
An official website of the United States government
Here’s how you know
Official websites use .gov A .gov website belongs to an official government organization in the United States.
Secure .gov websites use HTTPS A lock ( A locked padlock ) or https:// means you’ve safely connected to the .gov website. Share sensitive information only on official, secure websites.
- Heart-Healthy Living
- High Blood Pressure
- Sickle Cell Disease
- Sleep Apnea
- Information & Resources on COVID-19
- The Heart Truth®
- Learn More Breathe Better®
- Blood Diseases and Disorders Education Program
- Publications and Resources
- Blood Disorders and Blood Safety
- Sleep Science and Sleep Disorders
- Lung Diseases
- Health Disparities and Inequities
- Heart and Vascular Diseases
- Precision Medicine Activities
- Obesity, Nutrition, and Physical Activity
- Population and Epidemiology Studies
- Women’s Health
- Research Topics
- Clinical Trials
- All Science A-Z
- Grants and Training Home
- Policies and Guidelines
- Funding Opportunities and Contacts
- Training and Career Development
- Email Alerts
- NHLBI in the Press
- Research Features
- Past Events
- Upcoming Events
- Mission and Strategic Vision
- Divisions, Offices and Centers
- Advisory Committees
- Budget and Legislative Information
- Jobs and Working at the NHLBI
- Contact and FAQs
- NIH Sleep Research Plan
- < Back To Research Topics
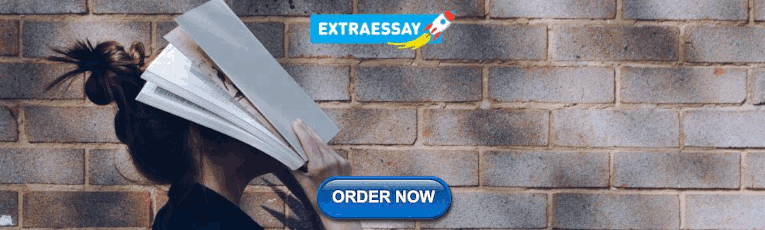
Coronary Heart Disease Research
Language switcher.
For almost 75 years, the NHLBI has been at the forefront of improving the nation’s health and reducing the burden of heart and vascular diseases . Heart disease, including coronary heart disease, remains the leading cause of death in the United States. However, the rate of heart disease deaths has declined by 70% over the past 50 years, thanks in part to NHLBI-funded research. Many current studies funded by the NHLBI focus on discovering genetic associations and finding new ways to prevent and treat the onset of coronary heart disease and associated medical conditions.

NHLBI research that really made a difference
The NHLBI supports a wide range of long-term studies to understand the risk factors of coronary heart disease. These ongoing studies, among others, have led to many discoveries that have increased our understanding of the causes of cardiovascular disease among different populations, helping to shape evidence-based clinical practice guidelines.
- Risk factors that can be changed: The NHLBI Framingham Heart Study (FHS) revealed that cardiovascular disease is caused by modifiable risk factors such as smoking, high blood pressure , obesity , high cholesterol levels, and physical inactivity. It is why, in routine physicals, healthcare providers check for high blood pressure, high cholesterol, unhealthy eating patterns, smoking, physical inactivity, and unhealthy weight. The FHS found that cigarette smoking increases the risk of heart disease. Researchers also showed that cardiovascular disease can affect people differently depending on sex or race, underscoring the need to address health disparities.
- Risk factors for Hispanic/Latino adults: The Hispanic Community Health Study/Study of Latinos (HCHS/SOL) found that heart disease risk factors are widespread among Hispanic/Latino adults in the United States , with 80% of men and 71% of women having at least one risk factor. Researchers also used HCHS/SOL genetic data to explore genes linked with central adiposity (the tendency to have excess body fat around the waist) in Hispanic/Latino adults. Before this study, genes linked with central adiposity, a risk factor for coronary heart disease, had been identified in people of European ancestry. These results showed that those genes also predict central adiposity for Hispanic/Latino communities. Some of the genes identified were more common among people with Mexican or Central/South American ancestry, while others were more common among people of Caribbean ancestry.
- Risk factors for African Americans: The Jackson Heart Study (JHS) began in 1997 and includes more than 5,300 African American men and women in Jackson, Mississippi. It has studied genetic and environmental factors that raise the risk of heart problems, especially high blood pressure, coronary heart disease, heart failure , stroke , and peripheral artery disease (PAD) . Researchers discovered a gene variant in African American individuals that doubles the risk of heart disease. They also found that even small spikes in blood pressure can lead to a higher risk of death. A community engagement component of the JHS is putting 20 years of the study’s findings into action by turning traditional gathering places, such as barbershops and churches, into health information hubs.
- Risk factors for American Indians: The NHLBI actively supports the Strong Heart Study , a long-term study that began in 1988 to examine cardiovascular disease and its risk factors among American Indian men and women. The Strong Heart Study is one of the largest epidemiological studies of American Indian people ever undertaken. It involves a partnership with 12 Tribal Nations and has followed more than 8,000 participants, many of whom live in low-income rural areas of Arizona, Oklahoma, and the Dakotas. Cardiovascular disease remains the leading cause of death for American Indian people. Yet the prevalence and severity of cardiovascular disease among American Indian people has been challenging to study because of the small sizes of the communities, as well as the relatively young age, cultural diversity, and wide geographic distribution of the population. In 2019, the NHLBI renewed its commitment to the Strong Heart Study with a new study phase that includes more funding for community-driven pilot projects and a continued emphasis on training and development. Read more about the goals and key findings of the Strong Heart Study.
Current research funded by the NHLBI
Within our Division of Cardiovascular Sciences , the Atherothrombosis and Coronary Artery Disease Branch of its Adult and Pediatric Cardiac Research Program and the Center for Translation Research and Implementation Science oversee much of our funded research on coronary heart disease.
Research funding
Find funding opportunities and program contacts for research on coronary heart disease.
Current research on preventing coronary heart disease
- Blood cholesterol and coronary heart disease: The NHLBI supports new research into lowering the risk of coronary heart disease by reducing levels of cholesterol in the blood. High levels of blood cholesterol, especially a type called low-density lipoprotein (LDL) cholesterol, raise the risk of coronary heart disease. However, even with medicine that lowers LDL cholesterol, there is still a risk of coronary heart disease due to other proteins, called triglyceride-rich ApoB-containing lipoproteins (ApoBCLs), that circulate in the blood. Researchers are working to find innovative ways to reduce the levels of ApoBCLs, which may help prevent coronary heart disease and other cardiovascular conditions.
- Pregnancy, preeclampsia, and coronary heart disease risk: NHLBI-supported researchers are investigating the link between developing preeclampsia during pregnancy and an increased risk for heart disease over the lifespan . This project uses “omics” data – such as genomics, proteomics, and other research areas – from three different cohorts of women to define and assess preeclampsia biomarkers associated with cardiovascular health outcomes. Researchers have determined that high blood pressure during pregnancy and low birth weight are predictors of atherosclerotic cardiovascular disease in women . Ultimately, these findings can inform new preventive strategies to lower the risk of coronary heart disease.
- Community-level efforts to lower heart disease risk among African American people: The NHLBI is funding initiatives to partner with churches in order to engage with African American communities and lower disparities in heart health . Studies have found that church-led interventions reduce risk factors for coronary heart disease and other cardiovascular conditions. NHLBI-supported researchers assessed data from more than 17,000 participants across multiple studies and determined that these community-based approaches are effective in lowering heart disease risk factors .
Find more NHLBI-funded studies on preventing coronary heart disease on the NIH RePORTER.

Learn about the impact of COVID-19 on your risk of coronary heart disease.
Current research on understanding the causes of coronary heart disease
- Pregnancy and long-term heart disease: NHLBI researchers are continuing the Nulliparous Pregnancy Outcomes Study: Monitoring Mothers-to-be (nuMoM2b) study to understand the relationship between pregnancy-related problems, such as gestational hypertension, and heart problems. The study also looks at how problems during pregnancy may increase risk factors for heart disease later in life. NuMoM2b launched in 2010, and long-term studies are ongoing, with the goal of collecting high-quality data and understanding how heart disease develops in women after pregnancy.
- How coronary artery disease affects heart attack risk: NHLBI-funded researchers are investigating why some people with coronary artery disease are more at risk for heart attacks than others. Researchers have found that people with coronary artery disease who have high-risk coronary plaques are more likely to have serious cardiac events, including heart attacks. However, we do not know why some people develop high-risk coronary plaques and others do not. Researchers hope that this study will help providers better identify which people are most at risk of heart attacks before they occur.
- Genetics of coronary heart disease: The NHLBI supports studies to identify genetic variants associated with coronary heart disease . Researchers are investigating how genes affect important molecular cascades involved in the development of coronary heart disease . This deeper understanding of the underlying causes for plaque buildup and damage to the blood vessels can inform prevention strategies and help healthcare providers develop personalized treatment for people with coronary heart disease caused by specific genetic mutations.
Find more NHLBI-funded studies on understanding the causes of coronary heart disease on the NIH RePORTER.

Recent findings suggest that cholesterol-lowering treatment can lower the risk of heart disease complications in people with HIV.
Current research on treatments for coronary heart disease
- Insight into new molecular targets for treatment: NHLBI-supported researchers are investigating the role of high-density lipoprotein (HDL) cholesterol in coronary heart disease and other medical conditions . Understanding how the molecular pathways of cholesterol affect the disease mechanism for atherosclerosis and plaque buildup in the blood vessels of the heart can lead to new therapeutic approaches for the treatment of coronary heart disease. Researchers have found evidence that treatments that boost HDL function can lower systemic inflammation and slow down plaque buildup . This mechanism could be targeted to develop a new treatment approach for coronary heart disease.
- Long-term studies of treatment effectiveness: The NHLBI is supporting the International Study of Comparative Health Effectiveness with Medical and Invasive Approaches (ISCHEMIA) trial EXTENDed Follow-up (EXTEND) , which compares the long-term outcomes of an initial invasive versus conservative strategy for more than 5,000 surviving participants of the original ISCHEMIA trial. Researchers have found no difference in mortality outcomes between invasive and conservative management strategies for patients with chronic coronary heart disease after more than 3 years. They will continue to follow up with participants for up to 10 years. Researchers are also assessing the impact of nonfatal events on long-term heart disease and mortality. A more accurate heart disease risk score will be constructed to help healthcare providers deliver more precise care for their patients.
- Evaluating a new therapy for protecting new mothers: The NHLBI is supporting the Randomized Evaluation of Bromocriptine In Myocardial Recovery Therapy for Peripartum Cardiomyopathy (REBIRTH) , for determining the role of bromocriptine as a treatment for peripartum cardiomyopathy (PPCM). Previous research suggests that prolactin, a hormone that stimulates the production of milk for breastfeeding, may contribute to the development of cardiomyopathy late in pregnancy or the first several months postpartum. Bromocriptine, once commonly used in the United States to stop milk production, has shown promising results in studies conducted in South Africa and Germany. Researchers will enroll approximately 200 women across North America who have been diagnosed with PPCM and assess their heart function after 6 months.
- Impact of mental health on response to treatment: NHLBI-supported researchers are investigating how mental health conditions can affect treatment effectiveness for people with coronary heart disease. Studies show that depression is linked to a higher risk for negative outcomes from coronary heart disease. Researchers found that having depression is associated with poor adherence to medical treatment for coronary heart disease . This means that people with depression are less likely to follow through with their heart disease treatment plans, possibly contributing to their chances of experiencing worse outcomes. Researchers are also studying new ways to treat depression in patients with coronary heart disease .
Find more NHLBI-funded studies on treating coronary heart disease on the NIH RePORTER.

Researchers have found no clear difference in patient survival or heart attack risk between managing heart disease through medication and lifestyle changes compared with invasive procedures.
Coronary heart disease research labs at the NHLBI
- Laboratory of Cardiac Physiology
- Laboratory of Cardiovascular Biology
- Minority Health and Health Disparities Population Laboratory
- Social Determinants of Obesity and Cardiovascular Risk Laboratory
- Laboratory for Cardiovascular Epidemiology and Genomics
- Laboratory for Hemostasis and Platelet Biology
Related coronary heart disease programs
- In 2002, the NHLBI launched The Heart Truth® , the first federally sponsored national health education program designed to raise awareness about heart disease as the leading cause of death in women. The NHLBI and The Heart Truth® supported the creation of the Red Dress® as the national symbol for awareness about women and heart disease, and also coordinate National Wear Red Day ® and American Heart Month each February.
- The Biologic Specimen and Data Repository Information Coordinating Center (BioLINCC) facilitates access to and maximizes the scientific value of NHLBI biospecimen and data collections. A main goal is to promote the use of these scientific resources by the broader research community. BioLINCC serves to coordinate searches across data and biospecimen collections and provide an electronic means for requesting additional information and submitting requests for collections. Researchers wanting to submit biospecimen collections to the NHLBI Biorepository to share with qualified investigators may also use the website to initiate the application process.
- Our Trans-Omics for Precision Medicine (TOPMed) Program studies the ways genetic information, along with information about health status, lifestyle, and the environment, can be used to predict the best ways to prevent and treat heart, lung, blood, and sleep disorders. TOPMed specifically supports NHLBI’s Precision Medicine Activities.
- NHLBI population and epidemiology studies in different groups of people, including the Atherosclerosis Risk in Communities (ARIC) Study , the Multi-Ethnic Study of Atherosclerosis (MESA) , and the Cardiovascular Health Study (CHS) , have made major contributions to understanding the causes and prevention of heart and vascular diseases, including coronary heart disease.
- The Cardiothoracic Surgical Trials Network (CTSN) is an international clinical research enterprise that studies heart valve disease , arrhythmias , heart failure, coronary heart disease, and surgical complications. The trials span all phases of development, from early translation to completion, and have more than 14,000 participants. The trials include six completed randomized clinical trials, three large observational studies, and many other smaller studies.

Learn how heart disease may be different for women than for men.
Explore more NHLBI research on coronary heart disease
The sections above provide you with the highlights of NHLBI-supported research on coronary heart disease. You can explore the full list of NHLBI-funded studies on the NIH RePORTER .
To find more studies:
- Type your search words into the Quick Search box and press enter.
- Check Active Projects if you want current research.
- Select the Agencies arrow, then the NIH arrow, then check NHLBI .
If you want to sort the projects by budget size — from the biggest to the smallest — click on the FY Total Cost by IC column heading.
- Research article
- Open access
- Published: 21 June 2021
A novel approach for heart disease prediction using strength scores with significant predictors
- Armin Yazdani 1 ,
- Kasturi Dewi Varathan ORCID: orcid.org/0000-0003-3421-4501 2 ,
- Yin Kia Chiam 1 ,
- Asad Waqar Malik 3 &
- Wan Azman Wan Ahmad 4
BMC Medical Informatics and Decision Making volume 21 , Article number: 194 ( 2021 ) Cite this article
12k Accesses
35 Citations
1 Altmetric
Metrics details
Cardiovascular disease is the leading cause of death in many countries. Physicians often diagnose cardiovascular disease based on current clinical tests and previous experience of diagnosing patients with similar symptoms. Patients who suffer from heart disease require quick diagnosis, early treatment and constant observations. To address their needs, many data mining approaches have been used in the past in diagnosing and predicting heart diseases. Previous research was also focused on identifying the significant contributing features to heart disease prediction, however, less importance was given to identifying the strength of these features.
This paper is motivated by the gap in the literature, thus proposes an algorithm that measures the strength of the significant features that contribute to heart disease prediction. The study is aimed at predicting heart disease based on the scores of significant features using Weighted Associative Rule Mining.
A set of important feature scores and rules were identified in diagnosing heart disease and cardiologists were consulted to confirm the validity of these rules. The experiments performed on the UCI open dataset, widely used for heart disease research yielded the highest confidence score of 98% in predicting heart disease.
This study managed to provide a significant contribution in computing the strength scores with significant predictors in heart disease prediction. From the evaluation results, we obtained important rules and achieved highest confidence score by utilizing the computed strength scores of significant predictors on Weighted Associative Rule Mining in predicting heart disease.
Peer Review reports
Introduction
Cardiovascular disease (CVD) is one of the most life-threatening diseases in the world. The World Health Organization (WHO) as well as the Global Burden of Disease (GBD) study reported cardiovascular disease as the main cause of death around the globe annually [ 40 , 56 ]. WHO revealed that CVD is expected to affect almost 23.6 million people by the year 2030. In some industrialized countries such as the United States of America, the rate is about 1 in 4 deaths [ 34 ]. The Middle East and North Africa (MENA) region has an even higher percentage, which is 39.2% of the mortality rate [ 20 ]. Hence, early and accurate diagnosis and the provision of appropriate treatments are keys to reducing the amount of death causing cardiovascular diseases. Availability of such services is essential for those who have a high risk of developing heart disease [ 29 ].
There are many features that contribute to heart disease prediction. Researchers in the past were more focused on identifying significant features to be used in their heart disease prediction models [ 8 ]. Less importance was given to determining the relationships between these features and to identifying their level of priority [ 32 , 32 ] within the prediction model. To address the issues which hinder early and accurate diagnosis, many data mining related studies were previously conducted [ 9 , 16 , 28 ].
Weighted Association Rule Mining (WARM) is one of the data mining techniques used to discover the relationships between features and to determine mining rules that lead to certain predictions [ 22 ]. The weight that is used in this mining technique provides users with a convenient way to indicate the importance of the features that contributes to heart disease and helps obtain more accurate rules [ 4 ]. In many prediction models, different features have different importance. Hence, different weights are assigned to different features based on their predicting capabilities [ 48 ]. The failure in determining the weight indicates the failure in determining the importance of the features.
Past research had used Weighted Associative Rule Mining (WARM) in heart disease prediction [ 18 , 31 , 46 , 48 , 50 ]. However, the prediction model reported in these studies still demands further exploration in terms of the number of features used, the strength of these features and the evaluation of scores obtained. In this research, we proposed an algorithm to compute the weight of each feature that contributes to heart disease prediction. We have experimented on all features as well as selected significant features using WARM. The results obtained showed that the significant features outperformed all features with the highest confidence score of 98% in predicting heart disease. To the best of our knowledge, this study is the first that used strength scores of significant predictors in WARM.
