Detecting earthquakes: a novel deep learning-based approach for effective disaster response
- Published: 01 April 2021
- Volume 51 , pages 8305–8315, ( 2021 )
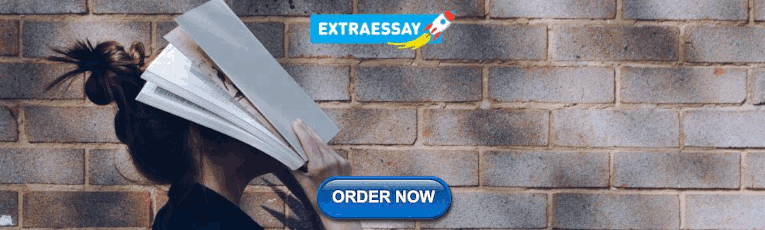
Cite this article
- Muhammad Shakeel ORCID: orcid.org/0000-0003-3822-0917 1 ,
- Katsutoshi Itoyama ORCID: orcid.org/0000-0002-7098-3896 1 ,
- Kenji Nishida ORCID: orcid.org/0000-0003-4214-4005 1 &
- Kazuhiro Nakadai ORCID: orcid.org/0000-0002-6134-4558 1 , 2
1228 Accesses
5 Citations
7 Altmetric
Explore all metrics
In the present study, we present an intelligent earthquake signal detector that provides added assistance to automate traditional disaster responses. To effectively respond in a crisis scenario, additional sensors and automation are always necessary. Deep learning has achieved success in various low signal-to-noise ratio tasks, which motivated us to propose a novel 3-dimensional (3D) CNN-RNN-based earthquake detector from a demonstration paradigm to real-time implementation. Data taken from the ST anford EA rthquake D ataset (STEAD) are used to train the network. After preprocessing the raw earthquake signals, features such as log-mel spectrograms are extracted. Once the model has learned spatial and temporal information from low-frequency earthquake waves, it can be employed in real time to distinguish small and large earthquakes from seismic noise with an accuracy, sensitivity, and specificity of 99.057%, 98.488%, and 99.621%, respectively. We also observe that the choice of filters in log-mel spectrogram impacts the results much more than the model complexity. Furthermore, we implement and test the model on data collected continuously over two months by a personal seismometer in the laboratory. The inference speed for a single prediction is 2.27 seconds, and the system delivers a stable detection of all 63 major earthquakes from November 2019 to December 2019 reported by the Japan Meteorological Agency.
This is a preview of subscription content, log in via an institution to check access.
Access this article
Price includes VAT (Russian Federation)
Instant access to the full article PDF.
Rent this article via DeepDyve
Institutional subscriptions
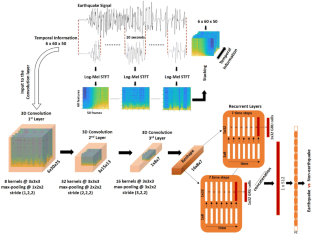
Similar content being viewed by others
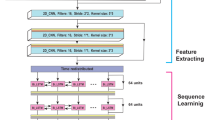
CRED: A Deep Residual Network of Convolutional and Recurrent Units for Earthquake Signal Detection
Research on Earthquake Detection Based on Machine Learning

Real-time earthquake magnitude estimation via a deep learning network based on waveform and text mixed modal
Given D D, Allen R M, Baltay A S, Bodin P, Cochran E S, Creager K, de Groot R M, Gee L S, Hauksson E, Heaton T H, Hellweg M, Murray J R, Thomas V I, Toomey D, Yelin T S (2018) Revised technical implementation plan for the shakealert system—an earthquake early warning system for the west coast of the united states. Technical Reprto, Open-File Report, Reston Survey US G (ed)
Murphy R R, Tadokoro S, Kleiner A (2016) Disaster robotics. Springer International Publishing
Zhu W, Beroza G C (2018) Phasenet: a deep-neural-network-based seismic arrival-time picking method. Geophys J Int 216(1):261–273. https://doi.org/10.1093/gji/ggy423
Google Scholar
Qu S, Guan Z, Verschuur E, Chen Y (2019) Automatic high-resolution microseismic event detection via supervised machine learning. Geophys J Int 218(3):2106–2121. https://doi.org/10.1093/gji/ggz273
Article Google Scholar
Wang J, Xiao Z, Liu C, Zhao D, Yao Z (2019) Deep learning for picking seismic arrival times. J Geophys Res Solid Earth 124(7):6612–6624. https://doi.org/10.1029/2019JB017536
Dokht R M H, Kao H, Visser R, Smith B (2019) Seismic event and phase detection using time–frequency representation and convolutional neural networks. Seismol Res Lett 90(2A):481–490. https://doi.org/10.1785/0220180308
Zhu L, Peng Z, McClellan J, Li C, Yao D, Li Z, Fang L (2019) Deep learning for seismic phase detection and picking in the aftershock zone of 2008 mw7.9 wenchuan earthquake. Phys Earth Planet Inter 293:106261. https://doi.org/10.1016/j.pepi.2019.05.004
Zhou Y, Yue H, Kong Q, Zhou S (2019) Hybrid event detection and phasepicking algorithm using convolutional and recurrent neural networks. Seismol Res Lett 90(3):1079–1087. https://doi.org/10.1785/0220180319
Pardo E, Garfias C, Malpica N (2019) Seismic phase picking using convolutional networks. IEEE Trans Geosci Remote Sens 57(9):7086–7092. https://doi.org/10.1109/TGRS.2019.2911402
Ross Z E, Meier M-A, Hauksson E (2018) P wave arrival picking and first-motion polarity determination with deep learning. Journal of Geophysical Research: Solid Earth 123(6):5120–5129. https://doi.org/10.1029/2017JB015251
Ross Z E, Meier MA, Hauksson E, Heaton T H (2018) Generalized seismic phase detection with deep learning. Bull Seismol Soc Am 108(5A):2894–2901. https://doi.org/10.1785/0120180080
Guo C, Zhu T, Gao Y, Wu S, Sun J (2020) Aenet: Automatic picking of p-wave first arrivals using deep learning. IEEE Trans Geosci Remote Sens:1–11. https://doi.org/10.1109/TGRS.2020.3010541
Yeck W L, Patton J M, Ross Z E, Hayes G P, Guy M R, Ambruz N B, Shelly D R, Benz H M, Earle P S (2020) Leveraging deep learning in global 24/7 realtime earthquake monitoring at the national earthquake information center. Seismol Res Lett 92(1):469–480. https://doi.org/10.1785/0220200178
Ku B, Kim G, Ahn J, Lee J, Ko H (2020) Attention-based convolutional neural network for earthquake event classification. IEEE Geosci Remote Sens Lett:1–5. https://doi.org/10.1109/LGRS.2020.3014418
Mousavi S M, Sheng Y, Zhu W, Beroza G C (2019) Stanford earthquake dataset (stead): A global data set of seismic signals for ai. IEEE Access 7:179464–179476. https://doi.org/10.1109/ACCESS.2019.2947848
Jung M, Chi S (2020) Human activity classification based on sound recognition and residual convolutional neural network. Autom Constr 114:103177. https://doi.org/10.1016/j.autcon.2020.103177
Li G, Zhang M, Li J, Lv F, Tong G (2021) Efficient densely connected convolutional neural networks. Pattern Recogn 109:107610. https://doi.org/10.1016/j.patcog.2020.107610
Lee H, Kwon H (2017) Going deeper with contextual cnn for hyperspectral image classification. IEEE Trans Image Process 26(10):4843–4855. https://doi.org/10.1109/TIP.2017.2725580
Article MathSciNet Google Scholar
Zhang X, Zou J, He K, Sun J (2016) Accelerating very deep convolutional networks for classification and detection. IEEE Trans Pattern Anal Mach Intell 38(10):1943–1955. https://doi.org/10.1109/TPAMI.2015.2502579
Sun Y, Xue B, Zhang M, Yen G G (2020) Evolving deep convolutional neural networks for image classification. IEEE Trans Evol Comput 24(2):394–407. https://doi.org/10.1109/TEVC.2019.2916183
Ren S, He K, Girshick R, Sun J (2017) Faster r-cnn: Towards real-time object detection with region proposal networks. IEEE Trans Pattern Anal Mach Intell 39(6):1137–1149. https://doi.org/10.1109/TPAMI.2016.2577031
Ding X, Li Q, Cheng Y, Wang J, Bian W, Jie B (2020) Local keypoint-based faster r-cnn. Appl Intell 50(10):3007–3022. https://doi.org/10.1007/s10489-020-01665-9
Diaz J, Schimmel M, Ruiz M, Carbonell R (February 2020) Seismometers within cities: A tool to connect earth sciences and society. Frontiers in Earth Science 8. https://doi.org/10.3389/feart.2020.00009
Vaezi Y, Van der Baan M (2015) Comparison of the STA/LTA and power spectral density methods for microseismic event detection. Geophys J Int 203(3):1896–1908. https://doi.org/10.1093/gji/ggv419
Li X, Shang X, Wang Z, Dong L, Weng L (2016) Identifying p-phase arrivals with noise: An improved kurtosis method based on dwt and sta/lta. J Appl Geophys 133:50–61. https://doi.org/10.1016/j.jappgeo.2016.07.022
Li Z, Zhan Z (2018) Pushing the limit of earthquake detection with distributed acoustic sensing and template matching: a case study at the Brady geothermal field. Geophys J Int 215(3):1583–1593. https://doi.org/10.1093/gji/ggy359
Dawei M, En-Jui L, Chen P (2017) Rapid earthquake detection through gpu-based template matching. Comput Geosci 109:305–314. https://doi.org/10.1016/j.cageo.2017.09.009
Perol T, Gharbi M, Denolle M (2018) Convolutional neural network for earthquake detection and location. Sci Adv 4(2). https://doi.org/10.1126/sciadv.1700578
Mousavi S M, Zhu W, Sheng Y, Beroza G C (2019) Cred: A deep residual network of convolutional and recurrent units for earthquake signal detection. Sci Rep 9(1):10267–10267. https://doi.org/10.1038/s41598-019-45748-1 . 31311942[pmid]
Deng Y, Wang L, Jia H, Tong X, Li F (2019) A sequence-to-sequence deep learning architecture based on bidirectional gru for type recognition and time location of combined power quality disturbance. IEEE Trans Ind Inf 15(8):4481–4493. https://doi.org/10.1109/TII.2019.2895054
Kim G, Ku B, Ko H (2020) Multifeature fusion-based earthquake event classification using transfer learning. IEEE Geosci Remote Sens Lett:1–5. https://doi.org/10.1109/LGRS.2020.2993302
Tous R, Alvarado L, Otero B, Cruz L, Rojas O (2020) Deep neural networks for earthquake detection and source region estimation in northcentral venezuela. Bull Seismol Soc Am 110(5):2519–2529. https://doi.org/10.1785/0120190172
Ji S, Xu W, Yang M, Yu K (2013) 3d convolutional neural networks for human action recognition. IEEE Trans Pattern Anal Mach Intell 35(1):221–231. https://doi.org/10.1109/TPAMI.2012.59
Torfi A, Iranmanesh S M, Nasrabadi N, Dawson J (2017) 3d convolutional neural networks for cross audio-visual matching recognition. IEEE Access 5:22081–22091. https://doi.org/10.1109/ACCESS.2017.2761539
Zhang Y, Shi L, Wu Y, Cheng K, Cheng J, Lu H (2020) Gesture recognition based on deep deformable 3d convolutional neural networks. Pattern Recogn 107:107416. https://doi.org/10.1016/j.patcog.2020.107416
Kubota T, Hino R, Inazu D, Suzuki S (2019) Fault model of the 2012 doublet earthquake, near the up-dip end of the 2011 tohoku-oki earthquake, based on a near-field tsunami: implications for intraplate stress state. Progress Earth Planet Sci 6(1):67. https://doi.org/10.1186/s40645-019-0313-y
Ravanelli M, Brakel P, Omologo M, Bengio Y (2018) Light gated recurrent units for speech recognition. IEEE Trans Emerging Top Comput Intell 2(2):92–102. https://doi.org/10.1109/TETCI.2017.2762739
Goodfellow I, Bengio Y, Courville A (2016) Deep learning. The MIT Press
Garbin C, Zhu X, Marques O (2020) Dropout vs. batch normalization: an empirical study of their impact to deep learning. Multimed Tools Appl 79(19):12777–12815. https://doi.org/10.1007/s11042-019-08453-9
Ribeiro D, Nascimento J C, Bernardino A, Carneiro G (2017) Improving the performance of pedestrian detectors using convolutional learning. Pattern Recogn 61:641–649. https://doi.org/10.1016/j.patcog.2016.05.027
Shearer P M (2009) Introduction to seismology. Cambridge Univ. Press
Noda K, Yamaguchi Y, Nakadai K, Okuno H G, Ogata T (2015) Audio-visual speech recognition using deep learning. Appl Intell 42(4):722–737. https://doi.org/10.1007/s10489-014-0629-7
Zhao Q, Guo F, Zu X, Li B, Yuan X (2019) An acoustic-based feature extraction method for the classification of moving vehicles in the wild. IEEE Access 7:73666–73674. https://doi.org/10.1109/ACCESS.2019.2920847
Wang Y, Liu J, Mišić J, Mišić V B, Lv S, Chang X (2019) Assessing optimizer impact on dnn model sensitivity to adversarial examples. IEEE Access 7:152766–152776. https://doi.org/10.1109/ACCESS.2019.2948658
Molaro J Parslee - the open source rover with rasberry shake on-board (2019 (accessed May 14, 2019)). https://raspberryshake.org/parslee-the-open-source-rover-with-raspberry-shake-on-board/
Download references
Acknowledgements
This work is supported by JSPS KAKENHI Grant No. 16H02884, 17K00365, and 19K12017.
Author information
Authors and affiliations.
Department of Systems and Control Engineering, School of Engineering, Tokyo Institute of Technology, 2-12-1 Ookayama, Meguro-ku, Tokyo, 152-8552, Japan
Muhammad Shakeel, Katsutoshi Itoyama, Kenji Nishida & Kazuhiro Nakadai
Honda Research Institute Japan Co., Ltd., 8-1, Honcho, Wako, Saitama, 351-0188, Japan
Kazuhiro Nakadai
You can also search for this author in PubMed Google Scholar
Corresponding author
Correspondence to Muhammad Shakeel .
Additional information
Publisher’s note.
Springer Nature remains neutral with regard to jurisdictional claims in published maps and institutional affiliations.
Rights and permissions
Reprints and permissions
About this article
Shakeel, M., Itoyama, K., Nishida, K. et al. Detecting earthquakes: a novel deep learning-based approach for effective disaster response. Appl Intell 51 , 8305–8315 (2021). https://doi.org/10.1007/s10489-021-02285-7
Download citation
Accepted : 18 February 2021
Published : 01 April 2021
Issue Date : November 2021
DOI : https://doi.org/10.1007/s10489-021-02285-7
Share this article
Anyone you share the following link with will be able to read this content:
Sorry, a shareable link is not currently available for this article.
Provided by the Springer Nature SharedIt content-sharing initiative
- Earthquake signal detection
- Log-Mel spectrogram
- Artificial intelligence
- Disaster response
- Find a journal
- Publish with us
- Track your research

An official website of the United States government
The .gov means it’s official. Federal government websites often end in .gov or .mil. Before sharing sensitive information, make sure you’re on a federal government site.
The site is secure. The https:// ensures that you are connecting to the official website and that any information you provide is encrypted and transmitted securely.
- Publications
- Account settings
Preview improvements coming to the PMC website in October 2024. Learn More or Try it out now .
- Advanced Search
- Journal List
- Sensors (Basel)

Earthquake Detection in a Static and Dynamic Environment Using Supervised Machine Learning and a Novel Feature Extraction Method
Irshad khan.
1 School of Computer Science, Kyungpook National University, Daegu 41566, Korea; [email protected]
Seonhwa Choi
2 National Disaster Management Research Institute, Ulsan 44538, Korea; rk.aerok@33iohchs
Young-Woo Kwon
Detecting earthquakes using smartphones or IoT devices in real-time is an arduous and challenging task, not only because it is constrained with the hard real-time issue but also due to the similarity of earthquake signals and the non-earthquake signals (i.e., noise or other activities). Moreover, the variety of human activities also makes it more difficult when a smartphone is used as an earthquake detecting sensor. To that end, in this article, we leverage a machine learning technique with earthquake features rather than traditional seismic methods. First, we split the detection task into two categories including static environment and dynamic environment. Then, we experimentally evaluate different features and propose the most appropriate machine learning model and features for the static environment to tackle the issue of noisy components and detect earthquakes in real-time with less false alarm rates. The experimental result of the proposed model shows promising results not only on the given dataset but also on the unseen data pointing to the generalization characteristics of the model. Finally, we demonstrate that the proposed model can be also used in the dynamic environment if it is trained with different dataset.
1. Introduction
Due to the nature of earthquakes, significant research efforts have been made to develop real-time earthquake detection systems for disaster management. Earthquake fatal levels of motion can cause fatalities and damage in populated regions [ 1 ]. Because typical human structures are unable to resist large magnitude earthquakes, possible ways to overcome such fatalities are to build earthquake-resistant buildings or to take advantage of an Earthquake Early Warning (EEW) system that provides seconds to minutes of warning in advance, thereby allowing people to move to safe areas or shut down dangerous machinery. However, it is not only costly to construct earthquake-resistant structures but also difficult to build a highly accurate nationwide EEW system.
In recent years, emerging computing technologies such as mobile computing and Internet-of-Thing (IoT) systems equipped with various MEMS (Micro Electro Mechanical Systems) sensors (e.g., accelerometers, gyroscopes, GPSs), Wi-Fi, bluetooth, etc., have been widely adopted in the following areas: smart healthcare, intelligent transportation systems, smart buildings, and earthquake early warning systems [ 2 , 3 , 4 , 5 ]. In particular, the MyShake project [ 6 ] leverages mobile technologies to develop an earthquake early warning system that combines a seismic method and a machine learning (ML) technology. The system is installed on a volunteer’s smartphone and then detects earthquakes using an Artificial Neural Network (ANN). It is the first global earthquake detection system using a smartphone and machine learning technique.
Based on the available literature, we can divide IoT-based earthquake detection into two parts by applicability. A mobile-based earthquake early warning system uses low-cost MEMS sensors in a smartphone or an IoT device as a seismic sensor in a dynamic environment, while the stationary sensor-based early warning system uses a dedicated device as a seismic sensor in static (i.e., fixed) environment. The non-earthquake data in a static environment include internal and external noises. The source of internal noises mainly come from a sensor in which an accelerometer continuously captures some vibratory signals. The external noises come from outside of a sensor because of constructions, heavy-traffic roads, etc., near the installed sensor. In a dynamic environment, the variety of human activities become a major part of the non-earthquake data, which significantly affects the system performance, and thus the earthquake detection task using a low-cost sensor is very challenging. In this environment, training a machine learning algorithm is critical because of the activities whose frequency and amplitude patterns look like earthquakes.
In traditional earthquake early warning systems, because acceleration data recorded from seismic sensors installed nationwide are sent to a centralized server for earthquake detection, network and processing delays are inevitable. Because there are a few seconds between a P-wave and an S-wave (e.g., 10 s [ 7 ]) depending on the distance from an hypocenter [ 8 , 9 ], to reduce the blind area of earthquake early warning, on-site or standalone earthquake detection devices have been recently introduced [ 10 , 11 , 24 ]. However, because of the real-time processing requirement and resource constraints of a detection device, heavy computational methods and deep neural networks cannot be applied at the sensor side. Nevertheless, the final detection can be performed at the server-side through advanced detection algorithms, a simple detection algorithm with a few features that require light computations at a client-side to complete the detection procedure as soon as possible is required. Because an earthquake detection device can be operated in either a static or a dynamic environment, trivial statistical amplitude and frequency features are not suitable for such environments.
As a result, our focus is to improve a machine learning model for an earthquake alert device that we developed in our prior work to detect earthquakes in static and dynamic environments [ 10 , 11 ]. The device not only detects an earthquake but also sends an alert with earthquake response information to nearby smart devices such as smartphones, smart TVs, etc. As the device operates independently, without any Internet connection or collaborations with other alert devices, it needs a highly accurate earthquake detection algorithm. Because traditional methods to detect earthquakes such as STA/LTA have high false alarm rates, it is risky to use only one earthquake detection method for a standalone device. Thus, we use both traditional earthquake detection methods and emerging technologies together to decrease the chance of false alarms and increase the overall earthquake detection ability. In this article, we systematically compare different earthquake features and datasets representing static and dynamic environments for the earthquake alert device, and then, based on our experimental results, we propose a new earthquake detection model that can be used in both static and dynamic environments.
The rest of the article is structured as follows. Section 2 introduces our prior work and compares relevant research efforts. Section 3 explains the methodology used in the proposed work, while Section 4 discusses (in detail) the experimental work done. Finally, Section 5 concludes this article.
2. Prior Work and Related Work
In this section, we introduce our prior work for earthquake detection using emerging technologies and then compare our work with related research efforts.
2.1. Prior Work
In our prior work [ 10 , 11 ], we developed an earthquake alert device that includes a 32 bit processor, Wi-Fi, bluetooth, a buzzer, an LED light, etc as shown in Figure 1 ; its hardware system is described in Table 1 . To detect an earthquake, the earthquake alert device uses a machine-learning-based algorithm and then sends out an alert message to nearby devices such as smartphones, smart watches, AI speakers and home automation devices, using Bluetooth or Wi-Fi.

