- Skip to main content
- Skip to primary sidebar
- Skip to footer
- QuestionPro

- Solutions Industries Gaming Automotive Sports and events Education Government Travel & Hospitality Financial Services Healthcare Cannabis Technology Use Case NPS+ Communities Audience Contactless surveys Mobile LivePolls Member Experience GDPR Positive People Science 360 Feedback Surveys
- Resources Blog eBooks Survey Templates Case Studies Training Help center

Home Market Research
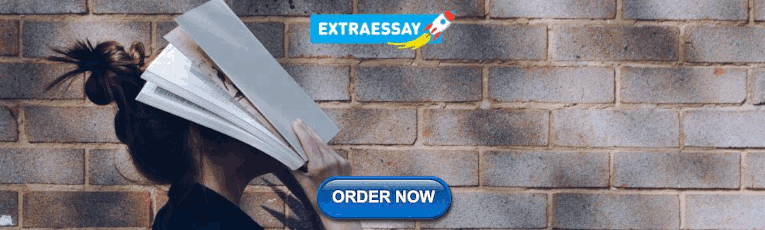
Empirical Research: Definition, Methods, Types and Examples
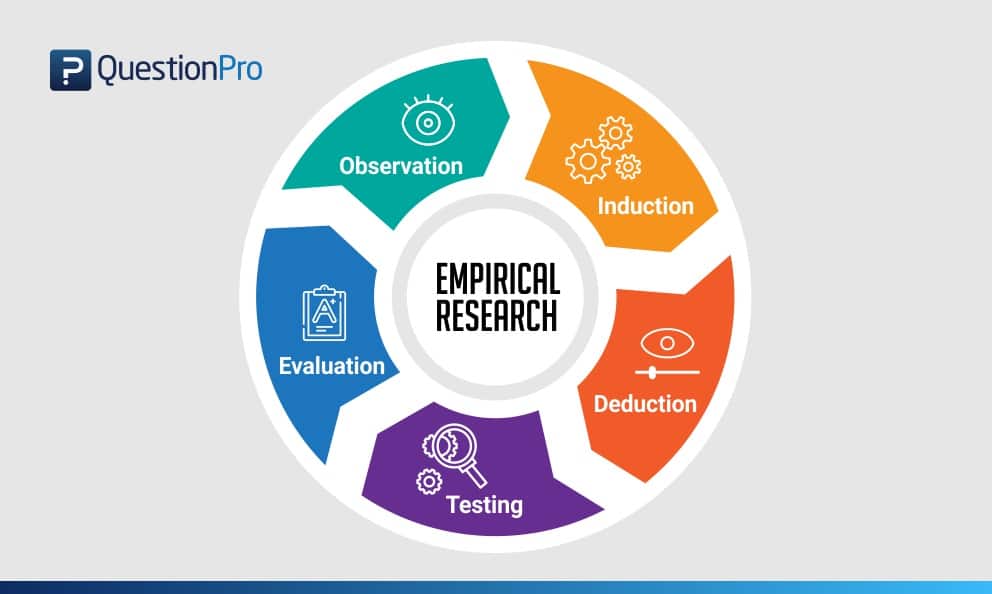
Content Index
Empirical research: Definition
Empirical research: origin, quantitative research methods, qualitative research methods, steps for conducting empirical research, empirical research methodology cycle, advantages of empirical research, disadvantages of empirical research, why is there a need for empirical research.
Empirical research is defined as any research where conclusions of the study is strictly drawn from concretely empirical evidence, and therefore “verifiable” evidence.
This empirical evidence can be gathered using quantitative market research and qualitative market research methods.
For example: A research is being conducted to find out if listening to happy music in the workplace while working may promote creativity? An experiment is conducted by using a music website survey on a set of audience who are exposed to happy music and another set who are not listening to music at all, and the subjects are then observed. The results derived from such a research will give empirical evidence if it does promote creativity or not.
LEARN ABOUT: Behavioral Research
You must have heard the quote” I will not believe it unless I see it”. This came from the ancient empiricists, a fundamental understanding that powered the emergence of medieval science during the renaissance period and laid the foundation of modern science, as we know it today. The word itself has its roots in greek. It is derived from the greek word empeirikos which means “experienced”.
In today’s world, the word empirical refers to collection of data using evidence that is collected through observation or experience or by using calibrated scientific instruments. All of the above origins have one thing in common which is dependence of observation and experiments to collect data and test them to come up with conclusions.
LEARN ABOUT: Causal Research
Types and methodologies of empirical research
Empirical research can be conducted and analysed using qualitative or quantitative methods.
- Quantitative research : Quantitative research methods are used to gather information through numerical data. It is used to quantify opinions, behaviors or other defined variables . These are predetermined and are in a more structured format. Some of the commonly used methods are survey, longitudinal studies, polls, etc
- Qualitative research: Qualitative research methods are used to gather non numerical data. It is used to find meanings, opinions, or the underlying reasons from its subjects. These methods are unstructured or semi structured. The sample size for such a research is usually small and it is a conversational type of method to provide more insight or in-depth information about the problem Some of the most popular forms of methods are focus groups, experiments, interviews, etc.
Data collected from these will need to be analysed. Empirical evidence can also be analysed either quantitatively and qualitatively. Using this, the researcher can answer empirical questions which have to be clearly defined and answerable with the findings he has got. The type of research design used will vary depending on the field in which it is going to be used. Many of them might choose to do a collective research involving quantitative and qualitative method to better answer questions which cannot be studied in a laboratory setting.
LEARN ABOUT: Qualitative Research Questions and Questionnaires
Quantitative research methods aid in analyzing the empirical evidence gathered. By using these a researcher can find out if his hypothesis is supported or not.
- Survey research: Survey research generally involves a large audience to collect a large amount of data. This is a quantitative method having a predetermined set of closed questions which are pretty easy to answer. Because of the simplicity of such a method, high responses are achieved. It is one of the most commonly used methods for all kinds of research in today’s world.
Previously, surveys were taken face to face only with maybe a recorder. However, with advancement in technology and for ease, new mediums such as emails , or social media have emerged.
For example: Depletion of energy resources is a growing concern and hence there is a need for awareness about renewable energy. According to recent studies, fossil fuels still account for around 80% of energy consumption in the United States. Even though there is a rise in the use of green energy every year, there are certain parameters because of which the general population is still not opting for green energy. In order to understand why, a survey can be conducted to gather opinions of the general population about green energy and the factors that influence their choice of switching to renewable energy. Such a survey can help institutions or governing bodies to promote appropriate awareness and incentive schemes to push the use of greener energy.
Learn more: Renewable Energy Survey Template Descriptive Research vs Correlational Research
- Experimental research: In experimental research , an experiment is set up and a hypothesis is tested by creating a situation in which one of the variable is manipulated. This is also used to check cause and effect. It is tested to see what happens to the independent variable if the other one is removed or altered. The process for such a method is usually proposing a hypothesis, experimenting on it, analyzing the findings and reporting the findings to understand if it supports the theory or not.
For example: A particular product company is trying to find what is the reason for them to not be able to capture the market. So the organisation makes changes in each one of the processes like manufacturing, marketing, sales and operations. Through the experiment they understand that sales training directly impacts the market coverage for their product. If the person is trained well, then the product will have better coverage.
- Correlational research: Correlational research is used to find relation between two set of variables . Regression analysis is generally used to predict outcomes of such a method. It can be positive, negative or neutral correlation.
LEARN ABOUT: Level of Analysis
For example: Higher educated individuals will get higher paying jobs. This means higher education enables the individual to high paying job and less education will lead to lower paying jobs.
- Longitudinal study: Longitudinal study is used to understand the traits or behavior of a subject under observation after repeatedly testing the subject over a period of time. Data collected from such a method can be qualitative or quantitative in nature.
For example: A research to find out benefits of exercise. The target is asked to exercise everyday for a particular period of time and the results show higher endurance, stamina, and muscle growth. This supports the fact that exercise benefits an individual body.
- Cross sectional: Cross sectional study is an observational type of method, in which a set of audience is observed at a given point in time. In this type, the set of people are chosen in a fashion which depicts similarity in all the variables except the one which is being researched. This type does not enable the researcher to establish a cause and effect relationship as it is not observed for a continuous time period. It is majorly used by healthcare sector or the retail industry.
For example: A medical study to find the prevalence of under-nutrition disorders in kids of a given population. This will involve looking at a wide range of parameters like age, ethnicity, location, incomes and social backgrounds. If a significant number of kids coming from poor families show under-nutrition disorders, the researcher can further investigate into it. Usually a cross sectional study is followed by a longitudinal study to find out the exact reason.
- Causal-Comparative research : This method is based on comparison. It is mainly used to find out cause-effect relationship between two variables or even multiple variables.
For example: A researcher measured the productivity of employees in a company which gave breaks to the employees during work and compared that to the employees of the company which did not give breaks at all.
LEARN ABOUT: Action Research
Some research questions need to be analysed qualitatively, as quantitative methods are not applicable there. In many cases, in-depth information is needed or a researcher may need to observe a target audience behavior, hence the results needed are in a descriptive analysis form. Qualitative research results will be descriptive rather than predictive. It enables the researcher to build or support theories for future potential quantitative research. In such a situation qualitative research methods are used to derive a conclusion to support the theory or hypothesis being studied.
LEARN ABOUT: Qualitative Interview
- Case study: Case study method is used to find more information through carefully analyzing existing cases. It is very often used for business research or to gather empirical evidence for investigation purpose. It is a method to investigate a problem within its real life context through existing cases. The researcher has to carefully analyse making sure the parameter and variables in the existing case are the same as to the case that is being investigated. Using the findings from the case study, conclusions can be drawn regarding the topic that is being studied.
For example: A report mentioning the solution provided by a company to its client. The challenges they faced during initiation and deployment, the findings of the case and solutions they offered for the problems. Such case studies are used by most companies as it forms an empirical evidence for the company to promote in order to get more business.
- Observational method: Observational method is a process to observe and gather data from its target. Since it is a qualitative method it is time consuming and very personal. It can be said that observational research method is a part of ethnographic research which is also used to gather empirical evidence. This is usually a qualitative form of research, however in some cases it can be quantitative as well depending on what is being studied.
For example: setting up a research to observe a particular animal in the rain-forests of amazon. Such a research usually take a lot of time as observation has to be done for a set amount of time to study patterns or behavior of the subject. Another example used widely nowadays is to observe people shopping in a mall to figure out buying behavior of consumers.
- One-on-one interview: Such a method is purely qualitative and one of the most widely used. The reason being it enables a researcher get precise meaningful data if the right questions are asked. It is a conversational method where in-depth data can be gathered depending on where the conversation leads.
For example: A one-on-one interview with the finance minister to gather data on financial policies of the country and its implications on the public.
- Focus groups: Focus groups are used when a researcher wants to find answers to why, what and how questions. A small group is generally chosen for such a method and it is not necessary to interact with the group in person. A moderator is generally needed in case the group is being addressed in person. This is widely used by product companies to collect data about their brands and the product.
For example: A mobile phone manufacturer wanting to have a feedback on the dimensions of one of their models which is yet to be launched. Such studies help the company meet the demand of the customer and position their model appropriately in the market.
- Text analysis: Text analysis method is a little new compared to the other types. Such a method is used to analyse social life by going through images or words used by the individual. In today’s world, with social media playing a major part of everyone’s life, such a method enables the research to follow the pattern that relates to his study.
For example: A lot of companies ask for feedback from the customer in detail mentioning how satisfied are they with their customer support team. Such data enables the researcher to take appropriate decisions to make their support team better.
Sometimes a combination of the methods is also needed for some questions that cannot be answered using only one type of method especially when a researcher needs to gain a complete understanding of complex subject matter.
We recently published a blog that talks about examples of qualitative data in education ; why don’t you check it out for more ideas?
Since empirical research is based on observation and capturing experiences, it is important to plan the steps to conduct the experiment and how to analyse it. This will enable the researcher to resolve problems or obstacles which can occur during the experiment.
Step #1: Define the purpose of the research
This is the step where the researcher has to answer questions like what exactly do I want to find out? What is the problem statement? Are there any issues in terms of the availability of knowledge, data, time or resources. Will this research be more beneficial than what it will cost.
Before going ahead, a researcher has to clearly define his purpose for the research and set up a plan to carry out further tasks.
Step #2 : Supporting theories and relevant literature
The researcher needs to find out if there are theories which can be linked to his research problem . He has to figure out if any theory can help him support his findings. All kind of relevant literature will help the researcher to find if there are others who have researched this before, or what are the problems faced during this research. The researcher will also have to set up assumptions and also find out if there is any history regarding his research problem
Step #3: Creation of Hypothesis and measurement
Before beginning the actual research he needs to provide himself a working hypothesis or guess what will be the probable result. Researcher has to set up variables, decide the environment for the research and find out how can he relate between the variables.
Researcher will also need to define the units of measurements, tolerable degree for errors, and find out if the measurement chosen will be acceptable by others.
Step #4: Methodology, research design and data collection
In this step, the researcher has to define a strategy for conducting his research. He has to set up experiments to collect data which will enable him to propose the hypothesis. The researcher will decide whether he will need experimental or non experimental method for conducting the research. The type of research design will vary depending on the field in which the research is being conducted. Last but not the least, the researcher will have to find out parameters that will affect the validity of the research design. Data collection will need to be done by choosing appropriate samples depending on the research question. To carry out the research, he can use one of the many sampling techniques. Once data collection is complete, researcher will have empirical data which needs to be analysed.
LEARN ABOUT: Best Data Collection Tools
Step #5: Data Analysis and result
Data analysis can be done in two ways, qualitatively and quantitatively. Researcher will need to find out what qualitative method or quantitative method will be needed or will he need a combination of both. Depending on the unit of analysis of his data, he will know if his hypothesis is supported or rejected. Analyzing this data is the most important part to support his hypothesis.
Step #6: Conclusion
A report will need to be made with the findings of the research. The researcher can give the theories and literature that support his research. He can make suggestions or recommendations for further research on his topic.
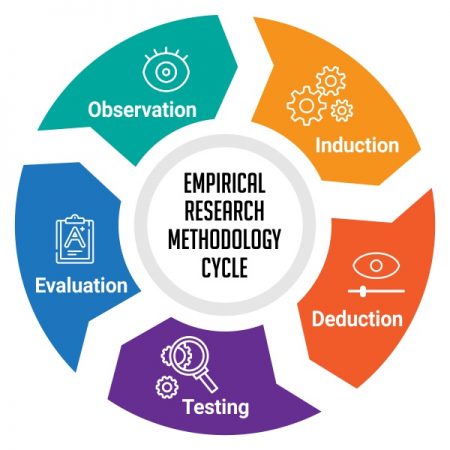
A.D. de Groot, a famous dutch psychologist and a chess expert conducted some of the most notable experiments using chess in the 1940’s. During his study, he came up with a cycle which is consistent and now widely used to conduct empirical research. It consists of 5 phases with each phase being as important as the next one. The empirical cycle captures the process of coming up with hypothesis about how certain subjects work or behave and then testing these hypothesis against empirical data in a systematic and rigorous approach. It can be said that it characterizes the deductive approach to science. Following is the empirical cycle.
- Observation: At this phase an idea is sparked for proposing a hypothesis. During this phase empirical data is gathered using observation. For example: a particular species of flower bloom in a different color only during a specific season.
- Induction: Inductive reasoning is then carried out to form a general conclusion from the data gathered through observation. For example: As stated above it is observed that the species of flower blooms in a different color during a specific season. A researcher may ask a question “does the temperature in the season cause the color change in the flower?” He can assume that is the case, however it is a mere conjecture and hence an experiment needs to be set up to support this hypothesis. So he tags a few set of flowers kept at a different temperature and observes if they still change the color?
- Deduction: This phase helps the researcher to deduce a conclusion out of his experiment. This has to be based on logic and rationality to come up with specific unbiased results.For example: In the experiment, if the tagged flowers in a different temperature environment do not change the color then it can be concluded that temperature plays a role in changing the color of the bloom.
- Testing: This phase involves the researcher to return to empirical methods to put his hypothesis to the test. The researcher now needs to make sense of his data and hence needs to use statistical analysis plans to determine the temperature and bloom color relationship. If the researcher finds out that most flowers bloom a different color when exposed to the certain temperature and the others do not when the temperature is different, he has found support to his hypothesis. Please note this not proof but just a support to his hypothesis.
- Evaluation: This phase is generally forgotten by most but is an important one to keep gaining knowledge. During this phase the researcher puts forth the data he has collected, the support argument and his conclusion. The researcher also states the limitations for the experiment and his hypothesis and suggests tips for others to pick it up and continue a more in-depth research for others in the future. LEARN MORE: Population vs Sample
LEARN MORE: Population vs Sample
There is a reason why empirical research is one of the most widely used method. There are a few advantages associated with it. Following are a few of them.
- It is used to authenticate traditional research through various experiments and observations.
- This research methodology makes the research being conducted more competent and authentic.
- It enables a researcher understand the dynamic changes that can happen and change his strategy accordingly.
- The level of control in such a research is high so the researcher can control multiple variables.
- It plays a vital role in increasing internal validity .
Even though empirical research makes the research more competent and authentic, it does have a few disadvantages. Following are a few of them.
- Such a research needs patience as it can be very time consuming. The researcher has to collect data from multiple sources and the parameters involved are quite a few, which will lead to a time consuming research.
- Most of the time, a researcher will need to conduct research at different locations or in different environments, this can lead to an expensive affair.
- There are a few rules in which experiments can be performed and hence permissions are needed. Many a times, it is very difficult to get certain permissions to carry out different methods of this research.
- Collection of data can be a problem sometimes, as it has to be collected from a variety of sources through different methods.
LEARN ABOUT: Social Communication Questionnaire
Empirical research is important in today’s world because most people believe in something only that they can see, hear or experience. It is used to validate multiple hypothesis and increase human knowledge and continue doing it to keep advancing in various fields.
For example: Pharmaceutical companies use empirical research to try out a specific drug on controlled groups or random groups to study the effect and cause. This way, they prove certain theories they had proposed for the specific drug. Such research is very important as sometimes it can lead to finding a cure for a disease that has existed for many years. It is useful in science and many other fields like history, social sciences, business, etc.
LEARN ABOUT: 12 Best Tools for Researchers
With the advancement in today’s world, empirical research has become critical and a norm in many fields to support their hypothesis and gain more knowledge. The methods mentioned above are very useful for carrying out such research. However, a number of new methods will keep coming up as the nature of new investigative questions keeps getting unique or changing.
Create a single source of real data with a built-for-insights platform. Store past data, add nuggets of insights, and import research data from various sources into a CRM for insights. Build on ever-growing research with a real-time dashboard in a unified research management platform to turn insights into knowledge.
