Nurse Selection Criteria + Example Responses
- Careers & Specialisations
- Wages, Salaries & Pay Rates
- Types of Nursing Courses
- ATAR for Nursing
- Scholarships
- Resume Tips & Template
- Nursing Cover Letter
- Interview Questions & Answers
- Selection Criteria Examples
- Tertiary Admissions Centres
- Nursing Agencies
- Nursing Jobs
When it comes to nursing positions, education and experience are important, but they are not the only factors employers take into consideration. Employers also look for certain key selection criteria that demonstrate a candidate’s ability to perform the role effectively. As with any interview, it’s often recommended that you follow the STAR method when provding a response. The STAR method is a structured manner of responding to behavioral-based interview questions by providing the specific Situation, Task, Action, and Result of the particular scenario. This article will go beyond education and experience, and provide insight into the key selection criteria for nursing positions, along with example STAR responses.
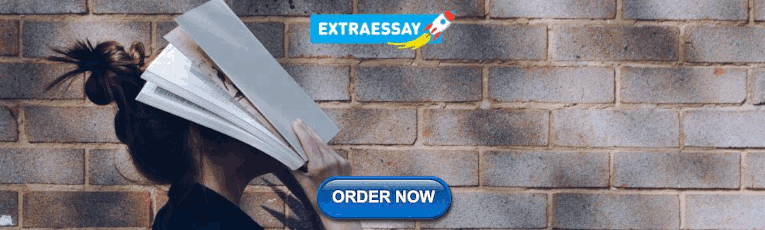
1. Communication & Interpersonal Skills
Effective communication and interpersonal skills are critical in the nursing profession. You need to be able to communicate with patients, their families, and other healthcare professionals in a clear and concise manner. Additionally, being able to form strong relationships with others is important in building trust and providing the best care for your patients. Employers will be looking for evidence of your communication and interpersonal skills during the interview.
Example response:
- Situation: During my time working as a nurse in a hospital, I was faced with a patient who was non-verbal and unable to communicate their needs.
- Task: I needed to assess their condition and administer medication.
- Action: I used non-verbal communication techniques, such as gestures and facial expressions, to understand their needs and communicate with them effectively. I also formed a rapport with the patient, by talking to them in a calm and reassuring tone.
- Result: The patient was able to receive the necessary treatment and was much more comfortable with the process. The patient and their family also expressed their gratitude for my compassionate and empathetic approach.
2. Compassion & Empathy
Compassion and empathy are key traits for nurses as they must be able to understand and connect with their patients. This requires an ability to listen, understand, and respond to the emotional and physical needs of patients. Nurses must be able to show compassion and empathy towards their patients and provide comfort and support.
Employers are looking for nurses who can demonstrate their compassion and empathy skills and show that they are able to connect with and understand their patients. They want to see that you have a genuine concern for the well-being of your patients and are able to provide comfort and support. They also want to know that you are able to maintain a professional demeanor and provide care in a respectful and empathetic manner.
By demonstrating your compassion and empathy skills, you show that you are a caring and empathetic nurse who is able to understand and connect with your patients. You also show that you are able to provide comfort and support to your patients, which is essential for providing high-quality patient care. Your compassion and empathy skills demonstrate your commitment to providing patient-centered care and helping your patients feel supported and understood.
- Situation: I was working in a hospice where a patient was in their final stages of life.
- Task: The patient was in a lot of pain and their family was upset and worried.
- Action: I listened to the patient’s concerns and provided comfort and reassurance to both the patient and their family. I also kept in close communication with the patient’s physician to ensure that their pain was managed appropriately.
- Result: The patient was able to pass away peacefully, and the family felt comforted knowing that their loved one was not alone. They also expressed their appreciation for my compassionate and empathetic approach.
3. Teamwork
Nursing is a team-oriented profession, and it is important to be able to work well with others. This involves being able to collaborate with other healthcare professionals, such as physicians and nursing assistants, to provide the best care for your patients. Nurses must be able to work towards a common goal and support their colleagues, while also being able to take initiative and lead when necessary.
Employers are looking for nurses who can demonstrate their teamwork skills and show that they are able to collaborate effectively with others. They want to see that you have a positive attitude, are supportive of your colleagues, and can work well under pressure. They also want to know that you have the ability to take initiative and lead when necessary, as this is essential for providing high-quality patient care.
By demonstrating your teamwork skills, you show that you are a collaborative and supportive nurse who is able to work well with others. You also show that you have the ability to take initiative and lead when necessary, which is essential for providing high-quality patient care. You demonstrate your commitment to teamwork and collaboration, which is essential for ensuring the best outcomes for your patients and the success of the healthcare team.
- Situation: I was working on a busy medical-surgical unit where the staff was stretched thin.
- Task: I needed to ensure that all of my patients received the care they needed in a timely manner.
- Action: I worked closely with my fellow nurses and nursing assistants to prioritize patient care, delegate tasks, and provide support when needed. I also kept open communication with the physician to ensure that everyone was on the same page.
- Result: We were able to provide the best care for our patients and maintain a positive and productive work environment. The unit received positive feedback from patients and their families for our teamwork and collaboration.
4. Quality Improvement
Quality improvement is an essential aspect of the nursing profession as it helps to ensure that patients receive the best care possible. It involves identifying areas for improvement and implementing changes to improve the quality of care. This could include improving patient outcomes, reducing errors, increasing patient satisfaction, or improving efficiency.
Quality improvement requires a systematic approach, collaboration, and an ongoing commitment to continuous improvement. Nurses play a vital role in this process as they are often on the front lines, working with patients and providing care. By being involved in quality improvement initiatives, nurses can make a positive impact on patient outcomes and contribute to the overall success of the healthcare organisation.
Employers will be looking for evidence of your ability to identify areas for improvement, implement changes, and monitor the results during the interview. They want to see that you have a commitment to providing the best care for your patients and are proactive in seeking ways to improve the quality of care.
- Situation: I was working in a hospital where the discharge process was taking longer than it should.
- Task: I needed to find a solution to improve the discharge process for patients.
- Action: I analyzed the current process, identified areas for improvement, and made suggestions for changes. I also collaborated with the rest of the nursing staff and physicians to implement the changes and monitor the results.
- Result: The discharge process was streamlined, and patients were able to be discharged faster, which improved their experience and satisfaction. The hospital also received positive feedback from patients and their families for the improved discharge process.
5. Continuous Professional Development (CPD)
Continuous professional development is important for nurses, as it helps them to stay up-to-date with the latest developments in the field and maintain their competency. Employers are looking for nurses who are committed to their ongoing professional development and have a strong desire to learn and grow in their careers. By demonstrating a commitment to CPD, nurses show that they are dedicated to providing the best care for their patients and are interested in staying current in their field.
- Situation: I was working as a nurse and wanted to further my knowledge in a specific area of nursing.
- Task: I needed to find ways to continue my professional development.
- Action: I researched and attended conferences, workshops, and courses related to my area of interest. I also sought out mentorship opportunities with experienced nurses.
- Result: I was able to expand my knowledge and skills in my area of interest, which helped me provide better care for my patients. I also received recognition from my peers and supervisors for my commitment to continuous professional development.
6. Problem-Solving
Problem-solving is a crucial skill for nurses as they often face complex and challenging situations in their daily work. It requires critical thinking, effective communication, and the ability to identify and analyse problems and find solutions. Nurses must be able to make informed decisions, prioritise tasks, and work effectively under pressure.
Employers are looking for nurses who can demonstrate their problem-solving skills and show that they can handle challenging situations in a calm and effective manner. They want to see that you can think creatively and come up with innovative solutions to problems. They also want to know that you have the ability to make decisions that benefit your patients, your team, and the organisation.
By demonstrating your problem-solving skills, you show that you are a competent nurse who can handle complex and challenging situations and make informed decisions. You also show that you have the ability to think critically and creatively, which is essential for providing high-quality patient care.
- Situation: I was working as a nurse in a busy emergency room where a patient was in critical condition.
- Task: I needed to find a solution to provide the best care for the patient in a limited amount of time.
- Action: I assessed the patient’s condition, gathered relevant information, and considered multiple options for treatment. I then collaborated with the physician to determine the best course of action.
- Result: The patient received the necessary treatment, and their condition stabilized. The patient and their family also expressed their gratitude for my quick thinking and effective problem-solving skills.
7. Legal Understanding
Legal understanding is an important aspect of nursing as nurses must be aware of and adhere to the laws and regulations that govern their practice. This includes understanding the laws and regulations related to patient privacy, informed consent, and medical ethics. Nurses must also be aware of the legal implications of their actions and understand how to handle difficult and complex legal situations.
Employers are looking for nurses who have a good understanding of the laws and regulations that govern their practice and who can demonstrate their ability to apply this knowledge in their daily work. They want to see that you have a commitment to upholding the ethical and legal standards of the nursing profession and are able to make informed decisions that are in line with these standards.
By demonstrating your legal understanding, you show that you are a responsible and ethical nurse who is committed to providing high-quality care to your patients. You also show that you are aware of the laws and regulations that govern your practice and have the ability to handle difficult and complex legal situations in a professional and responsible manner.
- Situation: I was working as a nurse and was faced with a situation where a patient’s privacy was in question.
- Task: I needed to ensure that the patient’s privacy was protected.
- Action: I consulted the relevant laws and regulations, and determined the appropriate course of action. I also kept the patient informed of the situation and their rights.
- Result: The patient’s privacy was protected, and the hospital was able to comply with the relevant laws and regulations. The patient also expressed their appreciation for my understanding of their rights and protection of their privacy.
In conclusion, education and experience are important factors when it comes to nursing positions, but they are not the only factors that employers take into consideration. Employers also look for evidence of key selection criteria such as communication and interpersonal skills, compassion and empathy, teamwork, quality improvement, continuous professional development, problem-solving, and legal knowledge.
It is essential for nursing candidates to understand these criteria and be able to provide examples of how they demonstrate them during the interview. By following the STAR method and being able to articulate your experiences and accomplishments, you can show the interviewer that you possess the skills and qualities necessary for a successful nursing career.
So, when preparing for a nursing interview , take the time to reflect on your experiences and think about how you can demonstrate these key selection criteria. Show the interviewer that you are a well-rounded and competent nurse who is committed to providing the best care for your patients. Good luck!
More information
- Nursing Schools
- Find Courses
- Diploma of Nursing
- Bachelor of Nursing
- Master of Nursing
- CPD Courses
- Nursing Courses Sydney
- Nursing Courses Melbourne
- Nursing Courses in QLD
- Nursing Courses Brisbane
- Nursing Courses Perth
- Nursing Courses Online
- Nursing Courses in Tasmania
- Nursing Courses in Darwin NT
- Nursing Courses in Adelaide SA
- Nursing Courses in Canberra ACT
- General Nursing
- Nursing Specialties
- Nursing Students
- United States Nursing
- World Nursing
- Boards of Nursing
- Breakroom / Clubs
- Nurse Q&A
- Student Q&A
- Fastest BSN
- Most Affordable BSN
- Fastest MSN
- Most Affordable MSN
- Best RN to BSN
- Fastest RN to BSN
- Most Affordable RN to BSN
- Best LPN/LVN
- Fastest LPN/LVN
- Most Affordable LPN/LVN
- Fastest DNP
- Most Affordable DNP
- Medical Assistant
- Best Online Medical Assistant
- Best Accelerated Medical Assistant
- Most Affordable Medical Assistant
- Nurse Practitioner
- Pediatric NP
- Neonatal NP
- Oncology NP
- Acute Care NP
- Aesthetic NP
- Women's Health NP
- Adult-Gerontology NP
- Emergency NP
- Best RN to NP
- Psychiatric-Mental Health NP
- RN Specialties
- Best RN Jobs and Salaries
- Aesthetic Nurse
- Nursing Informatics
- Nurse Case Manager
- Forensic Nurse
- Labor and Delivery Nurse
- Psychiatric Nurse
- Pediatric Nurse
- Travel Nurse
- Telemetry Nurse
- Dermatology Nurse
- Best NP Jobs and Salaries
- Family NP (FNP)
- Orthopedic NP
- Psychiatric-Mental Health NP (PMHNP)
- Nurse Educator
- Nurse Administrator
- Certified Nurse Midwife (CNM)
- Clinical Nurse Specialist (CNS)
- Certified Registered Nurse Anesthetist (CRNA)
- Best Free Online NCLEX-RN Study Guide
- The Nursing Process
- Question Leveling
- NCLEX-RN Question Identification
- Expert NCLEX-RN Test-Taking Strategies
- Best Scrubs for Nurses
- Best Shoes for Nurses
- Best Stethoscopes for Nurses
- Best Gifts for Nurses
- Undergraduate
- How to Become an LPN/LVN
- How to Earn an ADN
- Differences Between ADN, ASN, AAS
- How to Earn a BSN
- Best MSN Concentrations
- Is an MSN Worth It?
- How to Earn a DNP
- MSN vs. DNP
need help with selection criteria
Nursing Students Student Assist
Published Jun 27, 2014
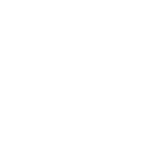
I am stacy. I am nearly graduate, I am applying transitional program at the moment. However, I found difficult in addressing selection criteria. Anyone could give me some help?
1. Demonstrated high level interpersonal, verbal and written communication skills
1. 2 Demonstrated clinical knowledge and clinical problem solving abilities
2. 3 An understanding of and ability to work within an interdisciplinary team
3. 4 An understanding of professional, ethical and legal requirements of registered nurse
4. 5 An understanding of EEO, Work health and safety, infection control and continuous quality improvement principle
6. 6 A demonstrated understanding of NSW health's core values- openness, collaboration, respect and empowerment
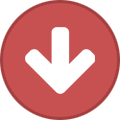
- + Add a Comment
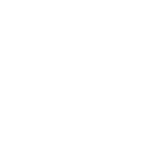
NotMyProblem MSN, ASN, BSN, MSN, LPN, RN
2,690 Posts
If I understand your question correctly, I will give you my thoughts on what I believe you are searching for. If you are hoping to be selected for a work position or a program seat, this is what the panel is looking for. I hope this helps you...
1. This area means that you are competent in written and spoken exchanges. You need to be able to understand information that you receive as well as making sure that others can understand what you are writing and saying. And you need to be able to do it in a professional, non-offensive manner at all times.
2. This means that you understand the information from your nursing training and are able to apply/use what you've learned so far to the work setting as it pertains to solving problems in patient care.
3. Working with an interdisciplinary team ( RN , LPN, CNA, MD, PT/OT, Case Manager, etc) means that you understand that other members of the healthcare team has a specific job to do with your patients as well, and you are able to use their suggestions to help get your patients healthy just as you would expect them to accept your suggestions (your clinical knowledge) that helps to keep your patients safe as they return to good health. In other words, you are all working together and understanding that everyone has his/her own job to do that brings the patient to the point of being ready for discharge.
4. This means that you understand your obligations and duties as a nurse, as well as knowing your limitations. You are expected to follow your state's Nurse Practice Act and the Code of Ethics set forth by the American Nurses Association. Basically, if you've not been trained to do it, don't do it! You must always work within your scope of practice.Also, you need to be able to act in the best interest of your patients. Believe it or not, that may even mean having the patient to be assigned another nurse. You see this more in ethical situations.
5. This section means that there are policies and procedures that you are expected to follow to keep everyone safe at that facility. That includes but is not limited to hand washing, fall prevention, following isolation procedures, etc., which includes protecting yourself, your patients, their families/visitors, or ANYONE you come in contact with while on duty.
6. If NSW is a facility that intends to employ you, this means that, like most places, they have a mission statement. This particular statement indicates that they (all team members) strive to be as open as possible about the care they provide (keep the patients and team members informed) and try to work WITH each other (collaboration) in a manner that shows that they treat each other and their customers as they should be treated in a way that improves and strengthens (empowerment) the facility as a whole.
Thanks for your help. That is very helpful.
You're very welcome!
(The last statement in my post should read "Good luck with the transition". This autocorrect on my iPad should be called auto-add. Sorry about the typos).
just wondering should I put some theory for the last question, it seems like more about understanding, so I should paraphase and express my own idea of openness, collaboration, repsect, and empowerment. however, they are all interrelated. I should explain them in all?
I would summarize it based my understanding and interpretation of the mission statement if I were you. No need to add theory.
As nurses,we use a range of communication skills, including verbal and written, to acquire, interpret and record their knowledge and understanding of people’s needs. The interpersonal skills that we use includes working with others, empathy with clients, family and colleagues, using sensitivity when dealing with people and relating to persons from differing cultural, spiritual, social and religious backgrounds. I have acquired and refined strong communication and interpersonal skills through my course and my working experience as AIN in St Vincent Private hospital. I have used active listening, asking for clarification and negotiating solutions to fulfill my roles as specified by SVPH. I also have demonstrated clear and precise written communication skills, using standard medical terminology and abbreviations. For example, I had written“ PAC”, “FASF”,”IDC”in the handover sheet for the other AIN and also provide a handover using the ISBAR. Moreover, I have correct record the patient’s FBC and utilized organisation protocols for electronic communication like adequately use the “web delacy” to order patients’ meal.
2. Demonstrated clinical knowledge and clinical problem solving abilities
A very important clinical nursing skill is knowing how to safely administer medications to patients. There are many routes for administration, and many different drugs, To prevent errors when giving drugs to patients, nurses follow "The 5 Rights of Medication Administration." The five rights refer to: the right patient, the right drug, the right dosage, the right time and the right route. The nurse repeats the "5 Rights" test three times before giving a drug to a patient, following the administration of medication policy according to the organization to ensure the correct administration such as verified by two RN , and understanding the nurse-initiated drugs.
My clinical problem solving approach incorporate the nursing process: assessing, interpreting, implementing and evaluating. I recently demonstrated my ability to utilise this process in my role as AIN in SVPH. When I addressed the constipation problem of my patient while I was doing my AIN special. I noticed he has a distended abdomen, and it hard likes a rock. In consideration of his surgery, and his documentation that he hasn’t open his bound. I suspected that he is constipated, so I told the RN and movical was given to the patient. Then I assess ed the patient for any bound movement and toileting in order to evaluate the interventions.
3. An understanding of and ability to work within an interdisciplinary team
Working within an interdisciplinary team means that each members of the health care team has contributed to patients’ health condition in different ways and roles. Nursing care also involves communicating with other health-care professionals, including physicians, speech therapists,physio therapists or social workers. Being a team player involves sharing insights, observations and concerns with relevant medical providers to make adjustments to patient care. Good teamwork could ensure the high quality of patient care, increased patient’s safety and ensured the person centered care is delivered effectively. I have developed effective teamwork through my several clinical placements in different facility and my work experience in SVPH. I worked together with an RN who guided me during my clinical practice in gastroenterology ward. We divided the work in regarding to the handover about 3 post ops would be coming. I prepared the bed,IVpole,Obs Machine, I was assigned to do the hourly OBS and IDC, and order the diet and referred the patient to the Diettion. Futhermore, In SVPH, when I was in the othopedic ward, I worked with one physiotherapist to help my patient to get up from the bed.
ensure the person centered care is delivered. Referred to the right person to allocate the care. Referrer to the social workers
4. An understanding of professional, ethical and legal requirements of registered nurse
I endeavor to provide quality and safety nursing care in accordance with ANMC competence standards, the Code of Ethics and the code of Professional Conduct. Understanding the legal requirement of a nurse, such as scope of practice, duty of care and informed consent. As professional, I treat personal information as private and confidentiality. This could be shown through the adequate disposal of the handover sheet. Moreover, maintaining a professional relationship with patients and be aware of the professional boundaries. Ethically, I respects the values,customs and spiritual beliefs of individuals. Also, respect patient’s privacy and dignity at all time by close the door or close the curten during procedure. Legally, perform my duty of care such as provide adequate obs assessment and relatively intervention. One of the essential legal requirement is informed consent, we relies on oral consent or implied consent for most nursing interventions. While I was perform my duty as AIN of providing post op wash for my patient, I always obtain consent to proceed the wash, always assess the patient’s condition before I roll the patient on the side and prevent any potential risk.
5. An understanding of EEO, Work health and safety, infection control and continuous quality improvement principle
There are policies and procedures that are expected to follow to keep everyone safe. The EEO refers to that a person cannot be against by their race, culture background or appearance and so on. A co-worker cannot be discriminated against due to his race. Work health and safety is about promote the health, safety and wellbeing of staff,patients and others in the workplace. Complies with the infection control standards in the nursing regulation. Using standard precautions at all times,comply with 5 moment of hand hygiene, adequately use of PPE and segregate waste into the correct waste management stream. I have clearly understanding of these principles by undertake annual mandatory training in work health and safety during my employment in SVPH. Assist in maintaining a clean and tidy unit, always double check the sharp bin to avoided any needle injured during my duty as AIN in SVPH.
Is this a class assignment? I thought maybe you were preparing for a job or college intake interview...
Yes, it is prepared for my job application, this is my draft. But like my brain just doesn't work properly. I feel like I couldn't write it down properly. Some questions request me to show that I understand that, so I just write out what it is.
Oh, I see. If that's the case, these questions/statements are requirements that are expected of the RN . I've seen this on many applications. All-in-all, they just expect you to behave and function as the professional you were trained to be in accordance to your state's nurse practice act combined with the facilities policies and procedures. Trying to memorize those sections is admiral but a bit of an overkill. Just follow the rules and demonstrate respect for others. Your preceptor will train you on what you need to know to get you started once you get the job. And remember, when there is something that you don't know, ASK.
