- Short Contribution
- Open access
- Published: 21 October 2019
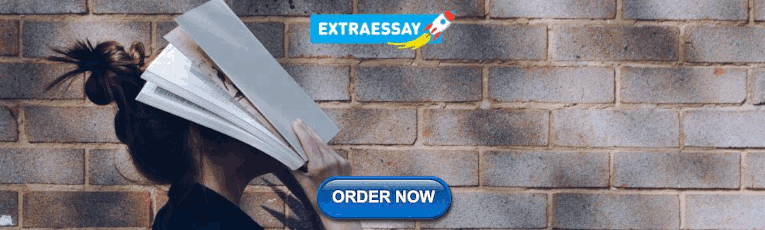
What about cyberspace (and cybercrime alongside it)? A reply to Farrell and Birks “Did cybercrime cause the crime drop?”
- Fernando Miró-Llinares 1 &
- Asier Moneva ORCID: orcid.org/0000-0002-2156-0213 1
Crime Science volume 8 , Article number: 12 ( 2019 ) Cite this article
16k Accesses
15 Citations
48 Altmetric
Metrics details
In this paper we question Farrell and Birks’ assertion of the emergence of cybercrime as an invalid explanation for the crime drop. Alternatively to the “cybercrime hypothesis”, we propose two non-exclusive hypotheses that highlight the essential role of cyberspace as an environment that has shifted criminal opportunities from physical to virtual space, which reflects on crime trends. The first hypothesis posits that the more time spent at home by many young people due to video games and online leisure activities, among other factors, could have had an impact on the juvenile crime drop. The second hypothesis states that the appearance of cyberspace has led to a shift in opportunities from physical space to cyberspace. This could have led to an increase in property-related criminal activity connected to the Internet to the detriment of physical crime which would not be reflected in the statistics. Both premises are supported by empirical evidence.
Introduction
In “Did cybercrime cause the crime drop”, Farrell and Birks ( 2018 ) refute the idea that “the international crime drop was the result of increased cybercrime” (p. 1) based on the lack of evidence provided, the temporal inconsistency of the causal conjecture, the lack of coherence with other explanatory frames for the crime drop (Farrell et al. 2014 ), such as the security hypothesis (Farrell et al. 2011 ), and the lack of an explanation of the micro mechanism. Through a selection of quotations that share online crime trends as a common denominator but have some variation in their emphasis (which is acknowledged by Farrell and Birks), they first proceed to coin a hypothesis (i.e., the Cybercrime Hypothesis) which they then reject by indicating the nonexistence of any causal relationship between the crime drop and the emergence of cyberspace as an area of opportunity that, in turn, has entailed an increase in cybercrime, pointing out that “the crime drop and rising cybercrime are independent trends caused by broad changes to crime opportunity structures” (Farrell and Birks 2018 , p. 3). In general, we believe that this thesis is overly bold and that there are sufficient arguments and evidence to support the inclusion of cyberspace and cybercrime in analyses of crime trends in recent decades. In particular, we believe that existing evidence suggests that the emergence of cyberspace as a new opportunity environment for cybercrime has contributed to the crime drop.
Evidence and argument
Drop or drops.
Farrell and Birks describe the international crime drop (Van Dijk et al. 2012 ) as: “the long-term decline in crimes including burglary, car theft and assault” (Farrell and Birks 2018 , p. 1). Generally, the literature recognizes this crime drop but acknowledges variations in the timing of different forms of crime drop in different locations (Eisner 2003 ; Zimring 2008 ). In addition to the fact that a specific cause may have influenced the crime drop at different times in different places, the literature typically points to multi-causality (Blumstein and Wallman 2006 ; Tonry 2014 ; Zimring 2008 ) or even to the concurrent and successive accumulation of different forms of crime drop caused by different concurrent and successive events within the overall crime drop context (Pinker 2011 ; Zimring 2008 ). We understand that a synchronous descent is meaningless as an explanatory element of a single factor in the crime drop, as there may be concurrent factors that interact and cause the timing of the descent to vary. So, it is possible that a cause may have contributed to the crime drop along with others subsequent to its appearance.
One of the main criticisms Farrell and Birks make of the Cybercrime Hypothesis is that there is not enough evidence to establish a causal relationship between the rise of cybercrime and the crime drop, but it is true that there is also no evidence to refute it. To do this, it would be necessary to demonstrate that the appearance of cyberspace and cyberspace-associated crime (cybercrime) had no impact on any form of crime drop at any given time. However, as we will demonstrate, consistent timing and evidence can be identified to address the inconsistencies and lack of evidence that Farrell and Birks find regarding their “cybercrime hypothesis” when the hypothesis is rephrased as follows: the onset of cyberspace and cybercrime have had an impact on certain forms of the so-called crime drop.
First hypothesis. Cyberspace: Information Technology (IT), online leisure, and the juvenile crime drop
Although the onset of the Internet occurred after the first signs of the crime drop, IT had already arrived. Computers and video games may be among the reasons that young people have spent more time at home since the mid-1990s (McCaffree and Proctor 2018 ). The increased presence of people at home could also explain the observed decrease in burglaries (Rosenfeld and Messner 2012 ). At this time, a decrease in vandalism-related arrests and other forms of crime associated with young people began to become evident in the United States (Fig. 1 a; see also Fernández-Molina and Bartolomé Gutiérrez ( 2018 ) for the Spanish case), and a similar trend was observed with respect to criminal damage in England and Wales (Fig. 1 b). Since the popularization of the Internet in the late 1990s, the number of households with Internet access and the use of digital platforms have increased (Fig. 1 c, d), and the use of video games among young people has continued to rise (The Nielsen Company 2018 ). In short, especially for young people, changes in crime rates could be explained within a Routine Activities framework (Cohen and Felson 1979 ) by trends regarding the use of leisure IT and at least partially by greater time spent at home (Aebi and Linde 2010 , 2014 ; Beerthuizen et al. 2017 ), with the consequent reduction in opportunities associated with physical space and increases in opportunities in cyberspace (Pyrooz et al. 2015 ). In fact, additional factors also played a role, such as innovations in security (Tilley et al. 2011 ). Thus, further evidence is needed to determine which factors had greater weight and the relationships among these factors, unless it is thought that a single mechanism triggered the crime drop.
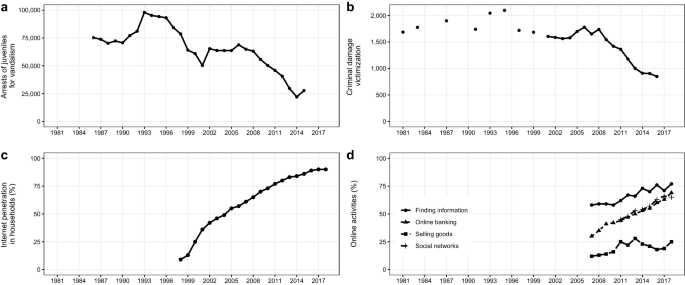
a Trend of juveniles under 18 arrested due to vandalism in the United States (1986–2015). Vandalism is defined as “To wilfully or maliciously destroy, injure, disfigure, or deface any public or private property, real or personal, without the consent of the owner or person having custody or control by cutting, tearing, breaking, marking, painting, drawing, covering with filth, or any other such means as may be specified by local law. Attempts are included”. Source: UCR. b Trend of criminal damage victimizations in England and Wales. This crime includes criminal damage to a vehicle, arson, and other criminal damage (1981–2016). Source: ONS. c Trend of estimated proportion of households with Internet access in GB (UK estimates from 1998 to 2004, GB estimates from 2005 to 2018) Source: ONS. d Trend of estimated proportion of the GB population using the Internet to perform different online activities: finding information about goods and services (2007–2018), online banking (2007–2018), selling goods or services (2007–2018), and social networking (2011–2018). Source: ONS
In their paper, Farrell and Birks ( 2018 ) discuss this claim in a paragraph under the subheading “It was not Internet-induced lifestyle or cultural change” (p. 3). Based on the evidence and arguments provided in the present paper, it cannot be denied that this was the case. In our opinion, there is insufficient evidence to support a claim that security is the only causal factor for the crime drop.
Second hypothesis. Cybercrime: switch of opportunities from physical space to cyberspace
Farrell and Birks ( 2018 ) argue that one of the main problems of the “cybercrime hypothesis” is the implausibility of the assumed causal mechanism. For these authors, a switch from traditional property crime to technological cyberfraud is barely conceivable. Farrell and Birks claim that cyberspace produces new opportunities, but these opportunities are unrelated to those found in physical space. The first critical observation regarding this form of expressing the “cybercrime hypothesis” relates to the generalization of the idea of cybercrime as a uniform and highly technological crime. In fact, there is not one single type of cybercrime or cyber fraud; instead, there are many forms of these crimes, some of which are barely technological (e.g., non-payment or non-delivery, romance fraud, Nigerian fraud) but are the most prevalent (Cross et al. 2014 ) and result in the greatest economic losses (Internet Crime Complaint Center 2017 ). In addition, the literature recognizes the existence of cyber-dependent crimes and cyber-enabled crimes (e.g., McGuire and Dowling 2013 ). In this sense, dual (Miró-Llinares 2012 ) or hybrid crimes (Caneppele and Aebi 2017 ) may be committed in physical space or in cyberspace; and relative to their physical variants, the cyber versions of such crimes normally require fewer skills but a different opportunity space.
This reasoning leads to the second critical observation: the impact of cybercrime on the physical crime drop is not necessarily associated with a shift in the activity of certain criminals but, rather, with a shift in criminal opportunities from physical space to cyberspace. This can lead to the commission of more crimes in the environment where the new opportunities emerge (Newman and Clarke 2003 ). Thus, the relevant issue is not the shift of people but the shift of opportunities, which has occurred because the popularization of the Internet and smartphones has resulted in a new area of criminal opportunity in cyberspace that has affected opportunities in physical space. This phenomenon of shifting opportunities can be observed in Fig. 2 a, b, which show a comparison between recorded offenses of two types of fraud and their economic cost. While traditional cheque frauds have decreased along with their associated economic loss, the data on online banking fraud, its cyber variant, show an opposite trend. Exchanges of physical money that resulted in fraud have decreased significantly, whereas e-commerce, online banking, and the use of credit cards have increased (Fig. 2 c, d; Button and Cross 2017 ). These phenomena have corresponded temporally with decreases in fraud-related arrests in the physical space and increases in online fraud (Fig. 2 e, f; see also Caneppele and Aebi 2017 ; Levi 2017 ). The mechanism is clearly evident: there are more opportunities in one place and fewer opportunities in another (Nuth 2008 ). The relationship between the dynamics of physical crime and cybercrime is not simply casual but may be causal in some cases. In other words, we are not claiming that the same individual who once stole bicycles now commits phishing (we ignore this, most likely that particular individual will not do so), but we do suggest that individuals who once found opportunities to steal bicycles now are finding more opportunities to commit fraud over the Internet through their daily activities (e.g., fraudulently offering bicycles that will never be sold to the buyer). Therefore, the point we are trying to make is not that people’s skills have changed, but that global opportunities have. This trend is shown in Leukfeldt’s research on criminal organizations (Leukfeldt et al. 2016 , 2017 ). Shifts in opportunities may lead criminals to spend more time attempting to engage in online fraud than in fraud in the physical space.
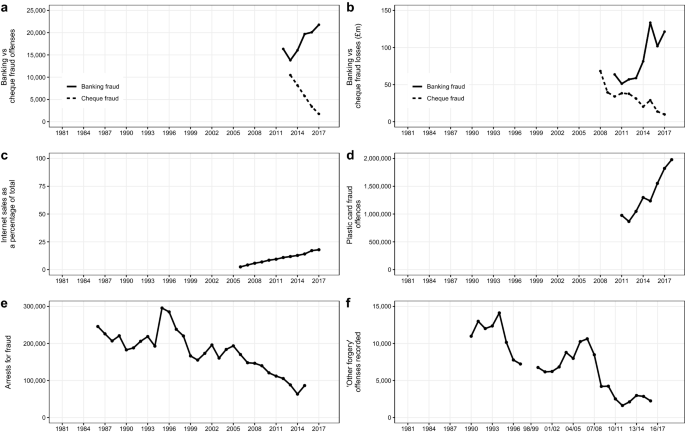
a Comparison between cheque and banking fraud offenses registered by UK Finance (2012–2017). Source: UK Finance. b Comparison between cheque and banking fraud losses in millions of pounds registered by UK Finance (2008–2017). Source: UK Finance. c Trend of arrests due to fraud in the United States (1986–2015). Fraud is defined as “The intentional perversion of the truth for the purpose of inducing another person or other entity in reliance upon it to part with something of value or to surrender a legal right. Fraudulent conversion, obtaining of money or property by false pretences, confidence games, and bad checks, except forgeries and counterfeiting, are included”. Source: UCR. d Trend of number of other forgery offences recorded by the police in England and Wales (1990–2015). We have selected this category from among all fraud offences since it is the only one with consistent values reported during the indicated period. The National Crime Recording Standard was introduced in 2002–2003, and data before and after that date are not directly comparable. Source: UK Home Office Official Statistics. e Trend of Internet sales as a percentage of total retail sales in England and Wales (2007–2017). Source: ONS. f Trend of plastic card fraud offences reported to the NFIB (2011–2018). Source: UK Finance
Considering the hypotheses further?
Considering the argument and evidence provided, the fact that the emergence of cyberspace and criminal opportunities, together with other causes, have had an impact on the decline in crime cannot be simply rejected. In fact, we firmly believe that there are sufficient arguments to further investigate this relationship in depth in future research. According to Farrell ( 2013 ), for a crime drop hypothesis to be seriously considered it must pass five tests. Our hypotheses on cyberspace and cybercrime pass the five tests proposed by the author because: (1) they have not previously been falsified and there are reasonable empirical reasons to take them into consideration; (2) their scope is cross-national by the very nature of cyberspace and the democratization of ICTs; (3) they are compatible with the previous increase in crime trends; (4) they are consistent with divergent trends of similar crimes that can be perpetrated in both cyberspace and physical space; and (5) differences in demographics and macro routine activities make them flexible enough to explain different timings and pace in the decline of crime trends.
Certainly, it is necessary to delve deeper into each of these points with more extensive research that cannot be conducted in a paper of these characteristics. However, in the words of Baumer et al. ( 2018 ), we believe “that the narrow conception of change adopted within criminology has hindered the field’s capacity to develop a stronger scientific understanding of crime trends” (p. 1). With this reply we intend to broaden that conception. Thus, claiming that cybercrime did not cause the crime drop deserves the following answer: “possibly, but the emergence of cyberspace and the crime that occurs within it has had an impact both in the progression of physical crime and in the spread of new forms of crime, all of which is being reflected in crime trends”.
It is difficult to believe that the IT revolution and the onset of cyberspace have not affected physical crime. Regarding the crime drop, we have provided counter-arguments to Farrell and Birks ( 2018 ) that support two hypotheses: (1) that the use of cyberspace for leisure activities at home, among other factors, has reduced the number of opportunities for certain crimes in the physical space, leading to certain forms of crime drop, especially for crimes associated with young people; and (2) that there have been increases in criminal opportunities in cyberspace that parallel decreases in criminal opportunities in physical space, particularly with respect to dual crimes (which can be conducted in both environments). These dynamics associated with the onset of cyberspace are coincident and, in certain cases, etiologically related. Cybercrime did not cause the crime drop. This was not singlehandedly induced by any one factor, but the onset of cyberspace as a new area of criminal opportunity and cybercrime impacted specific forms of the crime drop.
Availability of data and materials
The datasets analysed during the current study are publicly available in the UCR Publications repository, https://ucr.fbi.gov/ucr-publications ; and the Office for National Statistics repository, https://www.ons.gov.uk/ , https://www.gov.uk/government/statistics/historical-crime-data , https://www.ukfinance.org.uk/ .
Abbreviations
Great Britain
Information Technologies
National Fraud Intelligence Bureau
Office for National Statistics
Uniform Crime Reporting System
United Kingdom
United States
Aebi, M. F., & Linde, A. (2010). Is there a crime drop in Western Europe? European Journal on Criminal Policy and Research, 16 (4), 251–277. https://doi.org/10.1007/s10610-010-9130-y .
Article Google Scholar
Aebi, M. F., & Linde, A. (2014). The persistence of lifestyles: Rates and correlates of homicide in Western Europe from 1960 to 2010. European Journal of Criminology, 11 (5), 552–577. https://doi.org/10.1177/1477370814541178 .
Baumer, E. P., Vélez, M. B., & Rosenfeld, R. (2018). Bringing crime trends back into criminology: A critical assessment of the literature and a blueprint for future inquiry. Annual Review of Criminology, 1, 1–23. https://doi.org/10.1146/annurev-criminol-032317-092339 .
Beerthuizen, M. G., Weijters, G., & van der Laan, A. M. (2017). The release of grand theft auto V and registered juvenile crime in the Netherlands. European Journal of Criminology, 14 (6), 751–765. https://doi.org/10.1177/1477370817717070 .
Blumstein, A., & Wallman, J. (2006). The crime drop in America (2nd ed.). New York: Cambridge University Press.
Google Scholar
Button, M., & Cross, C. (2017). Technology and Fraud: The ‘Fraudogenic’ consequences of the Internet revolution. In M. R. McGuire & T. Holt (Eds.), The Routledge handbook of technology, crime and justice . London: Routledge.
Caneppele, S., & Aebi, M. F. (2017). Crime drop or police recording flop? On the relationship between the decrease of offline crime and the increase of online and hybrid crimes. Policing: A Journal of Policy and Practice . https://doi.org/10.1093/police/pax055 .
Cohen, L. E., & Felson, M. (1979). Social change and crime rate trends: a routine activity approach. American Sociological Review, 44, 588–608.
Cross, C., Smith, R. G., & Richards, K. (2014). Challenges of responding to online fraud victimisation in Australia. Trends & Issues in crime and criminal justice, 474 . Retrieved from: https://eprints.qut.edu.au/72186/1/tandi474.pdf .
Eisner, M. (2003). Long-term historical trends in violent crime. Crime and Justice, 30, 83–142.
Farrell, G. (2013). Five tests for a theory of the crime drop. Crime Science, 2 (5), 1–8. https://doi.org/10.1186/2193-7680-2-5 .
Farrell, G., & Birks, D. (2018). Did cybercrime cause the crime drop? Crime Science, 7 (8), 1–4. https://doi.org/10.1186/s40163-018-0082-8 .
Farrell, G., Tilley, N., & Tseloni, A. (2014). Why the crime drop? Crime and Justice, 43 (1), 421–490.
Farrell, G., Tseloni, A., Mailley, J., & Tilley, N. (2011). The crime drop and the security hypothesis. Journal of Research in Crime and Delinquency, 48 (2), 147–175.
Fernández-Molina, E., & Bartolomé Gutiérrez, R. (2018). Juvenile crime drop: What is happening with youth in Spain and why? European Journal of Criminology . https://doi.org/10.1177/1477370818792383 .
Internet Crime Complaint Center. (2017). 201 Internet crime report. Retrieved from: https://pdf.ic3.gov/2017_IC3Report.pdf .
Leukfeldt, E. R., Kleemans, E. R., & Stol, W. P. (2016). Cybercriminal networks, social ties and online forums: Social ties versus digital ties within phishing and malware networks. British Journal of Criminology, 57 (3), 704–722. https://doi.org/10.1093/bjc/azw009 .
Leukfeldt, E. R., Kleemans, E. R., & Stol, W. P. (2017). A typology of cybercriminal networks: From low-tech all-rounders to high-tech specialists. Crime, Law and Social Change, 67 (1), 21–37. https://doi.org/10.1007/s10611-016-9662-2 .
Levi, M. (2017). Assessing the trends, scale and nature of economic cybercrimes: Overview and issues. Crime, Law and Social Change, 67 (1), 3–20. https://doi.org/10.1007/s10611-016-9645-3 .
McCaffree, K., & Proctor, K. R. (2018). Cocooned from crime: The relationship between video games and crime. Society, 55 (1), 41–52. https://doi.org/10.1007/s12115-017-0211-0 .
McGuire, M., & Dowling, S. (2013). Cyber crime: A review of the evidence. Summary of key findings and implications. Home Office Research Report, 75 . Retrieved from: https://assets.publishing.service.gov.uk/government/uploads/system/uploads/attachment_data/file/246749/horr75-summary.pdf .
Miró-Llinares, F. (2012). El cibercrimen. Fenomenología y criminología de la delincuencia en el ciberespacio . Madrid: Marcial Pons.
Newman, G. R., & Clarke, R. V. (2003). Superhighway robbery . New York: Willan Publishing.
Nuth, M. S. (2008). Taking advantage of new technologies: For and against crime. Computer Law & Security Review, 24 (5), 437–446. https://doi.org/10.1016/j.clsr.2008.07.003 .
Pinker, S. (2011). The better angels of our nature: Why violence has declined . New York: Viking.
Pyrooz, D. C., Decker, S. H., & Moule, R. K., Jr. (2015). Criminal and routine activities in online settings: Gangs, offenders, and the Internet. Justice Quarterly, 32 (3), 471–499.
Rosenfeld, R., & Messner, S. F. (2012). The crime drop in comparative perspective: The impact of the economy and imprisonment on American and European burglary rates. In: The international crime drop (pp. 200–228). Palgrave Macmillan, London.
The Nielsen Company. (2018). U.S. games 360 report: 2018. Retrieved from: http://www.nielsen.com/us/en/insights/reports/2018/us-games-360-report-2018.html .
Tilley, N., Tseloni, A., & Farrell, G. (2011). Income disparities of burglary risk: Security availability during the crime drop. The British Journal of Criminology, 51 (2), 296–313. https://doi.org/10.1093/bjc/azr010 .
Tonry, M. (2014). Why crime rates are falling throughout the Western world. Crime and justice, 43 (1), 1–63.
Van Dijk, J. J. M., Tseloni, A., & Farrell, G. (Eds.). (2012). The international crime drop: New directions in research . New York: Palgrave Macmillan.
Zimring, F. E. (2008). The Great American crime decline . New York: Oxford University Press.
Download references
Acknowledgements
We thank Prof. Steven Kemp, University of Girona, for performing the English editing of the manuscript.
This research received funding from the Spanish Ministry of Economy, Industry, and Competitiveness (MINECO) under the Criminology, empirical evidence, and Criminal policy Project: on incorporating scientific evidence to decision-making regarding criminalization of conducts. Reference DER2017-86204-R. This research has been funded by the Spanish Ministry of Science, Innovation and Universities under FPU Grant reference FPU16/01671.
Author information
Authors and affiliations.
Crímina Research Centre for the Study and Prevention of Crime, Miguel Hernandez University, Elche, Spain
Fernando Miró-Llinares & Asier Moneva
You can also search for this author in PubMed Google Scholar
Contributions
FM developed the initial idea and drafted the contents of the present contribution. AM conducted the state-of-the-art literature review, retrieved and plotted the data used in the paper from public available repositories. Both authors wrote and reviewed the final version of the paper. Both authors read and approved the final manuscript.
Corresponding author
Correspondence to Asier Moneva .
Ethics declarations
Competing interests.
The authors declare that they have no competing interests.
Additional information
Publisher's note.
Springer Nature remains neutral with regard to jurisdictional claims in published maps and institutional affiliations.
Rights and permissions
Open Access This article is distributed under the terms of the Creative Commons Attribution 4.0 International License ( http://creativecommons.org/licenses/by/4.0/ ), which permits unrestricted use, distribution, and reproduction in any medium, provided you give appropriate credit to the original author(s) and the source, provide a link to the Creative Commons license, and indicate if changes were made. The Creative Commons Public Domain Dedication waiver (http://creativecommons.org/publicdomain/zero/1.0/) applies to the data made available in this article, unless otherwise stated.
Reprints and permissions
About this article
Cite this article.
Miró-Llinares, F., Moneva, A. What about cyberspace (and cybercrime alongside it)? A reply to Farrell and Birks “Did cybercrime cause the crime drop?”. Crime Sci 8 , 12 (2019). https://doi.org/10.1186/s40163-019-0107-y
Download citation
Received : 03 October 2018
Accepted : 10 October 2019
Published : 21 October 2019
DOI : https://doi.org/10.1186/s40163-019-0107-y
Share this article
Anyone you share the following link with will be able to read this content:
Sorry, a shareable link is not currently available for this article.
Provided by the Springer Nature SharedIt content-sharing initiative
- Security hypothesis
- Cybercrime hypothesis
- Information Technology
Crime Science
ISSN: 2193-7680
- General enquiries: [email protected]
- Open access
- Published: 21 April 2020
Review and insight on the behavioral aspects of cybersecurity
- Rachid Ait Maalem Lahcen 1 ,
- Bruce Caulkins 2 ,
- Ram Mohapatra 1 &
- Manish Kumar 3
Cybersecurity volume 3 , Article number: 10 ( 2020 ) Cite this article
45k Accesses
46 Citations
1 Altmetric
Metrics details
Stories of cyber attacks are becoming a routine in which cyber attackers show new levels of intention by sophisticated attacks on networks. Unfortunately, cybercriminals have figured out profitable business models and they take advantage of the online anonymity. A serious situation that needs to improve for networks’ defenders. Therefore, a paradigm shift is essential to the effectiveness of current techniques and practices. Since the majority of cyber incidents are human enabled, this shift requires expanding research to underexplored areas such as behavioral aspects of cybersecurity. It is more vital to focus on social and behavioral issues to improve the current situation. This paper is an effort to provide a review of relevant theories and principles, and gives insights including an interdisciplinary framework that combines behavioral cybersecurity, human factors, and modeling and simulation.
Introduction
Gary Warner delivered in March 1, 2014, a TEDX Birmingham presentation about our current approach to cybercrime. Warner, the Director of the Center for Information Assurance and Joint Forensics Research, at the University of Alabama, Birmingham, explained the challenges of protecting individuals and reporting cybercrimes. Benefits of making money and conducting low risk illegal acts drive cybercriminals. The Internet Security Threat Report ( Symantec 2017 ) shows that the average ransom was $373 in 2014 and it was $294 in 2015. It jumped to $1077 in 2016, and we surmise that it is due to the upsurge value of Bitcoin. A digital currency preferred by ransomware criminals because they can accept it globally without having to reveal their identities. The same report shows that the number of detection of ransomware increased to 463,841, in 2016; and more than 7.1 billion identities have been compromised in cyber attacks in the last 8 years. Malware attacks are on the rise, for instance, the recurrence of disk wiping malware "Shamoon" in the Middle East, and cyber attacks against Ukrainian targets involving the KillDisk Trojan. To show a historical damage that such malware can do, we give the example of the Ukranian power grid that suffered a cyber attack in December 2015. It caused an outage of around 225,000 customers. A modified KillDisk was used to delete the master boot record and logs of targeted systems’ organizations; consequently, it was used in stage two to amplify attacks by wiping off workstations, servers, and a Human Machine Interface card inside of a Remote Terminal Unit. Trojan Horse viruses are considered the third wave of malware that spreads across the Internet via malicious websites and emails ( Donaldson et al. 2015 ). There is no doubt that breaches of data are one of the most damaging cyber attacks ( Xu et al. 2018 ). Figure 1 depicts three main cyber targets, or their combination based on the work discussed in Donaldson et al. (2015) . They are usually referred to as CIA triad:
Confidentiality threat (Data Theft) that can target databases, backups, application servers, and system administrators.
Integrity threat (Alter Data) includes hijacking, changing financial data, stealing large amounts of money, reroute direct deposit, and damage of organization image.
Availability attacks (Denial Access) can be Distributed Denial of Service (DDoS), targeted denial of service, and physical destruction.
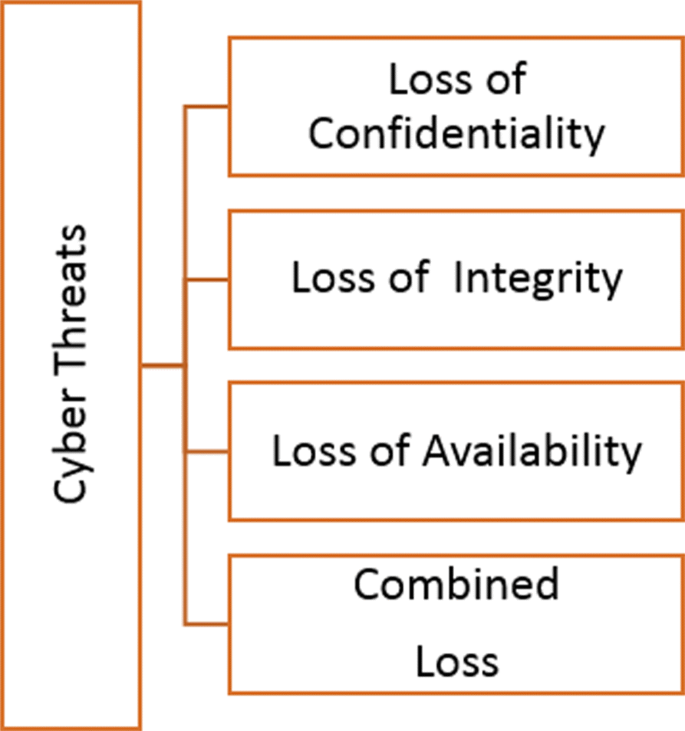
Losses caused by cyber threats, modified based on Donaldson et al. (2015)
Attackers will try to penetrate all levels of security defense system after they access the first level in the network. Therefore, the defender should be more motivated to analyze security at all levels using tools to find out vulnerabilities before the attackers do ( Lahcen et al. 2018 ). The 2018 Black Report pays particular attention to the period it takes intruders to hack organization’s cyber system, both by stages of the breach and by industry. The clear majority of respondents say that they can gain access to an organization’s system, to map and detect valuable data, to compromise it within 15 hours. Now, most industry reports say the average gap between a breach and its discovery is between 200 and 300 days ( Pogue 2018 ).
It is clear that cyber offenders or criminals still have an advantage over cyber defenders. Therefore, what are the deficiencies in current research and what areas need immediate attention or improvement? Thomas Holt at Michigan State University’s School of Criminal Justice argues that it is essential to situate a cybercrime threat in a multidisciplinary context ( Holt 2016 ). Hence, based on literature review described in “( Related work ”) section, we believe that the behavioral side of cybersecurity needs more research and can improve faster if it is integrated with human factors, and benefit from sophisticated modeling and simulation techniques. Our study emphasizes two necessary points:
(1) Interdisciplinary approach to cybersecurity is essential and it should be defined based on cyberspace understanding. We adopt a definition by the International Organization for Standardization of cyberspace, "the complex environment resulting from the interaction of people, software and services on the Internet by means of technology devices and networks connected to it, which does not exist in any physical form" ( Apvera 2018 ). This definition presents the cyberspace as a complex environment and initiates the interactions with people. Consequently, people’s biases and behaviors influence the interactions with software and technology, which affect the cyberspace. We believe that advancing this interdisciplinary research could bring more relevance and increase of cybercrimes’ manuscripts in top-tier journals. It is noticed that a low number of cyber-dependent crime manuscripts is due to a low number of criminologists who study cybercrime ( Payne and Hadzhidimova 2018 ). Thus, we address several behavioral and crime theories. Based on the proposed interdisciplinary approach, cyber teams have to include individuals with different backgrounds ranging from IT, criminology, psychology, and human factors.
(2) Enterprises must account for possibility of vulnerabilities including human error in the design of systems. Avoiding a vulnerability is a much better option than trying to patch it, or spend resources in guarding it. This may sound as a trivial proposition yet, in reality, many defenders and users often deal with security as a secondary task when their primary function is not security. The authors in Pfleeger and Caputo (2012) stated that security is barely the primary task of those who use the information infrastructure. Also, system developers focus on the user’s needs before integrating security into an architecture design. Afterwards, they add security tools that are easy to incorporate or meet some other system requirements. This is our rationale behind making modeling and simulation an essential component. The stakeholders such as users, managers, and developers, should be involved in building those models, and determine simulations that evaluate cognitive loads and response times to threats. Stakeholders can also use simulation to exercise real life scenarios of social engineering attacks. Furthermore, accounting for vulnerabilities may be affected by the budget. Enterprises keep cybersecurity’s budget to a minimum. A report by Friedman and Gokhale (2019) found that financial institutions’ on the average spending on cybersecurity is 10% of their IT spending or an average of 0.3% of revenue. Recently, some companies are spending more on cyber defense but in areas that may not maximize security. The report of Blackborrow and Christakis (2019) found that organizations are spending more on security but not wisely. This so called reactive security spending and results in widespread inefficiency. By all means, this status increases the complexity of the security problem. Therefore, the perceptions of various industries about their cybersecurity needs vary, in most cases, they lack.
Related work
We conducted a comprehensive literature review using different criteria to capture both a historical stand point and the latest findings. We started the search of theories, human factors, and decision making strategies from 1980. It is important to acknowledge their historical contributions and explore how they can be applied to cybercrimes. We started the search of cybercrime reports from 2014 to understand cybercrime trends and magnitudes. The search of other subjects such as insider threat, hacking, information security, cyber programs, etc. is from the past decade. Some of the search commands: (cybersecurity AND human factors), (cybersecurity AND behavioral aspects), (cybersecurity AND modeling and simulation), (interdisciplinary approach and cybersecurity), (cybersecurity AND crime theories). Some of the databases that were searched are EBSCO, IEEE Xplore, JSTOR, Science Direct, and Google Scholar. It is worthwhile to note that several search results that include interdisciplinary cybersecurity awareness are about educational undergraduate students. This explains the urgency in educating future cyber professionals who will work in interdisciplinary cyber teams. We observed in recent conferences that few speakers debate whether there is talent’s shortage or the problem is inadequate use of available tools. Nevertheless, our view is that the problem could be both. The two points mentioned in introduction (interdisciplinary approach and vulnerability in design) are used as criterion to decide related articles cited here.
It is acknowledged that human as the end user can be a critical backdoor into the network ( Ahram and Karwowski 2019 ). The research done by Addae et al. ( ) used behavioral science approach to determine the factors shaping cybersecurity behavioral decisions of users. The results suggest that security perceptions and general external factors affect individual cybersecurity adoptive behavior, and those factors are regulated by users traits (gender, age) and working environment. The authors in Maimon and Louderback (2019) conducted an interdisciplinary review reiterating that several criminological theories provide important frameworks that guide empirical investigations of different junctures within the cyber-dependent crime ecosystem. Also, they found that more research is needed and suspect that criminologists may not still bring cybercrime scholarship to the forefront of the criminological area. The authors in Payne and Hadzhidimova (2018) found that the most popular criminological explanations of cyber crime include learning theory, self-control theory, neutralization theory, and routine activities theory. In general, their finding reinforce the fact that integration of cybersecurity into criminal justice is not fast, probably because a few criminologists study cybercrimes. The work in Pfleeger and Caputo (2012) addresses the importance of involving human behavior when designing and building cyber technology. They presented two topics of behavioral aspects: (1) cognitive load that can contribute to inattentional blindness that prevents a team member to notice unexpected events when focusing on a primary task, and (2) biases that could help security designers and developers to anticipate perceptions and account for them in the designs. We will articulate more related work in the components’ sections of the proposed framework.
In summary, research has been consistent in acknowledging that behavioral aspects are still underexplored and the focus is more on the technology aspect. One of the challenges is the complexity of the models when addressing different theories. Our aim is to provide insights on current issues, for example, classifying insider threat under human error makes insider issue a design requirement. This insight makes our approach significant because it opens channels to use the best human factors practices found in healthcare, aviation and the chemical industry. It reinforces the idea of insider as a design requirement (prevention).
The rest of the paper proceeds as follows: “( Interdisciplinary framework )” section proposes the Interdisciplinary Framework, “( Behavioral cybersecurity )” section explains Behavioral Cybersecurity, “( Human factors )” section Human Factors is discussed, “( Modeling and simulation )” section deals with Modeling and Simulation component, and we mention Conclusion and Future Work in “( Conclusion and future work )” section.
Interdisciplinary framework
Because all partial solutions (Firewall, IDS/IPS, netflow, proxy, mail gateway, etc.) do not add up to a complete solution and the offenders still have the most latitude for variation at the network level ( Kemmerer 2016 ), it is necessary to invest in interdisciplinary frameworks. In this section, we propose an interdisciplinary framework that enables understanding of interconnectivity of relations and should serve as a background to improve research and maturity of security programs. We focus on three areas based on the work of Caulkins (2017) , depicted in a Venn diagram in Fig. 2 :
Behavioral cybersecurity is the main focus of our study. We address profiles and methods of hackers, insiders, behavioral, social, and crime theories. Weapons of influence that are largely used by the offenders and mostly ignored by the defenders will also be identified.
Integrate human factors discipline with behavioral cybersecurity. We give an insight on human factors that trigger human error. If we consider the insider problem as a human error, we can mitigate the risks by improving the environment, and plan it in the design requirement of future systems. The assumption is that system design enables insider risk because of the already existing vulnerabilities or conditions. The National Institute of Standards and Technology (NIST) recommends that the best method to involve everybody is to motivate everyone using incentives within the cyber economy ( Addae et al. ). Hence, it is worth integrating human factors to improve working environment, mitigate risks, and make the system’s probability of failure lower.
Using Modeling and simulation for researching, developing and implementing new techniques, tools and strategies is our recommendation. Modeling and simulation are useful for many reasons and can be extended to situations such as when real experimentation is not convenient, or dangerous, or not cost effective ( Niazi 2019 ). Simulation can test applications of human factors, for example, whether the real process may cause a cognitive load that will inhibit the security end-user to miss important information or threats. We review modeling and simulation in literature, and we provide insight in that section based on our focus on human error.
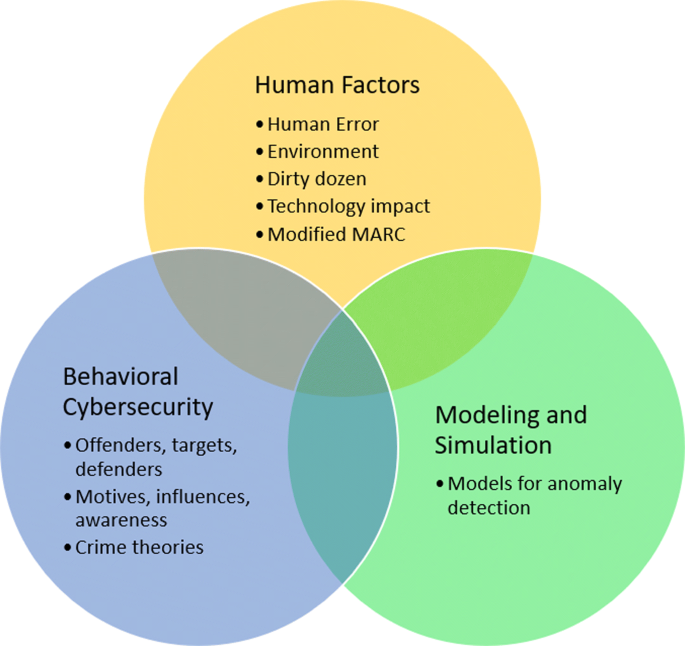
Venn diagram for the interdisciplinary framework, based on Caulkins (2017)
There is no doubt that behavioral cybersecurity is important, and it needs more research. We emphasize the three components of this proposed interdisciplinary framework because human performance is not affected solely by training, which is the main focus of cyber defenders. It is affected by the system itself, people’s biases, environment workload, administrative management, communication practices, human-computer interfaces, existing distractions, etc. Many factors still contribute to the slow research and implementation of interdisciplinary approaches. Unfortunately, many enterprises underestimate the severity of cyber incidents, or they pass the blame to one person when an incident occurs. For instance, Federal Trade Commission website reports that in September of 2017, Equifax announced a data breach that exposed the personal information of 147 million people and Equifax has agreed to a global settlement with the Federal Trade Commission, the Consumer Financial Protection Bureau, and 50 U.S. states and territories. The settlement includes up to $425 million to help people affected by the data breach ( FTC 2019 ). Yet, the settlement does little to those who file claims ($125 one time payout or credit monitoring for a number of years). Individuals cannot opt out of Equifax being their data steward which makes many persons nervous. Most of the online reports state that Equifax did not update a known vulnerability in the Apache Struts web-application software. Nevertheless, Equifax’s Chief Executive told members of Congress on October 3, 2017, that the massive breach happened because of a mistake by a single employee.
Behavioral cybersecurity
Cybercrime offenders: hackers, hackers’ techniques.
A hacker is a human that uses technical intellect to get unauthorized access to data to modify it, delete it or sell it by any means ( Pal and Anand 2018 ). Although a hacker may follow various steps to execute a successful attack, a usual network intrusion involves reconnaissance to collect information, scanning to set up a vulnerability profile, gaining access or penetrating an access point or level, maintaining access by accessing other levels or planting programs to keep access, and covering tracks to hide the trails ( Lahcen et al. 2018 ). The authors in Shetty et al. (2018) have surveyed hacking techniques:
The dictionary attack to crack vulnerable passwords. This is like brute force to defeat security. It takes advantage of users not being able to remember difficult passwords or the ones that do not make any sense so they use relevant or easy passwords. Often hackers find those users who adopt weak passwords such as 123456 or password . Currently, companies are enhancing passwords’ syntax and mandate specific changing procedures. Yet, users still use same passwords across websites.
Structured Query Language (SQL) injection of harmful code to modify the SQL query structure. It manipulates website’s database.
Cross Site Scripting (XSS) is an attack vector that injects malicious scripts into victim’s webpages.
Phishing is a social engineering attack in which a phisher fools the user to reveal secret information. Some examples are discussed in the weapons of influence “( Weapons of influence )” section.
Wireless hacking due to a weakness of some networks. Those networks do not even change vendor access point and default passwords. A Wi-Fi network can be hacked in wardriving if it has a vulnerable access point. A hacker uses port scanning and enumeration.
The Keylogger is a software that runs in the background and captures the user’s key strokes. With it, hackers can record credentials.
