Machine Learning
- Machine Learning Tutorial
- Machine Learning Applications
- Life cycle of Machine Learning
- Install Anaconda & Python
- AI vs Machine Learning
- How to Get Datasets
- Data Preprocessing
- Supervised Machine Learning
- Unsupervised Machine Learning
- Supervised vs Unsupervised Learning
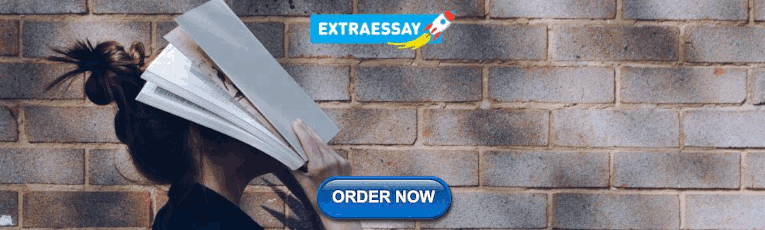
Supervised Learning
- Regression Analysis
- Linear Regression
- Simple Linear Regression
- Multiple Linear Regression
- Backward Elimination
- Polynomial Regression
Classification
- Classification Algorithm
- Logistic Regression
- K-NN Algorithm
- Support Vector Machine Algorithm
- Na�ve Bayes Classifier
Miscellaneous
- Classification vs Regression
- Linear Regression vs Logistic Regression
- Decision Tree Classification Algorithm
- Random Forest Algorithm
- Clustering in Machine Learning
- Hierarchical Clustering in Machine Learning
- K-Means Clustering Algorithm
- Apriori Algorithm in Machine Learning
- Association Rule Learning
- Confusion Matrix
- Cross-Validation
- Data Science vs Machine Learning
- Machine Learning vs Deep Learning
- Dimensionality Reduction Technique
- Machine Learning Algorithms
- Overfitting & Underfitting
- Principal Component Analysis
- What is P-Value
- Regularization in Machine Learning
- Examples of Machine Learning
- Semi-Supervised Learning
- Essential Mathematics for Machine Learning
- Overfitting in Machine Learning
- Types of Encoding Techniques
- Feature Selection Techniques in Machine Learning
- Bias and Variance in Machine Learning
- Machine Learning Tools
- Prerequisites for Machine Learning
- Gradient Descent in Machine Learning
- Machine Learning Experts Salary in India
- Machine Learning Models
- Machine Learning Books
- Linear Algebra for Machine learning
- Types of Machine Learning
- Feature Engineering for Machine Learning
- Top 10 Machine Learning Courses in 2021
- Epoch in Machine Learning
- Machine Learning with Anomaly Detection
- What is Epoch
- Cost Function in Machine Learning
- Bayes Theorem in Machine learning
- Perceptron in Machine Learning
- Entropy in Machine Learning
- Issues in Machine Learning
- Precision and Recall in Machine Learning
- Genetic Algorithm in Machine Learning
- Normalization in Machine Learning
- Adversarial Machine Learning
- Basic Concepts in Machine Learning
- Machine Learning Techniques
- Demystifying Machine Learning
- Challenges of Machine Learning
- Model Parameter vs Hyperparameter
- Hyperparameters in Machine Learning
- Importance of Machine Learning
- Machine Learning and Cloud Computing
- Anti-Money Laundering using Machine Learning
- Data Science Vs. Machine Learning Vs. Big Data
- Popular Machine Learning Platforms
- Deep learning vs. Machine learning vs. Artificial Intelligence
- Machine Learning Application in Defense/Military
- Machine Learning Applications in Media
- How can Machine Learning be used with Blockchain
- Prerequisites to Learn Artificial Intelligence and Machine Learning
- List of Machine Learning Companies in India
- Mathematics Courses for Machine Learning
- Probability and Statistics Books for Machine Learning
- Risks of Machine Learning
- Best Laptops for Machine Learning
- Machine Learning in Finance
- Lead Generation using Machine Learning
- Machine Learning and Data Science Certification
- What is Big Data and Machine Learning
- How to Save a Machine Learning Model
- Machine Learning Model with Teachable Machine
- Data Structure for Machine Learning
- Hypothesis in Machine Learning
- Gaussian Discriminant Analysis
- How Machine Learning is used by Famous Companies
- Introduction to Transfer Learning in ML
- LDA in Machine Learning
- Stacking in Machine Learning
- CNB Algorithm
- Deploy a Machine Learning Model using Streamlit Library
- Different Types of Methods for Clustering Algorithms in ML
- EM Algorithm in Machine Learning
- Machine Learning Pipeline
- Exploitation and Exploration in Machine Learning
- Machine Learning for Trading
- Data Augmentation: A Tactic to Improve the Performance of ML
- Difference Between Coding in Data Science and Machine Learning
- Data Labelling in Machine Learning
- Impact of Deep Learning on Personalization
- Major Business Applications of Convolutional Neural Network
- Mini Batch K-means clustering algorithm
- What is Multilevel Modelling
- GBM in Machine Learning
- Back Propagation through time - RNN
- Data Preparation in Machine Learning
- Predictive Maintenance Using Machine Learning
- NLP Analysis of Restaurant Reviews
- What are LSTM Networks
- Performance Metrics in Machine Learning
- Optimization using Hopfield Network
- Data Leakage in Machine Learning
- Generative Adversarial Network
- Machine Learning for Data Management
- Tensor Processing Units
- Train and Test datasets in Machine Learning
- How to Start with Machine Learning
- AUC-ROC Curve in Machine Learning
- Targeted Advertising using Machine Learning
- Top 10 Machine Learning Projects for Beginners using Python
- What is Human-in-the-Loop Machine Learning
- What is MLOps
- K-Medoids clustering-Theoretical Explanation
- Machine Learning Or Software Development: Which is Better
- How does Machine Learning Work