The developed earthquake alert device.
Specifications of the developed earthquake alert device.
The detection algorithm that we developed for the earthquake alert device is based on an artificial neural network (Artificial Neural Network) [ 12 ], which is a simple machine learning technique widely used in the last several decades. The used ANN model has three neurons in the input layer, five neurons in the hidden layer, and one neuron in the output layer as shown in Figure 2 .

The ANN model with three inputs with one hidden layer.
The detection algorithm consist of four phases including feature extraction, pre-processing, training, and testing of a machine learning model. To detect earthquakes, we use three features including inter-quartile range (IQR), zero crossing rate (ZC), and cumulative absolute velocity (CAV), which are the same features used in MyShake [ 6 ]. IQR is the amplitude between 25% and 75% of the acceleration vector sum. ZC is a frequency measure that indicates the number of time that the signal changes its sign. CAV is a cumulative measure of amplitude of the vector sum of three component acceleration. Then, we use 2 s of a sliding window with a 1.5 s overlap window on the acceleration data to calculate these three features in real-time.
After the extensive experiments, we installed devices in 29 locations of three different cities and operated them for two months. Even though the model showed a high accuracy of over 95% in our experiments, we found a few false alarms throughout the real operation. Therefore, in this article, we carefully assess the performance of the earthquake detection model and test its added features to determine the best features for earthquake detection in our operational environments. In the rest of the article, we present our efforts on the development of various features for earthquake detection and experimental results.
2.2. Related Work
Various monitoring systems leveraging mobile technologies have been proposed, such as eWatch, smartphones, and MEMS [ 13 ]. In particular, extensive research has been done on wearable IoT in healthcare. For example, eWatch [ 14 ] is an online activity recognition system that embeds four different sensors, i.e., an accelerometer, alight sensor, microphone, and thermometer. The system is very responsive and needs no wireless communication. Similarly, Kao et al. [ 15 ] have used a tri-axial accelerometer in a portable device that can be placed on the user’s dominant wrist to detect human activities, such as running, working, swinging, brushing teeth, knocking, walking, and hitting. The accelerometer of a smartphone has also been used for human activity recognition, such as walking, running, walking (fast and slow), climbing stairs (up and down), and excercising aerobatics [ 16 ]. In the literature, there are many applications that used a sensor-based monitoring system; however, these are beyond the scope of this article. Instead, we deal with the binary classification problem, and our goal is to detect earthquakes from the accelerometer data in which the rest of the data is the non-earthquake class, whether that includes human activity or noise.
Traditional seismic detection involves computational methods such as Short-Term Average/Long-Term Average(STA/LTA), cross-correlation, and template matching [ 17 , 18 , 19 , 20 , 21 ]. These methods are useful but have certain limitations. For example, STA/LTA can detect earthquakes without prior knowledge of the event but can also produce false positives when the situation is more challenging, such as when it involves a low signal to noise ratio (SNR), overlapping events, and some cultural noise. Similarly, cross-correlation detects earthquake signals but is computationally expensive, while template-based matching is a powerful computational method but requires prior information. The above methods are mostly operational in the central system. Moreover, the computational methods do not exhibit any intelligent behavior and operate only on the fixed threshold values.
Recently, there have been research efforts to use MEMS-based sensors as seismic sensors due to their low computational power and cost. Specifically, the NetQuakes project developed by the United States Geological Survey (USGS) installed MEMS sensors around the world but mostly in California [ 22 ] and began to collect seismic data from them. Similarly, the following projects developed around the world use MEMS sensors; Home Seismometer Network (HSN) developed by Japan Meteorological Agency (JMA), Palert system developed by NTU(National University Taiwan), Community Seismic Network and Quake-catcher Network (QCN) developed by California Institute of Technology and Standford University, respectively [ 23 , 24 , 25 , 26 ].
IoT systems for public safety are widely adopted, where the intelligence behavior of such sensors as MyShake, which combines machine learning with traditional STA/LTA algorithm, limit or exclude human intervention [ 27 ]. To our knowledge, this is the first globally used smartphone-based earthquake early warning system used in a dynamic environment. Besides, deep learning approaches have also been adopted to detect earthquakes offline or online at the server-side, such as searching seismic data, mining undetected earthquakes in the data archives, and finding the earthquake location [ 28 , 29 ]. In this article, our first goal is to improve the existing earthquake detection model’s performance in the static environment. The second goal is to evaluate the machine learning algorithms and feature sets (both existing and proposed) for sensor-side in the dynamic environment with a variety of human activities.
3. Proposed Methodology
This section will discuss the feature extraction and machine learning methodology. The proposed work follows the supervised machine learning methodology. The steps involved in our proposed methodology are feature extraction, preprocessing, training, testing, and validation.
3.1. Feature Extraction
In the context of ML-based earthquake detection, amplitude and frequency are the two key pieces of information among different statistics of the accelerometer signal. Therefore, based on these two statistics, we extracted features from X , Y , and Z components in the time and frequency domains. Time domain features include features used in MyShake and our proposed features. The MyShake features are the following.
- IQR (Interquartile Range): IQR is the interquartile range Q 3 – Q 1 of the 3 component vector sum V S ; V S = X 2 + Y 2 + Z 2 (1) where X , Y , and Z are the acceleration components.
- C A V (Cumulative Absolute Velocity): C A V feature is the cumulative measure of the V S in the time window and is calculated as C A V = ∫ 0 s | V S ( t ) | d t (2) where s is the total time period of the feature window in seconds, and t is the time. In this work, we used a two-second feature window.
- Z C (Zero-Crossing): Z C is the maximum zero-crossing rate of X , Y , and Z component and the zero-crossing rate of component X can be calculated as: Z C X = 1 N − 1 ∑ t = 1 N − 1 1 R < 0 ( X t X t − 1 ) (3) where N is the total length of the signal X and 1 R < 0 is indicator function.
IQR and CAV are the amplitude features, while ZC is the frequency feature, and these are proposed in [ 6 , 30 ]. These features detect earthquakes and can discriminate non-earthquake data, but through exhaustive experimental evaluations and also its implementation in the static environment as given in our previous work, we found that in a noisy environment (noisy sensors or external events), its performance can be degraded. Moreover, a dynamic environment—in which the variety of human activities that include some challenging activities whose signal patterns are similar earthquake patterns—can also degrade the performance of the model trained on these features. We observed that among the three features, zero-crossing is more sensitive to noise and creates false alarms even if there is wavering involving only one component. This is due to the fact that it counts the feature value for each component and then selects the maximum one. Hence, if there is a count at only one component, then it will select that value and discard the zero-crossing information of the other two components. We observe that earthquake motion has a zero-crossing rate at more than one component simultaneously, while other data—particularly noise data—have zero-crossing rates at only one component most of the time, as given in Table 2 . Two-second feature window with a 1-second sliding window is used to count ZC in both earthquake data and noise data, where, for the earthquake data, we selected 3 s of the strongest portion of the earthquake. Further details about datasets are given in the results section.
ZC count at only one component.
This sensitivity issue not only affects the performance of the machine learning model in a dynamic environment (when the sensors are assumed to be smartphones used in daily life) but also affects the model performance while in a fixed-sensors environment. Therefore, to overcome this issue, we tested different variations and statistical features of the amplitude and frequency characteristics of the signal. After extensive experiments, we proposed some variants of the zero-crossings, which are the following.
- Max ZC: Counts for that component whose maximum absolute amplitude value is greater than the other two components when there is more than one zero-crossings at a particular time t. Otherwise, it will behave like the ZC feature.
- Min ZC: Counts for the minimum one, which has lowest absolute amplitude value among the three, if there are zero-crossings in more than one component.
- Max Non ZC: This feature counts the maximum absolute amplitude component for non-zero-crossings when there is more than one non-zero-crossings simultaneously at a particular time.
These features are also based on the frequency and amplitude information of the signal; however, these are more specific and consider the other statistics, like multi-component zero-crossings and the frequency information, when there is no zero-crossing. The non-zero-crossing statistic is also important, because if the occurrences of ZC indicate the probability of an earthquake situation, then this feature indicates the probability of a normal situation. Similarly, the multi-component property of these features is also helpful to discriminate human activities from earthquake samples more efficiently.
Apart from the proposed features, we also tested features from the frequency domain, i.e., FFT (Fast Fourier Transform) [ 31 ]. In order to consider only one component of FFT, we used a Singular Value Decomposition (SVD) method to decompose multi-dimensional data into one dimension [ 32 ]. The SVD of an accelerometer matrix A of three components, X, Y, and Z.
where, A is an M x N matrix, where M represents two-second points, i.e., 200, and N is 3. SVD provides three new vectors U M x M , S M x N , a n d V N x N T , which, if linearly combined, give back the approximated original vector; where U is the set of singular vectors with singular values in vector S , V T is the primary direction. The new vectors are ordered, and the first vector explains most of the original acceleration amplitude and frequency information, as shown in Figure 3 . Figure 3 a depict almost the same structure; therefore, we select the first vector as a primary vector U [ : , 0 ] from the given SVD’s, along with the first value S [ 0 ] of S , which is a scaling factor (give amplitude information of the given vector). We extracted the following three additional features.

A two-second window of the strongest portion of the earthquake; ( a ) The vector sum of X, Y, and Z; ( b ) vector sum of the primary vector of SVD (center); ( c ) scree plot of the three components.

FFT of the primary vector of the SVD extracted from the two-second strongest portion of the earthquake window.
- SVD_Scale: The S [ 0 ] is considered the average amplitude feature.
- SVD_ZC: We also computed the zero-crossing rate of the primary vector i.e., U [ : , 0 ] .
We also analyzed the tsfresh [ 33 ], a time series feature-extraction python package for searching the computationally low and effective features such as c3, cid-ce, entropy, mean, and count-above-mean, etc. However, the feature space visualization was not more promising than the abovementioned features. Therefore, we selected only the above features for model training and testing.
3.2. Pre-Processing
In our methodology, the pre-processing involved balancing the dataset and scaling the features to range from 0 to 1. Balancing is required because the imbalanced datasets greatly affect the performance of the machine learning model [ 34 ]. In our case, the non-earthquake dataset (noise and human activities) is much larger than the earthquake dataset. Hence, we used the K-mean clustering algorithm to balance the non-earthquake dataset [ 35 ]. Using the K-Mean, clusters of the non-earthquake data are created according to the total number of earthquake data points, and we used centroids of the clusters to represent the non-earthquake data. As shown in Figure 5 , centroids represent the original data points in the IQR, ZC, and CAV feature space.

Centroids of the non-earthquake data (noise with some human activity).
Moreover, to improve the prediction performance and decrease the training time of the model, we also scaled data point d to the range of 0 to 1 using the min-max scaler as follows:
3.3. Machine Learning Model
The ANN (Artificial Neural Network) algorithm is designed to accomplish the detection task using both the existing and proposed features [ 12 ]. We used an X, 5, 1 layer network architecture for the training and testing of the ANN algorithm, as shown in Figure 6 , where X is the number of features input to the model. We kept the same five nodes of the hidden layer as proposed in [ 6 ], because the number of features input to the model is 3, 4, or 5, and through experimental results, the 5-node hidden layer is still good for the given number of features. For training the models, we used a multi-layer perceptron (MLP) with the stochastic gradient descent solver [ 36 , 37 , 38 ]. For the hidden layer and output layer, the inputs from the previous layer to each node will be first summed and then fed into an activation function as follows:
Here, w denotes the weights vector, d is the input vector, b is the bias, y is the output of the given node, and ϕ is the non-linear activation function. The logistic sigmoid function is used as the activation function for hidden and output layers, which is defined on input d as

The general structure of the ANN model.
4. Results and Discussion
To obtain a comprehensive comparison, we compared the proposed features with the existing features in both the static and dynamic environments. Accordingly, we trained ANN models with different non-earthquake datasets to distinguish the environments.
4.1. Dataset
The dataset that we used for training and testing the ANN models contains two classes of label data. One class of data is the time series earthquake dataset, which was download from the National Research Institute of Earth Science and Disaster Prevention (NIED) and USGS (United States Geological Survey) database [ 39 , 40 ]. A total of 385 earthquakes events with magnitudes ranging from 4 to 8, recorded between April 2009 and May 2019, were selected from the NIED database. Moreover, 120 stations’ data of three earthquakes, i.e., Tottori (2000) (magnitude 6.61), Niigata (2004) (magnitude 6.63) and Chuetsuoki (2007) (magnitude 6.8) were downloaded from the USGS database. The NIED earthquake data were pre-processed and converted into the unit (g). The sampling rate of all the earthquake data is 100 Hz. The data are presented in three columns titled EW, NS, and UD, respectively, where EW (East-West) and NS (North-South) are horizontal components, and UD (Up-Down) is a vertical component.
The second class of data is the time series of non-earthquake dataset recorded on mobile phones for several hours. In the experiments, we used two types of non-earthquake data, i.e., human activity data and noise data. Human activity data includes such activities as bicycle, bus, and car (in hand) riding, jump rope, running (hand, pocket), desk shaking (while mobile on top), climbing stairs (up-down) (bag, hand, pocket), walking (bag, hand, pocket), standing still, and working. Contrarily, noise data contain floor noises (e.g., different degrees of elevations) and machinery noises. These noise data are the external source data; and hence, to include sensor noise data, we also include the tail data of earthquake signals.
The models’ generalization characteristics are validated on the third dataset, which is earthquake data collected during shake table tests using different accelerometers (i.e., ADXL355 [ 41 ], LIS3DHH [ 42 ], MPU9250 [ 43 ], and MMA8452 [ 44 ]), which have different HW specifications and costs. Sensors were placed on the shake table located in Pusan National University to record two realistic earthquakes including Pohang [ 45 ] and El Centro [ 46 ], and we collected acceleration data from such low-cost accelerometers.
4.2. Performance Metrices
Different machine learning algorithms are evaluated with different performance matrices. The classification performance metrics are based on the confusion matrix [ 47 ], which gives a table of TP (True Positive), TN (True Negative), FP(False Positive), and FN (False Negative) as shown in Figure 7 .

Confusion Matrix.
Common performance measures of the classification such as accuracy, precision, and recall, are calculated from the confusion matrix. Accuracy is computed as
Accuracy is the ratio of predictive observations to the total observations; it is an intuitive measure to show the overall performance of the model.
Precision is computed as
The precision measure determines how accurate the model is out of those predictive positives. In other words, how many of them are actually (correctly) positive among all predictive positives. High precision means a low false-positive rate.
Recall is computed as
Recall determines the sensitivity of the model in terms of how many times it detects earthquakes from all the instances of the earthquakes.
The F1 score is a single score of precision and recall which is the harmonic mean of both. It takes both false positive and false negatives into account.
Finally, the classification model performance false and true positive rates can be visualized through a receiver operating characteristics (ROC) curve [ 48 ].
4.3. Evaluation
The evaluation is done in static and dynamic environments. In the static environment, the sensors are fixed (stationary) and, therefore, training a model with varieties of human activities is not required. Still, to train the model properly for the static environment, we used some instances of human activities like walking and waiting. This is because the model converges too quickly in the presence of only noisy data and thus cannot learn the underlying patterns of the data, especially earthquake patterns. We evaluated models based on different features and then, for the dynamic environment, we tested the model that showed the best results in the static environment to evaluate its full implementation applicability.
4.4. Static Environment
During the model evaluation in the static environment, we used a combination of different features discussed above. We trained the model using amplitude features combined with frequency features. Here, from the sets of different models, we will discuss six models, beginning with the existing MyShake model, i.e., IQR, ZC, and CAV (Model 1). The remaining five models with feature sets are given below.
- Model 2: IQR, FFT, CAV
- Model 3: IQR, ZC, FFT, CAV
- Model 4: IQR, SVD_ZC, CAV
- Model 5: IQR, ZC, CAV, SVD_Scale
- Model 6: IQR, CAV, MAX_ZC, MIN_ZC, MAX_NON_ZC
Through the experimental search, the five-nodes hidden-layer structure was used for training all the models, and the input layer nodes were determined according to the feature set. Table 3 provides details of the dataset used for training and testing the models in the static environment.
Dataset used for models training and testing in a static environment.
For training and testing the models, we split the data (earthquake and centroids of the non-earthquake) into 80% and 20%, respectively. In terms of testing the models, we first tested each model on the remaining 20% of the centroids (experiment 1), and then, in the second experiment (experiment 2), the models were tested on the original data (all the instances of the earthquake and non-earthquake class). For the receiving operating characteristics curves of the ANN models, 20% of the remaining data are shown in Figure 8 . All the models showed good results, where Model 6 shows the high AUROC of 0.9899 and rapid climb, which is close to the ideal case.