Literature review discusses several hacker profiles. They have various levels of education, they hold many certificates, and they are either self-employed or work for organizations. Hackers can be script kiddies who are the new and novice. Their intent is curiosity or notoriety. Cyber-punks such as virus writers, they have medium skill level and their intent could be notoriety with some financial gain. Insiders or previously called internals can be driven by many motives such as revenge or financial benefits. Insider’s skills are usually high. The intent of petty thieves, virus writers, grey hat or old guard hackers is curiosity or notoriety, but their skill levels are high. The motive of professional criminals or black hat hackers can be financial and they hold very high capabilities. The motive of information warriors who are cyber mercenaries is mainly espionage, and they are placed under Nation State groups. Political activist or hacktivists are ideologically motivated, and they manage to include members who posses high level of skills ( Hald and Pedersen 2012 ).
Insight on hackers’ techniques
It is important to understand that hacking techniques and hackers’ motives in order to anticipate hackers’ moves. All hackers do not think the same way as defenders or in a linear manner. Consequently, defenders need to be interdisciplinary in order to take in account various techniques and combat. We support this assumption with one of the real stories of exploitation by hackers that Mitnick and Simon discussed in Mitnick and Simon (2005) : Hackers changed firmware in the slot machines after hiring an insider or a casino employee. Their motive was money and their stimulus was that the programmers of the machines were human, hence, they most likely had a backdoor flaw in the programs. One hacker checked the patent office for a code since it was a requirement to include it for patent filing. The analysis of the code gave away its secret. The pseudo random generator in the machines was 32-bit random number generator and cracking it was trivial. The designers of the machine did not want real random number generation so they have some control over the odds and the game. The hackers in this story were programmers and their thinking was simple enough to find a sequence of instructions to reach their goal. At that time, casinos spend money in security guards and not in consulting with security sources. One hacker said that he did not even feel remorse because they are stealing from casinos who in return steal from people.
Therefore, we present some of the questions that should be answered periodically to predict hacker’s next move: Is the attack surface defined? Attack surface involves the sum of all the attack vectors where a hacker can attempt to exploit a vulnerability. What is a critical or a most vulnerable or a most damaging asset if exploited? How are the access points protected? How can hackers access crown jewels? An example of crown jewels is the most valued data. Where crown jewels are located (servers, network, backups, etc.)? Are the inventories of authorized and unauthorized devices known? Are operating systems well configured and updated? Is a system in place to identify stolen credentials or compromised user accounts? What type of malware defenses are used? How effective are training or awareness programs? Are employees aware of social media risks? How is the situation of employees in the working environment? How effective and robust are the used intrusion detection systems? Is the reporting system of a potential threat or breach clear? Is there a plan to combat insider threat? We should highlight that many companies see that emphasizing prevention increases cost and reduces productivity. The increase of cost is due to interaction with security control and incident response. Lost of productivity is due to granting permissions or re-certifying credentials or users’ accounts ( Donaldson et al. 2015 ). We think that they should analyze costs of different options: prevention driven program, incident response driven program, or a hybrid option.
Cybercrime offenders: insiders
Insiders’ threat.
An insider is a hacker from inside the organization; hence, this insider has access rights and is behind the firewalls. Insider threat is broadly recognized as an issue of highest importance for cybersecurity management ( Theoharidou et al. 2005 ). Several surveys have considered varying aspects of cybersecurity: The SANS Healthcare Cyber Security Survey ( Filkins 2014 ), The Insider Threat Spotlight 2015 Report ( Partners 2015 ), Department for Business Innovation and Skills, 2014 Information Security Breaches Survey ( Willetts 2014 ), etc. The Insider Threat Spotlight 2015 Report stated that companies were more concerned by inadvertent insider threat data leak breaches than malicious data breaches ( Partners 2015 ). However, their concerns do not surely translate to effective changes in cyber programs. According to the SANS Healthcare Cyber Security Survey, 51% considered careless insider as a main threat when it comes to human behavior as an aspect of cybersecurity ( Filkins 2014 ). Many theories can be applied to understand insider risk and motives, and can be applied to behavioral models. Often policies and risk management guidance are geared towards rational cyber-actors while rationalities of users and defenders represent cyber-system vulnerabilities ( Fineberg 2014 ). Irrational behavior can be dangerous and unpredictable, it builds on frustration or fury, and it can be motivated by lack of job satisfaction. Often cyber defenders do not verify irrational behaviors. The authors in Stanton et al. (2005) have concluded that end users’ behaviors that occur in organizations could be sited within these behavioral groups leading to intentional damage, harmful misuse, unsafe tinkering, naive mistakes, mindful assurance, simple hygiene, and using intentionality and technical expertise as criteria. Myers et al. (2009) have added automated insiders such as bots to unauthorized use of privileges. The authors in Azaria et al. (2014) have divided related works into six categories including psychological and social theories, anomaly based approaches, honeypot based approaches, graph based approaches, game theory approaches, and motivating studies. The authors in Greitzer and Hohimer (2011) have described a predictive modeling framework CHAMPION that integrates various data from cyber domain, to analyze psychological, and motivational factors that concern malicious exploitation by the insider. The ontologies in CHAMPION represent knowledge in the specialized domain to reason about data. The reifiers are used for the feeding of the ontologies’ primitive data types. The memory is used to store both the primitive data and the facts concluded by the reasoning system. In addition, the Auto-associative Memory Columns (AMCs) or reasoning components stacked in a hierarchy and are used for data’s interpretation and are used to infer new statements. The authors in Cappelli et al. (2014) have discussed the Management and Education of the Risk of Insider Threat (MERIT) models that can be implemented to communicate insider’s threat. They identified and validated seven observations after analyzing several insider IT sabotage cases. Those observations are insiders had personal predispositions, were disgruntled employees, were among those who suffered stressful events (sanctions), had behavioral precursors (drug use, aggressive, etc.), created unknown channels to attack after termination, or lacked physical and electronic access (exploited insufficient access). A limitation in dealing with insider threat research is the scarcity of data ( Stolfo et al. 2008 ).
Insight on insiders’ threat
We think that there is a confusion in classifying insider threat, and many organizations may not even have policies or controls addressing it. Another issue of concern is that organizations do not want to admit of having insider incidents, they choose firing the intruder, and protect their reputation. Our insight considers the insider as a human error to be addressed at the top level of any developed taxonomy. So we group all user errors and the insider into human error, summarized in Fig. 3 .
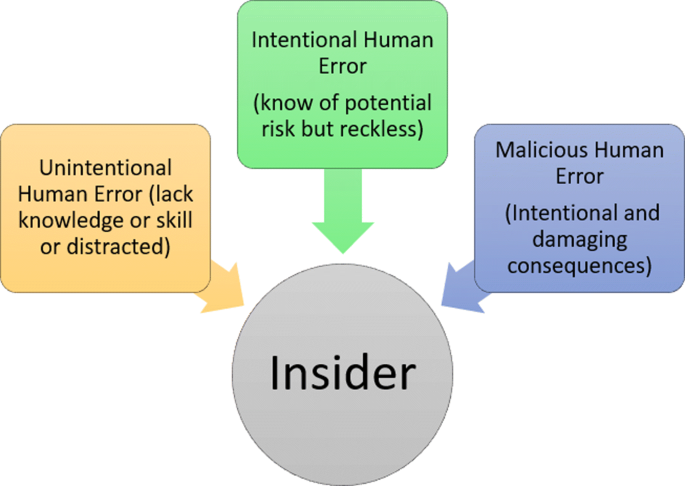
Proposed UIM human error as insider-anomaly concept
For this purpose, we adopt a definition of human error mentioned by the Center for Chemical Process Safety (AIChE) in Rodriguez et al. (2017) :
"Human error is any human action that exceeds some control limit as defined by the operating system."
We believe our insight is important because it simplifies this confusing issue to Unintentional - Intentional - Malicious or (UIM) instead of several categories. Moreover, it also allows to adopt lessons learned from industries that have a long history in applying human factors, and built mature programs. Besides, this insight allows to comprehend that failures happen at the management level, at the design level, or at the technical expert levels of the company; and they result in human error or failure ( Embrey et al. 1994 ). Obviously, UIM category is decided by its consequence or intent:
Unintentional human error can be due to lack of organized knowledge or operating skills. This error may remain unintentional or transforms to another type (intentional or malicious).
Intentional human error is caused by a user who knows of risky behavior but acts on it, or misuses assets. The wrong action may not necessarily bring a sudden harm to the organization, but it may still breach of existing laws or privacy.
Malicious human error is the worst error as it is intentional with specific and damaging consequences in mind.
This classification does not downgrade the insider threat. It brings it upfront in the system design, similar to human errors that are usually considered at the beginning of designs. It is easier to blame the human during a cyber incident instead of blaming the cyber program or the design of the systems. In fact, the system design that did not consider the human factor is also to blame. Often the user does not see the security policies in the same way as those who wrote them or want them implemented. It is imperative to realize that users often exhibit their own biases in decision making ( Fineberg 2014 ). This grouping can also be implemented in user’s training and help make awareness easier. We give few examples:
Unintentional error can happen from using a public Wi-Fi to access important accounts and not knowing about the risk. Or, while working, employee visits unsafe websites linked from social media.
Intentional error can occur if a user writes a password on a sticky note, leaves it near computer or in desk’s drawer and hoping no one else uses it.
Malicious error can occur with employee stealing confidential data (exfiltration).
As mentioned, a user error can change from a UIM category to another. For example, a user should not activate links or download attachments in emails without a verification. If a new employee is not aware of social engineering tactics, the employee may click on those links (unintentional). This employee’s clicking rate on those link should decrease with training, if not, employee’s action becomes intentional. Similarly, honeypots or decoys can be used to learn about user’s normal or deviant activities. Some companies implement programs to simulate real life scenarios such as phishing exercises. We suggest that they are transparent with employees about the use of phishing simulators or other awareness programs. The goal should be to improve the culture of cyber awareness and not adding stress to workloads.
We previously described the cyber targets (Fig. 1 ), and mentioned that the defender should consider them in the system design that usually inspects requirements. (1) To define confidentiality requirement, the organization should characterize data and its location. The user should differentiate whether one is dealing with public, confidential, or limited data. Compromising data may happen on the computer of the user, in transit across an open or close network, on a front-end server, or in storage ( Maiwald and Sieglein 2002 ). The user’s access to confidential data should be updated if data classification changes or a user’s status changes. Understanding that insider threat as a human error or anomaly within requirements of data security helps us to set up policies on credentials of persons who have access to confidential data. For example, to implement Just In Time (JIT) credentials. JIT helps to avoid permanent administrator (admin) privileges. It should in return mitigate the risk to steal admin credentials, and prevent admin data access outside the times in which there is no need to access confidential data. (2) Integrity is a system requirement. Data may be modified by the user, in transit across a closed or open network, a front-end server, or in storage ( Maiwald and Sieglein 2002 ). Considering user’s alteration of a system policy as an error helps to best treat integrity like confidentiality. Hence, the user’s access and impact on system integrity need to be examined. (3) Availability is also a system requirement. Because system’s components can be interconnected, a user who affects the availability of a part of a system can affect other parts. User’s error to make a system unavailable can easily happen intentionally or unintentionally if the system design did not identify failure points.
Behavior, social and crime theories
Computer scientists, security researchers, psychologists, social scientists have attempted to explain the behavior of users in relation to cybersecurity. There is insufficient knowledge about the behavior of the user toward information technologies that defend systems and data from troubles such as malware, spyware, and interruptions ( Dinev and Hu 2007 ). The authors in Greitzer and Hohimer (2011) have emphasized that the only way to be proactive in the cyber domain is to take behavioral or psycho-social data into account. At this point, we introduce theories that should help with such issues.
Theories: normative, planned behavior, social bond, and social cognition
There are questions about rationality when it comes to norms and the study of human cognition. The norms are essential to the study of informal argumentation, studies of judgment, and decision-making. Normative theories are studied in procedural theories forms and epistemic theories forms. It is difficult to resolve questions about suitable norms for a specific behavior without comprehending the origins of normativity ( Corner and Hahn 2013 ). It is recognized that playing a matching game between a particular behavior and some prescriptive standard is not enough to understand the concept of normativity. Hence, Corner and Han attempted to answer what makes something normative? It seems that there is a continuing debate on this subject. Our modest understanding is that a rational human behavior happens when the behavior matches some criterion, and logic is used to evaluate arguments. Yet, logic has limitations and may not be appropriate to judge arguments’ strength. Such limitations of logic encouraged the popularity to Bayesian probability as a calculating application for argument strength ( Corner and Hahn 2013 ). Therefore, the authors make a good argument that the Bayesian is suitable for the normativity’s requirements.
Another widely used theory is the Theory of Planned Behavior (TPB) depicted in Fig. 4 . It uses a predictive model that indicates that subjective norms and attitudes influence behavioral intention. The latter influences actual behavior. The TPB postulates that people’s behavioral intention is a good predictor of their real behavior. Another perception of behavior is the subjective norm. The ease or difficulty of performing behavior is the perceived behavioral control.
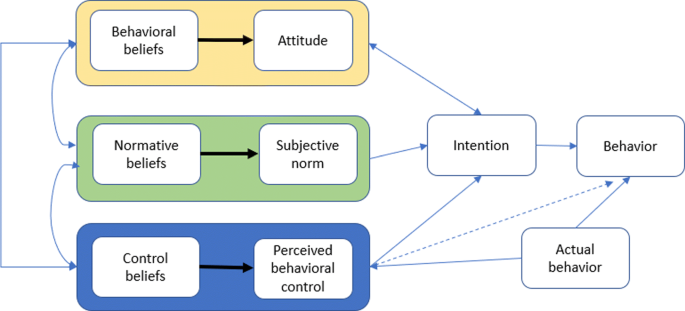
Theory of Planned Behavior diagram, from Icek (2019)
Generally, the greater is the attitude, subjective norm, and perceived behavioral control with respect to a behavior, the higher should be an individual’s intention to demonstrates the behavior under consideration. The attitude is connected to beliefs (behavioral, normative and control). In addition, multiple authors structure social pressure as a cause to normative beliefs. Until now, insufficient research is done on subjective norms regarding cybersecurity. An area in which TPB can be useful in the study of insider threat; as TPB is used successfully in predicting several health behaviors like smoking and substance use. It will be useful to understand the roles of various behavioral factors and learn which ones will have the highest predictive value in order to integrate it in a preventive plan, or an intrusion detection system. Similar to the work of Pabian and Vandebosch that studied cyberbullying using TPB; they found that cyberbullying intention is a predictor of self-reported cyberbullying behavior after six months ( Pabian and Vandebosch 2013 ). The attitude is the primary direct predictor of intention followed by the subjective norm. The authors in Dinev and Hu (2007) have integrated TPB and Technology Acceptance Model (TAM) and found that technology awareness is a predictor to a user behavioral intention to use anti-virus or anti-spyware. Technology awareness had the strong influence on attitudes toward behavior and behavioral intention. They also found that awareness is highly correlated with both TPB and TAM beliefs, and recommended that for managers to create social advocacy groups and networks. Their role is to advocate for cybercrime awareness. The authors of Burns and Roberts (2013) have used TPB to predict online protective behaviors. Their findings indicate a significant relationship between a subjective norm and intention. It also emphasizes that external parties influence the intention of the user to engage in cyber protective behavior.Social Cognition Theory (SCT) initiated as Social Learning Theory by Albert Bandura and became SCT in 1986. It postulates that cognitive factors are related to an environment and behavioral factors. Consequently, learning happens in a social context ( Hardy et al. 1980 ) with reciprocal determinism. Figure 5 depicts SCT basic diagram based on Hardy et al. (1980) . There is a reciprocal cause and effect between a person’s behavior and both the social world and personal characteristics. Hence, criminal or deviant behavior is a learned behavior just like any other behavior. Social Bond Theory makes the assumption that weaker social bonds can increase the chance of a person to be involved in a crime.
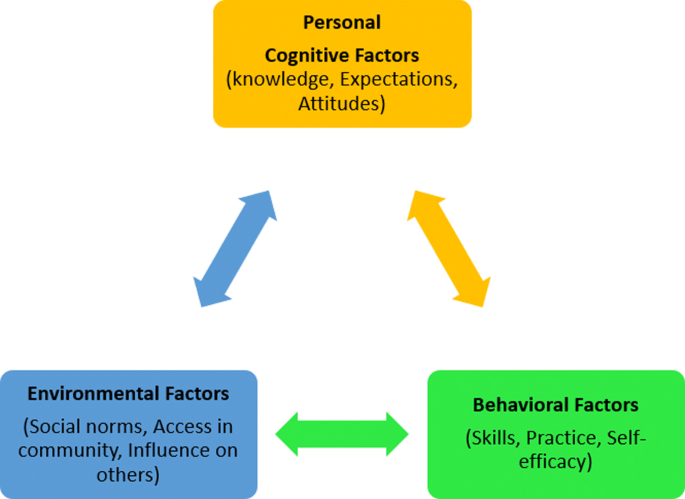
Social Cognition Theory basic diagram
The interesting part of SCT is that it tries to explain the maintenance of behavior, unlike other theories’ concern of initiating a behavior. SCT can be applied to the cyber domain to investigate decision support and behavior. It can probably support a robust security framework that studies practice behaviors of self-users. For example, studying the impact of self-efficacy is a cornerstone of SCT, on decision and cyber behavior. Self-efficacy is not self-esteem and it is kind of self-evaluation which is significant in individual behavior ( Hardy et al. 1980 ). Self-efficacy can influence the amount of effort, self-regulation, initiation of tasks, and handling of obstacles ( Hardy et al. 1980 ). Also, ill-defined circumstances and performance requirements can bring inconsistencies to self-efficacy expectation and performance ( Reardon 2011 ).
Theories: general deterrence, neutralization, self-control, and situational crime prevention
The authors of Theoharidou et al. (2005) have summarized criminology theories and security literature. It seems that all theories involve a motive and one theory is about the opportunity of a crime. Besides, General Deterrence Theory is based on a perpetrator committing a crime if the cost of sanction is less than the benefit of the crime. Hence, stiff punishment and awareness programs deter many potential perpetrators. Authors in Cheng et al. (2014) found that employees focus on the perceived benefits of personal internet use while, at the same time, finding justification for their behavior and keep less attention to the expected punishment. They are less worried about severity of punishment, and more worried about the likelihood of being caught. Those users try to justify their deviant behavior as excusable. This is a topic of neutralization theory. Hence, employees could use neutralization techniques to justify risky security behaviors. Neutralization is an excellent predictor of employees’ intention to violate information security policies ( Siponen and Vance 2010 ). They see it as an indicator of a motivational state that exists just prior to committing an act. Self-control Theory postulates that criminal acts attract low self-control people as these acts provide pleasure to them. A low self-control individual prefers immediately gratifying activities that involve risky behaviors, and shows little empathy for others. Self-control theory’s definition of crime is behaviors that provide momentary or immediate satisfactions and create negative consequences ( Gottfredson 2017 ). This theory can be applied to cybercrime and may be integrated with other stated theories. The theory of Situational Crime Prevention (SCP) makes the hypothesis that a perpetrator must have an opportunity in addition to a motive. A motive without an apportunity will not yield to a crime. Hence, it is different because it looks at the opportunities and the formation of motives to excite crimes ( Theoharidou et al. 2005 ). SCP framework includes rational choice, opportunity structure, specificity, and twenty-five techniques to reduce crime found in Freilich et al. ( ). The latest studies discussed complex issues in working with SCP, for instance, the competency and the responsibility to prevent a crime. Consequently, reducing cybercrime spike will depend on involving many parties such as law enforcement, government agencies, security companies, etc.
Multi-criteria decision-making
We should include Multi-criteria decision-making (MCDM) with above theories because conflicting ideas may arise and decisions need to be made to have good programs or models. MCDM is crucial for several real life problems including cybersecurity. However, the discussion on the usability of decision theory against cyber threats is limited, which indicates the existence of a gap ( Wilamowski et al. 2017 ). Often, challenges rise during the evaluation of alternatives in terms of a set of deciding measures. There is no doubt that decision making in this paper’s context cannot be easily modeled because of dealing with human element and judgement. A wide range of mathematical methods of MCDM for evaluation and validation of alternatives exist, and embedded in, linear programming, integer programming, design of experiments, Bayesian networks ( Wilamowski et al. 2017 ). MCDM usually involve three steps when using numerical analysis of the alternatives: (1) identify alternatives to criteria, (2) attach numerical measures to the criteria and impact of alternatives, and (3) rank each alternative after processing numerical values ( Triantaphyllou et al. 1997 ). The weighted sum model remains the simplest and the most widely used MCDM method. The authors of Triantaphyllou and Mann (1995) have used the analytical hierarchy of the process for decision making in engineering and found challenges. For instance, when some alternatives are similar or very close to each other, the decision-maker needs to be very careful. They suggest trying to consider additional decision making criteria to considerably discriminate among the alternatives. We can assume so far that decision making theories can easily give different answers to the same cybersecurity problem, yet they should be used as tools to back a decision as the authors of Triantaphyllou and Mann (1995) suggested. The authors of Wilamowski et al. (2017) have studied two theories in decision making: Analytical Hierarchy Process (AHP) and an Analytical Network Process (ANP). They determined that a generalized application benchmark framework could be employed to derive a Measure of Effectiveness (MOE) that relate to the overall operational success criteria (mission performance, safety, availability, and security). MOEs continuance are measured under specific environmental and operational conditions, from the users’ viewpoint. The AHP is an appropriate option if a situation requires rapid and effective decisions due to imminent threat. The ANP is appropriate if the time constraints are less important, and more far-reaching factors should be considered while constructing a defensive strategy. Their findings can provide cybersecurity policy makers a way to quantify the judgments of their technical team regarding cybersecurity policy.
The authors of Kabassi and Virvou (2015) have added Human Plausible Reasoning Theory (HPR) that is a cognitive theory to MCDM and provides more reasoning to a user interface. HPR depends on analyzing people’s answers to ordinary questions about the world. HPR theory assumes dynamic hierarchies to represent human knowledge. HPR defines parameters of certainty as a set of criteria that should be taken into account in order to select the best hypothesis. Nevertheless, HPR does not propose precise mathematical methods for combining these criteria. Indeed, MCDM compliments HPR and improves control in an intelligent user interface ( Kabassi and Virvou 2015 ).
Weapons of influence
We owe the credit, for this section’s title, to the first chapter title of Cialdini’s book "Influence - The Psychology of Persuasion" . Unfortunately, social engineers use weapons to influence and manipulates persons to disclose sensitive information or granting unauthorized access. Cialdini identified six principles of influence that guide human behavior ( Rodriguez et al. 2017 ): Reciprocity, scarcity, authority, consistency, liking and consensus. The authors in Haycock and Matthews (2016) have addressed them in their "Persuasive Advocacy" article. Based on their analysis, we give some examples in which social engineering can exploit and direct human actions with a view to understanding reason that motivates cybercrime:
Liking can give a false sense of credibility. Hackers can use it to build rapport, or encourage certain behaviors by generating fake likes, and artificially increasing the number of followers on social media to give the impression that other people are supporting that behavior.
Reciprocity is due to feeling of obligation to return favors. Hackers can offer free services or products and expect access or data in return.
Social proof or consensus summarizes how a person follows other’s lead. Hackers can use this type of validation to influence users and gain access to data. When people are not certain they may easily reply to other persons, especially peers.
Persuasion by peers. Hackers can persuade insiders to steal data for a cause that a peer or a role model is promoting.
Individuals who decree expertise or credentials try to harness the power of authority. Authority can bring phony claims and influence a user that is wary of job loss.
Consistency comes from the need to appear or to remain consistent. Hackers can find out about consistent actions and use them to distract a user prior to an attack.
Scarcity of resources makes a user vulnerable. It can influence a user to take an immediate action without thinking about consequences such as a data breach.
Researchers found that the effectiveness of each one of these principles is due to the victim’s personality characters. Examples from Uebelacker and Quiel (2014) and Caulkins (2017) about Cialdini principles’ work in social engineering: Agreeableness of a user has increased the vulnerability towards liking, authority, reciprocity, and social proof. Neuroticism indicates a user is less susceptible to most social engineering attacks. Conscientious user may not resist the principles of authority, reciprocity, and commitment and consistency, especially, when commitments are made public. Extraversion user may have greater vulnerability for the scarcity principle since the latter is considered as an excitement. Conscientiousness may decrease user’s susceptibility to cyber attacks. Yet, conscientiousness has a higher tendency to follow through commitments which may make the person susceptible to continuation of social engineering tactics. Agreeableness of a user may have increased susceptibility to phishing, and share passwords. Openness reduces social engineering vulnerability as more digitally literate users better detect social engineering attacks. Authors in Halevi et al. (2013) have found that women are more vulnerable to prize phishing attacks than men, and they found a high correlation between neurosis and responsiveness to phishing attacks. In addition to Cialdini’s work, researchers like Gragg and Stajano discussed what triggers of influence and scams. Table 1 is based on the work of Ferreira et al. (2015) and Caulkins (2017) , and it summarizes the principles of Cialdini, Gragg, and Stajano.
Those authors found that phishing emails use social engineering and depend on liking, deception, and similarity principles. Distraction is the second most commonly used principle. The combination of principles increase success of phishing attacks ( Ferreira et al. 2015 ). The elaboration likelihood model of persuasion in Cacioppo and Petty (2001) suggests that there are central (involve high elaboration) and peripheral (involve low elaboration) routes to persuasion. A person who is faced with a persuasive message will run through it using either a low or high elaboration.
Insight on discussed theories and principles
Applying described theories to cyber domains should help to identify targets by understanding opportunities of a crime. This can be a subject of asset management and risk assessment. What are the crown jewels? And what are their vulnerabilities? Should a company decoy offenders or harden the targets? Who may be interested in hacking them? A hacker type and technique are to be identified. A much better than a current situation in which those questions are asked during an incident response. Those theories can also explain an initiation of deviant behavior, maintenance of a behavior, and a motive of a cybercrime. They consider social and environmental factors that could be missed when preparing a prevention program. Little research is done in this field. One example is research can explore those theories’ use to develop simple models like Persona non Grata that identify adversaries who can be inside or outside security perimeters. Integrating different theories can further classify a deviant behavior as a misbehavior or a beginning of an imminent attack. It seems that creating a social advocacy group and cyber awareness can help improve users’ intentions and attitudes. Strong social bonds are much better than weaker social bonds. We also discussed decision making and understanding alternatives and norms. Weapons of influence are used by intruders, and the defenders lack the research to use them to defend confidentiality, integrity, and availability. The paper of Faklaris (2018) has suggestions on using weapons of influence to support IT professionals. The Commonly used attack vectors by social engineers are phishing (by email), vishing (phone call), impersonation and smishing (text message).
- Human factors
Relate human factors to cybersecurity
For the Human Factors, researchers can learn from the health and aviation industries since they have extensive work in this discipline. Human factors is the discipline that works to optimize the relationship between the humans and technology. We pick the Map-Assess-Recognize-Conclude (MARC) process shown in Fig. 6 and found in Parush et al. (2017) to address behavioral aspects and focus on human error.
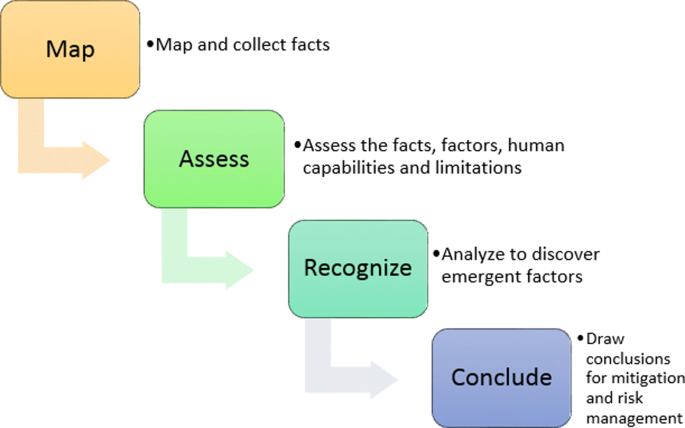
Interpretation of MARC process, based on Parush et al. (2017)
Mapping the user and the environment requires asking a set of questions on their characteristics, roles, knowledge, skills, experience, tasks, responsibility, personality traits, access points and locations, human machine interface, etc. Assessment can analyze known factors, collect facts on user capabilities and limitations, and the working environment. While assessing, one can recognize the emerging factors that were not initially included in the mapping and can cause a human error. The two types of emergent factors are environmental (physical and human) and human (psychological, physical). For example, fatigue or distraction can contribute to unintentional mistake, and loss of vigilance can cause intentional mistakes. Fatigue, distraction and loss of vigilance could be emergent factors. Norman argues that humans will make errors in the best designed systems so the systems should be designed to minimize the effect of the error ( Norman 1983 ). We agree with this view, as human errors are known to cause a variety of accidents in various industries and organizations. In aviation, twelve human errors or dirty dozen that lower people’s ability of performance and safety, which could lead to maintenance errors are: lack of communication, complacency, lack of knowledge, distraction, lack of teamwork, fatigue, lack of resources, pressure, lack of assertiveness, stress, lack of awareness, and norms ( Dupont 1997 ). We can easily relate those factors to cybersecurity.
Lack of communication is a problem for any organization. The survey by Ponemon Institute LLC (2014 ) found that 51% report lack of information from security solutions and are unsure if their solution can tell the cause of an attack. Lack of communication can certainly affect awareness negatively. Human factor integration can contribute to environmental situations involving work shifts, communication during emergencies, communication of concerns and risks to contractors, identification of tools, and communication of changes to procedures and plans. The main aim is to not miss important information, or create misunderstandings, or increase cost due to dealing with unhelpful information. Complacency can cause false confidence at both organizational level and at the user level. A user can feel confident because current behavior did not cause a breach, yet it does not mean that intentional wrong doing would not cause a future breach. Lack of knowledge can cause unintentional mistake such as not logging off accounts, or writing difficult to memorize password on a paper, etc. Distraction was already mentioned as a mistake and as a tactic of an attack. Lack of team work can cause a breach because hackers have an understanding on how IT teams work, and they can take advantage of their dysfunction. Fatigue was already mentioned as a problem factor. The environment in which the user is working can cause pressure and stress while it does not provide actionable policies or training to strengthen weaknesses. We discussed in SCT that environment affects behavioral factors. Lack of assertiveness can be connected to communication and self-efficacy. Lack of assertiveness can lead to not communicating directly with teammates potential concerns, or proposing possible solutions, or asking for a feedback. Lack of awareness can be caused by not being vigilant. Norms were discussed in Normative Behavior theory, and the user can conduct negative or unsafe behavior, or take a wrong action in ambiguous cases.
Insight based on chemical industry
Behavioral cybersecurity can benefit from the pitfalls recognized by human factors in other industries. We mention here our insight as an interpretation of human errors in cybersecurity based on common mistakes that happen in chemical industry sites, that are labeled as major hazard sites ( Noyes 2011 ). A parallel comparison of major vulnerable cyber environment to a major hazard site is the following:
Cyber defenders and users are not superhuman, and may not be able to intervene heroically in emergencies. The incident response team is formed by many members and its efficiency depends on many factors such as the team’s budget, training, whether teams are internal or external, available tools, etc. Actually, more research is needed on resilience and agility function of those response teams.
Not documenting assumptions or data sources when documenting probabilities of human failure. As mentioned previously, designs and plans are usually geared towards rational cyber-actors.
Assuming that a defender will always be present, detect a problem and immediately take an appropriate action.
Assuming that users and defenders are well-trained to respond to incidents. Note that training does not prevent violations.
Assuming that defenders and users will always follow procedures.
Assuming that defenders and users are highly motivated and thus not prone to unintentional errors or malicious violations.
Ignoring the human element, especially human performance as if the cyberspace is unmanned.
Inappropriate use of defense tools and losing sight of techniques or tools where they are the most effective.
Not knowing how to manage human error.
Moreover, we interpret three concerns that match with our literature review based on Noyes (2011) :
The focus is more on technology than human aspects.
Ignoring initial vulnerabilities in design and development of systems and focus on training.
Blame incidents on a user with or without investigating the system and management failures.
Modeling and simulation
Network security and all the tools associated with it do not provide perfect security. In fact, perfect security does not exist. Hence, there is a continuous need to develop new solutions and tools and test them. This is where modeling and simulation are helpful to save time and keep the cost down while creating test-beds or environments in which those new tools or strategies are tested. Several tools are already established for network simulation since the 1990s such as Network Simulation Testbed (NEST), Realistic and Large (REAL), OMNeT++, SSFNet, NS2, NS3, J-Sim, OPNET and QualNet ( Niazi 2019 ). Yet, not many of these tools are created to address the human element. The main challenge is to validate reliability and dependability of simulation in a comparison to real-life scenarios or data sets. The anonymity problem makes the challenge more difficult. The author in Cohen (1999) discussed the complexity issue in modeling; a simple model may not be as accurate, and the fully detailed models of every threat and defense mechanisms may have higher accuracy but are costly. Exploring answers to many questions about hackers’ or insiders’ behaviors could help research (or enterprises) to use modeling and simulation to detect anomalies and respond. For instance, what are all possible user behaviors? (Start an application, send a ping, open a file, etc.), what are acceptable or normal behaviors? (Open an authorized file, start an application, etc.), and what are unacceptable behaviors? (Open or attempt to open an unauthorized file, ping, send a bulk of pages to a printer, and browse irrelevant sites that probably can come from copying and pasting disable emails URLs, etc).
The theoretical models of human behavior have been developed and some examples are stated in Goerger (2004) :
(1) Baysian-networks are useful to reason from effects to causes or from causes to effects or by a mixed of inferences. Baysian networks are directed graphs and their models belong to the family of probabilistic graphical models. They can be used to simulate the impact of actions or motives, and build in action to mitigate the overall risk. Researchers have used Bayesian network models in intrusion detection systems. Those models have the flexibility to be combined with other techniques, yet authors in Xie et al. (2010) warn that the combination should be done with preserving Bayesian networks strength to identify and represent relevant uncertainties. Many of the behavioral theories can be tested by simulation. In Dutt et al. (2013) , Instance-Based Learning Theory predicts that both defender and adversary behaviors are likely to influence the defender’s accurate and timely detection of threats. The defender’s cyber awareness is affected by the defender’s cognitive abilities (experience and tolerance) and attacker’s strategy (timing of threats).
(2) A neural-network is a set of algorithms, that are designed to recognize patterns based on a cognitive model or try to mimic the properties of the human brain. Neural-network models are relatively fast, but require a training set to learn and apply learning in operating mode. There are several types of neural network and they are surveyed in Berman et al. (2019) and Parveen (2017) . They have useful applications in security and are already used in intrusion detection systems for anomaly detection ( Parveen 2017 ). Their work can be expanded in similar ways that banks currently using them to detect fraudulent transactions. Hence, they can be trained to detect abnormal behaviors. Yet, they still face the challenge of being used as a black box. The recommendation is to use them in combination with artificial intelligence or other models.
(3) While an agent based system could identify characteristics of the environment, it might be able to link user-based actions with their destructive impact on systems. Agent-based modeling is used by social scientists to analyze human behavior and social interactions. Those models are useful to study complex systems and the interaction of the networks can be shown using visualization methods.
(4) Multi-Agent System is a behavior model in which agents can act autonomously on behalf of their users. Agents can work individually or cooperatively. The Multi-Agent System is used recently in studying smart grid communication protocols.
(5) A rule-based or knowledge based system endeavors to imitate human behavior using an enumeration of steps with causal if/then association. Hence, there is precoding of possible situations. This causes a problem where rules are not determined before. Rule-based models are used in detecting anomalies in intrusion detection systems. In Chen and Mitchell (2015) , authors proposed a methodology to transform behavior rules used for intrusion detection to a state machine.
Conclusion and future work
Behavioral aspects of cybersecurity are becoming a vital area to research. The unpredictable nature of human behavior and actions make Human an important element and enabler of the level of cybersecurity. The goal from discussing reviewed theories is to underscore importance of social, behavior, environment, biases, perceptions, deterrence, intent, attitude, norms, alternatives, sanctions, decision making, etc. in understanding cybercrimes. Although those theories have some limitations, they can still collectively be used to strengthen a behavioral model. Both the user’s and the offender’s behaviors and intentions should be understood and modeled. Improving this area will definitely help improve readiness and prevent incidents. No system is 100% secure, but maximizing security cannot happen without considering the human element. The motto of Trust, but Verify mentioned by President Ronald Reagan applies to cybersecurity. There is a level of trust that is going to be put on a cyber domain in order to be able to work with it, however an ongoing verification is necessary. Employees have to be knowledgeable of the risks, and differentiate desired from undesired behaviors. Yet, some employees may not comply because of implementing techniques of neutralization. Cyber awareness training should be personalized because employees may have different credentials or levels of access and responsibilities. They also have their own biases to security. One size fits all awareness programs are not effective. There is a level of trust that needs to be put on employees, however, technology and cyber awareness must be taught, and a verification of compliance is necessary. More training is not always the solution. A conceptual framework that is interdisciplinary is proposed to bring together behavioral cybersecurity, human factors and modeling and simulation. Enterprises should be involved in research to make sure that models work the way they are intended. Using a model that is available for the sake of convenience without personalizing it may not be proper. George E. P. Box quote,
