
- SUGGESTED TOPICS
- The Magazine
- Newsletters
- Managing Yourself
- Managing Teams
- Work-life Balance
- The Big Idea
- Data & Visuals
- Reading Lists
- Case Selections
- HBR Learning
- Topic Feeds
- Account Settings
- Email Preferences
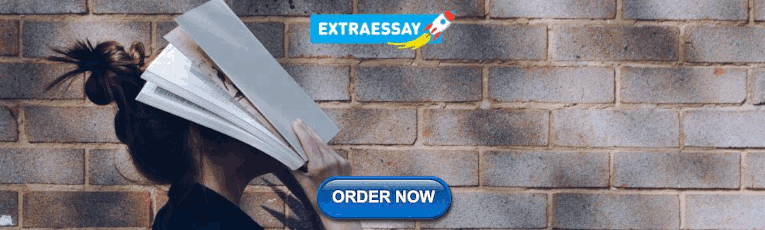
What Self-Awareness Really Is (and How to Cultivate It)
- Tasha Eurich

Although most people believe that they are self-aware, true self-awareness is a rare quality. In this piece, the author describes a recent large-scale investigation that shed light on some of the biggest roadblocks, myths, and truths about what self-awareness really is — and what it takes to cultivate it. Specifically, the study found that there are actually two distinct types of self-awareness, that experience and power can hinder self-awareness, and that introspection doesn’t always make you more self-aware. Understanding these key points can help leaders learn to see themselves more clearly.
It’s not just about introspection.
Self-awareness seems to have become the latest management buzzword — and for good reason. Research suggests that when we see ourselves clearly, we are more confident and more creative . We make sounder decisions , build stronger relationships , and communicate more effectively . We’re less likely to lie, cheat, and steal . We are better workers who get more promotions . And we’re more-effective leaders with more-satisfied employees and more-profitable companies .
- TE Tasha Eurich , PhD, is an organizational psychologist, researcher, and New York Times bestselling author. She is the principal of The Eurich Group, a boutique executive development firm that helps companies — from startups to the Fortune 100 — succeed by improving the effectiveness of their leaders and teams. Her newest book, Insight , delves into the connection between self-awareness and success in the workplace.
Partner Center
Measuring the Effects of Self-Awareness: Construction of the Self-Awareness Outcomes Questionnaire
Affiliation.
- 1 Business School, Manchester Metropolitan University, Manchester, United Kingdom.
- PMID: 27872672
- PMCID: PMC5114878
- DOI: 10.5964/ejop.v12i4.1178
Dispositional self-awareness is conceptualized in several different ways, including insight, reflection, rumination and mindfulness, with the latter in particular attracting extensive attention in recent research. While self-awareness is generally associated with positive psychological well-being, these different conceptualizations are also each associated with a range of unique outcomes. This two part, mixed methods study aimed to advance understanding of dispositional self-awareness by developing a questionnaire to measure its outcomes. In Study 1, expert focus groups categorized and extended an initial pool of potential items from previous research. In Study 2, these items were reduced to a 38 item self-report questionnaire with four factors representing three beneficial outcomes (reflective self-development, acceptance and proactivity) and one negative outcome (costs). Regression of these outcomes against self-awareness measures revealed that self-reflection and insight predicted beneficial outcomes, rumination predicted reduced benefits and increased costs, and mindfulness predicted both increased proactivity and costs. These studies help to refine the self-awareness concept by identifying the unique outcomes associated with the concepts of self-reflection, insight, reflection, rumination and mindfulness. It can be used in future studies to evaluate and develop awareness-raising techniques to maximize self-awareness benefits while minimizing related costs.
Keywords: insight; mindfulness; reflection; rumination; self-awareness; work.
Introduction: The Relational Self: Basic Forms of Self-Awareness
- Published: 20 January 2020
- Volume 39 , pages 501–507, ( 2020 )
Cite this article
- Anna Ciaunica 1
2 Citations
34 Altmetric
Explore all metrics
Avoid common mistakes on your manuscript.
Self-awareness, the feeling that our experiences are bound to the self—as a unitary entity distinct from others and the rest of the world—is a key aspect of the human mind. But how do we become aware of ourselves in a constantly changing and complex physical and social environment? How do we relate to others while keeping in touch with one’s self, with the fundamental feeling that there is an ‘I’ at the core of all our experiences and exchanges with the world and others? How is it possible to navigate such a dynamic environment without losing track of one’s self? What are the mechanisms underlying typical and atypical self-awareness and how can we spell them out within a coherent conceptual and empirical framework? What are the most basic or minimal forms of self-awareness? Is the minimal self relational or is it preferable to conceptualise it in an individualistic manner, as a fundamentally subjective sense of mineness? Is there is a ‘sense of we’, and if yes, what is its relationship with the minimal ‘individualistic’ self?
This special issue tackles these key questions by bringing together papers from philosophy, developmental and clinical psychology and theoretical neuroscience. The aim is to provide a multifaceted, interdisciplinary and rich perspective on the perennial and fundamental questions i) “what is a (minimal) self?” and ii) “how the self relates to the world and others?”
The papers in this special issue can be divided into three main groups. The first consists of four papers discussing the question whether (i) the minimal self is best understood and defined relationally, that is in terms of social interactions; or rather (ii) the minimal self is a tacit, non-socially grounded, experiential self-awareness. The second group contains three papers and combines resources from theoretical neuroscience (the Predictive Processing framework), experimental work (developmental studies), and philosophy in order to emphasise the fundamentally dynamic aspect of self-awareness. Finally, the last group consists in three papers bringing insights into these questions by focusing on atypical forms of self-awareness and social interactions, in particular schizophrenia and Autism Spectrum Condition. In what follows I provide a brief synopsis each of these contributions.
1 Minimal and Relational Self: Conceptual Issues
Hutto and Ilundáin‐Agurruza ’s “Selfless activity and experience: radicalizing minimal self-awareness” begins the special issue by offering a rich discussion of the key question “how best to characterize the most fundamental ways social creatures have of engaging and sharing experiences with others?”. They contrast relational and non-relational or individualistic views of the self. For example, relationalists hold that whatever awareness of the self exactly involves it is acquired through interactions with others. By contrast, the rival non-relationalist view of selfhood, individualists for short, assume that we possess a tacit sense or awareness of the self—a kind of non-socially grounded self- awareness—that is either already implicated in the way we act successfully in the world and in the ways that we experience the world and our actions in phenomenally charged ways. Pivotally, however, if such deflationary individualist theories of the self are accepted, then pre-reflexive self-awareness is at least one primitive, defining feature of who we are that would need to be in place before we are in a position to relate to and intersubjectively engage with others. They attempt to “give the individualists their due”, explicating how we might positively understand the distinctive, nonconceptual experience of our own actions and experiences, by drawing on insights from a radically enactive take on phenomenal experience. They also defend a late-developing relationalism about the emergence of explicit, conceptually based self-awareness, proposing that the latter develops in tandem with the mastery of self-reflective narrative practices. In doing so, they challenge Ciaunica’s ( 2017 ) developmental relationism which defends the possibility that there are quite basic forms of skin-to-skin sharing with others that precede rather than presuppose the exercise of empathetic abilities. They argue that our first conceptual, explicit sense of self is something that only arrives on the scene once we become able to hold our own—through the support of others—in discursive, narrative practices that give us a conceptual grip on what it is to be a temporally extended self that persists.
Bolis and Schilbach ’s “ ‘I interact therefore I am’: the self as a historical product of dialectical attunement” proposes a shift in perspective, i.e. moving from addressing the question of self-awareness from ‘being’ to ‘becoming’. They hold the radical claim that we construe the ‘self’ as a dynamic process rather than as a static entity. To this end they draw on dialectics and Bayesian accounts of cognition which allows us to holistically consider the ‘self’ as the interplay between internalization and externalization and the latter to operationalize our suggestion formally. Internalization is considered here as the co-construction of bodily hierarchical models of the (social) world and the organism, while externalization is taken as the collective transformation of the world. They suggest that the self is an historical product of dialectical attunement across multiple time scales, from species evolution and culture to individual development and everyday learning. Specifically, they describe concrete means for empirically testing their proposal in the form of two-person psychophysiology and multi-level analyses of intersubjectivity. In short, they argue that a fine-grained analysis of social interaction might allow us to reconsider the ‘self’ beyond the static individual, i.e. how it emerges and manifests itself in social relations. They make the case that such an approach could be relevant in multiple fields, from ethics and psychiatry to pedagogy and artificial intelligence.
Higgings ’s “The ‘we’ in ‘me’: an account of minimal relational selfhood” critically discusses the idea that selfhood involves a uniquely first-personal experiential dimension, which precedes any form of socially dependent selfhood (Gallagher 2005 ; Legrand 2007 ; Strawson 2009 ; Kriegel 2009 ; Nida-Rümelin 2017 ; Zahavi 1999 , 2011 , 2014 , 2016 , 2017a , b ). In his paper he draws attention against the temptation to view minimal experiential selfhood as “ontogenetically more primitive” (Zahavi 2014 , p. 14) than socially constituted selfhood. In other words, he argues that the ‘thinnest’ construal of minimal experiential selfhood fails to properly account for characteristics that are essential to human selfhood; namely, the intimate, embodied interactions that unfold at the incipient moments of human life. He argues that taking the ontogenesis of embodied human existence seriously involves accepting the de facto equiprimordiality of minimal experientialism with a ‘minimal’ form of relational selfhood, i.e. the co-constitution of experience through engagements with others. Specifically, he focuses on the dynamical and relational structure of consciousness, as a property of human beings considered holistically (i.e. as embodied beings) who are embedded in subject- dependent contexts and are therefore irreducible to descriptions that accord with some independent ‘objective’. In doing so he draws on work by Krueger ( 2013 , 2015 ) to show that early stages of human life, inclusive of the perspective of phenomenal consciousness, involve shared experiential states, in which one’s experiences are constitutively dependent on the modulatory role of others. One’s corporeality is thereby partly given over to another’s agency. Lastly, these kinds of experiential states are shown to be present at the most incipient moments of human life, thereby affirming that any naturalistically grounded notion of pre- reflective human minimal selfhood should be construed as ontogenetically equiprimordial with socially constituted experience. Such socially constituted experience amounts to a form of minimal relational selfhood, which is not preceded by any other dimension of selfhood within the manifestation of human life.
Leon ’s “For-me-ness, for-us-ness, and the we-relationship” investigates the relationship between for-me-ness and sociality. He draws very careful conceptual distinctions in order to point out some ambiguities in claims pursued by some of the critics that have recently pressed on the relationship between the two notions. He starts with the observation that the idea of for-me-ness or mineness raises a host of far-reaching questions, some of which concern its proper characterization, pervasiveness, phenomenal reality, and its relation to the prospects of providing a naturalistic account of consciousness. Leon notices that the proposal according to which there is a very tight link between subjectivity and sociality is certainly not new in the philosophical and psychological literature. However, a novel feature of some of the recent criticisms to the received understanding of for-me-ness is that they don’t appeal to language, narratives, social roles or cultural context to support this view, but rather to bottom-up approaches from developmental psychology (Ciaunica and Fotopoulou 2017 ; Ciaunica 2016 ). The main aim of his contribution is to discuss the relationship between for-me-ness and sociality, mainly in the context of recent criticisms to the received understanding of for-me-ness that have appealed to research in developmental psychology. He articulates a question concerning this relationship that builds on the idea that, occasionally at least, there is something it is like ‘for us’ to have an experience. This idea, he argues, has been explored in recent literature on shared experiences and collective intentionality (Schmid 2014a , b ), and it gestures towards the question of the extent to which some social interactions make a difference in the phenomenal character of their participants’ experiences. In the main part of his article, Leon proposes a construal of for-us-ness that complements the received understanding of for-me-ness, by drawing on Alfred Schutz’ concept of the “we-relationship” (Schutz 1962 , 1967 ; Schutz and Luckmann 1973 ), and on the idea of second-personal awareness, i.e. awareness of a ‘you’, as distinguished from awareness of a ‘she’ or he’. He concludes that this proposal provides a suitable account of basic forms of phenomenally manifest social connectedness, in a way that is cognitively undemanding and without incurring the theoretical costs of positing a sui generis plural pre-reflective self-awareness (Schmid 2014a ).
2 The Dynamic, Situated and Scaffolded Self
Kiverstein ’s “Free energy and the self: an ecological-enactive interpretation” addresses the interesting question: how did the feeling of being alive get started? One key observation is that not all living things feel alive. Plants almost certainly don’t. Bacteria are capable of a minimal form of purposive agency (Fulda 2017 ; Di Paolo et al 2017 ). However, since they lack a brain for regulating the changing internal state of their bodies, they probably also lack feeling (Thompson 2015 ). But what about insects that continue behaving in the same way when they have suffered severe injuries? They too most likely lack feeling insofar as their behaviour seems to be unaffected by damage to their bodies. Kiverstein give us an account about how subjectivity might have emerged early in evolutionary history out of processes of action control. In other words, subjectivity might be thought of as tied to processes of purposive agency on the one hand, and sensorimotor integration on the other. He defends the “phenomenological theory of selfhood” according to which any naturalistic explanation of phenomenal consciousness in the terms of psychology and the neurosciences will need to explain how mineness can be intrinsic to phenomenal consciousness. He gives a detailed account of a such naturalistic explanation that takes selfhood to emerge out of self-organising biological processes.
Kiverstein builds upon the free energy principle, according to which any living system will aim to minimise free energy in its sensory exchanges with the environment (Friston 2007 ). He shows how such a process of free energy minimisation is accomplished through a process referred to as “active inference”. He claims that active inference is best understood in ecological and enactive terms, with focus on the coupled dynamics of the animal in its eco-niche that lead towards dynamic equilibrium (Bruineberg and Rietveld 2014 ; Bruineberg et al. 2017 ). Importantly, active inference doesn’t suffice to explain mineness, since every living system will keep its own free energy to a minimum through a process of active inference. In order to address this key question, Kiverstein asks: what would need to be added to active inference to yield mineness? He suggests that recent theoretical work by Karl Friston may give us an answer to this question (Friston 2018 ). The basic idea is that once an organism reaches the level of complexity so that it can act to minimise its own expected free energy, mineness will emerge as intrinsic to the process of life itself. The author closes his paper by showing how the resulting account of mineness supports a relational theory of the self. This is because on this ecological and enactive reading of active inference the organism and its environment are co-specifying, and co-determining. The argument of Kiverstein’s paper is that any organism capable of action control of the right complexity will also be a self. Since organisms are best understood in relation to their environment, so also are selves.
Crucianelli and Filippetti ’s “Developmental perspectives on interpersonal affective touch” draws attention to a relatively overlooked aspect of interpersonal affective touch in shaping self-awareness and body ownership from the outset, in early life. They start with the basic observation that our first sensorial experiences, which provide us with information about our own body and the surrounding environment arise in the womb (Ciaunica 2016 , 2017 ; Ciaunica & Crucianelli 2019 ). In these early sensory interactions, touch is possibly the first route by which the developing body receives inputs from the external world and gradually shapes one’s body boundaries and its capabilities for action (e.g. Atkinson and Braddick 1982 ; Bernhardt 1987 ; Bremner and Lewkowicz 2012 ). The skin is the largest of our sensory organs and wraps our entire body surface (Serino and Haggard 2010 ; Gallace and Spence 2014 ); hence, evidence suggests that touch might play a pivotal role in developing a sense of self as separate from the other (see Field 2010 for a review). Somewhat paradoxically, touch is also our most social sense since it is fundamentally involved in how we explore the environment and engage in successful interactions (Gallace 2012 ; Ebisch et al. 2014 ; Rochat 2011 ; Gallace and Spence 2014 ), as well as how we bond with other people and form interpersonal attachments. In their paper they review and discuss recent developmental and adult findings, pointing to the central role of interpersonal affective touch in body awareness and social cognition in health and disorders. They propose that interpersonal affective touch, as an interoceptive modality invested of a social nature, can uniquely contribute to the ongoing debate in philosophy about the primacy of the relational nature of the minimal self. In the wake of these recent conceptualisations, Crucianelli and Filippetti review experimental and clinical evidence supporting the importance of interpersonal affective touch to the way we recognise and make sense of our body as our own. In line with previous work by Ciaunica ( 2017 ) and Ciaunica & Fotopoulou ( 2017 ) they defend the claim that affective touch constitutes a fundamental aspect of bodily self-consciousness (Blanke 2012 ; Dijkerman 2015 ) from the very first stages of human life.
Gallotti ’s “Shared and social discourse” discusses the scope and importance of claims about shared intentionality in social discourse. What is it for the capacity of sharing mental attitudes and contents to play an explanatory role in the study of the social mind? The motivation for addressing this question is originally tied to theoretical preoccupations about the tendency to make claims about shared and, especially, ‘we- intentionality’ in support of arguments about socially extended forms of mentality. The fact that evidence is interpreted as showing that shared intentionality works as a scaffolding for the development of full- blown, representational and normative, thinking, is often associated with claims about the conditions for the existence and identity of mental attitudes and contents being social all the way down. This argumentative line is present in several strands of social-cognitive research at the intersection of studies of shared intentionality and radically (i.e. en-active) externalist views of the mind, but it has not yet been articulated in a satisfactory manner. The key question is what human cognition could be like on the premise that humans are capable of creating cultures and institutions of unique complexity in the animal kingdom. Tomasello’s response is an account of the evolution of the human mind, which identifies the origin of species-specific forms of modern thinking in the emergence of a genetically evolved psychological adaptation for engaging in cognitively shared activities with co-specifics (Tomasello and Carpenter 2007 ; Call 2009 ). In a similar vein, Jane Heal has offered an authoritative formulation of the significance of shared cognitive activities in philosophical work on co-cognition (Heal 2013 ). Building upon earlier reflections on co-cognition in simulation theory (Heal 1998 ), Heal draws on insights about shared intentionality to advocate ‘Co-cognitivism’, the view that the logical structure and criteria of adequacy of psychological concepts are determined with a view to the sort of activities that individuals pursue together in everyday life. Co-cognitivism and the ‘Shared Intentionality Hypothesis' gesture to something like a general view of the function of shared intentionality, which has only begun to emerge in the philosophy of mind and society. The idea is that human psychology has evolved in accordance with the fact that novel routes to knowledge of things become available to interacting agents when they align their mental and bodily resources and act as a ‘we’ (Tollefsen and Dale 2012 ; Gallotti et al. 2017 ). Gallotti's goal is to develop a more balanced, if not cautious, assessment of the explanatory role of claims about shared intentionality in social discourse.
3 Minimal and Relational Self and its Disruptions
Krueger ’s “Schizophrenia and the scaffolded self” takes as a starting point a family of recent externalist approaches in philosophy of mind which argue that our psychological capacities are synchronically and diachronically “scaffolded” by external (i.e., beyond-the-brain) resources. Surprisingly, he writes, despite much recent interest in this topic, it has not yet found its way to philosophy of psychiatry in a substantive way. Since disturbances of affectivity figure so prominently in a wide variety of mental disorders, the topic seems like a fruitful place to bridge externalist paradigms and psychiatry. The main aim of his paper is an attempt to build such a bridge. Krueger considers how these “scaffolded” approaches might inform debates in phenomenological psychopathology. In doing so, he first introduces the idea of “affective scaffolding” and makes some taxonomic distinctions. More specifically, he uses schizophrenia as a case study to argue—along with others in phenomenological psychopathology—that schizophrenia is fundamentally a self- disturbance. Building upon previous existing work, he offers a subtle reconfiguration of these approaches. He claims that schizophrenia is not simply a disruption of ipseity or minimal self-consciousness but rather a disruption of the scaffolded self, established and regulated via its ongoing engagement with the world and others. He concludes that this way of thinking about the scaffolded self is potentially transformative both for our theoretical as well as practical understanding of the causes and character of schizophrenic experience, insofar as it suggests the need to consider new forms of intervention and treatment.
Constant , Bervoets , Hens and Van de Cruys ’s “Precise worlds for certain minds: An ecological perspective on the relational self in autism” addresses the question of how the various aspects of the relational self are experienced by people presented with clear challenges in social interaction, such as people with Autism Spectrum Condition (ASC). Indeed, relational and social accounts of the self posit that the sense of self depends on the entanglement of the individual with a significant, or generalized other, understood respectively as the representation of an individual profound significance in one’s life (Andersen and Chen 2002 ), and as the individually internalized ‘attitude of a whole community’ (Mead 1934 ). On that view, the self would heavily rest on the individual’s ability to coordinate a diversified repertoire of selves (cf. Zahavi 2010 ) accumulated over time through interpersonal relationships (Andersen and Chen 2002 ). However, ASC offers an interesting challenge for the relational views of the self precisely because it is broadly recognized as a disorder primarily impacting social relationships. Indeed, while they do not claim that autistic people completely lack a sense of self, many influential theories tend to suggest that the requirements for a sense of relational self are reduced or otherwise impaired. Yet, the abundance of autobiographical reports (Van Goidsenhoven and Masschelein 2016 ) by autistic people across the spectrum suggests that a desire for self-identification and recognition is particularly pronounced in people with ASC. Building upon the influential predictive processing (PP) paradigm, in explaining core behavioral traits of ASC (e.g., Lawson et al. 2014 ; Palmer et al. 2015 ; Pellicano and Burr 2012 ; Van de Cruys et al. 2014 ) PP approaches challenge the claims about the presence of specific neurocognitive dysfunctions in autistic cognition, as it traces back the symptoms of ASC to normal, though differently ‘tuned’, domain general neurocognitive mechanisms (cf. Bolis and Schilbach 2017 ).
