The Essex website uses cookies. By continuing to browse the site you are consenting to their use. Please visit our cookie policy to find out which cookies we use and why. View cookie policy.
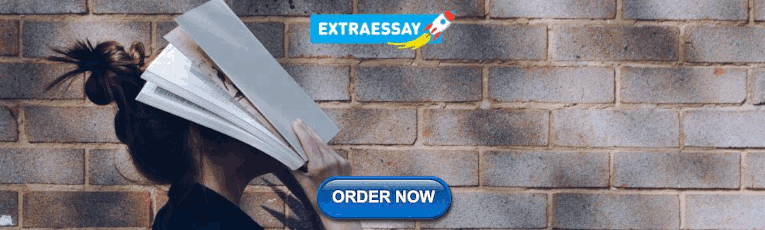
Research risk assessment
It's the responsibility of the principal investigators (PI) and researchers to identify reasonably foreseeable risks associated with their research and control the risks so far as is reasonably practicable.
All participants and research assistants have the right to expect protection from physical, psychological, social, legal and economic harm at all times during an investigation. Certain research may also present reputational, legal and / or economic risks to the University.
As part of the ethical approval process for research involving human participants you are required to identify potential risks associated with your research and the action you will take to mitigate risk. You may be asked to submit your risk assessment.
The risk assessment process is a careful examination of what could cause harm, who/what could be harmed and how. It will help you to determine what risk control measures are needed and whether you are doing enough.
Risk assessment responsibility
The PI and researchers need to take responsibility for all assessments associated with their projects. Occasionally you may need research workers or students to risk assess an aspect of the work and you will need to check the assessments are adequate and sign them off.
Risk assessors need to be competent and you’ll need to ensure they have adequate training and resource to do the assessments. There is risk assessment training available and help and advice help and advice help and advice from your Health and Safety adviser and safety specialists (for health and safety risks), or the REO Research Governance team for other risks. In some cases, the hazards are so unique to the research that the PI and their team might be the only people who know the work well enough to make valid judgements about the risk and justify their conclusions.
Risk assessment process
The risk assessment process is a careful examination of what could cause harm, who/what could be harmed and how. It will help you to determine what risk control measures are needed and whether you are doing enough.
To simplify the process you can use the health and safety risk assessment templates, risk estimation tool and guidance for all risks associated with your research project. Please refer to the research risk estimation guidance under how to carry out a risk assessment below to assist you.
Research risks
Typical risks that need to be considered as part of research ethics are:
- Social risks: disclosures that could affect participants standing in the community, in their family, and their job.
- Legal risks: activities that could result in the participant, researchers and / or University committing an offence; activities that might lead to a participant disclosing criminal activity to a researcher which would necessitate reporting to enforcement authorities; activities that could result in a civil claim for compensation.
- Economic harm: financial harm to participant, researcher and / or University through disclosure or other event.
- Reputational risk: damage to public perception of University or the University/researchers’ reputation in the eyes of funders, the research community and / or the general public.
- Safeguarding risks: Risk to young people, vulnerable adults and / or researcher from improper behaviour, abuse or exploitation. Risk to researcher of being in a comprising situation, in which there might be accusations of improper behaviour.
- Health and safety risks: risks of harm to health, physical injury or psychological harm to participants or the researcher. Further information on health and safety risks is given below.
Health and safety risks
The potential hazards and risks in research can be many and varied. You will need to be competent and familiar with the work or know where to obtain expert advice to ensure you have identified reasonably foreseeable risks. Here are some common research hazards and risks:
- Location hazards Location hazards Location hazards and risks are associated with where the research is carried out. For example: fire; visiting or working in participant’s homes; working in remote locations and in high crime areas; overseas travel; hot, cold or extreme weather conditions; working on or by water. Also hazardous work locations, such as construction sites, confined spaces, roofs or laboratories. For overseas travel, you will need to check country / city specific information, travel health requirements and consider emergency arrangements as part of your research planning, by following the University’s overseas travel health and safety standard .
- Activity hazards Activity hazards Activity hazards and risks associated with the tasks carried out. For example: potentially mentally harmful activities; distressing and stressful work and content; driving; tripping, or slipping; falling from height; physically demanding work; lifting, carrying, pushing and pulling loads; night time and weekend working.
- Machinery and equipment Machinery and equipment Machinery and equipment . For example: ergonomic hazards, including computer workstations and equipment; contact with electricity; contact with moving, rotating, ejecting or cutting parts in machinery and instruments; accidental release of energy from machines and instruments.
- Chemicals and other hazardous substances . The use, production, storage, waste, transportation and accidental release of chemicals and hazardous substances; flammable, dangerous and explosive substances; asphyxiating gases; allergens; biological agents, blood and blood products. You’ll need to gather information about the amount, frequency and duration of exposure and carry out a COSHH or DSEAR assessment which will inform whether you may need health surveillance for yourself and / or your research participants.
- Physical agents Physical agents Physical agents . For example: excessive noise exposure, hand-arm vibration and whole body vibration; ionising radiation; lasers; artificial optical radiation and electromagnetic fields. You’ll need to gather information about the amount, frequency and duration of exposure inform whether you may need health surveillance for yourself and / or your research participants.
When to carry out a risk assessment
Carrying out initial risk assessments as part of the planning process will help you identify whether existing resources and facilities are adequate to ensure risk control, or if the project needs to be altered accordingly. It will also help you to identify potential costs that need to be considered as part of the funding bid.
Once the project is approved, research specific risk assessments need to be carried out before work starts.
The research may need ethical approval if there is significant risk to participants, researchers or the University.
How to carry out a risk assessment
The University standard on risk assessments provides guidance, tips on getting it right, as well as resources and the forms to help you produce suitable and sufficient risk assessments and must be used.
- Risk assessment template (.dotx)
- Flow chart to research risk assessment (.pdf)
- Research risk assessment: Risk estimation tool (.pdf)
- Example of a Social Science research risk assessment (.pdf)
Refer to carrying out a risk assessment carrying out a risk assessment carrying out a risk assessment for step by step guidance.
Risk assessments must relate to the actual work and must be monitored by the PI. If there are significant changes to the activities, locations, equipment or substances used, the risk assessment will need to reviewed, updated and the old version archived. Risk assessments should also consider the end of projects, arrangements for waste disposal, equipment, controlled area decommission and emergencies.
Things to consider:
- The risks may be specialist in nature or general. Information can found from legislation, sector guidance, safety data sheets, manufacturers equipment information, research documents, forums and health and safety professionals.
- Practical research might involve less well-known hazards. Do you or your team have the expertise to assess the risk adequately? Do you know who to go to for expert advice?
- The capabilities, training, knowledge, skills and experience of the project team members. Are they competent or are there gaps?
- In fast changing research environments, is there a need to carry out dynamic risk assessments? Are they understood and recorded?
- The right personal protective equipment for the hazards identified and training in how to use it.
- Specific Occupational Health vaccinations, health surveillance and screening requirements identified and undertaken. With physical agents and substances you’ll need to make an informed decision about the amount, frequency and duration of exposure. If you need help with this contact Health and Safety.
- Associated activities: storage, transport/travel, cleaning, maintenance, foreseeable emergencies (eg spillages), decommissioning and disposal.
- The safe design, testing and maintenance of the facilities and equipment.
- Planned and preventative maintenance of general plant and specialist equipment.
These risk assessments relate to the actual work and must be monitored by the PI. If there are significant changes to the activities, locations, equipment or substances used, the risk assessment will need to reviewed, updated and the old version archived. Risk assessments should also consider the end of projects, arrangements for waste disposal, equipment and controlled area decommission and emergencies.
Training
If you would like training on completing a risk assessment, please book onto our Risk Assessment Essentials course via HR Organiser. If you are unable to access this, please email [email protected]
- Carrying out a risk assessment Carrying out a risk assessment Carrying out a risk assessment
- People especially at risk People especially at risk People especially at risk
- IOSH/USHA/UCEA guidance on managing health and safety in research (.pdf)
- Research governance: Ethical approval
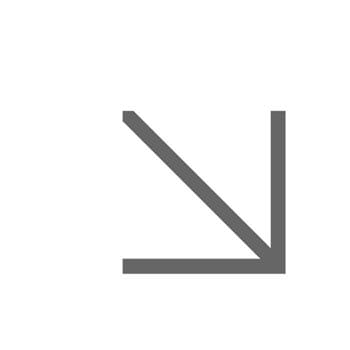
- For enquiries contact your Student Services Hub
- University of Essex
- Wivenhoe Park
- Colchester CO4 3SQ
- Accessibility
- Privacy and Cookie Policy

Data Science Journal
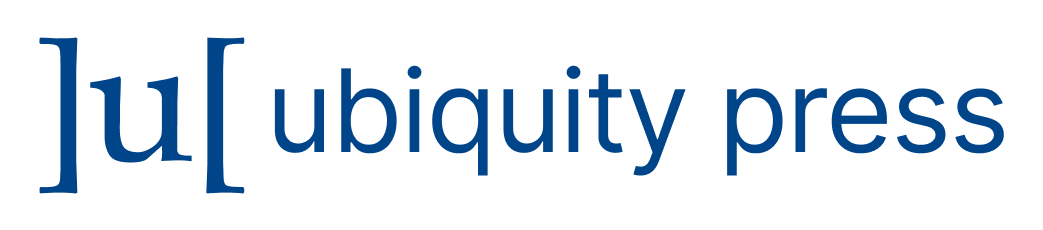
- Download PDF (English) XML (English)
- Alt. Display
Research Papers
Risk assessment for scientific data.
- Matthew S. Mayernik
- Kelsey Breseman
- Robert R. Downs
- Alexis Garretson
- Chung-Yi (Sophie) Hou
- Environmental Data Governance Initiative (EDGI) and Earth Science Information Partners (ESIP) Data Stewardship Committee
- risk assessment
- data preservation
- data stewardship
Introduction
At 1 the “The Rescue of Data At Risk” workshop held in Boulder, Colorado on September 8th and 9th, 2016, 2 participants were asked the following question: “How would you define ‘at risk’ data?” Discussions on this point ranged widely, and touched on several challenges, including lack of funding or personnel support for data management, natural and political disasters, and metadata loss. One participant’s organization’s definition of risk, however, stood out: “data were considered to be at risk unless they had a dedicated plan to not be at risk.” This simple statement vividly depicts how data’s default state is being in a state of risk. Thus, ongoing stewardship is required to keep data collections and archives in existence.
The risk factors that a given data collection or archive may face vary depending on the data’s characteristics, the data’s current environment, and the priorities and resources available at the time. Many risks can be reduced or eliminated by following best practices codified as certifications and guidelines, such as the CoreTrustSeal Data Repository Certification ( 2018 ) and the ISO 16363:2012. This ISO standard defines audit and certification procedures for trustworthy digital repositories ( ISO 2012b ). Both the CoreTrustSeal certification and ISO 16363:2012 are based on the ISO 14721:2012 standard that defines the Open Archival Information System (OAIS) Reference Model ( ISO 2012a ). But these certifications can be large and complex. Additionally, many of the organizations that hold valuable scientific data collections may not be aware of these standards, even if the organizations are potentially resourced to tackle the challenge ( Maemura, Moles & Becker 2017 ). Further, the attainment of such certifications does not necessarily reduce the risks to data that are outside of the scope of a particular certification instrument.
This paper presents an analysis of data risk factors that scientific data collections and archives may face, and a matrix to support data risk assessments to help ameliorate those risks. The three driving questions for this analysis are:
- How to assess what data are at risk?
- How to characterize what risk factors data collections and/or archives face?
- How to make risks more transparent, internally and/or externally?
The goals of this work are to inform and enable effective data risk assessment by: a) individuals and organizations who manage data collections, and b) individuals and organizations who want to help to reduce the risks associated with data preservation and stewardship. Stakeholders for these two activities include producers, stewards, sponsors, and users of data, as well as the management and staff of the institutions that employ them.
This project has been coordinated through the Data Stewardship Committee within the Earth Science Information Partners (ESIP), a non-profit organization that exists to support collection, stewardship, and use of Earth science data, information, and knowledge. 3 The immediate motivation for the project stemmed from the Data Stewardship Committee members engaging with groups who were undertaking grass-roots “data rescue” initiatives after the 2016 US presidential election. At that time, a number of loosely organized and coordinated efforts were initiated to duplicate data from US government organizations to prevent potential politically motivated data deletion or obfuscation (See for example Dennis 2016 ; Varinsky 2017 ). In many cases, these initiatives specifically focused on duplicating government-hosted Earth science data.
ESIP Data Stewardship Committee members wrote a white paper to provide the Earth science data centers’ perspective on these grass-roots “data rescue” activities ( Mayernik et al. 2017 ). That document described essential considerations within day-to-day work of existing federal and federally-funded Earth science data archiving organizations, including data centers’ constant focus on documentation, traceability, and persistence of scientific data. The white paper also provided suggestions for how the grass-roots efforts might productively engage with the data centers themselves.
One point that was emphasized in the white paper was that the actual risks faced by the data collections may not be transparent from the outside. In other words, “data rescue” activities may have in fact been duplicating data that were at minimal risk of being lost ( Lamdan 2018 ). This point, and the white paper in general, was well received by people inside and outside of these grass-roots initiatives ( Cornelius & Pasquetto 2017 ; McGovern 2017 ). Questions then came back to the ESIP Data Stewardship Committee about how to understand what data held by government agencies were actually at risk.
The analysis presented in this paper was initiated in response to these questions. Since then, these grass-roots “data rescue” initiatives have had mixed success in sustaining and formalizing their efforts ( Allen, Stewart & Wright 2017 ; Chodacki 2018 ; Janz 2018 ). The intention of our paper is to enable more effective data risk assessment broadly. Rescuing data after they have been corrupted, deleted, or lost can be time and effort intensive, and may be impossible ( Pienta & Lyle 2018 ). Thus, we aim to provide guidelines to any individual or organization that manages and provides access to scientific data. In turn, these individuals and organizations can better assess the risks that their data face, and characterize those risks.
When discussing risk and, in particular, data risk, it is useful to ask the question: what is the objective that is being challenged by the possible risk factors? With regard to data, in general, discussions of risk might presume that “risks” threaten the current or future access to data by the potential data users. Currently, continuing public access to and use of scientific data is particularly relevant in light of recent open data and open science initiatives. In this regard, risks for scientific data include factors that could hinder, constrain, or limit current or future data use. Identifying such risk factors to data use offers further analysis opportunities to prevent, mitigate, or eliminate the risks.
Data Risk Assessment
Risk assessment is a regular activity within many organizations. In a general sense, risk management plans are complementary to project management plans ( Cervone 2006 ). Organizational assessment of digital data and information collections is likewise not new ( Maemura, Moles & Becker 2017 ). The analysis presented in this paper builds on prior work in a number of areas: 1) research on data risks, 2) data rescue initiatives within government agencies & specific disciplines, 3) CODATA and RDA working groups & meetings, 4) trusted repository certifications, and 5) knowledge and experience of the ESIP Data Stewardship Committee members. Table 1 summarizes data risk factors that emerge from these knowledge bases. The list of risk factors shown in Table 1 is not meant to be exhaustive. Rather, it provides a useful illustration of the diverse ways in which data sets, collections, and archives might encounter risks to data usability and accessibility. The rest of this section details further key insights from the five areas of prior work noted above.
Risk factors for scientific data collections.
Research on data risks
A range of studies have explored the kinds of risks that scientific data may face, and potential ways to mitigate specific risk factors. Many of these studies touch on practices that are typical of scientific data archives. Metadata, for example, can be considered both a risk factor and a mitigation strategy. Insufficient metadata is itself a potential factor that can reduce the discoverability, usability, and preservability of data, particularly in situations where direct human knowledge of the data is absent ( Michener et al. 1997 ). In fact, many data rescue projects find that the “rescue” efforts must be targeted much more toward metadata than data (see Knapp, Bates & Barkstrom 2007 ; Hsu et al. 2015 ). This might be the case for a couple of reasons. First, insufficient or missing metadata might prevent data from being usable regardless of the condition of the data themselves. Examples include missing column headers in tabular data that prevent a user from knowing what the data are representing, and insufficient provenance metadata that prevent users from trusting the data due to lack of context about data collection and quality control. Second, metadata are also central to documenting and mitigating risks as they manifest while preventing risks from becoming problematic in the future ( Anderson et al. 2011 ). For example, documenting data ownership and usage rights is an essential step in mitigating the risk factor “Legal status for ownership and use” from Table 1 .
Different kinds of metadata might be necessary to reduce specific data risks. For example, specifications of file format structures are a critical type of metadata for mitigating risks associated with digital file format obsolescence. Open specifications complement other critical mitigation practices and tools related to file format obsolescence. As one example, keeping rendering software available is an important way to retain access to particular file formats, but this typically also requires maintaining documentation of how the rendering software works ( Ryan 2014 ).
Other risk factors (listed in Table 1 ) relate to the sustainability and transparency of the archiving organization. These factors are important in ensuring the accessibility of the data and the trustworthiness of the archive. As Yakel et al. ( 2013 ) note, “[t]rust in the repository is a separate and distinct factor from trust in the data” (pg. 154). For people outside of the repository, “institutional reputation appears to be the strongest structural assurance indicator of trust” (pg. 154). Effective communication about data risks and steps taken to eliminate problems is helpful in ensuring users that the archive is trustworthy ( Yoon 2017 ).
Data that face extreme or unusual risks, however, may not be manageable via typical data curation workflows. Downs and Chen ( 2017 ) note that dealing with some data risk factors “may well require divergence from regular data curation procedures as tradeoffs may be necessary” (pg. 273). For example, Gallaher et al. ( 2015 ) undertook an extensive project to recover, reconstruct, and reprocess data from early satellite missions into modern formats that are usable by modern scientists. This project involved dealing with degrading and fragile magnetic tapes, extracting data from the tapes’ unusual format, and recreating documentation for the data. Natural disasters, fires, and floods also present unpredictable risk factors to data collections of all kinds. While these kinds of events can be planned for and steps can be taken to prevent the occurrence of some of them (e.g. fires), they can still cause major data loss and/or require significant recovery effort.
Mitigating risks, of whatever kind, takes effort and resources. The time required to create metadata, re-format files, create contingency plans, and communicate these efforts to user communities can be considerable. This time investment can be the greatest barrier to performing risk assessment and mitigation activities ( Thompson, Robertson & Greenberg, 2014 ). Putting focus on assessment of data risk factors may mean that “certain priorities need to be re-ordered, new skills acquired and taught, resources redirected, and new networks constructed” ( Griffin 2015, pg. 93 ). It can be possible to automate some components of risk assessment ( Graf et al. 2017 ), but most of the steps require human effort. This intensive effort is vividly illustrated by the many data rescue initiatives that have taken place within government agencies and other kinds of organizations over the past few decades.
Data rescue initiatives within government agencies & specific disciplines
Legacy data are data collected in the past with different technologies and data formats than in use today. These data often face the largest numbers of risk factors that could lead to data loss. A wide range of government agencies and other organizations have conducted legacy data rescue initiatives to modernize data and make them more accessible and usable for today’s science. Each data rescue project typically faces many different kinds of data risks. For example, a recent satellite data rescue effort had to address the “loss of datasets, reconciliation of actual media contents with metadata available, deviation of the actual data format from expectations or documentation, and retiring expertise” ( Poli et al. 2017, pg. 1481 ). Data rescue projects typically involve work to prevent future risk factors from manifesting, in addition to modernizing data for accessibility and usability. For example, data rescue projects migrate data to less endangered data formats, and create new metadata and quality control documentation ( Levitus 2012 ).
CODATA/RDA working groups & meetings
Relevant professional organizations, including the International Council for Science (ICSU) Committee on Data for Science and Technology (CODATA) and the Research Data Alliance (RDA), also have been actively identifying improvements for data stewardship practices that can reduce potential risks to data. For example, the former Data At Risk Task Group (DAR-TG), of CODATA, raised awareness about the value of heritage data and described the benefits obtained from several data rescue projects ( Griffin 2015 ). This group also organized the 2016 “Rescue of Data At Risk” workshop mentioned in the introduction of this paper. That workshop led to a document titled, “Guidelines to the Rescue of Data At Risk” ( 2017 ). Subsequently, the Data Rescue Interest Group ( 2018 ) of the Research Data Alliance (RDA), spawned from the CODATA DAR-TG, also focuses on efforts to increase awareness of data rescue projects.
Repository certifications and maturity assessment
Many data repositories have conducted self-assessments and external assessments to document their compliance with the standards for trusted repositories and attain certification of their capabilities and practices for managing data. In addition to emphasizing organizational issues, repository certification instruments, such as ISO 16363 ( 2012b ) and CoreTrustSeal (2018) certification, also focus on digital object management and infrastructure capabilities. Engaging in such assessments offers benefits to repositories and their stakeholders. A key benefit is the identification of areas where improvements have been completed or need to be completed to reduce risks to data (CoreTrustSeal 2018). In an examination of perceptions of repository certification, Donaldson et al. ( 2017 ) found that process improvement was often reported by repository staff as a benefit of repository certification.
In addition to (or complementary to) formal certifications, data repositories may conduct data stewardship maturity assessment exercises to help in identifying data risks and informing data risk mitigation strategies ( Faundeen 2017 ). “Maturity” is used in the sense presented by Peng et al. ( 2015 ), and refers to the level of performance attained to ensure preservability, accessibility, usability, transparency/traceability, and sustainability of data, along with the level of performance in data quality assurance, data quality control/monitoring, data quality assessment, and data integrity checks. Maturity at the institutional (or archive) level in areas such as policy, funding, and infrastructure does not necessarily translate to comprehensive maturity at the dataset level ( Peng 2018 ). Data stewardship maturity assessment should therefore be performed both at the institutional level and at the dataset level. It is recognized that performing stewardship maturity assessments can be time consuming and resource intensive. However, the stewardship organizations are encouraged to perform self-assessment using “stage by stage” or “a la carte” approach (see example in Peng et al. 2019 ). Ultimately, both formal certifications and informal maturity assessments help organizations not only gain self-awareness, but also identify better solutions for their data that might be at risk of being lost or rendered unusable.
Developing a Data Risk Assessment Matrix
Risk assessment is a well-established field, with 30–40 years of history ( National Research Council 1983 ; Aven 2016 ). However, the practice of applying risk assessment methodologies to scientific data collections is less formally established, though regular audits and reviews of data management systems are common in some organizations ( Ramapriyan 2017 ).
The starting point for this project was to establish a process for categorizing the data risk factors shown in Table 1 . The initial idea of our effort was that if data risk factors could be categorized into a logical structure, it would allow data managers to assess the risks to their data collections via a set of predefined and consistent categories. To develop a logical categorization, we held a session to conduct a “card sorting” exercise at the 2018 ESIP Summer Meeting, which took place in July 2018 in Tucson, Arizona. “Card sorting” is an established method for developing categorizations of concepts, vocabulary terms, or web sites ( Zimmerman & Akerelrea 2002 ; Usability.gov 2019 ). Following the card sorting methodology, participants in the 2018 ESIP meeting session were provided the list of data risks in Table 1 , and asked to complete the following task: “Looking at the list of data risk factors, how would you group these factors, based on the categories you would define?”
Approximately 15 attendees engaged in the exercise. We used a combination of an online card sorting tool and hand-written recommendations to collect the completed card sorting categorizations. Following the completion of the exercise, the results were displayed in front of the session participants and a group discussion took place. The outcome of the card sorting exercise and subsequent discussion was a clear recognition that there could be many valid and useful ways of categorizing data risks. No single method for categorizing the risk factors would be sufficient to cover the diverse organizations and situations within which data collections exist. Depending on the situation(s), a data curation organization or individual is facing, they may need to categorize data risks in different ways. This characteristic is common in risk assessments generally, as risk prioritization and categorizations are dependent on the phenomena being assessed, the characteristics of the situation, and the goals of the organizations or people performing the assessment ( Slovic 1999 ).
Through subsequent discussion and analysis of the data risk assessment literature noted above, we identified at least ten different ways that data risk factors could be assessed. Many of these categorization methods are applicable to risk assessments of any kind ( Cervone 2006 ). The list below is not meant to be exhaustive, and some methods are likely related. Data risk factors could be categorized or prioritized according to the methods listed in Table 2 .
Methods for Categorizing Data Risks.
The lists shown in Tables 1 and 2 offer characteristics on which data risk assessments can be built. Combining the categorization methods from Table 2 with the selected risk factors from Table 1 leads to a risk assessment matrix, as shown in Table 3 . This figure shows an example of a selection of specific data risk factors and the categorization methods. Depending on the situation or data collection being assessed, different risk factors and/or categorization methods may be more applicable than the ones shown in Table 3 . Those conducting a data risk assessment can then use the matrix as a way to organize, prioritize, or potentially quantify the selected risks according to the categorization methods that are most relevant for the specific case at hand. The next section provides more detailed illustrations of the use of the data risk assessment matrix. Appendix I shows the full data risk assessment template, with all risks and categorization methods from Tables 1 and 2 .
Example of a blank data risk assessment matrix, after selection of specific risk factors and categorization methods of interest.
Application of the Data Risk Assessment Matrix
Three case studies are described below in which the data risk assessment matrix was used to develop a better understanding of data risks for particular resources. These cases enable evaluation of the data risk assessment framework presented in this paper, clarifying its strengths and weaknesses, and pinpointing the situations in which it can be most useful ( Becker, Maemura & Moles 2020 ).
Case 1 – NCAR Library Analog Data Collection
The National Center for Atmospheric Research (NCAR) Library maintains an analog data collection that consists of about 300 data sets in support of atmospheric and meteorological research conducted by NCAR scientists. These assets are largely compilations of measurements and statistics published by national and international meteorological services and other kinds of government entities. Many of these assets have been in the NCAR Library’s collections for decades, and most were minimally cataloged when they were first brought into the collection. As such, the current usage of the collection is minimal. A prior assessment done by the NCAR Library and a student assistant sought to identify individual assets that were of higher potential value and interest for current science. This assessment effort resulted in a modernization prioritization based on a geographic and temporal framework, and improved metadata records for about 5% of the collection ( Mayernik et al. 2018 ). This effort did not, however, include any kind of risk assessment related to the physical assets themselves.
The data risk assessment matrix was therefore helpful in doing a second-level priority analysis for these NCAR Library analog data assets. We used the matrix as a way to identify which risk factors were most important for these materials, and to characterize the mitigation efforts that were needed for each risk factor. In particular, we focused the risk assessment on the data assets that were previously identified as having high geospatial and temporal interest. The NCAR Library use of the matrix involved a series of steps:
- Step 1 – A number of risk factors listed in the matrix were identified as being of most importance, with the focus being on factors that prevented or impeded the use of these data within current scientific studies. The most immediate risk factors were identified to be the “lack of use” and the “lack of documentation/metadata” for these assets. Other risks that were secondary in immediacy, but still potentially important, were: Data mislabeling, the questionable legal status for ownership and use, media deterioration, lack of planning, and poor data governance.
- Step 2 – The second step was to identify which categorization methods shown in the matrix were most applicable/appropriate for the NCAR Library’s management and maintenance of this collection. The methods selected were: a) Length of recovery time, b) Who is responsible for addressing the problem, c) Nature of mitigation, and d) Resources required for mitigation.
- Step 3 – The third step was to fill in the boxes in the matrix for the risk factors and categorization methods. For example, for the “Length of recovery time” question, we used a simple 1–3 scale to indicate relative differences in how long it would take to mitigate the two most important risk factors: “lack of use” and the “lack of documentation/metadata”. As one example, some data assets were published by international agencies and therefore have title pages and documentation that are not in English. In turn, due to the lack of relevant foreign language expertise in the NCAR Library staff, developing new metadata for these resources will take more effort than for those assets that were published by English-speaking countries. For the “Resources required for mitigation” categorization method, a numerical scale was not as appropriate. Instead, we filled in the matrix with text descriptions of the resources required to mitigate the risk factors. An example entry under the “lack of documentation & metadata” risk factor was: “We would need to create new metadata for the library catalog, then transform to ISO for inclusion in NCAR DASH Search, with added challenge of needing to look at microfilm files (no current working reader in Library).”
In summary, the matrix was very useful as “something to think with.” In other words, it jump-started the process for doing the risk assessment because the NCAR Library staff did not need to spend time developing a comprehensive list of risk factors that may apply for these data, or brainstorm about how to categorize those risks. The risk factor matrix provided a ready-made starting point for the assessment. Because the matrix does not dictate how the cells should be filled in, the NCAR Library staff made decisions about how to apply the matrix for each categorization method that was chosen. The matrix structure could potentially be applied or customized to create a prioritization rubric, by supporting the creation of a numeric scoring process for categories where that is appropriate.
Case 2 – Mohonk Preserve Daniel Smiley Research Library
Mohonk Preserve is a land trust and nature preserve in New Paltz, New York covering more than 8,000 acres of a northern section of the Appalachian Mountains known as the Shawangunk Mountains. Mohonk Preserve’s conservation science division, the Daniel Smiley Research Center (DSCR), is affiliated with the Organization of Biological Field Stations (OBFS) and acts as a NOAA Climate Observation Center. DSRC staff and citizen scientists carry out a variety of long-term monitoring projects and manage an extensive archive of historical observations. The archive houses 60,000 physical items, 9,000 photographs, 86 years of natural history observations, 123 years of daily weather data, and a research library of legacy titles. The physical items include more than 3,000 herbarium specimens, 107 bird specimens, 140 butterfly specimens, 139 mammal specimens, 400 arthropod specimens, and over 14,000 index cards with handwritten and typed observations. The digitization process of the archive holdings is ongoing, but the packaging and publishing of datasets in the Environmental Data Initiative is a priority ( Mohonk Preserve et al. 2018a , 2018b , 2019 ). These data and natural history collections underpin the Mohonk Preserve’s land management and stewardship and have been crucial to an increasing number of scientific publications (e.g., Cook et al. 2009 ; Cook et al. 2008 ; Charifson et al. 2015 ; Richardson et al. 2016 ), but the collections remain underutilized.
The data rescue effort for the archives has largely consisted of digitization and cataloging. Hence, the data risk assessment matrix was used to guide the prioritization of datasets for publication and assess other data rescue needs and considerations for the archives. The most critical risk factors identified through the process were ‘lack of documentation & metadata’, ‘loss of knowledge’, and ‘lack of use.’ In order to address the lack of use, we collaboratively developed a prioritization of the data holdings for publication in a repository, particularly based around the value of data collected for scientific investigations, the temporal coverage of the dataset, and an assessment of the resources required for the digitization, packaging, and publishing of the relevant dataset.
We also realized through the data risk matrix process that many of our risk factors are interdependent – for example, the lack of documentation may not be because the documentation does not exist in the library, but rather that it may not be discoverable in the archives due to incomplete digitization or cataloging of the relevant records or field notes. For example, during the assessment process for our vernal pool monitoring dataset ( Mohonk Preserve et al. 2019 ), we discovered previously unknown environmental quality notes in narrative sections of an undigitized collection of field notes. This supported the current emphasis on the digitization and cataloging of the holdings and suggested areas of high importance, particularly the narrative sections of field notebooks. Additionally, the lack of documentation and metadata is directly related to the loss of knowledge through leadership transitions. Like many long-term ongoing collections projects, metadata and documentation– particularly related to data collection protocols– are held as tacit knowledge by key stakeholders who have been involved with the project for an extended period of time. The loss of those stakeholders or their knowledge, through retirement or employment changes, poses a significant risk to the long-term value of the associated data ( Michener et al. 1997 ).
Because the holdings largely consist of physical items, a subset of the risk factors in the matrix were not directly applicable to the collections but had corollaries in physical collections management. For example, bit rot and data corruption are not a concern for the physical items, but pests present a similar concern that needs mitigation in a physical archive setting. Additionally, storage hardware breakdown is not directly applicable to herbarium collections but ensuring that the mounting sheets are acid-free is key to ensuring the protection of the specimens and preventing deterioration over time. Considering physical risks to the collection media remains a crucial aspect of managing and planning for the future of physical specimen holdings. Through the data assessment process, one of the key risk areas identified through the assessment was the loss of knowledge and documentation due to retirement, so planning for mitigating this risk is ongoing. Overall, the matrix provided a helpful starting point for guiding conversations relating to the stewardship of the archives and proactively planning and allocating resources to make the data more accessible to scientists and researchers.
Case 3 – EDGI Response to the Deer Park Chemical Fire
On March 17 2019, tanks of chemicals at the Intercontinental Terminals Company (ITC) in Deer Park, Texas, caught fire and began a blaze that would last several days, emitting a chemical-laden plume of smoke over surrounding communities. The Environmental Data & Governance Initiative (EDGI) was approached for assistance in rapid-response archival backup of digital environmental data relevant to the fire in case of future tampering or loss of availability.
There were two major causes for concern: (1) evident tampering– the closest air quality monitor was taken down during the fire, and (2) potential conflict of interest– the entity furnishing the data might have some culpability in a future legal case using the data. The approaching organization hopes to use saved data as evidence in legal cases that may take several years (potentially due to the long timespan for benzene-related illnesses to surface in then-students at the local school and workers in nearby factories).
On a limited timeline and with little capacity, EDGI needed to downselect from hundreds to thousands of possibly relevant data sources (including air and water quality monitors affected by the fire’s plume, and plans and response documents surrounding handling of the fire). The primary mission: ensure the data of primary concern is backed up in a legally legitimate (traceable) format that will be usable in a decade or more.
The data risk matrix from this paper was not used at the time
Prioritization.
The approaching organization suggested a few directories of static data to archive. With additional investigation, EDGI also found some API-accessible structured data from the air monitors that was updated daily.
The information proposed for potential rescue included:
- Data from the Deer Park air quality monitor data that was taken down
- Data from other nearby air quality monitors
- Air quality monitors downstream of the plume (potentially very many of them, as the plume traveled more than 20 miles)
- Three years of back-data from any air quality monitors, to establish baseline
- Water quality monitors– local, downstream, and down-plume, in case relevant (no evidence of contamination yet, but the situation still developing), and three years of back data to establish baseline
- Future data from any monitors, to track the still-developing situation and archive it in case of any present risk
- Contextual information: air sampling plans, disaster response plans, air and water quality sampling maps, PDFs of additional air and water quality sampling from different entities than provide the API-callable data
There was no formal review process for deciding what to save. There was some brief discussion internally around technical feasibility and potential environmental justice-focused mapping efforts, but the major use anticipated for the saved data was the legal case. The whole process from request to data backup took just a few days. Ultimately, EDGI’s choices of data to save depended primarily on the abilities and assessment of the two volunteers available. The volunteers used the skills they had and their best intuitions– lacking a clear prioritization between different data that could be saved.
Applying the data risk matrix to this situation, the two major risk factors can be immediately identified as “catastrophe” and “political interference”. Both risk factors are relevant, likely, and potentially catastrophic in effect. This highlights the urgency and source of the risk.
The risk matrix is not as helpful in prioritizing which data to save under capacity and urgency constraints. The risk matrix identifies the type and intensity of risk, but since all of the data is equally high-risk in this use case, the context of the data and its use case (evidence in a far-future legal case) are necessary for the following tasks of identifying, locating, and prioritizing data to save. This was done based on the best assessment and abilities of the available volunteers.
Ultimately, EDGI saved:
- Structured data from the Deer Park and nearby Lynchburg Ferry air quality monitors: saved with metadata to IPFS via Qri ( qri.io ) with script to keep pulling updates
- All of the PDF data (primarily directories of 20–100 links, typically to PDFs, including maps, images, narratives, and tables of data): saved to the Internet Archive as a full site snapshot
Assessing risks to rescued data
Following the data rescue operation, this risk matrix was used to assess ongoing risks to the repositories of rescued data: (1) the PDF data saved to the Internet Archive and (2) the structured data from air quality monitors saved to IPFS. The risk matrix was very effective for identification of vulnerabilities and potential next steps to better secure the data.
The full matrix (all of the categories and all of the risk factors) was applied twice: to PDF data saved to the Internet Archive, and to structured data saved on the decentralized web (IPFS). A scale of numeric values (1 (low) to 3 (high)) was used to rate the category versus the risk factor. For example, the risk factor of Media Deterioration was rated 3 (high) for Severity of Risk, but 1 (low) for Likelihood of Occurrence. This numeric rating was important to use of the full matrix– instead of removing columns as irrelevant, they could be down-rated where the risk was low.
Use of the matrix immediately highlighted the difference in risks important to the data stored on IPFS versus the Internet Archive. For example, data on the Internet Archive is well-governed and reasonably easy to find, but much more susceptible to natural disaster and hardware deterioration than the data on IPFS. IPFS is a new technology designed to store data across many physical locations– so it’s very resilient to location-based risks, but its format may become obsolete as the technology develops.
The risk matrix is particularly useful when combined with spreadsheet tools. For example, a quick to-do list for EDGI as a data manager can be produced using a formula such as:
- Likelihood of occurrence > 1
- Resources for mitigation < 3
- Type of action: proactive
- Responsible party: EDGI
- Print mitigation action for rows where all of the above are true
Overall, the Risk Matrix outlined in this paper is a very useful tool for identifying risks to data and prioritizing next steps for mitigation– as long as the user has or can assume control over the data. However, in a data rescue use case, this risk matrix must be supplemented by additional context in order to prioritize which at-risk data should be saved when capacity is limited.
Conclusions and Lessons Learned
Risk assessments are instrumental for ensuring that existing data collections continue to be useful for scientific research and societal applications. Risk assessments are also an essential component of data rescue efforts in which interventions take place to prevent or minimize data loss. The data risk assessment framework presented in this paper provides a platform from which risk assessments can quickly begin.
To close out this paper, we discuss some observations and lessons learned in developing and applying the data risk assessment matrix. Data risk assessments can get significantly more in-depth and detailed than the basic template presented in Table 3 and Appendix I. As one example, the US Geological Survey (USGS) has undertaken a substantive project to create risk calculations for USGS-held data collections based on a number of criteria ( USGS 2019 ). The USGS process has involved the development of detailed formulas and weighting schemes to produce quantified assessments of data risk. The risk assessment matrix presented in this paper does not provide “out of the box” quantification measures or data risk prioritizations of the level of detail of the USGS project. The data risk matrix does, however, provide the foundations for an individual or organization to develop a more customized risk assessment rubric. The specifics of how risks were quantified or qualified, and how they were prioritized varied across the different uses of the matrix presented in the three case studies.
