Have a language expert improve your writing
Run a free plagiarism check in 10 minutes, automatically generate references for free.
- Knowledge Base
- Methodology
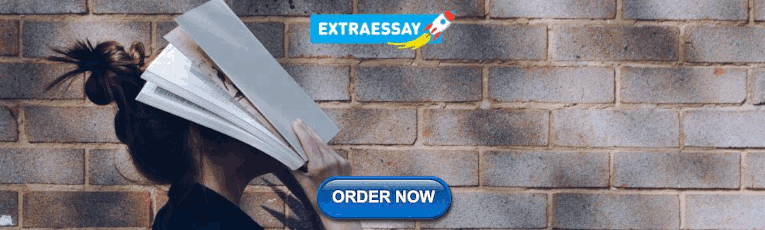
Research Methods | Definition, Types, Examples
Research methods are specific procedures for collecting and analysing data. Developing your research methods is an integral part of your research design . When planning your methods, there are two key decisions you will make.
First, decide how you will collect data . Your methods depend on what type of data you need to answer your research question :
- Qualitative vs quantitative : Will your data take the form of words or numbers?
- Primary vs secondary : Will you collect original data yourself, or will you use data that have already been collected by someone else?
- Descriptive vs experimental : Will you take measurements of something as it is, or will you perform an experiment?
Second, decide how you will analyse the data .
- For quantitative data, you can use statistical analysis methods to test relationships between variables.
- For qualitative data, you can use methods such as thematic analysis to interpret patterns and meanings in the data.
Table of contents
Methods for collecting data, examples of data collection methods, methods for analysing data, examples of data analysis methods, frequently asked questions about methodology.
Data are the information that you collect for the purposes of answering your research question . The type of data you need depends on the aims of your research.
Qualitative vs quantitative data
Your choice of qualitative or quantitative data collection depends on the type of knowledge you want to develop.
For questions about ideas, experiences and meanings, or to study something that can’t be described numerically, collect qualitative data .
If you want to develop a more mechanistic understanding of a topic, or your research involves hypothesis testing , collect quantitative data .
Qualitative | ||
---|---|---|
Quantitative | . |
You can also take a mixed methods approach, where you use both qualitative and quantitative research methods.
Primary vs secondary data
Primary data are any original information that you collect for the purposes of answering your research question (e.g. through surveys , observations and experiments ). Secondary data are information that has already been collected by other researchers (e.g. in a government census or previous scientific studies).
If you are exploring a novel research question, you’ll probably need to collect primary data. But if you want to synthesise existing knowledge, analyse historical trends, or identify patterns on a large scale, secondary data might be a better choice.
Primary | ||
---|---|---|
Secondary |
Descriptive vs experimental data
In descriptive research , you collect data about your study subject without intervening. The validity of your research will depend on your sampling method .
In experimental research , you systematically intervene in a process and measure the outcome. The validity of your research will depend on your experimental design .
To conduct an experiment, you need to be able to vary your independent variable , precisely measure your dependent variable, and control for confounding variables . If it’s practically and ethically possible, this method is the best choice for answering questions about cause and effect.
Descriptive | ||
---|---|---|
Experimental |
Prevent plagiarism, run a free check.
Research method | Primary or secondary? | Qualitative or quantitative? | When to use |
---|---|---|---|
Primary | Quantitative | To test cause-and-effect relationships. | |
Primary | Quantitative | To understand general characteristics of a population. | |
Interview/focus group | Primary | Qualitative | To gain more in-depth understanding of a topic. |
Observation | Primary | Either | To understand how something occurs in its natural setting. |
Secondary | Either | To situate your research in an existing body of work, or to evaluate trends within a research topic. | |
Either | Either | To gain an in-depth understanding of a specific group or context, or when you don’t have the resources for a large study. |
Your data analysis methods will depend on the type of data you collect and how you prepare them for analysis.
Data can often be analysed both quantitatively and qualitatively. For example, survey responses could be analysed qualitatively by studying the meanings of responses or quantitatively by studying the frequencies of responses.
Qualitative analysis methods
Qualitative analysis is used to understand words, ideas, and experiences. You can use it to interpret data that were collected:
- From open-ended survey and interview questions, literature reviews, case studies, and other sources that use text rather than numbers.
- Using non-probability sampling methods .
Qualitative analysis tends to be quite flexible and relies on the researcher’s judgement, so you have to reflect carefully on your choices and assumptions.
Quantitative analysis methods
Quantitative analysis uses numbers and statistics to understand frequencies, averages and correlations (in descriptive studies) or cause-and-effect relationships (in experiments).
You can use quantitative analysis to interpret data that were collected either:
- During an experiment.
- Using probability sampling methods .
Because the data are collected and analysed in a statistically valid way, the results of quantitative analysis can be easily standardised and shared among researchers.
Research method | Qualitative or quantitative? | When to use |
---|---|---|
Quantitative | To analyse data collected in a statistically valid manner (e.g. from experiments, surveys, and observations). | |
Meta-analysis | Quantitative | To statistically analyse the results of a large collection of studies. Can only be applied to studies that collected data in a statistically valid manner. |
Qualitative | To analyse data collected from interviews, focus groups or textual sources. To understand general themes in the data and how they are communicated. | |
Either | To analyse large volumes of textual or visual data collected from surveys, literature reviews, or other sources. Can be quantitative (i.e. frequencies of words) or qualitative (i.e. meanings of words). |
Quantitative research deals with numbers and statistics, while qualitative research deals with words and meanings.
Quantitative methods allow you to test a hypothesis by systematically collecting and analysing data, while qualitative methods allow you to explore ideas and experiences in depth.
In mixed methods research , you use both qualitative and quantitative data collection and analysis methods to answer your research question .
A sample is a subset of individuals from a larger population. Sampling means selecting the group that you will actually collect data from in your research.
For example, if you are researching the opinions of students in your university, you could survey a sample of 100 students.
Statistical sampling allows you to test a hypothesis about the characteristics of a population. There are various sampling methods you can use to ensure that your sample is representative of the population as a whole.
The research methods you use depend on the type of data you need to answer your research question .
- If you want to measure something or test a hypothesis , use quantitative methods . If you want to explore ideas, thoughts, and meanings, use qualitative methods .
- If you want to analyse a large amount of readily available data, use secondary data. If you want data specific to your purposes with control over how they are generated, collect primary data.
- If you want to establish cause-and-effect relationships between variables , use experimental methods. If you want to understand the characteristics of a research subject, use descriptive methods.
Methodology refers to the overarching strategy and rationale of your research project . It involves studying the methods used in your field and the theories or principles behind them, in order to develop an approach that matches your objectives.
Methods are the specific tools and procedures you use to collect and analyse data (e.g. experiments, surveys , and statistical tests ).
In shorter scientific papers, where the aim is to report the findings of a specific study, you might simply describe what you did in a methods section .
In a longer or more complex research project, such as a thesis or dissertation , you will probably include a methodology section , where you explain your approach to answering the research questions and cite relevant sources to support your choice of methods.
Is this article helpful?
More interesting articles.
- A Quick Guide to Experimental Design | 5 Steps & Examples
- Between-Subjects Design | Examples, Pros & Cons
- Case Study | Definition, Examples & Methods
- Cluster Sampling | A Simple Step-by-Step Guide with Examples
- Confounding Variables | Definition, Examples & Controls
- Construct Validity | Definition, Types, & Examples
- Content Analysis | A Step-by-Step Guide with Examples
- Control Groups and Treatment Groups | Uses & Examples
- Controlled Experiments | Methods & Examples of Control
- Correlation vs Causation | Differences, Designs & Examples
- Correlational Research | Guide, Design & Examples
- Critical Discourse Analysis | Definition, Guide & Examples
- Cross-Sectional Study | Definitions, Uses & Examples
- Data Cleaning | A Guide with Examples & Steps
- Data Collection Methods | Step-by-Step Guide & Examples
- Descriptive Research Design | Definition, Methods & Examples
- Doing Survey Research | A Step-by-Step Guide & Examples
- Ethical Considerations in Research | Types & Examples
- Explanatory Research | Definition, Guide, & Examples
- Explanatory vs Response Variables | Definitions & Examples
- Exploratory Research | Definition, Guide, & Examples
- External Validity | Types, Threats & Examples
- Extraneous Variables | Examples, Types, Controls
- Face Validity | Guide with Definition & Examples
- How to Do Thematic Analysis | Guide & Examples
- How to Write a Strong Hypothesis | Guide & Examples
- Inclusion and Exclusion Criteria | Examples & Definition
- Independent vs Dependent Variables | Definition & Examples
- Inductive Reasoning | Types, Examples, Explanation
- Inductive vs Deductive Research Approach (with Examples)
- Internal Validity | Definition, Threats & Examples
- Internal vs External Validity | Understanding Differences & Examples
- Longitudinal Study | Definition, Approaches & Examples
- Mediator vs Moderator Variables | Differences & Examples
- Mixed Methods Research | Definition, Guide, & Examples
- Multistage Sampling | An Introductory Guide with Examples
- Naturalistic Observation | Definition, Guide & Examples
- Operationalisation | A Guide with Examples, Pros & Cons
- Population vs Sample | Definitions, Differences & Examples
- Primary Research | Definition, Types, & Examples
- Qualitative vs Quantitative Research | Examples & Methods
- Quasi-Experimental Design | Definition, Types & Examples
- Questionnaire Design | Methods, Question Types & Examples
- Random Assignment in Experiments | Introduction & Examples
- Reliability vs Validity in Research | Differences, Types & Examples
- Reproducibility vs Replicability | Difference & Examples
- Research Design | Step-by-Step Guide with Examples
- Sampling Methods | Types, Techniques, & Examples
- Semi-Structured Interview | Definition, Guide & Examples
- Simple Random Sampling | Definition, Steps & Examples
- Stratified Sampling | A Step-by-Step Guide with Examples
- Structured Interview | Definition, Guide & Examples
- Systematic Review | Definition, Examples & Guide
- Systematic Sampling | A Step-by-Step Guide with Examples
- Textual Analysis | Guide, 3 Approaches & Examples
- The 4 Types of Reliability in Research | Definitions & Examples
- The 4 Types of Validity | Types, Definitions & Examples
- Transcribing an Interview | 5 Steps & Transcription Software
- Triangulation in Research | Guide, Types, Examples
- Types of Interviews in Research | Guide & Examples
- Types of Research Designs Compared | Examples
- Types of Variables in Research | Definitions & Examples
- Unstructured Interview | Definition, Guide & Examples
- What Are Control Variables | Definition & Examples
- What Is a Case-Control Study? | Definition & Examples
- What Is a Cohort Study? | Definition & Examples
- What Is a Conceptual Framework? | Tips & Examples
- What Is a Double-Barrelled Question?
- What Is a Double-Blind Study? | Introduction & Examples
- What Is a Focus Group? | Step-by-Step Guide & Examples
- What Is a Likert Scale? | Guide & Examples
- What is a Literature Review? | Guide, Template, & Examples
- What Is a Prospective Cohort Study? | Definition & Examples
- What Is a Retrospective Cohort Study? | Definition & Examples
- What Is Action Research? | Definition & Examples
- What Is an Observational Study? | Guide & Examples
- What Is Concurrent Validity? | Definition & Examples
- What Is Content Validity? | Definition & Examples
- What Is Convenience Sampling? | Definition & Examples
- What Is Convergent Validity? | Definition & Examples
- What Is Criterion Validity? | Definition & Examples
- What Is Deductive Reasoning? | Explanation & Examples
- What Is Discriminant Validity? | Definition & Example
- What Is Ecological Validity? | Definition & Examples
- What Is Ethnography? | Meaning, Guide & Examples
- What Is Non-Probability Sampling? | Types & Examples
- What Is Participant Observation? | Definition & Examples
- What Is Peer Review? | Types & Examples
- What Is Predictive Validity? | Examples & Definition
- What Is Probability Sampling? | Types & Examples
- What Is Purposive Sampling? | Definition & Examples
- What Is Qualitative Observation? | Definition & Examples
- What Is Qualitative Research? | Methods & Examples
- What Is Quantitative Observation? | Definition & Examples
- What Is Quantitative Research? | Definition & Methods
- What Is Quota Sampling? | Definition & Examples
- What is Secondary Research? | Definition, Types, & Examples
- What Is Snowball Sampling? | Definition & Examples
- Within-Subjects Design | Explanation, Approaches, Examples
News alert: UC Berkeley has announced its next university librarian
Secondary menu
- Log in to your Library account
- Hours and Maps
- Connect from Off Campus
- UC Berkeley Home
Search form
Research methods--quantitative, qualitative, and more: overview.
- Quantitative Research
- Qualitative Research
- Data Science Methods (Machine Learning, AI, Big Data)
- Text Mining and Computational Text Analysis
- Evidence Synthesis/Systematic Reviews
- Get Data, Get Help!
About Research Methods
This guide provides an overview of research methods, how to choose and use them, and supports and resources at UC Berkeley.
As Patten and Newhart note in the book Understanding Research Methods , "Research methods are the building blocks of the scientific enterprise. They are the "how" for building systematic knowledge. The accumulation of knowledge through research is by its nature a collective endeavor. Each well-designed study provides evidence that may support, amend, refute, or deepen the understanding of existing knowledge...Decisions are important throughout the practice of research and are designed to help researchers collect evidence that includes the full spectrum of the phenomenon under study, to maintain logical rules, and to mitigate or account for possible sources of bias. In many ways, learning research methods is learning how to see and make these decisions."
The choice of methods varies by discipline, by the kind of phenomenon being studied and the data being used to study it, by the technology available, and more. This guide is an introduction, but if you don't see what you need here, always contact your subject librarian, and/or take a look to see if there's a library research guide that will answer your question.
Suggestions for changes and additions to this guide are welcome!
START HERE: SAGE Research Methods
Without question, the most comprehensive resource available from the library is SAGE Research Methods. HERE IS THE ONLINE GUIDE to this one-stop shopping collection, and some helpful links are below:
- SAGE Research Methods
- Little Green Books (Quantitative Methods)
- Little Blue Books (Qualitative Methods)
- Dictionaries and Encyclopedias
- Case studies of real research projects
- Sample datasets for hands-on practice
- Streaming video--see methods come to life
- Methodspace- -a community for researchers
- SAGE Research Methods Course Mapping
Library Data Services at UC Berkeley
Library Data Services Program and Digital Scholarship Services
The LDSP offers a variety of services and tools ! From this link, check out pages for each of the following topics: discovering data, managing data, collecting data, GIS data, text data mining, publishing data, digital scholarship, open science, and the Research Data Management Program.
Be sure also to check out the visual guide to where to seek assistance on campus with any research question you may have!
Library GIS Services
Other Data Services at Berkeley
D-Lab Supports Berkeley faculty, staff, and graduate students with research in data intensive social science, including a wide range of training and workshop offerings Dryad Dryad is a simple self-service tool for researchers to use in publishing their datasets. It provides tools for the effective publication of and access to research data. Geospatial Innovation Facility (GIF) Provides leadership and training across a broad array of integrated mapping technologies on campu Research Data Management A UC Berkeley guide and consulting service for research data management issues
General Research Methods Resources
Here are some general resources for assistance:
- Assistance from ICPSR (must create an account to access): Getting Help with Data , and Resources for Students
- Wiley Stats Ref for background information on statistics topics
- Survey Documentation and Analysis (SDA) . Program for easy web-based analysis of survey data.
Consultants
- D-Lab/Data Science Discovery Consultants Request help with your research project from peer consultants.
- Research data (RDM) consulting Meet with RDM consultants before designing the data security, storage, and sharing aspects of your qualitative project.
- Statistics Department Consulting Services A service in which advanced graduate students, under faculty supervision, are available to consult during specified hours in the Fall and Spring semesters.
Related Resourcex
- IRB / CPHS Qualitative research projects with human subjects often require that you go through an ethics review.
- OURS (Office of Undergraduate Research and Scholarships) OURS supports undergraduates who want to embark on research projects and assistantships. In particular, check out their "Getting Started in Research" workshops
- Sponsored Projects Sponsored projects works with researchers applying for major external grants.
- Next: Quantitative Research >>
- Last Updated: Apr 25, 2024 11:09 AM
- URL: https://guides.lib.berkeley.edu/researchmethods
- Research Process
- Manuscript Preparation
- Manuscript Review
- Publication Process
- Publication Recognition
- Language Editing Services
- Translation Services
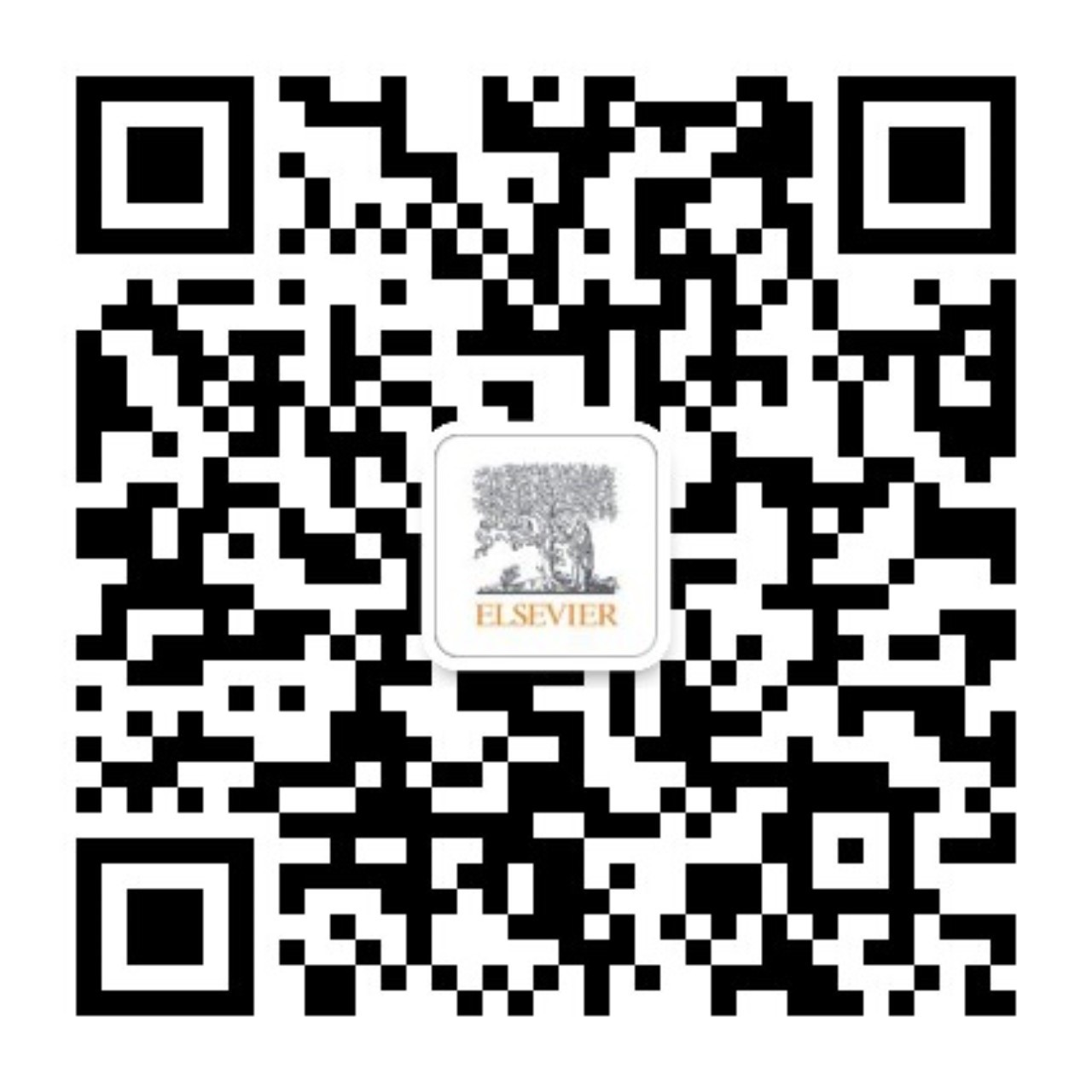
Choosing the Right Research Methodology: A Guide for Researchers
- 3 minute read
Table of Contents
Choosing an optimal research methodology is crucial for the success of any research project. The methodology you select will determine the type of data you collect, how you collect it, and how you analyse it. Understanding the different types of research methods available along with their strengths and weaknesses, is thus imperative to make an informed decision.
Understanding different research methods:
There are several research methods available depending on the type of study you are conducting, i.e., whether it is laboratory-based, clinical, epidemiological, or survey based . Some common methodologies include qualitative research, quantitative research, experimental research, survey-based research, and action research. Each method can be opted for and modified, depending on the type of research hypotheses and objectives.
Qualitative vs quantitative research:
When deciding on a research methodology, one of the key factors to consider is whether your research will be qualitative or quantitative. Qualitative research is used to understand people’s experiences, concepts, thoughts, or behaviours . Quantitative research, on the contrary, deals with numbers, graphs, and charts, and is used to test or confirm hypotheses, assumptions, and theories.
Qualitative research methodology:
Qualitative research is often used to examine issues that are not well understood, and to gather additional insights on these topics. Qualitative research methods include open-ended survey questions, observations of behaviours described through words, and reviews of literature that has explored similar theories and ideas. These methods are used to understand how language is used in real-world situations, identify common themes or overarching ideas, and describe and interpret various texts. Data analysis for qualitative research typically includes discourse analysis, thematic analysis, and textual analysis.
Quantitative research methodology:
The goal of quantitative research is to test hypotheses, confirm assumptions and theories, and determine cause-and-effect relationships. Quantitative research methods include experiments, close-ended survey questions, and countable and numbered observations. Data analysis for quantitative research relies heavily on statistical methods.
Analysing qualitative vs quantitative data:
The methods used for data analysis also differ for qualitative and quantitative research. As mentioned earlier, quantitative data is generally analysed using statistical methods and does not leave much room for speculation. It is more structured and follows a predetermined plan. In quantitative research, the researcher starts with a hypothesis and uses statistical methods to test it. Contrarily, methods used for qualitative data analysis can identify patterns and themes within the data, rather than provide statistical measures of the data. It is an iterative process, where the researcher goes back and forth trying to gauge the larger implications of the data through different perspectives and revising the analysis if required.
When to use qualitative vs quantitative research:
The choice between qualitative and quantitative research will depend on the gap that the research project aims to address, and specific objectives of the study. If the goal is to establish facts about a subject or topic, quantitative research is an appropriate choice. However, if the goal is to understand people’s experiences or perspectives, qualitative research may be more suitable.
Conclusion:
In conclusion, an understanding of the different research methods available, their applicability, advantages, and disadvantages is essential for making an informed decision on the best methodology for your project. If you need any additional guidance on which research methodology to opt for, you can head over to Elsevier Author Services (EAS). EAS experts will guide you throughout the process and help you choose the perfect methodology for your research goals.
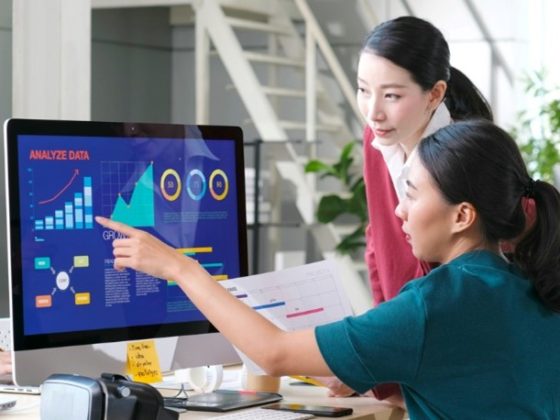
Why is data validation important in research?
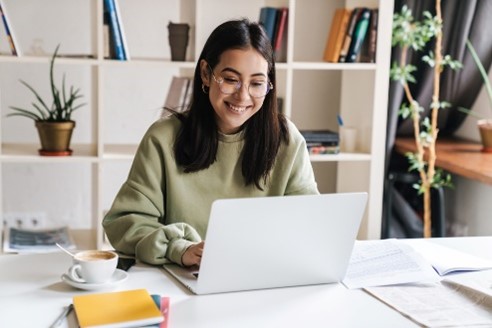
When Data Speak, Listen: Importance of Data Collection and Analysis Methods
You may also like.
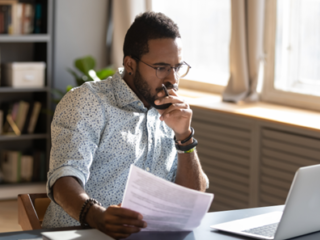
Descriptive Research Design and Its Myriad Uses
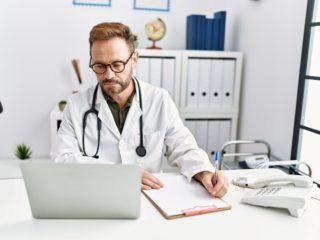
Five Common Mistakes to Avoid When Writing a Biomedical Research Paper
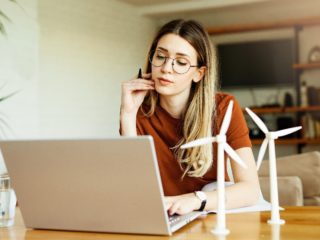
Making Technical Writing in Environmental Engineering Accessible
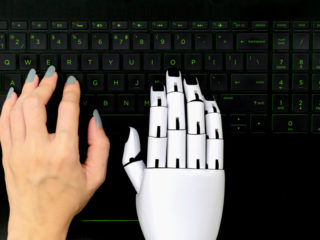
To Err is Not Human: The Dangers of AI-assisted Academic Writing
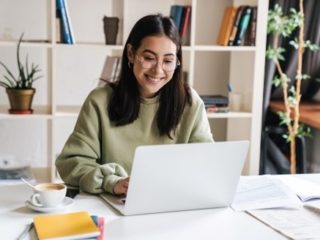
Writing a good review article
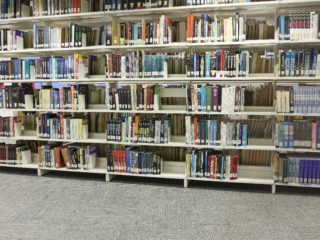
Scholarly Sources: What are They and Where can You Find Them?
Input your search keywords and press Enter.
- Skip to main content
- Skip to primary sidebar
- Skip to footer
- QuestionPro

- Solutions Industries Gaming Automotive Sports and events Education Government Travel & Hospitality Financial Services Healthcare Cannabis Technology Use Case NPS+ Communities Audience Contactless surveys Mobile LivePolls Member Experience GDPR Positive People Science 360 Feedback Surveys
- Resources Blog eBooks Survey Templates Case Studies Training Help center

