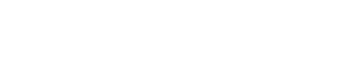
- Blockchains & Decentralized Systems
- Machine Learning & Statistics
- Computational Genomics
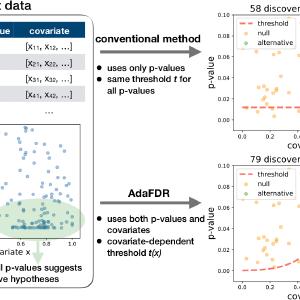
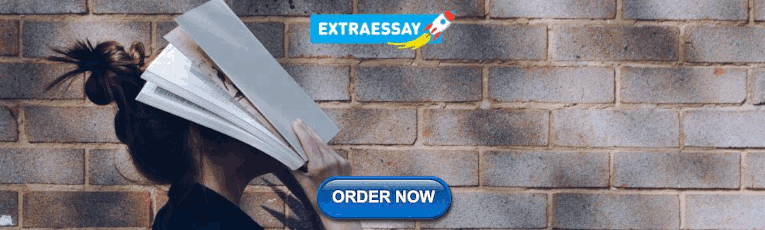
Covariate-adaptive multiple hypothesis testing
In multiple hypothesis testing, the data for each test boils down to a p-value while additional information is often available for each test, e.g., the functional annotation for genetic testing. Such information may inform how likely the small p-values are to be due to noise. Covariate-adaptive multiple testing methods utilize such information to improve detection power while controlling false positives.
Multiple hypothesis testing is an essential component of modern data science. Its goal is to maximize the number of discoveries while controlling the fraction of false discoveries. In many settings, in addition to the p-value, additional information/covariates for each hypothesis are available. For example, in eQTL studies, each hypothesis tests the correlation between a variant and the expression of a gene. We also have additional covariates such as the location, conservation, and chromatin status of the variant, which could inform how likely the association is to be due to noise. However, popular multiple hypothesis testing approaches, such as Benjamini-Hochberg procedure (BH) and independent hypothesis weighting (IHW), either ignore these covariates or assume the covariate to be univariate. We develop covariate-adaptive multiple testing methods, NeuralFDR and AdaFDR, to adaptively learn the optimal p-value threshold from covariates to significantly improve detection power while controlling the false discovery proportion.
Key Publications
- “AdaFDR: a Fast, Powerful and Covariate-Adaptive Approach to Multiple Hypothesis Testing”, Martin J. Zhang, Fei Xia, James Zou, 2018, bioRxiv 496372v1 , Best paper award in RECOMB 2019
- “NeuralFDR: Learning Discovery Thresholds from Hypothesis Features”, Fei Xia, Martin J. Zhang, James Y. Zou, David Tse, 2017, NIPS 2017
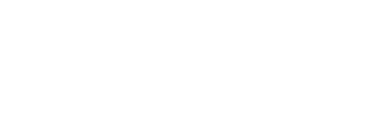
Principal Investigator
David Tse Room 264, Packard Building Electrical Engineering Department 350 Jane Stanford Way Stanford, CA 94305-9515
Helen Niu Room 310, Packard Building Electrical Engineering Department 350 Jane Stanford Way Stanford, CA 94305-9515
Tel: (650) 723-8121 Fax: (650) 723-9251 [email protected]
Combining Multiple Hypothesis Testing with Machine Learning Increases the Statistical Power of Genome-wide Association Studies
Affiliations.
- 1 Machine Learning Group, Technische Universität Berlin, Berlin, 10587, Germany.
- 2 Department of Computer Science, Humboldt University of Berlin, Berlin, 10099, Germany.
- 3 Institut de Biología Evolutiva (CSIC-UPF). Departament de Ciències Experimentals i de la Salut. Universitat Pompeu Fabra, Barcelona, 08003, Spain.
- 4 TomTom Research, Berlin, 12555, Germany.
- 5 School of Biology, Georgia Institute of Technology, Atlanta, 30332, GA, USA.
- 6 Department of Economics, Laboratory for Social and Neural Systems Research, University of Zurich, Zurich, 8006, Switzerland.
- 7 Institute for Statistics (FB 3), University of Bremen, Bremen, 28359, Germany.
- 8 Department of Mathematics, University of Potsdam, Potsdam, 14476, Germany.
- 9 Department of Economics, University of Mainz, Mainz, 55099, Germany.
- 10 Institució Catalana de Recerca i Estudis Avançats (ICREA), Barcelona, 08010, Spain.
- 11 Center for Genomic Regulation (CRG), Barcelona Institute of Science and Technology (BIST), Barcelona, 08003, Spain.
- 12 Department of Brain and Cognitive Engineering, Korea University, Seoul, Republic of Korea.
- PMID: 27892471
- PMCID: PMC5125008
- DOI: 10.1038/srep36671
The standard approach to the analysis of genome-wide association studies (GWAS) is based on testing each position in the genome individually for statistical significance of its association with the phenotype under investigation. To improve the analysis of GWAS, we propose a combination of machine learning and statistical testing that takes correlation structures within the set of SNPs under investigation in a mathematically well-controlled manner into account. The novel two-step algorithm, COMBI, first trains a support vector machine to determine a subset of candidate SNPs and then performs hypothesis tests for these SNPs together with an adequate threshold correction. Applying COMBI to data from a WTCCC study (2007) and measuring performance as replication by independent GWAS published within the 2008-2015 period, we show that our method outperforms ordinary raw p-value thresholding as well as other state-of-the-art methods. COMBI presents higher power and precision than the examined alternatives while yielding fewer false (i.e. non-replicated) and more true (i.e. replicated) discoveries when its results are validated on later GWAS studies. More than 80% of the discoveries made by COMBI upon WTCCC data have been validated by independent studies. Implementations of the COMBI method are available as a part of the GWASpi toolbox 2.0.
Publication types
- Research Support, Non-U.S. Gov't
Grants and funding
- 295642/ERC_/European Research Council/International
- Comprehensive Learning Paths
- 150+ Hours of Videos
- Complete Access to Jupyter notebooks, Datasets, References.

Hypothesis Testing – A Deep Dive into Hypothesis Testing, The Backbone of Statistical Inference
- September 21, 2023
Explore the intricacies of hypothesis testing, a cornerstone of statistical analysis. Dive into methods, interpretations, and applications for making data-driven decisions.

In this Blog post we will learn:
- What is Hypothesis Testing?
- Steps in Hypothesis Testing 2.1. Set up Hypotheses: Null and Alternative 2.2. Choose a Significance Level (α) 2.3. Calculate a test statistic and P-Value 2.4. Make a Decision
- Example : Testing a new drug.
- Example in python
1. What is Hypothesis Testing?
In simple terms, hypothesis testing is a method used to make decisions or inferences about population parameters based on sample data. Imagine being handed a dice and asked if it’s biased. By rolling it a few times and analyzing the outcomes, you’d be engaging in the essence of hypothesis testing.
Think of hypothesis testing as the scientific method of the statistics world. Suppose you hear claims like “This new drug works wonders!” or “Our new website design boosts sales.” How do you know if these statements hold water? Enter hypothesis testing.
2. Steps in Hypothesis Testing
- Set up Hypotheses : Begin with a null hypothesis (H0) and an alternative hypothesis (Ha).
- Choose a Significance Level (α) : Typically 0.05, this is the probability of rejecting the null hypothesis when it’s actually true. Think of it as the chance of accusing an innocent person.
- Calculate Test statistic and P-Value : Gather evidence (data) and calculate a test statistic.
- p-value : This is the probability of observing the data, given that the null hypothesis is true. A small p-value (typically ≤ 0.05) suggests the data is inconsistent with the null hypothesis.
- Decision Rule : If the p-value is less than or equal to α, you reject the null hypothesis in favor of the alternative.
2.1. Set up Hypotheses: Null and Alternative
Before diving into testing, we must formulate hypotheses. The null hypothesis (H0) represents the default assumption, while the alternative hypothesis (H1) challenges it.
For instance, in drug testing, H0 : “The new drug is no better than the existing one,” H1 : “The new drug is superior .”
2.2. Choose a Significance Level (α)
When You collect and analyze data to test H0 and H1 hypotheses. Based on your analysis, you decide whether to reject the null hypothesis in favor of the alternative, or fail to reject / Accept the null hypothesis.
The significance level, often denoted by $α$, represents the probability of rejecting the null hypothesis when it is actually true.
In other words, it’s the risk you’re willing to take of making a Type I error (false positive).
Type I Error (False Positive) :
- Symbolized by the Greek letter alpha (α).
- Occurs when you incorrectly reject a true null hypothesis . In other words, you conclude that there is an effect or difference when, in reality, there isn’t.
- The probability of making a Type I error is denoted by the significance level of a test. Commonly, tests are conducted at the 0.05 significance level , which means there’s a 5% chance of making a Type I error .
- Commonly used significance levels are 0.01, 0.05, and 0.10, but the choice depends on the context of the study and the level of risk one is willing to accept.
Example : If a drug is not effective (truth), but a clinical trial incorrectly concludes that it is effective (based on the sample data), then a Type I error has occurred.
Type II Error (False Negative) :
- Symbolized by the Greek letter beta (β).
- Occurs when you accept a false null hypothesis . This means you conclude there is no effect or difference when, in reality, there is.
- The probability of making a Type II error is denoted by β. The power of a test (1 – β) represents the probability of correctly rejecting a false null hypothesis.
Example : If a drug is effective (truth), but a clinical trial incorrectly concludes that it is not effective (based on the sample data), then a Type II error has occurred.
Balancing the Errors :