LEARN MORE FREE TRIAL
MORE LIKE THIS
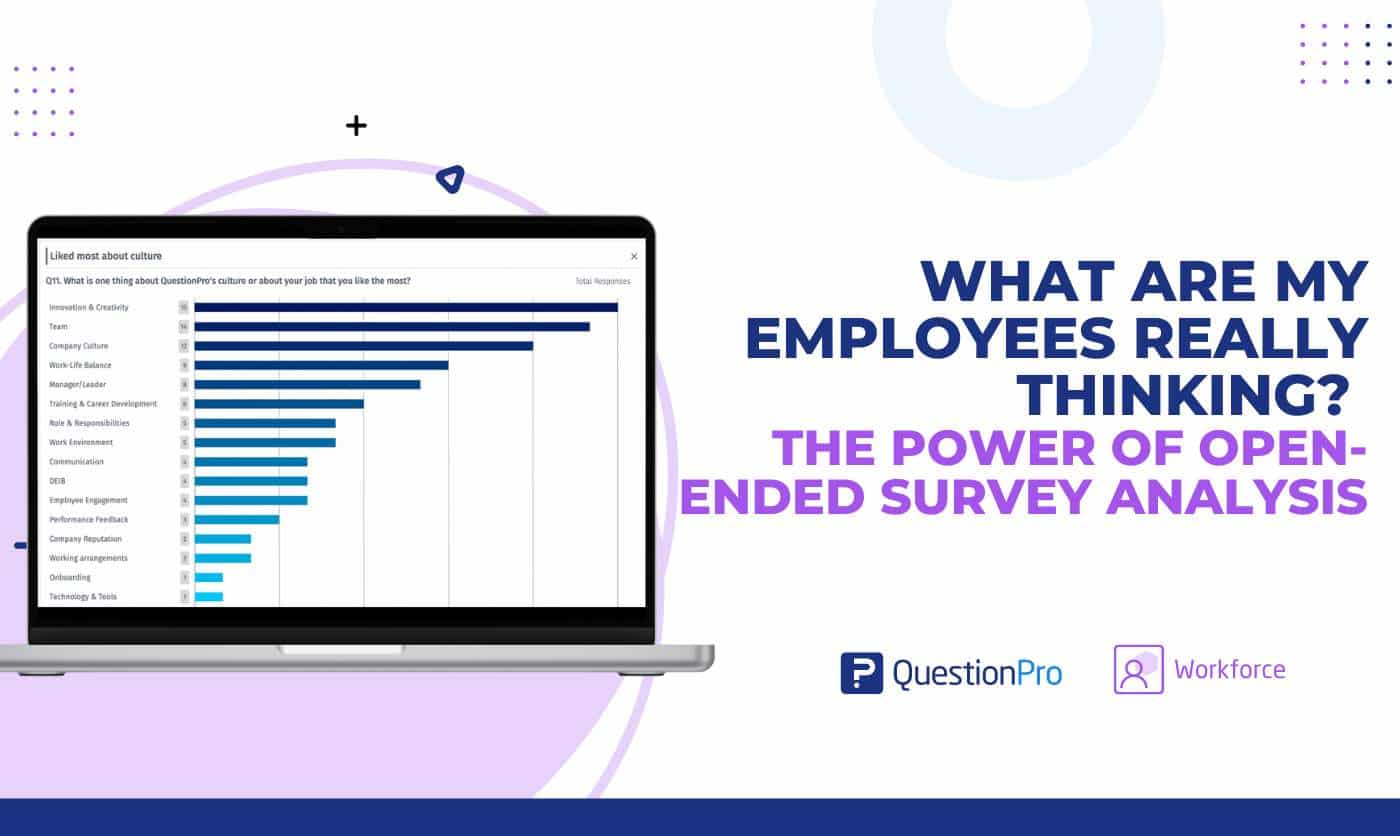
What Are My Employees Really Thinking? The Power of Open-ended Survey Analysis
May 24, 2024
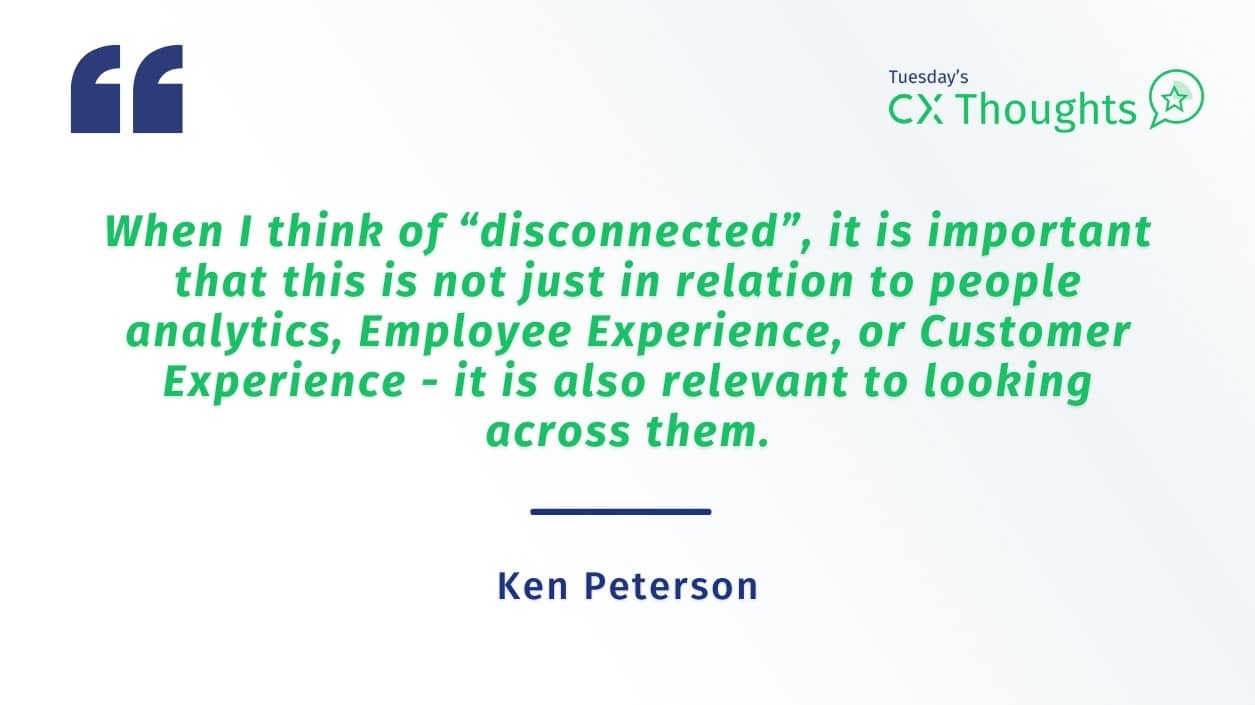
I Am Disconnected – Tuesday CX Thoughts
May 21, 2024
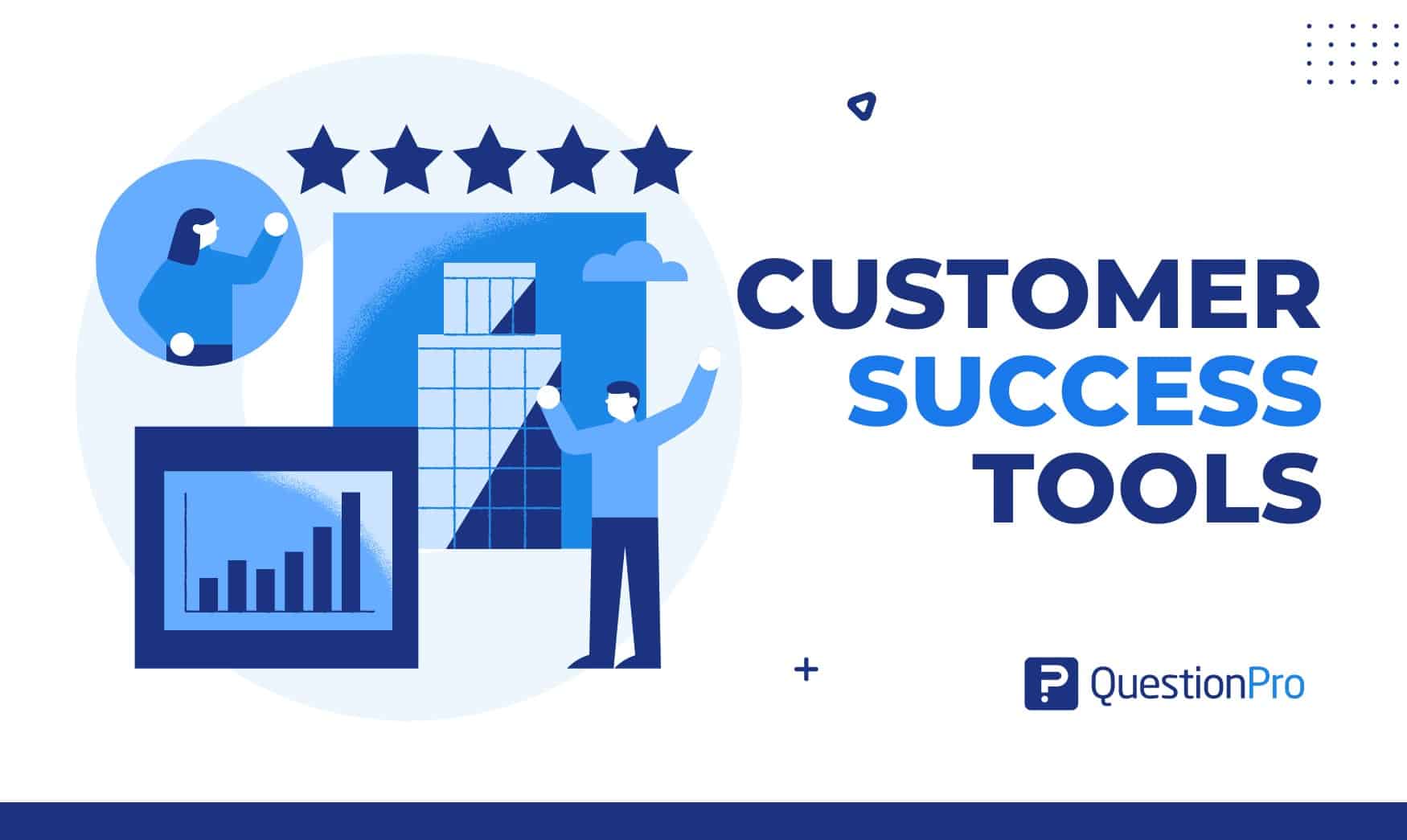
20 Best Customer Success Tools of 2024
May 20, 2024
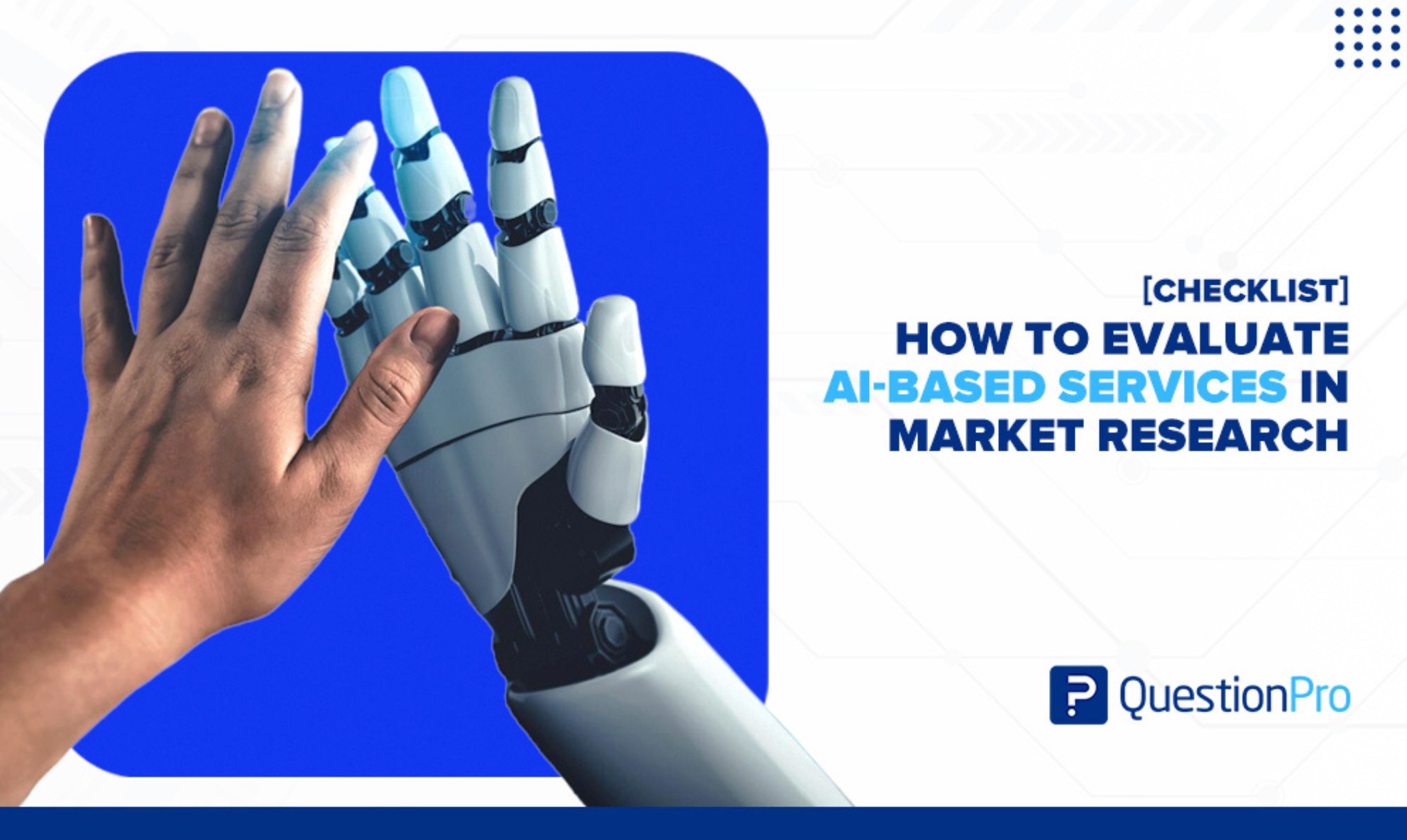
AI-Based Services Buying Guide for Market Research (based on ESOMAR’s 20 Questions)
Other categories.
- Academic Research
- Artificial Intelligence
- Assessments
- Brand Awareness
- Case Studies
- Communities
- Consumer Insights
- Customer effort score
- Customer Engagement
- Customer Experience
- Customer Loyalty
- Customer Research
- Customer Satisfaction
- Employee Benefits
- Employee Engagement
- Employee Retention
- Friday Five
- General Data Protection Regulation
- Insights Hub
- Life@QuestionPro
- Market Research
- Mobile diaries
- Mobile Surveys
- New Features
- Online Communities
- Question Types
- Questionnaire
- QuestionPro Products
- Release Notes
- Research Tools and Apps
- Revenue at Risk
- Survey Templates
- Training Tips
- Uncategorized
- Video Learning Series
- What’s Coming Up
- Workforce Intelligence
Have a language expert improve your writing
Run a free plagiarism check in 10 minutes, automatically generate references for free.
- Knowledge Base
- Methodology
Research Design | Step-by-Step Guide with Examples
Published on 5 May 2022 by Shona McCombes . Revised on 20 March 2023.
A research design is a strategy for answering your research question using empirical data. Creating a research design means making decisions about:
- Your overall aims and approach
- The type of research design you’ll use
- Your sampling methods or criteria for selecting subjects
- Your data collection methods
- The procedures you’ll follow to collect data
- Your data analysis methods
A well-planned research design helps ensure that your methods match your research aims and that you use the right kind of analysis for your data.
Table of contents
Step 1: consider your aims and approach, step 2: choose a type of research design, step 3: identify your population and sampling method, step 4: choose your data collection methods, step 5: plan your data collection procedures, step 6: decide on your data analysis strategies, frequently asked questions.
- Introduction
Before you can start designing your research, you should already have a clear idea of the research question you want to investigate.
There are many different ways you could go about answering this question. Your research design choices should be driven by your aims and priorities – start by thinking carefully about what you want to achieve.
The first choice you need to make is whether you’ll take a qualitative or quantitative approach.
Qualitative research designs tend to be more flexible and inductive , allowing you to adjust your approach based on what you find throughout the research process.
Quantitative research designs tend to be more fixed and deductive , with variables and hypotheses clearly defined in advance of data collection.
It’s also possible to use a mixed methods design that integrates aspects of both approaches. By combining qualitative and quantitative insights, you can gain a more complete picture of the problem you’re studying and strengthen the credibility of your conclusions.
Practical and ethical considerations when designing research
As well as scientific considerations, you need to think practically when designing your research. If your research involves people or animals, you also need to consider research ethics .
- How much time do you have to collect data and write up the research?
- Will you be able to gain access to the data you need (e.g., by travelling to a specific location or contacting specific people)?
- Do you have the necessary research skills (e.g., statistical analysis or interview techniques)?
- Will you need ethical approval ?
At each stage of the research design process, make sure that your choices are practically feasible.
Prevent plagiarism, run a free check.
Within both qualitative and quantitative approaches, there are several types of research design to choose from. Each type provides a framework for the overall shape of your research.
Types of quantitative research designs
Quantitative designs can be split into four main types. Experimental and quasi-experimental designs allow you to test cause-and-effect relationships, while descriptive and correlational designs allow you to measure variables and describe relationships between them.
With descriptive and correlational designs, you can get a clear picture of characteristics, trends, and relationships as they exist in the real world. However, you can’t draw conclusions about cause and effect (because correlation doesn’t imply causation ).
Experiments are the strongest way to test cause-and-effect relationships without the risk of other variables influencing the results. However, their controlled conditions may not always reflect how things work in the real world. They’re often also more difficult and expensive to implement.
Types of qualitative research designs
Qualitative designs are less strictly defined. This approach is about gaining a rich, detailed understanding of a specific context or phenomenon, and you can often be more creative and flexible in designing your research.
The table below shows some common types of qualitative design. They often have similar approaches in terms of data collection, but focus on different aspects when analysing the data.
Your research design should clearly define who or what your research will focus on, and how you’ll go about choosing your participants or subjects.
In research, a population is the entire group that you want to draw conclusions about, while a sample is the smaller group of individuals you’ll actually collect data from.
Defining the population
A population can be made up of anything you want to study – plants, animals, organisations, texts, countries, etc. In the social sciences, it most often refers to a group of people.
For example, will you focus on people from a specific demographic, region, or background? Are you interested in people with a certain job or medical condition, or users of a particular product?
The more precisely you define your population, the easier it will be to gather a representative sample.
Sampling methods
Even with a narrowly defined population, it’s rarely possible to collect data from every individual. Instead, you’ll collect data from a sample.
To select a sample, there are two main approaches: probability sampling and non-probability sampling . The sampling method you use affects how confidently you can generalise your results to the population as a whole.
Probability sampling is the most statistically valid option, but it’s often difficult to achieve unless you’re dealing with a very small and accessible population.
For practical reasons, many studies use non-probability sampling, but it’s important to be aware of the limitations and carefully consider potential biases. You should always make an effort to gather a sample that’s as representative as possible of the population.
Case selection in qualitative research
In some types of qualitative designs, sampling may not be relevant.
For example, in an ethnography or a case study, your aim is to deeply understand a specific context, not to generalise to a population. Instead of sampling, you may simply aim to collect as much data as possible about the context you are studying.
In these types of design, you still have to carefully consider your choice of case or community. You should have a clear rationale for why this particular case is suitable for answering your research question.
For example, you might choose a case study that reveals an unusual or neglected aspect of your research problem, or you might choose several very similar or very different cases in order to compare them.
Data collection methods are ways of directly measuring variables and gathering information. They allow you to gain first-hand knowledge and original insights into your research problem.
You can choose just one data collection method, or use several methods in the same study.
Survey methods
Surveys allow you to collect data about opinions, behaviours, experiences, and characteristics by asking people directly. There are two main survey methods to choose from: questionnaires and interviews.
Observation methods
Observations allow you to collect data unobtrusively, observing characteristics, behaviours, or social interactions without relying on self-reporting.
Observations may be conducted in real time, taking notes as you observe, or you might make audiovisual recordings for later analysis. They can be qualitative or quantitative.
Other methods of data collection
There are many other ways you might collect data depending on your field and topic.
If you’re not sure which methods will work best for your research design, try reading some papers in your field to see what data collection methods they used.
Secondary data
If you don’t have the time or resources to collect data from the population you’re interested in, you can also choose to use secondary data that other researchers already collected – for example, datasets from government surveys or previous studies on your topic.
With this raw data, you can do your own analysis to answer new research questions that weren’t addressed by the original study.
Using secondary data can expand the scope of your research, as you may be able to access much larger and more varied samples than you could collect yourself.
