Change Password
Your password must have 8 characters or more and contain 3 of the following:.
- a lower case character,
- an upper case character,
- a special character
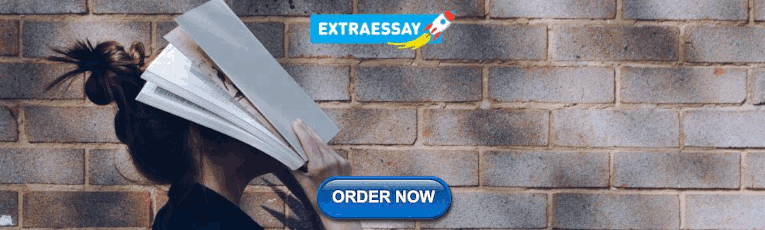
Password Changed Successfully
Your password has been changed
- Sign in / Register
Request Username
Can't sign in? Forgot your username?
Enter your email address below and we will send you your username
If the address matches an existing account you will receive an email with instructions to retrieve your username
To What Extent Do Study Habits Relate to Performance?
- Elise M. Walck-Shannon
- Shaina F. Rowell
- Regina F. Frey
*Address correspondence to: Elise M. Walck-Shannon ( E-mail Address: [email protected] ).
Biology Department, Washington University in St. Louis, St. Louis, MO 63130
Search for more papers by this author
Center for Integrative Research on Cognition, Learning, and Education (CIRCLE), Washington University in St. Louis, St. Louis, MO 63130
Department of Chemistry, University of Utah, Salt Lake City, UT 84112
Students’ study sessions outside class are important learning opportunities in college courses. However, we often depend on students to study effectively without explicit instruction. In this study, we described students’ self-reported study habits and related those habits to their performance on exams. Notably, in these analyses, we controlled for potential confounds, such as academic preparation, self-reported class absences, and self-reported total study time. First, we found that, on average, students used approximately four active strategies to study and that they spent about half of their study time using active strategies. In addition, both the number of active strategies and the proportion of their study time using active strategies positively predicted exam performance. Second, on average, students started studying 6 days before an exam, but how early a student started studying was not related to performance on in-term (immediate) or cumulative (delayed) exams. Third, on average, students reported being distracted about 20% of their study time, and distraction while studying negatively predicted exam performance. These results add nuance to lab findings and help instructors prioritize study habits to target for change.
INTRODUCTION
One of our goals in college courses is to help students develop into independent, self-regulated learners. This requires students to perform several metacognitive tasks on their own, including setting goals, choosing strategies, monitoring and reflecting on performance, and modifying those steps over time ( Zimmerman, 2002 ). There are many challenges that learners encounter in developing self-regulation. One such challenge is that students often misjudge their learning during the monitoring and reflection phases ( Kornell and Bjork, 2007 ). Often, students feel that they learn more from cognitively superficial tasks than from cognitively effortful tasks. As one example, students may feel that they have learned more if they reread a text passage multiple times than if they are quizzed on that same material ( Karpicke and Roediger, 2008 ). In contrast to students’ judgments, many effortful tasks are highly effective for learning. R. A. Bjork defines these effective, effortful tasks as desirable difficulties ( Bjork, 1994 ). In the present study, we investigated the frequency with which students reported carrying out effortful (active) or superficial (passive) study habits in a large introductory biology course. Additionally, we examined the relationship between study habits and performance on exams while controlling for prior academic preparation and total study time.
THEORETICAL FRAMEWORK
Why would difficulties be desirable.
During learning, the goal is to generate knowledge or skills that are robustly integrated with related knowledge and easily accessible. Desirable difficulties promote cognitive processes that either aid forming robust, interconnected knowledge or skills or retrieving that knowledge or skill ( Bjork, 1994 ; also see Marsh and Butler, 2013 , for a chapter written for educators). Learners employing desirable difficulties may feel that they put in more effort and make more mistakes, but they are actually realizing larger gains toward long-term learning than learners using cognitively superficial tasks.
Which Study Habits Are Difficult in a Desirable Way?
Study habits can include a wide variety of behaviors, from the amount of time that students study, to the strategies that they use while studying, to the environment in which they study. The desirable difficulties framework ( Bjork and Bjork, 2011 ), describes two main kinds of effective habits that apply to our study: 1) using effortful study strategies or techniques that prompt students to generate something or test themselves during studying and 2) distributing study time into multiple sessions to avoid “cramming” near the exam. In the following two paragraphs, we expand upon these study habits of interest.
The desirable difficulties framework suggests that study strategies whereby students actively generate a product or test themselves promote greater long-term learning than study strategies whereby students passively consume presentations. This is supported by strong evidence for the “generation effect,” in which new knowledge or skills are more robustly encoded and retrieved if you generate a solution, explanation, or summary, rather than looking it up ( Jacoby, 1978 ). A few generative strategies that are commonly reported among students—summarization, self-explanation, and practice testing—are compared below. Summarization is a learning strategy in which students identify key points and combine them into a succinct explanation in their own words. As predicted by the generation effect, evidence suggests that summarization is more effective than rewriting notes (e.g., laboratory study by Bretzing and Kulhavy, 1979 ) or reviewing notes (e.g., classroom study by King, 1992 ). Self-explanation is a learning strategy wherein students ask “how” and “why” questions for material as they are being exposed to the material or shortly after ( Berry, 1983 ). This is one form of elaborative interrogation, a robust memory technique in which learners generate more expansive details for new knowledge to help them remember that information ( Pressley et al. , 1987 ). Self-explanation requires little instruction and seems to be helpful for a broad array of tasks, including recall, comprehension, and transfer. Further, it is more effective than summarization (e.g., classroom study by King, 1992 ), perhaps because it prompts students to make additional connections between new and existing knowledge. Practice testing is supported by evidence of the “testing effect,” for which retrieving information itself actually promotes learning ( Karpicke and Roediger, 2008 ). The memory benefits of the “testing effect” can be achieved with any strategy in which students complete problems or practice retrieval without relying on external materials (quizzing, practice testing, flashcards, etc.). In this study, we refer to these strategies together as “self-quizzing.” Self-quizzing is especially effective at improving performance on delayed tests, even as long as 9–11 months after initial learning ( Carpenter, 2009 ). Additionally, in the laboratory, self-quizzing has been shown to be effective on a range of tasks from recall to inference ( Karpicke and Blunt, 2011 ). Overall, research suggests that active, more effortful strategies—such as self-quizzing, summarization, and self-explanation—are more effective for learning than passive strategies—such as rereading and rewriting notes. In this study, we asked whether these laboratory findings would extend to students’ self-directed study time, focusing especially on the effectiveness of effortful (herein, “active”) study strategies.
The second effective habit described by the desirable difficulties framework is to avoid cramming study time near exam time. The “spacing effect” describes the phenomenon wherein, when given equal study time, spacing study out into multiple sessions promotes greater long-term learning than massing (i.e., cramming) study into one study session. Like the “testing effect,” the “spacing effect” is especially pronounced for longer-term tests in the laboratory ( Rawson and Kintsch, 2005 ). Based on laboratory studies, we would expect that, in a course context, cramming study time into fewer sessions close to an exam would be less desirable for long-term learning than distributing study time over multiple sessions, especially if that learning is measured on a delay.
However, estimating spacing in practice is more complicated. Classroom studies have used two main methodologies to estimate spacing, either asking the students to report their study schedules directly ( Susser and McCabe, 2013 ) or asking students to choose whether they describe their pattern of study as spaced out or occurring in one session ( Hartwig and Dunlosky, 2012 ; Rodriguez et al. , 2018 ). The results from these analyses have been mixed; in some cases, spacing has been a significant, positive predictor of performance ( Rodriquez et al. , 2018 ; Susser and McCabe, 2013 ), but in other cases it has not ( Hartwig and Dunlosky, 2012 ).
In the present study, we do not claim measure spacing directly. Lab definitions of spacing are based on studying the same topic over multiple sessions. But, because our exams have multiple topics, some students who start studying early may not revisit the same topic in multiple sessions. Rather, in this study, we measure what we refer to as “spacing potential.” For example, if students study only on the day before the exam, there is little potential for spacing. If, instead, they are studying across 7 days, there is more potential for spacing. We collected two spacing potential measurements: (1) cramming , or the number of days in advance that a student began studying for the exam; and (2) consistency , or the number of days in the week leading up to an exam that a student studied. Based on our measurements, students with a higher spacing potential would exhibit less cramming and study more consistently than students with lower spacing potential. Because not every student with a high spacing potential may actually space out the studying of a single topic into multiple sessions, spacing potential is likely to underestimate the spacing effect; however, it is a practical way to indirectly estimate spacing in practice.
Importantly, not all difficult, or effortful, study tasks are desirable ( Bjork and Bjork, 2011 ). For example, in the present study, we examined students’ level of distraction while studying. Distraction can come in many forms, commonly “multitasking,” or splitting one’s attention among multiple tasks (e.g., watching lectures while also scrolling through social media). However, multitasking has been shown to decrease working memory for the study tasks at hand ( May and Elder, 2018 ). Thus, it may make a task more difficult, but in a way that interferes with learning rather than contributing to it.
In summary, available research suggests that active, effortful study strategies are more effective than passive ones; that cramming is less effective than distributing studying over time; and that focused study is more effective than distracted study. Whether students choose to use these more effective practices during their independent study time is a separate question.
How Do Students Actually Study for Their Courses?
There have been several studies surveying students’ general study habits. When asked free-response questions about their study strategies in general, students listed an average of 2.9 total strategies ( Karpicke et al. , 2009 ). In addition, few students listed active strategies, such as self-quizzing, but many students listed more passive strategies, such as rereading.
There have also been studies asking whether what students actually do while they are studying is related to their achievement. Hartwig and Dunlosky (2012) surveyed 324 college students about their general study habits and found that self-quizzing and rereading were positively correlated with grade point average (GPA). Other studies have shown that using Facebook or texting during study sessions was negatively associated with college GPA ( Junco, 2012 ; Junco and Cotten, 2012 ). While these findings are suggestive, we suspect that the use of study strategies and the relationship between study strategies and achievement may differ from discipline to discipline. The research we have reviewed thus far has been conducted for students’ “general” study habits, rather than for specific courses. To learn about how study habits relate to learning biology, it is necessary to look at study habits within the context of biology courses.
How Do Students Study for Biology Courses?
Several prior qualitative studies carried out within the context of specific biology courses have shown that students often report ineffective habits, such as favoring passive strategies or cramming. Hora and Oleson ( 2017 ) found that, when asked about study habits in focus groups, students in science, technology, engineering, and mathematics (STEM) courses (including biology) used predominantly passive strategies such as reviewing notes or texts, practices that in some cases were unchanged from high school. Tomanek and Montplaisir (2004) found that the majority of 13 interviewed students answered questions on old exams (100% of students) and reread lecture slides (92.3% of students) or the textbook (61.5% of students) to study for a biology exam, but only a small minority participated in deeper tasks such as explaining concepts to a peer (7.7% of students) or generating flashcards for retrieval practice (7.7% of students). We can also learn indirectly about students’ study habits by analyzing what they would change upon reflection. For example, in another study within an introductory biology classroom, Stanton and colleagues ( 2015 ) asked students what they would change about their studying for the next exam. In this context, 13.5% of students said that using active strategies would be more effective for learning, and 55.5% said that they wanted to spend more time studying, many of whom reported following through by studying earlier for the next exam ( Stanton et al. , 2015 ). In the current study, we extended prior research by exploring the prevalence of multiple study habits simultaneously, including the use of active study strategy and study timing, in a large sample of introductory biology students.
In addition to characterizing students’ study habits, we also aimed to show how those study habits were related to performance in a biology classroom. In one existing study, there were positive associations between exam performance and some (but not all) active strategies—such as completing practice exams and taking notes—but no significant associations between performance and some more passive strategies—such as reviewing notes/screencasts or reviewing the textbook ( Sebesta and Bray Speth, 2017 ). In another study, both self-reported study patterns (e.g., spacing studies into multiple sessions or one single session) and self-quizzing were positively related to overall course grade in a molecular biology course ( Rodriguez et al. , 2018 ). We build on this previous work by asking whether associations between performance and a wide variety of study habits still hold when controlling for confounding variables, such as student preparation and total study time.
In this study, we asked whether students actually use cognitive psychologists’ recommendations from the desirable difficulty framework in a specific biology course, and we investigated whether students who reported using those recommendations during studying performed differently on exams than those who did not. We wanted to focus on how students spend their study time, rather than the amount of time that they study, their level of preparation, or engagement. Therefore, we used regression analyses to hold preparation (i.e., ACT math and the course pretest scores), self-reported class absences, and overall study time equal. In this way, we estimated the relationship between particular study habit variables—including the strategies that students use, their timing of using those strategies, and their level of distraction while studying—and exam performance.
Students would use a combination of active and passive strategies, but those who used more active study strategies or who devoted more of their study time to active strategies would perform higher on their exams than those who used fewer active strategies or devoted less time to active strategies.
Students would vary in their study timing, but those with less spacing potential (e.g., crammed their study time or studied less consistently) would perform worse, especially on long-term tests (final exam and course posttest), than students with more spacing potential.
Students would report at least some distraction during their studying, but those who reported being distracted for a smaller percent of their study time would score higher on exams than students who reported being distracted for a larger percent of their study time.
Context and Participants
Data for this study were gathered from a large-enrollment introductory biology course (total class size was 623) during the Spring 2019 semester at a selective, private institution in the Midwest. This course covers basic biochemistry and molecular genetics. It is the first semester of a two-semester sequence. Students who take this course are generally interested in life science majors and/or have pre-health intentions. The data for this study came from an on-campus repository; both the repository and this study have been approved by our internal review board (IRB ID: 201810007 for this study; IRB ID: 201408004 for the repository). There were no exclusion criteria for the study. Anyone who gave consent and for whom all variables were available was considered for the analyses. However, because the variables were different in each analysis, the sample differed slightly from analysis to analysis. When we compared students who were included in the first hypothesis’s analyses to students who gave consent but were not included, we found no significant differences between participants and nonparticipants for ACT math score, pretest score, year in school, sex, or race (Supplemental Table 1). This suggested that our sample did not dramatically differ from the class as a whole.
Other than those analyses labeled “post hoc,” analyses were preplanned before data were retrieved.
Timeline of Assignments Used in This Study
Figure 1 shows a timeline of the assignments analyzed in this study, which included the exam 1 and 2 reflections (both online), exams 1 and 2 (both in person), the course pre and post knowledge tests (both online), and a cumulative final exam (in person). As shown in the text boxes within Figure 1 , the majority (85.7% [430/502] or greater) of students completed each of the assignments that were used in this study.
FIGURE 1. Timeline of assignments used in this study organized by mode of submission (online vs. in person) and grading (completion vs. accuracy). Exam days are indicated by thick lines. There were other course assignments (including a third exam), but they are not depicted here, because they were not analyzed in this study. Exam return is indicated by dotted lines. Light gray boxes represent weeks that class was in session. The number of consenting students who completed each assignment is indicated in the corresponding assignment box; the total number of consenting students was 502.
Exam Reflections
Students’ responses to exam 1 and 2 study habits reflections were central to all of our hypotheses. In these reflection assignments, students were asked to indicate their study habits leading up to the exam (see Supplemental Item 1 for prompts), including the timing of studying and type of study strategies. The list of strategies for students to choose from came from preliminary analysis of open-response questions in previous years. To increase the likelihood that students accurately remembered their study habits, we made the exercise available online immediately after each exam for 5 days. The reflection assignment was completed before exam grades were returned to students so that their performance did not bias their memory of studying. Students received 0.20% of the total course points for completion of each reflection.
Exams in this course contained both structured-response (multiple-choice, matching, etc.) and free-response questions. The exams were given in person and contained a mixture of lower-order cognitive level (i.e., recall and comprehension) and higher-order cognitive level (i.e., application, analysis, synthesis, or evaluation) questions. Two independent (A.B and G. Y.) raters qualitatively coded exam questions by cognitive level using a rubric slightly modified from Crowe et al. (2008) to bin lower-order and higher-order level questions. This revealed that 38% of exam points were derived from higher-order questions. Each in-term exam was worth 22.5% of the course grade, and the cumulative final exam was worth 25% of the course grade. To prepare for the exams, students were assigned weekly quizzes and were given opportunities for optional practice quizzing and in-class clicker questions as formative assessment. Students were also provided with weekly learning objectives and access to the previous year’s exams. None of the exam questions were identical to questions presented previously in problem sets, old exams, or quizzes. Additionally, in the first week of class, students were given a handout about effective study strategies that included a list of active study techniques along with content-specific examples. Further, on the first quiz, students were asked to determine the most active way to use a particular resource from a list of options. The mean and SD of these exams, and all other variables used in this analysis, can be found in Supplemental Table 2. Pairwise correlations for all variables can be found in Supplemental Table 3
Pre and Post Knowledge Test
As described previously ( Walck-Shannon et al. , 2019 ), the pre/posttest is a multiple-choice test that had been developed by the instructor team. The test contained 38 questions, but the percentage of questions correct is reported here for ease of interpretation. The same test was given online in the first week of classes and after class sessions had ended. One percent extra credit was given to students who completed both tests. To encourage students to participate fully, we presented the pre and posttests as learning opportunities in the course to foreshadow topics for the course (pretest) or review topics for the final (posttest). Additionally, we told students that “reasonable effort” was required for credit. Expressing this rationale seemed to be effective for participation rates. While others have found that participation is low when extra credit is offered as an incentive (38%, Padilla-Walker et al. , 2005 ), we found participation rates for the pre- and posttests to be high; 97.4% of students completed the pretest and 85.9% of students completed the posttest.
Statistical Analyses
To test our three hypotheses, we used hierarchical regression. We controlled for potential confounding variables in step 1 and factored in the study variable of interest at step 2 for each model. We performed the following steps to check that the assumptions of linear regression were met for each model: first, we made scatter plots and found that the relationship was roughly linear, rather than curved; second, we plotted the histogram of residuals and found that they were normally distributed and centered around zero; and finally, we checked for multicollinearity by verifying that no two variables in the model were highly correlated (greater than 0.8). All statistical analyses were performed in JMP Pro (SAS Institute).
Base Model Selection
The purpose of the base model was to account for potential confounding variables. Thus, we included variables that we theoretically expected to explain some variance in exam performance based on previous studies. First, based on a meta-analysis ( Westrick et al. , 2015 ) and our own previous study with a different cohort in this same course ( Walck-Shannon et al. , 2019 ), we expected academic preparation to predict performance. Therefore, we included ACT math and biology pretest scores in our base model. Second, the negative relationship between self-reported class absences and exam or course performance is well documented ( Gump, 2005 ; Lin and Chen, 2006 ; Credé et al. , 2010 ). Therefore, we included the number of class sessions missed in our base model. Finally, our research questions focus on how students use their study time, rather than the relationship between study time itself and performance. Because others have found a small but significant relationship between total study time and performance ( Credé and Kuncel, 2008 ), we controlled for the total number of hours spent studying in our base model. In summary, theoretical considerations of confounds prompted us to include ACT math score, biology pretest score, self-reported class absences, and self-reported exam study time as the base for each model.
Calculated Indices
In the following sections we provide descriptions of variables that were calculated from the reported data. If variables were used directly as input by the student (e.g., class absences, percent of study time distracted) or directly as reported by the registrar (e.g., ACT score), they are not listed below.
Total Exam Study Time.
In students’ exam reflections, they were asked to report both the number of hours that they studied each day in the week leading up to the exam and any hours that they spent studying more than 1 week ahead of the exam. The total exam study time was the sum of these study hours.
Number of Active Strategies Used.
To determine the number of active strategies used, we first had to define which strategies were active. To do so, all authors reviewed literature about desirable difficulties and effective study strategies (also reviewed in Bjork and Bjork, 2011 , and Dunlosky et al. , 2013 , respectively). Then, each author categorized the strategies independently. Finally, we met to discuss until agreement was reached. The resulting categorizations are given in Table 1 . Students who selected “other” and wrote a text description were recoded into existing categories. After the coding was in place, we summed the number of active strategies that each student reported to yield the number of active strategies variable.
a The classification of the strategy into active and passive is stated in “type.” Prevalences for exam 1 ( n = 424) and exam 2 ( n = 471) are reported.
Proportion of Study Time Using Active Strategies.
In addition to asking students which strategies they used, we also asked them to estimate the percentage of their study time they spent using each strategy. To calculate the proportion of study time using active strategies, we summed the percentages of time using each of the active strategies, then divided by the sum of the percentages for all strategies. For most students (90.0% for exam 1 and 92.8% for exam 2), the sum of all percentages was 100%. However, there were some students whose reported percentages did not add to 100%. If the summed percentages added to between 90 and 110%, they were still included in analyses. If, for example, the sum of all percentages was 90%, and 40% of that was using active strategies, this would become 0.44 (40/90). If the summed percentages were lower than 90% or higher than 110%, students were excluded from the analyses involving the proportion of active study time index.
Number of Days in Advance Studying Began.
In the exam 2 reflection, we asked students to report: 1) their study hours in the week leading up to the exam; and 2) if they began before this time, the total number of hours and date that they began studying. If students did not report any study hours earlier than the week leading up to the exam, we used their first reported study hour as the first day of study. If students did report study time before the week before the exam, we used the reported date that studying began as the first day of study. To get the number of days in advance variable, we counted the number of days between the first day of study and the day of the exam. If a student began studying on exam day, this would be recorded as 0. All students reported some amount of studying.
Number of Days Studied in Week Leading Up to the Exam.
As a measure of studying consistency, we counted the number of days that each student reported studying in the week leading up to exam 2. More specifically, the number of days with nonzero reported study hours were summed to give the number of days studied.
The study strategies that students selected, the timing with which they implemented those strategies, and the level of distraction they reported while doing so are described below. We depict the frequencies with which certain study variables were reported and correlate those study variables to exam 1 and exam 2 scores. For all performance analyses described in the Results section, we first controlled for a base model described below.
We attempted to control for some confounding variables using a base model, which included preparation (ACT math and course pretest percentage), self-reported class absences, and self-reported total study hours. For each analysis, we included all consenting individuals who responded to the relevant reflection questions for the model. Thus, the sample size and values for the variables in the base model differed slightly from analysis to analysis. For brevity, only the first base model is reported in the main text; the other base models included the same variables and are reported in Supplemental Tables 5A, 7A, and 8A.
The base model significantly predicted exam 1 score and exam 2 score for all analyses. Table 2 shows these results for the first analysis; exam 1: R 2 = 0.327, F (4, 419) = 51.010, p < 0.0001; exam 2: R 2 = 0.219, F(4, 466) = 32.751, p < 0.0001. As expected, all individual predictor terms were significant for both exams, with preparation and study time variables positively associated and absences negatively associated. For means and SDs of all continuous variables in this study, see Supplemental Table 2. We found that the preparatory variables were the most predictive, with the course pretest being more predictive than ACT math score. Total study time and class absences were predictive of performance to a similar degree. In summary, our base model accounted for a substantial proportion (32.7%) of the variance due to preparation, class absences, and study time, which allowed us to interpret the relationship between particular study habits and performance more directly.
aStandardized β values, unstandardized b values, and standard errors (SE) are reported. Each model’s R 2 is also reported. Ranges of possible values for each variable are shown in brackets. The values for the base models corresponding to Tables 4 – 6 vary slightly depending on the students included in that analysis (see Supplemental Table 4). The following symbols indicate significance: * p ≤ 0.05; ** p ≤ 0.01; *** p ≤ 0.001.
Did Students Who Used More Active Study Strategies Perform Better on Exams?
We first investigated the specific study strategies listed in Table 1 . Then, we examined the total amount of time spent on active strategies to test our hypothesis that students who spent more time actively studying performed better on exams. Further, we counted the number of different types of active strategies that students used to test whether students who used a more diverse set of active strategies performed better on exams than those who used fewer active strategies.
Study Strategies Differed in Their Frequency of Use and Effectiveness.
The frequency with which specific study strategies were employed is reported in Table 1 . Almost all students reported reading notes. The next most prevalent strategies were active in nature, including that students (in order of prevalence) completed problem sets, completed old exams, self-quizzed, synthesized notes, explained concepts, and made diagrams. Surprisingly, each of these active strategies was used by the majority of students (54.7–86.1%) for both exams 1 and 2 ( Table 1 ). Less frequently used strategies included those more passive in nature, including that students (in order of prevalence) watched lectures, reviewed online content, read the textbook, and rewrote notes. A relatively infrequent strategy was attending review sessions, office hours, and help sessions. Because student engagement varied dramatically in these different venues, we classified this category as mixed. In summary, our results showed that, after reading notes, the most frequently used strategies were active strategies.
Next, we wondered whether the types of strategies that students reported using were related to exam performance. For these analyses, we added whether a student used a specific strategy (0 or 1) into the model, after controlling for the base model reported in Table 2 . When holding preparation, class absences, and total study time equal, we found that, on average, students who reported having completed problem sets, explained concepts, self-quizzed, or attended review sessions earned 4.0–7.7% higher on average on both exams 1 and 2 than students who did not report using the strategy (see b unstd. in Table 3 ). Notably, these strategies were active in nature, except for the category attending review session, which was mixed in nature. The remaining active strategies were positively correlated to performance for only one of the exams. Additionally, we observed that the strategies categorized as passive were either nonsignificant or negatively related to performance on at least one exam. Together, these results suggest that active strategies tended to be positively related to exam performance. In our sample, each of these active strategies was used by the majority (more than half) of the students.
aThis table summarizes 12 two-step hierarchical models per exam. The first step adjusted for the base model (see Table 2 ), and the second step included one specific study strategy. Unstandardized b values, standard errors (SE), and the change in R 2 relative to the base model are reported. Full model outputs are reported in Supplemental Table 4. Unstandardized b coefficients correspond to the average difference in exam score between the people who did and did not use the strategy. The following symbols indicate significance: * p ≤ 0.05; ** p ≤ 0.01; *** p ≤ 0.001.
bRelative to a base model with R 2 = 0.327 that included ACT score, bio. pretest %, number of classes missed before exam 1, and total exam 1 study hours. See Table 2 for base model details.
cRelative to a base model with R 2 = 0.219 that included ACT score, bio. pretest %, number of classes missed between exams 1 and 2, and total exam 2 study hours. See Table 2 for base model details.
The Proportion of Time Spent Using Active Strategies Positively Predicted Exam Score.
To further understand how active strategies related to performance, we investigated the proportion of study time that students spent using active strategies. On average, students spent about half of their study time using active strategies for exam 1 (M = 0.524, SD = 0.244) and exam 2 (M = 0.548, SD = 0.243), though values varied from 0 to 1 ( Figure 2 ). Importantly, students who spent a larger proportion of their study time on active strategies tended to perform better on exams 1 and 2. More specifically, after accounting for our base model (Supplemental Table 5A), the proportion of time students spent using active strategies added significant additional predictive value for exam 1, F (1, 416) = 8.770, p = 0.003, Δ R 2 = 0.014; and exam 2, F (1, 450) = 14.848, p = 0.0001, Δ R 2 = 0.024. When holding preparation, class absences, and total study time equal, we found that students who spent all of their study time on active strategies scored 5.5% higher and 10.0% higher on exams 1 and 2, respectively, than those who spent none of their study time on active strategies ( Table 4 ). Overall, these two results suggested that, on average, students spent about half of their study time using active strategies and students who devoted more study time to active strategies tended to perform better on exams.
FIGURE 2. Distribution of the proportion of time that students devoted to active study for exam 1 ( n = 422) and exam 2 ( n = 456). Percentages of students in each bin are indicated.
aThis table summarizes two two-step hierarchical models per exam. The first step adjusted for the base model, and the second step included either proportion of active study time or the number of active strategies. Full model outputs are reported in Supplemental Table 5. Unstandardized b values, standard errors (SE), and the change in R 2 from the base model (see Supplemental Table 5A) are reported. Ranges of possible values for each variable are given in brackets. The following symbols indicate significance: * p ≤ 0.05; ** p ≤ 0.01; *** p ≤ 0.001.
bRelative to a base model with R 2 = 0.322 that included ACT score, bio. pretest %, number of classes missed before exam 1, and total exam 1 study hours. See Supplemental Table 5A for base model details.
cRelative to a base model with R 2 = 0.238 that included ACT score, bio. pretest %, number of classes missed between exams 1 and 2, and total exam 2 study hours. See Supplemental Table 5A for base model details.
The Number of Active Strategies Used Positively Predicted Exam Score.
We next investigated the number of active strategies used by each student. On average, students used approximately four active strategies for exam 1 (M = 4.212, SD = 1.510) and exam 2 (M = 4.239, SD = 1.501). Very few students used no active strategies and most students (73%) used four or more active strategies ( Figure 3 ). Further, those students who used more active strategies tended to perform higher on exams 1 and 2. More specifically, after accounting for our base model, the number of active strategies students used added significant additional predictive value for exam 1, ( F (1, 416) = 33.698, p < 0.0001 Δ R 2 = 0.024; and exam 2, F (1, 450) = 91.083, p < 0.0001, Δ R 2 = 0.066. When holding preparation, class absences, and total study time equal, we found that, for each additional active strategy used, students scored 1.9% and 2.8% higher on exams 1 and 2, respectively. Students who used all six active strategies scored 11.1% higher and 16.6% higher on exams 1 and 2, respectively, than those who used no active strategies ( Table 4 , See Supplemental Table 5A for base model). In summary, students who used a greater diversity of active strategies tended to perform better on exams.
FIGURE 3. Distribution of the number of active strategies that each student used for exam 1 ( n = 422) and exam 2 ( n = 456). Percentages of students in each bin are indicated.
Post Hoc Analysis 1: Are Certain Active Strategies Uniquely Predictive of Performance?
Though it was not part of our planned analyses, the previous finding that the number of active strategies is predictive of performance made us question whether certain active strategies are uniquely predictive or whether they each have overlapping benefits. To test this, we added all six of the active strategies into the model as separate variables in the same step. When doing so, we found that the following active strategies were distinctly predictive for both exams 1 and 2: explaining concepts, self-quizzing, and completing problem sets (Supplemental Table 6). In other words, the portion of exam-score variance explained by certain active strategies was non-overlapping.
Did Study Timing Predict Performance on Immediate or Delayed Exams?
We next characterized students’ spacing potential using two indices: 1) the number of days in advance that studying began (cramming) and 2) the number of days in the week leading up to the exam that a student studied (consistency). Notably, in these results, we adjusted for our base model, which included total study time. In this way, we addressed the timing of studying while holding the total amount of studying equal. We examined outcomes at two different times: exam 2, which came close after studying; and the cumulative final exam and the posttest, which came after about a 5-week delay.
Cramming Was Not a Significant Predictor of Exam 2, the Final Exam, or the Posttest.
While there was variation in the degree of cramming among students, this was not predictive of exam score on either immediate or delayed tests. On average, students began studying 5.842 d in advance of exam 2 (SD = 4.377). About a third of students began studying 0–3 days before the exam, and another third began studying 4–6 days before the exam ( Figure 4 A). When holding preparation, class absences, and total study time equal, we found that the number of days in advance that studying began was not a significant predictor of in-term exam 2, the posttest, or the cumulative final ( Table 5 ; see Supplemental Table 7A for base model).
FIGURE 4. Distributions of spacing potential variables for exam 2 ( n = 450). (A) The distribution of the days in advance that exam 2 studying began (cramming); (B) the distribution of the number of days studied in the week before exam 2 (consistency). Percentages of students in each bin are indicated.
aThis table summarizes two two-step hierarchical regression models per assessment. The first step adjusted for our base model, and second step included either days in advance, or number of days studied in week before exam. Full model outputs are reported in Supplemental Table 7. Unstandardized b values, standard errors (SE), and the change in R 2 from the base model (Supplemental Table 7A) are reported. Ranges of possible values for each variable are given in brackets. The following symbols indicate significance, * p ≤ 0.05; ** p ≤ 0.01; *** p ≤ 0.001.
bRelative to a base model with R 2 = 0.231 that included ACT score, bio. pretest %, number of classes missed before exam 2, and total exam 2 study hours. See Supplemental Table 7A for base model details.
cRelative to a base model with R 2 = 0.396 that included ACT score, bio. pretest %, number of classes missed before exam 2, and total exam 2 study hours. See Supplemental Table 7A for base model details.
dRelative to a base model with R 2 = 0.273 that included ACT score, bio. pretest %, number of classes missed before exam 2, and total exam 2 study hours. See Supplemental Table 7A for base model details.