In practice, there’s a trade-off between Type I and Type II errors. Reducing the risk of one typically increases the risk of the other. For example, if you want to decrease the probability of a Type I error (by setting a lower significance level), you might increase the probability of a Type II error unless you compensate by collecting more data or making other adjustments.
It’s essential to understand the consequences of both types of errors in any given context. In some situations, a Type I error might be more severe, while in others, a Type II error might be of greater concern. This understanding guides researchers in designing their experiments and choosing appropriate significance levels.
2.3. Calculate a test statistic and P-Value
Test statistic : A test statistic is a single number that helps us understand how far our sample data is from what we’d expect under a null hypothesis (a basic assumption we’re trying to test against). Generally, the larger the test statistic, the more evidence we have against our null hypothesis. It helps us decide whether the differences we observe in our data are due to random chance or if there’s an actual effect.
P-value : The P-value tells us how likely we would get our observed results (or something more extreme) if the null hypothesis were true. It’s a value between 0 and 1. – A smaller P-value (typically below 0.05) means that the observation is rare under the null hypothesis, so we might reject the null hypothesis. – A larger P-value suggests that what we observed could easily happen by random chance, so we might not reject the null hypothesis.
2.4. Make a Decision
Relationship between $α$ and P-Value
When conducting a hypothesis test:
We then calculate the p-value from our sample data and the test statistic.
Finally, we compare the p-value to our chosen $α$:
- If $p−value≤α$: We reject the null hypothesis in favor of the alternative hypothesis. The result is said to be statistically significant.
- If $p−value>α$: We fail to reject the null hypothesis. There isn’t enough statistical evidence to support the alternative hypothesis.
3. Example : Testing a new drug.
Imagine we are investigating whether a new drug is effective at treating headaches faster than drug B.
Setting Up the Experiment : You gather 100 people who suffer from headaches. Half of them (50 people) are given the new drug (let’s call this the ‘Drug Group’), and the other half are given a sugar pill, which doesn’t contain any medication.
- Set up Hypotheses : Before starting, you make a prediction:
- Null Hypothesis (H0): The new drug has no effect. Any difference in healing time between the two groups is just due to random chance.
- Alternative Hypothesis (H1): The new drug does have an effect. The difference in healing time between the two groups is significant and not just by chance.
Calculate Test statistic and P-Value : After the experiment, you analyze the data. The “test statistic” is a number that helps you understand the difference between the two groups in terms of standard units.
For instance, let’s say:
- The average healing time in the Drug Group is 2 hours.
- The average healing time in the Placebo Group is 3 hours.
The test statistic helps you understand how significant this 1-hour difference is. If the groups are large and the spread of healing times in each group is small, then this difference might be significant. But if there’s a huge variation in healing times, the 1-hour difference might not be so special.
Imagine the P-value as answering this question: “If the new drug had NO real effect, what’s the probability that I’d see a difference as extreme (or more extreme) as the one I found, just by random chance?”
For instance:
- P-value of 0.01 means there’s a 1% chance that the observed difference (or a more extreme difference) would occur if the drug had no effect. That’s pretty rare, so we might consider the drug effective.
- P-value of 0.5 means there’s a 50% chance you’d see this difference just by chance. That’s pretty high, so we might not be convinced the drug is doing much.
- If the P-value is less than ($α$) 0.05: the results are “statistically significant,” and they might reject the null hypothesis , believing the new drug has an effect.
- If the P-value is greater than ($α$) 0.05: the results are not statistically significant, and they don’t reject the null hypothesis , remaining unsure if the drug has a genuine effect.
4. Example in python
For simplicity, let’s say we’re using a t-test (common for comparing means). Let’s dive into Python:
Making a Decision : “The results are statistically significant! p-value < 0.05 , The drug seems to have an effect!” If not, we’d say, “Looks like the drug isn’t as miraculous as we thought.”
5. Conclusion
Hypothesis testing is an indispensable tool in data science, allowing us to make data-driven decisions with confidence. By understanding its principles, conducting tests properly, and considering real-world applications, you can harness the power of hypothesis testing to unlock valuable insights from your data.
More Articles
Correlation – connecting the dots, the role of correlation in data analysis, sampling and sampling distributions – a comprehensive guide on sampling and sampling distributions, law of large numbers – a deep dive into the world of statistics, central limit theorem – a deep dive into central limit theorem and its significance in statistics, skewness and kurtosis – peaks and tails, understanding data through skewness and kurtosis”, similar articles, complete introduction to linear regression in r, how to implement common statistical significance tests and find the p value, logistic regression – a complete tutorial with examples in r.
Subscribe to Machine Learning Plus for high value data science content
© Machinelearningplus. All rights reserved.

Machine Learning A-Z™: Hands-On Python & R In Data Science
Free sample videos:.


Evaluating Hypotheses in Machine Learning: A Comprehensive Guide
Learn how to evaluate hypotheses in machine learning, including types of hypotheses, evaluation metrics, and common pitfalls to avoid. Improve your ML model's performance with this in-depth guide.
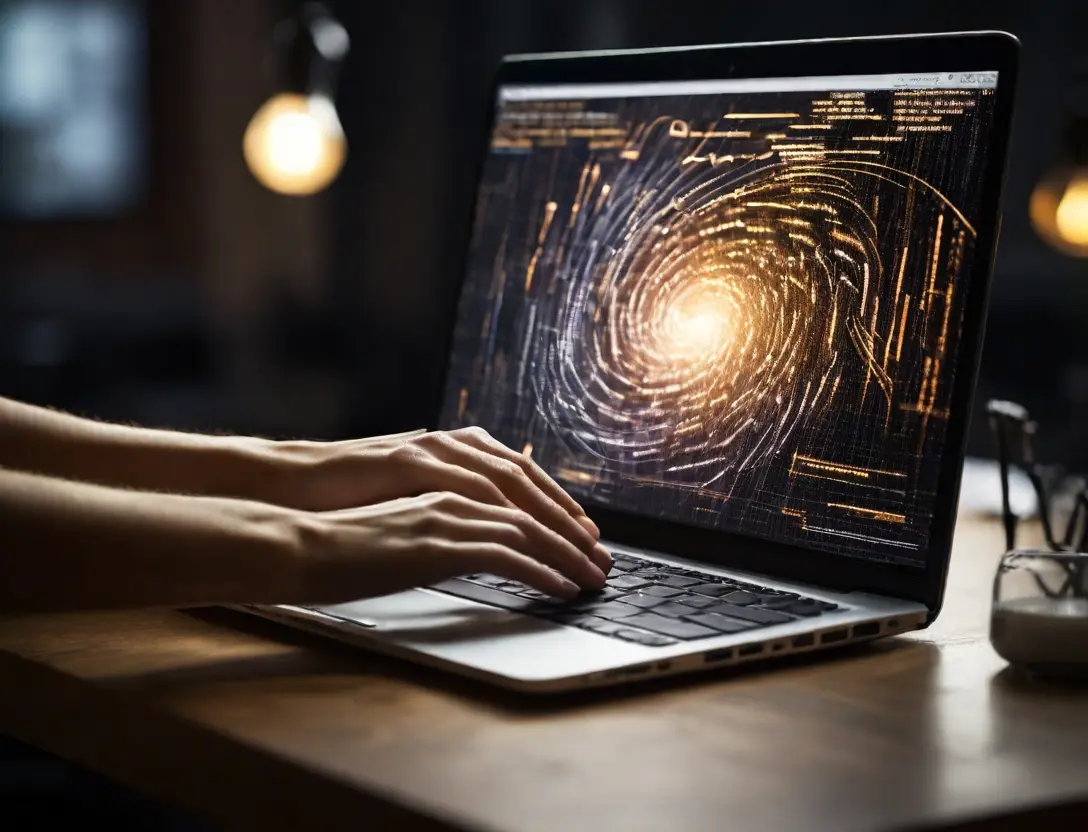
Create an image featuring JavaScript code snippets and interview-related icons or graphics. Use a color scheme of yellows and blues. Include the title '7 Essential JavaScript Interview Questions for Freshers'.
Introduction
Machine learning is a crucial aspect of artificial intelligence that enables machines to learn from data and make predictions or decisions. The process of machine learning involves training a model on a dataset, and then using that model to make predictions on new, unseen data. However, before deploying a machine learning model, it is essential to evaluate its performance to ensure that it is accurate and reliable. One crucial step in this evaluation process is hypothesis testing.
In this blog post, we will delve into the world of hypothesis testing in machine learning, exploring what hypotheses are, why they are essential, and how to evaluate them. We will also discuss the different types of hypotheses, common pitfalls to avoid, and best practices for hypothesis testing.
What are Hypotheses in Machine Learning?
In machine learning, a hypothesis is a statement that proposes a possible explanation for a phenomenon or a problem. It is a conjecture that is made about a population parameter, and it is used as a basis for further investigation. In the context of machine learning, hypotheses are used to define the problem that we are trying to solve.
For example, let's say we are building a machine learning model to predict the prices of houses based on their features, such as the number of bedrooms, square footage, and location. A possible hypothesis could be: "The price of a house is directly proportional to its square footage." This hypothesis proposes a possible relationship between the price of a house and its square footage.
Why are Hypotheses Essential in Machine Learning?
Hypotheses are essential in machine learning because they provide a framework for understanding the problem that we are trying to solve. They help us to identify the key variables that are relevant to the problem, and they provide a basis for evaluating the performance of our machine learning model.
Without a clear hypothesis, it is difficult to develop an effective machine learning model. A hypothesis helps us to:
- Identify the key variables that are relevant to the problem
- Develop a clear understanding of the problem that we are trying to solve
- Evaluate the performance of our machine learning model
- Refine our model and improve its accuracy
Types of Hypotheses in Machine Learning
There are two main types of hypotheses in machine learning: null hypotheses and alternative hypotheses.
Null Hypothesis
A null hypothesis is a hypothesis that proposes that there is no significant difference or relationship between variables. It is a hypothesis of no effect or no difference. For example, let's say we are building a machine learning model to predict the prices of houses based on their features. A null hypothesis could be: "There is no significant relationship between the price of a house and its square footage."
Alternative Hypothesis
An alternative hypothesis is a hypothesis that proposes that there is a significant difference or relationship between variables. It is a hypothesis of an effect or a difference. For example, let's say we are building a machine learning model to predict the prices of houses based on their features. An alternative hypothesis could be: "There is a significant positive relationship between the price of a house and its square footage."
Evaluating Hypotheses in Machine Learning
Evaluating hypotheses in machine learning involves testing the null hypothesis against the alternative hypothesis. This is typically done using statistical methods, such as t-tests, ANOVA, and regression analysis.
Here are the general steps involved in evaluating hypotheses in machine learning:
- Formulate the null and alternative hypotheses : Clearly define the null and alternative hypotheses that you want to test.
- Collect and prepare the data : Collect the data that you will use to test the hypotheses. Ensure that the data is clean, relevant, and representative of the population.
- Choose a statistical method : Select a suitable statistical method to test the hypotheses. This could be a t-test, ANOVA, regression analysis, or another method.
- Test the hypotheses : Use the chosen statistical method to test the null hypothesis against the alternative hypothesis.
- Interpret the results : Interpret the results of the hypothesis test. If the null hypothesis is rejected, it suggests that there is a significant relationship between the variables. If the null hypothesis is not rejected, it suggests that there is no significant relationship between the variables.
Common Pitfalls to Avoid in Hypothesis Testing
Here are some common pitfalls to avoid in hypothesis testing:
- Overfitting : Overfitting occurs when a model is too complex and performs well on the training data but poorly on new, unseen data. To avoid overfitting, use techniques such as regularization, early stopping, and cross-validation.
- Underfitting : Underfitting occurs when a model is too simple and fails to capture the underlying patterns in the data. To avoid underfitting, use techniques such as feature engineering, hyperparameter tuning, and model selection.
- Data leakage : Data leakage occurs when the model is trained on data that it will also be tested on. To avoid data leakage, use techniques such as cross-validation and walk-forward optimization.
- P-hacking : P-hacking occurs when a researcher selectively reports the results of multiple hypothesis tests to find a significant result. To avoid p-hacking, use techniques such as preregistration and replication.
Best Practices for Hypothesis Testing in Machine Learning
Here are some best practices for hypothesis testing in machine learning:
- Clearly define the hypotheses : Clearly define the null and alternative hypotheses that you want to test.
- Use a suitable statistical method : Choose a suitable statistical method to test the hypotheses.
- Use cross-validation : Use cross-validation to evaluate the performance of the model on unseen data.
- Avoid overfitting and underfitting : Use techniques such as regularization, early stopping, and feature engineering to avoid overfitting and underfitting.
- Document the results : Document the results of the hypothesis test, including the statistical method used, the results, and any conclusions drawn.
Evaluating hypotheses is a crucial step in machine learning that helps us to understand the problem that we are trying to solve and to evaluate the performance of our machine learning model. By following the best practices outlined in this blog post, you can ensure that your hypothesis testing is rigorous, reliable, and effective.
Remember to clearly define the null and alternative hypotheses, choose a suitable statistical method, and avoid common pitfalls such as overfitting, underfitting, data leakage, and p-hacking. By doing so, you can develop machine learning models that are accurate, reliable, and effective.
- [1] James, G., Witten, D., Hastie, T., & Tibshirani, R. (2013). An Introduction to Statistical Learning: With Applications in R. Springer.
- [2] Bishop, C. M. (2006). Pattern Recognition and Machine Learning. Springer.
- [3] Han, J., Pei, J., & Kamber, M. (2012). Data Mining: Concepts and Techniques. Morgan Kaufmann.
I hope this helps! Let me know if you need any further assistance.

