Have a language expert improve your writing
Run a free plagiarism check in 10 minutes, generate accurate citations for free.
- Knowledge Base
Methodology
- What is Secondary Research? | Definition, Types, & Examples
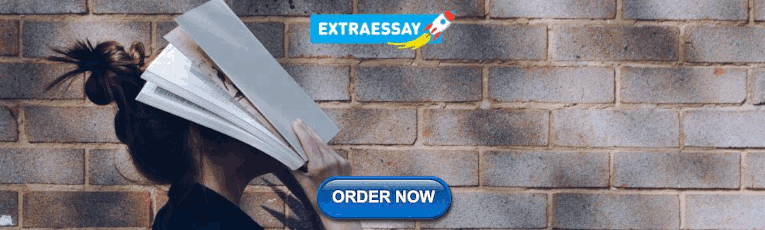
What is Secondary Research? | Definition, Types, & Examples
Published on January 20, 2023 by Tegan George . Revised on January 12, 2024.
Secondary research is a research method that uses data that was collected by someone else. In other words, whenever you conduct research using data that already exists, you are conducting secondary research. On the other hand, any type of research that you undertake yourself is called primary research .
Secondary research can be qualitative or quantitative in nature. It often uses data gathered from published peer-reviewed papers, meta-analyses, or government or private sector databases and datasets.
Table of contents
When to use secondary research, types of secondary research, examples of secondary research, advantages and disadvantages of secondary research, other interesting articles, frequently asked questions.
Secondary research is a very common research method, used in lieu of collecting your own primary data. It is often used in research designs or as a way to start your research process if you plan to conduct primary research later on.
Since it is often inexpensive or free to access, secondary research is a low-stakes way to determine if further primary research is needed, as gaps in secondary research are a strong indication that primary research is necessary. For this reason, while secondary research can theoretically be exploratory or explanatory in nature, it is usually explanatory: aiming to explain the causes and consequences of a well-defined problem.
Prevent plagiarism. Run a free check.
Secondary research can take many forms, but the most common types are:
Statistical analysis
Literature reviews, case studies, content analysis.
There is ample data available online from a variety of sources, often in the form of datasets. These datasets are often open-source or downloadable at a low cost, and are ideal for conducting statistical analyses such as hypothesis testing or regression analysis .
Credible sources for existing data include:
- The government
- Government agencies
- Non-governmental organizations
- Educational institutions
- Businesses or consultancies
- Libraries or archives
- Newspapers, academic journals, or magazines
A literature review is a survey of preexisting scholarly sources on your topic. It provides an overview of current knowledge, allowing you to identify relevant themes, debates, and gaps in the research you analyze. You can later apply these to your own work, or use them as a jumping-off point to conduct primary research of your own.
Structured much like a regular academic paper (with a clear introduction, body, and conclusion), a literature review is a great way to evaluate the current state of research and demonstrate your knowledge of the scholarly debates around your topic.
A case study is a detailed study of a specific subject. It is usually qualitative in nature and can focus on a person, group, place, event, organization, or phenomenon. A case study is a great way to utilize existing research to gain concrete, contextual, and in-depth knowledge about your real-world subject.
You can choose to focus on just one complex case, exploring a single subject in great detail, or examine multiple cases if you’d prefer to compare different aspects of your topic. Preexisting interviews , observational studies , or other sources of primary data make for great case studies.
Content analysis is a research method that studies patterns in recorded communication by utilizing existing texts. It can be either quantitative or qualitative in nature, depending on whether you choose to analyze countable or measurable patterns, or more interpretive ones. Content analysis is popular in communication studies, but it is also widely used in historical analysis, anthropology, and psychology to make more semantic qualitative inferences.
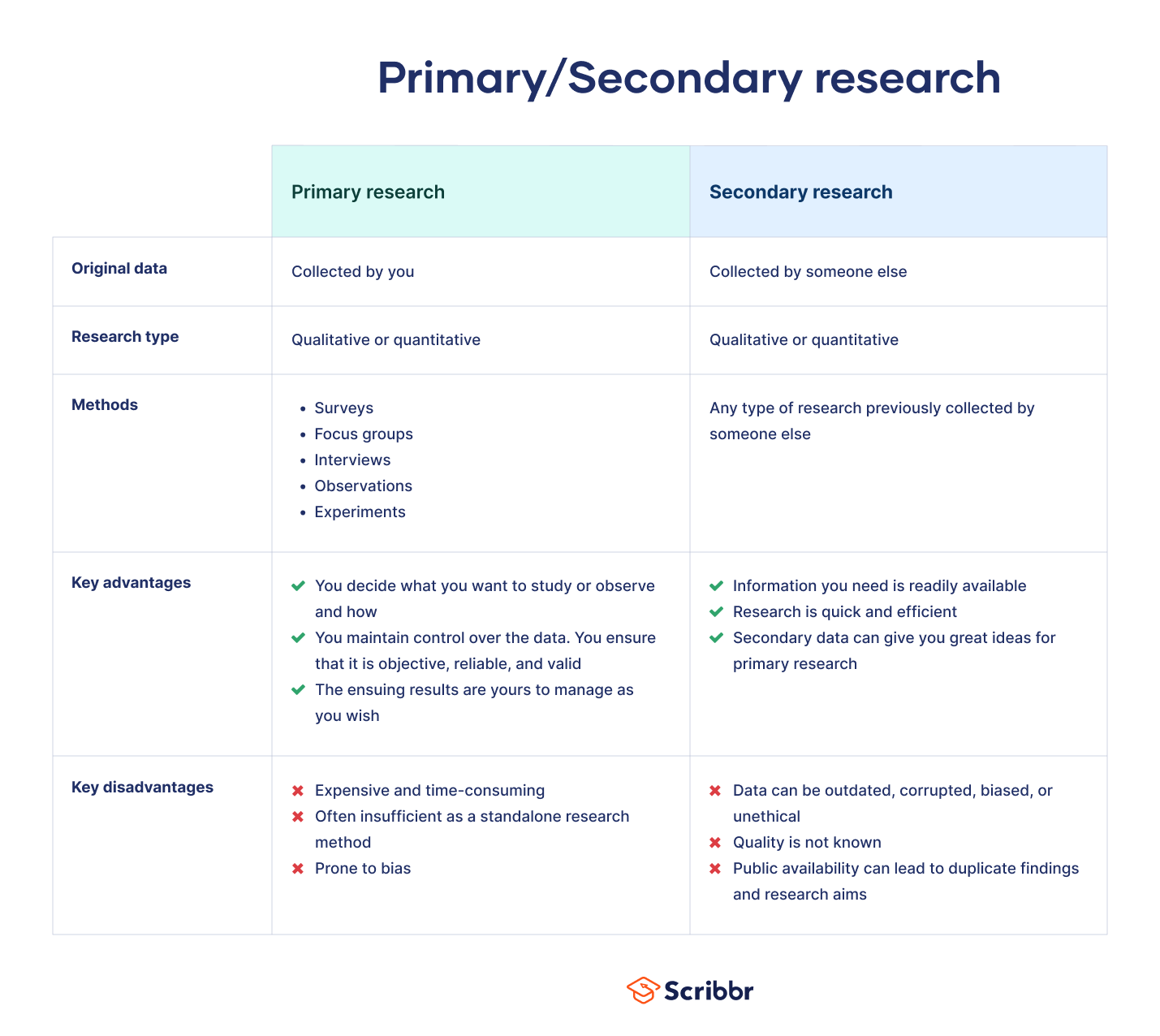
Secondary research is a broad research approach that can be pursued any way you’d like. Here are a few examples of different ways you can use secondary research to explore your research topic .
Secondary research is a very common research approach, but has distinct advantages and disadvantages.
Advantages of secondary research
Advantages include:
- Secondary data is very easy to source and readily available .
- It is also often free or accessible through your educational institution’s library or network, making it much cheaper to conduct than primary research .
- As you are relying on research that already exists, conducting secondary research is much less time consuming than primary research. Since your timeline is so much shorter, your research can be ready to publish sooner.
- Using data from others allows you to show reproducibility and replicability , bolstering prior research and situating your own work within your field.
Disadvantages of secondary research
Disadvantages include:
- Ease of access does not signify credibility . It’s important to be aware that secondary research is not always reliable , and can often be out of date. It’s critical to analyze any data you’re thinking of using prior to getting started, using a method like the CRAAP test .
- Secondary research often relies on primary research already conducted. If this original research is biased in any way, those research biases could creep into the secondary results.
Many researchers using the same secondary research to form similar conclusions can also take away from the uniqueness and reliability of your research. Many datasets become “kitchen-sink” models, where too many variables are added in an attempt to draw increasingly niche conclusions from overused data . Data cleansing may be necessary to test the quality of the research.
Here's why students love Scribbr's proofreading services
Discover proofreading & editing
If you want to know more about statistics , methodology , or research bias , make sure to check out some of our other articles with explanations and examples.
- Normal distribution
- Degrees of freedom
- Null hypothesis
- Discourse analysis
- Control groups
- Mixed methods research
- Non-probability sampling
- Quantitative research
- Inclusion and exclusion criteria
Research bias
- Rosenthal effect
- Implicit bias
- Cognitive bias
- Selection bias
- Negativity bias
- Status quo bias
A systematic review is secondary research because it uses existing research. You don’t collect new data yourself.
The research methods you use depend on the type of data you need to answer your research question .
- If you want to measure something or test a hypothesis , use quantitative methods . If you want to explore ideas, thoughts and meanings, use qualitative methods .
- If you want to analyze a large amount of readily-available data, use secondary data. If you want data specific to your purposes with control over how it is generated, collect primary data.
- If you want to establish cause-and-effect relationships between variables , use experimental methods. If you want to understand the characteristics of a research subject, use descriptive methods.
Quantitative research deals with numbers and statistics, while qualitative research deals with words and meanings.
Quantitative methods allow you to systematically measure variables and test hypotheses . Qualitative methods allow you to explore concepts and experiences in more detail.
Sources in this article
We strongly encourage students to use sources in their work. You can cite our article (APA Style) or take a deep dive into the articles below.
George, T. (2024, January 12). What is Secondary Research? | Definition, Types, & Examples. Scribbr. Retrieved June 11, 2024, from https://www.scribbr.com/methodology/secondary-research/
Largan, C., & Morris, T. M. (2019). Qualitative Secondary Research: A Step-By-Step Guide (1st ed.). SAGE Publications Ltd.
Peloquin, D., DiMaio, M., Bierer, B., & Barnes, M. (2020). Disruptive and avoidable: GDPR challenges to secondary research uses of data. European Journal of Human Genetics , 28 (6), 697–705. https://doi.org/10.1038/s41431-020-0596-x
Is this article helpful?
Tegan George
Other students also liked, primary research | definition, types, & examples, how to write a literature review | guide, examples, & templates, what is a case study | definition, examples & methods, what is your plagiarism score.

Library Guides
Dissertations 4: methodology: methods.
- Introduction & Philosophy
- Methodology
Primary & Secondary Sources, Primary & Secondary Data
When describing your research methods, you can start by stating what kind of secondary and, if applicable, primary sources you used in your research. Explain why you chose such sources, how well they served your research, and identify possible issues encountered using these sources.
Definitions
There is some confusion on the use of the terms primary and secondary sources, and primary and secondary data. The confusion is also due to disciplinary differences (Lombard 2010). Whilst you are advised to consult the research methods literature in your field, we can generalise as follows:
Secondary sources
Secondary sources normally include the literature (books and articles) with the experts' findings, analysis and discussions on a certain topic (Cottrell, 2014, p123). Secondary sources often interpret primary sources.
Primary sources
Primary sources are "first-hand" information such as raw data, statistics, interviews, surveys, law statutes and law cases. Even literary texts, pictures and films can be primary sources if they are the object of research (rather than, for example, documentaries reporting on something else, in which case they would be secondary sources). The distinction between primary and secondary sources sometimes lies on the use you make of them (Cottrell, 2014, p123).
Primary data
Primary data are data (primary sources) you directly obtained through your empirical work (Saunders, Lewis and Thornhill 2015, p316).
Secondary data
Secondary data are data (primary sources) that were originally collected by someone else (Saunders, Lewis and Thornhill 2015, p316).
Comparison between primary and secondary data
Primary data | Secondary data |
Data collected directly | Data collected from previously done research, existing research is summarised and collated to enhance the overall effectiveness of the research. |
Examples: Interviews (face-to-face or telephonic), Online surveys, Focus groups and Observations | Examples: data available via the internet, non-government and government agencies, public libraries, educational institutions, commercial/business information |
Advantages: •Data collected is first hand and accurate. •Data collected can be controlled. No dilution of data. •Research method can be customized to suit personal requirements and needs of the research. | Advantages: •Information is readily available •Less expensive and less time-consuming •Quicker to conduct |
Disadvantages: •Can be quite extensive to conduct, requiring a lot of time and resources •Sometimes one primary research method is not enough; therefore a mixed method is require, which can be even more time consuming. | Disadvantages: •It is necessary to check the credibility of the data •May not be as up to date •Success of your research depends on the quality of research previously conducted by others. |
Use
Virtually all research will use secondary sources, at least as background information.
Often, especially at the postgraduate level, it will also use primary sources - secondary and/or primary data. The engagement with primary sources is generally appreciated, as less reliant on others' interpretations, and closer to 'facts'.
The use of primary data, as opposed to secondary data, demonstrates the researcher's effort to do empirical work and find evidence to answer her specific research question and fulfill her specific research objectives. Thus, primary data contribute to the originality of the research.
Ultimately, you should state in this section of the methodology:
What sources and data you are using and why (how are they going to help you answer the research question and/or test the hypothesis.
If using primary data, why you employed certain strategies to collect them.
What the advantages and disadvantages of your strategies to collect the data (also refer to the research in you field and research methods literature).
Quantitative, Qualitative & Mixed Methods
The methodology chapter should reference your use of quantitative research, qualitative research and/or mixed methods. The following is a description of each along with their advantages and disadvantages.
Quantitative research
Quantitative research uses numerical data (quantities) deriving, for example, from experiments, closed questions in surveys, questionnaires, structured interviews or published data sets (Cottrell, 2014, p93). It normally processes and analyses this data using quantitative analysis techniques like tables, graphs and statistics to explore, present and examine relationships and trends within the data (Saunders, Lewis and Thornhill, 2015, p496).
Advantages | Disadvantages |
The study can be undertaken on a broader scale, generating large amounts of data that contribute to generalisation of results | Quantitative methods can be difficult, expensive and time consuming (especially if using primary data, rather than secondary data). |
Suitable when the phenomenon is relatively simple, and can be analysed according to identified variables. | Not everything can be easily measured. |
| Less suitable for complex social phenomena. |
| Less suitable for why type questions. |
Qualitative research
Qualitative research is generally undertaken to study human behaviour and psyche. It uses methods like in-depth case studies, open-ended survey questions, unstructured interviews, focus groups, or unstructured observations (Cottrell, 2014, p93). The nature of the data is subjective, and also the analysis of the researcher involves a degree of subjective interpretation. Subjectivity can be controlled for in the research design, or has to be acknowledged as a feature of the research. Subject-specific books on (qualitative) research methods offer guidance on such research designs.
Advantages | Disadvantages |
Qualitative methods are good for in-depth analysis of individual people, businesses, organisations, events. | The findings can be accurate about the particular case, but not generally applicable. |
Sample sizes don’t need to be large, so the studies can be cheaper and simpler. | More prone to subjectivity. |
Mixed methods
Mixed-method approaches combine both qualitative and quantitative methods, and therefore combine the strengths of both types of research. Mixed methods have gained popularity in recent years.
When undertaking mixed-methods research you can collect the qualitative and quantitative data either concurrently or sequentially. If sequentially, you can for example, start with a few semi-structured interviews, providing qualitative insights, and then design a questionnaire to obtain quantitative evidence that your qualitative findings can also apply to a wider population (Specht, 2019, p138).
Ultimately, your methodology chapter should state:
Whether you used quantitative research, qualitative research or mixed methods.
Why you chose such methods (and refer to research method sources).
Why you rejected other methods.
How well the method served your research.
The problems or limitations you encountered.
Doug Specht, Senior Lecturer at the Westminster School of Media and Communication, explains mixed methods research in the following video:
LinkedIn Learning Video on Academic Research Foundations: Quantitative
The video covers the characteristics of quantitative research, and explains how to approach different parts of the research process, such as creating a solid research question and developing a literature review. He goes over the elements of a study, explains how to collect and analyze data, and shows how to present your data in written and numeric form.
Link to quantitative research video
Some Types of Methods
There are several methods you can use to get primary data. To reiterate, the choice of the methods should depend on your research question/hypothesis.
Whatever methods you will use, you will need to consider:
why did you choose one technique over another? What were the advantages and disadvantages of the technique you chose?
what was the size of your sample? Who made up your sample? How did you select your sample population? Why did you choose that particular sampling strategy?)
ethical considerations (see also tab...)
safety considerations
validity
feasibility
recording
procedure of the research (see box procedural method...).
Check Stella Cottrell's book Dissertations and Project Reports: A Step by Step Guide for some succinct yet comprehensive information on most methods (the following account draws mostly on her work). Check a research methods book in your discipline for more specific guidance.
Experiments
Experiments are useful to investigate cause and effect, when the variables can be tightly controlled. They can test a theory or hypothesis in controlled conditions. Experiments do not prove or disprove an hypothesis, instead they support or not support an hypothesis. When using the empirical and inductive method it is not possible to achieve conclusive results. The results may only be valid until falsified by other experiments and observations.
For more information on Scientific Method, click here .
Observations
Observational methods are useful for in-depth analyses of behaviours in people, animals, organisations, events or phenomena. They can test a theory or products in real life or simulated settings. They generally a qualitative research method.
Questionnaires and surveys
Questionnaires and surveys are useful to gain opinions, attitudes, preferences, understandings on certain matters. They can provide quantitative data that can be collated systematically; qualitative data, if they include opportunities for open-ended responses; or both qualitative and quantitative elements.
Interviews
Interviews are useful to gain rich, qualitative information about individuals' experiences, attitudes or perspectives. With interviews you can follow up immediately on responses for clarification or further details. There are three main types of interviews: structured (following a strict pattern of questions, which expect short answers), semi-structured (following a list of questions, with the opportunity to follow up the answers with improvised questions), and unstructured (following a short list of broad questions, where the respondent can lead more the conversation) (Specht, 2019, p142).
This short video on qualitative interviews discusses best practices and covers qualitative interview design, preparation and data collection methods.
Focus groups
In this case, a group of people (normally, 4-12) is gathered for an interview where the interviewer asks questions to such group of participants. Group interactions and discussions can be highly productive, but the researcher has to beware of the group effect, whereby certain participants and views dominate the interview (Saunders, Lewis and Thornhill 2015, p419). The researcher can try to minimise this by encouraging involvement of all participants and promoting a multiplicity of views.
This video focuses on strategies for conducting research using focus groups.
Check out the guidance on online focus groups by Aliaksandr Herasimenka, which is attached at the bottom of this text box.
Case study
Case studies are often a convenient way to narrow the focus of your research by studying how a theory or literature fares with regard to a specific person, group, organisation, event or other type of entity or phenomenon you identify. Case studies can be researched using other methods, including those described in this section. Case studies give in-depth insights on the particular reality that has been examined, but may not be representative of what happens in general, they may not be generalisable, and may not be relevant to other contexts. These limitations have to be acknowledged by the researcher.
Content analysis
Content analysis consists in the study of words or images within a text. In its broad definition, texts include books, articles, essays, historical documents, speeches, conversations, advertising, interviews, social media posts, films, theatre, paintings or other visuals. Content analysis can be quantitative (e.g. word frequency) or qualitative (e.g. analysing intention and implications of the communication). It can detect propaganda, identify intentions of writers, and can see differences in types of communication (Specht, 2019, p146). Check this page on collecting, cleaning and visualising Twitter data.
Extra links and resources:
Research Methods
A clear and comprehensive overview of research methods by Emerald Publishing. It includes: crowdsourcing as a research tool; mixed methods research; case study; discourse analysis; ground theory; repertory grid; ethnographic method and participant observation; interviews; focus group; action research; analysis of qualitative data; survey design; questionnaires; statistics; experiments; empirical research; literature review; secondary data and archival materials; data collection.
Doing your dissertation during the COVID-19 pandemic
Resources providing guidance on doing dissertation research during the pandemic: Online research methods; Secondary data sources; Webinars, conferences and podcasts;
- Virtual Focus Groups Guidance on managing virtual focus groups
5 Minute Methods Videos
The following are a series of useful videos that introduce research methods in five minutes. These resources have been produced by lecturers and students with the University of Westminster's School of Media and Communication.
Case Study Research
Research Ethics
Quantitative Content Analysis
Sequential Analysis
Qualitative Content Analysis
Thematic Analysis
Social Media Research
Mixed Method Research
Procedural Method
In this part, provide an accurate, detailed account of the methods and procedures that were used in the study or the experiment (if applicable!).
Include specifics about participants, sample, materials, design and methods.
If the research involves human subjects, then include a detailed description of who and how many participated along with how the participants were selected.
Describe all materials used for the study, including equipment, written materials and testing instruments.
Identify the study's design and any variables or controls employed.
Write out the steps in the order that they were completed.
Indicate what participants were asked to do, how measurements were taken and any calculations made to raw data collected.
Specify statistical techniques applied to the data to reach your conclusions.
Provide evidence that you incorporated rigor into your research. This is the quality of being thorough and accurate and considers the logic behind your research design.
Highlight any drawbacks that may have limited your ability to conduct your research thoroughly.
You have to provide details to allow others to replicate the experiment and/or verify the data, to test the validity of the research.
Bibliography
Cottrell, S. (2014). Dissertations and project reports: a step by step guide. Hampshire, England: Palgrave Macmillan.
Lombard, E. (2010). Primary and secondary sources. The Journal of Academic Librarianship , 36(3), 250-253
Saunders, M.N.K., Lewis, P. and Thornhill, A. (2015). Research Methods for Business Students. New York: Pearson Education.
Specht, D. (2019). The Media And Communications Study Skills Student Guide . London: University of Westminster Press.
- << Previous: Introduction & Philosophy
- Next: Ethics >>
- Last Updated: Sep 14, 2022 12:58 PM
- URL: https://libguides.westminster.ac.uk/methodology-for-dissertations
CONNECT WITH US
Write Your Dissertation Using Only Secondary Research
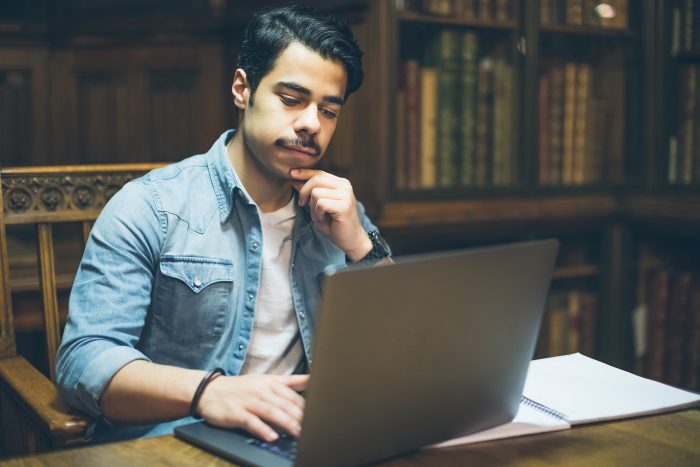
Writing a dissertation is already difficult to begin with but it can appear to be a daunting challenge when you only have other people’s research as a guide for proving a brand new hypothesis! You might not be familiar with the research or even confident in how to use it but if secondary research is what you’re working with then you’re in luck. It’s actually one of the easiest methods to write about!
Secondary research is research that has already been carried out and collected by someone else. It means you’re using data that’s already out there rather than conducting your own research – this is called primary research. Thankfully secondary will save you time in the long run! Primary research often means spending time finding people and then relying on them for results, something you could do without, especially if you’re in a rush. Read more about the advantages and disadvantages of primary research .
So, where do you find secondary data?
Secondary research is available in many different places and it’s important to explore all areas so you can be sure you’re looking at research you can trust. If you’re just starting your dissertation you might be feeling a little overwhelmed with where to begin but once you’ve got your subject clarified, it’s time to get researching! Some good places to search include:
- Libraries (your own university or others – books and journals are the most popular resources!)
- Government records
- Online databases
- Credible Surveys (this means they need to be from a reputable source)
- Search engines (google scholar for example).
The internet has everything you’ll need but you’ve got to make sure it’s legitimate and published information. It’s also important to check out your student library because it’s likely you’ll have access to a great range of materials right at your fingertips. There’s a strong chance someone before you has looked for the same topic so it’s a great place to start.
What are the two different types of secondary data?
It’s important to know before you start looking that they are actually two different types of secondary research in terms of data, Qualitative and quantitative. You might be looking for one more specifically than the other, or you could use a mix of both. Whichever it is, it’s important to know the difference between them.
- Qualitative data – This is usually descriptive data and can often be received from interviews, questionnaires or observations. This kind of data is usually used to capture the meaning behind something.
- Quantitative data – This relates to quantities meaning numbers. It consists of information that can be measured in numerical data sets.
The type of data you want to be captured in your dissertation will depend on your overarching question – so keep it in mind throughout your search!
Getting started
When you’re getting ready to write your dissertation it’s a good idea to plan out exactly what you’re looking to answer. We recommend splitting this into chapters with subheadings and ensuring that each point you want to discuss has a reliable source to back it up. This is always a good way to find out if you’ve collected enough secondary data to suit your workload. If there’s a part of your plan that’s looking a bit empty, it might be a good idea to do some more research and fill the gap. It’s never a bad thing to have too much research, just as long as you know what to do with it and you’re willing to disregard the less important parts. Just make sure you prioritise the research that backs up your overall point so each section has clarity.
Then it’s time to write your introduction. In your intro, you will want to emphasise what your dissertation aims to cover within your writing and outline your research objectives. You can then follow up with the context around this question and identify why your research is meaningful to a wider audience.
The body of your dissertation
Before you get started on the main chapters of your dissertation, you need to find out what theories relate to your chosen subject and the research that has already been carried out around it.
Literature Reviews
Your literature review will be a summary of any previous research carried out on the topic and should have an intro and conclusion like any other body of the academic text. When writing about this research you want to make sure you are describing, summarising, evaluating and analysing each piece. You shouldn’t just rephrase what the researcher has found but make your own interpretations. This is one crucial way to score some marks. You also want to identify any themes between each piece of research to emphasise their relevancy. This will show that you understand your topic in the context of others, a great way to prove you’ve really done your reading!
Theoretical Frameworks
The theoretical framework in your dissertation will be explaining what you’ve found. It will form your main chapters after your lit review. The most important part is that you use it wisely. Of course, depending on your topic there might be a lot of different theories and you can’t include them all so make sure to select the ones most relevant to your dissertation. When starting on the framework it’s important to detail the key parts to your hypothesis and explain them. This creates a good foundation for what you’re going to discuss and helps readers understand the topic.
To finish off the theoretical framework you want to start suggesting where your research will fit in with those texts in your literature review. You might want to challenge a theory by critiquing it with another or explain how two theories can be combined to make a new outcome. Either way, you must make a clear link between their theories and your own interpretations – remember, this is not opinion based so don’t make a conclusion unless you can link it back to the facts!
Concluding your dissertation
Your conclusion will highlight the outcome of the research you’ve undertaken. You want to make this clear and concise without repeating information you’ve already mentioned in your main body paragraphs. A great way to avoid repetition is to highlight any overarching themes your conclusions have shown
When writing your conclusion it’s important to include the following elements:
- Summary – A summary of what you’ve found overall from your research and the conclusions you have come to as a result.
- Recommendations – Recommendations on what you think the next steps should be. Is there something you would change about this research to improve it or further develop it?
- Show your contribution – It’s important to show how you’ve contributed to the current knowledge on the topic and not just repeated what other researchers have found.
Hopefully, this helps you with your secondary data research for your dissertation! It’s definitely not as hard as it seems, the hardest part will be gathering all of the information in the first place. It may take a while but once you’ve found your flow – it’ll get easier, promise! You may also want to read about the advantages and disadvantages of secondary research .
You may also like
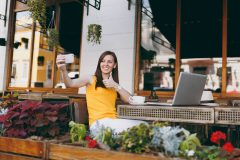
- +44 (0) 207 391 9032
Recent Posts
- The Complete Guide to Copy Editing: Roles, Rates, Skills, and Process
- How to Write a Paragraph: Successful Essay Writing Strategies
- Everything You Should Know About Academic Writing: Types, Importance, and Structure
- Concise Writing: Tips, Importance, and Exercises for a Clear Writing Style
- How to Write a PhD Thesis: A Step-by-Step Guide for Success
- How to Use AI in Essay Writing: Tips, Tools, and FAQs
- Copy Editing Vs Proofreading: What’s The Difference?
- How Much Does It Cost To Write A Thesis? Get Complete Process & Tips
- How Much Do Proofreading Services Cost in 2024? Per Word and Hourly Rates With Charts
- Academic Editing: What It Is and Why It Matters
- Academic News
- Custom Essays
- Dissertation Writing
- Essay Marking
- Essay Writing
- Essay Writing Companies
- Model Essays
- Model Exam Answers
- Oxbridge Essays Updates
- PhD Writing
- Significant Academics
- Student News
- Study Skills
- University Applications
- University Essays
- University Life
- Writing Tips

