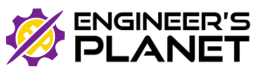
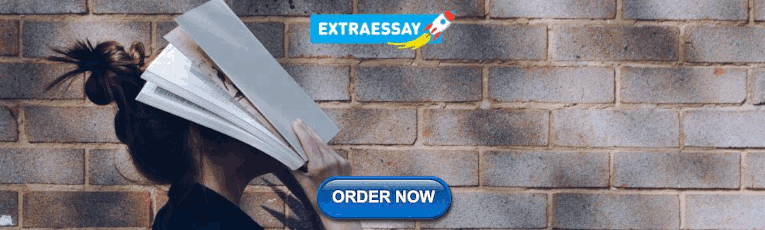
Embedded Systems Project Topics With Abstracts and Base Papers 2024
Embark on a transformative journey into the world of embedded systems with our meticulously curated selection of M.Tech project topics for 2024, thoughtfully complemented by trending IEEE base papers. These projects encapsulate the forefront of innovation and challenges in embedded systems, offering an indispensable resource for M.Tech students seeking to delve into the dynamic landscape of intelligent and interconnected devices .Our comprehensive compilation covers a diverse range of Embedded Systems project topics, each intricately paired with an associated base paper and a succinct abstract. From IoT applications and real-time systems to edge computing and hardware-software co-design, these projects mirror the current trends in embedded systems development. Stay ahead of the curve by exploring projects that align with the latest IEEE standards and technological breakthroughs. Whether you’re a student, researcher, or industry professional, our collection serves as a gateway to the cutting edge of embedded systems advancements. The project titles are strategically chosen to incorporate keywords that resonate with the latest trends in embedded systems , ensuring relevance and alignment with the evolving technological landscape. Delve into the abstracts to quickly grasp the scope, methodologies, and potential impacts of each project.
M.Tech Projects Topics List In Embedded Systems
1. Mobilenet-SSDv2: An Improved Object Detection Model for Embedded Systems. | ||
2. IoT-based smart monitoring and management system for fish farming. |
Shivam Kashyap
Machine learning in electrical engineering project topics with abstracts and base papers 2024, communication project topics with abstracts and base papers 2024, you may also like, how do electric cars work the science behind:..., how do mcbs work the engineering behind it, nikola tesla’s radio controlled boats: the science behind..., exploring transformative b.tech project topics in electrical engineering..., beyond the numbers: conquering percentage requirements for engineering..., effective note-taking strategies for engineers, top 10 questions with answers for engineering interview..., mtech | b.tech | ph.d project topics in..., matlab simulink project topics with abstracts and base..., optimization project topics with abstracts and base papers..., leave a reply cancel reply.
This site uses Akismet to reduce spam. Learn how your comment data is processed .
- Mentorship Sessions
- M.Tech Projects R&D
- Ph.D Projects R&D
- B.Tech Projects R&D
- 1. Internship
- 2. Workshops
- 3. Bootcamp
IMPORTANT LINKS
- Announcements New
- Project Downloads
- Career / Internship
- Privacy Policy
- Terms & Conditions
- MTech Projects Hot
- PhD Projects New
- BTech Projects
- AMIE Projects
- DBMS Projects Hot
- ECE & EEE Projects
- Research Paper
- Thesis Report
Fabrum Planet Solutions Pvt. Ltd.
- [email protected]
- +91 8882479344
- Delhi | Mumbai | Kolkata | Chennai
© 2024 All Rights Reserved Engineer’s Planet
Digital Media Partner #magdigit
- Terms & Conditions
This website uses cookies to improve your experience. We'll assume you're ok with this, but you can opt-out if you wish. OK Read More
A Review of a Research in Autonomous Vehicles with Embedded Systems
- Conference paper
- First Online: 20 February 2024
- Cite this conference paper
- Fulya Akdeniz 13 ,
- Mert Atay 13 ,
- Şule Vural 13 ,
- Burcu Kır Savaş 13 &
- Yaşar Becerikli 13
Part of the book series: Lecture Notes in Networks and Systems ((LNNS,volume 906))
Included in the following conference series:
- The Proceedings of the International Conference on Smart City Applications
229 Accesses
Designing an embedded system for practical applications needs objectives such as high accuracy, low power consumption and cost, and secure environment. NVIDIA’s Jetson is the most popular platform for embedded system applications which promises to achieve a balance between all these objectives. This paper focused on autonomous vehicles and embedded system studies evaluating the Jetson card features used in smart cars. The characteristics of autonomous vehicles and presents the data was compared for researchers categorized by author names, vehicle names, platform, camera, purpose and environment. In paper shows that most of studies had different purposes and tested area in different environments. We also developed a cost-effective lane detection model for developing and testing the autonomous vehicle JetRacer AI Kit and detail the overall structure of the system in this work. We tested the lane detection performance for system feasibility. In addition, we presented our ongoing work to further develop the features of this vehicle based on the proposed framework.
This is a preview of subscription content, log in via an institution to check access.
Access this chapter
Subscribe and save.
- Get 10 units per month
- Download Article/Chapter or eBook
- 1 Unit = 1 Article or 1 Chapter
- Cancel anytime
- Available as PDF
- Read on any device
- Instant download
- Own it forever
- Available as EPUB and PDF
- Compact, lightweight edition
- Dispatched in 3 to 5 business days
- Free shipping worldwide - see info
Tax calculation will be finalised at checkout
Purchases are for personal use only
Institutional subscriptions
Similar content being viewed by others
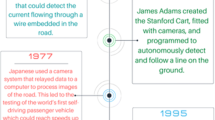
The perception systems used in fully automated vehicles: a comparative analysis
Autonomous Vehicles: Present Technological Traits and Scope for Future Innovation
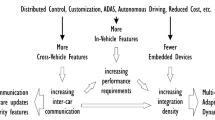
Smart mobility of the future – a challenge for embedded automotive systems
Akdeniz, F., Kayikçioğlu, İ., Kaya, İ., Kayikçioğlu, T.: Using WignerVille distribution in ECG arrhythmia detection for telemedicine applications. In: 2016 39th International Conference on Telecommunications and Signal Processing (TSP), pp. 409–412. IEEE (2016)
Google Scholar
DARPA Grand Challenge. https://en.wikipedia.org/wiki/DARPA_Grand_Challenge 2018–2021. Accessed 10 Jun 2022
Savaş, B.K., Becerikli, Y.: Real time driver fatigue detection system based on multi-task ConNN. IEEE Access 8 , 12491–12498 (2020)
Article Google Scholar
Stocco, A., Pulfer, B., Tonella, P.: Mind the Gap! A Study on the Transferability of Virtual vs Physical-world Testing of Autonomous Driving Systems. arXiv preprint arXiv:2112.11255 (2021)
Shi, W., Alawieh, M.B., Li, X., Yu, H.: Algorithm and hardware implementation for visual perception system in autonomous vehicle: a survey. Integration 59 , 148–156 (2017)
Bonnefon, J.F., Shariff, A., Rahwan, I.: The social dilemma of autonomous vehicles. Science 352 (6293), 1573–1576 (2016)
Akdeniz, F., Becerikli, Y.: Performance comparison of support vector machine, k-nearest-neighbor, artificial neural networks, and recurrent neural networks in gender recognition from voice signals. In: 2019 3rd International Symposium on Multidisciplinary Studies and Innovative Technologies (ISMSIT), pp. 1–4, IEEE
Kır Savaş, B., Becerikli, Y.: Behavior-based driver fatigue detection system with deep belief network. Neural Comput. Appl. 2022 (2019)
Karabulut, G.: Mini autonomous car architecture for urban driving scenarios (Master’s thesis, Middle East Technical University) (2019)
Castro, O., Céspedes, A., Ubaldo, R., Ramos, O.E.: Lane following with a duckiebot vehicle using visual feedback. In: 2019 IEEE Sciences and Humanities International Research Conference (SHIRCON), pp. 1–4, IEEE (2019)
Roberts, A., Maennel, O., Snetkov, N.: Cybersecurity test range for autonomous vehicle shuttles. In: 2021 IEEE European Symposium on Security and Privacy Workshops (EuroS&PW), pp. 239–248, IEEE (2021)
Kawakura, S., Shibasaki, R.: Deep learning-based self-driving car: JetBot with NVIDIA AI board to deliver items at agricultural workplace with object-finding and avoidance functions. Eur. J. Agric. Food Sci. 2 (3) (2020)
Bingöl, M.S., Kaymak, Ç., Ayşegül, U.Ç.A.R.: Derin Öğrenme Kullanarak Otonom Araçların İnsan Sürüşünden Öğrenmesi”. Fırat Üniversitesi Mühendislik Bilimleri Dergisi 31 (1), 177–185 (2019)
Yuhas, M., Feng, Y., Ng, D.J.X., Rahiminasab, Z., Easwaran, A.: Embedded out-of-distribution detection on an autonomous robot platform. In: Proceedings of the Workshop on Design Automation for CPS and IoT, pp. 13–18 (2021)
Loeminto, E.S., Khoswanto, H., Lim, R.: Pengenalan Traffic Light Pada Robot Mobil Duckietown. Teknika 7 (2), 89–93 (2018)
Kumar, C.S., Anjali, T., Rao, S.N.: A cost-effective framework for developing and testing autonomous RC cars. In: 2021 Sixth International Conference on Wireless Communications, Signal Processing and Networking (WiSPNET), pp. 26–29, IEEE (2021)
Seth, A., James, A., Mukhopadhyay, S.C.: 1/10th scale autonomous vehicle based on convolutional neural network. Int. J. Smart Sens. Intell. Syst. 13 (1), 1–17 (2020)
Sandha, S.S., Garcia, L., Balaji, B., Anwar, F.M., Srivastava, M.: Sim2Real transfer for deep reinforcement learning with stochastic state transition delays. In: 4th Conference on Robot Learning, Cambridge MA, USA.CoRL (2020)
Katyal, K.D., Polevoy, A., Moore, J., Knuth, C., Popek, K.M.: Highspeed robot navigation using predicted occupancy maps. In: 2021 IEEE International Conference on Robotics and Automation (ICRA), pp. 5476–5482, IEEE (2021)
O’Kelly, M., et al.: F1/10: An open-source autonomous cyber-physical platform (2019). arXiv preprint arXiv:1901.08567
O’Kelly, M., Zheng, H., Karthik, D., Mangharam, R.: F1tenth: an open-source evaluation environment for continuous control and reinforcement learning. Proc. Mach. Learn. Res. 123 , 77–89 (2020)
Liu, Y., Schofield, H., Shan, J.: Navigation of a self-driving vehicle using one fiducial marker. In: 2021 IEEE International Conference on Multisensor Fusion and Integration for Intelligent Systems (MFI), pp. 1–6, IEEE (2021)
Hu, J., Zhang, Y., Rakheja, S.: Adaptive trajectory tracking for car-like vehicles with input constraints. IEEE Trans. Ind. Electron. 69 (3), 2801–2810 (2021)
Sukhil, V., Behl, M.: Adaptive Lookahead Pure-Pursuit for Autonomous Racing (2021). arXiv preprint arXiv:2111.08873
Balaji, B., et al.: DeepRacer: educational autonomous racing platform for experimentation with sim2real reinforcement learning (2019). arXiv preprint arXiv:1911.01562
Chiba, S., Sasaoka, H.: Effectiveness of transfer learning in autonomous driving using model car. In: 2021 13th International Conference on Machine Learning and Computing, pp. 595–601 (2021)
Karaman, S., et al.: Project-based, collaborative, algorithmic robotics for high school students: programming self-driving race cars at MIT. In: 2017 IEEE Integrated STEM Education Conference (ISEC), pp. 195–203, IEEE (2017)
Scheffe, P., et al.: Networked and autonomous model-scale vehicles for experiments in research and education. IFAC-PapersOnLine 53 (2), 17332–17337 (2020)
Verschueren, R., De Bruyne, S., Zanon, M., Frasch, J.V., Diehl, M.: Towards time-optimal race car driving using nonlinear MPC in real-time. In: 53rd IEEE Conference on Decision and Control, pp. 2505–2510, IEEE (2014)
Hassanein, A.S., Mohammad, S., Sameer, M., Ragab, M.E.: A survey on Hough transform, theory, techniques and applications (2015). arXiv preprint arXiv:1502.02160
Chen, M.: Recognition and localization of target images for robot vision navigation control. J. Robot. 2022 , 8565913 (2022)
Macdonald, I.: Probabilistic Hough Transform. Notes, 1–4 (2016)
Stephens, R.S.: Probabilistic approach to the Hough transform. Image Vis. Comput. 9 (1), 66–71 (1991)
Cass, S.: Nvidia makes it easy to embed AI: the Jetson nano packs a lot of machine-learning power into DIY projects-[Hands on]. IEEE Spectr. 57 (7), 14–16 (2020)
Download references
Acknowledgements
The study was carried out in the Image Processing and Computer Vision Laboratory of the Computer Engineering Department of Kocaeli University, the study is supported by the BAP research and development center with Project ID 2337 and also supported by the TÜBİTAK 2209-A Project with project no:1919B012112773.