ROC curves of the models on 20% test data.
Despite the fact that all the models showed good results, other performance measures of the models should also be considered. Table 4 gives the performance score of the models on the two test datasets; the first test dataset is the remaining 20% of the centroids data and the second test dataset is the original non-earthquake data. Among all the models, Model 6 successfully classified the earthquake instances in both experiments (i.e., centroids and original data) with high accuracy, F1 score, and a low number of false positives. The false positive in the second experiment is comparatively high as compared to experiment 1 because there are more data points for a particular non-earthquake category with variations in the data. Still, the accuracy score of both experiments was very good. The accuracy and recall of the second experiment are slightly better than experiment 1, which indicates that the model is also trained well for unseen data to deal with the over-fitting problem. As a single frequency feature, FFT standalone cannot provide assistance to the model, as shown in the results of Model 2. However, with the ZC feature, it showed some improvement in the performance of Model 1, as seen in the Model 3 row. The new features of ZC_SVD and SVD_Scale can be used as a frequency feature and amplitude feature as suggested by Models 4 and 5, respectively.
Performance evaluation of the ANN models on test data.
The FP counts of Model 1 indicate that the model is sensitive to the noise due to the frequency feature of ZC, as discussed earlier. However, Model 6 has three different statistics for the frequency information, which gives more information to the machine learning model and contributes to the improved results of the model. It has been observed that, for the amplitude information, IQR and CAV are still good features, but the frequency feature is the most sensitive one since it is not only affected by the noise but also by the difference of the sampling rates.
Models Validation
As described in the dataset section, to further validate and compare these models on the unseen data, we used Pohang and El Centro earthquakes data. Here, we used 100% and 50% scale of both the earthquakes, where a 100% of Pohang and El Centro respectively represents approximate magnitudes of 5.4 and 6.9 earthquakes. Similarly, the 50% scale earthquake data represents moderate and low amplitude earthquake data, which allows us to evaluate the model performance on these low scale data.
During each test, these 100% and 50% scale data of both the earthquakes are input into the trained models. The duration of each earthquake data is 70–80 s, and the features are extracted from each sensor’s data according to the model feature set; for example, for Model 1, we extracted IQR, ZC, and CAV features. A two-second sliding window with a one-second overlap window was used on the raw acceleration to extract features.
The test results of the models on the Pohang and El Centro data are given in Figure 9 and Figure 10 , respectively. Figure 9 a shows the results of the detection process for Model 1 (right panel) on the normalized vector sum of three axes, along with the raw acceleration (left panel) of X, Y and Z components of the Pohang earthquake on the 100% scale. The sampling rate of the signal is 25 Hz and the threshold value is kept at 0.9 for the ANN models to detect earthquake triggers. The reason for choosing this threshold value is to decrease the FP rate of the model in the system implementation. As given in Table 5 , FP counts of the models’ on the original test data decreased, as a result, the precision of the models increased but the recall is also decreased. Here, we can see that the F1 score describes the overall performance of the models, and again Model 6’s F1 score is high compared to other models when the threshold value is set to 0.9. The cyan vertical line in the left panel represents the earthquake trigger when the ANN probability (wavy green line in the right panel) meets the threshold value (red line in the right panel).

Models’ test results on the Pohang earthquake at a scale of 100% (left) and 50% (right); the results are ordered from top to bottom for each subfigure’s sensor, namely ADXL355, LIS3DHH, MMA8452, and MPU9250.

Models’ test results on the El Centro earthquake at a scale of 100% (left) and 50% (right); the results are ordered from top to bottom for each subfigure’s sensor, namely ADXL355, LIS3DHH, MMA8452, and MPU9250.
Performance evaluation of the ANN models on the original test data using a 0.9 threshold value.
Model 1 detected earthquakes in both the 100% and 50% scales of the Pohang earthquakes, and did so at peak acceleration with very low false alarms, as shown in Figure 9 a,b. The ANN probability graph shows very smooth and stable probabilities in the LIS3DHH and MPU9250 cases during the non-earthquake portion, while the other two cases (i.e., ADXL355 and MMA8452 results, show high peaks due to sensor noise). In our previous publications [ 10 , 11 ], we observed that ADXL355 has noise on one component while MMA8452 has noise on all the components, which confused the model due to the zero-crossing feature. Model 1 produced similar results for the El Centro earthquake data across different scales, as shown in Figure 10 a,b. In the El Centro case, as we can see its signal pattern is different from the Pohang pattern, which resulted in more false triggers generated by Model 1.
Compared to Model 1, the proposed Model 6 showed promising results on the validation data of Pohang and El Centro earthquakes of different scales and sensors. as shown in Figure 9 k,l and Figure 10 k,l. The proposed Model 6 shows better performance on the accelerometer sensor data, where Model 1 produced high peaks in the probability graphs. Further, in the case of the ADXL355 accelerometer sensor, Model 6 was able to with the problem of noise on one component due to its extensive information about zero-crossing frequency. Multi-component noises can be a challenge for model performance, as the MMA8452 case reveals.
Model 2 shows poor results due to the lack of frequency information in the time domain, as shown in Figure 9 c,d and Figure 10 c,d. Despite having tested the FFT feature to provide the frequency information, through the experimental work, we observed that a single FFT feature is not enough to train the model. Then, although combine with the ZC feature it showed slightly better results than Model 1 in 20% test, the results of the Pohang and El Centro test data were poorer than those of Model 1, as shown in Figure 9 e,f and Figure 10 e,f. Similarly, the results of model 5 were also insignificant, as shown in Figure 9 i,j and Figure 10 i,j.
To Summarize, Models 1 and 6 show outstanding and consistent results in the unseen data, whereas other models show different performance results in different experimental scenarios. Therefore, we chose to test Models 1 and 6 in a dynamic environment.
4.5. Dynamic Environment
In the dynamic environment, we evaluate Model 6, which showed very good results as compared to other models, including the model used in MyShake. Therefore, to evaluate the model with the proposed features in the dynamic environment, we considered all the human activities recorded on smartphones for several hours. Due to the increase of non-earthquake data, we also include more earthquake data to keep the balance between earthquakes and non-earthquakes and then test the model performance on the larger datasets. The trained model is referred to as Dyn-model 6, and to compare the model with the state-of-the-art Myshake features, we also train Model 1 using the dynamic dataset and referred to it as Dyn-model 1. Table 6 provides details of the dataset used for training the models in the dynamic environment.
Datasets used for model training and testing in a dynamic environment.
We perform the same methodology used for the static data; that is, we first extracted features from the non-earthquake data then calculated centroids equal to the earthquake data points. To train the model, we split the data (earthquake and centroids) into 80% and 20% for training and testing, respectively. The best test results from a number of experiments are given in Table 7 . We can see that the accuracy of the Dyn-model 6 on the original data is higher than that of the centroids data, whereas the Dyn-model 6 accuracy on 20% is similar to its accuracy in the static test (i.e., approximately 94%). However, its accuracy on the original data in the dynamic test is lower than the static test due to the variation in the non-earthquake data. In the original data, the Dyn-model 6 falsely detects 1804 non-earthquake instances as earthquakes. We further investigate the Dyn-model 6 results on each activity and found that the FP of the Dyn-model 6 was mostly produced due to human activities such as bus riding, desk shaking, and walking, with the accuracy of 90.3%, 93.23%, and 91.65%, respectively.
Performance evaluation of the ANN models on the test data in the dynamic environment.
Like Dyn-model 6, Dyn-model 1 accuracy was also decreased due to the activities that can result in earthquake-like signals. Moreover, Dyn-model 6 results are better than those of Dyn-model 1 results due to the increasing frequency features and different recalculation of ZC to make it conditional to the maximum amplitude.
Model Validation
Similar to the validation for the static environmental model, we validated Dyn-model 6 on the unseen earthquake datasets generated during shake table tests on different sensors, i.e., El Centro and Pohang, as shown in Figure 11 . This was conducted because our ultimate goal was to see whether the trained model can detect earthquakes when the device is in a steady-state, as compared to the proposed Dyn-model 6, which was trained for the static environment and then retrained for the dynamic environment. The newly trained Dyn-model 6 showed almost the same detection results, but with fewer earthquake detection triggers during the earthquake windows for both the scales of the El Centro and Pohang earthquakes. The proposed Dyn-model 6 also detected some false triggers as can be shown in Figure 11 a. In particular, the proposed Dyn-model 6, when trained with dynamic data, showed far fewer detection triggers on the Pohang earthquakes; rather than detecting the earthquake, it only showed a peak below the given threshold of 0.9 for the data recorded on the MPU9250 scale 50%, as shown in Figure 11 d. These results indicate that the model learned differently, and the challenging non-earthquake data can affect the model performance, which can result in a false alarm, whether false positive or false negative.

Dyn-model 6 test results on the El Centro earthquake of scale 100% (left-top) and scale 50% (right-top); Model test results on the Pohang earthquake of scale 100% (left-bottom) and scale 50% (right-bottom); the results are ordered from top to bottom for each subfigure’s sensor, namely ADXL355, LIS3DHH, MMA8452, and MPU9250.
The validation results of Dyn-model 1 on the Pohang and El Centro datasets are given in Figure 12 . This time, Dyn-model 1 results are not as promising as those of the static environment in all the windows. In particular, it failed to detect the earthquake in the Pohang 50% scale. It showed the same probability peaks in the ADXL355 and MMA8452 sensors in all the windows, which support our claim discussed with regard to the static environment. Moreover, compared to the proposed model that is Dyn-model 6, again the performance of the existing model that is Dyn-model 1 is below in the dynamic environment too.