The three cases did demonstrate a common use pattern for the data risk matrix. The first step in each case was to review Tables 1 and 2 to determine which risk factors and categorization methods were most relevant. Clearly not all of the risk factors are applicable to all cases, and some of the risk factors are closely related, such as the “lack of documentation & metadata” and “lack of provenance information.” Once the risk factors and categorization methods have been filtered down into a smaller matrix, the next step is to determine how to fill in the matrix cells for particular datasets or collections. It may not be obvious how this would work for some data collections. Our cases involved using a mix of quantitative, qualitative, and ordinal rankings (such as using “high, medium, and low” designations for particular cells). This step may take some trial and error by the matrix user(s) to determine ranking approaches that are the most useful.
The third step is then to use the cell values in the matrix to guide conversations and decisions about risk mitigation priorities. In this sense, the matrix exercise can provide a high level overview of data collections, risks they may face, and the relative urgency and challenges that those risks present to the data stewards. The matrix can serve as a common reference point for discussions of resource allocations and stewardship priorities. However, as exemplified in the EDGI use case, prioritization in real-time, as would be required during catastrophic events such as disasters or wars particularly where there may be political interference, is difficult if not impossible. As such, preventing or minimizing data loss requires pre-planning at a scale rarely available.
The goal in creating this data risk assessment matrix has been to provide a light-weight way for data collections to be reviewed, documented, and evaluated against a set of known data risk factors. As the understanding of the value that scientific data have for research and societal uses increases, many initiatives recognize that “old data is the new data” ( NIWA 2019 ). Risk assessments are critical to ensure that “old data” can become “new data,” and are also critical to ensure that new data can continue to be newly useful into the future.
We list EDGI and the ESIP Data Stewardship Committee as authors due to the contributions of many individuals from both organizations to the work described in this paper. The named authors are the individuals involved in each organization who contributed directly to the paper’s text.
The workshop was organized under the auspices of the Research Data Alliance (RDA) and the Committee on Data (CODATA) within the International Science Council, http://www.codata.org/task-groups/data-at-risk/dar-workshops .
http://wiki.esipfed.org/index.php/Preservation_and_Stewardship .
Appendix I – Data Risk Assessment Template
Acknowledgements.
This project was organized and supported by the Data Stewardship Committee within the Earth Science Information Partners (ESIP). We thank ESIP and the committee participants for feedback on the project at numerous points in the past few years.
The work of Robert R. Downs was supported by the National Aeronautics and Space Administration under Contract 80GSFC18C0111 for the Socioeconomic Data and Applications Distributed Active Archive Center (DAAC).
Alexis Garretson acknowledges the support of the Environmental Data Initiative Summer Fellowship program and the Earth Science Information Partners Community Fellows Program. Alexis also acknowledges Mohonk Preserve staff, particularly the staff of the Daniel Smiley Research Center: Elizabeth C. Long, Megan Napoli, and Natalie Feldsine. This material is based upon work supported by the National Science Foundation Graduate Research Fellowship Program under Grant No. 1842191. Any opinions, findings, and conclusions or recommendations expressed in this material are those of the authors and do not necessarily reflect the views of the National Science Foundation.
The work of Chung-Yi (Sophie) Hou was supported by the National Center for Atmospheric Research.
The National Center for Atmospheric Research is sponsored by the U.S. National Science Foundation. Any opinions, findings, and conclusions or recommendations expressed in this publication are those of the author(s) and do not necessarily reflect the views of NCAR or the NSF.
Competing Interests
The authors have no competing interests to declare.
Allen, L, Stewart, C and Wright, S. 2017. Strategic open data preservation. College & Research Libraries News , 78(9). https://crln.acrl.org/index.php/crlnews/article/view/16771/18312 . DOI: https://doi.org/10.5860/crln.78.9.482
Anderson, WL, Faundeen, JL, Greenberg, J and Taylor, F. 2011. Metadata for data rescue and data at risk. In: Conference on Ensuring Long-Term Preservation in Adding Value to Scientific and Technical Data. http://hdl.handle.net/2152/20056
Aven, T. 2016. Risk assessment and risk management: Review of recent advances on their foundation. European Journal of Operational Research , 253(1): 1–13. DOI: https://doi.org/10.1016/j.ejor.2015.12.023
Becker, C, Maemura, E and Moles, N. 2020. The design and use of assessment frameworks in digital curation. Journal of the Association for Information Science and Technology (JASIST) , 71(1): 55–68. DOI: https://doi.org/10.1002/asi.24209
Cervone, HF. 2006. Project risk management. OCLC Systems & Services: International Digital Library Perspectives , 22(4): 256–62. DOI: https://doi.org/10.1108/10650750610706970
Charifson, DM, Huth, PC, Thompson, JE, Angyal, RK, Flaherty, MJ and Richardson, DC. 2015. History of Fish Presence and Absence Following Lake Acidification and Recovery in Lake Minnewaska, Shawangunk Ridge, NY. Northeastern Naturalist , 22: 762–781. DOI: https://doi.org/10.1656/045.022.0411
Chodacki, J. 2018. Data Mirror: Complementing data producers. Against the Grain , 29: 35. https://escholarship.org/uc/item/2n1715ff . DOI: https://doi.org/10.7771/2380-176X.7877
CoreTrustSeal Data Repository Certification. 2018. https://www.coretrustseal.org/ .
Cornelius, KB and Pasquetto, IV. 2018. “What data?” Records and data policy coordination during presidential transitions. In: Transforming Digital Worlds , 155–163. Springer International Publishing. DOI: https://doi.org/10.1007/978-3-319-78105-1_20
Cook, BI, Cook, ER, Anchukaitis, KJ, Huth, PC, Thompson, JE and Smiley, SF. 2009. A Homogeneous Record (1896–2006) of Daily Weather and Climate at Mohonk Lake, New York. Journal of Applied Meteorology and Climatology , 49: 544–555. DOI: https://doi.org/10.1175/2009JAMC2221.1
Cook, BI, Cook, ER, Huth, PC, Thompson, JE, Forster, A and Smiley, D. 2008. A cross-taxa phenological dataset from Mohonk Lake, NY and its relationship to climate. International Journal of Climatology , 28: 1369–1383. DOI: https://doi.org/10.1002/joc.1629
Data Rescue Interest Group. 2018. Research Data Alliance. https://rd-alliance.org/groups/data-rescue.html .
Dennis, B. 2016. Scientists are frantically copying U.S. climate data, fearing it might vanish under Trump. The Washington Post , Dec. 13, 2016. https://www.washingtonpost.com/news/energy-environment/wp/2016/12/13/scientists-are-frantically-copying-u-s-climate-data-fearing-it-might-vanish-under-trump/ .
Donaldson, DR, Dillo, I, Downs, R and Ramdeen, S. 2017. The perceived value of acquiring Data Seals of Approval. International Journal of Digital Curation , 12(1). DOI: https://doi.org/10.2218/ijdc.v12i1.481
Downs, RR and Chen, RS. 2017. Curation of scientific data at risk of loss: Data rescue and dissemination. In: Johnston, L (ed.), Curating Research Data. Volume One, Practical Strategies for Your Digital Repository . Association of College and Research Libraries. DOI: https://doi.org/10.7916/D8W09BMQ
Faundeen, J. 2017. Developing criteria to establish trusted digital repositories. Data Science Journal , 16: 22. DOI: https://doi.org/10.5334/dsj-2017-022
Gallaher, D, Campbell, GG, Meier, W, Moses, J and Wingo, D. 2015. The process of bringing dark data to light: The rescue of the early Nimbus satellite data. GeoResJ , 6: 124–134. DOI: https://doi.org/10.1016/j.grj.2015.02.013
Graf, R, Ryan, HM, Houzanme, T and Gordea, S. 2017. A decision support system to facilitate file format selection for digital preservation. Libellarium: Journal for the Research of Writing, Books, and Cultural Heritage Institutions , 9(2). DOI: https://doi.org/10.15291/libellarium.v9i2.274
Griffin, RE. 2015. When are old data new data? GeoResJ , 6: 92–97. DOI: https://doi.org/10.1016/j.grj.2015.02.004
Guidelines to the Rescue of Data At Risk. 2017. Research Data Alliance. https://www.rd-alliance.org/guidelines-rescue-data-risk .
Hsu, L, Lehnert, KA, Goodwillie, A, Delano, JW, Gill, JB, Tivey, MA, Ferrini, VL, Carbotte, SM and Arko, RA. 2015. Rescue of long-tail data from the ocean bottom to the Moon: IEDA Data Rescue Mini-Awards. GeoResJ , 6: 108–114. DOI: https://doi.org/10.1016/j.grj.2015.02.012
[ISO] International Organization for Standardization. 2012a. ISO 14721:2012 (CCSDS 650.0-M-2): Space data and information transfer systems — Open Archival Information System (OAIS) — Reference model. https://www.iso.org/standard/57284.html .
[ISO] International Organization for Standardization. 2012b. ISO 16363:2012 (CCSDS 652.0-R-1): Space data and information transfer systems — Audit and certification of trustworthy digital repositories. https://www.iso.org/standard/56510.html .
Janz, M. 2018. Maintaining access to public data: Lessons from Data Refuge. Against the Grain , 29: 30–33. DOI: https://doi.org/10.31229/osf.io/yavzh
Knapp, KR, Bates, JJ and Barkstrom, B. 2007. Scientific data stewardship: Lessons learned from a satellite–data rescue effort. Bulletin of the American Meteorological Society , 88(9): 1359–1362. DOI: https://doi.org/10.1175/BAMS-88-9-1359
Lamdan, S. 2018. Lessons from Datarescue: The limitations of grassroots climate change data preservation and the need for federal records law reform. University of Pennsylvania Law Review Online , 166(1): Article 12. https://scholarship.law.upenn.edu/penn_law_review_online/vol166/iss1/12 .
Levitus, S. 2012. The UNESCO-IOC-IODE “Global Oceanographic Data Archeology and Rescue” (GODAR) Project and “World Ocean Database” Project. Data Science Journal , 11: 46–71. DOI: https://doi.org/10.2481/dsj.012-014
Maemura, E, Moles, N and Becker, C. 2017. Organizational assessment frameworks for digital preservation: A literature review and mapping. Journal of the Association for Information Science and Technology , 68(7): 1619–1637. DOI: https://doi.org/10.1002/asi.23807
Mayernik, MS, Downs, RR, Duerr, R, Hou, C-Y, Meyers, N, Ritchey, N, Thomer, A and Yarmey, L. 2017. Stronger together: The case for cross-sector collaboration in identifying and preserving at-risk data. Figshare. DOI: https://doi.org/10.6084/m9.figshare.4816474.v1
Mayernik, MS, Huddle, J, Hou, C-Y and Phillips, J. 2018. Modernizing library metadata for historical weather and climate data collections. Journal of Library Metadata , 17(3/4): 219–239. DOI: https://doi.org/10.1080/19386389.2018.1440927
McGovern, NY. 2017. Data rescue. ACM SIGCAS Computers and Society , 47(2): 19–26. DOI: https://doi.org/10.1145/3112644.3112648
Michener, WK, et al. 1997. Nongeospatial metadata for the ecological sciences. Ecological Applications , 7(1): 330–342. DOI: https://doi.org/10.1890/1051-0761(1997)007[0330:NMFTES]2.0.CO;2
Mohonk Preserve, Belardo, C, Feldsine, N, Forester, A, Huth, P, Long, E, Morgan, V, Napoli, M, Pierce, E, Richardson, D, Smiley, D, Smiley, S and Thompson, J. 2018a. History of Acid Precipitation on the Shawangunk Ridge: Mohonk Preserve Precipitation Depths and pH, 1976 to Present. Environmental Data Initiative . DOI: https://doi.org/10.6073/pasta/734ea90749e78613452eacec489f419c
Mohonk Preserve, Forester, A, Huth, P, Long, E, Morgan, V, Napoli, M, Pierce, E, Smiley, D, Smiley, S and Thompson, J. 2018b. Mohonk Preserve Ground Water Springs Data, 1991 to Present. Environmental Data Initiative . DOI: https://doi.org/10.6073/pasta/928feed7ee748509ab065de7e3791966
Mohonk Preserve, Feldsine, N, Forester, A, Garretson, A, Huth, P, Long, E, Napoli, M, Pierce, E, Smiley, D, Smiley, S and Thompson, J. 2019. Mohonk Preserve Amphibian and Water Quality Monitoring Dataset at 11 Vernal Pools from 1931–Present. Environmental Data Initiative . DOI: https://doi.org/10.6073/pasta/864aea25998b73c5d1a5b5f36cb6583e
National Research Council. 1983. Risk Assessment in the Federal Government: Managing the Process. Washington, DC: The National Academies Press. DOI: https://doi.org/10.17226/366
NIWA. 2019. The week it snowed everywhere. NIWA Media Release , Nov. 21, 2019. https://niwa.co.nz/news/the-week-it-snowed-everywhere .
Peng, G. 2018. The state of assessing data stewardship maturity – An overview. Data Science Journal , 17: 7. DOI: https://doi.org/10.5334/dsj-2018-007
Peng, G, Milan, A, Ritchey, NA, Partee, RP, II, Zinn, S, McQuinn, E, Casey, KS, Lemieux, P, III, Ionin, R, Jones, P, Jakositz, A and Collins, D. 2019. Practical application of a data stewardship maturity matrix for the NOAA OneStop project. Data Science Journal , 18: 41. DOI: https://doi.org/10.5334/dsj-2019-041
Peng, G, Privette, JL, Kearns, EJ, Ritchey, NA and Ansari, S. 2015. A unified framework for measuring stewardship practices applied to digital environmental datasets. Data Science Journal , 13: 231–253. DOI: https://doi.org/10.2481/dsj.14-049
Pienta, AM and Lyle, J. 2018. Retirement in the 1950s: Rebuilding a longitudinal research database. IASSIST Quarterly , 42(1). DOI: https://doi.org/10.29173/iq19
Poli, P, Dee, DP, Saunders, R, John, VO, Rayer, P, Schulz, J, Bojinski, S, et al. 2017. Recent advances in satellite data rescue. Bulletin of the American Meteorological Society , 98(7): 1471–1484. DOI: https://doi.org/10.1175/BAMS-D-15-00194.1
Ramapriyan, HK. 2017. NASA’s EOSDIS: Trust and Certification. Presented at: 2017 ESIP Summer Meeting, Bloomington, IN. Figshare. DOI: https://doi.org/10.6084/m9.figshare.5258047.v1
Richardson, DC, Charifson, DM, Stanson, VJ, Stern, EM, Thompson, JE and Townley, LA. 2016. Reconstructing a trophic cascade following unintentional introduction of golden shiner to Lake Minnewaska, New York, USA. Inland Waters , 6: 29–33. DOI: https://doi.org/10.5268/IW-6.1.915
Ryan, H. 2014. Occam’s razor and file format endangerment factors. In: Proceedings of the 11th International Conference on Digital Preservation (iPres), October 6–10, 2014, Melbourne, Australia, 179–188. https://www.nla.gov.au/sites/default/files/ipres2014-proceedings-version_1.pdf .
Slovic, P. 1999. Trust, emotion, sex, politics, and science: Surveying the risk-assessment battlefield. Risk Analysis , 19(4): 689–701. DOI: https://doi.org/10.1023/A:1007041821623
Thompson, CA, Robertson, WD and Greenberg, J. 2014. Where have all the scientific data gone? LIS perspective on the data-at-risk predicament. College & Research Libraries , 75(6): 842–861. DOI: https://doi.org/10.5860/crl.75.6.842
Usability.gov . 2019. Card Sorting. US Department of Health & Human Services. https://www.usability.gov/how-to-and-tools/methods/card-sorting.html .
USGS. 2019. USGS Data at Risk: Expanding Legacy Data Inventory and Preservation Strategies. US Geological Survey. https://www.sciencebase.gov/catalog/item/58b5ddc3e4b01ccd54fde3fa .
Varinsky, D. 2017. Scientists across the US are scrambling to save government research in ‘Data Rescue’ events. Business Insider , Feb. 11, 2017. http://www.businessinsider.com/data-rescue-government-data-preservation-efforts-2017-2 .
Yakel, E, Faniel, I, Kriesberg, A and Yoon, A. 2013. Trust in digital repositories. International Journal of Digital Curation , 8(1). DOI: https://doi.org/10.2218/ijdc.v8i1.251
Yoon, A. 2017. Data reusers’ trust development. Journal of the Association for Information Science and Technology , 68(4): 946–956. DOI: https://doi.org/10.1002/asi.23730
Zimmerman, DE and Akerelrea, C. 2002. A group card sorting methodology for developing informational web sites. In: Proceedings IEEE International Professional Communication Conference, 437–445. IEEE. DOI: https://doi.org/10.1109/IPCC.2002.1049127
Thank you for visiting nature.com. You are using a browser version with limited support for CSS. To obtain the best experience, we recommend you use a more up to date browser (or turn off compatibility mode in Internet Explorer). In the meantime, to ensure continued support, we are displaying the site without styles and JavaScript.
- View all journals
- My Account Login
- Explore content
- About the journal
- Publish with us
- Sign up for alerts
- Open access
- Published: 08 April 2024
A case study on the relationship between risk assessment of scientific research projects and related factors under the Naive Bayesian algorithm
- Xuying Dong 1 &
- Wanlin Qiu 1
Scientific Reports volume 14 , Article number: 8244 ( 2024 ) Cite this article
Metrics details
- Computer science
- Mathematics and computing
This paper delves into the nuanced dynamics influencing the outcomes of risk assessment (RA) in scientific research projects (SRPs), employing the Naive Bayes algorithm. The methodology involves the selection of diverse SRPs cases, gathering data encompassing project scale, budget investment, team experience, and other pertinent factors. The paper advances the application of the Naive Bayes algorithm by introducing enhancements, specifically integrating the Tree-augmented Naive Bayes (TANB) model. This augmentation serves to estimate risk probabilities for different research projects, shedding light on the intricate interplay and contributions of various factors to the RA process. The findings underscore the efficacy of the TANB algorithm, demonstrating commendable accuracy (average accuracy 89.2%) in RA for SRPs. Notably, budget investment (regression coefficient: 0.68, P < 0.05) and team experience (regression coefficient: 0.51, P < 0.05) emerge as significant determinants obviously influencing RA outcomes. Conversely, the impact of project size (regression coefficient: 0.31, P < 0.05) is relatively modest. This paper furnishes a concrete reference framework for project managers, facilitating informed decision-making in SRPs. By comprehensively analyzing the influence of various factors on RA, the paper not only contributes empirical insights to project decision-making but also elucidates the intricate relationships between different factors. The research advocates for heightened attention to budget investment and team experience when formulating risk management strategies. This strategic focus is posited to enhance the precision of RAs and the scientific foundation of decision-making processes.
Similar content being viewed by others
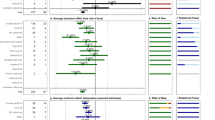
Systematic review and meta-analysis of ex-post evaluations on the effectiveness of carbon pricing
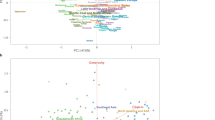
Principal component analysis
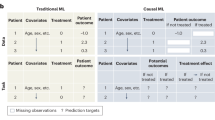
Causal machine learning for predicting treatment outcomes
Introduction.
Scientific research projects (SRPs) stand as pivotal drivers of technological advancement and societal progress in the contemporary landscape 1 , 2 , 3 . The dynamism of SRP success hinges on a multitude of internal and external factors 4 . Central to effective project management, Risk assessment (RA) in SRPs plays a critical role in identifying and quantifying potential risks. This process not only aids project managers in formulating strategic decision-making approaches but also enhances the overall success rate and benefits of projects. In a recent contribution, Salahuddin 5 provides essential numerical techniques indispensable for conducting RAs in SRPs. Building on this foundation, Awais and Salahuddin 6 delve into the assessment of risk factors within SRPs, notably introducing the consideration of activation energy through an exploration of the radioactive magnetohydrodynamic model. Further expanding the scope, Awais and Salahuddin 7 undertake a study on the natural convection of coupled stress fluids. However, RA of SRPs confronts a myriad of challenges, underscoring the critical need for novel methodologies 8 . Primarily, the intricate nature of SRPs renders precise RA exceptionally complex and challenging. The project’s multifaceted dimensions, encompassing technology, resources, and personnel, are intricately interwoven, posing a formidable challenge for traditional assessment methods to comprehensively capture all potential risks 9 . Furthermore, the intricate and diverse interdependencies among various project factors contribute to the complexity of these relationships, thereby limiting the efficacy of conventional methods 10 , 11 , 12 . Traditional approaches often focus solely on the individual impact of diverse factors, overlooking the nuanced relationships that exist between them—an inherent limitation in the realm of RA for SRPs 13 , 14 , 15 .
The pursuit of a methodology capable of effectively assessing project risks while elucidating the intricate interplay of different factors has emerged as a focal point in SRPs management 16 , 17 , 18 . This approach necessitates a holistic consideration of multiple factors, their quantification in contributing to project risks, and the revelation of their correlations. Such an approach enables project managers to more precisely predict and respond to risks. Marx-Stoelting et al. 19 , current approaches for the assessment of environmental and human health risks due to exposure to chemical substances have served their purpose reasonably well. Additionally, Awais et al. 20 highlights the significance of enthalpy changes in SRPs risk considerations, while Awais et al. 21 delve into the comprehensive exploration of risk factors in Eyring-Powell fluid flow in magnetohydrodynamics, particularly addressing viscous dissipation and activation energy effects. The Naive Bayesian algorithm, recognized for its prowess in probability and statistics, has yielded substantial results in information retrieval and data mining in recent years 22 . Leveraging its advantages in classification and probability estimation, the algorithm presents a novel approach for RA of SRPs 23 . Integrating probability analysis into RA enables a more precise estimation of project risks by utilizing existing project data and harnessing the capabilities of the Naive Bayesian algorithms. This method facilitates a quantitative, statistical analysis of various factors, effectively navigating the intricate relationships between them, thereby enhancing the comprehensiveness and accuracy of RA for SRPs.
This paper seeks to employ the Naive Bayesian algorithm to estimate the probability of risks by carefully selecting distinct research project cases and analyzing multidimensional data, encompassing project scale, budget investment, and team experience. Concurrently, Multiple Linear Regression (MLR) analysis is applied to quantify the influence of these factors on the assessment results. The paper places particular emphasis on exploring the intricate interrelationships between different factors, aiming to provide a more specific and accurate reference framework for decision-making in SRPs management.
This paper introduces several innovations and contributions to the field of RA for SRPs:
Comprehensive Consideration of Key Factors: Unlike traditional research that focuses on a single factor, this paper comprehensively considers multiple key factors, such as project size, budget investment, and team experience. This holistic analysis enhances the realism and thoroughness of RA for SRPs.
Introduction of Tree-Enhanced Naive Bayes Model: The naive Bayes algorithm is introduced and further improved through the proposal of a tree-enhanced naive Bayes model. This algorithm exhibits unique advantages in handling uncertainty and complexity, thereby enhancing its applicability and accuracy in the RA of scientific and technological projects.
Empirical Validation: The effectiveness of the proposed method is not only discussed theoretically but also validated through empirical cases. The analysis of actual cases provides practical support and verification, enhancing the credibility of the research results.
Application of MLR Analysis: The paper employs MLR analysis to delve into the impact of various factors on RA. This quantitative analysis method adds specificity and operability to the research, offering a practical decision-making basis for scientific and technological project management.
Discovery of New Connections and Interactions: The paper uncovers novel connections and interactions, such as the compensatory role of team experience for budget-related risks and the impact of the interaction between project size and budget investment on RA results. These insights provide new perspectives for decision-making in technology projects, contributing significantly to the field of RA for SRPs in terms of both importance and practical value.
The paper is structured as follows: “ Introduction ” briefly outlines the significance of RA for SRPs. Existing challenges within current research are addressed, and the paper’s core objectives are elucidated. A distinct emphasis is placed on the innovative aspects of this research compared to similar studies. The organizational structure of the paper is succinctly introduced, providing a brief overview of each section’s content. “ Literature review ” provides a comprehensive review of relevant theories and methodologies in the domain of RA for SRPs. The current research landscape is systematically examined, highlighting the existing status and potential gaps. Shortcomings in previous research are analyzed, laying the groundwork for the paper’s motivation and unique contributions. “ Research methodology ” delves into the detailed methodologies employed in the paper, encompassing data collection, screening criteria, preprocessing steps, and more. The tree-enhanced naive Bayes model is introduced, elucidating specific steps and the purpose behind MLR analysis. “ Results and discussion ” unfolds the results and discussions based on selected empirical cases. The representativeness and diversity of these cases are expounded upon. An in-depth analysis of each factor’s impact and interaction in the context of RA is presented, offering valuable insights. “ Discussion ” succinctly summarizes the entire research endeavor. Potential directions for further research and suggestions for improvement are proposed, providing a thoughtful conclusion to the paper.
Literature review
A review of ra for srps.
In recent years, the advancement of SRPs management has led to the evolution of various RA methods tailored for SRPs. The escalating complexity of these projects poses a challenge for traditional methods, often falling short in comprehensively considering the intricate interplay among multiple factors and yielding incomplete assessment outcomes. Scholars, recognizing the pivotal role of factors such as project scale, budget investment, and team experience in influencing project risks, have endeavored to explore these dynamics from diverse perspectives. Siyal et al. 24 pioneered the development and testing of a model geared towards detecting SRPs risks. Chen et al. 25 underscored the significance of visual management in SRPs risk management, emphasizing its importance in understanding and mitigating project risks. Zhao et al. 26 introduced a classic approach based on cumulative prospect theory, offering an optional method to elucidate researchers’ psychological behaviors. Their study demonstrated the enhanced rationality achieved by utilizing the entropy weight method to derive attribute weight information under Pythagorean fuzzy sets. This approach was then applied to RA for SRPs, showcasing a model grounded in the proposed methodology. Suresh and Dillibabu 27 proposed an innovative hybrid fuzzy-based machine learning mechanism tailored for RA in software projects. This hybrid scheme facilitated the identification and ranking of major software project risks, thereby supporting decision-making throughout the software project lifecycle. Akhavan et al. 28 introduced a Bayesian network modeling framework adept at capturing project risks by calculating the uncertainty of project net present value. This model provided an effective means for analyzing risk scenarios and their impact on project success, particularly applicable in evaluating risks for innovative projects that had undergone feasibility studies.
A review of factors affecting SRPs
Within the realm of SRPs management, the assessment and proficient management of project risks stand as imperative components. Consequently, a range of studies has been conducted to explore diverse methods and models aimed at enhancing the comprehension and decision support associated with project risks. Guan et al. 29 introduced a new risk interdependence network model based on Monte Carlo simulation to support decision-makers in more effectively assessing project risks and planning risk management actions. They integrated interpretive structural modeling methods into the model to develop a hierarchical project risk interdependence network based on identified risks and their causal relationships. Vujović et al. 30 provided a new method for research in project management through careful analysis of risk management in SRPs. To confirm the hypothesis, the study focused on educational organizations and outlined specific project management solutions in business systems, thereby improving the business and achieving positive business outcomes. Muñoz-La Rivera et al. 31 described and classified the 100 identified factors based on the dimensions and aspects of the project, assessed their impact, and determined whether they were shaping or directly affecting the occurrence of research project accidents. These factors and their descriptions and classifications made significant contributions to improving the security creation of the system and generating training and awareness materials, fostering the development of a robust security culture within organizations. Nguyen et al. concentrated on the pivotal risk factors inherent in design-build projects within the construction industry. Effective identification and management of these factors enhanced project success and foster confidence among owners and contractors in adopting the design-build approach 32 . Their study offers valuable insights into RA in project management and the adoption of new contract forms. Nguyen and Le delineated risk factors influencing the quality of 20 civil engineering projects during the construction phase 33 . The top five risks identified encompass poor raw material quality, insufficient worker skills, deficient design documents and drawings, geographical challenges at construction sites, and inadequate capabilities of main contractors and subcontractors. Meanwhile, Nguyen and Phu Pham concentrated on office building projects in Ho Chi Minh City, Vietnam, to pinpoint key risk factors during the construction phase 34 . These factors were classified into five groups based on their likelihood and impact: financial, management, schedule, construction, and environmental. Findings revealed that critical factors affecting office building projects encompassed both natural elements (e.g., prolonged rainfall, storms, and climate impacts) and human factors (e.g., unstable soil, safety behavior, owner-initiated design changes), with schedule-related risks exerting the most significant influence during the construction phase of Ho Chi Minh City’s office building projects. This provides construction and project management practitioners with fresh insights into risk management, aiding in the comprehensive identification, mitigation, and management of risk factors in office building projects.
While existing research has made notable strides in RA for SRPs, certain limitations persist. These studies limitations in quantifying the degree of influence of various factors and analyzing their interrelationships, thereby falling short of offering specific and actionable recommendations. Traditional methods, due to their inherent limitations, struggle to precisely quantify risk degrees and often overlook the intricate interplay among multiple factors. Consequently, there is an urgent need for a comprehensive method capable of quantifying the impact of diverse factors and revealing their correlations. In response to this exigency, this paper introduces the TANB model. The unique advantages of this algorithm in the RA of scientific and technological projects have been fully realized. Tailored to address the characteristics of uncertainty and complexity, the model represents a significant leap forward in enhancing applicability and accuracy. In comparison with traditional methods, the TANB model exhibits greater flexibility and a heightened ability to capture dependencies between features, thereby elevating the overall performance of RA. This innovative method emerges as a more potent and reliable tool in the realm of scientific and technological project management, furnishing decision-makers with more comprehensive and accurate support for RA.
Research methodology
This paper centers on the latest iteration of ISO 31000, delving into the project risk management process and scrutinizing the RA for SRPs and their intricate interplay with associated factors. ISO 31000, an international risk management standard, endeavors to furnish businesses, organizations, and individuals with a standardized set of risk management principles and guidelines, defining best practices and establishing a common framework. The paper unfolds in distinct phases aligned with ISO 31000:
Risk Identification: Employing data collection and preparation, a spectrum of factors related to project size, budget investment, team member experience, project duration, and technical difficulty were identified.
RA: Utilizing the Naive Bayes algorithm, the paper conducts RA for SRPs, estimating the probability distribution of various factors influencing RA results.
Risk Response: The application of the Naive Bayes model is positioned as a means to respond to risks, facilitating the formulation of apt risk response strategies based on calculated probabilities.
Monitoring and Control: Through meticulous data collection, model training, and verification, the paper illustrates the steps involved in monitoring and controlling both data and models. Regular monitoring of identified risks and responses allows for adjustments when necessary.
Communication and Reporting: Maintaining effective communication throughout the project lifecycle ensures that stakeholders comprehend the status of project risks. Transparent reporting on discussions and outcomes contributes to an informed project environment.
Data collection and preparation
In this paper, a meticulous approach is undertaken to select representative research project cases, adhering to stringent screening criteria. Additionally, a thorough review of existing literature is conducted and tailored to the practical requirements of SRPs management. According to Nguyen et al., these factors play a pivotal role in influencing the RA outcomes of SRPs 35 . Furthermore, research by He et al. underscored the significant impact of team members’ experience on project success 36 . Therefore, in alignment with our research objectives and supported by the literature, this paper identifies variables such as project scale, budget investment, team member experience, project duration, and technical difficulty as the focal themes. To ensure the universality and scientific rigor of our findings, the paper adheres to stringent selection criteria during the project case selection process. After preliminary screening of SRPs completed in the past 5 years, considering factors such as project diversity, implementation scales, and achieved outcomes, five representative projects spanning diverse fields, including engineering, medicine, and information technology, are ultimately selected. These project cases are chosen based on their capacity to represent various scales and types of SRPs, each possessing a typical risk management process, thereby offering robust and comprehensive data support for our study. The subsequent phase involves detailed data collection on each chosen project, encompassing diverse dimensions such as project scale, budget investment, team member experience, project cycle, and technical difficulty. The collected data undergo meticulous preprocessing to ensure data quality and reliability. The preprocessing steps comprised data cleaning, addressing missing values, handling outliers, culminating in the creation of a self-constructed dataset. The dataset encompasses over 500 SRPs across diverse disciplines and fields, ensuring statistically significant and universal outcomes. Particular emphasis is placed on ensuring dataset diversity, incorporating projects of varying scales, budgets, and team experience levels. This comprehensive coverage ensures the representativeness and credibility of the study on RA in SRPs. New influencing factors are introduced to expand the research scope, including project management quality (such as time management and communication efficiency), historical success rate, industry dynamics, and market demand. Detailed definitions and quantifications are provided for each new variable to facilitate comprehensive data processing and analysis. For project management quality, consideration is given to time management accuracy and communication frequency and quality among team members. Historical success rate is determined by reviewing past project records and outcomes. Industry dynamics are assessed by consulting the latest scientific literature and patent information. Market demand is gauged through market research and user demand surveys. The introduction of these variables enriches the understanding of RA in SRPs and opens up avenues for further research exploration.
At the same time, the collected data are integrated and coded in order to apply Naive Bayes algorithm and MLR analysis. For cases involving qualitative data, this paper uses appropriate coding methods to convert it into quantitative data for processing in the model. For example, for the qualitative feature of team member experience, numerical values are used to represent different experience levels, such as 0 representing beginners, 0 representing intermediate, and 2 representing advanced. The following is a specific sample data set example (Table 1 ). It shows the processed structured data, and the values in the table represent the specific characteristics of each project.
Establishment of naive Bayesian model
The Naive Bayesian algorithm, a probabilistic and statistical classification method renowned for its effectiveness in analyzing and predicting multi-dimensional data, is employed in this paper to conduct the RA for SRPs. The application of the Naive Bayesian algorithm to RA for SRPs aims to discern the influence of various factors on the outcomes of RA. The Naive Bayesian algorithm, depicted in Fig. 1 , operates on the principles of Bayesian theorem, utilizing posterior probability calculations for classification tasks. The fundamental concept of this algorithm hinges on the assumption of independence among different features, embodying the “naivety” hypothesis. In the context of RA for SRPs, the Naive Bayesian algorithm is instrumental in estimating the probability distribution of diverse factors affecting the RA results, thereby enhancing the precision of risk estimates. In the Naive Bayesian model, the initial step involves the computation of posterior probabilities for each factor, considering the given RA result conditions. Subsequently, the category with the highest posterior probability is selected as the predictive outcome.
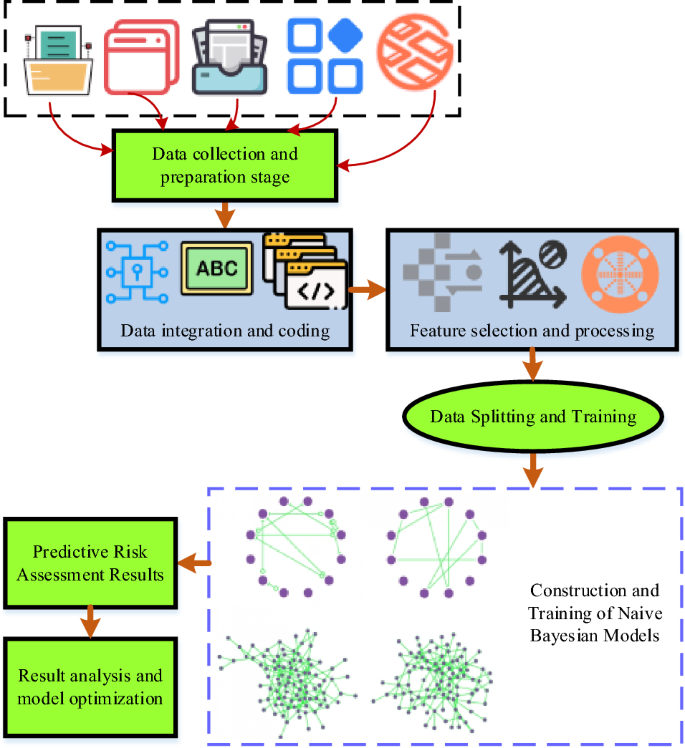
Naive Bayesian algorithm process.
In Fig. 1 , the data collection process encompasses vital project details such as project scale, budget investment, team member experience, project cycle, technical difficulty, and RA results. This meticulous collection ensures the integrity and precision of the dataset. Subsequently, the gathered data undergoes integration and encoding to convert qualitative data into quantitative form, facilitating model processing and analysis. Tailored to specific requirements, relevant features are chosen for model construction, accompanied by essential preprocessing steps like standardization and normalization. The dataset is then partitioned into training and testing sets, with the model trained on the former and its performance verified on the latter. Leveraging the training data, a Naive Bayesian model is developed to estimate probability distribution parameters for various features across distinct categories. Ultimately, the trained model is employed to predict new project features, yielding RA results.
Naive Bayesian models, in this context, are deployed to forecast diverse project risk levels. Let X symbolize the feature vector, encompassing project scale, budget investment, team member experience, project cycle, and technical difficulty. The objective is to predict the project’s risk level, denoted as Y. Y assumes discrete values representing distinct risk levels. Applying the Bayesian theorem, the posterior probability P(Y|X) is computed, signifying the probability distribution of projects falling into different risk levels given the feature vector X. The fundamental equation governing the Naive Bayesian model is expressed as:
In Eq. ( 1 ), P(Y|X) represents the posterior probability, denoting the likelihood of the project belonging to a specific risk level. P(X|Y) signifies the class conditional probability, portraying the likelihood of the feature vector X occurring under known risk level conditions. P(Y) is the prior probability, reflecting the antecedent likelihood of the project pertaining to a particular risk level. P(X) acts as the evidence factor, encapsulating the likelihood of the feature vector X occurring.