Home Market Research
Qualitative Data Analysis: What is it, Methods + Examples
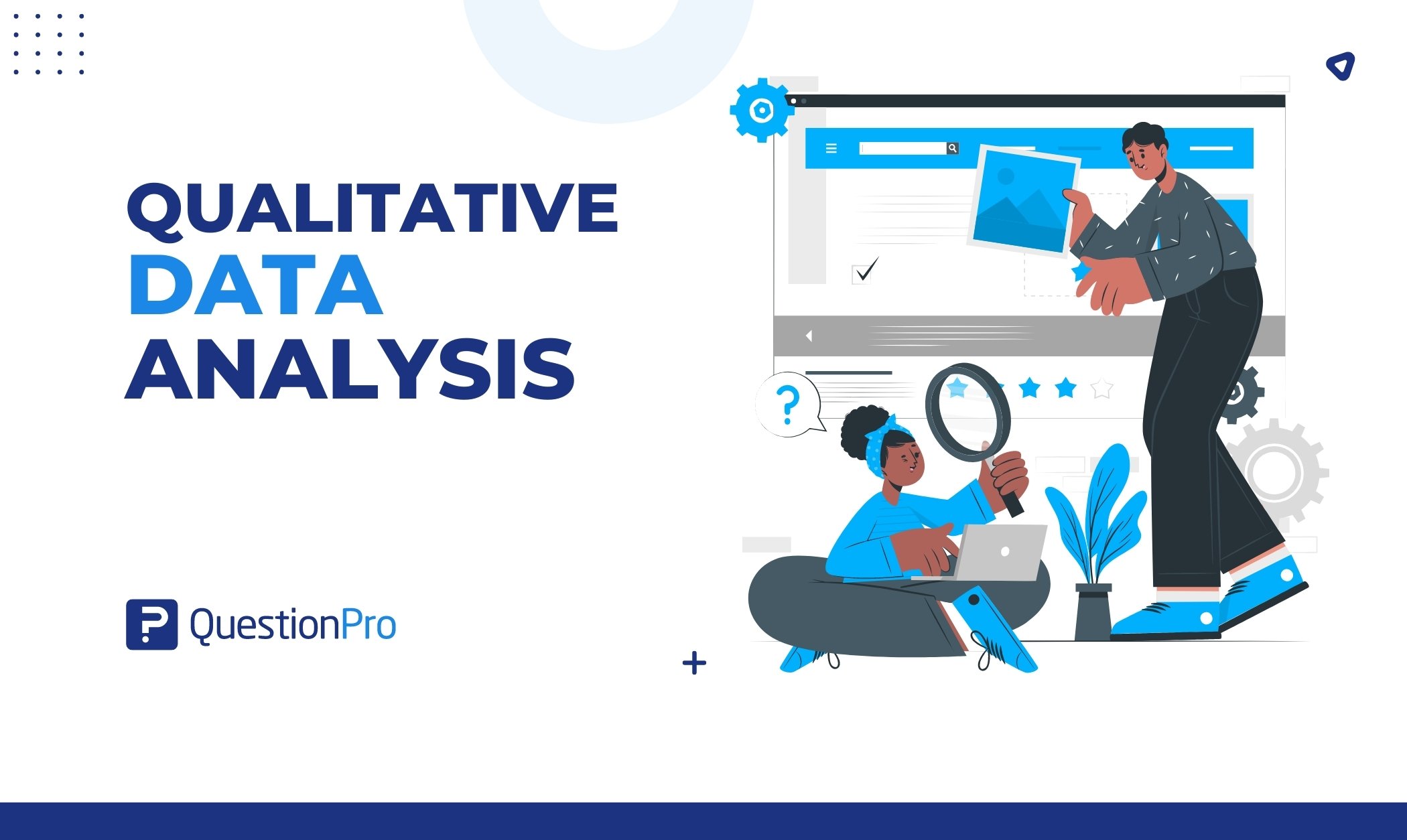
In a world rich with information and narrative, understanding the deeper layers of human experiences requires a unique vision that goes beyond numbers and figures. This is where the power of qualitative data analysis comes to light.
In this blog, we’ll learn about qualitative data analysis, explore its methods, and provide real-life examples showcasing its power in uncovering insights.
What is Qualitative Data Analysis?
Qualitative data analysis is a systematic process of examining non-numerical data to extract meaning, patterns, and insights.
In contrast to quantitative analysis, which focuses on numbers and statistical metrics, the qualitative study focuses on the qualitative aspects of data, such as text, images, audio, and videos. It seeks to understand every aspect of human experiences, perceptions, and behaviors by examining the data’s richness.
Companies frequently conduct this analysis on customer feedback. You can collect qualitative data from reviews, complaints, chat messages, interactions with support centers, customer interviews, case notes, or even social media comments. This kind of data holds the key to understanding customer sentiments and preferences in a way that goes beyond mere numbers.
Importance of Qualitative Data Analysis
Qualitative data analysis plays a crucial role in your research and decision-making process across various disciplines. Let’s explore some key reasons that underline the significance of this analysis:
In-Depth Understanding
It enables you to explore complex and nuanced aspects of a phenomenon, delving into the ‘how’ and ‘why’ questions. This method provides you with a deeper understanding of human behavior, experiences, and contexts that quantitative approaches might not capture fully.
Contextual Insight
You can use this analysis to give context to numerical data. It will help you understand the circumstances and conditions that influence participants’ thoughts, feelings, and actions. This contextual insight becomes essential for generating comprehensive explanations.
Theory Development
You can generate or refine hypotheses via qualitative data analysis. As you analyze the data attentively, you can form hypotheses, concepts, and frameworks that will drive your future research and contribute to theoretical advances.
Participant Perspectives
When performing qualitative research, you can highlight participant voices and opinions. This approach is especially useful for understanding marginalized or underrepresented people, as it allows them to communicate their experiences and points of view.
Exploratory Research
The analysis is frequently used at the exploratory stage of your project. It assists you in identifying important variables, developing research questions, and designing quantitative studies that will follow.
Types of Qualitative Data
When conducting qualitative research, you can use several qualitative data collection methods , and here you will come across many sorts of qualitative data that can provide you with unique insights into your study topic. These data kinds add new views and angles to your understanding and analysis.
Interviews and Focus Groups
Interviews and focus groups will be among your key methods for gathering qualitative data. Interviews are one-on-one talks in which participants can freely share their thoughts, experiences, and opinions.
Focus groups, on the other hand, are discussions in which members interact with one another, resulting in dynamic exchanges of ideas. Both methods provide rich qualitative data and direct access to participant perspectives.
Observations and Field Notes
Observations and field notes are another useful sort of qualitative data. You can immerse yourself in the research environment through direct observation, carefully documenting behaviors, interactions, and contextual factors.
These observations will be recorded in your field notes, providing a complete picture of the environment and the behaviors you’re researching. This data type is especially important for comprehending behavior in their natural setting.
Textual and Visual Data
Textual and visual data include a wide range of resources that can be qualitatively analyzed. Documents, written narratives, and transcripts from various sources, such as interviews or speeches, are examples of textual data.
Photographs, films, and even artwork provide a visual layer to your research. These forms of data allow you to investigate what is spoken and the underlying emotions, details, and symbols expressed by language or pictures.
When to Choose Qualitative Data Analysis over Quantitative Data Analysis
As you begin your research journey, understanding why the analysis of qualitative data is important will guide your approach to understanding complex events. If you analyze qualitative data, it will provide new insights that complement quantitative methodologies, which will give you a broader understanding of your study topic.
It is critical to know when to use qualitative analysis over quantitative procedures. You can prefer qualitative data analysis when:
- Complexity Reigns: When your research questions involve deep human experiences, motivations, or emotions, qualitative research excels at revealing these complexities.
- Exploration is Key: Qualitative analysis is ideal for exploratory research. It will assist you in understanding a new or poorly understood topic before formulating quantitative hypotheses.
- Context Matters: If you want to understand how context affects behaviors or results, qualitative data analysis provides the depth needed to grasp these relationships.
- Unanticipated Findings: When your study provides surprising new viewpoints or ideas, qualitative analysis helps you to delve deeply into these emerging themes.
- Subjective Interpretation is Vital: When it comes to understanding people’s subjective experiences and interpretations, qualitative data analysis is the way to go.
You can make informed decisions regarding the right approach for your research objectives if you understand the importance of qualitative analysis and recognize the situations where it shines.
Qualitative Data Analysis Methods and Examples
Exploring various qualitative data analysis methods will provide you with a wide collection for making sense of your research findings. Once the data has been collected, you can choose from several analysis methods based on your research objectives and the data type you’ve collected.
There are five main methods for analyzing qualitative data. Each method takes a distinct approach to identifying patterns, themes, and insights within your qualitative data. They are:
Method 1: Content Analysis
Content analysis is a methodical technique for analyzing textual or visual data in a structured manner. In this method, you will categorize qualitative data by splitting it into manageable pieces and assigning the manual coding process to these units.
As you go, you’ll notice ongoing codes and designs that will allow you to conclude the content. This method is very beneficial for detecting common ideas, concepts, or themes in your data without losing the context.
Steps to Do Content Analysis
Follow these steps when conducting content analysis:
- Collect and Immerse: Begin by collecting the necessary textual or visual data. Immerse yourself in this data to fully understand its content, context, and complexities.
- Assign Codes and Categories: Assign codes to relevant data sections that systematically represent major ideas or themes. Arrange comparable codes into groups that cover the major themes.
- Analyze and Interpret: Develop a structured framework from the categories and codes. Then, evaluate the data in the context of your research question, investigate relationships between categories, discover patterns, and draw meaning from these connections.
Benefits & Challenges
There are various advantages to using content analysis:
- Structured Approach: It offers a systematic approach to dealing with large data sets and ensures consistency throughout the research.
- Objective Insights: This method promotes objectivity, which helps to reduce potential biases in your study.
- Pattern Discovery: Content analysis can help uncover hidden trends, themes, and patterns that are not always obvious.
- Versatility: You can apply content analysis to various data formats, including text, internet content, images, etc.
However, keep in mind the challenges that arise:
- Subjectivity: Even with the best attempts, a certain bias may remain in coding and interpretation.
- Complexity: Analyzing huge data sets requires time and great attention to detail.
- Contextual Nuances: Content analysis may not capture all of the contextual richness that qualitative data analysis highlights.
Example of Content Analysis
Suppose you’re conducting market research and looking at customer feedback on a product. As you collect relevant data and analyze feedback, you’ll see repeating codes like “price,” “quality,” “customer service,” and “features.” These codes are organized into categories such as “positive reviews,” “negative reviews,” and “suggestions for improvement.”
According to your findings, themes such as “price” and “customer service” stand out and show that pricing and customer service greatly impact customer satisfaction. This example highlights the power of content analysis for obtaining significant insights from large textual data collections.
Method 2: Thematic Analysis
Thematic analysis is a well-structured procedure for identifying and analyzing recurring themes in your data. As you become more engaged in the data, you’ll generate codes or short labels representing key concepts. These codes are then organized into themes, providing a consistent framework for organizing and comprehending the substance of the data.
The analysis allows you to organize complex narratives and perspectives into meaningful categories, which will allow you to identify connections and patterns that may not be visible at first.
Steps to Do Thematic Analysis
Follow these steps when conducting a thematic analysis:
- Code and Group: Start by thoroughly examining the data and giving initial codes that identify the segments. To create initial themes, combine relevant codes.
- Code and Group: Begin by engaging yourself in the data, assigning first codes to notable segments. To construct basic themes, group comparable codes together.
- Analyze and Report: Analyze the data within each theme to derive relevant insights. Organize the topics into a consistent structure and explain your findings, along with data extracts that represent each theme.
Thematic analysis has various benefits:
- Structured Exploration: It is a method for identifying patterns and themes in complex qualitative data.
- Comprehensive knowledge: Thematic analysis promotes an in-depth understanding of the complications and meanings of the data.
- Application Flexibility: This method can be customized to various research situations and data kinds.
However, challenges may arise, such as:
- Interpretive Nature: Interpreting qualitative data in thematic analysis is vital, and it is critical to manage researcher bias.
- Time-consuming: The study can be time-consuming, especially with large data sets.
- Subjectivity: The selection of codes and topics might be subjective.
Example of Thematic Analysis
Assume you’re conducting a thematic analysis on job satisfaction interviews. Following your immersion in the data, you assign initial codes such as “work-life balance,” “career growth,” and “colleague relationships.” As you organize these codes, you’ll notice themes develop, such as “Factors Influencing Job Satisfaction” and “Impact on Work Engagement.”
Further investigation reveals the tales and experiences included within these themes and provides insights into how various elements influence job satisfaction. This example demonstrates how thematic analysis can reveal meaningful patterns and insights in qualitative data.
Method 3: Narrative Analysis
The narrative analysis involves the narratives that people share. You’ll investigate the histories in your data, looking at how stories are created and the meanings they express. This method is excellent for learning how people make sense of their experiences through narrative.
Steps to Do Narrative Analysis
The following steps are involved in narrative analysis:
- Gather and Analyze: Start by collecting narratives, such as first-person tales, interviews, or written accounts. Analyze the stories, focusing on the plot, feelings, and characters.
- Find Themes: Look for recurring themes or patterns in various narratives. Think about the similarities and differences between these topics and personal experiences.
- Interpret and Extract Insights: Contextualize the narratives within their larger context. Accept the subjective nature of each narrative and analyze the narrator’s voice and style. Extract insights from the tales by diving into the emotions, motivations, and implications communicated by the stories.
There are various advantages to narrative analysis:
- Deep Exploration: It lets you look deeply into people’s personal experiences and perspectives.
- Human-Centered: This method prioritizes the human perspective, allowing individuals to express themselves.
However, difficulties may arise, such as:
- Interpretive Complexity: Analyzing narratives requires dealing with the complexities of meaning and interpretation.
- Time-consuming: Because of the richness and complexities of tales, working with them can be time-consuming.
Example of Narrative Analysis
Assume you’re conducting narrative analysis on refugee interviews. As you read the stories, you’ll notice common themes of toughness, loss, and hope. The narratives provide insight into the obstacles that refugees face, their strengths, and the dreams that guide them.
The analysis can provide a deeper insight into the refugees’ experiences and the broader social context they navigate by examining the narratives’ emotional subtleties and underlying meanings. This example highlights how narrative analysis can reveal important insights into human stories.
Method 4: Grounded Theory Analysis
Grounded theory analysis is an iterative and systematic approach that allows you to create theories directly from data without being limited by pre-existing hypotheses. With an open mind, you collect data and generate early codes and labels that capture essential ideas or concepts within the data.
As you progress, you refine these codes and increasingly connect them, eventually developing a theory based on the data. Grounded theory analysis is a dynamic process for developing new insights and hypotheses based on details in your data.
Steps to Do Grounded Theory Analysis
Grounded theory analysis requires the following steps:
- Initial Coding: First, immerse yourself in the data, producing initial codes that represent major concepts or patterns.
- Categorize and Connect: Using axial coding, organize the initial codes, which establish relationships and connections between topics.
- Build the Theory: Focus on creating a core category that connects the codes and themes. Regularly refine the theory by comparing and integrating new data, ensuring that it evolves organically from the data.
Grounded theory analysis has various benefits:
- Theory Generation: It provides a one-of-a-kind opportunity to generate hypotheses straight from data and promotes new insights.
- In-depth Understanding: The analysis allows you to deeply analyze the data and reveal complex relationships and patterns.
- Flexible Process: This method is customizable and ongoing, which allows you to enhance your research as you collect additional data.
However, challenges might arise with:
- Time and Resources: Because grounded theory analysis is a continuous process, it requires a large commitment of time and resources.
- Theoretical Development: Creating a grounded theory involves a thorough understanding of qualitative data analysis software and theoretical concepts.
- Interpretation of Complexity: Interpreting and incorporating a newly developed theory into existing literature can be intellectually hard.
Example of Grounded Theory Analysis
Assume you’re performing a grounded theory analysis on workplace collaboration interviews. As you open code the data, you will discover notions such as “communication barriers,” “team dynamics,” and “leadership roles.” Axial coding demonstrates links between these notions, emphasizing the significance of efficient communication in developing collaboration.
You create the core “Integrated Communication Strategies” category through selective coding, which unifies new topics.
This theory-driven category serves as the framework for understanding how numerous aspects contribute to effective team collaboration. This example shows how grounded theory analysis allows you to generate a theory directly from the inherent nature of the data.
Method 5: Discourse Analysis
Discourse analysis focuses on language and communication. You’ll look at how language produces meaning and how it reflects power relations, identities, and cultural influences. This strategy examines what is said and how it is said; the words, phrasing, and larger context of communication.
The analysis is precious when investigating power dynamics, identities, and cultural influences encoded in language. By evaluating the language used in your data, you can identify underlying assumptions, cultural standards, and how individuals negotiate meaning through communication.
Steps to Do Discourse Analysis
Conducting discourse analysis entails the following steps:
- Select Discourse: For analysis, choose language-based data such as texts, speeches, or media content.
- Analyze Language: Immerse yourself in the conversation, examining language choices, metaphors, and underlying assumptions.
- Discover Patterns: Recognize the dialogue’s reoccurring themes, ideologies, and power dynamics. To fully understand the effects of these patterns, put them in their larger context.
There are various advantages of using discourse analysis:
- Understanding Language: It provides an extensive understanding of how language builds meaning and influences perceptions.
- Uncovering Power Dynamics: The analysis reveals how power dynamics appear via language.
- Cultural Insights: This method identifies cultural norms, beliefs, and ideologies stored in communication.
However, the following challenges may arise:
- Complexity of Interpretation: Language analysis involves navigating multiple levels of nuance and interpretation.
- Subjectivity: Interpretation can be subjective, so controlling researcher bias is important.
- Time-Intensive: Discourse analysis can take a lot of time because careful linguistic study is required in this analysis.
Example of Discourse Analysis
Consider doing discourse analysis on media coverage of a political event. You notice repeating linguistic patterns in news articles that depict the event as a conflict between opposing parties. Through deconstruction, you can expose how this framing supports particular ideologies and power relations.
You can illustrate how language choices influence public perceptions and contribute to building the narrative around the event by analyzing the speech within the broader political and social context. This example shows how discourse analysis can reveal hidden power dynamics and cultural influences on communication.
How to do Qualitative Data Analysis with the QuestionPro Research suite?
QuestionPro is a popular survey and research platform that offers tools for collecting and analyzing qualitative and quantitative data. Follow these general steps for conducting qualitative data analysis using the QuestionPro Research Suite:
- Collect Qualitative Data: Set up your survey to capture qualitative responses. It might involve open-ended questions, text boxes, or comment sections where participants can provide detailed responses.
- Export Qualitative Responses: Export the responses once you’ve collected qualitative data through your survey. QuestionPro typically allows you to export survey data in various formats, such as Excel or CSV.
- Prepare Data for Analysis: Review the exported data and clean it if necessary. Remove irrelevant or duplicate entries to ensure your data is ready for analysis.
- Code and Categorize Responses: Segment and label data, letting new patterns emerge naturally, then develop categories through axial coding to structure the analysis.
- Identify Themes: Analyze the coded responses to identify recurring themes, patterns, and insights. Look for similarities and differences in participants’ responses.
- Generate Reports and Visualizations: Utilize the reporting features of QuestionPro to create visualizations, charts, and graphs that help communicate the themes and findings from your qualitative research.
- Interpret and Draw Conclusions: Interpret the themes and patterns you’ve identified in the qualitative data. Consider how these findings answer your research questions or provide insights into your study topic.
- Integrate with Quantitative Data (if applicable): If you’re also conducting quantitative research using QuestionPro, consider integrating your qualitative findings with quantitative results to provide a more comprehensive understanding.
Qualitative data analysis is vital in uncovering various human experiences, views, and stories. If you’re ready to transform your research journey and apply the power of qualitative analysis, now is the moment to do it. Book a demo with QuestionPro today and begin your journey of exploration.
LEARN MORE FREE TRIAL
MORE LIKE THIS
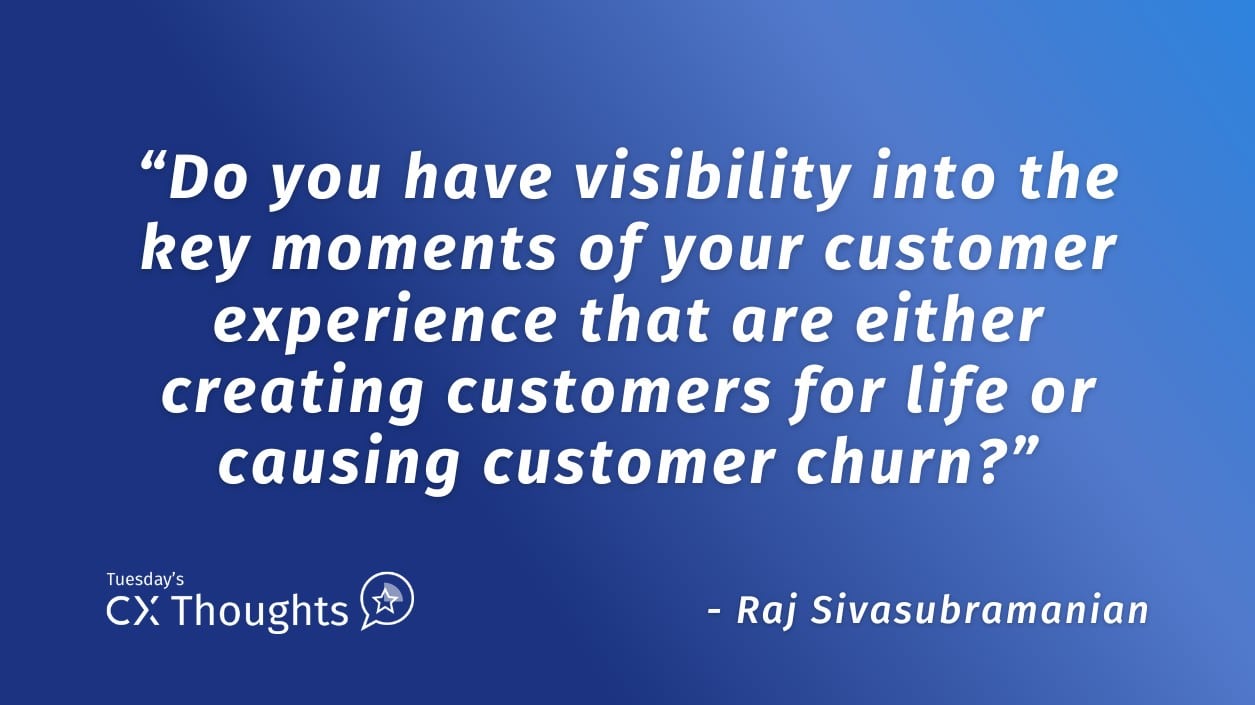
The Item I Failed to Leave Behind — Tuesday CX Thoughts
Jun 25, 2024
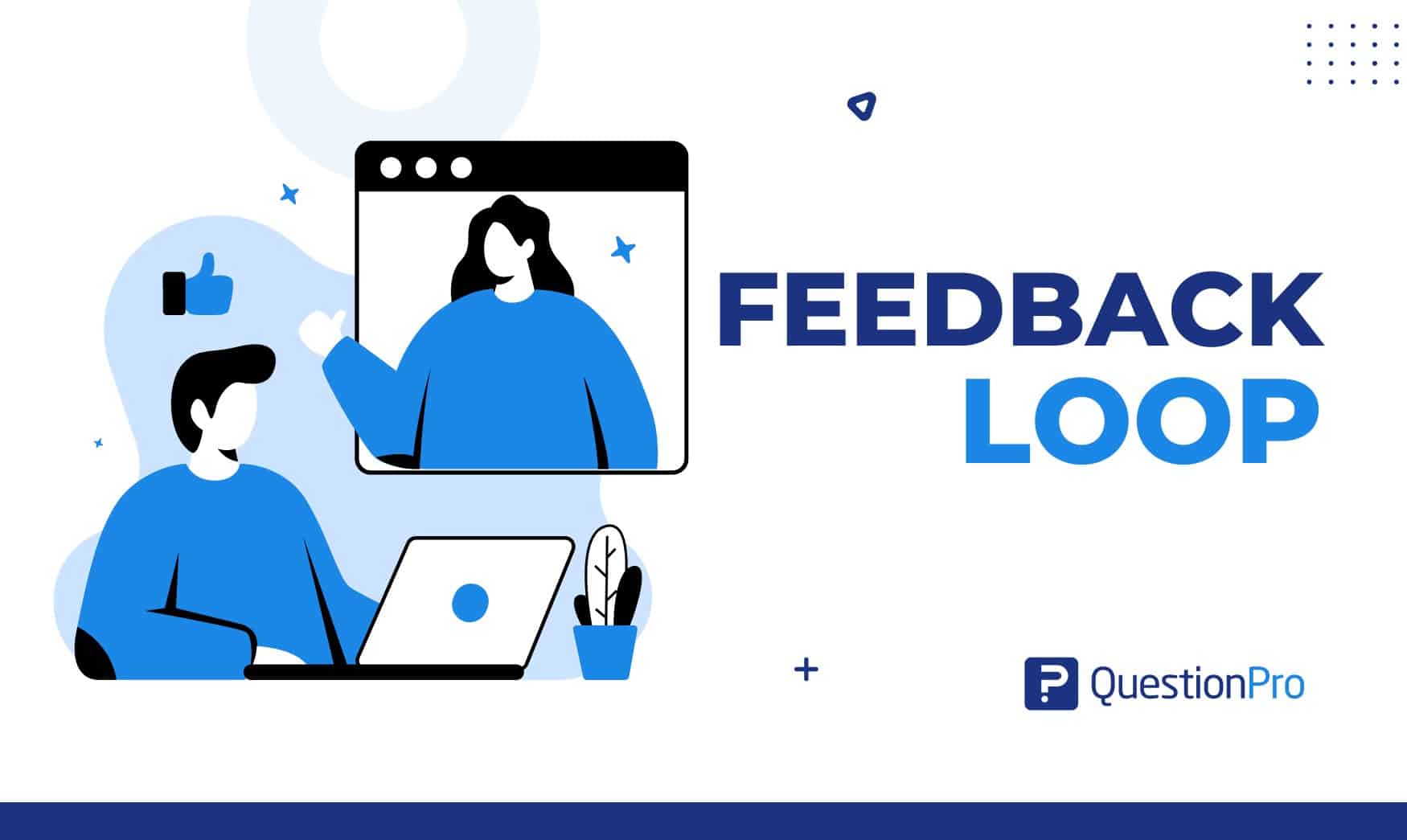
Feedback Loop: What It Is, Types & How It Works?
Jun 21, 2024
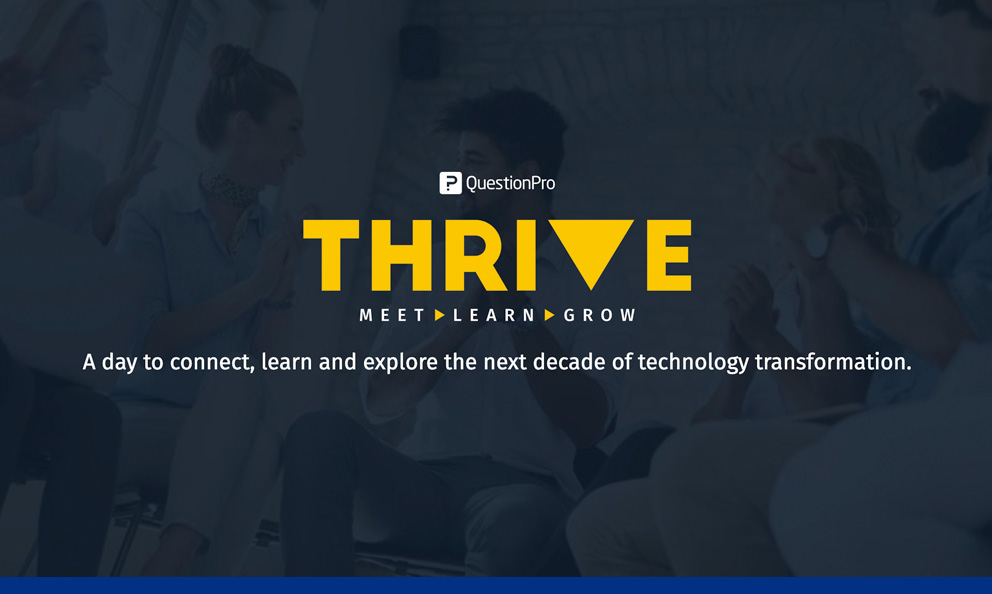
QuestionPro Thrive: A Space to Visualize & Share the Future of Technology
Jun 18, 2024
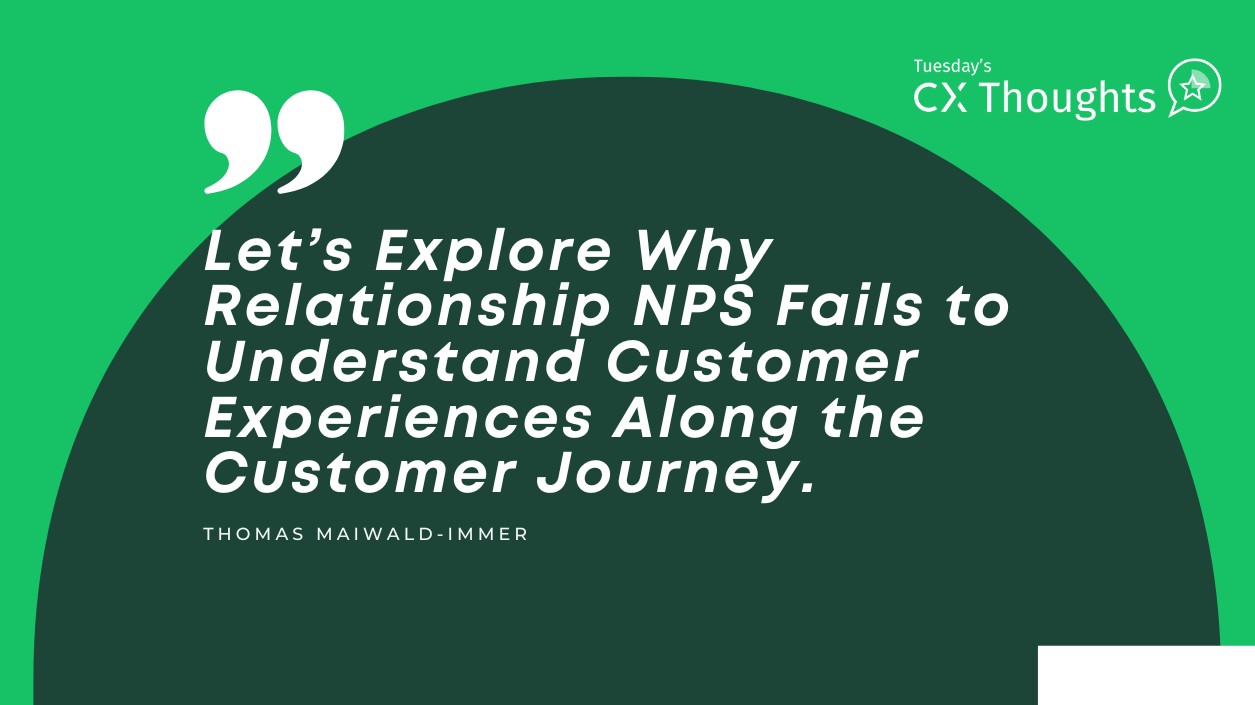
Relationship NPS Fails to Understand Customer Experiences — Tuesday CX
Other categories.
- Academic Research
- Artificial Intelligence
- Assessments
- Brand Awareness
- Case Studies
- Communities
- Consumer Insights
- Customer effort score
- Customer Engagement
- Customer Experience
- Customer Loyalty
- Customer Research
- Customer Satisfaction
- Employee Benefits
- Employee Engagement
- Employee Retention
- Friday Five
- General Data Protection Regulation
- Insights Hub
- Life@QuestionPro
- Market Research
- Mobile diaries
- Mobile Surveys
- New Features
- Online Communities
- Question Types
- Questionnaire
- QuestionPro Products
- Release Notes
- Research Tools and Apps
- Revenue at Risk
- Survey Templates
- Training Tips
- Tuesday CX Thoughts (TCXT)
- Uncategorized
- Video Learning Series
- What’s Coming Up
- Workforce Intelligence

Home » What are Research Methods?
What are Research Methods?
Imagine you’re starting on a journey of discovery, and research methods are your compass, map, and tools. These methods guide us in exploring the vast landscape of knowledge, ensuring our journey is structured, reliable, and fruitful.
Table of Contents
Research Methods