Dyn-model 1 test results on the El Centro earthquake of scale 100% (left-top) and scale 50% (right-top); Model test results on the Pohang earthquake of scale 100% (left-bottom) and scale 50% (right-bottom); the results are ordered from top to bottom for each subfigure’s sensor, namely ADXL355, LIS3DHH, MMA8452, and MPU9250.
4.6. Threats to Validity
The experimental results are subject to the following validity threats. Even though we deal with heterogeneous data recorded on different sensors, the models were trained on the data which were mostly recorded on the seismic sensors but we used low-cost accelerometers for validating their performance. Therefore, the experimental result may be different if the models are properly trained using earthquake data recorded on low-cost accelerometers. Furthermore, the datasets that we collected have different sampling rates. For instance, the models were trained with the earthquake data at a 100 Hz sampling rate and different sampling rate of non-earthquake data ranging from 50 to 100 Hz. Also, the sampling rate for the validation was 25 Hz. Despite above validity threats, the model showed outstanding performances, but the accuracy measurements may vary for different datasets and sampling rates.
5. Conclusions
In this article, we categorized seismic detection mechanisms into the static and dynamic environments and then evaluated different features using the ANN model in the static environment, which include new features and the existing features used in previous studies. Based on the experimental results performed in the static environment produced, the proposed features demonstrated more improved results than the existing features. For the dynamic environment, we used the same model tested for the static environment and then trained it with different datasets, which included various human activities. The selected model showed promising results with a lower possibility of false alarms than other models. As a result, our approach can be used for both a static and a dynamic environment without changing its model and features. As a future research direction, we will explore new features and models that require less computational power while maintaining a high detection ability against the challenging non-earthquake datasets.
Author Contributions
Conceptualization, Y.-W.K.; Data curation, I.K.; Funding acquisition, Y.-W.K.; Methodology, I.K.; Project administration, S.C. and Y.-W.K.; Software, I.K.; Supervision, S.C. and Y.-W.K.; Validation, I.K.; Writing—original draft, I.K.; Writing—review & editing, Y.-W.K. All authors have read and agreed to the published version of the manuscript.
This work was supported by National Disaster Management Research Institute (2019-02-02), Basic Science Research Program through the National Research Foundation of Korea(NRF) grant funded by the Ministry of Education(NRF-2017R1C1B5075658), and the BK21 Plus project (SW Human Resource Development Program for Supporting Smart Life) funded by the Ministry of Education, School of Computer Science and Engineering, Kyungpook National University, Korea (21A20131600005).
Conflicts of Interest
The authors declare no conflict of interest.
Thank you for visiting nature.com. You are using a browser version with limited support for CSS. To obtain the best experience, we recommend you use a more up to date browser (or turn off compatibility mode in Internet Explorer). In the meantime, to ensure continued support, we are displaying the site without styles and JavaScript.
- View all journals
- My Account Login
- Explore content
- About the journal
- Publish with us
- Sign up for alerts
- Open access
- Published: 06 August 2021
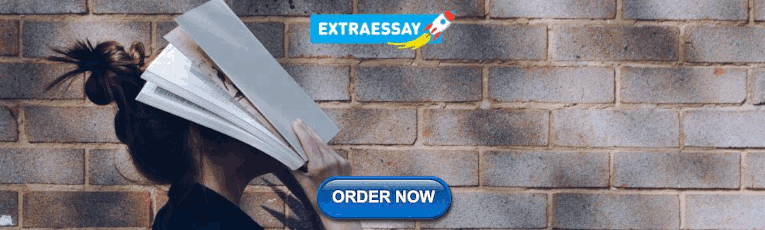
Machine learning and earthquake forecasting—next steps
- Gregory C. Beroza 1 ,
- Margarita Segou 2 &
- S. Mostafa Mousavi 1
Nature Communications volume 12 , Article number: 4761 ( 2021 ) Cite this article
20k Accesses
61 Citations
48 Altmetric
Metrics details
- Natural hazards
A new generation of earthquake catalogs developed through supervised machine-learning illuminates earthquake activity with unprecedented detail. Application of unsupervised machine learning to analyze the more complete expression of seismicity in these catalogs may be the fastest route to improving earthquake forecasting.
The past 5 years have seen a rapidly accelerating effort in applying machine learning to seismological problems. The serial components of earthquake monitoring workflows include: detection, arrival time measurement, phase association, location, and characterization. All of these tasks have seen rapid progress due to effective implementation of machine-learning approaches. They have proven opportune targets for machine learning in seismology mainly due to the large, labeled data sets, which are often publicly available, and that were constructed through decades of dedicated work by skilled analysts. These are the essential ingredient for building complex supervised models. Progress has been realized in research mode to analyze the details of seismicity well after the earthquakes being studied have occurred, and machine-learning techniques are poised to be implemented in operational mode for real-time monitoring. We will soon have a next generation of earthquake catalogs that contain much more information. How much more? These more complete catalogs typically feature at least a factor of ten more earthquakes (Fig. 1 ) and provide a higher-resolution picture of seismically active faults.
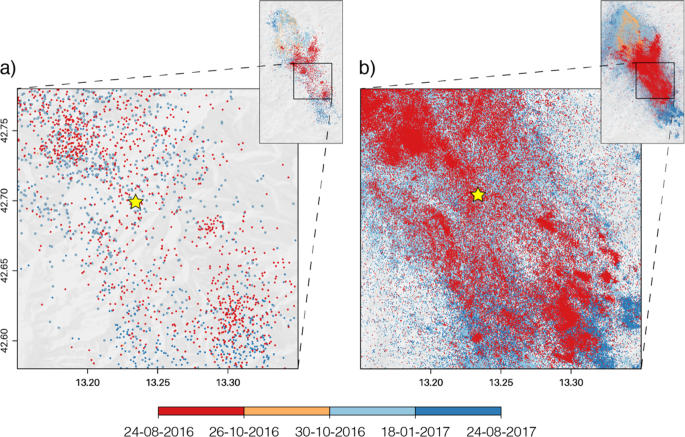
a Real-time catalog, available at http://cnt.rm.ingv.it/ and ( b ) machine-learning catalog 16 are shown for event magnitudes above their respective magnitude of completeness 12 , 16 Mc = 2.2 and Mc = 0.5.
This next generation of earthquake catalogs will not be the single, static objects seismologists are accustomed to working with. For example, less than 2 years after the 2019 Ridgecrest, California earthquake sequence there already exist four next-generation catalogs, each of which were developed with different enhanced detection techniques. Now, and in the future, this will be the norm, and earthquake catalogs will be updated and improved—potentially dramatically—with time. Second-generation deep learning models 1 that are specifically designed based on earthquake signal characteristics and that mimic the manual processing by analysts, can lead to performance increases beyond those offered by earlier models that adapted neural network architectures from other fields. Those interested in using earthquake catalogs for forecasting can anticipate a shifting landscape with continuing improvements.
While these improvements are impressive, the value of the extra information they provide is less clear. What will we learn about earthquake behavior from these deeper catalogs and how might it improve the prospects for the stubbornly difficult problem of earthquake forecasting?
Short-term deterministic earthquake prediction remains elusive and is perhaps impossible; however, probabilistic earthquake forecasting is another matter. It remains the subject of focused and sustained attention and it informs earthquake hazard characterization 2 and thus both policy and earthquake risk reduction. A key assumption is that what we learn from the newly uncovered small earthquakes in AI-based catalogs, will inform earthquake forecasting for events of all magnitudes. The observed scale invariance of earthquake behavior suggests this is a reasonable expectation.
Empirical seismological relationships have played a key role in the development of earthquake forecasting. These include Omori’s law 3 that describes the temporal decay of aftershock rate, the magnitude-frequency distribution, with the b-value describing the relative numbers of small vs. large earthquakes 4 , and the Epidemic Type Aftershock Sequence (ETAS) model 5 in which earthquakes are treated as a self-exciting process governed by Omori’s law for their frequency of occurrence and Gutenberg–Richter statistics for their magnitude. These empirical laws continue to prove their utility. Just in the past few years, the time dependence of the b-value has been used to try to anticipate the likelihood of large earthquakes during an ongoing earthquake sequence 6 and the ETAS model has been improved to better anticipate future large events 7 . So it appears that there is value in applying these longstanding relationships to improved earthquake catalogs, but our opinion is that much more needs to be done.
The relationships cited above date from 127, 77, and 33 years ago. The oldest of them, Omori’s Law, was developed based on felt reports without the benefit of instrumental measurements. We suggest that a fresh approach using more powerful techniques is warranted. Earthquake catalogs are complex, high-dimensional objects and as Fig. 1 makes clear, that is even more true for the deeper catalogs that are being developed through machine learning. Their high dimensionality makes them challenging for seismologists to explore, and the conventional approaches noted above seem unlikely to be taking advantage of the wealth of new information available in the new generation of deeper catalogs. We suggest that, having first enabled the development of these catalogs, the statistical-learning techniques of data science are now poised to play an important role in uncovering new relationships within them. The obvious next step is to apply the techniques of machine learning in discovery mode 8 to discern new relationships encoded in the seismicity.
There are tantalizing indications that such an approach may lead to new insights. In double-direct-shear experiments, background signals that were thought to be uninformative random noise have instead been shown to encode information on the state of friction and the eventual time of failure of faults in a laboratory setting 9 . Well-controlled laboratory analogs to faults lack the geologic complexity of the Earth, yet, weak natural background vibrations of a similar sort, that again were thought to be random noise, have been shown to embody information that can be used to predict the onset time of slow slip events in the Cascadia subduction zone 10 . Finally, unsupervised deep learning, in which algorithms are used to discern patterns in data without the benefit of prior labels, applied to seismic waveform data uncovered precursory signals preceding the large and damaging 2017 landslide and tsunami in Greenland 11 .
These examples are compelling but come with the caveat that they are not representative of the typical fast rupture velocity earthquakes on tectonic faults that are of societal concern. For such earthquakes, however, there are also indications from state-of-the-art forecasting approaches that next-generation earthquake catalogs may contain information that will lead to progress. Physics-based forecasting models, which account for changes in the Coulomb failure stress due to antecedent earthquakes that favor the occurrence of subsequent earthquakes, have shown increasing skill such that they are competitive with, and are beginning to outperform, statistical models. Coulomb failure models benefit particularly from deeper catalogs because they include many more small magnitude earthquakes. These small earthquakes add predictive power through their secondary triggering effects tracking the evolution of the fine-scale stress field that ultimately controls earthquake nucleation in foreshock and aftershock sequences. They can also be used to define the emerging active structures that comprise fault networks and by doing so clarify the relevant components of stress that would act to trigger earthquakes 12 . Secondary triggering and background stress heterogeneity were shown to improve stress triggering models 13 but were most effective when they incorporated near‐real‐time aftershock data from the sequence as it unfolded 14 . We note that there is no reason why more complete earthquake catalogs, developed with pre-trained neural network models, cannot be created in real time as an earthquake sequence unfolds. Finally, despite the disappointing history of the search for precursors, due diligence requires that seismologists consider the pursuit of signals that might be precursory.
We conclude that it is now possible to image the activity on active fault systems with unprecedented spatial resolution. This will enable experimentation with familiar hypotheses and enable the formulation of new hypotheses. It seems certain that the underlying processes that drive earthquake occurrence are encoded in this next generation of earthquake catalogs, but we may not find them unless we put new effort into searching for them. Unsupervised learning methods 15 are particularly well-suited tool for that effort.
Mousavi, S. M. et al. Earthquake Transformer - an attentive deep-learning model for simultaneous earthquake detection and phase picking. Nat. Commun. 11 , 3952, https://doi.org/10.1038/s41467-020-17591-w (2020).
Field, E. H. et al. A synoptic view of the third Uniform California Earthquake Rupture Forecast model. Seismological Res. Lett. 88 , 1259–1267, https://doi.org/10.1785/0220170045 (2017).
Omori, F. J. On the aftershocks of earthquakes. Coll. Sci. Imp. Univ. Tokyo 7 , 111–200 (1894).
Google Scholar
Gutenberg, B. & Richter, C. F. Frequency of earthquakes in California. Bull. Seismol. Soc. Am. 34 , 185–188 (1944).
Article Google Scholar
Ogata, Y. Statistical models for earthquake occurrences and residual analysis for point processes. J. Am. Stat. Assoc. 83 , 9–27 (1988).
Gulia, L. & Wiemer, S. Real-time discrimination of earthquake foreshocks and aftershocks. Nature 574 , 193–199, https://doi.org/10.1038/s41586-019-1606-4 (2019).
Shcherbakov, R. et al. Forecasting the magnitude of the largest expected earthquake. Nat. Commun. 10 , 4051, https://doi.org/10.1038/s41467-019-11958-4 (2019).
Bergen, K. J. et al. Machine learning for data-driven discovery in solid earth geoscience. Science 363 , eaau0323, https://doi.org/10.1126/science.aau0323 (2019).
Article CAS PubMed Google Scholar
Rouet-Leduc, B. et al. Machine learning predicts laboratory earthquakes. Geophys. Res. Lett . 44 , 9276–9282 https://doi.org/10.1002/2017GL074677 (2017).
Hulbert, C. et al. An exponential build-up in seismic energy suggests a months-long nucleation of slow slip in Cascadia. Nat. Commun. 11 , 4139, https://doi.org/10.1038/s41467-020-17754-9 (2020).
Article ADS CAS PubMed PubMed Central Google Scholar
Seydoux, L. et al. Clustering earthquake signals and background noises in continuous seismic data with unsupervised deep learning. Nat. Commun. 11 , 3972, https://doi.org/10.1038/s41467-020-17841-x (2020).
Mancini, S. et al. Improving physics-based aftershock forecasts during the 2016-2017 Central Italy earthquake cascade. J. Geophys. Res. Solid Earth 124 , https://doi.org/10.1029/2019JB017874 (2019).
Segou, M., & Parsons, T. A new technique to calculate earthquake stress transfer and to probe the physics of aftershocks. Bull. Seismol. Soc. Am . 110 , 863–873, https://doi.org/10.1785/0120190033 (2020).
Mancini, S. et al. The predictive skills of elastic Coulomb rate-and-state aftershock forecasts during the 2019 Ridgecrest, California, earthquake sequence. Bull. Seismol. Soc. Am. 110 , 1736–1751, https://doi.org/10.1785/0120200028 (2020).
Mousavi, S. M., et al. Unsupervised clustering of seismic signals using deep convolutional autoencoders. IEEE Trans. Geosci. Remote Sens. Lett. 16 , 1693–1697, https://doi.org/10.1109/LGRS.2019.2909218 (2019).
Article ADS Google Scholar
Tan, Y. J. et al. Machine-learning-based high-resolution catalog reveals how complex fault structures were activated during the 2016-2017 Central Italy sequence. Seismic Rec. 1 , 11–19, https://doi.org/10.1785/0320210001 (2021).
Download references
Acknowledgements
This work is supported by the NERC-NSFGEO 13 funded project The Central Apennines Earthquake Sequence under a New Microscrope (NE/R0000794/1). G.C.B. was supported by Department of Energy (Basic Energy Sciences; Award DE-SC0020445). Thanks to Dr. Simone Mancini for preparing the figure.
Author information
Authors and affiliations.
Department of Geophysics, Stanford University, Stanford, CA, USA
Gregory C. Beroza & S. Mostafa Mousavi
British Geological Survey, Research Avenue South, Lyell Centre, Edinburgh, UK
Margarita Segou
You can also search for this author in PubMed Google Scholar
Contributions
All authors contributed to the development of this comment, and all were involved in revising it.
Corresponding author
Correspondence to Gregory C. Beroza .
Ethics declarations
Competing interests.
The authors declare no competing interests.
Additional information
Publisher’s note Springer Nature remains neutral with regard to jurisdictional claims in published maps and institutional affiliations.
Rights and permissions
Open Access This article is licensed under a Creative Commons Attribution 4.0 International License, which permits use, sharing, adaptation, distribution and reproduction in any medium or format, as long as you give appropriate credit to the original author(s) and the source, provide a link to the Creative Commons license, and indicate if changes were made. The images or other third party material in this article are included in the article’s Creative Commons license, unless indicated otherwise in a credit line to the material. If material is not included in the article’s Creative Commons license and your intended use is not permitted by statutory regulation or exceeds the permitted use, you will need to obtain permission directly from the copyright holder. To view a copy of this license, visit http://creativecommons.org/licenses/by/4.0/ .
Reprints and permissions
About this article
Cite this article.
Beroza, G.C., Segou, M. & Mostafa Mousavi, S. Machine learning and earthquake forecasting—next steps. Nat Commun 12 , 4761 (2021). https://doi.org/10.1038/s41467-021-24952-6
Download citation
Received : 28 May 2021
Accepted : 12 July 2021
Published : 06 August 2021
DOI : https://doi.org/10.1038/s41467-021-24952-6
Share this article
Anyone you share the following link with will be able to read this content:
Sorry, a shareable link is not currently available for this article.
Provided by the Springer Nature SharedIt content-sharing initiative
This article is cited by
An all-in-one seismic phase picking, location, and association network for multi-task multi-station earthquake monitoring.
Communications Earth & Environment (2024)
Deep learning forecasting of large induced earthquakes via precursory signals
- Vincenzo Convertito
- Fabio Giampaolo
- Francesco Piccialli
Scientific Reports (2024)
Cascade and pre-slip models oversimplify the complexity of earthquake preparation in nature
- Patricia Martínez-Garzón
The Role of Machine Learning in Earthquake Seismology: A Review
- Anup Chitkeshwar
Archives of Computational Methods in Engineering (2024)
Machine learning-based multipath modeling in spatial domain applied to GNSS short baseline processing
- Yuanxin Pan
- Gregor Möller
- Benedikt Soja
GPS Solutions (2024)
Quick links
- Explore articles by subject
- Guide to authors
- Editorial policies
Sign up for the Nature Briefing newsletter — what matters in science, free to your inbox daily.