- Bookkeeping
- Financial Planning
- Animal Care
- Animal Science
- Dog Training
- Horse Breeding & Equine Studies
- Pet Grooming
- Veterinary Nursing
- Graphic Design
- Interior Design
- Photography
- User Experience Design
- Building & Construction
- Real Estate
- Business Administration
- Business Development
- Business Operations
- Change Management
- Customer Engagement
- Entrepreneurship
- Human Resources
- Leadership & Management
- Organisational Development
- Project Management
- Quality Management
- Small Business
- Supply Chain Operations
- Alcohol & Other Drugs
- Community Services
- Individual Support
- Education Support
- Training & Assessment
- Engineering
- Manufacturing
- Agriculture
- Conservation & Land Management
- Health Science
- Horticulture
- Social Science
- Sustainability
- Dermatology
- Eyebrow Specialist
- Eyelash Extension
- Hairdressing
- Nail Technology
- Allied Health
- Counselling
- Dental Assisting
- Health Administration
- Health Services Assistance
- Life Coaching
- Medical Administration
- Mental Health
- Natural Therapies
- Naturopathy
- Practice Management
- Sports & Fitness
- Culinary Arts
- Event Management
- Hospitality
- Wedding Planning
- Cloud Computing
- Cyber Security
- Data Science & Analytics
- Programming
- Systems Administration
- Web Development
- Compliance & Risk
- Criminal Justice & Psychology
- Work Health & Safety
- Advertising
- Digital Marketing
- Digital Media
- Social Media Marketing
- New South Wales
- Northern Territory
- Western Australia
- South Australia
- Graduate Diploma
- Advanced Diploma
- Associate Degree
- Graduate Certificate
- Undergraduate Certificate
- Certificate
- Certificate II
- Certificate III
- Certificate IV
- Courses by Provider
- Government Funded Courses
- Student Hub
- The Workforce Training Hub
Selection Criteria Response: Nursing Examples
In this post, 1. effective communication and interpersonal skills, 2. teamwork skills, 3. problem solving skills.

In this post Show
You will often be expected to respond to key selection criteria when applying for nursing jobs. Here are 3 examples to get you started.
While it might feel like your cover letter and resume should suffice when you’re submitting a job application, sometimes your recruiter expects a little more, depending on the field of work you’re in.
If you’re working in nursing, you’re often expected to respond to some key selection criteria in the application process.
This is an excellent opportunity to make your application stand out by matching your real-life experiences to your resume.
Here are some selection criteria examples for a registered nurse application in Australia, along with some answer examples to help you out:
Effective communication skills are essential for nursing. Show the recruiter that you’re good with people by explaining your methods, and reiterating your relevant skills.
Throughout my career in nursing care, I have proven my robust verbal communication skills when interacting one-on-one with my patients or their partners/spouses. I can effectively communicate medical terminology in digestible terms, making outcomes easier to understand by clearly indicating what any new information will mean for their overall diagnosis. I have also proven to be an effective communicator in group settings, with my patients’ larger family groups. One of my techniques is to direct my speech and eye contact between both my patient and their loved ones, to ensure that everyone feels included in the conversation. I know that I need to be conscious of the emotional impacts of what I am communicating to the group since health concerns often come hand-in-hand with hardship.
Teamwork is essential in any workplace, but especially in a hospital environment where you work with others every day. In your response to this prompt, don’t just exemplify your ability to work collaboratively – you should also prove that you’re a great team leader.
In my five years working at the Royal Melbourne Hospital as a registered nurse, I did a lot of shift work, meaning that I would not always be working with the same team. Through this experience, I thoroughly developed my teamwork skills and proved to be very adaptable as a team member. My team members were different every shift, but the outcomes of my work remained consistent, as a result of my effective interpersonal skills. I can slot into any working team and adapt my methods accordingly. Towards the end of my time at the RMH, I was occasionally rostered on with several junior staff members, meaning that I would have to step into a senior position and delegate tasks accordingly. My ability to perform as a team member or as a team leader, depending on the circumstances is one of my strongest assets.
In a nursing environment, you’re faced with new problems every day and need to be able to think on your feet, with an ability to act quickly. Provide examples of your skills in this area in your response.
When I first began my career in nursing, I primarily worked in aged care. A majority of my patients were living with degenerative brain conditions like Alzheimer’s disease. This meant that new problems arose all the time if a patient wandered off and got lost or was otherwise confused, having forgotten something significant. Patients would be lucid one second, and then stubborn and disoriented the next. This meant that I had to adapt to new circumstances quickly and efficiently, addressing new concerns every moment. It was also essential that while I solved each problem, that I had to remain calm and conscious of the patient’s vulnerability.
Overall, your key selection criteria response should come from your own real-life experience. Tailor your responses to the keywords of the position description, and try to use specific examples. Prove that your real experiences have earned you the skillset you have today.
How to Write Key Selection Criteria [+Templates]
Our Key Selection Criteria Hub can help you write the best possible answer that highlights your skillset to a potential employer.
Show Me How!
Latest Articles
How to apply for work placement: tips & resources.
Congratulations! You’re about to embark on an exciting journey where you will practise and develop t...
11 Hobbies That Can Make You Money: Use Your Passion for Profit
Hobbies often get a bad rap. They’re seen as just a way to kill time, a mindless escape from t...

Is The Job You Hate Killing You? Why You Need to Make a Change
Do you hate your job? Studies show that staying in a job you hate has negative health effects and ev...

Want to read more?
3 ways experiential learning can help you get your edge.
As the modern job market continues to shift and grow, employers are increasingly favouring experient...

How to Know When You Should Change Careers Over 50
From burnout, poor work/life balance, ageism, and lack of fulfillment, learn the signs that it’s tim...
How Technology is Affecting Career Choices (Infographic)
Technology is changing the way we work and affecting future career choices, see what areas are growi...

Subscribe to Our Newsletter
Get expert advice, insights, and explainers on tricky topics — designed to help you navigate your learning journey with confidence.
- Popular Subjects
- Qualification Type
- Courses by Location
- Business Admin
- Courses In ACT
- Courses In NSW
- Courses In NT
- Courses In QLD
- Courses In Tasmania
- Courses In VIC
- Courses In WA
Newsletter sign up
Newsletter sign up.
We’ll email you updates on job trends, career advice, study tips, news and more.
You are currently visiting our Australian website Training.com.au
Would you like to visit our New Zealand website instead?
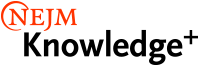
- Exploring the ACGME Core Competencies: Medical Knowledge (Part 5 of 7)

These Core Competencies define the basic skill sets and behavioral attributes deemed a requirement for every resident and practicing physician. Their success is measured in terms of the ability to: 1) administer a high level of care to diagnose and treat illness, 2) offer and implement strategies to improve patient health, 3) provide resources for disease prevention, and 4) support patients’ and families’ emotional needs while also treating their physical needs.
Therefore, these competencies are incorporated into the training and continuing education of almost every major medical education program. This includes the adoption by the American Board of Medical Specialties (ABMS), and, later, the integration of the ACGME Core Competencies into the Maintenance of Certification (MOC) program.
The ACGME Core Competencies are defined as:
- Practice-Based Learning and Improvement
- Patient Care and Procedural Skills
- Systems-Based Practice
Medical Knowledge
- Interpersonal and Communication Skills
- Professionalism
In Part 1 of this blog series, we listed the ACGME Core Competencies with a focus on EPAs and Milestones. Other articles in this series have addressed Practice-Based Learning and Improvement , Patient Care and Procedural Skills , and Systems-Based Practice . In this article, we break down the various components of the Medical Knowledge core competency. Each ACGME Core Competency represents a necessary skill set and attitude for training and practicing physicians to continually hone and develop. Medical Knowledge includes an understanding of all established and evolving biomedical, clinical, epidemiological, and social-behavioral sciences. However, resident physicians must also go beyond simply obtaining medical knowledge for themselves. They must also demonstrate the ability to apply it to patient care and appropriately and enthusiastically transfer that knowledge to others.
Medicine is constantly evolving, and even a seasoned physician with years of experience hasn’t “seen it all.” A desire for and an understanding of the need for a lifelong-learning approach to the practice of medicine is a requisite attribute for physicians providing quality health care. For this reason, the ACGME Core Competency of Medical Knowledge seeks to ensure that residents are trained to continually investigate, question, and seek new knowledge. But knowledge without application is fruitless. Sharing those best practices with medical colleagues and employing that knowledge in the diagnosis and treatment of patients is equally as important as obtaining it.
The ACGME Core Competencies: Sub-competencies for Medical Knowledge
The Medical Knowledge subcompetencies break down into manageable pieces—the skills and attributes that comprise this core competency. These include being able to demonstrate:
- An investigative and analytical approach to clinical problem solving and knowledge acquisition
- An ability to apply medical knowledge to clinical situations
- An ability to teach others
Investigative and Analytical Approach
First and foremost, residents must always maintain an open mind. They will demonstrate a willingness to never shy away from asking questions and searching out new and useful sources of information. They will consider alternative or additional diagnoses, seeking and initiating discussions with faculty. The continual search for medical knowledge includes identifying both universal and individualized goals and objectives for learning, at every stage in a resident’s career. This includes an awareness of areas needing improvement and a humility to absorb and process feedback on areas where growth is needed.
The lifelong-learner attribute is evidenced by the physician’s commitment to querying literature and texts on a regular basis, attending conferences, and critically evaluating new medical information and scientific evidence in order to modify their knowledge base accordingly.
Apply Medical Knowledge to Clinical Situations
The acquisition of medical knowledge must continually circulate back to the application of it and provide better and more relevant, quality patient care. Residents will demonstrate competence through the combination of a physical exam and interpretation of ancillary studies, such as laboratory work and imaging, to form a working diagnosis and initiate a therapeutic approach.
The application of medical knowledge should also be measured using Miller’s Pyramid, a framework for assessing clinical competence developed in 1990. According to it, a resident progresses from “Knows” to “Knows How” to “Shows How” to “Does.” The “Does” component is the key: what a resident may know and be able to demonstrate in a controlled setting should match how they perform in actual day-to-day interactions with patients on a regular basis.
Subsequently, a physician exemplifying the attributes of this core competency will apply the evidence-based medical skills and knowledge that they have obtained in a patient-centered approach, and do so consistently. It is a skill set that is evidenced and witnessed in all patient interactions and clinical situations, not just testing scenarios.
Finally, competent residents pay attention to the clinical outcomes first and foremost, but in a manner that takes into consideration the cost-effectiveness, the risk-benefit ratio, and patient preferences.
Ability to Teach Others
Obtaining and applying medical knowledge skills is essential for delivering quality health care. But they cannot survive in a vacuum. Practitioners must regularly participate in the act of sharing knowledge. Being a lifelong learner is only half of the equation. The other half requires the ability to teach and pass along to others the experiences and knowledge each individual has acquired through the years. To successfully embody the Medical Knowledge Core Competency, a resident needs to demonstrate the ability to educate others in an organized, enthusiastic, and effective manner.
Medical knowledge is where all medical education begins. And it never ends. Residents must understand that the continual process of investigating, questioning, and learning is an integral part of what makes residents and physicians successful, and contributes to the growth and improvement of the health care field as a whole.
Read more about the six ACGME Core Competencies:
- Exploring the ACGME Core Competencies (Part 1 of 7)
- Exploring the ACGME Core Competencies: Practice-Based Learning and Improvement (Part 2 of 7)
- Exploring the ACGME Core Competencies: Patient Care and Procedural Skills (Part 3 of 7)
- Exploring the ACGME Core Competencies: Systems-Based Practice (Part 4 of 7)
- Exploring the ACGME Core Competencies: Interpersonal and Communication Skills (Part 6 of 7)
- Exploring the ACGME Core Competencies: Professionalism (Part 7 of 7)
Share This Post!
i feel that if medical students entering residency do not recognize the role of the ACGME or understand the competencies by which they will be evaluated, it. is difficult to assess the efficacy, utility, and success of the Outcome Project
Medical knowledge is one competency that is most important to a physician’s practice. It is an ongoing activity that needs self commitment to be up-to-date with current concepts. It is a reality that the busier a physician is in his/her clinical practice, the less time he/she has for reading. This sounds contradictory but is true. It is also important to distinguish continuing competency from continuing education as one can be educated but not competent or vice versa both of which are dangerous.
Comments are closed.
- Research article
- Open access
- Published: 24 November 2016
Knowledge is not enough to solve the problems – The role of diagnostic knowledge in clinical reasoning activities
- Jan Kiesewetter ORCID: orcid.org/0000-0001-8165-402X 1 ,
- Rene Ebersbach 1 ,
- Nike Tsalas 2 ,
- Matthias Holzer 1 ,
- Ralf Schmidmaier 1 , 3 &
- Martin R. Fischer 1
BMC Medical Education volume 16 , Article number: 303 ( 2016 ) Cite this article
7911 Accesses
22 Citations
7 Altmetric
Metrics details
Clinical reasoning is a key competence in medicine. There is a lack of knowledge, how non-experts like medical students solve clinical problems. It is known that they have difficulties applying conceptual knowledge to clinical cases, that they lack metacognitive awareness and that higher level cognitive actions correlate with diagnostic accuracy. However, the role of conceptual, strategic, conditional, and metacognitive knowledge for clinical reasoning is unknown.
Medical students ( n = 21) were exposed to three different clinical cases and instructed to use the think-aloud method. The recorded sessions were transcribed and coded with regards to the four different categories of diagnostic knowledge (see above). The transcripts were coded using the frequencies and time-coding of the categories of knowledge. The relationship between the coded data and accuracy of diagnosis was investigated with inferential statistical methods.
The use of metacognitive knowledge is correlated with application of conceptual, but not with conditional and strategic knowledge. Furthermore, conceptual and strategic knowledge application is associated with longer time on task. However, in contrast to cognitive action levels the use of different categories of diagnostic knowledge was not associated with better diagnostic accuracy.
Conclusions
The longer case work and the more intense application of conceptual knowledge in individuals with high metacognitive activity may hint towards reduced premature closure as one of the major cognitive causes of errors in medicine. Additionally, for correct case solution the cognitive actions seem to be more important than the diagnostic knowledge categories.
Peer Review reports
Clinical experts need general and specific problem solving strategies in order to make adequate treatment decisions for their patients. Clinical problem solving (or clinical reasoning) as a skill involves different categories of knowledge as well as several cognitive abilities and is key for becoming a clinical expert [ 1 ]. Problem-solving occurs in well-known phases, described in models like the hypothetical-deductive model and pattern recognition, a process that requires the use of knowledge [ 2 – 4 ]. In university, the focus lies on teaching medical knowledge, in order to give the student a foundation for further clinical problem-solving when dealing with real patients [ 5 ]. According to recent studies [ 6 ] diagnostic knowledge can be categorised into three categories: Conceptual knowledge (“what information”), strategic knowledge (“how information”) and conditional knowledge (“why information”) [ 5 ]. Table 1 shows an overview of the definitions. These categories have been investigated in several studies regarding clinical reasoning of medical students and medical doctors [ 6 – 8 ].
The Revision of Bloom’s Taxonomy added a fourth category: Metacognitive knowledge, which “involves knowledge about cognition in general as well as awareness of one’s own knowledge about one’s own cognition” [ 9 , 10 ]. While handling a case, medical students or doctors are able to externalize their thoughts about the strategies of problem-solving or their application of knowledge [ 11 ]. Metacognition in this sense includes the judgements of how easily one believes one learns and whether one has the feeling of knowing something.
Surprisingly, little is known about the assessment and applicability of metacognition within the medical context and its relation to the knowledge categories in the situated learning contexts of medical students.
Whereas several methods are used to assess “classic” knowledge categories (e.g. multiple choice tests, key feature problems, interviews, questions, stimulated recall) it has proven difficult to measure and observe metacognition in a realistic setting [ 7 ]. Since metacognition cannot be observed directly in students [ 12 ], self-report methods like questionnaires, rating scales and stimulated recall are used. However, these self-reporting measures already reflect that, to be able to talk what one thinks, the student’s metacognitive activities and one’s verbal capacity are of importance [ 13 ]. When students are thinking aloud, registering the metacognitive activities without the student’s awareness is possible and the otherwise implicit cognitive processes can be observed [ 14 ].
In clinical problem solving research, traditionally only little parts of knowledge are investigated in relation to the correct diagnosis. Thus far, there is no model of clinical reasoning that, if applied, can explain how and why successful students come to the correct diagnosis, while unsuccessful students do not. However, it seems worthwhile to create evidence for such a holistic model of clinical problem solving of medical students that should include all knowledge categories. We therefore set out to observe all aforementioned knowledge categories simultaneously in order to identify the relationship between them. More specifically we wanted to answer the following research questions:
How are diagnostic knowledge categories interrelated?
The interplay of knowledge categories gives insight how students store clinical knowledge and whether some categories seem more important to them than others. Further, it has not been investigated how knowledge categories relate to previous knowledge.
How is the use of the diagnostic knowledge categories related to time on task?
It is important to understand how much time the application of the different knowledge categories takes.
How is the use of diagnostic knowledge categories related to diagnostic accuracy?
Especially, it seems interesting to identify the role each plays to solve a clinical case.
How are the knowledge categories divided over the course of a case solution?
It is interesting to see if some of the knowledge categories are used more frequently in the beginning and others are used more towards the end of the case solutions.
To answer this research questions we conducted a study where medical students first received a short knowledge training for clinical nephrology and a subsequent knowledge test to standardize previous knowledge. After that the students worked on paper-based, clinical case scenarios while thinking a-loud. The think-a-loud protocols were transcribed and coded according to the aforementioned knowledge categories. In the following paragraphs each step of the methodology is explained in detail.
Participants
Twenty-one medical students (female = 11) of two German medical faculties in their third, fourth and fifth year (M = 23.9 years; range 20–34) volunteered to take part in the study. These curricular years were chosen because the participants would have finished their internal medicine curriculum and should have enough prior knowledge to solve clinical problems but would not have experienced the final sixth clinical year of full-time electives that usually elevates students’ problem-solving substantially. This study was approved by the Ethical Committee of the Medical Faculty of LMU Munich. Written, informed consent was obtained from all participants and all participants received a small monetary compensation for participation.
Coding scheme
A coding scheme was established on the foundation of the knowledge type definitions [ 7 , 10 , 15 ]. The definition used in the coding scheme is illustrated in Table 2 . The coding scheme had an overall interrater reliability of k = .79; SD = .9 for the categories. One investigator (R.E.) coded all transcripts; a random 10% sample of the text was double coded.
Course of study
Students arrived and first filled out a pre-study questionnaire (see below), then students received a three hours of practicing a standardized learning unit in the field of clinical nephrology and upon completion, the students’ retention of content specific medical knowledge was tested using a multiple choice test. Then participants were instructed on the think-aloud method in a short practice exercise. Finally, students then solved three cases in clinical nephrology with the think-aloud method (see below).
Figure 1 shows the course of the study with knowledge training, a subsequent knowledge test, and work on the paper-based, clinical case scenarios.
Overview of the study
All students were recorded and recordings were transcribed and coded according to the defined knowledge categories. Codings were analysed for accuracy of the diagnosis.
Pre-study questionnaire
All participants completed a questionnaire containing items about their socio-demographic data, gender and age to control possible confounders. Further the participants were asked their overall grade of the preliminary medical examination. The reliability of this national multiple-choice exam is very high (Cronbachs α = .957) [ 16 ]. The performance of participants in this exam was used as an indicator for general prior knowledge in medicine. The results of the questionnaire and all other obtained data were pseudonymized.
Knowledge training and test
Although all participants had successfully passed their internal medicine curriculum a standardized learning tool was provided to refresh the textbook knowledge. Thirty flashcards were used containing 98 items with factual information on clinical nephrology and more precisely to acute renal failure and chronic renal insufficiency. This content matches with the pathomechanisms of the used cases. The content of the flashcards was previously published in another study (appendix S1 (online) of Schmidmaier et al. [ 17 ]). Within a 3 h electronic learning module it was ensured by testing that all participants could retrieve the contents of each flash card at least once. This was to help ensure that all students were able to show their problem-solving strategy and ability because they had the knowledge needed for application of strategies.
Clinical case scenarios
The three, paper-based case scenarios within the field of clinical nephrology were real cases from the department of internal medicine adapted by experts with anonymized, real supplemental material (i.e. lab values). After the transformation into paper-based scenarios, the cases were additionally reviewed by two content experts and one expert of medical education to ensure best possible authenticity of a paper-based case. All cases were structured the same way, containing two or three pages describing the patient’s symptoms and medical history. The results of the physical examination, blood tests, urine sample, ECG, and ultrasound scan were each described on separate pages.
The students’ task was to work on each case to show their problem-solving abilities with no instructions being given other than “Please work on this case”. They were not explicitly asked to state a diagnosis. Only one student and the test instructor were present in the room during the case elaboration. The test instructor sat behind the participant to avoid any diversion of thought [ 18 ]. The only interaction between the participant and instructor was when the instructor provided the next page of a case upon the participant’s request. Every case was interrupted after 10 min, independent of whether the case was solved or not. While participants were working on the cases using the think-aloud method, they were audio-recorded. All students did voluntarily state a diagnosis at the end of each case.
Data analysis
All audio recordings (total time of over 12 h) were transcribed and coded using the operationalized definitions of knowledge categories and metacognition described above. Data of three case sessions of 21 participants were evaluated and 63 sessions were analysed.
The standard qualitative content analysis [ 19 ] was used to assess, code, and analyse the process of thought, as it also yields very detailed quantitative data in consecutive analysis. It uses models with several categories for the coding of a text. In this study, the knowledge categories were used. The shortest section of text matching a particular knowledge category was determined as an episode. When different knowledge categories took place at the same time, one text section could be coded as more than one category. For examples see Table 2 .
Subsequently, the codings were marked as sections in the transcription software “f4” (f4 2011, Dr. T. Dresing, http://www.audiotranskription.de ) and exported to Microsoft Excel 2010 (Microsoft, 2010). For further analysis, the statistical environment “R” was used ( http://www.r-project.org/ ).
A predefined alpha level set at p < .05 was used for all tests of significance. If the data was used multiple times for comparisons we Bonferoni corrected for alpha error accumulation and report results as significant accordingly. Graphical illustrations were processed as the percentage of time spent on one knowledge category relative to the overall time. Although the categories of the model were described qualitatively, this was the basis for a quantitative analysis and graphical illustration of the results.
The frequencies of the categories and length of the episodes were analysed as quantitative dependent variables. The accuracy of diagnosis was established in a binary form ( correct or not correct ) as a dependent variable. Chi-squared tests were used to verify the relationship of dependent variables to all dichotomous socio-demographic participant variables (like gender), while Pearson correlation was used for all continuous dependent variables to correlate them to previously obtained participant data. Correlations between two dichotomous variables were calculated using crosstabs correlation coefficient ϕ. To gain insight how knowledge categories are divided over the course of time the cases were divided in 6 timewise equal parts. Frequencies of knowledge categories per sixth of the case were analysed as frequencies. As there a so many possible comparisons between the categories and sixth, we chose not to apply nonparametric statistical tests because of a to high alpha error accumulation.