Author information
Authors and affiliations.
Kocaeli University, Umuttepe Campus, Kocaeli, 41001, Turkey
Fulya Akdeniz, Mert Atay, Şule Vural, Burcu Kır Savaş & Yaşar Becerikli
You can also search for this author in PubMed Google Scholar
Corresponding author
Correspondence to Burcu Kır Savaş .
Editor information
Editors and affiliations.
Computer Science and Smart systems Laboratory, Faculty of Science and Techniques of Tangier, Abdelmalek Essaadi University, Tangier, Morocco
Mohamed Ben Ahmed
Anouar Abdelhakim Boudhir
École Spéciale des Travaux Publics, Paris, France
Rani El Meouche
Computer Engineering Department, Karabük University, Karabük, Türkiye
İsmail Rakıp Karaș
Rights and permissions
Reprints and permissions
Copyright information
© 2024 The Author(s), under exclusive license to Springer Nature Switzerland AG
About this paper
Cite this paper.
Akdeniz, F., Atay, M., Vural, Ş., Savaş, B.K., Becerikli, Y. (2024). A Review of a Research in Autonomous Vehicles with Embedded Systems. In: Ben Ahmed, M., Boudhir, A.A., El Meouche, R., Karaș, İ.R. (eds) Innovations in Smart Cities Applications Volume 7. SCA 2023. Lecture Notes in Networks and Systems, vol 906. Springer, Cham. https://doi.org/10.1007/978-3-031-53824-7_21
Download citation
DOI : https://doi.org/10.1007/978-3-031-53824-7_21
Published : 20 February 2024
Publisher Name : Springer, Cham
Print ISBN : 978-3-031-53823-0
Online ISBN : 978-3-031-53824-7
eBook Packages : Engineering Engineering (R0)
Share this paper
Anyone you share the following link with will be able to read this content:
Sorry, a shareable link is not currently available for this article.
Provided by the Springer Nature SharedIt content-sharing initiative
- Publish with us
Policies and ethics
- Find a journal
- Track your research
Information
- Author Services
Initiatives
You are accessing a machine-readable page. In order to be human-readable, please install an RSS reader.
All articles published by MDPI are made immediately available worldwide under an open access license. No special permission is required to reuse all or part of the article published by MDPI, including figures and tables. For articles published under an open access Creative Common CC BY license, any part of the article may be reused without permission provided that the original article is clearly cited. For more information, please refer to https://www.mdpi.com/openaccess .
Feature papers represent the most advanced research with significant potential for high impact in the field. A Feature Paper should be a substantial original Article that involves several techniques or approaches, provides an outlook for future research directions and describes possible research applications.
Feature papers are submitted upon individual invitation or recommendation by the scientific editors and must receive positive feedback from the reviewers.
Editor’s Choice articles are based on recommendations by the scientific editors of MDPI journals from around the world. Editors select a small number of articles recently published in the journal that they believe will be particularly interesting to readers, or important in the respective research area. The aim is to provide a snapshot of some of the most exciting work published in the various research areas of the journal.
Original Submission Date Received: .
- Active Journals
- Find a Journal
- Proceedings Series
- For Authors
- For Reviewers
- For Editors
- For Librarians
- For Publishers
- For Societies
- For Conference Organizers
- Open Access Policy
- Institutional Open Access Program
- Special Issues Guidelines
- Editorial Process
- Research and Publication Ethics
- Article Processing Charges
- Testimonials
- Preprints.org
- SciProfiles
- Encyclopedia
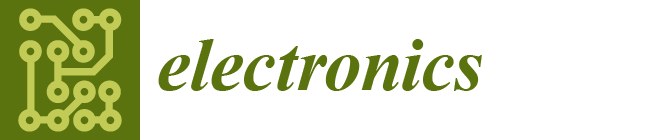
Journal Menu
- Electronics Home
- Aims & Scope
- Editorial Board
- Reviewer Board
- Topical Advisory Panel
- Instructions for Authors
- Special Issues
- Sections & Collections
- Article Processing Charge
- Indexing & Archiving
- Editor’s Choice Articles
- Most Cited & Viewed
- Journal Statistics
- Journal History
- Journal Awards
- Society Collaborations
- Conferences
- Editorial Office
Journal Browser
- arrow_forward_ios Forthcoming issue arrow_forward_ios Current issue
- Vol. 13 (2024)
- Vol. 12 (2023)
- Vol. 11 (2022)
- Vol. 10 (2021)
- Vol. 9 (2020)
- Vol. 8 (2019)
- Vol. 7 (2018)
- Vol. 6 (2017)
- Vol. 5 (2016)
- Vol. 4 (2015)
- Vol. 3 (2014)
- Vol. 2 (2013)
- Vol. 1 (2012)
Find support for a specific problem in the support section of our website.
Please let us know what you think of our products and services.
Visit our dedicated information section to learn more about MDPI.
Embedded IoT: System Design and Applications
- Print Special Issue Flyer
Special Issue Editors
Special issue information.
- Published Papers
A special issue of Electronics (ISSN 2079-9292). This special issue belongs to the section " Computer Science & Engineering ".
Deadline for manuscript submissions: closed (31 July 2021) | Viewed by 25076
Share This Special Issue
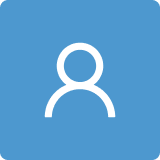
Dear Colleagues,
Embedded IoT systems combine a vast number of technological challenges related to embedded software, embedded computing, low-power communication protocols, embedded security protocols, embedded energy harvesting, near sensor data processing, embedded AI techniques, and integration in cloud and edge computing systems.
It is only by tackling all those challenges on their own or within a cross-layer optimization approach that the next generation of IoT-enabled embedded devices will become a reality and some emerging applications will find their way in our daily life and in industry. The impact of embedded IoT devices is starting to appear in many new domains like interconnected vehicles, Industry 4.0, smart buildings, smart cities, e-farming, e-health, and education. As such, it becomes necessary to reflect on the developed theories, engineering, and development techniques and the applications.
In this Special Issue, we aim at collecting high-quality submissions that include the theoretical aspects, the engineering aspects, and the application aspects of embedded IoT devices and systems. The topics of interest include but are not limited to:
- Embedded data visualization techniques for IoT-based instrumentation;
- Embedded AI techniques for IoT;
- Embedded energy harvesting for ultralow-power IoT devices;
- Low-power data processing techniques for energy-constrained IoT devices;
- Security aspects of IoT-based systems;
- Advanced IoT hardware platforms and innovative architectures;
- Cross platform software design techniques for embedded IoT;
- Embedded operating systems for IoT;
- Real-time operating systems for IoT;
- Communication strategies for embedded IoT;
- Innovative antenna design for embedded IoT systems;
- Edge computing techniques for IoT applications;
- Fog computing for IoT applications;
- Optimization techniques for embedded IoT infrastructure;
- Embedded IoT for Industry 4.0 applications;
- Embedded IoT for e-health applications;
- Embedded IoT for smart buildings;
- Embedded IoT for smart cities;
- Embedded IoT for smart mobility infrastructure;
- Embedded IoT for education;
- Embedded IoT for the green economy;
- Embedded IoT for the sharing economy.
Prof. Dr. Abdellah Touhafi Prof. Dr. Gianluca Cornetta Guest Editors
Manuscripts should be submitted online at www.mdpi.com by registering and logging in to this website . Once you are registered, click here to go to the submission form . Manuscripts can be submitted until the deadline. All submissions that pass pre-check are peer-reviewed. Accepted papers will be published continuously in the journal (as soon as accepted) and will be listed together on the special issue website. Research articles, review articles as well as short communications are invited. For planned papers, a title and short abstract (about 100 words) can be sent to the Editorial Office for announcement on this website.
Submitted manuscripts should not have been published previously, nor be under consideration for publication elsewhere (except conference proceedings papers). All manuscripts are thoroughly refereed through a single-blind peer-review process. A guide for authors and other relevant information for submission of manuscripts is available on the Instructions for Authors page. Electronics is an international peer-reviewed open access semimonthly journal published by MDPI.
Please visit the Instructions for Authors page before submitting a manuscript. The Article Processing Charge (APC) for publication in this open access journal is 2400 CHF (Swiss Francs). Submitted papers should be well formatted and use good English. Authors may use MDPI's English editing service prior to publication or during author revisions.
- embedded IoT
- embedded AI
- IoT hardware platforms
- IoT software platforms
- RTOS for IoT
- IoT security
- low-power IoT
- edge computing
- fog computing
- embedded energy harvesting
Published Papers (7 papers)
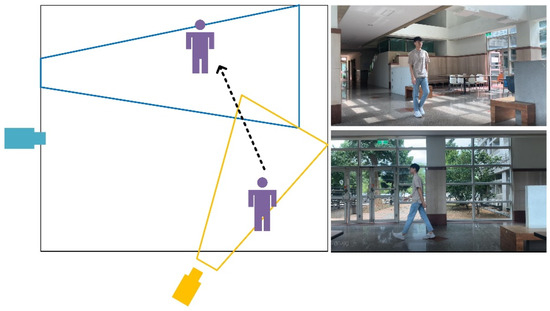
Further Information
Mdpi initiatives, follow mdpi.
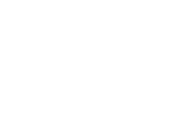
Subscribe to receive issue release notifications and newsletters from MDPI journals
Embedded Systems
Featured article, what are real-time embedded systems.
Real-time embedded systems combine features from both real-time systems and embedded systems, quickly delivering highly reliable responses to some external input.
The most cutting-edge real-time embedded systems are a key part of the future of semiconductors . As technology evolves beyond complementary metal-oxide semiconductors (CMOS), real-time embedded systems will become more powerful.
This is because as engineers develop digital logic technologies that go beyond CMOS scaling limits, chips will be able to provide greater computational power to digital devices. And more computational power means better real-time embedded systems.
What is a real-time system?
Real-time systems are computer systems that are designed to respond to an event or request from an external environment within a strictly defined time also known as a deadline. An important defining characteristic of these systems is that they are predictable and deterministic. This is essential for systems that need to respond in real time.
For some real-time applications, such as in medical devices sustaining human life, missing a deadline would be absolutely unacceptable. These systems are known as hard real-time systems. For other real-time applications, however, missing a deadline would be undesirable but not absolutely unacceptable. These are known as soft real-time systems.