The rest of the paper is organized as follows: Sect. 2 presents the background of the study followed by Sect. 3 on research objectives. Section 4 presents the methodology and Sect. 5 displays the results obtained by this research. Section 6 includes the discussions and Sect. 7 benchmarks this research against previous studies. Finally, Sect. 8 concludes the research with a summary of the findings and future work.
Related works
CVDs are disorders of the heart and blood vessels and include coronary heart disease, cerebrovascular disease and other conditions. Heart attacks and strokes are the main causes of mortality in cardiovascular disease in which the rate nears one out of three [ 6 ]. With the high rate of mortality, diagnosis and prevention measures need to be performed effectively and efficiently. Many data mining techniques have been used to help address these issues (Amin et al. [ 8 ]). Most of the past research looked into identifying features that contribute to better heart prediction accuracy [ 9 ]. However, very little researches looked into the relationships that exist between these features. The relationship between each feature that contributes to heart disease prediction can be obtained by using the Associative Rule Mining (ARM) technique [ 11 ]. The ARM technique is popular in transactional and relational datasets. The hidden knowledge in large datasets such as business transactions developed the interest of many business owners to understand the patterns that can help them to improve their business decisions (Agarwal and Mithal [ 1 ]). For instance, discovering the frequently bought items by customers in market basket analysis. This analysis looks at the various items found in customers’ shopping cart and identifies the associations between them. A good example would be if customers were looking to purchase milk, they were likely to purchase bread on the same trip to the supermarket. This approach is also widely used in the healthcare industry specifically in privacy preservation of healthcare data [ 15 ], predicting cancer associated protein interactions [ 12 ], predicting obstructive sleep apnea [ 43 ] and predicting co-diseases in Thyroid patients [ 23 ].
ARM is also used in heart disease prediction. Table 1 shows the studies that used ARM in heart disease prediction. Akbaş et al. [ 3 ], Shuriyaa and Rajendranb [ 42 ], Srinivas et al. [ 49 ], Khare and Gupta [ 24 ] and Lakshmi and Reddy [ 27 ] have used ARM on UCI dataset. Some of the studies listed in Table 1 used private datasets from hospitals and heart centres. Although the scores that were obtained from these datasets are high (99% by Sonet et al. [ 45 ]), 100% by Thanigaivel and Kumar [ 52 ], the studies have a limitation in terms of reproduction, as the datasets are not open for access. Akbaş et al. [ 3 ] on the other hand obtained a score of 97.8% in confidence using the UCI dataset. However, the confidence score obtained predicted people with no risk of heart disease.
Weighted Associative Rule Mining (WARM) is an extension of ARM, in which weights are assigned to differentiate the importance of the features mined. Let T be the training dataset in which contains T = {r 1 , r 2 , r 3 … r i } with a set of weight associated with each {attribute, attribute value} pair. Every ith record r i is a set of value and weight w i attached to each feature of r i tuple / record. In a weighted framework, each record is a set of triple {a i , v i , w i } where feature a i has a value of v i and weight of w i where 0 < w j < = 1.
Assigning a correct weight to each feature is a hard task. In various fields of studies, there are different ways of calculating the weights of features. For instance, according to Malarvizhi and Sathiyabhama [ 30 ] in web mining, visitor page dwelling time is a way of calculating weightage. WARM is widely used in research on shopping basket scenarios and in predicting customers’ behaviour. Chengis et al. [ 10 ] investigated on assigning weight before and after ARM. WARM was also used in predicting disease comorbidities using clinical as well as molecular data (Lakshmi and Vadivu 26 ). This technique is also used in predicting breast cancer [ 5 ]. Recent research by Park and Lim [ 39 ] used this technique to reduce design failures of pre-alarming systems in the shipbuilding industry.
However, not many researchers focused on applying WARM to cardiovascular disease. Table 2 shows studies on heart disease prediction using WARM. However, the weight of features was not precisely calculated (Jabbar et al. [ 21 ], Sundar et al. [ 50 ], Soni and Vyas [ 48 ]). Soni et al. [ 47 ] proposed a new framework, which was an associative classifier that used WARM. Different weights were assigned to different attributes based on their predicting capability. Their theoretical model yielded a confidence score of 79.5%. Soni and Vyas [ 48 ] also applied WARM and the confidence level they achieved was was 79.5%. Their research assigned weights based on age range, smoking habits, hypertension and BMI range. On the other hand, Soni et al. [ 46 ] assigned weights to each of the attributes based on the advice obtained from the medical experts. They presented an intelligent and effective heart attack prediction system using a weighted associative classifier by achieving a maximum score of 80% confidence. Meanwhile, Sundar et al. [ 50 ] developed a system using two data mining techniques, which are Naïve Bayes and WARM. Their experiments showed that WARM achieved a score of 84% on confidence score, outperforming Naïve Bayes, which obtained only 78%. Chauhan et al. [ 11 ] also used WARM in predicting heart disease. They obtained an accuracy score of 60.4%. Kharya et al. [ 25 ] used Weighted Bayesian Association Rule Mining Algorithm, which combines WARM with heart disease dataset. However, they failed to indicate the results obtained in their study. Ibrahim and Sivabalakrishnan [ 19 ] have used Random Walker Memetic algorithm-based WARM for predicting coronary disease. They obtained an accuracy of 95% using the UCI heart disease dataset.
Despite having research that is based on WARM in predicting heart disease, none of them was focused on identifying the important features to be used in heart disease prediction which would contribute to better prediction performance. The weight of each feature plays an equally important role in deciding which feature has the highest impact (strength) in predicting heart disease. The right weight of the significant features identified will yield an effective prediction model. Thus, this research is focused on identifying the weight of significant features and utilizing the generated score in predicting heart disease.
Research objectives
The main objectives of this research are as follows:
To compute the weight of significant features in heart disease prediction.
To predict heart disease using the computed weight of significant features (using WARM).To evaluate the performance of WARM in predicting heart disease.
Proposed methodology
This section describe in detail the methodology used as shown in Fig. 1 . It contains 5 main stages which are data pre-processing, feature selection, feature weight computation, apply WARM and model evaluation.
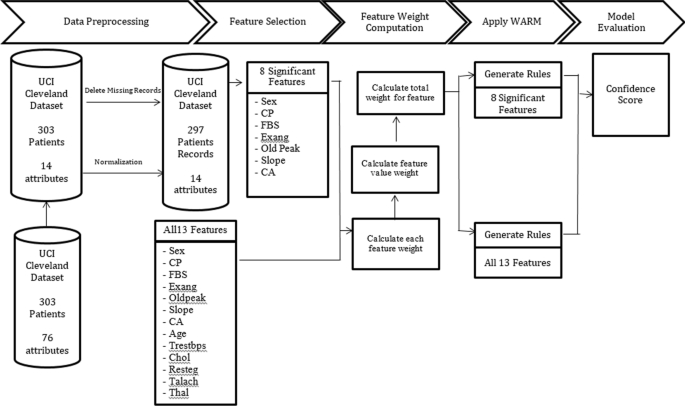
Methodology
This research uses the heart disease dataset that is obtained from UCI Machine Learning Repository [ 13 ]. UCI Machine Learning Repository is one of the largest available datasets, having over 417 various datasets. The Cleveland dataset from UCI Machine Learning Repository is one of the datasets on heart disease, which is widely used by researchers to date (Amin et al. [ 8 ]). This research will also use this dataset of which contains 303 rows. The dataset contains 76 features in which 14 attributes including class label are used. The 14 features together with their descriptions and data types are shown in Table 3 .
Experimental Setup
In this research, Weka 3.8 was used to conduct the experiments. The retrieved Cleveland dataset went through a pre-processing phase. The significant features were retrieved from a total of 14 factors from the Cleveland dataset (Amin [ 7 ]). Further, the weight of each significant feature was computed and assigned back to them accordingly. WARM was applied to the heart disease dataset to generate rules. Finally, evaluation was performed to obtain the confidence score of the best rules generated using WARM based on significant features. The detailed explanation of each process is explained in the following sections.
Data Pre-Processing
In the data pre-processing phase, all missing records were deleted from the dataset, which consists of 6 instances. Based on Table 3 , there are 13 normal attributes(age’, ‘sex’, ‘cp’, ‘trestbps’, ‘chol’, ‘fbs’, ‘restecg’, ‘thalach’, ‘exang’, ‘oldpeack’, ‘slope’, ‘ca’, ‘thal’) and 1 class label(‘goal’), which refers to the criticality level of heart disease in patients. It ranged from 0–4, in which 0 refers to’No Heart Disease’ and the other values indicates the presence of heart disease at different criticality levels. Since this research aims at predicting the presence of heart disease and not its criticality levels, the range from 1 to 4 is thus normalized to 1, which indicates the presence of heart disease, and 0 to represent the absence of heart disease. Data normalization is also performed as a part of the data transformation process that involved mounting data into nominal data. This is required, as WARM utilizes nominal data only. All the ranges formed for each features are indicated in Table 4 .
Feature Selection
Features were selected based on experiments conducted by Amin et al. [ 8 ] since they had used the same dataset (UCI). They performed a set of experiments that dealt with 8100 combinations of features with 7 different classification models (K-NN, Decision Tree, Naïve Bayes, Logistic Regression, Neural Network and Vote) to identify significant features. Table 5 shows the features obtained from the highest performance of each classification models. The highlighted columns indicate the features which appeared more than 10 times and thus were selected as significant features. The selected 8 features are sex, CP, Fbs, Exang, Oldpeak, Slope, CA, and Thal.
Feature weight computation
This section explains how the weight of the features was calculated. The fundamental of WARM states that different features in a dataset have different importance in predicting heart disease. The weight of each feature ranges from 0 to 1. Thus, a weight that is closer to 1 indicates a more significant feature. On the other hand, a weight that is closer to 0 is the least significant in heart disease prediction.
Calculate feature weight
The first step was to calculate the individual feature weights. Let R be the set of features R = {n 0 , n 1 , n 2 … n i } and (n > 0). In this experiment, the total number of features is 13 and after feature selection, it is reduced to 8 (Sex, CP, Fbs, Exang, Oldpeak, Slope, CA, and Thal). W (n) is the weight of each feature (W is the weight of each feature to be calculated and n represents a feature),
For example, the value of sex as displayed in Table 5 is’20’ and the sum of all the features will be’121’. The total value of significant features (Sex, CP, Fbs, Exang, Oldpeak, Slope, CA, andThal) is calculated as (20 + 18 + 12 + 12 + 14 + 12 + 19 + 14 = 121). Thus, to calculate the weight of ‘sex’ (weight of features, WOF):
Table 6 displays the calculated weights for each of the significant features. All weights were computed accordingly. From the distribution of the weights, CA has the greatest strength followed by Sex, CP, Oldpeak and Thal, Fbs, Exang and Slope has the similar weight of 0.09 each.
Calculate feature value weight
This section explains how feature values are computed. Feature values represent all the values that a feature contains. For instance, feature values for sex are male and female. Let A be the number of each feature value contained in the dataset and (A ∪ B) be the total number of records.
Table 7 shows the total sub value of each feature based on the UCI dataset. Male value is represented by 203 records and female by 94 records which gives a total of 297 records from the UCI dataset. To calculate the value of each feature weight, let A be the selected value and B be the rest of the features value,
Figure 2 shows the comparison of the percentage of males and females in the Cleveland heart disease dataset.
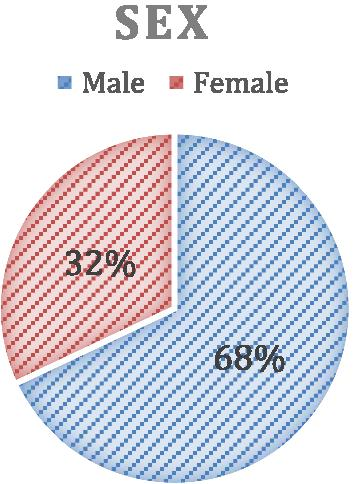
Comparison on the percentage of male and female in Cleveland heart disease dataset
Calculate total weight for feature
This section explains how the total weight for features is computed. The feature weight (W (n)) and feature value weight (W (value)) gives the total weight (W (t)) for the feature. The computation is shown below.
Example of calculating the total weight of feature W (t):
This section detailed out the algorithm to obtain the weighted score of each feature in predicting heart disease. The algorithm is stated as follows:
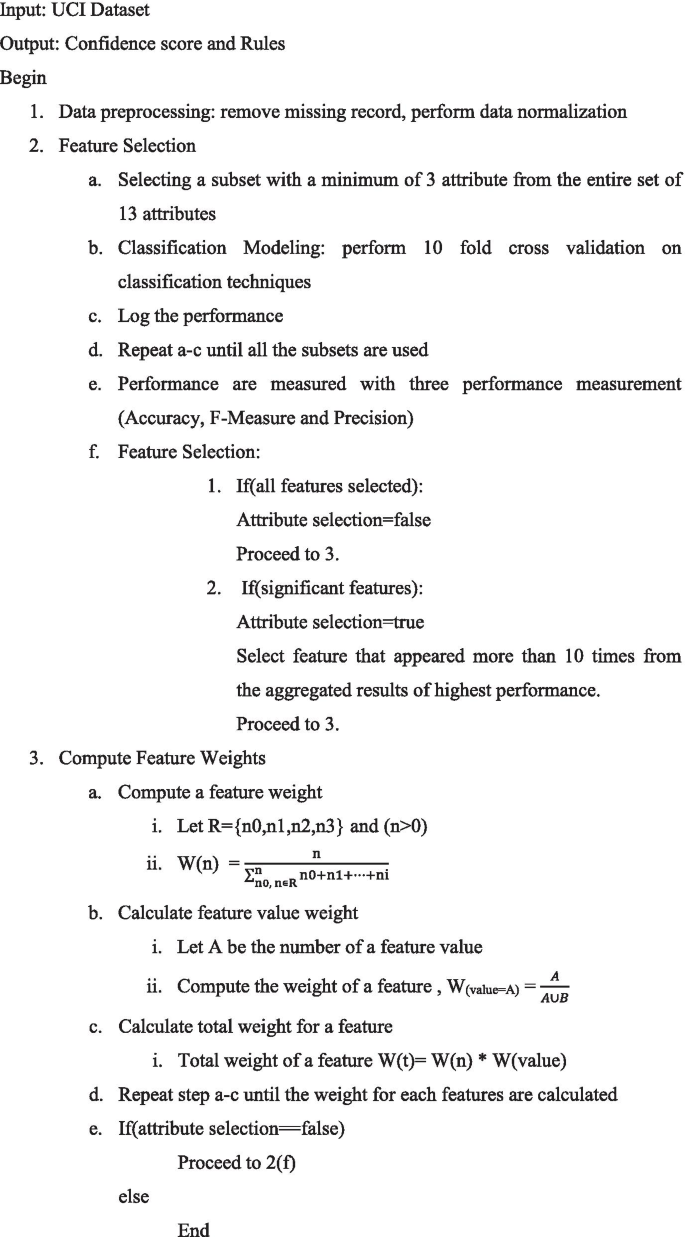
Not all features in the heart disease dataset have the same level of significance in predicting the risk of heart disease. Thus, different weights based on their prediction capability are assigned. These values are then imported into Weka 3.8 to experiment with WARM using Apriori Algorithm.
Apriori algorithm
The Apriori algorithm is a well-known approach in WARM. Apriori was first proposed by Agrawal and Srikant [ 2 ]. The algorithm starts with a dataset including transactions that wants to construct frequent item sets, having at least a user-specified threshold. In the algorithmic process of Apriori, an item set X of length k is frequent if and only if every subset of X, having length k—1, is also frequent. This consideration results in a substantial reduction of search space and allows rule discovery in a computationally feasible time. Apriori generates a rule of the form: s = > (f – s) if and only if the confidence of the rule is above the user-defined threshold. Confidence is essentially the accuracy of the rule and is used in Apriori to rank the rules (Agrawal & Srikant [ 2 ]; Mutter et al. [ 51 ]).
Weighted confidence
The confidence level is used in order to show how often the rule appears to be true. Let Y be the ‘goal’, then the weighted confidence of a rule X → Y can be calculated as the ratio of weighted support of \(\left( {X \cup Y} \right)\) over the weighted support of (X).
For instance, the rule {sex = Male, CA = 3} → {heart disease} has a confidence of 0.2/0.2 = 1.0. It means a patient who is a male and having 3 CA (major vessels coloured by fluoroscopy) has a 100% chance of having heart disease.
This phase generates rules based on the Apriori algorithm in Weighted Associative Rule Mining. Two sets of rules and confidence scores were generated for the followings:
All features—this includes all the 13 features.
Selected significant features (8 features).
The following section provides a detailed explanations of the results obtained which are the rules and confidence scores.
Results (rules and confidence level generated)
The rules and confidence level generated for all the (13) features and the selected significant features (8) are shown in this section.
All features
Table 8 shows the top 20 rules and confidence scores obtained for all the features using WARM. The rules were sorted by the highest confidence scores.
The highest confidence level achieved for predicting the risk of having heart disease is 96% and the number of features used to generate this rule is 3(CP, Slope and Thal). This can be clearly seen in Table 8 (Rule Number 7). The rule states that if the value of Chest Pain (CP) is asymptomatic, the slope is flat and the value of Thallium (Thal) is reversible, therefore, the patient has a very high tendency (confidence level = 96%) of having the risk of heart disease. All the highlighted rows in Table 8 show the rules that contributed to the prediction of the risk of having heart disease. Further, the Table 9 is the summary that shows the frequency of each features used in the rules, which were generated from Table 8 (which contains the rules that predicts heart disease). It shows the rule number and the features used in each of the top 20 rules. From the top 20 rules, only 6 rules predicts heart disease and others are non-sick rules which predicts no heart disease.