Descriptive data
Overall 983 distinct episodes of knowledge categories were be coded. Table 3 shows that the students’ reasoning consists mainly of conceptual and strategic knowledge. All cases contain these categories. Most often conceptual knowledge was used (CcK) with a 44% frequency, conditional knowledge (CdK) was used with a 36% frequency, strategic knowledge (SK) with a 21% frequency. Metacognition was identified most frequently (58%) but always in combination with other knowledge categories. Metacognition was used in every case with a mean of M = 9.02 per case (SD = 6.21). Figure 2 shows the time-line graphs of two participants, exemplifying little and extensive use of metacognition.
Time-line graph (Gantt-charts) of two participants of a session with a clinical case. The Gantt-chart shows the distribution of the use of different diagnostic knowledge categories over time. As metacognitive knowledge was only in use in combination with other knowledge categories its use is presented additively on top. The upper part of the figure shows a participant with only little use of the knowledge categories and the lower part of the figure a participant with much use of the knowledge categories
How are the diagnostic knowledge categories interrelated?
To answer this research question the frequency per case of the use of knowledge categories was correlated. Results show that conceptual and strategic knowledge are not significantly related (r CcK;SK = .23;n.s.; r CcK;CdK = .00;n.s.). Conceptual knowledge and metacognitive knowledge (r CcK;MK = .35) are significantly related, as are conditional and strategic knowledge (r CdK;SK = .27). The results are presented in Table 4 .
Interestingly prior knowledge (grades of PME and assessment of the learning phase in the field of clinical nephrology) was significantly correlated to metacognitive knowledge (r MK;PME = .41, r MK; LEARNING PHASE = .28).
How is the use of diagnostic knowledge categories related to time on task?
To answer this research question the time-on-task (TT) was correlated with the use of knowledge categories. In three cases the students had to be interrupted after 10 min. These students were included in the analysis with the maximum time. The overall time-on-task was not correlated with diagnostic accuracy (r TT; DIAGNOSTIC ACCURACY = -.13;n.s.). However, conceptual and strategic knowledge is significantly correlated to TT (see Table 5 ).
When correlating the use of the four knowledge categories to the correct solution none of them showed a significant result. As well, Chi squared tests of socio-demographic data of the participants (age, year of studies) and correct versus incorrect diagnosis yielded no significant result.
We found that frequencies of the used categories are not equally distributed over the case. Interestingly, in the first two sixth of the case the students used more conceptual and strategic knowledge. From the third sixth the students used more metacognition than any other category. Of course, metacognition could only be coded together with other categories, so there is a dependency of this category. However, the frequencies of conceptual and strategic knowledge decline in the fifth and sixth sixths. All frequencies over the course of the cases are depicted in Fig. 3 .
Diagnostic knowledge dimensions used by medical students over the course of the cases
We found the occurrence of a pattern of conditional and strategic knowledge right before the closure of cases, named sequence-at-closure (s@c). This sequence-at-closure appeared in 24 of the 63 case solutions (=38%) and is significantly correlated with the correct solution of the case (r ϕ . S@C; CORRECT SOLUTION = .37).
In this study the different knowledge categories including metacognition in case work of medical students were empirically coded and described. The diagnostic knowledge categories were applied for the first time to medical students problem-solving in a realistic environment. The result was application of conceptual, strategic and conditional knowledge throughout the cases. None of the knowledge categories on its own has a crucial role for good performance. Further, prior knowledge was not directly related to the correct diagnosis. These results supports the claim that it is not simply knowledge which solves clinical cases and more in this sense does not directly mean better. Instead, it is the goal-directed application of knowledge in a certain order that helps to solve cases. Over the course of the cases it seems that the application of conceptual and strategical knowledge declines, while the importance of metacognitive knowledge increases. We found that oftentimes the last two categories before a diagnostic decision was made by the participants consisted of a pattern of conditional and strategic knowledge at the closure of cases, named sequence-at-closure, which correlated with the correct solution of the case. This result relates to our previous findings regarding the so called higher loop of cognitive actions, which was associated with better diagnostic performance [ 20 ]. The higher loop consisted of the cognitive actions Evaluation, Representation and Integration. It seems that students who are ready to state a correct diagnosis evaluate and summarize their represented knowledge about the case with this final pattern of conditional and strategic knowledge before integrating into the correct solution. If students have a clear representation of the case in relation to their predefined clinical knowledge they know, why the patient’s symptoms and clinical findings occur and how to deal with them, then they have a very good chance to correctly diagnose the patient. This finding has direct implications for instructional medical education research, which we will discuss further below.
Metacognition could be coded in all participants. However, it always appeared in conjunction with other knowledge categories. This result seems plausible as the application of metacognition cannot be separated from the content of a case. The coding of metacognition was worthwhile; it significantly correlated with conceptual knowledge and with two distinct measures of prior knowledge. People who know more and scored better in their previous studies seem to have additional capacity to control and monitor their solution in a better way. Knowledge regularly is measured in assessment and learning research [ 6 ]. Thus far only a few studies take metacognitive knowledge into account. The few available studies take into regard interventional aspects, namely reflective practice [ 21 – 23 ]. There are many ways to assess metacognition. With our method, we tried to go one step beyond the current approaches to understand what is happening in the mind of medical students. It shows that high and low performers are not distinguished simply by their use of knowledge categories.
Limitations of the study
Our study has several limitations. First of all, aside from the correct solution of the case we did not code the performance within the knowledge categories. The knowledge could contain incorrect explanations and procedures. However, thus far there is no study that shows that the student who arrives at a correct diagnosis can necessarily only deduct it from correct knowledge.
The study included 21 participants and three cases per participant. We are aware that this sample is limited; this was necessary due to the elaborate data preparation process. On the other hand, qualitative research chooses to focus on the phenomenon of interest to unfold naturally, rather than a controlled influence of the interplay of variables [ 24 ]. The sample is relatively large considering it is a qualitative approach. The paper-based cases, while constructed with the most care and best possible authenticity, are still cases and not real patients with a real patient encounter with gestures and appearance and the possibility to ask the patient additional information. Thus the transferability to an authentic clinical environment might be limited. The think-aloud method limits our findings in a way that only verbal expressions can be analysed further coded and thus interpreted. Talking during the thought process requires metacognitive ability and this does confound with our dependent variable. Therefor, if some participants were more talkative than others they could possibly provide more information in all categories. However, we did not find any significant correlation between number of words expressed and number of categories coded.
The students who took part in our study volunteered and thus we cannot exclude a selection bias. The PME scores that we obtained, however are spread equally over the passing grades from 65 to 87% (M = 77.1%; SD = 6.6) and do not differ from the rest of the cohort of students.
The findings presented here show that the use of knowledge is not enough to distinguish between high and low performers. Further, it shows that the time students spent on the task is neither a positive nor a negative predictor for diagnostic accuracy. When medical educators design interventions to foster clinical reasoning it is important not to focus too much on the use of specific knowledge categories, but teach the use of the right sequences of knowledge at the right time, including the application of metacognition. This goes in line with a renewed conceptualization of the term “script” where students are supposed to go beyond the illness of the patient, but see diagnostic actions as a stereotypic process in which they learn the content of illnesses [ 25 ]. Studies investigating the interplay of cognitive actions and knowledge categories with instructional methods such as self-explanation prompts [ 8 , 21 , 26 , 27 ] are a promising next step in the endeavour to understand and foster clinical reasoning in medical students.
Boshuizen H, Schmidt HG. On the role of biomedical knowledge in clinical reasoning by experts, intermediates and novices. Cogn Sci. 1992;16(2):153–84.
Article Google Scholar
Elstein A, Shulman L, Sprafka S. Medical Problem Solving—An Analysis of Clinical Reasoning. 1978.
Book Google Scholar
Gräsel C. Problemorientiertes Lernen: Strategieanwendung und Gestaltungsmöglichkeiten: Hogrefe, Verl. für Psychologie. 1997.
Eva KW, Hatala RM, LeBlanc VR, Brooks LR. Teaching from the clinical reasoning literature: combined reasoning strategies help novice diagnosticians overcome misleading information. Med Educ. 2007;41(12):1152–8.
Kassirer JP. Teaching clinical reasoning: case-based and coached. Acad Med. 2010;85(7):1118.
Kopp V, Stark R, Kühne Eversmann L, Fischer MR. Do worked examples foster medical students’ diagnostic knowledge of hyperthyroidism? Med Educ. 2009;43(12):1210–7.
Schmidmaier R, Eiber S, Ebersbach R, Schiller M, Hege I, Holzer M, Fischer MR. Learning the facts in medical school is not enough: which factors predict successful application of procedural knowledge in a laboratory setting? BMC Med Educ. 2013;13(1):28.
Heitzmann N, Fischer F, Kühne-Eversmann L, Fischer MR. Enhancing Diagnostic Competence with Self-Explanation Prompts and Adaptable Feedback? Med Educ. 2015.
Pintrich PR. The role of metacognitive knowledge in learning, teaching, and assessing. Theory Pract. 2002;41(4):219–25.
Krathwohl DR. A revision of Bloom’s taxonomy: An overview. Theory Pract. 2002;41(4):212–8.
Flavell JH. Metacognition and cognitive monitoring: A new area of cognitive–developmental inquiry. Am Psychol. 1979;34(10):906.
Lai ER. Metacognition: A literature review. Always learning: Pearson research report 2011.
Sperling RA, Howard BC, Miller LA, Murphy C. Measures of children’s knowledge and regulation of cognition. Contemp Educ Psychol. 2002;27(1):51–79.
Whitebread D, Coltman P, Pasternak DP, Sangster C, Grau V, Bingham S, Almeqdad Q, Demetriou D. The development of two observational tools for assessing metacognition and self-regulated learning in young children. Metacognition Learn. 2009;4(1):63–85.
Van Gog T, Paas F, Van Merriënboer JJG. Process-oriented worked examples: Improving transfer performance through enhanced understanding. Instr Sci. 2004;32(1):83–98.
Google Scholar
Fischer MR, Herrmann S, Kopp V. Answering multiple‐choice questions in high‐stakes medical examinations. Med Educ. 2005;39(9):890–4.
Schmidmaier R, Ebersbach R, Schiller M, Hege I, Holzer M, Fischer MR. Using electronic flashcards to promote learning in medical students: retesting versus restudying. Med Educ. 2011;45(11):1101–10.
Ericsson KA, Simon HA. Verbal reports as data. Psychol Rev. 1980;87(3):215.
Mayring P. Qualitative content analysis. In: A companion to qualitative research. Thousand Oaks: Sage; 2004. p. 266–9.
Kiesewetter J, Ebersbach R, Görlitz A, Holzer M, Fischer MR, Schmidmaier R. Cognitive Problem Solving Patterns of Medical Students Correlate with Success in Diagnostic Case Solutions. PloS One. 2013;8(8).
Chamberland M, St‐Onge C, Setrakian J, Lanthier L, Bergeron L, Bourget A, Mamede S, Schmidt H, Rikers R. The influence of medical students’ self‐explanations on diagnostic performance. Med Educ. 2011;45(7):688–95.
Mamede S, van Gog T, Sampaio AM, de Faria RMD, Maria JP, Schmidt HG. How can students’ diagnostic competence benefit most from practice with clinical cases? The effects of structured reflection on future diagnosis of the same and novel diseases. Acad Med. 2014;89(1):121–7.
Mamede S, van Gog T, van den Berge K, Rikers RM, van Saase JL, van Guldener C, Schmidt HG. Effect of availability bias and reflective reasoning on diagnostic accuracy among internal medicine residents. JAMA. 2010;304(11):1198–203.
Patton MQ. Qualitative research: Wiley Online Library; 2005.
Berthold K, Eysink TH, Renkl A. Assisting self-explanation prompts are more effective than open prompts when learning with multiple representations. Instr Sci. 2009;37(4):345–63.
Chamberland M, Mamede S, St‐Onge C, Setrakian J, Bergeron L, Schmidt H. Self‐explanation in learning clinical reasoning: the added value of examples and prompts. Med Educ. 2015;49(2):193–202.
Kiesewetter J, Kollar I, Fernandez N, Lubarsky S, Kiessling C, Fischer MR, Charlin B. Crossing boundaries in interprofessional education: A call for instructional integration of two script concepts. J Interprof Care. 2016;1–4.
Download references
Acknowledgements
RS held a fellowship awarded by the private trust of Dr. med. Hildegard Hampp administered by LMU Munich, Germany during the project. The authors would like to acknowledge all students participating in the study. The authors would also like to thank Dorothea Lipp for her helpful comments on earlier versions of the manuscript.
Availability of data and materials
The datasets generated and/ analysed during the current study are not publicly available due to the large amount of raw written text but are available from the corresponding author on reasonable request. The Abstract was submitted to the 4th Research in Medical Education (RIME) Symposium 2015 and is available online.
Funds for this project were provided by Dr. med. Hildegard Hampp Trust administered by LMU Munich, Germany.
Authors’ contributions
JK contributed to the conceptual design of the study, the analysis and interpretation of data, as well as the drafting and revision of the paper. RE contributed to the conceptual design of the study, the analysis and interpretation of data, as well as the drafting and revision of the paper the acquisition and coding of data. NT contributed to the conceptual design and analysis of data and the revision of the paper. MH contributed to the conceptual design of the study, the analysis of data, and the revision of the paper. MRF contributed to the conceptual design of the study, to the interpretation of data, and the drafting and revision of the paper. RS contributed to the conceptual design of the study, to the interpretation of data, and the drafting and revision of the paper. All authors approved the final manuscript for submission.
Competing interests
Consent for publication.
Not applicable.
Ethics approval and consent to participate
This study was approved by the Ethical Committee of the Medical Faculty of LMU Munich. Written informed consent was obtained for all participants.
Author information
Authors and affiliations.
Institut für Didaktik und Ausbildungsforschung in der Medizin am Klinikum der Universität München, Ludwig-Maximilians-Universität, Munich, Germany
Jan Kiesewetter, Rene Ebersbach, Matthias Holzer, Ralf Schmidmaier & Martin R. Fischer
Lehrstuhl für Entwicklungspsychologie, Ludwig-Maximilians-Universität, Munich, Germany
Nike Tsalas
Medizinische Klinik und Poliklinik IV, Klinikum der Universität München, Ludwig-Maximilians-University, Munich, Germany
Ralf Schmidmaier
You can also search for this author in PubMed Google Scholar
Corresponding author
Correspondence to Jan Kiesewetter .
Rights and permissions
Open Access This article is distributed under the terms of the Creative Commons Attribution 4.0 International License ( http://creativecommons.org/licenses/by/4.0/ ), which permits unrestricted use, distribution, and reproduction in any medium, provided you give appropriate credit to the original author(s) and the source, provide a link to the Creative Commons license, and indicate if changes were made. The Creative Commons Public Domain Dedication waiver ( http://creativecommons.org/publicdomain/zero/1.0/ ) applies to the data made available in this article, unless otherwise stated.
Reprints and permissions
About this article
Cite this article.
Kiesewetter, J., Ebersbach, R., Tsalas, N. et al. Knowledge is not enough to solve the problems – The role of diagnostic knowledge in clinical reasoning activities. BMC Med Educ 16 , 303 (2016). https://doi.org/10.1186/s12909-016-0821-z
Download citation
Received : 18 December 2015
Accepted : 14 November 2016
Published : 24 November 2016
DOI : https://doi.org/10.1186/s12909-016-0821-z
Share this article
Anyone you share the following link with will be able to read this content:
Sorry, a shareable link is not currently available for this article.
Provided by the Springer Nature SharedIt content-sharing initiative
- Medical problem-solving
- Metacognition
- Knowledge categories
- Clinical reasoning
- Diagnostic reasoning
BMC Medical Education
ISSN: 1472-6920
- Submission enquiries: [email protected]
- General enquiries: [email protected]
- Search entire site
- Search for a course
- Browse study areas
Analytics and Data Science
- Data Science and Innovation
- Postgraduate Research Courses
- Business Research Programs
- Undergraduate Business Programs
- Entrepreneurship
- MBA Programs
- Postgraduate Business Programs
Communication
- Animation Production
- Business Consulting and Technology Implementation
- Digital and Social Media
- Media Arts and Production
- Media Business
- Media Practice and Industry
- Music and Sound Design
- Social and Political Sciences
- Strategic Communication
- Writing and Publishing
- Postgraduate Communication Research Degrees
Design, Architecture and Building
- Architecture
- Built Environment
- DAB Research
- Public Policy and Governance
- Secondary Education
- Education (Learning and Leadership)
- Learning Design
- Postgraduate Education Research Degrees
- Primary Education
Engineering
- Civil and Environmental
- Computer Systems and Software
- Engineering Management
- Mechanical and Mechatronic
- Systems and Operations
- Telecommunications
- Postgraduate Engineering courses
- Undergraduate Engineering courses
- Sport and Exercise
- Palliative Care
- Public Health
- Nursing (Undergraduate)
- Nursing (Postgraduate)
- Health (Postgraduate)
- Research and Honours
- Health Services Management
- Child and Family Health
- Women's and Children's Health
Health (GEM)
- Coursework Degrees
- Clinical Psychology
- Genetic Counselling
- Good Manufacturing Practice
- Physiotherapy
- Speech Pathology
- Research Degrees
Information Technology
- Business Analysis and Information Systems
- Computer Science, Data Analytics/Mining
- Games, Graphics and Multimedia
- IT Management and Leadership
- Networking and Security
- Software Development and Programming
- Systems Design and Analysis
- Web and Cloud Computing
- Postgraduate IT courses
- Postgraduate IT online courses
- Undergraduate Information Technology courses
- International Studies
- Criminology
- International Relations
- Postgraduate International Studies Research Degrees
- Sustainability and Environment
- Practical Legal Training
- Commercial and Business Law
- Juris Doctor
- Legal Studies
- Master of Laws
- Intellectual Property
- Migration Law and Practice
- Overseas Qualified Lawyers
- Postgraduate Law Programs
- Postgraduate Law Research
- Undergraduate Law Programs
- Life Sciences
- Mathematical and Physical Sciences
- Postgraduate Science Programs
- Science Research Programs
- Undergraduate Science Programs
Transdisciplinary Innovation
- Creative Intelligence and Innovation
- Diploma in Innovation
- Postgraduate Research Degree
- Transdisciplinary Learning
Graduate preparation
There are four key steps in registering and working as a nurse following your studies:
- applying for a position in the clinical sector;
- preparing for interviews;
- completing your studies, and;
- registering as a nurse with the national accrediting body.
Applying for a position in the clinical sector
The ideal next step in consolidating your undergraduate learning is to undertake an established ‘Transition to Practice’ program. These programs allow you to take a supported 12 to 18 months in the clinical sector, this usually includes rotation in two to three areas.
The availability of these positions has decreased over recent years due to several reasons including moves to better retain clinical staff, cuts to nursing positions generally and drops in the number of graduates hospitals are accepting into their transition to practice programs.
It is not essential to undertake a Transition to Practice program, many nurses enter the field without this type of program. If you want to work as a nurse, you will, it just may not take the pathway that people had in mind.
The key source of information for Transition to Practice programs in NSW is through the NSW Ministry of Health website . Access their handbook for a comprehensive guide to their application process.
Private Providers Some private health providers also have established Transition to Practice programs, for example Ramsey Health offers the GradPlus program, and HammondCare, St Vincent’s and Mater Health privates are also examples, be sure to check their application timelines.
Carefully read through the application process for each application site and be aware of the application timeline and how these relate to your study, clinical and exam periods.
Each year in May UTS welcomes a large selection of employers from the industry to meet with the best and the brightest future healthcare providers at the annual Nursing and Midwifery Fair. This is a fantastic opportunity to meet representative from health organisations and to learn more about career opportunities in the healthcare industry.
Clinical and personal references
Please read the NSW Transition to practice handbook (opens an external site) for details of the two required references. You will be required to produce one professional or personal reference and one clinical reference.
Clinical references can be obtained from your AIN work, your clinical facilitator, a CNE, a CNC or NUM.
- Remember they have every right to refuse. So make it easy for them and provide your Clinical Assessment Form
- You may also want to highlight nice comments that were made – this means they don’t have to go looking for what they said.
- Remember, they have every right to refuse so you need to be respectful of this – don’t leave this until the last minute!
- The 2016 template is availalbe at NSW Health Transition to Professional Practice , see Preparation for interview.
Creating your curriculum vitae
UTS Careers should be your first point of contact in developing your curriculum vitae. UTS Careers works closely with graduate recruiters and faculties to facilitate programs and events that equip UTS students with key employability skills, as well as the knowledge and the confidence vital for success. Their assistance includes assistance with developing resumes and applications, and importantly the interview and testing process.
Opportunities for international students
International students are considered given third priority in eligibility for NSW Transition to Practice positions. This is in line with other states and to prioritise NSW trained Australian Citizen and Permanent Residents.
While this must be taken into consideration, please be reassured that many international students received graduate positions last year under these requirements.
Give yourself the best opportunities but being the best candidate you can be on the day of interview. A very important part of this is ease with communication during interview, so use the opportunities such as HELPs conversations sessions .
Interview preparation
How to be the best candidate you can be on that day … “Selling yourself in interview as ‘confident but not cocky’”
The top three interview criteria are communication ability, clinical knowledge and problem solving and team working …
- You want your Curriculum Vitae and interview to show the interview panel that you are an engaged person, who communicates easily – they are employing you as part of their team – show them you want to be!
UTS Careers is your first point of contact for interview preparation and practice opportunities. The Careers Service works closely with graduate recruiters and faculties to facilitate programs and events that equip UTS students with key employability skills, as well as the knowledge and the confidence vital for success. Their assistance includes assistance with developing Resumes and applications, and importantly Interview Preparation and Interview testing
NSW TTP selection criteria
- Demonstrated high level interpersonal, verbal and written communication skills
- Demonstrated clinical knowledge and clinical problem solving abilities
- Demonstrated ability to work within an interdisciplinary team
- An understanding of the professional, ethical and legal requirements of the Registered Nurse or Midwife.