The Naive Bayes, serving as the most elementary Bayesian network classifier, operates under the assumption of attribute independence given the class label c , as expressed in Eq. ( 2 ):
The classification decision formula for Naive Bayes is articulated in Eq. ( 3 ):
The Naive Bayes model, rooted in the assumption of conditional independence among attributes, often encounters deviations from reality. To address this limitation, the Tree-Augmented Naive Bayes (TANB) model extends the independence assumption by incorporating a first-order dependency maximum-weight spanning tree. TANB introduces a tree structure that more comprehensively models relationships between features, easing the constraints of the independence assumption and concurrently mitigating issues associated with multicollinearity. This extension bolsters its efficacy in handling intricate real-world data scenarios. TANB employs conditional mutual information \(I(X_{i} ;X_{j} |C)\) to gauge the dependency between attributes \(X_{j}\) and \(X_{i}\) , thereby constructing the maximum weighted spanning tree. In TANB, any attribute variable \(X_{i}\) is permitted to have at most one other attribute variable as its parent node, expressed as \(Pa\left( {X_{i} } \right) \le 2\) . The joint probability \(P_{con} \left( {x,c} \right)\) undergoes transformation using Eq. ( 4 ):
In Eq. ( 4 ), \(x_{r}\) refers to the root node, which can be expressed as Eq. ( 5 ):
TANB classification decision equation is presented below:
In the RA of SRPs, normal distribution parameters, such as mean (μ) and standard deviation (σ), are estimated for each characteristic dimension (project scale, budget investment, team member experience, project cycle, and technical difficulty). This estimation allows the calculation of posterior probabilities for projects belonging to different risk levels under given feature vector conditions. For each feature dimension \({X}_{i}\) , the mean \({mu}_{i,j}\) and standard deviation \({{\text{sigma}}}_{i,j}\) under each risk level are computed, where i represents the feature dimension, and j denotes the risk level. Parameter estimation employs the maximum likelihood method, and the specific calculations are as follows.
In Eqs. ( 7 ) and ( 8 ), \({N}_{j}\) represents the number of projects belonging to risk level j . \({x}_{i,k}\) denotes the value of the k -th item in the feature dimension i . Finally, under a given feature vector, the posterior probability of a project with risk level j is calculated as Eq. ( 9 ).
In Eq. ( 9 ), d represents the number of feature dimensions, and Z is the normalization factor. \(P(Y=j)\) represents the prior probability of category j . \(P({X}_{i}\mid Y=j)\) represents the normal distribution probability density function of feature dimension i under category j . The risk level of a project can be predicted by calculating the posterior probabilities of different risk levels to achieve RA for SRPs.
This paper integrates the probability estimation of the Naive Bayes model with actual project risk response strategies, enabling a more flexible and targeted response to various risk scenarios. Such integration offers decision support to project managers, enhancing their ability to address potential challenges effectively and ultimately improving the overall success rate of the project. This underscores the notion that risk management is not solely about problem prevention but stands as a pivotal factor contributing to project success.
MLR analysis
MLR analysis is used to validate the hypothesis to deeply explore the impact of various factors on RA of SRPs. Based on the previous research status, the following research hypotheses are proposed.
Hypothesis 1: There is a positive relationship among project scale, budget investment, and team member experience and RA results. As the project scale, budget investment, and team member experience increase, the RA results also increase.
Hypothesis 2: There is a negative relationship between the project cycle and the RA results. Projects with shorter cycles may have higher RA results.
Hypothesis 3: There is a complex relationship between technical difficulty and RA results, which may be positive, negative, or bidirectional in some cases. Based on these hypotheses, an MLR model is established to analyze the impact of factors, such as project scale, budget investment, team member experience, project cycle, and technical difficulty, on RA results. The form of an MLR model is as follows.
In Eq. ( 10 ), Y represents the RA result (dependent variable). \({X}_{1}\) to \({X}_{5}\) represent factors, such as project scale, budget investment, team member experience, project cycle, and technical difficulty (independent variables). \({\beta }_{0}\) to \({\beta }_{5}\) are the regression coefficients, which represent the impact of various factors on the RA results. \(\epsilon\) represents a random error term. The model structure is shown in Fig. 2 .
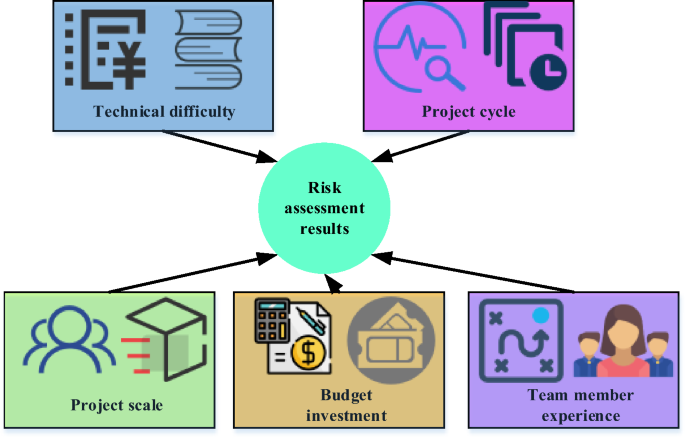
Schematic diagram of an MLR model.
In Fig. 2 , the MLR model is employed to scrutinize the influence of various independent variables on the outcomes of RA. In this specific context, the independent variables encompass project size, budget investment, team member experience, project cycle, and technical difficulty, all presumed to impact the project’s RA results. Each independent variable is denoted as a node in the model, with arrows depicting the relationships between these factors. In an MLR model, the arrow direction signifies causality, illustrating the influence of an independent variable on the dependent variable.
When conducting MLR analysis, it is necessary to estimate the parameter \(\upbeta\) in the regression model. These parameters determine the relationship between the independent and dependent variables. Here, the Ordinary Least Squares (OLS) method is applied to estimate these parameters. The OLS method is a commonly used parameter estimation method aimed at finding parameter values that minimize the sum of squared residuals between model predictions and actual observations. The steps are as follows. Firstly, based on the general form of an MLR model, it is assumed that there is a linear relationship between the independent and dependent variables. It can be represented by a linear equation, which includes regression coefficients β and the independent variable X. For each observation value, the difference between its predicted and actual values is calculated, which is called the residual. Residual \({e}_{i}\) can be expressed as:
In Eq. ( 11 ), \({Y}_{i}\) is the actual observation value, and \({\widehat{Y}}_{i}\) is the value predicted by the model. The goal of the OLS method is to adjust the regression coefficients \(\upbeta\) to minimize the sum of squared residuals of all observations. This can be achieved by solving an optimization problem, and the objective function is the sum of squared residuals.
Then, the estimated value of the regression coefficient \(\upbeta\) that minimizes the sum of squared residuals can be obtained by taking the derivative of the objective function and making the derivative zero. The estimated values of the parameters can be obtained by solving this system of equations. The final estimated regression coefficient can be expressed as:
In Eq. ( 13 ), X represents the independent variable matrix. Y represents the dependent variable vector. \(({X}^{T}X{)}^{-1}\) is the inverse of a matrix, and \(\widehat{\beta }\) is a parameter estimation vector.
Specifically, solving for the estimated value of regression coefficient \(\upbeta\) requires matrix operation and statistical analysis. Based on the collected project data, substitute it into the model and calculate the residual. Then, the steps of the OLS method are used to obtain parameter estimates. These parameter estimates are used to establish an MLR model to predict RA results and further analyze the influence of different factors.
The degree of influence of different factors on the RA results can be determined by analyzing the value of the regression coefficient β. A positive \(\upbeta\) value indicates that the factor has a positive impact on the RA results, while a negative \(\upbeta\) value indicates that the factor has a negative impact on the RA results. Additionally, hypothesis testing can determine whether each factor is significant in the RA results.
The TANB model proposed in this paper extends the traditional naive Bayes model by incorporating conditional dependencies between attributes to enhance the representation of feature interactions. While the traditional naive Bayes model assumes feature independence, real-world scenarios often involve interdependencies among features. To address this, the TANB model is introduced. The TANB model introduces a tree structure atop the naive Bayes model to more accurately model feature relationships, overcoming the limitation of assuming feature independence. Specifically, the TANB model constructs a maximum weight spanning tree to uncover conditional dependencies between features, thereby enabling the model to better capture feature interactions.
Assessment indicators
To comprehensively assess the efficacy of the proposed TANB model in the RA for SRPs, a self-constructed dataset serves as the data source for this experimental evaluation, as outlined in Table 1 . The dataset is segregated into training (80%) and test sets (20%). These indicators cover the accuracy, precision, recall rate, F1 value, and Area Under the Curve (AUC) Receiver Operating Characteristic (ROC) of the model. The following are the definitions and equations for each assessment indicator. Accuracy is the proportion of correctly predicted samples to the total number of samples. Precision is the proportion of Predicted Positive (PP) samples to actual positive samples. The recall rate is the proportion of correctly PP samples among the actual positive samples. The F1 value is the harmonic average of precision and recall, considering the precision and comprehensiveness of the model. The area under the ROC curve measures the classification performance of the model, and a larger AUC value indicates better model performance. The ROC curve suggests the relationship between True Positive Rate and False Positive Rate under different thresholds. The AUC value can be obtained by accumulating the area of each small rectangle under the ROC curve. The confusion matrix is used to display the prediction property of the model in different categories, including True Positive (TP), True Negative (TN), False Positive (FP), and False Negative (FN).
The performance of TANB in RA for SRPs can be comprehensively assessed to understand the advantages, disadvantages, and applicability of the model more comprehensively by calculating the above assessment indicators.
Results and discussion
Accuracy analysis of naive bayesian algorithm.
On the dataset of this paper, Fig. 3 reveals the performance of TANB algorithm under different assessment indicators.
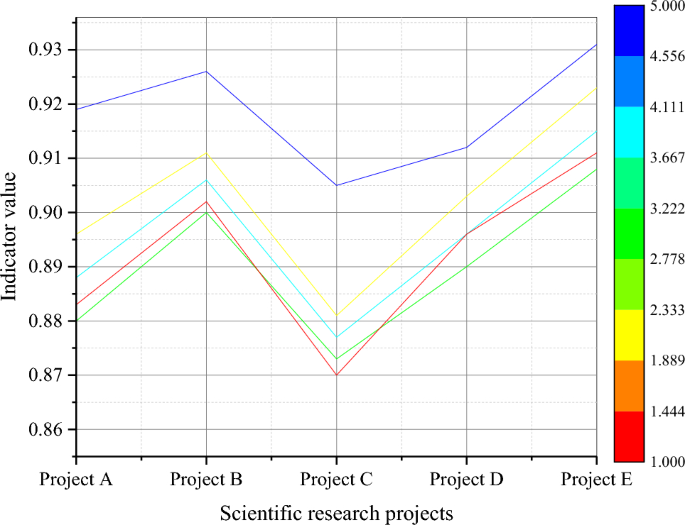
Performance assessment of TANB algorithm on different projects.
From Fig. 3 , the TANB algorithm performs well in various projects, ranging from 0.87 to 0.911 in accuracy. This means that the overall accuracy of the model in predicting project risks is quite high. The precision also maintains a high level in various projects, ranging from 0.881 to 0.923, indicating that the model performs well in classifying high-risk categories. The recall rate ranges from 0.872 to 0.908, indicating that the model can effectively capture high-risk samples. Meanwhile, the AUC values in each project are relatively high, ranging from 0.905 to 0.931, which once again emphasizes the effectiveness of the model in risk prediction. From multiple assessment indicators, such as accuracy, precision, recall, F1 value, and AUC, the TANB algorithm has shown good risk prediction performance in representative projects. The performance assessment results of the TANB algorithm under different feature dimensions are plotted in Figs. 4 , 5 , 6 and 7 .
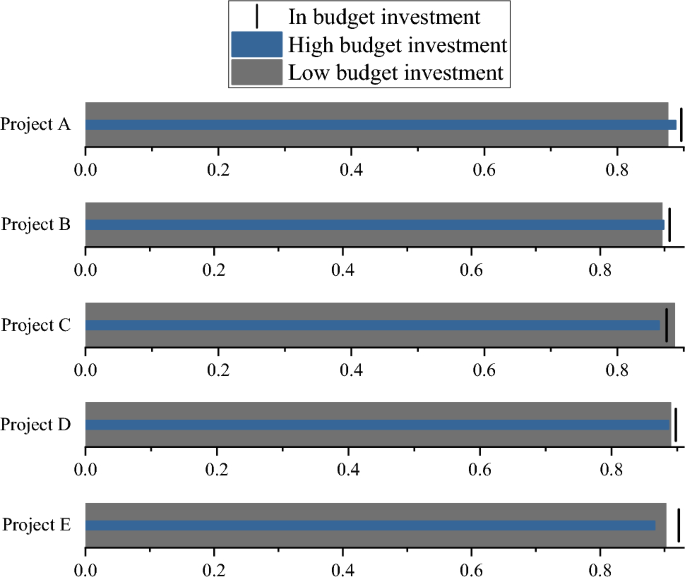
Prediction accuracy of TANB algorithm on different budget investments.
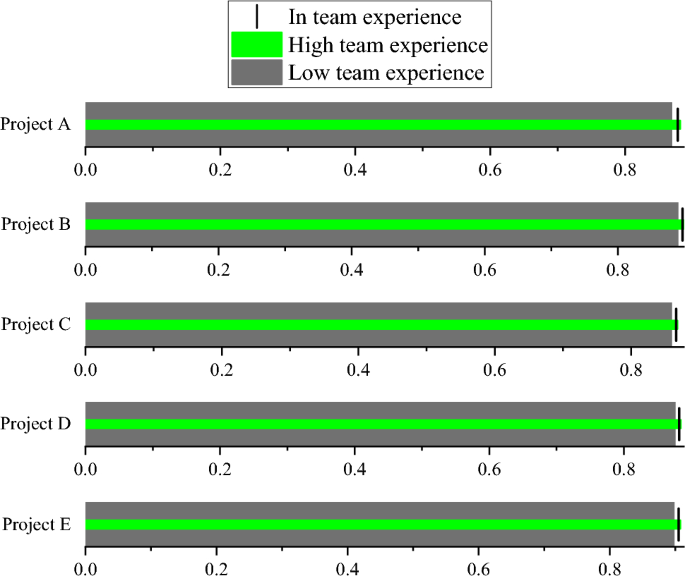
Prediction accuracy of TANB algorithm on different team experiences.
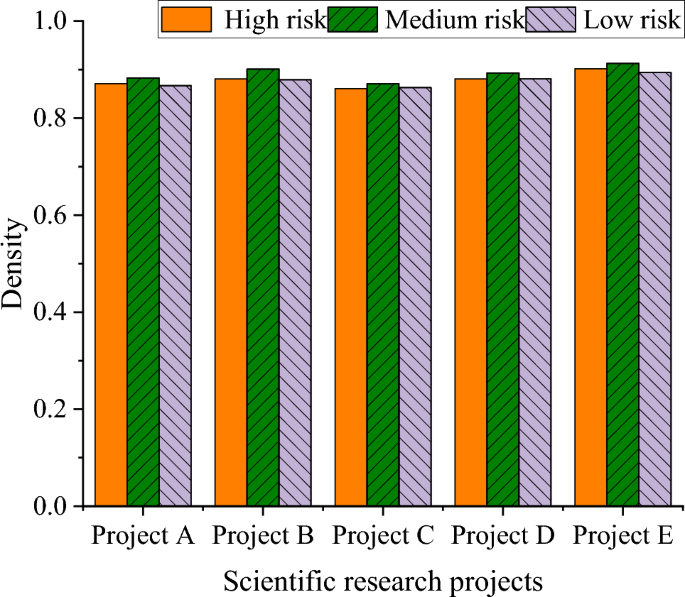
Prediction accuracy of TANB algorithm at different risk levels.
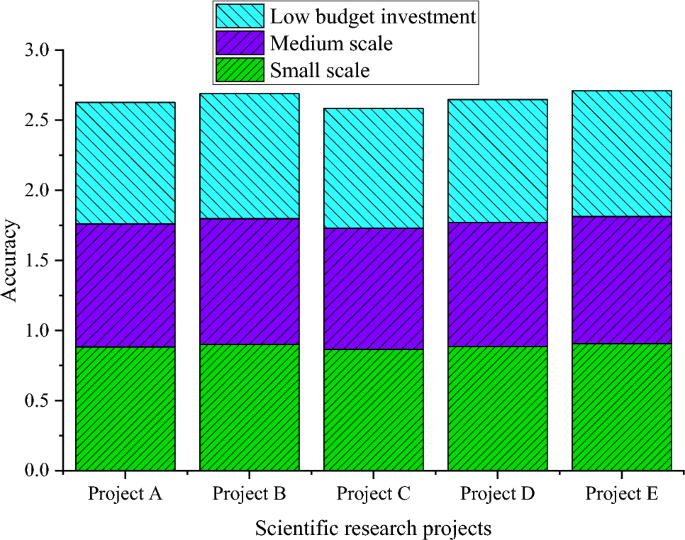
Prediction accuracy of TANB algorithm on different project scales.
From Figs. 4 , 5 , 6 and 7 , as the level of budget investment increases, the accuracy of most projects also shows an increasing trend. Especially in cases of high budget investment, the accuracy of the project is generally high. This may mean that a higher budget investment helps to reduce project risks, thereby improving the prediction accuracy of the TANB algorithm. It can be observed that team experience also affects the accuracy of the model. Projects with high team experience exhibit higher accuracy in TANB algorithms. This may indicate that experienced teams can better cope with project risks to improve the performance of the model. When budget investment and team experience are low, accuracy is relatively low. This may imply that budget investment and team experience can complement each other to affect the model performance.
There are certain differences in the accuracy of projects under different risk levels. Generally speaking, the accuracy of high-risk and medium-risk projects is relatively high, while the accuracy of low-risk projects is relatively low. This may be because high-risk and medium-risk projects require more accurate predictions, resulting in higher accuracy. Similarly, project scale also affects the performance of the model. Large-scale and medium-scale projects exhibit high accuracy in TANB algorithms, while small-scale projects have relatively low accuracy. This may be because the risks of large-scale and medium-scale projects are easier to identify and predict to promote the performance of the model. In high-risk and large-scale projects, accuracy is relatively high. This may indicate that the impact of project scale is more significant in specific risk scenarios.
Figure 8 further compares the performance of the TANB algorithm proposed here with other similar algorithms.
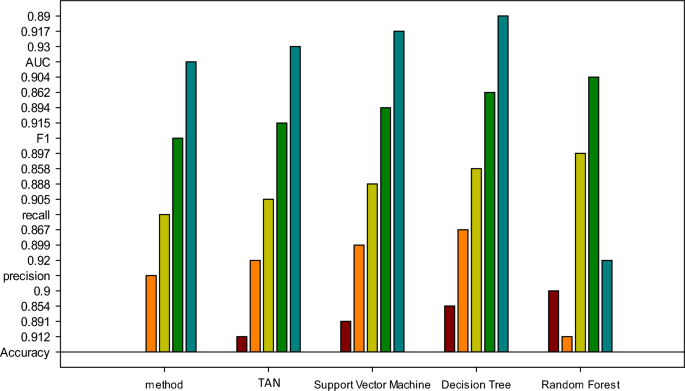
Performance comparison of different algorithms in RA of SRPs.
As depicted in Fig. 8 , the TANB algorithm attains an accuracy and precision of 0.912 and 0.920, respectively, surpassing other algorithms. It excels in recall rate and F1 value, registering 0.905 and 0.915, respectively, outperforming alternative algorithms. These findings underscore the proficiency of the TANB algorithm in comprehensively identifying high-risk projects while sustaining high classification accuracy. Moreover, the algorithm achieves an AUC of 0.930, indicative of its exceptional predictive prowess in sample classification. Thus, the TANB algorithm exhibits notable potential for application, particularly in scenarios demanding the recognition and comprehensiveness requisite for high-risk project identification. The evaluation results of the TANB model in predicting project risk levels are presented in Table 2 :
Table 2 demonstrates that the TANB model surpasses the traditional Naive Bayes model across multiple evaluation metrics, including accuracy, precision, and recall. This signifies that, by accounting for feature interdependence, the TANB model can more precisely forecast project risk levels. Furthermore, leveraging the model’s predictive outcomes, project managers can devise tailored risk mitigation strategies corresponding to various risk scenarios. For example, in high-risk projects, more assertive measures can be implemented to address risks, while in low-risk projects, risks can be managed more cautiously. This targeted risk management approach contributes to enhancing project success rates, thereby ensuring the seamless advancement of SRPs.
The exceptional performance of the TANB model in specific scenarios derives from its distinctive characteristics and capabilities. Firstly, compared to traditional Naive Bayes models, the TANB model can better capture the dependencies between attributes. In project RA, project features often exhibit complex interactions. The TANB model introduces first-order dependencies between attributes, allowing features to influence each other, thereby more accurately reflecting real-world situations and improving risk prediction precision. Secondly, the TANB model demonstrates strong adaptability and generalization ability in handling multidimensional data. SRPs typically involve data from multiple dimensions, such as project scale, budget investment, and team experience. The TANB model effectively processes these multidimensional data, extracts key information, and achieves accurate RA for projects. Furthermore, the paper explores the potential of using hybrid models or ensemble learning methods to further enhance model performance. By combining other machine learning algorithms, such as random forests and support vector regressors with sigmoid kernel, through ensemble learning, the shortcomings of individual models in specific scenarios can be overcome, thus improving the accuracy and robustness of RA. For example, in the study, we compared the performance of the TANB model with other algorithms in RA, as shown in Table 3 .
Table 3 illustrates that the TANB model surpasses other models in terms of accuracy, precision, recall, F1 value, and AUC value, further confirming its superiority and practicality in RA. Therefore, the TANB model holds significant application potential in SRPs, offering effective decision support for project managers to better evaluate and manage project risks, thereby enhancing the likelihood of project success.
Analysis of the degree of influence of different factors
Table 4 analyzes the degree of influence and interaction of different factors.
In Table 4 , the regression analysis results reveal that budget investment and team experience exert a significantly positive impact on RA outcomes. This suggests that increasing budget allocation and assembling a team with extensive experience can enhance project RA outcomes. Specifically, the regression coefficient for budget investment is 0.68, and for team experience, it is 0.51, both demonstrating significant positive effects (P < 0.05). The P-values are all significantly less than 0.05, indicating a significant impact. The impact of project scale is relatively small, at 0.31, and its P-value is also much less than 0.05. The degree of interaction influence is as follows. The impact of interaction terms is also significant, especially the interaction between budget investment and team experience and the interaction between budget investment and project scale. The P value of the interaction between budget investment and project scale is 0.002, and the P value of the interaction between team experience and project scale is 0.003. The P value of the interaction among budget investment, team experience, and project scale is 0.005. So, there are complex relationships and interactions among different factors, and budget investment and team experience significantly affect the RA results. However, the budget investment and project scale slightly affect the RA results. Project managers should comprehensively consider the interactive effects of different factors when making decisions to more accurately assess the risks of SRPs.
The interaction between team experience and budget investment
The results of the interaction between team experience and budget investment are demonstrated in Table 5 .
From Table 5 , the degree of interaction impact can be obtained. Budget investment and team experience, along with the interaction between project scale and technical difficulty, are critical factors in risk mitigation. Particularly in scenarios characterized by large project scales and high technical difficulties, adequate budget allocation and a skilled team can substantially reduce project risks. As depicted in Table 5 , under conditions of high team experience and sufficient budget investment, the average RA outcome is 0.895 with a standard deviation of 0.012, significantly lower than assessment outcomes under other conditions. This highlights the synergistic effects of budget investment and team experience in effectively mitigating risks in complex project scenarios. The interaction between team experience and budget investment has a significant impact on RA results. Under high team experience, the impact of different budget investment levels on RA results is not significant, but under medium and low team experience, the impact of different budget investment levels on RA results is significantly different. The joint impact of team experience and budget investment is as follows. Under high team experience, the impact of budget investment is relatively small, possibly because high-level team experience can compensate for the risks brought by insufficient budget to some extent. Under medium and low team experience, the impact of budget investment is more significant, possibly because the lack of team experience makes budget investment play a more important role in RA. Therefore, team experience and budget investment interact in RA of SRPs. They need to be comprehensively considered in project decision-making. High team experience can compensate for the risks brought by insufficient budget to some extent, but in the case of low team experience, the impact of budget investment on RA is more significant. An exhaustive consideration of these factors and their interplay is imperative for effectively assessing the risks inherent in SRPs. Merely focusing on budget allocation or team expertise may not yield a thorough risk evaluation. Project managers must scrutinize the project’s scale, technical complexity, and team proficiency, integrating these aspects with budget allocation and team experience. This holistic approach fosters a more precise RA and facilitates the development of tailored risk management strategies, thereby augmenting the project’s likelihood of success. In conclusion, acknowledging the synergy between budget allocation and team expertise, in conjunction with other pertinent factors, is pivotal in the RA of SRPs. Project managers should adopt a comprehensive outlook to ensure sound decision-making and successful project execution.
Risk mitigation strategies
To enhance the discourse on project risk management in this paper, a dedicated section on risk mitigation strategies has been included. Leveraging the insights gleaned from the predictive model regarding identified risk factors and their corresponding risk levels, targeted risk mitigation measures are proposed.
Primarily, given the significant influence of budget investment and team experience on project RA outcomes, project managers are advised to prioritize these factors and devise pertinent risk management strategies.
For risks stemming from budget constraints, the adoption of flexible budget allocation strategies is advocated. This may involve optimizing project expenditures, establishing financial reserves, or seeking additional funding avenues.
In addressing risks attributed to inadequate team experience, measures such as enhanced training initiatives, engagement of seasoned project advisors, or collaboration with experienced teams can be employed to mitigate the shortfall in expertise.
Furthermore, recognizing the impact of project scale, duration, and technical complexity on RA outcomes, project managers are advised to holistically consider these factors during project planning. This entails adjusting project scale as necessary, establishing realistic project timelines, and conducting thorough assessments of technical challenges prior to project commencement.
These risk mitigation strategies aim to equip project managers with a comprehensive toolkit for effectively identifying, assessing, and mitigating risks inherent in SRPs.
This paper delves into the efficacy of the TANB algorithm in project risk prediction. The findings indicate that the algorithm demonstrates commendable performance across diverse projects, boasting high precision, recall rates, and AUC values, thereby outperforming analogous algorithms. This aligns with the perspectives espoused by Asadullah et al. 37 . Particular emphasis was placed on assessing the impact of variables such as budget investment levels, team experience, and project size on algorithmic performance. Notably, heightened budget investment and extensive team experience positively influenced the results, with project size exerting a comparatively minor impact. Regression analysis elucidates the magnitude and interplay of these factors, underscoring the predominant influence of budget investment and team experience on RA outcomes, whereas project size assumes a relatively marginal role. This underscores the imperative for decision-makers in projects to meticulously consider the interrelationships between these factors for a more precise assessment of project risks, echoing the sentiments expressed by Testorelli et al. 38 .
In sum, this paper furnishes a holistic comprehension of the Naive Bayes algorithm’s application in project risk prediction, offering robust guidance for practical project management. The paper’s tangible applications are chiefly concentrated in the realm of RA and management for SRPs. Such insights empower managers in SRPs to navigate risks with scientific acumen, thereby enhancing project success rates and performance. The paper advocates several strategic measures for SRPs management: prioritizing resource adjustments and team training to elevate the professional skill set of team members in coping with the impact of team experience on risks; implementing project scale management strategies to mitigate potential risks by detailed project stage division and stringent project planning; addressing technical difficulty as a pivotal risk factor through assessment and solution development strategies; incorporating project cycle adjustment and flexibility management to accommodate fluctuations and mitigate associated risks; and ensuring the integration of data quality management strategies to bolster data reliability and enhance model accuracy. These targeted risk responses aim to improve the likelihood of project success and ensure the seamless realization of project objectives.
Achievements
In this paper, the application of Naive Bayesian algorithm in RA of SRPs is deeply explored, and the influence of various factors on RA results and their relationship is comprehensively investigated. The research results fully prove the good accuracy and applicability of Naive Bayesian algorithm in RA of science and technology projects. Through probability estimation, the risk level of the project can be estimated more accurately, which provides a new decision support tool for the project manager. It is found that budget input and team experience are the most significant factors affecting the RA results, and their regression coefficients are 0.68 and 0.51 respectively. However, the influence of project scale on the RA results is relatively small, and its regression coefficient is 0.31. Especially in the case of low team experience, the budget input has a more significant impact on the RA results. However, it should also be admitted that there are some limitations in the paper. First, the case data used is limited and the sample size is relatively small, which may affect the generalization ability of the research results. Second, the factors concerned may not be comprehensive, and other factors that may affect RA, such as market changes and policies and regulations, are not considered.
The paper makes several key contributions. Firstly, it applies the Naive Bayes algorithm to assess the risks associated with SRPs, proposing the TANB and validating its effectiveness empirically. The introduction of the TANB model broadens the application scope of the Naive Bayes algorithm in scientific research risk management, offering novel methodologies for project RA. Secondly, the study delves into the impact of various factors on RA for SRPs through MLR analysis, highlighting the significance of budget investment and team experience. The results underscore the positive influence of budget investment and team experience on RA outcomes, offering valuable insights for project decision-making. Additionally, the paper examines the interaction between team experience and budget investment, revealing a nuanced relationship between the two in RA. This finding underscores the importance of comprehensively considering factors such as team experience and budget investment in project decision-making to achieve more accurate RA. In summary, the paper provides crucial theoretical foundations and empirical analyses for SRPs risk management by investigating RA and its influencing factors in depth. The research findings offer valuable guidance for project decision-making and risk management, bolstering efforts to enhance the success rate and efficiency of SRPs.
This paper distinguishes itself from existing research by conducting an in-depth analysis of the intricate interactions among various factors, offering more nuanced and specific RA outcomes. The primary objective extends beyond problem exploration, aiming to broaden the scope of scientific evaluation and research practice through the application of statistical language. This research goal endows the paper with considerable significance in the realm of science and technology project management. In comparison to traditional methods, this paper scrutinizes project risk with greater granularity, furnishing project managers with more actionable suggestions. The empirical analysis validates the effectiveness of the proposed method, introducing a fresh perspective for decision-making in science and technology projects. Future research endeavors will involve expanding the sample size and accumulating a more extensive dataset of SRPs to enhance the stability and generalizability of results. Furthermore, additional factors such as market demand and technological changes will be incorporated to comprehensively analyze elements influencing the risks of SRPs. Through these endeavors, the aim is to provide more precise and comprehensive decision support to the field of science and technology project management, propelling both research and practice in this domain to new heights.
Limitations and prospects
This paper, while employing advanced methodologies like TANB models, acknowledges inherent limitations that warrant consideration. Firstly, like any model, TANB has its constraints, and predictions in specific scenarios may be subject to these limitations. Subsequent research endeavors should explore alternative advanced machine learning and statistical models to enhance the precision and applicability of RA. Secondly, the focus of this paper predominantly centers on the RA for SRPs. Given the unique characteristics and risk factors prevalent in projects across diverse fields and industries, the generalizability of the paper results may be limited. Future research can broaden the scope of applicability by validating the model across various fields and industries. The robustness and generalizability of the model can be further ascertained through the incorporation of extensive real project data in subsequent research. Furthermore, future studies can delve into additional data preprocessing and feature engineering methods to optimize model performance. In practical applications, the integration of research outcomes into SRPs management systems could provide more intuitive and practical support for project decision-making. These avenues represent valuable directions for refining and expanding the contributions of this research in subsequent studies.
Data availability
All data generated or analysed during this study are included in this published article [and its Supplementary Information files].
Moshtaghian, F., Golabchi, M. & Noorzai, E. A framework to dynamic identification of project risks. Smart and sustain. Built. Environ. 9 (4), 375–393 (2020).
Google Scholar
Nunes, M. & Abreu, A. Managing open innovation project risks based on a social network analysis perspective. Sustainability 12 (8), 3132 (2020).
Article Google Scholar
Elkhatib, M. et al. Agile project management and project risks improvements: Pros and cons. Mod. Econ. 13 (9), 1157–1176 (2022).
Fridgeirsson, T. V. et al. The VUCAlity of projects: A new approach to assess a project risk in a complex world. Sustainability 13 (7), 3808 (2021).
Salahuddin, T. Numerical Techniques in MATLAB: Fundamental to Advanced Concepts (CRC Press, 2023).
Book Google Scholar
Awais, M. & Salahuddin, T. Radiative magnetohydrodynamic cross fluid thermophysical model passing on parabola surface with activation energy. Ain Shams Eng. J. 15 (1), 102282 (2024).
Awais, M. & Salahuddin, T. Natural convection with variable fluid properties of couple stress fluid with Cattaneo-Christov model and enthalpy process. Heliyon 9 (8), e18546 (2023).
Article CAS PubMed PubMed Central Google Scholar
Guan, L., Abbasi, A. & Ryan, M. J. Analyzing green building project risk interdependencies using Interpretive Structural Modeling. J. Clean. Prod. 256 , 120372 (2020).
Gaudenzi, B. & Qazi, A. Assessing project risks from a supply chain quality management (SCQM) perspective. Int. J. Qual. Reliab. Manag. 38 (4), 908–931 (2021).
Lee, K. T., Park, S. J. & Kim, J. H. Comparative analysis of managers’ perception in overseas construction project risks and cost overrun in actual cases: A perspective of the Republic of Korea. J. Asian Archit. Build. Eng. 22 (4), 2291–2308 (2023).
Garai-Fodor, M., Szemere, T. P. & Csiszárik-Kocsir, Á. Investor segments by perceived project risk and their characteristics based on primary research results. Risks 10 (8), 159 (2022).
Senova, A., Tobisova, A. & Rozenberg, R. New approaches to project risk assessment utilizing the Monte Carlo method. Sustainability 15 (2), 1006 (2023).
Tiwari, P. & Suresha, B. Moderating role of project innovativeness on project flexibility, project risk, project performance, and business success in financial services. Glob. J. Flex. Syst. Manag. 22 (3), 179–196 (2021).
de Araújo, F., Lima, P., Marcelino-Sadaba, S. & Verbano, C. Successful implementation of project risk management in small and medium enterprises: A cross-case analysis. Int. J. Manag. Proj. Bus. 14 (4), 1023–1045 (2021).
Obondi, K. The utilization of project risk monitoring and control practices and their relationship with project success in construction projects. J. Proj. Manag. 7 (1), 35–52 (2022).
Atasoy, G. et al. Empowering risk communication: Use of visualizations to describe project risks. J. Constr. Eng. Manage. 148 (5), 04022015 (2022).
Dandage, R. V., Rane, S. B. & Mantha, S. S. Modelling human resource dimension of international project risk management. J. Global Oper. Strateg. Sourcing 14 (2), 261–290 (2021).
Wang, L. et al. Applying social network analysis to genetic algorithm in optimizing project risk response decisions. Inf. Sci. 512 , 1024–1042 (2020).
Marx-Stoelting, P. et al. A walk in the PARC: developing and implementing 21st century chemical risk assessment in Europe. Arch. Toxicol. 97 (3), 893–908 (2023).
Awais, M., Salahuddin, T. & Muhammad, S. Evaluating the thermo-physical characteristics of non-Newtonian Casson fluid with enthalpy change. Thermal Sci. Eng. Prog. 42 , 101948 (2023).
Article CAS Google Scholar
Awais, M., Salahuddin, T. & Muhammad, S. Effects of viscous dissipation and activation energy for the MHD Eyring-Powell fluid flow with Darcy-Forchheimer and variable fluid properties. Ain Shams Eng. J. 15 (2), 102422 (2024).
Yang, L., Lou, J. & Zhao, X. Risk response of complex projects: Risk association network method. J. Manage. Eng. 37 (4), 05021004 (2021).
Acebes, F. et al. Project risk management from the bottom-up: Activity Risk Index. Cent. Eur. J. Oper. Res. 29 (4), 1375–1396 (2021).
Siyal, S. et al. They can’t treat you well under abusive supervision: Investigating the impact of job satisfaction and extrinsic motivation on healthcare employees. Rationality Society 33 (4), 401–423 (2021).
Chen, D., Wawrzynski, P. & Lv, Z. Cyber security in smart cities: A review of deep learning-based applications and case studies. Sustain. Cities Soc. 66 , 102655 (2021).
Zhao, M. et al. Pythagorean fuzzy TODIM method based on the cumulative prospect theory for MAGDM and its application on risk assessment of science and technology projects. Int. J. Fuzzy Syst. 23 , 1027–1041 (2021).
Suresh, K. & Dillibabu, R. A novel fuzzy mechanism for risk assessment in software projects. Soft Comput. 24 , 1683–1705 (2020).
Akhavan, M., Sebt, M. V. & Ameli, M. Risk assessment modeling for knowledge based and startup projects based on feasibility studies: A Bayesian network approach. Knowl.-Based Syst. 222 , 106992 (2021).
Guan, L., Abbasi, A. & Ryan, M. J. A simulation-based risk interdependency network model for project risk assessment. Decis. Support Syst. 148 , 113602 (2021).
Vujović, V. et al. Project planning and risk management as a success factor for IT projects in agricultural schools in Serbia. Technol. Soc. 63 , 101371 (2020).
Muñoz-La Rivera, F., Mora-Serrano, J. & Oñate, E. Factors influencing safety on construction projects (FSCPs): Types and categories. Int. J. Environ. Res. Public Health 18 (20), 10884 (2021).
Article PubMed PubMed Central Google Scholar
Nguyen, P. T. & Nguyen, P. C. Risk management in engineering and construction: A case study in design-build projects in Vietnam. Eng. Technol. Appl. Sci. Res 10 , 5237–5241 (2020).
Nguyen PT, Le TT. Risks on quality of civil engineering projects-an additive probability formula approach//AIP Conference Proceedings. AIP Publishing, 2798(1) (2023).
Nguyen, P.T., Phu, P.C., Thanh, P.P., et al . Exploring critical risk factors of office building projects. 8 (2), 0309–0315 (2020).
Nguyen, H. D. & Macchion, L. Risk management in green building: A review of the current state of research and future directions. Environ. Develop. Sustain. 25 (3), 2136–2172 (2023).