Although all 13 features have been used for rules and confidence score generation as shown in Table 8 , only 9 features have been used for heart disease prediction based on the top 20 rules. The most significant feature in predicting heart disease is CP. This feature exist in all the 6 rules generated that predicts heart disease. Thal and Oldpeak exist in 4 rules out of the 6 rules in predicting heart disease.
Selected significant features
This section emphasizes on the rules and confidence scores obtained by the selected significant features. Table 10 shows the top 20 rules generated from the significant features using WARM. The confidence score obtained in predicting the risk of having heart disease using 8 selected significant features shows a comparatively high confidence level at 98%. The rule obtained for the top confidence score states as.
CP = asymptomatic, Exang = Yes, Oldpeak = greaterThanZero, Thal = reversible = = > class_HD = Heart Disease.
which means if Chest Pain (CP) is asymptomatic, exercise-induce angina (Exang) is present, Oldpeak (ST depression induced by exercise relative to rest) is present and, Thallium heart scan (Thal) is reversible then the patient is diagnosed as having heart disease. From the top 20 rules generated, 11 rules are meant for predicting heart disease as highlighted in Table 10 . Table 11 shows the summary of the frequency of existence of each features contained in the rules that predicts heart disease. There are a total of 11 rules out of 20 rules generated using significant features to predict the presence of heart disease. The most significant feature that exists in all the positive rules that predicts the Heart Disease is Chest pain (CP). Thallium heart scan (Thal) is seen in 9 out of 11 rules and Oldpeak (ST depression induced by exercise relative to rest) is seen in 7 rules.
Discussions
The implementation of WARM on selected significant features managed to achieve the highest confidence score in predicting heart disease which is 98% compared to 96% obtained from all features. It can be concluded that WARM predicts the risk of having heart disease well. From the top 20 rules generated, only 6 rules were based all features. On the other hand, 11 rules from the top 20 generated were based on the selected 8 features.
Studying the top 20 rules generated revealed some significant information. These findings were validated by a cardiologist:-
Asymptomatic chest pain, positive exercise-induced angina, Oldpeak > 0 and reversible thallium heart scan implies the presence of heart disease.
CP = asymptomatic, Exang = Yes, Oldpeak = greaterThanZero, Thal = reversible = = > class_HD = Heart Disease
Asymptomatic chest pain is one of the most important features as it appears in all the rules generated in detecting heart disease.
Reversible thallium heart scan and Oldpeak greater than zero are positively correlated with heart disease.
Males are more prone to have heart disease compared to females as all the sick rules stated sex as male and the healthy rules stated sex as female.
There is a strong negative correlation between CA and Thal for heart disease prediction.
The most common features that exist in healthy rules are Sex = Female, Exang (Exercise induce angina) = No and CA (Number of major vessels coloured by fluoroscopy) = Zero. A patient will be predicted as not having heart disease if the patient is female, angina is not induced by exercise and has no major vessels coloured by fluoroscopy.
Slope is not featured in any of the healthy rules.
This study managed to determine the processes involved in obtaining significant features and to devise a scoring mechanism to obtain the strength of each feature. This will enable for the correct weight to be imposed on each of the significant features to be used in WARM for predicting heart disease. The confidence score obtained in this study is the highest obtained in heart disease prediction using WARM based on the UCI dataset. This study can be used as a guide for computing thestrength scores of significant features found in other heart disease datasets.
Comparative analysis with existing work
This section performs comparison between the proposed work and existing works using WARM. The results obtained in this research proved that the weighted scores imposed on WARM for 8 significant features have the highest confidence score of 98% compared with other existing studies. Figure 3 shows the confidence score of all the existing studies on WARM that used the UCI Cleveland heart disease dataset in comparison with the proposed work. The confidence score obtained by both the experiments which includes all features and significant features in predicting heart disease using WARM achieved a significant difference in terms of the confidence score achieved compared to previous studies. The use of the significant features score in WARM provides the highest confidence of 98% predicting heart disease.
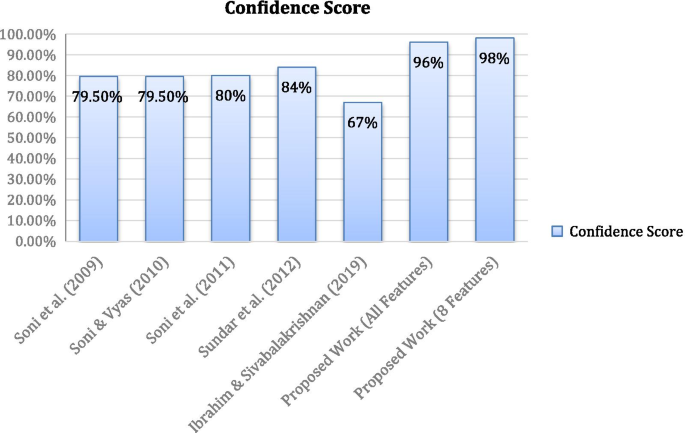
Result comparison on WARM using UCI Cleveland heart disease dataset
Table 12 presents a comparative analysis of WARM using significant features versus existing results of ARM in heart disease prediction. Rules that gave the highest confidence scores were retrieved and compared in this table. Research by Said et al. [ 41 ] and Khare and Gupta [ 24 ] showed lower confidence scores compared to this research. Although Sonet et al., [ 45 ] managed to obtain a confidence score of 99%, the rule generated for this score is questionable. The rule stated that if a patient has diabetes, then the patient will have heart disease. Although the risk of having heart disease is proven to be higher in diabetic patient, this rule cannot be generalized for all diabetic patients. This is the result of bias that might have existed in their dataset. The dataset used in their study is collected from 4 different medical institutions with a total of 131 records and is not an open dataset. Besides that, the dataset contained different features from the dataset used in this study.
This study also benchmarked the rules generated using the UCI dataset by past researches with the rules generated in our study. The extracted healthy rules are shown in Table 13 and sick rules are shown in Table 14 . Table 13 shows that our experiment with 8 significant features obtained the optimum confidence score of 100% for predicting healthy rules. The rules retrieved for this stated that if the sex is female, chest paint is non-angina and thallium heart scan is normal, this person is then predicted not to have heart disease.
Table 14 shows the sick rules together with the highest confidence scores of this research in comparison with other resesarch on associative and WARM for heart disease prediction. This study achieved a confidence score of 98% which is better than all the other predicted sick rules. To the best of our knowledge, the significant features’ weighted scores in our study managed to beat the scores obtained by all other research using ARM and WARM to predict heart disease.
This research contributed to obtaining the highest confidence score using significant features in WARM for heart disease prediction. Assigning appropriate weight scores have proven to improve the performance of confidence level in the prediction. A set of significant features with different weights to represent the strength of each of the features was used in heart disease prediction. To the best of our knowledge, this is the first study that made use of significant features in executing WARM. This research has also contributed to listing the top rules in predicting heart disease based on the UCI dataset. This is the first research that benchmarked the healthy rules and sick rules with the highest confidence scores. Future researches may look into predicting the risk levels of heart disease, as this will help medical practitioners and patients to gauge their heart disease severity. The algorithm used in this study for measuring weight can be further explored for use with other datasets to cater to other prediction models using the weighted approach. The machine learning techniques used in feature selection phase of this research is limited to the most popular techniques used in heart disease prediction research. Future researchers should look into exploring other machine learning techniques in selecting the significant features.
Data availability of materials
The datasets analysed during the current study are available as Cleveland Dataset in UCI Machine Learning Repository, [ https://www.kaggle.com/ronitf/heart-disease-uci ]
Agarwal R, Mittal M. Inventory classification using multi-level association rule mining. Int J Dec Supp Syst Technol. (IJDSST), 2019;11(2):1–12.
Agrawal R, Srikant R. Fast algorithms for mining association rules. In: Proceedings of 20th international conference very large data bases, VLDB. Vol. 1215, pp. 487–499; 1994.
Akbaş KE, Kivrak M, Arslan AK, Çolak C. Assessment of association rules based on certainty factor: an application on heart data set, in 2019 International artificial intelligence and data processing symposium (IDAP) (pp. 1–5). IEEE; 2019.
Altaf W, Shahbaz M, Guergachi A. Applications of association rule mining in health informatics: a survey. Artif Intell Rev. 2017;47(3):313–40.
Article Google Scholar
Alwidian J, Hammo BH, Obeid N. WCBA: weighted classification based on association rules algorithm for breast cancer disease. Appl Soft Comput. 2018;62:536–49.
American Heart Association. Heart disease and stroke statistics 2017 at-a-glance. Geraadpleegd van: https://healthmetrics.heart.org/wp-content/uploads/2017/06/Heart-Disease-and-Stroke-Statistics-2017-ucm_491265.pdf .
Amin MS. Identifying significant features and data mining techniques in predicting cardiovascular disease; 2018.
Amin MS, Chiam YK, Varathan KD Identification of significant features and data mining techniques in predicting heart disease. Telem Inform. 2019;36;82–93.
Bashir, S., Khan, Z. S., Khan, F. H., Anjum, A., & Bashir, K. (2019). Improving heart disease prediction using feature selection approaches. In 2019 16th International Bhurban Conference on Applied Sciences and Technology (IBCAST) (pp. 619–623). IEEE.
Cengiz AB, Birant KU, Birant D. Analysis of pre-weighted and post-weighted association rule mining, in 2019 Innovations in Intelligent Systems and Applications Conference (ASYU) (pp. 1–5). IEEE.
Chauhan A, Jain A, Sharma P, Deep V. Heart disease prediction using evolutionary rule learning, in 2018 4th International conference on computational intelligence & communication technology (CICT) (pp. 1–4). IEEE; 2018.
Dey L, Mukhopadhyay A. Biclustering-based association rule mining approach for predicting cancer-associated protein interactions. IET Syst Biol. 2019;13(5):234–42.
Dua, D., Graff, C. UCI machine learning repository [ http://archive.ics.uci.edu/ml ]. Irvine, CA: University of California, School of Information and Computer Science; 2019.
Domadiya N, Rao UP. Privacy-preserving association rule mining for horizontally partitioned healthcare data: a case study on the heart diseases. Sādhanā. 2018;43(8):1–9.
Domadiya N, Rao UP. Privacy preserving distributed association rule mining approach on vertically partitioned healthcare data. Procedia Comput Sci. 2019;148:303–12.
Fitriyani NL, Syafrudin M, Alfian G, Rhee J. HDPM: an effective heart disease prediction model for a clinical decision support system. IEEE Access. 2020;8:133034–50.
Han J, Pei J, Kamber M. Data mining: concepts and techniques. Elsevier; 2011.
Google Scholar
Ibrahim SP, Sivabalakrishnan M. An enhanced weighted associative classification algorithm without preassigned weight based on ranking hubs. Int J Adv Comput Sci Appl. 10(10); 2019.
Ibrahim SS, Sivabalakrishnan M. An evolutionary memetic weighted associative classification algorithm for heart disease prediction. In Recent Advances on Memetic Algorithms and its Applications in Image Processing (pp. 183–199). Springer, Singapore; 2020.
James SL, et al. Global, regional, and national incidence, prevalence, and yearslived with disability for 354 diseases and injuries for 195 countries and territories, 1990–2017: a systematic analysis for the Global Burden of Disease Study 2017. Lancet392 (10159), 1789–1858; 2018.
Jabbar MA, Deekshatulu BL, Chandra P. Graph based approach for heart disease prediction. In Proceedings of the third international conference on trends in information, telecommunication and computing. New York, NY: Springer. 2013. p. 465–474.
Kannan AG, Castro TARVC, BalaSubramanian R. A comprehensive study on various association rule mining techniques; 2018.
Khan SA, Yadav SK. Class-based associative classification using super subsets to predict the by-diseases in thyroid disorders. in International conference on advances in computational intelligence and informatics (pp. 301–308). Springer, Singapore; 2019.
Khare S, Gupta D. Association rule analysis in cardiovascular disease. In: Cognitive Computing and Information Processing (CCIP), 2016 Second International Conference on (pp. 1–6). IEEE; 2016.
Kharya S, Soni S, Swarnkar T. Weighted Bayesian association rule mining algorithm to construct Bayesian Belief network. In: 2019 International conference on applied machine learning (ICAML) (pp. 27–33). IEEE.
Lakshmi KS, Vadivu G. A novel approach for disease comorbidity prediction using weighted association rule mining. Journal of Ambient Intelligence and Humanized Computing , 1–8; 2019.
Lakshmi KP, Reddy CRK. Fast rule-based heart disease prediction using associative classification mining, in 2015 International conference on computer, communication and control (IC4) (pp. 1–5). IEEE; 2015.
Mahdi MA, Al-Janabi S. A novel software to improve healthcare base on predictive analytics and mobile services for cloud data centers, in International conference on big data and networks technologies (pp. 320–339). Springer, Cham; 2019.
Maji S, Arora S. Decision tree algorithms for prediction of heart disease. In Information and communication technology for competitive strategies (pp. 447–454). Springer, Singapore; 2019.
Malarvizhi SP, Sathiyabhama B. Frequent pagesets from web log by enhanced weighted association rule mining. Clust Comput. 2016;19(1):269–77.
Methaila A, Kansal P, Arya H, Kumar P. Early heart disease prediction using data mining techniques. Comput Sci Inf Technol J. 53–59; 2014.
Mohammed KI, Zaidan AA, Zaidan BB, Albahri OS, Albahri AS, Alsalem MA, Mohsin AH. Novel technique for reorganisation of opinion order to interval levels for solving several instances representing prioritisation in patients with multiple chronic diseases. Comput Methods Programs Biomed. 2020;185:105151.
Article CAS Google Scholar
Mohammed KI, Jaafar J, Zaidan AA, Albahri OS, Zaidan BB, Abdulkareem KH, Alamoodi AH. A uniform intelligent prioritisation for solving diverse and big data generated from multiple chronic diseases patients based on hybrid decision-making and voting method. IEEE Access. 2020;8:91521–30.
Murphy SL, Xu J, Kochanek KD, Arias E. Mortality in the United States, 2017. NCHS data brief, no 328. Hyattsville, MD: National Center for Health Statistics; 2018.
Mutter S, Hall M, Frank E. Using classification to evaluate the output of confidence-based association rule mining, in AI 2004: Advances in, Artificial Intelligence, 133–148; 2005.
Nahar J, Imam T, Tickle KS, Chen YPP. Association rule mining to detect factors which contribute to heart disease in males and females. Expert Syst Appl. 2013;40(4):1086–1093.
Nguyen T, et al. Classification of healthcare data using genetic fuzzy logic system and wavelets. Expert Syst Appl. 2015;42(4):2184–97.
Orphanou K, Dagliati A, Sacchi L, Stassopoulou A, Keravnou E, Bellazzi R. Incorporating repeating temporal association rules in naïve bayes classifiers for coronary heart disease diagnosis. J Biomed Inform. 2018;81:74–82.
Park HY, Lim DJ. A design failure pre-alarming system using score-and vote-based associative classification. Expert Syst Appl. 2021;164:113950.
Roth GA, et al. Global, regional, and national age-sex-specific mortality for 282 causes of death in 195 countries and territories, 1980–2017: a systematic analysisfor the Global Burden of Disease Study 2017. Lancet. 2018;392(10159):1736–88.
Said IU, Adam AH, Garko AB. Association rule mining on medical data to predict heart disease. Int J Sci Technol Manage. 2015. 26–35.
Shuriyaa B, Rajendranb A. Cardio vascular disease diagnosis using data mining techniques and ANFIS approach. Int J Appl Eng Res. 2018;13(21):15356–61.
Sim DYY, Teh CS, Ismail AI. Improved boosting algorithms by pre-pruning and associative rule mining on decision trees for predicting obstructive sleep apnea. Adv Sci Lett. 2017;23(11):11593–8.
Singh J, Kamra A, Singh H. Prediction of heart diseases using associative classification, in 2016 5th International conference on wireless networks and embedded systems (WECON) (pp. 1–7). IEEE; 2016.
Sonet, K. M. H., Rahman, M. M., Mazumder, P., Reza, A., & Rahman, R. M. (2017). Analyzing patterns of numerously occurring heart diseases using association rule mining. In 2017 Twelfth International Conference on Digital Information Management (ICDIM) (pp. 38–45). IEEE.
Soni J, Ansari U, Sharma D, Soni S. Intelligent and effective heart disease prediction system using weighted associative classifiers. International Journal on Computer Science and Engineering. 2011;3(6):2385–92.
Soni S, Pillai J, Vyas OP. An associative classifier using weighted association rule. In: 2009 World Congress on Nature & Biologically Inspired Computing (NaBIC). IEEE. 2009. p. 1492–1496.
Soni S, Vyas OP. Using associative classifiers for predictive analysis in health care data mining. Int J Comput Appl. 2010;4(5):33–7.
Srinivas K, Reddy BR, Rani BK, Mogili R. Hybrid Approach for prediction of cardiovascular disease using class association rules and MLP. Int J Electr Comput Eng. (2088–8708), 6(4); 2016.
Sundar NA, Latha PP, Chandra MR. Performance analysis of classification data mining techniques over heart disease database. Int J Eng Sci Adv Technol. 2012;2(3):470–8.
Taihua W, Fan G. Associating IDS alerts by an improved apriori algorithm. in Third international symposium on intelligent information technology and security informatics, 2010 Jinggangshan, China (pp. 478–482). IEEE; 2010.
Thanigaivel R, Kumar KR. Boosted apriori: an effective data mining association rules for heart disease prediction system. Middle-East J Sci Res. 2016;24(1):192–200.
UCI Machine Learning Repository: Heart Disease Data Set; 2010. http://archive.ics.uci.edu/ml/datasets/Heart+Disease
Vasanthanageswari S, Vanitha M. Predicting risk factor of congenital heart defect using association rule mining technique. Int J Pure Appl Math. 2018;118(7):399–404.
Wei-Jia L, Liang M, Hao C. Particle swarm optimisation-support vector machine optimised by association rules for detecting factors inducing heart diseases. J Intell Syst. 2017;26(3):573–83.
