A list of completed theses and new thesis topics from the Computer Vision Group.
Are you about to start a BSc or MSc thesis? Please read our instructions for preparing and delivering your work.
Below we list possible thesis topics for Bachelor and Master students in the areas of Computer Vision, Machine Learning, Deep Learning and Pattern Recognition. The project descriptions leave plenty of room for your own ideas. If you would like to discuss a topic in detail, please contact the supervisor listed below and Prof. Paolo Favaro to schedule a meeting. Note that for MSc students in Computer Science it is required that the official advisor is a professor in CS.
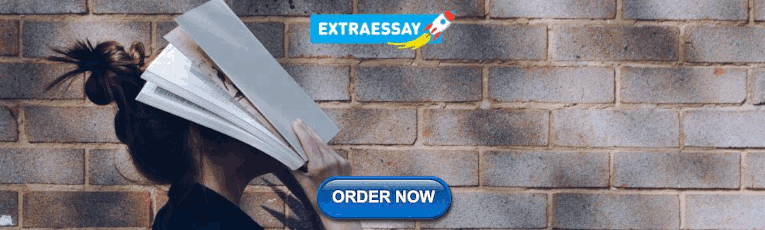
AI deconvolution of light microscopy images
Level: master.
Background Light microscopy became an indispensable tool in life sciences research. Deconvolution is an important image processing step in improving the quality of microscopy images for removing out-of-focus light, higher resolution, and beter signal to noise ratio. Currently classical deconvolution methods, such as regularisation or blind deconvolution, are implemented in numerous commercial software packages and widely used in research. Recently AI deconvolution algorithms have been introduced and being currently actively developed, as they showed a high application potential.
Aim Adaptation of available AI algorithms for deconvolution of microscopy images. Validation of these methods against state-of-the -art commercially available deconvolution software.
Material and Methods Student will implement and further develop available AI deconvolution methods and acquire test microscopy images of different modalities. Performance of developed AI algorithms will be validated against available commercial deconvolution software.

- Al algorithm development and implementation: 50%.
- Data acquisition: 10%.
- Comparison of performance: 40 %.
Requirements
- Interest in imaging.
- Solid knowledge of AI.
- Good programming skills.
Supervisors Paolo Favaro, Guillaume Witz, Yury Belyaev.
Institutes Computer Vison Group, Digital Science Lab, Microscopy imaging Center.
Contact Yury Belyaev, Microscopy imaging Center, [email protected] , + 41 78 899 0110.
Instance segmentation of cryo-ET images
Level: bachelor/master.
In the 1600s, a pioneering Dutch scientist named Antonie van Leeuwenhoek embarked on a remarkable journey that would forever transform our understanding of the natural world. Armed with a simple yet ingenious invention, the light microscope, he delved into uncharted territory, peering through its lens to reveal the hidden wonders of microscopic structures. Fast forward to today, where cryo-electron tomography (cryo-ET) has emerged as a groundbreaking technique, allowing researchers to study proteins within their natural cellular environments. Proteins, functioning as vital nano-machines, play crucial roles in life and understanding their localization and interactions is key to both basic research and disease comprehension. However, cryo-ET images pose challenges due to inherent noise and a scarcity of annotated data for training deep learning models.

Credit: S. Albert et al./PNAS (CC BY 4.0)
To address these challenges, this project aims to develop a self-supervised pipeline utilizing diffusion models for instance segmentation in cryo-ET images. By leveraging the power of diffusion models, which iteratively diffuse information to capture underlying patterns, the pipeline aims to refine and accurately segment cryo-ET images. Self-supervised learning, which relies on unlabeled data, reduces the dependence on extensive manual annotations. Successful implementation of this pipeline could revolutionize the field of structural biology, facilitating the analysis of protein distribution and organization within cellular contexts. Moreover, it has the potential to alleviate the limitations posed by limited annotated data, enabling more efficient extraction of valuable information from cryo-ET images and advancing biomedical applications by enhancing our understanding of protein behavior.
Methods The segmentation pipeline for cryo-electron tomography (cryo-ET) images consists of two stages: training a diffusion model for image generation and training an instance segmentation U-Net using synthetic and real segmentation masks.
1. Diffusion Model Training: a. Data Collection: Collect and curate cryo-ET image datasets from the EMPIAR database (https://www.ebi.ac.uk/empiar/). b. Architecture Design: Select an appropriate architecture for the diffusion model. c. Model Evaluation: Cryo-ET experts will help assess image quality and fidelity through visual inspection and quantitative measures 2. Building the Segmentation dataset: a. Synthetic and real mask generation: Use the trained diffusion model to generate synthetic cryo-ET images. The diffusion process will be seeded from either a real or a synthetic segmentation mask. This will yield to pairs of cryo-ET images and segmentation masks. 3. Instance Segmentation U-Net Training: a. Architecture Design: Choose an appropriate instance segmentation U-Net architecture. b. Model Evaluation: Evaluate the trained U-Net using precision, recall, and F1 score metrics.
By combining the diffusion model for cryo-ET image generation and the instance segmentation U-Net, this pipeline provides an efficient and accurate approach to segment structures in cryo-ET images, facilitating further analysis and interpretation.
References 1. Kwon, Diana. "The secret lives of cells-as never seen before." Nature 598.7882 (2021): 558-560. 2. Moebel, Emmanuel, et al. "Deep learning improves macromolecule identification in 3D cellular cryo-electron tomograms." Nature methods 18.11 (2021): 1386-1394. 3. Rice, Gavin, et al. "TomoTwin: generalized 3D localization of macromolecules in cryo-electron tomograms with structural data mining." Nature Methods (2023): 1-10.
Contacts Prof. Thomas Lemmin Institute of Biochemistry and Molecular Medicine Bühlstrasse 28, 3012 Bern ( [email protected] )
Prof. Paolo Favaro Institute of Computer Science Neubrückstrasse 10 3012 Bern ( [email protected] )
Adding and removing multiple sclerosis lesions with to imaging with diffusion networks
Background multiple sclerosis lesions are the result of demyelination: they appear as dark spots on t1 weighted mri imaging and as bright spots on flair mri imaging. image analysis for ms patients requires both the accurate detection of new and enhancing lesions, and the assessment of atrophy via local thickness and/or volume changes in the cortex. detection of new and growing lesions is possible using deep learning, but made difficult by the relative lack of training data: meanwhile cortical morphometry can be affected by the presence of lesions, meaning that removing lesions prior to morphometry may be more robust. existing ‘lesion filling’ methods are rather crude, yielding unrealistic-appearing brains where the borders of the removed lesions are clearly visible., aim: denoising diffusion networks are the current gold standard in mri image generation [1]: we aim to leverage this technology to remove and add lesions to existing mri images. this will allow us to create realistic synthetic mri images for training and validating ms lesion segmentation algorithms, and for investigating the sensitivity of morphometry software to the presence of ms lesions at a variety of lesion load levels., materials and methods: a large, annotated, heterogeneous dataset of mri data from ms patients, as well as images of healthy controls without white matter lesions, will be available for developing the method. the student will work in a research group with a long track record in applying deep learning methods to neuroimaging data, as well as experience training denoising diffusion networks..
Nature of the Thesis:
Literature review: 10%
Replication of Blob Loss paper: 10%
Implementation of the sliding window metrics:10%
Training on MS lesion segmentation task: 30%
Extension to other datasets: 20%
Results analysis: 20%
Fig. Results of an existing lesion filling algorithm, showing inadequate performance
Requirements:
Interest/Experience with image processing
Python programming knowledge (Pytorch bonus)
Interest in neuroimaging
Supervisor(s):
PD. Dr. Richard McKinley
Institutes: Diagnostic and Interventional Neuroradiology
Center for Artificial Intelligence in Medicine (CAIM), University of Bern
References: [1] Brain Imaging Generation with Latent Diffusion Models , Pinaya et al, Accepted in the Deep Generative Models workshop @ MICCAI 2022 , https://arxiv.org/abs/2209.07162
Contact : PD Dr Richard McKinley, Support Centre for Advanced Neuroimaging ( [email protected] )
Improving metrics and loss functions for targets with imbalanced size: sliding window Dice coefficient and loss.
Background The Dice coefficient is the most commonly used metric for segmentation quality in medical imaging, and a differentiable version of the coefficient is often used as a loss function, in particular for small target classes such as multiple sclerosis lesions. Dice coefficient has the benefit that it is applicable in instances where the target class is in the minority (for example, in case of segmenting small lesions). However, if lesion sizes are mixed, the loss and metric is biased towards performance on large lesions, leading smaller lesions to be missed and harming overall lesion detection. A recently proposed loss function (blob loss[1]) aims to combat this by treating each connected component of a lesion mask separately, and claims improvements over Dice loss on lesion detection scores in a variety of tasks.
Aim: The aim of this thesisis twofold. First, to benchmark blob loss against a simple, potentially superior loss for instance detection: sliding window Dice loss, in which the Dice loss is calculated over a sliding window across the area/volume of the medical image. Second, we will investigate whether a sliding window Dice coefficient is better corellated with lesion-wise detection metrics than Dice coefficient and may serve as an alternative metric capturing both global and instance-wise detection.
Materials and Methods: A large, annotated, heterogeneous dataset of MRI data from MS patients will be available for benchmarking the method, as well as our existing codebases for MS lesion segmentation. Extension of the method to other diseases and datasets (such as covered in the blob loss paper) will make the method more plausible for publication. The student will work alongside clinicians and engineers carrying out research in multiple sclerosis lesion segmentation, in particular in the context of our running project supported by the CAIM grant.

Fig. An annotated MS lesion case, showing the variety of lesion sizes
References: [1] blob loss: instance imbalance aware loss functions for semantic segmentation, Kofler et al, https://arxiv.org/abs/2205.08209
Idempotent and partial skull-stripping in multispectral MRI imaging
Background Skull stripping (or brain extraction) refers to the masking of non-brain tissue from structural MRI imaging. Since 3D MRI sequences allow reconstruction of facial features, many data providers supply data only after skull-stripping, making this a vital tool in data sharing. Furthermore, skull-stripping is an important pre-processing step in many neuroimaging pipelines, even in the deep-learning era: while many methods could now operate on data with skull present, they have been trained only on skull-stripped data and therefore produce spurious results on data with the skull present.
High-quality skull-stripping algorithms based on deep learning are now widely available: the most prominent example is HD-BET [1]. A major downside of HD-BET is its behaviour on datasets to which skull-stripping has already been applied: in this case the algorithm falsely identifies brain tissue as skull and masks it. A skull-stripping algorithm F not exhibiting this behaviour would be idempotent: F(F(x)) = F(x) for any image x. Furthermore, legacy datasets from before the availability of high-quality skull-stripping algorithms may still contain images which have been inadequately skull-stripped: currently the only solution to improve the skull-stripping on this data is to go back to the original datasource or to manually correct the skull-stripping, which is time-consuming and prone to error.
Aim: In this project, the student will develop an idempotent skull-stripping network which can also handle partially skull-stripped inputs. In the best case, the network will operate well on a large subset of the data we work with (e.g. structural MRI, diffusion-weighted MRI, Perfusion-weighted MRI, susceptibility-weighted MRI, at a variety of field strengths) to maximize the future applicability of the network across the teams in our group.
Materials and Methods: Multiple datasets, both publicly available and internal (encompassing thousands of 3D volumes) will be available. Silver standard reference data for standard sequences at 1.5T and 3T can be generated using existing tools such as HD-BET: for other sequences and field strengths semi-supervised learning or methods improving robustness to domain shift may be employed. Robustness to partial skull-stripping may be induced by a combination of learning theory and model-based approaches.

Dataset curation: 10%
Idempotent skull-stripping model building: 30%
Modelling of partial skull-stripping:10%
Extension of model to handle partial skull: 30%
Results analysis: 10%
Fig. An example of failed skull-stripping requiring manual correction
References: [1] Isensee, F, Schell, M, Pflueger, I, et al. Automated brain extraction of multisequence MRI using artificial neural networks. Hum Brain Mapp . 2019; 40: 4952– 4964. https://doi.org/10.1002/hbm.24750
Automated leaf detection and leaf area estimation (for Arabidopsis thaliana)
Correlating plant phenotypes such as leaf area or number of leaves to the genotype (i.e. changes in DNA) is a common goal for plant breeders and molecular biologists. Such data can not only help to understand fundamental processes in nature, but also can help to improve ecotypes, e.g., to perform better under climate change, or reduce fertiliser input. However, collecting data for many plants is very time consuming and automated data acquisition is necessary.
The project aims at building a machine learning model to automatically detect plants in top-view images (see examples below), segment their leaves (see Fig C) and to estimate the leaf area. This information will then be used to determine the leaf area of different Arabidopsis ecotypes. The project will be carried out in collaboration with researchers of the Institute of Plant Sciences at the University of Bern. It will also involve the design and creation of a dataset of plant top-views with the corresponding annotation (provided by experts at the Institute of Plant Sciences).

Contact: Prof. Dr. Paolo Favaro ( [email protected] )
Master Projects at the ARTORG Center
The Gerontechnology and Rehabilitation group at the ARTORG Center for Biomedical Engineering is offering multiple MSc thesis projects to students, which are interested in working with real patient data, artificial intelligence and machine learning algorithms. The goal of these projects is to transfer the findings to the clinic in order to solve today’s healthcare problems and thus to improve the quality of life of patients. Assessment of Digital Biomarkers at Home by Radar. [PDF] Comparison of Radar, Seismograph and Ballistocardiography and to Monitor Sleep at Home. [PDF] Sentimental Analysis in Speech. [PDF] Contact: Dr. Stephan Gerber ( [email protected] )
Internship in Computational Imaging at Prophesee
A 6 month intership at Prophesee, Grenoble is offered to a talented Master Student.
The topic of the internship is working on burst imaging following the work of Sam Hasinoff , and exploring ways to improve it using event-based vision.
A compensation to cover the expenses of living in Grenoble is offered. Only students that have legal rights to work in France can apply.
Anyone interested can send an email with the CV to Daniele Perrone ( [email protected] ).
Using machine learning applied to wearables to predict mental health
This Master’s project lies at the intersection of psychiatry and computer science and aims to use machine learning techniques to improve health. Using sensors to detect sleep and waking behavior has as of yet unexplored potential to reveal insights into health. In this study, we make use of a watch-like device, called an actigraph, which tracks motion to quantify sleep behavior and waking activity. Participants in the study consist of healthy and depressed adolescents and wear actigraphs for a year during which time we query their mental health status monthly using online questionnaires. For this masters thesis we aim to make use of machine learning methods to predict mental health based on the data from the actigraph. The ability to predict mental health crises based on sleep and wake behavior would provide an opportunity for intervention, significantly impacting the lives of patients and their families. This Masters thesis is a collaboration between Professor Paolo Favaro at the Institute of Computer Science ( [email protected] ) and Dr Leila Tarokh at the Universitäre Psychiatrische Dienste (UPD) ( [email protected] ). We are looking for a highly motivated individual interested in bridging disciplines.
Bachelor or Master Projects at the ARTORG Center
The Gerontechnology and Rehabilitation group at the ARTORG Center for Biomedical Engineering is offering multiple BSc- and MSc thesis projects to students, which are interested in working with real patient data, artificial intelligence and machine learning algorithms. The goal of these projects is to transfer the findings to the clinic in order to solve today’s healthcare problems and thus to improve the quality of life of patients. Machine Learning Based Gait-Parameter Extraction by Using Simple Rangefinder Technology. [PDF] Detection of Motion in Video Recordings [PDF] Home-Monitoring of Elderly by Radar [PDF] Gait feature detection in Parkinson's Disease [PDF] Development of an arthroscopic training device using virtual reality [PDF] Contact: Dr. Stephan Gerber ( [email protected] ), Michael Single ( [email protected]. ch )
Dynamic Transformer
Level: bachelor.
Visual Transformers have obtained state of the art classification accuracies [ViT, DeiT, T2T, BoTNet]. Mixture of experts could be used to increase the capacity of a neural network by learning instance dependent execution pathways in a network [MoE]. In this research project we aim to push the transformers to their limit and combine their dynamic attention with MoEs, compared to Switch Transformer [Switch], we will use a much more efficient formulation of mixing [CondConv, DynamicConv] and we will use this idea in the attention part of the transformer, not the fully connected layer.
- Input dependent attention kernel generation for better transformer layers.
Publication Opportunity: Dynamic Neural Networks Meets Computer Vision (a CVPR 2021 Workshop)
Extensions:
- The same idea could be extended to other ViT/Transformer based models [DETR, SETR, LSTR, TrackFormer, BERT]
Related Papers:
- Visual Transformers: Token-based Image Representation and Processing for Computer Vision [ViT]
- DeiT: Data-efficient Image Transformers [DeiT]
- Bottleneck Transformers for Visual Recognition [BoTNet]
- Tokens-to-Token ViT: Training Vision Transformers from Scratch on ImageNet [T2TViT]
- Outrageously Large Neural Networks: The Sparsely-Gated Mixture-of-Experts Layer [MoE]
- Switch Transformers: Scaling to Trillion Parameter Models with Simple and Efficient Sparsity [Switch]
- CondConv: Conditionally Parameterized Convolutions for Efficient Inference [CondConv]
- Dynamic Convolution: Attention over Convolution Kernels [DynamicConv]
- End-to-End Object Detection with Transformers [DETR]
- Rethinking Semantic Segmentation from a Sequence-to-Sequence Perspective with Transformers [SETR]
- End-to-end Lane Shape Prediction with Transformers [LSTR]
- TrackFormer: Multi-Object Tracking with Transformers [TrackFormer]
- BERT: Pre-training of Deep Bidirectional Transformers for Language Understanding [BERT]
Contact: Sepehr Sameni
Visual Transformers have obtained state of the art classification accuracies for 2d images[ViT, DeiT, T2T, BoTNet]. In this project, we aim to extend the same ideas to 3d data (videos), which requires a more efficient attention mechanism [Performer, Axial, Linformer]. In order to accelerate the training process, we could use [Multigrid] technique.
- Better video understanding by attention blocks.
Publication Opportunity: LOVEU (a CVPR workshop) , Holistic Video Understanding (a CVPR workshop) , ActivityNet (a CVPR workshop)
- Rethinking Attention with Performers [Performer]
- Axial Attention in Multidimensional Transformers [Axial]
- Linformer: Self-Attention with Linear Complexity [Linformer]
- A Multigrid Method for Efficiently Training Video Models [Multigrid]
GIRAFFE is a newly introduced GAN that can generate scenes via composition with minimal supervision [GIRAFFE]. Generative methods can implicitly learn interpretable representation as can be seen in GAN image interpretations [GANSpace, GanLatentDiscovery]. Decoding GIRAFFE could give us per-object interpretable representations that could be used for scene manipulation, data augmentation, scene understanding, semantic segmentation, pose estimation [iNeRF], and more.
In order to invert a GIRAFFE model, we will first train the generative model on Clevr and CompCars datasets, then we add a decoder to the pipeline and train this autoencoder. We can make the task easier by knowing the number of objects in the scene and/or knowing their positions.
Goals:
Scene Manipulation and Decomposition by Inverting the GIRAFFE
Publication Opportunity: DynaVis 2021 (a CVPR workshop on Dynamic Scene Reconstruction)
Related Papers:
- GIRAFFE: Representing Scenes as Compositional Generative Neural Feature Fields [GIRAFFE]
- Neural Scene Graphs for Dynamic Scenes
- pixelNeRF: Neural Radiance Fields from One or Few Images [pixelNeRF]
- NeRF: Representing Scenes as Neural Radiance Fields for View Synthesis [NeRF]
- Neural Volume Rendering: NeRF And Beyond
- GANSpace: Discovering Interpretable GAN Controls [GANSpace]
- Unsupervised Discovery of Interpretable Directions in the GAN Latent Space [GanLatentDiscovery]
- Inverting Neural Radiance Fields for Pose Estimation [iNeRF]
Quantized ViT
Visual Transformers have obtained state of the art classification accuracies [ViT, CLIP, DeiT], but the best ViT models are extremely compute heavy and running them even only for inference (not doing backpropagation) is expensive. Running transformers cheaply by quantization is not a new problem and it has been tackled before for BERT [BERT] in NLP [Q-BERT, Q8BERT, TernaryBERT, BinaryBERT]. In this project we will be trying to quantize pretrained ViT models.
Quantizing ViT models for faster inference and smaller models without losing accuracy
Publication Opportunity: Binary Networks for Computer Vision 2021 (a CVPR workshop)
Extensions:
- Having a fast pipeline for image inference with ViT will allow us to dig deep into the attention of ViT and analyze it, we might be able to prune some attention heads or replace them with static patterns (like local convolution or dilated patterns), We might be even able to replace the transformer with performer and increase the throughput even more [Performer].
- The same idea could be extended to other ViT based models [DETR, SETR, LSTR, TrackFormer, CPTR, BoTNet, T2TViT]
- Learning Transferable Visual Models From Natural Language Supervision [CLIP]
- Visual Transformers: Token-based Image Representation and Processing for Computer Vision [ViT]
- DeiT: Data-efficient Image Transformers [DeiT]
- BERT: Pre-training of Deep Bidirectional Transformers for Language Understanding [BERT]
- Q-BERT: Hessian Based Ultra Low Precision Quantization of BERT [Q-BERT]
- Q8BERT: Quantized 8Bit BERT [Q8BERT]
- TernaryBERT: Distillation-aware Ultra-low Bit BERT [TernaryBERT]
- BinaryBERT: Pushing the Limit of BERT Quantization [BinaryBERT]
- Rethinking Attention with Performers [Performer]
- End-to-End Object Detection with Transformers [DETR]
- Rethinking Semantic Segmentation from a Sequence-to-Sequence Perspective with Transformers [SETR]
- End-to-end Lane Shape Prediction with Transformers [LSTR]
- TrackFormer: Multi-Object Tracking with Transformers [TrackFormer]
- CPTR: Full Transformer Network for Image Captioning [CPTR]
- Bottleneck Transformers for Visual Recognition [BoTNet]
- Tokens-to-Token ViT: Training Vision Transformers from Scratch on ImageNet [T2TViT]
Multimodal Contrastive Learning
Recently contrastive learning has gained a lot of attention for self-supervised image representation learning [SimCLR, MoCo]. Contrastive learning could be extended to multimodal data, like videos (images and audio) [CMC, CoCLR]. Most contrastive methods require large batch sizes (or large memory pools) which makes them expensive for training. In this project we are going to use non batch size dependent contrastive methods [SwAV, BYOL, SimSiam] to train multimodal representation extractors.
Our main goal is to compare the proposed method with the CMC baseline, so we will be working with STL10, ImageNet, UCF101, HMDB51, and NYU Depth-V2 datasets.
Inspired by the recent works on smaller datasets [ConVIRT, CPD], to accelerate the training speed, we could start with two pretrained single-modal models and finetune them with the proposed method.
- Extending SwAV to multimodal datasets
- Grasping a better understanding of the BYOL
Publication Opportunity: MULA 2021 (a CVPR workshop on Multimodal Learning and Applications)
- Most knowledge distillation methods for contrastive learners also use large batch sizes (or memory pools) [CRD, SEED], the proposed method could be extended for knowledge distillation.
- One could easily extend this idea to multiview learning, for example one could have two different networks working on the same input and train them with contrastive learning, this may lead to better models [DeiT] by cross-model inductive biases communications.
- Self-supervised Co-training for Video Representation Learning [CoCLR]
- Learning Spatiotemporal Features via Video and Text Pair Discrimination [CPD]
- Audio-Visual Instance Discrimination with Cross-Modal Agreement [AVID-CMA]
- Self-Supervised Learning by Cross-Modal Audio-Video Clustering [XDC]
- Contrastive Multiview Coding [CPC]
- Contrastive Learning of Medical Visual Representations from Paired Images and Text [ConVIRT]
- A Simple Framework for Contrastive Learning of Visual Representations [SimCLR]
- Momentum Contrast for Unsupervised Visual Representation Learning [MoCo]
- Bootstrap your own latent: A new approach to self-supervised Learning [BYOL]
- Exploring Simple Siamese Representation Learning [SimSiam]
- Unsupervised Learning of Visual Features by Contrasting Cluster Assignments [SwAV]
- Contrastive Representation Distillation [CRD]
- SEED: Self-supervised Distillation For Visual Representation [SEED]
Robustness of Neural Networks
Neural Networks have been found to achieve surprising performance in several tasks such as classification, detection and segmentation. However, they are also very sensitive to small (controlled) changes to the input. It has been shown that some changes to an image that are not visible to the naked eye may lead the network to output an incorrect label. This thesis will focus on studying recent progress in this area and aim to build a procedure for a trained network to self-assess its reliability in classification or one of the popular computer vision tasks.
Contact: Paolo Favaro
Masters projects at sitem center
The Personalised Medicine Research Group at the sitem Center for Translational Medicine and Biomedical Entrepreneurship is offering multiple MSc thesis projects to the biomed eng MSc students that may also be of interest to the computer science students. Automated quantification of cartilage quality for hip treatment decision support. PDF Automated quantification of massive rotator cuff tears from MRI. PDF Deep learning-based segmentation and fat fraction analysis of the shoulder muscles using quantitative MRI. PDF Unsupervised Domain Adaption for Cross-Modality Hip Joint Segmentation. PDF Contact: Dr. Kate Gerber
Internships/Master thesis @ Chronocam
3-6 months internships on event-based computer vision. Chronocam is a rapidly growing startup developing event-based technology, with more than 15 PhDs working on problems like tracking, detection, classification, SLAM, etc. Event-based computer vision has the potential to solve many long-standing problems in traditional computer vision, and this is a super exciting time as this potential is becoming more and more tangible in many real-world applications. For next year we are looking for motivated Master and PhD students with good software engineering skills (C++ and/or python), and preferable good computer vision and deep learning background. PhD internships will be more research focused and possibly lead to a publication. For each intern we offer a compensation to cover the expenses of living in Paris. List of some of the topics we want to explore:
- Photo-realistic image synthesis and super-resolution from event-based data (PhD)
- Self-supervised representation learning (PhD)
- End-to-end Feature Learning for Event-based Data
- Bio-inspired Filtering using Spiking Networks
- On-the fly Compression of Event-based Streams for Low-Power IoT Cameras
- Tracking of Multiple Objects with a Dual-Frequency Tracker
- Event-based Autofocus
- Stabilizing an Event-based Stream using an IMU
- Crowd Monitoring for Low-power IoT Cameras
- Road Extraction from an Event-based Camera Mounted in a Car for Autonomous Driving
- Sign detection from an Event-based Camera Mounted in a Car for Autonomous Driving
- High-frequency Eye Tracking
Email with attached CV to Daniele Perrone at [email protected] .
Contact: Daniele Perrone
Object Detection in 3D Point Clouds
Today we have many 3D scanning techniques that allow us to capture the shape and appearance of objects. It is easier than ever to scan real 3D objects and transform them into a digital model for further processing, such as modeling, rendering or animation. However, the output of a 3D scanner is often a raw point cloud with little to no annotations. The unstructured nature of the point cloud representation makes it difficult for processing, e.g. surface reconstruction. One application is the detection and segmentation of an object of interest. In this project, the student is challenged to design a system that takes a point cloud (a 3D scan) as input and outputs the names of objects contained in the scan. This output can then be used to eliminate outliers or points that belong to the background. The approach involves collecting a large dataset of 3D scans and training a neural network on it.
Contact: Adrian Wälchli
Shape Reconstruction from a Single RGB Image or Depth Map
A photograph accurately captures the world in a moment of time and from a specific perspective. Since it is a projection of the 3D space to a 2D image plane, the depth information is lost. Is it possible to restore it, given only a single photograph? In general, the answer is no. This problem is ill-posed, meaning that many different plausible depth maps exist, and there is no way of telling which one is the correct one. However, if we cover one of our eyes, we are still able to recognize objects and estimate how far away they are. This motivates the exploration of an approach where prior knowledge can be leveraged to reduce the ill-posedness of the problem. Such a prior could be learned by a deep neural network, trained with many images and depth maps.
CNN Based Deblurring on Mobile
Deblurring finds many applications in our everyday life. It is particularly useful when taking pictures on handheld devices (e.g. smartphones) where camera shake can degrade important details. Therefore, it is desired to have a good deblurring algorithm implemented directly in the device. In this project, the student will implement and optimize a state-of-the-art deblurring method based on a deep neural network for deployment on mobile phones (Android). The goal is to reduce the number of network weights in order to reduce the memory footprint while preserving the quality of the deblurred images. The result will be a camera app that automatically deblurs the pictures, giving the user a choice of keeping the original or the deblurred image.
Depth from Blur
If an object in front of the camera or the camera itself moves while the aperture is open, the region of motion becomes blurred because the incoming light is accumulated in different positions across the sensor. If there is camera motion, there is also parallax. Thus, a motion blurred image contains depth information. In this project, the student will tackle the problem of recovering a depth-map from a motion-blurred image. This includes the collection of a large dataset of blurred- and sharp images or videos using a pair or triplet of GoPro action cameras. Two cameras will be used in stereo to estimate the depth map, and the third captures the blurred frames. This data is then used to train a convolutional neural network that will predict the depth map from the blurry image.
Unsupervised Clustering Based on Pretext Tasks
The idea of this project is that we have two types of neural networks that work together: There is one network A that assigns images to k clusters and k (simple) networks of type B perform a self-supervised task on those clusters. The goal of all the networks is to make the k networks of type B perform well on the task. The assumption is that clustering in semantically similar groups will help the networks of type B to perform well. This could be done on the MNIST dataset with B being linear classifiers and the task being rotation prediction.
Adversarial Data-Augmentation
The student designs a data augmentation network that transforms training images in such a way that image realism is preserved (e.g. with a constrained spatial transformer network) and the transformed images are more difficult to classify (trained via adversarial loss against an image classifier). The model will be evaluated for different data settings (especially in the low data regime), for example on the MNIST and CIFAR datasets.
Unsupervised Learning of Lip-reading from Videos
People with sensory impairment (hearing, speech, vision) depend heavily on assistive technologies to communicate and navigate in everyday life. The mass production of media content today makes it impossible to manually translate everything into a common language for assistive technologies, e.g. captions or sign language. In this project, the student employs a neural network to learn a representation for lip-movement in videos in an unsupervised fashion, possibly with an encoder-decoder structure where the decoder reconstructs the audio signal. This requires collecting a large dataset of videos (e.g. from YouTube) of speakers or conversations where lip movement is visible. The outcome will be a neural network that learns an audio-visual representation of lip movement in videos, which can then be leveraged to generate captions for hearing impaired persons.
Learning to Generate Topographic Maps from Satellite Images
Satellite images have many applications, e.g. in meteorology, geography, education, cartography and warfare. They are an accurate and detailed depiction of the surface of the earth from above. Although it is relatively simple to collect many satellite images in an automated way, challenges arise when processing them for use in navigation and cartography. The idea of this project is to automatically convert an arbitrary satellite image, of e.g. a city, to a map of simple 2D shapes (streets, houses, forests) and label them with colors (semantic segmentation). The student will collect a dataset of satellite image and topological maps and train a deep neural network that learns to map from one domain to the other. The data could be obtained from a Google Maps database or similar.
New Variables of Brain Morphometry: the Potential and Limitations of CNN Regression
Timo blattner · sept. 2022.
The calculation of variables of brain morphology is computationally very expensive and time-consuming. A previous work showed the feasibility of ex- tracting the variables directly from T1-weighted brain MRI images using a con- volutional neural network. We used significantly more data and extended their model to a new set of neuromorphological variables, which could become inter- esting biomarkers in the future for the diagnosis of brain diseases. The model shows for nearly all subjects a less than 5% mean relative absolute error. This high relative accuracy can be attributed to the low morphological variance be- tween subjects and the ability of the model to predict the cortical atrophy age trend. The model however fails to capture all the variance in the data and shows large regional differences. We attribute these limitations in part to the moderate to poor reliability of the ground truth generated by FreeSurfer. We further investigated the effects of training data size and model complexity on this regression task and found that the size of the dataset had a significant impact on performance, while deeper models did not perform better. Lack of interpretability and dependence on a silver ground truth are the main drawbacks of this direct regression approach.
Home Monitoring by Radar
Lars ziegler · sept. 2022.
Detection and tracking of humans via UWB radars is a promising and continuously evolving field with great potential for medical technology. This contactless method of acquiring data of a patients movement patterns is ideal for in home application. As irregularities in a patients movement patterns are an indicator for various health problems including neurodegenerative diseases, the insight this data could provide may enable earlier detection of such problems. In this thesis a signal processing pipeline is presented with which a persons movement is modeled. During an experiment 142 measurements were recorded by two separate radar systems and one lidar system which each consisted of multiple sensors. The models that were calculated on these measurements by the signal processing pipeline were used to predict the times when a person stood up or sat down. The predictions showed an accuracy of 72.2%.
Revisiting non-learning based 3D reconstruction from multiple images
Aaron sägesser · oct. 2021.
Arthroscopy consists of challenging tasks and requires skills that even today, young surgeons still train directly throughout the surgery. Existing simulators are expensive and rarely available. Through the growing potential of virtual reality(VR) (head-mounted) devices for simulation and their applicability in the medical context, these devices have become a promising alternative that would be orders of magnitude cheaper and could be made widely available. To build a VR-based training device for arthroscopy is the overall aim of our project, as this would be of great benefit and might even be applicable in other minimally invasive surgery (MIS). This thesis marks a first step of the project with its focus to explore and compare well-known algorithms in a multi-view stereo (MVS) based 3D reconstruction with respect to imagery acquired by an arthroscopic camera. Simultaneously with this reconstruction, we aim to gain essential measures to compare the VR environment to the real world, as validation of the realism of future VR tasks. We evaluate 3 different feature extraction algorithms with 3 different matching techniques and 2 different algorithms for the estimation of the fundamental (F) matrix. The evaluation of these 18 different setups is made with a reconstruction pipeline embedded in a jupyter notebook implemented in python based on common computer vision libraries and compared with imagery generated with a mobile phone as well as with the reconstruction results of state-of-the-art (SOTA) structure-from-motion (SfM) software COLMAP and Multi-View Environment (MVE). Our comparative analysis manifests the challenges of heavy distortion, the fish-eye shape and weak image quality of arthroscopic imagery, as all results are substantially worse using this data. However, there are huge differences regarding the different setups. Scale Invariant Feature Transform (SIFT) and Oriented FAST Rotated BRIEF (ORB) in combination with k-Nearest Neighbour (kNN) matching and Least Median of Squares (LMedS) present the most promising results. Overall, the 3D reconstruction pipeline is a useful tool to foster the process of gaining measurements from the arthroscopic exploration device and to complement the comparative research in this context.
Examination of Unsupervised Representation Learning by Predicting Image Rotations
Eric lagger · sept. 2020.
In recent years deep convolutional neural networks achieved a lot of progress. To train such a network a lot of data is required and in supervised learning algorithms it is necessary that the data is labeled. To label data there is a lot of human work needed and this takes a lot of time and money to be done. To avoid the inconveniences that come with this we would like to find systems that don’t need labeled data and therefore are unsupervised learning algorithms. This is the importance of unsupervised algorithms, even though their outcome is not yet on the same qualitative level as supervised algorithms. In this thesis we will discuss an approach of such a system and compare the results to other papers. A deep convolutional neural network is trained to learn the rotations that have been applied to a picture. So we take a large amount of images and apply some simple rotations and the task of the network is to discover in which direction the image has been rotated. The data doesn’t need to be labeled to any category or anything else. As long as all the pictures are upside down we hope to find some high dimensional patterns for the network to learn.
StitchNet: Image Stitching using Autoencoders and Deep Convolutional Neural Networks
Maurice rupp · sept. 2019.
This thesis explores the prospect of artificial neural networks for image processing tasks. More specifically, it aims to achieve the goal of stitching multiple overlapping images to form a bigger, panoramic picture. Until now, this task is solely approached with ”classical”, hardcoded algorithms while deep learning is at most used for specific subtasks. This thesis introduces a novel end-to-end neural network approach to image stitching called StitchNet, which uses a pre-trained autoencoder and deep convolutional networks. Additionally to presenting several new datasets for the task of supervised image stitching with each 120’000 training and 5’000 validation samples, this thesis also conducts various experiments with different kinds of existing networks designed for image superresolution and image segmentation adapted to the task of image stitching. StitchNet outperforms most of the adapted networks in both quantitative as well as qualitative results.
Facial Expression Recognition in the Wild
Luca rolshoven · sept. 2019.
The idea of inferring the emotional state of a subject by looking at their face is nothing new. Neither is the idea of automating this process using computers. Researchers used to computationally extract handcrafted features from face images that had proven themselves to be effective and then used machine learning techniques to classify the facial expressions using these features. Recently, there has been a trend towards using deeplearning and especially Convolutional Neural Networks (CNNs) for the classification of these facial expressions. Researchers were able to achieve good results on images that were taken in laboratories under the same or at least similar conditions. However, these models do not perform very well on more arbitrary face images with different head poses and illumination. This thesis aims to show the challenges of Facial Expression Recognition (FER) in this wild setting. It presents the currently used datasets and the present state-of-the-art results on one of the biggest facial expression datasets currently available. The contributions of this thesis are twofold. Firstly, I analyze three famous neural network architectures and their effectiveness on the classification of facial expressions. Secondly, I present two modifications of one of these networks that lead to the proposed STN-COV model. While this model does not outperform all of the current state-of-the-art models, it does beat several ones of them.
A Study of 3D Reconstruction of Varying Objects with Deformable Parts Models
Raoul grossenbacher · july 2019.
This work covers a new approach to 3D reconstruction. In traditional 3D reconstruction one uses multiple images of the same object to calculate a 3D model by taking information gained from the differences between the images, like camera position, illumination of the images, rotation of the object and so on, to compute a point cloud representing the object. The characteristic trait shared by all these approaches is that one can almost change everything about the image, but it is not possible to change the object itself, because one needs to find correspondences between the images. To be able to use different instances of the same object, we used a 3D DPM model that can find different parts of an object in an image, thereby detecting the correspondences between the different pictures, which we then can use to calculate the 3D model. To take this theory to practise, we gave a 3D DPM model, which was trained to detect cars, pictures of different car brands, where no pair of images showed the same vehicle and used the detected correspondences and the Factorization Method to compute the 3D point cloud. This technique leads to a completely new approach in 3D reconstruction, because changing the object itself was never done before.
Motion deblurring in the wild replication and improvements
Alvaro juan lahiguera · jan. 2019, coma outcome prediction with convolutional neural networks, stefan jonas · oct. 2018, automatic correction of self-introduced errors in source code, sven kellenberger · aug. 2018, neural face transfer: training a deep neural network to face-swap, till nikolaus schnabel · july 2018.
This thesis explores the field of artificial neural networks with realistic looking visual outputs. It aims at morphing face pictures of a specific identity to look like another individual by only modifying key features, such as eye color, while leaving identity-independent features unchanged. Prior works have covered the topic of symmetric translation between two specific domains but failed to optimize it on faces where only parts of the image may be changed. This work applies a face masking operation to the output at training time, which forces the image generator to preserve colors while altering the face, fitting it naturally inside the unmorphed surroundings. Various experiments are conducted including an ablation study on the final setting, decreasing the baseline identity switching performance from 81.7% to 75.8 % whilst improving the average χ2 color distance from 0.551 to 0.434. The provided code-based software gives users easy access to apply this neural face swap to images and videos of arbitrary crop and brings Computer Vision one step closer to replacing Computer Graphics in this specific area.
A Study of the Importance of Parts in the Deformable Parts Model
Sammer puran · june 2017, self-similarity as a meta feature, lucas husi · april 2017, a study of 3d deformable parts models for detection and pose-estimation, simon jenni · march 2015, accelerated federated learning on client silos with label noise: rho selection in classification and segmentation, irakli kelbakiani · may 2024.
Federated Learning has recently gained more research interest. This increased attention is caused by factors including the growth of decentralized data, privacy concerns, and new privacy regulations. In Federated Learning, remote servers keep training a model on local datasets independently, and subsequently, local models are aggregated into a global model, which achieves better overall performance. Sending local model weights instead of the entire dataset is a significant advantage of Federated Learning over centralized classical machine learning algorithms. Federated learning involves uploading and downloading model parameters multiple times, so there are multiple communication rounds between the global server and remote client servers, which imposes challenges. The high number of necessary communication rounds not only increases high-cost communication overheads but is also a critical limitation for servers with low network bandwidth, which leads to latency and a higher probability of training failures caused by communication breakdowns. To mitigate these challenges, we aim to provide a fast-convergent Federated Learning training methodology that decreases the number of necessary communication rounds. We found a paper about Reducible Holdout Loss Selection (RHO-Loss) batch selection methodology, which ”selects low-noise, task-relevant, non-redundant points for training” [1]; we hypothesize, if client silos employ RHO-Loss methodology and successfully avoid training their local models on noisy and non-relevant samples, clients may offer stable and consistent updates to the global server, which could lead to faster convergence of the global model. Our contribution focuses on investigating the RHO-Loss method in a simulated federated setting for the Clothing1M dataset. We also examine its applicability to medical datasets and check its effectiveness in a simulated federated environment. Our experimental results show a promising outcome, specifically a reduction in communication rounds for the Clothing1M dataset. However, as the success of the RHO-Loss selection method depends on the availability of sufficient training data for the target RHO model and for the Irreducible RHO model, we emphasize that our contribution applies to those Federated Learning scenarios where client silos hold enough training data to successfully train and benefit from their RHO model on their local dataset.
Amodal Leaf Segmentation
Nicolas maier · nov. 2023.
Plant phenotyping is the process of measuring and analyzing various traits of plants. It provides essential information on how genetic and environmental factors affect plant growth and development. Manual phenotyping is highly time-consuming; therefore, many computer vision and machine learning based methods have been proposed in the past years to perform this task automatically based on images of the plants. However, the publicly available datasets (in particular, of Arabidopsis thaliana) are limited in size and diversity, making them unsuitable to generalize to new unseen environments. In this work, we propose a complete pipeline able to automatically extract traits of interest from an image of Arabidopsis thaliana. Our method uses a minimal amount of existing annotated data from a source domain to generate a large synthetic dataset adapted to a different target domain (e.g., different backgrounds, lighting conditions, and plant layouts). In addition, unlike the source dataset, the synthetic one provides ground-truth annotations for the occluded parts of the leaves, which are relevant when measuring some characteristics of the plant, e.g., its total area. This synthetic dataset is then used to train a model to perform amodal instance segmentation of the leaves to obtain the total area, leaf count, and color of each plant. To validate our approach, we create a small dataset composed of manually annotated real images of Arabidopsis thaliana, which is used to assess the performance of the models.
Assessment of movement and pose in a hospital bed by ambient and wearable sensor technology in healthy subjects
Tony licata · sept. 2022.
The use of automated systems describing the human motion has become possible in various domains. Most of the proposed systems are designed to work with people moving around in a standing position. Because such system could be interesting in a medical environment, we propose in this work a pipeline that can effectively predict human motion from people lying on beds. The proposed pipeline is tested with a data set composed of 41 participants executing 7 predefined tasks in a bed. The motion of the participants is measured with video cameras, accelerometers and pressure mat. Various experiments are carried with the information retrieved from the data set. Two approaches combining the data from the different measure technologies are explored. The performance of the different carried experiments is measured, and the proposed pipeline is composed with components providing the best results. Later on, we show that the proposed pipeline only needs to use the video cameras, which make the proposed environment easier to implement in real life situations.
Machine Learning Based Prediction of Mental Health Using Wearable-measured Time Series
Seyedeh sharareh mirzargar · sept. 2022.
Depression is the second major cause for years spent in disability and has a growing prevalence in adolescents. The recent Covid-19 pandemic has intensified the situation and limited in-person patient monitoring due to distancing measures. Recent advances in wearable devices have made it possible to record the rest/activity cycle remotely with high precision and in real-world contexts. We aim to use machine learning methods to predict an individual's mental health based on wearable-measured sleep and physical activity. Predicting an impending mental health crisis of an adolescent allows for prompt intervention, detection of depression onset or its recursion, and remote monitoring. To achieve this goal, we train three primary forecasting models; linear regression, random forest, and light gradient boosted machine (LightGBM); and two deep learning models; block recurrent neural network (block RNN) and temporal convolutional network (TCN); on Actigraph measurements to forecast mental health in terms of depression, anxiety, sleepiness, stress, sleep quality, and behavioral problems. Our models achieve a high forecasting performance, the random forest being the winner to reach an accuracy of 98% for forecasting the trait anxiety. We perform extensive experiments to evaluate the models' performance in accuracy, generalization, and feature utilization, using a naive forecaster as the baseline. Our analysis shows minimal mental health changes over two months, making the prediction task easily achievable. Due to these minimal changes in mental health, the models tend to primarily use the historical values of mental health evaluation instead of Actigraph features. At the time of this master thesis, the data acquisition step is still in progress. In future work, we plan to train the models on the complete dataset using a longer forecasting horizon to increase the level of mental health changes and perform transfer learning to compensate for the small dataset size. This interdisciplinary project demonstrates the opportunities and challenges in machine learning based prediction of mental health, paving the way toward using the same techniques to forecast other mental disorders such as internalizing disorder, Parkinson's disease, Alzheimer's disease, etc. and improving the quality of life for individuals who have some mental disorder.
CNN Spike Detector: Detection of Spikes in Intracranial EEG using Convolutional Neural Networks
Stefan jonas · oct. 2021.
The detection of interictal epileptiform discharges in the visual analysis of electroencephalography (EEG) is an important but very difficult, tedious, and time-consuming task. There have been decades of research on computer-assisted detection algorithms, most recently focused on using Convolutional Neural Networks (CNNs). In this thesis, we present the CNN Spike Detector, a convolutional neural network to detect spikes in intracranial EEG. Our dataset of 70 intracranial EEG recordings from 26 subjects with epilepsy introduces new challenges in this research field. We report cross-validation results with a mean AUC of 0.926 (+- 0.04), an area under the precision-recall curve (AUPRC) of 0.652 (+- 0.10) and 12.3 (+- 7.47) false positive epochs per minute for a sensitivity of 80%. A visual examination of false positive segments is performed to understand the model behavior leading to a relatively high false detection rate. We notice issues with the evaluation measures and highlight a major limitation of the common approach of detecting spikes using short segments, namely that the network is not capable to consider the greater context of the segment with regards to its origination. For this reason, we present the Context Model, an extension in which the CNN Spike Detector is supplied with additional information about the channel. Results show promising but limited performance improvements. This thesis provides important findings about the spike detection task for intracranial EEG and lays out promising future research directions to develop a network capable of assisting experts in real-world clinical applications.
PolitBERT - Deepfake Detection of American Politicians using Natural Language Processing
Maurice rupp · april 2021.
This thesis explores the application of modern Natural Language Processing techniques to the detection of artificially generated videos of popular American politicians. Instead of focusing on detecting anomalies and artifacts in images and sounds, this thesis focuses on detecting irregularities and inconsistencies in the words themselves, opening up a new possibility to detect fake content. A novel, domain-adapted, pre-trained version of the language model BERT combined with several mechanisms to overcome severe dataset imbalances yielded the best quantitative as well as qualitative results. Additionally to the creation of the biggest publicly available dataset of English-speaking politicians consisting of 1.5 M sentences from over 1000 persons, this thesis conducts various experiments with different kinds of text classification and sequence processing algorithms applied to the political domain. Furthermore, multiple ablations to manage severe data imbalance are presented and evaluated.
A Study on the Inversion of Generative Adversarial Networks
Ramona beck · march 2021.
The desire to use generative adversarial networks (GANs) for real-world tasks such as object segmentation or image manipulation is increasing as synthesis quality improves, which has given rise to an emerging research area called GAN inversion that focuses on exploring methods for embedding real images into the latent space of a GAN. In this work, we investigate different GAN inversion approaches using an existing generative model architecture that takes a completely unsupervised approach to object segmentation and is based on StyleGAN2. In particular, we propose and analyze algorithms for embedding real images into the different latent spaces Z, W, and W+ of StyleGAN following an optimization-based inversion approach, while also investigating a novel approach that allows fine-tuning of the generator during the inversion process. Furthermore, we investigate a hybrid and a learning-based inversion approach, where in the former we train an encoder with embeddings optimized by our best optimization-based inversion approach, and in the latter we define an autoencoder, consisting of an encoder and the generator of our generative model as a decoder, and train it to map an image into the latent space. We demonstrate the effectiveness of our methods as well as their limitations through a quantitative comparison with existing inversion methods and by conducting extensive qualitative and quantitative experiments with synthetic data as well as real images from a complex image dataset. We show that we achieve qualitatively satisfying embeddings in the W and W+ spaces with our optimization-based algorithms, that fine-tuning the generator during the inversion process leads to qualitatively better embeddings in all latent spaces studied, and that the learning-based approach also benefits from a variable generator as well as a pre-training with our hybrid approach. Furthermore, we evaluate our approaches on the object segmentation task and show that both our optimization-based and our hybrid and learning-based methods are able to generate meaningful embeddings that achieve reasonable object segmentations. Overall, our proposed methods illustrate the potential that lies in the GAN inversion and its application to real-world tasks, especially in the relaxed version of the GAN inversion where the weights of the generator are allowed to vary.
Multi-scale Momentum Contrast for Self-supervised Image Classification
Zhao xueqi · dec. 2020.
With the maturity of supervised learning technology, people gradually shift the research focus to the field of self-supervised learning. ”Momentum Contrast” (MoCo) proposes a new self-supervised learning method and raises the correct rate of self-supervised learning to a new level. Inspired by another article ”Representation Learning by Learning to Count”, if a picture is divided into four parts and passed through a neural network, it is possible to further improve the accuracy of MoCo. Different from the original MoCo, this MoCo variant (Multi-scale MoCo) does not directly pass the image through the encoder after the augmented images. Multi-scale MoCo crops and resizes the augmented images, and the obtained four parts are respectively passed through the encoder and then summed (upsampled version do not do resize to input but resize the contrastive samples). This method of images crop is not only used for queue q but also used for comparison queue k, otherwise the weights of queue k might be damaged during the moment update. This will further discussed in the experiments chapter between downsampled Multi-scale version and downsampled both Multi-scale version. Human beings also have the same principle of object recognition: when human beings see something they are familiar with, even if the object is not fully displayed, people can still guess the object itself with a high probability. Because of this, Multi-scale MoCo applies this concept to the pretext part of MoCo, hoping to obtain better feature extraction. In this thesis, there are three versions of Multi-scale MoCo, downsampled input samples version, downsampled input samples and contrast samples version and upsampled input samples version. The differences between these versions will be described in more detail later. The neural network architecture comparison includes ResNet50 , and the tested data set is STL-10. The weights obtained in pretext will be transferred to self-supervised learning, and in the process of self-supervised learning, the weights of other layers except the final linear layer are frozen without changing (these weights come from pretext).
Self-Supervised Learning Using Siamese Networks and Binary Classifier
Dušan mihajlov · march 2020.
In this thesis, we present several approaches for training a convolutional neural network using only unlabeled data. Our autonomously supervised learning algorithms are based on connections between image patch i. e. zoomed image and its original. Using the siamese architecture neural network we aim to recognize, if the image patch, which is input to the first neural network part, comes from the same image presented to the second neural network part. By applying transformations to both images, and different zoom sizes at different positions, we force the network to extract high level features using its convolutional layers. At the top of our siamese architecture, we have a simple binary classifier that measures the difference between feature maps that we extract and makes a decision. Thus, the only way that the classifier will solve the task correctly is when our convolutional layers are extracting useful representations. Those representations we can than use to solve many different tasks that are related to the data used for unsupervised training. As the main benchmark for all of our models, we used STL10 dataset, where we train a linear classifier on the top of our convolutional layers with a small amount of manually labeled images, which is a widely used benchmark for unsupervised learning tasks. We also combine our idea with recent work on the same topic, and the network called RotNet, which makes use of image rotations and therefore forces the network to learn rotation dependent features from the dataset. As a result of this combination we create a new procedure that outperforms original RotNet.
Learning Object Representations by Mixing Scenes
Lukas zbinden · may 2019.
In the digital age of ever increasing data amassment and accessibility, the demand for scalable machine learning models effective at refining the new oil is unprecedented. Unsupervised representation learning methods present a promising approach to exploit this invaluable yet unlabeled digital resource at scale. However, a majority of these approaches focuses on synthetic or simplified datasets of images. What if a method could learn directly from natural Internet-scale image data? In this thesis, we propose a novel approach for unsupervised learning of object representations by mixing natural image scenes. Without any human help, our method mixes visually similar images to synthesize new realistic scenes using adversarial training. In this process the model learns to represent and understand the objects prevalent in natural image data and makes them available for downstream applications. For example, it enables the transfer of objects from one scene to another. Through qualitative experiments on complex image data we show the effectiveness of our method along with its limitations. Moreover, we benchmark our approach quantitatively against state-of-the-art works on the STL-10 dataset. Our proposed method demonstrates the potential that lies in learning representations directly from natural image data and reinforces it as a promising avenue for future research.
Representation Learning using Semantic Distances
Markus roth · may 2019, zero-shot learning using generative adversarial networks, hamed hemati · dec. 2018, dimensionality reduction via cnns - learning the distance between images, ioannis glampedakis · sept. 2018, learning to play othello using deep reinforcement learning and self play, thomas simon steinmann · sept. 2018, aba-j interactive multi-modality tissue sectionto-volume alignment: a brain atlasing toolkit for imagej, felix meyenhofer · march 2018, learning visual odometry with recurrent neural networks, adrian wälchli · feb. 2018.
In computer vision, Visual Odometry is the problem of recovering the camera motion from a video. It is related to Structure from Motion, the problem of reconstructing the 3D geometry from a collection of images. Decades of research in these areas have brought successful algorithms that are used in applications like autonomous navigation, motion capture, augmented reality and others. Despite the success of these prior works in real-world environments, their robustness is highly dependent on manual calibration and the magnitude of noise present in the images in form of, e.g., non-Lambertian surfaces, dynamic motion and other forms of ambiguity. This thesis explores an alternative approach to the Visual Odometry problem via Deep Learning, that is, a specific form of machine learning with artificial neural networks. It describes and focuses on the implementation of a recent work that proposes the use of Recurrent Neural Networks to learn dependencies over time due to the sequential nature of the input. Together with a convolutional neural network that extracts motion features from the input stream, the recurrent part accumulates knowledge from the past to make camera pose estimations at each point in time. An analysis on the performance of this system is carried out on real and synthetic data. The evaluation covers several ways of training the network as well as the impact and limitations of the recurrent connection for Visual Odometry.
Crime location and timing prediction
Bernard swart · jan. 2018, from cartoons to real images: an approach to unsupervised visual representation learning, simon jenni · feb. 2017, automatic and large-scale assessment of fluid in retinal oct volume, nina mujkanovic · dec. 2016, segmentation in 3d using eye-tracking technology, michele wyss · july 2016, accurate scale thresholding via logarithmic total variation prior, remo diethelm · aug. 2014, unsupervised object segmentation with generative models, adam jakub bielski · april 2024.
Advances in computer vision have transformed how we interact with technology, driven by significant breakthroughs in scalable deep learning and the availability of large datasets. These technologies now play a crucial role in various applications, from improving user experience through applications like organizing digital photo libraries, to advancing medical diagnostics and treatments. Despite these valuable applications, the creation of annotated datasets remains a significant bottleneck. It is not only costly and labor-intensive but also prone to inaccuracies and human biases. Moreover, it often requires specialized knowledge or careful handling of sensitive information. Among the tasks in computer vision, image segmentation particularly highlights these challenges, with its need for precise pixel-level annotations. This context underscores the need for unsupervised approaches in computer vision, which can leverage the large volumes of unlabeled images produced every day. This thesis introduces several novel methods for learning fully unsupervised object segmentation models using only collections of images. Unlike much prior work, our approaches are effective on complex real-world images and do not rely on any form of annotations, including pre-trained supervised networks, bounding boxes, or class labels. We identify and leverage intrinsic properties of objects – most notably, the cohesive movement of object parts – as powerful signals for driving unsupervised object segmentation. Utilizing innovative generative adversarial models, we employ this principle to either generate segmented objects or directly segment them in a manner that allows for realistic movement within scenes. Our work demonstrates how such generated data can train a segmentation model that effectively generalizes to realworld images. Furthermore, we introduce a method that, in conjunction with recent advances in self-supervised learning, achieves state-of-the-art results in unsupervised object segmentation. Our methods rely on the effectiveness of Generative Adversarial Networks, which are known to be challenging to train and exhibit mode collapse. We propose a new, more principled GAN loss, whose gradients encourage the generator model to explore missing modes in its distribution, addressing these limitations and enhancing the robustness of generative models.
Novel Techniques for Robust and Generalizable Machine Learning
Abdelhak lemkhenter · sept. 2023.
Neural networks have transcended their status of powerful proof-of-concept machine learning into the realm of a highly disruptive technology that has revolutionized many quantitative fields such as drug discovery, autonomous vehicles, and machine translation. Today, it is nearly impossible to go a single day without interacting with a neural network-powered application. From search engines to on-device photo-processing, neural networks have become the go-to solution thanks to recent advances in computational hardware and an unprecedented scale of training data. Larger and less curated datasets, typically obtained through web crawling, have greatly propelled the capabilities of neural networks forward. However, this increase in scale amplifies certain challenges associated with training such models. Beyond toy or carefully curated datasets, data in the wild is plagued with biases, imbalances, and various noisy components. Given the larger size of modern neural networks, such models run the risk of learning spurious correlations that fail to generalize beyond their training data. This thesis addresses the problem of training more robust and generalizable machine learning models across a wide range of learning paradigms for medical time series and computer vision tasks. The former is a typical example of a low signal-to-noise ratio data modality with a high degree of variability between subjects and datasets. There, we tailor the training scheme to focus on robust patterns that generalize to new subjects and ignore the noisier and subject-specific patterns. To achieve this, we first introduce a physiologically inspired unsupervised training task and then extend it by explicitly optimizing for cross-dataset generalization using meta-learning. In the context of image classification, we address the challenge of training semi-supervised models under class imbalance by designing a novel label refinement strategy with higher local sensitivity to minority class samples while preserving the global data distribution. Lastly, we introduce a new Generative Adversarial Networks training loss. Such generative models could be applied to improve the training of subsequent models in the low data regime by augmenting the dataset using generated samples. Unfortunately, GAN training relies on a delicate balance between its components, making it prone mode collapse. Our contribution consists of defining a more principled GAN loss whose gradients incentivize the generator model to seek out missing modes in its distribution. All in all, this thesis tackles the challenge of training more robust machine learning models that can generalize beyond their training data. This necessitates the development of methods specifically tailored to handle the diverse biases and spurious correlations inherent in the data. It is important to note that achieving greater generalizability in models goes beyond simply increasing the volume of data; it requires meticulous consideration of training objectives and model architecture. By tackling these challenges, this research contributes to advancing the field of machine learning and underscores the significance of thoughtful design in obtaining more resilient and versatile models.
Automated Sleep Scoring, Deep Learning and Physician Supervision
Luigi fiorillo · oct. 2022.
Sleep plays a crucial role in human well-being. Polysomnography is used in sleep medicine as a diagnostic tool, so as to objectively analyze the quality of sleep. Sleep scoring is the procedure of extracting sleep cycle information from the wholenight electrophysiological signals. The scoring is done worldwide by the sleep physicians according to the official American Academy of Sleep Medicine (AASM) scoring manual. In the last decades, a wide variety of deep learning based algorithms have been proposed to automatise the sleep scoring task. In this thesis we study the reasons why these algorithms fail to be introduced in the daily clinical routine, with the perspective of bridging the existing gap between the automatic sleep scoring models and the sleep physicians. In this light, the primary step is the design of a simplified sleep scoring architecture, also providing an estimate of the model uncertainty. Beside achieving results on par with most up-to-date scoring systems, we demonstrate the efficiency of ensemble learning based algorithms, together with label smoothing techniques, in both enhancing the performance and calibrating the simplified scoring model. We introduced an uncertainty estimate procedure, so as to identify the most challenging sleep stage predictions, and to quantify the disagreement between the predictions given by the model and the annotation given by the physicians. In this thesis we also propose a novel method to integrate the inter-scorer variability into the training procedure of a sleep scoring model. We clearly show that a deep learning model is able to encode this variability, so as to better adapt to the consensus of a group of scorers-physicians. We finally address the generalization ability of a deep learning based sleep scoring system, further studying its resilience to the sleep complexity and to the AASM scoring rules. We can state that there is no need to train the algorithm strictly following the AASM guidelines. Most importantly, using data from multiple data centers results in a better performing model compared with training on a single data cohort. The variability among different scorers and data centers needs to be taken into account, more than the variability among sleep disorders.
Learning Representations for Controllable Image Restoration
Givi meishvili · march 2022.
Deep Convolutional Neural Networks have sparked a renaissance in all the sub-fields of computer vision. Tremendous progress has been made in the area of image restoration. The research community has pushed the boundaries of image deblurring, super-resolution, and denoising. However, given a distorted image, most existing methods typically produce a single restored output. The tasks mentioned above are inherently ill-posed, leading to an infinite number of plausible solutions. This thesis focuses on designing image restoration techniques capable of producing multiple restored results and granting users more control over the restoration process. Towards this goal, we demonstrate how one could leverage the power of unsupervised representation learning. Image restoration is vital when applied to distorted images of human faces due to their social significance. Generative Adversarial Networks enable an unprecedented level of generated facial details combined with smooth latent space. We leverage the power of GANs towards the goal of learning controllable neural face representations. We demonstrate how to learn an inverse mapping from image space to these latent representations, tuning these representations towards a specific task, and finally manipulating latent codes in these spaces. For example, we show how GANs and their inverse mappings enable the restoration and editing of faces in the context of extreme face super-resolution and the generation of novel view sharp videos from a single motion-blurred image of a face. This thesis also addresses more general blind super-resolution, denoising, and scratch removal problems, where blur kernels and noise levels are unknown. We resort to contrastive representation learning and first learn the latent space of degradations. We demonstrate that the learned representation allows inference of ground-truth degradation parameters and can guide the restoration process. Moreover, it enables control over the amount of deblurring and denoising in the restoration via manipulation of latent degradation features.
Learning Generalizable Visual Patterns Without Human Supervision
Simon jenni · oct. 2021.
Owing to the existence of large labeled datasets, Deep Convolutional Neural Networks have ushered in a renaissance in computer vision. However, almost all of the visual data we generate daily - several human lives worth of it - remains unlabeled and thus out of reach of today’s dominant supervised learning paradigm. This thesis focuses on techniques that steer deep models towards learning generalizable visual patterns without human supervision. Our primary tool in this endeavor is the design of Self-Supervised Learning tasks, i.e., pretext-tasks for which labels do not involve human labor. Besides enabling the learning from large amounts of unlabeled data, we demonstrate how self-supervision can capture relevant patterns that supervised learning largely misses. For example, we design learning tasks that learn deep representations capturing shape from images, motion from video, and 3D pose features from multi-view data. Notably, these tasks’ design follows a common principle: The recognition of data transformations. The strong performance of the learned representations on downstream vision tasks such as classification, segmentation, action recognition, or pose estimation validate this pretext-task design. This thesis also explores the use of Generative Adversarial Networks (GANs) for unsupervised representation learning. Besides leveraging generative adversarial learning to define image transformation for self-supervised learning tasks, we also address training instabilities of GANs through the use of noise. While unsupervised techniques can significantly reduce the burden of supervision, in the end, we still rely on some annotated examples to fine-tune learned representations towards a target task. To improve the learning from scarce or noisy labels, we describe a supervised learning algorithm with improved generalization in these challenging settings.
Learning Interpretable Representations of Images
Attila szabó · june 2019.
Computers represent images with pixels and each pixel contains three numbers for red, green and blue colour values. These numbers are meaningless for humans and they are mostly useless when used directly with classical machine learning techniques like linear classifiers. Interpretable representations are the attributes that humans understand: the colour of the hair, viewpoint of a car or the 3D shape of the object in the scene. Many computer vision tasks can be viewed as learning interpretable representations, for example a supervised classification algorithm directly learns to represent images with their class labels. In this work we aim to learn interpretable representations (or features) indirectly with lower levels of supervision. This approach has the advantage of cost savings on dataset annotations and the flexibility of using the features for multiple follow-up tasks. We made contributions in three main areas: weakly supervised learning, unsupervised learning and 3D reconstruction. In the weakly supervised case we use image pairs as supervision. Each pair shares a common attribute and differs in a varying attribute. We propose a training method that learns to separate the attributes into separate feature vectors. These features then are used for attribute transfer and classification. We also show theoretical results on the ambiguities of the learning task and the ways to avoid degenerate solutions. We show a method for unsupervised representation learning, that separates semantically meaningful concepts. We explain and show ablation studies how the components of our proposed method work: a mixing autoencoder, a generative adversarial net and a classifier. We propose a method for learning single image 3D reconstruction. It is done using only the images, no human annotation, stereo, synthetic renderings or ground truth depth map is needed. We train a generative model that learns the 3D shape distribution and an encoder to reconstruct the 3D shape. For that we exploit the notion of image realism. It means that the 3D reconstruction of the object has to look realistic when it is rendered from different random angles. We prove the efficacy of our method from first principles.
Learning Controllable Representations for Image Synthesis
Qiyang hu · june 2019.
In this thesis, our focus is learning a controllable representation and applying the learned controllable feature representation on images synthesis, video generation, and even 3D reconstruction. We propose different methods to disentangle the feature representation in neural network and analyze the challenges in disentanglement such as reference ambiguity and shortcut problem when using the weak label. We use the disentangled feature representation to transfer attributes between images such as exchanging hairstyle between two face images. Furthermore, we study the problem of how another type of feature, sketch, works in a neural network. The sketch can provide shape and contour of an object such as the silhouette of the side-view face. We leverage the silhouette constraint to improve the 3D face reconstruction from 2D images. The sketch can also provide the moving directions of one object, thus we investigate how one can manipulate the object to follow the trajectory provided by a user sketch. We propose a method to automatically generate video clips from a single image input using the sketch as motion and trajectory guidance to animate the object in that image. We demonstrate the efficiency of our approaches on several synthetic and real datasets.
Beyond Supervised Representation Learning
Mehdi noroozi · jan. 2019.
The complexity of any information processing task is highly dependent on the space where data is represented. Unfortunately, pixel space is not appropriate for the computer vision tasks such as object classification. The traditional computer vision approaches involve a multi-stage pipeline where at first images are transformed to a feature space through a handcrafted function and then consequenced by the solution in the feature space. The challenge with this approach is the complexity of designing handcrafted functions that extract robust features. The deep learning based approaches address this issue by end-to-end training of a neural network for some tasks that lets the network to discover the appropriate representation for the training tasks automatically. It turns out that image classification task on large scale annotated datasets yields a representation transferable to other computer vision tasks. However, supervised representation learning is limited to annotations. In this thesis we study self-supervised representation learning where the goal is to alleviate these limitations by substituting the classification task with pseudo tasks where the labels come for free. We discuss self-supervised learning by solving jigsaw puzzles that uses context as supervisory signal. The rational behind this task is that the network requires to extract features about object parts and their spatial configurations to solve the jigsaw puzzles. We also discuss a method for representation learning that uses an artificial supervisory signal based on counting visual primitives. This supervisory signal is obtained from an equivariance relation. We use two image transformations in the context of counting: scaling and tiling. The first transformation exploits the fact that the number of visual primitives should be invariant to scale. The second transformation allows us to equate the total number of visual primitives in each tile to that in the whole image. The most effective transfer strategy is fine-tuning, which restricts one to use the same model or parts thereof for both pretext and target tasks. We discuss a novel framework for self-supervised learning that overcomes limitations in designing and comparing different tasks, models, and data domains. In particular, our framework decouples the structure of the self-supervised model from the final task-specific finetuned model. Finally, we study the problem of multi-task representation learning. A naive approach to enhance the representation learned by a task is to train the task jointly with other tasks that capture orthogonal attributes. Having a diverse set of auxiliary tasks, imposes challenges on multi-task training from scratch. We propose a framework that allows us to combine arbitrarily different feature spaces into a single deep neural network. We reduce the auxiliary tasks to classification tasks and the multi-task learning to multi-label classification task consequently. Nevertheless, combining multiple representation space without being aware of the target task might be suboptimal. As our second contribution, we show empirically that this is indeed the case and propose to combine multiple tasks after the fine-tuning on the target task.
Motion Deblurring from a Single Image
Meiguang jin · dec. 2018.
With the information explosion, a tremendous amount photos is captured and shared via social media everyday. Technically, a photo requires a finite exposure to accumulate light from the scene. Thus, objects moving during the exposure generate motion blur in a photo. Motion blur is an image degradation that makes visual content less interpretable and is therefore often seen as a nuisance. Although motion blur can be reduced by setting a short exposure time, an insufficient amount of light has to be compensated through increasing the sensor’s sensitivity, which will inevitably bring large amount of sensor noise. Thus this motivates the necessity of removing motion blur computationally. Motion deblurring is an important problem in computer vision and it is challenging due to its ill-posed nature, which means the solution is not well defined. Mathematically, a blurry image caused by uniform motion is formed by the convolution operation between a blur kernel and a latent sharp image. Potentially there are infinite pairs of blur kernel and latent sharp image that can result in the same blurry image. Hence, some prior knowledge or regularization is required to address this problem. Even if the blur kernel is known, restoring the latent sharp image is still difficult as the high frequency information has been removed. Although we can model the uniform motion deblurring problem mathematically, it can only address the camera in-plane translational motion. Practically, motion is more complicated and can be non-uniform. Non-uniform motion blur can come from many sources, camera out-of-plane rotation, scene depth change, object motion and so on. Thus, it is more challenging to remove non-uniform motion blur. In this thesis, our focus is motion blur removal. We aim to address four challenging motion deblurring problems. We start from the noise blind image deblurring scenario where blur kernel is known but the noise level is unknown. We introduce an efficient and robust solution based on a Bayesian framework using a smooth generalization of the 0−1 loss to address this problem. Then we study the blind uniform motion deblurring scenario where both the blur kernel and the latent sharp image are unknown. We explore the relative scale ambiguity between the latent sharp image and blur kernel to address this issue. Moreover, we study the face deblurring problem and introduce a novel deep learning network architecture to solve it. We also address the general motion deblurring problem and particularly we aim at recovering a sequence of 7 frames each depicting some instantaneous motion of the objects in the scene.
Towards a Novel Paradigm in Blind Deconvolution: From Natural to Cartooned Image Statistics
Daniele perrone · july 2015.
In this thesis we study the blind deconvolution problem. Blind deconvolution consists in the estimation of a sharp image and a blur kernel from an observed blurry image. Because the blur model admits several solutions it is necessary to devise an image prior that favors the true blur kernel and sharp image. Recently it has been shown that a class of blind deconvolution formulations and image priors has the no-blur solution as global minimum. Despite this shortcoming, algorithms based on these formulations and priors can successfully solve blind deconvolution. In this thesis we show that a suitable initialization can exploit the non-convexity of the problem and yield the desired solution. Based on these conclusions, we propose a novel “vanilla” algorithm stripped of any enhancement typically used in the literature. Our algorithm, despite its simplicity, is able to compete with the top performers on several datasets. We have also investigated a remarkable behavior of a 1998 algorithm, whose formulation has the no-blur solution as global minimum: even when initialized at the no-blur solution, it converges to the correct solution. We show that this behavior is caused by an apparently insignificant implementation strategy that makes the algorithm no longer minimize the original cost functional. We also demonstrate that this strategy improves the results of our “vanilla” algorithm. Finally, we present a study of image priors for blind deconvolution. We provide experimental evidence supporting the recent belief that a good image prior is one that leads to a good blur estimate rather than being a good natural image statistical model. By focusing the attention on the blur estimation alone, we show that good blur estimates can be obtained even when using images quite different from the true sharp image. This allows using image priors, such as those leading to “cartooned” images, that avoid the no-blur solution. By using an image prior that produces “cartooned” images we achieve state-of-the-art results on different publicly available datasets. We therefore suggests a shift of paradigm in blind deconvolution: from modeling natural image statistics to modeling cartooned image statistics.
New Perspectives on Uncalibrated Photometric Stereo
Thoma papadhimitri · june 2014.
This thesis investigates the problem of 3D reconstruction of a scene from 2D images. In particular, we focus on photometric stereo which is a technique that computes the 3D geometry from at least three images taken from the same viewpoint and under different illumination conditions. When the illumination is unknown (uncalibrated photometric stereo) the problem is ambiguous: different combinations of geometry and illumination can generate the same images. First, we solve the ambiguity by exploiting the Lambertian reflectance maxima. These are points defined on curved surfaces where the normals are parallel to the light direction. Then, we propose a solution that can be computed in closed-form and thus very efficiently. Our algorithm is also very robust and yields always the same estimate regardless of the initial ambiguity. We validate our method on real world experiments and achieve state-of-art results. In this thesis we also solve for the first time the uncalibrated photometric stereo problem under the perspective projection model. We show that unlike in the orthographic case, one can uniquely reconstruct the normals of the object and the lights given only the input images and the camera calibration (focal length and image center). We also propose a very efficient algorithm which we validate on synthetic and real world experiments and show that the proposed technique is a generalization of the orthographic case. Finally, we investigate the uncalibrated photometric stereo problem in the case where the lights are distributed near the scene. In this case we propose an alternating minimization technique which converges quickly and overcomes the limitations of prior work that assumes distant illumination. We show experimentally that adopting a near-light model for real world scenes yields very accurate reconstructions.
Subscribe to the PwC Newsletter
Join the community, computer vision, semantic segmentation.