However, it also means you don’t have any control over which variables to measure or how to measure them, so the conclusions you can draw may be limited.
As well as deciding on your methods, you need to plan exactly how you’ll use these methods to collect data that’s consistent, accurate, and unbiased.
Planning systematic procedures is especially important in quantitative research, where you need to precisely define your variables and ensure your measurements are reliable and valid.
Operationalisation
Some variables, like height or age, are easily measured. But often you’ll be dealing with more abstract concepts, like satisfaction, anxiety, or competence. Operationalisation means turning these fuzzy ideas into measurable indicators.
If you’re using observations , which events or actions will you count?
If you’re using surveys , which questions will you ask and what range of responses will be offered?
You may also choose to use or adapt existing materials designed to measure the concept you’re interested in – for example, questionnaires or inventories whose reliability and validity has already been established.
Reliability and validity
Reliability means your results can be consistently reproduced , while validity means that you’re actually measuring the concept you’re interested in.
For valid and reliable results, your measurement materials should be thoroughly researched and carefully designed. Plan your procedures to make sure you carry out the same steps in the same way for each participant.
If you’re developing a new questionnaire or other instrument to measure a specific concept, running a pilot study allows you to check its validity and reliability in advance.
Sampling procedures
As well as choosing an appropriate sampling method, you need a concrete plan for how you’ll actually contact and recruit your selected sample.
That means making decisions about things like:
- How many participants do you need for an adequate sample size?
- What inclusion and exclusion criteria will you use to identify eligible participants?
- How will you contact your sample – by mail, online, by phone, or in person?
If you’re using a probability sampling method, it’s important that everyone who is randomly selected actually participates in the study. How will you ensure a high response rate?
If you’re using a non-probability method, how will you avoid bias and ensure a representative sample?
Data management
It’s also important to create a data management plan for organising and storing your data.
Will you need to transcribe interviews or perform data entry for observations? You should anonymise and safeguard any sensitive data, and make sure it’s backed up regularly.
Keeping your data well organised will save time when it comes to analysing them. It can also help other researchers validate and add to your findings.
On their own, raw data can’t answer your research question. The last step of designing your research is planning how you’ll analyse the data.
Quantitative data analysis
In quantitative research, you’ll most likely use some form of statistical analysis . With statistics, you can summarise your sample data, make estimates, and test hypotheses.
Using descriptive statistics , you can summarise your sample data in terms of:
- The distribution of the data (e.g., the frequency of each score on a test)
- The central tendency of the data (e.g., the mean to describe the average score)
- The variability of the data (e.g., the standard deviation to describe how spread out the scores are)
The specific calculations you can do depend on the level of measurement of your variables.
Using inferential statistics , you can:
- Make estimates about the population based on your sample data.
- Test hypotheses about a relationship between variables.
Regression and correlation tests look for associations between two or more variables, while comparison tests (such as t tests and ANOVAs ) look for differences in the outcomes of different groups.
Your choice of statistical test depends on various aspects of your research design, including the types of variables you’re dealing with and the distribution of your data.
Qualitative data analysis
In qualitative research, your data will usually be very dense with information and ideas. Instead of summing it up in numbers, you’ll need to comb through the data in detail, interpret its meanings, identify patterns, and extract the parts that are most relevant to your research question.
Two of the most common approaches to doing this are thematic analysis and discourse analysis .
There are many other ways of analysing qualitative data depending on the aims of your research. To get a sense of potential approaches, try reading some qualitative research papers in your field.
A sample is a subset of individuals from a larger population. Sampling means selecting the group that you will actually collect data from in your research.
For example, if you are researching the opinions of students in your university, you could survey a sample of 100 students.
Statistical sampling allows you to test a hypothesis about the characteristics of a population. There are various sampling methods you can use to ensure that your sample is representative of the population as a whole.
Operationalisation means turning abstract conceptual ideas into measurable observations.
For example, the concept of social anxiety isn’t directly observable, but it can be operationally defined in terms of self-rating scores, behavioural avoidance of crowded places, or physical anxiety symptoms in social situations.
Before collecting data , it’s important to consider how you will operationalise the variables that you want to measure.
The research methods you use depend on the type of data you need to answer your research question .
- If you want to measure something or test a hypothesis , use quantitative methods . If you want to explore ideas, thoughts, and meanings, use qualitative methods .
- If you want to analyse a large amount of readily available data, use secondary data. If you want data specific to your purposes with control over how they are generated, collect primary data.
- If you want to establish cause-and-effect relationships between variables , use experimental methods. If you want to understand the characteristics of a research subject, use descriptive methods.
Cite this Scribbr article
If you want to cite this source, you can copy and paste the citation or click the ‘Cite this Scribbr article’ button to automatically add the citation to our free Reference Generator.
McCombes, S. (2023, March 20). Research Design | Step-by-Step Guide with Examples. Scribbr. Retrieved 21 May 2024, from https://www.scribbr.co.uk/research-methods/research-design/
Is this article helpful?
Shona McCombes
Research Methods
- Getting Started
- Literature Review Research
Research Design
- Research Design By Discipline
- SAGE Research Methods
- Teaching with SAGE Research Methods
Quantitative vs. Qualitative Research: The Differences Explained
From Scribbr
Empirical Research
What is empirical research.
"Empirical research is research that is based on observation and measurement of phenomena, as directly experienced by the researcher. The data thus gathered may be compared against a theory or hypothesis, but the results are still based on real life experience. The data gathered is all primary data, although secondary data from a literature review may form the theoretical background."
Characteristics of Empirical Research
Emerald Publishing's guide to conducting empirical research identifies a number of common elements to empirical research:
A research question , which will determine research objectives.
A particular and planned design for the research, which will depend on the question and which will find ways of answering it with appropriate use of resources.
The gathering of primary data , which is then analysed.
A particular methodology for collecting and analysing the data, such as an experiment or survey.
The limitation of the data to a particular group, area or time scale, known as a sample [emphasis added]: for example, a specific number of employees of a particular company type, or all users of a library over a given time scale. The sample should be somehow representative of a wider population.
The ability to recreate the study and test the results. This is known as reliability .
The ability to generalize from the findings to a larger sample and to other situations.
If you see these elements in a research article, you can feel confident that you have found empirical research. Emerald's guide goes into more detail on each element.
Emerald Publishing (n.d.). How to... conduct empirical research. https://www.emeraldgrouppublishing.com/how-to/research-methods/conduct-empirical-research-l
- Quantitative vs. Qualitative
- Data Collection Methods
- Analyzing Data
When collecting and analyzing data, quantitative research deals with numbers and statistics, while qualitative research deals with words and meanings. Both are important for gaining different kinds of knowledge.
Quantitative research
Common quantitative methods include experiments, observations recorded as numbers, and surveys with closed-ended questions.
Qualitative research
Common qualitative methods include interviews with open-ended questions, observations described in words, and literature reviews that explore concepts and theories.
Streefkerk, R. (2022, February 7). Qualitative vs. quantitative research: Differences, examples & methods. Scibbr. https://www.scribbr.com/methodology/qualitative-quantitative-research/
Quantitative and qualitative data can be collected using various methods. It is important to use a data collection method that will help answer your research question(s).
Many data collection methods can be either qualitative or quantitative. For example, in surveys, observations or case studies , your data can be represented as numbers (e.g. using rating scales or counting frequencies) or as words (e.g. with open-ended questions or descriptions of what you observe).
However, some methods are more commonly used in one type or the other.
Quantitative data collection methods
- Surveys : List of closed or multiple choice questions that is distributed to a sample (online, in person, or over the phone).
- Experiments : Situation in which variables are controlled and manipulated to establish cause-and-effect relationships.
- Observations: Observing subjects in a natural environment where variables can’t be controlled.
Qualitative data collection methods
- Interviews : Asking open-ended questions verbally to respondents.
- Focus groups: Discussion among a group of people about a topic to gather opinions that can be used for further research.
- Ethnography : Participating in a community or organization for an extended period of time to closely observe culture and behavior.
- Literature review : Survey of published works by other authors.
When to use qualitative vs. quantitative research
A rule of thumb for deciding whether to use qualitative or quantitative data is:
- Use quantitative research if you want to confirm or test something (a theory or hypothesis)
- Use qualitative research if you want to understand something (concepts, thoughts, experiences)
For most research topics you can choose a qualitative, quantitative or mixed methods approach . Which type you choose depends on, among other things, whether you’re taking an inductive vs. deductive research approach ; your research question(s) ; whether you’re doing experimental , correlational , or descriptive research ; and practical considerations such as time, money, availability of data, and access to respondents.
Streefkerk, R. (2022, February 7). Qualitative vs. quantitative research: Differences, examples & methods. Scibbr. https://www.scribbr.com/methodology/qualitative-quantitative-research/
Qualitative or quantitative data by itself can’t prove or demonstrate anything, but has to be analyzed to show its meaning in relation to the research questions. The method of analysis differs for each type of data.
Analyzing quantitative data
Quantitative data is based on numbers. Simple math or more advanced statistical analysis is used to discover commonalities or patterns in the data. The results are often reported in graphs and tables.
Applications such as Excel, SPSS, or R can be used to calculate things like:
- Average scores
- The number of times a particular answer was given
- The correlation or causation between two or more variables
- The reliability and validity of the results
Analyzing qualitative data
Qualitative data is more difficult to analyze than quantitative data. It consists of text, images or videos instead of numbers.
Some common approaches to analyzing qualitative data include:
- Qualitative content analysis : Tracking the occurrence, position and meaning of words or phrases
- Thematic analysis : Closely examining the data to identify the main themes and patterns
- Discourse analysis : Studying how communication works in social contexts
Comparison of Research Processes
Creswell, J. W., & Creswell, J. D. (2018). Research design : qualitative, quantitative, and mixed methods approaches (Fifth). SAGE Publications.
- << Previous: Literature Review Research
- Next: Research Design By Discipline >>
- Last Updated: Aug 21, 2023 4:07 PM
- URL: https://guides.lib.udel.edu/researchmethods
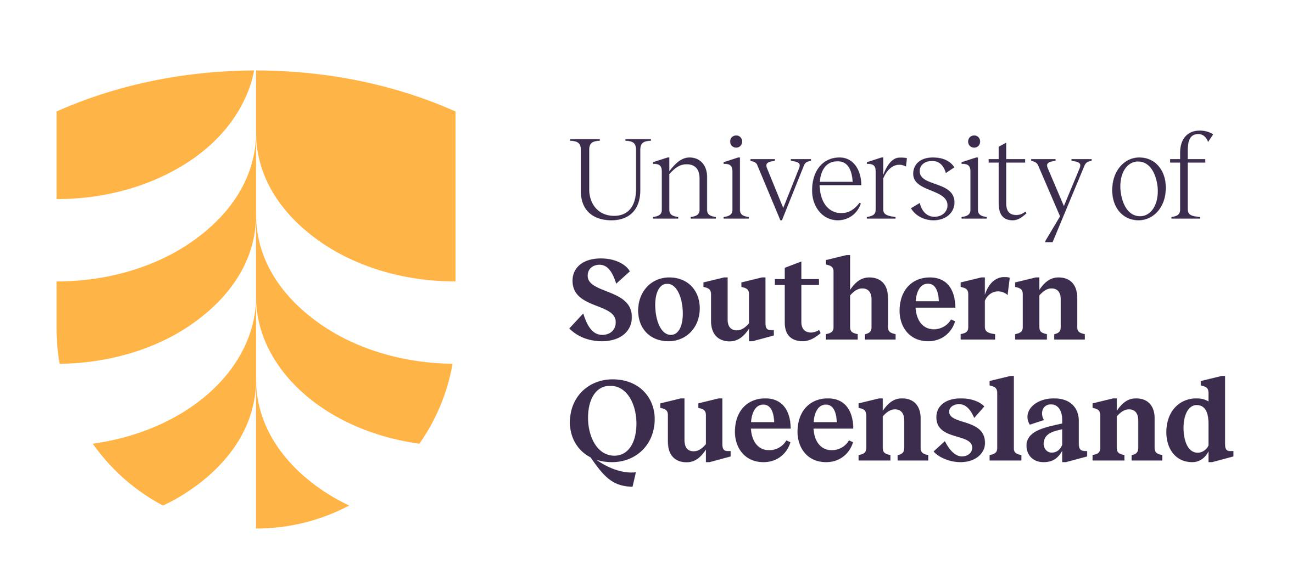
Want to create or adapt books like this? Learn more about how Pressbooks supports open publishing practices.
5 Research design
Research design is a comprehensive plan for data collection in an empirical research project. It is a ‘blueprint’ for empirical research aimed at answering specific research questions or testing specific hypotheses, and must specify at least three processes: the data collection process, the instrument development process, and the sampling process. The instrument development and sampling processes are described in the next two chapters, and the data collection process—which is often loosely called ‘research design’—is introduced in this chapter and is described in further detail in Chapters 9–12.
Broadly speaking, data collection methods can be grouped into two categories: positivist and interpretive. Positivist methods , such as laboratory experiments and survey research, are aimed at theory (or hypotheses) testing, while interpretive methods, such as action research and ethnography, are aimed at theory building. Positivist methods employ a deductive approach to research, starting with a theory and testing theoretical postulates using empirical data. In contrast, interpretive methods employ an inductive approach that starts with data and tries to derive a theory about the phenomenon of interest from the observed data. Often times, these methods are incorrectly equated with quantitative and qualitative research. Quantitative and qualitative methods refers to the type of data being collected—quantitative data involve numeric scores, metrics, and so on, while qualitative data includes interviews, observations, and so forth—and analysed (i.e., using quantitative techniques such as regression or qualitative techniques such as coding). Positivist research uses predominantly quantitative data, but can also use qualitative data. Interpretive research relies heavily on qualitative data, but can sometimes benefit from including quantitative data as well. Sometimes, joint use of qualitative and quantitative data may help generate unique insight into a complex social phenomenon that is not available from either type of data alone, and hence, mixed-mode designs that combine qualitative and quantitative data are often highly desirable.
Key attributes of a research design
The quality of research designs can be defined in terms of four key design attributes: internal validity, external validity, construct validity, and statistical conclusion validity.
Internal validity , also called causality, examines whether the observed change in a dependent variable is indeed caused by a corresponding change in a hypothesised independent variable, and not by variables extraneous to the research context. Causality requires three conditions: covariation of cause and effect (i.e., if cause happens, then effect also happens; if cause does not happen, effect does not happen), temporal precedence (cause must precede effect in time), and spurious correlation, or there is no plausible alternative explanation for the change. Certain research designs, such as laboratory experiments, are strong in internal validity by virtue of their ability to manipulate the independent variable (cause) via a treatment and observe the effect (dependent variable) of that treatment after a certain point in time, while controlling for the effects of extraneous variables. Other designs, such as field surveys, are poor in internal validity because of their inability to manipulate the independent variable (cause), and because cause and effect are measured at the same point in time which defeats temporal precedence making it equally likely that the expected effect might have influenced the expected cause rather than the reverse. Although higher in internal validity compared to other methods, laboratory experiments are by no means immune to threats of internal validity, and are susceptible to history, testing, instrumentation, regression, and other threats that are discussed later in the chapter on experimental designs. Nonetheless, different research designs vary considerably in their respective level of internal validity.
External validity or generalisability refers to whether the observed associations can be generalised from the sample to the population (population validity), or to other people, organisations, contexts, or time (ecological validity). For instance, can results drawn from a sample of financial firms in the United States be generalised to the population of financial firms (population validity) or to other firms within the United States (ecological validity)? Survey research, where data is sourced from a wide variety of individuals, firms, or other units of analysis, tends to have broader generalisability than laboratory experiments where treatments and extraneous variables are more controlled. The variation in internal and external validity for a wide range of research designs is shown in Figure 5.1.
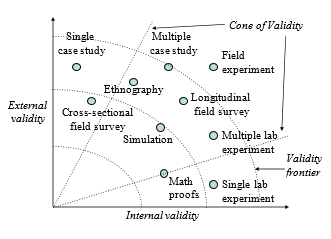
Some researchers claim that there is a trade-off between internal and external validity—higher external validity can come only at the cost of internal validity and vice versa. But this is not always the case. Research designs such as field experiments, longitudinal field surveys, and multiple case studies have higher degrees of both internal and external validities. Personally, I prefer research designs that have reasonable degrees of both internal and external validities, i.e., those that fall within the cone of validity shown in Figure 5.1. But this should not suggest that designs outside this cone are any less useful or valuable. Researchers’ choice of designs are ultimately a matter of their personal preference and competence, and the level of internal and external validity they desire.
Construct validity examines how well a given measurement scale is measuring the theoretical construct that it is expected to measure. Many constructs used in social science research such as empathy, resistance to change, and organisational learning are difficult to define, much less measure. For instance, construct validity must ensure that a measure of empathy is indeed measuring empathy and not compassion, which may be difficult since these constructs are somewhat similar in meaning. Construct validity is assessed in positivist research based on correlational or factor analysis of pilot test data, as described in the next chapter.
Statistical conclusion validity examines the extent to which conclusions derived using a statistical procedure are valid. For example, it examines whether the right statistical method was used for hypotheses testing, whether the variables used meet the assumptions of that statistical test (such as sample size or distributional requirements), and so forth. Because interpretive research designs do not employ statistical tests, statistical conclusion validity is not applicable for such analysis. The different kinds of validity and where they exist at the theoretical/empirical levels are illustrated in Figure 5.2.
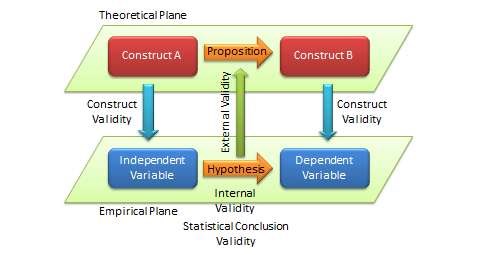
Improving internal and external validity
The best research designs are those that can ensure high levels of internal and external validity. Such designs would guard against spurious correlations, inspire greater faith in the hypotheses testing, and ensure that the results drawn from a small sample are generalisable to the population at large. Controls are required to ensure internal validity (causality) of research designs, and can be accomplished in five ways: manipulation, elimination, inclusion, and statistical control, and randomisation.
In manipulation , the researcher manipulates the independent variables in one or more levels (called ‘treatments’), and compares the effects of the treatments against a control group where subjects do not receive the treatment. Treatments may include a new drug or different dosage of drug (for treating a medical condition), a teaching style (for students), and so forth. This type of control is achieved in experimental or quasi-experimental designs, but not in non-experimental designs such as surveys. Note that if subjects cannot distinguish adequately between different levels of treatment manipulations, their responses across treatments may not be different, and manipulation would fail.
The elimination technique relies on eliminating extraneous variables by holding them constant across treatments, such as by restricting the study to a single gender or a single socioeconomic status. In the inclusion technique, the role of extraneous variables is considered by including them in the research design and separately estimating their effects on the dependent variable, such as via factorial designs where one factor is gender (male versus female). Such technique allows for greater generalisability, but also requires substantially larger samples. In statistical control , extraneous variables are measured and used as covariates during the statistical testing process.
Finally, the randomisation technique is aimed at cancelling out the effects of extraneous variables through a process of random sampling, if it can be assured that these effects are of a random (non-systematic) nature. Two types of randomisation are: random selection , where a sample is selected randomly from a population, and random assignment , where subjects selected in a non-random manner are randomly assigned to treatment groups.
Randomisation also ensures external validity, allowing inferences drawn from the sample to be generalised to the population from which the sample is drawn. Note that random assignment is mandatory when random selection is not possible because of resource or access constraints. However, generalisability across populations is harder to ascertain since populations may differ on multiple dimensions and you can only control for a few of those dimensions.
