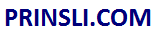
- [ July 15, 2024 ] What is Light Energy : प्रकाश क्या है? प्रकाश में कौन-कौन से गुण हैं? Knowledge
- [ June 20, 2024 ] Newton’s Laws of Motion : गति क्या है? न्यूटन के गति के नियम की व्याख्या Knowledge
- [ March 6, 2024 ] 369 Universe Number : 369 कोड क्या है? 369 नंबर का क्या रहस्य है? ब्लॉग
- [ March 4, 2024 ] Save Banana Peels : प्रदूषण से लड़ने में कमाल कर सकता है केला TOP 10
- [ December 6, 2023 ] Difference Between Nebula and Galaxy : निहारिका और आकाशगंगा में क्या अंतर है? Knowledge
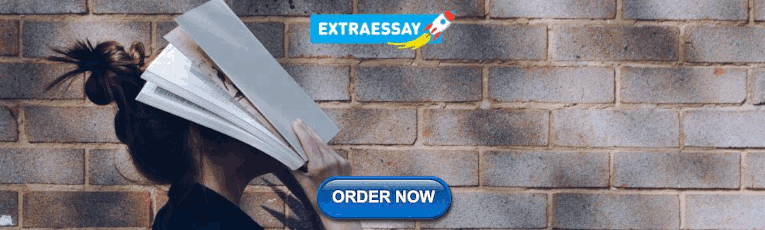
Objectives of Statistics
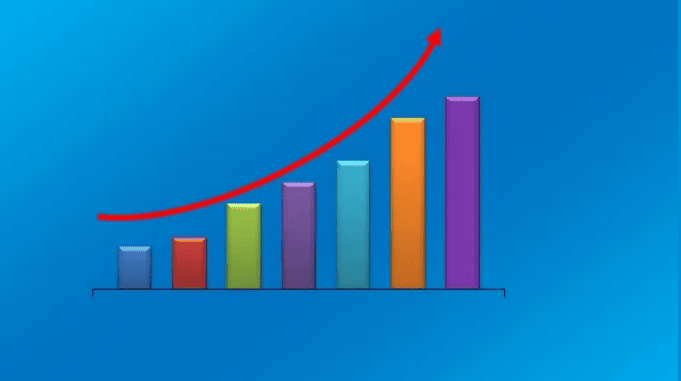
The Objectives of Statistics :
The main objective of statistics is to study the various facts and problems in the research field and to evaluate the causes and results of the changes occurring in them.
Statistics is the science of gaining knowledge from data. You can collect data in the correct manner, perform detailed analyses, and effectively present the results if you have statistical knowledge.
Statistics teaches people how to make intelligent and accurate conclusions about a larger population by analysing a small and limited sample of data . The following are the major objectives of statistics:
- To draw conclusions (inferences) about a population followed by an analysis of information contained in sample data. This includes assessing the degree of uncertainty in these inferences.
- To plan the process and the extent of sampling so that the observations can be used to draw valid conclusions.
- To determine the type and quantity of data that must be collected.
- To organise and summarise the information.
- To analyse and draw conclusions from the data.
- To assess the conclusions’ strengths and evaluate their uncertainty.
According to Prof. Boddington , “The main objective of statistical research is to enable comparison between past and present results to ascertain the reasons for changes, which have taken place and the effect of such changes in future.
According to Johnson and Jackson , “The real purpose of statistical methods is to make proper sense out of facts and figures, to make discoveries about unknown and to cost light upon the situation.”
According to Robert W. Buges , “The fundamental principle of statistics is to remove the domain of ignorance, prejudice, rule of thumb, arbitrary and premature decisions, traditions and dogmatism and to increase the domain where decisions are made and principles are formulated on the basis of analysed quantitative facts.”
Hence, it is clear that statistics is an integral stage of national development, which helps us in solving various complex problems.
(Source – Various books of college library)
Tags: the objective of statistics, objectives of statistics, objectives of studying statistics, aims and objectives of statistics, explain the objectives of statistics
Copyrighted Material © 2019 - 2024 Prinsli.com - All rights reserved
All content on this website is copyrighted. It is prohibited to copy, publish or distribute the content and images of this website through any website, book, newspaper, software, videos, YouTube Channel or any other medium without written permission. You are not authorized to alter, obscure or remove any proprietary information, copyright or logo from this Website in any way. If any of these rules are violated, it will be strongly protested and legal action will be taken.