- A demonstrated understanding of the role of the Registered Nurse or Midwife in applying continuous improvement and quality and safety.
- A demonstrated understanding of NSW Health’s CORE Values – Collaboration, Openness, Respect and Empowerment (students were asked to talk about these last year).
Concentrate on the ANMC competency domains (opens an external site), the UTS graduate attributes for nursing and midwifery and the NSW Health CORE strategy (opens an external site) – be able to talk about each of these and provide examples of how you demonstrate each of these qualities.
Practise, practise, practise – talk to yourself, your peers, your family and friends. It is best to practise this out loud so you have a go at talking about these important areas of nursing practice.
Resources to help you ace your interview
Discover how to effectively showcase your skills with our interview tips resources. We’ve compiled a range of resources designed to help you put your best foot forward in your interview.
1. Answering Interview Questions
Watch the examples below with interview questions and “good & bad” responses:
- Behavioural interview question examples
- Clinical Interview question examples
- Tips for interview
2. Learn from the experts
Q&A Panel - Recorded at the Nursing, Midwifery Fair on 15 May 2015 - representatives from NSW Local Health Districts, Ramsay and UTS Staff answer questions on application process, interviews and new graduate roles.
3. Mock Interviews
In May 2015 and 2016 we organised mock interviews conducted by the academics, local area health representatives from industry and UTS Careers. Check out what your fellow students thought of their mock interview .
Expert tip : Preparation is key! Work hard, know the selection criteria and practise answering interview questions to excel on the day.
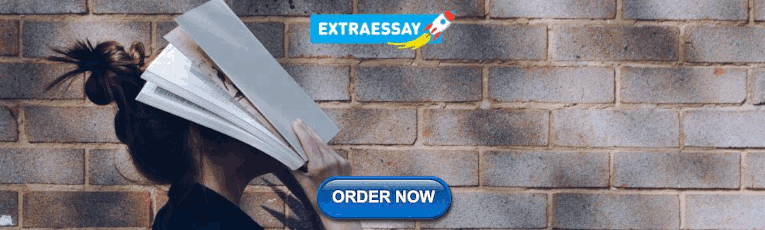
What’s next?
We have a range of important resources available to you:
- What is STAR? Learn more
- Get specialist help from UTS Careers. Make an appointment with Career Consultant Helen McNairney for help with preparation for applications and interviews.
- Practise your interview technique with targeted Health questions on Interview Stream via the UTS Careers website – these allow you to practise interview techniques from any location
Expert tip : Prepare and practise extensively –this will help you stand out from the pack and get ahead in your career.
AHPRA pathway and preparation
Application registration is a separate process from applying for graduate positions and is related to your course completion.
You can put through an online application for registration six weeks prior to completing your course: the final results ratification committee for Spring sits in mid December so you can put through an initial application at the start of November. (If you are finishing mid year, ratification occurs in the first week of July).
Academic transcript
You will need to provide a series of documents for registration. One of these documents is your academic transcript – while UTS will confirm your eligibility to complete as explained above, UTS does not provide your transcript to AHPRA, you will need to do this as part of the application process .
English language requirements for AHPRA registration – relevant to all students
All students, regardless of background, must provide evidence of English language skills that meet your profession’s English Language Skills Registration Standard to be eligible to be registered with AHPRA.
Please read your profession’s English Language Skills Standard carefully and provide the relevant evidence to AHPRA .
As outlined in this standard:
- An applicant for registration as a registered nurse and/ or a registered midwife who has provided evidence of completion of five (5) years* (full-time equivalent) of education taught and assessed in English, in any of the recognised countries listed in this registration standard, is considered to have demonstrated English language proficiency and has met the requirements of this standard;
- An applicant for registration as a registered nurse and/ or a registered midwife who has not completed five (5) years* (full-time equivalent) of education taught and assessed in English, in any of the recognised countries listed in this registration standard, will be required to demonstrate English language proficiency in accordance with Board-approved English language tests.
As per point a) above, domestic students will need to provide evidence of having completed five years of study, for example you might use your two final years of high school transcripts plus your three year Bachelor of Nursing degree transcript.
UTS process for final results confirmation
Once you have completed all subject assessments, your status will be converted to Approved to Complete and UTS will send a notification to AHPRA that you have met all requirements for completion of your degree.
For this to happen the following components must be in place:
- All subjects confirmed as passed and noted on transcript
- Signed by facilitator
- Submitted by you on time
- Reviewed and confirmed by subject coordinator
- Transferred to the clinical practice unit
- Uploaded into the clinical system
- All hours for your degree must be accounted for – if you are missing makeup hours this process cannot be finalised.
Clearly there are a number of elements that need to be completed so this process does take time.
The best ways to help this process is to make sure you:
- Submit your Clinical Assessment Forms on time
- Follow-up any outstanding results. If you see any ‘results pending’ on your transcript and you believe this should now be rectified, contact your subject coordinator to investigate further.
- Proactively arrange to complete any missed clinical practice hours by contacting the Clinical Practice Unit .
PLEASE NOTE: while UTS will confirm your eligibility to complete as explained above, UTS does not provide your transcript to AHPRA, you will need to do this as part of the application process .
Timeline of events
The following is a general guideline for the timeframes regarding TPP applications. The dates will change slightly from year to year. Other providers, such as Ramsay Health Care ( www.gradplus.com.au ) and private hospitals may have different timelines.

Postgraduate study
Join us in 2024
Applications to study postgraduate health at UTS are now open.
Postgraduate Info
Course guides
Diabetes Education and Management (PDF, 0.3MB)
High Performance Sport (PDF, 4.5MB)
Graduate Diploma Midwifery (PDF, 0.1MB)
Palliative Care (PDF, 1.9MB)
Graduate School of Health (PDF, 4.2MB)
Information sessions
Course enquiries
UTS acknowledges the Gadigal people of the Eora Nation, the Boorooberongal people of the Dharug Nation, the Bidiagal people and the Gamaygal people, upon whose ancestral lands our university stands. We would also like to pay respect to the Elders both past and present, acknowledging them as the traditional custodians of knowledge for these lands.


An official website of the United States government
The .gov means it's official. Federal government websites often end in .gov or .mil. Before sharing sensitive information, make sure you're on a federal government site.
The site is secure. The https:// ensures that you are connecting to the official website and that any information you provide is encrypted and transmitted securely.
- Publications
- Account settings
- Browse Titles
NCBI Bookshelf. A service of the National Library of Medicine, National Institutes of Health.
ten Cate O, Custers EJFM, Durning SJ, editors. Principles and Practice of Case-based Clinical Reasoning Education: A Method for Preclinical Students [Internet]. Cham (CH): Springer; 2018. doi: 10.1007/978-3-319-64828-6_3
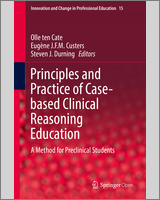
Principles and Practice of Case-based Clinical Reasoning Education: A Method for Preclinical Students [Internet].
Chapter 3 understanding clinical reasoning from multiple perspectives: a conceptual and theoretical overview.
Olle ten Cate and Steven J. Durning .
Affiliations
Published online: November 7, 2017.
Rather than a historical overview as in Chap. 2, this chapter provides the reader with insight into the various approaches that have been used to understand clinical reasoning. We review concepts and major scholars who have been involved in such investigations. Cognitive psychologists Newel and Simon theorized about problem-solving skills and artificial intelligence and initiated the use of computers as metaphors of thinking. Elstein and colleagues found that there is no such thing as a general problem-solving skill, independent of medical knowledge, and thus clinical reasoning is case specific. Reasoning then became analyzed in approaches, including forward reasoning from data to diagnosis; hypothetico-deductive reasoning with backward nature, from hypothesis to diagnosis; and abductive reasoning to understand early hypothesis generation that is so characteristic in clinical reasoning, elaborated by Patel and colleagues. Bordage introduced prototypes to characterize how physicians may remember illness presentations and semantic qualifiers to denote the shortened conceptual language and labels physicians use to store medical information systematically in memory. Illness scripts represent how encounters with diseases are remembered by physicians and were introduced by Feltovich and Barrows. Schmidt and Boshuizen elaborated the concept further and propose encapsulation of knowledge as a hypothetical process that happens when physicians regularly and routinely apply shortcuts in thinking typically ellaborated as pathophysiology. Reasoning ability appears not only to be case specific-- it is also situation or context specific . Clinicians with broad reasoning ability have extensive experience. Deliberate practice with many cases and in varying contexts is recommended by Ericsson to acquire reasoning expertise. To improve reasoning, some authors have focused on cognitive biases and error prevention. Norman, however, concludes that bias reduction strategies are unlikely to be successful but correcting knowledge deficiencies is likely to lead to reasoning success. Kahnemann promoted System 1 and System 2 thinking for instant pattern recognition (nonanalytic reasoning) and analytic reasoning, respectively . What actually happens in the brain during clinical reasoning is the domain of neuroscience, which may provide insights from research in the near future.
- Concepts and Definitions
This chapter is devoted to clarifying terminology and concepts that have been regularly cited and used in the last decades around clinical reasoning. Thus, this chapter represents a conceptual overview.
Success in clinical reasoning is essential to a physician’s performance. Clinical reasoning is both a process and an outcome (with the latter often being referred to as decision-making). While these decisions must be evidence based as much as possible, clearly decisions also involve patient perspectives, the relationship between the physician and the patient, and the system or environment where care is rendered. Definitions of clinical reasoning therefore must include these aspects. While definitions of clinical reasoning vary, they typically share the features that clinical reasoning entails: (i) the cognitive operations allowing physicians to observe, collect, and analyze information and (ii) the resulting decisions for actions that take into account a patient’s specific circumstances and preferences (Eva et al. 2007 ; Durning and Artino 2011 ).
The variety of definitions of clinical reasoning and the heterogeneity in research is likely in part due to the number of fields that have informed our understanding of clinical reasoning. In this chapter, a number of concepts from a broad spectrum of fields is presented to help the reader understand clinical reasoning and to assist the instruction of preclinical medical students. Many of these concepts reflect difficulties inherent to understanding how doctors think and how this type of thinking can be acquired by learners over time. Some provide hypotheses with more or less firm theoretical grounding, but a broad understanding of clinical reasoning requires an ongoing process of investigation.
Learning to Solve Problems in New Areas: Expanding the Learner Domain Space
Klahr and Dunbar proposed a model for scientific discovery (Klahr and Dunbar 1988 ) that may be helpful to understand how learners solve problems in unknown territory, such as what happens when a medical student starts learning to solve medical problems. The student has a learner domain space of knowledge that only partly overlaps, or not at all, with the expert domain space of knowledge, which is the space that contains all possible hypotheses a learner can generate about a problem. Knowledge building during inquiry learning can be considered as expanding the learner domain space to increase that overlap (Lazonder et al. 2008 ).
Early Thinking of Clinical Reasoning: The Computer Analogy
Building on the cognitive psychology work of Newell and Simon about problem-solving in the 1970s (Newell and Simon 1972 ), artificial intelligence (AI) computer models were created to resemble the clinical reasoning process, with programs like MYCIN and INTERNIST (Pauker et al. 1976 ). Analogies between cognitive functioning and the emerging computer capacities led to the assumption that both use algorithmic processes in the working memory, viewed as the central processing unit of the brain. Many predicted that like in chess, computer programs for medical diagnosis would quickly be developed and would perform superiorly to the practicing professional, outperforming the diagnostic accuracy of the best physician’s thinking. Four decades later, however, this has not yet happened and may be impossible. The emergence of self-driving cars as an analogy shows how humans can build highly complex machines, but at least this development in clinical reasoning has been much slower than many had thought it would (Wachter 2015 ; Clancey 1983 ). Robert Wachter, in a recent book about technology in health care, argues that, still better than computers, experienced physicians can distinguish between patients with similar signs and symptoms to determine that “that guy is sick, and the other is okay,” with the “the eyeball test” or intuition, which computers have not been able to capture so far (page 95), just as a computer cannot currently analyze nonverbal information that is so critical to communication in health care. Clinical decision support systems (CDSS, containing a large knowledge base and if-then rules for inferences) have been used with some success at the point of care to support clinicians in decision-making, particularly in medication decisions, but, integrated with electronic health records, they have not been shown to improve clinical outcome parameters as of yet (Moja et al. 2014 ).
Abandoning Clinical Reasoning as a General Problem-Solving Ability
Expertise in clinical reasoning was initially viewed as being synonymous with acquiring general problem-solving procedures (Newell and Simon 1972 ). However, in a groundbreaking study, published as a book in 1978 ( Medical Problem Solving ), Elstein and colleagues found few differences between expert (attending physicians) and novice diagnosticians (medical students) in the way they solve diagnostic problems (Elstein et al. 1978 ). The primary difference appeared to be in their knowledge and in particular the way it is structured as a consequence of experience. Thus while medical students and practicing physicians generated a similar number of diagnostic hypotheses differential diagnosis of similar length, practicing physicians were far more likely to list the correct diagnosis. This insight replaced the era that was marked by the belief that clinical reasoning could be measured as a distinct skill that would result in superior performance regardless of the specifics of a patient’s presentation. Content knowledge was shown to be very important but still does not guarantee success in clinical reasoning. Variation in clinical performance is a product of the expert’s integration of his or her knowledge of the signs and symptoms of disease with contextual factors in order to arrive at an adaptive solution.
Deconstructing the Reasoning Process
- Abstraction can be viewed as generalization from a finding to a conclusion (hemoglobin <12 gm/dl in an adult male is labeled as “anemia”).
- Abduction is a backward reasoning process to explain why this adult male should have anemia. “Abductive reasoning” was first coined as a term by logician C.S. Peirce in the nineteenth century to signify a common process when a surprising observation takes place that leads to a hypothesis (“The lawn is wet! Ergo, it has probably rained.”) and is based on knowledge of possible causations and must be tested (“but it could also be the neighbor’s sprinkler”). Abduction is considered to be a primary means of acquiring new ideas in clinical reasoning (Bolton 2015 ).
- Deduction is the process of testing the hypothesis (e.g., of anemia) through confirmation by expected other diagnostic findings: if conditions X and Y are met, inference Z must be true.
- Induction is the process of generalization from multiple cases and more applicable in research than in individual patient care: if multiple patients show similar signs and symptoms, general rules may be created to explain new cases.
Part of this process is forward-driven reasoning (hypothesis generation through data), and another part is backward-driven reasoning (hypothesis testing) (Patel et al. 2005 ).
Knowledge Representations to Support Reasoning
In a 1996 review, Custers and colleagues categorized the thinking about the way physician’s cognition is organized around clinical knowledge in three alternative frameworks and provided critical notes (Custers et al. 1996 ). These mental representations could have the form of prototypes , instances , or semantic networks . All three of these models have assets and drawbacks in their explanatory power for clinical reasoning. The prototype framework or prototype theory assumes that multiple encounters with related diseases lead physicians to remember the common denominators, resulting in single prototypes in long-term memory. The instances framework assumes that physicians actually remember the individual instances of patient encounters without abstraction, and context-specific (situation specific) information may be part of these instances. The semantic network theory posits the existence of nodes of information units, connected with other nodes in the network. The strength of the network and its nodes depends on the intensity of its use. Schemas and illness scripts are medically meaningful interconnected nodes that can be strengthened and adapted based on clinical experience.
Prototyping and Semantic Qualifiers
Georges Bordage introduced the term semantic qualifiers referring to the use of abstract, often binary, terms to help sort through and organize (e.g., chunk) patient information. They are “useful adjectives” that represent an abstraction of the situational clinical findings (Chang et al. 1998 ). A commonly cited example of the use of semantic qualifiers is translating a patient who is presenting with knee swelling and pain into a presentation of acute monarticular arthritis. Note three semantic qualifiers – “acute,” “monoarticular,” and “arthritis.” The reason why these qualifiers are important is that the structure of clinical knowledge in the clinician’s mind is organized with such qualifiers, as claimed by Bordage. To enable recognition and linkage, the clinician must first translate what she hears and sees into such terminology (Bordage 1994 ). An assumption is that the clinician’s memory contains prototypes of diseases (Bordage and Zacks 1984 ), generalizable representations that enable recognition. Bordage stresses how semantically rich discourses about patients are associated with greater diagnostic accuracy (Bordage 2007 ).
Illness Script Theory
Custers recently summarized scripts as high-level conceptual knowledge structures in long-term memory, representing general event sequences, in which the individual events are interconnected by temporal and often causal or hierarchical relationships (“usually diabetes type II occurs a older age, a overweight is associated; late symptoms might include vascular problems in the retina, in the lower limbs and in other places”). Scripts are activated as integral wholes in appropriate contexts that should contain relevant variables, including clinical findings in the patient. “Slots” in the reasoning process can be filled with information present in the actual situation, retrieved from memory, or inferred from the context (Custers 2015 ). Illness scripts, first introduced by Barrows and Feltovich, are believed to be chunks in long-term memory that contain three components, enabling conditions (past history and causes) , fault (pathophysiology), and consequences (signs and symptoms) (Feltovics and Barrows 1984 ), and are elaborated further by Schmidt and Boshuizen ( 1993 ). Illness scripts are stored in long-term memory as units with temporal (i.e., sequential) components, as a film script of unfolding events, and patients are remembered as instances of a script. With experience, physicians build a larger repertoire of illness scripts and more elaborated scripts.
Illness scripts are shaped by experience and continually refined throughout one’s clinical practice. When an experienced physician initially sees a patient, his or her verbal and nonverbal information is thought to immediately activate relevant illness scripts. This effortless, fast thinking, or nonanalytic process is referred to as script activation . In some cases, only one script is activated, and in these cases, one may arrive at the correct diagnosis (e.g., “type II diabetes mellitus”). In other cases, multiple scripts are activated, and then theory holds that we choose the most likely diagnosis by comparing and contrasting alternative illness scripts that were activated (through analytic or slow thinking). Early learners may not activate any scripts when they initially see a patient, and experts may activate one or several illness scripts.
Encapsulation of Knowledge and the Intermediate Effect
With increasing clinical information stored as illness scripts in the long-term memory of the physician, diagnostic reasoning should steadily become more accurate. However, studies have shown that more novice clinicians (e.g., those just out of training such as recent graduates from residency education) sometimes outperform physicians who have been in practice for some time (e.g., “experts”) on the recall of details from clinical cases seen. This finding was coined by Schmidt and Boshuizen as the intermediate effect (Schmidt and Boshuizen 1993 ). While inexperienced clinicians may consciously use pathophysiological thinking when solving clinical problems, the frequent use of similar thinking pathways leads to efficient shortcuts, and after a while it may no longer be possible to unfold these pathways. The pathophysiological knowledge about the disease becomes encapsulated into diagnostic labels or high-level simplified causal models that explain signs and symptoms (Schmidt and Mamede 2015 ).
System 1 and 2 Thinking as Dual Processes
Dual process theory refers to two processes that are thought to apply during reasoning (Croskerry et al. 2014 ). Briefly, dual process theory argues that we have two general thought processes. Fast thinking (sometimes called System I thinking or “nonanalytic” reasoning) is believed to be quick, subconscious, and typically effortless. An example of a fast thinking strategy is pattern recognition (Eva 2005 ). An example of pattern recognition in medicine would happen when a physician examines a patient with palpitations and immediately recognizes the cardinal features or “pattern” of Graves’ disease, when also observing exophthalmia, fine resting tremor, and thyromegaly. Slow or analytic thinking (System 2 thinking) on the other hand is effortful and conscious. An example of System 2 thinking would be working through a patient’s acid base status (e.g., calculating an anion gap, using Winter’s formula, and calculating a delta-delta gap). Dual process theory has recently been popularized in the book Thinking, Fast and Slow by Daniel Kahneman ( 2011 ). More recent work with dual process theory argues that both of these processes are used simultaneously, e.g., it’s not one or the other but rather one uses a combination of both fast and slow thinking in practice. In other words, fast and slow thinking can be viewed as a continuum (Custers 2013 ). Efficient clinical work requires fast thinking. The capacity of the working memory would be overloaded if analytic reasoning were required for all decisions in patient care (Young et al. 2014 ).
Case Specificity and Context Specificity
In Elstein and colleagues ’ seminal work on medical problem-solving (Elstein et al. 1978 ), researchers noted that physician performance on one patient or case did not predict performance on a subsequent content area or case, giving rise to the phenomenon of case specificity . These findings would be quite surprising if medical problem-solving were a general skill.
A second vexing problem in practice is the more recently highlighted phenomenon of context specificity . Context specificity refers to the finding that a physician can see two patients with the same chief complaint and the same (or nearly identical) symptoms and physical findings and have the same diagnosis, yet, in different contexts, arrive at different diagnoses (Durning et al. 2011 ). The context can be helpful to arrive at the correct diagnosis (Hobus et al. 1987 ) or harmful and lead to error (Eva 2005 ). In other words, something other than the “essential content” is driving the physician’s clinical reasoning. Durning and Artino hold that the outcome of clinical reasoning is driven by the context, which includes the physician, the patient, the system, and their interactions (Durning and Artino 2011 ). The notion of system includes appointment length, appointment location, support systems, and clinic staffing (Durning and Artino 2011 ) and stresses the importance of the situation. One example of “situativity” is situated cognition , which breaks down an activity like clinical reasoning into physician, patient, and environment as well as interactions between these components. Clinical reasoning is believed to emerge from these factors and their interactions. Another example of situativity, situated learning , stresses participation in an activity and identity formation as learning versus the acquisition of generalized facts.
Clinical Reasoning and the Development of Expert Performance
Despite the finding that clinical reasoning is content -dependent and context -dependent, expertise in diagnostic and therapeutic reasoning in general varies among physicians even with similar experience. Some internists are considered better diagnosticians and some surgeons better operators that others. It remains useful to think of what leads to superb performance, as education can be a part of it (Asch et al. 2014 ). Indeed, many scholars prefer the term expert performance as opposed to expertise when referring to clinical reasoning as the former acknowledges the many nuances to this ability that we have outlined in this chapter.