What is an embedded system?
Embedded systems combine hardware and embedded software for a specific function or functions within a larger system. A very simple and convenient definition of an embedded system is any computer system contained within a product that is not described as a computer.
While all embedded systems, or embedded applications, are computing systems, they have widely varying user interfaces. Some embedded systems are designed to perform a single task and do not require a user interface. Other embedded systems have complex graphical user interfaces.
Why are real-time embedded systems important?
Real-time embedded systems are important because they provide the means to achieve predictable responses to deadlines. This means that they can exert tight control over certain outcomes. They can produce results in real time, without a significant delay.
There are numerous examples of real-time systems in some of the most important places in contemporary commercial, governmental, military, medical, educational, and cultural infrastructures.
Examples include but aren’t limited to the following:
- Traffic control systems for highways, airspace, railway tracks, and shipping lanes
- Process control systems for power plants and chemical plants
- Medical systems for radiation therapy, patient monitoring, and defibrillation
- Military weapon systems
These examples demonstrate the concept of a system capable of responding predictably in real time. All of these systems have to do their jobs without significant time lags.
How a real-time embedded system works
A real-time embedded system works by combining real-time environmental interaction with embedded software processing, creating predictable, deterministic real-time computing systems that are capable of executing “mission critical” applications. Real-time embedded systems use input from the environment and generate real-time responses that affect outcomes.
Real-time embedded systems with embedded software differ from embedded Linux applications by being much more limited: embedded systems comprise static link libraries providing only task scheduling, interprocess communication, synchronization timing and interrupt services, and little else besides. This is in many ways an advantage compared to Linux, since their limited capabilities are geared toward fulfilling their functions.
How does an embedded system work?
An embedded system incorporates a rugged motherboard into an industrial enclosure, with an associated input and output (I/O) and software to fulfill the process that is the function of the system. The hardware component of an embedded system is broadly similar to that of other electronic systems. The hardware is based around a microprocessor or microcontroller, along with the elements of memory and I/O.
This hardware component is the central component of the system, responsible for carrying out computational tasks. To that end, embedded systems contain software known as firmware written for one particular application. The creators of these systems typically write the firmware in high-level formats and compile them to provide code that can be stored within a nonvolatile memory within the hardware.
How do reactive embedded systems work?
Many embedded systems are reactive embedded systems, meaning they must react continuously to any input from their environment by generating an output. Such systems are built around one crucial concern—safety. They embody real-time computing.
The basic structure of a reactive embedded system starts with a sensor, which continuously measures a physical quantity or input and converts it to an electrical signal. The system stores the signal in its memory and reads it with an external observer or analog-to-digital converter.
The processors acquire the digital signal, process it, and measure the output. They can then store the results to memory. A digital-to-analog converter converts the digital data back to analog data, and an actuator can compare the output with the actual expected output and store the approved output.
What are the hardware foundations of a real-time embedded system?
The hardware foundations of a real-time embedded system generally consist of either a microcontroller or a microprocessor. A microcontroller is basically a central processing unit (CPU), or a processor with integrated memory or peripheral devices.
A microprocessor, on the other hand, contains a CPU but makes use of external chips for memory and peripheral interfaces.
Pros and cons of using real-time embedded systems
The upsides and downsides to using real-time embedded systems largely stem from the same set of features. These systems are designed to perform a limited number of tasks quickly, reliably, and without error. As a result, they are prone to trade-offs such as limited function and being difficult to edit and program.
Embedded systems are small, relatively cheap, and specific to one particular task. Because of these features, they are highly reliable, load quickly, use system resources in a highly optimized and efficient manner, and consume low power.
This means that embedded systems are ideal for heterogeneous integration with other components. Thanks to sound embedded systems programming, an embedded system can reliably perform its single allotted task in a manner that enhances the functionality of the rest of the system.
Embedded systems are difficult to upgrade, and if one of them has a problem, it is necessary to reset the settings. Their hardware is very limited, troubleshooting them is difficult, and they do not make it easy to transfer data to other systems.
What are the advantages of using RTOS software architecture for an embedded system?
The advantages of using real-time operating system (RTOS) software architecture for the development of an embedded system include speed, efficiency, and reliability.
RTOS software architecture allows for priority-based scheduling , or real-time scheduling. Using an algorithm, a real-time operating system can act as a scheduler, deciding which tasks to execute at any point in time and which ones to suspend. For that matter, tasks can also suspend themselves.
These systems are fast and deterministic, making them ideal for integration with embedded systems in many ways. Their claims on memory usage are predictable, since each task is allocated defined stack space.
Code reuse is another benefit of RTOS architecture. Similar applications on similar platforms can use some of the same code, allowing developers to create and use a library of standard tasks. Real-time operating systems are also extremely reliable and have the capacity to deliver desired outcomes without error.
What are the disadvantages of using RTOS software architecture for an embedded system?
The disadvantages of using RTOS software architecture for an embedded system have a great deal to do with the same features that make these systems so capable.
For one thing, these systems possess limited task ability. They are designed to provide a fast, resource-efficient, highly reliable means of completing certain tasks. But part of the necessary trade-off is that they can run only a few tasks at the same time. This is necessary to avoid errors.
Another problem is editability. Real-time operating systems rely on complex algorithms, and the very complexity of these algorithms makes it difficult to edit them. A real-time operating system software architecture is not easy to program or edit.
These systems also need specific device drivers and interrupt signals to respond quickly to interrupts. They are not good at switching tasks.
Overall, these systems deliver speed and reliability in the handling of a few tasks at the expense of versatility and editability.
How to implement real-time embedded systems
From a software engineering perspective, how to implement real-time embedded systems is largely a question of choosing a design and installing the real-time operating system onto an embedded device.
What projects can take advantage of a real-time embedded system?
Any application that needs to respond to an external stimulus predictably, quickly, and without error can take advantage of a real-time embedded system. Examples include the following:
- Automobile airbag systems
- Traffic control systems
- Pacemaker systems
- Military weapons, tracking, and command-control systems
What is the best way to implement a real-time embedded system?
The best way to implement a real-time embedded system is to start with clear requirements so that you can be sure the design meets those requirements. Once you have a design, you can proceed to programming.
Object-oriented programming with message-passing ability offers some of the best ways to implement a real-time embedded system. The IEEE Xplore digital library includes numerous resources that offer guidance on implementing a wide range of real-time operating systems in the context of embedded devices.
How can you ensure a smooth implementation of a real-time embedded system?
Smoothly implementing a real-time embedded system starts with addressing the necessary elements of these systems. Their creators have to program them to meet given timing constraints; this is part of the software engineering design and the definition of a real-time system. These constraints include event response—that is, what the system is supposed to do when it encounters the stimulus it is designed to respond to—and task scheduling.
Task scheduling covers the ability of real-time embedded systems to respond to different inputs with different tasks, a task being a response in the form of a set of instructions. Common design patterns include round-robin scheduling, in which each system component takes a turn using shared resources, and queueing design, in which items take their turns.
Interested in becoming an IEEE member ? Joining this community of over 420,000 technology and engineering professionals will give you access to the resources and opportunities you need to keep on top of changes in technology, as well as help you get involved in standards development, network with other professionals in your local area or within a specific technical interest, mentor the next generation of engineers and technologists, and so much more.
Related topics
Top conferences on embedded systems, top videos on embedded systems.
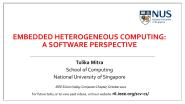
Xplore Articles related to Embedded Systems
Periodicals related to embedded systems, e-books related to embedded systems, courses related to embedded systems, top organizations on embedded systems, most published xplore authors for embedded systems.
embedded system engineering research papers IEEE PAPER
Embedded system 2020, embedded system 2019, embedded system 2018, embedded system 2016, embedded system 2015, embedded system research papers, fuzzy logic research papers, new embedded papers added june 2012, robotics engineering research papers.
- Free research papers and projects on robotics
- Peristaltic locomotion with antagonistic actuators in soft robotics
- On-chip robotics-Technical issues and future direction
- learning behaviours in mobile robotics by evolutionary fuzzy systems
- Integration of action and language knowledge-A roadmap for developmental robotics
- Learning Control in Robotics
- robotics platform for neuromorphic vision-Beobots
- Engineering the Evolution of Self-Organising Behaviours in Swarm Robotics
- Omni-directional Closed loop Walk for NAO
- robust speech recognition system for service-robotics applications
- A concept of dynamically reconfigurable real-time vision system for autonomous mobile robotics
- How the future of surgery is changing-Robotics tele surgery surgical simulators and other advanced technologies
- An insightful comparison between experiments in mobile robotics and in science
- Case Study in Assistive Robotics
- Spike-time robotics-a rapid response circuit for a robot that seeks temporally varying stimuli
- Towards motor skill learning for robotics
- ARGoS- a Modular Multi-Engine Simulator for Heterogeneous Swarm Robotics
- Molecubes-An open-source modular robotics kit
- soft-robotics
- planning-architecture-for-autonomous-robotics
- robotics-institute
- new-pathways-into-robotics-strategies-for-broadening-participation
More Robotics papers
Embedded system-recent papers.
- Design and implementation of an embedded home surveillance system by use of multiple ultrasonic sensors
- Development of Variable Rate Fertilizer Applicator Module Based on 8-bit Embedded System
- An operating system architecture for organic computing in embedded real-time systems
- Interactions around a contextually embedded system
- Generic Software Architecture for a Domain Specific Distributed Embedded System
- Patterns for distributed embedded control system software architecture
- Intelligent Car Control and Recognition Embedded System
- System-level development of embedded software
- Embedded Control System for High Power RF Amplifiers
- Fully Embedded EPICS-based Control of Low Level RF System for SuperKEKB
- Development of an embedded system and MATLAB-based GUI for online acquisition and analysis of ECG signal
- Design and implementation of an embedded monitor system for detection of a patient breath by double Web cams
- Dual Operating System Architecture for Real-Time Embedded Systems
embedded system-VLSI
- FPGA Based Design and Implementation of Embedded System for Tilt Measurement
- Towards an open embedded system on chip for network applications
- Design and implementation of real-time EP80579 based embedded system
- Design of USB interface of embedded system based on FPGA
- On-chip networks: A scalable, communication-centric embedded system design paradigm
- minimizing buffer capacities with throughput constraint for embedded system design
Military robotics
- Radio frequency controlled intelligent robot
- PackBot-A versatile platform for military robotics
- Determination of robot drop location for military path planning using GIS application
- Modelling and Simulation of a Military Urban Robot Using Working Model
Embedded system papers
- embedded system 34
- embedded system 33
- embedded system 32
- embedded system 31
IEEE PROJECTS 2022
Seminar reports, free ieee projects ieee papers.
- Open access
- Published: 06 August 2024
Factors influencing the research impact in cancer research: a collaboration and knowledge network analysis
- Shuang Liao ORCID: orcid.org/0000-0002-1016-6149 1 ,
- Christopher Lavender 1 &
- Huiwen Zhai 1
Health Research Policy and Systems volume 22 , Article number: 96 ( 2024 ) Cite this article
94 Accesses
Metrics details
Cancer is a major public health challenge globally. However, little is known about the evolution patterns of cancer research communities and the influencing factors of their research capacity and impact, which is affected not only by the social networks established through research collaboration but also by the knowledge networks in which the research projects are embedded.
The focus of this study was narrowed to a specific topic – 'synthetic lethality’ – in cancer research. This field has seen vibrant growth and multidisciplinary collaboration in the past decade. Multi-level collaboration and knowledge networks were established and analysed on the basis of bibliometric data from ‘synthetic lethality’-related cancer research papers. Negative binomial regression analysis was further applied to explore how node attributes within these networks, along with other potential factors, affected paper citations, which are widely accepted as proxies for assessing research capacity and impact.