He, S. et al. Risk assessment of oil and gas pipelines hot work based on AHP-FCE. Petroleum 9 (1), 94–100 (2023).
Asadullah, M. et al. Evaluation of machine learning techniques for hypertension risk prediction based on medical data in Bangladesh. Indones. J. Electr. Eng. Comput. Sci. 31 (3), 1794–1802 (2023).
Testorelli, R., de Araujo, F., Lima, P. & Verbano, C. Fostering project risk management in SMEs: An emergent framework from a literature review. Prod. Plan. Control 33 (13), 1304–1318 (2022).
Download references
Author information
Authors and affiliations.
Institute of Policy Studies, Lingnan University, Tuen Mun, 999077, Hong Kong, China
Xuying Dong & Wanlin Qiu
You can also search for this author in PubMed Google Scholar
Contributions
Xuying Dong and Wanlin Qiu played a key role in the writing of Risk Assessment of Scientific Research Projects and the Relationship between Related Factors Based on Naive Bayes Algorithm. First, they jointly developed clearly defined research questions and methods for risk assessment using the naive Bayes algorithm at the beginning of the research project. Secondly, Xuying Dong and Wanlin Qiu were responsible for data collection and preparation, respectively, to ensure the quality and accuracy of the data used in the research. They worked together to develop a naive Bayes algorithm model, gain a deep understanding of the algorithm, ensure the effectiveness and performance of the model, and successfully apply the model in practical research. In the experimental and data analysis phase, the collaborative work of Xuying Dong and Wanlin Qiu played a key role in verifying the validity of the model and accurately assessing the risks of the research project. They also collaborated on research papers, including detailed descriptions of methods, experiments and results, and actively participated in the review and revision process, ensuring the accuracy and completeness of the findings. In general, the joint contribution of Xuying Dong and Wanlin Qiu has provided a solid foundation for the success of this research and the publication of high-quality papers, promoted the research on the risk assessment of scientific research projects and the relationship between related factors, and made a positive contribution to the progress of the field.
Corresponding author
Correspondence to Wanlin Qiu .
Ethics declarations
Competing interests.
The authors declare no competing interests.
Additional information
Publisher's note.
Springer Nature remains neutral with regard to jurisdictional claims in published maps and institutional affiliations.
Supplementary Information
Supplementary information., rights and permissions.
Open Access This article is licensed under a Creative Commons Attribution 4.0 International License, which permits use, sharing, adaptation, distribution and reproduction in any medium or format, as long as you give appropriate credit to the original author(s) and the source, provide a link to the Creative Commons licence, and indicate if changes were made. The images or other third party material in this article are included in the article's Creative Commons licence, unless indicated otherwise in a credit line to the material. If material is not included in the article's Creative Commons licence and your intended use is not permitted by statutory regulation or exceeds the permitted use, you will need to obtain permission directly from the copyright holder. To view a copy of this licence, visit http://creativecommons.org/licenses/by/4.0/ .
Reprints and permissions
About this article
Cite this article.
Dong, X., Qiu, W. A case study on the relationship between risk assessment of scientific research projects and related factors under the Naive Bayesian algorithm. Sci Rep 14 , 8244 (2024). https://doi.org/10.1038/s41598-024-58341-y
Download citation
Received : 30 October 2023
Accepted : 27 March 2024
Published : 08 April 2024
DOI : https://doi.org/10.1038/s41598-024-58341-y
Share this article
Anyone you share the following link with will be able to read this content:
Sorry, a shareable link is not currently available for this article.
Provided by the Springer Nature SharedIt content-sharing initiative
- Naive Bayesian algorithm
- Scientific research projects
- Risk assessment
- Factor analysis
- Probability estimation
- Decision support
- Data-driven decision-making
By submitting a comment you agree to abide by our Terms and Community Guidelines . If you find something abusive or that does not comply with our terms or guidelines please flag it as inappropriate.
Quick links
- Explore articles by subject
- Guide to authors
- Editorial policies
Sign up for the Nature Briefing: AI and Robotics newsletter — what matters in AI and robotics research, free to your inbox weekly.

- Open access
- Published: 09 December 2020
Data of risk analysis management in university campuses
- Alireza Dehdashti 1 ,
- Farin Fatemi 1 ,
- Muhammadreza Janati 2 ,
- Fatemeh Asadi 2 &
- Marzieh Belji Kangarloo 2
BMC Research Notes volume 13 , Article number: 554 ( 2020 ) Cite this article
1 Citations
Metrics details
This data paper aims to provide the data set of a practical method to health, safety, and environmental risk assessment to assess and rank potential threats/hazards and to prevent and decrease the accidents and harmful consequences at an academic setting. Descriptive data on type of hazards, places, and persons at risk were collected. Quantitative data on risk probability and severity of identified hazards were determined. Additionally, the descriptive statistics and analytical tests were applied to create a concise perspective on health, safety and environmental hazards/threats situation in research location under study. The dataset further provides information on the prioritization of determined risks according to the relevant scores and levels for doing the relevant control measures to remove and mitigate the related risks.
Data description
This paper provides data of comprehensive risk assessment of health, safety and, environmental hazards of academic setting. For each identified hazard, the descriptive and numeric data are available. The information about the risk level and prevention or mitigation measure related to each hazard is provided. Additionally, the statistical tests are applied for determining the relations among the variables under study. The data and methodology on risk assessment in this article may be used to manage variety of risks in higher education institutions.
Risk assessment is the process of evaluating risks to persons’ safety and health from workplace hazards [ 1 ]. This data note aims to provide comprehensive information about the Health, Safety and Environment (HSE) risk assessment. Such these data sets are necessary for accident prevention and decrease the harmful impacts such as death, injuries and damages to structures and equipment at academic settings [ 2 , 3 ]. Also, such these risk assessment results promote information sharing across the university systems about best practices to mitigate the vulnerability of hazards [ 4 ]. Corresponding mitigation measures for each campus’s highest risks is beneficial to create a University-wide relative risk ranking of all threat events and to summarize the status of campus mitigation measures [ 5 ]. Taking steps to either eliminate or to reduce risks (as far as reasonably practicable) by introducing control measures should be done in the risk management phase [ 6 ]. To achieve these goals, we developed two checklists to collect descriptive and quantitative data based on the evidence review [ 7 , 8 ]. The laboratories, public offices, and peripheral areas of the academic setting were included in this study. Besides, we planned to determine the hazard mitigation strategies for each identified hazard in the assessed areas of the academic setting in the applied checklist [ 9 ]. In the current paper, we described the collected data during the HSE risk assessment in this study. A research paper was written up and published based on our data and methodology to further describe and prioritize risks in terms of varying risk impacts in the university environment [ 10 ]. These data may help researchers to assess probability, impact, and mitigation risks in detail and find a proactive approach toward risks in higher education institutions.
This cross-sectional study was conducted from June to July 2018 at the School of Public Health, Semnan, University of Medical Sciences, Semnan, Iran. For implementing a successful HSE risk assessment, we needed to identify the potential hazards into three individual domains of health, safety, and environment in different areas of the academic setting. First, a list of hazards in three sections of health, safety, and environment was developed in a checklist. The reviewed literature on concerning recent accidents or incidents within universities was much helpful in defining the list of HSE hazards. The hazards checklist was completed via on-site walking and interviews by three trained students in the 39 locations of the academic setting. Additionally, the persons at risk including students, staff, or faculties were determined for each hazard in the assessed location. The descriptive analysis indicated that the most frequency of hazards was related to health hazards (50.3%). The safety and environmental hazards were 44%, and 5.7%, respectively.
Second, the research team analyzed the risks based on the risk matrix in ISO 31000 [ 8 ] (see Table 1 ). Risk analysis was done by estimating the probability and severity of risks for identified hazards. The probability of occurrence metrics was measured on a five-point scale from “not applicable” to “inevitable”. The interpretation of each scale has been mentioned in data file 1 [ 11 ]. The novelty of this study is determining the severity rate in terms of human, equipment, and institution. To estimate the effect of each identified hazard on three mentioned terms, we applied two items with a five-point score response [ 12 ]. Responses were scored and averaged to obtain an overall severity score. The severity metrics were measured on a five-point scale from “very low” to “very high”. The final risk scores were calculated from multiplying probability by severity and categorized into 3 risk levels, risks rated in the top category (red) are larger than those rated in moderate category (yellow) and low category (green).
Based on the results, hazards with high risk level were belonged to safety category that they were included 5.7%, required immediate mitigation measures. From all identified health, safety and environmental hazards, 6.5%, 36% and 43% were categorized in moderate risk level, respectively. These hazards need to be corrected in the near future. The rest of identified HSE hazards had acceptable risk score and categorized in low risk hazards. Therefore, the mitigation measures were recommended in appropriate to determined risk levels for identified hazards.
In data file 2, in order to create a more detailed view on health, safety and environmental hazards/threats situation, the analytical tests were applied to examine associations among the main variables under study [ 13 ]. Type of analytical tests (Chi square or Kruskal–Wallis)was used based on type of variable that is qualitative or quantitative. The most important finding is related to significant relation between the calculated risk scores and the type of hazards. The safety-related hazards indicated a statistically higher contribution to the total risk score when compared to health and environmental hazards. The descriptive analysis and providing analytical information helps to withstand and cope with the adverse effects of accidents and emergencies [ 14 , 15 ].
Limitations
Although risk matrices can indeed be very useful in risk analysis, but can mistakenly assign higher qualitative ratings to smaller risks that leading to wrong risk management decision. It means that we might have quantitatively overestimated or underestimated the risk scores for identified hazards. Probably, applying new approaches to risk matrix such as new scaling and scoring methods to risk matrix extensions depends on the location of risk assessment in future studies would minimize the mentioned concern.
The data of this study were collected in a typical higher education institution with its exclusive hazards, which may limit its interpretation of safety, health, and environmental risks. However, the authors believe that an integrated approach for obtaining data will be beneficial to compare various domains of hazards in a workplace.
Availability of data and materials
The data described in this Data note can be freely and openly accessed via http://figshare.com . Please see Table 1 and Refs. [ 11 , 13 ] for details and links to the data.
Abbreviations
Health, safety, environment
Sarker MN, Yang B, Lv Y, Huq ME, Kamruzzaman MM. Climate change adaptation and resilience through big data. Int J Adv Comput Sci Appl. 2020;11:533–9. https://doi.org/10.14569/IJACSA.2020.0110368 .
Article Google Scholar
Farrokhi M, Khankeh H, Poursadeqiyan M. The necessity to establish health, safety and environment management major at the University of Social Welfare and Rehabilitation Sciences. Health Emerg Disasters Quarterly. 2019;4(3):119–26. http://hdq.uswr.ac.ir/article-1-205-en.html .
Salazar-Escoboza MA, Laborin-Alvarez JF, Alvarez-Chavez CR, Noriega-Orozco L, Borbon-Morales C. Safety climate perceived by users of academic laboratories in higher education institutes. Saf Sci. 2020;121:93–9. http://www.sciencedirect.com/science/article/pii/S0925753519306198 .
Skurka C, Quick BL, Reynolds-Tylus T, Short T, Bryan AL. An evaluation of a college campus emergency preparedness intervention. J Saf Res. 2018;65(67–72):000434745100008.
Google Scholar
Yang N, Wu YM. University public emergency plan formulation: Current situation and countermeasures. Jun H, Hong C, Jianbin J, editors 2010. 40–3 p.000289654800010.
Marincioni F, Fraboni R. A baseline assessment of emergency planning and preparedness in Italian universities. Disasters. 2012;36(2):291–315. https://doi.org/10.1111/j.1467-7717.2011.01259.x .
Article PubMed Google Scholar
Example risk assessments United Kingdom: Aberystwyth University; 2018. https://www.aber.ac.uk/en/hse/proc-prac/risk-assessment/#template-and-guidance .
Risk management framework. Second ed: Goverment of South Australia; 2012. https://dhs.sa.gov.au/data/assets/pdf_file/0008/9782/risk-management-framework.pdf .
Ilo PI, Izuagbe R, Mole AJC, Ekwueme L. Measuring disaster preparedness and response practices in university libraries in Nigeria: the role of disaster equipment. Int J Disaster Risk Reduct. 2018;31:85–91.
Dehdashti A, Fatemi F, Jannati M, Asadi F, Kangarloo MB. Applying health, safety, and environmental risk assessment at academic settings. BMC Public Health; 2020. https://bmcpublichealth.biomedcentral.com/articles/10.1186/s12889-020-09419-5 .
Fatemi F. Risk assessment data file. figshare. Dataset. 2020. https://doi.org/10.6084/m9figshare.13106825.v1 .
Nouri J, Mansouri N, Abbaspour M, Karbasi AR, Omidvari M. Designing a developed model for assessing the disaster induced vulnerability value in educational centers. Saf Sci. 2011;49(5):679–85. https://doi.org/10.1016/j.ssci.2011.01.002 .
Fatemi F. Analytical data. figshare. Dataset. 2020. https://doi.org/10.6084/m9.figshare.13106843.v1 .
Sarker MNI, Peng Y, Yiran C, Shouse RC. Disaster resilience through big data: Way to environmental sustainability. Int J Disaster Risk Reduct. 2020;51 March:101769. https://doi.org/10.1016/j.ijdrr.2020.101769 .
Tajdinan S, Afshari D. Checking of human errors in Ancoiler Device Control Room of Ahvaz Pipe Mill using SHERPA and HET methods at year 1390. Iran Occup Health. 2013;10(3). http://ioh.iums.ac.ir/article-1-771-en.html .
Download references
Acknowledgements
We thank all colleagues and staff of the School of Public Health who supported and assisted for conducting this research.
There is no funding source for this research.
Author information
Authors and affiliations.
Research Center for Health Sciences and Technologies, Semnan University of Medical Sciences, Semnan, Iran
Alireza Dehdashti & Farin Fatemi
Student Research Committee, Semnan University of Medical Siences, Semnan, Iran
Muhammadreza Janati, Fatemeh Asadi & Marzieh Belji Kangarloo
You can also search for this author in PubMed Google Scholar
Contributions
MJ, FA and MB collected data and contributed to entering data into dataset. FF and AD designed study, analyzed data, and prepared the manuscript. All authors read and approved the final manuscript.
Corresponding author
Correspondence to Farin Fatemi .
Ethics declarations
Ethics approval and consent to participate.
This study was approved by the Ethics Committee Review Board at Semnan university of Medical Sciences (IR.SEMUMS.REC.1398.131). All the participants signed a consent form and were informed on the purpose of the study prior to interview as per local protocol on research ethics.
Consent for publication
Not applicable.
Competing interests
The authors declare that they have no competing interests.
Additional information
Publisher's note.
Springer Nature remains neutral with regard to jurisdictional claims in published maps and institutional affiliations.
Rights and permissions
Open Access This article is licensed under a Creative Commons Attribution 4.0 International License, which permits use, sharing, adaptation, distribution and reproduction in any medium or format, as long as you give appropriate credit to the original author(s) and the source, provide a link to the Creative Commons licence, and indicate if changes were made. The images or other third party material in this article are included in the article's Creative Commons licence, unless indicated otherwise in a credit line to the material. If material is not included in the article's Creative Commons licence and your intended use is not permitted by statutory regulation or exceeds the permitted use, you will need to obtain permission directly from the copyright holder. To view a copy of this licence, visit http://creativecommons.org/licenses/by/4.0/ . The Creative Commons Public Domain Dedication waiver ( http://creativecommons.org/publicdomain/zero/1.0/ ) applies to the data made available in this article, unless otherwise stated in a credit line to the data.
Reprints and permissions
About this article
Cite this article.
Dehdashti, A., Fatemi, F., Janati, M. et al. Data of risk analysis management in university campuses. BMC Res Notes 13 , 554 (2020). https://doi.org/10.1186/s13104-020-05397-4
Download citation
Received : 17 October 2020
Accepted : 24 November 2020
Published : 09 December 2020
DOI : https://doi.org/10.1186/s13104-020-05397-4
Share this article
Anyone you share the following link with will be able to read this content:
Sorry, a shareable link is not currently available for this article.
Provided by the Springer Nature SharedIt content-sharing initiative
- Risk assessment
- Academic settings
BMC Research Notes
ISSN: 1756-0500
- Submission enquiries: [email protected]
- General enquiries: [email protected]
- Research article
- Open access
- Published: 12 April 2021
A risk assessment tool for resumption of research activities during the COVID-19 pandemic for field trials in low resource settings
- Suzanne M. Simkovich ORCID: orcid.org/0000-0003-2462-0856 1 , 2 , 3 ,
- Lisa M. Thompson 4 ,
- Maggie L. Clark 5 ,
- Kalpana Balakrishnan 6 ,
- Alejandra Bussalleu 7 , 8 ,
- William Checkley 1 , 2 ,
- Thomas Clasen 9 ,
- Victor G. Davila-Roman 10 ,
- Anaite Diaz-Artiga 11 ,
- Ephrem Dusabimana 12 ,
- Lisa de las Fuentes 10 ,
- Steven Harvey 2 , 13 ,
- Miles A. Kirby 14 ,
- Amy Lovvorn 9 ,
- Eric D. McCollum 15 ,
- Erick E. Mollinedo 16 ,
- Jennifer L. Peel 5 ,
- Ashlinn Quinn 17 ,
- Ghislaine Rosa 18 ,
- Lindsay J. Underhill 1 , 2 ,
- Kendra N. Williams 1 , 2 ,
- Bonnie N. Young 5 ,
- Joshua Rosenthal 17 &
HAPIN Investigators
BMC Medical Research Methodology volume 21 , Article number: 68 ( 2021 ) Cite this article
7 Citations
8 Altmetric
Metrics details
The spread of severe acute respiratory syndrome coronavirus-2 has suspended many non-COVID-19 related research activities. Where restarting research activities is permitted, investigators need to evaluate the risks and benefits of resuming data collection and adapt procedures to minimize risk.
In the context of the multicountry Household Air Pollution Intervention (HAPIN) trial conducted in rural, low-resource settings, we developed a framework to assess the risk of each trial activity and to guide protective measures. Our goal is to maximize the integrity of reseach aims while minimizing infection risk based on the latest scientific understanding of the virus.
We drew on a combination of expert consultations, risk assessment frameworks, institutional guidance and literature to develop our framework. We then systematically graded clinical, behavioral, laboratory and field environmental health research activities in four countries for both adult and child subjects using this framework. National and local government recommendations provided the minimum safety guidelines for our work.
Our framework assesses risk based on staff proximity to the participant, exposure time between staff and participants, and potential viral aerosolization while performing the activity. For each activity, one of four risk levels, from minimal to unacceptable, is assigned and guidance on protective measures is provided. Those activities that can potentially aerosolize the virus are deemed the highest risk.
Conclusions
By applying a systematic, procedure-specific approach to risk assessment for each trial activity, we were able to protect our participants and research team and to uphold our ability to deliver on the research commitments we have made to our staff, participants, local communities, and funders. This framework can be tailored to other research studies conducted in similar settings during the current pandemic, as well as potential future outbreaks with similar transmission dynamics.
The trial is registered with clinicaltrials.gov NCT02944682 on October 26. 2016 .
Peer Review reports
The spread of severe acute respiratory syndrome coronavirus-2 (SARS-CoV-2) and resulting coronavirus disease 2019 (COVID-19) has led to the temporary suspension of many non-COVID-19 related research activities worldwide. Where feasible, studies are considering remote data collection by telephone or web-based conferencing [ 1 , 2 , 3 ]. However, this approach is often not possible when performing anthropometric measurements, specimen collection, or when investigators need to make other direct observations. Even temporary suspension of research activities can potentially cause harm if investigators are evaluating an intervention that is hypothesized to be beneficial. Further, the suspension of data collection could result in loss of study power and potentially introduce bias. Every day, we are gaining a greater understanding of the transmissibility of SARS-CoV-2, and this knowledge increases our ability to safely resume a wide variety of non-COVID-19 related research activities [ 3 , 4 ]. Where local law or institutional regulations permit activities to restart, investigators need to evaluate the risks and benefits to both research staff and participants of resuming data collection. To safely conduct study activities, researchers need to develop standardized procedures that are based on realistic assessment of these risks, provide guidance on where and when they are manageable, as well as how to minimize the risk with physical distance measures and appropriate personal protective equipment (PPE).
Investigators in the Household Air Pollution Intervention Network (HAPIN) trial initially suspended data collection due to the pandemic in March 2020 and have since restarted collection of behavioural, environmental, biological and clinical measurements during the fifth year of a five-year, multi-country trial [ 5 , 6 , 7 , 8 ]. HAPIN is a randomized controlled trial in rural areas of Guatemala, India, Peru, and Rwanda that is assessing the health benefits of providing liquefied petroleum gas (LPG) stoves and an 18-month supply of free LPG to 3200 households that otherwise depend on solid biomass fuel (wood, animal dung, or crop residue) for cooking. Measurements of cooking behavior, personal and in-home exposure to air pollution, biological samples and clinical measurements are being collected longitudinally from pregnant women and their newborns in every household, along with an older, non-pregnant adult woman, if she resides in the house [ 5 , 6 , 7 , 8 ]. Our study involves home visits, as well as visits to health centers and hospitals during the woman’s pregnancy and the first year of the child’s life.
As SARS-CoV-2 spread globally, governments in all four countries implemented public safety restrictions that limited activities to those designated as essential. Essential activities varied across settings and during the initial period of restrictions. Research activities were not considered essential. However, LPG delivery for cooking was considered essential in all four countries. In Guatemala and Rwanda, our research teams were permitted to continue delivering LPG to study households without disruption. In India, the gas companies continued to deliver refill tanks to study participants. In Peru, our team was limited in its ability to deliver gas during the initial weeks of the restrictions, but we were later able to re-establish services with a local gas company for delivery.
With permission to continue delivery of the LPG intervention, we immediately implemented changes in our delivery protocols to minimize SARS-CoV-2 risk. Further, in anticipation of the additional easement of movement restrictions in countries around the world, we reviewed the literature for guidance on strategies researchers have used for assessing the risk of activities during COVID-19 or other pandemics, and found a dearth of available guidance. Perhaps the most relevant existing framework is that proposed by Lumeng and colleagues, which was designed for research focused in clinical settings in the U.S.A. Thus, we developed a risk assessment tool with the guiding principle of ethical research to minimize the potential risks to research staff, participants and rural communities participating in the HAPIN trial research settings. We wanted our risk assessment tool to allow researchers to assess the risk of each study activity utilizing the same general criteria to support management decisions across this large multinational, multi-disciplinary study with both competing and complementary activities. Although our risk assessment tool has been designed within the framework of specific activities of the HAPIN trial, we report here on our approach, which can be applied to other research contexts and questions in similar settings.
In developing our risk assessment tool, we drew on a combination of expert consultations, government regulations, national and local expertise, institutional guidance and review of emerging literature. We queried our multi-national panel of investigators and field team leaders from across the trial with expertise in the disciplines of clinical medicine and imaging, nursing, environmental science, epidemiology, behavioral science, community engagement and statistics, along with the trial funders who provide scientific guidance to the HAPIN trial. We sought input from local community leaders, the Ministries of Health, universities and non-governmental organizations regarding appropriate operations and safety concerns. We consulted with the Institutional Review Boards (IRBs) and Data and Safety Monitoring Board (DSMB) presiding over the trial regarding resumption of activities. We drew upon historical occupational health frameworks for infectious disease biosafety and risk assessment and the most recent peer-reviewed and grey literature about infection dynamics. We also considered staff experience. Using all of these inputs, we built a framework to evaluate risk of exposure to SARS-CoV-2 [ 3 ]. Our intent was to develop criteria that were clear, simple and actionable for field managers and staff to implement, and to recommend appropriate practices and materials, in accordance with the risk level of each procedure and perceived risk threshold.
While SARS-CoV2 research findings are still emerging, our assessment is based on the consensus that aerosolization and droplet carriage of virus, primarily from coughing, sneezing, singing, crying, talking, are the predominant modes of infection. It is unclear how long the virus remains in the air. Fomites from surface contact may also contribute to transmission, but are likely a smaller risk. Evidence of SARS-CoV-2 presence has been detected in urine, stool, breast milk, semen and blood, but we are not aware of documented transmission through these bodily fluids at the time of this publication. Furthermore, the risk of transmission is greatest in the two days preceding onset of symptoms and continues afterward for at least ten days, and up to twenty days in immunosuppressed patients. Because documented asymptomatic carriage has been widely reported, we assumed that any staff member, collaborator or community participant might be shedding the virus. Small children (especially infants) appear to be infected at the same rate as adults, but have more mild disease and thus may be unknowingly spreading disease. We agreed that viral transmissibility and the true prevalence of COVID-19 are not clearly known in any of our study sites due to limited testing. We also note that recent seroprevalence studies have reported that case burdens are likely underreported. As such, we chose to err on the side of caution and assume moderate to substantial incidence of disease in all our settings. Therefore, risk was defined as large-scale, uncontrolled community transmission. When widespread vaccination has been achieved in our settings and/or when there are other indications of lower prevalence of disease in our sites, we may adjust our risk levels accordingly.
We assessed each HAPIN data collection activity among each group of participants (pregnant woman /new mother, infant, or non-pregnant older adult woman) because the risks may vary with each participant group. Data collection activities were graded and agreed upon by our team of scientists. Local site investigators were asked to report perceived concerns by staff and participants in their communities. Risk factors and definitions were presented to the HAPIN steering committee, which met weekly, for feedback before adoption. Even with adoption by the steering committee, if local community risk factors at the sites did not allow continued trial activities, the activity was stopped until safety could be ensured. Standard Operating Procedures were developed for the resumption of study activities and included guidance on screening staff and participants for Covid-19 symptoms, transporting staff in project vehicles, cleaning equipment and surfaces, conducting home visits and health facility survelliance, and quarantining for suspected exposures to the virus. These documents are reviewed monthly by two assigned investigators on the trial to reflect they reflect the most up to date knowledge of transmission dynamics and local risk.
Evaluation of risk criteria for each procedure included the age of participant, location, required physical proximity, exposure time, aerosolization potential, and criteria for use of PPE (Table 1 ). Using these criteria we established a four level schedule that ranged from minimal to unacceptably high risk (Table 2 ). We then proceeded to assess each research activity according to the criteria outlined in Table 1 and assigned a risk level and appropriate PPE to each of these. We assessed research activities that included LPG fuel delivery, administration of tablet-based surveys (e.g. questionnaires asked of mothers about their children’s health), data downloads from environmental monitors, personal exposure assessment to household air pollution, biological sample collection (e.g. urine, nasal swabs, venous blood) and lab processing of biological samples in the field laboratories, clinical measures (e.g. newborn birth weight, lung ultrasound, blood pressure), observations in homes of pregnant women/new mothers, children, and vascular procedures in adults (Additional file 1 ).
Protective measures available in our settings were: a) where feasible, data collection was completed by telephone; b) where possible, face-to-face activities were conducted outside; c) when inside homes, clinics or offices, staff and participants minimized the number of people in the room; d) rigourous hygiene for staff, materials, equipment and surfaces were employed at all times; e) appropriate PPE was used based on the context and activity; f) under very high risk conditions, the visit or the procedure was suspended.
Using this assessment and taking necessary measures for protection, almost all of our research activities were deemed to pose potentially manageable risks. Biological sample collection spanned a range of assigned risk due to differences in participant-staff interaction. The activities with the highest level of risk were those that potentially aerosolize the virus during the procedure. For example, urine collection requires minimal contact (i.e., field workers instruct the participant to collect and store the urine sample until it can be retreived) resulting in low risk to both the participant and the study staff. However, dried blood spot collection from capillary blood draws from infants (who are unable to wear a mask and often cry during the procedure) could feasibly put field workers at high risk (examples of two procedures are provided in Table 1 ; all procedures are described in the Additional file 1 ). To illustrate Level 4 activities, we identified several activities that were not part of our protocol, but that could pose unmanageable risks (e.g. bronchoscopy, sputum inducting procedures, cardiopulmonary resusitation) for routine research in the pandemic context (Table 2 ).
Our risk assessment framework uses a four-level risk schedule that is flexible, allowing adjustment for changes to risk measures and definitions as new evidence emerges about virus transmission. The approach and risk assessment tool we present here can be adapted by other investigators who are assessing and managing the risk posed in their own research during the coronavirus pandemic. However, prior to the deployment of risk assessment tools such as ours, researchers, in association with community members, IRBs, DSMBs, and grant funders need to evaluate the importance of any activity related to the primary aims of a trial weighed against the associated risk of performing the research activity. Obviously, local health regulations related to mobility, home or clinic visits by researchers supersede any of these judgments.
The framework offers a way to systematically evaluate diverse research activities involving different disciplines using the same basic criteria and a scoring system to compare associated risk for a given procedure. It also provides clear guidance for field teams on the appropriate PPE and practices in the context of limited resource environments, and thus appropriately utilizes limited PPE where it may be scarce and expensive. Despite these strengths, there are limitations. Our framework does not make recommendations on whether or not to continue an activity – e.g. through an explict cost-benefit algorithm. Decisions on what research should be continued in the presence of risk also require a careful assessment of benefits. We chose to make the risk-benefit calculation and decisions regarding which activities to suspend an independent process from assessment of risk. In our context, an efficacy trial, we are in equipoise regarding the potential benefits of the intervention to participants. Thus, analysis of benefits can only be honestly assessed in terms of the potential benefit of a given activity to the integrity of the trial, not to trial participants as maybe the case for other kinds of clinical research. Among the criteria we used to examine potential benefits of risk in the HAPIN trial were whether or not the aim of any given procedure supports a primary, secondary or tertiary (exploratory) outcome of the trial protocol. This evaluation is made by the HAPIN Steering Committee.
Furthermore, we do not factor in specific local regulations into the matrix in an a priori fashion, and thus leave it to local investigators and study teams to adjust for these [ 9 ]. Because of this, our framework specifications may need to be adjusted to meet local institutional or government regulations regarding PPE or other safety practices. Finally, our framework is limited by the current evidence regarding transmission risk and should be reevaluated and updated as our understanding grows. Such updates will require evaluation by scientists who are up to date on the current literature and recommendations regarding transmission and appropriate PPE, and must be sensitive to changes in local practices. While the recently discovered variants of the SARS-COV-2 suggests higher transmission risk, we do not have experimental or observation evidence at this time that our framework should be significantly changed [ 10 ]. However, should evidence emerge that for example, cloth masks are less protective or residual survival of the virus on surfaces is greater, we would need to make changes to our protocols [ 11 ].
Of note, the most relevant existing framework we are aware of for resumption of research in the COVID-19 pandemic context is that published by Lumeng and colleagues designed for U.S. clinical research. Our framework was developed independently and for a different context, but their basic approach is similar to ours in that it provides for a high level set of principles, a tiered framework, and risk evaluation that includes factors such as duration and distance of contact between researchers and patients. Our framework adds a great detail to risk evaluation in more complex and varied environments, and outlines how these can apply to specific and diverse research tasks (See Additional file 1 : Tables 1–5). Our framework also differs from Lumeng et al’s in that we have not included an explict benefit analysis, as described in the preceding paragraphs.
This risk analysis takes place in the dynamic context of a global pandemic. We plan to reassess each activity using our tool at least monthly as more information about SARS-CoV-2 transmission and the local epidemics becomes available. While the pandemic has been disruptive to our research, we believe there may also be some benefits from the shift in some data collection methods. For example, collecting data via telephone instead of visiting in-person increases time use efficiency for staff and decreases the burden of household visits on participants. Costs are lower, with less fuel used to travel via truck or motorbike to distant participant homes. On the other hand, telephone surveys may introduce uncertainty, if questions are complex in nature and may lead to poor response rates or lower quality data [ 12 ]. We acknowledge that we have been able to resume study activities in some of our research sites, and attribute this to building relationships with participants over the past several years and the commitment of our local teams and collaborating institutions.
For researchers now facing the need to resume activities that may lead to risk of exposure to staff and participants, we offer the following advice. First, evaluate any scientific developments about risk of infection or severity of disease that might change the calculus fundamentally. Second, convene representatives of your research teams, IRB members, relevant patient groups, stakeholders, and infectious disease experts to evaluate research activities against our framework and risk schedule, and then adapt as necessary with broad input. Third, while the field, clinical and laboratory activities we presented (Tables 1-2, Additional file 1 ) may be similar in scope to other research activities, we have obviously not presented all of the potential scenarios. Research activities should be adapted to fit individual research needs, reviewed repeatedly by stakeholder groups until consensus is reached, and operationalized using Standard Operating Procedures for each activity stream.
Beyond the risk assessment tool we have outlined above, we offer the following brief description of how we deployed these rules for field teams that may have similar needs. At the beginning of the pandemic, we temporarily suspended all activities except for LPG fuel delivery until risk of the measurements could be assessed and procedures put into place to ensure safety. We continued collecting data by telephone when possible. When in-person contact was permitted by local authorities and local institutional IRBs, we used our framework to guide the appropriate protocols. If designated PPE was not available or could not be used properly at any time, we postponed the activity. Similarly, our rules required goggles or face shields for certain procedures, but participants (especially children) may find these terrifying, especially when combined with masks and gloves. In these situations, it may not be possible to complete the work as planned, and local staff had the autonomy and responsibility to decide whether any activity should proceed.
Finally, our guidance is based on expert opinion and has not been empirically verified at this time. Importantly, our framework does not substitute for the need for coordination and approval of IRBs when protocols are modified.
We are optimistic that by applying this systematic, procedure-specific approach to risk assessment for each research activity, we will minimize the disruption in our trial due to the pandemic and support the completion of our primary outcomes. Our framework can be applied to other field trials in low-resource settings to guide investigators in assessing the risk of each trial activity and implementing appropriate safety measures, where the level of risk is acceptable. While no activity in the current context is completely without risk of infection, utilizing a systematic approach is the optimal way to safeguard research activities, protect research staff and participants, and comply with the ethical obligations to those that have agreed to participate in trials, along with the communities and funders that have supported these efforts.
Availability of data and materials
Data sharing is not applicable to this article as no datasets were generated or analyzed during the current study.
Abbreviations
Severe acute respiratory syndrome coronavirus-2
Coronavirus disease 2019
Personal protective equipment
Household Air Pollution Intervention Network
Institutional Review Boards
Data and Safety Monitoring Board
Nicol GE, Piccirillo JF, Mulsant BH, Lenze EJ. Action at a distance: geriatric research during a pandemic. J Am Geriatr Soc. 2020;68(5):922–5.
Article Google Scholar
Bikson M, Hanlon CA, Woods AJ, Gillick BT, Charvet L, Lamm C, et al. Guidelines for TMS/tES clinical services and research through the COVID-19 pandemic. Brain Stimul. 2020;13(4):1124–49.
Padala PR, Jendro AM, Gauss CH, Orr LC, Dean KT, Wilson KB, et al. Participant and caregiver perspectives on clinical research during Covid-19 pandemic. J Am Geriatr Soc. 2020;68(6):E14–e8.
Coronavirus disease (COVID-2019) situation reports Geneva: World Health Organization; 2020 [Available from: http://www.who.int/emergencies/diseases/novel-coronavirus-2019/situation-reports/ . Accessed 20 Sept 2020.
Barr DB, Puttaswamy N, Jaacks LM, Steenland K, Rajkumar S, Gupton S, et al. Design and rationale of the biomarker Center of the Household air Pollution Intervention Network (HAPIN) trial. Environ Health Perspect. 2020;128(4):47010.
Article CAS Google Scholar
Clasen T, Checkley W, Peel JL, Balakrishnan K, McCracken JP, Rosa G, et al. Design and rationale of the HAPIN study: a multicountry randomized controlled trial to assess the effect of liquefied petroleum gas stove and continuous fuel distribution. Environ Health Perspect. 2020;128(4):47008.
Simkovich SM, Underhill LJ, Kirby MA, Goodman D, Crocker ME, Hossen S, et al. Design and conduct of facility-based surveillance for severe childhood pneumonia in the Household Air Pollution Intervention Network (HAPIN) trial. ERJ Open Res. 2020;6(1).
Johnson MA, Steenland K, Piedrahita R, Clark ML, Pillarisetti A, Balakrishnan K, et al. Air pollutant exposure and stove use assessment methods for the household air pollution intervention network (HAPIN) trial. Environ Health Perspect. 2020;128(4):47009.
Kigali, Rwanda: Rwanda Biomedical Centre (RBC); [Available from: https://www.rbc.gov.rw/index.php?id=188 . Accessed 1 Oct 2020
Emerging SARS-CoV-2 Variants: Centers for Disease Control and Prevention [updated January 15, 2021. Available from: http://www.gob.pe/minsa/ . Accessed 18 Jan 2021.
Leung K, Shum MH, Leung GM, Lam TT, Wu JT. Early transmissibility assessment of the N501Y mutant strains of SARS-CoV-2 in the United Kingdom, October to November 2020. Euro Surveill. 2021;26(1):2002106.
Carr ECJ, Worth A. The use of the telephone interview for research. NT Res. 2001;6(1):511–24.
Download references
Acknowledgements
A multidisciplinary, independent Data and Safety Monitoring Board (DSMB) appointed by the National Heart, Lung, and Blood Institute (NHLBI) monitors the quality of the data and protects the safety of patients enrolled in the HAPIN trial. NHLBI DSMB: Nancy R. Cook, Sc.D.; Stephen Hecht, Ph.D.; Catherine Karr, M.D., Ph.D.; Katie H. Kavounis, M.P.H.; Dong-Yun Kim, Ph.D.; Joseph Millum, Ph.D.; Lora A. Reineck, M.D., M.S.; Nalini Sathiakumar, M.D., Dr.P.H.; Paul K. Whelton, M.D.; Gail G. Weinmann, M.D.
Program Coordination: Gail Rodgers, M.D., Bill & Melinda Gates Foundation; Claudia L. Thompson, Ph.D., National Institute of Environmental Health Science (NIEHS); Mark J. Parascandola, Ph.D., M.P.H., National Cancer Institute (NCI); Danuta M. Krotoski, Ph.D., Eunice Kennedy Shriver National Institute of Child Health and Human Development (NICHD); Joshua P. Rosenthal, Ph.D., Fogarty International Center (FIC); Conception R. Nierras, Ph.D., NIH Office of Strategic Coordination Common Fund; Antonello Punturieri, M.D., Ph.D. and Barry S. Schmetter, B.S., National Heart, Lung, and Blood Institute (NHLBI).