- How to learn Machine Learning from Scratch
- Is Machine Learning Hard
- Face Recognition in Machine Learning
- Product Recommendation Machine Learning
- Designing a Learning System in Machine Learning
- Recommendation System - Machine Learning
- Customer Segmentation Using Machine Learning
- Detecting Phishing Websites using Machine Learning
- Hidden Markov Model in Machine Learning
- Sales Prediction Using Machine Learning
- Crop Yield Prediction Using Machine Learning
- Data Visualization in Machine Learning
- ELM in Machine Learning
- Probabilistic Model in Machine Learning
- Survival Analysis Using Machine Learning
- Traffic Prediction Using Machine Learning
- t-SNE in Machine Learning
- BERT Language Model
- Federated Learning in Machine Learning
- Deep Parametric Continuous Convolutional Neural Network
- Depth-wise Separable Convolutional Neural Networks
- Need for Data Structures and Algorithms for Deep Learning and Machine Learning
- Geometric Model in Machine Learning
- Machine Learning Prediction
- Scalable Machine Learning
- Credit Score Prediction using Machine Learning
- Extrapolation in Machine Learning
- Image Forgery Detection Using Machine Learning
- Insurance Fraud Detection -Machine Learning
- NPS in Machine Learning
- Sequence Classification- Machine Learning
- EfficientNet: A Breakthrough in Machine Learning Model Architecture
- focl algorithm in Machine Learning
- Gini Index in Machine Learning
- Rainfall Prediction using ML
- Major Kernel Functions in Support Vector Machine
- Bagging Machine Learning
- BERT Applications
- Xtreme: MultiLingual Neural Network
- History of Machine Learning
- Multimodal Transformer Models
- Pruning in Machine Learning
- ResNet: Residual Network
- Gold Price Prediction using Machine Learning
- Dog Breed Classification using Transfer Learning
- Cataract Detection Using Machine Learning
- Placement Prediction Using Machine Learning
- Stock Market prediction using Machine Learning
- How to Check the Accuracy of your Machine Learning Model
- Interpretability and Explainability: Transformer Models
- Pattern Recognition in Machine Learning
- Zillow Home Value (Zestimate) Prediction in ML
- Fake News Detection Using Machine Learning
- Genetic Programming VS Machine Learning
- IPL Prediction Using Machine Learning
- Document Classification Using Machine Learning
- Heart Disease Prediction Using Machine Learning
- OCR with Machine Learning
- Air Pollution Prediction Using Machine Learning
- Customer Churn Prediction Using Machine Learning
- Earthquake Prediction Using Machine Learning
- Factor Analysis in Machine Learning
- Locally Weighted Linear Regression
- Machine Learning in Restaurant Industry
- Machine Learning Methods for Data-Driven Turbulence Modeling
- Predicting Student Dropout Using Machine Learning
- Image Processing Using Machine Learning
- Machine Learning in Banking
- Machine Learning in Education
- Machine Learning in Healthcare
- Machine Learning in Robotics
- Cloud Computing for Machine Learning and Cognitive Applications
- Credit Card Approval Using Machine Learning
- Liver Disease Prediction Using Machine Learning
- Majority Voting Algorithm in Machine Learning
- Data Augmentation in Machine Learning
- Decision Tree Classifier in Machine Learning
- Machine Learning in Design
- Digit Recognition Using Machine Learning
- Electricity Consumption Prediction Using Machine Learning
- Data Analytics vs. Machine Learning
- Injury Prediction in Competitive Runners Using Machine Learning
- Protein Folding Using Machine Learning
- Sentiment Analysis Using Machine Learning
- Network Intrusion Detection System Using Machine Learning
- Titanic- Machine Learning From Disaster
- Adenovirus Disease Prediction for Child Healthcare Using Machine Learning
- RNN for Sequence Labelling
- CatBoost in Machine Learning
- Cloud Computing Future Trends
- Histogram of Oriented Gradients (HOG)
- Implementation of neural network from scratch using NumPy
- Introduction to SIFT( Scale Invariant Feature Transform)
- Introduction to SURF (Speeded-Up Robust Features)
- Kubernetes - load balancing service
- Kubernetes Resource Model (KRM) and How to Make Use of YAML
- Are Robots Self-Learning
- Variational Autoencoders
- What are the Security and Privacy Risks of VR and AR
- What is a Large Language Model (LLM)
- Privacy-preserving Machine Learning
- Continual Learning in Machine Learning
- Quantum Machine Learning (QML)
- Split Single Column into Multiple Columns in PySpark DataFrame
- Why should we use AutoML
- Evaluation Metrics for Object Detection and Recognition
- Mean Intersection over Union (mIoU) for image segmentation
- YOLOV5-Object-Tracker-In-Videos
- Predicting Salaries with Machine Learning
- Fine-tuning Large Language Models
- AutoML Workflow
- Build Chatbot Webapp with LangChain
- Building a Machine Learning Classification Model with PyCaret
- Continuous Bag of Words (CBOW) in NLP
- Deploying Scrapy Spider on ScrapingHub
- Dynamic Pricing Using Machine Learning
- How to Improve Neural Networks by Using Complex Numbers
- Introduction to Bayesian Deep Learning
- LiDAR: Light Detection and Ranging for 3D Reconstruction