Our study revealed that the synthetic lethality-based cancer research field is characterized by a knowledge network with high integration, alongside a collaboration network exhibiting some clustering. We found significant correlations between certain factors and citation counts. Specifically, a leading status within the nation-level international collaboration network and industry involvement were both found to be significantly related to higher citations. In the individual-level collaboration networks, lead authors’ degree centrality has an inverted U-shaped relationship with citations, while their structural holes exhibit a positive and significant effect. Within the knowledge network, however, only measures of structural holes have a positive and significant effect on the number of citations.
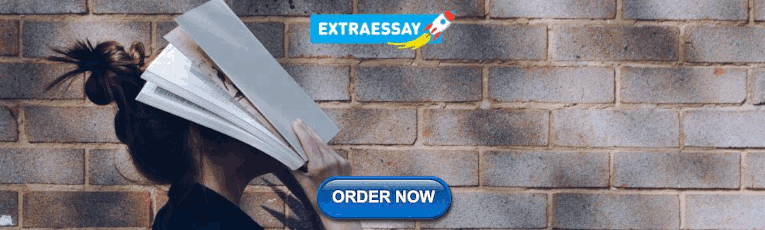
Conclusions
To enhance cancer research capacity and impact, non-leading countries should take measures to enhance their international collaboration status. For early career researchers, increasing the number of collaborators seems to be more effective. University–industry cooperation should also be encouraged, enhancing the integration of human resources, technology, funding, research platforms and medical resources. Insights gained through this study also provide recommendations to researchers or administrators in designing future research directions from a knowledge network perspective. Focusing on unique issues especially interdisciplinary fields will improve output and influence their research work.
Peer Review reports
Cancer is a major public health challenge globally [ 1 ]. According to WHO in 2019, cancer is estimated to be one of the top two leading causes of death in 112 out of 183 countries. To address this challenge, plenty of efforts have been made to accelerate progress and output in various field of cancer research, including cancer aetiology, vaccines and anti-cancer drug development, precision cancer medicine and treatment strategy innovations. However, little is known about the evolution patterns of cancer research communities and the influencing factors of their research capacity and impact, which is vital for establishing the reputation of cancer researchers and advancing their careers.
Although tumour mutational analysis has largely been exhausted for the identification of conventionally druggable targets, functional genomic screening based on synthetic lethality provides new avenues for discovering drug targets that were previously considered undruggable owing to their molecular structure or resulting functional loss [ 2 ]. In this study, we sought to reveal the factors that influence cancer research capacity, impact and the underlying logic through social network (research collaboration network) and knowledge network analysis based on synthetic lethality-related cancer research papers.
We focused our study in this area for several reasons. First, recent advances, such as CRISPR-based gene editing promoted the systematic screening for synthetic lethality-based cancer drug targets, resulting in unprecedented growth in this area. Second, an increasing number of researchers are contributing a wealth of knowledge elements in this area, along with the emergence of research achievements with high clinical value, such as the clinical use of PARP inhibitors in patients with BRCA mutant ovarian cancer. Third, there is a high level of collaboration in this field, including institutional, international and collaborations between academic institutions and industries, such as big pharmaceutical companies.
In the era of globalization, collaboration is the key to success in the fight against cancer, especially in the field of cancer research. Collaboration has been known to have an important influence in research capacity, output and impact [ 3 ]. Social networks, such as research collaboration networks, reflect the relationships and interactions among different levels of agents within the network – individuals, organizations or countries [ 4 ]. Their collaborative relationships serve as social capital [ 5 ]. According Li et al. [ 4 ], betweenness centrality, one of the structural social capital indicators, plays the most important role in leveraging resources in a co-authorship network. The positions of agents in the research collaboration network are vital for gaining and exchanging resources, ideas, knowledge and information from collaborating partners, thereby affecting their performance and innovative outputs [ 6 ]. Social network analysis is widely used to reveal the pattern of research collaboration and its impact on research citations.
Besides social capital, knowledge is also a vital resource for individuals and organizations in establishing competitiveness during innovation [ 7 ]. In addition to social networks, individuals and research organizations are also embedded in knowledge networks formed by the coupling of knowledge elements [ 8 ]. Formed through the combination of knowledge elements during the innovation process, knowledge networks serve as conduits for knowledge flow and influence future searches, the recombination of knowledge elements and, ultimately, the outcomes of innovation [ 9 ]. Therefore, the positions of individuals’ or organizations’ knowledge elements within the knowledge network will also impact their innovation outcomes.
In the fields of nano-energy and wind energy, studies have been conducted on the structural properties of both collaborative and knowledge networks, confirming the impact of node attributes in both networks on innovation performance, such as paper citation counts and patent counts [ 8 , 10 ]. To the best of our knowledge, no studies have been conducted on the structural properties of both social and knowledge networks as well as their impact on research capacity and performance in the field of cancer research.
In our study, negative binomial regression analysis was applied to explore how collaboration and knowledge networks, together with other potential factors, affected the research capacity and impact in the field of ‘synthetic lethality’-related cancer research. Regression model analysis was performed at the paper level, with citation number as the dependent variable. As greater research capacity and impact naturally leads to increased publication citations, citation-based measures are widely accepted as proxies for assessing the research capacity and impact of academic researchers [ 10 , 11 ]. The positive influence of international collaboration on the research impact of publications has been documented in former studies; although, the effects may vary across disciplines and countries [ 12 , 13 ]. We assume that the status of papers’ corresponding countries within the international collaboration network may also influence their research impact in general, as cooperation between scholars from specific countries may exhibit some clustering in the field of cancer research. Therefore, instead of simply categorizing the papers into ‘with’ or ‘without’ international collaboration, we further included the ‘country level international collaboration status’ as a potential influencing factor, based on metrics from country level collaboration networks.
In the preliminary analysis, we also established institutional level collaboration networks too, but they failed to reveal any significant correlation between their node attributes and paper citations. Then, we analysed the impact of institution types on citations and found that the existence of a company within the affiliation list was significantly related to citations, with P < 0.01. Therefore, industry involvement was further included in our final model.
Node attributes in both social and knowledge networks, as well as other potential factors, including journal impact factors (IF), country level international collaboration status, publication duration and industry involvement, were taken as the independent variables. Some of these factors have been proposed in previous studies as relevant to the citation impact of papers [ 12 , 14 , 15 ].
In the collaboration network, degree centrality indicated the number of direct ties (partners) one researcher had. A structural hole was originally considered to be a lack of linkage between any pair of nodes in the network [ 16 ]. Specifically, an author occupies structural holes in the collaboration/social network if they connect with other collaborators who are not connected themselves [ 17 ]. Therefore, structural hole values in the co-authoring networks in this study illustrate the degree to which an author’s partners are disconnected from each other, indicating non-redundant and efficient access to information for the focal author. Both metrics reflect an author’s degree of prestige among their collaboration partners for efficient access of information, knowledge and resources [ 18 ]. We reasoned that the average node attributes of a paper’s ‘research guarantors’ within the collaboration network would affect their research capacity and impact, making it an important factor for regression analysis.
Similarly, in the knowledge network, degree centrality indicates the number of direct linkages of a certain knowledge element, while structural holes represent the degree of disconnectedness among elements linked to a focal knowledge element, indicating information control advantages of the latter as researchers who study these uncombined elements are likely to use information in the focal element [ 19 ]. Besides, surprising combinations of research content from distant disciplines are also revealed as related to impactful research [ 20 ]. Differences in node attributes among knowledge elements of a certain paper reflect the author’s choice of research topic. A project with a frontier, multi-disciplinary research topic might have a higher measure in structural holes, while an ordinary and popular research topic might increase the measure of degree centrality.
For both networks, only local metrics such as degree centrality and structural holes were included. Global-level centrality metrics, such as closeness and betweenness centrality, were not considered to avoid a possible suppressor effect, as revealed in previous studies [ 4 , 21 ].
Data collection
The bibliographic data used in this study were extracted in March 2023 from Web of Science (WoS) and Journal Citation Report (JCR) databases. The following search terms were used in the WoS Core Collection to retrieve records of synthetic lethality-based cancer research papers:
1 : (((((((TS = (cancer*)) OR TS = (tumor*)) OR TS = (tumour*)) OR TS = (carcinoma*)) OR TS = (neoplasm*)) OR TS = (Glioblastoma)) OR TS = (Melanoma)) OR TS = (Adenocarcinoma).
2 : TS = ("Synthetic lethal*").
3 : #2 AND #1
4 : TS = ("correction:").
5 : (#3) NOT #4
Only documents classified as ‘articles’ were included. A total of 2074 papers were collected from WoS, along with the title, journal, abstract, authors and their affiliations, keywords, publication year, citation count and other information. The 5-year impact factors of the journals were downloaded from the Journal Citation Report (JCR) database. For all the journals listed in the dataset, the 5-year impact factors for the corresponding year were added manually.
Measurements
Collaboration and knowledge networks were created on the basis of 5-year moving windows (2009–2013, 2010–2014, 2011–2015, 2012–2016, 2013–2017), following previous approaches [ 10 , 22 ]. For example, if a paper was published in 2014, the metrics of its author and keywords were measured within the collaboration and knowledge networks for the years 2010–2014.
Dependent variables
The dependent variable was the number of citations for each paper in the sample, which was directly retrieved from the WoS dataset.
Independent variables
The Independent variables included degree centrality and structural holes in the collaboration and knowledge networks.
Construction of the collaboration and knowledge networks
In this study, the collaborative and knowledge networks were constructed on the basis of paper-level data following previous approaches [ 10 ].
The collaboration networks were established on the basis of co-author data of the papers included in each 5-year time frame, with authors serving as nodes and co-authorship experiences as the ties. Authors who co-published a paper were considered collaboration partners. Sci2 software was used for data cleaning and constructing the collaboration (co-author) network [ 23 ]. Manual unification of author names was conducted using the merge table function of Sci2 before the final construction of the network.
In addition to the individual level, a national-level international collaboration network was further constructed on the basis of bibliometric data to classify different countries based on their node attributes within the network.
Based on the papers included in each 5-year time frame, co-keyword networks were established in the study as the knowledge networks, with article keywords suggested by Web of Science (ISI keywords) considered as the knowledge elements and their co-appearance in the same paper served as a tie between them. Manual unification of keywords, including synonyms, singular and plural forms, abbreviations and so on, was performed on the extracted file before the final network construction.
Measurement of degree centrality and structural holes
For both the collaboration and knowledge networks, the measurement of degree centrality and structural holes followed previous approaches as adopted by Guan et al. [ 10 ]. The normalized degree centrality proposed by Freeman [ 24 ] employed in previous studies [ 10 , 21 , 25 ] was utilized to account for the size effect of different networks.
For the calculation of structural holes, we used Burt’s constraint measure to determine the network constraint C i . This measure indicates how strongly i can be constrained by its neighbours (Burt, 2009, 2004) [ 19 , 26 ]. Subsequently, the network constraint measure ( C i ) was subtracted from two to indicate the control advantage of each node in spanning structural holes, as based on previous studies [ 10 , 25 ]. Both metrics, degree centrality and structural holes, were calculated using Pajek software [ 27 ].
In this formula, i is the focal element, p ij indicates the proportion at which an element j accounts for element i ’s contacts. For instance, if i connects with j and five other elements, then p ij is 1/6. Additionally, k is the third element which connects with both i and j . Therefore, the focal element i would have lower p ij values if it connects with more elements, thereby being less constrained.