The main aim of their paper is to use the PP paradigm to attempt an ecological explanation of atypicalities of the relational self in ASC, specifically, those relating to its minimal, extended, and intersubjective aspects, though without positing major incapacities in social functioning. The main focus is in how these aspects become organized in patterns of sensory and social interactions with the body (e.g., interoception), the world (e.g., exteroception and material (environment), and other agents (e.g., social interactions). Specifically, they focus on a recent PP account of ASC called “HIPPEA”, the High, Inflexible Precision of Prediction Errors in Autism account (Van de Cruys et al. 2014 ). PP accounts of ASC emphasize different aspects of PP, even though they generally include a treatment of all basic mechanisms. The focus on HIPPEA is motivated by its interpretation of the mechanism of meta-learning. According to PP, meta-learning enables to detect learnable sensory cues, relevant for predicting future events with a certain level of reliability. It is a mechanism crucial to distinguish random sensory variability from the variability reporting causal regularities (e.g., recurrent causes of inputs). This new account enables a leverage the ecological and embodied implications of PP to discuss aspects of the relational self in ASC. The ecological implications of PP for understanding ASC are far-reaching (e.g., Bolis and Schilbach 2017 ; von der Lühe et al. 2016 ), but have so far not been exhaustively treated in the literature. This paper intends to fill this gap.
Kartner and Clowes ’s “The pre-reflective situational self” critically addresses the idea that pre-reflective self-awareness constitutes a minimal self. They argue that there are reasons to doubt this constituting role of mineness. Specifically, they claim that there are alternative possibilities and that the necessity for an adequate theory of the self within psychopathology gives us good reasons to believe that we need a thicker notion of the pre-reflective self. The authors propose instead the idea of a Pre-Reflective Situational Self. In a first step, they show how alternative conceptions of pre-reflective self-awareness point to philosophical problems with the standard phenomenological view. They claim that this is mainly due to fact that within the phenomenological account the mineness aspect is implicitly playing several roles. Consequently, they argue that a thin interpretation of pre-reflective self-awareness—based on a thin notion of mineness—cannot do its needed job within psychopathology. Rather a thicker conception of pre-reflective self is needed. In their proposal they carefully develop the notion of a pre-reflective situational self by analyzing the dynamical nature of the relation between self-awareness and the world, specifically through our interactive inhabitation of the social world. Specifically they discuss three alternative views which give reasons to question the standard phenomenological interpretation of pre-reflective self- awareness. They start by reviewing several of the most important of these, namely the cognitive view, the structure-awareness view and the relational view and show that each cast rather different light upon the interrelations between first-person givenness, mineness and the minimal self. They argue that the problematic nature of pre-reflective self-awareness this is especially revealed in the context of the Ipseity Hypothesis of Schizophrenia. Here, the thin notion of pre-reflective self does not appear to do what is required of it, namely to fulfil the necessary conceptual role in explaining what appears disrupted in this type of mental illness. This is problematic in their view, as the deployment of the notion of minimal self in “ipseity theories” of schizophrenia might be thought to be one place where the notion of pre-reflective self-awareness earns its status as a concept that has some empirical standing (Nordgaard and Parnas 2014 ; Parnas et al. 2005 ; Sass et al. 2013 ) In their paper they use this discussion to link it to related work on self-diminishment in schizophrenia, namely the self-diminishment view (Lysaker and Lysaker 2008 ) in order to argue that our sense of pre-reflective self is better understood as labile and situational through a thicker conception of self than is entertained in the more standard phenomenological views. On these grounds, they conclude that our sense of pre-reflective self in part must be dynamical in order to reflect our fluid embedding in shifting social contexts.
Andersen SM, Chen S (2002) The relational self: an interpersonal social-cognitive theory. Psychol Rev 109(4):619–645
Article Google Scholar
Atkinson J, Braddick O (1982) Sensory and perceptual capacities of the neonate. In: Stratton P (ed) Psychobiology of the human newborn. Wiley, London, pp 191–220
Google Scholar
Bernhardt J (1987) Sensory capabilities of the fetus. MCN Am J Matern Child Nurs 12(1):44–47
Blanke O (2012) Multisensory brain mechanisms of bodily selfconsciousness. Nat Rev Neurosci 13(8):556
Bolis D, Schilbach L (2017) Observing and participating in social interactions: action perception and action control across the autistic spectrum. Dev Cogn Neurosci 29:168–175
Bremner AJ, Lewkowicz DJ, Spence C (2012) The multisensory approach to development. In: Bremner AJ, Lewkowicz DJ, Spence C (eds) Multisensory development. Oxford University Press, Oxford, pp 1–26
Chapter Google Scholar
Bruineberg J, Rietveld E (2014) Self-organisation, free energy minimisation, and optimal grip on a field of affordances. Front Human Neurosci 8:599
Bruineberg J, Kiverstein J, Rietveld E (2017) The anticipating brain is not a scientist: the free energy principle from an ecological-enactive perspective. Synthese 195:2417–2444
Call J (2009) Contrasting the social cognition of humans and nonhuman apes: the shared intentionality hypothesis. Topics Cogn Sci 1:368–379
Ciaunica A (2016) Basic forms of pre-reflective self-consciousness: a developmental perspective. In: Miguens S, Preyer G, Bravo Morando C (eds) Pre-reflective consciousness: sartre and contemporary philosophy of mind. Routledge, London
Ciaunica A (2017) The meeting of bodies: basic forms of shared experiences. Topoi, Int J Philos. https://doi.org/10.1007/s11245-017-9500-x
Ciaunica A, Crucianelli L (2019) Minimal self-consciousness from within – a developmental perspective. J Conscious Stud 26(3–4):207–226
Ciaunica A, Fotopoulou A (2017) The touched self: psychological and philosophical perspectives on proximal intersubjectivity and the self. In: Durt C, Fuchs T, Tewes C (eds) Embodiment, enaction, and culture: investigating the constitution of the shared world. MIT Press, Cambridge.
Craig AD (2002) Opinion: how do you feel? Interoception: the sense of the physiological condition of the body. Nat Rev Neurosci 3(8):655
Craig AD, Craig AD (2009) How do you feel–now? The anterior insula and human awareness. Nat Rev Neurosci 10(1):59–70
Di Paolo E, Buhrmann T, Barandiaran XE (2017) Sensorimotor life: an enactive proposal. Oxford University Press, Oxford
Book Google Scholar
Dijkerman HC (2015) How do different aspects of self-consciousness interact? Trends Cogn Sci 19(8):427–428
Ebisch SJ, Ferri F, Gallese V (2014) Touching moments: desire modulates the neural anticipation of active romantic caress. Front Behav Neurosci 8:60
Field T (2010) Touch for socioemotional and physical well-being: a review. Dev Rev 30(4):367–383
Friston K (2007) Free energy and the brain. Synthese 159(3):417–458
Friston K (2018) (ms) Am I self-conscious. Manuscript under review, frontiers in theoretical and philosophical psychology philosophical and ethical aspects of a science of consciousness and the self
Fulda FC (2017) Natural agency: the case of bacterial cognition. J Am Philos Assoc 3:1–22
Gallace A (2012) Living with touch. Psychologist 25(12):896–899
Gallace A, Spence C (2014) In touch with the future: the sense of touch from cognitive neuroscience to virtual reality. Oxford University Press, Oxford
Gallagher S (2005) How the body shapes the mind. Oxford University Press, Oxford
Gallotti M, Fairhurst MT, Frith CD (2017) ‘Alignment in social interactions’. Conscious Cogn 48:253–261
Heal J (1998) Co-cognition and off-line simulation: two ways of understanding the simulation approach. Mind Lang 13:477–498
Heal J (2013) Social anti-individualism, co-cognitivism and second-person authority. Mind 122:340–371
Kriegel U (2009) Subjective consciousness: a self-representational theory. Oxford University Press, Oxford
Krueger J (2013) Merleau-ponty on shared emotions and the joint ownership thesis. Cont Philos Rev 46(4):509–531
Krueger J (2015) The affective 'we': self-regulation and shared emotions. In: Szanto T, Moran D (eds) The phenomenology of sociality: discovering the 'We'. Routledge, New York, pp 263–280
Lawson RP, Rees G, Friston KJ (2014) An aberrant precision account of autism. Front Hum Neurosci 8:302
Legrand D (2007) Pre-reflective self-as-subject from experiential and empirical perspectives. Conscious Cogn 16(3):583–599
Lysaker PH, Lysaker JT (2008) Schizophrenia and the fate of the self. Oxford University Press, Oxford
Mead HG (1934) Mind, self, and society. University of Chicago Press, Chicago
Nida-Rümelin M (2017) Self-awareness. Rev Philos Psychol 8(1):55–82
Nordgaard J, Parnas J (2014) Self-disorders and the schizophrenia spectrum: a study of 100 first hospital admissions. Schizophr Bull 40(6):1300–1307
Palmer CJ, Paton B, Enticott PG, Hohwy J (2015) “Subtypes” in the presentation of autistic traits in the general adult population. J Autism Dev Disord 45(5):1291–1301
Parnas J, Møller P, Kircher T, Thalbitzer J, Jansson L, Handest P, Zahavi D (2005) EASE: examination of anomalous self-experience. Psychopathology 38(5):236–258
Pellicano E, Burr D (2012) When the world becomes “too real”: a Bayesian explanation of autistic perception. Trends Cogn Sci 16(10):504–510
Rochat P (2011) Possession and morality in early development. New Dir Child Adolesc Dev 2011(132):23–38
Sass LA, Pienkos E, Nelson B, Medford N (2013) Anomalous self-experience in depersonalization and schizophrenia: a comparative investigation. Conscious Cogn 22(2):430–441
Schmid HB (2014a) Plural self-awareness. Phenomenol Cogn Sci 13(1):7–24
Schmid HB (2014b) The feeling of being a group. Corporate emotions and collective consciousness. In: von Scheve C, Salmela M (eds) Collective emotions: perspectives from psychology, philosophy, and sociology. Oxford University Press, Oxford
Schutz A (1962) Collected papers I the problem of social reality. M. Nijhoff, Hague
Schutz A (1967) Phenomenology of the social world. Northwestern University Press, Evanston
Schutz A, Luckmann T (1973) The structures of the life-world (Vol. I). Northwestern University Press, Evanston
Serino A, Haggard P (2010) Touch and the body. Neurosci Biobehav Rev 34(2):224–236
Strawson G (2009) Selves: an essay in revisionary metaphysics. Oxford University Press, Oxford
Thompson E (2015) Waking, dreaming, being: self and consciousness in neuroscience, meditation and philosophy. Columbia University Press, New York
Tollefsen D, Dale R (2012) Naturalizing joint action: a process-based approach. Philos Psychol 25:385–407
Tomasello M, Carpenter M (2007) Shared intentionality. Dev Sci 10:121–125
Van de Cruys S, Evers K, Van der Hallen R, Van Eylen L, Boets B, de-Wit L, Wagemans J (2014) Precise minds in uncertain worlds: predictive coding in autism. Psychol Rev 121(4):649–675
Van Goidsenhoven L, Masschelein A (2016) Donna Williams’s “triumph”: looking for “the place in the middle”at jessica kingsley publishers. Life Writ 2016:1–23
von der Lühe T, Manera V, Barisic I, Becchio C, Vogeley K, Schilbach L (2016) Interpersonal predictive coding, not action perception, is impaired in autism. Philos Trans R Soc Lond Ser B Biol Sci 371:20150373
Zahavi D (1999) Self-awareness and alterity: a phenomenological investigation. Northwestern University Press, Chicago
Zahavi D (2005) Subjectivity and selfhood: investigating the first-person perspective. MIT Press, Cambridge
Zahavi D (2010) Complexities of self. Autism 14(5):547–551
Zahavi D (2011) The experiential self: objections and clarifications. In: Sidertis M, Thompson E, Zahavi D (eds) Self, no self? Perspectives from analytical, phenomenological, and indian traditions. Oxford University Press, Oxford, p 5678
Zahavi D (2014) Self & other: exploring subjectivity, empathy, and shame. Oxford University Press, Oxford
Zahavi D (2016) Openness versus interdependence: a reply to Kyselo. Philos Psychol 29(7):1066–1067
Zahavi D (2017a) Thin, thinner, thinnest: defining the minimal self. In: Durt C, Fuchs T, Tewes C (eds) Embodiment, enaction, and culture: investigating the constitution of the shared world. MIT Press, Cambridge, pp 193–200
Zahavi D (2017b) ‘Consciousness and (minimal) selfhood: getting clearer on for-me-ness and mineness’. In: Kriegel U (ed.) The Oxford handbook of the philosophy of consciousness. Oxford University Press, Oxford
Download references
Author information
Authors and affiliations.
Senior Researcher Institute of Philosophy Porto, Portugal & Research Associate Institute of Cognitive Neuroscience, UCL, London, UK
Anna Ciaunica
You can also search for this author in PubMed Google Scholar
Corresponding author
Correspondence to Anna Ciaunica .
Additional information
Publisher's note.
Springer Nature remains neutral with regard to jurisdictional claims in published maps and institutional affiliations.
Rights and permissions
Reprints and permissions
About this article
Ciaunica, A. Introduction: The Relational Self: Basic Forms of Self-Awareness. Topoi 39 , 501–507 (2020). https://doi.org/10.1007/s11245-020-09689-z
Download citation
Published : 20 January 2020
Issue Date : July 2020
DOI : https://doi.org/10.1007/s11245-020-09689-z
Share this article
Anyone you share the following link with will be able to read this content:
Sorry, a shareable link is not currently available for this article.
Provided by the Springer Nature SharedIt content-sharing initiative
- Find a journal
- Publish with us
- Track your research

An official website of the United States government
The .gov means it’s official. Federal government websites often end in .gov or .mil. Before sharing sensitive information, make sure you’re on a federal government site.
The site is secure. The https:// ensures that you are connecting to the official website and that any information you provide is encrypted and transmitted securely.
- Publications
- Account settings
Preview improvements coming to the PMC website in October 2024. Learn More or Try it out now .
- Advanced Search
- Journal List
- Front Psychol
The Effect of Trait Self-Awareness, Self-Reflection, and Perceptions of Choice Meaningfulness on Indicators of Social Identity within a Decision-Making Context
Noam dishon.
1 Department of Psychological Sciences, Faculty of Health, Arts and Design, Swinburne University of Technology, Melbourne, VIC, Australia
Julian A. Oldmeadow
Christine critchley.
2 Department of Statistics, Data Science and Epidemiology, Faculty of Health, Arts and Design, Swinburne University of Technology, Melbourne, VIC, Australia
Jordy Kaufman
Theorists operating from within a narrative identity framework have suggested that self-reflective reasoning plays a central role in the development of the self. Typically, however, narrative identity researchers have investigated this relationship using correlational rather than experimental methods. In the present study, leveraging on a classic research paradigm from within the social identity literature we developed an experiment to test the extent to which self-reflection might have a causal impact on the self-concept within a decision-making context. In a minimal group paradigm participants were prompted to reflect on their painting choices either before or after allocating points to in-group∖ out-group members. As anticipated, self-reflection augmented social identification, but only when participants felt their choices were personally meaningful. Participants who reasoned about their choices and felt they were subjectively meaningful showed stronger similarity and liking for in-group members compared to those who did not reflect on their choices or found them to be subjectively meaningless. Hence, reflecting on and finding meaning in one’s choices may be an important step in linking behavior with in-group identification and thus the self-concept in turn. The absence of any effects on in-group favoritism (a third indicator of social identification measured) as well as implications of the study’s findings for self-perception, cognitive dissonance and social identity processes are also discussed.
Introduction
Psychological scientists have approached the issue of self and identity from a range of different positions. For example, some social and cultural psychologists have investigated self and identity using a social identity theory framework whereas other personality and developmental psychologists have pursued an approach informed by narrative identity theory (see, Tajfel and Turner, 1986 ; McAdams, 2001 ; Pasupathi et al., 2007 ; Miramontez et al., 2008 ). In the present paper, we synthesize aspects of both identity projects by utilizing an experimental paradigm associated with social identity theory (i.e., the minimal group paradigm), to investigate whether self-reflective reasoning, a cognitive process theorized to be central to narrative identity development, can have a causal effect on the self and identity. We also explore if such an effect could be impacted by the level of meaningfulness one associates with their self-reflective reasoning and modulated by individual differences in trait self-awareness.
Identity from a Narrative Identity Framework
McAdams (1985 , 2001 ) model of narrative identity postulates that our sense of identity is inextricably linked with the creation of a life story. According to this model, self-narratives have two primary functions. They facilitate our sense of self-continuity across time and they help us give context and meaning to the events of our lives so that we can make sense of who we are ( McAdams and McLean, 2013 ). Self-narratives, as McAdams and McLean (2013) , state facilitate meaning making because they allow the narrator to draw “…a semantic conclusion about the self from the episodic information that the story conveys” (pp. 236). Within the narrative identity literature the process of self-reflection coupled with the extraction of self-relevant meaning is referred to as autobiographical reasoning and it is theorized to be an essential cognitive process in narrative identity development and construction ( Singer et al., 2013 ). However, as Adler et al. (2016) note, within the narrative identity literature investigators have typically employed correlational research designs thereby rendering it difficult to draw causal conclusions. Adler et al. (2016) develop this idea further stating that given this paucity of experimental work “increasing methodological sophistication and variety in the study of narrative identity with an eye toward drawing causal inferences is vital” (pp. 29).
Self-Reflection, Meaning and the Self
Although research from within the narrative identity literature demonstrating a causal link between self-reflection and identity development remains scarce, several other lines of converging research also suggest that self-reflection should play an important role in self-concept development. For example within the clinical psychology literature, reflective functioning has been used to describe a persons ability to reflect on experiences, draw inferences about behavior from these reflections, and then use those inferences to construct and develop representations of the self ( Katznelson, 2014 ). Research which has investigated reflective functioning has demonstrated that changes in reflective functioning are linked to self-concept change. For example, in research with persons affected by borderline personality disorder, (a condition which is characterized by an unstable sense of self) Levy et al. (2006) found that improvements in reflective functioning were associated with improvements in self-representations and a more integrated sense of self.
Another reason for thinking that self-reflection should represent an important mechanism in self-concept construction and development comes from research which has utilized the self-referential memory paradigm. In a typical self-referential memory paradigm study, different word categories (i.e., traits and adjectives verse semantically and orthographically related words) are presented to participants who are instructed to remember them at exposure and then asked to recall them at a later time ( Rogers et al., 1977 ). The self-reference effect describes the tendency for participants to retrieve traits and adjectives that are self-related more successfully than words that are semantically or orthographically related ( Symons and Johnson, 1997 ). Schizophrenia is another condition of which an unstable sense of self represents a core feature (see Sass and Parnas, 2003 ), and research has demonstrated that persons affected by schizophrenia tend to display weaker self-reference effects compared to healthy controls which researchers have interpreted as an indication of reduced self-reflective capacity ( Harvey et al., 2011 ).
There are also several reasons for thinking that meaning-making tendencies should play an important role in self-concept construction and development in addition to the emphasis placed upon this process by narrative identity theorists as noted previously. Firstly, in a theoretical sense, influential thinkers such as Erikson (1963) , Frankl (1969) , and Bruner (1990) , have all argued strongly for the idea that meaning is likely to play an important role in self and identity development. At the same time, research from within the organizational psychology literature has demonstrated empirically that perceptions of meaningfulness are associated with a range of self-related outcomes. Psychological empowerment captures an employees cognitive-motivational stance toward their work and is comprised of four dimensions, impact , competence , autonomy , and of particular pertinence given the current investigation, meaning which reflects the degree to which one perceives their work as being personally meaningful ( Spreitzer, 1995 ; Holdsworth and Cartwright, 2003 ). The importance of perceptions of meaningfulness within the context of psychological empowerment is further highlighted by Spreitzer et al. ( 1997 , pp. 681) who argue that the dimension of meaning “serves as the ‘engine’ of empowerment.” Research exploring psychological empowerment at an individual factor level has noted that differences in meaning are positively associated with several self-related outcomes such as self-esteem and self-efficacy ( McAllister, 2016 ).