World Health Organization. Global action plan for the prevention and control of non-communicable diseases 214–2020. ISBN 978 92 4 150623 6. Geneva 2013; 2013.
Download references
Acknowledgements
The authors would like to thank Fundamental Research Grant Scheme (FRGS/1/2017/ICT01/UM/02/4, FP057-2017A) and Faculty Research Grant Scheme of Universiti Malaya (Project Code: GF011D-2019) for funding this research.
Project Code: Fundamental Research Grant Scheme (FRGS/1/2017/ICT01/UM/02/4, FP057-2017A) and Faculty Research Grant Scheme of Universiti Malaya (Project Code: 584 GF011D-2019).
Author information
Authors and affiliations.
Department of Software Engineering, Faculty of Computer Science and Information Technology, Universiti Malaya, Kuala Lumpur, Malaysia
Armin Yazdani & Yin Kia Chiam
Department of Information Systems, Faculty of Computer Science and Information Technology, Universiti Malaya, Kuala Lumpur, Malaysia
Kasturi Dewi Varathan
Department of Computing, National University of Sciences and Technology (NUST), Islamabad, Pakistan
Asad Waqar Malik
Department of Medicine, Faculty of Medicine, Universiti Malaya, Kuala Lumpur, Malaysia
Wan Azman Wan Ahmad
You can also search for this author in PubMed Google Scholar
Contributions
AY: Software, Performed Experiments, original draft preparation. KDV: Conceptualization, original draft preparation, supervision. YKC: original draft preparation, supervision. AWM: Visualization, reviewing and editing. WAWA: Cardiac expert for rules validation. All authors read and approved the final manuscript.
Corresponding author
Correspondence to Kasturi Dewi Varathan .
Ethics declarations
Ethics approval and consent to participate.
Not applicable.
Consent for publication
Not Applicable.
Competing interests
The authors declare no competing interests.
Additional information
Publisher's note.
Springer Nature remains neutral with regard to jurisdictional claims in published maps and institutional affiliations.
Rights and permissions
Open Access This article is licensed under a Creative Commons Attribution 4.0 International License, which permits use, sharing, adaptation, distribution and reproduction in any medium or format, as long as you give appropriate credit to the original author(s) and the source, provide a link to the Creative Commons licence, and indicate if changes were made. The images or other third party material in this article are included in the article's Creative Commons licence, unless indicated otherwise in a credit line to the material. If material is not included in the article's Creative Commons licence and your intended use is not permitted by statutory regulation or exceeds the permitted use, you will need to obtain permission directly from the copyright holder. To view a copy of this licence, visit http://creativecommons.org/licenses/by/4.0/ . The Creative Commons Public Domain Dedication waiver ( http://creativecommons.org/publicdomain/zero/1.0/ ) applies to the data made available in this article, unless otherwise stated in a credit line to the data.
Reprints and permissions
About this article
Cite this article.
Yazdani, A., Varathan, K.D., Chiam, Y.K. et al. A novel approach for heart disease prediction using strength scores with significant predictors. BMC Med Inform Decis Mak 21 , 194 (2021). https://doi.org/10.1186/s12911-021-01527-5
Download citation
Received : 18 September 2020
Accepted : 12 May 2021
Published : 21 June 2021
DOI : https://doi.org/10.1186/s12911-021-01527-5
Share this article
Anyone you share the following link with will be able to read this content:
Sorry, a shareable link is not currently available for this article.
Provided by the Springer Nature SharedIt content-sharing initiative
- Weighted associative rule mining
- Heart disease prediction
- Cardiovascular disease
- Weighted scores
BMC Medical Informatics and Decision Making
ISSN: 1472-6947
- General enquiries: [email protected]
Heart Disease Prediction using Machine Learning Techniques
- Original Research
- Published: 16 October 2020
- Volume 1 , article number 345 , ( 2020 )
Cite this article
- Devansh Shah 1 ,
- Samir Patel 1 &
- Santosh Kumar Bharti 1
33k Accesses
254 Citations
34 Altmetric
Explore all metrics
Heart disease, alternatively known as cardiovascular disease, encases various conditions that impact the heart and is the primary basis of death worldwide over the span of the past few decades. It associates many risk factors in heart disease and a need of the time to get accurate, reliable, and sensible approaches to make an early diagnosis to achieve prompt management of the disease. Data mining is a commonly used technique for processing enormous data in the healthcare domain. Researchers apply several data mining and machine learning techniques to analyse huge complex medical data, helping healthcare professionals to predict heart disease. This research paper presents various attributes related to heart disease, and the model on basis of supervised learning algorithms as Naïve Bayes, decision tree, K-nearest neighbor, and random forest algorithm. It uses the existing dataset from the Cleveland database of UCI repository of heart disease patients. The dataset comprises 303 instances and 76 attributes. Of these 76 attributes, only 14 attributes are considered for testing, important to substantiate the performance of different algorithms. This research paper aims to envision the probability of developing heart disease in the patients. The results portray that the highest accuracy score is achieved with K-nearest neighbor.
This is a preview of subscription content, log in via an institution to check access.
Access this article
Price includes VAT (Russian Federation)
Instant access to the full article PDF.
Rent this article via DeepDyve
Institutional subscriptions
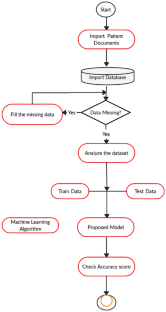
Similar content being viewed by others
Heart Disease Prediction Using Various Machine Learning Algorithms
Feature Selection and Prediction of Heart Disease Using Machine Learning Approaches
Heart Disease Prediction Using Modified Machine Learning Algorithm
Seckeler MD, Hoke TR. The worldwide epidemiology of acute rheumatic fever and rheumatic heart disease. Clin Epidemiol. 2011;3:67.
Article Google Scholar
Gaziano TA, Bitton A, Anand S, Abrahams-Gessel S, Murphy A. Growing epidemic of coronary heart disease in low-and middle-income countries. Curr Probl Cardiol. 2010;35(2):72–115.
Weng SF, Reps J, Kai J, Garibaldi JM, Qureshi N. Can machine-learning improve cardiovascular risk prediction using routine clinical data? PLoS ONE. 2017;12(4):e0174944.
Ramalingam VV, Dandapath A, Raja MK. Heart disease prediction using machine learning techniques: a survey. Int J Eng Technol. 2018;7(2.8):684–7.
Patel J, TejalUpadhyay D, Patel S. Heart disease prediction using machine learning and data mining technique. Heart Dis. 2015;7(1):129–37.
Google Scholar
Fatima M, Pasha M. Survey of machine learning algorithms for disease diagnostic. J Intell Learn Syst Appl. 2017;9:1–16. https://doi.org/10.4236/jilsa.2017.91001 .
Pahwa K, Kumar R. Prediction of heart disease using hybrid technique for selecting features. In: 2017 4th IEEE Uttar Pradesh section international conference on electrical, computer and electronics (UPCON). IEEE. p. 500–504.
Pouriyeh S, Vahid S, Sannino G, De Pietro G, Arabnia H, Gutierrez J. A comprehensive investigation and comparison of machine learning techniques in the domain of heart disease. In: 2017 IEEE symposium on computers and communications (ISCC). IEEE. p. 204–207.
Chauhan R, Bajaj P, Choudhary K, Gigras Y. Framework to predict health diseases using attribute selection mechanism. In: 2015 2nd international conference on computing for sustainable global development (INDIACom). IEEE. p. 1880–84.
Bouali H, Akaichi J. Comparative study of different classification techniques: heart disease use case. In: 2014 13th international conference on machine learning and applications. IEEE. p. 482–86.
Xu S, Zhang Z, Wang D, Hu J, Duan X, Zhu T. Cardiovascular risk prediction method based on CFS subset evaluation and random forest classification framework. In: 2017 IEEE 2nd international conference on big data analysis (ICBDA). IEEE. p. 228–32.
Otoom AF, Abdallah EE, Kilani Y, Kefaye A, Ashour M. Effective diagnosis and monitoring of heart disease. Int J Softw Eng Appl. 2015;9(1):143–56.
Vembandasamy K, Sasipriya R, Deepa E. Heart diseases detection using Naive Bayes algorithm. Int J Innov Sci Eng Technol. 2015;2(9):441–4.
Chaurasia V, Pal S. Data mining approach to detect heart diseases. Int J Adv Comput Sci Inf Technol (IJACSIT). 2014;2:56–66.
Parthiban G, Srivatsa SK. Applying machine learning methods in diagnosing heart disease for diabetic patients. Int J Appl Inf Syst (IJAIS). 2012;3(7):25–30.
Deepika K, Seema S. Predictive analytics to prevent and control chronic diseases. In: 2016 2nd international conference on applied and theoretical computing and communication technology (iCATccT). IEEE. p. 381–86.
Dwivedi AK. Performance evaluation of different machine learning techniques for prediction of heart disease. Neural Comput Appl. 2018;29(10):685–693.
Download references
Author information
Authors and affiliations.
Computer Engineering Department, School of Technology, Pandit Deendayal Petroleum University, Raisan, Gandhinagar, 382007, India
Devansh Shah, Samir Patel & Santosh Kumar Bharti
You can also search for this author in PubMed Google Scholar
Contributions
All authors have equal contribution to this study and all authors have read and approved the final manuscript.
Corresponding author
Correspondence to Devansh Shah .
Ethics declarations
Conflict of interest.
The authors declare that they have no competing interest.
Additional information
Publisher's note.
Springer Nature remains neutral with regard to jurisdictional claims in published maps and institutional affiliations.
This article is part of the topical collection “Advances in Computational Approaches for Artificial Intelligence, Image Processing, IoT and Cloud Applications” guest edited by Bhanu Prakash K N and M. Shivakumar”.
Rights and permissions
Reprints and permissions
About this article
Shah, D., Patel, S. & Bharti, S.K. Heart Disease Prediction using Machine Learning Techniques. SN COMPUT. SCI. 1 , 345 (2020). https://doi.org/10.1007/s42979-020-00365-y
Download citation
Received : 27 September 2020
Accepted : 02 October 2020
Published : 16 October 2020
DOI : https://doi.org/10.1007/s42979-020-00365-y
Share this article
Anyone you share the following link with will be able to read this content:
Sorry, a shareable link is not currently available for this article.
Provided by the Springer Nature SharedIt content-sharing initiative
- Heart disease prediction
- Data mining
- Decision tree
- Naïve Bayes
- Random forest
- Machine learning
Advertisement
- Find a journal
- Publish with us
- Track your research
Heart Disease Prediction Using Machine Learning
Ieee account.
- Change Username/Password
- Update Address
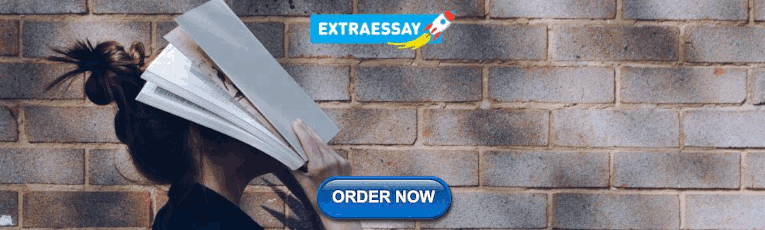
Purchase Details
- Payment Options
- Order History
- View Purchased Documents
Profile Information
- Communications Preferences
- Profession and Education
- Technical Interests
- US & Canada: +1 800 678 4333
- Worldwide: +1 732 981 0060
- Contact & Support
- About IEEE Xplore
- Accessibility
- Terms of Use
- Nondiscrimination Policy
- Privacy & Opting Out of Cookies
A not-for-profit organization, IEEE is the world's largest technical professional organization dedicated to advancing technology for the benefit of humanity. © Copyright 2024 IEEE - All rights reserved. Use of this web site signifies your agreement to the terms and conditions.
Thank you for visiting nature.com. You are using a browser version with limited support for CSS. To obtain the best experience, we recommend you use a more up to date browser (or turn off compatibility mode in Internet Explorer). In the meantime, to ensure continued support, we are displaying the site without styles and JavaScript.
- View all journals
Cardiovascular diseases articles from across Nature Portfolio
Cardiovascular diseases are pathological conditions affecting the heart and/or blood vessels that is, the cardiovascular system.
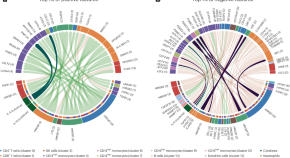
Immune signatures of disease stages and outcomes in myocardial infarction
A longitudinal multiomic dataset was assembled to characterize the immune landscape in myocardial infarction and chronic coronary syndromes. Multiomics factor analysis (MOFA) revealed immune signatures that associate with disease stage or treatment outcomes. This work opens new directions for future mechanistic and clinical studies on coronary artery disease and myocardial infarction.
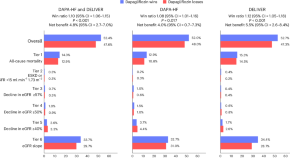
The efficacy of dapagliflozin in a hierarchical kidney outcome in heart failure
Dapagliflozin improved a hierarchical composite outcome, including death, a worsening kidney disease event, and estimated glomerular filtration rate slope, compared with placebo, in patients with heart failure. This hierarchical outcome — analyzed with win statistics — might provide the statistical power to evaluate the effect of treatments on kidney function in heart failure trials.
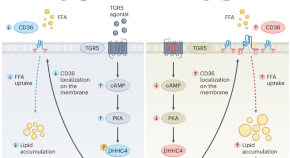
Bile acids for diabetic cardiomyopathy
Diabetes can cause heart failure by a toxic accumulation of lipids in cardiac myocytes, which impairs their function. This work shows that stimulation of the bile acid receptor TGR5 limits fatty acid uptake into cardiac myocytes and prevents the development of diabetic cardiomyopathy.
- David Weissman
- Christoph Maack
Related Subjects
- Acute coronary syndromes
- Arrhythmias
- Cardiomyopathies
- Congenital heart defects
- Dyslipidaemias
- Heart failure
- Hypertension
- Valvular disease
- Vascular diseases
Latest Research and Reviews
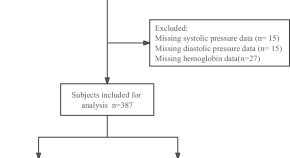
Association between high-altitude polycythemia and hypertension: a cross-sectional study in adults at Tibetan ultrahigh altitudes
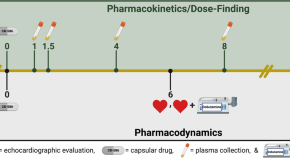
Cardiac myosin inhibitor, CK-586, minimally reduces systolic function and ameliorates obstruction in feline hypertrophic cardiomyopathy
- Victor N. Rivas
- Amanda E. Crofton
- Joshua A. Stern
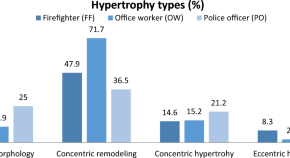
Structural and functional cardiac parameters across occupations: a cross-sectional study in differing work environments
- Roman Leischik
- Peter Foshag
- Markus Strauss
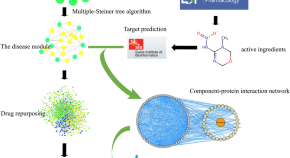
Exploring the pathways of drug repurposing and Panax ginseng treatment mechanisms in chronic heart failure: a disease module analysis perspective
- Chengzhi Xie
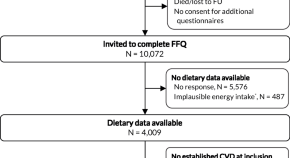
Dietary habits and compliance with dietary guidelines in patients with established cardiovascular disease
- Nadia E. Bonekamp
- Johanna M. Geleijnse
- F. L. J. Visseren
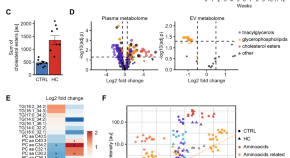
Effect of hypercholesterolemia on circulating and cardiomyocyte-derived extracellular vesicles
- Csenger Kovácsházi
- Szabolcs Hambalkó
- Zoltán Giricz
News and Comment
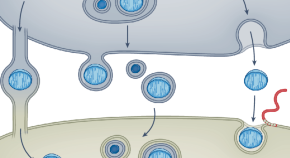
Artificially transplanted mitochondria in endothelial cells promote mitophagy
A study in Nature describes a single-cell-type strategy for vascular cell therapies that involves the artificial transplantation of mitochondria to endothelial cells, which promotes mitophagy and facilitates the formation of functional vessels in ischaemic tissue without the need for mesenchymal stromal cell support.
- Karina Huynh
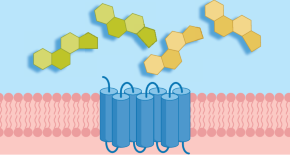
Bile acid receptor protects against diabetic cardiomyopathy
Activation of the bile acid receptor TGR5 inhibits CD36-mediated fatty acid uptake in cardiomyocytes and protects against cardiac lipotoxicity and the development of diabetic cardiomyopathy in mice, according to a new study.
- Irene Fernández-Ruiz
Multi-omic links between gut microbiome and cardiovascular disease
- Jordan Hindson
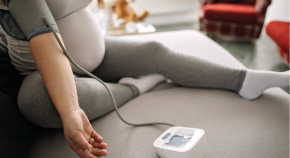
SASP in peripartum cardiomyopathy and preeclampsia
- Anna Kriebs
Quick links
- Explore articles by subject
- Guide to authors
- Editorial policies


A .gov website belongs to an official government organization in the United States.
A lock ( ) or https:// means you've safely connected to the .gov website. Share sensitive information only on official, secure websites.
- About Heart Disease
- Risk Factors
- Heart Disease Facts
- Healthy People 2030
- Heart Disease Communications Toolkit
- Publication
- Community-Clinical Linkages Health Equity Guide
- Emergency Medical Services (EMS) Home Rule State Law Fact Sheet
- American Heart Month Toolkits
- Healthy Eating Communications Kit
- About Stroke
- About High Blood Pressure
- About Cholesterol
What to know
Learn facts about how race, ethnicity, age, and other risk factors can contribute to heart disease risk. It’s important for everyone to know the facts about heart disease.