Research Methods are systematic strategies, steps, and tools that researchers use to gather, analyze, and interpret data about a particular topic. It’s like cooking a new recipe; you need the right ingredients (data), a good method (research design), and the proper tools (instruments like surveys or experiments) to create a delightful dish (knowledge).
Types of Research Methods
Qualitative research.
This is akin to painting a portrait. It focuses on understanding concepts, thoughts, and experiences through detailed, descriptive data. Imagine sitting down with someone and listening to their story to grasp the depth of their experiences. Tools for this might include interviews , observations , and textual analysis .
Quantitative Research
Now, imagine yourself counting stars in the sky. This method deals with numbers and statistical analysis. It seeks to quantify the problem by generating numerical data or data that can be transformed into usable statistics. Surveys with multiple-choice questions or experiments where you measure and compare are typical tools here.
Mixed Methods
Sometimes, a single perspective isn’t enough. Mixed methods blend the colors of both qualitative and quantitative research, offering a more comprehensive picture. It’s like using both a microscope and a telescope; you get the detail and the big picture.
Steps in the Research Process
Identifying the Problem : Every journey begins with recognizing where you want to go. What’s the question you’re burning to answer? This step involves defining the scope and purpose of your research.
Literature Review : Before you set out, you need to map the terrain by exploring what others have discovered before you. This involves reading and summarizing existing research on your topic.
Designing the Study : Here’s where you plan your route. Will you conduct interviews? Send out surveys? Observe behaviors? This step involves deciding on your research method, participants, and tools.
Collecting Data : Time to hit the road and gather your data. This is the hands-on part of your research, where you implement your chosen methods to collect information.
Analyzing Data : With your treasures in hand, you now sift through your findings, looking for patterns, themes, or statistical relationships. This step often involves software for qualitative or quantitative analysis.
Interpreting Results : What have you discovered? This stage is about making sense of your data, connecting the dots, and understanding what your findings mean in the context of your research question.
Reporting and Sharing Findings : The final step is to share your journey’s story. This could be a research paper, a presentation, or any format that communicates your discoveries to others.
Ethics in Research
Imagine you’re a guest in someone’s home; you must be respectful and considerate. Similarly, ethical considerations are paramount in research. This means ensuring confidentiality, obtaining informed consent, and treating all subjects (people, animals, the environment) with respect and dignity.
Research methods are the compass, map, and tools that guide us through the terrain of knowledge. They enable us to ask important questions, systematically gather and analyze data, and contribute valuable insights to our understanding of the world. As you start on your research journey, embrace the adventure, respect the process, and look forward to the discoveries that await you.
You may also like