- Open supplemental data
- Reference Manager
- Simple TEXT file
People also looked at
Original research article, feasibility study of an earthquake early warning system in eastern central italy.
- 1 Istituto Nazionale di Geofisica e Vulcanologia, Osservatorio Nazionale Terremoti, Ancona, Italy
- 2 Istituto Nazionale di Geofisica e Vulcanologia, Osservatorio Nazionale Terremoti, Roma, Italy
An earthquake early warning system (EEWS) is a monitoring infrastructure that allows alerting strategic points (targets) before the arrival of strong shaking waves during an earthquake. In a region like Central Italy, struck by recent and historical destructive earthquakes, the assessment of implementation of an EEWS is a significant challenge due to the proximity of seismic sources to many potential targets, such as historical towns, industrial plants, and hospitals. In order to understand the feasibility of an EEWS in such an area, we developed an original method of event declaration simulation (EDS), a tool for assessing the effectiveness of an EEWS for existing seismic networks, improving them with new stations, and designing new networks for EEW applications. Values of the time first alert (TFA), blind zone radius (BZ), and lead time (LT) have been estimated with respect to selected targets for different network configurations in the study region. Starting from virtual sources homogeneously arranged on regular mesh grids, the alert response was evaluated for actual and improved seismic networks operating in the area, taking into account the effects of the transmission and acquisition systems. In the procedure, the arrival times of the P wave picks, the association binder, the transmission latencies, and the computation times were used to simulate the configuration of PRESTo EEWS, simulating both real-time and playback elaborations of real earthquakes. The NLLOC software was used to estimate P and S arrival times, with a local velocity model also implemented in the PRESTo EEWS. Our results show that, although Italy’s main seismic sources are located close to urban areas, the lead times calculated with the EDS procedure, applied to actual and to improved seismic networks, encourage the implementation of EEWS in the study area. Considering actual delays due to data transmission and computation time, lead times of 5–10 s were obtained simulating real historical events striking some important targets of the region. We conclude that EEWSs are useful tools that can contribute to protecting people from the harmful effects of earthquakes in Italy.
1 Introduction
In the past twenty years, EEWSs have been implemented in different regions of the world and are considered a useful tool to reduce seismic risk ( Satriano et al., 2011b ). EEWSs were developed with different approaches, methodologies, and combining new experiences. At present, many countries have operational or prototype EEWSs. Allen et al. (2009b) described the status of EEW in the world and the principal operating systems at that time. Other examples include EEWS in Japan ( Odaka et al., 2003 ), Taiwan ( Wu and Teng, 2002 ; Hsiao et al., 2009 ), Mexico ( Suarez et al., 2009 ), Turkey ( Erdik et al., 2003 ; Alcik et al., 2009 ), and Romania ( Böse et al., 2007 ). The principal active systems are based on the software ElarmS ( Allen and Kanamori, 2003 ; Allen et al., 2009a ) and ShakeAlert ( Kohler et al., 2020 ) in California, on Virtual Seismologist in California and Switzerland ( Cua et al., 2009 ), in Europe ( Clinton et al., 2016 ), and in particular PRESTo in Italy ( Iannaccone et al., 2010 ; Satriano et al., 2011a ).
Major developments have led to two main types of systems: a regional alert system and an on-site system ( Satriano et al., 2011b ; Zollo et al., 2014 ). The regional system, based on the use of a regional network that records seismic events, aims to detect, locate, and determine the magnitude of an event starting from the analysis of a few seconds of the first arrivals of the P waves recorded at the stations ( Picozzi, 2012 ). The on-site system consists of a single sensor or more sensors near or inside the target structure to be alerted. In this system the P-wave recordings to the sensor are used to predict the peak ground motion at the site ( Colombelli et al., 2015 ). This approach could be considered useful for sites located within the BZ of a regional EEW system, allowing for a useful warning before the arrival of strong shaking waves. Caruso et al. (2017) proposed a P-wave-based EEW approach called on-site alert level (SAVE). Many studies combined the two EEW approaches ( Zollo et al., 2010 ; Colombelli et al., 2012a ); these systems combine local parameters and predicted ground motions at a regional scale to provide reliable and rapid estimates of the seismic source and the expected damage zone ( Colombelli et al., 2015 ).
The approaches for regional EEW can be classified as the “point-source” (simply the source as a concentrated volume) or “finite fault” (a more sophisticated and realistic characterization of the source, considering the entire fault area). Most studies have used the “point-source” demonstrating the reliability of this approach for the magnitude estimation of small to moderate events. However, it has been shown that this approach is not always accurate for strong earthquakes (magnitude> 6.5–7), due to the saturation of the P-wave parameters. Several authors (for example, Colombelli et al., 2012b ) estimated the magnitude over time windows longer than the recorded P-wave and/or the S-wave signal to obtain more accurate final values. These magnitude calculations are reliable at the cost of requiring more data and time ( Velazquez et al., 2020 ). In our study, the selected earthquakes have a moderate magnitude (≤ 6.5) and were considered as point sources.
Potentially, an EEWS can produce and transmit alert messages to different end-users to allow them to adopt several types of safety measures in a few seconds. The main benefits of an EEWS include public warning, first responder mobilization, and safety of health care and utility infrastructures, transit systems, and workplaces ( Allen and Melgar, 2019 ). Whereas in most cases evacuation of buildings is unrealistic, due to the short time available to act; a portion of the affected population can receive the alert and take safety measures in certain types of structures and infrastructures ( Iervolino et al., 2008 ).
Receiving an alert message increases personal situational awareness and yields a more rapid response, especially in well-trained people who can take precautionary and protective actions like “Drop-Cover-Hold on”, suspending delicate medical procedures, or slowing down a train ride. In shaking areas, a time of 10 s allows people to protect themselves and prepare for evacuation ( Fujinawa and Noda, 2013 ). A time interval of 5–7 s could be enough to trigger automatic mitigation actions ( Cauzzi et al., 2016 ) at power plants, energy sector grids, and utilities infrastructures to prevent explosions, combustions, loss of water, flooding, fatal collisions, and elevator interruptions. Social studies have demonstrated that receiving alert messages even a few seconds before the shaking occurs help people to prepare and react in the proper way ( Dunn et al., 2016 ; Becker et al., 2020a ).
The elongated shape of the Italian peninsula, combined with the small damage area for moderate, but often destructing Apenninic earthquakes (M6-7), determine small distances between sources and potential EEW targets. For this reason, in many cases the time to start safety actions may be too short. Therefore, an evaluation of the feasibility of an EEW implementation is needed in this area. A first theoretical evaluation was performed by Olivieri et al. (2008) with RSN (National Seismic Network, IV, INGV Seismological Data Center, 2006) and by Picozzi et al. (2015) using the RAN seismic network (Italian strong motion network) managed by the National Civil Protection ( Gorini et al., 2010 ), whose stations are mostly not connected in real-time.
Our study area extends for about 200 × 200 km in eastern central Italy and is characterized by the following two main seismic zones: 1) a NNW-SSE seismic zone elongated in the Appennines, where several moderate to strong earthquakes have occurred in the past and 2) a coastline-offshore seismic zone ( Figure 1a ), with less frequent and on average weaker seismicity. Figure 1b shows the target points chosen in the study compared to the individual and composite seismogenic sources from the DISS catalog ( DISS Working Group, 2018 ) . An individual seismogenic source (ISS) is a simplified, three-dimensional representation of a rectangular fault plane, whereas a composite seismogenic source (CSS) is a simplified, three-dimensional representation of a crustal fault containing an unspecified number of seismogenic sources that cannot be singled out. The area analyzed in our study is affected by different fault systems. We select as targets the cities with at least 40,000 inhabitants or with a significant cultural value. The selected cities are Ancona, Pesaro, Macerata, Ascoli Piceno, Fermo, Fabriano, Urbino, San Benedetto del Tronto, Civitanova Marche, Senigallia, Jesi, Perugia, Foligno, and Terni ( Table 1 ).
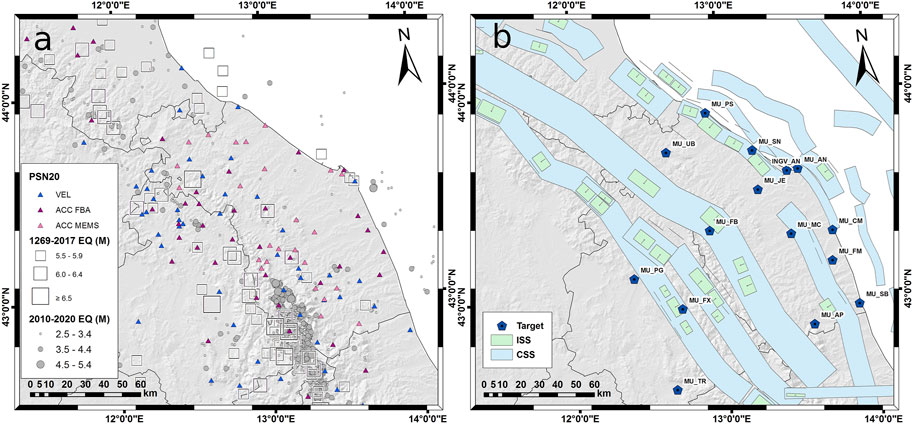
FIGURE 1 . Seismicity, seismic network, different fault zones, and selected targets of the study area. a) PSN20: Permanent Seismic Network in the year 2020. Blue triangles: velocimetric sensors. Purple triangles: FBA high performance accelerometer sensors. Pink triangles: MEMS accelerometer. Black squares: 1219–2019 EQ, moderate to strong earthquakes (M ≥ 5.5) extracted from CPTI15 database ( Rovida et al., 2021 ). Grey circles: earthquakes recorded by the seismic network from 2010 to 2020 in the magnitude range 2.5 ≤ M ≤ 5.4. b) Pentagon: Targets. ISS: individual seismogenic source. CSS: composite seismogenic source (see text for explanation, DISS Working Group (2018)) .
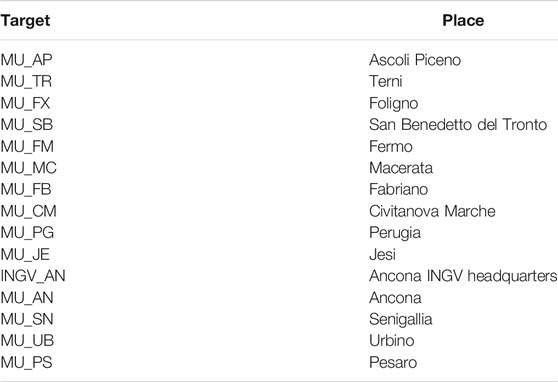
TABLE 1 . Target: city code. Place: extended name of cities.
In the study area, an EEWS based on PRESTo software has been operating since 2015. The system was based on permanent seismic networks managed by the INGV (National Institute of Geophysics and Vulcanology) composed by different sensors: velocimeters (short period and broad-band), high performance accelerometers, and MEMS. The seismic network in this area includes the RSN, a more dense local network (namely, the Alto Tiberina Near Fault Observatory—Taboo ( Chiaraluce et al., 2014 )), and some seismic stations installed for regional monitoring ( Cattaneo et al., 2017 ). A first evaluation of the performance of the EEWS was made analyzing the seismic sequence of 2016–2017 ( Festa et al., 2018 ). The system has been continuously operating over the years, without changing configuration, with some temporary interruptions.
In this work, the feasibility of a regional EEWS was evaluated by developing a procedure of event declaration simulation (EDS) for estimating the time useful to activate safety actions. The EDS procedure can be used for different applications: 1) to assess the feasibility of an EEWS in a specific area with an operating seismic network; 2) to plan the integration of new stations into an existing network; 3) to design a new network for an EEWS; and finally, 4) to assess the feasibility of an EEWS varying network density and trigger parameters. Regarding 3) and 4), the EDS can create virtual networks for the areas of interest and allow to plan investments and installations in advance.
The study was mainly based on the calculation of the time first alert (TFA)—the instant in which the event is declared starting from the coincidence of P phases at the stations, the blind zone radius (BZ)—the area in which no safety action can be carried out, and the lead time (LT)—the useful time to initiate safety actions on the targets. In the paper, we first propose a description of the method, of the parameters setting to obtain realistic simulations, and of the EDS validation with PRESTo EEWS. Then, an EDS application in eastern central Italy is showed, discussing the results of the TFA, BZ, and LT mapping in terms of feasibility and limits of the EEW implementation.
2 Event Declaration Simulation Method
The developed EDS is composed by a chain of subroutines including NonLinLoc modules (nonlinear location, or NLLoc; Lomax et al., 2009 ) in the preparatory phase and homemade python scripts in the core of the simulation that emulates some parameters similar to the PRESTo software. The NLLOC package is a well-known and widely used nonlinear inversion code, consisting of a set of programs and where it is possible to integrate an existing velocity model, travel-time calculation and probabilistic solution, for visualization of 3D volume data and location results ( http://alomax.free.fr/nlloc/ ).
The procedure of event declaration simulation needs the following inputs: arrival times of the P phases to the seismic stations, arrival time of the S phases to the targets, a binding configuration and latencies of the real-time data transmission vectors ( Figure 2 ). To obtain the arrival time of P and S phases, a velocity model, a seismic network, a seismic source, and target locations are required.
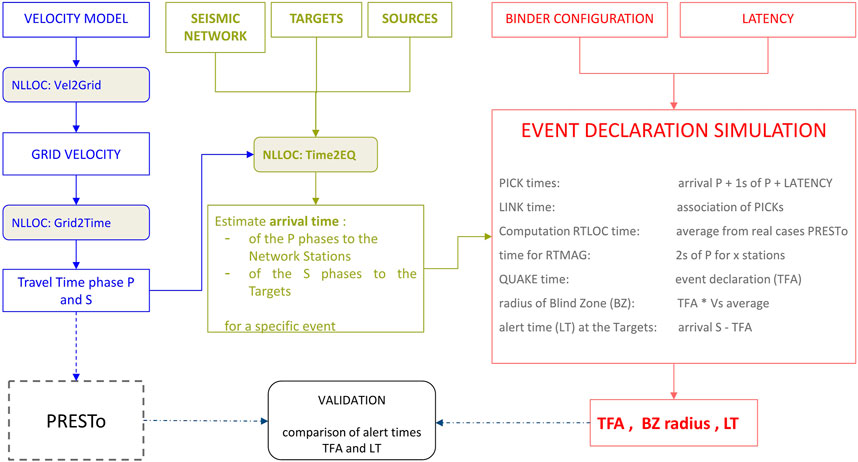
FIGURE 2 . Flow chart of the EDS method. Left (blue): P and S travel time calculation with NonLinLoc software modules ( Lomax et al., 2009 ). Middle (green): P and S arrival time relative at seismic stations and targets respectively. Right (red): input parameters and elements of the EDS core. Bottom : terms of the validation.
Figure 2 shows the most important steps of the simulation procedure, exemplifying the main blocks of the procedure from the inputs to the three outputs: TFA, BZ, and LT.
Starting from a velocity model and using the NLLoc Vel2Grid module, it is possible to create a defined grid of velocities, covering the volume of the study area. Then, the NLLoc Grid2Time module calculates the travel times from node points of the 3D velocity grid to the location of the seismic stations. The so obtained travel times are the same in use in the PRESTo system to locate the real seismic events.
The procedure estimates the arrival times of the P phases to the seismic stations and arrival times of the S phases to the targets, taking advantage of the NLLoc Time2EQ module, given the locations of seismic stations, seismic source and targets.
In the core of the simulation, the expected TFA, BZ, and LT are calculated starting from P and S arrival times, binder configuration (coincidences), and data latencies ( Figure 2 ).
The simulated parameters conceptually emulate some parameters of the PRESTo software.
“PICK time” is defined as the time of the P phase trigger at each seismic station, the sum of the estimated P phase arrival, the accumulation of 1 s of P waveform for the phase picker and the latency of the data packet. “LINK time” signs the moment when a certain number of PICKs are included in a relative small space and time interval and the binder declares an association. The simulation considers a “computation time” useful to locate the supposed event and the accumulation of 2 s of P waveform to compute the magnitude. The “computation time” and the time to compute the magnitude are inferred from real-time application of the PRESTo system implemented in the study area, collecting the log outputs relative to RTLOC and RTMAG modules ( Zollo et al., 2010 ; Satriano et al., 2011b ).
The integration of simulated parameters allows to calculate the “QUAKE time” (TFA) as the needed time to declare the event, summed to the “LINK time”. The BZ is estimated multiplying the TFA times the average of the S velocity in the travelled volume. Finally, the useful time (LT) to initiate actions to secure the targets is obtained from the difference between the arrival time of S phase at the targets and the TFA.
3 Event Declaration Simulation Configuration
The aim of this work is to assess the feasibility of an EEWS in eastern central Italy where a dense seismic network operates, and the main characteristics of the seismicity are well-known ( Figure 1a, b ). The study area is inhabited by about 2.5 million people, distributed in some main cities with population ranging from tens of thousands up to one hundred thousand inhabitants, and in several small historical villages where few hundred people live. Moreover, in most of the target towns and villages, both along the coasts and in the inner Umbria and Marche regions, residents increase dramatically during the summer and other vacation periods.
One of the starting points of the analysis is the velocity model of the volume crossed by seismic waves. A grid of 300 km × 300 km, 67 km thick, starting from 3 km above sea level was created, that could include all the seismicity of the region. A step of 1 km divides nodes of the grid and the central origin geographic point is 43.25 N – 13.00 E. To make the simulation more reliable, we chose a modified version of a 1D velocity model calculated for the region from an instrumental earthquakes catalog ( De Luca et al., 2009 ), preserving the Vp/Vs ratio equal to 1.85 and inserting a gradient between velocity layers ( Supplementary Table 1 ).
The configuration of the INGV seismic network has been evolving over the years. From 2015, when PRESTo software was installed for real-time monitoring and EEW testing, the number of stations has been increasing. Moreover, during the seismic sequence of the year 2016 ( Chiaraluce et al., 2017 ) an emergency temporary seismic network was installed to densify the permanent network ( Moretti et al., 2016 ; SISMIKO, 2020 ). Therefore, we set four seismic network configurations for EDS:
- PSN15: Permanent Seismic Network of the year 2015
- TSN16: Temporary Seismic Network of the 2016 seismic emergency (added to PSN15)
- PSN20: Permanent Seismic Network of the year 2020 (including PSN15)
- ASN20: Accelerometric Seismic Network of the year 2020.
The PSN15 is the same network configuration implemented in the real time PRESTo instance and contributed to validate EDS, to estimate data latencies and test performance of the PRESTo system in Festa et al. (2018) . TSN16 was used to test the response of the network with a significant increase of the density of the PSN15 up to a station inter-distance of about 5 km in the epicentral area of the 2016–2017 seismic sequence. PSN20 contributed to augment dataset of the EDS validation comparing results with a playback instance of the PRESTo software. The ASN20 helped to estimate the network response if we consider only accelerometric components, corresponding to a reduction of the network density, balanced by the certainty of unclipped records. A list of the seismic stations belonging to each network is inserted in Supplementary Table 2 .
The EDS is also able to manage arbitrary virtual networks, composed by scattered or equally spaced grid of stations to design network response in uncovered areas.
Also for the sources, we can input a single seismic source or a set of sources, scattered or equally spaced. The location of a single source, for example, is useful to reproduce the response of the seismic network in terms of TFA for an historical or a recent significant event. Furthermore, for the same event, the EDS returns LT relative to the main cities of the region. Extending the principle to a grid of equally spaced sources, the EDS can map the three output parameters (TFA, BZ, and LT) over the whole region. This approach allows classifying areas characterized by small or large TFA and BZ relative to the events’ epicenters. In this work, for a first mapping of TFA and BZ, we choose a grid of sources 5 km spaced at depth of 10 km. The LT was estimated for all the selected cities ( Table 1 ).
The number of triggered stations and the time interval for association are the main parameters for the set of the binder, the module that allows to declare an event. In the EDS, the binder configuration emulates the “Binder” of PRESTo software parameters ( PRESTo, 2013 ). We adopt the setup used in the real-time PRESTo instance for choosing the values of binder parameters in the EDS ( Table 2 ). The number of at least six stations in coincidence (STA_CO) inside a time window of 3 s (SEC_CO) is a good compromise between the heterogeneous density of the seismic network and the requirement of a rapid response of the system considered the distances of targets from the sources. The SEC_AS parameter is set to 10 s, a value that avoids the effect of the shift of a good location during grid search in the playback PRESTo instance with respect to the results of the real-time instance with SEC_AS = 15 s. The AVEL_MIN, AVEL_MAX, VEL_SPA, VEL_DIST parameters design a cone inside which the coincidence picks must fall.

TABLE 2 . Configuration Parameters of the EDS binder.
A key factor to take into account for an EEWS is the data latency due to the communication protocol ( Satriano et al., 2011a ). The latency affects the alert times of the EEWS which can only be activated when a good part of the data is available in nearly true real-time. The seismic data of the stations in eastern central Italy are transmitted by different types of transmission vectors: TCP/IP, WiFi, GPRS/UMTS, and Satellite (SAT-LIBRA and KA-SAT). At the time of the analysis, LTE routers were not available. Starting from the PRESTo log files, the real latency data for 86 seismic stations for the period 2015–2019 were collected. The long time window of the analysis allowed in some cases to assess the improved performance of the stations after the change of the transmission protocol. An average value of the latency for each type of transmission vectors was calculated by the geometric mean to exclude outliers, that is, values significantly out of the trend, referred to a malfunction of the station. The results of the latency classification are listed in Table 3 with the average values calculated and the number of stations used. Considering the main transmission vectors used in the network of this study, it is possible to make some considerations. As expected, the stations with a satellite time division multiple access carrier system (i.e., SAT-LIBRA) are those with the greatest latency and are not good for EEW application in small areas, but in our case few stations are equipped with this type of satellite transmission. Instead, another satellite system (i.e., KA-SAT) returns an average of 2.64 s, an acceptable value for EEW applications. The TCP/IP, WiFi, and GSM/UMTS latencies range between 1.86 and 2.83 s. The best value is for direct connection TCP/IP from remote stations. TCP/IP connections from other acquisition centers connected with a mixed copper-fiber line return a slight worsening. The transmission by WiFi backbone gives back a latency of 2.07 s, confirming suitable use for EEW systems. The WiFi system is not a public system, but a system dedicated to civil protection services, available thanks to the Regione Marche authority through an agreement with INGV. Each system used for data transmission (TCP/IP, WiFi, and GSM/UMTS, Satellite) could suffer temporary blackout of the communication lines. In case of a strong earthquake the redundancy of the transmission lines used in the network should reduce the risk of data blackout.
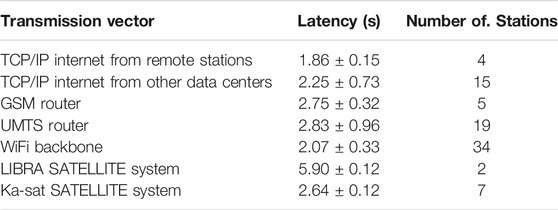
TABLE 3 . Transmission vector, mean and standard deviation of data latency, and number of stations used for each type.
Besides transmission times, another important parameter for an efficient EEWS is the computing time, defined as the difference between the association time of the stations and the computed TFA.
During this time, in the real-time system, the location and magnitude are estimated and the event is declared. The EDS does not simulate the earthquake location process and the magnitude estimate. So, the computing times inserted in our simulation are extracted from a statistics of the real-time PRESTo instance. The time difference between “QUAKE time” and “LINK time” was calculated and compared on a dataset of 91 events ( Supplementary Table 3 ) detected in real-time by the PRESTo system and re-simulated by EDS. The events, belonging to the INGV bulletin, were selected from August 2016 to May 2020, with magnitude 3.0 ≤ Mw ≤ 6.0. These events were detected by the system in real-time for the same period in the study area. The calculation times, taken from the system files in real-time, were distributed according to a lognormal curve ( Figure 3 ). The calculated values are mode equal to 0.29 s, median equal to 0.42 s, and average 0.51 s. Following these results, a value of 0.30 s was chosen as the average computation time for the simulation.
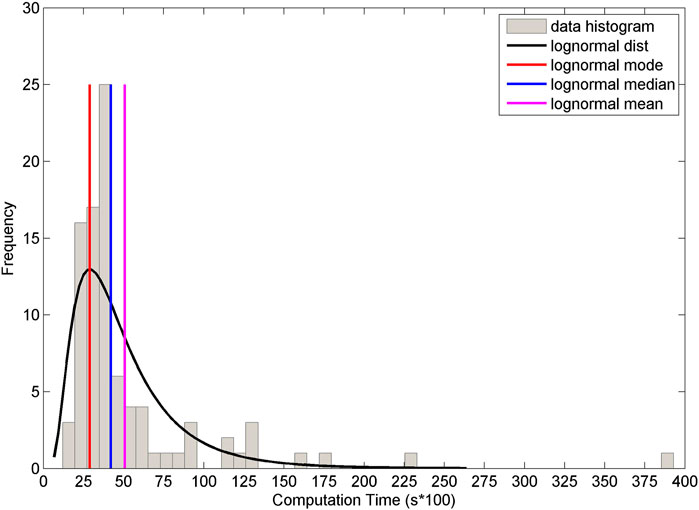
FIGURE 3 . Statistic of the computation time values.
4 Event Declaration Simulation Validation
The simulation procedure was validated comparing the first time of the alerts (TFA) of the EDS with first “QUAKE time” of the PRESTo instances. In the comparison with real time outputs of the PRESTo system, the values of mean latencies belonging to different transmission vectors are inserted in the EDS. Differently, for the comparison with PRESTo playback outputs, a zero latency was set in EDS. These two approaches help to increase the reliability of the validation, excluding that results are affected by a bad estimate of the latencies.
Figure 4 shows a detail of the processing of Mw 5.4 October 26, 2016 event and the comparison of arrival times relative to P phases of the triggered stations, the TFA and the arrival of S phases useful to calculate the LT at the target INGV_AN in the city of Ancona (epicentral distance 88 km). The black marks represent an operator review of the P phases at the triggered stations and the S phase at the target station (cT0). The black point represents the origin time of the located event, taken into account for the EDS test. The red marks are relative to PRESTo instances (cT1, cT3), while the blue marks are relative to EDS (cT2, cT4, cT5).
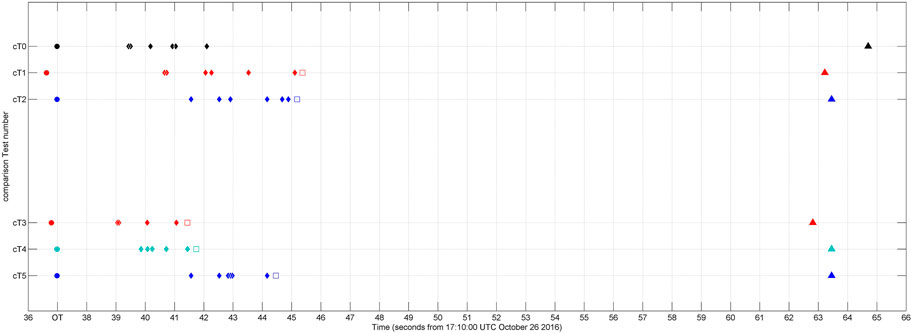
FIGURE 4 . Comparison of arrival times relative to Mw 5.4 October 26, 2016 earthquake. Circles: Origin Time. Diamonds: P phase arrival times of the triggered stations. Squares: TFA. Triangles: S phase arrival times at INGV_AN target in city of Ancona (88 km epicentral distance). cT0: arrival times of human event revision. cT1: PRESTo real time instance with PSN15. cT2: EDS with real latency and with PSN15. cT3: PRESTo playback instance with PSN15 and TSN16. cT4: EDS with zero latency and PSN15 + TSN16. cT5: EDS with real latency with PSN15 + TSN16.
The arrival times of operator reviewed P phases are lesser than the PRESTo and EDS real time tests where the data latencies are present. The reviewed arrival time is more similar for the tests in playback where zero latency is setting up but a 1 s of P waveform analysis remains.
The comparison of the TFA between PRESTo and EDS is very good for the two approaches, that is, real-time (cT1 vs cT2) and playback (cT3 vs cT4). The effect of the data latencies in the real time case imply a delay of about 3 s of the TFA with respect to the last P phase arrival time of the human reviewed case. This result is coherent with the values of the mean latencies calculated for the different transmission protocol ranging between 2 and 3 s inserted in the test, and 1 s needed to process the P waveform. The playback version of the test return a little early TFA respect to the last reviewed P phase, both for PRESTo and EDS, but the difference is small, 0.7 and 0.4 s respectively. Finally, the EDS allows to estimate a theoretical TFA adding emergency temporary seismic stations, active in epicentral area at the time of the earthquake, and stations data latencies (cT5). The effect on TFA is about 1 s of the advance respect the PRESTo real-time case (cT1), simply for the early achievement of the station coincidence determined by an augmented network density in the epicentral area. The values of S phase arrival times of PRESTo and EDS are coherent thanks to the same modeling of velocity volume used for travel time computation. From the good simulation of the TFA and S phase arrival at the target by EDS, a good estimate of the LT follows.
For a general validation of the TFA estimated with EDS, we selected 20 seismic events with 3.0 ≤ Mw ≤ 6.5, all elaborated also by PRESTo playback instance and 16 events by real-time instance. The selected events are scattered over the study area and over time, with the aim to insert in these tests different and unfavorable states of the network in terms of station inter-distances. The events belong to a time window from August 24, 2016, to January 28, 2020, with the hypocentral depth between 6.8 and 33.3 km ( Supplementary Table 4 ). The time window includes some major events of the 2016 Central Italy sequence, located in the southern sector of the region, while the other events were chosen in order to perform tests in the northern part of the region.
Table 4 summarizes the results of EDS estimates of TFA compared with real-time and playback PRESTo instances obtained by setting up the PSN15 configuration network. Moreover, the playback configuration (zero latency) was used to return a TFA mean difference, adding PSN16 emergency temporary station data relative to 2016–2017 events and the PSN20 network configuration for more recent events.