Multiple Hypothesis Testing
- First Online: 01 February 2019
Cite this chapter
- Michael Paluszek 3 &
- Stephanie Thomas 3
5214 Accesses
Tracking is the process of determining the position of other objects as their position changes with time. Air traffic control radar systems are used to track aircraft. Aircraft in flight must track all nearby objects to avoid collisions and to determine if they are threats. Automobiles with radar cruise control use their radar to track cars in front of them so that the car can maintain safe spacing and avoid a collision.
This is a preview of subscription content, log in via an institution to check access.
Access this chapter
- Available as PDF
- Read on any device
- Instant download
- Own it forever
- Available as EPUB and PDF
Tax calculation will be finalised at checkout
Purchases are for personal use only
Institutional subscriptions
S.S. Blackman and R.F. Popoli. Design and Analysis of Modern Tracking Systems. Artech House, 1999.
Google Scholar
D. B. Reid. An Algorithm for Tracking Multiple Targets. IEEE Transactions on Automatic Control, AC=24(6):843–854, December 1979.
L. D. Stone, C. A. Barlow, and T. L. Corwin. Bayesian Multiple Target Tracking. Artech House, 1999.
Download references
Author information
Authors and affiliations.
Plainsboro, NJ, USA
Michael Paluszek & Stephanie Thomas
You can also search for this author in PubMed Google Scholar
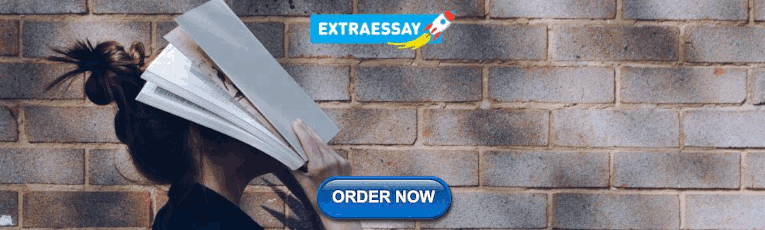
Rights and permissions
Reprints and permissions
Copyright information
© 2019 Michael Paluszek and Stephanie Thomas
About this chapter
Paluszek, M., Thomas, S. (2019). Multiple Hypothesis Testing. In: MATLAB Machine Learning Recipes. Apress, Berkeley, CA. https://doi.org/10.1007/978-1-4842-3916-2_12
Download citation
DOI : https://doi.org/10.1007/978-1-4842-3916-2_12
Published : 01 February 2019
Publisher Name : Apress, Berkeley, CA
Print ISBN : 978-1-4842-3915-5
Online ISBN : 978-1-4842-3916-2
eBook Packages : Professional and Applied Computing Apress Access Books Professional and Applied Computing (R0)
Share this chapter
Anyone you share the following link with will be able to read this content:
Sorry, a shareable link is not currently available for this article.
Provided by the Springer Nature SharedIt content-sharing initiative
- Publish with us
Policies and ethics
- Find a journal
- Track your research

An official website of the United States government
The .gov means it’s official. Federal government websites often end in .gov or .mil. Before sharing sensitive information, make sure you’re on a federal government site.
The site is secure. The https:// ensures that you are connecting to the official website and that any information you provide is encrypted and transmitted securely.
- Publications
- Account settings
Preview improvements coming to the PMC website in October 2024. Learn More or Try it out now .
- Advanced Search
- Journal List
- Scientific Reports

Combining Multiple Hypothesis Testing with Machine Learning Increases the Statistical Power of Genome-wide Association Studies
Bettina mieth.
1 Machine Learning Group, Technische Universität Berlin, Berlin, 10587, Germany
Marius Kloft
2 Department of Computer Science, Humboldt University of Berlin, Berlin, 10099, Germany
Juan Antonio Rodríguez
3 Institut de Biología Evolutiva (CSIC-UPF). Departament de Ciències Experimentals i de la Salut. Universitat Pompeu Fabra, Barcelona, 08003, Spain
Sören Sonnenburg
4 TomTom Research, Berlin, 12555, Germany
Robin Vobruba
Carlos morcillo-suárez, xavier farré, urko m. marigorta.
5 School of Biology, Georgia Institute of Technology, Atlanta, 30332, GA, USA
6 Department of Economics, Laboratory for Social and Neural Systems Research, University of Zurich, Zurich, 8006, Switzerland
Thorsten Dickhaus
7 Institute for Statistics (FB 3), University of Bremen, Bremen, 28359, Germany
Gilles Blanchard
8 Department of Mathematics, University of Potsdam, Potsdam, 14476, Germany
Daniel Schunk
9 Department of Economics, University of Mainz, Mainz, 55099, Germany
Arcadi Navarro
10 Institució Catalana de Recerca i Estudis Avançats (ICREA), Barcelona, 08010, Spain
11 Center for Genomic Regulation (CRG), Barcelona Institute of Science and Technology (BIST), Barcelona, 08003, Spain
Klaus-Robert Müller
12 Department of Brain and Cognitive Engineering, Korea University, Seoul, Republic of Korea
Associated Data
The standard approach to the analysis of genome-wide association studies (GWAS) is based on testing each position in the genome individually for statistical significance of its association with the phenotype under investigation. To improve the analysis of GWAS, we propose a combination of machine learning and statistical testing that takes correlation structures within the set of SNPs under investigation in a mathematically well-controlled manner into account. The novel two-step algorithm, COMBI, first trains a support vector machine to determine a subset of candidate SNPs and then performs hypothesis tests for these SNPs together with an adequate threshold correction. Applying COMBI to data from a WTCCC study (2007) and measuring performance as replication by independent GWAS published within the 2008–2015 period, we show that our method outperforms ordinary raw p -value thresholding as well as other state-of-the-art methods. COMBI presents higher power and precision than the examined alternatives while yielding fewer false ( i.e . non-replicated) and more true ( i.e . replicated) discoveries when its results are validated on later GWAS studies. More than 80% of the discoveries made by COMBI upon WTCCC data have been validated by independent studies. Implementations of the COMBI method are available as a part of the GWASpi toolbox 2.0.
The goal of genome-wide association studies (GWAS) (e.g. the WTCCC study 1 ) is to examine the relationship between genetic markers such as single-nucleotide polymorphisms (SNPs) and individual traits, which are usually complex diseases or behavioral characteristics. Generally, a large number of statistical tests are performed in parallel, each SNP being individually tested for association 2 , 3 , 4 . The standard approach consists of computing individual, SNP-specific p -values corresponding to a statistical association test and comparing these p -values against some given significance threshold (say t * ), meaning that precisely those SNPs with p -values smaller than t * are declared to be associated with the trait 4 , 5 , 6 . We refer to this approach as raw p -value thresholding (RPVT) and review some standard methods for choosing t * for the purpose of controlling multiple type I error rates (in particular, the family-wise error rate ( FWER ) and the expected number of false rejections ( ENFR )) in the Methods Section.
According to the GWAS catalog 7 , 8 (last accessed 03-07-2015), the more than 1,400 GWAS published so far have led to the identification of more than 11,000 SNPs associated with about 800 human diseases and anthropometric traits with p -values using t * = 1 × 10 −5 .
However, variants reported by GWAS tend to explain only small fractions of individual traits, and most of the heritability accounting for many complex diseases remains unexplained — a phenomenon usually referred to as the “mystery of missing heritability” 4 , 9 . There are several possible (not mutually exclusive) explanations for that phenomenon 10 , 11 , 12 , 13 . One frequently discussed possibility is that epistatic interactions between loci are ignored both in current heritability estimates and in usual testing procedures 12 , 14 . In addition to this issue, another shortcoming of current approaches based on testing each SNP independently is that they disregard any correlation structures among the set of SNPs under investigation that are introduced by both population genetics (linkage disequilibrium, LD) and biological relations ( e.g . functional relationships between genes). The latter issue by itself is likely to introduce confounding factors and artifacts, implying a loss in statistical power 15 and a lack of reliable insights about genotype-phenotype associations.
In this work, we propose a novel methodology — COMBI — that is a principled, reliable, and replicable method for identifying significant SNP-phenotype associations. The core idea is a two-step algorithm consisting of
- a machine learning and SNP selection step that drastically reduces the number of candidate SNPs by selecting only a small subset of the most predictive SNPs; and
- a statistical testing step where only the SNPs selected in step 1 are tested for association.
The main idea underlying COMBI is the use of the state-of-the-art machine learning technique support vector machine (SVM) 16 , 17 , 18 in the first step. Crucially, this method is tailored to predict the target output (here, the phenotype) from high-dimensional data with a possibly complex, unknown correlation structure. In our application, the SVM is trained using the complete SNP data of one chromosome. Thus, the first step acts as a filter, indicating SNPs that are relevant for phenotype classification with either high individual effects or effects in combination with the rest of SNPs, while discarding artifacts due to the correlation structure. The second step uses multiple statistical hypotheses testing for a quantitative assessment of individual relevance of the filtered SNPs. All in all, the two steps extract complementary types of information, which are combined in the final output. Importantly, the calibration of the method is such that a global statistical error criterion is controlled for the entire procedure consisting of steps 1 and 2.
The following section first introduces the methodology in a summary paragraph and in Fig. 1 ; then, the Methods Section continues to explain the method in more detail with some references to Supplementary Section 1 . An overview of related machine learning work is given in the Discussion Section. The performance of the COMBI method is reported in the Results Section, Supplementary Sections 2 and 3 ; where we also include and discuss the highly favorable comparisons with the algorithms that could potentially compete with the COMBI method. Note that COMBI yields better prediction with fewer false ( i.e . non-replicated) and more true ( i.e . replicated) discoveries when its results are validated on later, larger GWAS studies.