- Meta-Learning in Machine Learning
- Object Recognition in Medical Imaging
- Region-level Evaluation Metrics for Image Segmentation
- Sarcasm Detection Using Neural Networks
- SARSA Reinforcement Learning
- Single Shot MultiBox Detector (SSD) using Neural Networking Approach
- Stepwise Predictive Analysis in Machine Learning
- Vision Transformers vs. Convolutional Neural Networks
- V-Net in Image Segmentation
- Forest Cover Type Prediction Using Machine Learning
- Ada Boost algorithm in Machine Learning
- Continuous Value Prediction
- Bayesian Regression
- Least Angle Regression
- Linear Models
- DNN Machine Learning
- Why do we need to learn Machine Learning
- Roles in Machine Learning
- Clustering Performance Evaluation
- Spectral Co-clustering
- 7 Best R Packages for Machine Learning
- Calculate Kurtosis
- Machine Learning for Data Analysis
- What are the benefits of 5G Technology for the Internet of Things
- What is the Role of Machine Learning in IoT
- Human Activity Recognition Using Machine Learning
- Components of GIS
- Attention Mechanism
- Backpropagation- Algorithm
- VGGNet-16 Architecture
- Independent Component Analysis
- Nonnegative Matrix Factorization
- Sparse Inverse Covariance
- Accuracy, Precision, Recall or F1
- L1 and L2 Regularization
- Maximum Likelihood Estimation
- Kernel Principal Component Analysis (KPCA)
- Latent Semantic Analysis
- Overview of outlier detection methods
- Robust Covariance Estimation
- Spectral Bi-Clustering
- Drift in Machine Learning
- Credit Card Fraud Detection Using Machine Learning
- KL-Divergence
- Transformers Architecture
- Novelty Detection with Local Outlier Factor
- Novelty Detection
- Introduction to Bayesian Linear Regression
- Firefly Algorithm
- Keras: Attention and Seq2Seq
- A Guide Towards a Successful Machine Learning Project
- ACF and PCF
- Bayesian Hyperparameter Optimization for Machine Learning
- Random Forest Hyperparameter tuning in python
- Simulated Annealing
- Top Benefits of Machine Learning in FinTech
- Weight Initialisation
- Density Estimation
- Overlay Network
- Micro, Macro Weighted Averages of F1 Score
- Assumptions of Linear Regression
- Evaluation Metrics for Clustering Algorithms
- Frog Leap Algorithm
- Isolation Forest
- McNemar Test
- Stochastic Optimization
- Geomagnetic Field Using Machine Learning
- Image Generation Using Machine Learning
- Confidence Intervals
- Facebook Prophet
- Understanding Optimization Algorithms in Machine Learning
- What Are Probabilistic Models in Machine Learning
- How to choose the best Linear Regression model
- How to Remove Non-Stationarity From Time Series
- AutoEncoders
- Cat Classification Using Machine Learning
- AIC and BIC
- Inception Model
- Architecture of Machine Learning
- Business Intelligence Vs Machine Learning
- Guide to Cluster Analysis: Applications, Best Practices
- Linear Regression using Gradient Descent
- Text Clustering with K-Means
- The Significance and Applications of Covariance Matrix
- Stationarity Tests in Time Series
- Graph Machine Learning
- Introduction to XGBoost Algorithm in Machine Learning
- Bahdanau Attention
- Greedy Layer Wise Pre-Training
- OneVsRestClassifier
- Best Program for Machine Learning
- Deep Boltzmann machines (DBMs) in machine learning
- Find Patterns in Data Using Machine Learning
- Generalized Linear Models
- How to Implement Gradient Descent Optimization from Scratch
- Interpreting Correlation Coefficients
- Image Captioning Using Machine Learning
- fit() vs predict() vs fit_predict() in Python scikit-learn
- CNN Filters
- Shannon Entropy
- Time Series -Exponential Smoothing
- AUC ROC Curve in Machine Learning
- Vector Norms in Machine Learning
- Swarm Intelligence
- L1 and L2 Regularization Methods in Machine Learning
- ML Approaches for Time Series
- MSE and Bias-Variance Decomposition
- Simple Exponential Smoothing
- How to Optimise Machine Learning Model
- Multiclass logistic regression from scratch
- Lightbm Multilabel Classification
- Monte Carlo Methods
- What is Inverse Reinforcement learning
- Content-Based Recommender System
- Context-Awareness Recommender System
- Predicting Flights Using Machine Learning
- NTLK Corpus
- Traditional Feature Engineering Models
- Concept Drift and Model Decay in Machine Learning
- Hierarchical Reinforcement Learning
- What is Feature Scaling and Why is it Important in Machine Learning
- Difference between Statistical Model and Machine Learning
- Introduction to Ranking Algorithms in Machine Learning
- Multicollinearity: Causes, Effects and Detection
- Bag of N-Grams Model
- TF-IDF Model
Related Tutorials
- Tensorflow Tutorial
- PyTorch Tutorial
- Data Science Tutorial
- AI Tutorial
- NLP Tutorial
- Reinforcement Learning
Interview Questions
- Machine learning Interview
Latest Courses
We provides tutorials and interview questions of all technology like java tutorial, android, java frameworks
Contact info
G-13, 2nd Floor, Sec-3, Noida, UP, 201301, India
[email protected] .
Latest Post
PRIVACY POLICY
Online Compiler
Programmathically
Introduction to the hypothesis space and the bias-variance tradeoff in machine learning.