Heart disease in the United States
In the United States:
- Heart disease is the leading cause of death for men, women, and people of most racial and ethnic groups. 1
- One person dies every 33 seconds from cardiovascular disease. 1
- About 695,000 people died from heart disease in 2021—that's 1 in every 5 deaths . 1 2
- Heart disease costs about $239.9 billion each year from 2018 to 2019. 3 This includes the cost of health care services, medicines, and lost productivity due to death.
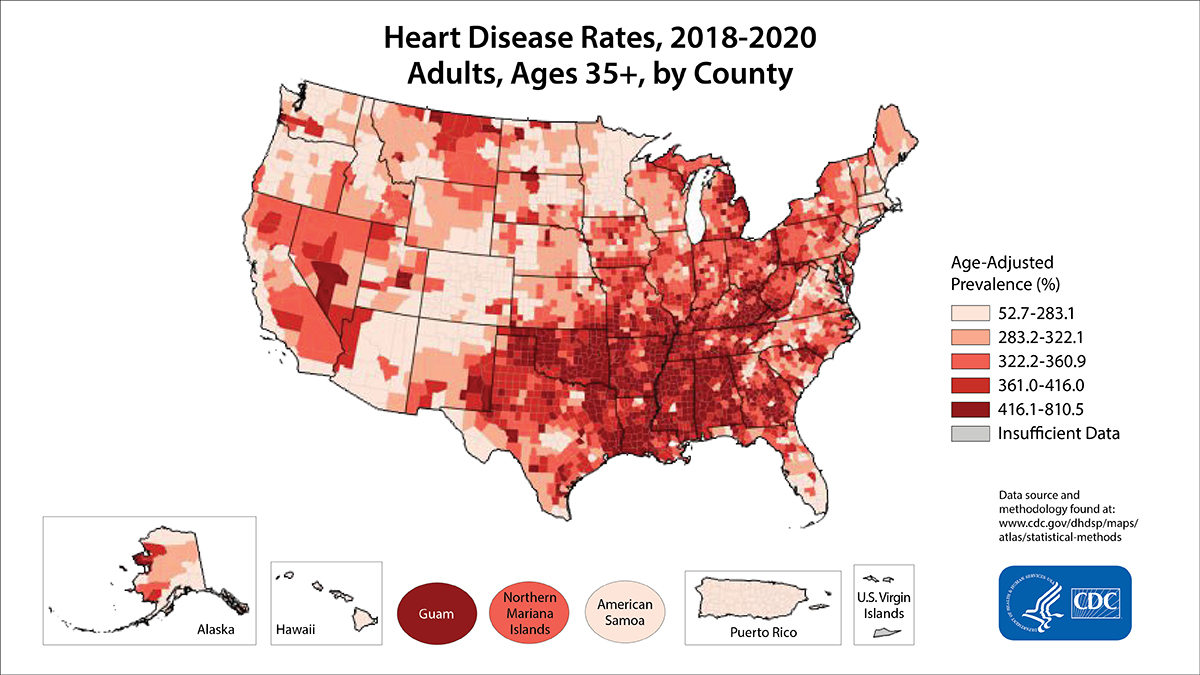
Coronary artery disease (CAD)
- Coronary heart disease is the most common type of heart disease, killing 375,476 people in 2021. 2
- About 1 in 20 adults age 20 and older have CAD (about 5%). 2
- In 2021, about 2 in 10 deaths from CAD happened in adults less than 65 years old. 1
Heart attack
- In the United States, someone has a heart attack every 40 seconds. 2
- 605,000 are a first heart attack. 2
- 200,000 happen to people who have already had a heart attack. 2
- About 1 in 5 heart attacks are silent—the damage is done, but the person is not aware of it. 2
Did you know?
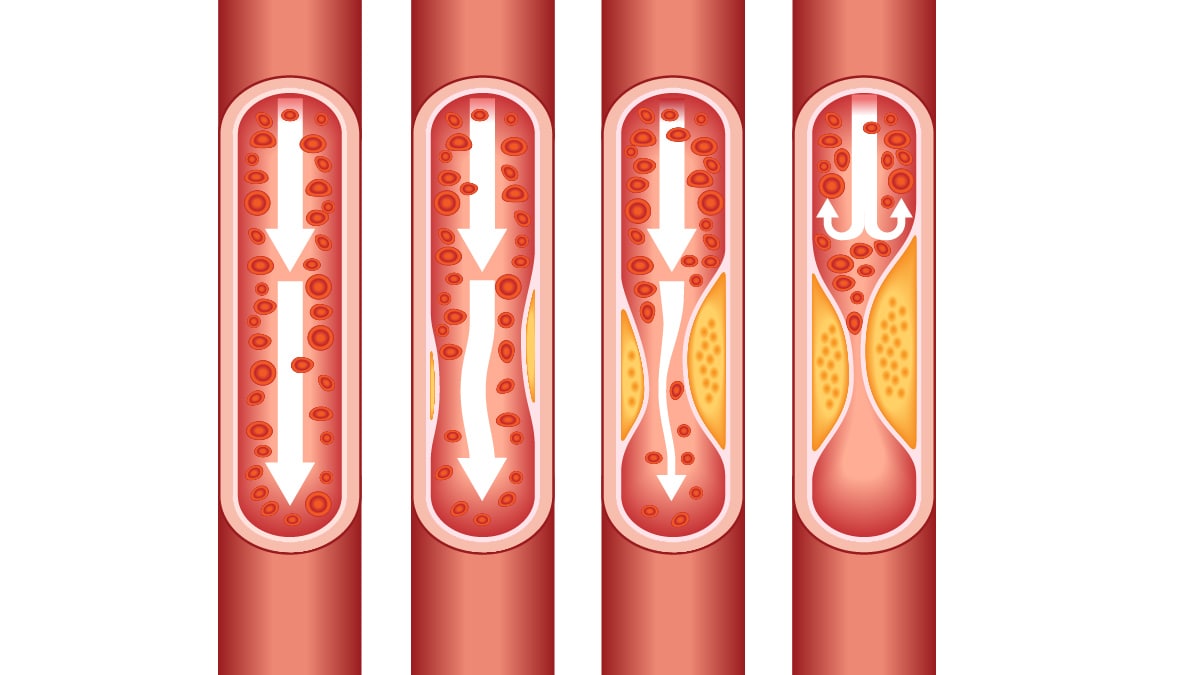
Who is affected
Heart disease deaths vary by sex, race, and ethnicity.
Heart disease is the leading cause of death for people of most racial and ethnic groups in the United States. These include African American, American Indian, Alaska Native, Hispanic, and White men. For women from the Pacific Islands and Asian American, American Indian, Alaska Native, and Hispanic women, heart disease is second only to cancer. 1
Below are the percentages of all deaths caused by heart disease in 2021, listed by ethnicity, race, and sex.
Race or Ethnic Group
% of Deaths
American Indian or Alaska Native
Black (Non-Hispanic)
Native Hawaiin or Other Pacific Islander
White (Non-Hispanic)
Americans at risk for heart disease
High blood pressure , high blood cholesterol , and smoking are key risk factors for heart disease.
Several other medical conditions and lifestyle choices can also put people at a higher risk for heart disease, including:
- Overweight and obesity
- Unhealthy diet
- Physical inactivity
- Excessive alcohol use
What CDC is doing
- Million Hearts ®
- CDC: Heart Disease Communications Kit
- National Heart, Lung, and Blood Institute
- National Center for Health Statistics. Multiple Cause of Death 2018–2021 on CDC WONDER Database. Accessed February 2, 2023. https://wonder.cdc.gov/mcd.html
- Tsao CW, Aday AW, Almarzooq ZI, et al. Heart Disease and Stroke Statistics—2023 Update: A Report From the American Heart Association. Circulation. 2023;147:e93–e621.
- National Center for Health Statistics. Percentage of coronary heart disease for adults aged 18 and over, United States, 2019—2021. National Health Interview Survey. Accessed February 17, 2023. https://wwwn.cdc.gov/NHISDataQueryTool/SHS_adult/index.html
Heart Disease
Heart disease is the leading cause of death in the United States.
For Everyone
Public health.
Featured Topics
Featured series.
A series of random questions answered by Harvard experts.
Explore the Gazette
Read the latest.
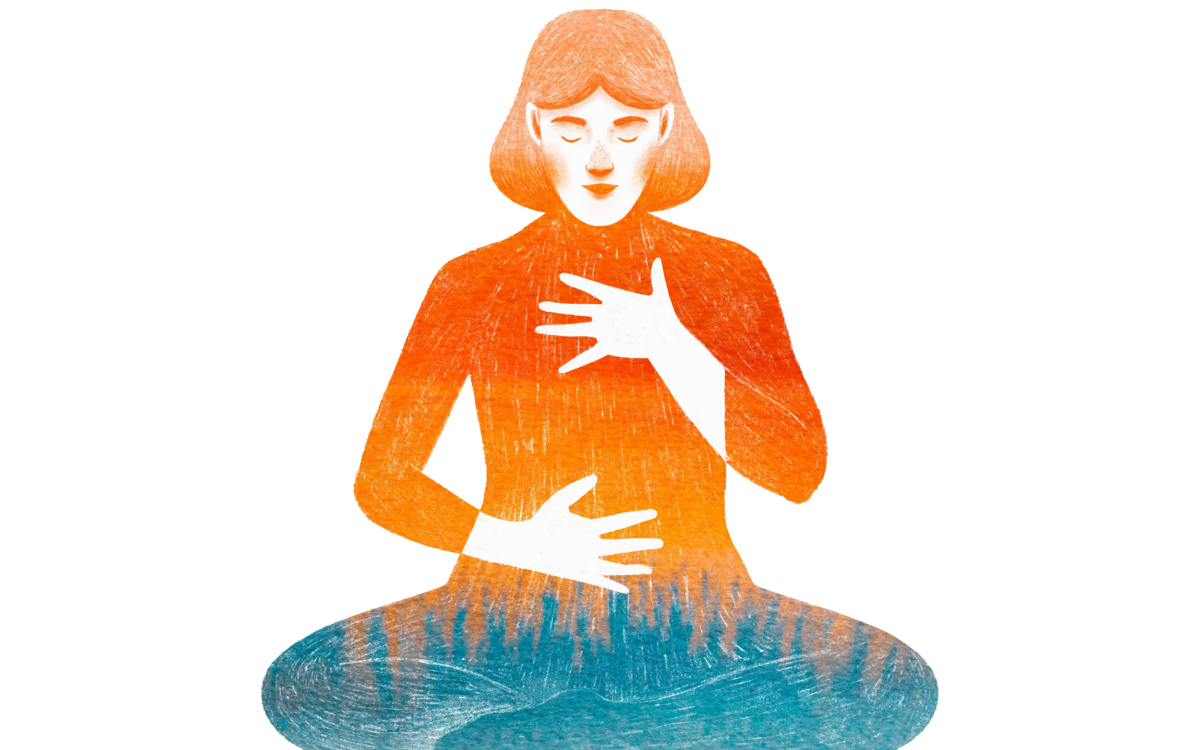
Had a bad experience meditating? You’re not alone.
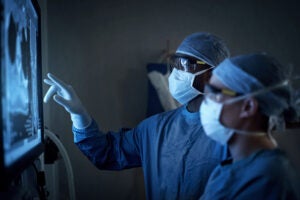
Families may remove brain-injured patients from life support too soon
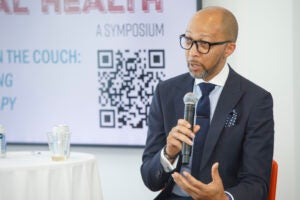
What to do about mental health crisis among Black males
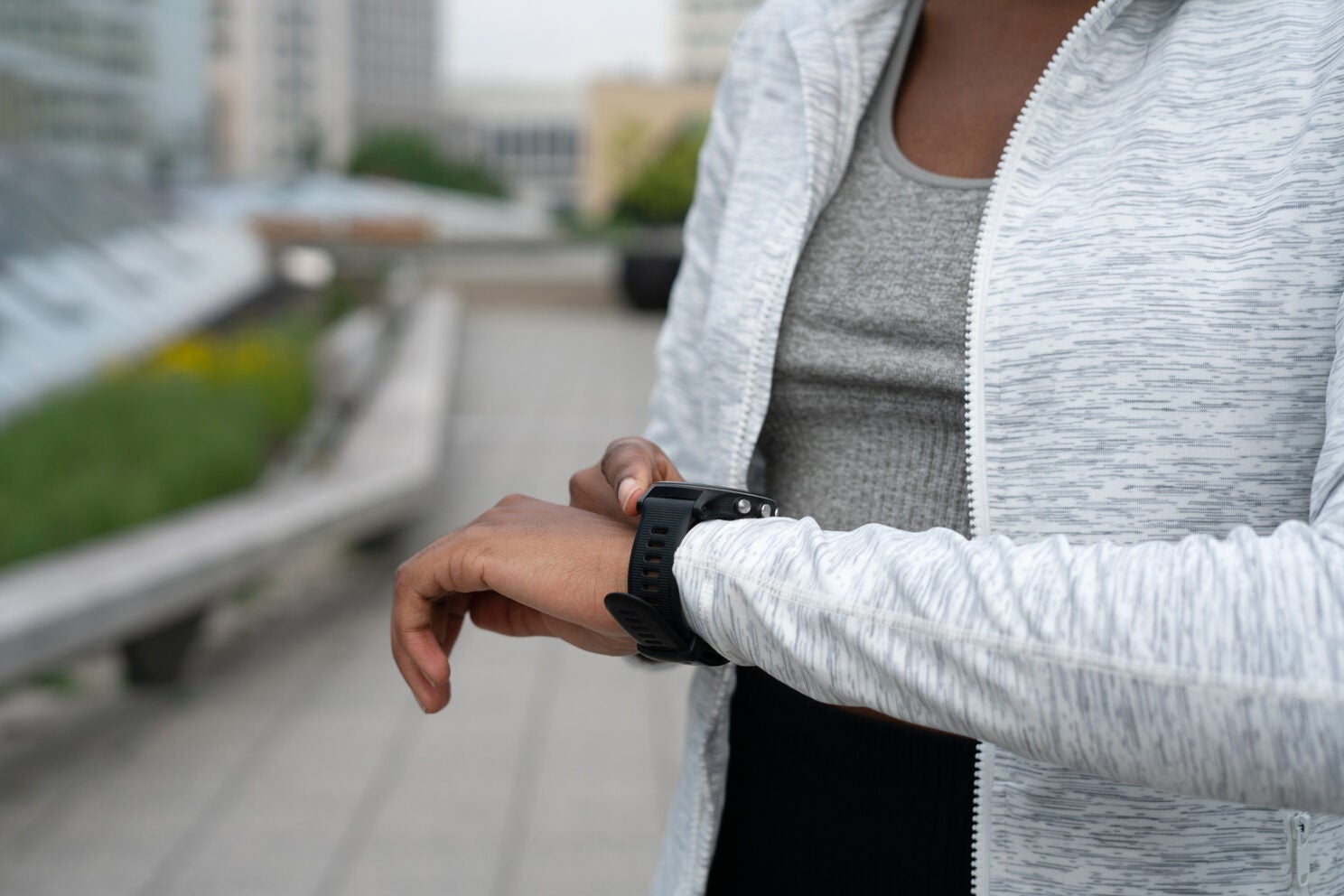
Everything counts!
Research finds step-count and time are equally valid in reducing health risks
Kira Sampson
BWH Communications
A new study suggests that both step-count and time-based exercise goals are equally effective in reducing risks of heart disease and early death.
Researchers from Harvard-affiliated Brigham and Women’s Hospital reviewed data on healthy women age 62+, who used wearable devices to record their physical activity, and then tracked their health outcomes. After a median follow-up of nine years, the researchers found higher levels of physical activity, whether in time of exercise or step counts, were associated with large risk reductions in mortality and cardiovascular disease. The most active quarter of women in the study had a 30 to 40 percent reduced risk compared to the least active quarter.
Results of the study are published in JAMA Internal Medicine .
“We recognized that existing physical activity guidelines focus primarily on activity duration and intensity but lack step-based recommendations,” said lead author Rikuta Hamaya, a researcher in the Division of Preventive Medicine at BWH. “With more people using smartwatches to measure their steps and overall health, we saw the importance of ascertaining how step-based measurements compare to time-based targets in their association with health outcomes — is one better than the other?”
“Movement looks different for everyone, and nearly all forms of movement are beneficial to our health.” Rikuta Hamaya
The current U.S. guidelines , last updated in 2018, recommend that adults engage in at least 150 minutes of moderate to vigorous physical activity (e.g., brisk walking) or 75 minutes of vigorous activity (e.g., jogging) per week. At that time, most of the existing evidence on health benefits came from studies where participants self-reported their physical activity. Few data points existed on the relationship between steps and health. Fast-forward to the present — with wearables being ubiquitous, step counts are now a popular metric among many fitness-tracking platforms. How do time-based goals stack up against step-based ones? Investigators sought to answer this question.
For this study, investigators collected data from 14,399 women who participated in the Women’s Health Study, and were healthy (free from cardiovascular disease and cancer). Between 2011 and 2015, participants aged 62 years and older were asked to wear research-grade wearables for seven consecutive days to record their physical activity levels, only removing the devices for sleep or water-related activities. Throughout the study period, annual questionnaires were administered to ascertain health outcomes of interest, in particular, death from any cause and cardiovascular disease. Investigators followed up with participants through the end of 2022.
At the time of device wear, researchers found that participants engaged in a median of 62 minutes of moderate-to-vigorous intensity physical activity per week and accumulated a median of 5,183 steps per day. During a median follow-up of nine years, approximately 9 percent of participants had passed and roughly 4 percent developed cardiovascular disease.