Tumor Segmentation

Panoptic Segmentation

3D Semantic Segmentation

Weakly-Supervised Semantic Segmentation
Representation learning.

Disentanglement
Graph representation learning, sentence embeddings.

Network Embedding
Classification.

Text Classification

Graph Classification

Audio Classification

Medical Image Classification
Object detection.

3D Object Detection

Real-Time Object Detection

RGB Salient Object Detection

Few-Shot Object Detection
Image classification.

Out of Distribution (OOD) Detection

Few-Shot Image Classification

Fine-Grained Image Classification

Semi-Supervised Image Classification
2d object detection.

Edge Detection
Thermal image segmentation.

Open Vocabulary Object Detection
Reinforcement learning (rl), off-policy evaluation, multi-objective reinforcement learning, 3d point cloud reinforcement learning, deep hashing, table retrieval, domain adaptation.

Unsupervised Domain Adaptation

Domain Generalization

Test-time Adaptation
Source-free domain adaptation, image generation.

Image-to-Image Translation

Text-to-Image Generation

Image Inpainting

Conditional Image Generation
Data augmentation.

Image Augmentation

Text Augmentation
Autonomous vehicles.

Autonomous Driving

Self-Driving Cars

Simultaneous Localization and Mapping

Autonomous Navigation

Image Denoising

Color Image Denoising

Sar Image Despeckling
Grayscale image denoising, meta-learning.

Few-Shot Learning

Sample Probing
Universal meta-learning, contrastive learning.

Super-Resolution

Image Super-Resolution

Video Super-Resolution

Multi-Frame Super-Resolution

Reference-based Super-Resolution
Pose estimation.

3D Human Pose Estimation

Keypoint Detection

3D Pose Estimation

6D Pose Estimation
Self-supervised learning.

Point Cloud Pre-training
Unsupervised video clustering, 2d semantic segmentation, image segmentation, text style transfer.

Scene Parsing

Reflection Removal
Visual question answering (vqa).

Visual Question Answering

Machine Reading Comprehension

Chart Question Answering

Embodied Question Answering

Depth Estimation

3D Reconstruction

Neural Rendering

3D Face Reconstruction
Anomaly detection.

Unsupervised Anomaly Detection

One-Class Classification
Supervised anomaly detection, anomaly detection in surveillance videos, sentiment analysis.

Aspect-Based Sentiment Analysis (ABSA)

Multimodal Sentiment Analysis

Aspect Sentiment Triplet Extraction

Twitter Sentiment Analysis

Temporal Action Localization

Video Understanding
Video generation.

Video Object Segmentation

Action Classification
Activity recognition.

Action Recognition

Human Activity Recognition
Egocentric activity recognition.

Group Activity Recognition
3d object super-resolution.

One-Shot Learning

Few-Shot Semantic Segmentation
Cross-domain few-shot.

Unsupervised Few-Shot Learning
Medical image segmentation.

Lesion Segmentation

Brain Tumor Segmentation

Cell Segmentation
Skin lesion segmentation, monocular depth estimation.

Stereo Depth Estimation
Depth and camera motion.

3D Depth Estimation
Exposure fairness, optical character recognition (ocr).

Active Learning

Handwriting Recognition
Handwritten digit recognition, irregular text recognition, instance segmentation.

Referring Expression Segmentation

3D Instance Segmentation

Real-time Instance Segmentation

Unsupervised Object Segmentation
Facial recognition and modelling.

Face Recognition

Face Swapping

Face Detection

Facial Expression Recognition (FER)

Face Verification
Object tracking.

Multi-Object Tracking

Visual Object Tracking

Multiple Object Tracking

Cell Tracking
Zero-shot learning.

Generalized Zero-Shot Learning

Compositional Zero-Shot Learning
Multi-label zero-shot learning, quantization, data free quantization, unet quantization, continual learning.

Class Incremental Learning
Continual named entity recognition, unsupervised class-incremental learning.

Action Recognition In Videos

3D Action Recognition
Self-supervised action recognition, few shot action recognition.
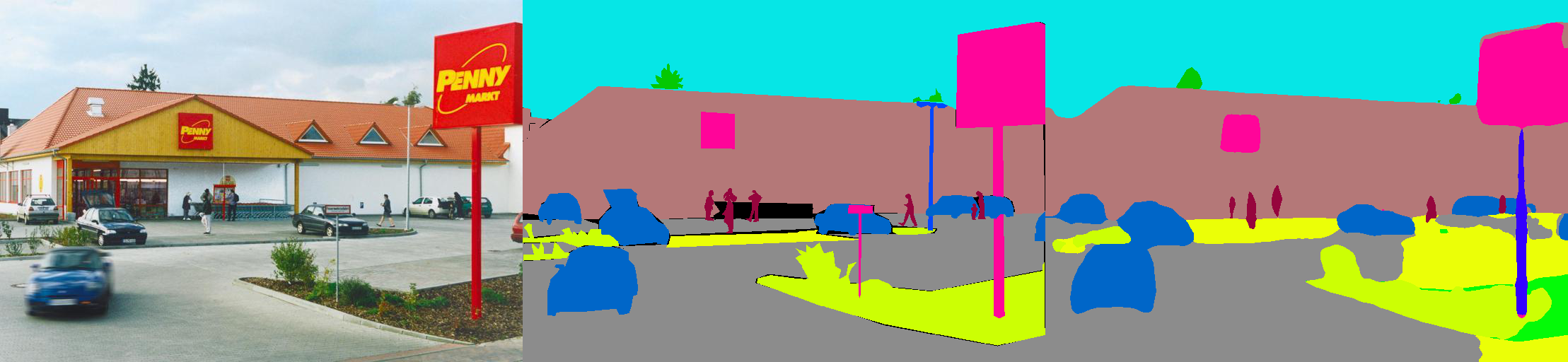
Scene Understanding
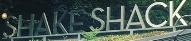
Scene Text Recognition

Scene Graph Generation

Scene Recognition
Adversarial attack.

Backdoor Attack

Adversarial Text
Adversarial attack detection, real-world adversarial attack, active object detection, image retrieval.

Sketch-Based Image Retrieval

Content-Based Image Retrieval

Composed Image Retrieval (CoIR)

Medical Image Retrieval
Dimensionality reduction.

Supervised dimensionality reduction
Online nonnegative cp decomposition, emotion recognition.
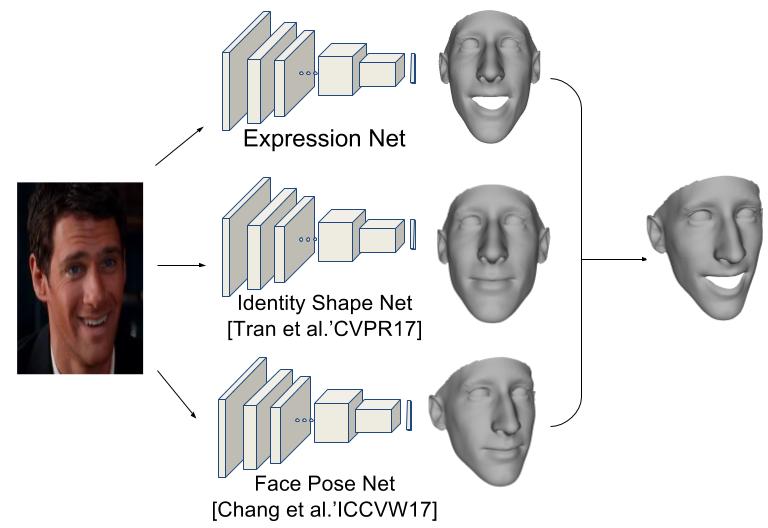
Speech Emotion Recognition

Emotion Recognition in Conversation

Multimodal Emotion Recognition
Emotion-cause pair extraction.

Monocular 3D Object Detection

3D Object Detection From Stereo Images

Multiview Detection
Robust 3d object detection, image reconstruction.

MRI Reconstruction

Film Removal
Optical flow estimation.

Video Stabilization
Style transfer.

Image Stylization
Font style transfer, style generalization, face transfer, action localization.

Action Segmentation
Spatio-temporal action localization, image captioning.

3D dense captioning
Controllable image captioning, aesthetic image captioning.

Relational Captioning
Person re-identification.

Unsupervised Person Re-Identification
Video-based person re-identification, generalizable person re-identification, cloth-changing person re-identification, image restoration.
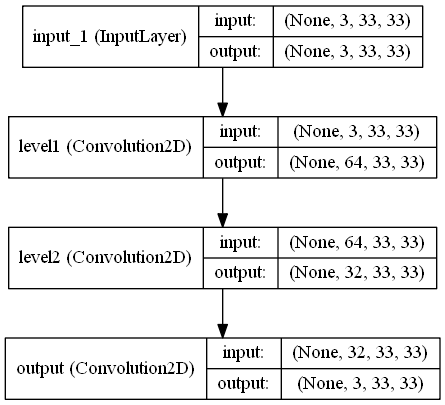
Demosaicking
Spectral reconstruction, underwater image restoration.

JPEG Artifact Correction
Visual relationship detection, lighting estimation.

3D Room Layouts From A Single RGB Panorama
Road scene understanding, action detection.

Skeleton Based Action Recognition

Online Action Detection
Audio-visual active speaker detection, metric learning.

Object Recognition

3D Object Recognition
Continuous object recognition.

Depiction Invariant Object Recognition

Monocular 3D Human Pose Estimation
Pose prediction.

3D Multi-Person Pose Estimation
3d human pose and shape estimation, image enhancement.

Low-Light Image Enhancement
Image relighting, de-aliasing, multi-label classification.

Missing Labels
Extreme multi-label classification, hierarchical multi-label classification, medical code prediction, continuous control.

Steering Control
Drone controller, 3d face modelling.

Semi-Supervised Video Object Segmentation

Unsupervised Video Object Segmentation

Referring Video Object Segmentation

Video Salient Object Detection
Trajectory prediction.

Trajectory Forecasting
Human motion prediction, out-of-sight trajectory prediction.

Multivariate Time Series Imputation
Image quality assessment, no-reference image quality assessment, blind image quality assessment.

Aesthetics Quality Assessment
Stereoscopic image quality assessment, object localization.

Weakly-Supervised Object Localization
Image-based localization, unsupervised object localization, monocular 3d object localization, novel view synthesis.

Novel LiDAR View Synthesis

Gournd video synthesis from satellite image

Blind Image Deblurring
Single-image blind deblurring, out-of-distribution detection, video semantic segmentation.

Camera shot segmentation
Cloud removal.

Facial Inpainting

Fine-Grained Image Inpainting
Instruction following, visual instruction following, change detection.

Semi-supervised Change Detection
Saliency detection.

Saliency Prediction

Co-Salient Object Detection
Video saliency detection, unsupervised saliency detection, image compression.

Feature Compression
Jpeg compression artifact reduction.

Lossy-Compression Artifact Reduction
Color image compression artifact reduction, prompt engineering.

Visual Prompting
Explainable artificial intelligence, explainable models, explanation fidelity evaluation, fad curve analysis, image registration.

Unsupervised Image Registration
Ensemble learning, visual reasoning.

Visual Commonsense Reasoning
Salient object detection, saliency ranking, visual tracking.

Point Tracking
Rgb-t tracking, real-time visual tracking.

RF-based Visual Tracking
3d point cloud classification.

3D Object Classification

Few-Shot 3D Point Cloud Classification
Supervised only 3d point cloud classification, zero-shot transfer 3d point cloud classification, 2d classification.
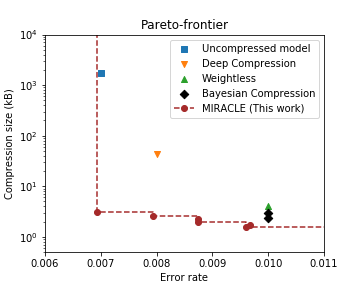
Neural Network Compression

Music Source Separation
Cell detection.

Plant Phenotyping
Open-set classification, motion estimation, image manipulation detection.

Zero Shot Skeletal Action Recognition
Generalized zero shot skeletal action recognition, whole slide images, visual grounding.

3D visual grounding
Person-centric visual grounding.

Phrase Extraction and Grounding (PEG)
Activity prediction, motion prediction, cyber attack detection, sequential skip prediction, gesture recognition.

Hand Gesture Recognition
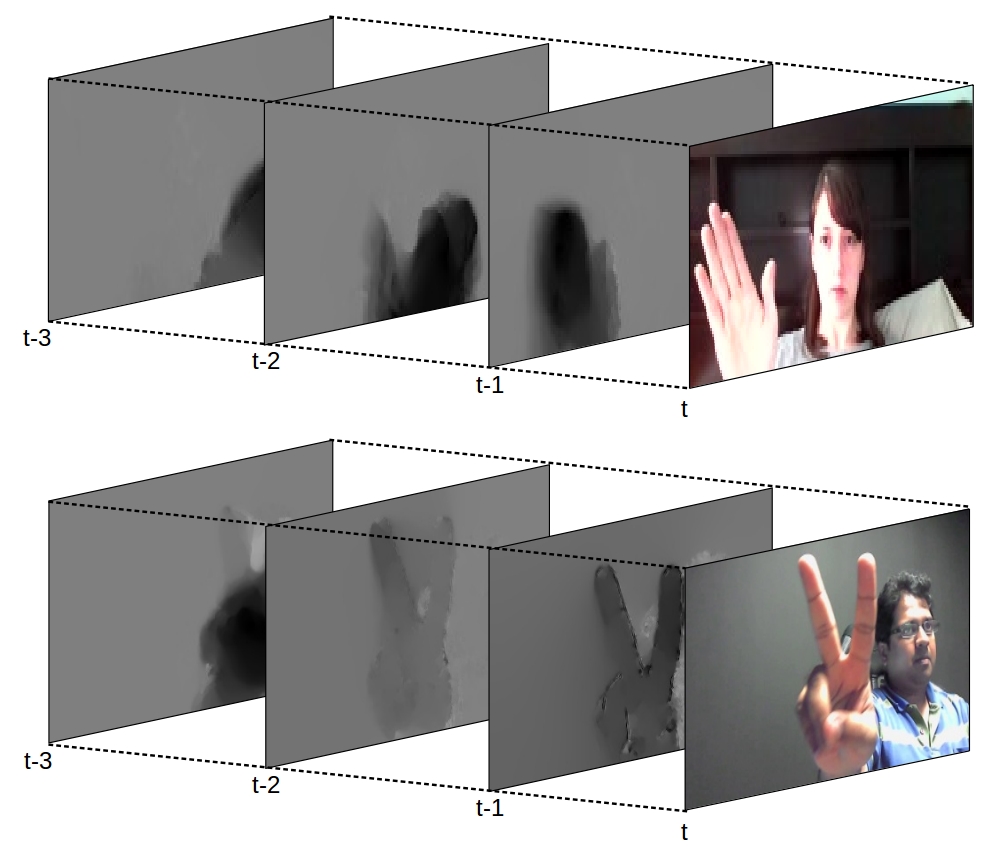
Hand-Gesture Recognition

RF-based Gesture Recognition

Robust 3D Semantic Segmentation

Real-Time 3D Semantic Segmentation

Unsupervised 3D Semantic Segmentation
Furniture segmentation, video question answering.
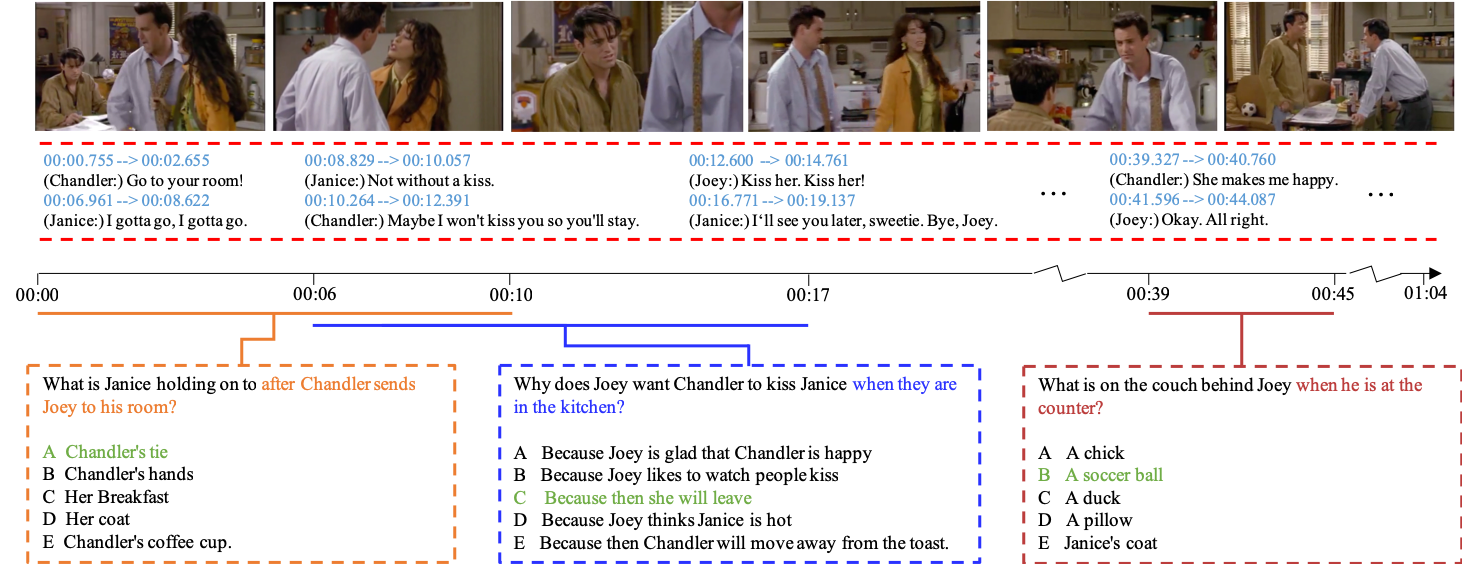
Zero-Shot Video Question Answer
Few-shot video question answering, text detection, video captioning.

Dense Video Captioning
Boundary captioning, visual text correction, audio-visual video captioning, point cloud registration.

Image to Point Cloud Registration
Medical diagnosis.

Alzheimer's Disease Detection

Retinal OCT Disease Classification
Blood cell count, thoracic disease classification, 3d point cloud interpolation, visual odometry.

Face Anti-Spoofing
Monocular visual odometry.

Hand Pose Estimation

Hand Segmentation
Gesture-to-gesture translation, rain removal.

Single Image Deraining
Image clustering.

Online Clustering

Face Clustering
Multi-view subspace clustering, multi-modal subspace clustering.

Image Dehazing

Single Image Dehazing
Colorization.

Line Art Colorization

Point-interactive Image Colorization

Color Mismatch Correction
Robot navigation.

PointGoal Navigation
Social navigation.

Sequential Place Learning
Image manipulation, conformal prediction.

Unsupervised Image-To-Image Translation

Synthetic-to-Real Translation

Multimodal Unsupervised Image-To-Image Translation

Cross-View Image-to-Image Translation

Fundus to Angiography Generation
Image editing, rolling shutter correction, shadow removal, multimodel-guided image editing, joint deblur and frame interpolation, multimodal fashion image editing, visual place recognition.

Indoor Localization
3d place recognition, visual localization.

DeepFake Detection

Synthetic Speech Detection
Human detection of deepfakes, multimodal forgery detection, stereo matching, object reconstruction.

3D Object Reconstruction

Crowd Counting

Visual Crowd Analysis
Group detection in crowds, human-object interaction detection.

Affordance Recognition
Image deblurring, low-light image deblurring and enhancement, earth observation, image matching.

Semantic correspondence
Patch matching, set matching.

Matching Disparate Images
Video quality assessment, video alignment, temporal sentence grounding, long-video activity recognition, point cloud classification, jet tagging, few-shot point cloud classification, hyperspectral.

Hyperspectral Image Classification
Hyperspectral unmixing, hyperspectral image segmentation, classification of hyperspectral images, document text classification.

Learning with noisy labels
Multi-label classification of biomedical texts, political salient issue orientation detection, 3d point cloud reconstruction.

Weakly Supervised Action Localization
Weakly-supervised temporal action localization.

Temporal Action Proposal Generation
Activity recognition in videos, scene classification.

2D Human Pose Estimation
Action anticipation.

3D Face Animation
Semi-supervised human pose estimation, point cloud generation, point cloud completion, referring expression, reconstruction, 3d human reconstruction.

Single-View 3D Reconstruction
4d reconstruction, single-image-based hdr reconstruction, compressive sensing, keyword spotting.

Small-Footprint Keyword Spotting
Visual keyword spotting, scene text detection.

Curved Text Detection
Multi-oriented scene text detection, boundary detection.

Junction Detection
Camera calibration, image matting.

Semantic Image Matting
Video retrieval, video-text retrieval, video grounding, video-adverb retrieval, replay grounding, composed video retrieval (covr), motion synthesis.

Motion Style Transfer
Temporal human motion composition, emotion classification.

Video Summarization

Unsupervised Video Summarization
Supervised video summarization, document ai, document understanding, point cloud segmentation, sensor fusion, superpixels.

Few-Shot Transfer Learning for Saliency Prediction

Aerial Video Saliency Prediction
3d anomaly detection, video anomaly detection, artifact detection, remote sensing.

Remote Sensing Image Classification
Change detection for remote sensing images, building change detection for remote sensing images.

Segmentation Of Remote Sensing Imagery

The Semantic Segmentation Of Remote Sensing Imagery
Document layout analysis.

Cross-Modal Retrieval
Image-text matching, cross-modal retrieval with noisy correspondence, multilingual cross-modal retrieval.

Zero-shot Composed Person Retrieval
Cross-modal retrieval on rsitmd.

Point cloud reconstruction

3D Semantic Scene Completion

3D Semantic Scene Completion from a single RGB image
Garment reconstruction, face generation.

Talking Head Generation
Talking face generation.

Face Age Editing
Facial expression generation, kinship face generation, video instance segmentation.

Privacy Preserving Deep Learning
Membership inference attack, human detection.

Generalized Few-Shot Semantic Segmentation

Generalized Referring Expression Segmentation
Virtual try-on, scene flow estimation.

Self-supervised Scene Flow Estimation
Video editing, video temporal consistency, 3d classification, depth completion.

Motion Forecasting

Multi-Person Pose forecasting

Multiple Object Forecasting
Face reconstruction, object discovery, carla map leaderboard, dead-reckoning prediction, gaze estimation.

Texture Synthesis

Text-based Image Editing
Text-guided-image-editing, concept alignment.

Zero-Shot Text-to-Image Generation
Conditional text-to-image synthesis, machine unlearning, continual forgetting, sign language recognition.

Image Recognition
Fine-grained image recognition, license plate recognition, material recognition, multi-view learning, incomplete multi-view clustering.

Breast Cancer Detection
Skin cancer classification.

Breast Cancer Histology Image Classification
Lung cancer diagnosis, classification of breast cancer histology images, gait recognition.

Multiview Gait Recognition
Gait recognition in the wild, weakly supervised segmentation, disease prediction, disease trajectory forecasting, human parsing.

Multi-Human Parsing
Pose tracking.

3D Human Pose Tracking
Interactive segmentation, scene generation.

3D Multi-Person Pose Estimation (absolute)

3D Multi-Person Pose Estimation (root-relative)

3D Multi-Person Mesh Recovery
Event-based vision.

Event-based Optical Flow

Event-Based Video Reconstruction
Event-based motion estimation, object counting, training-free object counting, open-vocabulary object counting, interest point detection, homography estimation.

3D Hand Pose Estimation
Facial landmark detection.

Unsupervised Facial Landmark Detection

3D Facial Landmark Localization
3d character animation from a single photo, scene segmentation.

Dichotomous Image Segmentation
Activity detection, inverse rendering, temporal localization.

Language-Based Temporal Localization
Temporal defect localization, multi-label image classification.

Multi-label Image Recognition with Partial Labels
3d object tracking.

3D Single Object Tracking
Template matching, text-to-video generation, text-to-video editing, subject-driven video generation, camera localization.

Camera Relocalization
Lidar semantic segmentation, visual dialog.

Motion Segmentation
Relation network, intelligent surveillance.

Vehicle Re-Identification
Text spotting.

Few-Shot Class-Incremental Learning
Class-incremental semantic segmentation, non-exemplar-based class incremental learning, disparity estimation.

Handwritten Text Recognition
Handwritten document recognition, unsupervised text recognition, knowledge distillation.

Data-free Knowledge Distillation
Self-knowledge distillation, moment retrieval.

Zero-shot Moment Retrieval
Text to video retrieval, partially relevant video retrieval, person search, decision making under uncertainty.

Uncertainty Visualization
Semi-supervised object detection.

Shadow Detection

Shadow Detection And Removal

Unconstrained Lip-synchronization
Mixed reality, video inpainting.

Cross-corpus
Micro-expression recognition, micro-expression spotting.

3D Facial Expression Recognition

Smile Recognition
Future prediction, human mesh recovery, video enhancement.

3D Multi-Object Tracking
Real-time multi-object tracking, multi-animal tracking with identification, trajectory long-tail distribution for muti-object tracking, grounded multiple object tracking.

Face Image Quality Assessment
Lightweight face recognition.

Age-Invariant Face Recognition
Synthetic face recognition, face quality assessement.

Burst Image Super-Resolution
Stereo image super-resolution, satellite image super-resolution, multispectral image super-resolution, image categorization, fine-grained visual categorization, video reconstruction.

Overlapped 10-1
Overlapped 15-1, overlapped 15-5, disjoint 10-1, disjoint 15-1, color constancy.

Few-Shot Camera-Adaptive Color Constancy
Hdr reconstruction, multi-exposure image fusion, open vocabulary semantic segmentation, zero-guidance segmentation, physics-informed machine learning, soil moisture estimation, deep attention, line detection, zero shot segmentation, visual recognition.

Fine-Grained Visual Recognition
Image cropping, sign language translation.

Stereo Matching Hand

3D Absolute Human Pose Estimation

Text-to-Face Generation
Image forensics, tone mapping, zero-shot action recognition, natural language transduction, video restoration.

Analog Video Restoration
Novel class discovery.

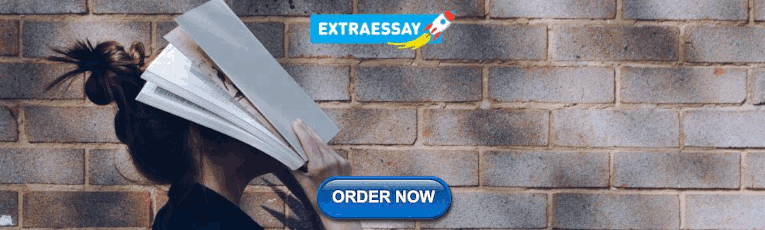
Breast Cancer Histology Image Classification (20% labels)
Transparent object detection, transparent objects, abnormal event detection in video.

Semi-supervised Anomaly Detection
Surface normals estimation.

Vision-Language Navigation

hand-object pose

Grasp Generation

3D Canonical Hand Pose Estimation
Cross-domain few-shot learning, texture classification, infrared and visible image fusion.

Image Animation

Image to 3D
Probabilistic deep learning, unsupervised few-shot image classification, generalized few-shot classification, pedestrian attribute recognition.

Steganalysis
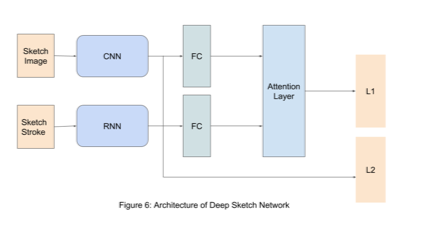
Sketch Recognition

Face Sketch Synthesis
Drawing pictures.

Photo-To-Caricature Translation
Spoof detection, face presentation attack detection, detecting image manipulation, cross-domain iris presentation attack detection, finger dorsal image spoof detection, computer vision techniques adopted in 3d cryogenic electron microscopy, single particle analysis, cryogenic electron tomography, action quality assessment, highlight detection, iris recognition, pupil dilation.

One-shot visual object segmentation

Unbiased Scene Graph Generation

Panoptic Scene Graph Generation
Image to video generation.

Unconditional Video Generation
Automatic post-editing.

Dense Captioning
Document image classification.

Image Stitching

Multi-View 3D Reconstruction
Person retrieval, universal domain adaptation, action understanding, blind face restoration.

Face Reenactment

Geometric Matching
Human action generation.

Action Generation
Object categorization, text based person retrieval, surgical phase recognition, online surgical phase recognition, offline surgical phase recognition, human dynamics.

3D Human Dynamics
Meme classification, hateful meme classification, severity prediction, intubation support prediction, cloud detection.

Text-To-Image
Story visualization, complex scene breaking and synthesis, diffusion personalization.

Diffusion Personalization Tuning Free

Efficient Diffusion Personalization
Image fusion, pansharpening, image deconvolution.

Image Outpainting

Table Recognition
Object segmentation.

Camouflaged Object Segmentation
Landslide segmentation, text-line extraction, point clouds, point cloud video understanding, point cloud rrepresentation learning.

Semantic SLAM

Object SLAM
Intrinsic image decomposition, line segment detection, situation recognition, grounded situation recognition, motion detection, multi-target domain adaptation, person identification, sports analytics, single-source domain generalization, evolving domain generalization, source-free domain generalization.

Robot Pose Estimation

Camouflaged Object Segmentation with a Single Task-generic Prompt
Image morphing, image shadow removal, visual prompt tuning, weakly-supervised instance segmentation, image smoothing, fake image detection.

GAN image forensics

Fake Image Attribution
Image steganography, rotated mnist, contour detection.

Face Image Quality
Lane detection.

3D Lane Detection
Layout design, license plate detection.

Occlusion Handling

Video Panoptic Segmentation
Viewpoint estimation.

Drone navigation
Drone-view target localization, value prediction, body mass index (bmi) prediction, crop classification, crop yield prediction, multi-object tracking and segmentation.

Zero-Shot Transfer Image Classification

3D Object Reconstruction From A Single Image

CAD Reconstruction
3d point cloud linear classification, person recognition.

Photo Retouching
Motion retargeting, shape representation of 3d point clouds, bird's-eye view semantic segmentation.

Dense Pixel Correspondence Estimation
Human part segmentation.
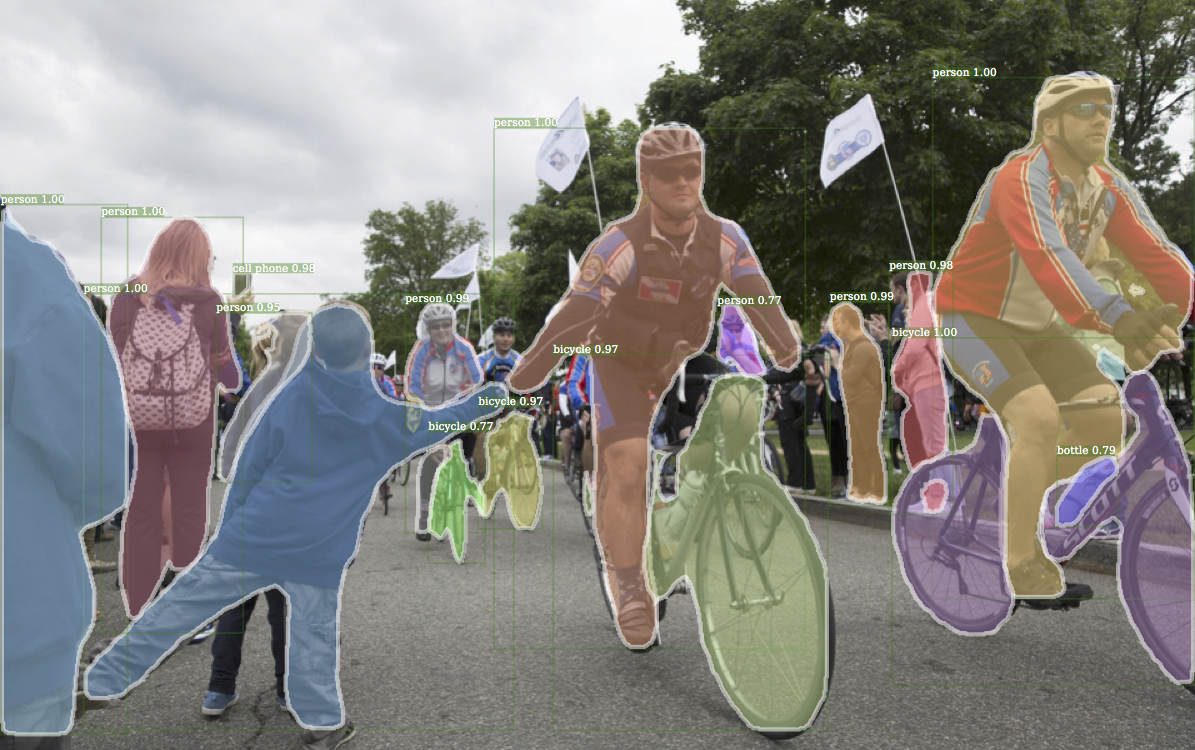
Multiview Learning

Document Shadow Removal
Symmetry detection, traffic sign detection, video style transfer, referring image matting.

Referring Image Matting (Expression-based)

Referring Image Matting (Keyword-based)

Referring Image Matting (RefMatte-RW100)
Referring image matting (prompt-based), human interaction recognition, one-shot 3d action recognition, mutual gaze, affordance detection.

Gaze Prediction
Image forgery detection, image instance retrieval, amodal instance segmentation, image quality estimation.

Image Similarity Search

Precipitation Forecasting
Referring expression generation, road damage detection.

Space-time Video Super-resolution
Video matting.

Open-World Semi-Supervised Learning
Semi-supervised image classification (cold start), hand detection, material classification.

Open Vocabulary Attribute Detection
Inverse tone mapping, image/document clustering, self-organized clustering, instance search.

Audio Fingerprint
3d shape modeling.

Action Analysis
Art analysis, facial editing.

Food Recognition

Holdout Set
Motion magnification, multispectral object detection, semi-supervised instance segmentation, binary classification, llm-generated text detection, cancer-no cancer per breast classification, cancer-no cancer per image classification, suspicous (birads 4,5)-no suspicous (birads 1,2,3) per image classification, cancer-no cancer per view classification, video segmentation, camera shot boundary detection, open-vocabulary video segmentation, open-world video segmentation, lung nodule classification, lung nodule 3d classification, lung nodule detection, lung nodule 3d detection, 3d scene reconstruction.

Zero-Shot Composed Image Retrieval (ZS-CIR)
Event segmentation, generic event boundary detection, image retouching, image-variation, jpeg artifact removal, point cloud super resolution, skills assessment.

Sensor Modeling
Handwriting verification, bangla spelling error correction, video prediction, earth surface forecasting, predict future video frames, ad-hoc video search, audio-visual synchronization, handwriting generation, pose retrieval, scanpath prediction, scene change detection.

Sketch-to-Image Translation
Skills evaluation, synthetic image detection, highlight removal, 3d shape reconstruction from a single 2d image.

Shape from Texture
Deception detection, deception detection in videos, 3d open-vocabulary instance segmentation.

3D Shape Representation

3D Dense Shape Correspondence
Birds eye view object detection.

Multiple People Tracking

Network Interpretation
Rgb-d reconstruction, seeing beyond the visible, semi-supervised domain generalization, unsupervised semantic segmentation.

Unsupervised Semantic Segmentation with Language-image Pre-training
2d pose estimation, category-agnostic pose estimation, open vocabulary keypoint detection, overlapping pose estimation, multiple object tracking with transformer.

Multiple Object Track and Segmentation
Constrained lip-synchronization, face dubbing, vietnamese visual question answering, explanatory visual question answering.

Video Visual Relation Detection
Human-object relationship detection, 3d shape reconstruction, defocus blur detection, event data classification, image comprehension, image manipulation localization, instance shadow detection, kinship verification, medical image enhancement, open vocabulary panoptic segmentation, single-object discovery, training-free 3d point cloud classification, video forensics.

Sequential Place Recognition
Autonomous flight (dense forest), autonomous web navigation.

Generative 3D Object Classification
Cube engraving classification, facial expression recognition, cross-domain facial expression recognition, zero-shot facial expression recognition, multimodal machine translation.