Receiving genotypes and corresponding phenotypes of a GWAS as input, the COMBI method first applies a machine learning step to select a set of candidate SNPs and then calculates p -values and corresponding significance thresholds in a statistical testing step.
Implementations of the COMBI method are available in R, MATLAB, and JAVA, as a part of the GWASpi toolbox 2.0 ( https://bitbucket.org/gwas_combi/gwaspi/ ).
Summary of The COMBI Method
Figure 1 shows a graphical representation of the COMBI method.
Input: a sample of observed genotypes { x i * } and corresponding phenotypes { y i }. We represent the j -th SNP of the i -th subject with a binary genotypic encoding, where x ij = (1, 0, 0), x ij = (0, 1, 0), or x ij = (0, 0, 1), depending on the number of minor alleles. We assume a binary phenotype, i.e., y i ∈ {+1, −1}.

- Statistical testing step (colored in blue). A hypothesis test (carried out as a χ 2 test) is performed for each of the selected SNPs. Those SNPs with p -value less than a significance threshold t* are returned. The threshold t* is calibrated using a permutation-based method over the whole procedure consisting of the machine learning selection and statistical testing steps. See Algorithm 2 for details.
Problem Setting and Methodology
In this section, we formally describe the statistical problem under investigation and propose a novel methodology for tackling it — based on a combination of machine learning and statistical testing techniques.
Problem Setting and Notation

Single SNP data are summarized in categories according to phenotypes (cases, Y = +1, and controls, Y = −1) and genotypes (A 1 A 1 , A 1 A 2 and A 2 A 2 ). The numbers n ik denote the numbers of individuals within the corresponding groups. n is the total number of subjects in the study.

Proposed workflow
The Bonferroni correction can only attain the prescribed FWER upper bound, and therefore have maximal power, if the p -values ( p j :1 ≤ j ≤ d ) do not exhibit strong (positive) dependencies, an assumption which is violated in GWAS due to strong LD in blocks of SNPs. An alternative way to calibrate the threshold t * for FWER control, taking the dependencies into account, is the Westfall-Young permutation procedure 23 , which controls the FWER under an assumption termed subset pivotality (see Westfall and Young 23 as well as Dickhaus and Stange 21 ). Furthermore, Meinshausen et al . 24 proved that this permutation procedure is asymptotically optimal in the class of RPVT procedures, provided that the subset pivotality condition is fulfilled. However, for RPVT the individual p -value for association of the j -th SNP only depends on x * j and thus ignores the possible correlations with the rest of the genotype – which could yield additional information. By contrast, machine learning approaches aimed at prediction try to take the information of the whole genotype into account at once, and thus implicitly consider all possible correlations, to strive for an optimal prediction of the phenotype. Based on this observation, we propose Algorithm 1 combining the advantages of the two techniques, consisting of the following two steps:
- the machine learning step, where an appropriate subset of candidate SNPs is selected, based on their relevance for prediction of the phenotype;
- the statistical testing step, where a hypothesis test is performed together with a Westfall-Young type threshold calibration for each SNP.
Additionally, a filter first processes the weight vector w output in the machine learning step before using it for the selection of candidate SNPs. The above steps are discussed in more detail in the following sections.
The machine learning and SNP selection step
The goal in machine learning is to determine, based on the sample, a function f ( x ) that predicts the unknown phenotype y based on the observation of genotype x . It is crucial to require such a function to not only capture the sample at hand, but to also generalize , as well as possible, to new and unseen measurements, i.e., the sign of f ( x ) is a good predictor for y for previously unseen patterns x and labels y . We consider linear models of the form f w , b ( x i * ) = w T x i * + b in this paper. A popular approach to learning such a model is given by the SVM 16 , 17 , 18 , which determines the parameter w of the model by solving, for C > 0 , the following optimization problem:

The problem above is similar to regression problems and can be interpreted as follows: we aim to minimize the trade-off (controlled by C ) between a vector w with small norm (the term on the left-hand side) and small errors on the data (the term on the right-hand side). Once a classification function f has been determined by solving the above optimization problem, it can be used to predict the phenotype of any genotype by putting

The above equation shows that the largest components (in absolute value) of the vector w (called SVM parameter or weight vector) also have the most influence on the predicted phenotype. Note that the weights vector contains three values for each position due to the feature embedding, which encodes each SNP with three binary variables. To convert the vector back to the original length, we simply take the average over the three weights. We also include an offset by including a constant feature that is all one.
Considering that the use of SVM weights as importance measures is a standard approach 25 , for each j the score abs ( w j ) can be interpreted as a measure for the importance of the j -th SNP for the phenotype prediction task. The main idea is to select only a small number k of candidate SNPs before statistical testing, namely those SNPs having the largest scores. Based on preliminary experiments, we noticed that the introduction of the following additional post-processing of the SVM parameter vector was beneficial before SNP selection: a p th-order moving average filter is applied as follows:

where l ∈ 1, …, d denotes a fixed filter length (required to be an odd number). The value p ∈ ]0, ∞[is a free parameter; in the case p = 1, a standard moving average filter is obtained.
The statistical testing step
In the statistical testing step (see Summary of the COMBI method and Fig. 1 ), we apply p -value thresholding only to the k p -values which correspond to the SNPs with largest filtered SVM weights. Calculation of these p -values is performed exactly as described above for RPVT, with the only modification that p -values for SNPs not ranked among the top k in terms of their filtered SVM weights are set to 1, without calculating a test statistic.

Our suggestion is to re-sample the entire workflow of Fig. 1 , thus following a Westfall and Young 23 type procedure, and to choose t* based on the permutation distribution of the re-sampled p -values.
In summary, the proposed methodology is formally stated as Algorithm 1.

Validation using simulated phenotypes
To assess the performance of the proposed COMBI method in comparison to other methods in a controlled environment, we conducted a number of simulation experiments with semi-real data. A block of 10,000 genotypes were taken from real WTCCC data 1 without breaking linkage, but the phenotypes were synthetically generated according to a known model. This ensures that the “basic truth” is known (allowing us to compute the number of true and false positives for each method in the comparison). We show that COMBI outperforms the most commonly used methods for GWAS on these data sets. For instance, it achieves higher true positive rates for all family-wise error levels than any other method that we have investigated 26 , 29 , 30 , including RPVT. In comparison to RPVT, the gain in true positive rate is up to 80%. For a detailed description and analysis of the semi-real data simulations, see Supplementary Section 2 .
Validation using WTCCC data
We then compared the performance of the COMBI method to that of other methods when applied to data from the 2007 WTCCC phase 1, consisting of 14,000 cases of seven common diseases and 3,000 shared controls (see Supplementary Section 3 for further information). In contrast to the simulations described above, the true underlying architecture of the traits under study is largely unknown. Hence, we used replicability in independent studies, one of the standards in the field, as a measure of performance. In summary, we proceeded as follows: the application of some method (for instance, COMBI or RPVT) to the 2007 WTCCC data results in a list of SNPs that are potentially associated with the trait (this is illustrated on the left-hand side of Fig. 2 ).

After producing a list of associated SNPs via an appropriate inference method (i.e. COMBI or RPVT), the GWAS catalog is used in an independent validation step to confirm or refute those candidate SNPs accessing the predictability of the used inference method.
We then evaluated this list of potentially associated SNPs for replicability on independent data to obtain the “List of confirmed associated SNPs” (illustrated on the right-hand side of Fig. 2 ). All studies for the WTCCC diseases included in the GWAS catalog by June 26, 2015 constituted the set of studies examined for replicability. Most of these studies were performed either with larger sample sizes or using meta-analysis techniques and were published after the original WTCCC paper. In a sense, we thus examined how well any particular method, when applied to the WTCCC dataset, is able to make discoveries in that dataset that were actually confirmed by later research using RPVT in independent publications.
Our validation procedure considers a physical window of 200kb around a certain SNP and selects all SNPs with strong LD (R 2 > 0.8) with the original SNP within that window. It queries the GWAS catalog for those SNPs to find out whether the selected SNPs have any entries. A hit indicates that a GWAS other than the original WTCCC study has since reported this SNP to be associated with the disease. Note that the GWAS catalog only contains SNPs with p -values < 10 −5 , meaning that we will miss some hits that are statistically weak but that might be biologically relevant, in the sense that they contribute to the classification of individuals according to phenotypes. For a detailed description of the automatic validation procedure, see Supplementary Section 3.2 . With this procedure, methods can be compared by counting the respective number of replicated and non-replicated reported associations.
Regarding significance levels, we aimed to stay as close in line with the original WTCCC study as possible, reporting not only the strong associations at the significance level of 5 × 10 −7 but also weak associations at 1 × 10 −5 . Within our validation pipeline we considered the full NHGRI GWAS Catalog 7 with the inclusion criterion of having achieved a p -value of 1×10 −5 in a GWAS. The “ somewhat liberal statistical threshold of p < 1 × 10 −5 was chosen to allow examination of borderline associations and to accommodate scans of various sizes while maintaining a consistent approach ” 7 .
We also ensured that the same statistical criterion (control of the FWER or the ENFR , respectively) was used for all methods, in order to have a fair comparison. This procedure is explained in detail in the Methods Section and Supplementary Section 1.1.1 .
Stability analysis
In addition, we established an “internal” validation by analyzing the stability of the reported associations (cf. Supplementary Section 3.4 for details); this stability measure indicates how well results can be reproduced on another independent sample.
Parameter selection
The analysis of WTCCC data required the selection of all free parameters of the COMBI method (e.g. the SVM optimization parameter C , the window size l of the moving average filter or the filter norm parameter p ). To this end, the semi-real datasets investigated in Supplementary Section 2 have been used to determine performance changes induced by varying those free parameters. Since our findings were in agreement with related literature and mostly biologically sensible, the optimal settings were assumed to be good choices for the application of the COMBI method to real data. For example, it was found that aggregating SNPs within the filtering step (See Summary of the COMBI method and Fig. 1 , the filtering step) based on a filter size of 35 is optimal, which is on the same magnitude as in Alexander and Lange 31 who find that grouping of SNPs into bins of size 40 helps the performance of their algorithm. The moving average filter of the COMBI method is designed to correct for non-independence of statistical tests within LD blocks. Given the SNP density in the arrays used by the original WTCCC study and LD patterns in the CEU population (1000 Genomes), we estimate that the average LD block (r 2 > 0.8) will harbor no more than 20–30 SNPs 32 , which supports our findings of setting the filter window size to 35 in the sense that we average-out blocks and conservatively add a bit of noise by potentially smoothing out signals across blocks.
See Supplementary Section 1.1 for a detailed description of the selection of all free parameters of the COMBI method.
Some parameters of the COMBI method could not be investigated within the simulation study, but had to be chosen manually for the WTCCC data. The decision to train the SVM separately on each chromosome was one of those tuning steps, as genome-wide training is very time and memory consuming on the one hand, and can only improve performance marginally on the other hand, as intergenic correlations between chromosomes are very rare.
Another parameter that was chosen manually was the number of active SNPs in one chromosome, i.e. the parameter k of The Screening Step presented in the Methods Section, which was set to 100 SNPs per chromosome after careful consideration. This choice is admittedly a wide, arbitrary upper bound for the number of SNPs that can present a detectable association with a given phenotype. Currently, the maximum total number of SNPs (not independent signals) associated with any phenotype is ~450 for human height and 180 for Crohn’s Disease (GWAS Catalog, accessed June 2015), so with k = 100 per chromosome one is well within what current evidence would support. After all, for future applications of COMBI k is a tuning parameter which has to be chosen by the researcher according to the assumed number of relevant loci.
The choice of exact values for all parameters will probably need to be adapted for each particular phenotype or disease under study, since they will have different genetic architectures and distribution of effect sizes 4 , 9 . For this manuscript and in order to provide a comprehensive and comparable set of results across many diseases we employed a unique set of parameter values supported by the results of our simulation study and other findings in related literature.
Manhattan plots and descriptive results
Figure 3 displays Manhattan plots for all seven diseases resulting from the standard RPVT approach (left) and the COMBI method (center) as well as the SVM weights (right). The center and right graph illustrates that the COMBI method discards SNPs with a low SVM score (cf. “The screening step” in Summary of the COMBI method and Fig. 1 ). Hence, the p -values for such SNPs are set to one without performing a statistical test, thereby drastically reducing the number of candidate associations. In contrast, the RPVT method results in p -values based on a formal significance test for every SNP, where many of these p -values are small and produce a lot of statistical noise. SNPs that show genome-wide statistical significance are highlighted in green in the left and right panel. For the standard RPVT, the threshold indicated by the horizontal dashed line is fixed a priori genome-wide. For the COMBI method, however, it was determined chromosome-wise via the permutation-based threshold over the whole COMBI procedure described in the Methods Section and Supplementary Section 1.1.1 to match the expected number of false rejections of RPVT.