For procedural performance, repetitive practice is key. Competence in colonoscopy requires experience with 150–200 colonoscopies under supervision (Ekkelenkamp et al. 2016 ). That competence improves with practice is not surprising and known from, for instance, in chess (De Groot 1978 ). Anecdotally, in the 1960s the Hungarian educational psychologist László Polgár was determined to raise his yet unborn children to become highly skilled in a specific domain and chose chess. All three daughters received careful, highly intensive training, from very young age on, and have become world-top chess players, two of which are currently considered the world’s best female chess players. Psychologist Ericsson has generalized the idea that, rather than innate talent, deliberate practice is key to expert performance (Ericsson et al. 1993 ). He distinguishes three subsequent mental representations: a planning phase with clear performance goals, a translation to execution, and a representation for monitoring how well one does. Applications in medical training have been described (Ericsson 2015 ) but have mainly focused on procedures. Clinical reasoning may benefit from deliberate practice, and the work of Mamede et al., using deliberate practice, shows how reasoning can benefit as well (Mamede et al. 2014 ).
Reflection During Diagnostic Thinking
Donald Schön coined the terminology of reflection in action and reflection on action , as a description of thinking of high-level professionals (Schön 1983 ). Knowing what to do when you do it may not require much effort if actions are routine, but professionals with nonroutine tasks may often face small problems or questions that require instant adaptive action. Schön maintains that reflection-in-action must be practiced by learners becoming professionals. Mamede and colleagues developed the method of “structured reflection” to improve students’ diagnostic reasoning (Mamede et al. 2010 , 2014 a, b). Structured reflection in the context of clinical reasoning means that problem-solvers explicitly match a patient’s presentation (case) against every diagnosis they consider for that case. Mamede et al. demonstrated a beneficial effect of this approach. Detailed comparison of a patient’s signs and symptoms with the already available and activated illness scripts and noticing similarities and discrepancies appears to be the mechanism behind this restructuring of knowledge as a consequence of structured reflection. The authors recommend deliberate reflection as a tool for learning clinical reasoning (Schmidt and Mamede 2015 ).
Bias and Error in Clinical Reasoning
- Availability bias . A differential diagnosis is influenced by what is easily recalled, creating a false sense of prevalence.
- Representative bias (or judging by similarity ). Clinical suspicion is influenced solely by signs and symptoms and neglects prevalence of competing diagnoses.
- Confirmation bias (or pseudodiagnosticity ). Additional testing confirms suspected diagnosis but fails to test competing hypotheses.
- Anchoring bias. Inadequate adjustment of a differential diagnosis in light of new data resulting in a final diagnosis unduly influenced by the starting point.
- Bounded rationality bias (or search satisficing ). Clinicians stop searching for additional diagnoses after the anticipated diagnosis is made leading to a premature closure of the reasoning process.
- Outcome bias . A clinical decision is judged on the outcome rather than on the logic and evidence supporting the decision.
A limitation of this approach is that when the reasoning is believed to be successful, biases are not typically recognized, and when looking at a case in hindsight, many mistakes can easily be labeled as caused by “bias.” Indeed, so-called biases actually may serve as heuristics to guide successful behavior (Gigerenzer and Gaissmaier 2011 ; Gigerenzer 2007 ). In a recent overview, Norman and colleagues conclude that interventions directed at error reduction through the identification of heuristics and biases have no effect on diagnostic errors. Instead, most errors seem to originate from a limited knowledge based of the clinician (Norman et al. 2017 ).
Neuroscience and Visual Expertise in Clinical Reasoning
While neuroscience is quickly uncovering many cognitive processes, clinical reasoning has hardly been subject of such studies. More recently however a new line of research has evolved which seeks to explore the biologic underpinnings of clinical reasoning. Indeed, an Achilles heel of clinical reasoning is that it is less subject to introspection or visualization, and thus these new methods such as functional magnetic resonance imaging (fMRI) and electroencephalogram (EEG) are emerging and show particular promise for enhancing our understanding of System 1 thinking. One of the first publications in this domain is from Durning et al. who studied brain process with functional MRI techniques in novices and experts solving clinical problems through vignette-based multiple choice questions. Many parts of the brain were activated. The researchers observed activity in various regions of the prefrontal cortex (Durning et al. 2015 ). While preliminary, fMRI may be a promising route of future investigation.
A new and related avenue of investigation is that of visual expertise (Bezemer 2017 ; van der Gijp et al. 2016 ). Medicine is a highly visual profession, not only for specific disciplines such as radiology, pathology, dermatology, surgery, and cardiology but also in primary care (Kok and Jarodzka 2017 ). Visually observing a patient, human tissue, or a representation of it, and recognizing abnormality, may not easily be expressed in words but can instantly lead to a System 1 recognition.
The intention of this chapter was to provide an overview of theoretical concepts, frequently used terms, and a number of significant thinkers and authors in this domain, all of which underlie our current understanding of clinical reasoning to support the teaching of students about clinical reasoning in the preclinical period and beyond.
While much of the cited literature appeared after the model of case-based clinical reasoning was first created in 1992 (ten Cate 1994 ), and some aspects apply to clinical rather than preclinical education, none of the recommendations that could be drawn for this chapter would conflict the CBCR approach.
Although it is apparent that there are still numerous gaps in our collective understanding of clinical reasoning, it is also clear that progress into a more thorough understanding of clinical reasoning is advancing.
- Asch, D. A., et al. (2014). How do you deliver a good obstetrician? Outcome-based evaluation of medical education. Academic Medicine, 89 (1), 24–26. [ PubMed : 24280859 ] [ CrossRef ]
- Bezemer, J. (2017). Visual research in clinical education. Medical Education, 51 (1), 105–113. [ PubMed : 27785819 ] [ CrossRef ]
- Bolton, J. W. (2015). Varieties of clinical reasoning. Journal of Evaluation in Clinical Practice , 21 , n/a–n/a. Available at: http://doi .wiley.com/10.1111/jep.12309 [ PubMed : 25652845 ]
- Bordage, G. (1994). Elaborated knowledge: A key to successful diagnostic thinking. Academic Medicine, 69 (11), 883–885. [ PubMed : 7945684 ] [ CrossRef ]
- Bordage, G. (2007). Prototypes and semantic qualifiers: From past to present. Medical Education, 41 (12), 1117–1121. [ PubMed : 18045363 ] [ CrossRef ]
- Bordage, G., & Zacks, R. (1984). The structure of medical knowledge in the memories of medical students and general practitioners: Categories and prototypes. Medical Education, 18 (11), 406–416. [ PubMed : 6503748 ] [ CrossRef ]
- Chang, R., Bordage, G., & Connell, K. (1998). The importance of early problem representation during case presentations. Academic Emergency Medicine: Official Journal of the Society for Academic Emergency Medicine, 73 (10), S109–S111. [ PubMed : 9795669 ]
- Clancey, W. J. (1983). The epistemology of a rule-based expert system – A framework for explanation. Artificial Intelligence, 20 (3), 215–251. [ CrossRef ]
- Croskerry, P., et al. (2014). Deciding about fast and slow decisions. Academic Medicine, 89 (2), 197–200. [ PubMed : 24362398 ] [ CrossRef ]
- Custers, E. J. F. M. (2013). Medical education and cognitive continuum theory: An alternative perspective on medical problem solving and clinical reasoning. Academic Medicine, 88 (8), 1074–1080. [ PubMed : 23807108 ] [ CrossRef ]
- Custers, E. J. F. M. (2015). Thirty years of illness scripts: Theoretical origins and practical applications. Medical Teacher, 37 (5), 457–462. [ PubMed : 25180878 ] [ CrossRef ]
- Custers, E., Regehr, G., & Norman, G. (1996). Mental representations of medical diagnostic knowledge: A review. Academic Medicine, 71 (10), S55–S61. [ PubMed : 8940935 ] [ CrossRef ]
- De Groot, A. (1978). Thought and choice in chess . The Hague: Mouton.
- Durning, S. J., & Artino, A. R. (2011). Situativity theory: A perspective on how participants and the environment can interact: AMEE guide no. 52. Medical Teacher, 33 (3), 188–199. [ PubMed : 21345059 ] [ CrossRef ]
- Durning, S., et al. (2011). Context and clinical reasoning: Understanding the perspective of the expert’s voice. Medical Education, 45 (9), 927–938. [ PubMed : 21848721 ] [ CrossRef ]
- Durning, S. J., et al. (2015). Neural basis of nonanalytical reasoning expertise during clinical evaluation. Brain and Behaviour, 309 , 1–10. [ PMC free article : PMC4356847 ] [ PubMed : 25798328 ]
- Ekkelenkamp, V. E., et al. (2016). Training and competence assessment in GI endoscopy: A systematic review. Gut, 65 (4), 607–615. Available at: http://gut .bmj.com/content/65/4/607 .abstract [ PubMed : 25636697 ]
- Elstein, A. S., Shulman, L. S., & Sprafka, S. A. (1978). Medical problem solving. In An analysis of clinical reasoning . Cambridge, MA: Harvard University Press.
- Ericsson, K. A. (2015). Acquisition and maintenance of medical expertise. Academic Medicine, 90 (11), 1–16. [ PubMed : 26375267 ] [ CrossRef ]
- Ericsson, K. A., et al. (1993). The role of deliberate practice in the acquisition of expert performance. Psychological Review, 100 (3), 363–406. [ CrossRef ]
- Eva, K. W. (2005). What every teacher needs to know about clinical reasoning. Medical Education, 39 (1), 98–106. [ PubMed : 15612906 ] [ CrossRef ]
- Eva, K. W., et al. (2007). Teaching from the clinical reasoning literature: Combined reasoning strategies help novice diagnosticians overcome misleading information. Medical Education, 41 (12), 1152–1158. [ PubMed : 18045367 ] [ CrossRef ]
- Feltovics, P. & Barrows, H. (1984). Issues of generality in medical problem solving. In H. Schmidt & M. De Volder (Eds), Tutorials in problem-based learning (pp. 128–142). Assen/Maastricht: Van Gorcum.
- Gigerenzer, G. (2007). Gut feelings. The intelligence of the unconscious . New York: Penguin Group.
- Gigerenzer, G., & Gaissmaier, W. (2011). Heuristic decision making. Annual Review of Psychology, 62 , 451–482. [ PubMed : 21126183 ] [ CrossRef ]
- Hobus, P. P. M., et al. (1987). Contextual factors in the activation of first diagnostic hypotheses: Expert-novice differences. Medical Education, 21 (6), 471–476. [ PubMed : 3696019 ] [ CrossRef ]
- Kahneman, D. (2011). Thinking, fast and slow . New York: Farrar, Straus and Giroux.
- Kempainen, R. R., Migeon, M. B., & Wolf, F. M. (2003). Understanding our mistakes: A primer on errors in clinical reasoning. Medical Teacher, 25 (2), 177–181. [ PubMed : 12745527 ] [ CrossRef ]
- Klahr, D., & Dunbar, K. (1988). Dual space search during scientific reasoning. Cognitive Science, 12 (1), 1–48. [ CrossRef ]
- Kok, E. M., & Jarodzka, H. (2017). Before your very eyes: The value and limitations of eye tracking in medical education. Medical Education, 51 (1), 114–122. [ PubMed : 27580633 ] [ CrossRef ]
- Lazonder, A. W., Wilhelm, P., & Hagemans, M. G. (2008). The influence of domain knowledge on strategy use during simulation-based inquiry learning. Learning and Instruction, 18 (6), 580–592. [ CrossRef ]
- Mamede, S., et al. (2010). Effect of availability bias and reflective reasoning on diagnostic accuracy among internal medicine residents. JAMA: The Journal of the American Medical Association, 304 (11), 1198–1203. [ PubMed : 20841533 ] [ CrossRef ]
- Mamede, S., van Gog, T., Sampaio, A. M., et al. (2014a). How can students’ diagnostic competence benefit most from practice with clinical cases? The effects of structured reflection on future diagnosis of the same and novel diseases. Academic Medicine: Journal of the Association of American Medical Colleges, 89 (1), 121–127. [ PubMed : 24280855 ] [ CrossRef ]
- Mamede, S., van Gog, T., van den Berge, K., et al. (2014b). Why do doctors make mistakes? A study of the role of salient distracting clinical features. Academic Medicine: Journal of the Association of American Medical Colleges, 89 (1), 114–120. [ PubMed : 24280846 ] [ CrossRef ]
- Miller, G. A. (1956). The magical number seven, plus or minus two: Some limits on our capacity for processing information. Psychological Review, 63 , 81–97. [ PubMed : 13310704 ] [ CrossRef ]
- Moja, L., et al. (2014). Effectiveness of computerized decision support systems linked to electronic health records: A systematic review and meta-analysis. American Journal of Public Health, 104 (12), e12–e22. [ PMC free article : PMC4232126 ] [ PubMed : 25322302 ] [ CrossRef ]
- Newell, A., & Simon, H. (1972). Human problem solving . Englewood Cliffs: Prentice-Hall.
- Norman, G. R., et al. (2017). The causes of errors in clinical reasoning: Cognitive biases, knowledge deficits, and dual process thinking. Academic Medicine, 92 (1), 23–30. [ PubMed : 27782919 ] [ CrossRef ]
- Patel, V., Arocha, J., & Zhang, J. (2005). Thinking and reasoning in medicine. In K. Holyoak & R. Morrison (Eds.), The Cambridge handbook of thinking and reasoning (pp. 727–750). Cambridge: Cambridge University Press.
- Pauker, S., et al. (1976). Towards the simulation of clinical cognition: Taking the present illness by computer. Americal Journal of Medicine, 60 , 981–996. [ PubMed : 779466 ] [ CrossRef ]
- Schmidt, H. G., & Boshuizen, H. P. A. (1993). On acquiring expertise in medicine. Educational Psychology Review, 5 (3), 205–221. [ CrossRef ]
- Schmidt, H. G., & Mamede, S. (2015). How to improve the teaching of clinical reasoning: A narrative review and a proposal. Medical Education, 49 (10), 961–973. [ PubMed : 26383068 ] [ CrossRef ]
- Schön, D. A. (1983). The reflective practitioner - how professionals think in action . New York: Basic Books.
- ten Cate, O. (1994). Training case-based clinical reasoning in small groups [Dutch]. Nederlands Tijdschrift voor Geneeskunde, 138 , 1238–1243. [ PubMed : 8015623 ]
- van der Gijp, A. et al. (2016). How visual search relates to visual diagnostic performance: A narrative systematic review of eye-tracking research in radiology. Advances in Health Sciences Education , 1–23. [ PMC free article : PMC5498587 ] [ PubMed : 27436353 ]
- Wachter, R. (2015). The digital doctor – hope, hype, harm at the Dawn of medicine’s computer age . New York: McGraw-Hill.
- Young, J. Q., et al. (2014). Cognitive load theory: Implications for medical education: AMEE guide no. 86. Medical Teacher, 36 (5), 371–384. [ PubMed : 24593808 ] [ CrossRef ]
Open Access This chapter is licensed under the terms of the Creative Commons Attribution 4.0 International License ( http://creativecommons.org/licenses/by/4.0/ ), which permits use, sharing, adaptation, distribution and reproduction in any medium or format, as long as you give appropriate credit to the original author(s) and the source, provide a link to the Creative Commons license and indicate if changes were made.
The images or other third party material in this chapter are included in the chapter’s Creative Commons license, unless indicated otherwise in a credit line to the material. If material is not included in the chapter’s Creative Commons license and your intended use is not permitted by statutory regulation or exceeds the permitted use, you will need to obtain permission directly from the copyright holder.
- Cite this Page ten Cate O, Durning SJ. Understanding Clinical Reasoning from Multiple Perspectives: A Conceptual and Theoretical Overview. 2017 Nov 7. In: ten Cate O, Custers EJFM, Durning SJ, editors. Principles and Practice of Case-based Clinical Reasoning Education: A Method for Preclinical Students [Internet]. Cham (CH): Springer; 2018. Chapter 3. doi: 10.1007/978-3-319-64828-6_3
- PDF version of this page (329K)
- PDF version of this title (3.4M)
In this Page
Related information.
- PMC PubMed Central citations
- PubMed Links to PubMed
Similar articles in PubMed
- A concept analysis of abductive reasoning. [J Adv Nurs. 2014] A concept analysis of abductive reasoning. Mirza NA, Akhtar-Danesh N, Noesgaard C, Martin L, Staples E. J Adv Nurs. 2014 Sep; 70(9):1980-1994. Epub 2014 Mar 13.
- Review Clinical reasoning in manual therapy. [Phys Ther. 1992] Review Clinical reasoning in manual therapy. Jones MA. Phys Ther. 1992 Dec; 72(12):875-84.
- Review Reasoning processes in clinical reasoning: from the perspective of cognitive psychology. [Korean J Med Educ. 2019] Review Reasoning processes in clinical reasoning: from the perspective of cognitive psychology. Shin HS. Korean J Med Educ. 2019 Dec; 31(4):299-308. Epub 2019 Nov 29.
- Management reasoning scripts: Qualitative exploration using simulated physician-patient encounters. [Perspect Med Educ. 2022] Management reasoning scripts: Qualitative exploration using simulated physician-patient encounters. Cook DA, Stephenson CR, Gruppen LD, Durning SJ. Perspect Med Educ. 2022 Aug; 11(4):196-206. Epub 2022 Jun 2.
- Review Research in clinical reasoning: past history and current trends. [Med Educ. 2005] Review Research in clinical reasoning: past history and current trends. Norman G. Med Educ. 2005 Apr; 39(4):418-27.
Recent Activity
- Understanding Clinical Reasoning from Multiple Perspectives: A Conceptual and Th... Understanding Clinical Reasoning from Multiple Perspectives: A Conceptual and Theoretical Overview - Principles and Practice of Case-based Clinical Reasoning Education
Your browsing activity is empty.
Activity recording is turned off.
Turn recording back on
Connect with NLM
National Library of Medicine 8600 Rockville Pike Bethesda, MD 20894
Web Policies FOIA HHS Vulnerability Disclosure
Help Accessibility Careers
- - Google Chrome
Intended for healthcare professionals
- Access provided by Google Indexer
- My email alerts
- BMA member login
- Username * Password * Forgot your log in details? Need to activate BMA Member Log In Log in via OpenAthens Log in via your institution

Search form
- Advanced search
- Search responses
- Search blogs
- Clinical problem...
Clinical problem solving and diagnostic decision making: selective review of the cognitive literature
- Related content
- Peer review
This article has a correction. Please see:
- Clinical problem solving and diagnostic decision making: selective review of the cognitive literature - November 02, 2006
- Arthur S Elstein , professor ( aelstein{at}uic.edu ) ,
- Alan Schwarz , assistant professor of clinical decision making.
- Department of Medical Education, University of Illinois College of Medicine, Chicago, IL 60612-7309, USA
- Correspondence to: A S Elstein
This is the fourth in a series of five articles
This article reviews our current understanding of the cognitive processes involved in diagnostic reasoning in clinical medicine. It describes and analyses the psychological processes employed in identifying and solving diagnostic problems and reviews errors and pitfalls in diagnostic reasoning in the light of two particularly influential approaches: problem solving 1 , 2 , 3 and decision making. 4 , 5 , 6 , 7 , 8 Problem solving research was initially aimed at describing reasoning by expert physicians, to improve instruction of medical students and house officers. Psychological decision research has been influenced from the start by statistical models of reasoning under uncertainty, and has concentrated on identifying departures from these standards.
Summary points
Problem solving and decision making are two paradigms for psychological research on clinical reasoning, each with its own assumptions and methods
The choice of strategy for diagnostic problem solving depends on the perceived difficulty of the case and on knowledge of content as well as strategy
Final conclusions should depend both on prior belief and strength of the evidence
Conclusions reached by Bayes's theorem and clinical intuition may conflict
Because of cognitive limitations, systematic biases and errors result from employing simpler rather than more complex cognitive strategies
Evidence based medicine applies decision theory to clinical diagnosis
Problem solving
Diagnosis as selecting a hypothesis.
The earliest psychological formulation viewed diagnostic reasoning as a process of testing hypotheses. Solutions to difficult diagnostic problems were found by generating a limited number of hypotheses early in the diagnostic process and using them to guide subsequent collection of data. 1 Each hypothesis can be used to predict what additional findings ought to be present if it were true, and the diagnostic process is a guided search for these findings. Experienced physicians form hypotheses and their diagnostic plan rapidly, and the quality of their hypotheses is higher than that of novices. Novices struggle to develop a plan and some have difficulty moving beyond collection of data to considering possibilities.
It is possible to collect data thoroughly but nevertheless to ignore, to misunderstand, or to misinterpret some findings, but also possible for a clinician to be too economical in collecting data and yet to interpret accurately what is available. Accuracy and thoroughness are analytically separable.
Pattern recognition or categorisation
Expertise in problem solving varies greatly between individual clinicians and is highly dependent on the clinician's mastery of the particular domain. 9 This finding challenges the hypothetico-deductive model of clinical reasoning, since both successful and unsuccessful diagnosticians use hypothesis testing. It appears that diagnostic accuracy does not depend as much on strategy as on mastery of content. Further, the clinical reasoning of experts in familiar situations frequently does not involve explicit testing of hypotheses. 3 10 , 11 , 12 Their speed, efficiency, and accuracy suggest that they may not even use the same reasoning processes as novices. 11 It is likely that experienced physicians use a hypothetico-deductive strategy only with difficult cases and that clinical reasoning is more a matter of pattern recognition or direct automatic retrieval. What are the patterns? What is retrieved? These questions signal a shift from the study of judgment to the study of the organisation and retrieval of memories.
Problem solving strategies
Hypothesis testing
Pattern recognition (categorisation)
By specific instances
By general prototypes
Viewing the process of diagnosis assigning a case to a category brings some other issues into clearer view. How is a new case categorised? Two competing answers to this question have been put forward and research evidence supports both. Category assignment can be based on matching the case to a specific instance (“instance based” or “exemplar based” recognition) or to a more abstract prototype. In the former, a new case is categorised by its resemblance to memories of instances previously seen. 3 11 This model is supported by the fact that clinical diagnosis is strongly affected by context—for example, the location of a skin rash on the body—even when the context ought to be irrelevant. 12
The prototype model holds that clinical experience facilitates the construction of mental models, abstractions, or prototypes. 2 13 Several characteristics of experts support this view—for instance, they can better identify the additional findings needed to complete a clinical picture and relate the findings to an overall concept of the case. These features suggest that better diagnosticians have constructed more diversified and abstract sets of semantic relations, a network of links between clinical features and diagnostic categories. 14
The controversy about the methods used in diagnostic reasoning can be resolved by recognising that clinicians approach problems flexibly; the method they select depends upon the perceived characteristics of the problem. Easy cases can be solved by pattern recognition: difficult cases need systematic generation and testing of hypotheses. Whether a diagnostic problem is easy or difficult is a function of the knowledge and experience of the clinician.