TABLE 4 . Results of the TFA comparison relative to real time and in playback PRESTo instances.
The TFA validation results return mean values lower than 1.0 s. The negative sign indicates an early TFA of the EDS, ascribable to non-simulation of the recursive recalculation of the location and magnitude that occurs in some real cases (PRESTo system) with the arrival of new data. The results of PSN15 real-time and playback cases (validation test 1 and 2) are similar, confirming a reliable simulation for both data latencies and station triggering, respectively. The last case (validation test 3) takes advantage of using 2016 temporary stations and latest installed stations, therefore the network density augmented in a part of analysis. The third test shows a better result, reducing the mean and the uncertainty of TFA difference.
The success of the EDS validation tests allows to perform simulations useful to quantify the EEW response of the actual seismic networks operating in eastern central Italy and to estimate the LT for the main cities in the region.
5 Event Declaration Simulation Applications, Results and Discussion
The EDS was developed to evaluate the EEW response of INGV seismic network in eastern central Italy in terms of TFA, BZ, and LD, but it is useful also to reproduce and design the feasibility of the system in different or not yet monitored areas.
The first possible application allowed by EDS is the response of a seismic network relative to one single seismic event with the aim of assessing LT for a set of targets.
Considering the hypocentral location of the Mw 6.0 August 24, 2016, event, we have simulated the response of four network configurations ( Table 5 ), assessing the LT for the main cities of the study area ( Figure 5 ). During the analysis, the calculation of the BZ is performed by multiplying the TFA by the average Vs estimated at the last station useful for coincidence. The TFA value is calculated as the arrival time of the P wave at the last station, added to the latency value and the estimated computing time of the system. Vs is calculated as the product of mean Vp at the last station for coincidence by the ratio Vs/Vp ( De Luca et al., 2009 , reported in Supplementary Table 1 ). In the first test (SST1), we used the PSN15 seismic network, the existing network at the time of the event. The second test (SST2) was performed inserting all stations of the TSN16, simulating the presence of the whole emergency network. This temporary seismic network was installed after the earthquake of August 24, 2016. The third test (SST3) represents the unfavorable case of a network consisting of only the current accelerometric INGV stations (ASN20). The last test (SST4) is an example of the design of a virtual arbitrary seismic network with the station inter-distance of 5 km. The calculated mean latency was associated to the PSN15 stations used for the latency statistics, a 2.75 s GSM/UMTS mean latency was associated to the TSN16 stations, and the mean latency was associated at new stations not present in PSN15, depending on their transmission vector. The virtual network with the inter-distance of 5 km was designed like a GSM/UMTS network and a mean latency of 2.75 s was applied to all virtual stations.