Higher levels of physical activity (whether assessed as step counts or time in moderate to vigorous activity) were associated with large risk reductions in death or cardiovascular disease — the most active quarter of women reduced their risk by 30-40 percent compared with the least-active quarter. Individuals in the top three quartiles of physical activity outlived those in the bottom quartile by an average of 2.22 and 2.36 months respectively, based on time and step-based measurements, at nine years of follow-up. This survival advantage persisted regardless of differences in body mass index (BMI).
While both metrics are useful in portraying health status, Hamaya explained that each has its advantages and downsides. For one, step counts may not account for differences in fitness levels. For example, if a 20-year-old and 80-year-old both walk for 30 minutes at moderate intensity, their step counts may differ significantly. Conversely, steps are straightforward to measure and less subject to interpretation compared to exercise intensity. Additionally, steps capture even sporadic movements of everyday life, not just exercise, and these kinds of daily life activities likely are those carried out by older individuals.
“For some, especially for younger individuals, exercise may involve activities like tennis, soccer, walking, or jogging, all of which can be easily tracked with steps. However, for others, it may consist of bike rides or swimming, where monitoring the duration of exercise is simpler,” said Hamaya. “That’s why it’s important for physical-activity guidelines to offer multiple ways to reach goals. Movement looks different for everyone, and nearly all forms of movement are beneficial to our health.”
The authors note that this study incorporates only a single assessment of time and step-based physical activity metrics. Further, most women included in the study were white and of higher socioeconomic status. Finally, this study was observational, and thus causal relations cannot be proven. In the future, Hamaya aims to collect more data via a randomized controlled trial to better understand the relationship between time and step-based exercise metrics and health.
“The next federal physical activity guidelines are planned for 2028,” said senior author I-Min Lee, an epidemiologist in the Division of Preventive Medicine at BWH. “Our findings further establish the importance of adding step-based targets, in order to accommodate flexibility of goals that work for individuals with differing preferences, abilities and lifestyles.”
Disclosures : Hamaya reported receiving consulting fees from DeSC Healthcare, Inc., outside of the submitted work. Co-authors Christopher Moore, Julie Buring, Kelly Evenson, and Lee reported receiving institutional support from the National Institutes of Health during the conduct of the study.
This research was supported in part by the National Institutes of Health (CA154647, CA047988, CA182913, HL043851, HL080467, and HL09935), the National Cancer Institute (5R01CA227122), Office of the Director, Office of Disease Prevention, and Office of Behavioral and Social Sciences Research; and by the extramural research program at the National Heart, Lung, and Blood Institute.
Get the best of the Gazette delivered to your inbox
By subscribing to this newsletter you’re agreeing to our privacy policy
Share this article
You might like.
Altered states of consciousness through yoga, mindfulness more common than thought and mostly beneficial, study finds — though clinicians ill-equipped to help those who struggle
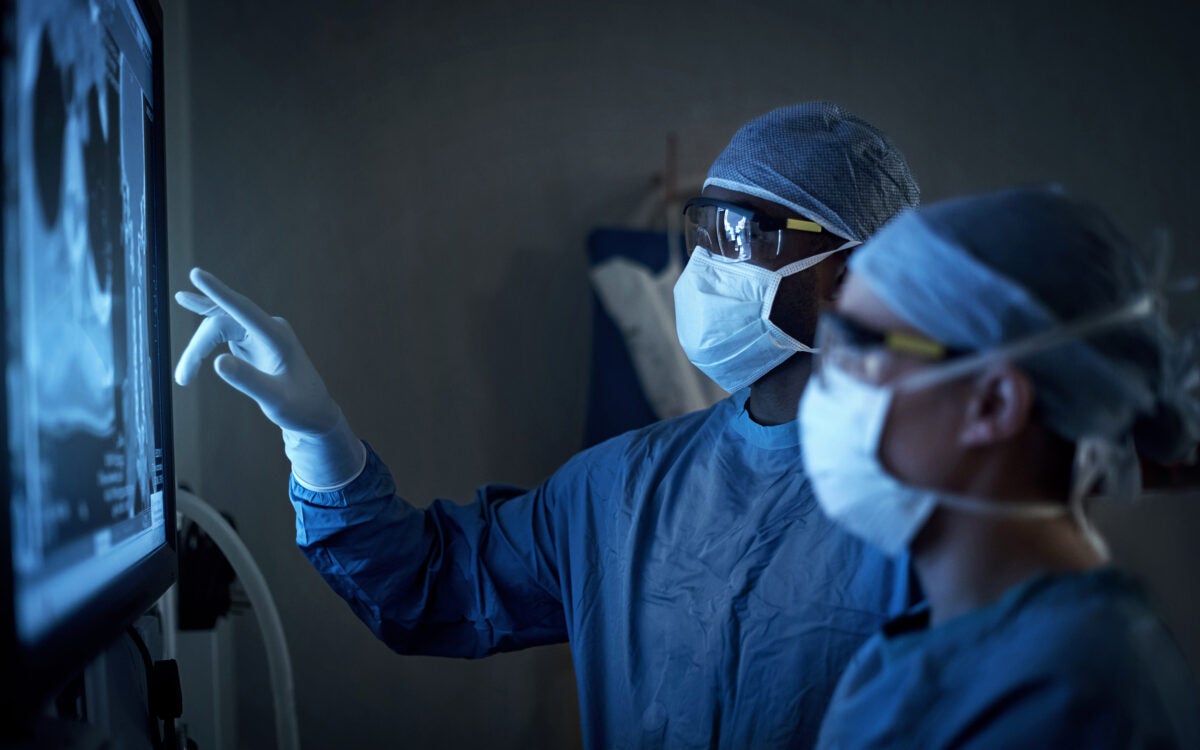
Of the survivors within one study group, more than 40% recovered at least some independence.
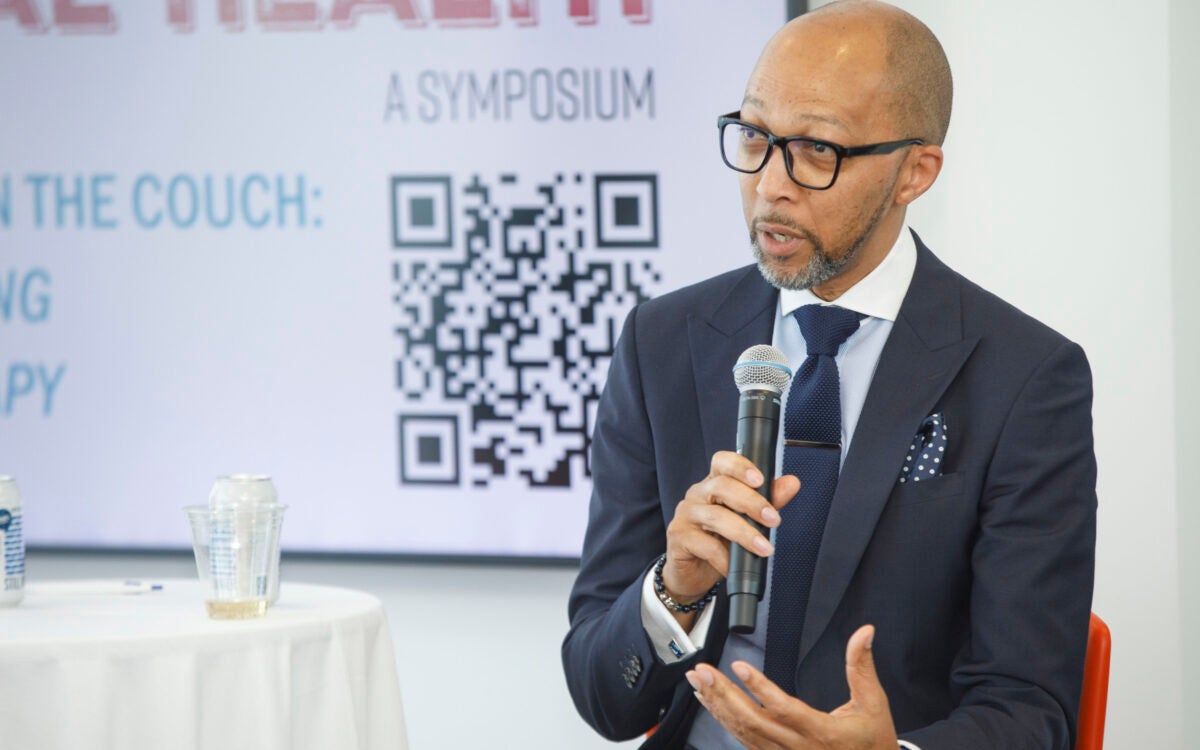
Symposium examines thorny, multifaceted dilemma from systemic racism in policing, healthcare to stigma attached to psychotherapy in community
Six receive honorary degrees
Harvard recognizes educator, conductor, theoretical physicist, advocate for elderly, writer, and Nobel laureate
Five alumni elected to the Board of Overseers
Six others join Alumni Association board
New study finds step-count and time are equally valid in reducing health risks

- Latest news
- UCL in the media
- Services for media
- Student news
- Tell us your story

Heart disease researchers at UCL awarded £3 million
24 May 2024
Researchers at the UCL Institute of Cardiovascular Science will receive £3 million over the next five years from the British Heart Foundation (BHF) as part of the charity’s Research Excellence Awards funding scheme.
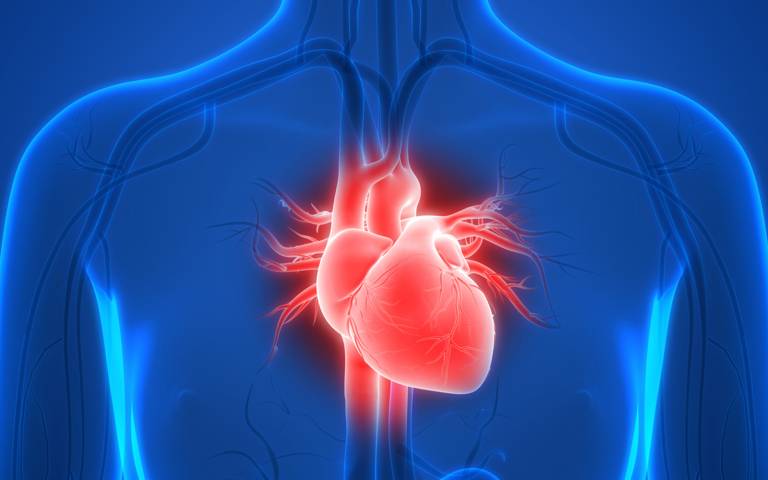
The new Centre of Research Excellence at UCL will enable cutting-edge research, innovative teaching and training, forward-looking policy development and advocacy with and for patients.
The centre will transform lives by trialling new treatments for rare diseases and cardiac arrythmia, delivering a simulation lab and robotics programme to develop devices for congenital and structural heart disorders, and enabling research to better understand ethnic differences in cardiovascular health.
Professor Perry Elliott, Head of the UCL Institute of Cardiovascular Science, said: “The award of a Centre of Research Excellence represents a landmark in our long-standing relationship with the BHF and is a substantial boost to our ambitions for cardiovascular science at UCL.
“The centre builds on an established track record of transformative science and benefits from a strategic network comprising three NIHR Biomedical Research Centres, the UCL Partners Academic Health Sciences Network, Barts Heart Centre, Royal Free Hospital, UCL Hospitals NHS Foundation Trust and Great Ormond Street Hospital.
“Over the next five years, we will use our Centre of Research Excellence to create a new diverse multidisciplinary workforce who will focus on transforming lives through innovation in therapeutics and targeted prevention.
The funding aims to support universities to cultivate world-class research environments that encourage collaboration, inclusion and innovation, and where visionary scientists can drive lifesaving breakthroughs.
The awards are part of a £35 million boost to UK cardiovascular disease research, supporting the Government’s ambition for the UK to become a science superpower. King’s College London and Imperial College London also received funding.
Professor Bryan Williams, Chief Scientific and Medical Officer at the British Heart Foundation, also a UCL professor of medicine and honorary consultant physician at UCLH, said: “We’re delighted to continue to support research at these three outstanding institutions to address the biggest challenges in cardiovascular disease. This funding recognises the incredible work happening in London and will help to cement its status as a leading destination for cardiovascular disease research.
“With generous donations from our supporters, this funding will power cutting-edge science and unlock lifesaving discoveries that can turn the tide on the devastation caused by heart and circulatory diseases.”
Research Excellence Awards offer researchers greater flexibility than traditional research funding, allowing scientists to quickly launch ambitious projects that can act as a springboard for larger, transformative funding applications.
The funding also aims to break down the silos that have traditionally existed in research, encouraging collaboration between experts from diverse fields. From clinicians to data scientists, biologists to engineers, the funding will support universities to attract the brightest minds, nurture new talent and foster collaboration to tackle the biggest questions in heart and circulatory disease research.
- UCL Institute of Cardiovascular Science
- Professor Perry Elliott’s academic profile
- UCL Population Health Sciences
- Credit: iStock / magicmine
Media contact
Mark greaves.
T: +44 (0)7990 675947
E: m.greaves [at] ucl.ac.uk


Understanding a broken heart
Recurrent heart failure linked to accumulated stress in immunity-forming stem cells.
The stress of heart failure is remembered by the body and appears to lead to recurrent failure, along with other related health issues, according to new research. Researchers have found that heart failure leaves a "stress memory" in the form of changes to the DNA modification of hematopoietic stem cells, which are involved in the production of blood and immune cells called macrophages. These immune cells play an important role in protecting heart health. However, a key signaling pathway (a chain of molecules which relays signals inside a cell), called transforming growth factor beta (TGF-β), in the hematopoietic stem cells was suppressed during heart failure, negatively affecting macrophage production. Improving TGF-β levels could be a new avenue for treating recurrent heart failure, while detecting accumulating stress memory could provide an early warning system before it occurs.
Healthier lives and improved well-being are among the United Nations' global Sustainable Development Goals. Positively, a recent study shows that life expectancy worldwide is projected to increase by about 4.5 years by 2050. Much of this is thanks to public health efforts to prevent disease and improved survival from illnesses, such as cardiovascular disorders. However, heart disease is still the leading cause of death worldwide, with 26 million people estimated to be affected by heart failure.
Once heart failure has occurred, it has a tendency to reoccur along with other health issues, such as kidney and muscle problems. Researchers in Japan wanted to understand what causes this recurrence and the deterioration of other organs, and whether it can be prevented.
"Based on our earlier research, we hypothesized that recurrence may be caused by stress experienced during heart failure accumulating in the body, particularly in hematopoietic stem cells," explained Project Professor Katsuhito Fujiu from the Graduate School of Medicine at the University of Tokyo. Hematopoietic stem cells are found in bone marrow and are the source of blood cells and a type of immune cell called macrophages, which help to protect heart health.
By studying mice with heart failure, the researchers found evidence of stress imprinting on the epigenome, that is, chemical changes occurred to the mice's DNA. An important signaling pathway, called the transforming growth factor beta, which is involved in regulating many cellular processes, was suppressed in the hematopoietic stem cells of mice with heart failure, leading to the production of dysfunctional immune cells.
This change persisted over an extended period of time, so when the team transplanted bone marrow from mice with heart failure into healthy mice, they found that the stem cells continued to produce dysfunctional immune cells. The latter mice later developed heart failure and became prone to organ damage.
"We termed this phenomenon stress memory because the stress from heart failure is remembered for an extended period and continues to affect the entire body. Although various other types of stress might also imprint this stress memory, we believe that the stress induced by heart failure is particularly significant," said Fujiu.
The good news is that by identifying and understanding these changes to the TGF-β signaling pathway, new avenues are now open for potential future treatments. "Completely new therapies could be considered to prevent the accumulation of this stress memory during hospitalization for heart failure," said Fujiu. "In animals with heart failure, supplementing additional active TGF-β has been shown to be a potential treatment. Correcting the epigenome of hematopoietic stem cells could also be a way to deplete stress memory."
Now that it has been identified, the team hopes to develop a system that can detect and prevent the accumulation of stress memory in humans, with a long-term goal of being able to not only prevent the recurrence of heart failure, but also catch the condition before it can fully develop.
- Heart Disease
- Stroke Prevention
- Immune System
- Mental Health
- Heart failure
- White blood cell
- Adult stem cell
- Embryonic stem cell
- Natural killer cell
Story Source:
Materials provided by University of Tokyo . Note: Content may be edited for style and length.
Journal Reference :
- Yukiteru Nakayama, Katsuhito Fujiu, Tsukasa Oshima, Jun Matsuda, Junichi Sugita, Takumi James Matsubara, Yuxiang Liu, Kohsaku Goto, Kunihiro Kani, Ryoko Uchida, Norifumi Takeda, Hiroyuki Morita, Yingda Xiao, Michiko Hayashi, Yujin Maru, Eriko Hasumi, Toshiya Kojima, Soh Ishiguro, Yusuke Kijima, Nozomu Yachie, Satoshi Yamazaki, Ryo Yamamoto, Fujimi Kudo, Mio Nakanishi, Atsushi Iwama, Ryoji Fujiki, Atsushi Kaneda, Osamu Ohara, Ryozo Nagai, Ichiro Manabe, Issei Komuro. Heart failure promotes multimorbidity through innate immune memory . Science Immunology , 2024; 9 (95) DOI: 10.1126/sciimmunol.ade3814
Cite This Page :
Explore More
- Future Climate Impacts Put Whale Diet at Risk
- Charge Your Laptop in a Minute?
- Caterpillars Detect Predators by Electricity
- 'Electronic Spider Silk' Printed On Human Skin
- Engineered Surfaces Made to Shed Heat
- Innovative Material for Sustainable Building
- Human Brain: New Gene Transcripts
- Epstein-Barr Virus and Resulting Diseases
- Origins of the Proton's Spin
- Symbiotic Bacteria Communicate With Plants
Trending Topics
Strange & offbeat.