Popular research designs
As noted earlier, research designs can be classified into two categories—positivist and interpretive—depending on the goal of the research. Positivist designs are meant for theory testing, while interpretive designs are meant for theory building. Positivist designs seek generalised patterns based on an objective view of reality, while interpretive designs seek subjective interpretations of social phenomena from the perspectives of the subjects involved. Some popular examples of positivist designs include laboratory experiments, field experiments, field surveys, secondary data analysis, and case research, while examples of interpretive designs include case research, phenomenology, and ethnography. Note that case research can be used for theory building or theory testing, though not at the same time. Not all techniques are suited for all kinds of scientific research. Some techniques such as focus groups are best suited for exploratory research, others such as ethnography are best for descriptive research, and still others such as laboratory experiments are ideal for explanatory research. Following are brief descriptions of some of these designs. Additional details are provided in Chapters 9–12.
Experimental studies are those that are intended to test cause-effect relationships (hypotheses) in a tightly controlled setting by separating the cause from the effect in time, administering the cause to one group of subjects (the ‘treatment group’) but not to another group (‘control group’), and observing how the mean effects vary between subjects in these two groups. For instance, if we design a laboratory experiment to test the efficacy of a new drug in treating a certain ailment, we can get a random sample of people afflicted with that ailment, randomly assign them to one of two groups (treatment and control groups), administer the drug to subjects in the treatment group, but only give a placebo (e.g., a sugar pill with no medicinal value) to subjects in the control group. More complex designs may include multiple treatment groups, such as low versus high dosage of the drug or combining drug administration with dietary interventions. In a true experimental design , subjects must be randomly assigned to each group. If random assignment is not followed, then the design becomes quasi-experimental . Experiments can be conducted in an artificial or laboratory setting such as at a university (laboratory experiments) or in field settings such as in an organisation where the phenomenon of interest is actually occurring (field experiments). Laboratory experiments allow the researcher to isolate the variables of interest and control for extraneous variables, which may not be possible in field experiments. Hence, inferences drawn from laboratory experiments tend to be stronger in internal validity, but those from field experiments tend to be stronger in external validity. Experimental data is analysed using quantitative statistical techniques. The primary strength of the experimental design is its strong internal validity due to its ability to isolate, control, and intensively examine a small number of variables, while its primary weakness is limited external generalisability since real life is often more complex (i.e., involving more extraneous variables) than contrived lab settings. Furthermore, if the research does not identify ex ante relevant extraneous variables and control for such variables, such lack of controls may hurt internal validity and may lead to spurious correlations.
Field surveys are non-experimental designs that do not control for or manipulate independent variables or treatments, but measure these variables and test their effects using statistical methods. Field surveys capture snapshots of practices, beliefs, or situations from a random sample of subjects in field settings through a survey questionnaire or less frequently, through a structured interview. In cross-sectional field surveys , independent and dependent variables are measured at the same point in time (e.g., using a single questionnaire), while in longitudinal field surveys , dependent variables are measured at a later point in time than the independent variables. The strengths of field surveys are their external validity (since data is collected in field settings), their ability to capture and control for a large number of variables, and their ability to study a problem from multiple perspectives or using multiple theories. However, because of their non-temporal nature, internal validity (cause-effect relationships) are difficult to infer, and surveys may be subject to respondent biases (e.g., subjects may provide a ‘socially desirable’ response rather than their true response) which further hurts internal validity.
Secondary data analysis is an analysis of data that has previously been collected and tabulated by other sources. Such data may include data from government agencies such as employment statistics from the U.S. Bureau of Labor Services or development statistics by countries from the United Nations Development Program, data collected by other researchers (often used in meta-analytic studies), or publicly available third-party data, such as financial data from stock markets or real-time auction data from eBay. This is in contrast to most other research designs where collecting primary data for research is part of the researcher’s job. Secondary data analysis may be an effective means of research where primary data collection is too costly or infeasible, and secondary data is available at a level of analysis suitable for answering the researcher’s questions. The limitations of this design are that the data might not have been collected in a systematic or scientific manner and hence unsuitable for scientific research, since the data was collected for a presumably different purpose, they may not adequately address the research questions of interest to the researcher, and interval validity is problematic if the temporal precedence between cause and effect is unclear.
Case research is an in-depth investigation of a problem in one or more real-life settings (case sites) over an extended period of time. Data may be collected using a combination of interviews, personal observations, and internal or external documents. Case studies can be positivist in nature (for hypotheses testing) or interpretive (for theory building). The strength of this research method is its ability to discover a wide variety of social, cultural, and political factors potentially related to the phenomenon of interest that may not be known in advance. Analysis tends to be qualitative in nature, but heavily contextualised and nuanced. However, interpretation of findings may depend on the observational and integrative ability of the researcher, lack of control may make it difficult to establish causality, and findings from a single case site may not be readily generalised to other case sites. Generalisability can be improved by replicating and comparing the analysis in other case sites in a multiple case design .
Focus group research is a type of research that involves bringing in a small group of subjects (typically six to ten people) at one location, and having them discuss a phenomenon of interest for a period of one and a half to two hours. The discussion is moderated and led by a trained facilitator, who sets the agenda and poses an initial set of questions for participants, makes sure that the ideas and experiences of all participants are represented, and attempts to build a holistic understanding of the problem situation based on participants’ comments and experiences. Internal validity cannot be established due to lack of controls and the findings may not be generalised to other settings because of the small sample size. Hence, focus groups are not generally used for explanatory or descriptive research, but are more suited for exploratory research.
Action research assumes that complex social phenomena are best understood by introducing interventions or ‘actions’ into those phenomena and observing the effects of those actions. In this method, the researcher is embedded within a social context such as an organisation and initiates an action—such as new organisational procedures or new technologies—in response to a real problem such as declining profitability or operational bottlenecks. The researcher’s choice of actions must be based on theory, which should explain why and how such actions may cause the desired change. The researcher then observes the results of that action, modifying it as necessary, while simultaneously learning from the action and generating theoretical insights about the target problem and interventions. The initial theory is validated by the extent to which the chosen action successfully solves the target problem. Simultaneous problem solving and insight generation is the central feature that distinguishes action research from all other research methods, and hence, action research is an excellent method for bridging research and practice. This method is also suited for studying unique social problems that cannot be replicated outside that context, but it is also subject to researcher bias and subjectivity, and the generalisability of findings is often restricted to the context where the study was conducted.
Ethnography is an interpretive research design inspired by anthropology that emphasises that research phenomenon must be studied within the context of its culture. The researcher is deeply immersed in a certain culture over an extended period of time—eight months to two years—and during that period, engages, observes, and records the daily life of the studied culture, and theorises about the evolution and behaviours in that culture. Data is collected primarily via observational techniques, formal and informal interaction with participants in that culture, and personal field notes, while data analysis involves ‘sense-making’. The researcher must narrate her experience in great detail so that readers may experience that same culture without necessarily being there. The advantages of this approach are its sensitiveness to the context, the rich and nuanced understanding it generates, and minimal respondent bias. However, this is also an extremely time and resource-intensive approach, and findings are specific to a given culture and less generalisable to other cultures.
Selecting research designs
Given the above multitude of research designs, which design should researchers choose for their research? Generally speaking, researchers tend to select those research designs that they are most comfortable with and feel most competent to handle, but ideally, the choice should depend on the nature of the research phenomenon being studied. In the preliminary phases of research, when the research problem is unclear and the researcher wants to scope out the nature and extent of a certain research problem, a focus group (for an individual unit of analysis) or a case study (for an organisational unit of analysis) is an ideal strategy for exploratory research. As one delves further into the research domain, but finds that there are no good theories to explain the phenomenon of interest and wants to build a theory to fill in the unmet gap in that area, interpretive designs such as case research or ethnography may be useful designs. If competing theories exist and the researcher wishes to test these different theories or integrate them into a larger theory, positivist designs such as experimental design, survey research, or secondary data analysis are more appropriate.
Regardless of the specific research design chosen, the researcher should strive to collect quantitative and qualitative data using a combination of techniques such as questionnaires, interviews, observations, documents, or secondary data. For instance, even in a highly structured survey questionnaire, intended to collect quantitative data, the researcher may leave some room for a few open-ended questions to collect qualitative data that may generate unexpected insights not otherwise available from structured quantitative data alone. Likewise, while case research employ mostly face-to-face interviews to collect most qualitative data, the potential and value of collecting quantitative data should not be ignored. As an example, in a study of organisational decision-making processes, the case interviewer can record numeric quantities such as how many months it took to make certain organisational decisions, how many people were involved in that decision process, and how many decision alternatives were considered, which can provide valuable insights not otherwise available from interviewees’ narrative responses. Irrespective of the specific research design employed, the goal of the researcher should be to collect as much and as diverse data as possible that can help generate the best possible insights about the phenomenon of interest.
Social Science Research: Principles, Methods and Practices (Revised edition) Copyright © 2019 by Anol Bhattacherjee is licensed under a Creative Commons Attribution-NonCommercial-ShareAlike 4.0 International License , except where otherwise noted.
Share This Book

- Ask a Librarian
Research: Overview & Approaches
- Getting Started with Undergraduate Research
- Planning & Getting Started
- Building Your Knowledge Base
- Locating Sources
- Reading Scholarly Articles
- Creating a Literature Review
- Productivity & Organizing Research
- Scholarly and Professional Relationships
Introduction to Empirical Research
Databases for finding empirical research, guided search, google scholar, examples of empirical research, sources and further reading.
- Interpretive Research
- Action-Based Research
- Creative & Experimental Approaches
Your Librarian

- Introductory Video This video covers what empirical research is, what kinds of questions and methods empirical researchers use, and some tips for finding empirical research articles in your discipline.

- Guided Search: Finding Empirical Research Articles This is a hands-on tutorial that will allow you to use your own search terms to find resources.

- Study on radiation transfer in human skin for cosmetics
- Long-Term Mobile Phone Use and the Risk of Vestibular Schwannoma: A Danish Nationwide Cohort Study
- Emissions Impacts and Benefits of Plug-In Hybrid Electric Vehicles and Vehicle-to-Grid Services
- Review of design considerations and technological challenges for successful development and deployment of plug-in hybrid electric vehicles
- Endocrine disrupters and human health: could oestrogenic chemicals in body care cosmetics adversely affect breast cancer incidence in women?
- << Previous: Scholarly and Professional Relationships
- Next: Interpretive Research >>
- Last Updated: May 23, 2024 11:51 AM
- URL: https://guides.lib.purdue.edu/research_approaches

- University of Memphis Libraries
- Research Guides
Empirical Research: Defining, Identifying, & Finding
Defining empirical research, what is empirical research, quantitative or qualitative.
- Introduction
- Database Tools
- Search Terms
- Image Descriptions
Calfee & Chambliss (2005) (UofM login required) describe empirical research as a "systematic approach for answering certain types of questions." Those questions are answered "[t]hrough the collection of evidence under carefully defined and replicable conditions" (p. 43).
The evidence collected during empirical research is often referred to as "data."
Characteristics of Empirical Research
Emerald Publishing's guide to conducting empirical research identifies a number of common elements to empirical research:
- A research question , which will determine research objectives.
- A particular and planned design for the research, which will depend on the question and which will find ways of answering it with appropriate use of resources.
- The gathering of primary data , which is then analysed.
- A particular methodology for collecting and analysing the data, such as an experiment or survey.
- The limitation of the data to a particular group, area or time scale, known as a sample [emphasis added]: for example, a specific number of employees of a particular company type, or all users of a library over a given time scale. The sample should be somehow representative of a wider population.
- The ability to recreate the study and test the results. This is known as reliability .
- The ability to generalize from the findings to a larger sample and to other situations.
If you see these elements in a research article, you can feel confident that you have found empirical research. Emerald's guide goes into more detail on each element.
Empirical research methodologies can be described as quantitative, qualitative, or a mix of both (usually called mixed-methods).
Ruane (2016) (UofM login required) gets at the basic differences in approach between quantitative and qualitative research:
- Quantitative research -- an approach to documenting reality that relies heavily on numbers both for the measurement of variables and for data analysis (p. 33).
- Qualitative research -- an approach to documenting reality that relies on words and images as the primary data source (p. 33).
Both quantitative and qualitative methods are empirical . If you can recognize that a research study is quantitative or qualitative study, then you have also recognized that it is empirical study.
Below are information on the characteristics of quantitative and qualitative research. This video from Scribbr also offers a good overall introduction to the two approaches to research methodology:
Characteristics of Quantitative Research
Researchers test hypotheses, or theories, based in assumptions about causality, i.e. we expect variable X to cause variable Y. Variables have to be controlled as much as possible to ensure validity. The results explain the relationship between the variables. Measures are based in pre-defined instruments.
Examples: experimental or quasi-experimental design, pretest & post-test, survey or questionnaire with closed-ended questions. Studies that identify factors that influence an outcomes, the utility of an intervention, or understanding predictors of outcomes.
Characteristics of Qualitative Research
Researchers explore “meaning individuals or groups ascribe to social or human problems (Creswell & Creswell, 2018, p3).” Questions and procedures emerge rather than being prescribed. Complexity, nuance, and individual meaning are valued. Research is both inductive and deductive. Data sources are multiple and varied, i.e. interviews, observations, documents, photographs, etc. The researcher is a key instrument and must be reflective of their background, culture, and experiences as influential of the research.
Examples: open question interviews and surveys, focus groups, case studies, grounded theory, ethnography, discourse analysis, narrative, phenomenology, participatory action research.
Calfee, R. C. & Chambliss, M. (2005). The design of empirical research. In J. Flood, D. Lapp, J. R. Squire, & J. Jensen (Eds.), Methods of research on teaching the English language arts: The methodology chapters from the handbook of research on teaching the English language arts (pp. 43-78). Routledge. http://ezproxy.memphis.edu/login?url=http://search.ebscohost.com/login.aspx?direct=true&db=nlebk&AN=125955&site=eds-live&scope=site .
Creswell, J. W., & Creswell, J. D. (2018). Research design: Qualitative, quantitative, and mixed methods approaches (5th ed.). Thousand Oaks: Sage.
How to... conduct empirical research . (n.d.). Emerald Publishing. https://www.emeraldgrouppublishing.com/how-to/research-methods/conduct-empirical-research .
Scribbr. (2019). Quantitative vs. qualitative: The differences explained [video]. YouTube. https://www.youtube.com/watch?v=a-XtVF7Bofg .
Ruane, J. M. (2016). Introducing social research methods : Essentials for getting the edge . Wiley-Blackwell. http://ezproxy.memphis.edu/login?url=http://search.ebscohost.com/login.aspx?direct=true&db=nlebk&AN=1107215&site=eds-live&scope=site .
- << Previous: Home
- Next: Identifying Empirical Research >>
- Last Updated: Apr 2, 2024 11:25 AM
- URL: https://libguides.memphis.edu/empirical-research
What is Empirical Research? Definition, Methods, Examples
Appinio Research · 09.02.2024 · 36min read
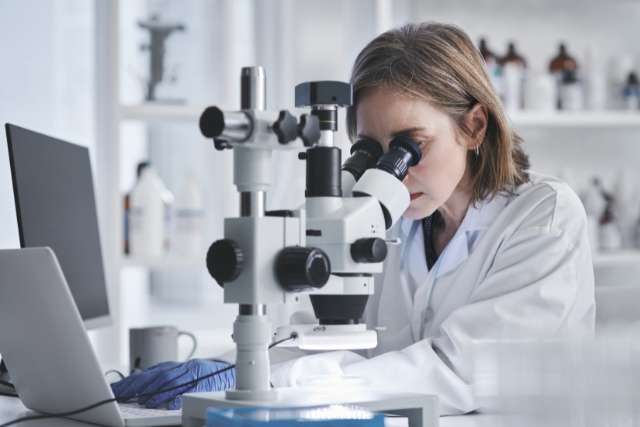
Ever wondered how we gather the facts, unveil hidden truths, and make informed decisions in a world filled with questions? Empirical research holds the key.
In this guide, we'll delve deep into the art and science of empirical research, unraveling its methods, mysteries, and manifold applications. From defining the core principles to mastering data analysis and reporting findings, we're here to equip you with the knowledge and tools to navigate the empirical landscape.
What is Empirical Research?
Empirical research is the cornerstone of scientific inquiry, providing a systematic and structured approach to investigating the world around us. It is the process of gathering and analyzing empirical or observable data to test hypotheses, answer research questions, or gain insights into various phenomena. This form of research relies on evidence derived from direct observation or experimentation, allowing researchers to draw conclusions based on real-world data rather than purely theoretical or speculative reasoning.
Characteristics of Empirical Research
Empirical research is characterized by several key features:
- Observation and Measurement : It involves the systematic observation or measurement of variables, events, or behaviors.
- Data Collection : Researchers collect data through various methods, such as surveys, experiments, observations, or interviews.
- Testable Hypotheses : Empirical research often starts with testable hypotheses that are evaluated using collected data.
- Quantitative or Qualitative Data : Data can be quantitative (numerical) or qualitative (non-numerical), depending on the research design.
- Statistical Analysis : Quantitative data often undergo statistical analysis to determine patterns , relationships, or significance.
- Objectivity and Replicability : Empirical research strives for objectivity, minimizing researcher bias . It should be replicable, allowing other researchers to conduct the same study to verify results.
- Conclusions and Generalizations : Empirical research generates findings based on data and aims to make generalizations about larger populations or phenomena.
Importance of Empirical Research
Empirical research plays a pivotal role in advancing knowledge across various disciplines. Its importance extends to academia, industry, and society as a whole. Here are several reasons why empirical research is essential:
- Evidence-Based Knowledge : Empirical research provides a solid foundation of evidence-based knowledge. It enables us to test hypotheses, confirm or refute theories, and build a robust understanding of the world.
- Scientific Progress : In the scientific community, empirical research fuels progress by expanding the boundaries of existing knowledge. It contributes to the development of theories and the formulation of new research questions.
- Problem Solving : Empirical research is instrumental in addressing real-world problems and challenges. It offers insights and data-driven solutions to complex issues in fields like healthcare, economics, and environmental science.
- Informed Decision-Making : In policymaking, business, and healthcare, empirical research informs decision-makers by providing data-driven insights. It guides strategies, investments, and policies for optimal outcomes.
- Quality Assurance : Empirical research is essential for quality assurance and validation in various industries, including pharmaceuticals, manufacturing, and technology. It ensures that products and processes meet established standards.
- Continuous Improvement : Businesses and organizations use empirical research to evaluate performance, customer satisfaction, and product effectiveness. This data-driven approach fosters continuous improvement and innovation.
- Human Advancement : Empirical research in fields like medicine and psychology contributes to the betterment of human health and well-being. It leads to medical breakthroughs, improved therapies, and enhanced psychological interventions.
- Critical Thinking and Problem Solving : Engaging in empirical research fosters critical thinking skills, problem-solving abilities, and a deep appreciation for evidence-based decision-making.
Empirical research empowers us to explore, understand, and improve the world around us. It forms the bedrock of scientific inquiry and drives progress in countless domains, shaping our understanding of both the natural and social sciences.
How to Conduct Empirical Research?
So, you've decided to dive into the world of empirical research. Let's begin by exploring the crucial steps involved in getting started with your research project.
1. Select a Research Topic
Selecting the right research topic is the cornerstone of a successful empirical study. It's essential to choose a topic that not only piques your interest but also aligns with your research goals and objectives. Here's how to go about it:
- Identify Your Interests : Start by reflecting on your passions and interests. What topics fascinate you the most? Your enthusiasm will be your driving force throughout the research process.
- Brainstorm Ideas : Engage in brainstorming sessions to generate potential research topics. Consider the questions you've always wanted to answer or the issues that intrigue you.
- Relevance and Significance : Assess the relevance and significance of your chosen topic. Does it contribute to existing knowledge? Is it a pressing issue in your field of study or the broader community?
- Feasibility : Evaluate the feasibility of your research topic. Do you have access to the necessary resources, data, and participants (if applicable)?