In this post, we introduce the hypothesis space and discuss how machine learning models function as hypotheses. Furthermore, we discuss the challenges encountered when choosing an appropriate machine learning hypothesis and building a model, such as overfitting, underfitting, and the bias-variance tradeoff.
The hypothesis space in machine learning is a set of all possible models that can be used to explain a data distribution given the limitations of that space. A linear hypothesis space is limited to the set of all linear models. If the data distribution follows a non-linear distribution, the linear hypothesis space might not contain a model that is appropriate for our needs.
To understand the concept of a hypothesis space, we need to learn to think of machine learning models as hypotheses.
The Machine Learning Model as Hypothesis
Generally speaking, a hypothesis is a potential explanation for an outcome or a phenomenon. In scientific inquiry, we test hypotheses to figure out how well and if at all they explain an outcome. In supervised machine learning, we are concerned with finding a function that maps from inputs to outputs.
But machine learning is inherently probabilistic. It is the art and science of deriving useful hypotheses from limited or incomplete data. Our functions are not axioms that explain the data perfectly, and for most real-life problems, we will never have all the data that exists. Accordingly, we will not find the one true function that perfectly describes the data. Instead, we find a function through training a model to map from known training input to known training output. This way, the model gradually approximates the assumed true function that describes the distribution of the data. So we treat our model as a hypothesis that needs to be tested as to how well it explains the output from a given input. We do this using a test or validation data set.
The Hypothesis Space
During the training process, we select a model from a hypothesis space that is subject to our constraints. For example, a linear hypothesis space only provides linear models. We can approximate data that follows a quadratic distribution using a model from the linear hypothesis space.
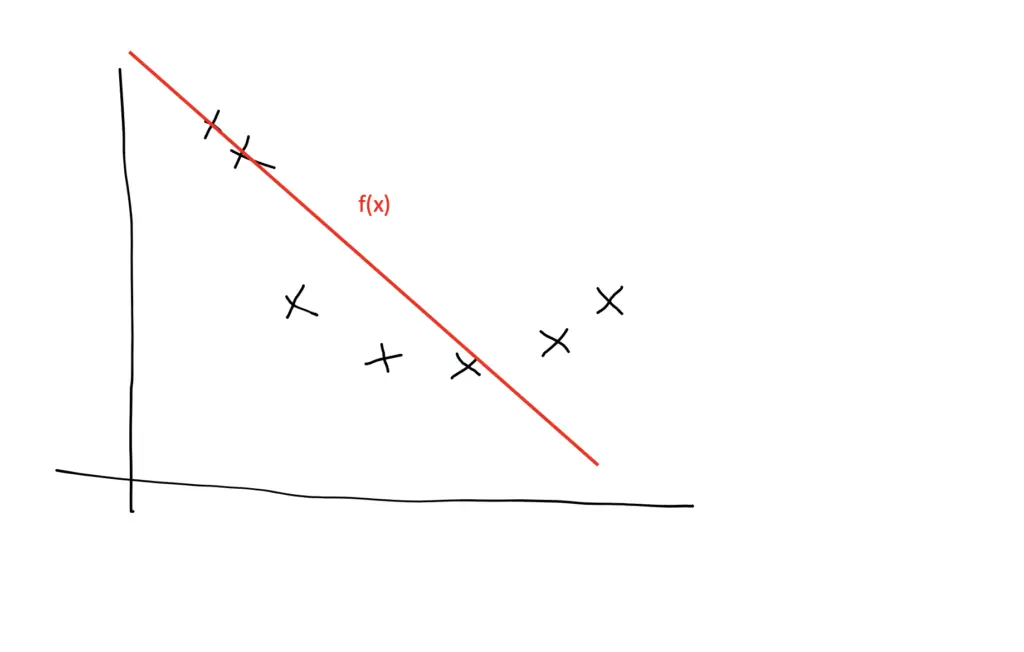
Of course, a linear model will never have the same predictive performance as a quadratic model, so we can adjust our hypothesis space to also include non-linear models or at least quadratic models.