Epigenetic Biomarkers of Socioeconomic Status are Associated with Age-Related Chronic Diseases and Mortality in Older Adults
- Find this author on Google Scholar
- Find this author on PubMed
- Search for this author on this site
- ORCID record for Lauren L. Schmitz
- For correspondence: [email protected] [email protected]
- ORCID record for Jennifer A. Smith
- Info/History
- Supplementary material
- Preview PDF
Later-life health is patterned by socioeconomic influences across the lifecourse. However, the pathways underlying the biological embedding of socioeconomic status (SES) and its consequences on downstream morbidity and mortality are not fully understood. Epigenetic markers like DNA methylation (DNAm) may be promising surrogates of underlying biological processes that can enhance our understanding of how SES shapes population health. Studies have shown that SES is associated with epigenetic aging measures, but few have examined relationships between early and later-life SES and DNAm sites across the epigenome. In this study, we trained and tested DNAm-based surrogates, or “biomarkers,” of childhood and adult SES in two large, multi-racial/ethnic samples of older adults—the Health and Retirement Study (HRS) (N=3,527) and the Multi-Ethnic Study of Atherosclerosis (MESA) (N=1,182). Both biomarkers were associated with downstream morbidity and mortality, and these associations persisted after controlling for measured SES, and in some cases, epigenetic aging clocks. Both childhood and adult SES biomarker CpG sites were enriched for genomic features that regulate gene expression (e.g., DNAse hypersensitivity sites and enhancers) and were implicated in prior epigenome-wide studies of inflammation, aging, and chronic disease. Distinct patterns also emerged between childhood CpGs and immune system dysregulation and adult CpGs and metabolic functioning, health behaviors, and cancer. Results suggest DNAm-based surrogate biomarkers of SES may be useful proxies for unmeasured social exposures that can augment our understanding of the biological mechanisms between social disadvantage and downstream health.
Significance Statement Information on DNA methylation (DNAm)—an epigenetic modification that plays a central role in regulating gene expression—is increasingly available in large epidemiological studies. Since DNAm is relatively stable but responsive to environmental influences, genome-wide signatures are promising surrogates or biomarkers of exposure that may both shed light on biological mechanisms between adverse environments and downstream health and/or act as proxies for unmeasured exposures. To better understand the biological embedding of social disadvantage, this study trained and tested DNAm-based surrogates of childhood and adult socioeconomic status (SES) in two US-based cohorts of older adults. Findings reveal distinct DNAm signatures of SES that connect social adversity across the lifecourse with dysregulated immune system responses, inflammatory pathways, poorer metabolic functioning, chronic diseases, and cancer.
Competing Interest Statement
The authors have declared no competing interest.
Funding Statement
This analysis was supported by the National Institute on Aging (NIA, R00 AG056599 (Schmitz)), the National Heart, Lung, and Blood Institute (NHLBI, R01 HL141292 (Smith)), and the National Human Genome Research Institute (NHGRI, T32 HG000040 (Opsasnick)). The NIA supports the Health and Retirement Study (U01AG009740) and its genotyping (RC2 AG0336495, RC4 AG039029). Research in MESA is supported by contracts 75N92020D00001, HHSN268201500003I, N01-HC-95159, 75N92020D00005, N01-HC-95160, 75N92020D00002, N01-HC-95161, 75N92020D00003, N01-HC-95162, 75N92020D00006, N01-HC-95163, 75N92020D00004, N01-HC-95164, 75N92020D00007, N01-HC-95165, N01-HC-95166, N01-HC-95167, N01-HC-95168 and N01-HC-95169 from the NHLBI and by grants UL1-TR-000040, UL1-TR-001079, and UL1-TR-001420 from the National Center for Advancing Translational Sciences (NCATS). The provision of genotyping data was supported in part by the National Center for Advancing Translational Sciences, CTSI grant UL1TR001881, and the National Institute of Diabetes and Digestive and Kidney Disease Diabetes Research Center (DRC) grant DK063491 to the Southern California Diabetes Endocrinology Research Center. Funding for SHARe genotyping was provided by NHLBI Contract N02-HL-64278. A full list of participating MESA investigators and institutions can be found at http://www.mesa-nhlbi.org [mesa-nhlbi.org]. Funding for MESA SHARe genotyping was provided by NHLBI Contract N02-HL-64278. Genotyping was performed at Affymetrix (Santa Clara, California, USA) and the Broad Institute of Harvard and MIT (Boston, Massachusetts, USA) using the Affymetrix Genome-Wide Human SNP Array 6.0. The MESA Epigenomics & Transcriptomics Studies were funded by NIH grants R01HL101250, R01HL119962, R01DK101921, R01HL135009, and 1RF1AG054474. This paper has been reviewed and approved by the MESA Publications and Presentations Committee. The content is solely the responsibility of the authors and does not necessarily represent the official views of the NIA, NHLBI, NHGRI, or NCATS.
Author Declarations
I confirm all relevant ethical guidelines have been followed, and any necessary IRB and/or ethics committee approvals have been obtained.
The details of the IRB/oversight body that provided approval or exemption for the research described are given below:
IRBs of University of Wisconsin-Madison and University of Michigan gave ethical approval for this work.
I confirm that all necessary patient/participant consent has been obtained and the appropriate institutional forms have been archived, and that any patient/participant/sample identifiers included were not known to anyone (e.g., hospital staff, patients or participants themselves) outside the research group so cannot be used to identify individuals.
I understand that all clinical trials and any other prospective interventional studies must be registered with an ICMJE-approved registry, such as ClinicalTrials.gov. I confirm that any such study reported in the manuscript has been registered and the trial registration ID is provided (note: if posting a prospective study registered retrospectively, please provide a statement in the trial ID field explaining why the study was not registered in advance).
I have followed all appropriate research reporting guidelines, such as any relevant EQUATOR Network research reporting checklist(s) and other pertinent material, if applicable.
Competing Interest Statement: The authors declare no conflicts of interest.
Data Availability
This study used restricted individual level information from the HRS, and our contractual agreement does not permit public dissemination of the data. Details on how to access restricted data for the HRS can be found at https://hrs.isr.umich.edu/data-products/restricted-data. Data used in this analysis from MESA can be obtained through the MESA Data Coordinating Center (https://www.mesanhlbi.org/) and through the database of Genotypes and Phenotypes (dbGaP; phs000209). Analysis code is posted on github: https://github.com/laurenschmitz/epigenetic-SES-biomarker.
View the discussion thread.
Supplementary Material
Thank you for your interest in spreading the word about medRxiv.
NOTE: Your email address is requested solely to identify you as the sender of this article.

Citation Manager Formats
- EndNote (tagged)
- EndNote 8 (xml)
- RefWorks Tagged
- Ref Manager
- Tweet Widget
- Facebook Like
- Google Plus One
Subject Area
- Public and Global Health
- Addiction Medicine (324)
- Allergy and Immunology (633)
- Anesthesia (168)
- Cardiovascular Medicine (2403)
- Dentistry and Oral Medicine (289)
- Dermatology (207)
- Emergency Medicine (381)
- Endocrinology (including Diabetes Mellitus and Metabolic Disease) (852)
- Epidemiology (11800)
- Forensic Medicine (10)
- Gastroenterology (705)
- Genetic and Genomic Medicine (3771)
- Geriatric Medicine (350)
- Health Economics (637)
- Health Informatics (2410)
- Health Policy (940)
- Health Systems and Quality Improvement (905)
- Hematology (342)
- HIV/AIDS (787)
- Infectious Diseases (except HIV/AIDS) (13348)
- Intensive Care and Critical Care Medicine (769)
- Medical Education (369)
- Medical Ethics (105)
- Nephrology (402)
- Neurology (3524)
- Nursing (199)
- Nutrition (529)
- Obstetrics and Gynecology (681)
- Occupational and Environmental Health (670)
- Oncology (1835)
- Ophthalmology (539)
- Orthopedics (222)
- Otolaryngology (287)
- Pain Medicine (234)
- Palliative Medicine (67)
- Pathology (447)
- Pediatrics (1038)
- Pharmacology and Therapeutics (426)
- Primary Care Research (424)
- Psychiatry and Clinical Psychology (3194)
- Public and Global Health (6185)
- Radiology and Imaging (1293)
- Rehabilitation Medicine and Physical Therapy (751)
- Respiratory Medicine (832)
- Rheumatology (380)
- Sexual and Reproductive Health (374)
- Sports Medicine (324)
- Surgery (406)
- Toxicology (50)
- Transplantation (172)
- Urology (147)

An official website of the United States government
The .gov means it's official. Federal government websites often end in .gov or .mil. Before sharing sensitive information, make sure you're on a federal government site.
The site is secure. The https:// ensures that you are connecting to the official website and that any information you provide is encrypted and transmitted securely.
- Publications
- Account settings
- Browse Titles
NCBI Bookshelf. A service of the National Library of Medicine, National Institutes of Health.
StatPearls [Internet]. Treasure Island (FL): StatPearls Publishing; 2024 Jan-.

StatPearls [Internet].
Cardiovascular disease.
Edgardo Olvera Lopez ; Brian D. Ballard ; Arif Jan .
Affiliations
Last Update: August 22, 2023 .
- Continuing Education Activity
The cardiovascular system consists of the heart and its blood vessels. A wide array of problems can arise within the cardiovascular system, a few of which include endocarditis, rheumatic heart disease, and conduction system abnormalities. Cardiovascular disease, also known as heart disease, refers to the following 4 entities: coronary artery disease (CAD) which is also referred to as coronary heart disease (CHD), cerebrovascular disease, peripheral artery disease (PAD), and aortic atherosclerosis. CAD results from decreased myocardial perfusion that causes angina due to ischemia and can result in myocardial infarction (MI), and/or heart failure. It accounts for one-third to one-half of all cases of cardiovascular disease. Cerebrovascular disease is the entity associated with strokes, also termed cerebrovascular accidents, and transient ischemic attacks (TIAs). Peripheral arterial disease (PAD) is arterial disease predominantly involving the limbs that may result in claudication. Aortic atherosclerosis is the entity associated with thoracic and abdominal aneurysms. This activity reviews the evaluation and treatment of cardiovascular disease and the role of the medical team in evaluating and treating these conditions.
- Review the cause of coronary artery disease.
- Describe the pathophysiology of atherosclerosis.
- Summarize the treatment options for heart disease.
- Outline the evaluation and treatment of cardiovascular disease and the role of the medical team in evaluating and treating this condition.
- Introduction
The cardiovascular system consists of the heart and blood vessels. [1] There is a wide array of problems that may arise within the cardiovascular system, for example, endocarditis, rheumatic heart disease, abnormalities in the conduction system, among others, cardiovascular disease (CVD) or heart disease refer to the following 4 entities that are the focus of this article [2] :
- Coronary artery disease (CAD): Sometimes referred to as Coronary Heart Disease (CHD), results from decreased myocardial perfusion that causes angina, myocardial infarction (MI), and/or heart failure. It accounts for one-third to one-half of the cases of CVD.
- Cerebrovascular disease (CVD): Including stroke and transient ischemic attack (TIA)
- Peripheral artery disease (PAD): Particularly arterial disease involving the limbs that may result in claudication
- Aortic atherosclerosis: Including thoracic and abdominal aneurysms
Although CVD may directly arise from different etiologies such as emboli in a patient with atrial fibrillation resulting in ischemic stroke, rheumatic fever causing valvular heart disease, among others, addressing risks factors associated to the development of atherosclerosis is most important because it is a common denominator in the pathophysiology of CVD.
The industrialization of the economy with a resultant shift from physically demanding to sedentary jobs, along with the current consumerism and technology-driven culture that is related to longer work hours, longer commutes, and less leisure time for recreational activities, may explain the significant and steady increase in the rates of CVD during the last few decades. Specifically, physical inactivity, intake of a high-calorie diet, saturated fats, and sugars are associated with the development of atherosclerosis and other metabolic disturbances like metabolic syndrome, diabetes mellitus, and hypertension that are highly prevalent in people with CVD. [3] [2] [4] [5]
According to the INTERHEART study that included subjects from 52 countries, including high, middle, and low-income countries, 9 modifiable risks factors accounted for 90% of the risk of having a first MI: smoking, dyslipidemia, hypertension, diabetes, abdominal obesity, psychosocial factors, consumption of fruits and vegetables, regular alcohol consumption, and physical inactivity. It is important to mention that in this study 36% of the population-attributable risk of MI was accounted to smoking. [6]
Other large cohort studies like the Framingham Heart Study [7] and the Third National Health and Nutrition Examination Survey (NHANES III) [5] have also found a strong association and predictive value of dyslipidemia, high blood pressure, smoking, and glucose intolerance. Sixty percent to 90% of CHD events occurred in subjects with at least one risk factor.
These findings have been translated into health promotion programs by the American Heart Association with emphasis on seven recommendations to decrease the risk of CVD: avoiding smoking, being physically active, eating healthy, and keeping normal blood pressure, body weight, glucose, and cholesterol levels. [8] [9]
On the other hand, non-modifiable factors as family history, age, and gender have different implications. [4] [7] Family history, particularly premature atherosclerotic disease defined as CVD or death from CVD in a first-degree relative before 55 years (in males) or 65 years (in females) is considered an independent risk factor. [10] There is also suggestive evidence that the presence of CVD risk factors may differently influence gender. [4] [7] For instance, diabetes and smoking more than 20 cigarettes per day had increased CVD risk in women compared to men. [11] Prevalence of CVD increases significantly with each decade of life. [12]
The presence of HIV (human immunodeficiency virus), [13] history of mediastinal or chest wall radiation, [14] microalbuminuria, [15] , increased inflammatory markers [16] [17] have also been associated with an increased rate and incidence of CVD.
Pointing out specific diet factors like meat consumption, fiber, and coffee and their relation to CVD remains controversial due to significant bias and residual confounding encountered in epidemiological studies. [18] [19]
- Epidemiology
Cardiovascular diseases (CVD) remain among the 2 leading causes of death in the United States since 1975 with 633,842 deaths or 1 in every 4 deaths, heart disease occupied the leading cause of death in 2015 followed by 595,930 deaths related to cancer. [2] CVD is also the number 1 cause of death globally with an estimated 17.7 million deaths in 2015, according to the World Health Organization (WHO). The burden of CVD further extends as it is considered the most costly disease even ahead of Alzheimer disease and diabetes with calculated indirect costs of $237 billion dollars per year and a projected increased to $368 billion by 2035. [20]
Although the age-adjusted rate and acute mortality from MI have been declining over time, reflecting the progress in diagnosis and treatment during the last couple of decades, the risk of heart disease remains high with a calculated 50% risk by age 45 in the general population. [7] [21] The incidence significantly increases with age with some variations between genders as the incidence is higher in men at younger ages. [2] The difference in incidence narrows progressively in the post-menopausal state. [2]
- Pathophysiology
Atherosclerosis is the pathogenic process in the arteries and the aorta that can potentially cause disease as a consequence of decreased or absent blood flow from stenosis of the blood vessels. [22]
It involves multiple factors dyslipidemia, immunologic phenomena, inflammation, and endothelial dysfunction. These factors are believed to trigger the formation of fatty streak, which is the hallmark in the development of the atherosclerotic plaque [23] ; a progressive process that may occur as early as in the childhood. [24] This process comprises intimal thickening with subsequent accumulation of lipid-laden macrophages (foam cells) and extracellular matrix, followed by aggregation and proliferation of smooth muscle cells constituting the formation of the atheroma plaque. [25] As this lesions continue to expand, apoptosis of the deep layers can occur, precipitating further macrophage recruitment that can become calcified and transition to atherosclerotic plaques. [26]
Other mechanisms like arterial remodeling and intra-plaque hemorrhage play an important role in the delay and accelerated the progression of atherosclerotic CVD but are beyond the purpose of this article. [27]
- History and Physical
The clinical presentation of cardiovascular diseases can range from asymptomatic (e.g., silent ischemia, angiographic evidence of coronary artery disease without symptoms, among others) to classic presentations as when patients present with typical anginal chest pain consistent of myocardial infarction and/or those suffering from acute CVA presenting with focal neurological deficits of sudden onset. [28] [29] [28]
Historically, coronary artery disease typically presents with angina that is a pain of substernal location, described as a crushing or pressure in nature, that may radiate to the medial aspect of the left upper extremity, to the neck or the jaw and that can be associated with nausea, vomiting, palpitations, diaphoresis, syncope or even sudden death. [30] Physicians and other health care providers should be aware of possible variations in symptom presentation for these patients and maintain a high index of suspicion despite an atypical presentation, for example, dizziness and nausea as the only presenting symptoms in patients having an acute MI [31] ), particularly in people with a known history of CAD/MI and for those with the presence of CVD risk factors. [32] [33] [34] [33] [32] Additional chest pain features suggestive of ischemic etiology are the exacerbation with exercise and or activity and resolution with rest or nitroglycerin. [35]
Neurologic deficits are the hallmark of cerebrovascular disease including TIA and stroke where the key differentiating factor is the resolution of symptoms within 24 hours for patients with TIA. [36] Although the specific symptoms depend on the affected area of the brain, the sudden onset of extremity weakness, dysarthria, and facial droop are among the most commonly reported symptoms that raise concern for a diagnosis of a stroke. [37] [38] Ataxia, nystagmus and other subtle symptoms as dizziness, headache, syncope, nausea or vomiting are among the most reported symptoms with people with posterior circulation strokes challenging to correlate and that require highly suspicion in patients with risks factors. [39]
Patients with PAD may present with claudication of the limbs, described as a cramp-like muscle pain precipitated by increased blood flow demand during exercise that typically subsides with rest. [40] Severe PAD might present with color changes of the skin and changes in temperature. [41]
Most patients with thoracic aortic aneurysm will be asymptomatic, but symptoms can develop as it progresses from subtle symptoms from compression to surrounding tissues causing cough, shortness of breath or dysphonia, to the acute presentation of sudden crushing chest or back pain due to acute rupture. [42] The same is true for abdominal aortic aneurysms (AAA) that cause no symptoms in early stages to the acute presentation of sudden onset of abdominal pain or syncope from acute rupture. [43]
A thorough physical examination is paramount for the diagnosis of CVD. Starting with a general inspection to look for signs of distress as in patients with angina or with decompensated heart failure, or chronic skin changes from PAD. Carotid examination with the patient on supine position and the back at 30 degrees for the palpation and auscultation of carotid pulses, bruits and to evaluate for jugular venous pulsations on the neck is essential. Precordial examination starting with inspection, followed by palpation looking for chest wall tenderness, thrills, and identification of the point of maximal impulse should then be performed before auscultating the precordium. Heart sounds auscultation starts in the aortic area with the identification of the S1 and S2 sounds followed by characterization of murmurs if present. Paying attention to changes with inspirations and maneuvers to correctly characterize heart murmurs is encouraged. Palpating peripheral pulses with bilateral examination and comparison when applicable is an integral part of the CVD examination. [44]
Thorough clinical history and physical exam directed but not limited to the cardiovascular system are the hallmarks for the diagnosis of CVD. Specifically, a history compatible with obesity, angina, decreased exercise tolerance, orthopnea, paroxysmal nocturnal dyspnea, syncope or presyncope, and claudication should prompt the clinician to obtain a more detailed history and physical exam and, if pertinent, obtain ancillary diagnostic test according to the clinical scenario (e.g., electrocardiogram and cardiac enzymes for patients presenting with chest pain).