How to do your dissertation secondary research in 4 steps
(Last updated: 12 May 2021)
Since 2006, Oxbridge Essays has been the UK’s leading paid essay-writing and dissertation service
We have helped 10,000s of undergraduate, Masters and PhD students to maximise their grades in essays, dissertations, model-exam answers, applications and other materials. If you would like a free chat about your project with one of our UK staff, then please just reach out on one of the methods below.
If you are reading this guide, it's very likely you may be doing secondary research for your dissertation, rather than primary. If this is indeed you, then here's the good news: secondary research is the easiest type of research! Congratulations!
In a nutshell, secondary research is far more simple. So simple, in fact, that we have been able to explain how to do it completely in just 4 steps (see below). If nothing else, secondary research avoids the all-so-tiring efforts usually involved with primary research. Like recruiting your participants, choosing and preparing your measures, and spending days (or months) collecting your data.
That said, you do still need to know how to do secondary research. Which is what you're here for. So, go make a decent-sized mug of your favourite hot beverage (consider a glass of water , too) then come back and get comfy.
Here's what we'll cover in this guide:
The basics: What's secondary research all about?
Understanding secondary research, advantages of secondary research, disadvantages of secondary research, methods and purposes of secondary research, types of secondary data, sources of secondary data, secondary research process in 4 steps, step 1: develop your research question(s), step 2: identify a secondary data set, step 3: evaluate a secondary data set, step 4: prepare and analyse secondary data.
To answer this question, let’s first recall what we mean by primary research . As you probably already know, primary research is when the researcher collects the data himself or herself. The researcher uses so-called “real-time” data, which means that the data is collected during the course of a specific research project and is under the researcher’s direct control.
In contrast, secondary research involves data that has been collected by somebody else previously. This type of data is called “past data” and is usually accessible via past researchers, government records, and various online and offline resources.
So to recap, secondary research involves re-analysing, interpreting, or reviewing past data. The role of the researcher is always to specify how this past data informs his or her current research.
In contrast to primary research, secondary research is easier, particularly because the researcher is less involved with the actual process of collecting the data. Furthermore, secondary research requires less time and less money (i.e., you don’t need to provide your participants with compensation for participating or pay for any other costs of the research).
Comparison basis | PRIMARY RESEARCH | SECONDARY RESEARCH |
---|---|---|
Definition | Involves collecting factual, first-hand data at the time of the research project | Involves the use of data that was collected by somebody else in the past |
Type of data | Real-time data | Past data |
Conducted by | The researcher himself/herself | Somebody else |
Needs | Addresses specific needs of the researcher | May not directly address the researcher’s needs |
Involvement | Researcher is very involved | Researcher is less involved |
Completion time | Long | Short |
Cost | High | Low |
One of the most obvious advantages is that, compared to primary research, secondary research is inexpensive . Primary research usually requires spending a lot of money. For instance, members of the research team should be paid salaries. There are often travel and transportation costs. You may need to pay for office space and equipment, and compensate your participants for taking part. There may be other overhead costs too.
These costs do not exist when doing secondary research. Although researchers may need to purchase secondary data sets, this is always less costly than if the research were to be conducted from scratch.
As an undergraduate or graduate student, your dissertation project won't need to be an expensive endeavour. Thus, it is useful to know that you can further reduce costs, by using freely available secondary data sets.
But this is far from the only consideration.
Most students value another important advantage of secondary research, which is that secondary research saves you time . Primary research usually requires months spent recruiting participants, providing them with questionnaires, interviews, or other measures, cleaning the data set, and analysing the results. With secondary research, you can skip most of these daunting tasks; instead, you merely need to select, prepare, and analyse an existing data set.
Moreover, you probably won’t need a lot of time to obtain your secondary data set, because secondary data is usually easily accessible . In the past, students needed to go to libraries and spend hours trying to find a suitable data set. New technologies make this process much less time-consuming. In most cases, you can find your secondary data through online search engines or by contacting previous researchers via email.
A third important advantage of secondary research is that you can base your project on a large scope of data . If you wanted to obtain a large data set yourself, you would need to dedicate an immense amount of effort. What's more, if you were doing primary research, you would never be able to use longitudinal data in your graduate or undergraduate project, since it would take you years to complete. This is because longitudinal data involves assessing and re-assessing a group of participants over long periods of time.
When using secondary data, however, you have an opportunity to work with immensely large data sets that somebody else has already collected. Thus, you can also deal with longitudinal data, which may allow you to explore trends and changes of phenomena over time.
With secondary research, you are relying not only on a large scope of data, but also on professionally collected data . This is yet another advantage of secondary research. For instance, data that you will use for your secondary research project has been collected by researchers who are likely to have had years of experience in recruiting representative participant samples, designing studies, and using specific measurement tools.
If you had collected this data yourself, your own data set would probably have more flaws, simply because of your lower level of expertise when compared to these professional researchers.
The first such disadvantage is that your secondary data may be, to a greater or lesser extent, inappropriate for your own research purposes. This is simply because you have not collected the data yourself.
When you collect your data personally, you do so with a specific research question in mind. This makes it easy to obtain the relevant information. However, secondary data was always collected for the purposes of fulfilling other researchers’ goals and objectives.
Thus, although secondary data may provide you with a large scope of professionally collected data, this data is unlikely to be fully appropriate to your own research question. There are several reasons for this. For instance, you may be interested in the data of a particular population, in a specific geographic region, and collected during a specific time frame. However, your secondary data may have focused on a slightly different population, may have been collected in a different geographical region, or may have been collected a long time ago.
Apart from being potentially inappropriate for your own research purposes, secondary data could have a different format than you require. For instance, you might have preferred participants’ age to be in the form of a continuous variable (i.e., you want your participants to have indicated their specific age). But the secondary data set may contain a categorical age variable; for example, participants might have indicated an age group they belong to (e.g., 20-29, 30-39, 40-49, etc.). Or another example: A secondary data set may contain too few ethnic categories (e.g., “White” and “Other”), while you would ideally want a wider range of racial categories (e.g., “White”, “Black or African American”, “American Indian”, and “Asian”). Differences such as these mean that secondary data may not be perfectly appropriate for your research.
The above two disadvantages may lead to yet another one: the existing data set may not answer your own research question(s) in an ideal way. As noted above, secondary data was collected with a different research question in mind, and this may limit its application to your own research purpose.
Unfortunately, the list of disadvantages does not end here. An additional weakness of secondary data is that you have a lack of control over the quality of data. All researchers need to establish that their data is reliable and valid. But if the original researchers did not establish the reliability and validity of their data, this may limit its reliability and validity for your research as well. To establish reliability and validity, you are usually advised to critically evaluate how the data was gathered, analysed, and presented.
But here lies the final disadvantage of doing secondary research: original researchers may fail to provide sufficient information on how their research was conducted. You might be faced with a lack of information on recruitment procedures, sample representativeness, data collection methods, employed measurement tools and statistical analyses, and the like. This may require you to take extra steps to obtain such information, if that is possible at all.
ADVANTAGES | DISADVANTAGES |
---|---|
Inexpensive: Conducting secondary research is much cheaper than doing primary research | Inappropriateness: Secondary data may not be fully appropriate for your research purposes |
Saves time: Secondary research takes much less time than primary research | Wrong format: Secondary data may have a different format than you require |
Accessibility: Secondary data is usually easily accessible from online sources. | May not answer your research question: Secondary data was collected with a different research question in mind |
Large scope of data: You can rely on immensely large data sets that somebody else has collected | Lack of control over the quality of data: Secondary data may lack reliability and validity, which is beyond your control |
Professionally collected data: Secondary data has been collected by researchers with years of experience | Lack of sufficient information: Original authors may not have provided sufficient information on various research aspects |

At this point, we should ask: “What are the methods of secondary research?” and “When do we use each of these methods?” Here, we can differentiate between three methods of secondary research: using a secondary data set in isolation , combining two secondary data sets, and combining secondary and primary data sets. Let’s outline each of these separately, and also explain when to use each of these methods.
Initially, you can use a secondary data set in isolation – that is, without combining it with other data sets. You dig and find a data set that is useful for your research purposes and then base your entire research on that set of data. You do this when you want to re-assess a data set with a different research question in mind.
Let’s illustrate this with a simple example. Suppose that, in your research, you want to investigate whether pregnant women of different nationalities experience different levels of anxiety during different pregnancy stages. Based on the literature, you have formed an idea that nationality may matter in this relationship between pregnancy and anxiety.
If you wanted to test this relationship by collecting the data yourself, you would need to recruit many pregnant women of different nationalities and assess their anxiety levels throughout their pregnancy. It would take you at least a year to complete this research project.
Instead of undertaking this long endeavour, you thus decide to find a secondary data set – one that investigated (for instance) a range of difficulties experienced by pregnant women in a nationwide sample. The original research question that guided this research could have been: “to what extent do pregnant women experience a range of mental health difficulties, including stress, anxiety, mood disorders, and paranoid thoughts?” The original researchers might have outlined women’s nationality, but weren’t particularly interested in investigating the link between women’s nationality and anxiety at different pregnancy stages. You are, therefore, re-assessing their data set with your own research question in mind.
Your research may, however, require you to combine two secondary data sets . You will use this kind of methodology when you want to investigate the relationship between certain variables in two data sets or when you want to compare findings from two past studies.
To take an example: One of your secondary data sets may focus on a target population’s tendency to smoke cigarettes, while the other data set focuses on the same population’s tendency to drink alcohol. In your own research, you may thus be looking at whether there is a correlation between smoking and drinking among this population.
Here is a second example: Your two secondary data sets may focus on the same outcome variable, such as the degree to which people go to Greece for a summer vacation. However, one data set could have been collected in Britain and the other in Germany. By comparing these two data sets, you can investigate which nation tends to visit Greece more.
Finally, your research project may involve combining primary and secondary data . You may decide to do this when you want to obtain existing information that would inform your primary research.
Let’s use another simple example and say that your research project focuses on American versus British people’s attitudes towards racial discrimination. Let’s say that you were able to find a recent study that investigated Americans’ attitudes of these kind, which were assessed with a certain set of measures. However, your search finds no recent studies on Britons’ attitudes. Let’s also say that you live in London and that it would be difficult for you to assess Americans’ attitudes on the topic, but clearly much more straightforward to conduct primary research on British attitudes.
In this case, you can simply reuse the data from the American study and adopt exactly the same measures with your British participants. Your secondary data is being combined with your primary data. Alternatively, you may combine these types of data when the role of your secondary data is to outline descriptive information that supports your research. For instance, if your project is focusing on attitudes towards McDonald’s food, you may want to support your primary research with secondary data that outlines how many people eat McDonald’s in your country of choice.
TABLE 3 summarises particular methods and purposes of secondary research:
METHOD | PURPOSE |
---|---|
Using secondary data set in isolation | Re-assessing a data set with a different research question in mind |
Combining two secondary data sets | Investigating the relationship between variables in two data sets or comparing findings from two past studies |
Combining secondary and primary data sets | Obtaining existing information that informs your primary research |
We have already provided above several examples of using quantitative secondary data. This type of data is used when the original study has investigated a population’s tendency to smoke or drink alcohol, the degree to which people from different nationalities go to Greece for their summer vacation, or the degree to which pregnant women experience anxiety.
In all these examples, outcome variables were assessed by questionnaires, and thus the obtained data was numerical.
Quantitative secondary research is much more common than qualitative secondary research. However, this is not to say that you cannot use qualitative secondary data in your research project. This type of secondary data is used when you want the previously-collected information to inform your current research. More specifically, it is used when you want to test the information obtained through qualitative research by implementing a quantitative methodology.
For instance, a past qualitative study might have focused on the reasons why people choose to live on boats. This study might have interviewed some 30 participants and noted the four most important reasons people live on boats: (1) they can lead a transient lifestyle, (2) they have an increased sense of freedom, (3) they feel that they are “world citizens”, and (4) they can more easily visit their family members who live in different locations. In your own research, you can therefore reuse this qualitative data to form a questionnaire, which you then give to a larger population of people who live on boats. This will help you to generalise the previously-obtained qualitative results to a broader population.
Importantly, you can also re-assess a qualitative data set in your research, rather than using it as a basis for your quantitative research. Let’s say that your research focuses on the kind of language that people who live on boats use when describing their transient lifestyles. The original research did not focus on this research question per se – however, you can reuse the information from interviews to “extract” the types of descriptions of a transient lifestyle that were given by participants.
TABLE 4 highlights the two main types of secondary data and their associated purposes:
TYPES | PURPOSES |
---|---|
Quantitative | Both can be used when you want to (a) inform your current research with past data, and (b) re-assess a past data set |
Qualitative | Both can be used when you want to (a) inform your current research with past data, and (b) re-assess a past data set |
Internal sources of data are those that are internal to the organisation in question. For instance, if you are doing a research project for an organisation (or research institution) where you are an intern, and you want to reuse some of their past data, you would be using internal data sources.
The benefit of using these sources is that they are easily accessible and there is no associated financial cost of obtaining them.
External sources of data, on the other hand, are those that are external to an organisation or a research institution. This type of data has been collected by “somebody else”, in the literal sense of the term. The benefit of external sources of data is that they provide comprehensive data – however, you may sometimes need more effort (or money) to obtain it.
Let’s now focus on different types of internal and external secondary data sources.
There are several types of internal sources. For instance, if your research focuses on an organisation’s profitability, you might use their sales data . Each organisation keeps a track of its sales records, and thus your data may provide information on sales by geographical area, types of customer, product prices, types of product packaging, time of the year, and the like.
Alternatively, you may use an organisation’s financial data . The purpose of using this data could be to conduct a cost-benefit analysis and understand the economic opportunities or outcomes of hiring more people, buying more vehicles, investing in new products, and so on.
Another type of internal data is transport data . Here, you may focus on outlining the safest and most effective transportation routes or vehicles used by an organisation.
Alternatively, you may rely on marketing data , where your goal would be to assess the benefits and outcomes of different marketing operations and strategies.
Some other ideas would be to use customer data to ascertain the ideal type of customer, or to use safety data to explore the degree to which employees comply with an organisation’s safety regulations.
The list of the types of internal sources of secondary data can be extensive; the most important thing to remember is that this data comes from a particular organisation itself, in which you do your research in an internal manner.
The list of external secondary data sources can be just as extensive. One example is the data obtained through government sources . These can include social surveys, health data, agricultural statistics, energy expenditure statistics, population censuses, import/export data, production statistics, and the like. Government agencies tend to conduct a lot of research, therefore covering almost any kind of topic you can think of.
Another external source of secondary data are national and international institutions , including banks, trade unions, universities, health organisations, etc. As with government, such institutions dedicate a lot of effort to conducting up-to-date research, so you simply need to find an organisation that has collected the data on your own topic of interest.
Alternatively, you may obtain your secondary data from trade, business, and professional associations . These usually have data sets on business-related topics and are likely to be willing to provide you with secondary data if they understand the importance of your research. If your research is built on past academic studies, you may also rely on scientific journals as an external data source.
Once you have specified what kind of secondary data you need, you can contact the authors of the original study.
As a final example of a secondary data source, you can rely on data from commercial research organisations. These usually focus their research on media statistics and consumer information, which may be relevant if, for example, your research is within media studies or you are investigating consumer behaviour.
INTERNAL SOURCES | EXTERNAL SOURCES |
---|---|
Definition: Internal to the organisation or research institution where you conduct your research | Definition: External to the organisation or research institution where you conduct your research |
Examples: • Sales data • Financial data • Transport data • Marketing data • Customer data • Safety data | Examples: |
At this point, you should have a clearer understanding of secondary research in general terms.
Now it may be useful to focus on the actual process of doing secondary research. This next section is organised to introduce you to each step of this process, so that you can rely on this guide while planning your study. At the end of this blog post, in Table 6 , you will find a summary of all the steps of doing secondary research.
For an undergraduate thesis, you are often provided with a specific research question by your supervisor. But for most other types of research, and especially if you are doing your graduate thesis, you need to arrive at a research question yourself.
The first step here is to specify the general research area in which your research will fall. For example, you may be interested in the topic of anxiety during pregnancy, or tourism in Greece, or transient lifestyles. Since we have used these examples previously, it may be useful to rely on them again to illustrate our discussion.
Once you have identified your general topic, your next step consists of reading through existing papers to see whether there is a gap in the literature that your research can fill. At this point, you may discover that previous research has not investigated national differences in the experiences of anxiety during pregnancy, or national differences in a tendency to go to Greece for a summer vacation, or that there is no literature generalising the findings on people’s choice to live on boats.
Having found your topic of interest and identified a gap in the literature, you need to specify your research question. In our three examples, research questions would be specified in the following manner: (1) “Do women of different nationalities experience different levels of anxiety during different stages of pregnancy?”, (2) “Are there any differences in an interest in Greek tourism between Germans and Britons?”, and (3) “Why do people choose to live on boats?”.
It is at this point, after reviewing the literature and specifying your research questions, that you may decide to rely on secondary data. You will do this if you discover that there is past data that would be perfectly reusable in your own research, therefore helping you to answer your research question more thoroughly (and easily).
But how do you discover if there is past data that could be useful for your research? You do this through reviewing the literature on your topic of interest. During this process, you will identify other researchers, organisations, agencies, or research centres that have explored your research topic.
Somewhere there, you may discover a useful secondary data set. You then need to contact the original authors and ask for a permission to use their data. (Note, however, that this happens only if you are relying on external sources of secondary data. If you are doing your research internally (i.e., within a particular organisation), you don’t need to search through the literature for a secondary data set – you can just reuse some past data that was collected within the organisation itself.)
In any case, you need to ensure that a secondary data set is a good fit for your own research question. Once you have established that it is, you need to specify the reasons why you have decided to rely on secondary data.
For instance, your choice to rely on secondary data in the above examples might be as follows: (1) A recent study has focused on a range of mental difficulties experienced by women in a multinational sample and this data can be reused; (2) There is existing data on Germans’ and Britons’ interest in Greek tourism and these data sets can be compared; and (3) There is existing qualitative research on the reasons for choosing to live on boats, and this data can be relied upon to conduct a further quantitative investigation.
Because such disadvantages of secondary data can limit the effectiveness of your research, it is crucial that you evaluate a secondary data set. To ease this process, we outline here a reflective approach that will allow you to evaluate secondary data in a stepwise fashion.
Step 3(a): What was the aim of the original study?
During this step, you also need to pay close attention to any differences in research purposes and research questions between the original study and your own investigation. As we have discussed previously, you will often discover that the original study had a different research question in mind, and it is important for you to specify this difference.
Let’s put this step of identifying the aim of the original study in practice, by referring to our three research examples. The aim of the first research example was to investigate mental difficulties (e.g., stress, anxiety, mood disorders, and paranoid thoughts) in a multinational sample of pregnant women.
How does this aim differ from your research aim? Well, you are seeking to reuse this data set to investigate national differences in anxiety experienced by women during different pregnancy stages. When it comes to the second research example, you are basing your research on two secondary data sets – one that aimed to investigate Germans’ interest in Greek tourism and the other that aimed to investigate Britons’ interest in Greek tourism.
While these two studies focused on particular national populations, the aim of your research is to compare Germans’ and Britons’ tendency to visit Greece for summer vacation. Finally, in our third example, the original research was a qualitative investigation into the reasons for living on boats. Your research question is different, because, although you are seeking to do the same investigation, you wish to do so by using a quantitative methodology.
Importantly, in all three examples, you conclude that secondary data may in fact answer your research question. If you conclude otherwise, it may be wise to find a different secondary data set or to opt for primary research.
Step 3(b): Who has collected the data?
Let’s say that, in our example of research on pregnancy, data was collected by the UK government; that in our example of research on Greek tourism, the data was collected by a travel agency; and that in our example of research on the reasons for choosing to live on boats, the data was collected by researchers from a UK university.
Let’s also say that you have checked the background of these organisations and researchers, and that you have concluded that they all have a sufficiently professional background, except for the travel agency. Given that this agency’s research did not lead to a publication (for instance), and given that not much can be found about the authors of the research, you conclude that the professionalism of this data source remains unclear.
Step 3(c): Which measures were employed?
Original authors should have documented all their sample characteristics, measures, procedures, and protocols. This information can be obtained either in their final research report or through contacting the authors directly.
It is important for you to know what type of data was collected, which measures were used, and whether such measures were reliable and valid (if they were quantitative measures). You also need to make a clear outline of the type of data collected – and especially the data relevant for your research.
Let’s say that, in our first example, researchers have (among other assessed variables) used a demographic measure to note women’s nationalities and have used the State Anxiety Inventory to assess women’s anxiety levels during different pregnancy stages, both of which you conclude are valid and reliable tools. In our second example, the authors might have crafted their own measure to assess interest in Greek tourism, but there may be no established validity and reliability for this measure. And in our third example, the authors have employed semi-structured interviews, which cover the most important reasons for wanting to live on boats.
Step 3(d): When was the data collected?
Ideally, you want your secondary data to have been collected within the last five years. For the sake of our examples, let’s say that all three original studies were conducted within this time-range.
Step 3(e): What methodology was used to collect the data?
We have already noted that you need to evaluate the reliability and validity of employed measures. In addition to this, you need to evaluate how the sample was obtained, whether the sample was large enough, if the sample was representative of the population, if there were any missing responses on employed measures, whether confounders were controlled for, and whether the employed statistical analyses were appropriate. Any drawbacks in the original methodology may limit your own research as well.
For the sake of our examples, let’s say that the study on mental difficulties in pregnant women recruited a representative sample of pregnant women (i.e., they had different nationalities, different economic backgrounds, different education levels, etc.) in maternity wards of seven hospitals; that the sample was large enough (N = 945); that the number of missing values was low; that many confounders were controlled for (e.g., education level, age, presence of partnership, etc.); and that statistical analyses were appropriate (e.g., regression analyses were used).
Let’s further say that our second research example had slightly less sufficient methodology. Although the number of participants in the two samples was high enough (N1 = 453; N2 = 488), the number of missing values was low, and statistical analyses were appropriate (descriptive statistics), the authors failed to report how they recruited their participants and whether they controlled for any confounders.
Let’s say that these authors also failed to provide you with more information via email. Finally, let’s assume that our third research example also had sufficient methodology, with a sufficiently large sample size for a qualitative investigation (N = 30), high sample representativeness (participants with different backgrounds, coming from different boat communities), and sufficient analyses (thematic analysis).
Note that, since this was a qualitative investigation, there is no need to evaluate the number of missing values and the use of confounders.
Step 3(f): Making a final evaluation
We would conclude that the secondary data from our first research example has a high quality. Data was recently collected by professionals, the employed measures were both reliable and valid, and the methodology was more than sufficient. We can be confident that our new research question can be sufficiently answered with the existing data. Thus, the data set for our first example is ideal.
The two secondary data sets from our second research example seem, however, less than ideal. Although we can answer our research questions on the basis of these recent data sets, the data was collected by an unprofessional source, the reliability and validity of the employed measure is uncertain, and the employed methodology has a few notable drawbacks.
Finally, the data from our third example seems sufficient both for answering our research question and in terms of the specific evaluations (data was collected recently by a professional source, semi-structured interviews were well made, and the employed methodology was sufficient).
The final question to ask is: “what can be done if our evaluation reveals the lack of appropriateness of secondary data?”. The answer, unfortunately, is “nothing”. In this instance, you can only note the drawbacks of the original data set, present its limitations, and conclude that your own research may not be sufficiently well grounded.

Your first sub-step here (if you are doing quantitative research) is to outline all variables of interest that you will use in your study. In our first example, you could have at least five variables of interest: (1) women’s nationality, (2) anxiety levels at the beginning of pregnancy, (3) anxiety levels at three months of pregnancy, (4) anxiety levels at six months of pregnancy, and (5) anxiety levels at nine months of pregnancy. In our second example, you will have two variables of interest: (1) participants’ nationality, and (2) the degree of interest in going to Greece for a summer vacation. Once your variables of interest are identified, you need to transfer this data into a new SPSS or Excel file. Remember simply to copy this data into the new file – it is vital that you do not alter it!
Once this is done, you should address missing data (identify and label them) and recode variables if necessary (e.g., giving a value of 1 to German participants and a value of 2 to British participants). You may also need to reverse-score some items, so that higher scores on all items indicate a higher degree of what is being assessed.
Most of the time, you will also need to create new variables – that is, to compute final scores. For instance, in our example of research on anxiety during pregnancy, your data will consist of scores on each item of the State Anxiety Inventory, completed at various times during pregnancy. You will need to calculate final anxiety scores for each time the measure was completed.
Your final step consists of analysing the data. You will always need to decide on the most suitable analysis technique for your secondary data set. In our first research example, you would rely on MANOVA (to see if women of different nationalities experience different stress levels at the beginning, at three months, at six months, and at nine months of pregnancy); and in our second example, you would use an independent samples t-test (to see if interest in Greek tourism differs between Germans and Britons).
The process of preparing and analysing a secondary data set is slightly different if your secondary data is qualitative. In our example on the reasons for living on boats, you would first need to outline all reasons for living on boats, as recognised by the original qualitative research. Then you would need to craft a questionnaire that assesses these reasons in a broader population.
Finally, you would need to analyse the data by employing statistical analyses.
Note that this example combines qualitative and quantitative data. But what if you are reusing qualitative data, as in our previous example of re-coding the interviews from our study to discover the language used when describing transient lifestyles? Here, you would simply need to recode the interviews and conduct a thematic analysis.
STEPS FOR DOING SECONDARY RESEARCH | EXAMPLE 1: USING SECONDARY DATA IN ISOLATION | EXAMPLE 2: COMBINING TWO SECONDARY DATA SETS | Outline all variables of interest; Transfer data to a new file; Address missing data; Recode variables; Calculate final scores; Analyse the data |
---|---|---|---|
1. Develop your research question | Do women of different nationalities experience different levels of anxiety during different stages of pregnancy? | Are there differences in an interest in Greek tourism between Germans and Britons? | Why do people choose to live on boats? |
2. Identify a secondary data set | A recent study has focused on a range of mental difficulties experienced by women in a multinational sample and this data can be reused | There is existing data on Germans’ and Britons’ interest in Greek tourism and these data sets can be compared | There is existing qualitative research on the reasons for choosing to live on boats, and this data can be relied upon to conduct a further quantitative investigation |
3. Evaluate a secondary data set | |||
(a) What was the aim of the original study? | To investigate mental difficulties (e.g., stress, anxiety, mood disorders, and paranoid thoughts) in a multinational sample of pregnant women | Study 1: To investigate Germans’ interest in Greek tourism; Study 2: To investigate Britons’ interest in Greek tourism | To conduct a qualitative investigation on reasons for choosing to live on boats |
(b) Who has collected the data? | UK government (professional source) | Travel agency (uncertain professionalism) | UK university (professional source) |
(c) Which measures were employed? | Demographic characteristics (nationality) and State Anxiety Inventory (reliable and valid) | Self-crafted measure to assess interest in Greek tourism (reliability and validity not established) | Semi-structured interviews (well-constructed) |
(d) When was the data collected? | 2015 (not outdated) | 2013 (not outdated) | 2014 (not outdated) |
(e) What methodology was used to collect the data? | Sample was representative (women from different backgrounds); large sample size (N = 975); low number of missing values; confounders controlled for (e.g., age, education, partnership status); analyses appropriate (regression) | Sample representativeness not reported; sufficient sample sizes (N1 = 453, N2 = 488); low number of missing values; confounders not controlled for; analyses appropriate (descriptive statistics) | Sample was representative (participants of different backgrounds, from different boat communities); sufficient sample size (N = 30); analyses appropriate (thematic analysis) |
(f) Making a final evaluation | Sufficiently developed data set | Insufficiently developed data set | Sufficiently developed data set |
4. Prepare and analyse secondary data | Outline all variables of interest; Transfer data to a new file; Address missing data; Recode variables; Calculate final scores; Analyse the data | Outline all variables of interest; Transfer data to a new file; Address missing data; Recode variables; Calculate final scores; Analyse the data | Outline all reasons for living on boats; Craft a questionnaire that assesses these reasons in a broader population; Analyse the data |
In summary…
^ Jump to top

A complete guide to dissertation primary research

How to write a dissertation proposal

Navigating tutorials with your dissertation supervisor

Planning a dissertation: the dos and don’ts

Dissertation research: how to find dissertation resources
- dissertation help
- dissertation primary research
- dissertation research
- dissertation tips
- study skills
Writing Services
- Essay Plans
- Critical Reviews
- Literature Reviews
- Presentations
- Dissertation Title Creation
- Dissertation Proposals
- Dissertation Chapters
- PhD Proposals
- CV Writing Service
- Business Proofreading Services
Editing Services
- Proofreading Service
- Editing Service
- Academic Editing Service
Additional Services
- Marking Services
- Consultation Calls
- Personal Statements
- Tutoring Services
Our Company
- Frequently Asked Questions
- Become a Writer
Terms & Policies
- Fair Use Policy
- Policy for Students in England
- Privacy Policy
- Terms & Conditions
- [email protected]
- Contact Form
Payment Methods
Cryptocurrency payments.
- What is Secondary Data? + [Examples, Sources, & Analysis]

- Data Collection
Aside from consulting the primary origin or source, data can also be collected through a third party, a process common with secondary data. It takes advantage of the data collected from previous research and uses it to carry out new research.
Secondary data is one of the two main types of data, where the second type is the primary data. These 2 data types are very useful in research and statistics, but for the sake of this article, we will be restricting our scope to secondary data.
We will study secondary data, its examples, sources, and methods of analysis.
What is Secondary Data?
Secondary data is the data that has already been collected through primary sources and made readily available for researchers to use for their own research. It is a type of data that has already been collected in the past.
A researcher may have collected the data for a particular project, then made it available to be used by another researcher. The data may also have been collected for general use with no specific research purpose like in the case of the national census.
Data classified as secondary for particular research may be said to be primary for another research. This is the case when data is being reused, making it primary data for the first research and secondary data for the second research it is being used for.
Sources of Secondary Data
Sources of secondary data include books, personal sources, journals, newspapers, websitess, government records etc. Secondary data are known to be readily available compared to that of primary data. It requires very little research and needs for manpower to use these sources.
With the advent of electronic media and the internet, secondary data sources have become more easily accessible. Some of these sources are highlighted below.
Books are one of the most traditional ways of collecting data. Today, there are books available for all topics you can think of. When carrying out research, all you have to do is look for a book on the topic being researched, then select from the available repository of books in that area. Books, when carefully chosen are an authentic source of authentic data and can be useful in preparing a literature review.
- Published Sources
There are a variety of published sources available for different research topics. The authenticity of the data generated from these sources depends majorly on the writer and publishing company.
Published sources may be printed or electronic as the case may be. They may be paid or free depending on the writer and publishing company’s decision.
- Unpublished Personal Sources
This may not be readily available and easily accessible compared to the published sources. They only become accessible if the researcher shares with another researcher who is not allowed to share it with a third party.
For example, the product management team of an organization may need data on customer feedback to assess what customers think about their product and improvement suggestions. They will need to collect the data from the customer service department, which primarily collected the data to improve customer service.
Journals are gradually becoming more important than books these days when data collection is concerned. This is because journals are updated regularly with new publications on a periodic basis, therefore giving to date information.
Also, journals are usually more specific when it comes to research. For example, we can have a journal on, “Secondary data collection for quantitative data ” while a book will simply be titled, “Secondary data collection”.
In most cases, the information passed through a newspaper is usually very reliable. Hence, making it one of the most authentic sources of collecting secondary data.
The kind of data commonly shared in newspapers is usually more political, economic, and educational than scientific. Therefore, newspapers may not be the best source for scientific data collection.
The information shared on websites is mostly not regulated and as such may not be trusted compared to other sources. However, there are some regulated websites that only share authentic data and can be trusted by researchers.
Most of these websites are usually government websites or private organizations that are paid, data collectors.
Blogs are one of the most common online sources for data and may even be less authentic than websites. These days, practically everyone owns a blog, and a lot of people use these blogs to drive traffic to their website or make money through paid ads.
Therefore, they cannot always be trusted. For example, a blogger may write good things about a product because he or she was paid to do so by the manufacturer even though these things are not true.
They are personal records and as such rarely used for data collection by researchers. Also, diaries are usually personal, except for these days when people now share public diaries containing specific events in their life.
A common example of this is Anne Frank’s diary which contained an accurate record of the Nazi wars.
- Government Records
Government records are a very important and authentic source of secondary data. They contain information useful in marketing, management, humanities, and social science research.
Some of these records include; census data, health records, education institute records, etc. They are usually collected to aid proper planning, allocation of funds, and prioritizing of projects.
Podcasts are gradually becoming very common these days, and a lot of people listen to them as an alternative to radio. They are more or less like online radio stations and are generating increasing popularity.
Information is usually shared during podcasts, and listeners can use it as a source of data collection.
Some other sources of data collection include:
- Radio stations
- Public sector records.
What are the Secondary Data Collection Tools?
Popular tools used to collect secondary data include; bots, devices, libraries, etc. In order to ease the data collection process from the sources of secondary data highlighted above, researchers use these important tools which are explained below.
There are a lot of data online and it may be difficult for researchers to browse through all these data and find what they are actually looking for. In order to ease this process of data collection, programmers have created bots to do an automatic web scraping for relevant data.
These bots are “ software robots ” programmed to perform some task for the researcher. It is common for businesses to use bots to pull data from forums and social media for sentiment and competitive analysis.
- Internet-Enabled Devices
This could be a mobile phone, PC, or tablet that has access to an internet connection. They are used to access journals, books, blogs, etc. to collect secondary data.
This is a traditional secondary data collection tool for researchers. The library contains relevant materials for virtually all the research areas you can think of, and it is accessible to everyone.
A researcher might decide to sit in the library for some time to collect secondary data or borrow the materials for some time and return when done collecting the required data.
Radio stations are one of the secondary sources of data collection, and one needs radio to access them. The advent of technology has even made it possible to listen to the radio on mobile phones, deeming it unnecessary to get a radio.
Secondary Data Analysis
Secondary data analysis is the process of analyzing data collected from another researcher who primarily collected this data for another purpose. Researchers leverage secondary data to save time and resources that would have been spent on primary data collection.
The secondary data analysis process can be carried out quantitatively or qualitatively depending on the kind of data the researcher is dealing with. The quantitative method of secondary data analysis is used on numerical data and is analyzed mathematically, while the qualitative method uses words to provide in-depth information about data.
How to Analyse Secondary Data
There are different stages of secondary data analysis, which involve events before, during, and after data collection. These stages include;
- Statement of Purpose
Before collecting secondary data for analysis, you need to know your statement of purpose. That is, a clear understanding of why you are collecting the data—the ultimate aim of the research work and how this data will help achieve it.
This will help direct your path towards collecting the right data, and choosing the best data source and method of analysis.
- Research Design
This is a written-down plan on how the research activities will be carried out. It describes the kind of data to be collected, the sources of data collection, method of data collection, tools, and even method of analysis.
A research design may also contain a timestamp of when each of these activities will be carried out. Therefore, serving as a guide for the secondary data analysis.
After identifying the purpose of the research, the researcher should design a research process that will guide the data analysis process.
- Developing the Research Questions
It is not enough to just know the research purpose, you need to develop research questions that will help in better identifying Secondary data. This is because they are usually a pool of data to choose from, and asking the right questions will assist in collecting authentic data.
For example, a researcher trying to collect data about the best fish feeds to enable fast growth in fishes will have to ask questions like, What kind of fish is considered? Is the data meant to be quantitative or qualitative? What is the content of the fish feed? The growth rate in fishes after feeding on it, and so on.
- Identifying Secondary Data
After developing the research questions, researchers use them as a guide to identifying relevant data from the data repository. For example, if the kind of data to be collected is qualitative, a researcher can filter out qualitative data.
The suitable secondary data will be the one that correctly answers the questions highlighted above. When looking for the solutions to a linear programming problem, for instance, the solutions will be numbers that satisfy both the objective and the constraints.
Any answer that doesn’t satisfy both, is not a solution.
- Evaluating Secondary Data
This stage is what many classify as the real data analysis stage because it is the point where analysis is actually performed. However, the stages highlighted above are a part of the data analysis process, because they influence how the analysis is performed.
Once a dataset that appears viable in addressing the initial requirements discussed above is located, the next step in the process is the evaluation of the dataset to ensure the appropriateness for the research topic. The data is evaluated to ensure that it really addresses the statement of the problem and answers the research questions.
After which it will now be analyzed either using the quantitative method or the qualitative method depending on the type of data it is.
Advantages of Secondary Data
- Ease of Access
Most of the sources of secondary data are easily accessible to researchers. Most of these sources can be accessed online through a mobile device. People who do not have access to the internet can also access them through print.
They are usually available in libraries, book stores, and can even be borrowed from other people.
- Inexpensive
Secondary data mostly require little to no cost for people to acquire them. Many books, journals, and magazines can be downloaded for free online. Books can also be borrowed for free from public libraries by people who do not have access to the internet.
Researchers do not have to spend money on investigations, and very little is spent on acquiring books if any.
- Time-Saving
The time spent on collecting secondary data is usually very little compared to that of primary data. The only investigation necessary for secondary data collection is the process of sourcing for necessary data sources.
Therefore, cutting the time that would normally be spent on the investigation. This will save a significant amount of time for the researcher
- Longitudinal and Comparative Studies
Secondary data makes it easy to carry out longitudinal studies without having to wait for a couple of years to draw conclusions. For example, you may want to compare the country’s population according to census 5 years ago, and now.
Rather than waiting for 5 years, the comparison can easily be made by collecting the census 5 years ago and now.
- Generating new insights
When re-evaluating data, especially through another person’s lens or point of view, new things are uncovered. There might be a thing that wasn’t discovered in the past by the primary data collector, that secondary data collection may reveal.
For example, when customers complain about difficulty using an app to the customer service team, they may decide to create a user guide teaching customers how to use it. However, when a product developer has access to this data, it may be uncovered that the issue came from and UI/UX design that needs to be worked on.
Disadvantages of Secondary Data
- Data Quality:
The data collected through secondary sources may not be as authentic as when collected directly from the source. This is a very common disadvantage with online sources due to a lack of regulatory bodies to monitor the kind of content that is being shared.
Therefore, working with this kind of data may have negative effects on the research being carried out.
- Irrelevant Data:
Researchers spend so much time surfing through a pool of irrelevant data before finally getting the one they need. This is because the data was not collected mainly for the researcher.
In some cases, a researcher may not even find the exact data he or she needs, but have to settle for the next best alternative.
- Exaggerated Data
Some data sources are known to exaggerate the information that is being shared. This bias may be some to maintain a good public image or due to a paid advert.
This is very common with many online blogs that even go a bead to share false information just to gain web traffic. For example, a FinTech startup may exaggerate the amount of money it has processed just to attract more customers.
A researcher gathering this data to investigate the total amount of money processed by FinTech startups in the US for the quarter may have to use this exaggerated data.
- Outdated Information
Some of the data sources are outdated and there are no new available data to replace the old ones. For example, the national census is not usually updated yearly.
Therefore, there have been changes in the country’s population since the last census. However, someone working with the country’s population will have to settle for the previously recorded figure even though it is outdated.
Secondary data has various uses in research, business, and statistics. Researchers choose secondary data for different reasons, with some of it being due to price, availability, or even needs of the research.
Although old, secondary data may be the only source of data in some cases. This may be due to the huge cost of performing research or due to its delegation to a particular body (e.g. national census).
In short, secondary data has its shortcomings, which may affect the outcome of the research negatively and also some advantages over primary data. It all depends on the situation, the researcher in question, and the kind of research being carried out.
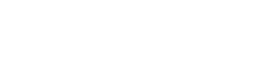
Connect to Formplus, Get Started Now - It's Free!
- advantages of secondary data
- secondary data analysis
- secondary data examples
- sources of secondary data
- busayo.longe
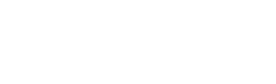
You may also like:
What is Numerical Data? [Examples,Variables & Analysis]
A simple guide on numerical data examples, definitions, numerical variables, types and analysis