The findings and conclusions in this report are those of the authors and do not necessarily represent the official position of the U.S. National Institutes of Health or Department of Health and Human Services.
HAPIN Investigators: Vigneswari Aravindalochanan, Kalpana Balakrishnan, Dana Boyd Barr, Vanessa Burrowes, Devan Campbell, Julia McPeek Campbell, Eduardo Canuz, Adly Castañaza, Howard Chang, William Checkley, Yunyun Chen, Marilú Chiang, Maggie L. Clark, Thomas Clasen, Rachel Craik, Mary Crocker, Victor Dávila-Román, Lisa de las Fuentes, Oscar De Léon, Anaité Diaz-Artiga, Ephrem Dusabimana, Lisa Elon, Juan Gabriel Espinoza, Irma Sayury Pineda Fuentes, Sarada Garg, Dina Goodman, Savannah Gupton, Meghan Hardison, Stella Hartinger, Steven A. Harvey, Mayari Hengstermann, Phabiola Herrera, Shakir Hossen, Penelope Howards, Lindsay Jaacks, Shirin Jabbarzadeh, Michael A. Johnson, Abigail Jones, Katherine Kearns, Miles Kirby, Jacob Kremer, Margaret Laws, Patricia M. Lenzen, Jiawen Liao, Amy Lovvorn, Fiona Majorin, Eric McCollum, John P. McCracken, Rachel M. Meyers, J. Jaime Miranda, Erick Mollinedo, Lawrence Moulton, Krishnendu Mukhopadhyay, Luke Naeher, Abidan Nambajimana, Florien Ndagijimana, Azhar Nizam, Jean de Dieu Ntivuguruzwa, Aris Papageorghiou, Jennifer Peel, Ricardo Piedrahita, Ajay Pillarisetti, Naveen Puttaswamy, Elisa Puzzolo, Ashlinn Quinn, Sarah Rajkumar, Usha Ramakrishnan, Davis Reardon, Ghislaine Rosa, Joshua Rosenthal, P. Barry Ryan, Zoe Sakas, Sankar Sambandam, Jeremy Sarnat, Suzanne Simkovich, Sheela Sinharoy, Kirk R. Smith, Kyle Steenland, Damien Swearing, Gurusamy Thangavel, Lisa M. Thompson, Ashley K. Toenjes, Lindsay Underhill, Jean Damascene Uwizeyimana, Viviane Valdes, Amit Verma, Lance Waller, Megan Warnock, Kendra Williams, Wenlu Ye, Bonnie N. Young.
The Healthcare Delivery Network at Medstar Health Research Institute supported the submission of this manuscript as Suzanne M. Simkovich transferred her affiliation.
Role of study sponsor
Program officials from all of the above listed organizations participated in regular conference calls, made recommendations about study design and participated in final decision-making on the trial study protocol for the overall HAPIN trial; program officials from the National Heart, Lung and Blood Institute, National Institute of Environmental Health Sciences, and the Bill & Melinda Gates Foundation commented on drafts of this substudy; however, no program officials had a role in the writing of this report or decision to submit it for publication. The corresponding authors share final responsibility for the decision to submit for publication.
This study is funded by the U.S. National Institutes of Health (cooperative agreement 1UM1HL134590) in collaboration with the Bill & Melinda Gates Foundation (OPP1131279). Participating NIH organizations include the National Heart, Lung and Blood Institute, National Institute of Environmental Health Sciences, National Cancer Institute, National Institute of Child Health and Human Development, Fogarty International Center, and the NIH Common Fund. Suzanne M. Simkovich was supported by funding from the National Heart, Lung and Blood Institute U., the National Heart, Lung, and Blood Institute 1F32HL143909–01, the National Heart, Lung, and Blood Institute K12HL137942. Lindsay J. Underhill and Kendra Williams were supported by Research Training Grant D43TW009340 (MPIs: Buekens, Checkley, Chi, Kondwani) funded by United States National Institutes of Health through the following Institutes and Centers: Fogarty International Center, National Institute of Neurological Disorders and Stroke, National Institute of Mental Health, National Heart, Lung, and Blood Institute and the National Institute of Environmental Health Sciences along with the National Heart, Lung, and Blood Institute 1F32HL143909–01.
Author information
Authors and affiliations.
Division of Pulmonary and Critical Care, School of Medicine, Johns Hopkins University, Baltimore, USA
Suzanne M. Simkovich, William Checkley, Lindsay J. Underhill & Kendra N. Williams
Center for Global Non-Communicable Disease Research and Training, School of Medicine, Johns Hopkins University, Baltimore, USA
Suzanne M. Simkovich, William Checkley, Steven Harvey, Lindsay J. Underhill & Kendra N. Williams
MedStar Health Research Institute, Hyattsville, USA
Suzanne M. Simkovich
Nell Hodgson Woodruff School of Nursing, Emory University, Atlanta, USA
Lisa M. Thompson
Department of Environmental and Radiological Health Sciences, Colorado State University, Fort Collins, USA
Maggie L. Clark, Jennifer L. Peel & Bonnie N. Young
Department of Environmental Health Engineering, ICMR Center for Advanced Research on Air Quality, Climate and Health, Sri Ramachandra Institute for Higher Education and Research (Deemed University), Chennai, India
Kalpana Balakrishnan
A.B. PRISMA, San Miguel, Peru
Alejandra Bussalleu
CLIMA – Latin American Center of Excellence in Climate Change and Health; and Intercultural Citizenship and Indigenous Health Unit, Faculty of Public Health and Administration, Universidad Peruana Cayetano Heredia, Lima, Peru
Gangarosa Department of Environmental Health, Rollins School of Public Health, Emory University, Atlanta, USA
Thomas Clasen & Amy Lovvorn
Cardiovascular Division, Department of Medicine, Washington University School of Medicine, St. Louis, USA
Victor G. Davila-Roman & Lisa de las Fuentes
Center for Health Studies, Universidad del Valle de Guatemala, Guatemala City, Guatemala
Anaite Diaz-Artiga
Eagle Research Center, Kigali, Rwanda
Ephrem Dusabimana
Department of International Health, Johns Hopkins Bloomberg School of Public Health, Johns Hopkins University, Baltimore, USA
Steven Harvey
Department of Global Health and Population, Harvard T.H. Chan School of Public Health, Boston, MA, USA
Miles A. Kirby
Global Program for Respiratory Sciences, Eudowood Division of Pediatric Respiratory Sciences, Department of Pediatrics, School of Medicine, Johns Hopkins University, Baltimore, USA
Eric D. McCollum
Department of Environmental Health Science, College of Public Health, University of Georgia, Athens, USA
Erick E. Mollinedo
Division of International Epidemiology and Population Studies, Fogarty International Center, National Institutes of Health, Bethesda, USA
Ashlinn Quinn & Joshua Rosenthal
Faculty of Infectious and Tropical Diseases, London School of Hygiene and Tropical Medicine, London, UK
Ghislaine Rosa
You can also search for this author in PubMed Google Scholar
- Vigneswari Aravindalochanan
- , Kalpana Balakrishnan
- , Dana Boyd Barr
- , Vanessa Burrowes
- , Devan Campbell
- , Julia Mc Peek Campbell
- , Eduardo Canuz
- , Adly Castañaza
- , Howard Chang
- , William Checkley
- , Yunyun Chen
- , Marilú Chiang
- , Maggie L. Clark
- , Thomas Clasen
- , Rachel Craik
- , Mary Crocker
- , Victor Dávila-Román
- , Lisa de las Fuentes
- , Oscar De Léon
- , Anaité Diaz-Artiga
- , Ephrem Dusabimana
- , Lisa Elon
- , Juan Gabriel Espinoza
- , Irma Sayury Pineda Fuentes
- , Sarada Garg
- , Dina Goodman
- , Savannah Gupton
- , Meghan Hardison
- , Stella Hartinger
- , Steven A. Harvey
- , Mayari Hengstermann
- , Phabiola Herrera
- , Shakir Hossen
- , Penelope Howards
- , Lindsay Jaacks
- , Shirin Jabbarzadeh
- , Michael A. Johnson
- , Abigail Jones
- , Katherine Kearns
- , Miles Kirby
- , Jacob Kremer
- , Margaret Laws
- , Patricia M. Lenzen
- , Jiawen Liao
- , Amy Lovvorn
- , Fiona Majorin
- , Eric McCollum
- , John P. McCracken
- , Rachel M. Meyers
- , J. Jaime Miranda
- , Erick Mollinedo
- , Lawrence Moulton
- , Krishnendu Mukhopadhyay
- , Luke Naeher
- , Abidan Nambajimana
- , Florien Ndagijimana
- , Azhar Nizam
- , Jean de Dieu Ntivuguruzwa
- , Aris Papageorghiou
- , Jennifer Peel
- , Ricardo Piedrahita
- , Ajay Pillarisetti
- , Naveen Puttaswamy
- , Elisa Puzzolo
- , Ashlinn Quinn
- , Sarah Rajkumar
- , Usha Ramakrishnan
- , Davis Reardon
- , Ghislaine Rosa
- , Joshua Rosenthal
- , P. Barry Ryan
- , Zoe Sakas
- , Sankar Sambandam
- , Jeremy Sarnat
- , Suzanne Simkovich
- , Sheela Sinharoy
- , Kirk R. Smith
- , Kyle Steenland
- , Damien Swearing
- , Gurusamy Thangavel
- , Lisa M. Thompson
- , Ashley K. Toenjes
- , Lindsay Underhill
- , Jean Damascene Uwizeyimana
- , Viviane Valdes
- , Amit Verma
- , Lance Waller
- , Megan Warnock
- , Kendra Williams
- & Bonnie N. Young
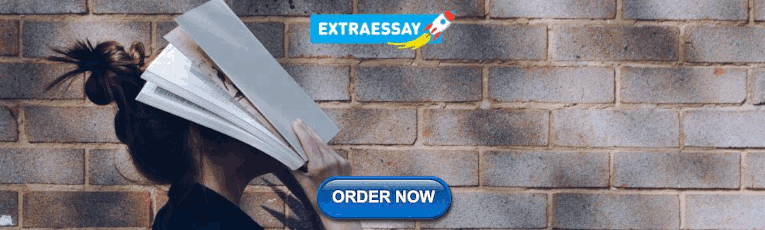
Contributions
SS & JR conceptualized design of the tool, built the tool, completed the risk assessment of each activity, provided scientific input to assess each activity and wrote the manuscript. LT and MC built the tool, provided scientific input to assess each activity and participated in the writing of the manuscript.TC, WC, AL, JP oversaw the trial’s decisions in risk and benefit and the building of the risk assessment tool and provided comments to the writing of the manuscript. KB, AB, WC, TC, VDR, ADA, LF, SH, MK, AL, EM, EM, ED, JP, AQ, GR, provided scientific input into the design of the tool, reviewed and provided input on each activity, and provided comments to the writing of the manuscript. LU, KW, BY provided scientific input into the design of the tool, completed the assessment of activities, provided scientific input on the tool and provided comments to the writing of the manuscript. All Authors have reviewed and approved the final manuscript.
Corresponding author
Correspondence to Suzanne M. Simkovich .
Ethics declarations
Ethics approval and consent to participate.
This project is a component of a larger clinical trial. The overall study protocol was reviewed and approved by institutional review boards (IRBs) or Ethics Committees at Emory University (00089799), Johns Hopkins University (00007403), Sri Ramachandra Institute of Higher Education and Research (IEC-N1/16/JUL/54/49) and the Indian Council of Medical Research – Health Ministry Screening Committee (5/8/4–30/(Env)/Indo-US/2016-NCD-I), Universidad del Valle de Guatemala (146–08-2016/11–2016) and Guatemalan Ministry of Health National Ethics Committee (11–2016), A.B. PRISMA (CE29841.17), the London School of Hygiene and Tropical Medicine (11664–5) and the Rwandan National Ethics Committee (No.357/RNEC/2018), and Washington University in St. Louis (201611159). Written consent was obtained from all participants.
Consent for publication
Not applicable.
Competing interests
No authors have competing interests.
Additional information
Publisher’s note.
Springer Nature remains neutral with regard to jurisdictional claims in published maps and institutional affiliations.
Suzanne M. Simkovich - Change in institution
Ashlinn Quinn and Joshua Rosenthal - the views expressed in this publication are those of the investigators and do not reflect official statements or policy of the National Institutes of Health.
Supplementary Information
Additional file 1., rights and permissions.
Open Access This article is licensed under a Creative Commons Attribution 4.0 International License, which permits use, sharing, adaptation, distribution and reproduction in any medium or format, as long as you give appropriate credit to the original author(s) and the source, provide a link to the Creative Commons licence, and indicate if changes were made. The images or other third party material in this article are included in the article's Creative Commons licence, unless indicated otherwise in a credit line to the material. If material is not included in the article's Creative Commons licence and your intended use is not permitted by statutory regulation or exceeds the permitted use, you will need to obtain permission directly from the copyright holder. To view a copy of this licence, visit http://creativecommons.org/licenses/by/4.0/ . The Creative Commons Public Domain Dedication waiver ( http://creativecommons.org/publicdomain/zero/1.0/ ) applies to the data made available in this article, unless otherwise stated in a credit line to the data.
Reprints and permissions
About this article
Cite this article.
Simkovich, S.M., Thompson, L.M., Clark, M.L. et al. A risk assessment tool for resumption of research activities during the COVID-19 pandemic for field trials in low resource settings. BMC Med Res Methodol 21 , 68 (2021). https://doi.org/10.1186/s12874-021-01232-x
Download citation
Received : 22 October 2020
Accepted : 17 February 2021
Published : 12 April 2021
DOI : https://doi.org/10.1186/s12874-021-01232-x
Share this article
Anyone you share the following link with will be able to read this content:
Sorry, a shareable link is not currently available for this article.
Provided by the Springer Nature SharedIt content-sharing initiative
- Risk assessment
BMC Medical Research Methodology
ISSN: 1471-2288
- General enquiries: [email protected]

An official website of the United States government
The .gov means it’s official. Federal government websites often end in .gov or .mil. Before sharing sensitive information, make sure you’re on a federal government site.
The site is secure. The https:// ensures that you are connecting to the official website and that any information you provide is encrypted and transmitted securely.
- Publications
- Account settings
Preview improvements coming to the PMC website in October 2024. Learn More or Try it out now .
- Advanced Search
- Journal List
- Int J Qual Health Care

A framework to support risk assessment in hospitals
Gulsum kubra kaya.
Engineering Design Centre, Department of Engineering, University of Cambridge, Trumpington Street, Cambridge, UK
James R Ward
P john clarkson, associated data, quality problem or issue.
A number of challenges have been identified with current risk assessment practice in hospitals, including: a lack of consultation with a sufficiently wide group of stakeholders; a lack of consistency and transparency; and insufficient risk assessment guidance. Consequently, risk assessment may not be fully effective as a means to ensure safety.
Initial assessment
We used a V system developmental model, in conjunction with mixed methods, including interviews and document analysis to identify user needs and requirements.
Choice of solution
One way to address current challenges is through providing good guidance on the fundamental aspects of risk assessment. We designed a risk assessment framework, comprising: a risk assessment model that depicts the main risk assessment steps; risk assessment explanation cards that provide prompts to help apply each step; and a risk assessment form that helps to systematize the risk assessment and document the findings.
Implementation
We conducted multiple group discussions to pilot the framework through the use of a representative scenario and used our findings for the user evaluation.
User evaluation was conducted with 10 participants through interviews and showed promising results.
Lessons learned
While the framework was recommended for use in practice, it was also proposed that it be adopted as a training tool. With its use in risk assessment, we anticipate that risk assessments would lead to more effective decisions being made and more appropriate actions being taken to minimize risks. Consequently, the quality and safety of care delivered could be improved.
Introduction
Across the world, healthcare has devoted substantial attention to ensuring safety [ 1 – 4 ]. A number of studies have been published, such as in relation to safety culture [ 4 ] and the reduction of harm [ 5 – 8 ]. Through continuing efforts to improve safety, reforms have been proposed that have been driven by safety–critical industries (e.g. nuclear and aviation), such as the implementation of risk management system [ 9 ]. So far, however, such reforms have largely prioritized the investigation of incidents over their prevention in the first place [ 3 ]. An approach which focuses on risk assessment [ 10 – 12 ] could complement this reactive practice. Risk assessment, as a part of the overarching process of risk management, aims to identify, analyse and evaluate risks that may have a negative influence on the quality and safety of the care delivered [ 11 , 13 – 16 ].
In the National Health Service in England (NHS England), hospitals assess a range of risks, including wrong medication, delayed discharge, patient claims and failure to comply with requirements. In so doing, hospitals provide risk assessment guidelines and training to support their staff—often frontline and risk management staff—and external authorities support and investigate hospitals to deliver safe care [ 15 , 17 – 19 ]. However, despite considerable efforts being made, a number of problems have been identified with current practice. For instance, patient safety-related risks can be ignored at the organizational level, and health information technology innovations can be assumed to be safe until something goes wrong [ 20 ]; risk assessment techniques are little-used, and if used, they may be used without training [ 12 , 21 ]; risk register systems can be used as bureaucratic data collection rather than to diagnose potential problems [ 19 ]; there can be a lack of consultation with a sufficiently wide group of stakeholders including patients [ 22 ]; risk assessment practice is criticized as lacking in consistency and transparency [ 17 ]; and the risk evaluation guidance provided is insufficient, which may lead to poor decisions being made [ 15 ]. Consequently, risk assessment may be underutilized when attempts are made to ensure safety.
Many of the problems stem from the foundations of risk assessment, including how to express risk, how to analyse it and how to use risk assessment as a tool to improve patient safety [ 14 ]. One way to address such problems is through providing good guidance on the fundamental aspects of risk assessment [ 10 , 23 ]. This paper, therefore, reports the design process for—and content of—a risk assessment framework (RAF). The RAF aims to guide healthcare staff on risk assessment as well as to address current challenges by learning from prescribed good risk assessment practice and the experience of healthcare staff (e.g. doctors, nurses and managers).
Study design
This study adopted a V system developmental model [ 24 , 25 ] (see Fig. Fig.1) 1 ) to design the proposed RAF through the consideration of user needs, requirements, multiple design concepts and the evaluation of the selected concept.

V system developmental model applied for the design of the RAF.
Semi-structured interviews were conducted with healthcare staff from different professions in multiple acute hospitals in NHS England to understand user needs in risk assessment (see Table Table1). 1 ). A purposive sampling strategy was used to ensure participants had sufficient experience of risk assessment. All potential participants were known to the research team, and we received permission to interview 12 individuals. The inclusion criterion was to select participants who had been involved in at least one risk assessment. Interview questions were developed based on the literature findings, with further input from the research team and were then piloted with a healthcare researcher. Participants were asked questions in relation to their understanding of risk assessment (e.g. why and how to assess risks), their practical experience in risk assessment (e.g. which methods to use and how to prioritize risks), their recommendations on how to improve risk assessment practice (e.g. participants’ views on the terminology and methods used), their views on their own organizational risk assessment guidelines, their views on good risk assessment practice (e.g. the accessibility and usability of the guidelines they use) and the challenges that they experience when undertaking risk assessment.
The characteristics of the participants in the user needs interview
T, telephone interviews; F, face-to-face interviews.
Requirements for the new RAF were elicited from the interviews, an extensive literature review and analysis of risk assessment-related documents from 100 hospitals and 35 risk assessment standards from other industries. The findings were reviewed by the authors to ensure that there were no conflicting or inconsistent requirements. From this, the authors identified 23 requirements to design the RAF (see Table Table2 2 ).
Requirements for the design of the RAF
Using the refined list of requirements, through multiple discussions the authors developed a range of design concepts, which were finally refined into a single concept. The authors then evaluated the framework through the use of a scenario as follows:
In a hospital setting, a neurorehabilitation unit will be moved from an old building to a new building, and the standards of the patient rooms will be changed. Since there is a change in the system, a risk assessment will be conducted to assess risks in the new neurorehabilitation unit before the move occurs. As a part of this, a risk assessment will be conducted to assess all risks in relation to the patient’s accommodation in a single-bed patient room.
User evaluation was conducted with 10 participants (8 were new participants) (see Table Table3). 3 ). The evaluation interviews comprised two parts. In the first part, the author (G.K.K.) explained the how the RAF could work with the risk assessment scenario given above. In the second part, each participant discussed their views on the 17 predetermined statements. A Likert scale was then used by the participants to rate their level of their agreement to each statement. The average rating was calculated by assigning a score to each Likert scale (i.e. ‘strongly agree = 5,’ ‘agree = 4, neutral = 3,’ ‘disagree = 2’ and ‘strongly disagree = 1’) in order to aid numerical analysis. In addition to the responses of the user evaluation statements, participants provided brief comments on two open-ended questions to improve the initial version of the framework: ‘what is familiar and what is new about the RAF?’ and ‘what changes would you recommend to improve the RAF?’ and were given additional space to add further comments.
The characteristics of the interview participants for the evaluation
a Participants who had also been involved in the previous interview process.
The design of the RAF was then developed iteratively to improve its usefulness and usability based on the comments given and discussions between the authors.
Risk assessment framework
Having considered all requirements, an RAF was designed by the authors, consisting of a risk assessment model, explanation cards and a risk assessment form.
The risk assessment model comprises four phases (identify, analyse, evaluate and manage), and each phase comprises four steps (see Fig. Fig.2 2 ).

Risk assessment model.
Each step is described on an A5 size double-sided explanation card (see Appendix 1 ), which provides a number of prompts. Table Table4 4 summarizes these prompts at each risk assessment step. These cards are designed to assist healthcare staff in undertaking risk assessment since they bring together the key principles of risk assessment. The risk assessment form is provided to document the risk assessment findings (see Fig. Fig.3 3 ).
A brief summary of the prompts provided for each assessment step

Risk assessment form.
In the identify phase, the system is described, potential undesired events are defined, their contributory factors are determined and their potential consequences are identified. This phase seeks to answer the question: ‘what might happen?’.
In the analyse phase, current controls are examined, and the severity of the consequence, the likelihood of occurrence and the level of risk are estimated. This step aims to address the question: ‘what is the level of risk?’.
In the evaluate phase, the estimated risk level is compared with the risk criteria (e.g. up to a risk score of 9 is generally tolerable) to decide whether or not the risk is tolerable and if there is any need to take any action. Any controls required are listed, and findings of the assessment are documented as well as shared. This phase, therefore, aims to address the question: ‘is there any need for action?’.
In the manage phase, which interacts with all the phases of risk assessment, a team is assembled, historical data are reviewed, techniques to be used in risk assessment are identified, and all activities related to these should be managed. Thus, this phase coordinates the management of all steps to conduct effective risk assessment by seeking to answer the question: ‘how to manage?’.
Evaluation of the RAF
The authors evaluated the framework by conducting a risk assessment through the use of the predetermined scenario. The authors identified 20 potential undesired events (e.g. patient falls); described a wide range of contributory factors (e.g. staff tiredness) and multiple consequences for each potential undesired event (e.g. treatment delay); examined current controls; estimated risk levels; evaluated their tolerability; listed required controls (e.g. replacement of the bed rails) and defined actions associated with the controls (e.g. responsibilities for implementation). This allowed the authors to crudely evaluate the usefulness of the framework, and, therefore, to develop the initial version of the framework iteratively. For instance, the initial version of the framework encouraged risk sources to be identified first, followed by risk scenarios. Subsequently, this was reversed, with the identification of undesired events coming first and then contributory factors, which are considered as risk sources. This was due to the fact that it was easier to identify the undesired event first. Indeed, undesired events are often known in healthcare [ 20 ] and it is more challenging to identify risk sources [ 10 ].
Each interview for the user evaluation lasted approximately 80 min. Results are shown in Table Table5 5 .
Results from the user evaluation statements (RAF = risk assessment framework)
RAF, risk assessment framework.
Regarding the open-ended questions, all participants responded to the first question. For example, I10 stated, ‘The general framework is familiar. However, it builds in a more robust and comprehensive approach to risk assessment and risk control.’ I5 highlighted the reduced jargon and technical terms and found it very useful. Similarly, I8 pointed out that ‘the methodology is presented in much more user-friendly terms than by experts such as ISO 31 000 and the Health and Safety Executive . ’ I9 did not find the RAF to be significantly different to current standards, but highlighted that the inclusion of the contributory factors was the part she found the most useful. She also added ‘I would see my primary use of the RAF as a training aid used during face to face training sessions, with staff then able to use the RAF as a post-training prompt to remind them of the steps they need to follow when carrying out a risk assessment.’ Other participants also claimed that the RAF is familiar to them in terms of its main steps (i.e. identify, analyse and evaluate), but they found the details to be different, systematic and helpful.
Seven of the participants responded to the second question. I9 provided three recommendations: to have stronger linkages between contributory factors and controls/actions, to consider estimating a target risk score as well as the actual risk score and to clarify to what extent to follow the RAF and when to do so. I8 recommended stronger links to objectives and that the framework should allow opportunities to be assessed as well as downside risks. I10 suggested there should be more explanation of what ‘system’ means. I7 suggested adding technique cards to explain a number of techniques to support risk assessment, whereas I4 claimed that adding such cards would make it too complicated. I2, I3 and I6 recommended developing specific cards for different users and use-cases (such as medical devices, and clinical and organizational risk assessments).
Seven participants provided further comments. I7 stated that the RAF closely follows their new risk management training handbook. I4 found the framework to be well presented and simple to understand and stated that it could be used as a teaching aid. I2 found it very accessible and easy to follow. I5 stated that they would like to implement it, I6 found it to be a useful tool, and I1 found the team approach necessary and found the RAF to be ‘really good’. I10 also appreciated the work by stating, ‘I think this is an excellent framework that will help many people’.
Based on the findings from the interviews, the authors further developed the initial design by providing additional guidance on the estimation of likelihood and consequence; developing a ‘abridged’ version of the explanation cards; colouring each phase with a different colour and numbering each card.
This study presented an RAF to guide healthcare staff in undertaking risk assessment. The framework was designed to be systematic and compatible with existing risk assessment practice. In essence, the framework simplifies the risk terminology used, and it brings together the principles of national and international risk assessment standards as well as a number of techniques (e.g. failure mode and effects analysis (FMEA), root cause analysis (RCA) and bow-ties). However, the framework should be tailored to the specific needs of the assessment. For example, local hospital requirements might require a slightly modified set of risk criteria or the use of a more specific contributory factors list. Additionally, we believe the framework could readily be used in different healthcare settings (e.g. primary care), in the UK and worldwide, since it provides guidance on the fundamental aspects of risk assessment.
The framework has the potential to identify a large number of risks through consideration of the system to be assessed, its parts and their interactions, and it has the potential to determine a wide range of contributory factors. In healthcare, contributory factors are often determined following an incident by the use of RCA. Yet, too often only a single cause is identified [ 26 ] despite the provision in the healthcare literature of a number of lists of multiple potential contributory factors [ 27 – 29 ]. Identifying many contributory factors not only helps to understand potential undesired events but also helps to set up effective controls in the system in order to prevent, detect or reduce the severity of the undesired events. However, it should also be noted that identifying many risks does not necessarily lead to better risk controls [ 30 ]. Even a good risk assessment does not lead to safe systems if the findings of the risk assessment are not implemented.
Furthermore, the framework suggests determining the tolerability of a risk through the consideration of multiple factors, including risk scores, organizational and regulatory requirements and the potential benefits of leaving the risk in place, and the framework urges its potential users to assemble a multidisciplinary team to undertake risk assessments. In the English NHS, organization-wide risks tend to be evaluated by individuals through the use of risk matrices in which consequence and likelihood axes are used and categorized, each with a score from 1 to 5 [ 15 ]. However, the use of risk matrices, and thus risk scores, has been criticized in the literature. To gain (or avoid) attention, lower risk scores can be artificially recategorized to a higher risk level (or vice versa), and risk scoring itself can be subjective [ 15 , 31 , 32 ] and can lead to biased judgements about the management of the risks [ 33 , 34 ]. However, taking into account multiple factors to determine the tolerability of a risk would help minimize the limitations of the use of risk matrices, and the involvement of a multidisciplinary team would help to minimize subjectivity in risk scoring.
While the RAF has been shown to offer great value in supporting effective risk assessment, there are some limitations to this study. First, the list of requirements is not intended to be exhaustive and constitutes only one approach to addressing the problems identified in this paper.
Second, the framework is built on the Safety I approach, whereas the Safety II approach might make a significant contribution to the current risk assessment practice, by considering success rather than only undesired events [ 35 , 36 ]. However, this approach has not yet been widely used and there are a number of criticisms regarding its usability and adoptability [ 37 – 39 ].
Third, the evaluation of the RAF was limited. The evaluation interviews were not designed to provide duplicating and reversing questions to establish the reliability of the responses. Additionally, participants’ views on the proposed RAF could have been biased since they evaluated the framework based on the explanations presented rather than on their experience of using it. While the authors of this paper aimed to minimize such limitations by conducting regular review meetings, a control study could be conducted to see the real impact of the framework in comparison to typical current risk assessment practice in hospitals.
Risk assessment supports decisions made in relation to potential undesired events. Despite significant efforts of healthcare professionals and organizations, there is a potential to improve current risk assessment practice in hospitals. In this paper, we designed an RAF to guide healthcare staff in risk assessment and to address existing challenges of risk assessment. This was subsequently evaluated to investigate its practical use.
The framework brings the principles of risk assessment together and learns from the experience of healthcare staff in risk assessment. It uses simplified risk terminology to minimize misconceptions and encourages: convening a multidisciplinary team, describing the system to be assessed, defining potential undesired events based on the system description, determining a wide range of contributory factors, considering all potential consequences, determining tolerability of a risks by considering multiple factors and considering control actions to minimize the potential undesired events defined.
The framework can be used as a training tool to guide in effective risk assessment as well as a tool to assess risks in healthcare settings. We believe that the framework can contribute to the quality and safety of care when it is used effectively and the assessment findings are implemented.
Supplementary Material
Supplementary data.
This work was funded by the National Institute for Health Research (NIHR) Collaboration for Leadership in Applied Health Research and Care (CLAHRC) East of England, at Cambridgeshire and Peterborough National Health Service Foundation Trust. The views expressed are those of the author(s) and not necessarily those of the NHS, the NIHR or the Department of Health and Social Care.
Risk assessment in supply chains: a state-of-the-art review of methodologies and their applications
- Original Research
- Published: 03 May 2022
- Volume 322 , pages 565–607, ( 2023 )
Cite this article
- Nishat Alam Choudhary 1 ,
- Shalabh Singh 1 ,
- Tobias Schoenherr ORCID: orcid.org/0000-0003-4958-189X 2 &
- M. Ramkumar 1
19 Citations
Explore all metrics
The year 2020 can be earmarked as the year of global supply chain disruption owing to the outbreak of the coronavirus (COVID-19). It is however not only because of the pandemic that supply chain risk assessment (SCRA) has become more critical today than it has ever been. With the number of supply chain risks having increased significantly over the last decade, particularly during the last 5 years, there has been a flurry of literature on supply chain risk management (SCRM), illustrating the need for further classification so as to guide researchers to the most promising avenues and opportunities. We therefore conduct a bibliometric and network analysis of SCRA publications to identify research areas and underlying themes, leading to the identification of three major research clusters for which we provide interpretation and guidance for future work. In doing so we focus in particular on the variety of parameters, analytical approaches, and characteristics of multi-criteria decision-making techniques for assessing supply chain risks. This offers an invaluable synthesis of the SCRA literature, providing recommendations for future research opportunities. As such, this paper is a formidable starting point for operations researchers delving into this domain, which is expected to increase significantly also due to the current pandemic.
Similar content being viewed by others
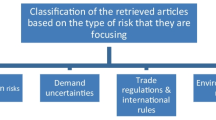
Sources of Uncertainty and Risk Quantification Methods in Supply Chain Management: A Literature Study
Supply Chain Risk Management: A Comprehensive Review
Categorizing Supply Chain Risks: Review, Integrated Typology and Future Research
Avoid common mistakes on your manuscript.
1 Introduction
In late 2019, the novel coronavirus emerged and within a period of a few months spread across the globe causing humanitarian, economic and supply chain crises. With many observers (McKinsey, 2020a; Mishra, 2020 ) considering it as a black swan event due to its unpredictability and severe impact, what is of concern is that such black swan events have been happening more frequently in the recent past. For example, in the timespan between 2000 and 2010 we witnessed several low-probability events that significantly impacted supply chains, including the 2008 recession, SARS, the Indonesian Tsunami, and the Swine Flu (McKinsey, 2020b). The occurrence of such events multiplied in the last decade, especially in the last 5 years. The zika virus, Brexit, hurricanes (e.g., Harvey, Maria and Irma), trade conflicts (e.g., between Japan and South Korea, and between the US and China), and the Australian wildfires are just some of the significant events that occurred. Researchers attribute this remarkable increase in supply chain risks and disruptions to the increasing uncertainty and complexity associated with global supply chains (Ivanov, 2020 ; Ivanov et al., 2017 ; Katsaliaki et al., 2021 ; Pournader et al., 2020 ; Sharma et al., 2021 ). As such, the business environment today is much different than that in the past (cf. Choi et al., 2016 ), and is destined to change at an even faster rate. A majority of associated potential risks can be classified as catastrophic (Wagner & Bode, 2008 ), and include natural catastrophes, political tensions, regional instabilities, and epidemics. What makes these risks particularly challenging is their interconnectedness with other supply chain risks. While the noted black swan events are unlikely but highly impactful, what cannot be neglected as part of a comprehensive supply chain risk management (SCRM) approach are risks of various types and flavours, ranging from the delivery of a wrong part from a supplier to a line shutdown—any of these has the potential to wreak havoc and affect other risks rendering careful risk assessment paramount. Along similar lines, the ‘Global Risk Report 2020’ (World Economic Forum, 2020 ), which identified 30 risks that can have a significant negative impact across countries, recognizes the inter-connectedness of risks. In an era of globalisation, this interconnectedness causes system complexities (cf. Sharma et al., 2021 ), which make the identification and assessment of supply chain risks a difficult undertaking. This is exacerbated by the fact that no disruption is likely the same, necessitating a nuanced and deliberate approach to truly understand how to manage a particular risk (DuHadway et al., 2019 ). One way to address these challenges is by considering various ways to classify, categorize, and assess risks (Sodhi et al., 2012 ).
The rapid rise and prevalence of global risks have led to a proliferation of the supply chain risk literature over the last decade, which has also resulted in various definitions of and perspectives on SCRM (Sodhi et al., 2012 ). As such, one of the ways SCRM can be approached is to determine risk sources and associated vulnerabilities, followed by their assessment and mitigation (Hermoso-Orzáez & Garzón-Moreno, 2021 ; Kleindorfer & Saad, 2009 ). Another view considers the link between SCRM and enterprise risk management (Sodhi et al., 2012 ), while yet another view takes the perspective of network structure (Kim et al., 2015 ; Wang et al., 2020). This existence of multiple perspectives is due to SCRM being multi-disciplinary in nature, and as such, this multifaceted view is needed for a thorough understanding of risk management. This however makes SCRM a complex domain that is not easily deducible, especially by scholars aiming to jump-start an SCRM research agenda. We believe that many may want to do so, given the criticality of SCRM that was brought to the fore again by the pandemic, and thus offering a synthesis of the literature, which we have done in this research, will be helpful.
The SCRM literature is broad, and no matter what type of classification is chosen, it will not be fully accurate. Based on the definition provided by Ho et al. ( 2015 ), SCRM is comprised of the stages of risk identification, risk assessment, risk mitigation, and risk recovery or resilience. Risk identification includes the recognition of events or activities that have the potential to cause a direct or indirect negative influence on supply chain performance (Ho et al., 2015 ). What needs to be noted in this vein is that the understanding of supply chain performance has widened, and expanded from a mere focus on economic parameters, like benefits and costs, to parameters capturing geopolitical, technological, social, and environmental dynamics (Chaudhuri et al., 2021 ; Ponomarov & Holcomb, 2009 ). Risk assessment refers to the analysis of risks based on stakeholder needs and the objectives of the firm. Risk mitigation then ensues, which involves the systematic deployment of resources to address the risks. Even if they cannot be mitigated, these plans may still be valuable in helping firms recover from the risk effects, making them more resilient. Resilience in the context of SCRM can be understood as an ability of a system to quickly bounce back and reach equilibrium again after a temporary disturbance (Ponomarov & Holcomb, 2009 ). This is also in line with Petit et al. (2010), who developed a conceptual framework for supply chain resilience, based on vulnerabilities and capabilities, highlighting supply chain risk assessment (SCRA) as a critical aspect of SCRM. Nevertheless, SCRA has been largely neglected in the literature compared to identification and mitigation. Further research in the area of SCRA is thus needed (Giannakis et al., 2022 ). We therefore focus on SCRA in this review, with a particular emphasis on the parameters, techniques and characteristics of decision-making methodologies.
In doing so, we build on prior literature surveys in SCRM. Specifically, while Ho et al. ( 2015 ) updated and compiled definitions of the above SCRM stages, Fahimnia et al. ( 2015 ) reviewed quantitative SCRM models. Both studies are comprehensive and serve as milestones for our discipline and foundation for our work. More recently, Xu et al. ( 2020 ) and Pournader et al. ( 2020 ) published SCRM reviews applying bibliometric and network analysis to develop literature clusters and discover underlying research themes. In addition, Katsaliaki et al. ( 2021 ) offered a content analysis of papers on supply chain disruptions and resilience, including an overview of some of the most popular modelling strategies. These papers approach SCRM from a holistic perspective and encourage a further focus on the assessment stage of the SCRM process. We respond to these calls with the present research. Such a focused review is needed, since despite the prevalence of literature reviews on risk management, it is undeniable that SCRM is a maturing topic. What is therefore needed are finer-grained reviews to capture the entirety of the field, highlighting SCRM angles that have not been covered extensively. We therefore focus on the assessment stage, which is a largely overlooked aspect within SCRM. We believe that such a more focused review enables a deeper classification of the literature that will subsequently help the field advance even more.