2. Formulate Research Questions
Once you've narrowed down your research topic, the next step is to formulate clear and precise research questions . These questions will guide your entire research process and shape your study's direction. To create effective research questions:
- Specificity : Ensure that your research questions are specific and focused. Vague or overly broad questions can lead to inconclusive results.
- Relevance : Your research questions should directly relate to your chosen topic. They should address gaps in knowledge or contribute to solving a particular problem.
- Testability : Ensure that your questions are testable through empirical methods. You should be able to gather data and analyze it to answer these questions.
- Avoid Bias : Craft your questions in a way that avoids leading or biased language. Maintain neutrality to uphold the integrity of your research.
3. Review Existing Literature
Before you embark on your empirical research journey, it's essential to immerse yourself in the existing body of literature related to your chosen topic. This step, often referred to as a literature review, serves several purposes:
- Contextualization : Understand the historical context and current state of research in your field. What have previous studies found, and what questions remain unanswered?
- Identifying Gaps : Identify gaps or areas where existing research falls short. These gaps will help you formulate meaningful research questions and hypotheses.
- Theory Development : If your study is theoretical, consider how existing theories apply to your topic. If it's empirical, understand how previous studies have approached data collection and analysis.
- Methodological Insights : Learn from the methodologies employed in previous research. What methods were successful, and what challenges did researchers face?
4. Define Variables
Variables are fundamental components of empirical research. They are the factors or characteristics that can change or be manipulated during your study. Properly defining and categorizing variables is crucial for the clarity and validity of your research. Here's what you need to know:
- Independent Variables : These are the variables that you, as the researcher, manipulate or control. They are the "cause" in cause-and-effect relationships.
- Dependent Variables : Dependent variables are the outcomes or responses that you measure or observe. They are the "effect" influenced by changes in independent variables.
- Operational Definitions : To ensure consistency and clarity, provide operational definitions for your variables. Specify how you will measure or manipulate each variable.
- Control Variables : In some studies, controlling for other variables that may influence your dependent variable is essential. These are known as control variables.
Understanding these foundational aspects of empirical research will set a solid foundation for the rest of your journey. Now that you've grasped the essentials of getting started, let's delve deeper into the intricacies of research design.
Empirical Research Design
Now that you've selected your research topic, formulated research questions, and defined your variables, it's time to delve into the heart of your empirical research journey – research design . This pivotal step determines how you will collect data and what methods you'll employ to answer your research questions. Let's explore the various facets of research design in detail.
Types of Empirical Research
Empirical research can take on several forms, each with its own unique approach and methodologies. Understanding the different types of empirical research will help you choose the most suitable design for your study. Here are some common types:
- Experimental Research : In this type, researchers manipulate one or more independent variables to observe their impact on dependent variables. It's highly controlled and often conducted in a laboratory setting.
- Observational Research : Observational research involves the systematic observation of subjects or phenomena without intervention. Researchers are passive observers, documenting behaviors, events, or patterns.
- Survey Research : Surveys are used to collect data through structured questionnaires or interviews. This method is efficient for gathering information from a large number of participants.
- Case Study Research : Case studies focus on in-depth exploration of one or a few cases. Researchers gather detailed information through various sources such as interviews, documents, and observations.
- Qualitative Research : Qualitative research aims to understand behaviors, experiences, and opinions in depth. It often involves open-ended questions, interviews, and thematic analysis.
- Quantitative Research : Quantitative research collects numerical data and relies on statistical analysis to draw conclusions. It involves structured questionnaires, experiments, and surveys.
Your choice of research type should align with your research questions and objectives. Experimental research, for example, is ideal for testing cause-and-effect relationships, while qualitative research is more suitable for exploring complex phenomena.
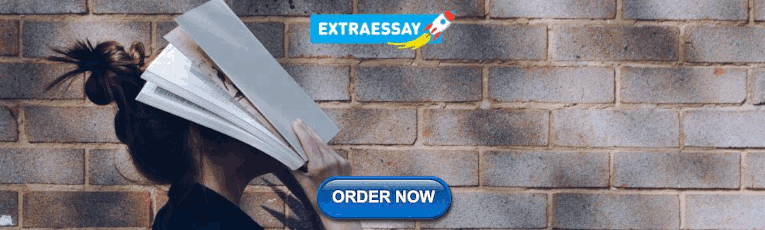
Experimental Design
Experimental research is a systematic approach to studying causal relationships. It's characterized by the manipulation of one or more independent variables while controlling for other factors. Here are some key aspects of experimental design:
- Control and Experimental Groups : Participants are randomly assigned to either a control group or an experimental group. The independent variable is manipulated for the experimental group but not for the control group.
- Randomization : Randomization is crucial to eliminate bias in group assignment. It ensures that each participant has an equal chance of being in either group.
- Hypothesis Testing : Experimental research often involves hypothesis testing. Researchers formulate hypotheses about the expected effects of the independent variable and use statistical analysis to test these hypotheses.
Observational Design
Observational research entails careful and systematic observation of subjects or phenomena. It's advantageous when you want to understand natural behaviors or events. Key aspects of observational design include:
- Participant Observation : Researchers immerse themselves in the environment they are studying. They become part of the group being observed, allowing for a deep understanding of behaviors.
- Non-Participant Observation : In non-participant observation, researchers remain separate from the subjects. They observe and document behaviors without direct involvement.
- Data Collection Methods : Observational research can involve various data collection methods, such as field notes, video recordings, photographs, or coding of observed behaviors.
Survey Design
Surveys are a popular choice for collecting data from a large number of participants. Effective survey design is essential to ensure the validity and reliability of your data. Consider the following:
- Questionnaire Design : Create clear and concise questions that are easy for participants to understand. Avoid leading or biased questions.
- Sampling Methods : Decide on the appropriate sampling method for your study, whether it's random, stratified, or convenience sampling.
- Data Collection Tools : Choose the right tools for data collection, whether it's paper surveys, online questionnaires, or face-to-face interviews.
Case Study Design
Case studies are an in-depth exploration of one or a few cases to gain a deep understanding of a particular phenomenon. Key aspects of case study design include:
- Single Case vs. Multiple Case Studies : Decide whether you'll focus on a single case or multiple cases. Single case studies are intensive and allow for detailed examination, while multiple case studies provide comparative insights.
- Data Collection Methods : Gather data through interviews, observations, document analysis, or a combination of these methods.
Qualitative vs. Quantitative Research
In empirical research, you'll often encounter the distinction between qualitative and quantitative research . Here's a closer look at these two approaches:
- Qualitative Research : Qualitative research seeks an in-depth understanding of human behavior, experiences, and perspectives. It involves open-ended questions, interviews, and the analysis of textual or narrative data. Qualitative research is exploratory and often used when the research question is complex and requires a nuanced understanding.
- Quantitative Research : Quantitative research collects numerical data and employs statistical analysis to draw conclusions. It involves structured questionnaires, experiments, and surveys. Quantitative research is ideal for testing hypotheses and establishing cause-and-effect relationships.
Understanding the various research design options is crucial in determining the most appropriate approach for your study. Your choice should align with your research questions, objectives, and the nature of the phenomenon you're investigating.
Data Collection for Empirical Research
Now that you've established your research design, it's time to roll up your sleeves and collect the data that will fuel your empirical research. Effective data collection is essential for obtaining accurate and reliable results.
Sampling Methods
Sampling methods are critical in empirical research, as they determine the subset of individuals or elements from your target population that you will study. Here are some standard sampling methods:
- Random Sampling : Random sampling ensures that every member of the population has an equal chance of being selected. It minimizes bias and is often used in quantitative research.
- Stratified Sampling : Stratified sampling involves dividing the population into subgroups or strata based on specific characteristics (e.g., age, gender, location). Samples are then randomly selected from each stratum, ensuring representation of all subgroups.
- Convenience Sampling : Convenience sampling involves selecting participants who are readily available or easily accessible. While it's convenient, it may introduce bias and limit the generalizability of results.
- Snowball Sampling : Snowball sampling is instrumental when studying hard-to-reach or hidden populations. One participant leads you to another, creating a "snowball" effect. This method is common in qualitative research.
- Purposive Sampling : In purposive sampling, researchers deliberately select participants who meet specific criteria relevant to their research questions. It's often used in qualitative studies to gather in-depth information.
The choice of sampling method depends on the nature of your research, available resources, and the degree of precision required. It's crucial to carefully consider your sampling strategy to ensure that your sample accurately represents your target population.
Data Collection Instruments
Data collection instruments are the tools you use to gather information from your participants or sources. These instruments should be designed to capture the data you need accurately. Here are some popular data collection instruments:
- Questionnaires : Questionnaires consist of structured questions with predefined response options. When designing questionnaires, consider the clarity of questions, the order of questions, and the response format (e.g., Likert scale , multiple-choice).
- Interviews : Interviews involve direct communication between the researcher and participants. They can be structured (with predetermined questions) or unstructured (open-ended). Effective interviews require active listening and probing for deeper insights.
- Observations : Observations entail systematically and objectively recording behaviors, events, or phenomena. Researchers must establish clear criteria for what to observe, how to record observations, and when to observe.
- Surveys : Surveys are a common data collection instrument for quantitative research. They can be administered through various means, including online surveys, paper surveys, and telephone surveys.
- Documents and Archives : In some cases, data may be collected from existing documents, records, or archives. Ensure that the sources are reliable, relevant, and properly documented.
To streamline your process and gather insights with precision and efficiency, consider leveraging innovative tools like Appinio . With Appinio's intuitive platform, you can harness the power of real-time consumer data to inform your research decisions effectively. Whether you're conducting surveys, interviews, or observations, Appinio empowers you to define your target audience, collect data from diverse demographics, and analyze results seamlessly.
By incorporating Appinio into your data collection toolkit, you can unlock a world of possibilities and elevate the impact of your empirical research. Ready to revolutionize your approach to data collection?
Book a Demo
Data Collection Procedures
Data collection procedures outline the step-by-step process for gathering data. These procedures should be meticulously planned and executed to maintain the integrity of your research.
- Training : If you have a research team, ensure that they are trained in data collection methods and protocols. Consistency in data collection is crucial.
- Pilot Testing : Before launching your data collection, conduct a pilot test with a small group to identify any potential problems with your instruments or procedures. Make necessary adjustments based on feedback.
- Data Recording : Establish a systematic method for recording data. This may include timestamps, codes, or identifiers for each data point.
- Data Security : Safeguard the confidentiality and security of collected data. Ensure that only authorized individuals have access to the data.
- Data Storage : Properly organize and store your data in a secure location, whether in physical or digital form. Back up data to prevent loss.
Ethical Considerations
Ethical considerations are paramount in empirical research, as they ensure the well-being and rights of participants are protected.
- Informed Consent : Obtain informed consent from participants, providing clear information about the research purpose, procedures, risks, and their right to withdraw at any time.
- Privacy and Confidentiality : Protect the privacy and confidentiality of participants. Ensure that data is anonymized and sensitive information is kept confidential.
- Beneficence : Ensure that your research benefits participants and society while minimizing harm. Consider the potential risks and benefits of your study.
- Honesty and Integrity : Conduct research with honesty and integrity. Report findings accurately and transparently, even if they are not what you expected.
- Respect for Participants : Treat participants with respect, dignity, and sensitivity to cultural differences. Avoid any form of coercion or manipulation.
- Institutional Review Board (IRB) : If required, seek approval from an IRB or ethics committee before conducting your research, particularly when working with human participants.
Adhering to ethical guidelines is not only essential for the ethical conduct of research but also crucial for the credibility and validity of your study. Ethical research practices build trust between researchers and participants and contribute to the advancement of knowledge with integrity.
With a solid understanding of data collection, including sampling methods, instruments, procedures, and ethical considerations, you are now well-equipped to gather the data needed to answer your research questions.
Empirical Research Data Analysis
Now comes the exciting phase of data analysis, where the raw data you've diligently collected starts to yield insights and answers to your research questions. We will explore the various aspects of data analysis, from preparing your data to drawing meaningful conclusions through statistics and visualization.
Data Preparation
Data preparation is the crucial first step in data analysis. It involves cleaning, organizing, and transforming your raw data into a format that is ready for analysis. Effective data preparation ensures the accuracy and reliability of your results.
- Data Cleaning : Identify and rectify errors, missing values, and inconsistencies in your dataset. This may involve correcting typos, removing outliers, and imputing missing data.
- Data Coding : Assign numerical values or codes to categorical variables to make them suitable for statistical analysis. For example, converting "Yes" and "No" to 1 and 0.
- Data Transformation : Transform variables as needed to meet the assumptions of the statistical tests you plan to use. Common transformations include logarithmic or square root transformations.
- Data Integration : If your data comes from multiple sources, integrate it into a unified dataset, ensuring that variables match and align.
- Data Documentation : Maintain clear documentation of all data preparation steps, as well as the rationale behind each decision. This transparency is essential for replicability.
Effective data preparation lays the foundation for accurate and meaningful analysis. It allows you to trust the results that will follow in the subsequent stages.
Descriptive Statistics
Descriptive statistics help you summarize and make sense of your data by providing a clear overview of its key characteristics. These statistics are essential for understanding the central tendencies, variability, and distribution of your variables. Descriptive statistics include:
- Measures of Central Tendency : These include the mean (average), median (middle value), and mode (most frequent value). They help you understand the typical or central value of your data.
- Measures of Dispersion : Measures like the range, variance, and standard deviation provide insights into the spread or variability of your data points.
- Frequency Distributions : Creating frequency distributions or histograms allows you to visualize the distribution of your data across different values or categories.
Descriptive statistics provide the initial insights needed to understand your data's basic characteristics, which can inform further analysis.
Inferential Statistics
Inferential statistics take your analysis to the next level by allowing you to make inferences or predictions about a larger population based on your sample data. These methods help you test hypotheses and draw meaningful conclusions. Key concepts in inferential statistics include:
- Hypothesis Testing : Hypothesis tests (e.g., t-tests, chi-squared tests) help you determine whether observed differences or associations in your data are statistically significant or occurred by chance.
- Confidence Intervals : Confidence intervals provide a range within which population parameters (e.g., population mean) are likely to fall based on your sample data.
- Regression Analysis : Regression models (linear, logistic, etc.) help you explore relationships between variables and make predictions.
- Analysis of Variance (ANOVA) : ANOVA tests are used to compare means between multiple groups, allowing you to assess whether differences are statistically significant.
Inferential statistics are powerful tools for drawing conclusions from your data and assessing the generalizability of your findings to the broader population.
Qualitative Data Analysis
Qualitative data analysis is employed when working with non-numerical data, such as text, interviews, or open-ended survey responses. It focuses on understanding the underlying themes, patterns, and meanings within qualitative data. Qualitative analysis techniques include:
- Thematic Analysis : Identifying and analyzing recurring themes or patterns within textual data.
- Content Analysis : Categorizing and coding qualitative data to extract meaningful insights.
- Grounded Theory : Developing theories or frameworks based on emergent themes from the data.
- Narrative Analysis : Examining the structure and content of narratives to uncover meaning.
Qualitative data analysis provides a rich and nuanced understanding of complex phenomena and human experiences.
Data Visualization
Data visualization is the art of representing data graphically to make complex information more understandable and accessible. Effective data visualization can reveal patterns, trends, and outliers in your data. Common types of data visualization include:
- Bar Charts and Histograms : Used to display the distribution of categorical data or discrete data .
- Line Charts : Ideal for showing trends and changes in data over time.
- Scatter Plots : Visualize relationships and correlations between two variables.
- Pie Charts : Display the composition of a whole in terms of its parts.
- Heatmaps : Depict patterns and relationships in multidimensional data through color-coding.
- Box Plots : Provide a summary of the data distribution, including outliers.
- Interactive Dashboards : Create dynamic visualizations that allow users to explore data interactively.
Data visualization not only enhances your understanding of the data but also serves as a powerful communication tool to convey your findings to others.
As you embark on the data analysis phase of your empirical research, remember that the specific methods and techniques you choose will depend on your research questions, data type, and objectives. Effective data analysis transforms raw data into valuable insights, bringing you closer to the answers you seek.
How to Report Empirical Research Results?
At this stage, you get to share your empirical research findings with the world. Effective reporting and presentation of your results are crucial for communicating your research's impact and insights.
1. Write the Research Paper
Writing a research paper is the culmination of your empirical research journey. It's where you synthesize your findings, provide context, and contribute to the body of knowledge in your field.
- Title and Abstract : Craft a clear and concise title that reflects your research's essence. The abstract should provide a brief summary of your research objectives, methods, findings, and implications.
- Introduction : In the introduction, introduce your research topic, state your research questions or hypotheses, and explain the significance of your study. Provide context by discussing relevant literature.
- Methods : Describe your research design, data collection methods, and sampling procedures. Be precise and transparent, allowing readers to understand how you conducted your study.
- Results : Present your findings in a clear and organized manner. Use tables, graphs, and statistical analyses to support your results. Avoid interpreting your findings in this section; focus on the presentation of raw data.
- Discussion : Interpret your findings and discuss their implications. Relate your results to your research questions and the existing literature. Address any limitations of your study and suggest avenues for future research.
- Conclusion : Summarize the key points of your research and its significance. Restate your main findings and their implications.
- References : Cite all sources used in your research following a specific citation style (e.g., APA, MLA, Chicago). Ensure accuracy and consistency in your citations.
- Appendices : Include any supplementary material, such as questionnaires, data coding sheets, or additional analyses, in the appendices.
Writing a research paper is a skill that improves with practice. Ensure clarity, coherence, and conciseness in your writing to make your research accessible to a broader audience.
2. Create Visuals and Tables
Visuals and tables are powerful tools for presenting complex data in an accessible and understandable manner.
- Clarity : Ensure that your visuals and tables are clear and easy to interpret. Use descriptive titles and labels.
- Consistency : Maintain consistency in formatting, such as font size and style, across all visuals and tables.
- Appropriateness : Choose the most suitable visual representation for your data. Bar charts, line graphs, and scatter plots work well for different types of data.
- Simplicity : Avoid clutter and unnecessary details. Focus on conveying the main points.
- Accessibility : Make sure your visuals and tables are accessible to a broad audience, including those with visual impairments.
- Captions : Include informative captions that explain the significance of each visual or table.
Compelling visuals and tables enhance the reader's understanding of your research and can be the key to conveying complex information efficiently.
3. Interpret Findings
Interpreting your findings is where you bridge the gap between data and meaning. It's your opportunity to provide context, discuss implications, and offer insights. When interpreting your findings:
- Relate to Research Questions : Discuss how your findings directly address your research questions or hypotheses.
- Compare with Literature : Analyze how your results align with or deviate from previous research in your field. What insights can you draw from these comparisons?
- Discuss Limitations : Be transparent about the limitations of your study. Address any constraints, biases, or potential sources of error.
- Practical Implications : Explore the real-world implications of your findings. How can they be applied or inform decision-making?
- Future Research Directions : Suggest areas for future research based on the gaps or unanswered questions that emerged from your study.
Interpreting findings goes beyond simply presenting data; it's about weaving a narrative that helps readers grasp the significance of your research in the broader context.
With your research paper written, structured, and enriched with visuals, and your findings expertly interpreted, you are now prepared to communicate your research effectively. Sharing your insights and contributing to the body of knowledge in your field is a significant accomplishment in empirical research.
Examples of Empirical Research
To solidify your understanding of empirical research, let's delve into some real-world examples across different fields. These examples will illustrate how empirical research is applied to gather data, analyze findings, and draw conclusions.
Social Sciences
In the realm of social sciences, consider a sociological study exploring the impact of socioeconomic status on educational attainment. Researchers gather data from a diverse group of individuals, including their family backgrounds, income levels, and academic achievements.
Through statistical analysis, they can identify correlations and trends, revealing whether individuals from lower socioeconomic backgrounds are less likely to attain higher levels of education. This empirical research helps shed light on societal inequalities and informs policymakers on potential interventions to address disparities in educational access.