In our own research we have found that individual differences in trait self-awareness are associated with perceptions of choice meaningfulness within a decision-making context (Dishon et al., under review). Based on pre-existing literature which has explored self-awareness more generally (e.g., Morin, 2011 ) we defined trait self-awareness as individual differences in the capacity to access knowledge, insight and understanding of internal self-related experiences. We found that participants with higher levels of trait self-awareness perceived significantly more meaning in a series of minor experimentally induced choices compared to those with lower levels of trait self-awareness. Moreover, this difference remained irrespective of whether or not participants were told that their choices were diagnostic of important personal characteristics. We concluded from this research that individuals high in trait self-awareness are more likely to reflect on their choices and more likely to find them meaningful than individuals low in trait self-awareness. Extending on this work and drawing upon the literature previously presented, in the present paper we propose and explore a theoretical model (see Figure Figure1 1 ) that articulates how self-reflection and perceptions of meaningfulness might affect the self within a choice context.

Self-reflection model.
Overview of the Self-Reflection Model
The assumptions underpinning this model are that when one is presented with a potential trigger event such as (but not limited to) a choice or behavior, the self will be affected (i.e., the choice/behavior will inform the self) as a consequence of (a) whether or not self-reflection takes place, and (b) the degree to which the choice is perceived to be personally meaningful. Moreover, (c) whether or not reflection takes place may be determined by individual or situational factors. For example, individuals with higher levels of trait self-awareness may be more predisposed to engage in self-reflective reasoning, whereas for others, situational cues such as an unexpected occurrence or a prompt from a third party might act as the catalyst for self-reflective reasoning. Several predictions arise from the model.
Prediction 1: If self-reflective reasoning does occur and the choice or behavior is perceived to be highly meaningful, then self-perception will occur (by which we mean the self-concept will be modified or changed as result of the behavior or action).
Prediction 2: If self-reflective reasoning does occur and the level of personal meaning associated with the choice or behavior is perceived to be low, its affect on the self will be weak or absent.
Prediction 3: If no self-reflective reasoning occurs there will be a weak effect on the self through an automatic self-perception process. Rather than predict no effect on the self in the absence of self-reflection, we allow for the possibility of an automatic or implicit self-perception process to occur because research has demonstrated that the self-concept can be impacted even in the absence of explicit reasoning. For example, in one demonstration of this type of effect, Klimmt et al. (2010) observed that exposing participants to different types of characters in video games led to automatic shifts in self-perception as measured in a follow up Implicit Association Test.
Prediction 4: Individuals high in trait self-awareness will be more likely to engage in self-reflective reasoning than individuals low in trait self-awareness 1 .
Prediction 5: Individuals low in trait self-awareness will engage in self-reflective reasoning only if prompted, or if some other situational cue triggers self-reflection.
Although narrative identity researchers have primarily looked at self-reflective reasoning in the context of autobiographical memories (see, Pasupathi, 2015 ), in the present study we sought to initially test the veracity of our self-reflection model on a smaller scale in a relatively minimal decision-making context. We did so for several reasons. First, decision-making lends itself well to experimental testing ( Carroll and Johnson, 1990 ). This is important because as noted earlier, to date, research investigating the relationship between self-reflective reasoning and the self has largely been correlational by design and attempts to test this possibility experimentally have been insufficient ( Adler et al., 2016 ). Second, consumer decision-making research has suggested that self-narratives often arise in every day decision-making contexts ( Phillips et al., 1995 ) and some narrative identity scholars have argued that day-to-day narratives which might not be overtly autobiographical nevertheless remain tightly linked to self and identity ( Bamberg, 2011 ; Pasupathi, 2015 ). Third, behaviorist and cognitive theories (i.e., self-perception theory and cognitive dissonance theory) suggest that the self is often informed by after-the-fact explanations for behaviors or post hoc reasoning for choices ( Brehm, 1956 ; Festinger, 1957 ; Bem, 1972 ). Another reason for thinking that self-reflection could impact self-perception stems from research by Wilson et al. (1993) which demonstrated that self-reflection can impact attitudes and post-choice satisfaction within a decision-making context.
Identity from a Social Identity Framework
From the view of social identity theory, our sense of identity is heavily influenced by the social groups that we belong to ( Tajfel and Turner, 1986 ). Social identity as originally conceptualized by Tajfel (1981) refers to “…that part of an individual’s self-concept which derives from his knowledge of his membership of a social group” (p. 255). According to the theory, we come to identify with certain social groups based upon the extent to which we think we share similarities with other group members. Then, in order to maintain a positive sense of our social identity we try to ensure that our group (the in-group) is favored over other out-groups. One way of doing this is by favoring one’s in-group and discriminating against the out-group. Within the social identity literature, the extent to which we feel similar to, like, or favor other in-group members is indicative of the extent to which our identification with that group has been incorporated into our self-concept ( Hogg, 1992 , 1993 ; Ellemers et al., 1999 ; Leach et al., 2008 ). The minimal group paradigm which facilitates the measurement of in-group favoritism and out-group discrimination is one way of measuring the extent to which group membership has been incorporated into the self-concept and therefore had an effect on social identity ( Otten, 2016 ).
In a typical minimal group paradigm experiment, participants are randomly allocated to a group and then asked to concurrently distribute resources to in-group and out-group members on allocation matrices specifically designed to measure allocation strategies that favor the in-group and∖or discriminate against an out-group ( Tajfel et al., 1971 ). Research in the field has consistently demonstrated that even when people are led to believe that their assignment to a group is for a trivial reason, such as their preferences for abstract artwork, they still tend to allocate resources more favorably to in-group members ( Otten, 2016 ). Whilst researchers have often been interested in using this methodology to investigate topics such as prejudice and discrimination, the allocation of resources within a minimal group paradigm environment need not be used exclusively for this end ( Bourhis et al., 1994 ). The allocation of resources within a minimal group paradigm context can also serve as a subtle and discreet measure of the degree to which group membership has been incorporated into the self-concept and one’s sense of social identity more generally ( Otten, 2016 ). Another way that social identity researchers have measured the extent to which commitment to a group can impact one’s self-concept and sense of identity is by measuring self-reported liking of, and similarity with, other anonymous in-group members (e.g., Hogg, 1992 , 1993 ; Ellemers et al., 1999 ; Leach et al., 2008 ). Ellemers et al. (1999) research is also important in the context of the current study because it demonstrates that social identification is more strongly affected when people are able to self-select into a group (as opposed to being assigned a group) and it would seem reasonable to think that self-reflective reasoning is a process that could be quite important for self-selection decisions.
The Current Study
In recent research in our lab we investigated the connection between self-reflective reasoning within a decision-making context and the self. We found that the degree of personal meaning that was given to a trivial choice was associated with individual differences in trait self-awareness (Dishon et al., under review). In the present study we sought to extend this research by investigating further if the cognitive process of engaging in self-reflective reasoning could affect one’s sense of identity. We also sought to explore whether an effect of this kind might be impacted by the extent to which one felt as though their reasoning had been personally meaningful and also moderated by individual differences in trait self-awareness. To test this model we developed an experiment that utilized and extended upon traditional minimal group paradigm work. Participants were randomly assigned to either an experimental or control condition. In the experimental condition participants were prompted to engage in self-reflective reasoning immediately after making painting choices whereas in the control condition participants went on to allocate resources immediately after selecting paintings. We used in-group∖out-group allocation strategies as one dependent measure of identity and we also used similarity and liking ratings with in-group∖out-group members as additional dependent measures of identity.
Based on the proposed model we hypothesized that participants who are relatively high in trait self-awareness would be more likely to spontaneously self-reflect on their choices and therefore be relatively unaffected by the self-reflection prompt manipulation. As such it was expected that for these participants, self-perception would be related to the perceived meaningfulness of their painting choices more so than condition. We also expected that participants who are relatively low in trait self-awareness would be less likely to spontaneously self-reflect on their choices and therefore more greatly affected by the self-reflection prompt manipulation. As such it was expected that for these participants, self-perception would be related to the perceived meaningfulness of their painting choices only in the experimental condition (i.e., when they have been prompted to self-reflect.)
Materials and Methods
Participants.
Two hundred and six undergraduate psychology students voluntarily participated in the study in exchange for course credit. During the procedure, a manipulation check was administered to ensure that participants had attended to feedback regarding group allocation (the details of which are explained further in the Procedure section below). The responses of 32 participants who failed the manipulation check were discarded leaving a remaining pool of 174 participants (139 female, 35 male) with a mean age of 33.06 years ( SD = 11.78). The difference in failure rates between conditions was not significant ( p = 0.518). Ethical approval for the study was provided by Swinburne University’s Human Research Ethics Committee (SUHREC).
Effects of the experimental manipulation on identity were inferred by, (a) the extent to which participants incorporated their in-group identification into their self-concept and measured by participant’s in-group favoritism when distributing resources to in-group∖out-group members on Tajfel matrices and, (b) participant’s self-identification with in-group∖out-group members which was assessed by measuring their liking of, and perceived similarity with, in-group∖out-group members.
Tajfel matrices
Tajfel matrices consist of six matrices in which participants are asked to allocate resources concurrently to an in-group member and out-group member along a spectrum of pre-determined in-group to out-group ratios. The six matrices comprise three pairs (one of each pair is a reversed version of the original).
There are four main allocation strategies that can be measured with Tajfel matrices. Parity is an allocation strategy whereby the participant distributes an equal amount of resources to both in-group and out-group recipients. Maximum In-Group Profit is an allocation strategy that sees the greatest possible amount of resources awarded to the in-group recipient irrespective of what is awarded to the out-group recipient. Maximum Difference reflects a strategy that optimizes the differential allocation of resources between recipients in favor of the in-group recipient at the expense, however, of absolute in-group profit. Maximum Joint Profit reflects a strategy in which overall allocation of resources is maximized across both in-group and out-group.
The matrices facilitated the calculation of pull scores which reflected participants’ gravitation toward particular allocation strategies. Matrix pair A compared the pull of Maximum In-Group Profit and Maximum Difference (i.e., in-group favoritism) against Maximum Joint Profit. Matrix pair B compared the pull of Maximum Difference against Maximum In-Group Profit and Maximum Joint Profit. Matrix C compared the pull of Parity against Maximum In-Group Profit and Maximum Difference [See Bourhis et al. ( 1994 ) for a comprehensive and in-depth account of the procedure involved in Tajfel matrix preparation, administration, and calculation].
Following a similar procedure to Grieve and Hogg (1999) we then conducted a factor analysis of the pull scores using principal axis factoring with promax rotation to examine the possibility of computing an overall in-group favoritism score. This revealed a single in-group favoritism factor which explained 48.9% of the variance (all loadings ≥ 0.63). The items were then summed and averaged to produced an overall measure of in-group favoritism with higher scores representing greater in-group favoritism (Cronbach’s α = 0.74).
In-group self-identification
As other researchers have done previously (e.g., Hains et al., 1997 ; Grieve and Hogg, 1999 ), participants’ liking of, and perceived similarity with, in-group∖out-group members were recorded to measure their level of self-identification with their in-group. To do so, after being presented with pairs of de-identified paintings by Paul Klee and Wassily Kandinsky and receiving feedback that their choices indicated a preference for the work of Klee irrespective of their actual choices, (see the Procedure section below for a more detailed account of the process involved,) participants were asked to imagine themselves meeting two people, one who had a preference for Klee and the other who had a preference for Kandinsky. Participants then rated on a seven-point scale which of these two people they thought they were most similar to in general (Q1), in artistic preferences (Q2), in painting preferences (Q3), in academic ability (Q4), and in political opinions (Q5). Using the same scenario, participants were also asked to rate who they thought they would like more (Q6), who they thought they would get along with more (Q7), and who they would like to meet more (Q8). Responses on questions 1–5 were summed and averaged to calculate an overall similarity score with higher scores representing a greater level of similarity with an in-group member (Cronbach’s α = 0.75). Response for questions 6–8 were summed and averaged to calculate an overall liking score with higher scores representing a greater level of liking for an in-group member (Cronbach’s α = 0.82).
Meaningfulness
Meaningfulness associated with self-reflective reasoning was measured by providing participants with a five-item Subjective Meaningfulness Scale which included items such as “I feel as though my choices were genuine” and, “My choices were meaningless.” Participants were asked to indicate their level of agreement with each statement on a 5-point scale (1 = strongly disagree, 5 = strongly agree). Responses were coded so that higher scores indicated greater level of meaningfulness. A factor analysis using principal axis factoring and promax rotation revealed that all five items loaded on a single factor which explained 32.6% of the variance (all loadings ≥ 0.43). Scores were then summed and averaged and an overall meaningfulness score was calculated (Cronbach’s α = 0.69; Guttman’s Lambda 2 = 0.70).
Trait Self-Awareness
Trait Self-Awareness was operationalized as function of participants’ scores on the Sense of Self Scale (SOSS; Flury and Ickes, 2007 ) which is a single factor 12-item measure designed to assess sense of self and self-understanding (Cronbach’s α = 0.86) and the Self-Reflection and Insight Scale (SRIS; Grant et al., 2002 ) which is a two factor 20-item measure of self-reflection and insight (Cronbach’s α = 0.88). In the present sample, using principal axis factoring and promax rotation, both measures retained their original factor structures with the SOSS exhibiting a single factor which accounted for 36.3% of the variance and the SRIS exhibiting two factors which accounted for a combined 53.6% of the variance (Factor 1 = 34.3%, Factor 2 = 19.3%). Both measures were scored so that higher scores indicated stronger sense of self and greater levels of self-reflection and insight and both measures were significantly correlated ( r = 0.35, p < 0.001). Scores on these scales were then summed to create an overall trait self-awareness score with higher scores representing greater levels of trait self-awareness (Cronbach’s α across the total 32-items = 0.77; principal axis factoring with promax rotation revealed three factors accounting for 50.6% of the variance [Factor 1 = 24.6%, Factor 2 = 21.8%, Factor 3 = 3.9%]).
Six pairs of images of paintings by Paul Klee and Wassily Kandinsky were utilized as the painting stimuli.
The experiment was administered online. Once consent to participate was provided, participants were informed they would be required to choose their preferred painting from six pairs of paintings which were then presented sequentially. All paintings were presented without the artists’ names attached to any of the works. After making their painting selections participants were randomly assigned to one of two conditions (a reasoning pre resource allocation, similarity and liking ratings condition or, a reasoning post resource allocation, similarity and liking ratings condition). Participants in both conditions were presented with all the same stimuli and experiences except the order of exposure was manipulated slightly between conditions as outlined below.
In the reasoning pre condition, after the initial painting selection phase, participants took part in the self-reflective reasoning phase. In the self-reflective reasoning phase participants were presented with and asked to reflect on a 15-item list of potential reasons for their painting selections and then presented with an open text box and asked to reflect further in their own words about their reasons for their painting choices. Following this participants were presented with and completed the subjective meaningfulness measure. Then although they remained unware to it at the time, irrespective of their actual choices participants were informed that their choices indicated that they preferred the works of Paul Klee 2 . Participants were then presented with instructions pertaining to the completion of the Tajfel matrices before moving on to complete them. Following this, participants were presented with the in-group∖out-group similarity and liking measure. Participants then completed the trait self-awareness measures before recording their gender (female, male, or other) and age. A manipulation check was then conducted whereby participants were asked to indicate who they had previously been informed that their painting choices indicated they preferred the works of (possible response were, Paul Klee, Wassily Kandinsky, or Don’t remember). Participants were then presented with a debriefing statement, informed the experiment was over and thanked for their participation.
In the reasoning post condition, the order of exposure was manipulated so that after making painting selections, participants were told their choices indicated a preference for Paul Klee 3 and were administered with the matrices and in-group∖out-group similarity and liking measures before the self-reflective reasoning phase. After completing the choice reasoning phase and the subjective meaningfulness measure, participants in this condition were also then presented with the same trait self-awareness 4 measures, demographic questions, manipulation check and debriefing as their counterparts in the alternate condition.
Outlier Analysis
Three multivariate outliers (1 in the control and 2 in the self-reflection condition) were detected and removed from the analysis thereby leaving a total sample of 171 (86 in the control condition and 85 in the self-reflection condition).
Descriptive Statistics
Descriptive statistics for trait self-awareness, choice meaningfulness, in-group similarity, in-group liking, and in-group favoritism as a function of self-reflection condition are presented in Table Table1 1 .
Means and standard deviations for trait self-awareness, choice meaningfulness, similarity, liking, and in-group favoritism by self-reflection condition.
Effect of Experimental Manipulation on IV’s
We conducted between groups analyses to investigate if the self-reflection and control groups differed on the IV’s of choice meaningfulness and trait self-awareness as a function of the self-reflection manipulation. Independent samples t -test’s revealed that there was no significant difference in choice meaningfulness ( p = 0.365) or trait self-awareness ( p = 0.218) between conditions thereby demonstrating the IV’s were robust to the self-reflection manipulation.
Multiple-Sample Path Analysis
We ran a multiple-sample path analysis using the structural equation modeling program MPLUS (v 7.4) to investigate if the main effects of meaningfulness and trait self-awareness as well as the interaction effects (i.e., choice meaningfulness × trait self-awareness) on the DV’s differed between the experimental self-reflection and control non-self-reflection conditions. The model tested three exogenous/independent variables all predicting the three endogenous/dependent variables, in-group favoritism, similarity and liking. The exogenous variables were the main effects of trait self-awareness and choice meaningfulness, and a trait self-awareness × choice meaningfulness interaction.
Because the parameter to case ratio was under the required minimum of 1 parameter to 5 cases (1:4.75 or 36:171) as suggested by Kline (2011) , we discreetly tested each section of the model. In other words, three independent models with each of the three endogenous dependant variables were examined separately thereby ensuring that the parameter to case ratio was sufficient (i.e., 1:17.1 or 10:171). In all models the Satorra–Bentler robust estimator was used to account for multivariate non-normality, and all parameters were free across the self-reflection and control conditions. Chi-square Wald tests were utilized on a fully unconstrained model to test significant differences in the effects across conditions given the expectation that there would be differences in regression weights across groups ( Muthén and Muthén, 1998–2017 ). There were no significant differences in the results of these separate models and the full model 5 . Given this, the parameters for the full model are presented in Table Table2 2 . Because the model was saturated with zero degrees of freedom fit indices are not reported.
Unstandardized regression weights and Wald Tests for the multi-sample path analysis.
Main Effects of Choice Meaningfulness and Trait Self-Awareness
The results in Table Table2 2 reveal that there was a significant main effect for choice meaningfulness in both the control and self-reflection conditions for similarity, however, Wald tests reveal that the difference in effects between conditions was not significant. This suggests that higher choice meaningfulness scores were associated with higher similarity scores in both the self-reflection and control conditions. The results in Table Table2 2 also demonstrate that there was a significant main effect of choice meaningfulness for liking in the self-reflection condition whereas the main effect of choice meaningfulness for liking in the control condition was not significant. The Wald test demonstrates that this difference in effects between conditions was significant, suggesting that higher choice meaningfulness scores were associated with higher liking scores in the self-reflection condition only. Whilst there was also a significant main effect of trait self-awareness on liking in the self-reflection condition, the Wald test demonstrates that this was not significantly different from the non-significant main effect of trait self-awareness in the control condition.
Trait Self-Awareness × Choice Meaningfulness Interaction Effects
As seen in Table Table2 2 , for liking, the interaction between trait self-awareness and choice meaningfulness was only significant in the self-reflection condition and as the significant Wald test demonstrates, the strength of this interaction effect was also significantly different between the control and self-reflection conditions (see Figure Figure2 2 ).

Predicted liking scores by trait self-awareness and choice meaningfulness groups across conditions. Groups were defined as 1 standard deviation above (high) and below (low) the mean (moderate).
The results in Figure Figure2 2 suggest that for the self-reflection group the relationship between choice meaningfulness and liking strengthens as trait self-awareness scores decrease. That is, higher choice meaningfulness scores appear to be strongly associated with higher liking scores for those with lower trait self-awareness scores. This demonstrates a stronger impact of the self-reflection manipulation on participants lower in trait self-awareness and a reduction in the impact of the manipulation as trait self-awareness levels increase. Parallel trends were observed for similarity though the Wald test was only marginally significant.
The present study explored whether engaging in self-reflective reasoning could affect in-group identification and thereby demonstrate an effect of self-reflection on indicators of social identity and the self-concept. The possibility that such an effect could be impacted by the perceived level of meaningfulness associated with reasoning, and modulated by individual differences in trait self-awareness was also explored. Based on previous research, we developed a model which predicted that participants with higher levels of trait self-awareness would be minimally affected by the self-reflection manipulation. It was therefore hypothesized that for these participants self-perception would be related to the perceived meaningfulness of their painting choices more so than condition. The model further predicted that the self-reflection manipulation would have a greater impact on participants lower in trait self-awareness. Consequently, it was further anticipated that for these participants, self-perception would be related to perceived meaningfulness of their painting choices only in the experimental condition (i.e., when they were prompted to self-reflect). Participants’ in-group similarity and liking ratings (but not in-group favoritism allocations) supported these predictions and provided general support for the theoretical model proposed earlier.