Brand vs Category Development Index: Formula & Template
In this article, we are going to break down the brand and category development index along with how it applies to all brands in the market.
Primary vs Secondary Data:15 Key Differences & Similarities
Simple guide on secondary and primary data differences on examples, types, collection tools, advantages, disadvantages, sources etc.
Categorical Data: Definition + [Examples, Variables & Analysis]
A simple guide on categorical data definitions, examples, category variables, collection tools and its disadvantages
Formplus - For Seamless Data Collection
Collect data the right way with a versatile data collection tool. try formplus and transform your work productivity today..
- How it works
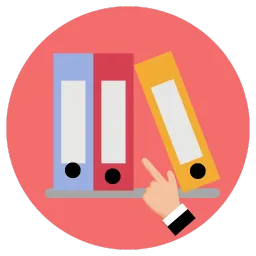
Primary vs Secondary Research – A Guide with Examples
Published by Alvin Nicolas at August 16th, 2021 , Revised On August 29, 2023
Introduction
Primary research or secondary research? How do you decide which is best for your dissertation paper?
As researchers, we need to be aware of the pros and cons of the two types of research methods to make sure their selected research method is the most appropriate, taking into account the topic of investigation .
The success of any dissertation paper largely depends on choosing the correct research design . Before you can decide whether you must base your research strategy on primary or secondary research; it is important to understand the difference between primary resources and secondary resources.
What is the Difference between Primary Sources and Secondary Sources?
What are primary sources.
According to UCL libraries, primary sources are articles, images, or documents that provide direct evidence or first-hand testimony about any given research topic.
Is it important that we have a clear understanding of the information resulting from actions under investigation ? Primary sources allow us to get close to those events to recognise their analysis and interpretation in scientific and academic communities.
Examples of Primary Sources
Classic examples of primary sources include;
- Original documents are prepared by the researcher investigating any given topic of research.
- Reporters witnessing an event and reporting news.
- Conducting surveys to collect data , such as primary elections and population census.
- Interviews , speeches, letters, and diaries – what the participants wrote or said during data collection.
- Audio, video, and image files were created to capture an event
What are Secondary Sources?
However, when the researcher wishes to analyse and understand information coming out of events or actions that have already occurred, their work is regarded as a secondary source.
In essence, no secondary source can be created without using primary sources. The same information source or evidence can be considered either primary or secondary, depending on who is presenting the information and where the information is presented.
Examples of Secondary Sources
Some examples of secondary sources are;
- Documentaries (Even though the images, videos, and audio are seen as primary sources by the developer of the documentary)
- Articles, publications, journals, and research documents are created by those not directly involved in the research.
- Dissertations , thesis, and essays .
- Critical reviews.
- Books presented as evidence.
Need help with getting started with your dissertation paper? Here is a comprehensive article on “ How to write a dissertation – Step by step guide “.
What Type of Research you Should Base your Dissertation on – Primary or Secondary?
Below you will find detailed guidelines to help you make an informed decision if you have been thinking of the question “Should I use primary or secondary research in my dissertation”.
Hire an Expert Writer
Proposal and dissertation orders completed by our expert writers are
- Formally drafted in academic style
- Plagiarism free
- 100% Confidential
- Never Resold
- Include unlimited free revisions
- Completed to match exact client requirements
Primary Research
Primary research includes an exhaustive analysis of data to answer research questions that are specific and exploratory in nature.
Primary research methods with examples include the use of various primary research tools such as interviews, research surveys , numerical data, observations, audio, video, and images to collect data directly rather than using existing literature.
Business organisations throughout the world have their employees or an external research agency conduct primary research on their behalf to address certain issues. On the other hand, undergraduate and postgraduate students conduct primary research as part of their dissertation projects to fill an obvious research gap in their respective fields of study.
As indicated above, primary data can be collected in a number of ways, and so we have also conducted in-depth research on the most common yet independent primary data collection techniques .
Sampling in Primary Research
When conducting primary research, it is vitally important to pay attention to the chosen sampling method which can be described as “ a specific principle used to select members of the population to participate in the research ”.
Oftentimes, the researcher might not be able to directly work with the targeted population because of its large size, and so it becomes indispensable to employ statistical sampling techniques where the researchers have no choice but to draw conclusions based on responses collected from the representative population.
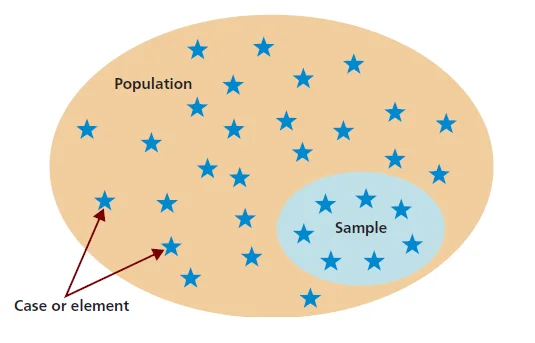
The process of sampling in primary data collection includes the following five steps;
- Identifying the target population.
- Selecting an appropriate sampling frame.
- Determining the sampling size.
- Choosing a sampling method .
- Practical application of the selected sampling technique.
The researcher can gather responses when conducting primary research, but nonverbal communication and gestures play a considerable role. They help the researcher identify the various hidden elements which cannot be identified when conducting the secondary research.
How to use Social Media Networks for Dissertation Research
Reasons Why you Should Use Primary Research
- As stated previously, the most prominent advantage of primary research over secondary research is that the researcher is able to directly collect the data from the respondents which makes the data more authentic and reliable.
- Primary research has room for customisation based on the personal requirements and/or limitations of the researcher.
- Primary research allows for a comprehensive analysis of the subject matter to address the problem at hand .
- The researcher will have the luxury to decide how to collect and use the data, which means that they will be able to make use of the data in whatever way deemed fit to them to gain meaningful insights.
- The results obtained from primary research are recognised as credible throughout academic and scientific communities.
Reasons Why you Should not Use Primary Research
- If you are considering primary research for your dissertation , you need to be aware of the high costs involved in the process of gathering primary data. Undergraduate and Masters’ students often do not have the financial resources to fund their own research work. Ph.D. students, on the other hand, are awarded a very limited research budget to work with. Thus, if you are on a low or limited budget, conducting primary research might not be the most suitable option.
- Primary research can be extremely time-consuming. Getting your target population to participate in online surveys and face-to-face or telephonic interviews requires patience and a lot of time. This is especially important for undergraduate and Masters’ students who are required to complete and submit their work within a certain timeframe.
- Primary research is well recognised only when it makes use of several methods of data collection . Having just one primary research method will undermine your research. Using more than one method of data collection will mean that you need more time and financial resources.
- There might be participants who wouldn’t be willing to disclose their information, thus this aspect is crucial and should be looked into carefully.
One important aspect of primary research that researchers should look into is research ethics. Keeping participants’ information confidential is a research responsibility that should never be overlooked.
How to Approach a Company for your Primary Study
What data collection method best suits your research?
- Find out by hiring an expert from ResearchProspect today!
- Despite how challenging the subject may be, we are here to help you.
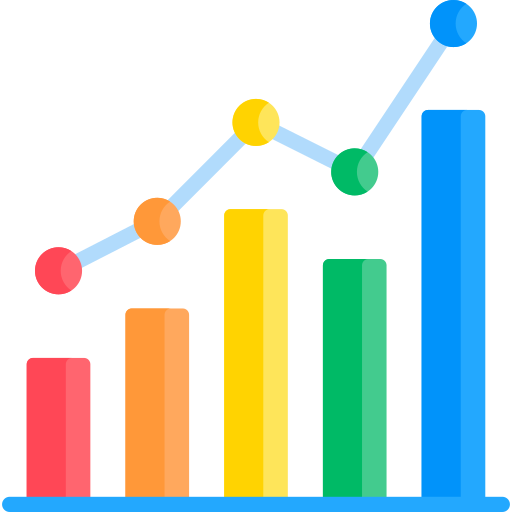
Secondary Research
Secondary research or desk-based research is the second type of research you could base your research methodology in a dissertation on. This type of research reviews and analyses existing research studies to improve the overall authenticity of the research.
Secondary research methods include the use of secondary sources of information including journal articles, published reports, public libraries, books, data available on the internet, government publications, and results from primary research studies conducted by other researchers in the past.
Unlike primary research, secondary research is cost-effective and less time-consuming simply because it uses existing literature and doesn’t require the researcher to spend time and financial resources to collect first-hand data.
Not all researchers and/or business organisations are able to afford a significant amount of money towards research, and that’s one of the reasons this type of research is the most popular in universities and organisations.
The Steps for Conducting Secondary Research
Secondary research involves the following five steps;
- Establishing the topic of research and setting up the research questions to be answered or the research hypothesis to be tested.
- Identifying authentic and reliable sources of information.
- Gather data relevant to the topic of research from various secondary sources such as books, journal articles, government publications, commercial sector reports.
- Combining the data in a suitable format so you can gain meaningful insights.
- Analysing the data to find a solution to a problem in hand
Reasons Why you Should Use Secondary Research
- Secondary sources are readily available with researchers facing little to no difficulty in accessing secondary data. Unlike primary data that involves a lengthy and complex process, secondary data can be collected by the researcher through a number of existing sources without having to leave the comfort of the desk.
- Secondary research is a simple process, and therefore the cost associated with it is almost negligible.
Reasons Why you Should Not Use Secondary Research
- Finding authentic and credible sources of secondary data is nothing less than a challenge. The internet these days is full of fake information, so it is important to exercise precaution when selecting and evaluating the available information.
- Secondary sources may not provide accurate and/or up-to-date numbers, so your research could be diluted if you are not including accurate statistics from recent timelines.
- Secondary research, in essence, is dependent on primary research and stems its findings from sets of primary data. The reliability of secondary research will, to a certain degree, depends on the quality of primary data used.
If you aren’t sure about the correct method of research for your dissertation paper, you should get help from an expert who can guide on whether you should use Primary or Secondary Research for your dissertation paper.
The Steps Involved in Writing a Dissertation
Key Differences between Primary and Secondary Research
Primary Research | Secondary Research |
---|---|
Research is conducted first hand to obtain data. Research “own” the data collected. | Research is based on data collected from previous researches. |
Primary research is based on raw data. | Secondary research is based on tried and tested data which is previously analysed and filtered. |
The data collected fits the needs of a researcher, it is customised. Data is collected based on the absolute needs of organisations or businesses. | Data may or may not be according to the requirement of a researcher. |
Researcher is deeply involved in research to collect data in primary research. | As opposed to primary research, secondary research is fast and easy. It aims at gaining a broader understanding of subject matter. |
Primary research is an expensive process and consumes a lot of time to collect and analyse data. | Secondary research is a quick process as data is already available. Researcher should know where to explore to get most appropriate data. |
Should I Use Primary or Secondary Research for my Dissertation Paper? – Conclusion
When choosing between primary and secondary research, you should always take into consideration the advantages and disadvantages of both types of research so you make an informed decision.
The best way to select the correct research strategy for your dissertation is to look into your research topic, research questions , aim and objectives – and of course the available time and financial resources.
Discussion pertaining to the two research techniques clearly indicates that primary research should be chosen when a specific topic, a case, organisation, etc. is to be researched about and the researcher has access to some financial resources.
Whereas secondary research should be considered when the research is general in nature and can be answered by analysing past researches and published data.
Not sure which research strategy you should apply, get in touch with us right away . At ResearchProspect, we have Masters and Ph.D. qualified writers in all academic subjects so you can be confident of having your research; completed to the highest academic standard and well-recognised in the academic world.
Check Prices Now
Frequently Asked Questions
What is the difference between primary vs secondary research.
Primary research involves collecting firsthand data from sources like surveys or interviews. Secondary research involves analyzing existing data, such as articles or reports. Primary is original data gathering, while secondary relies on existing information.
You May Also Like
Experimental research refers to the experiments conducted in the laboratory or under observation in controlled conditions. Here is all you need to know about experimental research.
This post provides the key disadvantages of secondary research so you know the limitations of secondary research before making a decision.
This article presents the key advantages and disadvantages of secondary research so you can select the most appropriate research approach for your study.
USEFUL LINKS
LEARNING RESOURCES
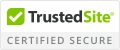
COMPANY DETAILS
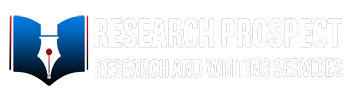
- How It Works
- Behavioral Psychology
- Behavioural Science
- Reinforcement (Psychology)
Secondary Data in Research – Uses and Opportunities
- October 2018
- Revista Ibero-Americana de Estratégia 17(04):01-04
- 17(04):01-04

- Universidade Presbiteriana Mackenzie

- Universidade Nove de Julho

Discover the world's research
- 25+ million members
- 160+ million publication pages
- 2.3+ billion citations

- Sayed Samiullah Saeedi
- Herlina Agustin
- Abdul Manan Sapi

- Lucian Blaga
- Sibiu Metologia
- Cercetării Științifice
- Sarika Tanwar

- Ramesha H. H
- Dilip Kumar
- Francis Ziwanai
- Vijaya Laxman Hake
- Rahul Sadashiv Mate

- Psihologie Metodologia
- Iordănescu Eugen
- Antonino Virgillito
- Federico Polidoro
- John Bohannon
- Sarah Boslaugh

- Recruit researchers
- Join for free
- Login Email Tip: Most researchers use their institutional email address as their ResearchGate login Password Forgot password? Keep me logged in Log in or Continue with Google Welcome back! Please log in. Email · Hint Tip: Most researchers use their institutional email address as their ResearchGate login Password Forgot password? Keep me logged in Log in or Continue with Google No account? Sign up
- Login to Survey Tool Review Center
Secondary Research Advantages, Limitations, and Sources
Summary: secondary research should be a prerequisite to the collection of primary data, but it rarely provides all the answers you need. a thorough evaluation of the secondary data is needed to assess its relevance and accuracy..
5 minutes to read. By author Michaela Mora on January 25, 2022 Topics: Relevant Methods & Tips , Business Strategy , Market Research
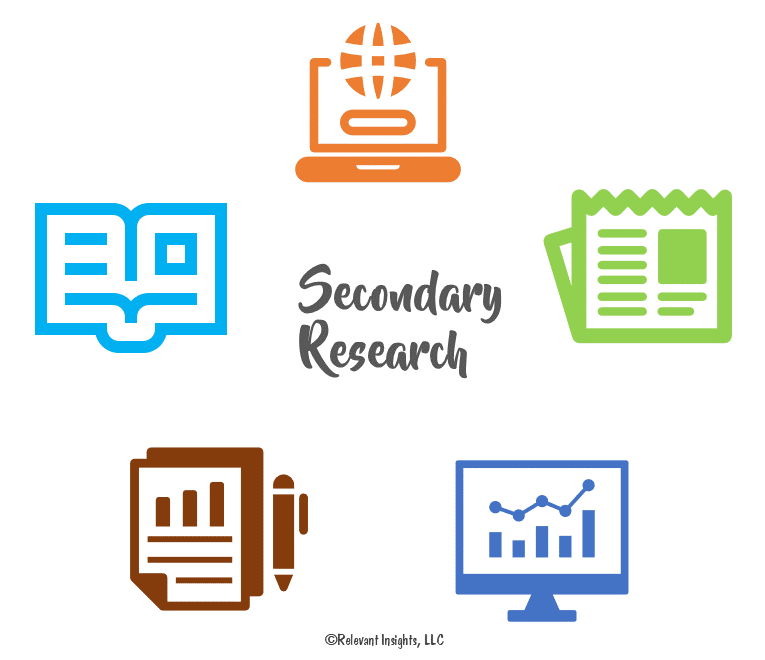
Secondary research is based on data already collected for purposes other than the specific problem you have. Secondary research is usually part of exploratory market research designs.
The connection between the specific purpose that originates the research is what differentiates secondary research from primary research. Primary research is designed to address specific problems. However, analysis of available secondary data should be a prerequisite to the collection of primary data.
Advantages of Secondary Research
Secondary data can be faster and cheaper to obtain, depending on the sources you use.
Secondary research can help to:
- Answer certain research questions and test some hypotheses.
- Formulate an appropriate research design (e.g., identify key variables).
- Interpret data from primary research as it can provide some insights into general trends in an industry or product category.
- Understand the competitive landscape.
Limitations of Secondary Research
The usefulness of secondary research tends to be limited often for two main reasons:
Lack of relevance
Secondary research rarely provides all the answers you need. The objectives and methodology used to collect the secondary data may not be appropriate for the problem at hand.
Given that it was designed to find answers to a different problem than yours, you will likely find gaps in answers to your problem. Furthermore, the data collection methods used may not provide the data type needed to support the business decisions you have to make (e.g., qualitative research methods are not appropriate for go/no-go decisions).
Lack of Accuracy
Secondary data may be incomplete and lack accuracy depending on;
- The research design (exploratory, descriptive, causal, primary vs. repackaged secondary data, the analytical plan, etc.)
- Sampling design and sources (target audiences, recruitment methods)
- Data collection method (qualitative and quantitative techniques)
- Analysis point of view (focus and omissions)
- Reporting stages (preliminary, final, peer-reviewed)
- Rate of change in the studied topic (slowly vs. rapidly evolving phenomenon, e.g., adoption of specific technologies).
- Lack of agreement between data sources.
Criteria for Evaluating Secondary Research Data
Before taking the information at face value, you should conduct a thorough evaluation of the secondary data you find using the following criteria:
- Purpose : Understanding why the data was collected and what questions it was trying to answer will tell us how relevant and useful it is since it may or may not be appropriate for your objectives.
- Methodology used to collect the data : Important to understand sources of bias.
- Accuracy of data: Sources of errors may include research design, sampling, data collection, analysis, and reporting.
- When the data was collected : Secondary data may not be current or updated frequently enough for the purpose that you need.
- Content of the data : Understanding the key variables, units of measurement, categories used and analyzed relationships may reveal how useful and relevant it is for your purposes.
- Source reputation : In the era of purposeful misinformation on the Internet, it is important to check the expertise, credibility, reputation, and trustworthiness of the data source.
Secondary Research Data Sources
Compared to primary research, the collection of secondary data can be faster and cheaper to obtain, depending on the sources you use.
Secondary data can come from internal or external sources.
Internal sources of secondary data include ready-to-use data or data that requires further processing available in internal management support systems your company may be using (e.g., invoices, sales transactions, Google Analytics for your website, etc.).
Prior primary qualitative and quantitative research conducted by the company are also common sources of secondary data. They often generate more questions and help formulate new primary research needed.
However, if there are no internal data collection systems yet or prior research, you probably won’t have much usable secondary data at your disposal.
External sources of secondary data include:
- Published materials
- External databases
- Syndicated services.
Published Materials
Published materials can be classified as:
- General business sources: Guides, directories, indexes, and statistical data.
- Government sources: Census data and other government publications.
External Databases
In many industries across a variety of topics, there are private and public databases that can bed accessed online or by downloading data for free, a fixed fee, or a subscription.
These databases can include bibliographic, numeric, full-text, directory, and special-purpose databases. Some public institutions make data collected through various methods, including surveys, available for others to analyze.
Syndicated Services
These services are offered by companies that collect and sell pools of data that have a commercial value and meet shared needs by a number of clients, even if the data is not collected for specific purposes those clients may have.
Syndicated services can be classified based on specific units of measurements (e.g., consumers, households, organizations, etc.).
The data collection methods for these data may include:
- Surveys (Psychographic and Lifestyle, advertising evaluations, general topics)
- Household panels (Purchase and media use)
- Electronic scanner services (volume tracking data, scanner panels, scanner panels with Cable TV)
- Audits (retailers, wholesalers)
- Direct inquiries to institutions
- Clipping services tracking PR for institutions
- Corporate reports
You can spend hours doing research on Google in search of external sources, but this is likely to yield limited insights. Books, articles journals, reports, blogs posts, and videos you may find online are usually analyses and summaries of data from a particular perspective. They may be useful and give you an indication of the type of data used, but they are not the actual data. Whenever possible, you should look at the actual raw data used to draw your own conclusion on its value for your research objectives. You should check professionally gathered secondary research.
Here are some external secondary data sources often used in market research that you may find useful as starting points in your research. Some are free, while others require payment.
- Pew Research Center : Reports about the issues, attitudes, and trends shaping the world. It conducts public opinion polling, demographic research, media content analysis, and other empirical social science research.
- Data.Census.gov : Data dissemination platform to access demographic and economic data from the U.S. Census Bureau.
- Data.gov : The US. government’s open data source with almost 200,00 datasets ranges in topics from health, agriculture, climate, ecosystems, public safety, finance, energy, manufacturing, education, and business.
- Google Scholar : A web search engine that indexes the full text or metadata of scholarly literature across an array of publishing formats and disciplines.
- Google Public Data Explorer : Makes large, public-interest datasets easy to explore, visualize and communicate.
- Google News Archive : Allows users to search historical newspapers and retrieve scanned images of their pages.
- Mckinsey & Company : Articles based on analyses of various industries.
- Statista : Business data platform with data across 170+ industries and 150+ countries.
- Claritas : Syndicated reports on various market segments.
- Mintel : Consumer reports combining exclusive consumer research with other market data and expert analysis.
- MarketResearch.com : Data aggregator with over 350 publishers covering every sector of the economy as well as emerging industries.
- Packaged Facts : Reports based on market research on consumer goods and services industries.
- Dun & Bradstreet : Company directory with business information.
Related Articles
- What Is Market Research?
- Step by Step Guide to the Market Research Process
- How to Leverage UX and Market Research To Understand Your Customers
- Why Your Business Needs Discovery Research
- Your Market Research Plan to Succeed As a Startup
- Top Reason Why Businesses Fail & What To Do About It
- What To Value In A Market Research Vendor
- Don’t Let The Budget Dictate Your Market Research Approach
- How To Use Research To Find High-Order Brand Benefits
- How To Prioritize What To Research
- Don’t Just Trust Your Gut — Do Research
- Understanding the Pros and Cons of Mixed-Mode Research
Subscribe to our newsletter to get notified about future articles
Subscribe and don’t miss anything!
Recent Articles
- How AI Can Further Remove Researchers in Search of Productivity and Lower Costs
- Re: Design/Growth Podcast – Researching User Experiences for Business Growth
- Why You Need Positioning Concept Testing in New Product Development
- Why Conjoint Analysis Is Best for Price Research
- The Rise of UX
- Making the Case Against the Van Westendorp Price Sensitivity Meter
- How to Future-Proof Experience Management and Your Business
- When Using Focus Groups Makes Sense
- How to Make Segmentation Research Actionable
- How To Integrate Market Research and UX Research for Desired Business Outcomes
Popular Articles
- Which Rating Scales Should I Use?
- What To Consider in Survey Design
- 6 Decisions To Make When Designing Product Concept Tests
- Write Winning Product Concepts To Get Accurate Results In Concept Tests
- How to Use Qualitative and Quantitative Research in Product Development
- The Opportunity of UX Research Webinar
- Myths & Misunderstandings About UX – MR Realities Podcast
- 12 Research Techniques to Solve Choice Overload
- Concept Testing for UX Researchers
- UX Research Geeks Podcast – Using Market Research for Better Context in UX
- A Researcher’s Path – Data Stories Leaders At Work Podcast
- How To Improve Racial and Gender Inclusion in Survey Design
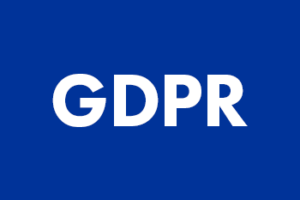
- Privacy Overview
- Strictly Necessary Cookies
This website uses cookies so that we can provide you with the best user experience possible. Cookie information is stored in your browser and performs functions such as recognising you when you return to our website and helping our team to understand which sections of the website you find most interesting and useful.
Strictly Necessary Cookie should be enabled at all times so that we can save your preferences for cookie settings.
If you disable this cookie, we will not be able to save your preferences. This means that every time you visit this website you will need to enable or disable cookies again.
Secondary data
Using secondary data can be a good alternative to collecting data directly from participants (primary data), removing the need for face-to-face contact.
Secondary data relating to living human subjects often requires ethical approval depending on the source and nature of the data. The extent to which the ethical review application form must be completed also depends on the source and nature of the data.
This guidance covers some of the ethical issues relating to use of secondary data and how this impacts the ethical application process.
Secondary data and ethical review
Ethical approval is required for projects where secondary data includes personal data - data that relates to identifiable living persons.
Data relating to the deceased
When data relates to deceased human subjects, ethical approval is required if the data includes either:
- sensitive personal data about living human subjects, or
- data relating to health or census information from the last 100 years.
And where this data identifies, or could identify, either the deceased individual or others.
Among the reasons ethical review is required is because:
- sensitive personal data can have implications for living relatives
- some data may be covered by Data Protection legislation.
Anonymised data
Data which are completely and robustly anonymised do not contain personal data and so ethical review and approval is usually not required.
For the avoidance of doubt, this means data that are already anonymised rather than data received in identifiable or pseudonymised form and then anonymised by the researcher.
However, there are scenarios involving anonymised data where ethical approval may be required (discuss with your School ethics committee if you are unsure):
Data from a source which requires assurances or additional approvals
If the data source requires assurances that the project has undergone ethical review or evidence that use of the data is legitimate, an ethical review application can be submitted.
If the data source requires a:
- Data Management Plan - contact Research Data Management .
- Data Protection Impact Assessment (DPIA) - contact Data Protection ( [email protected] ).
If the data involves or originates from the NHS or health and social care, see the Research involving the NHS page.
Data which risk re-identification of individuals
If the data could be used to re-identify individuals, then an ethical review application may be needed - consider the items of data you will be working with and whether this is a risk.
For example:
Combined data - combining data can lead to re-identification of individuals, particularly if data is linked at an individual level by matching unique reference numbers or data points.
Rare, unusual, or low number data – rare or unique data, such as that relating to unusual characteristics or rare health conditions, are difficult to truly anonymise as there often few individuals with those characteristics or conditions.
Reasonable means – GDPR suggests that the risk of identification, researchers should consider ‘means reasonably likely to be used’, accounting for factors such as costs and time involved and available technology.
Data with additional ethical considerations
If there are additional ethical considerations, an ethical review application can be submitted. For example, if data raises concerns around:
- the original participants’ consent for future use of the data
- the provenance of the data
- access to sensitive data not already in the public domain
- social profiling
- the research, data, or outcomes adversely impacting a particular group or community
See the section below on ethical considerations.
Secondary data types
Secondary data – internal datasets
Secondary datasets may sometimes be sourced from the within the University i.e. data collected as part of previous projects within a School. It is important to consider whether re-use of this data is in line with the original ethical approval and the consent given by participants. An ethical amendment may be required for both the original ethical approval to allow the data to be shared AND a new ethical review application for the new research project (if sufficiently different).
Internally sourced data should still be acknowledged and appropriately referenced, and the same considerations given as to other secondary data sources such as around access and permissions, data management and confidentiality. Researchers should also consider whether using this type of secondary data is appropriate for their needs (i.e. whether it meets the requirements for an academic research project).
Secondary data - large quantitative datasets
A commonly used source of secondary data are large quantitative data sets such as census data, health data, household surveys and market research.
There are several sources that can give access to these types of data and what is required to access them varies by source and by the nature of the data, for example:
- ‘open’ datasets where the data is freely available to download
- ‘closed’ datasets where users must register with the data source but that require minimal additional work
- datasets that contain more sensitive information and where users may have to complete paperwork such as a data management plan.
Sometimes more sensitive datasets can only be accessed via a secure web portal and no local copies retained.
Secondary data - qualitative and mixed-methods data
Secondary qualitative data is less common, largely due to the difficulty in anonymising qualitative data. However, there are sources of secondary qualitative data including the UK Data Service and library data such as oral histories, diaries and biographies.
Secondary data - biological data
There are several resources for access to biological data including human-related data. Use of biological data and bioinformatics is a wide are with several ethical concerns around confidentiality, implications of research into DNA and genomics, bias and profiling, the sensitivity of identifying risk levels related to disease. Researchers planning research involving biological data or bioinformatics should consult with disciplinary guidelines and organisations and colleagues with specific expertise. If using secondary data of this type, researchers must ensure they do so in accordance with the requirements of the data sources. Researchers should also ensure that they check if any NHS ethical approval, governance or R&D approvals are required .
Access, permissions and consent
Access to secondary data must always be used in accordance with the requirements of the data source, GDPR and the common law duty of confidentiality. Secondary data must always be appropriately referenced and acknowledged. Researchers should always act in accordance with the Principles of Good Research Conduct , even when working with secondary data.
Researchers should check whether their use is in line with the consent originally obtained from participants and seek assurances on this from the data source.
Where data is obtained in anonymous form, researchers should be conscious of the risk of de-anonymising data through triangulation of several data points or sets.
While there are open access datasets that are freely available, it is common that there are conditions and requirements put in place by the data source or controller around who can access the data and how it is used. For example, this might include:
- that researchers sign terms of use
- that researchers have a comprehensive data management plan
- that researchers can provide assurances around the security of the data once in their possession
- verification that the person accessing the data has a legitimate reason i.e. evidence that you are a researcher at a recognised institution
- that the data be accessed via a secure portal
- that no local copies are retained
- that any copies of the data be destroyed within a certain timescale (may require a destruction certificate)
- that the raw data be processed by the data source into an anonymised form before it is released
In the latter examples, where there is more complex requirements and the data source is providing a service such as preparing and moderating access, this may incur costs that would need to be factored into researchers plans and budgets.
Ethical considerations
Ethical issues to consider.
The ethical application form includes an early filter question on use of secondary sources. This means that if researchers are using secondary data with no additional ethical issues they can skip to the end of the form – the declarations section. If, however, there are ethical issues, researchers should describe these and how they will be mitigated in the ‘Ethical Considerations’ free text field later in the form.
If data are particularly sensitive, or it is required by the data source, researchers may wish to complete the Data Management section of the ethical review application form (Word) or a separate data management plan .
When making an application for ethical approval of research using secondary data, researchers should consider:
- Is the proposed research in line with the participants original consent? Can the data source provide assurances on participants original consent?
- How will the data be managed? If there is identifiable, personal or sensitive data how will confidentiality be maintained and data kept secure?
- Will the proposed research and use, management and storage of the data meet with the data sources requirements? Have all the appropriate documents been completed and permissions granted?
- Will the data source be acknowledged and referenced?
- Are there any copyright issues around the data?
- By pulling together several data sources is there any risk of de-anonymising participants?
- Will using this data or combining it with other data risk bias or ‘profiling’ of a particular group?
- How will you present the data or analysis? Will this ensure the confidentiality and anonymity of participants?
- Will the data identify individuals as being at risk of a condition or disease where they may have otherwise been unaware?
You may find parts of the UK Government's Data Ethics Framework useful for exploring some of the potential issues.
Resources - data sources
Data sources
The UK Data Service – this is one of the core UK sources of secondary data, including government data such as the Household Survey, plus an increasing amount of qualitative data and data collected as part of research funded by UK research councils https://www.ukdataservice.ac.uk/
The Office of National Statistics – this is the UK’s recognised national statistics institute and conducts the census in England and Wales amongst other large national and regional surveys https://www.ons.gov.uk/
The Scottish Governments statistics publications – this includes often aggregated statistics reporting regional level (rather than individual level) data, though some more detailed datasets are available for older data https://www.gov.scot/publications/?publicationTypes=statistics&page=1
NHS Digital data and statistics publications – this includes details about clinical indicators, health and social data, though again this is often aggregated and at a regional level rather than individual level data https://digital.nhs.uk/data-and-information/data-collections-and-data-sets/data-sets
Information Services Division (ISD) Scotland – this includes Scottish health and social dare data, often aggregated and at a regional level https://www.isdscotland.org/
Data.gov.uk – a new resource for ‘open’ UK government data https://data.gov.uk/
British Library – the British Library hold a number of collections including oral histories, biographies and newspaper articles. https://www.bl.uk/collection-guides/oral-history#
Qualitative Data Repository – a qualitative data repository hosted by Syracuse University https://qdr.syr.edu/ European Molecular Biology Laboratory – European Bioinformatics Institute (EMBL-EBI) https://www.ebi.ac.uk/
Health Informatics Centre (HIC) – local health informatics service linking health data https://www.dundee.ac.uk/hic/
Open access data directories
OpenAire.eu – A searchable directory of open access datasets such as those accompanying publications https://explore.openaire.eu/
JISC Directory of Open Access Repositories (OpenDOAR) – a searchable directory of open access repositories http://v2.sherpa.ac.uk/opendoar/
Resources - ethics
- Association of internet researchers – ethics guidance
- The European Commission (2018) – Use of previously collected data (‘secondary use’). Ethics and Data Protection , VII, 12-14
- Irwin, S. (2013). Qualitative secondary data analysis: Ethics, epistemology and context . Progress in development studies, 13(4), 295-306.
- Morrow, Virginia and Boddy, Janet and Lamb, Rowena (2014) The ethics of secondary data analysis . NCRM Working Paper. NOVELLA.
- Rodriquez, L. (2018) Secondary data analysis with young people. Some ethical and methodological considerations from practice. Children’s Research Digest Volume 4, Issue 3. The Childrens Research Network.
- Salerno, J., Knoppers, B. M., Lee, L. M., Hlaing, W. M., & Goodman, K. W. (2017). Ethics, big data and computing in epidemiology and public health . Annals of epidemiology, 27(5), 297-301.
- UK Data Service guidance on secondary analysis