Environmental Science
Environmental scientists often employ empirical research to assess the effects of environmental changes. For instance, researchers studying the impact of climate change on wildlife might collect data on animal populations, weather patterns, and habitat conditions over an extended period.
By analyzing this empirical data, they can identify correlations between climate fluctuations and changes in wildlife behavior, migration patterns, or population sizes. This empirical research is crucial for understanding the ecological consequences of climate change and informing conservation efforts.
Business and Economics
In the business world, empirical research is essential for making data-driven decisions. Consider a market research study conducted by a business seeking to launch a new product. They collect data through surveys , focus groups , and consumer behavior analysis.
By examining this empirical data, the company can gauge consumer preferences, demand, and potential market size. Empirical research in business helps guide product development, pricing strategies, and marketing campaigns, increasing the likelihood of a successful product launch.
Psychological studies frequently rely on empirical research to understand human behavior and cognition. For instance, a psychologist interested in examining the impact of stress on memory might design an experiment. Participants are exposed to stress-inducing situations, and their memory performance is assessed through various tasks.
By analyzing the data collected, the psychologist can determine whether stress has a significant effect on memory recall. This empirical research contributes to our understanding of the complex interplay between psychological factors and cognitive processes.
These examples highlight the versatility and applicability of empirical research across diverse fields. Whether in medicine, social sciences, environmental science, business, or psychology, empirical research serves as a fundamental tool for gaining insights, testing hypotheses, and driving advancements in knowledge and practice.
Conclusion for Empirical Research
Empirical research is a powerful tool for gaining insights, testing hypotheses, and making informed decisions. By following the steps outlined in this guide, you've learned how to select research topics, collect data, analyze findings, and effectively communicate your research to the world. Remember, empirical research is a journey of discovery, and each step you take brings you closer to a deeper understanding of the world around you. Whether you're a scientist, a student, or someone curious about the process, the principles of empirical research empower you to explore, learn, and contribute to the ever-expanding realm of knowledge.
How to Collect Data for Empirical Research?
Introducing Appinio , the real-time market research platform revolutionizing how companies gather consumer insights for their empirical research endeavors. With Appinio, you can conduct your own market research in minutes, gaining valuable data to fuel your data-driven decisions.
Appinio is more than just a market research platform; it's a catalyst for transforming the way you approach empirical research, making it exciting, intuitive, and seamlessly integrated into your decision-making process.
Here's why Appinio is the go-to solution for empirical research:
- From Questions to Insights in Minutes : With Appinio's streamlined process, you can go from formulating your research questions to obtaining actionable insights in a matter of minutes, saving you time and effort.
- Intuitive Platform for Everyone : No need for a PhD in research; Appinio's platform is designed to be intuitive and user-friendly, ensuring that anyone can navigate and utilize it effectively.
- Rapid Response Times : With an average field time of under 23 minutes for 1,000 respondents, Appinio delivers rapid results, allowing you to gather data swiftly and efficiently.
- Global Reach with Targeted Precision : With access to over 90 countries and the ability to define target groups based on 1200+ characteristics, Appinio empowers you to reach your desired audience with precision and ease.
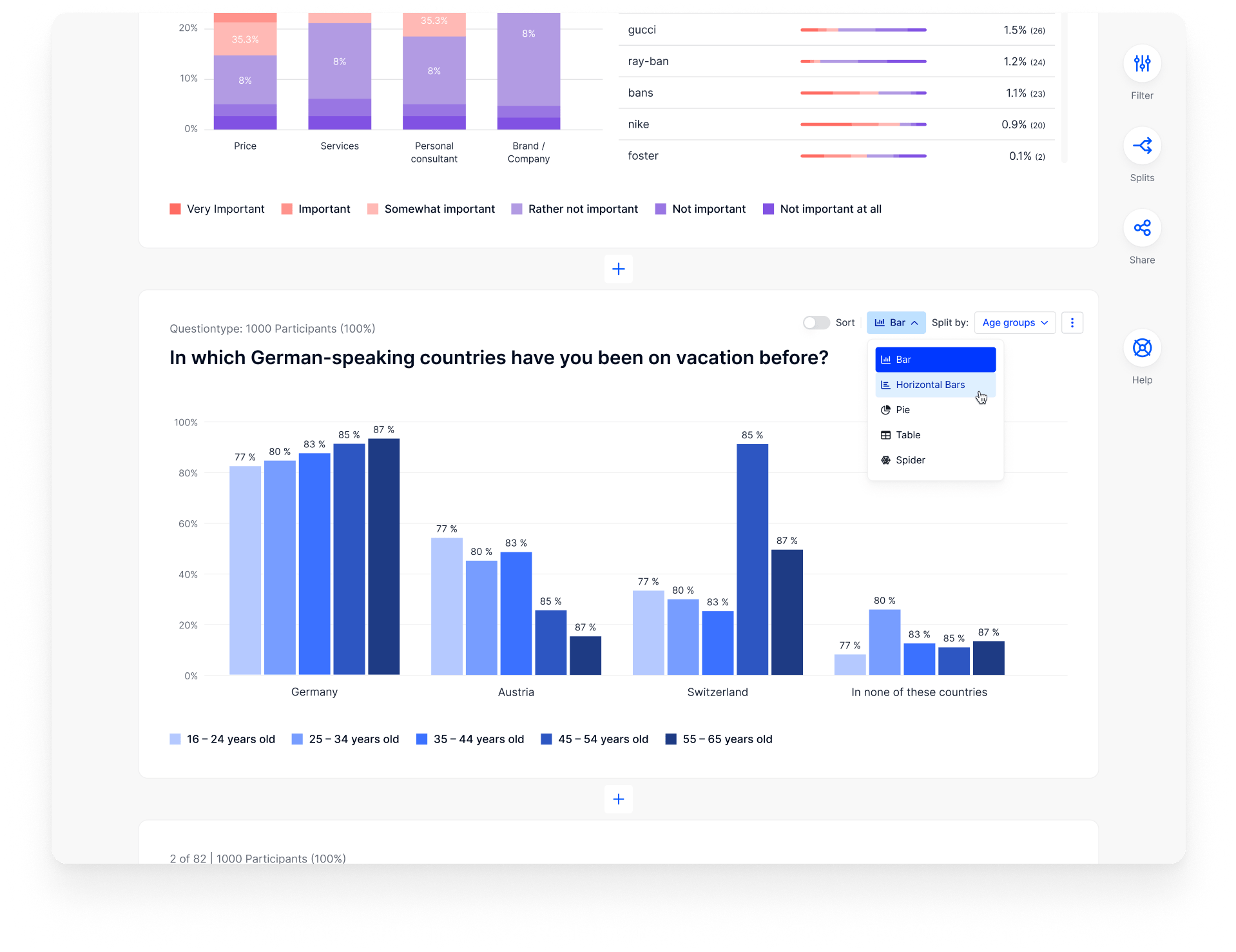
Get free access to the platform!
Join the loop 💌
Be the first to hear about new updates, product news, and data insights. We'll send it all straight to your inbox.
Get the latest market research news straight to your inbox! 💌
Wait, there's more
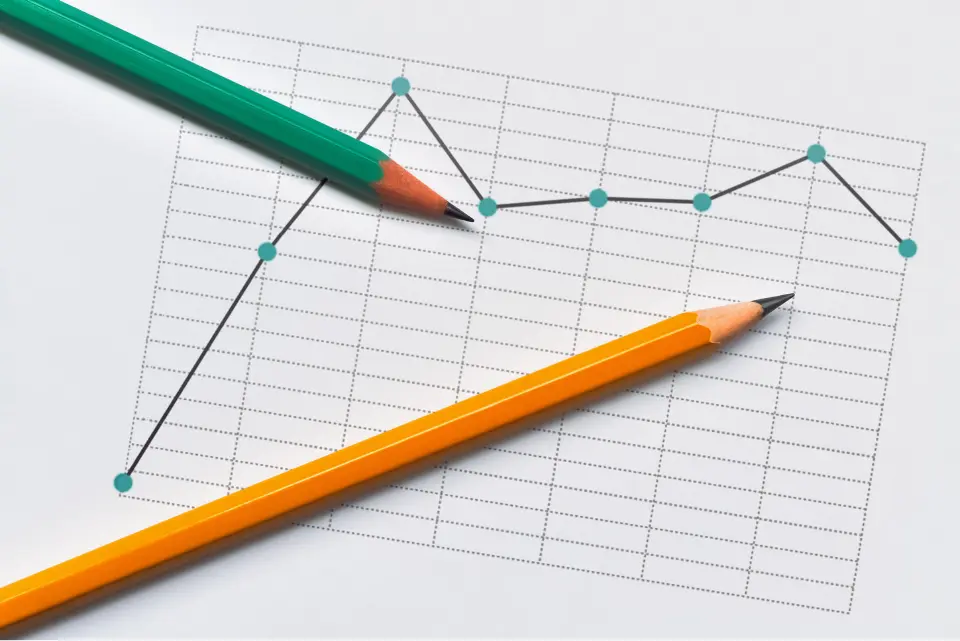
16.05.2024 | 30min read
Time Series Analysis: Definition, Types, Techniques, Examples
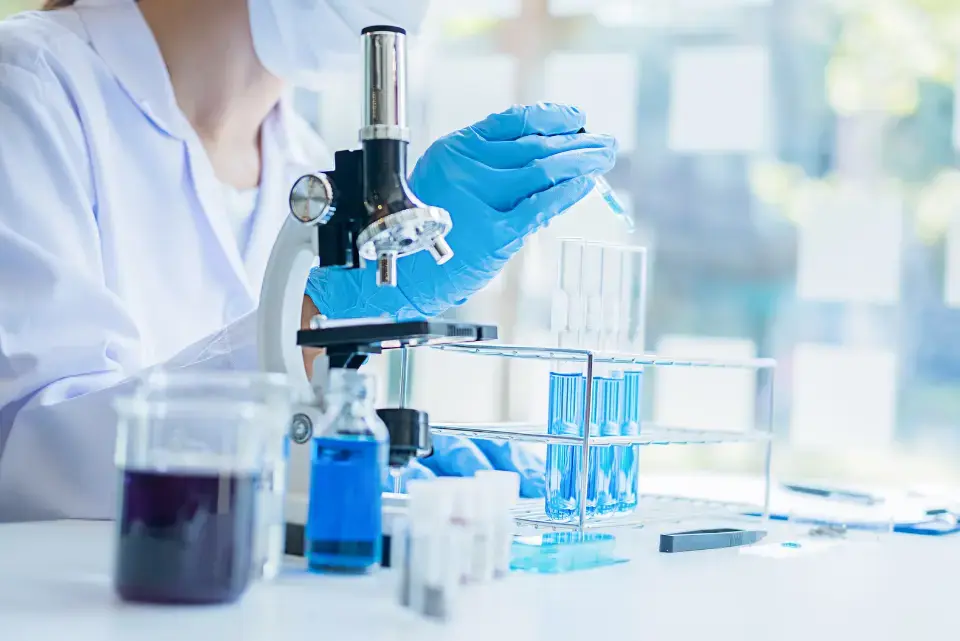
14.05.2024 | 31min read
Experimental Research: Definition, Types, Design, Examples
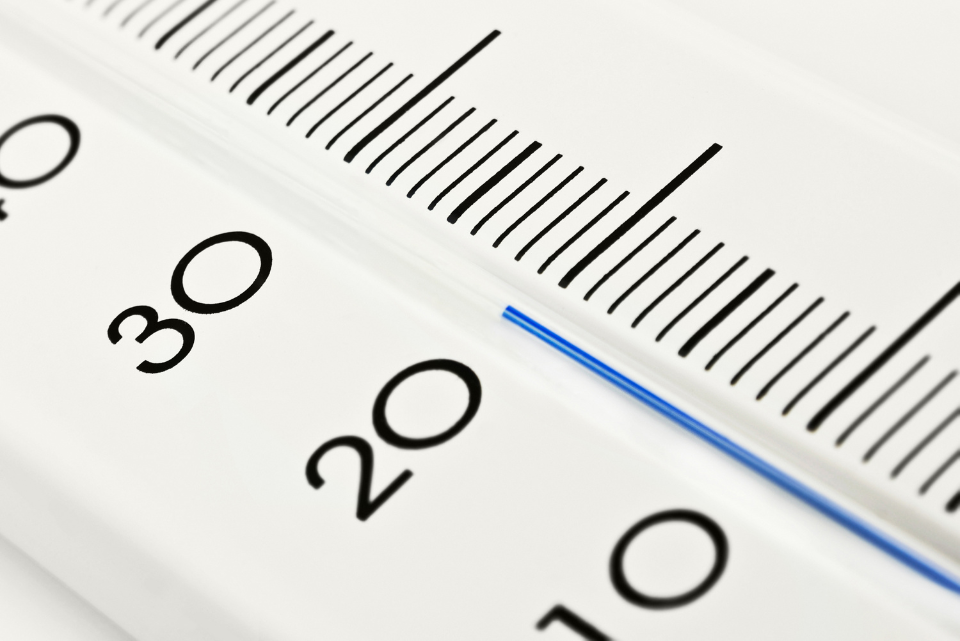
07.05.2024 | 29min read
Interval Scale: Definition, Characteristics, Examples
Penn State University Libraries
Empirical research in the social sciences and education.
- What is Empirical Research and How to Read It
- Finding Empirical Research in Library Databases
- Designing Empirical Research
- Ethics, Cultural Responsiveness, and Anti-Racism in Research
- Citing, Writing, and Presenting Your Work
Contact the Librarian at your campus for more help!

Introduction: What is Empirical Research?
Empirical research is based on observed and measured phenomena and derives knowledge from actual experience rather than from theory or belief.
How do you know if a study is empirical? Read the subheadings within the article, book, or report and look for a description of the research "methodology." Ask yourself: Could I recreate this study and test these results?
Key characteristics to look for:
- Specific research questions to be answered
- Definition of the population, behavior, or phenomena being studied
- Description of the process used to study this population or phenomena, including selection criteria, controls, and testing instruments (such as surveys)
Another hint: some scholarly journals use a specific layout, called the "IMRaD" format, to communicate empirical research findings. Such articles typically have 4 components:
- Introduction : sometimes called "literature review" -- what is currently known about the topic -- usually includes a theoretical framework and/or discussion of previous studies
- Methodology: sometimes called "research design" -- how to recreate the study -- usually describes the population, research process, and analytical tools used in the present study
- Results : sometimes called "findings" -- what was learned through the study -- usually appears as statistical data or as substantial quotations from research participants
- Discussion : sometimes called "conclusion" or "implications" -- why the study is important -- usually describes how the research results influence professional practices or future studies
Reading and Evaluating Scholarly Materials
Reading research can be a challenge. However, the tutorials and videos below can help. They explain what scholarly articles look like, how to read them, and how to evaluate them:
- CRAAP Checklist A frequently-used checklist that helps you examine the currency, relevance, authority, accuracy, and purpose of an information source.
- IF I APPLY A newer model of evaluating sources which encourages you to think about your own biases as a reader, as well as concerns about the item you are reading.
- Credo Video: How to Read Scholarly Materials (4 min.)
- Credo Tutorial: How to Read Scholarly Materials
- Credo Tutorial: Evaluating Information
- Credo Video: Evaluating Statistics (4 min.)
- Next: Finding Empirical Research in Library Databases >>
- Last Updated: Feb 18, 2024 8:33 PM
- URL: https://guides.libraries.psu.edu/emp
Empirical Design
- Living reference work entry
- First Online: 20 November 2018
- Cite this living reference work entry
- Xiafei Ma 4 &
- Wei Ping 4
99 Accesses
Concept design ; Contract design ; Design spiral ; Detailed design ; Optimal design ; Preliminary design ; Sequential engineering ; Technical design
Empirical design is a design method generally characterized by the collection of a large amount of data before much speculation as to their significance, or without much idea of what to expect, and is to be contrasted with more theoretical methods in which the collection of empirical data is guided largely by preliminary theoretical exploration of what to expect. The empirical method is necessary in entering hitherto completely unexplored fields and becomes less purely empirical as the acquired mastery of the field increases. Successful use of an exclusively empirical method demands a higher degree of intuitive ability in the practitioner (from Access Science @McGraw-Hill).
Scientific Fundamentals
What is empirical method.
The word “empirical” means “based only on observation or experiment.” So “empirical evidence” is evidence...
This is a preview of subscription content, log in via an institution to check access.
Access this chapter
Institutional subscriptions
Bei X, Chen N, Zhang S (2013) On the complexity of trial and error, STOC
Google Scholar
Bridgman PW, Holton G (2000) Empirical method. Access Science @ McGraw-Hill, New York. https://doi.org/10.1036/1097-8542.231000 . http://www.accessscience.com . Accessed 10 Apr 2000
https://en.wikipedia.org
Download references
Author information
Authors and affiliations.
Shanghai Jiao Tong University, Shanghai Jiao Tong University Underwater Engineering Insititute Co., Ltd., Shanghai, China
Xiafei Ma & Wei Ping
You can also search for this author in PubMed Google Scholar
Corresponding author
Correspondence to Xiafei Ma .
Editor information
Editors and affiliations.
Hadal Science and Technology Research Center, Shanghai Ocean University, Shanghai, China
Weicheng Cui
School of Naval Architecture, Ocean and Civil Engineering, Shanghai Jiao Tong University , Shanghai, China
School of Marine Science and Technology, Newcastle University , Newcastle, UK
Zhiqiang Hu
Section Editor information
Rights and permissions.
Reprints and permissions
Copyright information
© 2019 Springer Nature Singapore Pte Ltd.
About this entry
Cite this entry.
Ma, X., Ping, W. (2019). Empirical Design. In: Cui, W., Fu, S., Hu, Z. (eds) Encyclopedia of Ocean Engineering. Springer, Singapore. https://doi.org/10.1007/978-981-10-6963-5_62-1
Download citation
DOI : https://doi.org/10.1007/978-981-10-6963-5_62-1
Received : 20 August 2018
Accepted : 11 September 2018
Published : 20 November 2018
Publisher Name : Springer, Singapore
Print ISBN : 978-981-10-6963-5
Online ISBN : 978-981-10-6963-5
eBook Packages : Springer Reference Engineering Reference Module Computer Science and Engineering
- Publish with us
Policies and ethics
- Find a journal
- Track your research
Click through the PLOS taxonomy to find articles in your field.
For more information about PLOS Subject Areas, click here .
Loading metrics
Open Access
Peer-reviewed
Research Article
GIS for empirical research design: An illustration with georeferenced point data
Roles Conceptualization, Data curation, Formal analysis, Funding acquisition, Investigation, Methodology, Project administration, Resources, Software, Supervision, Validation, Visualization, Writing – original draft, Writing – review & editing
* E-mail: [email protected]
Affiliation Center for Southeast Asian Studies, Kyoto University, Kyoto, Japan

Roles Conceptualization, Funding acquisition, Investigation, Methodology, Project administration, Resources, Supervision, Validation, Visualization, Writing – original draft, Writing – review & editing
Affiliation Graduate School of Economics, University of Tokyo, Tokyo, Japan
- Katsuo Kogure,
- Yoshito Takasaki
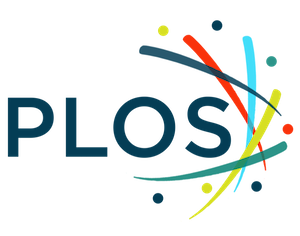
- Published: March 4, 2019
- https://doi.org/10.1371/journal.pone.0212316
- Reader Comments
This paper demonstrates how Geographic Information Systems (GIS) can be utilized to study the effects of spatial phenomena. Since experimental designs such as Randomized Controlled Trials are generally not feasible for spatial problems, researchers need to rely on quasi-experimental approaches using observational data. We provide a regression-based framework of the key procedures for GIS-based empirical research design using georeferenced point data for both spatial events of interest and subjects exposed to the events. We illustrate its utility and implementation through a case study on the impacts of the Cambodian genocide under the Pol Pot regime on post-conflict education.