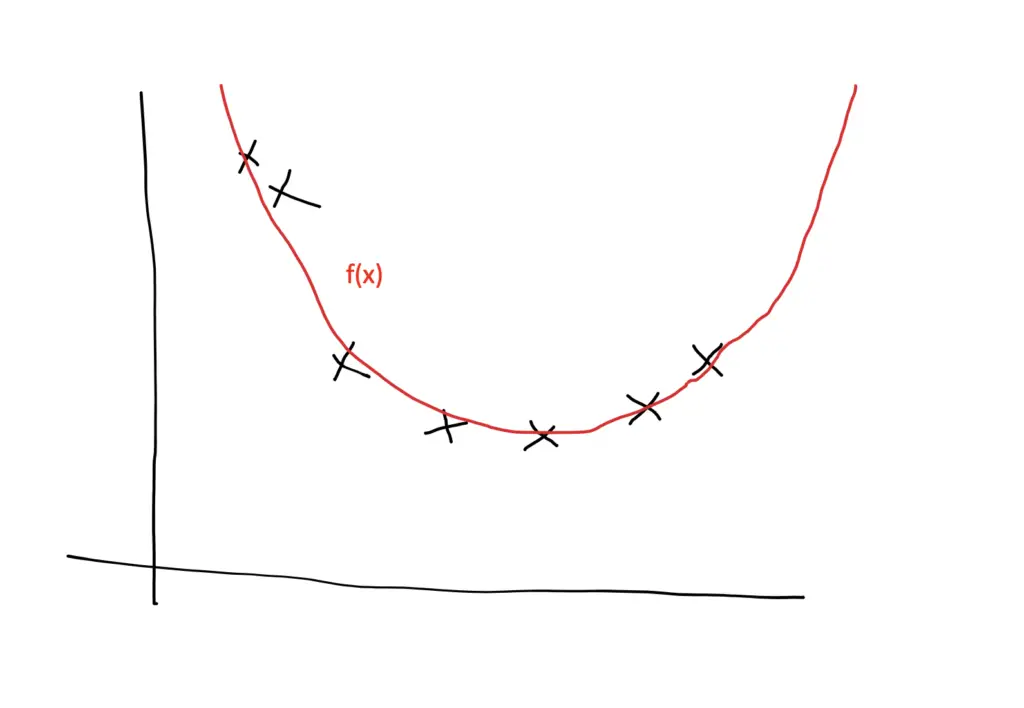
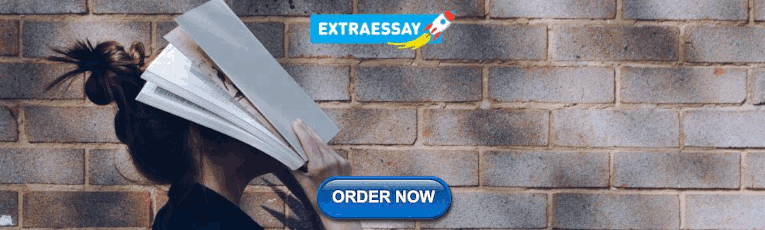
The Data Generating Process
The data generating process describes a hypothetical process subject to some assumptions that make training a machine learning model possible. We need to assume that the data points are from the same distribution but are independent of each other. When these requirements are met, we say that the data is independent and identically distributed (i.i.d.).
Independent and Identically Distributed Data
How can we assume that a model trained on a training set will perform better than random guessing on new and previously unseen data? First of all, the training data needs to come from the same or at least a similar problem domain. If you want your model to predict stock prices, you need to train the model on stock price data or data that is similarly distributed. It wouldn’t make much sense to train it on whether data. Statistically, this means the data is identically distributed . But if data comes from the same problem, training data and test data might not be completely independent. To account for this, we need to make sure that the test data is not in any way influenced by the training data or vice versa. If you use a subset of the training data as your test set, the test data evidently is not independent of the training data. Statistically, we say the data must be independently distributed .
Overfitting and Underfitting
We want to select a model from the hypothesis space that explains the data sufficiently well. During training, we can make a model so complex that it perfectly fits every data point in the training dataset. But ultimately, the model should be able to predict outputs on previously unseen input data. The ability to do well when predicting outputs on previously unseen data is also known as generalization. There is an inherent conflict between those two requirements.
If we make the model so complex that it fits every point in the training data, it will pick up lots of noise and random variation specific to the training set, which might obscure the larger underlying patterns. As a result, it will be more sensitive to random fluctuations in new data and predict values that are far off. A model with this problem is said to overfit the training data and, as a result, to suffer from high variance .
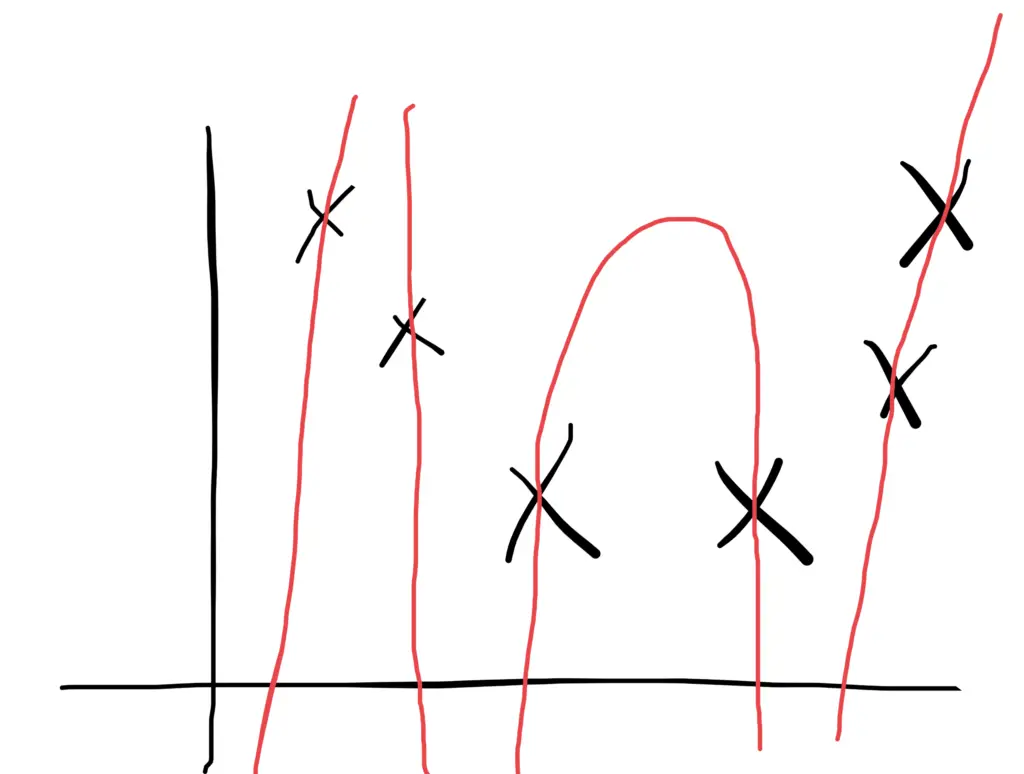
To avoid the problem of overfitting, we can choose a simpler model or use regularization techniques to prevent the model from fitting the training data too closely. The model should then be less influenced by random fluctuations and instead, focus on the larger underlying patterns in the data. The patterns are expected to be found in any dataset that comes from the same distribution. As a consequence, the model should generalize better on previously unseen data.