An official website of the United States government
The .gov means it’s official. Federal government websites often end in .gov or .mil. Before sharing sensitive information, make sure you’re on a federal government site.
The site is secure. The https:// ensures that you are connecting to the official website and that any information you provide is encrypted and transmitted securely.
- Publications
- Account settings
Preview improvements coming to the PMC website in October 2024. Learn More or Try it out now .
- Advanced Search
- Journal List
- HHS Author Manuscripts

Qualitative Secondary Analysis: A Case Exemplar
Judith ann tate.
The Ohio State University, College of Nursing
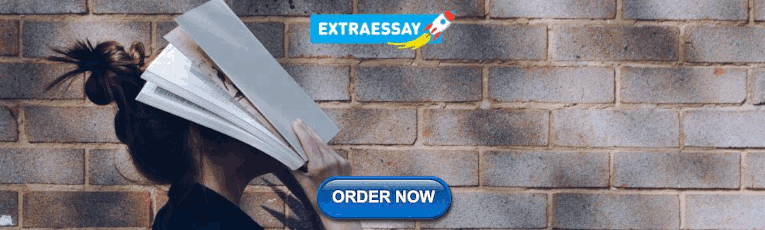
Mary Beth Happ
Qualitative secondary analysis (QSA) is the use of qualitative data collected by someone else or to answer a different research question. Secondary analysis of qualitative data provides an opportunity to maximize data utility particularly with difficult to reach patient populations. However, QSA methods require careful consideration and explicit description to best understand, contextualize, and evaluate the research results. In this paper, we describe methodologic considerations using a case exemplar to illustrate challenges specific to QSA and strategies to overcome them.
Health care research requires significant time and resources. Secondary analysis of existing data provides an efficient alternative to collecting data from new groups or the same subjects. Secondary analysis, defined as the reuse of existing data to investigate a different research question ( Heaton, 2004 ), has a similar purpose whether the data are quantitative or qualitative. Common goals include to (1) perform additional analyses on the original dataset, (2) analyze a subset of the original data, (3) apply a new perspective or focus to the original data, or (4) validate or expand findings from the original analysis ( Hinds, Vogel, & Clarke-Steffen, 1997 ). Synthesis of knowledge from meta-analysis or aggregation may be viewed as an additional purpose of secondary analysis ( Heaton, 2004 ).
Qualitative studies utilize several different data sources, such as interviews, observations, field notes, archival meeting minutes or clinical record notes, to produce rich descriptions of human experiences within a social context. The work typically requires significant resources (e.g., personnel effort/time) for data collection and analysis. When feasible, qualitative secondary analysis (QSA) can be a useful and cost-effective alternative to designing and conducting redundant primary studies. With advances in computerized data storage and analysis programs, sharing qualitative datasets has become easier. However, little guidance is available for conducting, structuring procedures, or evaluating QSA ( Szabo & Strang, 1997 ).
QSA has been described as “an almost invisible enterprise in social research” ( Fielding, 2004 ). Primary data is often re-used; however, descriptions of this practice are embedded within the methods section of qualitative research reports rather than explicitly identified as QSA. Moreover, searching or classifying reports as QSA is difficult because many researchers refrain from identifying their work as secondary analyses ( Hinds et al., 1997 ; Thorne, 1998a ). In this paper, we provide an overview of QSA, the purposes, and modes of data sharing and approaches. A unique, expanded QSA approach is presented as a methodological exemplar to illustrate considerations.
QSA Typology
Heaton (2004) classified QSA studies based on the relationship between the secondary and primary questions and the scope of data analyzed. Types of QSA included studies that (1) investigated questions different from the primary study, (2) applied a unique theoretical perspective, or (3) extended the primary work. Heaton’s literature review (2004) showed that studies varied in the choice of data used, from selected portions to entire or combined datasets.
Modes of Data Sharing
Heaton (2004) identified three modes of data sharing: formal, informal and auto-data. Formal data sharing involves accessing and analyzing deposited or archived qualitative data by an independent group of researchers. Historical research often uses formal data sharing. Informal data sharing refers to requests for direct access to an investigator’s data for use alone or to pool with other data, usually as a result of informal networking. In some instances, the primary researchers may be invited to collaborate. The most common mode of data sharing is auto-data, defined as further exploration of a qualitative data set by the primary research team. Due to the iterative nature of qualitative research, when using auto-data, it may be difficult to determine where the original study questions end and discrete, distinct analysis begins ( Heaton, 1998 ).
An Exemplar QSA
Below we describe a QSA exemplar conducted by the primary author of this paper (JT), a member of the original research team, who used a supplementary approach to examine concepts revealed but not fully investigated in the primary study. First, we describe an overview of the original study on which the QSA was based. Then, the exemplar QSA is presented to illustrate: (1) the use of auto-data when the new research questions are closely related to or extend the original study aims ( Table 1 ), (2) the collection of additional clinical record data to supplement the original dataset and (3) the performance of separate member checking in the form of expert review and opinion. Considerations and recommendations for use of QSA are reviewed with illustrations taken from the exemplar study ( Table 2 ). Finally, discussion of conclusions and implications is included to assist with planning and implementation of QSA studies.
Research question comparison
Primary study | QSA | |
---|---|---|
What is the process of care and communication in weaning LTMV patients from mechanical ventilation | What are the defining characteristics and cues of psychological symptoms such as anxiety and agitation exhibited by patients who are experiencing prolonged critical illness? | |
What interpersonal interactions (communication contacts, extent and content of communications) contribute to weaning success or are associated with inconsistent/plateau weaning patterns | How do clinicians discriminate between various psychological symptoms and behavioral signs? | |
What therapeutic strategies (e.g., medications/nutrients, use of instruction or comfort measures, rehabilitative treatments) contribute to weaning success or are associated with inconsistent/plateau weaning patterns | What therapeutic strategies (e.g., medications, non-pharmacologic methods) do clinicians undertake in response to patients’ anxiety and agitation? | |
What social (patient, family, clinician characteristics) and environmental factors (noise, lighting, room size/arrangement, work pattern, workload) contribute to weaning success or are associated with inconsistent/plateau weaning patterns | How do physiologic, social and behavioral characteristics of the patient influence the clinician’s interpretation and management of anxiety and agitation? What contextual factors influence interpretation and management of psychological symptoms and behavioral signs? |
Application of the Exemplar Qualitative Secondary Analysis (QSA)
QSA Example | |
---|---|
; ; ; ; ). | |
). |
Aitken, L. M., Marshall, A. P., Elliott, R., & McKinley, S. (2009). Critical care nurses' decision making: sedation assessment and management in intensive care. Journal of Clinical Nursing, 18 (1), 36–45.
Morse, J., & Field, P. (1995). Qualitative research methods for health professionals. (2nd ed.). Thousand Oaks, CA: Sage Publishing.
Patel, R. P., Gambrell, M., Speroff, T.,…Strength, C. (2009). Delirium and sedation in the intensive care unit: Survey of behaviors and attitudes of 1384 healthcare professionals. Critical Care Medicine, 37 (3), 825–832.
Shehabi, Y., Botha, J. A., Boyle, M. S., Ernest, D., Freebairn, R. C., Jenkins, I. R., … Seppelt, I. M. (2008). Sedation and delirium in the intensive care unit: an Australian and New Zealand perspective. Anaesthesia & Intensive Care, 36 (4), 570–578.
Tanios, M. A., de Wit, M., Epstein, S. K., & Devlin, J. W. (2009). Perceived barriers to the use of sedation protocols and daily sedation interruption: a multidisciplinary survey. Journal of Critical Care, 24 (1), 66–73.
Weinert, C. R., & Calvin, A. D. (2007). Epidemiology of sedation and sedation adequacy for mechanically ventilated patients in a medical and surgical intensive care unit. Critical Care Medicine , 35(2), 393–401.
The Primary Study
Briefly, the original study was a micro-level ethnography designed to describe the processes of care and communication with patients weaning from prolonged mechanical ventilation (PMV) in a 28-bed Medical Intensive Care Unit ( Broyles, Colbert, Tate, & Happ, 2008 ; Happ, Swigart, Tate, Arnold, Sereika, & Hoffman, 2007 ; Happ et al, 2007 , 2010 ). Both the primary study and the QSA were approved by the Institutional Review Board at the University of Pittsburgh. Data were collected by two experienced investigators and a PhD student-research project coordinator. Data sources consisted of sustained field observations, interviews with patients, family members and clinicians, and clinical record review, including all narrative clinical documentation recorded by direct caregivers.
During iterative data collection and analysis in the original study, it became apparent that anxiety and agitation had an effect on the duration of ventilator weaning episodes, an observation that helped to formulate the questions for the QSA ( Tate, Dabbs, Hoffman, Milbrandt & Happ, 2012 ). Thus, the secondary topic was closely aligned as an important facet of the primary phenomenon. The close, natural relationship between the primary and QSA research questions is demonstrated in the side-by-side comparison in Table 1 . This QSA focused on new questions which extended the original study to recognition and management of anxiety or agitation, behaviors that often accompany mechanical ventilation and weaning but occur throughout the trajectory of critical illness and recovery.
Considerations when Undertaking QSA ( Table 2 )
Practical advantages.
A key practical advantage of QSA is maximizing use of existing data. Data collection efforts represent a significant percentage of the research budget in terms of cost and labor ( Coyer & Gallo, 2005 ). This is particularly important in view of the competition for research funding. Planning and implementing a qualitative study involves considerable time and expertise not only for data collecting (e.g., interviews, participant observation or focus group), but in establishing access, credibility and relationships ( Thorne, 1994 ) and in conducting the analysis. The cost of QSA is often seen as negligible since the outlay of resources for data collection is assumed by the original study. However, QSA incurs costs related to storage, researcher’s effort for review of existing data, analysis, and any further data collection that may be necessary.
Another advantage of QSA is access to data from an assembled cohort. In conducting original primary research, practical concerns arise when participants are difficult to locate or reluctant to divulge sensitive details to a researcher. In the case of vulnerable critically ill patients, participation in research may seem an unnecessary burden to family members who may be unwilling to provide proxy consent ( Fielding, 2004 ). QSA permits new questions to be asked of data collected previously from these vulnerable groups ( Rew, Koniak-Griffin, Lewis, Miles, & O'Sullivan, 2000 ), or from groups or events that occur with scarcity ( Thorne, 1994 ). Participants’ time and effort in the primary study therefore becomes more worthwhile. In fact, it is recommended that data already collected from existing studies of vulnerable populations or about sensitive topics be analyzed prior to engaging new participants. In this way, QSA becomes a cumulative rather than a repetitive process ( Fielding, 2004 ).
Data Adequacy and Congruency
Secondary researchers must determine that the primary data set meets the needs of the QSA. Data may be insufficient to answer a new question or the focus of the QSA may be so different as to render the pursuit of a QSA impossible ( Heaton, 1998 ). The underlying assumptions, sampling plan, research questions, and conceptual framework selected to answer the original study question may not fit the question posed during QSA ( Coyer & Gallo, 2005 ). The researchers of the primary study may have selectively sampled participants and analyzed the resulting data in a manner that produced a narrow or uneven scope of data ( Hinds et al., 1997 ). Thus, the data needed to fully answer questions posed by the QSA may be inadequately addressed in the primary study. A critical review of the existing dataset is an important first step in determining whether the primary data fits the secondary questions ( Hinds et al., 1997 ).
Passage of Time
The timing of the QSA is another important consideration. If the primary study and secondary study are performed sequentially, findings of the original study may influence the secondary study. On the other hand, studies performed concurrently offer the benefit of access to both the primary research team and participants member checking ( Hinds et al., 1997 ).
The passage of time since the primary study was conducted can also have a distinct effect on the usefulness of the primary dataset. Data may be outdated or contain a historical bias ( Coyer & Gallo, 2005 ). Since context changes over time, characteristics of the phenomena of interest may have changed. Analysis of older datasets may not illuminate the phenomena as they exist today.( Hinds et al., 1997 ) Even if participants could be re-contacted, their perspectives, memories and experiences change. The passage of time also has an affect on the relationship of the primary researchers to the data – so auto-data may be interpreted differently by the same researcher with the passage of time. Data are bound by time and history, therefore, may be a threat to internal validity unless a new investigator is able to account for these effects when interpreting data ( Rew et al., 2000 ).
Researcher stance/Context involvement
Issues related to context are a major source of criticism of QSA ( Gladstone, Volpe, & Boydell, 2007 ). One of the hallmarks of qualitative research is the relationship of the researcher to the participants. It can be argued that removing active contact with participants violates this premise. Tacit understandings developed in the field may be difficult or impossible to reconstruct ( Thorne, 1994 ). Qualitative fieldworkers often react and redirect the data collection based on a growing knowledge of the setting. The setting may change as a result of external or internal factors. Interpretation of researchers as participants in a unique time and social context may be impossible to re-construct even if the secondary researchers were members of the primary team ( Mauthner, Parry, & Milburn, 1998 ). Because the context in which the data were originally produced cannot be recovered, the ability of the researcher to react to the lived experience may be curtailed in QSA ( Gladstone et al., 2007 ). Researchers utilize a number of tactics to filter and prioritize what to include as data that may not be apparent in either the written or spoken records of those events ( Thorne, 1994 ). Reflexivity between the researcher, participants and setting is impossible to recreate when examining pre-existing data.
Relationship of QSA Researcher to Primary Study
The relationship of the QSA researcher to the primary study is an important consideration. When the QSA researcher is not part of the original study team, contractual arrangements detailing access to data, its format, access to the original team, and authorship are required ( Hinds et al., 1997 ). The QSA researcher should assess the condition of the data, documents including transcripts, memos and notes, and clarity and flow of interactions ( Hinds et al., 1997 ). An outline of the original study and data collection procedures should be critically reviewed ( Heaton, 1998 ). If the secondary researcher was not a member of the original study team, access to the original investigative team for the purpose of ongoing clarification is essential ( Hinds et al., 1997 ).
Membership on the original study team may, however, offer the secondary researcher little advantage depending on their role in the primary study. Some research team members may have had responsibility for only one type of data collection or data source. There may be differences in involvement with analysis of the primary data.
Informed Consent of Participants
Thorne (1998) questioned whether data collected for one study purpose can ethically be re-examined to answer another question without participants’ consent. Many institutional review boards permit consent forms to include language about the possibility of future use of existing data. While this mechanism is becoming routine and welcomed by researchers, concerns have been raised that a generic consent cannot possibly address all future secondary questions and may violate the principle of full informed consent ( Gladstone et al., 2007 ). Local variations in study approval practices by institutional review boards may influence the ability of researchers to conduct a QSA.
Rigor of QSA
The primary standards for evaluating rigor of qualitative studies are trustworthiness (logical relationship between the data and the analytic claims), fit (the context within which the findings are applicable), transferability (the overall generalizability of the claims) and auditabilty (the transparency of the procedural steps and the analytic moves processes) ( Lincoln & Guba, 1991 ). Thorne suggests that standard procedures for assuring rigor can be modified for QSA ( Thorne, 1994 ). For instance, the original researchers may be viewed as sources of confirmation while new informants, other related datasets and validation by clinical experts are sources of triangulation that may overcome the lack of access to primary subjects ( Heaton, 2004 ; Thorne, 1994 ).
Our observations, derived from the experience of posing a new question of existing qualitative data serves as a template for researchers considering QSA. Considerations regarding quality, availability and appropriateness of existing data are of primary importance. A realistic plan for collecting additional data to answer questions posed in QSA should consider burden and resources for data collection, analysis, storage and maintenance. Researchers should consider context as a potential limitation to new analyses. Finally, the cost of QSA should be fully evaluated prior to making a decision to pursue QSA.
Acknowledgments
This work was funded by the National Institute of Nursing Research (RO1-NR07973, M Happ PI) and a Clinical Practice Grant from the American Association of Critical Care Nurses (JA Tate, PI).
Publisher's Disclaimer: This is a PDF file of an unedited manuscript that has been accepted for publication. As a service to our customers we are providing this early version of the manuscript. The manuscript will undergo copyediting, typesetting, and review of the resulting proof before it is published in its final citable form. Please note that during the production process errors may be discovered which could affect the content, and all legal disclaimers that apply to the journal pertain.
Disclosure statement: Drs. Tate and Happ have no potential conflicts of interest to disclose that relate to the content of this manuscript and do not anticipate conflicts in the foreseeable future.
Contributor Information
Judith Ann Tate, The Ohio State University, College of Nursing.
Mary Beth Happ, The Ohio State University, College of Nursing.
- Broyles L, Colbert A, Tate J, Happ MB. Clinicians’ evaluation and management of mental health, substance abuse, and chronic pain conditions in the intensive care unit. Critical Care Medicine. 2008; 36 (1):87–93. [ PubMed ] [ Google Scholar ]
- Coyer SM, Gallo AM. Secondary analysis of data. Journal of Pediatric Health Care. 2005; 19 (1):60–63. [ PubMed ] [ Google Scholar ]
- Fielding N. Getting the most from archived qualitative data: Epistemological, practical and professional obstacles. International Journal of Social Research Methodology. 2004; 7 (1):97–104. [ Google Scholar ]
- Gladstone BM, Volpe T, Boydell KM. Issues encountered in a qualitative secondary analysis of help-seeking in the prodrome to psychosis. Journal of Behavioral Health Services & Research. 2007; 34 (4):431–442. [ PubMed ] [ Google Scholar ]
- Happ MB, Swigart VA, Tate JA, Arnold RM, Sereika SM, Hoffman LA. Family presence and surveillance during weaning from prolonged mechanical ventilation. Heart & Lung: The Journal of Acute and Critical Care. 2007; 36 (1):47–57. [ PMC free article ] [ PubMed ] [ Google Scholar ]
- Happ MB, Swigart VA, Tate JA, Hoffman LA, Arnold RM. Patient involvement in health-related decisions during prolonged critical illness. Research in Nursing & Health. 2007; 30 (4):361–72. [ PubMed ] [ Google Scholar ]
- Happ MB, Tate JA, Swigart V, DiVirgilio-Thomas D, Hoffman LA. Wash and wean: Bathing patients undergoing weaning trials during prolonged mechanical ventilation. Heart & Lung: The Journal of Acute and Critical Care. 2010; 39 (6 Suppl):S47–56. [ PMC free article ] [ PubMed ] [ Google Scholar ]
- Heaton J. Secondary analysis of qualitative data. Social Research Update. 1998;(22) [ Google Scholar ]
- Heaton J. Reworking Qualitative Data. London: SAGE Publications; 2004. [ Google Scholar ]
- Hinds PS, Vogel RJ, Clarke-Steffen L. The possibilities and pitfalls of doing a secondary analysis of a qualitative data set. Qualitative Health Research. 1997; 7 (3):408–424. [ Google Scholar ]
- Lincoln YS, Guba EG. Naturalistic inquiry. Beverly Hills, CA: Sage Publishing; 1991. [ Google Scholar ]
- Mauthner N, Parry O, Milburn K. The data are out there, or are they? Implications for archiving and revisiting qualitative data. Sociology. 1998; 32 :733–745. [ Google Scholar ]
- Rew L, Koniak-Griffin D, Lewis MA, Miles M, O'Sullivan A. Secondary data analysis: new perspective for adolescent research. Nursing Outlook. 2000; 48 (5):223–229. [ PubMed ] [ Google Scholar ]
- Szabo V, Strang VR. Secondary analysis of qualitative data. Advances in Nursing Science. 1997; 20 (2):66–74. [ PubMed ] [ Google Scholar ]
- Tate JA, Dabbs AD, Hoffman LA, Milbrandt E, Happ MB. Anxiety and agitation in mechanically ventilated patients. Qualitative health research. 2012; 22 (2):157–173. [ PMC free article ] [ PubMed ] [ Google Scholar ]
- Thorne S. Secondary analysis in qualitative research: Issues and implications. In: Morse JM, editor. Critical Issues in Qualitative Research. Second. Thousand Oaks, CA: SAGE; 1994. [ Google Scholar ]
- Thorne S. Ethical and representational issues in qualitative secondary analysis. Qualitative Health Research. 1998; 8 (4):547–555. [ PubMed ] [ Google Scholar ]