Types of Mixed Research Methods

What is Mixed Research Methods

Types of Quantitative Research Methods

Quantitative Research Methods

Types of Qualitative Research Methods

Qualitative Research Methods

15 Types of Research Methods
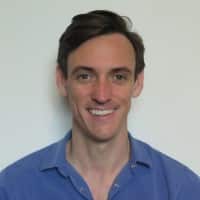
Chris Drew (PhD)
Dr. Chris Drew is the founder of the Helpful Professor. He holds a PhD in education and has published over 20 articles in scholarly journals. He is the former editor of the Journal of Learning Development in Higher Education. [Image Descriptor: Photo of Chris]
Learn about our Editorial Process
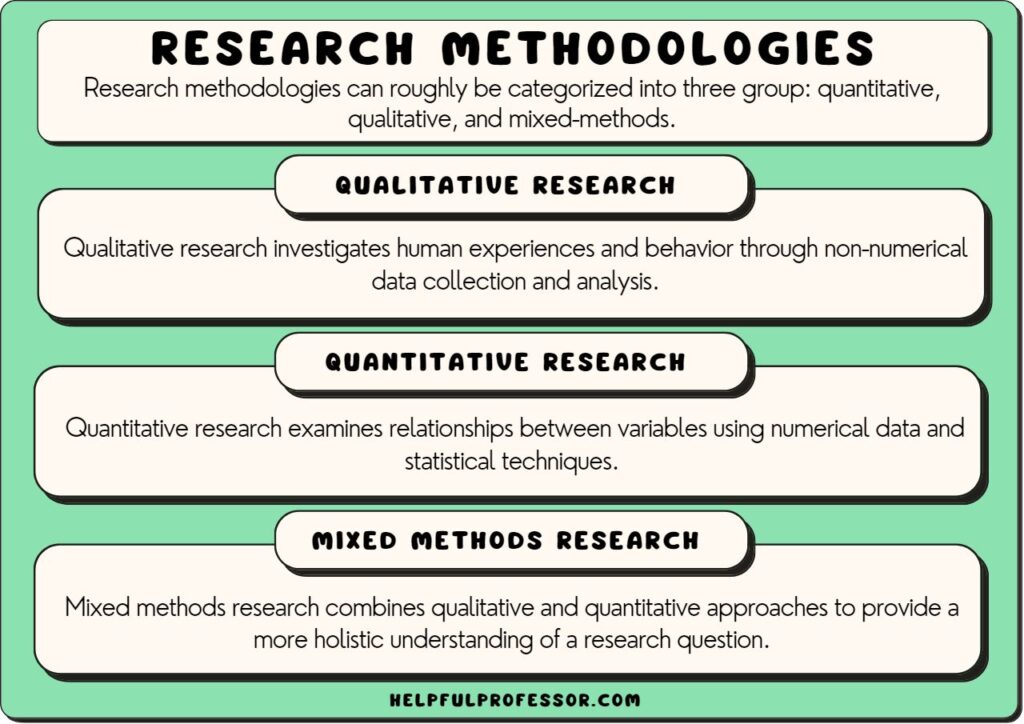
Research methods refer to the strategies, tools, and techniques used to gather and analyze data in a structured way in order to answer a research question or investigate a hypothesis (Hammond & Wellington, 2020).
Generally, we place research methods into two categories: quantitative and qualitative. Each has its own strengths and weaknesses, which we can summarize as:
- Quantitative research can achieve generalizability through scrupulous statistical analysis applied to large sample sizes.
- Qualitative research achieves deep, detailed, and nuance accounts of specific case studies, which are not generalizable.
Some researchers, with the aim of making the most of both quantitative and qualitative research, employ mixed methods, whereby they will apply both types of research methods in the one study, such as by conducting a statistical survey alongside in-depth interviews to add context to the quantitative findings.
Below, I’ll outline 15 common research methods, and include pros, cons, and examples of each .
Types of Research Methods
Research methods can be broadly categorized into two types: quantitative and qualitative.
- Quantitative methods involve systematic empirical investigation of observable phenomena via statistical, mathematical, or computational techniques, providing an in-depth understanding of a specific concept or phenomenon (Schweigert, 2021). The strengths of this approach include its ability to produce reliable results that can be generalized to a larger population, although it can lack depth and detail.
- Qualitative methods encompass techniques that are designed to provide a deep understanding of a complex issue, often in a specific context, through collection of non-numerical data (Tracy, 2019). This approach often provides rich, detailed insights but can be time-consuming and its findings may not be generalizable.
These can be further broken down into a range of specific research methods and designs:
Primarily Quantitative Methods | Primarily Qualitative methods |
---|---|
Experimental Research | Case Study |
Surveys and Questionnaires | Ethnography |
Longitudinal Studies | Phenomenology |
Cross-Sectional Studies | Historical research |
Correlational Research | Content analysis |
Causal-Comparative Research | Grounded theory |
Meta-Analysis | Action research |
Quasi-Experimental Design | Observational research |
Combining the two methods above, mixed methods research mixes elements of both qualitative and quantitative research methods, providing a comprehensive understanding of the research problem . We can further break these down into:
- Sequential Explanatory Design (QUAN→QUAL): This methodology involves conducting quantitative analysis first, then supplementing it with a qualitative study.
- Sequential Exploratory Design (QUAL→QUAN): This methodology goes in the other direction, starting with qualitative analysis and ending with quantitative analysis.
Let’s explore some methods and designs from both quantitative and qualitative traditions, starting with qualitative research methods.
Qualitative Research Methods
Qualitative research methods allow for the exploration of phenomena in their natural settings, providing detailed, descriptive responses and insights into individuals’ experiences and perceptions (Howitt, 2019).
These methods are useful when a detailed understanding of a phenomenon is sought.
1. Ethnographic Research
Ethnographic research emerged out of anthropological research, where anthropologists would enter into a setting for a sustained period of time, getting to know a cultural group and taking detailed observations.
Ethnographers would sometimes even act as participants in the group or culture, which many scholars argue is a weakness because it is a step away from achieving objectivity (Stokes & Wall, 2017).
In fact, at its most extreme version, ethnographers even conduct research on themselves, in a fascinating methodology call autoethnography .
The purpose is to understand the culture, social structure, and the behaviors of the group under study. It is often useful when researchers seek to understand shared cultural meanings and practices in their natural settings.
However, it can be time-consuming and may reflect researcher biases due to the immersion approach.
Pros of Ethnographic Research | Cons of Ethnographic Research |
---|---|
1. Provides deep cultural insights | 1. Time-consuming |
2. Contextually relevant findings | 2. Potential researcher bias |
3. Explores dynamic social processes | 3. May |
Example of Ethnography
Liquidated: An Ethnography of Wall Street by Karen Ho involves an anthropologist who embeds herself with Wall Street firms to study the culture of Wall Street bankers and how this culture affects the broader economy and world.
2. Phenomenological Research
Phenomenological research is a qualitative method focused on the study of individual experiences from the participant’s perspective (Tracy, 2019).
It focuses specifically on people’s experiences in relation to a specific social phenomenon ( see here for examples of social phenomena ).
This method is valuable when the goal is to understand how individuals perceive, experience, and make meaning of particular phenomena. However, because it is subjective and dependent on participants’ self-reports, findings may not be generalizable, and are highly reliant on self-reported ‘thoughts and feelings’.
Pros of Phenomenological Research | Cons of Phenomenological Research |
---|---|
1. Provides rich, detailed data | 1. Limited generalizability |
2. Highlights personal experience and perceptions | 2. Data collection can be time-consuming |
3. Allows exploration of complex phenomena | 3. Requires highly skilled researchers |
Example of Phenomenological Research
A phenomenological approach to experiences with technology by Sebnem Cilesiz represents a good starting-point for formulating a phenomenological study. With its focus on the ‘essence of experience’, this piece presents methodological, reliability, validity, and data analysis techniques that phenomenologists use to explain how people experience technology in their everyday lives.
3. Historical Research
Historical research is a qualitative method involving the examination of past events to draw conclusions about the present or make predictions about the future (Stokes & Wall, 2017).
As you might expect, it’s common in the research branches of history departments in universities.
This approach is useful in studies that seek to understand the past to interpret present events or trends. However, it relies heavily on the availability and reliability of source materials, which may be limited.
Common data sources include cultural artifacts from both material and non-material culture , which are then examined, compared, contrasted, and contextualized to test hypotheses and generate theories.
Pros of Historical Research | Cons of Historical Research |
---|---|
1. | 1. Dependent on available sources |
2. Can help understand current events or trends | 2. Potential bias in source materials |
3. Allows the study of change over time | 3. Difficult to replicate |
Example of Historical Research
A historical research example might be a study examining the evolution of gender roles over the last century. This research might involve the analysis of historical newspapers, advertisements, letters, and company documents, as well as sociocultural contexts.
4. Content Analysis
Content analysis is a research method that involves systematic and objective coding and interpreting of text or media to identify patterns, themes, ideologies, or biases (Schweigert, 2021).
A content analysis is useful in analyzing communication patterns, helping to reveal how texts such as newspapers, movies, films, political speeches, and other types of ‘content’ contain narratives and biases.
However, interpretations can be very subjective, which often requires scholars to engage in practices such as cross-comparing their coding with peers or external researchers.
Content analysis can be further broken down in to other specific methodologies such as semiotic analysis, multimodal analysis , and discourse analysis .
Pros of Content Analysis | Cons of Content Analysis |
---|---|
1. Unobtrusive data collection | 1. Lacks contextual information |
2. Allows for large sample analysis | 2. Potential coder bias |
3. Replicable and reliable if done properly | 3. May overlook nuances |
Example of Content Analysis
How is Islam Portrayed in Western Media? by Poorebrahim and Zarei (2013) employs a type of content analysis called critical discourse analysis (common in poststructuralist and critical theory research ). This study by Poorebrahum and Zarei combs through a corpus of western media texts to explore the language forms that are used in relation to Islam and Muslims, finding that they are overly stereotyped, which may represent anti-Islam bias or failure to understand the Islamic world.
5. Grounded Theory Research
Grounded theory involves developing a theory during and after data collection rather than beforehand.
This is in contrast to most academic research studies, which start with a hypothesis or theory and then testing of it through a study, where we might have a null hypothesis (disproving the theory) and an alternative hypothesis (supporting the theory).
Grounded Theory is useful because it keeps an open mind to what the data might reveal out of the research. It can be time-consuming and requires rigorous data analysis (Tracy, 2019).
Pros of Grounded Theory Research | Cons of Grounded Theory Research |
---|---|
1. Helps with theory development | 1. Time-consuming |
2. Rigorous data analysis | 2. Requires iterative data collection and analysis |
3. Can fill gaps in existing theories | 3. Requires skilled researchers |
Grounded Theory Example
Developing a Leadership Identity by Komives et al (2005) employs a grounded theory approach to develop a thesis based on the data rather than testing a hypothesis. The researchers studied the leadership identity of 13 college students taking on leadership roles. Based on their interviews, the researchers theorized that the students’ leadership identities shifted from a hierarchical view of leadership to one that embraced leadership as a collaborative concept.
6. Action Research
Action research is an approach which aims to solve real-world problems and bring about change within a setting. The study is designed to solve a specific problem – or in other words, to take action (Patten, 2017).
This approach can involve mixed methods, but is generally qualitative because it usually involves the study of a specific case study wherein the researcher works, e.g. a teacher studying their own classroom practice to seek ways they can improve.
Action research is very common in fields like education and nursing where practitioners identify areas for improvement then implement a study in order to find paths forward.
Pros of Action Research | Cons of Action Research |
---|---|
1. Addresses real-world problems and seeks to find solutions. | 1. It is time-consuming and often hard to implement into a practitioner’s already busy schedule |
2. Integrates research and action in an action-research cycle. | 2. Requires collaboration between researcher, practitioner, and research participants. |
3. Can bring about positive change in isolated instances, such as in a school or nursery setting. | 3. Complexity of managing dual roles (where the researcher is also often the practitioner) |
Action Research Example
Using Digital Sandbox Gaming to Improve Creativity Within Boys’ Writing by Ellison and Drew was a research study one of my research students completed in his own classroom under my supervision. He implemented a digital game-based approach to literacy teaching with boys and interviewed his students to see if the use of games as stimuli for storytelling helped draw them into the learning experience.
7. Natural Observational Research
Observational research can also be quantitative (see: experimental research), but in naturalistic settings for the social sciences, researchers tend to employ qualitative data collection methods like interviews and field notes to observe people in their day-to-day environments.
This approach involves the observation and detailed recording of behaviors in their natural settings (Howitt, 2019). It can provide rich, in-depth information, but the researcher’s presence might influence behavior.
While observational research has some overlaps with ethnography (especially in regard to data collection techniques), it tends not to be as sustained as ethnography, e.g. a researcher might do 5 observations, every second Monday, as opposed to being embedded in an environment.
Pros of Qualitative Observational Research | Cons of Qualitative Observational Research |
---|---|
1. Captures behavior in natural settings, allowing for interesting insights into authentic behaviors. | 1. Researcher’s presence may influence behavior |
2. Can provide rich, detailed data through the researcher’s vignettes. | 2. Can be time-consuming |
3. Non-invasive because researchers want to observe natural activities rather than interfering with research participants. | 3. Requires skilled and trained observers |
Observational Research Example
A researcher might use qualitative observational research to study the behaviors and interactions of children at a playground. The researcher would document the behaviors observed, such as the types of games played, levels of cooperation , and instances of conflict.
8. Case Study Research
Case study research is a qualitative method that involves a deep and thorough investigation of a single individual, group, or event in order to explore facets of that phenomenon that cannot be captured using other methods (Stokes & Wall, 2017).
Case study research is especially valuable in providing contextualized insights into specific issues, facilitating the application of abstract theories to real-world situations (Patten, 2017).
However, findings from a case study may not be generalizable due to the specific context and the limited number of cases studied (Walliman, 2021).
Pros of Case Study Research | Cons of Case Study Research |
---|---|
1. Provides detailed insights | 1. Limited generalizability |
2. Facilitates the study of complex phenomena | 2. Can be time-consuming |
3. Can test or generate theories | 3. Subject to observer bias |
See More: Case Study Advantages and Disadvantages
Example of a Case Study
Scholars conduct a detailed exploration of the implementation of a new teaching method within a classroom setting. The study focuses on how the teacher and students adapt to the new method, the challenges encountered, and the outcomes on student performance and engagement. While the study provides specific and detailed insights of the teaching method in that classroom, it cannot be generalized to other classrooms, as statistical significance has not been established through this qualitative approach.
Quantitative Research Methods
Quantitative research methods involve the systematic empirical investigation of observable phenomena via statistical, mathematical, or computational techniques (Pajo, 2022). The focus is on gathering numerical data and generalizing it across groups of people or to explain a particular phenomenon.
9. Experimental Research
Experimental research is a quantitative method where researchers manipulate one variable to determine its effect on another (Walliman, 2021).
This is common, for example, in high-school science labs, where students are asked to introduce a variable into a setting in order to examine its effect.
This type of research is useful in situations where researchers want to determine causal relationships between variables. However, experimental conditions may not reflect real-world conditions.
Pros of Experimental Research | Cons of Experimental Research |
---|---|
1. Allows for determination of causality | 1. Might not reflect real-world conditions |
2. Allows for the study of phenomena in highly controlled environments to minimize research contamination. | 2. Can be costly and time-consuming to create a controlled environment. |
3. Can be replicated so other researchers can test and verify the results. | 3. Ethical concerns need to be addressed as the research is directly manipulating variables. |
Example of Experimental Research
A researcher may conduct an experiment to determine the effects of a new educational approach on student learning outcomes. Students would be randomly assigned to either the control group (traditional teaching method) or the experimental group (new educational approach).
10. Surveys and Questionnaires
Surveys and questionnaires are quantitative methods that involve asking research participants structured and predefined questions to collect data about their attitudes, beliefs, behaviors, or characteristics (Patten, 2017).
Surveys are beneficial for collecting data from large samples, but they depend heavily on the honesty and accuracy of respondents.
They tend to be seen as more authoritative than their qualitative counterparts, semi-structured interviews, because the data is quantifiable (e.g. a questionnaire where information is presented on a scale from 1 to 10 can allow researchers to determine and compare statistical means, averages, and variations across sub-populations in the study).
Pros of Surveys and Questionnaires | Cons of Surveys and Questionnaires |
---|---|
1. Data can be gathered from larger samples than is possible in qualitative research. | 1. There is heavy dependence on respondent honesty |
2. The data is quantifiable, allowing for comparison across subpopulations | 2. There is limited depth of response as opposed to qualitative approaches. |
3. Can be cost-effective and time-efficient | 3. Static with no flexibility to explore responses (unlike semi- or unstrcutured interviewing) |
Example of a Survey Study
A company might use a survey to gather data about employee job satisfaction across its offices worldwide. Employees would be asked to rate various aspects of their job satisfaction on a Likert scale. While this method provides a broad overview, it may lack the depth of understanding possible with other methods (Stokes & Wall, 2017).
11. Longitudinal Studies
Longitudinal studies involve repeated observations of the same variables over extended periods (Howitt, 2019). These studies are valuable for tracking development and change but can be costly and time-consuming.
With multiple data points collected over extended periods, it’s possible to examine continuous changes within things like population dynamics or consumer behavior. This makes a detailed analysis of change possible.
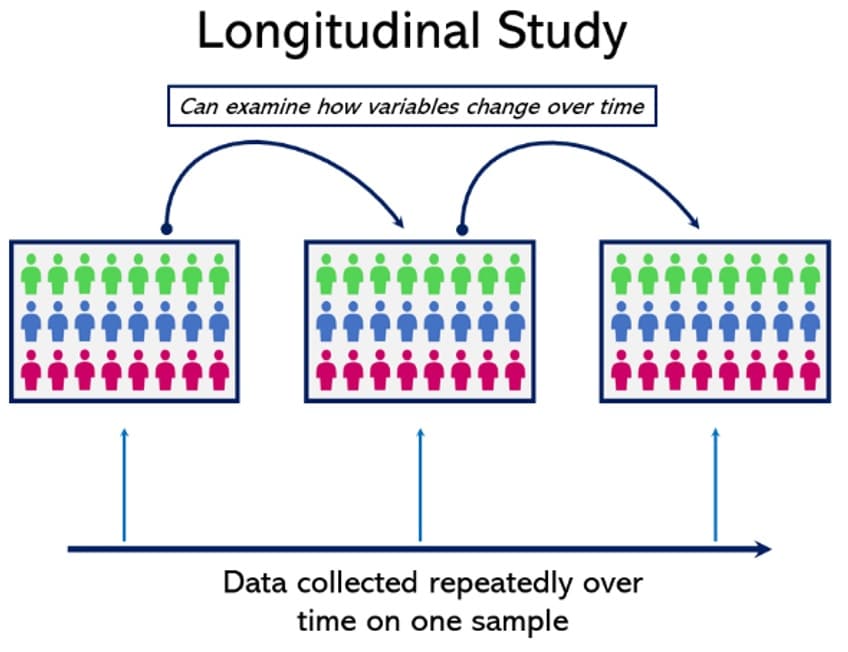
Perhaps the most relatable example of a longitudinal study is a national census, which is taken on the same day every few years, to gather comparative demographic data that can show how a nation is changing over time.
While longitudinal studies are commonly quantitative, there are also instances of qualitative ones as well, such as the famous 7 Up study from the UK, which studies 14 individuals every 7 years to explore their development over their lives.
Pros of Longitudinal Studies | Cons of Longitudinal Studies |
---|---|
1. Tracks changes over time allowing for comparison of past to present events. | 1. Is almost by definition time-consuming because time needs to pass between each data collection session. |
2. Can identify sequences of events, but causality is often harder to determine. | 2. There is high risk of participant dropout over time as participants move on with their lives. |
Example of a Longitudinal Study
A national census, taken every few years, uses surveys to develop longitudinal data, which is then compared and analyzed to present accurate trends over time. Trends a census can reveal include changes in religiosity, values and attitudes on social issues, and much more.
12. Cross-Sectional Studies
Cross-sectional studies are a quantitative research method that involves analyzing data from a population at a specific point in time (Patten, 2017). They provide a snapshot of a situation but cannot determine causality.
This design is used to measure and compare the prevalence of certain characteristics or outcomes in different groups within the sampled population.
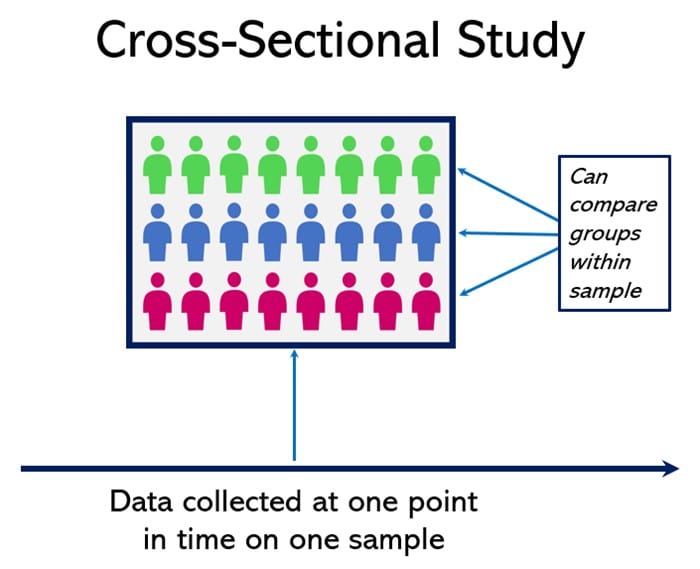
The major advantage of cross-sectional design is its ability to measure a wide range of variables simultaneously without needing to follow up with participants over time.
However, cross-sectional studies do have limitations . This design can only show if there are associations or correlations between different variables, but cannot prove cause and effect relationships, temporal sequence, changes, and trends over time.
Pros of Cross-Sectional Studies | Cons of Cross-Sectional Studies |
---|---|
1. Quick and inexpensive, with no long-term commitment required. | 1. Cannot determine causality because it is a simple snapshot, with no time delay between data collection points. |
2. Good for descriptive analyses. | 2. Does not allow researchers to follow up with research participants. |
Example of a Cross-Sectional Study
Our longitudinal study example of a national census also happens to contain cross-sectional design. One census is cross-sectional, displaying only data from one point in time. But when a census is taken once every few years, it becomes longitudinal, and so long as the data collection technique remains unchanged, identification of changes will be achievable, adding another time dimension on top of a basic cross-sectional study.
13. Correlational Research
Correlational research is a quantitative method that seeks to determine if and to what degree a relationship exists between two or more quantifiable variables (Schweigert, 2021).
This approach provides a fast and easy way to make initial hypotheses based on either positive or negative correlation trends that can be observed within dataset.
While correlational research can reveal relationships between variables, it cannot establish causality.
Methods used for data analysis may include statistical correlations such as Pearson’s or Spearman’s.
Pros of Correlational Research | Cons of Correlational Research |
---|---|
1. Reveals relationships between variables | 1. Cannot determine causality |
2. Can use existing data | 2. May be |
3. Can guide further experimental research | 3. Correlation may be coincidental |
Example of Correlational Research
A team of researchers is interested in studying the relationship between the amount of time students spend studying and their academic performance. They gather data from a high school, measuring the number of hours each student studies per week and their grade point averages (GPAs) at the end of the semester. Upon analyzing the data, they find a positive correlation, suggesting that students who spend more time studying tend to have higher GPAs.
14. Quasi-Experimental Design Research
Quasi-experimental design research is a quantitative research method that is similar to experimental design but lacks the element of random assignment to treatment or control.
Instead, quasi-experimental designs typically rely on certain other methods to control for extraneous variables.
The term ‘quasi-experimental’ implies that the experiment resembles a true experiment, but it is not exactly the same because it doesn’t meet all the criteria for a ‘true’ experiment, specifically in terms of control and random assignment.
Quasi-experimental design is useful when researchers want to study a causal hypothesis or relationship, but practical or ethical considerations prevent them from manipulating variables and randomly assigning participants to conditions.
Pros | Cons |
---|---|
1. It’s more feasible to implement than true experiments. | 1. Without random assignment, it’s harder to rule out confounding variables. |
2. It can be conducted in real-world settings, making the findings more applicable to the real world. | 2. The lack of random assignment may of the study. |
3. Useful when it’s unethical or impossible to manipulate the independent variable or randomly assign participants. | 3. It’s more difficult to establish a cause-effect relationship due to the potential for confounding variables. |
Example of Quasi-Experimental Design
A researcher wants to study the impact of a new math tutoring program on student performance. However, ethical and practical constraints prevent random assignment to the “tutoring” and “no tutoring” groups. Instead, the researcher compares students who chose to receive tutoring (experimental group) to similar students who did not choose to receive tutoring (control group), controlling for other variables like grade level and previous math performance.
Related: Examples and Types of Random Assignment in Research
15. Meta-Analysis Research
Meta-analysis statistically combines the results of multiple studies on a specific topic to yield a more precise estimate of the effect size. It’s the gold standard of secondary research .
Meta-analysis is particularly useful when there are numerous studies on a topic, and there is a need to integrate the findings to draw more reliable conclusions.
Some meta-analyses can identify flaws or gaps in a corpus of research, when can be highly influential in academic research, despite lack of primary data collection.
However, they tend only to be feasible when there is a sizable corpus of high-quality and reliable studies into a phenomenon.
Pros | Cons |
---|---|
Increased Statistical Power: By combining data from multiple studies, meta-analysis increases the statistical power to detect effects. | Publication Bias: Studies with null or negative findings are less likely to be published, leading to an overestimation of effect sizes. |
Greater Precision: It provides more precise estimates of effect sizes by reducing the influence of random error. | Quality of Studies: of a meta-analysis depends on the quality of the studies included. |
Resolving Discrepancies: Meta-analysis can help resolve disagreements between different studies on a topic. | Heterogeneity: Differences in study design, sample, or procedures can introduce heterogeneity, complicating interpretation of results. |
Example of a Meta-Analysis
The power of feedback revisited (Wisniewski, Zierer & Hattie, 2020) is a meta-analysis that examines 435 empirical studies research on the effects of feedback on student learning. They use a random-effects model to ascertain whether there is a clear effect size across the literature. The authors find that feedback tends to impact cognitive and motor skill outcomes but has less of an effect on motivational and behavioral outcomes.
Choosing a research method requires a lot of consideration regarding what you want to achieve, your research paradigm, and the methodology that is most valuable for what you are studying. There are multiple types of research methods, many of which I haven’t been able to present here. Generally, it’s recommended that you work with an experienced researcher or research supervisor to identify a suitable research method for your study at hand.
Hammond, M., & Wellington, J. (2020). Research methods: The key concepts . New York: Routledge.
Howitt, D. (2019). Introduction to qualitative research methods in psychology . London: Pearson UK.
Pajo, B. (2022). Introduction to research methods: A hands-on approach . New York: Sage Publications.
Patten, M. L. (2017). Understanding research methods: An overview of the essentials . New York: Sage
Schweigert, W. A. (2021). Research methods in psychology: A handbook . Los Angeles: Waveland Press.
Stokes, P., & Wall, T. (2017). Research methods . New York: Bloomsbury Publishing.
Tracy, S. J. (2019). Qualitative research methods: Collecting evidence, crafting analysis, communicating impact . London: John Wiley & Sons.
Walliman, N. (2021). Research methods: The basics. London: Routledge.
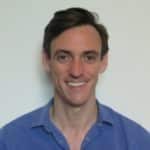
- Chris Drew (PhD) https://helpfulprofessor.com/author/chris-drew-phd/ 101 Class Group Name Ideas (for School Students)
- Chris Drew (PhD) https://helpfulprofessor.com/author/chris-drew-phd/ 19 Top Cognitive Psychology Theories (Explained)
- Chris Drew (PhD) https://helpfulprofessor.com/author/chris-drew-phd/ 119 Bloom’s Taxonomy Examples
- Chris Drew (PhD) https://helpfulprofessor.com/author/chris-drew-phd/ All 6 Levels of Understanding (on Bloom’s Taxonomy)
Leave a Comment Cancel Reply
Your email address will not be published. Required fields are marked *
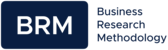
Regression Analysis
Regression analysis is a quantitative research method which is used when the study involves modelling and analysing several variables, where the relationship includes a dependent variable and one or more independent variables. In simple terms, regression analysis is a quantitative method used to test the nature of relationships between a dependent variable and one or more independent variables.
The basic form of regression models includes unknown parameters (β), independent variables (X), and the dependent variable (Y).
Regression model, basically, specifies the relation of dependent variable (Y) to a function combination of independent variables (X) and unknown parameters (β)
Y ≈ f (X, β)
Regression equation can be used to predict the values of ‘y’, if the value of ‘x’ is given, and both ‘y’ and ‘x’ are the two sets of measures of a sample size of ‘n’. The formulae for regression equation would be
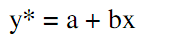
Do not be intimidated by visual complexity of correlation and regression formulae above. You don’t have to apply the formula manually, and correlation and regression analyses can be run with the application of popular analytical software such as Microsoft Excel, Microsoft Access, SPSS and others.
Linear regression analysis is based on the following set of assumptions:
1. Assumption of linearity . There is a linear relationship between dependent and independent variables.
2. Assumption of homoscedasticity . Data values for dependent and independent variables have equal variances.
3. Assumption of absence of collinearity or multicollinearity . There is no correlation between two or more independent variables.
4. Assumption of normal distribution . The data for the independent variables and dependent variable are normally distributed
My e-book, The Ultimate Guide to Writing a Dissertation in Business Studies: a step by step assistance offers practical assistance to complete a dissertation with minimum or no stress. The e-book covers all stages of writing a dissertation starting from the selection to the research area to submitting the completed version of the work within the deadline. John Dudovskiy
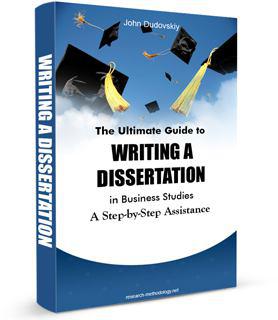
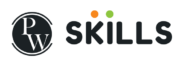
Data Analysis Techniques in Research – Methods, Tools & Examples
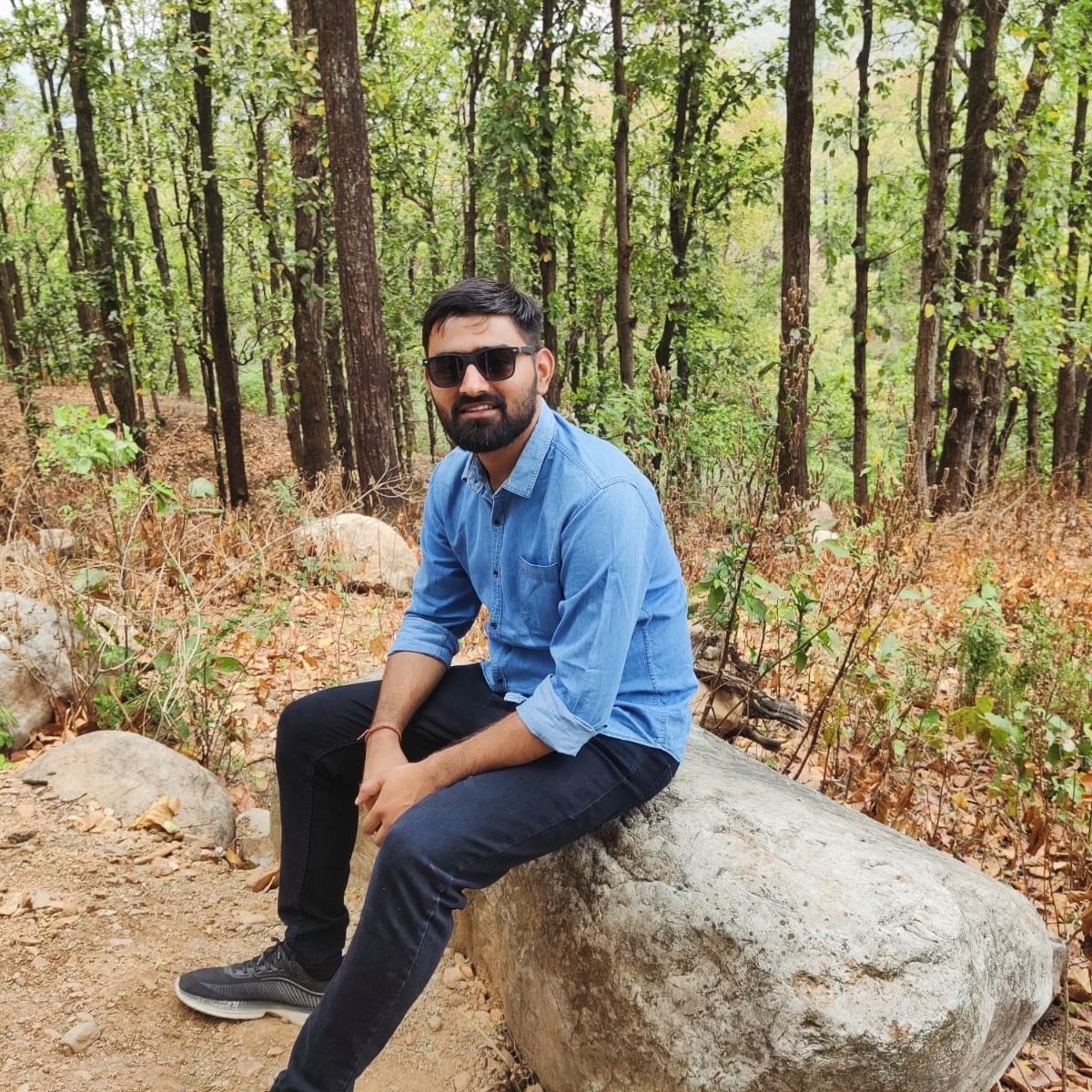
Varun Saharawat is a seasoned professional in the fields of SEO and content writing. With a profound knowledge of the intricate aspects of these disciplines, Varun has established himself as a valuable asset in the world of digital marketing and online content creation.
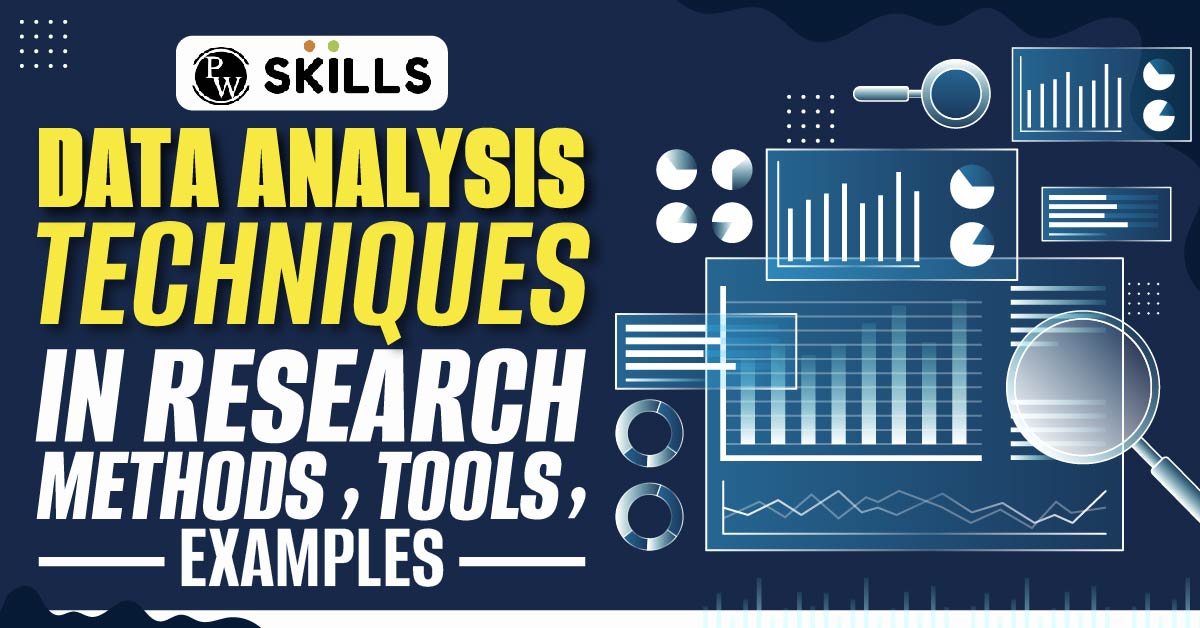
Data analysis techniques in research are essential because they allow researchers to derive meaningful insights from data sets to support their hypotheses or research objectives.
Data Analysis Techniques in Research : While various groups, institutions, and professionals may have diverse approaches to data analysis, a universal definition captures its essence. Data analysis involves refining, transforming, and interpreting raw data to derive actionable insights that guide informed decision-making for businesses.
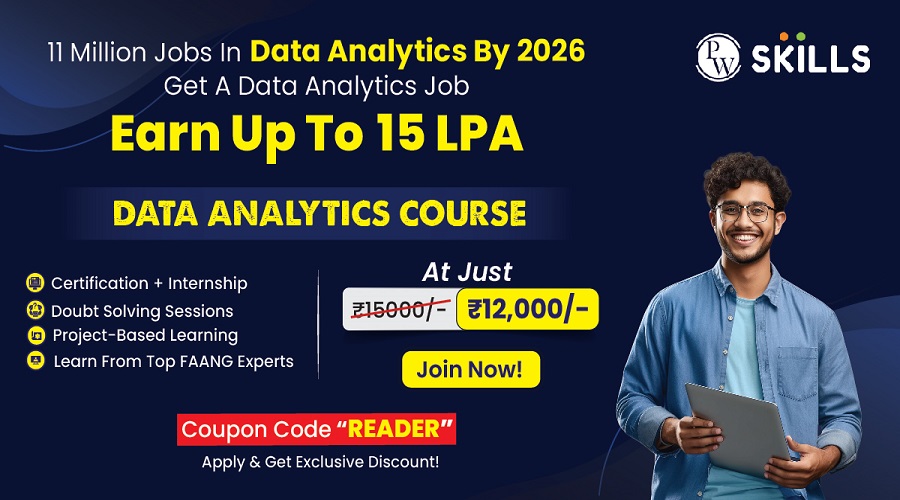
A straightforward illustration of data analysis emerges when we make everyday decisions, basing our choices on past experiences or predictions of potential outcomes.
If you want to learn more about this topic and acquire valuable skills that will set you apart in today’s data-driven world, we highly recommend enrolling in the Data Analytics Course by Physics Wallah . And as a special offer for our readers, use the coupon code “READER” to get a discount on this course.
Table of Contents
What is Data Analysis?
Data analysis is the systematic process of inspecting, cleaning, transforming, and interpreting data with the objective of discovering valuable insights and drawing meaningful conclusions. This process involves several steps:
- Inspecting : Initial examination of data to understand its structure, quality, and completeness.
- Cleaning : Removing errors, inconsistencies, or irrelevant information to ensure accurate analysis.
- Transforming : Converting data into a format suitable for analysis, such as normalization or aggregation.
- Interpreting : Analyzing the transformed data to identify patterns, trends, and relationships.
Types of Data Analysis Techniques in Research
Data analysis techniques in research are categorized into qualitative and quantitative methods, each with its specific approaches and tools. These techniques are instrumental in extracting meaningful insights, patterns, and relationships from data to support informed decision-making, validate hypotheses, and derive actionable recommendations. Below is an in-depth exploration of the various types of data analysis techniques commonly employed in research:
1) Qualitative Analysis:
Definition: Qualitative analysis focuses on understanding non-numerical data, such as opinions, concepts, or experiences, to derive insights into human behavior, attitudes, and perceptions.
- Content Analysis: Examines textual data, such as interview transcripts, articles, or open-ended survey responses, to identify themes, patterns, or trends.
- Narrative Analysis: Analyzes personal stories or narratives to understand individuals’ experiences, emotions, or perspectives.
- Ethnographic Studies: Involves observing and analyzing cultural practices, behaviors, and norms within specific communities or settings.
2) Quantitative Analysis:
Quantitative analysis emphasizes numerical data and employs statistical methods to explore relationships, patterns, and trends. It encompasses several approaches:
Descriptive Analysis:
- Frequency Distribution: Represents the number of occurrences of distinct values within a dataset.
- Central Tendency: Measures such as mean, median, and mode provide insights into the central values of a dataset.
- Dispersion: Techniques like variance and standard deviation indicate the spread or variability of data.
Diagnostic Analysis:
- Regression Analysis: Assesses the relationship between dependent and independent variables, enabling prediction or understanding causality.
- ANOVA (Analysis of Variance): Examines differences between groups to identify significant variations or effects.
Predictive Analysis:
- Time Series Forecasting: Uses historical data points to predict future trends or outcomes.
- Machine Learning Algorithms: Techniques like decision trees, random forests, and neural networks predict outcomes based on patterns in data.
Prescriptive Analysis:
- Optimization Models: Utilizes linear programming, integer programming, or other optimization techniques to identify the best solutions or strategies.
- Simulation: Mimics real-world scenarios to evaluate various strategies or decisions and determine optimal outcomes.
Specific Techniques:
- Monte Carlo Simulation: Models probabilistic outcomes to assess risk and uncertainty.
- Factor Analysis: Reduces the dimensionality of data by identifying underlying factors or components.
- Cohort Analysis: Studies specific groups or cohorts over time to understand trends, behaviors, or patterns within these groups.
- Cluster Analysis: Classifies objects or individuals into homogeneous groups or clusters based on similarities or attributes.
- Sentiment Analysis: Uses natural language processing and machine learning techniques to determine sentiment, emotions, or opinions from textual data.
Also Read: AI and Predictive Analytics: Examples, Tools, Uses, Ai Vs Predictive Analytics
Data Analysis Techniques in Research Examples
To provide a clearer understanding of how data analysis techniques are applied in research, let’s consider a hypothetical research study focused on evaluating the impact of online learning platforms on students’ academic performance.
Research Objective:
Determine if students using online learning platforms achieve higher academic performance compared to those relying solely on traditional classroom instruction.
Data Collection:
- Quantitative Data: Academic scores (grades) of students using online platforms and those using traditional classroom methods.
- Qualitative Data: Feedback from students regarding their learning experiences, challenges faced, and preferences.
Data Analysis Techniques Applied:
1) Descriptive Analysis:
- Calculate the mean, median, and mode of academic scores for both groups.
- Create frequency distributions to represent the distribution of grades in each group.
2) Diagnostic Analysis:
- Conduct an Analysis of Variance (ANOVA) to determine if there’s a statistically significant difference in academic scores between the two groups.
- Perform Regression Analysis to assess the relationship between the time spent on online platforms and academic performance.
3) Predictive Analysis:
- Utilize Time Series Forecasting to predict future academic performance trends based on historical data.
- Implement Machine Learning algorithms to develop a predictive model that identifies factors contributing to academic success on online platforms.
4) Prescriptive Analysis:
- Apply Optimization Models to identify the optimal combination of online learning resources (e.g., video lectures, interactive quizzes) that maximize academic performance.
- Use Simulation Techniques to evaluate different scenarios, such as varying student engagement levels with online resources, to determine the most effective strategies for improving learning outcomes.
5) Specific Techniques:
- Conduct Factor Analysis on qualitative feedback to identify common themes or factors influencing students’ perceptions and experiences with online learning.
- Perform Cluster Analysis to segment students based on their engagement levels, preferences, or academic outcomes, enabling targeted interventions or personalized learning strategies.
- Apply Sentiment Analysis on textual feedback to categorize students’ sentiments as positive, negative, or neutral regarding online learning experiences.
By applying a combination of qualitative and quantitative data analysis techniques, this research example aims to provide comprehensive insights into the effectiveness of online learning platforms.
Also Read: Learning Path to Become a Data Analyst in 2024
Data Analysis Techniques in Quantitative Research
Quantitative research involves collecting numerical data to examine relationships, test hypotheses, and make predictions. Various data analysis techniques are employed to interpret and draw conclusions from quantitative data. Here are some key data analysis techniques commonly used in quantitative research:
1) Descriptive Statistics:
- Description: Descriptive statistics are used to summarize and describe the main aspects of a dataset, such as central tendency (mean, median, mode), variability (range, variance, standard deviation), and distribution (skewness, kurtosis).
- Applications: Summarizing data, identifying patterns, and providing initial insights into the dataset.
2) Inferential Statistics:
- Description: Inferential statistics involve making predictions or inferences about a population based on a sample of data. This technique includes hypothesis testing, confidence intervals, t-tests, chi-square tests, analysis of variance (ANOVA), regression analysis, and correlation analysis.
- Applications: Testing hypotheses, making predictions, and generalizing findings from a sample to a larger population.
3) Regression Analysis:
- Description: Regression analysis is a statistical technique used to model and examine the relationship between a dependent variable and one or more independent variables. Linear regression, multiple regression, logistic regression, and nonlinear regression are common types of regression analysis .
- Applications: Predicting outcomes, identifying relationships between variables, and understanding the impact of independent variables on the dependent variable.
4) Correlation Analysis:
- Description: Correlation analysis is used to measure and assess the strength and direction of the relationship between two or more variables. The Pearson correlation coefficient, Spearman rank correlation coefficient, and Kendall’s tau are commonly used measures of correlation.
- Applications: Identifying associations between variables and assessing the degree and nature of the relationship.
5) Factor Analysis:
- Description: Factor analysis is a multivariate statistical technique used to identify and analyze underlying relationships or factors among a set of observed variables. It helps in reducing the dimensionality of data and identifying latent variables or constructs.
- Applications: Identifying underlying factors or constructs, simplifying data structures, and understanding the underlying relationships among variables.
6) Time Series Analysis:
- Description: Time series analysis involves analyzing data collected or recorded over a specific period at regular intervals to identify patterns, trends, and seasonality. Techniques such as moving averages, exponential smoothing, autoregressive integrated moving average (ARIMA), and Fourier analysis are used.
- Applications: Forecasting future trends, analyzing seasonal patterns, and understanding time-dependent relationships in data.
7) ANOVA (Analysis of Variance):
- Description: Analysis of variance (ANOVA) is a statistical technique used to analyze and compare the means of two or more groups or treatments to determine if they are statistically different from each other. One-way ANOVA, two-way ANOVA, and MANOVA (Multivariate Analysis of Variance) are common types of ANOVA.
- Applications: Comparing group means, testing hypotheses, and determining the effects of categorical independent variables on a continuous dependent variable.
8) Chi-Square Tests:
- Description: Chi-square tests are non-parametric statistical tests used to assess the association between categorical variables in a contingency table. The Chi-square test of independence, goodness-of-fit test, and test of homogeneity are common chi-square tests.
- Applications: Testing relationships between categorical variables, assessing goodness-of-fit, and evaluating independence.
These quantitative data analysis techniques provide researchers with valuable tools and methods to analyze, interpret, and derive meaningful insights from numerical data. The selection of a specific technique often depends on the research objectives, the nature of the data, and the underlying assumptions of the statistical methods being used.
Also Read: Analysis vs. Analytics: How Are They Different?
Data Analysis Methods
Data analysis methods refer to the techniques and procedures used to analyze, interpret, and draw conclusions from data. These methods are essential for transforming raw data into meaningful insights, facilitating decision-making processes, and driving strategies across various fields. Here are some common data analysis methods:
- Description: Descriptive statistics summarize and organize data to provide a clear and concise overview of the dataset. Measures such as mean, median, mode, range, variance, and standard deviation are commonly used.
- Description: Inferential statistics involve making predictions or inferences about a population based on a sample of data. Techniques such as hypothesis testing, confidence intervals, and regression analysis are used.
3) Exploratory Data Analysis (EDA):
- Description: EDA techniques involve visually exploring and analyzing data to discover patterns, relationships, anomalies, and insights. Methods such as scatter plots, histograms, box plots, and correlation matrices are utilized.
- Applications: Identifying trends, patterns, outliers, and relationships within the dataset.
4) Predictive Analytics:
- Description: Predictive analytics use statistical algorithms and machine learning techniques to analyze historical data and make predictions about future events or outcomes. Techniques such as regression analysis, time series forecasting, and machine learning algorithms (e.g., decision trees, random forests, neural networks) are employed.
- Applications: Forecasting future trends, predicting outcomes, and identifying potential risks or opportunities.
5) Prescriptive Analytics:
- Description: Prescriptive analytics involve analyzing data to recommend actions or strategies that optimize specific objectives or outcomes. Optimization techniques, simulation models, and decision-making algorithms are utilized.
- Applications: Recommending optimal strategies, decision-making support, and resource allocation.
6) Qualitative Data Analysis:
- Description: Qualitative data analysis involves analyzing non-numerical data, such as text, images, videos, or audio, to identify themes, patterns, and insights. Methods such as content analysis, thematic analysis, and narrative analysis are used.
- Applications: Understanding human behavior, attitudes, perceptions, and experiences.
7) Big Data Analytics:
- Description: Big data analytics methods are designed to analyze large volumes of structured and unstructured data to extract valuable insights. Technologies such as Hadoop, Spark, and NoSQL databases are used to process and analyze big data.
- Applications: Analyzing large datasets, identifying trends, patterns, and insights from big data sources.
8) Text Analytics:
- Description: Text analytics methods involve analyzing textual data, such as customer reviews, social media posts, emails, and documents, to extract meaningful information and insights. Techniques such as sentiment analysis, text mining, and natural language processing (NLP) are used.
- Applications: Analyzing customer feedback, monitoring brand reputation, and extracting insights from textual data sources.
These data analysis methods are instrumental in transforming data into actionable insights, informing decision-making processes, and driving organizational success across various sectors, including business, healthcare, finance, marketing, and research. The selection of a specific method often depends on the nature of the data, the research objectives, and the analytical requirements of the project or organization.
Also Read: Quantitative Data Analysis: Types, Analysis & Examples
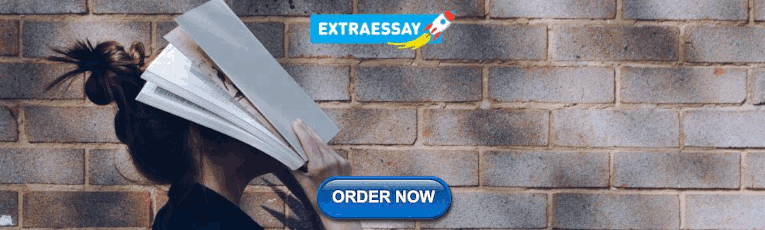
Data Analysis Tools
Data analysis tools are essential instruments that facilitate the process of examining, cleaning, transforming, and modeling data to uncover useful information, make informed decisions, and drive strategies. Here are some prominent data analysis tools widely used across various industries:
1) Microsoft Excel:
- Description: A spreadsheet software that offers basic to advanced data analysis features, including pivot tables, data visualization tools, and statistical functions.
- Applications: Data cleaning, basic statistical analysis, visualization, and reporting.
2) R Programming Language:
- Description: An open-source programming language specifically designed for statistical computing and data visualization.
- Applications: Advanced statistical analysis, data manipulation, visualization, and machine learning.
3) Python (with Libraries like Pandas, NumPy, Matplotlib, and Seaborn):
- Description: A versatile programming language with libraries that support data manipulation, analysis, and visualization.
- Applications: Data cleaning, statistical analysis, machine learning, and data visualization.
4) SPSS (Statistical Package for the Social Sciences):
- Description: A comprehensive statistical software suite used for data analysis, data mining, and predictive analytics.
- Applications: Descriptive statistics, hypothesis testing, regression analysis, and advanced analytics.
5) SAS (Statistical Analysis System):
- Description: A software suite used for advanced analytics, multivariate analysis, and predictive modeling.
- Applications: Data management, statistical analysis, predictive modeling, and business intelligence.
6) Tableau:
- Description: A data visualization tool that allows users to create interactive and shareable dashboards and reports.
- Applications: Data visualization , business intelligence , and interactive dashboard creation.
7) Power BI:
- Description: A business analytics tool developed by Microsoft that provides interactive visualizations and business intelligence capabilities.
- Applications: Data visualization, business intelligence, reporting, and dashboard creation.
8) SQL (Structured Query Language) Databases (e.g., MySQL, PostgreSQL, Microsoft SQL Server):
- Description: Database management systems that support data storage, retrieval, and manipulation using SQL queries.
- Applications: Data retrieval, data cleaning, data transformation, and database management.
9) Apache Spark:
- Description: A fast and general-purpose distributed computing system designed for big data processing and analytics.
- Applications: Big data processing, machine learning, data streaming, and real-time analytics.
10) IBM SPSS Modeler:
- Description: A data mining software application used for building predictive models and conducting advanced analytics.
- Applications: Predictive modeling, data mining, statistical analysis, and decision optimization.
These tools serve various purposes and cater to different data analysis needs, from basic statistical analysis and data visualization to advanced analytics, machine learning, and big data processing. The choice of a specific tool often depends on the nature of the data, the complexity of the analysis, and the specific requirements of the project or organization.
Also Read: How to Analyze Survey Data: Methods & Examples
Importance of Data Analysis in Research
The importance of data analysis in research cannot be overstated; it serves as the backbone of any scientific investigation or study. Here are several key reasons why data analysis is crucial in the research process:
- Data analysis helps ensure that the results obtained are valid and reliable. By systematically examining the data, researchers can identify any inconsistencies or anomalies that may affect the credibility of the findings.
- Effective data analysis provides researchers with the necessary information to make informed decisions. By interpreting the collected data, researchers can draw conclusions, make predictions, or formulate recommendations based on evidence rather than intuition or guesswork.
- Data analysis allows researchers to identify patterns, trends, and relationships within the data. This can lead to a deeper understanding of the research topic, enabling researchers to uncover insights that may not be immediately apparent.
- In empirical research, data analysis plays a critical role in testing hypotheses. Researchers collect data to either support or refute their hypotheses, and data analysis provides the tools and techniques to evaluate these hypotheses rigorously.
- Transparent and well-executed data analysis enhances the credibility of research findings. By clearly documenting the data analysis methods and procedures, researchers allow others to replicate the study, thereby contributing to the reproducibility of research findings.
- In fields such as business or healthcare, data analysis helps organizations allocate resources more efficiently. By analyzing data on consumer behavior, market trends, or patient outcomes, organizations can make strategic decisions about resource allocation, budgeting, and planning.
- In public policy and social sciences, data analysis is instrumental in developing and evaluating policies and interventions. By analyzing data on social, economic, or environmental factors, policymakers can assess the effectiveness of existing policies and inform the development of new ones.
- Data analysis allows for continuous improvement in research methods and practices. By analyzing past research projects, identifying areas for improvement, and implementing changes based on data-driven insights, researchers can refine their approaches and enhance the quality of future research endeavors.
However, it is important to remember that mastering these techniques requires practice and continuous learning. That’s why we highly recommend the Data Analytics Course by Physics Wallah . Not only does it cover all the fundamentals of data analysis, but it also provides hands-on experience with various tools such as Excel, Python, and Tableau. Plus, if you use the “ READER ” coupon code at checkout, you can get a special discount on the course.
For Latest Tech Related Information, Join Our Official Free Telegram Group : PW Skills Telegram Group
Data Analysis Techniques in Research FAQs
What are the 5 techniques for data analysis.
The five techniques for data analysis include: Descriptive Analysis Diagnostic Analysis Predictive Analysis Prescriptive Analysis Qualitative Analysis
What are techniques of data analysis in research?
Techniques of data analysis in research encompass both qualitative and quantitative methods. These techniques involve processes like summarizing raw data, investigating causes of events, forecasting future outcomes, offering recommendations based on predictions, and examining non-numerical data to understand concepts or experiences.
What are the 3 methods of data analysis?
The three primary methods of data analysis are: Qualitative Analysis Quantitative Analysis Mixed-Methods Analysis
What are the four types of data analysis techniques?
The four types of data analysis techniques are: Descriptive Analysis Diagnostic Analysis Predictive Analysis Prescriptive Analysis
- Why is Data Analytics Skills Important?
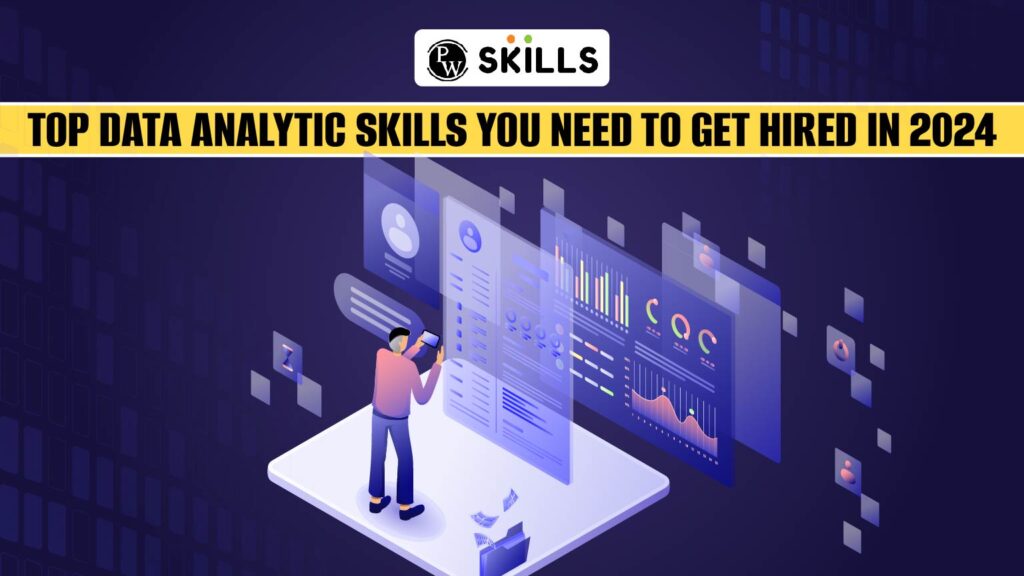
Data analytics skills are important for candidates to stand out from other candidates during a job interview and better their…
- What is ACL in Data Analytics? Types, Components, Roles, Benefits
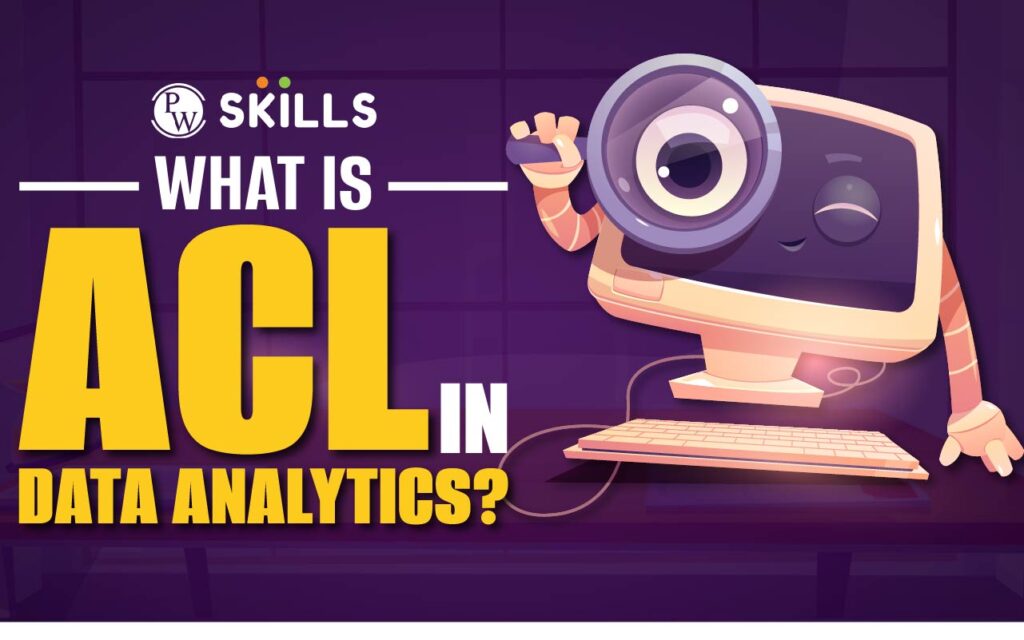
ACL in Data Analytics: In data analytics, ACL stands for “Access Control List,” a mechanism that regulates user access to…
- What Is Business Analytics Business Intelligence?
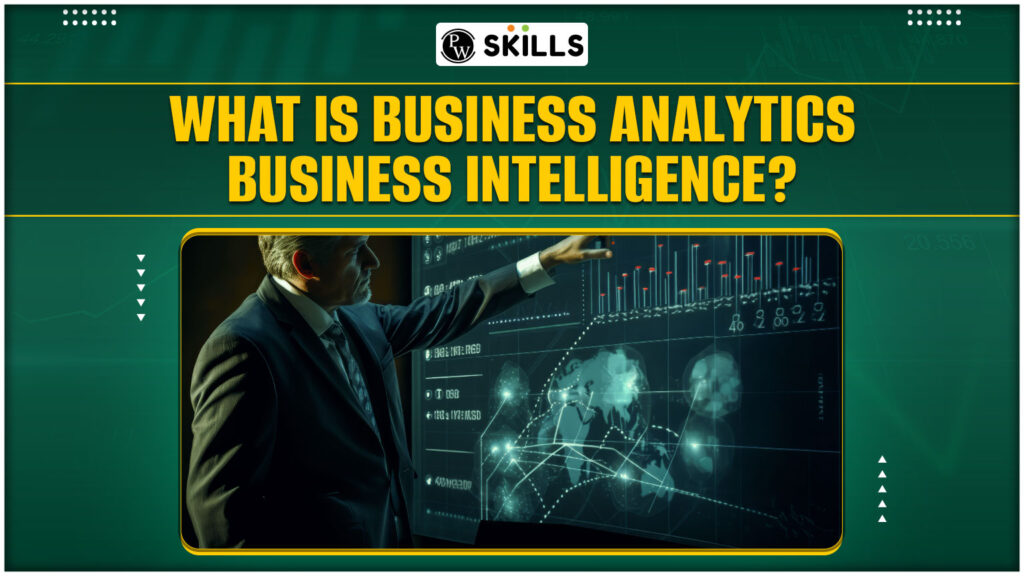
Want to learn what Business analytics business intelligence is? Reading this article will help you to understand all topics clearly,…
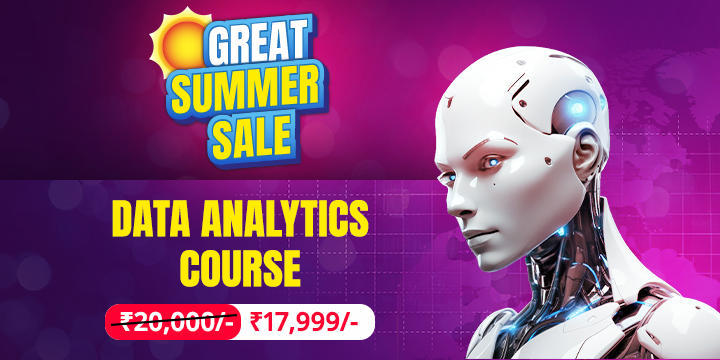
Related Articles
- Data Analytics Internships – What Does A Data Analyst Intern Do?
- What is Data Analytics in Database?
- Full Form Of OLAP
- Data Mining Architecture: Components, Types & Techniques
- What are Analytical Insights: Definition & Best Practices
- Storytelling with Data: Communicating Insights Effectively
- The Best Business Intelligence Software in 2024
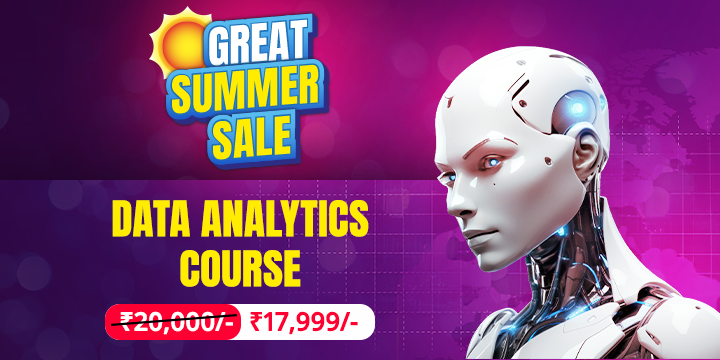
- About the Field
- Generalist MCM
- Marketing Communication
- Strategic and Organizational Communication
- Market Research and Analytics
- Admissions Overview
- Student Experience
- Career Overview
MASTER OF COMMUNICATION MANAGEMENT ONLINE
4 Research Methods for Audience Analysis
November 15, 2023
View all blog posts under Articles
Before communications professionals such as technical writers, public relations specialists, and others within the field launch a campaign or strategy, they often need to assess the target audience to make sure that all information provided is appropriate, appealing, and at the correct level.
The audience, also referred to as the end-user, will be the recipient of any items produced and shared, so it's crucial to make sure that all communications are targeted towards that group. In order to achieve this goal, many communications professionals will use research methods for audience analysis. As you work toward your master's in communications , it’s important to cultivate skills like these now that can help you at work later.
There are several commonly used methods; each offers its own insights into your target audience. Read on for four examples:
Primary Research
The first step when beginning audience analysis is gathering as much information as possible. If you have preconceived notions or ideas about your audience, avoid them and focus on the actual research performed instead. You should eliminate any information that has already been found by other researchers as part of your primary research phase, since this will allow you to start from scratch. You might choose to use qualitative or quantitative research methods during this process, but all information should come from your own work and findings.
Qualitative Research
Qualitative research focuses on the feelings and opinions of a consumer or member of the target audience. When performing this type of research, you should try to get into and understand the mindset of a customer to see which areas of the product or campaign are most appealing and which areas are lacking. Examples include interviews and focus groups.
Face-to-face or telephone interviews can provide a lot of insight into your target audience. This method of research can fall into either qualitative or quantitative, although most believe that it provides more information in the former category. An interview involves interaction between a single respondent and the moderator, or person asking the questions.
However, an interview doesn't have to follow a prescribed layout and format with a list of questions and time for answers. Instead, some researchers choose to make the interview process more comfortable and informal by simply engaging in a conversation with audience members. When conducting interviews, ask open-ended questions that allow for detailed responses instead of just "yes" or "no."
Focus Groups
One of the most common methods of qualitative research is hosting a focus group. In this method, you will organize a group of people and share information about your product or service, campaign and marketing ideas, and development. As the participants of the focus group view the information presented, you can gauge their reactions through body language, facial expressions, and actual feedback that they provide. Hosting focus groups in virtual settings is becoming more popular, thanks to technological advancements, but doing so can make it harder to see facial expressions and read body language.
When bringing people together to participate in a focus group, it's important to make sure that all participants have something in common. You might choose to divide groups by age, gender, or other factors. When you split them into more targeted groups, you can get more information that will help you better understand your audience . Most focus group hosts offer some type of compensation for participation, such as a free product, gift card, voucher, coupon, or even cash.
Quantitative Research
Quantitative research typically focuses on statistics and hard facts instead of opinions and feelings. For a better understanding of your audience, it's helpful to use methods that fall under this category when conducting both primary and secondary research. Examples of quantitative research include social media analytics, surveys/questionnaires, and trials with experimentation.
Social Media Analytics
In today's online world, social media is a critical area when researching your audience. Companies can reach millions of people with a single post, something that was never previously within reach. But simply sharing posts without following up on how they are performing is a waste of time and resources. Analytics are built into most social media platforms and allow users to view how many people viewed a post, as well as any interaction with the content, such as clicks and responses.
Using the information provided can help your company learn what type of content resonates with your target audience. By viewing what they choose to interact with, you can then create additional content that complements those topics. New jobs are popping up across the country , and they require experience in and an understanding of social media analytics.
Surveys and Questionnaires
Surveys and questionnaires are old-school methods of quantitative research that still work. Whether you conduct a follow-up survey by phone or email or slip a flyer into the bag of every customer who visits your store, you can get information from real buyers or users of your product. Using this information can help you improve the product or service as well as customer service techniques and the overall customer experience.
Secondary Research
Secondary research involves reviewing information presented from other sources. If your company has conducted primary research on other products or service, you might use that data for developing and improving new products and services. Some businesses also rely on external sources to present data.
If you're simply relying on the internet for market research, it may be time to reevaluate your strategy. The online world is certainly useful as a starting point but should not be the only method by which you conduct research. Talking to your customers and understanding their unique perspectives is critical to improving the way you speak to them and market your products. Keep in mind that it would be a mistake to rely on just one research method instead of employing several different ones.
If you are interested in understanding and working in market research for audience analysis, you may want to consider your advanced education options. The online Masters in Communication Management , offered by the Annenberg School for Communication and Journalism, includes courses that focus on research methods and how to improve audience engagement through understanding their thoughts and feelings.
Sources https://www.cleverism.com/market-research-techniques-primary-secondary/ https://www.entrepreneur.com/article/270131 http://study.com/academy/lesson/informal-and-formal-methods-of-audience-analysis.html http://study.com/academy/lesson/gathering-information-for-audience-analysis-research-observation.html
Get Program Details
Thank you for visiting nature.com. You are using a browser version with limited support for CSS. To obtain the best experience, we recommend you use a more up to date browser (or turn off compatibility mode in Internet Explorer). In the meantime, to ensure continued support, we are displaying the site without styles and JavaScript.
- View all journals
- Explore content
- About the journal
- Publish with us
- Sign up for alerts
- Published: 19 June 2024
Detection of microplastics in the human penis
- Jason Codrington ORCID: orcid.org/0009-0003-1490-4211 1 ,
- Alexandra Aponte Varnum 1 ,
- Lars Hildebrandt 2 ,
- Daniel Pröfrock 2 ,
- Joginder Bidhan 1 ,
- Kajal Khodamoradi ORCID: orcid.org/0000-0003-2951-4382 1 ,
- Anke-Lisa Höhme 3 ,
- Martin Held ORCID: orcid.org/0000-0003-1869-463X 3 ,
- Aymara Evans 1 ,
- David Velasquez ORCID: orcid.org/0009-0003-0475-4918 1 ,
- Christina C. Yarborough 1 ,
- Bahareh Ghane-Motlagh 4 ,
- Ashutosh Agarwal 1 , 5 ,
- Justin Achua ORCID: orcid.org/0000-0002-4159-439X 6 ,
- Edoardo Pozzi ORCID: orcid.org/0000-0002-0228-7039 1 , 7 , 8 ,
- Francesco Mesquita 1 ,
- Francis Petrella 1 ,
- David Miller 1 &
- Ranjith Ramasamy 1
International Journal of Impotence Research ( 2024 ) Cite this article
950 Accesses
860 Altmetric
Metrics details
- Medical research
- Sexual dysfunction
The proliferation of microplastics (MPs) represents a burgeoning environmental and health crisis. Measuring less than 5 mm in diameter, MPs have infiltrated atmospheric, freshwater, and terrestrial ecosystems, penetrating commonplace consumables like seafood, sea salt, and bottled beverages. Their size and surface area render them susceptible to chemical interactions with physiological fluids and tissues, raising bioaccumulation and toxicity concerns. Human exposure to MPs occurs through ingestion, inhalation, and dermal contact. To date, there is no direct evidence identifying MPs in penile tissue. The objective of this study was to assess for potential aggregation of MPs in penile tissue. Tissue samples were extracted from six individuals who underwent surgery for a multi-component inflatable penile prosthesis (IPP). Samples were obtained from the corpora using Adson forceps before corporotomy dilation and device implantation and placed into cleaned glassware. A control sample was collected and stored in a McKesson specimen plastic container. The tissue fractions were analyzed using the Agilent 8700 Laser Direct Infrared (LDIR) Chemical Imaging System (Agilent Technologies. Moreover, the morphology of the particles was investigated by a Zeiss Merlin Scanning Electron Microscope (SEM), complementing the detection range of LDIR to below 20 µm. MPs via LDIR were identified in 80% of the samples, ranging in size from 20–500 µm. Smaller particles down to 2 µm were detected via SEM. Seven types of MPs were found in the penile tissue, with polyethylene terephthalate (47.8%) and polypropylene (34.7%) being the most prevalent. The detection of MPs in penile tissue raises inquiries on the ramifications of environmental pollutants on sexual health. Our research adds a key dimension to the discussion on man-made pollutants, focusing on MPs in the male reproductive system.
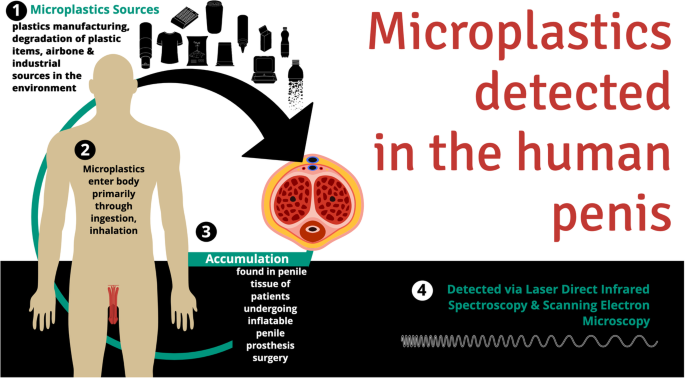
This is a preview of subscription content, access via your institution
Access options
Subscribe to this journal
Receive 8 print issues and online access
251,40 € per year
only 31,43 € per issue
Buy this article
- Purchase on Springer Link
- Instant access to full article PDF
Prices may be subject to local taxes which are calculated during checkout
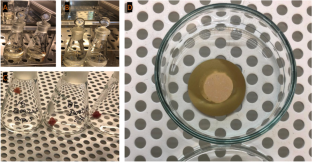
Similar content being viewed by others
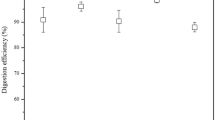
A novel method for extraction, quantification, and identification of microplastics in CreamType of cosmetic products
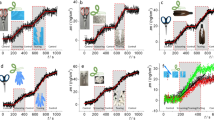
Microplastics generated when opening plastic packaging
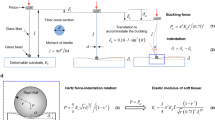
A portable pen-sized instrumentation to measure stiffness of soft tissues in vivo
Data availability.
All relevant data to the current study that was generated and analyzed is available upon reasonable request from the corresponding author.
Schwabl P, Köppel S, Königshofer P, Bucsics T, Trauner M, Reiberger T, et al. Detection of various microplastics in human stool: a prospective case series. Ann Intern Med. 2019;171:453–7.
Article PubMed Google Scholar
Zhu L, Zhu J, Zuo R, Xu Q, Qian Y, An L. Identification of microplastics in human placenta using laser direct infrared spectroscopy. Sci Total Environ. 2023;856:159060
Article CAS PubMed Google Scholar
Ragusa A, Svelato A, Santacroce C, Catalano P, Notarstefano V, Carnevali O, et al. Plasticenta: first evidence of microplastics in human placenta. Environ Int. 2021;146:106274.
Amato-Lourenço LF, Carvalho-Oliveira R, Júnior GR, Dos Santos Galvão L, Ando RA, Mauad T. Presence of airborne microplastics in human lung tissue. J Hazard Mater. 2021;416:126124.
Jenner LC, Rotchell JM, Bennett RT, Cowen M, Tentzeris V, Sadofsky LR. Detection of microplastics in human lung tissue using μFTIR spectroscopy. Sci Total Environ. 2022;831:154907.
Yang Y, Xie E, Du Z, Peng Z, Han Z, Li L, et al. Detection of various microplastics in patients undergoing cardiac surgery. Environ Sci Technol. 2023;57:10911–8.
Wang C, Zhao J, Xing B. Environmental source, fate, and toxicity of microplastics. J Hazard Mater. 2021;407:124357.
da Silva Brito WA, Mutter F, Wende K, Cecchini AL, Schmidt A, Bekeschus S. Consequences of nano and microplastic exposure in rodent models: the known and unknown. Part Fibre Toxicol. 2022;19:28.
Article PubMed PubMed Central Google Scholar
Wright SL, Kelly FJ. Plastic and human health: a micro issue? Environ Sci Technol. 2017;51:6634–47.
Ragusa A, Notarstefano V, Svelato A, Belloni A, Gioacchini G, Blondeel C, et al. Raman microspectroscopy detection and characterisation of microplastics in human breastmilk. Polymers. 2022;14:2700.
Article CAS PubMed PubMed Central Google Scholar
Cox KD, Covernton GA, Davies HL, Dower JF, Juanes F, Dudas SE. Human consumption of microplastics. Environ Sci Technol. 2019;53:7068–74.
Barceló D, Picó Y, Alfarhan AH. Microplastics: detection in human samples, cell line studies, and health impacts. Environ Toxicol Pharmacol. 2023;101:104204.
Gautam R, Jo J, Acharya M, Maharjan A, Lee D, KC PB. et al. Evaluation of potential toxicity of polyethylene microplastics on human derived cell lines. Sci Total Environ. 2022;838:156089
Sorci G, Loiseau C. Should we worry about the accumulation of microplastics in human organs? EBioMedicine. 2022;82:104191.
Wang W, Ge J, Yu X. Bioavailability and toxicity of microplastics to fish species: A review. Ecotoxicol Environ Saf. 2020;189:109913.
Yong CQY, Valiyaveettil S, Tang BL. Toxicity of microplastics and nanoplastics in mammalian systems. Int J Environ Res Public Health. 2020;17:1509.
D’Angelo S, Meccariello R. Microplastics: a threat for male fertility. Int J Environ Res Public Health. 2021;18:2392.
Hou B, Wang F, Liu T, Wang Z. Reproductive toxicity of polystyrene microplastics: In vivo experimental study on testicular toxicity in mice. J Hazard Mater. 2021;405:124028.
Jaeger VK, Walker UA. Erectile dysfunction in systemic sclerosis. Curr Rheumatol Rep. 2016;18:49.
Jung J, Jo HW, Kwon H, Jeong NY. Clinical neuroanatomy and neurotransmitter-mediated regulation of penile erection. Int Neurourol J. 2014;18:58–62.
Sopko NA, Hannan JL, Bivalacqua TJ. Understanding and targeting the Rho kinase pathway in erectile dysfunction. Nat Rev Urol. 2014;11:622–8.
Sorkhi S, Sanchez CC, Cho MC, Cho SY, Chung H, Park MG, et al. Transpelvic magnetic stimulation enhances penile microvascular perfusion in a rat model: a novel interventional strategy to prevent penile fibrosis after cavernosal nerve injury. World J Mens Health. 2022;40:501–8.
Hildebrandt L, Zimmermann T, Primpke S, Fischer D, Gerdts G, Pröfrock D. Comparison and uncertainty evaluation of two centrifugal separators for microplastic sampling. J Hazard Mater. 2021;414:125482.
Morgado V, Palma C, Bettencourt da Silva RJN. Bottom-up evaluation of the uncertainty of the quantification of microplastics contamination in sediment samples. Environ Sci Technol. 2022;56:11080–90.
Hildebrandt L, El Gareb F, Zimmermann T, Klein O, Kerstan A, Emeis KC, et al. Spatial distribution of microplastics in the tropical Indian Ocean based on laser direct infrared imaging and microwave-assisted matrix digestion. Environ Pollut Barking Essex 1987. 2022;307:119547.
CAS Google Scholar
Hansen J, Hildebrandt L, Zimmermann T, El Gareb F, Fischer EK, Pröfrock D. Quantification and characterization of microplastics in surface water samples from the Northeast Atlantic Ocean using laser direct infrared imaging. Mar Pollut Bull. 2023;190:114880.
Rani M, Ducoli S, Depero LE, Prica M, Tubić A, Ademovic Z, et al. A complete guide to extraction methods of microplastics from complex environmental matrices. Molecules. 2023;28:5710.
Enders K, Lenz R, Beer S, Stedmon CA. Extraction of microplastic from biota: recommended acidic digestion destroys common plastic polymers. ICES J Mar Sci. 2017;74:326–31.
Article Google Scholar
Lopes C, Fernández-González V, Muniategui-Lorenzo S, Caetano M, Raimundo J. Improved methodology for microplastic extraction from gastrointestinal tracts of fat fish species. Mar Pollut Bull. 2022;181:113911.
Barboza LGA, Dick Vethaak A, Lavorante BRBO, Lundebye AK, Guilhermino L. Marine microplastic debris: an emerging issue for food security, food safety and human health. Mar Pollut Bull. 2018;133:336–48.
Wang S, Lu W, Cao Q, Tu C, Zhong C, Qiu L, et al. Microplastics in the lung tissues associated with blood test index. Toxics. 2023;11:759.
Ribeiro VV, Nobre CR, Moreno BB, Semensatto D, Sanz-Lazaro C, Moreira LB, et al. Oysters and mussels as equivalent sentinels of microplastics and natural particles in coastal environments. Sci Total Environ. 2023;874:162468.
Ourgaud M, Phuong NN, Papillon L, Panagiotopoulos C, Galgani F, Schmidt N, et al. Identification and quantification of microplastics in the marine environment using the laser direct infrared (LDIR) technique. Environ Sci Technol. 2022;56:9999–10009.
Zhao Q, Zhu L, Weng J, Jin Z, Cao Y, Jiang H, et al. Detection and characterization of microplastics in the human testis and semen. Sci Total Environ. 2023;877:162713.
Wu P, Lin S, Cao G, Wu J, Jin H, Wang C, et al. Absorption, distribution, metabolism, excretion and toxicity of microplastics in the human body and health implications. J Hazard Mater. 2022;437:129361.
Urbanek AK, Rymowicz W, Mirończuk AM. Degradation of plastics and plastic-degrading bacteria in cold marine habitats. Appl Microbiol Biotechnol. 2018;102:7669–78.
Jin Y, Qiu J, Zhang L, Zhu M. [Biodegradation of polyethylene terephthalate: a review]. Sheng Wu Gong Cheng Xue Bao Chin J Biotechnol. 2023;39:4445–62.
Çaykara T, Sande MG, Azoia N, Rodrigues LR, Silva CJ. Exploring the potential of polyethylene terephthalate in the design of antibacterial surfaces. Med Microbiol Immunol. 2020;209:363–72.
Sharifinia M, Bahmanbeigloo ZA, Keshavarzifard M, Khanjani MH, Lyons BP. Microplastic pollution as a grand challenge in marine research: A closer look at their adverse impacts on the immune and reproductive systems. Ecotoxicol Environ Saf. 2020;204:111109.
Potential toxicity of polystyrene microplastic particles. Scientific Reports. Available from: https://www.nature.com/articles/s41598-020-64464-9
Zhang C, Chen J, Ma S, Sun Z, Wang Z. Microplastics may be a significant cause of male infertility. Am J Mens Health. 2022;16:15579883221096549.
Compa M, Capó X, Alomar C, Deudero S, Sureda A. A meta-analysis of potential biomarkers associated with microplastic ingestion in marine fish. Environ Toxicol Pharmacol. 2024;107:104414.
Hildebrandt L, Nack FL, Zimmermann T, Pröfrock D. Microplastics as a Trojan horse for trace metals. J Hazard Mater Lett. 2021;2:100035.
Article CAS Google Scholar
Download references
Author information
Authors and affiliations.
Desai Sethi Urology Institute, Miller School of Medicine, University of Miami, Miami, FL, USA
Jason Codrington, Alexandra Aponte Varnum, Joginder Bidhan, Kajal Khodamoradi, Aymara Evans, David Velasquez, Christina C. Yarborough, Ashutosh Agarwal, Edoardo Pozzi, Francesco Mesquita, Francis Petrella, David Miller & Ranjith Ramasamy
Institute of Coastal Environmental Chemistry, Department for Inorganic Environmental Chemistry, Helmholtz-Zentrum Hereon, Max-Planck-Str 1, 21502, Geesthacht, Germany
Lars Hildebrandt & Daniel Pröfrock
Institute of Membrane Research, Helmholtz-Zentrum Hereon, Max-Planck-Str 1, 21502, Geesthacht, Germany
Anke-Lisa Höhme & Martin Held
Dr. J.T. MacDonald Foundation BioNIUM, Miller School of Medicine, University of Miami, Miami, FL, USA
Bahareh Ghane-Motlagh
Department of Biomedical Engineering, University of Miami, Miami, FL, USA
Ashutosh Agarwal
University of Colorado, Anschutz Medical Campus, Aurora, CO, USA
Justin Achua
Vita-Salute San Raffaele University, Milan, Italy
Edoardo Pozzi
IRCCS Ospedale San Raffaele, Urology, Milan, Italy
You can also search for this author in PubMed Google Scholar
Contributions
Jason Codrington—conceptualization, methodology, investigation, project administration, data curation, visualization, writing—original draft, editing. Alexandra Aponte Varnum—investigation, writing—original draft, editing, data curation, visualization. Lars Hildebrandt—investigation, writing—original draft, validation, resources. Daniel Pröfrock—investigation, editing, validation, resources. Joginder Bidhan—resources, writing—original draft. Kajal Khodamoradi—project administration, resources. Anke-Lisa Höhme—investigation, visualization. Martin Held—writing—original draft, editing. Aymara Evans—writing—original draft. David Velasquez—writing—original draft. Christina C. Yarborough—writing—original draft. Bahareh Ghane-Motlagh—investigation. Ashutosh Agarwal—investigation. Justin Achua—writing—original draft. Edoardo Pozzi—editing. Francesco Mesquita—editing. Francis Petrella—writing—review. David Miller—writing—review. Ranjith Ramasamy—conceptualization, methodology, project administration, resources, supervision, editing, funding acquisition
Corresponding author
Correspondence to Ranjith Ramasamy .
Ethics declarations
Competing interests.
Dr. Edoardo Pozzi is currently an Associate Editor for the International Journal of Impotence Research.
Ethics approval
The study was approved by the Institutional Review Board of the University of Miami (Study # 20150740) and conducted following the Declaration of Helsinki. All patients provided written and informed consent to participate in the study.
Additional information
Publisher’s note Springer Nature remains neutral with regard to jurisdictional claims in published maps and institutional affiliations.
Rights and permissions
Springer Nature or its licensor (e.g. a society or other partner) holds exclusive rights to this article under a publishing agreement with the author(s) or other rightsholder(s); author self-archiving of the accepted manuscript version of this article is solely governed by the terms of such publishing agreement and applicable law.
Reprints and permissions
About this article
Cite this article.
Codrington, J., Varnum, A.A., Hildebrandt, L. et al. Detection of microplastics in the human penis. Int J Impot Res (2024). https://doi.org/10.1038/s41443-024-00930-6
Download citation
Received : 21 March 2024
Revised : 29 May 2024
Accepted : 04 June 2024
Published : 19 June 2024
DOI : https://doi.org/10.1038/s41443-024-00930-6
Share this article
Anyone you share the following link with will be able to read this content:
Sorry, a shareable link is not currently available for this article.
Provided by the Springer Nature SharedIt content-sharing initiative
Quick links
- Explore articles by subject
- Guide to authors
- Editorial policies