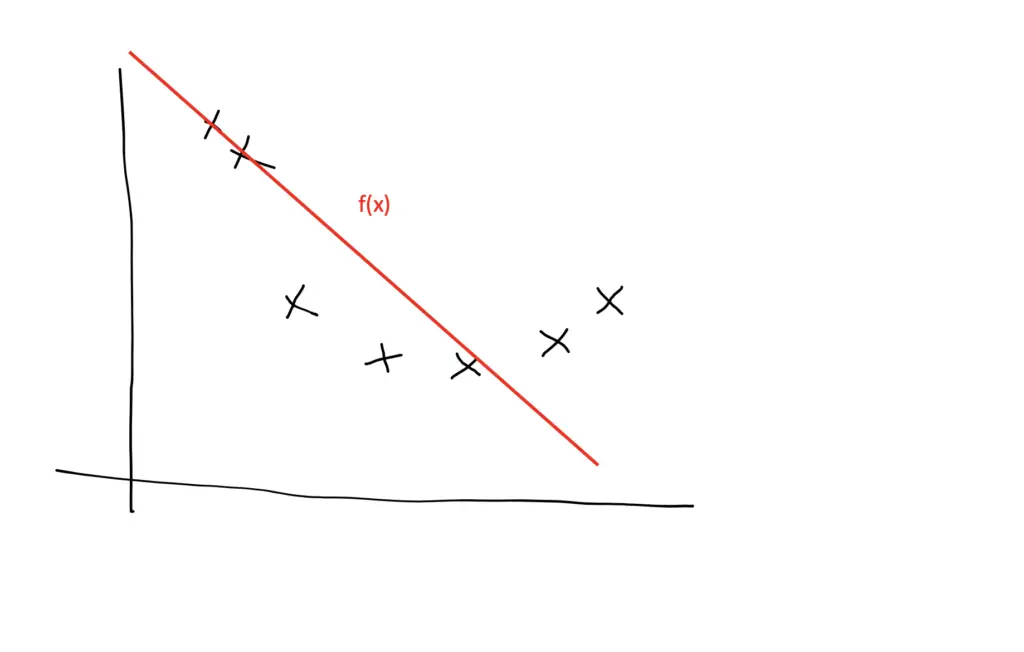
But if we go too far, the model might become too simple or too constrained by regularization to accurately capture the patterns in the data. Then the model will neither generalize well nor fit the training data well. A model that exhibits this problem is said to underfit the data and to suffer from high bias . If the model is too simple to accurately capture the patterns in the data (for example, when using a linear model to fit non-linear data), its capacity is insufficient for the task at hand.
When training neural networks, for example, we go through multiple iterations of training in which the model learns to fit an increasingly complex function to the data. Typically, your training error will decrease during learning the more complex your model becomes and the better it learns to fit the data. In the beginning, the training error decreases rapidly. In later training iterations, it typically flattens out as it approaches the minimum possible error. Your test or generalization error should initially decrease as well, albeit likely at a slower pace than the training error. As long as the generalization error is decreasing, your model is underfitting because it doesn’t live up to its full capacity. After a number of training iterations, the generalization error will likely reach a trough and start to increase again. Once it starts to increase, your model is overfitting, and it is time to stop training.
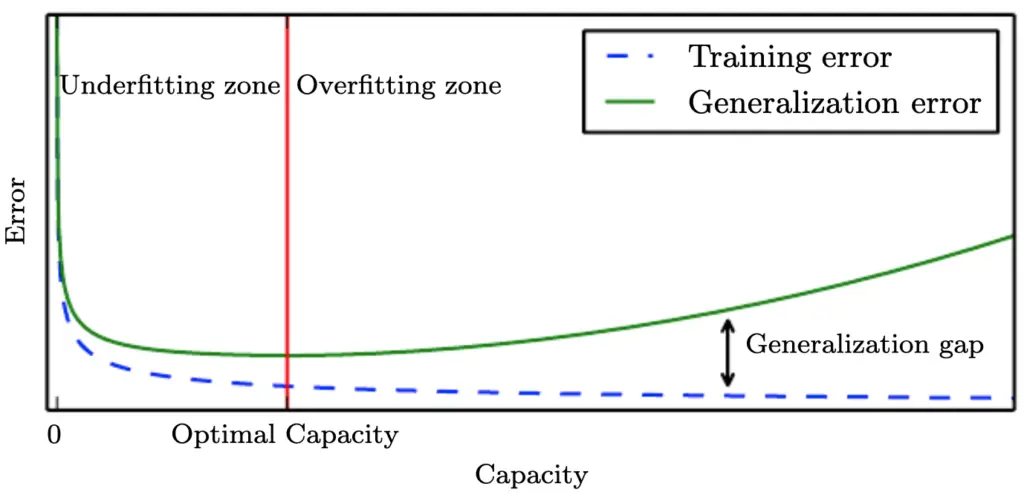
Ideally, you should stop training once your model reaches the lowest point of the generalization error. The gap between the minimum generalization error and no error at all is an irreducible error term known as the Bayes error that we won’t be able to completely get rid of in a probabilistic setting. But if the error term seems too large, you might be able to reduce it further by collecting more data, manipulating your model’s hyperparameters, or altogether picking a different model.