Studying Consistency Was Not a Significant Predictor of Exam 2, the Final Exam, or the Posttest.
While there was variation in how consistently students studied in the week leading up to exam 2, this consistency was not predictive of exam score either immediately or on delayed tests. On average, students studied 5 of the 8 days leading up to the exam (M = 5.082, SD = 1.810 ). Sixteen percent of students studied every day, and no students studied fewer than 2 days in the week leading up to the exam ( Figure 4 B). When holding preparation, class absences, and total study time equal, we found that the number of days studied in the week leading up to the exam was not a significant predictor of in-term exam 2, the posttest, or the cumulative final ( Table 5 ; see Supplemental Table 7A for base model).
In summary, our students varied in both the degree of cramming and the consistency of their studying. Even so, when holding preparation, class absences, and study time equal as part of our base model, neither of these spacing potential measures were predictive of performance on immediate or delayed tests.
Did Students Who Reported Being Less Distracted while Studying Perform Better on Exams?
In addition to the timing of studying, another factor that contextualizes the study strategies is how focused students are during study sessions. In the exam reflections, we asked students how distracted they were while studying. Here, we relate those estimates to exam scores while controlling for our base model of preparation, class absences, and total study time.
Distraction while Studying Was a Negative Predictor of Exam Score.
On average, students reported being distracted during 20% of their exam 1 and exam 2 study time (exam 1: M = 20.733, SD = 16.478; exam 2: M = 20.239, SD = 15.506) . Sixty-one percent of students reported being distracted during more than 10% of their study time ( Figure 5 ). Further, students who were more distracted while studying tended to perform lower on exams 1 and 2. After accounting for our base model, the percent of study time that students reported being distracted added significant additional predictive value for exam 1 and exam 2; exam 1: F (1, 429) = 12.365, p = 0.000, Δ R 2 = 0.019; exam 2: F (1, 467) = 8.942, p = 0.003, Δ R 2 = 0.015. When holding preparation, class absences, and total study time equal, we found that students who reported being distracted 10% more than other students scored about 1% lower on exams 1 and 2 ( Table 6 ; see Supplemental Table 8A for base model). In summary, this suggests that not only was it common for students to be distracted while studying, but this was also negatively related to exam performance.
FIGURE 5. Distribution of the percent of time students reported being distracted while studying for exam 1 ( n = 435) and exam 2 ( n = 473). Percentages of students in each bin are indicated.
aThis table summarizes one two-step hierarchical regression model per exam. The first step adjusted for our base model, and second step included the percent of study time that students reported being distracted. Full model outputs are reported in Supplemental Table 8. Unstandardized b values, standard errors (SE), and the change in R 2 from the base model (see Supplemental Table 8A) are reported. The range of possible values is given in brackets. The following symbols indicate significance: * p ≤ 0.05; ** p ≤ 0.01; *** p ≤ 0.001.
bRelative to a base model with R 2 = 0.328 that included ACT score, bio. pretest %, number of classes missed before exam 1, and total exam 1 study hours. See Supplemental Table 8A for base model details.
cRelative to a base model with R 2 = 0.219 that included ACT score, bio. pretest %, number of classes missed between exams 1 and 2, and total exam 2 study hours. See Supplemental Table 8A for base model details.
Students’ independent study behaviors are an important part of their learning in college courses. When holding preparation, class absences, and total study time equal, we found that students who spent more time on effortful, active study strategies and used a greater number of active strategies had higher scores for exams. Yet neither students who started studying earlier nor those who studied over more sessions scored differently than students who started later or studied over fewer sessions. Additionally, students who were more distracted while studying tended to perform worse than students who were less distracted. In other words, both the degree to which students employed desirably difficult strategies while studying and their level of focus when doing so were important for performance.
Specific Study Strategies (Hypothesis 1)
Our finding that more time and diversity of active study strategies were associated with higher exam grades was consistent with our hypothesis based on the desirable difficulties framework, laboratory, and classroom research studies ( Berry, 1983 ; King, 1992 ; Bjork, 1994 ; Karpicke and Roediger, 2008 ; Karpicke and Blunt, 2011 ; Hartwig and Dunlosky, 2012 ). Our study brought together lab research about effective strategies with what students did during self-directed study in an actual course. In doing so, we affirmed the lab findings that active strategies are generally effective, but also uncovered further nuances that highlight the value of investigating course-specific study strategies.
First, our study, when combined with other work, may have revealed that certain study strategies are more common than course-nonspecific surveys would predict. For example, compared with surveys of general study habits, our students reported relatively high use of active strategies. We found that the majority of students (73%) reported using four or more active strategies, which was more than the 2.9 average total strategies listed by students in a survey about general study habits at this same institution ( Karpicke et al. , 2009 ). In particular, we found that two-thirds of students reported the active study strategy of self-quizzing. This was considerably higher than what was found in a free-response survey about general habits not focused on a specific course at the same institution ( Karpicke et al. , 2009 ). In this survey, only 10.7% reported self-testing and 40.1% reported using flashcards. This higher frequency of self-quizzing behaviors may be due to a combination of factors in the course, the measures, and/or the students. In this course, we attempted to make self-quizzing easier by reopening the weekly quiz questions near exam time ( Walck-Shannon et al. , 2019 ). We also used a course-specific survey rather than the more general, course-nonspecific surveys used in the previous research. Additionally, it is possible that, in recent years, more students have become more aware of the benefits of self-testing and so are using this strategy with greater frequency. When we compared our frequencies of several categories to analogous categories from course-specific surveys of introductory biology students ( Sebesta and Bray Speth, 2017 ) and molecular biology students ( Rodriguez et al. , 2018 ), we saw similar results. Combined with our work, these studies suggest that when students focused on a particular course, they reported more active strategies than when prompted about studying in general.
Second, the opportunity to control for potential confounding variables in our study, including total study time, allowed us to better estimate the relationships between specific strategies and performance. This approach was important, given concerns raised by others that in classroom studies, benefits of certain strategies, such as explanation, could simply have been due to greater total study time ( Dunlosky et al. , 2013 ). Our results showed that, even when controlling for total study time, self-explanation and other strategies were still significant predictors of performance. This helped illustrate that the strategies themselves, and not just the time on task, were important considerations of students’ study habits.
Third, we were surprised by how predictive the diversity of active strategies was of performance. While we found that the proportion of active study time and the number of active strategies were both important predictors of performance, we found that the latter was a stronger predictor. This suggests that, if total study time was held equal, students who used a larger number of active strategies tended to perform better than those that used a smaller number of active strategies. This finding also deserves to be followed up in subsequent study to determine whether any of the active strategies that students use tend to co-occur in a “suite,” and whether any of those suites are particularly predictive of performance. We suspect that there is some limit to the benefit of using diverse strategies, as some strategies take a considerable amount of time to master ( Bean and Steenwyk, 1984 ; Armbruster et al. , 1987 ; Wong et al. , 2002 ), and students need to devote enough time to each strategy to learn how to use it well.
Additionally, we found that particular active study strategies—explanation, self-quizzing, and answering problem sets—were uniquely predictive of higher performance in a biology course context. Undergraduate biology courses introduce a large amount of discipline-specific terminology, in addition to requiring the higher-order prediction and application skills found among STEM courses ( Wandersee, 1988 ; Zukswert et al. , 2019 ). This is true for the course studied here, which covers biochemistry and molecular genetics, and the assessments that we used as our outcomes reflect this combination of terminology, comprehension, prediction, and application skills. Our results support the finding that active, effortful strategies can be effective on a variety of cognitive levels ( Butler, 2010 ; Karpicke and Blunt, 2011 ; Smith and Karpicke, 2014 ); and this work extends support of the desirable difficulties framework into biology by finding unique value for distinct generative or testing strategies.
Study Timing (Hypothesis 2)
Inconsistent with our second hypothesis that students with less spacing potential would perform worse than students with more spacing potential, we found no relationship between study timing and performance on in-term or cumulative exams. Because we knew that spacing was difficult to estimate, we analyzed two spacing potential indices, the degree of cramming (i.e., the number of days in advance that students started studying) and the consistency of studying (i.e., the number of days studied in the week leading up to the exam). We controlled for total study time, because the spacing effect is defined as identical study time spread over multiple sessions rather than fewer, massed sessions. When doing so, neither of these measures were significantly related to performance.
There are a few possible explanations why we may not have observed a “spacing effect.” First, as explained in the Introduction , we measured spacing potential. It could be that students with high spacing potential may have arranged their studies to mass studying each topic, rather than spacing it out, which would lead us to underestimate the spacing effect. Second, students likely studied again before our cumulative final. This delayed test is where we expected to see the largest effect, and restudying may have masked any spacing effect that did exist. Third, we asked students to directly report their study time, and some may have struggled to remember the exact dates that they studied. While this has the advantage that it results in more sensitive and direct measures of students’ spacing potential than asking students to interpret for themselves whether they binarily spaced their studies or crammed ( Hartwig and Dunlosky, 2012 ; Rodriguez et al. , 2018 ), students who did not remember their study schedules may have reported idealized study schedules with greater spacing, rather than realistic schedules with more cramming ( Susser and McCabe, 2013 ), thus minimizing the expected spacing effect.
Despite the lack of a spacing effect in our data, we certainly do not advocate that students cram their studying, as we find it likely that students who started studying earlier may also have tended to study more. Also, those same students who studied earlier may have felt less stressed and gotten more sleep. In other words, even though our estimation of spacing potential did not capture performance benefits, benefits of spacing for well-being may be multifaceted and not wholly captured by our study.
Distraction (Hypothesis 3)
Consistent with our third hypothesis, we found a negative relationship between distraction while studying and performance. This finding agreed with the few available studies that related distraction during self-directed out-of-class studying and grade, but differs in that our students reported a lower level of distraction than other published studies ( Junco, 2012 ; Junco and Cotten, 2012 ). One possible reason for our low distraction estimate may have been that students were inadvertently underestimating their distraction, as has been reported ( Kraushaar and Novak, 2010 ). In addition, some students may not have been including multitasking as a type of distraction, and this habit of multitasking while studying will likely be difficult to change, as students tend to underestimate how negatively it will affect performance ( Calderwood et al. , 2016 ).
Implications for Instruction
To encourage students to use more active study strategies, try asking students to turn in the output of the strategy as a low-stakes assignment. For example, to encourage self-explanation, you could ask students to turn in a short video of themselves verbally explaining a concept for credit. To encourage practice quizzing, try to publish or reopen quizzes near exam time ( Walck-Shannon et al. , 2019 ) and ask students to complete them for credit.
To encourage students to use active study strategies effectively, model those strategies during class. For example, when doing a clicker question, explicitly state your approach to answering the question and self-explain your reasoning out loud. This also gives you an opportunity to add the rationale for why certain strategies are effective or provide advice about carrying them out. In addition to modeling a strategy, remind students to do it often. Simply prompting students to explain their reasoning to their neighbors or themselves during a clicker question helps shifts students’ conversations toward explanation ( Knight et al. , 2013 ).
To encourage students to stay focused during studying, provide voluntary, structured study sessions. These could include highly structured peer-led team-learning sessions during which students work through a packet of new questions ( Hockings et al. , 2008 ; Snyder et al. , 2015 ) or more relaxed sessions during which students work through problems that have already been provided ( Kudish et al. , 2016 ).
Limitations and Future Directions
There are multiple caveats to these analyses, which may be addressed in future studies. First, our data about study behaviors were self-reported. While we opened the reflection exercise immediately after the exam to mitigate students forgetting their behaviors, some may still have misremembered. Further, some students may not have forgotten, but rather were unable to accurately self-report certain behaviors. As stated earlier, one behavior that is especially prone to this is distraction. But, similarly, we suspect that some students had trouble estimating the percent of study time that they spent using each strategy, while their binary report of whether they used it or not may be more accurate. This may be one reason why the number of active strategies has more explanatory power than the percent of time using an active strategy. Separately, although students were told that we would not analyze their responses until after the semester had ended, some may have conformed their responses to what they thought was desirable. However, there is not strong evidence that students conform their study habit responses to their beliefs about what is effective. For example, Blasiman and colleagues found that, even though students believed rereading was an ineffective strategy, they still reported using it more than other strategies ( Blasiman et al. , 2017 ). Another limitation due to self-reporting is that we lack knowledge of the exact, nuanced behaviors that a student carried out. Thus, a student who chose a strategy that we defined as active—such as “completing problem sets”—may have actually performed more passive behaviors. Specifically, while we did use verbal reminders and delay the release of a key when encouraging students to complete the problem sets and old exams before looking at the answers, some students may have looked up answers prematurely or may have read passively through portions of the key. These more passive behaviors may have underestimated the importance of active strategies. A second limitation is that these data were collected from a course at a selective research-intensive institution and may not be applicable to all student populations. A third limitation is that our analyses are correlational. While we have carefully selected potential confounds, there may be other important confounding variables that we did not account for. Finally, it was beyond the scope of this study to ask whether certain subgroups of students employed different strategies or whether strategies were more or less predictive of performance for different subgroups of students.
Despite these caveats, the main point is clear. Students’ course-specific study habits predict their performance. While many students in our sample reported using effective strategies, some students still had room to improve, especially with their level of distraction. One open question that remains is how we can encourage these students to change their study habits over time.
ACKNOWLEDGMENTS
We would like to thank April Bednarski, Kathleen Weston-Hafer, and Barbara Kunkel for their flexibility and feedback on the exam reflection exercises. We would also like to acknowledge Grace Yuan and Ashton Barber for their assistance categorizing exam questions. This research was supported in part by an internal grant titled “Transformational Initiative for Educators in STEM,” which aimed to foster the adoption of evidence-based teaching practices in science classrooms at Washington University in St. Louis.
- Armbruster, B. B., Anderson, T. H., & Ostertag, J. ( 1987 ). Does text structure/summarization instruction facilitate learning from expository text? Reading Research Quarterly , 22 (3), 331. Google Scholar
- Bean, T. W., & Steenwyk, F. L. ( 1984 ). The effect of three forms of summarization instruction on sixth graders’ summary writing and comprehension . Journal of Reading Behavior , 16 (4), 297–306. Google Scholar
- Berry, D. C. ( 1983 ). Metacognitive experience and transfer of logical reasoning . Quarterly Journal of Experimental Psychology Section A , 35 (1), 39–49. Google Scholar
- Bjork, E. L., & Bjork, R. A. ( 2011 ). Making things hard on yourself, but in a good way: Creating desirable difficulties to enhance learning . In Gernsbacher, M. A.Pew, R. W.Hough, L. M.Pomerantz, J. R. (Eds.) & FABBS Foundation, Psychology and the real world: Essays illustrating fundamental contributions to society (pp. 56–64). New York, NY: Worth Publishers. Google Scholar
- Bjork, R. A. ( 1994 ). Memory and metamemory considerations in the training of human beings . In Metcalfe, J.Shimamura, A. P. (Eds.), Metacognition: Knowing about knowing (pp. 185–205). Cambridge, MA: Worth Publishers. Google Scholar
- Blasiman, R. N., Dunlosky, J., & Rawson, K. A. ( 2017 ). The what, how much, and when of study strategies: Comparing intended versus actual study behaviour . Memory , 25 (6), 784–792. Medline , Google Scholar
- Bretzing, B. H., & Kulhavy, R. W. ( 1979 ). Notetaking and depth of processing . Contemporary Educational Psychology , 4 (2), 145–153. Google Scholar
- Butler, A. C. ( 2010 ). Repeated testing produces superior transfer of learning relative to repeated studying . Journal of Experimental Psychology: Learning, Memory, and Cognition , 36 (5), 1118–1133. Medline , Google Scholar
- Calderwood, C., Green, J. D., Joy-Gaba, J. A., & Moloney, J. M. ( 2016 ). Forecasting errors in student media multitasking during homework completion . Computers and Education , 94 , 37–48. Google Scholar
- Carpenter, S. K. ( 2009 ). Cue strength as a moderator of the testing effect: The benefits of elaborative retrieval . Journal of Experimental Psychology: Learning, Memory, and Cognition , 35 (6), 1563–1569. Medline , Google Scholar
- Credé, M., & Kuncel, N. R. ( 2008 ). Study habits, skills, and attitudes: The third pillar supporting collegiate academic performance . Perspectives on Psychological Science , 3 (6), 425–453. Medline , Google Scholar
- Credé, M., Roch, S. G., & Kieszczynka, U. M. ( 2010 ). Class attendance in college . Review of Educational Research , 80 (2), 272–295. Google Scholar
- Crowe, A., Dirks, C., & Wenderoth, M. P. ( 2008 ). Biology in Bloom: Implementing Bloom’s taxonomy to enhance student learning in biology . CBE—Life Sciences Education , 7 (4), 368–381. Link , Google Scholar
- Dunlosky, J., Rawson, K. A., Marsh, E. J., Nathan, M. J., & Willingham, D. T. ( 2013 ). Improving students’ learning with effective learning techniques . Psychological Science in the Public Interest , 14 (1), 4–58. Medline , Google Scholar
- Gump, S. E. ( 2005 ). The cost of cutting class: Attendance as a predictor of success . College Teaching , 53 (1), 21–26. Google Scholar
- Hartwig, M. K., & Dunlosky, J. ( 2012 ). Study strategies of college students: Are self-testing and scheduling related to achievement? Psychonomic Bulletin & Review , 19 (1), 126–134. Medline , Google Scholar
- Hockings, S. C., DeAngelis, K. J., & Frey, R. F. ( 2008 ). Peer-led team learning in general chemistry: Implementation and evaluation . Journal of Chemical Education , 85 (7), 990. Google Scholar
- Hora, M. T., & Oleson, A. K. ( 2017 ). Examining study habits in undergraduate STEM courses from a situative perspective . International Journal of STEM Education , 4 (1), 1. Google Scholar
- Jacoby, L. L. ( 1978 ). On interpreting the effects of repetition: Solving a problem versus remembering a solution . Journal of Verbal Learning and Verbal Behavior , 17 (6), 649–667. Google Scholar
- Junco, R. ( 2012 ). Too much face and not enough books: The relationship between multiple indices of Facebook use and academic performance . Computers in Human Behavior , 28 (1), 187–198. Google Scholar
- Junco, R., & Cotten, S. R. ( 2012 ). No A 4 U: The relationship between multitasking and academic performance . Computers & Education , 59 (2), 505–514. Google Scholar
- Karpicke, J. D., & Blunt, J. R. ( 2011 ). Retrieval practice produces more learning than elaborative studying with concept mapping . Science , 331 (6018), 772–775. Medline , Google Scholar
- Karpicke, J. D., Butler, A. C., & Roediger, H. L. ( 2009 ). Metacognitive strategies in student learning: Do students practise retrieval when they study on their own? Memory , 17 (4), 471–479. Medline , Google Scholar
- Karpicke, J. D., & Roediger, H. L. ( 2008 ). The critical importance of retrieval for learning . Science , 319 (5865), 966–968. Medline , Google Scholar
- King, A. ( 1992 ). Comparison of self-questioning, summarizing, and notetaking-review as strategies for learning from lectures . American Educational Research Journal , 29 (2), 303. Google Scholar
- Knight, J. K., Wise, S. B., & Southard, K. M. ( 2013 ). Understanding clicker discussions: Student reasoning and the impact of instructional cues . CBE—Life Sciences Education , 12 (4), 645–654. Link , Google Scholar
- Kornell, N., & Bjork, R. A. ( 2007 ). The promise and perils of self-regulated study . Psychonomic Bulletin & Review , 14 (2), 219–224. Medline , Google Scholar
- Kraushaar, J. M., & Novak, D. ( 2010 ). Examining the effects of student multitasking with laptops during the lecture . Journal of Information Systems Education , 21 (2), 241–251. Google Scholar
- Kudish, P., Shores, R., McClung, A., Smulyan, L., Vallen, E. A., & Siwicki, K. K. ( 2016 ). Active learning outside the classroom: Implementation and outcomes of peer-led team-learning workshops in introductory biology . CBE—Life Sciences Education , 15 (3), ar31. Link , Google Scholar
- Lin, T. F., & Chen, J. ( 2006 ). Cumulative class attendance and exam performance . Applied Economics Letters , 13 (14), 937–942. Google Scholar
- Marsh, E. J., & Butler, A. C. ( 2013 ). Memory in educational settings . In Reisberg, D. (Ed.), Oxford library of psychology. The Oxford handbook of cognitive psychology (pp. 299–317). Washington, DC: Oxford University Press. Google Scholar
- May, K. E., & Elder, A. D. ( 2018 ). Efficient, helpful, or distracting? A literature review of media multitasking in relation to academic performance . International Journal of Educational Technology in Higher Education , 15 (1), 13. Google Scholar
- Padilla-Walker, L. M., Thompson, R. A., Zamboanga, B. L., & Schmersal, L. A. ( 2005 ). Extra credit as incentive for voluntary research participation . Teaching of Psychology , 32 (3), 150–153. Google Scholar
- Pressley, M., McDaniel, M. A., Turnure, J. E., Wood, E., & Ahmad, M. ( 1987 ). Generation and precision of elaboration: Effects on intentional and incidental learning . Journal of Experimental Psychology: Learning, Memory, and Cognition , 13 (2), 291–300. Google Scholar
- Rawson, K. A., & Kintsch, W. ( 2005 ). Rereading effects depend on time of test . Journal of Educational Psychology , 97 (1), 70–80. Google Scholar
- Rodriquez, F., Rivas, M. J., Matsumura, L. H., Warschauer, M., & Sato, B. K. ( 2018 ). How do students study in STEM courses? Findings from a light-touch intervention and its relevance for underrepresented students . PLoS ONE , 13 (7), e0200767. Medline , Google Scholar
- Sebesta, A. J., & Bray Speth, E. ( 2017 ). How should I study for the exam? Self-regulated learning strategies and achievement in introductory biology . CBE—Life Sciences Education , 16 (2), ar30. Link , Google Scholar
- Smith, M. A., & Karpicke, J. D. ( 2014 ). Retrieval practice with short-answer, multiple-choice, and hybrid tests . Memory , 22 (7), 784–802. Medline , Google Scholar
- Snyder, J. J., Elijah Carter, B., & Wiles, J. R. ( 2015 ). Implementation of the peer-led team-learning instructional model as a stopgap measure improves student achievement for students opting out of laboratory . CBE—Life Sciences Education , 14 (1), ar2. Link , Google Scholar
- Stanton, J. D., Neider, X. N., Gallegos, I. J., & Clark, N. C. ( 2015 ). Differences in metacognitive regulation in introductory biology students: When prompts are not enough . CBE—Life Sciences Education , 14 (2), ar15. Link , Google Scholar
- Susser, J. A., & McCabe, J. ( 2013 ). From the lab to the dorm room: Metacognitive awareness and use of spaced study . Instructional Science , 41 (2), 345–363. Google Scholar
- Tomanek, D., & Montplaisir, L. ( 2004 ). Students’ studying and approaches to learning in introductory biology . Cell Biology Education , 3 (4), 253–262. Link , Google Scholar
- Walck-Shannon, E. M., Cahill, M. J., McDaniel, M. A., & Frey, R. F. ( 2019 ). Participation in voluntary re-quizzing is predictive of increased performance on cumulative assessments in introductory biology . CBE—Life Sciences Education , 18 (2), ar15. Link , Google Scholar
- Wandersee, J. H. ( 1988 ). The terminology problem in biology education: A reconnaissance . American Biology Teacher , 50 (2), 97–100. Google Scholar
- Westrick, P. A., Le, H., Robbins, S. B., Radunzel, J. M. R., & Schmidt, F. L. ( 2015 ). College performance and retention: A meta-analysis of the predictive validities of ACT® scores, high school grades, and SES . Educational Assessment , 20 (1), 23–45. Google Scholar
- Wong, R. M. F., Lawson, M. J., & Keeves, J. ( 2002 ). The effects of self-explanation training on students’ problem solving in high-school mathematics . Learning and Instruction , 12 (2), 233–262. Google Scholar
- Zimmerman, B. J. ( 2002 ). Becoming a self-regulated learner: An overview . Theory into Practice , 41 (2), 64–70. Google Scholar
- Zukswert, J. M., Barker, M. K., & McDonnell, L. ( 2019 ). Identifying troublesome jargon in biology: Discrepancies between student performance and perceived understanding . CBE—Life Sciences Education , 18 (1), ar6. Link , Google Scholar
- Causes and outcomes of at-risk underperforming pharmacy students: implications for policy and practice 19 April 2024 | BMC Medical Education, Vol. 24, No. 1
- Riding the wave towards flourishing in STEM education: Enhancing teaching efficacy through a K-12 training program Teaching and Teacher Education, Vol. 143
- Study strategy use among elementary school students: Is use of specific study strategies related to academic performance? 2 March 2024 | Metacognition and Learning, Vol. 39
- Exploring the link between perceived physical literacy and academic performance outcomes: insights from the EHDLA study 24 January 2024 | Frontiers in Sports and Active Living, Vol. 6
- Factors that influence general chemistry students’ decision making in study strategies 1 January 2024 | Chemistry Education Research and Practice
- Breaking the mold: Study strategies of students who improve their achievement on introductory biology exams 3 July 2023 | PLOS ONE, Vol. 18, No. 7
- The impact of effective study strategy use in an introductory anatomy and physiology class 31 May 2023 | Frontiers in Education, Vol. 8
- Assessing Learner Engagement and the Impact on Academic Performance within a Virtual Learning Environment 15 February 2023 | Pharmacy, Vol. 11, No. 1
- Which course resources and student approaches to learning are related to higher grades in introductory biology? Journal of Research in Science, Mathematics and Technology Education
- Sleep Deprivation and Study Habits Effects toward Medical Imaging Students, UiTM Puncak Alam 30 September 2022 | Environment-Behaviour Proceedings Journal, Vol. 7, No. 21
- Evaluating open-note exams: Student perceptions and preparation methods in an undergraduate biology class 18 August 2022 | PLOS ONE, Vol. 17, No. 8
- Eight Recommendations to Promote Effective Study Habits for Biology Students Enrolled in Online Courses Journal of Microbiology & Biology Education, Vol. 23, No. 1
- Analysis of Learning and Academic Performance of Education Students Before and During the Coronavirus Disease Pandemic 15 October 2021 | European Journal of Educational Research, Vol. volume-10-2021, No. volume-10-issue-4-october-2021
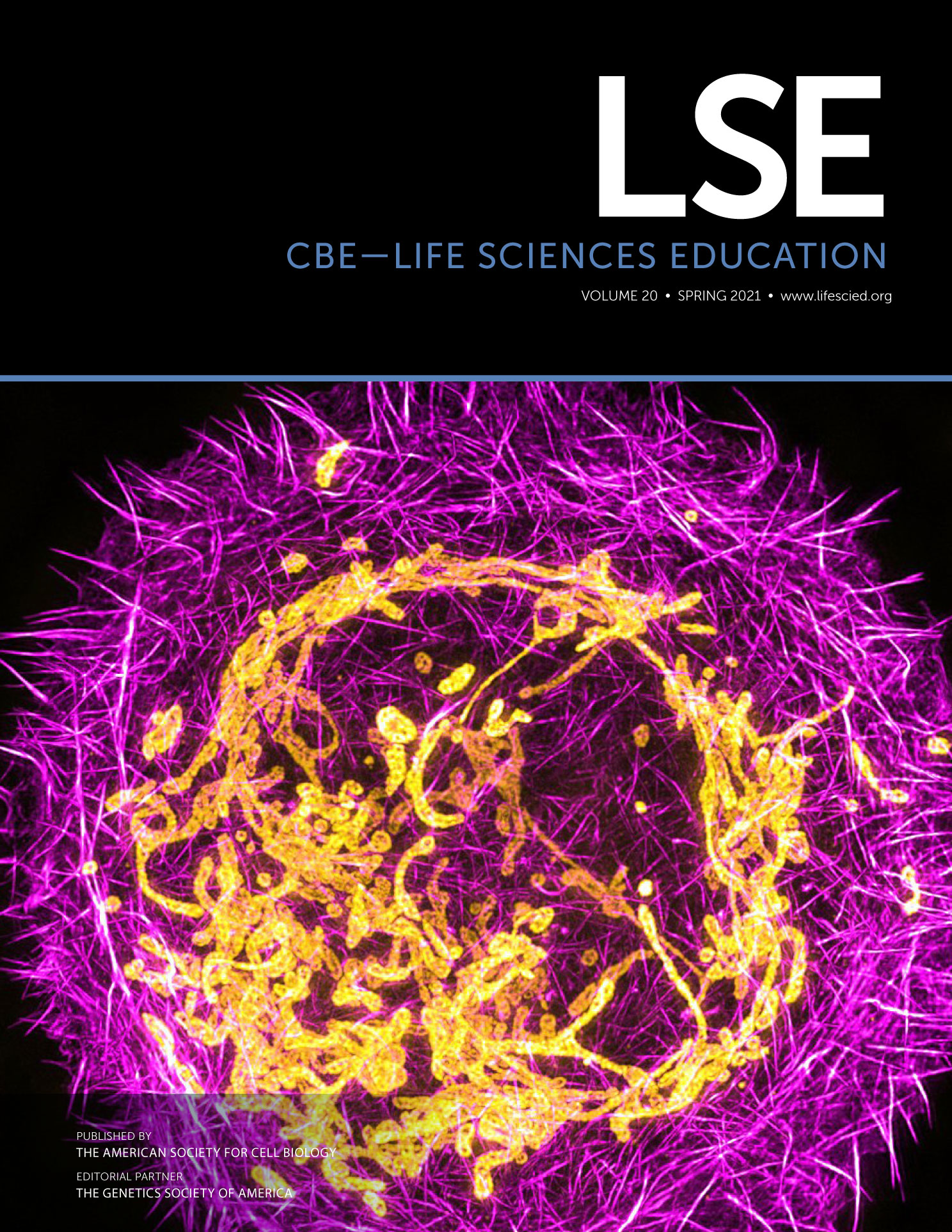
Submitted: 19 May 2020 Revised: 19 October 2020 Accepted: 22 October 2020
© 2021 E. M. Walck-Shannon et al. CBE—Life Sciences Education © 2021 The American Society for Cell Biology. This article is distributed by The American Society for Cell Biology under license from the author(s). It is available to the public under an Attribution–Noncommercial–Share Alike 3.0 Unported Creative Commons License (http://creativecommons.org/licenses/by-nc-sa/3.0).
- Open access
- Published: 01 February 2017
Examining study habits in undergraduate STEM courses from a situative perspective
- Matthew T. Hora 1 &
- Amanda K. Oleson 2
International Journal of STEM Education volume 4 , Article number: 1 ( 2017 ) Cite this article
42k Accesses
33 Citations
16 Altmetric
Metrics details
A growing body of research in cognitive psychology and education research is illuminating which study strategies are effective for optimal learning, but little descriptive research focuses on how undergraduate students in STEM courses actually study in real-world settings. Using a practice-based approach informed by situated cognition theory, we analyzed data from 61 STEM students about their study habits.
Results indicate that studying is a multi-faceted process that is initiated by instructor- or self-generated cues, followed by marshaling resources and managing distractions, and then implementing study behaviors that include selecting a social setting and specific strategies. Variations in some study behaviors are also evident according to the timing of their studying (e.g., cramming), course level, discipline, and social setting. Three cases of individual student practices reveal how studying is also shaped by how the course is designed and taught, students’ own beliefs about studying, and aspects of their personal lives.
Conclusions
The results indicate that studying involves various social, digital, and curricular resources, that many students persist in utilizing low-impact study strategies (e.g., re-reading text), and that the use of study strategies varies across different situations. We suggest that the focus on changing teaching behaviors that is dominant within STEM education be broadened to include a focus on instructional design that supports student self-regulatory behaviors and the adoption of high-impact study strategies.
Graphical abstract
The stages of studying across three cases: Brianna, Larry, and Angelica.