"All models are wrong, but some are useful"
should motivate researchers and organizations to ask more questions about the usefulness of a model, which in return promotes revising policies and approaches to security. Therefore, coordinating behavioral aspects and technical aspects of cybersecurity should be typical to each organization. Our future work will contribute to the three main concerns stated at the end of Section 3 . For instance, we will explore cyber incidents such as insider threat from the perspective of human error using the proposed framework. A concept model is depicted in Fig. 7 .
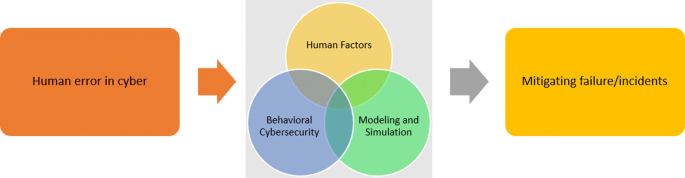
Mitigating human error concept model using proposed framework
The model can also support mitigating failure due to social engineering, or weapons of influence. Hence, future work will support a different kind of cyber ontologies. We will also study deception games using game theory with different attacker-defender scenarios. The final statement is remain vigilant and be prepared to expect the unexpectable.
Availability of data and materials
No data is used in this paper.
Abbreviations
Analytical hierarchy process
Analytical network process
Auto-associative memory columns
Cross site scripting
Distributed denial of service
Human plausible reasoning theory
Intrusion detection system
Just in time
Map-assess-recognize-conclude
Measure of effectiveness
National institute of standards and technology
Situational crime prevention
Social cognition theory
Structured query language
Technology acceptance model
Theory of planned behavior
Unintentional - intentional - malicious
Addae, JH, Sun X, Towey D, Radenkovic MExploring user behavioral data for adaptive cybersecurity. User Model User-Adap Inter 29(3):701–750. https://doi.org/10.1007/s11257-019-09236-5 .
Ahram, T, Karwowski W (2019) Advances in Human Factors in Cybersecurity In: AHFE: International Conference on Applied Human Factors and Ergonomics, 66–96.. Springer, Washington D.C.https://doi.org/10.4018/978-1-5225-9742-1.ch003.
Google Scholar
Apvera (2018) The Essential Guide to Risk Management & Compliance (GRC) 2018, Tech. rep.. Apvera.
Azaria, A, Richardson A, Kraus S, Subrahmanian VS (2014) Behavioral analysis of insider threat: A survey and bootstrapped prediction in imbalanced data. IEEE Trans Comput Soc Syst 1(2):135–155. https://doi.org/10.1109/TCSS.2014.2377811 .
Article Google Scholar
Berman, DS, Buczak AL, Chavis JS, Corbett CL (2019) A survey of deep learning methods for cyber security. Inf (Switzerland) 10(4). https://doi.org/10.3390/info10040122 .
Blackborrow, J, Christakis S (2019) Complexity In Cybersecurity Report 2019 - How Reducing Complexity Leads To Better Security Outcomes. Tech. Rep. May, Forrester’s Security & Risk research group.
Burns, S, Roberts L (2013) Applying the Theory of Planned Behaviour to predicting online safety behaviour. Crime Prev Community Saf 15(1):48–64. https://doi.org/10.1057/cpcs.2012.13 .
Cacioppo, JT, Petty RE (2001) The elaboration likelihood model of persuasion. Adv Exp Soc Psychol 19:673–676. https://doi.org/10.1558/ijsll.v14i2.309 .
Cappelli, D, Moore A, Trzeciak R (2014) The CERT Guide to Insider Threats How to Prevent, Detect, and Respond to Information Technology Crimes (Theft, Sabotage, Fraud) In: SEI Series in Software Engineering represents, 2nd edn.. Addison-Wesley, Westford, Massachusetts.
Caulkins, B (2017) Lecture title Modeling and Simulation of Behavioral Cybersecurity, Retrieved on December 26, 2018 from IDC 5602 Cybersecurity: A Multidisciplinary Approach.
Chen, IR, Mitchell R (2015) Behavior Rule Specification-Based Intrusion Detection for Safety Critical Medical Cyber Physical Systems. IEEE Trans Dependable Secure Comput 12(1). https://doi.org/10.1109/tdsc.2014.2312327 .
Cheng, L, Li W, Zhai Q, Smyth R (2014) Understanding personal use of the Internet at work: An integrated model of neutralization techniques and general deterrence theory. Comput Hum Behav 38:220–228. https://doi.org/10.1016/j.chb.2014.05.043 .
Cohen, F (1999) Simulating Cyber Attacks, Defences, and Consequences Modeling, Simulation, and Data Limitations in Information Protection. Comput Secur 18:479–518.
Corner, A, Hahn U (2013) Normative theories of argumentation: Are some norms better than others?Synthese 190(16):3579–3610. https://doi.org/10.1007/s11229-012-0211-y .
Dinev, T, Hu Q (2007) The Centrality of Awareness in the Formation of User Behavioral Intention toward Protective Information Technologies. J Assoc Inf Syst 8(7):386–408. https://doi.org/10.17705/1jais.00133 .
Donaldson, S, Siegel S, Williams CK, Aslam A (2015) Enterprise Cybersecurity - How to Build a Successful Cyberdefense Program Against Advanced Threats. Apress Media LLC, New York.
Dupont, G (1997) Human Error In Aviation Maintenance. https://nam02.safelinks.protection.outlook.com/?url=https%3A%2F%2Fwww.faa.gov%2Fabout%2Finitiatives%2Fmaintenance_hf%2Flibrary%2Fdocuments%2Fmedia%2Fhuman_factors_maintenance%2Fhuman_error_in_aviation_maintenance.pdf&data=02%7C01%7Crachid%40ucf.edu%7C12bb36a6d43b4079629208d7cc912306%7Cbb932f15ef3842ba91fcf3c59d5dd1f1%7C0%7C0%7C637202796057556002&sdata=xRO8qOMjAMovJLrEJMArj4%2B%2BYHTO6Pl9FdyDO9UQJR4%3D&reserved=0 . Accessed 28 Dec 2019.
Dutt, V, Ahn YS, Gonzalez C (2013) Cyber situation awareness: Modeling detection of cyber attacks with instance-based learning theory. Hum Factors 55(3):605–618. https://doi.org/10.1177/0018720812464045 .
Embrey, D, Kontogiannis T, Green M (1994) Guidelines for Preventing Human Error in Process Safety. Am Inst Chem Eng. https://doi.org/10.1002/9780470925096 .
Faklaris, C (2018) Social Cybersecurity and the Help Desk : New Ideas for IT Professionals to Foster Secure Workgroup Behaviors. Baltimore, MD: USENIX Symposium on Usable Privacy and Security.
Ferreira, A, Coventry L, Lenzini G (2015) Principles of Persuasion in Social Engineering and Their Use in Phishing. Springer International Publishing. https://doi.org/10.1007/978-3-319-20376-8_4 .
Filkins, B (2014) New Threats Drive Improved Practices: State of Cybersecurity in Health Care Organizations. Sans Inst. https://www.qualys.com/docs/sans-threats-drive-improved-practices-state-of-cybersecurity-health-care-organizations.pdf . Accessed 30 Mar 2020.
Fineberg, V (2014) BEC: Applying Behavioral Economics to Harden Cyberspace. J Cyber Secur Inf Syst 2(1):27–33.
Freilich, JD, Newman GR, Freilich JD, Newman GRSituational Crime Prevention In: Oxford Research Encyclopedia of Criminology and Criminal Justice, February 2020, 1–28. https://doi.org/10.1093/acrefore/9780190264079.013.3 .
Friedman, S, Gokhale N (2019) Pursuing cybersecurity maturity at financial institutions: Survey spotlights key traits among more advanced risk managers. Tech. rep. Deloitte Center for Financial Services analysis.
FTC (2019) Equifax Data Breach Settlement. https://www.ftc.gov/enforcement/cases-proceedings/refunds/equifax-data-breach-settlement . Accessed 27 Dec 2019.
Goerger, SR (2004) Validating human behavioral models for combat simulations using techniques for the evaluation of human performance. Tech. Rep. 3. Naval Postgraduate School, MOVES Institute, Monterey, CA.
Gottfredson, M (2017) Self-Control Theory and Crime. https://doi.org/10.1093/acrefore/9780190264079.013.252 .
Greitzer, FL, Hohimer RE (2011) Modeling Human Behavior to Anticipate Insider Attacks. J Strat Secur 4(2):25–48. https://doi.org/10.5038/1944-0472.4.2.2 . http://scholarcommons.usf.edu/jss/vol4/iss2/3/ .
Hald, SL, Pedersen JM (2012) An updated taxonomy for characterizing hackers according to their threat properties. Int Conf Adv Commun Technol ICACT:81–86.
Halevi, T, Lewis J, Memon N (2013) Phishing, Personality Traits and Facebook. https://doi.org/10.1111/j.1469-0691.2005.01161.x . http://arxiv.org/abs/1301.7643.
Hardy, AB, Howells G, Bandura A, Adams NE (1980) Tests of the generality of self-efficacy theory. Cogn Ther Res 4(1):39–66.
Haycock, K, Matthews JR (2016) Persuasive Advocacy. Public Libr Q 35(2):126–135. doi:10.1080/01616846.2016.1200362.
Holt, TJ (2016) Cybercrime through an interdisciplinary lens. Routledge Taylor & Francis Group. https://doi.org/10.4324/9781315618456 .
Icek, A (2019) Theory of Planned Behavior Diagram. http://people.umass.edu/aizen/tpb.diag.html . Accessed 7 Sept 2019.
Kabassi, K, Virvou M (2015) Combining decision-making theories with a cognitive theory for intelligent help: A comparison. IEEE Trans Hum Mach Syst 45(2):176–186. https://doi.org/10.1109/THMS.2014.2363467 .
Kemmerer, M (2016) Detecting the Adversary Post- Compromise with Threat Models and Behavioral Analytics. https://www.mitre.org/sites/default/files/publications/pr-16-3058-presentation-detecting-adversary-post-compromise.pdf . Accessed 27 Dec 2019.
Lahcen, RAM, Mohapatra R, Kumar M (2018) Cybersecurity: A survey of vulnerability analysis and attack graphs In: International Conference on Mathematics and Computing, 97–111.. Springer.
Maimon, D, Louderback ER (2019) Cyber-Dependent Crimes: An Interdisciplinary Review. Ann Rev Criminol 2(1):191–216. https://doi.org/10.1146/annurev-criminol-032317-092057 .
Maiwald, E, Sieglein W (2002) Security Planning & Disaster Recovery. Brandon A. Nordin, Berkeley, California.
Mitnick, KD, Simon WL (2005) The art of intrusion : the real stories behind the exploits of hackers, intruders, & deceivers. Wiley.
Myers, J, Grimaila MR, Mills RF (2009) Towards insider threat detection using web server logs In: Proceedings of the 5th Annual Workshop on Cyber Security and Information Intelligence Research Cyber Security and Information Intelligence Challenges and Strategies - CSIIRW ’09, 1. http://portal.acm.org/citation.cfm?doid=1558607.1558670 .
Niazi, MA (2019) Modeling and Simulation of Complex Communication Networks. Modeling and Simulation of Complex Communication Networks Edited by Muaz A. Niazi. The Institution of Engineering and Technology, London. https://doi.org/10.1049/pbpc018e .
Book Google Scholar
Norman, D (1983) Design Rules Based on Analyses of Human Error. Commun ACM 26(4):254–259.
Noyes, J (2011) The human factors toolkit Human factors in the management of major accident hazards. https://doi.org/10.1049/pbns032e_ch4 .
Pabian, S, Vandebosch H (2013) Using the theory of planned behaviour to understand cyberbullying. Eur J Dev Psychol 11(4):463–477. https://doi.org/10.1080/17405629.2013.858626. T4 - The importance of beliefs for developing interventions M4 - Citavi.
Pal, SK, Anand S (2018) InfoSec : A Comprehensive Study. IUP J Comput Sci XII:45–65.
Partners, CR (2015) Insider Threat Spotlight Report. Tech. rep. Crowd Research Partners.
Parush, A, Parush D, Ilan R (2017) Human factors in healthcare: a field guide to continuous improvement. Morgan & Claypool.
Parveen, J (2017) Neural Networks in Cyber Security. Int Res J Comput Sci 9(4):2015–2018.
MathSciNet Google Scholar
Payne, BK, Hadzhidimova L (2018) Cyber security and criminal justice programs in the United States: Exploring the intersections. Int J Crim Justice Sci 13(2):385–404.
Pfleeger, SL, Caputo DD (2012) Leveraging behavioral science to mitigate cyber security risk. Comput Secur 31(4):597–611. https://doi.org/10.1016/j.cose.2011.12.010 .
Pogue, C (2018) Decoding the minds of hackers. https://www.nuix.com/black-report/black-report-2018 .
Ponemon Institute LLC (2014) Exposing the Cybersecurity Cracks : A Global Perspective. Tech. Rep. April. Ponemon Institute LLC.
Reardon, S (2011) Antismoking drive tries cigarette ads, in reverse. Science 333(6038):23–24. https://doi.org/10.1126/science.333.6038.23 . https://science.sciencemag.org/content/333/6038/23 .
Rodriguez, MA, Bell J, Brown M, Carter D (2017) Integrating Behavioral Science with Human Factors to Address Process Safety. J Organ Behav Manag 37:301–315.
Shetty, SS, Shetty RR, Shetty TG, D’Souza DJ (2018) Survey of hacking techniques and it’s prevention. IEEE Int Conf Power Control Signals Instrum Eng ICPCSI 2017:1940–1945. https://doi.org/10.1109/ICPCSI.2017.8392053 .
Siponen, M, Vance A (2010) Neutralization: New insights into the problem of employee information systems security policy violations. MIS Q 34(3):487–502. https://doi.org/10.1038/174197b0 .
Stanton, JM, Stam KR, Mastrangelo P, Jolton J (2005) Analysis of end user security behaviors. Comput Secur 24(2):124–133. https://doi.org/10.1016/j.cose.2004.07.001 .
Stolfo, SJ, Bellovin SM, Hershkop S, Keromytis AD, Sinclair S, Smith SW (2008) Advances in information security: Insider attack and cyber security - Beyond the hacker. Springer, New York.
Symantec (2017) Internet Security Threat Report ISTR 22 Government Internet Security Threat Report. https://www.symantec.com/content/dam/symantec/docs/reports/istr-22-2017-en.pdf . Accesssed 27 Dec 2019.
Theoharidou, M, Kokolakis S, Karyda M, Kiountouzis E (2005) The insider threat to information systems and the effectiveness of iso17799. Comput Secur 24(6):472–484.
Triantaphyllou, E, Kovalerchuk B, Mann L, Knapp GM (1997) Determining the most important criteria in maintenance decision making. J Qual Maint Eng 3(1):16–28. https://doi.org/10.1108/13552519710161517 .
Triantaphyllou, E, Mann SH (1995) Using the analytic hierarchy process for decision making in engineering applications: some challenges. Int J Ind Eng Appl Pract 2(1):35–44.
Uebelacker, S, Quiel S (2014) The social engineering personality framework In: Proceedings - 4th Workshop on Socio-Technical Aspects in Security and Trust, STAST 2014 - Co-located with 27th IEEE Computer Security Foundations Symposium, CSF 2014 in the Vienna Summer of Logic 2014, January, 24–30. https://doi.org/10.1109/STAST.2014.12 .
Wilamowski, GC, Dever JR, Stuban SMF (2017) Using Analytical Hierarchy and Analytical Network Processes to Create CYBER SECURITY METRICS. Defense ARJ. https://doi.org/10.22594/dau.16-760.24.02 .
Willetts, D (2014) 2014 Information Security Breaches Survey: Technical Report. Tech. rep. Department of Business Innovation & Skills.
Xie, P, Li JH, Ou X, Liu P, Levy R (2010) Using Bayesian networks for cyber security analysis In: Proceedings of the International Conference on Dependable Systems and Networks, 211–220. https://doi.org/10.1109/DSN.2010.5544924 .
Xu, M, Schweitzer KM, Bateman RM, Xu S (2018) Modeling and Predicting Cyber Hacking Breaches. IEEE Trans Inf Forensic Secur 13(11):2856–2871. https://doi.org/10.1109/TIFS.2018.2834227 .
Download references
Acknowledgements
The authors would like to thank the journal for the opportunity to publish an open access paper, and many thanks to the outstanding reviewers for their hard work and feedback.
The authors declare that this work was not funded.
Author information
Authors and affiliations.
University of Central Florida, Mathematics Department, Orlando, 32816, FL, USA
Rachid Ait Maalem Lahcen & Ram Mohapatra
Institute for Simulation and Training, 3100 Technology Pkwy, Orlando, 32826, FL, USA
Bruce Caulkins
Birla Institute of Technology and Sciences - Pilani, Hyderabad Campus, Hyderabad, 500078, Telangana, India
Manish Kumar
You can also search for this author in PubMed Google Scholar
Contributions
Authors’ contributions.
All authors contributed to different parts of the manuscript. They participated in revising and approving revisions. The author(s) read and approved the final manuscript.
Authors’ information
Rachid Ait Maalem Lahcen is a Mathematics Instructor at University of Central Florida (UCF) Orlando Florida. He holds a Master of Sciences in Mechanical Engineering, a Master of Sciences in Modeling & Simulation, a graduate certificate in Mathematics, and a graduate certificate in Modeling and Simulation of Behavioral Cybersecurity. All from UCF. His research interests are cybersecurity, graph network, inverse problems, numerical methods and students’ learning.
Bruce Caulkins is a Research Assistant Professor and Director of the Modeling & Simulation (M&S) of Behavioral Cybersecurity Program at the Institute for Simulation & Training (IST) at the University of Central Florida (UCF). He is a retired Army Colonel with over 28 years of experience in tactical, operational, and strategic communications and cyberspace operations. In his last military assignment, he was the Chief of the Cyber Strategy, Plans, Policy, and Exercises Division (J65) within the U.S. Pacific Command. In this capacity, he gained extensive insight into cyber capabilities, operational requirements, combatant command requirements, coalition and partner cyber/communications interoperability, and human factor requirements. He also led over a dozen coalition and partner interoperability exercises, to include the HADR-focused PACIFIC ENDEAVOR. Bruce previously taught at and ran several communications and cyber-related schools within the Army’s Training and Doctrine Command. He earned his Ph.D. in Modeling and Simulation at the University of Central Florida, focusing on anomaly detection within intrusion-detection systems. His research interests include behavioral aspects of cybersecurity; threat modeling; cyber workforce development; anomaly detection; cyber security and analysis; cyber education and training methodologies; predictive modeling; data mining; cyber strategy; and, cyber policy.
Ram Mohapatra received his Ph.D. from Jabalpur University India, and taught in American University of Beirut, University of Alberta, Edmonton, York University, Downsview, and at the University of Central Florida, Orlando from 1984where he serves as a Professor of Mathematics. His research interests are in Summability Theory and Sequence Spaces, Fourier Analysis and wavelets, Frame and Approximation Theory, Variational Inequalities and Optimization Theory, Harmonic Functions and Complex Analysis. He has written over 150 research papers in refereed journals. His current research interest is Cyber Security and Graph Theory. In addition to the journal papers, he has written many book chapters, edited seven monographs/ proceedings of conferences, and written two books: one on Fuzzy Differential Equations and the other on Biomedical Statistics with computing. He serves as a member of the editorial Board of five journals in Mathematics.
Manish Kumar is presently working as assistant professor in the Department of Mathematics at the Birla Institute of Technology and Science, Pilani at Hyderabad campus, Hyderabad, Telangana, India. Dr. Kumar obtained his Master of Science in Mathematics from Banaras Hindu University, Varanasi, Ph. D. in Department of Applied Mathematics at Indian School of Mines, Dhanbad, and received various awards. Dr. Kumar is guiding several undergraduate students and published various research papers in national and international journals of repute. Dr. Kumar had chaired a session at the International Congress in Honor in Faculty of Arts and Science, Department of Mathematics in Bursa, Turkey, and also organized a Symposium in ICNAAM 2013 at Rhodes in Greece. Dr. Kumar is member of several national and international professional bodies and societies. Dr. Kumar has visited and delivered invited talks in several national and international conferences, including his recent talk on “Two stage hyper-chaotic system based image encryption in wavelet packet domain for wireless communication systems” at ICM 2018 in Rio de Janeiro, Brazil. Dr. Kumar research areas are pseudo-differential operators, distribution theory, wavelet analysis and its applications, digital image processing, and cryptography.
Corresponding author
Correspondence to Rachid Ait Maalem Lahcen .
Ethics declarations
Competing interests.
The authors declare that they have no competing interests.
Additional information
Publisher’s note.
Springer Nature remains neutral with regard to jurisdictional claims in published maps and institutional affiliations.
Rights and permissions
Open Access This article is licensed under a Creative Commons Attribution 4.0 International License, which permits use, sharing, adaptation, distribution and reproduction in any medium or format, as long as you give appropriate credit to the original author(s) and the source, provide a link to the Creative Commons licence, and indicate if changes were made. The images or other third party material in this article are included in the article’s Creative Commons licence, unless indicated otherwise in a credit line to the material. If material is not included in the article’s Creative Commons licence and your intended use is not permitted by statutory regulation or exceeds the permitted use, you will need to obtain permission directly from the copyright holder. To view a copy of this licence, visit http://creativecommons.org/licenses/by/4.0/ .
Reprints and permissions
About this article
Cite this article.
Maalem Lahcen, R.A., Caulkins, B., Mohapatra, R. et al. Review and insight on the behavioral aspects of cybersecurity. Cybersecur 3 , 10 (2020). https://doi.org/10.1186/s42400-020-00050-w
Download citation
Received : 06 October 2019
Accepted : 10 March 2020
Published : 21 April 2020
DOI : https://doi.org/10.1186/s42400-020-00050-w
Share this article
Anyone you share the following link with will be able to read this content:
Sorry, a shareable link is not currently available for this article.
Provided by the Springer Nature SharedIt content-sharing initiative
- Cybersecurity
- Behavioral aspects
- Crime theories
Did cybercrime cause the crime drop?
Affiliation.
- 1 School of Law, University of Leeds, Leeds, LS2 9JT UK.
- PMID: 30956932
- PMCID: PMC6428390
- DOI: 10.1186/s40163-018-0082-8
Recent studies have hypothesised that the international crime drop was the result of the rise in cybercrime. We subject this 'cybercrime hypothesis' to critical assessment. We find significant evidence and argument indicating that cybercrime could not have caused the crime drop, and so we reject the cybercrime hypothesis.
Keywords: Crime decline; Crime drop; Cybercrime; Displacement; Internet fraud; Offender adaptation; Security hypothesis.
Advertisement
Cybercrime, Differential Association, and Self-Control: Knowledge Transmission Through Online Social Learning
- Published: 08 November 2021
- Volume 46 , pages 935–955, ( 2021 )
Cite this article
- Thomas E. Dearden ORCID: orcid.org/0000-0003-0549-927X 1 &
- Katalin Parti ORCID: orcid.org/0000-0002-8484-3237 1
2310 Accesses
8 Citations
1 Altmetric
Explore all metrics
In an increasingly digital world, our social interactions are increasingly moving online. Differential association and social learning theories suggest that we learn both moral definitions and the how-to of crime from those we associate with. In this paper we examine whether online or offline social learning leads to more self-disclosed forms of cyber-offending. Using a national online sample of 1,109 participants, we find both online and offline social learning are important correlates to cyber-offending. In addition, we predict that lower self-control will interact with social learning to further increase the likelihood of cyber-offending. Overall, we find that both social learning and self-control, individually and as an interaction, have a large effect-size in predicting cyber-offending.
This is a preview of subscription content, log in via an institution to check access.
Access this article
Price includes VAT (Russian Federation)
Instant access to the full article PDF.
Rent this article via DeepDyve
Institutional subscriptions
Similar content being viewed by others
Social Identity Theory
Advances in social media research: past, present and future.
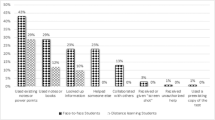
Cheating is in the Eye of the Beholder: an Evolving Understanding of Academic Misconduct
Acar, A. (2008). Antecedents and consequences of online social networking behavior: The case of Facebook. Journal of Website Promotion, 3 (1-2), 62–83. https://doi.org/10.1080/15533610802052654
Article Google Scholar
Akers, R. L. (2009). Social learning and social structure: A general theory of crime and deviance. New Brunswick, NJ: Transaction.
Akers, R.L. (1998). Social learning and social structure: A general theory of crime and deviance. Boston, MA: Northeastern University Press.
Baek, H. (2018). Computer-specific parental management and online deviance across gender in South Korea: A test of self-control theory. International Journal of Cyber Criminology, 12 (1), 68–83. https://doi.org/10.5281/zenodo.1467844
Bettencourt, A. (2014). Empirical Assessment of Risk Factors: How Online and Offline Lifestyle, Social Learning, And Social Networking Sites Influence Crime Victimization. In BSU Master’s Theses and Projects. Item 10. Available at http://vc.bridgew.edu/theses/10 . Accessed 13 November 2020.
Brinker, D. L., Gastil, J., & Richards, R. C. (2015). Inspiring and informing citizens online: A media richness analysis of varied civic education modalities. Journal of Computer-Mediated Communication, 20 (5), 504–519. https://doi.org/10.1111/jcc4.12128
Boeringer, S., Shehan, C. L., & Akers, R. L. (1991). Social contexts and social learning in sexual coercion and aggression: Assessing the contribution of fraternity membership. Family Relations, 40 (1), 558–564.
Buker, H. (2011). Formation of self-control: Gottfredson and Hirschi's general theory of crime and beyond. Aggression and Violent Behavior, 16 (3), 265–276. https://doi.org/10.1016/j.avb.2011.03.005
Burgess, R. L., & Akers, R. L. (1966). A differential association reinforcement theory of criminal behavior. Social Problems, 14 (2), 128–147. https://doi.org/10.1525/sp.1966.14.2.03a00020
Burt, C. H., Simons, R. L., & Simons, L. G. (2006). A longitudinal test of the effects of parenting and the stability of self-control: Negative evidence for the general theory of crime. Criminology, 44 (2), 353–396. https://doi.org/10.1111/j.1745-9125.2006.00052.x
Burton Jr., V. S., Cullen, F. T., Evans, D. T., & Dunaway, R. G. (1994). Reconsidering strain theory: Operationalization, rival theories, and adult criminality. Journal of Quantitative Criminology, 10 (3), 213–239.
Burruss, G. W., Bossler, A. M., & Holt, T. J. (2012). Assessing the mediation of a fuller social learning model on low self-control’s influence on software piracy. Crime and Delinquency, 59 (8), 1157–1184. https://doi.org/10.1177/0011128712437915
Clevenger, S. L., Navarro, J. N., & Jasinski, J. L. (2014). A matter of low self-control? Exploring differences between child pornography possessors and child pornography producers/distributers using self-control theory. Sexual Abuse, 28 (6), 555–571. https://doi.org/10.1177/1079063214557173
Cohran, J. K., Sellers, C. S., Wiesbrock, V., & Palacios, W. R. (2011). Repetitive intimate partner victimization: An exploratory application of social learning theory. Deviant Behavior, 32 (9), 790–817. https://doi.org/10.1080/01639625.2010.538342
Daft, R. L., & Lengel, R. H. (1986). Organizational information requirements, media richness and structural design. Management Science, 32 (5), 554–571. https://doi.org/10.1287/mnsc.32.5.554
Daft, R. L., Lengel, R. H., & Trevino, L. K. (1987). Message equivocality, media selection and manager performance: Implications for information systems. Management Information Systems Quarterly, 11 , 355–366. https://doi.org/10.2307/248682
Davenport, T.H., & Beck, J.C. (2002). The Attention Economy. Understanding the New Currency of Business. United Kingdom: Harvard Business School Press
Donner, C. M., Marcum, C. D., Jennings, W. G., Higgins, G. E., & Banfield, J. (2014). Low Self-Control and Cybercrime: Exploring the Utility of the General Theory of Crime beyond Digital Piracy. Computers in Human Behavior, 34 , 165–172. https://doi.org/10.1016/j.chb.2014.01.040
Duckworth, A. L., Gendler, T. S., & Gross, J. J. (2016). Situational strategies for self-control. Perspectives on Psychological Science, 11 (1), 35–55. https://doi.org/10.1177/1745691615623247
Duckworth, A. L., Gendler, T. S., & Gross, J. J. (2014). Self-control in school-age children. Educational Psychologist, 49 , 199–217. https://doi.org/10.1080/00461520.2014.926225
Evans, J., & Mathur, A. (2005). The value of online surveys. Internet Research, 15 (2), 195–219.
Fox, K. A., Nobles, M. R., & Akers, R. L. (2011). Is stalking a learned phenomenon? An empirical test of social learning theory. Journal of Criminal Justice, 39 (1), 39–47. https://doi.org/10.1016/j.jcrimjus.2010.10.002
Gagnon, A. (2018). Extending Social Learning Theory to Explain Victimization Among Gang and Ex-Gang Offenders. International Journal of Offender Therapy and Comparative Criminology, 62 (13), 4124–4141. https://doi.org/10.1177/0306624X18763761
GlobalWebIndex (2020). Social. GlobalWebIndex’s Flagship Report on the Latest Trends in Social Media. Globalwebindex.com, https://www.globalwebindex.com/reports/social. Accessed November 13, 2020.
Gottfredson, M., & Hirschi, T. (1990). A General Theory of Crime. Stanford, CA: Stanford University Press.
Hay, C., & Forrest, W. (2006). The development of self-control: Examining self-control theory’s stability thesis. Criminology, 44 (4), 739–774. https://doi.org/10.1111/j.1745-9125.2006.00062.x
Hawdon, J. (2012). Applying differential association theory to online hate groups: A theoretical statement. Research on Finnish Society, 5 , 39–47.
Higgins, G. E., Fell, B. D., & Wilson, A. L. (2007). Low self-control and social learning in understanding students’ intentions to pirate movies in the United States. Social Science Computer Review, 25 (3), 339–357. https://doi.org/10.1177/0894439307299934
Higgins, G. E., Fell, B. D., & Wilson, A. L. (2006). Digital piracy: Assessing the contributions of an integrated self-control theory and social learning theory using structural equation modeling. Criminal Justice Studies, 19 (1), 3–22. https://doi.org/10.1080/14786010600615934
Higgins, G. E., & Makin, D. A. (2004a). Does social learning theory condition the effects of low self-control on college students’ software piracy? Journal of Economic Crime Management, 2 (2), 1–22.
Google Scholar
Higgins, G. E., & Makin, D. A. (2004b). Self-control, deviant peers, and software piracy. Psychological Reports, 95 (3), 921–931. https://doi.org/10.2466/pr0.95.3.921-931
Higgins, G. E., & Wilson, A. L. (2006). Low self-control, moral beliefs, and social learning theory in university students’ intentions to pirate software. Security Journal, 19 (2), 75–92. https://doi.org/10.1057/palgrave.sj.8350002
Higgins, G. E., Wolfe, S. E., & Ricketts, M. L. (2009). Digital piracy: A latent class analysis. Social Science Computer Review, 27 (1), 24–40. https://doi.org/10.1177/0894439308321350
Hinduja, S., & Ingram, J. (2009). Social learning theory and music piracy: the differential role of online and offline peer influences. Criminal Justice Studies, 22 (4), 405–420. https://doi.org/10.1080/14786010903358125
Hinduja, S., & Ingram, J. (2008). Self-control and ethical beliefs on the social learning of intellectual property theft. Western Criminological Review, 9 (2), 52–72.
Hollinger, R. C. (1993). Crime by computer: Correlates of software piracy and unauthorized account access. Security Journal, 4 (1), 2–12.
Holt, T. J., Burruss, G. W., & Bossler, A. M. (2010). Social learning and cyber- deviance: Examining the importance of a full social learning model in the virtual world. Journal of Crime and Justice, 33 (2), 31–61. https://doi.org/10.1080/0735648X.2010.9721287
Hope, T. L., Grasmick, H. G., & Pointon, L. J. (2012). The family in Gottfredson and Hirschi's General Theory of Crime: Structure, parenting, and self-control. Sociological Focus, 36 (4), 291–311. https://doi.org/10.1080/00380237.2003.10571226
Hutchings, A., & Clayton, R. (2016). Exploring the Provision of Online Booter Services. Deviant Behavior, 37 (10), 1163–1178. https://doi.org/10.1080/01639625.2016.1169829
Ingram, J. R., & Hinduja, S. (2008). Neutralizing music piracy: An empirical examination. Deviant Behavior, 29 (4), 334–366. https://doi.org/10.1080/01639620701588131
Internetworldstats (2020). Internet usage statistics: The internet big picture, https://www.internetworldstats.com/stats.htm . Accessed April 20, 2021.
Ishii, K., Lyons, M. M., & Carr, S. A. (2019). Revisiting media richness theory for today and future. Human Behavior and Emerging Technologies, 1 , 124–131. https://doi.org/10.1002/hbe2.138
Kemp, S. (2020). Digital 2020. Global Digital Overview. We Are Social, Hootsuite, January 30, 2020, https://datareportal.com/reports/digital-2020-global-digital-overview . Accessed November 13, 2020.
Lanza-Kaduce, L., & Klug, M. (1986). Learning to cheat: The interaction of moral development and social learning theories. Deviant Behavior, 7 (3), 243–259. https://doi.org/10.1080/01639625.1986.9967710
Laub, J. H. & Sampson, R.J. (2003). Shared Beginnings, Divergent Lives: Delinquent Boys at Age 70. Boston, MA: Harvard University Press.
Lehdonvirta, V., Oksanen, A., Räsänen, P., & Blank, G. (2020). Social media, web, and panel surveys: using non-probability samples in social and policy research. Policy & Internet. https://doi.org/10.1002/poi3.238
Lehdonvirta, V., & Räsänen, P. (2011). How do young people identify with online and offline peer groups? A comparison between UK, Spain and Japan. Journal of Youth Studies, 14 (1), 91–108. https://doi.org/10.1080/13676261.2010.506530
Li, C. K., Holt, T. J., Bossler, A. M., & May, D. C. (2016). Examining the mediating effects of social learning on the low self-control—Cyber bullying relationship in a youth sample. Deviant Behavior, 37 (2), 126–138. https://doi.org/10.1080/01639625.2014.1004023
Lodge, J. M., & Harrison, W. J. (2019). The role of attention in learning in the digital age. Yale Journal of Biological Medicine, 92 (1), 21–28.
MacInnis, B., Krosnick, J. A., Ho, A. S., & Cho, M. J. (2018). The accuracy of measurements with probability and nonprobability survey samples: Replication and extension. Public Opinion Quarterly, 82 (4), 707–744. https://doi.org/10.1093/poq/nfy038
Malin, J., & Fowers, B. J. (2009). Adolescent self-control and music and movie piracy. Computers in Human Behavior, 25 (3), 718–722. https://doi.org/10.1016/j.chb.2008.12.029
Marcum, C. D., Higgins, G. E., Ricketts, M. L., & Wolfe, S. E. (2014). Hacking in high school: Cybercrime perpetration by juveniles. Deviant Behavior, 35 (7), 581–591. https://doi.org/10.1080/01639625.2013.867721
McCuddy, T., & Vogel, M. (2014). More than just friends: Online social networks and offending. Criminal Justice Review, 40 (2), 169–189. https://doi.org/10.1177/0734016814557010
McGloin, J. M., & O'Neill Shermer, L. (2009). Self-control and deviant peer network structure. Journal of Research in Crime and Delinquency, 46 (1), 35–72. https://doi.org/10.1177/0022427808326585
Meldrum, R. & Clark, J. (2013). Adolescent virtual time spent socializing with peers, substance use, and delinquency. Crime & Delinquency, 61 (8), 1104–1126., https://doi.org/10.1177/0011128713492499
Meldrum, R. C., Young, J. T., & Weerman, F. M. (2009). Reconsidering the effect of self-control and delinquent peers: Implications of measurement for theoretical significance. Journal of Research in Crime and Delinquency, 46 (3), 353–376. https://doi.org/10.1177/0022427809335171
Merkovity, N., Imre, R., & Owen, S. (2015). Homogenizing social media: Affect/effect and globalization of media and the public sphere. In Media and Globalization: Different Cultures, Societies, Political Systems (pp. 59-71) New York: Marie Curie-Sklodowska University Press (under Columbia University Press)
Miller, B., & Morris, R. G. (2016). Virtual peer effects in social learning theory. Crime and Delinquency, 62 (12), 1543–1569. https://doi.org/10.1177/0011128714526499
Moon, B., McCluskey, J. D., & McCluskey, C. P. (2010). A General Theory of Crime and computer crime: An empirical test. Journal of Criminal Justice, 38 (4), 767–772. https://doi.org/10.1016/j.jcrimjus.2010.05.003
Morris, R., & Blackburn, A. (2009). Cracking the code: An empirical exploration of social learning theory and computer crime. Journal of Crime and Justice, 32 (1), 2–32. https://doi.org/10.1080/0735648X.2009.9721260
Morris, R. G., & Higgins, G. E. (2010). Criminological theory in the digital age: The case of social learning theory and digital piracy. Journal of Criminal Justice, 38 (4), 470–480. https://doi.org/10.1016/j.jcrimjus.2010.04.016
Morris, R. G., & Higgins, G. E. (2009). Neutralizing potential and self-reported digital piracy: A multitheoretical exploration among college undergraduates. Criminal Justice Review, 34 (2), 173–195. https://doi.org/10.1177/0734016808325034
Na, C., & Paternoster, R. (2012). Can self-control change substantially over time? Rethinking the relationship between self- and social control. Criminology, 50 (2), 427–462. https://doi.org/10.1111/j.1745-9125.2011.00269.x
Nicolaides, A. (2012). Globalisation and Americanisation – The hijacking of indigenous African culture. Global Advanced Research Journal of History, Political Science and International Relations, 1 (6), 118–131.
Nodeland, B., & Morris, R. (2020). A test of social learning theory and self-control on cyber offending. Deviant Behavior, 41 (1), 41–56. https://doi.org/10.1080/01639625.2018.1519135
Osgood, D., & Anderson, A. (2004). Unstructured socialization and rates of delinquency. Criminology, 42 (3), 519–550. https://doi.org/10.1111/j.1745-9125.2004.tb00528.x
Osgood, D., Wilson, J., O’Malley, P., Bachman, J., & Johnston, L. (1996). Routine activities and individual deviant behavior. American Sociological Review, 61 (4), 635–655. https://doi.org/10.2307/2096397
Pempek, T., Yermolayeva, Y., & Calvert, S. (2009). College students’ social networking experiences on Facebook. Journal of Applied Developmental Psychology, 30 (3), 227–238. https://doi.org/10.1016/j.appdev.2008.12.010
Peng, M., Chen, X., Zhao, Q., & Zhou, Z. (2018). Attentional scope is reduced by Internet use: A behavior and ERP study. PLoS ONE, 13 (6), e0198543. https://doi.org/10.1371/journal.pone.0198543
Pratt, T. C., Cullen, F. T., Sellers, C. S., Winfree Jr., L. T., Madensen, T. D., Daigle, L. E., et al. (2010). The empirical status of social learning theory: A meta-analysis. Justice Quarterly, 27 (6), 765–802. https://doi.org/10.1080/07418820903379610
Restubog, S. L. D., Garcia, P. R. J. M., Toledano, L. S., Amarnani, R. K., Tolentino, L. R., & Tang, R. L. (2011). Yielding to (cyber)-temptation: Exploring the buffering role of selfcontrol in the relationship between organizational justice and cyberloafing behavior in the workplace. Journal of Research in Personality, 45 (2), 247–251. https://doi.org/10.1016/j.jrp.2011.01.006
Rogers, M.K. (2001). A Social Learning Theory and Moral Disengagement Analysis of Criminal Computer Behavior: An Exploratory Study. Unpublished doctoral dissertation, University of Manitoba, Winnipeg.
Schroeder, R. D., & Ford, J. A. (2012). Prescription drug misuse: A test of three competing criminological theories. Journal of Drug Issues, 42 (1), 11–27. https://doi.org/10.1177/0022042612436654
Sellers, C. S., Cochran, J. K., & Branch, K. A. (2005). Social learning theory and partner violence: A research note. Deviant Behavior, 26 (4), 379–395. https://doi.org/10.1080/016396290931669
Sellers, C. S., Cochran, J. K., & Winfree, L. T., Jr. (2003). Social learning theory and courtship violence: An empirical test. In R. L. Akers & G. F. Jensen (Eds.), Advances In Criminological Theory: Vol. 11. Social Learning Theory And The Explanation of Crime: A Guide For The New Century (pp. 109-128). New Brunswick, NJ: Transaction.
Shadmanfaat, S. M., Howell, C. J., Muniz, C. N., Cochran, J. K., & Kabiri, S. (2018). The predictive ability of self-control and differential association on sports fans’ decision to engage in cyber bullying perpetration against rivals. International Journal of Cyber Criminology, 12 (2), 362–375. https://doi.org/10.5281/zenodo.3365618
Simmons, A. D., & Bobo, L. D. (2015). Can non-full-probability internet surveys yield useful data? A comparison with full-probability face-to-face surveys in the domain of race and social inequality attitudes. Sociological Methodology, 45 (1), 357–387. https://doi.org/10.1177/0081175015570096
Skinner, W. F., & Fream, A. M. (1997). A social learning theory analysis of computer crime among college students. Journal of Research in Crime and Delinquency, 34 (4), 495–518. https://doi.org/10.1177/0022427897034004005
Steger, M. (2013). Globalization: A Very Short Introduction (3 rd ed.). Oxford (UK): Oxford University Press.
Sutherland, E. H. (1947). Principles of Criminology (4th ed.). Philadelphia, PA: Lippincott.
Sutherland, E.H., Cressey, D.R., & Luckenbill, D. (1995). The theory of differential association. In N.J. Herman (ed.) Deviance. A Symbolic Interactionist Approach. (pp. 64—71). Lanham, MD: General Hall
Tasheuras, O. N. (2019). Fostering resiliency and preventing re-victimization: A proposed social learning theory intervention for adult survivors of childhood sexual abuse. Crisis, Stress and Human Resilience: An International Journal, 1 (1), 22–27.
U.S. Census Bureau (2019). https://www.census.gov/quickfacts/fact/table/US/RHI125218#RHI125218 . Accessed November 13, 2020.
Van Ouytsel, J., Ponnet, K., & Walrave, M. (2017). Cyber dating abuse: Investigating digital monitoring behaviors among adolescents from a social learning perspective. Journal of Interpersonal Violence, 37 (23-24), 5157–5178. https://doi.org/10.1177/0886260517719538
Van Zoonen, L. (2013). From identity to identification: fixating the fragmented self. Media, Culture and Society, 35 (1), 44–51. https://doi.org/10.1177/0163443712464557
Vazsonyi, A. T., Machackova, H., Sevcikova, A., Smahel, D., & Cerna, A. (2012). Cyber bullying in context: Direct and indirect effects by low self-control across 25 European countries. European Journal of Developmental Psychology, 9 (2), 210–227. https://doi.org/10.1080/17405629.2011.644919
Walters, G. (2020). Explaining the drug-crime connection with peers, proactive criminal thinking, and victimization: Systemic, cognitive social learning, and person proximity mechanisms. Psychology of Addictive Behaviors. https://doi.org/10.1037/adb0000606
Wansink, B. (2001). Editorial: The Power of Panels. Journal of Database Marketing & Customer Strategy Management, 8 (3), 190–194.
Warr, M. (2002). Companions in Crime: The Social Aspects of Criminal Conduct . Cambridge University Press.
Book Google Scholar
Weerman, F., Bernasco, W., Bruinsma, G., & Pauwels, L. (2013). When is spending time with peers related to delinquency? The importance of where, what, and with whom. Crime & Delinquency, 61 (10), 1–28. https://doi.org/10.1177/0011128713478129
Weinberg, J. D., Freese, J., & McElhattan, D. (2014). Comparing data characteristics and results of an online factorial survey between a population-based and a crowdsource-recruited sample. Sociological Science , 1 , 292—310. DOI 10.15195/v1.a19
White, H. R., Pandina, R. J., & LaGrange, R. L. (1987). Longitudinal predictors of serious substance use and delinquency. Criminology, 25 (3), 715–740. https://doi.org/10.1111/j.1745-9125.1987.tb00816.x
Winfree Jr., L. T., Mays, G. L., & Vigil-Backstrom, T. (1994). Youth gangs and incarcerated delinquents: Exploring the ties between gang membership, delinquency, and social learning. Justice Quarterly, 11 (2), 229–256.
Download references
This research was funded by the Center for Peace Studies and Violence Prevention at Virginia Tech. Grant number 105-19.
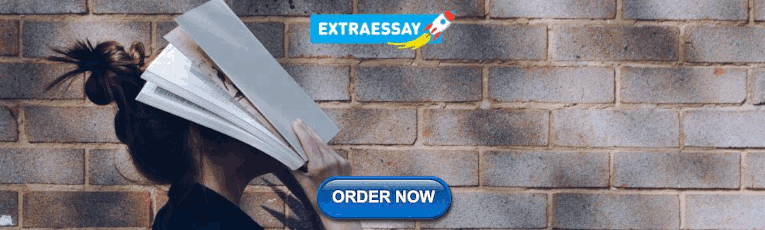
Author information
Authors and affiliations.
Virginia Tech, Blacksburg, VA, USA
Thomas E. Dearden & Katalin Parti
You can also search for this author in PubMed Google Scholar
Corresponding author
Correspondence to Thomas E. Dearden .
Additional information
Publisher’s note.
Springer Nature remains neutral with regard to jurisdictional claims in published maps and institutional affiliations.
Rights and permissions
Reprints and permissions
About this article
Dearden, T.E., Parti, K. Cybercrime, Differential Association, and Self-Control: Knowledge Transmission Through Online Social Learning. Am J Crim Just 46 , 935–955 (2021). https://doi.org/10.1007/s12103-021-09655-4
Download citation
Received : 14 December 2020
Accepted : 22 June 2021
Published : 08 November 2021
Issue Date : December 2021
DOI : https://doi.org/10.1007/s12103-021-09655-4
Share this article
Anyone you share the following link with will be able to read this content:
Sorry, a shareable link is not currently available for this article.
Provided by the Springer Nature SharedIt content-sharing initiative
- Social Learning
- Differential Association
- Online Crime
- Find a journal
- Publish with us
- Track your research