Citation: Kogure K, Takasaki Y (2019) GIS for empirical research design: An illustration with georeferenced point data. PLoS ONE 14(3): e0212316. https://doi.org/10.1371/journal.pone.0212316
Editor: Roee Gutman, Brown University, UNITED STATES
Received: May 15, 2018; Accepted: January 31, 2019; Published: March 4, 2019
Copyright: © 2019 Kogure, Takasaki. This is an open access article distributed under the terms of the Creative Commons Attribution License , which permits unrestricted use, distribution, and reproduction in any medium, provided the original author and source are credited.
Data Availability: All relevant data are within the paper and its Supporting Information files.
Funding: This study is supported by Grants-in-Aid for Scientific Research No. 15K17044, No. 25257106, and No. 18H05312, Japan Society for the Promotion of Science ( https://kaken.nii.ac.jp/ja/grant/KAKENHI-PROJECT-15K17044/ , https://kaken.nii.ac.jp/ja/grant/KAKENHI-PROJECT-25257106/ , https://kaken.nii.ac.jp/ja/grant/KAKENHI-PROJECT-18H05312/ ). The funders had no role in study design, data collection and analysis, decision to publish, or preparation of the manuscript.
Competing interests: The authors have declared that no competing interests exist.
Introduction
With the growing availability of spatial data from Global Positioning Systems (GPS) and remote sensing, Geographic Information Systems (GIS)—computer systems designed to gather, store, manage, display, and analyze spatial data—have become an important tool in geographical, environmental, health, and social science research [ 1 , 2 ]. Although GIS has been used to visualize, process, create, and analyze spatial data across disciplines, its full potential has yet to be realized [ 3 , 4 ]. This paper demonstrates how GIS can help in designing empirical research to study the effects of spatial phenomena. Although investigating causal questions is one of the most important research themes across disciplines [ 5 ], it has received limited attention in spatial contexts [ 6 , 7 ]. The gold standard for answering causal questions is Randomized Controlled Trials, which can identify the causal effects of interest through random variation in treatment variables generated by researchers. In spatial contexts, this experimental approach is generally not feasible, so researchers need to rely on a quasi-experimental approach using observational data to approximate an experimental study [ 8 ].
Spatial data can be classified into three types: (1) geostatistical data collected over a continuous spatial domain (e.g., temperature), (2) area/lattice data collected on a regular or irregular lattice with well-defined boundaries (e.g., population density in an administrative area), and (3) point pattern data consisting of the observed locations of events/objects of interest (e.g., schools) [ 9 ]. Focusing on point pattern data, we consider the occurrence of particular ‘events’ as ‘treatments’ in the literature of causal analysis. This way of formulating an empirical question is a new perspective in the literature that enables empirical researchers to connect spatial statistics with causal analysis.
Our general goal is to evaluate the impacts of the occurrence of spatial events (treatments) during a specific period on local subjects. We consider the following situation/setting where researchers can use GIS to design credible and transparent empirical research. First, the impacts of spatial events are limited to the surrounding local areas. Such events may include the placement of local public goods (e.g., schools, health facilities, clean water facilities) and the occurrence of environmental, epidemiological, political, social, and cultural events (e.g., point source pollution, contagious disease, local conflicts, local beliefs/norms). Second, point pattern data of both events of interest and subjects exposed to the events are available. Although this presumption may not be satisfied in many cases at the moment, more and more household and field surveys have collected georeferenced information using a smartphone or tablet with an integrated GPS chip-set [ 10 ]. Spatial point data will become even more widely available in the near future, despite continuing privacy concerns [ 11 ]. Regarding the two sets of spatial point data, we assume that while the data-generating process of events follows some form of stochastic mechanism, the locations of subjects are fixed: Local residents do not move/relocate in response to the spatial events of interest (or the migration patterns of subjects are similar around the locations of events if migration is prevalent). Although how reasonable this assumption is depends on empirical contexts, it serves as a benchmark setting.
The key question in estimating the treatment effects in spatial contexts is how to address the threat of unobserved spatial confounding factors. Following the potential outcomes framework [ 12 ] for a binary treatment variable, extant studies use covariates and spatial (geographic) information—as a proxy for unobserved spatial confounding factors—to construct matched pairs of treated and control units. Specifically, one study [ 13 ] proposes an integer programing method that matches directly on covariates and spatial proximity (distance) and other study [ 14 ] proposes distance adjusted propensity score that combines spatial proximity with propensity score.
Following the strategy of exploiting spatial proximity for comparison, we (1) limit a global sample to spatially limited (local) samples using point buffers around the locations of events processed by GIS (spatial clusters) and (2) adjust for unobserved factors that affect or are correlated with both outcomes of interest and treatments and are shared within spatial clusters by controlling for spatial cluster fixed effects in a linear regression model. We further limit the sample to subjects within selected spatial clusters where the locations of events are plausibly exogenous (according to the statistical significance test discussed below). Our approach shares the same objective of adjusting for unobserved spatial confounding factors in the literature.
Our approach follows one of Fisher’s three principles of experimental design, “blocking” (“local control”), with the aim of comparing outcomes among more homogeneous groups, with different levels of treatments within blocks, to reduce omitted variable bias systematically [ 15 ]. GIS can flexibly implement this principle in spatial contexts. We do not specifically consider/model spatially correlated residuals addressed in some related literature [ 16 , 17 ]. Rather, we restrict local samples so that residual spatial variability within small spatial clusters is plausibly assumed to be nonsignificant. Since a linear regression model is a primary workhorse in empirical studies across disciplines, our regression-based approach, which considers both binary and continuous treatment variables, should have significant practical merits.
The reminder of this paper is divided into two sections: We first provide a regression-based framework of the key procedures for GIS-based empirical research design and then implement the framework in a case study on historical political events—the Cambodian genocide.
A framework of GIS-based empirical research
The key procedures for GIS-based empirical research design consist of three stages: diagnosis , design , and analysis .
Stage 1: Diagnosis
The diagnosis stage examines how the events of interest occur. Based on theories, findings in the literature, and/or anecdotal evidence, researchers must understand the potential determinants of the locations of the events. Visualizing spatial patterns using GIS can facilitate this process.
Stage 2: Design
The design stage consists of two main tasks: creating treatment variables and constructing a credible analysis sample to approximate an experimental study.
Treatment variables.
We consider a general case where exactly how each subject is affected (treated) by the events is unknown (if it is known, it directly informs the creation of treatment variables). We assume that subjects living in closer proximity to the locations of events are more strongly treated by the events: The degree to which subjects are exposed to the events depends on the distance from the locations of events. This assumption should be reasonable for various spatial events. By calculating distance between two spatial points using GIS, we can create distance-based treatment variables. If information about the attributes of points (“marks”) is available, it is possible to make more flexible treatment variables. Treatment variables can be binary, discrete, or continuous.
Credible sample.
This central task consists of two or three steps of constructing a credible analysis sample from a given global sample which represents the population of interest (global sample). The procedure is the same for binary, discrete, and continuous treatment variables.
Step 1—Global sample.
The first step assesses the exogeneity of locations of events/treatment variables (binary, discrete, or continuous) for the global sample. As commonly done in empirical studies based on a regression-based framework, we statistically examine the joint significance of observed covariates on treatment variables. Rejecting the exogeneity implies that adjusting for their systematic difference is indispensable. This exogeneity check cannot assess the significance of unobserved factors affecting or correlated with both the outcomes of interest and treatments (“confounders”). If such unobserved confounders exist, the global sample fails to capture the treatment effects of interest: The estimates suffer from omitted variable bias.
Step 2—Spatial clusters.
The second step deals with this potential omitted variable bias. While instrumental variables methods are conventional, it is often difficult to find valid instrumental variables that are correlated with the locations of events (treatments), but not with the outcome of interest. We employ an alternative approach, blocking, by considering point buffers around the locations of events processed by GIS as neighborhoods, which we call spatial clusters , and compare outcomes among subjects, with different levels of exposure to the events, within spatial clusters. Since unobserved confounders (e.g., socioeconomic environments) should be similar within small spatial clusters, omitted variable bias can be reduced systematically. Specifically, for each subject, we create a dummy variable for each spatial cluster that takes the value of 1 if the subject belongs to the spatial cluster and 0 otherwise. If the subject belongs to more than one spatial cluster, corresponding more than one dummy variable takes 1 (an example is given in our case study). Adjusting for these dummy variables ( spatial cluster fixed effects ) in the regression model can eliminate unobserved confounders that are constant within spatial clusters. Prior to the analysis stage, we reassess the exogeneity of the treatment variables for this spatially limited (local) sample, adding spatial cluster fixed effects.
In this second step (and the third step discussed below), the size of spatial clusters is a key auxiliary parameter. Although with smaller cluster size, the number of balanced spatial clusters (described below) becomes larger and socioeconomic characteristics within spatial clusters should be more similar, the number of observations within spatial clusters becomes smaller and the variation in the exposure to the events becomes more limited, thus constraining the creation of treatment variables. This bias-variance trade-off needs to be carefully taken into account by researchers when they choose cluster size. Choosing the optimal size of spatial clusters based on data-driven approaches is beyond the scope of this paper.
Step 3—Balanced spatial clusters.
If concerns about omitted variable bias still remain in this local sample, the third step is called for. Although omitted variable bias cannot be directly assessed, it may be indirectly assessed through the correlations between the treatment variable and observed covariates (which are relevant according to theories, contextual knowledge, and/or findings in related literature) as well as their joint significance, because unobserved confounders about which researchers are typically concerned are those related to observed confounders [ 18 ]: Their significant correlations may imply that some unobserved confounders (that are related to observed confounders) vary within spatial clusters. Then, the estimates based on the local sample may still suffer from omitted variable bias. With information of the location determinants examined in the first diagnosis stage (that affects the occurrence of events), GIS can be utilized to alleviate the remaining concerns about omitted variable bias, as follows. Here we assume that a key binary location determinant is available.
We employ the following procedure for each spatial cluster. First, we additionally create small buffers around the location of the event and classify subjects into the buffers. Second, we create a crosstabulation on the distribution of the key binary location determinant across the buffers. Lastly, we examine the homogeneity of the distribution based on Fisher’s exact test, a statistical significance test for independence between two categorical variables [ 19 ]. Based on the results, we further limit the subjects to those within selected spatial clusters with the homogeneous distribution of the location determinant, which we call balanced spatial clusters . Since the locations of events within balanced spatial clusters are plausibly assumed to be exogenous (unobserved confounders are arguably more similar within selected spatial clusters), we can further reduce bias. The choice of buffer size for Fisher’s exact test involves a trade-off: Although with narrower buffer bandwidth, the homogeneity of the distribution of the location determinant is tested more strictly, the number of observations within buffers becomes smaller, thus weakening statistical power.
Stage 3: Analysis
The analysis stage examines the impacts of the treatment variables on the outcomes of interest based on the samples constructed in the second design stage. We estimate a regression equation controlling for spatial cluster fixed effects by ordinary least squares (OLS). We assume that within spatial clusters, there is no residual spatial variability and neighborhood spillover effects, if any, are constant. Then, in our regression model, spatial cluster fixed effects control for local spillover effects within spatial clusters. We assume that there are no spillover effects across spatial clusters. We check the sensitivity of estimation results to potential omitted variable bias due to remaining unobserved confounders within balanced spatial clusters. We do so by employing coefficient stability approaches (e.g., [ 18 , 20 ]) corresponding to our regression-based framework.
Internal and external validity.
Of critical importance in empirical studies is the validation of empirical findings [ 21 ]. Internal validity is the degree to which the findings for the (sub)population being studied are credible; external validity is the degree to which the findings can be extrapolated to other populations and settings [ 22 ]. Our framework aims to improve internal validity: The estimates based on the local sample within balanced spatial clusters have higher internal validity than those based on the global sample. On the other hand, the former estimates are prone to limited external validity because the local sample may not represent the population of interest. In quasi-experimental designs, such a trade-off always exists. Although the subpopulation being studied can differ distinctly from the population of interest, in our approach, well-defined subpopulations represented by the local sample are identified and thus assessing the credibility of findings for the population of interest is possible. This is not possible in standard instrumental variables methods because subpopulations affected by instruments are not identified.
A case study: Impacts of the Cambodian genocide
Our case study examines how the Cambodian genocide under the Pol Pot regime (1975-1979) altered people’s post-conflict behavior, parental investment in child education in particular. The locations of events and subjects of our interest are those of execution sites (“killing sites”) and households, respectively. The relationship between violence and behavior is an important topic in psychology and social sciences [ 23 – 25 ]. The availability of the two types of georeferenced point data enables our framework; in contrast, finding valid instrumental variables is difficult in this context. After providing brief historical background, the motivation, and the data, we implement our GIS-based empirical research design.
Historical background.
The Khmer Rouge (officially the Communist Party of Kampuchea) led by Pol Pot ruled Cambodia in a form of primitive communism from 1975 to 1979. Its communist revolution completely denied any right to private property, not only material private properties, but also one’s own family: Spouses and children were treated as collective property owned by the state [ 26 ]. People were forced to conform with the ideologies of the Pol Pot regime; those who disobeyed Khmer Rouge’s rules were treated as enemies of the society, being sent to reeducation camps and/or executed. During the Pol Pot era, approximately two million people died of execution, disease, starvation, or exhaustion [ 27 ].
Motivation.
Our case study is motivated by the following social contexts. Under the Pol Pot regime, intellectuals were persecuted and many of them were executed [ 28 ]; formal school education was also denied and abolished. After its collapse in 1979, the remnants of the Khmer Rouge continued guerilla warfare against the new government army until the 1990s; thus, the threat of violence against people by the Khmer Rouge persisted. Indeed, survivors often suffered from long-term mental health disorders, such as post-traumatic stress disorder (PTSD) [ 29 ]. Although formal school education resumed soon after the regime’s collapse [ 30 ], parental investment in child education may have been influenced negatively by the Khmer Rouge’s rules, particularly for those who were strongly exposed to the genocidal violence.
We utilize two data sets: the Khmer Rouge historical database and the complete count 1998 Cambodian Population Census microdata. The former data contain comprehensive information on the genocide during the Pol Pot era (events) with geocoded locations of more than 500 killing sites (e.g., burials, prisons) and the number of victims (marks). The latter data contain the basic information of individual and household socioeconomic characteristics (subjects’ outcomes) and geocoded locations of villages in the country. Since no location information is available at the household level, we substitute the point locations of villages for those of households; thus, all households residing in the same village share the same location information. Section 1 in S1 Supplementary Material provides a detailed description of the data. All data used in the paper were fully anonymized before we accessed them. The IRB approval for this study was received from the University of Tokyo (Approval number: 16-80, Date: August 10, 2016).
We first examine how killing sites were established. According to anecdotal evidence provided by international organizations and historians, the Khmer Rouge used schools, universities, and government buildings as prisons and reeducation camps and trucks to transport prisoners to killing sites [ 31 ]. This suggests that the locations of killing sites were relatively developed areas. Using GIS, we plot the locations of 514 killing sites, along with information about victims, as well as the locations of villages on the base map of the 1977 administrative divisions ( Fig 1 ). We also overlay the major roads (national and provincial road networks) in 1973 and the district mean education level of non-migrant women aged 36-50 (a cohort that should have finished primary school education, if receiving any education, before 1975; see Section 2 in S1 Supplementary Material for a detailed discussion) to capture the level of regional development before the Pol Pot era. This GIS map reveals that killing sites were commonly located near major roads and in districts with relatively high education levels (though in the eastern and western zones many sites were also located relatively far from major roads). These relationships are also confirmed empirically (Table B in S1 Supplementary Material ). These results suggest that the level of regional development prior to the Pol Pot era is a key determinant of the placement of killing sites.
- PPT PowerPoint slide
- PNG larger image
- TIFF original image
The geographic distribution of 514 killing sites and their number of victims in districts surveyed by DC-Cam are depicted (districts not surveyed have a white background). The 1977 administrative zones of the Pol Pot regime (DK zones (1977)) and the 1998 districts are depicted. For information on education and major roads, see the text and Section 2 in S1 Supplementary Material .
https://doi.org/10.1371/journal.pone.0212316.g001
We first describe the population of interest and the sample, then create treatment variables, and after that follow the three steps of constructing a credible sample.
Population.
In 1975, urban people, who were persecuted under the Pol Pot regime, were forced to migrate to the countryside to engage in forced agricultural work [ 28 ]. During the Pol Pot era, many of them experienced forced migration several times. Because our treatment variables (defined below) are based on the locations of killing sites and villages where couples lived in 1998, we can construct treatment variables only for non-migrant couples. The population of our interest is thus non-migrant rural couples (who had their first child during and after the Pol Pot era, as detailed below). We exclude urban couples and rural couples with migration experience (about 57% of the couples in rural areas) from the scope of our study.
Our global sample consists of non-migrant rural couples in the areas surveyed about the mass killings who had their first child in 1977-1982 (see Section 3.1 in S1 Supplementary Material for details). Because the couples who had their first child during and after the Pol Pot regime had distinct institutional experiences (the former were controlled as family organizations and the latter were not), we divide the sample into two groups: couples whose first child was born in 1977-1979 and couples whose first child was born in 1980-1982. Taking into account a transition period, we further divide the latter into two groups: couples whose first child was born in 1980 and those whose first child was born in 1981-1982. We examine the genocide impacts on children’s educational outcomes (defined below) for these three subsamples separately. We assume that the timing of having the first child is unrelated to potential genocide impacts (Section 3.2 in S1 Supplementary Material provides evidence for this assumption). This assumption implies that for example, if couples whose first child was born in 1980 or 1981-1982 had had their first child in 1977-1979, their estimated genocide impacts would be identical to those for couples whose first child was actually born in 1977-1979. For illustrative purposes and the sake of brevity, we highlight the results for the subsample of couples whose first child was born in 1977-1979 in the text and report those for other two subsamples in S1 Supplementary Material .
Using GIS, we create two treatment variables of the genocidal violence. A binary measure ( Genocidal Violence I ) takes the value of 1 if the points of villages where couples resided are within 3.0 km of at least one killing site and 0 otherwise. A continuous measure ( Genocidal Violence II ) is the logarithmic value of the inverse-distance weighted sum of the number of victims for all killing sites located within 6.0 km of villages where couples resided (this corresponds to 6.0 km spatial clusters defined below) (we use log because the original value has right-skewed distribution, S1 Fig ). Since 184 killing sites lack information about the number of victims ( Fig 1 ), the continuous measure is defined only among villages with complete victim information for all killing sites located within 6.0 km of the villages. For this reason, we focus on the binary treatment variable in the design stage for the analysis of both the binary and continuous treatment variables. Section 4 in S1 Supplementary Material conducts robustness checks for alternative cluster size and continuous measures.