As concerns mount regarding the quality of undergraduate education, particularly in the science, technology, engineering, and mathematics (STEM) fields, policymakers, educators, and student affairs professionals are increasingly focusing on how to support student learning throughout their academic careers. Given that students’ academic success is shaped by a complex matrix of psychological, cultural, and organizational factors, scholars are investigating a variety of issues that may impact student success including underlying psychological attributes such as engagement (Carini et al. 2006 ) and perseverance or “grit” (Duckworth et al. 2007 ), what instructors believe about teaching and learning (Hativa and Goodyear 2002 ), and the types of teaching methods used in the classroom (Freeman et al. 2014 ). However, while these areas of research shed light on key aspects of student learning, these foci overlook a key piece of the student learning puzzle—what students actually do when they leave the classroom and study.
A considerable body of literature exists on college student study skills and habits, with foci on students’ cognitive styles and approaches to learning (Biggs 1987 ; Riding and Cheema 1991 ), the use of specific study techniques (Karpicke et al. 2009 ) and the role of study habits and time spent studying on overall student achievement (Nonis and Hudson 2010 ; Robbins et al 2004 ). Investigating the nature of study habits is important because factors related to studying such as motivation and specific study techniques have been linked to academic success. In a meta-analysis of 72, 431 students, Credé and Kuncel ( 2008 ) found that motivation and study skills (e.g., time management) were positively associated with grade point average and grades in individual courses. Furthermore, a comprehensive review of research on specific study strategies found that some (e.g., distributed practice) led to learning gains whereas others (e.g., re-reading text) did not (Dunlosky et al. 2013 ) and that many college students are not employing these study habits (Hartwig and Dunlosky 2012 ), and understanding why students persist in using ineffective study practices and how to change this state of affairs, from a situative perspective, is of particular importance to the field of STEM education. Thus, knowing whether or not (and why) students are using these practices is important information for instructors and student affairs/academic advising professionals.
Yet for the field of postsecondary education in general, and STEM education in particular, relatively little is known about student study habits, largely due to the lack of robust descriptive research that accounts for students’ behaviors in real-world settings. The gaps in the literature are twofold. First, much of the research on studying is based on survey research or experimental studies of specific study strategies, with few qualitative, descriptive studies of how students actually study in real-world situations. Such an approach to research, that focuses on descriptive accounts of naturalistic behaviors in order to inform educational programming and reforms, is becoming increasingly important in research on reform implementation in both K-12 and postsecondary contexts (Hora 2016 ; Coburn and Turner 2012 ; Spillane et al. 2002 ). Second is view of study habits as decontextualized, not shaped by social, curricular, situation; given insights from situated cognition research on how activity and learning itself is “distributed, stretched over (and) not divided among” mind, tools, and social and organizational contexts (Lave 1988 , p. 1), and that decision-making and behavior cannot be properly understood without close attention to the naturalistic settings in which they unfold (Klein 2008 ), the reliance on decontextualized survey research for insights into study habits is no longer tenable.
In this exploratory study, we utilize a practice-based approach to focus on the actual study behaviors of 61 undergraduates at three research universities in the USA and Canada who were enrolled in biology, physics, earth science, and mechanical engineering courses. Drawing upon situated cognition theory to conceptualize studying as a behavior that encompasses individual study strategies as they unfold in specific social, technological, and institutional contexts, we analyze data using inductive thematic analysis from 22 focus groups, and these students provided detailed information about their study habits that allowed us to answer the following research questions: (1) What behaviors do students taking undergraduate STEM courses engage in when studying? (2) What underlying contextual factors, if any, influence these behaviors?
We pursued this line of research because while the question “How can we teach students if we do not know how they learn?” (Coffield et al. 2004 , p. 1) is important, we also wonder “How can we best support student success if we do not understand how they study?” Insights gleaned from the data presented in this paper, which indicate that studying is a complex, multi-dimensional practice that implicates cues, social resources, artifacts, and study strategies, can provide faculty and student affairs professionals with a new way to think about studying that extends the prior focus on specific, decontextualized study strategies.
Discussions regarding the state of undergraduate education in the early twenty-first century often focus on the role of the instructor and their pedagogical acumen in the classroom (e.g., Bok 2009 ). Indeed, much of the focus in the STEM education literature is on how to affect changes in faculty teaching practices and philosophies about student learning (PCAST 2012 ). While instructors certainly play an important role in facilitating student learning by crafting experiences that engage students in these ways (or not), researchers have long questioned whether enough attention has been placed on the other actor involved in the learning enterprise—the student. As Entwistle and Tait ( 1990 , p. 170) observed, student behaviors are “part of a broader academic environment which affects learning probably as much as, if not more than, the classroom skills of the lecturer.” According to this view, the student as an agent actively engaged in his or her own learning and overall experience in college is a central, if not primary, part of the teaching and learning equation that is too often overlooked.
Psychological approaches to understanding study habits and academic success
In early research on the change processes that young people undergo while in college (Pascarella and Terenzini 2005 ) and the factors that contribute to students’ lack of persistence (Tinto 1993 ), higher education scholars have paid particularly close attention to the psychological factors that shape students’ experiences and ultimate success (or lack thereof). For instance, attributes associated with academic success such as involvement (Astin 1984 ) and engagement have been used to explain students’ relative success in their academic coursework (Carini et al. 2006 ). An underlying assumption in this literature is that students’ mental stances or psychological attributes play a major role in their academic outcomes and that higher education professionals should support them by facilitating higher degrees of involvement and engagement to increase their prospects for success.
Another line of inquiry has focused on subconscious psychological traits associated with student learning including cognitive styles and approaches to learning (see Coffield et al. 2004 for a review). For example, researchers have argued that people have stable cognitive styles or “typical or habitual mode(s) of problem solving, thinking, perceiving, and remembering” that shape how they think and learn (Riding and Cheema 1991 , p. 194). Another commonly used construct is that of approaches to studying, which refers to more elastic, changeable approaches and preferences that learners have for studying and learning (Entwistle and Tait 1990 ). Early work in this area argued for the existence of two distinct approaches to learning whose basic outlines persist to the present time: deep approaches to learning that involve searching for meaning and surface approaches that involve rote memorization (Marton and Säljö 1976 ; Biggs 1987 ). While such approaches to learning are theorized as being relatively stable within an individual, they can change over time with concerted effort. Furthermore, these psychological attributes should not be considered as operating independently from the context in which studying occurs (Ramsden 1979 ). In fact, early research in this area found that some students actively sought information in the environment (e.g., textbooks, lecture content) and then studied using what was called “cue-seeking” behavior, whereas others were more “cue-deaf” or worked to succeed without seeking hints about exams (Miller and Parlett 1974 ).
This focus on the origins of student’s motivation to initiate studying is similar to a long-standing line of inquiry that examines the degree to which learners are able and willing to assume control of their own learning process or what is known as self-regulated learning (Zimmerman and Schunk 2001 ). A self-regulated learner engages in a process of initiating the learning process on their own, setting goals, identifying appropriate strategies, and reflecting on his or her own task performance—all of which ultimately leads to a decision to enact changes in future behaviors or to maintain current practices (Cassidy 2011 ). Self-regulated learning is a particularly valuable idea in college student success, with empirical research in this area indicating that students who exhibit high degrees of self regulation have higher rates of academic achievement as measured by persistence and grades (Boekaerts and Corno 2005 ).
Research on study habits and skills
Another body of literature that examines studying focuses directly on the study habits and skills that students utilize during the act of studying itself. However, what at first glance may appear to be a straightforward, easily defined term is operationalized in a variety of ways in the literature. For instance, Robbins et al. ( 2004 , p. 276) define study skills as “activities necessary to organize and complete schoolwork tasks and to prepare for and take tests” and operationalize the construct using measures including time management, leadership skills, communication skills, and the un-defined category of “study skills and habits” (see also Credé and Kuncel 2008 ; Lotkowski et al. 2004 ). Other scholars have defined study habits in different ways, including the ability to concentrate, the scheduling of regular review sessions, and hours spend studying (Nonis and Hudson 2010 ). Conceptualizing study habits in terms of time spent studying is rather common, and a widely cited report by Babcock and Marks ( 2010 ) found that hours spent studying has declined from 24 h a week in 1961 to 14 h a week in 2003. In 2009, the picture was bleaker, with over half of freshmen who took the Your First College Year Survey and over half of seniors who took the College Senior Survey spending 10 h or less per week studying or doing homework (Ruiz et al. 2010 ; Franke et al. 2010 ).
While these studies capture important facets of studying and the role that they play in student achievement and persistence, the specific strategies and actions students actually engage in during their study sessions remain obscured. Providing more clarity on specific study habits, Karpicke et al. ( 2009 ) found that the preferred study strategy of 84% of the surveyed undergraduates was re-reading textbooks and lecture notes. Unfortunately, a study examining the utility of 10 learning techniques in the empirical literature found that habits such as these considered low utility in regard their impact on student learning, in contrast to high-utility techniques such as practice testing and distributed practice (i.e., taking tests over time), thus suggesting that many undergraduates utilize study habits that are ineffective (Dunlosky et al 2013 ).
Given the ubiquity of the Internet and digital media in many people’s lives, researchers are also investigating how these artifacts are being used as study aids. In one study exploring student utilization of digital and “traditional” resources, researchers found that 39 and 44% of students search Wikipedia and Google, respectively, if they need help with coursework, with only 36% seeking out a faculty member (Morgan et al. 2012 ). Similarly, a 2010 study of 36,950 undergraduates found that 33% used wikis, 24% used video-sharing websites, and 12% used blogging tools (Smith and Caruso 2010 ). Besides these more traditional digital media, including course websites hosted on institutional learning management systems, some argue that other tools that facilitate personalized learning (Dabbagh and Kitsantas 2012 ) and digitally mediated social learning via open Internet-based resources (Seely Brown and Adler 2008 ) are under-utilized in higher education. Researchers are also examining how digital media can inhibit studying, however, and Rosen et al. ( 2013 ) found middle-school, high-school, and undergraduate students were unable to remain on task for even 6 min before being tempted by Facebook or texting when studying at home.
However, the literature on study skills, strategies, and habits is limited by a tendency to reduce the complex and multi-faceted behaviors that comprise studying to metrics that cannot capture how and why students study (i.e., hours spent studying) or focus on strategies (e.g., re-reading) at the expense of other possible behaviors or choices students make. Perhaps the single largest limitation, however, is the lack of attention paid to the contexts within which students actually study. While some scholars have focused on the environmental contexts of studying (Kuo et al. 2004 ) and the interaction among study habits and social factors (Treisman 1992 ; Robbins et al 2004 ), few recent studies have attempted to describe studying behaviors as a multi-faceted process that includes not only study strategies but also how situations and resources are implicated in these practices.
This is important because educational practice, whether a group of undergraduates studying for a biology course or an administrator finalizing a budget, should not be thought of solely in terms of an individual making decisions in isolation, as the context of decision-making as well as tools and other artifacts utilized as part of the process is critically important. In studying the practices of principals in K-12 settings, for example, researchers have utilized theoretical frameworks from situated and distributed cognition which assert that the institutional context is not a mere backdrop for activity but is instead an integral feature of individual cognition and decision-making as well as task performance itself (Halverson 2003 ; Hora 2012 ; Spillane et al 2002 ). In this study, we draw upon these frameworks to conceptualize studying as the discrete behaviors of individuals (e.g., reviewing notes) as they unfold within specific contexts and that implicate particular artifacts and resources.
Why does the lack of descriptive research on student study habits that adopt a situative perspective matter? Because fine-grained descriptions of people’s behaviors in specific contexts and situations illuminates the specific steps people take when solving problems or performing tasks—information that can then be used by instructors and educational leaders to improve their practices and design more locally attuned interventions (Coburn and Turner 2012 ; Spillane et al. 2001 ). Educational researchers across the K-16 spectrum have argued that more practice-based research should be conducted on the various behaviors associated with teaching and learning, so that how and why educators and students make decisions in “the wild” of schools, colleges, and universities can illuminate barriers and supports to effective practice, rather than simply prescribing how people should think and act regardless of the situation (Bastedo 2012 ). With such a comprehensive and multi-faceted approach to describing studying, we set out to document the study habits of 61 undergraduates taking STEM courses as a corrective to the focus on both teaching and study strategies alone, in the hopes that such accounts could inform ways that educators can improve student learning and academic success.
Exploratory research is intended to examine poorly understood phenomena and generate new insights and hypotheses that can guide future research on the topic (Slavin 2002 ; Stebbins 2001 ). In this exploratory study, we examine the study habits of a group of STEM students, with a focus on describing the lived experiences and subjective interpretations of individuals and groups or what cultural anthropologists call an “emic” account of social life (Merriam 2014 ). The study took place at three large, public research universities in the USA and Canada that had similar undergraduate populations (approximately 25,000 students). These sites were selected due to the presence of instructional reform initiatives, which was a criterion for the larger study on STEM instructors’ data driven decision-making upon which this analysis is based. The disciplines included in this study are biology, geology, physics, and mechanical engineering based on the STEM-related focus of the larger study. For this study, a non-random purposive sampling procedure was used to identify faculty study participants. Faculty were included in the study population if they were listed as instructors in each institution’s course listings for the 2013 spring semester. We contacted 165 instructors via email requesting their participation in the study, and 59 participated (36% response rate). Thus, the faculty whose classes were recruited into the study were unique in that they were self-selected and taught undergraduate STEM courses at large research universities.
These instructors represented the initial pool of courses from which we selected student participants for the focus groups. We selected the focus group technique in order to collect a large amount of qualitative, in-depth data in a shorter amount of time than would be possible with individual interviews (Bernard 2011 ). Of the 59 faculty who participated in the larger study, we asked 30 instructors they would recruit students for participation in focus groups, of which 22 instructors agreed. The 30 courses (and instructors) selected for recruitment represented the largest courses across all four of the disciplines included in the study, which increased the prospects of recruiting sufficient numbers of students. Those instructors sent email requests to their classes, and students contacted the research team if they were interested in participation. There was a $20 incentive, and 61 students participated (see Table 1 ).
Data collection
A team of four researchers conducted the student focus group interviews using a semi-structured interview protocol, with each group led by one or two moderators depending upon scheduling constraints. The key question posed to participants in the focus groups was: “Please imagine for a moment how you typically study for this course —can you describe in as much detail as possible your study situation?” This question was followed by probes regarding the types of materials used for studying, whether participants studied alone or with others, and any additional details not yet described. While the open-ended nature of the questions resulted in detailed observations about study practices, it also led to idiosyncratic accounts that were not always comparable across individuals. We also did not provide a definition for the act of “studying” during the focus groups, which was based on our goal of capturing students’ own unique perceptions about what behaviors and situations constituted a study session. Each focus group included between two and six students and lasted approximately 45 min. These focus groups were audio recorded and transcribed.
Data analysis
Transcripts were entered into NVivo qualitative analysis software and then segmented into manageable units or discrete statements by participants that encapsulated a single thought or idea (Gee 1986 ). First, a code list was created to segment the data that aligned with the research questions guiding the analysis. We were interested in segments related to “study strategies” and “study situations,” and thus, any utterances pertaining to these two categories were sought out. Both analysts reviewed five transcripts with these two codes in mind and highlighted text fragments related to both codes and then met to ensure a common understanding of the relationship between the codes and the raw data. Upon ensuring that the codes were being applied similarly, the second author then segmented the remainder of the dataset. Second, we followed a structured approach to grounded theory that involved using a combination of a pre-existing “coding paradigm” and the inductive analysis of transcripts to develop a code list with which to analyze the entire dataset. The second author developed a preliminary code list using an inductive open-coding approach where terms or ideas mentioned by study participants themselves (e.g., re-reading textbooks) were used to create code names (Glaser and Strauss 1967 ) while the research questions and theoretical framework were also kept in mind (Strauss and Corbin 1990 ). After developing the initial code list, we met to discuss the codes and revised them while reviewing text fragments and discussing the applicability of codes to the data. During this process, we attempted to derive codes that maintained as much fidelity to participants’ own language and descriptions of study behaviors as possible.
The second author then developed the final code list using the constant comparative method, where each occurrence of a code was compared to each previous instance of that code in order to confirm or alter the code and/or its definition (Glaser and Strauss 1967 ), after which the final code list was applied to the entire dataset. At this point in the analytic process, qualitative researchers have the option of reporting recurrent themes with or without numeric counts of their prevalence. In this paper, where all study participants responded to questions in a similar fashion (e.g., specific study strategies), we elected to report the number of times a code was applied to the raw data in order to convey to readers the frequency with which a particular behavior or observation was identified in the data. In other cases where responses were more ambiguous and/or where different respondents interpreted questions differently, we report recurrent themes instead of numeric counts.
The data were also entered into a data matrix with subjects as rows and study cues, resources, and strategies as columns. These data were analyzed using exploratory data reduction methods (i.e., hierarchical cluster analysis and multi-dimensional scaling) to see if patterns across the data could be discerned. Clear patterns were not discernable, so these data were then organized to report the frequency with which particular strategies were used according to different groups of students (e.g., discipline, social situation). The results reported in this paper depict the percentage of students within each group reporting each strategy, with results weighted according to the size of each respective group.
Next, we analyzed two students and one entire focus group who provided particularly rich details about their study habits in order to depict how studying unfolds in real-world settings at the individual level. These subjects were selected because of the level of detail they provided when self-reporting their conceptions of what studying means, the contexts in which their studying occurred, and their actual study behaviors. These case studies also highlight the situated nature of studying in the influence of peers, curricular artifacts, and other features of the environment on their study habits. Finally, we examined the resulting themes to explore any patterns in the data and identified a new way of thinking about studying that is reported in this paper.
Limitations to this study include the self-selected nature of the sample, both of the participating instructors and students, that limits generalizability of the findings to broader populations of undergraduates. Such limitations to generalizability are an inherent part of research using small, non-randomly selected samples, but their strength is in illuminating behaviors at a fine-grained level. While future research involving larger samples will be necessary to assess how widespread the behaviors reported in this paper truly are, the data do raise questions and considerations about studying that can be applied to different institutions. Another limitation is that the focus group method may introduce an element of self-censoring and social desirability bias by participants due to the public nature of the setting, which can result in incomplete or inaccurate answers to the facilitator’s questions. Finally, because participants discussed their studying with varying degrees of specificity it was difficult at times to ascertain whether similar behaviors were being reported. The limitations associated with social desirability and the veracity of students’ accounts could not be overcome with the current study, though future work should consider incorporating an observational component to corroborate self-reported behaviors with actual practice.
Before reporting data addressing the research questions guiding the study, we first discuss how respondents had differing notions of what activities constituted “studying.” For some, it meant any exposure to course material such as attending a class, whereas for others, studying implied completing assigned tasks. In yet other cases studying referred to activities that were not assigned and took place outside of class. As one student said, “I see studying more as something that I do separate from any assigned material.” In addition to these task-oriented conceptions, some reported “folk” theories of the learning or ideas about phenomena that are not necessarily grounded in evidence. For example, one student stated, “Studying to me means stressing out your brain so that it realizes that the information is significant.”
Thus, for the students in this study, “studying” was not easily distilled into a set of discrete strategies such as re-reading the textbook or hours spent engaged in discrete strategies. Further, as we discuss below, students’ views of studying also implicate a variety of strategies, social and physical settings, and resources as being involved in the studying process (Greeno 1998 ; Halverson 2003 ; Robbins et al 2004 ). Future research should delve more deeply into what students consider to be studying in terms of its physical, artifactual, and temporal boundaries. To maintain a consistent definition for this analysis, however, we defined studying as any interaction with course material outside of the classroom.
Cues to initiate studying and timing of study strategies
Prior to engaging in particular study activities, students frequently discussed why they started studying, which centered on the core idea of “cues” that trigger study behaviors. These cues were either provided by the instructor or were internally generated. While students were not explicitly asked about what cued their study sessions, descriptions of the study processes for many students provided information for this analysis. Another important aspect of these preliminary stages of studying is when students choose to study—either throughout the semester, several days before an assessment, or the day before a test or exam (i.e., cramming).
Instructor-generated cues
Throughout a given semester, 40 students reported that instructors often provided cues regarding when and what they should study. The most important cue for students tended to be the announcement of an upcoming assessment, thus initiating the process of studying. For some, an impending assessment was the only reason for studying. Similarly, instructors’ discussions about assessments (e.g., topics that would be covered) served as a primary rationale for some students to attend class. One participant said, “I go to class to (hear) the professor say this week on the exam you will see this subject or that subject.” Consequently, for some students, the classroom becomes a venue in which cues pertaining to assessments are sought and then applied to their studying.
Self-generated cues
Fewer (four) participants also discussed another cue for studying, that of recognizing that they were not sufficiently prepared or familiar with the course material. One participant explained that he studied after realizing that he did not understand a concept, which then set in motion a series of study behaviors that lasted until he felt conversant with the material. He said, “…and then I realize, ‘Oh man, I don’t understand pulleys so well,’ so last week I studied pulleys until I understood them.” Others reported a strong desire to learn certain skills and material so that they could reach their career goals.
Next, we discuss findings regarding when students reported engaging in study activities. For 11 respondents studying took place several days before an exam or test, while 14 reported waiting until the last day or even night before, popularly known as “cramming.” While the literature indicates that cramming is an ineffective way to study (e.g., Kornell 2009 ), and some students recognize its limitations (e.g., one student reported that after cramming “[the information] is not still in my brain”), this mode of preparation remains a common method. Finally, 15 respondents discussed studying throughout the term. In some cases, this practice was instigated by course-specific factors such as an instructor’s use of weekly quizzes, whereas in others, the student established a regular schedule of studying on their own.
Marshaling resources for studying
After discussing cues and timing for studying, the respondents then discussed collecting and utilizing a variety of resources with which to study. In describing students’ use of resources, we included references to commonly used tools such as course websites and textbooks as well as human resources that learners draw upon when studying. This represents a broader view of resources within organizations than is commonly used but captures knowledge and capabilities of instructors and staff within an educational organization (Gamoran et al. 2003 ). Understanding the resources used during studying is important because digital, print, and human resources and tools are used to enhance or even shape the studying act itself.
The resources discussed by the respondents included digital tools and media, print resources, and human resources, and those most commonly reported are depicted in Table 2 .
Digital resources
While the most commonly reported digital tool included laptops or desktop computers, we focus here instead on the applications used by students on these now ubiquitous resources for college students. The most widely reported resource was the course website (27 students), which operated on various learning management system (LMS) platforms. These websites were developed by instructors who posted a variety of learning resources including videotaped lectures, readings, practice exams, and course syllabi. One student described her professor’s course website as such, “So basically like any way you learn you can find it on [course website name] through all her resources and find a good way to study for you.”
The next most widely used digital resource included websites for seeking out new information including Google (24) and Wikipedia (13). These websites helped students expand upon lecture notes or clarify concepts or steps in solving problems. For example, one participant noted that in lecture, he listened for key words that could be included on exams and then looked them up online, because “With the Internet and Wikipedia you just need to know a few keywords and you can learn about anything.” Other resources included Facebook (9) which was used as an organizing tool and Youtube (5) for informational purposes. These results support prior research that found college students regularly utilize these online resources, even more so than their own instructors (e.g., Morgan et al. 2012 ).
However, the evidence suggests that technology also acts as a disruptive force in some students’ study habits. Nineteen respondents reported that some digital resources, usually cell phones and Facebook, regularly disrupted their studying yet they had no strategy for managing these distractions. One student noted, “[When studying] I look up sports stuff, any excuse not to be studying….at a computer I can just click on whatever I want.” To mitigate the potential distractions of the Internet or a buzzing phone, 21 students reported having developed strategies for managing distractions, often by deliberately removing them from their study “space.” The optimal studying situation for one student was in an isolated cubicle in the library basement with no cell phone reception, and he would turn off his laptop’s wireless Internet signal. In another case, a student went to her parent’s house on the weekends for a self-imposed “no devices zone” where her phone was confiscated so she could concentrate. Thus, digital resources can both enhance and detract from an individual’s studying, and students have varying degrees of success when it comes to managing the detrimental aspect of digital devices and media.
Print-based resources
Another type of resource that respondents regularly used was print-based resources such as textbooks (34) and lecture notes (33). Lecture notes took many forms including notes taken by student in class as well as notes and/or PowerPoint slides provided by the instructor, both of which were reported as important resources for studying. Another less utilized print resource discussed by five respondents was cue cards, which were mostly used to memorize key facts and formulas.
Human resources
The last type of resource reported by students pertained to the knowledge and content-expertise of people within their courses and/or departments. These included instructors (8) as well as teaching assistants (8) and tutors (4). In some cases, the participants reported approaching instructors or teaching assistants outside of class to obtain assistance with homework, upcoming or previous exams, and challenging concepts or problems. For students who were especially struggling with the course, tutors provided expertise and one-on-one instruction that these students viewed as an especially important form of academic support.
Setting and strategies
Next, we report data that speak to the studying process itself, particularly with whom students study and the specific strategies they employ.
The social setting in which studying occurs
When describing their actual study sessions, the respondents noted whether or not they studied alone or with others. For 39 respondents, studying was often a solitary affair. Some students noted that studying alone was an explicit strategy to reduce distracting conversations with others, while others stated that it was simply a habit. In contrast, 35 students described studying in groups. In these cases, the respondents stated that group-based studying was useful because peers could provide new insights or solutions. However, because 24 students reported studying both alone and in groups, depending on the proximity to an exam or the nature of the assignment, it is clear that for some students in the sample, the social setting in which studying occurred was rather flexible and not a fixed criterion or preference.
Employing specific study strategies
The studying process next involves the selection of specific strategies or techniques. While the participants often described these strategies using imprecise or idiosyncratic terminology such that it was often not possible to align them with those discussed in the literature (e.g., Dunlosky et al. 2013 ), it was possible to identify several core strategies utilized by this group of undergraduate students. In this section, we elaborate on the most commonly referenced strategies (see Table 3 ).
Thirty-eight participants re-read or reviewed course material or notes taken in class. This strategy was discussed as both a general practice that took place throughout the term as well as an initial step in preparing for exams. For example, one participant said that he re-read all of his lecture notes before working with old test materials “to try to understand what the professor had said fully” before attempting to take practice tests.
Given the broad conception of studying used in this analysis (i.e., any interaction students have with course material outside of class), we include the strategy of “doing homework” which 25 participants reported. As one participant put it: “My method of studying is pretty much to do any homework or review questions…” Homework also provided a litmus test of understanding—one participant explained how he learned a lot in class, but it really became clear when he answered the homework questions correctly.
While students reported reviewing lecture notes from class, this particular strategy involved 22 students creating their own artifacts such as cue cards, consolidating notes from different sources (some instructor-provided, others self-procured) into one set of notes, and so on. For example, one participant explained, “I write myself notes and everything is in my notes, including the textbook material and the prof’s slides and what the prof said or the stuff I found in Wikipedia or everything.” Others created study aids (e.g., games or cheat sheets) that were used throughout the term for study sessions.
Twenty participants reported reading the textbook in some capacity, either in full or in part, either assigned or unassigned, or they consulted the book when confronted with unfamiliar material. Often, the participants did not specify if they were re-reading, reading it for the first time, or if they were skimming. Importantly, the depth with which students read textbooks appeared to vary based on their intentions. In one case, a student explained, “Sometimes I just go through the chapter we’re going to go through in class and I just read all the captions for the images (to prepare for the lecture) so I know what we’re going to talk about and then afterwards I’ll read through the chapter.” In most cases, however, students spoke more ambiguously about reading.
Nineteen participants reported working with test materials provided by the instructor or students who had previously taken the course. One participant reported her routine as taking practice exams in a simulated test-taking environment, followed by an item-by-item analysis of her performance. Another talked about reviewing tests from previous years and randomly selecting problems to complete for practice. In both cases, the materials provided the students with an opportunity to monitor their level of understanding (or lack thereof) while also becoming attuned to the test-maker’s approach.
Working on problems was a strategy reported by 17 participants. Although ambiguous, the specific nature of the term “problems” likely refers to mathematical or computational problems given that many of these participants were enrolled in science or engineering courses. As one participant said, “I just find every single practice problem that I can get my hands on and do it.”
Eleven participants reported working on a variety of questions while studying. In one class, students worked on study questions or short essay prompts that review that day’s lecture. Further, instead of relying on practice exams, one student in that class reported, “I’ve found the best way to do well on the test is not to do all of her practice exams, but do (the) study questions.” Others reported working on end-of-chapter questions and completing discussion questions as an effective study strategy.
Taking quizzes related to course material outside of class was another method of studying reported by 11 participants. Sometimes the instructor provided the quiz to test comprehension after a reading assignment. One student who takes bi-weekly extra-credit quizzes provided by her instructor said, “I take them pretty seriously, I’ll prep a little bit before them even though they’re only five questions and if I get something wrong I’ll read (about it).”
Other factors influencing the study process
In addition to specific cues, resources, and study strategies, respondents also discussed various situations or factors that influenced their study behaviors.
Role of instructor in providing resources for studying
Student’s use of resources during their studying depends, in part, on the instructor and his/her provision of particular resources within the course. For example, some instructors provided their students with a variety of modalities and tools for learning (e.g., podcasts, supplementary readings, online lecture notes) that other students might not have had access to in other courses or with other instructors. These can be offered as in-class resources, or more commonly, embedded within the course’s website or LMS. Students can then select from the resources made available by their instructors, as well as resources that they find on their own, to construct their own unique study situation.
Course characteristics and discipline
The participants described how disciplinary content and course structure also influenced the strategies and resources they used. Some students perceived that different disciplines required different approaches to studying. One participant said, “You can’t study math how you would study biology, right?” The student followed up this observation by describing how studying for a math course entailed doing numerous problem sets, while a biology course required extensive reading, memorization, and understanding laboratory assignments. Other course characteristics that influenced teaching were the assessments and teaching methods used in the course. For instance, one student explained how her approach to preparing for multiple-choice exams emphasized a surface knowledge of selected topics: “Instead of looking at a topic and being able to discuss it for paragraphs at a time in like an essay format, I’ll try to memorize details that I feel are important.” Another respondent student noted that his studying “tends to match the style of the class” so that in a class taught with PowerPoint slides, his studying entails “a lot of time looking at slides,” whereas a more interactive class involves focusing on concepts and hands-on activities. This student’s approach to studying suggests that an instructor’s teaching style may have consequences for student learning not only through in-class comprehension of material but also by sending messages to students regarding the best way to study.
Personal situations and dispositions
The participants also alluded to personal factors that influenced their studying such as the lack of time due to heavy course loads and/or work schedules, family situations, and health-related issues. Additionally, the participants brought to a course pre-existing dispositions and experiences that influenced their approach to studying. One of these pertains to historic study habits from high school, where some students attempted to alter their “old” study behaviors to fit with the “new” expectations and demands of the university, while others simply continued using what had worked for them previously. Finally, student’s personal reasons for taking a course (e.g., to satisfy degree requirement, curiosity) also shaped how participants approached their studying.
Patterns in cues, resources, and strategies
Next, we sought to explore whether or not patterns in the data existed in regard to how cues, resources, and strategies were inter-related or not. Preliminary analyses using exploratory data techniques did not reveal discernable patterns, and no clear links were evident across the three primary components of studying identified in the data (i.e., cues, resource use, strategies). Instead, we chose to examine patterns in the use of study strategies considered effective in the literature (e.g., Dunlosky et al. 2013 ) according to two aspects of study behaviors (i.e., study timing, social setting) and two variables related to subject characteristics (i.e., course level and discipline). All analyses include weighted averages.
First, when looking at when studying occurs according to three groups of students (i.e., less than 1 week prior, cramming, throughout term), some data points stand out (see Fig. 1 ). Crammers review notes more often than others (94%), while those studying less than 1 week prior to exams use more textbooks (73%), study questions (55%), and video (36%) than other groups and those studying throughout the term or semester use problem sets (53%) more often than others. These data indicate that some variation in study strategies is evident depending on when students choose to study.