Aggregating the measures into paper level
Since this study was conducted at the paper level, we needed to aggregate the degree centrality and structural holes values for each publication. For the knowledge network, the values of degree centrality and structural holes of all the keywords of a specific paper were averaged to obtain its paper-level measure. However, in the collaboration network, two different approaches were initially adopted for data aggregation. The first approach, termed the ‘lead author’ approach, entailed averaging only the measures of the first author and the corresponding/co-corresponding authors for each paper. The other approach, termed the ‘all-author’ approach, involved averaging the measures of all authors for the regression analysis. The ‘lead author’ approach is based on the academic consensus regarding the order of authorship in the field of biomedical research and the concept of a ‘research guarantor’. We assume that the first and corresponding authors contribute the most to these papers, and therefore, their node attributes might be more relevant to the research impact of the publications than those of the other authors. A similar approach of allocating credits to corresponding authors, institutions or countries has also been adopted and proven to be useful in previous studies [ 28 , 29 , 30 ]. Regression results for the two models are compared in the results section of this article.
Control variables
The following variables were controlled for in the regression analysis: a journal’s 5-year impact factor, year since publication, industry involvement and status in international collaboration (for countries). Dummy variables were created for the last two variables.
To classify different countries according to their status in international collaboration, country-level collaboration networks were further created on the basis of co-author data from the papers included in each 5-year network. In these networks, countries served as nodes, and co-authorship experiences formed the ties. Degree centrality and structural holes were calculated for each country, using the same approaches adopted in individual networks.
In the preliminary analysis, no significant relationship was found between country level degree centrality/structural holes (both as continuous variables) and paper citations. This is unsurprising, as significant variations exist in the number of citations among papers from the same country. To improve statistical power, countries were subsequently ranked and classified into two categories. Leading countries were defined as those with structural holes ranked in the top 20%, while the remaining countries were categorized as non-leading countries. The 20% cutoff was selected to ensure the stability of relevant metric values for leading countries over time, as well as good discrimination power between the leading countries and others. When calculating the international collaboration status for each paper, only the corresponding country was considered Table 1 .
Structural characteristics and evolution patterns of collaboration and knowledge networks
The structural characteristics of the collaboration network and knowledge network in the synthetic lethality-based cancer research field are presented in Table 2 by time period and visualized in Fig. 1 and Fig. 2 , to illustrate the evolution pattern and parameter changes over time. Both networks are weighted, with edge attributes also presented in Table 2 .
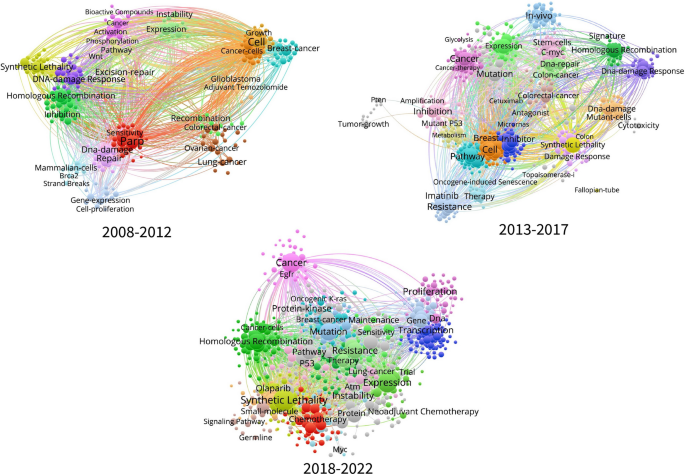
Evolution and pattern of the knowledge network in synthetic lethality-based cancer research papers (2008–2022). The main component of the knowledge network in synthetic lethality-based cancer research papers (2008–2022) are presented for each 5-year duration. The nodes represent keywords, which are further clustered according to relatedness. Nodes from the same cluster are presented in the same colour. The size of each node indicates the frequency of occurrence. Only items with a total link strength above 10 are included. VOSviewer was used for visualization [ 31 ]
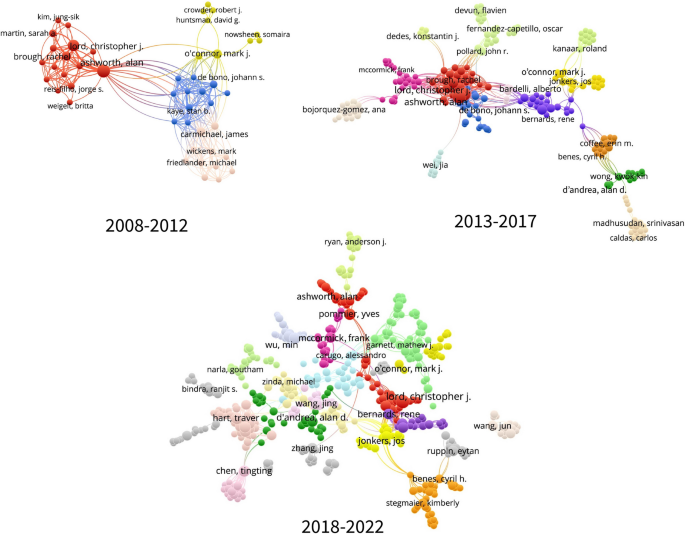
Evolution and pattern of the collaboration network in synthetic lethality-based cancer research papers (2008–2022). The main component of the collaboration network of authors in synthetic lethality-based cancer research papers (2008–2022) are presented for each 5-year duration. The nodes represent authors, who are further clustered according to relatedness. Nodes from the same cluster are presented in the same colour. The size of each node indicates the number of publications by the author, and the strongest collaboration links are represented by lines. Only the largest set of connected items (authors) is included. VOSviewer was used for visualization
In the knowledge network, a rapid increase in network size and edge number was observed in both period 2 and period 3 (as presented in Table 2 ), indicating fast growth and high integration of knowledge in this area. A decreasing trend was observed in clustering coefficients in both period 2 and period 3, revealing an evolution pattern of the knowledge network towards lower integration and clustering (as shown in Fig. 1 ), which may be due to the expansion of knowledge fields involved and the growing importance of interdisciplinary research.
For the collaboration network, period 2 and period 3 also saw a dramatic increase in network size and edge number, revealing fast growth of the co-authoring network. Despite a moderate increase in period 2, the typical number of co-authoring relationships (average degree) and frequency (mean weight) remained relatively stable across the three periods compared to network growth, resulting in a drop in network density from 0.009 to 0.002. High network clustering coefficients were observed in all three periods, with the highest in period 2 (0.948), confirming vigorous and tight research cooperation in this field, as shown in Fig. 2 .
When examining the country-level collaboration network (Fig. 3 ), the USA is undoubtedly at the centre of international cooperation, taking top priority. The most robust links are found between the USA and China, the USA and England, the USA and Germany and the USA and Canada, which align precisely with previous studies on global scientific collaboration networks [ 32 ]. European countries have also developed an important cluster within the network, with high density and integration, which is unsurprising considering their scientific advancements and the short geographic distances between them.
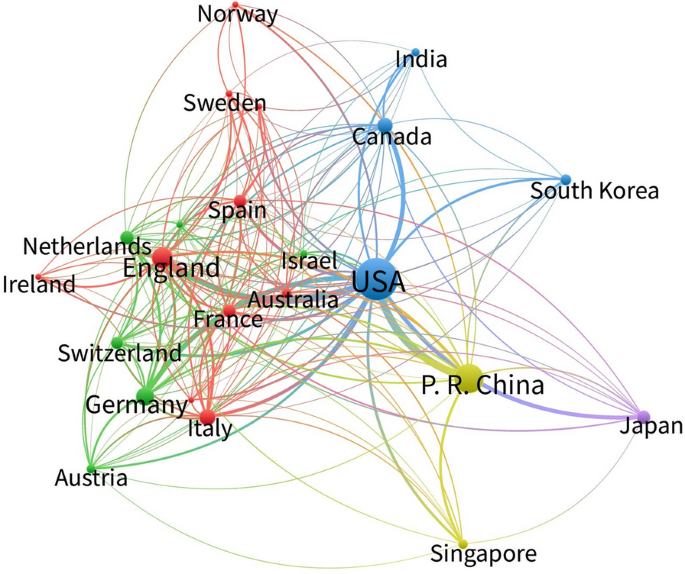
Main component of the collaboration network of countries in synthetic lethality-based cancer research papers (2018–2022). The nodes represent countries, and the size of each node indicates the number of publications from that country. Nodes from the same cluster are presented in the same colour. Only countries with a total link strength above 10 are included. VOSviewer was used for visualization
Regression analysis and results
Table 3 displays the descriptive statistics of the variables and their correlation data.
To address the issue of over-dispersion, a negative binomial regression model was used in this study for data analysis. VIF (variance inflation factor) values of all the variables were found to be below 2, indicating the absence of significant multicollinearity. Five regression models were constructed: model 1 includes only the control variables and models 2 and 3 incorporate the independent variables from the collaboration networks based on the lead author approach and the all-author approach, respectively. Models 4 and 5 encompass all the variables, with collaboration networks based on the lead author approach and the all-author approach, respectively. Table 4 presents the regression results for the number of citations for each paper.
We first compared the regression results between different aggregation approaches for collaboration network parameters, one based on lead author data (model 2 and model 4) and one on all author data (model 3 and model 5). The regression results based on ‘lead author’ and ‘all-author’ approaches are quite consistent regarding the influence of the author’s degree centrality on citations. However, a notable difference was observed in the coefficient significance for structural holes. In the lead author models, the author’s structural holes value is significantly related to the citation number, regardless of whether knowledge network parameters are included. However, no significant relationship was found between the author’s structural holes value and the citation number in either of the all-author models. We believe that the all-author aggregation method might have smoothed out the significant correlation between lead author structure holes and citation number. Therefore, we chose the lead author aggregation method over the all-author one for the final model (model 4).
As shown in model 2 and model 4, the coefficient for degree centrality in the collaboration network is positive and significant ( P < 0.05 in model 2, P < 0.1 in model 4). Conversely, the coefficient for (degree centrality in the collaboration network) 2 is negative at the significance level of P < 0.05 in both models. According to an analysis on this phenomenon in a previous study in the field of wind energy [ 10 ], this indicates that the average degree centrality of the lead authors in the collaboration network has an inverted U-shaped relationship with the number of citations for the paper. To assess the validity of this relationship, we set all other variables to their average values and presented the inverted U-shaped relationship between author degree centrality and citation number in Fig 4 , following a previous approach. Utilizing the delta method, we calculated the turning point to be 0.0418 (95% confidence interval [CI] 0.0211–0.0626), which falls within our normalized degree centrality data range of 0.0000–0.0743. Consequently, a moderate number of ties among the lead authors in a collaboration network could increase the paper’s citation count (the academic influence) to some extent, while degree centrality beyond a certain threshold can have a negative impact on citations.
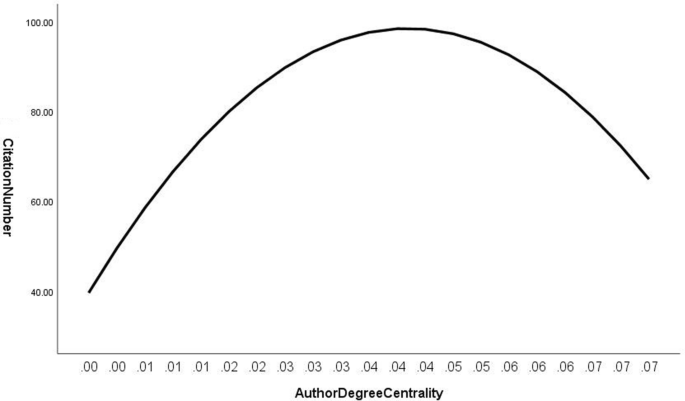
The relationship between author degree centrality and citation number
Furthermore, the coefficient for structural holes in the collaboration network is positive and significant (adjusted odds ratio 1.32, 95% CI 0.96–1.81; P = 0.09 in model 2 and adjusted odds ratio 1.32, 95% CI 0.97–1.81; P = 0.08 in model 4). This suggests that the average structural holes of the lead authors in the collaboration network are positively associated with the number of citations for the paper.