Considering first the main effects of meaningfulness across conditions, the data demonstrated that whilst greater levels of meaning were associated with greater in-group similarity scores in both conditions, greater levels of meaning were only associated with in-group liking scores in the self-reflection condition. Taken together these results suggest that compared to the control condition, in the self-reflection condition stronger perceptions of meaningfulness led to stronger in-group identification. This is in line with predictions 1 and 2 relating to the self-reflection pathway in the theoretical model. Additionally, in line with prediction 3 of the theoretical model relating to the no self-reflection pathway, the effect of meaning on in-group perceptions in the control condition was smaller relative to the self-reflection condition. Moreover, the fact that there was no interaction between trait self-awareness and choice meaningfulness in the control group is suggestive of the possibility that in this condition, the main effect of meaning on in-group liking was the product of automatic or implicit processing because it occurred in the absence of any situational prompting and was not also impacted by pre-existing individual dispositions toward spontaneous self-reflection.
At the same time, the interaction between trait self-awareness and choice meaningfulness in the experimental condition indicates that the relationship between perceptions of choice meaningfulness and in-group liking strengthens as trait self-awareness levels decrease. This result suggests that the situational self-reflection prompt exhibited a stronger impact on participants who were less inclined (in terms of individual disposition) to engage in spontaneous self-reflection, and had less of an impact on participants with greater levels of individual disposition toward spontaneous self-reflection. This provides support for the hypothesis presented earlier and is also in line with predictions 4 and 5 of the theoretical model. A similar trend was also noted for in-group similarity, however, the difference in the strength of the effect between conditions was only marginally significant.
Limitations and Future Directions
One aspect of the study that could be viewed as both a limitation and strength is the way in which identity was measured. In the present study utilizing an already established experimental paradigm we developed a subtle way of testing the effect of self-reflective reasoning on identity. However, the cognitive process we were investigating was a process theorized from a narrative identity theory perspective, and the methodology used was born out of the social identity theory literature. On the one hand this approach represented a strength of the design in that it facilitated a discreet measurement of the effect of self-reflective reasoning on identity. At the same time, however, there are differences in the way that identity is conceptualized across both projects. To provide a stronger test of the hypothesis that self-reflective reasoning can affect narrative identity, experimental work with a more traditional dependent measure of narrative identity would be useful.
Another limitation was the way in which trait self-awareness was operationalized. In the present study trait self-awareness was operationalized as a function of participants’ scores on the SOSS and the SRIS. Whilst we had good reason to combine and operationalize these measures as a means of measuring trait self-awareness, future research aimed at the development of a dedicated measure of trait self-awareness would be worthwhile.
Though we were able to ensure that the modeling we conducted was sufficiently powered the present study could have benefited from a larger sample size. In the present study the parameter to cases ratio for the overall model was less then recommended (e.g., Kline, 2011 ). Therefore as outlined in the results section, to ensure that our modeling was sufficiently powered we initially computed three discreet models, one for each dependent variable. Although there was no significant difference in the outcomes between the individual and the combined models, in future to avoid the necessity of running independent models for each dependent variable it would be beneficial to recruit a larger sample which meets the parameter to cases ratio for the entire model in the first instance.
It is also possible that the deception that we engaged in (i.e., providing all participants with feedback that they preferred the work of Klee, irrespective of their actual choices) could have raised suspicions amongst participants who may have actually had some pre-existing knowledge of Klee and∖or Kandinsky (i.e., the artists whose works were used as the choice stimuli). Whilst we did include a manipulation check to ensure that the deception had had its intended effect, in future research, to address this issue more comprehensively it would be beneficial if participants were also directly questioned about their pre-existing knowledge of the artists whose works are used as the choice stimuli. Another way that this issue could be controlled for in the future would be to use the works of unknown artists as the choice stimuli.
Implications
The results of the present study may be of value to researchers who are interested in the developmental trajectory of narrative identity and autobiographical reasoning. Previous research looking at the development of narrative identity has suggested that the ability to cultivate a life-story tends to arise on average by about 14 years of age and that this is preceded by autobiographical reasoning for memorable life events which tends to first arise between the ages of nine and ten ( Bohn and Berntsen, 2008 ). Little is known, however, about the antecedents to the onset of autobiographical reasoning processes. Whilst it could be the case that development of autobiographical reasoning processes occurs in a stepwise fashion with little preceding them, the results of this study which demonstrate that reasoning about a trivial choice can effect the self and identity, beg the question that perhaps autobiographical reasoning processes develop as a continuous extension of more basic self-reflective reasoning processes which develop earlier in childhood. Perhaps it is the practice of more basic self-reflective reasoning which lays the cognitive foundations for, and facilitates the development of, more advanced autobiographical reasoning. One piece of recent research which dovetails with this idea comes from Bryan et al. (2014) who found in their work that children between the ages of three and six are already engaging in everyday decision making behaviors that are motivated by their developing sense of self and identity.
The fact that we observed significant effects for in-group identification and no effects on in-group favoritism has implications for researchers interested in intergroup discrimination and self-categorization. Specifically, the effects that we observed for in-group liking and in-group similarity suggest that self-categorization is likely to be influenced by both self-reflection and the level of subjective meaningfulness associated with choices or behaviors on which self-categorization is based. The absence of any effect on in-group favoritism suggests that intergroup discrimination is unlikely to be substantially impacted by self-reflection or choice meaningfulness. Research which has investigated positive-negative asymmetry within a minimal group paradigm context may help explain the discrepancy in effects between the attitudinal and behavioral measures. Positive-negative asymmetry research (see, Buhl, 1999 ; Mummendey et al., 2000 ) has demonstrated that group members tend to display stronger in-group preferences on positive stimuli compared to negative stimuli (i.e., evaluations of well regarded attributes such as creativity or intelligence, verses allocations of aversive noise). Given this research, one possibility that exists then is that in the current study, the attitudinal in-group liking and similarity measures which required participants to evaluate group members along positive dimensions were perceived more favorably compared to the behavioral measure of in-group favoritism which required participants to make allocation choices that had the potential to disadvantage out-group members.
Another possible explanation, however, for the absence of an effect on in-group favoritism could be due to aspects of the wider cultural climate within which participants were located at the time. Specifically, when this experiment took place Australia remained in the midst of a nation-wide debate regarding the legalization of same-sex marriage. Within the context of this debate university students have had strong messages of social justice and fairness directed at them at a cultural level. For example, the National Union of Students, which is the nations peak student representative body strongly advocated for students to support marriage equality ( Barlow, 2017 ). Given the cultural climate and the strong messages of social justice and fairness directed at students during the period in which this experiment took place, it is possible that in the allocation matrix tasks participants felt more compelled to engage in resource allocations which emphasized parity rather than discrimination. At the same time, in-group similarity and in-group liking ratings may have remained relatively immune to the impact of these cultural messages because perceptions of in-group identification do not necessarily equate to out-group discrimination and therefore do not have the same kinds of implications for one’s sense of fairness or social justice.
The present study may also have some implications for researchers whose work is informed by self-perception and cognitive dissonance theories. The results of the present study suggest that the application of self-reflection theory could be useful in some contexts in which cognitive dissonance and self-perception theories are not well positioned to explain the effect of choice or behavior on the self. According to self-perception theory ( Bem, 1972 ), after-the-fact explanations for behavior are generally limited to attributions about the internal (dispositional) or external (situational) cause of a behavior and are also only likely to occur in circumstances in which there is a weak or non-existent pre-existing explanation for the behavior. From the view of cognitive dissonance theory ( Festinger, 1957 ), post hoc reasoning about choices is limited to choices that induce dissonance and are motivated by a desire to reduce dissonance. Our model, however, suggests that choice or behavior is likely to effect the self as a consequence of whether it was actually perceived to be personally meaningful and that this needn’t be exclusive to dissonance inducing choices, nor to behaviors for which one does not have a pre-existing explanation.
Within the narrative identity literature, reflecting on life events in a personally meaningful way has been conceptualized as one of the key psychological mechanisms underpinning our sense of identity. To date, however, research on this issue has been largely correlational with little causal evidence available to confirm or disconfirm this claim. In the present study we sought to test experimentally if this cognitive process theorized to be so vital for identity development, could have a causal effect on self and identity. We also sought to explore the possibility that such an effect could be impacted by the level of meaningfulness associated with self-reflective reasoning, and modulated by individual differences in trait self-awareness. The results of this study largely supported our hypothesis and the proposed model from which those predictions were derived. For participants who were high in trait self-awareness, being prompted to engage in self-reflective reasoning mattered little. For this group of participants, in-group liking and similarity was related to perceptions of subjective meaningfulness relatively equally across conditions. At the same time, however, for participants low in trait self-awareness, being prompted to engage in self-reflection mattered a great deal. For these participants, subjective meaningfulness moderated in-group liking and similarity only when they had been prompted to engage in self-reflection. Overall the results of this study provide evidence to suggest that engaging in self-reflective reasoning can affect the self and identity and that this effect is impacted by both choices meaningfulness and individual differences in trait self-awareness.
Author Contributions
ND, JAO, CC, and JK all contributed to the paper and approved it for publication.
Conflict of Interest Statement
The authors declare that the research was conducted in the absence of any commercial or financial relationships that could be construed as a potential conflict of interest.
Funding. ND was supported by an Australian Government funded Research Training Program Scholarship.
1 Even though we predict that those high in trait self-awareness will be more likely to engage in self-reflection, given the magnitude of the relationship between trait self-awareness and perceptions of choice meaningfulness noted in our previous study (Dishon et al., under review) there is still scope for those high in trait self-awareness to find their choices meaningless.
2 Participants remained unware of this deception until they were debriefed at the end of the study.
3 As was the case in the reasoning pre condition, participants in this condition also remained unaware to the fact that they had received this feedback irrespective of their actual choices up until they were debriefed at the end of the study.
4 Even though individual differences in trait self-awareness represent a starting point in the theoretical model, in both conditions the trait self-awareness measure was administered after the self-reflection manipulation and in-group∖out-group ratings had taken place because we wanted to avoid potentially priming self-reflection processes in participants prior to their exposure to the self-reflection manipulation.
5 We also unpacked the in-group favoritism factor and ran the model on each of the individual pull scores. There was no significant difference between these models and the models using the in-group favoritism factor.
- Adler J. M., Lodi-Smith J., Philippe F. L., Houle I. (2016). The incremental validity of narrative identity in predicting well-being a review of the field and recommendations for the future. Pers. Soc. Psychol. Rev. 20 142–175. 10.1177/1088868315585068 [ PubMed ] [ CrossRef ] [ Google Scholar ]
- Bamberg M. (2011). Who am I? Big or small—shallow or deep? Theory Psychol. 21 122–129. 10.1177/0959354309357646 [ CrossRef ] [ Google Scholar ]
- Barlow K. (2017). The Massive Drive to Get Students to Enroll for Same-Sex Marriage Vote. The Huffington Post Australia. Available at: http://www.huffingtonpost.com.au [accessed August 22 2017]. [ Google Scholar ]
- Bem D. J. (1972). Self-perception theory. Adv. Exp. Soc. Psychol. 6 1–62. 10.1016/S0065-2601(08)60024-6 [ CrossRef ] [ Google Scholar ]
- Bohn A., Berntsen D. (2008). Life story development in childhood: the development of life story abilities and the acquisition of cultural life scripts from late middle childhood to adolescence. Dev. Psychol. 44 1135–1147. 10.1037/0012-1649.44.4.1135 [ PubMed ] [ CrossRef ] [ Google Scholar ]
- Bourhis R. Y., Sachdev I., Gagnon A. (1994). “Intergroup research with the Tajfel matrices: methodological notes,” in The Psychology of Prejudice: the Ontario Symposium on Personality and Social Psychology , eds Zanna M., Olson J. (Hillsdale, NJ: Lawrence Erlbaum; ), 209–232. [ Google Scholar ]
- Brehm J. W. (1956). Postdecision changes in the desirability of alternatives. J. Abnorm. Soc. Psychol. 52 384–389. 10.1037/h0041006 [ PubMed ] [ CrossRef ] [ Google Scholar ]
- Bruner J. S. (1990). Acts of Meaning. Cambridge, MA: Harvard University Press. [ Google Scholar ]
- Bryan C. J., Master A., Walton G. M. (2014). “Helping” versus “being a helper”: invoking the self to increase helping in young children. Child Dev. 85 1836–1842. 10.1111/cdev.12244 [ PubMed ] [ CrossRef ] [ Google Scholar ]
- Buhl T. (1999). Positive-negative asymmetry in social discrimination: meta-analytical evidence. Group Process. Intergroup Relat. 2 51–58. 10.1177/1368430299021004 [ CrossRef ] [ Google Scholar ]
- Carroll J. S., Johnson E. J. (1990). Decision Research: A Field Guide. Thousand Oaks, CA: Sage Publications, Inc. [ Google Scholar ]
- Ellemers N., Kortekaas P., Ouwerkerk J. W. (1999). Self-categorisation, commitment to the group and group self-esteem as related but distinct aspects of social identity. Eur. J. Soc. Psychol. 29 371–389. 10.1002/(SICI)1099-0992(199903/05)29:2/3<371::AID-EJSP932>3.0.CO;2-U [ CrossRef ] [ Google Scholar ]
- Erikson E. H. (1963). Childhood and Society. New York, NY: Norton. [ Google Scholar ]
- Festinger L. (1957). A Theory of Cognitive Dissonance. Standford, CA: Stanford University Press. [ Google Scholar ]
- Flury J. M., Ickes W. (2007). Having a weak versus strong sense of self: the sense of self scale (SOSS). Self Identity 6 281–303. 10.1080/15298860601033208 [ CrossRef ] [ Google Scholar ]
- Frankl V. E. (1969). The Will to Meaning: Principles and Application of Logotherapy. New York, NY: World Publishing. [ Google Scholar ]
- Grant A. M., Franklin J., Langford P. (2002). The self-reflection and insight scale: a new measure of private self-consciousness. Soc. Behav. Pers. 30 821–835. 10.2224/sbp.2002.30.8.821 [ CrossRef ] [ Google Scholar ]
- Grieve P. G., Hogg M. A. (1999). Subjective uncertainty and intergroup discrimination in the minimal group situation. Pers. Soc. Psychol. Bull. 25 926–940. 10.1177/01461672992511002 [ CrossRef ] [ Google Scholar ]
- Hains S. C., Hogg M. A., Duck J. M. (1997). Self-categorization and leadership: effects of group prototypicality and leader stereotypicality. Pers. Soc. Psychol. Bull. 23 1087–1100. 10.1177/01461672972310009 [ CrossRef ] [ Google Scholar ]
- Harvey P. O., Lee J., Horan W. P., Ochsner K., Green M. F. (2011). Do patients with schizophrenia benefit from a self-referential memory bias? Schizophr. Res. 127 171–177. 10.1016/j.schres.2010.11.011 [ PMC free article ] [ PubMed ] [ CrossRef ] [ Google Scholar ]
- Hogg M. A. (1992). The Social Psychology of Group Cohesiveness: From Attraction to Social Identity. London: Harvester Wheatsheaf. [ Google Scholar ]
- Hogg M. A. (1993). Group cohesiveness: a critical review and some new directions. Eur. Rev. Soc. Psychol. 4 85–111. 10.1080/14792779343000031 [ CrossRef ] [ Google Scholar ]
- Holdsworth L., Cartwright S. (2003). Empowerment, stress and satisfaction: an exploratory study of a call centre. Leadersh. Organ. Dev. J. 24 131–140. 10.1108/01437730310469552 [ CrossRef ] [ Google Scholar ]
- Katznelson H. (2014). Reflective functioning: a review. Clin. Psychol. Rev. 34 107–117. 10.1016/j.cpr.2013.12.003 [ PubMed ] [ CrossRef ] [ Google Scholar ]
- Klimmt C., Hefner D., Vorderer P., Roth C., Blake C. (2010). Identification with video game characters as automatic shift of self-perceptions. Media Psychol. 13 323–338. 10.1080/15213269.2010.524911 [ CrossRef ] [ Google Scholar ]
- Kline R. B. (2011). Principles and Practice of Structural Equation Modeling. New York, NY: Guilford Press. [ Google Scholar ]
- Leach C. W., Van Zomeren M., Zebel S., Vliek M. L., Pennekamp S. F., Doosje B., et al. (2008). Group-level self-definition and self-investment: a hierarchical (multicomponent) model of in-group identification. J. Pers. Soc. Psychol. 95 144–165. 10.1037/0022-3514.95.1.144 [ PubMed ] [ CrossRef ] [ Google Scholar ]
- Levy K. N., Clarkin J. F., Yeomans F. E., Scott L. N., Wasserman R. H., Kernberg O. F. (2006). The mechanisms of change in the treatment of borderline personality disorder with transference focused psychotherapy. J. Clin. Psychol. 62 481–501. 10.1002/jclp.20239 [ PubMed ] [ CrossRef ] [ Google Scholar ]
- McAdams D. P. (1985). Power, Intimacy, and the Life Story: Personological Inquiries into Identity. New York, NY: Guilford Press. [ Google Scholar ]
- McAdams D. P. (2001). The psychology of life stories. Rev. Gen. Psychol. 5 100–122. 10.1037/1089-2680.5.2.100 [ CrossRef ] [ Google Scholar ]
- McAdams D. P., McLean K. C. (2013). Narrative identity. Curr. Dir. Psychol. Sci. 22 233–238. 10.1177/0963721413475622 [ CrossRef ] [ Google Scholar ]
- McAllister I. (2016). The Mediating Role of Psychological Empowerment on the Relationship between Job and Personal Resources and Employee Engagement. Master’s thesis, San Jose State University, San Jose, CA. [ Google Scholar ]
- Miramontez D. R., Benet-Martinez V., Nguyen A.-M. D. (2008). Bicultural identity and self/group personality perceptions. Self Identity 7 430–445. 10.1080/15298860701833119 [ CrossRef ] [ Google Scholar ]
- Morin A. (2011). Self-awareness part 1: definition, measures, effects, functions, and antecedents. Soc. Pers. Psychol. Compass 5 807–823. 10.1111/j.1751-9004.2011.00387.x [ CrossRef ] [ Google Scholar ]
- Mummendey A., Otten S., Berger U., Kessler T. (2000). Positive-negative asymmetry in social discrimination: valence of evaluation and salience of categorization. Pers. Soc. Psychol. Bull. 26 1258–1270. 10.1177/0146167200262007 [ CrossRef ] [ Google Scholar ]
- Muthén L. K., Muthén B. O. (1998–2017). Mplus User’s Guide. 8th Edn Los Angeles, CA: Muthén & Muthén. [ Google Scholar ]
- Otten S. (2016). The minimal group paradigm and its maximal impact in research on social categorization. Curr. Opin. Psychol. 11 85–89. 10.1016/j.copsyc.2016.06.010 [ CrossRef ] [ Google Scholar ]
- Pasupathi M. (2015). “Autobiographical reasoning and my discontent: alternative paths from narrative to identity,” in The Oxford Handbook of Identity Development , eds McLean K. C., Syed M. (New York, NY: Oxford; ), 166–181. [ Google Scholar ]
- Pasupathi M., Mansour E., Brubaker J. R. (2007). Developing a life story: constructing relations between self and experience in autobiographical narratives. Hum. Dev. 50 85–110. 10.1159/000100939 [ CrossRef ] [ Google Scholar ]
- Phillips D. M., Olson J. C., Baumgartner H. (1995). Consumption visions in consumer decision making. Adv. Consum. Res. 22 280–284. [ Google Scholar ]
- Rogers T. B., Kuiper N. A., Kirker W. S. (1977). Self-reference and the encoding of personal information. J. Pers. Soc. Psychol. 35 677–688. 10.1037/0022-3514.35.9.677 [ PubMed ] [ CrossRef ] [ Google Scholar ]
- Sass L. A., Parnas J. (2003). Schizophrenia, consciousness, and the self. Schizophr. Bull. 29 427–444. 10.1093/oxfordjournals.schbul.a007017 [ PubMed ] [ CrossRef ] [ Google Scholar ]
- Singer J. A., Blagov P., Berry M., Oost K. M. (2013). Self-defining memories, scripts, and the life story: narrative identity in personality and psychotherapy. J. Pers. 81 569–582. 10.1111/jopy.12005 [ PubMed ] [ CrossRef ] [ Google Scholar ]
- Spreitzer G. M. (1995). Psychological empowerment in the workplace: dimensions, measurement, and validation. Acad. Manage. J. 38 1442–1465. 10.2307/256865 [ CrossRef ] [ Google Scholar ]
- Spreitzer G. M., Kizilos M. A., Nason S. W. (1997). A dimensional analysis of the relationship between psychological empowerment and effectiveness, satisfaction, and strain. J. Manag. 23 679–704. [ Google Scholar ]
- Symons C. S., Johnson B. T. (1997). The self-reference effect in memory: a meta- analysis. Psychol. Bull. 121 371–394. 10.1037/0033-2909.121.3.371 [ PubMed ] [ CrossRef ] [ Google Scholar ]
- Tajfel H. (1981). Human Groups and Social Categories: Studies in Social Psychology. Cambridge, MA: Cambridge University Press. [ Google Scholar ]
- Tajfel H., Billig M. G., Bundy R. P., Flament C. (1971). Social categorization and intergroup behaviour. Eur. J. Soc. Psychol. 1 149–178. 10.1002/ejsp.2420010202 [ CrossRef ] [ Google Scholar ]
- Tajfel H., Turner J. C. (1986). “The social identity theory of intergroup behavior,” in The Psychology of Intergroup Relations , eds Worchel S., Austin W. (Chicago, IL: Nelson-Hall; ), 7–24. [ Google Scholar ]
- Wilson T. D., Lisle D. J., Schooler J. W., Hodges S. D., Klaaren K. J., LaFleur S. J. (1993). Introspecting about reasons can reduce post-choice satisfaction. Pers. Soc. Psychol. Bull. 19 331–339. 10.1177/0146167293193010 [ CrossRef ] [ Google Scholar ]
ORIGINAL RESEARCH article
Know yourself: physical and psychological self-awareness with lifelog.