Selected study strategies by timing of study practices and social setting
Second, when organizing the data according to two groups (i.e., studying alone or studying in groups) differences in study strategies are also evident (see Fig. 2 ). Note that some students reporting doing both, hence, the large numbers in both groups that do not sum to 60 (42 and 36, respectively). Students studying alone tend to review notes (64%) and textbooks (52%) and also do practice tests (24%) and quizzes (19%) more than those studying in groups. In contrast, those studying in groups create study artifacts (47%), do problems (31%) and questions (31%), and use online materials such as video (14%) and the Mastering Physics/Anatomy videos (19%).
Third, when the data are organized according to the discipline of the course students were enrolled in at the time of data collection, additional points of variation are evident (see Fig. 3 ). Again, students may or may not be majors in these fields but discussed their study habits in relation to these disciplines. Students taking biology courses (26) report reviewing notes (69%) and textbooks (46%), doing practice tests (31%) and questions (35%), and reviewing videos (27%) more than students taking courses in other fields. Students in physics courses (11) reported creating artifacts (64%), doing problem sets (82%), and mastering resources (45%) more than others. Mechanical engineers and geology students did not report any study strategies more than other groups.
Selected study strategies by discipline and course level
Finally, the data indicate that study habits vary by course level, with students in upper division courses (18) reporting using certain study strategies more than those in lower division courses (43), including practice tests (33%), questions (39%), video (28%), and mastering physics or anatomy resources (33%). The students in lower division courses reported reviewing notes (58%), creating artifacts (37%), reviewing textbooks (51%), and doing problems (42%) and quizzes (16%) more than the students in upper division courses (see Fig. 4 ).
Case examples
Finally, to illustrate how each of these sets of findings is evident in students’ own real-world experiences, we present three in-depth analyses of students’ actual studying practices. The first two cases are those of individual students—Larry and Brianna—whose study behaviors reflect different sequences of decisions that link particular cues, resource use, and strategies. The final case is that of a group of five students in a single course (i.e., upper division anatomy and physiology). These cases illustrate how studying is a complex, idiosyncratic practice, while also being shaped by the social, institutional, and technological milieu in which students operate.
Larry. When we spoke with Larry he was studying for an upper level biology course that was required for his major. He first talked about his personal view of what studying means, stating that:
Studying to me means stressing out your brain so that it realizes that the information is significant. Basically, your brain can be lazy when it doesn’t think that something’s important and stressing it is what makes it retain information. So studying for exams is a lot about stressing your brain out.
This theory of how the brain and learning work thus set the stage for Larry’s subsequent study habits, which was a process that began in the classroom. There, he “frantically” wrote on the instructor’s PowerPoint slides that he printed off before class, labeling images, drawing arrows, and identifying mechanisms for cell signaling that were being discussed in class. Larry said that he did not necessarily understand the concepts at the time but took the notes down to reference later. In fact, it is not until he finds the time to sit in the library and read the relevant sections of the textbook that “it all comes together and finally makes sense.” While reading, he writes down key terms and their definitions in a notebook. For Larry, the library represented an important resource in his education because he does not own the book because he cannot afford it. Thus, he spent a lot of time in the library reading one of two copies on reserve. He also attended the optional recitation section for the course where he was able to speak with the instructor one-on-one and earn extra credit.
While he tried to study throughout the term, with his demanding course load and work schedule, he often only had time to study 3 or 4 days prior to an exam. Describing his study habits as “messy” and comprised of “lots of big stages,” Larry first gathered his notes from classroom sessions and his review of the textbook and then made flashcards for key concepts from the course. He also completed the end-of-chapter quizzes in the text and reviewed (and retakes) any old quizzes or exams from the course. The day before the exam, he tells himself “Wow Larry, you really have to get to it now,” and he sequestered himself at the library to review his notes and difficult concepts in the textbook and to re-watch videotaped lectures from the course website. All along, he deliberately studied alone because he had to maximize the limited time available for studying, such that he “cannot afford to sit around and have people talk about other stuff.” After several hours in the library reviewing these materials, Larry generally felt ready for the exam. Altogether, in Larry’s case, studying is an act that is instigated by instructor’s cues (i.e., upcoming exam), informed by a folk theory of the mind, involves a variety of curricular resources, and is strongly influenced by his personal situation.
Brianna. Next, we consider the case of Brianna who was enrolled in a lower division physics course when we met with her group. Her general approach to studying was to hope that the instructor was direct about expectations and guidelines because then, “the ball is in (her) court and (she) either learns the material or not.” Thus, Brianna was relatively self-motivated but relied on instructors to provide cues regarding when and how hard to study. This motivation is also sparked by her aspiration to attend medical school, which requires doing well on the Medical College Admission Test (MCAT). Brianna observed that “even if I get an A on a test but have no idea what is going on, it wouldn’t set me up to be in a good place for studying the MCAT in the future.”
In addition, she relied heavily on what she called her own preferred “learning style,” which centered on reading and re-reading text, whether it be the textbook or notes taken in class. Given her reliance on text and notes, Brianna observed that, “I pretty much show up to lecture just to write down what he’s saying.” The notes she took in class then became an important artifact for later studying, as she used them to create flashcards from her notes (and the textbook) and an outline for the course that is added to throughout the term. During her actual study sessions, Brianna either studied alone, reviewing her notes, scanning various digital resources, and doing practice problems, or with a group of friends in the library where she typically had on her headphones while surrounded by classmates who intermittently helped one another out on difficult problems.
Brianna’s use of digital technology is notable because she described online videos, the course website, the Internet, and social media as the “majority” of the resources she used to study. For example, she consulted free online tutoring videos (especially videos featuring one tutor in particular at the University of California at Berkeley), which helped her fill out her notes and summaries from the class and textbook. Overall she described the Internet as a “great resource” for finding course materials (e.g., slides, notes, exams, and videos) from other instructors teaching the same course at other colleges or universities. The questions these other instructors ask their students provides insights into what Brianna perceives her professor may ask, so she values their outsider’s perspective. Further, when doing her homework problems, she looked up the solutions online, even when she was confident about her answer. She does this to ensure that she is “approaching [the problem] in the right way” or to see if there are alternative methods to solving the problem. This, in turn, gives her a more “holistic grasp of the question.” Finally, in this and other courses, social media sites such as Facebook provided a place where she and her friends posted questions and shared approaches to different problems. Notably, most of the digital resources Brianna utilized were not part of the official course materials organized by her instructor and posted on the course website.
Thus, for Brianna studying involves a process of re-reading course materials and tools such as cue cards and digital media, largely in response to instructor’s cues about upcoming exams or homework. Driven by the desire to attend medical school introduced an element of motivation that made her take studying rather seriously.
Dr. Wells’ course. The final case is that of five female students taking an upper division anatomy and physiology course with Dr. Wells. In this course, which had an enrollment of 525 students across three sections, Dr. Wells had provided a rich array of learning resources on the course website that included weekly postings of videotaped lectures and PowerPoint slides, weekly practice questions, old exams, and links to other online resources. The students in the focus group noted that Dr. Wells did not simply post these resources and let students figure out how to utilize them but instead discussed in class how to use each tool and study with them. As Angelica noted, “She just does a really great job of giving us a lot of different ways to study.” Jacquie concurred, saying that while an online course she was taking was similarly well-resourced, “Dr. Wells provides more alternative methods to study which is what makes her course stand out.” Ultimately, in providing such a variety of resources for studying, Dr. Wells had crafted a learning experience that stood out for these students. For Bailey, who had little experience with the material, this was particularly important because “it’s really hard to stick your fingers in and get going,” and if you only have a textbook to work with, the entry points to the material are limited, often inaccessible and not particularly engaging.
In many ways, Dr. Wells was running a partially flipped classroom, in that students watched videotaped lectures online and came to a class that was highly interactive and engaging. During the class, Dr. Wells was constantly in motion, using her iPad to project slides on the screen while also writing and drawing using a stylus pen. Many questions were asked of students, including peer-based activities and small group discussions. Linda noted that Dr. Wells also emphasized important ideas across various formats such as clicker questions, practice tests, and study questions, such that “the repetition is awesome…even if you’re tired or distracted, eventually you’ll still get it.”
In terms of how these students actually studied in the course, weekly study questions (i.e., short essay questions that recap entire lectures) provided by Dr. Wells played an important role. For Jacquie, who said that she essentially crams before the exam—saying “well yeah, that’s why we study, for the exams”—her lecture notes, study questions, the mastering anatomy online resources, and old test materials were all utilized during study sessions. After discussing the course with her friends, however, she concluded that the best way to succeed in the course was “not to do all of her practice exams but to do those freaking (weekly) study questions.” Angelica said that “I noticed when I don’t do the study questions I don’t do very well.” This approach is similar to the technique of distributed practice, or regularly spaced testing of material over time, which is one of the high-impact study strategies identified by Dunlosky and colleagues ( 2013 ). Robin also spent 3–4 h after each class doing the study questions, along with a variety of other tools including the mastering anatomy activities, notes, lecture videotapes, and old test materials. In fact, while the group varied on the timing of their studying, all were cued by the instructor, used a variety of digital and print resources and between four and six study strategies. This reliance on multiple resources and study strategies is unsurprising given how Dr. Wells structured her course and guided her students in regard to studying. Within this learning environment, students then developed their own approach to studying but in ways strongly shaped by the resources and strategies Dr. Wells had embedded in the course structure.
The field of higher education in general and STEM education in particular continues to grapple with how to best facilitate learning, persistence, and retention throughout students’ postsecondary careers. Does the answer lie in changing teacher behaviors alone, such as the adoption of active learning techniques, structural responses such as reducing student debt and dealing with the rising price of college, or is success also dependent upon student attributes such as engagement and motivation? What these questions reveal is that students’ experiences in college are shaped by a variety of influences and that the intersection among policy, economics, organizations, and instruction provides a more accurate frame for thinking about student success than a search for a single “magic bullet” solution.
The same idea applies to thinking about the role that effective study strategies play in student learning. While the use of high-impact practices such as distributed practice is certainly a key ingredient in leading to student learning (Dunlosky et al. 2013 ), it is important to recognize that students’ adoption of these practices requires several antecedent conditions to be in place before this can happen. These include knowledge of these methods, time to study, access to the resources required to study in this manner, and so on. Similarly, studying is not simply about using strategies such as re-reading text or doing practice problems but is a process that involves cues about when to study, the timing of their actual study sessions, which resources to utilize, where to study, and which strategies to employ. How these stages unfold in practice are also shaped by a variety of factors such as a students’ personal life, the course material, and how instructors structure courses and make learning resources available. This is not to diminish the importance of high-impact study strategies but instead to point out that there are many steps taken by students to get to the point where they can sit down and utilize them with some regularity.
In the remainder of this paper, we discuss how this exploratory study contributes to the literature on college student study habits, particularly through the articulation of a multi-dimensional conception of studying that can provide instructors and administrators with a more nuanced account of how students engage in studying. In combination with the data reported in this paper as well as developments in educational technology and research, such an account also highlights the importance of instructional design that facilitates students’ use of high-impact strategies, diversified learning tools, and self-regulatory capabilities.
A new approach for thinking about undergraduates’ study behaviors
The results reported in this paper confirm and extend prior research on college student study habits. The data reinforce prior research that some of the most dominant study strategies utilized by students include reviewing notes and re-reading textbooks (Karpicke et al. 2009 ), utilize a variety of digital resources (Smith and Caruso 2010 ), and also rely on instructors to provide cues to begin studying (Miller and Parlett 1974 ). While the study described here is limited by a small sample size and lack of data on the impact of various study habits on learning outcomes, it was designed to shed light on fine-grained behaviors among a small group of students in order to advance our understanding of decision-making and action in specific social, organizational, technological contexts. In doing so, we extend the prior literature by offering an integrative multi-stage approach for thinking about study behaviors.
When interpreting the results from this exploratory study, we observed that students discussed their studying in terms of stages that began with cues to study and ended with their use of specific strategies. Along the way, they made decisions about who to study with and which resources to use, an account consistent with a situative theory of cognition, which posits that mental activity and social action is situated within specific socio-cultural and organizational contexts while also being distributed among mind, tool, and activity (Greeno 1998 ; Spillane et al. 2001 ). In other words, studying is not solely a matter of a “mind” sequestered with a book and highlighter pen, or a behavior that could be distilled into hours spent studying or the prevalence of a particular strategy, but instead involves people interacting with one another and various tools in specific situations (Seely Brown and Adler 2008 ). This is not to diminish the value of experimental work that does hone in on specific aspects of studying such as how students self-pace their study or time spent on specific tasks (Bjork, Dunlosky and Kornell 2013 ) but instead is an argument that a broader perspective of the act of studying itself is also useful.
Consequently, based on the data presented in this paper, we suggest that a new way of thinking about studying is warranted that includes the following components: (1) recognizing the situation and detecting cues to initiate studying, (2) marshaling resources and managing distractions (or not), (3) selecting a time and social setting to study, and then selecting specific strategies, and (4) engaging in a period of self-reflection. We illustrate this approach using the three cases reported earlier in the paper (see Fig. 1 ).
Some caveats are necessary when interpreting this figure. First, while the stages of cue detection and timing, resource use, and strategies are based on data from this paper, the self-regulative period is not. Instead, it is included as a post-assessment phase of reflection and commitment that the literature indicates is an important aspect of learning (Zimmerman and Schunk 2001 ). Second, we do not claim that all 61 participants in the study progressed through each of these steps, but instead that this conception of studying captures the broad range of behaviors and experiences students reported engaging in during a recent study session. As a result, we are not suggesting that this account of studying is generalizable to all students but is a heuristic device for thinking about studying in a more multi-dimensional manner than is common at the present time.
Thus, we argue that conceptions of the act of "studying" extend beyond a focus on discrete, decontextualized factors such as hours spent studying or the use of specific strategies (e.g., re-reading text). In making this argument, we highlight the importance of ecological validity when thinking about study habits in general and interpreting laboratory-based research in particular. In other words, understanding how findings from the literature about "effective" study habits may vary according to disciplinary, social, institutional, or personal situations will be important for future work in the area. We also suggest that a more multi-dimensional conception of studying can also be a useful interpretive framework for educators, instructional designers, and administrators to begin thinking more broadly and strategically about how their courses are designed (or not) to foster effective study habits. By recognizing that studying involves multiple states, resources, strategies and actors, it becomes necessary to move beyond simply providing “how-to” guides for studying or recommendations for students to use high-impact practices to instead think about the role that cue-seeking, resource acquisition, and distraction management play in shaping students’ study habits. With a more situative view of studying in mind, it is possible to consider how the course as a whole creates an environment that prompts particular study behaviors, such as Dr. Wells’ provision of various learning tools via her LMS that prompted students to study with them. Thinking of studying in these terms, in the remainder of this paper we highlight ways that educators can facilitate or support effective studying and learning practices: fostering self-regulated learning and using principles from instructional design to encourage high-impact studying.
Fostering self-regulated learners
One of the most pressing issues facing educators is the fact that many students continue to utilize ineffective study practices, such as re-reading textbooks or cramming the night before an exam. Informing some low-impact practices are “faulty mental models” (p. 417) about how memory and learning work, such as the view that information can be recalled and played back like a recording (Bjork, Dunlosky and Kornell 2013 ). Instead, the retrieval process involves reconstructing knowledge from various stored memories, is heavily dependent on specific cues, and that upon cueing information in memory becomes reinforced. Essentially, students need to understand that in order to create a library of information in their minds that is easily accessed and retained over the long term requires a “meaningful encoding of that information” which involves integrating information into a network of connected ideas and then regularly practicing retrieval of that information (Dunlosky et al. 2013 ).
Besides becoming more sophisticated learners and theorists about how the mind works, it is clear that students can also benefit from more guidance about how to more effectively study and learn. Educational psychologists argue that becoming a more adept learner is not simply about amassing tips and strategies about how to study but is based on becoming what is known as a self-regulated learner, which is the “self-directive process by which learners transform their mental abilities into academic skills” (Zimmerman 2002 , p. 65). Self-regulation is not just a quality or personal aptitude, however, but is best thought of as a sequence of states that include forethought (i.e., plans for studying), performance, and self-reflection. Motivation to initiate studying on one’s own is important, but perhaps more critical is the self-monitoring of performance, especially the ability to scrutinize and interpret failure and make corrections (Boekaerts and Corno 2005 ). Furthermore, while considerable barriers exist for students to develop self-regulative habits, such as a belief that intelligence is “fixed” and not malleable (Yeager and Dweck 2012 ) and assumptions that learning should be simple and unproblematic (Bjork, Dunlosky and Kornell, 2013 ), helping students to develop this aptitude is critical because it is a core aspect of success not only in school but also in life and the workplace (Pellegrino and Hilton 2012 ).
So how, if at all, can STEM educators embed self-regulatory skills into their courses? Setting aside for the moment the extent to which self-control, goal setting, and responsibility should be learned in the home, grade school, or various other cultural fields during childhood, the fact remains that it is possible to teach some aspect of self-regulatory competencies in the college classroom (Nilson 2013 ). One strategy is to create a classroom environment with high expectations and a low- to zero-tolerance policy for irresponsible behavior or late assignments, thereby encouraging if not forcing students to set goals for themselves and achieve them. Another strategy is the widely used instructional wrapper, which refers to prompts for students to reflect on their performance before and after an assignment or activity, which trains students to regularly reflect on their study habits and approach to learning (Lovett 2013 ). Other ideas include modeling learning strategies such as self-monitoring and summarizing in front of students in what is known as a “cognitive apprenticeship” (Palincsar and Brown 1984 ), using small group work tasks designed to spark self-regulation (Fitch et al. 2012 ) and assigning open-ended tasks and assessments requiring students to choose strategies and take control of their learning (Boekaerts and Corno 2005 ).
We conclude this discussion about self-regulation with a note about digital media. While online resources and digital devices can play an important and productive role in facilitating student learning (Dabbagh and Kitsantas 2012 ; Smith and Caruso 2010 ), it is evident from the data that they can detract from focused study. Thus, another aspect about self-regulation is the willingness and ability to remove digital distractions when they are not serving a productive purpose, such as the students in this study who deliberately went to libraries without wireless Internet or parents’ homes where devices were confiscated. As will be discussed in the next section, technology can and should be part of educators’ instructional toolkit, but students would be well served by adopting more self-regulated stances when it comes to the presence of technology in their study sessions.
Encouraging personalized learning and high-impact studying through course structure
Next, we turn to issue of how instructors, through the deliberate design of their courses, can facilitate effective study habits. Here, we focus on two aspects of effective studying: the use of multiple representations and modalities and the use of high-impact strategies. In both cases, we can look to the example of Dr. Wells’ course where she embedded within the structure of the course itself opportunities for students to draw upon various learning tools while also imposing a high-impact study habit (i.e., distributed practice) via weekly practice questions.
First, providing students with a variety of learning resources and tools offers them a variety of entry points with which to explore the material. The rationale for doing so is not to support students’ distinct learning styles, an idea that is popular but unsupported by the empirical evidence (Pashler et al. 2008 ), but instead is based on the fact that learners that engage with varied representations of an idea or concept demonstrate improved learning outcomes (Pellegrino and Hilton 2012 ). In addition, the provision of various learning tools is useful because today’s learners are broadly proficient in developing personalized learning pathways, whether for academic or personal purposes, using online resources and social media (Dabbagh and Kitsantas 2012 ). In doing so, many create social learning environments, or what some call “participatory cultures,” where people develop online learning communities where they collectively create, share, and learn from each other (Jenkins et al. 2006 ). Essentially, the idea is to make available a repertoire of learning tools and media for students that they can then select from to approach the material from multiple perspective and according to their own unique way of engaging with different learning modalities.
The second approach for facilitating effective study habits pertains to the structure of a course, from the timing and nature of assessments to the types of learning activities students are required to do. Again, consider the example of Dr. Wells, who embedded within her course an assessment strategy that forced her students to engage in the high-impact study habit of distributed practice. Through weekly practice questions, students were required to regularly take mini-exams on different topics (Dunlosky et al. 2013 ). Spacing out study sessions on distinct topics enhances learning through the spacing effect and also by introducing comparisons or “interference” across topics, which results in higher-order representations or complex mental models that not only are repositories of information but also facilitate transfer and retention (Bjork, Dunlosky, and Kornell 2013 ).
Similarly, the role of course structure in facilitating student learning has been well documented in STEM education, where pre-class reading quizzes and weekly practice exams have been linked to improved student learning (Freeman et al. 2011 ), and even in reducing the achievement gap between white and under-represented minority students, because highly structured courses with regular practice may introduce study and learning skills to students with little experience from high school (Haak et al. 2011 ). The takeaway here is that as educators, we can design our courses and teach our classes with explicit attention towards creating (and mandating) situations for students to engage in certain study habits.
The attention currently being placed on STEM instructors and their pedagogical acumen as key facilitators of student learning is well-placed, but the relationship between teaching and learning is anything but direct, linear, and unproblematic. What students decide to do in terms of when and how to study act as critical intermediaries between what instructors do in the classroom and students’ ultimate performance in college. As Entwistle and Tait suggested over 25 years ago, ( 1990 , p. 170), students’ behaviors and strategies “affects learning probably as much as, if not more than, the classroom skills of the lecturer.” On this point, there are both promising and troubling signs. While students are increasingly utilizing varied resources and media in a deliberate and creative manner to advance their studies, some study methods that are demonstrably ineffective continue to be widely used. The exploratory study reported in this paper offers a new, multi-dimensional way to think about studying that suggests future research directions exploring undergraduate study habits including similar descriptive research with larger samples and additional disciplines, experimental research focusing on specific strategies under different conditions (e.g., resource use, cues for studying), and examining the relationship between course structure and studying.
Ultimately, students must take responsibility for their learning and strategize ways to create situations—whether in a quiet library basement or a group study session online—where they can effectively study and learn. However, postsecondary educators must also be cognizant of the pressures facing today’s college students and the fact that many have not been taught how to engage in high-impact study habits but instead rely on re-reading highlighted text. One of the guiding principles for instructional design should be the idea that it is no longer tenable to assume that students have been taught how to effectively study and learn prior to their matriculation into a college or university. While students bring a wealth of new learning habits and technological acumen to the twenty-first century classroom—whether online or face-to-face—they still need guidance in how to study. This conclusion, however, should not lead to complaints about unprepared students or a failed K-12 sector but instead needs to spark postsecondary educators to carefully design of rich and engaging learning environments that sparks self-regulatory habits of mind and encourages high-impact studying, so that students are well positioned to succeed.
Astin, A. W. (1984). Student involvement: a developmental theory for higher education. Journal of College Student Development, 40 (5), 518–529.
Google Scholar
Babcock, P., & Marks, M. (2010). Leisure college, USA: the decline in student study time. American Enterprise Institute for Public Policy Research, 7 , 1–7.
Bastedo, M. N. (2012). Organizing higher education: a manifesto. In M. N. Bastedo (Ed.), The organization of higher education: managing colleges for a new era (pp. 3–17). Baltimore: The Johns Hopkins University Press.
Bernard, H. R. (2011). Research methods in anthropology: qualitative and quantitative approaches (5th ed.). Lanham: Altamira Press.
Biggs, J. (1987). Student approaches to learning . Hawthorn: Australian Council for Educational Research.
Bjork, R. A., Dunlosky, J., & Kornell, N. (2013). Self-regulated learning: Beliefs, techniques, and illusions. Annual review of psychology, 64, 417–444.
Article Google Scholar
Boekaerts, M., & Corno, L. (2005). Self-regulation in the classroom: a perspective on assessment and intervention. Applied Psychology, 54 (2), 199–231.
Bok, D. (2009). Our underachieving colleges: a candid look at how much students learn and why they should be learning more . Princeton: Princeton University Press.
Carini, R. M., Kuh, G. D., & Klein, S. P. (2006). Student engagement and student learning: testing the linkages. Research in Higher Education, 47 (1), 1–32.
Cassidy, S. (2011). Self-regulated learning in higher education: identifying key component processes. Studies in Higher Education, 36 (8), 989–1000.
Coburn, C. E., & Turner, E. O. (2012). The practice of data use: an introduction. American Journal of Education, 118 (2), 99–111.
Coffield, F., Moseley, D., Hall, E., & Ecclestone, K. (2004). Learning styles and pedagogy in post-16 learning: a systematic and critical review . London: Learning Skills Network.
Credé, M., & Kuncel, N. R. (2008). Study habits, skills, and attitudes: the third pillar supporting collegiate academic performance. Perspectives on Psychological Science, 3 (6), 425–453.
Dabbagh, N., & Kitsantas, A. (2012). Personal learning environments, social media, and self-regulated learning: a natural formula for connecting formal and informal learning. The Internet and higher education, 15 (1), 3–8.
Duckworth, A. L., Peterson, C., Matthews, M. D., & Kelly, D. R. (2007). Grit: perseverance and passion for long-term goals. Journal of Personality and Social Psychology, 92 (6), 1087–1101.
Dunlosky, J., Rawson, K. A., Marsh, E. J., Nathan, M. J., & Willingham, D. T. (2013). Improving students’ learning with effective learning techniques: promising directions from cognitive and educational psychology. Psychological Science in the Public Interest, 14 (1), 4–58.
Entwistle, N. J., & Tait, H. (1990). Approaches to learning, evaluations of teaching, and preferences for contrasting academic environments. Higher Education, 19 (2), 169–194.
Fitch, T., Marshall, J., & McCarthy, W. (2012). The effect of solution-focused groups on self-regulated learning. Journal of College Student Development, 53 (4), 586–595.
Franke, R., Ruiz, S., Sharkness, J., DeAngelo, L., & Pryor, J. (2010). Findings from the 2009 administration of the College Senior Survey (CSS): National aggregates. Chicago: Higher Education Research Institute at the University of California Los Angeles.
Freeman, S., Haak, D., & Wenderoth, M. P. (2011). Increased course structure improves performance in introductory biology. CBE-Life Sciences Education, 10 (2), 175–186.
Freeman, S., Eddy, S. L., McDonough, M., Smith, M. K., Okoroafor, N., Jordt, H., & Wenderoth, M. P. (2014). Active learning increases student performance in science, engineering, and mathematics. Proceedings of the National Academy of Sciences, 111 (23), 8410–8415.
Gamoran, A., Anderson, C. W., Quiroz, P. A., Secada, W. G., Williams, T., & Ashman, S. (2003). Transforming teaching in math and science: how schools and districts can support change . New York: Teachers College Press.
Gee, J. P. (1986). Units in the production of narrative discourse. Discourse Processes, 9 , 391–422.
Glaser, B. G., & Strauss, A. L. (1967). The discovery of grounded theory: strategies for qualitative research . New Brunswick: Aldine Transaction.
Greeno, J. G. (1998). The situativity of knowing, learning, and research. American psychologist, 53 (1), 5–26.
Haak, D. C., HilleRisLambers, J., Pitre, E., & Freeman, S. (2011). Increased structure and active learning reduce the achievement gap in introductory biology. Science, 332 (6034), 1213–1216.
Halverson, R. (2003). Systems of practice: how leaders use artifacts to create professional community in schools. Educational Policy Analysis Archives, 11 (37), 1–35.
Hartwig, M. K., & Dunlosky, J. (2012). Study strategies of college students: are self-testing and scheduling related to achievement? Psychonomic Bulletin & Review, 19 (1), 126–134.
Hativa, N., & Goodyear, P. (Eds.). (2002). Teacher thinking, beliefs, and knowledge in higher education . Norwell: Kluwer Academic Publishers.
Hora, M. T. (2012). Organizational factors and instructional decision-making: A cognitive perspective. The Review of Higher Education, 35(2), 207–235.
Hora, M. T. (2016). Navigating the problem space of academic work: How workload and curricular affordances shape STEM faculty decisions about teaching and learning. AERA Open, 2(1), 1–19.
Jenkins, H., Clinton, K., Purushotma, R., Robison, A. J., and Weigel, M. (2006). Confronting the challenges of participatory culture: media education for the 21 st century. Chicago: The John D. and Catherine T. MacArthur Foundation.
Karpicke, J. D., Butler, A. C., & Roediger, H. L., III. (2009). Metacognitive strategies in student learning: do students practice retrieval when they study on their own? Memory, 17 (4), 471–479.
Klein, G. (2008). Naturalistic decision making. Human Factors, 50 (3), 456–460.
Kornell, N. (2009). Optimising learning using flashcards: spacing is more effective than cramming. Applied Cognitive Psychology, 23 , 1297–1317.
Kuo, J., Hagie, C., & Miller, M. T. (2004). Encouraging college student success: the instructional challenges, response strategies, and study skills of contemporary undergraduates. Journal of Instructional Psychology, 31 (1), 60–67.
Lave, J. (1988). Cognition in practice: mind, mathematics and culture in everyday life . Cambridge: Cambridge University Press.
Book Google Scholar
Lotkowski, V. A., Robbins, S. B., & Noeth, R. J. (2004). The role of academic and non-academic factors in improving college retention . ACT Policy Report, Retrieved from http://ww.cfder.org/uploads/3/0/4/9/3049955/the_role_of_academic_and_non_academic_factors_in_improving_college_retention.pdf .
Lovett, M.C. (2013). Make exams worth more than the grade: using exam wrappers to promote metacognition (pp. 18-52), in Using reflection and metacognition to improve student learning: across the disciplines, across the academy, ed. Kaplan, M, Silver, N., LaVague-Manty, D., & Meizlish, D. Sterling, VA: Stylus Publishing.
Marton, F., & Säljö, R. (1976). On qualitative differences in learning: I. Outcome and process. British Journal of Educational Psychology, 46 , 4–11.
Merriam, S. B. (2014). Qualitative research: a guide to design and implementation . San Francisco: John Wiley & Sons.
Miller, C. M., & Parlett, M. R. (1974). Up to the mark: a study of the examination game . London: SRHE.
Morgan, G., Moskal, P., Wolf, A., Dziuban, C., McMartin, F., & Morrill, J. (2012). Understanding student use of digital learning resources. [Powerpoint slides]. Retrieved from http://bit.ly/1vjZRsY
Nilson, L. (2013). Creating self-regulated learners: strategies to strengthen students self-awareness and learning skills . Sterling: Stylus Publishing.
Nonis, S. A., & Hudson, G. I. (2010). Performance of college students: impact of study time and study habits. The Journal of Education for Business, 85 , 229–238.
Palincsar, A. S., & Brown, A. L. (1984). Reciprocal teaching of comprehension-fostering and comprehension-monitoring activities. Cognition and Instruction, 1 , 117–175.
Pascarella, E., & Terenzini, P. (2005). How college affects students: a third decade of research . San Francisco: Jossey-Bass.
Pashler, H., McDaniel, M., Rohrer, D., & Bjork, R. (2008). Learning styles concepts and evidence. Psychological science in the public interest, 9 (3), 105–119.
Pellegrino, J. W., & Hilton, M. L. (Eds.). (2012). Education for life and work: developing transferable knowledge and skills in the 21 st century . Washington, DC: National Academies Press.
President’s Council of Advisors on Science and Technology (2012). Report to the President. Engage to excel: Producing one million additional college graduates with degrees in science, technology, engineering and mathematics. Washington, D.C.: Executive Office of the President.
Ramsden, P. (1979). Student learning and perceptions of the academic environment. Higher Education, 8 (4), 411–427.
Riding, R., & Cheema, I. (1991). Cognitive styles—an overview and integration. Educational Psychology, 11 (3-4), 193–215.
Robbins, S. B., Lauver, K., Le, H., Davis, D., Langley, R., & Carlstrom, A. (2004). Do psychosocial and study skill factors predict college outcomes? A meta-analysis. Psychological Bulletin, 130 (2), 261–288.
Rosen, L. D., Carrier, L. M., & Cheever, N. A. (2013). Facebook and texting made me do it: media-induced task-switching while studying. Computers in Human Behavior, 29 , 948–958.
Ruiz, S., Sharkness, J., Kelly, K., DeAngelo, L., & Pryor, J. (2010). Findings from the 2009 administration of the Your First College Year (YFCY): national aggregates. Los Angeles: Higher Education Research Institute at the University of California Los Angeles.
Seely Brown, J., & Adler, R. P. (2008). Open education, the long tail, and learning 2.0. Educause Review, 43 (1), 16–20.
Slavin, R. E. (2002). Evidence-based education policies: transforming educational practice and research. Educational researcher, 31 (7), 15–21.