Unlike in the collaboration network, the coefficients for degree centrality in knowledge networks are negative and non-significant in model 4, suggesting that there is no significant relationship between a paper’s knowledge element’s average degree centrality in the knowledge network and its number of citations. However, positive and significant coefficients are found for structural holes in knowledge network in model 4 (adjusted odds ratio 4.37, 95% CI 1.28–14.96; P = 0.02 in model 4). This indicates that a paper’s knowledge element’s average structural holes in the knowledge network are positively related to its number of citations.
It is worth noting that the Akaike information criterion (AIC) for model 4 is the lowest among all models. Therefore, the addition of the independent variables based on the paper’s degree centrality and structural holes in both the collaboration and knowledge networks successfully improved the model.
All the control variables included in this study are significantly associated with the number of paper citations, indicating the important influence of journal reputation, citation duration, the international collaboration status of the corresponding country and collaboration with pharmaceutical companies.
It has been well-documented that academic collaboration in the form of co-authorship may influence research capacity and impact [ 33 ], but the extent varies across countries, research fields and even research topics [ 34 ]. To eliminate the potential influence of ‘hot’ or ‘cold’ research topics, this study narrowed its focus to a specific topic within the field of cancer research – synthetic lethality-based cancer research.
Regression model establishment
Based on the regression results, the inclusion of node attributes of lead authors in the collaboration network and knowledge elements in the knowledge network effectively improved the regression model. Therefore, we have demonstrated that centrality and structural holes in these two networks are highly influential factors for the research impact of synthetic lethality-based cancer research papers. Additionally, all of the control variables in the regression model are statistically significant. Aside from journal IF and publication year, which have long been recognized as vital factors affecting a paper’s citation count, this study also reveals a strong relationship between international collaboration status and industry involvement with paper citations.
Initially, no significant variance was observed between papers with or without international collaboration in the regression models (data not shown). We attribute this to the intricate nature of the relationship between international collaboration, specifically international co-authorship and cancer research impact, in terms of citation count, for different entities. At the national level, the proportion of internationally co-authored cancer research papers by a country may be influenced by factors such as its stage of scientific development, country size, economic development level, funding policies and medical resource availability [ 11 ].
More importantly, it is crucial to consider the different roles of the collaborating institutions/countries when analysing the impact of international collaboration, especially the role of the lead collaborators (research guarantors) [ 35 ]. We found that papers authored by leading countries (according to the corresponding country), which ranked high in structural holes ranking, not degree centrality, in the international collaboration network, exhibited significantly higher citation counts compared with others. Therefore, bridging structural holes can increase a country’s paper citation count. It is the status of a country within the cancer research network that matters, not the number of collaborators.
As shown in Fig 3 , in the field of synthetic lethality-based cancer research, the collaboration network at the country level exhibits a pattern with noticeable clustering. We speculate that leading countries prioritize obtaining non-redundant cancer research information, ideas and resources across these clusters, enhancing their overall research capacity and influence more effectively. Despite having some collaborators, non-leading countries may have limited access to resources and information beyond their own cluster due to barriers such as geographical distance, language difficulties or a lack of cooperation history. To improve overall research capacity and influence by enhancing positions in the cancer research network, governments should consider offering more international research fund supporting leadership in cancer research or taking a more active role in international health/cancer research organizations, such as the World Health Organization and the Union of International Cancer Control (UICC).
Moreover, the significant relationship between industry involvement and citations underscores the importance of integrating industry and academic researchers in cancer research. Since most companies involved in synthetic lethality-based cancer research are pharmaceutical or biotechnology companies, we conducted literature searches on university-industry collaborations in related fields.
Pharmaceutical involvement not only signifies the profound integration of human resources, knowledge, technology, funding, research platforms and medical resources but also serves as a robust predictor of the clinical experimentation of basic research findings [ 36 ]. In a study on university–industry collaborations within the Irish pharmaceutical industry, the significance of government funding was emphasized in motivating collaborations during the initiation phase, while the establishment of intellectual property (IP) agreements was identified as the impetus for knowledge sharing in the engagement phase [ 37 ]. This is supported by a survey of 105 university–industry collaborations within the US biotechnology industry, where researchers found that transparent IP policies enabled the formation of trust, which is vital for effective knowledge transfer and achieving success in university–industry collaborations [ 38 ]. To encourage university–industry cooperation in synthetic lethality-based cancer research, attention should be paid to the establishment of reasonable research policies and efficient processes, such as those for the establishment of intellectual property rights protection. Government investment is also crucial, with more national funds for university–industry led cooperation needed.
The relationship between lead authors’ average degree centrality in the collaboration network and paper citations
The observed inverted U-shaped relationship between the average degree centrality of lead authors in the collaboration network and the number of paper citations is consistent with previous research [ 10 ]. Central authors typically have numerous connections with others, enabling them priority in acquiring ideas, the latest information and resources, which can effectively promote their own research [ 39 ]. As indicated from the rising part of the inverted U-shaped curve before reaching the vertex, engaging in cancer research collaboration, whether in a leading or supporting role, enhances an author's academic research capacity, influence and reputation, ultimately contributing to increased paper citations.
However, when the inverted U-shaped curve reaches its peak, citations will start to decline as degree centrality increases. As in our study, if an author’s centrality exceeds a certain threshold, the surplus of information can overwhelm them, resulting in a decrease in the quality of knowledge, which, in turn, negatively impacts research output and influence. The above result again emphasizes the importance of encouraging cancer research collaboration, especially for young cancer researchers at an early stage of their careers.
The relationship between lead authors’ average structural holes in the collaboration network and paper citations
Based on the regression results, a positive relationship between the average structural holes of lead authors in the collaboration network and paper citation counts was found in both model 2 and model 4, although at a lower significant level compared with degree centrality. This suggests that by connecting with unique and diverse collaborators, central authors bridging structural holes can enhance the efficiency of their information acquisition, when conducting research on synthetic lethality-related cancer projects. Authors who bridge more structural holes have access to more non-redundant information and gain more control benefits [ 40 ], resulting in increased research capacity and impact and higher citation rates for their papers, as found in our study.
A significant difference was also observed between country and individual level collaboration networks. While degree centrality is the most important factor for individuals, the value of structural holes seems to be more influential at the country level. This reflects differences in the impact of social networks at different dimensions. At the macro level, the overall international collaboration status of a country is more important than the number of cooperating partners, while at the individual level, especially for young researchers, the number of collaborators directly determines the quality of resources and information obtained, thereby affecting the building of their research capabilities and the influence of research achievements.
The relationship between structural holes in the knowledge network and paper citations
This study revealed a positive relationship between the average structural holes of the knowledge elements in the knowledge network and paper citations, at the significance level of P < 0.05 in model 4. However, degree centrality showed no significant influence on citation counts. We posit that knowledge elements bridging rich structural holes in the knowledge network offer non-redundant information to cancer researchers who explore them. Additionally, they also create combinatorial opportunities between unconnected knowledge elements during the searching process, ultimately increasing citations from researchers across various disciplines. Therefore, elements bridging structural holes enjoy advantages in controlling the flow of knowledge during searches, thereby enhancing the citation opportunities for the papers involved. The above results provide suggestions for the selection of cancer research directions and keywords. Focusing on unique issues that connect with diverse information in cancer, especially interdisciplinary fields, will improve citations and the influence of research work.
These finding aligns with a previous study that analysed the influence of collaboration and knowledge network structures on organizational exploratory innovations in the field of nano-energy [ 8 ]. Although the two studies differ in terms of research field, level of analysis, variables and regression model design, they both reveal that in the context of exploratory research practices, the centrality of researchers/organizations in collaboration networks and the structural holes in their knowledge elements have a significant positive impact on their academic achievements and influence.
There are several innovations of this study.
A new method in aggregating collaboration network measures at the paper level was adopted in this paper. In previous studies, the ‘all-author’ approach was typically used for data aggregation at the paper level in social network analysis [ 10 , 41 , 42 ]. As a result, an inverted U-shaped relationship was revealed between authors’ centrality and paper citations, while the effects from the structural holes value remained non-significant [ 10 ].
After comparing the regression results of the ‘all-author’ and ‘lead author’ approaches, this study focused solely on the degree centrality and structural holes values of the lead authors, specifically the first and corresponding authors. Our regression results confirm the significant relationship between the authors’ structural holes value and paper citations, which might have been smoothed out by the ‘all-author’ approach. This choice reflects a distinctive characteristic of the biomedical research field, where the first and corresponding authors typically make core contributions to the article and better represent the research strengths compared with the other authors. This method has broad generalizability to other bibliometric studies based on biomedical data.
Multi-level collaboration networks were established, including individual-level collaboration networks based on authors and national-level collaboration networks. To account for the influence of a corresponding country’s international collaboration status, countries were ranked and categorized on the basis of their structural holes values.
We revealed the vital importance of collaboration and knowledge networks, international collaboration status and university–industry cooperation in influencing cancer research capacity and impact, as well as the differences in their contributing factors. For non-leading countries, measures should be taken to enhance the international collaboration status – to become connected with unique collaborators across various clusters. For individuals, especially early career researchers, increasing the number of their collaborators seems to be sufficiently effective. University–industry cooperation should also be encouraged, enhancing the integration of human resources, technology, funding, research platforms and medical resources in the fight against cancer.
Insights gained through this study provide recommendations to research workers or administrators for the design of research directions through a knowledge network perspective. Focusing on unique issues especially interdisciplinary fields, will ultimately improve output and the influence of their research work.
Limitations and follow-up research
This study is based on a relatively limited number of articles published on one specific topic within the field of cancer research: synthetic lethality, which is characterized by rapid growth and multi-disciplinary interaction from around the world. Future research may explore other cancer research fields or topics and the various patterns of collaboration and knowledge networks, as well as other potential factors affecting the impact of cancer research, such as unexpected combinations of keywords[ 20 ].
Analyses may also be conducted to explore the availability of funding and its impact on citations. Considering the rise of gold open access publishing, it may also be necessary to consider the impact of access modality while carrying-out regression analysis based on citation data.
Finally, this study averaged only the measures of the first and corresponding authors while calculating the collaboration network metrics for each publication, emphasizing their significant contributions and impact on the related article. Future studies may further analyse the impact of other authors in different positions on the author list.
Availability of data and materials
The datasets used and/or analyzed during the current study are available from the corresponding author upon reasonable request.
Abbreviations
Impact factor
Deo SVS, Sharma J, Kumar S. GLOBOCAN 2020 report on global cancer burden: challenges and opportunities for surgical oncologists. Ann Surg Oncol. 2022;29:6497–500.
Article CAS PubMed Google Scholar
Huang A, Garraway LA, Ashworth A, Weber B. Synthetic lethality as an engine for cancer drug target discovery. Nat Rev Drug Discovery. 2020;19:23–38.
Bailie J, Matous P, Bailie R, Passey ME. Patterns of collaboration and knowledge generated by an Australian rural research centre over 20 years: a co-authorship network analysis. Health Res Policy Syst. 2023;21:87.
Article PubMed PubMed Central Google Scholar
Li EY, Liao CH, Yen HR. Co-authorship networks and research impact: a social capital perspective. Res Policy. 2013;42:1515–30.