- Open access
- Published: 24 June 2024
Effects of memory and attention on the association between video game addiction and cognitive/learning skills in children: mediational analysis
- Amani Ali Kappi 1 ,
- Rania Rabie El-Etreby 2 ,
- Ghada Gamal Badawy 3 ,
- Gawhara Ebrahem 3 &
- Warda El Shahat Hamed 2
BMC Psychology volume 12 , Article number: 364 ( 2024 ) Cite this article
144 Accesses
Metrics details
Video games have become a prevalent source of entertainment, especially among children. Furthermore, the amount of time spent playing video games has grown dramatically. The purpose of this research was to examine the mediation effects of attention and child memory on the relationship between video games addiction and cognitive and learning abilities in Egyptian children.
A cross-sectional research design was used in the current study in two schools affiliated with Dakahlia District, Egypt. The study included 169 children aged 9 to 13 who met the inclusion criteria, and their mothers provided the questionnaire responses. The data collection methods were performed over approximately four months from February to May. Data were collected using different tools: Socio-demographic Interview, Game Addiction Scale for Children (GASC), Children’s Memory Questionnaire (CMQ), Clinical Attention Problems Scale, Learning, Executive, and Attention Functioning (LEAF) Scale.
There was a significant indirect effect of video game addiction on cognitive and learning skills through attention, but not child memory. Video game addiction has a significant impact on children’s attention and memory. Both attention and memory have a significant impact on a child’s cognitive and learning skills.
Conclusions
These results revealed the significant effect of video game addiction on cognitive and learning abilities in the presence of mediators. It also suggested that attention-focused therapies might play an important role in minimizing the harmful effects of video game addiction on cognitive and learning abilities.
Peer Review reports
Introduction
The use of video games has increased significantly in recent years. Historically, such games are used more often by children. Despite the positive impacts of video games on socialization and enjoyment, empirical and clinical research has consistently demonstrated that many children can become addicted due to excessive use. Among Arab children and adolescents, the prevalence of video game addiction is 62% of 393 adolescents in Saudi Arabia, 5% in Jordan, 6% in Syria, and 7.8% in Kuwait [ 1 , 2 ]. The varying incidence rates can be attributable to variations in the research population, cultural determinants, and evaluation or diagnostic standards.
In addition, video games, the internet, and other new technologies have become children’s top leisure pursuits. Today, they comprise a virtual environment in which thousands of gamers simultaneously participate worldwide; rather than being a personal or lonely leisure activity, they are often a group activity that establishes new social networks [ 3 ]. Although playing video games in moderation can have many positive effects, their exploitation may lead to addictions and societal issues [ 4 ]. The Diagnostic and Statistical Manual of Mental Disorders, Fifth Edition (DSM-5), identifies repetitive and persistent behavior related to online video games as the core element of addiction. This behavior should persist for at least 12 months and result in significant impairment. Additionally, addiction should be accompanied by psychological and social symptoms, as well as tolerance and withdrawal symptoms [ 5 ].
Different studies have examined the impact of video games on children’s cognitive abilities and school performance [ 6 , 7 ]. The recent literature has shown how video games affect the brain and alter its functioning while being played. It demonstrates how specific cortical and subcortical structures are involved [ 8 , 9 , 10 ]. Research indicates that excessive play of the same typees of games might negatively impact school-age children’s cognitive and academic skills as well as their capacity to maintain and enhance memories [ 7 ]. Possible consequences of video game addiction may include memory and attention-related difficulties [ 4 , 6 , 11 ]. For instance, children’s memory scores negatively correlated with greater levels of video game addiction in Lebanon [ 6 ]. Furthermore, studies show that action-game players are more likely to succeed at short-term concentration tests while they perform below average in long-term, less exciting activities. At the point of game addiction, difficulties with focus are likely to become much more apparent [ 12 ]. Studies show a substantial association between gaming addiction and inattention, even after controlling other variables such as personality factors, anxiety and depression symptoms, and attention deficit hyperactivity disorder [ 13 , 14 ].
Prior studies have illustrated the association between video game addiction and psychiatric disorders, social phobia, mental well-being, and risky health behaviors [ 15 , 16 , 17 ]. Another study shows an association between video game addiction and memory, attention, cognitive, and learning abilities among Lebanese children [ 18 ]. However, all of these studies explain the association without controlling for any history of mental or behavioral disorders such as ADHD, anxiety, or depression. However, to the best of our knowledge, a few studies have specifically investigated the effect of attention and child memory on the relationship between video game addiction and cognitive and learning abilities in Egyptian children. Therefore, this study aimed to explore the mediation effect of attention and child memory on the association between addiction to video game and cognitive and learning abilities among Egyptian children. Our hypotheses were: (1) child attention mediates the relationship between video game addiction and cognitive and learning abilities among Egyptian children; and (2) child memory mediates the relationship between video game addiction and cognitive and learning abilities among Egyptian children.
Literature review
Video games have transformed into complex experiences that embody principles recognized by psychologists, neuroscientists, and educators as crucial for behavior, learning, and cognitive functions. While video games offer social and entertainment benefits, extensive research indicates that their excessive use can lead to adverse psychological consequences and even addiction in a minority of players. Symptoms like impaired control over gaming and prioritizing games over daily responsibilities may signify gaming addiction [ 19 ].
The Diagnostic and Statistical Manual of Mental Disorders (DSM-5) acknowledged video game addiction as an internet gaming disorder in its fifth edition, highlighting the need for further research [ 20 ]. Similarly, the 11th edition of the International Classification of Diseases (ICD-11) classified gaming disorder as a recurrent pattern of gaming behavior that encompasses both online and offline gaming [ 21 ]. Scientific evidence indicates that addictions can develop due to a combination of genetic susceptibility and repeated exposure to specific stimuli [ 22 ].
Growing public concerns have emerged regarding the potential negative impacts of video games, notably on children’s memory [ 23 ]. Individuals with various behavioral disorders and those with addictive tendencies often find their memory, crucial for comprehension and cognitive abilities like memory updating and working memory, compromised [ 24 ]. Although some research delves into video games’ effects on cognitive functions and academic achievement in children [ 25 , 26 ], the impact on memory remains a contentious topic.
Despite being a leisure activity, video gaming can pose issues for certain children, impacting their ability to focus. Meta-analysis and systematic reviews by Ho et al. and Carli et al. indicated a link between inattention and addiction to the internet and gaming [ 27 ]. Additionally, numerous studies corroborated this connection, demonstrating a robust correlation between the severity of inattention in ADHD and addiction to the internet or gaming. This correlation persisted even after controlling for factors such as depression and anxiety symptoms, as well as personality traits [ 27 ].
Study design and sample
This study has a cross-sectional descriptive design. It was conducted in two convienient selected preparatory schools, Emam Mohamed Abdo Preparatory and Omar Ibn Elkhatab Preparatory School. The two schools are affiliated with xxx. The participants were selected at random from the list of school principals. The research was open to all students between the ages of 9 and 13 with no history of physical, mental, or cognitive disorders. Each student’s parents provided the questionnaire responses. Using the G-power software 3.1.9.2, the study’s sample size was determined. Based on an average effect size of f = 0.15, a 2-sides test at alpha = 0.05, a statistical power (1-β) of 0.95, and eight predictors (age, gender, educational level of the child and mother, video game addiction, memory, attention, and learning abilities), power analysis was performed. A minimum of 166 participants were required based on these criteria.
Ethical consideration
The study approved by the Research Ethics Committee (REC) of Mansoura University’s Faculty of Nursing (IRB P0506/9/8/2023). The study’s purpose, methodology, duration, and benefits were also explained to the directors of the two selected institutions. Mothers’ consents obtained after explaining the study’s objective and the data kept confidential. The participants were informed that they had their right to withdraw from the study at any time.
Data Collection
The following tools were utilized in the study:
Socio-demographic questionnaire
Child and mother’s information was collected, such as age, sex, number of children, and level of education.
- Video game addiction
We used the Game Addiction Scale for Children (GASC) to measure children’s video game addiction. The GASC developed by Yılmaz, Griffiths [ 28 ] according to DSM criteria to evaluate gaming addiction. It includes 21 self-reported items rated on five-point Likert scale (from 1 = never to 5 = very frequently), where higher score shows more hazardous online gaming usage. An individual’s total score can range from a minimum of 21 to a maximum of 105; a score above 90 may be a sign of a video game addiction. It is also emphasized that this is not a diagnostic tool, however, but merely an indicator that a child may have a gaming addiction. Such a diagnosis could only be made by a comprehensive clinical evaluation. Seven criteria for video game addiction are determined by the scale: salience, tolerance, mood modification, withdrawal, relapse, conflict, and issues. The scale shows an acceptable internal consistency reliability ( r = 0.89, p < 0.001) [ 19 ].
Children’s memory
We used the Children’s Memory questionnaire (CMQ) to assess children’s memory rated by their parents. The CMQ developed by Drysdale, Shores [ 29 ]. It included 34 items that rated on a five-point Likert scale ranging from 1 = never or almost never, to 5 = more than once a day. Higher scores indicate a more significant reduction in the cognitive domain. The scale is divided into three subscales: working memory and attention, visual memory, and episodic memory. The Cronbach alpha value for the episodic memory subscale was 0.88, the visual memory is 0.77, and the working memory is 0.84 [ 29 ].
Attention of children
The Clinical Attention Problems Scale was used to measure children’s attention level in the morning and afternoon. This scale was developed by Edelbrock and Rancurello [ 30 ] and includes 12 items. The possible responses are 0 = not true, 1 = somewhat or sometimes true, and 2 = very often or often true. The higher the scores, the more attention there is. The Cronbach alpha values for the clinical attention problem in the morning is 0.84 and for the afternoon is 0.83.
Cognitive and learning skills
We used the Learning, Executive, and Attention Functioning (LEAF) scale to measure children’s cognitive and learning skills. The LEAF scale is a self-reported 55 items scale developed by Castellanos, Kronenberger [ 31 ]. The scale assesses core cognitive abilities and related academic and learning abilities. The LEAF assesses cognitive skills such as attention, processing speed, working memory, sustained sequential processing to accomplish goals (such as planning and carrying out goal-directed tasks), and new problem-solving. Moreover, the LEAF approach takes into account academic functioning, declarative/factual memory, and understanding and concept formulation.
The LEAF includes 55 items, with 11 academic subscales that rate a person’s reading, writing, and mathematics proficiency. The LEAF is divided into subscales that measure comprehension and conceptual learning, factual memory, attention, processing speed, visual-spatial organization, sustained sequential processing, working memory, new problem-solving, mathematics, basic reading, and written expression skills. Each subscale has the same number of items. The responses were rated on a three-point scale ranging from 0 to 3. Higher scores indicate more significant issues with cognition. The five component items are added to provide the subscale score for each of the 11 subject areas. Three criterion-referenced ranges are established for the interpretation of LEAF subscale raw scores. Out of nine, a score of five to nine is classified as the “borderline problem range,” a score of less than five as the “no problem range,” and a score of nine or above as the “problem range.” The Cronbach alpha value for the LEAF scale is 0.96.
Validity and reliability
Study tools were translated into Arabic by the researchers. Five pediatric nursing and psychiatric and mental health nursing experts tested them for content validity. At first, the scales were translated into Arabic using a forward and backward translation method. The translated questionnaires were then adapted to fit Arabic cultural norms. Two highly proficient native Arabic speakers who are accomplished academics in the fields of psychiatry and mental health nursing, and hold the academic status of Full Professor translated the questionnaire from English to Arabic. An English-language expert who is fluent in Arabic back translated the Arabic version. Native Arabic speakers who were not involved in the translation process verified the final translation. The forward-to-back translation process was repeated until the comparative findings matched exactly. The questionnaires were then given to three Arabic psychiatric nursing professionals, who provided their opinions on its importance, relevance, and simplicity. The tools’ reliability was tested using Cronbach’s alpha test (tool I α = 0.86, tool II α = 0.81, tool III α = 0.95, and tool IV α = 0.95, respectively). Additionally, a confirmatory factor analysis were carried out to validate the content of the four scales after translation. The data collection methods were performed over approximately four months from February to May. Also, a pilot study was conducted to assess the study tools’ feasibility and determine the time required to complete the tools. 10% of the initial participants were randomly selected from the same schools. Minimal modifications were then made to the tools. Mothers of students who participated in the pilot study were excluded from the primary study. The data was collected for four months (February to May). An online Google form was created to collect data. The link was then shared with selected student parents through WhatsApp groups. The link outlined the study’s purpose and methods, and participants signed a consent form.
Data collection procedure
We obtained permission to translate the study scales into Arabic. We collected data from February to May using an online Google Form for four months. The Google Form included full details regarding the study’s aims and processes to ensure transparency and establish participants’ trust. An extensive description of the response process additionally supports the Attention Problems Scale. For instance, mothers are required to respond to the items and their relevance to their children in the morning and afternoon. We distributed the survey link to the selected students’ mothers through WhatsApp groups as it was convenient and widespread among the target demographic. Before proceeding to the survey questions, participants were required to read and sign this consent form to ensure that participants received information about the study and voluntarily consented.
Statistical analysis
We employed the Statistical Package for Social Science version 26 [ 23 ] to analyze the data. We analyzed the demographic data using descriptive statistics such as means, standard deviations, frequency, and percentages. In order to evaluate the mediator effects of memory and attention on the relationship between cognitive, academic, and learning skills and video gaming addiction, we ran the multiple regression PROCESS macro with 5,000 bootstraps in SPSS version 3.4 [ 24 ]. We also included confounding variables, such as the age of the child, gender, the age of the mother, education, and job status, as covariates in the mediation model.
Sample characteristics
There were 169 children their mothers responded to the study surveys. The children’s mean age was 13 (SD = 3.9), while the mothers’ mean age was 41 (SD = 7.1). According to mothers, the children were ranked third in their household. Most mothers (72%) said they lived in rural areas. About 61% of the families had at least three children. Half of the mothers had high school or less education, and more than half were unemployed. Most children were in middle school (72%), see Table 1 .
Study variables description
The mean scores for all scales are presented in Table 2 . The mean score of the video gaming addiction total scale was 61 ± 19.3, indicating a moderate level of addiction. The attention total scale mean was 9 ± 6.50, indicating moderate attention problems. The mean score on the total scale for child memory was 80 ± 31,4, indicating moderate memory issues. Eight subscales of the LEAF had mean scores of 5: factual memory, processing speed, visual-spatial organization, sustained sequential processing, working memory, novel problem-solving, mathematics skills, and written expression skills. These mean scores indicate that a borderline problem exists. However, the mean scores for the comprehension and conceptual learning subscale, attention subscale, and basic reading skills subscale were below five, indicating that there was no problem.
Mediating effect of memory, attention problem on the association between video gaming addiction and cognitive, learning, and academic skills
Video game addiction had a significant impact on attention problems (b = 0.34, p < 0.001; a1), and child memory (b = 0.18, p < 0.001; a2). In turn, both attention problems (b = 0.48, p < 0.001; b1) and child memory (b = 0.38, p < 0.001; b2) had significant impact on cognitive and learning skills. The results reveal a significant indirect effect of video game addiction on cognitive and learning skills through attention problems (b = 0.17, CI: 0.82, 0.25; c ’ 1). However, there was no significant indirect effect of video game addiction on cognitive and learning skills through child memory (b = 0.07, CI: -0.01, 0.16; c ’ 2). The analysis revealed that confounding variables had no significant effect on the direct or indirect pathways linking video game addiction to cognitive and learning skills. The direct effect of video game addiction on cognitive and learning skills in the presence of the mediators was also found to be significant (b = 0.11, CI: 0.008, 0.401; c ’ -c). Figure 1 displays the mediation analysis findings.
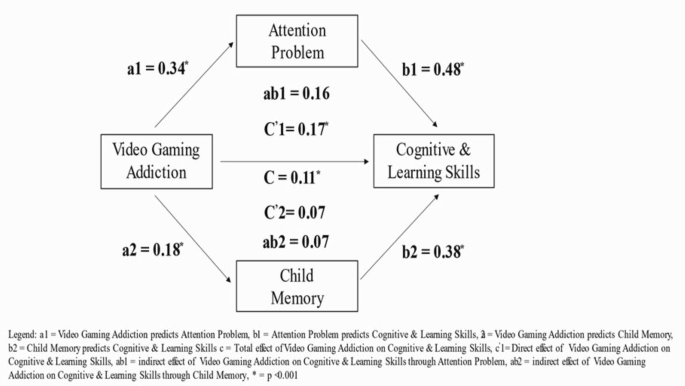
Mediation effect of attention problem and child memory on the association between video gaming addiction and cognitive and learning skills
Previous research has explored the relationship between video game addiction, attention, and memory. Some studies have focused on the relationship between video game addiction and cognitive and learning skills. Others have examined the association between video gaming addiction and all other variables (attention, memory, learning, and cognitive skills). However, no study has explicitly examined the direct and indirect effect of video gaming addiction on learning and cognitive skills through the mediation effect of attention and memory.
This study was done on a sample of Egyptian school children to evaluate the mediation effect of attention and memory on the relationship between video game addiction and cognitive and learning abilities in children. The present study reveals that a gaming addiction can significantly impact attention and memory. This result agrees with Farchakh, Haddad [ 6 ], who conducted a study on a group of Lebanese school children aged 9 to 13 to investigate the association between gaming addiction, attention, memory, cognitive, and learning skills. They found that a greater degree of addiction to video gaming was significantly associated with worse attention scores and worse memory scores. An earlier study suggests that the link between inattention and video game addiction could be described by game genres’ immediate response and reward system. Alrahili, Alreefi [ 2 ] suggest that this may alleviate the boredom typically reported by inattentive users while simultaneously introducing a lack of responsiveness to real-world rewards. Another study on Turkish schoolchildren aged 10 to 16 years old revealed that the total recall scores of the subject group (children who regularly play video games) are significantly lower than those of the control group (children who do not regularly play video games; [ 7 ]).
The current study demonstrates that attention and child memory significantly impacted cognitive and learning skills. This agrees with the opinion of, Gallen, Anguera [ 32 ], who argues that children and young people process information differently, affecting the performance of various cognitive tasks. Additionally, this result disagrees with the findings of Ellah, Achor, and Enemarie [ 26 ], who have stated that students’ working memory has no statistically significant correlation with learning and problem-solving skills. Moreover, their same study showed that different measures of working memory can be attributed to a small variation in low-ability students’ problem-solving skills.
The results revealed a significant indirect effect of video game addiction on cognitive and learning skills through attention. This could be related to the relationship between attention and learning skills. Attention is an essential factor in the learning process because it helps a person make efficient use of data by directing their learning to relevant components and relationships in the input material. If a student can pay attention, they may be able to better retain and understand this material; if not, a lack of attention may lead to difficulties in learning and academic performance. As video gaming addiction affects students’ attention, it may directly affect learning skills [ 33 ]. Another study agrees with the current result, revealing that video game addiction negatively affects adolescents’ learning skills and grade point average [ 34 ].
A child’s memory has an effect on their cognitive and learning skills. Encoding, consolidating, and retrieving experiences and information are the foundation for learning new skills and knowledge [ 35 ]. Video game addiction affects children’s memory. Hence, the expectation is that video game addiction directly affects cognitive and learning skills. However, the present study reveals no significant indirect effect of video game addiction on cognitive and learning skills through child memory. For example, perceptual attention to the exterior world and reflective attention to interior memories need modification of shared representational components in the occipitotemporal cortex. This is shown in episodic memory by recovering an experience from memory, which includes reactivating some of the same sensory areas used during encoding. Furthermore, the prefrontal cortex involves continuous and reflecting attention [ 36 ]. The prefrontal cortex controls memory recall by choosing target memories and filtering or suppressing competing memories [ 36 ].
Another aspect that may be responsible for the absence of a mediating effect of memory on the association between video game addiction and cognitive and learning skills is the presence of the many factors that affect learning and cognitive skills besides memory alone. Life circumstances can affect learning skills rather than memory itself, for example. Problem solving (one of the learning skills) requires a brain that works effectively. Therefore, it is critical to address needs such as physical health, which is influenced by self-care needs such as diet, sleep, and relaxation, as well as children’s social and emotional needs. Furthermore, learning experiences that use all the senses, rather than only hearing or seeing information, result in effective and straightforward information retrieval from memory during problem-solving processes. Such abilities are supposed to be acquired by active participation in learning activities by children [ 37 ]. Finally, long-term focus on online gaming may eventually lead to neglect in learning, leading to a deterioration in learning performance [ 38 ].
Limitations
Our study has some limitations. First, we administered the Clinical Attention Problems Scale only once per student rather than conducting repeated measurements in the morning and afternoon. This approach overlooks potential daytime variations in attention levels, limiting our understanding of each child’s attentional profile. This choice was driven by practical considerations such as reducing the testing burden and participant fatigue. Future research could address this limitation by implementing repeated assessments to comprehend better daytime patterns in children’s attention levels and their implications for learning and behavior. Causality analysis was not possible due to the use of a cross-sectional sample. In addition, some results may be attributable to the small sample size. To fully understand the complex interplay between video game addiction and cognitive outcomes, longitudinal studies and controlled experiments are necessary to provide more conclusive insights into the relationship. It was difficult to include both parents in the study, as most of the fathers said they were too busy to participate. Hence, mothers were the subjects of the study. Certain differences (or lack thereof) are probably artifacts of the sample size. As a result, our findings must be validated by analyzing larger samples. Despite these limitations, this work has the potential to provide insights and open new research avenues.
Implications
Healthcare professionals should be aware of how much children participate in these games and be willing to engage in in-depth conversations with parents about the impact these games may have on children’s health. Therefore, periodical workshops should be held by pediatric and community mental health nurses to enhance student awareness of the effects of video games on their memory, attention, and academic performance. In addition, teaching programs should be held at schools to improve students’ attention, memory, learning, and cognitive skills.
Video game addiction has a significant impact on children’s attention and memory. Both attention and memory have a significant impact on a child’s cognitive and learning skills. These results reveal a significant indirect effect of video game addiction on cognitive and learning skills through attention. However, video game addiction had no significant indirect effect on cognitive and learning skills through child memory. In the presence of the mediators, the direct impact of video game addiction on cognitive and learning skills was also significant.
Data availability
No datasets were generated or analysed during the current study.
Almutairi TA et al. Prevalence of internet gaming disorder and its association with psychiatric comorbidities among a sample of adults in three arab countries. Middle East Curr Psychiatry. 2023;30(1).
Alrahili N, et al. The prevalence of video game addiction and its relation to anxiety, depression, and attention deficit hyperactivity disorder (ADHD) in children and adolescents in Saudi Arabia: a cross-sectional study. Cureus. 2023;15(8):e42957–42957.
PubMed PubMed Central Google Scholar
Johannes N, Vuorre M, Przybylski AK. Video game play is positively correlated with well-being. Royal Soc open Sci. 2021;8(2):202049–202049.
Article Google Scholar
Esposito MR, et al. An investigation into video game addiction in pre-adolescents and adolescents: a cross-sectional study. Med (Kaunas Lithuania). 2020;56(5):221.
Google Scholar
American Psychiatric Association, D., &, Association AP. Diagnostic and statistical manual of mental disorders: DSM-5 (Vol. 5). American psychiatric association Washington. DC; 2013.
Farchakh Y, et al. Video gaming addiction and its association with memory, attention and learning skills in Lebanese children. Child Adolesc Psychiatry Mental Health. 2020;14(1):46–46.
Özçetin M, et al. The relationships between video game experience and cognitive abilities in adolescents. Neuropsychiatr Dis Treat. 2019;15:1171–80.
Article PubMed PubMed Central Google Scholar
Kwak KH, et al. Comparison of behavioral changes and brain activity between adolescents with internet gaming disorder and student pro-gamers. Int J Environ Res Public Health. 2020;17(2):441.
Lee D, et al. Gray Matter differences in the anterior cingulate and orbitofrontal cortex of young adults with internet gaming disorder: surface-based morphometry. J Behav Addictions. 2018;7(1):21–30.
Mondéjar T, et al. Analyzing EEG waves to support the design of serious games for cognitive training. J Ambient Intell Humaniz Comput. 2019;10:2161–74.
Bediou B, et al. Meta-analysis of action video game impact on perceptual, attentional, and cognitive skills. Psychol Bull. 2018;144(1):77–110.
Article PubMed Google Scholar
García-Redondo P, et al. Serious games and their effect improving attention in students with Learning Disabilities. Int J Environ Res Public Health. 2019;16(14):2480.
Evren C, et al. Relationships of Internet addiction and internet gaming disorder symptom severities with probable attention deficit/hyperactivity disorder, aggression and negative affect among university students. ADHD Atten Deficit Hyperactivity Disorders. 2019;11(4):413–21.
Stavropoulos V, et al. Associations between attention deficit hyperactivity and internet gaming disorder symptoms: is there consistency across types of symptoms, gender and countries? Addict Behav Rep. 2019;9:100158–100158.
Ahmed GK, et al. Relation between internet gaming addiction and comorbid psychiatric disorders and emotion avoidance among adolescents: a cross-sectional study. Psychiatry Res. 2022;312:114584.
Raouf SYA, et al. Video game disorder and mental wellbeing among university students: a cross-sectional study. Pan Afr Med J. 2022;41:89–89.
Purwaningsih E, Nurmala I. The impact of online game addiction on adolescent mental health: a systematic review and meta-analysis. Open Access Macedonian J Med Sci (OAMJMS). 2021;9(F):260–74.
Farchakh Y, et al. Video gaming addiction and its association with memory, attention and learning skills in Lebanese children. Child Adolesc Psychiatry Mental Health. 2020;14(1):1–11.
Nogueira M, et al. Addictive video game use: an emerging pediatric problem? Acta Med Port. 2019;32(3):183–8.
Christakis DA. The challenges of defining and studying digital addiction in children. JAMA. 2019;321(23):2277–8.
Kök Eren H, Örsal Ö. Computer game addiction and loneliness in children. Iran J Public Health. 2018;47(10):1504–10.
Choi BY, et al. Transitions in problematic internet use: a one-year longitudinal study of boys. Psychiatry Investig. 2019;16(6):433–42.
Du X, et al. Compensatory increase of functional connectivity density in adolescents with internet gaming disorder. Brain Imaging Behav. 2017;11(6):1901–9.
Lim JA, et al. Changes of quality of life and cognitive function in individuals with internet gaming disorder: a 6-month follow-up. Med (Baltim). 2016;95(50):e5695.
Teng Z, et al. A longitudinal study of link between exposure to violent video games and aggression in Chinese adolescents: the mediating role of moral disengagement. Dev Psychol. 2019;55(1):184–95.
van den Eijnden R, et al. The impact of heavy and disordered use of games and social media on adolescents’ psychological, social, and school functioning. J Behav Addict. 2018;7(3):697–706.
Evren C, et al. Relationships of Internet addiction and internet gaming disorder symptom severities with probable attention deficit/hyperactivity disorder, aggression and negative affect among university students. Atten Defic Hyperact Disord. 2019;11(4):413–21.
Yılmaz E, Griffiths MD, Kan A. Development and validation of videogame addiction scale for children (VASC). Int J Mental Health Addict. 2017;15(4):869–82.
Drysdale K, Shores A, Levick W. Use of the everyday memory questionnaire with children. Child Neuropsychol. 2004;10(2):67–75.
Edelbrock C, Rancurello MD. Childhood hyperactivity: an overview of rating scales and their applications. Clin Psychol Rev. 1985;5(5):429–45.
Castellanos I, Kronenberger WG, Pisoni DB. Questionnaire-based assessment of executive functioning: psychometrics applied neuropsychology. Child. 2018;7(2):93–109.
Gallen CL, et al. Enhancing neural markers of attention in children with ADHD using a digital therapeutic. PLoS ONE. 2021;16(12):e0261981–0261981.
Lindsay GW. Attention in psychology, neuroscience, and machine learning. Front Comput Neurosci. 2020;14:29–29.
van den Eijnden R, et al. The impact of heavy and disordered use of games and social media on adolescents’ psychological, social, and school functioning. J Behav Addictions. 2018;7(3):697–706.
Scerif G, et al. Making the executive ‘function’for the foundations of mathematics: the need for explicit theories of change for early interventions. Educational Psychol Rev. 2023;35(4):110.
Miller EK, Lundqvist M, Bastos AM. Working memory 2.0. Neuron. 2018;100(2):463–75.
Aydoğan Y, Özyürek A. The relationship between problem-solving skills and memory development in preschool children. J History Cult Art Res. 2020;9(3):43.
Jin Y, et al. Social Factors Associated with Video Game Addiction among teenagers: School, Family and Peers, in advances in Social Science, Education and Humanities Research. Atlantis; 2021.
Download references
Acknowledgements
The authors extend their heartfelt appreciation and gratitude to all parents who willingly participated in the study.
The authors gratefully acknowledge the funding of the Deanship of Graduate Studies and Scientific Research, Jazan University, Saudi Arabia, through Project Number: GSSRD-24.
Author information
Authors and affiliations.
Department of Nursing, College of Nursing, Jazan University, Jazan, Kingdom of Saudi Arabia
Amani Ali Kappi
Psychiatric and Mental Health Nursing Department, College of Nursing, Mansoura University, Mansoura, Egypt
Rania Rabie El-Etreby & Warda El Shahat Hamed
Pediatric Nursing Department, College of Nursing, Mansoura University, Mansoura, Egypt
Ghada Gamal Badawy & Gawhara Ebrahem
You can also search for this author in PubMed Google Scholar
Contributions
Amany Ali Kappi contributed to the project by designing the methodology, performing formal analysis, analyzing the data, and writing both the original draft and the manuscript. Rania Rabie El-Etreby contributed to conceptualizing, methodology, conducting, drafting, reviewing, and editing the manuscript. Ghada Gamal Badawy, was responsible for designing, executing, and documenting the investigation, including methodology, and manuscript preparation. Gawhara Ebrahem was responsible for designing, executing, and documenting the investigation, including methodology, and manuscript preparation Warda El Shahat Hamed conceptualized and prepared the methodology and investigation and contributed to writing the original draft. She also reviewed and edited the document. All authors read and approved the final manuscript.
Corresponding author
Correspondence to Warda El Shahat Hamed .
Ethics declarations
Ethics approval and consent to participate.
The researchers obtained approval for this study and data collection in this study from the Research Ethical Committee (REC) of Mansoura University’s Faculty of Nursing (IRB P0506/9/8/2023). All procedures were conducted in accordance with ethical standards outlined by the responsible committee on human experimentation and the Helsinki Declaration of 2008. Consent forms were obtained from all participants. Informed consent was obtained from all the participants in this study (from the mothers of the participant children).
Consent for publication
Not applicable.
Patient or public contributions
No patient or public contributions.
Competing interests
The authors declare no competing interests.
Conflict of interest
The authors declare no conflict of interest.
Additional information
Publisher’s note.
Springer Nature remains neutral with regard to jurisdictional claims in published maps and institutional affiliations.
Rights and permissions
Open Access This article is licensed under a Creative Commons Attribution 4.0 International License, which permits use, sharing, adaptation, distribution and reproduction in any medium or format, as long as you give appropriate credit to the original author(s) and the source, provide a link to the Creative Commons licence, and indicate if changes were made. The images or other third party material in this article are included in the article’s Creative Commons licence, unless indicated otherwise in a credit line to the material. If material is not included in the article’s Creative Commons licence and your intended use is not permitted by statutory regulation or exceeds the permitted use, you will need to obtain permission directly from the copyright holder. To view a copy of this licence, visit http://creativecommons.org/licenses/by/4.0/ . The Creative Commons Public Domain Dedication waiver ( http://creativecommons.org/publicdomain/zero/1.0/ ) applies to the data made available in this article, unless otherwise stated in a credit line to the data.
Reprints and permissions
About this article
Cite this article.
Kappi, A.A., El-Etreby, R.R., Badawy, G.G. et al. Effects of memory and attention on the association between video game addiction and cognitive/learning skills in children: mediational analysis. BMC Psychol 12 , 364 (2024). https://doi.org/10.1186/s40359-024-01849-9
Download citation
Received : 16 April 2024
Accepted : 10 June 2024
Published : 24 June 2024
DOI : https://doi.org/10.1186/s40359-024-01849-9
Share this article
Anyone you share the following link with will be able to read this content:
Sorry, a shareable link is not currently available for this article.
Provided by the Springer Nature SharedIt content-sharing initiative
- Child memory
- Learning skills
BMC Psychology
ISSN: 2050-7283
- General enquiries: [email protected]
Have a language expert improve your writing
Run a free plagiarism check in 10 minutes, generate accurate citations for free.
- Knowledge Base
Methodology
- How to Do Thematic Analysis | Step-by-Step Guide & Examples
How to Do Thematic Analysis | Step-by-Step Guide & Examples
Published on September 6, 2019 by Jack Caulfield . Revised on June 22, 2023.
Thematic analysis is a method of analyzing qualitative data . It is usually applied to a set of texts, such as an interview or transcripts . The researcher closely examines the data to identify common themes – topics, ideas and patterns of meaning that come up repeatedly.
There are various approaches to conducting thematic analysis, but the most common form follows a six-step process: familiarization, coding, generating themes, reviewing themes, defining and naming themes, and writing up. Following this process can also help you avoid confirmation bias when formulating your analysis.
This process was originally developed for psychology research by Virginia Braun and Victoria Clarke . However, thematic analysis is a flexible method that can be adapted to many different kinds of research.
Table of contents
When to use thematic analysis, different approaches to thematic analysis, step 1: familiarization, step 2: coding, step 3: generating themes, step 4: reviewing themes, step 5: defining and naming themes, step 6: writing up, other interesting articles.
Thematic analysis is a good approach to research where you’re trying to find out something about people’s views, opinions, knowledge, experiences or values from a set of qualitative data – for example, interview transcripts , social media profiles, or survey responses .
Some types of research questions you might use thematic analysis to answer:
- How do patients perceive doctors in a hospital setting?
- What are young women’s experiences on dating sites?
- What are non-experts’ ideas and opinions about climate change?
- How is gender constructed in high school history teaching?
To answer any of these questions, you would collect data from a group of relevant participants and then analyze it. Thematic analysis allows you a lot of flexibility in interpreting the data, and allows you to approach large data sets more easily by sorting them into broad themes.
However, it also involves the risk of missing nuances in the data. Thematic analysis is often quite subjective and relies on the researcher’s judgement, so you have to reflect carefully on your own choices and interpretations.
Pay close attention to the data to ensure that you’re not picking up on things that are not there – or obscuring things that are.
Here's why students love Scribbr's proofreading services
Discover proofreading & editing
Once you’ve decided to use thematic analysis, there are different approaches to consider.
There’s the distinction between inductive and deductive approaches:
- An inductive approach involves allowing the data to determine your themes.
- A deductive approach involves coming to the data with some preconceived themes you expect to find reflected there, based on theory or existing knowledge.
Ask yourself: Does my theoretical framework give me a strong idea of what kind of themes I expect to find in the data (deductive), or am I planning to develop my own framework based on what I find (inductive)?
There’s also the distinction between a semantic and a latent approach:
- A semantic approach involves analyzing the explicit content of the data.
- A latent approach involves reading into the subtext and assumptions underlying the data.
Ask yourself: Am I interested in people’s stated opinions (semantic) or in what their statements reveal about their assumptions and social context (latent)?
After you’ve decided thematic analysis is the right method for analyzing your data, and you’ve thought about the approach you’re going to take, you can follow the six steps developed by Braun and Clarke .
The first step is to get to know our data. It’s important to get a thorough overview of all the data we collected before we start analyzing individual items.
This might involve transcribing audio , reading through the text and taking initial notes, and generally looking through the data to get familiar with it.
Next up, we need to code the data. Coding means highlighting sections of our text – usually phrases or sentences – and coming up with shorthand labels or “codes” to describe their content.
Let’s take a short example text. Say we’re researching perceptions of climate change among conservative voters aged 50 and up, and we have collected data through a series of interviews. An extract from one interview looks like this:
Interview extract | Codes |
---|---|
Personally, I’m not sure. I think the climate is changing, sure, but I don’t know why or how. People say you should trust the experts, but who’s to say they don’t have their own reasons for pushing this narrative? I’m not saying they’re wrong, I’m just saying there’s reasons not to 100% trust them. The facts keep changing – it used to be called global warming. |
In this extract, we’ve highlighted various phrases in different colors corresponding to different codes. Each code describes the idea or feeling expressed in that part of the text.
At this stage, we want to be thorough: we go through the transcript of every interview and highlight everything that jumps out as relevant or potentially interesting. As well as highlighting all the phrases and sentences that match these codes, we can keep adding new codes as we go through the text.
After we’ve been through the text, we collate together all the data into groups identified by code. These codes allow us to gain a a condensed overview of the main points and common meanings that recur throughout the data.
Next, we look over the codes we’ve created, identify patterns among them, and start coming up with themes.
Themes are generally broader than codes. Most of the time, you’ll combine several codes into a single theme. In our example, we might start combining codes into themes like this:
Codes | Theme |
---|---|
Uncertainty | |
Distrust of experts | |
Misinformation |
At this stage, we might decide that some of our codes are too vague or not relevant enough (for example, because they don’t appear very often in the data), so they can be discarded.
Other codes might become themes in their own right. In our example, we decided that the code “uncertainty” made sense as a theme, with some other codes incorporated into it.
Again, what we decide will vary according to what we’re trying to find out. We want to create potential themes that tell us something helpful about the data for our purposes.
Now we have to make sure that our themes are useful and accurate representations of the data. Here, we return to the data set and compare our themes against it. Are we missing anything? Are these themes really present in the data? What can we change to make our themes work better?
If we encounter problems with our themes, we might split them up, combine them, discard them or create new ones: whatever makes them more useful and accurate.
For example, we might decide upon looking through the data that “changing terminology” fits better under the “uncertainty” theme than under “distrust of experts,” since the data labelled with this code involves confusion, not necessarily distrust.
Now that you have a final list of themes, it’s time to name and define each of them.
Defining themes involves formulating exactly what we mean by each theme and figuring out how it helps us understand the data.
Naming themes involves coming up with a succinct and easily understandable name for each theme.
For example, we might look at “distrust of experts” and determine exactly who we mean by “experts” in this theme. We might decide that a better name for the theme is “distrust of authority” or “conspiracy thinking”.
Finally, we’ll write up our analysis of the data. Like all academic texts, writing up a thematic analysis requires an introduction to establish our research question, aims and approach.
We should also include a methodology section, describing how we collected the data (e.g. through semi-structured interviews or open-ended survey questions ) and explaining how we conducted the thematic analysis itself.
The results or findings section usually addresses each theme in turn. We describe how often the themes come up and what they mean, including examples from the data as evidence. Finally, our conclusion explains the main takeaways and shows how the analysis has answered our research question.
In our example, we might argue that conspiracy thinking about climate change is widespread among older conservative voters, point out the uncertainty with which many voters view the issue, and discuss the role of misinformation in respondents’ perceptions.
If you want to know more about statistics , methodology , or research bias , make sure to check out some of our other articles with explanations and examples.
- Normal distribution
- Measures of central tendency
- Chi square tests
- Confidence interval
- Quartiles & Quantiles
- Cluster sampling
- Stratified sampling
- Discourse analysis
- Cohort study
- Peer review
- Ethnography
Research bias
- Implicit bias
- Cognitive bias
- Conformity bias
- Hawthorne effect
- Availability heuristic
- Attrition bias
- Social desirability bias
Cite this Scribbr article
If you want to cite this source, you can copy and paste the citation or click the “Cite this Scribbr article” button to automatically add the citation to our free Citation Generator.
Caulfield, J. (2023, June 22). How to Do Thematic Analysis | Step-by-Step Guide & Examples. Scribbr. Retrieved June 28, 2024, from https://www.scribbr.com/methodology/thematic-analysis/
Is this article helpful?
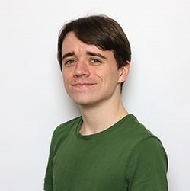
Jack Caulfield
Other students also liked, what is qualitative research | methods & examples, inductive vs. deductive research approach | steps & examples, critical discourse analysis | definition, guide & examples, get unlimited documents corrected.
✔ Free APA citation check included ✔ Unlimited document corrections ✔ Specialized in correcting academic texts