Our review was guided by the framework displayed in Fig. 1 . Specifically, in a first stage, a review of prior relevant literature reviews aided us in the identification of prominent authors and SCRA literature streams, as well as the pinpointing of missing aspects in need of further investigation. For example, Ho et al. ( 2015 ) focused on the application of SCRA in different contexts, without however capturing the various decision-making techniques. Similarly, Fahimnia et al. ( 2015 ) concentrated on broader aspects surrounding quantitative SCRM models, and also Xu et al. ( 2020 ) did not cover the intricacies of decision-making techniques. These are however critical, especially in risk assessment, to help decision makers focus on the most relevant risks (see Table 1 for a comparative analysis). In this vein, we complement the review of Katsaliaki et al. ( 2021 ) by our focus on modelling approaches and the provision of citation analyses. As such, we for instance uncover that the chosen decision-making technique must also be aligned with the context (which was not always done)–an issue we identified as an area in need of further investigation in the planning stage of our research. This led us to the development of the following three research questions that guided our study:
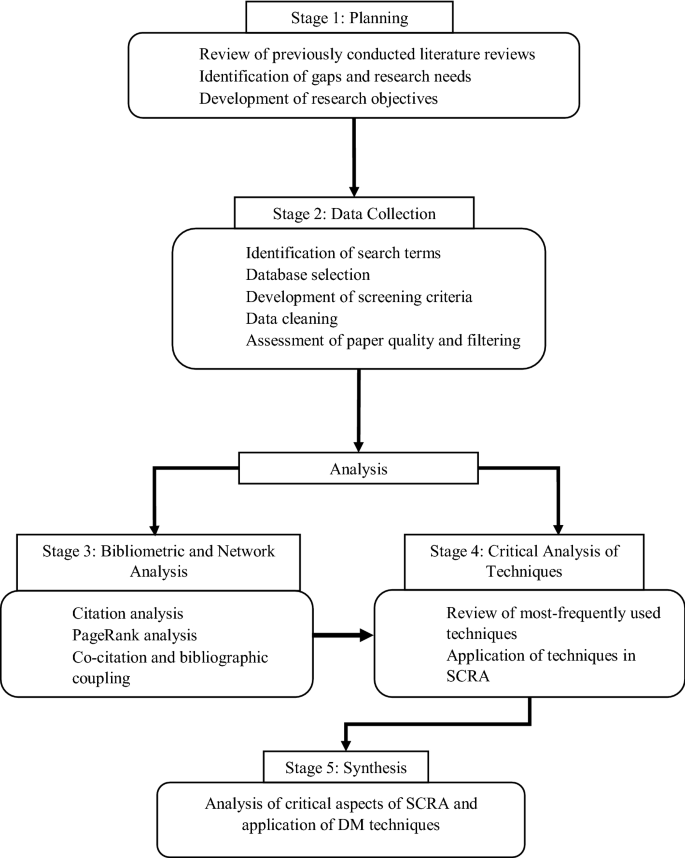
Review framework
What are the major themes in SCRA?
What are the key parameters on which supply chain risks are assessed?
What are the major decision-making techniques applied, and what are their major characteristics?
To answer these research questions developed in the first stage of our review, we compiled in the second stage our repository of relevant papers on SCRA. The third stage focused on the bibliometric and visual network analysis of the papers, followed by a critical analysis of the multi-criteria decision-making (MCDM) techniques in stage four. The fifth stage then served as a synthesis of these findings, assimilating a holistic understanding of SCRA and its applied decision-making techniques. Instrumental in this step was in particular the visual network analysis, which is a form of exploratory data analysis (Venturini et al., 2019 ) that led to the generation of network clusters. Since the foundation of the network is based on citations, studies deploying similar decision-making techniques are likely grouped together as they either cite each other or are foundational studies for the specific technique. Exploring these topics by cluster allows us to better understand the application scope of the decision-making techniques, offering an invaluable foundation for operations researchers to further advance the domain of SCRA. Within this context, our contributions are three-fold. First, we enhance the overall SCRM literature by concentrating on its early stage of risk assessment . Second, we identify common parameters applied in SCRA decision-making. And third, we critically review the decision-making tools and techniques and map them based on the SCRA characteristics.
The remainder of the paper is structured as follows. Section 2 describes the methodology, with Sects. 3 and 4 presenting the bibliometric and network analyses, respectively. Section 5 provides a review of the parameters on which the risks are assessed, Sect. 6 offers an overview of the predominant SCRA techniques, and Sect. 7 of some of the most common SCRA characteristics. Section 8 provides suggestions for a future research agenda, and Sect. 9 concludes.
2 Research methodology
Literature reviews synthesize evidence available in a specific domain, provide the foundation for a cohesive understanding and can serve as a starting point for scholars to focus on the most pressing issues. As such, a synthesis of the literature helps us find out what we know and what we do not, as well as what works and what does not (Booth et al., 2016 ). Methods applied for carrying out literature syntheses include critical reviews, citation analyses, content analyses, integrative reviews, meta-analyses, mixed-methods reviews, state-of-the-art reviews, and systematic literature reviews (Booth et al., 2016 ). Systematic literature reviews differ from traditional narrative reviews in that they employ a reproducible, scientific, and transparent process that minimizes selection bias while carrying out the literature search (Petticrew & Roberts, 2008 ). Some of the review techniques applied in the SCRM literature are captured in Table 1 .
In our structured literature review, we followed a three-step iterative process:
Identification of appropriate Search Terms and Timeframe
It was rather difficult to identify focused SCRA literature using only keyword searches since many SCRA papers do not include “risk assessment” as a specific keyword. As such, we sought guidance from previous review papers listed in Table 1 and commenced with the following first set of keywords: (1) “supply chain risk”; (2) “supply chain” and “risk”; and (3) “supply risk”. While we also used a few additional keywords like “uncertainty”, “uncertain”, and “disruption”, since they are closely associated with the risk management domain, their inclusion did not yield any further results. We focused on the literature since 2010 to ensure recency and applicability of the derived conclusions.
(II) Search Process
We searched for the selected keywords in the title, topic and the keywords of articles found in Google Scholar, EBSCO, PROQUEST, JSTOR, Wiley, Emerald, INFORMS, Taylor and Francis, and Elsevier. This yielded a total of 1357 articles.
(III) Screening and Finalizing
To identify the set of papers that focuses on SCRA, we further narrowed down the results (using Microsoft Excel) by ensuring the title contained one or more of the following terms: (1) “assess”, (2) “identify”, or (3) “analys*”. This resulted in a total of 216 articles.
We then scrutinized these articles by reading the abstracts to ensure that the article is indeed about SCRA, while at the same time ensuring that the journals in which the articles are published are part of the Australian Business Dean Council (ABDC) or the Association of Business Schools (ABS) (here, we focused on journals with a rank of 2 or greater) lists. This kind of filtering criterion allows researchers to narrow down the scope without compromising on the quality of the studies. For instance, in their review of the analytic hierarchy process (AHP), Ho and Ma ( 2018 ) included only A/A*-ranked journals from the ABDC list and only 3/4/4*-ranked journals from the ABS journal lists. This selection approach yielded a set of 161 articles in our sample. We further cross-checked these identified papers in the Web of Science database, which is considered a reliable source of high-quality journals (Govindan et al., 2015 ). Removing papers not listed in this database reduced the total number of articles to 136. We now continue with bibliometric and network analyses, applying both inductive and deductive approaches (Fahimnia et al., 2015 ; Seuring & Müller, 2008 ).
3 Bibliometric analysis
We commence our categorization and review with a bibliometric analysis, which has as its objective the assessment of the research’s impact. This has been a common approach in literature reviews (Pournader et al., 2020 ; Xu et al., 2020 ) to identify emerging areas. Citation counts, publication trends, authors’ influence, and frequency of publications in top journals are frequently-used variables.
The distribution of SCRA publications across years is shown in Fig. 2 . As can be seen, the number of publications increased steadily until 2016. A short decline between 2014 and 2018 is followed by a rise in publications again in 2019. With our search concluding in September 2020, the already high number of publications on the topic indicates increasing interest. Our review set contains 58 journals, with the top four journals capturing around 33% of the literature. These four journals are the International Journal of Production Research , the International of Production Economics , Journal of Cleaner Production , and the European Journal of Operational Research . Table 2 presents the publication frequencies across the 15 journals with the most papers in our sample.
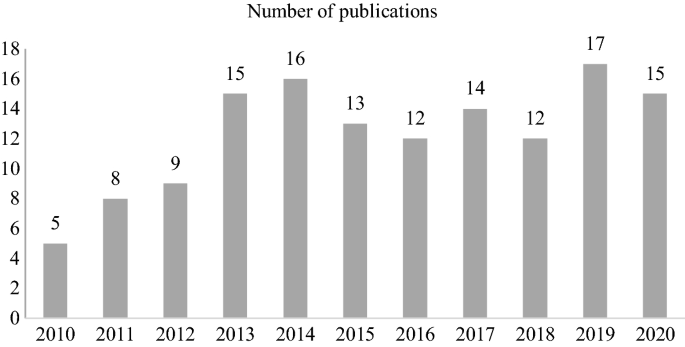
Publication trend
Table 3 offers some further insight by providing an overview of the literature based on the context in which SCRA was investigated. Some of the articles where the context was ambiguous or difficult to identify were excluded. As can be seen, the context involving a typical supplier–manufacturer–retailer environment is the most commonly investigated setting. Most of these works appeared in Computers and Industrial Engineering , the European Journal of Operational Research , the International Journal of Production Economics , and the International Journal of Production Research . What also stood out was that the context of sustainable supply chains. The dominant journal for this theme was the Journal of Cleaner Production .
4 Network analysis
Network analysis aims to capture social network dynamics and relationships. Actors in the network are represented by nodes and their relationships as lines. The software package ‘Gephi 0.9.2’ was used to conduct the network analysis and its graphical visualization. The package uses a 3D render engine to display large real-time networks and presents dynamic visualizations (Bastian et al., 2009 ). Since Gephi requires graph form datasets in the ‘.NET’ format, we relied on ‘BibExcel’ to convert the literature data from the ‘.txt’ format (extracted from Web of Science) to the required ‘.NET’ format. This data contained information like authors, cited references, and keywords. Utilizing datasets from Web of Science is standard practice for network analysis (Fahimnia et al., 2015 ; Pournader et al., 2020 ; Xu et al., 2020 ). In addition, we utilize ‘VOSviewer’ to visualize the networks.
4.1 Citation analysis
Citation analysis is one of many ways for measuring the significance of a publication. By capturing the frequency with which a publication is cited by other publications, a measure of ‘popularity’ can be generated (Ding & Cronin, 2011 ). Out of the 136 articles identified, a total of 107 articles are connected to each other through ‘undirected’ citations, which means that they are either cited by, or have been cited, by the connected article. Figure 3 captures the derived citation network with the nodes representing the papers. The size of the node indicates the citation strength, while the lines connected to other publications indicate the undirected citations; Table 4 lists the most highly cited papers in the network. Papers in the network are evaluated based on their local citation score, which indicates how many times an article has been cited by other papers in the network. In contrast, the global citation scores indicate how many times the papers are cited in the Web of Science database.

Citation analysis network
4.2 PageRank analysis
Brin and Page ( 1998 ) developed a measure to capture the equivalence of a webpage’s ‘importance’ through the capturing of its citations. As such, while high citations may imply popularity, this measure does not provide any indication about the paper’s importance. Such assessment can however be done via the PageRank measure, which is a standard practice in literature reviews (Fahimnia et al., 2015 ; Pournader et al., 2020 ; Xu et al., 2020 ). The PageRank measure can be computed as follows:
where A is the paper under consideration, cited by papers T 1 , T 2 ,…,T n that have citations C(T 1 ), C(T 2 ),…,C(T n ). The parameter d is a damping factor between 0 and 1, representing the fraction of random walks that continue to propagate along the citations (Brin & Page, 1998 ). Brin and Page ( 1998 ) used d = 0.85, assuming an individual surfer would randomly follow about six hyperlinks giving a leakage probability of 1/6 = 1 − d (for d = 0.85) before beginning a new search. For citation networks, d = 0.5 is considered appropriate, assuming an average path length of 2 (Fahimnia et al., 2015 ; Pournader et al., 2020 ). The PageRank forms a probability distribution, thereby producing a score between 0 and 1. For a detailed overview of this metric, the interested reader is referred to Brin and Page ( 1998 ).
Our PageRank scores, which are obtained using Gephi, range between 0.004209 and 0.011594. Table 5 displays the top 10 papers based on their PageRank score. When comparing Tables 4 and 5 it becomes evident that citations alone cannot measure the importance of a paper, as there is no overlap in publications between the two tables. What may also explain this observation is that citations require time to accumulate, as indicated by the consistent earlier publication years of the papers presented in Table 4 .
4.3 Co-citation analysis and bibliographic coupling
While citation analysis can identify the growth of an article’s popularity over time (Pilkington & Meredith, 2009 ), it has also limitations. An alternative is co-citation analysis, which is a type of network analysis that captures the network’s groups, their relationship, and their evolution over time (Pilkington & Meredith, 2009 ). Co-citation analysis is widely used in bibliometric literature analysis within the field of operations and supply chain management (Ben-Daya et al., 2019 ; Fahimnia et al., 2015 ; Pournader et al., 2020 ; Xu et al., 2020 ). With co-citation analysis, any two references can be identified that are commonly cited together by another publication (Pilkington & Meredith, 2009 ). A total of 49 out of the 5504 references in our paper set were co-cited more than 10 times by the publications in our sample. The low number is not surprising, since co-citation analysis, although useful, primarily focuses on influential literature beyond the selected sample. In addition, the approach disadvantages more recent publications, which is why we complemented this analysis with bibliographic coupling (Boyack & Klavans, 2010 ). Bibliographic coupling considers the relatedness of two publications based on the number of references they share. A bibliographic coupling of our sample using Gephi identifies 123 nodes (publications) and 3180 edges (citations). Gephi, by default, randomly generates positions for the nodes in a cartesian coordinate system, with the nodes being of identical sizes. In doing so, we apply the Force Atlas algorithm, which is based on attraction and repulsive strength of the linked and non-linked nodes respectively (Bastian et al., 2009 ). The resulting visualization is shown in Fig. 4 . As can be seen, two outliers were identified—Bouwknegt et al. ( 2015 ) and Ortúzar et al. ( 2020 ). Outliers are weakly connected nodes, suggesting dissimilar characteristics from the densely connected nodes, thereby not aiding in cluster identification. After removing these outliers, the Force Atlas algorithm was applied to generate the visualization in Fig. 5 .
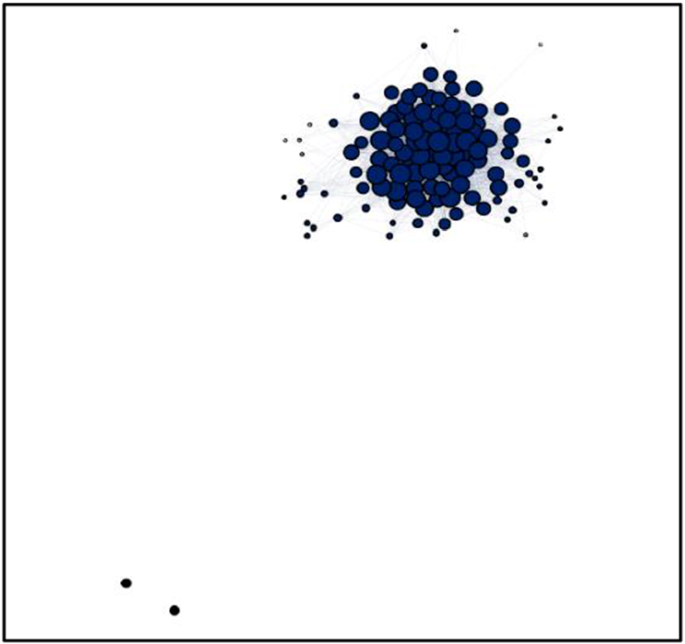
Force algorithm visualization with outliers (136 nodes)
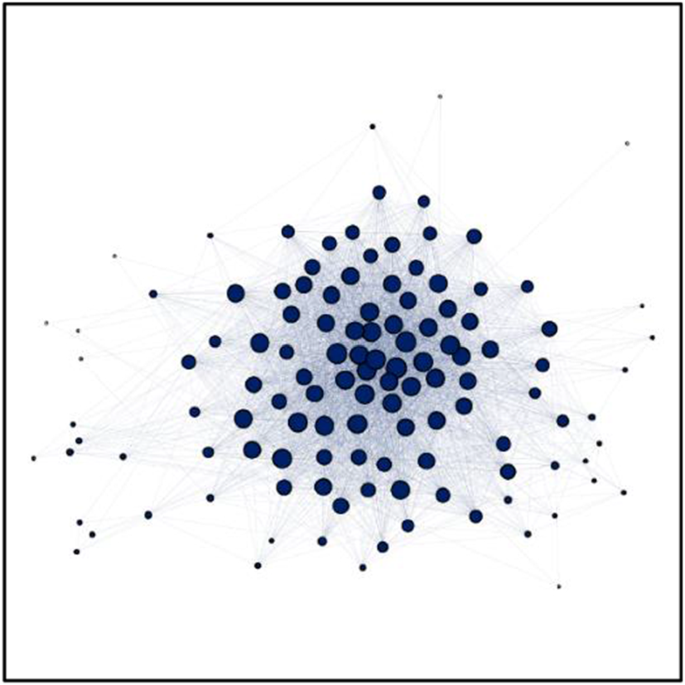
Force algorithm visualization without outliers
4.4 Literature classification
We further classified the literature via data clustering using the modularity function based on Blondel et al. ( 2008 ) in Gephi (cf. Fahimnia et al., 2015 ), which yielded a total of six clusters (Fig. 6 ). The bibliographic network’s modularity index of our sample is 0.105 (modularity index values range between − 1 and + 1), indicating a moderate connection between the nodes. Three clusters (clusters 1–3) comprise 77% of our literature sample, which is why we focus in our discussion and interpretation on these three clusters. Papers in clusters 4–6 do not reveal any identifiable theme, but rather have characteristics similar to either clusters 1, 2 or 3. Therefore, the papers of clusters 4–6 are discussed within the scope of clusters 1–3. Apart from these, there are 15 papers which did not conform to any specific theme. Figure 7 shows a different visualization of the cluster analysis using VOSviewer applying the lin-log modularity algorithm, with the top 20 publications based on PageRank scores of the three clusters listed in Table 6 . We now proceed with a descriptive overview of the clusters.
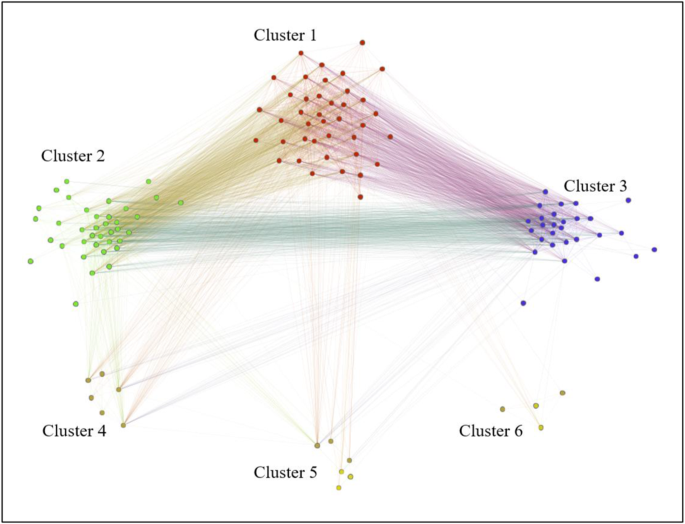
Literature visualization
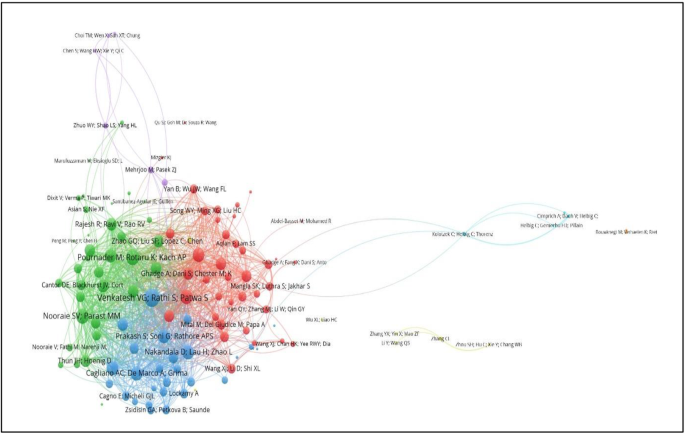
Literature visualization using VOSviewer
4.4.1 Cluster 1: Sustainability and related risks
Cluster 1 has an average PageRank score of 0.0090 and contains 40 publications. The use of MCDM techniques stands out in this cluster: out of the top 20 publications listed in Table 6 , 16 use MCDM techniques to assess supply chain risks. The techniques are either in the form of a combination of or integration with multiple MCDM techniques. Contextually, the cluster contains publications related to sustainability (Mangla et al., 2016 , 2018 ; Rostamzadeh et al., 2018 ; Wu et al., 2017 , 2019 ; Yan et al., 2020 ; Zimmer et al., 2017 ), most of them having appeared in the Journal of Cleaner Production . With sustainable SCRM being in an early phase, conceptual frameworks with strong methodological foundations are lacking, positioning this as an intriguing area to investigate further. Supply chains moving agricultural products and food also represent a large part of this cluster (Mohib & Deif, 2020 ; Wang, Li, et al., 2012 ; Yan et al., 2019 ; Zhao et al., 2020 ). This carries promise, since food crises rank high in ‘The Global Risk Report 2020’ (World Economic Forum, 2020 ) on both impact and likelihood of occurrence. Risks inherent to food supply chains therefore represent a promising research domain going forward.
Further themes of this cluster are approaches to reduce industry-specific risks. In addition, uncertainty plays a large role in this cluster, and hence the use of fuzzy logic is predominant. What is further striking is that most of the literature in this group considers risk hierarchies, which are however not reflective of a holistic approach to SCRM. A further pattern was the use of the two dimensions of impact and likelihood of occurrence, and the empirical model testing involving single case studies.
4.4.2 Cluster 2: Risk assessment at the network level
The average PageRank score of cluster 2 is 0.0075, which is lower than the scores of clusters 1 and 3. Cluster 2 contains 37 articles, with relatively few papers including MCDM techniques. Only eight publications in the top 20 articles of cluster 2 deploy some form of MCDM technique (Atwater et al., 2014 ; Garvey et al., 2015 ; Kwak et al., 2018 ; Nooraie et al., 2020 ; Pournader et al., 2016 ; Rajesh & Ravi, 2015 ; Rajesh et al., 2015 ; Talluri et al., 2013 ). Publications in this cluster are characterized by risk assessments at the network level, rather than tier-level risks. As such, supply chains are studied on the general, rather than on an industry-specific or category level (Nooraie & Parast, 2015 ; Pournader et al., 2016 ; Sherwin et al., 2016 ). The few industry-specific studies included are within the automobile and electronics supply chains. What is also striking is that low probability disruptive risks, like natural disasters, form a dominant theme in this group (Cantor et al., 2014 ; Dixit et al., 2020 ; Simchi-Levi et al., 2015 ). Various approaches to deal with multiple aspects of supply chain risk are used, with methods applied including vignette-based field experiments (Cantor et al., 2014 ), genetic algorithms (Nooraie et al., 2020 ), and Branch and Reduce algorithms (Huang & Goetschalckx, 2014 ). Further approaches include simulation and predictive modelling considering different scenarios and multiple objectives. Another feature of this group is that there are very few articles that assess risks based on impact and likelihood, contrary to the other clusters. However, this cluster acknowledges to a greater degree the effect of risk propagation in the network (Dixit et al., 2020 ; Garvey et al., 2015 ; Rajesh et al., 2015 ). A further theme is risk assessment via supply chain network optimization. An interesting observation was also that a large proportion of this cluster’s work was published in 2017 and prior.
4.4.3 Cluster 3: Assessment of operational risks
The average PageRank score of cluster 3 is 0.0093. The 28 articles in this cluster contain both MCDM techniques and more empirically-focused qualitative analyses of supply chain risks. Supply chain risks are studied in general as well as with a specific focus. Dominant industries in this cluster are the food, fashion and automobile industries (Diabat et al., 2012 ; Lockamy, 2014 ; Nakandala et al., 2017 ; Thun et al., 2011 ). With most of the articles in this group having been published between 2010 and 2015, a further theme within this group is the application of cause-and-effect analysis to risks. Interpretive structural modelling (ISM) is commonly used (Diabat et al., 2012 ; Pfohl et al., 2011 ; Prakash et al., 2017 ; Venkatesh et al., 2015 ), as are empirical analyses and matrix-based assessment tools. We also observe a diverse set of SCRA factors, including not only impact and likelihood, but also safety, dependence, quality and efficiency parameters on which risks are assessed. A further characteristic of this cluster was that articles studied risks associated with multiple modes of transportation (Vilko & Hallikas, 2012 ; Zhang et al., 2012 ), and a major theme included the assessment of risks found in product-based supply chains. Thus, in most cases, risks were related to demand, processes, quality, logistics, new product development and procurement. These risks are not new, and hence the assessment of such risks was primarily done in the earlier years in our review timeframe.
5 SCRA parameters
We now delve deeper into the various parameters on which risks are assessed. Risks come in various shapes and forms, and thus need to be assessed for instance based on their origin (e.g., whether they are supply- or demand-based) or their type (e.g., environmental, social, political). Clearly, there is no one-size-fits-all approach that would allow assessment to be conducted in a standard, optimal way due to the various dynamics and influences at play. For example, risks related to the food supply chain are different to those of the automotive supply chain, while risks in product-based industries are dissimilar to those in service-oriented industries. Based on our analysis on the prevalence of certain parameters in our sample paper set, we integrated common approaches into a framework presented in Fig. 8 and elaborate on each of them in the following. While risk probability and severity are key parameters, there are a multitude of other parameters that have been explored.
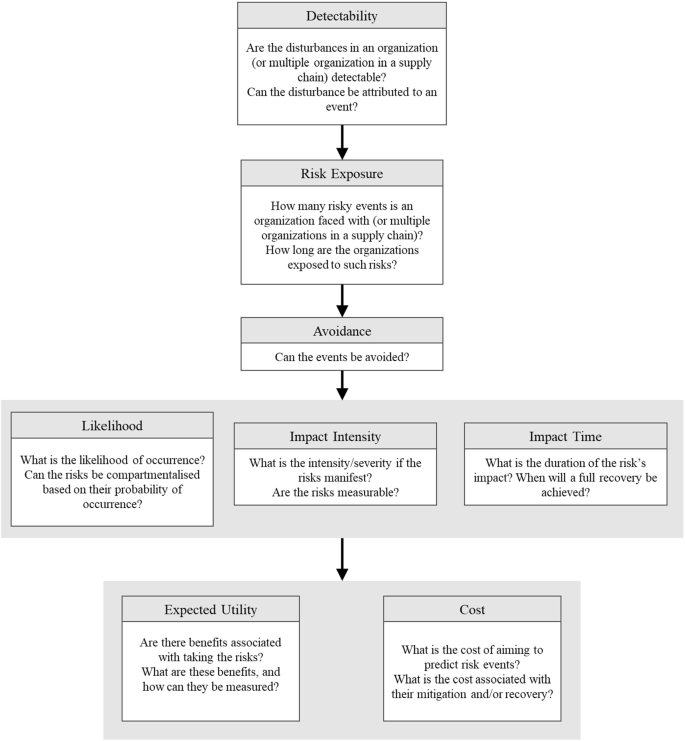
SCRA decision-making parameters
One of these is the detectability of the risks. Wang, Li, et al. ( 2012 ) and Kumar et al. ( 2013 ) considered detectability as an important parameter when organizations consider business continuity as a strategy along with efficiency. Early detection of risks fosters faster reaction, and better preparedness from severe consequences. Another parameter is risk exposure, which captures how many risk events or potential sources of risks the focal organization or the supply chain is being exposed to, and to what extent. For example, Tabrizi and Razmi ( 2013 ) developed a mathematical model managing potential sources of risks the supply chain network is exposed to using fuzzy logic. Another parameter is a firm’s strategy to avoid the effect of risks when they are exposed to them. For instance, Thun and Hoenig ( 2011 ) empirically analysed SCRM in the German automobile industry and categorized it into reactive and preventive approaches. This categorization stems from the fact that certain risks are avoidable while some are not. Analysing the extent to which risks can be avoided should help in resource allocation and efficiency. The above three parameters—detectability, risk exposure and avoidance—streamline the approach of risk assessment and further quantification of the risks in terms of their likelihood and impact, aiding in the capture of underlying complexities. Another parameter that offers value is the duration of the risk’s impact as demonstrated by Simchi-Levi et al. ( 2015 ) and their engagement with Ford Motor Company. A final dimension of risk assessment identified in our review is the expected utility, which is usually measured in terms of costs and benefits (Mehrjoo & Pasek, 2016 ; Pournader et al., 2016 ; Xingli & Liao, 2020 ).
5.1 Detectability
Detectability, which refers to the likelihood with which risks can be detected before they manifest, should not be confused with likelihood of occurrence, which indicates how probably a risk is to manifest (Giannakis & Papadopoulos, 2016 ). It is frequently measured on a ratio scale and is an integral component of the Failure Mode and Effect Analysis (FMEA; Ghadge et al., 2017 ; Kara et al., 2020 ).
5.2 Risk exposure
Risk exposure is often a combination of several risk-related indicators, reflecting an assessment of multiple entities at one point in time or the assessment of a single entity over a span of time. This assessment can then be captured in risk exposure indices (Kara et al., 2020 ; Ojha et al., 2018 ; Roehrich et al., 2014 ). The use of such indices is practical as multiple factors can be incorporated, alleviating decision-makers to assess risks on a continuous basis.
5.3 Avoidance
SCRA models have either a preventive or a reactive orientation (Thun & Hoenig, 2011 ). For the former, a crucial factor is how easily risks can be avoided. For instance, risks related to demand and supply can be avoided by developing capabilities, such as information sharing or the strategic selection of suppliers, locations, or customers. Risk avoidance as a strategy is however not always a viable option (Viswanadham & Samvedi, 2013 ), which is where reactive approaches come into play that aim to reduce a risk’s impact while it is manifesting. Both serve as important elements in the assessment of risks, and the degree to which risks can be avoided can be considered a means to capture the resulting level of risk exposure.
5.4 Impact intensity
Impact intensity captures the ‘severity’ associated with the risk and is the most common factor on which risks are assessed. However, the measurability of impact lacks a common standard, with most studies relying on experts’ judgement to evaluate and understand risks (Ma & Wong, 2018 ; Samvedi et al., 2013 ); in many of these instances, risk is rated on Likert scales anchored at negligible/no-impact and catastrophic/crisis. Another practice of identifying impact or severity is to categorize levels of severity as done by Tummala and Schoenherr ( 2011 ). In their illustrative assessment, severity is negligible if the service level is not affected due to sufficient safety stock, severity is marginal if service levels decrease with depleting stocks, severity is critical if the plant is shut down for a week, and severity is catastrophic if the plant is shut down for a month. Such pre-determined thresholds can serve as guidance to trigger certain actions once risks manifest.
5.5 Impact time
Supply chain risks can also be assessed based on the duration of a risk’s impact, which cannot only include the actual time during which the risk manifests, but also include the recovery time. This is indeed a critical dimension, since supply delays tend to propagate through the supply chain, resulting organizations to lose significant market share long after the risk event has ended. Even for low-probability, high-impact events, the time-to-recovery and time-to-service are valuable parameters to assess risks (Simchi-Levi et al., 2014 ).
5.6 Likelihood of occurrence
Likelihood of occurrence is an important factor in SCRA since it assesses the probability of the risk manifesting. Likelihood of occurrence is often measured on a Likert scale using expert judgments (Aqlan & Lam, 2015 ; Tazelaar & Snijders, 2013 ). The probability can also be obtained through simulation (Qazi et al., 2018 ).
Cost plays an important role in SCRA, since risks manifesting themselves generally result in higher costs, with risk avoidance and prevention mechanisms also being associated with costs (Nooraie & Parast, 2016 ). Decision-makers are responsible to find the right balance between the cost associated with a disruption versus the costs incurred for mechanisms aimed at preventing or mitigating such a disruption. Cost (or the avoidance of costs by preventing a risk from happening) can be used as a motivation to analyse risks and work towards their prevention (Brun et al., 2006 ). As a measure of efficiency, cost is an important factor and is widely used in supplier selection studies (Viswanadham & Samvedi, 2013 ), both from the perspective of manufacturing (Xu et al., 2013 ) and procurement (Zhuo et al., 2018 ).
5.8 Expected utility
Utility theory motivates this factor, with the expected utility capturing the value obtained conditional on the risk’s influence. While the risk level may be high, taking these risks may be associated with a potential higher return (as the saying goes—high risks, high rewards—which however also entails potential risk of failure). For example, investing in an innovative but uncertain technology may be risky, but if the venture is successful, it may yield significant returns. Decision-makers’ behaviours play a significant role within this context (Qazi et al., 2018 ; Xu et al., 2013 ; Yan et al., 2019 ).
6 SCRA techniques
We now proceed with a review of the various SCRA techniques covered in our literature sample. Our analysis captured more than 100 techniques to assess supply chain risks, with a majority of them being quantitative. Table 7 presents some of the most frequently applied techniques in our sample, Fig. 9 captures them across the years, indicating a general increasing trend of MCDM techniques, and Table 8 presents their distribution across the eight journals with the highest frequencies. Some of the most commonly applied techniques are further assessed in the remainder of this section.
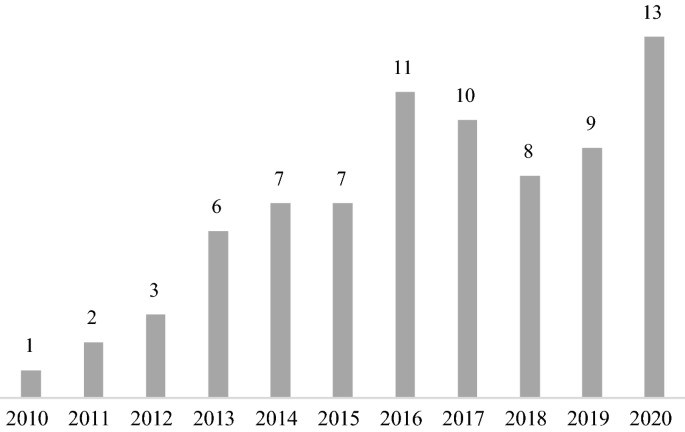
Trend of MCDM Techniques in the SCRA Literature
6.1 Critical analysis of commonly-used techniques
6.1.1 fuzzy sets.
Lofti A. Zadeh conceptualized fuzzy sets in 1965 (Zadeh, 1988 ), with the notion that fuzzy logic is a matter of degree that can describe everything. It is a multi-valued logic that deals with imprecise reasoning. This thus seems to be a fitting application for assessing the impact or severity of supply chain risks due to the inherent subjectivity and unpredictability of the associated values. As Ma and Wong ( 2018 ) suggested, determining a precise value for these dimensions is both infeasible and impractical. The application of fuzzy sets has thus received significant attention by researchers when quantifying and assessing risks. In fact, risk is not the only parameter that is commonly captured by a fuzzy set. Some additional settings in which fuzzy sets were applied include performance (Rostamzadeh et al., 2018 ), criteria weight determination (Samvedi et al., 2013 ), the influence on sustainability (Wu et al., 2017 ), and risky events’ likelihood of occurrence (Nakandala et al., 2017 ).
There are different ways to develop a fuzzy set. Normally, a three-set number is denoted as a fuzzy set. To illustrate, Pournader et al. ( 2016 ) developed a triangular fuzzy numbered set to identify the influence of risks as follows: “Extremely low” (1, 1, 3), “Low” (1, 3, 5), “Fair” (3, 5, 7), “High” (5, 7, 9), and “Extremely high” (7, 9, 9). A four-number fuzzy set representing a trapezoidal fuzzy number is also commonly used. These fuzzy values are then de-fuzzified to obtain a single value. To develop a better understanding of fuzzy set theory’s applications, we refer the interested reader to Zimmermann ( 1985 ). Although fuzzy sets are helpful in quantifying uncertain, imprecise variables, they alone are not sufficient to assess risks. In the SCRA literature, fuzzy set theory is therefore used in combination with other methods.
6.1.2 Analytic hierarchy process and its extensions
One of the most commonly MCDM techniques is the AHP. Saaty ( 2004 ) developed the AHP that consists of three main steps, i.e., hierarchy construction, priority analysis, and consistency verification. The hierarchy captures the goal, the criteria, and the alternatives. Once the hierarchy is constructed, the alternatives are compared against each other based on the criteria, with the weights then being developed through pairwise comparisons. The criteria are also compared against each other, and weights are developed in a similar fashion. A consistency ratio is calculated based on the pair-wise comparison, which provides a validity check for the results obtained. Illustrative studies include Viswanadham and Samvedi ( 2013 ) and Wang, Chan, et al. ( 2012 ), who used performance attributes as criteria to identify risky suppliers, and Schaefer et al. ( 2019 ), who developed a ranking of water risks based on AHP. It is interesting to note that AHP has received various extensions in the SCRA literature. Consider for instance fuzzy AHP, Monte Carlo AHP, DEA-AHP, Grey-AHP, and Delphi AHP (Duleba et al., 2021 ; Mital et al., 2018 ; Rathore et al., 2017 ; Salehi Heidari et al., 2018 ; Samvedi et al., 2013 ; Viswanadham & Samvedi, 2013 ; Wang, Li, et al., 2012 ; Zimmer et al., 2017 ). The interested reader aiming to find out more about the various integrated forms of AHP and its applications is referred to Ho ( 2008 ) and Ho and Ma ( 2018 ).