- Department of Computer Science and Technology, Institute for Artificial Intelligence, Beijing National Research Center for Information Science and Technology, Tsinghua University, Beijing, China
Self-awareness is an essential concept in physiology and psychology. Accurate overall self-awareness benefits the development and well being of an individual. The previous research studies on self-awareness mainly collect and analyze data in the laboratory environment through questionnaires, user study, or field research study. However, these methods are usually not real-time and unavailable for daily life applications. Therefore, we propose a new direction of utilizing lifelog for self-awareness. Lifelog records about daily activities are used for analysis, prediction, and intervention on individual physical and psychological status, which can be automatically processed in real-time. With the help of lifelog, ordinary people are able to understand their condition more precisely, get effective personal advice about health, and even discover physical and mental abnormalities at an early stage. As the first step on using lifelog for self-awareness, we learn from the traditional machine learning problems, and summarize a schema on data collection, feature extraction, label tagging, and model learning in the lifelog scenario. The schema provides a flexible and privacy-protected method for lifelog applications. Following the schema, four topics were conducted: sleep quality prediction, personality detection, mood detection and prediction, and depression detection. Experiments on real datasets show encouraging results on these topics, revealing the significant relation between daily activity records and physical and psychological self-awareness. In the end, we discuss the experiment results and limitations in detail and propose an application, Lifelog Recorder , for multi-dimensional self-awareness lifelog data collection.
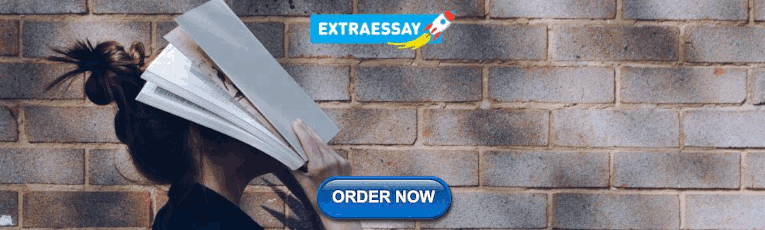
1. Introduction
As an important concept in biological and psychological studies, self-awareness is the experience of own personality or individuality of an individual ( 1 ). It describes how an individual consciously understands their character, feelings, and desires. The previous studies on self-awareness mainly explored the physical and mental status of people with human effort, such as field research study ( 2 ), user study ( 3 ), and questionnaires ( 4 ). However, these methods are time-consuming and limited to the laboratory environment, which is impossible for large-scale applications in daily life.
With the development of portable devices and storage techniques, recording daily life with digital sensors, such as wristbands and smartphones, becomes increasingly popular. As a result, lifelog, as the data reflection on life experience passively gathered and processed with multimedia sensors ( 5 ), has drawn attention in academy and industry. The lifelog provides a new possibility for self-awareness detection, as it has been proved that daily activity reflects the subjective health status of people ( 6 ).
Current lifelog analysis mainly focuses on the objective reflection of lifelog for a specific short-term goal, such as scene searching, diet monitoring, and item recommendation. However, the ultimate goal of lifelog should be to record detailed memories in the daily activities of an individual and help people better understand and live their life ( 5 ). Therefore, it is necessary to move from objective analysis to subjective understanding of lifelog, and connect lifelog with the lifelogger more tightly.
Having noticed this tendency in lifelogging research studies, we propose to improve the life-long self-awareness of people on the physical and mental status with the help of lifelog records. In this study, we integrate a series of our recent research studies about applying lifelogging to detect subjective self-awareness, revealing the great potential of lifelog on self-exploring for ordinary people.
To be specific, four topics about life-long self-awareness exploration based on lifelog data are introduced, from physical analysis on sleep quality prediction , to psychological exploration about static personality detection , real-time mood detection and prediction , and further depression mood detection . Generally, experiments on all topics follow the same schema: 1) lifelog data collection, 2) activity features extraction, 3) label tagging, and 4) prediction/detection with popular machine learning models. All four topics give insight into the relation between lifelog and the physical/psychological status of lifeloggers, and the experiments show that lifelog does reflect abundant information for self-understanding. However, due to the limitation of dataset collection, cross-task analyses are not applied in these experiments. To formalize lifelog data collection and achieve the goal of long-term stable records for ordinary people, we build an application for semi automatic multi-dimensional dataset collection, which frees users from integrating heterogeneous data, helpful for the more in-depth research studies on lifelog and psychology in the future.
The main contributions of this study are as follows: 1) We reveal a new inter-disciplinary direction for lifelog and psychology. A relation from objective data to subjective self-awareness is established. Moreover, four new topics were proposed about sleep, personality, and mood. To the best of our knowledge, this is the first study to conduct these subjective understanding tasks via lifelog records. Traditionally, psychological experts assist people in detecting their status. With lifelog, ordinary people can make daily detection by themselves, and early awareness of physical and mental diseases (e.g., depression) is possible. 2) We summarize a schema for lifelog-based analysis, prediction, and intervention, providing a flexible and general framework for practical applications on multiple scenarios. Different from traditional machine learning problems, lifelog data are usually highly heterogeneous and personalized, based on a self-collected dataset. So the data collection, feature processing, and label tagging occupy a large chunk in the experiments. 3) Experiments on real data show encouraging results on multiple self-awareness tasks, demonstrating the capacity of lifelog for automatic perception on self-awareness. Moreover, we provide a novel light-weight method and application for collecting lifelog data. 4) Active or passive data by simple portable devices are supported in experiments. Meanwhile, the applied models are light and possible to deploy on personal devices such as smartphones. This means the data collection and analysis can be dealt with locally, which helps protect privacy for users.
In the rest of this study, we will review the related work in section 2, and give an overview of the schema and topics in section 3. Then, how we adopt the four topics about lifelog-based self-awareness are introduced, respectively, in sections 4–7. After that, in section 8, we will summarize and discuss the results and limitations of our experiments. Based on the discussions, the data collection method and application are shown in section 9. Finally, conclusions and possible future directions are present in section 10.
2. Related Work
2.1. personalized lifelog research study.
With the development of portable devices, lifelog collection and storage have become convenient. Therefore, lifelog-based research study has drawn much attention, especially personalized applications.
One of the most fundamental and essential tasks for lifelog is to build search engines on the recorded data ( 7 , 8 ). As lifelog includes details about personal daily life, it can serve as memory assistance, helping people recall events in the past. Various search engines are built for searching lifelog in an interactive manner ( 9 – 11 ), where users can scan the lifelog records and give real-time feedback to search engines. The lifelog records are also used for self-monitoring on daily activities such as diet ( 12 ), smoking cessation ( 13 ), and exercise ( 14 ). These studies utilize active or passive logging records to help users adjust behaviors. However, the self-monitoring research studies focus on the objective collection and recording of daily activities without in-depth analysis and understanding of the data. Further, some researchers pay attention to enhance traditional retrieval tasks with lifelog information. For example, Kumar et al. ( 15 ) give suggestions for activities from history timeline records in lifelog. Uno et al. ( 16 ) recommend music based on contextual phenomena and the historical preference of users. Nakamura et al. ( 17 ) transform lifelog into text and recommend similar TV programs for users.
These previous research studies either focus on the objective reflection of lifelog or design for an external short-term goal. However, they ignore that lifelog data are powerful for exploring the overall status of a recorder, especially mental status. The most distinctive feature of lifelog is that it can record information about the passively and thoroughly. Therefore, lifelog can help reflect the physical and mental status of user over a long period. It is valuable and possible to utilize lifelog for real-time and life-long self-awareness for ordinary people without professional intervention.
2.2. Machine Learning in Psychology
As the fastest-growing application of computer science, machine learning is widely used for various traditional scenarios, developing interesting topics in inter-disciplines ( 18 ). Psychology is one representative example. Since more and more precise sensors are used for research studies on psychology, it becomes difficult for the manual effort to deal with the large-scale data. Algorithms can help detect phenomena and features in the records, and mine the underlying information about human activity.
On one hand, machine learning algorithms are used for modeling human behavioral patterns in traditional psychological laboratory studies. One typical example is that Koelstra et al. ( 19 ) proposed a well-known dataset in emotion analysis, Deap, which includes electroencephalogram (EEG), facial expression, and other biometric data of 32 participants watching emotional music videos. Based on the dataset, a large amount of machine learning methods were proposed to improve the emotion prediction accuracy or find in-depth information about emotion and physiological feature, including support vector machine (SVM) ( 20 , 21 ), convolutional neural network (CNN) ( 22 ), and residual long short-term memory (LSTM) ( 23 ). On the other hand, the development of portable devices and the internet has provided new resources for revealing the mental status of people. Machine learning, especially deep learning methods, is helpful for dealing with big data on the internet. Lin et al. ( 24 ) detected the stress level of users based on their tweet text and social relationship with the CNN model. Tweeter information is also used for suicide probability prediction with a deep learning model ( 25 ). Machine learning methods are also applied for digital personal information, such as phone use and smartphone sensor records, for stress and mood detection ( 26 ).
Compared with the laboratory studies and internet-based psychological research studies, lifelog-based research studies try to solve similar problems with entirely different information and records. Different from laboratory study data, lifelog is collected in an open environment, with complex and personalized simulations. So the lifelog records contain more noise and personal data. The lifelog-based study is also diverse from the large-scale internet research studies. Lifelog is more personalized, with more data for each user, but much fewer users in total. Therefore, more issues about privacy and individuation should be taken into consideration when designing machine learning algorithms in lifelog. There are already some research studies considering daily records and psychology, such as prediction of next mood record with history mood sequence using LSTM network ( 27 ), or filling missing data in heart rate with CNN encoder-decoder structure ( 28 ). However, they usually focused on one or two kinds of data in daily life. In this study, we try to consider the integration of multi-modal sensor data about user and environment to detect the mental status of user with machine learning methods, bridging the gap between daily records and mental health status.
3. Overview of Schema and Topics
First, we summarize a schema for applying lifelog to understanding self-awareness, as shown in Figure 1B . The schema consists of four steps:
• Step 1: Lifelog collection . Since there is no widely acknowledged public dataset about lifelog, we need to collect daily activities for each experiment with portable sensors passively and actively.
• Step 2: Feature extraction . After removing noise and invalid data, features are extracted and calculated from the lifelog by categories such as diet, activity, biometrics, and environment.
• Step 3: Label tagging . Labels are usually tagged manually with participants and then used for supervised learning of prediction or detection model.
• Step 4: Model learning . Many machine learning models can be used for training. However, we suggest not to use too complex models, such as deep learning methods. Because of privacy concerns, it is better to make predictions and even training on local mobile terminals. So light models are preferred for our lifelog analysis schema.
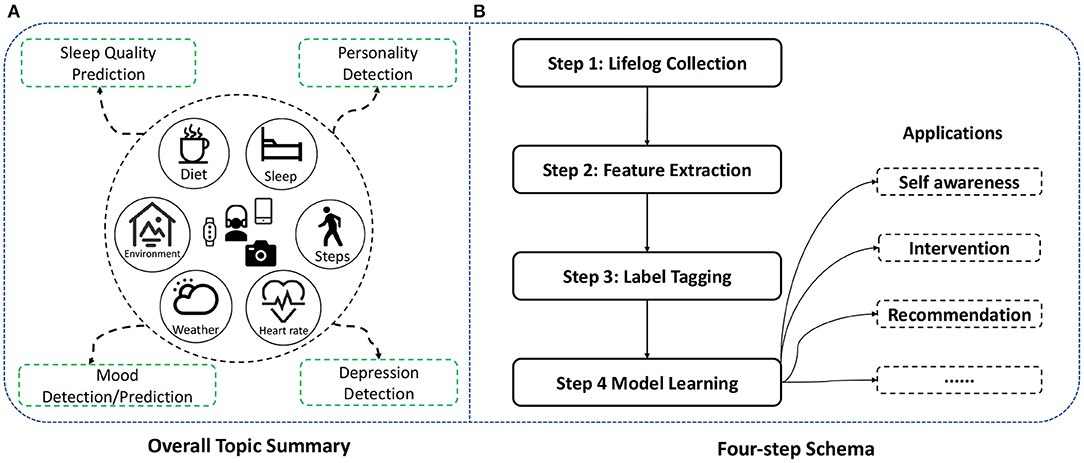
Figure 1 . An illustration for data and topic generation and the lifelog analysis schema. (A) Lifelog is recorded automatically with wristbands, smartphones, and cameras and then used for the four topics. (B) Four-step schema is proposed for analyzing each topic, and the model can be further used for various applications, such as self-awareness, status intervention, and activity recommendation.
Following the schema, the lifelog is first collected for each user, as shown in the middle of Figure 1 . Smartphones, wristbands, and wearable cameras are used for recording data automatically. Generally, various multi-modal data are gathered, including diet records, sleep quality, activity (e.g., steps), biometrics (e.g., heart rate), context information (e.g., weather), and environment. It is worth noting that not all types of data are compulsory for each task. Actually, the selection of records and features is quite flexible. According to the experiment condition or existing dataset, one or more categories of features can be used for experiments.
Based on the dataset collection, four topics are selected to explore the life-long physical and psychological self-awareness with lifelog. We start from the physical understanding, sleep quality prediction from lifelog. Instead of traditional monitoring methods during sleep, we study how to predict sleep quality in advance based on daytime activities. Then, we move to the psychological scenario, detecting the ability for personality detection of people with daily life records, which provides a time-saving method for predicting Big-Five personality. A more in-depth study on psychological application pays attention to real-time mood detection and prediction . Unlike stable personality, the mood is more flexible, so the prediction is more challenging. The mood detection and prediction are also quite meaningful, as mood awareness is essential for mental health. Finally, we take a further step about mood status, conducting depression detection for patients and healthy people. The abnormal mood detection task makes real-time mental intervention possible in daily life.
Life-long detection and intervention of self status are the focus for all four topics. By experiments on real datasets, we show that ordinary people can gain physical and mental self-awareness more fully and deeply with the help of lifelog.
With these self-awareness tasks based on lifelog, there can be many exciting and essential applications. When an explainable system for sleep quality prediction is conducted, the system can give suggestions at bedtime if poor sleep is predicted, such as drinking milk if the user is too nervous or doing some sports if the user lacks exercise at day. Based on real-time mood detection, the system can intervene when an abnormal mood is detected. For instance, it can play some peaceful music for the user or show suggestions about mood adjustment. If the user tends to self-harm or suicide, the system may also help contact family and doctors. These applications all focus on long-period detection of users and provide life-long benefits.
In experiments, the cold-start problem is especially considered. Information of some users is shown only in the test phase for models to evaluate the capacity of the system to deal with new users.
When analyzing the experiment results, we find it difficult to conduct cross-task comparison due to inconsistent dataset collection. Since lifelog collection is onerous work, it is hard to collect overall multi-dimensional data at a time. In order to free the lifeloggers from annoying work of labeling and integrating data, we provide an application Lifelog Recorder , and application (APP)-centered lifelog collection method, which is helpful for long-term data collection for non-professional users.
4. Sleep Quality Prediction
4.1. background and task definition.
First, we propose the sleep quality prediction task, a physical analysis of lifelog to predict daily sleep quality with the lifelog in about 12 h before sleep.
With the help of portable devices, we are able to measure sleep quality in real time. For instance, cardiotachometer and actigraphy are used in commercial smart wristbands to record heart rate and acceleration while users are sleeping. Based on the data, the analysis algorithm can calculate energy expenditure, sleep onset time, sleep efficiency, and others, and assess an overall score to reflect sleep quality.
High-quality sleep has positive effects on mood, productivity, and mental health and, therefore, is essential for daily life ( 29 ). Some researchers paid attention to monitoring physical status based on data during sleep ( 30 ). However, on the one hand, intervention is usually not available if low sleep quality is detected while the user is sleeping. On the other hand, psychological studies show that activities during the daytime have a significant relationship with sleep quality ( 31 ). Therefore, we investigate how to predict sleep quality in advance with daytime lifelog, so that intervention can be provided before sleep.
For user u at day d t , given daytime lifelog records l t at d t , and historical sleep quality s → = [ s 0 , s 1 , … , s t - 1 ] (recorded with sleep monitor or user questionnaires in terms of score/rating.), we try to extract features f → t and further predict his/her sleep quality score s t at d t :
where F and M represent the feature extraction process and the prediction model, respectively. F consists of various methods to obtain information from the multi-modal raw lifelog records.
4.2. Methods
In our study in the NII Testbeds and Community for Information access Research (NTCIR13) ( 32 ), we explore the sleep prediction task preliminarily. We follow the dataset and feature extraction in the study and conduct more experiments and in-depth analysis about sleep quality prediction. To be specific, we add more accurate models and try a different combination of weak classifier and ensemble models for overall prediction. We also add a discussion about the cold-start problem and feature ablation study. The new experiments are discussed in the next section. In this study, we first re describe the methods in the way of four-step schema:
1) Lifelog collection : Overall data collection is conducted with multiple wearable sensors, including wearable cameras, wristbands, and smartphones. Four categories of lifelog are recorded: biometrics, activities, external environment, and diet.
2) Feature extraction : For feature extraction process F , data are shift according to sleep time so that the last hour of the day is always the 24th h in order to ensure data consistency. Since activities closer to sleep time should be more important for prediction, we consider sum/average values all lifelog data in periods 23rd–24th, 19th–22nd, 11th–18th h as features. Given the lifelog records, statistic features are extracted, calculated, and min-max normalized in these three periods. Then, all features are concatenated to form the feature vector f → t .
3) Label tagging : The label for this task is the sleep quality. It can be scores calculated with smartphones and smart wristbands or ratings manually labeled by the user. A single score/rating is tagged for one night, and the historical sleep quality sequence is also concatenated with f → t and used for prediction.
4) Model learning : M can be conducted with any classifier. To balance the accuracy and efficiency, weak classifiers (e.g., decision tree and Bayes net) with ensemble learning algorithms (such as boosting and bagging) can constitute a classifier, which is then trained by supervised learning.
4.3. Experimental Settings
Dataset . We use data of two lifeloggers from NTCIR13 ( 33 ) and five from our own data collection. We only count in the data with sleep quality labels, which consist of 31 days on user 1, 22 days on user 2–4, 14 days on user 5, 55 days on user 6, and 134 days on user 7. In all, 300 instances (i.e., days) from 7 lifeloggers are collected in total.
Lifelog in five categories are recorded: Biometrics include heart rate and active/rest/total calorie burn; activities include steps and active/rest time; external environment is represented with indoor/outdoor time, weather, temperature, sun-rise and sunset time, and weekday/weekend; diets include nutrition/calorie consumption; sleep timing is the time for sleep.
Most of the features are records from commercial portable devices directly, such as smart phone, smart watch, and wristbands. Diet information is collected with a fitness application on smart-phone, where users enter the barcodes or types of their meals and snack manually, and the application will convert them into calorie intake. The only post-process feature is the active/rest time , which is decided by the percentage of active calories in total calories. If active calories take up more than 50% of total calories in duration, it will be determined as active time, otherwise as rest time.