How to... Use secondary data & archival material
Find out what secondary data is – as opposed to primary data – and how to go about collecting and using it.
On this page
What is secondary data & archival material, using published data sets, using archival data, secondary data as part of the research design, gaining access to, and using, archives, primary & secondary data.
All research will involve the collection of data. Much of this data will be collected directly through some form of interaction between the researcher and the people or organisation concerned, using such methods as interviews, focus groups, surveys and participant observation. Such methods involve the collection of primary data, and herein lies the opportunity for the researcher to develop and demonstrate the greatest skill.
However sometimes the researcher will use data which has already been collected for other purposes – in other words, he or she is going to an existing source rather than directly interacting with people. The data may have been:
- Deliberately collected and analysed, for example for some official survey such as the UK Labour Market Trends (now published as Economic & Labour Market Review (ELMR) ) or General Household Survey .
- Created in a more informal sense as a record of people's activities, for example, letters or other personal items, household bills, company records, etc. At some point, they may have been deliberately collected and organised into an archive.
Either way, such material is termed secondary data.
Rather confusingly, the latter form of secondary data is also referred to as primary source material.
"Primary resources are sources that are usually created at the time of an event. Primary resources are the direct evidence or first hand accounts of historical events without secondary analysis or interpretation." (York University Libraries Archival Research Tutorial)
This distinguishes them from secondary sources which describe, analyse and refer to the primary sources.
The above definitions and distinctions can be described diagrammatically as follows:
Types of secondary data
Secondary data is found in print or electronic form, if the latter, on CD-ROM, as an online computer database, or on the Internet. Furthermore, it can be in the form of statistics collected by governments, trade associations, organisations that exist to collect and sell statistical data, or just as plain documents in archives or company records.
A crucial distinction is whether or not the data has been interpreted, or whether it exists in raw form.
- Raw data, also referred to as documentary or archival data, will exist in the form in which it was originally intended, for example meeting minutes, staff records, reports on new markets, accounts of sales of goods/services etc.
- Interpreted data, which may also be referred to as survey data, will have been collected for a particular purpose, for example, to analyse spending patterns.
Because interpreted data will have been collected deliberately, the plan behind its collection and interpretation will also have been deliberate – that is, it will have been subjected to a particular research design.
By contrast, raw data will not have been processed, and will exist in its original form. (See " Using archival data " section in this guide.)
When and why to use secondary data
There are various reasons for using secondary data:
- A particularly good collection of data already exists.
- You are doing a historical study – that is, your study begins and ends at a particular point in time.
- You are covering an extended period, and analysing development over that period – a longitudinal study.
- The unit that you are studying may be difficult, or simply too large, to study directly.
- You are doing a case study of a particular organisation/industry/area, and it is important to look at the relevant documents.
You should pay particular attention to the place of secondary documents within your research design. How prominent a role you give to this method may depend on your subject: for example, if you are researching in the area of accounting, finance or business history, secondary documentary sources are likely to play an important part. Otherwise, use of secondary data is likely to play a complementary part in your research design. For example, if you are studying a particular organisation, you would probably want to supplement observation/interviews with a look at particular documents produced by that organisation.
In " Learning lessons? The registration of lobbyists at the Scottish parliament " ( Journal of Communication Management , Vol. 10 No. 1), the author uses archival research at the Scottish parliament as a supplementary research method (along with the media and focus groups), his main method being interviews and participant observation of meetings.
This point is further developed in the " Secondary data as part of the research design " section of this guide. Reasons for using the different types of secondary data are further developed in the individual sections.
NB If you are doing a research project/dissertation/thesis, check your organisation's view of secondary data. Some organisations may require you to use primary data as your principle research method.
Advantages and disadvantages of secondary data collection
The advantages of using secondary data are:
- The fact that much information exists in documented form – whether deliberately processed or not – means that such information cannot be ignored by the researcher, and generally saves time and effort collecting data which would otherwise have to be collected directly. In particular:
- Many existing data sets are enormous, and far greater than the researcher would be able to collect him or herself, with a far larger sample.
- The data may be particularly good quality, which can apply both to archival data (e.g. a complete collection of records on a particular topic) and to published data sets, particularly those which come from a government source, or from one of the leading commercial providers of data and statistics.
- You can access information which you may otherwise have had to secure in a more obtrusive manner.
- Existence of a large amount of data can facilitate different types of analysis, such as:
- longitudinal or international analysis of information which would have otherwise been difficult to collect due to scale.
- manipulation of data within the particular data set, including the comparison of particular subsets.
- Unforseen discoveries can be made – for example, the link between smoking and lung cancer was made by analysing medical records.
The disadvantages of secondary data collection are:
- There may be a cost to acquiring the data set.
- You will need to familiarise yourself with the data, and if you are dealing with a large and complex data set, it will be hard to manage.
- The data may not match the research question: there may be too much data, or there may be gaps, or the data may have been collected for a completely different purpose.
- The measures, for example between countries/states/historical periods, may not be directly comparable. (See the " Secondary data as part of the research design " section of this guide for a further development of this topic.)
- The researcher has no control over the quality of the data, which may not be seen as rigorous and reliable as data which are specifically collected by the researcher, who has adopted a specific research design for the question.
- Collecting primary data builds up more research skills than collecting secondary data.
- Company data particularly may be seen as commercially sensitive, and it may be difficult to gain access to company archives, which may be stored in different departments or on the company intranet, to which access may be difficult.
What are they?
As discussed in the previous section, these are sources of data which have already been collected and worked on by someone else, according to a particular research design. Other points to note are:
- Mostly they will have been collect by means of a survey, which may be:
- a census, which is an "official count", normally carried out by the government, with obligatory participation, for example the UK population censuses carried out every ten years
- a repeated survey, which involves collecting information at regular intervals, for example government surveys about household expenditure
- an ad hoc survey, done just once for a particular purpose, such as for example a market research survey.
- Interpreted data as referring to a particular social unit is termed a data set.
- A database is a structured data set, produced as a matrix with each social unit having a row, and each variable a column.
- Sometimes, different data sets are combined to produce multiple source secondary data: for example, the publication Business Statistics of the United States: Patterns of Economic Change contains data on virtually all aspects of the US economy from 1929 onwards. Such multiple source data sets may have been compiled on:
- a time series basis, that is they are based on repeated surveys (see above) or on comparable variables from different surveys to provide longitudinal data
- a geographical basis, providing information on different areas.
Key considerations
There are a number of points to consider when using data sets, some practical and others associated with the research design (yours and theirs).
Practical considerations relate to cost and use:
- Whilst much data is freely available, there may be a charge. For example, Business Statistics of the United States: Patterns of Economic Change is priced US$147. So, when deciding what data to use it's a good idea to check what's already in your library.
- Is the data available in computerised form, or will you have to enter it manually? If it is available in computerised form, is it in a form suitable to your research design (see below) or will you have to tabulate the data in a different form?
Research considerations include:
- Is the data set so important to your research that you cannot ignore it? For example, if you were doing a project which involved top corporations, you could not afford to ignore the publications which provided data and statistics, such as Europe's 15,000 Largest Companies 2006 .
- Does the data generally cover the research question?
- Is the coverage relevant, or does it leave out areas (e.g. only Asia as opposed to Australasia) or time periods (e.g. only starting in 1942 when you wanted data from 1928)?
- Are the variables relevant, for example if you are interested in household expenditure does it break down the households in ways relevant to your project?
- Are the measures used the same, for example, is growth in sales expressed as an amount or a percentage?
- In the case of data from different countries, has the data been collected in the same way? For example, workers affected by strikes may include those directly affected in one country, and those indirectly affected in another.
- Is the data reliable, and current? Note that data from government, and reputable commercial sources, is likely to be trustworthy but you should be wary of information on the Internet unless you know its source. Data from trustworthy sources is likely to have been collected by a team of experts, with good quality research design and instruments.
- The advantage of survey data in particular is that you have access to a far larger sample than you would otherwise have been able to collect yourself.
- There is an obvious advantage to using a large data source, however you need to allow for the time needed to extract what you want, and to re-tabulate the data in a form suitable for your research.
- How has the data been collected, for example it it longitudinal or geographical? This will affect the type of research question it can help with, for example, if you were comparing France and Germany, you would obviously want geographical data.
- How intrinsic to your research design will the use of secondary data be? Beware of relying on it entirely, but it may be a useful way of triangulating other research, for example if you have done a survey of shopping habits, you can assess how generalisable your findings are by looking at a census.
- While use of secondary data sets may not be seen as rigorous as collecting data yourself, the big advantage is that they are in a permanently available form and can be checked by others, which is an important point for validity.
And finally...
- Will the benefits you gain from using secondary data sets as a research methods outweigh the costs of acquiring the data, and the time spent sorting out what is relevant?
Producers of published secondary data include:
- Governments and intergovermental organisations, who produce a wide variety of data. For example, from the US Government come such titles as Budget of the United States Government , Business Statistics of the United States: Patterns of Economic Change , County and City Extra (source of data for every state), and Handbook of U.S. Labor Statistics .
- Trade associations and organisations representing particular interests, such as for example the American Marketing Association. These may have data and information relevant to their particular interest group.
- company information: for example AMADEUS provides pan European information on companies that includes balance sheets, profit and loss, ratios, descriptive etc., while FAME does a similar job for companies in the UK and Ireland.
- market research: for example, Mintel specialises in consumer, media and market research and published reports into particular market sectors, whilst Key Note "boasts one of the most comprehensive databases available to corporations in the UK", having published almost 1,000 reports spanning 30 industry sectors.
Where to find such information? The key is to have a very clear idea of what it is you are trying to find: what particular aspects of the research question are you attempting to answer?
You may well find sources listed in your literature review, or your tutor may point you in certain directions, but at some point you will need to consult the tertiary literature, which will point you in the direction of archives, indexes, catalogues and gateways. Your library will probably have Subject Guides covering your areas of interest. The following is a very basic list:
- UK Economic and Social Data Services (ESDS) . Contains links to: UK Data Archive (University of Essex); Institute for Social and Economic Research (University of Essex); Manchester Information and Associated Services (University of Manchester); and Cathie Marsh Centre for Census and Survey Research (University of Manchester). These contain access to a wide range of national and international data sets.
- http://epp.eurostat.ec.europa.eu . Statistics of the European Union.
- University of Michigan . Gateway to statistical resources on the Web.
- D&B Hoovers . Company information on US and international companies.
Archival, or documentary secondary data, are documentary records left by people as a by product of their eveyday activity. They may be formally deposited in an archive or they may just exist as company records.
Historians make considerable use of archival material as a key research technique, using a wide range of personal documents such as letters, diaries, household bills, which are often stored in some sort of formal "archive".
Business researchers talk about "archival research" because they use many of the same techniques for recording and analysing information. Companies, by their very nature, tend to create records, both officially in the form of annual reports, declarations of share value etc., and unofficially in the e-mails, letters, meeting minutes and agendas, sales data, employee records etc. which are the by-product of their daily activities.
If you are studying a business and management related subject, you may make use of archival material for a number of reasons:
- Your research takes a historical perspective, and you want to gain insight into management decisions outside the memories of those whom you interview.
- Archival research is an important tool in your particular discipline – for example, finance and accounting.
- You wish to undertake archival research as part of qualitative research in order to triangulate with interviews, focus groups etc., or perhaps as exploratory research prior to the main research.
- You may be undertaking a case study, or basing your research project on your own organisation; in either case, you should look at company documents as part of this research.
In " Financial reporting and local government reform – a (mis)match? " ( Qualitative Research in Accounting & Management , Vol. 2 No. 2), Robyn Pilcher uses archival research – "Data was obtained from annual reports provided electronically to the DLG and checked against hard copies of these reports and supporting notes" – and interviews as exploratory research to investigate use of flawed financial figures by political parties, before carrying out a detailed examination of a few councils.
" Coalport Bridge Tollhouse, 1793-1995 " ( Structural Survey , Vol. 14 No. 4) is a historical study of this building drawing on such documents as maps, plans, photos, account books, meeting minutes, legal opinions and census records.
As distinct from published data sets, you will have to record and process the data yourself, in order to create your own data set.
Sometimes this archival material will be stored in "official" archives, such as the UK Public Record Office. Mostly however, it will be company specific, stored in official company archives or perhaps in smaller collections in individual departments or business units. Records can exist in physical or electronic form – the latter commonly on the company intranet.
Whatever the company's archiving policy, there is no doubt that businesses provide a rich source of data. Here is a (non exhaustive) list of the forms that data can take:
- Organisational records – for example HR, accounts, pay roll data etc.
- Data referring to the sales of goods or services
- Project files
- Organisation charts
- Meeting minutes and agendas
- Sales literature: catalogues, copies of adverts, brochures etc.
- Annual reports
- Reports to shareholders
- Transcripts of speeches
- Non textual material: maps and plans, videos, tapes, photographs.
Management Information Systems can hold a considerable amount of data. For example, the following HR records may be held:
- data on recruitment, e.g. details of vacancies, dates, job details and criteria
- staff employment details, for example job analysis and evaluation, salary grades, terms and conditions of employment, job objectives, job competencies, performance appraisals
- data relevant to succession and career planning, e.g. the effects of not filling jobs
- management training and development, e.g. training records showing types of training.
Source: Peter Kingsbury (1997), IT Answers to HR Questions , CIPD.
The media (newspapers, magazines, advertisements, television and radio programmes, books, the Internet) can also throw valuable light on events, and media sources should not be ignored.
There are a number of points to consider when using archival material:
- You will need to gain access to the company, and this may prove difficult (see the " Gaining access to, and using, archives " section in this guide). On the other hand, if you are doing a report/project on your own organisation, access may be a lot easier, although even here you should gain agreement to access and use of material.
- Even if you are successful in gaining access to the company, it may be difficult and time-consuming to locate all the information you need, especially if the company does not have a clear archiving policy, and you may need to go through a vast range of documents.
- The data may be incomplete, and may not answer your research question – for example, there may be a gap in records, correspondence may be one-sided and not include responses.
- The data may be biased, in other words it will be written by people who have a particular view. For example, meeting minutes are the "official" version and often things go on in meetings which are not recorded; profitability in annual reports may be reported in such a way as to show a positive rather than a true picture.
- Informal and verbal interactions cannot be captured.
- Archival research is time-consuming, both in locating and in recording documents, so for that reason may not be feasible for smaller projects.
- You will also need to decide how to record data: historians are used to laboriously copying out documents considered too frail to photocopy, and business researchers may need to resort to this if (as is likely) company documents are considered confidential, although in such cases, note-taking may also be out. You will also need to find a suitable way of coding and referring to particular documents.
- Finally, you will need to construct your own data set, for which you will need to have a particular research method.
In " Participatory group observation – a tool to analyse strategic decision-making " ( Qualitative Market Research , Vol. 5 No. 1), Christine Vallaster and Oliver Koll highlight the benefit of multiple methods for studying complex issues, it being thus possible to supplement the weaknesses of one method with the strengths of another and study a phenomenon from a diversity of views, and achieve a high degree of validity. In the case in question, archival research was used to analyse documents (organisation charts, company reports, memos, meeting minutes), and whilst the limitations in terms of incompleteness, selectivity, and not being authored by interviewees were acknowledged, so was their supporting value to interviews, and the same textual analysis method was used for both methods.
We have already mentioned, as part of our discussion of the two main types of secondary data, some considerations in respect to how they are used as part of the research. In this section, we shall look more generally at how secondary data can fit in to the overall research design.
Theoretical framework
Researchers take different views of the facts they are researching. For some, facts exist as independent reality; others admit the possibility of interpretation by the actors concerned. The two views, and their implication for the documents and data concerned, can be summed up as follows:
- Positivists see facts as existing independently of interpretation, so documents are an objective reflection of reality.
- Interpretivists , and even more so realists, see reality as influenced by the social environment, open to manipulation by those who are part of it. A document must be seen in its social context, and an attempt to make sense of that context.
Some examples would be:
- minutes of a sales meeting the purpose of which was to monitor sales, with sales being affected by external influences
- brochure or flyer which was created for a particular item, and designed to appeal to current fashions
- training records of people doing National Vocational Qualifications (used in the UK to acknowledge the value of existing skills).
Reliability and validity
Reliability and validity is important to any research design, and an important consideration with secondary data is the extent to which it relates to the research question, in other words how reliably it can answer it. You need to consider the fit very carefully before deciding to proceed. Some questions which may help here are:
How reliable is the data?
In the case of published data, you will be able to make a judgement by looking at its provenance: does it come from the government, or from a reputable commercial source? The same applies to the Internet – what is the source? Look for publisher information and copyright statements. How up to date is the material?
You also need to make intrinsic judgements, however: what is the methodology behind the survey, and how robust is it? How large was the sample and what was the response rate?
There are fewer obvious external measures you can use to check unpublished, archival material: that from businesses can be notoriously inconsistent and inaccurate. Records can be incomplete with some documents missing; sometimes, whole archives can disappear when companies are taken over. In addition, some documents such as letters, reports, e-mails, meeting minutes etc. have a subjective element, reflecting the view of the author, or the perceived wishes of the recipient. For example, meeting minutes may not reflect a controversial discussion that took place but only the agreed action points; a report on sales may be intended to put a positive spin on a situation and disguise its real seriousness. It helps when assessing reliability to consider who the intended audience is.
If you are using media reports, be aware that these may only include what they consider to be the most pertinent points.
Measurement validity
One of the biggest problems with secondary data is to do with the measurements involved. These may just not be the same as the ones you want (e.g. sales given in revenue rather than quantity), they may deliberately be distorted (e.g. non recording of minor accidents, sick leave etc.), or they may be different for different countries. If the measures are inexact, you need to take a view as to how serious the problem is and how you can address it.
Does the data cover the time frame, geographical area, and variable in which you are interested? For example, if you are studying a particular period in a company, do you have meeting minutes to cover that period, or do they stop/start at a time within the boundaries of that period? Do you have the sales figures for all the countries your are interested in, and all the product types?
You can greatly increase the validity and reliability of your use of secondary data if you triangulate with another research method. For example if you are seeking insights into a period of change within a company, you can use documentary records to compare with interviews with key informants.
" Leading beyond tragedy: the balance of personal identity and adaptability " ( Leadership & Organization Development Journal , Vol. 26 No. 6) is a case study of the Norwegian company Wilhelmson's Lines loss of key employees in a plane crash, and uses archival research along with on-site interviews and participant observation as the tools of case study analysis.
" The human resource management practice of retail branding: an ethnography within Oxfam Trading Division " ( International Journal of Retail & Distribution Management , Vol. 33 No. 7) uses an ethnographic approach and includes scanning the company intranet along with participant observation and interviews.
Quantitative or qualitative?
Documentary data can be used as part of a qualitative or quantitative research design.
Much data, whether from company archives or from published data sets, is statistical, and can therefore be used as part of a quantitative design, for example how many sales were made of a particular item, what were reasons for absenteeism, company profitability etc.
One way of using secondary data in quantitative research is to compare it with data you have collected yourself, probably by a survey. For example, you can compare your own survey data with that from a census or other published survey, which will inevitably have a much larger sample, thereby helping you generalise, and/or triangulate, your findings.
Textual data can also be used qualitatively, for example marketing literature can be used to as backup information on marketing campaigns, and e-mails, letters, meeting minutes etc. can throw additional light on management decisions.
Content analysis is often quoted as a method of analysis: this involves analysing occurrence of key concepts and ideas and either draw statistical inferences or carry out a qualitative assessment, looking at the main themes that emerge.
Archives may be found in national collections, such as the UK's Public Record Office, or as smaller collections associated with national, local or federal government organisations, academic libraries, professional or trade associations, or charities; they may also be found in companies. The latter are generally closely controlled; the former are most likely to be publically available. This page gives a brief overview of how to gain access to archival collections, and what you can expect when you get there.
Preparation
An archival collection, even an open one, is not like a library where you can just turn up. You need to establish opening hours, and then make arrangements to visit.
It is best to write ahead explaining:
- Your project
- Precisely what it is you are looking for.
In order to be clear about point 2, you will need to know not only the precise scope of your research but also how this particular collection can help you. You will therefore need to spend time researching (perhaps more than one) collection, so make sure that this is allowed for in your research plan.
You also need to understand the key difference between libraries and archives:
- Archives are collections of unpublished material, housed in closed stacks, organised according to the principles of the original collector. You can only access the material in situ, and you will need to handle the collection with special care.
- Libraries contain published material, in open stacks, classified according to a particular system, and you may be able to take the material out on loan.
Locating sources
Bibliographic databases are good sources for finding archival collections: you can search by subject, keyword, personal or geographical name. Whilst not containing records of each item, catalogue records of archival collections are generally lengthier than for published materials and may include a summary of materials contained in the collection.
More detailed information about the collection, usually at the level of the box or folder, is found in Finding Aids .
You can find suitable databases through your library's Subject Guides.
Gaining access to commercial collections
As indicated above, commercial archival or document collections are more tightly controlled than public ones, access to which will depend upon a clearly stated request and proof of identity.
Commercial sources, by contrast, may require more negotiation, and more convincing, because of the perceived sensitivity of their material and the fact that they exist for their customers and shareholders, and not as an archival collection. Companies understandably count the opportunity cost of time spent "helping a researcher with their enquiries", not to mentioning opening up possibly sensitive documents to the prying eyes of an outsider.
This can cause problems to the researcher because if the research project is based on one or a few companies, if access is denied then the overall validity of the research will be prejudiced. Given the likelihood that other research methods, such as interview, survey etc. are also being used, it is best to approach access in the widest sense, and stress the benefits to the organisation, the credibility of the researcher, and assurance of confidentiality.
What Is Secondary Data? A Complete Guide
What is secondary data, and why is it important? Find out in this post.
Within data analytics, there are many ways of categorizing data. A common distinction, for instance, is that between qualitative and quantitative data . In addition, you might also distinguish your data based on factors like sensitivity. For example, is it publicly available or is it highly confidential?
Probably the most fundamental distinction between different types of data is their source. Namely, are they primary, secondary, or third-party data? Each of these vital data sources supports the data analytics process in its own way. In this post, we’ll focus specifically on secondary data. We’ll look at its main characteristics, provide some examples, and highlight the main pros and cons of using secondary data in your analysis.
We’ll cover the following topics:
What is secondary data?
- What’s the difference between primary, secondary, and third-party data?
- What are some examples of secondary data?
- How to analyse secondary data
- Advantages of secondary data
- Disadvantages of secondary data
- Wrap-up and further reading
Ready to learn all about secondary data? Then let’s go.
1. What is secondary data?
Secondary data (also known as second-party data) refers to any dataset collected by any person other than the one using it.
Secondary data sources are extremely useful. They allow researchers and data analysts to build large, high-quality databases that help solve business problems. By expanding their datasets with secondary data, analysts can enhance the quality and accuracy of their insights. Most secondary data comes from external organizations. However, secondary data also refers to that collected within an organization and then repurposed.
Secondary data has various benefits and drawbacks, which we’ll explore in detail in section four. First, though, it’s essential to contextualize secondary data by understanding its relationship to two other sources of data: primary and third-party data. We’ll look at these next.
2. What’s the difference between primary, secondary, and third-party data?
To best understand secondary data, we need to know how it relates to the other main data sources: primary and third-party data.
What is primary data?
‘Primary data’ (also known as first-party data) are those directly collected or obtained by the organization or individual that intends to use them. Primary data are always collected for a specific purpose. This could be to inform a defined goal or objective or to address a particular business problem.
For example, a real estate organization might want to analyze current housing market trends. This might involve conducting interviews, collecting facts and figures through surveys and focus groups, or capturing data via electronic forms. Focusing only on the data required to complete the task at hand ensures that primary data remain highly relevant. They’re also well-structured and of high quality.
As explained, ‘secondary data’ describes those collected for a purpose other than the task at hand. Secondary data can come from within an organization but more commonly originate from an external source. If it helps to make the distinction, secondary data is essentially just another organization’s primary data.
Secondary data sources are so numerous that they’ve started playing an increasingly vital role in research and analytics. They are easier to source than primary data and can be repurposed to solve many different problems. While secondary data may be less relevant for a given task than primary data, they are generally still well-structured and highly reliable.
What is third-party data?
‘Third-party data’ (sometimes referred to as tertiary data) refers to data collected and aggregated from numerous discrete sources by third-party organizations. Because third-party data combine data from numerous sources and aren’t collected with a specific goal in mind, the quality can be lower.
Third-party data also tend to be largely unstructured. This means that they’re often beset by errors, duplicates, and so on, and require more processing to get them into a usable format. Nevertheless, used appropriately, third-party data are still a useful data analytics resource. You can learn more about structured vs unstructured data here .
OK, now that we’ve placed secondary data in context, let’s explore some common sources and types of secondary data.
3. What are some examples of secondary data?
External secondary data.
Before we get to examples of secondary data, we first need to understand the types of organizations that generally provide them. Frequent sources of secondary data include:
- Government departments
- Public sector organizations
- Industry associations
- Trade and industry bodies
- Educational institutions
- Private companies
- Market research providers
While all these organizations provide secondary data, government sources are perhaps the most freely accessible. They are legally obliged to keep records when registering people, providing services, and so on. This type of secondary data is known as administrative data. It’s especially useful for creating detailed segment profiles, where analysts hone in on a particular region, trend, market, or other demographic.
Types of secondary data vary. Popular examples of secondary data include:
- Tax records and social security data
- Census data (the U.S. Census Bureau is oft-referenced, as well as our favorite, the U.S. Bureau of Labor Statistics )
- Electoral statistics
- Health records
- Books, journals, or other print media
- Social media monitoring, internet searches, and other online data
- Sales figures or other reports from third-party companies
- Libraries and electronic filing systems
- App data, e.g. location data, GPS data, timestamp data, etc.
Internal secondary data
As mentioned, secondary data is not limited to that from a different organization. It can also come from within an organization itself.
Sources of internal secondary data might include:
- Sales reports
- Annual accounts
- Quarterly sales figures
- Customer relationship management systems
- Emails and metadata
- Website cookies
In the right context, we can define practically any type of data as secondary data. The key takeaway is that the term ‘secondary data’ doesn’t refer to any inherent quality of the data themselves, but to how they are used. Any data source (external or internal) used for a task other than that for which it was originally collected can be described as secondary data.
4. How to analyse secondary data
The process of analysing secondary data can be performed either quantitatively or qualitatively, depending on the kind of data the researcher is dealing with. The quantitative method of secondary data analysis is used on numerical data and is analyzed mathematically. The qualitative method uses words to provide in-depth information about data.
There are different stages of secondary data analysis, which involve events before, during, and after data collection. These stages include:
- Statement of purpose: Before collecting secondary data, you need to know your statement of purpose. This means you should have a clear awareness of the goal of the research work and how this data will help achieve it. This will guide you to collect the right data, then choosing the best data source and method of analysis.
- Research design: This is a plan on how the research activities will be carried out. It describes the kind of data to be collected, the sources of data collection, the method of data collection, tools used, and method of analysis. Once the purpose of the research has been identified, the researcher should design a research process that will guide the data analysis process.
- Developing the research questions: Once you’ve identified the research purpose, an analyst should also prepare research questions to help identify secondary data. For example, if a researcher is looking to learn more about why working adults are increasingly more interested in the “gig economy” as opposed to full-time work, they may ask, “What are the main factors that influence adults decisions to engage in freelance work?” or, “Does education level have an effect on how people engage in freelance work?
- Identifying secondary data: Using the research questions as a guide, researchers will then begin to identify relevant data from the sources provided. If the kind of data to be collected is qualitative, a researcher can filter out qualitative data—for example.
- Evaluating secondary data: Once relevant data has been identified and collates, it will be evaluated to ensure it fulfils the criteria of the research topic. Then, it is analyzed either using the quantitative or qualitative method, depending on the type of data it is.
You can learn more about secondary data analysis in this post .
5. Advantages of secondary data
Secondary data is suitable for any number of analytics activities. The only limitation is a dataset’s format, structure, and whether or not it relates to the topic or problem at hand.
When analyzing secondary data, the process has some minor differences, mainly in the preparation phase. Otherwise, it follows much the same path as any traditional data analytics project.
More broadly, though, what are the advantages and disadvantages of using secondary data? Let’s take a look.
Advantages of using secondary data
It’s an economic use of time and resources: Because secondary data have already been collected, cleaned, and stored, this saves analysts much of the hard work that comes from collecting these data firsthand. For instance, for qualitative data, the complex tasks of deciding on appropriate research questions or how best to record the answers have already been completed. Secondary data saves data analysts and data scientists from having to start from scratch.
It provides a unique, detailed picture of a population: Certain types of secondary data, especially government administrative data, can provide access to levels of detail that it would otherwise be extremely difficult (or impossible) for organizations to collect on their own. Data from public sources, for instance, can provide organizations and individuals with a far greater level of population detail than they could ever hope to gather in-house. You can also obtain data over larger intervals if you need it., e.g. stock market data which provides decades’-worth of information.
Secondary data can build useful relationships: Acquiring secondary data usually involves making connections with organizations and analysts in fields that share some common ground with your own. This opens the door to a cross-pollination of disciplinary knowledge. You never know what nuggets of information or additional data resources you might find by building these relationships.
Secondary data tend to be high-quality: Unlike some data sources, e.g. third-party data, secondary data tends to be in excellent shape. In general, secondary datasets have already been validated and therefore require minimal checking. Often, such as in the case of government data, datasets are also gathered and quality-assured by organizations with much more time and resources available. This further benefits the data quality , while benefiting smaller organizations that don’t have endless resources available.
It’s excellent for both data enrichment and informing primary data collection: Another benefit of secondary data is that they can be used to enhance and expand existing datasets. Secondary data can also inform primary data collection strategies. They can provide analysts or researchers with initial insights into the type of data they might want to collect themselves further down the line.
6. Disadvantages of secondary data
They aren’t always free: Sometimes, it’s unavoidable—you may have to pay for access to secondary data. However, while this can be a financial burden, in reality, the cost of purchasing a secondary dataset usually far outweighs the cost of having to plan for and collect the data firsthand.
The data isn’t always suited to the problem at hand: While secondary data may tick many boxes concerning its relevance to a business problem, this is not always true. For instance, secondary data collection might have been in a geographical location or time period ill-suited to your analysis. Because analysts were not present when the data were initially collected, this may also limit the insights they can extract.
The data may not be in the preferred format: Even when a dataset provides the necessary information, that doesn’t mean it’s appropriately stored. A basic example: numbers might be stored as categorical data rather than numerical data. Another issue is that there may be gaps in the data. Categories that are too vague may limit the information you can glean. For instance, a dataset of people’s hair color that is limited to ‘brown, blonde and other’ will tell you very little about people with auburn, black, white, or gray hair.
You can’t be sure how the data were collected: A structured, well-ordered secondary dataset may appear to be in good shape. However, it’s not always possible to know what issues might have occurred during data collection that will impact their quality. For instance, poor response rates will provide a limited view. While issues relating to data collection are sometimes made available alongside the datasets (e.g. for government data) this isn’t always the case. You should therefore treat secondary data with a reasonable degree of caution.
Being aware of these disadvantages is the first step towards mitigating them. While you should be aware of the risks associated with using secondary datasets, in general, the benefits far outweigh the drawbacks.
7. Wrap-up and further reading
In this post we’ve explored secondary data in detail. As we’ve seen, it’s not so different from other forms of data. What defines data as secondary data is how it is used rather than an inherent characteristic of the data themselves.
To learn more about data analytics, check out this free, five-day introductory data analytics short course . You can also check out these articles to learn more about the data analytics process:
- What is data cleaning and why is it important?
- What is data visualization? A complete introductory guide
- 10 Great places to find free datasets for your next project