Want to create or adapt books like this? Learn more about how Pressbooks supports open publishing practices.
Criminological Explanations of Cybercrime
Module 3 provides an overview of several theories of crime causation derived from a multidisciplinary academic discipline that encompasses research from various fields such as Criminology, Victimology, Sociology, Internet Science, Computer Science, etc., and considers how the now existing body of cybercriminological research is used to understand the underlying conditions and motivations for individual and entities who commit cybercrime.
Learning Objectives
After completing this module, you should be able to:
- categorize criminological theories of crime causation.
- summarize assumptions for the most substantive categories of criminological theory.
- discuss the significance of theory as it relates causation.
- explain the connection between theory and public policy as related to policy and practice within the private and public sphere.
- describe the similarities and differences between criminology and cybercriminology.
Computer crime, also known as cybercrime, refers to criminal activities that are conducted through the use of computers or other digital technologies. Criminologists have developed a number of theoretical domains to explain why individuals engage in computer crime, including:
1. Rational Choice Theory – This theory suggests that individuals engage in computer crime because they believe it is a profitable and low-risk activity. In other words, they weigh the potential benefits of committing a crime against the potential risks of getting caught and punished.
2. Social Learning Theory – This theory argues that individuals learn to engage in computer crime through observing the behaviors of others, particularly those who are close to them. They may also be influenced by media portrayals of hackers as glamorous and successful.
3. Strain Theory – This theory posits that individuals engage in computer crime when they experience strain or pressure in their lives, such as economic hardship or social exclusion. Computer crime may provide a way for them to alleviate their stress or gain a sense of power and control.
4. Routine Activities Theory – This theory suggests that computer crime occurs when there is a convergence of three factors: a motivated offender, a suitable target (such as a vulnerable computer system), and the absence of capable guardians (such as effective cybersecurity measures).
5. Self-Control Theory – This theory proposes that individuals who engage in computer crime have low levels of self-control, which makes them more likely to act impulsively and make decisions without considering the consequences.
Overall, these criminological theories help us understand the various motives, opportunities, and situational factors that contribute to computer crime. By better understanding the underlying causes of this type of criminal behavior, public and private sectors can develop more effective strategies for preventing and responding to cybercrime.
Cybercriminology as an outgrowth of traditional criminological teachings and is important for several reasons. The following provides a preliminary list of reasons that necessitate a peer-reviewed supported examination of the computer crime.
Rising Cybercrime Rates – With the increasing reliance on technology and the internet, cybercrime has become a significant threat. Cybercriminals engage in activities such as hacking, identity theft, phishing, ransomware attacks, and more. Understanding the motivations, methods, and trends of cybercriminals is crucial for developing effective countermeasures.
Economic Impact – Cybercrimes can cause significant financial losses for individuals, businesses, and governments. These losses include expenses related to data breaches, theft of intellectual property, disruption of services, and costs associated with recovery and prevention. By studying cybercriminology, researchers and practitioners can work to mitigate these economic impacts.
Technological Advancements – As technology continues to evolve, so do the techniques and tools used by cybercriminals. By studying cybercriminology, experts can stay updated on the latest tactics employed by cybercriminals and develop strategies to defend against them.
Privacy and Data Protection – Cybercrimes often involve breaches of personal and sensitive information, leading to concerns about privacy and data protection. Studying cybercriminology helps to identify vulnerabilities in data systems, improve encryption methods, and develop effective security protocols to safeguard sensitive information.
Global Reach – Cybercrimes transcend geographical boundaries. A cybercriminal from one part of the world can easily target victims in another. This global reach makes it necessary to have a comprehensive understanding of cybercriminal behavior, legal frameworks, and international cooperation to combat cybercrime effectively.
Legal and Regulatory Challenges – Cybercrimes can be complex in terms of jurisdiction, making it challenging for law enforcement to apprehend and prosecute cybercriminals. Cybercriminology helps legal experts understand the intricacies of cybercrime and develop relevant laws and regulations to address these challenges.
Public Awareness and Education – Cybercriminology research can contribute to public awareness and education about online threats and how to protect oneself from cybercrime. Educating individuals and organizations about best practices for online safety can help reduce the risk of falling victim to cybercrimes.
Cybersecurity Workforce Development – As cybercrimes become more sophisticated, there’s a growing need for skilled professionals in the field of cybersecurity. Studying cybercriminology can provide insights into the skill sets required to counter cyber threats and contribute to the development of a well-trained cybersecurity workforce.
Policy Formulation – Policymakers need accurate information to develop effective strategies to combat cybercrime. Cybercriminology research provides valuable insights into the motivations and behaviors of cybercriminals, helping policymakers make informed decisions about legislation, regulations, and international cooperation.
Prevention and Detection – Understanding the psychology and techniques of cybercriminals can help in early detection and prevention of cybercrimes. By analyzing patterns of cybercriminal behavior, experts can create better predictive models to identify potential threats before they escalate.
In essence, cybercriminology plays a critical role in enhancing our understanding of cybercriminal behavior, devising effective countermeasures, protecting sensitive information, and maintaining the overall security of digital systems and networks.
Key Takeaways
- Cyber criminology is a sub – discipline of criminology that studies cybercrimes, cyber criminals, cyber victims, cyber laws, and cyber policies from a social science perspective.
- Professor K. Jaishankar founded the field of cyber criminology in 2007 with the launch of the International Journal of Cyber Criminology and the proposal of the Space Transition Theory of Cyber Crimes.
- Space Transition Theory of Cyber Crimes is a theoretical framework that explains how people behave differently when they move from physical space to cyber space and how this affects their criminal propensity and victimization risk.
- Contribution and impact of cyber criminology include advancing the scholarship, teaching, and professionalization of the field, as well as informing cyber policy and prevention strategies.
- Challenges of cyber criminology include the lack of empirical data, the marginalization by mainstream criminology, the need for holistic and interdisciplinary approach, and the creation of jobs and careers.
Key Terms/Concepts
Applied Criminology Behavioral Theory Classical School of Criminology Correlation Criminal Justice Criminologist Cybercriminology Cyberspace Cybervictims Cybercrime Determinism Positivism Rational Choice Routine Activities Theory Space Transition Theory Social Theory Theoretical Criminology Theory
Modern Example
An excellent resource filled with cybercriminology related discussions that advance the field and reinforce the necessity and role of criminology within the examination and response to all forms of digital crime.
“Cybercrimeology is a podcast about cybercrime, its research and its researchers. We talk to top researchers from around the world to learn about different forms of cybercrime and their research. We learn about cybercrime theory, organized crime online, Darknet drug markets, cybercrime awareness and crime prevention, technology-facilitated intimate partner violence and much more. The podcast has been running since November of 2019 and there is still so much to learn. I am happy to have you along for the journey into this fascinating subject.” (Cybercriminology, 2024) [last accessed, February 2024]
Access the Cybercriminology podcast by clicking HERE
Read, review, watch and listen
- Read Cyber Criminology: Evolution, Contribution and Impact ( Karuppannan, 2018 ) [click Download file PDF] (pp. 1-16)
- Review TEDx Talks Cyber Crime Isn’t About Computers: It’s About Behavior (Anderson, 2017) [also embedded below]
- Watch How criminal investigations have evolved along with technology (THV11, 2012) [also embedded below / last accessed February 2024]
- Watch Careers in criminology (UniSC University of the Sunshine Coast, Aug. 30, 2021) [also embedded below]
- Watch Kahan Academy’s Rational Choice-Exchange Theory ( Brown, S. 2014 )
- Watch Kahan Academy’s Behavioral Theory ( Desai, 2019 )
- Watch Social Theories Overview ( Brown, 2015 )
- Watch Social Theories Overview (part 2) ( Brown, 2015 )
- Listen to Cybercrimeology most recent episode and voluntarily explore others (last accessed February 2024)
Activity 3 – Criminological Explanations of Cybercrime
Students should review the course syllabus to determine the assignment of this activity.
This is a copy of the module’s activity that students find within Blackboard. For that reason, refer to the Activities page to submit your work for review.
The purpose of this activity is to introduce traditional theories of crime causation that serve as the basis of examining social phenomena.
Within the framework of classical/choice theory, perceptions of opportunities to commit crime are very important. Routine activities theory builds on this foundation by claiming that crimes are more likely to be committed by motivated offenders who have suitable targets in the absence of capable guardians. Routine activities of perpetrators, as well as those of potential victims and other actors, thus result in opportunities for committing and preventing cybercrimes with technology. As such, routine activities theory has important implications for understanding crimes committed with or prevented with computers, other electronic IT devices, or information systems.
One measure of situational crime prevention is target hardening. Target hardening makes it more difficult or increases the amount of effort needed for offenders to carry out crimes on specific targets. The use of locked doors, windows, alarm systems, watchdogs, and community crime watch programs are all examples of target hardening–making it harder to become a victim of crime.
Instructions
- Locate a computer crime or IT-enabled abuse news story using a search engine of your choice, e.g., Google, or an electronic COD library resource.
- Review the tenets of routine activities theory (RAT) as introduced within Chapter 3 (see pp. 50-51)
- Watch Cybercrime isn’t about computers” It’s about behavior by Adam Anderson ( https://youtu.be/c_2Ja-OTmGc )
- As a byproduct of rational choice theory (i.e., routine activities), review Arizona State University Center for Problem-Oriented Policing’s Twenty-Five Techniques of Situational Prevention [ https://popcenter.asu.edu/sites/default/files/library/25%20techniques%20grid.pdf ] (University, 2021)
Answer the following questions:
- Think about the crime example that you located and explain how that might have been prevented. Incorporate techniques of situational prevention into the explanation.
- In your own words, explain how technology (specifically cyber-space) has changed behavior (if at all) and explain what methods of reducing opportunity are necessary to reduce the prevalence of cybercrime.
AND Answer one of the following three questions:
- Use rational choice exchange theory to explain “Why?” cybercrime happens.
- Use behavioral theory to explain “Why?” cybercrime happens.
- Use social theory to explain “Why?” cybercrime happens.
Behavioral theory or behaviorism is the second major psychological theory. This theory maintains that human behavior is developed through learning experiences. The hallmark of behavioral theory is the notion that people alter or change their behavior according to the reactions this behavior elicits in other people (Bandura, 1978).
Cybercriminology combines coursework in Criminal Justice and Computer Science to study the growing problem of computer crime.
Rational choice in criminology adopts a utilitarian belief that humans are reasoning actors who weigh means and ends, costs and benefits, in order to make a rational choice. This method was designed by Cornish and Clarke to assist in thinking about situational crime prevention.
Routine Activities Theory – According to Cohen and Felson, crime occurs when there is a convergence in time and space of three factors: (1) motivated offender, (2) suitable target, and (3) the absence of a capable guardian, e.g., inadequate software protection.
Social theory is an empirically tested set of ideas within the social sciences, e.g., social process, that views criminality as a function of people’s interactions with various organizations, institutions, and processes in society; people in all walks of life have the potential to become criminals if they maintain destructive social relationships.
Theory is an attempt to answer the question of “Why?” within the framework of established propositions that provide the basis for an empirical examination.
Refer to the course learning management system (LMS); that is Blackboard (BB), for the correct due date. In addition, submit your work via BB for grading.
Supplemental Resources
- On-line Activities Guardianship and Malware Infection-An Examination of Routine Activities Theory (A. Bossler & T. Hold, 2009 International Journal of Cyber Criminology (IJCC) ISSN: 0974 – 2891 January – June 2009, Vol 3 (1): 400–420.
- International Journal of Cybercriminology (last accessed, February 2024)
- Specialize in Cyber Criminology at UQ – UQ Humanities, Arts and Social Sciences (last accessed, February 2024)
- Enhancing relationships between criminology and cybersecurity (Dupont, Benoit & Whelan, Chad. (2021). Enhancing relationships between criminology and cybersecurity. Journal of Criminology. 54. 000486582110039. 10.1177/00048658211003925.)
Read, Review, Watch and Listen to all listed materials by the due date listed within the course LMS site.
Click HERE to report any needed updates, e.g., broken links.
A multidisciplinary field that utilizes theories, research methods, and practical knowledge to address and solve real-world problems related to crime, criminal behavior, and the criminal justice system. It involves the practical application of criminological theories and concepts to develop strategies and interventions that can prevent crime, reduce criminal behavior, and improve the functioning of the criminal justice system.
Applied criminology draws from various disciplines such as sociology, psychology, law, anthropology, and public policy. Its primary focus is on practical outcomes and the implementation of evidence-based practices to create safer communities, enhance the effectiveness of law enforcement agencies, and promote social justice.
Professionals working in applied criminology may engage in a range of activities, including conducting research to evaluate the effectiveness of crime prevention programs, analyzing crime patterns and trends, developing policies and interventions to address specific crime issues, providing expertise and guidance to law enforcement agencies, advocating for criminal justice reform, and working with communities to implement crime prevention strategies.
Overall, applied criminology aims to bridge the gap between theoretical knowledge and real-world application, with the goal of reducing crime, improving the criminal justice system, and creating safer societies.
Also known as behaviorism is the second major psychological theory. This theory maintains that human behavior is developed through learning experiences. The hallmark of behavioral theory is the notion that people alter or change their behavior according to the reactions this behavior elicits in other people (Bandura, 1978).
A theory of crime and punishment that originated in the 18th century and was developed by various Enlightenment thinkers, most notably Cesare Beccaria and Jeremy Bentham. It is considered one of the foundational theories of criminology. The classical school of criminology is based on the idea of free will and rational choice. It posits that individuals are rational beings who weigh the potential benefits and costs of their actions before engaging in criminal behavior. According to this perspective, people choose to commit crimes when the perceived benefits outweigh the potential risks or punishments.
Refers to a statistical measure that quantifies the relationship or association between two or more variables. It describes the extent to which changes in one variable are related to changes in another variable. Correlation does not imply causation, meaning that a correlation between two variables does not necessarily indicate that one variable causes the other to change. Correlation analysis is widely used in various fields, including statistics, social sciences, economics, and medical research. It helps researchers and analysts understand the degree and direction of association between variables, identify patterns, make predictions, and guide decision-making. However, it is important to note that correlation alone does not establish a cause-and-effect relationship between variables, as other factors or variables may be involved.
Refers to the system of practices and institutions established by governments to maintain social order, deter, and control crime, and administer justice to those who violate the law. It encompasses a broad range of processes, organizations, and individuals involved in the detection, investigation, prosecution, and punishment of criminal offenses.
A professional who studies the causes, consequences, prevention, and control of criminal behavior. Criminology is a multidisciplinary field that draws from various disciplines, including sociology, psychology, law, anthropology, and criminal justice. Criminologists apply scientific methods and theories to analyze and understand crime patterns, criminal behavior, and the functioning of the criminal justice system.
The work of a criminologist can vary depending on their specialization and the context in which they operate. Some common roles and responsibilities of criminologists include:
1. Criminologists conduct empirical research to examine crime trends, identify risk factors for criminal behavior, and evaluate the effectiveness of crime prevention programs and policies. They collect and analyze data, design research studies, and interpret findings to contribute to the knowledge and understanding of crime and its implications. 2. Criminologists develop and assess strategies and interventions aimed at preventing crime and reducing recidivism. They work with communities, law enforcement agencies, policymakers, and other stakeholders to implement evidence-based practices and policies that promote public safety and crime reduction. 3. Criminologists analyze existing laws, policies, and practices within the criminal justice system to assess their impact and effectiveness. They provide recommendations for policy reform and improvements based on their research findings and understanding of criminological theories. 4. Some criminologists specialize in the field of criminal profiling, where they use psychological and behavioral analysis to create profiles of unknown criminals based on crime scene evidence and patterns. They assist law enforcement agencies in investigations by providing insights into the likely characteristics and motivations of offenders. 5. Criminologists often work in academic institutions, teaching criminology courses and mentoring students. They contribute to the education and training of future professionals in the field of criminal justice. Additionally, criminologists may engage in public outreach and advocacy, promoting evidence-based policies and raising awareness about criminal justice issues. Overall, criminologists play a crucial role in understanding, analyzing, and addressing issues related to crime, criminal behavior, and the criminal justice system. Their work aims to inform policy, improve crime prevention strategies, and contribute to the development of effective and fair criminal justice practices.
Combines coursework within the behavioral sciences and Computer Science to study the growing problem of computer crime.
The virtual environment created by interconnected computers and networks, where information and communication take place.
The individuals or groups who suffer harm or loss as a result of cyber crimes, such as identity theft, cyber bullying, online fraud, etc.
Is any criminal offense (e.g., fraud, theft, or distribution of child sexual abuse material [CSAM]) committed using a computer specially to access without authorization, transmit, or manipulate data via the Internet or otherwise aided by various forms of computer technology, such as the use of online social networks to bully others or sending sexually explicit digital photos with a smart phone.
A philosophical concept that posits that every event or phenomenon, including human actions and choices, is causally determined by preceding events and conditions. It suggests that there is a fixed chain of cause and effect in the universe, and given the same circumstances, the same outcome will always occur.
According to determinism, free will is an illusion, and human behavior is ultimately governed by factors beyond individual control, such as genetics, environment, upbringing, and societal influences. It suggests that individuals do not have true autonomy or the ability to make choices that are independent of causal factors.
Within criminology refers to a theoretical approach that emphasizes the application of scientific methods and empirical observation in the study of crime and criminal behavior. It emerged in the late 19th century as a response to the limitations of earlier philosophical and moralistic explanations of crime.
In criminology adopts a utilitarian belief that humans are reasoning actors who weigh means and ends, costs and benefits, in order to make a rational choice. This method was designed by Cornish and Clarke to assist in thinking about situational crime prevention.
According to Cohen and Felson, crime occurs when there is a convergence in time and space of three factors: (1) motivated offender, (2) suitable target, and (3) the absence of a capable guardian, e.g., inadequate software protection.
A theoretical framework proposed by Jaishankar in 2008 to explain the causation of cyber crimes. It argues that people behave differently when they move from one space to another, such as from physical space to cyber space.
Is an empirically tested set of ideas within the social sciences, e.g., social process, that views criminality as a function of people's interactions with various organizations, institutions, and processes in society; people in all walks of life have the potential to become criminals if they maintain destructive social relationships.
Refers to the study of crime and criminal behavior through the lens of various theoretical perspectives. It seeks to understand the causes, patterns, and dynamics of crime by developing and applying theoretical frameworks. Theoretical criminology plays a crucial role in shaping our understanding of crime, informing policy and interventions, and guiding research in the field of criminology.
Theoretical criminology encompasses a wide range of perspectives and theories, each offering different explanations and insights into criminal behavior.
An attempt to answer the question of "Why?" within the framework of established propositions that provide the basis for an empirical examination.
Computers and Criminal Justice Copyright © 2021 by Eric R. Ramirez-Thompson, PhD is licensed under a Creative Commons Attribution-NonCommercial-ShareAlike 4.0 International License , except where otherwise noted.
Share This Book