Smith, S. D., & Caruso, J. B. (2010). Key findings: the ECAR study of undergraduate students and information technology . Boulder: EDUCAUSE Center for Applied Research.
Spillane, J. P., Halverson, R., & Diamond, J. B. (2001). Investigating school leadership practice: a distributed perspective. Educational Researcher, 30 (3), 23–28.
Spillane, J. P., Reiser, B. J., & Reimer, T. (2002). Policy implementation and cognition: reframing and refocusing implementation research. Review of Educational Research, 72 (3), 387–431.
Stebbins, R. A. (2001). Exploratory research in the social sciences. Qualitative research methods in the social sciences, 48 . Thousand Oaks: Sage Publications.
Strauss, A., & Corbin, J. M. (1990). Basics of qualitative research: grounded theory procedures and techniques . Thousand Oaks: Sage Publications, Inc.
Tinto, V. (1993). Leaving college: Rethinking the causes and cures of student attrition. (2nd ed.). Chicago: University of Chicago Press.
Treisman, U. (1992). Studying students studying calculus: a look at the lives of minority mathematics students in college. The College Mathematics Journal, 23 (5), 362–372.
Yeager, D. S., & Dweck, C. S. (2012). Mindsets that promote resilience: when students believe that personal characteristics can be developed. Educational Psychologist, 47 (4), 302–314.
Zimmerman, B. J. (2002). Becoming a self-regulated learner: an overview. Theory into Practice, 41 (2), 64–70.
Zimmerman, B. J., & Schunk, D. H. (Eds.). (2001). Self-regulated learning and academic achievement: theoretical perspectives . Hillsdale: Lawrence Erlbaum Associates.
Download references
Acknowledgements
The authors would also like to thank Jana Bouwma-Gearhart and Jennifer Collins for their involvement in this study and collecting data reported in this paper.
This research was supported by a grant from the National Science Foundation (DUE#1224624) for the Tracking the Processes of Data Driven Decision-Making Study ( http://tpdm.wceruw.org ).
Authors’ contributions
AO participated in the design of the study and data collection, led the data analysis, and collaborated with MH to draft the manuscript. MH conceived of the study, led the design, participated in data collection and analysis, and finalized the manuscript. Both authors read and approved the final manuscript.
Competing interests
The authors declare that they have no competing interests.
Author information
Authors and affiliations.
Department of Liberal Arts and Applied Studies, University of Wisconsin-Madison, 21 N. Park St., Madison, WI, 53715, USA
Matthew T. Hora
Wisconsin Center for Education Research, University of Wisconsin-Madison, Madison, USA
Amanda K. Oleson
You can also search for this author in PubMed Google Scholar
Corresponding author
Correspondence to Matthew T. Hora .
Rights and permissions
Open Access This article is distributed under the terms of the Creative Commons Attribution 4.0 International License ( http://creativecommons.org/licenses/by/4.0/ ), which permits unrestricted use, distribution, and reproduction in any medium, provided you give appropriate credit to the original author(s) and the source, provide a link to the Creative Commons license, and indicate if changes were made.
Reprints and permissions
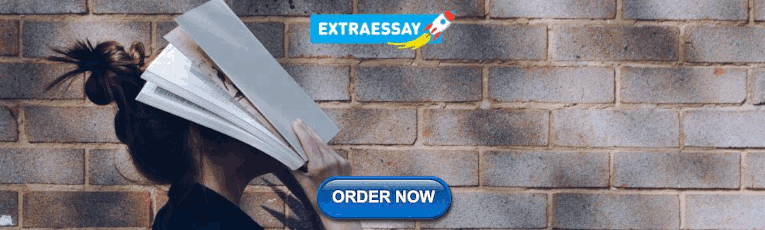
About this article
Cite this article.
Hora, M.T., Oleson, A.K. Examining study habits in undergraduate STEM courses from a situative perspective. IJ STEM Ed 4 , 1 (2017). https://doi.org/10.1186/s40594-017-0055-6
Download citation
Received : 01 August 2016
Accepted : 06 January 2017
Published : 01 February 2017
DOI : https://doi.org/10.1186/s40594-017-0055-6
Share this article
Anyone you share the following link with will be able to read this content:
Sorry, a shareable link is not currently available for this article.
Provided by the Springer Nature SharedIt content-sharing initiative
- Learn Management System
- Study Strategy
- Study Session
- Digital Resource
- Medical College Admission Test

An official website of the United States government
The .gov means it’s official. Federal government websites often end in .gov or .mil. Before sharing sensitive information, make sure you’re on a federal government site.
The site is secure. The https:// ensures that you are connecting to the official website and that any information you provide is encrypted and transmitted securely.
- Publications
- Account settings
Preview improvements coming to the PMC website in October 2024. Learn More or Try it out now .
- Advanced Search
- Journal List
- Adv Med Educ Pract
Study Habits of Highly Effective Medical Students
Khalid a bin abdulrahman.
1 Department of Medical Education, College of Medicine, Imam Mohammad Ibn Saud Islamic University (IMSIU), Riyadh, Saudi Arabia
Ahmad M Khalaf
Fahad b bin abbas, omran t alanazi.
Study habits have been the most significant indicator of academic performance and play a unique role in students’ academic accomplishment. The study is aiming to determine the most common study habits of highly successful medical students and their relation to academic achievement.
A cross-sectional observational study was conducted from September to December 2019 among medical students of both gender from six medical colleges in Saudi Arabia. The students answered the standardized questionnaires to study the different learning habits among medical students, including learning prioritization, knowledge retention strategies, motivation, daily hours of studying, study learning resources.
Six hundred and seventy-five medical students enrolled themselves electively into the study. The results showed a significant correlation between study habits and students’ academic accomplishments. The top ten study habits of highly effective medical students are managing their time effectively, they get rid of interruptions (phone, family, friends) that disrupt their daily work, they use goal-setting to determine their most important activities, their daily study hours is ranging between 3 and 4 hours, they study alone for knowledge retention of medical information, learn from multiple sources and invest in technology with high efficiency, they contribute to the teaching of their peers, they study the main lecture slides with notes when no exam is coming, and they study lecture slides with notes and previous exam questions when preparing for upcoming exams; finally, they maintained motivation for self-gratification and fulfillment of their family dreams.
This study’s outcomes consolidate general study practices that can be credited to learning achievement and expand recognition to inspire less accomplished students by investigating and exploring factors that have enhanced and worked for many accomplished students.
Introduction
Every year, tens of thousands of elite high school graduates compete for limited places in Saudi medical schools. There is no doubt that most of those admitted to medical colleges are students who have proven their ability and willingness to adapt to the study of medicine and overcome the challenges of successive exams and long study hours, especially since the medium of instruction in Saudi medical colleges in the English language. 1–3 Although many Saudi medical colleges have developed their curricula, some have even adopted modern curricula and methods in medical education that focus on active learning, problem-based learning, vertical and horizontal integration. It also allows the student to learn and participate in solving community health problems. 4–7 All this to maximize and improve the student’s experience and ease the tension and pressures students complain about in the old curricula. Some studies have indicated that successful students in medical schools can define their learning styles and use them in a way that makes them adapt to different circumstances, manage their time effectively, learn from multiple sources, invest in technology with high efficiency, and contribute to the education of their peers. 8–16
Medical college presents a specific difficult task to undergraduates because of the patent volume and broadness of data students who recently performed scholastically in college are confronted with. They are therefore compelled to devise better approaches to study adequately and advantageously. Deciding on powerful techniques to study in medical school is of utmost significance. 17
Subsequently, a comprehension of the kinds of study habits that are best in medical school is imperative, as the early realization of the study habits that are associated with progress can assist students in reaching their maximum capability and accomplishing proficiency during the pre-clinical and clinical years, which will help them in the residency selection program. 18 , 19
One of the core academic aims has always been the enhancement of student success. Several studies have been conducted to classify factors that affect students’ performance and achievement (positive or negative). It is a very complex process defining these variables and the connection between them. 20 It has been observed that participant attributes, behavior, learning environment, and educational activities affect their performance and achievement. 16 It has also been found that there are associations between academic performance and study skills, study habits, research attitudes, and motivation.
A study showed that medical students were significantly affected by academic burnout and engrossed in a literary adaptation. Medical students with significant intellectual flexibility will experience less academic burnout, more engrossment in learning, and better educational performance. Examining academic adaptability and approaches to upgrade such habits may help medical schools improve such skills and commitment. 21 A previous study showed that most medical students prefer to study lecture handouts containing what the teacher says. 15 Moreover, high GPA students enjoy learning more than lower GPA students (85.52–83.80%) and use textbooks more often (12.50–6.66%). 15 Nonetheless, another study on medical students elucidated that internal motivation is an essential stimulus for self-regulation strategies and better academic performance. 22
This study aims to determine the everyday study habits of highly effective medical students and examine their correlation with their academic achievement.
Materials and Methods
Study design.
This was a cross-sectional institutional-based observational study conducted among medical students at various medical colleges in Saudi Arabia. The study was conducted after approval from the IMSIU IRB committee number 68–2019 dated 17 November 2019.
Participants and Sampling
All medical students of either gender with a high GPA (4 and above out of 5) of the pre-clinical and clinical years were invited by email through the vice-dean of academic affairs of the six medical colleges in Saudi Arabia. Two reminder email messages were sent to enhance the response rate. The data was collected from the responded medical students in the six medical colleges, namely Imam Mohammad Ibn Saud Islamic University (IMSIU), King Abdulaziz University, King Saud Bin Abdulaziz University for Health Sciences (KSAU-HS), Qassim University, Alfaisal University, and King Saud University.
Study Questionnaire
The questionnaire was designed to study medical students’ learning habits, including time management and study resources. The students were informed about the purpose of the study. Instructions regarding the questionnaires were provided to volunteering students. The confidentiality of information was also ensured. Once students voluntarily signed the informed consent, they were requested to fill the study questionnaires. The questionnaire was adapted from different resources and manuscripts, which made it suitable for our study. The questionnaire was subjected to piloting testing by 25 students; some questions were modified accordingly. All students were emailed to participate and been reminded by emails and via an SMS web link. The Institute Review Board at Imam Mohammad Ibn Saud Islamic University had approved the study and it was according to the declaration of Helsinki.
The medical students’ prioritization was measured employing five indicators. However, the students’ overall perceived prioritization was calculated using the first four items’ mean. The multiple response dichotomy analysis was employed to describe the students’ preferred methods for memorization.
Statistical Data Analysis
The means and standard deviations were employed to describe the continuous variables, frequency, and percentages for categorical variables. Cronbach’s alpha test was used to evaluate the reliability of medical students’ measured prioritization indicators. One item among the four indicators (measuring the students’ procrastination) was reversed before computing the total prioritization mean score. The SPSS IBM V20 was employed for data analysis, and the statistical significance alpha level was considered at a level of 0.050. Microsoft Excel Program was used for creating figures and depictions.
Six hundred and seventy-five medical students enrolled themselves electively into the study. Table 1 summarizes the demographic characteristics and the daily study hours of medical students who participated in the study. The descriptive analysis of the medical students’ perceived indicators of prioritization of studies and activities are shown in Table 2 . The students’ mean collective prioritization was rated as 3.41/5, SD=0.78 points, denoting that the students prioritized between medium and high on average; if expressed as a percentage, it is 3.41/5 X 100= 68.2% out of hundred percent prioritization ability in general. The commonest used study method was studying alone according to 85.3% of the medical students, followed by group study according to 34% of the medical students, followed by teaching another student according to 26.4% of the medical students, and discussion with the course teacher according to 12% of the medical students Table 3 .
The Medical Students’ Sociodemographic and Academic Characteristics N=675
The Descriptive Analysis of the Medical Students’ Perceived Indicators of Prioritization of Studies and Activities
The Medical Student’s Used Methods of Knowledge Retention of Medical Information N=675
The students were also asked to select from a list of all the study methods they used when no exams approached Table 4 . More than 83.0% of the medical students used main lecture slides with notes, 76.1% used video software like YouTube and Osmosis.
The Studying Methods Used by Medical Students When Exams Were Not Expected N=675
Medical students were asked to indicate their best methods of studying when they had exams approaching. The most used study method by the students was main lectures according to 92.4% of the students, 74.8% of the student’s used previous exam questions, 34.4% used multiple-choice questions (MCQ) exam review books, 17.9% of the students also used self-assessment MCQ tests from the internet, 62.1% of the students used video software like YouTube and Osmosis, 35.7% used reference textbooks, 14.4% of the students used additional relevant medical books. Furthermore, the students were asked to suggest their best sources of motivation for success. The highest motivation according to the medical students was self-gratification and fulfillment of their family dreams, followed by maintaining a high level of educational status according to 62.8% of the students, followed by getting prepared to satisfy the requirements for joining the competitive medical residency program according to 52.9% of the medical students, and recognition of the fact that being a doctor may bring high income according to 43.6% of the medical students. However, 22.6% of the medical students were motivated by the reward of being highly distinctive students. Figure 1 summarizes the top ten study habits of highly effective medical students. Regarding the social status of the students, high GPA students were introverted primarily (66.2%), and the low GPA group was also predominantly introverted (62.2%) (p=0.330). The high GPA students also indicated that they were somewhat satisfied with their social life (44.9%) than 40.8% in the low GPA group. 37.8% of high GPA students were satisfied than 36.7% in the low GPA group (p=0.286).

The top ten study habits of highly effective medical students.
Decent time management strategies and skills improve academic achievement. 10 Students who do not design their time adequately use up all available time to overcome and master the content. In this regard, giving assistance and aid to students in time management should help them utilize their study time more productively and adequately. This measure ought to improve their educational performance. 13 In our study, most participants preferred to study alone (85.3%) as it is the most common method of knowledge retention regardless of their total GPA. Other students also used different medical information retention techniques like peer tutoring (26.4%) and group study (24%). This finding may indicate that most of the students’ overall study method is studying alone, which was also the same result obtained in a study that compared male and female medical students’ study habits. 15 When there were no exams expected, the majority of participants used the main lecture slides with their notes (83.4%), followed by videos such as YouTube and Osmosis (76.1%) and reference textbooks (46.1%). Similarly, when exams were close, the studying methods did not change drastically. Most of the study members used main lecture slides with their personalized notes (92.4%) and videos (62.1%). Surprisingly, most students utilized previous exam questions as a studying method (74.8%). Homogeneous results have also shown that most medical students prefer to study lecture handouts containing what the teacher says. 15 It is striking that most students rely on lecture slides and student notes in their daily study, especially to prepare for the various exams, and they ignore reading from reference books. Perhaps the explanation for this is that students focus on the sources that revolve around the content of the exams. This is undoubtedly an unhealthy phenomenon that students avoid reading from reference books, and their biggest concern is to get the highest scores on exams only. Here, we advise professors of medical colleges to devise methods and strategies that direct and motivate students to read from reference books and selective medical articles. Most students’ concept of motivation was to satisfy themselves and their family (75.4%), other students opined that their motivation was to maintain a high level of educational status (62.8%), having a good income as doctors (43.6%), and getting rewarded for being one of the top students in the class (22.6%). Students with superior study techniques have more active and dynamic learning styles and are more engaged in educational subjects; they will also have better retaining and memory capacities. 23 Furthermore, high GPA students enjoy studying more than lower GPA students (85.52–83.80%) and use textbooks more often (12.50–6.66%). 15 Regarding the study hours per day, students with higher GPAs studied on average 3–4 hours daily (45.5%) than lower GPA students (38.3%) regardless of whether the exam is close or not, with no statistical difference detected. Another comparable study on 257 medical students showed that 43% of students with high GPA study 10–14 hours close to the final exam, and 50% in the lower GPA group. Furthermore, 47% of female students preferred studying 10–14 hours close to the final assessments compared to 44% of the male group. For study hours not close to the final exam, 75% of students with high GPAs chose to study 1–4 hours daily compared to 65% of the lower GPA group. Also, 64% of female students favored studying 1–4 hours each day compared to 78% (p=0.050). 15 It appears that their students, whether in the high or low GPA group, either male or female, invested only the energy required to meet the least requirements, which implies that they express more surface learning. 24 Although this does not apply to our students due to the increase in study hours. Regarding the social status of the students, high GPA students were introverted primarily (66.2%), and the low GPA group was also predominantly introverted (62.2%) (p=0.330). The high GPA students also indicated that they were somewhat satisfied with their social life (44.9%) than 40.8% in the low GPA group. 37.8% of high GPA students were satisfied than 36.7% in the low GPA group (p=0.286). Another study that was conducted on 640 Latino adolescents to find out if there is any relationship between academic performance and loneliness revealed that just about a fourth of the Latino adolescents struggled to find their ideal position, thus, suffering from chronic or increasing loneliness, which had impending ramifications for their future educational achievement. 25 This study’s outcomes consolidate general study practices that can be credited to learning achievement and expand recognition to inspire less accomplished students by investigating and exploring factors that have enhanced and worked for many accomplished students.
Limitations
The current study has a few limitations. For instance, factors investigated here were of potential associations and not intended to imply causation implicitly. Consequently, the investigation report provided here was established on the bivariate comparison (high versus low GPA). Another limitation is that a student may comprehend each question distinctly, and it should be noted that the GPA and other components of the questionnaire were self-reported. It is also crucial to recall that what works for one student may not work for another, so students should also be urged to discover study habits that work efficiently and effectively for them. The habits introduced here are intended to be a guide that might be of aid to students whose current study habits are not working efficiently and effectively.
The top ten study habits of highly effective medical students are; managing their time effectively, they get rid of interruptions from (phone, family, friends) that disrupt their daily work, they use goal-setting to determine their most important activities, their daily study hours is ranging between 3–4 hours, they study alone for knowledge retention of medical information, learn from multiple sources and invest in technology with high efficiency, they contribute to the teaching of their peers, they study the main lecture slides with notes when no exam is coming, and they study lecture slides with notes and previous exam questions when preparing for upcoming exams; finally, they maintained motivation for self-gratification and fulfillment of their family dreams.
The authors reported no conflicts of interest for this work.
Academia.edu no longer supports Internet Explorer.
To browse Academia.edu and the wider internet faster and more securely, please take a few seconds to upgrade your browser .
Enter the email address you signed up with and we'll email you a reset link.
- We're Hiring!
- Help Center
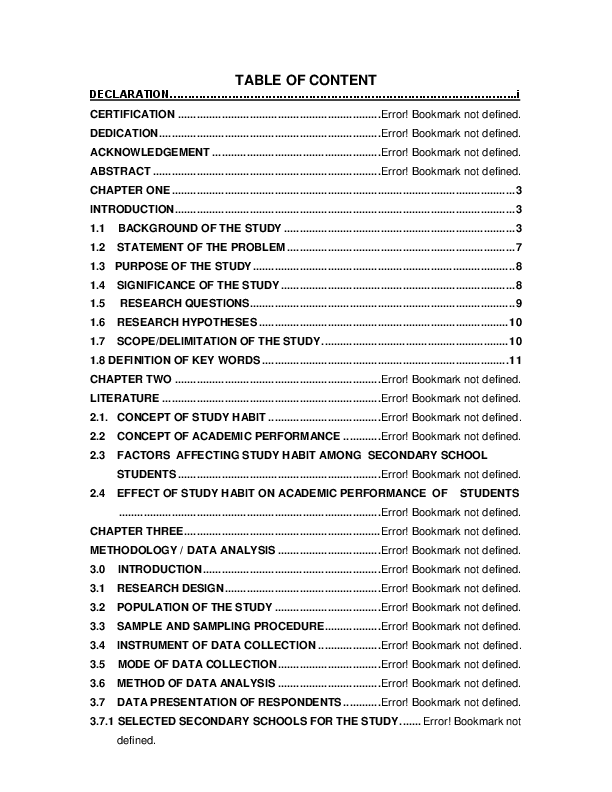
THE IMPACT OF STUDY HABITS ON THE ACADEMIC PERFORMANCE OF STUDENTS

Related Papers
ariel ochea
Impact Factor(JCC): 1.7843-This article can be downloaded from www.impactjournals.us ABSTRACT The development of a Country relies mostly on the levels of education among the people. Without education human race would have remained but as another animal race. Education is a process towards development. The term study habit can be as the students' way of study whether systematic, efficient or inefficient. Academic achievement refers to what and how an individual has learnt qualitatively and quantitatively after a period of instruction given. A habit is something that is done on a scheduled, regular, planned basis and that is not relegated to a second place or optional place in one's life. It is simply done, no reservations, no excuses, and no expectations. Study habits keep the learner perfect in getting knowledge and developing attitude towards things necessary for achievement in different field of human endeavour. Students who develop good study habits at school increase the potential to complete their assignments successfully and to learn the material they are studying. They also reduce the possibility of not knowing what is expected and of having to spend time studying at home. Study habits are the ways that your study habits that you have formed during your school years. Study habits can be good ones, or bad ones. Good study habits include being organized, keeping good notes, reading your textbooks, listening in class, and working every day. Bad study habits include skipping class, not doing your work, etc.
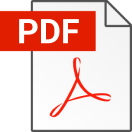
The Impact of Good Study Habit on Academic Achievement of Secondary School Students
Awolesi Oluwadamilola
INTRODUCTION The present day educational sector is becoming increasingly dynamic. The determination of every individual is to attain success and this success affects the personal and social dimensions of life. In this regard, academic performance is one of the major factors that influence individual's success in any educational setting. It is any body's guess that good habits and skills will help us to promote efficiency in our tasks. In education, proper study habits and skills requires proficiency as well as optimum learning quality (Dehghani & Soltanalgharaei, 2014). Productive study requires conceptualization and intention. It could include some skills such as note-taking, observation, asking question, listening, thinking and presenting idea with respect to new discoveries. Thus, students are expected to be interested in learning and must be able to apply requisite skills. On the other hand, inefficient study leads to waste of time and learner's energy (Hashemian & Hashemian, 2014). Study habits and skills like other skills can be taught and learnt. The interplay among motivation, habits, attitudes and behaviors has great impact on the academic performance of students. Study habit is buying out a dedicated scheduled and uninterrupted time to apply one's self to the task of learning. Studying is the procedure of getting information from prints that is information stored in written materials (magazines, newspapers, books). It is an organized gaining of intelligence and an interpretation of information and ideologies that calls for memorizing and usage. Studying can be expressed as the utilization of one's intellectual ability to the gaining, comprehending and arrangement of information; doing it over and over again entails some method of formal learning. Habit is a thing that one does often and almost without thinking; especially something that is hard to stop. A person's habit consists of plethora of ways an individual performs specific and general activity. Habit is relative to person or people. Each human being acts in a unique way. This is so because nature made things
Epoh Sedruol
The nature of study habits of elementary pupils inferred effectiveness and efficiency in school management. Many of the learning activities in school required fully brained pupils with excellent study habit skills. Pupils with good study habit skills dominated and sought to be great leaders of tomorrow. Formation of good study habits especially at the early years had immense implication towards academe. The researcher determined the study habits of the Grade V pupils in Cabalasan Elementary School, Division of Baybay City during the school year 2014-2015 with an end view of recommending ideas for an intervention in improving pupil’s study habits. The study employed normative survey method using checklist of Ralph C. Preston and Morton Botel. Interpretation of data was based on the use of simple percentage, mean and Fischer’s t-test. Results showed that less than half of the time lead in most frequent response in Grade V pupils of Cabalasan Elementary School for the school year 2014-2015 wherein 4 out of 5 descriptive responses showed that there was no significant difference between male and female grade five pupils in study habit contexts such as previewing, reading, note taking while reading, remembering, report writing, listening & taking class notes, preparing for examination, taking examination, planning time and arranging physical setting. The study implied that aside from collaborating with all the stakeholders, continuous learning must be offered in school through provision of time in searching, collecting, organizing and interpreting ideas in a learning hub with available reference books and study equipment for a more relevant and fruitful integration of knowledge. Stressing the importance of macro skills such as listening, reading, writing and speaking in language was of upmost necessity in pupil’s study habits and learning development.
Paul Ryan Cabase
Study habits is how one studies. That is, the habits which students form during their school years. Without good study habits, a student cannot succeed. Thus, this study investigated the impact of study habits on secondary school students' academic performance in the Federal Capital Territory, Abuja. The study was guided by one null hypothesis. The study adopted a descriptive survey research design as its plan. The sample of the study constituted of 1050 senior secondary school students drawn from the Federal Capital Territory, Abuja. The instrument used for data collection was questionnaire. Chi-square was used for data analysis. The finding of the study revealed that there is significant relationship between study habits and students' academic performance. It was recommended that teachers and school guidance counselors should collaboratively guide students on how to develop good study habits; thereby enhancing their academic success.
Ifeoma Obidile
The paper examined study habits as necessary tools for examination success among secondary school students. It listed the major generally accepted patterns of study students can make use of in order to succeed in their studies and examinations with ease. The following recommendations for helping students acquire good study habits are made. These include: policy makers on educational curriculum should ensure that seminar or a course on good study habits should be included in the secondary school curriculum so that students will be helped to inculcate a good study habits to enhancing their examination performances, qualified guidance counsellors should be employed by government and proprietors of secondary schools to be helping students come out of the difficulties they are experiencing in their studies, school authorities should be releasing examination time table on time for proper revision of class work and classrooms and places of study should be devoid of noise, will be well ventilated and lighted, studying environment must be kept clean to avoid unnecessary distractions and infections, Guidance Counsellors and teachers should try to be friendly with the students so that the students will not have any barrier in approaching any of them for clarifications and help and then conclusion.
Dr Yashpal D Netragaonkar
Education is regarded as an agent of nation development so factors that promote academic performance such as effective study habits should be encouraged among students. According to him, the promotion of effective study habits among students should be of profound interest to all stakeholders in the field of education. In India ineffective study habits lead to poor career performance, inefficiency on job, lack of job satisfaction, low productivity, and gross under development and retrogression of the nation’s intellectual advancement. Good study habits make student’s days in school rich, productive and enjoyable. Keyword: Study Habits , effective ,education , academic performance
American Journal of Educational Research
The pattern of behaviour adopted by a student in his/her study is his/her study habits, which reveals his/her personality. Study habits serve as the vehicle of learning and may be seen as both means and ends to learning. This study, thus, investigated the study habits of secondary school science students in the Jalingo metropolis, Taraba State, Nigeria. The study which was guided by three research questions, employed a descriptive correlational research design. The sample of the study is made up of 199 students selected from 5 secondary schools through simple random sampling. Data for the study were generated through a questionnaire, tagged “Science Students’ Study Habit Questionnaire”. Descriptive statistics of frequency counts, mean, and product-moment coefficient of correlation were used for data analysis. The study revealed that the secondary school science students in the Jalingo metropolis have poor study habits and weak academic performance. The study also found a strong posi...
IJARW Research Publication , Rah Rayo
Study habits are at the core of a learner's academic success. It is an action like reading, taking notes, conducting study groups that students perform frequently, and regularly accomplishing the learning goals. It can be defined as effective or counterproductive based on whether it serves the students well. Thus, the study's primary purpose was to determine the relationship between study habits and the students' academic performance. The descriptive-correlation design was utilized to describe the respondents' profile regarding their study habits and academic performance. A total of one hundred twenty-six (126) Grade 11 senior high school learners participated in this study. Moreover, the main research instrument utilized in the study was the Palsane and Sharma Study Habit Inventory. Its eight sub-scales are budgeting time, physical condition, reading ability, note-taking, learning motivation, memory, taking examinations, and health. The findings showed that the respondents' study habits are at a relatively average level. The result revealed no significant relationship between study habits and academic performance. Also, the results showed that the study habits of the students are at a relatively average level. Additionally, enhancing students' study habits are relevant, especially in note-taking, reading ability, and health, thus improving their academic performance.
RELATED PAPERS
Tehnologii informatice şi de comunicaţie în domeniul muzical
Scandinavian Journal of History
Sverrir Jakobsson
Ceria Cesar
Romer Castillo
Jussara Rêgo
Arum Soesanti
Markus Loecher
Nuevas de Indias. Anuario del CEAC
Cristina Gimeno-Maldonado
Physical Review E
Miguel Rodriguez
Erling Birkedal
Wosinsky Mór Múzeum Évkönyve 34.
Sándor Balázs Kovács
Spectroscopy Letters
Raul Alejandro Ucañani Morales
The ANNALS of the American Academy of Political and Social Science
Barbara J Robles, PhD
Proceedings of the National Academy of Sciences of the United States of America
Joseph Bryant
Life Sciences
Nuran Ercal
Lecture Notes in Computer Science
Sofia Leiva Paniagua
Ulises Arredondo
hkjtgh hgjthg
Cultura, Direito e Sociedade eBooks
Cássius G . Chai
Proceedings IX Simpósio Brasileiro de Sistemas Elétricos
Vanildo Neves
Cancer research
Alexandre Mignon
SUNIL KUTTY
Continental Shelf Research
Robert Lafite
Dennis Hansell
RELATED TOPICS
- We're Hiring!
- Help Center
- Find new research papers in:
- Health Sciences
- Earth Sciences
- Cognitive Science
- Mathematics
- Computer Science
- Academia ©2024
A quantitative study examining the effects of sleep quality on construction workers’ performance in the city of Jeddah, Saudi Arabia
- Original Article
- Open access
- Published: 14 May 2024
Cite this article
You have full access to this open access article
- Ahmad Baghdadi ORCID: orcid.org/0009-0009-1734-1390 1
Poor sleep and related sleep disorders have been linked to subpar performance in sectors like health and education. Such sleep issues in the workforce negatively affect individual and organisational productivity. Despite this, the high-stress environment of the construction sector has been largely overlooked. This study delves into the role of sleep in construction field management and human resource practices, examining how a better understanding of workers' sleep patterns could enhance job performance. The research involved an online survey of 119 construction workers in the city of Jeddah in Saudi Arabia, focusing on their sleep duration and its impact on job performance, with data analysis conducted using SPSS software. The findings indicate that inadequate sleep influenced by factors such as dietary habits is significantly related to poor performance. The majority of workers are affected by poor sleep quality. The study suggests that construction management should implement health awareness campaigns to improve workers' responsiveness and awareness regarding sleep. It emphasises the need for management to develop strategies to increase sleep awareness and education in the construction industry, aiming to improve overall job performance.
Similar content being viewed by others
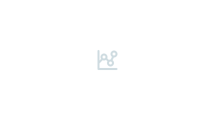
Sleep Quality Among Latino Farmworkers in North Carolina: Examination of the Job Control-Demand-Support Model
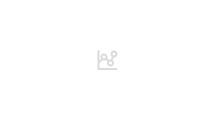
Work intensity and workers’ sleep: A case of working Australians
The Influence of Shift Workers Sleeping Quality upon Job Performance
Avoid common mistakes on your manuscript.
1 Introduction
The construction sector offers a significant platform for establishing sleep quality impacts among workers. It requires both physical energy and emotional concentration to perform. Thus, any lapse in sleep quality can disrupt the performance and engagement levels among the individual workers or their respective teams [ 1 ]. Construction workers experience the challenges of sleep deprivation, owing to the nature of their workplace and the responsibilities expected of them. This study explores the relevance or impacts of quality sleep on construction workers' performance and engagement levels in the city of Jeddah, Saudi Arabia. Through the assessment of the research on related subjects, it is evident that the construction sector is briefly explored. There needs to be more in the perspective of construction field management on how leadership can improve performance by targeting the aspects of quality sleep. Therefore, this study seeks to explore the components of sleep science, its relevance, and its significance in enhancing the performances of construction field workers. An ideal literature review reveals the contemporary issues, research gaps, implications and future studies that can be bridged to achieve the core expectations and goals. Achieving the objectives requires critically assessing past scholarly views, including theoretical frameworks and the sleep science that connect the variables.
Thus, this study aims to survey construction workers in the city of Jeddah, Saudi Arabia, to understand the impact of sleep quality on their performance. Thus, the purpose is to correlate sleep quality to the performances of the construction sector workers in light of whether they experience sleep deprivation or the right quality and the interventions the management can undertake. Specifically, this is an online survey of male construction workers to assess their sleep deprivation situation, which enables policy shift and management approaches towards improving performances. Similar studies have been conducted in the health, education, and related sectors to underscore the significance of ample and quality sleep on the performance of the employees. However, the main research question is: “ What are the specific impacts of sleep quality on the performance of construction workers in Jeddah, Saudi Arabia? ” Other questions include:
What are the specific activities, addictions, and patterns in your lifestyle or behaviour that contribute to sleep deprivation?