Article Google Scholar
Nahapiet J, Ghoshal S. Social capital, intellectual capital, and the organizational advantage. Academy Manag Rev. 1998;23:242–66.
Phelps C, Heidl R, Wadhwa A. Knowledge, networks, and knowledge networks: a review and research agenda. J Manag. 2012;38:1115–66.
Google Scholar
Moorthy S, Polley DE. Technological knowledge breadth and depth: performance impacts. J Knowl Manag. 2010;14:359–77.
Guan JC, Liu N. Exploitative and exploratory innovations in knowledge network and collaboration network: a patent analysis in the technological field of nano-energy. Res Policy. 2016;45:97–112.
Yayavaram S, Ahuja G. Decomposability in knowledge structures and its impact on the usefulness of inventions and knowledge-base malleability. Adm Sci Q. 2008;53:333–62.
Guan JC, Yan Y, Zhang JJ. The impact of collaboration and knowledge networks on citations. J Informet. 2017;11:407–22.
de Moya-Anegon F, Guerrero-Bote VP, Lopez-Illescas C, Moed HF. Statistical relationships between corresponding authorship, international co-authorship and citation impact of national research systems. J Informet. 2018;12:1251–62.
Khor KA, Yu LG. Influence of international co-authorship on the research citation impact of young universities. Scientometrics. 2016;107:1095–110.
Article CAS PubMed PubMed Central Google Scholar
Moed HF. Citation analysis in research evaluation. Berlin: Springer; 2005.
Tahamtan I, Afshar AS, Ahamdzadeh K. Factors affecting number of citations: a comprehensive review of the literature. Scientometrics. 2016;107:1195–225.
Wang L, Thijs B, Glänzel W. Characteristics of international collaboration in sport sciences publications and its influence on citation impact. Scientometrics. 2015;105:843–62.
Abbasi A, Wigand RT, Hossain L. Measuring social capital through network analysis and its influence on individual performance. Libr Inf Sci Res. 2014;36:66–73.
Burt RS. The contingent value of social capital. Adm Sci Q. 1997;42:339–65.
Tsai W. Knowledge transfer in intraorganizational networks: effects of network position and absorptive capacity on business unit innovation and performance. Acad Manag J. 2001;44:996–1004.
Burt RS. Structural holes: the social structure of competition. Cambridge: Harvard University Press; 2009.
Shi F, Evans J. Surprising combinations of research contents and contexts are related to impact and emerge with scientific outsiders from distant disciplines. Nat Commun. 2023. https://doi.org/10.1038/s41467-023-36741-4 .
Abbasi A, Altmann J, Hossain L. Identifying the effects of co-authorship networks on the performance of scholars: a correlation and regression analysis of performance measures and social network analysis measures. J Informet. 2011;5:594–607.
Wang J. Knowledge creation in collaboration networks: effects of tie configuration. Res Policy. 2016;45:68–80.
Science of Science (Sci2) Tool [ http://sci.slis.indiana.edu ]
Freeman LC. Centrality in social networks conceptual clarification. Soc Networks. 1978;1:215–39.
Wang CL, Rodan S, Fruin M, Xu XY. Knowledge networks, collaboration networks, and exploratory innovation. Acad Manag J. 2014;57:484–514.
Burt RS. Structural holes and good ideas. Am J Sociol. 2004;110:349–99.
Exploratory social network analysis with Pajek (2nd ed.) [ http://mrvar.fdv.uni-lj.si/pajek/ ]
Gazni A, Ghaseminik Z. Author practices in citing other authors, institutions, and journals. J Asso Info Sci Technol. 2016;67:2536–49.
Article CAS Google Scholar
Cova T, Jarmelo S, Formosinho SJ, de Melo JSS, Pais A. Unsupervised characterization of research institutions with task-force estimation. J Informet. 2015;9:59–68.
Guerrero-Bote VP, Olmeda-Gómez C, Moya-Anegón F. Atlas of scientific institutions in food science (Scopus, 2003–2013). Lwt-Food Science And Technology. 2016;67:133–42.
van Eck NJ, Waltman L. Software survey: VOSviewer, a computer program for bibliometric mapping. Scientometrics. 2010;84:523–38.
Article PubMed Google Scholar
Isfandyari-Moghaddam A, Saberi MK, Tahmasebi-Limoni S, Mohammadian S, Naderbeigi F. Global scientific collaboration: a social network analysis and data mining of the co-authorship networks. J Inf Sci. 2023;49:1126–41.
Polyakov M, Polyakov S, Iftekhar MS. Does academic collaboration equally benefit impact of research across topics? the case of agricultural, resource, environmental and ecological economics. Scientometrics. 2017;113:1385–405.
Sud P, Thelwall M. Not all international collaboration is beneficial: the mendeley readership and citation impact of biochemical research collaboration. J Asso Infor Sci Technol. 2016;67:1849–57.
Moya-Anegón F, Guerrero-Bote VP, Bornmann L, Moed HF. The research guarantors of scientific papers and the output counting: a promising new approach. Scientometrics. 2013;97:421–34.
Contopoulos-Ioannidis DG, Ntzani EE, Ioannidis JPA. Translation of highly promising basic science research into clinical applications. Am J Med. 2003;114:477–84.
O’Dwyer M, Filieri R, O’Malley L. Establishing successful university-industry collaborations: barriers and enablers deconstructed. J Technol Transfer. 2023;48:900–31.
Bstieler L, Hemmert M, Barczak G. Trust formation in university-industry collaborations in the us biotechnology industry: ip policies, shared governance, and champions. J Prod Innov Manag. 2015;32:111–21.
Abbasi A, Hossain L, Leydesdorff L. Betweenness centrality as a driver of preferential attachment in the evolution of research collaboration networks. J Informet. 2012;6:403–12.
Rodan S, Galunic C. More than network structure: how knowledge heterogeneity influences managerial performance and innovativeness. Strateg Manag J. 2004;25:541–62.
Zhang Y, Ding JL, Yan H, He M, Wang W. A study of the influence of collaboration networks and knowledge networks on the citations of papers in sports industry in china. Complexity. 2022. https://doi.org/10.1155/2022/9236743 .
Colladon AF, D’Angelo CA, Gloor PA. Predicting the future success of scientific publications through social network and semantic analysis. Scientometrics. 2020;124:357–77.
Download references
Acknowledgements
Not applicable.
This work was supported by the Department of Science and Technology of Guangdong Province of China under the grant 2020A1414010027.
Author information
Authors and affiliations.
State Key Laboratory of Oncology in South China, Guangdong Provincial Clinical Research Center for Cancer, Sun Yat-sen University Cancer Center, No. 651 Dongfeng East Road, Guangzhou, 510060, Guangdong, People’s Republic of China
Shuang Liao, Christopher Lavender & Huiwen Zhai
You can also search for this author in PubMed Google Scholar
Contributions
S.L. conceived of and designed the study and contributed to the study design, data analysis, data interpretation and writing of the manuscript. C.L. contributed to writing of the manuscript. H.W.Z. contributed to data acquisition.
Corresponding author
Correspondence to Shuang Liao .
Ethics declarations
Ethics approval and consent to participate.
Ethics approval was not required as the material is publicly available.
Consent for publication
Competing interests.
The authors declare no competing interests.
Additional information
Publisher’s note.
Springer Nature remains neutral with regard to jurisdictional claims in published maps and institutional affiliations.
Rights and permissions
Open Access This article is licensed under a Creative Commons Attribution-NonCommercial-NoDerivatives 4.0 International License, which permits any non-commercial use, sharing, distribution and reproduction in any medium or format, as long as you give appropriate credit to the original author(s) and the source, provide a link to the Creative Commons licence, and indicate if you modified the licensed material. You do not have permission under this licence to share adapted material derived from this article or parts of it. The images or other third party material in this article are included in the article’s Creative Commons licence, unless indicated otherwise in a credit line to the material. If material is not included in the article’s Creative Commons licence and your intended use is not permitted by statutory regulation or exceeds the permitted use, you will need to obtain permission directly from the copyright holder. To view a copy of this licence, visit http://creativecommons.org/licenses/by-nc-nd/4.0/ .
Reprints and permissions
About this article
Cite this article.
Liao, S., Lavender, C. & Zhai, H. Factors influencing the research impact in cancer research: a collaboration and knowledge network analysis. Health Res Policy Sys 22 , 96 (2024). https://doi.org/10.1186/s12961-024-01205-8
Download citation
Received : 13 March 2024
Accepted : 30 July 2024
Published : 06 August 2024
DOI : https://doi.org/10.1186/s12961-024-01205-8
Share this article
Anyone you share the following link with will be able to read this content:
Sorry, a shareable link is not currently available for this article.
Provided by the Springer Nature SharedIt content-sharing initiative
- Research capacity and impact
- Collaboration network
- Knowledge network
- Paper citations
- Synthetic lethality
- International collaboration
- Cancer research
Health Research Policy and Systems
ISSN: 1478-4505
- Submission enquiries: Access here and click Contact Us
- General enquiries: [email protected]

How to cite ChatGPT
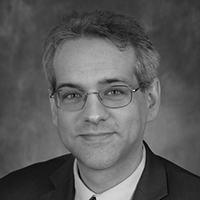
Use discount code STYLEBLOG15 for 15% off APA Style print products with free shipping in the United States.
We, the APA Style team, are not robots. We can all pass a CAPTCHA test , and we know our roles in a Turing test . And, like so many nonrobot human beings this year, we’ve spent a fair amount of time reading, learning, and thinking about issues related to large language models, artificial intelligence (AI), AI-generated text, and specifically ChatGPT . We’ve also been gathering opinions and feedback about the use and citation of ChatGPT. Thank you to everyone who has contributed and shared ideas, opinions, research, and feedback.
In this post, I discuss situations where students and researchers use ChatGPT to create text and to facilitate their research, not to write the full text of their paper or manuscript. We know instructors have differing opinions about how or even whether students should use ChatGPT, and we’ll be continuing to collect feedback about instructor and student questions. As always, defer to instructor guidelines when writing student papers. For more about guidelines and policies about student and author use of ChatGPT, see the last section of this post.
Quoting or reproducing the text created by ChatGPT in your paper
If you’ve used ChatGPT or other AI tools in your research, describe how you used the tool in your Method section or in a comparable section of your paper. For literature reviews or other types of essays or response or reaction papers, you might describe how you used the tool in your introduction. In your text, provide the prompt you used and then any portion of the relevant text that was generated in response.
Unfortunately, the results of a ChatGPT “chat” are not retrievable by other readers, and although nonretrievable data or quotations in APA Style papers are usually cited as personal communications , with ChatGPT-generated text there is no person communicating. Quoting ChatGPT’s text from a chat session is therefore more like sharing an algorithm’s output; thus, credit the author of the algorithm with a reference list entry and the corresponding in-text citation.
When prompted with “Is the left brain right brain divide real or a metaphor?” the ChatGPT-generated text indicated that although the two brain hemispheres are somewhat specialized, “the notation that people can be characterized as ‘left-brained’ or ‘right-brained’ is considered to be an oversimplification and a popular myth” (OpenAI, 2023).
OpenAI. (2023). ChatGPT (Mar 14 version) [Large language model]. https://chat.openai.com/chat
You may also put the full text of long responses from ChatGPT in an appendix of your paper or in online supplemental materials, so readers have access to the exact text that was generated. It is particularly important to document the exact text created because ChatGPT will generate a unique response in each chat session, even if given the same prompt. If you create appendices or supplemental materials, remember that each should be called out at least once in the body of your APA Style paper.