An official website of the United States government
The .gov means it’s official. Federal government websites often end in .gov or .mil. Before sharing sensitive information, make sure you’re on a federal government site.
The site is secure. The https:// ensures that you are connecting to the official website and that any information you provide is encrypted and transmitted securely.
- Publications
- Account settings
Preview improvements coming to the PMC website in October 2024. Learn More or Try it out now .
- Advanced Search
- Journal List
- Springer Nature - PMC COVID-19 Collection

Cybercrime Victimization and Problematic Social Media Use: Findings from a Nationally Representative Panel Study
Eetu marttila.
Economic Sociology, Department of Social Research, University of Turku, Assistentinkatu 7, 20014 Turku, Finland
Aki Koivula
Pekka räsänen, associated data.
The survey data used in this study will be made available through via Finnish Social Science Data Archive (FSD, http://www.fsd.uta.fi/en/ ) after the manuscript acceptance. The data are also available from the authors on scholarly request.
Analyses were run with Stata 16.1. The code is also available from the authors on request for replication purposes.
According to criminological research, online environments create new possibilities for criminal activity and deviant behavior. Problematic social media use (PSMU) is a habitual pattern of excessive use of social media platforms. Past research has suggested that PSMU predicts risky online behavior and negative life outcomes, but the relationship between PSMU and cybercrime victimization is not properly understood. In this study, we use the framework of routine activity theory (RAT) and lifestyle-exposure theory (LET) to examine the relationship between PSMU and cybercrime victimization. We analyze how PSMU is linked to cybercrime victimization experiences. We explore how PSMU predicts cybercrime victimization, especially under those risky circumstances that generally increase the probability of victimization. Our data come from nationally representative surveys, collected in Finland in 2017 and 2019. The results of the between-subjects tests show that problematic PSMU correlates relatively strongly with cybercrime victimization. Within-subjects analysis shows that increased PSMU increases the risk of victimization. Overall, the findings indicate that, along with various confounding factors, PSMU has a notable cumulative effect on victimization. The article concludes with a short summary and discussion of the possible avenues for future research on PSMU and cybercrime victimization.
Introduction
In criminology, digital environments are generally understood as social spaces which open new possibilities for criminal activity and crime victimization (Yar, 2005 ). Over the past decade, social media platforms have established themselves as the basic digital infrastructure that governs daily interactions. The rapid and vast adaptation of social media technologies has produced concern about the possible negative effects, but the association between social media use and decreased wellbeing measures appears to be rather weak (Appel et al., 2020 ; Kross et al., 2020 ). Accordingly, researchers have proposed that the outcomes of social media use depend on the way platforms are used, and that the negative outcomes are concentrated among those who experience excessive social media use (Kross et al., 2020 ; Wheatley & Buglass, 2019 ). Whereas an extensive body of research has focused either on cybercrime victimization or on problematic social media use, few studies have focused explicitly on the link between problematic use and victimization experiences (e.g., Craig et al., 2020 ; Longobardi et al., 2020 ).
As per earlier research, the notion of problematic use is linked to excessive and uncontrollable social media usage, which is characterized by compulsive and routinized thoughts and behavior (e.g., Kuss & Griffiths, 2017 ). The most frequently used social scientific and criminological accounts of risk factors of victimization are based on routine activity theory (RAT) (Cohen & Felson, 1979 ) and lifestyle-exposure theory (LET) (Hindelang et al., 1978 ). Although RAT and LET were originally developed to understand how routines and lifestyle patterns may lead to victimization in physical spaces, they have been applied in online environments (e.g., Milani et al., 2020 ; Räsänen et al., 2016 ).
As theoretical frameworks, RAT and LET presume that lifestyles and routine activities are embedded in social contexts, which makes it possible to understand behaviors and processes that lead to victimization. The excessive use of social media platforms increases the time spent in digital environments, which, according to lifestyle and routine activities theories, tends to increase the likelihood of ending up in dangerous situations. Therefore, we presume that problematic use is a particularly dangerous pattern of use, which may increase the risk of cybercrime victimization.
In this study, we employ the key elements of RAT and LET to focus on the relationship between problematic social media use and cybercrime victimization. Our data come from high quality, two-wave longitudinal population surveys, which were collected in Finland in 2017 and 2019. First, we examine the cross-sectional relationship between problematic use and victimization experiences at Wave 1, considering the indirect effect of confounding factors. Second, we test for longitudinal effects by investigating whether increased problematic use predicts an increase in victimization experiences at Wave 2.
Literature Review
Problematic social media use.
Over the last few years, the literature on the psychological, cultural, and social effects of social media has proliferated. Prior research on the topic presents a nuanced view of social media and its consequences (Kross et al., 2020 ). For instance, several studies have demonstrated that social media use may produce positive outcomes, such as increased life satisfaction, social trust, and political participation (Kim & Kim, 2017 ; Valenzuela et al., 2009 ). The positive effects are typically explained to follow from use that satisfy individuals’ socioemotional needs, such as sharing emotions and receiving social support on social media platforms (Pang, 2018 ; Verduyn et al., 2017 ).
However, another line of research associates social media use with several negative effects, including higher stress levels, increased anxiety and lower self-esteem (Kross et al., 2020 ). Negative outcomes, such as depression (Shensa et al., 2017 ), decreased subjective well-being (Wheatley & Buglass, 2019 ) and increased loneliness (Meshi et al., 2020 ), are also commonly described in the research literature. The most common mechanisms that are used to explain negative outcomes of social media use are social comparison and fear of missing out (Kross et al., 2020 ). In general, it appears that the type of use that does not facilitate interpersonal connection is more detrimental to users’ health and well-being (Clark et al., 2018 ).
Even though the earlier research on the subject has produced somewhat contradictory results, the researchers generally agree that certain groups of users are at more risk of experiencing negative outcomes of social media use. More specifically, the researchers have pointed out that there is a group of individuals who have difficulty controlling the quantity and intensity of their use of social media platforms (Kuss & Griffiths, 2017 ). Consequently, new concepts, such as problematic social media use (Bányai et al., 2017 ) and social networking addiction (Griffiths et al., 2014 ) have been developed to assess excessive use. In this research, we utilize the concept of problematic social media use (PSMU), which is applied broadly in the literature. In contrast to evidence of social media use in general, PSMU consistently predicts negative outcomes in several domains of life, including decreased subjective well-being (Kross et al., 2013 ; Wheatley & Buglass, 2019 ), depression (Hussain & Griffiths, 2018 ), and loneliness (Marttila et al., 2021 ).
To our knowledge, few studies have focused explicitly on the relationship between PSMU and cybercrime victimization. One cross-national study of young people found that PSMU is consistently and strongly associated with cyberbullying victimization across countries (Craig et al., 2020 ) and another one of Spanish adolescents returned similar results (Martínez-Ferrer et al., 2018 ). Another study of Italian adolescents found that an individual’s number of followers on Instagram was positively associated with experiences of cybervictimization (Longobardi et al., 2020 ). A clear limitation of the earlier studies is that they focused on adolescents and often dealt with cyberbullying or harassment. Therefore, the results are not straightforwardly generalizable to adult populations or to other forms of cybercrime victimization. Despite this, there are certain basic assumptions about cybercrime victimization that must be considered.
Cybercrime Victimization, Routine Activity, and Lifestyle-Exposure Theories
In criminology, the notion of cybercrime is used to refer to a variety of illegal activities that are performed in online networks and platforms through computers and other devices (Yar & Steinmetz, 2019 ). As a concept, cybercrime is employed in different levels of analysis and used to describe a plethora of criminal phenomena, ranging from individual-level victimization to large-scale, society-wide operations (Donalds & Osei-Bryson, 2019 ). In this study, we define cybercrime as illegal activity and harm to others conducted online, and we focus on self-reported experiences of cybercrime victimization. Therefore, we do not address whether respondents reported an actual crime victimization to the authorities.
In Finland and other European countries, the most common types of cybercrime include slander, hacking, malware, online fraud, and cyberbullying (see Europol, 2019 ; Meško, 2018 ). Providing exact estimates of cybercrime victims has been a challenge for previous criminological research, but 1 to 15 percent of the European population is estimated to have experienced some sort of cybercrime victimization (Reep-van den Bergh & Junger, 2018 ). Similarly, it is difficult to give a precise estimate of the prevalence of social media-related criminal activity. However, as a growing proportion of digital interactions are mediated by social media platforms, we can expect that cybercrime victimization on social media is also increasing. According to previous research, identity theft (Reyns et al., 2011 ), cyberbullying (Lowry et al., 2016 ), hate speech (Räsänen et al., 2016 ), and stalking (Marcum et al., 2017 ) are all regularly implemented on social media. Most of the preceding studies have focused on cybervictimization of teenagers and young adults, which are considered the most vulnerable population segments (e.g., Hawdon et al., 2017 ; Keipi et al., 2016 ).
One of the most frequently used conceptual frameworks to explain victimization is routine activity theory (RAT) (Cohen & Felson, 1979 ). RAT claims that the everyday routines of social actors place individuals at risk for victimization by exposing them to dangerous people, places, and situations. The theory posits that a crime is more likely to occur when a motivated offender, a suitable target, and a lack of capable guardians converge in space and time (Cohen & Felson, 1979 ). RAT is similar to lifestyle-exposure theory (LET), which aims to understand the ways in which lifestyle patterns in the social context allow different forms of victimization (Hindelang et al., 1978 ).
In this study, we build our approach on combining RAT and LET in order to examine risk-enhancing behaviors and characteristics fostered by online environment. Together, these theories take the existence of motivated offenders for granted and therefore do not attempt to explain their involvement in crime. Instead, we concentrate on how routine activities and lifestyle patterns, together with the absence of a capable guardian, affect the probability of victimization.
Numerous studies have investigated the applicability of LET and RAT for cybercrime victimization (e.g., Holt & Bosser, 2008 , 2014 ; Leukfeldt & Yar, 2016 ; Näsi et al., 2017 ; Vakhitova et al., 2016 , 2019 ; Yar, 2005 ). The results indicate that different theoretical concepts are operationalizable to online environments to varying degrees, and that some operationalizations are more helpful than others (Näsi et al., 2017 ). For example, the concept of risk exposure is considered to be compatible with online victimization, even though earlier studies have shown a high level of variation in how the risk exposure is measured (Vakhitova et al., 2016 ). By contrast, target attractiveness and lack of guardianship are generally considered to be more difficult to operationalize in the context of technology-mediated victimization (Leukfeldt & Yar, 2016 ).
In the next section, we will take a closer look at how the key theoretical concepts LET and RAT have been operationalized in earlier studies on cybervictimization. Here, we focus solely on factors that we can address empirically with our data. Each of these have successfully been applied to online environments in prior studies (e.g., Hawdon et al., 2017 ; Keipi et al., 2016 ).
Confounding Elements of Lifestyle and Routine Activities Theories and Cybercrime Victimization
Exposure to risk.
The first contextual component of RAT/LET addresses the general likelihood of experiencing risk situations. Risk exposure has typically been measured by the amount of time spent online or the quantity of different online activities – the hours spent online, the number of online accounts, the use of social media services (Hawdon et al., 2017 ; Vakhitova et al., 2019 ). The studies that have tested the association have returned mixed results, and it seems that simply the time spent online does not predict increased victimization (e.g., Ngo & Paternoster, 2011 ; Reyns et al., 2011 ). On the other hand, the use of social media platforms (Bossler et al., 2012 ; Räsänen et al., 2016 ) and the number of accounts in social networks are associated with increased victimization (Reyns et al., 2011 ).
Regarding the association between the risk of exposure and victimization experiences, previous research has suggested that specific online activities may increase the likelihood of cybervictimization. For example, interaction with other users is associated with increased victimization experiences, whereas passive use may protect from cybervictimization (Holt & Bossler, 2008 ; Ngo & Paternoster, 2011 ; Vakhitova et al., 2019 ). In addition, we assume that especially active social media use, such as connecting with new people, is a risk factor and should be taken into account by measuring the proximity to offenders in social media.
Proximity to Offenders
The second contextual component of RAT/LET is closeness to the possible perpetrators. Previously, proximity to offenders was typically measured by the amount of self-disclosure in online environments, such as the number of followers on social media platforms (Vakhitova et al., 2019 ). Again, earlier studies have returned inconsistent results, and the proximity to offenders has mixed effects on the risk victimization. For example, the number of online friends does not predict increased risk of cybercrime victimization (Näsi et al., 2017 ; Räsänen et al., 2016 ; Reyns et al., 2011 ). By contrast, a high number of social media followers (Longobardi et al., 2020 ) and online self-disclosures are associated with higher risk of victimization (Vakhitova et al., 2019 ).
As in the case of risk exposure, different operationalizations of proximity to offenders may predict victimization more strongly than others. For instance, compared to interacting with friends and family, contacting strangers online may be much riskier (Vakhitova et al., 2016 ). Earlier studies support this notion, and allowing strangers to acquire sensitive information about oneself, as well as frequent contact with strangers on social media, predict increased risk for cybervictimization (Craig et al., 2020 ; Reyns et al., 2011 ). Also, compulsive online behavior is associated with a higher probability of meeting strangers online (Gámez-Guadix et al., 2016 ), and we assume that PSMU use may be associated with victimization indirectly through contacting strangers.
Target Attractiveness
The third contextual element of RAT/LET considers the fact that victimization is more likely among those who share certain individual and behavioral traits. Such traits can be seen to increase attractiveness to offenders and thereby increase the likelihood of experiencing risk situations. Earlier studies on cybercrime victimization have utilized a wide selection of measures to operationalize target attractiveness, including gender and ethnic background (Näsi et al., 2017 ), browsing risky content (Räsänen et al., 2016 ), financial status (Leukfeldt & Yar, 2016 ) or relationship status, and sexual orientation (Reyns et al., 2011 ).
In general, these operationalizations do not seem to predict victimization reliably or effectively. Despite this, we suggest that certain operationalizations of target attractiveness may be valuable. Past research on the different uses of social media has suggested that provocative language or expressions of ideological points of view can increase victimization. More specifically, political activity is a typical behavioral trait that tends to provoke reactions in online discussions (e.g. , Lutz & Hoffmann, 2017 ). In studies of cybervictimization, online political activity is associated with increased victimization (Vakhitova et al., 2019 ). Recent studies have also emphasized how social media have brought up and even increased political polarization (van Dijk & Hacker, 2018 ).
In Finland, the main division has been drawn between the supporters of the populist right-wing party, the Finns, and the supporters of the Green League and the Left Alliance (Koiranen et al., 2020 ). However, it is noteworthy that Finland has a multi-party system based on socioeconomic cleavages represented by traditional parties, such as the Social Democratic Party of Finland, the National Coalition Party, and the Center Party (Koivula et al., 2020 ). Indeed, previous research has shown that there is relatively little affective polarization in Finland (Wagner, 2021 ). Therefore, in the Finnish context it is unlikely that individuals would experience large-scale victimization based on their party preference.
Lack of Guardianship
The fourth element of RAT/LET assesses the role of social and physical guardianship against harmful activity. The lack of guardianship is assumed to increase victimization, and conversely, the presence of capable guardianship to decrease the likelihood victimization (Yar, 2005 ). In studies of online activities and routines, different measures of guardianship have rarely acted as predictors of victimization experiences (Leukfeldt & Yar, 2016 ; Vakhitova et al., 2016 ).
Regarding social guardianship, measures such as respondents’ digital skills and online risk awareness have been used, but with non-significant results (Leukfeldt & Yar, 2016 ). On the other hand, past research has indicated that victims of cyber abuse in general are less social than non-victims, which indicates that social networks may protect users from abuse online (Vakhitova et al., 2019 ). Also, younger users, females, and users with low educational qualifications are assumed to have weaker social guardianship against victimization and therefore are in more vulnerable positions (e.g., Keipi et al., 2016 ; Pratt & Turanovic, 2016 ).
In terms of physical guardianship, several technical measures, such as the use of firewalls and virus scanners, have been utilized in past research (Leukfeldt & Yar, 2016 ). In a general sense, technical security tools function as external settings in online interactions, similar to light, which may increase the identifiability of the aggressor in darkness. Preceding studies, however, have found no significant connection between technical guardianship and victimization (Vakhitova et al., 2016 ). Consequently, we decided not to address technical guardianship in this study.
Based on the preceding research findings discussed above, we stated the following two hypotheses:
- H1: Increased PSMU associates with increased cybercrime victimization.
- H2: The association between PSMU and cybercrime victimization is confounded by factors assessing exposure to risk, proximity to offenders, target attractiveness, and lack of guardianship.
Research Design
Our aim was to analyze how problematic use of social media is linked to cybercrime victimization experiences. According to RAT and LET, cybercrime victimization relates to how individuals’ lifestyles expose them to circumstances that increase the probability of victimization (Hindelang et al., 1978 ) and how individuals behave in different risky environments (Engström, 2020 ). Our main premise is that PSMU exposes users more frequently to environments that increase the likelihood of victimization experiences.
We constructed our research in two separate stages on the basis of the two-wave panel setting. In the first stage, we approached the relationship between PSMU and cybercrime victimization cross-sectionally by using a large and representative sample of the Finnish population aged 18–74. We also analyzed the extent to which the relationship between PSMU and cybercrime victimization was related to the confounders. In the second stage of analysis, we paid more attention to longitudinal effects and tested for the panel effects, examining changes in cybercrime victimization in relation to changes in PSMU.
Participants
We utilized two-wave panel data that were derived from the first and second rounds of the Digital Age in Finland survey. The cross-sectional study was based on the first round of the survey, organized in December 2017, for a total of 3,724 Finns. In this sample, two-thirds of the respondents were randomly sampled from the Finnish population register, and one-third were supplemented from a demographically balanced online respondent pool organized by Taloustutkimus Inc. We analyzed social media users ( N = 2,991), who accounted for 77% of the original data. The data over-represented older citizens, which is why post-stratifying weights were applied to correspond with the official population distribution of Finns aged 18–74 (Sivonen et al., 2019 ).
To form a longitudinal setting, respondents were asked whether they were willing to participate in the survey a second time about a year after the first data collection. A total of 1,708 participants expressed willingness to participate in the follow-up survey that was conducted 15 months after the first round, in March 2019. A total of 1,134 people participated in the follow-up survey, comprising a response rate of 67% in the second round.
The question form was essentially the same for both rounds of data collection.
The final two-wave data used in the second-stage of analysis mirrored on population characteristics in terms of gender (males 50.8%) and age (M = 49.9, SD = 16.2) structures. However, data were unrepresentative in terms of education and employment status when compared to the Finnish population: tertiary level education was achieved by 44.5% of participants and only 50.5% of respondents were employed. The data report published online shows a more detailed description of the data collection and its representativeness (Sivonen et al., 2019 ).
Our dependent variable measured whether the participants had been a target of cybercrime. Cybercrime was measured with five dichotomous questions inquiring whether the respondent had personally: 1) been targeted by threat or attack on social media, 2) been falsely accused online, 3) been targeted with hateful or degrading material on the Internet, 4) experienced sexual harassment on social media, and 5) been subjected to account stealing. 1 In the first round, 159 respondents (14.0%) responded that they had been the victim of cybercrime. In the second round, the number of victimization experiences increased by about 6 percentage points, as 71 respondents had experienced victimization during the observation period.
Our main independent variable was problematic social media use (PSMU). Initially, participants’ problematic and excessive social media usage was measured through an adaptation of the Compulsive Internet Use Scale (CIUS) , which consists of 14 items ratable on a 5-point Likert scale (Meerkerk et al., 2009 ). Our measure included five items on a 4-point scale scored from 1 (never) to 4 (daily) based on how often respondents: 1) “Have difficulties with stopping social media use,” 2)”'Have been told by others you should use social media less,” 3) “Have left important work, school or family related things undone due to social media use,” 4) “Use social media to alleviate feeling bad or stress,” and 5) “Plan social media use beforehand.”
For our analysis, all five items were used to create a new three-level variable to assess respondents’ PSMU at different intensity levels. If the respondent was experiencing daily or weekly at least one of the signs of problematic use daily, PSMU was coded as at least weekly . Second, if the respondent was experiencing less than weekly at least one of the signs of problematic use, PSMU was coded as occasionally. Finally, if the respondent was not experiencing any signs of problematic use, PSMU was coded to none.
To find reliable estimates for the effects of PSMU, we controlled for general social media use , including respondents’ activity on social networking sites and instant messenger applications. We combined two items to create a new four-level variable to measure respondents’ social media use (SMU). If a respondent reported using either social media platforms (e.g., Facebook, Twitter), instant messengers (e.g., WhatsApp, Facebook Messenger) or both many hours per day, we coded their activity as high . We coded activity as medium , if respondents reported using social media daily . Third, we coded activity as low for those respondents who reported using social media only on a weekly basis. Finally, we considered activity as very low if respondents reported using platforms or instant messengers less than weekly.
Confounding variables were related to participants’ target attractiveness, proximity to offenders, and potential guardianship factors.
Target attractiveness was measured by online political activity . Following previous studies (Koiranen et al., 2020 ; Koivula et al., 2019 ), we formed the variable based on four single items: following political discussions, participating in political discussions, sharing political content, and creating political content. Participants’ activity was initially determined by means of a 5-point scale (1 = Never, 2 = Sometimes, 3 = Weekly, 4 = Daily, and 5 = Many times per day). For analysis purposes, we first separated “politically inactive” users, who reported never using social media for political activities. Second, we coded as “followers” participants who only followed but never participated in the political discussions in social media. Third, we classified as “occasional participants” those who at least sometimes participated in political activities on social media. Finally, those participants who at least weekly used social media to participate in political activities were classified as “active participants.”
Proximity to offenders was considered by analyzing contacting strangers on social media . Initially, the question asked the extent to which respondents were in contact with strangers on social media, evaluated with a 5-point interval scale, from 1 ( Not at all ) to 5 ( Very much ). For the analysis, we merged response options 1 and 2 to form value 1, and 4 and 5 to form 3. Consequently, we used a three-level variable to measure respondents’ tendency to contact strangers on social media, in which 1 = Low, 2 = Medium, and 3 = High intensity.
Lack of guardianship was measured by gender, age, education, and main activity. Respondent’s gender (1 = Male , 2 = Female ), age (in years), level of education, and main activity were measured. While these variables could also be placed under target attractiveness, we placed them here. This is because background characteristics the variables measure are often invisible in online environments and exist only in terms of expressed behavior (e.g., Keipi et al., 2016 ). For statistical analysis, we classified education and main activity into binary variables. Education was measured with a binary variable that implied whether the respondent had achieved at least tertiary level education or not. The dichotomization can be justified by relatively high educational levels in Finland, where tertiary education is often considered as cut-off point between educated and non-educated citizens (Leinsalu et al., 2020 ). Main activity was measured with a binary variable that differentiated unemployed respondents from others (working, retirees, and full-time students). Regarding the lack of guardianship, unemployed people are less likely to relate to informal peer-networks occurring at workplaces or educational establishments, a phenomenon that also takes place in many senior citizens’ activities. Descriptive statistics for all measurements are provided in (Table (Table1 1 ).
Descriptive statistics for the applied variables
Analytic techniques
The analyses were performed in two different stages with STATA 16. In the cross-sectional approach we analyzed the direct and indirect associations between PSMU and cybercrime victimization. We reported average marginal effects and their standard errors with statistical significances (Table (Table2.). 2 .). The main effect of PSMU was illustrated in Fig. 1 by utilizing a user-written coefplot package (Jann, 2014 ).
The likelihood of cybercrime victimization according to confounding and control variables. Average marginal effects (AME) with standard errors estimated from the logit models
Standard errors in parentheses
*** p < 0.001, ** p < 0.01, * p < 0.05