Manhattan plots for all seven diseases resulting from the standard RPVT approach and the COMBI method as well as the SVM weights. We plot −log 10 of the χ 2 trend test p -values for both COMBI and RPVT and the corresponding SVM weights against position on each chromosome. Chromosomes are shown in alternating colours for clarity, with significant p -values highlighted in green. Please note that for the RPVT, the threshold indicated by the horizontal dashed line is fixed a priori genome-wide. For the COMBI method, it was determined chromosome-wise via the permutation-based threshold over the whole COMBI procedure. All panels are truncated at −log 10 ( p -value) = 15, although some markers exceed this significance threshold.
In Table 2 , we present all significant associations reported by the COMBI method. Associations with a raw p -value > 10 −5 were not reported in studies using only RPVT. If they are selected by the COMBI method, we consider them to be new findings and highlight them in grey. The last column of Table 2 indicates whether the reported associations were validated (i.e., were reported as significant in at least one independent study published after the WTCCC). The COMBI method finds 46 significant locations. 34 of these 46 significant locations have a p -value below 10 −5 and were thus also found by the RPVT approach.
For all seven diseases we present SNPs reaching genome-wide significance along with their rs-identifier, corresponding chromosome, χ 2 trend test p -value, SVM weight and the result of the validation pipeline indicating whether the SNP has been found significant with a p -value < 10 −5 in at least one external GWAS or meta-analysis. PMID references of those studies are given in the last column. SNPs that do not show genome-wide significance in the case of RPVT are highlighted in bold case.
Crucially, our COMBI method found 12 additional SNPs. Out of these, ten (>83%) have already been replicated in later GWAS or meta analyses. The COMBI discoveries that have been replicated independently using individual SNP testing are for bipolar disorder rs2989476 (Chr. 1), rs1344484 (Chr. 16), rs4627791 (Chr. 3), and rs1375144 (Chr. 2); for coronary artery disease rs6907487 (Chr. 6) and rs383830 (Chr. 5); for Crohn’s disease rs12037606 (Chr. 1), rs10228407 (Chr. 7), and rs4263839 (Chr. 9) and for type 2 diabetes rs6718526 (Chr. 2). Given the current debate on the replicability of GWAS findings obtained by single-SNP analyses 33 , it is remarkable that GWAS studies published later had already replicated more than 83% of novel SNPs the COMBI method detected by reanalyzing data published in 2007.
Two out of the 12 SNPs with p -values exceeding 10 −5 had not yet been reported in any GWAS or meta analyses as being associated with the corresponding diseases. Those are rs11110912 (Chr. 12) for hypertension and rs6950410 (Chr. 7) for type 1 diabetes. SNP rs11110912 was included in the original WTCCC analysis, but a p -value higher than 10 −5 was obtained (1.94 × 10 −5 ) 1 , so it was not collected in the GWAS Catalog. SNP rs6950410 has been detected as associated to multiple complex diseases 34 . Regarding the biological plausibility of these two SNPs, we examined a number of functional indicators to assess their potential role in disease. In particular, we explored the genomic regions in which they map and their potential roles as regulatory SNPs, status as eQTLs, and role in Mendelian disease. Overall, there is no strong evidence of functional roles (see Supplementary Section 3.5 ) but SNP rs11110912 (Chr. 12), for which COMBI suggested a link to hypertension, is an intronic SNP mapping on a gene, MYBPC1, that has been previously linked to familial hypertrophic cardiomyopathy, suggesting that COMBI has given rise to another interesting true positive finding.
GWAS catalog validation results – results obtained by the COMBI method are better replicated than those obtained by RPVT
The COMBI method also outperforms the RPVT approach for different type 1 error levels. Figure 4 shows the receiver operator characteristic (ROC) and precision-recall (PR) curves that have been generated based on the replication of SNPs according to the GWAS catalog (here, due to absence of basic truth knowledge, replicated reported associations are counted as true positives, and non-replicated associations as false positives). As the dark blue lines are consistently above the light blue lines, the COMBI method achieves both higher numbers of true positives ( i.e . higher true positive rate (TPR)) as well as a higher precision (proportion of replicated associations amongst the SNPs classified as associated with the trait) for given numbers of false and true positives ( i.e . lower false positive rate (FPR)) than RPVT for almost all levels of error. For comparison, we show also the result achieved when selecting SNP based on the highest SVM weights in absolute value (after filtering). The results show that discarding either one of the two steps in the COMBI method (machine learning or statistical testing step) will lead to a decrease in performance.