Are you aware of the impacts of quality sleep on your workplace performance? Does quality sleep matter to you at the workplace?
What recommendations can you give management to ensure policy change and a shift in management to address sleep deprivation among employees?
2 Literature review
Correlating sleep deprivation or quality to the performance and output of the workforce has been studied in the past, especially in the health and education sectors. In these areas, the primary motivation has been the performance of healthcare providers and students when subjected to sleep deprivation or inadequate sleep. Thus, a range of literature is available that offers background information on how this research can be contextualised, including the research gaps to be addressed through the execution of this study. Therefore, relevant literature that connects to the variables is researched through suitable databases, libraries, and sites that provide the relevant information, patterns, trends, and contemporaries for achieving the expected outcomes. The expectation is that these studies can help give the correct information, address the gap, and help with discussion regarding the impacts of sleep deprivation on the performance of construction sector workers.
2.1 Sleep deprivation and performances
Sleep deprivation is an expanding problem affecting general workers and construction workers. Sleep deprivation affects a worker's alertness, attention, and other cognitive functions. Nearly 93% of Indians are sleep-deprived, 58% of the people believe that their work is affected due to lack of sleep, and 87% of Indians accept that their health is affected due to lack of sleep. In the survey, the researcher found that 70% of college workers are affected by sleep deprivation, particularly chronic sleep deprivation, which has more negative consequences. However, some studies explored the subject of sleep deprivation in the context of establishing the risk factors or the behavioural patterns that cause it. For instance, sleep deprivation includes alcohol consumption and food intake as a worldwide problem, as evident in the study on college workers [ 2 ].
Signs and symptoms of sleep deprivation are often manifested in the workplace. For instance, the imminent results of sleep deprivation are biased feelings of fatigue [ 3 ]. There are connections between these symptoms and poor performance, based on the science of sleep, which is explored in many studies. For instance, a comparative analysis of the research findings in [ 4 , 5 ] shows the similarities in the context of alcohol consumption and how they impact the concentration levels among workers and create sleep deprivation. The two authors appreciate that alcoholism and substance and drug use are indeed risk factors and have the relevant symptoms to ascertain the claims. However, authors in [ 6 ] prioritised the behaviours such as taking stimulants to ensure people stay awake.
Sleep quality is directly correlated to the performances and well-being of workers, irrespective of their sectorial affiliations [ 7 ]. For instance, studies conducted in China and South Korea established that about 30% and 63% of the construction workers in these countries struggle with sleep quality [ 7 ]. Besides, the impacts of these trends on the scope of sleep routines, including the higher chances of workplace injuries and under-performances, are correlated. Work-related juries and possible fatalities are attributed to the poor or inadequate sleep quality that characterises workers in the construction sector. Similarly, construction workers and the relationship between sleep quality and their performances can be connected to their mental, emotional, and behavioural routines. Critical components of such studies demonstrate that construction workers who experience low or poor sleep quality are more likely to experience cognitive behaviour and rational abilities challenges resulting from continued sleep deprivation [ 8 ]. These outcomes underline the holistic impacts of sleep quality on performance and the health and wellness of the construction workers, which translate to their commitment and output.
2.2 Theoretical framework
This study is anchored on the significance of ample and quality sleep on workers' performance. Thus, sleep science is a crucial part of the study when exploring the available literature. For instance, Oswald's Restoration Theory of Sleep underlines the significance of getting the right amount of sleep in the workforce. This individual approach is widely applied in various sectors to underline the need for the consciousness of sleep routines and the science and studies that support the assumptions. For instance, Adeyanju explored the relevance of Oswald's theoretical framework in ascertaining students' academic performance [ 9 ]. The author used the theory's core tenets and characteristics, including that sleep is crucial for restoring the mind, body, and physical and emotional wellness. Sleep serves as a reset session, hence refreshing and restarting the mind to achieve the expected goals and objectives of learning and performance [ 9 ]. Moreover, Himashree et al. [ 10 ] correlated sleep and performance to understand the trends and how the workers are impacted [ 10 ]. The outcomes included a direct correlation, in which those with sleep deprivation showed little interest, physical synergy, and mental fatigue related to the loss of psychological capital to perform.
Restoration theory underlines the key activities individuals should take in a personal capacity to ensure optimal sleep routines. Oswald's view was that any disruption of the restoration process could have overarching mental and emotional health implications on the workers, impacting their engagement levels and performances [ 9 ]. Oswald demonstrated that disruptions to the restoration process when sleeping could have negative implications, including interference with people's ability to sleep, workers becoming more impulsive and emotional in decisions and behaviours, and having a health risk hazard as part of this strategic failure [ 10 ]. Oswald's view of sleep forms an integral part of this study as it explores how the disruptions to the restorative roles of sleep can impact the holistic nature of humans and their ultimate performances in the workplace.
2.3 Sleep deprivation and health risks
Poor sleep quality is a risk factor for mental fatigue. Studies have connected these variables to establish how inadequate sleep leads to low daytime job performance [ 11 ]. Such studies demonstrate the significance of quality sleep in daytime performances, including alertness, memory, learning, critical thinking, and effectiveness in task execution. A secondary data analysis in [ 12 ] demonstrates the factors impacting sleep quality among construction workers and how they generally lead to low performance, commitment, and motivation in their daytime commitments. Other factors explored include occupational injuries, decline in job competencies, and poor intergroup and team dynamics to accomplish tasks. This is because the affected workforce uses specific and personal methods to address the sleepiness they experience. These studies were evident in the findings across Southern India, which demonstrated the severity of inadequate sleep in the construction sector and their implications on the workforce [ 1 ]. As mentioned in other studies, quality sleep leads to higher employee self-efficacy, which translates into confidence, commitment, and performance. These factors interrelate to achieve the expected results and outcomes that directly impact the employees' commitment and the possible solutions for the workforce.
Sleep deprivation is a serious health risk factor that cuts across many sectors and areas of wellness. In the context of performances, some of the risk factors that lead to sleep deprivation and the low-quality trends beyond optimal are excessive alcohol and drug intakes, which continue to hurt the concentration and physical and mental abilities of the employees. These elements make it challenging for the workforce to gain the right mindset and physical abilities to complete construction tasks [ 4 ]. Besides, the nature of the construction workplace, including associated noise and disturbances from machine operations, can impact mental peace, which leads to sleep deprivation. According to a study on this subject matter among college workers in China, the devastating impacts of the sound environment in the construction fields can deter achieving proper sleep routines [ 2 ].
In some studies, sleep deprivation is associated with severe instances of worker frustration, limitations, and absenteeism [ 13 ]. These research works significantly impact the understanding of sleep quality and how they align with commitment and performance. Some studies have also explored the impact of overtime work routines on standard sleep patterns. For instance, Parkes' article established the implications of the overtime work model among offshore day workers, including those having sleep disorders that have long-term impacts [ 14 ]. The findings demonstrate the challenges in adjusting to the overtime work routines for either day or night-shift employees and how the administrators can address the implications on the employees' performances.
3 Methodology
Researching sleep and its implications in construction field management requires assessing workers' responsiveness to tasks. This research employs an online descriptive survey method to collect data on sleep deprivation or the quality of workers' performance in construction. Previous applications in similar aspects of the sleep domain and the project management or health contexts inform the choice and rationale for this method.
In this context, the online descriptive survey methodology is crucial in correlating sleep patterns and quality to the workers' performance or output in construction projects. This is relevant for project management and human resources to understand how to design training and awareness routines for employees to improve their sleep. In this regard, the construction workers within the city of Jeddah are sampled using stratified random sampling. They participated in this online survey to assess their responses to the correlation between the two variables. These methods are consistent and help assess the correlation based on the behaviour and responses of the construction workers.
3.1 Questionnaire and sample size
The data collected from this survey contains six sections, which the participants fill in. The initial part of the survey gathers basic respondent details such as gender, location, and age to validate the study's credibility. The second part collects demographic data on trends, behaviours, and personal characteristics, including height, weight, and lifestyle habits, aiding in categorising participants for sleep disorder research. The third section examines socio-demographic factors affecting bedtime, including time spent on social media and online activities. The fourth section queries educational background to assess awareness of sleep's impact. The fifth section probes health issues, including insomnia and mental and physical health status. Finally, the sixth section explores respondents' understanding of sleep quality, patterns, and quantity to identify trends.
These are meant to include as much reliable information as possible for the qualitative and quantitative analysis. The expectation is that the data collected directly answers the question of the sleep routines and performance of the construction workers at their sites based on the project management analytics and metrics.
3.2 Survey details and participants
The study was conducted in 2023 in Jeddah, Saudi Arabia. A stratified random sampling method was used to ensure that only the eligible participants were selected. This included the construction workers in the identified site, who were male members of this workforce. The rationale for choosing this sampling method is to ensure that only the eligible participants are selected and that the workers are included across age, gender, related variables and stratification elements. A total of 119 male participants working at the identified site were selected. The researcher then distributed the forms through the online platforms. The recruitment process of the participants included approaching their workplace, which included the human resource department for voluntary participation.
Consequently, the willing participants were informed, and their emails were given for the engagement. Despite the progressive and effective engagement with the employers, trust was challenged, especially with the job emails they considered private. However, this was a voluntary participation process, and upon explanation of the reasons for the job email, it was possible to convince the 119 to participate voluntarily.
The data is collected through the survey questionnaires that are administered online to enhance the efficiency of reaching all the participants. Besides, it gives them the flexibility of taking the study within their comfort before sending it back. The collected primary data across the six questionnaire sections are analysed through logistics regression to link sleep deprivation and construction worker performance outcomes. Besides, the socio-economic lines are used as the parameters of sleep that improve their responsiveness and suitability in understanding the outcomes. These methods are justified within the context of online surveys and how they apply to this study that investigates sleep patterns and construction worker performances. Significant themes are identified from the patterns of the responses, including how the mean, median, and regressions are contextualised to make sense of the variables and correlation.
Ethical considerations, including consent, voluntary participation, practical elaboration and explanation of the scope and methodologies of the study, and privacy and confidentiality elements are considered for this study. Moreover, the data collected from the participants were secured, especially as they were stored in the personal computer using unique passwords and access keys. Besides, an assurance of not using the responses for any other purpose rather than the study and deleting the emails after the completion was implemented. The data collection process, including filling and delivering the survey questionnaire, responded to the needs of the ethical considerations, including safety, privacy, and security.
4 Results and findings
4.1 data analysis.
This research aimed to investigate the undulations of construction workers' performance due to sleep deprivation or varying elements of sleep routines. Besides, the study sought the risk factors that impact sleep, especially among construction workers. Consequently, this research collected primary data from the participants, including those aligned to critical variables. Data analysis was carried out on demographic, socio-demographic, and sleep data. The statistical analysis used a statistical package for the social science software. Thus, the study employed SPSS (Statistical Package for the Social Sciences) based on its effectiveness as a software for social science and strategic statistical analysis methods. Consequently, the choice is based on the convenience of the tool in advancing data management, performing analysis, and improving data visualisation for comparative review.
The statistical analysis enables the identification of the relevant themes that connect the two variables, sleep deprivation and the performance of the construction workers. The statistical analysis began with descriptive analysis. All the regressions were started with demographic data and then gradually added up with socio-demographic data and sleeping problems before exams. The diversity of approaches employed in this study demonstrates the specificity of the subject matter in correlating the study's variables to understand performance and other aspects of construction worker's engagement.
4.2 Descriptive statistics
Table 1 shows the descriptive results of age and time spent on social media. This smoking habit is considered as a baseline for the results. The regression technique is used to identify the link between sleep deprivation and worker performance. The co-relation technique was used before regression analysis to filter the factors, as shown in Fig. 1 . The binary logistic regression was carried out in this study to identify the specific relationship between two variables. The descriptive statistics in Table 1 demonstrate the essential aspects, including the respondents' age, behaviour, browsing before sleeping routines, missing the workplace, substances use such as smoking, social media, and stress. The data collected are analysed in the context of statistics, standard error, and related contexts to get the right co-efficient for decision-making routines on sleep and construction workers' performances. These are represented in the statistical components, including the mean, standard deviation, and variance.
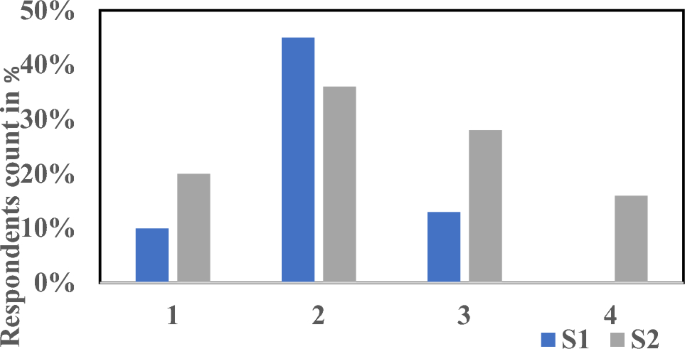
Relation between food intake and sleep timing
4.3 Regression analysis results
Table 2 represents the constructed demographic variables. The comparison between sleep factors and workers' performance includes coefficients, standard errors, Wald statistics, and degrees of freedom for the factors filtered in the co-relation technique. Where B = constant, S.E = standard error, W = Wald Statistics, p = Significance (P < 0.05). Table 2 summarises these outcomes to showcase the patterns, findings, and responses in the context of demographic variables applied in the study. These include age, gender, height, weight, smoking, and food intake time. These variables help in understanding the correlation between sleep routines and the performance of employees, especially in areas considered deprived.
Food intake is numbered in binary logistic regression. Figure 1 represents the relation between food intake and sleep: S1—food intake timing, S2 —sleeping time. S1 is coded as [(1) before 8 pm, (2) 8 pm–9 pm, (3) 9 pm–10 pm], and S2 as [(1) 4h–5h, (2) 6h–7h, (3) > 8h]. Table 3 shows that sleep deprivation was associated with worker outcomes. It also demonstrates the sleep deprivation variables, which inform crucial determinants of sleep deprivation as used in the study. However, Fig. 1 showcases the respondents' views on food intake time and sleeping routines. This is relevant in how variables relate to eating habits and their impacts on sleep time.
5 Discussion
The research findings and the literature review attempt to correlate sleep deprivation to the worker's performance in construction projects. The primary motivation is that this information is crucial for the project managers and construction personnel administrators to inform and influence the workers to adopt healthy sleep patterns. In this regard, the findings demonstrate the consistency with most of the literature reviewed in this study to establish the patterns. For instance, the descriptive statistics in Table 1 indicate the investigated variables to showcase the correlation. Consequently, the participant's age, behaviour, browsing before sleep patterns, missed workplace, smoking, social media, and stress are all responsible for an employee's performance. Generally, those with sleep challenges or deprivation tend to have negative connotations that align with their state of physical, mental, and emotional health. From the results, the study established that improper food intake and social media networking, impact construction workers' performances. These are the risk factors for creating the right environment for understanding sleep routines and their impacts on performance [ 15 ]. These results demonstrate the factors that can lead to sleep deprivation among construction workers, with the participants showcasing their implications on sleep quality.
5.1 Risk factors to sleep deprivation among workers
The findings highlight the impacts of issues with mental and emotional health risk factors, including substance use such as smoking, browsing and social media activities, and other patterns. Table 1 indicates the descriptive statistics on the key variables and the coefficient values on the implications of these aspects regarding the standard deviation, variance, and mean for each variable. Ideally, people's sleep challenges emerge from the behavioural patterns mentioned here, which hinder their sleep quality. This result underscores the increased sleep health promotion patterns that most projects and institutions use in their management practices to optimise the performances of their employees [ 16 ]. The primary motivation for using these aspects is the high illiteracy of the risk factors that impact quality sleep among the employees. Besides, Oswald's Restoration theory of sleep underscores its significance, informing the project management best practices [ 17 ]. The findings in Table 1 showcase statistical coefficients and their values that connect these variables and how the critical tenets of sleep theories can improve awareness and knowledge among construction workers. Similarly, the theoretical frameworks and the necessity for improving the rationale for knowledge and the significance of sleep are embedded here [ 10 ].
In the era of social media, online gaming, and other addictive mobile and digital apps, most people spend large chunks of their time engaged actively in these entertaining products. Consequently, most of them play till midnight or beyond, establishing the addictive nature of social media use. Thus, the socio-demographic variables indicate that most participants cited that social media use takes their time for sleeping, resulting in deprivation. These are also indicated in Table 3 , showing the impacts of social media use and related activities such as online gaming. These findings are unsurprising in the context of the literature reviewed on the subject. Sleep quality versus the quality of life domains has been studied, and these aspects are evident, including how the higher-education workers respond to these dynamics [ 18 ]. Only a tiny segment of the participants (P < 0.05) demonstrates the implications of social media use, mobile phones, and gaming as not impacting their lives and sleep routines. However, the construction human and personnel management team must develop the right educational tools to give the employees the right and necessary awareness of how their social media use at home or work might impact their sleep and restoration phases.
5.2 Behavioural and lifestyle patterns and their implications to sleep deprivation
In the context of binary logistics regression, Fig. 1 demonstrates how eating patterns can lead to sleeping challenges and the eventual performance implications. The primary purpose of this question on diet and eating habits and routines is to establish the correlation, including whether it impacts sleeping time and quality. For instance, the food intake timing can be early or late, which gives varying time to responsiveness and suitability. The correlation in these variables demonstrates that participants could take their food before 8 pm, 8–9 pm, or 9–10 pm, while S2 includes sleeping for 4–5 h, 6–7 h, or more than 8 h. These findings, as evident in Fig. 1 , demonstrate that the food intake timing, especially those who take their food late at night, have less sleep and are thus deprived. These aspects are further explored in assessing sleep deprivation in the context of curriculum exam performances and outcomes in Indian society [ 19 ]. The results show the implications of eating routines and timing of the workers in the construction sector.
The human resource management practices in the construction sector should devise a mechanism for monitoring the sleep patterns among the workers. This will help create the learning manuals and training guides to eradicate the risk factors, including the sleeping routines and associated outcomes. Specifically, Fig. 1 shows the implications of late eating patterns and engaging in disruptive activities such as social media use. Thus, despite the presence of workers or lack of absenteeism, they still show the aspects of daytime sleepiness, which hurt their engagement, concentration, and participation [ 20 ]. Performances are brain-based. Thus, any factor that impedes these aspects can easily ruin the performance of the construction workers in their respective responsibilities. The labour force in the present situation is often submerged in disruptive behaviours, especially the youths, which limits their productivity. This study demonstrates these factors' alignment with long-term sleepiness and implications [ 21 ]. The age, gender, and experience are crucial in defining their sleep routines and the understanding of the risk factors that characterise such situations. They are anticipated to have the adaptive mechanisms of ample and quality time that freshens their minds before they report to their respective workplaces.
5.3 Workplace environment as a risk to quality sleep
The nature of the workplace can impact sleep. Typically, operating the machines in construction work and the risk involved can be demanding and exhausting, which subjects the employees to being tired even with the available sleep time. Despite the evident significance of technology in improving workers' performances, there can be impediments. It takes time to learn, while the addictive trends of some apps, including mobile phones, can impact youthful employees. These components lead to sleep disorders and deprivation, while people tend to align with the technological needs and innovations in their areas. These include operating the machines and having safety patterns, which can impact the time found for resting [ 21 ]. The expectation is that the construction workers are impacted by the technological exposure that they have, their eating habits and patterns, and substances use such as smoking that directly impact their sleep times and cause deprivation [ 22 ].
5.4 Impacts of sleep and interventions by individual employs to overcome sleepiness
The findings show disparities in the appreciation of sleeping risk factors that impact individual work routines. Thus, most people appreciate the presence of such risk factors differently, calling for standardised mechanisms for human resource practitioners in the construction sector to embrace. There are varying degrees of alertness, woke, mood swings, and related manifestations of sleep disorder that different employees experience, which underline the need to use practical solutions. The analytics shown in Table 3 show these differences, showing the need for the stakeholders to have the right metrics and approaches to control the manifestation of sleep disorder in the workers and how it impacts their performances. These indicate the glaring challenges in having a uniform method of appreciating the presence of deprivation as a performance hindrance factor and developing an organisational level or sectorial approach to overcoming the outcomes. Dong and Zhang [ 23 ] defines these issues in the context of how each participant had their approaches to appreciating sleep disorders, even when working in the same sector or industry.
6 Conclusion and recommendations
This study primarily investigates the relationship between sleep deprivation and the performance of construction sector workers in Jeddah, Saudi Arabia. Utilising a sample of 119 construction workers, the study employs. Participants were surveyed online and divided into five categories to collect comprehensive data regarding their sleep patterns and their impact on work performance. This method aligns with established research standards and policies, ensuring the study's conclusions are robust and reliable. The study provides a comprehensive analysis of the impact of sleep quality on the performance of construction workers in Jeddah, Saudi Arabia. The main findings from the research indicate a significant correlation between poor sleep quality and decreased job performance among construction workers. The data analysis, particularly through binary logistic regression, highlighted specific lifestyle and behavioural patterns that contribute to sleep deprivation, such as irregular dietary habits, excessive use of social media, and engagement in shift work. These factors not only disrupt sleep patterns but also affect the workers' daily functioning and safety on the job site. The results underscore the critical need for targeted interventions that address these modifiable risk factors to enhance sleep quality among construction workers. The findings confirm a clear correlation between sleep deprivation and reduced performance among construction workers. The study underscores the importance of management strategies that assess and educate workers on optimising sleep routines to enhance overall job performance. Future research should focus on developing and implementing these management strategies effectively.
The research also delves into how personal behaviours, such as excessive engagement with social media and online gaming, can adversely affect sleep patterns. Management often unrecognises these factors, but they significantly affect worker performance. The study's results are significant for the construction sector's human resource management (HRM). They provide insights into how HRM can integrate sleep consciousness into their practices, enhancing worker performance through better sleep habits. Thus, recommendations can be applied to ensure that the study's main aspects and implications are introduced at the construction sector sites to achieve the expected outcomes, come as follows:
Improving sleep awareness among employees
Most employees are victims of their behavioural and lifestyle routines, which subject them to sleeping disorders without their awareness. This lack of appreciation for the significance of sleep is a significant risk factor for their performance, as established in the study's findings. Consequently, management should devise effective mechanisms that create a dedicated learning niche for the employees. This includes training them on the core aspects of sleep awareness and consciousness, enhancing their overall well-being and productivity.
Promote personal behaviour changes towards sleep quality
These elements directly impact the employees' performances, yet it is often considered a private matter whether they sleep late or early. Thus, despite its controversial appeal, this recommendation seeks to identify the individual challenges among the workforce and enforce the behavioural or lifestyle changes that will make them appreciate the outcomes. By addressing these personal habits, the initiative aims to enhance overall sleep quality and improve work performance and health.
Implementing regular health and safety audits
Regular health and safety audits should be conducted to enhance workplace productivity further and ensure the health and safety of construction workers. These audits will help identify potential hazards that could lead to accidents or exacerbate sleep deprivation issues among workers. By systematically assessing the work environment and practices, management can implement necessary changes to mitigate risks. This proactive approach ensures compliance with safety regulations and demonstrates a commitment to worker welfare, which can boost morale and productivity.
Development of a comprehensive wellness program
Another recommendation is developing and implementing a comprehensive wellness program focusing on sleep health. This program should offer resources and support for various aspects of health, including mental health, physical fitness, nutritional advice, and sleep education. Workshops and seminars can be organised to educate workers on the importance of maintaining a healthy lifestyle and how it impacts their work performance. Regular health screenings can also help detect any underlying issues affecting sleep, such as sleep apnea or chronic stress. By investing in employees' overall well-being, companies can improve productivity and reduce absenteeism and turnover rates.
6.1 Limitations and recommendations for future research
The study's main limitation is using male-only participants, creating a discriminative framework. A men-only survey gives insights from their perspective in an industry with female workers and administrators. These limitations can adversely affect the study's generalisation and its long-term implications. The reason behind the selection of male workers is that most of the construction in Saudi Arabia are men. This is particularly true for the projects selected for this study. However, future studies should address the roles of HRM in influencing sleep consciousness and awareness through organisational learning to impact performances. This should be a priority area for the construction work administrators to ensure they inform and educate the workers on the various aspects of sleep science and its importance.
Availability of data and materials
All data and materials are available as required.
Sathvik S, Krishnaraj L, Awuzie BO (2023) An assessment of prevalence of poor sleep quality among construction workers in Southern India. Built Environ Project Asset Manag 13(2):290–305
Article Google Scholar
Meng Q et al (2020) Effects of the sound environment on the sleep of college workers in China. Sci Total Environ 705:135794. https://doi.org/10.1016/j.cptl.2019.11.004
Patte KA, Qian W, Leatherdale ST (2017) Sleep duration trends and trajectories among youth in the COMPASS study. Sleep Health 3(5):309–316. https://doi.org/10.1016/j.sleh.2017.06.006
Hu N et al (2020) Alcohol consumption and incidence of sleep disorder: a systematic review and meta-analysis of cohort studies. Drug Alcohol Depend. https://doi.org/10.1016/j.drugalcdep.2020.108259
Kenney SR et al (2012) Global sleep quality as a moderator of alcohol consumption and consequences in college workers. Addict Behav 37(4):507–512. https://doi.org/10.1016/j.addbeh.2012.01.006
Article MathSciNet Google Scholar
Faber NS, Häusser JA, Kerr NL (2017) Sleep deprivation impairs and caffeine enhances my performance, but not always our performance: how acting in a group can change the effects of impairments and enhancements. Pers Soc Psychol Rev 21(1):3–28
Wendimu DE, Meshesha SG (2023) Factors associated with poor sleep quality among construction workers in Arba Minch town, Ethiopia: a cross-sectional study. Health Sci Rep 6(11):e1715
Chandra SS, Loganathan K, Awuzie BO, Wang F (2023) A longitudinal study examining the association between cognitive behavior and rational abilities and the effect of sleep quality on construction laborers. Sustainability 15(7):6257
Adeyanju JO (2023) Ian Oswald’s theory of sleep as a strategy for promoting excellent students’ academic performance. Shodh Sari Int Multidiscip J 02(02):04–13. https://doi.org/10.59231/SARI7570
Himashree G, Banerjee PK, Selvamurthy W (2002) Sleep and performance-recent trends. Indian J Physiol Pharmacol 46(1):6–24
Google Scholar
Shi J, Long L (2018) The depletion effects of sleep deprivation among employees: a new topic in organisation and management research. Adv Psychol Sci 26(5):896
Kim Y, Lee S, Lim J, Park S, Seong S, Cho Y, Kim H (2021) Factors associated with poor quality of sleep-in construction workers: a secondary data analysis. Int J Environ Res Public Health 18(5):2279
Parkes KR (2015) Sleep patterns of offshore day-workers in relation to overtime work and age. Appl Ergon 48:232–239. https://doi.org/10.1016/j.apergo.2014.12.004
Reis C et al (2020) Social timing influences sleep quality in patients with sleep disorders. Sleep Med 71:8–17. https://doi.org/10.1016/j.sleep.2020.02.019
Mnatzaganian CL, Atayee RS, Namba JM, Brandl K, Lee KC (2020) The effect of sleep quality, sleep components, and environmental sleep factors on core curriculum exam scores among pharmacy students. Curr Pharm Teach Learn 12(2):119–126
Levenson JC et al (2016) Pilot study of a sleep health promotion program for college workers. Sleep Health 2(2):167–174. https://doi.org/10.1016/j.sleh.2016.03.006
SONU Sleep (2022) Oswald's restoration theory of sleep: a brief summary. SONU Sleep. https://sonusleep.com/blogs/news/oswalds-restoration-theory-of-sleep-a-brief-summary . Accessed 29 Feb 2024
Marques DR et al (2017) Associations between sleep quality and domains of quality of life in a non-clinical sample: results from higher education workers. Sleep Health 3(5):348–356. https://doi.org/10.1016/j.sleh.2017.07.004
Mnatzaganian CL et al (2020) The effect of sleep quality, sleep components, and environmental sleep factors on core curriculum exam scores among pharmacy workers. Curr Pharm Teach Learn 12(2):119–126. https://doi.org/10.1016/j.cptl.2019.11.004
Mirghani HO, Ahmed MA, Elbadawi AS (2015) Daytime sleepiness and chronic sleep deprivation effects on academic performance among the Sudanese medical workers. J Taibah Univ Med Sci 10(4):467–470. https://doi.org/10.1016/j.jtumed.2015.05.003
Ose SO, Jensen C (2017) Youth outside the labour force—perceived barriers by service providers and service users: a mixed method approach. Children Youth Serv Rev 81:148–156. https://doi.org/10.1016/j.childyouth.2017.08.002
Phegley K (2017) Consequences of fatigue and sleep deficiency in the workplace: implications for the construction industry. Wright State University. https://corescholar.libraries.wright.edu/cgi/viewcontent.cgi?article=1211&context=mph . Accessed 15 Feb 2024
Dong Y, Zhang X (2020) Investigation of the effects of awakening daylight on the morning alertness, mood, and sleep quality of male college workers. Build Environ 180:106989. https://doi.org/10.1016/j.buildenv.2020.106989
Download references
No funds, grants, or other support was received.
Author information
Authors and affiliations.
Department of Civil and Environmental Engineering, College of Engineering and Computing in Al-Qunfudhah, Umm Al-Qura University, Mecca, Saudi Arabia
Ahmad Baghdadi
You can also search for this author in PubMed Google Scholar
Corresponding author
Correspondence to Ahmad Baghdadi .
Ethics declarations
Conflict of interest.
The author declared that that there is no competing interest involved.
Ethical standards
Ethical considerations were meticulously adhered to throughout the research process. Participants were fully informed about the study's scope and procedures, ensuring their consent was informed and voluntary.
Additional information
Publisher's note.
Springer Nature remains neutral with regard to jurisdictional claims in published maps and institutional affiliations.
Rights and permissions
Open Access This article is licensed under a Creative Commons Attribution 4.0 International License, which permits use, sharing, adaptation, distribution and reproduction in any medium or format, as long as you give appropriate credit to the original author(s) and the source, provide a link to the Creative Commons licence, and indicate if changes were made. The images or other third party material in this article are included in the article's Creative Commons licence, unless indicated otherwise in a credit line to the material. If material is not included in the article's Creative Commons licence and your intended use is not permitted by statutory regulation or exceeds the permitted use, you will need to obtain permission directly from the copyright holder. To view a copy of this licence, visit http://creativecommons.org/licenses/by/4.0/ .
Reprints and permissions
About this article
Baghdadi, A. A quantitative study examining the effects of sleep quality on construction workers’ performance in the city of Jeddah, Saudi Arabia. J. Umm Al-Qura Univ. Eng.Archit. (2024). https://doi.org/10.1007/s43995-024-00065-1
Download citation
Received : 19 April 2024
Accepted : 02 May 2024
Published : 14 May 2024
DOI : https://doi.org/10.1007/s43995-024-00065-1
Share this article
Anyone you share the following link with will be able to read this content:
Sorry, a shareable link is not currently available for this article.
Provided by the Springer Nature SharedIt content-sharing initiative
- Construction workplace
- Sleep deprivation
- And workplace performances
- Find a journal
- Publish with us
- Track your research
Consumer sentiment and behavior continue to reflect the uncertainty of the COVID-19 crisis
Heading into the fall season, there is a renewed sense of caution as coronavirus cases soar in parts of the world. While the uncertainty from COVID-19 persists throughout the globe, its impact is felt differently across countries. As a result, there is significant variance in how consumers respond to the crisis and adapt to the next normal.
About our surveys
While these themes hold true across the 45 countries we have tracked through the crisis (see sidebar, “About our surveys”), the following exhibits focus on a subset of 13 core countries, selected because of their economic significance and the impact that COVID-19 has had on their populations.
1. Shift to value and essentials
Consumer sentiment varies greatly across countries impacted by COVID-19. Consumers in China, India, and Indonesia consistently report higher optimism than the rest of the world, while those in Europe and Japan remain less optimistic about their countries’ economic conditions after COVID-19. Except for Italy, optimism has declined throughout European countries, in line with the rise in confirmed cases since late July.