Likelihood of cybercrime victimization according to the level of problematic social media use. Predicted probabilities with 95% confidence intervals
When establishing the indirect effects, we used the KHB-method developed by Karlson et al. ( 2012 ) and employed the khb command in Stata (Kohler et al., 2011 ). The KHB method decomposes the total effect of an independent variable into direct and indirect via a confounding / mediating variable (Karlson et al., 2012 ). Based on decomposition analysis, we reported logit coefficients for the total effect, direct effects, and indirect effects with statistical significances and confounding percentages (Table (Table3 3 .).
The decomposition of effect of PSMU on online victimization with respect to confounding factors. The logit coefficients estimated using the KHB method
In the second stage, we analyzed the panel effects. We used hybrid mixed models to distinguish two time-varying factors: between-person effects and within-person effects, and predicted changes in cybercrime victimization with respect to changes in problematic social media use. We also tested how the relationship between cybercrime victimization and other time-varying variables changed over the observation period. The hybrid models were performed by using the xthybrid command (Schunck & Perales, 2017 ).
The results for our first hypothesis are presented in Fig. 1 . The likelihood of becoming a victim of cybercrime increased significantly as PSMU increased. Respondents who reported problematic use on a daily basis experienced cybercrime with a probability of more than 40%. The probability of becoming a victim was also high, 30%, if problematic use occurred weekly.
The models predicting cybercrime victimization are shown in Table Table2. 2 . In the first model (M1), PSMU significantly predicted the risk of victimization if a participant reported even occasional problematic use (AME 0.06; p < 0.001). If the respondent reported problematic use weekly (AME 0.17; p < 0.001) or daily (AME 0.33; p < 0.001), his or her probability of becoming a victim was significantly higher.
The next three models (M2-M4) were constructed on the basis of variables measuring risk exposure, proximity to offenders, and target attractiveness. The second model (M2) indicates that highly intensive social media use (AME 0.19, p < 0.001) was related to cybercrime victimization. The third (M3) model presents that those who reported low intensity of meeting strangers online had lower probability of being victims (AME -0.11, p < 0.001) and those who reported high intensity had higher probability (AME 0.12, p < 0.05). Finally, the fourth (M4) model suggests that political activity was related to victimization: those who reported participating occasionally (AME 0.07, p < 0.01) and actively (AME 0.14, p < 0.001) had higher probability of being a victim.
Next, we evaluated how different guardianship factors were related to victimization. The fifth model (M5) indicates that age, gender, and economic activity were identified as significant protective factors. According to the results, older (AME -0.01, p < 0.001) and male (AME -0.04, p < 0.001) participants were less likely to be targets of cybercrime. Interestingly, higher education or unemployment was not related to victimization. Finally, the fifth model also suggests that the effect of PSMU remained significant even after controlling for confounding and control variables.
We decomposed the fifth model to determine how different confounding and control variables affected the relationship between PSMU and victimization. The results of the decomposition analysis are shown in Table Table3. First, 3 . First, the factors significantly influenced the association between PSMU and victimization ( B = 0.38, p < 0.001), which means that the confounding percentage of background factors was 58.7%. However, the total effect of PSMU remained significant ( B = 0.27, p < 0.001). Age was the most significant factor in the association between PSMU and victimization ( B = 0.14; p < 0.001), explaining 36% of the total confounding percentage. Political activity was also a major contributing factor ( B = 0.12, p < 0.001) that explained 31.2% of the total confounding percentage. The analysis also revealed that meeting strangers online significantly confounded the relationship between PSMU and victimization ( B = 0.7, p < 0.001).
In the second stage, we examined the longitudinal effects of PSMU on cybercrime victimization using panel data from Finnish social media users. We focused on the factors varying in short term, that is why we also analyzed the temporal effects of SMU, contacting strangers online, and online political activity on victimization. The demographic factors that did not change over time or for which temporal variability did not vary across clusters (such as age) were not considered in the second stage.
Table Table4 4 shows the hybrid models predicting each variable separately. The within-effects revealed that increased PSMU increased individuals’ probability of being victimized during the observation period ( B = 0.77, p = 0.02). Moreover, the between-effects of PSMU was significant ( B = 2.00, p < 0.001), indicating that increased PSMU was related to individuals’ higher propensity to be victimized over the observation period.
Unadjusted logit coefficients of cybercrime victimization according to PSMU and confounding variables from hybrid generalized mixed models
Each variable modelled separately
We could not find significant within-subject effects in terms of other factors. However, the between-effects indicated that SMU ( B = 2.00, p < 0.001), low intensity of meeting strangers online ( B = -3.27, p < 0.001), and online political participation ( B = 2.08, p < 0.001) distinguished the likelihood of individuals being victimized.
Over the last decade, social media has revolutionized the way people communicate and share information. As the everyday lives of individuals are increasingly mediated by social media technologies, some users may experience problems with excessive use. In prior studies, problematic use has been associated with many negative life outcomes, ranging from psychological disorders to economic consequences.
The main objective of this study was to determine whether PSMU is also linked to increased cybercrime victimization. First, we examined how PSMU associates with cybercrime victimization and hypothesized that increased PSMU associates with increased cybercrime victimization (H1). Our findings from the cross-sectional study indicated that PSMU is a notable predictor of victimization. In fact, daily reported problematic use increased the likelihood of cybercrime victimization by more than 30 percentage points. More specifically, the analysis showed that more than 40% of users who reported experiencing problematic use daily reported being victims of cybercrime, while those who never experienced problematic use had a probability of victimization of slightly over 10%.
We also examined how PSMU captures other risk factors contributing to cybercrime victimization. Here, we hypothesized that the association between PSMU and cybercrime victimization is mediated by exposure to risk, proximity to offenders, target attractiveness, and lack of guardianship (H2). The decomposition analysis indicated that confounding factors explained over 50 percent of the total effect of PSMU. A more detailed analysis showed that the association between PSMU and cybercrime victimization was related to respondents’ young age, online political activity, activity to meet strangers online, and intensity of general social media use. This means that PSMU and victimization are linked to similar factors related to routine activities and lifestyle that increase the target's attractiveness, proximity to offenders and lack of guardianship. Notably, the effect of PSMU remained significant even after controlling for the confounding factors.
In the longitudinal analysis, we confirmed the first hypothesis and found that increased PSMU was associated with increased cybercrime victimization in both within- and between-subject analyses. The result indicated a clear link between problematic use and cybercrime experiences during the observation period: as problematic use increases, so does the individual’s likelihood of becoming a victim of cybercrime. At the same time, according to the between-subject analysis, it also appears that cybercrime experiences are generally more likely to increase for those who experience more problematic use. Interestingly, we could not find within-subject effects in terms of other factors. This means, for example, that individuals' increased encounters with strangers or increased online political activity were not directly reflected in the likelihood of becoming a victim during the observation period. The between-subject analyses, however, indicated that an individual’s increased propensity to be victimized is related to higher level of social media activity, intensity of meeting strangers online, and online political activity over time.
Our findings are consistent with those of preceding research pointing to the fact that cybervictimization is indeed a notable threat, especially to those already in vulnerable circumstances (Keipi et al., 2016 ). The probabilities of cybercrime risk vary in online interactional spaces, depending on the absence and presence of certain key components suggested in our theoretical framework. Despite the seriousness of our findings, recent statistics indicate that cybercrime victimization is still relatively rare in Finland. In 2020, seven percent of Finnish Internet users had experienced online harassment, and 13 percent reported experiencing unwelcome advances during the previous three months (OSF, 2020 ). However, both forms of cybercrime victimization are clearly more prevalent among younger people and those who use social media frequently.
Cybercrime is becoming an increasingly critical threat as social media use continues to spread throughout segments of the population. Certain online activities and routinized behaviors can be considered to be particularly risky and to increase the probability of cybercrime victimization. In our study, we have identified problematic social media use as a specific behavioral pattern or lifestyle that predicts increased risk of becoming a victim of cybercrime.
Although the overall approach of our study was straightforward, the original theoretical concepts are ambiguously defined and alternative meanings have been given to them. It follows that the empirical operationalization of the concepts was not in line with some studies looking at the premises of RAT and LET framework. Indeed, different empirical measures have been employed to address the basic elements associating with risks of victimization (e.g., Hawdon et al., 2017 ; Pratt & Turanovic, 2016 ). In our investigation, we focused on selected online activities and key socio-demographic background factors.
Similarly, we need to be cautious when discussing the implications of our findings. First, our study deals with one country alone, which means that the findings cannot be generalized beyond Finland or beyond the timeline 2017 to 2019. This means that our findings may not be applicable to the highly specific time of the COVID-19 pandemic when online activities have become more versatile than ever before. In addition, although our sample was originally drawn from the national census database, some response bias probably exists in the final samples. Future research should use longitudinal data that better represent, for example, different socio-economic groups. We also acknowledge that we did not control for the effect of offline social relations on the probability of cybercrime risk. Despite these limitations, we believe our study has significance for contemporary cybercrime research.
Our study shows that PSMU heightens the risk of cybercrime victimization. Needless to say, future research should continue to identify specific activities that comprise “dangerous” lifestyles online, which may vary from one population group to another. In online settings, there are a variety of situations and circumstances that are applicable to different forms of cybercrime. For instance, lack of basic online skills regarding cybersecurity can work like PSMU.
In general, our findings contribute to the assumption that online and offline victimization should not necessarily be considered distinct phenomena. Therefore, our theoretical framework, based on RAT and LET, seems highly justified. Our observations contribute to an increasing body of research that demonstrates how routine activities and lifestyle patterns of individuals can be applied to crimes committed in the physical world, as well as to crimes occurring in cyberspace.
Biographies
is a PhD student at the Unit of Economic Sociology, University of Turku, Finland. Marttila is interested in the use of digital technologies, risks, and well-being.
is a University Lecturer at the Unit of Economic Sociology, University of Turku, Finland. Koivula’s research deals with political preferences, consumer behavior and use of online platforms.
is Professor of Economic Sociology at University of Turku, Finland. His current research interests are in digital inequalities and online hate speech in platform economy.
Open Access funding provided by University of Turku (UTU) including Turku University Central Hospital. This study was funded by the Strategic Research Council of the Academy of Finland (decision number 314171).
Data Availability
Code availability, declarations.
The authors declare no conflicts of interest.
All procedures performed in studies involving human participants were in accordance with the ethical standards of the institutional and/or national research committee and with the 1964 Helsinki declaration and its later amendments or comparable ethical standards.
2) Have you been falsely accused online?
3) Have you been targeted with hateful or degrading material on the Internet?
4) Have you experienced sexual harassment social media?
5) Has your online account been stolen or a new account made with your name without your permission?
Publisher's Note
Springer Nature remains neutral with regard to jurisdictional claims in published maps and institutional affiliations.
- Appel M, Marker C, Gnambs T. Are social media ruining our lives? A review of meta-analytic evidence. Review of General Psychology. 2020; 24 (1):60–74. doi: 10.1177/1089268019880891. [ CrossRef ] [ Google Scholar ]
- Bányai, F., Zsila, Á., Király, O., Maraz, A., Elekes, Z., Griffiths, M. D., et al. (2017). Problematic social media use: Results from a large-scale nationally representative adolescent sample. PLoS ONE , 12 (1). 10.1371/journal.pone.0169839 [ PMC free article ] [ PubMed ]
- Bossler AM, Holt TJ, May DC. Predicting online harassment victimization among a juvenile population. Youth & Society. 2012; 44 (4):500–523. doi: 10.1177/0044118X11407525. [ CrossRef ] [ Google Scholar ]
- Clark JL, Algoe SB, Green MC. Social network sites and well-being: The role of social connection. Current Directions in Psychological Science. 2018; 9 :44–49. doi: 10.1016/j.copsyc.2015.10.006. [ CrossRef ] [ Google Scholar ]
- Cohen LE, Felson M. Social change and crime rate trends: A routine activity approach. American Sociological Review. 1979; 44 (4):588–608. doi: 10.2307/2094589. [ CrossRef ] [ Google Scholar ]
- Craig W, Boniel-Nissim M, King N, Walsh SD, Boer M, Donnelly PD, et al. Social media use and cyber-bullying: A cross-national analysis of young people in 42 countries. Journal of Adolescent Health. 2020; 66 (6):S100–S108. doi: 10.1016/j.jadohealth.2020.03.006. [ PubMed ] [ CrossRef ] [ Google Scholar ]
- Donalds C, Osei-Bryson KM. Toward a cybercrime classification ontology: A knowledge-based approach. Computers in Human Behavior. 2019; 92 :403–418. doi: 10.1016/j.chb.2018.11.039. [ CrossRef ] [ Google Scholar ]
- Engström A. Conceptualizing lifestyle and routine activities in the early 21st century: A systematic review of self-report measures in studies on direct-contact offenses in young populations. Crime & Delinquency. 2020; 67 (5):737–782. doi: 10.1177/0011128720937640. [ CrossRef ] [ Google Scholar ]
- Europol (2019). European Union serious and organised crime threat assessment. Online document, available at: https://ec.europa.eu/home-affairs/what-we-do/policies/cybercrime_en
- Gámez-Guadix M, Borrajo E, Almendros C. Risky online behaviors among adolescents: Longitudinal relations among problematic Internet use, cyberbullying perpetration, and meeting strangers online. Journal of Behavioral Addictions. 2016; 5 (1):100–107. doi: 10.1556/2006.5.2016.013. [ PMC free article ] [ PubMed ] [ CrossRef ] [ Google Scholar ]
- Griffiths, M. D., Kuss, D. J., & Demetrovics, Z. (2014). Social networking addiction: An overview of preliminary findings. In K. P. Rosenberg & L. C. B. T.-B. A. Feder (Eds.), Behavioral addictions: Criteria, evidence, and treatment (pp. 119–141). San Diego: Academic Press. 10.1016/B978-0-12-407724-9.00006-9
- Hawdon J, Oksanen A, Räsänen P. Exposure to online hate in four nations: A cross-national consideration. Deviant Behavior. 2017; 38 (3):254–266. doi: 10.1080/01639625.2016.1196985. [ CrossRef ] [ Google Scholar ]
- Hindelang MJ, Gottfredson MR, Garofalo J. Victims of personal crime: An empirical foundation for a theory of personal victimization. Ballinger Publishing Co; 1978. [ Google Scholar ]
- Holt TJ, Bossler AM. Examining the applicability of lifestyle-routine activities theory for cybercrime victimization. Deviant Behavior. 2008; 30 (1):1–25. doi: 10.1080/01639620701876577. [ CrossRef ] [ Google Scholar ]
- Holt TJ, Bossler AM. An assessment of the current state of cybercrime scholarship. Deviant Behavior. 2014; 35 (1):20–40. doi: 10.1080/01639625.2013.822209. [ CrossRef ] [ Google Scholar ]
- Hussain, Z., & Griffiths, M. D. (2018). Problematic social networking site use and comorbid psychiatric disorders: A systematic review of recent large-scale studies. Frontiers in Psychiatry , 9 (686). 10.3389/fpsyt.2018.00686 [ PMC free article ] [ PubMed ]
- Jann, B. (2014). Plotting regression coefficients and other estimates . The Stata Journal , 14 (4), 708–737. 10.1177%2F1536867X1401400402
- Karlson, K. B., Holm, A., & Breen, R. (2012). Comparing regression coefficients between same-sample nested models using logit and probit: A new method. Sociological methodology, 42 (1), 286–313. 10.1177%2F0081175012444861
- Keipi, T., Näsi, M., Oksanen, A., & Räsänen, P. (2016). Online hate and harmful content: Cross-national perspectives. Taylor & Francis. http://library.oapen.org/handle/20.500.12657/22350
- Kim B, Kim Y. College students’ social media use and communication network heterogeneity: Implications for social capital and subjective well-being. Computers in Human Behavior. 2017; 73 :620–628. doi: 10.1016/j.chb.2017.03.033. [ CrossRef ] [ Google Scholar ]
- Kohler, U., Karlson, K. B., & Holm, A. (2011). Comparing coefficients of nested nonlinear probability models. The Stata Journal, 11 (3), 420–438. 10.1177/1536867X1101100306
- Koivula A, Kaakinen M, Oksanen A, Räsänen P. The role of political activity in the formation of online identity bubbles. Policy & Internet. 2019; 11 (4):396–417. doi: 10.1002/poi3.211. [ CrossRef ] [ Google Scholar ]
- Koivula A, Koiranen I, Saarinen A, Keipi T. Social and ideological representativeness: A comparison of political party members and supporters in Finland after the realignment of major parties. Party Politics. 2020; 26 (6):807–821. doi: 10.1177/1354068818819243. [ CrossRef ] [ Google Scholar ]
- Koiranen I, Koivula A, Saarinen A, Keipi T. Ideological motives, digital divides, and political polarization: How do political party preference and values correspond with the political use of social media? Telematics and Informatics. 2020; 46 :101322. doi: 10.1016/j.tele.2019.101322. [ CrossRef ] [ Google Scholar ]
- Kross E, Verduyn P, Demiralp E, Park J, Lee DS, Lin N, et al. Facebook use predicts declines in subjective well-being in young adults. PLoS ONE. 2013; 8 (8):e69841. doi: 10.1371/journal.pone.0069841. [ PMC free article ] [ PubMed ] [ CrossRef ] [ Google Scholar ]
- Kross E, Verduyn P, Sheppes G, Costello CK, Jonides J, Ybarra O. Social media and well-being: Pitfalls, progress, and next steps. Trends in Cognitive Sciences. 2020; 25 (1):55–66. doi: 10.1016/j.tics.2020.10.005. [ PubMed ] [ CrossRef ] [ Google Scholar ]
- Kuss D, Griffiths M. Social networking sites and addiction: Ten lessons learned. International Journal of Environmental Research and Public Health. 2017; 14 (3):311. doi: 10.3390/ijerph14030311. [ PMC free article ] [ PubMed ] [ CrossRef ] [ Google Scholar ]
- Leinsalu M, Baburin A, Jasilionis D, Krumins J, Martikainen P, Stickley A. Economic fluctuations and urban-rural differences in educational inequalities in mortality in the Baltic countries and Finland in 2000–2015: A register-based study. International Journal for Equity in Health. 2020; 19 (1):1–6. doi: 10.1186/s12939-020-01347-5. [ PMC free article ] [ PubMed ] [ CrossRef ] [ Google Scholar ]
- Leukfeldt ER, Yar M. Applying routine activity theory to cybercrime: A theoretical and empirical analysis. Deviant Behavior. 2016; 37 (3):263–280. doi: 10.1080/01639625.2015.1012409. [ CrossRef ] [ Google Scholar ]
- Longobardi C, Settanni M, Fabris MA, Marengo D. Follow or be followed: Exploring the links between Instagram popularity, social media addiction, cyber victimization, and subjective happiness in Italian adolescents. Children and Youth Services Review. 2020; 113 :104955. doi: 10.1016/j.childyouth.2020.104955. [ CrossRef ] [ Google Scholar ]
- Lowry PB, Zhang J, Wang C, Siponen M. Why do adults engage in cyberbullying on social media? An integration of online disinhibition and deindividuation effects with the social structure and social learning model. Information Systems Research. 2016; 27 (4):962–986. doi: 10.1287/isre.2016.0671. [ CrossRef ] [ Google Scholar ]
- Lutz C, Hoffmann CP. The dark side of online participation: Exploring non-, passive and negative participation. Information, Communication & Society. 2017; 20 (6):876–897. doi: 10.1080/1369118X.2017.1293129. [ CrossRef ] [ Google Scholar ]
- Marcum CD, Higgins GE, Nicholson J. I’m watching you: Cyberstalking behaviors of university students in romantic relationships. American Journal of Criminal Justice. 2017; 42 (2):373–388. doi: 10.1007/s12103-016-9358-2. [ CrossRef ] [ Google Scholar ]
- Martínez-Ferrer B, Moreno D, Musitu G. Are adolescents engaged in the problematic use of social networking sites more involved in peer aggression and victimization? Frontiers in Psychology. 2018; 9 :801. doi: 10.3389/fpsyg.2018.00801. [ PMC free article ] [ PubMed ] [ CrossRef ] [ Google Scholar ]
- Marttila E, Koivula A, Räsänen P. Does excessive social media use decrease subjective well-being? A longitudinal analysis of the relationship between problematic use, loneliness and life satisfaction. Telematics and Informatics. 2021; 59 :101556. doi: 10.1016/j.tele.2020.101556. [ CrossRef ] [ Google Scholar ]
- Meerkerk GJ, Van Den Eijnden RJJM, Vermulst AA, Garretsen HFL. The Compulsive Internet Use Scale (CIUS): Some psychometric properties. Cyberpsychology and Behavior. 2009; 12 (1):1–6. doi: 10.1089/cpb.2008.0181. [ PubMed ] [ CrossRef ] [ Google Scholar ]
- Meshi D, Cotten SR, Bender AR. Problematic social media use and perceived social isolation in older adults: A cross-sectional study. Gerontology. 2020; 66 (2):160–168. doi: 10.1159/000502577. [ PubMed ] [ CrossRef ] [ Google Scholar ]
- Meško G. On some aspects of cybercrime and cybervictimization. European Journal of Crime, Criminal Law and Criminal Justice. 2018; 26 (3):189–199. doi: 10.1163/15718174-02603006. [ CrossRef ] [ Google Scholar ]
- Milani R, Caneppele S, Burkhardt C. Exposure to cyber victimization: Results from a Swiss survey. Deviant Behavior. 2020 doi: 10.1080/01639625.2020.1806453. [ CrossRef ] [ Google Scholar ]
- Näsi M, Räsänen P, Kaakinen M, Keipi T, Oksanen A. Do routine activities help predict young adults’ online harassment: A multi-nation study. Criminology and Criminal Justice. 2017; 17 (4):418–432. doi: 10.1177/1748895816679866. [ CrossRef ] [ Google Scholar ]
- Ngo FT, Paternoster R. Cybercrime victimization: An examination of individual and situational level factors. International Journal of Cyber Criminology. 2011; 5 (1):773–793. [ Google Scholar ]
- Official Statistics of Finland (OSF) (2020). Väestön tieto- ja viestintätekniikan käyttö [online document]. ISSN=2341–8699. 2020, Liitetaulukko 29. Vihamielisten viestien näkeminen, häirinnän kokeminen ja epäasiallisen lähestymisen kohteeksi joutuminen sosiaalisessa mediassa 2020, %-osuus väestöstä. Helsinki: Tilastokeskus. Available at: http://www.stat.fi/til/sutivi/2020/sutivi_2020_2020-11-10_tau_029_fi.html
- Pang H. How does time spent on WeChat bolster subjective well-being through social integration and social capital? Telematics and Informatics. 2018; 35 (8):2147–2156. doi: 10.1016/j.tele.2018.07.015. [ CrossRef ] [ Google Scholar ]
- Pratt TC, Turanovic JJ. Lifestyle and routine activity theories revisited: The importance of “risk” to the study of victimization. Victims & Offenders. 2016; 11 (3):335–354. doi: 10.1080/15564886.2015.1057351. [ CrossRef ] [ Google Scholar ]
- Reep-van den Bergh CMM, Junger M. Victims of cybercrime in Europe: A review of victim surveys. Crime Science. 2018; 7 (1):1–15. doi: 10.1186/s40163-018-0079-3. [ CrossRef ] [ Google Scholar ]
- Reyns BW, Henson B, Fisher BS. Being pursued online. Criminal Justice and Behavior. 2011; 38 (11):1149–1169. doi: 10.1177/0093854811421448. [ CrossRef ] [ Google Scholar ]
- Räsänen P, Hawdon J, Holkeri E, Keipi T, Näsi M, Oksanen A. Targets of online hate: Examining determinants of victimization among young Finnish Facebook users. Violence and Victims. 2016; 31 (4):708–725. doi: 10.1891/0886-6708.vv-d-14-00079. [ PubMed ] [ CrossRef ] [ Google Scholar ]
- Schunck, R., & Perales, F. (2017). Within- and between-cluster effects in generalized linear mixed models: A discussion of approaches and the xthybrid command. The Stata Journal , 17(1), 89–115. 10.1177%2F1536867X1701700106
- Shensa A, Escobar-Viera CG, Sidani JE, Bowman ND, Marshal MP, Primack BA. Problematic social media use and depressive symptoms among U.S. young adults: A nationally-representative study. Social Science and Medicine. 2017; 182 :150–157. doi: 10.1016/j.socscimed.2017.03.061. [ PMC free article ] [ PubMed ] [ CrossRef ] [ Google Scholar ]
- Sivonen, J., Kuusela, A., Koivula, A., Saarinen, A., & Keipi, T. (2019). Working papers in economic sociology: Research Report on Finland in the Digital Age Round 2 Panel-survey . Turku.
- Wagner M. Affective polarization in multiparty systems. Electoral Studies. 2021; 69 :102199. doi: 10.1016/j.electstud.2020.102199. [ CrossRef ] [ Google Scholar ]
- Vakhitova ZI, Alston-Knox CL, Reynald DM, Townsley MK, Webster JL. Lifestyles and routine activities: Do they enable different types of cyber abuse? Computers in Human Behavior. 2019; 101 :225–237. doi: 10.1016/j.chb.2019.07.012. [ CrossRef ] [ Google Scholar ]
- Vakhitova ZI, Reynald DM, Townsley M. Toward the adaptation of routine activity and lifestyle exposure theories to account for cyber abuse victimization. Journal of Contemporary Criminal Justice. 2016; 32 (2):169–188. doi: 10.1177/1043986215621379. [ CrossRef ] [ Google Scholar ]
- Valenzuela S, Park N, Kee KF. Is there social capital in a social network site?: Facebook use and college student’s life satisfaction, trust, and participation. Journal of Computer-Mediated Communication. 2009; 14 (4):875–901. doi: 10.1111/j.1083-6101.2009.01474.x. [ CrossRef ] [ Google Scholar ]
- Van Dijk JA, Hacker KL. Internet and democracy in the network society. Routledge. 2018 doi: 10.4324/9781351110716. [ CrossRef ] [ Google Scholar ]
- Verduyn P, Ybarra O, Résibois M, Jonides J, Kross E. Do social network sites enhance or undermine subjective well-being? A critical review. Social Issues and Policy Review. 2017; 11 (1):274–302. doi: 10.1111/sipr.12033. [ CrossRef ] [ Google Scholar ]
- Wheatley D, Buglass SL. Social network engagement and subjective well-being: A life-course perspective. The British Journal of Sociology. 2019; 70 (5):1971–1995. doi: 10.1111/1468-4446.12644. [ PubMed ] [ CrossRef ] [ Google Scholar ]
- Yar M. The novelty of ‘Cybercrime’ European Journal of Criminology. 2005; 2 (4):407–427. doi: 10.1177/147737080556056. [ CrossRef ] [ Google Scholar ]
- Yar, M., & Steinmetz, K. F. (2019). Cybercrime and society . SAGE Publications Limited.
Hypotheses and Theories of Cybercrime
Cybercrime has multiple similarities and differences from traditional crime. Firstly, there are generally the same motivations for committing crimes and performing violations. People choose to get involved in criminal activity due to the desire for financial and material goods, forbidden sexual interests and perversions, or even harassment. Although despite all the resemblances, cybercrimes differ from the real ones in a way that sometimes is harder to detect, and it gives more freedom for people to commit them. For instance, on the Internet, it is much easier to bully and harass people with impunity due to the distance that separates the offender and victim (Lowry et al., 2016). It is crucial to add that there is a possibility of staying anonymous and not revealing the true identity so that it is possible to avoid direct exposure. Thus, this paper aims to compare and contrast different theories connected with cybercrimes and identify the hypotheses with the main reasons for committing an offense.
The first theory is built around the subcultures, the groups with their specific features and norms. While it may be harmless and serve to unite people with similar interests or life perceptions, subcultures can also unite those with unacceptable preferences in general society (Holt et al., 2017). Since the Internet provides some sort of anonymity, people can find each other and have conversations about their subjects of interest in a safe environment without being afraid of judgment.
It is connected with the social learning theory because the members of a certain subgroup are affected by the common molars and outlooks characterized specifically for this circle. Often, it brings together individuals with deviant sexual preferences and desires, so they can exchange their thoughts and even materials to find satisfaction in the webspace (Holt et al., 2017). Thus, the subcultures and the social learning theory have the same behavioral patterns based on observing other people’s actions online.
There is Agnew’s General Strain Theory, which also has some foundations for cybercrimes. The theory implies that when a person fails to achieve financial success or avoids losses and adequate risks, they are more likely to be engaged in criminal behavior (Holt et al., 2017). The frustration the individual feels when they fail to accomplish something can stimulate them to find new ways of achieving it and stimulates them to take illegal actions.
In addition, Deterrence theory has several main principles about criminology and why people commit crimes. The hypothesis comes from the classical school of criminology that, in the first place, views the individual as a rational creature with free will and the ability to make decisions (Holt et al., 2017). Since the person consciously decided to commit a crime knowing that it will have negative consequences for someone and for themselves in the first place if they are caught. Moreover, when no one pushes them to perform a violation, it demonstrates that the individual chose the easiest way.
Therefore, without considering certain exceptions and mental illnesses, those who committed the crime were perfectly aware of its illegibility and the possible outcomes. It aligns with the example of digital pirates who know that they will take no responsibility for their actions, and they are more stimulated to pirate games, films, series, and many more (Holt et al., 2017). Deterrence theory has several similarities with Agnew’s General Strain Theory, and in some way, they complement each other. Since the person has committed a crime, even if they may feel like it was necessary and their only chance to get what they want, they acted according to their free will and purposely made a choice.
Techniques of neutralization aim to identify how personal morals and perceptions influence the decision of whether to commit a crime or not. For example, the student may have conformal beliefs and respect the law but download pirated music and books without considering it a criminal act. Moreover, many people seem to be perceived cybercrime differently and find reasons why it has nothing to do with criminality (Holt et al., 2017). It resembles Agnew’s General Strain Theory because people have justifications for their actions in both cases. In the first case, individuals might not see anything wrong with it. In the other case, they might claim that they barely had any other choice.
However, neutralization and Deterrence theory techniques seem to be the main reasons offenders commit crimes on the Internet. The crucial fact is that there is a lack of physical interactions since it is happening online, which gives the feeling of action insignificance. That is why some people can genuinely believe that pirating materials is not an actual crime or that watching and sharing child pornography is improper because it can be done safely without external judgment.
In conclusion, different cybercrimes can be easier to perform due to the possibility of staying anonymous and the distance separating the victims and the offenders. Therefore, the individuals may bully people, spread viruses, and exchange illegal materials with a minimized feeling of danger and impunity. The subculture and social learning theory imply that online behavior can be influenced by watching other people from the same community or group, which can be a foundation for criminal actions. Deterrence and the techniques of neutralization seem to contain the most explanation of why people commit cybercrime. Agnew’s General Strain Theory compliments them in a way that offenders act out of their free will and choice to get something the easier way and gain more pleasure out of it.
Holt, T. J., Bossler, A. M., & Seigfried-Spellar, K. C. (2017). Cybercrime and digital forensics: An introduction . Routledge.
Lowry, P. B., Zhang, J., Wang, C., & Siponen, M. (2016). Why do adults engage in cyberbullying on social media? Integration of online disinhibition and deindividuation effects with the social structure and social learning model. Information Systems Research , 27 (4), 962-986.
- Chicago (A-D)
- Chicago (N-B)
IvyPanda. (2023, March 19). Hypotheses and Theories of Cybercrime. https://ivypanda.com/essays/hypotheses-and-theories-of-cybercrime/
"Hypotheses and Theories of Cybercrime." IvyPanda , 19 Mar. 2023, ivypanda.com/essays/hypotheses-and-theories-of-cybercrime/.
IvyPanda . (2023) 'Hypotheses and Theories of Cybercrime'. 19 March.
IvyPanda . 2023. "Hypotheses and Theories of Cybercrime." March 19, 2023. https://ivypanda.com/essays/hypotheses-and-theories-of-cybercrime/.
1. IvyPanda . "Hypotheses and Theories of Cybercrime." March 19, 2023. https://ivypanda.com/essays/hypotheses-and-theories-of-cybercrime/.
Bibliography
IvyPanda . "Hypotheses and Theories of Cybercrime." March 19, 2023. https://ivypanda.com/essays/hypotheses-and-theories-of-cybercrime/.
- Gender and Crime Correlation in Strain Theory
- Satire as a Tool for Fighting Political Impunity
- Criminology Models Overview and Analysis
- Criminal Justice: Burglary, Theft, and Criminal Trespass
- Trial by Jury vs. Trial by Bench
- Aspects of Criminal Litigation
- Phishing as Type of Cybercrime
- Current Trends in Globalization of Crime
Whoops! You have to login to access the Reading Center functionalities!
Username or Email Address
Remember Me
Search the site...
- All categories
- Research & Threat Intelligence
- Product News & Tutorials