The strategies reviewed are neither proof against error nor always consistent with statistical rules of inference. Errors that can occur in difficult cases in internal medicine include failure to generate the correct hypothesis; misperception or misreading the evidence, especially visual cues; and misinterpretations of the evidence. 15 16 Many diagnostic problems are so complex that the correct solution is not contained in the initial set of hypotheses. Restructuring and reformulating should occur as data are obtained and the clinical picture evolves. However, a clinician may quickly become psychologically committed to a particular hypothesis, making it more difficult to restructure the problem.
Decision making
Diagnosis as opinion revision.
From the point of view of decision theory, reaching a diagnosis means updating opinion with imperfect information (the clinical evidence). 8 17 The standard rule for this task is Bayes's theorem. The pretest probability is either the known prevalence of the disease or the clinician's subjective impression of the probability of disease before new information is acquired. The post-test probability, the probability of disease given new information, is a function of two variables, pretest probability and the strength of the evidence, measured by a “likelihood ratio.”
Bayes's theorem tells us how we should reason, but it does not claim to describe how opinions are revised. In our experience, clinicians trained in methods of evidence based medicine are more likely than untrained clinicians to use a Bayesian approach to interpreting findings. 18 Nevertheless, probably only a minority of clinicians use it in daily practice and informal methods of opinion revision still predominate. Bayes's theorem directs attention to two major classes of errors in clinical reasoning: in the assessment of either pretest probability or the strength of the evidence. The psychological study of diagnostic reasoning from this viewpoint has focused on errors in both components, and on the simplifying rules or heuristics that replace more complex procedures. Consequently, this approach has become widely known as “heuristics and biases.” 4 19
Errors in estimation of probability
Availability —People are apt to overestimate the frequency of vivid or easily recalled events and to underestimate the frequency of events that are either very ordinary or difficult to recall. Diseases or injuries that receive considerable media attention are often thought of as occurring more commonly than they actually do. This psychological principle is exemplified clinically in the overemphasis of rare conditions, because unusual cases are more memorable than routine problems.
Representativeness —Representativeness refers to estimating the probability of disease by judging how similar a case is to a diagnostic category or prototype. It can lead to overestimation of probability either by causing confusion of post-test probability with test sensitivity or by leading to neglect of base rates and implicitly considering all hypotheses equally likely. This is an error, because if a case resembles disease A and disease B equally, and A is much more common than B, then the case is more likely to be an instance of A. Representativeness is associated with the “conjunction fallacy”—incorrectly concluding that the probability of a joint event (such as the combination of findings to form a typical clinical picture) is greater than the probability of any one of these events alone.
Heuristics and biases
Availability
Representativeness
Probability transformations
Effect of description detail
Conservatism
Anchoring and adjustment
Order effects
Decision theory assumes that in psychological processing of probabilities, they are not transformed from the ordinary probability scale. Prospect theory was formulated as a descriptive account of choices involving gambling on two outcomes, 20 and cumulative prospect theory extends the theory to cases with multiple outcomes. 21 Both prospect theory and cumulative prospect theory propose that, in decision making, small probabilities are overweighted and large probabilities underweighted, contrary to the assumption of standard decision theory. This “compression” of the probability scale explains why the difference between 99% and 100% is psychologically much greater than the difference between, say, 60% and 61%. 22
Support theory
Support theory proposes that the subjective probability of an event is inappropriately influenced by how detailed the description is. More explicit descriptions yield higher probability estimates than compact, condensed descriptions, even when the two refer to exactly the same events. Clinically, support theory predicts that a longer, more detailed case description will be assigned a higher subjective probability of the index disease than a brief abstract of the same case, even if they contain the same information about that disease. Thus, subjective assessments of events, while often necessary in clinical practice, can be affected by factors unrelated to true prevalence. 23
Errors in revision of probability
In clinical case discussions, data are presented sequentially, and diagnostic probabilities are not revised as much as is implied by Bayes's theorem 8 ; this phenomenon is called conservatism. One explanation is that diagnostic opinions are revised up or down from an initial anchor, which is either given in the problem or subjectively formed. Final opinions are sensitive to the starting point (the “anchor”), and the shift (“adjustment”) from it is typically insufficient. 4 Both biases will lead to collecting more information than is necessary to reach a desired level of diagnostic certainty.
It is difficult for everyday judgment to keep separate accounts of the probability of a disease and the benefits that accrue from detecting it. Probability revision errors that are systematically linked to the perceived cost of mistakes show the difficulties experienced in separating assessments of probability from values, as required by standard decision theory. There is a tendency to overestimate the probability of more serious but treatable diseases, because a clinician would hate to miss one. 24
Bayes's theorem implies that clinicians given identical information should reach the same diagnostic opinion, regardless of the order in which information is presented. However, final opinions are also affected by the order of presentation of information. Information presented later in a case is given more weight than information presented earlier. 25
Other errors identified in data interpretation include simplifying a diagnostic problem by interpreting findings as consistent with a single hypothesis, forgetting facts inconsistent with a favoured hypothesis, overemphasising positive findings, and discounting negative findings. From a Bayesian standpoint, these are all errors in assessing the diagnostic value of clinical evidence—that is, errors in implicit likelihood ratios.
Educational implications
Two recent innovations in medical education, problem based learning and evidence based medicine, are consistent with the educational implications of this research. Problem based learning can be understood as an effort to introduce the formulation and testing of clinical hypotheses into the preclinical curriculum. 26 The theory of cognition and instruction underlying this reform is that since experienced physicians use this strategy with difficult problems, and since practically any clinical situation selected for instructional purposes will be difficult for students, it makes sense to provide opportunities for students to practise problem solving with cases graded in difficulty. The finding of case specificity showed the limits of teaching a general problem solving strategy. Expertise in problem solving can be separated from content analytically, but not in practice. This realisation shifted the emphasis towards helping students acquire a functional organisation of content with clinically usable schemas. This goal became the new rationale for problem based learning. 27
Evidence based medicine is the most recent, and by most standards the most successful, effort to date to apply statistical decision theory in clinical medicine. 18 It teaches Bayes's theorem, and residents and medical students quickly learn how to interpret diagnostic studies and how to use a computer based nomogram to compute post-test probabilities and to understand the output. 28
We have selectively reviewed 30 years of psychological research on clinical diagnostic reasoning. The problem solving approach has focused on diagnosis as hypothesis testing, pattern matching, or categorisation. The errors in reasoning identified from this perspective include failure to generate the correct hypothesis; misperceiving or misreading the evidence, especially visual cues; and misinterpreting the evidence. The decision making approach views diagnosis as opinion revision with imperfect information. Heuristics and biases in estimation and revision of probability have been the subject of intense scrutiny within this research tradition. Both research paradigms understand judgment errors as a natural consequence of limitations in our cognitive capacities and of the human tendency to adopt short cuts in reasoning.
Both approaches have focused more on the mistakes made by both experts and novices than on what they get right, possibly leading to overestimation of the frequency of the mistakes catalogued in this article. The reason for this focus seems clear enough: from the standpoint of basic research, errors tell us a great deal about fundamental cognitive processes, just as optical illusions teach us about the functioning of the visual system. From the educational standpoint, clinical instruction and training should focus more on what needs improvement than on what learners do correctly; to improve performance requires identifying errors. But, in conclusion, we emphasise, firstly, that the prevalence of these errors has not been established; secondly, we believe that expert clinical reasoning is very likely to be right in the majority of cases; and, thirdly, despite the expansion of statistically grounded decision supports, expert judgment will still be needed to apply general principles to specific cases.
Series editor J A Knottnerus
Preparation of this review was supported in part by grant RO1 LM5630 from the National Library of Medicine.
Competing interests None declared.
“The Evidence Base of Clinical Diagnosis,” edited by J A Knottnerus, can be purchased through the BMJ Bookshop ( http://www.bmjbookshop.com/ )
- Elstein AS ,
- Shulman LS ,
- Bordage G ,
- Schmidt HG ,
- Norman GR ,
- Boshuizen HPA
- Kahneman D ,
- Sox HC Jr . ,
- Higgins MC ,
- Mellers BA ,
- Schwartz A ,
- Chapman GB ,
- Sonnenberg F
- Glasziou P ,
- Pliskin J ,
- Brooks LR ,
- Coblentz CL ,
- Lemieux M ,
- Kassirer JP ,
- Kopelman RI
- Sackett DL ,
- Haynes RB ,
- Guyatt GH ,
- Richardson WS ,
- Rosenberg W ,
- Tversky A ,
- Fischhoff B ,
- Bostrom A ,
- Quadrell M J
- Redelmeier DA ,
- Koehler DJ ,
- Liberman V ,
- Wallsten TS
- Bergus GR ,
Basic science knowledge underlies clinical science knowledge and clinical problem solving: evidence from veterinary medicine
- Open access
- Published: 16 May 2024
Cite this article
You have full access to this open access article
- Jared A. Danielson ORCID: orcid.org/0000-0002-3657-3412 1 ,
- Rebecca G. Burzette 2 ,
- Misty R. Bailey ORCID: orcid.org/0000-0002-6104-6980 3 ,
- Linda M. Berent 4 ,
- Heather Case 5 ,
- Anita Casey-Reed 5 ,
- John Dascanio ORCID: orcid.org/0000-0003-0796-2555 6 , 8 ,
- Richard A. Feinberg ORCID: orcid.org/0000-0003-1386-9867 7 ,
- Tamara S. Hancock ORCID: orcid.org/0000-0002-6453-6248 4 &
- Claudia A. Kirk ORCID: orcid.org/0000-0003-4157-6607 3
145 Accesses
Explore all metrics
Medical sciences education emphasizes basic science learning as a prerequisite to clinical learning. Studies exploring relationships between achievement in the basic sciences and subsequent achievement in the clinical sciences generally suggest a significant positive relationship. Basic science knowledge and clinical experience are theorized to combine to form encapsulated knowledge– a dynamic mix of information that is useful for solving clinical problems. This study explores the relationship between basic science knowledge (BSK), clinical science knowledge (CSK), and clinical problem-solving ability, as measured within the context of four veterinary colleges using both college-specific measures and professionally validated, standardized measures of basic and clinical science knowledge and problem-solving ability. Significant correlations existed among all variables. Structural equation modeling and confirmatory factor analysis were used to produce models showing that newly acquired BSK directly and significantly predicted BSK retained over time and newly acquired CSK, as well as indirectly predicted clinical problem-solving ability (mediated by newly acquired CSK and BSK retained over time). These findings likely suggest a gradual development of schema (encapsulated knowledge) and not an isolated development of biomedical versus clinical knowledge over time. A broader implication of these results is that explicitly teaching basic science knowledge positively and durably affects subsequent clinical knowledge and problem-solving ability independent of instructional strategy or curricular approach. Furthermore, for veterinary colleges specifically, student performance as measured by both course-level and standardized tests are likely to prove useful for predicting subsequent academic achievement in classroom and clinical settings, licensing examination performance, and/or for identifying students likely in need of remediation in clinical knowledge.
Similar content being viewed by others
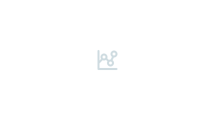
How Much Content Knowledge Do Graduating Medical Students Retain from Their Pre-Clinical and Clinical Courses?
Integrating foundational sciences in a clinical context in the post-clerkship curriculum, how do physicians become medical experts a test of three competing theories: distinct domains, independent influence and encapsulation models.
Avoid common mistakes on your manuscript.
Introduction
Research across multiple disciplines supports the notion that conceptual, verbal, and principle-based, discipline-specific knowledge is foundational for problem solving in any knowledge domain (Jonassen, 2000 ). This assumption is inherent in many current curricular approaches to medical sciences education, which emphasize basic science learning as a prerequisite to clinical learning (e.g. Finnerty et al., 2010 ). Despite the general assumption that basic science knowledge undergirds clinical learning and/or proficiency, research in the medical sciences has produced a variety of perspectives regarding the relationship between basic science knowledge and subsequent clinical proficiency, which is manifested in tasks such as making an accurate diagnosis and treatment plan. Furthermore, answering the question of how most effectively to integrate basic science knowledge into curricular programs meant to produce clinical proficiency remains elusive (Kulasegaram et al., 2013 ). This challenge is not unique to training human physicians, and is increasingly receiving attention in veterinary medical education as well. Particularly as Competency Based Veterinary Medical Education (AAVMC et al., 2018a , 2018b ; Salisbury et al., 2019 ) has received increased attention, those who teach topics in the basic sciences have struggled to define their role in competency based educational models.
Researchers have employed three broad strategies to explore how basic science knowledge relates to clinical science proficiency: (1) Observing experts to determine their clinical reasoning processes, or comparing clinical reasoning between novices and experts (Rikers et al., 2005 ; Rikers, Schmidt et al., 2005 ); (2) Teaching novices in a variety of ways, some of which rely on basic science knowledge, and some of which do not (Baghdady et al., 2009 , 2013 ; Woods et al., 2005 , 2006 , 2006b , 2007 ); and (3) Analyzing the relationship between basic and clinical science knowledge across time as clinical proficiency grows (Schauber et al., 2013 ).
Multiple studies show that medical experts employ more biomedical knowledge than less experienced practitioners, even though that knowledge may be encapsulated within their clinical knowledge, and, therefore, difficult to detect (de Bruin et al., 2005 ; Norman et al., 1989 ; Rikers et al., 2004 ; Rikers, Loyens, Rikers et al., 2005a ; Rikers, Schmidt et al., 2005 ). For instance, de Bruin et al. ( 2005 ) administered tests of clinical diagnosis ability, basic science knowledge, and clinical knowledge to family physicians and medical students at various points in their training. They found that, for both students and physicians, clinical knowledge mediated the predictive relationship between basic science knowledge and diagnostic performance, meaning that basic science knowledge predicted diagnostic performance when it also predicted clinical knowledge. This mediated relationship can be interpreted to mean that basic science knowledge contributed to clinical problem solving ability particularly for those individuals for whom it had also contributed to clinical knowledge.
Similarly, in studies exploring the relationship between the extent to which instruction employs basic science concepts and subsequent proficiency, educational strategies that integrate basic science and clinical concepts produce greater learning gains than those that do not (Baghdady et al., 2009 ; Kulasegaram et al., 2013 , 2017 ; Lisk et al., 2016 ; Woods et al., 2005 , 2006 , 2006b , 2007 ). For example, Baghdady and colleagues ( 2009 ), found that when pre-dental students were taught basic science concepts, they performed diagnostic tasks more accurately than students who were taught structured algorithms or feature lists, even though all students performed similarly on a simple memory test of the facts that had been taught.
Studies exploring relationships between achievement in the basic sciences and subsequent achievement in the clinical sciences generally, but not universally, suggest a significant positive relationship. Cianciolo and colleagues ( 2013 ) found a low-moderate positive relationship between biomedical knowledge and clinical information gathering and interpretation in medical students over time. Similarly, studies have shown that basic science knowledge during veterinary school (Danielson et al., 2011 ) and prior to veterinary admission (Danielson & Burzette, 2020 ) significantly and positively predicted clinical problem-solving ability as measured by licensing examination scores. In contrast, Schauber et al. ( 2013 ) found a negative relationship between students’ early levels of basic science knowledge and subsequent gains in clinical knowledge. They speculated that this unexpected finding could be due to individual differences in student motivation or cognitive characteristics, interference from irrelevant information, or inappropriate transfer.
One complexity associated with discussions of basic science and clinical science knowledge are the variety of labels that have been employed to refer to the many intellectual skills that contribute to clinical proficiency. Labels such as “knowledge,” “reasoning,” “critical thinking,” and “problem solving” can all be found in the literature, but researchers do not use these labels equivalently. Furthermore, there is a paucity of studies establishing psychometrically measurable distinctions between closely associated intellectual skills such as “diagnostic reasoning,” “clinical reasoning,” “critical thinking,”, “diagnostic problem solving” and “clinical problem solving.” The present study does not seek to resolve this dilemma or to provide a typology of intellectual skills in the medical sciences. However, in order to provide definitional clarity to constructs measured in the present study, we define those constructs as follows, borrowing from Smith and Ragan’s ( 2005 ) taxonomy of learning outcomes, which were adapted from Gagné et al’s ( 1992 ) varieties of learning:
Basic science knowledge
We define basic science knowledge as all of the declarative knowledge, concepts, principles and procedures associated with the disciplines of anatomy, physiology, pathology, microbiology, and pharmacology that underly a veterinary medical education. As specified by the AVMA COE accreditation standards, this knowledge provides “an understanding of the central biological principles and mechanisms that underlie animal health and disease from the molecular and cellular level to organismal and population manifestations” and “scientific, discipline-based instruction in an orderly and concise manner so that students gain an understanding of normal function, homeostasis, pathophysiology, mechanisms of health/disease, and the natural history and manifestations of important animal diseases...” (AVMA-COE, 2023 p. 25).
Clinical science knowledge
We define clinical science knowledge as all of the declarative knowledge, concepts, principles, procedures, and problem-solving ability associated with the theory and practice of medicine. As defined by the AVMA COE accreditation standards, this knowledge includes “principles and hands-on experiences in physical and laboratory diagnostic methods and interpretation (including diagnostic imaging, diagnostic pathology, and necropsy), disease prevention, biosecurity, therapeutic intervention (including surgery and dentistry), and patient management and care (including intensive care, emergency medicine and isolation procedures) involving clinical diseases of individual animals and populations,” and emphasizing “problem solving that results in making and applying medical judgments” (AVMA-COE, 2023 pp. 25–26).
Clinical problem-solving ability
Smith and Ragan define problem solving as the ability to “select from a number of possible rules, whether relational or procedural, and apply those rules in a unique sequence and combination to solve a previously unencountered problem” (2005 p. 81). For the purpose of the present study, scores on the North American Veterinary Licensing Examination (NAVLE®) were used as a measure of clinical problem solving. The NAVLE® provides realistic clinical scenarios that require examinees to synthesize their existing knowledge and select an appropriate diagnosis or next step related to diagnosis or treatment. Thus, the content of NAVLE is focused on clinical knowledge, but the item format often requires problem solving skills that go beyond factual recall to correctly respond.
Theoretically, basic science knowledge contributes to clinical knowledge and/or clinical problem-solving ability in two ways. First, as noted above, multiple studies have established that when students learn clinical concepts in the context of underlying basic science knowledge, they are better able to solve clinical problems (Baghdady et al., 2009 , 2013 ; Kulasegaram et al., 2013 , 2017 ; Lisk et al., 2016 ; Woods et al., 2005 , 2006 2006b , 2007 ). In the studies cited, this effect was evident within a week of initial exposure to the content.
Second, underlying biomedical knowledge has been shown to be inherent to clinical problem solving in experts. Castillo et al. summarize the contribution of basic science knowledge to clinical ability in experts as follows:
“For the experienced physician, biomedical knowledge is sometimes described as encapsulated with clinical knowledge in mental representations of diseases (Schmidt & Rikers, 2007 ). This clustering of symptoms into meaningful patterns based on basic science knowledge (Schmidt & Boshuizen, 1992 ) provides a way of explaining symptoms simultaneously, thus facilitating clinical problem solving (de Bruin et al., 2005 ; Schmidt & Boshuizen, 1993 ). Therefore, the value of the basic sciences in clinical reasoning goes beyond the development of static knowledge structures. Rather, basic science knowledge should also serve as the foundation for the development of dynamic mental structures to support medical problem solving” (Castillo et al., 2018 p. 593).
From this perspective, the expert’s dynamic, encapsulated knowledge comprises both biomedical knowledge and clinical knowledge (characterized by exemplars encountered in practice)– and is accessed dynamically when clinical problems are encountered (Rikers, Schmidt, et al., 2005 ).
If basic science knowledge contributes to clinical knowledge in the ways described above, measures of basic science knowledge should predict measures of clinical ability for at least two reasons. First, basic science knowledge, independent of clinical experience or encapsulated knowledge, provides a foundational set of concepts and principles upon which learners draw as they solve clinical problems; therefore, measures of basic science knowledge should directly predict clinical science knowledge and/or ability. Second, basic science knowledge and clinical experience are theorized to combine to form encapsulated knowledge– a dynamic mix of information that is useful for solving clinical problems. Therefore, as learners grow in knowledge and experience, their maturing knowledge structures begin to resemble, even if only modestly at first, the encapsulated knowledge of experts. Such knowledge structures would begin to form as students first encounter clinical cases and should be reflected by grades in clinical courses. That being the case, the relationship between foundational basic science and clinical problem-solving ability should also be mediated by intermediate measures of clinical science knowledge and ability. Finally, if basic science knowledge persists over time, independently of encapsulated knowledge, a strong correlation should exist between basic science knowledge when it is first acquired and basic science knowledge that is retained over a period of months to years. Furthermore, because memories fade over time (Anderson, 2000 ), basic science knowledge that has been retained over time, either because it has been rehearsed, or because it has been encapsulated in clinical science knowledge, should predict subsequent clinical proficiency more powerfully than basic science knowledge when it is first acquired.
It is important to note that these relationships between basic science knowledge and subsequent clinical science knowledge and proficiency do not infer a particular curricular strategy or sequence. For example, some curricula introduce basic science principles early on with little discussion of clinical application. Other curricula integrate basic science instruction into clinical instruction very early in the educational process. Hypothesized relationships between basic science knowledge and clinical science knowledge and proficiency would, theoretically, be consistent across a variety of curricular approaches.
The present study explores the relationship between basic and clinical science knowledge as measured within the context of four veterinary colleges using both college-specific measures and professionally-validated, standardized measures of basic science knowledge, and clinical problem solving.