In the NTCIR13 dataset, the sleep quality score is calculated with smart wristbands and recorded as an integer s between 0 and 100. Since we formalize the task as a classification problem, the numeric sleep scores are grouped into four nominal categories: “Good” for s ∈ [80, 100], “Borderline” for s ∈ [60, 80], “Poor” for s ∈ [40, 60], and “Sleepless” for s ∈ [0, 40].
After feature process F , feature vector f → t of four categories and the historical sleep quality are extracted.
For the prediction model, some popular classifiers, such as BayesNet, locally weighted regression (LWL), C4.5 decision tree, logistic model trees (LMT), random forest (RF), and decision table (DT), are chosen as basic weak classifiers. Ensemble algorithms AdaBoost and Bagging are used to aggregate the weak classifiers.
Evaluation metric . Prediction accuracy is used as the metric to evaluate classifier performance.
4.4. Experiments and Results
We conduct experiments on overall prediction performance, cold-start user prediction, and ablation study on features. Compared with our previous publication ( 32 ), we add more algorithms for classification in overall prediction, and the cold-start and ablation study tasks are both new.
4.4.1. Overall Prediction
We conduct 10-fold cross-validation on 300 instances of all seven users, to evaluate the performance of the various combinations of week classifiers and ensemble algorithm. At each fold, 10% (30 instances) of the dataset is split for the test set, and the others for the training set. Average accuracy among 10-fold is recorded as the final results.
The results of overall sleep quality prediction with lifelog are shown in Table 1 . Among all the methods, the combination of the C4.5 decision tree and bagging performs the best, reaching an accuracy of 77.44%. This demonstrates that activity records in the daytime can predict sleep quality. Tree-structured weak classifiers (i.e., C4.5, LMT, RF, and DT) all have good performance, while the linear model (LWL) performs worse, which indicates the relationship between lifelog features and sleep quality may be complex non linear relation. For most classifiers, ensemble algorithms will improve performance. However, random forest (RF) is an exception since RF itself is an ensemble model.
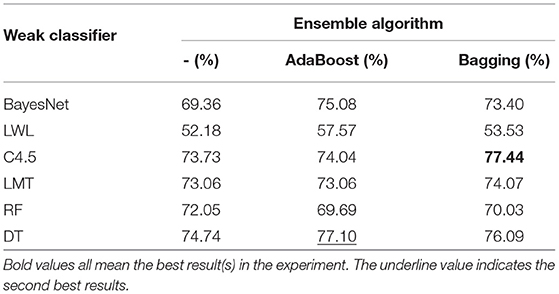
Table 1 . Results (in terms of accuracy) for overall sleep quality prediction of various classifiers with different ensemble algorithm.
4.4.2. Cold-Start User Prediction
Furthermore, we conduct cold-start user prediction to explore whether pre trained classifiers can be used for new users in sleep quality prediction. Moreover, we test the ability to make a prediction on a user without any history data by removing historical sleep features. At each time, we keep the instances of each user as the test set and train the models on the data of other six users.
The prediction accuracy results are shown in Figure 2 . It can be observed that training with data from strangers also gains encouraging results on the sleep quality prediction for cold-start users. Moreover, although user 7 have much more instances than the other 6 users, the models trained with user 1–6 still have good performance on user 7, and the other prediction performance does not skew as well. On the one hand, it illustrates that the prediction is rather trustworthy even if we have an imbalanced dataset. On the other hand, prediction accuracy varies with users. User 4 and user 6 have a good performance, while prediction on user 3 is less accurate. This results from the personalization of lifelog, and we speculate that user 3 may have different sleep habits from others. Surprisingly, removing sleep history features results in accuracy improvement for some users. The reason might be that some users have a large fluctuation in sleep status, which introduces noise if we consider the historical sleep records.
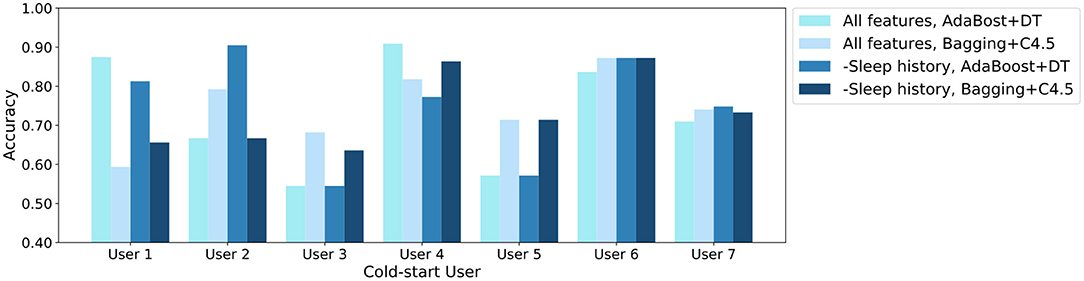
Figure 2 . The cold-start user sleep quality prediction accuracy for seven users. The experiments are conducted with all features and features except historical sleep ( - Sleep History ), respectively.
4.4.3. Ablation Study
We repeat the experiments using 10-fold cross-validation and remove one category of the feature at a time to analyze the effect of each feature category on our predictions.
As shown in Table 2 , sleep timing seems to have a big share in accurate prediction, while diet data do not have a significant effect on the validation result. The small impact of diet features might be because data on calorie intake are only available for a part of user 7 lifelog. Another important point is that some of the categories contain overlapped information, such as calories burned in the biometrics category and steps in the activity category and time spent outdoors in environmental features, and time spent at home or work in the activities category. This might reduce the effect of the removal of some categories.
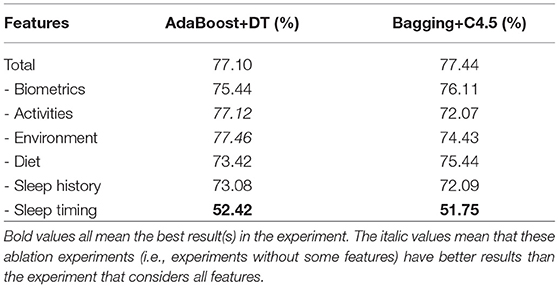
Table 2 . Results (in terms of accuracy) for ablation study on different features.
From the above experiments, it can be concluded that lifelog at daytime can help predict the sleep quality of the user in advance, and the prediction is efficient, even for cold-start users. The prediction of sleep quality is essential in the life-long term and can help people better understand their physical status.
In the future, recommendations on activities at bedtime can be a further research topic for better sleep quality.
5. Personality Detection
5.1. task definition.
In this section, we move from physical awareness to subjective psychological self-understanding with lifelog. This task aims to evaluate Big Five personality based on daily activities and context as the start of psychological study since personality is usually stable and essential for self-awareness.
Big Five personality ( 34 ), also known as the five-factor model (FFM), is a widely-used professional evaluation in psychology, consisting of five dimensions: Openness to Experience, Conscientiousness, Extraversion, Agreeableness, and Neuroticism. The traditional way to measure Big Five personality is by filling questionnaires, which is time-consuming and labor-intensive. Therefore, we propose a new idea to conduct personality evaluation with lifelog. In this way, a large amount of data can be collected and analyzed automatically to form Big Five personality results, available for large-scale applications.
To formalize, given lifelog records l in a period of time, we collect features f → and predict personality scores in five dimensions S → = { s 1 , s 2 , s 3 , s 4 , s 5 } with a prediction model M .
5.2. Findings
We proposed the personality detection task in our previous study ( 35 ). Following the dataset processing and experiment settings in that publication, we re conduct the experiments with LR (published before) and two more models (SVM and GBDT). We also report new results about performances on each user. For completeness, we summarize the training target and then show the new results and findings. Detailed information about dataset collection and feature selection can be found in the original study ( 35 ).
Target . We predict each of the five dimensions in the Big Five Personality as a classification problem. To be specific, the Neuroticism-Extraversion-Openness Five-Factor Inventory (NEO-FFI) questionnaire ( 36 ) is applied to test Big Five personality as the ground truth. The output includes five dimensions of personality, of which each dimension is a score between 0 and 5, and then binarized with threshold 3.
Results and Main Findings . Three traditional classifiers are used in our experiments, LR, SVM, GBDT. To evaluate the overall performance of personality prediction, we conduct five fold cross-validation and report average accuracy in Table 3 . Though only six features are taken into consideration, the performance of personality prediction is surprisingly excellent, with almost all prediction results approaching 100% for SVM and GBDT. Moreover, we perform the leave-one-participant-out experiment, i.e., predict on each user, with all the others as the training set, and the average accuracy of five dimensions is shown in Figure 3 . GBDT gains 100% accuracy on all users except user 29, and the simple LR also achieves 100% accuracy on more than half of the users. The promising results show that it is highly convincing to predict personality based on daily activities and habits.
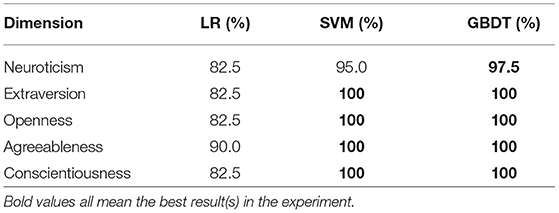
Table 3 . Personality prediction results (in terms of average accuracy of five fold cross-validation) on five dimensions of Big-Five personality.
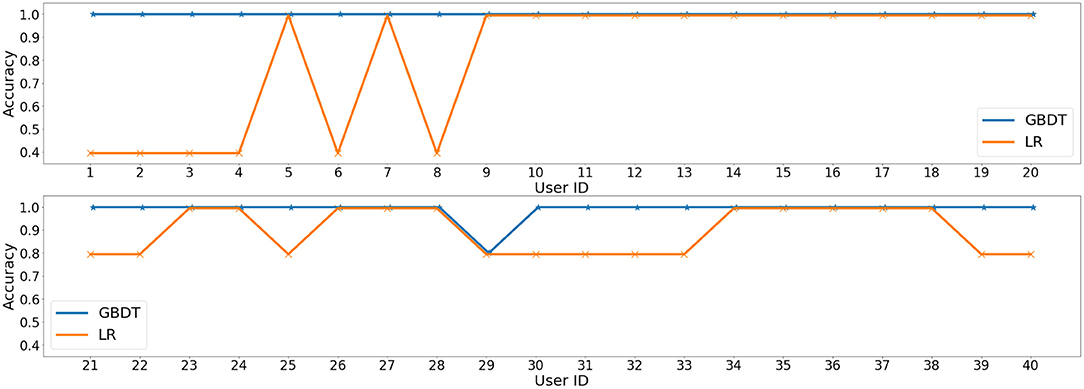
Figure 3 . Average accuracy on five dimensions for each participant with Logistic Regression (LR) and Gradient Boosting Decision Tree (GBDT), respectively.
Limitations . As the first attempt to predict personality, the present task is still quite simplified, with all personality scores binarized, and life records of all participants clear and intact. More complex processing and predicting methods should be considered in large-scale practical applications for personality detection.
6. Mood Detection
6.1. task definition.
We consider a more complex topic in psychological self-awareness, mood detection in daily life ( 37 ). As we discuss in section 5, lifelog can be used to deduce the mental status (i.e., personality) of people. However, personality is relatively stable and predictable. However, the mood is much more dynamic and complex, so it is valuable to explore whether mood changes are detectable and even predictable in advance with daily activity records.
The mood is the background feeling that can influence the thoughts and actions of a person ( 38 ). Since mental health is getting more attention, mood detection is an essential task for both health care and self-understanding. In previous research studies, interview, survey, or self-report activities have been used for mood detection, which can be biased and inaccurate ( 39 , 40 ). To capture mood changes and give real-time insights about personalized mood, we propose to detect and predict mood with automatically collected lifelog data.
For user u i at day d t , given daytime lifelog records l t , we define two tasks as follow:
• Mood detection : Detect u i mood at the wake-up time of day d t in two dimensions: Valence (Stress) and Arousal (Energy).
• Mood prediction : Predict u i mood at the wake-up time of day d t +1 in two dimensions: Valence (Stress) and Arousal (Energy).
6.2. Findings
We explore the mood detection and prediction tasks in our study in first AAAC Asian Conference on Affective Computing and Intelligent Interaction (ACII Asia 2018) ( 37 ). In this study, we list the target, main findings, and limitations of the study. Detailed information about the experiments, including dataset collection, feature extraction, and model training, can be found at Soleimaninejadian et al. ( 37 ).
Target . In the dataset, users choose mood labels every morning from a list of four kinds of mood, including happy, content, anxious, and depressed. Following the mood model of Thayer (41) , four kinds of mood are binarized into two dimensions, negative valence and arousal, that is: Happy : (0,1), Content : (0,0), Anxious : (1,1), and Depressed : (1,0). Accurate prediction on each dimension is set as the target of prediction and detection task.
Main findings . In the publication in Soleimaninejadian et al. ( 37 ), traditional machine learning methods show encouraging results on both detection and prediction tasks on two-dimensional mood. The performance of mood detection demonstrates that activities in the following day can reflect mood at wake-up time. Mood prediction result indicates that activities may influence mood status the next morning. In all, the experiment shows a close and significant relationship between mood states and daily activity, and the relation can be reflected by lifelog, which confirm that it is possible to track life-long mental health with daily records and timely.
Limitations . In this study, mood is recorded and predicted/detected daily. However, the mood is dynamically changing with time, recording once a day is too rough for mood detection. Moreover, mood rhythms exist in both short and long term ( 42 ). Therefore, in the future, more fine-grained labels and periodic changing should be considered for mood prediction and detection.
7. Depression Detection and Intervention
7.1. task definition.
In this section, we pay attention to detecting a kind of abnormal mood, depression, based on mood detection and prediction in the previous section.
Depression mood, as a reflection of the common psychological illness of the same name, is increasingly becoming an essential factor affecting the happiness of people. It makes people feel unpleasant and will endanger the life of the patients in severe cases ( 43 ). Therefore, in-time detection and intervention of depression mood are of great significance for mental health and life-long well-being. However, depression detection is more challenging than detection of normal mood. Since depression appears irregularly and unexpectedly ( 44 ), conventional methods like surveys and questionnaires are not able to detect the changes in time.
Lifelog is appropriate for detecting depression since it records activities automatically, and the machine learning model is fast enough to respond to unexpected depression mood. Therefore, we explore the competence of lifelog for depression detection:
Given lifelog l in a period of time and the corresponding series of mood records M → = ( m t 0 , m t 1 , … , m t n ) , depression detection is formulated as a classification problem: predicting m t i belongs to which type of mood with l t ( t < t i ) (0 ≤ i ≤ n ).
7.2. Methods
The depression detection task is a new task, and we have not published the results before. We describe the methods following the four-step schema for lifelog analysis:
1) Lifelog collection : Considering the convenience of data collection, a single wristband is used for lifelog collection, which can record activity intensity, activity type, and some primary biometrics data per minute.
2) Feature extraction : After removing invalid data, two groups of features are considered in data processing: activities and biometrics data. Min-max normalization is conducted on all features.
3) Label tagging : For mood labels, participants choose one of the 12 moods to describe their current status as shown in Table 4 . Participants are asked to label their mood once per hour. The categories are set according to the mood model of Thayer, as shown in Figure 4 . At the same time, participants should also record the activity they are doing. If they are engaged in multiple tasks, they only need to record the most important one. As we are interested in the intensity and valence of emotion, the 12 moods are classified into five categories (0–4), and experiments are evaluated on these categories.

Table 4 . Twelve types of mood labels for depression detection task and their categories according to Thayer's two-dimensional mood model.
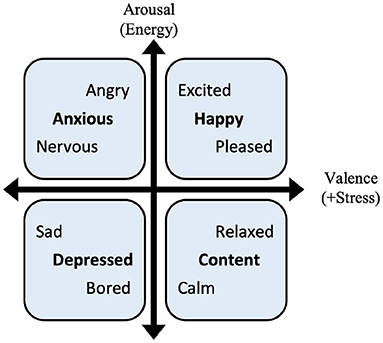
Figure 4 . Thayer's two-dimensional mood model ( 32 ).
Since mood labels are sparse, we then fill in the mood labels with two methods: 1) activity-based padding, when a user is engaged in the same activity, if the mood labels at the beginning and end of the activity are consistent, and the labels during the activity is padded the same as the beginning and end of the activity. If the labels are inconsistent at the beginning and the end of the activity, the data during the activity will be abandoned. We have to make a trade-off between label accuracy and data scale, since the mood labels are too sparse, while lifelog data are recorded per minute. 2) Label-based padding, since labels are usually recorded at the time when the mood is intense, and the change of emotion takes time, it can be considered that the labels of adjacent periods to the original mood label are consistent. In our experiment, we set the duration of adjacent periods as 2 min before and after the label.
4) Model learning : Same as the previous tasks, any classifier can work for depression detection. We choose two ensemble models, GBDT and random forest (RF), in experiments.
7.3. Experimental Settings
We collect lifelog records with wristbands from 14 healthy participants and 2 patients with depression. The patients are both clinically diagnosed, one as mild depression, and the other as moderate depression. They are well informed about the experiment. The collection includes activity type, activity intensity, steps, and heart rate, with a frequency of once a minute. During the experiment, three healthy participants dropped out. One healthy participant and one depression patient did not record any mood labels, so they are also removed from the dataset. In the end, we have 10 healthy participants and one depression patient in our data. In the process of data collection, due to the inevitable accidental occurrence of bad contact when wearing the wristbands, some of the records have missing heart rate or invalid activity intensity (labeled with 0 in the data). There are 31.3% invalid data in our collection. After removing the invalid data, the dataset contains 218,330 records during 241 days from 11 participants, varying from less than a week to more than a month per participant. The participants all report their data on consecutive days, and the concrete value of days for each user is shown in Table 5 .

Table 5 . Days of records for each user in our experiment. User 1-10 are ten healthy participants, and User 11 is the patient.
Four features in two categories are extracted: 1) Activities: activity intensity, activity type, and walking steps; 2) Biometrics: heart rate. The frequency of all the features is once per minute. Mood labels are complemented in each minute with the activity-based method and label-based method, respectively. Then, depression detection task is conducted on the 1-min features to predict the label in the minute.
For mood detection, the features within a minute are used for predicting the mood label in the minute. Since the short-term mood change is considered, the bound between detection and prediction is indistinct. In the following statement, we mix the statements of “mood detection” and “mood prediction.”
Since our lifelog dataset is not a large-scale collection, while simple classifiers are not able to achieve good performance, ensemble models are suitable for improving prediction performance. Therefore, two mainstream ensemble models, GBDT and Random Forest (RF), are applied for mood detection. In overall prediction and self-data prediction tasks, five-fold cross-validation is conducted for evaluation. As for the cold-start prediction, each user is taken as the test set to evaluate the model trained on 10 other users.
Accuracy on twelve-label classification is used as the evaluation metric.
7.4. Experiments and Results
7.4.1. overall prediction.
In overall prediction, five-fold cross-validation is conducted, and average accuracy is shown in Table 6 . It can be concluded that GBDT performs better than RF in our experiment and that the activity-based padding method for mood labels is more accurate in mood detection. Compared with the mood detection and prediction experiments in section 6, the detection accuracy is lower in this task. There are two main reasons. On the one hand, the mood labels are more complex and dense in the depression detection task, and the available lifelog data are less, so the depression detection task is more challenging. On the other hand, our system may reveal some mood changes that users themselves have ignored, as mood tags are labeled with subjective judgment. For instance, psychological research studies have shown that about 90% of people with depression often do not realize they may be depressed in the early stages of illness ( 45 ). Therefore, our detection model may help discover some early symptoms for abnormal mood and remind users to seek professional help and early intervention.
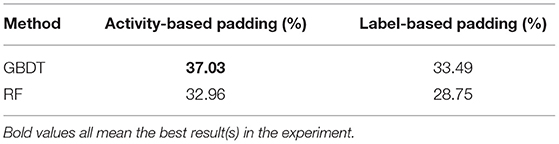
Table 6 . Overall results (in terms of accuracy) on depression prediction with activity-based and label-based label padding methods.
The prediction accuracy for each user is presented in the second column of Table 7 . Prediction accuracy is quite diverse for different users. For instance, user 5 gains quite high accuracy, while the accuracy is low for user 1, 2, 3, and 7. This indicates that mood awareness is quite personalized and motivates us to conduct self-data and cold-start user detection tasks.
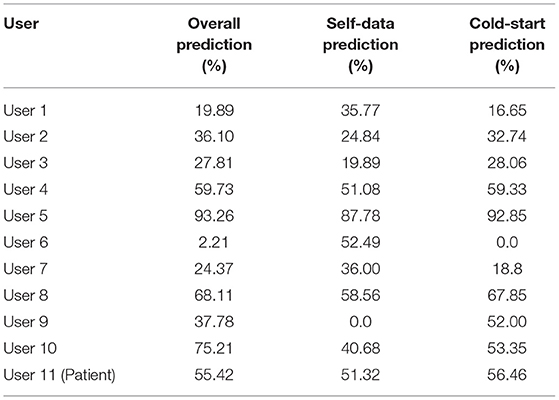
Table 7 . Depression detection results (in terms of accuracy) with GBDT and activity-based padding for each user.