Bias Variance Tradeoff
We’ve talked about bias and variance in the previous section. Now it is time to clarify what we actually mean by these terms.
Understanding Bias and Variance
In a nutshell, bias measures if there is any systematic deviation from the correct value in a specific direction. If we could repeat the same process of constructing a model several times over, and the results predicted by our model always deviate in a certain direction, we would call the result biased.
Variance measures how much the results vary between model predictions. If you repeat the modeling process several times over and the results are scattered all across the board, the model exhibits high variance.
In their book “Noise” Daniel Kahnemann and his co-authors provide an intuitive example that helps understand the concept of bias and variance. Imagine you have four teams at the shooting range.
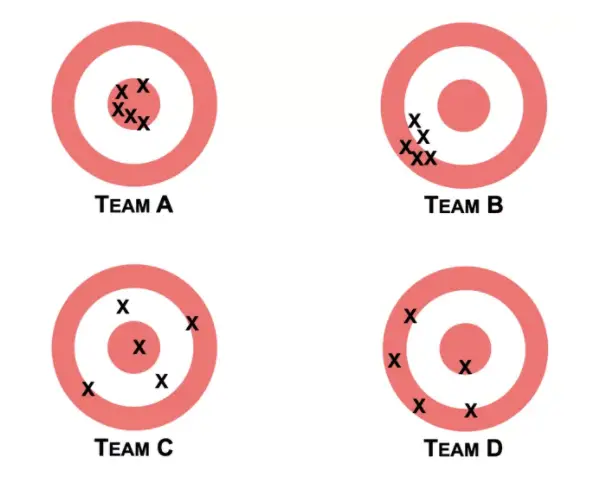
Team B is biased because the shots of its team members all deviate in a certain direction from the center. Team B also exhibits low variance because the shots of all the team members are relatively concentrated in one location. Team C has the opposite problem. The shots are scattered across the target with no discernible bias in a certain direction. Team D is both biased and has high variance. Team A would be the equivalent of a good model. The shots are in the center with little bias in one direction and little variance between the team members.
Generally speaking, linear models such as linear regression exhibit high bias and low variance. Nonlinear algorithms such as decision trees are more prone to overfitting the training data and thus exhibit high variance and low bias.
A linear model used with non-linear data would exhibit a bias to predict data points along a straight line instead of accomodating the curves. But they are not as susceptible to random fluctuations in the data. A nonlinear algorithm that is trained on noisy data with lots of deviations would be more capable of avoiding bias but more prone to incorporate the noise into its predictions. As a result, a small deviation in the test data might lead to very different predictions.
To get our model to learn the patterns in data, we need to reduce the training error while at the same time reducing the gap between the training and the testing error. In other words, we want to reduce both bias and variance. To a certain extent, we can reduce both by picking an appropriate model, collecting enough training data, selecting appropriate training features and hyperparameter values. At some point, we have to trade-off between minimizing bias and minimizing variance. How you balance this trade-off is up to you.
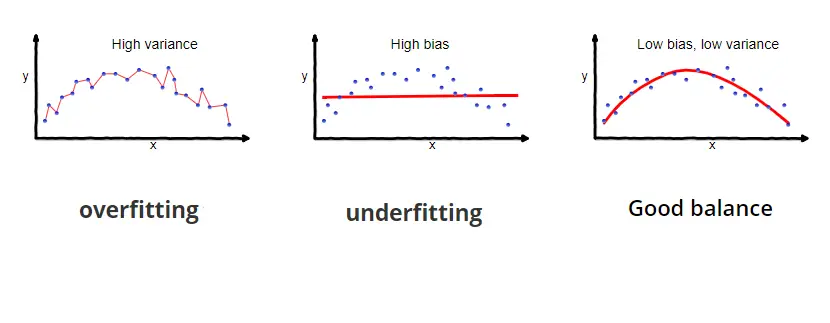
The Bias Variance Decomposition
Mathematically, the total error can be decomposed into the bias and the variance according to the following formula.
Remember that Bayes’ error is an error that cannot be eliminated.
Our machine learning model represents an estimating function \hat f(X) for the true data generating function f(X) where X represents the predictors and y the output values.
Now the mean squared error of our model is the expected value of the squared difference of the output produced by the estimating function \hat f(X) and the true output Y.
The bias is a systematic deviation from the true value. We can measure it as the squared difference between the expected value produced by the estimating function (the model) and the values produced by the true data-generating function.
Of course, we don’t know the true data generating function, but we do know the observed outputs Y, which correspond to the values generated by f(x) plus an error term.
The variance of the model is the squared difference between the expected value and the actual values of the model.
Now that we have the bias and the variance, we can add them up along with the irreducible error to get the total error.
A machine learning model represents an approximation to the hypothesized function that generated the data. The chosen model is a hypothesis since we hypothesize that this model represents the true data generating function.
We choose the hypothesis from a hypothesis space that may be subject to certain constraints. For example, we can constrain the hypothesis space to the set of linear models.
When choosing a model, we aim to reduce the bias and the variance to prevent our model from either overfitting or underfitting the data. In the real world, we cannot completely eliminate bias and variance, and we have to trade-off between them. The total error produced by a model can be decomposed into the bias, the variance, and irreducible (Bayes) error.