TABLE 5 . Results of tests with single source and different network configurations.
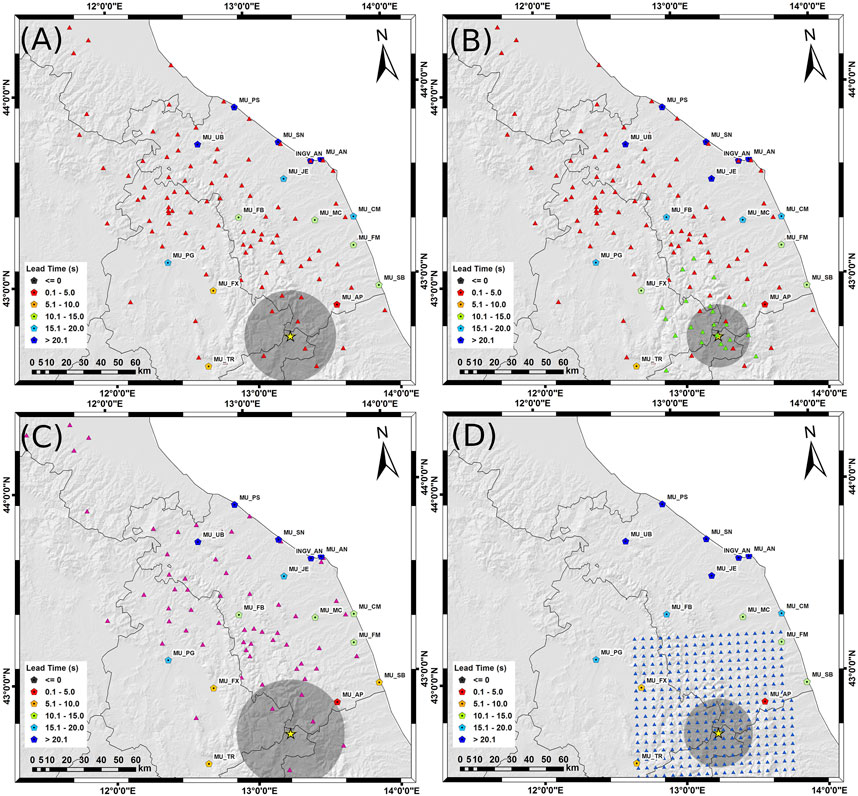
FIGURE 5 . EDS of the EEW response relative to a single source. Yellow star: location of the Mw 6.0 August 24, 2016 earthquake. Gray circle: Blind Zone. Pentagon: Targets. Legend: color map of the LT (s) linked to the targets. (A) SST1 test. Red triangles: PSN15 network. (B) SST2 test. Red triangles: PSN15 network; green triangles: TSN16. (C) SST3 test. Purple triangles: ASN20. (D) SST4 test. Blue triangles: 5 km inter-distance virtual network.
The network response in terms of TFA varies in dependence of the seismic station distribution and of network density around the epicentral area ( Figure 5 ). The addition of TSN16 to PSN15 around the seismic event causes a reduction of TFA from 8.7 to 6.3 s (gain of 2.4 s) and a reduction of the blind zone (BZ) radius from 26.5 to 18.3 km ( Table 5 ). In the third case, the sparse distribution of the accelerometric stations around the epicentral area causes a worsening of the network response with respect to the other two tests and TFA and BZ jump to 9.8 s and 31.4 km respectively. In the SST3 test, the MU_AP target has not safely time for activate protection actions, being on the BZ border, and MU_SB, MU_FX, and MU_TR targets have less than 10 s of LT ( Figure 5C and Table 6 ). The sample SST4 returns similar values of the SST2 test ( Table 5 and Table 6 ), strengthening the idea that the EDS can represent a tool for design an improvement of the EEWS starting from an existent seismic network or a new EEWS imaging an entirely new network in uncovered areas.
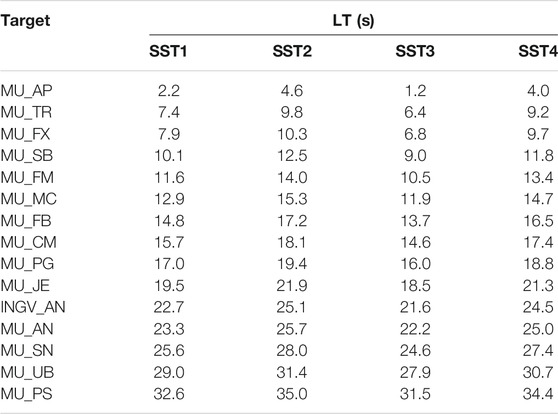
TABLE 6 . Results of LT for selected targets. Target: city code. Place: extended name of cities. LT: Values of LT in seconds referred to the test in Table 4 .
The EDS is also a tool to map the TFA, BZ, and LT over the territory. Indeed, it is possible to configure the procedure including a set of sources, every combined with a TFA and a BZ value. Moreover, it is possible to obtain LT linked to each epicentral location referred to a single target.
The example to explain the use of EDS to mapping EEW parameters is showed in Figure 6 , where the PSN20 is applied. In the left column, a set of locations referred to the main historical seismic events of Mw ≥ 5.5 from 1269 to 2017 AD that hit the region is presented ( Figure 6A ), extracted from CPTI15 catalog ( Rovida et al., 2021 ). In the right column, a grid of sources with inter-distance of 5 km, depth of 10 km and covering the whole region is depicted ( Figure 6B ). The example of historical events answers the question of which TFA and BZ would occur with the actual seismic network if seismic events repeat in the same locations. Each historical epicentral location is mapped in terms of TFA and BZ ( Figures 6C, E ). Smaller TFA (red points) are mapped where the network is denser in inland areas at the center of the network. For southern inland events and coastal events (yellow dots), the EEWS provides less protection which is significantly reduced for northern events (green dots) where TFA greater than 10 s results in BZ with a radius greater than 30 km.
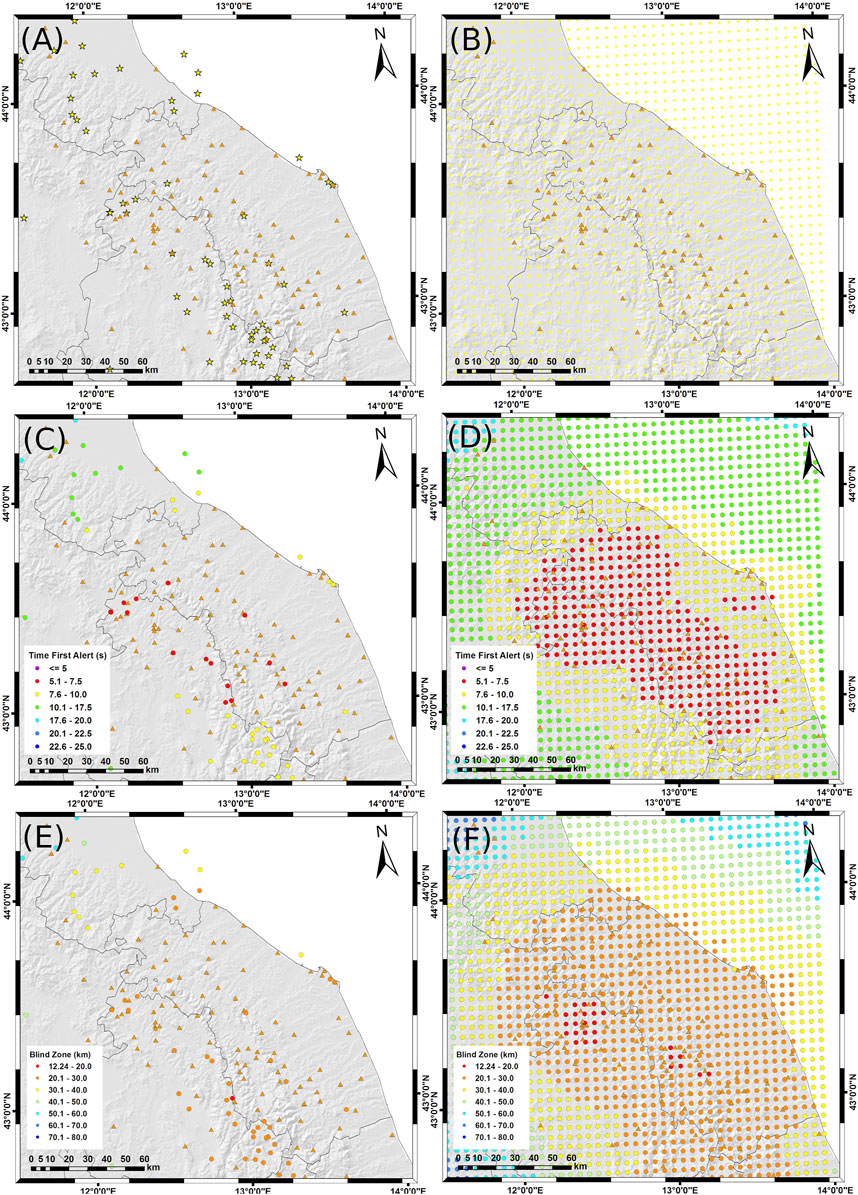
FIGURE 6 . TFA and BZ mapping. (A) : historical events from CPTI15 v3.0 Mw ≥ 5.5 from1269 to 2017 AD, depth 10 km before 1997. (B) : virtual grid of sources distant of 5 km and depth of 10 km. (C) : mapping of TFA with historical events. (D) : mapping of TFA with virtual grid. (E) : mapping of BZ with historical events. (F) : mapping of BZ with virtual grid.
The same principle can be extended to the whole territory, mapping TFA ( Figure 6D ), and BZ ( Figure 6F ) with the grid of sources. The map of the TFA depicts the edges of the areas within which, if a seismic event occurs, the EEWS responds with a TFA threshold. In particular, for the eastern part of central Italy, with the actual INGV network, an EEWS could produce TFA less than 7.5 s for a large inland area that includes part of the most active seismic zones. Around the Adriatic coast, the seismic network is less dense and an earthquake that occurs off-shore is out of the network. Therefore, the coastal and off-shore TFAs are shorter than those in the inland zones. Besides, the elongated distribution of the seismic stations next to the coast could cause bad locations and estimate of the magnitude by the EEWS, worsening even more the protection provided by the alarm. Also, the northern part of the region is lacking stations and the resulting TFA are similar to the off-shore values. For the largest part of the region, a BZ ranging between 20 and 30 km from the epicenter would not be protected by a warning. The BZ radius increases up to over 30–40 km for the off-shore locations and the EEW system could not produce a TFA for coastal cities. To overcome this problem, offshore seismometers would be extremely useful.
The EDS allows exporting results of the TFA values to map LT referred to a single target. The LT map helps to link places of hypothetical epicenters and the time available for safety actions at the target. For example, the map in Figure 7 shows the LT available for the city of Fabriano (in particular for the location of the city Hall, MU_FB target). The color map shows the values of LT in equally spaced sources, located at 10 km depth. The black dots limit the epicenters for which the city of Fabriano falls in the BZ. Indeed, in this zone the LT is negative (≤0 s). The area marked with red dots corresponds to a 0 s < LT ≤ 5 s where an alarm could be provided but security actions are unlikely. In the orange area (5 s < LT ≤ 10 s) triggering automatic actions would be possible ( Cauzzi et al., 2016 ). Besides the orange area (LT > 10 s), trained people can take precautionary and protective actions ( Fujinawa and Noda, 2013 ) and the probability of a successful alert increases significantly ( Becker et al., 2020b ).
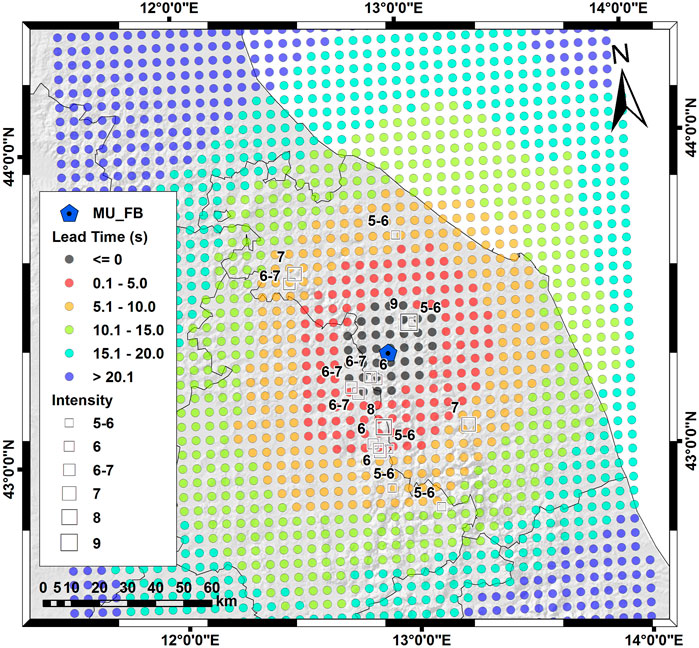
FIGURE 7 . LT mapping for city of Fabriano and historical destructive earthquake. Blue pentagon: place of city of Fabriano (MU_FB target). Colored circles: values of LT in seconds. Squares: CPTI15 v3.0 historical earthquakes and macroseismic intensity (DBMI15 v3.0, Locati et al., 2021 ) estimated for the city of Fabriano.
This argument makes sense in particular for those earthquakes that could damage the target. Therefore, the destructive earthquakes for the city of Fabriano, extracted from CPTI15 catalog ( Rovida et al., 2021 ), overlap the LT map in Figure 7 . Clearly, most of the events fall in black and red areas, since the MU_FB target is placed close to the inland active seismic zone ( Figure 1 ) and the EEWS would be almost useless. However, some historical events that caused damages with a macroseismic intensity from five to six to 7, fall in the orange area where automatic actions are possible. For the southernmost event (Mw 6.5 on October 30, 2016), an EEWS could have provided an alert 10 s before the arrival of the first S seismic waves to the city of Fabriano, a good LT to take several safety actions.
6 Conclusion
The feasibility of an EEWS derives from the design of a seismic network with respect to the seismic sources located within or around it. EEWSs are today an effective contribution to the problem of seismic risk mitigation, but few countries have operational systems ( Satriano et al., 2011b ). Implementation of EEWSs in Italy is a challenge. Italy is an elongated peninsula, with its central part crossed by a mountain chain that is seismically active and runs very close to urbanized areas. Therefore, the simulations of seismic networks contribute in the field of territorial safety by identifying the time in which some types of protection actions could be activated.
In this work, we have developed a simulation procedure (EDS), useful to estimate the feasibility of an EEWS and showed some applications in eastern central Italy, where INGV manages a dense seismic network. A validation process was performed by comparing results of simulations with real-time and playback instances of the EEW PRESTo system implemented in the same region ( Festa et al., 2018 ).
EDS is a tool to simulate different seismic network responses reproducing the physical contest of a specific historical or recent earthquake; moreover, EDS is a tool to map EEW parameters (TFA, BZ, and LT) to classify the whole territory in terms of areas where it is possible to activate safety actions. With EDS, it is possible to model real seismic networks inserting actual or theoretical data latencies due to different transmission vectors and to design new seismic networks in uncovered areas.
As expected, the results of the EDS application in eastern central Italy highlights short, but still useful, alert times for innermost land and coastal areas overlooking the active seismic zones. However, we have shown that most of the currently used transmission vectors have latencies between 2 and 3 s, an acceptable value for seismic early warnings. The quantitative estimate of TFA, BZ, and LT supplies useful information to project an improvement of the EEWS with the aim to reduce as much as possible the TFA. Modern approaches include the use of low-cost sensors for a greater diffusion of the seismic monitoring throughout the territory, and the development of networks oriented toward smart cities using fast protocols and connectivity ( Ladina et al., 2016 ; Pierleoni et al., 2018 ; D’Alessandro et al., 2019 ). In the area of the 2016 Central Italy sequence, we have estimated a gain of about 2.5 s of LT, adding the 2016 emergency temporary stations (station inter-distance about 5 km) over the permanent network, with a data latency similar to GPRS connections. The result was repeated setting a virtual network in a station grid with a constant inter-distance of 5 km and a mean data latency of 2.6 s to demonstrate the ability to project an EEWS in uncovered areas and to obtain realistic times of the first alert.
Although the Italian territory is mostly characterized by seismic sources next to urbanized areas, the lead times calculated with EDS procedure encourage the opportunity of implementing an EEWS for several interesting targets. Simulating historical events, a LT between 5 and 10 s was reproduced for some of these targets. We conclude that automatic safety actions, situational awareness and a more rapid response by well-trained people are a realistic goal in eastern central Italy.
We are aware that the study presented here has some limitations, first of all the system performance is evaluated analyzing mainly the rapidity of an EWS, whereas no evaluation assessment is made on the reliability of the earthquake impact prediction. This implies that the evaluation of the shaking could be inaccurate or even lead to false or missed alerts. However, the goal of our analysis was to determine whether an EWS could be a viable solution to reduce seismic exposure in certain regions of Italy, and in which conditions (network geometry, transmission times, etc.). Our results are encouraging provided that some technological issues are considered and that people’s awareness of seismic risk is increased. Further studies will be dedicated to a more thorough assessment of EWS.
The development of a widespread monitoring infrastructure near the main seismic sources, the massive training of citizens, and the collaboration with civil protection authorities could improve the scenarios simulated in this work, making an EEWS really effective in protecting people from the harmful effects of earthquakes in Italy.
Data Availability Statement
The raw data supporting the conclusions of this article will be made available by the authors, without undue reservation.
Author Contributions
Conceptualization; methodology; validation; data analysis and curation: CL, SM; data analysis supervision: AA, MC; writing-original draft preparation; writing-review and editing: CL, SM, AA, MC. All authors contributed to manuscript, read and approved the submitted version.
This work has been carried out within the Project ART-IT (Allerta Rapida Terremoti in Italia), funded by the Italian Ministry of University and Research (Progetto Premiale 2015, DM. 850/2017), and supported also by the Agreement between Civil Protection Department of the Regione Marche and INGV.
Conflict of Interest
The authors declare that the research was conducted in the absence of any commercial or financial relationships that could be construed as a potential conflict of interest.
Publisher’s Note
All claims expressed in this article are solely those of the authors and do not necessarily represent those of their affiliated organizations, or those of the publisher, the editors and the reviewers. Any product that may be evaluated in this article, or claim that may be made by its manufacturer, is not guaranteed or endorsed by the publisher.
Acknowledgments
The authors thank Mario Locati for support with DBMI15 v2.0, Luca Elia for suggestion and explanations with PRESTo configuration, and Simona Colombelli for the suggestions about the details of the work.
Supplementary Material
The Supplementary Material for this article can be found online at: https://www.frontiersin.org/articles/10.3389/feart.2021.685751/full#supplementary-material
Alcik, H., Ozel, O., Apaydin, N., and Erdik, M. (2009). A Study on Warning Algorithms for Istanbul Earthquake Early Warning System. Geophys. Res. Lett. 36, L00B05. doi:10.1029/2008GL036659
CrossRef Full Text | Google Scholar
Allen, R. M., Brown, H., Hellweg, M., Khainovski, O., Lombard, P., and Neuhauser, D. (2009a). Real-time Earthquake Detection and hazard Assessment by ElarmS across California. Geophys. Res. Lett. 36, L00B08. doi:10.1029/2008GL036766
Allen, R. M., Gasparini, P., Kamigaichi, O., and Böse, M. (2009b). The Status of Earthquake Early Warning Around the World: An Introductory Overview. Seismological Res. Lett. 80 (5), 682–693. doi:10.1785/gssrl.80.5.682
Allen, R. M., and Kanamori, H. (2003). The Potential for Earthquake Early Warning in Southern California. Science 300, 786–789. doi:10.1126/science.1080912
PubMed Abstract | CrossRef Full Text | Google Scholar
Allen, R. M., and Melgar, D. (2019). Earthquake Early Warning: Advances, Scientific Challenges, and Societal Needs. Annu. Rev. Earth Planet. Sci. 47, 361–388. doi:10.1146/annurev-earth-053018-060457
Becker, J. S., Potter, S. H., Prasanna, R., Tan, M. L., Payne, B. A., Holden, C., et al. (2020b). Scoping the Potential for Earthquake Early Warning in Aotearoa New Zealand: A Sectoral Analysis of Perceived Benefits and Challenges. Int. J. Disaster Risk Reduction 51, 101765. doi:10.1016/j.ijdrr.2020.101765
Becker, J. S., Potter, S. H., Vinnell, L. J., Nakayachi, K., McBride, S. K., and Johnston, D. M. (2020a). Earthquake Early Warning in Aotearoa New Zealand: a Survey of Public Perspectives to Guide Warning System Development. Humanit Soc. Sci. Commun. 7. doi:10.1057/s41599-020-00613-9
Böse, M., Ionescu, C., and Wenzel, F. (2007). Earthquake Early Warning for Bucharest, Romania: Novel and Revised Scaling Relations. Geophys. Res. Lett. 34, L07302. doi:10.1029/2007GL029396
Caruso, A., Colombelli, S., Elia, L., Picozzi, M., and Zollo, A. (2017). An On-Site Alert Level Early Warning System for Italy. J. Geophys. Res. Solid Earth 122. doi:10.1002/2016JB013403
Cattaneo, M., Frapiccini, M., Ladina, C., Marzorati, S., and Monachesi, G. (2017). A Mixed Automatic-Manual Seismic Catalog for Central-Eastern Italy: Analysis of Homogeneity. Ann. Geophys. 60, 6. doi:10.4401/ag-7333
Cauzzi, C., Behr, Y., Le Guenan, T., Douglas, J., Auclair, S., Woessner, J., et al. (2016). Earthquake Early Warning and Operational Earthquake Forecasting as Real-Time hazard Information to Mitigate Seismic Risk at Nuclear Facilities. Bull. Earthquake Eng. 14, 2495–2512. doi:10.1007/s10518-016-9864-0
Chiaraluce, L., Amato, A., Carannante, S., Castelli, V., Cattaneo, M., Cocco, M., et al. (2014). The Alto Tiberina Near Fault Observatory (Northern Apennines, Italy). Ann. Geophys. 57, 3. doi:10.4401/ag-6426
Chiaraluce, L., Di Stefano, R., Tinti, E., Scognamiglio, L., Michele, M., Casarotti, E., et al. (2017). The 2016 Central Italy Seismic Sequence: A First Look at the Mainshocks, Aftershocks, and Source Models. Seismological Res. Lett. 88 (3), 757–771. doi:10.1785/0220160221
Clinton, J., Zollo, A., Marmureanu, A., Zulfikar, C., and Parolai, S. (2016). State-of-the Art and Future of Earthquake Early Warning in the European Region. Bull. Earthquake Eng. 14, 2441–2458. doi:10.1007/s10518-016-9922-7
Colombelli, S., Amoroso, O., Zollo, A., and Kanamori, H. (2012a). Test of a Threshold-Based Earthquake Early-Warning Method Using Japanese Data. Bull. Seismological Soc. America 102, 1266–1275. doi:10.1785/0120110149
Colombelli, S., Caruso, A., Zollo, A., Festa, G., and Kanamori, H. (2015). A P Wave‐based, On‐site Method for Earthquake Early Warning. Geophys. Res. Lett. 42, 1390–1398. doi:10.1002/2014GL063002
Colombelli, S., Zollo, A., Festa, G., and Kanamori, H. (2012b). Early Magnitude and Potential Damage Zone Estimates for the Great Mw 9 Tohoku-Oki Earthquake. Geophys. Res. Lett. 39, a–n. doi:10.1029/2012gl053923
Cua, G., Fischer, M., Heaton, T., and Wiemer, S. (2009). Real-time Performance of the Virtual Seismologist Earthquake Early Warning Algorithm in Southern California. Seismological Res. Lett. 80, 740–747. Number 5. doi:10.1785/gssrl.80.5.740
D’Alessandro, A., Costanzo, A., Ladina, C., Buongiorno, F., Cattaneo, M., Falcone, S., et al. (2019). Urban Seismic Networks, Structural Health and Cultural Heritage Monitoring: The National Earthquakes Observatory (INGV Ltaly) Experience. Front. Built Environ. doi:10.3389/fbuil.2019.00127
De Luca, G., Cattaneo, M., Monachesi, G., and Amato, A. (2009). Seismicity in Central and Northern Apennines Integrating the Italian National and Regional Networks. Tectonophysics 476, 121–135. doi:10.1016/j.tecto.2008.11.032
DISS Working Group (2018). Database of Individual Seismogenic Sources (DISS), Version 3.2.1: A Compilation of Potential Sources for Earthquakes Larger Than M 5.5 in Italy and Surrounding Areas. Istituto Nazionale di Geofisica e Vulcanologia. Available at: http://diss.rm.ingv.it/diss/ . doi:10.6092/INGV.IT-DISS3.2.1
Dunn, P. T., Ahn, A. Y. E., Bostrom, A., and Vidale, J. E. (2016). Perceptions of Earthquake Early Warnings on the U.S. West Coast. Int. J. Disaster Risk Reduction 20, 112–122. doi:10.1016/j.ijdrr.2016.10.019
Erdik, M., Fahjan, Y., Ozel, O., Alcik, H., Mert, A., and Gul, M. (2003). Instabul Earthquake Rapid Response and the Early Warning System. Bull. Earthquake Eng. 1 (1), 157–163. doi:10.1023/A:1024813612271
Festa, G., Picozzi, M., Caruso, A., Colombelli, S., Cattaneo, M., Chiaraluce, L., et al. (2018). Performance of Earthquake Early Warning Systems during the 2016-2017 Mw 5-6.5 Central Italy Sequence. Seismol. Res. Lett. 89, 1–12. doi:10.1785/0220170150
Fujinawa, Y., and Noda, Y. (2013). Japan's Earthquake Early Warning System on 11 March 2011: Performance, Shortcomings, and Changes. Earthquake Spectra 29 (S1), S341–S368. doi:10.1193/1.4000127
Gorini, A., Nicoletti, M., Marsan, P., Bianconi, R., De Nardis, R., Filippi, L., et al. (2010). The Italian strong Motion Network. Bull. Earthquake Eng. 8, 1075–1090. doi:10.1007/s10518-009-9141-6
Hsiao, N.-C., Wu, Y.-M., Shin, T.-C., Zhao, L., and Teng, T.-L. (2009). Development of Earthquake Early Warning System in Taiwan. Geophys. Res. Lett. 36, L00B02. doi:10.1029/2008GL036596
Iannaccone, G., Zollo, A., Elia, L., Convertito, V., Satriano, C., Martino, C., et al. (2010). A Prototype System for Earthquake Early-Warning and Alert Management in Southern Italy. Bull. Earthquake Eng. 8, 1105–1129. doi:10.1007/s10518-009-9131-8
Iervolino, I., Galasso, C., and Manfredi, G. (2008). “Information-dependent lead-time Maps for Earthquake Early Warning in the Campania Region,” in The 14th World Conference on Earthquake Engineering , Beijing, China , October 12-17, 2008 .
Google Scholar
INGV Seismological Data Centre (2006). Rete Sismica Nazionale (RSN) . Italy: Istituto Nazionale di Geofisica e Vulcanologia (INGV) . doi:10.13127/sd/x0fxnh7qfy
CrossRef Full Text
Kohler, M. D., Smith, D. E., Andrews, J., Chung, A. I., Hartog, R., Henson, I., et al. (2020). Earthquake Early Warning ShakeAlert 2.0: Public Rollout. Seismol. Res. Lett. 91 (3), 1763–1775. doi:10.1785/0220190245
Ladina, C., Marzorati, S., Monachesi, G., Cattaneo, M., Frapiccini, M., and Castelli, V. (2016). Strong-motion Observations Recorded in Strategic Public Buildings during the 24 August 2016 Mw 6.0 Amatrice (central Italy) Earthquake. Ann. Geophys. 59. Fast Track 5 (2016): The Amatrice seismic sequence: preliminary data and results. doi:10.4401/ag-7194
Locati, M., Camassi, R., Rovida, A., Ercolani, E., Bernardini, F., Castelli, V., et al. (2021). Database Macrosismico Italiano (DBMI15), versione 3.0 . Istituto Nazionale di Geofisica e Vulcanologia (INGV) . doi:10.13127/DBMI/DBMI15.3
Lomax, A., Michelini, A., and Curtis, A. (2009). “Earthquake LocationEarthquake Location, Direct, Global-Search Methods,” in Encyclopedia of Complexity and System Science (New York: Springer ), 2449–2473. doi:10.1007/978-0-387-30440-3_150
Moretti, M., Pondrelli, S., Margheriti, L., Abruzzese, L., Anselmi, M., Arroucau, P., et al. (2016). SISMIKO: Emergency Network Deployment and Data Sharing for the 2016 central Italy Seismic Sequence. Ann. Geophys. 59.Fast Track 5. doi:10.4401/ag-7212
Odaka, T., Ashiya, K., Tsukada, S., Sato, S., Ohtake, K., and Nozaka, D. (2003). A New Method of Quickly Estimating Epicentral Distance and Magnitude from a Single Seismic Record. Bull. Seismological Soc. America 93 (1), 526–532. doi:10.1785/0120020008
Olivieri, M., Allen, R. M., and Wurman, G. (2008). The potential for earthquake early warning in Italy using ElarmS. Bull. Seismol. Soc. Am. 98 (1), 495–503. doi:10.1785/0120070054
Picozzi, M. (2012). An Attempt of Real-Time Structural Response Assessment by an Interferometric Approach: A Tailor-Made Earthquake Early Warning for Buildings. Soil Dyn. Earthquake Eng. 38, 109–118. doi:10.1016/j.soildyn.2012.02.003
Picozzi, M., Zollo, A., Brondi, P., Colombelli, S., Elia, L., and Martino, C. (2015). Exploring the Feasibility of a Nationwide Earthquake Early Warning System in Italy. J. Geophys. Res. Solid Earth 120, 2446–2465. doi:10.1002/2014JB011669
Pierleoni, P., Marzorati, S., Ladina, C., Raggiunto, S., Belli, A., Palma, L., et al. (2018). Perfarmance Evaluation of a Low-Cost Sensing Unit for Seismic Applications: Field Testing during Seismic Events of 2016-2017 in Central Ltaly. IEEE Sensor Joumal 18, 16. doi:10.11O9/JSEN.2018.285006510.1109/jsen.2018.2850065
PRESTo, (2013). Probabilistic and Evolutionary Early Warning SysTem. Available at: http://www.prestoews.org (Accessed March 25, 2021).
Rovida, A., Locati, M., Camassi, R., Lolli, B., Gasperini, P., and Antonucci, A. (2021). Catalogo Parametrico dei Terremoti Italiani (CPTI15), versione 3.0 . Istituto Nazionale di Geofisica e Vulcanologia (INGV) . doi:10.13127/CPTI/CPTI15.3
Satriano, C., Elia, L., Martino, C., Lancieri, M., Zollo, A., and Iannaccone, G. (2011a). PRESTo, the Earthquake Early Warning System for Southern Italy: Concepts, Capabilities and Future Perspectives. Soil Dyn. Earthquake Eng. 31, 137–153. doi:10.1016/j.soildyn.2010.06.008
Satriano, C., Wu, Y.-M., Zollo, A., and Kanamori, H. (2011b). Earthquake Early Warning: Concepts, Methods and Physical Grounds. Soil Dyn. Earthquake Eng. 31, 106–118. doi:10.1016/j.soildyn.2010.07.007
SISMIKO (2020). Coordinamento Delle Reti Sismiche mobile in Emergenza. Available at: http://sismiko.ingv.it (Accessed March 25, 2021).
Suarez, G., Novelo, D., and Mansilla, E. (2009). Performance Evaluation of the Seismic Alert System (SAS) in Mexico City: A Seismological and a Social Perspective. Seismological Res. Lett. 80 (5), 707–716. doi:10.1785/gssrl.80.5.707
Velazquez, O., Pescaroli, G., Cremen, G., and Galasso, C. (2020). A Review of the Technical and Socio-Organizational Components of Earthquake Early Warning Systems. Front. Earth Sci. 8, 533498. doi:10.3389/feart.2020.533498
Wu, Y.-M., and Teng, T. L. (2002). A Virtual Subnetwork Approach to Earthquake Early Warning. Bull. Seismological Soc. America 92 (5), 2008–2018. doi:10.1785/0120010217
Zollo, A., Amoroso, O., Lancieri, M., Wu, Y.-M., and Kanamori, H. (2010). A Threshold-Based Earthquake Early Warning Using Dense Accelerometer Networks. Geophys. J. Int. 183, 963–974. doi:10.1111/j.1365-246X.2010.04765.x
Zollo, A., Colombelli, S., Elia, L., Emolo, A., Festa, G., Iannaccone, G., et al. (2014). “An Integrated Regional and On-Site Earthquake Early Warning System for Southern Italy: Concepts, Methodologies and Performances,” in Book: Early Warning for Geological Disasters, Advanced Technologies in Earth Sciences , 117–137. doi:10.1007/978-3-642-12233-0_7
Keywords: earthquakes, early warning, seismic networks, seismic risk reduction, simulation
Citation: Ladina C, Marzorati S, Amato A and Cattaneo M (2021) Feasibility Study of an Earthquake Early Warning System in Eastern Central Italy. Front. Earth Sci. 9:685751. doi: 10.3389/feart.2021.685751
Received: 25 March 2021; Accepted: 05 July 2021; Published: 20 August 2021.
Reviewed by:
Copyright © 2021 Ladina, Marzorati, Amato and Cattaneo. This is an open-access article distributed under the terms of the Creative Commons Attribution License (CC BY). The use, distribution or reproduction in other forums is permitted, provided the original author(s) and the copyright owner(s) are credited and that the original publication in this journal is cited, in accordance with accepted academic practice. No use, distribution or reproduction is permitted which does not comply with these terms.
*Correspondence: Chiara Ladina, [email protected]
This article is part of the Research Topic
The New Frontiers of Earthquake Early Warning Systems
Earthquake monitoring and warning system
Ieee account.
- Change Username/Password
- Update Address
Purchase Details
- Payment Options
- Order History
- View Purchased Documents
Profile Information
- Communications Preferences
- Profession and Education
- Technical Interests
- US & Canada: +1 800 678 4333
- Worldwide: +1 732 981 0060
- Contact & Support
- About IEEE Xplore
- Accessibility
- Terms of Use
- Nondiscrimination Policy
- Privacy & Opting Out of Cookies
A not-for-profit organization, IEEE is the world's largest technical professional organization dedicated to advancing technology for the benefit of humanity. © Copyright 2024 IEEE - All rights reserved. Use of this web site signifies your agreement to the terms and conditions.
Academia.edu no longer supports Internet Explorer.
To browse Academia.edu and the wider internet faster and more securely, please take a few seconds to upgrade your browser .
Enter the email address you signed up with and we'll email you a reset link.
- We're Hiring!
- Help Center
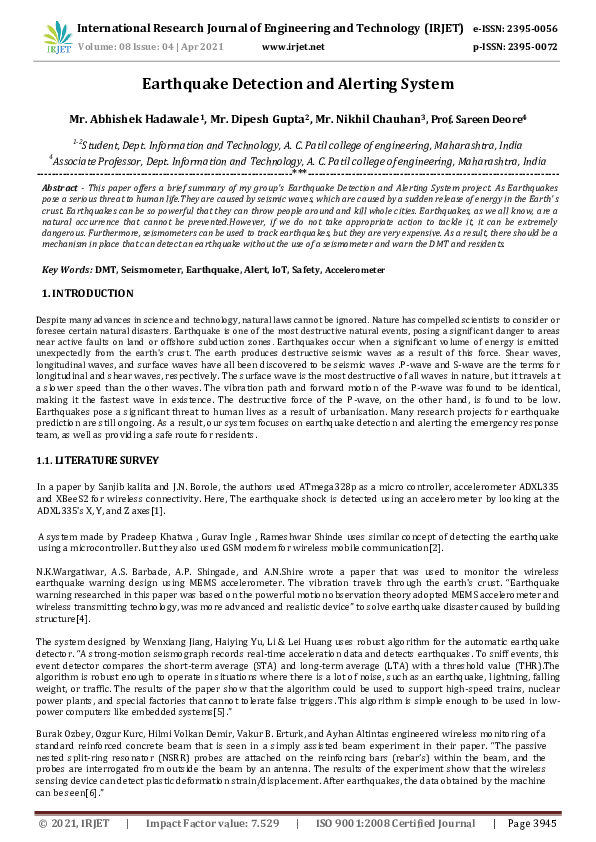
Earthquake Detection and Alerting System