An official website of the United States government
Here’s how you know
The .gov means it’s official. Federal government websites often end in .gov or .mil. Before sharing sensitive information, make sure you’re on a federal government site.
The site is secure. The https:// ensures that you are connecting to the official website and that any information you provide is encrypted and transmitted securely.
Take action
- Report an antitrust violation
- File adjudicative documents
- Find banned debt collectors
- View competition guidance
- Competition Matters Blog
Slow the Roll-up: Help Shine a Light on Serial Acquisitions
View all Competition Matters Blog posts
We work to advance government policies that protect consumers and promote competition.
View Policy
Search or browse the Legal Library
Find legal resources and guidance to understand your business responsibilities and comply with the law.
Browse legal resources
- Find policy statements
- Submit a public comment
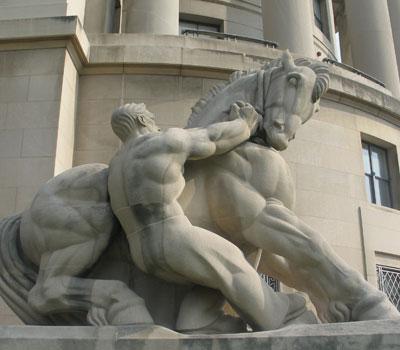
Vision and Priorities
Memo from Chair Lina M. Khan to commission staff and commissioners regarding the vision and priorities for the FTC.
Technology Blog
Global perspectives from the international competition network tech forum.
View all Technology Blog posts
Advice and Guidance
Learn more about your rights as a consumer and how to spot and avoid scams. Find the resources you need to understand how consumer protection law impacts your business.
- Report fraud
- Report identity theft
- Register for Do Not Call
- Sign up for consumer alerts
- Get Business Blog updates
- Get your free credit report
- Find refund cases
- Order bulk publications
- Consumer Advice
- Shopping and Donating
- Credit, Loans, and Debt
- Jobs and Making Money
- Unwanted Calls, Emails, and Texts
- Identity Theft and Online Security
- Business Guidance
- Advertising and Marketing
- Credit and Finance
- Privacy and Security
- By Industry
- For Small Businesses
- Browse Business Guidance Resources
- Business Blog
Servicemembers: Your tool for financial readiness
Visit militaryconsumer.gov
Get consumer protection basics, plain and simple
Visit consumer.gov
Learn how the FTC protects free enterprise and consumers
Visit Competition Counts
Looking for competition guidance?
- Competition Guidance
News and Events
Latest news, ftc data shows major increases in cash payments to government impersonation scammers.
View News and Events
Upcoming Event
Closed commission meeting - june 20, 2024.
View more Events
Sign up for the latest news
Follow us on social media
--> --> --> --> -->
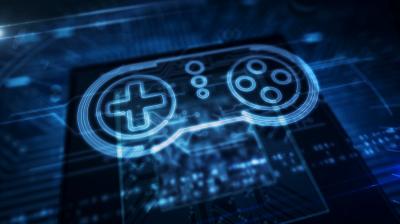
Playing it Safe: Explore the FTC's Top Video Game Cases
Learn about the FTC's notable video game cases and what our agency is doing to keep the public safe.
Latest Data Visualization
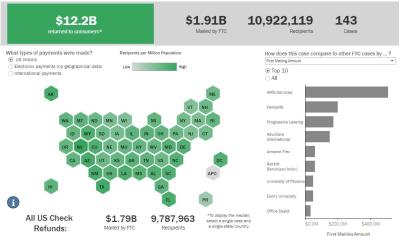
FTC Refunds to Consumers
Explore refund statistics including where refunds were sent and the dollar amounts refunded with this visualization.
About the FTC
Our mission is protecting the public from deceptive or unfair business practices and from unfair methods of competition through law enforcement, advocacy, research, and education.
Learn more about the FTC
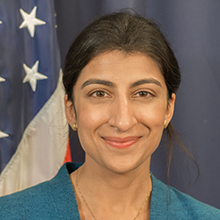
Meet the Chair
Lina M. Khan was sworn in as Chair of the Federal Trade Commission on June 15, 2021.
Chair Lina M. Khan
Looking for legal documents or records? Search the Legal Library instead.
- Cases and Proceedings
- Premerger Notification Program
- Merger Review
- Anticompetitive Practices
- Competition and Consumer Protection Guidance Documents
- Warning Letters
- Consumer Sentinel Network
- Criminal Liaison Unit
- FTC Refund Programs
- Notices of Penalty Offenses
- Advocacy and Research
- Advisory Opinions
- Cooperation Agreements
- Federal Register Notices
- Public Comments
- Policy Statements
- International
- Office of Technology Blog
- Military Consumer
- Consumer.gov
- Bulk Publications
- Data and Visualizations
- Stay Connected
- Commissioners and Staff
- Bureaus and Offices
- Budget and Strategy
- Office of Inspector General
- Careers at the FTC
FTC Warns About Misuses of Biometric Information and Harm to Consumers
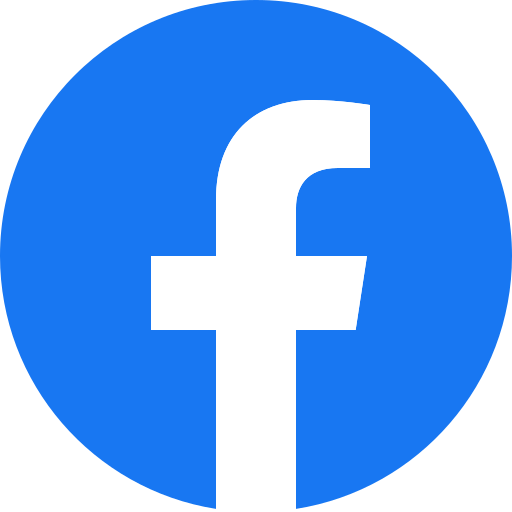
- Consumer Protection
- Bureau of Consumer Protection
- Consumer Privacy
- Artificial Intelligence
The Federal Trade Commission today issued a warning that the increasing use of consumers’ biometric information and related technologies, including those powered by machine learning, raises significant consumer privacy and data security concerns and the potential for bias and discrimination.
Biometric information refers to data that depict or describe physical, biological, or behavioral traits, characteristics, or measurements of or relating to an identified or identifiable person’s body.
“In recent years, biometric surveillance has grown more sophisticated and pervasive, posing new threats to privacy and civil rights,” said Samuel Levine, Director of the FTC’s Bureau of Consumer Protection. “Today’s policy statement makes clear that companies must comply with the law regardless of the technology they are using.”
In a policy statement , the Commission said the agency is committed to combatting unfair or deceptive acts and practices related to the collection and use of consumers’ biometric information and the marketing and use of biometric information technologies.
Recent years have seen a proliferation of biometric information technologies. For instance, facial, iris, or fingerprint recognition technologies collect and process biometric information to identify individuals. Other biometric information technologies use or claim to use biometric information in order to determine characteristics of individuals, ranging from the individuals’ age, gender, or race to the individuals’ personality traits, aptitudes, or demeanor.
Consumers face new and increasing risks associated with the collection and use of biometric information. For example, using biometric information technologies to identify consumers in certain locations could reveal sensitive personal information about them such as whether they accessed particular types of healthcare, attended religious services, or attended political or union meetings. Large databases of biometric information could also be attractive targets for malicious actors who could misuse such information. Additionally, some technologies using biometric information, such as facial recognition technology, may have higher rates of error for certain populations than for others.
In recent years, the FTC has brought enforcement actions against photo app maker Everalbum and Facebook , charging they misrepresented their uses of facial recognition technology. The FTC also issued a report about facial recognition in 2012 that recommended best practices to protect consumers’ privacy.
Today’s policy statement warns that false or unsubstantiated claims about the accuracy or efficacy of biometric information technologies or about the collection and use of biometric information may violate the FTC Act. The policy statement also notes that it will consider several factors in determining whether a business’s use of biometric information or biometric information technology could be unfair in violation of the FTC Act including:
- Failing to assess foreseeable harms to consumers before collecting biometric information;
- Failing to promptly address known or foreseeable risks and identify and implement tools for reducing or eliminating those risks;
- Engaging in surreptitious and unexpected collection or use of biometric information;
- Failing to evaluate the practices and capabilities of third parties, including affiliates, vendors, and end users, who will be given access to consumers’ biometric information or will be charged with operating biometric information technologies;
- Failing to provide appropriate training for employees and contractors whose job duties involve interacting with biometric information or technologies that use such information; and
- Failing to conduct ongoing monitoring of technologies that the business develops, offers for sale, or uses, in connection with biometric information to ensure that the technologies are functioning as anticipated and that the technologies are not likely to harm consumers
The Commission voted 3 -0 during an open Commission meeting to adopt the policy statement.
FTC staff who worked on this matter include Robin Wetherill and Amanda Koulousias.
The Federal Trade Commission works to promote competition and protect and educate consumers . The FTC will never demand money, make threats, tell you to transfer money, or promise you a prize. Learn more about consumer topics at consumer.ftc.gov , or report fraud, scams, and bad business practices at ReportFraud.ftc.gov . Follow the FTC on social media , read consumer alerts and the business blog , and sign up to get the latest FTC news and alerts .
Contact Information
Media contact.
Juliana Gruenwald Henderson Office of Public Affairs 202-326-2924
- Get 7 Days Free
Electra Therapeutics Presents Late-Breaking Clinical Data at EHA2024 from Ongoing Phase 1b Study of ELA026 in Secondary Hemophagocytic Lymphohistiocytosis (sHLH)
ELA026 demonstrated a high overall response rate (ORR) across a range of sHLH patients and a favorable safety profile in this life-threatening hyperinflammatory condition
In patients with the poorest prognosis, those with malignancy-associated HLH, early treatment with ELA026 resulted in 100% ORR and improved survival
Electra Therapeutics, Inc. , a clinical stage biotechnology company developing antibody therapies against novel targets for immunological diseases and cancer, announced the presentation of clinical data for ELA026 in secondary hemophagocytic lymphohistiocytosis (sHLH), a life-threatening hyperinflammatory condition. The results were presented today as one of six abstracts selected for oral presentation in a late-breaking session at the European Hematology Association (EHA) Congress in Madrid, Spain.
Results were reported for sHLH patients in the ongoing Phase 1b clinical study, which has enrolled a majority of patients with the deadliest sHLH subtype, malignancy-associated HLH (mHLH). In treatment‑naive mHLH patients, ELA026 achieved a 100% overall response rate (ORR) by week 4 and improved survival at two months compared to natural history studies. ELA026 also demonstrated a high response rate across a range of sHLH patients and a favorable safety profile in this patient population.
ELA026 is a first-in-class monoclonal antibody that targets signal regulatory protein (SIRP)-α/β1/γ on the cell surface of myeloid cells and T lymphocytes, the pathological immune cells that induce hyperinflammation in sHLH. The Phase 1b study is an ongoing open-label, multi-dose, single-arm, multicenter study designed to evaluate the safety and efficacy of ELA026, assess biomarkers, and identify a dose for Phase 2/3 testing (ClinicalTrials.gov identifier: NCT05416307 ).
“These data show very promising results for ELA026 as a potential treatment for sHLH, which is a challenging disease that is devastating for patients and has no approved treatment options,” said Swaminathan P. Iyer, MD, Professor in the Department of Lymphoma/Myeloma at The University of Texas MD Anderson Cancer Center. “Notably, the analysis reveals improved survival was achieved with ELA026 in treatment-naïve mHLH patients, suggesting benefit from early treatment intervention for patients with this rapidly progressing disease.”
Study Results Presented at EHA 2024 Congress
The oral presentation at EHA2024, entitled “ELA026 Targeting of SIRP(+) Immune Cells Results in a High Response Rate and Improved 2-Month Survival of Treatment-naïve Malignancy-associated Hemophagocytic Lymphohistiocytosis in a Phase 1 Study,” was presented by the lead author, Abhishek Maiti, MD, Assistant Professor in the Department of Leukemia at The University of Texas MD Anderson Cancer Center. The data describe analysis of sHLH patients in three cohorts of the ongoing Phase 1b clinical study, including the following highlights:
- A majority of enrolled patients had malignancy-associated HLH (mHLH) which has the poorest prognosis, with a mortality rate of approximately 50% at two months. 1
- ELA026 was observed to have a favorable safety profile, with manageable adverse events, in this patient population.
- In Cohorts 1 and 2, which have completed enrollment (n=12), ELA026 achieved an overall response rate (ORR) of 75% by week 4. Enrollment in Cohort 3 is ongoing (n=5 as of April 17, 2024).
- Across all cohorts, 8 patients had treatment-naïve mHLH; in this group, ELA026 achieved an ORR of 100% by week 4 and survival was 88% at two months.
- In the study, pharmacodynamic and biomarker responses correlated with clinical responses.
- The Phase 1b study has expanded to include up to 20 patients in Cohort 3 and will continue to evaluate ELA026 in frontline treatment settings in patients with various subtypes of sHLH.
“We are delighted that the results of the clinical study of ELA026 in sHLH were recognized and selected as a late-breaking presentation at EHA. This interim data is extremely encouraging, particularly with the high response rates and improved survival at two months achieved in treatment-naïve mHLH patients, and suggests ELA026’s promise as a first-line treatment. Survival at two months is a clinically meaningful benefit for this patient population and demonstrates the ability of ELA026 to rapidly extinguish the hyperinflammation in sHLH, enabling mHLH patients to pursue curative therapies for their underlying cancer,” said Kim‑Hien Dao, DO, PhD, Chief Medical Officer at Electra. “We look forward to continuing enrollment in this study and advancing the clinical program to further assess the safety and efficacy of ELA026 in sHLH patients who currently have no approved therapies.”
About Secondary Hemophagocytic Lymphohistiocytosis (sHLH)
Secondary hemophagocytic lymphohistiocytosis (sHLH) is a rare, life-threatening inflammatory disease for which there is no approved treatment. It can be triggered by cancer (malignancy-associated HLH, or mHLH), infection, autoimmune disease, or immunotherapy. sHLH is associated with a systemic inflammatory response for which patients require immediate intervention. Without treatment, patients may experience multiple organ failure and death. sHLH has a high mortality rate during the first months of diagnosis, with mHLH patients having the poorest outcomes.
About Electra Therapeutics
Electra Therapeutics is a clinical stage biotechnology company developing therapies against novel targets for immunological diseases and cancer. The company’s lead product candidate, ELA026, is a first-in-class monoclonal antibody that targets SIRP on the cell surface of myeloid cells and T lymphocytes, and precisely depletes pathological immune cells. ELA026 is in clinical development for secondary hemophagocytic lymphohistiocytosis (sHLH), a rare, life-threatening hyperinflammatory condition for which there is no approved treatment, as well as additional disease indications. For more information, please visit www.electra-therapeutics.com and follow us on LinkedIn .
1 Löfstedt A, et al. Blood. 2024 Jan 18;143(3):233-242.
Kathryn Morris, The Yates Network LLC 914-204-6412 [email protected]
View source version on businesswire.com: https://www.businesswire.com/news/home/20240616038888/en/
Market Updates
Is it time to ditch your money market fund for longer-term bonds, what’s happening in the markets this week, 4 reasons why today’s stock market is delivering impressive performance, what does nvidia’s stock split mean for investors, 5 undervalued stocks to buy as their stories play out, markets brief: return of the meme stocks, it’s been a terrible time for bonds. here’s why you should own them, which ai stocks are turning hype into revenue, stock picks, tesla: shareholder vote reduces key person risk, after earnings, is crowdstrike stock a buy, a sell, or fairly valued, adobe’s strong quarterly results drive share gains, what does broadcom’s stock split mean for investors, 5 ultracheap stocks to buy with the best returns on investment, broadcom earnings: ai sales growth accelerates, oracle earnings: iaas signings more than make up for miss, this undervalued stock is a buy after its dividend increase, sponsor center.
Copernicus: May 2024, streak of global records for surface air and ocean temperatures continues
- 1. May 2024 – Surface air temperature and sea surface temperature highlights:
- 2. May 2024 – Hydrological highlights
- 3. May 2024 – Sea Ice highlights
- 4. 2024 Boreal Spring Seasonal Highlights
- 5. More Information
- 6. About Copernicus and ECMWF
Bonn, 06/06/2024

Daily sea surface temperature (°C) averaged over the extra-polar global ocean (60°S–60°N) for all 12-month periods spanning June to May of the following year. The last 12 months (June 2023 to May 2024) are shown with a thick red line, the period from June 2015 to May 2016 with a blue line, and the period from June 2022 to May 2023 with an orange line. All other years are shown with thin grey lines. The light-red colour shading highlights the margin by which daily values in 2023–2024 exceeded previous daily records. Data source: ERA5. Credit: Copernicus Climate Change Service/ECMWF. ACCESS TO DATA | DOWNLOAD THE ORIGINAL IMAGE
The Copernicus Climate Change Service (C3S) , implemented by the European Centre for Medium-Range Weather Forecasts on behalf of the European Commission with funding from the EU, routinely publishes monthly climate bulletins reporting on the changes observed in global surface air and sea temperatures, sea ice cover and hydrological variables. Additionally, the bulletin also includes highlights regarding the boreal spring (March-April-May). Most of the reported findings are based on the ERA5 reanalysis dataset, using billions of measurements from satellites, ships, aircraft and weather stations around the world.
May 2024 – Surface air temperature and sea surface temperature highlights:
May 2024 was warmer globally than any previous May in the data record, with an average ERA5 surface air temperature of 15.91°C, 0.65°C above the 1991-2020 average for May and 0.19°C above the previous high set in May 2020.
This is the twelfth month in a row that is the warmest in the ERA5 data record for the respective month of the year. While unusual, a similar streak of monthly global temperature records happened previously in 2015/2016.
The month was 1.52°C above the estimated May average for 1850-1900, the designated pre-industrial reference period.
The global-average temperature for the past 12 months (June 2023 – May 2024) is the highest on record, at 0.75°C above the 1991-2020 average and 1.63°C above the 1850-1900 pre-industrial average.
The average European temperature for May 2024 was 0.88°C above the 1991-2020 average for May, and the third warmest May on record for the continent.
Temperatures were below average over the eastern equatorial Pacific, indicating a developing La Niña, but air temperatures over the ocean remained at an unusually high level over many regions.
The sea surface temperature (SST) averaged for May 2024 over 60°S–60°N was 20.93°C, the highest value on record for the month.

According to Samantha Burgess, Deputy Director of the Copernicus Climate Change Service (C3S): " The climate continues to alarm us - the last 12 months have broken records like never before - caused primarily by our greenhouse gas emissions and an added boost from the El Niño event in the tropical Pacific. Until we reach net-zero global emissions the climate will continue to warm, will continue to break records, and will continue to produce even more extreme weather events. If we choose to continue to add greenhouse gases to the atmosphere then 2023/4 will soon look like a cool year, in a similar way to how 2015/6 now appears ."

Anomalies and extremes in surface air temperature for the 12-month period from June 2023 to May 2024. Colour categories refer to the percentiles of the temperature distributions for the 1991–2020 reference period. The extreme (“Coolest” and “Warmest”) categories are based on rankings for the period 1979–2024. Percentiles and rankings are relative to all 12-month averages between January 1979 and May 2024. Data source: ERA5. Credit: Copernicus Climate Change Service/ECMWF. ACCESS TO DATA | DOWNLOAD THE ORIGINAL IMAGE
For additional graphics and data on May temperature, please visit the statement released along with the World Meteorological Organisation (WMO) and the United Nations Secretary General (UNSG) on Wednesday 5th here .
May 2024 – Hydrological highlights
May 2024 was wetter than average over much of Iceland, UK and Ireland, central and most of south-eastern Europe, north of Iberian Peninsula and western Russia. Heavy rainfall led to widespread flooding and associated damage over Germany, the Benelux, and Italy, amongst other regions.
Much of the Iberian Peninsula, southwest Türkiye and a large region across Eastern Europe, including southern Fennoscandia and the Baltic Countries, were drier than average.
In May 2024, it was wetter than average over parts of USA and Canada. In southwestern Asia, Afghanistan experienced exceptional precipitation and flooding. Typhoons affected Japan, northern Philippines and southern China. Further wetter-than-average regions include part of Australia and southeastern Africa. Southern Brazil saw heavy precipitation aggravating April’s severe floods.
Drier-than-average conditions were seen in northern Mexico, and regions of the USA, and Canada, which saw wildfires, as well as across Asia, over much of Australia, southern Africa and South America.
May 2024 – Sea Ice highlights
Arctic sea ice extent was only slightly below average, as it was in May 2022 and 2023.
Antarctic sea ice extent was 8% below average, the 6th lowest extent for May in the satellite data record, markedly smaller in magnitude than the record -17% observed in May 2023.
2024 Boreal Spring Seasonal Highlights
The global average temperature for March-May 2024 was a record 0.68°C above the 1991-2020 average for these three months.
The European average temperature for spring (March-May) 2024 was the highest on record for the season, 1.50°C warmer than the 1991-2020 average for the season and 0.36°C warmer than the previous warmest European spring, in 2014.
European spring 2024 was wetter than average over much of western Europe, Italy, westernmost Russia and part of the southern Caucasus as well as parts of the Iberian Peninsula and southern Fennoscandia. Record seasonal precipitation was recorded in parts of France, Italy, the Netherlands, Belgium, and Ireland.
Conversely, it was drier than average in northern Scandinavia, most of Eastern Europe and eastern Spain.
Beyond Europe, March to May 2024 was wetter than average in parts of North America, over the Arabian Peninsula, parts of southwest and central Asia, Japan, and eastern China. Austral autumn was wetter than average over most of Australia, eastern southern Africa and southern Brazil.
Drier-than-average regions include south-western and parts of inland USA and Canada, west of the Caspian Sea, across central Asia and southernmost China, regions of Australia, most of South America and southern Africa. - End -
More Information
More information about climate variables in May and climate updates of previous months as well as high-resolution graphics can be downloaded here . You can read more about the streak of monthly temperature records in this article.
Study " Indicators of Global Climate Change 2023” just released and co-authored by scientists of the Copernicus Climate Change Service here .
Answers to frequently asked questions regarding temperature monitoring can be found here.
Temperature monitoring FAQs
Follow near-real-time data for the globe on Climate Pulse here.
More on trends and projections on Climate Atlas here.
Information about the C3S data set and how it is compiled:
Temperature and hydrological maps and data are from ECMWF Copernicus Climate Change Service’s ERA5 dataset.
Sea ice maps and data are from a combination of information from ERA5, as well as from the EUMETSAT OSI SAF Sea Ice Index v2.1, Sea Ice Concentration CDR/ICDR v2 and fast-track data provided upon request by OSI SAF.
Regional area averages quoted here are the following longitude/latitude bounds:
Globe, 180W-180E, 90S-90N, over land and ocean surfaces.
Europe, 25W-40E, 34N-72N, over land surfaces only.
About the Data and Analysis
Information on national records and impacts:
Information on national records and impacts are based on national and regional reports. For details see the respective temperature and hydrological C3S climate bulletin for the month.
C3S has followed the recommendation of the World Meteorological Organization (WMO) to use the most recent 30-year period for calculating climatological averages and changed to the reference period of 1991-2020 for its C3S Climate Bulletins covering January 2021 onward. Figures and graphics for both the new and previous period (1981-2010) are provided for transparency.
More information on the reference period
About Copernicus and ECMWF
Copernicus is a component of the European Union’s space programme, with funding by the EU, and is its flagship Earth observation programme, which operates through six thematic services: Atmosphere, Marine, Land, Climate Change, Security and Emergency. It delivers freely accessible operational data and services providing users with reliable and up-to-date information related to our planet and its environment. The programme is coordinated and managed by the European Commission and implemented in partnership with the Member States, the European Space Agency (ESA), the European Organisation for the Exploitation of Meteorological Satellites (EUMETSAT), the European Centre for Medium-Range Weather Forecasts (ECMWF), EU Agencies and Mercator Océan, amongst others.
ECMWF operates two services from the EU’s Copernicus Earth observation programme: the Copernicus Atmosphere Monitoring Service (CAMS) and the Copernicus Climate Change Service (C3S). They also contribute to the Copernicus Emergency Management Service (CEMS), which is implemented by the EU Joint Research Centre (JRC). The European Centre for Medium-Range Weather Forecasts (ECMWF) is an independent intergovernmental organisation supported by 35 states. It is both a research institute and a 24/7 operational service, producing and disseminating numerical weather predictions to its Member States. This data is fully available to the national meteorological services in the Member States. The supercomputer facility (and associated data archive) at ECMWF is one of the largest of its type in Europe and Member States can use 25% of its capacity for their own purposes.
ECMWF has expanded its location across its Member States for some activities. In addition to an HQ in the UK and Computing Centre in Italy, offices with a focus on activities conducted in partnership with the EU, such as Copernicus, are in Bonn, Germany.
The Copernicus Atmosphere Monitoring Service website can be found at http://atmosphere.copernicus.eu/
The Copernicus Climate Change Service website can be found at https://climate.copernicus.eu/
More information on Copernicus: www.copernicus.eu
The ECMWF website can be found at https://www.ecmwf.int/
- Open access
- Published: 15 June 2024
Safety and efficacy analysis of neoadjuvant pertuzumab, trastuzumab and standard chemotherapy for HER2–positive early breast cancer: real–world data from NeoPowER study
- Fabio Canino 1 ,
- Monica Barbolini 2 ,
- Ugo De Giorgi 3 ,
- Tommaso Fontana 4 ,
- Valeria Gaspari 5 ,
- Caterina Gianni 3 ,
- Lorenzo Gianni 5 ,
- Antonio Maestri 6 ,
- Santino Minichillo 6 ,
- Luca Moscetti 2 ,
- Antonella Mura 6 ,
- Stefania Vittoria Luisa Nicoletti 5 ,
- Claudia Omarini 2 ,
- Rachele Pagani 4 ,
- Samanta Sarti 3 ,
- Angela Toss 1 , 2 ,
- Claudio Zamagni 4 ,
- Riccardo Cuoghi Costantini 7 ,
- Federica Caggia 1 ,
- Giuseppina Antonelli 1 ,
- Federica Baglio 1 ,
- Lorenzo Belluzzi 1 ,
- Giulio Martinelli 1 ,
- Salvatore Natalizio 1 ,
- Ornella Ponzoni 1 ,
- Massimo Dominici 1 , 2 &
- Federico Piacentini 1 , 2
BMC Cancer volume 24 , Article number: 735 ( 2024 ) Cite this article
Metrics details
The addition of pertuzumab (P) to trastuzumab (H) and standard chemotherapy (CT) as neoadjuvant treatment (NaT) for patients with HER2 + breast cancer (BC), has shown to increase the pathological complete response (pCR) rate, without main safety concerns. The aim of NeoPowER trial is to evaluate safety and efficacy of P + H + CT in a real–world population.
We retrospectively reviewed the medical records of stage II–III, HER2 + BC patients treated with NaT: who received P + H + CT (neopower group) in 5 Emilia Romagna institutions were compared with an historical group who received H + CT (control group). The primary endpoint was the safety, secondary endpoints were pCR rate, DRFS and OS and their correlation to NaT and other potential variables.
260 patients were included, 48% received P + H + CT, of whom 44% was given anthraciclynes as part of CT, compared to 83% in the control group. The toxicity profile was similar, excluding diarrhea more frequent in the neopower group (20% vs. 9%). Three patients experienced significant reductions in left ventricular ejection fraction (LVEF), all receiving anthracyclines. The pCR rate was 46% ( P + H + CT) and 40% (H + CT) ( p = 0.39). The addition of P had statistically correlation with pCR only in the patients receiving anthra-free regimens (OR = 3.05, p = 0.047). Preoperative use of anthracyclines (OR = 1.81, p = 0.03) and duration of NaT (OR = 1.18, p = 0.02) were statistically related to pCR. 12/21 distant-relapse events and 14/17 deaths occurred in the control group. Patients who achieve pCR had a significant increase in DRFS (HR = 0.23, p = 0.009).
Conclusions
Adding neoadjuvant P to H and CT is safe. With the exception of diarrhea, rate of adverse events of grade > 2 did not differ between the two groups. P did not increase the cardiotoxicity when added to H + CT, nevertheless in our population all cardiac events occurred in patients who received anthracycline-containing regimens. Not statistically significant, higher pCR rate is achievable in patients receiving neoadjuvant P + H + CT. The study did not show a statistically significant correlation between the addition of P and long-term outcomes.
Peer Review reports
Breast cancer is the most frequently diagnosed malignancy and the leading cause of cancer death in women in Italy, with about 55,000 new diagnoses and 12,500 deaths annually [ 1 ].
About 15–20% of invasive breast cancers overexpress human epidermal growth factor 2 (HER2). HER2 positive (HER2+) breast cancer is independently associated with high grade, aggressive phenotype, and poorer prognosis, compared to HER2 negative (HER2−) counterpart [ 2 ].
The development of anti-HER2 agents has resulted in a deep improvement in the outcome of patients with this type of disease. In particular, the addition of the monoclonal antibody trastuzumab (H) to standard neoadjuvant chemotherapy (CT) regimens increased pathological complete response (pCR) rates, reducing the risk of relapse [ 3 ].
pCR is defined as the absence of residual invasive disease in the breast and axillary lymph nodes (excluding carcinoma in situ) after preoperative treatment. pCR has long been used as a surrogate for long-term efficacy outcomes in neoadjuvant studies. In a pooled analysis, Cortazar et al. [ 4 ] demonstrated that patients who obtained pCR after preoperatory treatment for breast cancer, have an improvement in event-free survival (EFS) and overall survival (OS) compared to those who obtained a no-pCR. This correlation is strongest for the most aggressive breast cancer phenotypes, triple negative and HER2+.
Subsequent studies have shown that adding pertuzumab (P) to H and neoadjuvant CT further increased pCR rates. The greater effectiveness of the dual HER2 blockade is due to the synergistic action of the two monoclonal antibodies, which bind different epitopes of the HER2 receptor: H inhibits ligand-independent signaling and induces antibody dependent cellular cytotoxicity - ADCC; on the other hand, P inhibits ligand-dependent heterodimerization with other members of the HER family. The final effect is a more powerful inhibition of the proliferation of cancer cells and an increase in apoptosis [ 5 ].
Neosphere [ 6 , 7 ], TRYPHAENA [ 8 , 9 ] and Berenice [ 10 ] trials evaluated the efficacy and safety of adding P to H and neoadjuvant chemotherapy, showing higher rates of pCR that correlated with improved long-term outcomes (progression free survival – PFS, disease free survival – DFS), without worsening treatment tolerability and potential cardiotoxicity.
In particular, the results of NeoSphere and TRYPHAENA guaranteed the accelerated approval for the use of P in the neoadjuvant setting by the FDA and EMA in 2013. Nevertheless, the drug is refundable by the Italian healthcare system in this setting only from November 2023, so to prescribe it was necessary to require nominal use for each patient.
The aim of the NeoPower study was to collect and analyze the data of patients with HER2 + early breast cancer (eBC) treated in the neoadjuvant setting with P, H and chemotherapy in different cancer centers in Emilia Romagna, in order to evaluate the tolerability and efficacy of the treatment in real life.
Patients and methods
Study design and participants.
NeoPowER was an observational, retrospective, multicenter study that involved patients treated at the following cancer centers in Emilia Romagna: AOU Policlinico di Modena; AUSL Bologna, Ospedale Bellaria; IRCCS Istituto Romagnolo per lo Studio dei Tumori (IRST) Dino Amadori di Meldola; AUSL della Romagna, Ospedale Infermi di Rimini; AOU Bologna, IRCCS Policlinico Sant’Orsola-Malpighi.
The study included: patients aged 18 years or older and baseline Eastern Cooperative Oncology Group (ECOG) performance status of 0 or 1; with operable (T2-3, N0-1, M0), locally advanced or inflammatory (T2 3, N2-3, M0 or T4a-d, any N, M0) breast cancer; HER2 overexpression confirmed by immunohistochemistry (IHC) 3 + or 2 + and amplified in situ hybridization (ISH), as per local laboratory assessment; who received at least one and no more than eight course of NaT with anti HER2 agents as clinical practice (patients enrolled in any clinical trials were excluded), followed by adequate surgical treatment on T and N.
Main exclusion criteria were: metastatic disease (stage IV) at diagnosis; HER2 negative breast cancer (HER2 score 0, 1 + or 2 + and ISH negative); neoadjuvant treatment other than that considered in this study; failure to perform surgery after neoadjuvant treatment due to patient refusal, evidence of metastatic disease or other reasons.
Patients who received pertuzumab, trastuzumab and chemotherapy formed the P + H + CT (or Neopower) group, while those treated at Modena cancer centre with trastuzumab and chemotherapy constituted the H + CT (or control) group.
The primary endpoint was the safety of neoadjuvant treatment. The main adverse events were graded according to the National Cancer Institute Common Terminology Criteria for Adverse Events (CTCAE) version 5.0.
Secondary endpoints were: pCR rate (pCR defined as absence of residual invasive neoplastic cells at microscopic examination of the breast and axillary lymph nodes after surgery. The presence of isolated tumour cells – ITCs, was not considered pCR); distant relapse free survival – DRFS (the time from the first date of no disease [i.e date of surgery] to the first documentation of distant relapsed disease / last follow-up); overall survival – OS (the time from the date of diagnosis to death / last follow-up).
Data collection and procedures
Clinicopathological data were acquired from electronic medical records of each centres and included: patient demographics; tumor size “T” (determined preferably with magnetic resonance imaging - RMI, alternatively with ultrasound and/or mammography), nodal status “N”, stage (according to TNM classification, 8th edition), grade, biological characteristics including hormone receptor expression (estrogen and progesterone receptors – ER/PgR – positivity was defined as ≥ 1% cells staining by IHC), Ki67 and HER2score before and after neoadjuvant treatment and surgery; type of chemotherapy used, duration and main adverse events of neoadjuvant treatment; type of surgery and adjuvant treatments performed according to clinical practice (anti HER2 agents, chemotherapy, endocrine therapy, radiotherapy).
Pertuzumab was administred at loading dose of 840 mg, followed by 420 mg every 21 days; trastuzumab loading dose was 8 mg/kg, followed by 6 mg/kg every 21 days (or 4 mg/kg loading dose followed by 2 mg/kg weekly). The choice of the taxane-based (docetaxel or paclitaxel) chemotherapy regimen was at the physician’s discretion. Changes in dose, schedule and drugs for toxicities were carried out according to standard guidelines.
As per clinical practice, all patients underwent echocardiogram at the beginning, before anthacycline therapy and at the end of neoadjuvant treatment. The values of left ventricular ejection fraction (LVEF) by the echocardiograms at each timepoint were registered to analyze cardiac safety. In patients without specific symptoms, we considered a decrease in LVEF of 10–15% from baseline and < 50% or ≥ 16% from baseline (regardless of the value achieved) to be significant.
This observational research was reported according to STROBE guidelines ( https://www.strobe-statement.org/ ), while the checklists were reported as supplemental materials.
Ethical committee
This study was performed in line with the principles of the Declaration of Helsinki. Approval was granted by the Ethics Committee of the Area Vasta Emilia Nord (approval date 05/03/2019, approval code 1133/2018). All individual participants included in the study accepted and signed the informed consent form for the treatment and publication of their anonymized clinical data. Data were analysed in aggregate and anonymous form.
Statistical analysis
Continuous variables were reported as median value with interquartile range (IQR) or mean and standard deviation (SD), while categorical variables were reported as absolute and percentage frequencies.
Comparative assessments were performed by applying Pearson’s χ2 test or Fisher exact test for categorical data and Student t test or Wilcoxon-Mann-Whitney test for continuous variables.
Univariable and multivariable logistic regression models were used to assess the impact of study arms and covariates on pCR.
DRFS and OS was calculated using Kaplan-Meier estimators and comparisons between curves were performed with the Mantel-Cox log-rank test.
Cox proportional hazard regression models were used to estimate hazard ratios (HR) and their 95% CIs and p -values. Multivariable Cox regression models have also been defined in order to take into account the possible effect of other covariates.
The covariates inclusion in all multivariables regression models was driven by both their clinical relevance and the imbalances emerged from the univariable analysis.
For all analyses, the results were considered statistically significant when associated with a p -value below the significance level alpha 0.05.
All analyses were carried out using R statistical software version 4.2.1 (The R Foundation for Statistical Computing, 2022).
Patient and treatment characteristics
The study included 260 elegible patients. We retrospectively reviewed the electronic medical records of 126 patients (48%) who received pertuzumab, trastuzumab and chemotherapy ( P + H + CT or neopower group) in 5 Emilia Romagna oncology centers (Modena, Bologna Bellaria, Bologna S. Orsola, Meldola, Rimini) from May 2016 to October 2022. The data of 134 patients (52%) who received trastuzumab and chemotherapy (H + CT or control group) at Modena Cancer Center between January 2007 and July 2021, were collected as control group.
The characteristics of patients (Table 1 ) were well balanced. Median age was 52 years in both groups, cN0 at diagnosis in about 36–40% of patients, stage II in 73% and hormone receptors positive (HR+) in 61%. In contrast, 62% of patients in the P + H + CT cohort had a Ki67 ≥ 30%, compared to 43% in H + CT group.
All the patients received standard taxanes based neoadjuvant CT associated to anti-HER2 agents. CT backbones were as follow: in the P + H + CT cohort, 63% of patients received docetaxel (D) and 44% sequential anthracyclines. In the control group, weekly paclitaxel (wPtx) was administered in 93% of cases, anthracycline-containing regimens was given to 83% of patients, and among them, 44% also received 5-fluorouracil (Table 2 ).
The median time to surgery and the number of mastectomies performed were similar in the two cohorts. Instead, more axillary lymph node dissections – ALND – were performed in the control group, 69% vs. 34%.
With regard to post-operative treatment, 7% (H + CT) vs. 29% ( P + H + CT) of patients received anthracyclines as adjuvant chemotherapy. In the Neopower group, 12% and 28% of patients received H + P and trastuzumab-emtansine (TDM1) respectively as post-neoadjuvant treatment, while more than 87% recevived H alone in the control group (Table 3 ).
Safety analysis
252 patients were included in the safety analysis, 123 (49%) received P. There were 729 treatment-related adverse events (AEs) of any grade (G), 328 and 401 in neopower and control respectively. Overall, 88% AEs were G1-2 in both groups.
In the P + H + CT cohort the most common AEs of any G were diarrhea 20%, anemia 13% and neutropenia 12%; conversely, in the control were anemia 17%, neutropenia 15% and nausea 12%. Figure 1 resume in detail incidence of AEs, by comparing the two treatment group.
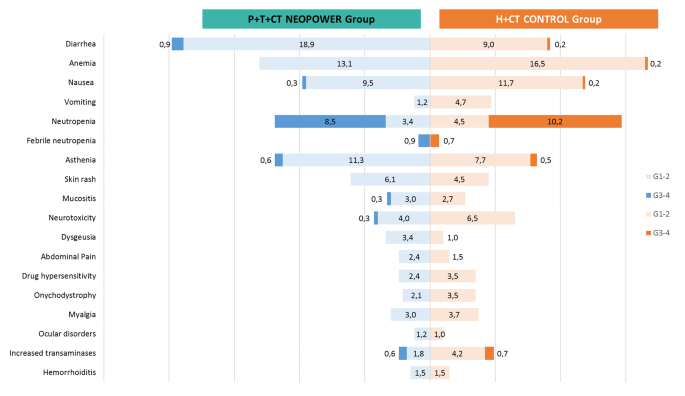
Overall AEs in P + H + CT and H + CT groups
The most frequent AE of G3-4 was neutropenia, 8.5% and 10% in the neopower and control cohort respectively. Of these, 6 events were febrile neutropenias, 3 for each group, 4 related to D. Twenty-three patients who were receiving D in the P + H + CT cohort, received granulocyte colony-stimulating factor (G-CSF) to prevent febrile neutropenia. None of the patients who had febrile neutropenia received prophylaxis with G-CSF.
Forty neurotoxicity (G1 = 77.5%, G2 = 20% and G3 = 2.5%) and 22 drug hypersensitivity events (G1 = 32% and G2 = 68%) were observed, 90% and 73% respectively associated with wPtx.
We recorded 3 serious adverse events (SAEs): 1 urinary tract infection ( P + H + CT), 1 typhlitis and 1 sepsis (H + CT).
Drug related AEs led to a similar rate of dose reductions and drug-switch (D→wPtx) in both groups: 25% and 7% vs. 22% and 6% in the P + H + CT and control cohort respectively. In patients in the H + CT group more drug discontinuations were observed (9% vs. 2%).
Higher rates of diarrhea of anyG occurred in patients of P + H + CT group compared to control, 20% vs. 9%, less than 1% were diarrhea of G3-4.
Patients who received anthracyclines-containing regimens had higher rates of vomiting (4% vs. 1%) and nausea (13% vs. 7%).
Cardiac safety analysis
At least two timepoints were needed to assess the cardiac safety and 205 patients were evaluable, 111 (54%) in the P + H + CT cohort. Data on any risk factors and concomitant drugs concerning the cardiovascular system were collected (Figs. 2 and 3 ).
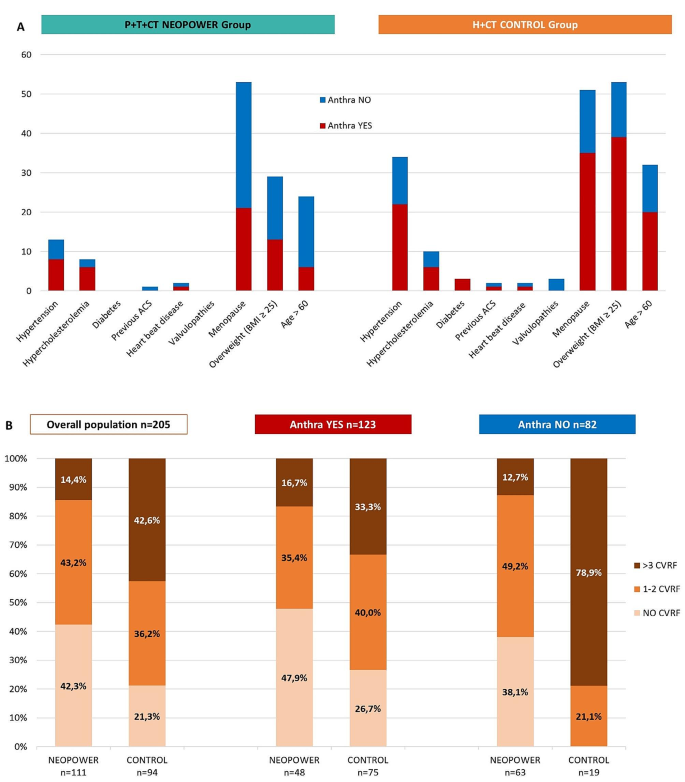
( a ) Patients’ cardiovascular risk factors (CVRF) at diagnosis, distributed according to the treatment arm and the use of neoadjuvant anthracycline. ( b ) Number of CVRF per patient at diagnosis, distributed according to the treatment arm and the use of neoadjuvant anthracycline
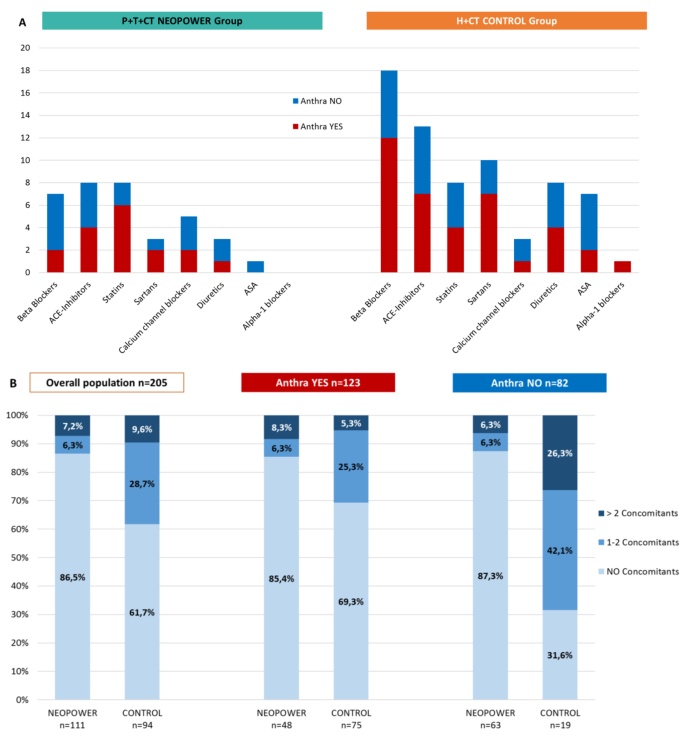
( a ) Patients’ concomitant cardiovascular drugs at diagnosis, distributed according to the treatment arm and the use of neoadjuvant anthracycline. ( b ) Number of concomitant cardiovascular drugs per patient at diagnosis, distributed according to the treatment arm and the use of neoadjuvant anthracycline
The H + CT group had more patients with at least one CVRF (79%) and taking at least one concomitants at diagnosis (38%), compared to the neopower group, 58% at 13% respectively.
The median variation in LVEF pre- and after-neoadjuvant CT was − 5% in overall population and control group, -4% in neopower cohort.
After preoperative treatment, there were 3 (1.5%) significant LVEF reduction events, of which 2 (2%) occurred in the control group. All three patients were symptomatic and received anthracycline as part of neoadjuvant chemotherapy. Everyone had at least one CVRF, but only one of them already took concomitant medications. After temporary discontinuation of antineoplastics and cardioprotective therapy, we observed the recovery of LVEF in 2 patients. However, one of them required permanent treatments discontinuation. Figure 4 show LVEF trends during neoadjuvant treatment in each group, according to the use of neodiuvant anthracycline.