The model shown in Fig. 1 tests the hypothesized relationships discussed above as measured in the present study. The measures, described in more detail in the Materials and Methods section, were as follows: (1) Measures of basic science knowledge included grades in basic science courses and the Veterinary Educational Assessment (VEA®); (2) Grades in clinical science courses were used to measure clinical science knowledge; and (3) Scores on the North American Veterinary Licensing Examination (NAVLE®) were used as measures of clinical problem-solving ability. We hypothesized that pooling basic sciences grades would produce a valid basic science knowledge construct, pooling clinical science grades would produce a valid clinical science knowledge construct, and that the sub scores of the VEA would also form a valid construct representing basic science knowledge retained over time. We proposed testing these hypotheses with confirmatory factor analysis.
Given the documented relationship between basic science knowledge and clinical ability, regardless of expertise level, we hypothesized a positive predictive relationship between measures of basic science knowledge and subsequent measures of clinical knowledge and problem-solving ability (direct effects). Given the assumption that encapsulated basic science knowledge contributes to problem-solving ability, we hypothesized that the relationship between basic science knowledge and clinical problem-solving ability would be mediated by clinical science knowledge (specific indirect effects). Finally, we theorized a significant positive relationship between basic science knowledge shortly after it was acquired and basic science knowledge that was retained over time (direct effect). We proposed using structural equation modeling to test these hypotheses.
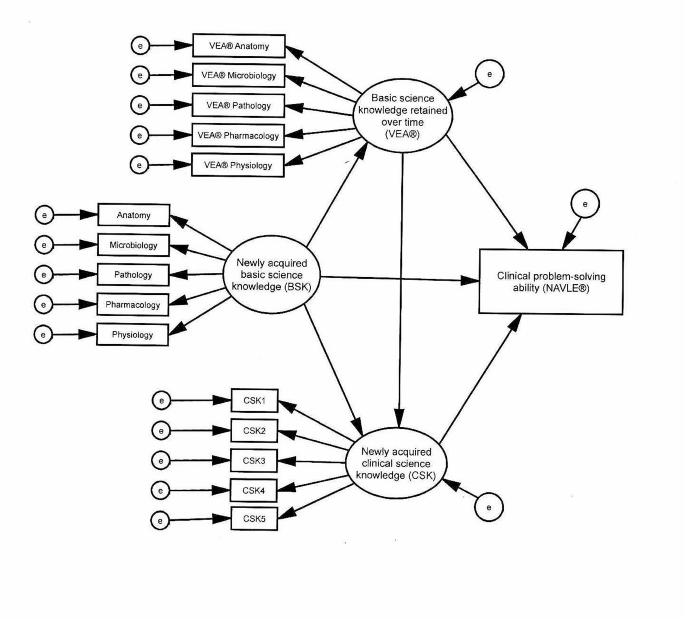
Hypothesized model. Newly acquired basic science knowledge (BSK) represented course grades in the subjects shown. Newly acquired clinical science knowledge (CSK) was measured with student achievement in clinical courses. e = error, VEA® = Veterinary Educational Assessment, NAVLE® = North American Veterinary Licensing Examination
Materials and methods
Participants.
Students from four AVMA-COE accredited colleges of veterinary medicine, two located in the midwest and two in the southeast of the United States were study participants. All participating students graduated between 2016 and 2019. During the period of the study, one of the participating institutions administered the VEA® four times, two administered it three times, and one administered it twice, with 1,310 students total taking both the VEA® and NAVLE® exams. Of the students taking both exams, 1,161 (88.6%) consented to have their NAVLE® scores released to their college, and were included in the study. Participants were 79.9% female and 20.1% male, in response to a male/female binary questionnaire item; ages at the time of the VEA® administration ranged from 22 to 53 years ( M = 25.8, SD = 2.71).
This study was approved by the Iowa State University Institutional Review Board (exempt; IRB #18-438-01). The data were collected from academic records for students who graduated between 2016 and 2019 from one of four U.S.-based veterinary programs accredited by the American Veterinary Medical Association Council on Education (AVMA-COE). All students participated in the curriculum of one of the four participating colleges. Two of the colleges employed a traditional, discipline-based curriculum in which students participated in predominantly lecture and laboratory instruction for 3 years, with early semesters focusing on the basic sciences and later semesters focusing more on the clinical sciences (such as medicine and surgery). During the fourth year, students learned in clinical rotations where they spent most of their time working directly with client-owned animals under the direct supervision of veterinary faculty within a veterinary teaching hospital. The curriculum of the third institution was similar, except that didactic instruction was completed in 2 years (including summers) and followed by 2 years of clinical instruction. The curriculum at the fourth institution was similar to the first two, except that the fourth-year clinical instruction was offered in preceptorships in a variety of privately-owned clinics, rather than in an institutionally-owned teaching hospital.
Evaluation practices differ among instructors, with some basing grades exclusively on individuals’ exam scores, and others including other factors such as quiz scores, group participation, assignments, or extra credit experiences. Therefore, in order to minimize the potential effect of factors that influenced student grades, but did not reflect achievement, for basic or clinical science courses, instructors were asked to select the best indicator of overall student achievement, with some choosing the course grade, and others choosing one or multiple pooled exam scores. For each course, the instructor’s chosen student achievement measure functioned as the grade for the purposes of this study. Course grades were designated as either basic science (courses in anatomy, physiology, pathology, microbiology, and pharmacology) or clinical science (courses emphasizing the practice of medicine or surgery). For all participating students, grades in basic and clinical sciences courses were collected, as well as VEA® and NAVLE® scores. A more detailed description of each measure or category of measures is provided below.
Newly acquired basic science knowledge (BSK) Basic science grades were computed for each content area that mirrored those assessed in the VEA® (anatomy, microbiology, pathology, pharmacology, and physiology). For instance, if a student took two courses in anatomy, one course in pharmacology, three microbiology-related courses, and two courses in pathology, the average grade for each cluster of courses would represent that student’s mean score for that content area. We refer to these scores as newly acquired basic science knowledge (BSK) because they measure what students recalled directly after studying for a course in which they were enrolled. Certainly, much of the knowledge that contributed to such grades had been acquired weeks or even months prior to the assessments that produced them. However, tested/graded knowledge is almost invariably reviewed by examinees shortly before an assessment.
For each college, we computed mean grades for each basic science course area, based on the areas assessed in the VEA® (anatomy, microbiology, pathology, pharmacology, and physiology). These variables served as indicators for newly acquired BSK. Note that these indicators were not identical across colleges.
Basic science knowledge retained over time (VEA®) The VEA®, developed by the International Council for Veterinary Assessment (ICVA), is a 240-item, multiple-choice examination that was designed to assess knowledge in the areas of veterinary anatomy, physiology, pharmacology, microbiology, and pathology (ICVA, 2022 ). At the time it was administered to the participants in this study, the VEA® included 200 items. As is standard for commercially available standardized examinations, the VEA® was administered in multiple forms, with different forms used each time the examination was administered.
For the present study, VEA® scores are considered to represent knowledge retained over time rather than newly acquired knowledge because the VEA® measured knowledge that had persisted well beyond the point at which it was assessed for a grade. All participants took the VEA® in the third year of their veterinary training, months to years (depending on the topic) after completing all, or the overwhelming majority, of their basic science curriculum.
Newly acquired clinical science knowledge (CSK) Each institution provided grades for all core clinical science (e.g., medicine or surgery) courses (approximately 20 per institution), including both didactic courses and clinical rotations. For each institution, all clinical science courses were randomly assigned to one of five clinical science indicators (CSK1– CSK5), with each indicator comprising scores from four to five courses, and each indicator’s value being the average grade for the randomly-assigned courses. As was the case with basic science course grades, these scores are considered to represent newly acquired knowledge because they were earned directly following study. While the participating colleges all offered similar clinical science courses, such as medicine and surgery, all of their courses were unique to their colleges, so none of the indicators were identical across colleges, however, they were hypothesized to measure equivalent constructs, and therefore, were treated equivalently in the data modeling. Their hypothesized equivalence was tested by determining whether or not they produced consistent results in the model.
Clinical problem-solving ability (NAVLE®) The NAVLE® is a 360-item, multiple-choice examination administered by the ICVA that is required for veterinary licensure in the United States and Canada. Like the VEA®, the NAVLE® was professionally developed adhering to rigorous psychometric standards. Students are not required to release their NAVLE® scores to their schools. Approximately 91% of students released their NAVLE® scores to the four colleges in the study timeframe (A. Casey-Reed, personal communication, August 13, 2021).
Analytic strategy and data modeling
Within each college, the grade-based indicators as well as the VEA® and NAVLE® scores were standardized, then collapsed across colleges.
Correlations were calculated among all variables to show zero-order relationships in order to allow subsequent replication of the analysis, and to aid in interpretation of the subsequent structural equation model.
We used structural equation modeling (SEM) to test relationships among the variables of interest. Structural Equation Modeling was used because it allows for exploration of mediated relationships among variables, and we hypothesized mediated relationships as students’ knowledge increased over time. Confirmatory factor analysis (CFA) was used to verify the measurement quality of the latent constructs used in the model, with standardized and unstandardized coefficients, standard error, probability value, and squared multiple correlation all being calculated and reported. As part of the SEM, Mahalanobis distances were calculated to identify multivariate outliers, which can lead to spurious results. Inspection of Mahalanobis distances in the initial sample of 1,177 identified 16 cases that were multivariate outliers ( p <.001). These cases were excluded from further analysis, leaving 1,161 cases. We then examined the distributions of the indicators; no variable showed excessive skewness (< 2.1) or kurtosis (< 7.0) (see Table 1 ).
Goodness of fit indices were examined. Thresholds for a good fit were root mean square error of approximation (RMSEA) < 0.08, comparative fit index (CFI) > 0.95, Tucker-Lewis fit index (TLI) > 0.95, and standardized root mean square residual (SRMR) < 0.08 (Hu & Bentler, 1999 ). The maximum likelihood method was used to estimate parameters because the data were normally distributed.
All analyses were conducted using IBM SPSS 27 and IBM SPSS Amos 27.
Correlations
Table 1 summarizes correlations. We expected correlations among measures of basic science skills and among measures of clinical science skills to be higher than those between measures of basic science skills and clinical science skills. Inspection of Table 1 shows that correlations among the basic science measures ranged from 0.721 to 0.815; correlations among the clinical science measures ranged from 0.508 to 0.616; correlations among the VEA® measures ranged from 0.437 to 0.673. Correlations between the basic science measures and the clinical science measures ranged from 0.521 to 0.678; correlations between the basic science measures and the VEA® measures ranged from 0.330 to 0.501; correlations between the clinical science measures and the VEA® measures ranged from 0.243 to 0.366; correlations among the basic science measures and the NAVLE® ranged from 0.567 to 0.626; correlations among the clinical science measures and the NAVLE® ranged from 0.469 to 0.579; correlations among the VEA® measures and the NAVLE® ranged from 0.463 to 0.580.
Confirmatory factor analysis (CFA)
As is common to SEM, CFA was conducted to verify the measurement quality of the latent constructs used in the model. Inspection of fit indices for the model presented in Fig. 2 showed that the model provided a good fit for the data, χ 2 (83) = 358.430, p <.001; RMSEA = 0.053, 90% CI [0.048, 0.059], p =.151; CFI = 0.978; TLI = 0.972; SRMR = 0.026. Table 2 presents the unstandardized and standardized coefficients, as well as the squared multiple correlation (SMC). As seen in Table 2 , all indicators demonstrated significant loadings (e.g. standardized regression coefficients) on the expected factors. Additionally, inspection of the SMCs showed that BSK accounted for 75–81% of the variance in the indicators; CSK accounted for 51–67% of the variance in the indicators and VEA® accounted for 47–74% of the variance in the indicators. The composite reliability / McDonald omega coefficient (ω) for BSK was 0.943, 95% CI [0.935, 0.949], demonstrating excellent reliability. For CSK, ω = 0.876, 95% CI [0.863, 0.878], demonstrating good reliability. For VEA®, ω = 0.867, 95% CI [0.854, 0.878], also demonstrating good reliability.
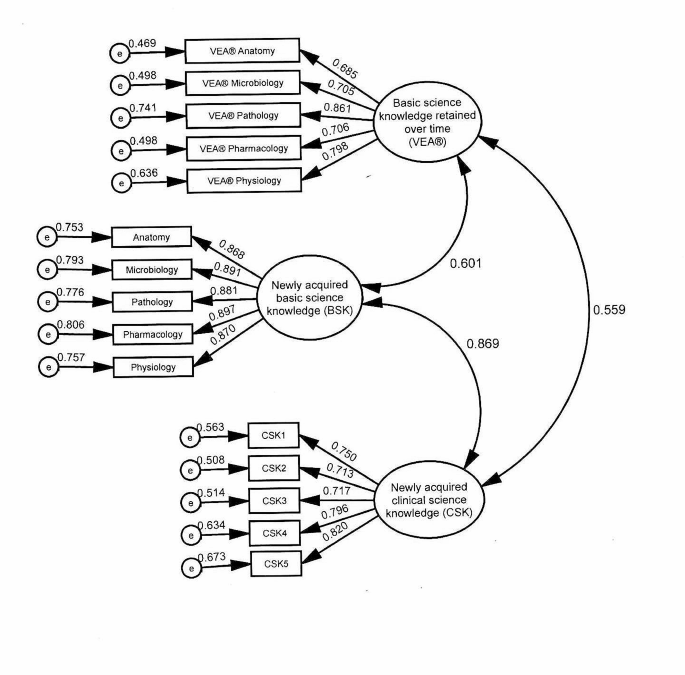
Confirmatory factor analysis. Newly acquired basic science knowledge (BSK) represented course grades in the subjects shown. Newly acquired clinical science knowledge (CSK) was measured with student achievement in clinical courses. e = error, VEA® = Veterinary Educational Assessment
Structural equation models (SEM)
Direct effects Fig. 3 shows the model tested. In this model, BSK was hypothesized to predict CSK, VEA®, and NAVLE®. VEA® was hypothesized to predict CSK and NAVLE®. CSK was hypothesized to predict NAVLE®. Inspection of fit indexes for this model showed that the model provided a good fit for the data, χ 2 (99) = 509.651, p <.001; RMSEA = 0.060, 90% CI [0.055, 0.065], p =.001; CFI = 0.970; TLI = 0.963; SRMR = 0.026). Examination of the standardized residual covariance matrix showed that no covariance was greater than 2, indicating a good fit by conventional standards. Squared multiple correlations demonstrated that 37% of the variance in VEA®, 59% of the variance in NAVLE®, and 75% of variance in CSK were accounted for by the model.
Table 3 presents the unstandardized and standardized coefficients for the direct effects in the hypothesized model. As hypothesized, BSK predicted CSK, β = 0.829, and BSK predicted VEA®, β = 0.607. VEA® predicted CSK, β = 0.059, and NAVLE®, β = 0.396. CSK predicted NAVLE®, β = 0.393. BSK did not predict NAVLE®, β = 0.082, p =.120.
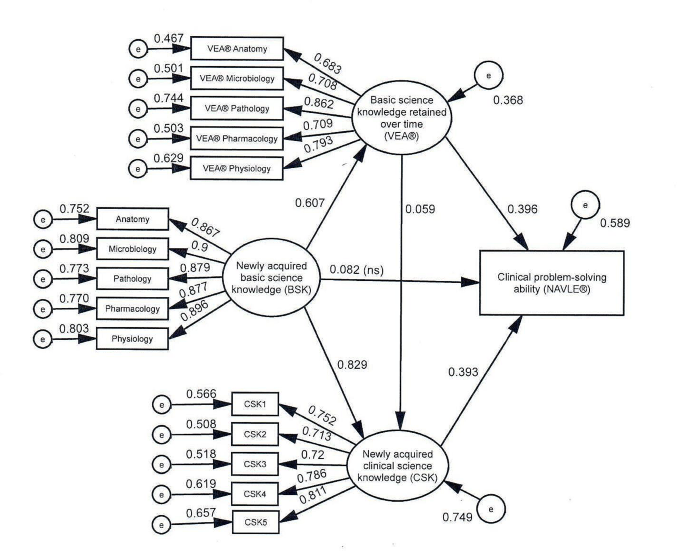
Structural equation model tested. Newly acquired basic science knowledge (BSK) represented course grades in the subjects shown. Newly acquired clinical science knowledge (CSK) was measured with student achievement in clinical courses. e = error, VEA® = Veterinary Educational Assessment, NAVLE® = North American Veterinary Licensing Examination
Specific indirect effects Table 4 contains the specific indirect effects (SIE) of mediated variables. BSK’s effect on NAVLE® is mediated by VEA®, SIE = 0.269. BSK’s effect on NAVLE® is also mediated by CSK, SIE = 0.364. Finally, BSK’s effect on NAVLE® is mediated by both VEA® and CSK combined (see Fig. 3 ), SIE = 0.027.
Individual school models To explore the possibility that the tested model was a good fit for the multi-institution pooled data set, but not a good fit for one or more of the participating colleges, the same model was applied individually to each college. The significance of the coefficients for the direct and indirect relationships were identical in each of the individual college models with two exceptions. First, the direct relationship between VEA® and CSK was not significant in any of the four individual school models, and second, the direct relationship between CSK and NAVLE® was not significant for one of the individual school models. In both cases, because the underlying coefficients were similar across individual schools, we hypothesize that the relatively smaller institution samples provided inadequate statistical power to detect significant differences in the smaller single institution samples.
All of the hypotheses were supported by the findings, except the hypothesis of a direct positive relationship between newly acquired basic science knowledge and clinical problem-solving ability (NAVLE).
Based on prior research, we expected both measures of basic science knowledge to have a positive association with subsequent clinical science knowledge (Cianciolo et al., 2013 ; Danielson & Burzette, 2020 ; Danielson et al., 2011 ). The present study reinforces those findings and supports Schauber and colleagues’ ( 2013 ) hypothesis that their findings of a negative association were atypical.
In the present study, newly acquired basic science knowledge directly and significantly predicted both basic science knowledge retained over time and newly acquired clinical science knowledge, but did not directly predict clinical problem-solving ability. However, its association with clinical problem-solving ability was measurable when mediated by both CSK and VEA® independently, as well as by those two measures combined. This suggests three independent knowledge mechanisms by which BSK might be hypothesized to influence clinical problem solving. First, BSK’s direct association with CSK as well as its indirect association with NAVLE® (mediated by CSK) may represent the encapsulated basic science knowledge hypothesized to be integrated into clinical knowledge (de Bruin et al., 2005 ). Second, BSK’s direct association with VEA®, as well as VEA®’s association with CSK and NAVLE® likely represents the explicit knowledge of basic science concepts and principles that remain accessible to learners long after initial learning, and even after expertise has developed. The strength of these associations suggests that explicit basic science knowledge might be hypothesized to continue positively to affect clinical science knowledge, independent of encapsulated knowledge. The effect of BSK on NAVLE® that is mediated by both CSK and VEA® might be explained by some more general phenomenon that would contribute equally to both basic and clinical science knowledge, such as general intelligence or scientific reasoning. General scholastic ability, measured by instruments such as the ACT, SAT and GRE has been shown to be strongly related to general cognitive ability (Frey & Detterman, 2004 ; Koenig et al., 2008 ), and to scientific reasoning (Sternberg et al., 2019 ) when the latter was measured using a multiple choice format. Furthermore, within veterinary medicine, general scholastic ability, as measured by GRE verbal scores, have been shown to be significantly related to both VEA® and NAVLE® scores (Danielson & Burzette, 2020 ). Therefore, it is reasonable to hypothesize that general cognitive ability might contribute to achievement in both the basic and clinical sciences, and could explain the variance in NAVLE® scores identified in our study that is not explicitly linked to either basic or clinical science knowledge.
While these findings support the hypothesized relationships among basic and clinical science knowledge and clinical problem-solving ability, it would be naïve to suppose that any given measure of medical science ability reflects only basic science knowledge or clinical science knowledge. Most medical science learners have at least some exposure to both biomedical science and clinical knowledge very early in their educational process, and some veterinary learners have years of exposure to clinical cases prior to even enrolling in a veterinary program. Therefore, these findings likely suggest a gradual development of schema (encapsulated knowledge), and not the isolated development of biomedical vs. clinical knowledge over time, nor the point at which biomedical science ceases to contribute to achievement and clinical knowledge begins to contribute to achievement (see Rikers, Schmidt, et al., 2005 ). The fact that the predictive relationships were similar across multiple colleges with variability in learners, instructors, courses, assessments, and instructional/curricular approaches suggests that the relationships among variables, and any resulting theoretical implications, are resistant to such variability.
Implications for instruction
A number of studies (Baghdady et al., 2009 , 2013 ; Woods et al., 2005 , 2006 , 2006b , 2007 ) have identified a significant positive relationship between teaching strategies that explicitly teach relevant basic science concepts and subsequent clinical knowledge over a relatively short time frame (such as a week.) Given the results of the present study, such strategies may also have positive effects in the longer term. However, the present findings emerged from four institutions employing different curricular approaches, and from many instructors employing a broad variety of instructional and assessment approaches. Therefore, the broader implication is that explicitly teaching basic science knowledge might be hypothesized to positively and durably affect subsequent clinical knowledge independent of instructional strategy or curricular approach. Furthermore, for veterinary colleges specifically, student performance as measured by both course-level and standardized tests (such as the VEA®) are likely to prove useful for predicting subsequent academic achievement in both classroom and clinical settings, as well as licensing examination performance) and/or for identifying students likely in need of remediation in clinical knowledge.
Limitations
This study was conducted at four veterinary colleges in North America. While those colleges’ curricular approaches varied, they were all inherently discipline based, and traditional in their approach; other common broad curricular approaches, such as Problem Based Learning (PBL), an organ-system based model or a clinical presentation-based model, were not represented. It is possible that these findings might not generalize to institutions that use substantially different curricular models, particularly models that seek to integrate basic and clinical science knowledge throughout the curriculum. Nonetheless, ample evidence suggests that curricular approach is a relatively weak factor for explaining student achievement, when compared with other factors, such as student or teacher effects (Hattie, 2015 ; Hecker & Violato, 2008 ), so implications regarding the generalizability of these findings due to curricular approach are likely to be modest. One strength of this study, the broad sampling of scores across several institutions and multiple courses, is also a potential weakness. While it allows us to conclude that basic science knowledge is important for clinical science knowledge, it does not provide detail regarding which instructional approaches may be more effective or less effective, or how much basic science knowledge is needed for subsequent clinical proficiency. Additionally, the present study was not able to partition general intelligence or aptitude prior to any of the basic knowledge instruction. Such factors have the potential to contribute to initial clinical or basic science knowledge or could influence the acquisition of knowledge or skills. Finally, clinical knowledge and problem solving, as defined in this study, do not include many important elements of a graduate veterinarian’s portfolio of abilities and attributes, including technical and other procedural skills, communication ability, professionalism, ethical conduct, time management and many context-specific abilities. Further research is recommended to address these limitations.