Face to Face Translation
Multimodal lexical translation, 10-shot image generation, 2d semantic segmentation task 3 (25 classes), document enhancement, 4d panoptic segmentation, action assessment, bokeh effect rendering, drivable area detection, face anonymization, font recognition, horizon line estimation, image imputation.

Long Video Retrieval (Background Removed)
Medical image denoising.

Occlusion Estimation
Physiological computing.

Lake Ice Monitoring
Short-term object interaction anticipation, spatio-temporal video grounding, unsupervised 3d point cloud linear evaluation, wireframe parsing, single-image-generation, unsupervised anomaly detection with specified settings -- 30% anomaly, root cause ranking, anomaly detection at 30% anomaly, anomaly detection at various anomaly percentages.

Unsupervised Contextual Anomaly Detection
Landmark tracking, muscle tendon junction identification, 3d object captioning, 3d semantic occupancy prediction, animated gif generation, generalized referring expression comprehension, image deblocking, infrared image super-resolution, motion disentanglement, persuasion strategies, scene text editing, image to sketch recognition, traffic accident detection, accident anticipation, unsupervised landmark detection, visual speech recognition, lip to speech synthesis, continual anomaly detection, gaze redirection, weakly supervised action segmentation (transcript), weakly supervised action segmentation (action set)), calving front delineation in synthetic aperture radar imagery, calving front delineation in synthetic aperture radar imagery with fixed training amount.

Handwritten Line Segmentation
Handwritten word segmentation.

General Action Video Anomaly Detection
Physical video anomaly detection, monocular cross-view road scene parsing(road), monocular cross-view road scene parsing(vehicle).

Transparent Object Depth Estimation
3d scene editing, age and gender estimation, data ablation.

Occluded Face Detection
Gait identification, historical color image dating, stochastic human motion prediction, image retargeting, image and video forgery detection, motion captioning, personality trait recognition, personalized segmentation, scene-aware dialogue, spatial relation recognition, spatial token mixer, steganographics, story continuation.

Unsupervised Anomaly Detection with Specified Settings -- 0.1% anomaly
Unsupervised anomaly detection with specified settings -- 1% anomaly, unsupervised anomaly detection with specified settings -- 10% anomaly, unsupervised anomaly detection with specified settings -- 20% anomaly, vehicle speed estimation, visual analogies, visual social relationship recognition, zero-shot text-to-video generation, text-guided-generation, video frame interpolation, 3d video frame interpolation, unsupervised video frame interpolation.

eXtreme-Video-Frame-Interpolation
Continual semantic segmentation, overlapped 5-3, overlapped 25-25, micro-expression generation, micro-expression generation (megc2021), mistake detection, online mistake detection, period estimation, art period estimation (544 artists), unsupervised panoptic segmentation, unsupervised zero-shot panoptic segmentation, 3d rotation estimation, camera auto-calibration, defocus estimation, derendering, fingertip detection, hierarchical text segmentation, human-object interaction concept discovery.

One-Shot Face Stylization
Speaker-specific lip to speech synthesis, multi-person pose estimation, neural stylization.

Part-aware Panoptic Segmentation

Population Mapping
Pornography detection, prediction of occupancy grid maps, raw reconstruction, repetitive action counting, svbrdf estimation, semi-supervised video classification, spectrum cartography, supervised image retrieval, synthetic image attribution, training-free 3d part segmentation, unsupervised image decomposition, video propagation, vietnamese multimodal learning, weakly supervised 3d point cloud segmentation, weakly-supervised panoptic segmentation, drone-based object tracking, brain visual reconstruction, brain visual reconstruction from fmri.

Human-Object Interaction Generation
Image-guided composition, fashion understanding, semi-supervised fashion compatibility.

intensity image denoising
Lifetime image denoising, observation completion, active observation completion, boundary grounding.

Video Narrative Grounding
3d inpainting, 3d scene graph alignment, 4d spatio temporal semantic segmentation.

Age Estimation

Few-shot Age Estimation
Brdf estimation, camouflage segmentation, clothing attribute recognition, damaged building detection, depth image estimation, detecting shadows, dynamic texture recognition.

Disguised Face Verification
Few shot open set object detection, gaze target estimation, generalized zero-shot learning - unseen, hd semantic map learning, human-object interaction anticipation, image deep networks, keypoint detection and image matching, manufacturing quality control, materials imaging, micro-gesture recognition, multi-person pose estimation and tracking.

Multi-modal image segmentation
Multi-object discovery, neural radiance caching.

Parking Space Occupancy

Partial Video Copy Detection

Multimodal Patch Matching
Perpetual view generation, procedure learning, prompt-driven zero-shot domain adaptation, jersey number recognition, single-shot hdr reconstruction, on-the-fly sketch based image retrieval, thermal image denoising, trademark retrieval, unsupervised instance segmentation, unsupervised zero-shot instance segmentation, vehicle key-point and orientation estimation.

Video Individual Counting
Video-adverb retrieval (unseen compositions), video-to-image affordance grounding.

Vietnamese Scene Text
Visual sentiment prediction, human-scene contact detection, localization in video forgery, video classification, student engagement level detection (four class video classification), multi class classification (four-level video classification), 3d canonicalization, 3d surface generation.

Visibility Estimation from Point Cloud
Amodal layout estimation, blink estimation, camera absolute pose regression, change data generation, constrained diffeomorphic image registration, continuous affect estimation, deep feature inversion, document image skew estimation, earthquake prediction, fashion compatibility learning.

Displaced People Recognition
Finger vein recognition, flooded building segmentation.

Future Hand Prediction
Generative temporal nursing, grounded multimodal named entity recognition, house generation, human fmri response prediction, hurricane forecasting, ifc entity classification, image declipping, image similarity detection.

Image Text Removal
Image-to-gps verification.

Image-based Automatic Meter Reading
Dial meter reading, indoor scene reconstruction, jpeg decompression.

Kiss Detection
Laminar-turbulent flow localisation.

Landmark Recognition
Brain landmark detection, corpus video moment retrieval, mllm evaluation: aesthetics, medical image deblurring, mental workload estimation, meter reading, motion expressions guided video segmentation, natural image orientation angle detection, multi-object colocalization, multilingual text-to-image generation, video emotion detection, nwp post-processing, occluded 3d object symmetry detection, open set video captioning, pso-convnets dynamics 1, pso-convnets dynamics 2, partial point cloud matching.

Partially View-aligned Multi-view Learning

Pedestrian Detection

Thermal Infrared Pedestrian Detection
Personality trait recognition by face, physical attribute prediction, point cloud semantic completion, point cloud classification dataset, point- of-no-return (pnr) temporal localization, pose contrastive learning, potrait generation, procedure step recognition, prostate zones segmentation, pulmorary vessel segmentation, pulmonary artery–vein classification, reference expression generation, safety perception recognition, interspecies facial keypoint transfer, specular reflection mitigation, specular segmentation, state change object detection, surface normals estimation from point clouds, train ego-path detection.

Transform A Video Into A Comics
Transparency separation, typeface completion.

Unbalanced Segmentation

Unsupervised Long Term Person Re-Identification
Video correspondence flow.

Key-Frame-based Video Super-Resolution (K = 15)
Zero-shot single object tracking, yield mapping in apple orchards, lidar absolute pose regression, opd: single-view 3d openable part detection, self-supervised scene text recognition, spatial-aware image editing, video narration captioning, spectral estimation, spectral estimation from a single rgb image, 3d prostate segmentation, aggregate xview3 metric, atomic action recognition, composite action recognition, calving front delineation from synthetic aperture radar imagery, computer vision transduction, crosslingual text-to-image generation, zero-shot dense video captioning, document to image conversion, frame duplication detection, geometrical view, hyperview challenge.

Image Operation Chain Detection
Kinematic based workflow recognition, logo recognition.

MLLM Aesthetic Evaluation
Motion detection in non-stationary scenes, open-set video tagging, satellite orbit determination.

Segmentation Based Workflow Recognition
2d particle picking, small object detection.

Rice Grain Disease Detection
Sperm morphology classification, video & kinematic base workflow recognition, video based workflow recognition, video, kinematic & segmentation base workflow recognition, animal pose estimation.
Research Topics
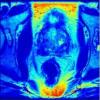
Biomedical Imaging
The current plethora of imaging technologies such as magnetic resonance imaging (MR), computed tomography (CT), position emission tomography (PET), optical coherence tomography (OCT), and ultrasound provide great insight into the different anatomical and functional processes of the human body.
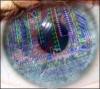
Computer Vision
Computer vision is the science and technology of teaching a computer to interpret images and video as well as a typical human. Technically, computer vision encompasses the fields of image/video processing, pattern recognition, biological vision, artificial intelligence, augmented reality, mathematical modeling, statistics, probability, optimization, 2D sensors, and photography.
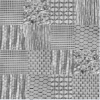
Image Segmentation/Classification
Extracting information from a digital image often depends on first identifying desired objects or breaking down the image into homogenous regions (a process called 'segmentation') and then assigning these objects to particular classes (a process called 'classification'). This is a fundamental part of computer vision, combining image processing and pattern recognition techniques.
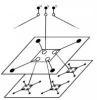
Multiresolution Techniques
The VIP lab has a particularly extensive history with multiresolution methods, and a significant number of research students have explored this theme. Multiresolution methods are very broad, essentially meaning than an image or video is modeled, represented, or features extracted on more than one scale, somehow allowing both local and non-local phenomena.
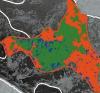
Remote Sensing
Remote sensing, or the science of capturing data of the earth from airplanes or satellites, enables regular monitoring of land, ocean, and atmosphere expanses, representing data that cannot be captured using any other means. A vast amount of information is generated by remote sensing platforms and there is an obvious need to analyze the data accurately and efficiently.
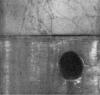
Scientific Imaging
Scientific Imaging refers to working on two- or three-dimensional imagery taken for a scientific purpose, in most cases acquired either through a microscope or remotely-sensed images taken at a distance.
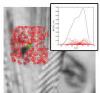
Stochastic Models
In many image processing, computer vision, and pattern recognition applications, there is often a large degree of uncertainty associated with factors such as the appearance of the underlying scene within the acquired data, the location and trajectory of the object of interest, the physical appearance (e.g., size, shape, color, etc.) of the objects being detected, etc.
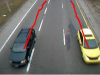
Video Analysis
Video analysis is a field within computer vision that involves the automatic interpretation of digital video using computer algorithms. Although humans are readily able to interpret digital video, developing algorithms for the computer to perform the same task has been highly evasive and is now an active research field.
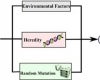
Evolutionary Deep Intelligence
Deep learning has shown considerable promise in recent years, producing tremendous results and significantly improving the accuracy of a variety of challenging problems when compared to other machine learning methods.
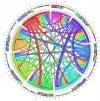
Discovery Radiomics
Radiomics, which involves the high-throughput extraction and analysis of a large amount of quantitative features from medical imaging data to characterize tumor phenotype in a quantitative manner, is ushering in a new era of imaging-driven quantitative personalized cancer decision support and management.
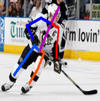
Sports Analytics
Sports Analytics is a growing field in computer vision that analyzes visual cues from images to provide statistical data on players, teams, and games. Want to know how a player's technique improves the quality of the team? Can a team, based on their defensive position, increase their chances to the finals? These are a few out of a plethora of questions that are answered in sports analytics.

- Contact Waterloo
- Maps & Directions
- Accessibility
The University of Waterloo acknowledges that much of our work takes place on the traditional territory of the Neutral, Anishinaabeg and Haudenosaunee peoples. Our main campus is situated on the Haldimand Tract, the land granted to the Six Nations that includes six miles on each side of the Grand River. Our active work toward reconciliation takes place across our campuses through research, learning, teaching, and community building, and is co-ordinated within the Office of Indigenous Relations .

- Advisers & Contacts
- Bachelor of Arts & Bachelor of Science in Engineering
- Prerequisites
- Declaring Computer Science for AB Students
- Declaring Computer Science for BSE Students
- Class of '25, '26 & '27 - Departmental Requirements
- Class of 2024 - Departmental Requirements
- COS126 Information
- Important Steps and Deadlines
- Independent Work Seminars
- Guidelines and Useful Information
Undergraduate Research Topics
- AB Junior Research Workshops
- Undergraduate Program FAQ
- How to Enroll
- Requirements
- Certificate Program FAQ
- Interdepartmental Committee
- Minor Program
- Funding for Student Group Activities
- Mailing Lists and Policies
- Study Abroad
- Jobs & Careers
- Admissions Requirements
- Breadth Requirements
- Pre-FPO Checklist
- FPO Checklist
- M.S.E. Track
- M.Eng. Track
- Departmental Internship Policy (for Master's students)
- General Examination
- Fellowship Opportunities
- Travel Reimbursement Policy
- Communication Skills
- Course Schedule
- Course Catalog
- Research Areas
- Interdisciplinary Programs
- Technical Reports
- Computing Facilities
- Researchers
- Technical Staff
- Administrative Staff
- Graduate Students
- Undergraduate Students
- Graduate Alumni
- Climate and Inclusion Committee
- Resources for Undergraduate & Graduate Students
- Outreach Initiatives
- Resources for Faculty & Staff
- Spotlight Stories
- Job Openings
- Undergraduate Program
- Independent Work & Theses
Suggested Undergraduate Research Topics
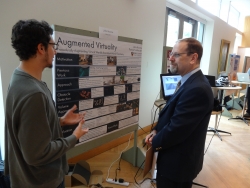
How to Contact Faculty for IW/Thesis Advising
Send the professor an e-mail. When you write a professor, be clear that you want a meeting regarding a senior thesis or one-on-one IW project, and briefly describe the topic or idea that you want to work on. Check the faculty listing for email addresses.
Parastoo Abtahi, Room 419
Available for single-semester IW and senior thesis advising, 2024-2025
- Research Areas: Human-Computer Interaction (HCI), Augmented Reality (AR), and Spatial Computing
- Input techniques for on-the-go interaction (e.g., eye-gaze, microgestures, voice) with a focus on uncertainty, disambiguation, and privacy.
- Minimal and timely multisensory output (e.g., spatial audio, haptics) that enables users to attend to their physical environment and the people around them, instead of a 2D screen.
- Interaction with intelligent systems (e.g., IoT, robots) situated in physical spaces with a focus on updating users’ mental model despite the complexity and dynamicity of these systems.
Ryan Adams, Room 411
Research areas:
- Machine learning driven design
- Generative models for structured discrete objects
- Approximate inference in probabilistic models
- Accelerating solutions to partial differential equations
- Innovative uses of automatic differentiation
- Modeling and optimizing 3d printing and CNC machining
Andrew Appel, Room 209
Available for Fall 2024 IW advising, only
- Research Areas: Formal methods, programming languages, compilers, computer security.
- Software verification (for which taking COS 326 / COS 510 is helpful preparation)
- Game theory of poker or other games (for which COS 217 / 226 are helpful)
- Computer game-playing programs (for which COS 217 / 226)
- Risk-limiting audits of elections (for which ORF 245 or other knowledge of probability is useful)
Sanjeev Arora, Room 407
- Theoretical machine learning, deep learning and its analysis, natural language processing. My advisees would typically have taken a course in algorithms (COS423 or COS 521 or equivalent) and a course in machine learning.
- Show that finding approximate solutions to NP-complete problems is also NP-complete (i.e., come up with NP-completeness reductions a la COS 487).
- Experimental Algorithms: Implementing and Evaluating Algorithms using existing software packages.
- Studying/designing provable algorithms for machine learning and implementions using packages like scipy and MATLAB, including applications in Natural language processing and deep learning.
- Any topic in theoretical computer science.
David August, Room 221
Not available for IW or thesis advising, 2024-2025
- Research Areas: Computer Architecture, Compilers, Parallelism
- Containment-based approaches to security: We have designed and tested a simple hardware+software containment mechanism that stops incorrect communication resulting from faults, bugs, or exploits from leaving the system. Let's explore ways to use containment to solve real problems. Expect to work with corporate security and technology decision-makers.
- Parallelism: Studies show much more parallelism than is currently realized in compilers and architectures. Let's find ways to realize this parallelism.
- Any other interesting topic in computer architecture or compilers.
Mark Braverman, 194 Nassau St., Room 231
- Research Areas: computational complexity, algorithms, applied probability, computability over the real numbers, game theory and mechanism design, information theory.
- Topics in computational and communication complexity.
- Applications of information theory in complexity theory.
- Algorithms for problems under real-life assumptions.
- Game theory, network effects
- Mechanism design (could be on a problem proposed by the student)
Sebastian Caldas, 221 Nassau Street, Room 105
- Research Areas: collaborative learning, machine learning for healthcare. Typically, I will work with students that have taken COS324.
- Methods for collaborative and continual learning.
- Machine learning for healthcare applications.
Bernard Chazelle, 194 Nassau St., Room 301
- Research Areas: Natural Algorithms, Computational Geometry, Sublinear Algorithms.
- Natural algorithms (flocking, swarming, social networks, etc).
- Sublinear algorithms
- Self-improving algorithms
- Markov data structures
Danqi Chen, Room 412
- My advisees would be expected to have taken a course in machine learning and ideally have taken COS484 or an NLP graduate seminar.
- Representation learning for text and knowledge bases
- Pre-training and transfer learning
- Question answering and reading comprehension
- Information extraction
- Text summarization
- Any other interesting topics related to natural language understanding/generation
Marcel Dall'Agnol, Corwin 034
- Research Areas: Theoretical computer science. (Specifically, quantum computation, sublinear algorithms, complexity theory, interactive proofs and cryptography)
- Research Areas: Machine learning
Jia Deng, Room 423
- Research Areas: Computer Vision, Machine Learning.
- Object recognition and action recognition
- Deep Learning, autoML, meta-learning
- Geometric reasoning, logical reasoning
Adji Bousso Dieng, Room 406
- Research areas: Vertaix is a research lab at Princeton University led by Professor Adji Bousso Dieng. We work at the intersection of artificial intelligence (AI) and the natural sciences. The models and algorithms we develop are motivated by problems in those domains and contribute to advancing methodological research in AI. We leverage tools in statistical machine learning and deep learning in developing methods for learning with the data, of various modalities, arising from the natural sciences.
Robert Dondero, Corwin Hall, Room 038
- Research Areas: Software engineering; software engineering education.
- Develop or evaluate tools to facilitate student learning in undergraduate computer science courses at Princeton, and beyond.
- In particular, can code critiquing tools help students learn about software quality?
Zeev Dvir, 194 Nassau St., Room 250
- Research Areas: computational complexity, pseudo-randomness, coding theory and discrete mathematics.
- Independent Research: I have various research problems related to Pseudorandomness, Coding theory, Complexity and Discrete mathematics - all of which require strong mathematical background. A project could also be based on writing a survey paper describing results from a few theory papers revolving around some particular subject.
Benjamin Eysenbach, Room 416
- Research areas: reinforcement learning, machine learning. My advisees would typically have taken COS324.
- Using RL algorithms to applications in science and engineering.
- Emergent behavior of RL algorithms on high-fidelity robotic simulators.
- Studying how architectures and representations can facilitate generalization.
Christiane Fellbaum, 1-S-14 Green
- Research Areas: theoretical and computational linguistics, word sense disambiguation, lexical resource construction, English and multilingual WordNet(s), ontology
- Anything having to do with natural language--come and see me with/for ideas suitable to your background and interests. Some topics students have worked on in the past:
- Developing parsers, part-of-speech taggers, morphological analyzers for underrepresented languages (you don't have to know the language to develop such tools!)
- Quantitative approaches to theoretical linguistics questions
- Extensions and interfaces for WordNet (English and WN in other languages),
- Applications of WordNet(s), including:
- Foreign language tutoring systems,
- Spelling correction software,
- Word-finding/suggestion software for ordinary users and people with memory problems,
- Machine Translation
- Sentiment and Opinion detection
- Automatic reasoning and inferencing
- Collaboration with professors in the social sciences and humanities ("Digital Humanities")
Adam Finkelstein, Room 424
- Research Areas: computer graphics, audio.
Robert S. Fish, Corwin Hall, Room 037
- Networking and telecommunications
- Learning, perception, and intelligence, artificial and otherwise;
- Human-computer interaction and computer-supported cooperative work
- Online education, especially in Computer Science Education
- Topics in research and development innovation methodologies including standards, open-source, and entrepreneurship
- Distributed autonomous organizations and related blockchain technologies
Michael Freedman, Room 308
- Research Areas: Distributed systems, security, networking
- Projects related to streaming data analysis, datacenter systems and networks, untrusted cloud storage and applications. Please see my group website at http://sns.cs.princeton.edu/ for current research projects.
Ruth Fong, Room 032
- Research Areas: computer vision, machine learning, deep learning, interpretability, explainable AI, fairness and bias in AI
- Develop a technique for understanding AI models
- Design a AI model that is interpretable by design
- Build a paradigm for detecting and/or correcting failure points in an AI model
- Analyze an existing AI model and/or dataset to better understand its failure points
- Build a computer vision system for another domain (e.g., medical imaging, satellite data, etc.)
- Develop a software package for explainable AI
- Adapt explainable AI research to a consumer-facing problem
Note: I am happy to advise any project if there's a sufficient overlap in interest and/or expertise; please reach out via email to chat about project ideas.
Tom Griffiths, Room 405
Available for Fall 2024 single-semester IW advising, only
Research areas: computational cognitive science, computational social science, machine learning and artificial intelligence
Note: I am open to projects that apply ideas from computer science to understanding aspects of human cognition in a wide range of areas, from decision-making to cultural evolution and everything in between. For example, we have current projects analyzing chess game data and magic tricks, both of which give us clues about how human minds work. Students who have expertise or access to data related to games, magic, strategic sports like fencing, or other quantifiable domains of human behavior feel free to get in touch.
Aarti Gupta, Room 220
- Research Areas: Formal methods, program analysis, logic decision procedures
- Finding bugs in open source software using automatic verification tools
- Software verification (program analysis, model checking, test generation)
- Decision procedures for logical reasoning (SAT solvers, SMT solvers)
Elad Hazan, Room 409
- Research interests: machine learning methods and algorithms, efficient methods for mathematical optimization, regret minimization in games, reinforcement learning, control theory and practice
- Machine learning, efficient methods for mathematical optimization, statistical and computational learning theory, regret minimization in games.
- Implementation and algorithm engineering for control, reinforcement learning and robotics
- Implementation and algorithm engineering for time series prediction
Felix Heide, Room 410
- Research Areas: Computational Imaging, Computer Vision, Machine Learning (focus on Optimization and Approximate Inference).
- Optical Neural Networks
- Hardware-in-the-loop Holography
- Zero-shot and Simulation-only Learning
- Object recognition in extreme conditions
- 3D Scene Representations for View Generation and Inverse Problems
- Long-range Imaging in Scattering Media
- Hardware-in-the-loop Illumination and Sensor Optimization
- Inverse Lidar Design
- Phase Retrieval Algorithms
- Proximal Algorithms for Learning and Inference
- Domain-Specific Language for Optics Design
Peter Henderson , 302 Sherrerd Hall
- Research Areas: Machine learning, law, and policy
Kyle Jamieson, Room 306
- Research areas: Wireless and mobile networking; indoor radar and indoor localization; Internet of Things
- See other topics on my independent work ideas page (campus IP and CS dept. login req'd)
Alan Kaplan, 221 Nassau Street, Room 105
Research Areas:
- Random apps of kindness - mobile application/technology frameworks used to help individuals or communities; topic areas include, but are not limited to: first response, accessibility, environment, sustainability, social activism, civic computing, tele-health, remote learning, crowdsourcing, etc.
- Tools automating programming language interoperability - Java/C++, React Native/Java, etc.
- Software visualization tools for education
- Connected consumer devices, applications and protocols
Brian Kernighan, Room 311
- Research Areas: application-specific languages, document preparation, user interfaces, software tools, programming methodology
- Application-oriented languages, scripting languages.
- Tools; user interfaces
- Digital humanities
Zachary Kincaid, Room 219
- Research areas: programming languages, program analysis, program verification, automated reasoning
- Independent Research Topics:
- Develop a practical algorithm for an intractable problem (e.g., by developing practical search heuristics, or by reducing to, or by identifying a tractable sub-problem, ...).
- Design a domain-specific programming language, or prototype a new feature for an existing language.
- Any interesting project related to programming languages or logic.
Gillat Kol, Room 316
- Research area: theory
Aleksandra Korolova, 309 Sherrerd Hall
- Research areas: Societal impacts of algorithms and AI; privacy; fair and privacy-preserving machine learning; algorithm auditing.
Advisees typically have taken one or more of COS 226, COS 324, COS 423, COS 424 or COS 445.
Pravesh Kothari, Room 320
- Research areas: Theory
Amit Levy, Room 307
- Research Areas: Operating Systems, Distributed Systems, Embedded Systems, Internet of Things
- Distributed hardware testing infrastructure
- Second factor security tokens
- Low-power wireless network protocol implementation
- USB device driver implementation
Kai Li, Room 321
- Research Areas: Distributed systems; storage systems; content-based search and data analysis of large datasets.
- Fast communication mechanisms for heterogeneous clusters.
- Approximate nearest-neighbor search for high dimensional data.
- Data analysis and prediction of in-patient medical data.
- Optimized implementation of classification algorithms on manycore processors.
Xiaoyan Li, 221 Nassau Street, Room 104
- Research areas: Information retrieval, novelty detection, question answering, AI, machine learning and data analysis.
- Explore new statistical retrieval models for document retrieval and question answering.
- Apply AI in various fields.
- Apply supervised or unsupervised learning in health, education, finance, and social networks, etc.
- Any interesting project related to AI, machine learning, and data analysis.
Lydia Liu, Room 414
- Research Areas: algorithmic decision making, machine learning and society
- Theoretical foundations for algorithmic decision making (e.g. mathematical modeling of data-driven decision processes, societal level dynamics)
- Societal impacts of algorithms and AI through a socio-technical lens (e.g. normative implications of worst case ML metrics, prediction and model arbitrariness)
- Machine learning for social impact domains, especially education (e.g. responsible development and use of LLMs for education equity and access)
- Evaluation of human-AI decision making using statistical methods (e.g. causal inference of long term impact)
Wyatt Lloyd, Room 323
- Research areas: Distributed Systems
- Caching algorithms and implementations
- Storage systems
- Distributed transaction algorithms and implementations
Alex Lombardi , Room 312
- Research Areas: Theory
Margaret Martonosi, Room 208
- Quantum Computing research, particularly related to architecture and compiler issues for QC.
- Computer architectures specialized for modern workloads (e.g., graph analytics, machine learning algorithms, mobile applications
- Investigating security and privacy vulnerabilities in computer systems, particularly IoT devices.
- Other topics in computer architecture or mobile / IoT systems also possible.
Jonathan Mayer, Sherrerd Hall, Room 307
Available for Spring 2025 single-semester IW, only
- Research areas: Technology law and policy, with emphasis on national security, criminal procedure, consumer privacy, network management, and online speech.
- Assessing the effects of government policies, both in the public and private sectors.
- Collecting new data that relates to government decision making, including surveying current business practices and studying user behavior.
- Developing new tools to improve government processes and offer policy alternatives.
Mae Milano, Room 307
- Local-first / peer-to-peer systems
- Wide-ares storage systems
- Consistency and protocol design
- Type-safe concurrency
- Language design
- Gradual typing
- Domain-specific languages
- Languages for distributed systems
Andrés Monroy-Hernández, Room 405
- Research Areas: Human-Computer Interaction, Social Computing, Public-Interest Technology, Augmented Reality, Urban Computing
- Research interests:developing public-interest socio-technical systems. We are currently creating alternatives to gig work platforms that are more equitable for all stakeholders. For instance, we are investigating the socio-technical affordances necessary to support a co-op food delivery network owned and managed by workers and restaurants. We are exploring novel system designs that support self-governance, decentralized/federated models, community-centered data ownership, and portable reputation systems. We have opportunities for students interested in human-centered computing, UI/UX design, full-stack software development, and qualitative/quantitative user research.
- Beyond our core projects, we are open to working on research projects that explore the use of emerging technologies, such as AR, wearables, NFTs, and DAOs, for creative and out-of-the-box applications.
Christopher Moretti, Corwin Hall, Room 036
- Research areas: Distributed systems, high-throughput computing, computer science/engineering education
- Expansion, improvement, and evaluation of open-source distributed computing software.
- Applications of distributed computing for "big science" (e.g. biometrics, data mining, bioinformatics)
- Software and best practices for computer science education and study, especially Princeton's 126/217/226 sequence or MOOCs development
- Sports analytics and/or crowd-sourced computing
Radhika Nagpal, F316 Engineering Quadrangle
- Research areas: control, robotics and dynamical systems
Karthik Narasimhan, Room 422
- Research areas: Natural Language Processing, Reinforcement Learning
- Autonomous agents for text-based games ( https://www.microsoft.com/en-us/research/project/textworld/ )
- Transfer learning/generalization in NLP
- Techniques for generating natural language
- Model-based reinforcement learning
Arvind Narayanan, 308 Sherrerd Hall
Research Areas: fair machine learning (and AI ethics more broadly), the social impact of algorithmic systems, tech policy
Pedro Paredes, Corwin Hall, Room 041
My primary research work is in Theoretical Computer Science.
* Research Interest: Spectral Graph theory, Pseudorandomness, Complexity theory, Coding Theory, Quantum Information Theory, Combinatorics.
The IW projects I am interested in advising can be divided into three categories:
1. Theoretical research
I am open to advise work on research projects in any topic in one of my research areas of interest. A project could also be based on writing a survey given results from a few papers. Students should have a solid background in math (e.g., elementary combinatorics, graph theory, discrete probability, basic algebra/calculus) and theoretical computer science (226 and 240 material, like big-O/Omega/Theta, basic complexity theory, basic fundamental algorithms). Mathematical maturity is a must.
A (non exhaustive) list of topics of projects I'm interested in: * Explicit constructions of better vertex expanders and/or unique neighbor expanders. * Construction deterministic or random high dimensional expanders. * Pseudorandom generators for different problems. * Topics around the quantum PCP conjecture. * Topics around quantum error correcting codes and locally testable codes, including constructions, encoding and decoding algorithms.
2. Theory informed practical implementations of algorithms Very often the great advances in theoretical research are either not tested in practice or not even feasible to be implemented in practice. Thus, I am interested in any project that consists in trying to make theoretical ideas applicable in practice. This includes coming up with new algorithms that trade some theoretical guarantees for feasible implementation yet trying to retain the soul of the original idea; implementing new algorithms in a suitable programming language; and empirically testing practical implementations and comparing them with benchmarks / theoretical expectations. A project in this area doesn't have to be in my main areas of research, any theoretical result could be suitable for such a project.
Some examples of areas of interest: * Streaming algorithms. * Numeric linear algebra. * Property testing. * Parallel / Distributed algorithms. * Online algorithms. 3. Machine learning with a theoretical foundation
I am interested in projects in machine learning that have some mathematical/theoretical, even if most of the project is applied. This includes topics like mathematical optimization, statistical learning, fairness and privacy.
One particular area I have been recently interested in is in the area of rating systems (e.g., Chess elo) and applications of this to experts problems.
Final Note: I am also willing to advise any project with any mathematical/theoretical component, even if it's not the main one; please reach out via email to chat about project ideas.
Iasonas Petras, Corwin Hall, Room 033
- Research Areas: Information Based Complexity, Numerical Analysis, Quantum Computation.
- Prerequisites: Reasonable mathematical maturity. In case of a project related to Quantum Computation a certain familiarity with quantum mechanics is required (related courses: ELE 396/PHY 208).
- Possible research topics include:
1. Quantum algorithms and circuits:
- i. Design or simulation quantum circuits implementing quantum algorithms.
- ii. Design of quantum algorithms solving/approximating continuous problems (such as Eigenvalue problems for Partial Differential Equations).
2. Information Based Complexity:
- i. Necessary and sufficient conditions for tractability of Linear and Linear Tensor Product Problems in various settings (for example worst case or average case).
- ii. Necessary and sufficient conditions for tractability of Linear and Linear Tensor Product Problems under new tractability and error criteria.
- iii. Necessary and sufficient conditions for tractability of Weighted problems.
- iv. Necessary and sufficient conditions for tractability of Weighted Problems under new tractability and error criteria.
3. Topics in Scientific Computation:
- i. Randomness, Pseudorandomness, MC and QMC methods and their applications (Finance, etc)
Yuri Pritykin, 245 Carl Icahn Lab
- Research interests: Computational biology; Cancer immunology; Regulation of gene expression; Functional genomics; Single-cell technologies.
- Potential research projects: Development, implementation, assessment and/or application of algorithms for analysis, integration, interpretation and visualization of multi-dimensional data in molecular biology, particularly single-cell and spatial genomics data.
Benjamin Raphael, Room 309
- Research interests: Computational biology and bioinformatics; Cancer genomics; Algorithms and machine learning approaches for analysis of large-scale datasets
- Implementation and application of algorithms to infer evolutionary processes in cancer
- Identifying correlations between combinations of genomic mutations in human and cancer genomes
- Design and implementation of algorithms for genome sequencing from new DNA sequencing technologies
- Graph clustering and network anomaly detection, particularly using diffusion processes and methods from spectral graph theory
Vikram Ramaswamy, 035 Corwin Hall
- Research areas: Interpretability of AI systems, Fairness in AI systems, Computer vision.
- Constructing a new method to explain a model / create an interpretable by design model
- Analyzing a current model / dataset to understand bias within the model/dataset
- Proposing new fairness evaluations
- Proposing new methods to train to improve fairness
- Developing synthetic datasets for fairness / interpretability benchmarks
- Understanding robustness of models
Ran Raz, Room 240
- Research Area: Computational Complexity
- Independent Research Topics: Computational Complexity, Information Theory, Quantum Computation, Theoretical Computer Science
Szymon Rusinkiewicz, Room 406
- Research Areas: computer graphics; computer vision; 3D scanning; 3D printing; robotics; documentation and visualization of cultural heritage artifacts
- Research ways of incorporating rotation invariance into computer visiontasks such as feature matching and classification
- Investigate approaches to robust 3D scan matching
- Model and compensate for imperfections in 3D printing
- Given a collection of small mobile robots, apply control policies learned in simulation to the real robots.
Olga Russakovsky, Room 408
- Research Areas: computer vision, machine learning, deep learning, crowdsourcing, fairness&bias in AI
- Design a semantic segmentation deep learning model that can operate in a zero-shot setting (i.e., recognize and segment objects not seen during training)
- Develop a deep learning classifier that is impervious to protected attributes (such as gender or race) that may be erroneously correlated with target classes
- Build a computer vision system for the novel task of inferring what object (or part of an object) a human is referring to when pointing to a single pixel in the image. This includes both collecting an appropriate dataset using crowdsourcing on Amazon Mechanical Turk, creating a new deep learning formulation for this task, and running extensive analysis of both the data and the model
Sebastian Seung, Princeton Neuroscience Institute, Room 153
- Research Areas: computational neuroscience, connectomics, "deep learning" neural networks, social computing, crowdsourcing, citizen science
- Gamification of neuroscience (EyeWire 2.0)
- Semantic segmentation and object detection in brain images from microscopy
- Computational analysis of brain structure and function
- Neural network theories of brain function
Jaswinder Pal Singh, Room 324
- Research Areas: Boundary of technology and business/applications; building and scaling technology companies with special focus at that boundary; parallel computing systems and applications: parallel and distributed applications and their implications for software and architectural design; system software and programming environments for multiprocessors.
- Develop a startup company idea, and build a plan/prototype for it.
- Explore tradeoffs at the boundary of technology/product and business/applications in a chosen area.
- Study and develop methods to infer insights from data in different application areas, from science to search to finance to others.
- Design and implement a parallel application. Possible areas include graphics, compression, biology, among many others. Analyze performance bottlenecks using existing tools, and compare programming models/languages.
- Design and implement a scalable distributed algorithm.
Mona Singh, Room 420
- Research Areas: computational molecular biology, as well as its interface with machine learning and algorithms.
- Whole and cross-genome methods for predicting protein function and protein-protein interactions.
- Analysis and prediction of biological networks.
- Computational methods for inferring specific aspects of protein structure from protein sequence data.
- Any other interesting project in computational molecular biology.
Robert Tarjan, 194 Nassau St., Room 308
- Research Areas: Data structures; graph algorithms; combinatorial optimization; computational complexity; computational geometry; parallel algorithms.
- Implement one or more data structures or combinatorial algorithms to provide insight into their empirical behavior.
- Design and/or analyze various data structures and combinatorial algorithms.
Olga Troyanskaya, Room 320
- Research Areas: Bioinformatics; analysis of large-scale biological data sets (genomics, gene expression, proteomics, biological networks); algorithms for integration of data from multiple data sources; visualization of biological data; machine learning methods in bioinformatics.
- Implement and evaluate one or more gene expression analysis algorithm.
- Develop algorithms for assessment of performance of genomic analysis methods.
- Develop, implement, and evaluate visualization tools for heterogeneous biological data.
David Walker, Room 211
- Research Areas: Programming languages, type systems, compilers, domain-specific languages, software-defined networking and security
- Independent Research Topics: Any other interesting project that involves humanitarian hacking, functional programming, domain-specific programming languages, type systems, compilers, software-defined networking, fault tolerance, language-based security, theorem proving, logic or logical frameworks.
Shengyi Wang, Postdoctoral Research Associate, Room 216
Available for Fall 2024 single-semester IW, only
- Independent Research topics: Explore Escher-style tilings using (introductory) group theory and automata theory to produce beautiful pictures.
Kevin Wayne, Corwin Hall, Room 040
- Research Areas: design, analysis, and implementation of algorithms; data structures; combinatorial optimization; graphs and networks.
- Design and implement computer visualizations of algorithms or data structures.
- Develop pedagogical tools or programming assignments for the computer science curriculum at Princeton and beyond.
- Develop assessment infrastructure and assessments for MOOCs.
Matt Weinberg, 194 Nassau St., Room 222
- Research Areas: algorithms, algorithmic game theory, mechanism design, game theoretical problems in {Bitcoin, networking, healthcare}.
- Theoretical questions related to COS 445 topics such as matching theory, voting theory, auction design, etc.
- Theoretical questions related to incentives in applications like Bitcoin, the Internet, health care, etc. In a little bit more detail: protocols for these systems are often designed assuming that users will follow them. But often, users will actually be strictly happier to deviate from the intended protocol. How should we reason about user behavior in these protocols? How should we design protocols in these settings?
Huacheng Yu, Room 310
- data structures
- streaming algorithms
- design and analyze data structures / streaming algorithms
- prove impossibility results (lower bounds)
- implement and evaluate data structures / streaming algorithms
Ellen Zhong, Room 314
Opportunities outside the department.
We encourage students to look in to doing interdisciplinary computer science research and to work with professors in departments other than computer science. However, every CS independent work project must have a strong computer science element (even if it has other scientific or artistic elements as well.) To do a project with an adviser outside of computer science you must have permission of the department. This can be accomplished by having a second co-adviser within the computer science department or by contacting the independent work supervisor about the project and having he or she sign the independent work proposal form.
Here is a list of professors outside the computer science department who are eager to work with computer science undergraduates.
Maria Apostolaki, Engineering Quadrangle, C330
- Research areas: Computing & Networking, Data & Information Science, Security & Privacy
Branko Glisic, Engineering Quadrangle, Room E330
- Documentation of historic structures
- Cyber physical systems for structural health monitoring
- Developing virtual and augmented reality applications for documenting structures
- Applying machine learning techniques to generate 3D models from 2D plans of buildings
- Contact : Rebecca Napolitano, rkn2 (@princeton.edu)
Mihir Kshirsagar, Sherrerd Hall, Room 315
Center for Information Technology Policy.
- Consumer protection
- Content regulation
- Competition law
- Economic development
- Surveillance and discrimination
Sharad Malik, Engineering Quadrangle, Room B224
Select a Senior Thesis Adviser for the 2020-21 Academic Year.
- Design of reliable hardware systems
- Verifying complex software and hardware systems
Prateek Mittal, Engineering Quadrangle, Room B236
- Internet security and privacy
- Social Networks
- Privacy technologies, anonymous communication
- Network Science
- Internet security and privacy: The insecurity of Internet protocols and services threatens the safety of our critical network infrastructure and billions of end users. How can we defend end users as well as our critical network infrastructure from attacks?
- Trustworthy social systems: Online social networks (OSNs) such as Facebook, Google+, and Twitter have revolutionized the way our society communicates. How can we leverage social connections between users to design the next generation of communication systems?
- Privacy Technologies: Privacy on the Internet is eroding rapidly, with businesses and governments mining sensitive user information. How can we protect the privacy of our online communications? The Tor project (https://www.torproject.org/) is a potential application of interest.
Ken Norman, Psychology Dept, PNI 137
- Research Areas: Memory, the brain and computation
- Lab: Princeton Computational Memory Lab
Potential research topics
- Methods for decoding cognitive state information from neuroimaging data (fMRI and EEG)
- Neural network simulations of learning and memory
Caroline Savage
Office of Sustainability, Phone:(609)258-7513, Email: cs35 (@princeton.edu)
The Campus as Lab program supports students using the Princeton campus as a living laboratory to solve sustainability challenges. The Office of Sustainability has created a list of campus as lab research questions, filterable by discipline and topic, on its website .
An example from Computer Science could include using TigerEnergy , a platform which provides real-time data on campus energy generation and consumption, to study one of the many energy systems or buildings on campus. Three CS students used TigerEnergy to create a live energy heatmap of campus .
Other potential projects include:
- Apply game theory to sustainability challenges
- Develop a tool to help visualize interactions between complex campus systems, e.g. energy and water use, transportation and storm water runoff, purchasing and waste, etc.
- How can we learn (in aggregate) about individuals’ waste, energy, transportation, and other behaviors without impinging on privacy?
Janet Vertesi, Sociology Dept, Wallace Hall, Room 122
- Research areas: Sociology of technology; Human-computer interaction; Ubiquitous computing.
- Possible projects: At the intersection of computer science and social science, my students have built mixed reality games, produced artistic and interactive installations, and studied mixed human-robot teams, among other projects.
David Wentzlaff, Engineering Quadrangle, Room 228
Computing, Operating Systems, Sustainable Computing.
- Instrument Princeton's Green (HPCRC) data center
- Investigate power utilization on an processor core implemented in an FPGA
- Dismantle and document all of the components in modern electronics. Invent new ways to build computers that can be recycled easier.
- Other topics in parallel computer architecture or operating systems