Besides a diagnosis prompted by clinical suspicion, most of the efforts should be oriented for primary prevention by targeting people with the presence of risk factors and treat modifiable risk factors by all available means. All patient starting at age 20 should be engaged in the discussion of CVD risk factors and lipid measurement. [9] Several calculators that use LDL-cholesterol and HDL-cholesterol levels and the presence of other risk factors calculate a 10-year or 30-year CVD score to determine if additional therapies like the use of statins and aspirin are indicated for primary prevention, generally indicated if such risk is more than ten percent. [10] Like other risk assessment tools, the use of this calculators have some limitations, and it is recommended to exert precaution when assessing patients with diabetes and familial hypercholesterolemia as their risk can be underestimated. Another limitation to their use is that people older than 79 were usually excluded from the cohorts where these calculators were formulated, and individualized approach for these populations is recommended by discussing risk and benefits of adjunctive therapies and particular consideration of life expectancy. Some experts recommend a reassessment of CVD risk every 4 to 6 years. [9]
Preventative measures like following healthy food habits, avoiding overweight and following an active lifestyle are pertinent in all patients, particularly for people with non-modifiable risk factors such as family history of premature CHD or post-menopause. [9] [8]
The use of inflammatory markers and other risk assessment methods as coronary artery calcification score (CAC) are under research and have limited applications that their use should not replace the identification of people with known risk factors, nonetheless these resources remain as promising tools in the future of primary prevention by detecting people with subclinical atherosclerosis at risk for CVD. [45]
- Treatment / Management
Management of CVD is very extensive depending on the clinical situation (catheter-directed thrombolysis for acute ischemic stroke, angioplasty for peripheral vascular disease, coronary stenting for CHD); however, patients with known CVD should be strongly educated on the need for secondary prevention by risk factor and lifestyle modification. [9] [46]
- Differential Diagnosis
- Acute pericarditis
- Angina pectoris
- Artherosclerosis
- Coronary artery vasospasm
- Dilated cardiomyopathy
- Giant cell arteritis
- Hypertension
- Hypertensive heart disease
- Kawasaki disease
- Myocarditis
The prognosis and burden of CVD have been discussed in other sections.
- Complications
The most feared complication from CVD is death and, as explained above, despite multiple discoveries in the last decades CVD remains in the top leading causes of death all over the world owing to the alarming prevalence of CVD in the population. [2] Other complications as the need for longer hospitalizations, physical disability and increased costs of care are significant and are the focus for health-care policymakers as it is believed they will continue to increase in the coming decades. [20]
For people with heart failure with reduced ejection fraction (HFreEF) of less than 35%, as the risk of life-threatening arrhythmias is exceedingly high in these patients, current guidelines recommend the implantation of an implantable-cardioverter defibrillator (ICD) for those with symptoms equivalent to a New York Heart Association (NYHA) Class II-IV despite maximal tolerated medical therapy. [47]
Strokes can leave people with severe disabling sequelae like dysarthria or aphasia, dysphagia, focal or generalized muscle weakness or paresis that can be temporal or cause permanent physical disability that may lead to a complete bedbound state due to hemiplegia with added complications secondary to immobility as is the higher risk of developing urinary tract infections and/or risk for thromboembolic events. [48] [49]
There is an increased risk of all-cause death for people with PAD compared to those without evidence of peripheral disease. [50] Chronic wounds, physical limitation, and limb ischemia are among other complications from PAD. [51]
- Consultations
An interprofessional approach that involves primary care doctors, nurses, dietitians, cardiologists, neurologists, and other specialists is likely to improve outcomes. This has been shown to be beneficial in patients with heart failure, [52] coronary disease, [53] and current investigations to assess the impact on other forms of CVD are under planning and promise encouraging results.
- Deterrence and Patient Education
Efforts should be directed toward primary prevention by leading a healthy lifestyle, and an appropriate diet starting as early as possible with the goal of delay or avoid the initiation of atherosclerosis as it relates to the future risk of CVD. The AHA developed the concept of "ideal cardiovascular health" defined by the presence of [8] :
- Ideal health behaviors: Nonsmoking, body mass index less than 25 kg/m2, physical activity at goal levels, and the pursuit of a diet consistent with current guideline recommendations
- Ideal health factors: Untreated total cholesterol less than 200 mg/dL, untreated blood pressure less than 120/80 mm Hg, and fasting blood glucose less than 100 mg/dL) with the goal to improve the health of all Americans with an expected decrease in deaths from CVD by 20%
Specific attention should be made to people at higher risk for CVD as are people with diabetes, hypertension, hyperlipidemia, smokers, and obese patients. Risk factors modification by controlling their medical conditions, avoiding smoking, taking appropriate measures to lose weight and maintaining an active lifestyle is of extreme importance. [8] [9] [10] The recommendations on the use of statins and low-dose aspirin for primary and secondary prevention has been discussed in other sections.
- Pearls and Other Issues
Cardiovascular disease generally refers to 4 general entities: CAD, CVD, PVD, and aortic atherosclerosis.
CVD is the main cause of death globally.
Measures aimed to prevent the progression of atherosclerosis are the hallmark for primary prevention of CVD.
Risk factor and lifestyle modification are paramount in the prevention of CVD.
- Enhancing Healthcare Team Outcomes
An interprofessional and patient-oriented approach can help to improve outcomes for people with cardiovascular disease as shown in patients with heart failure (HF) who had better outcomes when the interprofessional involvement of nurses, dietitians, pharmacists, and other health professionals was used (Class 1A). [52]
Similarly, positive results were obtained in people in an intervention group who were followed by an interprofessional team comprised of pharmacists, nurses and a team of different physicians. This group had a reduction in all-cause mortality associated with CAD by 76% compared to the control group. [53] Healthcare workers should educate the public on lifestyle changes and reduce the modifiable risk factors for heart disease to a minimum.
- Review Questions
- Access free multiple choice questions on this topic.
- Comment on this article.
Atherosclerosis as a result of coronary heart disease. Contributed by National Heart, Lung and Blood Institute (NIH)
Coronary Artery Disease Pathophysiology. Coronary artery disease is usually caused by an atherosclerotic plaque that blocks the lumen of a coronary artery, typically the left anterior descending artery. Contributed by S Bhimji, MD
Disclosure: Edgardo Olvera Lopez declares no relevant financial relationships with ineligible companies.
Disclosure: Brian Ballard declares no relevant financial relationships with ineligible companies.
Disclosure: Arif Jan declares no relevant financial relationships with ineligible companies.
This book is distributed under the terms of the Creative Commons Attribution-NonCommercial-NoDerivatives 4.0 International (CC BY-NC-ND 4.0) ( http://creativecommons.org/licenses/by-nc-nd/4.0/ ), which permits others to distribute the work, provided that the article is not altered or used commercially. You are not required to obtain permission to distribute this article, provided that you credit the author and journal.
- Cite this Page Olvera Lopez E, Ballard BD, Jan A. Cardiovascular Disease. [Updated 2023 Aug 22]. In: StatPearls [Internet]. Treasure Island (FL): StatPearls Publishing; 2024 Jan-.
In this Page
Bulk download.
- Bulk download StatPearls data from FTP
Related information
- PMC PubMed Central citations
- PubMed Links to PubMed
Similar articles in PubMed
- Review De-risking primary prevention: role of imaging. [Ther Adv Cardiovasc Dis. 2021] Review De-risking primary prevention: role of imaging. Shafter AM, Shaikh K, Johanis A, Budoff MJ. Ther Adv Cardiovasc Dis. 2021 Jan-Dec; 15:17539447211051248.
- Shared and non-shared familial susceptibility of coronary heart disease, ischemic stroke, peripheral artery disease and aortic disease. [Int J Cardiol. 2013] Shared and non-shared familial susceptibility of coronary heart disease, ischemic stroke, peripheral artery disease and aortic disease. Calling S, Ji J, Sundquist J, Sundquist K, Zöller B. Int J Cardiol. 2013 Oct 3; 168(3):2844-50. Epub 2013 Apr 30.
- Stenting for peripheral artery disease of the lower extremities: an evidence-based analysis. [Ont Health Technol Assess Ser....] Stenting for peripheral artery disease of the lower extremities: an evidence-based analysis. Medical Advisory Secretariat. Ont Health Technol Assess Ser. 2010; 10(18):1-88. Epub 2010 Sep 1.
- Impact of cardiovascular disease on health care economic burden and resource utilization: a retrospective cohort study in adults in the United States with type 2 diabetes with or without stroke, myocardial infarction, and peripheral arterial disease. [Curr Med Res Opin. 2022] Impact of cardiovascular disease on health care economic burden and resource utilization: a retrospective cohort study in adults in the United States with type 2 diabetes with or without stroke, myocardial infarction, and peripheral arterial disease. King A, Rajpura J, Liang Y, Paprocki Y, Uzoigwe C. Curr Med Res Opin. 2022 Nov; 38(11):1831-1840. Epub 2022 Sep 29.
- Review Prevention of ventricular fibrillation, acute myocardial infarction (myocardial necrosis), heart failure, and mortality by bretylium: is ischemic heart disease primarily adrenergic cardiovascular disease? [Am J Ther. 2004] Review Prevention of ventricular fibrillation, acute myocardial infarction (myocardial necrosis), heart failure, and mortality by bretylium: is ischemic heart disease primarily adrenergic cardiovascular disease? Bacaner M, Brietenbucher J, LaBree J. Am J Ther. 2004 Sep-Oct; 11(5):366-411.
Recent Activity
- Cardiovascular Disease - StatPearls Cardiovascular Disease - StatPearls
Your browsing activity is empty.
Activity recording is turned off.
Turn recording back on
Connect with NLM
National Library of Medicine 8600 Rockville Pike Bethesda, MD 20894
Web Policies FOIA HHS Vulnerability Disclosure
Help Accessibility Careers
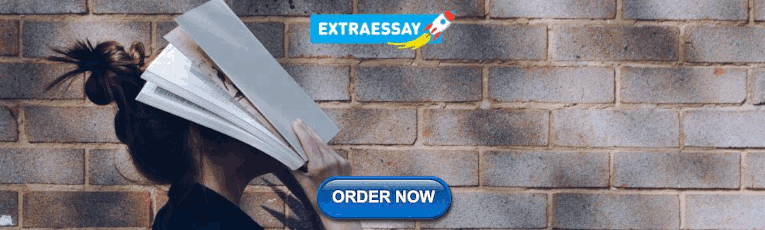
IMAGES
VIDEO
COMMENTS
Background: The American Heart Association, in conjunction with the National Institutes of Health, annually reports the most up-to-date statistics related to heart disease, stroke, and cardiovascular risk factors, including core health behaviors (smoking, physical activity, diet, and weight) and health factors (cholesterol, blood pressure, and glucose control) that contribute to cardiovascular ...
Congenital Heart Disease 10; Mechanical Circulatory Support 7; Venous Disorders 3; By Article Category. Research 3315; Commentary 1372; Other 1306; Clinical Cases 1263; Review 1215; Media 442 ...
Heart disease is a fatal human disease, rapidly increases globally in both developed and undeveloped countries and consequently, causes death. ... research study recommends that which feature ...
Introduction and background. Atherosclerosis is the process of formation of plaque composed of cholesterol, fat, calcium, and other substances in the wall of large and medium-sized arteries causing diminished blood flow to an area of the body [1,2].Moreover, atherosclerotic vascular disease can be classified into two arenas: a cardiovascular disease affecting the heart and peripheral blood ...
Global Burden of Disease Study Methods. GBD 2019 is a multinational collaborative research study that estimates disease burden for every country in the world (1,4).The study is an ongoing effort, updated annually, and is designed to allow for consistent comparison over time from 1990 to 2019, by age and sex, and across locations.
Goerlich et al. review the current knowledge of the cardiovascular complications of the post-COVID condition, including postural orthostatic tachycardia syndrome, myocardial injury, heart failure ...
Cardiovascular disease (CVD) is an umbrella term for a number of linked pathologies, commonly defined as coronary heart disease (CHD), cerebrovascular disease, peripheral arterial disease, rheumatic and congenital heart diseases and venous thromboembolism. Globally CVD accounts for 31% of mortality, the majority of this in the form of CHD and ...
The science of heart health focuses on several related biological phenomena, including inflammation, cholesterol level and a lingering form of immune response that tends to exacerbate the risk of ...
This paper aims to provide a comprehensive overview of recent research on heart disease diagnosis by reviewing articles published by reputable sources between 2014 and 2022.
Heart disease, including coronary heart disease, remains the leading cause of death in the United States. However, the rate of heart disease deaths has declined by 70% over the past 50 years, thanks in part to NHLBI-funded research. Many current studies funded by the NHLBI focus on discovering genetic associations and finding new ways to ...
This paper is motivated by the gap in the literature, thus proposes an algorithm that measures the strength of the significant features that contribute to heart disease prediction. ... The experiments performed on the UCI open dataset, widely used for heart disease research yielded the highest confidence score of 98% in predicting heart disease ...
This research aims to foresee the odds of having heart disease as probable cause of computerized prediction of heart disease that is helpful in the medical field for clinicians and patients [].To accomplish the aim, we have discussed the use of various machine learning algorithms on the data set and dataset analysis is mentioned in this research paper.
Cardiovascular disease refers to any critical condition that impacts the heart. Because heart diseases can be life-threatening, researchers are focusing on designing smart systems to accurately diagnose them based on electronic health data, with the aid of machine learning algorithms. This work presents several machine learning approaches for predicting heart diseases, using data of major ...
Fig. 2 shows the number of papers published on heart disease using ML approaches over the past ten years based on our selected 451 papers. From 2012 to 2020, the steady growth of the publication is observed, while there is an exponential growth in 2021. ... Fig. 9 demonstrates the number of research papers related to funded projects. The number ...
The use of analytics in healthcare improves care by facilitating preventive care and EDA is a vital step while analysing data. In this paper, the risk factors that causes heart disease is considered and predicted using K-means algorithm and the analysis is carried out using a publicly available data for heart disease.
In order to predict heart disease, a random forest algorithm is used for data visualization as well as data analytics. Along with that, Dogan et al. [38] have described that this research paper discusses classification performances, pre-processing methods, and evaluation metrics. Furthermore, the result of the visualized data shows that the ...
All the participants had a low-to-moderate risk of atherosclerotic cardiovascular disease, as determined by the score on the American Heart Association and American College of Cardiology 2013 ...
Documents within this cluster include research on ischaemic heart disease and arrhythmias. Haemodynamics and biomechanics are another emerging area that includes documents on atherosclerosis and vascular diseases such as aneurysms, but also heart failure and assist devices. ... In their analysis of poorly cited papers covering 165 000 papers in ...
Cardiovascular diseases are pathological conditions affecting the heart and/or blood vessels that is, the cardiovascular system. Featured Immune signatures of disease stages and outcomes in ...
One person dies every 33 seconds from cardiovascular disease. 1. About 695,000 people died from heart disease in 2021—that's 1 in every 5 deaths. 1 2. Heart disease costs about $239.9 billion each year from 2018 to 2019. 3 This includes the cost of health care services, medicines, and lost productivity due to death. View Larger.
May 20, 2024 5 min read. A new study suggests that both step-count and time-based exercise goals are equally effective in reducing risks of heart disease and early death. Researchers from Harvard-affiliated Brigham and Women's Hospital reviewed data on healthy women age 62+, who used wearable devices to record their physical activity, and ...
Mark Greaves. T: +44 (0)7990 675947. E: m.greaves [at] ucl.ac.uk. Researchers at the UCL Institute of Cardiovascular Science will receive £3 million over the next five years from the British Heart Foundation (BHF) as part of the charity's Research Excellence Awards funding scheme.
The stress of heart failure is remembered by the body and appears to lead to recurrent failure, along with other related health issues, according to new research. Researchers have found that heart ...
Scientists do know that asthma and cardiovascular disease share a common bond: inflammation. The lungs add oxygen to blood, which the heart then pumps to the rest of the body. It's possible, Jarjour said, that inflammation in the lungs "gets carried by the blood and spills over to the rest of the body and (impacts) brain health and ...
In fact, the new study found that people with existing heart disease at the beginning of the research had a 15% lower risk of progressing from atrial fibrillation to a heart attack and a 9% lower ...
Competing Interest Statement. The authors have declared no competing interest. Funding Statement. This analysis was supported by the National Institute on Aging (NIA, R00 AG056599 (Schmitz)), the National Heart, Lung, and Blood Institute (NHLBI, R01 HL141292 (Smith)), and the National Human Genome Research Institute (NHGRI, T32 HG000040 (Opsasnick)).
The cardiovascular system consists of the heart and blood vessels.[1] There is a wide array of problems that may arise within the cardiovascular system, for example, endocarditis, rheumatic heart disease, abnormalities in the conduction system, among others, cardiovascular disease (CVD) or heart disease refer to the following 4 entities that are the focus of this article[2]: