driven organization
- Offload common tasks
- Provide information at the speed of operation needs
- Use personnel where they’re needed most
- Understand the vast amount of data that the organization uses and produces every day
Developing the Data Strategy
For McLean, it became clear that developing a Data Strategy was not going to happen over a weekend, especially within the context of a government agency with longstanding bureaucratic norms and entrenched ways of doing things. It would be a multi-year endeavor, requiring organization, patience, and support. The first step was to create a vision: What was the realm of possibility for the organization? What did it want to do?
“You need to ensure that your organization can achieve it. But don’t be so short-sighted that it’s too easy. Make it a challenge, make it difficult,” said McLean.
If the vision is the “to-be” state, the next step is to define the “as-is” state. In the case of USTRANSCOM, there was a great deal of legacy infrastructure that worked well at the time of development – before cloud computing was a widespread option – but now led to unhelpful silos that threw up barriers to enterprise interoperability.
Key exercises during this period included a gap analysis that looked at what the organization needed to accomplish to go from the “as-is” state to the “to-be” state, a consideration of organization priorities, and a SWOT analysis considering strengths, weaknesses, opportunities, and threats.
A major challenge at this point is to bring about cultural change. “You’re potentially uprooting the very processes and legacy knowledge and skills that brought people to their career pinnacle,” said McLean.
People will be highly motivated to defend legacy programs that have worked in the past. The value proposition must be equally defensible, with clear benefits outlined, in order to overcome that resistance. On the other side of the coin, overselling the vision can feed into the hype cycle, where inflated expectations deflate into disillusionment. So, the vision has to be broken down into clear, digestible chunks, to give people some early clear “wins” in what will inevitably be a multi-year process.
Properly selling your vision is key, and that means engaging at all levels of the organization: the C-suite, middle management, and the grassroots level of personnel who do the frontline work. There has to be a sense of transparency and partnership, so that the program isn’t seen as something merely for the data people, but rather something that will make everyone in the company function better. There has to some marketing of the vision to create buy-in at all levels. “People support what they help create,” said McLean.
However, one advantage the organization did have was strong support for a Data Strategy from the commander of USTRANSCOM. There was a standing monthly meeting with the commander to keep him updated on how they were moving forward with strategy and what their achievements were, and this created strong forward momentum and buy-in from management.
Executing the Data Strategy
After the strategy is developed, it’s time to make it a reality. The first step, McLean said, is establishing a foundation by investing in people, technology, and processes. USTRANSCOM established a new chief data officer (CDO) position and a new Data Management team, added people to the Data Architecture team, and hired new data scientists and data engineers.
As training materials, the organization used DATAVERSITY courses, sent personnel to conferences, and brought in noted speakers. For technology investment, the organization bought into Plateau as an integrator and installed IBM’s Cloud Pak for data.
In terms of processes, the team first defined terms in a common lexicon to create a business glossary , which was sent out for peer review, and created a DataOps team. They identified data sources, tables, and elements, then created data profiles and meta-tagged data for quality standards.
Next, they ingested the data, making system connections and security adjustments and optimizing the portfolio. After that came data enrichment and quality review, and that led finally to the creation of data visualizations, expanded analytics, and data services.
The key was to approach the execution from both the “top down” and “bottom up.” Approaching from the top down meant finding ways to immediately show value through tangible results, such as by reducing IT portfolio costs. That reduction in costs led to greater funding to do even more for the organization. The bottom-up approach was to start populating the environment with trusted, valuable data. Although that approach is not glamorous and results are not so immediately tangible, it’s still crucial.
Since starting the process of executing a Data Strategy, USTRANSCOM has embarked on a number of ambitious data projects. The organization currently has 10 active data analytics use cases in motion, and they are working on building a data environment – one project alone has 1,425 tables of reference data that need to be moved out of silos. The current goal of the Data Science team is to build out reusable analytics to be used for future endeavors.
While there have already been notable achievements, there’s still a long way to go. Thinking in the long-term is, ultimately, one of the biggest factors in successfully executing a Data Strategy.
“The real takeaway here is it’s always a multi-year plan,” said McLean. “There’s nothing that you’re going to solve in the first year or two. These are things that you have to be committed to as an organization.”
Want to learn more about DATAVERSITY’s upcoming events? Check out our current lineup of online and face-to-face conferences here .

Here is the video of the Enterprise Data World Presentation:
Image used under license from Shutterstock.com
Leave a Reply Cancel reply
You must be logged in to post a comment.

Data Analytics Case Study: Complete Guide in 2024
What are data analytics case study interviews.
When you’re trying to land a data analyst job, the last thing to stand in your way is the data analytics case study interview.
One reason they’re so challenging is that case studies don’t typically have a right or wrong answer.
Instead, case study interviews require you to come up with a hypothesis for an analytics question and then produce data to support or validate your hypothesis. In other words, it’s not just about your technical skills; you’re also being tested on creative problem-solving and your ability to communicate with stakeholders.
This article provides an overview of how to answer data analytics case study interview questions. You can find an in-depth course in the data analytics learning path .
How to Solve Data Analytics Case Questions
Check out our video below on How to solve a Data Analytics case study problem:
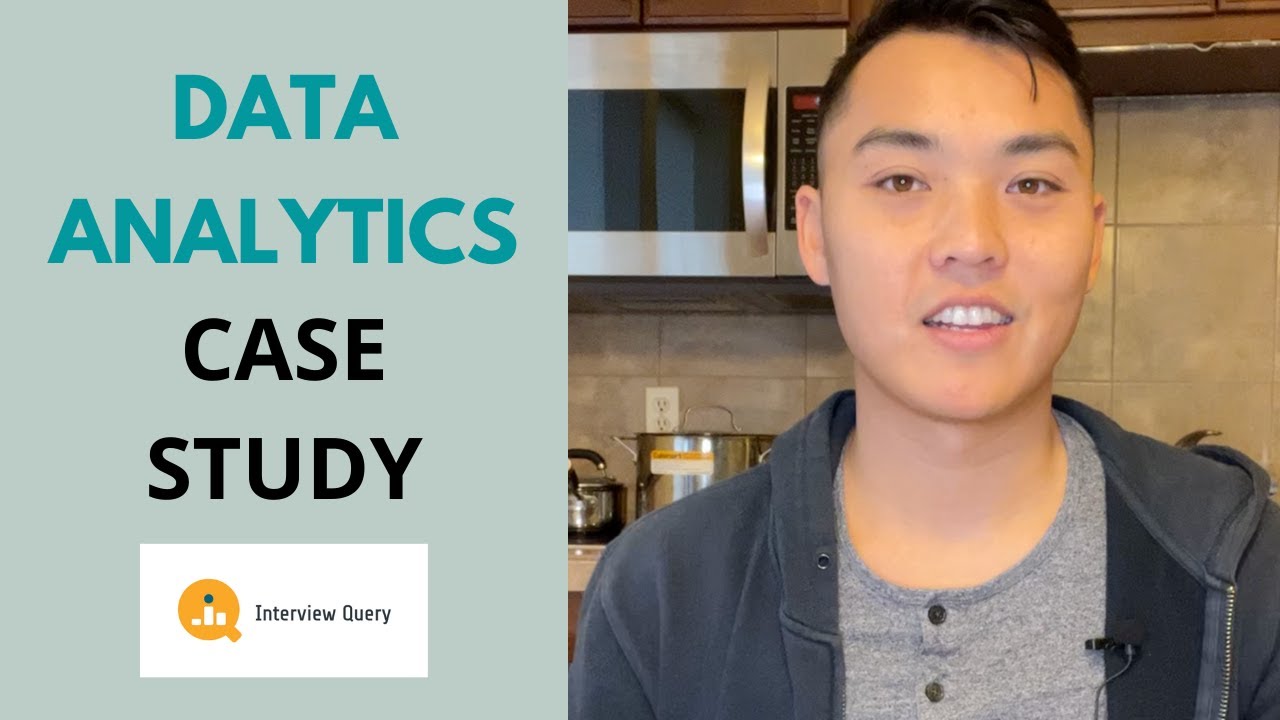
With data analyst case questions, you will need to answer two key questions:
- What metrics should I propose?
- How do I write a SQL query to get the metrics I need?
In short, to ace a data analytics case interview, you not only need to brush up on case questions, but you also should be adept at writing all types of SQL queries and have strong data sense.
These questions are especially challenging to answer if you don’t have a framework or know how to answer them. To help you prepare , we created this step-by-step guide to answering data analytics case questions.
We show you how to use a framework to answer case questions, provide example analytics questions, and help you understand the difference between analytics case studies and product metrics case studies .
Data Analytics Cases vs Product Metrics Questions
Product case questions sometimes get lumped in with data analytics cases.
Ultimately, the type of case question you are asked will depend on the role. For example, product analysts will likely face more product-oriented questions.
Product metrics cases tend to focus on a hypothetical situation. You might be asked to:
Investigate Metrics - One of the most common types will ask you to investigate a metric, usually one that’s going up or down. For example, “Why are Facebook friend requests falling by 10 percent?”
Measure Product/Feature Success - A lot of analytics cases revolve around the measurement of product success and feature changes. For example, “We want to add X feature to product Y. What metrics would you track to make sure that’s a good idea?”
With product data cases, the key difference is that you may or may not be required to write the SQL query to find the metric.
Instead, these interviews are more theoretical and are designed to assess your product sense and ability to think about analytics problems from a product perspective. Product metrics questions may also show up in the data analyst interview , but likely only for product data analyst roles.
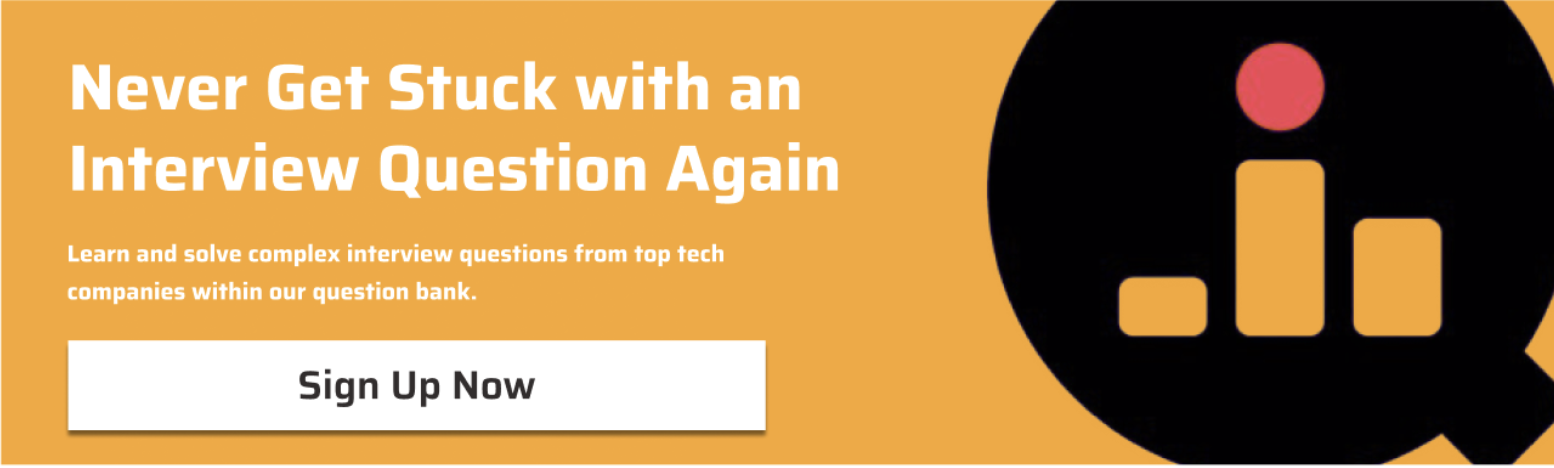
TRY CHECKING: Marketing Analytics Case Study Guide
Data Analytics Case Study Question: Sample Solution
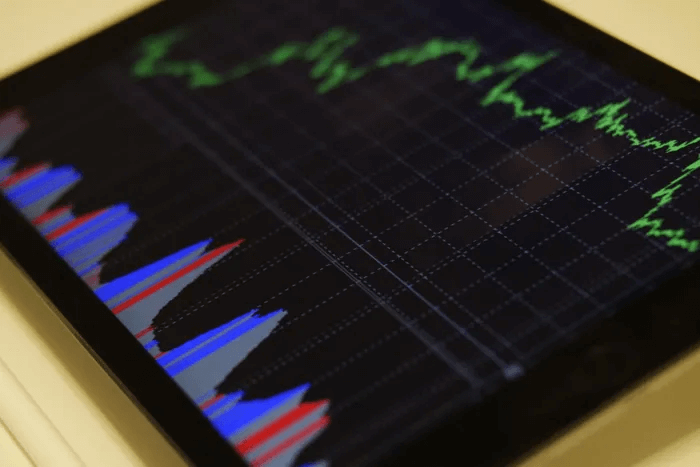
Let’s start with an example data analytics case question :
You’re given a table that represents search results from searches on Facebook. The query column is the search term, the position column represents each position the search result came in, and the rating column represents the human rating from 1 to 5, where 5 is high relevance, and 1 is low relevance.
Each row in the search_events table represents a single search, with the has_clicked column representing if a user clicked on a result or not. We have a hypothesis that the CTR is dependent on the search result rating.
Write a query to return data to support or disprove this hypothesis.
search_results table:
Column | Type |
---|---|
VARCHAR | |
INTEGER | |
INTEGER | |
INTEGER |
search_events table
Column | Type |
---|---|
INTEGER | |
VARCHAR | |
BOOLEAN |
Step 1: With Data Analytics Case Studies, Start by Making Assumptions
Hint: Start by making assumptions and thinking out loud. With this question, focus on coming up with a metric to support the hypothesis. If the question is unclear or if you think you need more information, be sure to ask.
Answer. The hypothesis is that CTR is dependent on search result rating. Therefore, we want to focus on the CTR metric, and we can assume:
- If CTR is high when search result ratings are high, and CTR is low when the search result ratings are low, then the hypothesis is correct.
- If CTR is low when the search ratings are high, or there is no proven correlation between the two, then our hypothesis is not proven.
Step 2: Provide a Solution for the Case Question
Hint: Walk the interviewer through your reasoning. Talking about the decisions you make and why you’re making them shows off your problem-solving approach.
Answer. One way we can investigate the hypothesis is to look at the results split into different search rating buckets. For example, if we measure the CTR for results rated at 1, then those rated at 2, and so on, we can identify if an increase in rating is correlated with an increase in CTR.
First, I’d write a query to get the number of results for each query in each bucket. We want to look at the distribution of results that are less than a rating threshold, which will help us see the relationship between search rating and CTR.
This CTE aggregates the number of results that are less than a certain rating threshold. Later, we can use this to see the percentage that are in each bucket. If we re-join to the search_events table, we can calculate the CTR by then grouping by each bucket.
Step 3: Use Analysis to Backup Your Solution
Hint: Be prepared to justify your solution. Interviewers will follow up with questions about your reasoning, and ask why you make certain assumptions.
Answer. By using the CASE WHEN statement, I calculated each ratings bucket by checking to see if all the search results were less than 1, 2, or 3 by subtracting the total from the number within the bucket and seeing if it equates to 0.
I did that to get away from averages in our bucketing system. Outliers would make it more difficult to measure the effect of bad ratings. For example, if a query had a 1 rating and another had a 5 rating, that would equate to an average of 3. Whereas in my solution, a query with all of the results under 1, 2, or 3 lets us know that it actually has bad ratings.
Product Data Case Question: Sample Solution
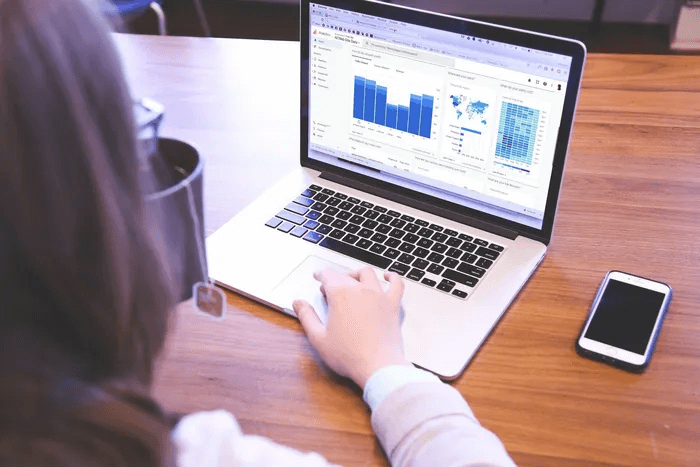
In product metrics interviews, you’ll likely be asked about analytics, but the discussion will be more theoretical. You’ll propose a solution to a problem, and supply the metrics you’ll use to investigate or solve it. You may or may not be required to write a SQL query to get those metrics.
We’ll start with an example product metrics case study question :
Let’s say you work for a social media company that has just done a launch in a new city. Looking at weekly metrics, you see a slow decrease in the average number of comments per user from January to March in this city.
The company has been consistently growing new users in the city from January to March.
What are some reasons why the average number of comments per user would be decreasing and what metrics would you look into?
Step 1: Ask Clarifying Questions Specific to the Case
Hint: This question is very vague. It’s all hypothetical, so we don’t know very much about users, what the product is, and how people might be interacting. Be sure you ask questions upfront about the product.
Answer: Before I jump into an answer, I’d like to ask a few questions:
- Who uses this social network? How do they interact with each other?
- Has there been any performance issues that might be causing the problem?
- What are the goals of this particular launch?
- Has there been any changes to the comment features in recent weeks?
For the sake of this example, let’s say we learn that it’s a social network similar to Facebook with a young audience, and the goals of the launch are to grow the user base. Also, there have been no performance issues and the commenting feature hasn’t been changed since launch.
Step 2: Use the Case Question to Make Assumptions
Hint: Look for clues in the question. For example, this case gives you a metric, “average number of comments per user.” Consider if the clue might be helpful in your solution. But be careful, sometimes questions are designed to throw you off track.
Answer: From the question, we can hypothesize a little bit. For example, we know that user count is increasing linearly. That means two things:
- The decreasing comments issue isn’t a result of a declining user base.
- The cause isn’t loss of platform.
We can also model out the data to help us get a better picture of the average number of comments per user metric:
- January: 10000 users, 30000 comments, 3 comments/user
- February: 20000 users, 50000 comments, 2.5 comments/user
- March: 30000 users, 60000 comments, 2 comments/user
One thing to note: Although this is an interesting metric, I’m not sure if it will help us solve this question. For one, average comments per user doesn’t account for churn. We might assume that during the three-month period users are churning off the platform. Let’s say the churn rate is 25% in January, 20% in February and 15% in March.
Step 3: Make a Hypothesis About the Data
Hint: Don’t worry too much about making a correct hypothesis. Instead, interviewers want to get a sense of your product initiation and that you’re on the right track. Also, be prepared to measure your hypothesis.
Answer. I would say that average comments per user isn’t a great metric to use, because it doesn’t reveal insights into what’s really causing this issue.
That’s because it doesn’t account for active users, which are the users who are actually commenting. A better metric to investigate would be retained users and monthly active users.
What I suspect is causing the issue is that active users are commenting frequently and are responsible for the increase in comments month-to-month. New users, on the other hand, aren’t as engaged and aren’t commenting as often.
Step 4: Provide Metrics and Data Analysis
Hint: Within your solution, include key metrics that you’d like to investigate that will help you measure success.
Answer: I’d say there are a few ways we could investigate the cause of this problem, but the one I’d be most interested in would be the engagement of monthly active users.
If the growth in comments is coming from active users, that would help us understand how we’re doing at retaining users. Plus, it will also show if new users are less engaged and commenting less frequently.
One way that we could dig into this would be to segment users by their onboarding date, which would help us to visualize engagement and see how engaged some of our longest-retained users are.
If engagement of new users is the issue, that will give us some options in terms of strategies for addressing the problem. For example, we could test new onboarding or commenting features designed to generate engagement.
Step 5: Propose a Solution for the Case Question
Hint: In the majority of cases, your initial assumptions might be incorrect, or the interviewer might throw you a curveball. Be prepared to make new hypotheses or discuss the pitfalls of your analysis.
Answer. If the cause wasn’t due to a lack of engagement among new users, then I’d want to investigate active users. One potential cause would be active users commenting less. In that case, we’d know that our earliest users were churning out, and that engagement among new users was potentially growing.
Again, I think we’d want to focus on user engagement since the onboarding date. That would help us understand if we were seeing higher levels of churn among active users, and we could start to identify some solutions there.
Tip: Use a Framework to Solve Data Analytics Case Questions
Analytics case questions can be challenging, but they’re much more challenging if you don’t use a framework. Without a framework, it’s easier to get lost in your answer, to get stuck, and really lose the confidence of your interviewer. Find helpful frameworks for data analytics questions in our data analytics learning path and our product metrics learning path .
Once you have the framework down, what’s the best way to practice? Mock interviews with our coaches are very effective, as you’ll get feedback and helpful tips as you answer. You can also learn a lot by practicing P2P mock interviews with other Interview Query students. No data analytics background? Check out how to become a data analyst without a degree .
Finally, if you’re looking for sample data analytics case questions and other types of interview questions, see our guide on the top data analyst interview questions .

Study Case Data Analysis: Strategy to Improve Sales Bakery

Introduction
Data analysts or scientists are pivotal professionals whose skills are applicable across various industries, regardless of scale. It is not always about machine learning model that sounds cool but about how to use various mathematical methods to help solve problems. In this article, I want to share a sample study case about how to optimize the sales of a bakery during peak seasons. I don’t have any experience and also know much about a bakery but with common knowledge and good data, we can solve this problem.
In this article, I want to split the article into the following sections:
Understanding the Problem
Methodology.
Also, I try to make this article concise but easy to understand especially for ordinary people. Hope you enjoy this article.
As I mention before, the problem about this case is how to optimize the sales of a bakery during peak seasons. To answer this question, we need to know when the peak seasons occurs and what affects profit. Remember that how detailed we are in answering this question is determined by how much data we have.
We need at least data transaction (that must include transaction date and products sold) so we can see the pattern of sales. Since it’s common knowledge that to increase profits the industry will increase sales, reduce costs, or enhance operational efficiency we can use the mining results to propose strategy of business. Please note that it is a minimum data required for this case, if we get more detailed data we can serve a more complex strategy.
The dataset in this case comes from kaggle . To solve this problem, I use data mining procedure with Python and visualize it using Looker Studio. Python programming helps me to apply data mining while Looker Studio helps me to provide sales dashboard and executive summary.
The result can be seen by clicking this link . The executive summary from the finding results are shown in here:
According to the result, I propose the big idea:
- Since we know when the peak season occurs, we can improve the efficiency of storing raw materials and bread production according to the existing pattern. We need more analysis based on the supply chain of this store.
- We have to prepare more bread from 8 a.m. to 12 a.m. than at any other time. Also, Monday and Sunday are two days that more people like to buy bread. The plan for the number of breads to be sold needs further discussion.
- To increase our sales, we can do a promotion bundling strategy. Since Traditional Baguette is the favorite product, we can bundle it with Pain AU Chocolat and Croissant. Also, since there are many high confidence products bundled with Coupe, we can take advantage of this opportunity for bundling strategy.

Written by Baha Tegar
Text to speech
More From Forbes
Moving beyond analysis paralysis: data for strategic decision making.
- Share to Facebook
- Share to Twitter
- Share to Linkedin
Andrew Glor is a Partner at Foresight Strategy , an analytics consultancy that helps brands achieve growth through evidence-based frameworks.
In today’s digital age, the proliferation of touchpoints has generated a tidal wave of data, offering businesses an unprecedented understanding of their customers and markets. Coupled with advancements in analytics tools and algorithms, this has fueled the rise of data-driven brand strategies.
But the sheer volume of information can be overwhelming, leading to the dreaded analysis paralysis, especially when making higher-level strategic decisions.
Too often, businesses get bogged down with dashboards and reporting the news, lacking the critical thinking and foresight needed to use data to illuminate future opportunities and pathways to growth.
What Is “Data-Driven Strategy?"
A data-driven strategy involves a robust understanding of the current market landscape and what drives brand, competitor and category performance. It includes assessing future investment opportunities based on current insights and projections, focusing on the most significant leverage points for brand growth. This means modeling clear building blocks with specific KPIs and realistic financial assumptions. Success depends on understanding target consumer segments and how the brand will source volume from within or outside the category.
Today’s NYT Mini Crossword Clues And Answers For Sunday, August 25th
Giovanni ribisi on his character’s debut in kevin costner’s ‘horizon’, the best golf shoes for wide feet are roomy, breathable and supportive, mapping the market: where to play.
One of the most significant strategic decisions is determining where to play. This involves mapping the market to identify potential revenue pools. Once you’ve established where to play, you can dive deeper into the data for the specific segment you’ve chosen to analyze and focus on what factors are important to winning that segment. Here are a few options for mapping the market.
Product-based segmentation can help identify categories and quality tiers, while place-based segmentation looks at geographical regions and distribution channels.
People-based segmentation considers demographic and behavioral factors and “purpose”-based (sometimes called demand space) segmentation examines consumer occasions and motivations.
Identifying Critical Data Sources
Whichever option you choose, the key to avoiding analysis paralysis is to use data and modeling to size the opportunity offered by each segment, allowing you to create "revenue maps.” For instance, category data sources offer a broad view of market sizes, trends and forecasts, providing valuable context for strategic decisions.
Complementing this, household panel data allows for demographic and behavioral segmentation, shedding light on penetration and purchase patterns. Point of sale data adds another layer by providing detailed insights into products across various channels and retailers, which is essential for understanding sales, pricing and distribution dynamics. Finally, brand health data from research providers highlights brand equity and consumer perceptions, which are crucial for positioning strategies and capturing trends that can help project the opportunity into the future.
Despite all this, sometimes the only way to know is to ask! Custom survey data can fill in any gaps, offering direct consumer insights that other sources might miss.
Evaluating Strategic Opportunities
As I said, the basic goal of strategic analysis is to identify and prioritize investment opportunities. To determine what makes your efforts worthwhile, consider several factors.
1. Assess the size of the segment. Is it a large market? Evaluate its lifetime value implications. For example, if we take diapers, although the smallest sizes represent a smaller absolute value, they are strategically important for retaining consumers as they progress through the category.
2. Examine the growth potential of the segment. Is it expanding? Consider how quickly and sustainably it is growing and where it sits in the product lifecycle. Hard seltzer had explosive growth, but the market became saturated, and there were natural limits to how long that wave could continue.
3. Identify share gaps. Are there segments where your share is below the “fair share” you would expect based on your total market position? Segments where you are not playing at all? Or has your share fallen below a previous high-water mark? Understanding these gaps can reveal valuable opportunities.
4. Consider uniqueness. Does the segment have distinct behaviors or attributes that set it apart? And how well does it fit with what you know about your brand—either strengths to exploit or gaps to close? These differences can often be identified through survey data.
5. Evaluate the return on investment (ROI). Is the segment profitable? This involves triangulating your financials and expected margins for playing in a segment with its market size. After thoroughly analyzing these aspects, you can make strategic decisions that drive growth for the top and bottom lines.
Practical Considerations: What's Realistic?
While you don’t want to let constraints cloud your assessment of the market and key opportunities, thinking practically for realistic strategic planning can be helpful. Consider benchmarking when identifying an opportunity; assess the size and growth of market leaders within a specific timeframe to gauge the realism of your ambitions.
Opportunities don't exist in isolation, either. Through scenario modeling, you can calculate and understand the interaction effects of pricing elasticity and cannibalization, using historical data to predict the potential impacts of the moves you make.
And don’t forget to set KPIs. This process helps define the goals your brand needs to achieve over a given period, allowing for necessary adjustments along the way and ensuring strategic objectives are met through regular progress checks.
The Human Element
Remember: Data is just the beginning. Use your qualitative judgment, business experience and market expertise as a critical filter on the strategic opportunities you have. There are always factors that won’t show up in the data but will make or break the success of a strategy, such as competitor or partner responses, cultural attitudes and ingrained category behaviors, and your organization’s capacity to execute.
Despite the rise of synthetic data and calls for synthetic strategy, human interpretation is still (and will always be) needed. A brand strategy is ultimately a human decision, ensuring expertise guides the process rather than algorithms. While statistical methodologies can provide valuable input, they rarely offer definitive strategic answers. And, honestly, are there ever truly definitive answers in strategy? Or only different choices with different potential implications for the future?
The balance of data-driven insights and human-centric storytelling is essential for creating compelling, effective brand strategies in a fast-moving market landscape.
Forbes Business Council is the foremost growth and networking organization for business owners and leaders. Do I qualify?
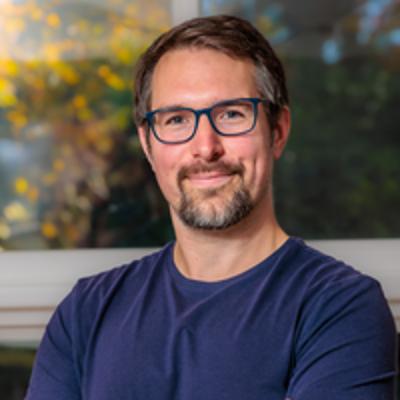
- Editorial Standards
- Reprints & Permissions
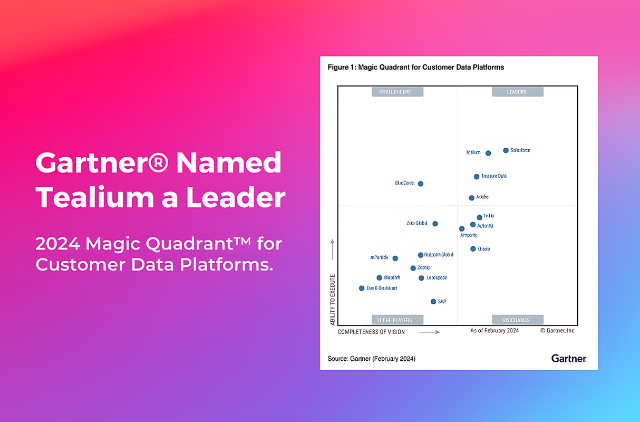
What Is Real-Time Data? What It Means, Best Practices, The Benefits of Real-Time Data and More
Real-time data is a game changer for businesses looking to get an edge for superior customer experiences. Access to real-time data supports informed decision making across teams. Buyers expect prompt, personalized experiences, whether they’re interacting with customer support or managing their banking on a mobile device. The era of ‘batch and blast’ campaigns and fragmented experiences is no longer acceptable.
But what is real-time data, and why is it so important? In this blog post, we will explore the concept of real-time data, its significance, and how real-time data collection can transform your business strategies.
What Is Real-Time Data?
The definition of real-time data.
Real-time data is information that becomes accessible immediately after it’s generated. Think of it like in-the-moment data! Real-time data is crucial for time-sensitive applications like customer interactions and enables real-time analytics, providing instant insights and allowing for rapid responses to changing conditions. Unlike historical data, which reflects past events, real-time data provides current insights that can drive immediate context and appropriate action in the moment.
Key Characteristics of Real-Time Data
- Immediate Availability : Data is accessible as soon as it is generated.
- Continuous Flow : Information is constantly updated, providing a stream of current data.
- Time-Sensitive : Real-time data is valuable for making decisions that depend on the most recent information.
What Is An Example of Real-Time Data?
Real-time data example for healthcare.
Real-time data empowers organizations to seize critical moments and can help provide the tools to act upon them. Consider this scenario: A patient visits a healthcare website and fills out a form to learn more about knee replacement surgery. When they call to schedule a doctor’s visit, the information they provided online is instantly accessible to the doctor’s office, eliminating the need for the patient to repeat themselves.
This streamlined experience is far superior to a healthcare experience without real-time data. What happens when you don’t use real-time data? You risk losing a patient! The ability to respond promptly and with greater relevance to customer actions leads to increased satisfaction and improved business outcomes. To get tools to support real-time data for healthcare, see our product Tealium for Healthcare .
What Is Real-Time Data Collection?
Real-time data collection is the process of gathering information instantly as events occur, without any delay. This method contrasts with traditional data collection techniques, which involve delays between the generation and processing of data. Real-time data collection ensures that information is immediately available for analysis and action, enabling businesses to make timely and informed decisions. This approach is essential for applications requiring immediate insights, such as customer behavior analysis and operational monitoring.
What Is Real-Time Data Processing?
Real-time data processing involves the continuous and immediate handling of data as it is generated, typically within milliseconds (at least for us at Tealium – see our page on real-time data collection and data quality ). Processing of data is commonly done to maintain high data quality or enrich the data with additional insights. By processing the data as it is captured, you can save time by reducing the need for data clean-up and ad hoc reporting, while also driving more intelligent customer engagement in the moment.
What Is Real-Time Data Analysis?
Real-time data analysis is the process of examining and interpreting data as soon as it becomes available, allowing you to gain immediate insights and activate the results. Real-time analysis is important to reduce any downtime that might impact results, and also to better understand what’s happening in customer experience.
What Is Real-Time Data Activation?
Real-time data activation means that you’re using data as soon as it’s collected. With Tealium , real-time data activation means harnessing data the moment it’s generated, empowering your business with instant insights and the ability to take immediate action in response to live events or user behaviors.
Real-time activation isn’t typically a capability for reverse ETL vendors because their primary focus is on extracting and syncing data from warehouses to external systems, which often involves batch processing rather than instant data flow. Additionally, reverse ETL tools are designed to work with structured data that has already been stored and processed, making real-time updates challenging due to latency and data pipeline complexities.
To harness real-time data power, you’ll want a tool that helps you use all parts of the data supply chain (collection, processing, analysis, and activation). Your access request is processed in real-time, but the data won’t be up-to-the-minute unless the entire supply chain operates in real-time.
What Are The Benefits of Real-Time Data?
4 key benefits of real-time data.
Utilizing real-time data offers numerous advantages for businesses across various industries:
- Competitive Advantage : Staying ahead of trends and market changes with real-time insights can provide a significant edge over competitors. For more on how to use real-time data for a competitive advantage, see our ebook, Era of Data Differentiation .
- Enhanced Decision Making : Access to up-to-date information allows businesses to make better and faster decisions.
- Increased Efficiency : Real-time data helps teams identify and address issues promptly, reducing downtime and improving operational efficiency.
- Improved Customer Experience : By automating your understanding of customer behavior in real-time, businesses can deliver more personalized and timely responses.
What Is A Real-Time Customer Data Platform (CDP)?
A real-time customer data platform (CDP) is one central hub, where you can collect customer data, support high data quality, and activate the results. This platform integrates data from multiple sources to create a unified and comprehensive customer profile, allowing businesses to deliver personalized experiences at the right moment.
Key Features Of A Real-Time Customer Data Platform (CDP)
- Unified Customer Profiles : Combines data from various channels to create a comprehensive view of each customer.
- Real-Time Data Processing : Processes and updates customer data instantly as new information is collected.
- Segmentation and Targeting : Enables precise segmentation of customers for targeted marketing campaigns.
- Data Activation : Activates data across marketing, sales, and customer service platforms for seamless customer interactions.
At Tealium , we know customer data comes from many sources (web, mobile applications, IoT, servers, kiosks, and offline sources). Tealium gives you one place to collect it, bring it together, and activate it across all the marketing and analytics tools (see our Integrations page ) you use to power customer-first experiences.
What Is The Importance of a Real-Time Customer Data Platform (CDP)?
5 reasons why a customer data platform is important.
The importance of a real-time CDP lies in its capabilities for personalization at scale, insights, efficiency, better customer experience, and data-driven decision-making.
- Personalization at Scale : Real-time CDPs allow businesses to deliver highly personalized experiences to customers at scale, increasing engagement and satisfaction.
- Timely Insights : Provides immediate insights into customer behavior, enabling businesses to respond quickly to changing customer needs and preferences.
- Operational Efficiency : Streamlines data management processes and reduces the time spent on data integration and analysis.
- Consistent Customer Experience : Ensures that customer interactions are consistent and relevant across all touchpoints.
- Data-Driven Decision Making : Empowers businesses with real-time data to make informed decisions and optimize marketing strategies.
The Best Tools For Real-Time Data Collection
What are the best tools for real-time data collection.
When it comes to the best tool for real-time data collection and activation, we believe you should consider a tool that places privacy and consent at the core when collecting first-party data. Tealium does this in order to assist you in creating trusted customer relationships! With Tealium, you can be confident in complying with evolving, global privacy regulations while you deliver trusted and relevant customer experiences.
Real-Time Data Case Studies
Top real-time use cases.
Elevating Experiences: The Power of Real-Time Data in Building Customer Trust : How can you harness real-time data to build stronger customer relationships? Industry leaders from BBVA, KVIK, and ImmoScout24 provide valuable insights on crafting personalized experiences that truly connect, helping you create lasting customer bonds.
Why Real-time Data Matters For Realizing True Customer Lifetime Value: We sat down with guest speaker, Rusty Warner, from Forrester, to discuss about the incredible power of real-time data and for shaping role in CX and driving strategic business outcomes.
The Total Economic Impact of CDP: Real-Time Personalisation through Right-Time Data Activation : We explore how to measure and quantify the impact of a CDP by examining the economic value of data as a new commodity.
Key Takeaways On Real-Time
Real-time data is a game-changer for businesses looking to stay competitive and responsive in a dynamic market. By understanding what real-time data is and how to effectively collect and utilize it, businesses can make informed decisions, improve operational efficiency, and deliver superior customer experiences.
Ready to leverage the power of real-time data for your business? Get a demo and explore how how our real-time solutions can help you harness data to drive your business forward.
Post Author
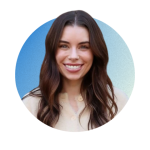
Sign Up for Our Blog
Related content, want a cdp that works with your tech stack, talk to a cdp expert and see if tealium is the right fit to help drive roi for your business..
- Growth & Acquisition
- Loyalty & Retention
- Customer Experience & Personalization
- Predictive Insights & Customer Analytics
- Data Collection & Privacy
- Single View of the Customer
- Real-Time Data Collection & Quality
- Real-Time CDP & Predictive Insights
- Data Management and Storage
- Integrations Overview
- Tealium Integrations Marketplace
- Tealium’s Suite of Conversions API (CAPI) Integrations
- Resource Library
- CDP RFP Template
- Events & Webinars
- Customer Case Studies
- See All Industries
- Financial Services
- Sports & Entertainment
- Travel & Hospitality
- Find a Partner
- Tealium Partner Network
- Developer Overview
- Product Guides
- Tealium Support Desk
- Tealium Education
- Privacy at Tealium
- Privacy Settings
- Service Terms
- Terms of Use
- Security & Compliance
Information
- Author Services
Initiatives
You are accessing a machine-readable page. In order to be human-readable, please install an RSS reader.
All articles published by MDPI are made immediately available worldwide under an open access license. No special permission is required to reuse all or part of the article published by MDPI, including figures and tables. For articles published under an open access Creative Common CC BY license, any part of the article may be reused without permission provided that the original article is clearly cited. For more information, please refer to https://www.mdpi.com/openaccess .
Feature papers represent the most advanced research with significant potential for high impact in the field. A Feature Paper should be a substantial original Article that involves several techniques or approaches, provides an outlook for future research directions and describes possible research applications.
Feature papers are submitted upon individual invitation or recommendation by the scientific editors and must receive positive feedback from the reviewers.
Editor’s Choice articles are based on recommendations by the scientific editors of MDPI journals from around the world. Editors select a small number of articles recently published in the journal that they believe will be particularly interesting to readers, or important in the respective research area. The aim is to provide a snapshot of some of the most exciting work published in the various research areas of the journal.
Original Submission Date Received: .
- Active Journals
- Find a Journal
- Proceedings Series
- For Authors
- For Reviewers
- For Editors
- For Librarians
- For Publishers
- For Societies
- For Conference Organizers
- Open Access Policy
- Institutional Open Access Program
- Special Issues Guidelines
- Editorial Process
- Research and Publication Ethics
- Article Processing Charges
- Testimonials
- Preprints.org
- SciProfiles
- Encyclopedia
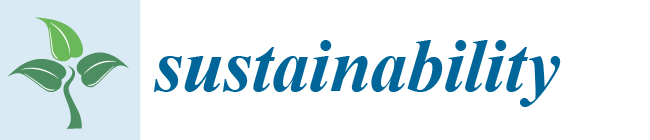
Article Menu

- Subscribe SciFeed
- Recommended Articles
- Google Scholar
- on Google Scholar
- Table of Contents
Find support for a specific problem in the support section of our website.
Please let us know what you think of our products and services.
Visit our dedicated information section to learn more about MDPI.
JSmol Viewer
Investigation of perception differences in shared mobility between driver’s license holders and nonholders: a case study of seoul, gyeonggi, and incheon in south korea.
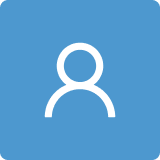
1. Introduction
2. data description, 2.1. classification of sm services based on their purpose, 2.2. survey overview and site introduction, 2.3. sample characteristics, 2.4. shared mobility awareness and user experience, 2.5. reasons for using shared mobility, 3. methodology, 3.1. two-proportion z-test.
- The two populations must be normal or approximately normal.
- The two samples must be randomly sampled from the two populations.
- The two proportions must be independent.
- The first step is to calculate the standard error of the difference between the two population proportions.
- The second step is to calculate the Z-test statistic by taking the difference between the two population proportions and dividing it by the standard error of the difference.
- Set the significance level, e.g., as 0.01 or 0.05. If a significance level of 0.05 is chosen, the null hypothesis is rejected for a p -value less than <0.05.
3.2. Logistic Regression Analysis
3.3. evaluating user satisfaction: comparative analysis and two-sample t-test, 4.1. impact of driving experience on shared mobility service usage, 4.2. shared mobility satisfaction depending on driver’s license possession, 5. discussion, 6. conclusions, author contributions, institutional review board statement, informed consent statement, data availability statement, acknowledgments, conflicts of interest.
- Vega-Gonzalo, M.; Gomez, J.; Christidis, P.; Vassallo, J.M. The role of shared mobility in reducing perceived private car dependency. Transp. Res. Part D Transp. Environ. 2024 , 126 , 104023. [ Google Scholar ] [ CrossRef ]
- Machado, C.A.S.; de Salles Hue, N.P.M.; Berssaneti, F.T.; Quintanilha, J.A. An overview of shared mobility. Sustainability 2018 , 10 , 4342. [ Google Scholar ] [ CrossRef ]
- Martínez-Díaz, M.; Soriguera, F.; Pérez, I. Technology: A necessary but not sufficient condition for future personal mobility. Sustainability 2018 , 10 , 4141. [ Google Scholar ] [ CrossRef ]
- Yoon, H.R.; Ki, H.K. Introduction of Seoul Integrated Transportation Service (MaaS) ; Research Institute in Seoul: Seoul, Republic of Korea, 2019; pp. 1–23. [ Google Scholar ]
- Dhinakaran, K.; Srinath, S.; Sriram, S.; Venkateshwar, R. GPS based tracking system for transit objects. In Proceedings of the 2017 Third International Conference on Science Technology Engineering & Management (ICONSTEM), Chennai, India, 23–24 March 2017; pp. 194–201. [ Google Scholar ]
- Park, Y.; Akar, G. Why do bicyclists take detours? A multilevel regression model using smartphone GPS data. J. Transp. Geogr. 2019 , 74 , 191–200. [ Google Scholar ] [ CrossRef ]
- Yi, W.; Yan, J. Energy consumption and emission influences from shared mobility in China: A national level annual data analysis. Appl. Energy 2020 , 277 , 115549. [ Google Scholar ] [ CrossRef ]
- Eisele, W.L.; Fossett, T.; Schrank, D.L.; Farzaneh, M.; Meier, P.J.; Williams, S.P. Greenhouse Gas Emissions and Urban Congestion: Incorporation of Carbon Dioxide Emissions and Associated Fuel Consumption into Texas A&M Transportation Institute Urban Mobility Report. Transp. Res. Rec. 2014 , 2427 , 73–82. [ Google Scholar ]
- Shapiro, R.J.; Hassett, K.A.; Arnold, F.S. Conserving Energy and Preserving the Environment: The Role of Public Transportation ; American Public Transportation Association: Washington, DC, USA, 2002. [ Google Scholar ]
- Giesel, F.; Nobis, C. The impact of carsharing on car ownership in German cities. Transp. Res. Procedia 2016 , 19 , 215–224. [ Google Scholar ] [ CrossRef ]
- Martin, E.; Cohen, A.; Botha, J.L.; Shaheen, S. Bikesharing and Bicycle Safety ; MINETA(MTI): San Jose, CA, USA, 2016. [ Google Scholar ]
- Wappelhorst, S.; Sauer, M.; Hinkeldein, D.; Bocherding, A.; Glaß, T. Potential of electric carsharing in urban and rural areas. Transp. Res. Procedia 2014 , 4 , 374–386. [ Google Scholar ] [ CrossRef ]
- Nijland, H.; van Meerkerk, J. Mobility and environmental impacts of car sharing in the Netherlands. Environ. Innov. Soc. Transit. 2017 , 23 , 84–91. [ Google Scholar ] [ CrossRef ]
- Heineke, K.; Kloss, B.; Scurtu, D. The Future of Micromobility: Ridership and Revenue after a Crisis ; McKinsey: New York, NY, USA, 2020. [ Google Scholar ]
- Cheng, R.; Zeng, W.; Wu, X.; Chen, F.; Miao, B. Exploring the Influence of the Built Environment on the Demand for Online Car-Hailing Services Using a Multi-Scale Geographically and Temporally Weighted Regression Model. Sustainability 2024 , 16 , 1794. [ Google Scholar ] [ CrossRef ]
- Efthymiou, D.; Antoniou, C.; Waddell, P. Factors affecting the adoption of vehicle sharing systems by young drivers. Transp. Policy 2013 , 29 , 64–73. [ Google Scholar ] [ CrossRef ]
- Zhong, J.; Lin, Y.; Yang, S. The impact of ride-hailing services on private car use in urban areas: An examination in Chinese cities. J. Adv. Transp. 2020 , 2020 , 8831674. [ Google Scholar ] [ CrossRef ]
- Kim, K.; Baek, C.; Lee, J.-D. Creative destruction of the sharing economy in action: The case of Uber. Transp. Res. Part A Policy Pract. 2018 , 110 , 118–127. [ Google Scholar ] [ CrossRef ]
- Mitropoulos, L.; Kortsari, A.; Ayfantopoulou, G. A systematic literature review of ride-sharing platforms, user factors and barriers. Eur. Transp. Res. Rev. 2021 , 13 , 61. [ Google Scholar ] [ CrossRef ] [ PubMed ]
- Campisi, T.; Akgün, N.; Ticali, D.; Tesoriere, G. Exploring public opinion on personal mobility vehicle use: A case study in Palermo, Italy. Sustainability 2020 , 12 , 5460. [ Google Scholar ] [ CrossRef ]
- Mohn, T. The Good News/Bad News for E-Scooters and Speed. Available online: https://www.forbes.com/sites/tanyamohn/2023/05/17/the-good-newsbad-news-for-e-scooters-and-speed/?sh=4692305b30e4 (accessed on 5 December 2023).
- Castillo, A. Available online: https://www.americancityandcounty.com/2023/05/22/research-highlights-safety-tradeoffs-of-electric-scooter-speed-limiters/ (accessed on 5 March 2024).
- Zagorskas, J.; Burinskienė, M. Challenges caused by increased use of e-powered personal mobility vehicles in European cities. Sustainability 2019 , 12 , 273. [ Google Scholar ] [ CrossRef ]
- Lee, M.; Chow, J.; Yoon, G.; He, B. Forecasting e-scooter competition with direct and access trips by mode and distance in New York City. arXiv 2019 , arXiv:1908.08127. [ Google Scholar ]
- Pham, T.Q.; Nakagawa, C.; Shintani, A.; Ito, T. Evaluation of the effects of a personal mobility vehicle on multiple pedestrians using personal space. IEEE Trans. Intell. Transp. Syst. 2015 , 16 , 2028–2037. [ Google Scholar ] [ CrossRef ]
- Howe, E.; Bock, B. Global Scootersharing Market Report 2018 ; InnoZ-Innovation Centre for Mobility and Societal Change (InnoZ) GmbH: Berlin, Germany, 2018. [ Google Scholar ]
- Clercq, G.D. Paris Considers Electric Scooter Ban over Safety Concerns. Available online: https://www.reuters.com/world/europe/paris-considers-electric-scooter-ban-over-safety-concerns-2022-11-15/ (accessed on 10 November 2023).
- Ko, E.; Kim, H.; Lee, J. Survey data analysis on intention to use shared mobility services. J. Adv. Transp. 2021 , 2021 , 5585542. [ Google Scholar ] [ CrossRef ]
- Ko, E.; Kwon, Y.; Son, W.; Kim, J.; Kim, H. Factors Influencing Intention to Use Mobility as a Service: Case Study of Gyeonggi Province, Korea. Sustainability 2021 , 14 , 218. [ Google Scholar ] [ CrossRef ]
- Sherwin, H.; Chatterjee, K.; Jain, J. An exploration of the importance of social influence in the decision to start bicycling in England. Transp. Res. Part A Policy Pract. 2014 , 68 , 32–45. [ Google Scholar ] [ CrossRef ]
- Roukouni, A.; Homem de Almeida Correia, G. Evaluation methods for the impacts of shared mobility: Classification and critical review. Sustainability 2020 , 12 , 10504. [ Google Scholar ] [ CrossRef ]
- Fazio, M.; Giuffrida, N.; Le Pira, M.; Inturri, G.; Ignaccolo, M. Planning suitable transport networks for e-scooters to foster micromobility spreading. Sustainability 2021 , 13 , 11422. [ Google Scholar ] [ CrossRef ]
- Ignaccolo, M.; Inturri, G.; Cocuzza, E.; Giuffrida, N.; Le Pira, M.; Torrisi, V. Developing micromobility in urban areas: Network planning criteria for e-scooters and electric micromobility devices. Transp. Res. Procedia 2022 , 60 , 448–455. [ Google Scholar ] [ CrossRef ]
- Krenn, P.J.; Oja, P.; Titze, S. Development of a bikeability index to assess the bicycle-friendliness of urban environments. Open J. Civ. Eng. 2015 , 5 , 451–459. [ Google Scholar ] [ CrossRef ]
- Correia, G.; Viegas, J.M. Carpooling and carpool clubs: Clarifying concepts and assessing value enhancement possibilities through a Stated Preference web survey in Lisbon, Portugal. Transp. Res. Part A Policy Pract. 2011 , 45 , 81–90. [ Google Scholar ] [ CrossRef ]
- Prieto, M.; Baltas, G.; Stan, V. Car sharing adoption intention in urban areas: What are the key sociodemographic drivers? Transp. Res. Part A Policy Pract. 2017 , 101 , 218–227. [ Google Scholar ] [ CrossRef ]
- Ho, C.Q.; Mulley, C.; Hensher, D.A. Public preferences for mobility as a service: Insights from stated preference surveys. Transp. Res. Part A Policy Pract. 2020 , 131 , 70–90. [ Google Scholar ] [ CrossRef ]
- Kim, D.; Park, Y.; Ko, J. Factors underlying vehicle ownership reduction among carsharing users: A repeated cross-sectional analysis. Transp. Res. Part D Transp. Environ. 2019 , 76 , 123–137. [ Google Scholar ] [ CrossRef ]
- Institute, N.G.I. Available online: https://map.ngii.go.kr/ms/map/NlipMap.do (accessed on 11 November 2023).
- Korea, S. KOSIS Korean Statistical Service. Available online: https://kosis.kr/visual/populationKorea/PopulationDashBoardMain.do (accessed on 5 October 2023).
- Agency, K.N.P. Status of Driver’s License Holders. Available online: https://kosis.kr/statHtml/statHtml.do?orgId=132&tblId=DT_13201_A002 (accessed on 29 July 2023).
- Korea, M.o.t.I.a.S.o. Population Status by Age 20–60. Available online: https://jumin.mois.go.kr/ageStatMonth.do (accessed on 30 July 2023).
- Kim, J. Smart Mobility Service Issue and Policy Implication ; Gyeonggi Research Institute: Suwon, Republic of Korea, 2020. [ Google Scholar ]
- Kim, Y.R.C.; Ji, W.S.; Song, J.R. Smart Mobility Services: Issue and Policy Implications ; Kyunggi Policy Research Center: Suwon, Republic of Korea, 2020; Volume 1–184. [ Google Scholar ]
- Ma, Q.; Yang, H.; Mayhue, A.; Sun, Y.; Huang, Z.; Ma, Y. E-Scooter safety: The riding risk analysis based on mobile sensing data. Accid. Anal. Prev. 2021 , 151 , 105954. [ Google Scholar ] [ CrossRef ]
- LaValley, M.P. Logistic regression. Circulation 2008 , 117 , 2395–2399. [ Google Scholar ] [ CrossRef ]
- Li, W.; Kamargianni, M. An integrated choice and latent variable model to explore the influence of attitudinal and perceptual factors on shared mobility choices and their value of time estimation. Transp. Sci. 2020 , 54 , 62–83. [ Google Scholar ] [ CrossRef ]
- Basu, R.; Ferreira, J. Planning car-lite neighborhoods: Does bikesharing reduce auto-dependence? Transp. Res. Part D Transp. Environ. 2021 , 92 , 102721. [ Google Scholar ] [ CrossRef ]
- Le Vine, S.; Polak, J. The impact of free-floating carsharing on car ownership: Early-stage findings from London. Transp. Policy 2019 , 75 , 119–127. [ Google Scholar ] [ CrossRef ]
- Jain, T.; Rose, G.; Johnson, M. Changes in private car ownership associated with car sharing: Gauging differences by residential location and car share typology. Transportation 2022 , 49 , 503–527. [ Google Scholar ] [ CrossRef ]
- Hinkeldein, D.; Schoenduwe, R.; Graff, A.; Hoffmann, C. Who would use integrated sustainable mobility services–and why? In Sustainable Urban Transport ; Emerald Group Publishing Limited: Bradford, UK, 2015; Volume 7, pp. 177–203. [ Google Scholar ]
Click here to enlarge figure
Service | Concept | Use | |
---|---|---|---|
| Car sharing | A short-period rental service for members | (1) Search for available vehicles near the parking lot using a smartphone application |
(2) Pay and reserve a vehicle with a smartphone application | |||
(3) After use, park at the designated place | |||
Car-hailing | A service that books transportation | (1) Reserve vehicle departure point and destination point in real-time using a smartphone application | |
(2) Take the vehicle to the departure point | |||
| Bike sharing | A sharing service for single-person transportation modes | (1) Search for available electric bikes or scooters using a smartphone application |
Scooter sharing (e-scooter) | A sharing service for single-person transportation modes powered by electric batteries | (2) Pay and reserve PM with a smartphone application | |
(3) After use, park freely on the street |
Sample Characteristics | Driving License Holder | Driving License Nonholder | Number of Samples | % | |
---|---|---|---|---|---|
Gender | Male | 503 | 23 | 526 | 50.21% |
Female | 415 | 100 | 515 | 49.79% | |
Age | 20s | 172 | 63 | 235 | 22.57% |
30s | 227 | 22 | 249 | 23.91% | |
40s | 262 | 19 | 281 | 26.99% | |
50s | 257 | 19 | 276 | 26.51% |
Republic of Korea | % | Seoul, Kyunggi, Incheon | % | |
---|---|---|---|---|
Driving license holder | 1779 | 89% | 918 | 88% |
Driving license Nonholder | 221 | 11% | 123 | 12% |
Total | 2000 | 100% | 1041 | 100% |
Service | Number of Samples | Gender | Age | Area | ||||||
---|---|---|---|---|---|---|---|---|---|---|
Male | Female | 20s | 30s | 40s | 50s | Seoul | Gyeonggi Province | Incheon | ||
799 76.8% | 386 (73.4%) | 413 (80.2%) | 215 (91.5%) | 186 (74.7%) | 203 (72.2%) | 195 (70.7%) | 329 (83.7%) | 386 (72.0%) | 84 (75%) | |
798 76.7% | 391 (74.3%) | 407 (79.0%) | 209 (88.9%) | 179 (71.9%) | 204 (72.6%) | 206 (74.6%) | 319 (81.2%) | 407 (75.9%) | 72 (64.3%) | |
817 78.5% | 426 (81.0%) | 391 (75.9%) | 207 (88.1%) | 190 (76.3%) | 202 (71.9%) | 218 (79%) | 351 (89.3%) | 384 (71.6%) | 82 (73.2%) | |
468 45.0% | 228 (43.3%) | 240 (46.6%) | 138 (53.7%) | 117 (47%) | 110 (39.1%) | 103 (37.3%) | 210 (53.4%) | 210 (39.2%) | 48 (42.9%) | |
663 63.7% | 337 (64.1%) | 326 (63.6%) | 84 (35.7%) | 179 (71.9%) | 196 (69.8%) | 204 (73.9%) | 218 (55.5%) | 378 (70.5%) | 67 (59.8%) | |
| 208 20.0% | 128 (24.3%) | 80 (15.5%) | 50 (21.3%) | 62 (24.9%) | 56 (19.9%) | 40 (14.5%) | 96 (24.4%) | 92 (17.2%) | 20 (17.9%) |
1041 | 526 | 515 | 235 | 249 | 281 | 276 | 393 | 536 | 112 |
Purpose for Usage | Total | Ages | Seoul | Kyunggi | Incheon | |||||
---|---|---|---|---|---|---|---|---|---|---|
20s | 30s | 40s | 50s | |||||||
Shared Car | Car-Sharing | Need a car to travel to destination | 340 (26.7%) | 125 (27.5%) | 96 (26.3%) | 71 (30.7%) | 48 (21.4%) | 160 (27.4%) | 155 (26.3%) | 25 (24.5%) |
Need a car urgently | 277 (21.7%) | 92 (20.2%) | 89 (24.3%) | 48 (20.5%) | 48 (21.4%) | 128 (21.8%) | 125 (21.1%) | 24 (24.0%) | ||
Hard to use personal vehicle | 173 (13.5%) | 46 (10.1%) | 56 (15.3%) | 37 (!5.8%) | 34 (15.1%) | 72 (12.1%) | 88 (14.9%) | 13 (13%) | ||
Other reason | 486 (38.2%) | 192 (29.7%) | 124 (25.4%) | 77 (24.8%) | 93 (29.6%) | 226 (38.7%) | 221 (37.6%) | 39 (38.5%) | ||
Total Responses | 1272 (100%) | 455 (100%) | 365 (100%) | 233 (100%) | 223 (100%) | 586 (100%) | 589 (100%) | 101 (100%) | ||
Car-Hailing | When too early or too late to use transportation | 659 (22.4%) | 171 (17.4%) | 184 (17.9%) | 159 (14.1%) | 145 (13.6%) | 258 (22.8%) | 340 (23.1%) | 61 (18.3%) | |
Urgent movement during a limited time | 644 (21.9%) | 206 (21.0%) | 161 (15.7%) | 151 (13.48%) | 126 (11.8%) | 234 (20.7%) | 316 (21.5%) | 94 (28.2%) | ||
Hard to use public transportation | 520 (17.7%) | 99 (10.1%) | 157 (15.3%) | 132 (11.7%) | 132 (12.3%) | 177 (15.6%) | 295 (20.0%) | 48 (14.3%) | ||
Other reasons | 1114 (37.9%) | 314 (39.8%) | 301 (37.5%) | 271 (38.0%) | 228 (36.2%) | 463 (40.9%) | 520 (35.4%) | 130 (39.2%) | ||
Total Responses | 2934 (100%) | 790 (100%) | 803 (100%) | 713 (100%) | 631 (100%) | 1132 (100%) | 1471 (100%) | 333 (100%) | ||
Personal Mobility | Bike Sharing | When it is an uncertain distance to walk | 177 (21.0%) | 65 (20.6%) | 51 (21.3%) | 38 (24.7%) | 23 (17.3%) | 94 (20.3%) | 72 (22.5%) | 11 (18.8%) |
No special reason, but want to use bicycle | 167 (19.8%) | 71 (22.3%) | 51 (21.0%) | 23 (15.1%) | 22 (16.5%) | 88 (19.1%) | 60 (18.9%) | 18 (29.9%) | ||
Need exercise with bike | 161 (18.1%) | 71 (22.3%) | 36 (15.0%) | 26 (17.1%) | 28 (21.2%) | 103 (22.2%) | 52 (16.4%) | 6 (9.4%) | ||
Other reasons | 338 (41.2%) | 110 (34.7%) | 103 (42.7%) | 66 (43.1%) | 59 (45.0%) | 177 (38.4%) | 135 (42.3%) | 25 (41.9%) | ||
Total Responses | 838 (100%) | 317 (100%) | 241 (100%) | 153 (100%) | 132 (100%) | 462 (100%) | 319 (100%) | 60 (100%) | ||
e-scooter | When it is an uncertain distance to walk | 79 (29.4%) | 35 (29.0%) | 21 (27.2%) | 18 (38.5%) | 5 (20.8%) | 32 (28.8%) | 36 (32.6%) | 10 (22.7%) | |
Hard to use public transportation | 49 (17.9%) | 25 (20.3%) | 14 (17.9%) | 8 (17.6%) | 2 (6.3%) | 23 (20.7%) | 14 (12.7%) | 11 (23.9%) | ||
Commuting period | 31 (11.7%) | 12 (10.0%) | 6 (7.9%) | 6 (13.2%) | 7 (29.2%) | 15 (13.6%) | 15 (13.6%) | 2 (3.4%) | ||
Other reasons | 110 (36.3%) | 49 (40.7%) | 36 (47.0%) | 14 (30.8%) | 11 (43.8%) | 42 (37.4%) | 46 (37.6%) | 22 (25.0%) | ||
Total Responses | 266 (100%) | 121 (100%) | 77 (100%) | 46 (100%) | 25 (100%) | 112 (100%) | 111 (100%) | 45 (100%) |
Sample | No. with Driving License | No. of Total Samples | % of Driving License | Z Statistic | p-Value | ||
---|---|---|---|---|---|---|---|
Car-Hailing | p1 (Deselected) | 216 | 246 | 0.8780 (88%) | −0.20818 | 0.4175 | |
p2 (Selected) | 702 | 795 | 0.8830 (88%) | ||||
Car Sharing | p1 (Deselected) | 603 | 696 | 0.8643 (86%) | −2.08296 | 0.0186 * | |
p2 (Selected) | 315 | 345 | 0.9130 (91%) | ||||
Bike Sharing | p1 (Deselected) | 735 | 824 | 0.8919 (89%) | 2.05512 | 0.0199 * | |
p2 (Selected) | 183 | 217 | 0.8433 (84%) | ||||
Shared e-Scooter | p1 (Deselected) | 852 | 970 | 0.8783 (88%) | −1.27467 | 0.1012 | |
p2 (Selected) | 66 | 71 | 0.9295 (92%) | ||||
Total Sample | - | 918 | 1041 | 0.8818 (88%) |
Car-hailing | Variable | Intercept | License | Car sharing | E-scooter | Shared bike |
Coefficient | 0.7379 | −0.0124 | 0.3658 | 0.0381 | 0.1827 | |
p-value | 0.001 | 0.957 | <0.001 | 0.711 | 0.002 | |
Car sharing | Variable | Intercept | License | Car-hailing | E-scooter | Shared bike |
Coefficient | −2.390 | 0.547 | 0.296 | 0.406 | 0.238 | |
p-value | <0.001 | 0.019 | <0.001 | <0.001 | <0.001 | |
Bike sharing | Variable | Intercept | License | Car-hailing | Car sharing | E-scooter |
Coefficient | −1.684 | −0.609 | 0.158 | 0.262 | 0.088 | |
p-value | <0.001 | 0.007 | 0.004 | <0.001 | 0.244 | |
Shared e-scooter | Variable | Intercept | License | Car-hailing | Car sharing | Bike sharing |
Coefficient | −3.708 | 0.462 | −0.028 | 0.366 | 0.103 | |
p-value | <0.001 | 0.338 | 0.740 | <0.001 | 0.124 |
Gender | Age | ||||||
---|---|---|---|---|---|---|---|
Male | Female | 20s | 30s | 40s | 50s | ||
3.582 | 3.693 | 3.579 | 3.622 | 3.661 | 3.688 | ||
3.616 | 3.707 | 3.719 | 3.650 | 3.628 | 3.638 | ||
| 3.982 | 3.971 | 3.988 | 4.016 | 3.923 | 3.940 | |
| 3.480 | 3.714 | 3.625 | 3.550 | 3.250 | 3.636 |
Driver’s License Holder | Driver’s license Nonholder | t-Statistic | p-Value | |||
---|---|---|---|---|---|---|
Mean | SD | Mean | SD | |||
3.66 | 0.696 | 3.68 | 0.71 | −0.251 | 0.401 | |
3.64 | 0.727 | 3.43 | 0.971 | 1.471 | 0.071 * | |
3.98 | 0.741 | 3.97 | 0.834 | 0.054 | 0.479 | |
| 3.58 | 0.823 | 3.20 | 0.447 | 1.676 | 0.071 * |
Correlation | Hailing | Sharing | Bike Sharing | Shared e-Scooter |
---|---|---|---|---|
Car-Hailing | 1.0000 | 0.2443 * | 0.1406 * | 0.0586 |
Car Sharing | 1.0000 | 0.2282 * | 0.2072 * | |
Bike sharing | 1.0000 | 0.0798 * | ||
Shared e-Scooter | 1.0000 |
The statements, opinions and data contained in all publications are solely those of the individual author(s) and contributor(s) and not of MDPI and/or the editor(s). MDPI and/or the editor(s) disclaim responsibility for any injury to people or property resulting from any ideas, methods, instructions or products referred to in the content. |
Share and Cite
Baek, J.; Shin, J.-Y. Investigation of Perception Differences in Shared Mobility between Driver’s License Holders and Nonholders: A Case Study of Seoul, Gyeonggi, and Incheon in South Korea. Sustainability 2024 , 16 , 7225. https://doi.org/10.3390/su16167225
Baek J, Shin J-Y. Investigation of Perception Differences in Shared Mobility between Driver’s License Holders and Nonholders: A Case Study of Seoul, Gyeonggi, and Incheon in South Korea. Sustainability . 2024; 16(16):7225. https://doi.org/10.3390/su16167225
Baek, Jiin, and Ju-Young Shin. 2024. "Investigation of Perception Differences in Shared Mobility between Driver’s License Holders and Nonholders: A Case Study of Seoul, Gyeonggi, and Incheon in South Korea" Sustainability 16, no. 16: 7225. https://doi.org/10.3390/su16167225
Article Metrics
Article access statistics, further information, mdpi initiatives, follow mdpi.
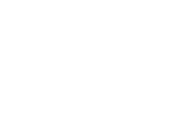
Subscribe to receive issue release notifications and newsletters from MDPI journals
- Open access
- Published: 21 August 2024
Exploring social activity patterns among community-dwelling older adults in South Korea: a latent class analysis
- Jiyoung Shin 1 , 4 ,
- Hun Kang 2 ,
- Seongmi Choi 1 &
- JiYeon Choi ORCID: orcid.org/0000-0003-1947-7952 1 , 3
BMC Geriatrics volume 24 , Article number: 697 ( 2024 ) Cite this article
60 Accesses
Metrics details
With the trend of digitalization, social activities among the older population are becoming more diverse as they increasingly adopt technology-based alternatives. To gain a comprehensive understanding of social activities, this study aimed to identify the patterns of digital and in-person social activities among community-dwelling older adults in South Korea, examine the associated factors, and explore the difference in depressive symptoms by the identified latent social activity patterns.
Data were extracted from a nationwide survey conducted with 1,016 community-dwelling older adults (mean age 68.0 ± 6.5 years, 47.8% male). The main variables assessed were digital social activities (eight items), in-person social activities (six items), and depressive symptoms (20 items). Data were analyzed using latent class analysis, multinomial logistic regression, and multiple linear regression.
We identified four distinct social activity patterns: “minimal in both digital and in-person” (22.0%), “moderate in both digital and in-person” (46.7%), “moderate in digital & very high in in-person” (14.5%), and “high in both digital and in-person” (16.8%). Younger age, living in multi-generational households, and higher digital literacy were associated with a higher likelihood of being in the “moderate in both digital and in-person” than the “minimal in both digital and in-person” group. Younger age, male, living in multi-generational households, residing in metropolitan areas, no dependency on IADL items, doing daily physical exercise, and higher digital literacy were associated with a higher likelihood of being in the “moderate in digital & very high in in-person” than the “minimal in both digital and in-person” group. Younger age, living in multi-generational households, no dependency on IADL items, doing daily physical exercise, and higher digital literacy were associated with a higher likelihood of being in the “high in both digital and in-person” than the “minimal in both digital and in-person” group. Depressive symptoms were significantly higher in the group with minimal engagement in both digital and in-person activities, compared to other three groups.
Conclusions
This study highlights distinct patterns of social activities among Korean community-dwelling older adults. Since older adults with minimal social activity engagement can be more vulnerable to depressive symptoms, interventions that address modifiable attributes, such as supporting digital literacy and facilitating physical activity of older adults, could serve as potential strategies to enhance their social activity engagement and, consequently, their mental well-being.
Peer Review reports
With increasing digitalization, social activities among the older population are becoming diverse. More older adults increasingly adopt technology-based alternatives [ 1 ]. Notably, older adults in South Korea show high rates of smartphone usage (90%) [ 1 ] and internet usage rate (98%), surpassing those of other developed countries such as Japan, Sweden, and the Netherlands [ 1 , 2 ]. Current social activities now encompass digital interactions including social media use [ 3 ], complementing in-person social interactions [ 4 ]. Therefore, to gain a deeper understanding of social activities among older individuals in our rapidly digitizing society, it is crucial to explore both traditional, predominantly in-person social activities and digital-based remote interactions.
Studies have consistently demonstrated that engaging in social activities can help prevent and alleviate depressive symptoms in older adults [ 5 , 6 , 7 , 8 , 9 , 10 , 11 ]. In a large cohort study in the United States, perceived social activity level was identified as the social determinant most strongly associated with depression among older adults [ 12 ]. Additionally, social participation, including social connections, informal social participation, and volunteering, has been linked to promoting healthy aging and reducing depressive symptoms in older adults [ 13 ]. However, the direction and strength of the association between social activity and depression may vary depending on the types of social activity [ 12 ].
While previous studies have mainly focused on exploring the effect of each social activity on depression individually [ 14 , 15 , 16 , 17 ], only a few studies classified social activities and compared the characteristics based on patterns of social activities [ 18 , 19 ]. Since we tend to engage in social activities in a complex manner rather than exclusively choosing one, we need to consider the possibility that their individual effects may offset each other [ 15 ]. Considering all, it is valuable to examine the factors associated with social activities to gain specific and personalized insights into modifiable interventions.
Furthermore, despite the increasing digitalization, most previous studies investigating the relationship between social activities and depressive symptoms have primarily focused on either digital or in-person modes of social activities [ 5 , 6 , 7 , 8 , 10 ]. To gain a comprehensive understanding of how older adults participate in various social activities, including both digital and in-person activities, it is essential to explore the combined engagement in these diverse forms of social activities. This is particularly important within the context of an aging population in a rapidly digitalizing world. Therefore, this study aimed to (1) identify the patterns of digital and in-person social activities among community-dwelling older adults in South Korea, (2) identify the associated factors, and (3) explore the difference in depressive symptoms by the identified latent social activity patterns.
Data source and sample
Data were extracted from a nationwide cross-sectional survey conducted to understand the digital literacy status and associated factors among community-dwelling older adults in South Korea [ 20 ]. The survey was conducted from October to November 2022, using proportional stratified sampling based on region, sex, and age groups to match South Korea’s registered population in June 2022. Participants who met the following criteria were selected: (1) aged 60 or older, (2) achieved a minimum score of 22 on the Korean version of the Mini-Mental State Examination (2nd edition) [ 21 ], and (3) proficient in the Korean language. A total of 1,016 older adults participated and provided information on their sociodemographic characteristics, health status, health behavior, social activities, and social support.
Ethics approval and consent to participate
This study was reviewed and approved by the Institutional Review Board of Yonsei University (ref no.: 4-2023-0983). Participants provided informed consent before the survey and received gift vouchers worth 10,000 Korean won as compensation upon completing survey.
Social activities
Social activities encompassed both traditional in-person social activities and digital social activities. In-person social activities were evaluated via questions adapted from the Korea Longitudinal Study of Ageing (KLoSA) [ 22 ]. Participants were asked regarding the frequency of their involvement in various activities over the past year, such as religious gatherings, social gatherings, leisure/culture/sports activities, alumni meetings, volunteer work, political/civic/interest group activities, and others. Responses that indicated “not at all” were recoded as non-participation (0), while other responses were categorized as participation (1) for six social activities, which excluded the “others” category.
To assess digital social activities—which included phone calls, text messages, messengers, information search, e-mails, blogs, online education, and app use—, participants were asked regarding the purpose of their digital device use which included desktops and laptops, mobile phones, tablets, e-books, and wearable devices. Options included phone calls, text messages, messengers, information search, e-mail, blogs, online education, other, or not using digital devices. Variables for digital social activities were coded as participation (1) or non-participation (0) for each activity, which excluded the “other” and “not using digital devices.”
Depressive symptoms
The frequency and severity of depressive symptoms were measured via the Center for Epidemiological Studies-Depression (CES-D) scale which consists of 20 items [ 23 ]. We used the integrated Korean version of the CES-D developed by Jeon et al. [ 24 ]. Participants rated their experience of depressive symptoms over the past week on a 0–3 scale for each item, which ranged from “rarely or never (< 1 day)” to “most or all of the time (5–7 days).” After four positively worded items were reverse-coded, we aggregated the scores. The total scores ranged between 0 and 60, and higher scores indicated more severe depressive symptoms [ 23 ]. We also categorized respondents as either at risk for clinical depression or not, using the most widely recommended threshold (≥ 16) [ 25 ]. The original CES-D and Korean version had Cronbach’s alphas of 0.85 and 0.91, respectively [ 23 , 24 ]. In our sample, the Cronbach’s alpha was 0.89.
Variables associated with social activity
Socio-demographic factors.
Based on previous studies [ 19 , 26 , 27 , 28 , 29 ], participants’ sociodemographic factors included age, sex, educational level, living arrangements, region, and economic activities. Age was recorded in years, and sex was coded as male (1) or female (0). Educational levels were categorized as below middle school (0), below high school (1), below college (2), and college or higher (3). Living arrangements were categorized as living alone (0), with a spouse (1), or in a multigenerational household (2). Region was divided into metropolitan areas (1), which included Seoul, Incheon, and Gyeonggi provinces, and non-metropolitan areas (0), which included cities and provinces outside the metropolitan area. Economic activities were coded as “Yes” (2) when currently engaged in income-generating work, “Used to” (1) when previously employed but not currently, and “Never” (0) when there has been no lifetime work experience.
Health factors
Variables that may be associated with social activity were selected based on previous studies [ 27 , 28 , 29 , 30 ]. Health status variables included diagnosed disabilities, chronic diseases, functional status, and health-related quality of life. Participants indicated whether they had been diagnosed with a disability (1) or not (0). To assess older adults’ objective health status, participants reported whether they had been diagnosed with a chronic condition that lasted over three months [ 31 ]. The number of chronic diseases was categorized as 0, 1, and 2 or more.
Functional status was assessed using the Korean Activities of Daily Living (K-ADL) and Korean Instrumental Activities of Daily Living (K-IADL). The K-ADL and K-IADL consist of seven items (dressing, washing face and hands, bathing, eating, transfer, toileting, and continence) and 10 items (decorating, housework, preparing meals, laundry, going out for a short distance, using transportation, shopping, handling money, using the telephone, and taking medicine), respectively [ 32 ]. Participants answered each item based on the extent to which they required assistance. The Cronbach’s alpha values for the K-ADL and K-IADL at the time of development were 0.94 [ 33 ] and 0.94 [ 34 ], respectively. In our sample, the Cronbach’s alpha values were 0.75 and 0.78, respectively. For analysis, we categorized the number of items that required assistance into three groups: 0, 1, and 2 or more.
Health-related quality of life was assessed via the 12-item Short-Form Health Survey (SF-12), a concise version of the 36-item Short-Form Health Survey [ 35 ], version 2 (SF-12v2). The SF-12 measures eight health domains: physical functioning, role-physical, bodily pain, general health, vitality, social functioning, role-emotional, and mental health. The scores for each domain contributed to the Physical Component Summary (PCS) and Mental Component Summary (MCS) scores. Scores for the PCS (six items) and MCS (six items) were calculated and standardized based on published algorithms for the SF-12v2 [ 35 ]. On a range of 0–100, higher scores indicated a higher quality of life. In the original version of the SF-12, the Cronbach’s alpha values for PCS and MCS were 0.89 and 0.86 for the United States and 0.76 and 0.77 for the United Kingdom, respectively [ 35 ]. In our sample, the Cronbach’s alpha values were 0.81 for PCS and 0.72 for MCS.
Health behaviors included smoking, alcohol consumption, and physical activity. Based on their smoking status, participants were categorized as non-smoker (0), ex-smoker (1), or current smoker (2). Regarding alcohol consumption, participants answered by specifying whether they had consumed alcohol at least once during the past year (1) or not (0). Physical activity was assessed based on whether participants engaged in continuous physical activity for 10 min or more (1) or not (0).
Social factors
Digital literacy and social support were chosen as potential social factors associated with social activity, based on prior findings [ 5 ]. Digital literacy was assessed via the Everyday Digital Literacy Questionnaire (EDLQ) developed from the survey of our data source. The EDLQ was created with reference to the European Commission’s Digital Competence (DigComp) framework and consists of three domains: information and communication (nine items), content creation and management (four items), and safety and security (nine items) [ 20 ]. Participants responded to each item on a 5-point Likert scale that ranged from 1 (not at all) to 5 (very much so). Higher scores indicated higher levels of digital literacy. The EDLQ exhibited a high level of reliability with a Cronbach’s alpha value of 0.98 [ 20 ].
Social support was assessed via the Multidimensional Scale for Perceived Social Support (MSPSS), which evaluated perceived social support from family, friends, and significant others on 12 items [ 36 ]. The original instrument was structured with a 7-point Likert scale, which ranged from 1 (very strongly disagree) to 7 (very strongly agree) [ 36 ]. For this present study, we opted to use the Korean translated version, which employed a 5-point Likert scale that ranged from “strongly disagree” to “strongly agree” [ 37 ], in consideration of both the translated version we referenced and our participants’ characteristics. A higher mean score indicated a greater level of perceived social support. The Cronbach’s alpha values were 0.88, 0.89, and 0.94 for the original version, Korean translated version, and our sample, respectively.
Data analysis
Latent Class Analysis (LCA) is a person-centered modeling approach that relies on the response patterns of observed variables to identify latent subpopulations within a sample [ 38 ]. This approach can be particularly valuable to identify multiple subgroups within a sample that share common characteristics and could benefit from similar interventions [ 38 ]. We employed a LCA to identify patterns of social activity among community-dwelling older adults, using six in-person activities and eight digital social activities as indicator variables for social activities. The sample size was considered adequate, aligning with the recommendations of numerous prior studies that suggested the inclusion of 300 or more participants [ 39 ].
Model fit was assessed via three information criteria: Bayesian Information Criterion (BIC), Akaike Information Criterion (AIC), and Sample-Size Adjusted Bayesian Information Criterion (SSABIC). Lower values indicated a better fit [ 38 ]. To determine how accurately the model defined the classes, we employed entropy [ 40 ]. An entropy value of 0.8 or higher was recommended as an acceptable threshold, with values closer to 1 considered ideal [ 41 ]. Class solutions were evaluated via three relative fit indices: Lo-Mendell-Rubin Likelihood Ratio Test (LMR-LRT), Adjusted Lo-Mendell-Rubin Likelihood Ratio Test (Adj. LMR-LRT), and Bootstrapped Likelihood Ratio Test (BLRT). These indices assessed whether a model with k classes significantly improved the fit compared with a model with k-1 classes [ 42 ]. If the improvement was not statistically significant ( p > .05), the model with k-1 classes was selected [ 42 ].
Next, variables associated with social activities were introduced as auxiliary variables to minimize classification errors among classes, which resulted in the creation of the most probable class variables [ 43 ]. After then, we identified variables that differentiated the classes via a multinomial logistic regression model. We estimated the odds ratio (OR) for the likelihood of belonging to a specific class membership compared with the reference group, along with their corresponding 95% confidence intervals (CI). Additionally, we conducted an analysis of variance (ANOVA) to compare depressive symptoms among classes derived from LCA. Finally, we examined the association between depressive symptoms and social activity classes using multiple linear regression. In our analysis, we controlled for covariates known to influence depressive symptoms in older adults, as identified in previous reviews [ 44 , 45 , 46 , 47 , 48 ]. The LCA and multinomial logistic regression were conducted using Mplus 8.8 (Muthén & Muthén) [ 43 ] and subsequent analyses were performed using IBM SPSS Statistics for Windows version 26.0 (IBM Corp., Armonk, NY, USA).
Sample characteristics
Table 1 shows participants’ characteristics. The mean age of 1,016 participants was 68.0 years (SD = 6.5). More than half of the participants were female (52.2%, n = 530), had completed education beyond high school graduation (54.2%, n = 551), lived with spouse (51.6%, n = 524), and resided in non-metropolitan areas (53.8%, n = 547). Regarding physical health, 95.2% ( n = 967) reported having no disability, and 69.3% ( n = 704) reported having diagnosis of one or more chronic condition.
The results of K-ADL and K-IADL indicate that 93.7% ( n = 952) had no dependence in ADL and 82.6% ( n = 839) had no dependence in any of IADL. More than half of the participants reported being engaged in daily physical exercise for more than 10 min per day (58.5%, n = 594), were non-drinkers (50.8%, n = 516) and non-smokers (61.8%, n = 628). Health related quality of life scores for physical and mental health were 50.1 ± 7.3 and 49.4 ± 8.4 respectively. Digital literacy had a mean score of 57.1 ± 24.0, and perceived social support had a mean score of 3.9 ± 0.7. The mean score for depressive symptoms across our sample was 12.0 ± 7.6 and 26.2% ( n = 266) were at risk for clinical depression.
Identification of social activity patterns
Model selection: 4-class model.
Table 2 summarizes the model fit indices for the selectable 2 to 6 latent classes. Decreases in the AIC, BIC, and SSABIC values were less pronounced after the 3-class point. The entropy values, which indicated the quality of the class classification, satisfied the recommended thresholds across all the models. The p -values for the LMR-LRT and Adj. LMR-LRT were not statistically significant ( p < .05) in the 5-class and 6-class scenarios. Considering the strong statistical evidence of the goodness-of-fit measures and the theoretical interpretability, the 4-class model was chosen for our sample.
Characterization of social activity patterns
The characteristics of social activity patterns in the 4-class model can be explored based on the probabilities (ranging from 0 to 1) of respondents indicating participation in each social activity (see Table 3 ). Final class frequencies and proportions for the latent classes were described based on their most likely latent class membership. Class 1, the second-largest group (22.0%, n = 224), showed the lowest level of participation in overall social activities among the four classes. Approximately half participated in phone calls (49.2%) and about a quarter used text messages (24.0%), while the likelihood of participation in other digital device activities was very limited. For in-person social activities, over half had a probability of participating in social gatherings (55.1%); however, the probability of participation in other activities remained at the lowest level among the four classes.
Class 2, the largest group (46.7%, n = 474), displayed notable variation in participation rates across different types of social activities. Regarding digital social activities, majority had participated in phone calls (99.5%), text messages (98.2%), messenger apps (95.8%), and information search (80.2%). However, participation in other activities remained low (< 20%). Regarding in-person social activities, apart from social gatherings (75.1%) and alumni meetings (28.8%), participation rates were consistently below 20%.
Class 3, the smallest group (14.5%, n = 147), exhibited similar likelihoods of participating in digital social activities compared to Class 2, while displaying the highest likelihood of participating in in-person social activities among the four groups. Respondents in Class 3 showed a high likelihood of participating not only in social gatherings (100.0%) but also in alumni meetings (91.7%) and leisure/culture/sports activities (80.1%).
Class 4, the third largest group (16.8%, n = 171), showed the highest likelihood of engagement in digital social activities among the four groups, and also demonstrated the second highest level of participation in in-person social activities. Particularly, the majority of respondents in Class 4 indicated a likelihood of participation in phone calls (100.0%), information search (100.0%), messenger apps (98.9%), and text messages (98.8%), with over 50% indicating participation in the remaining digital activities as well.
Figure 1 illustrates the probability of social activity for each class. The x-axis and y-axis display indicators of social activities and a probability scale from 0 to 1, indicating the probability of engaging in each activity. Based on the distribution of the 14 social activities, classes were labeled as “minimal in both digital and in-person” (Class 1, 22.0%, n = 224), “moderate in both digital and in-person” (Class 2, 46.7%, n = 474), “moderate in digital & very high in in-person” (Class 3, 14.5%, n = 147), and “high in both digital and in-person” (Class 4, 16.8%, n = 171).

Probability of the social activities among the four latent classes
Characteristics associated with social activity patterns
Table 4 presents the results of multinomial logistic regression examining the role of characteristics that may be associated with social activity patterns. Younger age, multi-generational households compared to living alone, and higher digital literacy were associated with a higher likelihood of being in the “moderate in both digital and in-person” social activity group rather than the “minimal in both digital and in-person” group. In addition, younger age, male, multi-generational households, residing in metropolitan areas, no dependency on IADL items, doing daily physical exercise for more than 10 min, and higher digital literacy were associated with a higher likelihood of being in the “moderate in digital & very high in in-person” social activity group rather than the “minimal in both digital and in-person” group. Similarly, younger age, multi-generational households, no dependency on IADL items, doing daily physical exercise for more than 10 min, and higher digital literacy were associated with a higher likelihood of being in the “high in both digital and in-person” social activity group rather than the “minimal in both digital and in-person” group.
Comparison of depressive symptoms by social activity patterns
Table 5 shows the results of the ANOVA conducted to explore the differences in depressive symptoms by identified social activity patterns. The mean CES-D scores were observed as follows: minimal in both digital and in-person (14.64 ± 8.52), moderate in both digital and in-person (11.67 ± 7.24), moderate in digital & very high in in-person (11.21 ± 7.41), and high in both digital and in-person (10.03 ± 6.83), with significant differences among the groups.
Subsequently, we examined the association between depressive symptoms and social activity patterns, controlling for sex, chronic diseases, disabilities, functional status, physical activity, and alcohol consumption. There was no multicollinearity among the variables included in the model (variance inflation factor < 10). After accounting for covariates, the association between social activity patterns and depressive symptoms remained significant (see Table 6 ). Compared to the group with minimal engagement in both digital and in-person activities, all three other groups exhibited disparities in the CES-D scores.
Principal findings
We investigated social activity patterns among a nationwide sample of community-dwelling older adults in South Korea. Highlighting the heterogeneity and diversity of social activity within the older adult population, we examined the patterns of social activities encompassing both digital and in-person interactions. Four distinct groups emerged: “minimal in both digital and in-person,” “moderate in both digital and in-person,” “moderate in digital & very high in in-person,” and “high in both digital and in-person” social activity groups. Older adults in the minimal social activity group showed significantly higher levels of depressive symptoms compared to the other three groups, while accounting for covariates. This finding supports previous studies indicating an inverse association between older adults’ social activity and depressive symptoms [ 5 , 6 , 7 , 8 , 9 , 10 , 11 , 12 ]. This study builds upon prior research by identifying a subgroup of older adults characterized by significant inactivity, near isolation, and heightened levels of depressive symptoms compared to other groups within the population. The lessons learned from this study, which focuses on older adults in South Korea – where aging and digitalization are progressing at a pace unmatched elsewhere – can serve as valuable references for future digital policies and support programs for older adults in other regions worldwide experiencing similar demographic and technological shifts.
The four latent classes showed more pronounced distinctions in digital activities compared to in-person activities. While in-person social activities consistently demonstrated a high probability of participation across classes, in the order of social gatherings, alumni meetings, leisure/culture/sports activities, or religious gatherings, digital social activities displayed distinct characteristics and variations across the classes.
Interestingly, digital social activity of the two “moderate in digital” groups, comprising 61.2% of the sample, predominantly consisted of individual communication methods, such as phone calls, text messages, and messengers, as well as online information search. However, their participation rates in activities such as email, blogs, online education, and other app use were very low, like the minimally active group. This characterization is consistent with findings from a nationally representative sample from South Korea in 2020, indicating that over 89% of the sample engaged in digital social activities related to individual communication (e.g., receiving/sending messages), while involvement in more advanced activities (e.g., online commerce, app use, financial activities) remained low, at less than 20% [ 49 ]. On the other hand, the minimal group, which exhibited the highest depressive symptoms, showed notably low levels of engagement even in individual communication via mobile phones. In the minimal group, it was discovered that one out of two individuals may not participate in phone calls, and only one out of five may engage in text messaging activities. These findings suggest that despite the high smartphone use rate among Korean older adults [ 1 ] and the country’s leading global internet usage rate [ 2 ], approximately 20% of older adults may remain digitally isolated. To address this issue and assist the minimally active group reaching at least a moderately active level, interventions should be tailored to cater the diverse needs of older adults. Factors such as health status, socioeconomic status, and resource availability, which are linked to social activity, should be considered.
Our results highlight two modifiable attributes – digital literacy and physical activity – that could facilitate social activities among older adults. Specifically, in our sample, older adults with higher digital literacy were more inclined to belong to the moderately or highly active group rather than the minimal social activity group. This finding aligns with a previous study that reported a positive association between older adults’ engagement in social activities and their use of information and communications technology (ICT), particularly in relation to digital literacy [ 50 ]. In our study, it is noteworthy that older adults with high digital literacy were more likely to belong to the other moderately or highly social active group than the minimally active group, regardless of their age or living arrangements. This finding underscores the importance of developing strategies to support digital literacy and encourage the use of digital device to facilitate social activities among older adults. For example, providing digital literacy education tailored for older adults [ 51 , 52 ] may enhance their ability to use digital devices for social activities. This can create opportunities for older adults to engage in social interactions, even in the absence of in-person interactions [ 53 , 54 ]. Importantly, interventions targeting older adults should be tailored to their unique needs. Digital literacy training programs can be structured in tiers or personalized to individual capacities [ 52 ]. When working with older adults, it is crucial to recognize that they may require more time, patience, and frequent reminders to grasp digital skills effectively [ 55 ]. Additionally, efforts should focus on fostering positive perceptions and experiences of ICT among older adults. By accumulating positive experiences with ICT, older adults can develop a deeper understanding and curiosity about technology, seamlessly integrate it into their daily routines, and enhance their overall digital literacy [ 56 , 57 ]. Such interventions can be especially valuable in unforeseen circumstances where in-person social interactions are not possible, such as during the COVID-19 pandemic. It can aid older adults in staying connected and reducing feeling of isolation [ 58 ]. Moreover, since older adults may face limitations in participating in in-person social activities due reduced physical capabilities [ 59 ], providing them with the skills to engage in digital social interactions could prove beneficial for maintaining their social and psychological well-being.
Based on our findings, physical activity also emerges as a contributing factor in fostering social activity among older adults [ 60 , 61 ]. Older adults who engaged in more than 10 min of daily physical activity were more inclined to belong to the “moderate in digital & very high in in-person” group and “high in both digital and in-person” group compared to the “minimal in both digital and in-person” group. Previous studies have similarly highlighted the association between physical activity and social activity [ 29 , 30 ]. This association may be partially attributed to the social aspects inherent in physical activity, where participants interact with others; hence, physical activity itself serves as a form of social activity [ 62 , 63 ]. Especially, group-based physical activity classes, such as aerobic exercise, walking, and strength training [ 64 ], inherently promote social interactions among group members. Such programs are likely to sustain older adults’ involvement in social activities and, additionally, promote their psychosocial well-being and mental health. In situations where in-person gatherings are not feasible, such as during a pandemic, exchanging and discussing physical activity experiences via social networking services can also foster a sense of social connectedness [ 65 ].
Furthermore, our research indicates that older adults who are older or living alone are more inclined to be categorized into a minimally socially active group rather than the other three groups, corroborating the findings of previous studies. While further research is warranted to investigate the socioeconomic and health-related characteristics influencing older adults’ social activity participation more comprehensively, individuals with these characteristics should be given priority attention, especially considering limited community resources.
Limitations
This study has several limitations. First, we analyzed cross-sectional data; thus, we could not consider time variables, which made it challenging to establish causal relationships among the relevant variables. Additional longitudinal studies are needed to better understand how social activities among older adults evolve over time, whether changes in depressive symptoms are associated with different patterns of social activity, and which factors influence these changes. Second, although we included six indicators of in-person social activities and eight indicators of digital social activities, it is possible that other meaningful activities were missed. Nevertheless, to our knowledge, this study is significant as one of the first comprehensive investigations on expected social activities among older adults in a digital society. Third, due to the characteristics of our analytical methodology, we dichotomized each social activity into participation and non-participation, which limited our understanding of the extent of participation in each social activity. Future research should adopt a more detailed approach by clustering social activities among older adults based on their frequency, intensity, and quality. Fourth, although we emphasize the importance of preventing depressive symptoms by promoting social activities among older adults, our study did not directly examine factors associated with depressive symptoms, as our primary focus was on social activity patterns. In our study, the social activity patterns identified through latent class analysis are unique to our sample and are not established concepts. Future studies could concentrate on analyzing the impact of more well-defined social activity patterns on depressive symptoms. This approach has the potential to provide in-depth insights into effective strategies for preventing and addressing depressive symptoms among older adults.
Our findings suggest that distinct patterns of social activity can be observed among community-dwelling older adults. Furthermore, these patterns may have varying implications for the risk of depressive symptoms. Notably, older adults with limited social activity were more susceptible to depressive symptoms. Therefore, interventions that address modifiable factors, such as supporting digital literacy of older adults, could serve as a potential strategy to enhance their social engagement and, consequently, their mental well-being. Furthermore, promoting physical activity may be a promising interventional approach to encourage older adults to actively participate in social activities.
Data availability
The datasets used and/or analyzed during the current study are available from the corresponding author on reasonable request.
Abbreviations
Adjusted Lo-Mendell-Rubin Likelihood Ratio Test
Activities of daily living
Akaike Information Criterion
Analysis of variance
Bayesian Information Criterion
Bootstrapped Likelihood Ratio Test
Center for Epidemiological Studies-Depression scale
Confidence interval
Digital Competence
Everyday Digital Literacy Questionnaire
Instrumental activities of daily living
Information and communication technology
Korea Longituinal Study of Ageing
- Latent class analysis
Lo-Mendell-Rubin Likelihood Ratio Test
Mental Component Summary
Multidimensional Scale for Perceived Social Support
Physical Component Summary
12-item Short-Form Health Survey
12-item Short-Form Health Survey—version 2
Sample-Size Adjusted Bayesian Information Criterion
Gallup, Report. The 2012–2022 report on smartphone use & brand, smart watch, and wireless earphone. Gallup. 2022. https://www.gallup.co.kr/gallupdb/reportContent.asp?seqNo=1309 Accessed 12 Apr 2024.
Wike R, Silver L, Fetterolf J, Huang C, Austin S, Clancy L, Gubbala S. Internet, smartphone and social media use. Pew Research Center. 2022. https://www.pewresearch.org/global/2022/12/06/internet-smartphone-and-social-media-use-in-advanced-economies-2022 / Accessed 12 Apr 2024.
Hunsaker A, Hargittai E. A review of internet use among older adults. New Media Soc. 2018;20(10):3937–54.
Article Google Scholar
Cornejo R, Tentori M, Favela J. Enriching in-person encounters through social media: a study on family connectedness for the elderly. Int J Hum Comput Stud. 2013;71(9):889–99.
Choi E, Han KM, Chang J, Lee YJ, Choi KW, Han C, Ham BJ. Social participation and depressive symptoms in community-dwelling older adults: emotional social support as a mediator. J Psychiatr Res. 2021;137:589–96.
Article PubMed Google Scholar
Hao G, Bishwajit G, Tang S, Nie C, Ji L, Huang R. Social participation and perceived depression among elderly population in South Africa. Clin Interv Aging. 2017;12:971–6.
Article PubMed PubMed Central Google Scholar
Jeon GS, Choi KW, Jang KS. Social networking site usage and its impact on depressive symptoms among older men and women in South Korea. Int J Environ Res Public Health. 2020;17(8).
Liu Q, Pan H, Wu Y. Migration Status, Internet Use, and Social Participation among Middle-aged and older adults in China: consequences for Depression. Int J Environ Res Public Health. 2020;17(16).
Miller LM, Steele JS, Wu CY, Kaye J, Dodge HH, Gonzales MM, Lyons KS. Depressive symptoms in older adult couples: associations with dyadic physical health, social engagement, and close friends. Front Psychiatry. 2022;13:989182.
Wu HY, Chiou AF. Social media usage, social support, intergenerational relationships, and depressive symptoms among older adults. Geriatr Nurs. 2020;41(5):615–21.
Yuen HK, Huang P, Burik JK, Smith TG. Impact of participating in volunteer activities for residents living in long-term-care facilities. Am J Occup Ther. 2008;62(1):71–6.
Ryu E, Jenkins GD, Wang Y, Olfson M, Talati A, Lepow L, Coombes BJ, Charney AW, Glicksberg BS, Mann JJ, et al. The importance of social activity to risk of major depression in older adults. Psychol Med. 2023;53(6):2634–42.
Douglas H, Georgiou A, Westbrook J. Social participation as an indicator of successful aging: an overview of concepts and their associations with health. Aust Health Rev. 2017;41(4):455–62.
Croezen S, Avendano M, Burdorf A, van Lenthe FJ. Social participation and depression in old age: a fixed-effects analysis in 10 European countries. Am J Epidemiol. 2015;182(2):168–76.
Hofer M, Hargittai E. Online social engagement, depression, and anxiety among older adults. New Media Soc. 2024;26(1):113–30.
Min J, Ailshire J, Crimmins EM. Social engagement and depressive symptoms: do baseline depression status and type of social activities make a difference? Age Ageing. 2016;45(6):838–43.
Won S, Kim H. Social participation, health-related behavior, and depression of older adults living alone in Korea. Asian Soc Work Policy Rev. 2020;14(1):61–71.
Hong SI, Hasche L, Bowland S. Structural relationships between social activities and longitudinal trajectories of depression among older adults. Gerontologist. 2009;49(1):1–11.
Article CAS PubMed PubMed Central Google Scholar
van Hees SGM, van den Borne BHP, Menting J, Sattoe JNT. Patterns of social participation among older adults with disabilities and the relationship with well-being: a latent class analysis. Arch Gerontol Geriatr. 2020;86:103933.
Choi J, Choi S, Song K, Baek J, Kim H, Choi M, Kim Y, Chu SH, Shin J. Everyday digital literacy questionnaire for older adults: Instrument Development and Validation Study. J Med Internet Res. 2023;25:e51616.
Kang YW, Jahng SM, Kim SY. Korean Dementia Association: Korean-Mini Mental State Examination, 2nd Edition (K-MMSE ~ 2) user’s guide. 2020.
Korea Employment Information Service: Korean Longitudinal Study of Ageing (KLoSA). Korea Employment Information Service: Chungcheongbuk-do Republic of Korea. 2020. https://survey.keis.or.kr/klosa/klosaque/List.jsp Accessed 12 Apr 2024.
Radloff LS. The CES-D scale: a self-report depression scale for research in the general population. Appl Psychol Meas. 1977;1(3):385–401.
Jeon G, Choi S, Yang B. Integrated Korean version of CES-D development. Kor J Psychol: Health. 2001;6(1):59–76.
Google Scholar
Vilagut G, Forero CG, Barbaglia G, Alonso J. Screening for Depression in the General Population with the Center for epidemiologic studies Depression (CES-D): a systematic review with Meta-analysis. PLoS ONE. 2016;11(5):e0155431.
Chan E, Procter-Gray E, Churchill L, Cheng J, Siden R, Aguirre A, Li W. Associations among living alone, social support and social activity in older adults. AIMS Public Health. 2020;7(3):521–34.
Chen J, Zeng Y, Fang Y. Effects of social participation patterns and living arrangement on mental health of Chinese older adults: a latent class analysis. Front Public Health. 2022;10:915541.
Jang Y, Chiriboga DA. Social activity and depressive symptoms in Korean American older adults: the conditioning role of acculturation. J Aging Health. 2011;23(5):767–81.
Richard L, Gauvin L, Gosselin C, Laforest S. Staying connected: neighbourhood correlates of social participation among older adults living in an urban environment in Montreal, Quebec. Health Promot Int. 2009;24(1):46–57.
Buchman AS, Boyle PA, Wilson RS, Fleischman DA, Leurgans S, Bennett DA. Association between late-life social activity and motor decline in older adults. Arch Intern Med. 2009;169(12):1139–46.
Ministry of Health and Welfare. 2020 National survey of Korean older adults. Ministry of Health and Welfare, Korea Institute for Health and Social Affairs: Sejong, Republic of Korea. 2020.
Won CW, Yang KY, Rho YG, Kim S, Lee E-J, Yoon J, Cho K, Shin H, Cho BR, Oh J, et al. The development of Korean activities of daily living (K-ADL) and Korean instrumental activities of daily living (K-IADL) scale. Ann Geriatr Med Res. 2002;6(2):107–20.
Won CW, Rho YG, Kim SY, Cho BR, Lee YS. The validity and reliability of Korean activities of Daily Living (K-ADL) scale. Ann Geriatr Med Res. 2002;6(2):98–106.
Won CW, Rho YG, Sunwoo D, Lee YS. The validity and reliability of Korean Instrumental activities of Daily Living (K-IADL) scale. Ann Geriatr Med Res. 2002;6(4):273–80.
Ware J Jr., Kosinski M, Keller SD. A 12-Item short-form Health Survey: construction of scales and preliminary tests of reliability and validity. Med Care. 1996;34(3):220–33.
Zimet GD, Dahlem NW, Zimet SG, Farley GK. The multidimensional scale of perceived social support. J Pers Assess. 1988;52(1):30–41.
Shin JS, Lee YB. The effects of social supports on psychosocial well-being of the unemployed. Korean J Soc Welf. 1999;37:241–69.
Weller BE, Bowen NK, Faubert SJ. Latent class analysis: a guide to best practice. J Black Psychol. 2020;46(4):287–311.
Nylund-Gibson K, Choi AY. Ten frequently asked questions about latent class analysis. Transl Issues Psychol Sci. 2018;4(4):440–61.
Wang MC, Deng Q, Bi X, Ye H, Yang W. Performance of the entropy as an index of classification accuracy in latent profile analysis: a Monte Carlo simulation study. Acta Psychol Sin. 2017;49(11):1473–82.
Celeux G, Soromenho G. An entropy criterion for assessing the number of clusters in a mixture model. J Classif. 1996;13:195–212.
Williams GA, Kibowski F. Latent class analysis and latent profile analysis. In: Handbook of methodological approaches to community-based research: Qualitative, quantitative, and mixed methods 2016: 143–151.
Asparouhov T, Muthén B. Auxiliary variables in mixture modeling: a 3-step approach using M plus. Struct Equ Model. 2014;21(3):329–41.
Cole MG, Dendukuri N. Risk factors for depression among elderly community subjects: a systematic review and meta-analysis. Am J Psychiatry. 2003;160(6):1147–56.
Djernes JK. Prevalence and predictors of depression in populations of elderly: a review. Acta Psychiatr Scand. 2006;113(5):372–87.
Article CAS PubMed Google Scholar
Vink D, Aartsen MJ, Schoevers RA. Risk factors for anxiety and depression in the elderly: a review. J Affect Disord. 2008;106(1–2):29–44.
Catalan-Matamoros D, Gomez-Conesa A, Stubbs B, Vancampfort D. Exercise improves depressive symptoms in older adults: an umbrella review of systematic reviews and meta-analyses. Psychiatry Res. 2016;244:202–9.
Maier A, Riedel-Heller SG, Pabst A, Luppa M. Risk factors and protective factors of depression in older people 65+. A systematic review. PLoS ONE. 2021;16(5):e0251326.
Jeon GS, Choi K. Purposes of Internet Use and its impacts on physical and psychological health of Korean older adults. Healthc (Basel) 2024, 12(2).
Kim J, Lee HY, Christensen MC, Merighi JR. Technology Access and Use, and their associations with Social Engagement among older adults: do women and men Differ? J Gerontol B Psychol Sci Soc Sci. 2017;72(5):836–45.
PubMed Google Scholar
Lee H, Lim JA, Nam HK. Effect of a Digital Literacy Program on Older Adults’ Digital Social Behavior: A Quasi-Experimental Study. Int J Environ Res Public Health 2022, 19(19).
Ngiam NHW, Yee WQ, Teo N, Yow KS, Soundararajan A, Lim JX, Lim HA, Tey A, Tang KWA, Tham CYX, et al. Building Digital Literacy in older adults of low socioeconomic status in Singapore (Project Wire Up): Nonrandomized Controlled Trial. J Med Internet Res. 2022;24(12):e40341.
Zapletal A, Wells T, Russell E, Skinner MW. On the triple exclusion of older adults during COVID-19: technology, digital literacy and social isolation. Soc Sci Humanit Open. 2023;8(1):100511.
PubMed PubMed Central Google Scholar
Zhao W, Kelly RM, Rogerson MJ, Waycott J. Understanding older adults’ participation in Online Social activities: lessons from the COVID-19 pandemic. Proc ACM Hum Comput Interact. 2022;6(CSCW2):1–26.
Mubarak F, Suomi R. Elderly Forgotten? Digital Exclusion in the information age and the Rising Grey Digital divide. Inquiry. 2022;59:469580221096272.
Kania-Lundholm M, Torres S. The divide within: older active ICT users position themselves against different ‘Others’. J Aging Stud. 2015;35:26–36.
Schreuers K, Quan-Haase A, Martin K. Problematizing the digital literacy paradox in the context of older adults’ ICT use: aging, media discourse, and self-determination. Can J Commun. 2017;42(2):1–34.
Rolandi E, Vaccaro R, Abbondanza S, Casanova G, Pettinato L, Colombo M, Guaita A. Loneliness and Social Engagement in older adults based in Lombardy during the COVID-19 lockdown: the Long-Term effects of a course on Social networking sites Use. Int J Environ Res Public Health 2020, 17(21).
Leung AY, Molassiotis A, Carino DA. A challenge to healthy aging: limited social participation in Old Age. Aging Dis. 2021;12(7):1536–8.
King AC. Interventions to promote physical activity by older adults. J Gerontol Biol Sci Med Sci. 2001;56(Spec 2):36–46.
Valdes-Badilla PA, Gutierrez-Garcia C, Perez-Gutierrez M, Vargas-Vitoria R, Lopez-Fuenzalida A. Effects of physical activity Governmental Programs on Health Status in Independent older adults: a systematic review. J Aging Phys Act. 2019;27(2):265–75.
Fern AK. Benefits of physical activity in older adults: programming modifications to enhance the exercise experience. ACSM Health Fit J. 2009;13(5):12–6.
Gill K, Overdorf V. Incentives for exercise in younger and older women. J Sport Behav. 1994;17(2):87–98.
King AC, Rejeski WJ, Buchner DM. Physical activity interventions targeting older adults. A critical review and recommendations. Am J Prev Med. 1998;15(4):316–33.
Zuo Y, Ma Y, Zhang M, Wu X, Ren Z. The impact of sharing physical activity experience on social network sites on residents’ social connectedness:a cross-sectional survey during COVID-19 social quarantine. Global Health. 2021;17(1):10.
Download references
Acknowledgements
Not applicable.
This work was supported by the Basic Science Research Program through the National Research Foundation of Korea (NRF), funded by the Ministry of Education (No.2020R1A6A1A03041989).
Author information
Authors and affiliations.
Mo-Im Kim Nursing Research Institute, Yonsei University College of Nursing, 50-1 Yonsei-ro, Seodaemun-gu, Seoul, 03722, South Korea
Jiyoung Shin, Seongmi Choi & JiYeon Choi
Department of Social and Behavioral Sciences, Yale University School of Public Health, 60 College Street, New Haven, CT, 06510, USA
Yonsei University Institute for Innovation in Digital Healthcare, 50-1 Yonsei-ro, Seodaemun-gu, Seoul, 03722, South Korea
JiYeon Choi
Health Insurance Research Institute, National Health Insurance Service, 2, Segye-ro, Wonju-si, Gangwon-Do, 26464, South Korea
Jiyoung Shin
You can also search for this author in PubMed Google Scholar
Contributions
JC conceptualized and supervised the study. JC, JS, and SC developed the study and analytical design. SC prepared dataset and JS performed data analysis. HK and JS wrote the draft and JC reviewed and edited the manuscript. All the authors have read and agreed to the published version of the manuscript.
Corresponding author
Correspondence to JiYeon Choi .
Ethics declarations
This study was reviewed and approved by the Institutional Review Board (ref no.: 4-2023-0983). This study is not derived from a clinical trial, and a clinical trial number is not applicable in this case.
Consent for publication
Competing interests.
The authors declare no competing interests.
Additional information
Publisher’s note.
Springer Nature remains neutral with regard to jurisdictional claims in published maps and institutional affiliations.
Rights and permissions
Open Access This article is licensed under a Creative Commons Attribution 4.0 International License, which permits use, sharing, adaptation, distribution and reproduction in any medium or format, as long as you give appropriate credit to the original author(s) and the source, provide a link to the Creative Commons licence, and indicate if changes were made. The images or other third party material in this article are included in the article’s Creative Commons licence, unless indicated otherwise in a credit line to the material. If material is not included in the article’s Creative Commons licence and your intended use is not permitted by statutory regulation or exceeds the permitted use, you will need to obtain permission directly from the copyright holder. To view a copy of this licence, visit http://creativecommons.org/licenses/by/4.0/ . The Creative Commons Public Domain Dedication waiver ( http://creativecommons.org/publicdomain/zero/1.0/ ) applies to the data made available in this article, unless otherwise stated in a credit line to the data.
Reprints and permissions
About this article
Cite this article.
Shin, J., Kang, H., Choi, S. et al. Exploring social activity patterns among community-dwelling older adults in South Korea: a latent class analysis. BMC Geriatr 24 , 697 (2024). https://doi.org/10.1186/s12877-024-05287-5
Download citation
Received : 01 December 2023
Accepted : 07 August 2024
Published : 21 August 2024
DOI : https://doi.org/10.1186/s12877-024-05287-5
Share this article
Anyone you share the following link with will be able to read this content:
Sorry, a shareable link is not currently available for this article.
Provided by the Springer Nature SharedIt content-sharing initiative
- Older adults
- Social activity
- Digital activity
- Digital and in-person
- Mental health
- South Korea
BMC Geriatrics
ISSN: 1471-2318
- General enquiries: [email protected]

Protocol for Systematic Review and Meta-Analysis of Prehospital Large Vessel Occlusion Screening Scales
- Find this author on Google Scholar
- Find this author on PubMed
- Search for this author on this site
- ORCID record for Hidetaka Suzuki
- For correspondence: [email protected]
- ORCID record for Yohei Okada
- ORCID record for Yamagami Hiroshi
- ORCID record for Hitoshi Kobata
- Info/History
- Preview PDF
Background Large Vessel Occlusion (LVO) is a serious condition that causes approximately 24-46% of acute ischemic strokes (AIS). LVO strokes tend to have higher mortality rates and result in more severe longterm disabilities compared to non LVO ischemic strokes. Early intervention with endovascular therapy (EVT) is recommended; however, EVT is limited to tertiary care hospitals with specialized facilities. Therefore, identifying patients with a high probability of LVO in prehospital settings and ensuring their rapid transfer to appropriate hospitals is crucial. While LVO diagnosis typically requires advanced imaging like MRI or CT scans, various scoring systems based on neurological symptoms have been developed for prehospital use. Although previous systematic reviews have addressed some of these scales, recent studies have introduced new scales and additional data on their accuracy. This systematic review and meta-analysis aim to summarize the current evidence on the diagnostic accuracy of these prehospital LVO screening scales.
Methods This systematic review and meta-analysis will be conducted in accordance with the PRISMA-DTA Statement and the Cochrane Handbook for Systematic Reviews of Diagnostic Test Accuracy. We will include observational studies and randomized controlled trials that assess the utility of LVO scales in suspected stroke patients in prehospital settings. Eligible studies must provide sufficient data to calculate sensitivity and specificity, and those lacking such data or being case reports will be excluded. The literature search will cover CENTRAL, MEDLINE, and Ichushi databases, including studies in English and Japanese. Bias will be assessed using QUADAS-2, and meta-analysis will be conducted using a random effects model, with subgroup and sensitivity analyses to explore heterogeneity.
Competing Interest Statement
The authors have declared no competing interest.
Funding Statement
This study did not receive any funding
Author Declarations
I confirm all relevant ethical guidelines have been followed, and any necessary IRB and/or ethics committee approvals have been obtained.
The details of the IRB/oversight body that provided approval or exemption for the research described are given below:
We will search the following databases CENTRAL, MEDLINE, and Ichushi.
I confirm that all necessary patient/participant consent has been obtained and the appropriate institutional forms have been archived, and that any patient/participant/sample identifiers included were not known to anyone (e.g., hospital staff, patients or participants themselves) outside the research group so cannot be used to identify individuals.
I understand that all clinical trials and any other prospective interventional studies must be registered with an ICMJE-approved registry, such as ClinicalTrials.gov. I confirm that any such study reported in the manuscript has been registered and the trial registration ID is provided (note: if posting a prospective study registered retrospectively, please provide a statement in the trial ID field explaining why the study was not registered in advance).
I have followed all appropriate research reporting guidelines, such as any relevant EQUATOR Network research reporting checklist(s) and other pertinent material, if applicable.
Data Availability
All data produced in the present study are available upon reasonable request to the authors.
View the discussion thread.
Thank you for your interest in spreading the word about medRxiv.
NOTE: Your email address is requested solely to identify you as the sender of this article.

Citation Manager Formats
- EndNote (tagged)
- EndNote 8 (xml)
- RefWorks Tagged
- Ref Manager
- Tweet Widget
- Facebook Like
- Google Plus One
Subject Area
- Addiction Medicine (342)
- Allergy and Immunology (665)
- Anesthesia (180)
- Cardiovascular Medicine (2625)
- Dentistry and Oral Medicine (314)
- Dermatology (222)
- Emergency Medicine (397)
- Endocrinology (including Diabetes Mellitus and Metabolic Disease) (930)
- Epidemiology (12175)
- Forensic Medicine (10)
- Gastroenterology (756)
- Genetic and Genomic Medicine (4064)
- Geriatric Medicine (385)
- Health Economics (676)
- Health Informatics (2625)
- Health Policy (997)
- Health Systems and Quality Improvement (979)
- Hematology (360)
- HIV/AIDS (845)
- Infectious Diseases (except HIV/AIDS) (13659)
- Intensive Care and Critical Care Medicine (790)
- Medical Education (398)
- Medical Ethics (109)
- Nephrology (430)
- Neurology (3832)
- Nursing (209)
- Nutrition (570)
- Obstetrics and Gynecology (734)
- Occupational and Environmental Health (690)
- Oncology (2008)
- Ophthalmology (581)
- Orthopedics (238)
- Otolaryngology (304)
- Pain Medicine (250)
- Palliative Medicine (73)
- Pathology (471)
- Pediatrics (1107)
- Pharmacology and Therapeutics (459)
- Primary Care Research (447)
- Psychiatry and Clinical Psychology (3400)
- Public and Global Health (6499)
- Radiology and Imaging (1390)
- Rehabilitation Medicine and Physical Therapy (806)
- Respiratory Medicine (869)
- Rheumatology (400)
- Sexual and Reproductive Health (407)
- Sports Medicine (338)
- Surgery (441)
- Toxicology (52)
- Transplantation (185)
- Urology (165)
Drivers of land use land cover change and livelihood coping strategies in Sheka biosphere reserve; a case of Shato forest, Southwest Ethiopia
- Open access
- Published: 21 August 2024
- Volume 5 , article number 208 , ( 2024 )
Cite this article
You have full access to this open access article
- Workaferahu Ameneshewa 1 ,
- Yechale Kebede 1 ,
- Abiyot Legesse 2 &
- Dikaso Unbushe 3
73 Accesses
Explore all metrics
It is evident that the means of subsistence of the community have a significant impact on the management of natural resources. This study examined the socio-economic drivers of LULCC and assessed the impacts of such changes on rural livelihoods in Shato forest, southwest Ethiopia. To map the land use and land cover, supervised classifications were used. The data were collected from 358 household heads through semi-structured questionnaires. A logistic regression model was employed to investigate the dependence of rural households on forest resources. LULC analysis results showed that about 308.29 ha of wetland and 3215.6 ha of natural forest were converted to other land use types during the last 30 years. The findings reveal that a household’s education level, household size, distance from the market, total land owned, skills and social network significantly affect their dependency on forest resources. Respondents gave high rankings to covariates such as erratic rainfall (1.70), market price (1.53), low crop output (1.28), and inadequate infrastructure (1.24). These covariates force rural communities of the study area into two major livelihood diversification strategies. These were crop and income diversification. The study comes to conclude that, the extensive and imprudent use of natural resources is a result of changes in livelihood strategies to cope up with the aforementioned shocks. Thus, the decreasing nature of forest resources results in covariate shocks in the study area and needs serious intervention mechanisms to tackle this trajectory of catastrophe.
Similar content being viewed by others

Household Dependence on Forest Resources in the Luki Biosphere Reserve, Democratic Republic of CONGO
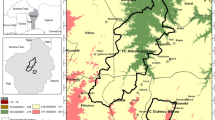
Identifying the land use and land cover change drivers: methods and case studies of two forest reserves in Northern Benin
Impacts of community-based forest management policies implemented by a local forest institution: a case study from bayombong, nueva vizcaya, philippines.
Avoid common mistakes on your manuscript.
1 Introduction
Throughout human history, the interaction between humans and nature has been described and conceptualized in a variety of ways, with significant cultural variation continuing to this day [ 1 , 2 , 3 ]. Land-use and land-cover change (LULC) is a complex process driven by social, economic, and environmental factors [ 4 ]. These changes can have significant impacts on ecosystems, biodiversity, and human livelihoods [ 5 , 6 ].
In recent years, there has been increasing concern about the conservation of ecosystems in semiarid regions [ 7 ], which resulted in the creation of conservation units [ 8 ]. However, in some situations, the creation of reserve areas can affect the livelihoods of local populations, who must find new ways to survive [ 9 ]. In addition, when natural resources are banned from being used, those rural residents who depend on them may become more socioeconomically vulnerable, which could lead to conflicts between the environment and social institutions [ 10 ].
In developing countries like Ethiopia, where a large portion of the population relies on agriculture and natural resources for subsistence, understanding the drivers of LULC is crucial [ 11 ]. Therefore, a more thorough comprehension of the intricate relationships between changing land use and land cover, rural livelihoods, and coping mechanisms is essential for making decisions [ 12 ]. High dependence on the forest may affect the strategies that the residents execute to collect resources. In humid tropical forests, local people usually prefer to use plant species from forests near their settlements [ 13 ].
Accurate mapping and measurements of land cover conversions are necessary to quantify the dynamics of land use and land cover, and doing so also helps in understanding the contribution of terrestrial ecosystems to the global carbon pool [ 5 ]. To understand the impact of changes in land use and cover on biodiversity, fine-resolution, spatially explicit data on landscape fragmentation were required [ 14 , 15 ]. The impact of land use changes on biodiversity, the feedback on livelihood strategies from dynamics of LULC, and the susceptibility of places and people to changes in land use and cover all depend on a thorough understanding of the dynamic human–environment interactions related to land use change [ 16 , 17 ].
The multitude of issues contributing to the intricate interplay between social, political, economic, technological, and biophysical factors can contribute to LULCC [ 4 ]. As a result, extensive studies have been done to determine the factors contributing to LULCC: from land use change processes in urban areas [ 18 ], to deforestation in tropical regions [ 19 , 20 ], and to Changes in land use and expansion of agriculture in mountainous regions [ 21 , 22 ].
In developing nations like Africa, a majority of population rely on agriculture both commercial and subsistence farming and the production of charcoal to make a living [ 11 , 23 ]. The main drivers of LULC change in Ethiopia were the expansion of agriculture and settlement into forested areas, logging, the production of charcoal, and the collection of fuel wood [ 24 ]. And, the socioeconomic drivers of LULC dynamics are multi faced and intricate in space and time, demanding more investigation in Ethiopia [ 25 , 26 ]. The degree to which households rely on forests is determined by several factors, including the distance between the household's homestead and the forest patch, the distance between the household’s homestead and market, skill, income, household size, and the head of the household's educational attainment [ 27 ].
Ethiopia is among the countries characterized by diverse vegetation zones [ 28 ]. But the country’s forests are deteriorating and becoming less plentiful as a result of the increased demand for agricultural land brought on by population growth [ 29 , 30 ]. A decrease in natural vegetation, such as forests, shrub lands, and woods, has been shown in most recent studies. This drop is attributed to the conversion of forests into agricultural and grazing lands, which has released settlement areas around the nation [ 31 , 32 ].
To alleviate poverty and improve living standards, rural households in developing countries engage in a variety of activities [ 33 ]. As defined by [ 34 ], livelihood diversification: is the process by which households combine a variety of activities and social support systems to mitigate shocks and improve their welfare. It's acknowledged as a strategy for dealing with the diverse idiosyncratic shocks people face [ 33 ]. Research on livelihood diversification in the past has primarily focused on measuring the extent and drivers as well as analyzing the primary sources of income for various sets of livelihood activities [ 33 , 35 , 36 ]. Several studies have been carried out to determine the livelihood diversification activities pursued by rural communities [ 36 , 37 , 38 ]. However, more recent research has gone beyond measurement and analysis to distinguish between two main types of livelihood diversification; distressed diversification, which encourages impoverished families to fend off shocks brought on by LULC dynamics [ 34 , 37 ], activities frequently linked to gathering or making use of natural resources like wild foods, fisheries, or hunting [ 39 ]. During difficult times, people turn to low-skill nonfarm activities in an attempt to secure their financial future [ 40 ]. Progressive diversification, is usually thought of as an ex-ante strategy implemented by merely well-off households [ 41 ], also contribute to the total income of richer households [ 42 ]
Rural communities in forested regions rely on forest resources for their livelihoods, including food, medicine, and construction materials [ 43 ]. Alterations in land use patterns can disrupt these livelihood strategies by limiting access to essential resources and undermining traditional practices [ 44 ]. The influence of the community on natural resource management is displayed through their interaction with the environment which can be clear from an analysis of their livelihood. Increasing dependence on forest resources could put additional pressure on it, which would result in excessive use, and ultimately lead to deforestation and land degradation [ 45 ].
LSAI is abbreviation for large scale agricultural investment. There isn’t a single, widely recognized definition for the term “LSAI” because, it can be determined a variety of contextual factors as well as the parties’ interests [ 46 ]. In this article, we conceptualized LSAI as a commercial agricultural investment that is mechanized and undertaken on a land area larger than 200 hectares by either domestic or foreign investors [ 47 ].
In Southwestern Ethiopia, Masha district ( Shato area in particular), where this study was conducted, human-induced changes in land use/land cover are often observed [ 48 , 49 ]. Major land uses in the area included wetlands, natural forests, tea and coffee plantations, crops, and settlements [ 50 ].
The researcher was observed that, in addition to the efforts made by various researchers on the causes of LULCC that have been done in the Sheka zone; high deforestation rates of 36% for tea plantations had also been observed between the periods of 1987 and 2005 in four areas in Sheka zone [ 51 , 52 , 53 ]. There were trends of increasing tea and coffee plantations, rural settlement, and decreasing forest land in the area. The result showed that from the year 1991–2021, about 20% of natural forest in the study area was converted to other LU/LC types while plantation and rural settlement increased by 2, 234.3 ha and 1289.6 ha. Shato core area covers 5023.3 ha (25.5%) of the study area, and currently, only 3541 ha (18%) are left [ 54 ]. The major proximate drivers of LU/LCC were an expansion of subsistence and commercial agriculture and unsustainable exploitations of forest products.
The primary source of income for the local community of Masha district's are cattle production and crop farming [ 49 ]. Furthermore, non-farm pursuits like beekeeping and non-timber forest products provide additional revenue streams and means of subsistence for the locals [ 55 ]. One of the main drivers of the LULC changes in the Masha district ( Shato core area), was the expansion of large-scale commercial coffee production and tea plantations.
Analyses of the Shakichos livelihood or means of subsistence indicated how a current change in their ecological resource management contributes to environmental degradation [ 48 ]. Little is known about the impacts of changes in land use and land cover as a function of rural livelihood coping strategies in the communities found around Shato forest of Sheka biosphere reserve. This is even though the region is one of the most favorable for large-scale agriculture particularly cash crop cultivation in Ethiopia.
In addition, the livelihood of the rural population in the area around the forest depends on the forests near their homes, forest-related items, and traditional small-scale farming practices [ 56 ]. Therefore, the degradation of the natural forest of Masha woreda , in addition to its global impact has had a more prominent negative impact on the livelihoods of residents living in the area or adjacent areas [ 27 ]. However, no studies have been undertaken to explore socioeconomic drivers of LULC dynamics: identifying short-term idiosyncratic shocks induced by LULC dynamics, and analyzing rural communities’ decision to pursue different livelihood strategies in Sheka Zone, Southwest Ethiopia. The results of the research serve to foster the development of sound policies and management strategies for the sustainable use and management of the natural resources of the area [ 26 ]. Therefore, this study examined the socio-economic drivers of land use and land cover change and assesses the relationship between socio-economic drivers and rural livelihoods in Sheka Biosphere Reserve, A case of Shato forest, Southwest Ethiopia.
2 Theoretical framework
We address the study of the link between nature and society from a more balanced perspective by concentrating on “cultural-human ecology.” To do this, we utilize systems theory and ecology to offer thorough explanations of the intricate relationships that exist between individuals and their biophysical surroundings [ 57 ], and to analyze the ways in which human communities and cultures adapt to the particulars of their local ecosystem through livelihood patterns [ 58 ].
The idea of adaptation, which refers to the continuous process of adjustment humans go through to deal with both internal and external impulses, is significant in the majority of studies [ 59 ]. The basic function of adaptation is ‘to maintain a balance between population, resources and productivity’ [ 60 ]. It is acknowledged that our theoretical explanation of land-use change has certain limitations. Researchers argue, for example, that cultural-human ecology falls in explaining the social transformation processes that have an impact on the environment and in missing to consider the power dynamics that both facilitate and propel social struggles against traditional social categories [ 61 , 62 ]. Also, it does not take into consideration the class-based disparities that result in unequal choices for all members of a system, particularly those related to the environment. Additionally, the viewpoint of cultural-human ecology is “historical and does not account for the fact that decisions made in specific social systems and locational settings are the product of environmental transformations.” [ 63 ]. In the study context, the drivers of land-use change and community perceptions of land-use change are explained using the theoretical paradigm discussed above.
3 Methods and materials
3.1 description of the study area.
This study (Fig. 1 ) was conducted in four Kebeles of Masha Woreda which share a common boundary with Shato forest (core area), Sheka zone, southwest Ethiopia. It extends 7° 45′ 0″ to 7° 52′ 30″ N and 35° 25′ 0″ to 35° 35′ 0″ E. Rugged topography conditions from gentle to very steep slopes and its elevation ranging from 1639 to 2376 m. The primary source of income for about 90% of the households in the subject area is a mixed agricultural and livestock system used for subsistence.
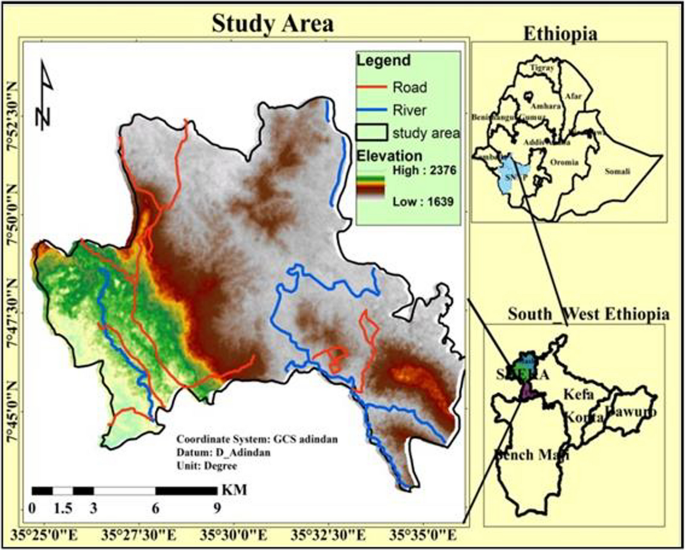
Map of the study area
3.2 Research approach and data sources
The study used an inductive approach and employed a mixed research design dominantly quantitative method supplemented by qualitative data (QUAN/Qual). And, investigated the socioeconomic drivers of LULC dynamics on the livelihoods of rural communities and the households cope up the consequence of land use/land cover change. The researcher analyzed data generated from satellite images, questionnaires, focus group discussions, participant observation, and document review. Responses by the respondents regarding the socioeconomic drivers of LULC dynamics and coping strategies were ranked using a Likert scale. The responses of respondents were coded and analyzed in SPSS V. 23. A thematic analysis was also conducted during FGDs and key informant interviews. It is led by a skilled facilitator and involves six to twelve participants who have shared characteristics relevant to the specific discussion topic [ 64 ].
Common characteristics may be related to a specific issue, occupation, age, socioeconomic class, place of residence, or experience adopting or not adopting a behavior that a project has advocated. Scholars discern recurring themes or trends within the conversations pertaining to a particular subject matter or research. This allows for a deeper understanding of the insights gained from FGDs. In order to uncover and interpret themes in qualitative data that was gathered, such as interviews, thematic analysis was also used. Data collected through FGDs and key informant interviews were qualitatively analyzed in alignment with the quantitative techniques.
3.3 Data sources
Primary and secondary data were the two major categories of data sources widely used in this study. Primary data instances were from questionnaires, focus group discussions and in-depth interviews with key informants, while secondary data were acquired from both published and unpublished materials.
3.4 Sampling procedure and sample size determination
Due to the nature of vulnerability to changes in cultural practice, livelihood system, and land use land cover change, Masha Woreda was selected purposively. This was confirmed during the field observation, and supported by a study of [ 65 , 66 ], Masha Woreda was exposed to all causes of land use land cover change ranging from forest conversion to agriculture by smallholder farmers to a large-scale coffee and tea plantations of their first kind in the country. Four kebeles ( Keja-chewaqa, Welo, Beto, and Yepho ) were selected as a sample of the study from a total of 19 kebeles, based on four reasons; its vicinity to huge investment (i.e. covering over 3000 ha) and number of Agri-investments available in the woreda (pilot survey result) , population size [ 67 ], indigenous cultural practices of forest conservation and management and high vulnerability for LULC dynamics during the last 30 years (1991–2021). Four FGDs were held having eight members each (inclusion in the group was based on role, gender, and age).
To select the sampled households, the total list of households living within the sampled kebeles was obtained from the local administration office. According to projected demographic estimates from the Sheka zone Finance and Economic Development Department (2022), the total population of sampled kebeles were 11,404 with household size of 3427. The sample size of the respondents was determined using the method developed by Yamane [ 68 ] and [ 69 ]. The formula was reasonably selected because, a population is finite and whose size can be determined [ 70 ].
The researcher employed a simple random sampling technique to reduce the risk of bias or inaccuracy within the data being collected [ 71 ].
where n is the sample size, N is the population size, e is the precision level (5–10%). The precision level in this research is 5% (0.05). The total sample size of the respondents was 358. The number of sample respondents from each kebele was selected by using proportional allocation formula [ 72 ]. By using the formula (Eq. 2 ), 119, 93, 76, and 70 respondents were selected for Welo, Keja-Chewaqa, Yepho and Beto Kebeles respectively.
ni is the sample size for Kebele I; Ni is the population size of sample Kebele I; N is the total population size of all sample kebele; n is the total sample size.
According to methodological flow chart (Fig. 2 ), landsat images of the study area from 1991, 2006, and 2021 as well as socioeconomic data from sample households were used in the research. After classifying the Landsat images, maps of land use and cover were generated and 358 sample households were selected randomly for the study. These sample respondents, along with four focus group participants, identified and ranked livelihood shocks, coping mechanisms, and socioeconomic drivers of LULCC. Lastly, a binary regression model was utilized to examine the relationship between socioeconomic factor causes LULC dynamics and the livelihoods of the rural community of the research area.
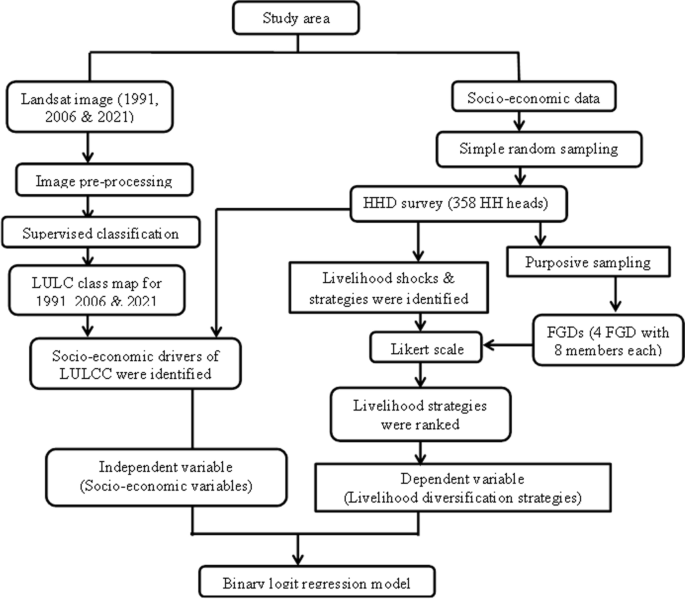
(Source: Author, 2023/24)
Methodological flow chart of drivers of LULCC and rural livelihoods
3.4.1 Method of data collection and analysis
Data for socioeconomic drivers of LULCC and livelihood coping strategies were collected through semi-structured questionnaires both open and close-ended. This was done to meet the requirements of the main drivers of land use and land cover change, socioeconomic data should be integrated [ 73 ].
The questionnaires were developed based on literature and expert discussions. By using the methods, we were able to employ cross-reference and confirm the answers, which ultimately increased the validity and consistency of the results. Questionnaires were distributed to 358 households identified through simple random sampling. Questionnaire data were processed and coded using the SPSS V.20 and subjected to further analysis. Likert scale was also used to measure (rank) the major socioeconomic drivers and shocks as a response to land use land cover dynamics. Pearson’s Chi-square analysis was used to determine associations between socioeconomic variables and diversification strategies.
A series of logistic regression was fitted to determine the effect of the predictors on the livelihood coping strategies, from which the estimated odds ratios (y) were generated. This allowed for the identification of variables that were independent predictors (socio-economic variable) for main coping strategies (dependent variable). Odds ratios were used to measure the magnitude of the strength of association between two binary data values. In this case, we quantitatively assessed the relative importance of several predictor variables. Content analysis was used to analyze qualitative data, whereby the discussions from the focus group were objectively and subjectively analyzed [ 74 ]. Based on similarities, codes are grouped into categories, which are then further examined and integrated to find overarching themes that reflect key ideas, patterns, or meanings in the data related with study objectives.
For land use land cover classification MSS Landsat imagers for the years 1991, 2006, and 2021 were used (selection of band resampling). After doing all image pre-processing steps, the researchers used maximum likelihood classification techniques in conjunction with supervised image classification using the Non-Parametric Rule. The primary benefit of supervised learning is that it enables the involvement of experts who can gather data or generate data output based on prior knowledge and who have a precise understanding of the classes present in the training data [ 75 ]. LULC classification and layout were done in ERDAS imagine V.2015 and Arc gis v10.8, respectively. Finally accuracy assessment was made to cross-check LULC classes prepared in software with spatial features found on the ground using ground truth data.
3.4.2 Measurement of variables
This section was aimed at collecting data to characterize rural livelihoods that are impacted by changes in land use and land cover in the study area. The questionnaires were pretested and revised before the actual interviews of the sampled households. The standardized questionnaire was written in English and was translated into Amharic languages and then Shekinonoo (local language) in which respondents were conversant during the interview. Respondents to the survey were not identified either by name or by location to maintain confidentiality (i.e., the researchers made respondents name and location anonymous). In each household, data on the socio-demographics, shocks, and livelihood strategies were collected using a questionnaire. The survey was administered in the period March–April and September 2023.
3.4.3 Model specification
Logistic regression has been widely used in several studies to examine the relationship between rural livelihood and forest resources dependency [ 76 , 77 , 78 ]. When analyzing dichotomous outcome variables, the binary logit has an advantage over the probit due to its great flexibility and ease of use, even though both produce equal parameter estimates [ 79 ]. Moreover, it changes the focus from forecasting probabilities inside the interval (0, 1) to predict the odds of an event occurring inside the real line [ 80 ]. Thus, a binary logit regression model was applied to show the relationship between independents (Socioeconomic variable that aggravates LULC dynamics) and outcome or dependent variables (livelihoods coping strategies of communities) of the biosphere reserve, a case of Shato forest (core area).
To identify the determinants of the rural household decision to employ various livelihood coping strategies to the changes in land use and land cover. It was assumed that a rational rural household could choose among the two mutually exclusive livelihood coping strategies that offer the maximum utility. For each of the coping options, the households were categorized as either equal to 1 if that household had opted for the livelihood coping option and 0 if that household had not opted for the livelihood coping option. Therefore, in this study, each indicator was taken as a binary outcome and used logistic regression to model several explanatory variables including (1) gender; (2) age; (3) education; (4) social network (yes;1, no;0). The age variables were categorized as follows: age: 0–29, 30–45 and above 45 [ 81 , 82 ]. And, education: illiterate (not able to read & write) and literate (able to read and write) [ 83 ]; the distance between the household and the forest in Km; 0–3; 4–8 and 9–12 and the distance between the household and market place in km; 0–3; 4–8, 9–12. The same standardizations were done for the rest continuous variables (total land owned, family size, and annual income).
In the logistic regression analyses, dummy variables were constructed for these categories with the last category used as a reference (Table 1 ). Before that, the response of the respondents was classified into dummy responses (Are you diversifying your livelihoods to cope up with shocks?), and the responses were, yes (1) and no (2). The respondents who said “yes” were further asked (i.e. which one is their major livelihood diversification strategy? The Chi-square test at a = 0.05 significance level was used to assess the goodness of fit of the models. Coping strategies such as selling fuel wood did nothing and other was not included in the logistic regression model because they were mentioned by few respondents, which made them insufficient for inclusion in the model.
The functional form of the binary logistic regression model used in the present study for estimation was specified following [ 84 ], as:
For ease of exposition, the probability that a given household will be apply livelihood diversification strategy to shocks (environmental vulnerability) is expressed as
where βo is an intercept and β 1 , β 2 …and β 11 are slopes of the equation in the model, and X 1 , X 2 … and X 11 are vectors of relevant household characteristics.
4 Results and discussion
4.1 results, 4.1.1 land use land cover dynamics.
Five major LULC categories, namely Forest, Rural settlement, settlement, wetland, and plantation were analyzed based on Landsat imagery for the years 1991, 2006, and 2021. The accuracy assessment is performed by using the random sampling method, a total of 155 points were selected from different LULC classes in ArcGIS v10.8.
The most terrible disaster that happened to natural forests in the study area was almost all deforestation occurred in and around the core area of a biosphere reserve. The following map (Fig. 4 ) shows clearly the severity of forest cover change in Shato core area. This study has quantified the dynamics of LU/LCC and its drivers in the Shato forest (core area) of the Sheka Biosphere Reserve southwestern Ethiopia. The result showed that about 20% of natural forest in the study area was converted to other LU/LC types while plantation and rural settlement increased by 2, 234.3 ha (10.2%) and 1289.6 ha (6.6%). Shato core area covers 5023.3 ha (25.5%) of the study area, and currently, only 3,541 ha (18%) are left. The rapid decline in forest resources occurred between 1991 and 2021 (Figs. 3 and 4 ).
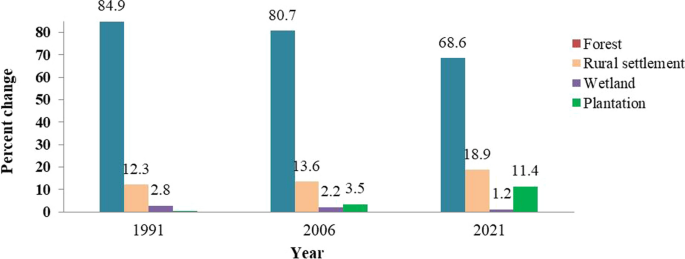
LULCC of the study area for 1991, 2006 & 2021. Time period from 1991 to 2021
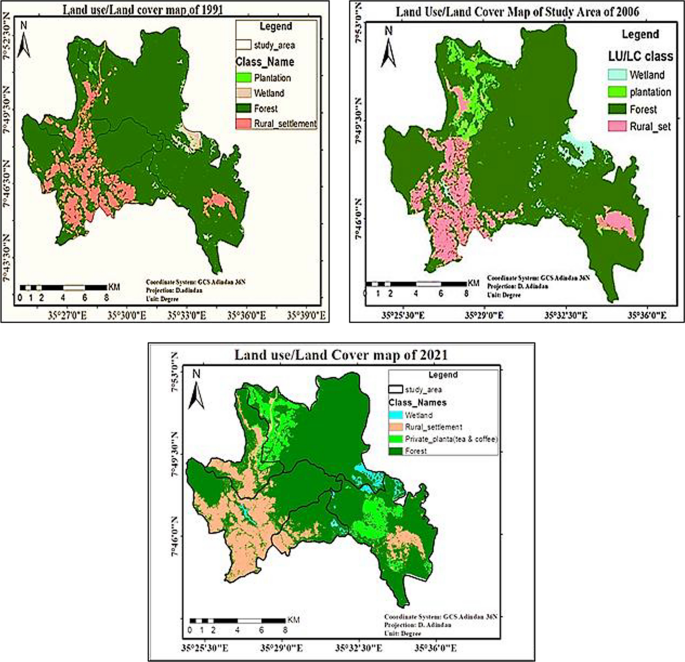
LULC map of the study area for the year 1991, 2006 & 2021. Time period from 1991 to 2021
The accuracy assessment of the finding was 74%, 81%, and 81.2%, respectively, for the images from 1991, 2006, and 2021, according to the data acquired using the random sample technique.
The producer's accuracy ranged from 60 to 91%, whereas the user's accuracy varied from 60 to 88% for all classifications. An accuracy value greater than 70% is considered to be acceptable and the Kappa value ranging from 0.40 to 0.85 represents good correspondence [ 85 ]. Because, of the quality of + ETM 1991 image and cloud coverage, the kappa coefficient result of the 1991 accuracy assessment is 0.74 (which falls in good), while MSS 2006 & 2021 accuracy assessment results are 0.81 and 0.812 respectively.
4.1.2 Demographic and socioeconomic characteristics of the respondents
The sample survey result indicates an average rural household in the study area consists of 358 households. Of the sample respondents, 12.3% (n = 44) of Household heads were female while males accounted for 87.7% (n = 314) of the sample respondents. Based on the responses of households, 69.8% (n = 250) can read and write.
However, 30.2% (n = 108) of the respondents never attended school. Almost, 78.2% (n = 280) respondents (heads of households) were between 30–45 years and the rest 21.8% (n = 78) household heads were between 0–29 and above 45 years. Most heads of households 89.7% (n = 321) reportedly use firewood for cooking and heating and the rest 10.3% (n = 37) use charcoal. The majority of the supply of fuelwood for heating and cooking was covered by females and children 80.4% (n = 288), self-15.4% (n = 55), and purchase 4.2% (n = 15). The role of heads of households 80.4% (n = 288) were farmers, 4.2% (n = 15) were engaged in fuelwood collection, 5.3% (n = 19) worked at private investment, 3.9% (n = 14) engaged in marketing products; 3.1% (n = 11) also involved in weeding & herding, and the rest 3.1% (n = 11) had involved in sowing and herding. Regarding mitigation measures for forest degradation, 59.8% (n = 214) responded that they apply customary forest management and conservation measures whereas 40.2% (n = 144) regularly plant trees. The survey result has also shown that major causes for deforestation in the study area were the need for fertile land 185(51.7%); the need for additional farmland 88 (24.6%) and illegal expansions of investment responded by 85 (23.7%) respectively.
4.1.3 Driving forces of land use/land cover dynamics
Land use and land cover change is a complex phenomenon that directly and indirectly is influenced by multiple socioeconomic and biophysical driving forces that operate over different scales. A checklist of possible drivers of LULC Changes was developed from the literature [ 86 ]. FGDs, analysis discovered that the main factors that aggravate LULCC (deforestation) were extensive agriculture, specifically the development of coffee and tea plantations in and around the core area; population growth and settlement expansion were the dominant drivers. Using the Relative Importance Index (RII) in Likert scale analysis from survey responses discovered that, from ten socioeconomic variables identified, and ranked by respondents, five major socioeconomic variables analyzed and interpreted as follows respectively: land size (0.86), family size (0.82), educational status (0.79), skills (0.70) and age (0.62) which directly or indirectly linked with the drivers of LULC dynamics in the study area (Table 2 ).
The above mean ranks were further elaborated by FGDs conducted in September 2023 at Welo and Yepho Kebele with communities; they responded that because of large-scale agricultural investment in the area, new villages were established in and around the core area, which is not recommended for agricultural activities. And, subsequently, the settlement expansion took place toward forest (induced by LSAI in the study area forces households to expand and increase the size of the land (86% of respondents listed as primary drivers). Followed by family size (82%), the increment of family size leads to the establishment of new settlements in and around forest areas, which leads to LULC change.
Educational status ranked as the third major driver with a mean rank of (0.79), majority of respondents replied that land (forest) is major source on which their livelihood depends. The more members of the household engage in formal education, the more they tend to diversify their livelihood strategy and the less the family relies on forest resources. Education can also influence the ability of households to cope with changes in land use and livelihood opportunities [ 87 , 88 ]. Skills related to forest product extraction with a particular emphasis on the widespread problem of illegal logging, beehive preparation, collecting fuelwood, and producing charcoal scored 0.70.
Besides, the area’s LULC dynamics (declining forest resources) can be attributed to several reasons, including age (ranked at 0.54), distance to market (0.53), and distance to the forest (rated at 0.50) (Table 2 ). Older age is also expected to reduce dependence on forests (that is, older adults spend less time and physical energy on forestry activities [ 89 ]. However, according to [ 83 ], the majority of household heads (respondents) in the study area found between 30–45 years (active age for agricultural activities).
For the first analysis of the relationship between dependent variables (livelihood coping strategies) and socioeconomic variables (age, educational status, family size, land size, distance from biosphere reserve, distance to market, social network, skills, land question, and annual income), correlation analysis was applied before modeling continued (Table 3 ).
From eleven socioeconomic variables, seven of them (Table 3 ) show significant correlations with dependent variables (i.e., educational status, land-holding size, family size, distance to market(km), skills, social network, and annual income) were analyzed to determine the relationship with livelihood coping strategies.
According to [ 90 ], Pearson correlation analysis can be performed to describe the relationship between the dependent variable and the independent socioeconomic variables before modeling analysis. Similarly, the Pearson correlation coefficient indicates positive and negative correlations. While a negative correlation suggests that the dependent variable decreases as the value of the independent socioeconomic variable rises, a positive correlation shows that the dependent variable (in this case, livelihood diversification) increases with the value of the independent socioeconomic variable.
The independent variable distance to market negatively correlated with the livelihood diversification strategies. The correlation result depicts that distance to market increases, the livelihood diversification strategy decreases. As furthest the market place; the less they diversify their livelihoods. According to our research, market distance has an impact on the livelihood possibilities for rural HHDs and is well substantiated by [ 91 ], but differs from the results of [ 92 ], market distance had a positive influence on the choice of non-farm activities. In our discussion with the FGDs, there is no transportation access between village and nearby market place, majority of items were transported by human power (bare foot) this impedes the frequents movement of HHDs to market place to sell their products and decrease their income source(decrease livelihood diversification). This was supported by [ 93 ], revealed that access to infrastructure would help households to diversify to more remunerative strategies. However, it was discovered that certain goods, like charcoal and chat, may have brought in more money when they were sold directly to buyers in distant marketplaces [ 94 ].
In the case of landholding size, as the number of landless respondent’s increases, the drivers of land use/land cover dynamics increase, while as the number of landless respondent’s decreases, the drivers of land use/land cover dynamics also decrease.
Shocks are an important source of the vulnerability of rural households and were reported for the previous 5 consecutive years during the study (according to ranked survey results and FGDs). In the present study, covariate shocks (exist for a short period) such as irregular rainfall (1.70), market price (1.53), low crop yield (1.28%), and poor infrastructure (1.24) were the most highly ranked shocks by respondents (Fig. 5 ).
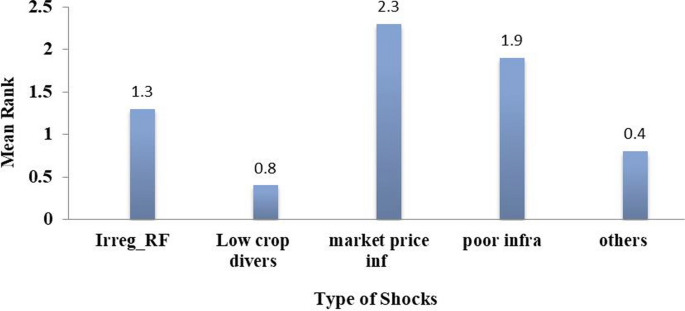
Types of shocks ranked by Community. NB: Irreg_RF: Irregular rainfall, Low crop divers: low crop diversification; market price inf: market price inflation, Poor infra: Poor infrastructure. Time period from 2019 to 2024
4.2.1 Livelihood coping strategies
Rural households in the study area engage in various livelihood coping strategies for short-term shocks. Virtually, all households rely on a combination of these livelihood coping strategies as a means of survival. Depending on the survey results obtained the prominent coping strategies were crop and income diversification. The rural communities from the study area were engaged in different livelihood coping strategies to counter the shocks they faced due to LULC changes during the study period.
The results have revealed that the most prominent coping strategies used in response to LULC change-related shocks included crop diversification (planting inset), income diversification (selling livestock, crop stock, forest products, borrowing from relatives), and others. Rural communities in the study area tend to employ the following major Livelihood coping strategies to cope up with shocks driven by socioeconomic-related LULC dynamics. Crop diversification ranks first with 0.516 followed by income diversification with a mean value of 0.41 and the rest of the respondents (0.07) did nothing in response to shocks induced by LULC dynamics.
The independent variables that explain the diversification of rural families to irregular shocks (vulnerability) were family size, age, educational status, land size, social network, distance to market, distance to forest, skills, source of income, land acquisitions, and annual income (Table 4 ). Concerning the tendency of respondents to choose a diversity of income sources, the influence of gender was significant (p\0.05).
About 29% of female respondents ranked agriculture as the priority source of income compared to their male counterparts (71%). The results further revealed that livestock rearing is an important source of income for men (71%) and less important for women (29%). In addition, 63% of women respondents considered off-farm activities as an important source of income compared to their male counterparts (37%). The dominant off-farm activities in the study area include selling charcoal & firewood, collecting honey, preparing and selling beehives, collecting traditional medicine, selling poles…etc.
Table 5 displays the correlation between the predictor variables and the outcome as well as the regression coefficients (B), Wald statistics (for assessing statistical significance), and the most significant odds ratios (Exp (B) for each variable category. The result for educational status is very significant at (Wald = 5.696, df = 2, p < 0 0.005), The variable family size has an Exp (b) of 0.621 and exp (b) of 0.621 times more likely than a households with no family. People with a large family size have a 62.1% higher chance of diversifying their livelihood to participate in LULC dynamics than households without a family.
With more family members, households may have the workforce needed to pursue diverse income-generating activities simultaneously, such as farming, off-farm employment, or small-scale businesses [ 95 ].
B (Beta) is the expected change in log chances for a unit change in the ratio between the odds (Table 5 ). The regression model considers the response of the survey result (i.e. dummy) “Is household apply livelihood coping strategy for covariates (shocks) caused by LULC dynamics in your study area?” The outcome variable of the model is categorical and takes a value equal to 1 if a rural household uses a livelihood diversification strategy, 0 if not, and is included in the model (Table 5 ). Compared to households with no education (illiterate), households having an education are significantly more likely to use crop diversification and income diversification. The output of the logistic regression model revealed that after adjusting for the effect of the factors, there is a significant influence of education and coping strategies (p\0.01) and was associated with high uncertainty (y [1]).
The age of the head of the household showed no significant influence when choosing a coping strategy (p[0.5) and had a negative impact on livelihood diversification. Compared to households with a market distance of above 3 km, households with a distance of below 3 are more likely to use both crop and income diversification.
4.2.2 Discussion
Rural communities in the study area have traditional practices and cultural ties to forests that are integral to their way of life. Changes in forest cover disrupted these practices, leading to a loss of cultural identity and traditional knowledge, which can have a profound impact on the livelihoods and well-being of local communities. A decrease in forest cover can lead to a reduction in the availability of these resources, affecting the livelihoods of communities that depend on them for their income and sustenance [ 96 ]. The remote sensing, rural household interview, and FGD results confirmed that land use and land cover changes have been ongoing during the study period, but reached a noticeable peak. The magnitude of the changes in land use and land cover reported here is likely to be influenced by the highly heterogeneous, mosaic, and complex spatio-temporal characteristics of land use and land cover of the study area.
LULC analysis results showed that about 308.29 ha (56.7%) of wetland and 3215.6 ha (19.6%) of natural forest were converted to other land use types in the last 30 years. Plantation and rural settlement increased by 2234.3 ha (10.2%) and 1289.6 ha (6.6%) respectively from 1991–2021(Fig. 4 ). While these responses do not identify specific changes in the day-to-day activities of focus group members, they point to changes in their livelihood coping strategies among their households as a result of the changes in land use and land cover. One of the female respondents said ‘‘If my community has no availability of natural resources, I will need to take up other companies to ensure my children's food and education”. The perceived expansion of forest land also confirms the findings from the analysis of satellite images. A set of predictor variables and the extent of rural household livelihood diversification were compared using the binary logistic regression model (Table 3 ). It was selected because it can be employed with mixed continuous, discrete, and dichotomous variables [ 97 ]. Rural household livelihood diversification status is a dichotomous outcome variable of the binary logistic regression model used in this analysis. It is assigned a value of 1 if a rural household has diversified its livelihood and 0 otherwise.
The likelihood ratio tests (Table 5 ) of this study measure how the log-likelihood improves if a predictor is added as an explanatory variable. Of the total 11 variables included in the study, six were significant at 5% and 10%. These results reveal that family size, education, the distance to the market in kilometers, total land, social network, and household skills are all six strongly significantly contributing to the explanation of variation in livelihood diversification strategies (**). Age is weakly significantly contributing to the explanation of the variation in livelihood diversification strategies (*).
Distance to the forest, land acquisitions, annual income, and source of income do not significantly contribute to the explanation of the variation in livelihood diversification strategies. The significant variables were education (p = 0.014, at 5% significant), family size (p = 0.035, at 5%, significant), distance from the market place in km (p = 0.025 at 5%), total land owned (p = 0.054, at 10% significant), skills (p = 0.049, 5% significant) and social network (P = 0.04, 5% significant) (Table 5 ). Even if land size and distance from the market were significant in the regression model, both have a negative relationship with outcome (dependent variables).
In this analysis, land size was found to have a negative impact on rural household livelihood diversification. Its influence on livelihood diversification was statistically significant at p < 0.05 probability level. In relation to rural households with smaller land sizes, the negative coefficient shows that rural families with relatively larger land sizes are less likely to diversify their livelihood options. Assuming all other factors remain unchanged, an increase of one unit in the land-holding sizes of rural households results in 0.841 reductions in the odds ratio favoring the diversification of rural livelihood options. The probability of a rural household pursuing varied livelihood strategies decreases as the amount of their land holding grows. This is because households with relatively larger land holdings are more willing to attempt new ventures such as extra farming [ 97 , 98 ]. This finding is in agreement with that of [ 38 , 98 , 99 , 100 ], because households cannot sustain themselves solely from agricultural produce on a smaller plot of cultivated land, they must engage in additional non-farm income-generating ventures. Diversification reduces risk by distributing it among several crops or enterprises, but it also creates uncertainty. Due to their lower risks, smaller landowners might be more open to trying out new crops or pursuits [ 101 ]. Furthermore, rather than pursuing diversification of their means of subsistence, rural families with larger land holdings are forced to pursue extensification of agriculture [ 102 ].
The variable educational status has an exp (b) of 1.72 times more likely than an uneducated person. Education people have a 72% higher chance of choosing a livelihood diversification strategy than those illiterate (income diversification). Education is regarded as one of the most important contributors to more remunerative salaried and skilled employment in rural Africa [ 103 , 104 ]. Therefore, education opens up the potential for better-paying jobs that demand formal education. According to our study, regular employment prospects that provide a steady monthly income were not regarded as major sources of livelihood. Based on the logistic regression results, the odds ratio increased for education level. This is so that people can learn how to cope and diversify their sources of income to live better lives. One explanation for this might be that the sample households' average level of education is insufficient to qualify them for formal employment. This is consistent with the findings by [ 91 ] for all of sub-Saharan Africa.
The study revealed a statistically significant and inverse relationship between the distance traveled to reach the closest marketing area and the diversification of livelihood strategies, with a significance level of less than 5%. This indicates a rural family’s chances of diversifying livelihood decreases with distance from the marketing area increase. All other variables being equal, for every one unit increase in market distance, the odds ratio in favor of diversifying rural livelihoods decreases by a factor of 0.331. The study’s findings are consistent with those of [ 36 , 105 ], who revealed that the distance traveled to reach the marketing place had an adverse impact on the state of rural households’ ability to diversify their livelihoods.
However, Age, distance from the nearest biosphere reserve, land acquisitions, and major source of income were not significant in explaining those dependent variables. Moreover, results shown in (Table 5 ) also illustrate that the independent variables of the study have a significant association with livelihood strategies. Similarly, 0.75 Pseudo R2 was formed by the model; moreover, 75% correct prediction percentage signifies the rationality of the study model’s (logistic regression model) descriptive power. The likelihood ratio test (Table 5 ), reveals that the model with explanatory variables is significantly better able to predict the variation in livelihood diversification strategy than the model without explanatory variables (intercept only, Chi-square (11 = 17.69, p = 0.049). The insignificant Pearson’s Goodness-of-Fit test means that predicted probabilities are in line with the observed probabilities, as the binomial distribution predicts. This implies a good model fit and the result of Goodness-of-Fit model of this study was good. The Hosmer and Lemeshow test statistic indicates a poor fit if the significance value is less than 0.05 [ 106 ]. In this test, the Hosmer and Lemeshow model has a good fit, as the chi-square value is 9.2, p = 0.31, which is greater than 0.05. The model states there is a relationship between socio-economic variables and livelihood diversification strategies. LULC dynamics in the study area have created socioeconomic impacts on rural livelihoods and the community tends to diversify their livelihoods to overcome short-term shocks.
In order to maximize benefits and minimize negative effects, the study result is expected to facilitate formulation of better policies, programs and intervention mechanisms for sustainable management of natural resource and sustainable livelihood options in the study area. It helps to bridge the knowledge gaps by creating awareness on the concerned to prioritize the issue of Shato forest (core area), Sheka Biosphere Reserve for intervention planning. Thus, this study undoubtedly viable to serve as source of information for further research activities wider both in scope and depth.
5 Conclusions and recommendations
Land is the major natural resource that economic, social, infrastructure, and other human activities are undertaken. Hence, changes in land use/land cover have occurred at all times in the past, are presently ongoing, and are likely to continue in the future. However, changes in the condition and composition of land use/land cover affect the livelihood of rural communities directly or indirectly. The Sheka Zone has greater potential for Large-scale agricultural investment (LSAI) and has attracted many LSAIs such as the East African Tea Plantation farm project and the Haile and Alem coffee plantation. In total, both companies have received above 5000 hectares of land in and around Shato forest of Sheka Biosphere Reserve. In Ethiopia, especially in the Southwest Ethiopia regional state, the impact of large scale agri-investment on the improvement of local people's livelihoods has not received the attention it deserves due to a lack of reliable data. Hence, the majority of the local community in the study area depends on small-scale and family agriculture for survival.
LULC analysis results showed that decrease in wetland and natural forests, and an increase in LSAI (Plantation) and rural settlement from the year 1991–2021. LSAI in general and LSAI-induced rural settlement expansions, in particular, were the trigger factors for the land use land cover dynamics in the study area. Additionally, the livelihoods of the locals have been significantly influenced by LULC dynamics, which has forced rural communities to search for various diversification options. The FGDs and survey questions responses reveal that LULC dynamics lead to the occurrence of covariate shocks (existing for a short period); such as irregular rainfall, market price, low crop yield, and poor infrastructure were the most highly ranked shocks by respondents. The socioeconomic drivers of land use and land cover dynamics in the study area were also identified by this study; the most prevalent ones were an expansion of land owned by household, a lack of formal education, and an increase in family sizes, and skills.
The binary logistic regression model examined the relationship between the livelihood diversification strategy (dependent) and the socioeconomic (independent) variables. As demonstrated by the likelihood ratio test, the model with explanatory variables considerably outperforms the model without explanatory factors in predicting the variation in livelihood diversification strategy. The model showed that the educational status of the household head, land size in the household, family size, social network, distance traveled to arrive at the nearest marketing area, and skills were among the critical factors determining rural livelihood diversification. Policymakers and stakeholders should consider these variables while designing intervention mechanisms. Only a few variables were examined in this study due to financial and time constraints; other factors, such as agricultural income, on-farm income, and off-farm income, should be taken into account in future research. In order to promote equitable development and livelihood diversification strategies, sustainable land management techniques that put the interests and rights of rural communities need to be taken as crucial agenda. Future studies ought to focus on the interaction between the agricultural value chain and the LSAI, as well as the implications of the LSAI for gender. Future studies are advised to dealt on the functioning of the biotic and ecosystem of the biosphere reserve. Our analysis was restricted to the relationship between LULC dynamics and livelihoods of the rural community.
Data availability
Data is provided within the manuscript.
Russell R, Guerry AD, Balvanera P, Gould RK, Basurto X, Chan KM, et al. Humans and nature: How knowing and experiencing nature affect well-being. Annu Rev Environ Resour. 2013;38:473–502.
Article Google Scholar
Vasseur L, Horning D, Thornbush M, Cohen-Shacham E, Andrade A, Barrow E, et al. Complex problems and unchallenged solutions: bringing ecosystem governance to the forefront of the UN sustainable development goals. Ambio. 2017;46:731–42.
Yeung HW. Rethinking relational economic geography. Trans Inst Br Geogr. 2005;30(1):37–51.
Geist HJ, Lambin EF. Proximate causes and underlying driving forces of tropical deforestation: Tropical forests are disappearing as the result of many pressures, both local and regional, acting in various combinations in different geographical locations. Bioscience. 2002;52(2):143–50.
Lambin EF, Geist HJ, Lepers E. Dynamics of land-use and land-cover change in tropical regions. Annu Rev Environ Resour. 2003;28(1):205–41.
Maitima JM, Olson J, Mugatha S, Mugisha S, Mutie I. Land use changes, impacts and options for sustaining productivity and livelihoods in the basin of lake Victoria. J Sustain Dev Afr. 2010;12(3):1520–5509.
Google Scholar
Cao S. Impact of China’s large-scale ecological restoration program on the environment and society in arid and semiarid areas of China: achievements, problems, synthesis, and applications. Crit Rev Environ Sci Technol. 2011;41(4):317–35.
Article CAS Google Scholar
Neri M, Jameli D, Bernard E, Melo FP. Green versus green? Adverting potential conflicts between wind power generation and biodiversity conservation in Brazil. Perspect Ecol Conserv. 2019;17(3):131–5.
Gonçalves PHS, de Cunha Melo CVS, de Assis Andrade C, de Oliveira DVB, de Moura Brito Junior V, Rito KF, et al. Livelihood strategies and use of forest resources in a protected area in the Brazilian semiarid. Environ Dev Sustain. 2021;24:1–21.
Schwarz AM, Béné C, Bennett G, Boso D, Hilly Z, Paul C, et al. Vulnerability and resilience of remote rural communities to shocks and global changes: empirical analysis from Solomon Islands. Glob Environ Change. 2011;21(3):1128–40.
Sedano F, Mizu-Siampale A, Duncanson L, Liang M. Influence of charcoal production on forest degradation in Zambia: a remote sensing perspective. Remote Sens. 2022;14(14):3352.
Scoones I. Livelihoods perspectives and rural development. In: Scoones I, editor. Critical perspectives in rural development studies. Routledge; 2013. p. 159–84.
Angelsen A, Wunder S. Exploring the forest-poverty link. CIFOR Occas Pap. 2003;40:1–20.
De Chazal J, Rounsevell MD. Land-use and climate change within assessments of biodiversity change: a review. Glob Environ Change. 2009;19(2):306–15.
Castillo CP, Jacobs-Crisioni C, Diogo V, Lavalle C. Modelling agricultural land abandonment in a fine spatial resolution multi-level land-use model: an application for the EU. Environ Model Softw. 2021;136: 104946.
Moges DM, Bhat HG. An insight into land use and land cover changes and their impacts in Rib watershed, north-western highland Ethiopia. Land Degrad Dev. 2018;29(10):3317–30.
Munthali M, Mustak S, Adeola A, Botai J, Singh S, Davis N. Modelling land use and land cover dynamics of Dedza district of Malawi using hybrid Cellular Automata and Markov model. Remote Sens Appl Soc Environ. 2020;17: 100276.
Seto KC, Kaufmann RK. Modeling the drivers of urban land use change in the Pearl River Delta, China: integrating remote sensing with socioeconomic data. Land Econ. 2003;79(1):106–21.
DeFries RS, Rudel T, Uriarte M, Hansen M. Deforestation driven by urban population growth and agricultural trade in the twenty-first century. Nat Geosci. 2010;3(3):178–81.
Houghton R. Carbon emissions and the drivers of deforestation and forest degradation in the tropics. Curr Opin Environ Sustain. 2012;4(6):597–603.
Alexander P, Rounsevell MD, Dislich C, Dodson JR, Engström K, Moran D. Drivers for global agricultural land use change: the nexus of diet, population, yield and bioenergy. Glob Environ Change. 2015;35:138–47.
Mottet A, Ladet S, Coqué N, Gibon A. Agricultural land-use change and its drivers in mountain landscapes: a case study in the Pyrenees. Agric Ecosyst Environ. 2006;114(2–4):296–310.
Wang J, Bretz M, Dewan MAA, Delavar MA. Machine learning in modelling land-use and land cover-change (LULCC): current status, challenges and prospects. Sci Total Environ. 2022;822: 153559.
Negassa MD, Mallie DT, Gemeda DO. Forest cover change detection using Geographic Information Systems and remote sensing techniques: a spatio-temporal study on Komto Protected forest priority area, East Wollega Zone, Ethiopia. Environ Syst Res. 2020;9:1–14.
Reid RS, Kruska RL, Muthui N, Taye A, Wotton S, Wilson CJ, et al. Land-use and land-cover dynamics in response to changes in climatic, biological and socio-political forces: the case of southwestern Ethiopia. Landsc Ecol. 2000;15:339–55.
Tolessa T, Dechassa C, Simane B, Alamerew B, Kidane M. Land use/land cover dynamics in response to various driving forces in Didessa sub-basin. Ethiopia GeoJournal. 2020;85:747–60.
Seyoum A. Economic value of afromontane natural forest in Sheka Zone, southwest Ethiopia. Forests of Sheka. MELCA Mahiber Afr Biodivers Netw. 2007;183–218.