An official website of the United States government
Here's how you know
Official websites use .gov A .gov website belongs to an official government organization in the United States.
Secure .gov websites use HTTPS A lock ( Lock A locked padlock ) or https:// means you’ve safely connected to the .gov website. Share sensitive information only on official, secure websites.
- Quarterly Roundup
- Science Findings
- Scientific Journals
- Station Reports
- Digital Subscriptions
- Order Publications
- Data and Tools
2024 Urban Inventory of Portland, Oregon and San Diego, California
Published: | April 1, 2024 |
Type: | Guide |
This document describes the standards, codes, methods, and definitions for Forest Inventory and Analysis (FIA) field data items. The objective is to describe URBAN FIA field procedures that are consistent and uniform across all FIA units. This URBAN FIA guide will serve as the framework for regional FIA programs; individual programs may add regional variables that support their CORE program, but may not change or add to the nationally standard URBAN FIA requirements. Unless otherwise noted, the items in this field guide are considered standard URBAN FIA, that is, the information will be collected by all FIA units as specified. Items or codes specified as URBAN OPTIONAL are not required by individual units; however, if the item is collected or coded, it will be done as specified in this field guide. It is expected that on average all items in this guide can be measured by a one or two-person field crew in less than one day, including travel time to and from the plot. URBAN FIA plots will be referred to as Urban plots throughout the field procedures. URBAN FIA variables that are optional are referred to as URBAN OPTIONAL.
Log in using your username and password
- Search More Search for this keyword Advanced search
- Latest content
- Current issue
- Browse by collection
- BMJ Journals
You are here
- Online First
- Intracoronary thrombolysis in ST-elevation myocardial infarction: a systematic review and meta-analysis
- Article Text
- Article info
- Citation Tools
- Rapid Responses
- Article metrics