While AHP is a powerful decision-support tool, its limitations must be realized. To obtain realistic outcomes, an imprecise real-life understanding must first be transformed into a precise mathematical one—as such, it represents an approximation of reality. This requires expertise in understanding the concerned subject, which in our case is supply chain risk. Also, the criteria and alternatives along with their respective weights must be consistent to yield acceptable results. Another limitation is the non-consideration of feedback or interdependency between criteria and alternatives. If these limitations are however kept in mind, then AHP offers a formidable approach and process to facilitate decision-making.
6.1.3 Analytic Network Process
The Analytic Network Process (ANP), also attributed to Saaty ( 2004 ), is an adaptation of the general AHP structure and considers feedback or dependency of the alternatives and criteria, the absence of which we noted as a major limitation of AHP. As the choice of alternatives is determined by the presence of criteria, the alternatives also affect the decision-makers criteria evaluation. The SCRA literature using MCDM techniques considers risks as criteria as well as alternatives. In the former, alternatives are the solutions for different risk criteria, whereas in the latter, risks are evaluated based on different parameters as criteria. In both cases there is a dependency between alternatives and criteria. ANP is thus well suited for SCRA. For example, Chand et al. ( 2017 ) considered four risk types as criteria and four supply chain types as solutions, capturing green supply chains, agile supply chains, lean supply chains and reverse supply chains. The risks are dependent on the supply chain type an organization is adopting, a scenario that can effectively leverage ANP to assess the risks. Similarly, Martino et al. ( 2017 ) developed risk ranks in the retail fashion industry based on multiple supply chain objectives. Although the use of ANP solves the feedback or dependency of criteria and alternatives, risks in supply chains form complex networks where risks percolate from one level to another. ANP does not consider such flow or cause-and-effect analysis of risks, illustrating that all approaches have some drawbacks that need to be considered.
6.1.4 Bayesian Networks
A Bayesian model is an acyclic graphical depiction of uncertain events (Heckerman et al., 1995 ). Specifically, in a Bayesian network, an event is represented by a child node that is triggered by another event representing a parent node. All nodes have associated probabilities, with the Bayes Rule of conditional probability indicating the child node’s occurrence. With a disruption being triggered by previous events, Bayesian networks can utilize either deterministic or probabilistic data. What makes them especially amenable to the area of SCRA is that risk assessment can be done using either historical data or expert judgment. For example, Lawrence et al. ( 2020 ) developed a Bayesian Network to analyse supplier disruption following extreme weather risks. Similarly, Kumar Sharma and Sharma ( 2015 ) proposed a model to predict disruption risks in a supply chain, and Zheng and Zhang ( 2020 ) developed a Bayesian Network model to assess supply chain risk parameters. The latter paper concludes that the probability of a risk occurring becomes stable after a certain time. Further, Garvey et al. ( 2015 ) studied disruption effects on multiple firms in a supply chain and suggested that a Bayesian Network is an appropriate tool to measure risk propagation through a supply chain. It should also be noted that Bayesian Network models are highly useful when the assessment criteria are primarily based on the likelihood of events. Interested readers are advised to consult Heckerman et al. ( 1995 ) for a thorough introduction to Bayesian Networks and their applications.
6.1.5 Failure Mode and Effect Analysis
FMEA is a popular approach to analyse risks (Schneider, 1996 ). Traditional FMEA is based on three criteria: the likelihood of occurrence, the impact of the risk, and its detectability. Events are rated based on these three criteria, which are multiplied to form a Risk Priority Number (RPN). This facilitates decisions on which risk to focus on first—namely that with the highest RPN. While the technique is widely used in project management (Carbone & Tippett, 2004 ), it is also frequently applied in the SCRA literature (Chen & Wu, 2013 ; Kumar et al., 2013 ). It is easy to apply, but generally has to be integrated with other techniques, like Fuzzy sets or Grey theory, to capture the inherent uncertainty. A further limitation is that the interdependencies of the events are not accounted for.
6.1.6 Grey theory
Julong Deng proposed the grey theory concept in 1982 by combining principles of system theory, space theory, and control theory. The approach provides a measure to analyse relationships between discrete quantitative and qualitative series that are independent by nature (Chang et al., 1999 ). Grey theory has the advantage to be able to deal with partial information and uncertainty when multi-attribute decision-making problems arise (Chang et al., 1999 ; Rahimnia et al., 2011 ). It has thus been widely accepted in the SCRA literature (Ali et al., 2019 ; Rajesh & Ravi, 2015 ; Rajesh et al., 2015 ; Rao et al., 2017 ), as having only partial information is inherent to risks.
6.1.7 Conditional Value at Risk
Conditional Value at Risk (CVaR) is a measure that is helpful when dealing with low-probability, high-impact risks (Dixit et al., 2020 ). The concept of CVaR is borrowed from the finance literature (Rockafellar & Uryasev, 2002 ) where an expected loss is estimated for a given event. The amount of losses is the financial risk determined through a statistical measure called Value-at-Risk. If the probability distribution of the losses due to risky events are known, the losses at the tail can be identified though VaR, and the events causing these losses can be highlighted (Mehrjoo & Pasek, 2016 ). This concept is frequently applied in the SCRA literature, especially when considering risks with low probabilities (Mehrjoo & Pasek, 2016 ; Xu et al., 2013 ; Yan et al., 2019 ).
6.1.8 Interpretive structural modelling
When assessing supply chain risks it is difficult to determine their interdependencies, which is however important as risks can influence each other, yielding complex network structures. ISM is used to identify those complex structures (Kwak et al., 2018 ). For instance, Prakash et al. ( 2017 ) and Diabat et al. ( 2012 ) used ISM to identify risks in the food supply chain, while Venkatesh et al. ( 2015 ) used ISM to identify risks in the fashion supply chain. Specifically, Diabat et al. ( 2012 ) categorized forecasting-based risk in the top of the hierarchy, and Venkatesh et al. ( 2015 ) integrated fuzzy logic to deal with uncertainty in experts’ decision-making. The study recognized globalization, behavioural aspects of employees, and safety and security as risks that have highest dependencies.
Further, Kwak et al. ( 2018 ) developed an empirical model to identify risks and determine how certain risks in supply chains cause other risks. The study found that conflicts between trade partners, dependency upon logistics service providers, failure in logistics control, and failure in information exchange play pivotal roles in propagating risks in the supply chain. These risks are low in probability of occurrence but severe in their impact.
In a further study, Prakash et al. ( 2017 ) used ISM to evaluated risks in a perishable food supply chain providing a hierarchy of risks. The ISM technique is often followed by Matrice d’Impacts Croisés-Multiplication Appliquée à un Classement (MICMAC) analysis, an approach that allows the categorization of risks based on dependence and driving power. The categorization identifies for instance natural disasters and terrorist attacks as high-level risks that are beyond the control of the organization. In this vein, Pfohl et al. ( 2011 ) confirmed natural disasters and terrorist attacks as risks having high driving power. In contrast, lower-level risks can be in the form of supplier capabilities, transportation issues, forecast errors, and point-of-sales data errors. The study further integrated both the RPN and the Risk Mitigation Number (RMN), with the RMN representing the products of the RPNs and the Risk Mitigation Indices (RMIs) of all risks—the RMI captures feasible mitigation strategies, with its value ranging from 1 to 0 (and 1 representing an effective risk mitigation strategy). Overall, we note that ISM is widely used as an exploratory research approach, necessitating the validation of the model via Structural Equation Modelling.
6.1.9 Delphi technique
The Delphi technique was originally developed by Dalkey and Helmer to forecast future events (Murry & Hammons, 1995 ). However, it has seen wide application in qualitative research in areas such as problem solving and decision-making. The strength of the method lies in its assumptions that group decisions are better than decisions made by a single person. To avoid confrontations possible in face-to-face meetings, it employs anonymous, controlled feedback procedures from experts whose responses are validated statistically. The minimum number of experts acceptable for this technique varies. Amongst our literature sample reviewed, Moktadir et al. ( 2018 ) considered ten experts, Markmann et al. ( 2013 ) included 80 experts, Vilko and Hallikas ( 2012 ) had 22 experts, and Kwak et al. ( 2018 ) solicited input from 36 experts. It should be noted that as a decision-making tool, the Delphi method is restricted to the initial identification of risks. It can however be easily integrated with other techniques, like AHP, FMEA, ISM, and DEMATEL, for further assessment and analysis.
6.1.10 TOPSIS
The Technique for Order of Preference by Similarity to Ideal Solution (TOPSIS) is a MCDM technique for a finite set of alternatives (Lai et al., 1994 ; Yoon & Hwang, 1995 ). It defines both a positive ideal solution and a negative ideal solution from the list of alternatives, and then compares the distance of the alternatives to them. The alternative that is the closest to the positive ideal solution and the farthest from the negative ideal solution is considered the best solution. The technique is highly suitable for SCRA decision-making as it is easy to implement. For example, Abdel-Basset and Mohamed ( 2020 ) considered six risk areas with 33 underlying risks as sub-criteria, to identify the best telecommunication equipment segment in a Chinese telecommunication company for sustainable SCRM. Rostamzadeh et al. ( 2018 ) developed 7 sustainable risks as criteria, with 44 underlying risks as sub-criteria to evaluate the best petrochemical complex location in Iran. Common risks considered in both studies are financial, organizational, supply-related, environmental and information-technology related risks.
It should also be noted that the Criteria Importance Through Inter-criteria Correlation (CRITIC) method can be integrated to determine the objective weights of the criteria (i.e., the risks). Fuzzy sets are also used to extract the uncertainty of the decision-makers’ choice. For instance, Rathore et al. ( 2017 ) used TOPSIS to rank the risks in a food supply chain based on criteria like impact, probability, risk type, cost, and duration, with Fuzzy AHP then being used to allocate criteria weights. A very similar technique was adopted by Samvedi et al. ( 2013 ) and Viswanadham and Samvedi ( 2013 ) for a holistic view on SCRM and supplier selection, respectively. The use of TOPSIS has not been that prevalent in the literature and requires integration with other techniques to yield substantial conclusions.
6.1.11 DEMATEL
Decision-making and trial evaluation laboratory (DEMATEL) was developed by the Geneva Research Centre of the Battelle Memorial Institute (Gabus & Fontela, 1972 ). It is a method of structural modelling that aims to identify the strength of relationships between system elements, which is thus a formidable approach when it comes to supply chain risks, as they are interconnected and interdependent. There are obvious advantages associated with considering the independencies of risks rather than assessing them in isolation. The approach was applied for instance by Ali et al. ( 2019 ), who used it to investigate the cause-and-effect relations among supply chain risks. The study identified food supply chain risks related to a lack of skilled personnel, man-made disruptions, IT system failures, legal and regulatory risks, and capacity to be in the ‘cause’ group, while risks related to poor customer relationships, poor-quality products, supplier bankruptcy, change in customer taste, and poor leaderships are in the ‘effect’ group. A similar study was conducted by Song et al. ( 2017 ), who identified the relationship strength between risks in a sustainable supply chain, categorizing them into cause and effect groups. Rajesh and Ravi ( 2015 ) also modelled enablers of supply chain risk mitigation using DEMATEL. An approach that is commonly integrated with DEMATEL is Grey theory, leading to the development of Grey-DEMATEL. Grey theory, as discussed above, can deal with imperfect information. Therefore, such combination of approaches ensures a better ability to identify structural dependencies between supply chain risks.
6.1.12 Mean–Variance method
Mean–Variance analysis in SCRA is derived from portfolio risk management theory in finance, developed by Nobel Laureate Harry Markowitz. The idea behind the theory is to obtain an expected value of the payoff given by the mean, and an expected variation of the payoff given by the variance. Tsan-Ming Choi is a prominent author who has worked extensively using this method (Choi et al., 2008a , 2008b , 2019 ). For instance, Choi et al. ( 2019 ) explained that the Mean–Variance method can be applied as an analytical measure for risk aversion, can be included in an optimization objective, and as a performance measure to capture the risk of operations. When assessing supply chain risk, there are two approaches with which this can be done. The first one is to maximise the expected profit and subjecting the variance to some constraints, with the second one minimising the risk and subjecting the expected profit and other variables to some constraints. Unlike the previous techniques that considered certain criteria to assess risks, or use risk as a criterion to assess alternatives, mean–variance models in SCRA take into consideration the attitude of the decision-maker, which can be categorized into being risk-averse, risk-neutral or risk-seeking. As such, model assumptions change based on the decision makers. It is also important to note that in most studies using the Mean–Variance approach, risk is primarily captured in the form of financial losses to stakeholders. For example, Zhuo et al. ( 2018 ) analysed an option contract in a decentralized supply chain considering a retailer and a supplier. Chen et al. ( 2014 ) studied the risk sharing behaviour when Radio Frequency Identification (RFID) technology is used to reduce misplaced inventory, and Choi et al. ( 2019 ) analysed the supply chain risk with air logistics considering the application of blockchain technology. What makes the Mean–Variance approach so powerful in supply chain risk analysis is that the attitude of the decision-maker is considered. The Mean–Variance method can be applied as an analytical measure within the context of risk aversion, but can also be integrated in the optimization objective or as a performance measure to capture the risk of profit or loss. For a more detailed background and technical aspects of the Mean–Variance approach, the interested reader is referred to Chiu and Choi ( 2016 ).
6.1.13 Fault-Tree Analysis
Fault Tree Analysis (FTA) is another tool that uses logic diagrams from a directed graph of the system’s model being analysed and describes relationships between variables and events in the system. It was developed by Bell Telephone Laboratories in 1961. Our review of SCRA literature detected several studies deploying this technique. For example, Sherwin et al. ( 2016 ) formulated a fault-tree to assess supply chain disruption risk caused by the delay in delivery. Lei and MacKenzie ( 2019 ) studied risks in different types of supply chains using FTA. The types are categorized based on competition and cooperation between material suppliers. The use of Boolean Logic gates like AND, OR etc., makes the formulation of the system easy to comprehend. It must be noted though that FTA does not capture the causes of failures. However, FTA can be integrated with other techniques to consider a comparison of different risks or risky events in a system. In this vein, Mangla et al. ( 2016 ) for example developed a logic diagram of risks in sustainable supply chains using FTA and integrated it with fuzzy sets and AHP to rank them. The application of FTA is limited in SCRA as the logic diagram is based on some form of causality, which the method does not establish.
7 Discussion: SCRA characteristics
Our bibliometric and network analysis of the literature helped in disintegrating themes and uncovering layers of supply chain risks, and—combined with a critical analysis of decision-making techniques—helped us in identifying dominant characteristics of SCRA. These include uncertainty, hierarchy, propagation, expected impact and cause–effect relationships. SCRA literature is largely framed by these five aspects, with various decision-making techniques being incorporated and modified to analyse one or more of these aspects (Table 9 ). This section focuses on these SCRA characteristics and suggests promising analysis techniques for their investigation.
7.1 Uncertainty
The term uncertainty is used in a variety of contexts among our sample. In fact, it is one of the most frequently used keywords in the SCR literature. Other terms closely associated with uncertainty are volatility, instability, and lack of clarity. The probabilistic nature of future events is one of the major contexts in which uncertainty is studied. Unpredictable customer demands and unreliable supply reflect uncertainty. There are however also other variables leading to uncertainty, including material prices, capacity availability, lack of information, and political instability. All of these can lead to decision-making under uncertainty. Two of the most commonly used techniques to address this context are Fuzzy Logic and Grey Theory.
7.1.1 Fuzzy logic and Grey theory
Most SCRA studies involve decisions made by experts, reflecting the fact that most decisions cannot be reduced to a deterministic value. As such, experts may be able to most accurately capture the attributes associated with risks, but when studied in diverse contexts, converting these into measurable values can contain imprecision, uncertainty, or vagueness. Nevertheless, reflecting on the risk is valuable as it allows to uncover underlying phenomena. The uncertainty that is still inherent is captured by using Fuzzy Set and Grey Theory. Grey Theory closely resembles Fuzzy Logic, with the difference being in their properties. Grey Theory generally has an upper and a lower limit for the imprecise value, covering the range of imprecision. In contrast, fuzzy sets define a membership function, thus accounting for the imprecision. Both theories have their own technique of converting the imprecise value to a precise (crisp) value. The evaluation procedure steps for grey relations are less than those for fuzzy logic (Hsu & Wen, 2000 ). However, there are no significant advantages of one technique over the other.
7.2 Hierarchy
One of the outcomes of risk assessment is the generation of risk priorities in a supply chain. These priorities are not easy to identify until the right criteria are defined. The criteria on which risks are evaluated can be hierarchical, with such hierarchy generally identified by experts. Popular tools in this regard include the AHP and the ANP.
7.2.1 AHP and ANP
AHP and ANP are two frequently used MCDM techniques for SCRA. The use of both implies a priori knowledge of all elements (goal, criteria and alternatives). Since it is commonly accepted that supply chain risks are interconnected, it would be naïve to develop assessments models without an understanding of risk interactions. Nevertheless, both AHP and ANP are great approaches to prioritize resources and develop capabilities.
7.3 Propagation
Supply chains function in a highly interdependent network of organizations, rendering the effect of any event or activity difficult to be analysed in isolation. Risks also do not happen in isolation, but are rather interactive (Qazi et al., 2018 ) and propagate in a complex network (Mizgier, 2017 ). The Global Risk Report (World Economic Forum, 2020 ) presents a diagrammatic network of how experts believe risks are interconnected. However, the network is developed by identifying pairs of interconnected risks, and a higher level of sophistication is required to understand the propagation of risk effects in a complex supply chain network. A simple hierarchical network developed through AHP and ANP is insufficient, which is why studies have used Bayesian Belief Networks and FTA to understand risk propagation.
7.3.1 Bayesian belief network and fault tree analysis
Assessment studies relating to a network of events focus on their conditional occurrence probabilities. As such, Bayesian Network Analysis and FTA are good techniques to assess the dependency of supply chain events. Flows are a fundamental part of supply chain networks, captured for instance by the flow of materials, the flow of information, or the flow of money. In this vein, Sherwin et al. ( 2016 ) noted the risks of delay in material flow using FTA. In most cases, FTAs are static and assume independent probabilities. Lei and MacKenzie ( 2019 ) developed a dynamic fault-tree where these assumptions are removed to reflect a realistic network. The ease of developing a fault-tree is a great advantage for decision-makers, as the relationships are represented though Boolean Logic. However, the ease comes with a costly assumption of statistical independence of underlying events. Bayesian Networks somewhat alleviate this issue (Garvey et al., 2015 ; Lawrence et al., 2020 ; Lockamy, 2014 ; Qazi et al., 2018 ; Sharma & Routroy, 2016 ; Zheng & Zhang, 2020 ). Two advantages in particular need to be noted. First, expert opinions can be integrated when a risky event cannot solely be treated based on historical events. And second, conditionalities of risky events on other events can be incorporated. This is highlighted by ‘The Global Risk Report (World Economic Forum, 2020 ), which captures how experts around the globe think about one global risk triggering another. An alternate technique similar to Bayesian Networks is the use of Artificial Neural Networks, which however require extensive data sets, thus making predictive models for low-probability, high-impact risks difficult.
7.4 Expected impact
A consideration of SCRA is incomplete without analysing the impact the risk under study can have. MCDM techniques like AHP, TOPSIS and ANP are capable to rank and categorize risks based on their impact, but techniques like CVaR and Mean–Variance are better in their quantitative assessment of the expected risk outcome.
7.4.1 CVaR and Mean–Variance
In the above discussion we identified studies using techniques revolving around the probability of occurrence and the severity of impact, often quantified in terms of financial losses. As such, tools from finance were relied on to advance the SCRM field, which led to the development of the Mean–Variance approach and CVaR. While the application of CVaR has shown more predominance in the literature of SCRA than Mean–Variance, both approaches hold great promise for SCRA.
7.5 Cause-and-effect analysis
In our view, the SCRA literature has not applied cause-and-effect analysis to its fullest potential. This can be explained by the fact that developing a causal, generalized structural network is difficult, and often does not make sense as the uncertainty aspect of risks poses different challenges across industries. Narrowing it down to specific industry or case-based approaches helps identify certain cause-based and effect-based events separately. ISM and DEMATEL are two techniques frequently used in SCRA in this regard.
7.5.1 ISM and DEMATEL
SCRA includes the identification of structural interrelationships between elements in a supply chain. This involves the dependencies, interactions, and strengths of the elements. Both ISM and DEMATEL are unique in their way while at the same time having some similarities. As such, both involve expert judgements to compare a pair of elements, and both involve developing a diagraph representing the sequence of elements, with most of the elements being in the form of risks (Diabat et al., 2012 ; Kwak et al., 2018 ; Prakash et al., 2017 ; Song et al., 2017 ; Venkatesh et al., 2015 ). The use of ISM provides a hierarchy of risks that cannot be obtained in DEMATEL, which however is able to obtain relative interaction strengths between the risks. Nevertheless, both methods come with limitations, including the bias that may be inherent in the experts’ decision-making, and the lack of a relative importance weight for the variables. While the first limitation can be overcome by using Structural Equation Modelling, the second limitation can be overcome by integrating the approaches with other techniques like AHP or ANP.
8 Future research agenda
Future research opportunities in SCRA are abundant. While earlier work has developed ways to identify and classify risks, the assessment aspect has received lesser attention in review studies. We addressed this shortcoming in this review, which we believe was critical as risks can be multifarious and guidance was needed on appropriate assessment approaches. The multitude of contexts was also illustrated in Table 3 . We observed a shift in studies starting in 2015 to focus more on risks related to a specific industry rather than the supply chain in general. Furthermore, it is also observable that service industries, like healthcare, tourism, and retailing, do not have adequate representation from a supply chain context perspective. In this vein, Ho et al. ( 2015 ) conceded that service supply chain risks are largely unexplored in SCRM literature, and our review suggests that not much has changed since then. As such, service supply chains consider risks primarily as a deviation in service levels, in addition to risky behaviours of both buyers and suppliers (Wang et al., 2015 ); however, what has received very limited attention is the identification of a broader set of risks and their assessment.
We identified sustainability-related supply chain risks and operational risks as two clusters in the SCRA literature. The former is an emerging area while the latter is a perennial topic that however is in needs of constant revision and updating. Sustainability-related risks associated with the social dimension have a wider scope due to their rise in awareness. For example, Ahmadi et al. ( 2017 ) highlighted social sustainable criteria that affect supply chains, stressing the importance of contractual stakeholders’ influence, work health and safety, and training and community influence. Assessment approaches towards social risks in the supply chain may require additional parameters than already discussed. For example, risks posed by involuntary mass migration of workers, social instability, inter-state conflict, and infectious diseases, are characteristically unique and so are their repercussions in the supply chain. Further research exploring these risks will be an intriguing area of research. Certain environmental risks are also yet to be addressed within the purview of the supply chain, such as biodiversity loss and climate action failure. For example, Levner and Ptuskin ( 2018 ) addressed the adverse ecological effects due to supply chain activities, conceptualizing the ripple effect and utilizing entropy theory. Alternatively, environmental issues can also be a source of supply chain risks. We recommend future researchers to delve into these domains.
The network perspective, discussed in the second cluster, has also been getting particular attention in the recent past. Multiple objectives, along with the propagation of risks, increase the difficulty to model uncertainty and its effects. Bayesian Belief Networks can be useful in this regard as they are able to capture these complexities. The use of Network Theory, which is commonly used in the analysis of supply network idiosyncrasies, can also be integrated with the above discussed techniques.
Furthermore, the techniques discussed have an abundant scope of further development through their integration. While we have highlighted the rising trend in the application of MCDM techniques within the SCRA literature, we encourage researchers to explore the integration of inter-disciplinary tools and decision-making techniques to capture real-life contexts with limited assumptions. The use of CVaR, a financial concept, as a measure of resilience integrated with network modelling techniques (Dixit et al., 2020 ) is one such example. Our analysis and discussion should guide researchers to map their objectives with such techniques. In this vein, we also highlight a limitation of the articles we reviewed, i.e., their lack of application of the models in real life cases. The application of models in real life cases should be encouraged, enabling practitioners to easily incorporate and validate them.
9 Conclusion
We reviewed 136 articles from top international journals from 2010 to 2020 in the specific area of SCRA, focusing on the decision-making techniques applied. With our review, we make several contributions to the field of SCRM. First, we provide more specificity for the area of SCRA. To the best of our knowledge, while SCRA is of critical importance, there exists no comprehensive and focused review in this domain. Through both a bibliometric and a network analysis, we demonstrated the influence of certain SCRA aspects. As such, our analysis and interpretation now provide a clearer and more holistic understanding of the subject, highlighting the major themes in SCRA. This overview is thus invaluable for researchers in designing impactful studies on SCRA by considering the assumptions and variables outlined in this review paper. Second, we highlighted eight important parameters to be considered when assessing risks in supply chains: avoidance, cost, impact intensity, impact time, detectability, likelihood, risk exposure and expected utility. Risk assessment based on these factors covers almost all risk aspects and should aid researchers and practitioners in the risk management process. Third, a critical evaluation of the decision-making techniques used in the SCRA literature and their characteristics was provided, offering researchers a clear and concise understanding of technique application areas. With the help of this review, researchers can easily narrow down their research area and the most appropriate technique to study SCRA. Finally, the five SCRA aspects identified provide crucial guidance for researchers, since the decision-making techniques and methods all have limitations, and thus their use must be carefully weighted. To overcome this shortcoming, an integration of multiple techniques is an accepted practice. Robust decision-making techniques can be developed to incorporate all five aspects. However, the more approaches that are integrated, the greater the ensuing complexity, which may prevent practitioners from adopting the approach.
Through this study we uncovered many promising research directions. Specifically, we found that risk assessment related to sustainability is underrepresented in mainstream operations and supply chain literature. Especially the social and environmental aspects are under-researched. Yet, the importance of these risks is clear, as demonstrated in the ‘The Global Risk Report’ (World Economic Forum, 2020 ). As such, climate risks are widely regarded as the risks that can have high impact. Researchers may thus find it compelling to assess climate risks for the supply chain of a specific industry. Research on mitigation models can be a successive step for the achievement of this objective. In addition, our review did not lead to the identification of many health-related risks, representing a pressing research opportunity for our discipline, as illustrated by the havoc that COVID-19 has wreaked on global supply chains.
While we believe this review to be providing an invaluable foundation for scholars to get a “head-start” in the SCRA literature, enabling them to jump-start their SCRA research agenda, we acknowledge its limitations. As such, while our selection procedure for narrowing down the articles helped us to derive a focused set of papers within the domain of SCRA, other relevant works may have been missed, such as conference papers, theses, dissertations, and books. Also, with the review being targeted to the academic community, we did not review practitioner journals. Nevertheless, we believe that our exposition of the variety of techniques and their application can be invaluable for practitioners as well. Another angle that the review took was its focus on decision-making techniques. While this provides a unique perspective, it is also limited in that other angles were not considered. We however hope that the review provided offers motivation and inspiration for scholars to delve deeper into the fascinating domain of SCRA, interest in which is expected to increase as triggered by the pandemic.
Abdel-Basset, M., & Mohamed, R. (2020). A novel plithogenic TOPSIS–CRITIC model for sustainable supply chain risk management. Journal of Cleaner Production, 247 , 119586.
Google Scholar
Ahmadi, H. B., Kusi-Sarpong, S., & Rezaei, J. (2017). Assessing the social sustainability of supply chains using Best Worst Method. Resources, Conservation and Recycling, 126 , 99–106.
Ali, S. M., Moktadir, M. A., Kabir, G., Chakma, J., Rumi, M. J. U., & Islam, M. T. (2019). Framework for evaluating risks in food supply chain: Implications in food wastage reduction. Journal of Cleaner Production, 228 , 786–800.
Aqlan, F., & Lam, S. S. (2015). A fuzzy-based integrated framework for supply chain risk assessment. International Journal of Production Economics, 161 , 54–63.
Asian, S., & Nie, X. (2014). Coordination in supply chains with uncertain demand and disruption risks: Existence, analysis, and insights. IEEE Transactions on Systems, Man, and Cybernetics: Systems, 44 (9), 1139–1154.
Atwater, C., Gopalan, R., Lancioni, R., & Hunt, J. (2014). Measuring supply chain risk: Predicting motor carriers’ ability to withstand disruptive environmental change using conjoint analysis. Transportation Research Part c: Emerging Technologies, 48 , 360–378.
Bastian, M., Heymann, S., & Jacomy, M. (2009). Gephi: An open source software for exploring and manipulating networks visualization and exploration of large graphs .
Behzadi, G., O’Sullivan, M. J., Olsen, T. L., & Zhang, A. (2018). Agribusiness supply chain risk management: A review of quantitative decision models. Omega, 79 , 21–42.
Ben-Daya, M., Hassini, E., & Bahroun, Z. (2019). Internet of things and supply chain management: A literature review. International Journal of Production Research, 57 (15–16), 4719–4742.
Berle, Ø., Norstad, I., & Asbjørnslett, B. E. (2013). Optimization, risk assessment and resilience in LNG transportation systems. Supply Chain Management: An International Journal, 18 (3), 253–264.
Blondel, V. D., Guillaume, J. L., Lambiotte, R., & Lefebvre, E. (2008). Fast unfolding of communities in large networks. Journal of Statistical Mechanics: Theory and Experiment, 2008 (10), P10008.
Booth, A., Sutton, A., & Papaioannou, D. (2016). Systematic approaches to a successful literature review . Sage.
Bouwknegt, M., Verhaelen, K., Rzezutka, A., Kozyra, I., Maunula, L., von Bonsdorff, C. H., Vantarakis, A., Kokkinos, P., Petrovic, T., Lazic, S., Pavlik, I., Vasickova, P., Willems, K. A., Havelaar, A. H., Rutjes, S. A., & de Roda Husman, A. M. (2015). Quantitative farm-to-fork risk assessment model for norovirus and hepatitis A virus in European leafy green vegetable and berry fruit supply chains. International Journal of Food Microbiology, 198 , 50–58.
Boyack, K. W., & Klavans, R. (2010). Co-citation analysis, bibliographic coupling, and direct citation: Which citation approach represents the research front most accurately? Journal of the American Society for Information Science and Technology, 61 (12), 2389–2404.
Brin, S., & Page, L. (1998). The anatomy of a large-scale hypertextual web search engine. Computer Networks and ISDN Systems, 30 (1–7), 107–117.
Brun, A., Caridi, M., Fahmy Salama, K., & Ravelli, I. (2006). Value and risk assessment of supply chain management improvement projects. International Journal of Production Economics, 99 (1–2), 186–201.
Cagliano, A. C., De Marco, A., Grimaldi, S., & Rafele, C. (2012). An integrated approach to supply chain risk analysis. Journal of Risk Research, 15 (7), 817–840.
Cagno, E., & Micheli, G. J. L. (2011). Enhancing EPC supply chain competitiveness through procurement risk management. Risk Management, 13 (3), 147–180.
Cantor, D. E., Blackhurst, J. V., & Cortes, J. D. (2014). The clock is ticking: The role of uncertainty, regulatory focus, and level of risk on supply chain disruption decision making behavior. Transportation Research Part e: Logistics and Transportation Review, 72 , 159–172.
Carbone, T. A., & Tippett, D. D. (2004). Project risk management using the project risk FMEA. Engineering Management Journal, 16 (4), 28–35.
Chand, M., Raj, T., Shankar, R., & Agarwal, A. (2017). Select the best supply chain by risk analysis for Indian industries environment using MCDM approaches. Benchmarking, 24 (5), 1400–1413.
Chang, C. L., Wei, C. C., & Lee, Y. H. (1999). Failure mode and effects analysis using fuzzy method and grey theory. Kybernetes, 28 (8–9), 1072–1080.
Chaudhuri, A., Bhatia, M. S., Kayikci, Y., Fernandes, K. J., & Fosso-Wamba, S. (2021). Improving social sustainability and reducing supply chain risks through blockchain implementation: Role of outcome and behavioural mechanisms. Annals of Operations Research . https://doi.org/10.1007/s10479-021-04307-6
Article Google Scholar
Chari, F., & Ngcamu, B. S. (2017). An assessment of the impact of disaster risks on dairy supply chain performance in Zimbabwe. Cogent Engineering, 4 (1), 1409389.
Chaudhuri, A., Mohanty, B. K., & Singh, K. N. (2013). Supply chain risk assessment during new product development: A group decision making approach using numeric and linguistic data. International Journal of Production Research, 51 (10), 2790–2804.
Chen, P. S., & Wu, M. T. (2013). A modified failure mode and effects analysis method for supplier selection problems in the supply chain risk environment: A case study. Computers and Industrial Engineering, 66 (4), 634–642.
Chen, S., Wang, H., Xie, Y., & Qi, C. (2014). Mean-risk analysis of radio frequency identification technology in supply chain with inventory misplacement: Risk-sharing and coordination. Omega (united Kingdom), 46 , 86–103.
Chen, T. K., Liao, H. H., & Kuo, H. J. (2013). Internal liquidity risk, financial bullwhip effects, and corporate bond yield spreads: Supply chain perspectives. Journal of Banking and Finance, 37 (7), 2434–2456.
Chiu, C. H., & Choi, T. M. (2016). Supply chain risk analysis with mean–variance models: A technical review. Annals of Operations Research, 240 (2), 489–507.
Choi, T. M., Li, D., & Yan, H. (2008a). Mean–variance analysis of a single supplier and retailer supply chain under a returns policy. European Journal of Operational Research, 184 (1), 356–376.
Choi, T. M., Li, D., Yan, H., & Chiu, C. H. (2008b). Channel coordination in supply chains with agents having mean–variance objectives. Omega, 36 (4), 565–576.
Choi, T. M., Wang, M., & Yue, X. (2016). Emerging production optimization issues in supply chain systems. Annals of Operations Research, 240 (2), 381–393.
Choi, T. M., Wen, X., Sun, X., & Chung, S. H. (2019). The mean–variance approach for global supply chain risk analysis with air logistics in the blockchain technology era. Transportation Research Part e: Logistics and Transportation Review, 127 , 178–191.
Cimprich, A., Bach, V., Helbig, C., Thorenz, A., Schrijvers, D., Sonnemann, G., Young, S. B., Sonderegger, T., & Berger, M. (2019). Raw material criticality assessment as a complement to environmental life cycle assessment: Examining methods for product-level supply risk assessment. Journal of Industrial Ecology, 23 (5), 1226–1236.
Diabat, A., Govindan, K., & Panicker, V. V. (2012). Supply chain risk management and its mitigation in a food industry. International Journal of Production Research, 50 (11), 3039–3050.
Ding, Y., & Cronin, B. (2011). Popular and/or prestigious? Measures of scholarly esteem. Information Processing and Management, 47 (1), 80–96.
Dixit, V., Verma, P., & Tiwari, M. K. (2020). Assessment of pre and post-disaster supply chain resilience based on network structural parameters with CVaR as a risk measure. International Journal of Production Economics, 227 , 107655.
Dong, Q., & Cooper, O. (2016). An orders-of-magnitude AHP supply chain risk assessment framework. International Journal of Production Economics, 182 , 144–156.
dos Santos, S. F., Brandi, H. S., Borschiver, S., & de Souza, V. (2017). Estimating vulnerability to risks: An application in a biofuel supply chain. Clean Technologies and Environmental Policy, 19 (5), 1257–1269.
DuHadway, S., Carnovale, S., & Hazen, B. (2019). Understanding risk management for intentional supply chain disruptions: Risk detection, risk mitigation, and risk recovery. Annals of Operations Research, 283 (1), 179–198.
Duleba, S., Alkharabsheh, A., & Gündoğdu, F. K. (2021). Creating a common priority vector in intuitionistic fuzzy AHP: A comparison of entropy-based and distance-based models. Annals of Operations Research . https://doi.org/10.1007/s10479-021-04491-5
Ekwall, D., & Lantz, B. (2016). Supply chain risk analysis and assessment: Cargo Theft. Transportation Journal, 55 (4), 400–419.
Elleuch, H., Hachicha, W., & Chabchoub, H. (2014). A combined approach for supply chain risk management: Description and application to a real hospital pharmaceutical case study. Journal of Risk Research, 17 (5), 641–663.
Esterman, M., Palekar, S., Conway, F., Ehmann, J., & Limcharoen, V. (2020). Toward robust concurrent product development across the supply chain: A risk assessment framework. Journal of Engineering Design, 31 (3), 150–177.
Fahimnia, B., Tang, C. S., Davarzani, H., & Sarkis, J. (2015). Quantitative models for managing supply chain risks: A review. European Journal of Operational Research, 247 (1), 1–15.
Gabus, A., & Fontela, E. (1972). World problems, an invitation to further thought within the framework of DEMATEL (pp. 1–8). Battelle Geneva Research Center.
Garvey, M. D., Carnovale, S., & Yeniyurt, S. (2015). An analytical framework for supply network risk propagation: A Bayesian network approach. European Journal of Operational Research, 243 (2), 618–627.
Ge, H., Nolan, J., & Gray, R. (2015). Identifying strategies to mitigate handling risks in the Canadian grain supply chain. Canadian Journal of Agricultural Economics/revue Canadienne D’agroeconomie, 63 (1), 101–128.
Ghadge, A., Dani, S., Chester, M., & Kalawsky, R. (2013). A systems approach for modelling supply chain risks. Supply Chain Management, 18 (5), 523–538.
Ghadge, A., Fang, X., Dani, S., & Antony, J. (2017). Supply chain risk assessment approach for process quality risks. International Journal of Quality and Reliability Management, 34 (7), 940–954.
Giannakis, M., Dubey, R., Yan, S., Spanaki, K., & Papadopoulos, T. (2022). Social media and sensemaking patterns in new product development: Demystifying the customer sentiment. Annals of Operations Research, 308 , 145–175.
Giannakis, M., & Papadopoulos, T. (2016). Supply chain sustainability: A risk management approach. International Journal of Production Economics, 171 , 455–470.