Mallox affiliate leverages PureCrypter in MS-SQL exploitation campaigns

This report was originally published for our customers on 2 May 2024.
As part of our critical vulnerabilities monitoring routine, Sekoia’s Threat & Detection Research (TDR) team deploys and supervises honeypots in different locations around the world to identify potential exploitations.
Table of contents
Introduction, initial access, exploitation, post exploitation, anti-analysis, next stage execution, mallox ransomware deployment, internal structure, raas operation, double extortion.
- Victimology
Payload overview
- Identified affiliates
Focus maestro
Focus vampire, focus hiervos, infrastructure.
- Xhost-overview
Recently, our team observed an incident involving our MS-SQL (Microsoft SQL) honeypot. It was targeted by an intrusion set leveraging brute-force tactics, aiming to deploy the Mallox ransomware via PureCrypter through several MS-SQL exploitation techniques.
Our investigation of Mallox samples led us to identify two affiliates with distinct modus operandi. The first focuses on exploiting vulnerable assets, while the second aims at broader compromises of information systems on a larger scale.
This blogpost report aims at presenting a comprehensive technical analysis of the techniques used to compromise the MS-SQL server we deployed. Additionally, it delves into the behaviour observed, with a focus on Mallox ransomware and its affiliates. Finally, we offer insights into detection opportunities to mitigate such threats in the future.
Infection flow
Our MS-SQL honeypot was deployed online on 15 April 2024 8am UTC and monitored throughout the following week. It exposes the MS-SQL port, the authentication is configured as mixed and the sa (SQL Administrator) account is associated with a weak password.
The initial access occurred through a brute-force attack targeting the MS-SQL server. As illustrated in the graph below, the attacker primarily targeted the “ sa ” account. The account was compromised at 8.50 am, less than an hour after it went online. We observed approximately 320 attempts per minute during this timeframe.

Figure 1. A breakdown of the accounts targeted by bruteforce .
All of the attacking IPs addresses belong to AS208091, which is owned by the hosting provider XHost Internet Solution. Despite a successful compromise of the account, the attacker persisted to brute-force throughout the entire observation window.
The first attempt of exploitation was observed on 15 April 2024, at 2.17 p.m, several hours after the account was compromised. All of the exploitation attempts (connection, payload hosting) can be traced back to AS208091. Based on the collected IOCs and the spotted TTPs, we attribute all of the exploitation attempts to the same intrusion set.
The MS-SQL logs provide detailed information about the attacker’s actions, revealing two distinct exploitation schemes. Based on the timestamps, it is likely that the attacker utilised scripts or tools in both cases.
From numerous exploitation attempts we observed, 19 of them allowed us to identify two distinct, recurring operating patterns. The commands are systematically aimed at dropping and executing the same payload. Sections dedicated to this threat are included later in this report.
The observed exploitation attempts are detailed below.
Exploitation Pattern 1:
- The attacker enabled the “ TRUSTWORTHY ” parameters for the master database which is disabled by default. These parameters allow database users to impersonate other users by using the EXECUTE AS statement.
- It enabled the clr enabled parameter, which allows the SQL Server to execute user assemblies. Activating both the “ clr enabled “ and “ TRUSTWORTHY ” parameters is a prerequisite for exploiting CLR Assembly.
The attacker created an assembly named “ shell” and stored it on the “ msdb ” database with “ Unsafe ” permission. This assembly is a .NET DLL containing a class called StoredProcedure which includes a cmd_exec function. This function executes commands passed to it as parameters via cmd.exe . This assembly corresponds to a CLR SqlShell malware, which has been documented by Asec in connection with the compromise of an MS-SQL server by the Trigona ransomware.

Figure 2. cmd_exec function from shell assembly .
- The attacker created a stored procedure named cmd_exec that calls the SqlShell malware.
- Using echo and redirect , it creates a PowerShell script that downloads a binary and saves it to the ProgramData folder;
- It then calls PowerShell to execute the script;
- Finally, It uses WMIC to execute the binary.
Further execution is blocked by Microsoft Defender. At this stage, it is unclear whether the following actions are executed iteratively by the script or executed because the previous command has been blocked.
- The attacker enabled xp_cmdshell parameters to allow SQL Server to spawn a Windows command shell and pass in a string for execution. This is a well known technique used by attackers to compromise MS-SQL servers.
- It used xp_cmdshell to execute the same command that was observed in case 1
and also enabled Ole Automation Procedures parameters to allow the SQL Server to leverage OLE objects to interact with other COM objects.
- Finally, it used sp_oacreate to create the OLE object wscript.shell , and then called this object via sp_oamethod to execute arbitrary commands on the underlying operating system.
Exploitation Pattern 2:
In this case, based on MS-SQL log analysis and more specifically the client_app_name field, a relevant pattern emerges: vYMiFrYR . This application name appears several times and is systematically associated with the same action sequence. It is most certainly an exploitation tool.
Note that the CrackMapExec MS-SQL tool leaves a fairly similar trace: a random application name of 8 characters long. This is also the case for the Metasploit exploit module.

Figure 3. MS-SQL logs extract related to exploitation .
In this instance, we see the same sequence as in the previous case, but without the attempt to deploy the assembly and the associated stored procedure.
The payloads dropped through MS-SQL exploitation correspond to PureCrypter . The behaviour observed is very similar to the analysis of ANY RUN .
The infection chain is as follows:
- The payload downloads a file from the Internet. The file has a random name and a multimedia file extension ( e.g. mp4, wav, pdf). As documented by Any Run, this behaviour is specific for Purecrypter;
- The downloaded file contains encrypted data via 3DES;
- A .NET library is obtained after decryption. It is executed using the Reflective Code Loading technique by the previous payload. This DLL corresponds to PureCrypter’s stage2. Its first action is to load a third-party payload from the resources;
- This third-party payload is the Mallox ransomware.