The results of all seven diseases have been pooled. The curves have been generated based on the replication of SNPs according to the GWAS catalog. Replicated reported associations are counted as true positives, and non-replicated associations as false positives. Note that the COMBI lines end at some point and the RPVT and the raw SVM lines continue. At the endpoint of the COMBI curve all SNPs selected in the SVM step are also significant in the statistical testing step; i.e . if one wanted to add just one more SNP to the list of reported associations, all other SNPs would also become significant, as they have a p -value of 1. The points on the RPVT and COMBI lines represent the final results of the two methods when applying the corresponding significance thresholds and are described in more detail in Table 3 .
We now investigate the points on the curves that correspond to the application of t* = 10 −5 in the case of RPVT and to the value of t * resulting from the permutation-based method in the case of the COMBI method (described in the Methods Section) in more detail. See Table 3 for the numbers corresponding to those points. A total of 78 SNPs were found to be significant with RPVT, since it only performs the statistical testing step, and 46 with the COMBI method, which has the additional layer of the machine learning screening step prior to the statistical testing.
The table represents the information given by the points on the RPVT and COMBI lines in Fig. 4 . The final results of the two methods when applying the corresponding significance thresholds are shown. At significance threshold t* = 10 −5 , COMBI achieves 28 SNPs recall at precision 61%, while RPVT achieves a recall of only 24 SNPs at precision 32%.
Although the COMBI method finds fewer SNPs, the number of replicated SNPs is greater (28 in contrast to 24 of RPVT). The COMBI method also classifies only 18 of the unreplicated SNPs as associated with the trait (yielding a precision of 61%). This is in contrast to RPVT, which classifies 52 of the unreplicated SNPs as associated with the trait (yielding a precision of only 32%). In other words, if both methods are calibrated with respect to the same type I error criterion, the COMBI method reports significantly more replicated associations (Fisher’s exact test p -value of 0.0014).
Stability results – COMBI method is more stable than RPVT
From simulations considering internal stability, we found that the COMBI method produces more stable results than RPVT; cf. Supplementary Section 3.4 for details.
Runtime analysis and implementation details
The COMBI method is implemented in Matlab/Octave, R and Java as a part of the GWASpi toolbox 2.0 ( https://bitbucket.org/gwas_combi/gwaspi/ ). The complete method is available in all these programming languages. The implementation for Matlab/Octave is cluster oriented and uses libLinear 35 . The Java implementation is desktop computer oriented and makes use of the following packages: libLinear 35 , libSVM 36 and apache commons math 37 . Finally, the R implementation requires LiblineaR 38 , qqman 39 , data.table 40 , gtools 41 and snpStats 42 .
The runtime of the method depends on a variety of factors such as available cluster memory, hardware resources and operating system. For this analysis we have run the method with the Matlab/Octave implementation on the following technical platform: 40 * Intel(R) Xeon(R) CPU E5-2650 v3 @ 2.30GHz 64bit, 128GB RAM, Ubuntu 14.04.4 LTS (GNU/Linux 3.13.0–79-generic x86_64), GNU Octave version 3.8.1. The analysis of WTCCC’s data on Crohn’s disease chromosome 18 (assuming calculations on more chromosomes can be computed in parallel if necessary) took 9h 15min and 24s. See Supplementary Section 3.7 for a more detailed runtime analysis.
Several related machine learning methods have been successfully used in the context of statistical genomics. These approaches can be classified into two groups:
- Methods that construct a model from genetic data in order to carry out accurate predictions on a phenotype 43 , 44 , 45 , 46 , 47 , 48 , 49 , 50 , 51 , 52 , 53 , 54 , 55 , 56 .
- Methods that use machine learning to construct a statistical association test or rank genetic markers according to their predicted association with a phenotype 30 , 31 , 57 , 58 , 59 , 60 , 61 , 62 , 63 , 64 , 65 , 66 , 67 .
The set of papers that fall into the first category study the predictive performance of penalized regression and classification models including support vector machines 16 , 17 , 18 , random forests 68 , and sparsity-inducing methods such as the elastic net 69 on various complex diseases (including the ones studied here), showing that machine learning methods such as SVMs – if appropriately applied - can perform well at predicting disease risks. See Supplementary Section 3.3 , where we compare the prediction performance of various methods on the WTCCC data.
However, the main point of interest of the present contribution does not lie in risk prediction but rather in the identification of regions associated with diseases. The COMBI method should thus be compared to true alternative methods that stem from the second category, some of which include two-stage approaches first performing statistical testing and then machine learning to refine the set of predicted associations 30 , 57 . These approaches, however, are unable to identify correlation structures of SNPs that have been excluded in the first step and neither method is validated on real data in terms of a comparison to the GWAS database. Similarly, Pahikkala et al . 59 and He and Lin 60 develop methods for ranking genetic markers based on the sure independence screening strategy 70 and stability selection analyzing only one SNP at a time. Recently the approach has been extended to detect gene-to-gene interactions by Li et al . 71 , but neither of the methods have been validated on independent external studies.
Another approach is by Alexander and Lange 31 , who apply the stability selection method of Meinshausen and Bühlmann 58 to the WTCCC data set to rank SNPs according to their predicted association with a phenotype. The authors find that stability selection effectively controls the FWER when applied to GWAS data but suffers a loss of power, while at the same time rendering conservative results.
The work that is probably most closely related to the present research is the two-step algorithm by Wasserman and Roeder 27 (and the extension by Meinshausen et al . 26 ), who split the data into two equal parts performing marker selection on the first part and then testing the selected markers on the second part. See Supplementary Section 2.2.4 for a detailed description of this approach.
In order to investigate and compare performance of the COMBI method to other machine learning approaches, the work of Roshan et al . 30 , Wasserman and Roeder 27 and Meinshausen et al . 26 are selected as representative baseline methods. In Supplementary Section 2.2.4 , we show that the COMBI approach outperforms all of these methods on semi-real data.
An important and very closely related recent method by Lippert et al . 14 , 72 aims to identify putative significant disease-marker associations using two approaches based on linear mixed models (LMMs): a univariate test and a test for pairwise epistatic interactions. LMMs, like COMBI, address the issue of population stratification in GWAS, cf. Mimno et al . 73 . However, in contrast to COMBI, they still test SNPs (or pairs of SNPs) individually one after the other and thus potentially lose detection power. Another possible shortcoming of LMMs and related methods over SVMs is that they are more tailored for regression and not binary classification. For a comparison of COMBI with Lippert et al . 14 , 72 on real WTCCC data see Supplementary Section 3.6 . Recently their approach has been extended for disease risk prediction (Rakitsch et al . 56 ) and related approaches have been proposed by Loh et al . 74 and Song et al . 75 suffering the same drawbacks as discussed above.
An extension of LMMs to multivariate cases was developed by Zhou and Stephens 76 , but has not yet been applied to WTCCC. Fitting LMMs to multiple phenotypes provides no novel insight into analyzing multiple genotypes/SNPs at once, which is the issue COMBI addresses.
Our approach can be extended to explore a number of different research directions by substituting one of the two steps of the algorithm with other suitable procedures. Thus, one could either apply other machine learning prediction methods (as mentioned above) instead of training an SVM in the first step of the COMBI method. For example, the SVM training could be replaced by a SNP selection by random forests or component-wise boosting. Alternatively, one could perform a different statistical test in the final step of the COMBI method, such as procedures correcting for population structures or other confounding factors 72 , 77 . These alternatives are possible options for future research (and some have been implemented in the literature), however, COMBI performs better than any of the other machine learning methods we compared it to ( Supplementary Section 2.2.4 ).
COMBI also seems to perform better than other state-of-the-art methods for univariate analyses. For instance, a recent method by Lippert et al . 14 aims to identify putative significant disease-marker associations from the WTCCC data using two approaches based on linear mixed models: an univariate test and a test for pairwise epistatic interactions. When their univariate method results are checked against the same validation criteria that we used for COMBI, it turns out that our method reports 17 more true positives (4.4 times more positives) for the three diseases for which their univariate method reports at least one hit ( Supplementary Section 3.6 ).
The COMBI method also holds great potential for testing pairwise SNP-trait associations, as it drastically reduces the number of candidate associations by selecting a subset of the most predictive SNPs in the machine learning step. Again, a comparison against the method Lippert et al . 14 propose for detecting epistatic interactions, is favorable to COMBI (see Supplementary Section 3.6 ). In future work we will extend the COMBI method to a regression setup where the phenotype is not binary.
To summarize, we proposed a novel and powerful method for analyzing GWAS data that is based on applying a carefully designed machine learning step that is tailored to the GWAS data before applying a classical multiple testing step. Certain machine learning models, in particular appropriately designed linear SVMs, take high-dimensional correlation structures into account and thus implicitly incorporate interactions between different loci. A subset of predictive candidate SNPs is extracted within the machine learning step. The p -values corresponding to association tests are then thresholded for these candidate SNPs in a subsequent statistical testing step. The COMBI method was shown to outperform the RPVT approach both on controlled, semi-real data and on data from the WTCCC 2007 study, for which reported associations were validated by their replicability in external later studies. The empirical analysis showed a significant increase in detection power for replicated SNPs, while yielding fewer unconfirmed discoveries. Two new (as yet unreplicated) candidate associations were reported.
Additional Information
How to cite this article : Mieth, B. et al . Combining Multiple Hypothesis Testing with Machine Learning Increases the Statistical Power of Genome-wide Association Studies. Sci. Rep . 6 , 36671; doi: 10.1038/srep36671 (2016).
Publisher's note: Springer Nature remains neutral with regard to jurisdictional claims in published maps and institutional affiliations.
Supplementary Material
Acknowledgments.
