What is Distance Education? Its Benefits & Examples
By Teach Educator
Published on: July 24, 2024
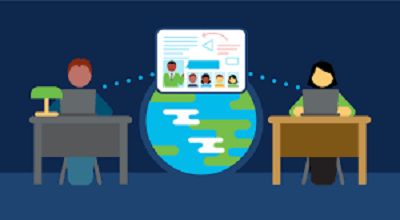
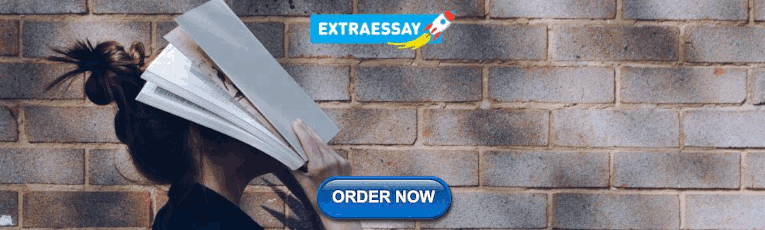
Distance Education
Distance Education , also known as online education or e-learning, refers to a mode of education. Where students and instructors are physically separated and use technology to facilitate learning. It allows individuals to pursue educational opportunities without being present in a traditional classroom setting.
Distance education can take various forms, including online courses, virtual classrooms, video lectures, and other digital methods of content delivery. Here are some key aspects, benefits, and examples of distance edu:
Key Aspects of Distance Education:
- Remote Learning: Distance education enables students to access educational resources. And engage with course materials from remote locations, typically through the Internet.
- Flexible Schedules: Students can often choose when and where they study. Making it suitable for those with busy schedules, work commitments, or other responsibilities.
- Diverse Course Offerings: Distance edu programs cover a wide range of subjects and levels. From K-12 to higher education and professional development courses.
- Digital Resources: Learning materials are often delivered in digital formats. Such as videos, text, interactive quizzes, and forums, accessible through computers, tablets, or smartphones.
- Communication Tools: Online platforms provide various tools for communication between students. And instructors, including email, discussion boards, chat, and video conferencing.
Benefits of Distance Education:
- Flexibility: Students can balance education with work, family, and other commitments due to the flexibility of online learning.
- Accessibility: It allows individuals from different geographical locations to access education and pursue their goals without the need to relocate.
- Cost-Efficiency: Distance education often reduces costs associated with commuting, accommodation, and traditional course materials.
- Personalized Learning: Some online platforms offer adaptive learning tools. Allowing students to progress at their own pace and receive tailored content.
- Global Networking: Students can interact with peers and instructors from around the world. Leading to diverse perspectives and global networking opportunities.
Examples of Distance Education:
- Online Universities: Institutions like the University of Phoenix, and Southern New Hampshire University. Western Governors University offers fully online degree programs.
- Massive Open Online Courses (MOOCs): Platforms like Coursera, edX, and Udacity offer free. And paid courses from top universities and organizations worldwide.
- Virtual High Schools: Many K-12 schools offer online courses for high school students. Allowing them to earn credits and diplomas remotely.
- Corporate Training: Companies often use distance edu for employee training and development, using platforms like LinkedIn Learning or Skillsoft.
- Language Learning Apps: Apps like Duolingo and Rosetta Stone provide language learning through digital platforms.
- Professional Certifications: Organizations like CompTIA and Cisco offer online certification courses for IT professionals.
- Online Tutoring and Homework Help: Websites like Khan Academy and Chegg offer educational resources, including video lessons and homework assistance.
- Remote Workshops and Webinars: Many industries and professionals use webinars and online workshops for skill development and knowledge sharing.
In summary, distance education leverages technology to provide flexible and accessible learning opportunities. Making it a valuable option for individuals seeking education and professional development in various fields.
Related Post
What is the mode in mathematics with examples.
Mode in Mathematics with Examples Mode in Mathematics is a subject that encompasses various concepts, one of which is statistics. When working with sets of numbers or data, ...
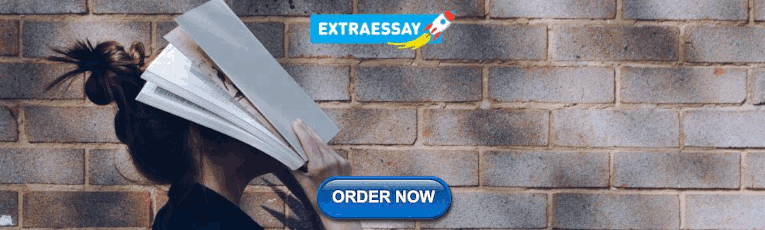
What are Functional Skills? Latest 2024
Functional Skills Functional skills are practical abilities that are essential for navigating daily life, academic pursuits, and the workplace. These skills enable individuals to solve problems, communicate effectively, ...
What is the definition of schooling? & meaning of happy schooling
Schooling Definition Schooling refers to the organized and structured process of acquiring knowledge, skills, and education through a formal system, typically within institutions. Such as schools, colleges, and ...
What is the purpose of the chronicle of higher education?
chronicle of higher education The Chronicle of Higher Education is a source of news, opinion, advice, and jobs for people in higher education. It covers topics such as ...
Leave a Comment Cancel reply
Save my name, email, and website in this browser for the next time I comment.
Latest Post
Best astrologer in united states – latest 2025, emis-gb app free download (latest), teach educator.
"Teach Educator aims to empower learners and educators alike through its comprehensive services. Dedicated to bridging educational gaps, it offers a range of resources designed to enhance teaching methodologies, provide updated curriculum insights, and foster professional development. With a commitment to accessibility, Teach Educator ensures that educational tools and information are readily available to all, promoting inclusivity in learning.
© Teach Educator 2021 - 2024 | All Rights Reserved
Privacy policy
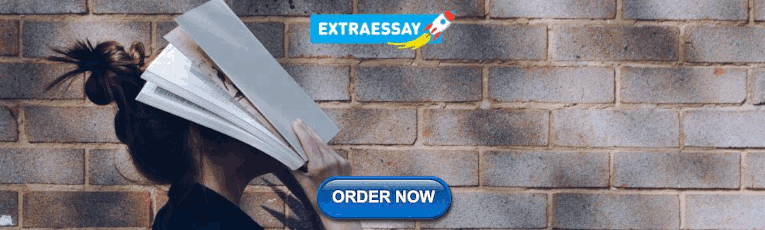
IMAGES
VIDEO