Figure 4. Mallox deployment workflow .
It is worth noting that during these last attempts, the attacker tried to deploy Mallox without PureCrypter. Since previous attempts involving PureCrypter have failed, the attacker likely attempted to spread its ransomware directly. This was possibly done to ensure that the failures were not related to the crypter.
PureCrypter payload analysis
PureCrypter is a loader developed in .NET whose main capability is to download and execute a payload.
It is developed and sold as a Malware-as-a-Service (MaaS) by a threat actor operating under the alias PureCoder (aka PureTeam). PureCoder operates on various Russian-speaking cybercrime forums such as XSS, UfoLabs and CrackedIO, where it offers a wide range of malware from the Pure family, alongside PureCrypter ( e.g. PureMiner, PureLogs, PureClipper, etc. )
PureCoder customers subscribe for either monthly or lifetime licences. The malware allows customised PureCrypter payloads by choosing the injection, anti-analysis and persistence methods. Intrusion sets such as 8220 gang and the Mallox ransomware operators were previously reported leveraging PureCrypter in lucrative campaigns.
PureCrypter employs various techniques to evade detection and analysis by security software and researchers. By using these techniques, the first stage of the infection attempts to be stealthy, allowing it to carry out its malicious activities unimpeded.
The loader performs a series of environment detection and anti-analysis techniques that are listed below:
- The malware lists all running processes and searches for the module name SbieDll.dll, which is the DLL used by Sandboxie .
- The malware retrieves the Win32_BIOS using a WMI query (`select * from Win32_BIOS`) to check if it is running in a virtual environment. It looks for values such as VMWare, Virtual, A M I, or Xen. A similar test is performed on the computer manufacturer model using another WMI query (select * from Win32_ComputerSystem), with tested values including Microsoft, VMWare, and Virtual.
- The malware also checks the monitor size. If the monitor size is 1440×900 or if the width is below 1024 and the height is below 768, the malware stops its execution.
- The malware checks the username as well. If the username is `john`, `anna`, or `xxxxxxxx`, the malware exits.
- A network test is performed using the following commands: `ipconfig /renew and ipconfig /release`.
- The malware uses a technique detailed by The Red Team Vade Mecum called EtwEventWrite Patching to avoid system logging events.

Figure 5. Patching EtwEventWrite .

Figure 6. Amsi ScanBuffer patching .
- The malware prepares for the execution of the next payload by adding `MpPreference -Exclusion` to Windows Defender and ExclusionProcess for itself and the dropped payload.
- The malware ensures its persistence on the infected host by adding a registry key in the current user hive under `Software\Microsoft\Windows\CurrentVersion\Run\`.
- Finally, the malware looks at its processus privileges in order to elevate them with the SeDebugPrivilege that might be used by the dropped payload.
Prior to the series of environment detection and privilege adjustment checks, the loader loads a resource with a specific structure . The first four bytes of the resource indicate the size of the data to be deflated . The loader then uses a memory stream object to read the correct number of bytes from the resource, which is then gunzipped .

Figure 7. Function to read the compressed resource .
This resource is a protobuf definition, which aligns with some of our previous observations regarding the imported libraries . The definition, however, is incomplete and is as follows:
Where the “Ydxhjxwf.exe” is the name under which the Mallox ransomware is executed, the long entry is the PE stored encrypted using AES in CBC mode. Purecrypter executes its next-stage payload, the Mallox ransomware with the filename “Ydxhjxwf.exe”.
NB: The long entry in the protobuf definition is Mallox PE stored encrypted using AES in CBC mode.
Mallox is a Ransomware-as-a-Service (RaaS) operation distributing the namesake ransomware. The Mallox ransomware is distributed since at least June 2021 and is also known as Fargo, TargetCompany, Mawahelper, etc . Several variants of the ransomware are simultaneously leveraged by Mallox operators.The attack volume accelerated in late 2022 and continued to increase throughout 2023, likely due to the RaaS launchment and the adoption of the double extortion technique as detailed in the next sections of this part. Moreover, Mallox was reported to be the most distributed ransomware in early 2023 based on AhnLab data .
The intrusion set is reported to mainly exploit vulnerable MS-SQL Servers to gain access. Also, it was previously reported compromising victims’ networks through brute-force and dictionary attacks targeting accounts protected with weak credentials. Alternatively, Mallox operators exploit known, unpatched vulnerabilities.
Mallox operators deploying the Xollam variant were also reported leveraging OneNote for phishing campaigns aiming to gain access to victims’ systems.
The Mallox ransomware representatives are likely former members of tier ransomware operations. Of note, they declared having acquired the Mallox project from another threat group.
Although the Mallox internal organisation and its structure remain undocumented, their negotiation website introduces several categories of “staff” people, which we observed evolving over time. Notably, we identified the presence of the following usernames: Admin , Support , Maestro , Team , Neuroframe , Panda and Grindr .

Figure 8. Screenshot from Mallox .onion website with Staff section.
As detailed later in this report, we were also able to identify these usernames, in addition to Hiervos and Vampire , in Mallox ransomware samples collected in the wild in April 2024. Therefore, TDR analysts assess that these names correspond to Mallox operators and/or affiliates of their private RaaS. As of April 2024, Sekoia is not able to establish any direct link between these usernames and known personas operating on cybercrime forums that we monitor.
We observed the Mallox ransomware operation transitioning into the Ransomware-as-a-Service distribution model from mid-2022.
TDR analysts identified two online personas – “Mallx” and “RansomR” (aka “Mallox”) – operating on multiple underground forums and actively recruiting affiliates (referred to as “pentesters” in the ransomware-related slang) for distributing the Mallox ransomware.
It is possible that RansomR and Mallx are the same individual or two different individuals sharing the role of administrator of the Mallox RaaS program.
Our observations reveal that the RaaS recruitment campaigns launched by the RansomR persona on numerous cybercrime forums were only maintained for a short time, and the threat actor ceased to be active in mid-2023. On the contrary, the Mallx persona persisted in recruiting affiliates for the Mallox RaaS, also acquiring initial accesses on the RAMP forum and conducting other cybercrime-related activities ( e.g. selling 0day vulnerabilities) until at least March 2024. Of note, RAMP is currently a top-tier forum and marketplace dedicated to cybercrime activities among which Ransomware-as-a-Service is a major component.
In January 2023, Mallox representatives stated they are a small, closed ransomware group operating from the European region. This is consistent with their recruiting ads on the RAMP forum posted throughout 2023, where Mallx seeked to partially expand its private affiliate program. The threat actor was looking to partner with advanced, Russian-speaking threat actors able to establish initial access on victims’ networks either for sale to Mallox operators or for direct participation in their private RaaS if the obtained accesses proved to be of significant interest.
As illustrated below, the Mallox RaaS operation focuses on the exploitation of Fortinet, Cisco and VPN accesses for ransomware propagation. It leverages the Big Game Hunting (BGH) strategy, as it targets entities with a high revenue (over $10M) primarily in the United States, the United Kingdom, Canada, Australia and Germany. Mallox’ victims selection seems consistent with those of most opportunistic ransomware, sparing government and educational assets from attacks.

Figure 9. Mallox RaaS advertisement on the RAMP forum.
Based on our observations, Mallox was distributed in simple extortion campaigns centred around data encryption which persisted until early 2022.
This tactic evolved by mid-year 2022, when Mallox transitioned to leveraging the double extortion strategy by exfiltrating victims’ data in addition to encrypting it, further threatening to publish stolen data. Initially, they used dedicated Twitter,Telegram and cybercrime forums accounts for data leakage.
From October 2022 onwards, Mallox started to use dedicated TOR resources for double extortion, urging victims to engage negotiations via a dedicated TOR page using provided personal IDs, or by sending the IDs to a specific email address. Based on the evidence gathered by TDR, Mallox operators exclusively communicate in English on their negotiation portal with victims.
In separate cases, ransomware operators leverage the triple extortion tactic by threatening to contact the victims’ partners to discreditate them, and also warn victims based in Europe that they are at risk of contravening the GDPR principles if the stolen data ends up being publicly released.
The group abuses the AnonFiles file-sharing service to upload and share exfiltrated data.
Ransom demands associated with Mallox compromises vary widely, being reported to range from $1000 to $60,000. TDR found that in one case involving a Colombian-based victim, the ransom amount was reduced from $50,000 to $20,000 within a two-week period.
Victimology of Mallox ransomware
Mallox is almost certainly an opportunistic intrusion set impacting organisations in various verticals, notably the manufacturing, the retail and the technology ones.
Although Mallox representatives actively seek high-revenue targets (as indicated in recruitment posts on cybercrime forums), most of the ransomware’s victims known in open-source are small and middle size enterprises. However, a few big names, such as the Federation of Indian Chambers of Commerce and Industry or Garuda Indonesia airline company. No casualties were observed in Eastern Europe, in line with the group’s previous announcements about avoiding attacking entities from Kazakhstan, Russia, Qatar, and Ukraine. Based on Trend Micro telemetry data from 2022 and 2023, Mallox campaigns notably impacted Asian countries.
The victims identified by Sekoia in open source ranged from $5M to over $780M in annual revenue.
On the Mallox Data Leak Site (DLS), stolen data from over 35 victims was released between 21 October 2022 and April 2024. It is noteworthy that the real number of all Mallox compromises is expected to be much higher.

Figure 10. Countries affected by Mallox since January 2023 based on claims on the Mallox Data Leak Site .
Mallox ransomware is developed in C++, the malware does not have any anti-analysis nor environment detection. This aligns with the use of PureCrypter as an initial payload in its campaign.
One of the first actions of the ransomware is to check the default language to ensure that it is not executed in a russian-speaking environment.

Figure 11. Countries not affected by Mallox since january 2023 .
The ransomware then adjusts its privileges to leverage the SeTakeOwnershipPrivilege and SeDebugPrivilege privileges.
Whereafter, the Mallox begins its destructive activities by starting a thread that disables certain recovery options and ignores all failures at boot time. This thread is also responsible for stopping a set of services.
- bcdedit /set {current} bootstatuspolicy ignoreallfailures
- bcdedit /set {current} recoveryenabled no
- Stop services ( See figure 12 and 13):

Figure 12. Extract of Service that the ransomware attempt to stop .

Figure 13. Mallox function used to stop services .
The malware deletes shadow copies using the infamous command: vssadmin.exe delete shadows /all /quiet. It also deletes links to tools such as wmic.exe, powershell.exe, bcdedit.exe, etc.
The main function of the ransomware iterates through the disks and drives of the infected host to encrypt files.
Once the files are encrypted, the malware registers the new victim with its Command and Control server by sending a host fingerprint over an HTTP POST request. The fingerprint includes five pieces of information:
- A field “ user ” that contains the ransomware operator’s name;
- A field “ TargetID ” that contains the victim’s identifier;
- A field “ max_size_of_file ” that contains the largest file;
- A field “ SystemInformation ” that contains the OS version and architecture, the default language, the public IP address and username;
- A field “ size_of_hdd ” that contains the size of the hard drive disk.

Figure 14. HTTP POST request to register new victim .
Before ending its activity, the ransomware displays the following message to the victim: “ Do NOT shutdown OR reboot your PC: this might damage your files permanently! ” Additionally, it alters some registry keys to hide the Shutdown, Restart, and Signout buttons in the Windows GUI menu. These changes are made in the hive “SOFTWARE\\Microsoft\\PolicyManager\\default\\Start\\” with the following keys:
- HideShutDown
- HideRestart
- HideSignOut
Mallox ransomware affiliates identified
Reversing and sandbox execution revealed data being sent via HTTP Post to the URL hxxp://91.215.85[.]142/QWEwqdsvsf/ap.php . Pivoting on this URI path takes us back to whyers[.]io , which is also associated with Mallox. This URI path is therefore a helpfulmonitoring pattern.
As detailed previously, data sent via POST corresponds to the host fingerprint.

Figure 15. Data exchange between a victim and Mallox C2 .

Figure 16. Username sent to the C2 .
System information can be viewed in the Mallox onion page and as this network communication is the only one observed, Sekoia assesses with high confidence that this URL serves as a relay to the Mallox .onion site.
As detailed above, maestro is identified as a Mallox “staff member”. It is also possibly a ransomware operator. TDR assumes that the username would be the affiliate’s or operator’s ID attribute. To confirm this hypothesis, we analysed the public sandbox execution associated with Mallox from ANY.RUN and Triage. In the around twenty cases investigated, the above-mentioned URL was presented in 19, and for the remaining cases, the data was sent to hxxps://whyers[.]io .
As a result, five different users were identified: maestro , hiervos , admin , vampire and panda.
Based on the infection IDs associated with these usernames, it was possible to obtain information on the ransomware operations conducted by some of them. It was also found that Mallox creates unique payment addresses (Bitcoin and Tether) for each infection ID.
Maestro is the user to whom the most recent of the collected samples are linked. The ransom fixed by Maestro is always $5,000. An infection ID is generated each time the ransomware is run. Maestro seems to target vulnerable servers, but does not appear to seek to lateralise itself in the victims’ information systems.
Since March 2024, it has been using PureCrypter to load Mallox. This is the only affiliate observed to use this combination of malware.
Few samples are linked to this user. The ransom demanded is usually high, as $3,000,000. It also leverages the double extortion technique, with a bot sending a daily message in the Mallox .onion victim chat to pressure the victims by reminding the number of days left to pay before the data is released.
Unlike Maestro , in vampire -related campaigns the infection ID is associated with the sample. It does not vary between each ransomware execution.
Based on this information, Sekoia assumes that vampire is more likely to target a company’s entire IT system than isolated servers.
Based on the samples analysed, this user appeared to be one of the most active operators/affiliates in 2023. In most cases, a different ID is generated each time the ransomware is executed. The ransom demanded was 4,500$ in 2023 and 3,000$ in 2024. Hiervos operates in the same way as Maestro , targeting independent servers.
A case was also found where the ransomware was associated with a fixed ID. The ransom demand was also higher, reaching 15,000$.
All the attacks (bruteforce and exploitation) conducted by maestro are carried out from IP addresses in AS208091 and owned by Xhost. A Shodan search on these IP addresses shows very similar profiles, they are systematically OS Windows 2012 servers exposing the same ports, in particular Netbios. By pivoting on the Netbios names associated with these servers, new IP addresses are identified. They always belong to the same AS and have the same characteristics.
Various threat intelligence reports, particularly relating to ransomware activity, previously referred to this AS. Research into the related IP addresses also shows that most of them are known to the intelligence community and are associated with brute force attacks targeting MS-SQL, RDPs and VPNs. VirusTotal shows that many of them are associated with hosting malware, in particular PureCrypter.
Xhost overview
AS208091 is owned by the company XHOST INTERNET SOLUTIONS LP, registered in the United Kingdom on 31 January 2022. According to information from the English House Registry, the company’s office is registered at Suite 6060 128 Aldersgate Street, Barbican, London, England, EC1A 4AE. It is a virtual office address belonging to Mail Boxes ETC. Xhost Internet Solutions which is a Limited Partnership (LP); the partners are two companies domiciled in the Seychelles that appear in various open-source articles covering financial controversies. Establishing Limited Partnerships (LPs) or Limited Liability Partnerships (LLPs) in the UK recognised as a common method exploited for money laundering.
The Xhost website hxxps://www.isxhost[.]uk/ is static, does not display any customer interface – only a contact page points to an email address that does not respond to the solicitation. The abusive email address returns a 550 Mail error (Mailbox is full / Blocks limit exceeded / Inode limit exceeded). It is a kind of empty shell.
Xhost presents the profile of a shell company whose website serves to legitimise its business. Sekoia continues its investigation to determine who manages the company’s assets (range of IP addresses and AS).
MS-SQL logs are not natively collected in a Windows event log. However, they do contain information that is useful for detecting a compromise. It is recommended to include them into the SOC perimeter. Based on MS-SQL logs:
- Track connections to the MS-SQL server, particularly from public IP addresses. Monitor IP addresses that manage to connect after several failed authentications.
- Check parameter changes, in particular the activation of xp_cmdshell, clr or Ole Automation.
The execution of drop commands and payload execution via the MS-SQL server can be detected based on the process tree. This type of rule works very well on a honeypot, but in production it runs the risk of generating false positives linked to the use of advanced stored procedures for sysadmin or dbadmin.
WMI is abused by attackers, in this case WMIC is called to execute the payload. This behaviour is relevant and could be detected with this rule.
Mallox use bcedit to inhibit system recovery. This technique could be caught with this rule
Although it has an mp4, mp3 or wav extension, the mime type of the downloaded file does not correspond to a multimedia file. If the proxy logs the real mime type of the file, by comparing the extension name with the mime type, it is possible to detect this masquerade.
The Mallox ransomware operation has been active since June 2021, and enhanced its reach over time with the adoption of the (private) RaaS model and the double extortion technique.
The MS-SQL exploitation operations detailed in this report are consistent with the previously documented initial access methods attributed to the Mallox group.
Our recent investigations on Mallox-related compromises provided valuable insights into its business model. Of particular interest is the use of two distinct operating methods. The first involves the targeting of vulnerable servers in a singular operation, which makes it possible to remain discreet in return for relatively low revenues. The second method involves a broader compromise of information systems coupled with double extortion tactics, resulting in significantly higher income.
Our analysis also highlights various users of this RaaS, including Maestro, who appears to be one of the staff and a ransomware operator. The investigation reveals the common TTPs leveraged by this operator that focuses on targeting MS-SQL servers, and details the techniques used to exploit vulnerable servers. The usage of Xhost IPs addresses also stands out as a significant behavioural pattern associated with Maestro.
When investigating the hosting company Xhost Internet linked to AS208091, suspicions arise. While formal links with cybercrime-related activities remain unproven, the involvement of this AS previous instances of ransomware compromise and the longevity of the IP address monitoring is intriguing. Sekoia.io analysts will continue to monitor activities associated with this AS and to investigate the related operations.
The list of IoCs is available on Sekoia GitHub repository .
Thank you for reading this blogpost. We welcome any reaction, feedback or critics about this analysis. Please contact us on tdr[at]sekoia.io .
- Sekoia.io Mid-2022 Ransomware Threat Landscape
- Adversary infrastructures tracked in 2023
- Log4Shell: the defender’s worst nightmare ?
- MSDT abused to achieve RCE on Microsoft Office
- ActiveMQ CVE-2023-46604 Exploited by Kinsing: Threat Analysis
Share this post:
What's next
Elevating cybersecurity: the sekoia.io methodology for advanced detection engineering.
In the constantly evolving cybersecurity landscape, Sekoia.io is at the forefront of crafting sophisticated detection engineering strategies. This blog...
How to empower the MSSP business with the Sekoia SOC platform?
The managed security service market is blooming. Statista states it’s projected to reach 65.53 billion U.S. dollars in 2028....

Guarding Democracy: Assessing Cyber Threats to 2024 Worldwide Elections
Executive Summary Introduction 2024 marks a pivotal moment in global politics as an unusual number of elections have and...

Comments are closed.
Trending topics.

Special Features
Vendor voice.
Personal Tech

Aghast iOS users report long-deleted photos back from the dead after update
Apple might be hanging on to nuked iphone snaps for a while.
Some iPhone users are reportedly seeing photos they had previously deleted resurface on their devices ever since updating to the latest version of iOS.
The user reports originate from Reddit , and it's not just a couple of Apple users experiencing issues. By our count, 16 people who deleted their photos say they've come back. The deleted photos are apparently marked as recently added, making it very obvious which have made a comeback.
One user says that even photos from 2010 reappeared, and that they have "deleted them repeatedly."
The Register was able to find a handful of instances of X users reporting the same problem. However, if the bug (assuming it does exist) was widespread, there would likely be far more reports across social media in general.
The recent complaints were preceded by a different Reddit thread where three users reported the exact same thing happening in the beta version of iOS 17.5.
The users connect the return of deleted photos to updating to the latest iOS version 17.5, and there are all sorts of theories on what causes the issue. The basic premise for all theories is that the photos aren't entirely deleted, and that they stick around either through local storage or within iCloud.
- Samsung sole winner as US smartphone market hits sixth quarterly decline in a row
Meta, Spotify break Apple's device fingerprinting rules – new claim
Apple confirms ipados will fall under its alternative business terms in the eu, apple sales slip, but investors offered bite of $110b stock buyback.
The local storage theory hinges on the fact that the act of clicking delete on iOS and other operating systems usually doesn't destroy data because actually deleting the information for good requires overwriting it. Instead of doing the intensive process of overwriting files with zeroes every time someone wants to delete something, most of the time the area where such code is stored is marked as free to use, and the data will be overwritten over time by newly made files.
The other idea is that Apple is keeping user photos in the iCloud storage service for a very, very long time, and that it's the source of the revived photos. This theory has some credibility, if we can believe the user that said photos from 2010 re-emerged, which is questionable through local storage since the user must have gone through at least a couple different devices in the last 14 years.
What actually causes the photos to get added back to users' devices is also unclear, although some suggest it's due to a bug fix that went too far. Some users previously reported disappearing photos on older versions of iOS 17, and the fix may have resulted in both accidentally and purposefully deleted photos being brought back to life.
If the issue is genuine, it wouldn't be the first time iCloud has kept its hands on data after it was supposedly deleted, despite Apple's emphasis on the privacy of its users. Back in 2017, iCloud was patched to fix a glitch where user browser history was retained for up to a year or so.
The Register asked Apple to comment. ®
Narrower topics
- Privacy Sandbox
Broader topics
- Cloud Computing
- Operating System
- Steve Wozniak
Send us news
Other stories you might like
Brit publishers beg apple not to hurt online ad revenue, an attorney says she saw her library reading habits reflected in mobile ads. that's not supposed to happen, easing the cloud migration journey.
Google, Apple gear to raise tracking tag stalker alarm
Apple's 'incredibly private' safari is not so private in europe, apple geofences third-party browser engine work for eu devices, apple on track for quarter of all iphones to be made in india by 2028, china 'the most competitive market in the world' for the iphone says tim cook, miss your morning iphone alarm it's not just you, and apple is looking into it.

- Advertise with us
Our Websites
- The Next Platform
- Blocks and Files
Your Privacy
- Cookies Policy
- Privacy Policy
- Ts & Cs

Copyright. All rights reserved © 1998–2024

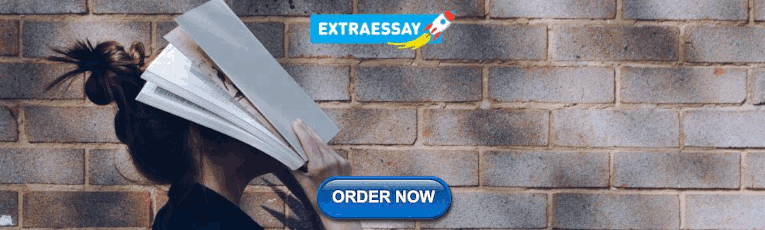
IMAGES
VIDEO
COMMENTS
We recently rejected the cybercrime hypothesis as an explanation for the international crime drop (Farrell and Birks 2018).For the sake of clarity, here we reiterate this hypothesis—which we derived from the work of varying scholars quoted in our original manuscript (Farrell and Birks 2018: p1).By our definition, the cybercrime hypothesis remains a matter of substitution—such that, as ...
Abstract. Several criminological and psychological theories and their empirical support for explaining cybercrime are reviewed. Social learning theory, self-control theory, and subcultural theories have garnered much empirical attention and support. Lack of moral qualms, association with deviant peers and neutralizations have consistently been ...
First, they highlight the international nature of cybercrime research. The authors hail from the United States, Canada, Israel, the Netherlands, and Germany. Second, they demonstrate that theory is at the heart of all criminology research, even when the focus is on complex forms of cybercrime.
prominent rational choice theories in criminology are deterrence theory and routine activity theory. 2.1 Deterrence Theory Beccaria (1764) argued that crime in society reflected ineffective law rather than the presence of evil, which was contrary to some of the early origins of criminological thought based on religion and spirituality.
Moreover, cybercrime theory has focused heavily on individual-level variation in cybercrime and largely ignored group-level variation, which is a shortcoming because understanding differences across, for example, different nations or political or religious groups, is an important part of the study of crime in the physical world.
Abstract. 'Cybercrime' is an umbrella concept used by criminologists to refer to traditional crimes that are enhanced via the use of networked technologies (i.e. cyber-enabled crimes) and newer forms of crime that would not exist without networked technologies (i.e. cyber-dependent crimes). Cybersecurity is similarly a very broad concept ...
Cybercrime theory and Discerning if there is a Crime 101 Transglobal Digital World A momentous event in the 20th century was when Neil Armstrong first set foot on the moon and declared it "one small step for man, one giant leap for mankind." The world existed in a physical realm, and while daunting, it was, well, "real." We had nation-states ...
It is widely accepted that cybercrime is highly prevalent and increasing. A recent report suggests that Internet Service Providers (ISPs) record around 80 billion automated scans daily by online perpetrators with the aim of identifying targets for cybercrime (Lewis, 2018), and in the year ending September 2019, 1 million 'computer misuse' crimes were reportedly committed against households ...
Abstract. This volume highlights the central role of the human factor in cybercrime and the need to develop a more interdisciplinary research agenda to understand better the constant evolution of online harms and craft more effective responses. The term "human factor" is understood very broadly and encompasses individual, institutional, and ...
In "Did cybercrime cause the crime drop", Farrell and Birks refute the idea that "the international crime drop was the result of increased cybercrime" (p.1) based on the lack of evidence provided, the temporal inconsistency of the causal conjecture, the lack of coherence with other explanatory frames for the crime drop (Farrell et al. 2014), such as the security hypothesis (Farrell et ...
1. Introduction. The development of information and communication technology (ICT) makes modern life more convenient [1, 2].However, increases in cybercrimes exploiting such technology have emerged as a severe social issue [1, 3].Since the onset of the COVID-19 pandemic, more internet users worldwide have become dependent on the internet in all areas, including education, financial ...
The central question of this article is whether routine activity theory (RAT) can be used as an analytical framework to study cybercrimes. Both a theoretical analysis and an analysis of empirical studies have thus far failed to provide a clear answer. The multivariate analysis presented in this article tries to avoid some of the limitations of ...
This theory can be applied to cybercrime and may be integrated with other stated theories. The theory of Situational Crime Prevention (SCP) makes the hypothesis that a perpetrator must have an opportunity in addition to a motive. A motive without an apportunity will not yield to a crime.
Abstract. Recent studies have hypothesised that the international crime drop was the result of the rise in cybercrime. We subject this 'cybercrime hypothesis' to critical assessment. We find significant evidence and argument indicating that cybercrime could not have caused the crime drop, and so we reject the cybercrime hypothesis.
In an increasingly digital world, our social interactions are increasingly moving online. Differential association and social learning theories suggest that we learn both moral definitions and the how-to of crime from those we associate with. In this paper we examine whether online or offline social learning leads to more self-disclosed forms of cyber-offending. Using a national online sample ...
Cybercrime, or the use of computer technology or online networks to commit crimes, ranges from fraud and identity theft to threats and intimidation. ... To use the familiar language of Routine Activity Theory (Cohen & Felson, 1979), we have a lot more suitable targets in insufficiently guarded space being victimized by an increasing number ...
Computer crime, also known as cybercrime, refers to criminal activities that are conducted through the use of computers or other digital technologies. Criminologists have developed a number of theoretical domains to explain why individuals engage in computer crime, including: 1. Rational Choice Theory - This theory suggests that individuals ...
Cybercrime is crime that is mediated by networked technology (Wall, Citation 2007). Old crimes such as theft, fraud, and harassment find new forms in cyberspace and information technologies. ... and proposes the space transition theory. Another focus in the field is the extent to which offenders in the real world have a greater propensity to ...
Cyber criminology is a multidisciplinary field that encompasses practices from multiple fields such as criminology, victimology, sociology, information assurance, and computer information systems (Jaishankar, 2007). Cyber criminology focuses on why individuals commit cybercrimes and why they engage in certain criminal behavior in cyberspace.
In the longitudinal analysis, we confirmed the first hypothesis and found that increased PSMU was associated with increased cybercrime victimization in both within- and between-subject analyses. The result indicated a clear link between problematic use and cybercrime experiences during the observation period: as problematic use increases, so ...
Hypotheses and Theories of Cybercrime. Cybercrime has multiple similarities and differences from traditional crime. Firstly, there are generally the same motivations for committing crimes and performing violations. People choose to get involved in criminal activity due to the desire for financial and material goods, forbidden sexual interests ...
In the past two decades, the incidence and prevalence of cybercrime within organizations has increased substantially worldwide (Bissell et al., 2019; Furnell, 2002; Mickelberg et al., 2014), while the rate of in-person crimes has declined on average across the United States (Sharkey et al., 2017).The 2019 Cost of Cybercrime Study of 355 large companies in 11 nations found that the average ...
Mrs. Alito was reportedly angered by this, and flew her flag upside-down in response. It is very hard to tell what intentions were behind one single gesture, reportedly not even done by the ...
To confirm this hypothesis, we analysed the public sandbox execution associated with Mallox from ANY.RUN and Triage. In the around twenty cases investigated, the above-mentioned URL was presented in 19, and for the remaining cases, ... While formal links with cybercrime-related activities remain unproven, the involvement of this AS previous ...
Wed 15 May 2024 // 19:30 UTC. Some iPhone users are reportedly seeing photos they had previously deleted resurface on their devices ever since updating to the latest version of iOS. The user reports originate from Reddit, and it's not just a couple of Apple users experiencing issues. By our count, 16 people who deleted their photos say they've ...