- AI - General
- Computer Vision - General
- Academic Paper(s)
- Facial Recognition
- Image enhancing
- Object Detection/Tracking
- Phone Technology
- How Stuff Works
- Controversies
- Book Reviews
Be an Optimist Prime in the world of Computer Vision and AI
How to find a good thesis topic in computer vision.
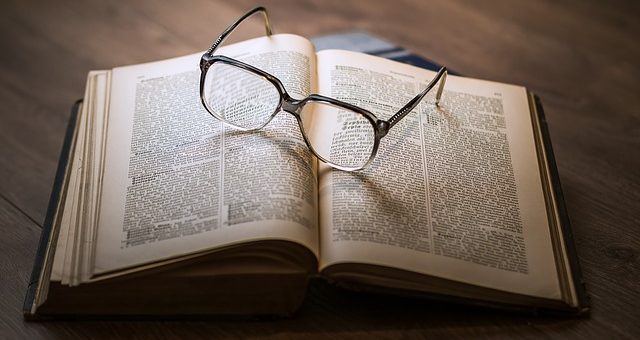
“What are some good thesis topics in Computer Vision?”
This is a common question that people ask in forums – and it’s an important question to ask for two reasons:
- There’s nothing worse than starting over in research because the path you decided to take turned out to be a dead end.
- There’s also nothing worse than being stuck with a generally good topic but one that doesn’t interest you at all. A “good” thesis topic has to be one that interests you and will keep you involved and stimulated for as long as possible.
For these reasons, it’s best to do as much research as you can to avoid the above pitfalls or your days of research will slowly become torturous for you – and that would be a shame because computer vision can truly be a lot of fun 🙂
So, down to business.
The purpose of this post is to propose ways to find that one perfect topic that will keep you engaged for months (or years) to come – and something you’ll be proud to talk about amongst friends and family.
I’ll start the discussion off by saying that your search strategy for topics depends entirely on whether you’re preparing for a Master’s thesis or a PhD. The former can be more general, the latter is (nearly always) very fine-grained specific. Let’s start with undergraduate topics first.
Undergraduate Studies
I’ll propose here three steps you can take to assist in your search: looking at the applications of computer vision, examining the OpenCV library, and talking to potential supervisors.
Applications of Computer Vision
Computer Vision has so many uses in the world. Why not look through a comprehensive list of them and see if anything on that list draws you in? Here’s one such list I collected from the British Machine Vision Association :
- agriculture
- augmented reality
- autonomous vehicles (big one nowadays!)
- character recognition
- industrial quality inspection
- face recognition
- gesture analysis
- image restoration
- medical image analysis
- pollution monitoring
- process control
- remote sensing
- robotics (e.g. navigation)
- security and surveillance
Go through this list and work out if something stands out for you. Perhaps your family is involved in agriculture? Look up how computer vision is helping in this field! The Economist wrote a fascinating article entitled “ The Future of Agriculture ” in which they discuss, among other things, the use of drones to monitor crops, create contour maps of fields, etc. Perhaps Computer Vision can assist with some of these tasks? Look into this!
OpenCV is the best library out there for image and video processing (I’ll be writing a lot more about it on this blog). Other libraries do exist that do certain specific things a little better, e.g. Tracking.js , which performs things like tracking inside the browser, but generally speaking, there’s nothing better than OpenCV.
On the topic of searching for thesis topics, I recall once reading a suggestion of going through the functions that OpenCV has to offer and seeing if anything sticks out at you there. A brilliant idea. Work down the list of the OpenCV documentation . Perhaps face recognition interests you? There are so many interesting projects where this can be utilised!
Talk to potential supervisors
You can’t go past this suggestion. Every academic has ideas constantly buzzing around his head. Academics are immersed in their field of research and are always to talking to people in the industry to look for interesting projects that they could get funding for. Go and talk to the academics at your university that are involved in Computer Vision. I’m sure they’ll have at least one project proposal ready to go for you.
You should also run any ideas of yours past them that may have emerged from the two previous steps. Or at least mention things that stood out for you (e.g. agriculture). They may be able to come up with something themselves.
PhD Studies
Well, if you’ve reached this far in your studies then chances are you have a fairly good idea of how this all works now. I won’t patronise you too much, then. But I will mention three points that I wish someone had told me prior to starting my PhD adventure:
- You should be building your research topic around a supervisor . They’ve been in the field for a long time and know where the niches and dead ends are. Use their experience! If there’s a supervisor who is constantly publishing in object tracking, then doing research with them in this area makes sense.
- If your supervisor has a ready-made topic for you, CONSIDER TAKING IT . I can’t stress this enough. Usually the first year of your PhD involves you searching (often blindly) around various fields in Computer Vision and then just going deeper and deeper into one specific area to find a niche. If your supervisor has a topic on hand for you, this means that you are already one year ahead of the crowd. And that means one year saved of frustration because searching around in a vast realm of publications can be daunting – believe me, I’ve been there.
- Avoid going into trending topics. For example, object recognition using Convolutional Neural Networks is a topic that currently everyone is going crazy about in the world of Computer Vision. This means that in your studies, you will be competing for publications with big players (e.g. Google) who have money, manpower, and computer power at their disposal. You don’t want to enter into this war unless you are confident that your supervisor knows what they’re doing and/or your university has the capabilities to play in this big league also.
Spending time looking for a thesis topic is time worth spending. It could save you from future pitfalls. With respect to undergraduate thesis topics looking at Computer Vision applications is one place to start. The OpenCV library is another. And talking to potential supervisors at your university is also a good idea.
With respect to PhD thesis topics, it’s important to take into consideration what the fields of expertise of your potential supervisors are and then searching for topics in these areas. If these supervisors have ready-made topics for you, it is worth considering them to save you a lot of time and stress in the first year or so of your studies. Finally, it’s usually good to avoid trending topics because of the people you will be competing against for publications.
But the bottom line is, devote time to finding a topic that truly interests you . It’ll be the difference between wanting to get out of bed to do more and more research in your field or dreading each time you have to walk into your Computer Science building in the morning.
To be informed when new content like this is posted, subscribe to the mailing list:
Related posts
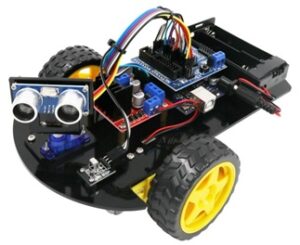
Arduino – Rotor Sweeping for Obstacle Detection Code
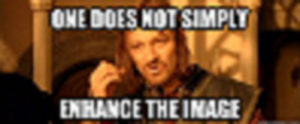
Security Film or Image Enhancing is Possible
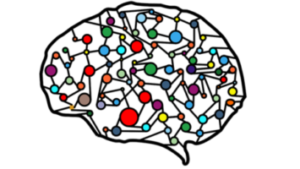
How Deep Learning Works – The Very Basics
One reply to “how to find a good thesis topic in computer vision”.
- Pingback: My Top 5 Posts So Far |
Leave a Reply Cancel reply
Your email address will not be published. Required fields are marked *
Save my name, email, and website in this browser for the next time I comment.
- DSpace@MIT Home
- MIT Libraries
- Doctoral Theses
Learning to solve problems in computer vision with synthetic data
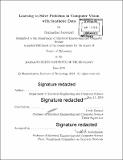
Other Contributors
Terms of use, description, date issued, collections.
Master's theses in Computer Vision
If you want to do your master's thesis project within the field of Computer Vision, there are several options:
- Internal Master's thesis at the Computer Vision Lab (CVL) Internal master's theses are normally connected to a research project, and explore a specific research idea. Some project suggestions are listed here: CVL Master's thesis proposal repository . If you already have an idea for a project, you may also contact one of the CVL examiners directly. See the list of examiners below.
- External Master's thesis at a company We maintain a list of research projects defined by external partners. These project proposals are found here: External Master's thesis project proposals . Future external projects may ** also be posted here: LiU exjobbsportal . If you do not find an interesting project on the page above, you may also contact companies/organizations directly. Oftent they have plans for projects, or are able to create a new one for you. A list of suitable companies can be found here: Computer Vision oriented companies/organisations .
If you have tried the possibilities above and still not found any interesting project, you can also directly contact one of the examiners at CVL, see list of examiners below.
Assignment of examiner and internal supervisor
Examiners for a Master's thesis in computer vision:
- Per-Erik Forssén (CVL Master's thesis coordinator)
- Michael Felsberg
- Maria Magnusson
- Mårten Wadenbäck
- Bastian Wandt
- Jörgen Ahlberg
- Amanda Berg
- Leif Haglund
- Lasse Alfredsson
Assignment of examiner is made after you contact the coordinator or an examiner (you will not necessarily get the one you contact). When contacting an examiner, you should provide the following information:
- Your name and personal number (we need to check your qualifications in Ladok)
- Name of the company and email to a contact person (for external Master's projects)
- Whether it is a master's thesis or bachelor's thesis
- When you want to start
- A project description (e.g. the ad from the company).
- Suggested course code for the project, corresponding to your main field of study (Sv:huvudområde) (e.g. TQET33, TQDT33, TQME33, TQMD33, TQTM33).
Thesis presentation in Swedish
- English to Swedish translations for Computer Vision (in Swedish) .
- Swedish Optical Terminology (in Swedish).
- Statistiktermer på Svenska (in Swedish).
Scientific publication of a master's thesis work
It is not uncommon that master's theses in computer vision are of such quality that they can be turned into scientific publications. This usually requires substantial amount of extra work, but could be a good acheivement to put in your CV. If you are interested in submitting your work for peer review at a conference or in a journal, check with your examiner or university supervisor for hints on how to frame the work and where to submit it. If you feel that your university supervisor has helped you substantially, also consider inviting him/her for co-authorship.
Other information sources
- University regulations regarding Master's thesis projects are definied in Studieinfo .
- There are also department spectific rules and practical information .
- Information about master's theses from LiTH. (will soon be moved)
- An attendance form for master's thesis presentations (Framläggningsblankett).
- Publishing your student thesis page at LiU Electronic Press. We recommend that the defence is announced one week in advance, at the vision-seminars mailing list. List subscribers may do so by sending an email to: vision-seminars.isy AT lists.liu.se .
- Session 1 (Klas Nordberg)
- Session 2 (Marcus Wallenberg)
- Automatic grammar checking tools for the English language are highly recommented. One such tool is Grammarly.
- Help with writing in English can also be had from Academic English Support at IKK .
- ** : This is contingent on this site being fixed to: (i) allow a proposal to be categorized as more than one "Main field of study" , as all Computer Vision projects fall under 2-4 "Main fields of study" (ii) allow easier inclusion of PDF attachments. Right now the proposal has to first be added, then removed, then found, then the attachment added, then the proposal should be added again.
You are using an outdated browser. Please upgrade your browser .
T4Tutorials.com
Computer vision research topics ideas.
List of Computer vision Research Topics Ideas for MS and PH.D. 1. Deep learning-enabled medical computer vision – Research questions 2. Deep learning, computer vision, and entomology – Research questions 3. Exploring human–nature interactions in national parks with social media photographs and computer vision 4. Assessing the potential for deep learning and computer vision to identify bumble bee species from images 6. Duplicate detection of images using computer vision techniques – Research questions 7. Noncontact cable force estimation with unmanned aerial vehicle and computer vision 8. Computer vision based two-stage waste recognition-retrieval algorithm for waste classification 9. A survey on generative adversarial networks for imbalance problems in computer vision tasks 10. Deception in the eyes of deceiver: A computer vision and machine learning based automated deception detection 11. A computer vision approach based on deep learning for the detection of dairy cows in free stall barn 12. Classification of fermented cocoa beans (cut test) using computer vision 13. Real-time water level monitoring using live cameras and computer vision techniques 14. Aeroelastic Vibration Measurement Based on Laser and Computer Vision Technique 15. Individualized SAR calculations using computer vision-based MR segmentation and a fast electromagnetic solver 16. Crop Nutrition and Computer Vision Technology 17. Advancing Eosinophilic Esophagitis Diagnosis and Phenotype Assessment with Deep Learning Computer Vision 18. Computer Vision-Based Bridge Damage Detection Using Deep Convolutional Networks with Expectation Maximum Attention Module 19. Analysis of ultrasonic vocalizations from mice using computer vision and machine learning 20. Developing a mold-free approach for complex glulam production with the assist of computer vision technologies 21. Decoding depressive disorder using computer vision 22. Assessment of Computer Vision Syndrome and Personal Risk Factors among Employees of Commercial Bank of Ethiopia in Addis Ababa, Ethiopia 23. One Label, One Billion Faces: Usage and Consistency of Racial Categories in Computer Vision 24. A survey of image labelling for computer vision applications 25. Development of Kid Height Measurement Application based on Image using Computer Vision 26. Computer vision AC-STEM automated image analysis for 2D nanopore applications 27. Displacement Identification by Computer Vision for Condition Monitoring of Rail Vehicle Bearings 28. An Open-Source Computer Vision Tool for Automated Vocal Fold Tracking From Videoendoscopy 29. Computer Vision and Human Behaviour, Emotion and Cognition Detection: A Use Case on Student Engagement 30. Computer vision-based tree trunk and branch identification and shaking points detection in Dense-Foliage canopy for automated harvesting of apples 31. Computer Vision–Based Estimation of Flood Depth in Flooded-Vehicle Images 32. An automated light trap to monitor moths (Lepidoptera) using computer vision-based tracking and deep learning 33. The Use of Saliency in Underwater Computer Vision: A Review 34. Computer vision for liquid samples in hospitals and medical labs using hierarchical image segmentation and relations prediction 35. Computer vision syndrome prevalence according to individual and video display terminal exposure characteristics in Spanish university students 36. Computer vision and unsupervised machine learning for pore-scale structural analysis of fractured porous media 37. Research on computer vision enhancement in intelligent robot based on machine learning and deep learning 38. Deformable Scintillation Dosimeter I: Challenges and Implementation using Computer Vision Techniques 39. Use of Computer Vision to Identify the Frequency and Magnitude of Insulin Syringe Preparation Errors 40. Action recognition of dance video learning based on embedded system and computer vision image 41. Frontiers of computer vision technologies on real estate property photographs and floorplans 42. Analysis of UAV-Acquired Wetland Orthomosaics Using GIS, Computer Vision, Computational Topology and Deep Learning 43. Computer vision applied to dual-energy computed tomography images for precise calcinosis cutis quantification in patients with systemic sclerosis 44. Human Motion Gesture Recognition Based on Computer Vision 45. Application of computer vision in fish intelligent feeding system—A review 46. Application of Computer Vision in 3D Film 47. WiCV 2020: The Seventh Women In Computer Vision Workshop 48. Computer vision based obstacle detection and target tracking for autonomous vehicles 49. Evaluating Congou black tea quality using a lab-made computer vision system coupled with morphological features and chemometrics 50. Research on Key Technologies in the Field of Computer Vision Based on Deep Learning 51. Online detection of naturally DON contaminated wheat grains from China using Vis-NIR spectroscopy and computer vision 52. A Computer Vision-Based Occupancy and Equipment Usage Detection Approach for Reducing Building Energy Demand 53. Application of Computer Vision Technology in Agricultural Products and Food Inspection 54. Automatic Evaluation of Wheat Resistance to Fusarium Head Blight Using Dual Mask-RCNN Deep Learning Frameworks in Computer Vision 55. A computer vision algorithm for locating and recognizing traffic signal control light status and countdown time 56. Microplastic abundance quantification via a computer-vision-based chemometrics-assisted approach 57. Computer Vision for Dietary Assessment 58. Determinants of computer vision system’s technology acceptance to improve incoming cargo receiving at Eastern European and Central Asian transportation … 59. CONSTRUCTION OF A SOMATOSENSORY INTERACTIVE SYSTEM BASED ON COMPUTER VISION AND AUGMENTED REALITY TECHNIQUES USI… 60. Estimating California’s Solar and Wind Energy Production using Computer Vision Deep Learning Techniques on Weather Images 61. Leaf disease segmentation and classification of Jatropha Curcas L. and Pongamia Pinnata L. biofuel plants using computer vision based approaches 62. Automated correlation of petrographic images of sandstones to a textural properties database extracted with computer vision techniques 63. Computer Vision-based Intelligent Bookshelf System 64. Computer Vision Techniques for Crowd Density and Motion Direction Analysis 65. Computer Vision System for Landing Platform State Assessment Onboard of Unmanned Aerial Vehicle in Case of Input Visual Information Distortion 66. Research on Bridge Deck Health Assessment System Based on BIM and Computer Vision Technology 67. Computer Vision for Dynamic Student Data Management in Higher Education Platform. 68. Tulipp and ClickCV: How the Future Demands of Computer Vision Can Be Met Using FPGAs 69. Stripenn detects architectural stripes from chromatin conformation data using computer vision 70. Having Fun with Computer Vision 71. Application of Computer Vision in Pipeline Inspection Robot 72. Design of Digital Museum Narrative Space Based on Perceptual Experience Data Mining and Computer Vision 73. Study on Pipelined Parallel Processing Architectures for Imaging and Computer Vision 74. Research on fire inspection robot based on computer vision 75. ActiveNet: A computer-vision based approach to determine lethargy 76. Individual Wave Detection and Tracking within a Rotating Detonation Engine through Computer Vision Object Detection applied to High-Speed Images 77. Human Thorax Parametric Reconstruction Using Computer Vision 78. Advancing Eosinophilic Esophagitis Diagnosis and Phenotype Assessment with Deep Learning Computer Vision 79. LANE DETECTION USING COMPUTER VISION FOR SELF-DRIVING CARS 80. Automatic Gear Sorting Using Wireless PLC Based on Computer Vision 81. Computer Vision-based Marker-less Real Time Motion Analysis for Rehabilitation–An Interdisciplinary Research Project 82. Computer vision in surgery 83. GUIs for Computer Vision 84. Surgical navigation technology based on computer vision and vr towards iot 85. A web-based survey on various symptoms of computer vision syndrome and the genetic understanding based on a multi-trait genome-wide association study 86. Automated Classification and Detection of Malaria Cell Using Computer Vision 87. Computer-Assisted Self-Training for Kyudo Posture Rectification Using Computer Vision Methods 88. Comparison of Computer Vision Techniques for Drowsiness Detection While Driving 89. A Real-Time Computer Vision System for Workers’ PPE and Posture Detection in Actual Construction Site Environment 90. Embedded Computer Vision System Applied to a Four-Legged Line Follower Robot 91. Computer Vision in Industry, Practice in the Czech Republic 92. Computer vision for microscopic skin cancer diagnosis using handcrafted and non-handcrafted features 93. Deep nets: What have they ever done for vision? 94. Analysis of Traditional Computer Vision Techniques Used for Hemp Leaf Water Stress Detection and Classification 95. Embedded Computer Vision System Applied to a Four-Legged Line Follower Robot 96. Deep Learning and Computer Vision Strategies for Automated Gene Editing with a Single-Cell Electroporation Platform 97. A computer vision-based approach for behavior recognition of gestating sows fed different fiber levels during high ambient temperature 98. Swin transformer: Hierarchical vision transformer using shifted windows 99. GEJALA COMPUTER VISION SYNDROME YANG DIALAMI OLEH KARYAWAN BUMN SEKTOR KEUANGAN KOTA TASIKMALAYA 100. Sistem Identifikasi Tingkat Kematangan Buah Nanas Secara Non-Destruktif Berbasis Computer Vision 101. Field-programmable gate arrays in a low power vision system 102. SiT: Self-supervised vIsion Transformer 103. SISTEM PENDETEKSI MASKER WAJAH DAN SUHU TUBUH MENGGUNAKAN TEKNIK COMPUTER VISION DAN SENSOR INFRARED NON-CONTACT 104. Do we really need explicit position encodings for vision transformers? 105. Cvt: Introducing convolutions to vision transformers 106. Pyramid vision transformer: A versatile backbone for dense prediction without convolutions 107. Training vision transformers for image retrieval 108. Transformers in Vision: A Survey 109. Uncertainty-assisted deep vision structural health monitoring 110. Twins: Revisiting spatial attention design in vision transformers 111. Crossvit: Cross-attention multi-scale vision transformer for image classification 112. Smart Computer Laboratory: IoT Based Smartphone Application 113. A Dense Tensor Accelerator with Data Exchange Mesh for DNN and Vision Workloads 114. Physics-based vision meets deep learning 115. Neural vision-based semantic 3D world modeling 116. Scaling up visual and vision-language representation learning with noisy text supervision 117. Deep Learning–Based Scene Simplification for Bionic Vision 118. Techniques To Improve Machine Vision In Robots 119. Future Vision Exhibition: Artificial Landscapes 120. Enabling energy efficient machine learning on a Ultra-Low-Power vision sensor for IoT 121. Tokens-to-token vit: Training vision transformers from scratch on imagenet 122. Vision-based Sensors for Production Control 123. Machine Learning and Deep Learning Applications-A Vision 124. The quiet revolution in machine vision-a state-of-the-art survey paper, including historical review, perspectives, and future directions 125. Synthesizing Pose Sequences from 3D Assets for Vision-Based Activity Analysis 126. Applying Mobile Intelligent API Vision Kit and Normalized Features for Face Recognition Using Live Cameras 127. A New Approach for Fire Pixel Detection in Building Environment Using Vision Sensor 128. A Vision-Based Parameter Estimation for an Aircraft in Approach Phase 129. ViLT: Vision-and-Language Transformer Without Convolution or Region Supervision 130. Analysis of Target Detection and Tracking for Intelligent Vision System 131. Test Automation with Grad-CAM Heatmaps-A Future Pipe Segment in MLOps for Vision AI? 132. Investigating the Vision Transformer Model for Image Retrieval Tasks 133. Vision-based adjusting of a digital model to real-world conditions for wire insertion tasks 134. Combining brief and ad for edge-preserved dense stereo matching 135. Elf: accelerate high-resolution mobile deep vision with content-aware parallel offloading 136. Shallow Convolution Neural Network for an Industrial Robot Real Time Vision System 137. Research Status of Gesture Recognition Based on Vision: A Review 138. Multi-scale vision longformer: A new vision transformer for high-resolution image encoding 139. Road Peculiarities Detection using Deep Learning for Vehicle Vision System 140. Reinforcement learning applied to machine vision: state of the art 141. Vision based inspection system for leather surface defect detection using fast convergence particle swarm optimization ensemble classifier approach 142. Evaluation of visual complications among professional computer users 143. Brain Tumor Segmentation: A Comparative Analysis 144. Detection of Atlantic salmon bone residues using machine vision technology 145. Understanding Perceptual Bias in Machine Vision Systems 146. The MVTec anomaly detection dataset: a comprehensive real-world dataset for unsupervised anomaly detection 147. Scene text detection and recognition: The deep learning era 148. Vision-based continuous sign language recognition using multimodal sensor fusion 149. Egocentric Vision for Dog Behavioral Analysis 150. Vision-based Docking of a Mobile Robot 151. VLGrammar: Grounded Grammar Induction of Vision and Language 152. Mask-aware photorealistic facial attribute manipulation 153. Real-time plant phenomics under robotic farming setup: A vision-based platform for complex plant phenotyping tasks 154. VTGAN: Semi-supervised Retinal Image Synthesis and Disease Prediction using Vision Transformers 155. Deep Vision Based Surveillance System to Prevent Train-Elephant Collisions 156. Boosting High-Level Vision with Joint Compression Artifacts Reduction and Super-Resolution 157. Autonomous, onboard vision-based trash and litter detection in low altitude aerial images collected by an unmanned aerial vehicle 158. Research on the algorithm of painting image style feature extraction based on intelligent vision 159. Fast semantic segmentation method for machine vision inspection based on a fewer-parameters atrous convolution neural network 160. Investigating Bi-Level Optimization for Learning and Vision from a Unified Perspective: A Survey and Beyond 161. Vision-Based Full-Field Sensing for Condition Assessment of Structural Systems 162. New method of traffic flow forecasting based on quantum particle swarm optimization strategy for intelligent transportation system 163. Same-different conceptualization: a machine vision perspective 164. Toward high-quality magnetic data survey using UAV: development of a magnetic-isolated vision-based positioning system 165. Urban landscape ecological design and stereo vision based on 3D mesh simplification algorithm and artificial intelligence 166. Transformer in transformer 167. A Robotic grinding station based on an industrial manipulator and vision system 168. Applications of Internet of Things (IoT) in Agriculture-The Potential and Challenges in Smart Farm in Uganda 169. Vision-Based Patient Monitoring and Management in Mental Health Settings 170. Mitigating Demographic Bias in Facial Datasets with Style-Based Multi-attribute Transfer 171. Dynamic tree branch tracking for aerial canopy sampling using stereo vision 172. Smart Office Model Based on Internet of Things 173. How to construct low-altitude aerial image datasets for deep learning [J] 174. Pretraining boosts out-of-domain robustness for pose estimation 175. A benchmark and evaluation of non-rigid structure from motion 176. The devil is in the boundary: Exploiting boundary representation for basis-based instance segmentation 177. Vision based collision detection for a safe collaborative industrial manipulator 178. Eden: Multimodal synthetic dataset of enclosed garden scenes 179. Pixel-wise crowd understanding via synthetic data 180. Vision-Based Framework for Automatic Progress Monitoring of Precast Walls by Using Surveillance Videos during the Construction Phase 181. LSPnet: A 2D Localization-oriented Spacecraft Pose Estimation Neural Network 182. Transfer of Learning from Vision to Touch: A Hybrid Deep Convolutional Neural Network for Visuo-Tactile 3D Object Recognition 183. UAV Use Case: Real-Time Obstacle Avoidance System for Unmanned Aerial Vehicles Based on Stereo Vision 184. Improving grain size analysis using computer vision techniques and implications for grain growth kinetics 185. Comparison of full-reference image quality models for optimization of image processing systems 186. High Precision Medicine Bottles Vision Online Inspection System and Classification Based on Multi-Features and Ensemble Learning via Independence Test 187. Efficient attention: Attention with linear complexities 188. Fusion Learning Using Semantics and Graph Convolutional Network for Visual Food Recognition 189. The ikea asm dataset: Understanding people assembling furniture through actions, objects and pose 190. DualSR: Zero-Shot Dual Learning for Real-World Super-Resolution 191. Modelling and Analysis of Facial Expressions Using Optical Flow Derived Divergence and Curl Templates 192. Barlow twins: Self-supervised learning via redundancy reduction 193. Facial expression recognition in the wild via deep attentive center loss 194. Facial Beauty Prediction and Analysis Based on Deep Convolutional Neural Network: A Review 195. Rodnet: Radar object detection using cross-modal supervision 196. Using open-source computer vision software for identification and tracking of convective storms 197. Improving Robustness and Uncertainty Modelling in Neural Ordinary Differential Equations 198. Vision and Inertial Sensor Fusion for Terrain Relative Navigation 199. Domain-Aware Unsupervised Hyperspectral Reconstruction for Aerial Image Dehazing 200. A method for classifying citrus surface defects based on machine vision 201. Transgan: Two transformers can make one strong gan 202. Improving Point Cloud Semantic Segmentation by Learning 3D Object Detection 203. Information Systems Integration to Enhance Operational Customer Relationship Management in the Pharmaceutical Industry 204. Attentional feature fusion 205. The Isowarp: The Template-Based Visual Geometry of Isometric Surfaces 206. Vision-Based Diagnosis and Location of Insulator Self-Explosion Defects 207. Activity Recognition with Moving Cameras and Few Training Examples: Applications for Detection of Autism-Related Headbanging 208. Development and Validation of an Unsupervised Feature Learning System for Leukocyte Characterization and Classification: A Multi-Hospital Study 209. DualSANet: Dual Spatial Attention Network for Iris Recognition 210. Long-Range Attention Network for Multi-View Stereo 211. Towards visually explaining video understanding networks with perturbation 212. MinkLoc3D: Point Cloud Based Large-Scale Place Recognition 213. p-RT: A Runtime Framework to Enable Energy-Efficient Real-Time Robotic Vision Applications on Heterogeneous Architectures 214. JOLO-GCN: Mining Joint-Centered Light-Weight Information for Skeleton-Based Action Recognition 215. Binarized neural architecture search for efficient object recognition 216. Learning transferable visual models from natural language supervision 217. Improved techniques for training single-image gans 218. Machine Vision 219. SSDMNV2: A real time DNN-based face mask detection system using single shot multibox detector and MobileNetV2 220. Pervasive label errors in test sets destabilize machine learning benchmarks 221. MPRNet: Multi-Path Residual Network for Lightweight Image Super Resolution 222. Fuzzy-aided solution for out-of-view challenge in visual tracking under IoT-assisted complex environment 223. Deep Learning in X-ray Testing 224. Tresnet: High performance gpu-dedicated architecture 225. ATM: Attentional Text Matting 226. Classmix: Segmentation-based data augmentation for semi-supervised learning 227. Videossl: Semi-supervised learning for video classification 228. Stratified rule-aware network for abstract visual reasoning 229. A hierarchical privacy-preserving IoT architecture for vision-based hand rehabilitation assessment 230. Adversarial reinforcement learning for unsupervised domain adaptation 231. Visual question answering model based on graph neural network and contextual attention 232. Towards Balanced Learning for Instance Recognition 233. The automatic detection of pedestrians under the high-density conditions by deep learning techniques 234. RGB-D salient object detection: A survey 235. A deep active learning system for species identification and counting in camera trap images 236. Domain Impression: A Source Data Free Domain Adaptation Method 237. Robust feature learning for adversarial defense via hierarchical feature alignment 238. HADEM-MACS: a hybrid approach for detection and extraction of objects in movement by multimedia autonomous computer systems 239. Vision-Based Method Integrating Deep Learning Detection for Tracking Multiple Construction Machines 240. Bottleneck transformers for visual recognition 241. Single Image Human Proxemics Estimation for Visual Social Distancing 242. SoFA: Source-Data-Free Feature Alignment for Unsupervised Domain Adaptation 243. Contrastive learning of general-purpose audio representations 244. Knowledge distillation: A survey 245. Let’s Get Dirty: GAN Based Data Augmentation for Camera Lens Soiling Detection in Autonomous Driving 246. Video Semantic Analysis: The Sparsity Based Locality-Sensitive Discriminative Dictionary Learning Factor 247. Vision-Based Tactile Sensor Mechanism for the Estimation of Contact Position and Force Distribution Using Deep Learning 248. A Discriminative Model for Multiple People Detection 249. Generative adversarial networks and their application to 3D face generation: A survey 250. Semantic hierarchy emerges in deep generative representations for scene synthesis 251. Fake face detection via adaptive manipulation traces extraction network 252. Accuracy of smartphone video for contactless measurement of hand tremor frequency 253. Video Captioning of Future Frames 254. Disentangled Contour Learning for Quadrilateral Text Detection 255. Visual Structure Constraint for Transductive Zero-Shot Learning in the Wild 256. CNN features with bi-directional LSTM for real-time anomaly detection in surveillance networks 257. CityFlow-NL: Tracking and Retrieval of Vehicles at City Scaleby Natural Language Descriptions 258. 3D Head Pose Estimation through Facial Features and Deep Convolutional Neural Networks 259. Rescuenet: Joint building segmentation and damage assessment from satellite imagery 260. Alleviating over-segmentation errors by detecting action boundaries 261. Multiresolution Adaptive Threshold Based Segmentation of Real-Time Vision-Based Database for Human Motion Estimation 262. Simplifying dependent reductions in the polyhedral model 263. Multi-camera traffic scene mosaic based on camera calibration 264. Novel View Synthesis via Depth-guided Skip Connections 265. Self-Supervised Pretraining Improves Self-Supervised Pretraining 266. Weakly Supervised Multi-Object Tracking and Segmentation 267. Proposal learning for semi-supervised object detection 268. You only look yourself: Unsupervised and untrained single image dehazing neural network 269. Algorithm for epipolar geometry and correcting monocular stereo vision based on a plane mirror 270. Mutual Information Maximization on Disentangled Representations for Differential Morph Detection 271. Route planning methods in indoor navigation tools for vision impaired persons: a systematic review 272. Subject Guided Eye Image Synthesis with Application to Gaze Redirection 273. Roles of artificial intelligence in construction engineering and management: A critical review and future trends 274. PI-Net: Pose Interacting Network for Multi-Person Monocular 3D Pose Estimation 275. Swag: Superpixels weighted by average gradients for explanations of cnns 276. Impact diagnosis in stiffened structural panels using a deep learning approach 277. X-ray Testing 278. Computer Assisted Classification Framework for Detection of Acute Myeloid Leukemia in Peripheral Blood Smear Images 279. IGSSTRCF: Importance Guided Sparse Spatio-Temporal Regularized Correlation Filters for Tracking 280. A Unified Learning Approach for Hand Gesture Recognition and Fingertip Detection 281. EventAnchor: Reducing Human Interactions in Event Annotation of Racket Sports Videos 282. An Evolution of CNN Object Classifiers on Low-Resolution Images 283. A vector-based representation to enhance head pose estimation 284. Automatic Defect Detection of Print Fabric Using Convolutional Neural Network 285. Multi-Scale Voxel Class Balanced ASPP for LIDAR Pointcloud Semantic Segmentation 286. Non-Destructive Quality Inspection of Potato Tubers Using Automated Vision System 287. Layering Defects Detection in Laser Powder Bed Fusion using Embedded Vision System 288. Label-Free Robustness Estimation of Object Detection CNNs for Autonomous Driving Applications 289. Convolutional Neural Networks and Transfer Learning for Quality Inspection of Different Sugarcane Varieties 290. Vision-Based Suture Tensile Force Estimation in Robotic Surgery 291. FEANet: Foreground-edge-aware network with DenseASPOC for human parsing 292. Application of a convolutional neural network for detection of ignition sources and smoke 293. Image matching across wide baselines: From paper to practice 294. Towards Annotation-free Instance Segmentation and Tracking with Adversarial Simulations 295. Self-Supervised Learning for Domain Adaptation on Point Clouds 296. Ontology-driven event type classification in images 297. Apple Ripeness Identification Using Deep Learning 298. Deep sparse transfer learning for remote smart tongue diagnosis [J] 299. CONVERSATION OF REAL IMAGES INTO CARTOONIZE IMAGE FORMAT USING GENERATIVE ADVERSARIAL NETWORK 300. RICORD: A Precedent for Open AI in COVID-19 Image Analytics 301. CapGen: A Neural Image Caption Generator with Speech Synthesis 302. Computer-Aided Diagnosis of Alzheimer’s Disease through Weak Supervision Deep Learning Framework with Attention Mechanism 303. A novel and intelligent vision-based tutor for Yogasana: e-YogaGuru 304. Plant Trait Estimation and Classification Studies in Plant Phenotyping Using Machine Vision-A Review 305. Iranis: A Large-scale Dataset of Farsi License Plate Characters 306. A numerical framework for elastic surface matching, comparison, and interpolation 307. Sign language recognition from digital videos using deep learning methods 308. Enhanced Information Fusion Network for Crowd Counting 309. This Face Does Not Exist… But It Might Be Yours! Identity Leakage in Generative Models 310. Defense-friendly Images in Adversarial Attacks: Dataset and Metrics for Perturbation Difficulty 311. Guided attentive feature fusion for multispectral pedestrian detection 312. Vision-Based Guidance for Tracking Dynamic Objects 313. Single-shot fringe projection profilometry based on Deep Learning and Computer Graphics 314. Improving Video Captioning with Temporal Composition of a Visual-Syntactic Embedding 315. Adversarial deepfakes: Evaluating vulnerability of deepfake detectors to adversarial examples 316. Vision-Aided 6G Wireless Communications: Blockage Prediction and Proactive Handoff 317. FACEGAN: Facial Attribute Controllable rEenactment GAN 318. Benchmarking the robustness of semantic segmentation models with respect to common corruptions 319. Vision-based egg quality prediction in Pacific bluefin tuna (Thunnus orientalis) by deep neural network 320. Diagnosing colorectal abnormalities using scattering coefficient maps acquired from optical coherence tomography 321. Recovering Trajectories of Unmarked Joints in 3D Human Actions Using Latent Space Optimization 322. Only time can tell: Discovering temporal data for temporal modeling 323. Deep Learning applications for COVID-19 324. PointCutMix: Regularization Strategy for Point Cloud Classification 325. A Survey on the Usage of Pattern Recognition and Image Analysis Methods for the Lifestyle Improvement on Low Vision and Visually Impaired People 326. Mechanical System Control by RGB-D Device 327. DACS: Domain Adaptation via Cross-domain Mixed Sampling 328. Vision-Based Vibration Monitoring of Structures and Infrastructures: An Overview of Recent Applications 329. CycleSegNet: Object Co-segmentation with Cycle Refinement and Region Correspondence 330. Explainable Fingerprint ROI Segmentation Using Monte Carlo Dropout 331. Self-supervised pretraining of visual features in the wild 332. Optimized Z-Buffer Using Divide and Conquer 333. Efficient and robust unsupervised inverse intensity compensation for stereo image registration under radiometric changes 334. Zero-shot text-to-image generation 335. PoseRBPF: A Rao–Blackwellized Particle Filter for 6-D Object Pose Tracking 336. MobiSamadhaan—Intelligent Vision-Based Smart City Solution 337. A Training Method for Low Rank Convolutional Neural Networks Based on Alternating Tensor Compose-Decompose Method 338. Individual Sick Fir Tree (Abies mariesii) Identification in Insect Infested Forests by Means of UAV Images and Deep Learning 339. Fighting against COVID-19: A novel deep learning model based on YOLO-v2 with ResNet-50 for medical face mask detection 340. 3D Image Conversion of a Scene from Multiple 2D Images with Background Depth Profile 341. Falsification of a Vision-based Automatic Landing System 342. Generating Physically Sound Training Data for Image Recognition of Additively Manufactured Parts 343. Shelf Auditing Based on Image Classification Using Semi-Supervised Deep Learning to Increase On-Shelf Availability in Grocery Stores 344. Continuous 3D Multi-Channel Sign Language Production via Progressive Transformers and Mixture Density Networks 345. Research on Detection Method of Sheet Surface Defects Based on Machine Vision 346. Low-Resolution LiDAR Upsampling Using Weighted Median Filter 347. RGB-D Human Action Recognition of Deep Feature Enhancement and Fusion Using Two-Stream ConvNet 348. Breaking Shortcuts by Masking for Robust Visual Reasoning 349. Correlation filter tracking based on superpixel and multifeature fusion 350. Segmentation of body parts of cows in RGB-depth images based on template matching 351. Electric Scooter and Its Rider Detection Framework Based on Deep Learning for Supporting Scooter-Related Injury Emergency Services 352. A Model of Diameter Measurement Based on the Machine Vision 353. Transreid: Transformer-based object re-identification 354. LightLayers: Parameter Efficient Dense and Convolutional Layers for Image Classification 355. Residual Dual Scale Scene Text Spotting by Fusing Bottom-Up and Top-Down Processing 356. Pedestrian Detection on Multispectral Images in Different Lighting Conditions 357. CovidSens: a vision on reliable social sensing for COVID-19 358. An Improved Approach for Face Detection 359. Classification and Measuring Accuracy of Lenses Using Inception Model V3 360. Nighttime image dehazing based on Retinex and dark channel prior using Taylor series expansion 361. Multiple Object Tracking Using Convolutional Neural Network on Aerial Imagery Sequences 362. Piano Skills Assessment 363. Automatic recognition of surface cracks in bridges based on 2D-APES and mobile machine vision 364. Real-time Navigation for Drogue-Type Autonomous Aerial Refueling Using Vision-Based Deep Learning Detection 365. Robust 3D Reconstruction Through Noise Reduction of Ultra-Fast Images 366. Survey of Occluded and Unoccluded Face Recognition 367. Cell tracking in time-lapse microscopy image sequences 368. Depth Estimation Using Blob Detection for Stereo Vision Images 369. Fast human activity recognition 370. Single Shot Multitask Pedestrian Detection and Behavior Prediction 371. The Vision of Digital Surgery 372. Modeling of Potato Slice Drying Process in a Microwave Dryer using Artificial Neural Network and Machine Vision 373. VinVL: Making Visual Representations Matter in Vision-Language Models 374. Quality safety monitoring of LED chips using deep learning-based vision inspection methods 375. Machine Vision Based Phenotype Recognition of Plant and Animal 376. Towards manufacturing robotics accuracy degradation assessment: A vision-based data-driven implementation 377. Attention guided low-light image enhancement with a large scale low-light simulation dataset 378. Human action identification by a quality-guided fusion of multi-model feature 379. Optimal quantization using scaled codebook 380. A Robust Illumination-Invariant Camera System for Agricultural Applications 381. Real-Time Gait-Based Age Estimation and Gender Classification From a Single Image 382. Counting and Tracking of Vehicles and Pedestrians in Real Time Using You Only Look Once V3 383. Style Normalization and Restitution for DomainGeneralization and Adaptation 384. A Machine Vision-Based Method Optimized for Restoring Broiler Chicken Images Occluded by Feeding and Drinking Equipment 385. Face Recognition for Surveillance Systems using SRGAN 386. FACIAL RECOGNITION AND ATTENDANCE SYSTEM USING DLIB AND FACE RECOGNITION LIBRARIES 387. Deep Preset: Blending and Retouching Photos with Color Style Transfer 388. Transunet: Transformers make strong encoders for medical image segmentation 389. List-wise learning-to-rank with convolutional neural networks for person re-identification 390. Intra-Camera Supervised Person Re-Identification 391. Generating Masks from Boxes by Mining Spatio-Temporal Consistencies in Videos 392. A Deep Convolutional Encoder-Decoder Architecture Approach for Sheep Weight Estimation 393. Automatic Borescope Damage Assessments for Gas Turbine Blades via Deep Learning 394. Foreground-aware Semantic Representations for Image Harmonization 395. A Learning-Based Approach to Parametric Rotoscoping of Multi-Shape Systems 396. Energy-efficient cluster-based unmanned aerial vehicle networks with deep learning-based scene classification model 397. Mobile-Aware Deep Learning Algorithms for Malaria Parasites and White Blood Cells Localization in Thick Blood Smears 398. SChISM: Semantic Clustering via Image Sequence Merging for Images of Human-Decomposition 399. Multilingual Multimodal Pre-training for Zero-Shot Cross-Lingual Transfer of Vision-Language Models 400. Locate Globally, Segment Locally: A Progressive Architecture With Knowledge Review Network for Salient Object Detection 401. Using Feature Selection Based on Multi-view for Rice Seed Images Classification 402. Road images augmentation with synthetic traffic signs using neural networks 403. Java Tools For Image Understanding The Java Imaging and Vision Environment (JIVE) 404. Improved ECO Algorithm Based on Residual Neural Network 405. Viewpoint and Scale Consistency Reinforcement for UAV Vehicle Re-Identification 406. Automated Surveillance Model for Video-Based Anomalous Activity Detection Using Deep Learning Architecture 407. Real-Time, YOLO-Based Intelligent Surveillance and Monitoring System Using Jetson TX2 408. Countering Inconsistent Labelling by Google’s Vision API for Rotated Images 409. Towards Accurate Camouflaged Object Detection with Mixture Convolution and Interactive Fusion 410. Effectiveness of arbitrary transfer sets for data-free knowledge distillation 411. Accelerated High-Level Synthesis Feature Detection for FPGAs Using HiFlipVX 412. Supervised deep learning of elastic SRV distances on the shape space of curves 413. Evaluating GAN-Based Image Augmentation for Threat Detection in Large-Scale Xray Security Images 414. Where to Start Your Deep Learning 415. Real-Time Detection and Spatial Localization of Insulators for UAV Inspection Based on Binocular Stereo Vision 416. Excitation dropout: Encouraging plasticity in deep neural networks 417. The Edge Computing Cloud Architecture Based on 5G Network for Industrial Vision Detection 418. Estimating Galactic Distances From Images Using Self-supervised Representation Learning 419. Real-Time Hair Segmentation Using Mobile-Unet 420. Driving among Flatmobiles: Bird-Eye-View occupancy grids from a monocular camera for holistic trajectory planning 421. Attention-based context aggregation network for monocular depth estimation 422. A Large-Scale, Time-Synchronized Visible and Thermal Face Dataset 423. Identification of Suitable Contrast Enhancement Technique for Improving the Quality of Astrocytoma Histopathological Images. 424. Run-Time Monitoring of Machine Learning for Robotic Perception: A Survey of Emerging Trends 425. Prevalence and risk factor assessment of digital eye strain among children using online e-learning during the COVID-19 pandemic: Digital eye strain among … 426. Pedestrian Detection: Unification of Global and Local Features 427. A comprehensive analysis of weakly-supervised semantic segmentation in different image domains 428. Deep learning assisted vision inspection of resistance spot welds 429. Identifying centres of interest in paintings using alignment and edge detection: Case studies on works by Luc Tuymans 430. Going deeper with image transformers 431. Spike-thrift: Towards energy-efficient deep spiking neural networks by limiting spiking activity via attention-guided compression 432. Adversarial feature distribution alignment for semi-supervised learning 433. G2d: Generate to detect anomaly 434. Anomaly Detection in Crowded Scenes Using Motion Influence Map and Convolutional Autoencoder 435. A study on attention-based LSTM for abnormal behavior recognition with variable pooling 436. Deep convolutional neural network based autonomous drone navigation 437. A survey on contrastive self-supervised learning 438. Independent Learning of Motion Parameters for Deep Visual Odometry 439. Image segmentation using deep learning: A survey 440. Using Interaction Protocols to Control Vision Systems 441. TGCN: Time Domain Graph Convolutional Network for Multiple Objects Tracking 442. A Survey on Crowd Counting Methods and Datasets 443. RGSR: A two-step lossy JPG image super-resolution based on noise reduction 444. Image processing effects on the deep face recognition system [J] 445. A weakly supervised consistency-based learning method for covid-19 segmentation in ct images 446. Improving Few-Shot Learning using Composite Rotation based Auxiliary Task 447. Investigating large-scale graphs for community detection 448. Estimating Galactic Distances From Images Using Self-supervised Representation Learning 449. Pig Breed Detection Using Faster R-CNN 450. A Vision-Based System For Non-Intrusive Posture Correction Notifications 451. Inception recurrent convolutional neural network for object recognition 452. A Meta-Q-Learning Approach to Discriminative Correlation Filter based Visual Tracking 453. SWD: Low-Compute Real-Time Object Detection Architecture 454. An empirical study of multi-scale object detection in high resolution UAV images 455. Vision Guided Robots. Calibration and Motion Correction 456. Deep-emotion: Facial expression recognition using attentional convolutional network 457. Single-Object Tracking Algorithm Based on Two-Step Spatiotemporal Deep Feature Fusion in a Complex Surveillance Scenario 458. A Focus-Measurement Based 3D Surface Reconstruction System for Dimensional Metrology 459. Image Pre-Processing Method of Machine Learning for Edge Detection with Image Signal Processor Enhancement 460. Distress Recognition in Unpaved Roads Using Unmanned Aerial Systems and Deep Learning Segmentation 461. Noise density range sensitive mean-median filter for impulse noise removal 462. A Novel Fusion of Deep Learning and Android Application for Real-Time Mango Fruits Disease Detection 463. Deep Gaussian Denoiser Epistemic Uncertainty and Decoupled Dual-Attention Fusion 464. Patient Emotion Recognition in Human Computer Interaction System Based on Machine Learning Method and Interactive Design Theory 465. Real-Time Hair Segmentation Using Mobile-Unet. Electronics 2021, 10, 99 466. Diagonal-kernel convolutional neural networks for image classification 467. Dense-Resolution Network for Point Cloud Classification and Segmentation 468. U2-ONet: A Two-Level Nested Octave U-Structure Network with a Multi-Scale Attention Mechanism for Moving Object Segmentation 469. Anonymous Person Tracking Across Multiple Camera Using Color Histogram and Body Pose Estimation 470. On-line three-dimensional coordinate measurement of dynamic binocular stereo vision based on rotating camera in large FOV 471. Image Compression and Reconstruction Using Encoder–Decoder Convolutional Neural Network 472. Extracting Effective Image Attributes with Refined Universal Detection 473. Self-supervised training for blind multi-frame video denoising 474. On the Tightness of Semidefinite Relaxations for Rotation Estimation 475. Poly Scale Space Technique for Feature Extraction in Lip Reading: A New Strategy 476. Influence of phosphate concentration on amine, amide, and hydroxyl CEST contrast 477. Controlling biases and diversity in diverse image-to-image translation 478. A New Feature Fusion Network for Student Behavior Recognition in Education 479. Localizing License Plates in Real Time with RetinaNet Object Detector 480. Application of a Vision-Based Single Target on Robot Positioning System 481. A predictive machine learning application in agriculture: Cassava disease detection and classification with imbalanced dataset using convolutional neural … 482. Threat Detection in Social Media Images Using the Inception-v3 Model 483. Augmenting Crop Detection for Precision Agriculture with Deep Visual Transfer Learning—A Case Study of Bale Detection 484. Evolving Smooth Manifolds of Arbitrary Codimen-sion in Rn 485. A Unified Learning Approach for Hand Gesture Recognition and Fingertip Detection 486. CNN-Based RGB-D Salient Object Detection: Learn, Select, and Fuse 487. A multi-platform comparison of local feature description methods 488. Smart Vehicle Tracker for Parking System 489. Knowledge distillation for incremental learning in semantic segmentation 490. MSLp: Deep Superresolution for Meteorological Satellite Image 491. Improving Object Detection Quality by Incorporating Global Contexts via Self-Attention 492. Self-Supervised Pretraining of 3D Features on any Point-Cloud 493. Beyond covariance: Sice and kernel based visual feature representation 494. Attention-based VGG-16 model for COVID-19 chest X-ray image classification 495. High-performance large-scale image recognition without normalization 496. Learning data augmentation with online bilevel optimization for image classification 497. DEEP LEARNING IN LANDCOVER CLASSIFICATION 498. Cobot User Frame Calibration: Evaluation and Comparison between Positioning Repeatability Performances Achieved by Traditional and Vision-Based Methods 499. A Survey of Image Enhancement and Object Detection Methods 500. Multi-object Tracking with a Hierarchical Single-branch Network 501. A Hybrid Approach Based on Lp1 Norm-Based Filters and Normalized Cut Segmentation for Salient Object Detection 502. Skin Lesion Classification Using Deep Learning 503. S-VVAD: Visual Voice Activity Detection by Motion Segmentation 504. Spatio-temporal attention on manifold space for 3D human action recognition 505. Mixup Without Hesitation 506. Deep learning for real-time semantic segmentation: Application in ultrasound imaging 507. Self-supervised monocular depth estimation with direct methods 508. Small object detection using context and attention 509. Resolution invariant person reid based on feature transformation and self-weighted attention 510. Deep learning enables accurate diagnosis of novel coronavirus (COVID-19) with CT images 511. Steel bridge corrosion inspection with combined vision and thermographic images 512. Instance Segmentation for Direct Measurements of Satellites in Metal Powders and Automated Microstructural Characterization from Image Data 513. Minimal solution for estimating fundamental matrix under planar motion 514. Component-level Script Classification Benchmark with CNN on AUTNT Dataset 515. Jaa-net: Joint facial action unit detection and face alignment via adaptive attention 516. Machine Learning Techniques for Predicting Crop Production in India 517. Introduction to Natural Language Processing 518. Structured Scene Memory for Vision-Language Navigation 519. Comparison-Based Study to Predict Breast Cancer: A Survey 520. Aadhaar-Based Authentication and Authorization Scheme for Remote Healthcare Monitoring 521. Nonlinear Approximation and (Deep) ReLU Networks 522. A Workflow Allocation Strategy Under Precedence Constraints for IaaS Cloud Environment 523. Person Identification Using Histogram of Gradient and Support Vector Machine on GEI 524. Adaptive streaming of 360-degree videos with reinforcement learning 525. Video Tagging and Recommender System Using Deep Learning 526. Scale variance minimization for unsupervised domain adaptation in image segmentation 527. Convolutional Elman Jordan Neural Network for Reconstruction and Classification Using Attention Window 528. Channel Capacity in Psychovisual Deep-Nets: Gaussianization Versus Kozachenko-Leonenko 529. Assessing the Viability of Visual Vibrometry for Use in Structural Engineering 530. A Deep Learning-Based Hotel Image Classifier for Online Travel Agencies 531. UVCE-IIITT@ DravidianLangTech-EACL2021: Tamil Troll Meme Classification: You need to Pay more Attention 532. Through-Wall Human Pose Reconstruction via UWB MIMO Radar and 3D CNN 533. Novel Assessments of Technical and Nontechnical Cardiac Surgery Quality: Protocol for a Mixed Methods Study 534. Biologically inspired visual computing: the state of the art 535. A Robust Surf-Based Online Human Tracking Algorithm Using Adaptive Object Model 536. Attention based pruning for shift networks 537. Rectifying pseudo label learning via uncertainty estimation for domain adaptive semantic segmentation 538. Hexacopter design for carrying payload for warehouse applications 539. Implementation of Recommender System Using Neural Networks and Deep Learning 540. A multi-scale and multi-level feature aggregation network for crowd counting 541. Foodborne Disease Outbreak Prediction Using Deep Learning 542. LRA-Net: local region attention network for 3D point cloud completion 543. Partial Domain Adaptation Using Selective Representation Learning For Class-Weight Computation 544. Estimating Body Pose from A Single Image 545. Hybrid Feature Selection Method for Predicting the Kidney Disease Membranous Nephropathy 546. Prognosis of Breast Cancer by Implementing Machine Learning Algorithms Using Modified Bootstrap Aggregating 547. Robust local binary descriptor in rotation change using polar location 548. A Multimodal Biometric System Based on Finger Knuckle Print, Fingerprint, and Palmprint Traits 549. Efficientps: Efficient panoptic segmentation 550. ShadingNet: image intrinsics by fine-grained shading decomposition 551. Perception of Plant Diseases in Color Images Through Adaboost 552. Data Mining in Cloud Computing: Survey 553. Artificial Neural Network Analysis for Predicting Spatial Patterns of Urbanization in India 554. Navier–Stokes-Based Image Inpainting for Restoration of Missing Data Due to Clouds 555. An Attribute-Based Break-Glass Access Control Framework for Medical Emergencies 556. Detection of Life Threatening ECG Arrhythmias Using Morphological Patterns and Wavelet Transform Method 557. Improvement of Identity Recognition with Occlusion Detection-Based Feature Selection 558. Generative Video Compression as Hierarchical Variational Inference 559. SLiKER: Sparse loss induced kernel ensemble regression 560. Is Space-Time Attention All You Need for Video Understanding? 561. Cityguide: A seamless indoor-outdoor wayfinding system for people with vision impairments 562. An Efficient Multimodal Biometric System Integrated with Liveness Detection Technique 563. Microscopic brain tumor detection and classification using 3D CNN and feature selection architecture 564. A Survey of Brain-Inspired Intelligent Robots: Integration of Vision, Decision, Motion Control, and Musculoskeletal Systems 565. Scale selection 566. Automated detection and classification of spilled loads on freeways based on improved YOLO network 567. Information Granules and Granular Computing 568. DAN : Breast Cancer Classification from High-Resolution Histology Images Using Deep Attention Network 569. Image Segmentation of MR Images with Multi-directional Region Growing Algorithm 570. Satellite Radar Interferometry for DEM Generation Using Sentinel-1A Imagery 571. On the Effect of Training Convolution Neural Network for Millimeter-Wave Radar-Based Hand Gesture Recognition 572. Synthetic Vision for Virtual Character Guidance 573. Evaluation of AOMDV Routing Protocol for Optimum Transmitted Power in a Designed Ad-hoc Wireless Sensor Network 574. Evaluation metrics for conditional image generation 575. Image Inpainting Using Double Discriminator Generative Adversarial Networks 576. Crowd counting method based on the self-attention residual network 577. Cyber Espionage—An Ethical Analysis 578. Analysis of Gait and Face Biometric Traits from CCTV Streams for Forensics 579. A facial expression recognition model using hybrid feature selection and support vector machines 580. Predicting the Big-Five Personality Traits from Handwriting 581. Image engineering 582. Learning efficient text-to-image synthesis via interstage cross-sample similarity distillation 583. Faster and Secured Web Services Communication Using Modified IDEA and Custom-Level Security 584. Improved Image Deblurring Using GANs 585. Hand-Based Person Identification using Global and Part-Aware Deep Feature Representation Learning 586. Identification for Recycling Polyethylene Terephthalate (PET) Plastic Bottles by Polarization Vision 587. Holistic filter pruning for efficient deep neural networks 588. Vision-based vehicle speed estimation: A survey 589. Learning Temporal Dynamics from Cycles in Narrated Video 590. Analysis of MRI Image Compression Using Compressive Sensing 591. Analysis of PQ Disturbances in Renewable Grid Integration System Using Non-parametric Spectral Estimation Approach 592. A Porcine Abdomen Cutting Robot System Using Binocular Vision Techniques Based on Kernel Principal Component Analysis 593. Arc Length method for extracting crack pattern characteristics 594. AF-EMS Detector: Improve the Multi-Scale Detection Performance of the Anchor-Free Detector 595. A Transfer Learning Approach for Drowsiness Detection from EEG Signals 596. Deep audio-visual learning: A survey 597. MAFNet: Multi-style attention fusion network for salient object detection 598. HAVANA: Hierarchical and Variation-Normalized Autoencoder for Person Re-identification 599. An Empirical Comparison of Generative Adversarial Network (GAN) Measures 600. An enhanced 3DCNN-ConvLSTM for spatiotemporal multimedia data analysis
More Research Topics on Image processing
- Image Based Rendering Research Topics
- Hyperspectral image analysis Research Topics
- Medical Imaging Research Topics
- Computer vision Research Topics
Related Posts:
- Research Questions - Deep learning-enabled medical computer vision
- Research Questions - Deep learning, computer vision, and entomology
- Research questions - Duplicate detection of images using computer vision techniques
- What is PAO 11/82 of vision?
- How to Write a Vision Statement?
- List of Journals on Digital image Processing, Usability and Vision
Javascript is disabled in your browser. Please enable it for full functionality and experience.
- Direkt zur Navigation springen
- Direkt zur Suche springen
- Direkt zum Inhalt springen
- Fraunhofer HHI
- Departments
- Start page >
- Departments >
- Vision and Imaging Technologies >
- Research Groups >
- Computer Vision & Graphics >
- CVG Research Overview
- AI-Based Building Digitalization
- Portrait Relighting
- Neural Speech-Driven Face Animation
- Video-driven Facial Animation
- Publications
- Student Opportunities
- IMC Research Overview
- Research Topics
- Pose and gesture analysis
- Behaviour analysis for human-computer interaction
- Contact-free Human-Computer Interaction
- Image Quality Estimation
- Subjective Tests
- Birgit Nierula
Research Topics of the Computer Vision & Graphics Group
Seeing, modelling and animating humans.
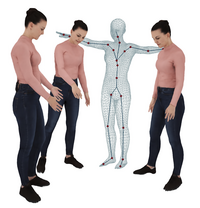
Realistic human modelling is a challenging task in Computer Vision and Graphics. We investigate new methods for capturing and analyzing human bodies and faces in images and videos as well as new compact models for the representation of facial expressions as well as human bodies and their motion. We combine model-based and image-and video based representations with generative AI models as well as neural rendering.
Read more about current research projects in this field.
Scenes, Structure and Motion
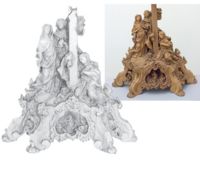
We have a long tradition in 3D scene analysis and continuously perform innovative research in 3D capturing as well as 3D reconstruction, ranging from highly detailed stereo as well as multi-view images of static objects and scenes, addressing even complex surface and shape properties, over monocular shape-from-X methods, to analyzing deforming objects in monocular video.
Computational Imaging and Video
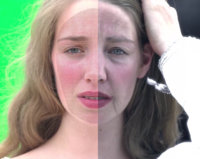
We perform innovative research in the field of video processing and computational video opening up new opportunities for how dynamic scenes can be analyzed and video footage can be represented, edited and seamlessly augmented with new content.
Learning and Inference
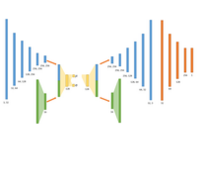
Our research combines computer vision, computer graphics, and machine learning to understand images and video data. In our research, we focus on the combination of deep learning with strong models or physical constraints in order to combine the advantages of model-based and data-driven methods.
Augmented and Mixed Reality
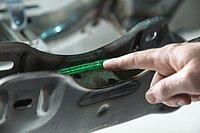
Our experience in tracking dynamic scenes and objects as well as photorealistic rendering enables new augmented reality solutions where virtual content is seamlessly blended into real video footage with applications e.g. multi-media, industry or medicine.
Previous Research Projects

We have performed various research projects in the above fields over the years.
Read more about older research projects here.
- Zur Metanavigation
- Zur Hauptnavigation
- Zur Subnavigation
- Zum Seitenfuss
Photo: © NYU Depth Dataset V2/Nathan Silberman/Ge Gao
If you are a student at UHH interested in a thesis with our group, you can contact Dr. Christian Wilms (B.Sc. theses) or Dr. Ehsan Yaghoubi (M.Sc. theses). Below is a list with selected titles of B.Sc. and M.Sc. theses completed in our group to orient you towards potential topics. You can also see our recent papers for possible directions for a thesis.
Selection of titles of complete Master theses: - A Deep Learning Approach for Top-down Attention with Attribute Preference - Salient object detection with AttentionMask - 3D Segmentation in the Context of Inscriptions - Active Visual Object Search Using Reinforcement Learning - Saliency-Guided Sign Language Recognition - Object Discovery in 3D Scenes via Shape Analysis using Adapted PCLV - Learning Efficient Deep Feature Representations for Indoor Visual Positioning
Selection of titles of completed Bachelor theses: - Weakly Supervised Object Detection in RoboCup Scenarios - Lokalisierung von Flugzeug-Leitwerken - IoU Predictions for Segmentation Mask Propsals - Klassifikation von malignen Melanomen mittels konditionierten ConvNets - Segmentierung von Schienenbildern zur automatisierten Wartung - Bildklassifikation von Flugzeugtypen - Segmentation of numerical weather prediction data for characterization of atmospheric airmasses - Object Detection in Remote Sensing Image Data Using AttentionMask - TileAttention: Entdeckung sehr kleiner Objekte - Superpixel Pooling for Instance Segmentation
Necessary requirements: You should have some pre-knowledge in computer vision before starting a thesis. For a B.Sc. thesis you should have at least attended the lecture "Einführung in die Bildverarbeitung", the lab course "Praktikum Computer Vision", or have equivalent knowledge. For a M.Sc. thesis, you should have attended the lectures "Computer Vision 1" and "Computer Vision 2", and ideally also the master project, or have equivalent knowledge. Pre-knowledge in machine learning is also helpful.
Computer Science Thesis Topics
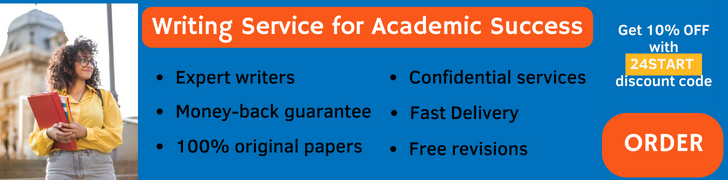
This page provides a comprehensive list of computer science thesis topics , carefully curated to support students in identifying and selecting innovative and relevant areas for their academic research. Whether you are at the beginning of your research journey or are seeking a specific area to explore further, this guide aims to serve as an essential resource. With an expansive array of topics spread across various sub-disciplines of computer science, this list is designed to meet a diverse range of interests and academic needs. From the complexities of artificial intelligence to the intricate designs of web development, each category is equipped with 40 specific topics, offering a breadth of possibilities to inspire your next big thesis project. Explore our guide to find not only a topic that resonates with your academic ambitions but also one that has the potential to contribute significantly to the field of computer science.
1000 Computer Science Thesis Topics and Ideas