This paper is part of a larger project on the genetics of social and economic behavior. The idea for this paper arose in the workshop that regularly takes place in the context of this project at the University of Zurich, and which is based on the collaboration of teams at universities in Berlin, Barcelona, Mainz, and Zurich. EF acknowledges support from the advanced ERC grant (ERC-2011-AdG 295642-FEP) on the Foundation of Economic Preferences. MK, BM, and KRM were supported by the German National Science Foundation (DFG) under the grants MU 987/6-1 and RA 1894/1-1. TD and DS were supported by the German National Science Foundation (DFG) under the grants DI 1723/3-1 und SCHU 2828/2-1. GB and TS acknowledge support of the German National Science Foundation (DFG) under the research group grant FOR 1735. MK, DT, KRM, and GB acknowledge financial support by the FP7-ICT Programme of the European Community, under the PASCAL2 Network of Excellence. MK acknowledges a postdoctoral fellowship by the German Research Foundation (DFG), award KL 2698/2-1, and from the Federal Ministry of Science and Education (BMBF) awards 031L0023A and 031B0187B. AN acknowledges support from the Spanish Multiple Sclerosis Network (REEM), of the Instituto de Salud Carlos III (RD12/0032/0011), the Spanish National Institute for Bioinformatics (PT13/0001/0026) the Spanish Government Grant BFU2012-38236 and from FEDER. This project has received funding from the European Union’s Horizon 2020 research and innovation programme under grant agreement No. 634143 (MedBioinformatics). MK and KRM were financially supported by the Ministry of Education, Science, and Technology, through the National Research Foundation of Korea under Grant R31-10008 (MK, KRM) and BK21 (KRM).
The authors declare no competing financial interests.
Author Contributions E.F., T.D., G.B., D.S., A.N. and K.-R.M. designed and directed research; B.M., M.K., J.A.R., S.S., R.V., C M.-S., X.F., U.M.M. and D.S. performed research and analyzed data; and B.M., M.K., J.A.R., C M.-S., E.F., T.D., G.B., D.S., A.N. and K.-R.M. wrote the paper.
- The Wellcome Trust Case Control Consortium. Genome-wide association study of 14,000 cases of seven common diseases and 3,000 shared controls . Nature 447 , 661–678 (2007). [ PMC free article ] [ PubMed ] [ Google Scholar ]
- Wray N. R. et al. Pitfalls of predicting complex traits from SNPs . Nat. Rev. Genet. 14 , 507–515 (2013). [ PMC free article ] [ PubMed ] [ Google Scholar ]
- Edwards S. L., Beesley J., French J. D. & Dunning A. M. Beyond GWASs: illuminating the dark road from association to function . Am. J. Hum. Genet. 93 , 779–797 (2013). [ PMC free article ] [ PubMed ] [ Google Scholar ]
- Visscher P. M., Brown M. A., McCarthy M. I. & Yang J. Five years of GWAS discovery . Am. J. Hum. Genet. 90 , 7–24 (2012). [ PMC free article ] [ PubMed ] [ Google Scholar ]
- Ripke S. et al. Genome-wide association analysis identifies 13 new risk loci for schizophrenia . Nat. Genet. 45 , 1150–1159 (2013). [ PMC free article ] [ PubMed ] [ Google Scholar ]
- Beecham A. H. et al. Analysis of immune-related loci identifies 48 new susceptibility variants for multiple sclerosis . Nat. Genet. 45 , 1353–1360 (2013). [ PMC free article ] [ PubMed ] [ Google Scholar ]
- Hindorff L. A. et al. Potential etiologic and functional implications of genome-wide association loci for human diseases and traits . Proc. Natl. Acad. Sci. 106 , 9362–9367 Catalog of Published Genome-Wide Association Studies at www.genome.gov/gwastudies (2009). [ PMC free article ] [ PubMed ] [ Google Scholar ]
- Welter D. et al. The NHGRI GWAS Catalog, a curated resource of SNP-trait associations . Nucleic Acids Res. 42 , D1001–D1006 (2014). [ PMC free article ] [ PubMed ] [ Google Scholar ]
- Manolio T. a. et al. Finding the missing heritability of complex diseases . Nature 461 , 747–753 (2009). [ PMC free article ] [ PubMed ] [ Google Scholar ]
- Lee S. H., Wray N. R., Goddard M. E. & Visscher P. M. Estimating missing heritability for disease from genome-wide association studies . Am. J. Hum. Genet. 88 , 294–305 (2011). [ PMC free article ] [ PubMed ] [ Google Scholar ]
- Gibson G. Rare and common variants: twenty arguments . Nat. Rev. Genet. 13 , 135–145 (2012). [ PMC free article ] [ PubMed ] [ Google Scholar ]
- Zuk O., Hechter E., Sunyaev S. R. & Lander E. S. The mystery of missing heritability: Genetic interactions create phantom heritability . Proc. Natl. Acad. Sci. 109 , 1193–1198 (2012). [ PMC free article ] [ PubMed ] [ Google Scholar ]
- Mackay T. F. C. Epistasis and quantitative traits: using model organisms to study gene-gene interactions . Nat. Rev. Genet. 15 , 22–33 (2014). [ PMC free article ] [ PubMed ] [ Google Scholar ]
- Lippert C. et al. An exhaustive epistatic SNP association analysis on expanded Wellcome Trust data . Sci. Rep. 3 , 1099 (2013). [ PMC free article ] [ PubMed ] [ Google Scholar ]
- Van de Geer S., Bühlmann P., Ritov Y. & Dezeure R. On asymptotically optimal confidence regions and tests for high-dimensional models . Ann. Stat. 42 , 1166–1202 (2014). [ Google Scholar ]
- Boser B. E., Guyon I. M. & Vapnik V. N. A Training Algorithm for Optimal Margin Classifiers . In Fifth Annual Workshop on Computational Learning Theory 144–152 (ACM Press, 1992). [ Google Scholar ]
- Cortes C. & Vapnik V. Support-vector networks . Mach. Learn. 20 , 273–297 (1995). [ Google Scholar ]
- Müller K. R., Mika S., Rätsch G., Tsuda K. & Schölkopf B. An introduction to kernel-based learning algorithms . IEEE Trans. neural networks 12 , 181–201 (2001). [ PubMed ] [ Google Scholar ]
- Agresti A. Categorical Data Analysis. (Wiley, 2002). [ Google Scholar ]
- Moskvina V. & Schmidt K. M. On multiple-testing correction in genome-wide association studies . Genet. Epidemiol. 32 , 567–573 (2008). [ PubMed ] [ Google Scholar ]
- Dickhaus T. & Stange J. Multiple point hypothesis test problems and effective numbers of tests for control of the family-wise error rate . Calcutta Stat. Assoc. Bull. 65 , 123–144 (2013). [ Google Scholar ]
- Dickhaus T. Simultaneous Statistical Inference with Applications in the Life Sciences. (Springer, 2014). [ Google Scholar ]
- Westfall P. & Young S. Resampling-Based Multiple Testing: Examples and Methods for p-Value Adjustment. (Wiley, 1993). [ Google Scholar ]
- Meinshausen N., Maathuis M. H. & Bühlmann P. Asymptotic optimality of the Westfall-Young permutation procedure for multiple testing under dependence . Ann. Stat. 39 , 3369–3391 (2011). [ Google Scholar ]
- Guyon I. & Elisseeff A. An introduction to variable and feature selection . Journal of machine learning research 3 , 1157–1182 (2003). [ Google Scholar ]
- Meinshausen N., Meier L. & Bühlmann P. p-Values for High-Dimensional Regression . J. Am. Stat. Assoc. 104 , 1671 (2009). [ Google Scholar ]
- Wasserman L. & Roeder K. High-dimensional variable selection . Ann. Stat. 37 , 2178–2201 (2009). [ PMC free article ] [ PubMed ] [ Google Scholar ]
- Dudoit S. & van der Laan M. Multiple Testing Procedures with Applications to Genomics. (Springer Science & Business Media, 2008). [ Google Scholar ]
- Roeder K. & Wasserman L. Genome-Wide Significance Levels and Weighted Hypothesis Testing . Stat. Sci. 24 , 398–413 (2009). [ PMC free article ] [ PubMed ] [ Google Scholar ]
- Roshan U., Chikkagoudar S., Wei Z., Wang K. & Hakonarson H. Ranking causal variants and associated regions in genome-wide association studies by the support vector machine and random forest . Nucleic Acids Res. 39 , e62 (2011). [ PMC free article ] [ PubMed ] [ Google Scholar ]
- Alexander D. H. & Lange K. Stability selection for genome-wide association . Genet. Epidemiol. 35 , 722–728 (2011). [ PubMed ] [ Google Scholar ]
- The HapMap International Consortium. A haplotype map of the human genome . Nature 437 , 1299–1320 (2005). [ PMC free article ] [ PubMed ] [ Google Scholar ]
- Marigorta U. M. & Navarro A. High Trans-ethnic Replicability of GWAS Results Implies Common Causal Variants . PLoS Genet 9 , e1003566 (2013). [ PMC free article ] [ PubMed ] [ Google Scholar ]
- Preuss C., Riemenschneider M., Wiedmann D. & Stoll M. Evolutionary dynamics of co-segregating gene clusters associated with complex diseases . PLoS One 7 , e36205 (2012). [ PMC free article ] [ PubMed ] [ Google Scholar ]
- Fan R.-E., Chang K.-W., Hsieh C.-J., Wang X.-R. & Lin C.-J. LIBLINEAR: A Library for Large Linear Classification . Journal of Machine Learning Research 9 , 1871–1874. Software available at http://www.csie.ntu.edu.tw/~cjlin/liblinear (2008). [ Google Scholar ]
- Chang C.-C. & Lin C.-L. LIBSVM: a library for support vector machines . ACM Trans. Intell. Syst. Technol. 2 (27), 1–27. Software available at http://www.csie.ntu.edu.tw/~cjlin/libsvm (2011). [ Google Scholar ]
- The Apache Software Foundation. Commons Math: The Apache Commons Mathematics Library. Java version 1.7. Software available at http://commons.apache.org/proper/commons-math/ (2016).
- Helleputte T. & Gramme P. LiblineaR: Linear Predictive Models Based on the LIBLINEAR C/C++ Library. R package version 1.94-2 from http://dnalytics.com/liblinear/ (2015).
- Turner S. D. qqman: an R package for visualizing GWAS results using Q-Q and manhattan plots. biorXiv doi: 10.1101/005165. R package version 0.1.2 from http://cran.r-project.org/web/packages/qqman/ (2014). [ CrossRef ]
- Dowle M., Srinivasan A., Short T. & Lianoglou S. with contributions from Saporta, R. & Antonyan, E. data.table: Extension of Data.frame. R package version 1.9.6. from https://CRAN.R-project.org/package=data.table (2015).
- Warnes G. R., Bolker B. & Lumley T. gtools: Various R Programming Tools. R package version 3.5.0. from https://CRAN.R-project.org/package=gtools (2015).
- Clayton D. snpStats: SnpMatrix and XSnpMatrix classes and methods. R package version 1.22.0 from http://bioconductor.org/packages/release/bioc/html/snpStats.html (2015).
- Mittag F. et al. Use of support vector machines for disease risk prediction in genome-wide association studies: Concerns and opportunities . Hum. Mutat. 33 , 1708–1718 (2012). [ PMC free article ] [ PubMed ] [ Google Scholar ]
- Davies R. W. et al. Improved Prediction of Cardiovascular Disease Based on a Panel of Single Nucleotide Polymorphisms Identified Through Genome-Wide Association Studies . Circ. Cardiovasc. Genet. 3 , 468–474 (2010). [ PMC free article ] [ PubMed ] [ Google Scholar ]
- Evans D. M., Visscher P. M. & Wray N. R. Harnessing the information contained within genome-wide association studies to improve individual prediction of complex disease risk . Hum. Mol. Genet. 18 , 3525–3531 (2009). [ PubMed ] [ Google Scholar ]
- Ioannidis J. P. A. Prediction of Cardiovascular Disease Outcomes and Established Cardiovascular Risk Factors by Genome-Wide Association Markers . Circ. Cardiovasc. Genet. 2 , 7–15 (2009). [ PubMed ] [ Google Scholar ]
- Kooperberg C., LeBlanc M. & Obenchain V. Risk prediction using genome-wide association studies . Genet. Epidemiol. 34 , 643–652 (2010). [ PMC free article ] [ PubMed ] [ Google Scholar ]
- Quevedo J. R., Bahamonde A., Perez-Enciso M. & Luaces O. Disease Liability Prediction from Large Scale Genotyping Data Using Classifiers with a Reject Option . IEEE/ACM Trans. Comput. Biol. Bioinforma. 9 , 88–97 (2012). [ PubMed ] [ Google Scholar ]
- Wei Z. et al. From Disease Association to Risk Assessment: An Optimistic View from Genome-Wide Association Studies on Type 1 Diabetes . PLoS Genet. 5 , e1000678 (2009). [ PMC free article ] [ PubMed ] [ Google Scholar ]
- Wei Z. et al. Large Sample Size, Wide Variant Spectrum, and Advanced Machine-Learning Technique Boost Risk Prediction for Inflammatory Bowel Disease . Am. J. Hum. Genet. 92 , 1008–1012 (2013). [ PMC free article ] [ PubMed ] [ Google Scholar ]
- Wray N. R., Yang J., Goddard M. E. & Visscher P. M. The genetic interpretation of area under the ROC curve in genomic profiling . PLoS Genet. 6 , e1000864 (2010). [ PMC free article ] [ PubMed ] [ Google Scholar ]
- Austin E., Pan W. & Shen X. Penalized regression and risk prediction in genome-wide association studies . Stat. Anal. Data Min. 6 , 315–328 (2013). [ PMC free article ] [ PubMed ] [ Google Scholar ]
- Okser S. et al. Regularized machine learning in the genetic prediction of complex traits . PLoS Genet. 10 , e1004754 (2014). [ PMC free article ] [ PubMed ] [ Google Scholar ]
- Wu Q., Ye Y., Liu Y. & Ng M. K. SNP selection and classification of genome-wide SNP data using stratified sampling random forests . IEEE Trans. Nanobiosci. 11 , 216–227 (2012). [ PubMed ] [ Google Scholar ]
- Schwarz D. F., König I. R. & Ziegler A. On safari to Random Jungle: a fast implementation of Random Forests for high-dimensional data . Bioinformatics 26 , 1752–1758 (2010). [ PMC free article ] [ PubMed ] [ Google Scholar ]
- Rakitsch B., Lippert C., Stegle O. & Borgwardt K. A Lasso multi-marker mixed model for association mapping with population structure correction . Bioinformatics 29 , 206–214 (2013). [ PubMed ] [ Google Scholar ]