When given a follow-up prompt of “What is a more accurate representation?” the ChatGPT-generated text indicated that “different brain regions work together to support various cognitive processes” and “the functional specialization of different regions can change in response to experience and environmental factors” (OpenAI, 2023; see Appendix A for the full transcript).
Creating a reference to ChatGPT or other AI models and software
The in-text citations and references above are adapted from the reference template for software in Section 10.10 of the Publication Manual (American Psychological Association, 2020, Chapter 10). Although here we focus on ChatGPT, because these guidelines are based on the software template, they can be adapted to note the use of other large language models (e.g., Bard), algorithms, and similar software.
The reference and in-text citations for ChatGPT are formatted as follows:
- Parenthetical citation: (OpenAI, 2023)
- Narrative citation: OpenAI (2023)
Let’s break that reference down and look at the four elements (author, date, title, and source):
Author: The author of the model is OpenAI.
Date: The date is the year of the version you used. Following the template in Section 10.10, you need to include only the year, not the exact date. The version number provides the specific date information a reader might need.
Title: The name of the model is “ChatGPT,” so that serves as the title and is italicized in your reference, as shown in the template. Although OpenAI labels unique iterations (i.e., ChatGPT-3, ChatGPT-4), they are using “ChatGPT” as the general name of the model, with updates identified with version numbers.
The version number is included after the title in parentheses. The format for the version number in ChatGPT references includes the date because that is how OpenAI is labeling the versions. Different large language models or software might use different version numbering; use the version number in the format the author or publisher provides, which may be a numbering system (e.g., Version 2.0) or other methods.
Bracketed text is used in references for additional descriptions when they are needed to help a reader understand what’s being cited. References for a number of common sources, such as journal articles and books, do not include bracketed descriptions, but things outside of the typical peer-reviewed system often do. In the case of a reference for ChatGPT, provide the descriptor “Large language model” in square brackets. OpenAI describes ChatGPT-4 as a “large multimodal model,” so that description may be provided instead if you are using ChatGPT-4. Later versions and software or models from other companies may need different descriptions, based on how the publishers describe the model. The goal of the bracketed text is to briefly describe the kind of model to your reader.
Source: When the publisher name and the author name are the same, do not repeat the publisher name in the source element of the reference, and move directly to the URL. This is the case for ChatGPT. The URL for ChatGPT is https://chat.openai.com/chat . For other models or products for which you may create a reference, use the URL that links as directly as possible to the source (i.e., the page where you can access the model, not the publisher’s homepage).
Other questions about citing ChatGPT
You may have noticed the confidence with which ChatGPT described the ideas of brain lateralization and how the brain operates, without citing any sources. I asked for a list of sources to support those claims and ChatGPT provided five references—four of which I was able to find online. The fifth does not seem to be a real article; the digital object identifier given for that reference belongs to a different article, and I was not able to find any article with the authors, date, title, and source details that ChatGPT provided. Authors using ChatGPT or similar AI tools for research should consider making this scrutiny of the primary sources a standard process. If the sources are real, accurate, and relevant, it may be better to read those original sources to learn from that research and paraphrase or quote from those articles, as applicable, than to use the model’s interpretation of them.
We’ve also received a number of other questions about ChatGPT. Should students be allowed to use it? What guidelines should instructors create for students using AI? Does using AI-generated text constitute plagiarism? Should authors who use ChatGPT credit ChatGPT or OpenAI in their byline? What are the copyright implications ?
On these questions, researchers, editors, instructors, and others are actively debating and creating parameters and guidelines. Many of you have sent us feedback, and we encourage you to continue to do so in the comments below. We will also study the policies and procedures being established by instructors, publishers, and academic institutions, with a goal of creating guidelines that reflect the many real-world applications of AI-generated text.
For questions about manuscript byline credit, plagiarism, and related ChatGPT and AI topics, the APA Style team is seeking the recommendations of APA Journals editors. APA Style guidelines based on those recommendations will be posted on this blog and on the APA Style site later this year.
Update: APA Journals has published policies on the use of generative AI in scholarly materials .
We, the APA Style team humans, appreciate your patience as we navigate these unique challenges and new ways of thinking about how authors, researchers, and students learn, write, and work with new technologies.
American Psychological Association. (2020). Publication manual of the American Psychological Association (7th ed.). https://doi.org/10.1037/0000165-000
Related and recent
Comments are disabled due to your privacy settings. To re-enable, please adjust your cookie preferences.
APA Style Monthly
Subscribe to the APA Style Monthly newsletter to get tips, updates, and resources delivered directly to your inbox.
Welcome! Thank you for subscribing.
APA Style Guidelines
Browse APA Style writing guidelines by category
- Abbreviations
- Bias-Free Language
- Capitalization
- In-Text Citations
- Italics and Quotation Marks
- Paper Format
- Punctuation
- Research and Publication
- Spelling and Hyphenation
- Tables and Figures
Full index of topics
IEEE Account
- Change Username/Password
- Update Address
Purchase Details
- Payment Options
- Order History
- View Purchased Documents
Profile Information
- Communications Preferences
- Profession and Education
- Technical Interests
- US & Canada: +1 800 678 4333
- Worldwide: +1 732 981 0060
- Contact & Support
- About IEEE Xplore
- Accessibility
- Terms of Use
- Nondiscrimination Policy
- Privacy & Opting Out of Cookies
A not-for-profit organization, IEEE is the world's largest technical professional organization dedicated to advancing technology for the benefit of humanity. © Copyright 2024 IEEE - All rights reserved. Use of this web site signifies your agreement to the terms and conditions.
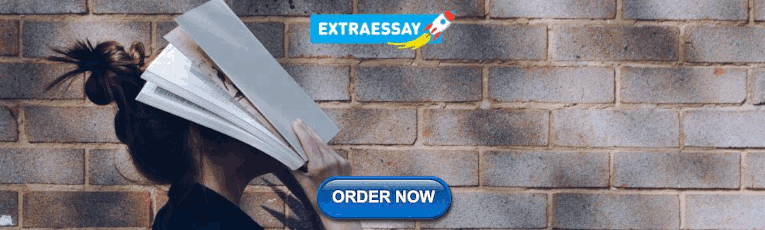
IMAGES
COMMENTS
Electronics, Embedded Systems, and Microcontrollers, Hardware in the Loop | Explore the latest full-text research PDFs, articles, conference papers, preprints and more on EMBEDDED SYSTEMS.
Embark on a transformative journey into the world of embedded systems with our meticulously curated selection of M.Tech project topics for 2024, thoughtfully complemented by trending IEEE base papers. These projects encapsulate the forefront of innovation and challenges in embedded systems, offering an indispensable resource for M.Tech students seeking to delve into the dynamic landscape of ...
Explore the latest full-text research PDFs, articles, conference papers, preprints and more on EMBEDDED SYSTEMS PROGRAMMING. Find methods information, sources, references or conduct a literature ...
A Literature Review on Embedded Systems. Abstract: Embedded system design is crucial for the development of industry, technology, and science, and it is an area that has significantly grown in recent years throughout Latin America, both in academia and in industry. Embedded System (ES) refers to electronic equipment with a computing core which ...
This paper proposed an Actor-oriented modeling and designing methodology for complex real time embedded system. Embedded system can be divided into multiple single computing model subsystems according to different computation model and different abstract hierarchy by this method. Then, specialist can construct this subsystem according to their previous experiences and knowledge, and the ...
We investigate essential technologies for development of IoT systems, existing trends, and its distinguishing properties. By discussing the key characteristics, main application domains, and major research issues in IoT, this paper provides a comprehensive IoT perspective for embedded system design.
Introduction. Real-time and networked embedded systems are important bidirectional bridges between the physical and the information worlds. Embedded intelligence increasingly pervades industry, infrastructure, and public and private spaces, being identified as a society and economy emerging "neural system" that supports both societal ...
forms used in our vehicles, smart appliances, wearables, mobile devices, and computers. The need to train the next generation of embedded systems designers and engineers with relevant skills across hardware, software, and their co-design remains pressing today. This paper describes the evolution of embedded systems education over the past two decades and challenges facing the designers and ...
This paper was the first comprehensive review of research in autonomous vehicles with embedded systems such as JetRacer AI Kit. We developed an end-to-end lane detection system for autonomous vehicles. We performed image processing and lane detection processes using a HOG Transform model.
The objective of this Special Issue is to invite original and high-quality papers that describe research, technical aspects, or developments in real-time embedded systems architecture, software, and applications. The research on real-time embedded systems has gained great interest in the last years as a result of the ever-increasing utilization of such systems in the IoT (Internet of Things ...
Embedded systems are increasingly becoming a key technological component of all kinds of complex technical systems, ranging from vehicles, telephones, audio-video equipment, aircraft, toys, security systems, medical diagnostics, to weapons, pacemakers, climate control systems, manufacturing systems, intelligent power systems etc. IJES addresses the state of the art of all aspects of embedded ...
This Special Issue (SI) invites papers on research achievements in emerging and new technologies in embedded systems. Embedded systems have matured as a discriminating technology for a wide variety of applications in our daily life. However, this immense impact is unfortunately confined by the power and cost constraints of hardware devices.
PDF | Embedded system design is crucial for the development of industry, technology, and science, and it is an area that has significantly grown in... | Find, read and cite all the research you ...
This paper describes the evolution of embedded systems education over the past two decades and challenges facing the designers and instructors of embedded systems curricula in the 2020s. Reflections from over a decade of teaching the design of embedded computing systems are presented, with insights on strategies that show promise to address ...
An embedded system need to run only authorized firmware. In this paper, we present some basic types of code protection together with a hardware based implementation that can be easily applied for an appropriate level of cybersecurity in embedded projects.
As such, it becomes necessary to reflect on the developed theories, engineering, and development techniques and the applications. In this Special Issue, we aim at collecting high-quality submissions that include the theoretical aspects, the engineering aspects, and the application aspects of embedded IoT devices and systems.
An embedded system incorporates a rugged motherboard into an industrial enclosure, with an associated input and output (I/O) and software to fulfill the process that is the function of the system. The hardware component of an embedded system is broadly similar to that of other electronic systems.
embedded system engineering research papers IEEE PAPER. Embedded systems are computer systems that are part of larger systems and they perform some of the requirements of these systems. Some examples of such systems are auto mobile control systems, industrial processes control systems, mobile phones, or small sensor controllers.
Comprehensive project ideas for mastering beginner to advanced-level topics related to embedded systems.
Abstract In this research paper the concept of embedded systems, its applications as well as the economic importance in the modern technology was reviewed. Embedded systems are not only one of the ...
The purpose of this paper is to present our approach in learning embedded systems at our University. It is based on combination of traditional (face-to-face) learning and PBL. Our PBL represents an interdisciplinary project based on wireless sensor monitoring of real-world environment (greenhouse). The students use UML that was shown as an excellent tool for developing such a projects. From ...
Courses in embedded systems can approach the subject from a variety of perspectives, ranging from emphasis on hardware, emphasis on software, or emphasis on system design. This paper describes a course in embedded systems that requires students to develop software to control a system that is physically available in the laboratory. The hardware models a rapid transit system that is controlled ...
Cancer is a major public health challenge globally. However, little is known about the evolution patterns of cancer research communities and the influencing factors of their research capacity and impact, which is affected not only by the social networks established through research collaboration but also by the knowledge networks in which the research projects are embedded.
This post outlines how to create references for large language model AI tools like ChatGPT and how to present AI-generated text in a paper.
The Internet of Things (IoT)-centric concepts like augmented reality, high-resolution video streaming, self-driven cars, smart environment, e-health care, etc. have a ubiquitous presence now. These applications require higher data-rates, large bandwidth, increased capacity, low latency and high throughput. In light of these emerging concepts, IoT has revolutionized the world by providing ...