Would you like to learn more about our Marketing & Sales Practice ?
Divergent sentiment is also reflected in spending intent across categories. In most countries, consumers intend to continue shifting their spending to essentials, while cutting back on most discretionary categories. However, in China and India, spending is bouncing back beyond grocery and household supplies. Chinese consumers plan to increase spending on discretionary categories such as travel and apparel, suggesting that the country is further along the path to recovery than other countries. In India, consumers report a higher intent to spend across categories as they prepare for upcoming festivals (Diwali, for example) and the wedding season, which runs from October to December.
2. Shock to loyalty
Consumers across the globe have responded to the crisis and its associated disruption to normal consumer behaviors by trying different shopping behaviors and expressing a high intent (65 percent or more) to incorporate these behaviors going forward. However, the change has been less pronounced in countries with a moderate degree of economic shock, such as Germany and Japan.
Given consumers’ price sensitivity, value remains the primary reason for consumers to try new brands as well as new places to shop. Aside from value, convenience and availability are most often cited as top drivers of consumers’ decisions about where to shop, while quality and purpose (desire to support local businesses, for example) are the more important considerations when choosing new brands.
3. Homebody economy
Consumers around the globe are at vastly different stages of resuming out-of-home activities. In China, more than 80 percent of consumers report engaging regularly with out-of-home activities. Countries with stricter government lockdown measures, such as Mexico and Brazil, have the lowest percentage of consumers reporting they have resumed “normal” activities.
Consumers’ intent to engage with out-of-home activities varies by category and country. Most intend to leave home to shop for necessities but maintain low engagement in shared services. Consistent with other indicators of recovery, China is again an outlier with most consumers intending to engage in more than half of the activities tracked.
4. Flight to digital
The shift to digital persists across countries and categories as consumers in most parts of the world keep low out-of-home engagement. Food and household categories have seen an average of over 30 percent growth in online customer base across countries. Online growth for China seems more moderate, as the country had a high level of online penetration prior to the pandemic.

Global surveys of consumer sentiment during the coronavirus crisis
5. holiday outlook.
Compared to last year, consumers across the globe plan to reduce holiday spending, even in countries that have exhibited signs of recovery in spending intent in the next few weeks. China stands out as the only exception, with more consumers reporting increased spending than decreased spending.
The flight to digital and omnichannel will be prevalent during the holiday season, with 30 to 60 percent of consumers across countries reporting an intent to shift online for holiday shopping.
We will continue tracking consumer sentiment to gauge how people’s expectations, perceptions, and behaviors change throughout the pandemic. In addition to the exhibits embedded here, please see the country-level survey data, which will be updated regularly.
Or click directly to see survey results from these countries: Argentina , Australia , Belgium , Brazil , Canada , Central America , Chile , China , Colombia , Denmark , Dominican Republic , France , Germany , India , Indonesia , Italy , Japan , Korea , Mexico , the Netherlands , New Zealand , Nigeria , Peru , Poland , Portugal , Qatar , Saudi Arabia , South Africa , Spain , Sweden , Switzerland , Turkey , the United Arab Emirates , the United Kingdom , or the United States .
Tamara Charm is a senior expert in McKinsey’s Boston office; Anne Grimmelt is a senior expert in the Stamford office; Hyunjin Kim is a consultant in the San Francisco office, where Kelsey Robinson is a partner; Nancy Lu is a consultant in the New York office; Mayank is a specialist in the Chicago office; Mianne Ortega is a director in the Madrid office; Yvonne Staack is a senior expert in the Hamburg office; and Naomi Yamakawa is a partner in the Tokyo office.
The authors wish to thank Reinhold Barchet, Shruti Bhargava, Marco Catena, Jeremy Carlson, Resil Das, Gizem Gunday, Karina Huerta, Julia Katharina Schmidt, Elaine Lo, Emily Lu, John-Michael Maas, Jorge Omeñaca, Sebastian Pflumm, Denny Praseco, Sidhika Ramlakan, Roberta Romeo, Peter Saffert, Claudia Zaroni, and Cherie Zhang for their contributions to this article.
Explore a career with us
- Open supplemental data
- Reference Manager
- Simple TEXT file
People also looked at
Original research article, consumption of fruits and vegetables and its association with sleep duration among finnish adult population: a nationwide cross-sectional study.
- 1 Doctoral School of Health Sciences, University of Helsinki, Helsinki, Finland
- 2 Finnish Institute for Health and Welfare, Helsinki, Finland
- 3 Master School, Health and Wellbeing, Turku University of Applied Sciences, Turku, Finland
Introduction: Sleep and diet are crucial determinants of overall health and wellbeing, with the potential to mutually influence each other. This study examined the association between sleep duration and fruits and vegetables (FV) consumption among Finnish adults.
Methods: The study analyzed data from the National FinHealth 2017 Study involving 5,043 adults aged 18 years and above. Participants reported their habitual sleep duration, and dietary consumption through a validated self-administered questionnaire. Confounders such as demographic, socio-economic factors, and chronotype were considered. A sensitivity analysis, which excluded energy under-reporters, was conducted to validate the findings.
Results: Mean dietary consumption was compared across three sleep duration categories (short, normal, long), revealing that short sleepers consumed 37 g/d fewer FV, and long sleepers consumed 73 g/d fewer FV than normal sleepers. Binary logistic regression analyses consistently demonstrated significant negative association between FV consumption and both short and long sleep duration across all models, even when adjusted for a range of covariates. Linear regression analyses revealed a positive but non-significant association between sleep duration and FV consumption that became significant when excluding energy under-reporters, particularly in model 1.
Discussion: This study suggests a consistent pattern where deviation from normal sleep duration was associated with decreased FV consumption, suggesting the need for considering sleep patterns in dietary intervention. The substantial role of accurate energy reporting in explaining these associations is highlighted. Further research, including longitudinal studies, is needed to better understand the mechanisms underlying these associations.
Introduction
Sleep and diet are critical determinants of overall health and wellbeing ( 1 ). It is recommended that a healthy adult should sleep between 7 to 9 h a night ( 2 , 3 ). However, the modern landscape, shaped by lifestyle dynamics and environmental triggers such as sedentary habits and dietary choices, has led to a concerning surge in adult sleep deprivation ( 4 , 5 ). Finland, for instance, has witnessed an upswing in sporadic insomnia symptoms ( 6 ) accompanied by reduced sleep duration in adult population ( 7 ). This emerging public health concern is amplified by the link between inadequate sleep and adverse health outcomes, including cardiovascular diseases ( 8 ), cognitive malfunction ( 9 ), and increased all-cause mortality ( 10 ).
Recognizing the intricate relationship between health outcomes and dietary choices ( 11 ), substantial efforts have been directed toward enhancing people’s food-related knowledge ( 12 – 14 ). However, certain aspects of modern life, such as time constraints, easily accessible fast foods, stress, and inadequate sleep, collectively contribute to unhealthy food choices ( 15 , 16 ). The World Health Organization (WHO) recommends a daily consumption of at least 400 g of fruits and vegetables, a guideline underpinned by the well-established benefits of substantial FV intake in mitigating the risk of chronic diseases ( 17 – 19 ). The recently updated Nordic Nutrition Recommendations for 2023 advocate an even higher daily consumption of at least 500 to 800 g of vegetables, fruits, and berries, with half of the consumption coming from vegetables ( 20 ). Unfortunately, data from many countries, including Finland shows non-compliance ( 21 – 24 ). In Finland, for example, only 14% of men and 22% of women met the current national recommendation of consuming a minimum of 500 g of vegetables, fruits, and berries per day ( 25 , 26 ).
While the significance of adequate sleep and consumption of FV for overall health is becoming more evident through numerous studies, a notable gap exists in comprehending the potential interplay between these factors within the general population. Existing research has primarily concentrated on specific demographic segments, including pregnant women, adolescents, children, young adults, and the elderly, often with limited sample sizes ( 27 – 33 ). Consequently, a comprehensive understanding of these associations across a broader spectrum of the population remains elusive. To bridge this gap, our study embarked on a nationwide cross-sectional analysis encompassing a larger and more diverse adult cohort.
Chronotype, which reflects an individual’s preference for timing of activities during the day (morning or evening preference), has demonstrated its influence on both sleep patterns and dietary choices in prior research ( 34 – 37 ). However, previous studies investigating the link between sleep duration and FV consumption have often overlooked chronotype as a potential confounder. Including chronotype in the analysis allows us to delve into how an individual’s innate preference for activity timing interacts with their sleep duration and dietary behaviors. This aspect of our study is pivotal as it contributes an additional layer of understanding when examining the association between sleep duration and dietary behaviors.
Our study provides a holistic perspective on the interactions between sleep duration and fruits and vegetable consumption. We aim to specifically explore how sleep duration influence fruits and vegetable consumption and vice-versa . Additionally, we will investigate the role of chronotype as a potential confounder in the associations between sleep duration and fruits and vegetables consumption.
The data for this study were derived from the National FinHealth 2017 Study, which is a population-based cross-sectional health survey. It comprises of a representative sample of individuals aged 18 and older residing in Finland. Participants were selected through stratified random sampling methods based on gender, age, and geographical location. Out of the eligible sample ( n = 10,247) who received an invitation letter to a health examination and to complete a self-administered questionnaire by mail, 58.1% participated in the health examination. During this examination, participants were provided with additional questionnaires, including the Food Frequency Questionnaire (FFQ), which could also be completed electronically. Of those who took part in the health examination 86.1% returned the FFQ. Exclusions from the study were made for various reasons: incomplete FFQs (110 cases), duplicate responses (9 cases) and withdrawal of consent (7 cases). To ensure the credibility of the dietary data, FFQs that reported extreme and implausible energy intake values were also excluded (51 FFQs). This was based on daily energy intake thresholds that marked the lowest and highest 0.5% of sex-specific energy intake for monitoring dietary intake in large cohort studies ( 38 , 39 ). After these exclusions, the total sample size was narrowed to 5,125 participants. A further exclusion was made due to missing information on sleep duration (80 cases), resulting in a final analytical sample of 5,043 adults for this study. The pattern of missing data was evaluated using Little’s MCAR test, which yielded a Chi-Square statistic of 2.167 with one degree of freedom ( p = 0.14), suggesting that the missingness was random. As a result, listwise deletion was employed to handle missing data in the analysis.
The study protocol and method of the FinHealth 2017 study is described in detail elsewhere ( 40 , 41 ). Ethical approval for the FinHealth 2017 Study was obtained from the Coordinating Ethics Committee for the Hospital District of Helsinki and Uusimaa (Reference 37/13/03/00/2016), and all participants provided written informed consent. In addition, for this study a permission was acquired from the institutional review board at the Finnish Institute for Health and Welfare (THL) to further analyze the dataset (Reference FT2019_025). All procedures were performed in accordance with the valid guidelines and regulations as well as the Declaration of Helsinki and its amendments.
Study variables
This study examined two pivotal variables: sleep duration and total fruit and vegetable consumption (TFVC). Sleep duration was assessed through a self-administered questionnaire. Participants were asked to report their habitual sleep duration with the question, “How many hours do you sleep in 24 h?” They were instructed to provide their response in hours and minutes, reflecting their average sleep pattern. For subsequent analysis, minutes were converted into hours, and the total sleep duration was then computed. The resulting total sleep duration was further classified into three categories: short sleepers (less than 7 h per day), normal sleepers (7–9 h per day, the reference group), and long sleepers (more than 9 h per day).
The primary dietary variable of interest was the consumption of fruits (including citrus fruits, apple, berries, other fresh and canned fruits) and vegetables (including green leafy vegetables, root-vegetables, cabbages, mushrooms, legumes, fruit vegetables, other fresh and canned vegetables). The dietary information was gathered using a validated 134-item self-administered semi-quantitative food frequency questionnaire (FFQ) ( 42 – 44 ). Participants reported their habitual diet over the past 12 months, indicating the average consumption of each food item with a scale of 10 frequency categories ranging from none to six or more times a day (none, less than once a month, 1–3 times a month, once a week, 2–4 times a week, 5–6 times a week, once a day, 2–3 times a day, 4–5 times a day, and 6 or more times a day). The portion sizes were predefined and expressed in common household and natural units (e.g., glass, or slice). The average daily food consumption (g/day) and energy intake were calculated using the FINESSI software of THL and the Finnish National Food Composition Database (FINELI) ( 39 ).
Background variables
The FinHealth 2017 Study included information on background variables including gender, age, education, employment, household income, cohabitation, the number of household members, the number of living children, Body Mass Index (BMI), physical activity level, smoking, alcohol consumption, total energy intake and chronotype. These variables were included in the statistical regression models as control variables, reflecting their established associations with sleep duration in previous literature.
For further analysis, age, education, employment, household income, cohabitation, body-mass index (BMI), physical activity level and chronotypes were grouped into different categories. Participants were categorized by age into younger adults (18–34 years), middle-aged adults (35–64 years), or older adults (65 years or older). BMI was grouped in four categories as follows: BMI < 18.5 (underweight), BMI 18.5–24.9 (normal weight), BMI 25.0–29.9 (overweight), or BMI > 30.0 (obese).
Physical activity levels over the previous 12 months were assessed by four categories: inactive (light activities such as reading and watching television); moderately active (walking, gardening or other activities ≥ 4 h/week); active (running, swimming or other physically demanding activities ≥ 3 h/week); or very active (competition or other heavy sports several times/week). The categories “active” and “very active” were combined for the present study as only a few participants were classified as “very active.”
Chronotype was assessed using self-evaluation questions with four available options: “definitely a morning type,” “rather more a morning than an evening type,” “rather more an evening than a morning type” and “definitely an evening type.” Participant’s response was further categorized into morning type, intermediate type (combining “rather more a morning than an evening type” and “rather more an evening than a morning type,” and evening type during analysis).
Education levels were expressed as basic (elementary/basic/lower secondary education), intermediate (vocational/upper secondary/high school/non-university lower education), or higher (college/university education). Working status was categorized as employed (paid job/self-employed/unpaid employment in a family-owned business, apprenticeship, and paid internship), or outside of work (unemployed/student/unpaid internship/retired/on family leave/stay-at-home mother/father).
Household income was grouped based on annual income before tax reduction as low (lowest through 35,000 €), middle (35,001 to 60,000 €), or high (60,001 € and higher). Participants in married/cohabitated/registered partnership were grouped as living together, whereas those who were single/separated/divorced/widowed were grouped as living alone.
Statistical analyses
Descriptive statistics were calculated, and FV consumption compared between sleep categories using analyses of covariance, followed by the Bonferroni multiple comparisons post-hoc test with adjustments for age, gender and energy intake were made where relevant.
Linear regression analysis was employed to explore the potential influence of sleep duration on FV consumption, employing three models with increasing levels of covariate adjustment. Model 1 adjusted for basic covariates such as age, gender and total energy intake. Model 2 further adjusted for an expanded set of covariates, including BMI, education, employment, marital status, household income, the number of household members, the number of living children, smoking, and alcohol intake. Model 3 included all the covariates from Model 2, along with chronotype as an additional covariate.
Binary logistic regression analysis was undertaken to explore the potential influence of FV consumption across different sleep categories: short sleep vs. normal sleep, and long sleep vs. normal sleep, adjusting for the same set of covariates used in the linear regression analysis. To estimate the magnitude of differences between normal sleepers and short or long sleepers, effect sizes were calculated using Cohen’s d and the effect-size correlation rYλ.
A sensitivity analysis was conducted by repeating both the linear and logistic regression analyses after excluding participants identified as energy under-reporters. Energy misreporting was assessed by calculating the ratio of reported energy intake to predicted Basal Metabolic Rate (BMR). Participants with an energy intake to BMR ratio (EI:BMR) of 1.14 or less were considered under-reporters and were excluded from this secondary analysis ( 39 ).
All statistical analyses were performed using the IBM Statistical Package for Social Sciences (SPSS) Statistics, Version 28 (International Business Machines Corporation, Armonk, NY, United States).
Table 1 depicts the distribution of the study population across different sleep duration categories. Of the 5,043 participants, 21% were short sleepers, 76.1% were normal sleepers, and the remaining 2.9% were long sleepers. The mean sleep duration for short sleepers was 6.0 h (SD = 0.6), for normal sleepers 7.7 h (SD = 0.6), and for long sleepers 10.1 h (SD = 0.7). The majority of the participants identified themselves as intermediate types (61.7%), while 22.4% identified as morning types and 15.9% as evening types. The mean age of the participants was 55 years (SD = 16.0), with 55.9% being female, and 71.2% being in marital, cohabiting, or registered relationships. The education levels showed diversity: 19.2% held basic education, 49.3% intermediate, and 31.5% higher education. Regarding employment, half of the participants were employed, and the other half were not part of the workforce. The participants’ income levels varied, with 36.4% falling within the low-income bracket, 32.0% within middle income, and 312.6% within higher income bracket. Household configurations displayed variety, with 71.6% having 1–2 members, 26.8% having 3–5 members, and 1.7% exceeding 5 members. In terms of children in the household, 22.5% had none, 69.2% had 1–3 children, and 8.3% had more than 3 children. Regarding substance use, 17.1% smoked daily, and 20.9% consumed alcohol at a risk level. Notably, nearly half of the participants were overweight (40.4%) or obese (25.1%). Physical activity levels varied, with 46.9% of participants reporting a moderately active level followed by 29.0% who were active and 24.1% who were inactive.
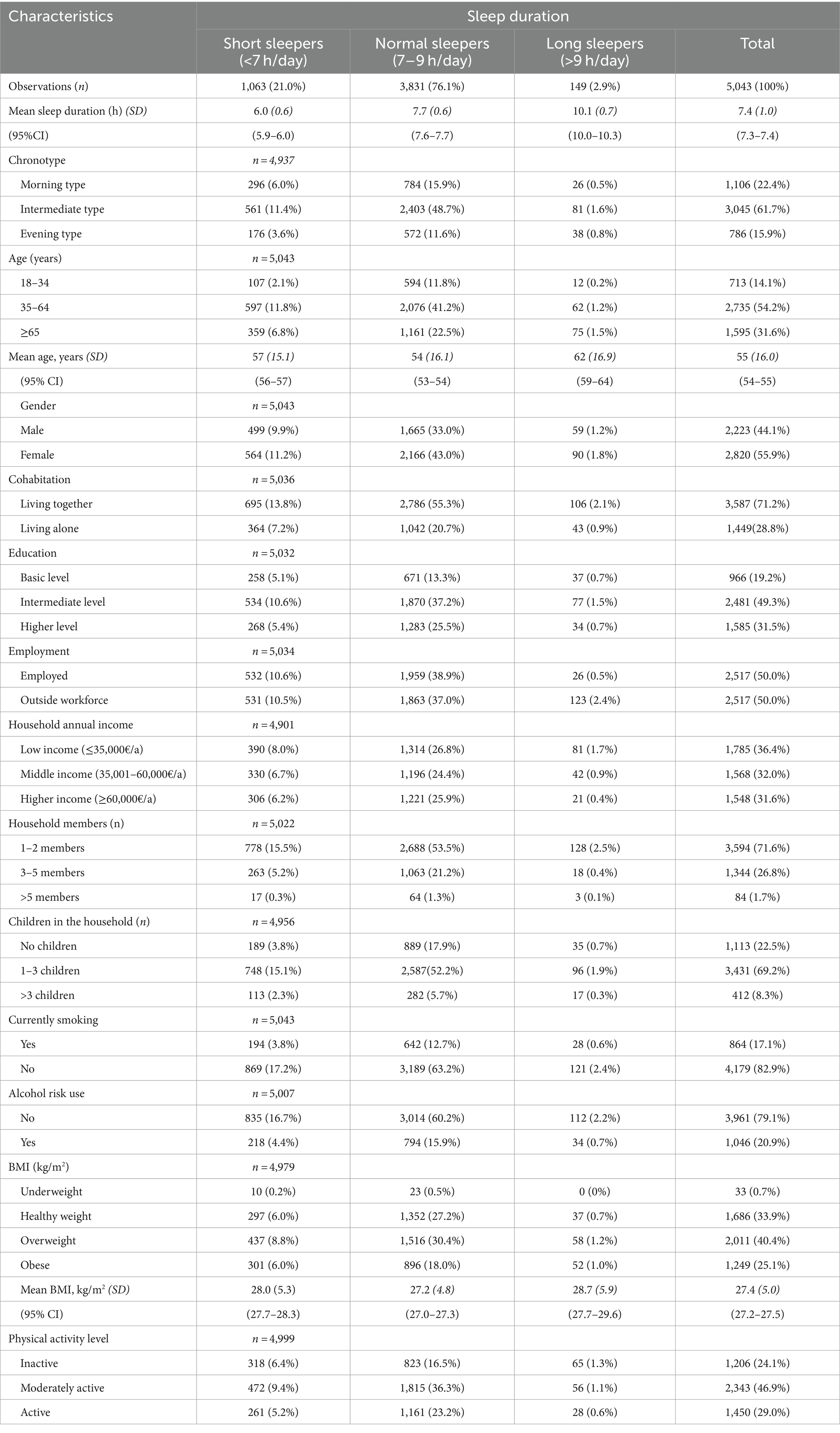
Table 1 . Study population by sleep categories.
Table 2 displays the mean differences in the consumption of various FV across different sleep duration categories. It was observed that normal sleepers had higher consumption of FV including all sub-groups when compared to both short and long sleepers. These differences were statistically significant after adjusting for covariates including age, gender, and energy intake with significance maintained at the 0.05 level following Bonferroni correction for multiple comparisons.
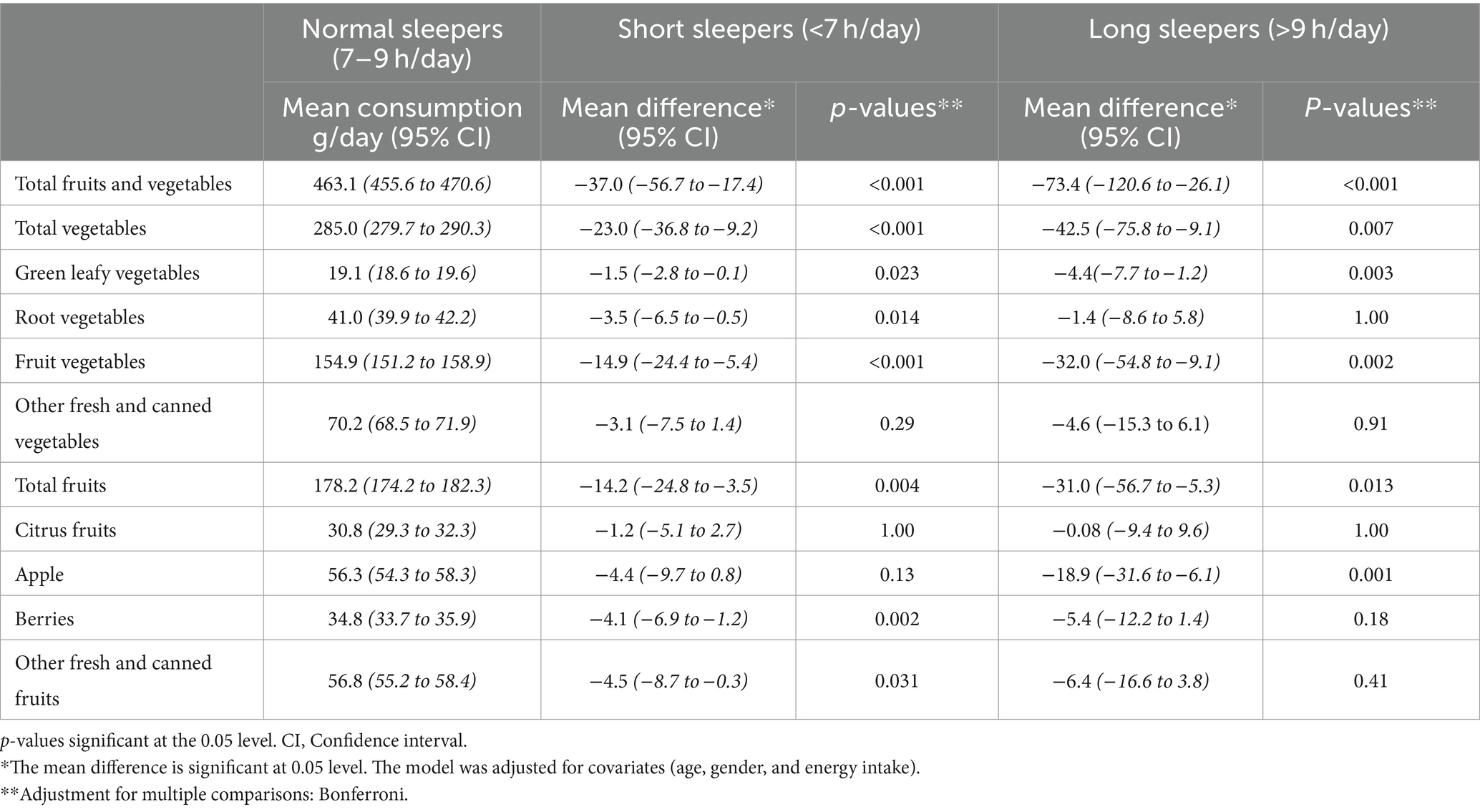
Table 2 . Mean difference in consumption of fruits and vegetables across sleep duration categories.
Effect sizes measured by Cohen’s d, were small but notable. For normal vs. short sleepers, Cohen’s d was 0.16 and for normal vs. long sleepers, it was 0.21. Corresponding effect-size correlations were 0.08 and 0.10, respectively, suggesting a small yet positive association between sleep duration and FVs consumption.
In the vegetable sub-group, significant differences were observed in the consumption of green leafy vegetables, root vegetables and fruit vegetables (e.g., tomatoes, cucumbers), between normal and short sleepers. Similarly, for normal vs. long sleepers, significant differences were again noted for green leafy vegetables and fruit vegetables. However, other fresh and canned vegetables such as cabbage, mushroom, onion, peas and beans did not exhibit significant differences.
In the fruit sub-groups, a significant mean difference was observed in the consumption of berries and other fresh and canned fruits between normal and short sleepers. Conversely, for normal vs. long sleepers, the only significant difference was observed in apple consumption.
Table 3 presents the results of linear regression analyses exploring the association between sleep duration and TFVC, along with its sub-groups. Initially, the association between sleep duration and TFVC was observed to be positive across all models, though it did not reach statistical significance. This trend persisted even with the inclusion of additional covariates with slight decrease in the strength of the association in Models 2 and 3, which did not significantly impact the associations.
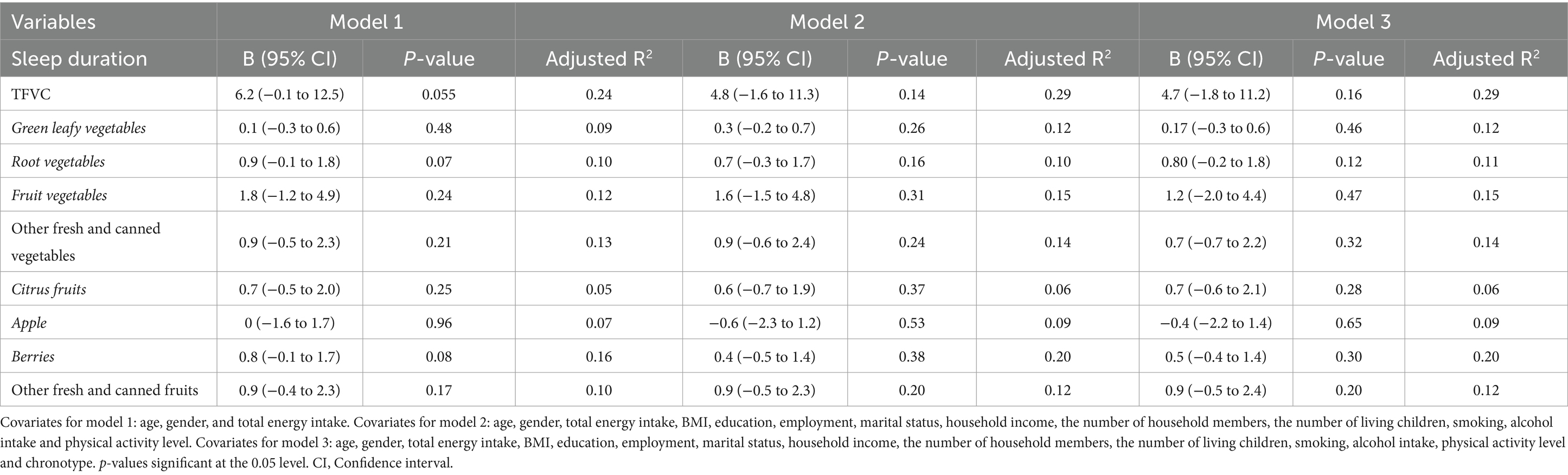
Table 3 . Association between sleep duration and FV consumption.
However, when a sensitivity analysis was conducted by excluding energy under reporters ( n = 1,926)-the association between sleep duration and TFVC in Model 1 became statistically significant (B = 11.9, 95% CI [2.6, 21.3], p = 0.012), indicating a stronger relationship after removing potential bias from underreporting. Although this significance was nearly reached in Models 2 and 3 ( p = 0.06), the proximity to significance suggests that energy underreporting might be attenuating the true magnitude of the observed relationships.
In the subgroup analysis, the pattern was somewhat similar, with certain food groups such as root vegetables and other fresh and canned vegetables showing a significant increase in the strength of association in the sensitivity analysis compared to the full cohort. For instance, root vegetables showed a significant association with sleep duration in Model 1 after excluding underreporters (B = 1.6, p = 0.029). The association for other fresh and canned vegetables also became significant (B = 2.6, p = 0.015 in Model 1) in the sensitivity analysis.
Conversely, no significant associations were observed for green leafy vegetables, fruit vegetables, citrus fruits, apples, berries, and other fresh and canned fruits after excluding energy underreporters, similar to the original analysis.
Table 4 presents the outcomes of binary logistic regression models that examined how the consumption of various fruits and vegetables is associated with the likelihood of short or long sleep durations, using normal sleep as the reference category.
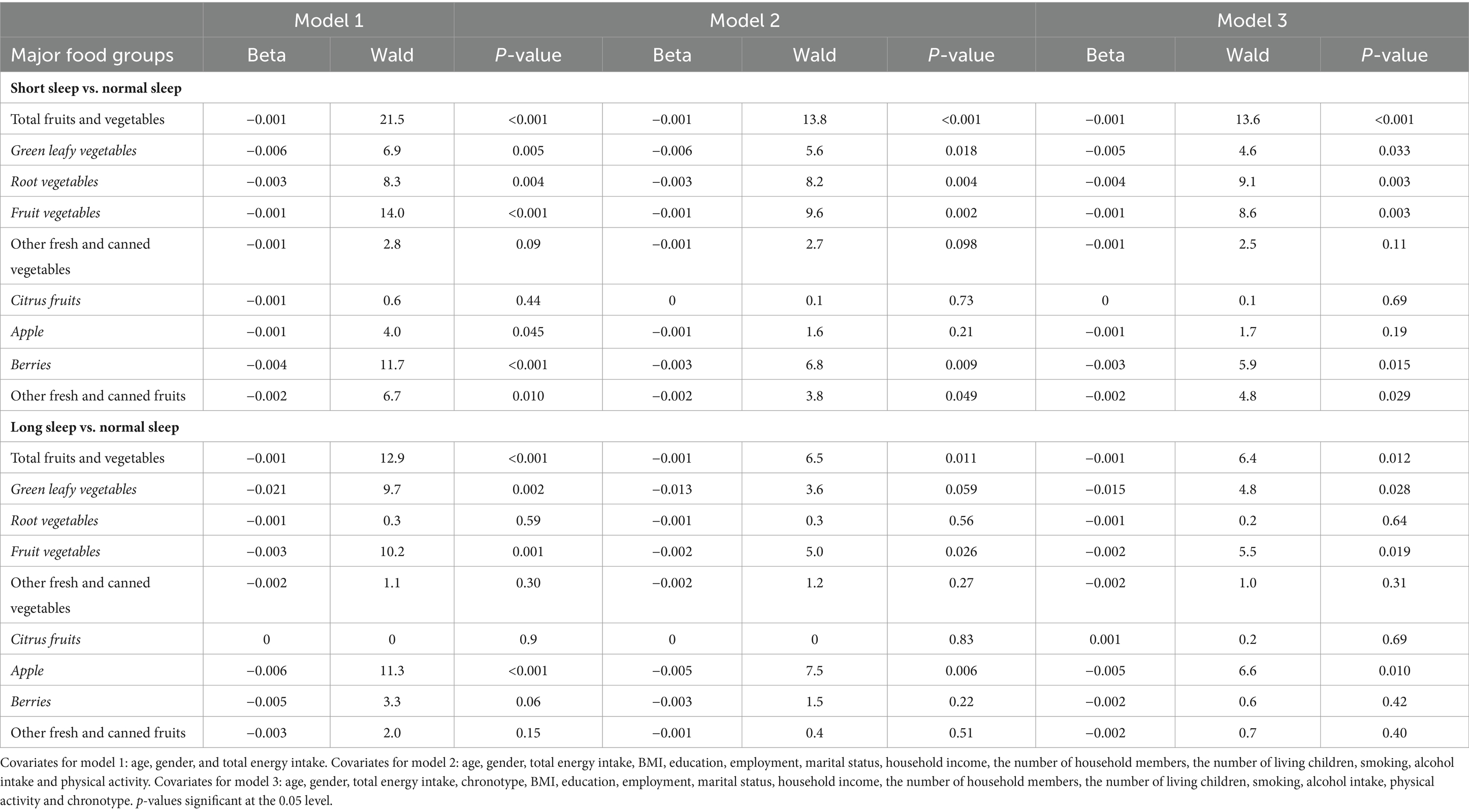
Table 4 . Association between FV consumption and sleep duration categories.