- http://orcid.org/0000-0002-3678-2855 Rajan Rehan 1 , 2 ,
- http://orcid.org/0000-0002-7235-3919 Sohaib Virk 3 ,
- http://orcid.org/0000-0002-0180-0072 Christopher C Y Wong 4 , 5 ,
- Freda Passam 6 ,
- Jamie Layland 7 ,
- Anthony Keech 8 ,
- Andy Yong 4 ,
- http://orcid.org/0000-0001-7712-6750 Harvey D White 9 ,
- William Fearon 10 ,
- Martin Ng 1 , 11
- 1 Royal Prince Alfred Hospital , Camperdown , New South Wales , Australia
- 2 Concord Hospital , Concord , New South Wales , Australia
- 3 Systematic Reviews , CORE Group , Sydney , New South Wales , Australia
- 4 Cardiology , Concord Repatriation General Hospital , Concord , New South Wales , Australia
- 5 Stanford Hospital , Stanford , California , USA
- 6 Department of Hematology , University of Sydney , Sydney , New South Wales , Australia
- 7 Monash University , Melbourne , Victoria , Australia
- 8 NHMRC Clinical Trials Centre , The University of Sydney , Sydney , New South Wales , Australia
- 9 Cardiology Department , Green Lane Cardiovascular Service and Green Lane Cardiovascular Research Unit, Auckland City Hospital , Auckland , New Zealand
- 10 Stanford University , Stanford , California , USA
- 11 Department of Cardiology , The University of Sydney , Sydney , New South Wales , Australia
- Correspondence to Professor Martin Ng, Department of Cardiology, The University of Sydney, Sydney, New South Wales, Australia; Martin.ng{at}sydney.edu.au
Background Despite restoration of epicardial blood flow in acute ST-elevation myocardial infarction (STEMI), inadequate microcirculatory perfusion is common and portends a poor prognosis. Intracoronary (IC) thrombolytic therapy can reduce microvascular thrombotic burden; however, contemporary studies have produced conflicting outcomes.
Objectives This meta-analysis aims to evaluate the efficacy and safety of adjunctive IC thrombolytic therapy at the time of primary percutaneous coronary intervention (PCI) among patients with STEMI.
Methods Comprehensive literature search of six electronic databases identified relevant randomised controlled trials. The primary outcome was major adverse cardiac events (MACE). The pooled risk ratio (RR) and weighted mean difference (WMD) with a 95% CI were calculated.
Results 12 studies with 1915 patients were included. IC thrombolysis was associated with a significantly lower incidence of MACE (RR=0.65, 95% CI 0.51 to 0.82, I 2 =0%, p<0.0004) and improved left ventricular ejection fraction (WMD=1.87; 95% CI 1.07 to 2.67; I 2 =25%; p<0.0001). Subgroup analysis demonstrated a significant reduction in MACE for trials using non-fibrin (RR=0.39, 95% CI 0.20 to 0.78, I 2 =0%, p=0.007) and moderately fibrin-specific thrombolytic agents (RR=0.62, 95% CI 0.47 to 0.83, I 2 =0%, p=0.001). No significant reduction was observed in studies using highly fibrin-specific thrombolytic agents (RR=1.10, 95% CI 0.62 to 1.96, I 2 =0%, p=0.75). Furthermore, there were no significant differences in mortality (RR=0.91; 95% CI 0.48 to 1.71; I 2 =0%; p=0.77) or bleeding events (major bleeding, RR=1.24; 95% CI 0.47 to 3.28; I 2 =0%; p=0.67; minor bleeding, RR=1.47; 95% CI 0.90 to 2.40; I 2 =0%; p=0.12).
Conclusion Adjunctive IC thrombolysis at the time of primary PCI in patients with STEMI improves clinical and myocardial perfusion parameters without an increased rate of bleeding. Further research is needed to optimise the selection of thrombolytic agents and treatment protocols.
- Acute Coronary Syndrome
- Myocardial Infarction
- Meta-Analysis
- Atherosclerosis
Data availability statement
All data relevant to the study are included in the article or uploaded as supplemental information.
This is an open access article distributed in accordance with the Creative Commons Attribution Non Commercial (CC BY-NC 4.0) license, which permits others to distribute, remix, adapt, build upon this work non-commercially, and license their derivative works on different terms, provided the original work is properly cited, appropriate credit is given, any changes made indicated, and the use is non-commercial. See: http://creativecommons.org/licenses/by-nc/4.0/ .
https://doi.org/10.1136/heartjnl-2024-324078
Statistics from Altmetric.com
Request permissions.
If you wish to reuse any or all of this article please use the link below which will take you to the Copyright Clearance Center’s RightsLink service. You will be able to get a quick price and instant permission to reuse the content in many different ways.
WHAT IS ALREADY KNOWN ON THIS TOPIC
ST-elevation myocardial infarction (STEMI) is a significant cause of morbidity and mortality worldwide. Microvascular obstruction affects about half of patients with STEMI, leading to adverse outcomes. Previous studies on adjunctive intracoronary thrombolysis have shown inconsistent results.
WHAT THIS STUDY ADDS
This meta-analysis demonstrates that adjunctive intracoronary thrombolysis during primary percutaneous coronary intervention (PCI) significantly reduces major adverse cardiac events and improves left ventricular ejection fraction. Furthermore, it significantly improves myocardial perfusion parameters without increasing bleeding risk.
HOW THIS STUDY MIGHT AFFECT RESEARCH, PRACTICE OR POLICY
Adjunctive intracoronary thrombolysis in patients with STEMI undergoing primary PCI shows promise for clinical benefit. Future studies should identify high-risk patients for microcirculatory dysfunction to optimise treatment strategies and clinical outcomes.
Introduction
Ischaemic heart disease remains a leading cause of morbidity and mortality worldwide. 1 2 ST-elevation myocardial infarction (STEMI) occurs due to coronary vessel occlusion causing transmural myocardial ischaemia and subsequent necrosis. 3 The cornerstone of contemporary management involves prompt reopening of the occluded coronary artery with percutaneous coronary intervention (PCI). 4 5 Despite restoring epicardial blood flow, roughly 50% of patients fail to achieve adequate microvascular perfusion. 6 This phenomenon, known as microvascular obstruction (MVO), is predictive of a poor cardiac prognosis driven by left ventricular remodelling and larger infarct size. 7–9
In patients with STEMI, MVO is characterised by distal embolisation of atherothrombotic debris and fibrin-rich microvascular thrombi. 10 A growing body of evidence supports the efficacy of adjunctive low-dose intracoronary (IC) thrombolysis in this population. Sezer et al performed the first randomised controlled trial (RCT), demonstrating an improvement in myocardial perfusion with low-dose IC streptokinase post-PCI. 11 Subsequent studies focused on newer fibrin-specific agents with a lower propensity for systemic bleeding. 12 Despite encouraging results, many studies were inadequately powered and yielded conflicting outcomes. This meta-analysis aims to evaluate the efficacy and safety of adjunctive IC thrombolytic therapy at the time of primary PCI in patients with STEMI.
The present study was conducted in accordance with the Preferred Reporting Items for Systematic Reviews and Meta-Analyses (PRISMA) statement. 13
Search strategy and study selection
Electronic searches were performed using PubMed, Ovid Medline, Cochrane Library, ProQuest, ACP Journal Club and Google Scholar from their dates of inception to January 2022. The search terms “STEMI” AND “intracoronary” AND (“thrombolysis” OR “tenecteplase” OR “alteplase” OR “prourokinase” OR “urokinase” OR “streptokinase”) were combined as both keywords and Medical Subject Headings terms, with filters for RCTs. This was supplemented by hand searching the bibliographies of review articles and all potentially relevant studies.
Two reviewers (RR and SV) independently screened the title and abstracts of articles identified in the search. Full-text publications were subsequently reviewed separately if either reviewer considered the manuscript as being potentially eligible. Any disagreements regarding final study inclusion were resolved by discussion and consensus with a third reviewer (CCYW).
Eligibility criteria
Studies were included if they met following inclusion criteria: (1) RCT, (2) STEMI population, (3) IC thrombolysis given to treatment group with comparison with a control group (CG) receiving no thrombolytic therapy, (4) major adverse cardiovascular event (MACE) was an outcome reported.
All publications were limited to those involving human subjects and no restrictions were based on language. Reviews, meta-analyses, abstracts, case reports, conference presentations, editorials and expert opinions were excluded. When institutions published duplicate studies with accumulating numbers of patients or increased lengths of follow-up, only the most complete reports were included for assessment.
Data extraction and quality assessment
Two investigators (RR and SV) independently extracted data from text, tables and figures. Any discrepancies were resolved by discussion and consensus with a third reviewer (CCYW). For each of the included trials, the following data were extracted: publication year, number of patients, baseline characteristics of participants, treatment details (including specific agents administered), follow-up duration and endpoints.
Study quality and risk of bias were critically appraised using the updated Cochrane Collaboration Risk-of-Bias Tool V.2. 14 Five domains of bias were evaluated: (1) randomisation process, (2) deviations from study protocol, (3) missing outcome data, (4) outcome measurement and (5) selective reporting of results.
The predetermined primary endpoint was MACE, which represented a composite outcome as defined by each individual study. While the individual components of MACE were generally consistent across studies, minor discrepancies existed ( online supplemental table 1 ). Secondary outcomes included clinical endpoints (mortality, heart failure (HF), major and minor bleeding), myocardial perfusion endpoints (thrombolysis in myocardial infarction (TIMI) flow grade 3, TIMI myocardial perfusion grade (TMPG), corrected TIMI frame count (CTFC), ST-resolution (STR)) and echocardiographic parameters (left ventricular ejection fraction (LVEF)). Subgroup analysis for MACE was conducted based on fibrin specificity of the thrombolytic agent. This classification comprised non-fibrin-specific agents (streptokinase and urokinase), moderately fibrin-specific agents (prourokinase) and highly fibrin-specific agents (alteplase and tenectaplase). Clinical outcomes were assessed at the end of the follow-up period, which ranged from 1 to 12 months, while echocardiographic parameters were evaluated within a time frame of 1–6 months.
Supplemental material
Statistical analysis.
The mean difference (MD) or relative risk (RR) was used as summary statistics and reported with 95% CIs. Meta-analyses were performed using random-effects models to take into account the anticipated clinical and methodological diversity between studies. The I 2 statistic was used to estimate the percentage of total variation across studies due to heterogeneity rather than chance, with values exceeding 50% indicative of considerable heterogeneity. For meta-analysis of continuous data, values presented as median and IQR were converted to mean and SD using the quantile method previously described by Wan et al . 15 For subgroup analyses, a standard test of heterogeneity was used to assess for significant difference between subgroups with p<0.05 considered statistically significant.
Meta-regression analyses were performed to explore potential heterogeneity with the following moderator variables individually assessed for significance: publication year, mean age, proportion of male participants, percentage of left anterior descending artery infarcts, proportion of smokers, as well as baseline prevalence of diabetes, hypertension and dyslipidaemia.
Publication bias was assessed for the primary endpoint of MACE using funnel plots comparing log of point estimates with their SE. Egger’s linear regression method and Begg’s rank correlation test were used to detect funnel plot asymmetry. 16 17 Statistical analysis was conducted with Review Manager V.5.3.5 (Cochrane Collaboration, Oxford, UK) and Comprehensive Meta-Analysis V.3.0 (Biostat, Englewood, New Jersey, USA). All p values were two sided, and values <0.05 were considered statistically significant.
A total of 245 unique records were identified through electronic searches using six online databases, from which 85 duplicates were removed. Of these, 120 were excluded based on title and abstract alone. After screening the full text of the remaining 40 articles, 12 studies 18–29 were found to meet the inclusion criteria, as summarised on the PRISMA flow chart in figure 1 .
- Download figure
- Open in new tab
- Download powerpoint
Preferred Reporting Items for Systematic Reviews and Meta-Analyses flow chart of literature search and study selection.
IC thrombolysis was examined in 12 studies (n=1030 received IC thrombolysis and 885 no IC thrombolysis). Included studies used non-fibrin-specific (streptokinase, urokinase), moderately fibrin-specific (prourokinase) and highly fibrin-specific thrombolytic (alteplase, tenecteplase) agents. The timing and delivery of IC thrombolytic therapy varied between studies. A complete summary of study characteristics and baseline participant characteristics is presented in tables 1 and 2 , respectively. Primary and secondary outcomes are summarised in online supplemental table 2 . According to the revised Cochrane tool, the overall risk of bias assessment for procedural measures was judged to be ‘low risk’ in two studies, ‘some concerns’ in eight studies and ‘high risk’ in two studies ( online supplemental figure 1 ).
- View inline
Summary of studies investigating intracoronary thrombolysis for patients with STEMI
Summary of baseline patient characteristics in studies investigating intracoronary thrombolysis for patients with STEMI
Clinical outcomes
All 12 RCTs reported the incidence of MACE. Compared with the CG, IC thrombolysis treatment significantly improved the occurrence of MACE at the end of follow-up (RR=0.65, 95% CI 0.51 to 0.82, I 2 =0%, p<0.0004; figure 2 ). Subgroup analysis demonstrated a significant reduction in MACE for trials using non-fibrin (RR=0.39, 95% CI 0.20 to 0.78, I 2 =0%, p=0.007) and moderately fibrin-specific thrombolysis (RR=0.62, 95% CI 0.47 to 0.83, I 2 =0%, p=0.001). MACE was observed at a similar rate in studies using highly fibrin-specific thrombolysis (RR=1.10, 95% CI 0.62 to 1.96, I 2 =0%, p=0.75). Test for subgroup difference was not significant (p=0.07). Furthermore, IC thrombolysis was associated with an improvement of LVEF (weighted MD (WMD)=1.87; 95% CI, 1.07 to 2.67; I 2 =25%; p<0.0001; online supplemental figure 2 ). There was a trend towards lower incidence of HF hospitalisation (RR=0.66; 95% CI 0.42 to 1.05; I 2 =0%; p=0.08; online supplemental figure 3 ), though not statistically significant. No significant differences were observed in mortality (RR=0.95; 95% CI 0.50 to 1.81; I 2 =0%; p=0.88; online supplemental figure 4 ), major bleeding (RR=1.24; 95% CI 0.47 to 3.28; I 2 =0%; p=0.67; online supplemental figure 5 ) and minor bleeding events (RR=1.47; 95% CI 0.90 to 2.40; I 2 =0%; p=0.12; online supplemental figure 6 ) between the two groups.
Forest plot displaying relative risk for major adverse cardiovascular events with intracoronary (IC) thrombolysis (stratified by fibrin-specific and non-fibrin-specific agents) or placebo in ST-elevation myocardial infarction. Squares and diamonds=risk ratios. Lines=95% CIs.
Myocardial perfusion outcomes
In patients with STEMI, IC thrombolysis significantly improved TIMI flow grade 3 (RR=1.09; 95% CI 1.02 to 1.15; I 2 =63%; p=0.006), TMPG (RR=1.38; 95% CI 1.13 to 1.68; I 2 =54%; p=0.001), complete STR (RR=1.20; 95% CI 1.10 to 1.31; I 2 =51%; p<0.0001) and CTFC (WMD=−4.58; 95% CI −6.23 to –2.72; I 2 =41%; p<0.0001) when compared with the CG ( figure 3 ).
Forest plots of myocardial perfusion outcomes with intracoronary (IC) thrombolysis or placebo in ST-elevation myocardial infarction. (A) Thrombolysis in myocardial infarction (TIMI) flow grade 3. (B) TIMI myocardial perfusion grade 3. (C) ST-segment resolution. (D) Corrected TIMI frame count. Squares and diamonds=risk ratios/weighted mean difference. Lines=95% CIs.
Meta-regression results
For primary endpoint of MACE, meta-regression analyses did not identify the following moderator variables as significant effect modifiers: publication year (p=0.97), proportion of male (p=0.23), prevalence of diabetes (p=0.44), proportion of smokers (p=0.68), prevalence of dyslipidaemia (p=0.44) and prevalence of hypertension (p=0.21).
Publication bias
Both Egger’s linear regression method (p=0.73) and Begg’s rank correlation test (p=0.63) suggested that publication bias was not an influencing factor when MACE was selected as the primary endpoint.
The present meta-analysis examined 12 RCTs that included 1915 patients with STEMI undergoing primary PCI. All trials evaluated the efficacy and safety of IC thrombolytic agents compared with a CG. The main findings were that patients administered IC thrombolysis had: (1) significantly lower incidence of MACE, (2) improvement in LVEF and (3) superior myocardial perfusion parameters (TIMI flow grade 3, TMPG, CTFC and complete STR). Notably, there were no significant differences observed in mortality and bleeding events in both groups.
Mortality rates following STEMI remain high, with 30-day mortality rates ranging from 5.4% to 14% and 1-year mortality rates ranging from 6.6% to 17.5%. 30 Despite the increased availability of primary PCI facilities and advancements in reperfusion strategies, there has been limited improvement in STEMI mortality rates. 31 Moreover, complications such as HF, arrhythmia, repeat revascularisation and reinfarction continue to be prevalent. 32–34 Despite restoring epicardial blood flow through PCI, MVO is evident in almost half of patients with STEMI. 6 It is characterised by distal embolisation of atherothrombotic debris, de novo microvascular thrombosis formation and plugging of circulating blood cells. 35 Furthermore, the upregulation of inflammatory mediators leads to intramyocardial haemorrhage and further microvascular necrosis. 36 37 These mechanistic pathways contribute to a larger infarct size, adverse myocardial remodelling and worse prognosis. 7 8 38
Thrombolytic therapy is an effective treatment for acute coronary thrombosis. 39 It inhibits red blood cell aggregation and dissolves thrombi to facilitate adequate microvascular perfusion. 40 41 Thrombolytic agents are commonly classified based on their affinity for fibrin. Streptokinase and urokinase lack fibrin specificity, indiscriminately activating both circulating and clot-bound plasminogen. Prourokinase has moderate fibrin specificity with a propensity for activation on fibrin surfaces, although systemic fibrinogen degradation has been observed. Alteplase and tenectaplase are highly fibrin specific, activating fibrin-bound plasminogen with minimal impact on circulating free plasminogen.
Utilisation of a facilitated PCI strategy with adjunctive intravenous thrombolysis improves coronary flow acutely, 42 however, causes paradoxical activation of thrombin, leading to increased bleeding. 43 44 As a result, clinicians considered the administration of IC thrombolytic therapy. Encouraging results from an open-chest animal model 45 led to the first randomised trial using adjunctive IC streptokinase in 41 patients with STEMI undergoing primary PCI. 11 In the IC streptokinase group, patients demonstrated improved coronary flow reserve, index of microcirculatory resistance (IMR) and CTFC 2 days after primary PCI. 11 Further RCTs with moderately fibrin-specific thrombolytic agents (prourokinase) demonstrated similar results with improved myocardial perfusion parameters. 19 20 22 23 26–28 Notably, the T-TIME Study, a large RCT of 440 patients comparing a highly fibrin-specific thrombolytic agent (alteplase) against placebo, reported different outcomes. At 3-month follow-up, there were no significant differences in rates of death or HF hospitalisation between groups. In addition, microvascular obstruction (% left ventricular mass) on cardiac magnetic resonance (CMR) between groups at 2–7 days did not differ. The ICE T-TIMI trial, which also used a highly fibrin-specific thrombolytic agent (tenecteplase), investigated its efficacy in 40 patients. This small study administered two fixed doses of 4 mg of IC tenecteplase and evaluated the primary endpoint of culprit lesion per cent diameter stenosis after the first bolus of tenecteplase or placebo. The results indicated no significant difference in the primary endpoint between the two groups.
In an initial meta-analysis of six RCTs investigating the use of IC thrombolysis in patients with STEMI compared with placebo, findings revealed a reduction in MVO but no impact on MACE. 46 Subsequent analyses, including studies with larger sample sizes or focusing on specific thrombolytic agents, have since been conducted with varied results. 47 48 Our meta-analysis, which is the largest to date, demonstrates that adjunctive IC thrombolysis in patients with STEMI improves both clinical and microcirculation outcomes. Although bleeding events did not significantly increase, it is plausible that a tradeoff may exist for reducing MACE. Notably, subgroup analysis for MACE demonstrated no significant benefit for highly fibrin-specific agents ( figure 2 ).
Intuitively, fibrin-specific thrombolytics are presumed to offer inherent advantages over their less fibrin-specific counterparts. In vivo studies have revealed that administration of alteplase in patients with STEMI induced shorter periods of thrombin and kallikrein activation, less reduction in fibrinogen, and a decrease in D-dimer and plasmin–antiplasmin complexes compared with streptokinase. 49 In this regard, tenecteplase demonstrates superior performance relative to alteplase with almost no paradoxical procoagulant effect due to reduced activation of thrombin and the kallikrein–factor XII system. 50
Nonetheless, other variables may diminish the significance of fibrin specificity. It has been argued that administration of IC alteplase, a short-acting thrombolytic with a half-life of 4–6 min, before flow optimisation with stenting may have contributed to the negative results seen in T-TIME. Although prourokinase has a similarly short half-life and was also given before stenting in multiple studies, it was associated with better results. 19 20 22 23 26–28 The therapeutic efficacy of prourokinase predominantly relies on its conversion to urokinase, a non-fibrin-specific direct plasminogen activator, potentially resulting in a prolonged duration of action. Furthermore, inducing a systemic fibrinolytic state with a non-selective agent may be paradoxically desirable in patients receiving adjunctive IC thrombolytics during primary PCI. This approach can potentially prevent further thrombus reaccumulation and embolisation to the microcirculation, especially in a highly thrombogenic environment. In contrast, fibrin-specific agents may heighten the risk of rethrombosis and reocclusion due to their limited impact on systemic fibrinogen depletion. Nevertheless, such varied outcomes across these studies could be attributed to the heterogeneous methodologies used.
Despite encouraging results, future studies targeting patients at the highest risk of MVO with appropriately powered sample sizes are required. The ongoing RESTORE-MI (Restoring Microcirculatory Perfusion in STEMI) trial ( NCT03998319 ) has a unique approach in which all study participants will undergo assessment of microvascular integrity after primary PCI prior to inclusion. Only patients with objective evidence of microvascular dysfunction (IMR value >32) following reperfusion will be randomised to treatment with IC tenecteplase or placebo. The primary endpoint measured will be cardiovascular mortality and rehospitalisation for HF at 24 months, in addition to infarct size on CMR at 6 months post-PCI. This study may potentially support a novel therapeutic approach towards treating MVO in patients with STEMI in the future.
Limitations
Several key limitations should be considered when interpreting the findings of the present meta-analysis. First, several studies were subject to bias due to issues in randomisation and blinding, leading to an increased chance of type 1 (false-positive) error. In addition, the sample size of individual studies, except for the T-TIME trial, was relatively small. Second, inconsistencies in the duration of follow-up and the definition of clinical outcomes, such as MACE, were observed among the studies. Third, interventional protocols varied between RCTs. For example, IC thrombolytic therapy differed in agent, dosage, timing and route of administration. Initial studies used non-fibrin-specific agents, while contemporary studies moved towards newer fibrin-specific therapy. Besides Sezer et al , 25 all other studies administered IC thrombolysis therapy prior to stent implantation. 18–24 26–29 Within the latter group, some delivered before flow restoration, 19 21 29 though most did so after balloon dilation or thrombus aspiration. 18 20 22–24 26–28 Similarly, the methods of IC administration of the agents varied between non-selective delivery through guiding catheters 24 25 to selective delivery via IC catheters. 18–24 26–29 Furthermore, antiplatelet, anticoagulant and glycoprotein IIb/IIIa inhibitors (GPI) regimens also differed ( table 1 ). Finally, patient selection was diverse between studies. Though regression analysis did not detect any significant effect modifiers, total ischaemic time was omitted due to significant heterogeneity in reporting.
Impaired myocardial perfusion remains a clinical challenge in patients with STEMI. Despite its limitations, this meta-analysis favours the use of IC thrombolytic therapy during primary PCI. Overall, IC thrombolysis reduced the incidence of MACE and improved myocardial perfusion markers without increasing the risk of bleeding. Future clinical trials should be appropriately powered for clinical outcomes and focus on patients at high risk of microcirculatory dysfunction.
Ethics statements
Patient consent for publication.
Not applicable.
Ethics approval
- Chierchia S ,
- Lawton JS ,
- Tamis-Holland JE ,
- Bangalore S , et al
- Agewall S , et al
- Sorajja P ,
- Costantini C , et al
- van de Hoef TP ,
- Meuwissen M , et al
- Regenfus M ,
- Schlundt C ,
- Krähner R , et al
- Fearon WF ,
- Ng M , et al
- Holmes DR ,
- Herrmann J , et al
- Gören T , et al
- Liberati A ,
- Tetzlaff J , et al
- Sterne JAC ,
- Savović J ,
- Page MJ , et al
- Liu J , et al
- Davey Smith G ,
- Schneider M , et al
- Hao GZ , et al
- Gibson CM ,
- Gopalakrishnan L , et al
- Liu Z , et al
- Du X , et al
- McCartney PJ ,
- Maznyczka AM , et al
- Aslanger E , et al
- Wu H , et al
- Feng Q , et al
- Wang Y , et al
- Pelliccia F ,
- Tanzilli G , et al
- Mozaffarian D ,
- Benjamin EJ ,
- Go AS , et al
- Chandra M , et al
- Melenovský V ,
- Stehlik J , et al
- Windecker S ,
- Myat A , et al
- Rathor P , et al
- Konijnenberg LSF ,
- Duncker DJ , et al
- Betgem RP ,
- de Waard GA ,
- Nijveldt R , et al
- Yellon DM ,
- Hausenloy DJ
- Messalli G ,
- Dymarkowski S , et al
- ↵ Randomised trial of intravenous streptokinase, oral aspirin, both, or neither among 17,187 cases of suspected acute myocardial infarction: ISIS-2. ISIS-2 (Second international study of infarct survival) collaborative group . Lancet 1988 ; 2 : 349 – 60 . doi:10.1016/S0140-6736(88)92833-4 OpenUrl PubMed Web of Science
- Schwartz RS ,
- Farb A , et al
- Zalewski J ,
- Godlewski J , et al
- Hillis WS ,
- Been M , et al
- ↵ Primary versus Tenecteplase-facilitated percutaneous coronary intervention in patients with ST-segment elevation acute myocardial infarction (ASSENT-4 PCI): randomised trial . The Lancet 2006 ; 367 : 569 – 78 . doi:10.1016/S0140-6736(06)68147-6 OpenUrl
- Tendera M ,
- de Belder MA , et al
- Armiger LC ,
- White HD , et al
- Alyamani M ,
- Campbell S ,
- Navarese E , et al
- Tian W , et al
- Li ZP , et al
- Hoffmeister HM ,
- Kastner C , et al
- Ehlers R , et al
Supplementary materials
Supplementary data.
This web only file has been produced by the BMJ Publishing Group from an electronic file supplied by the author(s) and has not been edited for content.
- Data supplement 1
X @RajanRehan23
Contributors RR—conceptualisation, methodology, data analysis, writing (original draft preparation), reviewing and editing the final manuscript. SV—methodology, data analysis. CCYW—conceptualisation, methodology, data analysis. FP—supervision, writing (reviewing and editing). JL—supervision, writing (reviewing and editing). AK—supervision, writing (reviewing and editing). AY—conceptualisation, methodology, writing (reviewing and editing). HDW—conceptualisation, methodology, writing (reviewing and editing). WF—conceptualisation, methodology, writing (reviewing and editing). MN—conceptualisation, methodology, supervision, writing (reviewing and editing), guarantor.
Funding This study is funded by the National Health and Medical Research Council (2022150).
Competing interests JL has received minor honoraria from Abbott Vascular, Boehringer Ingelheim and Bayer. AY has received minor honoraria and research support from Abbot Vascular and Philips Healthcare. WF has received research support from Abbott Vascular and Medtronic; and has minor stock options with HeartFlow. MN has received research support from Abbot Vascular. HDW has received grant support paid to the institution and fees for serving on Steering Committees of the ODYSSEY trial from Sanofi and Regeneron Pharmaceuticals, the ISCHEMIA and MINT Study from the National Institutes of Health, the STRENGTH trial from Omthera Pharmaceuticals, the HEART-FID Study from American Regent, the DAL-GENE Study from DalCor Pharma UK, the AEGIS-II Study from CSL Behring, the CLEAR OUTCOMES Study from Esperion Therapeutics, and the SOLIST-WHF and SCOREDS trials from Sanofi Aventis Australia. The remaining authors have nothing to disclose.
Patient and public involvement Patients and/or the public were not involved in the design, or conduct, or reporting, or dissemination plans of this research.
Provenance and peer review Not commissioned; externally peer reviewed.
Supplemental material This content has been supplied by the author(s). It has not been vetted by BMJ Publishing Group Limited (BMJ) and may not have been peer-reviewed. Any opinions or recommendations discussed are solely those of the author(s) and are not endorsed by BMJ. BMJ disclaims all liability and responsibility arising from any reliance placed on the content. Where the content includes any translated material, BMJ does not warrant the accuracy and reliability of the translations (including but not limited to local regulations, clinical guidelines, terminology, drug names and drug dosages), and is not responsible for any error and/or omissions arising from translation and adaptation or otherwise.
Read the full text or download the PDF:
- Privacy Policy