Teketay D, Lemenih M, Bekele T, Yemshaw Y, Feleke S, Tadesse W, et al. Forest resources and challenges of sustainable forest management and conservation in Ethiopia. In: Degraded forests in Eastern Africa. Routledge; 2010. p. 31–75.
Berhanu A, Woldu Z, Demissew S, Melesse S. Temporal vegetation cover dynamics in Northwestern Ethiopia: status and trends. Ethiop J Biol Sci. 2019;18(2):123–43.
Zegeye H. Major drivers and consequences of deforestation in Ethiopia: implications for forest conservation. Asian J Sci Technol. 2017;8(8):5166–75.
Mesfin D, Simane B, Belay A, Recha JW, Taddese H. Woodland cover change in the Central Rift Valley of Ethiopia. Forests. 2020;11(9):916.
Wassie SB. Natural resource degradation tendencies in Ethiopia: a review. Environ Syst Res. 2020;9:1–29.
Alobo LS. Rural livelihood diversification in sub-Saharan Africa: a literature review. J Dev Stud. 2015;51(9):1125–38.
Ellis F. Rural livelihoods and diversity in developing countries. Oxford University Press; 2000.
Book Google Scholar
Babatunde R, Olagunju F, Fakayode S, Adejobi A. Determinants of participation in off-farm employment among small-holder farming households in Kwara State. Nigeria Prod Agric Technol. 2010;6(2):1–14.
Kassie GW, Kim S, Fellizar FP Jr. Determinant factors of livelihood diversification: evidence from Ethiopia. Cogent Soc Sci. 2017;3(1):1369490.
Ellis F, Freeman HA. Rural livelihoods and poverty reduction strategies in four African countries. J Dev Stud. 2004;40(4):1–30.
Abera A, Yirgu T, Uncha A. Determinants of rural livelihood diversification strategies among Chewaka resettlers’ communities of Southwestern Ethiopia. Agric Food Secur. 2021;10(1):30.
Rubiyanto CW, Hirota I. A review on livelihood diversification: dynamics, measurements and case studies in Montane mainland Southeast Asia. Rev Agric Sci. 2021;9:128–42.
Mcelwee PD. Forest environmental income in Vietnam: household socioeconomic factors influencing forest use. Environ Conser. 2008;35(2):147–59.
Assan JK, Beyene FR. Livelihood impacts of environmental conservation programmes in the Amhara region of Ethiopia. J Sustain Dev. 2013;6(10):87.
Vedeld P, Angelsen A, Sjaastad E, Kobugabe Berg G. Counting on the environment: forest incomes and the rural poor. World Bank; 2004.
Rasmussen LV, Watkins C, Agrawal A. Forest contributions to livelihoods in changing agriculture-forest landscapes. For Policy Econ. 2017;84:1–8.
Wunder S, Angelsen A, Belcher B. Forests, livelihoods, and conservation: broadening the empirical base. World Dev. 2014;64:S1-11.
Kamwi JM, Chirwa PW, Manda SO, Graz PF, Kätsch C. Livelihoods, land use and land cover change in the Zambezi Region, Namibia. Popul Environ. 2015;37:207–30.
Guyalo AK, Alemu EA, Degaga DT. Impact of large-scale agricultural investments on the food security status of local community in Gambella region, Ethiopia. Agric Food Secur. 2022;11(1):43.
Matrix L. Land Matrix: Ethiopia. Ethiop Land Matrix. 2016.
Woldemariam T, Fetene M. Forests of Sheka: Ecological, social, legal and economic dimensions of recent land use/land cover changes, overview and synthesis. For Sheka Multidiscip Case Stud Impacts Land UseLand Cover Chang Melca-Mahiber Addis Ababa Viii 231 pp. 2007;1–21.
Berhanu Y, Dalle G, Sintayehu DW, Kelboro G, Nigussie A. Woody species dynamics in Sheka Forest Biosphere Reserve, Southwest Ethiopia. For Ecol Manag. 2022;519: 120313.
Ameneshewa W. Spatio-Temporal forest cover change detection using remote sensing and GIS techniques: in the case of Masha Woreda, Sheka Zone, SNNPRS of Ethiopia; 2015;
Bedru S. Land-use/land-cover changes in Andracha and Masha Woredas of Sheka Zone, SNNP Regional State. For Sheka Ecol Soc Leg Econ Dimens Recent Land-UseLand-Cover Chang Overv Synth Multidiscip Case Stud Impact Land-UseLand-Cover Chang South West Ethiop. 2007;21–56.
Mahiber M. Communal forest ownership: an option to address the underlying causes of deforestation and forest degradation in Ethiopia; 2008.
Girma HM, Hassan RM, Hertzler G. Forest conservation versus conversion under uncertain market and environmental forest benefits in Ethiopia: the case of Sheka forest. For Policy Econ. 2012;21:101–7.
Ameneshewa W, Kebede Y, Unbushe D, Legesse A. Correction: Trends of land use land cover dynamics of sheka biosphere reserve, a case of Shato Core Area, Southwest Ethiopia. PLoS ONE. 2023;18(12): e0296112.
Belay A. Sheka forest biosphere reserve beekeeping practices and characteristics of Schefflera abyssinica honey Ethiopia. Environ Dev Sustain. 2021;23:11818–36.
Fekadu A, Soromessa T, Warkineh DB. Role of forest provisioning services to local livelihoods: based on relative forest income (RFI) approach in southwest Ethiopia coffee forest. Environ Syst Res. 2021;10:1–15.
Galaz V, Olsson P, Hahn T, Folke C, Svedin U. The problem of fit among biophysical systems, environmental and resource regimes, and broader governance systems: insights and emerging challenges. 2008.
Steward JH. The concept and method of cultural ecology. Anthropol Theory Issues Epistemol. 2005;Sep 12:100–6.
Smith CA, Lazarus RS. Emotion and adaptation. Handb Personal Theory Res. 1990;21:609–37.
Briassoulis H. Analysis of land use change: theoretical and modeling approaches. Regional Research Institute, West Virginia University; 2020.
Campbell B. Beyond cultural models of the environment: linking subjectivities of dwelling and power. In: Culture and the environment in the Himalaya. Routledge; 2009. p. 204–21.
Campbell EK. Beyond anthropocentrism. J Hist Behav Sci. 1983;19(1):54–67.
Raphael John L, Hambati H, Ato AF. An intensity analysis of land-use and land-cover change in Karatu District, Tanzania: community perceptions and coping strategies. Afr Geogr Rev. 2014;33(2):150–73.
Scheelbeek PF, Hamza YA, Schellenberg J, Hill Z. Improving the use of focus group discussions in low income settings. BMC Med Res Methodol. 2020;20:1–10.
Girma A. Plant communities, species diversity, seedling bank and resprouting in Nandi forests, Kenya. 2012.
Taddesse YY. Mathematical modeling on assessing rate of deforestation in the Sheka Forest South West Ethiopia. Int J Sci Acad Res. 2022;2(2):1–7.
Genet A. Population growth and land use land cover change scenario in Ethiopia. Int J Environ Prot Policy. 2020;8(4):77–85.
Yamane T. Statistics: An introductory analysis 1973.
Nanjundeswaraswamy T, Divakar S. Determination of sample size and sampling methods in applied research. Proc Eng Sci. 2021;3(1):25–32.
Singh AS, Masuku MB. Sampling techniques & determination of sample size in applied statistics research: an overview. Int J Econ Commer Manag. 2014;2(11):1–22.
Burns TJ, Kick EL, Davis BL. Theorizing and rethinking linkages between the natural environment and the modern world-system: deforestation in the late 20th century. J World-Syst Res. 2003. https://doi.org/10.5195/jwsr.2003.237 .
Nguyen T, Vojnovic M. Weighted proportional allocation. ACM SIGMETRICS Perform Eval Rev. 2011;39(1):133–44.
Hailu A, Mammo S, Kidane M. Dynamics of land use, land cover change trend and its drivers in Jimma Geneti District, Western Ethiopia. Land Use Policy. 2020;99: 105011.
Hsieh HF, Shannon SE. Three approaches to qualitative content analysis. Qual Health Res. 2005;15(9):1277–88.
Richards JA. Supervised classification techniques. In: Remote sensing digital image analysis. Springer; 2013. p. 247–318.
Chapter Google Scholar
Shakibaie A, Firozjaie EL, Firozjaie HL, Khatami S. Estimated value of forest conservation of Gazu forest in Mazandaran. Am-Eur J Agric Environ Sci. 2013;13(7):1007–11.
Hussain J, Zhou K, Akbar M, Raza G, Ali S, Hussain A, et al. Dependence of rural livelihoods on forest resources in Naltar Valley, a dry temperate mountainous region, Pakistan. Glob Ecol Conserv. 2019;20: e00765.
Ali N, Hu X, Hussain J. The dependency of rural livelihood on forest resources in Northern Pakistan’s Chaprote Valley. Glob Ecol Conserv. 2020;22: e01001.
Menard SW. Logistic regression: from introductory to advanced concepts and applications. Sage; 2010.
Hosmer DW Jr, Lemeshow S, May S. Applied survival analysis: regression modeling of time-to-event data, vol. 618. John Wiley & Sons; 2008.
Yeboah FK, Jayne TS, Muyanga M, Chamberlin J. Youth access to land, migration and employment opportunities: evidence from sub-Saharan Africa. 2019.
Yigezu WG. The challenges and prospects of Ethiopian agriculture. Cogent Food Agric. 2021;7(1):1923619.
Gidey E, Dikinya O, Sebego R, Segosebe E, Zenebe A, Mussa S, et al. Land use and land cover change determinants in Raya Valley, Tigray, Northern Ethiopian Highlands. Agriculture. 2023;13(2):507.
Bekabil UT, Bedemo A. Dynamics of farmers’ participation in conservation agriculture: binary logistic regression analysis. Dynamics. 2015;13:74–83.
Congalton RG. A review of assessing the accuracy of classifications of remotely sensed data. Remote Sens Environ. 1991;37(1):35–46.
Lambin EF, Geist HJ. Land-use and land-cover change: local processes and global impacts. Springer Science & Business Media; 2008.
Paavola J. Livelihoods, vulnerability and adaptation to climate change in Morogoro, Tanzania. Environ Sci Policy. 2008;11(7):642–54.
Uberhuaga P, Smith-Hall C, Helles F. Forest income and dependency in lowland Bolivia. Environ Dev Sustain. 2012;14(1):3–23.
Lepetu J, Alavalapati J, Nair P. Forest dependency and its implication for protected areas management: a case study from Kasane Forest Reserve, Botswana. Int J Environ Res. 2009;3(4):525–36.
Chen P, Shi X. Dynamic evaluation of China’s ecological civilization construction based on target correlation degree and coupling coordination degree. Environ Impact Assess Rev. 2022;93: 106734.
Ellis F, Bahiigwa G. Livelihoods and rural poverty reduction in Uganda. World Dev. 2003;31(6):997–1013.
Kung JK, Fai LY. So what if there is income inequality? The distributive consequence of nonfarm employment in rural China. Econ Dev Cult Change. 2001;50(1):19–46.
Jiao X, Pouliot M, Walelign SZ. Livelihood strategies and dynamics in rural Cambodia. World Dev. 2017;97:266–78.
Teshager Abeje M, Tsunekawa A, Adgo E, Haregeweyn N, Nigussie Z, Ayalew Z, et al. Exploring drivers of livelihood diversification and its effect on adoption of sustainable land management practices in the Upper Blue Nile Basin, Ethiopia. Sustainability. 2019;11(10):2991.
Reardon T, Berdegué J, Escobar G. Rural nonfarm employment and incomes in Latin America: overview and policy implications. World Dev. 2001;29(3):395–409.
Vedeld P, Angelsen A, Bojö J, Sjaastad E, Berg GK. Forest environmental incomes and the rural poor. For Policy Econ. 2007;9(7):869–79.
Gecho Y, Ayele G, Lemma T, Alemu D. Rural household livelihood strategies: Options and determinants in the case of Wolaita Zone, Southern Ethiopia. Soc Sci. 2014;3(3):92–104.
Tamerat T. Livelihood resources and determinants in Tigray Region of Ethiopia. Int J Lean Think. 2016;7(2):57–66.
Adugna E. Livelihood strategies and food security in Wolayta, Southern Ethiopia: the case of Boloso Sore district. MSc Thesis Submitt Sch Grad Stud Haramaya Univ; 2008.
Yizengaw YS, Okoyo EN, Beyene F. Determinants of livelihood diversification strategies: the case of smallholder rural farm households in Debre Elias Woreda, East Gojjam Zone, Ethiopia. Afr J Agric Res. 2015;10(19):1998–2013.
Adnan KM, Ying L, Ayoub Z, Sarker SA, Menhas R, Chen F, et al. Risk management strategies to cope catastrophic risks in agriculture: the case of contract farming, diversification and precautionary savings. Agriculture. 2020;10(8):351.
Anshiso D, Shiferaw M. Determinants of rural livelihood diversification: The case of rural households in Lemmo district, Hadiyya Zone of Southern Ethiopia. J Econ Sustain Dev. 2016;7(5):32–9.
Barrett CB, Bezuneh M, Clay DC, Reardon T. Heterogeneous Contraints, Incentives, and Income Diversification Strategies in Rural Africa. 2001.
Belay Wondim G. Livelihood Diversification among pastoralists and its effect on poverty: the case of Amibara District, Zone Three of Afar National Regional State. 2017.
Debele B, Desta G. Livelihood diversification: strategies, determinants and challenges for pastoral and agro-pastoral communities of Bale Zone, Ethiopia. Int Rev Soc Sci Humanit. 2016;11(2):37–51.
Nattino G, Pennell ML, Lemeshow S. Assessing the goodness of fit of logistic regression models in large samples: a modification of the Hosmer-Lemeshow test. Biometrics. 2020;76(2):549–60.
Download references
Author information
Authors and affiliations.
Department of Geography and Environmental Studies, College of Social Science, Arbaminch University, Arbaminch, Ethiopia
Workaferahu Ameneshewa & Yechale Kebede
Department of Geography and Environmental Studies, College of Social Science, Dilla University, Dilla, Ethiopia
Abiyot Legesse
Department of Biology, College of Natural and Computational Science, Wolaita Sodo University, Sodo, Ethiopia
Dikaso Unbushe
You can also search for this author in PubMed Google Scholar
Contributions
The first author and corresponding author did analysis, map, graph, table preparation, edition, and overall write up. Co-authors supports with supervision and edition.
Corresponding author
Correspondence to Workaferahu Ameneshewa .
Ethics declarations
Ethics approval and consent to participate.
This study involved a questionnaire-based survey of households and the study protocol was conducted in accordance with the 1964 Helsinki Declaration and approved by Arbaminch University, research and extension office based on Helsinki guidelines. Informed consent was obtained from all individual participants included in the study.
Competing interests
The authors declare no competing interests.
Additional information
Publisher's note.
Springer Nature remains neutral with regard to jurisdictional claims in published maps and institutional affiliations.
Rights and permissions
Open Access This article is licensed under a Creative Commons Attribution-NonCommercial-NoDerivatives 4.0 International License, which permits any non-commercial use, sharing, distribution and reproduction in any medium or format, as long as you give appropriate credit to the original author(s) and the source, provide a link to the Creative Commons licence, and indicate if you modified the licensed material. You do not have permission under this licence to share adapted material derived from this article or parts of it. The images or other third party material in this article are included in the article’s Creative Commons licence, unless indicated otherwise in a credit line to the material. If material is not included in the article’s Creative Commons licence and your intended use is not permitted by statutory regulation or exceeds the permitted use, you will need to obtain permission directly from the copyright holder. To view a copy of this licence, visit http://creativecommons.org/licenses/by-nc-nd/4.0/ .
Reprints and permissions
About this article
Ameneshewa, W., Kebede, Y., Legesse, A. et al. Drivers of land use land cover change and livelihood coping strategies in Sheka biosphere reserve; a case of Shato forest, Southwest Ethiopia. Discov Sustain 5 , 208 (2024). https://doi.org/10.1007/s43621-024-00415-y
Download citation
Received : 09 June 2024
Accepted : 12 August 2024
Published : 21 August 2024
DOI : https://doi.org/10.1007/s43621-024-00415-y
Share this article
Anyone you share the following link with will be able to read this content:
Sorry, a shareable link is not currently available for this article.
Provided by the Springer Nature SharedIt content-sharing initiative
- Shato Forest
- Crop diversification
- Sheka biosphere
- Find a journal
- Publish with us
- Track your research
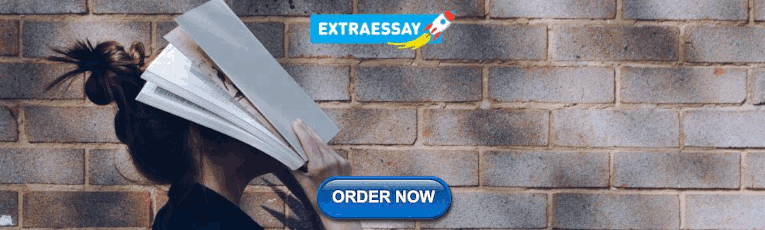
IMAGES
COMMENTS
The specific strategies for analysis in these stages centred on the work of Miles and Huberman ( 1994 ), which has been successfully used in case study research. The data were managed using NVivo software. Review methods: Literature examining qualitative data analysis was reviewed and strategies illustrated by the case study example provided ...
Case study protocol is a formal document capturing the entire set of procedures involved in the collection of empirical material . It extends direction to researchers for gathering evidences, empirical material analysis, and case study reporting . This section includes a step-by-step guide that is used for the execution of the actual study.
136 CASE STUDY RESEARCH data, and rival explanations. All four strategies underlie the analytic techniques to be described below. Without such strategies (or alternatives to them), case study analysis will proceed with difficulty. The remainder of this chapter covers the specific analytic techniques, to be
Humana's Automated Data Analysis Case Study. The key thing to note here is that the approach to creating a successful data program varies from industry to industry. Let's start with one to demonstrate the kind of value you can glean from these kinds of success stories. Humana has provided health insurance to Americans for over 50 years.
Researchers offer different levels to fulfill the research using a case study strategy. For example, Yin (1994) proposes four levels: design the case study, conduct the case study, ... - Cross-case analysis- Data reduction (open coding) Innovation in start-ups: ideas filling the void of resources and capabilities (Paradkar et al., 2015)
A case study is a research method that involves an in-depth examination and analysis of a particular phenomenon or case, such as an individual, organization, community, event, or situation. It is a qualitative research approach that aims to provide a detailed and comprehensive understanding of the case being studied.
These insights empower data-driven strategies, aiding in more effective resource allocation, product development, and marketing efforts. Ultimately, case studies bridge the gap between data science and business decision-making, enhancing a company's ability to thrive in a competitive landscape.
A case study is one of the most commonly used methodologies of social research. This article attempts to look into the various dimensions of a case study research strategy, the different epistemological strands which determine the particular case study type and approach adopted in the field, discusses the factors which can enhance the effectiveness of a case study research, and the debate ...
ve as a brief refresher to the case study method. As a refresher, the chapter does not fully cover all the options or nuances that you might encounter when customizing your own case study (refer to Yin, 2009a, to obtain a full rendition of the entire method).Besides discussing case study design, data collection, and analysis, the refresher addr.
Using case studies as a research strategy depends mainly on the nature of the research question and the researcher's access to the data. ... Data analysis. Analyzing case study research involves making sense of the rich, detailed data to answer the research question. This process can be challenging due to the volume and complexity of case study ...
In case-control studies we can calculate: • The odds ratio to measure association between disease and exposure: The odds of being exposed for a case is a/c The odds of being exposed for a control is b/d. The odds ratio of exposure for cases vs controls is ORExp = (a/c)/(b/d) = (a x d)/(b x c) Exposed. Non-exposed.
Sentiment analysis in action: 5 Real-world sentiment analysis case studies. 4. The data analysis process. In order to gain meaningful insights from data, data analysts will perform a rigorous step-by-step process.
A case study in data science is an in-depth analysis of a real-world problem using data-driven approaches. It involves collecting, cleaning, and analyzing data to extract insights and solve challenges, offering practical insights into how data science techniques can address complex issues across various industries.
propose an approach to the analysis of case study data by logically linking the data to a series of propositions and then interpreting the subsequent information. Like the Yin (1994) strategy, the Miles and Huberman (1994) process of analysis of case study data, although quite detailed, may still be insufficient to guide the novice researcher.
Sales Analytics. Improving their online sales by understanding user pre-purchase behaviour. New line of designs in the website contributed to 6% boost in sales. 60% increase in checkout to the payment page. Google Analytics. Enhanced Ecommerce. *. Marketing Automation. Marketing.
Having an effective Data Strategy allows the government to make better strategic and tactical decisions. "Especially in our military and government world, we need to make decisions quickly, and they need to be accurate," said McLean. Having an effective Data Strategy also helps organizations reap the benefits of data in the first place ...
Step 1: With Data Analytics Case Studies, Start by Making Assumptions. Hint: Start by making assumptions and thinking out loud. With this question, focus on coming up with a metric to support the hypothesis. If the question is unclear or if you think you need more information, be sure to ask.
In conclusion, data analytics case studies serve as invaluable tools for businesses seeking growth and innovation. By harnessing the power of data, organizations can make informed decisions ...
Please note that it is a minimum data required for this case, if we get more detailed data we can serve a more complex strategy. Methodology. The dataset in this case comes from kaggle. To solve ...
The scope of study is often determined by project budget constraints. Data Analysis: Analyzing Data - Case StudiesAccountability Modules. Data Analysis: Analyzing Data - Case Studies - 2Texas State Auditor's Office, Methodology Manual, rev. 5/95. Design the case study, taking care to select the most relevant event(s) for examination.
The balance of data-driven insights and human-centric storytelling is essential for creating compelling, effective brand strategies in a fast-moving market landscape. Subscribe To Newsletters BETA
Home | Agency for Healthcare Research and Quality
Jessica Nina Lester is an associate professor of Counseling and Educational Psychology at Indiana University. She received her PhD from the University of Tennessee, Knoxville. Her research strand focuses on the study and development of qualitative research methodologies and methods at a theoretical, conceptual, and technical level.
Note: Sources include all reports and peer-reviewed publications (*).The data source indicates the document that provided fatality rates used in the meta-analysis. a See Supporting Information for methods used to calculate fatality rates from raw data.; b Data presented for comparison purposes only. Data are not used in the meta-analysis due to unique study design that involves acoustic ...
Real-Time Data Collection & Quality Collect customer data, ensure data quality, and send it anywhere. Real-Time CDP & Predictive Insights Deliver relevant and trusted experiences based on real-time customer data. Data Management & Storage Own and access your most important enterprise asset, your customer data. Tealium for AI Power AI initiatives with consented, filtered, and enriched data in ...
Vaccination was the single most effective measure in mitigating the impact of the COVID-19 pandemic. Our study aims to quantify the impact of vaccination programmes during this initial year of vaccination by estimating the number of case fatalities avoided, having Sweden as a case study. Using Swedish data on age-specific reported incidence, vaccination uptake, and contact structures, along ...
This study will serve as foundational data for researching strategies to reduce personal car ownership through the promotion of public transportation and SM services. ... Ko, E.; Kim, H.; Lee, J. Survey data analysis on intention to use shared mobility services. J. Adv. Transp ... A Case Study of Seoul, Gyeonggi, and Incheon in South Korea ...
Background With the trend of digitalization, social activities among the older population are becoming more diverse as they increasingly adopt technology-based alternatives. To gain a comprehensive understanding of social activities, this study aimed to identify the patterns of digital and in-person social activities among community-dwelling older adults in South Korea, examine the associated ...
Eligible studies must provide sufficient data to calculate sensitivity and specificity, and those lacking such data or being case reports will be excluded. ... This systematic review and meta-analysis will be conducted in accordance with the PRISMA-DTA Statement and the Cochrane Handbook for Systematic Reviews of Diagnostic Test Accuracy ...
It is evident that the means of subsistence of the community have a significant impact on the management of natural resources. This study examined the socio-economic drivers of LULCC and assessed the impacts of such changes on rural livelihoods in Shato forest, southwest Ethiopia. To map the land use and land cover, supervised classifications were used. The data were collected from 358 ...