The present study supports prior research documenting the positive predictive relationship between basic science knowledge and clinical problem solving. Specifically, basic science knowledge appears to contribute to clinical problem-solving ability both directly and mediated through subsequent clinical knowledge, where it is theorized to become encapsulated or schematized. While no specific instructional approaches are indicated based on the present study, this relationship between basic science and clinical science knowledge appears sufficiently robust to be evident across a variety of institutions, courses, instructors, and assessment processes.
Further research into the relationship between basic science knowledge and subsequent achievement is warranted. First, while this paper has documented the relationship between basic science knowledge, clinical science knowledge and clinical problem solving as defined narrowly, associations between these constructs and measures of workplace based clinical proficiency, including abilities such as procedural knowledge and skill, communication ability, time management, patient handling, and so forth, remain largely unexplored. Additional research to establish valid measures of workplace based clinical proficiency, and to associate those measures with predictive measures such as those discussed in this paper, will be critical to a growing understanding of how best to adapt educational practices to the needs of clinical practitioners.
AAVMC Working Group on Competency-Based Veterinary Education, Molgaard, L. K., Hodgson, J. L., Bok, H. G. J., Chaney, K. P., Ilkiw, J. E., Matthew, S. M., May, S. A., Read, E. K., Rush, B. R., & Salisbury, S. K. (2018a). Competency-based Veterinary Education: Part 1 - CBVE Framework . Association of American Veterinary Medical Colleges.
AAVMC Working Group on Competency-Based Veterinary Education, Molgaard, L. K., Hodgson, J. L., Bok, H. G. J., Chaney, K. P., Ilkiw, J. E., Matthew, S. M., May, S. A., Read, E. K., Rush, B. R., & Salisbury, S. K. (2018b). Competency-based Veterinary Education: Part 2 - Entrustable Professional activities . Association of American Veterinary Medical Colleges.
Anderson, J. R. (2000). Retention of Memories. Learning and memory: An integrated approach (pp. 226–264). John Wiley & Sons, Inc.
AVMA-COE (2023). Accreditation Policies and Procedures of the AVMA Council on Education: June 2023 . https://www.avma.org/sites/default/files/2023-10/coe-pp-Jun-2023.pdf .
Baghdady, M. T., Pharoah, M. J., Regehr, G., Lam, E. W. N., & Woods, N. N. (2009). The role of Basic sciences in diagnostic oral Radiology. Journal of Dental Education , 73 (10), 1187–1193. https://doi.org/10.1002/j.0022-0337.2009.73.10.tb04810.x .
Article Google Scholar
Baghdady, M. T., Carnahan, H., Lam, E. W. N., & Woods, N. N. (2013). Integration of Basic Sciences and Clinical Sciences in oral Radiology Education for Dental Students. Journal of Dental Education , 77 (6), 757–763. https://doi.org/10.1002/j.0022-0337.2013.77.6.tb05527.x .
Castillo, J. M., Park, Y. S., Harris, I., Cheung, J. J. H., Sood, L., Clark, M. D., Kulasegaram, K., Brydges, R., Norman, G., & Woods, N. (2018). A critical narrative review of transfer of basic science knowledge in health professions education. Medical Education , 52 (6), 592–604. https://doi.org/10.1111/medu.13519 .
Cianciolo, A. T., Williams, R. G., Klamen, D. L., & Roberts, N. K. (2013). Biomedical knowledge, clinical cognition and diagnostic justification: A structural equation model. Medical Education , 47 (3), 309–316. https://doi.org/10.1111/medu.12096 .
Danielson, J. A., & Burzette, R. G. (2020). GRE and Undergraduate GPA as predictors of Veterinary Medical School Grade Point Average, VEA scores and NAVLE scores while Accounting for Range Restriction. Frontiers in Veterinary Science , 7 , 576354–576354. https://doi.org/10.3389/fvets.2020.576354 .
Danielson, J. A., Wu, T. F., Molgaard, L. K., & Preast, V. A. (2011). Relationships among common measures of student performance and scores on the North American Veterinary Licensing Examination. Journal of the American Veterinary Medical Association , 238 (4), 454–461. https://doi.org/10.2460/javma.238.4.454 .
de Bruin, A. B. H., Schmidt, H. G., & Rikers, R. M. J. P. (2005). The role of Basic Science Knowledge and Clinical Knowledge in Diagnostic reasoning: A structural equation modeling Approach. Academic Medicine , 80 (8), 765–773. https://journals.lww.com/academicmedicine/Fulltext/2005/08000/The_Role_of_Basic_Science_Knowledge_and_Clinical.14.aspx .
Finnerty, E. P., Chauvin, S., Bonaminio, G., Andrews, M., Carroll, R. G., & Pangaro, L. N. (2010). Flexner Revisited: The role and value of the Basic sciences in Medical Education. Academic Medicine , 85 (2), 349–355. https://doi.org/10.1097/ACM.0b013e3181c88b09 .
Frey, M. C., & Detterman, D. K. (2004). Scholastic Assessment or g? The Relationship between the Scholastic Assessment Test and General Cognitive ability. Psychological Science , 15 (6), 373–378. https://doi.org/10.1111/j.0956-7976.2004.00687.x .
Gagné, R. M., Briggs, L. J., & Wager, W. W. (1992). Principles of instructional design (4th ed.). Harcourt Brace Jovanovich College.
Hattie, J. (2015). The applicability of visible learning to higher education. Scholarship of Teaching and Learning in Psychology , 1 (1), 79–91.
Hecker, K., & Violato, C. (2008). How much do differences in medical schools influence student performance? A longitudinal study employing hierarchical linear modeling. Teaching and Learning in Medicine , 20 (2), 104–113. https://doi.org/10.1080/10401330801991915 .
Hu, L., & Bentler, P. M. (1999). Cutoff criteria for fit indexes in covariance structure analysis: Conventional criteria versus new alternatives. Structural Equation Modeling: A Multidisciplinary Journal , 6 (1), 1–55. https://doi.org/10.1080/10705519909540118 .
ICVA (2022). Veterinary Educational Assessment . International Council for Veterinary Assessment. Retrieved 16 Feb from https://www.icva.net/other-exams/qualifying-examination-vea/ .
Jonassen, D. H. (2000). Toward a design theory of Problem solving. Educational Technology Research and Development , 48 (4), 63–85.
Koenig, K. A., Frey, M. C., & Detterman, D. K. (2008). ACT and general cognitive ability. Intelligence , 36 (2), 153–160.
Kulasegaram, K. M., Martimianakis, M. A., Mylopoulos, M., Whitehead, C. R., & Woods, N. N. (2013). Cognition before Curriculum: Rethinking the integration of Basic Science and Clinical Learning. Academic Medicine , 88 (10), 1578–1585. https://doi.org/10.1097/ACM.0b013e3182a45def .
Kulasegaram, K. M., Chaudhary, Z., Woods, N., Dore, K., Neville, A., & Norman, G. (2017). Contexts, concepts and cognition: Principles for the transfer of basic science knowledge. Medical Education , 51 (2), 184–195. https://doi.org/10.1111/medu.13145 .
Lisk, K., Agur, A. M. R., & Woods, N. N. (2016). Exploring cognitive integration of basic science and its effect on diagnostic reasoning in novices. Perspectives on Medical Education , 5 (3), 147–153. https://doi.org/10.1007/s40037-016-0268-2 .
Norman, G. R., Brooks, L. R., & Allen, S. W. (1989). Recall by expert medical practitioners and novices as a record of processing attention. Journal of Experimental Psychology: Learning Memory and Cognition , 15 (6), 1166–1174. https://doi.org/10.1037/0278-7393.15.6.1166 .
Rikers, R. M., Loyens, S. M., & Schmidt, H. G. (2004). The role of encapsulated knowledge in clinical case representations of medical students and family doctors. Med Educ , 38 (10), 1035–1043. http://www.ncbi.nlm.nih.gov/entrez/query.fcgi?cmd=Retrieve&db=PubMed&dopt=Citation&list_uids=15461648
Rikers, R. M. J. P., Schmidt, H. G., & Moulaert, V. (2005). Biomedical knowledge: Encapsulated or two worlds apart? Applied Cognitive Psychology , 19 (2), 223–231. https://doi.org/10.1002/acp.1107 .
Rikers, R. M. J. P., Loyens, S., te Winkel, W., Schmidt, H. G., & Sins, P. H. M. (2005a). The role of Biomedical Knowledge in clinical reasoning: A lexical decision study. Academic Medicine , 80 (10), 945–949. https://journals.lww.com/academicmedicine/Fulltext/2005/10000/The_Role_of_Biomedical_Knowledge_in_Clinical.15.aspx .
Salisbury, S. K., Chaney, K. P., Ilkiw, J. E., Read, E. K., Rush, B. R., Bok, H. G. J., Danielson, J. A., Hodgson, J. L., Matthew, S. M., May, S. A., & Molgaard, L. K. (2019). Compentency-based Veterinary Education: Part 3 - milestones. In A. o. A. V. M. Colleges . AAVMC.
Schauber, S. K., Hecht, M., Nouns, M., Z., & Dettmer, S. (2013). On the role of biomedical knowledge in the acquisition of clinical knowledge. Medical Education , 47 (12), 1223–1235. https://doi.org/10.1111/medu.12229 .
Schmidt, H. G., & Boshuizen, H. (1992). Encapsulation of biomedical knowledge. Advanced models of cognition for medical training and practice (pp. 265–282). Springer.
Schmidt, H. G., & Boshuizen, H. (1993). On the origin of intermediate effects in clinical case recall. Memory & Cognition , 21 (3), 338–351.
Schmidt, H. G., & Rikers, R. M. (2007). How expertise develops in medicine: Knowledge encapsulation and illness script formation. Medical Education , 41 (12), 1133–1139.
Google Scholar
Smith, P. L., & Ragan, T. J. (2005). Instructional analysis: Analyzing the learning task. Instructional Design (3rd ed.). Wiley.
Sternberg, R. J., Wong, C. H., & Sternberg, K. (2019). The relation of tests of scientific reasoning to each other and to tests of general intelligence. Journal of Intelligence , 7 (3), 20.
Woods, N. N., Brooks, L. R., & Norman, G. R. (2005). The value of basic science in clinical diagnosis: Creating coherence among signs and symptoms. Medical Education , 39 (1), 107–112. https://doi.org/10.1111/j.1365-2929.2004.02036.x .
Woods, N. N., Howey, E. H. A., Brooks, L. R., & Norman, G. R. (2006). Speed kills? Speed, accuracy, encapsulations and causal understanding. Medical Education , 40 (10), 973–979. https://doi.org/10.1111/j.1365-2929.2006.02556.x
Woods, N. N., Neville, A. J., Levinson, A. J., Howey, E. H. A., Oczkowski, W. J., & Norman, G. R. (2006b). The value of Basic Science in clinical diagnosis. Academic Medicine , 81 (10), S124–S127. https://journals.lww.com/academicmedicine/Fulltext/2006/10001/The_Value_of_Basic_Science_in_Clinical_Diagnosis.31.aspx .
Woods, N. N., Brooks, L. R., & Norman, G. R. (2007). It all make sense: Biomedical knowledge, causal connections and memory in the novice diagnostician. Advances in Health Sciences Education , 12 (4), 405. https://doi.org/10.1007/s10459-006-9055-x .
Download references
Acknowledgements
We appreciate valuable insight from Dr. Kent Hecker, who reviewed an early manuscript draft.
Author information
Authors and affiliations.
Department of Veterinary Pathology, Iowa State University, Ames, IA, USA
Jared A. Danielson
College of Veterinary Medicine, Iowa State University, Ames, IA, USA
Rebecca G. Burzette
College of Veterinary Medicine, University of Tennessee, Knoxville, TN, USA
Misty R. Bailey & Claudia A. Kirk
Department of Veterinary Pathobiology, University of Missouri, Columbia, MO, USA
Linda M. Berent & Tamara S. Hancock
International Council for Veterinary Assessment, Bismarck, ND, USA
Heather Case & Anita Casey-Reed
College of Veterinary Medicine, Lincoln Memorial University, Harrogate, TN, USA
John Dascanio
National Board of Medical Examiners, Philadelphia, PA, USA
Richard A. Feinberg
School of Veterinary Medicine, Texas Tech University, Amarillo, TX, USA
You can also search for this author in PubMed Google Scholar
Contributions
J.D. led overall manuscript preparation, and drafted the Introduction, Discussion, Limitations, and Conclusion sections. R.B. drafted the Methods and Results sections, and prepared figures and tables. All authors contributed to the study design and participated in overall manuscript review and revision.
Corresponding author
Correspondence to Jared A. Danielson .
Ethics declarations
Ethical approval.
This study was approved by the Iowa State University Institutional Review Board (exempt; IRB #18-438-01).
Competing interests
Authors HC and AC-R receive a salary from the International Council for Veterinary Assessment, where they are the chief executive officer and the program manager, respectively. ICVA is the veterinary medicine equivalent to the MCC and NBME. A number of MCC and NBME staff are lead and co-authors on various measurement manuscripts with colleagues in academia, for example the recent Montierro et al. manuscript from McMaster where Debra Pugh (MCC) was listed as an author. This is an equivalent situation, to provide validity evidence for ICVA assessment methods. Furthermore, neither HC nor AC-R were involved in collecting or analyzing the data; their role was to help ensure the accuracy of portrayals of the ICVA measures, and to participate in overall manuscript review and revision. Therefore, their conflict of interest had no influence on the present study. No other authors declare financial interests. No funding was received for conducting this study.
Additional information
Publisher’s note.
Springer Nature remains neutral with regard to jurisdictional claims in published maps and institutional affiliations.
Rights and permissions
Open Access This article is licensed under a Creative Commons Attribution 4.0 International License, which permits use, sharing, adaptation, distribution and reproduction in any medium or format, as long as you give appropriate credit to the original author(s) and the source, provide a link to the Creative Commons licence, and indicate if changes were made. The images or other third party material in this article are included in the article’s Creative Commons licence, unless indicated otherwise in a credit line to the material. If material is not included in the article’s Creative Commons licence and your intended use is not permitted by statutory regulation or exceeds the permitted use, you will need to obtain permission directly from the copyright holder. To view a copy of this licence, visit http://creativecommons.org/licenses/by/4.0/ .
Reprints and permissions
About this article
Danielson, J.A., Burzette, R.G., Bailey, M.R. et al. Basic science knowledge underlies clinical science knowledge and clinical problem solving: evidence from veterinary medicine. Adv in Health Sci Educ (2024). https://doi.org/10.1007/s10459-024-10334-2
Download citation
Received : 16 May 2023
Accepted : 14 April 2024
Published : 16 May 2024
DOI : https://doi.org/10.1007/s10459-024-10334-2
Share this article
Anyone you share the following link with will be able to read this content:
Sorry, a shareable link is not currently available for this article.
Provided by the Springer Nature SharedIt content-sharing initiative
- Clinical learning
- Encapsulated knowledge
- Standardized exams
- Find a journal
- Publish with us
- Track your research
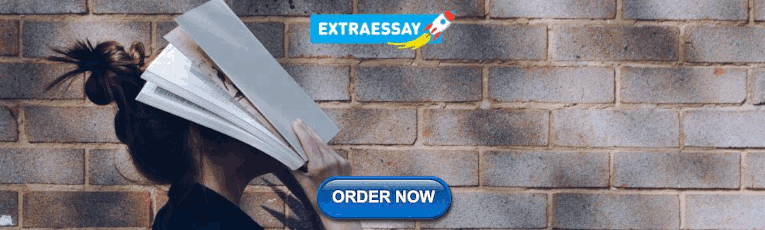
IMAGES
VIDEO
COMMENTS
This article will go beyond education and experience, and provide insight into the key selection criteria for nursing positions, along with example STAR responses. 1. Communication & Interpersonal Skills. Effective communication and interpersonal skills are critical in the nursing profession. You need to be able to communicate with patients ...
1. Demonstrated high level interpersonal, verbal and written communication skills. 1. 2 Demonstrated clinical knowledge and clinical problem solving abilities. 2. 3 An understanding of and ability to work within an interdisciplinary team. 3. 4 An understanding of professional, ethical and legal requirements of registered nurse
Learning to provide safe and quality health care requires technical expertise, the ability to think critically, experience, and clinical judgment. The high-performance expectation of nurses is dependent upon the nurses' continual learning, professional accountability, independent and interdependent decisionmaking, and creative problem-solving abilities.
Here are some selection criteria examples for a registered nurse application in Australia, along with some answer examples to help you out: 1. Effective Communication and Interpersonal Skills. Effective communication skills are essential for nursing. Show the recruiter that you're good with people by explaining your methods, and reiterating ...
• Demonstrated clinical knowledge and clinical problem solving abilities. • Demonstrated ability to work within a team. • An understanding of the professional, ethical and legal requirements of the Registered Nurse or Registered Midwife. • A demonstrated understanding of the role of the Registered Nurse or Midwife in applying continuous
In reflection, a deterioration of the patient was detected and effective, appropriate interventions were used to improve the status of the patient. I have indicated the ability to use clinical knowledge and problem- solving skills to implement safe and effective interventions. Demonstrate your ability to work within a team.
Take an Individualized Approach to Clinical Knowledge in Nursing. According to Academic Medicine, "Clinical knowledge entails information about relations of particular signs and symptoms with specific diseases.". A clinician's expertise in this area is developed over time through patient encounters, where his or her "knowledge base is ...
An investigative and analytical approach to clinical problem solving and knowledge acquisition; An ability to apply medical knowledge to clinical situations; An ability to teach others; Investigative and Analytical Approach. First and foremost, residents must always maintain an open mind. They will demonstrate a willingness to never shy away ...
This chapter introduces the concept of clinical reasoning. It attempts to define what clinical reasoning is and what its features are. Solving clinical problems involves the ability to reason about causality of pathological processes, requiring knowledge of anatomy and the working and pathology of organ systems, and it requires the ability to compare patient problems as patterns with instances ...
Abstract. Clinical reasoning assessment is an essential component of determining a health professional's competence. Clinical reasoning cannot be assessed directly. It must be gleaned from a health professional's choices and decisions. Clinical knowledge and knowledge organization, rather than a general problem solving process, serve as the ...
In summary, the ability to clinically reason using information and evidence available, the ability to draw on one's knowledge base in context including theoretical education and knowledge and clinical experience in context and the practice of employing reflective process to nursing actions that include consideration of patient/family ...
Problem-solving: To investigate how training problem-solving skills affected the rate of self-handicapping among nursing students. 33: Svellingen et al., 2021 : n = 146: 4 days in 3 years: Clinical simulation: To evaluate the impact of multiple simulations on students' self-reported clinical decision-making skills and self-confidence. 33
Clinical experts need general and specific problem solving strategies in order to make adequate treatment decisions for their patients. Clinical problem solving (or clinical reasoning) as a skill involves different categories of knowledge as well as several cognitive abilities and is key for becoming a clinical expert [].Problem-solving occurs in well-known phases, described in models like the ...
Demonstrated clinical knowledge and clinical problem solving abilities; Demonstrated ability to work within an interdisciplinary team; An understanding of the professional, ethical and legal requirements of the Registered Nurse or Midwife. A demonstrated understanding of the role of the Registered Nurse or Midwife in applying continuous ...
The article provides a description of a method of teaching a clinical problem-solving process to primary health care nurses/clinical nurse practitioners (PHC nurses). The process was developed in the Soweto PHC Nurse Training Unit over the past 30 years as a result of the changing availability and role of nurse and doctor teaching staff.
Demonstrated Clinical Knowledge And Clinical Problem Solving Abilities ... Nurse Practitioners Mike Walsh 2006 This is a comprehensive text for nurse practitioners that integrates the required clinical skills with professional and theoretical issues in one book. It uses a systems approach to cover the most frequently presenting problems that ...
In general, for hypertensive emergencies, blood-pressure targets are below 180/120 mm Hg within the first hour and then below 160/110 mm Hg over the subsequent 23 hours, unless there is an acute ...
McWhinney argued that although general principles of medical problem solving are common, each discipline has its own way of applying them [Citation 5]. He demonstrated how specific clinical strategies have been elaborated in primary care, corresponding to the particular morbidity pattern with undifferentiated conditions, illness presenting in ...
Rather than a historical overview as in Chap. 2, this chapter provides the reader with insight into the various approaches that have been used to understand clinical reasoning. We review concepts and major scholars who have been involved in such investigations. Cognitive psychologists Newel and Simon theorized about problem-solving skills and artificial intelligence and initiated the use of ...
The complex nature of clinical problem-solving skills and the rich interplay between the implicit and explicit forms of knowledge must be taken into consideration when we want to explain 'acquisition' of clinical skills. ... neurological terms appear in the model only when Dreyfus gestures toward artificial neural networks to demonstrate ...
This is the fourth in a series of five articles This article reviews our current understanding of the cognitive processes involved in diagnostic reasoning in clinical medicine. It describes and analyses the psychological processes employed in identifying and solving diagnostic problems and reviews errors and pitfalls in diagnostic reasoning in the light of two particularly influential ...
Students who master the contents of this book will take a long step forward in acquiring the diagnostic skills that are the hallmark of the experienced physician. The transformation from medical student to physician is a gradual one, requiring the assimilation of vast amounts of knowledge as well as the development of the ability to "think like a doctor."
Research across multiple disciplines supports the notion that conceptual, verbal, and principle-based, discipline-specific knowledge is foundational for problem solving in any knowledge domain (Jonassen, 2000).This assumption is inherent in many current curricular approaches to medical sciences education, which emphasize basic science learning as a prerequisite to clinical learning (e.g ...