In the three subsamples of the global sample ( Global Sample ), we examine the joint significance of pre-treatment village characteristics (distance to major roads (km), the proportion of non-migrant women aged 36-50 with grade 1-5 of primary education and the proportion of non-migrant women aged 36-50 with grade 6 or above) and parental characteristics (mother’s and father’s age, and a set of dummy variables for mother’s and father’s educational attainment (grade 1-5 and grade 6 or above)). The dependent variable is the binary treatment variable defined above (i.e., Genocidal Violence I ). We estimate all regression equations by OLS with zone and district fixed effects controlled for. Logistic regression with many dummy variables can have a well-known incidental parameter problem [ 32 ]. The results reported in column 1 of Table 1 (and columns 1 and 2 of Table J in S1 Supplementary Material ) show that villages (couples) located (living) near killing sites are more likely to have been developed (educated) in the subsample of couples whose first child was born in 1977-1979 (the results for other two subsamples reported in columns 1 and 2 of Table J in S1 Supplementary Material are similar). Thus, controlling for these pre-treatment factors is indispensable. With limited relevant pre-treatment variables in our data, however, unobserved confounders are a major concern. It is likely that our estimates based on Global Sample suffer from omitted variable bias.
https://doi.org/10.1371/journal.pone.0212316.t001
Using GIS, we create 6.0 km spatial clusters around the locations of killing sites and identify village points within the spatial clusters ( Fig 2 ). We then limit the sample to couples living within the spatial clusters. We assess this local sample ( Local Sample I ) in the same way as we do Global Sample above, except that spatial cluster fixed effects are additionally controlled (433 spatial clusters in total): Unobserved confounders common within spatial clusters are now fully controlled for (see S2 Fig for the distribution of the number of spatial clusters to which villages in the three subsamples of all local samples belong). The results reported in column 2 of Table 1 (and columns 3 and 4 of Table J in S1 Supplementary Material ) show that although some significant differences disappear, substantial significant differences remain; the listed variables are all jointly significant in the three subsamples (in both panels A and B). These results raise a concern that our estimates in Local Sample I are likely to still suffer from omitted variable bias because unobserved confounders that are related to the observed covariates may vary within the spatial clusters.
The number of villages within 0-2.0 km, 2.0-4.0 km, and 4.0-6.0 km buffers of Killing Site 474 is 9, 15, and 23, respectively.
https://doi.org/10.1371/journal.pone.0212316.g002
We further limit the sample to couples living within selected spatial clusters with similar levels of regional development according to Fisher’s exact test. Since it is not feasible to implement Fisher’s exact test for the two measures depicted in Fig 1 , we use the proportion of migrant households as a proxy for the level of regional development (see Section 3.3 in S1 Supplementary Material for details); in-migration is generally strongly correlated with regional development [ 33 ]. With the lack of historical migration data, we use data from the 1998 Census to calculate the migrant proportion. We confirm that the migrant proportion is positively correlated with the locations of killing sites (Table B in S1 Supplementary Material ). We assume that this measure captures the level of regional development within spatial clusters well.
Within each 6.0 km spatial cluster, we create smaller buffers using a 2.0 km bandwidth which is narrower than the 3.0 km bandwidth determining the treatment status ( Fig 2 ). For each of the three subsamples of Local Sample I, we conduct Fisher’s exact test on the homogeneity in the proportion of migrant households across the three (0-2.0 km, 2.0-4.0 km, and 4.0-6.0 km) buffers. We define spatial clusters as balanced if the null hypothesis of no association in the proportion of migrant households across the three buffers cannot be rejected for all three subsamples. To be conservative, we also conduct the same exercise for 4.0 km spatial clusters.
To provide a specific example, Table 2 reports the results of Fisher’s exact tests for Killing Site 474 depicted in Fig 2 (see S3 Fig for the location of Killing Site 474). In all three subsamples, the number of non-migrant households is larger than that of migrant households within the three buffers. As none of the three subsamples can reject the null hypothesis at conventional levels, the spatial cluster of Killing Site 474 is balanced (the same results hold for the 4.0 km spatial cluster (not reported)). S3 Fig shows the results of the same Fisher’s exact tests for all 514 killing sites. There are 115 balanced spatial clusters (see Section 3.3 in S1 Supplementary Material for details). Although the sample is limited to households within 115 balanced spatial clusters, we also adjust for spatial cluster fixed effects for unbalanced spatial clusters to which they belong, if any, in our regression model (see panel B of S2 Fig ).
https://doi.org/10.1371/journal.pone.0212316.t002
Almost all significant differences found in Global Sample and Local Sample I vanish in this further selected sample ( Local Sample II ) based on these 115 balanced spatial clusters ( Table 1 column 3 and Table J columns 5 and 6). The magnitude of many coefficients becomes smaller. In addition, the listed variables are jointly insignificant at conventional levels in all regressions. These results suggest that the observed covariates and unobserved covariates related to the observed ones, if any, are similar within the balanced spatial clusters; thus, Local Sample II should yield less biased estimates than Global Sample and Local Sample I. At the same time, relative to the population of interest, Local Sample II (and Local Sample I) contain households and villages with favorable characteristics because they focus on villages around killing sites, which tend to have been located in relatively developed areas (see Section 3.1 in S1 Supplementary Material for a more detailed consideration). Therefore, the results should be taken with some caution regarding external validity.
We construct outcome variables, specify regression models, and present the estimation results.
We consider educational outcomes for children aged 15-21 and 6-14. In the 1998 Cambodian education system, the former cohort had already finished the nine-year compulsory education (due to delayed entry, temporary dropout, or grade retention, some were still receiving it though), and the latter cohort were still receiving it, if they were receiving any education. Most of these children were born after the Pol Pot regime. The exposure of couples to the genocidal violence may have affected their fertility decisions and thus the existence of most of these children. Thus, we use household, not child, as a unit of analysis. The household-level outcome measures of interest are the average years of schooling ( Years of schooling ) for children aged 15-21 and the average grade progression ( Grade progression ) for children aged 6-14, where the grade progression of each child is given by Grade −( Age −5), which takes 0 if the child progresses from any grade to the next higher grade and negative values otherwise. Table D in S1 Supplementary Material provides the descriptive statistics of these outcome measures.
Empirical specification.
Fig 3 plots the point estimates and 95% confidence intervals of the impacts of the binary genocide measure for children aged 15-21 (panel A) and 6-14 (panel B) of the couples whose first child was born in 1977-1979. For comparison, we present all results in Global Sample and Local Samples I and II; the smaller the sample size, the wider the confidence intervals. Although the point estimates are mostly positive in Global Sample and Local Sample I, estimates become negative in Local Sample II. For example, in Local Sample II, although the couples’ exposure to the genocidal violence increased the years of schooling of children aged 15-21 by 0.136 years in Global Sample, it rather decreased their years of schooling by 0.355 years (7.9% of the mean among those with no exposure). Such adverse impacts are found for children aged both 15-21 and 6-14. In contrast, among couples whose first child was born in 1980 or 1981-1982, none of the estimates in Local Sample II are statistically significantly different from 0 (see S4 Fig ).
https://doi.org/10.1371/journal.pone.0212316.g003
Fig 4 (and S5 Fig ) show the results for the continuous genocide measure in Local Sample I with victim information ( Local Sample III ) and Local Sample II with victim information ( Local Sample IV ), which contain 289 spatial clusters and 83 balanced spatial clusters, respectively (Table A in S1 Supplementary Material ). To construct Local Sample IV from Local Sample III , we employ the same procedure of Fisher’s exact test discussed above. The results are qualitatively the same as those for the binary genocide measure.
https://doi.org/10.1371/journal.pone.0212316.g004
Regression diagnostics based on Local Samples II and IV suggest that our models are appropriate (see S6 and S7 Figs). It is also noted that we use robust standard errors adjusted for clustering by village for conservative statistical inference.
To address remaining threats to internal validity, we assess the sensitivity of the results to potential omitted variable bias due to unobserved confounders that vary within balanced spatial clusters for Local Samples II and IV, using the Oster’s coefficient stability approach [ 18 ] (see Section 5 in S1 Supplementary Material for details). The results confirm that the estimated negative impacts are robust to omitted variable bias even under conservative assumptions.
Thus, we conclude that the genocidal violence had adverse impacts on child education among the couples who had their first child during the Pol Pot era. The analysis and discussion of potential mechanisms underlying these patterns are provided elsewhere [ 34 ].
Concluding remarks
This paper provided a regression-based framework for GIS-based empirical research design using georeferenced point data for both spatial events of interest and subjects exposed to the events and illustrated its utility and implementation through an empirical case study from Cambodia. GIS is particularly useful in understanding the locational determinants of spatial events, creating treatment variables, constructing credible samples, and implementing blocking (local control). GIS can potentially play a key role in designing credible and transparent empirical research as spatial point data become more widely available. Much work is needed to overcome the limitations of our approach. Promising avenues include designing the optimal choice of the size of spatial clusters and the bandwidth of buffers, allowing a change in subject locations, capturing local spillover effects, and analyzing dynamic treatment effects. Ultimately, our approach needs to be extended to one in the potential outcomes framework for causal analysis. These works are left for future research.
Supporting information
S1 fig. distribution of continuous genocide measures..
Kernel density of the distribution of the continuous genocide measures based on the first-, second-, and third-order polynomials in distance is shown for each subsample in Global Sample.
https://doi.org/10.1371/journal.pone.0212316.s001
S2 Fig. Distribution of number of spatial clusters to which villages belong.
The figure provides the distribution of the number of spatial clusters to which villages in each subsample of Local Samples I (panel A), II (panel B), III (panel C), and IV (panel D) belong.
https://doi.org/10.1371/journal.pone.0212316.s002
S3 Fig. Results of Fisher’s exact tests.
The figure provides the results of Fisher’s exact tests. The location of killing site 474 analyzed in Fig 2 and Table 2 is depicted.
https://doi.org/10.1371/journal.pone.0212316.s003
S4 Fig. Estimation results (binary genocide measure)—Other subsamples.
The figure provides point estimates and 95% confidence intervals of genocide impacts on children’s educational outcomes based on binary genocide measure.
https://doi.org/10.1371/journal.pone.0212316.s004
S5 Fig. Estimation results (continuous genocide measure)—Other subsamples.
The figure provides point estimates and 95% confidence intervals of genocide impacts on children’s educational outcomes based on continuous genocide measure.
https://doi.org/10.1371/journal.pone.0212316.s005
S6 Fig. Regression diagnostics—Local Sample II (binary genocide measure).
Regression diagnostics are presented for each subsample of Local Sample II. Each of the three figures depicts the following: the distribution of residuals, along with a normal density (green) (left); a normal quantile-quantile plot of residuals (middle); a residual plot, along with a locally weighted scatterplot smoothing curve (bandwidth = 0.8) (green) (right).
https://doi.org/10.1371/journal.pone.0212316.s006
S7 Fig. Regression diagnostics—Local Sample IV (continuous genocide measure).
Regression diagnostics are presented for each subsample of Local Sample IV. See the notes to S6 Fig for each figure.
https://doi.org/10.1371/journal.pone.0212316.s007
S1 Supplementary Material. PDF file containing the Supplementary Material.
This file provides the detailed descriptions of data, diagnosis, design, and analysis (Section 1: Data details; 2: Diagnosis details; 3: Design details; 4: Robustness checks; 5: Sensitivity analysis), including 18 tables (Tables A–R).
https://doi.org/10.1371/journal.pone.0212316.s008
S1 File. Replication files.
This zip file contains the dataset and Stata do-file to replicate the empirical analyses presented in the case study.
https://doi.org/10.1371/journal.pone.0212316.s009
Acknowledgments
We thank the editor and three anonymous referees for comments that greatly improved the paper. An earlier version of this paper benefited significantly from the comments and suggestions of Oliver T. Coomes, Keisuke Hirano, Kosuke Imai, Da-Wei Kuan, and seminar participants at Chengchi University and GIScience 2018 (RMIT University). We also thank H.E. San Sy Than, H.E. Hang Lina, and Fumihiko Nishi for providing us with the 100% count 1998 Cambodia Population Census microdata.
- 1. Alam BM. Application of Geographic Information Systems. InTech; 2012.
- 2. Ballas D, Clarke G, Franklin RS, Newing A. GIS and the Social Sciences: Theory and Applications. 1st ed. Oxford: Routledge; 2017.
- View Article
- PubMed/NCBI
- Google Scholar
- 5. Angrist JD, Pischke J. Mostly Harmless Econometrics: An Empiricist’s Companion. Princeton: Princeton University Press; 2009.
- 6. Anselin L. Spatial Econometrics: Methods and Models. Boston: Kluwer; 1988.
- 8. Rosenbaum PR. Design of Observational Studies. New York: Springer; 2010.
- 9. Cressie NAC. Statistics for Spatial Data. Revised ed. New York: Wiley; 1993.
- 11. Eldawy A, Mokbel MF. The Era of Big Spatial Data: A Survey. Hanover, MA: Now Publishers; 2016.
- 14. Papadogeorgou G, Choirat C, Zigler CM. Adjusting for Unmeasured Spatial Confounding with Distance Adjusted Propensity Score Matching. Biostatistics (forthcoming).
- 15. Fisher RA. Design of Experiments. 1st ed. London: Oliver and Boyd; 1935.
- 18. Emily Oster. Unobservable Selection and Coefficient Stability: Theory and Evidence. Journal of Business & Economic Statistics (forthcoming).
- 19. Fisher RA. Statistical Methods for Research Workers. 1st ed. London: Oliver and Boyd; 1925.
- 21. Shadish WR, Cook TD, Campbell DT. Experimental and Quasi-Experimental Designs for Generalized Causal Inference. Belmont, CA: Wadsworth Cengage Learning; 2002.
- 22. Stock J, Watson M. Introduction to Econometrics. 3rd ed. Boston: Pearson; 2011.
- 26. Short P. Pol Pot: Anatomy of a Nightmare. New York: Henry Holt and Company; 2004.
- 27. Dy K. A History of Democratic Kampuchea (1975-1979). Phnom Penh: Documentation Center of Cambodia; 2007.
- 28. Kiernan B. The Pol Pot Regime: Race, Power, and Genocide in Cambodia under the Khmer Rouge, 1975-79. 3rd ed. New Haven, CT: Yale University Press; 2008.
- 29. Beth VS, Daryn R, Youk C. Cambodia’s Hidden Scars: Trauma Psychology in the Wake of the Khmer Rouge. Phnom Penh: Documentation Center of Cambodia; 2011.
- 30. Vickery M. Kampuchea: Politics, Economics and Society. London: Frances Pinter; 1986.
- 31. Chandler D. Voices from S-21. Berkeley: University of California Press; 1999.
- 33. Mazumdar D. Rural-Urban Migration in Developing Countries. In: Mills ES, editor. Handbook of Regional and Urban Economics: Urban Economics. vol. 2. New York: North Holland; 1987. p. 1097–1128.
- 34. Kogure K, Takasaki Y. Conflict, Institutions, and Economic Behavior: Legacies of the Cambodian Genocide; 2016. Unpublished manuscript, CIRJE-F-1034, University of Tokyo.
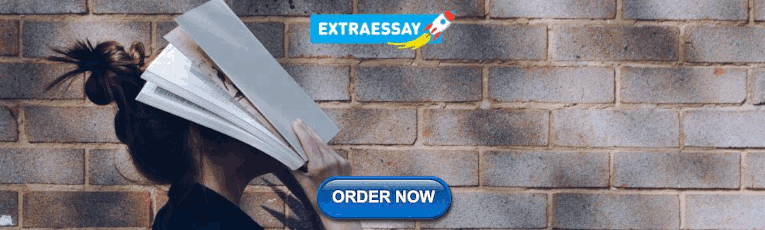
IMAGES
VIDEO
COMMENTS
Learn how to design a research strategy using empirical data. Compare different types of qualitative and quantitative research designs, and choose the best methods for your aims and objectives.
Empirical research is defined as any research where conclusions of the study is strictly drawn from concretely empirical evidence, and therefore "verifiable" evidence. ... The type of research design used will vary depending on the field in which it is going to be used. Many of them might choose to do a collective research involving ...
Learn how to design a research strategy using empirical data. This guide covers the steps, types, and methods of qualitative and quantitative research designs with examples.
Emerald Publishing's guide to conducting empirical research identifies a number of common elements to empirical research: A research question, which will determine research objectives. A particular and planned design for the research, which will depend on the question and which will find ways of answering it with appropriate use of resources.
Research design is a comprehensive plan for data collection in an empirical research project. It is a 'blueprint' for empirical research aimed at answering specific research questions or testing specific hypotheses, and must specify at least three processes: the data collection process, the instrument development process, and the sampling process.
Hence, empirical research is a method of uncovering empirical evidence. Through the process of gathering valid empirical data, scientists from a variety of fields, ranging from the social to the natural sciences, have to carefully design their methods. This helps to ensure quality and accuracy of data collection and treatment.
We define research design as a combination of decisions within a research process. These decisions enable us to make a specific type of argument by answering the research question. It is the implementation plan for the research study that allows reaching the desired (type of) conclusion. Different research designs make it possible to draw ...
Empirical research is research using empirical evidence. It is also a way of gaining knowledge by means of direct and indirect observation or experience. ... Some Key Concepts for the Design and Review of Empirical Research Archived 2021-04-16 at the Wayback Machine This page was last edited on 8 March 2024, at ...
Strategies for Empirical Research in Writing is a particularly accessible approach to both qualitative and quantitative empirical research methods, helping novices appreciate the value of empirical research in writing while easing their fears about the research process. ... advocating rigorous design and implementation of empirical research ...
The Vice President for Research is a university-wide post responsible for overseeing all aspects of research at Tulane University. Priorities for the office include fostering interdisciplinary collaboration and building the research portfolio in a manner compliant with government regulation.
Empirical research methodologies can be described as quantitative, qualitative, or a mix of both (usually called mixed-methods). Ruane (2016) (UofM login required) gets at the basic differences in approach between quantitative and qualitative research: Quantitative research -- an approach to documenting reality that relies heavily on numbers both for the measurement of variables and for data ...
Types of Research Designs Compared | Guide & Examples. Published on June 20, 2019 by Shona McCombes.Revised on June 22, 2023. When you start planning a research project, developing research questions and creating a research design, you will have to make various decisions about the type of research you want to do.. There are many ways to categorize different types of research.
Abstract. Design research brings together influences from the whole gamut of social, psychological, and more technical sciences to create a tradition of empirical study stretching back over 50 years (Horvath 2004; Cross 2007 ). A growing part of this empirical tradition is experimental, which has gained in importance as the field has matured.
Typically, empirical research embodies the following elements: A research question, which will determine research objectives. A particular and planned design for the research, which will depend on the question and which will find ways of answering it with appropriate use of resources. The gathering of primary data, which is then analysed.
Empirical Research Design. Now that you've selected your research topic, formulated research questions, and defined your variables, it's time to delve into the heart of your empirical research journey - research design. This pivotal step determines how you will collect data and what methods you'll employ to answer your research questions.
Another hint: some scholarly journals use a specific layout, called the "IMRaD" format, to communicate empirical research findings. Such articles typically have 4 components: Introduction: ... Methodology: sometimes called "research design" -- how to recreate the study -- usually describes the population, research process, ...
Historically, empirical research traditions based on phenomenological philosophy have been developed and used across several disciplines and fields of knowledge, including nursing and the broader field of healthcare research (K. Dahlberg et al., 2008; van Manen, 2014).Since 1990 phenomenological research is included as a subject heading in the database Cumulative Index to Nursing and Allied ...
While many books and articles guide various qualitative research methods and analyses, there is currently no concise resource that explains and differentiates among the most common qualitative approaches. We believe novice qualitative researchers, students planning the design of a qualitative study or taking an introductory qualitative research course, and faculty teaching such courses can ...
Second, to suggest a defense of this empirical development, as if properly understood, it would assist empirically grounded contemporary analytic political theory to achieve its own stated goals. Third, to provide a template for a research design in empirical contemporary analytic political theory.
Empirical design method is a learning method purely based on experience. By the response of a test black box due to changing the parameters of the black box system intermittently or continuously, the subjects of the applied empirical design seek a way to reach their goal. The success or failure of the subject's behavior is evaluated by its ...
Under the qualitative research design, empirical studies had evolved to test the conventional concepts of evidence and truth while still observing the fundamental principles of recognizing the subjects beings studied as empirical (Powner, 2015). This method can be semi-structured or unstructured. Results from this research type are more ...
Concluding remarks. This paper provided a regression-based framework for GIS-based empirical research design using georeferenced point data for both spatial events of interest and subjects exposed to the events and illustrated its utility and implementation through an empirical case study from Cambodia.
for validity and reliability. Design is basically concerned with the aims, uses, purposes, intentions and plans within the. pr actical constraint of location, time, money and the researcher's ...