Academic Writing, Editing, Proofreading, And Problem Solving Services
Get 10% off with 24start discount code, browse computer science thesis topics:, artificial intelligence thesis topics, augmented reality thesis topics, big data analytics thesis topics, bioinformatics thesis topics, blockchain technology thesis topics, cloud computing thesis topics, computer engineering thesis topics, computer vision thesis topics, cybersecurity thesis topics, data science thesis topics, digital transformation thesis topics, distributed systems and networks thesis topics, geographic information systems (gis) thesis topics, human-computer interaction (hci) thesis topics, image processing thesis topics, information system thesis topics, information technology thesis topics.
- Internet Of Things (IoT) Thesis Topics
Machine Learning Thesis Topics
Neural networks thesis topics, programming thesis topics, quantum computing thesis topics, robotics thesis topics, software engineering thesis topics, web development thesis topics.
- Ethical Implications of AI in Decision-Making Processes
- The Role of AI in Personalized Medicine: Opportunities and Challenges
- Advances in AI-Driven Predictive Analytics in Retail
- AI in Autonomous Vehicles: Safety, Regulation, and Technology Integration
- Natural Language Processing: Improving Human-Machine Interaction
- The Future of AI in Cybersecurity: Threats and Defenses
- Machine Learning Algorithms for Real-Time Data Processing
- AI and the Internet of Things: Transforming Smart Home Technology
- The Impact of Deep Learning on Image Recognition Technologies
- Reinforcement Learning: Applications in Robotics and Automation
- AI in Finance: Algorithmic Trading and Risk Assessment
- Bias and Fairness in AI: Addressing Socio-Technical Challenges
- The Evolution of AI in Education: Customized Learning Experiences
- AI for Environmental Conservation: Tracking and Predictive Analysis
- The Role of Artificial Neural Networks in Weather Forecasting
- AI in Agriculture: Predictive Analytics for Crop and Soil Management
- Emotional Recognition AI: Implications for Mental Health Assessments
- AI in Space Exploration: Autonomous Rovers and Mission Planning
- Enhancing User Experience with AI in Video Games
- AI-Powered Virtual Assistants: Trends, Effectiveness, and User Trust
- The Integration of AI in Traditional Industries: Case Studies
- Generative AI Models in Art and Creativity
- AI in LegalTech: Document Analysis and Litigation Prediction
- Healthcare Diagnostics: AI Applications in Radiology and Pathology
- AI and Blockchain: Enhancing Security in Decentralized Systems
- Ethics of AI in Surveillance: Privacy vs. Security
- AI in E-commerce: Personalization Engines and Customer Behavior Analysis
- The Future of AI in Telecommunications: Network Optimization and Service Delivery
- AI in Manufacturing: Predictive Maintenance and Quality Control
- Challenges of AI in Elderly Care: Ethical Considerations and Technological Solutions
- The Role of AI in Public Safety and Emergency Response
- AI for Content Creation: Impact on Media and Journalism
- AI-Driven Algorithms for Efficient Energy Management
- The Role of AI in Cultural Heritage Preservation
- AI and the Future of Public Transport: Optimization and Management
- Enhancing Sports Performance with AI-Based Analytics
- AI in Human Resources: Automating Recruitment and Employee Management
- Real-Time Translation AI: Breaking Language Barriers
- AI in Mental Health: Tools for Monitoring and Therapy Assistance
- The Future of AI Governance: Regulation and Standardization
- AR in Medical Training and Surgery Simulation
- The Impact of Augmented Reality in Retail: Enhancing Consumer Experience
- Augmented Reality for Enhanced Navigation Systems
- AR Applications in Maintenance and Repair in Industrial Settings
- The Role of AR in Enhancing Online Education
- Augmented Reality in Cultural Heritage: Interactive Visitor Experiences
- Developing AR Tools for Improved Sports Coaching and Training
- Privacy and Security Challenges in Augmented Reality Applications
- The Future of AR in Advertising: Engagement and Measurement
- User Interface Design for AR: Principles and Best Practices
- AR in Automotive Industry: Enhancing Driving Experience and Safety
- Augmented Reality for Emergency Response Training
- AR and IoT: Converging Technologies for Smart Environments
- Enhancing Physical Rehabilitation with AR Applications
- The Role of AR in Enhancing Public Safety and Awareness
- Augmented Reality in Fashion: Virtual Fitting and Personalized Shopping
- AR for Environmental Education: Interactive and Immersive Learning
- The Use of AR in Building and Architecture Planning
- AR in the Entertainment Industry: Games and Live Events
- Implementing AR in Museums and Art Galleries for Interactive Learning
- Augmented Reality for Real Estate: Virtual Tours and Property Visualization
- AR in Consumer Electronics: Integration in Smart Devices
- The Development of AR Applications for Children’s Education
- AR for Enhancing User Engagement in Social Media Platforms
- The Application of AR in Field Service Management
- Augmented Reality for Disaster Management and Risk Assessment
- Challenges of Content Creation for Augmented Reality
- Future Trends in AR Hardware: Wearables and Beyond
- Legal and Ethical Considerations of Augmented Reality Technology
- AR in Space Exploration: Tools for Simulation and Training
- Interactive Shopping Experiences with AR: The Future of Retail
- AR in Wildlife Conservation: Educational Tools and Awareness
- The Impact of AR on the Publishing Industry: Interactive Books and Magazines
- Augmented Reality and Its Role in Automotive Manufacturing
- AR for Job Training: Bridging the Skill Gap in Various Industries
- The Role of AR in Therapy: New Frontiers in Mental Health Treatment
- The Future of Augmented Reality in Sports Broadcasting
- AR as a Tool for Enhancing Public Art Installations
- Augmented Reality in the Tourism Industry: Personalized Travel Experiences
- The Use of AR in Security Training: Realistic and Safe Simulations
- The Role of Big Data in Improving Healthcare Outcomes
- Big Data and Its Impact on Consumer Behavior Analysis
- Privacy Concerns in Big Data: Ethical and Legal Implications
- The Application of Big Data in Predictive Maintenance for Manufacturing
- Real-Time Big Data Processing: Tools and Techniques
- Big Data in Financial Services: Fraud Detection and Risk Management
- The Evolution of Big Data Technologies: From Hadoop to Spark
- Big Data Visualization: Techniques for Effective Communication of Insights
- The Integration of Big Data and Artificial Intelligence
- Big Data in Smart Cities: Applications in Traffic Management and Energy Use
- Enhancing Supply Chain Efficiency with Big Data Analytics
- Big Data in Sports Analytics: Improving Team Performance and Fan Engagement
- The Role of Big Data in Environmental Monitoring and Sustainability
- Big Data and Social Media: Analyzing Sentiments and Trends
- Scalability Challenges in Big Data Systems
- The Future of Big Data in Retail: Personalization and Customer Experience
- Big Data in Education: Customized Learning Paths and Student Performance Analysis
- Privacy-Preserving Techniques in Big Data
- Big Data in Public Health: Epidemiology and Disease Surveillance
- The Impact of Big Data on Insurance: Tailored Policies and Pricing
- Edge Computing in Big Data: Processing at the Source
- Big Data and the Internet of Things: Generating Insights from IoT Data
- Cloud-Based Big Data Analytics: Opportunities and Challenges
- Big Data Governance: Policies, Standards, and Management
- The Role of Big Data in Crisis Management and Response
- Machine Learning with Big Data: Building Predictive Models
- Big Data in Agriculture: Precision Farming and Yield Optimization
- The Ethics of Big Data in Research: Consent and Anonymity
- Cross-Domain Big Data Integration: Challenges and Solutions
- Big Data and Cybersecurity: Threat Detection and Prevention Strategies
- Real-Time Streaming Analytics in Big Data
- Big Data in the Media Industry: Content Optimization and Viewer Insights
- The Impact of GDPR on Big Data Practices
- Quantum Computing and Big Data: Future Prospects
- Big Data in E-Commerce: Optimizing Logistics and Inventory Management
- Big Data Talent: Education and Skill Development for Data Scientists
- The Role of Big Data in Political Campaigns and Voting Behavior Analysis
- Big Data and Mental Health: Analyzing Patterns for Better Interventions
- Big Data in Genomics and Personalized Medicine
- The Future of Big Data in Autonomous Driving Technologies
- The Role of Bioinformatics in Personalized Medicine
- Next-Generation Sequencing Data Analysis: Challenges and Opportunities
- Bioinformatics and the Study of Genetic Diseases
- Computational Models for Understanding Protein Structure and Function
- Bioinformatics in Drug Discovery and Development
- The Impact of Big Data on Bioinformatics: Data Management and Analysis
- Machine Learning Applications in Bioinformatics
- Bioinformatics Approaches for Cancer Genomics
- The Development of Bioinformatics Tools for Metagenomics Analysis
- Ethical Considerations in Bioinformatics: Data Sharing and Privacy
- The Role of Bioinformatics in Agricultural Biotechnology
- Bioinformatics and Viral Evolution: Tracking Pathogens and Outbreaks
- The Integration of Bioinformatics and Systems Biology
- Bioinformatics in Neuroscience: Mapping the Brain
- The Future of Bioinformatics in Non-Invasive Prenatal Testing
- Bioinformatics and the Human Microbiome: Health Implications
- The Application of Artificial Intelligence in Bioinformatics
- Structural Bioinformatics: Computational Techniques for Molecular Modeling
- Comparative Genomics: Insights into Evolution and Function
- Bioinformatics in Immunology: Vaccine Design and Immune Response Analysis
- High-Performance Computing in Bioinformatics
- The Challenge of Proteomics in Bioinformatics
- RNA-Seq Data Analysis and Interpretation
- Cloud Computing Solutions for Bioinformatics Data
- Computational Epigenetics: DNA Methylation and Histone Modification Analysis
- Bioinformatics in Ecology: Biodiversity and Conservation Genetics
- The Role of Bioinformatics in Forensic Analysis
- Mobile Apps and Tools for Bioinformatics Research
- Bioinformatics and Public Health: Epidemiological Studies
- The Use of Bioinformatics in Clinical Diagnostics
- Genetic Algorithms in Bioinformatics
- Bioinformatics for Aging Research: Understanding the Mechanisms of Aging
- Data Visualization Techniques in Bioinformatics
- Bioinformatics and the Development of Therapeutic Antibodies
- The Role of Bioinformatics in Stem Cell Research
- Bioinformatics and Cardiovascular Diseases: Genomic Insights
- The Impact of Machine Learning on Functional Genomics in Bioinformatics
- Bioinformatics in Dental Research: Genetic Links to Oral Diseases
- The Future of CRISPR Technology and Bioinformatics
- Bioinformatics and Nutrition: Genomic Insights into Diet and Health
- Blockchain for Enhancing Cybersecurity in Various Industries
- The Impact of Blockchain on Supply Chain Transparency
- Blockchain in Healthcare: Patient Data Management and Security
- The Application of Blockchain in Voting Systems
- Blockchain and Smart Contracts: Legal Implications and Applications
- Cryptocurrencies: Market Trends and the Future of Digital Finance
- Blockchain in Real Estate: Improving Property and Land Registration
- The Role of Blockchain in Managing Digital Identities
- Blockchain for Intellectual Property Management
- Energy Sector Innovations: Blockchain for Renewable Energy Distribution
- Blockchain and the Future of Public Sector Operations
- The Impact of Blockchain on Cross-Border Payments
- Blockchain for Non-Fungible Tokens (NFTs): Applications in Art and Media
- Privacy Issues in Blockchain Applications
- Blockchain in the Automotive Industry: Supply Chain and Beyond
- Decentralized Finance (DeFi): Opportunities and Challenges
- The Role of Blockchain in Combating Counterfeiting and Fraud
- Blockchain for Sustainable Environmental Practices
- The Integration of Artificial Intelligence with Blockchain
- Blockchain Education: Curriculum Development and Training Needs
- Blockchain in the Music Industry: Rights Management and Revenue Distribution
- The Challenges of Blockchain Scalability and Performance Optimization
- The Future of Blockchain in the Telecommunications Industry
- Blockchain and Consumer Data Privacy: A New Paradigm
- Blockchain for Disaster Recovery and Business Continuity
- Blockchain in the Charity and Non-Profit Sectors
- Quantum Resistance in Blockchain: Preparing for the Quantum Era
- Blockchain and Its Impact on Traditional Banking and Financial Institutions
- Legal and Regulatory Challenges Facing Blockchain Technology
- Blockchain for Improved Logistics and Freight Management
- The Role of Blockchain in the Evolution of the Internet of Things (IoT)
- Blockchain and the Future of Gaming: Transparency and Fair Play
- Blockchain for Academic Credentials Verification
- The Application of Blockchain in the Insurance Industry
- Blockchain and the Future of Content Creation and Distribution
- Blockchain for Enhancing Data Integrity in Scientific Research
- The Impact of Blockchain on Human Resources: Employee Verification and Salary Payments
- Blockchain and the Future of Retail: Customer Loyalty Programs and Inventory Management
- Blockchain and Industrial Automation: Trust and Efficiency
- Blockchain for Digital Marketing: Transparency and Consumer Engagement
- Multi-Cloud Strategies: Optimization and Security Challenges
- Advances in Cloud Computing Architectures for Scalable Applications
- Edge Computing: Extending the Reach of Cloud Services
- Cloud Security: Novel Approaches to Data Encryption and Threat Mitigation
- The Impact of Serverless Computing on Software Development Lifecycle
- Cloud Computing and Sustainability: Energy-Efficient Data Centers
- Cloud Service Models: Comparative Analysis of IaaS, PaaS, and SaaS
- Cloud Migration Strategies: Best Practices and Common Pitfalls
- The Role of Cloud Computing in Big Data Analytics
- Implementing AI and Machine Learning Workloads on Cloud Platforms
- Hybrid Cloud Environments: Management Tools and Techniques
- Cloud Computing in Healthcare: Compliance, Security, and Use Cases
- Cost-Effective Cloud Solutions for Small and Medium Enterprises (SMEs)
- The Evolution of Cloud Storage Solutions: Trends and Technologies
- Cloud-Based Disaster Recovery Solutions: Design and Reliability
- Blockchain in Cloud Services: Enhancing Transparency and Trust
- Cloud Networking: Managing Connectivity and Traffic in Cloud Environments
- Cloud Governance: Managing Compliance and Operational Risks
- The Future of Cloud Computing: Quantum Computing Integration
- Performance Benchmarking of Cloud Services Across Different Providers
- Privacy Preservation in Cloud Environments
- Cloud Computing in Education: Virtual Classrooms and Learning Management Systems
- Automation in Cloud Deployments: Tools and Strategies
- Cloud Auditing and Monitoring Techniques
- Mobile Cloud Computing: Challenges and Future Trends
- The Role of Cloud Computing in Digital Media Production and Distribution
- Security Risks in Multi-Tenancy Cloud Environments
- Cloud Computing for Scientific Research: Enabling Complex Simulations
- The Impact of 5G on Cloud Computing Services
- Federated Clouds: Building Collaborative Cloud Environments
- Managing Software Dependencies in Cloud Applications
- The Economics of Cloud Computing: Cost Models and Pricing Strategies
- Cloud Computing in Government: Security Protocols and Citizen Services
- Cloud Access Security Brokers (CASBs): Security Enforcement Points
- DevOps in the Cloud: Strategies for Continuous Integration and Deployment
- Predictive Analytics in Cloud Computing
- The Role of Cloud Computing in IoT Deployment
- Implementing Robust Cybersecurity Measures in Cloud Architecture
- Cloud Computing in the Financial Sector: Handling Sensitive Data
- Future Trends in Cloud Computing: The Role of AI in Cloud Optimization
- Advances in Microprocessor Design and Architecture
- FPGA-Based Design: Innovations and Applications
- The Role of Embedded Systems in Consumer Electronics
- Quantum Computing: Hardware Development and Challenges
- High-Performance Computing (HPC) and Parallel Processing
- Design and Analysis of Computer Networks
- Cyber-Physical Systems: Design, Analysis, and Security
- The Impact of Nanotechnology on Computer Hardware
- Wireless Sensor Networks: Design and Optimization
- Cryptographic Hardware: Implementations and Security Evaluations
- Machine Learning Techniques for Hardware Optimization
- Hardware for Artificial Intelligence: GPUs vs. TPUs
- Energy-Efficient Hardware Designs for Sustainable Computing
- Security Aspects of Mobile and Ubiquitous Computing
- Advanced Algorithms for Computer-Aided Design (CAD) of VLSI
- Signal Processing in Communication Systems
- The Development of Wearable Computing Devices
- Computer Hardware Testing: Techniques and Tools
- The Role of Hardware in Network Security
- The Evolution of Interface Designs in Consumer Electronics
- Biometric Systems: Hardware and Software Integration
- The Integration of IoT Devices in Smart Environments
- Electronic Design Automation (EDA) Tools and Methodologies
- Robotics: Hardware Design and Control Systems
- Hardware Accelerators for Deep Learning Applications
- Developments in Non-Volatile Memory Technologies
- The Future of Computer Hardware in the Era of Quantum Computing
- Hardware Solutions for Data Storage and Retrieval
- Power Management Techniques in Embedded Systems
- Challenges in Designing Multi-Core Processors
- System on Chip (SoC) Design Trends and Challenges
- The Role of Computer Engineering in Aerospace Technology
- Real-Time Systems: Design and Implementation Challenges
- Hardware Support for Virtualization Technology
- Advances in Computer Graphics Hardware
- The Impact of 5G Technology on Mobile Computing Hardware
- Environmental Impact Assessment of Computer Hardware Production
- Security Vulnerabilities in Modern Microprocessors
- Computer Hardware Innovations in the Automotive Industry
- The Role of Computer Engineering in Medical Device Technology
- Deep Learning Approaches to Object Recognition
- Real-Time Image Processing for Autonomous Vehicles
- Computer Vision in Robotic Surgery: Techniques and Challenges
- Facial Recognition Technology: Innovations and Privacy Concerns
- Machine Vision in Industrial Automation and Quality Control
- 3D Reconstruction Techniques in Computer Vision
- Enhancing Sports Analytics with Computer Vision
- Augmented Reality: Integrating Computer Vision for Immersive Experiences
- Computer Vision for Environmental Monitoring
- Thermal Imaging and Its Applications in Computer Vision
- Computer Vision in Retail: Customer Behavior and Store Layout Optimization
- Motion Detection and Tracking in Security Systems
- The Role of Computer Vision in Content Moderation on Social Media
- Gesture Recognition: Methods and Applications
- Computer Vision in Agriculture: Pest Detection and Crop Analysis
- Advances in Medical Imaging: Machine Learning and Computer Vision
- Scene Understanding and Contextual Inference in Images
- The Development of Vision-Based Autonomous Drones
- Optical Character Recognition (OCR): Latest Techniques and Applications
- The Impact of Computer Vision on Virtual Reality Experiences
- Biometrics: Enhancing Security Systems with Computer Vision
- Computer Vision for Wildlife Conservation: Species Recognition and Behavior Analysis
- Underwater Image Processing: Challenges and Techniques
- Video Surveillance: The Evolution of Algorithmic Approaches
- Advanced Driver-Assistance Systems (ADAS): Leveraging Computer Vision
- Computational Photography: Enhancing Image Capture Techniques
- The Integration of AI in Computer Vision: Ethical and Technical Considerations
- Computer Vision in the Gaming Industry: From Design to Interaction
- The Future of Computer Vision in Smart Cities
- Pattern Recognition in Historical Document Analysis
- The Role of Computer Vision in the Manufacturing of Customized Products
- Enhancing Accessibility with Computer Vision: Tools for the Visually Impaired
- The Use of Computer Vision in Behavioral Research
- Predictive Analytics with Computer Vision in Sports
- Image Synthesis with Generative Adversarial Networks (GANs)
- The Use of Computer Vision in Remote Sensing
- Real-Time Video Analytics for Public Safety
- The Role of Computer Vision in Telemedicine
- Computer Vision and the Internet of Things (IoT): A Synergistic Approach
- Future Trends in Computer Vision: Quantum Computing and Beyond
- Advances in Cryptography: Post-Quantum Cryptosystems
- Artificial Intelligence in Cybersecurity: Threat Detection and Response
- Blockchain for Enhanced Security in Distributed Networks
- The Impact of IoT on Cybersecurity: Vulnerabilities and Solutions
- Cybersecurity in Cloud Computing: Best Practices and Tools
- Ethical Hacking: Techniques and Ethical Implications
- The Role of Human Factors in Cybersecurity Breaches
- Privacy-preserving Technologies in an Age of Surveillance
- The Evolution of Ransomware Attacks and Defense Strategies
- Secure Software Development: Integrating Security in DevOps (DevSecOps)
- Cybersecurity in Critical Infrastructure: Challenges and Innovations
- The Future of Biometric Security Systems
- Cyber Warfare: State-sponsored Attacks and Defense Mechanisms
- The Role of Cybersecurity in Protecting Digital Identities
- Social Engineering Attacks: Prevention and Countermeasures
- Mobile Security: Protecting Against Malware and Exploits
- Wireless Network Security: Protocols and Practices
- Data Breaches: Analysis, Consequences, and Mitigation
- The Ethics of Cybersecurity: Balancing Privacy and Security
- Regulatory Compliance and Cybersecurity: GDPR and Beyond
- The Impact of 5G Technology on Cybersecurity
- The Role of Machine Learning in Cyber Threat Intelligence
- Cybersecurity in Automotive Systems: Challenges in a Connected Environment
- The Use of Virtual Reality for Cybersecurity Training and Simulation
- Advanced Persistent Threats (APT): Detection and Response
- Cybersecurity for Smart Cities: Challenges and Solutions
- Deep Learning Applications in Malware Detection
- The Role of Cybersecurity in Healthcare: Protecting Patient Data
- Supply Chain Cybersecurity: Identifying Risks and Solutions
- Endpoint Security: Trends, Challenges, and Future Directions
- Forensic Techniques in Cybersecurity: Tracking and Analyzing Cyber Crimes
- The Influence of International Law on Cyber Operations
- Protecting Financial Institutions from Cyber Frauds and Attacks
- Quantum Computing and Its Implications for Cybersecurity
- Cybersecurity and Remote Work: Emerging Threats and Strategies
- IoT Security in Industrial Applications
- Cyber Insurance: Risk Assessment and Management
- Security Challenges in Edge Computing Environments
- Anomaly Detection in Network Security Using AI Techniques
- Securing the Software Supply Chain in Application Development
- Big Data Analytics: Techniques and Applications in Real-time
- Machine Learning Algorithms for Predictive Analytics
- Data Science in Healthcare: Improving Patient Outcomes with Predictive Models
- The Role of Data Science in Financial Market Predictions
- Natural Language Processing: Emerging Trends and Applications
- Data Visualization Tools and Techniques for Enhanced Business Intelligence
- Ethics in Data Science: Privacy, Fairness, and Transparency
- The Use of Data Science in Environmental Science for Sustainability Studies
- The Impact of Data Science on Social Media Marketing Strategies
- Data Mining Techniques for Detecting Patterns in Large Datasets
- AI and Data Science: Synergies and Future Prospects
- Reinforcement Learning: Applications and Challenges in Data Science
- The Role of Data Science in E-commerce Personalization
- Predictive Maintenance in Manufacturing Through Data Science
- The Evolution of Recommendation Systems in Streaming Services
- Real-time Data Processing with Stream Analytics
- Deep Learning for Image and Video Analysis
- Data Governance in Big Data Analytics
- Text Analytics and Sentiment Analysis for Customer Feedback
- Fraud Detection in Banking and Insurance Using Data Science
- The Integration of IoT Data in Data Science Models
- The Future of Data Science in Quantum Computing
- Data Science for Public Health: Epidemic Outbreak Prediction
- Sports Analytics: Performance Improvement and Injury Prevention
- Data Science in Retail: Inventory Management and Customer Journey Analysis
- Data Science in Smart Cities: Traffic and Urban Planning
- The Use of Blockchain in Data Security and Integrity
- Geospatial Analysis for Environmental Monitoring
- Time Series Analysis in Economic Forecasting
- Data Science in Education: Analyzing Trends and Student Performance
- Predictive Policing: Data Science in Law Enforcement
- Data Science in Agriculture: Yield Prediction and Soil Health
- Computational Social Science: Analyzing Societal Trends
- Data Science in Energy Sector: Consumption and Optimization
- Personalization Technologies in Healthcare Through Data Science
- The Role of Data Science in Content Creation and Media
- Anomaly Detection in Network Security Using Data Science Techniques
- The Future of Autonomous Vehicles: Data Science-Driven Innovations
- Multimodal Data Fusion Techniques in Data Science
- Scalability Challenges in Data Science Projects
- The Role of Digital Transformation in Business Model Innovation
- The Impact of Digital Technologies on Customer Experience
- Digital Transformation in the Banking Sector: Trends and Challenges
- The Use of AI and Robotics in Digital Transformation of Manufacturing
- Digital Transformation in Healthcare: Telemedicine and Beyond
- The Influence of Big Data on Decision-Making Processes in Corporations
- Blockchain as a Driver for Transparency in Digital Transformation
- The Role of IoT in Enhancing Operational Efficiency in Industries
- Digital Marketing Strategies: SEO, Content, and Social Media
- The Integration of Cyber-Physical Systems in Industrial Automation
- Digital Transformation in Education: Virtual Learning Environments
- Smart Cities: The Role of Digital Technologies in Urban Planning
- Digital Transformation in the Retail Sector: E-commerce Evolution
- The Future of Work: Impact of Digital Transformation on Workplaces
- Cybersecurity Challenges in a Digitally Transformed World
- Mobile Technologies and Their Impact on Digital Transformation
- The Role of Digital Twin Technology in Industry 4.0
- Digital Transformation in the Public Sector: E-Government Services
- Data Privacy and Security in the Age of Digital Transformation
- Digital Transformation in the Energy Sector: Smart Grids and Renewable Energy
- The Use of Augmented Reality in Training and Development
- The Role of Virtual Reality in Real Estate and Architecture
- Digital Transformation and Sustainability: Reducing Environmental Footprint
- The Role of Digital Transformation in Supply Chain Optimization
- Digital Transformation in Agriculture: IoT and Smart Farming
- The Impact of 5G on Digital Transformation Initiatives
- The Influence of Digital Transformation on Media and Entertainment
- Digital Transformation in Insurance: Telematics and Risk Assessment
- The Role of AI in Enhancing Customer Service Operations
- The Future of Digital Transformation: Trends and Predictions
- Digital Transformation and Corporate Governance
- The Role of Leadership in Driving Digital Transformation
- Digital Transformation in Non-Profit Organizations: Challenges and Benefits
- The Economic Implications of Digital Transformation
- The Cultural Impact of Digital Transformation on Organizations
- Digital Transformation in Transportation: Logistics and Fleet Management
- User Experience (UX) Design in Digital Transformation
- The Role of Digital Transformation in Crisis Management
- Digital Transformation and Human Resource Management
- Implementing Change Management in Digital Transformation Projects
- Scalability Challenges in Distributed Systems: Solutions and Strategies
- Blockchain Technology: Enhancing Security and Transparency in Distributed Networks
- The Role of Edge Computing in Distributed Systems
- Designing Fault-Tolerant Systems in Distributed Networks
- The Impact of 5G Technology on Distributed Network Architectures
- Machine Learning Algorithms for Network Traffic Analysis
- Load Balancing Techniques in Distributed Computing
- The Use of Distributed Ledger Technology Beyond Cryptocurrencies
- Network Function Virtualization (NFV) and Its Impact on Service Providers
- The Evolution of Software-Defined Networking (SDN) in Enterprise Environments
- Implementing Robust Cybersecurity Measures in Distributed Systems
- Quantum Computing: Implications for Network Security in Distributed Systems
- Peer-to-Peer Network Protocols and Their Applications
- The Internet of Things (IoT): Network Challenges and Communication Protocols
- Real-Time Data Processing in Distributed Sensor Networks
- The Role of Artificial Intelligence in Optimizing Network Operations
- Privacy and Data Protection Strategies in Distributed Systems
- The Future of Distributed Computing in Cloud Environments
- Energy Efficiency in Distributed Network Systems
- Wireless Mesh Networks: Design, Challenges, and Applications
- Multi-Access Edge Computing (MEC): Use Cases and Deployment Challenges
- Consensus Algorithms in Distributed Systems: From Blockchain to New Applications
- The Use of Containers and Microservices in Building Scalable Applications
- Network Slicing for 5G: Opportunities and Challenges
- The Role of Distributed Systems in Big Data Analytics
- Managing Data Consistency in Distributed Databases
- The Impact of Distributed Systems on Digital Transformation Strategies
- Augmented Reality over Distributed Networks: Performance and Scalability Issues
- The Application of Distributed Systems in Smart Grid Technology
- Developing Distributed Applications Using Serverless Architectures
- The Challenges of Implementing IPv6 in Distributed Networks
- Distributed Systems for Disaster Recovery: Design and Implementation
- The Use of Virtual Reality in Distributed Network Environments
- Security Protocols for Ad Hoc Networks in Emergency Situations
- The Role of Distributed Networks in Enhancing Mobile Broadband Services
- Next-Generation Protocols for Enhanced Network Reliability and Performance
- The Application of Blockchain in Securing Distributed IoT Networks
- Dynamic Resource Allocation Strategies in Distributed Systems
- The Integration of Distributed Systems with Existing IT Infrastructure
- The Future of Autonomous Systems in Distributed Networking
- The Integration of GIS with Remote Sensing for Environmental Monitoring
- GIS in Urban Planning: Techniques for Sustainable Development
- The Role of GIS in Disaster Management and Response Strategies
- Real-Time GIS Applications in Traffic Management and Route Planning
- The Use of GIS in Water Resource Management
- GIS and Public Health: Tracking Epidemics and Healthcare Access
- Advances in 3D GIS: Technologies and Applications
- GIS in Agricultural Management: Precision Farming Techniques
- The Impact of GIS on Biodiversity Conservation Efforts
- Spatial Data Analysis for Crime Pattern Detection and Prevention
- GIS in Renewable Energy: Site Selection and Resource Management
- The Role of GIS in Historical Research and Archaeology
- GIS and Machine Learning: Integrating Spatial Analysis with Predictive Models
- Cloud Computing and GIS: Enhancing Accessibility and Data Processing
- The Application of GIS in Managing Public Transportation Systems
- GIS in Real Estate: Market Analysis and Property Valuation
- The Use of GIS for Environmental Impact Assessments
- Mobile GIS Applications: Development and Usage Trends
- GIS and Its Role in Smart City Initiatives
- Privacy Issues in the Use of Geographic Information Systems
- GIS in Forest Management: Monitoring and Conservation Strategies
- The Impact of GIS on Tourism: Enhancing Visitor Experiences through Technology
- GIS in the Insurance Industry: Risk Assessment and Policy Design
- The Development of Participatory GIS (PGIS) for Community Engagement
- GIS in Coastal Management: Addressing Erosion and Flood Risks
- Geospatial Analytics in Retail: Optimizing Location and Consumer Insights
- GIS for Wildlife Tracking and Habitat Analysis
- The Use of GIS in Climate Change Studies
- GIS and Social Media: Analyzing Spatial Trends from User Data
- The Future of GIS: Augmented Reality and Virtual Reality Applications
- GIS in Education: Tools for Teaching Geographic Concepts
- The Role of GIS in Land Use Planning and Zoning
- GIS for Emergency Medical Services: Optimizing Response Times
- Open Source GIS Software: Development and Community Contributions
- GIS and the Internet of Things (IoT): Converging Technologies for Advanced Monitoring
- GIS for Mineral Exploration: Techniques and Applications
- The Role of GIS in Municipal Management and Services
- GIS and Drone Technology: A Synergy for Precision Mapping
- Spatial Statistics in GIS: Techniques for Advanced Data Analysis
- Future Trends in GIS: The Integration of AI for Smarter Solutions
- The Evolution of User Interface (UI) Design: From Desktop to Mobile and Beyond
- The Role of HCI in Enhancing Accessibility for Disabled Users
- Virtual Reality (VR) and Augmented Reality (AR) in HCI: New Dimensions of Interaction
- The Impact of HCI on User Experience (UX) in Software Applications
- Cognitive Aspects of HCI: Understanding User Perception and Behavior
- HCI and the Internet of Things (IoT): Designing Interactive Smart Devices
- The Use of Biometrics in HCI: Security and Usability Concerns
- HCI in Educational Technologies: Enhancing Learning through Interaction
- Emotional Recognition and Its Application in HCI
- The Role of HCI in Wearable Technology: Design and Functionality
- Advanced Techniques in Voice User Interfaces (VUIs)
- The Impact of HCI on Social Media Interaction Patterns
- HCI in Healthcare: Designing User-Friendly Medical Devices and Software
- HCI and Gaming: Enhancing Player Engagement and Experience
- The Use of HCI in Robotic Systems: Improving Human-Robot Interaction
- The Influence of HCI on E-commerce: Optimizing User Journeys and Conversions
- HCI in Smart Homes: Interaction Design for Automated Environments
- Multimodal Interaction: Integrating Touch, Voice, and Gesture in HCI
- HCI and Aging: Designing Technology for Older Adults
- The Role of HCI in Virtual Teams: Tools and Strategies for Collaboration
- User-Centered Design: HCI Strategies for Developing User-Focused Software
- HCI Research Methodologies: Experimental Design and User Studies
- The Application of HCI Principles in the Design of Public Kiosks
- The Future of HCI: Integrating Artificial Intelligence for Smarter Interfaces
- HCI in Transportation: Designing User Interfaces for Autonomous Vehicles
- Privacy and Ethics in HCI: Addressing User Data Security
- HCI and Environmental Sustainability: Promoting Eco-Friendly Behaviors
- Adaptive Interfaces: HCI Design for Personalized User Experiences
- The Role of HCI in Content Creation: Tools for Artists and Designers
- HCI for Crisis Management: Designing Systems for Emergency Use
- The Use of HCI in Sports Technology: Enhancing Training and Performance
- The Evolution of Haptic Feedback in HCI
- HCI and Cultural Differences: Designing for Global User Bases
- The Impact of HCI on Digital Marketing: Creating Engaging User Interactions
- HCI in Financial Services: Improving User Interfaces for Banking Apps
- The Role of HCI in Enhancing User Trust in Technology
- HCI for Public Safety: User Interfaces for Security Systems
- The Application of HCI in the Film and Television Industry
- HCI and the Future of Work: Designing Interfaces for Remote Collaboration
- Innovations in HCI: Exploring New Interaction Technologies and Their Applications
- Deep Learning Techniques for Advanced Image Segmentation
- Real-Time Image Processing for Autonomous Driving Systems
- Image Enhancement Algorithms for Underwater Imaging
- Super-Resolution Imaging: Techniques and Applications
- The Role of Image Processing in Remote Sensing and Satellite Imagery Analysis
- Machine Learning Models for Medical Image Diagnosis
- The Impact of AI on Photographic Restoration and Enhancement
- Image Processing in Security Systems: Facial Recognition and Motion Detection
- Advanced Algorithms for Image Noise Reduction
- 3D Image Reconstruction Techniques in Tomography
- Image Processing for Agricultural Monitoring: Crop Disease Detection and Yield Prediction
- Techniques for Panoramic Image Stitching
- Video Image Processing: Real-Time Streaming and Data Compression
- The Application of Image Processing in Printing Technology
- Color Image Processing: Theory and Practical Applications
- The Use of Image Processing in Biometrics Identification
- Computational Photography: Image Processing Techniques in Smartphone Cameras
- Image Processing for Augmented Reality: Real-time Object Overlay
- The Development of Image Processing Algorithms for Traffic Control Systems
- Pattern Recognition and Analysis in Forensic Imaging
- Adaptive Filtering Techniques in Image Processing
- Image Processing in Retail: Customer Tracking and Behavior Analysis
- The Role of Image Processing in Cultural Heritage Preservation
- Image Segmentation Techniques for Cancer Detection in Medical Imaging
- High Dynamic Range (HDR) Imaging: Algorithms and Display Techniques
- Image Classification with Deep Convolutional Neural Networks
- The Evolution of Edge Detection Algorithms in Image Processing
- Image Processing for Wildlife Monitoring: Species Recognition and Behavior Analysis
- Application of Wavelet Transforms in Image Compression
- Image Processing in Sports: Enhancing Broadcasts and Performance Analysis
- Optical Character Recognition (OCR) Improvements in Document Scanning
- Multi-Spectral Imaging for Environmental and Earth Studies
- Image Processing for Space Exploration: Analysis of Planetary Images
- Real-Time Image Processing for Event Surveillance
- The Influence of Quantum Computing on Image Processing Speed and Security
- Machine Vision in Manufacturing: Defect Detection and Quality Control
- Image Processing in Neurology: Visualizing Brain Functions
- Photogrammetry and Image Processing in Geology: 3D Terrain Mapping
- Advanced Techniques in Image Watermarking for Copyright Protection
- The Future of Image Processing: Integrating AI for Automated Editing
- The Evolution of Enterprise Resource Planning (ERP) Systems in the Digital Age
- Information Systems for Managing Distributed Workforces
- The Role of Information Systems in Enhancing Supply Chain Management
- Cybersecurity Measures in Information Systems
- The Impact of Big Data on Decision Support Systems
- Blockchain Technology for Information System Security
- The Development of Sustainable IT Infrastructure in Information Systems
- The Use of AI in Information Systems for Business Intelligence
- Information Systems in Healthcare: Improving Patient Care and Data Management
- The Influence of IoT on Information Systems Architecture
- Mobile Information Systems: Development and Usability Challenges
- The Role of Geographic Information Systems (GIS) in Urban Planning
- Social Media Analytics: Tools and Techniques in Information Systems
- Information Systems in Education: Enhancing Learning and Administration
- Cloud Computing Integration into Corporate Information Systems
- Information Systems Audit: Practices and Challenges
- User Interface Design and User Experience in Information Systems
- Privacy and Data Protection in Information Systems
- The Future of Quantum Computing in Information Systems
- The Role of Information Systems in Environmental Management
- Implementing Effective Knowledge Management Systems
- The Adoption of Virtual Reality in Information Systems
- The Challenges of Implementing ERP Systems in Multinational Corporations
- Information Systems for Real-Time Business Analytics
- The Impact of 5G Technology on Mobile Information Systems
- Ethical Issues in the Management of Information Systems
- Information Systems in Retail: Enhancing Customer Experience and Management
- The Role of Information Systems in Non-Profit Organizations
- Development of Decision Support Systems for Strategic Planning
- Information Systems in the Banking Sector: Enhancing Financial Services
- Risk Management in Information Systems
- The Integration of Artificial Neural Networks in Information Systems
- Information Systems and Corporate Governance
- Information Systems for Disaster Response and Management
- The Role of Information Systems in Sports Management
- Information Systems for Public Health Surveillance
- The Future of Information Systems: Trends and Predictions
- Information Systems in the Film and Media Industry
- Business Process Reengineering through Information Systems
- Implementing Customer Relationship Management (CRM) Systems in E-commerce
- Emerging Trends in Artificial Intelligence and Machine Learning
- The Future of Cloud Services and Technology
- Cybersecurity: Current Threats and Future Defenses
- The Role of Information Technology in Sustainable Energy Solutions
- Internet of Things (IoT): From Smart Homes to Smart Cities
- Blockchain and Its Impact on Information Technology
- The Use of Big Data Analytics in Predictive Modeling
- Virtual Reality (VR) and Augmented Reality (AR): The Next Frontier in IT
- The Challenges of Digital Transformation in Traditional Businesses
- Wearable Technology: Health Monitoring and Beyond
- 5G Technology: Implementation and Impacts on IT
- Biometrics Technology: Uses and Privacy Concerns
- The Role of IT in Global Health Initiatives
- Ethical Considerations in the Development of Autonomous Systems
- Data Privacy in the Age of Information Overload
- The Evolution of Software Development Methodologies
- Quantum Computing: The Next Revolution in IT
- IT Governance: Best Practices and Standards
- The Integration of AI in Customer Service Technology
- IT in Manufacturing: Industrial Automation and Robotics
- The Future of E-commerce: Technology and Trends
- Mobile Computing: Innovations and Challenges
- Information Technology in Education: Tools and Trends
- IT Project Management: Approaches and Tools
- The Role of IT in Media and Entertainment
- The Impact of Digital Marketing Technologies on Business Strategies
- IT in Logistics and Supply Chain Management
- The Development and Future of Autonomous Vehicles
- IT in the Insurance Sector: Enhancing Efficiency and Customer Engagement
- The Role of IT in Environmental Conservation
- Smart Grid Technology: IT at the Intersection of Energy Management
- Telemedicine: The Impact of IT on Healthcare Delivery
- IT in the Agricultural Sector: Innovations and Impact
- Cyber-Physical Systems: IT in the Integration of Physical and Digital Worlds
- The Influence of Social Media Platforms on IT Development
- Data Centers: Evolution, Technologies, and Sustainability
- IT in Public Administration: Improving Services and Transparency
- The Role of IT in Sports Analytics
- Information Technology in Retail: Enhancing the Shopping Experience
- The Future of IT: Integrating Ethical AI Systems
Internet of Things (IoT) Thesis Topics
- Enhancing IoT Security: Strategies for Safeguarding Connected Devices
- IoT in Smart Cities: Infrastructure and Data Management Challenges
- The Application of IoT in Precision Agriculture: Maximizing Efficiency and Yield
- IoT and Healthcare: Opportunities for Remote Monitoring and Patient Care
- Energy Efficiency in IoT: Techniques for Reducing Power Consumption in Devices
- The Role of IoT in Supply Chain Management and Logistics
- Real-Time Data Processing Using Edge Computing in IoT Networks
- Privacy Concerns and Data Protection in IoT Systems
- The Integration of IoT with Blockchain for Enhanced Security and Transparency
- IoT in Environmental Monitoring: Systems for Air Quality and Water Safety
- Predictive Maintenance in Industrial IoT: Strategies and Benefits
- IoT in Retail: Enhancing Customer Experience through Smart Technology
- The Development of Standard Protocols for IoT Communication
- IoT in Smart Homes: Automation and Security Systems
- The Role of IoT in Disaster Management: Early Warning Systems and Response Coordination
- Machine Learning Techniques for IoT Data Analytics
- IoT in Automotive: The Future of Connected and Autonomous Vehicles
- The Impact of 5G on IoT: Enhancements in Speed and Connectivity
- IoT Device Lifecycle Management: From Creation to Decommissioning
- IoT in Public Safety: Applications for Emergency Response and Crime Prevention
- The Ethics of IoT: Balancing Innovation with Consumer Rights
- IoT and the Future of Work: Automation and Labor Market Shifts
- Designing User-Friendly Interfaces for IoT Applications
- IoT in the Energy Sector: Smart Grids and Renewable Energy Integration
- Quantum Computing and IoT: Potential Impacts and Applications
- The Role of AI in Enhancing IoT Solutions
- IoT for Elderly Care: Technologies for Health and Mobility Assistance
- IoT in Education: Enhancing Classroom Experiences and Learning Outcomes
- Challenges in Scaling IoT Infrastructure for Global Coverage
- The Economic Impact of IoT: Industry Transformations and New Business Models
- IoT and Tourism: Enhancing Visitor Experiences through Connected Technologies
- Data Fusion Techniques in IoT: Integrating Diverse Data Sources
- IoT in Aquaculture: Monitoring and Managing Aquatic Environments
- Wireless Technologies for IoT: Comparing LoRa, Zigbee, and NB-IoT
- IoT and Intellectual Property: Navigating the Legal Landscape
- IoT in Sports: Enhancing Training and Audience Engagement
- Building Resilient IoT Systems against Cyber Attacks
- IoT for Waste Management: Innovations and System Implementations
- IoT in Agriculture: Drones and Sensors for Crop Monitoring
- The Role of IoT in Cultural Heritage Preservation: Monitoring and Maintenance
- Advanced Algorithms for Supervised and Unsupervised Learning
- Machine Learning in Genomics: Predicting Disease Propensity and Treatment Outcomes
- The Use of Neural Networks in Image Recognition and Analysis
- Reinforcement Learning: Applications in Robotics and Autonomous Systems
- The Role of Machine Learning in Natural Language Processing and Linguistic Analysis
- Deep Learning for Predictive Analytics in Business and Finance
- Machine Learning for Cybersecurity: Detection of Anomalies and Malware
- Ethical Considerations in Machine Learning: Bias and Fairness
- The Integration of Machine Learning with IoT for Smart Device Management
- Transfer Learning: Techniques and Applications in New Domains
- The Application of Machine Learning in Environmental Science
- Machine Learning in Healthcare: Diagnosing Conditions from Medical Images
- The Use of Machine Learning in Algorithmic Trading and Stock Market Analysis
- Machine Learning in Social Media: Sentiment Analysis and Trend Prediction
- Quantum Machine Learning: Merging Quantum Computing with AI
- Feature Engineering and Selection in Machine Learning
- Machine Learning for Enhancing User Experience in Mobile Applications
- The Impact of Machine Learning on Digital Marketing Strategies
- Machine Learning for Energy Consumption Forecasting and Optimization
- The Role of Machine Learning in Enhancing Network Security Protocols
- Scalability and Efficiency of Machine Learning Algorithms
- Machine Learning in Drug Discovery and Pharmaceutical Research
- The Application of Machine Learning in Sports Analytics
- Machine Learning for Real-Time Decision-Making in Autonomous Vehicles
- The Use of Machine Learning in Predicting Geographical and Meteorological Events
- Machine Learning for Educational Data Mining and Learning Analytics
- The Role of Machine Learning in Audio Signal Processing
- Predictive Maintenance in Manufacturing Through Machine Learning
- Machine Learning and Its Implications for Privacy and Surveillance
- The Application of Machine Learning in Augmented Reality Systems
- Deep Learning Techniques in Medical Diagnosis: Challenges and Opportunities
- The Use of Machine Learning in Video Game Development
- Machine Learning for Fraud Detection in Financial Services
- The Role of Machine Learning in Agricultural Optimization and Management
- The Impact of Machine Learning on Content Personalization and Recommendation Systems
- Machine Learning in Legal Tech: Document Analysis and Case Prediction
- Adaptive Learning Systems: Tailoring Education Through Machine Learning
- Machine Learning in Space Exploration: Analyzing Data from Space Missions
- Machine Learning for Public Sector Applications: Improving Services and Efficiency
- The Future of Machine Learning: Integrating Explainable AI
- Innovations in Convolutional Neural Networks for Image and Video Analysis
- Recurrent Neural Networks: Applications in Sequence Prediction and Analysis
- The Role of Neural Networks in Predicting Financial Market Trends
- Deep Neural Networks for Enhanced Speech Recognition Systems
- Neural Networks in Medical Imaging: From Detection to Diagnosis
- Generative Adversarial Networks (GANs): Applications in Art and Media
- The Use of Neural Networks in Autonomous Driving Technologies
- Neural Networks for Real-Time Language Translation
- The Application of Neural Networks in Robotics: Sensory Data and Movement Control
- Neural Network Optimization Techniques: Overcoming Overfitting and Underfitting
- The Integration of Neural Networks with Blockchain for Data Security
- Neural Networks in Climate Modeling and Weather Forecasting
- The Use of Neural Networks in Enhancing Internet of Things (IoT) Devices
- Graph Neural Networks: Applications in Social Network Analysis and Beyond
- The Impact of Neural Networks on Augmented Reality Experiences
- Neural Networks for Anomaly Detection in Network Security
- The Application of Neural Networks in Bioinformatics and Genomic Data Analysis
- Capsule Neural Networks: Improving the Robustness and Interpretability of Deep Learning
- The Role of Neural Networks in Consumer Behavior Analysis
- Neural Networks in Energy Sector: Forecasting and Optimization
- The Evolution of Neural Network Architectures for Efficient Learning
- The Use of Neural Networks in Sentiment Analysis: Techniques and Challenges
- Deep Reinforcement Learning: Strategies for Advanced Decision-Making Systems
- Neural Networks for Precision Medicine: Tailoring Treatments to Individual Genetic Profiles
- The Use of Neural Networks in Virtual Assistants: Enhancing Natural Language Understanding
- The Impact of Neural Networks on Pharmaceutical Research
- Neural Networks for Supply Chain Management: Prediction and Automation
- The Application of Neural Networks in E-commerce: Personalization and Recommendation Systems
- Neural Networks for Facial Recognition: Advances and Ethical Considerations
- The Role of Neural Networks in Educational Technologies
- The Use of Neural Networks in Predicting Economic Trends
- Neural Networks in Sports: Analyzing Performance and Strategy
- The Impact of Neural Networks on Digital Security Systems
- Neural Networks for Real-Time Video Surveillance Analysis
- The Integration of Neural Networks in Edge Computing Devices
- Neural Networks for Industrial Automation: Improving Efficiency and Accuracy
- The Future of Neural Networks: Towards More General AI Applications
- Neural Networks in Art and Design: Creating New Forms of Expression
- The Role of Neural Networks in Enhancing Public Health Initiatives
- The Future of Neural Networks: Challenges in Scalability and Generalization
- The Evolution of Programming Paradigms: Functional vs. Object-Oriented Programming
- Advances in Compiler Design and Optimization Techniques
- The Impact of Programming Languages on Software Security
- Developing Programming Languages for Quantum Computing
- Machine Learning in Automated Code Generation and Optimization
- The Role of Programming in Developing Scalable Cloud Applications
- The Future of Web Development: New Frameworks and Technologies
- Cross-Platform Development: Best Practices in Mobile App Programming
- The Influence of Programming Techniques on Big Data Analytics
- Real-Time Systems Programming: Challenges and Solutions
- The Integration of Programming with Blockchain Technology
- Programming for IoT: Languages and Tools for Device Communication
- Secure Coding Practices: Preventing Cyber Attacks through Software Design
- The Role of Programming in Data Visualization and User Interface Design
- Advances in Game Programming: Graphics, AI, and Network Play
- The Impact of Programming on Digital Media and Content Creation
- Programming Languages for Robotics: Trends and Future Directions
- The Use of Artificial Intelligence in Enhancing Programming Productivity
- Programming for Augmented and Virtual Reality: New Challenges and Techniques
- Ethical Considerations in Programming: Bias, Fairness, and Transparency
- The Future of Programming Education: Interactive and Adaptive Learning Models
- Programming for Wearable Technology: Special Considerations and Challenges
- The Evolution of Programming in Financial Technology
- Functional Programming in Enterprise Applications
- Memory Management Techniques in Programming: From Garbage Collection to Manual Control
- The Role of Open Source Programming in Accelerating Innovation
- The Impact of Programming on Network Security and Cryptography
- Developing Accessible Software: Programming for Users with Disabilities
- Programming Language Theories: New Models and Approaches
- The Challenges of Legacy Code: Strategies for Modernization and Integration
- Energy-Efficient Programming: Optimizing Code for Green Computing
- Multithreading and Concurrency: Advanced Programming Techniques
- The Impact of Programming on Computational Biology and Bioinformatics
- The Role of Scripting Languages in Automating System Administration
- Programming and the Future of Quantum Resistant Cryptography
- Code Review and Quality Assurance: Techniques and Tools
- Adaptive and Predictive Programming for Dynamic Environments
- The Role of Programming in Enhancing E-commerce Technology
- Programming for Cyber-Physical Systems: Bridging the Gap Between Digital and Physical
- The Influence of Programming Languages on Computational Efficiency and Performance
- Quantum Algorithms: Development and Applications Beyond Shor’s and Grover’s Algorithms
- The Role of Quantum Computing in Solving Complex Biological Problems
- Quantum Cryptography: New Paradigms for Secure Communication
- Error Correction Techniques in Quantum Computing
- Quantum Computing and Its Impact on Artificial Intelligence
- The Integration of Classical and Quantum Computing: Hybrid Models
- Quantum Machine Learning: Theoretical Foundations and Practical Applications
- Quantum Computing Hardware: Advances in Qubit Technology
- The Application of Quantum Computing in Financial Modeling and Risk Assessment
- Quantum Networking: Establishing Secure Quantum Communication Channels
- The Future of Drug Discovery: Applications of Quantum Computing
- Quantum Computing in Cryptanalysis: Threats to Current Cryptography Standards
- Simulation of Quantum Systems for Material Science
- Quantum Computing for Optimization Problems in Logistics and Manufacturing
- Theoretical Limits of Quantum Computing: Understanding Quantum Complexity
- Quantum Computing and the Future of Search Algorithms
- The Role of Quantum Computing in Climate Science and Environmental Modeling
- Quantum Annealing vs. Universal Quantum Computing: Comparative Studies
- Implementing Quantum Algorithms in Quantum Programming Languages
- The Impact of Quantum Computing on Public Key Cryptography
- Quantum Entanglement: Experiments and Applications in Quantum Networks
- Scalability Challenges in Quantum Processors
- The Ethics and Policy Implications of Quantum Computing
- Quantum Computing in Space Exploration and Astrophysics
- The Role of Quantum Computing in Developing Next-Generation AI Systems
- Quantum Computing in the Energy Sector: Applications in Smart Grids and Nuclear Fusion
- Noise and Decoherence in Quantum Computers: Overcoming Practical Challenges
- Quantum Computing for Predicting Economic Market Trends
- Quantum Sensors: Enhancing Precision in Measurement and Imaging
- The Future of Quantum Computing Education and Workforce Development
- Quantum Computing in Cybersecurity: Preparing for a Post-Quantum World
- Quantum Computing and the Internet of Things: Potential Intersections
- Practical Quantum Computing: From Theory to Real-World Applications
- Quantum Supremacy: Milestones and Future Goals
- The Role of Quantum Computing in Genetics and Genomics
- Quantum Computing for Material Discovery and Design
- The Challenges of Quantum Programming Languages and Environments
- Quantum Computing in Art and Creative Industries
- The Global Race for Quantum Computing Supremacy: Technological and Political Aspects
- Quantum Computing and Its Implications for Software Engineering
- Advances in Humanoid Robotics: New Developments and Challenges
- Robotics in Healthcare: From Surgery to Rehabilitation
- The Integration of AI in Robotics: Enhanced Autonomy and Learning Capabilities
- Swarm Robotics: Coordination Strategies and Applications
- The Use of Robotics in Hazardous Environments: Deep Sea and Space Exploration
- Soft Robotics: Materials, Design, and Applications
- Robotics in Agriculture: Automation of Farming and Harvesting Processes
- The Role of Robotics in Manufacturing: Increased Efficiency and Flexibility
- Ethical Considerations in the Deployment of Robots in Human Environments
- Autonomous Vehicles: Technological Advances and Regulatory Challenges
- Robotic Assistants for the Elderly and Disabled: Improving Quality of Life
- The Use of Robotics in Education: Teaching Science, Technology, Engineering, and Math (STEM)
- Robotics and Computer Vision: Enhancing Perception and Decision Making
- The Impact of Robotics on Employment and the Workforce
- The Development of Robotic Systems for Environmental Monitoring and Conservation
- Machine Learning Techniques for Robotic Perception and Navigation
- Advances in Robotic Surgery: Precision and Outcomes
- Human-Robot Interaction: Building Trust and Cooperation
- Robotics in Retail: Automated Warehousing and Customer Service
- Energy-Efficient Robots: Design and Utilization
- Robotics in Construction: Automation and Safety Improvements
- The Role of Robotics in Disaster Response and Recovery Operations
- The Application of Robotics in Art and Creative Industries
- Robotics and the Future of Personal Transportation
- Ethical AI in Robotics: Ensuring Safe and Fair Decision-Making
- The Use of Robotics in Logistics: Drones and Autonomous Delivery Vehicles
- Robotics in the Food Industry: From Production to Service
- The Integration of IoT with Robotics for Enhanced Connectivity
- Wearable Robotics: Exoskeletons for Rehabilitation and Enhanced Mobility
- The Impact of Robotics on Privacy and Security
- Robotic Pet Companions: Social Robots and Their Psychological Effects
- Robotics for Planetary Exploration and Colonization
- Underwater Robotics: Innovations in Oceanography and Marine Biology
- Advances in Robotics Programming Languages and Tools
- The Role of Robotics in Minimizing Human Exposure to Contaminants and Pathogens
- Collaborative Robots (Cobots): Working Alongside Humans in Shared Spaces
- The Use of Robotics in Entertainment and Sports
- Robotics and Machine Ethics: Programming Moral Decision-Making
- The Future of Military Robotics: Opportunities and Challenges
- Sustainable Robotics: Reducing the Environmental Impact of Robotic Systems
- Agile Methodologies: Evolution and Future Trends
- DevOps Practices: Improving Software Delivery and Lifecycle Management
- The Impact of Microservices Architecture on Software Development
- Containerization Technologies: Docker, Kubernetes, and Beyond
- Software Quality Assurance: Modern Techniques and Tools
- The Role of Artificial Intelligence in Automated Software Testing
- Blockchain Applications in Software Development and Security
- The Integration of Continuous Integration and Continuous Deployment (CI/CD) in Software Projects
- Cybersecurity in Software Engineering: Best Practices for Secure Coding
- Low-Code and No-Code Development: Implications for Professional Software Development
- The Future of Software Engineering Education
- Software Sustainability: Developing Green Software and Reducing Carbon Footprints
- The Role of Software Engineering in Healthcare: Telemedicine and Patient Data Management
- Privacy by Design: Incorporating Privacy Features at the Development Stage
- The Impact of Quantum Computing on Software Engineering
- Software Engineering for Augmented and Virtual Reality: Challenges and Innovations
- Cloud-Native Applications: Design, Development, and Deployment
- Software Project Management: Agile vs. Traditional Approaches
- Open Source Software: Community Engagement and Project Sustainability
- The Evolution of Graphical User Interfaces in Application Development
- The Challenges of Integrating IoT Devices into Software Systems
- Ethical Issues in Software Engineering: Bias, Accountability, and Regulation
- Software Engineering for Autonomous Vehicles: Safety and Regulatory Considerations
- Big Data Analytics in Software Development: Enhancing Decision-Making Processes
- The Future of Mobile App Development: Trends and Technologies
- The Role of Software Engineering in Artificial Intelligence: Frameworks and Algorithms
- Performance Optimization in Software Applications
- Adaptive Software Development: Responding to Changing User Needs
- Software Engineering in Financial Services: Compliance and Security Challenges
- User Experience (UX) Design in Software Engineering
- The Role of Software Engineering in Smart Cities: Infrastructure and Services
- The Impact of 5G on Software Development and Deployment
- Real-Time Systems in Software Engineering: Design and Implementation Challenges
- Cross-Platform Development Challenges: Ensuring Consistency and Performance
- Software Testing Automation: Tools and Trends
- The Integration of Cyber-Physical Systems in Software Engineering
- Software Engineering in the Entertainment Industry: Game Development and Beyond
- The Application of Machine Learning in Predicting Software Bugs
- The Role of Software Engineering in Cybersecurity Defense Strategies
- Accessibility in Software Engineering: Creating Inclusive and Usable Software
- Progressive Web Apps (PWAs): Advantages and Implementation Challenges
- The Future of Web Accessibility: Standards and Practices
- Single-Page Applications (SPAs) vs. Multi-Page Applications (MPAs): Performance and Usability
- The Impact of Serverless Computing on Web Development
- The Evolution of CSS for Modern Web Design
- Security Best Practices in Web Development: Defending Against XSS and CSRF Attacks
- The Role of Web Development in Enhancing E-commerce User Experience
- The Use of Artificial Intelligence in Web Personalization and User Engagement
- The Future of Web APIs: Standards, Security, and Scalability
- Responsive Web Design: Techniques and Trends
- JavaScript Frameworks: Vue.js, React.js, and Angular – A Comparative Analysis
- Web Development for IoT: Interfaces and Connectivity Solutions
- The Impact of 5G on Web Development and User Experiences
- The Use of Blockchain Technology in Web Development for Enhanced Security
- Web Development in the Cloud: Using AWS, Azure, and Google Cloud
- Content Management Systems (CMS): Trends and Future Developments
- The Application of Web Development in Virtual and Augmented Reality
- The Importance of Web Performance Optimization: Tools and Techniques
- Sustainable Web Design: Practices for Reducing Energy Consumption
- The Role of Web Development in Digital Marketing: SEO and Social Media Integration
- Headless CMS: Benefits and Challenges for Developers and Content Creators
- The Future of Web Typography: Design, Accessibility, and Performance
- Web Development and Data Protection: Complying with GDPR and Other Regulations
- Real-Time Web Communication: Technologies like WebSockets and WebRTC
- Front-End Development Tools: Efficiency and Innovation in Workflow
- The Challenges of Migrating Legacy Systems to Modern Web Architectures
- Microfrontends Architecture: Designing Scalable and Decoupled Web Applications
- The Impact of Cryptocurrencies on Web Payment Systems
- User-Centered Design in Web Development: Methods for Engaging Users
- The Role of Web Development in Business Intelligence: Dashboards and Reporting Tools
- Web Development for Mobile Platforms: Optimization and Best Practices
- The Evolution of E-commerce Platforms: From Web to Mobile Commerce
- Web Security in E-commerce: Protecting Transactions and User Data
- Dynamic Web Content: Server-Side vs. Client-Side Rendering
- The Future of Full Stack Development: Trends and Skills
- Web Design Psychology: How Design Influences User Behavior
- The Role of Web Development in the Non-Profit Sector: Fundraising and Community Engagement
- The Integration of AI Chatbots in Web Development
- The Use of Motion UI in Web Design: Enhancing Aesthetics and User Interaction
- The Future of Web Development: Predictions and Emerging Technologies
We trust that this comprehensive list of computer science thesis topics will serve as a valuable starting point for your research endeavors. With 1000 unique and carefully selected topics distributed across 25 key areas of computer science, students are equipped to tackle complex questions and contribute meaningful advancements to the field. As you proceed to select your thesis topic, consider not only your personal interests and career goals but also the potential impact of your research. We encourage you to explore these topics thoroughly and choose one that will not only challenge you but also push the boundaries of technology and innovation.
The Range of Computer Science Thesis Topics
Computer science stands as a dynamic and ever-evolving field that continuously reshapes how we interact with the world. At its core, the discipline encompasses not just the study of algorithms and computation, but a broad spectrum of practical and theoretical knowledge areas that drive innovation in various sectors. This article aims to explore the rich landscape of computer science thesis topics, offering students and researchers a glimpse into the potential areas of study that not only challenge the intellect but also contribute significantly to technological progress. As we delve into the current issues, recent trends, and future directions of computer science, it becomes evident that the possibilities for research are both vast and diverse. Whether you are intrigued by the complexities of artificial intelligence, the robust architecture of networks and systems, or the innovative approaches in cybersecurity, computer science offers a fertile ground for developing thesis topics that are as impactful as they are intellectually stimulating.
Current Issues in Computer Science
One of the prominent current issues in computer science revolves around data security and privacy. As digital transformation accelerates across industries, the massive influx of data generated poses significant challenges in terms of its protection and ethical use. Cybersecurity threats have become more sophisticated, with data breaches and cyber-attacks causing major concerns for organizations worldwide. This ongoing battle demands continuous improvements in security protocols and the development of robust cybersecurity measures. Computer science thesis topics in this area can explore new cryptographic methods, intrusion detection systems, and secure communication protocols to fortify digital defenses. Research could also delve into the ethical implications of data collection and use, proposing frameworks that ensure privacy while still leveraging data for innovation.
Another critical issue facing the field of computer science is the ethical development and deployment of artificial intelligence (AI) systems. As AI technologies become more integrated into daily life and critical infrastructure, concerns about bias, fairness, and accountability in AI systems have intensified. Thesis topics could focus on developing algorithms that address these ethical concerns, including techniques for reducing bias in machine learning models and methods for increasing transparency and explainability in AI decisions. This research is crucial for ensuring that AI technologies promote fairness and do not perpetuate or exacerbate existing societal inequalities.
Furthermore, the rapid pace of technological change presents a challenge in terms of sustainability and environmental impact. The energy consumption of large data centers, the carbon footprint of producing and disposing of electronic waste, and the broader effects of high-tech innovations on the environment are significant concerns within computer science. Thesis research in this domain could focus on creating more energy-efficient computing methods, developing algorithms that reduce power consumption, or innovating recycling technologies that address the issue of e-waste. This research not only contributes to the field of computer science but also plays a crucial role in ensuring that technological advancement does not come at an unsustainable cost to the environment.
These current issues highlight the dynamic nature of computer science and its direct impact on society. Addressing these challenges through focused research and innovative thesis topics not only advances the field but also contributes to resolving some of the most pressing problems facing our global community today.
Recent Trends in Computer Science
In recent years, computer science has witnessed significant advancements in the integration of artificial intelligence (AI) and machine learning (ML) across various sectors, marking one of the most exciting trends in the field. These technologies are not just reshaping traditional industries but are also at the forefront of driving innovations in areas like healthcare, finance, and autonomous systems. Thesis topics within this trend could explore the development of advanced ML algorithms that enhance predictive analytics, improve automated decision-making, or refine natural language processing capabilities. Additionally, AI’s role in ethical decision-making and its societal impacts offers a rich vein of inquiry for research, focusing on mitigating biases and ensuring that AI systems operate transparently and justly.
Another prominent trend in computer science is the rapid growth of blockchain technology beyond its initial application in cryptocurrencies. Blockchain is proving its potential in creating more secure, decentralized, and transparent networks for a variety of applications, from enhancing supply chain logistics to revolutionizing digital identity verification processes. Computer science thesis topics could investigate novel uses of blockchain for ensuring data integrity in digital transactions, enhancing cybersecurity measures, or even developing new frameworks for blockchain integration into existing technological infrastructures. The exploration of blockchain’s scalability, speed, and energy consumption also presents critical research opportunities that are timely and relevant.
Furthermore, the expansion of the Internet of Things (IoT) continues to be a significant trend, with more devices becoming connected every day, leading to increasingly smart environments. This proliferation poses unique challenges and opportunities for computer science research, particularly in terms of scalability, security, and new data management strategies. Thesis topics might focus on optimizing network protocols to handle the massive influx of data from IoT devices, developing solutions to safeguard against IoT-specific security vulnerabilities, or innovative applications of IoT in urban planning, smart homes, or healthcare. Research in this area is crucial for advancing the efficiency and functionality of IoT systems and for ensuring they can be safely and effectively integrated into modern life.
These recent trends underscore the vibrant and ever-evolving nature of computer science, reflecting its capacity to influence and transform an array of sectors through technological innovation. The continual emergence of new research topics within these trends not only enriches the academic discipline but also provides substantial benefits to society by addressing practical challenges and enhancing the capabilities of technology in everyday life.
Future Directions in Computer Science
As we look toward the future, one of the most anticipated areas in computer science is the advancement of quantum computing. This emerging technology promises to revolutionize problem-solving in fields that require immense computational power, such as cryptography, drug discovery, and complex system modeling. Quantum computing has the potential to process tasks at speeds unachievable by classical computers, offering breakthroughs in materials science and encryption methods. Computer science thesis topics might explore the theoretical underpinnings of quantum algorithms, the development of quantum-resistant cryptographic systems, or practical applications of quantum computing in industry-specific scenarios. Research in this area not only contributes to the foundational knowledge of quantum mechanics but also paves the way for its integration into mainstream computing, marking a significant leap forward in computational capabilities.
Another promising direction in computer science is the advancement of autonomous systems, particularly in robotics and vehicle automation. The future of autonomous technologies hinges on improving their safety, reliability, and decision-making processes under uncertain conditions. Thesis topics could focus on the enhancement of machine perception through computer vision and sensor fusion, the development of more sophisticated AI-driven decision frameworks, or ethical considerations in the deployment of autonomous systems. As these technologies become increasingly prevalent, research will play a crucial role in addressing the societal and technical challenges they present, ensuring their beneficial integration into daily life and industry operations.
Additionally, the ongoing expansion of artificial intelligence applications poses significant future directions for research, especially in the realm of AI ethics and policy. As AI systems become more capable and widespread, their impact on privacy, employment, and societal norms continues to grow. Future thesis topics might delve into the development of guidelines and frameworks for responsible AI, studies on the impact of AI on workforce dynamics, or innovations in transparent and fair AI systems. This research is vital for guiding the ethical evolution of AI technologies, ensuring they enhance societal well-being without diminishing human dignity or autonomy.
These future directions in computer science not only highlight the field’s potential for substantial technological advancements but also underscore the importance of thoughtful consideration of their broader implications. By exploring these areas in depth, computer science research can lead the way in not just technological innovation, but also in shaping a future where technology and ethics coexist harmoniously for the betterment of society.
In conclusion, the field of computer science is not only foundational to the technological advancements that characterize the modern age but also crucial in solving some of the most pressing challenges of our time. The potential thesis topics discussed in this article reflect a mere fraction of the opportunities that lie in the realms of theory, application, and innovation within this expansive field. As emerging technologies such as quantum computing, artificial intelligence, and blockchain continue to evolve, they open new avenues for research that could potentially redefine existing paradigms. For students embarking on their thesis journey, it is essential to choose a topic that not only aligns with their academic passions but also contributes to the ongoing expansion of computer science knowledge. By pushing the boundaries of what is known and exploring uncharted territories, students can leave a lasting impact on the field and pave the way for future technological breakthroughs. As we look forward, it’s clear that computer science will continue to be a key driver of change, making it an exciting and rewarding area for academic and professional growth.
Thesis Writing Services by iResearchNet
At iResearchNet, we specialize in providing exceptional thesis writing services tailored to meet the diverse needs of students, particularly those pursuing advanced topics in computer science. Understanding the pivotal role a thesis plays in a student’s academic career, we offer a suite of services designed to assist students in crafting papers that are not only well-researched and insightful but also perfectly aligned with their academic objectives. Here are the key features of our thesis writing services:
- Expert Degree-Holding Writers : Our team consists of writers who hold advanced degrees in computer science and related fields. Their academic and professional backgrounds ensure that they bring a wealth of knowledge and expertise to your thesis.
- Custom Written Works : Every thesis we produce is tailor-made to meet the specific requirements and guidelines provided by the student. This bespoke approach ensures that each paper is unique and of the highest quality.
- In-depth Research : We pride ourselves on conducting thorough and comprehensive research for every thesis. Our writers utilize the latest resources, databases, and scholarly articles to gather the most relevant and up-to-date information.
- Custom Formatting : Each thesis is formatted according to academic standards and the specific requirements of the student’s program, whether it’s APA, MLA, Chicago/Turabian, or Harvard style.
- Top Quality : Quality is at the core of our services. From language clarity to factual accuracy, each thesis is crafted to meet the highest academic standards.
- Customized Solutions : Recognizing that every student’s needs are different, we offer customized solutions that cater to individual preferences and requirements.
- Flexible Pricing : We provide a range of pricing options to accommodate students’ different budgets, ensuring that our services are accessible to everyone.
- Short Deadlines : Our services are designed to accommodate even the tightest deadlines, with the ability to handle requests that require a turnaround as quick as 3 hours.
- Timely Delivery : We guarantee timely delivery of all our papers, helping students meet their submission deadlines without compromising on quality.
- 24/7 Support : Our customer support team is available around the clock to answer any questions and provide assistance whenever needed.
- Absolute Privacy : We maintain a strict privacy policy to ensure that all client information is kept confidential and secure.
- Easy Order Tracking : Our client portal allows for easy tracking of orders, giving students the ability to monitor the progress of their thesis writing process.
- Money-Back Guarantee : We offer a money-back guarantee to ensure that all students are completely satisfied with our services.
At iResearchNet, we are dedicated to supporting students by providing them with high-quality, reliable, and professional thesis writing services. By choosing us, students can be confident that they are receiving expert help that not only meets but exceeds their expectations. Whether you are tackling complex topics in computer science or any other academic discipline, our team is here to help you achieve academic success.
Order Your Custom Thesis Paper Today!
Are you ready to take the next step towards academic excellence in computer science? At iResearchNet, we are committed to helping you achieve your academic goals with our premier thesis writing services. Our team of expert writers is equipped to handle the most challenging topics and tightest deadlines, ensuring that you receive a top-quality, custom-written thesis that not only meets but exceeds your academic requirements.
Don’t let the stress of thesis writing hold you back. Whether you’re grappling with complex algorithms, innovative software solutions, or groundbreaking data analysis, our custom thesis papers are crafted to provide you with the insights and depth needed to excel. With flexible pricing, personalized support, and guaranteed confidentiality, you can trust iResearchNet to be your partner in your academic journey.
Act now to secure your future! Visit our website to place your order or speak with one of our representatives to learn more about how we can assist you. Remember, when you choose iResearchNet, you’re not just getting a thesis paper; you’re investing in your success. Order your custom thesis paper today and take the first step towards standing out in the competitive field of computer science. With iResearchNet, you’re one step closer to not only completing your degree but also making a significant impact in the world of technology.
ORDER HIGH QUALITY CUSTOM PAPER