7.4.2. Self-Data Detection
The data of each user are used for training a specific classifier for detection. Five fold classification is conducted for each user, and average accuracy is reported in the third column of Table 7 . Detection accuracy declines for users with good performance in the overall experiment, such as user 4, 5, 8, and 10. This is because less data is used for training the classifier. However, user 1, 6, and 7 have better performance in self-data prediction task. They may have strong specificity in mood patterns, which can be learned better with their own data.
We also notice that user 9 has zero accuracy in this task. This user has non-stable mood records, which is hard to be predicted with his/her data only.
7.4.3. Cold-Start Detection
We further investigate the cold-start problem by setting each user as the test set and all the other 10 users as the training set. The results are shown in the fourth columns in Table 7 . Cold-start results keep with overall prediction accuracy, which illustrates our classifier is suitable for new users in the depression detection task. Meanwhile, as the mood label is marked with the users, we conjecture that physical activity and mood awareness may be inconsistent for some users. The most classic example is user 6, for whom overall prediction and cold-start prediction tasks both fail, but self-data prediction gains good performance. After observing the data, we find that with almost the same activity intensity, user 6 has many labels in categories 1, while other participants stay calm or relaxed most time, which may explain the inaccuracy.
Furthermore, the cold-start prediction result (accuracy of 56.56%) of user 11 demonstrates that models trained with the data of healthy people can help predict the mood of the patients. This observation is encouraging as it reveals the similarity of mood awareness between healthy and. Therefore, we are able to design systems for patients with depression with data from healthy people or with historical data when the patient has not caught up with depression, which is of great value for practical applications.
In this task, we further investigate the relationship between psychological status and daily activity. The experiments on lifelog from healthy people and depression patients focus on more flexible and real-time detection of mood. The results show that it is possible to detect mood changes in real-time with lifelog records, demonstrating the power of lifelog on self-awareness in the psychological scenario.
It is worth mentioning that our system is a supplement and assistance for professional diagnosis, but can not replace the medical treatment by any means. In the future, deeper insight into the mechanism of emotion changes and carefully designed models for mood detection are two possible directions to improve the detection accuracy.
8. Discussion
Inspecting the experiments for four topics on the new direction of self-awareness with lifelog, we argue that the relation between objective lifelog data and subjective self-awareness is established efficiently. The novel direction gains insight into automatic self-tracking for life-long health and well being. We believe that it is a step toward the ultimate goal for lifelog study: to help people better understand and live their life ( 5 ).
8.1. Lifelog Information for Automatic Self-Awareness
In Table 8 , we summarize the categories of lifelog information used in each of the four topics: sleep quality (prediction), personality (detection), mood (prediction and detection), and depression (detection). It can be observed that biometrics data (e.g., heart rate and calorie burn) are used in all topics. Activity records, environment data, and mood records are used in most topics. These categories are exactly the main focus of lifelog at present, which can be captured with state-of-the-art wearable sensors easily.

Table 8 . A summary of lifelog information categories used in each of the four topics.
On the one hand, as we mentioned, the choice for categories is quite flexible, which means that the category combination shown in Table 8 is not compulsive for each topic but can adjust with the change of devices and record form.
On the other hand, the unused information is not meaningless for the tasks. For instance, daily activities can reflect some characteristics of personality. Sleep quality is definitely influenced by mood status. Due to the limitation of dataset collection design, we are not able to include all multi-dimensional data in our experiments. More in-depth research studies and analyses about the heterogeneous information are left for further analysis. We propose a method and application for collecting information in section 9, providing a paradigm of lifelog collection.
8.2. Direct and Indirect Information Extraction and Application
Both direct and indirect information are extracted from the multi-modal lifelog records. For instance, the environment category in sleep quality prediction and mood detection and prediction topics includes direct records such as weather and indoor/outdoor time, which can be captured easily. Besides, indirect features of room tidiness and decoration are contained in the external environment for personality detection topic based on reprocessing of raw image data.
The extraction process for both direct and indirect information indicates that lifelog contains abundant information for user status of great potential to explore in the future. Besides categories mentioned in Table 8 , more detailed features are possible to deploy in the lifelog research studies, such as micro-expression, computer usage, and smartphone usage.
Moreover, the lifelog feature extraction provides new tasks for traditional and inter disciplinary research studies. For example, in the personality detection topic, room tidiness level and decoration level are used as features, which are extracted from photographs taken by the user. However, the requirement of tidiness and decoration index can not be satisfied with traditional object detection task or image sentiment task in computer vision scenario but needs more knowledge about psychology. Therefore, it provides new challenges and opportunities for computer vision research studies.
8.3. Relationship Among Tasks
The encouraging results for experiments show a significant relationship between lifelog records and physical and mental status. Moreover, there are relations between different tasks, as shown in Figure 5 . As the most stable concept, personality provides the base for mood and the possibility of depression of an individual ( 46 ). Personality also has an influence on sleep quality. For instance, people with high neuroticism may have more problems falling asleep. Moreover, long-term bad mood or tension will cause depression, so mood detection can help discover depression at an early stage. Apparently, the daytime mood has the influence on sleep quality. On the one hand, feeling too excited or too sad will both lead to low sleep quality. On the other hand, having a good sleep helps people more energetic, so sleep quality is also important for mood detection. A similar relation also exists between depression and sleep quality.
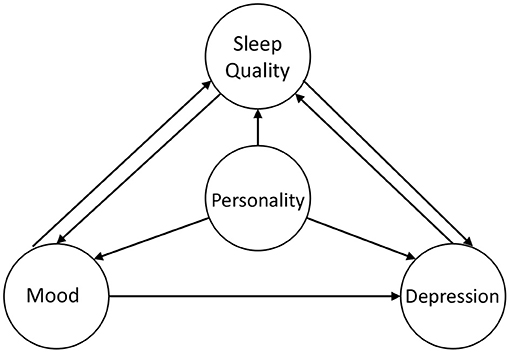
Figure 5 . An illustration of the relationship between the four topics involved in this study.
Because the dataset collection and experiments are conducted in a progressive way, the participants of each task differ from each other, and thus, it is difficult to analyse the overall cross-task status of an individual. For future studies, it is of great value to integrate all self-awareness tasks into a framework. Joint learning methods are possible to conduct to leverage multi-task prediction accuracy. With this framework, we hope to provide an overview of the wellbeing of users and find cross-task insight into the status of the user.
8.4. Data Scale
Dataset collection is an important but difficult task in lifelog research study, as it includes long-term participant engagement, and multi-dimensional data should be collected at the same time. Since data recording is volunteer and users can quit the experiment at any point, data imbalance a cross dimensions and users is common in lifelog records. In the above experiments, we also suffer from data imbalance problems. From the cold-start experiments, we verify that model transfer between users is available. However, more in-depth analysis is required for data scale and data diversity. For example, we want to know how much data are enough for each task and cross-task analysis, the performance of our methods for short-term and long-term data in each task. Moreover, the consistency among tasks and participants is also an interesting problem, for instance, whether sleep quality prediction is more accurate for participants with higher mood prediction accuracy. Analysis and discussions like these are important for the practical user of lifelog research studies.
In the future, we will collect more data soundly in long term to support in-depth analysis.
8.5. Privacy Issues
In lifelog-related research studies, privacy is always an essential problem ( 5 ), as the lifelog collection is usually all-day and much personal information is collected during the process, such as the Global Positioning System (GPS) location and personal photographs. In our schema, a new perspective is provided for protecting privacy in lifelog analysis. The light-training models used in these tasks can be deployed on personal terminals, which means the lifelog data can be processed locally without uploading to the cloud or sharing with others. It solves the privacy problem fundamentally.
Our cold-start experiments on sleep quality detection, personality detection, and depression detection show that most methods work well for new users. Therefore, a well-trained model can be deployed directly for new users. If the user is not willing to record his/her own information, he/she can choose to use the general model in the application.
Under these settings, training and updating the models at a personal terminal are essential for higher accuracy and better user experience. Moreover, user feedback should also be considered in the interactive dynamic update of the framework.
Moreover, participants in our experiments are all informed of the details of what data we collect and how we will use the data.
8.6. Limitations
As the first step to link lifelog and psychology, we take some preliminary attempts in experiments. In this study, we discuss some limitations in our work.
Conventional feature extraction strategies and machine learning models are used in the experiments. Since our main purpose is to explore the possibility of reflecting psychological status from daily life records, we keep the applied strategies simple. It is helpful for calculation on the personal terminals as we argued in the privacy discussions. But some distinctive features of lifelog are ignored, such as sequential patterns, time-related features, and context information between labels. We believe including more features, as well as designing domain-specific models to deal with the lifelog features, can improve the performance of the tasks.
In this study, we review a series of progressive experiments, which are conducted on different datasets over years. As we are exploring the lifelog research scenario, the collected information is different between tasks, as shown in Table 8 . Therefore, we admit that the interesting and meaningful topic of cross-task comparison and analysis on the same participants is unavailable in our study. In order to standardize the lifelog collection in the future research studies, we propose a method and application for collecting multi-dimensional data as follows.
9. Lifelog Recorder for Multi-Dimensional Self-Awareness Data Collection
As we discussed in the last section, the previous experiments on self-awareness were conducted on different participants with multi-dimensional information (as shown in Table 8 ). This experiment settings makes it difficult to evaluate overall self-awareness for one particular user. However, collecting multi-dimensional lifelog dataset is a time-consuming and laborious task. The commercially available applications are not suitable for collecting data from multiple sources (e.g., wristbands, smart-phone, and manual input) nor do most of them support the export of original data. The previous lifelog collection process for research studies highly depended on the participants, and the lifeloggers needed to collect, integrate, and upload multi-dimensional data themselves, which include heavy tasks and professional operations during the period. This process makes it hard to conduct experiments on many users for a long period. Therefore, it is necessary to develop a new application customized for psychology-related lifelog research studies.
Following the four-step schema, we develop a new APP in the Android environment, Lifelog Recorder , for collecting heterogeneous information as shown in Table 8 . The data flow for Lifelog Recorder is shown in Figure 6 . Centered at the Lifelog Recorder , lifelog data about the user and environment are collected, and uploaded to the Server .
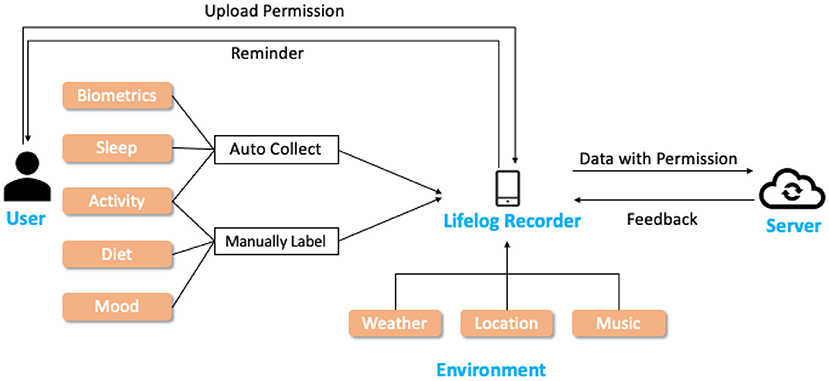
Figure 6 . Data flow for Lifelog Recorder application. The application takes on the role of collecting lifelog and exchanging data with the server, which ease the burden of users.
During the data collection experiment, we will provide smart wrist bands for the user, and the Lifelog Recorder needed to be installed on the smartphone of the user. The biometrics (heart rate and intensity), sleep quality, and activities (activity type and steps) are collected with wrist-band and uploaded to Lifelog Recorder automatically. The user is not only asked to record their mood and activity on a regular basis but also take photographs of his/her diets. To further free the users, notifications can be customized for each user to remind them to record mood and diet in time. Environmental information, such as weather, music, and location, are recorded by the APP. In the end, with the permission of the user, Lifelog Recorder communicates with the server, uploads lifelog records, and is ready to receive feedback data for extended tasks such as music or news recommendation.
With the help of Lifelog Recorder , we are able to collect multi-dimensional data in an easier way, moving from traditional user-centered methods to an APP-centered collection method, and free the user from the onerous task of annotating and collecting lifelog. Therefore, besides researchers, non professional participants can be involved in long-period data collection tasks, which can enlarge the lifelog dataset significantly. Actually, we are collecting multi-dimensional self-awareness data in a field study with the Lifelog Recorder , which include all information categories in Table 8 . The data will be made publically available after we finish collecting and removing the sensible personalized information.
Based on the data, we are able to explore interesting problems, such as cross-task performance.
10. Conclusions and Future Studies
In this study, we propose a new direction of investigating the capacity of lifelog records for tracking physical and psychological self-awareness in the life-long term. We summarize a four-step paradigm for lifelog analysis and application, including lifelog collection, feature extraction, label tagging, and model selection. Following the paradigm, four topics are proposed for insight into physical and psychological health: sleep quality prediction, personality detection, mood detection and prediction, and depression mood detection. Among the topics, some tasks based on short-term lifelog, while others need longer periods of records. But they all focus on life-long analysis and understanding of people. Experiments on real-world data show encouraging results on all tasks, revealing the great potential of lifelog for long-term self-tracking. On discussing the concerned issues and limitations of our experiments, we further put forward a method and an application for lifelog data collection.
In conclusion, we reveal a new direction of employing objective lifelog data for subjective self-awareness detection. The experiments show that lifelog is a good reflection of the physical and mental status of people. Analysis, detection, and prediction with lifelog records can help people better understand their status.
In the end, we present some directions for further study. First, prediction and detection are just the primary understanding for well being. Based on the accurate prediction, recommendation and intervention methods can be further explored. Second, as we mentioned, the light-training framework can be deployed on personal devices. Therefore, local update of the models is important in application. Moreover, we will try domain-specific features and new methods for improving the task performance. Finally, based on the Lifelog Recorder application, we will design an overall system to integrate all tasks automatically, and conduct cross-task in-depth analysis about task performance, data scale, and model feasibility.
Data Availability Statement
The datasets presented in this article are not readily available because The dataset contains lifelog records of participants, such as photos and location information. Due to privacy concern, the dataset can not be made publicly accessable. Requests to access the datasets should be directed to Min Zhang, z-m@tsinghua.edu.cn .
Ethics Statement
The studies involving human participants were reviewed and approved by Jing Qian, Tsinghua University. The patients/participants provided their written informed consent to participate in this study. Written informed consent was obtained from the individual(s) for the publication of any potentially identifiable images or data included in this article.
Author Contributions
JL contributed to data analysis and writing and editing of the manuscript. WM contributed to the design of methods. MZ contributed to guidance and method design through the research study. PW contributed to the data collection and experiments on depression detection topic. YL and SM contributed to the discussion in the experiment and manuscript writing. All authors contributed to the article and approved the submitted version.
This work is supported by the National Key Research and Development Program of China (2018YFC0831900), the Natural Science Foundation of China (grant nos. 61672311, 61532011), and the Tsinghua University Guoqiang Research Institute.
Conflict of Interest
The authors declare that the research was conducted in the absence of any commercial or financial relationships that could be construed as a potential conflict of interest.
Publisher's Note
All claims expressed in this article are solely those of the authors and do not necessarily represent those of their affiliated organizations, or those of the publisher, the editors and the reviewers. Any product that may be evaluated in this article, or claim that may be made by its manufacturer, is not guaranteed or endorsed by the publisher.
Acknowledgments
We thank all the participants in lifelog collection, and all partners in the THUIR laboratory for their support, assistance, and input throughout the research.
1. Duval S, Wicklund RA. A Theory of Objective Self Awareness . Academic Press (1972).
2. Rochat P. Five levels of self-awareness as they unfold early in life. Conscious Cogn . (2003) 12:717–31. doi: 10.1016/S1053-8100(03)00081-3
PubMed Abstract | CrossRef Full Text | Google Scholar
3. Lou H, Changeux JP, Rosenstand A. Towards a cognitive neuroscience of self-awareness. Neurosci Biobeh Rev . (2017) 83:765–73. doi: 10.1016/j.neubiorev.2016.04.004
CrossRef Full Text | Google Scholar
4. Killian KD. Development and validation of the emotional Self-awareness questionnaire: a measure of emotional intelligence. J Marital Family Ther . (2012) 38:502–14. doi: 10.1111/j.1752-0606.2011.00233.x
5. Gurrin C, Smeaton AF, Doherty AR. Lifelogging: personal big data. Found Trends Inf Retrieval . (2014) 8:1–125. doi: 10.1561/1500000033
6. Bouchard C, Blair SN, Haskell WL. Physical activity and health. Hum Kinet . (2012) 3–20. doi: 10.5040/9781492595717
7. Gurrin C, Le TK, Ninh VT, Dang-Nguyen DT, Jónsson B, Lokoš J, et al. Introduction to the third annual lifelog search challenge (LSC'20). In: Proceedings of the 2020 International Conference on Multimedia Retrieval . Dublin (2020). p. 584–5.
8. Silva A, Pinho S, Macedo L, Moulin C. Does SenseCam improve general cognitive performance. Am J Prev Med . (2013) 44:302–7. doi: 10.1016/j.amepre.2012.11.005
9. Zhou L, Hinbarji Z, Dang-Nguyen DT, Gurrin C. Lifer: an interactive lifelog retrieval system. In: Proceedings of the 2018 ACM Workshop on The Lifelog Search Challenge . Yokohama (2018). p. 9–14.
10. Li J, Zhang M, Ma W, Liu Y, Ma S. A multi-level interactive lifelog search engine with user feedback. In: Proceedings of the Third Annual Workshop on Lifelog Search Challenge . Dublin (2020). p. 29–35.
11. Duane A, Gurrin C, Huerst W. Virtual reality lifelog explorer: lifelog search challenge at ACM ICMR (2018). In: Proceedings of the 2018 ACM Workshop on the Lifelog Search Challenge . Yokohama (2018). p. 20–23.
12. Bilal HSM, Khan WA, Lee S. Unhealthy dietary behavior based user life-log monitoring for wellness services. In: International Conference on Smart Homes and Health Telematics . (Paris: Springer) (2017). p. 73–84.
13. Kawamoto K, Tanaka T, Kuriyama H. Your activity tracker knows when you quit smoking. In: Proceedings of the 2014 ACM International Symposium on Wearable Computers . Seattle, WA (2014). p. 107–10.
14. Nishiyama Y, Okoshi T, Yonezawa T, Nakazawa J, Takashio K, Tokuda H. Toward health exercise behavior change for teams using lifelog sharing models. IEEE J Biomed Health Inf . (2015) 20:775–86. doi: 10.1109/JBHI.2015.2478903
15. Kumar G, Jerbi H, Gurrin C, O'Mahony MP. Towards activity recommendation from lifelogs. In: Proceedings of the 16th International Conference on Information Integration and Web-Based Applications & Services . Hanoi (2014) p. 87–96.
16. Uno A, Itoh T. MALL: A life log based music recommendation system and portable music player. In: Proceedings of the 29th Annual ACM Symposium on Applied Computing . Gyeongju (2014). p. 939–44.
17. Nakamura Y, Itou T, Tezuka H, Ishihara T, Abe M. Personalized TV-program recommendations based on life log. In: 2010 Digest of Technical Papers International Conference on Consumer Electronics (ICCE) . Las Vegas, NV: IEEE (2010). p. 143–4.
18. Holzinger A. Interactive machine learning for health informatics: when do we need the human-in-the-loop? Brain Inf . (2016) 3:119–31. doi: 10.1007/s40708-016-0042-6
19. Koelstra S, Muhl C, Soleymani M, Lee JS, Yazdani A, Ebrahimi T, et al. Deap: a database for emotion analysis; using physiological signals. IEEE Trans Affect Comput . (2011) 3:18–31. doi: 10.1109/T-AFFC.2011.15
20. Samara A, Menezes MLR, Galway L. Feature extraction for emotion recognition and modelling using neurophysiological data. In: 2016 15th International Conference on Ubiquitous Computing and Communications and 2016 International Symposium on Cyberspace and Security (IUCC-CSS) . Granada: IEEE (2016). p. 138–44.
21. Shin D, Shin D, Shin D. Development of emotion recognition interface using complex EEG/ECG bio-signal for interactive contents. Multimedia Tools Appl . (2017) 76:11449–70. doi: 10.1007/s11042-016-4203-7
22. Tripathi S, Acharya S, Sharma RD, Mittal S, Bhattacharya S. Using deep and convolutional neural networks for accurate emotion classification on DEAP dataset. In: Twenty-Ninth IAAI Conference . San Francisco, CA (2017).
23. Ma J, Tang H, Zheng WL, Lu BL. Emotion recognition using multimodal residual LSTM network. In: Proceedings of the 27th ACM International Conference on Multimedia . Nice (2019). p. 176–83.