- Shi G. et al. Mining gold dust under the genome wide significance level: a two-stage approach to analysis of GWAS . Genet. Epidemiol. 35 , 111–118 (2011). [ PMC free article ] [ PubMed ] [ Google Scholar ]
- Meinshausen N. & Bühlmann P. Stability selection . J. R. Stat. Soc. Ser. B Statistical Methodol. 72 , 417–473 (2010). [ Google Scholar ]
- Pahikkala T., Okser S., Airola A., Salakoski T. & Aittokallio T. Wrapper-based selection of genetic features in genome-wide association studies through fast matrix operations . Algorithms Mol. Biol. 7 , 11 (2012). [ PMC free article ] [ PubMed ] [ Google Scholar ]
- He Q. & Lin D. Y. Y. A variable selection method for genome-wide association studies . Bioinformatics 27 , 1–8 (2011). [ PMC free article ] [ PubMed ] [ Google Scholar ]
- Zhou H., Sehl M. E., Sinsheimer J. S. & Lange K. Association screening of common and rare genetic variants by penalized regression . Bioinformatics 26 , 2375–2382 (2010). [ PMC free article ] [ PubMed ] [ Google Scholar ]
- Minnier J., Yuan M., Liu J. S. & Cai T. Risk classification with an adaptive naive Bayes Kernel machine model . J. Am. Stat. Assoc. 110 , 393–404 (2015). [ PMC free article ] [ PubMed ] [ Google Scholar ]
- Nguyen T. T., Huang J. Z., Wu Q., Nguyen T. T. & Li M. J. Genome-wide association data classification and SNPs selection using two-stage quality-based Random Forests . BMC Genomics 16 , S5 (2015). [ PMC free article ] [ PubMed ] [ Google Scholar ]
- Tsai M. Y. Variable selection in Bayesian generalized linear-mixed models: An illustration using candidate gene case-control association studies . Biometrical Journal 57 , 234–253 (2015). [ PubMed ] [ Google Scholar ]
- Manor O. & Segal E. Predicting disease risk using bootstrap ranking and classification algorithms . PLoS Comput. Biol. 9 , e1003200 (2013). [ PMC free article ] [ PubMed ] [ Google Scholar ]
- Hoffman G. E., Logsdon B. A. & Mezey J. G. PUMA: a unified framework for penalized multiple regression analysis of GWAS data . PLoS Comput. Biol. 9 , e1003101 (2013). [ PMC free article ] [ PubMed ] [ Google Scholar ]
- Fisher C. K. & Mehta P. Bayesian feature selection for high-dimensional linear regression via the Ising approximation with applications to genomics . Bioinformatics 11 , 1754–1761 (2015). [ PubMed ] [ Google Scholar ]
- Breiman L. Random forests . Machine learning 45 , 5–32 (2001). [ Google Scholar ]
- Zou H. & Hastie T. Regularization and variable selection via the elastic net . J. R. Stat. Soc. Ser. B Statistical Methodol. 67 , 301–320 (2005). [ Google Scholar ]
- Fan J. & Lv J. Sure independence screening for ultrahigh dimensional feature space . J. R. Stat. Soc. Ser. B Statistical Methodol. 70 , 849–911 (2008). [ PMC free article ] [ PubMed ] [ Google Scholar ]
- Li J., Zhong W., Li R. & Wu R. A fast algorithm for detecting gene–gene interactions in genome-wide association studies . The annals of applied statistics 8 , 2292 (2014). [ PMC free article ] [ PubMed ] [ Google Scholar ]
- Lippert C. et al. FaST linear mixed models for genome-wide association studies . Nat. Methods 8 , 833–835 (2011). [ PubMed ] [ Google Scholar ]
- Mimno D., Blei D. M. & Engelhardt B. E. Posterior predictive checks to quantify lack-of-fit in admixture models of latent population structure . Proc. Natl. Acad. Sci. 112 , 3441–3450 (2015). [ PMC free article ] [ PubMed ] [ Google Scholar ]
- Loh P. R. et al. Efficient Bayesian mixed-model analysis increases association power in large cohorts . Nat. Genet. 47 , 284–290 (2015). [ PMC free article ] [ PubMed ] [ Google Scholar ]
- Song M., Hao W. & Storey J. D. Testing for genetic associations in arbitrarily structured populations . Nat. Genet. 47 , 550–554 (2015). [ PMC free article ] [ PubMed ] [ Google Scholar ]
- Zhou X. & Stephens M. Efficient multivariate linear mixed model algorithms for genome-wide association studies . Nat. Methods 11 , 407–409 (2014). [ PMC free article ] [ PubMed ] [ Google Scholar ]
- Kang H. M. et al. Efficient control of population structure in model organism association mapping . Genetics 178 , 1709–1723 (2008). [ PMC free article ] [ PubMed ] [ Google Scholar ]
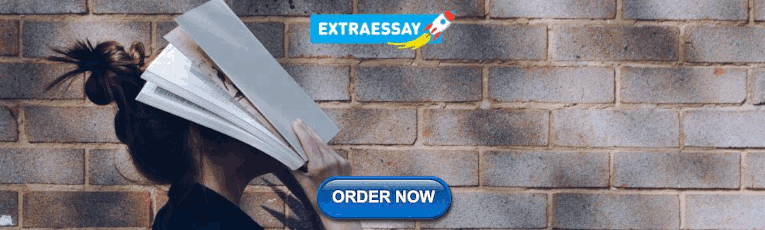
IMAGES
VIDEO
COMMENTS
Combining Multiple Hypothesis Testing with Machine Learning Increases the Statistical Power of Genome-wide Association Studies Bettina Mieth 1 na1 na2 , Marius Kloft 2 na1 na2 ,
Often case that we use hypothesis testing to select which features are useful for our prediction model; for example, there are 20 features you are interested in as independent (predictor) features to create your machine learning model. You might think to test each feature using hypothesis testing separately with some level of significance α 0.05.
A hypothesis test (carried out as a χ 2 test) is performed for each of the selected SNPs. Those SNPs with p-value less than a significance threshold t* are returned. The threshold t* is calibrated using a permutation-based method over the whole procedure consisting of the machine learning selection and statistical testing steps. See Algorithm 2
Keywords: FDR control, false discovery rate, sequential hypothesis testing, sequential experimentation, p-values 1. Introduction ... Although it is not a focus of research in machine learning, multiple decision-making has been promi-nent during the past two decades in statistics, in the wake of seminal research by Benjamini and Hochberg
Covariate-adaptive multiple testing methods utilize such information to improve detection power while controlling false positives. Multiple hypothesis testing is an essential component of modern data science. Its goal is to maximize the number of discoveries while controlling the fraction of false discoveries. In many settings, in addition to ...
Online multiple hypothesis testing David S. Robertsona, James M. S. Wasonb, and Aaditya Ramdasc aMRC Biostatistics Unit, University of Cambridge, UK bPopulation Health Sciences Institute, Newcastle University, Newcastle, UK cDepartments of Statistics and Machine Learning, Carnegie Mellon University, Pittsburgh, Pennsylvania, USA Abstract Modern data analysis frequently involves large-scale ...
Combining Multiple Hypothesis Testing with Machine Learning Increases the Statistical Power of Genome-wide Association Studies Sci Rep . 2016 Nov ... we propose a combination of machine learning and statistical testing that takes correlation structures within the set of SNPs under investigation in a mathematically well-controlled manner into ...
Greedy RLS is the first known implementation of a machine learning based method with the capability to conduct a wrapper-based feature selection on an entire GWAS containing several thousand examples and over 400,000 variants and has better classification performance on independent test data than a classifier trained using features selected by ...
The null hypothesis represented as H₀ is the initial claim that is based on the prevailing belief about the population. The alternate hypothesis represented as H₁ is the challenge to the null hypothesis. It is the claim which we would like to prove as True. One of the main points which we should consider while formulating the null and alternative hypothesis is that the null hypothesis ...
The process of hypothesis testing is to draw inferences or some conclusion about the overall population or data by conducting some statistical tests on a sample. The same inferences are drawn for different machine learning models through T-test which I will discuss in this tutorial. For drawing some inferences, we have to make some assumptions ...
In this investigation, a synthesis of Convolutional Neural Networks (CNNs) and Bayesian inference is presented, leading to a novel approach to the problem of Multiple Hypothesis Testing (MHT). Diverging from traditional paradigms, this study introduces a sequence-based uncalibrated Bayes factor approach to test many hypotheses using the same family of sampling parametric models. A two-step ...
Hypothesis testing is an indispensable tool in data science, allowing us to make data-driven decisions with confidence. By understanding its principles, conducting tests properly, and considering real-world applications, you can harness the power of hypothesis testing to unlock valuable insights from your data.
Differential privacy (DP) is by far the most widely accepted framework for mitigating privacy risks in machine learning. However, exactly how small the privacy parameter $ε$ needs to be to protect against certain privacy risks in practice is still not well-understood. In this work, we study data reconstruction attacks for discrete data and analyze it under the framework of multiple hypothesis ...
Evaluating hypotheses in machine learning involves testing the null hypothesis against the alternative hypothesis. This is typically done using statistical methods, such as t-tests, ANOVA, and regression analysis. ... P-hacking occurs when a researcher selectively reports the results of multiple hypothesis tests to find a significant result. To ...
Summarize the framework for using statistical hypothesis tests in a machine learning project presented in Thomas Dietterich's 1998 paper. ... and away from hypothesis test. Yes, ensemble multiple runs, it's a default in most DL+CV papers, even GAN papers these days. Reply. Jan Brauner May 26, 2019 at 5:53 am # Alright, thanks again. Both ...
and research models, machine learning is far less frequently used than inferential statistics. Additionally, statistics calls for improving the test of theory by showing the magnitude of the phenomena being studied. This article extends current XAI methods and develops a model agnostic hypothesis testing framework for machine learning.
where . 1. M in the denominator of the third formula is the measurement dimension. 2. V C is the measurement volume. 3. S = HPT T + R the measurement residual covariance matrix. 4. d 2 = y T S −1 y is the normalized statistical distance for the measurement. The statistical distance is defined by the residual y, the difference between the measurement and the estimated measurement, and the ...
Combining Multiple Hypothesis Testing with Machine Learning ... investigation and propose a novel methodology for tackling it — based on a combination of machine learning and statistical testing ...
that utilizes deep learning techniques for this recalibration. The way we use this technique is also known as transfer learning in the machine learning language. Transfer learning is meant as a statistical technique in machine learning where a model developed for a particular task (i.e., analyzing an MHT problem where the truth is known) is ...
In this tutorial, you will discover how to use statistical hypothesis tests for comparing machine learning algorithms. After completing this tutorial, you will know: Performing model selection based on the mean model performance can be misleading. The five repeats of two-fold cross-validation with a modified Student's t-Test is a good ...
Hypothesis testing and binary classification are rooted in two different cultures: inference and prediction, each of which has been extensively studied in statistics and machine learning, respectively, in the historical development of data sciences. 9 In brief, an inferential task aims to infer an unknown truth from observed data, and ...
However, there is very little theoretical understanding of this phenomenon. In this paper, we analyze privacy leakage in connection to the multiple hypothesis testing problem in information the-ory, and show that the empirical privacy protection conferred by DP with high ε may be more than previously thought.
Statistical testing step (colored in blue). A hypothesis test (carried out as a χ 2 test) is performed for each of the selected SNPs. Those SNPs with p-value less than a significance threshold t* are returned. The threshold t* is calibrated using a permutation-based method over the whole procedure consisting of the machine learning selection and statistical testing steps.
The Leishmania life cycle alternates between promastigotes, found in the sandfly, and amastigotes, found in mammals. When an infected sandfly bites a host, promastigotes are engulfed by phagocytes (i.e., neutrophils, dendritic cells, and macrophages) to establish infection. When these phagocytes die or break down, amastigotes must be re-internalized to survive within the acidic phagolysosome ...