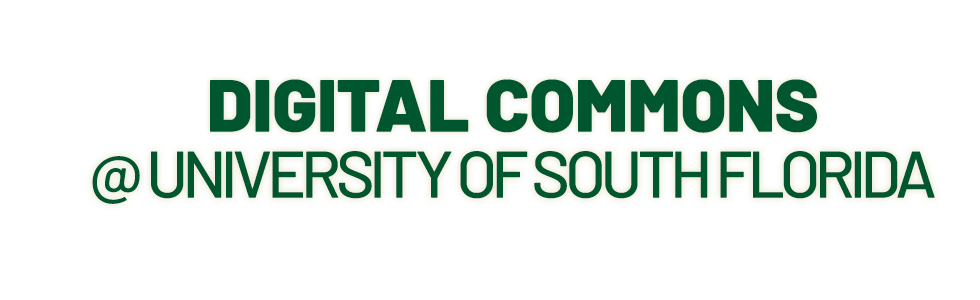
- USF Research
- USF Libraries
Digital Commons @ USF > College of Engineering > Computer Science and Engineering > Theses and Dissertations
Computer Science and Engineering Theses and Dissertations
Theses/dissertations from 2023 2023.
Refining the Machine Learning Pipeline for US-based Public Transit Systems , Jennifer Adorno
Insect Classification and Explainability from Image Data via Deep Learning Techniques , Tanvir Hossain Bhuiyan
Brain-Inspired Spatio-Temporal Learning with Application to Robotics , Thiago André Ferreira Medeiros
Evaluating Methods for Improving DNN Robustness Against Adversarial Attacks , Laureano Griffin
Analyzing Multi-Robot Leader-Follower Formations in Obstacle-Laden Environments , Zachary J. Hinnen
Secure Lightweight Cryptographic Hardware Constructions for Deeply Embedded Systems , Jasmin Kaur
A Psychometric Analysis of Natural Language Inference Using Transformer Language Models , Antonio Laverghetta Jr.
Graph Analysis on Social Networks , Shen Lu
Deep Learning-based Automatic Stereology for High- and Low-magnification Images , Hunter Morera
Deciphering Trends and Tactics: Data-driven Techniques for Forecasting Information Spread and Detecting Coordinated Campaigns in Social Media , Kin Wai Ng Lugo
Deciphering Trends and Tactics: Data-driven Techniques for Forecasting Information Spread and Detecting Coordinated Campaigns in Social Media , Kin Wai NG Lugo
Automated Approaches to Enable Innovative Civic Applications from Citizen Generated Imagery , Hye Seon Yi
Theses/Dissertations from 2022 2022
Towards High Performing and Reliable Deep Convolutional Neural Network Models for Typically Limited Medical Imaging Datasets , Kaoutar Ben Ahmed
Task Progress Assessment and Monitoring Using Self-Supervised Learning , Sainath Reddy Bobbala
Towards More Task-Generalized and Explainable AI Through Psychometrics , Alec Braynen
A Multiple Input Multiple Output Framework for the Automatic Optical Fractionator-based Cell Counting in Z-Stacks Using Deep Learning , Palak Dave
On the Reliability of Wearable Sensors for Assessing Movement Disorder-Related Gait Quality and Imbalance: A Case Study of Multiple Sclerosis , Steven Díaz Hernández
Securing Critical Cyber Infrastructures and Functionalities via Machine Learning Empowered Strategies , Tao Hou
Social Media Time Series Forecasting and User-Level Activity Prediction with Gradient Boosting, Deep Learning, and Data Augmentation , Fred Mubang
A Study of Deep Learning Silhouette Extractors for Gait Recognition , Sneha Oladhri
Analyzing Decision-making in Robot Soccer for Attacking Behaviors , Justin Rodney
Generative Spatio-Temporal and Multimodal Analysis of Neonatal Pain , Md Sirajus Salekin
Secure Hardware Constructions for Fault Detection of Lattice-based Post-quantum Cryptosystems , Ausmita Sarker
Adaptive Multi-scale Place Cell Representations and Replay for Spatial Navigation and Learning in Autonomous Robots , Pablo Scleidorovich
Predicting the Number of Objects in a Robotic Grasp , Utkarsh Tamrakar
Humanoid Robot Motion Control for Ramps and Stairs , Tommy Truong
Preventing Variadic Function Attacks Through Argument Width Counting , Brennan Ward
Theses/Dissertations from 2021 2021
Knowledge Extraction and Inference Based on Visual Understanding of Cooking Contents , Ahmad Babaeian Babaeian Jelodar
Efficient Post-Quantum and Compact Cryptographic Constructions for the Internet of Things , Rouzbeh Behnia
Efficient Hardware Constructions for Error Detection of Post-Quantum Cryptographic Schemes , Alvaro Cintas Canto
Using Hyper-Dimensional Spanning Trees to Improve Structure Preservation During Dimensionality Reduction , Curtis Thomas Davis
Design, Deployment, and Validation of Computer Vision Techniques for Societal Scale Applications , Arup Kanti Dey
AffectiveTDA: Using Topological Data Analysis to Improve Analysis and Explainability in Affective Computing , Hamza Elhamdadi
Automatic Detection of Vehicles in Satellite Images for Economic Monitoring , Cole Hill
Analysis of Contextual Emotions Using Multimodal Data , Saurabh Hinduja
Data-driven Studies on Social Networks: Privacy and Simulation , Yasanka Sameera Horawalavithana
Automated Identification of Stages in Gonotrophic Cycle of Mosquitoes Using Computer Vision Techniques , Sherzod Kariev
Exploring the Use of Neural Transformers for Psycholinguistics , Antonio Laverghetta Jr.
Secure VLSI Hardware Design Against Intellectual Property (IP) Theft and Cryptographic Vulnerabilities , Matthew Dean Lewandowski
Turkic Interlingua: A Case Study of Machine Translation in Low-resource Languages , Jamshidbek Mirzakhalov
Automated Wound Segmentation and Dimension Measurement Using RGB-D Image , Chih-Yun Pai
Constructing Frameworks for Task-Optimized Visualizations , Ghulam Jilani Abdul Rahim Quadri
Trilateration-Based Localization in Known Environments with Object Detection , Valeria M. Salas Pacheco
Recognizing Patterns from Vital Signs Using Spectrograms , Sidharth Srivatsav Sribhashyam
Recognizing Emotion in the Wild Using Multimodal Data , Shivam Srivastava
A Modular Framework for Multi-Rotor Unmanned Aerial Vehicles for Military Operations , Dante Tezza
Human-centered Cybersecurity Research — Anthropological Findings from Two Longitudinal Studies , Anwesh Tuladhar
Learning State-Dependent Sensor Measurement Models To Improve Robot Localization Accuracy , Troi André Williams
Human-centric Cybersecurity Research: From Trapping the Bad Guys to Helping the Good Ones , Armin Ziaie Tabari
Theses/Dissertations from 2020 2020
Classifying Emotions with EEG and Peripheral Physiological Data Using 1D Convolutional Long Short-Term Memory Neural Network , Rupal Agarwal
Keyless Anti-Jamming Communication via Randomized DSSS , Ahmad Alagil
Active Deep Learning Method to Automate Unbiased Stereology Cell Counting , Saeed Alahmari
Composition of Atomic-Obligation Security Policies , Yan Cao Albright
Action Recognition Using the Motion Taxonomy , Maxat Alibayev
Sentiment Analysis in Peer Review , Zachariah J. Beasley
Spatial Heterogeneity Utilization in CT Images for Lung Nodule Classication , Dmitrii Cherezov
Feature Selection Via Random Subsets Of Uncorrelated Features , Long Kim Dang
Unifying Security Policy Enforcement: Theory and Practice , Shamaria Engram
PsiDB: A Framework for Batched Query Processing and Optimization , Mehrad Eslami
Composition of Atomic-Obligation Security Policies , Danielle Ferguson
Algorithms To Profile Driver Behavior From Zero-permission Embedded Sensors , Bharti Goel
The Efficiency and Accuracy of YOLO for Neonate Face Detection in the Clinical Setting , Jacqueline Hausmann
Beyond the Hype: Challenges of Neural Networks as Applied to Social Networks , Anthony Hernandez
Privacy-Preserving and Functional Information Systems , Thang Hoang
Managing Off-Grid Power Use for Solar Fueled Residences with Smart Appliances, Prices-to-Devices and IoT , Donnelle L. January
Novel Bit-Sliced In-Memory Computing Based VLSI Architecture for Fast Sobel Edge Detection in IoT Edge Devices , Rajeev Joshi
Edge Computing for Deep Learning-Based Distributed Real-time Object Detection on IoT Constrained Platforms at Low Frame Rate , Lakshmikavya Kalyanam
Establishing Topological Data Analysis: A Comparison of Visualization Techniques , Tanmay J. Kotha
Machine Learning for the Internet of Things: Applications, Implementation, and Security , Vishalini Laguduva Ramnath
System Support of Concurrent Database Query Processing on a GPU , Hao Li
Deep Learning Predictive Modeling with Data Challenges (Small, Big, or Imbalanced) , Renhao Liu
Countermeasures Against Various Network Attacks Using Machine Learning Methods , Yi Li
Towards Safe Power Oversubscription and Energy Efficiency of Data Centers , Sulav Malla
Design of Support Measures for Counting Frequent Patterns in Graphs , Jinghan Meng
Automating the Classification of Mosquito Specimens Using Image Processing Techniques , Mona Minakshi
Models of Secure Software Enforcement and Development , Hernan M. Palombo
Functional Object-Oriented Network: A Knowledge Representation for Service Robotics , David Andrés Paulius Ramos
Lung Nodule Malignancy Prediction from Computed Tomography Images Using Deep Learning , Rahul Paul
Algorithms and Framework for Computing 2-body Statistics on Graphics Processing Units , Napath Pitaksirianan
Efficient Viewshed Computation Algorithms On GPUs and CPUs , Faisal F. Qarah
Relational Joins on GPUs for In-Memory Database Query Processing , Ran Rui
Micro-architectural Countermeasures for Control Flow and Misspeculation Based Software Attacks , Love Kumar Sah
Efficient Forward-Secure and Compact Signatures for the Internet of Things (IoT) , Efe Ulas Akay Seyitoglu
Detecting Symptoms of Chronic Obstructive Pulmonary Disease and Congestive Heart Failure via Cough and Wheezing Sounds Using Smart-Phones and Machine Learning , Anthony Windmon
Toward Culturally Relevant Emotion Detection Using Physiological Signals , Khadija Zanna
Theses/Dissertations from 2019 2019
Beyond Labels and Captions: Contextualizing Grounded Semantics for Explainable Visual Interpretation , Sathyanarayanan Narasimhan Aakur
Empirical Analysis of a Cybersecurity Scoring System , Jaleel Ahmed
Phenomena of Social Dynamics in Online Games , Essa Alhazmi
A Machine Learning Approach to Predicting Community Engagement on Social Media During Disasters , Adel Alshehri
Interactive Fitness Domains in Competitive Coevolutionary Algorithm , ATM Golam Bari
Measuring Influence Across Social Media Platforms: Empirical Analysis Using Symbolic Transfer Entropy , Abhishek Bhattacharjee
A Communication-Centric Framework for Post-Silicon System-on-chip Integration Debug , Yuting Cao
Authentication and SQL-Injection Prevention Techniques in Web Applications , Cagri Cetin
Multimodal Emotion Recognition Using 3D Facial Landmarks, Action Units, and Physiological Data , Diego Fabiano
Robotic Motion Generation by Using Spatial-Temporal Patterns from Human Demonstrations , Yongqiang Huang
Advanced Search
- Email Notifications and RSS
- All Collections
- USF Faculty Publications
- Open Access Journals
- Conferences and Events
- Theses and Dissertations
- Textbooks Collection
Useful Links
- Rights Information
- SelectedWorks
- Submit Research
Home | About | Help | My Account | Accessibility Statement | Language and Diversity Statements
Privacy Copyright
Computer Vision, ML, and AI in the Study of Fine Art
Ongoing research in the analysis of art is building upon the vast store of algorithms and knowledge from mainstream computer vision, deep learning, and artificial intelligence.
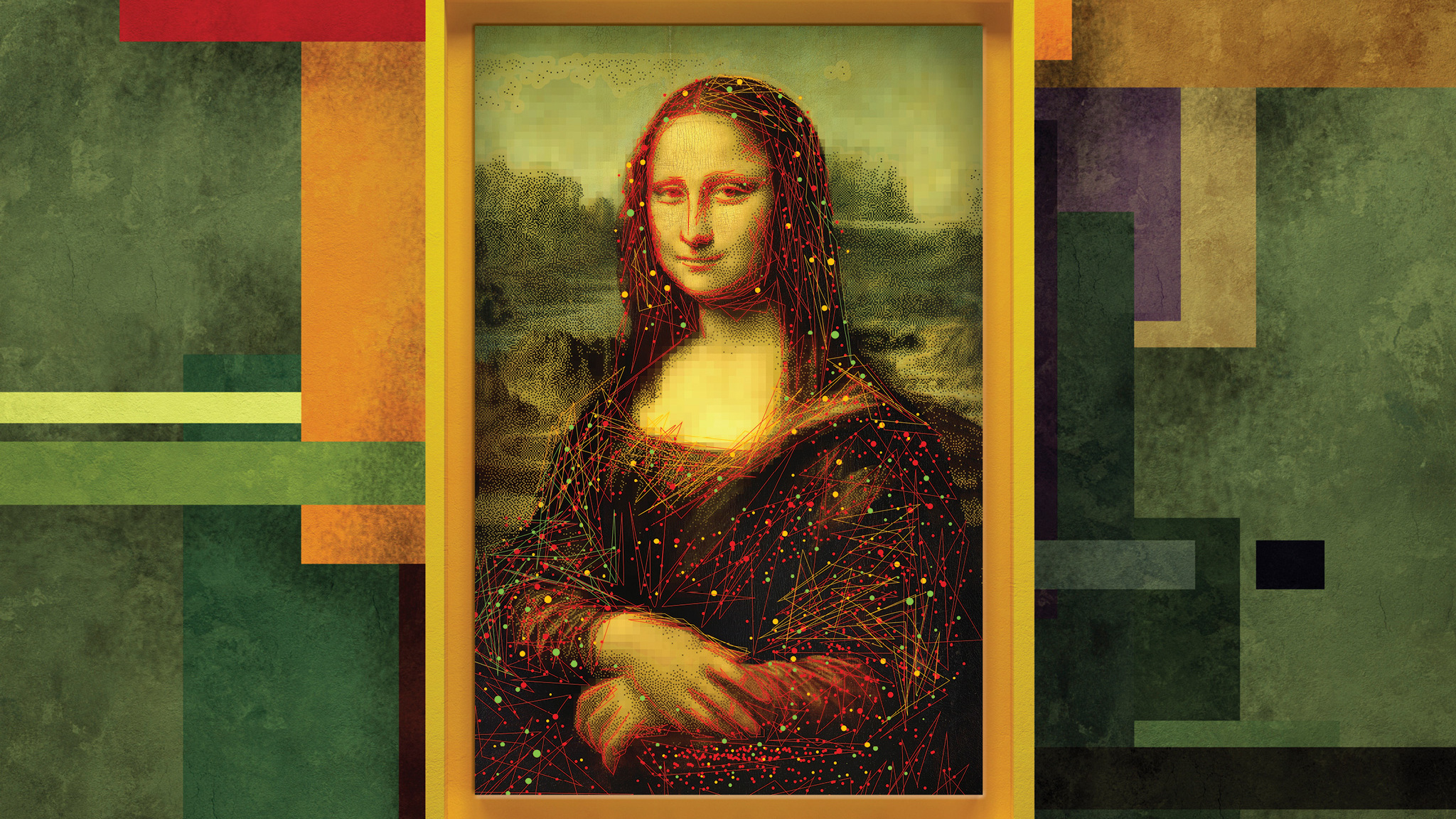
- Hacker News
- Download PDF
- Join the Discussion
- View in the ACM Digital Library
Computer Vision in the Study of Fine-Art Paintings and Drawings
Computer-based tools for image analysis of art, computer methods in resolving debates in art analysis, problems in art analysis that resist techniques from mainstream ai research, conclusions, opportunities, and future directions, acknowledgments.
In the past decade, computer vision (CV), machine learning (ML), and artificial intelligence (AI) have been applied to problems in the history and interpretation of fine-art paintings and drawings. Such automated methods provide new tools for connoisseurs and art scholars and have resolved art-historical debates that proved resistant to traditional art-historical methods. Nevertheless, immense challenges and opportunities remain for the application of AI in the study of art, specifically on problems that are inadequately addressed by mainstream AI research. For this reason, art analysis serves as a grand challenge to image-based AI.
Key Insights
Fine-art images are some of the most sophisticated, complex and valuable images ever created.
Such images present entire classes of problems not well addressed by mainstream AI research.
Fine-art paintings and drawings thus serve as a grand challenge to AI.
The computational tools, used with deep understanding of the relevant art-historical context, empower art scholars to answer outstanding questions, pose new classes of questions, and develop richer interpretive strategies.
Advances in imaging technology and especially CV and AI have, for decades, benefited nearly every scientific and engineering discipline, including medicine, geology, biology, chemistry, and psychology. Consider that works of art bear the most memorable and important images ever created by humans, and many works themselves are exceedingly valuable—not just financially but culturally. It is natural, then, that computer methods, properly guided by scholars’ knowledge of history and context, should be of service in the humanistic studies of art as well. In fact, in the past few years, rigorous automated image analysis has assisted some art historians, critics, and connoisseurs in their scholarly studies of fine-art paintings and drawings.
Such rigorous computer image analysis of fine art is rather different from traditional “digital humanities,” which has generally concentrated on digital methods of capture and display but where the fundamental analyses and interpretations are still performed by human scholars and connoisseurs. 38 As we shall see, however, artworks pose several profound problems that require sophisticated methods beyond those in traditional digital humanities. These represent a grand challenge to AI, well beyond what is generally addressed in research in digital humanities programs and even mainstream artificial intelligence.
This article explores three manifestations of research into computer image-based analysis of fine art. First, the article offers an example of how computer image analysis can help art scholars by expanding traditional non-automatic approaches. Then, we refer to some debates and issues in art scholarship that have been aided significantly—and in some cases solved—thanks in large part to computer methods. The article then turns to broad problems in image-based intelligence that arise in art analysis that are inadequately addressed by mainstream AI research and hence present great opportunities for research. The article concludes with thoughts about future directions.
Computer image analysis has automated several tasks traditionally performed by connoisseurs and art historians, such as the analysis of composition in landscapes, 22 of brushstrokes and other marks, 23 of canvas weave, 24 lighting, 21 and general properties of style. 9 Such tools do not replace the connoisseur but instead empower art scholars and extend the analyses they can perform, including at scales impractical for efforts solely “by eye.” These digital tools, when used with an awareness of the art historical context, can provide rigor and consistency to analyses and enable the analyses of large-scale trends.
Consider just one example, the analysis of pose in portraiture—a formal property that artists set for numerous compositional and expressive ends. 39 Art scholars have traditionally used rather subjective, informal, and coarse descriptions of portrait poses—such as frontal, profile, or three-quarters view—which they determine entirely by eye. Such traditional methods scale poorly and preclude detailed analyses of thousands of portraits, as might reveal trends in portraiture over centuries or even over a single prolific portraitist’s career.
Computer image analysis offers a powerful tool for portrait pose analysis. Figure 1 shows how a pose can be described by three rotation angles as well as a “production model” of the projection of a generic head onto the artist’s picture plane. Two deep neural network (DNN) methods—Perspective-n-point 17 and Fine-grained Structured Aggregation network (FSA-net) 40 —can estimate these pose angles automatically based on the locations of extracted visual keypoints, such as the corners of the eyes, mouth, tip of the nose, and so on. These algorithms prove remarkably robust to variations in artistic style.
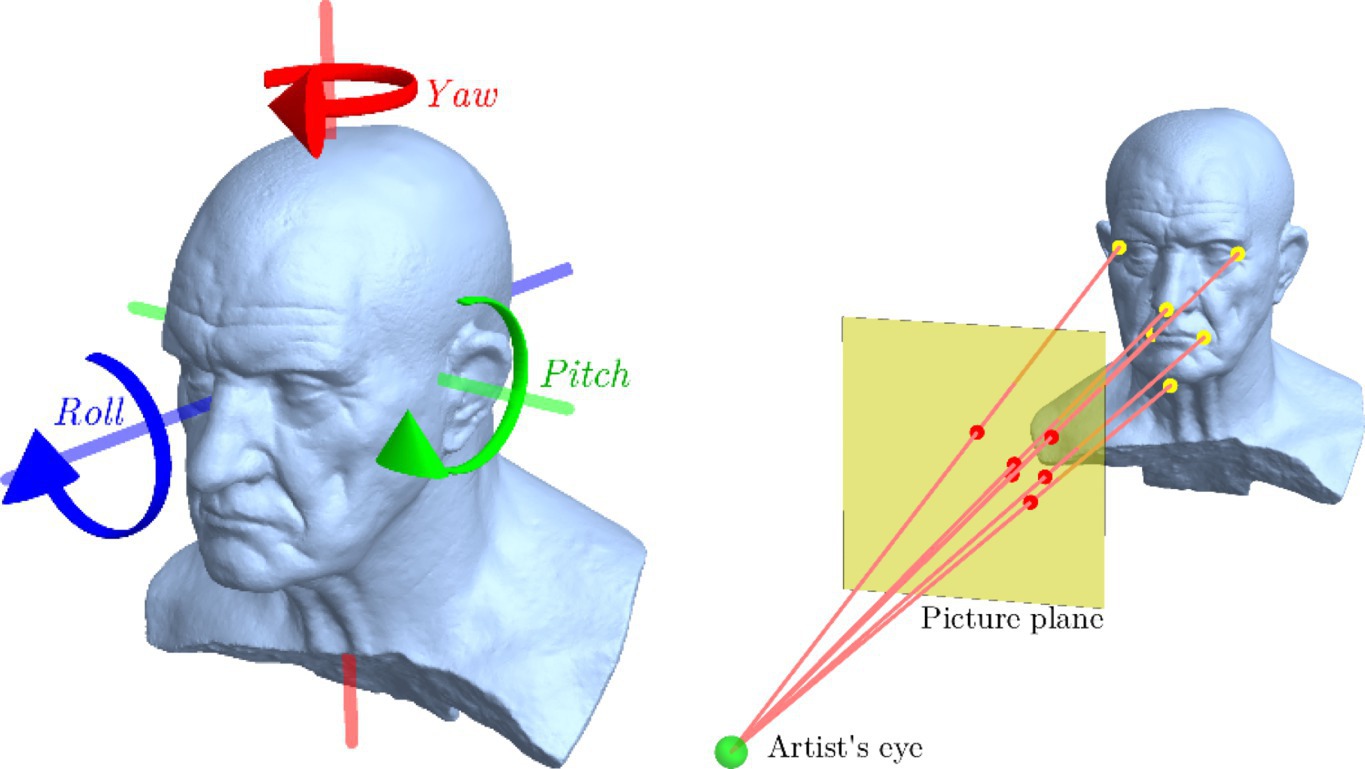
Such automated tools permit the rapid and accurate analysis of large corpora of portraits. Figure 2 shows computed distributions of the absolute value of the roll angle of 11,000 portraits from a half millennium of Western canon and Japanese art, here grouped by art movement. Yaw, tilt, and measures such as the location of the head within the frame of the artwork can be similarly estimated, all within four minutes on a desktop computer. 4
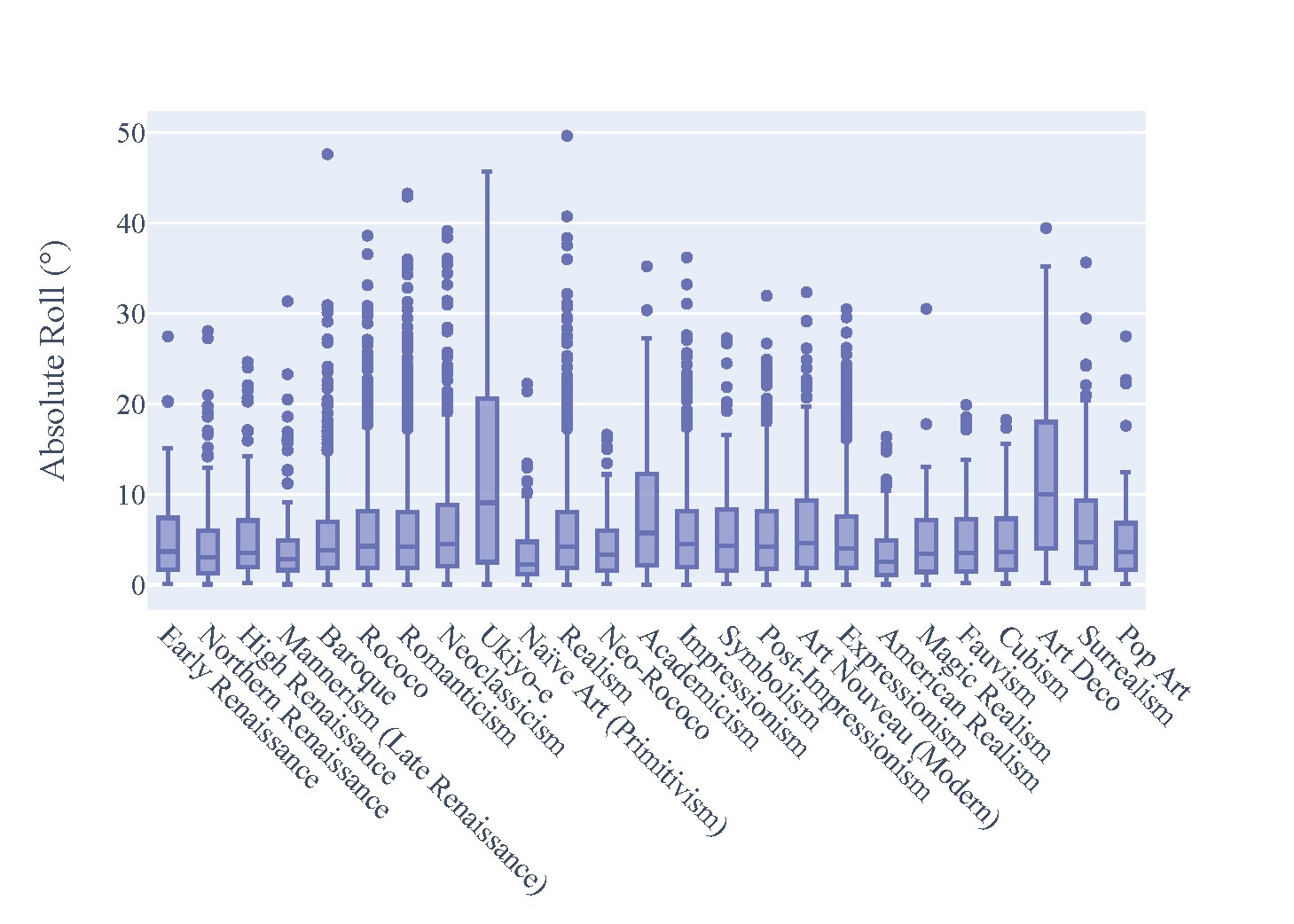
There is rich information to be interpreted in this, and related plots, but consider just the differences between Japanese Ukiyo-e (“Floating world”) art and so-called Naïve or Primitivist art. The box-whisker plots in Figure 2 confirm that Ukiyo-e portraits often exhibit large rotation angles associated with the mie poses of kabuki actors in dramatic moments in plays, or of geishas in seductive poses. By contrast, in Naïve or Primitivist portraits, poses are rather “simple,” favoring direct forward gaze. Such pronounced differences are evident in the representative works in Figure 3 .
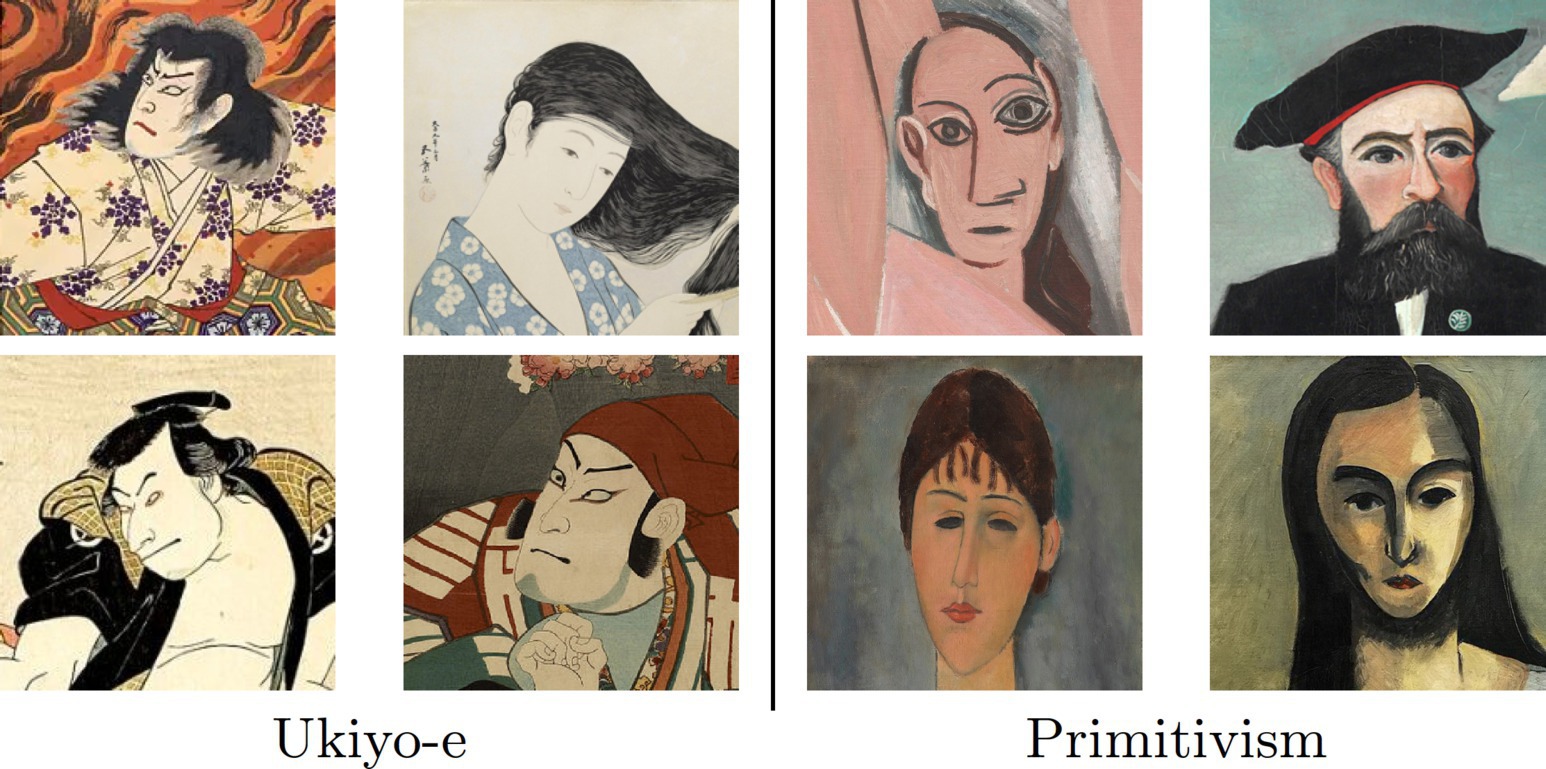
Artists often take characteristic bodily stances in the studio when executing self-portraits. For instance, a right-handed artist will place the easel to the right of the plane mirror so as to better reach it with the dominant hand, and the artist’s head is often rotated somewhat toward the canvas. The head of a left-handed artist will be rotated in the opposite direction. The distribution of (signed) yaw angles will differ between right- and left-handed self-portraitists; indeed, such differences are evident in the computational data. That is, the computational results reveal which artists were likely left-handed. 4
The automated pose-estimation algorithms extend previous work, providing a foundation for a range of future studies in art analysis. 18 For instance, one can incorporate face-based gender recognition as a pre-processing step to explore gender-specific trends in poses. 25 Likewise, with possible style-based tailoring of face-recognition algorithms, one might explore trends in non-Western art, particularly Asian art, such as portraits from the Song Dynasty 41 or from the Renaissance. 13
Deep neural networks for art analysis. Deep networks developed for natural photographs have been modified through their architectures and transfer training to perform well on segmentation of art images throughout a wealth of styles and media—a task vastly more difficult than in natural images. 14 Deep networks trained with large corpora of art images and contributed textual summaries of human responses can accurately predict human emotional responses to novel artworks. 1 Deep networks have been applied to the extremely challenging and important task of art authentication. 8 , 28 Most of these methods address just image information and thus have not been accepted by the art community because authentication also rests on studies of materials (pigment, canvas, and so on), provenance (documentary record of ownership and display), iconography, and more. As such, there are deep challenges to AI for incorporating such diverse forms of information for authentication, which will be discussed again later in this article.
Rigorous computer image analysis has not merely served as a tool easing traditional interpretive tasks but has also resolved art historical debates for which methods from traditional art scholarship proved incomplete or inadequate. In 2000, the celebrated British and American artist David Hockney, later aided by thin-film physicist Charles Falco, claimed to have “proven” that some artists as early as 1420 secretly used optical devices during the execution of some of their works, and more broadly that the use of optics led to an enhanced realism or “optical look” of the “ars nova” or new art of that time. 15 , 16
Rigorous computer image analysis has not merely served as a tool easing traditional interpretive tasks but has also resolved art historical debates.
Part of the evidence they adduced rested on Hockney’s claim that the complex chandelier in Jan van Eyck’s Arnolfini portrait was “in perfect perspective,” which (Hockney claimed) implied van Eyck used optics to draw it. Sophisticated homographic analysis of the image of the chandelier, based on an ACM Dissertation Award-winning thesis, 6 showed that in fact the chandelier deviated significantly from “perfect perspective,” thereby rebutting the optical proposal, at least for that painting. 5 , 6 , 29
Hockney also adduced Georges de la Tour’s Christ in the Carpenter’s Shop as evidence that this artist used optical projections. In brief, his claim rests on his reading of the work that the light source was “outside the [frame of the] picture.” Application of sophisticated and rigorous occluding-contour algorithms and maximum-likelihood estimation of the location of the source of illumination based on five classes of image information showed definitively that Hockney’s claim was false and that instead the source of the illumination was at the depicted candle. Such rigorous methods thus refuted Hockney’s argument. 36
Hockney and Falco claimed their most definitive evidence for Lorenzo Lotto’s putative use of optics arose in Husband and Wife , where they claimed geometric and other “anomalies” in the depicted carpet “proved” that Lotto used a small, concave mirror to project an image during the execution of this work. 16 Rigorous analysis using computer ray-tracing software showed instead that the proposed setup simply could not work as claimed. Moreover, when the required corrections to their setup were made, the computer ray-tracing evidence contradicted the optical claim. 27
Broadly speaking, computer image analysis supported and confirmed the unanimous independent scholarly rejection of Hockney’s theory. 35 Indeed, partially in response to the rigorous computer-assisted analyses, Hockney himself has retreated from his projection claim. Similarly, engineer and self-described non-artist Tim Jenison argued that Johannes Vermeer secretly used a catadioptric telescope during the creation of his sublime interior genre paintings, 20 but computer-enhanced analyses (and facts of historical and material culture) refuted Jenison’s claim. 31 , 32 , 37
Most traditional AI algorithms have been developed for analyzing natural photographs, videos, and specialized images such as medical x-radiographs. These images have properties that, at base, derive from the laws governing the natural world, be they everyday physics of objects and light, medical or remote images governed by electromagnetic radiation, and so on. Art images differ in numerous ways from those just listed and present several deep challenges that are inadequately addressed by current mainstream research, including the following:
Style. There is no unanimous agreement concerning the definition of style in fine-art paintings. Nevertheless, style certainly refers to formal properties of color, composition, marks, brush strokes, and so on considered as distinct from the nominal subject matter. Portraits, to take just one genre, can be rendered in styles that are highly realistic, expressive, or abstract, in unnatural colors executed in a wide variety of marks and brush strokes and innumerable personal styles. The variety of styles found in paintings is vastly greater than that found in the natural photographs that have commanded the attention of the AI community.
Style presents several challenges to computer analysis, for instance the recognition of style and identifying an artist (“author”) by the style of a work: image segmentation, object recognition, and scene analysis. Works such as shown in Figure 4 present great challenges in these regards—challenges to human viewers and algorithms alike.
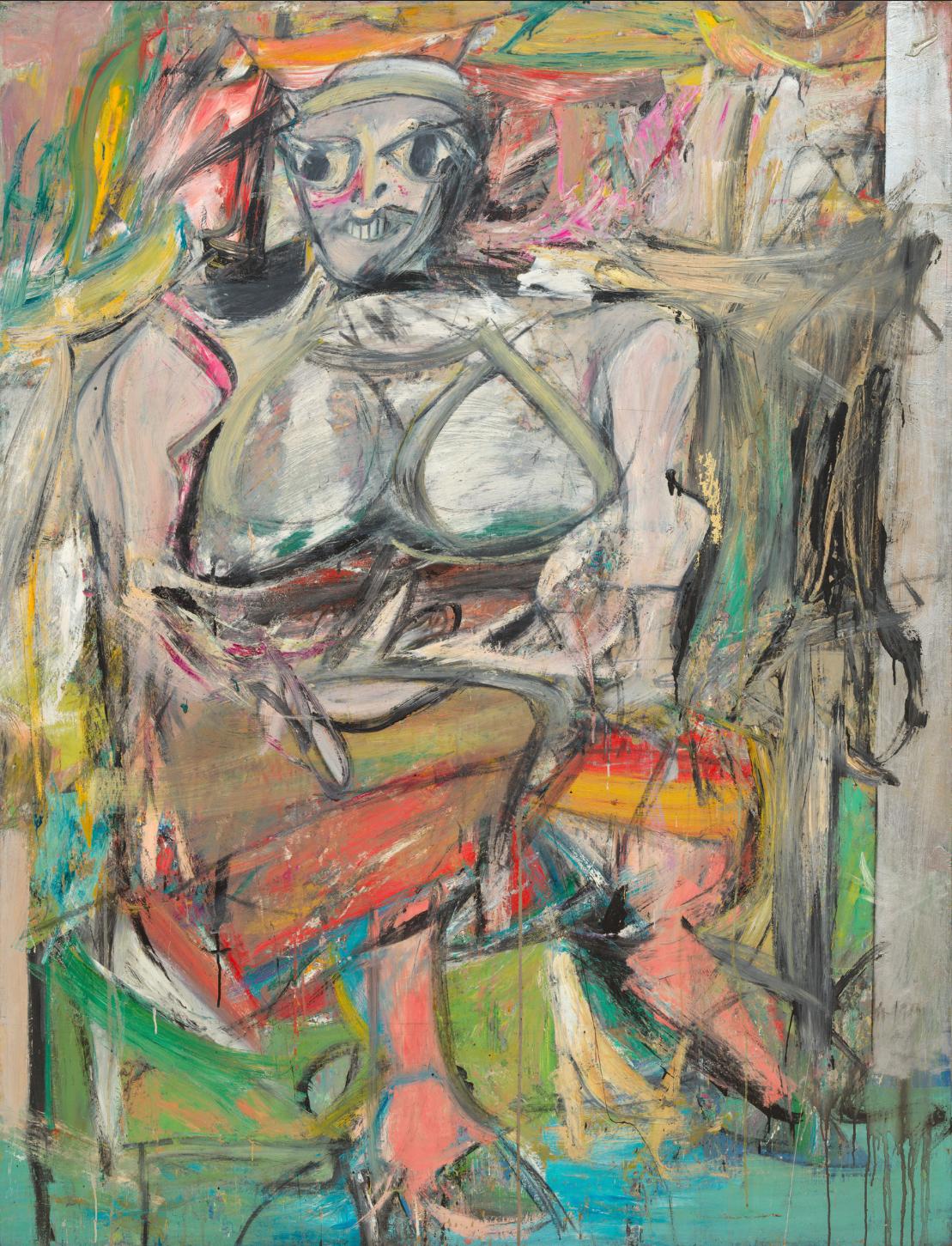
Small data sets. As mentioned earlier in this article, an important recent development in image analysis is the use of DNNs trained with hundreds of millions or more natural photographs. Such approaches have provided human-level or superhuman-level performance on several image-analysis tasks. Unfortunately, such systems generally perform quite poorly on stylized paintings and drawings, for instance the work in Figure 4 .
The rather obvious approach would be to train deep networks with large corpora of representative art images. Alas, this approach generally cannot be followed directly because there simply are not enough representative art images. After all, the Spanish master Pablo Picasso—one of the most prolific artists of all time—leaves us merely 13,500 paintings. Johannes Vermeer leaves us just 33, each considered a distinctive masterpiece, such as shown in Figure 5 .
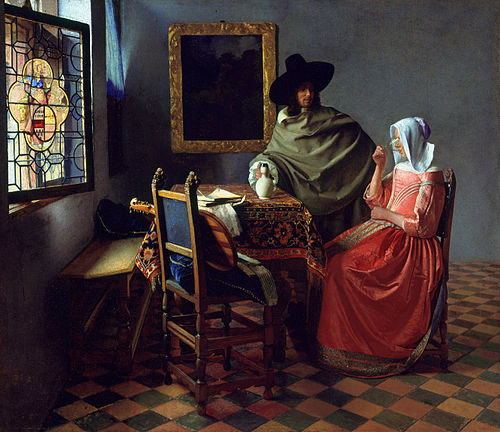
One way to overcome the relative paucity of art images is to transfer-train a DNN, that is, present art images as additional training patterns to a network previously trained with a very large number of photographs. This approach has proven of only modest value on problems such as image segmentation and is unlikely to provide significant benefit on more challenging problems, such as scene analysis. An alternate approach has shown success for the problem of image segmentation in artworks. We used deep networks to map artistic style onto photographs to thereby produce a large corpus of images of modern subjects rendered in artistic styles of artists. Training with this large corpus of “surrogate artworks” yields highly accurate performance on tasks such as segmentation. 14 Nevertheless, much research remains to be done.
Imaginary objects. Non-realist artists frequently depict imaginary objects or creatures, such as angels, dragons, and so on. Artists from the Dada Movement of the early 20 th century, such as Salvador Dalí, Marcel Duchamp, Man Ray, and René Magritte, frequently altered the properties of objects for surprise. The fact that these objects are unusual or even unique is often central to the artist’s expressive goals, of course. The fact that such objects are rare or unique presents a great challenge to AI systems for recognizing such objects and interpreting their associated artworks. These objects do not appear in large corpora of photographs typically used for training networks for image analysis. Perhaps one approach would be to develop modular deep networks that can flexibly decompose an image passage into components and styles that are rarely found in images.
Nonphysical conventions. Because artists are not constrained to slavishly depict the physical world, they can employ nonphysical conventions in service of their artistic goals. Thus, they can depict figures floating into the heavens, bulls that swim, infants that glow, and so on, as exemplified in Figure 6. The computational problem here is to recognize the non-physical convention given that training sets of natural photographs do not depict scenes in such conventions.
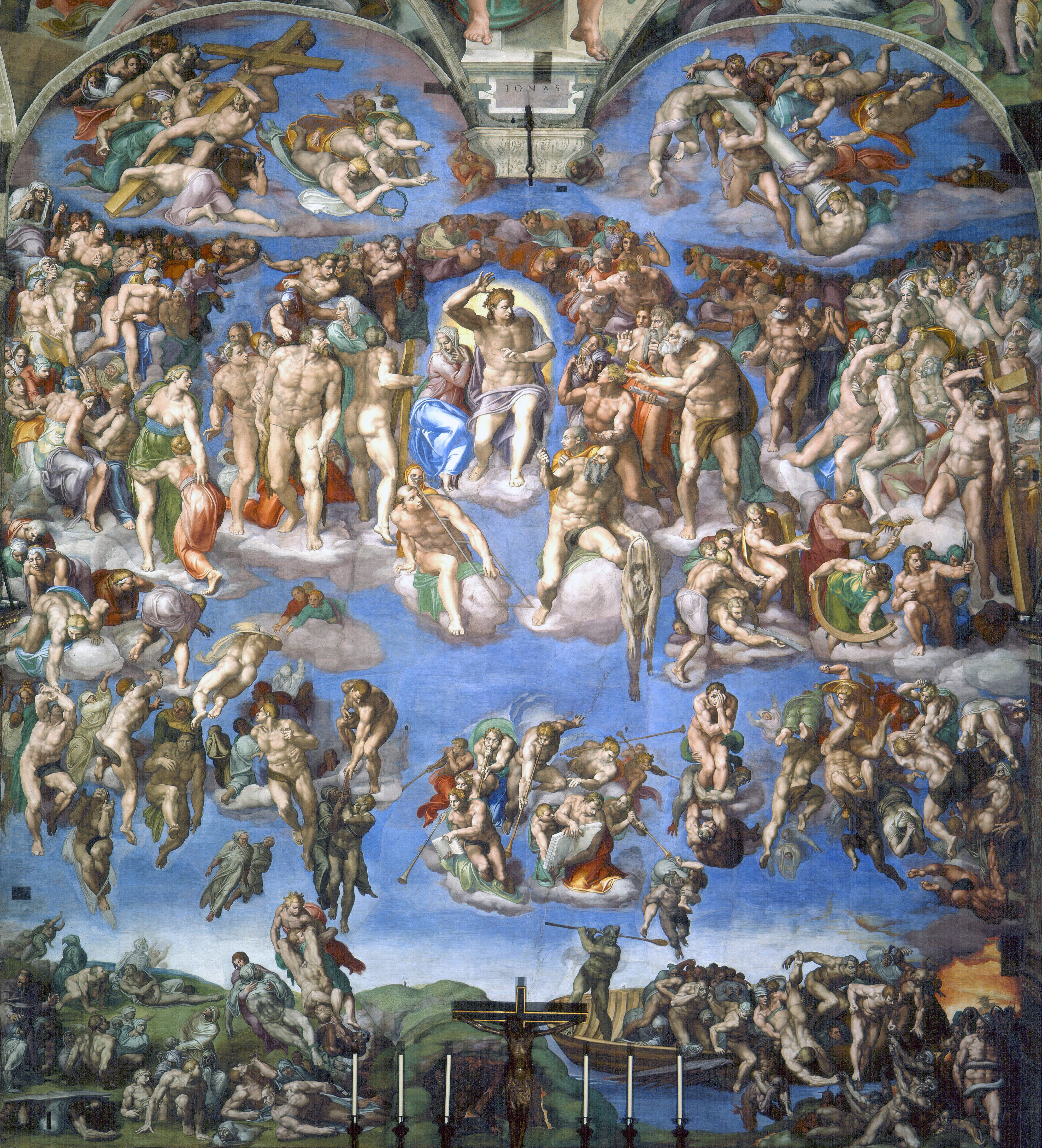
The development of a work, as revealed by its multiple layers. Many works of art, in particular Old Master easel paintings, were developed through underdrawings, revisions, and so on. These hidden layers are revealed through X-ray, infrared, and other imaging methods. Art scholars seek to understand an artist’s praxis and artistic intent by studying the changes in composition and other formal properties. For example, Figure 7 shows an X-ray and the visible image in the central passage in Rembrandt’s The Night Watch . Careful examination reveals numerous differences—large and small—between these two versions, and these are studied closely by art scholars crafting interpretations.
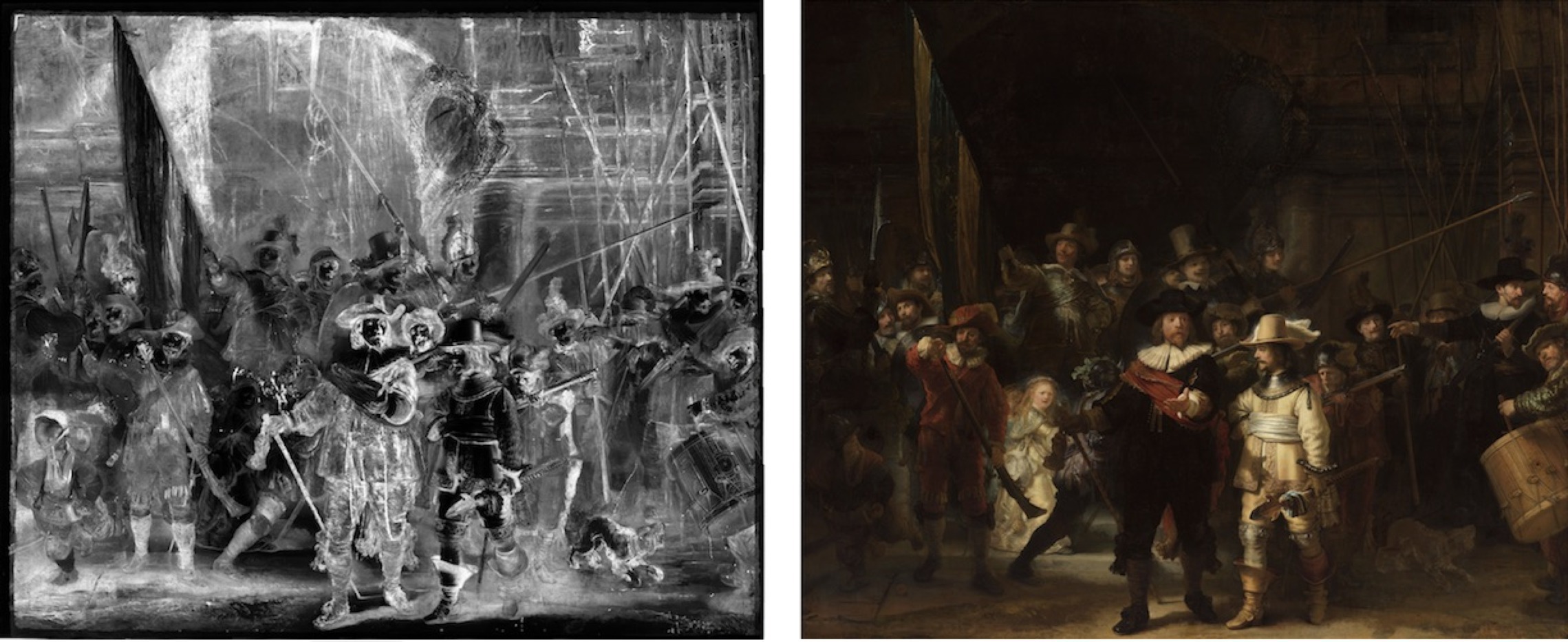
The computational task is to detect, represent, analyze, and ultimately interpret such changes, which have no counterpart in the vast number of natural photographs that dominate traditional AI research.
Abstraction. Abstraction is an extremely important genre of art, spanning a great variety of forms and styles (See Figure 8), and one for which natural photographs have little or no relevance. The challenges to AI research include recognizing artists (“authors”) by their abstract works, 19 , 30 tracing the development of abstract styles throughout an artist’s career (and thus helping to establish the execution date of works), and related problems.
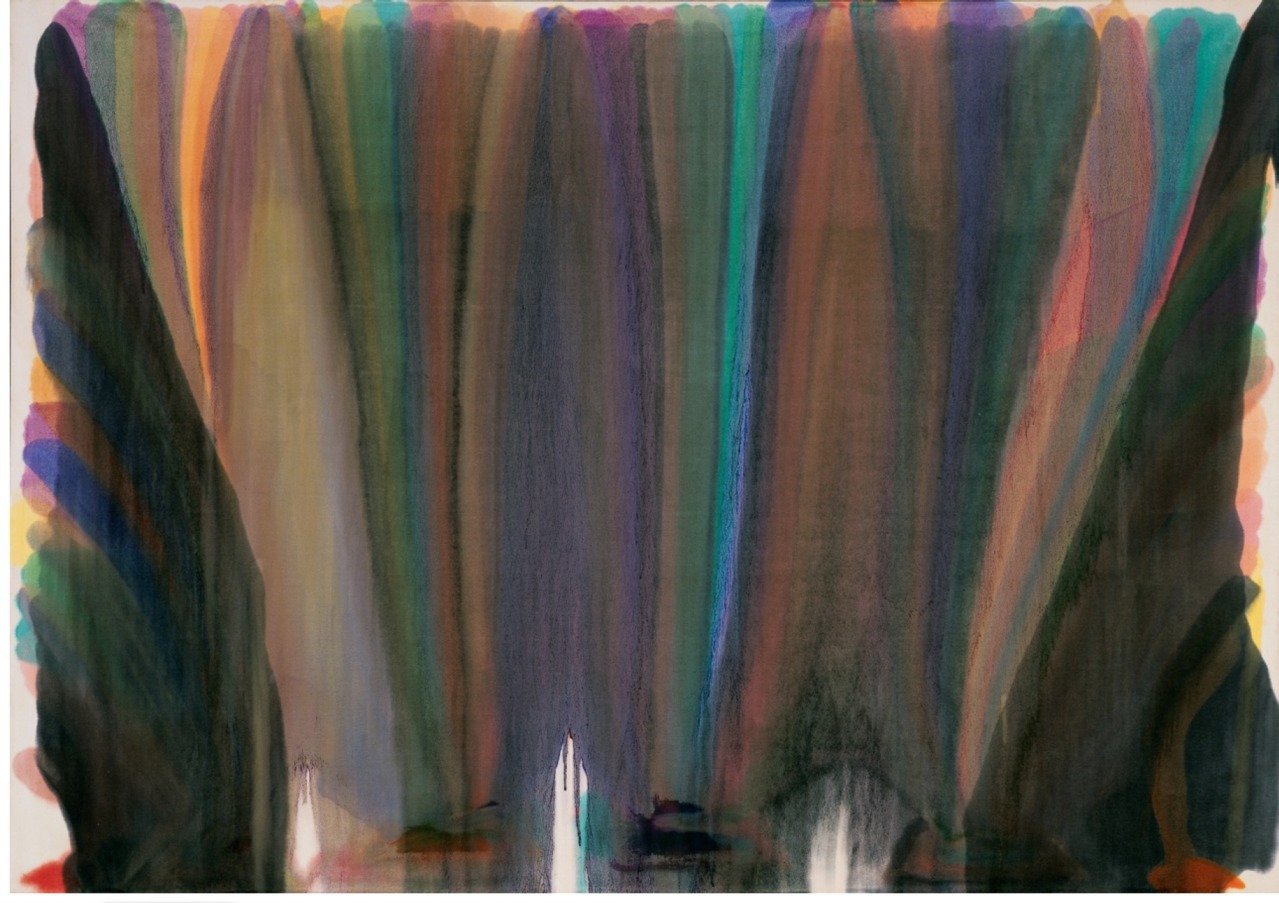
Recovering lost works. A great deal of fine art, including some of the most important and influential artworks ever created, has been lost to war, fire, flood, iconoclasm, theft, and other reasons. 3 For example, Diego Velázquez’s Las Meninas is often considered one of the most important paintings of the Western canon; it barely escaped a fire in the Alcazar Palace in 1743. Alas, this artist’s Expulsion of the Moriscos , which in his day was even more highly praised than Las Meninas , was completely burned in that same fire. Recovering the image of such a work would be of immense value to art history and to our cultural heritage more generally. It would provide deeper insight into Velázquez and his oeuvre, the cultural environment of the Spanish Golden Age, provide insights into artists who were influenced by the lost work, and much more. 7
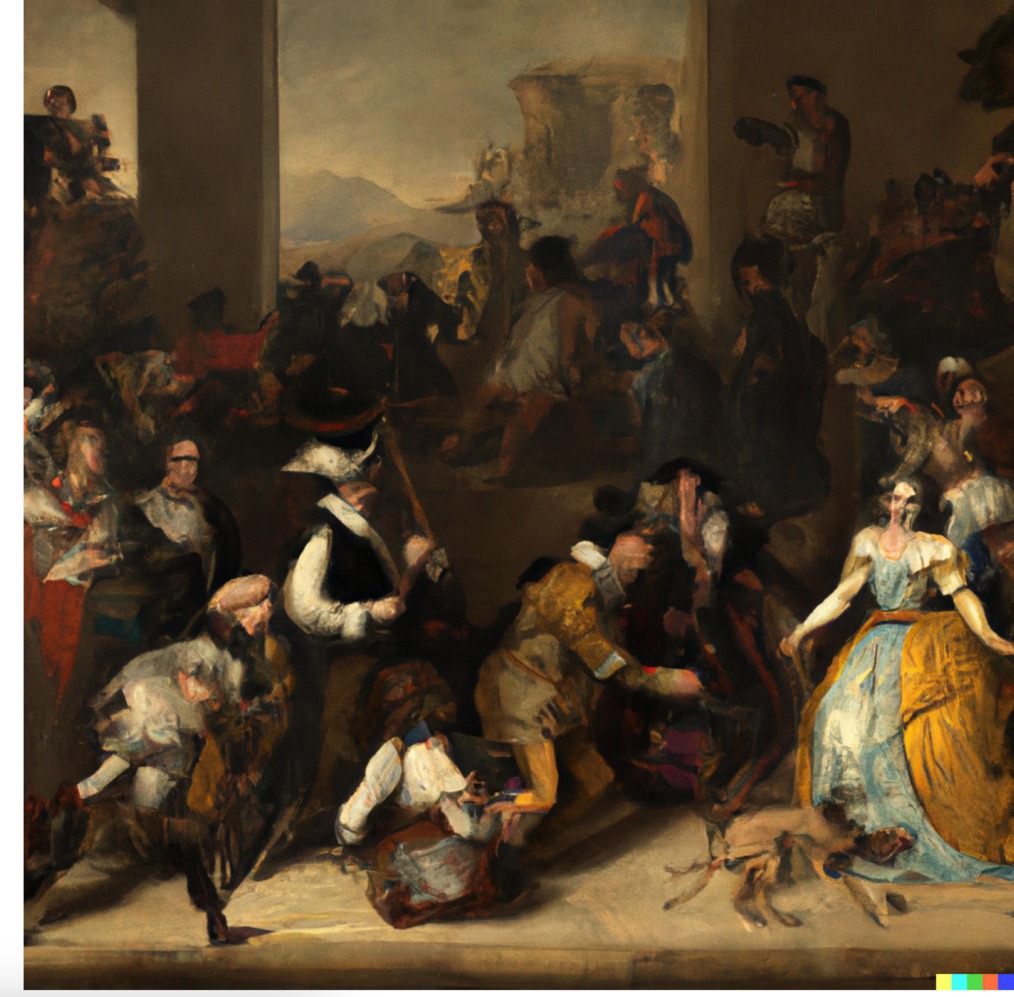
Computational methods based on DNNs have shown promise in recovering properties or portions of lost artwork, and thus show such as the colors in paintings by the Austrian artist Gustav Klimt, 26 and of ghost paintings 2 and missing passages of Rembrandt’s The Night Watch . 10 Computational reconstruction of a full image of a lost artwork would require a sophisticated integration of information in diverse forms: preparatory sketches and other works by the artist, copies by other artists, textual descriptions of the work, knowledge of the author’s working methods and media (available pigments and drawing tools), the likely date of execution, and more, as shown in the proof-of-concept computational reconstruction in Figure 9. 11
Semantics. Much of human communications is indirect or non-explicit, and so only relatively narrow forms of intelligence can be captured in datasets of real images, for instance. While one of the core purposes of artwork was for the artist to convey a complex and indirect meaning, verbose descriptions for which accompany virtually every masterpiece.
As a result, perhaps the deepest and most challenging class of problems posed by art that is not adequately addressed by current traditional AI research concerns semantics, that is, deriving coherent “meanings” associated with works.
Mainstream AI approaches to semantic image analysis seek to form text summaries, such as captions, of a photographic image. The semantic problems arising in art are rather different. Here the goal is to infer the artist’s meaning or intention, for example what message, moral, story, or abstract idea the artist seeks to convey. Consider, for instance, René Magritte’s celebrated T he Treachery of Images in Figure 10. Mainstream semantic analysis would recognize the pipe, and the text, and presumably detect the contradiction, but not infer why the artist created this work and how this relates to the fact that this is a painting of a pipe, a painting of text, and much more. Preliminary results using deep networks extract components of meaning in religious art. 34
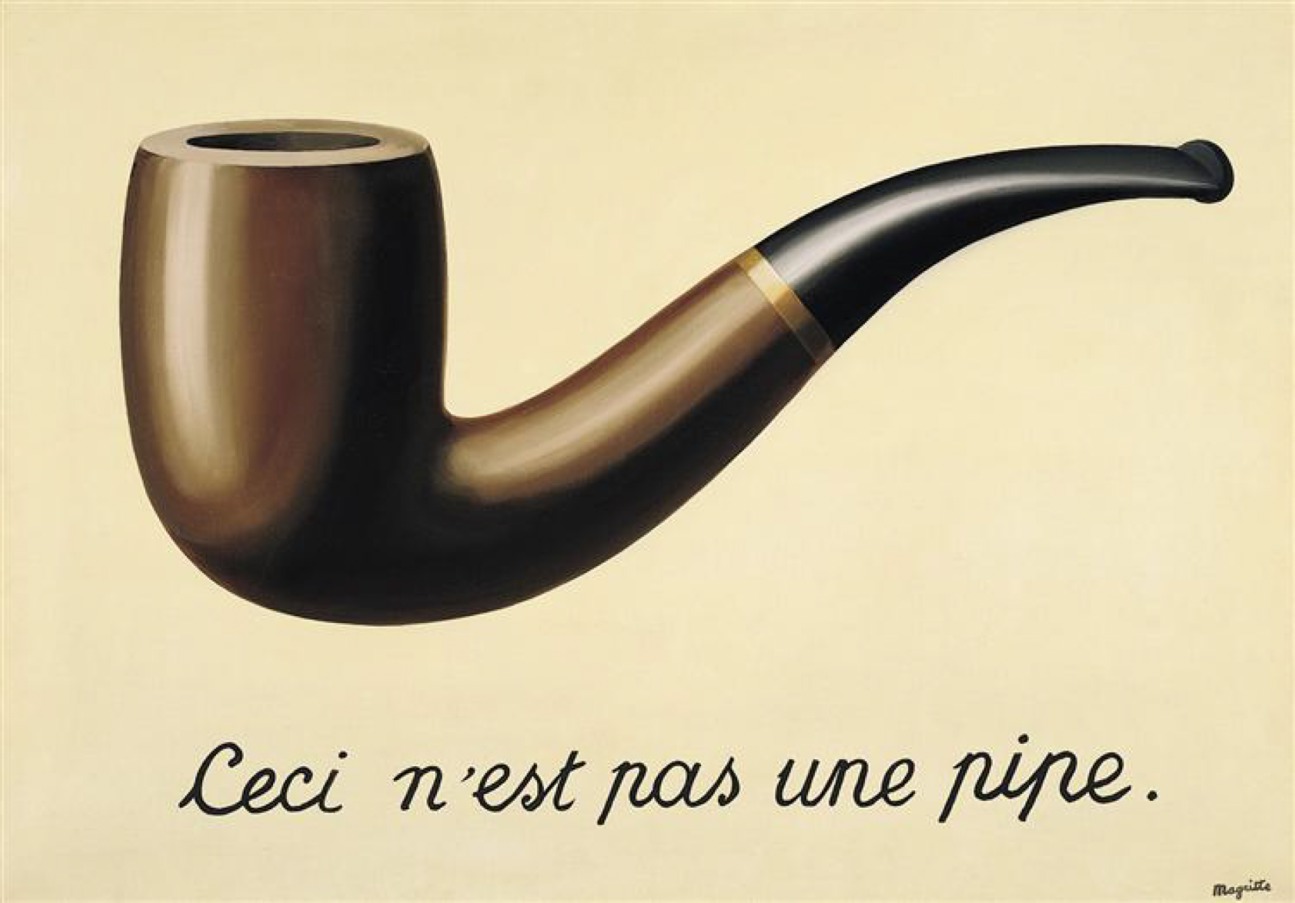
Fine art paintings and drawings represent some of the most carefully constructed, memorable, complex, and important images of any form, and they present deep challenges to computer vision and artificial intelligence that in many cases are not addressed by mainstream research focused on natural photographs, medical and remote sensed images, and others.
Ongoing research in the analysis of art is building upon the vast store of algorithms and knowledge from mainstream computer vision, deep learning, and artificial intelligence. Continued progress demands an integration of humanists’ knowledge of art historical facts and contexts as well as on computer scientists’ knowledge of algorithms, and creativity in tailoring them to problems in art. Such a research program promises to empower humanist scholars and shed light upon some of the most sophisticated and fascinating aspects of intelligence—human and machine. 12 , 33
The author wrote much of this article while an External Reader at the Library at the Getty Research Institute, Los Angeles and as a Leonardo@Djerassi Fellow in Residency at the Djerassi Foundation, Woodside, CA, and would like to thank these institutions for their support.
Submit an Article to CACM
CACM welcomes unsolicited submissions on topics of relevance and value to the computing community.
You Just Read
© 2024 Copyright held by the owner/author(s). Publication rights licensed to ACM.
May 2024 Issue
Vol. 67 No. 5
Pages: 68-75
Related Reading
To PiM or Not to PiM
Architecture and Hardware
Research and Advances
Democratizing Domain-Specific Computing
Advertisement
Join the Discussion (0)
Become a member or sign in to post a comment, the latest from cacm.
How Does Life Change Post Academic Tenure?
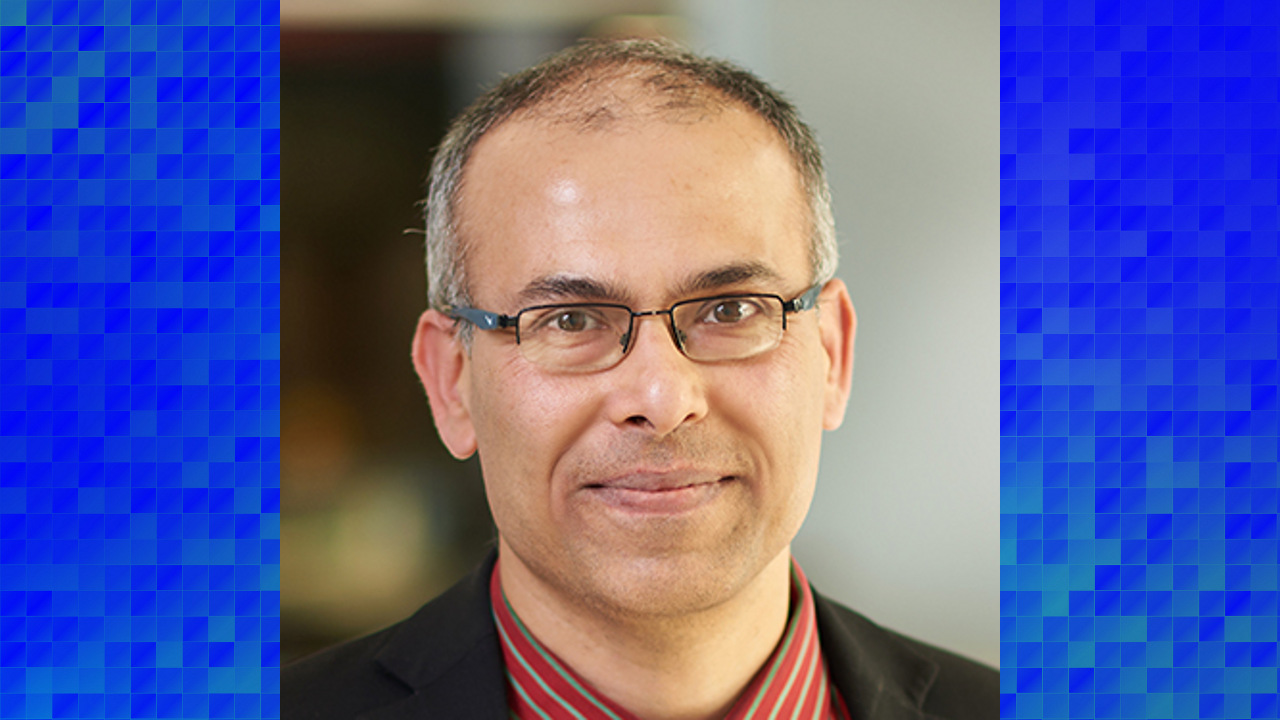
LLM Hallucinations: A Bug or A Feature?
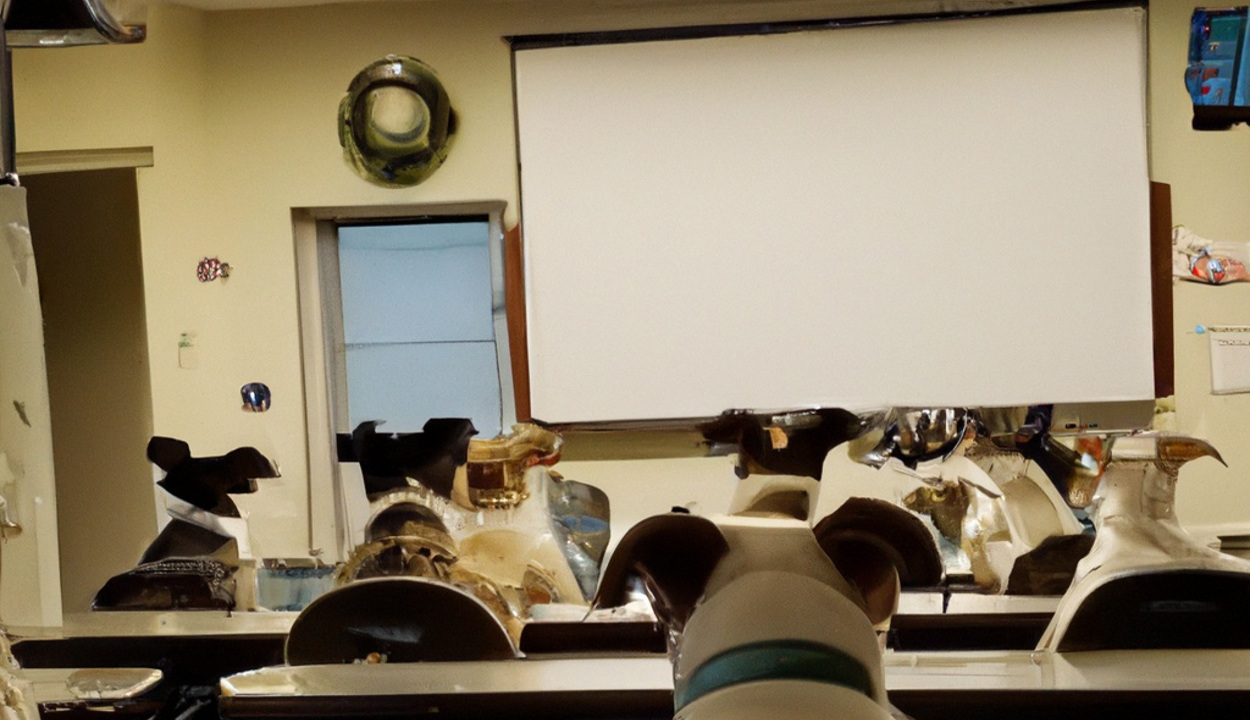
When Colorless Green DNNs Sleep Furiously in an Unexplainable Fantasy
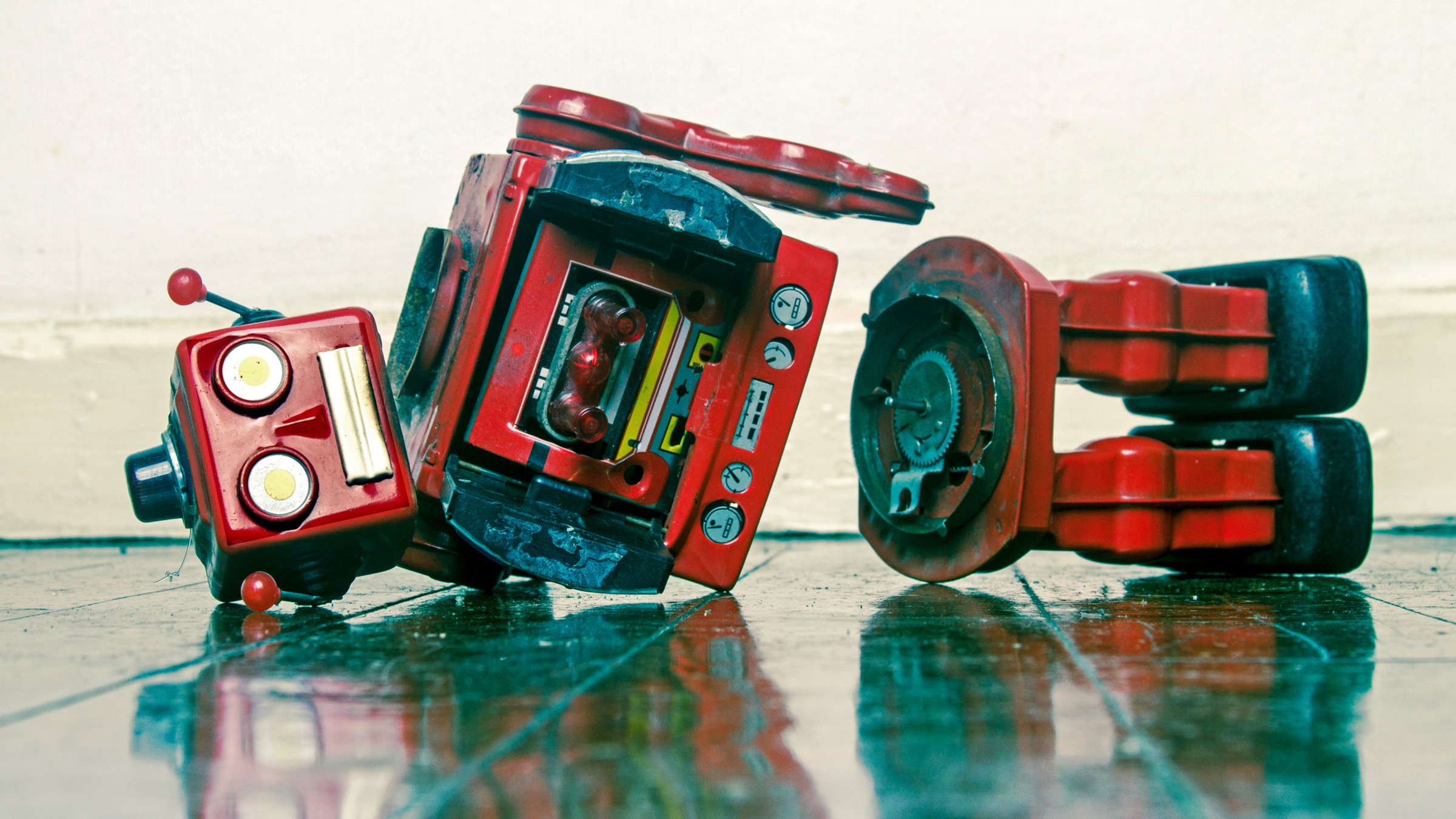
Shape the Future of Computing
ACM encourages its members to take a direct hand in shaping the future of the association. There are more ways than ever to get involved.
Communications of the ACM (CACM) is now a fully Open Access publication.
By opening CACM to the world, we hope to increase engagement among the broader computer science community and encourage non-members to discover the rich resources ACM has to offer.
Advanced Topics in Computer Vision
- © 2013
- Giovanni Maria Farinella ORCID: https://orcid.org/0000-0002-6034-0432 0 ,
- Sebastiano Battiato ORCID: https://orcid.org/0000-0001-6127-2470 1 ,
- Roberto Cipolla 2
Dipartimento di Matematica e Informatica, Università di Catania, Catania, Italy
You can also search for this editor in PubMed Google Scholar
Department of Engineering, University of Cambridge, Cambridge, United Kingdom
- Presents a broad selection of cutting-edge research from internationally-recognized computer vision groups
- Covers both theoretical and practical aspects of reconstruction, registration, and recognition
- Provides an overview of challenging areas, and describes novel algorithms designed to infer the semantic content of images and videos
- Includes supplementary material: sn.pub/extras
Part of the book series: Advances in Computer Vision and Pattern Recognition (ACVPR)
44k Accesses
53 Citations
3 Altmetric
This is a preview of subscription content, log in via an institution to check access.
Access this book
- Available as EPUB and PDF
- Read on any device
- Instant download
- Own it forever
- Compact, lightweight edition
- Dispatched in 3 to 5 business days
- Free shipping worldwide - see info
- Durable hardcover edition
Tax calculation will be finalised at checkout
Other ways to access
Licence this eBook for your library
Institutional subscriptions
Table of contents (14 chapters)
Front matter, visual features—from early concepts to modern computer vision.
- Martin Weinmann
Where Next in Object Recognition and how much Supervision Do We Need?
- Sandra Ebert, Bernt Schiele
Recognizing Human Actions by Using Effective Codebooks and Tracking
- Lamberto Ballan, Lorenzo Seidenari, Giuseppe Serra, Marco Bertini, Alberto Del Bimbo
Evaluating and Extending Trajectory Features for Activity Recognition
- Ross Messing, Atousa Torabi, Aaron Courville, Chris Pal
Co-recognition of Images and Videos: Unsupervised Matching of Identical Object Patterns and Its Applications
- Minsu Cho, Young Min Shin, Kyoung Mu Lee
Stereo Matching—State-of-the-Art and Research Challenges
- Michael Bleyer, Christian Breiteneder
Visual Localization for Micro Aerial Vehicles in Urban Outdoor Environments
- Andreas Wendel, Horst Bischof
Moment Constraints in Convex Optimization for Segmentation and Tracking
- Maria Klodt, Frank Steinbrücker, Daniel Cremers
Large Scale Metric Learning for Distance-Based Image Classification on Open Ended Data Sets
- Thomas Mensink, Jakob Verbeek, Florent Perronnin, Gabriela Csurka
Top–Down Bayesian Inference of Indoor Scenes
- Luca Del Pero, Kobus Barnard
Efficient Loopy Belief Propagation Using the Four Color Theorem
- Radu Timofte, Luc Van Gool
Boosting k -Nearest Neighbors Classification
- Paolo Piro, Richard Nock, Wafa Bel Haj Ali, Frank Nielsen, Michel Barlaud
Learning Object Detectors in Stationary Environments
- Peter M. Roth, Sabine Sternig, Horst Bischof
Video Temporal Super-resolution Based on Self-similarity
- Mihoko Shimano, Takahiro Okabe, Imari Sato, Yoichi Sato
Back Matter
- 3D Reconstruction
- Computer Vision
- Convex Optimization
- Event and Activity Recognition
- Image Segmentation
- Image and Video Analysis
- Large Scale Image Classification
- Learning Objects
- Loopy Belief Propagation
- Message Parsing
- Metric Learning
- Object Detection
- Scene Understanding
- Stereo Matching
- Super Resolution
- Visual Features
About this book
From the book reviews:
“The goal of this book is to provide an overview of recent works in computer vision. … The book is intended more for engineers and researchers who will use it as a relevant source of knowledge in the computer vision field, and benefit from the presence of recent and representative methods that are among the best existing solutions to solve the problems reviewed in the book.” (Sebastien Lefevre, Computing Reviews, June, 2014)
Editors and Affiliations
Giovanni Maria Farinella, Sebastiano Battiato
Roberto Cipolla
About the editors
Bibliographic information.
Book Title : Advanced Topics in Computer Vision
Editors : Giovanni Maria Farinella, Sebastiano Battiato, Roberto Cipolla
Series Title : Advances in Computer Vision and Pattern Recognition
DOI : https://doi.org/10.1007/978-1-4471-5520-1
Publisher : Springer London
eBook Packages : Computer Science , Computer Science (R0)
Copyright Information : Springer-Verlag London 2013
Hardcover ISBN : 978-1-4471-5519-5 Published: 07 October 2013
Softcover ISBN : 978-1-4471-7025-9 Published: 23 August 2016
eBook ISBN : 978-1-4471-5520-1 Published: 24 September 2013
Series ISSN : 2191-6586
Series E-ISSN : 2191-6594
Edition Number : 1
Number of Pages : XIV, 433
Number of Illustrations : 38 b/w illustrations, 180 illustrations in colour
Topics : Computer Imaging, Vision, Pattern Recognition and Graphics
- Publish with us
Policies and ethics
- Find a journal
- Track your research
- Bibliography
- More Referencing guides Blog Automated transliteration Relevant bibliographies by topics
- Automated transliteration
- Relevant bibliographies by topics
- Referencing guides
Dissertations / Theses on the topic 'Computer vision;Machine learning'
Create a spot-on reference in apa, mla, chicago, harvard, and other styles.
Consult the top 50 dissertations / theses for your research on the topic 'Computer vision;Machine learning.'
Next to every source in the list of references, there is an 'Add to bibliography' button. Press on it, and we will generate automatically the bibliographic reference to the chosen work in the citation style you need: APA, MLA, Harvard, Chicago, Vancouver, etc.
You can also download the full text of the academic publication as pdf and read online its abstract whenever available in the metadata.
Browse dissertations / theses on a wide variety of disciplines and organise your bibliography correctly.
Chen, Zhilu. "Computer Vision and Machine Learning for Autonomous Vehicles." Digital WPI, 2017. https://digitalcommons.wpi.edu/etd-dissertations/488.
Öberg, Filip. "Football analysis using machine learning and computer vision." Thesis, Luleå tekniska universitet, Institutionen för system- och rymdteknik, 2021. http://urn.kb.se/resolve?urn=urn:nbn:se:ltu:diva-85276.
Yang, Chen. "Machine Learning and Computer Vision for PCB Verification." Thesis, KTH, Skolan för elektroteknik och datavetenskap (EECS), 2020. http://urn.kb.se/resolve?urn=urn:nbn:se:kth:diva-290370.
Arteta, Carlos Federico. "Computer vision and machine learning for microscopy image analysis." Thesis, University of Oxford, 2015. https://ora.ox.ac.uk/objects/uuid:62a03c45-2616-49a4-8976-fb1ff481915f.
Landecker, Will. "Interpretable Machine Learning and Sparse Coding for Computer Vision." PDXScholar, 2014. https://pdxscholar.library.pdx.edu/open_access_etds/1937.
Burns, James Ian. "Agricultural Crop Monitoring with Computer Vision." Thesis, Virginia Tech, 2014. http://hdl.handle.net/10919/52563.
Mairal, Julien. "Sparse coding for machine learning, image processing and computer vision." Phd thesis, École normale supérieure de Cachan - ENS Cachan, 2010. http://tel.archives-ouvertes.fr/tel-00595312.
Jonsson, Erik. "Channel-Coded Feature Maps for Computer Vision and Machine Learning." Doctoral thesis, Linköping : Department of Electrical Engineering, Linköpings universitet, 2008. http://urn.kb.se/resolve?urn=urn:nbn:se:liu:diva-11040.
Kendall, Alex Guy. "Geometry and uncertainty in deep learning for computer vision." Thesis, University of Cambridge, 2019. https://www.repository.cam.ac.uk/handle/1810/287944.
Borngrund, Carl. "Machine vision for automation of earth-moving machines : Transfer learning experiments with YOLOv3." Thesis, Luleå tekniska universitet, Institutionen för system- och rymdteknik, 2019. http://urn.kb.se/resolve?urn=urn:nbn:se:ltu:diva-75169.
La, Alex W. "Eigenblades: Application of Computer Vision and Machine Learning for Mode Shape Identification." BYU ScholarsArchive, 2017. https://scholarsarchive.byu.edu/etd/7228.
Yu, Haiyue. "Quantitative analysis of TMA images using computer vision and machine learning approaches." Thesis, University of Oxford, 2016. https://ora.ox.ac.uk/objects/uuid:e4cc472e-8c01-4121-b044-f3b4b19a8742.
Kulkarni, Amruta Kiran. "Classification of Faults in Railway Ties Using Computer Vision and Machine Learning." Thesis, Virginia Tech, 2017. http://hdl.handle.net/10919/86522.
Lan, Xiangyuan. "Multi-cue visual tracking: feature learning and fusion." HKBU Institutional Repository, 2016. https://repository.hkbu.edu.hk/etd_oa/319.
Cao, Qiong. "Some topics on similarity metric learning." Thesis, University of Exeter, 2015. http://hdl.handle.net/10871/18662.
Miller, Erik G. (Erik Gundersen). "Learning from one example in machine vision by sharing probability densities." Thesis, Massachusetts Institute of Technology, 2002. http://hdl.handle.net/1721.1/29902.
Kulkarni, Sanjeev R. (Sanjeev Ramesh). "Problems of computational and informational complexity in machine vision and learning." Thesis, Massachusetts Institute of Technology, 1991. http://hdl.handle.net/1721.1/13878.
Billings, Rachel Mae. "On Efficient Computer Vision Applications for Neural Networks." Thesis, Virginia Tech, 2021. http://hdl.handle.net/10919/102957.
Stoddart, Evan. "Computer Vision Techniques for Automotive Perception Systems." The Ohio State University, 2019. http://rave.ohiolink.edu/etdc/view?acc_num=osu1555357244145006.
Thomure, Michael David. "The Role of Prototype Learning in Hierarchical Models of Vision." PDXScholar, 2014. https://pdxscholar.library.pdx.edu/open_access_etds/1665.
Papaioannou, Athanasios. "Component analysis of complex-valued data for machine learning and computer vision tasks." Thesis, Imperial College London, 2017. http://hdl.handle.net/10044/1/49235.
Niemi, Mikael. "Machine Learning for Rapid Image Classification." Thesis, Linköpings universitet, Institutionen för medicinsk teknik, 2013. http://urn.kb.se/resolve?urn=urn:nbn:se:liu:diva-97375.
Beale, Dan. "Autonomous visual learning for robotic systems." Thesis, University of Bath, 2012. https://ethos.bl.uk/OrderDetails.do?uin=uk.bl.ethos.558886.
Cleland, Andrew Lewis. "Bounding Box Improvement with Reinforcement Learning." PDXScholar, 2018. https://pdxscholar.library.pdx.edu/open_access_etds/4438.
Mohan, Vandana. "Computer vision and machine learning methods for the analysis of brain and cardiac imagery." Diss., Georgia Institute of Technology, 2010. http://hdl.handle.net/1853/39628.
Kaloskampis, Ioannis. "Recognition of complex human activities in multimedia streams using machine learning and computer vision." Thesis, Cardiff University, 2013. http://orca.cf.ac.uk/59377/.
Harekoppa, Pooja Puttaswamygowda. "Application of Computer Vision Techniques for Railroad Inspection using UAVs." Thesis, Virginia Tech, 2016. http://hdl.handle.net/10919/72273.
Arthur, Richard B. "Vision-Based Human Directed Robot Guidance." Diss., CLICK HERE for online access, 2004. http://contentdm.lib.byu.edu/ETD/image/etd564.pdf.
Serafini, Sara. "Machine Learning applied to OCR tasks." Master's thesis, Alma Mater Studiorum - Università di Bologna, 2019.
Annavarjula, Vaishnavi. "Computer-Vision Based Retinal Image Analysis for Diagnosis and Treatment." Thesis, Blekinge Tekniska Högskola, Institutionen för datalogi och datorsystemteknik, 2017. http://urn.kb.se/resolve?urn=urn:nbn:se:bth-14979.
Ding, Lei. "From Pixels to People: Graph Based Methods for Grouping Problems in Computer Vision." The Ohio State University, 2010. http://rave.ohiolink.edu/etdc/view?acc_num=osu1289845859.
Nichols, Scott A. "Improvement of the camera calibration through the use of machine learning techniques." [Gainesville, Fla.] : University of Florida, 2001. http://etd.fcla.edu/etd/uf/2001/anp1587/nichols%5Fthesis.pdf.
Kuang, Zhanghui, and 旷章辉. "Learning structural SVMs and its applications in computer vision." Thesis, The University of Hong Kong (Pokfulam, Hong Kong), 2014. http://hdl.handle.net/10722/206663.
Agarwal, Ankur. "Machine Learning for Image Based Motion Capture." Phd thesis, Grenoble INPG, 2006. http://tel.archives-ouvertes.fr/tel-00390301.
Erlandsson, Niklas. "Utilizing machine learning in wildlife camera traps for automatic classification of animal species : An application of machine learning on edge devices." Thesis, Linnéuniversitetet, Institutionen för datavetenskap och medieteknik (DM), 2021. http://urn.kb.se/resolve?urn=urn:nbn:se:lnu:diva-104952.
Stewart, Kendall Lee. "The Performance of Random Prototypes in Hierarchical Models of Vision." Thesis, Portland State University, 2015. http://pqdtopen.proquest.com/#viewpdf?dispub=1605894.
I investigate properties of HMAX, a computational model of hierarchical processing in the primate visual cortex. High-level cortical neurons have been shown to respond highly to particular natural shapes, such as faces. HMAX models this property with a dictionary of natural shapes, called prototypes, that respond to the presence of those shapes. The resulting set of similarity measurements is an effective descriptor for classifying images. Curiously, prior work has shown that replacing the dictionary of natural shapes with entirely random prototypes has little impact on classification performance. This work explores that phenomenon by studying the performance of random prototypes on natural scenes, and by comparing their performance to that of sparse random projections of low-level image features.
Pradeep, Subramanian. "A Computer-Aided Framework for Cell Phenotype Identification, Analysis and Classification." Thesis, Virginia Tech, 2017. http://hdl.handle.net/10919/78859.
Komodakis, Nikos. "Graphical Model Inference and Learning for Visual Computing." Habilitation à diriger des recherches, Université Paris-Est, 2013. http://tel.archives-ouvertes.fr/tel-00866078.
Wallenberg, Marcus. "Embodied Visual Object Recognition." Doctoral thesis, Linköpings universitet, Datorseende, 2017. http://urn.kb.se/resolve?urn=urn:nbn:se:liu:diva-132762.
Zhu, Yuehan. "Automated Supply-Chain Quality Inspection Using Image Analysis and Machine Learning." Thesis, Högskolan Kristianstad, Fakulteten för naturvetenskap, 2019. http://urn.kb.se/resolve?urn=urn:nbn:se:hkr:diva-20069.
Bartoli, Giacomo. "Edge AI: Deep Learning techniques for Computer Vision applied to embedded systems." Master's thesis, Alma Mater Studiorum - Università di Bologna, 2018. http://amslaurea.unibo.it/16820/.
Almin, Fredrik. "Detection of Non-Ferrous Materials with Computer Vision." Thesis, Linköpings universitet, Datorseende, 2020. http://urn.kb.se/resolve?urn=urn:nbn:se:liu:diva-175519.
Minakshi, Mona. "A Machine Learning Framework to Classify Mosquito Species from Smart-phone Images." Scholar Commons, 2018. https://scholarcommons.usf.edu/etd/7340.
Tydén, Amanda, and Sara Olsson. "Edge Machine Learning for Animal Detection, Classification, and Tracking." Thesis, Linköpings universitet, Reglerteknik, 2020. http://urn.kb.se/resolve?urn=urn:nbn:se:liu:diva-166572.
Han, Ji Wan. "A multi-level machine learning system for attention-based object recognition." Thesis, University of Hertfordshire, 2011. http://hdl.handle.net/2299/6377.
Jan, Asim. "Deep learning based facial expression recognition and its applications." Thesis, Brunel University, 2017. http://bura.brunel.ac.uk/handle/2438/15944.
Bohg, Jeannette. "Multi-Modal Scene Understanding for Robotic Grasping." Doctoral thesis, KTH, Datorseende och robotik, CVAP, 2011. http://urn.kb.se/resolve?urn=urn:nbn:se:kth:diva-49062.
QC 20111125
Häggström, Frida. "/Maybe/Probably/Certainly." Thesis, Konstfack, Grafisk design & illustration, 2020. http://urn.kb.se/resolve?urn=urn:nbn:se:konstfack:diva-7400.
Mohapatra, Akrit. "Natural Language Driven Image Edits using a Semantic Image Manipulation Language." Thesis, Virginia Tech, 2018. http://hdl.handle.net/10919/83452.
Cogswell, Michael Andrew. "Understanding Representations and Reducing their Redundancy in Deep Networks." Thesis, Virginia Tech, 2016. http://hdl.handle.net/10919/78167.
Home > FACULTIES > Computer Science > CSD-ETD
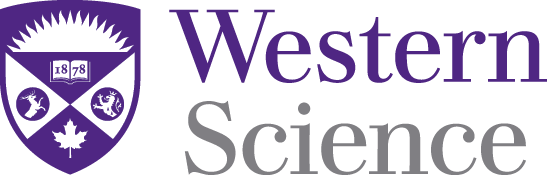
Computer Science Theses and Dissertations
This collection contains theses and dissertations from the Department of Computer Science, collected from the Scholarship@Western Electronic Thesis and Dissertation Repository
Theses/Dissertations from 2024 2024
A Target-Based and A Targetless Extrinsic Calibration Methods for Thermal Camera and 3D LiDAR , Farhad Dalirani
Investigating Tree- and Graph-based Neural Networks for Natural Language Processing Applications , Sudipta Singha Roy
Theses/Dissertations from 2023 2023
Classification of DDoS Attack with Machine Learning Architectures and Exploratory Analysis , Amreen Anbar
Multi-view Contrastive Learning for Unsupervised Domain Adaptation in Brain-Computer Interfaces , Sepehr Asgarian
Improved Protein Sequence Alignments Using Deep Learning , Seyed Sepehr Ashrafzadeh
INVESTIGATING IMPROVEMENTS TO MESH INDEXING , Anurag Bhattacharjee
Algorithms and Software for Oligonucleotide Design , Qin Dong
Framework for Assessing Information System Security Posture Risks , Syed Waqas Hamdani
De novo sequencing of multiple tandem mass spectra of peptide containing SILAC labeling , Fang Han
Local Model Agnostic XAI Methodologies Applied to Breast Cancer Malignancy Predictions , Heather Hartley
A Quantitative Analysis Between Software Quality Posture and Bug-fixing Commit , Rongji He
A Novel Method for Assessment of Batch Effect on single cell RNA sequencing data , Behnam Jabbarizadeh
Dynamically Finding Optimal Kernel Launch Parameters for CUDA Programs , Taabish Jeshani
Citation Polarity Identification From Scientific Articles Using Deep Learning Methods , Souvik Kundu
Denoising-Based Domain Adaptation Network for EEG Source Imaging , Runze Li
Decoy-Target Database Strategy and False Discovery Rate Analysis for Glycan Identification , Xiaoou Li
DpNovo: A DEEP LEARNING MODEL COMBINED WITH DYNAMIC PROGRAMMING FOR DE NOVO PEPTIDE SEQUENCING , Yizhou Li
Developing A Smart Home Surveillance System Using Autonomous Drones , Chongju Mai
Look-Ahead Selective Plasticity for Continual Learning , Rouzbeh Meshkinnejad
The Two Visual Processing Streams Through The Lens Of Deep Neural Networks , Aidasadat Mirebrahimi Tafreshi
Source-free Domain Adaptation for Sleep Stage Classification , Yasmin Niknam
Data Heterogeneity and Its Implications for Fairness , Ghazaleh Noroozi
Enhancing Urban Life: A Policy-Based Autonomic Smart City Management System for Efficient, Sustainable, and Self-Adaptive Urban Environments , Elham Okhovat
Evaluating the Likelihood of Bug Inducing Commits Using Metrics Trend Analysis , Parul Parul
On Computing Optimal Repairs for Conditional Independence , Alireza Pirhadi
Open-Set Source-Free Domain Adaptation in Fundus Images Analysis , Masoud Pourreza
Migration in Edge Computing , Arshin Rezazadeh
A Modified Hopfield Network for the K-Median Problem , Cody Rossiter
Predicting Network Failures with AI Techniques , Chandrika Saha
Toward Building an Intelligent and Secure Network: An Internet Traffic Forecasting Perspective , Sajal Saha
An Exploration of Visual Analytic Techniques for XAI: Applications in Clinical Decision Support , Mozhgan Salimiparsa
Attention-based Multi-Source-Free Domain Adaptation for EEG Emotion Recognition , Amir Hesam Salimnia
Global Cyber Attack Forecast using AI Techniques , Nusrat Kabir Samia
IMPLEMENTATION OF A PRE-ASSESSMENT MODULE TO IMPROVE THE INITIAL PLAYER EXPERIENCE USING PREVIOUS GAMING INFORMATION , Rafael David Segistan Canizales
A Computational Framework For Identifying Relevant Cell Types And Specific Regulatory Mechanisms In Schizophrenia Using Data Integration Methods , Kayvan Shabani
Weakly-Supervised Anomaly Detection in Surveillance Videos Based on Two-Stream I3D Convolution Network , Sareh Soltani Nejad
Smartphone Loss Prevention System Using BLE and GPS Technology , Noshin Tasnim
A Hybrid Continual Machine Learning Model for Efficient Hierarchical Classification of Domain-Specific Text in The Presence of Class Overlap (Case Study: IT Support Tickets) , Yasmen M. Wahba
Reducing Negative Transfer of Random Data in Source-Free Unsupervised Domain Adaptation , Anthony Wong
Deep Neural Methods for True/Pseudo- Invasion Classification in Colorectal Polyp Whole-Slide Images , Zhiyuan Yang
Developing a Relay-based Autonomous Drone Delivery System , Muhammad Zakar
Learning Mortality Risk for COVID-19 Using Machine Learning and Statistical Methods , Shaoshi Zhang
Machine Learning Techniques for Improved Functional Brain Parcellation , Da Zhi
Theses/Dissertations from 2022 2022
The Design and Implementation of a High-Performance Polynomial System Solver , Alexander Brandt
Defining Service Level Agreements in Serverless Computing , Mohamed Elsakhawy
Algorithms for Regular Chains of Dimension One , Juan P. Gonzalez Trochez
Towards a Novel and Intelligent e-commerce Framework for Smart-Shopping Applications , Susmitha Hanumanthu
Multi-Device Data Analysis for Fault Localization in Electrical Distribution Grids , Jacob D L Hunte
Towards Parking Lot Occupancy Assessment Using Aerial Imagery and Computer Vision , John Jewell
Potential of Vision Transformers for Advanced Driver-Assistance Systems: An Evaluative Approach , Andrew Katoch
Psychological Understanding of Textual journals using Natural Language Processing approaches , Amirmohammad Kazemeinizadeh
Driver Behavior Analysis Based on Real On-Road Driving Data in the Design of Advanced Driving Assistance Systems , Nima Khairdoost
Solving Challenges in Deep Unsupervised Methods for Anomaly Detection , Vahid Reza Khazaie
Developing an Efficient Real-Time Terrestrial Infrastructure Inspection System Using Autonomous Drones and Deep Learning , Marlin Manka
Predictive Modelling For Topic Handling Of Natural Language Dialogue With Virtual Agents , Lareina Milambiling
Improving Deep Entity Resolution by Constraints , Soudeh Nilforoushan
Respiratory Pattern Analysis for COVID-19 Digital Screening Using AI Techniques , Annita Tahsin Priyoti
Extracting Microservice Dependencies Using Log Analysis , Andres O. Rodriguez Ishida
False Discovery Rate Analysis for Glycopeptide Identification , Shun Saito
Towards a Generalization of Fulton's Intersection Multiplicity Algorithm , Ryan Sandford
An Investigation Into Time Gazed At Traffic Objects By Drivers , Kolby R. Sarson
Exploring Artificial Intelligence (AI) Techniques for Forecasting Network Traffic: Network QoS and Security Perspectives , Ibrahim Mohammed Sayem
A Unified Representation and Deep Learning Architecture for Persuasive Essays in English , Muhammad Tawsif Sazid
Towards the development of a cost-effective Image-Sensing-Smart-Parking Systems (ISenSmaP) , Aakriti Sharma
Advances in the Automatic Detection of Optimization Opportunities in Computer Programs , Delaram Talaashrafi
Reputation-Based Trust Assessment of Transacting Service Components , Konstantinos Tsiounis
Fully Autonomous UAV Exploration in Confined and Connectionless Environments , Kirk P. Vander Ploeg
Three Contributions to the Theory and Practice of Optimizing Compilers , Linxiao Wang
Developing Intelligent Routing Algorithm over SDN: Reusable Reinforcement Learning Approach , Wumian Wang
Predicting and Modifying Memorability of Images , Mohammad Younesi
Theses/Dissertations from 2021 2021
Generating Effective Sentence Representations: Deep Learning and Reinforcement Learning Approaches , Mahtab Ahmed
A Physical Layer Framework for a Smart City Using Accumulative Bayesian Machine Learning , Razan E. AlFar
Load Balancing and Resource Allocation in Smart Cities using Reinforcement Learning , Aseel AlOrbani
Contrastive Learning of Auditory Representations , Haider Al-Tahan
Cache-Friendly, Modular and Parallel Schemes For Computing Subresultant Chains , Mohammadali Asadi
Protein Interaction Sites Prediction using Deep Learning , Sourajit Basak
Predicting Stock Market Sector Sentiment Through News Article Based Textual Analysis , William A. Beldman
Improving Reader Motivation with Machine Learning , Tanner A. Bohn
A Black-box Approach for Containerized Microservice Monitoring in Fog Computing , Shi Chang
Visualization and Interpretation of Protein Interactions , Dipanjan Chatterjee
A Framework for Characterising Performance in Multi-Class Classification Problems with Applications in Cancer Single Cell RNA Sequencing , Erik R. Christensen
Exploratory Search with Archetype-based Language Models , Brent D. Davis
Evolutionary Design of Search and Triage Interfaces for Large Document Sets , Jonathan A. Demelo
Building Effective Network Security Frameworks using Deep Transfer Learning Techniques , Harsh Dhillon
A Deep Topical N-gram Model and Topic Discovery on COVID-19 News and Research Manuscripts , Yuan Du
Automatic extraction of requirements-related information from regulatory documents cited in the project contract , Sara Fotouhi
Developing a Resource and Energy Efficient Real-time Delivery Scheduling Framework for a Network of Autonomous Drones , Gopi Gugan
A Visual Analytics System for Rapid Sensemaking of Scientific Documents , Amirreza Haghverdiloo Barzegar
Calibration Between Eye Tracker and Stereoscopic Vision System Employing a Linear Closed-Form Perspective-n-Point (PNP) Algorithm , Mohammad Karami
Fuzzy and Probabilistic Rule-Based Approaches to Identify Fault Prone Files , Piyush Kumar Korlepara
Parallel Arbitrary-precision Integer Arithmetic , Davood Mohajerani
A Technique for Evaluating the Health Status of a Software Module Using Process Metrics , . Ria
Visual Analytics for Performing Complex Tasks with Electronic Health Records , Neda Rostamzadeh
Predictive Model of Driver's Eye Fixation for Maneuver Prediction in the Design of Advanced Driving Assistance Systems , Mohsen Shirpour
A Generative-Discriminative Approach to Human Brain Mapping , Deepanshu Wadhwa
WesternAccelerator:Rapid Development of Microservices , Haoran Wei
A Lightweight and Explainable Citation Recommendation System , Juncheng Yin
Mitosis Detection from Pathology Images , Jinhang Zhang
Theses/Dissertations from 2020 2020
Visual Analytics of Electronic Health Records with a focus on Acute Kidney Injury , Sheikh S. Abdullah
Towards the Development of Network Service Cost Modeling-An ISP Perspective , Yasmeen Ali
- Accessible Formats
Advanced Search
- Notify me via email or RSS
- Expert Gallery
- Online Journals
- eBook Collections
- Reports and Working Papers
- Conferences and Symposiums
- Electronic Theses and Dissertations
- Digitized Special Collections
- All Collections
- Disciplines
Author Corner
- Submit Thesis/Dissertation
Home | About | FAQ | My Account | Accessibility Statement | Privacy | Copyright
©1878 - 2016 Western University
ScholarWorks@UMass Amherst
Home > CICS > CS > CS_DISS
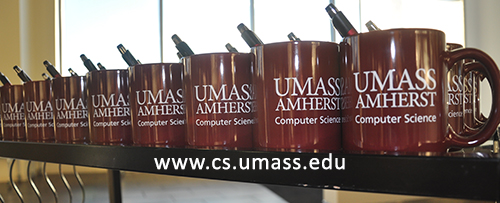
Computer Science Department Dissertations Collection
Dissertations from 2024 2024.
Enabling Privacy and Trust in Edge AI Systems , Akanksha Atrey, Computer Science
Generative Language Models for Personalized Information Understanding , Pengshan Cai, Computer Science
Towards Automatic and Robust Variational Inference , Tomas Geffner, Computer Science
Multi-SLAM Systems for Fault-Tolerant Simultaneous Localization and Mapping , Samer Nashed, Computer Science
Policy Gradient Methods: Analysis, Misconceptions, and Improvements , Christopher P. Nota, Computer Science
Data to science with AI and human-in-the-loop , Gustavo Perez Sarabia, Computer Science
Question Answering By Case-Based Reasoning With Textual Evidence , Dung N. Thai, Computer Science
Dissertations from 2023 2023
An Introspective Approach for Competence-Aware Autonomy , Connor Basich, Computer Science
Foundations of Node Representation Learning , Sudhanshu Chanpuriya, Computer Science
Learning to See with Minimal Human Supervision , Zezhou Cheng, Computer Science
IMPROVING USER EXPERIENCE BY OPTIMIZING CLOUD SERVICES , Ishita Dasgupta, Computer Science
Automating the Formal Verification of Software , Emily First, Computer Science
Learning from Sequential User Data: Models and Sample-efficient Algorithms , Aritra Ghosh, Computer Science
Human-Centered Technologies for Inclusive Collection and Analysis of Public-Generated Data , Mahmood Jasim, Computer Science
Rigorous Experimentation For Reinforcement Learning , Scott M. Jordan, Computer Science
Towards Robust Long-form Text Generation Systems , Kalpesh Krishna, Computer Science
Emerging Trustworthiness Issues in Distributed Learning Systems , Hamid Mozaffari, Computer Science
TOWARDS RELIABLE CIRCUMVENTION OF INTERNET CENSORSHIP , Milad nasresfahani, Computer Science
Evidence Assisted Learning for Clinical Decision Support Systems , Bhanu Pratap Singh Rawat, Computer Science
DESIGN AND ANALYSIS OF CONTENT CACHING SYSTEMS , Anirudh Sabnis, Computer Science
Quantifying and Enhancing the Security of Federated Learning , Virat Vishnu Shejwalkar, Computer Science
Effective and Efficient Transfer Learning in the Era of Large Language Models , Tu Vu, Computer Science
Data-driven Modeling and Analytics for Greening the Energy Ecosystem , John Wamburu, Computer Science
Bayesian Structural Causal Inference with Probabilistic Programming , Sam A. Witty, Computer Science
LEARNING TO RIG CHARACTERS , Zhan Xu, Computer Science
GRAPH REPRESENTATION LEARNING WITH BOX EMBEDDINGS , Dongxu Zhang, Computer Science
Dissertations from 2022 2022
COMBINATORIAL ALGORITHMS FOR GRAPH DISCOVERY AND EXPERIMENTAL DESIGN , Raghavendra K. Addanki, Computer Science
MEASURING NETWORK INTERFERENCE AND MITIGATING IT WITH DNS ENCRYPTION , Seyed Arian Akhavan Niaki, Computer Science
Few-Shot Natural Language Processing by Meta-Learning Without Labeled Data , Trapit Bansal, Computer Science
Communicative Information Visualizations: How to make data more understandable by the general public , Alyxander Burns, Computer Science
REINFORCEMENT LEARNING FOR NON-STATIONARY PROBLEMS , Yash Chandak, Computer Science
Modeling the Multi-mode Distribution in Self-Supervised Language Models , Haw-Shiuan Chang, Computer Science
Nonparametric Contextual Reasoning for Question Answering over Large Knowledge Bases , Rajarshi Das, Computer Science
Languages and Compilers for Writing Efficient High-Performance Computing Applications , Abhinav Jangda, Computer Science
Controllable Neural Synthesis for Natural Images and Vector Art , Difan Liu, Computer Science
Probabilistic Commonsense Knowledge , Xiang Li, Computer Science
DISTRIBUTED LEARNING ALGORITHMS: COMMUNICATION EFFICIENCY AND ERROR RESILIENCE , Raj Kumar Maity, Computer Science
Practical Methods for High-Dimensional Data Publication with Differential Privacy , Ryan H. McKenna, Computer Science
Incremental Non-Greedy Clustering at Scale , Nicholas Monath, Computer Science
High-Quality Automatic Program Repair , Manish Motwani, Computer Science
Unobtrusive Assessment of Upper-Limb Motor Impairment Using Wearable Inertial Sensors , Brandon R. Oubre, Computer Science
Mixture Models in Machine Learning , Soumyabrata Pal, Computer Science
Decision Making with Limited Data , Kieu My Phan, Computer Science
Neural Approaches for Language-Agnostic Search and Recommendation , Hamed Rezanejad Asl Bonab, Computer Science
Low Resource Language Understanding in Voice Assistants , Subendhu Rongali, Computer Science
Enabling Daily Tracking of Individual’s Cognitive State With Eyewear , Soha Rostaminia, Computer Science
LABELED MODULES IN PROGRAMS THAT EVOLVE , Anil K. Saini, Computer Science
Reliable Decision-Making with Imprecise Models , Sandhya Saisubramanian, Computer Science
Data Scarcity in Event Analysis and Abusive Language Detection , Sheikh Muhammad Sarwar, Computer Science
Representation Learning for Shape Decomposition, By Shape Decomposition , Gopal Sharma, Computer Science
Metareasoning for Planning and Execution in Autonomous Systems , Justin Svegliato, Computer Science
Approximate Bayesian Deep Learning for Resource-Constrained Environments , Meet Prakash Vadera, Computer Science
ANSWER SIMILARITY GROUPING AND DIVERSIFICATION IN QUESTION ANSWERING SYSTEMS , Lakshmi Nair Vikraman, Computer Science
Dissertations from 2021 2021
Neural Approaches to Feedback in Information Retrieval , Keping Bi, Computer Science
Sociolinguistically Driven Approaches for Just Natural Language Processing , Su Lin Blodgett, Computer Science
Enabling Declarative and Scalable Prescriptive Analytics in Relational Data , Matteo Brucato, Computer Science
Neural Methods for Answer Passage Retrieval over Sparse Collections , Daniel Cohen, Computer Science
Utilizing Graph Structure for Machine Learning , Stefan Dernbach, Computer Science
Enhancing Usability and Explainability of Data Systems , Anna Fariha, Computer Science
Algorithms to Exploit Data Sparsity , Larkin H. Flodin, Computer Science
3D Shape Understanding and Generation , Matheus Gadelha, Computer Science
Robust Algorithms for Clustering with Applications to Data Integration , Sainyam Galhotra, Computer Science
Improving Evaluation Methods for Causal Modeling , Amanda Gentzel, Computer Science
SAFE AND PRACTICAL MACHINE LEARNING , Stephen J. Giguere, Computer Science
COMPACT REPRESENTATIONS OF UNCERTAINTY IN CLUSTERING , Craig Stuart Greenberg, Computer Science
Natural Language Processing for Lexical Corpus Analysis , Abram Kaufman Handler, Computer Science
Social Measurement and Causal Inference with Text , Katherine A. Keith, Computer Science
Concentration Inequalities in the Wild: Case Studies in Blockchain & Reinforcement Learning , A. Pinar Ozisik, Computer Science
Resource Allocation in Distributed Service Networks , Nitish Kumar Panigrahy, Computer Science
History Modeling for Conversational Information Retrieval , Chen Qu, Computer Science
Design and Implementation of Algorithms for Traffic Classification , Fatemeh Rezaei, Computer Science
SCALING DOWN THE ENERGY COST OF CONNECTING EVERYDAY OBJECTS TO THE INTERNET , Mohammad Rostami, Computer Science
Deep Learning Models for Irregularly Sampled and Incomplete Time Series , Satya Narayan Shukla, Computer Science
Traffic engineering in planet-scale cloud networks , Rachee Singh, Computer Science
Video Adaptation for High-Quality Content Delivery , Kevin Spiteri, Computer Science
Learning from Limited Labeled Data for Visual Recognition , Jong-Chyi Su, Computer Science
Human Mobility Monitoring using WiFi: Analysis, Modeling, and Applications , Amee Trivedi, Computer Science
Geometric Representation Learning , Luke Vilnis, Computer Science
Understanding of Visual Domains via the Lens of Natural Language , Chenyun Wu, Computer Science
Towards Practical Differentially Private Mechanism Design and Deployment , Dan Zhang, Computer Science
Audio-driven Character Animation , Yang Zhou, Computer Science
Dissertations from 2020 2020
Noise-Aware Inference for Differential Privacy , Garrett Bernstein, Computer Science
Motion Segmentation - Segmentation of Independently Moving Objects in Video , Pia Katalin Bideau, Computer Science
An Empirical Assessment of the Effectiveness of Deception for Cyber Defense , Kimberly J. Ferguson-Walter, Computer Science
Integrating Recognition and Decision Making to Close the Interaction Loop for Autonomous Systems , Richard Freedman, Computer Science
Improving Reinforcement Learning Techniques by Leveraging Prior Experience , Francisco M. Garcia, Computer Science
Optimization and Training of Generational Garbage Collectors , Nicholas Jacek, Computer Science
Understanding the Dynamic Visual World: From Motion to Semantics , Huaizu Jiang, Computer Science
Improving Face Clustering in Videos , SouYoung Jin, Computer Science
Reasoning About User Feedback Under Identity Uncertainty in Knowledge Base Construction , Ariel Kobren, Computer Science
Learning Latent Characteristics of Data and Models using Item Response Theory , John P. Lalor, Computer Science
Higher-Order Representations for Visual Recognition , Tsung-Yu Lin, Computer Science
Learning from Irregularly-Sampled Time Series , Steven Cheng-Xian Li, Computer Science
Dynamic Composition of Functions for Modular Learning , Clemens GB Rosenbaum, Computer Science
Improving Visual Recognition With Unlabeled Data , Aruni Roy Chowdhury, Computer Science
Deep Neural Networks for 3D Processing and High-Dimensional Filtering , Hang Su, Computer Science
Towards Optimized Traffic Provisioning and Adaptive Cache Management for Content Delivery , Aditya Sundarrajan, Computer Science
The Limits of Location Privacy in Mobile Devices , Keen Yuun Sung, Computer Science
ALGORITHMS FOR MASSIVE, EXPENSIVE, OR OTHERWISE INCONVENIENT GRAPHS , David Tench, Computer Science
System Design for Digital Experimentation and Explanation Generation , Emma Tosch, Computer Science
Advanced Search
- Notify me via email or RSS
- Collections
- Disciplines
Author Corner
- Login for Faculty Authors
- Faculty Author Gallery
- Expert Gallery
- University Libraries
- Computer Science Website
- UMass Amherst
This page is sponsored by the University Libraries.
© 2009 University of Massachusetts Amherst • Site Policies
Privacy Copyright