24. Lin H, Jia J, Qiu J, Zhang Y, Shen G, Xie L, et al. Detecting stress based on social interactions in social networks. IEEE Trans Knowl Data Eng . (2017) 29:1820–33. doi: 10.1109/TKDE.2017.2686382
25. Du J, Zhang Y, Luo J, Jia Y, Wei Q, Tao C, et al. Extracting psychiatric stressors for suicide from social media using deep learning. BMC Med Inf Decis Making . (2018) 18:77–87. doi: 10.1186/s12911-018-0632-8
26. Kołakowska A, Szwoch W, Szwoch M. A review of emotion recognition methods based on data acquired via smartphone sensors. Sensors . (2020) 20:6367. doi: 10.3390/s20216367
27. Spathis D, Servia-Rodriguez S, Farrahi K, Mascolo C, Rentfrow J. Sequence multi-task learning to forecast mental wellbeing from sparse self-reported data. In: Proceedings of the 25th ACM SIGKDD International Conference on Knowledge Discovery & Data Mining . Anchorage, AK (2019). p. 2886–94.
28. Lin S, Wu X, Martinez G, Chawla NV. Filling missing values on wearable-sensory time series data. In: Proceedings of the 2020 SIAM International Conference on Data Mining . (Cincinnati, OH: SIAM) (2020). p. 46–54.
29. Horne J. Why we Sleep . Oxford: Oxford University Press (1992).
30. Sathyanarayana A, Srivastava J, Fernandez-Luque L. The science of sweet dreams: predicting sleep efficiency from wearable device data. Computer . (2017) 50:30–38. doi: 10.1109/MC.2017.91
31. Fishman EI, Steeves JA, Zipunnikov V, Koster A, Berrigan D, Harris TA, et al. Association between objectively measured physical activity and mortality in NHANES. Med Sci Sports Exerc . (2016) 48:1303. doi: 10.1249/MSS.0000000000000885
32. Soleimaninejadian P, Wang Y, Tong H, Feng Z, Zhang M, Liu Y, et al. THIR2 at the NTCIR-13 lifelog-2 task: bridging technology and psychology through the lifelog personality, mood and sleep quality. In: Proceedings of the NTCIR-13 Conference . Tokyo (2017). p. 20.
33. Kato MP, Liu Y. Overview of NTCIR-13. In: Proceedings of the NTCIR-13 Conference . Tokyo (2017).
34. Goldberg LR. An alternative “description of personality”: the big-five factor structure. J Pers Soc Psychol . (1990) 59:1216. doi: 10.1037/0022-3514.59.6.1216
35. Wang Y, Zhang M, Soleimaninejadian P, Tong H, Feng Z. Big five personality measurement based on lifelog. In: Proceedings of the 2nd Workshop on Lifelogging Tools and Applications . Mountain View, CA (2017). p. 25–8.
36. McCrae RR, Costa PT Jr. A contemplated revision of the NEO Five-Factor Inventory. Pers Individ Diff . (2004) 36:587–96. doi: 10.1016/S0191-8869(03)00118-1
37. Soleimaninejadian P, Zhang M, Liu Y, Ma S. Mood detection and prediction based on user daily activities. In: 2018 First Asian Conference on Affective Computing and Intelligent Interaction (ACII Asia) . Beijing: IEEE (2018). p. 1–6.
38. Poon JM. Mood: a review of its antecedents and consequences. Int J Organ Theory Behav . (2001) 4:357–88. doi: 10.1108/IJOTB-04-03-04-2001-B008
39. Roshanaei M, Han R, Mishra S. Having fun?: Personalized activity-based mood prediction in social media. In: Prediction and Inference from Social Networks and Social Media . Springer (2017). p. 1–18.
40. Park K, Lee S, Kim E, Park M, Park J, Cha M. Mood and weather: Feeling the heat? In: Proceedings of the International AAAI Conference on Web and Social Media. Vol. 7 . Cambridge, MA (2013).
41. Thayer RE. The Biopsychology of Mood and Arousal . Oxford University Press (1990).
42. McClung CA. How might circadian rhythms control mood? Let me count the ways. Biol Psychiatry . (2013) 74:242–9. doi: 10.1016/j.biopsych.2013.02.019
43. Beck AT, Alford BA. Depression: Causes and Treatment . University of Pennsylvania Press. (2009).
44. Alghowinem S, Goecke R, Wagner M, Parker G, Breakspear M. Eye movement analysis for depression detection. In: 2013 IEEE International Conference on Image Processing . Melbourne, VIC: IEEE (2013). p. 4220–4.
45. Karp DA. Living with depression: Illness and identity turning points. Qual Health Res . (1994) 4:6–30. doi: 10.1177/104973239400400102
46. Hirschfeld RM, Klerman GL, Clayton PJ, Keller MB. Personality and depression: Empirical findings. Arch Gen Psychiatry . (1983) 40:993–8. doi: 10.1001/archpsyc.1983.01790080075010
Keywords: lifelog, data mining, machine laerning, sleep quality, personality, mood, depression
Citation: Li J, Ma W, Zhang M, Wang P, Liu Y and Ma S (2021) Know Yourself: Physical and Psychological Self-Awareness With Lifelog. Front. Digit. Health 3:676824. doi: 10.3389/fdgth.2021.676824
Received: 06 March 2021; Accepted: 09 July 2021; Published: 11 August 2021.
Reviewed by:
Copyright © 2021 Li, Ma, Zhang, Wang, Liu and Ma. This is an open-access article distributed under the terms of the Creative Commons Attribution License (CC BY) . The use, distribution or reproduction in other forums is permitted, provided the original author(s) and the copyright owner(s) are credited and that the original publication in this journal is cited, in accordance with accepted academic practice. No use, distribution or reproduction is permitted which does not comply with these terms.
*Correspondence: Min Zhang, z-m@tsinghua.edu.cn
Disclaimer: All claims expressed in this article are solely those of the authors and do not necessarily represent those of their affiliated organizations, or those of the publisher, the editors and the reviewers. Any product that may be evaluated in this article or claim that may be made by its manufacturer is not guaranteed or endorsed by the publisher.
Academia.edu no longer supports Internet Explorer.
To browse Academia.edu and the wider internet faster and more securely, please take a few seconds to upgrade your browser .
- We're Hiring!
- Help Center
Self-awareness
- Most Cited Papers
- Most Downloaded Papers
- Newest Papers
- Save to Library
- Last »
- Brain Science Follow Following
- Perubahan Follow Following
- Sosial Follow Following
- Inductive Reasoning Follow Following
- Mahasiswa Follow Following
- Representations Follow Following
- Cognitive Development (Psychology) Follow Following
- Self Consciousness Follow Following
- Deductive reasoning Follow Following
- Clinical Psychology Follow Following
Enter the email address you signed up with and we'll email you a reset link.
- Academia.edu Publishing
- We're Hiring!
- Help Center
- Find new research papers in:
- Health Sciences
- Earth Sciences
- Cognitive Science
- Mathematics
- Computer Science
- Academia ©2024
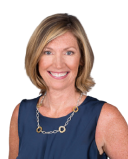
- Emotional Intelligence
How to Become More Self-Aware and Why You Should
Self-awareness contributes to healthy decisions and relationships..
Posted May 28, 2024 | Reviewed by Michelle Quirk
- Research shows that self-awareness wards off depression and anxiety and improves your physical health.
- There are 3 key areas of self-awareness: relational, emotional, and physical.
- You can only know your important personal qualities by paying close attention to yourself.
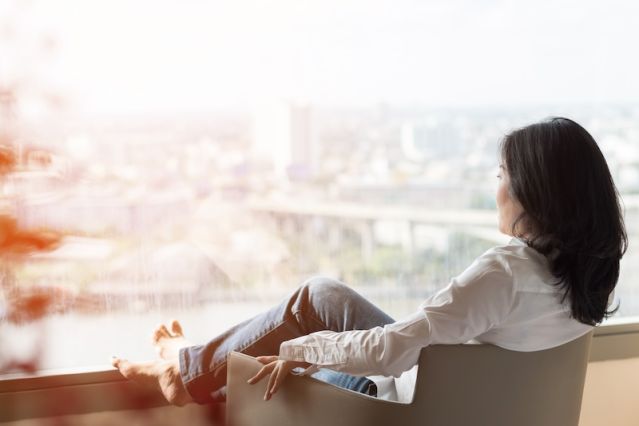
What does it mean to be self-aware? It means having what therapists call an “observing ego.” The observing ego is a part of yourself that is able to observe yourself with some amount of objectivity. When your observing ego is healthy, you can, for example, look back on a way that you acted or spoke and accurately say, “I think I was a bit harsh there” or “I should have stood up for myself more.”
Your observing ego is able to watch your actions, your choices, your feelings, your needs, and your wants. This makes you a reliable reporter when you need to share any of that information with others. The key to a healthy observing ego is simply paying attention . And it’s vital to have this to live a healthy life and thrive.
How can you seek what you truly want, choose options that will bring you joy, and make decisions that are healthy for you if you are not quite sure what you want, enjoy, or need? You can only know these important personal qualities by paying close attention to yourself.
The 3 Key Areas of Self-Awareness
- Physical: This involves being aware of your body and what it needs to be healthy and strong. This includes the amount of sleep you need, the amount and type of food, rest, recreation, and exercise. It also means being aware of your body’s cues to you about what’s wrong or what it needs.
- Relational: How do others see you and experience you? What are your strengths, weaknesses, foibles, and flaws when it comes to your relationships? Being aware of your habits and qualities and how you relate to others enables you to manage your own reactions and responses and also understand others’ reactions to you.
- Emotional: This type of self-awareness is the most important. It involves being able to notice when you are experiencing a feeling and being able to identify what that feeling is. Knowing when you are angry, hurt, or lonely , for example, allows you to attend to the feeling and use it to motivate, inform, guide, and enrich you.
Knowing yourself well and being aware of your physical, relational, and emotional needs and rewards pays off in substantial ways. A 2023 study by Sung and Rubens et al. found that self-awareness is a buffer that prevents life stressors, even trauma , from becoming depression and anxiety .
Higher emotional self-awareness has been found to improve your overall health. Loucks and Britton (2015) found that people who are more aware of what they are thinking and feeling in the moment have lower BMI (body mass index), lower fasting glucose, less smoking , and higher levels of physical activity.
It is true that some people have higher levels of natural self-awareness than others. One of the biggest obstacles to self-awareness comes from childhood . Those who grew up in a household where their emotions were ignored or discouraged by their parents tend to push their emotions and emotional needs away and try to make them disappear. This is the definition of childhood emotional neglect.
Those who grew up with childhood emotional neglect are going through their adult lives unaware that they are squelching a deeply vital resource from within: the natural fount of emotions that is wired into all human beings at birth.
Living your life without full access to your own feelings can leave you with a sense of emptiness and purposelessness. With your emotions tamped down, you may lack enough of the very substance that should be keeping you connected to your body, feeding your relationships, and stoking your energy. You may end up feeling disconnected from yourself and your life in a way that eventually becomes your new norm.
How to Increase Your Self-Awareness
- Start thinking of your body as something to take care of. Pay attention to your body’s needs, day after day, and collect data. What’s the optimal amount of sleep it needs? What foods make it feel the best? How much of what kinds of exercise give it energy? Track your answers, write them down, and try to live by them.
- Pay closer attention to the feedback you receive from the people who know you best. The people around you may have observed more about you than you have observed about yourself. You may be missing lots of valuable information about how others see you, especially if this is not a concept you’ve thought about before. By simply paying attention to how others react to you and what they say about you, you can gather, and file away, loads of useful data about how others perceive you and how you are in your relationships with others.
- Begin to pay more attention to what you are feeling. This is the single most powerful way for you to build your self-awareness. If you grew up with your emotions neglected, you are probably continuing to neglect them. Becoming aware of your emotions is becoming aware of the most deeply personal, biological expression of who you are. It is also a giant stride toward healing the emotional neglect you grew up with, which comes with a whole host of other life advantages.
Becoming more aware of your body, your relationships, and your emotions all combine in a wonderful way to make a profound difference in your life. Knowing who you are, what you need, what you feel, and how you affect others gradually helps you to feel more grounded, more fulfilled, more connected, and more alive.

© Jonice Webb, Ph.D.
To determine if you might be living with the effects of childhood emotional neglect, you can take the free Emotional Neglect Questionnaire. You'll find the link in my bio.
Sung, Angelina, Rubens, Sonia L., Soliemannjad, Rose R., & Zeringue, Megan M. Socioemotional health, trauma exposure, and internalizing symptoms in students attending an alternative high school. American Journal of Orthopsychiatry, Vol 93(3), 2023, 269–278.
Loucks, E.B., Britton, W.B., Howe, C.J. et al. Positive Associations of Dispositional Mindfulness with Cardiovascular Health: the New England Family Study. Int J Behav Med. 22, 540–550 (2015).
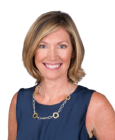
Jonice Webb, Ph.D., is a licensed psychologist and author of two books, Running On Empty: Overcome Your Childhood Emotional Neglect and Running On Empty No More: Transform Your Relationships.
- Find a Therapist
- Find a Treatment Center
- Find a Psychiatrist
- Find a Support Group
- Find Online Therapy
- United States
- Brooklyn, NY
- Chicago, IL
- Houston, TX
- Los Angeles, CA
- New York, NY
- Portland, OR
- San Diego, CA
- San Francisco, CA
- Seattle, WA
- Washington, DC
- Asperger's
- Bipolar Disorder
- Chronic Pain
- Eating Disorders
- Passive Aggression
- Personality
- Goal Setting
- Positive Psychology
- Stopping Smoking
- Low Sexual Desire
- Relationships
- Child Development
- Self Tests NEW
- Therapy Center
- Diagnosis Dictionary
- Types of Therapy
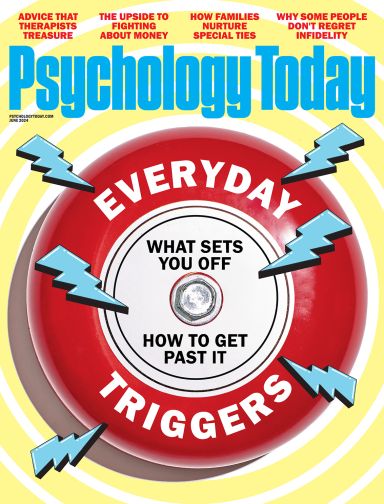
At any moment, someone’s aggravating behavior or our own bad luck can set us off on an emotional spiral that threatens to derail our entire day. Here’s how we can face our triggers with less reactivity so that we can get on with our lives.
- Gaslighting
- Affective Forecasting
- Neuroscience
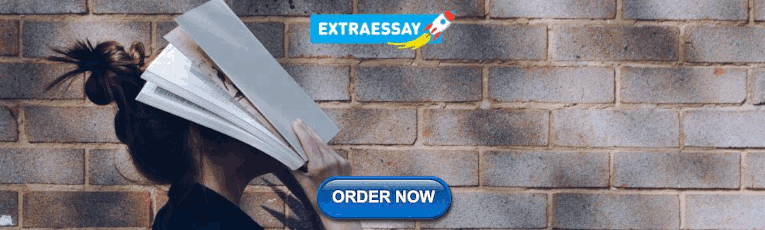
IMAGES
VIDEO
COMMENTS
The literature on self-awareness is characterized by multiple definitions (Sutton, 2016; Williams, 2008), and rarely does the literature recognize the complexity of the construct (Sutton et al., 2015).Self-awareness is frequently confused with concepts such as self-consciousness and self-knowledge, both of which are regularly discussed and explored interchangeably alongside self-awareness ...
The final result of this study was a comprehensive list of 83 items representing the outcomes of self-awareness as reported by experts in the field and previous research evaluations of self-awareness training. Further, the themes and categories identified in this study provided context for interpretation of statistical results in Study 2.
Self-awareness seems to have become the latest management buzzword — and for good reason. Research suggests that when we see ourselves clearly, we are more confident and more creative. We make ...
Developmental Psychology. Psychology. Self-Awareness. ArticlePDF Available. Developing Self-Awareness: Learning Processes for Self- and Interpersonal Growth. November 2022. Annual Review of ...
1 INTRODUCTION. Self-awareness can be defined as the ability to take oneself as the object of awareness (Morin, 2011).Although this definition may suggest the idea of a unitary self that acts as both observer and object of awareness, in reality there is a multiplicity of self-processes included in this self-model, with different features being at the focus of awareness at any given time ...
Furthermore, beyond research on mindfulness (which is inherently accepting), some research also suggests that self-acceptance may play a role in well-being. Most relevant to self-connection is research that conceptualizes self-acceptance as unconditional and less evaluative, as compared to positive self-evaluations (e.g., Ryff & Keyes, 1995).
Self-awareness is an essential concept in physiology and psychology. ... The previous research studies on self-awareness mainly collect and analyze data in the laboratory environment through questionnaires, user study, or field research study. ... 2010 Digest of Technical Papers International Conference on Consumer Electronics (ICCE). Las Vegas ...
Self-awareness represents the capacity of becoming the object of one's own attention. In this state. one actively identifies, processes, and stores information about the self. This paper ...
In Study 2, these items were reduced to a 38 item self-report questionnaire with four factors representing three beneficial outcomes (reflective self-development, acceptance and proactivity) and one negative outcome (costs). Regression of these outcomes against self-awareness measures revealed that self-reflection and insight predicted ...
Self-awareness is not only a gift, but it is a responsibility. Mufti James Hannush Many years have passed since existential-humanistic ideas were developed and empirically tested by research on Self-Determination Theory (SDT). Bloom's Taxonomy (Bloom, 1956; Bloom, Engelhart, Furst, Hill, & Krathwohl, 1956), an
The papers in this special issue can be divided into three main groups. The first consists of four papers discussing the question whether (i) the minimal self is best understood and defined relationally, that is in terms of social interactions; or rather (ii) the minimal self is a tacit, non-socially grounded, experiential self-awareness.
PDF | On Jun 3, 2018, Benjamin M Basile and others published Self-Awareness | Find, read and cite all the research you need on ResearchGate
This framework of self-awareness, -regulation, and -transcendence (S-ART) illustrates a method for becoming aware of the conditions that cause (and remove) distortions or biases. The development of S-ART through meditation is proposed to modulate self-specifying and narrative self-networks through an integrative fronto-parietal control network ...
Purpose: This research paper aims to follow a line of research that examines the impact of elements of emotional intelligence (EI), particularly those related to self-awareness, on self-other agreement and performance. Design/methodology/approach: This is a quantitative study that employs the same methodology as Sosik and Megerian to analyze survey data gathered from a matched sample of 146 ...
shrouded by moods and emotions, is self-awareness, which marks the mainstay of emotional intelligence (Goleman, 1995). Despite its seemingly obvious importance, self-awareness has received little credit insubordinates, but also leadership research so far, with the author's own research only yielding 127 results. The picture looks even more dire
Trait Self-Awareness was operationalized as function of participants' scores on the Sense of Self Scale (SOSS; Flury and Ickes, 2007) which is a single factor 12-item measure designed to assess sense of self and self-understanding (Cronbach's α = 0.86) and the Self-Reflection and Insight Scale (SRIS; Grant et al., 2002) which is a two ...
positive state (Silvia & Duval, 2001) is one that was adopted for this research. The Role of Self-Awareness in Management Education Self-awareness is frequently included in management programmes, such as MBAs (Lawrence et al., 2018), in leadership development programs (Mirvis, 2008; Svalgaard, 2018) and in courses aimed at initiating and developing
Although the selfawareness component of self-connection resembles mindfulness in that both constructs rely on a person's awareness (Kabat-Zinn, 1994;Lindsay & Creswell, 2017), self-awareness only ...
Self-awareness is an essential concept in physiology and psychology. Accurate overall self-awareness benefits the development and well being of an individual. The previous research studies on self-awareness mainly collect and analyze data in the laboratory environment through questionnaires, user study, or field research study.
Use of first person pronouns as a function of increased objective self-awareness and performance feedback. A recent integrating formulation in social psychology, the Duval-Wicklund theory of "objective self-awareness," has the core assumption that attention focused on the self is always aversive and, hence, avoided.
Key points. Research shows that self-awareness wards off depression and anxiety and improves your physical health. There are 3 key areas of self-awareness: relational, emotional, and physical.
The present research is a summary of three studies designed to develop and begin validation for a scale to measure self-awareness in the context of leadership and leader development. The result of ...
In the pursuit of sustainable energy solutions, the integration of renewable energy sources and hydrogen technologies has emerged as a promising avenue. This paper introduces the Integrated Renewable Energy-Driven Hydrogen System as a holistic approach to achieve energy independence and self-sufficiency. Seamlessly integrating renewable energy sources, hydrogen production, storage, and ...