change in LVEF after neoadjuvant: ( a ) P + H + CT, Anthra YES; ( b ) P + H + CT, Anthra NO; ( c ) H + CT, Anthra YES; ( d ) H + CT, Anthra NO
pCR analysis
pCR analysis included 259 eligible patients. One patient in the neopower group prematurely stopped preoperative treatment because of adverse events and was not considered.
In overall population, pCR rates were 46% in P + H + CT cohort, slightly higher compared to 40% of the control group. The addition of P had no statistically significant correlation with pCR (OR = 1.24, 95%CI [0.76–2.03], p = 0.390), even after adjusting for imbalanced parameters between groups (OR = 1.63, 95%CI [0.92-3.00], p = 0.120).
At univariate analysis, HR negative (OR = 3.79, 95%CI [2.24–6.44], p < 0.001), estrogen – ER and progesterone receptors – PgR expressions (OR = 0.98, 95%CI [0.98–0.99], p < 0.001), Ki67 ≥ 30% (OR = 1.69, 95%CI [1.02–2.79], p = 0.040), the use of preoperative anthracyclines (OR = 1.81, 95%CI [1.07–3.07], p = 0.030) and neoadjuvant treatment duration (OR = 1.18, 95%CI [1.03–1.36], p = 0.020) resulted statistically related to pCR (Fig. 5 ).
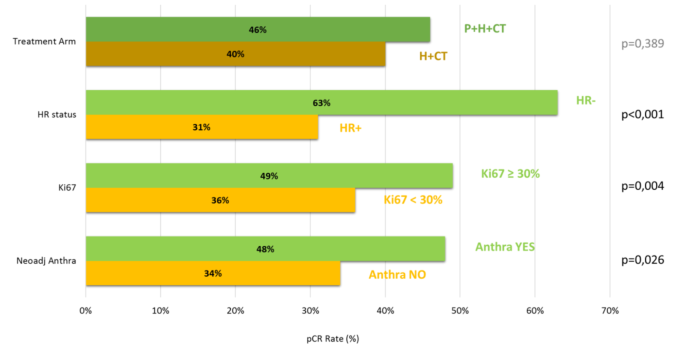
Differences in pCR rate in overall population according to statistically significant variables and treatment arm
By performing the same analysis in the subpopulation that received preoperative anthracyclines, results were similar: HR– disease (OR = 4.37, 95%CI [2.23–8.57], p < 0.001), ER (OR = 0.98, 95%CI [0.97–0.99], p < 0.001) and PgR (OR = 0.98, 95%CI [0.97–0.99], p = 0.003) expression, and Ki67 (OR = 1.02, 95%CI [1.00-1.05], p = 0.020) were statistically associated to pCR, P use was not (OR = 1.41, 95%CI [0.74–2.68], p = 0.300).
ER expression was found to be an independent factor associated with pCR in multivariate analysis, both in overall population (OR: 0.98, 95%CI [0.97–0.99], p = 0.005), and in the subpopulation receiving neoadjuvant anthracyclines (OR: 0.97, 95%CI [0.95–0.99], p = 0.001).
In the subgroup treated with anthracycline-free regimens, the addition of P was found to be statistically related to the pCR rate (OR = 3.05, 95%CI [0,94 − 9,95], p = 0.047), even in the analysis adjusted for unbalanced parameters between groups (OR = 5.65, 95%CI [1,04–30,65], p = 0.045). Once again HR– disease (OR = 3.31, 95%CI [1.34–8.14], p = 0.009), ER (OR = 0.99, 95%CI [0.98–0.99], p = 0.010) and PgR (OR = 0.98, 95%CI [0.97–0.99], p = 0.004) expression were associated with the pCR. None of them confirmed a statistically significant correlation in multivariate analysis. Table 4 shows the results of uni- and multi-variate analysis. Table 5 shows the analysis adjusted for imbalanced parameters between groups.
Survival analysis
Median follow up duration was 36,5 [range 5–77] and 71 [10–176] months in neopower and control group respectively.
DRFS analysis included 257 of the 260 eligible patients. Three patients were excluded due to incomplete data. Twentyone distant relapse events occurred: 9 in the P + H + CT cohort and 12 in the control; 3-years DRFS rate was 89.7% (95%CI 82.6–96.8%) and 93.8 (95%CI 89.7–97.9%) respectively.
OS analysis included 258 of the 260 eligible patients. A total of 17 deaths occurred: 3 in neopower and 14 in the control group; 3-years OS rate was 100% and 96.1 (95%CI 92.7–99.5%) respectively.
The Cox proportional hazard model, adjusted for unbalanced parameters between groups, showed that the addition of P was not statistically related to an improvement in DRFS (HR = 1.44, 95%CI 0.52–3.99, p = 0.490) and OS (HR = 0.41, 95%CI 0.09–1.83, p = 0.240). In this model, stage III at diagnosis was the only prognostic variable correlated with statistical significance to survival (HR = 2.95, 95%CI 1.19–7.30, p = 0.019 for DRFS; HR 5.74, 95%CI 1.94–17.02, p = 0.002 for OS). Figures 6 and 7 shows the Kaplan Meier curves and the forest plots that represent these relationships for DRFS and OS respectively.
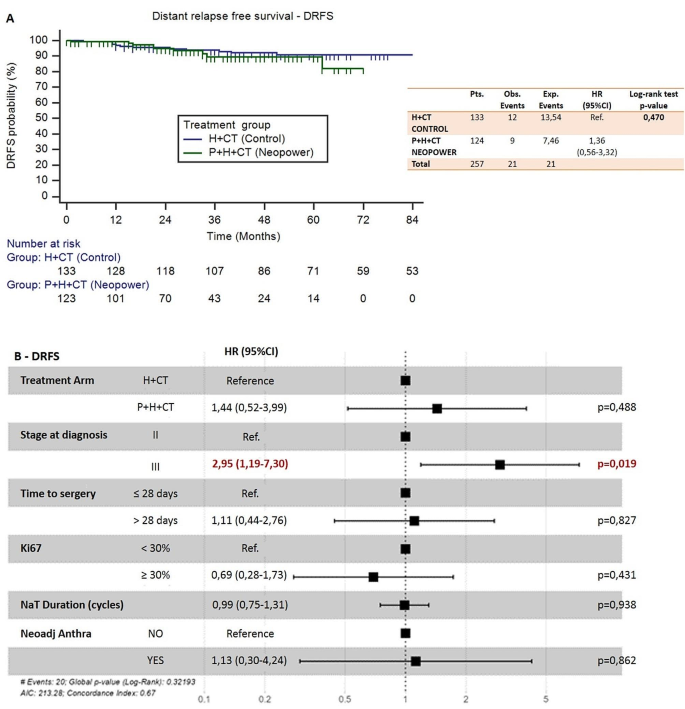
( a ) Kaplan Meier curves for distant relapse free survival – DRFS; ( b ) Forest plot representing Cox proportional hazard model adjusted for unbalanced parameters related to DRFS (Neopower vs. Control)
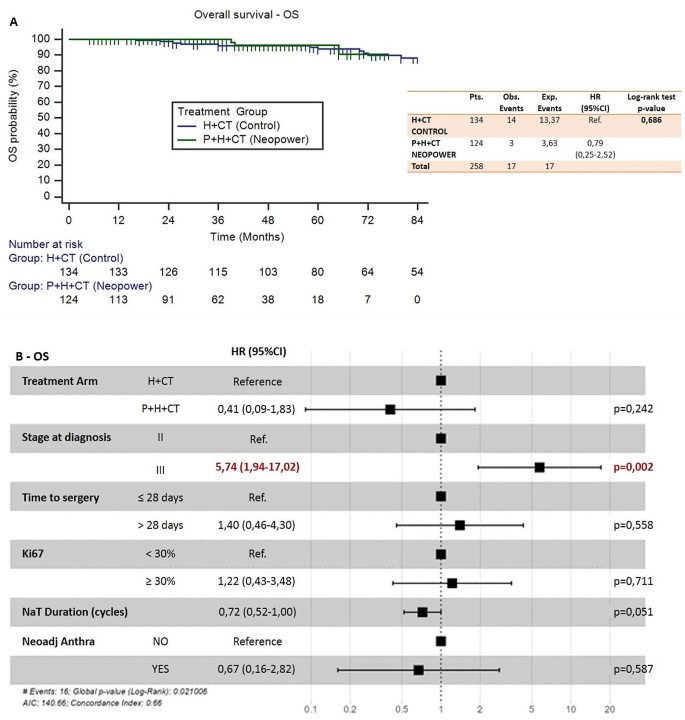
( a ) Kaplan Meier curves for overall survival - OS; ( b ) Forest plot representing Cox proportional hazard model adjusted for unbalanced parameters related to OS (Neopower vs. Control)
The same analysis was performed by comparing patients who achieved the pCR and those who had residual invasive disease after preoperative treatment (no-pCR).
Of the 21 distant relapse events and 17 deaths, 18 and 12 respectively occurred in the no-pCR group. Compared to those with residual invasive disease, patients who achieve pCR had a significant increase in DRFS (HR 0.23, 95%CI [0.10–0.54], p = 0.009), but not in OS (HR 0.60, 95%CI [0.23–1.57], p = 0.323). Figures 8 and 9 shows the Kaplan Meier curves that represent these relationships for DRFS and OS respectively.
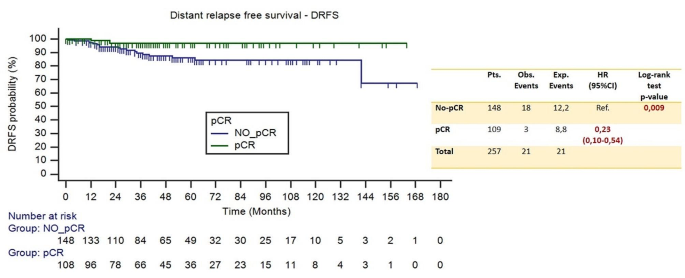
Kaplan Meier curves for distant relapse free survival – DRFS (pCR vs. no-pCR)

Kaplan Meier curves for overall survival – OS (pCR vs. no-pCR)
Based on the results shown, the NeoPowER study reaches its primary endpoint, safety: the addition of P to H and CT, as neoadjuvant treatment in stage II-III HER2 + BC patients, is confirmed to be safe. The AEs rate of G3-4 (about 12%) and toxicity profile, including cardiac events that occurred in about 2% of patients, were overlapping in both groups. Diarrhea was the only AE significantly more frequent in the P + H + CT group than control (20% vs. 9%), although of G ≤ 2 in almost all cases (only 3 out of 65 events were of G3).
It should be noted that even the rate of severe neutropenias and febrile neutropenias were similar between groups, despite the wider use of D in the neopower cohort compared to control (63% vs. 6%). This data is probably related to the use of G-CSF as primary prevention for febrile neutropenia. All patients who received this prophylaxis belonged to the P + H + CT cohort, none developed febrile neutropenia, 3 of them developed neutropenia of G3 between the sixth and eighth course of preoperative treatment, in the anthracycline phase.
Interpretation of results is more complex for secondary endpoints. In patients who received anthracycline-free regimens, the addition of P correlated significantly with pCR. Those receiving H and taxane alone achieved the lowest rates of pCR (17% vs. ≥ 40% adding P and/or anthracyclines), despite more favorable clinical-pathological factors than the overall population (G3 68% vs. 78%, median Ki67 25% vs. 30%). To date, neoadjuvant treatment with single cytotoxic agent (taxane) and H is to be considered sub-optimal in patients with stage II-III HER2-positive breast cancer.
However in our study, the addition of P was not statistically related to pCR in overall population. Conversely, in univariate analysis, use of neadjuvant anthracyclines and duration of preoperative treatment were related to pCR with statistical significance, as well as HR negative and high proliferative index (Ki67 ≥ 30%) disease, these findings wer not confirmed at multivariate analysis.
While not achieving statistical significance on this secondary endpoint, some considerations need to be made. Although we observed a limited use of anthracycline (44% vs. 83%) and a consequent shorter median duration of neoadjuvant treatment (119 vs. 153 days) in P + H + CT cohort compared to the control, the first saw a numerically higher pCR rate (46% vs. 40%). In our population all the three cardiac events occurred in patients who received both anthracyclines and HER2-inhibitors, even if in a sequential strategy. Moreover, the use of anthracyclines were related to a higher rate of nausea and vomiting, well manageable AEs, but which could significantly affect the patient’s quality of life. These data should be taken into account, especially considering evidence from randomized controlled trials, such as TRYPHAENA [ 8 , 9 ] and TRAIN-2 [ 11 , 12 ], which showed that HER2 dual blockade associated with anthra-free chemotherapy (carboplatin-taxane) compared to anthracycline-contaning regimens, allows to achieve similar pCR rates and long-term outcomes with a more favourable toxicity profile.
The overall pCR rate observed in the neopower group (46%) was lower than those obtained in other real world experiences (range 51–68%) carried out by several authors in the same setting [ 13 , 14 , 15 , 16 , 17 , 18 , 19 ]. The heterogeneity of cytotoxic treatments associated to HER2 dual blockade in our study may have contributed to the difference observed. In the P + H + CT group, patients treated with neoadjuvant anthracycline obtained a pCR rate of 54%, similar to other real-world studies. The pCR rate dropped to 39% in patients who received an anthra-free regimen. Of these, 70% received 4 courses of P + H + taxane and 48% adjuvant anthracyclines. This is the same treatment scheme used in the NeoSphere trial, in which patients in the P + H + Docetaxel arm achieved a comparable “total pCR” rate (complete pathological response on breast and axillary lymph-nodes) of 39% [ 6 ]. Thus, over two-third of this subpopulation received shorter neoadjuvant CT (4 cycles) than used in both current clinical practice and most of the previously mentioned real world and clinical trials (range 6–9 cycles). Note that the treatment scheme of the NeoSphere trial has been the reference in our centers for some time. It should also be noted that, in our study, 85% of patients received this treatment scheme between 2016 and 2019, prior to the results of the KATHERINE trial [ 20 ] and the availability of adjuvant trastuzumab-emtansine (TDM1) for patients with residual invasive disease after NaT. This change in clinical practice, together with the increasing amount of data showing better results in pcr rate using longer preoperative treatments, probably led clinicians to anticipate anthracycline more frequently in the neoadjuvant phase, diverging from the scheme used in the NeoSphere trial. Finally, only 1 out of 69 patients received carboplatin associated to P + H and taxanes. In the period analyzed, it was not our daily clinical practice to add carboplatin instead of anthracyclines for the pre-operative treatment of these patients. The addition of carboplatin to anthra-free regimens and double HER2 blockade has been correlated with a numerical increase in pCR rates [ 21 , 22 , 23 ] and event-free survival (EFS), at the expense of an increased incidence of thrombocytopenia of G > 2 (13%) [ 24 ]. It could be therefore assumed that both the shorter duration of the NaT and the lack of carboplatin in the anthra-free regimens contributed to different results compared to similar real world experiences.
Although pCR retains a leading role as a surrogate for long-term efficacy results in neoadjuvant studies, the “invasive residual disease” may be a limited concept today. In other settings, many experiences showed that preoperative treatments can have very different long-term outcomes on the individual patient, depending on the burden of invasive residual disease [ 25 ]. For this reason, the use of residual cancer burden (RCB) to stratify individual risk is becoming increasingly widespread [ 26 ]. The efforts of future research should not be limited to modulating the pre-operative treatment, which represents a crucial phase for the treatment of these patients, but also the post-operative according to this risk, in order to get closer and closer to the concept of personalized medicine. Some ongoing trials are heading in this direction (CompassHER2 pCR - NCT04266249–2020/02/12, CompassHER2 RD - NCT04457596–2020/07/07, Decrescendo - NCT04675827–2020/12/19).
The strengths of NeoPowER study were the multicentric design, which allowed to reach an adequate sample size despite the objective difficulties to use neoadjuvant P in Italy before november 2023; and the comparison with the control arm, although indirect, performed only in a limited number of other real-world experiences. A limitation was the retrospective design, involving a time frame during which clinical practice changed as previously argued; this period was particularly long for the control group (2007–2021), while it was shorter for the NEOPOWER group (2016–2022). This was related to the decision to include in the historical control group only patients treated at the Modena centre: a longer time frame was necessary to obtain an adequate sample size, while being aware of the potential bias this could have introduced. For an in-depth discussion of this topic, see the supplementary Table S1 . The heterogeneity of chemotherapy schemes associated to HER2 dual blockade were also a limitation of the study. These factors may have affected efficacy outcomes.
NeoPowER real world trial confirm that adding neoadjuvant P to H and chemotherapy is safe, even when compared to H + CT alone. With the exception of diarrhea, toxicity profile does not differ between the two groups.
Moreover P doesn’t increase the cardiotoxicity when added to H + CT, nevertheless in our population all cardiac events occurred in patients who received anthracycline-containing regimens.
The study did not show a statistically significant difference in pCR rates in patients receiving neoadjuvant P + H + CT, when compared to H + CT. HR negative disease, Ki67 ≥ 30%, the use of preoperative anthracyclines and neoadjuvant treatment duration resulted statistically related to pCR rate.
The study did not show a statistically significant correlation between the addition of P and long-term outcomes (DRFS and OS). Residual invasive disease remains a negative prognostic factor.
It could be assumed that both the shorter duration of NaT and the lack of carboplatin in the anthra-free regimens received by most patients in the NEOPOWER group, contributed to the failure to reach the secondary endpoints of efficacy of our study.
Data availability
The data that support the findings of this study are not openly available due to reasons of sensitivity and are available from the corresponding author upon reasonable request. Data are located in controlled access data storage at University Hospital of Modena.
Abbreviations
Trastuzumab
Chemotherapy
- Neoadjuvant treatment
Pathological complete response
Human epidermal growth factor 2
HER2 positive
HER2 negative
Event-free survival
Overall survival
Progression free survival
Disease free survival
- Early breast cancer
Eastern Cooperative Oncology Group
Immunohistochemistry
In situ hybridization
Common Terminology Criteria for Adverse Events
Isolated tumor cell
Distant relapse free survival
Estrogen receptors
Progesterone receptors
Left ventricular ejection fraction
Interquartile range
Standard deviation
Hazard ratios
Weekly paclitaxel
Carboplatinum
Anthracycline
Epirubicin-Cyclophosphamide
Trastuzumab-emtansine
Sentinel Node Biopsy
Axillary lymph node dissections
Adverse events
Serious adverse events
Cardiovascular risk factors
Hormone receptors
Linee Guida AIOM. Carcinoma Mammario in Stadio Precoce. 2023.
Kreutzfeldt J, Rozeboom B, Dey N, De P. The trastuzumab era: current and upcoming targeted HER2 + breast cancer therapies. 2020;23.
Gianni L, Eiermann W, Semiglazov V, Manikhas A, Lluch A, Tjulandin S, Zambetti M, Vazquez F, Byakhow M, Lichinitser M, et al. Neoadjuvant chemotherapy with trastuzumab followed by adjuvant trastuzumab versus neoadjuvant chemotherapy alone, in patients with HER2-Positive locally advanced breast cancer (the NOAH Trial): a randomised controlled superiority trial with a parallel HER2-Negative cohort. 2010;375:8.
Cortazar P, Zhang L, Untch M, Mehta K, Costantino JP, Wolmark N, Bonnefoi H, Cameron D, Gianni L, Valagussa P, et al. Pathological complete response and long-term clinical benefit in breast Cancer: the CTNeoBC Pooled Analysis. Lancet. 2014;384:164–72. https://doi.org/10.1016/S0140-6736(13)62422-8
Article PubMed Google Scholar
Matthews CM, Nymberg K, Berger M, Vargo CA, Dempsey J, Li J, Ramaswamy B, Reinbolt R, Sardesai S, Wesolowski R, et al. Pathological complete response rates with pertuzumab-based neoadjuvant chemotherapy in breast cancer: a single-center experience. J Oncol Pharm Pract. 2020;26:572–9. https://doi.org/10.1177/1078155219857800
Article CAS PubMed Google Scholar
Gianni L, Pienkowski T, Im Y-H, Roman L, Tseng L-M, Liu M-C, Lluch A, Staroslawska E, Haba-Rodriguez J. Efficacy and safety of neoadjuvant pertuzumab and trastuzumab in women with locally advanced, inflammatory, or early HER2-Positive breast cancer (NeoSphere): a randomised multicentre, open-label, phase 2 trial. Lancet Oncol. 2012;13:25–32. https://doi.org/10.1016/S1470-2045(11)70336-9 . ImS.-A.
Gianni L, Pienkowski T, Im Y-H, Tseng L-M, Liu M-C, Lluch A, Starosławska E, de la Haba-Rodriguez J, Im S-A, Pedrini JL, et al. 5-Year analysis of neoadjuvant pertuzumab and trastuzumab in patients with locally advanced, inflammatory, or early-stage HER2-Positive breast cancer (NeoSphere): a multicentre, open-label, phase 2 randomised trial. Lancet Oncol. 2016;17:791–800. https://doi.org/10.1016/S1470-2045(16)00163-7
Schneeweiss A, Chia S, Hickish T, Harvey V, Eniu A, Hegg R, Tausch C, Seo JH, Tsai Y-F, Ratnayake J, et al. Pertuzumab plus trastuzumab in combination with standard neoadjuvant anthracycline-containing and anthracycline-free chemotherapy regimens in patients with HER2-Positive early breast cancer: a randomized phase II cardiac safety study (TRYPHAENA). Ann Oncol. 2013;24:2278–84. https://doi.org/10.1093/annonc/mdt182
Schneeweiss A, Chia S, Hickish T, Harvey V, Eniu A, Waldron-Lynch M, Eng-Wong J, Kirk S, Cortés J. Long-term efficacy analysis of the randomised, phase II TRYPHAENA cardiac safety study: evaluating pertuzumab and trastuzumab plus standard neoadjuvant anthracycline-containing and anthracycline-free chemotherapy regimens in patients with HER2-Positive early breast cancer. Eur J Cancer. 2018;89:27–35. https://doi.org/10.1016/j.ejca.2017.10.021
Swain SM, Ewer MS, Viale G, Delaloge S, Ferrero J-M, Verrill M, Colomer R, Vieira C, Werner TL, Douthwaite H, et al. Pertuzumab, trastuzumab, and standard anthracycline- and taxane-based chemotherapy for the neoadjuvant treatment of patients with HER2-Positive localized breast cancer (BERENICE): a phase II, open-label, multicenter, multinational cardiac safety study. Ann Oncol. 2018;29:646–53. https://doi.org/10.1093/annonc/mdx773
van Ramshorst MS, van der Voort A, van Werkhoven ED, Mandjes IA, Kemper I, Dezentjé VO, Oving IM, Honkoop AH, Tick LW, van de Wouw AJ, et al. Neoadjuvant chemotherapy with or without anthracyclines in the presence of dual HER2 blockade for HER2-Positive breast cancer (TRAIN-2): a multicentre, open-label, randomised, phase 3 trial. Lancet Oncol. 2018;19:1630–40. https://doi.org/10.1016/S1470-2045(18)30570-9
van der Voort A, van Ramshorst MS, van Werkhoven ED, Mandjes IA, Kemper I, Vulink AJ, Oving IM, Honkoop AH, Tick LW, van de Wouw AJ, et al. Three-year follow-up of neoadjuvant chemotherapy with or without anthracyclines in the presence of dual ERBB2 blockade in patients with ERBB2-Positive breast cancer. JAMA Oncol. 2021;7:1–7. https://doi.org/10.1001/jamaoncol.2021.1371
Article PubMed Central Google Scholar
Fasching PA, Hartkopf AD, Gass P, Häberle L, Akpolat-Basci L, Hein A, Volz B, Taran F-A, Nabieva N, Pott B, et al. Efficacy of neoadjuvant pertuzumab in addition to chemotherapy and trastuzumab in routine clinical treatment of patients with primary breast cancer: a multicentric analysis. Breast Cancer Res Treat. 2019;173:319–28. https://doi.org/10.1007/s10549-018-5008-3
González-Santiago S, Saura C, Ciruelos E, Alonso JL, de la Morena P, Santisteban Eslava M, Gallegos Sancho MI, de Luna A, Dalmau E, Servitja S, et al. Real-world effectiveness of dual HER2 blockade with pertuzumab and trastuzumab for neoadjuvant treatment of HER2-Positive early breast cancer (the NEOPETRA Study). Breast Cancer Res Treat. 2020;184:469–79. https://doi.org/10.1007/s10549-020-05866-1
Berg T, Jensen M-B, Jakobsen EH, Al-Rawi S, Kenholm J, Andersson M. Neoadjuvant chemotherapy and HER2 dual blockade including biosimilar trastuzumab (SB3) for HER2-Positive early breast cancer: population based real world data from the Danish breast cancer group (DBCG). Breast. 2020;54:242–7. https://doi.org/10.1016/j.breast.2020.10.014
Article PubMed PubMed Central Google Scholar
Boér K, Kahán Z, Landherr L, Csőszi T, Máhr K, Ruzsa Á, Horváth Z, Budai B, Rubovszky G. Pathologic complete response Rates after neoadjuvant pertuzumab and trastuzumab with chemotherapy in early stage HER2-Positive breast cancer - increasing rates of breast conserving surgery: a real-world experience. Pathol Oncol Res. 2021;27:1609785. https://doi.org/10.3389/pore.2021.1609785
Irelli A, Parisi A, D’Orazio C, Sidoni T, Rotondaro S, Patruno L, Pavese F, Bafile A, Resta V, Pizzorno L, et al. Anthracycline-free neoadjuvant treatment in patients with HER2-Positive breast cancer: real-life use of pertuzumab, trastuzumab and taxanes association with an exploratory analysis of PIK3CA mutational status. Cancers (Basel). 2022;14:3003. https://doi.org/10.3390/cancers14123003
van der Voort A, Liefaard MC, van Ramshorst MS, van Werkhoven E, Sanders J, Wesseling J, Scholten A, Vrancken Peeters MJTFD, de Munck L, Siesling S, et al. Efficacy of neoadjuvant treatment with or without pertuzumab in patients with stage II and III HER2-Positive breast cancer: a nationwide cohort analysis of pathologic response and 5-Year survival. Breast. 2022;65:110–5. https://doi.org/10.1016/j.breast.2022.07.005
Fabbri A, Nelli F, Botticelli A, Giannarelli D, Marrucci E, Fiore C, Virtuoso A, Scagnoli S, Pisegna S, Alesini D, et al. Pathologic response and survival after neoadjuvant chemotherapy with or without pertuzumab in patients with HER2-Positive breast cancer: the neopearl nationwide collaborative study. Frontiers in Oncology. 2023;13.
von Minckwitz G, Huang C-S, Mano MS, Loibl S, Mamounas EP, Untch M, Wolmark N, Rastogi P, Schneeweiss A, Redondo A, et al. Trastuzumab emtansine for residual invasive HER2-Positive breast cancer. N Engl J Med. 2019;380:617–28. https://doi.org/10.1056/NEJMoa1814017
Article Google Scholar
Spring L, Niemierko A, Haddad S, Yuen M, Comander A, Reynolds K, Shin J, Bahn A, Brachtel E, Specht M, et al. Effectiveness and tolerability of neoadjuvant pertuzumab containing regimens for HER2-Positive localized breast cancer. Breast Cancer Res Treat. 2018;172:733–40. https://doi.org/10.1007/s10549-018-4959-8
Article CAS PubMed PubMed Central Google Scholar
Ma X, Zhang X, Zhou X, Ren X, Ma X, Zhang W, Yang R, Song T, Liu Y. Real-world study of trastuzumab and pertuzumab combined with chemotherapy in neoadjuvant treatment for patients with HER2-Positive breast cancer. Med (Baltim). 2022;101:e30892. https://doi.org/10.1097/MD.0000000000030892
Article CAS Google Scholar
de Pinho IS, Luz P, Alves L, Lopes-Brás R, Patel V, Esperança-Martins M, Gonçalves L, Freitas R, Simão D, Galnares MR, et al. Anthracyclines versus no anthracyclines in the neoadjuvant strategy for HER2 + breast cancer: real-world evidence. Clin Drug Investig. 2023. https://doi.org/10.1007/s40261-023-01291-6
Villacampa G, Matikas A, Oliveira M, Prat A, Pascual T, Papakonstantinou A. Landscape of neoadjuvant therapy in HER2-Positive breast cancer: a systematic review and network meta-analysis. Eur J Cancer. 2023;190. https://doi.org/10.1016/j.ejca.2023.03.042
Squifflet P, Saad ED, Loibl S, van Mackelenbergh MT, Untch M, Rastogi P, Gianni L, Schneeweiss A, Conte P, Piccart M, et al. Re-evaluation of pathologic complete response as a surrogate for event-free and overall survival in human epidermal growth factor receptor 2-Positive, early breast cancer treated with neoadjuvant therapy including anti-human epidermal growth factor receptor 2 therapy. J Clin Oncol. 2023;41:2988–97. https://doi.org/10.1200/JCO.22.02363
Symmans WF, Yau C, Chen Y-Y, Balassanian R, Klein ME, Pusztai L, Nanda R, Parker BA, Datnow B, Krings G, et al. Assessment of residual cancer burden and event-free survival in neoadjuvant treatment for high-risk breast cancer: an analysis of Data from the I-SPY2 randomized clinical trial. JAMA Oncol. 2021;7:1654–63. https://doi.org/10.1001/jamaoncol.2021.3690
Download references
Acknowledgements
Not applicable.
No funding was received for conducting this study.
Author information
Authors and affiliations.
Division of Medical Oncology, Department of Medical and Surgical Sciences for Children and Adults, University Hospital of Modena, Largo del Pozzo 71, Modena, 41124, Italy
Fabio Canino, Angela Toss, Federica Caggia, Giuseppina Antonelli, Federica Baglio, Lorenzo Belluzzi, Giulio Martinelli, Salvatore Natalizio, Ornella Ponzoni, Massimo Dominici & Federico Piacentini
Division of Medical Oncology, Department of Oncology and Hematology, University Hospital of Modena, Modena, Italy
Monica Barbolini, Luca Moscetti, Claudia Omarini, Angela Toss, Massimo Dominici & Federico Piacentini
Department of Medical Oncology, IRCCS Istituto Romagnolo per lo Studio dei Tumori (IRST) “Dino Amadori”, Meldola, Italy
Ugo De Giorgi, Caterina Gianni & Samanta Sarti
Istituto di Ricovero e Cura a Carattere Scientifico (IRCCS) Azienda Ospedaliero-Universitaria di Bologna, Bologna, Italy
Tommaso Fontana, Rachele Pagani & Claudio Zamagni
Department of Medical Oncology, Infermi Hospital, AUSL della Romagna, Rimini, Italy
Valeria Gaspari, Lorenzo Gianni & Stefania Vittoria Luisa Nicoletti
Department of Medical Oncology, AUSL di Bologna, Bologna, Italy
Antonio Maestri, Santino Minichillo & Antonella Mura
Unit of Clinical Statistics, University Hospital of Modena, Modena, Italy
Riccardo Cuoghi Costantini
You can also search for this author in PubMed Google Scholar
Contributions
FP, CO, FC, MD contributed to conceptualize and design the work. FC, TF, VG, CG, AM, SVLN, RP, SS, GA, FB, LB, GM, SN, OP contributed to filter and collect data. MB, UDG, LG, SM, AM, LM, AT, CZ, MD contributed to reviewing data and overseeing the progress of work. RCC, FC, FP, FC contributed to analyze data and interpret results. FC, TF, VG, CG, SM, AM, SVLN, RP, SS, GA, FB, LB, GM, SN, OP, FP contributed to the drafting of the single sections of the manuscript and the rendering of tables and figures. UDG, LG, AM, CZ, LM, AT, CO, MB, FP, MD contributed to the final work review. All authors read and approved the final manuscript.
Corresponding author
Correspondence to Fabio Canino .
Ethics declarations
Ethics approval and consent to participate.
This study was performed in line with the principles of the Declaration of Helsinki. Approval was granted by the Ethics Committee of the Area Vasta Emilia Nord (approval date 05/03/2019, approval code 1133/2018). All individual participants included in the study accepted and signed the informed consent form for the treatment and publication of their anonymized clinical data.
Consent for publication
Competing interests.
The authors declare no competing interests.
Additional information
Publisher’s note.
Springer Nature remains neutral with regard to jurisdictional claims in published maps and institutional affiliations.
Electronic supplementary material
Below is the link to the electronic supplementary material.
Supplementary Material 1
Supplementary material 2, rights and permissions.
Open Access This article is licensed under a Creative Commons Attribution 4.0 International License, which permits use, sharing, adaptation, distribution and reproduction in any medium or format, as long as you give appropriate credit to the original author(s) and the source, provide a link to the Creative Commons licence, and indicate if changes were made. The images or other third party material in this article are included in the article’s Creative Commons licence, unless indicated otherwise in a credit line to the material. If material is not included in the article’s Creative Commons licence and your intended use is not permitted by statutory regulation or exceeds the permitted use, you will need to obtain permission directly from the copyright holder. To view a copy of this licence, visit http://creativecommons.org/licenses/by/4.0/ . The Creative Commons Public Domain Dedication waiver ( http://creativecommons.org/publicdomain/zero/1.0/ ) applies to the data made available in this article, unless otherwise stated in a credit line to the data.
Reprints and permissions
About this article
Cite this article.
Canino, F., Barbolini, M., De Giorgi, U. et al. Safety and efficacy analysis of neoadjuvant pertuzumab, trastuzumab and standard chemotherapy for HER2–positive early breast cancer: real–world data from NeoPowER study. BMC Cancer 24 , 735 (2024). https://doi.org/10.1186/s12885-024-12506-0
Download citation
Received : 03 March 2024
Accepted : 11 June 2024
Published : 15 June 2024
DOI : https://doi.org/10.1186/s12885-024-12506-0
Share this article
Anyone you share the following link with will be able to read this content:
Sorry, a shareable link is not currently available for this article.
Provided by the Springer Nature SharedIt content-sharing initiative
- HER2 dual blockade
- Real world data
ISSN: 1471-2407
- Submission enquiries: [email protected]
- General enquiries: [email protected]
A generative AI reset: Rewiring to turn potential into value in 2024
It’s time for a generative AI (gen AI) reset. The initial enthusiasm and flurry of activity in 2023 is giving way to second thoughts and recalibrations as companies realize that capturing gen AI’s enormous potential value is harder than expected .
With 2024 shaping up to be the year for gen AI to prove its value, companies should keep in mind the hard lessons learned with digital and AI transformations: competitive advantage comes from building organizational and technological capabilities to broadly innovate, deploy, and improve solutions at scale—in effect, rewiring the business for distributed digital and AI innovation.
About QuantumBlack, AI by McKinsey
QuantumBlack, McKinsey’s AI arm, helps companies transform using the power of technology, technical expertise, and industry experts. With thousands of practitioners at QuantumBlack (data engineers, data scientists, product managers, designers, and software engineers) and McKinsey (industry and domain experts), we are working to solve the world’s most important AI challenges. QuantumBlack Labs is our center of technology development and client innovation, which has been driving cutting-edge advancements and developments in AI through locations across the globe.
Companies looking to score early wins with gen AI should move quickly. But those hoping that gen AI offers a shortcut past the tough—and necessary—organizational surgery are likely to meet with disappointing results. Launching pilots is (relatively) easy; getting pilots to scale and create meaningful value is hard because they require a broad set of changes to the way work actually gets done.
Let’s briefly look at what this has meant for one Pacific region telecommunications company. The company hired a chief data and AI officer with a mandate to “enable the organization to create value with data and AI.” The chief data and AI officer worked with the business to develop the strategic vision and implement the road map for the use cases. After a scan of domains (that is, customer journeys or functions) and use case opportunities across the enterprise, leadership prioritized the home-servicing/maintenance domain to pilot and then scale as part of a larger sequencing of initiatives. They targeted, in particular, the development of a gen AI tool to help dispatchers and service operators better predict the types of calls and parts needed when servicing homes.
Leadership put in place cross-functional product teams with shared objectives and incentives to build the gen AI tool. As part of an effort to upskill the entire enterprise to better work with data and gen AI tools, they also set up a data and AI academy, which the dispatchers and service operators enrolled in as part of their training. To provide the technology and data underpinnings for gen AI, the chief data and AI officer also selected a large language model (LLM) and cloud provider that could meet the needs of the domain as well as serve other parts of the enterprise. The chief data and AI officer also oversaw the implementation of a data architecture so that the clean and reliable data (including service histories and inventory databases) needed to build the gen AI tool could be delivered quickly and responsibly.