Home > School, College, or Department > MCECS > Computer Science > Dissertations and Theses
Computer Science Dissertations and Theses
Theses/dissertations from 2024 2024.
MmWave RAT Optimization: MAC Layer Initial Access Design and Transport Layer Integration , Suresh Srinivasan (Dissertation)
Theses/Dissertations from 2023 2023
Seeing in the Dark: Towards Robust Pedestrian Detection at Nighttime , Afnan Althoupety (Dissertation)
A Deep Hierarchical Variational Autoencoder for World Models in Complex Reinforcement Learning Environments , Sriharshitha Ayyalasomayajula (Thesis)
Toward Efficient Rendering: A Neural Network Approach , Qiqi Hou (Dissertation)
Energy Auction with Non-Relational Persistence , Michael Ramez Howard (Thesis)
Implementing a Functional Logic Programming Language via the Fair Scheme , Andrew Michael Jost (Dissertation)
Multi-Agent Deep Reinforcement Learning for Radiation Localization , Benjamin Scott Totten (Thesis)
Theses/Dissertations from 2022 2022
Using Intrinsically-Typed Definitional Interpreters to Verify Compiler Optimizations in a Monadic Intermediate Language , Dani Barrack (Thesis)
An Automated Zoom Class Session Analysis Tool to Improve Education , Jack Arlo Cannon II (Thesis)
Scaling EPA-RIMM with Multicore System Management Interrupt Handlers , Alexander K. Freed (Thesis)
Unpaired Style Transfer Conditional Generative Adversarial Network for Scanned Document Generation , David Jonathan Hawbaker (Thesis)
Toward Analyzing the Diversity of Extractive Summaries , Aaron David Hudson (Thesis)
Making Curry with Rice: An Optimizing Curry Compiler , Steven Libby (Dissertation)
Domain Knowledge as Motion-Aware Inductive Bias for Deep Video Synthesis: Two Case Studies , Long Mai (Dissertation)
Theses/Dissertations from 2021 2021
Efficient Neuromorphic Algorithms for Gamma-Ray Spectrum Denoising and Radionuclide Identification , Merlin Phillip Carson (Thesis)
Storing Intermediate Results in Space and Time: SQL Graphs and Block Referencing , Basem Ibrahim Elazzabi (Dissertation)
Automated Test Generation for Validating SystemC Designs , Bin Lin (Dissertation)
Forecasting Optimal Parameters of the Broken Wing Butterfly Option Strategy Using Differential Evolution , David Munoz Constantine (Thesis)
Situate: An Agent-Based System for Situation Recognition , Max Henry Quinn (Dissertation)
Theses/Dissertations from 2020 2020
Multiple Diagram Navigation , Hisham Benotman (Dissertation)
Smart Contract Vulnerabilities on the Ethereum Blockchain: a Current Perspective , Daniel Steven Connelly (Thesis)
Extensible Performance-Aware Runtime Integrity Measurement , Brian G. Delgado (Dissertation)
Novel View Synthesis - a Neural Network Approach , Hoang Le (Dissertation)
Exploring the Potential of Sparse Coding for Machine Learning , Sheng Yang Lundquist (Dissertation)
Workflow Critical Path: a Data-Oriented Path Metric for Holistic HPC Workflows , Daniel D. Nguyen (Thesis)
Novel View Synthesis in Time and Space , Simon Niklaus (Dissertation)
Balancing Security, Performance and Deployability in Encrypted Search , David Joel Pouliot (Dissertation)
Theses/Dissertations from 2019 2019
A Secure Anti-Counterfeiting System using Near Field Communication, Public Key Cryptography, Blockchain, and Bayesian Games , Naif Saeed Alzahrani (Dissertation)
Spectral Clustering for Electrical Phase Identification Using Advanced Metering Infrastructure Voltage Time Series , Logan Blakely (Thesis)
Local Radiance , Scott Peter Britell (Dissertation)
Correct-by-Construction Typechecking with Scope Graphs , Katherine Imhoff Casamento (Thesis)
Versatile Binary-level Concolic Testing , Bo Chen (Dissertation)
Crumpled and Abraded Encryption: Implementation and Provably Secure Construction , Scott Sherlock Griffy (Thesis)
Knowing Without Knowing: Real-Time Usage Identification of Computer Systems , Leila Mohammed Hawana (Thesis)
Design and Experimental Evaluation of DeepMarket: an Edge Computing Marketplace with Distributed TensorFlow Execution Capability , Soyoung Kim (Thesis)
Localizing Little Landmarks with Transfer Learning , Sharad Kumar (Thesis)
Context-Aware Wi-Fi Infrastructure-based Indoor Positioning Systems , Huy Phuong Tran (Dissertation)
Theses/Dissertations from 2018 2018
Bounding Box Improvement with Reinforcement Learning , Andrew Lewis Cleland (Thesis)
Sensing Building Structure Using UWB Radios for Disaster Recovery , Jeong Eun Lee (Dissertation)
Annotation-Enabled Interpretation and Analysis of Time-Series Data , Niveditha Venugopal (Thesis)
EPA-RIMM-V: Efficient Rootkit Detection for Virtualized Environments , Tejaswini Ajay Vibhute (Thesis)
Theses/Dissertations from 2017 2017
Improved Scoring Models for Semantic Image Retrieval Using Scene Graphs , Erik Timothy Conser (Thesis)
Refining Bounding-Box Regression for Object Localization , Naomi Lynn Dickerson (Thesis)
Fully Generic Programming Over Closed Universes of Inductive-Recursive Types , Larry Diehl (Dissertation)
Communicating at Terahertz Frequencies , Farnoosh Moshirfatemi (Dissertation)
Designing In-Headset Authoring Tools for Virtual Reality Video , Cuong Nguyen (Dissertation)
Certifying Loop Pipelining Transformations in Behavioral Synthesis , Disha Puri (Dissertation)
Power-Aware Datacenter Networking and Optimization , Qing Yi (Dissertation)
Theses/Dissertations from 2016 2016
Identifying Relationships between Scientific Datasets , Abdussalam Alawini (Dissertation)
Information Representation and Computation of Spike Trains in Reservoir Computing Systems with Spiking Neurons and Analog Neurons , Amin Almassian (Thesis)
Investigations of an "Objectness" Measure for Object Localization , Lewis Richard James Coates (Thesis)
Image Stitching: Handling Parallax, Stereopsis, and Video , Fan Zhang (Dissertation)
Theses/Dissertations from 2015 2015
Novel Methods for Learning and Adaptation in Chemical Reaction Networks , Peter Banda (Dissertation)
Post-silicon Functional Validation with Virtual Prototypes , Kai Cong (Dissertation)
Novel Cryptographic Primitives and Protocols for Censorship Resistance , Kevin Patrick Dyer (Dissertation)
Hardware/Software Interface Assurance with Conformance Checking , Li Lei (Dissertation)
Leveraging Contextual Relationships Between Objects for Localization , Clinton Leif Olson (Thesis)
The Performance of Random Prototypes in Hierarchical Models of Vision , Kendall Lee Stewart (Thesis)
Tweakable Ciphers: Constructions and Applications , Robert Seth Terashima (Dissertation)
Scalable Equivalence Checking for Behavioral Synthesis , Zhenkun Yang (Dissertation)
Theses/Dissertations from 2014 2014
The Nax Language: Unifying Functional Programming and Logical Reasoning in a Language based on Mendler-style Recursion Schemes and Term-indexed Types , Ki Yung Ahn (Dissertation)
Using Spammers' Computing Resources for Volunteer Computing , Thai Le Quy Bui (Thesis)
Towards Constructing Interactive Virtual Worlds , Francis Chang (Dissertation)
System-wide Performance Analysis for Virtualization , Deron Eugene Jensen (Thesis)
Advances in Piecewise Smooth Image Reconstruction , Ralf Juengling (Dissertation)
Interpretable Machine Learning and Sparse Coding for Computer Vision , Will Landecker (Dissertation)
Optimizing Data Movement in Hybrid Analytic Systems , Patrick Michael Leyshock (Dissertation)
Ranked Similarity Search of Scientific Datasets: An Information Retrieval Approach , Veronika Margaret Megler (Dissertation)
Using GIST Features to Constrain Search in Object Detection , Joanna Browne Solmon (Thesis)
The Role of Prototype Learning in Hierarchical Models of Vision , Michael David Thomure (Dissertation)
Theses/Dissertations from 2013 2013
Object Detection and Recognition in Natural Settings , George William Dittmar (Thesis)
Trust-but-Verify: Guaranteeing the Integrity of User-generated Content in Online Applications , Akshay Dua (Dissertation)
Equivalence Checking for High-Assurance Behavioral Synthesis , Kecheng Hao (Dissertation)
Type Classes and Instance Chains: A Relational Approach , John Garrett Morris (Dissertation)
Theses/Dissertations from 2012 2012
Using Dataflow Optimization Techniques with a Monadic Intermediate Language , Justin George Bailey (Thesis)
A Survey and Analysis of Solutions to the Oblivious Memory Access Problem , Erin Elizabeth Chapman (Thesis)
A Data-Descriptive Feedback Framework for Data Stream Management Systems , Rafael J. Fernández Moctezuma (Dissertation)
Extending Relativistic Programming to Multiple Writers , Philip William Howard (Dissertation)
The Basic Scheme for the Evaluation of Functional Logic Programs , Arthur Peters (Thesis)
The Link Between Image Segmentation and Image Recognition , Karan Sharma (Thesis)
Relativistic Causal Ordering A Memory Model for Scalable Concurrent Data Structures , Josh Triplett (Dissertation)
Theses/Dissertations from 2011 2011
Conceptual Modeling of Data with Provenance , David William Archer (Dissertation)
Low-latency Estimates for Window-Aggregate Queries over Data Streams , Amit Bhat (Thesis)
Information Processing in Two-Dimensional Cellular Automata , Martin Cenek (Dissertation)
Scalable and Efficient Tasking for Dynamic Sensor Networks , Thanh Xuan Dang (Dissertation)
On the Effect of Topology on Learning and Generalization in Random Automata Networks , Alireza Goudarzi (Thesis)
HOLCF '11: A Definitional Domain Theory for Verifying Functional Programs , Brian Charles Huffman (Dissertation)
A Functional Approach to Memory-Safe Operating Systems , Rebekah Leslie (Dissertation)
Factoring Semiprimes Using PG2N Prime Graph Multiagent Search , Keith Eirik Wilson (Thesis)
High Speed Wireless Networking for 60GHz , Candy Yiu (Dissertation)
Theses/Dissertations from 2010 2010
Extensible Scheduling in a Haskell-based Operating System , Kenneth William Graunke (Thesis)
Addressing Automated Adversaries of Network Applications , Edward Leo Kaiser (Dissertation)
An Automata-Theoretic Approach to Hardware/Software Co-verification , Juncao Li (Dissertation)
Practical Type Inference for the GADT Type System , Chuan-kai Lin (Dissertation)
Scalable event tracking on high-end parallel systems , Kathryn Marie Mohror (Dissertation)
Performance Analysis of Hybrid CPU/GPU Environments , Michael Shawn Smith (Thesis)
Theses/Dissertations from 2009 2009
Computational Techniques for Reducing Spectra of the Giant Planets in Our Solar System , Holly L. Grimes (Thesis)
Programmer Friendly Refactoring Tools , Emerson Murphy-Hill (Dissertation)
A Framework for Superimposed Applications : Techniques to Represent, Access, Transform, and Interchange Bi-level Information , Sudarshan Srivivasa Murthy (Dissertation)
Graphical User Interfaces as Updatable Views , James Felger Terwilliger (Dissertation)
Advanced Search
- Notify me via email or RSS
- Featured Collections
- All Authors
- Schools & Colleges
- Dissertations & Theses
- PDXOpen Textbooks
- Conferences
- Collections
- Disciplines
- Faculty Expert Gallery
- Submit Research
- Faculty Profiles
- Terms of Use
- Feedback Form
Home | About | My Account | Accessibility Statement | Portland State University
Privacy Copyright