- Click to share on Facebook (Opens in new window)
- Click to share on Twitter (Opens in new window)
- Click to share on LinkedIn (Opens in new window)
- Click to share on WhatsApp (Opens in new window)
- Click to share on Pinterest (Opens in new window)
- Click to share on Telegram (Opens in new window)
- Click to share on Tumblr (Opens in new window)
- Business Statistics
- Introduction of Statistics
- Objective of Statistics
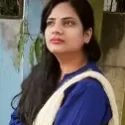
Related Articles
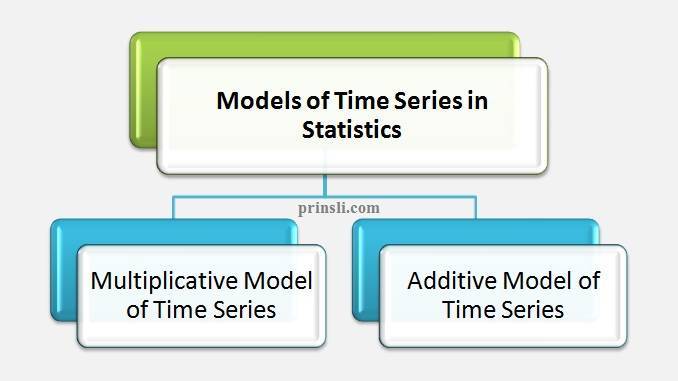
Models of Time Series in Statistics
Models of Time Series: Additive model of time series, Multiplicative model of time series, Difference between Multiplicative and Additive models… […]
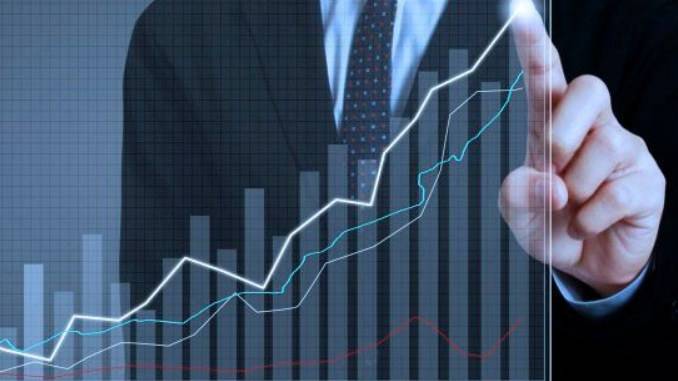
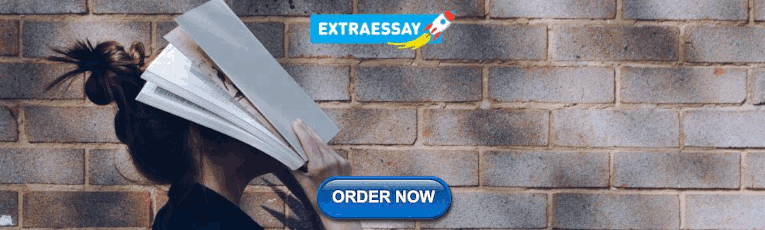
Features and Importance of Index Number in Statistics
Features of Index Numbers in Statistics – Index Number has the following features in statistics: (i) Index numbers are specialized averages that are capable of being expressed in percentages. (ii) Index numbers measure the changes […]
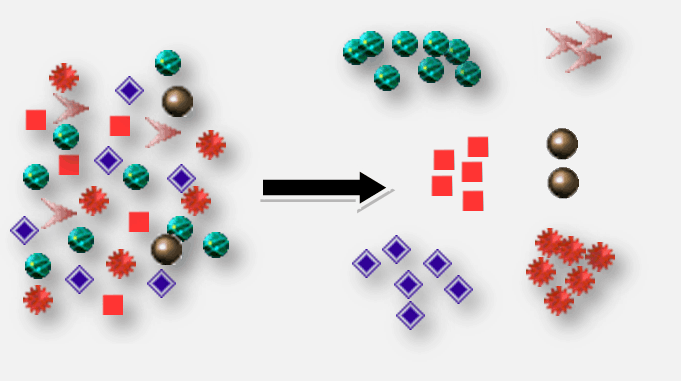
Functions of Classification of Data in Statistics
Makes data more understandable by simplifying it. Condenses information. Prioritizes the collected key data while separating the optional elements. […]
Be the first to comment
Leave a reply cancel reply.
Your email address will not be published.
Save my name, email, and website in this browser for the next time I comment.
Notify me of follow-up comments by email.
Notify me of new posts by email.
Copyright © 2019 - 2024 Prinsli.com - All rights reserved
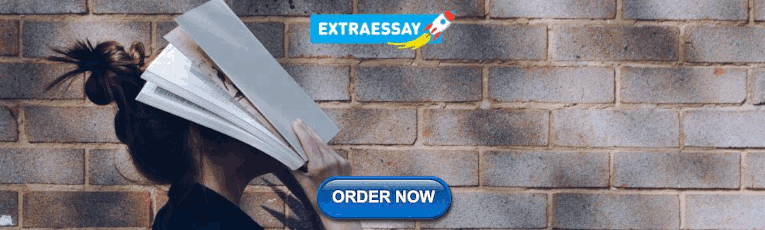
IMAGES
VIDEO
COMMENTS
Lots of resources will recommend the SMART approach for developing and writing your research objectives. SMART means that your research objectives are. S pecific. M easurable. A chievable. R elevant. T ime-bound. Here’s an example of a poorly framed …
Research objectives are concise statements that describe what the research is aiming to achieve. They define the scope and direction of the research and maintain focus. The objectives should be SMART—specific, …
When designing a research study: Research objectives guide the design and implementation of a research study. They help to identify the appropriate research methods, …
Objectives. Upon completion of this lesson, you should be able to: Identify outcomes that are continuous, binary, event times, counts, ordered or unordered categories and repeated …
Research objectives act as a guide to determin e suitable research design, problem statement, data collection procedure, analyze and interpret data and variables of the …
Research objectives describe concisely what the research is trying to achieve. They summarize the accomplishments a researcher wishes to achieve through the project and provides direction to the study.
In summary, quantitative research offers a structured, objective framework geared for hypothesis testing and generalizable insights, while non-quantitative research provides a …