Home » Research Design – Types, Methods and Examples
Research Design – Types, Methods and Examples
Table of Contents

Research Design
Definition:
Research design refers to the overall strategy or plan for conducting a research study. It outlines the methods and procedures that will be used to collect and analyze data, as well as the goals and objectives of the study. Research design is important because it guides the entire research process and ensures that the study is conducted in a systematic and rigorous manner.
Types of Research Design
Types of Research Design are as follows:
Descriptive Research Design
This type of research design is used to describe a phenomenon or situation. It involves collecting data through surveys, questionnaires, interviews, and observations. The aim of descriptive research is to provide an accurate and detailed portrayal of a particular group, event, or situation. It can be useful in identifying patterns, trends, and relationships in the data.
Correlational Research Design
Correlational research design is used to determine if there is a relationship between two or more variables. This type of research design involves collecting data from participants and analyzing the relationship between the variables using statistical methods. The aim of correlational research is to identify the strength and direction of the relationship between the variables.
Experimental Research Design
Experimental research design is used to investigate cause-and-effect relationships between variables. This type of research design involves manipulating one variable and measuring the effect on another variable. It usually involves randomly assigning participants to groups and manipulating an independent variable to determine its effect on a dependent variable. The aim of experimental research is to establish causality.
Quasi-experimental Research Design
Quasi-experimental research design is similar to experimental research design, but it lacks one or more of the features of a true experiment. For example, there may not be random assignment to groups or a control group. This type of research design is used when it is not feasible or ethical to conduct a true experiment.
Case Study Research Design
Case study research design is used to investigate a single case or a small number of cases in depth. It involves collecting data through various methods, such as interviews, observations, and document analysis. The aim of case study research is to provide an in-depth understanding of a particular case or situation.
Longitudinal Research Design
Longitudinal research design is used to study changes in a particular phenomenon over time. It involves collecting data at multiple time points and analyzing the changes that occur. The aim of longitudinal research is to provide insights into the development, growth, or decline of a particular phenomenon over time.
Structure of Research Design
The format of a research design typically includes the following sections:
- Introduction : This section provides an overview of the research problem, the research questions, and the importance of the study. It also includes a brief literature review that summarizes previous research on the topic and identifies gaps in the existing knowledge.
- Research Questions or Hypotheses: This section identifies the specific research questions or hypotheses that the study will address. These questions should be clear, specific, and testable.
- Research Methods : This section describes the methods that will be used to collect and analyze data. It includes details about the study design, the sampling strategy, the data collection instruments, and the data analysis techniques.
- Data Collection: This section describes how the data will be collected, including the sample size, data collection procedures, and any ethical considerations.
- Data Analysis: This section describes how the data will be analyzed, including the statistical techniques that will be used to test the research questions or hypotheses.
- Results : This section presents the findings of the study, including descriptive statistics and statistical tests.
- Discussion and Conclusion : This section summarizes the key findings of the study, interprets the results, and discusses the implications of the findings. It also includes recommendations for future research.
- References : This section lists the sources cited in the research design.
Example of Research Design
An Example of Research Design could be:
Research question: Does the use of social media affect the academic performance of high school students?
Research design:
- Research approach : The research approach will be quantitative as it involves collecting numerical data to test the hypothesis.
- Research design : The research design will be a quasi-experimental design, with a pretest-posttest control group design.
- Sample : The sample will be 200 high school students from two schools, with 100 students in the experimental group and 100 students in the control group.
- Data collection : The data will be collected through surveys administered to the students at the beginning and end of the academic year. The surveys will include questions about their social media usage and academic performance.
- Data analysis : The data collected will be analyzed using statistical software. The mean scores of the experimental and control groups will be compared to determine whether there is a significant difference in academic performance between the two groups.
- Limitations : The limitations of the study will be acknowledged, including the fact that social media usage can vary greatly among individuals, and the study only focuses on two schools, which may not be representative of the entire population.
- Ethical considerations: Ethical considerations will be taken into account, such as obtaining informed consent from the participants and ensuring their anonymity and confidentiality.
How to Write Research Design
Writing a research design involves planning and outlining the methodology and approach that will be used to answer a research question or hypothesis. Here are some steps to help you write a research design:
- Define the research question or hypothesis : Before beginning your research design, you should clearly define your research question or hypothesis. This will guide your research design and help you select appropriate methods.
- Select a research design: There are many different research designs to choose from, including experimental, survey, case study, and qualitative designs. Choose a design that best fits your research question and objectives.
- Develop a sampling plan : If your research involves collecting data from a sample, you will need to develop a sampling plan. This should outline how you will select participants and how many participants you will include.
- Define variables: Clearly define the variables you will be measuring or manipulating in your study. This will help ensure that your results are meaningful and relevant to your research question.
- Choose data collection methods : Decide on the data collection methods you will use to gather information. This may include surveys, interviews, observations, experiments, or secondary data sources.
- Create a data analysis plan: Develop a plan for analyzing your data, including the statistical or qualitative techniques you will use.
- Consider ethical concerns : Finally, be sure to consider any ethical concerns related to your research, such as participant confidentiality or potential harm.
When to Write Research Design
Research design should be written before conducting any research study. It is an important planning phase that outlines the research methodology, data collection methods, and data analysis techniques that will be used to investigate a research question or problem. The research design helps to ensure that the research is conducted in a systematic and logical manner, and that the data collected is relevant and reliable.
Ideally, the research design should be developed as early as possible in the research process, before any data is collected. This allows the researcher to carefully consider the research question, identify the most appropriate research methodology, and plan the data collection and analysis procedures in advance. By doing so, the research can be conducted in a more efficient and effective manner, and the results are more likely to be valid and reliable.
Purpose of Research Design
The purpose of research design is to plan and structure a research study in a way that enables the researcher to achieve the desired research goals with accuracy, validity, and reliability. Research design is the blueprint or the framework for conducting a study that outlines the methods, procedures, techniques, and tools for data collection and analysis.
Some of the key purposes of research design include:
- Providing a clear and concise plan of action for the research study.
- Ensuring that the research is conducted ethically and with rigor.
- Maximizing the accuracy and reliability of the research findings.
- Minimizing the possibility of errors, biases, or confounding variables.
- Ensuring that the research is feasible, practical, and cost-effective.
- Determining the appropriate research methodology to answer the research question(s).
- Identifying the sample size, sampling method, and data collection techniques.
- Determining the data analysis method and statistical tests to be used.
- Facilitating the replication of the study by other researchers.
- Enhancing the validity and generalizability of the research findings.
Applications of Research Design
There are numerous applications of research design in various fields, some of which are:
- Social sciences: In fields such as psychology, sociology, and anthropology, research design is used to investigate human behavior and social phenomena. Researchers use various research designs, such as experimental, quasi-experimental, and correlational designs, to study different aspects of social behavior.
- Education : Research design is essential in the field of education to investigate the effectiveness of different teaching methods and learning strategies. Researchers use various designs such as experimental, quasi-experimental, and case study designs to understand how students learn and how to improve teaching practices.
- Health sciences : In the health sciences, research design is used to investigate the causes, prevention, and treatment of diseases. Researchers use various designs, such as randomized controlled trials, cohort studies, and case-control studies, to study different aspects of health and healthcare.
- Business : Research design is used in the field of business to investigate consumer behavior, marketing strategies, and the impact of different business practices. Researchers use various designs, such as survey research, experimental research, and case studies, to study different aspects of the business world.
- Engineering : In the field of engineering, research design is used to investigate the development and implementation of new technologies. Researchers use various designs, such as experimental research and case studies, to study the effectiveness of new technologies and to identify areas for improvement.
Advantages of Research Design
Here are some advantages of research design:
- Systematic and organized approach : A well-designed research plan ensures that the research is conducted in a systematic and organized manner, which makes it easier to manage and analyze the data.
- Clear objectives: The research design helps to clarify the objectives of the study, which makes it easier to identify the variables that need to be measured, and the methods that need to be used to collect and analyze data.
- Minimizes bias: A well-designed research plan minimizes the chances of bias, by ensuring that the data is collected and analyzed objectively, and that the results are not influenced by the researcher’s personal biases or preferences.
- Efficient use of resources: A well-designed research plan helps to ensure that the resources (time, money, and personnel) are used efficiently and effectively, by focusing on the most important variables and methods.
- Replicability: A well-designed research plan makes it easier for other researchers to replicate the study, which enhances the credibility and reliability of the findings.
- Validity: A well-designed research plan helps to ensure that the findings are valid, by ensuring that the methods used to collect and analyze data are appropriate for the research question.
- Generalizability : A well-designed research plan helps to ensure that the findings can be generalized to other populations, settings, or situations, which increases the external validity of the study.
Research Design Vs Research Methodology
Research Design | Research Methodology |
---|---|
The plan and structure for conducting research that outlines the procedures to be followed to collect and analyze data. | The set of principles, techniques, and tools used to carry out the research plan and achieve research objectives. |
Describes the overall approach and strategy used to conduct research, including the type of data to be collected, the sources of data, and the methods for collecting and analyzing data. | Refers to the techniques and methods used to gather, analyze and interpret data, including sampling techniques, data collection methods, and data analysis techniques. |
Helps to ensure that the research is conducted in a systematic, rigorous, and valid way, so that the results are reliable and can be used to make sound conclusions. | Includes a set of procedures and tools that enable researchers to collect and analyze data in a consistent and valid manner, regardless of the research design used. |
Common research designs include experimental, quasi-experimental, correlational, and descriptive studies. | Common research methodologies include qualitative, quantitative, and mixed-methods approaches. |
Determines the overall structure of the research project and sets the stage for the selection of appropriate research methodologies. | Guides the researcher in selecting the most appropriate research methods based on the research question, research design, and other contextual factors. |
Helps to ensure that the research project is feasible, relevant, and ethical. | Helps to ensure that the data collected is accurate, valid, and reliable, and that the research findings can be interpreted and generalized to the population of interest. |
About the author
Muhammad Hassan
Researcher, Academic Writer, Web developer
You may also like

Future Research – Thesis Guide

Literature Review – Types Writing Guide and...

How to Publish a Research Paper – Step by Step...

Delimitations in Research – Types, Examples and...

Research Paper Title Page – Example and Making...

Figures in Research Paper – Examples and Guide
Leave a comment x.
Save my name, email, and website in this browser for the next time I comment.
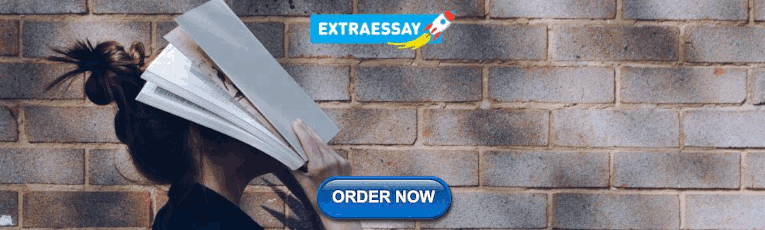
COMMENTS
Research methods are specific procedures for collecting and analyzing data. Developing your research methods is an integral part of your research design. When planning your methods, there are two key decisions you will make. First, decide how you will collect data. Your methods depend on what type of data you need to answer your research question:
This research method is used to gather information on attitudes, beliefs, and behaviors of individuals. Researchers use survey research to collect data quickly and efficiently from a large sample size. Survey research can be conducted through various methods such as online, phone, mail, or in-person interviews. Quantitative Research Analysis ...
Definition of research in data analysis: According to LeCompte and Schensul, research data analysis is a process used by researchers to reduce data to a story and interpret it to derive insights. The data analysis process helps reduce a large chunk of data into smaller fragments, which makes sense. Three essential things occur during the data ...
Quantitative research methods are used to collect and analyze numerical data. This type of research is useful when the objective is to test a hypothesis, determine cause-and-effect relationships, and measure the prevalence of certain phenomena. Quantitative research methods include surveys, experiments, and secondary data analysis.
Step 1: Explain your methodological approach. Step 2: Describe your data collection methods. Step 3: Describe your analysis method. Step 4: Evaluate and justify the methodological choices you made. Tips for writing a strong methodology chapter. Other interesting articles.
Research methods are specific procedures for collecting and analysing data. Developing your research methods is an integral part of your research design. When planning your methods, there are two key decisions you will make. ... For quantitative data, you can use statistical analysis methods to test relationships between variables. For ...
The research methodology is an important section of any research paper or thesis, as it describes the methods and procedures that will be used to conduct the research. It should include details about the research design, data collection methods, data analysis techniques, and any ethical considerations.
About Research Methods. This guide provides an overview of research methods, how to choose and use them, and supports and resources at UC Berkeley. As Patten and Newhart note in the book Understanding Research Methods, "Research methods are the building blocks of the scientific enterprise. They are the "how" for building systematic knowledge.
Abstract. A perennial bestseller since 1997, this updated tenth edition of Understanding Research Methods provides a detailed overview of all the important concepts traditionally covered in a ...
The SAGE Handbook of. tive Data AnalysisUwe FlickMapping the FieldData analys. s is the central step in qualitative research. Whatever the data are, it is their analysis that, in a de. isive way, forms the outcomes of the research. Sometimes, data collection is limited to recording and docu-menting naturally occurring ph.
Qualitative research involves collecting and analyzing non-numerical data (e.g., text, video, or audio) to understand concepts, opinions, or experiences. It can be used to gather in-depth insights into a problem or generate new ideas for research. Qualitative research is the opposite of quantitative research, which involves collecting and ...
These methods are used to understand how language is used in real-world situations, identify common themes or overarching ideas, and describe and interpret various texts. Data analysis for qualitative research typically includes discourse analysis, thematic analysis, and textual analysis. Quantitative research methodology:
Qualitative Data Analysis Methods and Examples. Exploring various qualitative data analysis methods will provide you with a wide collection for making sense of your research findings. Once the data has been collected, you can choose from several analysis methods based on your research objectives and the data type you've collected.
Research methods are the compass, map, and tools that guide us through the terrain of knowledge. They enable us to ask important questions, systematically gather and analyze data, and contribute valuable insights to our understanding of the world. As you start on your research journey, embrace the adventure, respect the process, and look ...
Research methods refer to the strategies, tools, and techniques used to gather and analyze data in a structured way in order to answer a research question or investigate a hypothesis (Hammond & Wellington, 2020). Generally, ... Content analysis is a research method that involves systematic and objective coding and interpreting of text or media ...
Regression analysis is a quantitative research method which is used when the study involves modelling and analysing several variables, where the relationship includes a dependent variable and one or more independent variables. In simple terms, regression analysis is a quantitative method used to test the nature of relationships between a dependent variable and one or more independent variables.
Data Analysis. Definition: Data analysis refers to the process of inspecting, cleaning, transforming, and modeling data with the goal of discovering useful information, drawing conclusions, and supporting decision-making. It involves applying various statistical and computational techniques to interpret and derive insights from large datasets.
Data analysis techniques in research are categorized into qualitative and quantitative methods, each with its specific approaches and tools. These techniques are instrumental in extracting meaningful insights, patterns, and relationships from data to support informed decision-making, validate hypotheses, and derive actionable recommendations.
Textual analysis is a broad term for various research methods used to describe, interpret and understand texts. All kinds of information can be gleaned from a text - from its literal meaning to the subtext, symbolism, assumptions, and values it reveals. The methods used to conduct textual analysis depend on the field and the aims of the ...
In order to achieve this goal, many communications professionals will use research methods for audience analysis. As you work toward your master's in communications, it's important to cultivate skills like these now that can help you at work later. There are several commonly used methods; each offers its own insights into your target audience.
QA/QC. Research focusing on particulate plastics in the micro- and nanometer size range is still in an adolescent stage and related data comes along with significant analytical uncertainties [23 ...
Our trend analysis shows the global age-standardised prevalence of insufficient physical ... we were able to revise modelling methods to estimate trends in insufficient physical activity in all 197 countries. ... but true prevalence during strict limitations on movement might have deviated from this. Some research suggests that lockdowns, on ...
Background Video games have become a prevalent source of entertainment, especially among children. Furthermore, the amount of time spent playing video games has grown dramatically. The purpose of this research was to examine the mediation effects of attention and child memory on the relationship between video games addiction and cognitive and learning abilities in Egyptian children. Methods A ...
Qualitative Research. Qualitative research is a type of research methodology that focuses on exploring and understanding people's beliefs, attitudes, behaviors, and experiences through the collection and analysis of non-numerical data. It seeks to answer research questions through the examination of subjective data, such as interviews, focus ...
Presented in part at the 2022 American Society of Clinical Oncology (ASCO) Annual Meeting; June 3-7, 2022; Chicago, Illinois; the Society of Integrative Oncology (SIO) 2022 International Conference; October 20-22, 2022; Scottsdale, Arizona; and the 2023 Society for Acupuncture Research (SAR) International Research Conference; May 18-21, 2023; New York, New York.
How to Do Thematic Analysis | Step-by-Step Guide & Examples. Published on September 6, 2019 by Jack Caulfield.Revised on June 22, 2023. Thematic analysis is a method of analyzing qualitative data.It is usually applied to a set of texts, such as an interview or transcripts.The researcher closely examines the data to identify common themes - topics, ideas and patterns of meaning that come up ...
This document describes the standards, codes, methods, and definitions for Forest Inventory and Analysis (FIA) field data items. The objective is to describe URBAN FIA field procedures that are consistent and uniform across all FIA units. This URBAN FIA guide will serve as the framework for regional FIA programs; individual programs may add regional variables that support their CORE program ...
Egger's linear regression method and Begg's rank correlation test were used to detect funnel plot asymmetry.16 17 Statistical analysis was conducted with Review Manager V.5.3.5 (Cochrane Collaboration, Oxford, UK) and Comprehensive Meta-Analysis V.3.0 (Biostat, Englewood, New Jersey, USA). All p values were two sided, and values <0.05 were ...
Research design should be written before conducting any research study. It is an important planning phase that outlines the research methodology, data collection methods, and data analysis techniques that will be used to investigate a research question or problem.
the collection method for the other forms listed. In addition to web-based electronic forms, information may be collected through email or telephone. These methods enable individuals, private sector entities, personnel working at other federal or state agencies, and international entities, including individuals, companies and