Related Papers
IRJET Journal
This paper offers a brief summary of my group's Earthquake Detection and Alerting System project. As Earthquakes pose a serious threat to human life.They are caused by seismic waves, which are caused by a sudden release of energy in the Earth's crust. Earthquakes can be so powerful that they can throw people around and kill whole cities. Earthquakes, as we all know, are a natural occurrence that cannot be prevented.However, if we do not take appropriate action to tackle it, it can be extremely dangerous. Furthermore, seismometers can be used to track earthquakes, but they are very expensive. As a result, there should be a mechanism in place that can detect an earthquake without the use of a seismometer and warn the DMT and residents.
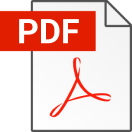
Internets of Things-enabled Intelligent Transportation Systems (ITS) are gaining significant attention in academic literature and industry, and are seen as an answer to enhancing road safety in smart cities. Due to the ever increasing number of vehicles, a big rise within the number of road accidents has been observed. Vehicles embedded with a plethora of sensors enable us to not only monitor the present situation of the vehicle and its surroundings but also facilitates the detection of incidents. Significant research, for instance, has been conducted on accident rescue, particularly on the utilization of data and Communication Technologies (ICT) for efficient and prompt rescue operations. The majority of such works provide sophisticated solutions that specialise in reducing response times. However, such solutions are often expensive and aren't available altogether sorts of vehicles. Given this, we present a completely unique Internet of Things-based accident detection and reporting system for a sensible city environment. The proposed approach aims to require advantage of advanced specifications of smartphones to style and develop a low-cost solution for enhanced transportation systems that's deployable in legacy vehicles. In this context, a customized Android application is developed to collect information regarding speed, gravity, pressure, sound, and site. The speed is a factor that is used to help improve the identification of accidents. It arises due to clear differences in environmental conditions (e.g., noise, deceleration rate) that arise in low speed collisions, versus higher speed collisions).The information acquired is further processed to detect road incidents. Furthermore, a navigation system is additionally developed to report the incident to the closest hospital. The proposed approach is validated through simulations and comparison with a true data set of road accidents acquired from Road Safety Open Repository, and shows promising leads to terms of accuracy.
Natural disasters such as earth quake and flood etc have very harmful effects lively hood and even loss of life. There are lot of incident took place in past decades one of such incident which as took place in recent time in India Up flood due over flow of Ganga river which has effected severely and many people lost their life the incident of Jharkhand earth quake also took place in recent times so to avoid such situation we implemented a system where the location is displayed on Google map and data is recorded in blynk app and alerting is done through buzzer here we can see demonstration of both earth quake and flood when the sensor observes or senses the vibrations the earth quake is detected notification is sent to blynk app and water level rises from the dam the sensor senses up to the level the water level has been increased which is recorded in graph form in blynk app which we can demonstrate using glass by pouring water level at different level hence the alerting is done prior to the event so there is less chances of risk to the life and whole system works on Wi-Fi model. The proposed system which we have implemented in this course of time consist of the two sensors MEMS and ultrasonic sensors most commonly called as flood sensors, GPS, Wi-Fi module, LCD display and Arduino
One of the prime reasons for vehicular accidents is due to undetected potholes and road humps. Well maintained roads contribute a major portion to the country's economy. The importance of road infrastructure society could be compared with importance of blood vessels for humans. To ensure road surface quality it should be monitored continuously and repaired as necessary In this paper ultrasonic sensor are used to identify potholes and humps to measure their depth and height respectively. This provides a prototype of an IoT based potholes and hump detection system that can be integrated with the vehicle and provide timely information to maintenance authorities so that necessary steps can be taken for safety of drivers.
In the present time internet becomes an essential need and without it we can't imagine our lifestyle. This paper talks about a smart military base which is design to upgrade the defence security of our military. It collects data from various sensors fitted inside it and send it to the cloud storage having IP address 184.106.153.149. We use ESP8266 WIFI module for uploading the data on the cloud in real time and Arduino uno microcontroller board to control all the sensors and modules. We can observe the movement ,temperature, flammable or toxic gases present in the air and humidity inside the base from any corner of the world. This project is based on the concept of internet of things(IoT).
The objective of the smart helmet is to provide a means and apparatus for detecting and reporting accidents. Sensors, and cloud computing infrastructures are utilised for building the system. The accident detection system communicates the accelerometer values to the processor which continuously monitors for erratic variations. When an accident occurs, the related details are sent to the emergency contacts by utilizing a cloud based service. The vehicle location is obtained by making use of the global positioning system. The system promises a reliable and quick delivery of information relating to the accident in real time and up dated to cloud which are accessed by IOT. Thus, by making use of the ubiquitous connectivity which is a salient feature for the smart cities, a smart helmet for accident detection is built.
The Internet of Things (IoT) is assuming an imperative part in our day by day lives all together to accomplish assignments by in corporating the utilization of sensor networks including our current circumstance. The frameworks created to notice the actual inclination that makes information furthermore, the made information is put away in the cloud. The put away data is then used for planning applications for controlling important activities. This paper describes the application and experimentation of a framework made out of sensors for checking temperature and stickiness of the zone encompassing. This noticed data is used to perform transient activities, for example, controlling the electronic contraptions for warming or cooling that takes additional time. The recorded information is stacked to cloud for capacity and further accessed through an Android application and showcases the results to the versatile clients. The system introduced in this paper utilized Arduino UNO board, DHT11 sensor, soil moisture sensor, ESP8266 Wi-Fi module, which makes data to open IoT based API organization Thing Speak through which it is examined and kept aside. An Android application is made which gets to the cloud also, shows results for end-customers through REST API Web organization. The exploratory outcomes shown demonstrate the adequacy of the framework.
The loss of properties and living population is getting enhanced by every year due to the dynamic alterations in weather conditions which results in heavy floods. Therefore, implementation of an intelligent analysis of flood risk is necessitated for the field of research in Disaster management. This project implements an intelligent IoT-based flood monitoring and alerting system using Raspberry Pi model, where water sensors and rain sensors are utilized to alert the authorities regarding the heaviness of rain and monitoring of water level in a lake or river. This system alerts the people in nearby villages since it utilizes IoT system for notifying the village people.
Healthcare is given the major importance now a-days in all the countries with the advent of the chronic diseases. This work provides better solution for remote health monitoring with affordable cost using latest technologies. IoT is the fast-growing technology in every sector. With the integration of IoT and sensors healthcare monitoring can be brought to such a pace. Sensor readout are carried out using LabVIEW; a virtual instrumentation software. The results will be copied in the IoT server and then it will be analyzed. If it is in normal condition the report will be forwarded to the patient or if it is critical the results will be sent to the healthcare immediately, also ambulance can be called automatically without human intervention, if necessary. This ensures that proper treatment is provided to the patient at the early stage. The health will be monitored continuously and properly diagnosed of the state of patient health. It reduces the complexity, the cost, the waiting time and the death rates. These devices can be installed at any remote location, even at work environment, and can be operated without the help of medical personal. This work focuses on fast-track generation of report and reaching the doctor even before the patient arrive.
There are a couple of spots that are more disposed to flooding than various spots; the execution of flood-prepared systems near any critical water an area or stream gives fundamental data that can ensure property and save lives. Clearly, the best flood alerted techniques are extreme and need high help, and require incredibly qualified delegates to work it. These days, there is no thought regarding when floods will happen, so there is a need to caution individuals who are close to the overwhelmed region. Hence, we are arranging this system to enlighten people about the approaching flood through notice and prepared messages. Sending real time data to the users who have installed the app and also providing a direction details to the users within the app. For that reason, we will utilize a few sensors that will assist with giving data about the flood. This framework gives genuine execution to affiliations, associations, and people excited about creating and working flood seeing and cautioning structures. This undertaking likewise incorporates a Sensor-Based Water Quality Monitoring System, which is used for assessing physical and substance boundaries of the water. The boundaries, for instance, Temperature, pH, flex sensor and water level, and nature of the water, can be assessed. This sensor data is essential for quality monitoring of the flood alerting system
RELATED PAPERS
Rinku Bhagat
- We're Hiring!
- Help Center
- Find new research papers in:
- Health Sciences
- Earth Sciences
- Cognitive Science
- Mathematics
- Computer Science
- Academia ©2024
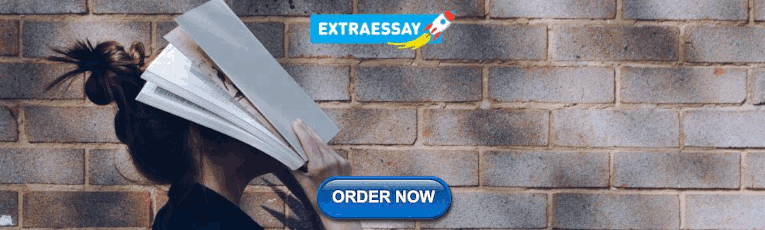
IMAGES
VIDEO
COMMENTS
In the present study, we present an intelligent earthquake signal detector that provides added assistance to automate traditional disaster responses. To effectively respond in a crisis scenario, additional sensors and automation are always necessary. Deep learning has achieved success in various low signal-to-noise ratio tasks, which motivated us to propose a novel 3-dimensional (3D) CNN-RNN ...
Earthquake Early Warning System (EEWS) is a system for early detection of strong earthquakes. based on the predicted arrival t ime of the S waves, w hich can cause significant shocks and even ...
In this paper, we present a multi-task network for simultaneous earthquake detection and phase picking based on a hierarchical attentive model. Our network consists of one deep encoder and three ...
1 Joint Centre for Disaster Research, Massey University, Wellington, New Zealand; 2 Department of Civil and Environmental Engineering, The University of Auckland, Auckland, New Zealand; Earthquake early warning system (EEWS) plays an important role in detecting ground shaking during an earthquake and alerting the public and authorities to take appropriate safety measures, reducing possible ...
Earthquake early warning systems (EEWS) are crucial for saving lives in earthquake-prone areas. In this study, we explore the potential of IoT and cloud infrastructure in realizing a sustainable EEWS that is capable of providing early warning to people and coordinating disaster response efforts. To achieve this goal, we provide an overview of the fundamental concepts of seismic waves and ...
In the standalone approach, straightforward earthquake detection algorithms can be easily implemented on less powerful mobile devices, but there is a possibility of false alarms. To address this limitation, in this paper, we present a collaborative approach that detects an earthquake using multiple smartphones located in a near area so as to ...
The SCSN has been producing local earthquake catalogs for a magnitude of completeness of M3.25 from 1932, presently reaching as low as M1.7.The present routine detection method is short-term-average/long-term average (STA/LTA) (Allen, 1982) combined with manual review.STA/LTA only tracks amplitude changes without completely utilizing the complex waveform features, thereby typically failing at ...
showed far fewer detection triggers on the Pohang earthquakes; rather than detecting the earthquake, it only showed a peak below the given threshold of 0.9 for the data recorded on the MPU9250 ...
1 Introduction. Earthquake early warning systems (EEWSs) are shifting earthquake science toward real-time event detection and data processing [Kuyuk and Allen, 2013a, 2013b; Kuyuk et al., 2014; Satriano et al., 2010].These systems are minimizing the time needed to calculate source parameters of earthquakes (essentially, location, and magnitude) to within a few seconds of their occurrence.
Earthquake is one of the natural disasters that have a big impact on society. Currently, there are many studies on earthquake detection. However, the vibrations that were detected by sensors were ...
Due to the nature of earthquakes, significant research efforts have been made to develop real-time earthquake detection systems for disaster management. Earthquake fatal levels of motion can cause fatalities and damage in populated regions . Because typical human structures are unable to resist large magnitude earthquakes, possible ways to ...
This paper proposes the use of earthquake detector sensors in building columns and foundation footings to detect seismic activity and provide early warning to building occupants.
Metrics. A new generation of earthquake catalogs developed through supervised machine-learning illuminates earthquake activity with unprecedented detail. Application of unsupervised machine ...
An earthquake early warning system (EEWS) is a monitoring infrastructure that allows alerting strategic points (targets) before the arrival of strong shaking waves during an earthquake. In a region like Central Italy, struck by recent and historical destructive earthquakes, the assessment of implementation of an EEWS is a significant challenge due to the proximity of seismic sources to many ...
The project aims at designing an earthquake monitoring and warning system that is capable of detecting earthquakes as well as warning people to take necessary precautions. This system will not only attempt to save human lives, but will also store the data for later use by professionals working at this sector. The objective of this project is to design a LabVIEW based control system by ...
The aims of review paper are: i). Review the vary keywords used in research. ii). Studies the different types of earthquakes detector that are appropriate. iii). Review the effectiveness of earthquake detectors and the effects of installation on environment. iv). Examination the output produced by the earthquake detectors through the created or ...
1.2. Research motivation. The current use of IoT technology has been recognized as an emergent study subject in every domain of science and engineering in response to earthquake-related losses (Bandyopadhyay and Sen, 2011, Gubbi et al., 2013).Small, low-cost sensors are utilized to collect seismic data for IoT-enabled earthquake monitoring and prediction (Abbasi et al., 2021).
Ipin Prasojo, The Design of Earthquake Detector Using Pen dulum Swing Based on ATMega328. microcontroller activ ates the photodiode senso r and LCD. Once activated, the LCD will display the text ...
Published 2019. Engineering, Environmental Science. An Earthquake detector using Arduino board and sensitive vibration device that can detect minute vibrations is presented in this paper. The components are able to provide immediate alert and helps to take preventive measures against earthquakes accordingly. ijsdr.org.
IRJET Journal. This paper offers a brief summary of my group's Earthquake Detection and Alerting System project. As Earthquakes pose a serious threat to human life.They are caused by seismic waves, which are caused by a sudden release of energy in the Earth's crust. Earthquakes can be so powerful that they can throw people around and kill whole ...
As the initial step of a research project which aims to explore the best use of P-waves to generate earthquake alerts, this Work in Progress paper (WiPe) presents the initial partial findings from ...
Conclusion. A deep learning-based integrated earthquake risk-mapping model for NE India using a complete earthquake catalog, DEM and shapefile data, and spatial analysis is proposed in this research. The chosen area is NE of India, which is characterized by 262,230 km 2 and falls under the Indian government.
Regression analysis is a statistical method. used for finding relationships between independent and de-. pendent variables. Regression analysis can be used for. prediction and forecasting. In ...