About Author

Related Posts
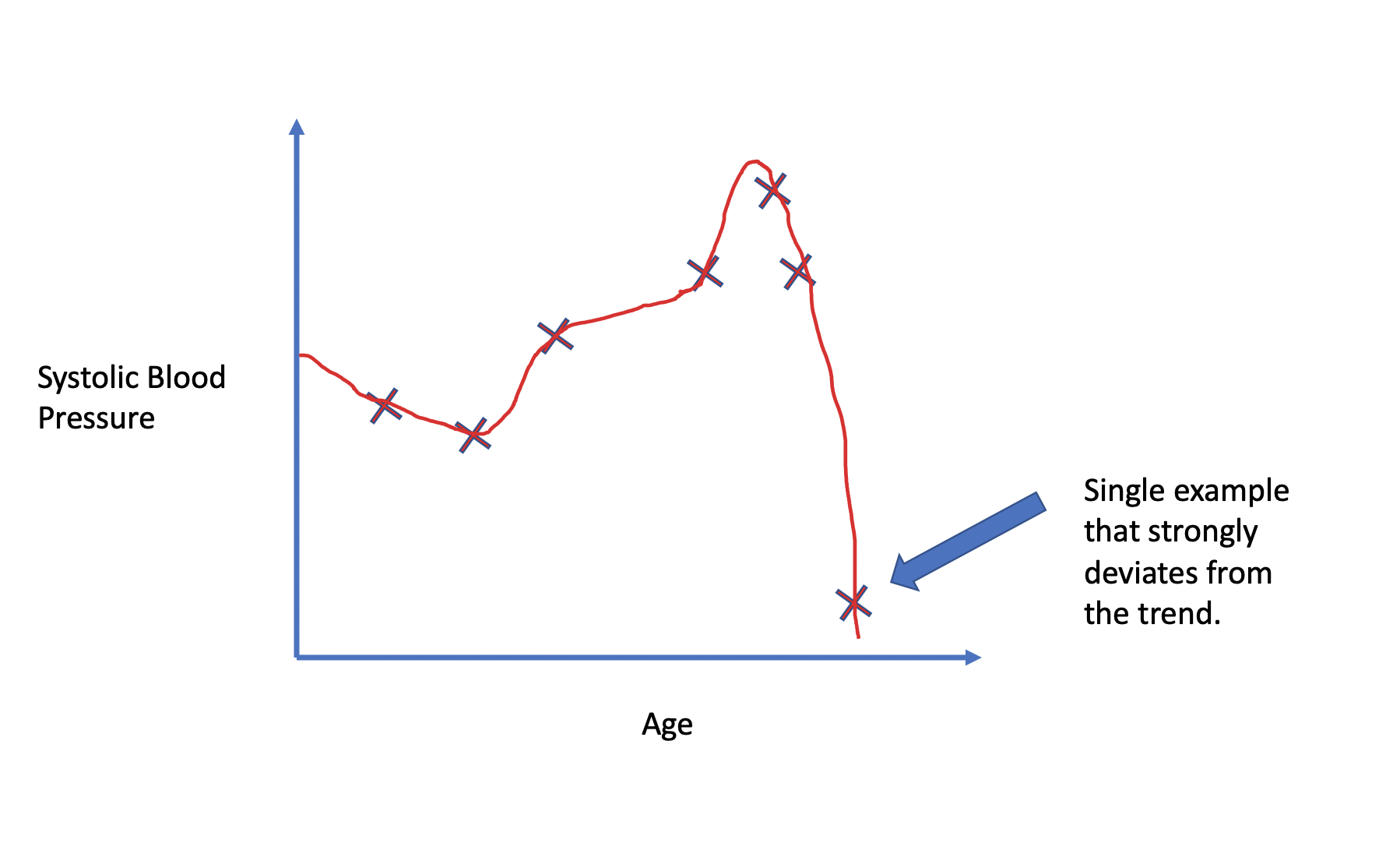
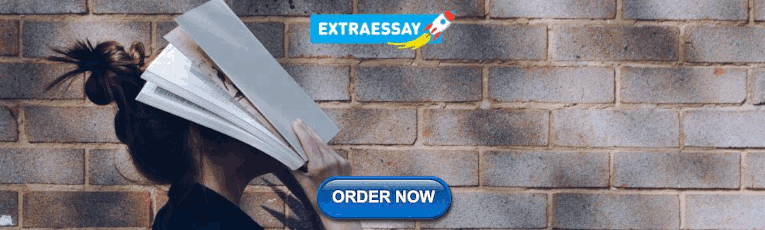
IMAGES
VIDEO
COMMENTS
A hypothesis in machine learningis the model’s presumption regarding the connection between the input features and the result. It is an illustration of the mapping function that the algorithm is attempting to discover using the training set. To minimize the discrepancy between the expected and actual …
In this article, we talked about hypotheses spaces in machine learning. An algorithm’s hypothesis space contains all the models it can learn from any dataset. The algorithms with too expressive spaces can generalize …
The hypothesis space is $2^{2^4}=65536$ because for each set of features of the input space two outcomes (0 and 1) are possible. The ML algorithm helps us to find one function , sometimes also referred as …
Hypothesis space is defined as a set of all possible legal hypotheses; hence it is also known as a hypothesis set. It is used by supervised machine learning algorithms to determine the best possible hypothesis to describe the target …
The hypothesis space used by a machine learning system is the set of all hypotheses that might possibly be returned by it. It is typically de ned by a hypothesis language, possibly in …
The hypothesis space in machine learning is a set of all possible models that can be used to explain a data distribution given the limitations of that space. A linear hypothesis space is limited to the set of all linear models.
An example of a model that approximates the target function and performs mappings of inputs to outputs is called a hypothesis in machine learning. The choice of algorithm (e.g. neural network) and the configuration …