Govindan, K., Soleimani, H., & Kannan, D. (2015). Reverse logistics and closed-loop supply chain: A comprehensive review to explore the future. European Journal of Operational Research, 240 (3), 603–626.
Hansen, J. K., Roni, M. S., Nair, S. K., Hartley, D. S., Griffel, L. M., Vazhnik, V., & Mamun, S. (2019). Setting a baseline for Integrated Landscape Design: Cost and risk assessment in herbaceous feedstock supply chains. Biomass and Bioenergy, 130 , 105388.
Ηatzisymeon, M., Kamenopoulos, S., & Tsoutsos, T. (2019). Risk assessment of the life-cycle of the used cooking oil-to-biodiesel supply chain. Journal of Cleaner Production, 217 , 836–843.
Heckerman, D., Geiger, D., & Chickering, D. M. (1995). Learning Bayesian networks: The combination of knowledge and statistical data . Springer.
Heckmann, I., Comes, T., & Nickel, S. (2015). A critical review on supply chain risk—Definition, measure and modeling. Omega, 52 , 119–132.
Helbig, C., Gemechu, E. D., Pillain, B., Young, S. B., Thorenz, A., Tuma, A., & Sonnemann, G. (2016). Extending the geopolitical supply risk indicator: Application of life cycle sustainability assessment to the petrochemical supply chain of polyacrylonitrile-based carbon fibers. Journal of Cleaner Production, 137 , 1170–1178.
Hermoso-Orzáez, M. J., & Garzón-Moreno, J. (2021). Risk management methodology in the supply chain: A case study applied. Annals of Operations Research . https://doi.org/10.1007/s10479-021-04220-y
Ho, W. (2008). Integrated analytic hierarchy process and its applications—A literature review. European Journal of Operational Research, 186 (1), 211–228.
Ho, W., & Ma, X. (2018). The state-of-the-art integrations and applications of the analytic hierarchy process. European Journal of Operational Research, 267 (2), 399–414.
Ho, W., Zheng, T., Yildiz, H., & Talluri, S. (2015). Supply chain risk management: A literature review. International Journal of Production Research, 53 (16), 5031–5069.
Hsieh, C. C., & Lu, Y. T. (2010). Manufacturer’s return policy in a two-stage supply chain with two risk-averse retailers and random demand. European Journal of Operational Research, 207 (1), 514–523.
Hsu, C. I., & Wen, Y. H. (2000). Application of Grey theory and multiobjective programming towards airline network design. European Journal of Operational Research, 127 (1), 44–68.
Huang, E., & Goetschalckx, M. (2014). Strategic robust supply chain design based on the Pareto-optimal tradeoff between efficiency and risk. European Journal of Operational Research, 237 (2), 508–518.
Hult, G. T. M., Craighead, C. W., & Ketchen, D. J. (2010). Risk uncertainty and supply chain decisions: A real options perspective. Decision Sciences, 41 (3), 435–458.
Ivanov, D. (2020). Predicting the impacts of epidemic outbreaks on global supply chains: A simulation-based analysis on the coronavirus outbreak (COVID-19/SARS-CoV-2) case. Transportation Research Part e: Logistics and Transportation Review, 136 , 101922.
Ivanov, D., Dolgui, A., Sokolov, B., & Ivanova, M. (2017). Literature review on disruption recovery in the supply chain*. International Journal of Production Research, 55 (20), 6158–6174.
Jiang, B., Li, J., & Shen, S. (2018). Supply chain risk assessment and control of port enterprises: Qingdao port as case study. Asian Journal of Shipping and Logistics, 34 (3), 198–208.
Johnson, E. C., Jr. (2015). Business lawyers are in a unique position to help their clients identify supply-chain risks involving labor trafficking and child labor. The Business Lawyer, 70 (4), 1083–1122.
Kara, M. E., Oktay Fırat, S. Ü., & Ghadge, A. (2020). A data mining-based framework for supply chain risk management. Computers and Industrial Engineering, 139 , 105570.
Katsaliaki, K., Galetsi, P., & Kumar, S. (2021). Supply chain disruptions and resilience: A major review and future research agenda. Annals of Operations Research . https://doi.org/10.1007/s10479-020-03912-1
Kim, Y., Chen, Y. S., & Linderman, K. (2015). Supply network disruption and resilience: A network structural perspective. Journal of Operations Management, 33–34 , 43–59.
Kleindorfer, P. R., & Saad, G. H. (2009). Managing disruption risks in supply chains. Production and Operations Management, 14 (1), 53–68.
Klibi, W., & Martel, A. (2012). Scenario-based Supply Chain Network risk modeling. European Journal of Operational Research, 223 (3), 644–658.
Kolotzek, C., Helbig, C., Thorenz, A., Reller, A., & Tuma, A. (2018). A company-oriented model for the assessment of raw material supply risks, environmental impact and social implications. Journal of Cleaner Production, 176 , 566–580.
Kumar Sharma, S., & Sharma, S. (2015). Developing a Bayesian network model for supply chain risk assessment. Supply Chain Forum: An International Journal, 16 (4), 50–72.
Kumar, S., & Schmitz, S. (2011). Managing recalls in a consumer product supply chain—Root cause analysis and measures to mitigate risks. International Journal of Production Research, 49 (1), 235–253.
Kumar, S., Boice, B. C., & Shepherd, M. J. (2013). Risk assessment and operational approaches to manage risk in global supply chains. Transportation Journal, 52 (3), 391–411.
Kwak, D. W., Rodrigues, V. S., Mason, R., Pettit, S., & Beresford, A. (2018). Risk interaction identification in international supply chain logistics: Developing a holistic model. International Journal of Operations and Production Management, 38 (2), 372–389.
Lai, Y.-J., Liu, T.-Y., & Hwang, C.-L. (1994). TOPSIS for MODM. European Journal of Operational Research, 76 (3), 486–500.
Lavastre, O., Gunasekaran, A., & Spalanzani, A. (2012). Supply chain risk management in French companies. Decision Support Systems, 52 (4), 828–838.
Lawrence, J. M., Ibne Hossain, N. U., Jaradat, R., & Hamilton, M. (2020). Leveraging a Bayesian network approach to model and analyze supplier vulnerability to severe weather risk: A case study of the U.S. pharmaceutical supply chain following Hurricane Maria. International Journal of Disaster Risk Reduction, 49 , 101607.
Lei, X., & MacKenzie, C. A. (2019). Assessing risk in different types of supply chains with a dynamic fault tree. Computers and Industrial Engineering, 137 , 106061.
Levner, E., & Ptuskin, A. (2018). Entropy-based model for the ripple effect: Managing environmental risks in supply chains. International Journal of Production Research, 56 (7), 2539–2551.
Li, Y., & Wang, Q. (2019). Research on hotel supply chain risk assessment with dual generalized triangular fuzzy Bonferroni mean operators. Journal of Intelligent and Fuzzy Systems, 37 (2), 1953–1965.
Liu, Z., Hua, S., & Zhai, X. (2020). Supply chain coordination with risk-averse retailer and option contract: Supplier-led vs Retailer-led. International Journal of Production Economics, 223 , 107518.
Lockamy, A. (2014). Assessing disaster risks in supply chains. Industrial Management and Data Systems, 114 (5), 755–777.
Ma, H. L., & Wong, W. H. C. (2018). A fuzzy-based House of Risk assessment method for manufacturers in global supply chains. Industrial Management and Data Systems, 118 (7), 1463–1476.
Mangla, S. K., Kumar, P., & Barua, M. K. (2016). An integrated methodology of FTA and fuzzy AHP for risk assessment in green supply chain. International Journal of Operational Research, 25 (1), 77–99.
Mangla, S. K., Luthra, S., & Jakhar, S. (2018). Benchmarking the risk assessment in green supply chain using fuzzy approach to FMEA: Insights from an Indian case study. Benchmarking, 25 (8), 2660–2687.
Markmann, C., Darkow, I. L., & von der Gracht, H. (2013). A Delphi-based risk analysis—Identifying and assessing future challenges for supply chain security in a multi-stakeholder environment. Technological Forecasting and Social Change, 80 (9), 1815–1833.
Martino, G., Fera, M., Iannone, R., & Miranda, S. (2017). Supply chain risk assessment in the fashion retail industry: An analytic network process approach. International Journal of Applied Engineering Research, 12 , 140–154.
Marufuzzaman, M., Eksioglu, S. D., Li, X., & Wang, J. (2014). Analyzing the impact of intermodal-related risk to the design and management of biofuel supply chain. Transportation Research Part e: Logistics and Transportation Review, 69 , 122–145.
Mckinsey.com. (2020a). COVID-19: Implications for business. https://www.mckinsey.com/business-functions/risk/our-insights/covid-19-implications-for-business
Mckinsey.com. (2020b). Why now is the time to stress-test your industrial supply chain . https://www.mckinsey.com/business-functions/operations/our-insights/why-now-is-the-time-to-stress-test-your-industrial-supply-chain
Mckinsey.com. (2020c). Supply chain risk management is back. https://www.mckinsey.com/business-functions/operations/our-insights/supply-chain-risk-management-is-back
Mehrjoo, M., & Pasek, Z. J. (2016). Risk assessment for the supply chain of fast fashion apparel industry: A system dynamics framework. International Journal of Production Research, 54 (1), 28–48.
Meng, Q., Liu, X., Song, Y., & Wang, W. (2019). An extended generalized TODIM method for risk assessment of supply chain in social commerce under interval type-2 fuzzy environment. Journal of Intelligent and Fuzzy Systems, 37 (6), 8551–8565.
Min, H., Park, H., & Ahn, S. B. (2017). Measuring the supply chain risk in offshoring countries using data envelopment analysis and the analytic hierarchy process. Benchmarking, 24 (7), 1977–1994.
Mishra, P. K. (2020). COVID-19, Black Swan events and the future of disaster risk management in India. Progress in Disaster Science, 8 , 100137.
Mital, M., Del Giudice, M., & Papa, A. (2018). Comparing supply chain risks for multiple product categories with cognitive mapping and Analytic Hierarchy Process. Technological Forecasting and Social Change, 131 , 159–170.
Mizgier, K. J. (2017). Global sensitivity analysis and aggregation of risk in multi-product supply chain networks. International Journal of Production Research, 55 (1), 130–144.
Mohib, A. M. N., & Deif, A. M. (2020). Supply chain multi-state risk assessment using universal generating function. Production Planning and Control, 31 (9), 699–708.
Moktadir, M. A., Ali, S. M., Mangla, S. K., Sharmy, T. A., Luthra, S., Mishra, N., & Garza-Reyes, J. A. (2018). Decision modeling of risks in pharmaceutical supply chains. Industrial Management and Data Systems, 118 (7), 1388–1412.
Murry, J. W., & Hammons, J. O. (1995). Delphi: A versatile methodology for conducting qualitative research. The Review of Higher Education, 18 (4), 423–436.
Nakandala, D., Lau, H., & Zhao, L. (2017). Development of a hybrid fresh food supply chain risk assessment model. International Journal of Production Research, 55 (14), 4180–4195.
Nooraie, S. V., & Parast, M. M. (2015). A multi-objective approach to supply chain risk management: Integrating visibility with supply and demand risk. International Journal of Production Economics, 161 , 192–200.
Nooraie, S. V., & Parast, M. M. (2016). Mitigating supply chain disruptions through the assessment of trade-offs among risks, costs and investments in capabilities. International Journal of Production Economics, 171 , 8–21.
Nooraie, S. V., Fathi, M., Narenji, M., Parast, M. M., Pardalos, P. M., & Stanfield, P. M. (2020). A multi-objective model for risk mitigating in supply chain design. International Journal of Production Research, 58 (5), 1338–1361.
Ojha, R., Ghadge, A., Tiwari, M. K., & Bititci, U. S. (2018). Bayesian network modelling for supply chain risk propagation. International Journal of Production Research, 56 (17), 5795–5819.
Olson, D. L., & Wu, D. (2011). Risk management models for supply chain: A scenario analysis of outsourcing to China. Supply Chain Management, 16 (6), 401–408.
Ortúzar, J. E., Dogan, O. B., Sotomayor, G., Jiménez, C., Clarke, J., Flores, R. A., Gray, G. M., Rupnow, J. H., & Wang, B. (2020). Quantitative assessment of microbial quality and safety risk: A preliminary case study of strengthening raspberry supply system in Chile. Food Control, 113 , 107166.
Park, S., Lee, T. E., & Sung, C. S. (2010). A three-level supply chain network design model with risk-pooling and lead times. Transportation Research Part e: Logistics and Transportation Review, 46 (5), 563–581.
Peng, M., Peng, Y., & Chen, H. (2014). Post-seismic supply chain risk management: A system dynamics disruption analysis approach for inventory and logistics planning. Computers and Operations Research, 42 , 14–24.
Petticrew, M., & Roberts, H. (2008). Systematic reviews in the social sciences: A practical guide . Wiley.
Pettit, T. J., Fiksel, J., & Croxton, K. L. (2010). Ensuring supply chain resilience: Development of a conceptual framework. Journal of Business Logistics, 31 (1), 1–21.
Pfohl, H. C., Gallus, P., & Thomas, D. (2011). Interpretive structural modeling of supply chain risks. International Journal of Physical Distribution and Logistics Management, 41 (9), 839–859.
Pilkington, A., & Meredith, J. (2009). The evolution of the intellectual structure of operations management-1980–2006: A citation/co-citation analysis. Journal of Operations Management, 27 (3), 185–202.
Ponomarov, S. Y., & Holcomb, M. C. (2009). Understanding the concept of supply chain resilience. The International Journal of Logistics Management, 20 (1), 124–143.
Pournader, M., Kach, A., & Talluri, S. (2020). A review of the existing and emerging topics in the supply chain risk management literature. Decision Sciences, 51 (4), 867–919.
Pournader, M., Rotaru, K., Kach, A. P., & Razavi Hajiagha, S. H. (2016). An analytical model for system-wide and tier-specific assessment of resilience to supply chain risks. Supply Chain Management, 21 (5), 589–609.
Prakash, S., Soni, G., Rathore, A. P. S., & Singh, S. (2017). Risk analysis and mitigation for perishable food supply chain: A case of dairy industry. Benchmarking, 24 (1), 2–23.
Qazi, A., Dickson, A., Quigley, J., & Gaudenzi, B. (2018). Supply chain risk network management: A Bayesian belief network and expected utility based approach for managing supply chain risks. International Journal of Production Economics, 196 , 24–42.
Qu, S. J., Goh, M., De Souza, R., & Wang, T.-N. (2014). Proximal point algorithms for convex multi-criteria optimization with applications to supply chain risk management. Journal of Optimization Theory and Applications, 163 , 949–956.
Rahimnia, F., Moghadasian, M., & Mashreghi, E. (2011). Application of grey theory approach to evaluation of organizational vision. Grey Systems: Theory and Application, 1 (1), 33–46.
Rajagopal, V., Venkatesan, S. P., & Goh, M. (2017). Decision-making models for supply chain risk mitigation: A review. Computers and Industrial Engineering, 113 , 646–682.
Rajesh, R., & Ravi, V. (2015). Modeling enablers of supply chain risk mitigation in electronic supply chains: A Grey-DEMATEL approach. Computers and Industrial Engineering, 87 , 126–139.
Rajesh, R., Ravi, V., & Venkata Rao, R. (2015). Selection of risk mitigation strategy in electronic supply chains using grey theory and digraph-matrix approaches. International Journal of Production Research, 53 (1), 238–257.
Rangel, D. A., de Oliveira, T. K., & Leite, M. S. A. (2015). Supply chain risk classification: Discussion and proposal. International Journal of Production Research, 53 (22), 6868–6887.
Rao, C., Xiao, X., Goh, M., Zheng, J., & Wen, J. (2017). Compound mechanism design of supplier selection based on multi-attribute auction and risk management of supply chain. Computers and Industrial Engineering, 105 , 63–75.
Rathore, R., Thakkar, J. J., & Jha, J. K. (2017). A quantitative risk assessment methodology and evaluation of food supply chain. International Journal of Logistics Management, 28 (4), 1272–1293.
Rayas, V. M., & Serrato, M. A. (2017). A framework of the risk assessment for the supply chain of hazardous materials. NETNOMICS: Economic Research and Electronic Networking, 18 (2–3), 215–226.
Rockafellar, R. T., & Uryasev, S. (2002). Conditional value-at-risk for general loss distributions. Journal of Banking and Finance, 26 (7), 1443–1471.
Roehrich, J. K., Grosvold, J., & Hoejmose, S. U. (2014). Reputational risks and sustainable supply chain management: Decision making under bounded rationality. International Journal of Operations and Production Management, 34 (5), 695–719.
Rostamzadeh, R., Ghorabaee, M. K., Govindan, K., Esmaeili, A., & Nobar, H. B. K. (2018). Evaluation of sustainable supply chain risk management using an integrated fuzzy TOPSIS–CRITIC approach. Journal of Cleaner Production, 175 , 651–669.
Rotaru, K., Wilkin, C., & Ceglowski, A. (2014). Analysis of SCOR’s approach to supply chain risk management. International Journal of Operations and Production Management, 34 (10), 1246–1268.
Saaty, T. L. (2004). Decision making—The analytic hierarchy and network processes (AHP/ANP). Journal of Systems Science and Systems Engineering, 13 (1), 1–35.
Sahay, N., & Ierapetritou, M. (2015). Flexibility assessment and risk management in supply chains. AIChE Journal, 61 (12), 4166–4178.
Salehi Heidari, S., Khanbabaei, M., & Sabzehparvar, M. (2018). A model for supply chain risk management in the automotive industry using fuzzy analytic hierarchy process and fuzzy TOPSIS. Benchmarking, 25 (9), 3831–3857.
Samvedi, A., Jain, V., & Chan, F. T. S. (2013). Quantifying risks in a supply chain through integration of fuzzy AHP and fuzzy TOPSIS. International Journal of Production Research, 51 (8), 2433–2442.
Santibañez-Aguilar, J. E., Guillen-Gosálbez, G., Morales-Rodriguez, R., Jiménez-Esteller, L., Castro-Montoya, A. J., & María Ponce-Ortega, J. (2015). Financial risk assessment and optimal planning of biofuels supply chains under uncertainty. BioEnergy Research, 9 (4), 1053–1069.
Sawik, T. (2013). Integrated selection of suppliers and scheduling of customer orders in the presence of supply chain disruption risks. International Journal of Production Research, 51 (23–24), 7006–7022.
Sawik, T. (2014). Optimization of cost and service level in the presence of supply chain disruption risks: Single vs. multiple sourcing. Computers and Operations Research, 51 , 11–20.
Schaefer, T., Udenio, M., Quinn, S., & Fransoo, J. C. (2019). Water risk assessment in supply chains. Journal of Cleaner Production, 208 , 636–648.
Schauer, S., Polemi, N., & Mouratidis, H. (2019). MITIGATE: A dynamic supply chain cyber risk assessment methodology. Journal of Transportation Security, 12 (1–2), 1–35.
Schmitt, A. J., & Singh, M. (2012). A quantitative analysis of disruption risk in a multi-echelon supply chain. International Journal of Production Economics, 139 (1), 22–32.
Schneider, H. (1996). Failure mode and effect analysis: FMEA from theory to execution. Technometrics, 38 (1), 80–80.
Seuring, S., & Müller, M. (2008). From a literature review to a conceptual framework for sustainable supply chain management. Journal of Cleaner Production, 16 (15), 1699–1710.
Sharma, S., & Routroy, S. (2016). Modeling information risk in supply chain using Bayesian networks. Journal of Enterprise Information Management, 29 (2), 238–254.
Sharma, S. K., Srivastava, P. R., Kumar, A., Jindal, A., & Gupta, S. (2021). Supply chain vulnerability assessment for manufacturing industry. Annals of Operations Research . https://doi.org/10.1007/s10479-021-04155-4
Shenoi, V. V., Dath, T. N. S., Rajendran, C., & Shahabudeen, P. (2018). Strategic action grids: A study on supply chain risk management in manufacturing industries in India. Benchmarking: an International Journal, 25 (8), 3045–3061.
Sherwin, M. D., Medal, H., & Lapp, S. A. (2016). Proactive cost-effective identification and mitigation of supply delay risks in a low volume high value supply chain using fault-tree analysis. International Journal of Production Economics, 175 , 153–163.
Simchi-Levi, D., Schmidt, W., & Wei, Y. (2014). From superstorms to factory fires: Managing unpredictable supply-chain disruptions. Harvard Business Review . https://hbr.org/2014/01/from-superstorms-to-factory-fires-managing-unpredictable-supply-chain-disruptions
Simchi-Levi, D., Schmidt, W., Wei, Y., Zhang, P. Y., Combs, K., Ge, Y., Gusikhin, O., Sanders, M., & Zhang, D. (2015). Identifying risks and mitigating disruptions in the automotive supply chain. Interfaces, 45 (5), 375–390.
Sodhi, M. S., Son, B.-G., & Tang, C. S. (2012). Researchers’ perspectives on supply chain risk management. Production and Operations Management, 21 (1), 1–13.
Song, W., Ming, X., & Liu, H. C. (2017). Identifying critical risk factors of sustainable supply chain management: A rough strength-relation analysis method. Journal of Cleaner Production, 143 , 100–115.
Tabrizi, B. H., & Razmi, J. (2013). Introducing a mixed-integer non-linear fuzzy model for risk management in designing supply chain networks. Journal of Manufacturing Systems, 32 (2), 295–307.
Talluri, S. S., Kull, T. J., Yildiz, H., & Yoon, J. (2013). Assessing the efficiency of risk mitigation strategies in supply chains. Journal of Business Logistics, 34 (4), 253–269.
Tazelaar, F., & Snijders, C. (2013). Operational risk assessments by supply chain professionals: Process and performance. Journal of Operations Management, 31 (1–2), 37–51.
Thun, J. H., & Hoenig, D. (2011). An empirical analysis of supply chain risk management in the German automotive industry. International Journal of Production Economics, 131 (1), 242–249.
Thun, J. H., Drüke, M., & Hoenig, D. (2011). Managing uncertainty—An empirical analysis of supply chain risk management in small and medium-sized enterprises. International Journal of Production Research, 49 (18), 5511–5525.
Tummala, R., & Schoenherr, T. (2011). Assessing and managing risks using the Supply Chain Risk Management Process (SCRMP). Supply Chain Management, 16 (6), 474–483.
Tuncel, G., & Alpan, G. (2010). Risk assessment and management for supply chain networks: A case study. Computers in Industry, 61 (3), 250–259.
van den Brink, S., Kleijn, R., Sprecher, B., & Tukker, A. (2020). Identifying supply risks by mapping the cobalt supply chain. Resources, Conservation and Recycling, 156 , 104743.
Venkatesh, V. G., Rathi, S., & Patwa, S. (2015). Analysis on supply chain risks in Indian apparel retail chains and proposal of risk prioritization model using interpretive structural modeling. Journal of Retailing and Consumer Services, 26 , 153–167.
Venturini, T., Jacomy, M., & Jensen, P. (2019). What do we see when we look at networks. In An introduction to visual network analysis and force-directed layouts , April 26, 2019.
Vilko, J. P. P., & Hallikas, J. M. (2012). Risk assessment in multimodal supply chains. International Journal of Production Economics, 140 (2), 586–595.
Viswanadham, N., & Samvedi, A. (2013). Supplier selection based on supply chain ecosystem, performance and risk criteria. International Journal of Production Research, 51 (21), 6484–6498.
Wagner, S. M., & Bode, C. (2008). An empirical examination of supply chain performance along several dimensions of risk. Journal of Business Logistics, 29 (1), 307–325.
Wagner, S. M., & Neshat, N. (2012). A comparison of supply chain vulnerability indices for different categories of firms. International Journal of Production Research, 50 (11), 2877–2891.
Wan, C., Yan, X., Zhang, D., Qu, Z., & Yang, Z. (2019). An advanced fuzzy Bayesian-based FMEA approach for assessing maritime supply chain risks. Transportation Research Part e: Logistics and Transportation Review, 125 , 222–240.
Wang, X., Chan, H. K., Yee, R. W. Y., & Diaz-Rainey, I. (2012). A two-stage fuzzy-AHP model for risk assessment of implementing green initiatives in the fashion supply chain. International Journal of Production Economics, 135 (2), 595–606.
Wang, X., Chen, X., Durugbo, C., & Cai, Z. (2018). Manage risk of sustainable product–service systems: A case-based operations research approach. Annals of Operations Research, 291 , 897–920.
Wang, X., Li, D., & Shi, X. (2012). A fuzzy model for aggregative food safety risk assessment in food supply chains. Production Planning and Control, 23 (5), 377–395.
Wang, Y., Wallace, S. W., Shen, B., & Choi, T. M. (2015). Service supply chain management: A review of operational models. European Journal of Operational Research, 247 (3), 685–698.
Wiengarten, F., Pagell, M., & Fynes, B. (2013). The importance of contextual factors in the success of outsourcing contracts in the supply chain environment: The role of risk and complementary practices. Supply Chain Management, 18 (6), 630–643.
World Economic Forum. (2020). The Global Risk Report . http://www3.weforum.org/docs/WEF_Global_Risk_Report_2020.pdf
Wu, D. D., Zhang, Y., Wu, D., & Olson, D. L. (2010). Fuzzy multi-objective programming for supplier selection and risk modeling: A possibility approach. European Journal of Operational Research, 200 (3), 774–787.
Wu, K. J., Liao, C. J., Tseng, M. L., Lim, M. K., Hu, J., & Tan, K. (2017). Toward sustainability: Using big data to explore the decisive attributes of supply chain risks and uncertainties. Journal of Cleaner Production, 142 , 663–676.
Wu, Y., Jia, W., Li, L., Song, Z., Xu, C., & Liu, F. (2019). Risk assessment of electric vehicle supply chain based on fuzzy synthetic evaluation. Energy, 182 , 397–411.
Xingli, W., & Liao, H. (2020). Utility-based hybrid fuzzy axiomatic design and its application in supply chain finance decision making with credit risk assessments. Computers in Industry, 114 , 103144.
Xu, M., Cui, Y., Hu, M., Xu, X., Zhang, Z., Liang, S., & Qu, S. (2019). Supply chain sustainability risk and assessment. Journal of Cleaner Production, 225 , 857–867.
Xu, S., Zhang, X., Feng, L., & Yang, W. (2020). Disruption risks in supply chain management: A literature review based on bibliometric analysis. International Journal of Production Research, 58 (11), 3508–3526.
Xu, X., Meng, Z., & Shen, R. (2013). A tri-level programming model based on Conditional Value-at-Risk for three-stage supply chain management. Computers and Industrial Engineering, 66 (2), 470–475.
Yan, B., Wu, J., & Wang, F. (2019). CVaR-based risk assessment and control of the agricultural supply chain. Management Decision, 57 (7), 1496–1510.
Yan, Q., Zhang, M., Li, W., & Qin, G. (2020). Risk assessment of new energy vehicle supply chain based on variable weight theory and cloud model: A case study in China. Sustainability, 12 (8), 3150.
Yoon, K. P., & Hwang, C.-L. (1995). Multiple attribute decision making: An introduction (Vol. 104). Sage.
Yu, M. C., & Goh, M. (2014). A multi-objective approach to supply chain visibility and risk. European Journal of Operational Research, 233 (1), 125–130.
Zadeh, L. A. (1988). Fuzzy logic. Computer, 21 (4), 83–93.
Zhang, C. L. (2016). Risk assessment of supply chain finance with intuitionistic fuzzy information. Journal of Intelligent and Fuzzy Systems, 31 (3), 1967–1975.
Zhang, D. Y., Cao, X., Wang, L., & Zeng, Y. (2012). Mitigating the risk of information leakage in a two-level supply chain through optimal supplier selection. Journal of Intelligent Manufacturing, 23 (4), 1351–1364.
Zhang, Y. X., Yin, X., & Mao, Z. F. (2019). Study on risk assessment of pharmaceutical distribution supply chain with bipolar fuzzy information. Journal of Intelligent and Fuzzy Systems, 37 (2), 2009–2017.
Zhao, G., Liu, S., Lopez, C., Chen, H., Lu, H., Mangla, S. K., & Elgueta, S. (2020). Risk analysis of the agri-food supply chain: A multi-method approach. International Journal of Production Research, 58 (16), 4851–4876.
Zheng, X., & Zhang, L. (2020). Risk assessment of supply-chain systems: A probabilistic inference method. Enterprise Information Systems, 14 (6), 858–877.
Zhou, Z. Z., & Johnson, M. E. (2014). Quality risk ratings in global supply chains. Production and Operations Management, 23 (12), 2152–2162.
Zhu, Y., Zhou, L., Xie, C., Wang, G. J., & Nguyen, T. V. (2019). Forecasting SMEs’ credit risk in supply chain finance with an enhanced hybrid ensemble machine learning approach. International Journal of Production Economics, 211 , 22–33.
Zhuo, W., Shao, L., & Yang, H. (2018). Mean–variance analysis of option contracts in a two-echelon supply chain. European Journal of Operational Research, 271 (2), 535–547.
Zimmer, K., Fröhling, M., Breun, P., & Schultmann, F. (2017). Assessing social risks of global supply chains: A quantitative analytical approach and its application to supplier selection in the German automotive industry. Journal of Cleaner Production, 149 , 96–109.
Zimmermann, H. J. (1985). Applications of fuzzy set theory to mathematical programming. Information Sciences, 36 (1–2), 29–58.
Zsidisin, G. A., Petkova, B., Saunders, L. W., & Bisseling, M. (2016). Identifying and managing supply quality risk. International Journal of Logistics Management, 27 (3), 908–930.
Download references
Author information
Authors and affiliations.
Operations Management & Quantitative Techniques Group, Indian Institute of Management Raipur, Atal Nagar, Raipur, 493661, India
Nishat Alam Choudhary, Shalabh Singh & M. Ramkumar
Department of Supply Chain Management, Broad College of Business, Michigan State University, 632 Bogue St., East Lansing, MI, USA
Tobias Schoenherr
You can also search for this author in PubMed Google Scholar
Corresponding author
Correspondence to Tobias Schoenherr .
Ethics declarations
Conflict of interest.
The authors declare that they have no conflict of interest.
Additional information
Publisher's note.
Springer Nature remains neutral with regard to jurisdictional claims in published maps and institutional affiliations.
Rights and permissions
Reprints and permissions
About this article
Choudhary, N.A., Singh, S., Schoenherr, T. et al. Risk assessment in supply chains: a state-of-the-art review of methodologies and their applications. Ann Oper Res 322 , 565–607 (2023). https://doi.org/10.1007/s10479-022-04700-9
Download citation
Accepted : 04 April 2022
Published : 03 May 2022
Issue Date : March 2023
DOI : https://doi.org/10.1007/s10479-022-04700-9
Share this article
Anyone you share the following link with will be able to read this content:
Sorry, a shareable link is not currently available for this article.
Provided by the Springer Nature SharedIt content-sharing initiative
- Risk assessment
- Supply chain management
- Decision-making techniques
- Literature review
- Find a journal
- Publish with us
- Track your research
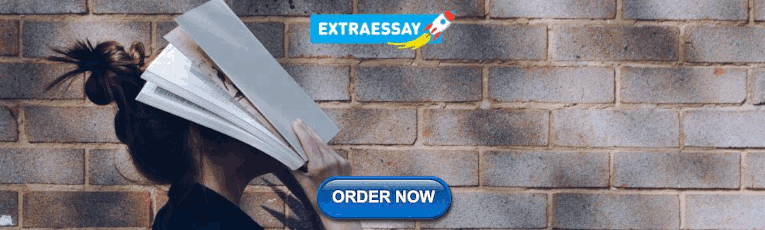
IMAGES
VIDEO
COMMENTS
Introduction. The concept of risk and risk assessments has a long history. More than 2400 years ago the Athenians offered their capacity of. assessing risk before making decisions ( Bernstein ...
Review. 1. Introduction. The concept of risk and risk assessments has a long history. More than 2400 years ago the Athenians offered their capacity of assessing risk before making decisions ( Bernstein, 1996 ). However, risk assessment and risk management as a scientific field is young, not more than 30-40 years old.
Research risk assessment. It's the responsibility of the principal investigators (PI) and researchers to identify reasonably foreseeable risks associated with their research and control the risks so far as is reasonably practicable. All participants and research assistants have the right to expect protection from physical, psychological, social ...
Introduction. Risk is an essential part of everyday life and risks are unavoidable in any complex program. 1 A common definition of risk is "the chance of something happening that will have an impact on the achievement of the stated organizational objectives". 2 Risk management is defined in the literature as "all the activities connected with hazard identification, assessment, selection ...
This paper presents an analysis of data risk factors that scientific data collections may face, and a data risk assessment matrix to support data risk assessments to help ameliorate those risks. The goals of this work are to inform and enable effective data risk assessment by: a) individuals and organizations who manage data collections, and b ...
A risk assessment determines the likelihood, consequences and tolerances of possible incidents. "Risk assessment is an inherent part of a broader risk management strategy to introduce control measures to eliminate or reduce any potential risk- related consequences." 1 The main purpose of risk assessment is to avoid negative consequences related to risk or to evaluate possible opportunities.
This paper delves into the nuanced dynamics influencing the outcomes of risk assessment (RA) in scientific research projects (SRPs), employing the Naive Bayes algorithm. The methodology involves ...
Objectives. This data paper aims to provide the data set of a practical method to health, safety, and environmental risk assessment to assess and rank potential threats/hazards and to prevent and decrease the accidents and harmful consequences at an academic setting. Descriptive data on type of hazards, places, and persons at risk were collected.
8. [A] Carcinogenic risk from paper is close to being acceptable (7.2 × 10 −6 ), but risk from PAH in ink (1 × 10 −3) exceeds de minimis by 1000 times. [B] As the two are combined in the same material, we consider only ink risk. For acceptable risk, divide 200 tons/1000 = 0.2 ton (200 kg) per acre application.
Rationale The spread of severe acute respiratory syndrome coronavirus-2 has suspended many non-COVID-19 related research activities. Where restarting research activities is permitted, investigators need to evaluate the risks and benefits of resuming data collection and adapt procedures to minimize risk. Objectives In the context of the multicountry Household Air Pollution Intervention (HAPIN ...
Risk communication is a fundamental piece of the risk examination, both between risk. assessors and risk directors and between assessors, administrators, and different partners. Risk assessment ...
This paper, therefore, reports the design process for—and content of—a risk assessment framework (RAF). The RAF aims to guide healthcare staff on risk assessment as well as to address current challenges by learning from prescribed good risk assessment practice and the experience of healthcare staff (e.g. doctors, nurses and managers).
This paper aims to. present a tool for assessment and analysis of these risks within. the healthcare system and the probability-impact matrix is the. proposed tool used to prioritise and develop ...
E. Examples of Research Studies with Suggested IRB Decisions . F. Additional Resources . A. Introduction to Risk Assessment and Reduction and the Role of the IRB. Risk Assessment involves the consideration of physical and psychological risks along with the protection of privacy. The student researcher, adult sponsor and qualified scientist must ...
The year 2020 can be earmarked as the year of global supply chain disruption owing to the outbreak of the coronavirus (COVID-19). It is however not only because of the pandemic that supply chain risk assessment (SCRA) has become more critical today than it has ever been. With the number of supply chain risks having increased significantly over the last decade, particularly during the last 5 ...
Conclusions. The prepared systematic literature review was aimed at providing a general overview of related research to risk in mining sector. After a search process that yielded 736 results, 94 papers were selected. These papers were strictly related to the area of analysis, assessment and risk management in mining.
completed and submitted where relevant (see questions 43.2 and 44.1 on the Application Form for Research Ethics Approval). Prior to completion, if there is any aspect of the risks or risk management process associated with your proposed research that you feel unsure about then it is your responsibility (as the researcher) to seek further guidance.
Risk Management―The Revealing Hand. by Robert S. Kaplan and Anette Mikes. This article explores the role, organization, and limitations of risk identification and risk management, especially in situations that are not amenable to quantitative risk modeling. It argues that firms can avoid the artificial choice between quantitative and ...
Total Risk - The risk of an event occurring without consideration for internal controls Manageable Risk - The risk that can be mitigated through internal controls • Not static • Based on a multitude of factors Remaining Risk-The risk that remains after considering current controls • Not static • Based on a multitude of factors 5
Conducting a Risk Assessment . A risk assessment can be a valuable tool to help your unit identify, evaluate and prioritize its risks in order to improve decision-making and resource allocation. Harvard's Institutional Risk Management (IRM) program recommends the following process for c onducting risk assessments. We are here to consult with
46 Research Ethics Support and Review in Research Organisations NOTES: The assessment of risk may be one of those areas where some degree of overlap between research governance and independent ethics review might occur. Institutions might themselves allocate a clear division of labour between governance and
Sample Risk Assessment Templates and Forms. There are multiple ways to record risk assessment reports. Manual risk assessments, such as paper-based forms, are common but can take too much time. This option is prone to erroneous data input and loss of reports. Digital templates and forms are examples of efficient recordkeeping.
Below are the 5 steps on how to efficiently perform risk assessments: 1. Identify hazards. Survey the workplace and look at what could reasonably be expected to cause harm. Identify common workplace hazards. Check the manufacturer's or suppliers' instructions or data sheets for any obvious hazards.
Template. You can use a risk assessment template to help you keep a simple record of: who might be harmed and how. what you're already doing to control the risks. what further action you need to take to control the risks. who needs to carry out the action. when the action is needed by. Risk assessment template (Word Document Format) (.docx)
At the same time, carrying out earthquake disaster risk assessment research is not only the basis for national and regional medium- and long-term planning and development, but also an important decision-making basis for earthquake emergencies. ... this paper conducts a comprehensive assessment of earthquake disaster risk in Qinghai Province ...
Binge watching and sleep and dream issues are important factors to consider in the assessment of suicide risk, particularly among emerging adults who are already at a higher risk. ... Self-report questionnaires were administered to a sample of 1012 emerging adults (50% girls) aged 18-25 (M = 21.73, SD = 2.28). ... Feature papers represent the ...