Creating value beyond the hype
Let’s deliver on the promise of technology from strategy to scale.
Our book Rewired: The McKinsey Guide to Outcompeting in the Age of Digital and AI (Wiley, June 2023) provides a detailed manual on the six capabilities needed to deliver the kind of broad change that harnesses digital and AI technology. In this article, we will explore how to extend each of those capabilities to implement a successful gen AI program at scale. While recognizing that these are still early days and that there is much more to learn, our experience has shown that breaking open the gen AI opportunity requires companies to rewire how they work in the following ways.
Figure out where gen AI copilots can give you a real competitive advantage
The broad excitement around gen AI and its relative ease of use has led to a burst of experimentation across organizations. Most of these initiatives, however, won’t generate a competitive advantage. One bank, for example, bought tens of thousands of GitHub Copilot licenses, but since it didn’t have a clear sense of how to work with the technology, progress was slow. Another unfocused effort we often see is when companies move to incorporate gen AI into their customer service capabilities. Customer service is a commodity capability, not part of the core business, for most companies. While gen AI might help with productivity in such cases, it won’t create a competitive advantage.
To create competitive advantage, companies should first understand the difference between being a “taker” (a user of available tools, often via APIs and subscription services), a “shaper” (an integrator of available models with proprietary data), and a “maker” (a builder of LLMs). For now, the maker approach is too expensive for most companies, so the sweet spot for businesses is implementing a taker model for productivity improvements while building shaper applications for competitive advantage.
Much of gen AI’s near-term value is closely tied to its ability to help people do their current jobs better. In this way, gen AI tools act as copilots that work side by side with an employee, creating an initial block of code that a developer can adapt, for example, or drafting a requisition order for a new part that a maintenance worker in the field can review and submit (see sidebar “Copilot examples across three generative AI archetypes”). This means companies should be focusing on where copilot technology can have the biggest impact on their priority programs.
Copilot examples across three generative AI archetypes
- “Taker” copilots help real estate customers sift through property options and find the most promising one, write code for a developer, and summarize investor transcripts.
- “Shaper” copilots provide recommendations to sales reps for upselling customers by connecting generative AI tools to customer relationship management systems, financial systems, and customer behavior histories; create virtual assistants to personalize treatments for patients; and recommend solutions for maintenance workers based on historical data.
- “Maker” copilots are foundation models that lab scientists at pharmaceutical companies can use to find and test new and better drugs more quickly.
Some industrial companies, for example, have identified maintenance as a critical domain for their business. Reviewing maintenance reports and spending time with workers on the front lines can help determine where a gen AI copilot could make a big difference, such as in identifying issues with equipment failures quickly and early on. A gen AI copilot can also help identify root causes of truck breakdowns and recommend resolutions much more quickly than usual, as well as act as an ongoing source for best practices or standard operating procedures.
The challenge with copilots is figuring out how to generate revenue from increased productivity. In the case of customer service centers, for example, companies can stop recruiting new agents and use attrition to potentially achieve real financial gains. Defining the plans for how to generate revenue from the increased productivity up front, therefore, is crucial to capturing the value.

McKinsey Live Event: Unlocking the full value of gen AI
Join our colleagues Jessica Lamb and Gayatri Shenai on April 8, as they discuss how companies can navigate the ever-changing world of gen AI.
Upskill the talent you have but be clear about the gen-AI-specific skills you need
By now, most companies have a decent understanding of the technical gen AI skills they need, such as model fine-tuning, vector database administration, prompt engineering, and context engineering. In many cases, these are skills that you can train your existing workforce to develop. Those with existing AI and machine learning (ML) capabilities have a strong head start. Data engineers, for example, can learn multimodal processing and vector database management, MLOps (ML operations) engineers can extend their skills to LLMOps (LLM operations), and data scientists can develop prompt engineering, bias detection, and fine-tuning skills.
A sample of new generative AI skills needed
The following are examples of new skills needed for the successful deployment of generative AI tools:
- data scientist:
- prompt engineering
- in-context learning
- bias detection
- pattern identification
- reinforcement learning from human feedback
- hyperparameter/large language model fine-tuning; transfer learning
- data engineer:
- data wrangling and data warehousing
- data pipeline construction
- multimodal processing
- vector database management
The learning process can take two to three months to get to a decent level of competence because of the complexities in learning what various LLMs can and can’t do and how best to use them. The coders need to gain experience building software, testing, and validating answers, for example. It took one financial-services company three months to train its best data scientists to a high level of competence. While courses and documentation are available—many LLM providers have boot camps for developers—we have found that the most effective way to build capabilities at scale is through apprenticeship, training people to then train others, and building communities of practitioners. Rotating experts through teams to train others, scheduling regular sessions for people to share learnings, and hosting biweekly documentation review sessions are practices that have proven successful in building communities of practitioners (see sidebar “A sample of new generative AI skills needed”).
It’s important to bear in mind that successful gen AI skills are about more than coding proficiency. Our experience in developing our own gen AI platform, Lilli , showed us that the best gen AI technical talent has design skills to uncover where to focus solutions, contextual understanding to ensure the most relevant and high-quality answers are generated, collaboration skills to work well with knowledge experts (to test and validate answers and develop an appropriate curation approach), strong forensic skills to figure out causes of breakdowns (is the issue the data, the interpretation of the user’s intent, the quality of metadata on embeddings, or something else?), and anticipation skills to conceive of and plan for possible outcomes and to put the right kind of tracking into their code. A pure coder who doesn’t intrinsically have these skills may not be as useful a team member.
While current upskilling is largely based on a “learn on the job” approach, we see a rapid market emerging for people who have learned these skills over the past year. That skill growth is moving quickly. GitHub reported that developers were working on gen AI projects “in big numbers,” and that 65,000 public gen AI projects were created on its platform in 2023—a jump of almost 250 percent over the previous year. If your company is just starting its gen AI journey, you could consider hiring two or three senior engineers who have built a gen AI shaper product for their companies. This could greatly accelerate your efforts.
Form a centralized team to establish standards that enable responsible scaling
To ensure that all parts of the business can scale gen AI capabilities, centralizing competencies is a natural first move. The critical focus for this central team will be to develop and put in place protocols and standards to support scale, ensuring that teams can access models while also minimizing risk and containing costs. The team’s work could include, for example, procuring models and prescribing ways to access them, developing standards for data readiness, setting up approved prompt libraries, and allocating resources.
While developing Lilli, our team had its mind on scale when it created an open plug-in architecture and setting standards for how APIs should function and be built. They developed standardized tooling and infrastructure where teams could securely experiment and access a GPT LLM , a gateway with preapproved APIs that teams could access, and a self-serve developer portal. Our goal is that this approach, over time, can help shift “Lilli as a product” (that a handful of teams use to build specific solutions) to “Lilli as a platform” (that teams across the enterprise can access to build other products).
For teams developing gen AI solutions, squad composition will be similar to AI teams but with data engineers and data scientists with gen AI experience and more contributors from risk management, compliance, and legal functions. The general idea of staffing squads with resources that are federated from the different expertise areas will not change, but the skill composition of a gen-AI-intensive squad will.
Set up the technology architecture to scale
Building a gen AI model is often relatively straightforward, but making it fully operational at scale is a different matter entirely. We’ve seen engineers build a basic chatbot in a week, but releasing a stable, accurate, and compliant version that scales can take four months. That’s why, our experience shows, the actual model costs may be less than 10 to 15 percent of the total costs of the solution.
Building for scale doesn’t mean building a new technology architecture. But it does mean focusing on a few core decisions that simplify and speed up processes without breaking the bank. Three such decisions stand out:
- Focus on reusing your technology. Reusing code can increase the development speed of gen AI use cases by 30 to 50 percent. One good approach is simply creating a source for approved tools, code, and components. A financial-services company, for example, created a library of production-grade tools, which had been approved by both the security and legal teams, and made them available in a library for teams to use. More important is taking the time to identify and build those capabilities that are common across the most priority use cases. The same financial-services company, for example, identified three components that could be reused for more than 100 identified use cases. By building those first, they were able to generate a significant portion of the code base for all the identified use cases—essentially giving every application a big head start.
- Focus the architecture on enabling efficient connections between gen AI models and internal systems. For gen AI models to work effectively in the shaper archetype, they need access to a business’s data and applications. Advances in integration and orchestration frameworks have significantly reduced the effort required to make those connections. But laying out what those integrations are and how to enable them is critical to ensure these models work efficiently and to avoid the complexity that creates technical debt (the “tax” a company pays in terms of time and resources needed to redress existing technology issues). Chief information officers and chief technology officers can define reference architectures and integration standards for their organizations. Key elements should include a model hub, which contains trained and approved models that can be provisioned on demand; standard APIs that act as bridges connecting gen AI models to applications or data; and context management and caching, which speed up processing by providing models with relevant information from enterprise data sources.
- Build up your testing and quality assurance capabilities. Our own experience building Lilli taught us to prioritize testing over development. Our team invested in not only developing testing protocols for each stage of development but also aligning the entire team so that, for example, it was clear who specifically needed to sign off on each stage of the process. This slowed down initial development but sped up the overall delivery pace and quality by cutting back on errors and the time needed to fix mistakes.
Ensure data quality and focus on unstructured data to fuel your models
The ability of a business to generate and scale value from gen AI models will depend on how well it takes advantage of its own data. As with technology, targeted upgrades to existing data architecture are needed to maximize the future strategic benefits of gen AI:
- Be targeted in ramping up your data quality and data augmentation efforts. While data quality has always been an important issue, the scale and scope of data that gen AI models can use—especially unstructured data—has made this issue much more consequential. For this reason, it’s critical to get the data foundations right, from clarifying decision rights to defining clear data processes to establishing taxonomies so models can access the data they need. The companies that do this well tie their data quality and augmentation efforts to the specific AI/gen AI application and use case—you don’t need this data foundation to extend to every corner of the enterprise. This could mean, for example, developing a new data repository for all equipment specifications and reported issues to better support maintenance copilot applications.
- Understand what value is locked into your unstructured data. Most organizations have traditionally focused their data efforts on structured data (values that can be organized in tables, such as prices and features). But the real value from LLMs comes from their ability to work with unstructured data (for example, PowerPoint slides, videos, and text). Companies can map out which unstructured data sources are most valuable and establish metadata tagging standards so models can process the data and teams can find what they need (tagging is particularly important to help companies remove data from models as well, if necessary). Be creative in thinking about data opportunities. Some companies, for example, are interviewing senior employees as they retire and feeding that captured institutional knowledge into an LLM to help improve their copilot performance.
- Optimize to lower costs at scale. There is often as much as a tenfold difference between what companies pay for data and what they could be paying if they optimized their data infrastructure and underlying costs. This issue often stems from companies scaling their proofs of concept without optimizing their data approach. Two costs generally stand out. One is storage costs arising from companies uploading terabytes of data into the cloud and wanting that data available 24/7. In practice, companies rarely need more than 10 percent of their data to have that level of availability, and accessing the rest over a 24- or 48-hour period is a much cheaper option. The other costs relate to computation with models that require on-call access to thousands of processors to run. This is especially the case when companies are building their own models (the maker archetype) but also when they are using pretrained models and running them with their own data and use cases (the shaper archetype). Companies could take a close look at how they can optimize computation costs on cloud platforms—for instance, putting some models in a queue to run when processors aren’t being used (such as when Americans go to bed and consumption of computing services like Netflix decreases) is a much cheaper option.
Build trust and reusability to drive adoption and scale
Because many people have concerns about gen AI, the bar on explaining how these tools work is much higher than for most solutions. People who use the tools want to know how they work, not just what they do. So it’s important to invest extra time and money to build trust by ensuring model accuracy and making it easy to check answers.
One insurance company, for example, created a gen AI tool to help manage claims. As part of the tool, it listed all the guardrails that had been put in place, and for each answer provided a link to the sentence or page of the relevant policy documents. The company also used an LLM to generate many variations of the same question to ensure answer consistency. These steps, among others, were critical to helping end users build trust in the tool.
Part of the training for maintenance teams using a gen AI tool should be to help them understand the limitations of models and how best to get the right answers. That includes teaching workers strategies to get to the best answer as fast as possible by starting with broad questions then narrowing them down. This provides the model with more context, and it also helps remove any bias of the people who might think they know the answer already. Having model interfaces that look and feel the same as existing tools also helps users feel less pressured to learn something new each time a new application is introduced.
Getting to scale means that businesses will need to stop building one-off solutions that are hard to use for other similar use cases. One global energy and materials company, for example, has established ease of reuse as a key requirement for all gen AI models, and has found in early iterations that 50 to 60 percent of its components can be reused. This means setting standards for developing gen AI assets (for example, prompts and context) that can be easily reused for other cases.
While many of the risk issues relating to gen AI are evolutions of discussions that were already brewing—for instance, data privacy, security, bias risk, job displacement, and intellectual property protection—gen AI has greatly expanded that risk landscape. Just 21 percent of companies reporting AI adoption say they have established policies governing employees’ use of gen AI technologies.
Similarly, a set of tests for AI/gen AI solutions should be established to demonstrate that data privacy, debiasing, and intellectual property protection are respected. Some organizations, in fact, are proposing to release models accompanied with documentation that details their performance characteristics. Documenting your decisions and rationales can be particularly helpful in conversations with regulators.
In some ways, this article is premature—so much is changing that we’ll likely have a profoundly different understanding of gen AI and its capabilities in a year’s time. But the core truths of finding value and driving change will still apply. How well companies have learned those lessons may largely determine how successful they’ll be in capturing that value.

The authors wish to thank Michael Chui, Juan Couto, Ben Ellencweig, Josh Gartner, Bryce Hall, Holger Harreis, Phil Hudelson, Suzana Iacob, Sid Kamath, Neerav Kingsland, Kitti Lakner, Robert Levin, Matej Macak, Lapo Mori, Alex Peluffo, Aldo Rosales, Erik Roth, Abdul Wahab Shaikh, and Stephen Xu for their contributions to this article.
This article was edited by Barr Seitz, an editorial director in the New York office.
Explore a career with us
Related articles.

The economic potential of generative AI: The next productivity frontier
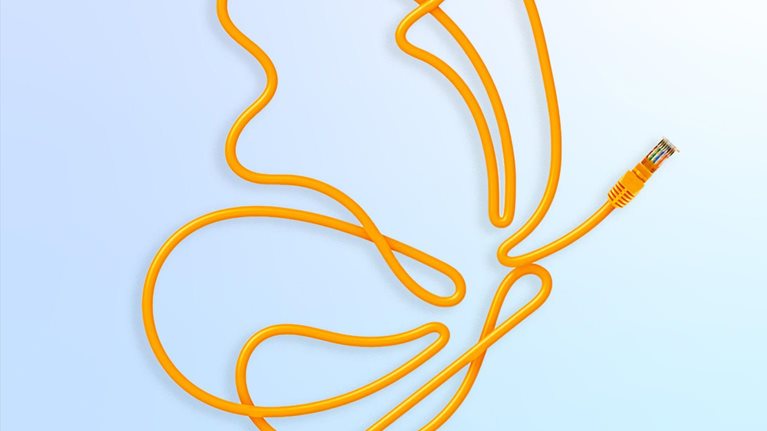
Rewired to outcompete

Meet Lilli, our generative AI tool that’s a researcher, a time saver, and an inspiration
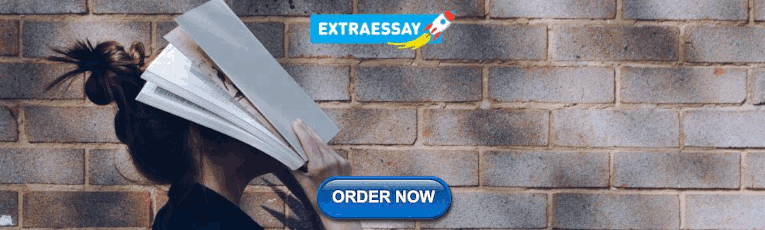
IMAGES
VIDEO
COMMENTS
The process of data analysis in secondary research. Secondary analysis (i.e., the use of existing data) is a systematic methodological approach that has some clear steps that need to be followed for the process to be effective. In simple terms there are three steps: Step One: Development of Research Questions. Step Two: Identification of dataset.
This document provides guidance to investigators conducting research involving the secondary use of existing data. Should you need additional assistance please contact the Office for Protection of Human Subjects (OPHS) at 510-642-7461 or at [email protected]. Table of Contents: Scope. When does the secondary use of existing data not require review?
Types of secondary data are as follows: Published data: Published data refers to data that has been published in books, magazines, newspapers, and other print media. Examples include statistical reports, market research reports, and scholarly articles. Government data: Government data refers to data collected by government agencies and departments.
When to use secondary research. Secondary research is a very common research method, used in lieu of collecting your own primary data. It is often used in research designs or as a way to start your research process if you plan to conduct primary research later on.. Since it is often inexpensive or free to access, secondary research is a low-stakes way to determine if further primary research ...
Secondary research plays a crucial role in dissertation writing, providing a foundation for your primary research. By leveraging existing data, you can gain valuable insights, identify research gaps, and enhance the credibility of your study. Unlike primary research, which involves collecting original data directly through experiments, surveys ...
The use of primary data, as opposed to secondary data, demonstrates the researcher's effort to do empirical work and find evidence to answer her specific research question and fulfill her specific research objectives. ... Dissertations and project reports: a step by step guide. Hampshire, England: Palgrave Macmillan. Lombard, E. (2010). Primary ...
Write Your Dissertation Using Only Secondary Research. November 2020 by Keira Bennett. Writing a dissertation is already difficult to begin with but it can appear to be a daunting challenge when you only have other people's research as a guide for proving a brand new hypothesis! You might not be familiar with the research or even confident in ...
SDA involves investigations where data collected for a previous study is analyzed - either by the same researcher(s) or different researcher(s) - to explore new questions or use different analysis strategies that were not a part of the primary analysis (Szabo and Strang, 1997).For research involving quantitative data, SDA, and the process of sharing data for the purpose of SDA, has become ...
Introduction. Secondary data analysis is a valuable research approach that can be used to advance knowledge across many disciplines through the use of quantitative, qualitative, or mixed methods data to answer new research questions (Polit & Beck, 2021).This research method dates to the 1960s and involves the utilization of existing or primary data, originally collected for a variety, diverse ...
Initially, you can use a secondary data set in isolation - that is, without combining it with other data sets. You dig and find a data set that is useful for your research purposes and then base your entire research on that set of data. You do this when you want to re-assess a data set with a different research question in mind.
Sources of Secondary Data. Sources of secondary data include books, personal sources, journals, newspapers, websitess, government records etc. Secondary data are known to be readily available compared to that of primary data. It requires very little research and needs for manpower to use these sources.
strategies for obtaining access to secondary data via the Internet and offers some advice regarding the use of secondary data. The Pros and Cons of Accessing Secondary Data via the Internet. Conducting secondary analyses of data downloaded from a website is . becoming commonplace for all researchers, not just students engaged in dissertation ...
Primary Research. Primary research includes an exhaustive analysis of data to answer research questions that are specific and exploratory in nature. Primary research methods with examples include the use of various primary research tools such as interviews, research surveys, numerical data, observations, audio, video, and images to collect data directly rather than using existing literature.
Step 3: Design your research process. After defining your statement of purpose, the next step is to design the research process. For primary data, this involves determining the types of data you want to collect (e.g. quantitative, qualitative, or both) and a methodology for gathering them. For secondary data analysis, however, your research ...
In simple terms, secondary data is every. dataset not obtained by the author, or "the analysis. of data gathered b y someone else" (Boslaugh, 2007:IX) to be more sp ecific. Secondary data may ...
Compared to primary research, the collection of secondary data can be faster and cheaper to obtain, depending on the sources you use. Secondary data can come from internal or external sources. Internal sources of secondary data include ready-to-use data or data that requires further processing available in internal management support systems ...
However, the use of secondary data has a number of potential limitations and their implications that need to be noted and be mitigated for (Chauvette et al., 2019; ... (Unpublished doctoral dissertation). Imperial College London. Google Scholar. Ehrlinger L., Wöß W. (2022). A survey of data quality measurement and monitoring tools.
Secondary data analysis. Secondary analysis refers to the use of existing research data to find answer to a question that was different from the original work ( 2 ). Secondary data can be large scale surveys or data collected as part of personal research. Although there is general agreement about sharing the results of large scale surveys, but ...
Secondary data. Using secondary data can be a good alternative to collecting data directly from participants (primary data), removing the need for face-to-face contact. Secondary data relating to living human subjects often requires ethical approval depending on the source and nature of the data. The extent to which the ethical review ...
Qualitative secondary analysis (QSA) is the use of qualitative data collected by someone else or to answer a different research question. Secondary analysis of qualitative data provides an opportunity to maximize data utility particularly with difficult to reach patient populations. However, QSA methods require careful consideration and ...
In this case study, the author discusses the use of secondary data in completing a dissertation that explores the impact of cultural competence on treatment outcomes for depressed youth of color. The challenges faced in the PhD process, as well as the pros and cons of the use of secondary data, are also discussed.
NB If you are doing a research project/dissertation/thesis, check your organisation's view of secondary data. Some organisations may require you to use primary data as your principle research method. ... While use of secondary data sets may not be seen as rigorous as collecting data yourself, the big advantage is that they are in a permanently ...
5. Advantages of secondary data. Secondary data is suitable for any number of analytics activities. The only limitation is a dataset's format, structure, and whether or not it relates to the topic or problem at hand. When analyzing secondary data, the process has some minor differences, mainly in the preparation phase.
The Federal Trade Commission today issued a warning that the increasing use of consumers' biometric information and related technologies, including those powered by machine learning, raises significant consumer privacy and data security concerns and the potential for bias and discrimination. Biometric information refers to data that depict or ...
Business Wire Electra Therapeutics Presents Late-Breaking Clinical Data at EHA2024 from Ongoing Phase 1b Study of ELA026 in Secondary Hemophagocytic Lymphohistiocytosis (sHLH)
The global average temperature for March-May 2024 was a record 0.68°C above the 1991-2020 average for these three months. The European average temperature for spring (March-May) 2024 was the highest on record for the season, 1.50°C warmer than the 1991-2020 average for the season and 0.36°C warmer than the previous warmest European spring, in 2014.
The primary endpoint was the safety, secondary endpoints were pCR rate, DRFS and OS and their correlation to NaT and other potential variables. 260 patients were included, 48% received P + H + CT, of whom 44% was given anthraciclynes as part of CT, compared to 83% in the control group. ... (63% vs. 6%). This data is probably related to the use ...
It's time for a generative AI (gen AI) reset. The initial enthusiasm and flurry of activity in 2023 is giving way to second thoughts and recalibrations as companies realize that capturing gen AI's enormous potential value is harder than expected.. With 2024 shaping up to be the year for gen AI to prove its value, companies should keep in mind the hard lessons learned with digital and AI ...