Special Features
Vendor voice.
Personal Tech

Two big computer vision papers boost prospect of safer self-driving vehicles
New chip and camera technology bring closer potential of hands-free road time.
Like nuclear fusion and jet-packs, the self-driving car is a long-promised technology that has stalled for years - yet armed with research, boffins think they have created potential improvements.
Citizens of Phoenix, San Francisco, and Los Angeles are able to take one of Waymo's self-driving taxis, first introduced to the public in December 2020. But they have not been without their glitches. Just last month in San Francisco, for example, one of the taxi service's autonomous vehicles drove down the wrong side of the street to pass a unicycle. In December last year, a Waymo vehicle hit a backwards-facing pickup truck , resulting in a report with the US National Highway Traffic Safety Administration (NHTSA) and a software update.
But this week, not one but two groups of researchers bidding to improve the performance of self-driving cars and other autonomous vehicles have published papers in the international science journal Nature.
A design for a new chip geared towards autonomous vehicles has arrived from China. Tsinghua University's Luping Shi and colleagues have taken inspiration from the human visual system by both combining low-accuracy, fast event-based detection with more accurate, but slower visualization of an image.
The researchers were able to show the chip — dubbed Tianmouc — could process pixel arrays quickly and robustly in an automotive driving perception system.
In a paper published today, the authors said: "We demonstrate the integration of a Tianmouc chip into an autonomous driving system, showcasing its abilities to enable accurate, fast and robust perception, even in challenging corner cases on open roads. The primitive-based complementary sensing paradigm helps in overcoming fundamental limitations in developing vision systems for diverse open-world applications."
In a separate paper, Davide Scaramuzza, University of Zurich robotics and perception professor, and his colleagues adopt a similar hybrid approach but apply it to camera technologies.
Youtube Video
Cameras for self-driving vehicles navigate a trade-off between bandwidth and latency. While high-res color cameras have good resolution, they require high bandwidth to detect rapid changes. Conversely, reducing the bandwidth increases latency, affecting the timely processing of data for potentially life-saving decision making.
To get out of this bind, the Swiss-based researchers developed a hybrid camera combining event processing with high-bandwidth image processing. Events cameras only record intensity changes, and report them as sparse measurements, meaning the system does not suffer from the bandwidth/latency trade-off.
The event camera is used to detect changes in the blind time between image frames using events. Event data converted into a graph, which changes over time and connects nearby points, is computed locally. The resulting hybrid object detector reduces the detection time in dangerous high-speed situations, according to an explanatory video.
- Among AI infrastructure hopefuls, Qualcomm has become an unlikely ally
- GhostStripe attack haunts self-driving cars by making them ignore road signs
- Boston Dynamics' humanoid Atlas is dead, long live the ... new commercial Atlas
- US military pulls the trigger, uses AI to target air strikes
In their paper , the authors say: "Our method exploits the high temporal resolution and sparsity of events and the rich but low temporal resolution information in standard images to generate efficient, high-rate object detections, reducing perceptual and computational latency."
They argue their use of a 20 frames per second RGB camera plus an event camera can achieve the same latency as a 5,000-fps camera with the bandwidth of a 45-fps camera without compromising accuracy.
"Our approach paves the way for efficient and robust perception in edge-case scenarios by uncovering the potential of event cameras," the authors write.
With a hybrid approach to both cameras and data processing in the offing, more widespread adoption of self-driving vehicles may be just around the corner. ®
Narrower topics
- Large Language Model
- Machine Learning
- Neural Networks
- Tensor Processing Unit
Broader topics
- Self-driving Car
Send us news
Other stories you might like
Ai smartphones must balance promise against hype and privacy concerns, will windows drive a pc refresh everyone's talking about ai, ai chip sales predicted to jump by a third this year – then cool off, time for a fresh approach to compute architecture.
Prepare your audits: EU Commission approves first-of-its-kind AI Act
Hpe q2 sales rise was brought to you by the letters a and i, using ai in science can add to reproducibility woes, say boffins, raspberry pi unveils hailo-powered ai kit to make the model 5 smarter, google finally addresses those bizarre ai search results, 'building ai co-workers going to be largest opportunity of tech in our lifetime', mit professor hoses down predictions ai will put a rocket under the economy, by 2030, software developers will be using ai to cut their workload 'in half'.

- Advertise with us
Our Websites
- The Next Platform
- Blocks and Files
Your Privacy
- Cookies Policy
- Privacy Policy
- Ts & Cs

Copyright. All rights reserved © 1998–2024

What does the Apple Vision Pro do? Here's everything you need to know after WWDC reveal.
No doubt you’ve heard Apple officially took the wraps off Apple Vision Pro, its first “spatial computing” device which debuted at this week’s Worldwide Developer Conference (WWDC) at Apple Park in Cupertino, California.
Not only is this ski goggle-shaped mixed-reality headset Apple’s biggest product since Apple Watch debuted in 2015, but it’s easily the most ambitious.
In development for multiple years and tied to more than 5,000 patents, Apple Vision Pro – simply referred to as “Vision Pro,” henceforth in this article – will be available in early 2024 for an astounding $3,499 to start.
Even if you could afford it, is it any good?
I had a chance to wear and use Vision Pro for about 30 minutes and run through several applications that fused augmented reality (where you can see digital information overlaid on top of the real world around you) with virtual reality (realistic imagery that fully envelopes your field of vision, and is tied to head-tracking too).
Here's what you need to know and my impressions of the Vision Pro:
What is the Apple Vision Pro price?
Pricing for the Vision Pro starts at $3,499.
When is the Apple Vision Pro available?
It will be available in early 2024 – in the U.S. only. Other countries will follow at a later date.
Apple Vision Pro preorder
Apple has not disclosed when you can preorder Vision Pro, so more details to come on that.
What does the Apple Vision Pro do?
It’s a wearable headset, with twin micro‑OLED displays – one for each eye – and featuring 23 million pixels, which is more than a 4K TV.
The result is incredible clarity.
The new visionOS operating system features a fully three-dimensional user interface controlled with your eyes (tracked with inward-facing sensors), voice (including Siri support, which I did not try), and hands, whether it’s via finger gestures in the air or by twisting a Digital Crown knob on the side of the headset (similar to the dial on Apple Watch). There’s also support for an optional wireless Magic Keyboard or Trackpad but was not part of my demo.
Is Apple Vision Pro augmented reality?
It’s mixed reality, sometimes referred to as XR, which combines augmented reality with virtual reality.
When opening the Photos app, for example, I viewed a number of high-resolution images and the room automatically dimmed around them for added immersion (including panoramic shots of Iceland and Oregon’s coast).
One virtual reality-like experience is called Environments, which are full-screen relaxing videos tied to head-tracking (so you can “look around”), but when the Apple employees in my room talked with me, their images gradually appeared in my scene (called “breakthrough,” as to stay connected with those around you). So, like augmented reality, but shifts between the two smoothly.
Is the Vision Pro worth it?
I’ve seen a lot of VR in my day, but not with this much clarity and depth. The user interface was graceful and intuitive, and the applications are seemingly endless (for work and play). I found it comfortable, but not as light as PlayStation VR2 (PSVR2).
One of the most impressive parts of the demo by far was seeing “spatial" photos and videos, captured by Vision Pro headset, including scenes of kids blowing out a birthday cake and another with a few friends hanging outside by a fire, sipping drinks, telling stories and laughing. It truly looked 3D, like really being there, which lets you then relive memories long after you captured them with the same realistic depth.
No VR headset I’ve tried can do anything like this.
Requiring an external battery (that only lasts up to two hours) isn’t ideal, but remember, this is a Gen 1 product, and if any company can figure out how to improve products, including battery management, it’s Apple. Think of how much the iPhone has evolved over the years. This category is in its infancy.
Yes, the concerns over price are real, and Apple no doubt knows this isn’t a mass-market product. The company isn't tone-deaf by working on something most people can’t afford. Instead, it is clearly investing in what’s possible for the future.
I also get that some people think technology like this is disconcerting as it may encourage less human interaction (similar to the concerns over the metaverse), so it won’t be for everyone.
Will it flop? Who knows. But Apple isn’t just dipping its toe in the spatial computing space, it's diving in head first.
As someone who has been reviewing tech for nearly 30 years, consider me super excited about Apple Vision Pro.
Skeptics, and there are many, should at least reserve judgment until trying it out for themselves – though no word yet when it may be available for the general public to give it a spin. Otherwise, deep-pocketed Apple fans who consider themselves “early adopters” may need to take a leap of faith.
Follow Marc on Twitter for his “Tech Tip of the Day” posts: @marc_saltzman . Email him or subscribe to his Tech It Out podcast .
The views and opinions expressed in this column are the author's and do not necessarily reflect those of USA TODAY.
share this!
June 5, 2024
This article has been reviewed according to Science X's editorial process and policies . Editors have highlighted the following attributes while ensuring the content's credibility:
fact-checked
peer-reviewed publication
trusted source
Cutting-edge vision chip brings human eye-like perception to machines
by Li Han, Guo Lili, Tsinghua University
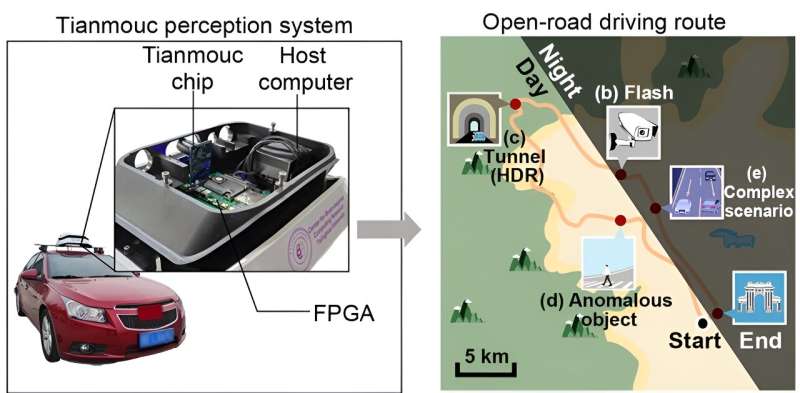
With the rapid advancement of artificial intelligence, unmanned systems such as autonomous driving and embodied intelligence are continuously being promoted and applied in real-world scenarios, leading to a new wave of technological revolution and industrial transformation. Visual perception, a core means of information acquisition, plays a crucial role in these intelligent systems. However, achieving efficient, precise, and robust visual perception in dynamic, diverse, and unpredictable environments remains an open challenge.
In open-world scenarios, intelligent systems must not only process vast amounts of data but also handle various extreme events, such as sudden dangers, drastic light changes at tunnel entrances, and strong flash interference at night in driving scenarios.
Traditional visual sensing chips, constrained by the "power wall" and "bandwidth wall," often face issues of distortion, failure, or high latency when dealing with these scenarios, severely impacting the stability and safety of the system.
To address these challenges, the Center for Brain Inspired Computing Research (CBICR) at Tsinghua University has focused on brain-inspired vision sensing technologies, and proposed an innovative complementary sensing paradigm comprising a primitive-based representation and two complementary visual pathways.
The research paper based on these results, " A Vision Chip with Complementary Pathways for Open-world Sensing ," was featured as the cover article of Nature in the May 30, 2024 issue.
Inspired by the fundamental principles of the human visual system, this approach decomposes visual information into primitive-based visual representations. By combining these primitives, it mimics the features of the human visual system, forming two complementary and information-complete visual perception pathways.
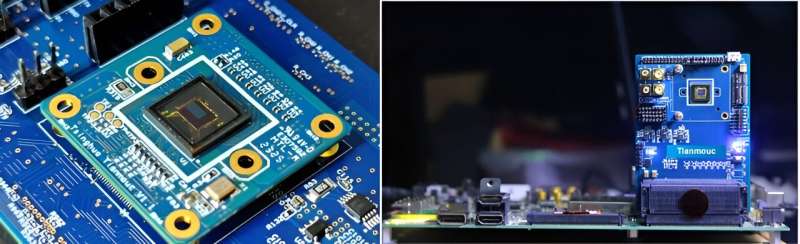
Based on this new paradigm, CBICR has developed the world's first brain-inspired complementary vision chip, Tianmouc. This chip achieves high-speed visual information acquisition at 10,000 frames per second, 10-bit precision, and a high dynamic range of 130 dB, all while reducing bandwidth by 90% and maintaining low power consumption . It not only overcomes the performance bottlenecks of traditional visual sensing paradigms but also efficiently handles various extreme scenarios, ensuring system stability and safety.
Leveraging the Tianmouc chip , the team has developed high-performance software and algorithms, and validated their performance on a vehicle-mounted perception platform running in open environments. In various extreme scenarios, the system demonstrated low-latency, high-performance real-time perception, showcasing its immense potential for applications in the field of intelligent unmanned systems.
The successful development of Tianmouc is a significant breakthrough in the field of visual sensing chips. It not only provides strong technological support for the advancement of the intelligent revolution but also opens new avenues for crucial applications such as autonomous driving and embodied intelligence.
Combined with CBICR's established technological foundation in brain-inspired computing chips like Tianjic, toolchains, and brain-inspired robotics, the addition of Tianmouc will further enhance the brain-inspired intelligence ecosystem, powerfully driving the progress of artificial general intelligence.
Explore further
Feedback to editors

Scientists develop electrified charcoal 'sponge' that can soak up CO₂ directly from the air
2 hours ago

Future-self chatbot gives users a glimpse of the life ahead of them

Researchers debut novel manifold design theory

Using fill factors to prevent loss of resolution in bendable video screens
3 hours ago

Researchers create an autonomously navigating wheeled-legged robot
6 hours ago

Cognitive psychology tests show AIs are irrational—just not in the same way that humans are
17 hours ago

Study introduces improved plutonium production with enhanced efficiency and reduced costs
20 hours ago

New energy source powers subsea robots indefinitely

AI and robotics enhance design of sustainable aerogels for wearable tech

Know your source: RAGE tool unveils ChatGPT's sources
21 hours ago
Related Stories

Integration propels machine vision: Research reviews in-sensor visual perception and inference
Sep 27, 2023

Advancing brain-inspired computing with hybrid neural networks
Apr 10, 2024

Bio-inspired cameras and AI help drivers detect pedestrians and obstacles faster
May 29, 2024

Self-powered and broadband opto-sensor with bionic visual adaptation function
Jul 25, 2023

Researchers develop biomimetic olfactory chips to enable advanced gas sensing and odor detection
Mar 28, 2024

A multi-camera differential binocular vision sensor for robots and autonomous systems
Feb 19, 2024
Recommended for you

Model uses mathematical psychology to help computers understand human emotions

Using AI to decode dog vocalizations
Jun 4, 2024

Supercomputer helps retrain AIs to avoid creating offensive pictures for specific cultures
23 hours ago
Let us know if there is a problem with our content
Use this form if you have come across a typo, inaccuracy or would like to send an edit request for the content on this page. For general inquiries, please use our contact form . For general feedback, use the public comments section below (please adhere to guidelines ).
Please select the most appropriate category to facilitate processing of your request
Thank you for taking time to provide your feedback to the editors.
Your feedback is important to us. However, we do not guarantee individual replies due to the high volume of messages.
E-mail the story
Your email address is used only to let the recipient know who sent the email. Neither your address nor the recipient's address will be used for any other purpose. The information you enter will appear in your e-mail message and is not retained by Tech Xplore in any form.
Your Privacy
This site uses cookies to assist with navigation, analyse your use of our services, collect data for ads personalisation and provide content from third parties. By using our site, you acknowledge that you have read and understand our Privacy Policy and Terms of Use .
E-mail newsletter
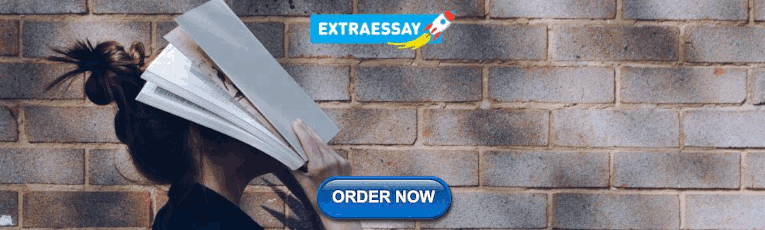
COMMENTS
A list of completed theses and new thesis topics from the Computer Vision Group. Are you about to start a BSc or MSc thesis? Please read our instructions for preparing and delivering your work. PhD Theses Master Theses Bachelor Theses Thesis Topics. Novel Techniques for Robust and Generalizable Machine Learning. PDF Abstract.
Computer Vision. 4668 benchmarks • 1438 tasks • 3033 datasets • 47987 papers with code Semantic Segmentation Semantic Segmentation. 307 benchmarks 5327 papers with code Tumor Segmentation. 3 benchmarks ...
Computer Vision. Computer vision is the science and technology of teaching a computer to interpret images and video as well as a typical human. Technically, computer vision encompasses the fields of image/video processing, pattern recognition, biological vision, artificial intelligence, augmented reality, mathematical modeling, statistics, probability, optimization, 2D sensors, and photography.
How to Contact Faculty for IW/Thesis Advising. Send the professor an e-mail. When you write a professor, be clear that you want a meeting regarding a senior thesis or one-on-one IW project, and briefly describe the topic or idea that you want to work on. ... Computer vision. Independen Work Topics: Constructing a new method to explain a model ...
With respect to undergraduate thesis topics looking at Computer Vision applications is one place to start. The OpenCV library is another. And talking to potential supervisors at your university is also a good idea. With respect to PhD thesis topics, it's important to take into consideration what the fields of expertise of your potential ...
This thesis considers the use of synthetic data to allow the use of DNN to solve problems in computer vision. First, we consider using synthetic data for problems where collection of real data is not feasible. We focus on the problem of magnifying small motion in videos. Using synthetic data allows us to train DNN models that magnify motion ...
Internal Master's thesis at the Computer Vision Lab (CVL) Internal master's theses are normally connected to a research project, and explore a specific research idea. Some project suggestions are listed here: CVL Master's thesis proposal repository. If you already have an idea for a project, you may also contact one of the CVL examiners directly.
The features of big data could be captured by DL automatically and efficiently. The current applications of DL include computer vision (CV), natural language processing (NLP), video/speech recognition (V/SP), and finance and banking (F&B). Chai and Li (2019) provided a survey of DL on NLP and the advances on V/SP. The survey emphasized the ...
The dissertation is focused on trying to answer these key questions in the context of Computer Vision and, in particular, Object Recognition, a task that has been heavily revolutionized by recent ...
List of Computer vision Research Topics Ideas for MS and PH.D. 1. Deep learning-enabled medical computer vision - Research questions. 2. Deep learning, computer vision, and entomology - Research questions. 3. Exploring human-nature interactions in national parks with social media photographs and computer vision. 4.
MASTER THESIS DISSERTATION, MASTER IN COMPUTER VISION, SEPTEMBER 2021 3 Fig. 3. Different teams can use different formations in the same season, as can be seen above for a team like FC Barcelona that has recently been changing its style and formation of play quite regularly. Modelling the motion of players of a team becomes difficult when you ...
Our research combines computer vision, computer graphics, and machine learning to understand images and video data. In our research, we focus on the combination of deep learning with strong models or physical constraints in order to combine the advantages of model-based and data-driven methods. Read more about current research projects in this ...
Below is a list with selected titles of B.Sc. and M.Sc. theses completed in our group to orient you towards potential topics. You can also see our recent papers for possible directions for a thesis. You should have some pre-knowledge in computer vision before starting a thesis. For a B.Sc. thesis you should have at least attended the lecture ...
This section offers a well-organized and extensive list of 1000 computer science thesis topics, designed to illuminate diverse pathways for academic inquiry and innovation. Whether your interest lies in the emerging trends of artificial intelligence or the practical applications of web development, this assortment spans 25 critical areas of ...
Your thesis could be based on UI and computer vision as they really are changing the land scape and help an open source project in the process. We also want to add image homography and feature tracking to the next release (1.3). We have quick release cycles as well (about every 3 months).
Video (online) Consult the top 50 dissertations / theses for your research on the topic 'Computer Science. Computer vision.'. Next to every source in the list of references, there is an 'Add to bibliography' button. Press on it, and we will generate automatically the bibliographic reference to the chosen work in the citation style you need: APA ...
Design, Deployment, and Validation of Computer Vision Techniques for Societal Scale Applications, Arup Kanti Dey. PDF. AffectiveTDA: Using Topological Data Analysis to Improve Analysis and Explainability in Affective Computing, Hamza Elhamdadi. PDF. Automatic Detection of Vehicles in Satellite Images for Economic Monitoring, Cole Hill. PDF
View in the ACM Digital Library. In the past decade, computer vision (CV), machine learning (ML), and artificial intelligence (AI) have been applied to problems in the history and interpretation of fine-art paintings and drawings. Such automated methods provide new tools for connoisseurs and art scholars and have resolved art-historical debates ...
From the book reviews: "The goal of this book is to provide an overview of recent works in computer vision. … The book is intended more for engineers and researchers who will use it as a relevant source of knowledge in the computer vision field, and benefit from the presence of recent and representative methods that are among the best existing solutions to solve the problems reviewed in ...
Our job is to interpret the cues! Depth cues: Linear perspective. Depth cues: Aerial perspective. Depth ordering cues: Occlusion. Shape cues: Texture gradient. Shape and lighting cues: Shading. Position and lighting cues: Cast shadows. Grouping cues: Similarity (color, texture, proximity) Grouping cues: "Common fate".
As an important and active research topic in computer vision community, visual tracking is a key component in many applications ranging from video surveillance and robotics to human computer. In this thesis, we propose new appearance models based on multiple visual cues and address several research issues in feature learning and fusion for ...
Theses/Dissertations from 2022. PDF. The Design and Implementation of a High-Performance Polynomial System Solver, Alexander Brandt. PDF. Defining Service Level Agreements in Serverless Computing, Mohamed Elsakhawy. PDF. Algorithms for Regular Chains of Dimension One, Juan P. Gonzalez Trochez. PDF.
Geometric Representation Learning, Luke Vilnis, Computer Science. PDF. Understanding of Visual Domains via the Lens of Natural Language, Chenyun Wu, Computer Science. PDF. Towards Practical Differentially Private Mechanism Design and Deployment, Dan Zhang, Computer Science. PDF. Audio-driven Character Animation, Yang Zhou, Computer Science
Theses/Dissertations from 2019. PDF. A Secure Anti-Counterfeiting System using Near Field Communication, Public Key Cryptography, Blockchain, and Bayesian Games, Naif Saeed Alzahrani (Dissertation) PDF. Spectral Clustering for Electrical Phase Identification Using Advanced Metering Infrastructure Voltage Time Series, Logan Blakely (Thesis) PDF.
Like nuclear fusion and jet-packs, the self-driving car is a long-promised technology that has stalled for years - yet armed with research, boffins think they have created potential improvements. Citizens of Phoenix, San Francisco, and Los Angeles are able to take one of Waymo's self-driving taxis, first introduced to the public in December 2020.
I had a chance to wear and use Vision Pro for about 30 minutes and run through several applications that fused augmented reality (where you can see digital information overlaid on top of the real ...
This groundbreaking multimodal model integrates text, vision, and in the future, audio capabilities, setting a new standard for generative and conversational AI experiences. GPT-4o is available now in the Azure OpenAI Service API and Azure AI Studio with support for text and images. We believe that multimodal AI will enable new and exciting use ...
Based on this new paradigm, CBICR has developed the world's first brain-inspired complementary vision chip, Tianmouc. This chip achieves high-speed visual information acquisition at 10,000 frames per second, 10-bit precision, and a high dynamic range of 130 dB, all while reducing bandwidth by 90% and maintaining low power consumption.It not only overcomes the performance bottlenecks of ...