For individuals with short sleep duration, a consistent negative association with TFVC was observed across all models. This pattern suggests that short sleepers tend to consume fewer FV compared to those with normal sleep duration ( p < 0.001). A similar negative trend was observed for specific sub-groups such as green leafy vegetables, root vegetables, fruit vegetables, and berries, though the strength and significance of association varied across the models. Conversely, no significant associations were found for the consumption of other fresh and canned vegetables and citrus fruits ( p > 0.05), except for apple consumption, which showed a negative association with short sleep only in Model 1.
Among long sleepers, similar negative associations were reported with TFVC that were significant in Models 1 and 3. The consumption of green leafy vegetables showed a notable reduction in model 1, and TFVC showed a consistent negative association across all models. However, no significant associations were found for root vegetables, other fresh and canned vegetables, and citrus fruits in any models. The relationship with apple consumption remained significantly negative across all models for long sleepers, while the associations for berries and other fresh and canned fruits did not maintain significance in Models 2 and 3.
Unlike linear regression, in the sensitivity analysis, the logistic regression revealed a persistent negative association between short sleep duration and total fruit and vegetable consumption (Beta = −0.001, p < 0.001 across all models), indicating that the findings were stable even when potentially biased self-reported energy intake was accounted for. Similarly, the negative associations for specific sub-groups such as green leafy vegetables, root vegetables, and fruit vegetables remained significant, with minor variations in Beta coefficients and Wald statistics. For long sleepers, the sensitivity analysis generally showed a slight decrease in the strength of associations, yet the negative trend in total fruit and vegetable consumption remained significant in Models 1 and 3, with similar patterns observed for specific sub-groups. This sensitivity analysis result confirms the validity of our original findings, suggesting that the associations between TFVC and sleep duration are not substantially altered when controlling for energy underreporting. The detailed outcomes of the sensitivity analysis are presented in Supplementary Table 2 .
Overall, the results indicate that both short and long sleep durations were associated with a decrease in the consumption of certain fruits and vegetables when compared to normal sleep durations.
This study examined the associations between FV consumption and sleep duration in a population-based cohort of Finnish adults, revealing a significant association between sleep duration and FV consumption. This included various sub-groups such as green leaf, root and fruit vegetables. Our findings are in line with existing literature, which consistently demonstrates reduced FV consumption among individuals with either inadequate or excessive sleep durations ( 27 , 29 , 30 , 32 , 33 , 45 ). A closely related study by Noorwali et al. ( 31 ), focused on similar exposure and outcome variables in UK adults aged 19–65. Both studies categorized sleep duration into short, normal, and long sleepers, enabling direct comparisons. However, distinctions in dietary assessment methods exist. Noorwali et al. using a 4-day food diary validated with biomarkers, while our study employed a 12-month FFQ, assessing habitual diet and providing insights into long-term dietary patterns. Despite these methodological differences, both studies consistently reported reduced FV consumption among individuals with inadequate or excessive sleep durations.
One possible explanation for the observed negative association between short and long sleepers and FV consumption, as supported by previous studies, is that individuals with normal sleep durations are more likely to adopt healthy lifestyles. These lifestyles are often characterized by high FV consumption, regular physical activity, and better quality of life ( 46 ). Hormonal mechanisms might also play a role. Certain FVs such as cherries, kiwi, tomatoes, and cucumbers contain high levels of melatonin, a hormone vital for regulating circadian rhythms and sleep patterns ( 47 ). The significant associations we observed between sleep duration and specific FV sub-groups, such as root, fruit, and green leafy vegetables, further support these notions.
Moreover, fruits and vegetables serve as abundant sources of a variety of micronutrients and non-nutrient bioactive compounds, including vitamins, phytochemicals like (poly) phenolic compounds and carotenoids, vital minerals such as potassium, calcium, and magnesium, and dietary fiber. Their impact on human health is significant, attributed to their medicinal properties such as anti-inflammatory, antimicrobial, antioxidant, anticancer, and their preventive effects against various chronic diseases ( 48 ). It is plausible to suggest that the broader spectrum of health-promoting properties inherent in various fruits and vegetables, as outlined by their bioactive components, may have influenced the observed associations between sleep duration and specific FV-subgroups.
In our analysis, we observed that including a comprehensive set of covariates, did not significantly change the observed association between sleep duration and FV consumption. This suggests that the relationship between sleep duration and FV consumption is robust and remains consistent across different models. In our study, we expanded beyond the conventional covariates, such as age, gender, BMI, physical activity level and socioeconomic status, by incorporating chronotype as a covariate in Model 3. This addition did not significantly alter the associations, indicating that these dietary behaviors are associated with sleep duration independently of the chronotype. The inclusion of chronotype as a covariate was informed by emerging research indicating its influence on dietary patterns. Studies have shown that evening chronotypes are often associated with unhealthy dietary behaviors, including a propensity for obesity-related eating habits ( 34 , 49 , 50 ). However, our findings suggest that while chronotype may play a role in general dietary preferences and behaviors, its impact on the specific relationship between sleep duration and FV consumption is minimal. This is evident from the consistency of the association between sleep duration and various types of FV consumption, as indicated by the similar B values and p -values observed across Models 2 and 3 in both linear and logistic regression analyses.
Furthermore, we examined the likelihood of individuals falling into specific sleep duration categories based on their dietary habits, providing insights into the predictive value of sleep patterns for FV consumption. Our finding highlights that sleep duration categories can serve as predictive factors for FV consumption, albeit with relatively small effect sizes. A similar finding was observed in another study, which reported that shorter sleep duration at night was associated with lower FV consumption the following day ( 33 ). This reinforces the idea that sleep patterns may have relevance in understanding dietary choices, as well as in formulating dietary interventions. Additional research including longitudinal studies is essential to understand these complex relationship and to ascertain its implications on public health and lifestyle intervention.
Strengths and limitations
A notable strength of our study is the utilization of a large, randomly selected population-based sample, which enhances the statistical power and potential generalizability of our findings. Nonetheless, it is important to acknowledge certain limitations. One key aspect is our approach to managing missing data. We employed listwise deletion based on the assumption, supported by Little’s MCAR test (Chi-Square = 1.400, df = 2, p = 0.497) that the data was missing completely at random (MCAR). However, the potential for non-random missingness cannot be entirely ruled out. While listwise deletion is a valid approach under the MCAR condition, the potential for non-random missingness remains, and if the missing data are not MCAR, it could introduce bias and affect the generalizability of our results.
Furthermore, the reliance on self-reported data for sleep duration and food consumption may introduce recall and reporting biases such as under-or over-reporting. To address this issue, our statistical analyses included adjustments for factors like age, gender, and total energy intake. These adjustments, particularly for energy intake, help to some extent in mitigating biases related to dietary reporting. However, it is important to note that such adjustments cannot fully eliminate the inherent limitations of self-reported dietary data. Additionally, the Finnish Food Frequency Questionnaire (FFQ), though widely validated and ensuring comprehensive coverage, presents inherent limitations. Its length, comprising 134-items, may result in participant fatigue and consequent reporting errors influenced by participant characteristics.
These limitations, combined with the cross-sectional nature of our study, imply that we can identify associations, but causality cannot be inferred.
In summary, this study highlights a significant link between sleep duration and FV consumption among adults. The associations persisted across most FV subgroups, even after conducting sensitivity analysis, suggesting a strong and consistent relationship. While the findings indicate modest effect sizes, they carry substantial statistical and practical implications. Targeted interventions focusing on FV sub-groups with pronounced associations, such as green leafy vegetables and fruit vegetables can lead to impactful behavior change. Additional research, particularly longitudinal studies, is needed to better understand these associations and their public health implications, especially in regions with similar population structures and dietary patterns to Finland.
Data availability statement
The data analyzed in this study is subject to the following licenses/restrictions: this study used data from National FinHealth Study conducted by THL Finland. Data can be made available from THL upon reasonable request. Requests to access these datasets should be directed to [email protected] .
Ethics statement
The studies involving humans were approved by THL Ethics Committee [email protected] . The studies were conducted in accordance with the local legislation and institutional requirements. The participants provided their written informed consent to participate in this study.
Author contributions
AT: Conceptualization, Data curation, Formal analysis, Investigation, Methodology, Software, Visualization, Writing – original draft, Writing – review & editing. TL: Conceptualization, Supervision, Writing – review & editing. MM: Methodology, Validation, Writing – review & editing. TP: Conceptualization, Methodology, Resources, Supervision, Writing – review & editing.
The author(s) declare financial support was received for the research, authorship, and/or publication of this article. Open access publication was funded by Helsinki University Library.
Conflict of interest
The authors declare that the research was conducted in the absence of any commercial or financial relationships that could be construed as a potential conflict of interest.
Publisher’s note
All claims expressed in this article are solely those of the authors and do not necessarily represent those of their affiliated organizations, or those of the publisher, the editors and the reviewers. Any product that may be evaluated in this article, or claim that may be made by its manufacturer, is not guaranteed or endorsed by the publisher.
Supplementary material
The Supplementary material for this article can be found online at: https://www.frontiersin.org/articles/10.3389/fnut.2024.1319821/full#supplementary-material
1. Colten, HR, and Altevogt, BM. Sleep Disorders and Sleep Deprivation: An Unmet Public Health Problem . Washington (DC): National Academies Press (2006).
Google Scholar
2. Watson, NF, Badr, MS, Belenky, G, Bliwise, DL, Buxton, OM, Buysse, D, et al. Recommended amount of sleep for a healthy adult: a joint consensus statement of the American Academy of sleep medicine and Sleep Research Society. Sleep . (2015) 38:843–4. doi: 10.5665/sleep.4716
PubMed Abstract | Crossref Full Text | Google Scholar
3. World Health Organization . WHO Technical Meeting on Sleep and Health; (2004). Available at: https://www.euro.who.int/__data/assets/pdf_file/0008/114101/E84683.pdf .
4. Altun, I, Cinar, N, and Dede, C. The contributing factors to poor sleep experiences in according to the university students: a cross-sectional study. J Res Med Sci . (2012) 17:557–61.
PubMed Abstract | Google Scholar
5. Shochat, T . Impact of lifestyle and technology developments on sleep. Nat Sci Sleep . (2012) 4:19–31. doi: 10.2147/NSS.S18891
6. Hublin, C, Haasio, L, and Kaprio, J. Changes in self-reported sleep duration with age – a 36-year longitudinal study of Finnish adults. BMC Public Health . (2020) 20:4–11. doi: 10.1186/s12889-020-09376-z
7. Kronholm, E, Partonen, T, Härmä, M, Hublin, C, Lallukka, T, Peltonen, M, et al. Prevalence of insomnia-related symptoms continues to increase in the Finnish working-age population. J Sleep Res . (2016) 25:454–7. doi: 10.1111/jsr.12398
8. Sabanayagam, C, and Shankar, A. Sleep duration and cardiovascular disease: results from the National Health Interview Survey. Sleep . (2010) 33:1037–42. doi: 10.1093/sleep/33.8.1037
9. Ma, Y, Liang, L, Zheng, F, Shi, L, Zhong, B, and Xie, W. Association between sleep duration and cognitive decline. JAMA Netw Open . (2020) 3:e2013573. doi: 10.1001/jamanetworkopen.2020.13573
10. Yin, J, Jin, X, Shan, Z, Li, S, Huang, H, Li, P, et al. Relationship of sleep duration with all-cause mortality and cardiovascular events: a systematic review and dose-response meta-analysis of prospective cohort studies. J Am Heart Assoc . (2017) 6:5947. doi: 10.1161/JAHA.117.005947
11. Meier, T, Gräfe, K, Senn, F, Sur, P, Stangl, GI, Dawczynski, C, et al. Cardiovascular mortality attributable to dietary risk factors in 51 countries in the WHO European region from 1990 to 2016: a systematic analysis of the global burden of disease study. Eur J Epidemiol . (2019) 34:37–55. doi: 10.1007/s10654-018-0473-x
12. Locally Driven Collaborative Project (LDCP) Healthy Eating Team . A call to action for healthy eating: using a food literacy framework for public health program planning, policy, and evaluation. Ontario Dietitians in Public Health. (2018). Available at: https://www.odph.ca/upload/membership/document/2018-11/food-lit-call-to-action-booklet-sept-final.pdf
13. Vidgen, HA, and Gallegos, D. Defining food literacy and its components. Appetite . (2014) 76:50–9. doi: 10.1016/j.appet.2014.01.010
Crossref Full Text | Google Scholar
14. West, EG, Lindberg, R, Ball, K, and McNaughton, SA. The role of a food literacy intervention in promoting food security and food literacy—ozharvest’s nest program. Nutrients . (2020) 12:1–24. doi: 10.3390/nu12082197
15. Jabs, J, and Devine, CM. Time scarcity and food choices: an overview. Appetite . (2006) 47:196–204. doi: 10.1016/j.appet.2006.02.014
16. Kern, DM, Auchincloss, AH, Stehr, MF, Roux, AVD, Moore, LV, Kanter, GP, et al. Neighborhood prices of healthier and unhealthier foods and associations with diet quality: evidence from the multi-ethnic study of atherosclerosis. Int J Environ Res Public Health . (2017) 14:1–14. doi: 10.3390/ijerph14111394
17. World Health Organization . Diet, nutrition and the prevention of chronic diseases. Report of a Joint WHO/FAO Expert Consultation. WHO Technical Report Series 916. Geneva: WHO. (2003). Available: https://iris.who.int/bitstream/handle/10665/42665/WHO_TRS_916.pdf
18. He, FJ, Nowson, CA, Lucas, M, and MacGregor, GA. Increased consumption of fruit and vegetables is related to a reduced risk of coronary heart disease: Meta-analysis of cohort studies. J Hum Hypertens . (2007) 21:717–28. doi: 10.1038/sj.jhh.1002212
19. Woodside, JV, Young, IS, and McKinley, MC. Fruits and vegetables: measuring intake and encouraging increased consumption. Proc Nutr Soc . (2013) 72:236–45. doi: 10.1017/S0029665112003059
20. Helsedirektoratet . Nordic nutrition recommendations 2022. (2022). Available at: https://www.helsedirektoratet.no/english/nordic-nutrition-recommendations-2022 (Accessed 04 June 2023)
21. Diethelm, K, Jankovic, N, Moreno, LA, Huybrechts, I, de Henauw, S, de Vriendt, T, et al. Food intake of European adolescents in the light of different food-based dietary guidelines: results of the HELENA (healthy lifestyle in Europe by nutrition in adolescence) study. Public Health Nutr . (2012) 15:386–98. doi: 10.1017/S1368980011001935
22. Kim, SY, Freeland-Graves, JH, and Kim, HJ. Twenty year trends in vegetable consumption by preparation method and eating location for Korean population from 1998 to 2017. Br J Nutr . (2020) 126:747–56. doi: 10.1017/S0007114520004511
23. Lynch, C, Kristjansdottir, AG, te Velde, SJ, Lien, N, Roos, E, Thorsdottir, I, et al. Fruit and vegetable consumption in a sample of 11-year-old children in ten European countries—the PRO GREENS cross-sectional survey. Public Health Nutr . (2013) 17:2436–44. doi: 10.1017/S1368980014001347
24. Yip, CSC, Yip, YC, and Chan, W. Time-trend analysis of fruit and vegetable intake in Hong Kong, 2004–2016. Public Health . (2019) 177:102–11. doi: 10.1016/j.puhe.2019.08.012
25. Valsta, L, Kaartinen, N, Tapanainen, H, Mannisto, S, and Sääksjärvi, K Ravitsemus Suomessa—FinRavinto 2017-tutkimus (nutrition in Finland—the national FinDiet 2017 survey). Finnish Institute for Health and Welfare (THL). Report 12/2018, 239 (2018).
26. Kaartinen, NE, Tapanainen, H, Männistö, S, Reinivuo, H, Virtanen, SM, Jousilahti, P, et al. Aikuisväestönruoankäytön ja ravintoaineiden saannin muutokset vuosina 1997-2017: kansallinenFinRavinto-tutkimus [Changes in food consumption and nutrient intake in Finnishadults 1997-2017: the National FinDiet Survey]. Finnish Med J . (2021) 76:273–280.
27. Börnhorst, C, Wijnhoven, TMA, Kunešová, M, Yngve, A, Rito, AI, Lissner, L, et al. WHO European childhood obesity surveillance initiative: associations between sleep duration, screen time and food consumption frequencies. BMC Public Health . (2015) 15:442. doi: 10.1186/s12889-015-1793-3
28. Francisca, V, Córdova, SB, and Pablo, E. Consequences of short sleep duration on the dietary intake in children: a systematic review and metanalysis. Sleep Med Rev . (2018) 42:68–84. doi: 10.1016/j.smrv.2018.05.006
29. Jansen, EC, Prather, A, and Leung, CW. Associations between sleep duration and dietary quality: results from a nationally-representative survey of US adults. Appetite . (2019) 153:104748. doi: 10.1016/j.appet.2020.104748
30. Jansen, EC, She, R, Rukstalis, M, and Alexander, GL. Changes in fruit and vegetable consumption in relation to changes in sleep characteristics over a 3-month period among young adults. Sleep Health . (2021) 7:345–52. doi: 10.1016/j.sleh.2021.02.005
31. Noorwali, EA, Hardie, LJ, and Cade, JE. Recommended sleep duration is associated with higher consumption of fruits and vegetables; cross-sectional and prospective analyses from the UK Women’s cohort study. Sleep Sci Pract . (2018b) 2:1–14. doi: 10.1186/s41606-018-0032-0
32. Pengpid, S, and Peltzer, K. Fruit and vegetable consumption is protective from short sleep and poor sleep quality among university students from 28 countries. Nat Sci Sleep . (2020) 12:627–33. doi: 10.2147/NSS.S263922
33. Winpenny, EM, Rowthorn, H, Hollidge, S, Westgate, K, Goodyer, IM, Brage, S, et al. Shorter sleep among adolescents is associated with lower fruit and vegetable consumption the following day. Int J Behav Nutr Phys Act . (2023) 20:12. doi: 10.1186/s12966-023-01420-6
34. Teixeira, GP, Guimarães, KC, Soares, AGNS, Marqueze, EC, Moreno, CRC, Mota, MC, et al. Role of chronotype in dietary intake, meal timing, and obesity: a systematic review. Nutr Rev . (2023) 81:75–90. doi: 10.1093/nutrit/nuac044
35. Vitale, JA, Roveda, E, Montaruli, A, Galasso, L, Weydahl, A, Caumo, A, et al. Chronotype influences activity circadian rhythm and sleep: differences in sleep quality between weekdays weekend. Chronobiol Int . (2015) 32:405415:405–15. doi: 10.3109/07420528.2014.986273
36. Mota, MC, Waterhouse, J, De-Souza, DA, Rossato, LT, Silva, CM, Araújo, MBJ, et al. Association between chronotype, food intake and physical activity in medical residents. Chronobiol Int . (2016) 33:730–9. doi: 10.3109/07420528.2016.1167711
37. Roepke, SE, and Duffy, JF. Differential impact of chronotype on weekday and weekend sleep timing and duration. Nat Sci Sleep . (2010) 2010:213–20. doi: 10.2147/NSS.S12572
38. Meltzer, HM, Brantsaeter, AL, Ydersbond, TA, Alexander, J, and Haugen, M. Methodological challenges when monitoring the diet of pregnant women in a large study: experiences from the Norwegian mother and child cohort study (MoBa). Matern Child Nutr . (2008) 4:14–27. doi: 10.1111/j.1740-8709.2007.00104.x
39. Goldberg, GR, Black, AE, Jebb, SA, Cole, TJ, Murgatroyd, PR, Coward, WA, et al. Critical evaluation of energy intake data using fundamental principles of energy physiology: 1. Derivation of cut-off limits to identify under-recording. Eur J Clin Nutr . (1991) 45:569–81.
40. Borodulin, K, and Sääksjärvi, K. FinHealth 2017 study-methods. The Finnish Institute of Health and Welfare (2019). Available at: http://urn.fi/URN:ISBN:978-952-343-449-3
41. Paalanen, L, Männistö, S, Virtanen, MJ, Knekt, P, Räsänen, L, Montonen, J, et al. Validity of a food frequency questionnaire varied by age and body mass index. J Clin Epidemiol . (2006) 59:994–1001. doi: 10.1016/j.jclinepi.2006.01.002
42. Kaartinen, NE, Tapanainen, H, Valsta, LM, Similä, ME, Reinivuo, H, Korhonen, T, et al. Relative validity of a FFQ in measuring carbohydrate fractions, dietary glycaemic index and load: exploring the effects of subject characteristics. Br J Nutr . (2012) 107:1367–75. doi: 10.1017/S0007114511004296
43. Männistö, S, Virtanen, M, Mikkonen, T, and Pietinen, P. Reproducibility and validity of a food frequency questionnaire in a case-control study on breast cancer. J Clin Epidemiol . (1996) 49:401–9. doi: 10.1016/0895-4356(95)00551-X
44. Reinivuo, H, Hirvonen, T, Ovaskainen, ML, Korhonen, T, and Valsta, LM. Dietary survey methodology of FINDIET 2007 with a risk assessment perspective. Public Health Nutr . (2010) 13:915–9. doi: 10.1017/S1368980010001096
45. Noorwali, EA, Cade, JE, Burley, VJ, and Hardie, LJ. The relationship between sleep duration and fruit/vegetable intakes in UK adults: a cross-sectional study from the National Diet and nutrition survey. BMJ Open . (2018a) 8:1–9. doi: 10.1136/bmjopen-2017-020810
46. Meng, X, Li, Y, Li, S, Zhou, Y, Gan, RY, Xu, DP, et al. Dietary sources and bioactivities of melatonin. Nutrients . (2017) 9:1–64. doi: 10.3390/nu9040367
47. Jyväkorpi, SK, Urtamo, A, Kivimäki, M, and Strandberg, TE. Associations of sleep quality, quantity and nutrition in oldest-old men the Helsinki businessmen study (HBS). Eur Geriatr Med . (2021) 12:117–22. doi: 10.1007/s41999-020-00421-z
48. Karasawa, MMG, and Mohan, C. Fruits as prospective reserves of bioactive compounds: a review. Nat Prod Bioprospect . (2018) 8:335–46. doi: 10.1007/s13659-018-0186-6
49. Maukonen, M, Kanerva, N, Partonen, T, Kronholm, E, Tapanainen, H, Kontto, J, et al. Chronotype diffences in timing of energy and macronutrieint intakes: a population-based study in adults. Obes Soc . (2017) 25:608–15. doi: 10.1002/oby.21747
50. Mazri, FH, Manaf, ZA, Shahar, S, and Mat Ludin, AF. The association between Chronotype and dietary pattern among adults: a scoping review. Int J Environ Res Public Health . (2019) 17:68. doi: 10.3390/ijerph17010068
Keywords: fruits and vegetable consumption, sleep duration, chronotype, dietary habits, public health nutrition
Citation: Thapa A, Lahti T, Maukonen M and Partonen T (2024) Consumption of fruits and vegetables and its association with sleep duration among Finnish adult population: a nationwide cross-sectional study. Front. Nutr . 11:1319821. doi: 10.3389/fnut.2024.1319821
Received: 11 October 2023; Accepted: 09 April 2024; Published: 16 May 2024.
Reviewed by:
Copyright © 2024 Thapa, Lahti, Maukonen and Partonen. This is an open-access article distributed under the terms of the Creative Commons Attribution License (CC BY) . The use, distribution or reproduction in other forums is permitted, provided the original author(s) and the copyright owner(s) are credited and that the original publication in this journal is cited, in accordance with accepted academic practice. No use, distribution or reproduction is permitted which does not comply with these terms.
*Correspondence: Anupa Thapa, [email protected]
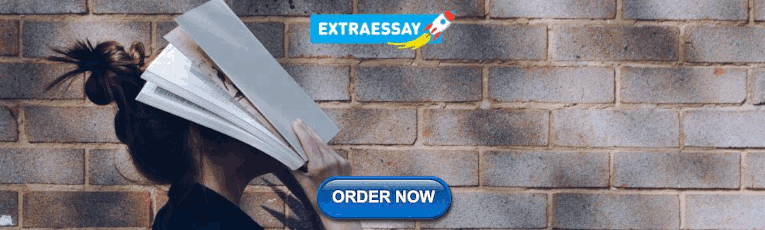
IMAGES
VIDEO
COMMENTS
Study Habits Survey The purpose of this questionnaire is to help you get information about how well you study right now. As you examine the results, you will discover your areas of strength and of weakness. The results will have meaning only if you are honest and respond as accurately as possible. If the statement is true about you, circle Y ...
The conceptual model, shown in. Figure 1. , assumes that the influence of Study Skill Habits on academic procrastination is mediated by Study Self-Efficacy. The SSH construct is specified as a formative latent construct, whereas SSE and procrastination are specified as reflective latent constructs.
dents to adopt study habits associated with better perfor-mance)? 3.2 Study Habits Survey The survey questions were designed based on the findings from our own prior work that explored study habits of higher- and lower-performing students in CS1 [18]. In that work, we interviewed 19 CS1 students in an North American research-intensive institution,
Many first-year college students are unprepared for the academic rigors of college, with as few as 27% of American high school students demonstrating proficiency in English, reading, mathematics, and science on the ACT college entrance exam ().College students may rely on study habits they have developed throughout their elementary and secondary education which served them sufficiently in the ...
Study Habits Questionnair e for college students: validation and r eliability. Abstract: This study presents and discusses the construction of an instrument to evaluate study habits. The ...
relationship between study habits and academic performance of secondary school students in Mathematics. To carry out this study, three research questions and three null hypotheses were raised to guide the study. The population of 1128 Senior Secondary School Two (SS2) students with a sample size of 200 respondents was
1.) You are given an assignment that requires some resource materials (dictionary, encyclopedia, atlas, almanac, etc.). How likely are you to: a.) Ask the Librarian for help. b.) Ask the learning disability specialist or your resource room teacher for help. c.) Ask a parent for help. d.)
Study habits questionnaire This questionnaire is designed to record your use of technology and learning tools. You are expected to complete this questionnaire regularly, and your answers should reflect the last 24 hours prior to completion of the questionnaire. Please answer all of the questions honestly - remember that the data
An investigation to determine if a positive correlation exists between student scores on the Survey of Study Habits and Attitudes and students' grade point average. Unpublished doctoral dissertation, Nova University, FL. ... The prediction of academic achievement by a British Study Habits Inventory. Research in Higher Education, 5, 365-372 ...
The first questionnaire concerning study habits and attitudes was created in 1954 and it was called the "Survey of Study Habits and Attitudes Inventory" (Holtzman, Brown, ... The current research study was urged by the information collected in tutorial action plans from students of the four courses (course means the year of study (first ...
It isanaction like reading, taking notes, conducting study groups that students perform. frequently, and regularly accomplishing the. learning goals. It can be defined as effective or ...
Study habits are the most important predictor of academic performance and global research has revealed that study habits affect academic performance. 8 In this regard, ... PSSHI has 45 questions and measures the study habits of students in eight areas, including time management (five items), eg, "I study at a specific time of the day ...
8 general effective study habits to boost your grades. Adopt the right study mindset. Know the class expectations. Choose an effective study location. Have the right study materials. Use helpful ...
This study examined students' attitudes, study habits, and academic performance in science using self-learning modules. This type of research is a descriptive-correlational design utilizing a ...
Students' study sessions outside class are important learning opportunities in college courses. However, we often depend on students to study effectively without explicit instruction. In this study, we described students' self-reported study habits and related those habits to their performance on exams. Notably, in these analyses, we controlled for potential confounds, such as academic ...
Exploratory research is intended to examine poorly understood phenomena and generate new insights and hypotheses that can guide future research on the topic (Slavin 2002; Stebbins 2001).In this exploratory study, we examine the study habits of a group of STEM students, with a focus on describing the lived experiences and subjective interpretations of individuals and groups or what cultural ...
Study habits is a well planned and deliberate pattern of study, which has attained a form of consistency on the ... The study adopted a descriptive correlation survey design Type of research This research is an applied research. It aims at improving students as products of education system by developing generalization
Good study habits include being organized, keeping good notes, reading your textbooks, listening in class, and working every day. However, research has shown that Facebook, Yahoo, Twitter, MySpace, moves, English Premier League, European Football Leagues among other things have taken over the time students could have used for reading and studying.
Study Questionnaire. The questionnaire was designed to study medical students' learning habits, including time management and study resources. The students were informed about the purpose of the study. Instructions regarding the questionnaires were provided to volunteering students. The confidentiality of information was also ensured.
The researcher determined the study habits of the Grade V pupils in Cabalasan Elementary School, Division of Baybay City during the school year 2014-2015 with an end view of recommending ideas for an intervention in improving pupil's study habits. The study employed normative survey method using checklist of Ralph C. Preston and Morton Botel.
In order to study habits, we must be able to reliably measure them. Therefore, the current investigation aimed to develop a validated, representative scale to measure individual variation in dependence on habits: the Habitual Tendencies Questionnaire (HTQ). ... Paul Matthews and Chessie Broadhurst for research assistance and help in developing ...
The research involved an online survey of 119 construction workers in the city of Jeddah in Saudi Arabia, focusing on their sleep duration and its impact on job performance, with data analysis conducted using SPSS software. ... The findings indicate that inadequate sleep influenced by factors such as dietary habits is significantly related to ...
Since mid-March, McKinsey has fielded consumer surveys across the globe to understand the impact of COVID-19 on consumer sentiment and stated behavior. The surveys, now fielded in 45 countries, are conducted online in local languages on a weekly, bi-weekly, or monthly basis, depending on the region. In each country, results are sampled and ...
How do study habits affect the academic performance of senior high school students in Cagraray Island, Philippines? This quantitative study investigates this question using a survey of 120 ...
IntroductionSleep and diet are crucial determinants of overall health and wellbeing, with the potential to mutually influence each other. This study examined the association between sleep duration and fruits and vegetables (FV) consumption among Finnish adults.MethodsThe study analyzed data from the National FinHealth 2017 Study involving 5,043 adults aged 18 years and above. Participants ...
Study habits. Association. Academic performance. Family background. Study habits contribute significantly in the development of knowledge and perceptual. capacities. Study habits tell a person ...
A survey was then developed online to collect data for the study. Once the research was created, the authors invited researchers and industry experts to test the research. This was performed to ensure the face validity, readability, and comprehensibility of the scales, in addition to ensuring that key informants could answer all survey questions.